Deformable DETR
Overview
変形可能 DETR モデルは、Xizhou Zhu、Weijie Su、Lewei Lu、Bin Li、Xiaogang Wang, Jifeng Dai によって Deformable DETR: Deformable Transformers for End-to-End Object Detection で提案されました 変形可能な DETR は、参照周囲の少数の主要なサンプリング ポイントのみに注目する新しい変形可能なアテンション モジュールを利用することにより、収束の遅さの問題と元の DETR の制限された特徴の空間解像度を軽減します。
論文の要約は次のとおりです。
DETR は、優れたパフォーマンスを実証しながら、物体検出における多くの手作業で設計されたコンポーネントの必要性を排除するために最近提案されました。ただし、画像特徴マップの処理における Transformer アテンション モジュールの制限により、収束が遅く、特徴の空間解像度が制限されるという問題があります。これらの問題を軽減するために、私たちは Deformable DETR を提案しました。この DETR のアテンション モジュールは、参照周囲の少数の主要なサンプリング ポイントのみに注目します。変形可能な DETR は、10 分の 1 のトレーニング エポックで、DETR よりも優れたパフォーマンス (特に小さなオブジェクトの場合) を達成できます。 COCO ベンチマークに関する広範な実験により、私たちのアプローチの有効性が実証されました。
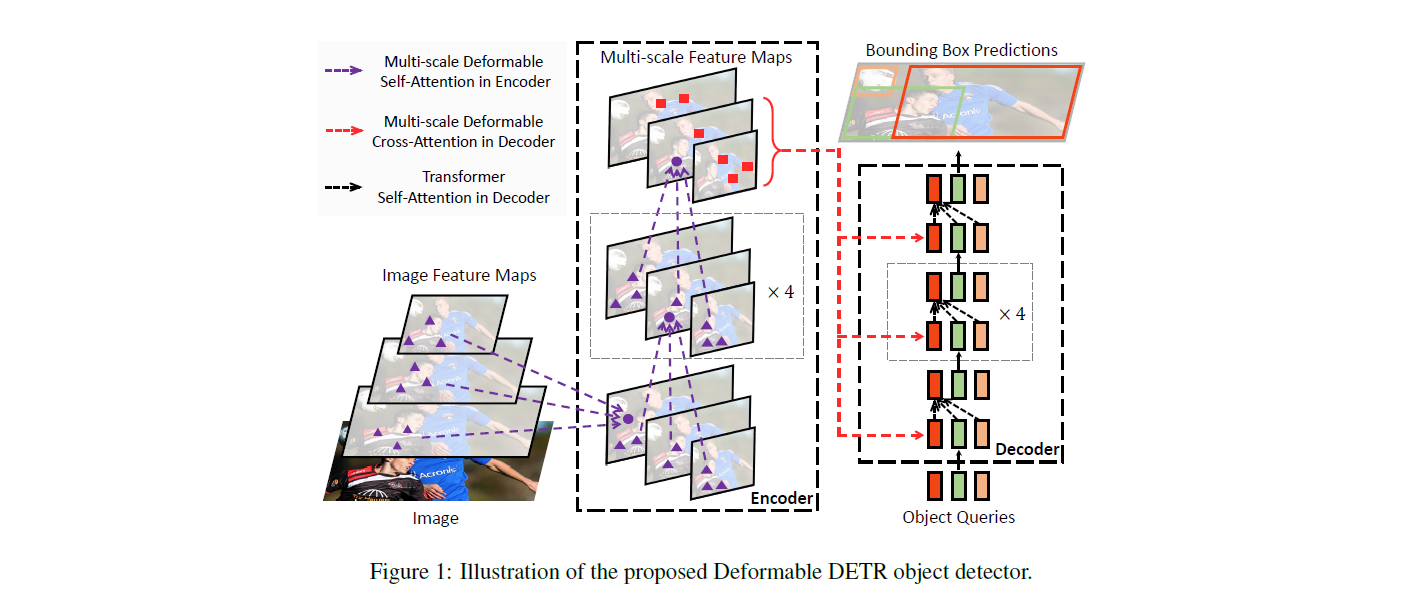
このモデルは、nielsr によって提供されました。元のコードは ここ にあります。
Usage tips
Resources
Deformable DETR の使用を開始するのに役立つ公式 Hugging Face およびコミュニティ (🌎 で示される) リソースのリスト。
- DeformableDetrForObjectDetection のカスタム データセットでの推論と微調整に関するデモ ノートブックは、こちら にあります。
- 物体検出タスクガイド も参照してください。
ここに含めるリソースの送信に興味がある場合は、お気軽にプル リクエストを開いてください。審査させていただきます。リソースは、既存のリソースを複製するのではなく、何か新しいものを示すことが理想的です。
DeformableDetrImageProcessor
class transformers.DeformableDetrImageProcessor
< source >( format: Union = <AnnotationFormat.COCO_DETECTION: 'coco_detection'> do_resize: bool = True size: Dict = None resample: Resampling = <Resampling.BILINEAR: 2> do_rescale: bool = True rescale_factor: Union = 0.00392156862745098 do_normalize: bool = True image_mean: Union = None image_std: Union = None do_convert_annotations: Optional = None do_pad: bool = True pad_size: Optional = None **kwargs )
Parameters
- format (
str
, optional, defaults to"coco_detection"
) — Data format of the annotations. One of “coco_detection” or “coco_panoptic”. - do_resize (
bool
, optional, defaults toTrue
) — Controls whether to resize the image’s (height, width) dimensions to the specifiedsize
. Can be overridden by thedo_resize
parameter in thepreprocess
method. - size (
Dict[str, int]
optional, defaults to{"shortest_edge" -- 800, "longest_edge": 1333}
): Size of the image’s(height, width)
dimensions after resizing. Can be overridden by thesize
parameter in thepreprocess
method. Available options are:{"height": int, "width": int}
: The image will be resized to the exact size(height, width)
. Do NOT keep the aspect ratio.{"shortest_edge": int, "longest_edge": int}
: The image will be resized to a maximum size respecting the aspect ratio and keeping the shortest edge less or equal toshortest_edge
and the longest edge less or equal tolongest_edge
.{"max_height": int, "max_width": int}
: The image will be resized to the maximum size respecting the aspect ratio and keeping the height less or equal tomax_height
and the width less or equal tomax_width
.
