File size: 4,244 Bytes
2ec36d0 11e9781 7eb703e 11e9781 00344c3 11e9781 2118454 69964ca 7eb703e 9632a6b 1b9d89a 9632a6b 1b9d89a b974e77 9632a6b 1b9d89a 0a5ce0a 1b9d89a 0a5ce0a 1b9d89a cceed7c 11e9781 cceed7c 11e9781 |
1 2 3 4 5 6 7 8 9 10 11 12 13 14 15 16 17 18 19 20 21 22 23 24 25 26 27 28 29 30 31 32 33 34 35 36 37 38 39 40 41 42 43 44 45 46 47 48 49 50 51 52 53 54 55 56 57 58 59 60 61 62 63 64 65 66 67 68 69 70 71 72 73 74 75 76 77 78 79 80 81 82 83 84 85 86 87 88 89 90 91 92 93 94 95 96 97 98 99 100 101 102 103 104 105 106 107 108 109 110 111 112 113 114 115 116 117 118 119 120 121 122 123 124 125 126 127 128 129 130 131 132 133 134 135 136 137 138 139 140 141 142 143 144 145 146 147 148 149 150 151 152 153 154 155 156 157 158 159 160 161 162 163 164 165 166 167 168 169 170 171 172 173 174 175 176 177 178 179 180 181 182 183 184 185 186 187 188 189 190 191 192 193 194 195 196 197 198 199 200 201 202 203 204 205 206 207 208 209 210 211 212 213 214 215 216 217 218 219 220 221 222 223 224 225 226 227 228 229 230 |
---
license: mit
datasets:
- espnet/yodas
- facebook/voxpopuli
- facebook/multilingual_librispeech
- google/fleurs
library_name: espnet
tags:
- espnet
- audio
- speech
- multilingual
language:
- multilingual
- ab
- af
- ak
- am
- ar
- as
- av
- ay
- az
- ba
- bm
- be
- bn
- bi
- bo
- sh
- br
- bg
- ca
- cs
- ce
- cv
- ku
- cy
- da
- de
- dv
- dz
- el
- en
- eo
- et
- eu
- ee
- fo
- fa
- fj
- fi
- fr
- fy
- ff
- ga
- gl
- gn
- gu
- zh
- ht
- ha
- he
- hi
- sh
- hu
- hy
- ig
- ia
- ms
- is
- it
- jv
- ja
- kn
- ka
- kk
- kr
- km
- ki
- rw
- ky
- ko
- kv
- lo
- la
- lv
- ln
- lt
- lb
- lg
- mh
- ml
- mr
- ms
- mk
- mg
- mt
- mn
- mi
- my
- zh
- nl
- 'no'
- 'no'
- ne
- ny
- oc
- om
- or
- os
- pa
- pl
- pt
- ms
- ps
- qu
- ro
- rn
- ru
- sg
- sk
- sl
- sm
- sn
- sd
- so
- es
- sq
- su
- sv
- sw
- ta
- tt
- te
- tg
- tl
- th
- ti
- ts
- tr
- uk
- ms
- vi
- wo
- xh
- ms
- yo
- ms
- zu
- za
---
[XEUS - A Cross-lingual Encoder for Universal Speech]()
XEUS is a large-scale multilingual speech encoder by Carnegie Mellon University's [WAVLab]() that covers over **4000** languages. It is pre-trained on over 1 million hours of publicly available speech datasets. It can be requires fine-tuning to be used in downstream tasks such as Speech Recognition or Translation. XEUS uses the [E-Branchformer]() architecture and is trained using [HuBERT]()-style masked prediction of discrete speech tokens extracted from [WavLabLM](). During training, the input speech is also augmented with acoustic noise and reverberation, making XEUS more robust. The total model size is 577M parameters.
XEUS tops the [ML-SUPERB]() multilingual speech recognition leaderboard, outperforming [MMS](), [w2v-BERT 2.0](), and [XLS-R](). XEUS also sets a new state-of-the-art on 4 tasks in the monolingual [SUPERB]() benchmark.
