lol thanks! I’ve always wondered why HF posts don’t support markdown.
Huang Liang Hsun PRO
lianghsun
AI & ML interests
Founder of Twinkle AI. Focused on applying deep learning in legal and scientific domains, with expertise in NLP and model fine-tuning.
Recent Activity
updated
a model
2 days ago
twinkle-ai/Llama-3.2-3B-F1-Instruct
updated
a dataset
3 days ago
twinkle-ai/tw-reasoning-instruct-50k
replied to
their
post
4 days ago
With the arrival of Twinkle April — Twinkle AI’s annual open-source celebration held every April — our community is excited to unveil its very first project:
📊 Twinkle Eval (https://github.com/ai-twinkle/Eval), a next-generation evaluation tool led by our contributor @tedslin .
Unlike traditional evaluation tools like iKala’s ievals (https://github.com/ikala-ai/ievals), which can only evaluate language models (LMs) one sample at a time, Twinkle Eval is designed with Large Reasoning Models (LRMs) in mind. As reasoning time increases with more complex models, traditional tools become increasingly inefficient 😲 — for example, evaluating LRMs on the https://huggingface.co/datasets/ikala/tmmluplus benchmark could take *
half a day without finishing.
One question we were especially curious about:
Does shuffling multiple-choice answer order impact model accuracy? 🤔
→ See: "Change Answer Order Can Decrease MMLU Accuracy" – arXiv:2406.19470v1
To address these challenges, Twinkle Eval brings three key innovations to the table:
1️⃣ Parallelized evaluation of samples
2️⃣ Multi-round testing for stability
3️⃣ Randomized answer order to test robustness
After running experiments, we observed that Twinkle Eval can speed up evaluation by up to 15× 🚀🚀. Interestingly, most models scored slightly lower under the 2️⃣3️⃣ test settings compared to their claimed performance — suggesting further benchmarking is needed.
This framework also comes with additional tunable parameters and detailed logging of LM behavior per question — perfect for those who want to dive deeper. 😆
If you find Twinkle Eval useful, please ⭐ the project and help spread the word 🤗
Organizations
lianghsun's activity
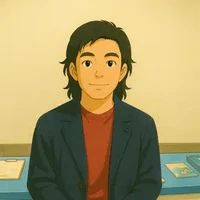
replied to
their
post
4 days ago
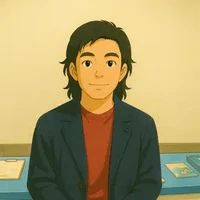
posted
an
update
4 days ago
Post
2034
With the arrival of Twinkle April — Twinkle AI’s annual open-source celebration held every April — our community is excited to unveil its very first project:
📊 Twinkle Eval (https://github.com/ai-twinkle/Eval), a next-generation evaluation tool led by our contributor @tedslin .
Unlike traditional evaluation tools like iKala’s ievals (https://github.com/ikala-ai/ievals), which can only evaluate language models (LMs) one sample at a time, Twinkle Eval is designed with Large Reasoning Models (LRMs) in mind. As reasoning time increases with more complex models, traditional tools become increasingly inefficient 😲 — for example, evaluating LRMs on the ikala/tmmluplus benchmark could take *
half a day without finishing.
One question we were especially curious about:
Does shuffling multiple-choice answer order impact model accuracy? 🤔
→ See: "Change Answer Order Can Decrease MMLU Accuracy" – arXiv:2406.19470v1
To address these challenges, Twinkle Eval brings three key innovations to the table:
1️⃣ Parallelized evaluation of samples
2️⃣ Multi-round testing for stability
3️⃣ Randomized answer order to test robustness
After running experiments, we observed that Twinkle Eval can speed up evaluation by up to 15× 🚀🚀. Interestingly, most models scored slightly lower under the 2️⃣3️⃣ test settings compared to their claimed performance — suggesting further benchmarking is needed.
This framework also comes with additional tunable parameters and detailed logging of LM behavior per question — perfect for those who want to dive deeper. 😆
If you find Twinkle Eval useful, please ⭐ the project and help spread the word 🤗
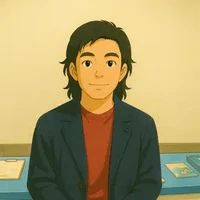
posted
an
update
3 months ago
Post
2409
🖖 Let me introduce the work I've done over the past three months: 𝗟𝗹𝗮𝗺𝗮-𝟯.𝟮-𝗧𝗮𝗶𝘄𝗮𝗻-𝟯𝗕 and 𝗟𝗹𝗮𝗺𝗮-𝟯.𝟮-𝗧𝗮𝗶𝘄𝗮𝗻-𝟯𝗕-𝗜𝗻𝘀𝘁𝗿𝘂𝗰𝘁, now open-sourced on 🤗 Hugging Face.
