modelId
stringlengths 4
122
| author
stringlengths 2
42
| last_modified
unknown | downloads
int64 0
392M
| likes
int64 0
6.56k
| library_name
stringclasses 368
values | tags
sequencelengths 1
4.05k
| pipeline_tag
stringclasses 51
values | createdAt
unknown | card
stringlengths 1
1M
|
---|---|---|---|---|---|---|---|---|---|
google/owlvit-base-patch32 | google | "2023-12-12T13:47:41Z" | 552,847 | 123 | transformers | [
"transformers",
"pytorch",
"safetensors",
"owlvit",
"zero-shot-object-detection",
"vision",
"arxiv:2205.06230",
"license:apache-2.0",
"region:us"
] | zero-shot-object-detection | "2022-07-05T06:30:01Z" | ---
license: apache-2.0
tags:
- vision
- zero-shot-object-detection
inference: false
---
# Model Card: OWL-ViT
## Model Details
The OWL-ViT (short for Vision Transformer for Open-World Localization) was proposed in [Simple Open-Vocabulary Object Detection with Vision Transformers](https://arxiv.org/abs/2205.06230) by Matthias Minderer, Alexey Gritsenko, Austin Stone, Maxim Neumann, Dirk Weissenborn, Alexey Dosovitskiy, Aravindh Mahendran, Anurag Arnab, Mostafa Dehghani, Zhuoran Shen, Xiao Wang, Xiaohua Zhai, Thomas Kipf, and Neil Houlsby. OWL-ViT is a zero-shot text-conditioned object detection model that can be used to query an image with one or multiple text queries.
OWL-ViT uses CLIP as its multi-modal backbone, with a ViT-like Transformer to get visual features and a causal language model to get the text features. To use CLIP for detection, OWL-ViT removes the final token pooling layer of the vision model and attaches a lightweight classification and box head to each transformer output token. Open-vocabulary classification is enabled by replacing the fixed classification layer weights with the class-name embeddings obtained from the text model. The authors first train CLIP from scratch and fine-tune it end-to-end with the classification and box heads on standard detection datasets using a bipartite matching loss. One or multiple text queries per image can be used to perform zero-shot text-conditioned object detection.
### Model Date
May 2022
### Model Type
The model uses a CLIP backbone with a ViT-B/32 Transformer architecture as an image encoder and uses a masked self-attention Transformer as a text encoder. These encoders are trained to maximize the similarity of (image, text) pairs via a contrastive loss. The CLIP backbone is trained from scratch and fine-tuned together with the box and class prediction heads with an object detection objective.
### Documents
- [OWL-ViT Paper](https://arxiv.org/abs/2205.06230)
### Use with Transformers
```python3
import requests
from PIL import Image
import torch
from transformers import OwlViTProcessor, OwlViTForObjectDetection
processor = OwlViTProcessor.from_pretrained("google/owlvit-base-patch32")
model = OwlViTForObjectDetection.from_pretrained("google/owlvit-base-patch32")
url = "http://images.cocodataset.org/val2017/000000039769.jpg"
image = Image.open(requests.get(url, stream=True).raw)
texts = [["a photo of a cat", "a photo of a dog"]]
inputs = processor(text=texts, images=image, return_tensors="pt")
outputs = model(**inputs)
# Target image sizes (height, width) to rescale box predictions [batch_size, 2]
target_sizes = torch.Tensor([image.size[::-1]])
# Convert outputs (bounding boxes and class logits) to COCO API
results = processor.post_process_object_detection(outputs=outputs, threshold=0.1, target_sizes=target_sizes)
i = 0 # Retrieve predictions for the first image for the corresponding text queries
text = texts[i]
boxes, scores, labels = results[i]["boxes"], results[i]["scores"], results[i]["labels"]
# Print detected objects and rescaled box coordinates
for box, score, label in zip(boxes, scores, labels):
box = [round(i, 2) for i in box.tolist()]
print(f"Detected {text[label]} with confidence {round(score.item(), 3)} at location {box}")
```
## Model Use
### Intended Use
The model is intended as a research output for research communities. We hope that this model will enable researchers to better understand and explore zero-shot, text-conditioned object detection. We also hope it can be used for interdisciplinary studies of the potential impact of such models, especially in areas that commonly require identifying objects whose label is unavailable during training.
#### Primary intended uses
The primary intended users of these models are AI researchers.
We primarily imagine the model will be used by researchers to better understand robustness, generalization, and other capabilities, biases, and constraints of computer vision models.
## Data
The CLIP backbone of the model was trained on publicly available image-caption data. This was done through a combination of crawling a handful of websites and using commonly-used pre-existing image datasets such as [YFCC100M](http://projects.dfki.uni-kl.de/yfcc100m/). A large portion of the data comes from our crawling of the internet. This means that the data is more representative of people and societies most connected to the internet. The prediction heads of OWL-ViT, along with the CLIP backbone, are fine-tuned on publicly available object detection datasets such as [COCO](https://cocodataset.org/#home) and [OpenImages](https://storage.googleapis.com/openimages/web/index.html).
### BibTeX entry and citation info
```bibtex
@article{minderer2022simple,
title={Simple Open-Vocabulary Object Detection with Vision Transformers},
author={Matthias Minderer, Alexey Gritsenko, Austin Stone, Maxim Neumann, Dirk Weissenborn, Alexey Dosovitskiy, Aravindh Mahendran, Anurag Arnab, Mostafa Dehghani, Zhuoran Shen, Xiao Wang, Xiaohua Zhai, Thomas Kipf, Neil Houlsby},
journal={arXiv preprint arXiv:2205.06230},
year={2022},
}
``` |
facebook/detr-resnet-50 | facebook | "2024-04-10T13:56:31Z" | 546,019 | 727 | transformers | [
"transformers",
"pytorch",
"safetensors",
"detr",
"object-detection",
"vision",
"dataset:coco",
"arxiv:2005.12872",
"license:apache-2.0",
"endpoints_compatible",
"region:us"
] | object-detection | "2022-03-02T23:29:05Z" | ---
license: apache-2.0
tags:
- object-detection
- vision
datasets:
- coco
widget:
- src: https://huggingface.co/datasets/mishig/sample_images/resolve/main/savanna.jpg
example_title: Savanna
- src: https://huggingface.co/datasets/mishig/sample_images/resolve/main/football-match.jpg
example_title: Football Match
- src: https://huggingface.co/datasets/mishig/sample_images/resolve/main/airport.jpg
example_title: Airport
---
# DETR (End-to-End Object Detection) model with ResNet-50 backbone
DEtection TRansformer (DETR) model trained end-to-end on COCO 2017 object detection (118k annotated images). It was introduced in the paper [End-to-End Object Detection with Transformers](https://arxiv.org/abs/2005.12872) by Carion et al. and first released in [this repository](https://github.com/facebookresearch/detr).
Disclaimer: The team releasing DETR did not write a model card for this model so this model card has been written by the Hugging Face team.
## Model description
The DETR model is an encoder-decoder transformer with a convolutional backbone. Two heads are added on top of the decoder outputs in order to perform object detection: a linear layer for the class labels and a MLP (multi-layer perceptron) for the bounding boxes. The model uses so-called object queries to detect objects in an image. Each object query looks for a particular object in the image. For COCO, the number of object queries is set to 100.
The model is trained using a "bipartite matching loss": one compares the predicted classes + bounding boxes of each of the N = 100 object queries to the ground truth annotations, padded up to the same length N (so if an image only contains 4 objects, 96 annotations will just have a "no object" as class and "no bounding box" as bounding box). The Hungarian matching algorithm is used to create an optimal one-to-one mapping between each of the N queries and each of the N annotations. Next, standard cross-entropy (for the classes) and a linear combination of the L1 and generalized IoU loss (for the bounding boxes) are used to optimize the parameters of the model.

## Intended uses & limitations
You can use the raw model for object detection. See the [model hub](https://huggingface.co/models?search=facebook/detr) to look for all available DETR models.
### How to use
Here is how to use this model:
```python
from transformers import DetrImageProcessor, DetrForObjectDetection
import torch
from PIL import Image
import requests
url = "http://images.cocodataset.org/val2017/000000039769.jpg"
image = Image.open(requests.get(url, stream=True).raw)
# you can specify the revision tag if you don't want the timm dependency
processor = DetrImageProcessor.from_pretrained("facebook/detr-resnet-50", revision="no_timm")
model = DetrForObjectDetection.from_pretrained("facebook/detr-resnet-50", revision="no_timm")
inputs = processor(images=image, return_tensors="pt")
outputs = model(**inputs)
# convert outputs (bounding boxes and class logits) to COCO API
# let's only keep detections with score > 0.9
target_sizes = torch.tensor([image.size[::-1]])
results = processor.post_process_object_detection(outputs, target_sizes=target_sizes, threshold=0.9)[0]
for score, label, box in zip(results["scores"], results["labels"], results["boxes"]):
box = [round(i, 2) for i in box.tolist()]
print(
f"Detected {model.config.id2label[label.item()]} with confidence "
f"{round(score.item(), 3)} at location {box}"
)
```
This should output:
```
Detected remote with confidence 0.998 at location [40.16, 70.81, 175.55, 117.98]
Detected remote with confidence 0.996 at location [333.24, 72.55, 368.33, 187.66]
Detected couch with confidence 0.995 at location [-0.02, 1.15, 639.73, 473.76]
Detected cat with confidence 0.999 at location [13.24, 52.05, 314.02, 470.93]
Detected cat with confidence 0.999 at location [345.4, 23.85, 640.37, 368.72]
```
Currently, both the feature extractor and model support PyTorch.
## Training data
The DETR model was trained on [COCO 2017 object detection](https://cocodataset.org/#download), a dataset consisting of 118k/5k annotated images for training/validation respectively.
## Training procedure
### Preprocessing
The exact details of preprocessing of images during training/validation can be found [here](https://github.com/google-research/vision_transformer/blob/master/vit_jax/input_pipeline.py).
Images are resized/rescaled such that the shortest side is at least 800 pixels and the largest side at most 1333 pixels, and normalized across the RGB channels with the ImageNet mean (0.485, 0.456, 0.406) and standard deviation (0.229, 0.224, 0.225).
### Training
The model was trained for 300 epochs on 16 V100 GPUs. This takes 3 days, with 4 images per GPU (hence a total batch size of 64).
## Evaluation results
This model achieves an AP (average precision) of **42.0** on COCO 2017 validation. For more details regarding evaluation results, we refer to table 1 of the original paper.
### BibTeX entry and citation info
```bibtex
@article{DBLP:journals/corr/abs-2005-12872,
author = {Nicolas Carion and
Francisco Massa and
Gabriel Synnaeve and
Nicolas Usunier and
Alexander Kirillov and
Sergey Zagoruyko},
title = {End-to-End Object Detection with Transformers},
journal = {CoRR},
volume = {abs/2005.12872},
year = {2020},
url = {https://arxiv.org/abs/2005.12872},
archivePrefix = {arXiv},
eprint = {2005.12872},
timestamp = {Thu, 28 May 2020 17:38:09 +0200},
biburl = {https://dblp.org/rec/journals/corr/abs-2005-12872.bib},
bibsource = {dblp computer science bibliography, https://dblp.org}
}
``` |
ibm-granite/granite-timeseries-ttm-r1 | ibm-granite | "2024-11-07T10:18:15Z" | 542,791 | 218 | transformers | [
"transformers",
"safetensors",
"tinytimemixer",
"time series",
"forecasting",
"pretrained models",
"foundation models",
"time series foundation models",
"time-series",
"time-series-forecasting",
"arxiv:2401.03955",
"license:apache-2.0",
"endpoints_compatible",
"region:us"
] | time-series-forecasting | "2024-04-05T03:20:10Z" | ---
license: apache-2.0
pipeline_tag: time-series-forecasting
tags:
- time series
- forecasting
- pretrained models
- foundation models
- time series foundation models
- time-series
---
# Granite-TimeSeries-TTM-R1 Model Card
<p align="center" width="100%">
<img src="ttm_image.webp" width="600">
</p>
TinyTimeMixers (TTMs) are compact pre-trained models for Multivariate Time-Series Forecasting, open-sourced by IBM Research.
**With less than 1 Million parameters, TTM (accepted in NeurIPS 24) introduces the notion of the first-ever “tiny” pre-trained models for Time-Series Forecasting.**
TTM outperforms several popular benchmarks demanding billions of parameters in zero-shot and few-shot forecasting. TTMs are lightweight
forecasters, pre-trained on publicly available time series data with various augmentations. TTM provides state-of-the-art zero-shot forecasts and can easily be
fine-tuned for multi-variate forecasts with just 5% of the training data to be competitive. Refer to our [paper](https://arxiv.org/pdf/2401.03955.pdf) for more details.
**The current open-source version supports point forecasting use-cases specifically ranging from minutely to hourly resolutions
(Ex. 10 min, 15 min, 1 hour.).**
**Note that zeroshot, fine-tuning and inference tasks using TTM can easily be executed in 1 GPU machine or in laptops too!!**
**New updates:** TTM-R1 comprises TTM variants pre-trained on 250M public training samples. We have another set of TTM models released recently under TTM-R2 trained on a much larger pretraining
dataset (~700M samples) which can be accessed from [here](https://huggingface.co/ibm-granite/granite-timeseries-ttm-r2). In general, TTM-R2 models perform better than
TTM-R1 models as they are trained on larger pretraining dataset. However, the choice of R1 vs R2 depends on your target data distribution. Hence requesting users to
try both R1 and R2 variants and pick the best for your data.
## Model Description
TTM falls under the category of “focused pre-trained models”, wherein each pre-trained TTM is tailored for a particular forecasting
setting (governed by the context length and forecast length). Instead of building one massive model supporting all forecasting settings,
we opt for the approach of constructing smaller pre-trained models, each focusing on a specific forecasting setting, thereby
yielding more accurate results. Furthermore, this approach ensures that our models remain extremely small and exceptionally fast,
facilitating easy deployment without demanding a ton of resources.
Hence, in this model card, we plan to release several pre-trained
TTMs that can cater to many common forecasting settings in practice. Additionally, we have released our source code along with
our pretraining scripts that users can utilize to pretrain models on their own. Pretraining TTMs is very easy and fast, taking
only 3-6 hours using 6 A100 GPUs, as opposed to several days or weeks in traditional approaches.
Each pre-trained model will be released in a different branch name in this model card. Kindly access the required model using our
getting started [notebook](https://github.com/IBM/tsfm/blob/main/notebooks/hfdemo/ttm_getting_started.ipynb) mentioning the branch name.
## Model Releases (along with the branch name where the models are stored):
- **512-96:** Given the last 512 time-points (i.e. context length), this model can forecast up to next 96 time-points (i.e. forecast length)
in future. This model is targeted towards a forecasting setting of context length 512 and forecast length 96 and
recommended for hourly and minutely resolutions (Ex. 10 min, 15 min, 1 hour, etc). This model refers to the TTM-Q variant used in the paper. (branch name: main) [[Benchmark Scripts]](https://github.com/ibm-granite/granite-tsfm/blob/main/notebooks/hfdemo/tinytimemixer/ttm-r1_benchmarking_512_96.ipynb)
- **1024-96:** Given the last 1024 time-points (i.e. context length), this model can forecast up to next 96 time-points (i.e. forecast length)
in future. This model is targeted towards a long forecasting setting of context length 1024 and forecast length 96 and
recommended for hourly and minutely resolutions (Ex. 10 min, 15 min, 1 hour, etc). (branch name: 1024-96-v1) [[Benchmark Scripts]](https://github.com/ibm-granite/granite-tsfm/blob/main/notebooks/hfdemo/tinytimemixer/ttm-r1_benchmarking_1024_96.ipynb)
We can also use the [[get_model]](https://github.com/ibm-granite/granite-tsfm/blob/main/tsfm_public/toolkit/get_model.py) utility to automatically select the required model based on your input context length and forecast length requirement.
For more variants (till forecast length 720), refer to our new model card [here](https://huggingface.co/ibm-granite/granite-timeseries-ttm-r2)
## Model Capabilities with example scripts
The below model scripts can be used for any of the above TTM models. Please update the HF model URL and branch name in the `from_pretrained` call appropriately to pick the model of your choice.
- Getting Started [[colab]](https://colab.research.google.com/github/ibm-granite/granite-tsfm/blob/main/notebooks/hfdemo/ttm_getting_started.ipynb)
- Zeroshot Multivariate Forecasting [[Example]](https://github.com/ibm-granite/granite-tsfm/blob/main/notebooks/hfdemo/ttm_getting_started.ipynb)
- Finetuned Multivariate Forecasting:
- Channel-Independent Finetuning [[Example 1]](https://github.com/ibm-granite/granite-tsfm/blob/main/notebooks/hfdemo/ttm_getting_started.ipynb) [[Example 2]](https://github.com/ibm-granite/granite-tsfm/blob/main/notebooks/hfdemo/tinytimemixer/ttm_m4_hourly.ipynb)
- Channel-Mix Finetuning [[Example]](https://github.com/ibm-granite/granite-tsfm/blob/main/notebooks/tutorial/ttm_channel_mix_finetuning.ipynb)
- **New Releases (extended features released on October 2024)**
- Finetuning and Forecasting with Exogenous/Control Variables [[Example]](https://github.com/ibm-granite/granite-tsfm/blob/main/notebooks/tutorial/ttm_with_exog_tutorial.ipynb)
- Finetuning and Forecasting with static categorical features [Example: To be added soon]
- Rolling Forecasts - Extend forecast lengths beyond 96 via rolling capability [[Example]](https://github.com/ibm-granite/granite-tsfm/blob/main/notebooks/hfdemo/ttm_rolling_prediction_getting_started.ipynb)
- Helper scripts for optimal Learning Rate suggestions for Finetuning [[Example]](https://github.com/ibm-granite/granite-tsfm/blob/main/notebooks/tutorial/ttm_with_exog_tutorial.ipynb)
## Benchmarks
TTM outperforms popular benchmarks such as TimesFM, Moirai, Chronos, Lag-Llama, Moment, GPT4TS, TimeLLM, LLMTime in zero/fewshot forecasting while reducing computational requirements significantly.
Moreover, TTMs are lightweight and can be executed even on CPU-only machines, enhancing usability and fostering wider
adoption in resource-constrained environments. For more details, refer to our [paper](https://arxiv.org/pdf/2401.03955.pdf) TTM-Q referred in the paper maps to the `512-96` model
uploaded in the main branch. For other variants (TTM-B, TTM-E and TTM-A) please refer [here](https://huggingface.co/ibm-granite/granite-timeseries-ttm-r2). For more details, refer to the paper.
<p align="center" width="100%">
<img src="benchmarks.webp" width="600">
</p>
## Recommended Use
1. Users have to externally standard scale their data independently for every channel before feeding it to the model (Refer to [TSP](https://github.com/IBM/tsfm/blob/main/tsfm_public/toolkit/time_series_preprocessor.py), our data processing utility for data scaling.)
2. The current open-source version supports only minutely and hourly resolutions(Ex. 10 min, 15 min, 1 hour.). Other lower resolutions (say weekly, or monthly) are currently not supported in this version, as the model needs a minimum context length of 512 or 1024.
3. Enabling any upsampling or prepending zeros to virtually increase the context length for shorter-length datasets is not recommended and will
impact the model performance.
## Model Details
For more details on TTM architecture and benchmarks, refer to our [paper](https://arxiv.org/pdf/2401.03955.pdf).
TTM-1 currently supports 2 modes:
- **Zeroshot forecasting**: Directly apply the pre-trained model on your target data to get an initial forecast (with no training).
- **Finetuned forecasting**: Finetune the pre-trained model with a subset of your target data to further improve the forecast.
**Since, TTM models are extremely small and fast, it is practically very easy to finetune the model with your available target data in few minutes
to get more accurate forecasts.**
The current release supports multivariate forecasting via both channel independence and channel-mixing approaches.
Decoder Channel-Mixing can be enabled during fine-tuning for capturing strong channel-correlation patterns across
time-series variates, a critical capability lacking in existing counterparts.
In addition, TTM also supports exogenous infusion and categorical data infusion.
### Model Sources
- **Repository:** https://github.com/ibm-granite/granite-tsfm/tree/main/tsfm_public/models/tinytimemixer
- **Paper:** https://arxiv.org/pdf/2401.03955.pdf
### Blogs and articles on TTM:
- Refer to our [wiki](https://github.com/ibm-granite/granite-tsfm/wiki)
## Uses
```
# Load Model from HF Model Hub mentioning the branch name in revision field
model = TinyTimeMixerForPrediction.from_pretrained(
"https://huggingface.co/ibm/TTM", revision="main"
)
# Do zeroshot
zeroshot_trainer = Trainer(
model=model,
args=zeroshot_forecast_args,
)
)
zeroshot_output = zeroshot_trainer.evaluate(dset_test)
# Freeze backbone and enable few-shot or finetuning:
# freeze backbone
for param in model.backbone.parameters():
param.requires_grad = False
finetune_forecast_trainer = Trainer(
model=model,
args=finetune_forecast_args,
train_dataset=dset_train,
eval_dataset=dset_val,
callbacks=[early_stopping_callback, tracking_callback],
optimizers=(optimizer, scheduler),
)
finetune_forecast_trainer.train()
fewshot_output = finetune_forecast_trainer.evaluate(dset_test)
```
## Training Data
The original r1 TTM models were trained on a collection of datasets from the Monash Time Series Forecasting repository. The datasets used include:
- Australian Electricity Demand: https://zenodo.org/records/4659727
- Australian Weather: https://zenodo.org/records/4654822
- Bitcoin dataset: https://zenodo.org/records/5122101
- KDD Cup 2018 dataset: https://zenodo.org/records/4656756
- London Smart Meters: https://zenodo.org/records/4656091
- Saugeen River Flow: https://zenodo.org/records/4656058
- Solar Power: https://zenodo.org/records/4656027
- Sunspots: https://zenodo.org/records/4654722
- Solar: https://zenodo.org/records/4656144
- US Births: https://zenodo.org/records/4656049
- Wind Farms Production data: https://zenodo.org/records/4654858
- Wind Power: https://zenodo.org/records/4656032
## Citation
Kindly cite the following paper, if you intend to use our model or its associated architectures/approaches in your
work
**BibTeX:**
```
@inproceedings{ekambaram2024tinytimemixersttms,
title={Tiny Time Mixers (TTMs): Fast Pre-trained Models for Enhanced Zero/Few-Shot Forecasting of Multivariate Time Series},
author={Vijay Ekambaram and Arindam Jati and Pankaj Dayama and Sumanta Mukherjee and Nam H. Nguyen and Wesley M. Gifford and Chandra Reddy and Jayant Kalagnanam},
booktitle={Advances in Neural Information Processing Systems (NeurIPS 2024)},
year={2024},
}
```
## Model Card Authors
Vijay Ekambaram, Arindam Jati, Pankaj Dayama, Wesley M. Gifford, Sumanta Mukherjee, Chandra Reddy and Jayant Kalagnanam
## IBM Public Repository Disclosure:
All content in this repository including code has been provided by IBM under the associated
open source software license and IBM is under no obligation to provide enhancements,
updates, or support. IBM developers produced this code as an
open source project (not as an IBM product), and IBM makes no assertions as to
the level of quality nor security, and will not be maintaining this code going forward. |
sentence-transformers/stsb-xlm-r-multilingual | sentence-transformers | "2024-11-05T19:56:54Z" | 540,827 | 37 | sentence-transformers | [
"sentence-transformers",
"pytorch",
"tf",
"onnx",
"safetensors",
"openvino",
"xlm-roberta",
"feature-extraction",
"sentence-similarity",
"transformers",
"arxiv:1908.10084",
"license:apache-2.0",
"autotrain_compatible",
"text-embeddings-inference",
"endpoints_compatible",
"region:us"
] | sentence-similarity | "2022-03-02T23:29:05Z" | ---
license: apache-2.0
library_name: sentence-transformers
tags:
- sentence-transformers
- feature-extraction
- sentence-similarity
- transformers
pipeline_tag: sentence-similarity
---
# sentence-transformers/stsb-xlm-r-multilingual
This is a [sentence-transformers](https://www.SBERT.net) model: It maps sentences & paragraphs to a 768 dimensional dense vector space and can be used for tasks like clustering or semantic search.
## Usage (Sentence-Transformers)
Using this model becomes easy when you have [sentence-transformers](https://www.SBERT.net) installed:
```
pip install -U sentence-transformers
```
Then you can use the model like this:
```python
from sentence_transformers import SentenceTransformer
sentences = ["This is an example sentence", "Each sentence is converted"]
model = SentenceTransformer('sentence-transformers/stsb-xlm-r-multilingual')
embeddings = model.encode(sentences)
print(embeddings)
```
## Usage (HuggingFace Transformers)
Without [sentence-transformers](https://www.SBERT.net), you can use the model like this: First, you pass your input through the transformer model, then you have to apply the right pooling-operation on-top of the contextualized word embeddings.