- resample (
PILImageResampling
, optional, defaults toPILImageResampling.BILINEAR
) — Resampling filter to use if resizing the image. - do_rescale (
bool
, optional, defaults toTrue
) — Controls whether to rescale the image by the specified scalerescale_factor
. Can be overridden by thedo_rescale
parameter in thepreprocess
method. - rescale_factor (
int
orfloat
, optional, defaults to1/255
) — Scale factor to use if rescaling the image. Can be overridden by therescale_factor
parameter in thepreprocess
method. do_normalize — Controls whether to normalize the image. Can be overridden by thedo_normalize
parameter in thepreprocess
method. - image_mean (
float
orList[float]
, optional, defaults toIMAGENET_DEFAULT_MEAN
) — Mean values to use when normalizing the image. Can be a single value or a list of values, one for each channel. Can be overridden by theimage_mean
parameter in thepreprocess
method. - image_std (
float
orList[float]
, optional, defaults toIMAGENET_DEFAULT_STD
) — Standard deviation values to use when normalizing the image. Can be a single value or a list of values, one for each channel. Can be overridden by theimage_std
parameter in thepreprocess
method. - do_convert_annotations (
bool
, optional, defaults toTrue
) — Controls whether to convert the annotations to the format expected by the DETR model. Converts the bounding boxes to the format(center_x, center_y, width, height)
and in the range[0, 1]
. Can be overridden by thedo_convert_annotations
parameter in thepreprocess
method. - do_pad (
bool
, optional, defaults toTrue
) — Controls whether to pad the image. Can be overridden by thedo_pad
parameter in thepreprocess
method. IfTrue
, padding will be applied to the bottom and right of the image with zeros. Ifpad_size
is provided, the image will be padded to the specified dimensions. Otherwise, the image will be padded to the maximum height and width of the batch. - pad_size (
Dict[str, int]
, optional) — The size{"height": int, "width" int}
to pad the images to. Must be larger than any image size provided for preprocessing. Ifpad_size
is not provided, images will be padded to the largest height and width in the batch.
Constructs a Deformable DETR image processor.
preprocess
< source >( images: Union annotations: Union = None return_segmentation_masks: bool = None masks_path: Union = None do_resize: Optional = None size: Optional = None resample = None do_rescale: Optional = None rescale_factor: Union = None do_normalize: Optional = None do_convert_annotations: Optional = None image_mean: Union = None image_std: Union = None do_pad: Optional = None format: Union = None return_tensors: Union = None data_format: Union = <ChannelDimension.FIRST: 'channels_first'> input_data_format: Union = None pad_size: Optional = None **kwargs )
Parameters
- images (
ImageInput
) — Image or batch of images to preprocess. Expects a single or batch of images with pixel values ranging from 0 to 255. If passing in images with pixel values between 0 and 1, setdo_rescale=False
. - annotations (
AnnotationType
orList[AnnotationType]
, optional) — List of annotations associated with the image or batch of images. If annotation is for object detection, the annotations should be a dictionary with the following keys:- “image_id” (
int
): The image id. - “annotations” (
List[Dict]
): List of annotations for an image. Each annotation should be a dictionary. An image can have no annotations, in which case the list should be empty. If annotation is for segmentation, the annotations should be a dictionary with the following keys: - “image_id” (
int
): The image id. - “segments_info” (
List[Dict]
): List of segments for an image. Each segment should be a dictionary. An image can have no segments, in which case the list should be empty. - “file_name” (
str
): The file name of the image.
- “image_id” (
- return_segmentation_masks (
bool
, optional, defaults to self.return_segmentation_masks) — Whether to return segmentation masks. - masks_path (
str
orpathlib.Path
, optional) — Path to the directory containing the segmentation masks. - do_resize (
bool
, optional, defaults to self.do_resize) — Whether to resize the image. - size (
Dict[str, int]
, optional, defaults to self.size) — Size of the image’s(height, width)
dimensions after resizing. Available options are:{"height": int, "width": int}
: The image will be resized to the exact size(height, width)
. Do NOT keep the aspect ratio.{"shortest_edge": int, "longest_edge": int}
: The image will be resized to a maximum size respecting the aspect ratio and keeping the shortest edge less or equal toshortest_edge
and the longest edge less or equal tolongest_edge
.{"max_height": int, "max_width": int}
: The image will be resized to the maximum size respecting the aspect ratio and keeping the height less or equal tomax_height
and the width less or equal tomax_width
.
