Transformers documentation
Image-to-Image 작업 가이드
Image-to-Image 작업 가이드
Image-to-Image 작업은 애플리케이션이 이미지를 입력받아 또 다른 이미지를 출력하는 작업입니다. 여기에는 이미지 향상(초고해상도, 저조도 향상, 빗줄기 제거 등), 이미지 복원 등 다양한 하위 작업이 포함됩니다.
이 가이드에서는 다음을 수행하는 방법을 보여줍니다.
- 초고해상도 작업을 위한 image-to-image 파이프라인 사용,
- 파이프라인 없이 동일한 작업을 위한 image-to-image 모델 실행
이 가이드가 발표된 시점에서는, image-to-image
파이프라인은 초고해상도 작업만 지원한다는 점을 유의하세요.
필요한 라이브러리를 설치하는 것부터 시작하겠습니다.
pip install transformers
이제 Swin2SR 모델을 사용하여 파이프라인을 초기화할 수 있습니다. 그런 다음 이미지와 함께 호출하여 파이프라인으로 추론할 수 있습니다. 현재 이 파이프라인에서는 Swin2SR 모델만 지원됩니다.
from transformers import pipeline
device = torch.device('cuda' if torch.cuda.is_available() else 'cpu')
pipe = pipeline(task="image-to-image", model="caidas/swin2SR-lightweight-x2-64", device=device)
이제 이미지를 불러와 봅시다.
from PIL import Image
import requests
url = "https://huggingface.co/datasets/huggingface/documentation-images/resolve/main/transformers/tasks/cat.jpg"
image = Image.open(requests.get(url, stream=True).raw)
print(image.size)
# (532, 432)
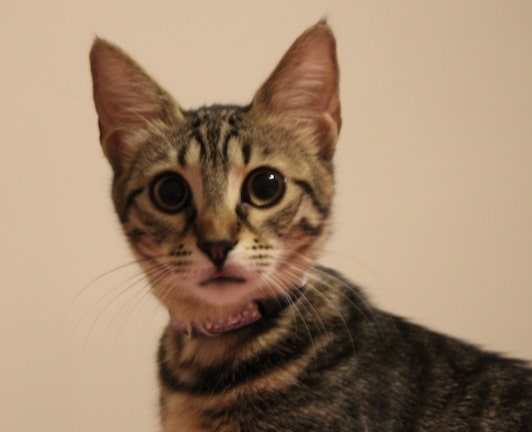
이제 파이프라인으로 추론을 수행할 수 있습니다. 고양이 이미지의 업스케일된 버전을 얻을 수 있습니다.
upscaled = pipe(image)
print(upscaled.size)
# (1072, 880)
파이프라인 없이 직접 추론을 수행하려면 Transformers의 Swin2SRForImageSuperResolution
및 Swin2SRImageProcessor
클래스를 사용할 수 있습니다. 이를 위해 동일한 모델 체크포인트를 사용합니다. 모델과 프로세서를 초기화해 보겠습니다.
from transformers import Swin2SRForImageSuperResolution, Swin2SRImageProcessor
model = Swin2SRForImageSuperResolution.from_pretrained("caidas/swin2SR-lightweight-x2-64").to(device)
processor = Swin2SRImageProcessor("caidas/swin2SR-lightweight-x2-64")
pipeline
우리가 직접 수행해야 하는 전처리와 후처리 단계를 추상화하므로, 이미지를 전처리해 보겠습니다. 이미지를 프로세서에 전달한 다음 픽셀값을 GPU로 이동시키겠습니다.
pixel_values = processor(image, return_tensors="pt").pixel_values
print(pixel_values.shape)
pixel_values = pixel_values.to(device)
이제 픽셀값을 모델에 전달하여 이미지를 추론할 수 있습니다.
import torch
with torch.no_grad():
outputs = model(pixel_values)
출력은 아래와 같은 ImageSuperResolutionOutput
유형의 객체입니다 👇
(loss=None, reconstruction=tensor([[[[0.8270, 0.8269, 0.8275, ..., 0.7463, 0.7446, 0.7453],
[0.8287, 0.8278, 0.8283, ..., 0.7451, 0.7448, 0.7457],
[0.8280, 0.8273, 0.8269, ..., 0.7447, 0.7446, 0.7452],
...,
[0.5923, 0.5933, 0.5924, ..., 0.0697, 0.0695, 0.0706],
[0.5926, 0.5932, 0.5926, ..., 0.0673, 0.0687, 0.0705],
[0.5927, 0.5914, 0.5922, ..., 0.0664, 0.0694, 0.0718]]]],
device='cuda:0'), hidden_states=None, attentions=None)
reconstruction
를 가져와 시각화를 위해 후처리해야 합니다. 어떻게 생겼는지 살펴봅시다.