```python
from transformers import AutoTokenizer, AutoModel
import torch
#Mean Pooling - Take attention mask into account for correct averaging
def mean_pooling(model_output, attention_mask):
token_embeddings = model_output[0] #First element of model_output contains all token embeddings
input_mask_expanded = attention_mask.unsqueeze(-1).expand(token_embeddings.size()).float()
return torch.sum(token_embeddings * input_mask_expanded, 1) / torch.clamp(input_mask_expanded.sum(1), min=1e-9)
# Sentences we want sentence embeddings for
sentences = ['This is an example sentence', 'Each sentence is converted']
# Load model from HuggingFace Hub
tokenizer = AutoTokenizer.from_pretrained('sentence-transformers/stsb-xlm-r-multilingual')
model = AutoModel.from_pretrained('sentence-transformers/stsb-xlm-r-multilingual')
# Tokenize sentences
encoded_input = tokenizer(sentences, padding=True, truncation=True, return_tensors='pt')
# Compute token embeddings
with torch.no_grad():
model_output = model(**encoded_input)
# Perform pooling. In this case, max pooling.
sentence_embeddings = mean_pooling(model_output, encoded_input['attention_mask'])
print("Sentence embeddings:")
print(sentence_embeddings)
```
## Evaluation Results
For an automated evaluation of this model, see the *Sentence Embeddings Benchmark*: [https://seb.sbert.net](https://seb.sbert.net?model_name=sentence-transformers/stsb-xlm-r-multilingual)
## Full Model Architecture
```
SentenceTransformer(
(0): Transformer({'max_seq_length': 128, 'do_lower_case': False}) with Transformer model: XLMRobertaModel
(1): Pooling({'word_embedding_dimension': 768, 'pooling_mode_cls_token': False, 'pooling_mode_mean_tokens': True, 'pooling_mode_max_tokens': False, 'pooling_mode_mean_sqrt_len_tokens': False})
)
```
## Citing & Authors
This model was trained by [sentence-transformers](https://www.sbert.net/).
If you find this model helpful, feel free to cite our publication [Sentence-BERT: Sentence Embeddings using Siamese BERT-Networks](https://arxiv.org/abs/1908.10084):
```bibtex
@inproceedings{reimers-2019-sentence-bert,
title = "Sentence-BERT: Sentence Embeddings using Siamese BERT-Networks",
author = "Reimers, Nils and Gurevych, Iryna",
booktitle = "Proceedings of the 2019 Conference on Empirical Methods in Natural Language Processing",
month = "11",
year = "2019",
publisher = "Association for Computational Linguistics",
url = "http://arxiv.org/abs/1908.10084",
}
``` |
diffusers/stable-diffusion-xl-1.0-inpainting-0.1 | diffusers | "2023-09-03T16:36:39Z" | 539,552 | 293 | diffusers | [
"diffusers",
"safetensors",
"stable-diffusion-xl",
"stable-diffusion-xl-diffusers",
"text-to-image",
"inpainting",
"arxiv:2112.10752",
"base_model:stabilityai/stable-diffusion-xl-base-1.0",
"base_model:finetune:stabilityai/stable-diffusion-xl-base-1.0",
"license:openrail++",
"diffusers:StableDiffusionXLInpaintPipeline",
"region:us"
] | text-to-image | "2023-09-01T14:07:10Z" |
---
license: openrail++
base_model: stabilityai/stable-diffusion-xl-base-1.0
tags:
- stable-diffusion-xl
- stable-diffusion-xl-diffusers
- text-to-image
- diffusers
- inpainting
inference: false
---
# SD-XL Inpainting 0.1 Model Card

SD-XL Inpainting 0.1 is a latent text-to-image diffusion model capable of generating photo-realistic images given any text input, with the extra capability of inpainting the pictures by using a mask.
The SD-XL Inpainting 0.1 was initialized with the `stable-diffusion-xl-base-1.0` weights. The model is trained for 40k steps at resolution 1024x1024 and 5% dropping of the text-conditioning to improve classifier-free classifier-free guidance sampling. For inpainting, the UNet has 5 additional input channels (4 for the encoded masked-image and 1 for the mask itself) whose weights were zero-initialized after restoring the non-inpainting checkpoint. During training, we generate synthetic masks and, in 25% mask everything.
## How to use
```py
from diffusers import AutoPipelineForInpainting
from diffusers.utils import load_image
import torch
pipe = AutoPipelineForInpainting.from_pretrained("diffusers/stable-diffusion-xl-1.0-inpainting-0.1", torch_dtype=torch.float16, variant="fp16").to("cuda")
img_url = "https://raw.githubusercontent.com/CompVis/latent-diffusion/main/data/inpainting_examples/overture-creations-5sI6fQgYIuo.png"
mask_url = "https://raw.githubusercontent.com/CompVis/latent-diffusion/main/data/inpainting_examples/overture-creations-5sI6fQgYIuo_mask.png"
image = load_image(img_url).resize((1024, 1024))
mask_image = load_image(mask_url).resize((1024, 1024))
prompt = "a tiger sitting on a park bench"
generator = torch.Generator(device="cuda").manual_seed(0)
image = pipe(
prompt=prompt,
image=image,
mask_image=mask_image,
guidance_scale=8.0,
num_inference_steps=20, # steps between 15 and 30 work well for us
strength=0.99, # make sure to use `strength` below 1.0
generator=generator,
).images[0]
```
**How it works:**
`image` | `mask_image`
:-------------------------:|:-------------------------:|
<img src="https://raw.githubusercontent.com/CompVis/latent-diffusion/main/data/inpainting_examples/overture-creations-5sI6fQgYIuo.png" alt="drawing" width="300"/> | <img src="https://raw.githubusercontent.com/CompVis/latent-diffusion/main/data/inpainting_examples/overture-creations-5sI6fQgYIuo_mask.png" alt="drawing" width="300"/>
`prompt` | `Output`
:-------------------------:|:-------------------------:|
<span style="position: relative;bottom: 150px;">a tiger sitting on a park bench</span> | <img src="https://huggingface.co/datasets/valhalla/images/resolve/main/tiger.png" alt="drawing" width="300"/>
## Model Description
- **Developed by:** The Diffusers team
- **Model type:** Diffusion-based text-to-image generative model
- **License:** [CreativeML Open RAIL++-M License](https://huggingface.co/stabilityai/stable-diffusion-xl-base-1.0/blob/main/LICENSE.md)
- **Model Description:** This is a model that can be used to generate and modify images based on text prompts. It is a [Latent Diffusion Model](https://arxiv.org/abs/2112.10752) that uses two fixed, pretrained text encoders ([OpenCLIP-ViT/G](https://github.com/mlfoundations/open_clip) and [CLIP-ViT/L](https://github.com/openai/CLIP/tree/main)).
## Uses
### Direct Use
The model is intended for research purposes only. Possible research areas and tasks include
- Generation of artworks and use in design and other artistic processes.
- Applications in educational or creative tools.
- Research on generative models.
- Safe deployment of models which have the potential to generate harmful content.
- Probing and understanding the limitations and biases of generative models.
Excluded uses are described below.
### Out-of-Scope Use
The model was not trained to be factual or true representations of people or events, and therefore using the model to generate such content is out-of-scope for the abilities of this model.
## Limitations and Bias
### Limitations
- The model does not achieve perfect photorealism
- The model cannot render legible text
- The model struggles with more difficult tasks which involve compositionality, such as rendering an image corresponding to “A red cube on top of a blue sphere”
- Faces and people in general may not be generated properly.
- The autoencoding part of the model is lossy.
- When the strength parameter is set to 1 (i.e. starting in-painting from a fully masked image), the quality of the image is degraded. The model retains the non-masked contents of the image, but images look less sharp. We're investing this and working on the next version.
### Bias
While the capabilities of image generation models are impressive, they can also reinforce or exacerbate social biases.
|
facebook/mms-1b-all | facebook | "2023-06-15T10:45:44Z" | 536,378 | 112 | transformers | [
"transformers",
"pytorch",
"safetensors",
"wav2vec2",
"automatic-speech-recognition",
"mms",
"ab",
"af",
"ak",
"am",
"ar",
"as",
"av",
"ay",
"az",
"ba",
"bm",
"be",
"bn",
"bi",
"bo",
"sh",
"br",
"bg",
"ca",
"cs",
"ce",
"cv",
"ku",
"cy",
"da",
"de",
"dv",
"dz",
"el",
"en",
"eo",
"et",
"eu",
"ee",
"fo",
"fa",
"fj",
"fi",
"fr",
"fy",
"ff",
"ga",
"gl",
"gn",
"gu",
"zh",
"ht",
"ha",
"he",
"hi",
"hu",
"hy",
"ig",
"ia",
"ms",
"is",
"it",
"jv",
"ja",
"kn",
"ka",
"kk",
"kr",
"km",
"ki",
"rw",
"ky",
"ko",
"kv",
"lo",
"la",
"lv",
"ln",
"lt",
"lb",
"lg",
"mh",
"ml",
"mr",
"mk",
"mg",
"mt",
"mn",
"mi",
"my",
"nl",
"no",
"ne",
"ny",
"oc",
"om",
"or",
"os",
"pa",
"pl",
"pt",
"ps",
"qu",
"ro",
"rn",
"ru",
"sg",
"sk",
"sl",
"sm",
"sn",
"sd",
"so",
"es",
"sq",
"su",
"sv",
"sw",
"ta",
"tt",
"te",
"tg",
"tl",
"th",
"ti",
"ts",
"tr",
"uk",
"vi",
"wo",
"xh",
"yo",
"zu",
"za",
"dataset:google/fleurs",
"arxiv:2305.13516",
"license:cc-by-nc-4.0",
"endpoints_compatible",
"region:us"
] | automatic-speech-recognition | "2023-05-27T11:43:21Z" | ---
tags:
- mms
language:
- ab
- af
- ak
- am
- ar
- as
- av
- ay
- az
- ba
- bm
- be
- bn
- bi
- bo
- sh
- br
- bg
- ca
- cs
- ce
- cv
- ku
- cy
- da
- de
- dv
- dz
- el
- en
- eo
- et
- eu
- ee
- fo
- fa
- fj
- fi
- fr
- fy
- ff
- ga
- gl
- gn
- gu
- zh
- ht
- ha
- he
- hi
- sh
- hu
- hy
- ig
- ia
- ms
- is
- it
- jv
- ja
- kn
- ka
- kk
- kr
- km
- ki
- rw
- ky
- ko
- kv
- lo
- la
- lv
- ln
- lt
- lb
- lg
- mh
- ml
- mr
- ms
- mk
- mg
- mt
- mn
- mi
- my
- zh
- nl
- 'no'
- 'no'
- ne
- ny
- oc
- om
- or
- os
- pa
- pl
- pt
- ms
- ps
- qu
- qu
- qu
- qu
- qu
- qu
- qu
- qu
- qu
- qu
- qu
- qu
- qu
- qu
- qu
- qu
- qu
- qu
- qu
- qu
- qu
- qu
- ro
- rn
- ru
- sg
- sk
- sl
- sm
- sn
- sd
- so
- es
- sq
- su
- sv
- sw
- ta
- tt
- te
- tg
- tl
- th
- ti
- ts
- tr
- uk
- ms
- vi
- wo
- xh
- ms
- yo
- ms
- zu
- za
license: cc-by-nc-4.0
datasets:
- google/fleurs
metrics:
- wer
---
# Massively Multilingual Speech (MMS) - Finetuned ASR - ALL
This checkpoint is a model fine-tuned for multi-lingual ASR and part of Facebook's [Massive Multilingual Speech project](https://research.facebook.com/publications/scaling-speech-technology-to-1000-languages/).
This checkpoint is based on the [Wav2Vec2 architecture](https://huggingface.co/docs/transformers/model_doc/wav2vec2) and makes use of adapter models to transcribe 1000+ languages.
The checkpoint consists of **1 billion parameters** and has been fine-tuned from [facebook/mms-1b](https://huggingface.co/facebook/mms-1b) on 1162 languages.
## Table Of Content
- [Example](#example)
- [Supported Languages](#supported-languages)
- [Model details](#model-details)
- [Additional links](#additional-links)
## Example
This MMS checkpoint can be used with [Transformers](https://github.com/huggingface/transformers) to transcribe audio of 1107 different
languages. Let's look at a simple example.
First, we install transformers and some other libraries
```
pip install torch accelerate torchaudio datasets
pip install --upgrade transformers
````
**Note**: In order to use MMS you need to have at least `transformers >= 4.30` installed. If the `4.30` version
is not yet available [on PyPI](https://pypi.org/project/transformers/) make sure to install `transformers` from
source:
```
pip install git+https://github.com/huggingface/transformers.git
```
Next, we load a couple of audio samples via `datasets`. Make sure that the audio data is sampled to 16000 kHz.
```py
from datasets import load_dataset, Audio
# English
stream_data = load_dataset("mozilla-foundation/common_voice_13_0", "en", split="test", streaming=True)
stream_data = stream_data.cast_column("audio", Audio(sampling_rate=16000))
en_sample = next(iter(stream_data))["audio"]["array"]
# French
stream_data = load_dataset("mozilla-foundation/common_voice_13_0", "fr", split="test", streaming=True)
stream_data = stream_data.cast_column("audio", Audio(sampling_rate=16000))
fr_sample = next(iter(stream_data))["audio"]["array"]
```
Next, we load the model and processor
```py
from transformers import Wav2Vec2ForCTC, AutoProcessor
import torch
model_id = "facebook/mms-1b-all"
processor = AutoProcessor.from_pretrained(model_id)
model = Wav2Vec2ForCTC.from_pretrained(model_id)
```
Now we process the audio data, pass the processed audio data to the model and transcribe the model output, just like we usually do for Wav2Vec2 models such as [facebook/wav2vec2-base-960h](https://huggingface.co/facebook/wav2vec2-base-960h)
```py
inputs = processor(en_sample, sampling_rate=16_000, return_tensors="pt")
with torch.no_grad():
outputs = model(**inputs).logits
ids = torch.argmax(outputs, dim=-1)[0]
transcription = processor.decode(ids)
# 'joe keton disapproved of films and buster also had reservations about the media'
```
We can now keep the same model in memory and simply switch out the language adapters by calling the convenient [`load_adapter()`]() function for the model and [`set_target_lang()`]() for the tokenizer. We pass the target language as an input - "fra" for French.
```py
processor.tokenizer.set_target_lang("fra")
model.load_adapter("fra")
inputs = processor(fr_sample, sampling_rate=16_000, return_tensors="pt")
with torch.no_grad():
outputs = model(**inputs).logits
ids = torch.argmax(outputs, dim=-1)[0]
transcription = processor.decode(ids)
# "ce dernier est volé tout au long de l'histoire romaine"
```
In the same way the language can be switched out for all other supported languages. Please have a look at:
```py
processor.tokenizer.vocab.keys()
```
For more details, please have a look at [the official docs](https://huggingface.co/docs/transformers/main/en/model_doc/mms).
## Supported Languages
This model supports 1162 languages. Unclick the following to toogle all supported languages of this checkpoint in [ISO 639-3 code](https://en.wikipedia.org/wiki/ISO_639-3).
You can find more details about the languages and their ISO 649-3 codes in the [MMS Language Coverage Overview](https://dl.fbaipublicfiles.com/mms/misc/language_coverage_mms.html).
<details>
<summary>Click to toggle</summary>
- abi
- abk
- abp
- aca
- acd
- ace
- acf
- ach
- acn
- acr
- acu
- ade
- adh
- adj
- adx
- aeu
- afr
- agd
- agg
- agn
- agr
- agu
- agx
- aha
- ahk
- aia
- aka
- akb
- ake
- akp
- alj
- alp
- alt
- alz
- ame
- amf
- amh
- ami
- amk
- ann
- any
- aoz
- apb
- apr
- ara
- arl
- asa
- asg
- asm
- ast
- ata
- atb
- atg
- ati
- atq
- ava
- avn
- avu
- awa
- awb
- ayo
- ayr
- ayz
- azb
- azg
- azj-script_cyrillic
- azj-script_latin
- azz
- bak
- bam
- ban
- bao
- bas
- bav
- bba
- bbb
- bbc
- bbo
- bcc-script_arabic
- bcc-script_latin
- bcl
- bcw
- bdg
- bdh
- bdq
- bdu
- bdv
- beh
- bel
- bem
- ben
- bep
- bex
- bfa
- bfo
- bfy
- bfz
- bgc
- bgq
- bgr
- bgt
- bgw
- bha
- bht
- bhz
- bib
- bim
- bis
- biv
- bjr
- bjv
- bjw
- bjz
- bkd
- bkv
- blh
- blt
- blx
- blz
- bmq
- bmr
- bmu
- bmv
- bng
- bno
- bnp
- boa
- bod
- boj
- bom
- bor
- bos
- bov
- box
- bpr
- bps
- bqc
- bqi
- bqj
- bqp
- bre
- bru
- bsc
- bsq
- bss
- btd
- bts
- btt
- btx
- bud
- bul
- bus
- bvc
- bvz
- bwq
- bwu
- byr
- bzh
- bzi
- bzj
- caa
- cab
- cac-dialect_sanmateoixtatan
- cac-dialect_sansebastiancoatan
- cak-dialect_central
- cak-dialect_santamariadejesus
- cak-dialect_santodomingoxenacoj
- cak-dialect_southcentral
- cak-dialect_western
- cak-dialect_yepocapa
- cap
- car
- cas
- cat
- cax
- cbc
- cbi
- cbr
- cbs
- cbt
- cbu
- cbv
- cce
- cco
- cdj
- ceb
- ceg
- cek
- ces
- cfm
- cgc
- che
- chf
- chv
- chz
- cjo
- cjp
- cjs
- ckb
- cko
- ckt
- cla
- cle
- cly
- cme
- cmn-script_simplified
- cmo-script_khmer
- cmo-script_latin
- cmr
- cnh
- cni
- cnl
- cnt
- coe
- cof
- cok
- con
- cot
- cou
- cpa
- cpb
- cpu
- crh
- crk-script_latin
- crk-script_syllabics
- crn
- crq
- crs
- crt
- csk
- cso
- ctd
- ctg
- cto
- ctu
- cuc
- cui
- cuk
- cul
- cwa
- cwe
- cwt
- cya
- cym
- daa
- dah
- dan
- dar
- dbj
- dbq
- ddn
- ded
- des
- deu
- dga
- dgi
- dgk
- dgo
- dgr
- dhi
- did
- dig
- dik
- dip
- div
- djk
- dnj-dialect_blowowest
- dnj-dialect_gweetaawueast
- dnt
- dnw
- dop
- dos
- dsh
- dso
- dtp
- dts
- dug
- dwr
- dyi
- dyo
- dyu
- dzo
- eip
- eka
- ell
- emp
- enb
- eng
- enx
- epo
- ese
- ess
- est
- eus
- evn
- ewe
- eza
- fal
- fao
- far
- fas
- fij
- fin
- flr
- fmu
- fon
- fra
- frd
- fry
- ful
- gag-script_cyrillic
- gag-script_latin
- gai
- gam
- gau
- gbi
- gbk
- gbm
- gbo
- gde
- geb
- gej
- gil
- gjn
- gkn
- gld
- gle
- glg
- glk
- gmv
- gna
- gnd
- gng
- gof-script_latin
- gog
- gor
- gqr
- grc
- gri
- grn
- grt
- gso
- gub
- guc
- gud
- guh
- guj
- guk
- gum
- guo
- guq
- guu
- gux
- gvc
- gvl
- gwi
- gwr
- gym
- gyr
- had
- hag
- hak
- hap
- hat
- hau
- hay
- heb
- heh
- hif
- hig
- hil
- hin
- hlb
- hlt
- hne
- hnn
- hns
- hoc
- hoy
- hrv
- hsb
- hto
- hub
- hui
- hun
- hus-dialect_centralveracruz
- hus-dialect_westernpotosino
- huu
- huv
- hvn
- hwc
- hye
- hyw
- iba
- ibo
- icr
- idd
- ifa
- ifb
- ife
- ifk
- ifu
- ify
- ign
- ikk
- ilb
- ilo
- imo
- ina
- inb
- ind
- iou
- ipi
- iqw
- iri
- irk
- isl
- ita
- itl
- itv
- ixl-dialect_sangasparchajul
- ixl-dialect_sanjuancotzal
- ixl-dialect_santamarianebaj
- izr
- izz
- jac
- jam
- jav
- jbu
- jen
- jic
- jiv
- jmc
- jmd
- jpn
- jun
- juy
- jvn
- kaa
- kab
- kac
- kak
- kam
- kan
- kao
- kaq
- kat
- kay
- kaz
- kbo
- kbp
- kbq
- kbr
- kby
- kca
- kcg
- kdc
- kde
- kdh
- kdi
- kdj
- kdl
- kdn
- kdt
- kea
- kek
- ken
- keo
- ker
- key
- kez
- kfb
- kff-script_telugu
- kfw
- kfx
- khg
- khm
- khq
- kia
- kij
- kik
- kin
- kir
- kjb
- kje
- kjg
- kjh
- kki
- kkj
- kle
- klu
- klv
- klw
- kma
- kmd
- kml
- kmr-script_arabic
- kmr-script_cyrillic
- kmr-script_latin
- kmu
- knb
- kne
- knf
- knj
- knk
- kno
- kog
- kor
- kpq
- kps
- kpv
- kpy
- kpz
- kqe
- kqp
- kqr
- kqy
- krc
- kri
- krj
- krl
- krr
- krs
- kru
- ksb
- ksr
- kss
- ktb
- ktj
- kub
- kue
- kum
- kus
- kvn
- kvw
- kwd
- kwf
- kwi
- kxc
- kxf
- kxm
- kxv
- kyb
- kyc
- kyf
- kyg
- kyo
- kyq
- kyu
- kyz
- kzf
- lac
- laj
- lam
- lao
- las
- lat
- lav
- law
- lbj
- lbw
- lcp
- lee
- lef
- lem
- lew
- lex
- lgg
- lgl
- lhu
- lia
- lid
- lif
- lin
- lip
- lis
- lit
- lje
- ljp
- llg
- lln
- lme
- lnd
- lns
- lob
- lok
- lom
- lon
- loq
- lsi
- lsm
- ltz
- luc
- lug
- luo
- lwo
- lww
- lzz
- maa-dialect_sanantonio
- maa-dialect_sanjeronimo
- mad
- mag
- mah
- mai
- maj
- mak
- mal
- mam-dialect_central
- mam-dialect_northern
- mam-dialect_southern
- mam-dialect_western
- maq
- mar
- maw
- maz
- mbb
- mbc
- mbh
- mbj
- mbt
- mbu
- mbz
- mca
- mcb
- mcd
- mco
- mcp
- mcq
- mcu
- mda
- mdf
- mdv
- mdy
- med
- mee
- mej
- men
- meq
- met
- mev
- mfe
- mfh
- mfi
- mfk
- mfq
- mfy
- mfz
- mgd
- mge
- mgh
- mgo
- mhi
- mhr
- mhu
- mhx
- mhy
- mib
- mie
- mif
- mih
- mil
- mim
- min
- mio
- mip
- miq
- mit
- miy
- miz
- mjl
- mjv
- mkd
- mkl
- mkn
- mlg
- mlt
- mmg
- mnb
- mnf
- mnk
- mnw
- mnx
- moa
- mog
- mon
- mop
- mor
- mos
- mox
- moz
- mpg
- mpm
- mpp
- mpx
- mqb
- mqf
- mqj
- mqn
- mri
- mrw
- msy
- mtd
- mtj
- mto
- muh
- mup
- mur
- muv
- muy
- mvp
- mwq
- mwv
- mxb
- mxq
- mxt
- mxv
- mya
- myb
- myk
- myl
- myv
- myx
- myy
- mza
- mzi
- mzj
- mzk
- mzm
- mzw
- nab
- nag
- nan
- nas
- naw
- nca
- nch
- ncj
- ncl
- ncu
- ndj
- ndp
- ndv
- ndy
- ndz
- neb
- new
- nfa
- nfr
- nga
- ngl
- ngp
- ngu
- nhe
- nhi
- nhu
- nhw
- nhx
- nhy
- nia
- nij
- nim
- nin
- nko
- nlc
- nld
- nlg
- nlk
- nmz
- nnb
- nno
- nnq
- nnw
- noa
- nob
- nod
- nog
- not
- npi
- npl
- npy
- nso
- nst
- nsu
- ntm
- ntr
- nuj
- nus
- nuz
- nwb
- nxq
- nya
- nyf
- nyn
- nyo
- nyy
- nzi
- obo
- oci
- ojb-script_latin
- ojb-script_syllabics
- oku
- old
- omw
- onb
- ood
- orm
- ory
- oss
- ote
- otq
- ozm
- pab
- pad
- pag
- pam
- pan
- pao
- pap
- pau
- pbb
- pbc
- pbi
- pce
- pcm
- peg
- pez
- pib
- pil
- pir
- pis
- pjt
- pkb
- pls
- plw
- pmf
- pny
- poh-dialect_eastern
- poh-dialect_western
- poi
- pol
- por
- poy
- ppk
- pps
- prf
- prk
- prt
- pse
- pss
- ptu
- pui
- pus
- pwg
- pww
- pxm
- qub
- quc-dialect_central
- quc-dialect_east
- quc-dialect_north
- quf
- quh
- qul
- quw
- quy
- quz
- qvc
- qve
- qvh
- qvm
- qvn
- qvo
- qvs
- qvw
- qvz
- qwh
- qxh
- qxl
- qxn
- qxo
- qxr
- rah
- rai
- rap
- rav
- raw
- rej
- rel
- rgu
- rhg
- rif-script_arabic
- rif-script_latin
- ril
- rim
- rjs
- rkt
- rmc-script_cyrillic
- rmc-script_latin
- rmo
- rmy-script_cyrillic
- rmy-script_latin
- rng
- rnl
- roh-dialect_sursilv
- roh-dialect_vallader
- rol
- ron
- rop
- rro
- rub
- ruf
- rug
- run
- rus
- sab
- sag
- sah
- saj
- saq
- sas
- sat
- sba
- sbd
- sbl
- sbp
- sch
- sck
- sda
- sea
- seh
- ses
- sey
- sgb
- sgj
- sgw
- shi
- shk
- shn
- sho
- shp
- sid
- sig
- sil
- sja
- sjm
- sld
- slk
- slu
- slv
- sml
- smo
- sna
- snd
- sne
- snn
- snp
- snw
- som
- soy
- spa
- spp
- spy
- sqi
- sri
- srm
- srn
- srp-script_cyrillic
- srp-script_latin
- srx
- stn
- stp
- suc
- suk
- sun
- sur
- sus
- suv
- suz
- swe
- swh
- sxb
- sxn
- sya
- syl
- sza
- tac
- taj
- tam
- tao
- tap
- taq
- tat
- tav
- tbc
- tbg
- tbk
- tbl
- tby
- tbz
- tca
- tcc
- tcs
- tcz
- tdj
- ted
- tee
- tel
- tem
- teo
- ter
- tes
- tew
- tex
- tfr
- tgj
- tgk
- tgl
- tgo
- tgp
- tha
- thk
- thl
- tih
- tik
- tir
- tkr
- tlb
- tlj
- tly
- tmc
- tmf
- tna
- tng
- tnk
- tnn
- tnp
- tnr
- tnt
- tob
- toc
- toh
- tom
- tos
- tpi
- tpm
- tpp
- tpt
- trc
- tri
- trn
- trs
- tso
- tsz
- ttc
- tte
- ttq-script_tifinagh
- tue
- tuf
- tuk-script_arabic
- tuk-script_latin
- tuo
- tur
- tvw
- twb
- twe
- twu
- txa
- txq
- txu
- tye
- tzh-dialect_bachajon
- tzh-dialect_tenejapa
- tzj-dialect_eastern
- tzj-dialect_western
- tzo-dialect_chamula
- tzo-dialect_chenalho
- ubl
- ubu
- udm
- udu
- uig-script_arabic
- uig-script_cyrillic
- ukr
- umb
- unr
- upv
- ura
- urb
- urd-script_arabic
- urd-script_devanagari
- urd-script_latin
- urk
- urt
- ury
- usp
- uzb-script_cyrillic
- uzb-script_latin
- vag
- vid
- vie
- vif
- vmw
- vmy
- vot
- vun
- vut
- wal-script_ethiopic
- wal-script_latin
- wap
- war
- waw
- way
- wba
- wlo
- wlx
- wmw
- wob
- wol
- wsg
- wwa
- xal
- xdy
- xed
- xer
- xho
- xmm
- xnj
- xnr
- xog
- xon
- xrb
- xsb
- xsm
- xsr
- xsu
- xta
- xtd
- xte
- xtm
- xtn
- xua
- xuo
- yaa
- yad
- yal
- yam
- yao
- yas
- yat
- yaz
- yba
- ybb
- ycl
- ycn
- yea
- yka
- yli
- yor
- yre
- yua
- yue-script_traditional
- yuz
- yva
- zaa
- zab
- zac
- zad
- zae
- zai
- zam
- zao
- zaq
- zar
- zas
- zav
- zaw
- zca
- zga
- zim
- ziw
- zlm
- zmz
- zne
- zos
- zpc
- zpg
- zpi
- zpl
- zpm
- zpo
- zpt
- zpu
- zpz
- ztq
- zty
- zul
- zyb
- zyp
- zza
</details>
## Model details
- **Developed by:** Vineel Pratap et al.