- resample (
PILImageResampling
, optional, defaults to self.resample) — Resampling filter to use when resizing the image. - do_rescale (
bool
, optional, defaults to self.do_rescale) — Whether to rescale the image. - rescale_factor (
float
, optional, defaults to self.rescale_factor) — Rescale factor to use when rescaling the image. - do_normalize (
bool
, optional, defaults to self.do_normalize) — Whether to normalize the image. - do_convert_annotations (
bool
, optional, defaults to self.do_convert_annotations) — Whether to convert the annotations to the format expected by the model. Converts the bounding boxes from the format(top_left_x, top_left_y, width, height)
to(center_x, center_y, width, height)
and in relative coordinates. - image_mean (
float
orList[float]
, optional, defaults to self.image_mean) — Mean to use when normalizing the image. - image_std (
float
orList[float]
, optional, defaults to self.image_std) — Standard deviation to use when normalizing the image. - do_pad (
bool
, optional, defaults to self.do_pad) — Whether to pad the image. IfTrue
, padding will be applied to the bottom and right of the image with zeros. Ifpad_size
is provided, the image will be padded to the specified dimensions. Otherwise, the image will be padded to the maximum height and width of the batch. - format (
str
orAnnotationFormat
, optional, defaults to self.format) — Format of the annotations. - return_tensors (
str
orTensorType
, optional, defaults to self.return_tensors) — Type of tensors to return. IfNone
, will return the list of images. - data_format (
ChannelDimension
orstr
, optional, defaults toChannelDimension.FIRST
) — The channel dimension format for the output image. Can be one of:"channels_first"
orChannelDimension.FIRST
: image in (num_channels, height, width) format."channels_last"
orChannelDimension.LAST
: image in (height, width, num_channels) format.- Unset: Use the channel dimension format of the input image.
- input_data_format (
ChannelDimension
orstr
, optional) — The channel dimension format for the input image. If unset, the channel dimension format is inferred from the input image. Can be one of:"channels_first"
orChannelDimension.FIRST
: image in (num_channels, height, width) format."channels_last"
orChannelDimension.LAST
: image in (height, width, num_channels) format."none"
orChannelDimension.NONE
: image in (height, width) format.
- pad_size (
Dict[str, int]
, optional) — The size{"height": int, "width" int}
to pad the images to. Must be larger than any image size provided for preprocessing. Ifpad_size
is not provided, images will be padded to the largest height and width in the batch.
Preprocess an image or a batch of images so that it can be used by the model.
post_process_object_detection
< source >( outputs threshold: float = 0.5 target_sizes: Union = None top_k: int = 100 ) → List[Dict]
Parameters
- outputs (
DetrObjectDetectionOutput
) — Raw outputs of the model. - threshold (
float
, optional) — Score threshold to keep object detection predictions. - target_sizes (
torch.Tensor
orList[Tuple[int, int]]
, optional) — Tensor of shape(batch_size, 2)
or list of tuples (Tuple[int, int]
) containing the target size (height, width) of each image in the batch. If left to None, predictions will not be resized. - top_k (
int
, optional, defaults to 100) — Keep only top k bounding boxes before filtering by thresholding.
Returns
List[Dict]
A list of dictionaries, each dictionary containing the scores, labels and boxes for an image in the batch as predicted by the model.
Converts the raw output of DeformableDetrForObjectDetection into final bounding boxes in (top_left_x, top_left_y, bottom_right_x, bottom_right_y) format. Only supports PyTorch.
DeformableDetrFeatureExtractor
Preprocess an image or a batch of images.
post_process_object_detection
< source >( outputs threshold: float = 0.5 target_sizes: Union = None top_k: int = 100 ) → List[Dict]
Parameters
- outputs (
DetrObjectDetectionOutput
) — Raw outputs of the model. - threshold (
float
, optional) — Score threshold to keep object detection predictions. - target_sizes (
torch.Tensor
orList[Tuple[int, int]]
, optional) — Tensor of shape(batch_size, 2)
or list of tuples (Tuple[int, int]
) containing the target size (height, width) of each image in the batch. If left to None, predictions will not be resized. - top_k (
int
, optional, defaults to 100) — Keep only top k bounding boxes before filtering by thresholding.
Returns
List[Dict]
A list of dictionaries, each dictionary containing the scores, labels and boxes for an image in the batch as predicted by the model.
Converts the raw output of DeformableDetrForObjectDetection into final bounding boxes in (top_left_x, top_left_y, bottom_right_x, bottom_right_y) format. Only supports PyTorch.