More information about XEUS, including ***download links for our crawled 4000-language dataset***, can be found in the [project page]().
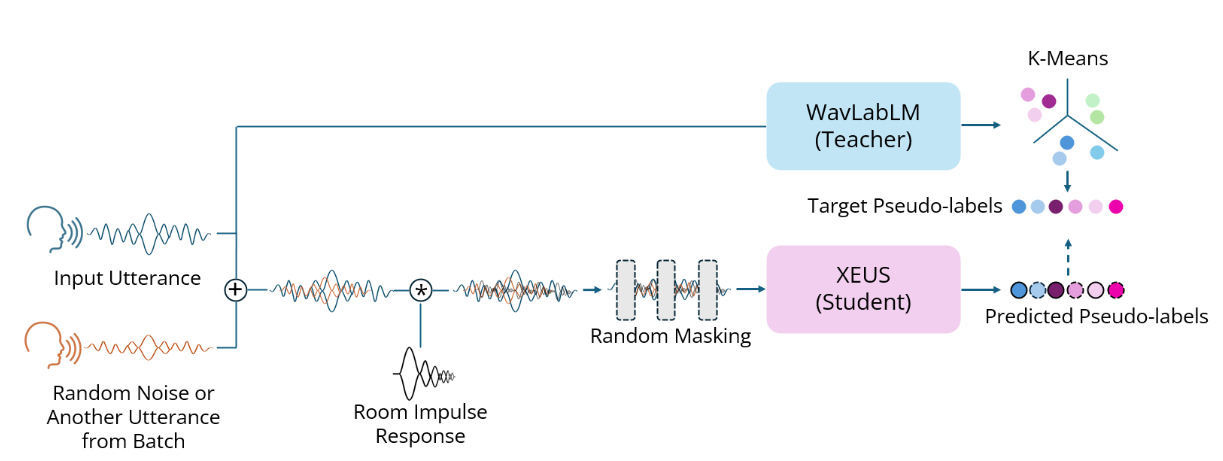
## Requirements
The code for XEUS is still in progress of being merged into the main ESPnet repo. It can instead be used from the following fork:
```
pip install -e git+git://github.com/wanchichen/espnet.git@ssl
```
XEUS supports [Flash Attention](), which can be installed as follows:
```
pip install flash-attn --no-build-isolation
```
## Usage
```python
from torch.nn.utils.rnn import pad_sequence
from espnet2.tasks.ssl import SSLTask
import soundfile as sf
device = "cuda" if torch.cuda.is_available() else "cpu"
xeus_model, xeus_train_args = SSLTask.build_model_from_file(
config = None,
ckpt = '/path/to/checkpoint/here/checkpoint.pth',
device,
)
wavs, sampling_rate = sf.read('/path/to/audio.wav') # sampling rate should be 16000
wav_lengths = torch.LongTensor([len(wav) for wav in [wavs]]).to(device)
wavs = pad_sequence([wavs], batch_first=True).to(device)
# we recommend use_mask=True during fine-tuning
feats = xeus_model.encode(wavs, wav_lengths, use_mask=False, use_final_output=False)[0][-1] # take the output of the last layer
```
With Flash Attention:
```python
[layer.use_flash_attn = True for layer in xeus_model.encoder.encoders]
with torch.cuda.amp.autocast(dtype=torch.bfloat16):
feats = xeus_model.encode(wavs, wav_lengths, use_mask=False, use_final_output=False)[0][-1]
```
Tune the masking settings:
```python
xeus_model.masker.mask_prob = 0.65 # default 0.8
xeus_model.masker.mask_length = 20 # default 10
xeus_model.masker.mask_selection = 'static' # default 'uniform'
xeus_model.train()
feats = xeus_model.encode(wavs, wav_lengths, use_mask=True, use_final_output=False)[0][-1]
```
## Results


|