𝗹𝗶𝗮𝗻𝗴𝗵𝘀𝘂𝗻/𝗟𝗹𝗮𝗺𝗮-𝟯.𝟮-𝗧𝗮𝗶𝘄𝗮𝗻-𝟯𝗕: This model is built on top of 𝗺𝗲𝘁𝗮-𝗹𝗹𝗮𝗺𝗮/𝗟𝗹𝗮𝗺𝗮-𝟯.𝟮-𝟯𝗕 with continual pretraining. The training dataset consists of a mixture of Traditional Chinese and multilingual texts in specific proportions, including 20B tokens of Traditional Chinese text.
𝗹𝗶𝗮𝗻𝗴𝗵𝘀𝘂𝗻/𝗟𝗹𝗮𝗺𝗮-𝟯.𝟮-𝗧𝗮𝗶𝘄𝗮𝗻-𝟯𝗕-𝗜𝗻𝘀𝘁𝗿𝘂𝗰𝘁: This is a fine-tuned conversational model based on the foundation model.
This Llama-3.2-Taiwan open-source project is currently a one-person effort (yes, I did everything from text preparation — so exhausting!). If you're interested, feel free to join the Discord server for discussions.
🅱🅴🅽🅲🅷🅼🅰🆁🅺🅸🅽🅶
The evaluation was conducted using ikala/tmmluplus, though the README page does not yet reflect the latest results. The performance is close to the previous versions, indicating that further improvements might require adding more specialized knowledge in the datasets.
🅰 🅲🅰🅻🅻 🅵🅾🆁 🆂🆄🅿🅿🅾🆁🆃
If anyone is willing to provide compute resources, it would be greatly appreciated to help this project continue and grow. 💪
---
🏔️ Foundation model: lianghsun/Llama-3.2-Taiwan-3B
🤖 Instruction model: lianghsun/Llama-3.2-Taiwan-3B-Instruct
⚡ GGUF: lianghsun/Llama-3.2-Taiwan-3B-Instruct-GGUF
𝗹𝗶𝗮𝗻𝗴𝗵𝘀𝘂𝗻/𝗟𝗹𝗮𝗺𝗮-𝟯.𝟮-𝗧𝗮𝗶𝘄𝗮𝗻-𝟯𝗕: This model is built on top of 𝗺𝗲𝘁𝗮-𝗹𝗹𝗮𝗺𝗮/𝗟𝗹𝗮𝗺𝗮-𝟯.𝟮-𝟯𝗕 with continual pretraining. The training dataset consists of a mixture of Traditional Chinese and multilingual texts in specific proportions, including 20B tokens of Traditional Chinese text.
𝗹𝗶𝗮𝗻𝗴𝗵𝘀𝘂𝗻/𝗟𝗹𝗮𝗺𝗮-𝟯.𝟮-𝗧𝗮𝗶𝘄𝗮𝗻-𝟯𝗕-𝗜𝗻𝘀𝘁𝗿𝘂𝗰𝘁: This is a fine-tuned conversational model based on the foundation model.
This Llama-3.2-Taiwan open-source project is currently a one-person effort (yes, I did everything from text preparation — so exhausting!). If you're interested, feel free to join the Discord server for discussions.
🅱🅴🅽🅲🅷🅼🅰🆁🅺🅸🅽🅶
The evaluation was conducted using ikala/tmmluplus, though the README page does not yet reflect the latest results. The performance is close to the previous versions, indicating that further improvements might require adding more specialized knowledge in the datasets.
🅰 🅲🅰🅻🅻 🅵🅾🆁 🆂🆄🅿🅿🅾🆁🆃
If anyone is willing to provide compute resources, it would be greatly appreciated to help this project continue and grow. 💪
---
🏔️ Foundation model: lianghsun/Llama-3.2-Taiwan-3B
🤖 Instruction model: lianghsun/Llama-3.2-Taiwan-3B-Instruct
⚡ GGUF: lianghsun/Llama-3.2-Taiwan-3B-Instruct-GGUF