DeformableDetrConfig
class transformers.DeformableDetrConfig
< source >( use_timm_backbone = True backbone_config = None num_channels = 3 num_queries = 300 max_position_embeddings = 1024 encoder_layers = 6 encoder_ffn_dim = 1024 encoder_attention_heads = 8 decoder_layers = 6 decoder_ffn_dim = 1024 decoder_attention_heads = 8 encoder_layerdrop = 0.0 is_encoder_decoder = True activation_function = 'relu' d_model = 256 dropout = 0.1 attention_dropout = 0.0 activation_dropout = 0.0 init_std = 0.02 init_xavier_std = 1.0 return_intermediate = True auxiliary_loss = False position_embedding_type = 'sine' backbone = 'resnet50' use_pretrained_backbone = True backbone_kwargs = None dilation = False num_feature_levels = 4 encoder_n_points = 4 decoder_n_points = 4 two_stage = False two_stage_num_proposals = 300 with_box_refine = False class_cost = 1 bbox_cost = 5 giou_cost = 2 mask_loss_coefficient = 1 dice_loss_coefficient = 1 bbox_loss_coefficient = 5 giou_loss_coefficient = 2 eos_coefficient = 0.1 focal_alpha = 0.25 disable_custom_kernels = False **kwargs )
Parameters
- use_timm_backbone (
bool
, optional, defaults toTrue
) — Whether or not to use thetimm
library for the backbone. If set toFalse
, will use theAutoBackbone
API. - backbone_config (
PretrainedConfig
ordict
, optional) — The configuration of the backbone model. Only used in caseuse_timm_backbone
is set toFalse
in which case it will default toResNetConfig()
. - num_channels (
int
, optional, defaults to 3) — The number of input channels. - num_queries (
int
, optional, defaults to 300) — Number of object queries, i.e. detection slots. This is the maximal number of objects DeformableDetrModel can detect in a single image. In casetwo_stage
is set toTrue
, we usetwo_stage_num_proposals
instead. - d_model (
int
, optional, defaults to 256) — Dimension of the layers. - encoder_layers (
int
, optional, defaults to 6) — Number of encoder layers. - decoder_layers (
int
, optional, defaults to 6) — Number of decoder layers. - encoder_attention_heads (
int
, optional, defaults to 8) — Number of attention heads for each attention layer in the Transformer encoder. - decoder_attention_heads (
int
, optional, defaults to 8) — Number of attention heads for each attention layer in the Transformer decoder. - decoder_ffn_dim (
int
, optional, defaults to 1024) — Dimension of the “intermediate” (often named feed-forward) layer in decoder. - encoder_ffn_dim (
int
, optional, defaults to 1024) — Dimension of the “intermediate” (often named feed-forward) layer in decoder. - activation_function (
str
orfunction
, optional, defaults to"relu"
) — The non-linear activation function (function or string) in the encoder and pooler. If string,"gelu"
,"relu"
,"silu"
and"gelu_new"
are supported. - dropout (
float
, optional, defaults to 0.1) — The dropout probability for all fully connected layers in the embeddings, encoder, and pooler. - attention_dropout (
float
, optional, defaults to 0.0) — The dropout ratio for the attention probabilities. - activation_dropout (
float
, optional, defaults to 0.0) — The dropout ratio for activations inside the fully connected layer. - init_std (
float
, optional, defaults to 0.02) — The standard deviation of the truncated_normal_initializer for initializing all weight matrices. - init_xavier_std (
float
, optional, defaults to 1) — The scaling factor used for the Xavier initialization gain in the HM Attention map module. - encoder_layerdrop (
float
, optional, defaults to 0.0) — The LayerDrop probability for the encoder. See the [LayerDrop paper](see https://arxiv.org/abs/1909.11556) for more details. - auxiliary_loss (
bool
, optional, defaults toFalse
) — Whether auxiliary decoding losses (loss at each decoder layer) are to be used. - position_embedding_type (
str
, optional, defaults to"sine"
) — Type of position embeddings to be used on top of the image features. One of"sine"
or"learned"
. - backbone (
str
, optional, defaults to"resnet50"
) — Name of backbone to use whenbackbone_config
isNone
. Ifuse_pretrained_backbone
isTrue
, this will load the corresponding pretrained weights from the timm or transformers library. Ifuse_pretrained_backbone
isFalse
, this loads the backbone’s config and uses that to initialize the backbone with random weights. - use_pretrained_backbone (
bool
, optional, defaults toTrue
) — Whether to use pretrained weights for the backbone. - backbone_kwargs (
dict
, optional) — Keyword arguments to be passed to AutoBackbone when loading from a checkpoint e.g.{'out_indices': (0, 1, 2, 3)}
. Cannot be specified ifbackbone_config
is set. - dilation (
bool
, optional, defaults toFalse
) — Whether to replace stride with dilation in the last convolutional block (DC5). Only supported whenuse_timm_backbone
=True
. - class_cost (
float
, optional, defaults to 1) — Relative weight of the classification error in the Hungarian matching cost. - bbox_cost (
float
, optional, defaults to 5) — Relative weight of the L1 error of the bounding box coordinates in the Hungarian matching cost. - giou_cost (
float
, optional, defaults to 2) — Relative weight of the generalized IoU loss of the bounding box in the Hungarian matching cost. - mask_loss_coefficient (
float
, optional, defaults to 1) — Relative weight of the Focal loss in the panoptic segmentation loss. - dice_loss_coefficient (
float
, optional, defaults to 1) — Relative weight of the DICE/F-1 loss in the panoptic segmentation loss. - bbox_loss_coefficient (
float
, optional, defaults to 5) — Relative weight of the L1 bounding box loss in the object detection loss. - giou_loss_coefficient (
float
, optional, defaults to 2) — Relative weight of the generalized IoU loss in the object detection loss. - eos_coefficient (