- **Model type:** Multi-Lingual Automatic Speech Recognition model
- **Language(s):** 1000+ languages, see [supported languages](#supported-languages)
- **License:** CC-BY-NC 4.0 license
- **Num parameters**: 1 billion
- **Audio sampling rate**: 16,000 kHz
- **Cite as:**
@article{pratap2023mms,
title={Scaling Speech Technology to 1,000+ Languages},
author={Vineel Pratap and Andros Tjandra and Bowen Shi and Paden Tomasello and Arun Babu and Sayani Kundu and Ali Elkahky and Zhaoheng Ni and Apoorv Vyas and Maryam Fazel-Zarandi and Alexei Baevski and Yossi Adi and Xiaohui Zhang and Wei-Ning Hsu and Alexis Conneau and Michael Auli},
journal={arXiv},
year={2023}
}
## Additional Links
- [Blog post](https://ai.facebook.com/blog/multilingual-model-speech-recognition/)
- [Transformers documentation](https://huggingface.co/docs/transformers/main/en/model_doc/mms).
- [Paper](https://arxiv.org/abs/2305.13516)
- [GitHub Repository](https://github.com/facebookresearch/fairseq/tree/main/examples/mms#asr)
- [Other **MMS** checkpoints](https://huggingface.co/models?other=mms)
- MMS base checkpoints:
- [facebook/mms-1b](https://huggingface.co/facebook/mms-1b)
- [facebook/mms-300m](https://huggingface.co/facebook/mms-300m)
- [Official Space](https://huggingface.co/spaces/facebook/MMS)
|
xiaxy/elastic-bert-chinese-ner | xiaxy | "2022-11-24T01:07:03Z" | 535,350 | 4 | transformers | [
"transformers",
"pytorch",
"bert",
"token-classification",
"license:apache-2.0",
"autotrain_compatible",
"endpoints_compatible",
"region:us"
] | token-classification | "2022-11-23T10:18:47Z" | ---
license: apache-2.0
---
用于适配elastic8的中文ner模型,支持人名、地名、组织机构名识别
|
jbetker/wav2vec2-large-robust-ft-libritts-voxpopuli | jbetker | "2022-02-25T19:07:57Z" | 534,537 | 8 | transformers | [
"transformers",
"pytorch",
"wav2vec2",
"automatic-speech-recognition",
"endpoints_compatible",
"region:us"
] | automatic-speech-recognition | "2022-03-02T23:29:05Z" | This checkpoint is a wav2vec2-large model that is useful for generating transcriptions with punctuation. It is intended for use in building transcriptions for TTS models, where punctuation is very important for prosody.
This model was created by fine-tuning the `facebook/wav2vec2-large-robust-ft-libri-960h` checkpoint on the [libritts](https://research.google/tools/datasets/libri-tts/) and [voxpopuli](https://github.com/facebookresearch/voxpopuli) datasets with a new vocabulary that includes punctuation.
The model gets a respectable WER of 4.45% on the librispeech validation set. The baseline, `facebook/wav2vec2-large-robust-ft-libri-960h`, got 4.3%.
Since the model was fine-tuned on clean audio, it is not well-suited for noisy audio like CommonVoice (though I may upload a checkpoint for that soon too). It still does pretty good, though.
The vocabulary is uploaded to the model hub as well `jbetker/tacotron_symbols`.
Check out my speech transcription script repo, [ocotillo](https://github.com/neonbjb/ocotillo) for usage examples: https://github.com/neonbjb/ocotillo |
davebulaval/MeaningBERT | davebulaval | "2024-03-24T01:17:22Z" | 534,118 | 2 | transformers | [
"transformers",
"safetensors",
"bert",
"text-classification",
"autotrain_compatible",
"endpoints_compatible",
"region:us"
] | text-classification | "2023-11-14T01:15:53Z" | ---
title: MeaningBERT
emoji: 🦀
colorFrom: purple
colorTo: indigo
sdk: gradio
sdk_version: 4.2.0
app_file: app.py
pinned: false
---
# Here is MeaningBERT
MeaningBERT is an automatic and trainable metric for assessing meaning preservation between sentences. MeaningBERT was
proposed in our
article [MeaningBERT: assessing meaning preservation between sentences](https://www.frontiersin.org/articles/10.3389/frai.2023.1223924/full).
Its goal is to assess meaning preservation between two sentences that correlate highly with human judgments and sanity
checks. For more details, refer to our publicly available article.
> This public version of our model uses the best model trained (where in our article, we present the performance results
> of an average of 10 models) for a more extended period (500 epochs instead of 250). We have observed later that the
> model can further reduce dev loss and increase performance. Also, we have changed the data augmentation technique used
> in the article for a more robust one, that also includes the commutative property of the meaning function. Namely, Meaning(Sent_a, Sent_b) = Meaning(Sent_b, Sent_a).
- [HuggingFace Model Card](https://huggingface.co/davebulaval/MeaningBERT)
- [HuggingFace Metric Card](https://huggingface.co/spaces/davebulaval/meaningbert)
## Sanity Check
Correlation to human judgment is one way to evaluate the quality of a meaning preservation metric.
However, it is inherently subjective, since it uses human judgment as a gold standard, and expensive since it requires
a large dataset
annotated by several humans. As an alternative, we designed two automated tests: evaluating meaning preservation between
identical sentences (which should be 100% preserving) and between unrelated sentences (which should be 0% preserving).
In these tests, the meaning preservation target value is not subjective and does not require human annotation to
be measured. They represent a trivial and minimal threshold a good automatic meaning preservation metric should be able to
achieve. Namely, a metric should be minimally able to return a perfect score (i.e., 100%) if two identical sentences are
compared and return a null score (i.e., 0%) if two sentences are completely unrelated.
### Identical Sentences
The first test evaluates meaning preservation between identical sentences. To analyze the metrics' capabilities to pass
this test, we count the number of times a metric rating was greater or equal to a threshold value X∈[95, 99] and divide
It is calculated by the number of sentences to create a ratio of the number of times the metric gives the expected rating. To account
for computer floating-point inaccuracy, we round the ratings to the nearest integer and do not use a threshold value of
100%.
### Unrelated Sentences
Our second test evaluates meaning preservation between a source sentence and an unrelated sentence generated by a large
language model.3 The idea is to verify that the metric finds a meaning preservation rating of 0 when given a completely
irrelevant sentence mainly composed of irrelevant words (also known as word soup). Since this test's expected rating is
0, we check that the metric rating is lower or equal to a threshold value X∈[5, 1].
Again, to account for computer floating-point inaccuracy, we round the ratings to the nearest integer and do not use
a threshold value of 0%.
## Use MeaningBERT
You can use MeaningBERT as a [model](https://huggingface.co/davebulaval/MeaningBERT) that you can retrain or use for
inference using the following with HuggingFace
```python
# Load model directly
from transformers import AutoTokenizer, AutoModelForSequenceClassification
tokenizer = AutoTokenizer.from_pretrained("davebulaval/MeaningBERT")
model = AutoModelForSequenceClassification.from_pretrained("davebulaval/MeaningBERT")
```
or you can use MeaningBERT as a metric for evaluation (no retrain) using the following with HuggingFace
```python
import torch
from transformers import AutoTokenizer, AutoModelForSequenceClassification
tokenizer = AutoTokenizer.from_pretrained("davebulaval/MeaningBERT")
scorer = AutoModelForSequenceClassification.from_pretrained("davebulaval/MeaningBERT")
scorer.eval()
documents = ["He wanted to make them pay.", "This sandwich looks delicious.", "He wants to eat."]
simplifications = ["He wanted to make them pay.", "This sandwich looks delicious.",
"Whatever, whenever, this is a sentence."]
# We tokenize the text as a pair and return Pytorch Tensors
tokenize_text = tokenizer(documents, simplifications, truncation=True, padding=True, return_tensors="pt")
with torch.no_grad():
# We process the text
scores = scorer(**tokenize_text)
print(scores.logits.tolist())
```
or using our HuggingFace Metric module
```python
import evaluate
documents = ["He wanted to make them pay.", "This sandwich looks delicious.", "He wants to eat."]
simplifications = ["He wanted to make them pay.", "This sandwich looks delicious.",
"Whatever, whenever, this is a sentence."]
meaning_bert = evaluate.load("davebulaval/meaningbert")
print(meaning_bert.compute(documents=documents, simplifications=simplifications))
```
------------------
## Cite
Use the following citation to cite MeaningBERT
```
@ARTICLE{10.3389/frai.2023.1223924,
AUTHOR={Beauchemin, David and Saggion, Horacio and Khoury, Richard},
TITLE={MeaningBERT: assessing meaning preservation between sentences},
JOURNAL={Frontiers in Artificial Intelligence},
VOLUME={6},
YEAR={2023},
URL={https://www.frontiersin.org/articles/10.3389/frai.2023.1223924},
DOI={10.3389/frai.2023.1223924},
ISSN={2624-8212},
}
```
------------------
## Contributing to MeaningBERT
We welcome user input, whether it regards bugs found in the library or feature propositions! Make sure to have a
look at our [contributing guidelines](https://github.com/GRAAL-Research/MeaningBERT/blob/main/.github/CONTRIBUTING.md)
for more details on this matter.
## License
MeaningBERT is MIT licensed, as found in
the [LICENSE file](https://github.com/GRAAL-Research/risc/blob/main/LICENSE).
------------------
|
Salesforce/blip2-opt-2.7b | Salesforce | "2024-03-22T11:58:17Z" | 533,780 | 314 | transformers | [
"transformers",
"pytorch",
"safetensors",
"blip-2",
"visual-question-answering",
"vision",
"image-to-text",
"image-captioning",
"en",
"arxiv:2301.12597",
"license:mit",
"endpoints_compatible",
"region:us"
] | image-to-text | "2023-02-06T16:21:49Z" | ---
language: en
license: mit
tags:
- vision
- image-to-text
- image-captioning
- visual-question-answering
pipeline_tag: image-to-text
---
# BLIP-2, OPT-2.7b, pre-trained only
BLIP-2 model, leveraging [OPT-2.7b](https://huggingface.co/facebook/opt-2.7b) (a large language model with 2.7 billion parameters).
It was introduced in the paper [BLIP-2: Bootstrapping Language-Image Pre-training with Frozen Image Encoders and Large Language Models](https://arxiv.org/abs/2301.12597) by Li et al. and first released in [this repository](https://github.com/salesforce/LAVIS/tree/main/projects/blip2).
Disclaimer: The team releasing BLIP-2 did not write a model card for this model so this model card has been written by the Hugging Face team.
## Model description
BLIP-2 consists of 3 models: a CLIP-like image encoder, a Querying Transformer (Q-Former) and a large language model.
The authors initialize the weights of the image encoder and large language model from pre-trained checkpoints and keep them frozen
while training the Querying Transformer, which is a BERT-like Transformer encoder that maps a set of "query tokens" to query embeddings,
which bridge the gap between the embedding space of the image encoder and the large language model.
The goal for the model is simply to predict the next text token, giving the query embeddings and the previous text.
<img src="https://huggingface.co/datasets/huggingface/documentation-images/resolve/main/transformers/model_doc/blip2_architecture.jpg"
alt="drawing" width="600"/>
This allows the model to be used for tasks like:
- image captioning
- visual question answering (VQA)
- chat-like conversations by feeding the image and the previous conversation as prompt to the model
## Direct Use and Downstream Use
You can use the raw model for conditional text generation given an image and optional text. See the [model hub](https://huggingface.co/models?search=Salesforce/blip) to look for
fine-tuned versions on a task that interests you.
## Bias, Risks, Limitations, and Ethical Considerations
BLIP2-OPT uses off-the-shelf OPT as the language model. It inherits the same risks and limitations as mentioned in Meta's model card.
> Like other large language models for which the diversity (or lack thereof) of training
> data induces downstream impact on the quality of our model, OPT-175B has limitations in terms
> of bias and safety. OPT-175B can also have quality issues in terms of generation diversity and
> hallucination. In general, OPT-175B is not immune from the plethora of issues that plague modern
> large language models.
>
BLIP2 is fine-tuned on image-text datasets (e.g. [LAION](https://laion.ai/blog/laion-400-open-dataset/) ) collected from the internet. As a result the model itself is potentially vulnerable to generating equivalently inappropriate content or replicating inherent biases in the underlying data.
BLIP2 has not been tested in real world applications. It should not be directly deployed in any applications. Researchers should first carefully assess the safety and fairness of the model in relation to the specific context they’re being deployed within.
### How to use
For code examples, we refer to the [documentation](https://huggingface.co/docs/transformers/main/en/model_doc/blip-2#transformers.Blip2ForConditionalGeneration.forward.example).
### Memory requirements
The memory requirements differ based on the precision one uses. One can use 4-bit inference using [Bitsandbytes](https://huggingface.co/blog/4bit-transformers-bitsandbytes), which greatly reduce the memory requirements.
| dtype | Largest Layer or Residual Group | Total Size | Training using Adam |
|-------------------|---------------------------------|------------|----------------------|
| float32 | 490.94 MB | 14.43 GB | 57.72 GB |
| float16/bfloat16 | 245.47 MB | 7.21 GB | 28.86 GB |
| int8 | 122.73 MB | 3.61 GB | 14.43 GB |
| int4 | 61.37 MB | 1.8 GB | 7.21 GB |
#### Running the model on CPU
<details>
<summary> Click to expand </summary>
```python
import requests
from PIL import Image
from transformers import Blip2Processor, Blip2ForConditionalGeneration
processor = Blip2Processor.from_pretrained("Salesforce/blip2-opt-2.7b")
model = Blip2ForConditionalGeneration.from_pretrained("Salesforce/blip2-opt-2.7b")
img_url = 'https://storage.googleapis.com/sfr-vision-language-research/BLIP/demo.jpg'
raw_image = Image.open(requests.get(img_url, stream=True).raw).convert('RGB')
question = "how many dogs are in the picture?"
inputs = processor(raw_image, question, return_tensors="pt")
out = model.generate(**inputs)
print(processor.decode(out[0], skip_special_tokens=True).strip())
```
</details>
#### Running the model on GPU
##### In full precision
<details>
<summary> Click to expand </summary>
```python
# pip install accelerate
import requests
from PIL import Image
from transformers import Blip2Processor, Blip2ForConditionalGeneration
processor = Blip2Processor.from_pretrained("Salesforce/blip2-opt-2.7b")
model = Blip2ForConditionalGeneration.from_pretrained("Salesforce/blip2-opt-2.7b", device_map="auto")
img_url = 'https://storage.googleapis.com/sfr-vision-language-research/BLIP/demo.jpg'
raw_image = Image.open(requests.get(img_url, stream=True).raw).convert('RGB')
question = "how many dogs are in the picture?"
inputs = processor(raw_image, question, return_tensors="pt").to("cuda")
out = model.generate(**inputs)
print(processor.decode(out[0], skip_special_tokens=True).strip())
```
</details>
##### In half precision (`float16`)
<details>
<summary> Click to expand </summary>
```python
# pip install accelerate
import torch
import requests
from PIL import Image
from transformers import Blip2Processor, Blip2ForConditionalGeneration
processor = Blip2Processor.from_pretrained("Salesforce/blip2-opt-2.7b")
model = Blip2ForConditionalGeneration.from_pretrained("Salesforce/blip2-opt-2.7b", torch_dtype=torch.float16, device_map="auto")
img_url = 'https://storage.googleapis.com/sfr-vision-language-research/BLIP/demo.jpg'
raw_image = Image.open(requests.get(img_url, stream=True).raw).convert('RGB')
question = "how many dogs are in the picture?"
inputs = processor(raw_image, question, return_tensors="pt").to("cuda", torch.float16)
out = model.generate(**inputs)
print(processor.decode(out[0], skip_special_tokens=True).strip())
```
</details>
##### In 8-bit precision (`int8`)
<details>
<summary> Click to expand </summary>
```python
# pip install accelerate bitsandbytes
import torch
import requests
from PIL import Image
from transformers import Blip2Processor, Blip2ForConditionalGeneration
processor = Blip2Processor.from_pretrained("Salesforce/blip2-opt-2.7b")
model = Blip2ForConditionalGeneration.from_pretrained("Salesforce/blip2-opt-2.7b", load_in_8bit=True, device_map="auto")
img_url = 'https://storage.googleapis.com/sfr-vision-language-research/BLIP/demo.jpg'
raw_image = Image.open(requests.get(img_url, stream=True).raw).convert('RGB')
question = "how many dogs are in the picture?"
inputs = processor(raw_image, question, return_tensors="pt").to("cuda", torch.float16)
out = model.generate(**inputs)
print(processor.decode(out[0], skip_special_tokens=True).strip())
```
</details> |
microsoft/trocr-base-handwritten | microsoft | "2024-05-27T20:09:41Z" | 532,647 | 329 | transformers | [
"transformers",
"pytorch",
"safetensors",
"vision-encoder-decoder",
"image-text-to-text",
"trocr",
"image-to-text",
"arxiv:2109.10282",
"endpoints_compatible",
"region:us"
] | image-to-text | "2022-03-02T23:29:05Z" | ---
tags:
- trocr
- image-to-text
widget:
- src: https://fki.tic.heia-fr.ch/static/img/a01-122-02.jpg
example_title: Note 1
- src: https://encrypted-tbn0.gstatic.com/images?q=tbn:ANd9GcSoolxi9yWGAT5SLZShv8vVd0bz47UWRzQC19fDTeE8GmGv_Rn-PCF1pP1rrUx8kOjA4gg&usqp=CAU
example_title: Note 2
- src: https://encrypted-tbn0.gstatic.com/images?q=tbn:ANd9GcRNYtTuSBpZPV_nkBYPMFwVVD9asZOPgHww4epu9EqWgDmXW--sE2o8og40ZfDGo87j5w&usqp=CAU
example_title: Note 3
---
# TrOCR (base-sized model, fine-tuned on IAM)
TrOCR model fine-tuned on the [IAM dataset](https://fki.tic.heia-fr.ch/databases/iam-handwriting-database). It was introduced in the paper [TrOCR: Transformer-based Optical Character Recognition with Pre-trained Models](https://arxiv.org/abs/2109.10282) by Li et al. and first released in [this repository](https://github.com/microsoft/unilm/tree/master/trocr).
Disclaimer: The team releasing TrOCR did not write a model card for this model so this model card has been written by the Hugging Face team.
## Model description
The TrOCR model is an encoder-decoder model, consisting of an image Transformer as encoder, and a text Transformer as decoder. The image encoder was initialized from the weights of BEiT, while the text decoder was initialized from the weights of RoBERTa.
Images are presented to the model as a sequence of fixed-size patches (resolution 16x16), which are linearly embedded. One also adds absolute position embeddings before feeding the sequence to the layers of the Transformer encoder. Next, the Transformer text decoder autoregressively generates tokens.
## Intended uses & limitations
You can use the raw model for optical character recognition (OCR) on single text-line images. See the [model hub](https://huggingface.co/models?search=microsoft/trocr) to look for fine-tuned versions on a task that interests you.
### How to use
Here is how to use this model in PyTorch:
```python
from transformers import TrOCRProcessor, VisionEncoderDecoderModel
from PIL import Image
import requests
# load image from the IAM database
url = 'https://fki.tic.heia-fr.ch/static/img/a01-122-02-00.jpg'
image = Image.open(requests.get(url, stream=True).raw).convert("RGB")
processor = TrOCRProcessor.from_pretrained('microsoft/trocr-base-handwritten')
model = VisionEncoderDecoderModel.from_pretrained('microsoft/trocr-base-handwritten')
pixel_values = processor(images=image, return_tensors="pt").pixel_values
generated_ids = model.generate(pixel_values)
generated_text = processor.batch_decode(generated_ids, skip_special_tokens=True)[0]
```
### BibTeX entry and citation info
```bibtex
@misc{li2021trocr,
title={TrOCR: Transformer-based Optical Character Recognition with Pre-trained Models},
author={Minghao Li and Tengchao Lv and Lei Cui and Yijuan Lu and Dinei Florencio and Cha Zhang and Zhoujun Li and Furu Wei},
year={2021},
eprint={2109.10282},
archivePrefix={arXiv},
primaryClass={cs.CL}
}
``` |
timm/vit_base_patch16_224.augreg2_in21k_ft_in1k | timm | "2023-05-06T00:00:25Z" | 531,601 | 7 | timm | [
"timm",
"pytorch",
"safetensors",
"image-classification",
"dataset:imagenet-1k",
"dataset:imagenet-21k",
"arxiv:2106.10270",
"arxiv:2010.11929",
"license:apache-2.0",
"region:us"
] | image-classification | "2022-12-22T07:24:28Z" | ---
tags:
- image-classification
- timm
library_name: timm
license: apache-2.0
datasets:
- imagenet-1k
- imagenet-21k
---
# Model card for vit_base_patch16_224.augreg2_in21k_ft_in1k
A Vision Transformer (ViT) image classification model. Trained on ImageNet-21k by paper authors and (re) fine-tuned on ImageNet-1k with additional augmentation and regularization by Ross Wightman.
