genbio-ai/proteinMoE-16b-Petal
genbio-ai/proteinMoE-16b-Petal is a fine-tuned version of genbio-ai/proteinMoE-16b, specifically designed for protein structure prediction. This model uses amino acid sequences as input to predict tokens that can be decoded into 3D structures by genbio-ai/petal-decoder. It surpasses existing state-of-the-art models, such as ESM3-open, in structure prediction tasks, demonstrating its robustness and capability in this domain.
Model Architecture Details
This model retains the architecture of AIDO.Protein 16B, a transformer encoder-only architecture with dense MLP layers replaced by sparse Mixture of Experts (MoE) layers. Each token activates 2 experts using a top-2 routing mechanism. A visual summary of the architecture is provided below:
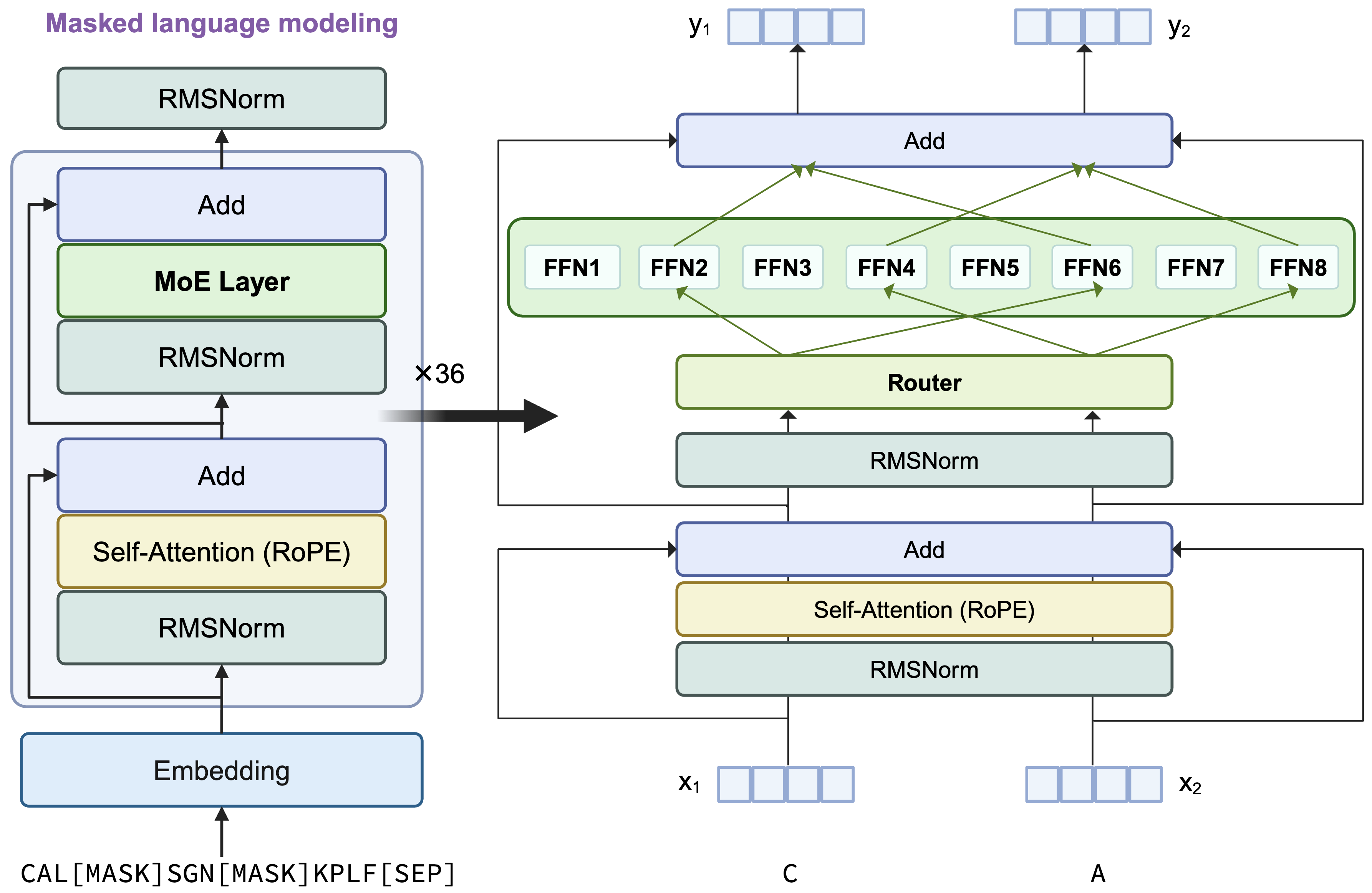
Key Differences
The final output linear layer has been adapted to support a new vocabulary size:
- Input Vocabulary Size: 44 (amino acids + special tokens)
- Output Vocabulary Size: 512 (structure tokens without special tokens)
Architecture Parameters
Component | Value |
---|---|
Number of Attention Heads | 36 |
Number of Hidden Layers | 36 |
Hidden Size | 2304 |
Number of MoE Layers per Block | 8 |
Number of MoE Layers per Token | 2 |
Input Vocabulary Size | 44 |
Output Vocabulary Size | 512 |
Context Length | 1024 |
Training Details
The fine-tuning process used 0.4 trillion tokens, using AlphaFold database with 170M samples and PDB database with 0.4M samples, making it highly specialized for structure prediction. The training took around 20 days on 64 A100 GPUs.
- Batch Size: Global batch size of 2048
- Context Length: 1024
- Precision: FP16
- Hardware: 64 NVIDIA A100 80GB GPUs
- Learning Rate: Max learning rate of 1e-4
- Scheduler: Cosine decay with 2.5% warmup
- Tokens Trained: 4T tokens
- Training steps: 200k steps
Tokenization
The input sequence should be single-chain amino acid sequences.
- Input Tokenization: The sequences are tokenized at the amino acid level and terminated with a
[SEP]
token (id=34). - Output Tokenization: Each input token is converted into a structure token. The output can be decoded into 3D structures in PDB format using genbio-ai/petal-decoder.
Results
TODO