float
, optional, defaults to 0.1) — Relative classification weight of the ‘no-object’ class in the object detection loss. - num_feature_levels (
int
, optional, defaults to 4) — The number of input feature levels. - encoder_n_points (
int
, optional, defaults to 4) — The number of sampled keys in each feature level for each attention head in the encoder. - decoder_n_points (
int
, optional, defaults to 4) — The number of sampled keys in each feature level for each attention head in the decoder. - two_stage (
bool
, optional, defaults toFalse
) — Whether to apply a two-stage deformable DETR, where the region proposals are also generated by a variant of Deformable DETR, which are further fed into the decoder for iterative bounding box refinement. - two_stage_num_proposals (
int
, optional, defaults to 300) — The number of region proposals to be generated, in casetwo_stage
is set toTrue
. - with_box_refine (
bool
, optional, defaults toFalse
) — Whether to apply iterative bounding box refinement, where each decoder layer refines the bounding boxes based on the predictions from the previous layer. - focal_alpha (
float
, optional, defaults to 0.25) — Alpha parameter in the focal loss. - disable_custom_kernels (
bool
, optional, defaults toFalse
) — Disable the use of custom CUDA and CPU kernels. This option is necessary for the ONNX export, as custom kernels are not supported by PyTorch ONNX export.
This is the configuration class to store the configuration of a DeformableDetrModel. It is used to instantiate a Deformable DETR model according to the specified arguments, defining the model architecture. Instantiating a configuration with the defaults will yield a similar configuration to that of the Deformable DETR SenseTime/deformable-detr architecture.
Configuration objects inherit from PretrainedConfig and can be used to control the model outputs. Read the documentation from PretrainedConfig for more information.
Examples:
>>> from transformers import DeformableDetrConfig, DeformableDetrModel
>>> # Initializing a Deformable DETR SenseTime/deformable-detr style configuration
>>> configuration = DeformableDetrConfig()
>>> # Initializing a model (with random weights) from the SenseTime/deformable-detr style configuration
>>> model = DeformableDetrModel(configuration)
>>> # Accessing the model configuration
>>> configuration = model.config
DeformableDetrModel
class transformers.DeformableDetrModel
< source >( config: DeformableDetrConfig )
Parameters
- config (DeformableDetrConfig) — Model configuration class with all the parameters of the model. Initializing with a config file does not load the weights associated with the model, only the configuration. Check out the from_pretrained() method to load the model weights.
The bare Deformable DETR Model (consisting of a backbone and encoder-decoder Transformer) outputting raw hidden-states without any specific head on top.
This model inherits from PreTrainedModel. Check the superclass documentation for the generic methods the library implements for all its model (such as downloading or saving, resizing the input embeddings, pruning heads etc.)
This model is also a PyTorch torch.nn.Module subclass. Use it as a regular PyTorch Module and refer to the PyTorch documentation for all matter related to general usage and behavior.
forward
< source >( pixel_values: FloatTensor pixel_mask: Optional = None decoder_attention_mask: Optional = None encoder_outputs: Optional = None inputs_embeds: Optional = None decoder_inputs_embeds: Optional = None output_attentions: Optional = None output_hidden_states: Optional = None return_dict: Optional = None ) → transformers.models.deformable_detr.modeling_deformable_detr.DeformableDetrModelOutput
or tuple(torch.FloatTensor)
Parameters
- pixel_values (
torch.FloatTensor
of shape(batch_size, num_channels, height, width)
) — Pixel values. Padding will be ignored by default should you provide it.Pixel values can be obtained using AutoImageProcessor. See DeformableDetrImageProcessor.call() for details.
- pixel_mask (
torch.LongTensor
of shape(batch_size, height, width)
, optional) — Mask to avoid performing attention on padding pixel values. Mask values selected in[0, 1]
:- 1 for pixels that are real (i.e. not masked),
- 0 for pixels that are padding (i.e. masked).
- decoder_attention_mask (
torch.FloatTensor
of shape(batch_size, num_queries)
, optional) — Not used by default. Can be used to mask object queries. - encoder_outputs (
tuple(tuple(torch.FloatTensor)
, optional) — Tuple consists of (last_hidden_state
, optional:hidden_states
, optional:attentions
)last_hidden_state
of shape(batch_size, sequence_length, hidden_size)
, optional) is a sequence of hidden-states at the output of the last layer of the encoder. Used in the cross-attention of the decoder. - inputs_embeds (
torch.FloatTensor
of shape(batch_size, sequence_length, hidden_size)
, optional) — Optionally, instead of passing the flattened feature map (output of the backbone + projection layer), you can choose to directly pass a flattened representation of an image. - decoder_inputs_embeds (
torch.FloatTensor
of shape(batch_size, num_queries, hidden_size)
, optional) — Optionally, instead of initializing the queries with a tensor of zeros, you can choose to directly pass an embedded representation. - output_attentions (
bool
, optional) — Whether or not to return the attentions tensors of all attention layers. Seeattentions
under returned tensors for more detail. - output_hidden_states (
bool
, optional) — Whether or not to return the hidden states of all layers. Seehidden_states
under returned tensors for more detail. - return_dict (
bool
, optional) — Whether or not to return a ModelOutput instead of a plain tuple.