## Model Details
- **Model Type:** Image classification / feature backbone
- **Model Stats:**
- Params (M): 86.6
- GMACs: 16.9
- Activations (M): 16.5
- Image size: 224 x 224
- **Papers:**
- How to train your ViT? Data, Augmentation, and Regularization in Vision Transformers: https://arxiv.org/abs/2106.10270
- An Image is Worth 16x16 Words: Transformers for Image Recognition at Scale: https://arxiv.org/abs/2010.11929v2
- **Dataset:** ImageNet-1k
- **Pretrain Dataset:** ImageNet-21k
- **Original:** https://github.com/google-research/vision_transformer
## Model Usage
### Image Classification
```python
from urllib.request import urlopen
from PIL import Image
import timm
img = Image.open(urlopen(
'https://huggingface.co/datasets/huggingface/documentation-images/resolve/main/beignets-task-guide.png'
))
model = timm.create_model('vit_base_patch16_224.augreg2_in21k_ft_in1k', pretrained=True)
model = model.eval()
# get model specific transforms (normalization, resize)
data_config = timm.data.resolve_model_data_config(model)
transforms = timm.data.create_transform(**data_config, is_training=False)
output = model(transforms(img).unsqueeze(0)) # unsqueeze single image into batch of 1
top5_probabilities, top5_class_indices = torch.topk(output.softmax(dim=1) * 100, k=5)
```
### Image Embeddings
```python
from urllib.request import urlopen
from PIL import Image
import timm
img = Image.open(urlopen(
'https://huggingface.co/datasets/huggingface/documentation-images/resolve/main/beignets-task-guide.png'
))
model = timm.create_model(
'vit_base_patch16_224.augreg2_in21k_ft_in1k',
pretrained=True,
num_classes=0, # remove classifier nn.Linear
)
model = model.eval()
# get model specific transforms (normalization, resize)
data_config = timm.data.resolve_model_data_config(model)
transforms = timm.data.create_transform(**data_config, is_training=False)
output = model(transforms(img).unsqueeze(0)) # output is (batch_size, num_features) shaped tensor
# or equivalently (without needing to set num_classes=0)
output = model.forward_features(transforms(img).unsqueeze(0))
# output is unpooled, a (1, 197, 768) shaped tensor
output = model.forward_head(output, pre_logits=True)
# output is a (1, num_features) shaped tensor
```
## Model Comparison
Explore the dataset and runtime metrics of this model in timm [model results](https://github.com/huggingface/pytorch-image-models/tree/main/results).
## Citation
```bibtex
@article{steiner2021augreg,
title={How to train your ViT? Data, Augmentation, and Regularization in Vision Transformers},
author={Steiner, Andreas and Kolesnikov, Alexander and and Zhai, Xiaohua and Wightman, Ross and Uszkoreit, Jakob and Beyer, Lucas},
journal={arXiv preprint arXiv:2106.10270},
year={2021}
}
```
```bibtex
@article{dosovitskiy2020vit,
title={An Image is Worth 16x16 Words: Transformers for Image Recognition at Scale},
author={Dosovitskiy, Alexey and Beyer, Lucas and Kolesnikov, Alexander and Weissenborn, Dirk and Zhai, Xiaohua and Unterthiner, Thomas and Dehghani, Mostafa and Minderer, Matthias and Heigold, Georg and Gelly, Sylvain and Uszkoreit, Jakob and Houlsby, Neil},
journal={ICLR},
year={2021}
}
```
```bibtex
@misc{rw2019timm,
author = {Ross Wightman},
title = {PyTorch Image Models},
year = {2019},
publisher = {GitHub},
journal = {GitHub repository},
doi = {10.5281/zenodo.4414861},
howpublished = {\url{https://github.com/huggingface/pytorch-image-models}}
}
```
|
facebook/wav2vec2-base | facebook | "2021-12-28T12:44:31Z" | 529,671 | 76 | transformers | [
"transformers",
"pytorch",
"wav2vec2",
"pretraining",
"speech",
"en",
"dataset:librispeech_asr",
"arxiv:2006.11477",
"license:apache-2.0",
"endpoints_compatible",
"region:us"
] | null | "2022-03-02T23:29:05Z" | ---
language: en
datasets:
- librispeech_asr
tags:
- speech
license: apache-2.0
---
# Wav2Vec2-Base
[Facebook's Wav2Vec2](https://ai.facebook.com/blog/wav2vec-20-learning-the-structure-of-speech-from-raw-audio/)
The base model pretrained on 16kHz sampled speech audio. When using the model make sure that your speech input is also sampled at 16Khz.
**Note**: This model does not have a tokenizer as it was pretrained on audio alone. In order to use this model **speech recognition**, a tokenizer should be created and the model should be fine-tuned on labeled text data. Check out [this blog](https://huggingface.co/blog/fine-tune-wav2vec2-english) for more in-detail explanation of how to fine-tune the model.
[Paper](https://arxiv.org/abs/2006.11477)
Authors: Alexei Baevski, Henry Zhou, Abdelrahman Mohamed, Michael Auli
**Abstract**
We show for the first time that learning powerful representations from speech audio alone followed by fine-tuning on transcribed speech can outperform the best semi-supervised methods while being conceptually simpler. wav2vec 2.0 masks the speech input in the latent space and solves a contrastive task defined over a quantization of the latent representations which are jointly learned. Experiments using all labeled data of Librispeech achieve 1.8/3.3 WER on the clean/other test sets. When lowering the amount of labeled data to one hour, wav2vec 2.0 outperforms the previous state of the art on the 100 hour subset while using 100 times less labeled data. Using just ten minutes of labeled data and pre-training on 53k hours of unlabeled data still achieves 4.8/8.2 WER. This demonstrates the feasibility of speech recognition with limited amounts of labeled data.
The original model can be found under https://github.com/pytorch/fairseq/tree/master/examples/wav2vec#wav2vec-20.
# Usage
See [this notebook](https://colab.research.google.com/drive/1FjTsqbYKphl9kL-eILgUc-bl4zVThL8F?usp=sharing) for more information on how to fine-tune the model. |
intfloat/multilingual-e5-base | intfloat | "2024-02-15T07:12:22Z" | 526,904 | 232 | sentence-transformers | [
"sentence-transformers",
"pytorch",
"onnx",
"safetensors",
"xlm-roberta",
"mteb",
"Sentence Transformers",
"sentence-similarity",
"multilingual",
"af",
"am",
"ar",
"as",
"az",
"be",
"bg",
"bn",
"br",
"bs",
"ca",
"cs",
"cy",
"da",
"de",
"el",
"en",
"eo",
"es",
"et",
"eu",
"fa",
"fi",
"fr",
"fy",
"ga",
"gd",
"gl",
"gu",
"ha",
"he",
"hi",
"hr",
"hu",
"hy",
"id",
"is",
"it",
"ja",
"jv",
"ka",
"kk",
"km",
"kn",
"ko",
"ku",
"ky",
"la",
"lo",
"lt",
"lv",
"mg",
"mk",
"ml",
"mn",
"mr",
"ms",
"my",
"ne",
"nl",
"no",
"om",
"or",
"pa",
"pl",
"ps",
"pt",
"ro",
"ru",
"sa",
"sd",
"si",
"sk",
"sl",
"so",
"sq",
"sr",
"su",
"sv",
"sw",
"ta",
"te",
"th",
"tl",
"tr",
"ug",
"uk",
"ur",
"uz",
"vi",
"xh",
"yi",
"zh",
"arxiv:2402.05672",
"arxiv:2108.08787",
"arxiv:2104.08663",
"arxiv:2210.07316",
"license:mit",
"model-index",
"autotrain_compatible",
"text-embeddings-inference",
"endpoints_compatible",
"region:us"
] | sentence-similarity | "2023-05-19T10:26:40Z" | ---
tags:
- mteb
- Sentence Transformers
- sentence-similarity
- sentence-transformers
model-index:
- name: multilingual-e5-base
results:
- task:
type: Classification
dataset:
type: mteb/amazon_counterfactual
name: MTEB AmazonCounterfactualClassification (en)
config: en
split: test
revision: e8379541af4e31359cca9fbcf4b00f2671dba205
metrics:
- type: accuracy
value: 78.97014925373135
- type: ap
value: 43.69351129103008
- type: f1
value: 73.38075030070492
- task:
type: Classification
dataset:
type: mteb/amazon_counterfactual
name: MTEB AmazonCounterfactualClassification (de)
config: de
split: test
revision: e8379541af4e31359cca9fbcf4b00f2671dba205
metrics:
- type: accuracy
value: 71.7237687366167
- type: ap
value: 82.22089859962671
- type: f1
value: 69.95532758884401
- task:
type: Classification
dataset:
type: mteb/amazon_counterfactual
name: MTEB AmazonCounterfactualClassification (en-ext)
config: en-ext
split: test
revision: e8379541af4e31359cca9fbcf4b00f2671dba205
metrics:
- type: accuracy
value: 79.65517241379312
- type: ap
value: 28.507918657094738
- type: f1
value: 66.84516013726119
- task:
type: Classification
dataset:
type: mteb/amazon_counterfactual
name: MTEB AmazonCounterfactualClassification (ja)
config: ja
split: test
revision: e8379541af4e31359cca9fbcf4b00f2671dba205
metrics:
- type: accuracy
value: 73.32976445396146
- type: ap
value: 20.720481637566014
- type: f1
value: 59.78002763416003
- task:
type: Classification
dataset:
type: mteb/amazon_polarity
name: MTEB AmazonPolarityClassification
config: default
split: test
revision: e2d317d38cd51312af73b3d32a06d1a08b442046
metrics:
- type: accuracy
value: 90.63775
- type: ap
value: 87.22277903861716
- type: f1
value: 90.60378636386807
- task:
type: Classification
dataset:
type: mteb/amazon_reviews_multi
name: MTEB AmazonReviewsClassification (en)
config: en
split: test
revision: 1399c76144fd37290681b995c656ef9b2e06e26d
metrics:
- type: accuracy
value: 44.546
- type: f1
value: 44.05666638370923
- task:
type: Classification
dataset:
type: mteb/amazon_reviews_multi
name: MTEB AmazonReviewsClassification (de)
config: de
split: test
revision: 1399c76144fd37290681b995c656ef9b2e06e26d
metrics:
- type: accuracy
value: 41.828
- type: f1
value: 41.2710255644252
- task:
type: Classification
dataset:
type: mteb/amazon_reviews_multi
name: MTEB AmazonReviewsClassification (es)
config: es
split: test
revision: 1399c76144fd37290681b995c656ef9b2e06e26d
metrics:
- type: accuracy
value: 40.534
- type: f1
value: 39.820743174270326
- task:
type: Classification
dataset:
type: mteb/amazon_reviews_multi
name: MTEB AmazonReviewsClassification (fr)
config: fr
split: test
revision: 1399c76144fd37290681b995c656ef9b2e06e26d
metrics:
- type: accuracy
value: 39.684
- type: f1
value: 39.11052682815307
- task:
type: Classification
dataset:
type: mteb/amazon_reviews_multi
name: MTEB AmazonReviewsClassification (ja)
config: ja
split: test
revision: 1399c76144fd37290681b995c656ef9b2e06e26d
metrics:
- type: accuracy
value: 37.436
- type: f1
value: 37.07082931930871
- task:
type: Classification
dataset:
type: mteb/amazon_reviews_multi
name: MTEB AmazonReviewsClassification (zh)
config: zh
split: test
revision: 1399c76144fd37290681b995c656ef9b2e06e26d
metrics:
- type: accuracy
value: 37.226000000000006
- type: f1
value: 36.65372077739185
- task:
type: Retrieval
dataset:
type: arguana
name: MTEB ArguAna
config: default
split: test
revision: None
metrics:
- type: map_at_1
value: 22.831000000000003
- type: map_at_10
value: 36.42
- type: map_at_100
value: 37.699
- type: map_at_1000
value: 37.724000000000004
- type: map_at_3
value: 32.207
- type: map_at_5
value: 34.312
- type: mrr_at_1
value: 23.257
- type: mrr_at_10
value: 36.574
- type: mrr_at_100
value: 37.854
- type: mrr_at_1000
value: 37.878
- type: mrr_at_3
value: 32.385000000000005
- type: mrr_at_5
value: 34.48
- type: ndcg_at_1
value: 22.831000000000003
- type: ndcg_at_10
value: 44.230000000000004
- type: ndcg_at_100
value: 49.974000000000004
- type: ndcg_at_1000
value: 50.522999999999996
- type: ndcg_at_3
value: 35.363
- type: ndcg_at_5
value: 39.164
- type: precision_at_1
value: 22.831000000000003
- type: precision_at_10
value: 6.935
- type: precision_at_100
value: 0.9520000000000001
- type: precision_at_1000
value: 0.099
- type: precision_at_3
value: 14.841
- type: precision_at_5
value: 10.754
- type: recall_at_1
value: 22.831000000000003
- type: recall_at_10
value: 69.346
- type: recall_at_100
value: 95.235
- type: recall_at_1000
value: 99.36
- type: recall_at_3
value: 44.523
- type: recall_at_5
value: 53.769999999999996
- task:
type: Clustering
dataset:
type: mteb/arxiv-clustering-p2p
name: MTEB ArxivClusteringP2P
config: default
split: test
revision: a122ad7f3f0291bf49cc6f4d32aa80929df69d5d
metrics:
- type: v_measure
value: 40.27789869854063
- task:
type: Clustering
dataset:
type: mteb/arxiv-clustering-s2s
name: MTEB ArxivClusteringS2S
config: default
split: test
revision: f910caf1a6075f7329cdf8c1a6135696f37dbd53
metrics:
- type: v_measure
value: 35.41979463347428
- task:
type: Reranking
dataset:
type: mteb/askubuntudupquestions-reranking
name: MTEB AskUbuntuDupQuestions
config: default
split: test
revision: 2000358ca161889fa9c082cb41daa8dcfb161a54
metrics:
- type: map
value: 58.22752045109304
- type: mrr
value: 71.51112430198303
- task:
type: STS
dataset:
type: mteb/biosses-sts
name: MTEB BIOSSES
config: default
split: test
revision: d3fb88f8f02e40887cd149695127462bbcf29b4a
metrics:
- type: cos_sim_pearson
value: 84.71147646622866
- type: cos_sim_spearman
value: 85.059167046486
- type: euclidean_pearson
value: 75.88421613600647
- type: euclidean_spearman
value: 75.12821787150585
- type: manhattan_pearson
value: 75.22005646957604
- type: manhattan_spearman
value: 74.42880434453272
- task:
type: BitextMining
dataset:
type: mteb/bucc-bitext-mining
name: MTEB BUCC (de-en)
config: de-en
split: test
revision: d51519689f32196a32af33b075a01d0e7c51e252
metrics:
- type: accuracy
value: 99.23799582463465
- type: f1
value: 99.12665274878218
- type: precision
value: 99.07098121085595
- type: recall
value: 99.23799582463465
- task:
type: BitextMining
dataset:
type: mteb/bucc-bitext-mining
name: MTEB BUCC (fr-en)
config: fr-en
split: test
revision: d51519689f32196a32af33b075a01d0e7c51e252
metrics:
- type: accuracy
value: 97.88685890380806
- type: f1
value: 97.59336708489249
- type: precision
value: 97.44662117543473
- type: recall
value: 97.88685890380806
- task:
type: BitextMining
dataset:
type: mteb/bucc-bitext-mining
name: MTEB BUCC (ru-en)
config: ru-en
split: test
revision: d51519689f32196a32af33b075a01d0e7c51e252
metrics:
- type: accuracy
value: 97.47142362313821
- type: f1
value: 97.1989377670015
- type: precision
value: 97.06384944001847
- type: recall
value: 97.47142362313821
- task:
type: BitextMining
dataset:
type: mteb/bucc-bitext-mining
name: MTEB BUCC (zh-en)
config: zh-en
split: test
revision: d51519689f32196a32af33b075a01d0e7c51e252
metrics:
- type: accuracy
value: 98.4728804634018
- type: f1
value: 98.2973494821836
- type: precision
value: 98.2095839915745
- type: recall
value: 98.4728804634018
- task:
type: Classification
dataset:
type: mteb/banking77
name: MTEB Banking77Classification
config: default
split: test
revision: 0fd18e25b25c072e09e0d92ab615fda904d66300
metrics:
- type: accuracy
value: 82.74025974025975
- type: f1
value: 82.67420447730439
- task:
type: Clustering
dataset:
type: mteb/biorxiv-clustering-p2p
name: MTEB BiorxivClusteringP2P
config: default
split: test
revision: 65b79d1d13f80053f67aca9498d9402c2d9f1f40
metrics:
- type: v_measure
value: 35.0380848063507
- task:
type: Clustering
dataset:
type: mteb/biorxiv-clustering-s2s
name: MTEB BiorxivClusteringS2S
config: default
split: test
revision: 258694dd0231531bc1fd9de6ceb52a0853c6d908
metrics:
- type: v_measure
value: 29.45956405670166
- task:
type: Retrieval
dataset:
type: BeIR/cqadupstack
name: MTEB CQADupstackAndroidRetrieval
config: default
split: test
revision: None
metrics:
- type: map_at_1
value: 32.122
- type: map_at_10
value: 42.03
- type: map_at_100
value: 43.364000000000004
- type: map_at_1000
value: 43.474000000000004
- type: map_at_3
value: 38.804
- type: map_at_5
value: 40.585
- type: mrr_at_1
value: 39.914
- type: mrr_at_10
value: 48.227
- type: mrr_at_100
value: 49.018
- type: mrr_at_1000
value: 49.064
- type: mrr_at_3
value: 45.994
- type: mrr_at_5
value: 47.396
- type: ndcg_at_1
value: 39.914
- type: ndcg_at_10
value: 47.825
- type: ndcg_at_100
value: 52.852
- type: ndcg_at_1000
value: 54.891
- type: ndcg_at_3
value: 43.517
- type: ndcg_at_5
value: 45.493
- type: precision_at_1
value: 39.914
- type: precision_at_10
value: 8.956
- type: precision_at_100
value: 1.388
- type: precision_at_1000
value: 0.182
- type: precision_at_3
value: 20.791999999999998
- type: precision_at_5
value: 14.821000000000002
- type: recall_at_1
value: 32.122
- type: recall_at_10
value: 58.294999999999995
- type: recall_at_100
value: 79.726
- type: recall_at_1000
value: 93.099
- type: recall_at_3
value: 45.017
- type: recall_at_5
value: 51.002
- task:
type: Retrieval
dataset:
type: BeIR/cqadupstack
name: MTEB CQADupstackEnglishRetrieval
config: default
split: test
revision: None
metrics:
- type: map_at_1
value: 29.677999999999997
- type: map_at_10
value: 38.684000000000005
- type: map_at_100
value: 39.812999999999995
- type: map_at_1000
value: 39.945
- type: map_at_3
value: 35.831
- type: map_at_5
value: 37.446
- type: mrr_at_1
value: 37.771
- type: mrr_at_10
value: 44.936
- type: mrr_at_100
value: 45.583
- type: mrr_at_1000
value: 45.634
- type: mrr_at_3
value: 42.771
- type: mrr_at_5
value: 43.994
- type: ndcg_at_1
value: 37.771
- type: ndcg_at_10
value: 44.059
- type: ndcg_at_100
value: 48.192
- type: ndcg_at_1000
value: 50.375
- type: ndcg_at_3
value: 40.172000000000004
- type: ndcg_at_5
value: 41.899
- type: precision_at_1
value: 37.771
- type: precision_at_10
value: 8.286999999999999
- type: precision_at_100
value: 1.322
- type: precision_at_1000
value: 0.178
- type: precision_at_3
value: 19.406000000000002
- type: precision_at_5
value: 13.745
- type: recall_at_1
value: 29.677999999999997
- type: recall_at_10
value: 53.071
- type: recall_at_100
value: 70.812
- type: recall_at_1000
value: 84.841
- type: recall_at_3
value: 41.016000000000005
- type: recall_at_5
value: 46.22
- task:
type: Retrieval
dataset:
type: BeIR/cqadupstack
name: MTEB CQADupstackGamingRetrieval
config: default
split: test
revision: None
metrics:
- type: map_at_1
value: 42.675000000000004
- type: map_at_10
value: 53.93599999999999
- type: map_at_100
value: 54.806999999999995
- type: map_at_1000
value: 54.867
- type: map_at_3
value: 50.934000000000005
- type: map_at_5
value: 52.583
- type: mrr_at_1
value: 48.339
- type: mrr_at_10
value: 57.265
- type: mrr_at_100
value: 57.873
- type: mrr_at_1000
value: 57.906
- type: mrr_at_3
value: 55.193000000000005
- type: mrr_at_5
value: 56.303000000000004
- type: ndcg_at_1
value: 48.339
- type: ndcg_at_10
value: 59.19799999999999
- type: ndcg_at_100
value: 62.743
- type: ndcg_at_1000
value: 63.99399999999999
- type: ndcg_at_3
value: 54.367
- type: ndcg_at_5
value: 56.548
- type: precision_at_1
value: 48.339
- type: precision_at_10
value: 9.216000000000001
- type: precision_at_100
value: 1.1809999999999998
- type: precision_at_1000
value: 0.134
- type: precision_at_3
value: 23.72
- type: precision_at_5
value: 16.025
- type: recall_at_1
value: 42.675000000000004
- type: recall_at_10
value: 71.437
- type: recall_at_100
value: 86.803
- type: recall_at_1000
value: 95.581
- type: recall_at_3
value: 58.434
- type: recall_at_5
value: 63.754
- task:
type: Retrieval
dataset:
type: BeIR/cqadupstack
name: MTEB CQADupstackGisRetrieval
config: default
split: test
revision: None
metrics:
- type: map_at_1
value: 23.518
- type: map_at_10
value: 30.648999999999997
- type: map_at_100
value: 31.508999999999997
- type: map_at_1000
value: 31.604
- type: map_at_3
value: 28.247
- type: map_at_5
value: 29.65
- type: mrr_at_1
value: 25.650000000000002
- type: mrr_at_10
value: 32.771
- type: mrr_at_100
value: 33.554
- type: mrr_at_1000
value: 33.629999999999995
- type: mrr_at_3
value: 30.433
- type: mrr_at_5
value: 31.812
- type: ndcg_at_1
value: 25.650000000000002
- type: ndcg_at_10
value: 34.929
- type: ndcg_at_100
value: 39.382
- type: ndcg_at_1000
value: 41.913
- type: ndcg_at_3
value: 30.292
- type: ndcg_at_5
value: 32.629999999999995
- type: precision_at_1
value: 25.650000000000002
- type: precision_at_10
value: 5.311
- type: precision_at_100
value: 0.792
- type: precision_at_1000
value: 0.105
- type: precision_at_3
value: 12.58
- type: precision_at_5
value: 8.994
- type: recall_at_1
value: 23.518
- type: recall_at_10
value: 46.19
- type: recall_at_100
value: 67.123
- type: recall_at_1000
value: 86.442
- type: recall_at_3
value: 33.678000000000004
- type: recall_at_5
value: 39.244
- task:
type: Retrieval
dataset:
type: BeIR/cqadupstack
name: MTEB CQADupstackMathematicaRetrieval
config: default
split: test
revision: None
metrics:
- type: map_at_1
value: 15.891
- type: map_at_10
value: 22.464000000000002
- type: map_at_100
value: 23.483
- type: map_at_1000
value: 23.613
- type: map_at_3
value: 20.080000000000002
- type: map_at_5
value: 21.526
- type: mrr_at_1
value: 20.025000000000002
- type: mrr_at_10
value: 26.712999999999997
- type: mrr_at_100
value: 27.650000000000002
- type: mrr_at_1000
value: 27.737000000000002
- type: mrr_at_3
value: 24.274
- type: mrr_at_5
value: 25.711000000000002
- type: ndcg_at_1
value: 20.025000000000002
- type: ndcg_at_10
value: 27.028999999999996
- type: ndcg_at_100
value: 32.064
- type: ndcg_at_1000
value: 35.188
- type: ndcg_at_3
value: 22.512999999999998
- type: ndcg_at_5
value: 24.89
- type: precision_at_1
value: 20.025000000000002
- type: precision_at_10
value: 4.776
- type: precision_at_100
value: 0.8500000000000001
- type: precision_at_1000
value: 0.125
- type: precision_at_3
value: 10.531
- type: precision_at_5
value: 7.811
- type: recall_at_1
value: 15.891
- type: recall_at_10
value: 37.261
- type: recall_at_100
value: 59.12
- type: recall_at_1000
value: 81.356
- type: recall_at_3
value: 24.741
- type: recall_at_5
value: 30.753999999999998
- task:
type: Retrieval
dataset:
type: BeIR/cqadupstack
name: MTEB CQADupstackPhysicsRetrieval
config: default
split: test
revision: None
metrics:
- type: map_at_1
value: 27.544
- type: map_at_10
value: 36.283
- type: map_at_100
value: 37.467
- type: map_at_1000
value: 37.574000000000005
- type: map_at_3
