Datasets:
language:
- tr
license:
- cc-by-sa-4.0
multilinguality:
- monolingual
size_categories:
- 1K<n<10K
task_categories:
- token-classification
task_ids:
- named-entity-recognition
pretty_name: Vitamins and Supplements NER Dataset
Dataset Card for turkish-nlp-suite/vitamins-supplements-NER
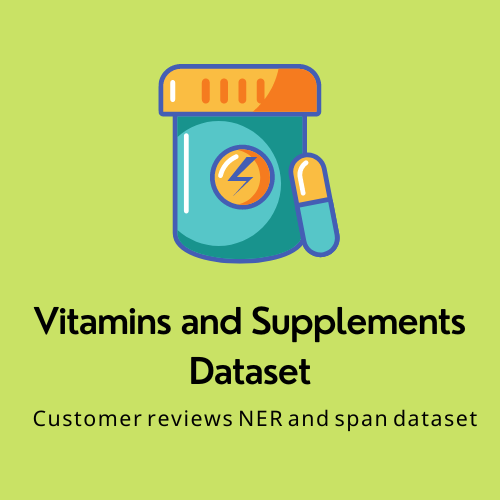
Dataset Description
- Repository: Vitamins and Supplements NER Dataset
- Paper: ACL link
- Dataset: Vitamins and Supplements NER Dataset
- Domain: E-commerce, customer reviews, medical
Dataset Summary
The Vitamins and Supplements NER Dataset is a NER dataset containing customer reviews with entity and span annotations. User reviews were collected from a popular supplement products e- commerce website Vitaminler.com. Each customer review in the Vitamins and Supplements NER Dataset describes a customer’s experience with a supplement product in terms of that product’s effectiveness, side effects, taste and smell, as well as comments on supplement usage frequency and dosage, active ingredients, brand, and similar products by other brands. An example review from the dataset with entity and span annotations looks like this:

The customer praises a biotin supplement; in their review they stated that they suffer from Thyroiditis and as a result they're experiencing hair loss. They purchased the biotin product to prevent the hair fall and they described the effectiveness of the product as "their hair loss reduced noticably". Visual is created by displaCy.
Tagset
For this dataset we annotated both entities and spans. Span annotations are common in medical NLP datasets, spans capture the information about "what happens with the entity", i.e. more semantics about the entities in the text. NER tags and their distribution are in the dataset are as follows:
Tag | Count |
---|---|
Disease | 1.875 |
Biomolecule | 859 |
User | 634 |
Other_product | 543 |
Recommender | 436 |
Dosage | 471 |
Brand | 275 |
User_demographics | 192 |
Ingredient | 175 |
Other_brand | 121 |
Distribution of span tags:
Tag | Count |
---|---|
Effect | 2.562 |
Side_effect | 608 |
Taste_smell | 558 |
Health_complaints | 858 |
All annotations are done by Co-one. many thanks to them for their contributions.
Dataset Instances
The dataset includes around 2.5K annotated reviews with annotations. Each dataset instance contains
- customer review text
- entities and spans annotated
Here's an example for you:
{
"text": "Bu zamana kadar kullandığım en iyi B12 takviyesi. Doktorum saç dökülmem için verdi ama aç karnına dil altına bir fıs kullanınca KABIZLIK sorunumu çözdü. çok mutlu oldum. Indirimde gördüğünüz an kaçırmayın derim."
"spans": [
{ "val": "saç dökülmem", "label": "HASTALIK", "start": 59, "end": 71 },
{ "val": " KABIZLIK", "label": "HASTALIK", "start": 127, "end": 136 },
{ "val": "B12", "label": "BİYOMOLEKÜL", "start": 35, "end": 38 },
{ "val": " Doktorum", "label": "TAVSİYE_EDEN", "start": 49, "end": 58 },
{ "val": "bir fıs", "label": "DOZ", "start": 109, "end": 116 }
]
}
If you're rather interested in a big JSON, you can find the dataset as a single JSON in dataset's Github repo.
Data Split
name | train | validation | test |
---|---|---|---|
Vitamins and Supplements NER Dataset | 2072 | 200 | 200 |
Citation
This work is supported by Google Developer Experts Program. Part of Duygu 2022 Fall-Winter collection, "Turkish NLP with Duygu"/ "Duygu'yla Türkçe NLP". All rights reserved. If you'd like to use this dataset in your own work, please kindly cite A Diverse Set of Freely Available Linguistic Resources for Turkish :
@inproceedings{altinok-2023-diverse,
title = "A Diverse Set of Freely Available Linguistic Resources for {T}urkish",
author = "Altinok, Duygu",
booktitle = "Proceedings of the 61st Annual Meeting of the Association for Computational Linguistics (Volume 1: Long Papers)",
month = jul,
year = "2023",
address = "Toronto, Canada",
publisher = "Association for Computational Linguistics",
url = "https://aclanthology.org/2023.acl-long.768",
pages = "13739--13750",
abstract = "This study presents a diverse set of freely available linguistic resources for Turkish natural language processing, including corpora, pretrained models and education material. Although Turkish is spoken by a sizeable population of over 80 million people, Turkish linguistic resources for natural language processing remain scarce. In this study, we provide corpora to allow practitioners to build their own applications and pretrained models that would assist industry researchers in creating quick prototypes. The provided corpora include named entity recognition datasets of diverse genres, including Wikipedia articles and supplement products customer reviews. In addition, crawling e-commerce and movie reviews websites, we compiled several sentiment analysis datasets of different genres. Our linguistic resources for Turkish also include pretrained spaCy language models. To the best of our knowledge, our models are the first spaCy models trained for the Turkish language. Finally, we provide various types of education material, such as video tutorials and code examples, that can support the interested audience on practicing Turkish NLP. The advantages of our linguistic resources are three-fold: they are freely available, they are first of their kind, and they are easy to use in a broad range of implementations. Along with a thorough description of the resource creation process, we also explain the position of our resources in the Turkish NLP world.",
}