Returns
transformers.models.deformable_detr.modeling_deformable_detr.DeformableDetrModelOutput
or tuple(torch.FloatTensor)
A transformers.models.deformable_detr.modeling_deformable_detr.DeformableDetrModelOutput
or a tuple of
torch.FloatTensor
(if return_dict=False
is passed or when config.return_dict=False
) comprising various
elements depending on the configuration (DeformableDetrConfig) and inputs.
- init_reference_points (
torch.FloatTensor
of shape(batch_size, num_queries, 4)
) — Initial reference points sent through the Transformer decoder. - last_hidden_state (
torch.FloatTensor
of shape(batch_size, num_queries, hidden_size)
) — Sequence of hidden-states at the output of the last layer of the decoder of the model. - intermediate_hidden_states (
torch.FloatTensor
of shape(batch_size, config.decoder_layers, num_queries, hidden_size)
) — Stacked intermediate hidden states (output of each layer of the decoder). - intermediate_reference_points (
torch.FloatTensor
of shape(batch_size, config.decoder_layers, num_queries, 4)
) — Stacked intermediate reference points (reference points of each layer of the decoder). - decoder_hidden_states (
tuple(torch.FloatTensor)
, optional, returned whenoutput_hidden_states=True
is passed or whenconfig.output_hidden_states=True
) — Tuple oftorch.FloatTensor
(one for the output of the embeddings + one for the output of each layer) of shape(batch_size, num_queries, hidden_size)
. Hidden-states of the decoder at the output of each layer plus the initial embedding outputs. - decoder_attentions (
tuple(torch.FloatTensor)
, optional, returned whenoutput_attentions=True
is passed or whenconfig.output_attentions=True
) — Tuple oftorch.FloatTensor
(one for each layer) of shape(batch_size, num_heads, num_queries, num_queries)
. Attentions weights of the decoder, after the attention softmax, used to compute the weighted average in the self-attention heads. - cross_attentions (
tuple(torch.FloatTensor)
, optional, returned whenoutput_attentions=True
is passed or whenconfig.output_attentions=True
) — Tuple oftorch.FloatTensor
(one for each layer) of shape(batch_size, num_queries, num_heads, 4, 4)
. Attentions weights of the decoder’s cross-attention layer, after the attention softmax, used to compute the weighted average in the cross-attention heads. - encoder_last_hidden_state (
torch.FloatTensor
of shape(batch_size, sequence_length, hidden_size)
, optional) — Sequence of hidden-states at the output of the last layer of the encoder of the model. - encoder_hidden_states (
tuple(torch.FloatTensor)
, optional, returned whenoutput_hidden_states=True
is passed or whenconfig.output_hidden_states=True
) — Tuple oftorch.FloatTensor
(one for the output of the embeddings + one for the output of each layer) of shape(batch_size, sequence_length, hidden_size)
. Hidden-states of the encoder at the output of each layer plus the initial embedding outputs. - encoder_attentions (
tuple(torch.FloatTensor)
, optional, returned whenoutput_attentions=True
is passed or whenconfig.output_attentions=True
) — Tuple oftorch.FloatTensor
(one for each layer) of shape(batch_size, num_queries, num_heads, 4, 4)
. Attentions weights of the encoder, after the attention softmax, used to compute the weighted average in the self-attention heads. - enc_outputs_class (
torch.FloatTensor
of shape(batch_size, sequence_length, config.num_labels)
, optional, returned whenconfig.with_box_refine=True
andconfig.two_stage=True
) — Predicted bounding boxes scores where the topconfig.two_stage_num_proposals
scoring bounding boxes are picked as region proposals in the first stage. Output of bounding box binary classification (i.e. foreground and background). - enc_outputs_coord_logits (
torch.FloatTensor
of shape(batch_size, sequence_length, 4)
, optional, returned whenconfig.with_box_refine=True
andconfig.two_stage=True
) — Logits of predicted bounding boxes coordinates in the first stage.
The DeformableDetrModel forward method, overrides the __call__
special method.
Although the recipe for forward pass needs to be defined within this function, one should call the Module
instance afterwards instead of this since the former takes care of running the pre and post processing steps while
the latter silently ignores them.
Examples:
>>> from transformers import AutoImageProcessor, DeformableDetrModel
>>> from PIL import Image
>>> import requests
>>> url = "http://images.cocodataset.org/val2017/000000039769.jpg"
>>> image = Image.open(requests.get(url, stream=True).raw)
>>> image_processor = AutoImageProcessor.from_pretrained("SenseTime/deformable-detr")
>>> model = DeformableDetrModel.from_pretrained("SenseTime/deformable-detr")
>>> inputs = image_processor(images=image, return_tensors="pt")
>>> outputs = model(**inputs)
>>> last_hidden_states = outputs.last_hidden_state
>>> list(last_hidden_states.shape)
[1, 300, 256]
DeformableDetrForObjectDetection
class transformers.DeformableDetrForObjectDetection
< source >( config: DeformableDetrConfig )
Parameters
- config (DeformableDetrConfig) — Model configuration class with all the parameters of the model. Initializing with a config file does not load the weights associated with the model, only the configuration. Check out the from_pretrained() method to load the model weights.