value: 33.528999999999996
- type: map_at_5
value: 35.028999999999996
- type: mrr_at_1
value: 34.166999999999994
- type: mrr_at_10
value: 41.866
- type: mrr_at_100
value: 42.666
- type: mrr_at_1000
value: 42.716
- type: mrr_at_3
value: 39.541
- type: mrr_at_5
value: 40.768
- type: ndcg_at_1
value: 34.166999999999994
- type: ndcg_at_10
value: 41.577
- type: ndcg_at_100
value: 46.687
- type: ndcg_at_1000
value: 48.967
- type: ndcg_at_3
value: 37.177
- type: ndcg_at_5
value: 39.097
- type: precision_at_1
value: 34.166999999999994
- type: precision_at_10
value: 7.420999999999999
- type: precision_at_100
value: 1.165
- type: precision_at_1000
value: 0.154
- type: precision_at_3
value: 17.291999999999998
- type: precision_at_5
value: 12.166
- type: recall_at_1
value: 27.544
- type: recall_at_10
value: 51.99399999999999
- type: recall_at_100
value: 73.738
- type: recall_at_1000
value: 89.33
- type: recall_at_3
value: 39.179
- type: recall_at_5
value: 44.385999999999996
- task:
type: Retrieval
dataset:
type: BeIR/cqadupstack
name: MTEB CQADupstackProgrammersRetrieval
config: default
split: test
revision: None
metrics:
- type: map_at_1
value: 26.661
- type: map_at_10
value: 35.475
- type: map_at_100
value: 36.626999999999995
- type: map_at_1000
value: 36.741
- type: map_at_3
value: 32.818000000000005
- type: map_at_5
value: 34.397
- type: mrr_at_1
value: 32.647999999999996
- type: mrr_at_10
value: 40.784
- type: mrr_at_100
value: 41.602
- type: mrr_at_1000
value: 41.661
- type: mrr_at_3
value: 38.68
- type: mrr_at_5
value: 39.838
- type: ndcg_at_1
value: 32.647999999999996
- type: ndcg_at_10
value: 40.697
- type: ndcg_at_100
value: 45.799
- type: ndcg_at_1000
value: 48.235
- type: ndcg_at_3
value: 36.516
- type: ndcg_at_5
value: 38.515
- type: precision_at_1
value: 32.647999999999996
- type: precision_at_10
value: 7.202999999999999
- type: precision_at_100
value: 1.1360000000000001
- type: precision_at_1000
value: 0.151
- type: precision_at_3
value: 17.314
- type: precision_at_5
value: 12.145999999999999
- type: recall_at_1
value: 26.661
- type: recall_at_10
value: 50.995000000000005
- type: recall_at_100
value: 73.065
- type: recall_at_1000
value: 89.781
- type: recall_at_3
value: 39.073
- type: recall_at_5
value: 44.395
- task:
type: Retrieval
dataset:
type: BeIR/cqadupstack
name: MTEB CQADupstackRetrieval
config: default
split: test
revision: None
metrics:
- type: map_at_1
value: 25.946583333333333
- type: map_at_10
value: 33.79725
- type: map_at_100
value: 34.86408333333333
- type: map_at_1000
value: 34.9795
- type: map_at_3
value: 31.259999999999998
- type: map_at_5
value: 32.71541666666666
- type: mrr_at_1
value: 30.863749999999996
- type: mrr_at_10
value: 37.99183333333333
- type: mrr_at_100
value: 38.790499999999994
- type: mrr_at_1000
value: 38.85575000000001
- type: mrr_at_3
value: 35.82083333333333
- type: mrr_at_5
value: 37.07533333333333
- type: ndcg_at_1
value: 30.863749999999996
- type: ndcg_at_10
value: 38.52141666666667
- type: ndcg_at_100
value: 43.17966666666667
- type: ndcg_at_1000
value: 45.64608333333333
- type: ndcg_at_3
value: 34.333000000000006
- type: ndcg_at_5
value: 36.34975
- type: precision_at_1
value: 30.863749999999996
- type: precision_at_10
value: 6.598999999999999
- type: precision_at_100
value: 1.0502500000000001
- type: precision_at_1000
value: 0.14400000000000002
- type: precision_at_3
value: 15.557583333333334
- type: precision_at_5
value: 11.020000000000001
- type: recall_at_1
value: 25.946583333333333
- type: recall_at_10
value: 48.36991666666666
- type: recall_at_100
value: 69.02408333333334
- type: recall_at_1000
value: 86.43858333333331
- type: recall_at_3
value: 36.4965
- type: recall_at_5
value: 41.76258333333334
- task:
type: Retrieval
dataset:
type: BeIR/cqadupstack
name: MTEB CQADupstackStatsRetrieval
config: default
split: test
revision: None
metrics:
- type: map_at_1
value: 22.431
- type: map_at_10
value: 28.889
- type: map_at_100
value: 29.642000000000003
- type: map_at_1000
value: 29.742
- type: map_at_3
value: 26.998
- type: map_at_5
value: 28.172000000000004
- type: mrr_at_1
value: 25.307000000000002
- type: mrr_at_10
value: 31.763
- type: mrr_at_100
value: 32.443
- type: mrr_at_1000
value: 32.531
- type: mrr_at_3
value: 29.959000000000003
- type: mrr_at_5
value: 31.063000000000002
- type: ndcg_at_1
value: 25.307000000000002
- type: ndcg_at_10
value: 32.586999999999996
- type: ndcg_at_100
value: 36.5
- type: ndcg_at_1000
value: 39.133
- type: ndcg_at_3
value: 29.25
- type: ndcg_at_5
value: 31.023
- type: precision_at_1
value: 25.307000000000002
- type: precision_at_10
value: 4.954
- type: precision_at_100
value: 0.747
- type: precision_at_1000
value: 0.104
- type: precision_at_3
value: 12.577
- type: precision_at_5
value: 8.741999999999999
- type: recall_at_1
value: 22.431
- type: recall_at_10
value: 41.134
- type: recall_at_100
value: 59.28600000000001
- type: recall_at_1000
value: 78.857
- type: recall_at_3
value: 31.926
- type: recall_at_5
value: 36.335
- task:
type: Retrieval
dataset:
type: BeIR/cqadupstack
name: MTEB CQADupstackTexRetrieval
config: default
split: test
revision: None
metrics:
- type: map_at_1
value: 17.586
- type: map_at_10
value: 23.304
- type: map_at_100
value: 24.159
- type: map_at_1000
value: 24.281
- type: map_at_3
value: 21.316
- type: map_at_5
value: 22.383
- type: mrr_at_1
value: 21.645
- type: mrr_at_10
value: 27.365000000000002
- type: mrr_at_100
value: 28.108
- type: mrr_at_1000
value: 28.192
- type: mrr_at_3
value: 25.482
- type: mrr_at_5
value: 26.479999999999997
- type: ndcg_at_1
value: 21.645
- type: ndcg_at_10
value: 27.306
- type: ndcg_at_100
value: 31.496000000000002
- type: ndcg_at_1000
value: 34.53
- type: ndcg_at_3
value: 23.73
- type: ndcg_at_5
value: 25.294
- type: precision_at_1
value: 21.645
- type: precision_at_10
value: 4.797
- type: precision_at_100
value: 0.8059999999999999
- type: precision_at_1000
value: 0.121
- type: precision_at_3
value: 10.850999999999999
- type: precision_at_5
value: 7.736
- type: recall_at_1
value: 17.586
- type: recall_at_10
value: 35.481
- type: recall_at_100
value: 54.534000000000006
- type: recall_at_1000
value: 76.456
- type: recall_at_3
value: 25.335
- type: recall_at_5
value: 29.473
- task:
type: Retrieval
dataset:
type: BeIR/cqadupstack
name: MTEB CQADupstackUnixRetrieval
config: default
split: test
revision: None
metrics:
- type: map_at_1
value: 25.095
- type: map_at_10
value: 32.374
- type: map_at_100
value: 33.537
- type: map_at_1000
value: 33.634
- type: map_at_3
value: 30.089
- type: map_at_5
value: 31.433
- type: mrr_at_1
value: 29.198
- type: mrr_at_10
value: 36.01
- type: mrr_at_100
value: 37.022
- type: mrr_at_1000
value: 37.083
- type: mrr_at_3
value: 33.94
- type: mrr_at_5
value: 35.148
- type: ndcg_at_1
value: 29.198
- type: ndcg_at_10
value: 36.729
- type: ndcg_at_100
value: 42.114000000000004
- type: ndcg_at_1000
value: 44.592
- type: ndcg_at_3
value: 32.644
- type: ndcg_at_5
value: 34.652
- type: precision_at_1
value: 29.198
- type: precision_at_10
value: 5.970000000000001
- type: precision_at_100
value: 0.967
- type: precision_at_1000
value: 0.129
- type: precision_at_3
value: 14.396999999999998
- type: precision_at_5
value: 10.093
- type: recall_at_1
value: 25.095
- type: recall_at_10
value: 46.392
- type: recall_at_100
value: 69.706
- type: recall_at_1000
value: 87.738
- type: recall_at_3
value: 35.303000000000004
- type: recall_at_5
value: 40.441
- task:
type: Retrieval
dataset:
type: BeIR/cqadupstack
name: MTEB CQADupstackWebmastersRetrieval
config: default
split: test
revision: None
metrics:
- type: map_at_1
value: 26.857999999999997
- type: map_at_10
value: 34.066
- type: map_at_100
value: 35.671
- type: map_at_1000
value: 35.881
- type: map_at_3
value: 31.304
- type: map_at_5
value: 32.885
- type: mrr_at_1
value: 32.411
- type: mrr_at_10
value: 38.987
- type: mrr_at_100
value: 39.894
- type: mrr_at_1000
value: 39.959
- type: mrr_at_3
value: 36.626999999999995
- type: mrr_at_5
value: 38.011
- type: ndcg_at_1
value: 32.411
- type: ndcg_at_10
value: 39.208
- type: ndcg_at_100
value: 44.626
- type: ndcg_at_1000
value: 47.43
- type: ndcg_at_3
value: 35.091
- type: ndcg_at_5
value: 37.119
- type: precision_at_1
value: 32.411
- type: precision_at_10
value: 7.51
- type: precision_at_100
value: 1.486
- type: precision_at_1000
value: 0.234
- type: precision_at_3
value: 16.14
- type: precision_at_5
value: 11.976
- type: recall_at_1
value: 26.857999999999997
- type: recall_at_10
value: 47.407
- type: recall_at_100
value: 72.236
- type: recall_at_1000
value: 90.77
- type: recall_at_3
value: 35.125
- type: recall_at_5
value: 40.522999999999996
- task:
type: Retrieval
dataset:
type: BeIR/cqadupstack
name: MTEB CQADupstackWordpressRetrieval
config: default
split: test
revision: None
metrics:
- type: map_at_1
value: 21.3
- type: map_at_10
value: 27.412999999999997
- type: map_at_100
value: 28.29
- type: map_at_1000
value: 28.398
- type: map_at_3
value: 25.169999999999998
- type: map_at_5
value: 26.496
- type: mrr_at_1
value: 23.29
- type: mrr_at_10
value: 29.215000000000003
- type: mrr_at_100
value: 30.073
- type: mrr_at_1000
value: 30.156
- type: mrr_at_3
value: 26.956000000000003
- type: mrr_at_5
value: 28.38
- type: ndcg_at_1
value: 23.29
- type: ndcg_at_10
value: 31.113000000000003
- type: ndcg_at_100
value: 35.701
- type: ndcg_at_1000
value: 38.505
- type: ndcg_at_3
value: 26.727
- type: ndcg_at_5
value: 29.037000000000003
- type: precision_at_1
value: 23.29
- type: precision_at_10
value: 4.787
- type: precision_at_100
value: 0.763
- type: precision_at_1000
value: 0.11100000000000002
- type: precision_at_3
value: 11.091
- type: precision_at_5
value: 7.985
- type: recall_at_1
value: 21.3
- type: recall_at_10
value: 40.782000000000004
- type: recall_at_100
value: 62.13999999999999
- type: recall_at_1000
value: 83.012
- type: recall_at_3
value: 29.131
- type: recall_at_5
value: 34.624
- task:
type: Retrieval
dataset:
type: climate-fever
name: MTEB ClimateFEVER
config: default
split: test
revision: None
metrics:
- type: map_at_1
value: 9.631
- type: map_at_10
value: 16.634999999999998
- type: map_at_100
value: 18.23
- type: map_at_1000
value: 18.419
- type: map_at_3
value: 13.66
- type: map_at_5
value: 15.173
- type: mrr_at_1
value: 21.368000000000002
- type: mrr_at_10
value: 31.56
- type: mrr_at_100
value: 32.58
- type: mrr_at_1000
value: 32.633
- type: mrr_at_3
value: 28.241
- type: mrr_at_5
value: 30.225
- type: ndcg_at_1
value: 21.368000000000002
- type: ndcg_at_10
value: 23.855999999999998
- type: ndcg_at_100
value: 30.686999999999998
- type: ndcg_at_1000
value: 34.327000000000005
- type: ndcg_at_3
value: 18.781
- type: ndcg_at_5
value: 20.73
- type: precision_at_1
value: 21.368000000000002
- type: precision_at_10
value: 7.564
- type: precision_at_100
value: 1.496
- type: precision_at_1000
value: 0.217
- type: precision_at_3
value: 13.876
- type: precision_at_5
value: 11.062
- type: recall_at_1
value: 9.631
- type: recall_at_10
value: 29.517
- type: recall_at_100
value: 53.452
- type: recall_at_1000
value: 74.115
- type: recall_at_3
value: 17.605999999999998
- type: recall_at_5
value: 22.505
- task:
type: Retrieval
dataset:
type: dbpedia-entity
name: MTEB DBPedia
config: default
split: test
revision: None
metrics:
- type: map_at_1
value: 8.885
- type: map_at_10
value: 18.798000000000002
- type: map_at_100
value: 26.316
- type: map_at_1000
value: 27.869
- type: map_at_3
value: 13.719000000000001
- type: map_at_5
value: 15.716
- type: mrr_at_1
value: 66
- type: mrr_at_10
value: 74.263
- type: mrr_at_100
value: 74.519
- type: mrr_at_1000
value: 74.531
- type: mrr_at_3
value: 72.458
- type: mrr_at_5
value: 73.321
- type: ndcg_at_1
value: 53.87499999999999
- type: ndcg_at_10
value: 40.355999999999995
- type: ndcg_at_100
value: 44.366
- type: ndcg_at_1000
value: 51.771
- type: ndcg_at_3
value: 45.195
- type: ndcg_at_5
value: 42.187000000000005
- type: precision_at_1
value: 66
- type: precision_at_10
value: 31.75
- type: precision_at_100
value: 10.11
- type: precision_at_1000
value: 1.9800000000000002
- type: precision_at_3
value: 48.167
- type: precision_at_5
value: 40.050000000000004
- type: recall_at_1
value: 8.885
- type: recall_at_10
value: 24.471999999999998
- type: recall_at_100
value: 49.669000000000004
- type: recall_at_1000
value: 73.383
- type: recall_at_3
value: 14.872
- type: recall_at_5
value: 18.262999999999998
- task:
type: Classification
dataset:
type: mteb/emotion
name: MTEB EmotionClassification
config: default
split: test
revision: 4f58c6b202a23cf9a4da393831edf4f9183cad37
metrics:
- type: accuracy
value: 45.18
- type: f1
value: 40.26878691789978
- task:
type: Retrieval
dataset:
type: fever
name: MTEB FEVER
config: default
split: test
revision: None
metrics:
- type: map_at_1
value: 62.751999999999995
- type: map_at_10
value: 74.131
- type: map_at_100
value: 74.407
- type: map_at_1000
value: 74.423
- type: map_at_3
value: 72.329
- type: map_at_5
value: 73.555
- type: mrr_at_1
value: 67.282
- type: mrr_at_10
value: 78.292
- type: mrr_at_100
value: 78.455
- type: mrr_at_1000
value: 78.458
- type: mrr_at_3
value: 76.755
- type: mrr_at_5
value: 77.839
- type: ndcg_at_1
value: 67.282
- type: ndcg_at_10
value: 79.443
- type: ndcg_at_100
value: 80.529
- type: ndcg_at_1000
value: 80.812
- type: ndcg_at_3
value: 76.281
- type: ndcg_at_5
value: 78.235
- type: precision_at_1
value: 67.282
- type: precision_at_10
value: 10.078
- type: precision_at_100
value: 1.082
- type: precision_at_1000
value: 0.11199999999999999
- type: precision_at_3
value: 30.178
- type: precision_at_5
value: 19.232
- type: recall_at_1
value: 62.751999999999995
- type: recall_at_10
value: 91.521
- type: recall_at_100
value: 95.997
- type: recall_at_1000
value: 97.775
- type: recall_at_3
value: 83.131
- type: recall_at_5
value: 87.93299999999999
- task:
type: Retrieval
dataset:
type: fiqa
name: MTEB FiQA2018
config: default
split: test
revision: None
metrics:
- type: map_at_1
value: 18.861
- type: map_at_10
value: 30.252000000000002
- type: map_at_100
value: 32.082
- type: map_at_1000
value: 32.261
- type: map_at_3
value: 25.909
- type: map_at_5
value: 28.296
- type: mrr_at_1
value: 37.346000000000004
- type: mrr_at_10
value: 45.802
- type: mrr_at_100
value: 46.611999999999995
- type: mrr_at_1000
value: 46.659
- type: mrr_at_3
value: 43.056
- type: mrr_at_5
value: 44.637
- type: ndcg_at_1
value: 37.346000000000004
- type: ndcg_at_10
value: 38.169
- type: ndcg_at_100
value: 44.864
- type: ndcg_at_1000
value: 47.974
- type: ndcg_at_3
value: 33.619
- type: ndcg_at_5
value: 35.317
- type: precision_at_1
value: 37.346000000000004
- type: precision_at_10
value: 10.693999999999999
- type: precision_at_100
value: 1.775
- type: precision_at_1000
value: 0.231
- type: precision_at_3
value: 22.325
- type: precision_at_5
value: 16.852
- type: recall_at_1
value: 18.861
- type: recall_at_10
value: 45.672000000000004
- type: recall_at_100
value: 70.60499999999999
- type: recall_at_1000
value: 89.216
- type: recall_at_3
value: 30.361
- type: recall_at_5
value: 36.998999999999995
- task:
type: Retrieval
dataset:
type: hotpotqa
name: MTEB HotpotQA
config: default
split: test
revision: None
metrics:
- type: map_at_1
value: 37.852999999999994
- type: map_at_10
value: 59.961
- type: map_at_100
value: 60.78
- type: map_at_1000
value: 60.843
- type: map_at_3
value: 56.39999999999999
- type: map_at_5
value: 58.646
- type: mrr_at_1
value: 75.70599999999999
- type: mrr_at_10
value: 82.321
- type: mrr_at_100
value: 82.516
- type: mrr_at_1000
value: 82.525
- type: mrr_at_3
value: 81.317
- type: mrr_at_5
value: 81.922
- type: ndcg_at_1
value: 75.70599999999999
- type: ndcg_at_10
value: 68.557
- type: ndcg_at_100
value: 71.485
- type: ndcg_at_1000
value: 72.71600000000001
- type: ndcg_at_3
value: 63.524
- type: ndcg_at_5
value: 66.338
- type: precision_at_1
value: 75.70599999999999
- type: precision_at_10
value: 14.463000000000001
- type: precision_at_100
value: 1.677
- type: precision_at_1000
value: 0.184
- type: precision_at_3
value: 40.806
- type: precision_at_5
value: 26.709
- type: recall_at_1
value: 37.852999999999994
- type: recall_at_10
value: 72.316
- type: recall_at_100
value: 83.842
- type: recall_at_1000
value: 91.999
- type: recall_at_3
value: 61.209
- type: recall_at_5
value: 66.77199999999999
- task:
type: Classification
dataset:
type: mteb/imdb
name: MTEB ImdbClassification
config: default
split: test
revision: 3d86128a09e091d6018b6d26cad27f2739fc2db7
metrics:
- type: accuracy
value: 85.46039999999999
- type: ap
value: 79.9812521351881
- type: f1
value: 85.31722909702084
- task:
type: Retrieval
dataset:
type: msmarco
name: MTEB MSMARCO
config: default
split: dev
revision: None
metrics:
- type: map_at_1
value: 22.704
- type: map_at_10
value: 35.329
- type: map_at_100
value: 36.494
- type: map_at_1000
value: 36.541000000000004
- type: map_at_3
value: 31.476
- type: map_at_5
value: 33.731
- type: mrr_at_1
value: 23.294999999999998
- type: mrr_at_10
value: 35.859
- type: mrr_at_100
value: 36.968
- type: mrr_at_1000
value: 37.008
- type: mrr_at_3
value: 32.085
- type: mrr_at_5
value: 34.299
- type: ndcg_at_1
value: 23.324
- type: ndcg_at_10
value: 42.274
- type: ndcg_at_100
value: 47.839999999999996
- type: ndcg_at_1000
value: 48.971
- type: ndcg_at_3
value: 34.454
- type: ndcg_at_5
value: 38.464
- type: precision_at_1
value: 23.324
- type: precision_at_10
value: 6.648
- type: precision_at_100
value: 0.9440000000000001
- type: precision_at_1000
value: 0.104
- type: precision_at_3
value: 14.674999999999999
- type: precision_at_5
value: 10.850999999999999
- type: recall_at_1
value: 22.704
- type: recall_at_10
value: 63.660000000000004
- type: recall_at_100
value: 89.29899999999999
- type: recall_at_1000
value: 97.88900000000001
- type: recall_at_3
value: 42.441
- type: recall_at_5
value: 52.04
- task:
type: Classification
dataset:
type: mteb/mtop_domain
name: MTEB MTOPDomainClassification (en)
config: en
split: test
revision: d80d48c1eb48d3562165c59d59d0034df9fff0bf
metrics:
- type: accuracy
value: 93.1326949384405
- type: f1
value: 92.89743579612082
- task:
type: Classification
dataset:
type: mteb/mtop_domain
name: MTEB MTOPDomainClassification (de)
config: de
split: test
revision: d80d48c1eb48d3562165c59d59d0034df9fff0bf
metrics:
- type: accuracy
value: 89.62524654832347
- type: f1
value: 88.65106082263151
- task:
type: Classification
dataset:
type: mteb/mtop_domain
name: MTEB MTOPDomainClassification (es)
config: es
split: test
revision: d80d48c1eb48d3562165c59d59d0034df9fff0bf
metrics:
- type: accuracy
value: 90.59039359573046
- type: f1
value: 90.31532892105662
- task:
type: Classification
dataset:
type: mteb/mtop_domain
name: MTEB MTOPDomainClassification (fr)
config: fr
split: test
revision: d80d48c1eb48d3562165c59d59d0034df9fff0bf
metrics:
- type: accuracy
value: 86.21046038208581
- type: f1
value: 86.41459529813113
- task:
type: Classification
dataset:
type: mteb/mtop_domain
name: MTEB MTOPDomainClassification (hi)
config: hi
split: test
revision: d80d48c1eb48d3562165c59d59d0034df9fff0bf
metrics:
- type: accuracy
value: 87.3180351380423
- type: f1
value: 86.71383078226444
- task:
type: Classification
dataset:
type: mteb/mtop_domain
name: MTEB MTOPDomainClassification (th)
config: th
split: test
revision: d80d48c1eb48d3562165c59d59d0034df9fff0bf
metrics:
- type: accuracy
value: 86.24231464737792
- type: f1
value: 86.31845567592403
- task:
type: Classification
dataset:
type: mteb/mtop_intent
name: MTEB MTOPIntentClassification (en)
config: en
split: test
revision: ae001d0e6b1228650b7bd1c2c65fb50ad11a8aba
metrics:
- type: accuracy
value: 75.27131782945736
- type: f1
value: 57.52079940417103
- task:
type: Classification
dataset:
type: mteb/mtop_intent
name: MTEB MTOPIntentClassification (de)
config: de
split: test
revision: ae001d0e6b1228650b7bd1c2c65fb50ad11a8aba
metrics:
- type: accuracy
value: 71.2341504649197
- type: f1
value: 51.349951558039244
- task:
type: Classification
dataset:
type: mteb/mtop_intent
name: MTEB MTOPIntentClassification (es)
config: es
split: test
revision: ae001d0e6b1228650b7bd1c2c65fb50ad11a8aba
metrics:
- type: accuracy
value: 71.27418278852569
- type: f1
value: 50.1714985749095
- task:
type: Classification
dataset:
type: mteb/mtop_intent
name: MTEB MTOPIntentClassification (fr)
config: fr
split: test
revision: ae001d0e6b1228650b7bd1c2c65fb50ad11a8aba
metrics:
- type: accuracy
value: 67.68243031631694
- type: f1
value: 50.1066160836192
- task:
type: Classification
dataset:
type: mteb/mtop_intent
name: MTEB MTOPIntentClassification (hi)
config: hi
split: test
revision: ae001d0e6b1228650b7bd1c2c65fb50ad11a8aba
metrics:
- type: accuracy
value: 69.2362854069559
- type: f1
value: 48.821279948766424
- task:
type: Classification
dataset:
type: mteb/mtop_intent
name: MTEB MTOPIntentClassification (th)
config: th
split: test
revision: ae001d0e6b1228650b7bd1c2c65fb50ad11a8aba
metrics:
- type: accuracy
value: 71.71428571428571
- type: f1
value: 53.94611389496195
- task:
type: Classification
dataset:
type: mteb/amazon_massive_intent
name: MTEB MassiveIntentClassification (af)
config: af
split: test
revision: 31efe3c427b0bae9c22cbb560b8f15491cc6bed7
metrics:
- type: accuracy
value: 59.97646267652992
- type: f1
value: 57.26797883561521
- task:
type: Classification