outputs.reconstruction.data.shape
# torch.Size([1, 3, 880, 1072])
출력 텐서의 차원을 축소하고 0번째 축을 제거한 다음, 값을 클리핑하고 NumPy 부동소수점 배열로 변환해야 합니다. 그런 다음 [1072, 880] 모양을 갖도록 축을 재정렬하고 마지막으로 출력을 0과 255 사이의 값을 갖도록 되돌립니다.
import numpy as np
# 크기를 줄이고, CPU로 이동하고, 값을 클리핑
output = outputs.reconstruction.data.squeeze().cpu().clamp_(0, 1).numpy()
# 축을 재정렬
output = np.moveaxis(output, source=0, destination=-1)
# 값을 픽셀값 범위로 되돌리기
output = (output * 255.0).round().astype(np.uint8)
Image.fromarray(output)
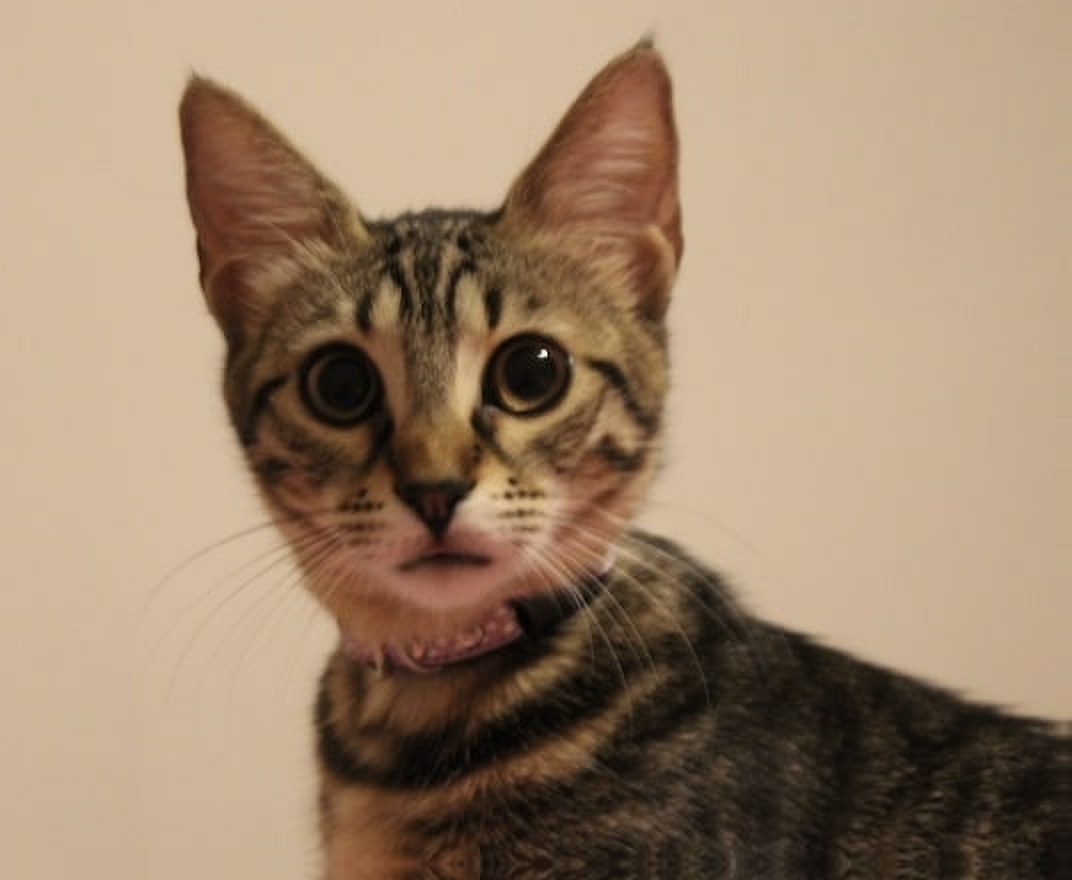