Deformable DETR Model (consisting of a backbone and encoder-decoder Transformer) with object detection heads on top, for tasks such as COCO detection.
This model inherits from PreTrainedModel. Check the superclass documentation for the generic methods the library implements for all its model (such as downloading or saving, resizing the input embeddings, pruning heads etc.)
This model is also a PyTorch torch.nn.Module subclass. Use it as a regular PyTorch Module and refer to the PyTorch documentation for all matter related to general usage and behavior.
forward
< source >( pixel_values: FloatTensor pixel_mask: Optional = None decoder_attention_mask: Optional = None encoder_outputs: Optional = None inputs_embeds: Optional = None decoder_inputs_embeds: Optional = None labels: Optional = None output_attentions: Optional = None output_hidden_states: Optional = None return_dict: Optional = None ) → transformers.models.deformable_detr.modeling_deformable_detr.DeformableDetrObjectDetectionOutput
or tuple(torch.FloatTensor)
Parameters
- pixel_values (
torch.FloatTensor
of shape(batch_size, num_channels, height, width)
) — Pixel values. Padding will be ignored by default should you provide it.Pixel values can be obtained using AutoImageProcessor. See DeformableDetrImageProcessor.call() for details.
- pixel_mask (
torch.LongTensor
of shape(batch_size, height, width)
, optional) — Mask to avoid performing attention on padding pixel values. Mask values selected in[0, 1]
:- 1 for pixels that are real (i.e. not masked),
- 0 for pixels that are padding (i.e. masked).
- decoder_attention_mask (
torch.FloatTensor
of shape(batch_size, num_queries)
, optional) — Not used by default. Can be used to mask object queries. - encoder_outputs (
tuple(tuple(torch.FloatTensor)
, optional) — Tuple consists of (last_hidden_state
, optional:hidden_states
, optional:attentions
)last_hidden_state
of shape(batch_size, sequence_length, hidden_size)
, optional) is a sequence of hidden-states at the output of the last layer of the encoder. Used in the cross-attention of the decoder. - inputs_embeds (
torch.FloatTensor
of shape(batch_size, sequence_length, hidden_size)
, optional) — Optionally, instead of passing the flattened feature map (output of the backbone + projection layer), you can choose to directly pass a flattened representation of an image. - decoder_inputs_embeds (
torch.FloatTensor
of shape(batch_size, num_queries, hidden_size)
, optional) — Optionally, instead of initializing the queries with a tensor of zeros, you can choose to directly pass an embedded representation. - output_attentions (
bool
, optional) — Whether or not to return the attentions tensors of all attention layers. Seeattentions
under returned tensors for more detail. - output_hidden_states (
bool
, optional) — Whether or not to return the hidden states of all layers. Seehidden_states
under returned tensors for more detail. - return_dict (
bool
, optional) — Whether or not to return a ModelOutput instead of a plain tuple. - labels (
List[Dict]
of len(batch_size,)
, optional) — Labels for computing the bipartite matching loss. List of dicts, each dictionary containing at least the following 2 keys: ‘class_labels’ and ‘boxes’ (the class labels and bounding boxes of an image in the batch respectively). The class labels themselves should be atorch.LongTensor
of len(number of bounding boxes in the image,)
and the boxes atorch.FloatTensor
of shape(number of bounding boxes in the image, 4)
.
Returns
transformers.models.deformable_detr.modeling_deformable_detr.DeformableDetrObjectDetectionOutput
or tuple(torch.FloatTensor)
A transformers.models.deformable_detr.modeling_deformable_detr.DeformableDetrObjectDetectionOutput
or a tuple of
torch.FloatTensor
(if return_dict=False
is passed or when config.return_dict=False
) comprising various
elements depending on the configuration (DeformableDetrConfig) and inputs.