dataset:
type: mteb/amazon_massive_intent
name: MTEB MassiveIntentClassification (am)
config: am
split: test
revision: 31efe3c427b0bae9c22cbb560b8f15491cc6bed7
metrics:
- type: accuracy
value: 53.65501008742435
- type: f1
value: 50.416258382177034
- task:
type: Classification
dataset:
type: mteb/amazon_massive_intent
name: MTEB MassiveIntentClassification (ar)
config: ar
split: test
revision: 31efe3c427b0bae9c22cbb560b8f15491cc6bed7
metrics:
- type: accuracy
value: 57.45796906523201
- type: f1
value: 53.306690547422185
- task:
type: Classification
dataset:
type: mteb/amazon_massive_intent
name: MTEB MassiveIntentClassification (az)
config: az
split: test
revision: 31efe3c427b0bae9c22cbb560b8f15491cc6bed7
metrics:
- type: accuracy
value: 62.59246805648957
- type: f1
value: 59.818381969051494
- task:
type: Classification
dataset:
type: mteb/amazon_massive_intent
name: MTEB MassiveIntentClassification (bn)
config: bn
split: test
revision: 31efe3c427b0bae9c22cbb560b8f15491cc6bed7
metrics:
- type: accuracy
value: 61.126429051782104
- type: f1
value: 58.25993593933026
- task:
type: Classification
dataset:
type: mteb/amazon_massive_intent
name: MTEB MassiveIntentClassification (cy)
config: cy
split: test
revision: 31efe3c427b0bae9c22cbb560b8f15491cc6bed7
metrics:
- type: accuracy
value: 50.057162071284466
- type: f1
value: 46.96095728790911
- task:
type: Classification
dataset:
type: mteb/amazon_massive_intent
name: MTEB MassiveIntentClassification (da)
config: da
split: test
revision: 31efe3c427b0bae9c22cbb560b8f15491cc6bed7
metrics:
- type: accuracy
value: 66.64425016812375
- type: f1
value: 62.858291698755764
- task:
type: Classification
dataset:
type: mteb/amazon_massive_intent
name: MTEB MassiveIntentClassification (de)
config: de
split: test
revision: 31efe3c427b0bae9c22cbb560b8f15491cc6bed7
metrics:
- type: accuracy
value: 66.08944182918628
- type: f1
value: 62.44639030604241
- task:
type: Classification
dataset:
type: mteb/amazon_massive_intent
name: MTEB MassiveIntentClassification (el)
config: el
split: test
revision: 31efe3c427b0bae9c22cbb560b8f15491cc6bed7
metrics:
- type: accuracy
value: 64.68056489576328
- type: f1
value: 61.775326758789504
- task:
type: Classification
dataset:
type: mteb/amazon_massive_intent
name: MTEB MassiveIntentClassification (en)
config: en
split: test
revision: 31efe3c427b0bae9c22cbb560b8f15491cc6bed7
metrics:
- type: accuracy
value: 72.11163416274377
- type: f1
value: 69.70789096927015
- task:
type: Classification
dataset:
type: mteb/amazon_massive_intent
name: MTEB MassiveIntentClassification (es)
config: es
split: test
revision: 31efe3c427b0bae9c22cbb560b8f15491cc6bed7
metrics:
- type: accuracy
value: 68.40282447881641
- type: f1
value: 66.38492065671895
- task:
type: Classification
dataset:
type: mteb/amazon_massive_intent
name: MTEB MassiveIntentClassification (fa)
config: fa
split: test
revision: 31efe3c427b0bae9c22cbb560b8f15491cc6bed7
metrics:
- type: accuracy
value: 67.24613315400134
- type: f1
value: 64.3348019501336
- task:
type: Classification
dataset:
type: mteb/amazon_massive_intent
name: MTEB MassiveIntentClassification (fi)
config: fi
split: test
revision: 31efe3c427b0bae9c22cbb560b8f15491cc6bed7
metrics:
- type: accuracy
value: 65.78345662407531
- type: f1
value: 62.21279452354622
- task:
type: Classification
dataset:
type: mteb/amazon_massive_intent
name: MTEB MassiveIntentClassification (fr)
config: fr
split: test
revision: 31efe3c427b0bae9c22cbb560b8f15491cc6bed7
metrics:
- type: accuracy
value: 67.9455279085407
- type: f1
value: 65.48193124964094
- task:
type: Classification
dataset:
type: mteb/amazon_massive_intent
name: MTEB MassiveIntentClassification (he)
config: he
split: test
revision: 31efe3c427b0bae9c22cbb560b8f15491cc6bed7
metrics:
- type: accuracy
value: 62.05110961667788
- type: f1
value: 58.097856564684534
- task:
type: Classification
dataset:
type: mteb/amazon_massive_intent
name: MTEB MassiveIntentClassification (hi)
config: hi
split: test
revision: 31efe3c427b0bae9c22cbb560b8f15491cc6bed7
metrics:
- type: accuracy
value: 64.95292535305985
- type: f1
value: 62.09182174767901
- task:
type: Classification
dataset:
type: mteb/amazon_massive_intent
name: MTEB MassiveIntentClassification (hu)
config: hu
split: test
revision: 31efe3c427b0bae9c22cbb560b8f15491cc6bed7
metrics:
- type: accuracy
value: 64.97310020174848
- type: f1
value: 61.14252567730396
- task:
type: Classification
dataset:
type: mteb/amazon_massive_intent
name: MTEB MassiveIntentClassification (hy)
config: hy
split: test
revision: 31efe3c427b0bae9c22cbb560b8f15491cc6bed7
metrics:
- type: accuracy
value: 60.08069939475453
- type: f1
value: 57.044041742492034
- task:
type: Classification
dataset:
type: mteb/amazon_massive_intent
name: MTEB MassiveIntentClassification (id)
config: id
split: test
revision: 31efe3c427b0bae9c22cbb560b8f15491cc6bed7
metrics:
- type: accuracy
value: 66.63752521856085
- type: f1
value: 63.889340907205316
- task:
type: Classification
dataset:
type: mteb/amazon_massive_intent
name: MTEB MassiveIntentClassification (is)
config: is
split: test
revision: 31efe3c427b0bae9c22cbb560b8f15491cc6bed7
metrics:
- type: accuracy
value: 56.385339609952936
- type: f1
value: 53.449033750088304
- task:
type: Classification
dataset:
type: mteb/amazon_massive_intent
name: MTEB MassiveIntentClassification (it)
config: it
split: test
revision: 31efe3c427b0bae9c22cbb560b8f15491cc6bed7
metrics:
- type: accuracy
value: 68.93073301950234
- type: f1
value: 65.9884357824104
- task:
type: Classification
dataset:
type: mteb/amazon_massive_intent
name: MTEB MassiveIntentClassification (ja)
config: ja
split: test
revision: 31efe3c427b0bae9c22cbb560b8f15491cc6bed7
metrics:
- type: accuracy
value: 68.94418291862812
- type: f1
value: 66.48740222583132
- task:
type: Classification
dataset:
type: mteb/amazon_massive_intent
name: MTEB MassiveIntentClassification (jv)
config: jv
split: test
revision: 31efe3c427b0bae9c22cbb560b8f15491cc6bed7
metrics:
- type: accuracy
value: 54.26025554808339
- type: f1
value: 50.19562815100793
- task:
type: Classification
dataset:
type: mteb/amazon_massive_intent
name: MTEB MassiveIntentClassification (ka)
config: ka
split: test
revision: 31efe3c427b0bae9c22cbb560b8f15491cc6bed7
metrics:
- type: accuracy
value: 48.98789509078682
- type: f1
value: 46.65788438676836
- task:
type: Classification
dataset:
type: mteb/amazon_massive_intent
name: MTEB MassiveIntentClassification (km)
config: km
split: test
revision: 31efe3c427b0bae9c22cbb560b8f15491cc6bed7
metrics:
- type: accuracy
value: 44.68728984532616
- type: f1
value: 41.642419349541996
- task:
type: Classification
dataset:
type: mteb/amazon_massive_intent
name: MTEB MassiveIntentClassification (kn)
config: kn
split: test
revision: 31efe3c427b0bae9c22cbb560b8f15491cc6bed7
metrics:
- type: accuracy
value: 59.19300605245461
- type: f1
value: 55.8626492442437
- task:
type: Classification
dataset:
type: mteb/amazon_massive_intent
name: MTEB MassiveIntentClassification (ko)
config: ko
split: test
revision: 31efe3c427b0bae9c22cbb560b8f15491cc6bed7
metrics:
- type: accuracy
value: 66.33826496301278
- type: f1
value: 63.89499791648792
- task:
type: Classification
dataset:
type: mteb/amazon_massive_intent
name: MTEB MassiveIntentClassification (lv)
config: lv
split: test
revision: 31efe3c427b0bae9c22cbb560b8f15491cc6bed7
metrics:
- type: accuracy
value: 60.33960995292536
- type: f1
value: 57.15242464180892
- task:
type: Classification
dataset:
type: mteb/amazon_massive_intent
name: MTEB MassiveIntentClassification (ml)
config: ml
split: test
revision: 31efe3c427b0bae9c22cbb560b8f15491cc6bed7
metrics:
- type: accuracy
value: 63.09347679892402
- type: f1
value: 59.64733214063841
- task:
type: Classification
dataset:
type: mteb/amazon_massive_intent
name: MTEB MassiveIntentClassification (mn)
config: mn
split: test
revision: 31efe3c427b0bae9c22cbb560b8f15491cc6bed7
metrics:
- type: accuracy
value: 58.75924680564896
- type: f1
value: 55.96585692366827
- task:
type: Classification
dataset:
type: mteb/amazon_massive_intent
name: MTEB MassiveIntentClassification (ms)
config: ms
split: test
revision: 31efe3c427b0bae9c22cbb560b8f15491cc6bed7
metrics:
- type: accuracy
value: 62.48486886348352
- type: f1
value: 59.45143559032946
- task:
type: Classification
dataset:
type: mteb/amazon_massive_intent
name: MTEB MassiveIntentClassification (my)
config: my
split: test
revision: 31efe3c427b0bae9c22cbb560b8f15491cc6bed7
metrics:
- type: accuracy
value: 58.56422326832549
- type: f1
value: 54.96368702901926
- task:
type: Classification
dataset:
type: mteb/amazon_massive_intent
name: MTEB MassiveIntentClassification (nb)
config: nb
split: test
revision: 31efe3c427b0bae9c22cbb560b8f15491cc6bed7
metrics:
- type: accuracy
value: 66.18022864828512
- type: f1
value: 63.05369805040634
- task:
type: Classification
dataset:
type: mteb/amazon_massive_intent
name: MTEB MassiveIntentClassification (nl)
config: nl
split: test
revision: 31efe3c427b0bae9c22cbb560b8f15491cc6bed7
metrics:
- type: accuracy
value: 67.30329522528581
- type: f1
value: 64.06084612020727
- task:
type: Classification
dataset:
type: mteb/amazon_massive_intent
name: MTEB MassiveIntentClassification (pl)
config: pl
split: test
revision: 31efe3c427b0bae9c22cbb560b8f15491cc6bed7
metrics:
- type: accuracy
value: 68.36919973100201
- type: f1
value: 65.12154124788887
- task:
type: Classification
dataset:
type: mteb/amazon_massive_intent
name: MTEB MassiveIntentClassification (pt)
config: pt
split: test
revision: 31efe3c427b0bae9c22cbb560b8f15491cc6bed7
metrics:
- type: accuracy
value: 68.98117014122394
- type: f1
value: 66.41847559806962
- task:
type: Classification
dataset:
type: mteb/amazon_massive_intent
name: MTEB MassiveIntentClassification (ro)
config: ro
split: test
revision: 31efe3c427b0bae9c22cbb560b8f15491cc6bed7
metrics:
- type: accuracy
value: 65.53799596503026
- type: f1
value: 62.17067330740817
- task:
type: Classification
dataset:
type: mteb/amazon_massive_intent
name: MTEB MassiveIntentClassification (ru)
config: ru
split: test
revision: 31efe3c427b0bae9c22cbb560b8f15491cc6bed7
metrics:
- type: accuracy
value: 69.01815736381977
- type: f1
value: 66.24988369607843
- task:
type: Classification
dataset:
type: mteb/amazon_massive_intent
name: MTEB MassiveIntentClassification (sl)
config: sl
split: test
revision: 31efe3c427b0bae9c22cbb560b8f15491cc6bed7
metrics:
- type: accuracy
value: 62.34700739744452
- type: f1
value: 59.957933424941636
- task:
type: Classification
dataset:
type: mteb/amazon_massive_intent
name: MTEB MassiveIntentClassification (sq)
config: sq
split: test
revision: 31efe3c427b0bae9c22cbb560b8f15491cc6bed7
metrics:
- type: accuracy
value: 61.23402824478815
- type: f1
value: 57.98836976018471
- task:
type: Classification
dataset:
type: mteb/amazon_massive_intent
name: MTEB MassiveIntentClassification (sv)
config: sv
split: test
revision: 31efe3c427b0bae9c22cbb560b8f15491cc6bed7
metrics:
- type: accuracy
value: 68.54068594485541
- type: f1
value: 65.43849680666855
- task:
type: Classification
dataset:
type: mteb/amazon_massive_intent
name: MTEB MassiveIntentClassification (sw)
config: sw
split: test
revision: 31efe3c427b0bae9c22cbb560b8f15491cc6bed7
metrics:
- type: accuracy
value: 55.998655010087425
- type: f1
value: 52.83737515406804
- task:
type: Classification
dataset:
type: mteb/amazon_massive_intent
name: MTEB MassiveIntentClassification (ta)
config: ta
split: test
revision: 31efe3c427b0bae9c22cbb560b8f15491cc6bed7
metrics:
- type: accuracy
value: 58.71217215870882
- type: f1
value: 55.051794977833026
- task:
type: Classification
dataset:
type: mteb/amazon_massive_intent
name: MTEB MassiveIntentClassification (te)
config: te
split: test
revision: 31efe3c427b0bae9c22cbb560b8f15491cc6bed7
metrics:
- type: accuracy
value: 59.724277067921996
- type: f1
value: 56.33485571838306
- task:
type: Classification
dataset:
type: mteb/amazon_massive_intent
name: MTEB MassiveIntentClassification (th)
config: th
split: test
revision: 31efe3c427b0bae9c22cbb560b8f15491cc6bed7
metrics:
- type: accuracy
value: 65.59515803631473
- type: f1
value: 64.96772366193588
- task:
type: Classification
dataset:
type: mteb/amazon_massive_intent
name: MTEB MassiveIntentClassification (tl)
config: tl
split: test
revision: 31efe3c427b0bae9c22cbb560b8f15491cc6bed7
metrics:
- type: accuracy
value: 60.860793544048406
- type: f1
value: 58.148845819115394
- task:
type: Classification
dataset:
type: mteb/amazon_massive_intent
name: MTEB MassiveIntentClassification (tr)
config: tr
split: test
revision: 31efe3c427b0bae9c22cbb560b8f15491cc6bed7
metrics:
- type: accuracy
value: 67.40753194351043
- type: f1
value: 63.18903778054698
- task:
type: Classification
dataset:
type: mteb/amazon_massive_intent
name: MTEB MassiveIntentClassification (ur)
config: ur
split: test
revision: 31efe3c427b0bae9c22cbb560b8f15491cc6bed7
metrics:
- type: accuracy
value: 61.52320107599194
- type: f1
value: 58.356144563398516
- task:
type: Classification
dataset:
type: mteb/amazon_massive_intent
name: MTEB MassiveIntentClassification (vi)
config: vi
split: test
revision: 31efe3c427b0bae9c22cbb560b8f15491cc6bed7
metrics:
- type: accuracy
value: 66.17014122394083
- type: f1
value: 63.919964062638925
- task:
type: Classification
dataset:
type: mteb/amazon_massive_intent
name: MTEB MassiveIntentClassification (zh-CN)
config: zh-CN
split: test
revision: 31efe3c427b0bae9c22cbb560b8f15491cc6bed7
metrics:
- type: accuracy
value: 69.15601882985878
- type: f1
value: 67.01451905761371
- task:
type: Classification
dataset:
type: mteb/amazon_massive_intent
name: MTEB MassiveIntentClassification (zh-TW)
config: zh-TW
split: test
revision: 31efe3c427b0bae9c22cbb560b8f15491cc6bed7
metrics:
- type: accuracy
value: 64.65030262273034
- type: f1
value: 64.14420425129063
- task:
type: Classification
dataset:
type: mteb/amazon_massive_scenario
name: MTEB MassiveScenarioClassification (af)
config: af
split: test
revision: 7d571f92784cd94a019292a1f45445077d0ef634
metrics:
- type: accuracy
value: 65.08742434431743
- type: f1
value: 63.044060042311756
- task:
type: Classification
dataset:
type: mteb/amazon_massive_scenario
name: MTEB MassiveScenarioClassification (am)
config: am
split: test
revision: 7d571f92784cd94a019292a1f45445077d0ef634
metrics:
- type: accuracy
value: 58.52387357094821
- type: f1
value: 56.82398588814534
- task:
type: Classification
dataset:
type: mteb/amazon_massive_scenario
name: MTEB MassiveScenarioClassification (ar)
config: ar
split: test
revision: 7d571f92784cd94a019292a1f45445077d0ef634
metrics:
- type: accuracy
value: 62.239408204438476
- type: f1
value: 61.92570286170469
- task:
type: Classification
dataset:
type: mteb/amazon_massive_scenario
name: MTEB MassiveScenarioClassification (az)
config: az
split: test
revision: 7d571f92784cd94a019292a1f45445077d0ef634
metrics:
- type: accuracy
value: 63.74915938130463
- type: f1
value: 62.130740689396276
- task:
type: Classification
dataset:
type: mteb/amazon_massive_scenario
name: MTEB MassiveScenarioClassification (bn)
config: bn
split: test
revision: 7d571f92784cd94a019292a1f45445077d0ef634
metrics:
- type: accuracy
value: 65.00336247478144
- type: f1
value: 63.71080635228055
- task:
type: Classification
dataset:
type: mteb/amazon_massive_scenario
name: MTEB MassiveScenarioClassification (cy)
config: cy
split: test
revision: 7d571f92784cd94a019292a1f45445077d0ef634
metrics:
- type: accuracy
value: 52.837928715534645
- type: f1
value: 50.390741680320836
- task:
type: Classification
dataset:
type: mteb/amazon_massive_scenario
name: MTEB MassiveScenarioClassification (da)
config: da
split: test
revision: 7d571f92784cd94a019292a1f45445077d0ef634
metrics:
- type: accuracy
value: 72.42098184263618
- type: f1
value: 71.41355113538995
- task:
type: Classification
dataset:
type: mteb/amazon_massive_scenario
name: MTEB MassiveScenarioClassification (de)
config: de
split: test
revision: 7d571f92784cd94a019292a1f45445077d0ef634
metrics:
- type: accuracy
value: 71.95359784801613
- type: f1
value: 71.42699340156742
- task:
type: Classification
dataset:
type: mteb/amazon_massive_scenario
name: MTEB MassiveScenarioClassification (el)
config: el
split: test
revision: 7d571f92784cd94a019292a1f45445077d0ef634
metrics:
- type: accuracy
value: 70.18157363819772
- type: f1
value: 69.74836113037671
- task:
type: Classification
dataset:
type: mteb/amazon_massive_scenario
name: MTEB MassiveScenarioClassification (en)
config: en
split: test
revision: 7d571f92784cd94a019292a1f45445077d0ef634
metrics:
- type: accuracy
value: 77.08137188971082
- type: f1
value: 76.78000685068261
- task:
type: Classification
dataset:
type: mteb/amazon_massive_scenario
name: MTEB MassiveScenarioClassification (es)
config: es
split: test
revision: 7d571f92784cd94a019292a1f45445077d0ef634
metrics:
- type: accuracy
value: 71.5030262273033
- type: f1
value: 71.71620130425673
- task:
type: Classification
dataset:
type: mteb/amazon_massive_scenario
name: MTEB MassiveScenarioClassification (fa)
config: fa
split: test
revision: 7d571f92784cd94a019292a1f45445077d0ef634
metrics:
- type: accuracy
value: 70.24546065904505
- type: f1
value: 69.07638311730359
- task:
type: Classification
dataset:
type: mteb/amazon_massive_scenario
name: MTEB MassiveScenarioClassification (fi)
config: fi
split: test
revision: 7d571f92784cd94a019292a1f45445077d0ef634
metrics:
- type: accuracy
value: 69.12911903160726
- type: f1
value: 68.32651736539815
- task:
type: Classification
dataset:
type: mteb/amazon_massive_scenario
name: MTEB MassiveScenarioClassification (fr)
config: fr
split: test
revision: 7d571f92784cd94a019292a1f45445077d0ef634
metrics:
- type: accuracy
value: 71.89307330195025
- type: f1
value: 71.33986549860187
- task:
type: Classification
dataset:
type: mteb/amazon_massive_scenario
name: MTEB MassiveScenarioClassification (he)
config: he
split: test
revision: 7d571f92784cd94a019292a1f45445077d0ef634
metrics:
- type: accuracy
value: 67.44451916610626
- type: f1
value: 66.90192664503866
- task:
type: Classification
dataset:
type: mteb/amazon_massive_scenario
name: MTEB MassiveScenarioClassification (hi)
config: hi
split: test
revision: 7d571f92784cd94a019292a1f45445077d0ef634
metrics:
- type: accuracy
value: 69.16274377942166
- type: f1
value: 68.01090953775066
- task:
type: Classification
dataset:
type: mteb/amazon_massive_scenario
name: MTEB MassiveScenarioClassification (hu)
config: hu
split: test
revision: 7d571f92784cd94a019292a1f45445077d0ef634
metrics:
- type: accuracy
value: 70.75319435104237
- type: f1
value: 70.18035309201403
- task:
type: Classification
dataset:
type: mteb/amazon_massive_scenario
name: MTEB MassiveScenarioClassification (hy)
config: hy
split: test
revision: 7d571f92784cd94a019292a1f45445077d0ef634
metrics:
- type: accuracy
value: 63.14391392064559
- type: f1
value: 61.48286540778145
- task:
type: Classification
dataset:
type: mteb/amazon_massive_scenario
name: MTEB MassiveScenarioClassification (id)
config: id
split: test
revision: 7d571f92784cd94a019292a1f45445077d0ef634
metrics:
- type: accuracy
value: 70.70275722932078
- type: f1
value: 70.26164779846495
- task:
type: Classification
dataset:
type: mteb/amazon_massive_scenario
name: MTEB MassiveScenarioClassification (is)
config: is
split: test
revision: 7d571f92784cd94a019292a1f45445077d0ef634
metrics:
- type: accuracy
value: 60.93813046402153
- type: f1
value: 58.8852862116525
- task:
type: Classification
dataset:
type: mteb/amazon_massive_scenario
name: MTEB MassiveScenarioClassification (it)
config: it
split: test
revision: 7d571f92784cd94a019292a1f45445077d0ef634
metrics:
- type: accuracy
value: 72.320107599193
- type: f1
value: 72.19836409602924
- task:
type: Classification
dataset:
type: mteb/amazon_massive_scenario
name: MTEB MassiveScenarioClassification (ja)
config: ja
split: test
revision: 7d571f92784cd94a019292a1f45445077d0ef634
metrics:
- type: accuracy
value: 74.65366509751176
- type: f1
value: 74.55188288799579
- task:
type: Classification
dataset:
type: mteb/amazon_massive_scenario
name: MTEB MassiveScenarioClassification (jv)
config: jv
split: test
revision: 7d571f92784cd94a019292a1f45445077d0ef634
metrics:
- type: accuracy
value: 59.694014794889036
- type: f1
value: 58.11353311721067
- task:
type: Classification
dataset:
type: mteb/amazon_massive_scenario
name: MTEB MassiveScenarioClassification (ka)
config: ka
split: test
revision: 7d571f92784cd94a019292a1f45445077d0ef634
metrics:
- type: accuracy
value: 54.37457969065231
- type: f1
value: 52.81306134311697
- task:
type: Classification
dataset:
type: mteb/amazon_massive_scenario
name: MTEB MassiveScenarioClassification (km)
config: km
split: test
revision: 7d571f92784cd94a019292a1f45445077d0ef634
metrics:
- type: accuracy
value: 48.3086751849361
- type: f1
value: 45.396449765419376
- task:
type: Classification
dataset:
type: mteb/amazon_massive_scenario
name: MTEB MassiveScenarioClassification (kn)
config: kn
split: test
revision: 7d571f92784cd94a019292a1f45445077d0ef634
metrics:
- type: accuracy
value: 62.151983860121064
- type: f1
value: 60.31762544281696
- task:
type: Classification
dataset:
type: mteb/amazon_massive_scenario
name: MTEB MassiveScenarioClassification (ko)
config: ko
split: test
revision: 7d571f92784cd94a019292a1f45445077d0ef634
metrics:
- type: accuracy
value: 72.44788164088769
- type: f1
value: 71.68150151736367
- task:
type: Classification
dataset:
type: mteb/amazon_massive_scenario
name: MTEB MassiveScenarioClassification (lv)
config: lv
split: test
revision: 7d571f92784cd94a019292a1f45445077d0ef634
metrics:
- type: accuracy
value: 62.81439139206455
- type: f1
value: 62.06735559105593
- task:
type: Classification
dataset:
type: mteb/amazon_massive_scenario
name: MTEB MassiveScenarioClassification (ml)
config: ml
split: test
revision: 7d571f92784cd94a019292a1f45445077d0ef634
metrics:
- type: accuracy
value: 68.04303967720242
- type: f1
value: 66.68298851670133
- task:
type: Classification
dataset:
type: mteb/amazon_massive_scenario
name: MTEB MassiveScenarioClassification (mn)
config: mn
split: test
revision: 7d571f92784cd94a019292a1f45445077d0ef634
metrics:
- type: accuracy
value: 61.43913920645595
- type: f1
value: 60.25605977560783
- task:
type: Classification
dataset:
type: mteb/amazon_massive_scenario
name: MTEB MassiveScenarioClassification (ms)
config: ms
split: test
revision: 7d571f92784cd94a019292a1f45445077d0ef634
metrics:
- type: accuracy
value: 66.90316072629456
- type: f1
value: 65.1325924692381
- task:
type: Classification
dataset:
type: mteb/amazon_massive_scenario
name: MTEB MassiveScenarioClassification (my)
config: my
split: test
revision: 7d571f92784cd94a019292a1f45445077d0ef634
metrics:
- type: accuracy
value: 61.63752521856086
- type: f1
value: 59.14284778039585
- task:
type: Classification
dataset:
type: mteb/amazon_massive_scenario
name: MTEB MassiveScenarioClassification (nb)
config: nb
split: test
revision: 7d571f92784cd94a019292a1f45445077d0ef634
metrics:
- type: accuracy
value: 71.63080026899797
- type: f1
value: 70.89771864626877
- task:
type: Classification
dataset:
type: mteb/amazon_massive_scenario
name: MTEB MassiveScenarioClassification (nl)
config: nl
split: test
revision: 7d571f92784cd94a019292a1f45445077d0ef634
metrics:
- type: accuracy
value: 72.10827168796234
- type: f1
value: 71.71954219691159
- task:
type: Classification
dataset:
type: mteb/amazon_massive_scenario
name: MTEB MassiveScenarioClassification (pl)
config: pl
split: test
revision: 7d571f92784cd94a019292a1f45445077d0ef634
metrics:
- type: accuracy
value: 70.59515803631471
- type: f1
value: 70.05040128099003
- task:
type: Classification
dataset:
type: mteb/amazon_massive_scenario
name: MTEB MassiveScenarioClassification (pt)
config: pt
split: test
revision: 7d571f92784cd94a019292a1f45445077d0ef634
metrics:
- type: accuracy
value: 70.83389374579691
- type: f1
value: 70.84877936562735
- task:
type: Classification
dataset:
type: mteb/amazon_massive_scenario
name: MTEB MassiveScenarioClassification (ro)
config: ro
split: test
revision: 7d571f92784cd94a019292a1f45445077d0ef634
metrics:
- type: accuracy
value: 69.18628110289173
- type: f1
value: 68.97232927921841
- task:
type: Classification
dataset:
type: mteb/amazon_massive_scenario
name: MTEB MassiveScenarioClassification (ru)
config: ru
split: test
revision: 7d571f92784cd94a019292a1f45445077d0ef634
metrics:
- type: accuracy
value: 72.99260255548083
- type: f1
value: 72.85139492157732
- task:
type: Classification
dataset:
type: mteb/amazon_massive_scenario
name: MTEB MassiveScenarioClassification (sl)
config: sl
split: test
revision: 7d571f92784cd94a019292a1f45445077d0ef634
metrics:
- type: accuracy
value: 65.26227303295225
- type: f1
value: 65.08833655469431
- task:
type: Classification
dataset:
type: mteb/amazon_massive_scenario
name: MTEB MassiveScenarioClassification (sq)
config: sq
split: test
revision: 7d571f92784cd94a019292a1f45445077d0ef634
metrics:
- type: accuracy
value: 66.48621385339611
- type: f1
value: 64.43483199071298
- task:
type: Classification
dataset:
type: mteb/amazon_massive_scenario
name: MTEB MassiveScenarioClassification (sv)
config: sv
split: test
revision: 7d571f92784cd94a019292a1f45445077d0ef634
metrics:
- type: accuracy
value: 73.14391392064559
- type: f1
value: 72.2580822579741
- task:
type: Classification
dataset:
type: mteb/amazon_massive_scenario
name: MTEB MassiveScenarioClassification (sw)
config: sw
split: test
revision: 7d571f92784cd94a019292a1f45445077d0ef634
metrics:
- type: accuracy
value: 59.88567585743107
- type: f1
value: 58.3073765932569
- task:
type: Classification
dataset:
type: mteb/amazon_massive_scenario
name: MTEB MassiveScenarioClassification (ta)
config: ta
split: test
revision: 7d571f92784cd94a019292a1f45445077d0ef634
metrics:
- type: accuracy
value: 62.38399462004034
- type: f1
value: 60.82139544252606
- task:
type: Classification
dataset:
type: mteb/amazon_massive_scenario
name: MTEB MassiveScenarioClassification (te)
config: te
split: test
revision: 7d571f92784cd94a019292a1f45445077d0ef634
metrics:
- type: accuracy
value: 62.58574310692671
- type: f1
value: 60.71443370385374
- task:
type: Classification
dataset:
type: mteb/amazon_massive_scenario
name: MTEB MassiveScenarioClassification (th)
config: th
split: test
revision: 7d571f92784cd94a019292a1f45445077d0ef634
metrics:
- type: accuracy
value: 71.61398789509079
- type: f1
value: 70.99761812049401
- task:
type: Classification
dataset:
type: mteb/amazon_massive_scenario
name: MTEB MassiveScenarioClassification (tl)
config: tl
split: test
revision: 7d571f92784cd94a019292a1f45445077d0ef634
metrics:
- type: accuracy
value: 62.73705447209146
- type: f1
value: 61.680849331794796
- task:
type: Classification
dataset:
type: mteb/amazon_massive_scenario
name: MTEB MassiveScenarioClassification (tr)
config: tr
split: test
revision: 7d571f92784cd94a019292a1f45445077d0ef634
metrics:
- type: accuracy
value: 71.66778749159381
- type: f1
value: 71.17320646080115
- task:
type: Classification
dataset:
type: mteb/amazon_massive_scenario
name: MTEB MassiveScenarioClassification (ur)
config: ur
split: test
revision: 7d571f92784cd94a019292a1f45445077d0ef634
metrics:
- type: accuracy
value: 64.640215198386
- type: f1
value: 63.301805157015444
- task:
type: Classification
dataset:
type: mteb/amazon_massive_scenario
name: MTEB MassiveScenarioClassification (vi)
config: vi
split: test
revision: 7d571f92784cd94a019292a1f45445077d0ef634
metrics:
- type: accuracy
value: 70.00672494956288
- type: f1
value: 70.26005548582106
- task:
type: Classification
dataset:
type: mteb/amazon_massive_scenario
name: MTEB MassiveScenarioClassification (zh-CN)
config: zh-CN
split: test
revision: 7d571f92784cd94a019292a1f45445077d0ef634
metrics:
- type: accuracy
value: 75.42030934767989
- type: f1
value: 75.2074842882598
- task:
type: Classification
dataset:
type: mteb/amazon_massive_scenario
name: MTEB MassiveScenarioClassification (zh-TW)
config: zh-TW
split: test
revision: 7d571f92784cd94a019292a1f45445077d0ef634
metrics:
- type: accuracy
value: 70.69266980497646
- type: f1
value: 70.94103167391192
- task:
type: Clustering
dataset:
type: mteb/medrxiv-clustering-p2p
name: MTEB MedrxivClusteringP2P
config: default
split: test
revision: e7a26af6f3ae46b30dde8737f02c07b1505bcc73
metrics:
- type: v_measure
value: 28.91697191169135
- task:
type: Clustering
dataset:
type: mteb/medrxiv-clustering-s2s
name: MTEB MedrxivClusteringS2S
config: default
split: test
revision: 35191c8c0dca72d8ff3efcd72aa802307d469663
metrics:
- type: v_measure
value: 28.434000079573313
- task:
type: Reranking
dataset:
type: mteb/mind_small
name: MTEB MindSmallReranking
config: default
split: test
revision: 3bdac13927fdc888b903db93b2ffdbd90b295a69
metrics:
- type: map
value: 30.96683513343383
- type: mrr
value: 31.967364078714834
- task:
type: Retrieval
dataset:
type: nfcorpus
name: MTEB NFCorpus
config: default
split: test
revision: None
metrics:
- type: map_at_1
value: 5.5280000000000005
- type: map_at_10
value: 11.793
- type: map_at_100
value: 14.496999999999998
- type: map_at_1000
value: 15.783
- type: map_at_3
value: 8.838
- type: map_at_5
value: 10.07
- type: mrr_at_1
value: 43.653
- type: mrr_at_10
value: 51.531000000000006
- type: mrr_at_100
value: 52.205
- type: mrr_at_1000
value: 52.242999999999995
- type: mrr_at_3
value: 49.431999999999995
- type: mrr_at_5
value: 50.470000000000006
- type: ndcg_at_1
value: 42.415000000000006
- type: ndcg_at_10
value: 32.464999999999996
- type: ndcg_at_100
value: 28.927999999999997
- type: ndcg_at_1000
value: 37.629000000000005
- type: ndcg_at_3
value: 37.845
- type: ndcg_at_5
value: 35.147
- type: precision_at_1
value: 43.653
- type: precision_at_10
value: 23.932000000000002
- type: precision_at_100
value: 7.17
- type: precision_at_1000
value: 1.967
- type: precision_at_3
value: 35.397
- type: precision_at_5
value: 29.907
- type: recall_at_1
value: 5.5280000000000005
- type: recall_at_10
value: 15.568000000000001
- type: recall_at_100
value: 28.54
- type: recall_at_1000
value: 59.864
- type: recall_at_3
value: 9.822000000000001
- type: recall_at_5
value: 11.726
- task:
type: Retrieval
dataset:
type: nq
name: MTEB NQ
config: default
split: test
revision: None
metrics:
- type: map_at_1
value: 37.041000000000004
- type: map_at_10
value: 52.664
- type: map_at_100
value: 53.477
- type: map_at_1000
value: 53.505
- type: map_at_3
value: 48.510999999999996
- type: map_at_5
value: 51.036
- type: mrr_at_1
value: 41.338
- type: mrr_at_10
value: 55.071000000000005
- type: mrr_at_100
value: 55.672
- type: mrr_at_1000
value: 55.689
- type: mrr_at_3
value: 51.82
- type: mrr_at_5
value: 53.852
- type: ndcg_at_1
value: 41.338
- type: ndcg_at_10
value: 60.01800000000001
- type: ndcg_at_100
value: 63.409000000000006
- type: ndcg_at_1000
value: 64.017
- type: ndcg_at_3
value: 52.44799999999999
- type: ndcg_at_5
value: 56.571000000000005
- type: precision_at_1
value: 41.338
- type: precision_at_10
value: 9.531
- type: precision_at_100
value: 1.145
- type: precision_at_1000
value: 0.12
- type: precision_at_3
value: 23.416
- type: precision_at_5
value: 16.46
- type: recall_at_1
value: 37.041000000000004
- type: recall_at_10
value: 79.76299999999999
- type: recall_at_100
value: 94.39
- type: recall_at_1000
value: 98.851
- type: recall_at_3
value: 60.465
- type: recall_at_5
value: 69.906
- task:
type: Retrieval
dataset:
type: quora
name: MTEB QuoraRetrieval
config: default
split: test
revision: None
metrics:
- type: map_at_1
value: 69.952
- type: map_at_10
value: 83.758
- type: map_at_100
value: 84.406
- type: map_at_1000
value: 84.425
- type: map_at_3
value: 80.839
- type: map_at_5
value: 82.646
- type: mrr_at_1
value: 80.62
- type: mrr_at_10
value: 86.947
- type: mrr_at_100
value: 87.063
- type: mrr_at_1000
value: 87.064
- type: mrr_at_3
value: 85.96000000000001
- type: mrr_at_5
value: 86.619
- type: ndcg_at_1
value: 80.63
- type: ndcg_at_10
value: 87.64800000000001
- type: ndcg_at_100
value: 88.929
- type: ndcg_at_1000
value: 89.054
- type: ndcg_at_3
value: 84.765
- type: ndcg_at_5
value: 86.291
- type: precision_at_1
value: 80.63
- type: precision_at_10
value: 13.314
- type: precision_at_100
value: 1.525
- type: precision_at_1000
value: 0.157
- type: precision_at_3
value: 37.1
- type: precision_at_5
value: 24.372
- type: recall_at_1
value: 69.952
- type: recall_at_10
value: 94.955
- type: recall_at_100
value: 99.38
- type: recall_at_1000
value: 99.96000000000001
- type: recall_at_3
value: 86.60600000000001
- type: recall_at_5
value: 90.997
- task:
type: Clustering
dataset:
type: mteb/reddit-clustering
name: MTEB RedditClustering
config: default
split: test
revision: 24640382cdbf8abc73003fb0fa6d111a705499eb
metrics:
- type: v_measure
value: 42.41329517878427
- task:
type: Clustering
dataset:
type: mteb/reddit-clustering-p2p
name: MTEB RedditClusteringP2P
config: default
split: test
revision: 282350215ef01743dc01b456c7f5241fa8937f16
metrics:
- type: v_measure
value: 55.171278362748666
- task:
type: Retrieval
dataset:
type: scidocs
name: MTEB SCIDOCS
config: default
split: test
revision: None
metrics:
- type: map_at_1
value: 4.213
- type: map_at_10
value: 9.895
- type: map_at_100
value: 11.776
- type: map_at_1000
value: 12.084
- type: map_at_3
value: 7.2669999999999995
- type: map_at_5
value: 8.620999999999999
- type: mrr_at_1
value: 20.8
- type: mrr_at_10
value: 31.112000000000002
- type: mrr_at_100
value: 32.274
- type: mrr_at_1000
value: 32.35
- type: mrr_at_3
value: 28.133000000000003
- type: mrr_at_5
value: 29.892999999999997
- type: ndcg_at_1
value: 20.8
- type: ndcg_at_10
value: 17.163999999999998
- type: ndcg_at_100
value: 24.738
- type: ndcg_at_1000
value: 30.316
- type: ndcg_at_3
value: 16.665
- type: ndcg_at_5
value: 14.478
- type: precision_at_1
value: 20.8
- type: precision_at_10
value: 8.74
- type: precision_at_100
value: 1.963
- type: precision_at_1000
value: 0.33
- type: precision_at_3
value: 15.467
- type: precision_at_5
value: 12.6
- type: recall_at_1
value: 4.213
- type: recall_at_10
value: 17.698
- type: recall_at_100
value: 39.838
- type: recall_at_1000
value: 66.893
- type: recall_at_3
value: 9.418
- type: recall_at_5
value: 12.773000000000001
- task:
type: STS
dataset:
type: mteb/sickr-sts
name: MTEB SICK-R
config: default
split: test
revision: a6ea5a8cab320b040a23452cc28066d9beae2cee
metrics:
- type: cos_sim_pearson
value: 82.90453315738294
- type: cos_sim_spearman
value: 78.51197850080254
- type: euclidean_pearson
value: 80.09647123597748
- type: euclidean_spearman
value: 78.63548011514061
- type: manhattan_pearson
value: 80.10645285675231
- type: manhattan_spearman
value: 78.57861806068901
- task:
type: STS
dataset:
type: mteb/sts12-sts
name: MTEB STS12
config: default
split: test
revision: a0d554a64d88156834ff5ae9920b964011b16384
metrics:
- type: cos_sim_pearson
value: 84.2616156846401
- type: cos_sim_spearman
value: 76.69713867850156
- type: euclidean_pearson
value: 77.97948563800394
- type: euclidean_spearman
value: 74.2371211567807
- type: manhattan_pearson
value: 77.69697879669705
- type: manhattan_spearman
value: 73.86529778022278
- task:
type: STS
dataset:
type: mteb/sts13-sts
name: MTEB STS13
config: default
split: test
revision: 7e90230a92c190f1bf69ae9002b8cea547a64cca
metrics:
- type: cos_sim_pearson
value: 77.0293269315045
- type: cos_sim_spearman
value: 78.02555120584198
- type: euclidean_pearson
value: 78.25398100379078
- type: euclidean_spearman
value: 78.66963870599464
- type: manhattan_pearson
value: 78.14314682167348
- type: manhattan_spearman
value: 78.57692322969135
- task:
type: STS
dataset:
type: mteb/sts14-sts
name: MTEB STS14
config: default
split: test
revision: 6031580fec1f6af667f0bd2da0a551cf4f0b2375
metrics:
- type: cos_sim_pearson
value: 79.16989925136942
- type: cos_sim_spearman
value: 76.5996225327091
- type: euclidean_pearson
value: 77.8319003279786
- type: euclidean_spearman
value: 76.42824009468998
- type: manhattan_pearson
value: 77.69118862737736
- type: manhattan_spearman
value: 76.25568104762812
- task:
type: STS
dataset:
type: mteb/sts15-sts
name: MTEB STS15
config: default
split: test
revision: ae752c7c21bf194d8b67fd573edf7ae58183cbe3
metrics:
- type: cos_sim_pearson
value: 87.42012286935325
- type: cos_sim_spearman
value: 88.15654297884122
- type: euclidean_pearson
value: 87.34082819427852
- type: euclidean_spearman
value: 88.06333589547084
- type: manhattan_pearson
value: 87.25115596784842
- type: manhattan_spearman
value: 87.9559927695203
- task:
type: STS
dataset:
type: mteb/sts16-sts
name: MTEB STS16
config: default
split: test
revision: 4d8694f8f0e0100860b497b999b3dbed754a0513
metrics:
- type: cos_sim_pearson
value: 82.88222044996712
- type: cos_sim_spearman
value: 84.28476589061077
- type: euclidean_pearson
value: 83.17399758058309
- type: euclidean_spearman
value: 83.85497357244542
- type: manhattan_pearson
value: 83.0308397703786
- type: manhattan_spearman
value: 83.71554539935046
- task:
type: STS
dataset:
type: mteb/sts17-crosslingual-sts
name: MTEB STS17 (ko-ko)
config: ko-ko
split: test
revision: af5e6fb845001ecf41f4c1e033ce921939a2a68d
metrics:
- type: cos_sim_pearson
value: 80.20682986257339
- type: cos_sim_spearman
value: 79.94567120362092
- type: euclidean_pearson
value: 79.43122480368902
- type: euclidean_spearman
value: 79.94802077264987
- type: manhattan_pearson
value: 79.32653021527081
- type: manhattan_spearman
value: 79.80961146709178
- task:
type: STS
dataset:
type: mteb/sts17-crosslingual-sts
name: MTEB STS17 (ar-ar)
config: ar-ar
split: test
revision: af5e6fb845001ecf41f4c1e033ce921939a2a68d
metrics:
- type: cos_sim_pearson
value: 74.46578144394383
- type: cos_sim_spearman
value: 74.52496637472179
- type: euclidean_pearson
value: 72.2903807076809
- type: euclidean_spearman
value: 73.55549359771645
- type: manhattan_pearson
value: 72.09324837709393
- type: manhattan_spearman
value: 73.36743103606581
- task:
type: STS
dataset:
type: mteb/sts17-crosslingual-sts
name: MTEB STS17 (en-ar)
config: en-ar
split: test
revision: af5e6fb845001ecf41f4c1e033ce921939a2a68d
metrics:
- type: cos_sim_pearson
value: 71.37272335116
- type: cos_sim_spearman
value: 71.26702117766037
- type: euclidean_pearson
value: 67.114829954434
- type: euclidean_spearman
value: 66.37938893947761
- type: manhattan_pearson
value: 66.79688574095246
- type: manhattan_spearman
value: 66.17292828079667
- task:
type: STS
dataset:
type: mteb/sts17-crosslingual-sts
name: MTEB STS17 (en-de)
config: en-de
split: test
revision: af5e6fb845001ecf41f4c1e033ce921939a2a68d
metrics:
- type: cos_sim_pearson
value: 80.61016770129092
- type: cos_sim_spearman
value: 82.08515426632214
- type: euclidean_pearson
value: 80.557340361131
- type: euclidean_spearman
value: 80.37585812266175
- type: manhattan_pearson
value: 80.6782873404285
- type: manhattan_spearman
value: 80.6678073032024
- task:
type: STS
dataset:
type: mteb/sts17-crosslingual-sts
name: MTEB STS17 (en-en)
config: en-en
split: test
revision: af5e6fb845001ecf41f4c1e033ce921939a2a68d
metrics:
- type: cos_sim_pearson
value: 87.00150745350108
- type: cos_sim_spearman
value: 87.83441972211425
- type: euclidean_pearson
value: 87.94826702308792
- type: euclidean_spearman
value: 87.46143974860725
- type: manhattan_pearson
value: 87.97560344306105
- type: manhattan_spearman
value: 87.5267102829796
- task:
type: STS
dataset:
type: mteb/sts17-crosslingual-sts
name: MTEB STS17 (en-tr)
config: en-tr
split: test
revision: af5e6fb845001ecf41f4c1e033ce921939a2a68d
metrics:
- type: cos_sim_pearson
value: 64.76325252267235
- type: cos_sim_spearman
value: 63.32615095463905
- type: euclidean_pearson
value: 64.07920669155716
- type: euclidean_spearman
value: 61.21409893072176
- type: manhattan_pearson
value: 64.26308625680016
- type: manhattan_spearman
value: 61.2438185254079
- task:
type: STS
dataset:
type: mteb/sts17-crosslingual-sts
name: MTEB STS17 (es-en)
config: es-en
split: test
revision: af5e6fb845001ecf41f4c1e033ce921939a2a68d
metrics:
- type: cos_sim_pearson
value: 75.82644463022595
- type: cos_sim_spearman
value: 76.50381269945073
- type: euclidean_pearson
value: 75.1328548315934
- type: euclidean_spearman
value: 75.63761139408453
- type: manhattan_pearson
value: 75.18610101241407
- type: manhattan_spearman
value: 75.30669266354164
- task:
type: STS
dataset:
type: mteb/sts17-crosslingual-sts
name: MTEB STS17 (es-es)
config: es-es
split: test
revision: af5e6fb845001ecf41f4c1e033ce921939a2a68d
metrics:
- type: cos_sim_pearson
value: 87.49994164686832
- type: cos_sim_spearman
value: 86.73743986245549
- type: euclidean_pearson
value: 86.8272894387145
- type: euclidean_spearman
value: 85.97608491000507
- type: manhattan_pearson
value: 86.74960140396779
- type: manhattan_spearman
value: 85.79285984190273
- task:
type: STS
dataset:
type: mteb/sts17-crosslingual-sts
name: MTEB STS17 (fr-en)
config: fr-en
split: test
revision: af5e6fb845001ecf41f4c1e033ce921939a2a68d
metrics:
- type: cos_sim_pearson
value: 79.58172210788469
- type: cos_sim_spearman
value: 80.17516468334607
- type: euclidean_pearson
value: 77.56537843470504
- type: euclidean_spearman
value: 77.57264627395521
- type: manhattan_pearson
value: 78.09703521695943
- type: manhattan_spearman
value: 78.15942760916954
- task:
type: STS
dataset:
type: mteb/sts17-crosslingual-sts
name: MTEB STS17 (it-en)
config: it-en
split: test
revision: af5e6fb845001ecf41f4c1e033ce921939a2a68d
metrics:
- type: cos_sim_pearson
value: 79.7589932931751
- type: cos_sim_spearman
value: 80.15210089028162
- type: euclidean_pearson
value: 77.54135223516057
- type: euclidean_spearman
value: 77.52697996368764
- type: manhattan_pearson
value: 77.65734439572518
- type: manhattan_spearman
value: 77.77702992016121
- task:
type: STS
dataset:
type: mteb/sts17-crosslingual-sts
name: MTEB STS17 (nl-en)
config: nl-en
split: test
revision: af5e6fb845001ecf41f4c1e033ce921939a2a68d
metrics:
- type: cos_sim_pearson
value: 79.16682365511267
- type: cos_sim_spearman
value: 79.25311267628506
- type: euclidean_pearson
value: 77.54882036762244
- type: euclidean_spearman
value: 77.33212935194827
- type: manhattan_pearson
value: 77.98405516064015
- type: manhattan_spearman
value: 77.85075717865719
- task:
type: STS
dataset:
type: mteb/sts22-crosslingual-sts
name: MTEB STS22 (en)
config: en
split: test
revision: 6d1ba47164174a496b7fa5d3569dae26a6813b80
metrics:
- type: cos_sim_pearson
value: 59.10473294775917
- type: cos_sim_spearman
value: 61.82780474476838
- type: euclidean_pearson
value: 45.885111672377256
- type: euclidean_spearman
value: 56.88306351932454
- type: manhattan_pearson