- loss (
torch.FloatTensor
of shape(1,)
, optional, returned whenlabels
are provided)) — Total loss as a linear combination of a negative log-likehood (cross-entropy) for class prediction and a bounding box loss. The latter is defined as a linear combination of the L1 loss and the generalized scale-invariant IoU loss. - loss_dict (
Dict
, optional) — A dictionary containing the individual losses. Useful for logging. - logits (
torch.FloatTensor
of shape(batch_size, num_queries, num_classes + 1)
) — Classification logits (including no-object) for all queries. - pred_boxes (
torch.FloatTensor
of shape(batch_size, num_queries, 4)
) — Normalized boxes coordinates for all queries, represented as (center_x, center_y, width, height). These values are normalized in [0, 1], relative to the size of each individual image in the batch (disregarding possible padding). You can use~DeformableDetrProcessor.post_process_object_detection
to retrieve the unnormalized bounding boxes. - auxiliary_outputs (
list[Dict]
, optional) — Optional, only returned when auxilary losses are activated (i.e.config.auxiliary_loss
is set toTrue
) and labels are provided. It is a list of dictionaries containing the two above keys (logits
andpred_boxes
) for each decoder layer. - last_hidden_state (
torch.FloatTensor
of shape(batch_size, num_queries, hidden_size)
, optional) — Sequence of hidden-states at the output of the last layer of the decoder of the model. - decoder_hidden_states (
tuple(torch.FloatTensor)
, optional, returned whenoutput_hidden_states=True
is passed or whenconfig.output_hidden_states=True
) — Tuple oftorch.FloatTensor
(one for the output of the embeddings + one for the output of each layer) of shape(batch_size, num_queries, hidden_size)
. Hidden-states of the decoder at the output of each layer plus the initial embedding outputs. - decoder_attentions (
tuple(torch.FloatTensor)
, optional, returned whenoutput_attentions=True
is passed or whenconfig.output_attentions=True
) — Tuple oftorch.FloatTensor
(one for each layer) of shape(batch_size, num_heads, num_queries, num_queries)
. Attentions weights of the decoder, after the attention softmax, used to compute the weighted average in the self-attention heads. - cross_attentions (
tuple(torch.FloatTensor)
, optional, returned whenoutput_attentions=True
is passed or whenconfig.output_attentions=True
) — Tuple oftorch.FloatTensor
(one for each layer) of shape(batch_size, num_queries, num_heads, 4, 4)
. Attentions weights of the decoder’s cross-attention layer, after the attention softmax, used to compute the weighted average in the cross-attention heads. - encoder_last_hidden_state (
torch.FloatTensor
of shape(batch_size, sequence_length, hidden_size)
, optional) — Sequence of hidden-states at the output of the last layer of the encoder of the model. - encoder_hidden_states (
tuple(torch.FloatTensor)
, optional, returned whenoutput_hidden_states=True
is passed or whenconfig.output_hidden_states=True
) — Tuple oftorch.FloatTensor
(one for the output of the embeddings + one for the output of each layer) of shape(batch_size, sequence_length, hidden_size)
. Hidden-states of the encoder at the output of each layer plus the initial embedding outputs. - encoder_attentions (
tuple(torch.FloatTensor)
, optional, returned whenoutput_attentions=True
is passed or whenconfig.output_attentions=True
) — Tuple oftorch.FloatTensor
(one for each layer) of shape(batch_size, sequence_length, num_heads, 4, 4)
. Attentions weights of the encoder, after the attention softmax, used to compute the weighted average in the self-attention heads. - intermediate_hidden_states (
torch.FloatTensor
of shape(batch_size, config.decoder_layers, num_queries, hidden_size)
) — Stacked intermediate hidden states (output of each layer of the decoder). - intermediate_reference_points (
torch.FloatTensor
of shape(batch_size, config.decoder_layers, num_queries, 4)
) — Stacked intermediate reference points (reference points of each layer of the decoder). - init_reference_points (
torch.FloatTensor
of shape(batch_size, num_queries, 4)
) — Initial reference points sent through the Transformer decoder. - enc_outputs_class (
torch.FloatTensor
of shape(batch_size, sequence_length, config.num_labels)
, optional, returned whenconfig.with_box_refine=True
andconfig.two_stage=True
) — Predicted bounding boxes scores where the topconfig.two_stage_num_proposals
scoring bounding boxes are picked as region proposals in the first stage. Output of bounding box binary classification (i.e. foreground and background). - enc_outputs_coord_logits (
torch.FloatTensor
of shape(batch_size, sequence_length, 4)
, optional, returned whenconfig.with_box_refine=True
andconfig.two_stage=True
) — Logits of predicted bounding boxes coordinates in the first stage.
The DeformableDetrForObjectDetection forward method, overrides the __call__
special method.
Although the recipe for forward pass needs to be defined within this function, one should call the Module
instance afterwards instead of this since the former takes care of running the pre and post processing steps while
the latter silently ignores them.
Examples:
>>> from transformers import AutoImageProcessor, DeformableDetrForObjectDetection
>>> from PIL import Image
>>> import requests
>>> url = "http://images.cocodataset.org/val2017/000000039769.jpg"
>>> image = Image.open(requests.get(url, stream=True).raw)
>>> image_processor = AutoImageProcessor.from_pretrained("SenseTime/deformable-detr")
>>> model = DeformableDetrForObjectDetection.from_pretrained("SenseTime/deformable-detr")
>>> inputs = image_processor(images=image, return_tensors="pt")
>>> outputs = model(**inputs)
>>> # convert outputs (bounding boxes and class logits) to Pascal VOC format (xmin, ymin, xmax, ymax)
>>> target_sizes = torch.tensor([image.size[::-1]])
>>> results = image_processor.post_process_object_detection(outputs, threshold=0.5, target_sizes=target_sizes)[
... 0
... ]
>>> for score, label, box in zip(results["scores"], results["labels"], results["boxes"]):
... box = [round(i, 2) for i in box.tolist()]
... print(
... f"Detected {model.config.id2label[label.item()]} with confidence "
... f"{round(score.item(), 3)} at location {box}"
... )
Detected cat with confidence 0.8 at location [16.5, 52.84, 318.25, 470.78]
Detected cat with confidence 0.789 at location [342.19, 24.3, 640.02, 372.25]
Detected remote with confidence 0.633 at location [40.79, 72.78, 176.76, 117.25]