value: 46.101218127323186
- type: manhattan_spearman
value: 56.80953694186333
- task:
type: STS
dataset:
type: mteb/sts22-crosslingual-sts
name: MTEB STS22 (de)
config: de
split: test
revision: 6d1ba47164174a496b7fa5d3569dae26a6813b80
metrics:
- type: cos_sim_pearson
value: 45.781923079584146
- type: cos_sim_spearman
value: 55.95098449691107
- type: euclidean_pearson
value: 25.4571031323205
- type: euclidean_spearman
value: 49.859978118078935
- type: manhattan_pearson
value: 25.624938455041384
- type: manhattan_spearman
value: 49.99546185049401
- task:
type: STS
dataset:
type: mteb/sts22-crosslingual-sts
name: MTEB STS22 (es)
config: es
split: test
revision: 6d1ba47164174a496b7fa5d3569dae26a6813b80
metrics:
- type: cos_sim_pearson
value: 60.00618133997907
- type: cos_sim_spearman
value: 66.57896677718321
- type: euclidean_pearson
value: 42.60118466388821
- type: euclidean_spearman
value: 62.8210759715209
- type: manhattan_pearson
value: 42.63446860604094
- type: manhattan_spearman
value: 62.73803068925271
- task:
type: STS
dataset:
type: mteb/sts22-crosslingual-sts
name: MTEB STS22 (pl)
config: pl
split: test
revision: 6d1ba47164174a496b7fa5d3569dae26a6813b80
metrics:
- type: cos_sim_pearson
value: 28.460759121626943
- type: cos_sim_spearman
value: 34.13459007469131
- type: euclidean_pearson
value: 6.0917739325525195
- type: euclidean_spearman
value: 27.9947262664867
- type: manhattan_pearson
value: 6.16877864169911
- type: manhattan_spearman
value: 28.00664163971514
- task:
type: STS
dataset:
type: mteb/sts22-crosslingual-sts
name: MTEB STS22 (tr)
config: tr
split: test
revision: 6d1ba47164174a496b7fa5d3569dae26a6813b80
metrics:
- type: cos_sim_pearson
value: 57.42546621771696
- type: cos_sim_spearman
value: 63.699663168970474
- type: euclidean_pearson
value: 38.12085278789738
- type: euclidean_spearman
value: 58.12329140741536
- type: manhattan_pearson
value: 37.97364549443335
- type: manhattan_spearman
value: 57.81545502318733
- task:
type: STS
dataset:
type: mteb/sts22-crosslingual-sts
name: MTEB STS22 (ar)
config: ar
split: test
revision: 6d1ba47164174a496b7fa5d3569dae26a6813b80
metrics:
- type: cos_sim_pearson
value: 46.82241380954213
- type: cos_sim_spearman
value: 57.86569456006391
- type: euclidean_pearson
value: 31.80480070178813
- type: euclidean_spearman
value: 52.484000620130104
- type: manhattan_pearson
value: 31.952708554646097
- type: manhattan_spearman
value: 52.8560972356195
- task:
type: STS
dataset:
type: mteb/sts22-crosslingual-sts
name: MTEB STS22 (ru)
config: ru
split: test
revision: 6d1ba47164174a496b7fa5d3569dae26a6813b80
metrics:
- type: cos_sim_pearson
value: 52.00447170498087
- type: cos_sim_spearman
value: 60.664116225735164
- type: euclidean_pearson
value: 33.87382555421702
- type: euclidean_spearman
value: 55.74649067458667
- type: manhattan_pearson
value: 33.99117246759437
- type: manhattan_spearman
value: 55.98749034923899
- task:
type: STS
dataset:
type: mteb/sts22-crosslingual-sts
name: MTEB STS22 (zh)
config: zh
split: test
revision: 6d1ba47164174a496b7fa5d3569dae26a6813b80
metrics:
- type: cos_sim_pearson
value: 58.06497233105448
- type: cos_sim_spearman
value: 65.62968801135676
- type: euclidean_pearson
value: 47.482076613243905
- type: euclidean_spearman
value: 62.65137791498299
- type: manhattan_pearson
value: 47.57052626104093
- type: manhattan_spearman
value: 62.436916516613294
- task:
type: STS
dataset:
type: mteb/sts22-crosslingual-sts
name: MTEB STS22 (fr)
config: fr
split: test
revision: 6d1ba47164174a496b7fa5d3569dae26a6813b80
metrics:
- type: cos_sim_pearson
value: 70.49397298562575
- type: cos_sim_spearman
value: 74.79604041187868
- type: euclidean_pearson
value: 49.661891561317795
- type: euclidean_spearman
value: 70.31535537621006
- type: manhattan_pearson
value: 49.553715741850006
- type: manhattan_spearman
value: 70.24779344636806
- task:
type: STS
dataset:
type: mteb/sts22-crosslingual-sts
name: MTEB STS22 (de-en)
config: de-en
split: test
revision: 6d1ba47164174a496b7fa5d3569dae26a6813b80
metrics:
- type: cos_sim_pearson
value: 55.640574515348696
- type: cos_sim_spearman
value: 54.927959317689
- type: euclidean_pearson
value: 29.00139666967476
- type: euclidean_spearman
value: 41.86386566971605
- type: manhattan_pearson
value: 29.47411067730344
- type: manhattan_spearman
value: 42.337438424952786
- task:
type: STS
dataset:
type: mteb/sts22-crosslingual-sts
name: MTEB STS22 (es-en)
config: es-en
split: test
revision: 6d1ba47164174a496b7fa5d3569dae26a6813b80
metrics:
- type: cos_sim_pearson
value: 68.14095292259312
- type: cos_sim_spearman
value: 73.99017581234789
- type: euclidean_pearson
value: 46.46304297872084
- type: euclidean_spearman
value: 60.91834114800041
- type: manhattan_pearson
value: 47.07072666338692
- type: manhattan_spearman
value: 61.70415727977926
- task:
type: STS
dataset:
type: mteb/sts22-crosslingual-sts
name: MTEB STS22 (it)
config: it
split: test
revision: 6d1ba47164174a496b7fa5d3569dae26a6813b80
metrics:
- type: cos_sim_pearson
value: 73.27184653359575
- type: cos_sim_spearman
value: 77.76070252418626
- type: euclidean_pearson
value: 62.30586577544778
- type: euclidean_spearman
value: 75.14246629110978
- type: manhattan_pearson
value: 62.328196884927046
- type: manhattan_spearman
value: 75.1282792981433
- task:
type: STS
dataset:
type: mteb/sts22-crosslingual-sts
name: MTEB STS22 (pl-en)
config: pl-en
split: test
revision: 6d1ba47164174a496b7fa5d3569dae26a6813b80
metrics:
- type: cos_sim_pearson
value: 71.59448528829957
- type: cos_sim_spearman
value: 70.37277734222123
- type: euclidean_pearson
value: 57.63145565721123
- type: euclidean_spearman
value: 66.10113048304427
- type: manhattan_pearson
value: 57.18897811586808
- type: manhattan_spearman
value: 66.5595511215901
- task:
type: STS
dataset:
type: mteb/sts22-crosslingual-sts
name: MTEB STS22 (zh-en)
config: zh-en
split: test
revision: 6d1ba47164174a496b7fa5d3569dae26a6813b80
metrics:
- type: cos_sim_pearson
value: 66.37520607720838
- type: cos_sim_spearman
value: 69.92282148997948
- type: euclidean_pearson
value: 40.55768770125291
- type: euclidean_spearman
value: 55.189128944669605
- type: manhattan_pearson
value: 41.03566433468883
- type: manhattan_spearman
value: 55.61251893174558
- task:
type: STS
dataset:
type: mteb/sts22-crosslingual-sts
name: MTEB STS22 (es-it)
config: es-it
split: test
revision: 6d1ba47164174a496b7fa5d3569dae26a6813b80
metrics:
- type: cos_sim_pearson
value: 57.791929533771835
- type: cos_sim_spearman
value: 66.45819707662093
- type: euclidean_pearson
value: 39.03686018511092
- type: euclidean_spearman
value: 56.01282695640428
- type: manhattan_pearson
value: 38.91586623619632
- type: manhattan_spearman
value: 56.69394943612747
- task:
type: STS
dataset:
type: mteb/sts22-crosslingual-sts
name: MTEB STS22 (de-fr)
config: de-fr
split: test
revision: 6d1ba47164174a496b7fa5d3569dae26a6813b80
metrics:
- type: cos_sim_pearson
value: 47.82224468473866
- type: cos_sim_spearman
value: 59.467307194781164
- type: euclidean_pearson
value: 27.428459190256145
- type: euclidean_spearman
value: 60.83463107397519
- type: manhattan_pearson
value: 27.487391578496638
- type: manhattan_spearman
value: 61.281380460246496
- task:
type: STS
dataset:
type: mteb/sts22-crosslingual-sts
name: MTEB STS22 (de-pl)
config: de-pl
split: test
revision: 6d1ba47164174a496b7fa5d3569dae26a6813b80
metrics:
- type: cos_sim_pearson
value: 16.306666792752644
- type: cos_sim_spearman
value: 39.35486427252405
- type: euclidean_pearson
value: -2.7887154897955435
- type: euclidean_spearman
value: 27.1296051831719
- type: manhattan_pearson
value: -3.202291270581297
- type: manhattan_spearman
value: 26.32895849218158
- task:
type: STS
dataset:
type: mteb/sts22-crosslingual-sts
name: MTEB STS22 (fr-pl)
config: fr-pl
split: test
revision: 6d1ba47164174a496b7fa5d3569dae26a6813b80
metrics:
- type: cos_sim_pearson
value: 59.67006803805076
- type: cos_sim_spearman
value: 73.24670207647144
- type: euclidean_pearson
value: 46.91884681500483
- type: euclidean_spearman
value: 16.903085094570333
- type: manhattan_pearson
value: 46.88391675325812
- type: manhattan_spearman
value: 28.17180849095055
- task:
type: STS
dataset:
type: mteb/stsbenchmark-sts
name: MTEB STSBenchmark
config: default
split: test
revision: b0fddb56ed78048fa8b90373c8a3cfc37b684831
metrics:
- type: cos_sim_pearson
value: 83.79555591223837
- type: cos_sim_spearman
value: 85.63658602085185
- type: euclidean_pearson
value: 85.22080894037671
- type: euclidean_spearman
value: 85.54113580167038
- type: manhattan_pearson
value: 85.1639505960118
- type: manhattan_spearman
value: 85.43502665436196
- task:
type: Reranking
dataset:
type: mteb/scidocs-reranking
name: MTEB SciDocsRR
config: default
split: test
revision: d3c5e1fc0b855ab6097bf1cda04dd73947d7caab
metrics:
- type: map
value: 80.73900991689766
- type: mrr
value: 94.81624131133934
- task:
type: Retrieval
dataset:
type: scifact
name: MTEB SciFact
config: default
split: test
revision: None
metrics:
- type: map_at_1
value: 55.678000000000004
- type: map_at_10
value: 65.135
- type: map_at_100
value: 65.824
- type: map_at_1000
value: 65.852
- type: map_at_3
value: 62.736000000000004
- type: map_at_5
value: 64.411
- type: mrr_at_1
value: 58.333
- type: mrr_at_10
value: 66.5
- type: mrr_at_100
value: 67.053
- type: mrr_at_1000
value: 67.08
- type: mrr_at_3
value: 64.944
- type: mrr_at_5
value: 65.89399999999999
- type: ndcg_at_1
value: 58.333
- type: ndcg_at_10
value: 69.34700000000001
- type: ndcg_at_100
value: 72.32
- type: ndcg_at_1000
value: 73.014
- type: ndcg_at_3
value: 65.578
- type: ndcg_at_5
value: 67.738
- type: precision_at_1
value: 58.333
- type: precision_at_10
value: 9.033
- type: precision_at_100
value: 1.0670000000000002
- type: precision_at_1000
value: 0.11199999999999999
- type: precision_at_3
value: 25.444
- type: precision_at_5
value: 16.933
- type: recall_at_1
value: 55.678000000000004
- type: recall_at_10
value: 80.72200000000001
- type: recall_at_100
value: 93.93299999999999
- type: recall_at_1000
value: 99.333
- type: recall_at_3
value: 70.783
- type: recall_at_5
value: 75.978
- task:
type: PairClassification
dataset:
type: mteb/sprintduplicatequestions-pairclassification
name: MTEB SprintDuplicateQuestions
config: default
split: test
revision: d66bd1f72af766a5cc4b0ca5e00c162f89e8cc46
metrics:
- type: cos_sim_accuracy
value: 99.74653465346535
- type: cos_sim_ap
value: 93.01476369929063
- type: cos_sim_f1
value: 86.93009118541033
- type: cos_sim_precision
value: 88.09034907597535
- type: cos_sim_recall
value: 85.8
- type: dot_accuracy
value: 99.22970297029703
- type: dot_ap
value: 51.58725659485144
- type: dot_f1
value: 53.51351351351352
- type: dot_precision
value: 58.235294117647065
- type: dot_recall
value: 49.5
- type: euclidean_accuracy
value: 99.74356435643564
- type: euclidean_ap
value: 92.40332894384368
- type: euclidean_f1
value: 86.97838109602817
- type: euclidean_precision
value: 87.46208291203236
- type: euclidean_recall
value: 86.5
- type: manhattan_accuracy
value: 99.73069306930694
- type: manhattan_ap
value: 92.01320815721121
- type: manhattan_f1
value: 86.4135864135864
- type: manhattan_precision
value: 86.32734530938124
- type: manhattan_recall
value: 86.5
- type: max_accuracy
value: 99.74653465346535
- type: max_ap
value: 93.01476369929063
- type: max_f1
value: 86.97838109602817
- task:
type: Clustering
dataset:
type: mteb/stackexchange-clustering
name: MTEB StackExchangeClustering
config: default
split: test
revision: 6cbc1f7b2bc0622f2e39d2c77fa502909748c259
metrics:
- type: v_measure
value: 55.2660514302523
- task:
type: Clustering
dataset:
type: mteb/stackexchange-clustering-p2p
name: MTEB StackExchangeClusteringP2P
config: default
split: test
revision: 815ca46b2622cec33ccafc3735d572c266efdb44
metrics:
- type: v_measure
value: 30.4637783572547
- task:
type: Reranking
dataset:
type: mteb/stackoverflowdupquestions-reranking
name: MTEB StackOverflowDupQuestions
config: default
split: test
revision: e185fbe320c72810689fc5848eb6114e1ef5ec69
metrics:
- type: map
value: 49.41377758357637
- type: mrr
value: 50.138451213818854
- task:
type: Summarization
dataset:
type: mteb/summeval
name: MTEB SummEval
config: default
split: test
revision: cda12ad7615edc362dbf25a00fdd61d3b1eaf93c
metrics:
- type: cos_sim_pearson
value: 28.887846011166594
- type: cos_sim_spearman
value: 30.10823258355903
- type: dot_pearson
value: 12.888049550236385
- type: dot_spearman
value: 12.827495903098123
- task:
type: Retrieval
dataset:
type: trec-covid
name: MTEB TRECCOVID
config: default
split: test
revision: None
metrics:
- type: map_at_1
value: 0.21
- type: map_at_10
value: 1.667
- type: map_at_100
value: 9.15
- type: map_at_1000
value: 22.927
- type: map_at_3
value: 0.573
- type: map_at_5
value: 0.915
- type: mrr_at_1
value: 80
- type: mrr_at_10
value: 87.167
- type: mrr_at_100
value: 87.167
- type: mrr_at_1000
value: 87.167
- type: mrr_at_3
value: 85.667
- type: mrr_at_5
value: 87.167
- type: ndcg_at_1
value: 76
- type: ndcg_at_10
value: 69.757
- type: ndcg_at_100
value: 52.402
- type: ndcg_at_1000
value: 47.737
- type: ndcg_at_3
value: 71.866
- type: ndcg_at_5
value: 72.225
- type: precision_at_1
value: 80
- type: precision_at_10
value: 75
- type: precision_at_100
value: 53.959999999999994
- type: precision_at_1000
value: 21.568
- type: precision_at_3
value: 76.667
- type: precision_at_5
value: 78
- type: recall_at_1
value: 0.21
- type: recall_at_10
value: 1.9189999999999998
- type: recall_at_100
value: 12.589
- type: recall_at_1000
value: 45.312000000000005
- type: recall_at_3
value: 0.61
- type: recall_at_5
value: 1.019
- task:
type: BitextMining
dataset:
type: mteb/tatoeba-bitext-mining
name: MTEB Tatoeba (sqi-eng)
config: sqi-eng
split: test
revision: 9080400076fbadbb4c4dcb136ff4eddc40b42553
metrics:
- type: accuracy
value: 92.10000000000001
- type: f1
value: 90.06
- type: precision
value: 89.17333333333333
- type: recall
value: 92.10000000000001
- task:
type: BitextMining
dataset:
type: mteb/tatoeba-bitext-mining
name: MTEB Tatoeba (fry-eng)
config: fry-eng
split: test
revision: 9080400076fbadbb4c4dcb136ff4eddc40b42553
metrics:
- type: accuracy
value: 56.06936416184971
- type: f1
value: 50.87508028259473
- type: precision
value: 48.97398843930635
- type: recall
value: 56.06936416184971
- task:
type: BitextMining
dataset:
type: mteb/tatoeba-bitext-mining
name: MTEB Tatoeba (kur-eng)
config: kur-eng
split: test
revision: 9080400076fbadbb4c4dcb136ff4eddc40b42553
metrics:
- type: accuracy
value: 57.3170731707317
- type: f1
value: 52.96080139372822
- type: precision
value: 51.67861124382864
- type: recall
value: 57.3170731707317
- task:
type: BitextMining
dataset:
type: mteb/tatoeba-bitext-mining
name: MTEB Tatoeba (tur-eng)
config: tur-eng
split: test
revision: 9080400076fbadbb4c4dcb136ff4eddc40b42553
metrics:
- type: accuracy
value: 94.3
- type: f1
value: 92.67333333333333
- type: precision
value: 91.90833333333333
- type: recall
value: 94.3
- task:
type: BitextMining
dataset:
type: mteb/tatoeba-bitext-mining
name: MTEB Tatoeba (deu-eng)
config: deu-eng
split: test
revision: 9080400076fbadbb4c4dcb136ff4eddc40b42553
metrics:
- type: accuracy
value: 97.7
- type: f1
value: 97.07333333333332
- type: precision
value: 96.79500000000002
- type: recall
value: 97.7
- task:
type: BitextMining
dataset:
type: mteb/tatoeba-bitext-mining
name: MTEB Tatoeba (nld-eng)
config: nld-eng
split: test
revision: 9080400076fbadbb4c4dcb136ff4eddc40b42553
metrics:
- type: accuracy
value: 94.69999999999999
- type: f1
value: 93.2
- type: precision
value: 92.48333333333333
- type: recall
value: 94.69999999999999
- task:
type: BitextMining
dataset:
type: mteb/tatoeba-bitext-mining
name: MTEB Tatoeba (ron-eng)
config: ron-eng
split: test
revision: 9080400076fbadbb4c4dcb136ff4eddc40b42553
metrics:
- type: accuracy
value: 92.9
- type: f1
value: 91.26666666666667
- type: precision
value: 90.59444444444445
- type: recall
value: 92.9
- task:
type: BitextMining
dataset:
type: mteb/tatoeba-bitext-mining
name: MTEB Tatoeba (ang-eng)
config: ang-eng
split: test
revision: 9080400076fbadbb4c4dcb136ff4eddc40b42553
metrics:
- type: accuracy
value: 34.32835820895522
- type: f1
value: 29.074180380150533
- type: precision
value: 28.068207322920596
- type: recall
value: 34.32835820895522
- task:
type: BitextMining
dataset:
type: mteb/tatoeba-bitext-mining
name: MTEB Tatoeba (ido-eng)
config: ido-eng
split: test
revision: 9080400076fbadbb4c4dcb136ff4eddc40b42553
metrics:
- type: accuracy
value: 78.5
- type: f1
value: 74.3945115995116
- type: precision
value: 72.82967843459222
- type: recall
value: 78.5
- task:
type: BitextMining
dataset:
type: mteb/tatoeba-bitext-mining
name: MTEB Tatoeba (jav-eng)
config: jav-eng
split: test
revision: 9080400076fbadbb4c4dcb136ff4eddc40b42553
metrics:
- type: accuracy
value: 66.34146341463415
- type: f1
value: 61.2469400518181
- type: precision
value: 59.63977756660683
- type: recall
value: 66.34146341463415
- task:
type: BitextMining
dataset:
type: mteb/tatoeba-bitext-mining
name: MTEB Tatoeba (isl-eng)
config: isl-eng
split: test
revision: 9080400076fbadbb4c4dcb136ff4eddc40b42553
metrics:
- type: accuracy
value: 80.9
- type: f1
value: 76.90349206349207
- type: precision
value: 75.32921568627451
- type: recall
value: 80.9
- task:
type: BitextMining
dataset:
type: mteb/tatoeba-bitext-mining
name: MTEB Tatoeba (slv-eng)
config: slv-eng
split: test
revision: 9080400076fbadbb4c4dcb136ff4eddc40b42553
metrics:
- type: accuracy
value: 84.93317132442284
- type: f1
value: 81.92519105034295
- type: precision
value: 80.71283920615635
- type: recall
value: 84.93317132442284
- task:
type: BitextMining
dataset:
type: mteb/tatoeba-bitext-mining
name: MTEB Tatoeba (cym-eng)
config: cym-eng
split: test
revision: 9080400076fbadbb4c4dcb136ff4eddc40b42553
metrics:
- type: accuracy
value: 71.1304347826087
- type: f1
value: 65.22394755003451
- type: precision
value: 62.912422360248435
- type: recall
value: 71.1304347826087
- task:
type: BitextMining
dataset:
type: mteb/tatoeba-bitext-mining
name: MTEB Tatoeba (kaz-eng)
config: kaz-eng
split: test
revision: 9080400076fbadbb4c4dcb136ff4eddc40b42553
metrics:
- type: accuracy
value: 79.82608695652173
- type: f1
value: 75.55693581780538
- type: precision
value: 73.79420289855072
- type: recall
value: 79.82608695652173
- task:
type: BitextMining
dataset:
type: mteb/tatoeba-bitext-mining
name: MTEB Tatoeba (est-eng)
config: est-eng
split: test
revision: 9080400076fbadbb4c4dcb136ff4eddc40b42553
metrics:
- type: accuracy
value: 74
- type: f1
value: 70.51022222222223
- type: precision
value: 69.29673599347512
- type: recall
value: 74
- task:
type: BitextMining
dataset:
type: mteb/tatoeba-bitext-mining
name: MTEB Tatoeba (heb-eng)
config: heb-eng
split: test
revision: 9080400076fbadbb4c4dcb136ff4eddc40b42553
metrics:
- type: accuracy
value: 78.7
- type: f1
value: 74.14238095238095
- type: precision
value: 72.27214285714285
- type: recall
value: 78.7
- task:
type: BitextMining
dataset:
type: mteb/tatoeba-bitext-mining
name: MTEB Tatoeba (gla-eng)
config: gla-eng
split: test
revision: 9080400076fbadbb4c4dcb136ff4eddc40b42553
metrics:
- type: accuracy
value: 48.97466827503016
- type: f1
value: 43.080330405420874
- type: precision
value: 41.36505499593557
- type: recall
value: 48.97466827503016
- task:
type: BitextMining
dataset:
type: mteb/tatoeba-bitext-mining
name: MTEB Tatoeba (mar-eng)
config: mar-eng
split: test
revision: 9080400076fbadbb4c4dcb136ff4eddc40b42553
metrics:
- type: accuracy
value: 89.60000000000001
- type: f1
value: 86.62333333333333
- type: precision
value: 85.225
- type: recall
value: 89.60000000000001
- task:
type: BitextMining
dataset:
type: mteb/tatoeba-bitext-mining
name: MTEB Tatoeba (lat-eng)
config: lat-eng
split: test
revision: 9080400076fbadbb4c4dcb136ff4eddc40b42553
metrics:
- type: accuracy
value: 45.2
- type: f1
value: 39.5761253006253
- type: precision
value: 37.991358436312
- type: recall
value: 45.2
- task:
type: BitextMining
dataset:
type: mteb/tatoeba-bitext-mining
name: MTEB Tatoeba (bel-eng)
config: bel-eng
split: test
revision: 9080400076fbadbb4c4dcb136ff4eddc40b42553
metrics:
- type: accuracy
value: 89.5
- type: f1
value: 86.70333333333333
- type: precision
value: 85.53166666666667
- type: recall
value: 89.5
- task:
type: BitextMining
dataset:
type: mteb/tatoeba-bitext-mining
name: MTEB Tatoeba (pms-eng)
config: pms-eng
split: test
revision: 9080400076fbadbb4c4dcb136ff4eddc40b42553
metrics:
- type: accuracy
value: 50.095238095238095
- type: f1
value: 44.60650460650461
- type: precision
value: 42.774116796477045
- type: recall
value: 50.095238095238095
- task:
type: BitextMining
dataset:
type: mteb/tatoeba-bitext-mining
name: MTEB Tatoeba (gle-eng)
config: gle-eng
split: test
revision: 9080400076fbadbb4c4dcb136ff4eddc40b42553
metrics:
- type: accuracy
value: 63.4
- type: f1
value: 58.35967261904762
- type: precision
value: 56.54857142857143
- type: recall
value: 63.4
- task:
type: BitextMining
dataset:
type: mteb/tatoeba-bitext-mining
name: MTEB Tatoeba (pes-eng)
config: pes-eng
split: test
revision: 9080400076fbadbb4c4dcb136ff4eddc40b42553
metrics:
- type: accuracy
value: 89.2
- type: f1
value: 87.075
- type: precision
value: 86.12095238095239
- type: recall
value: 89.2
- task:
type: BitextMining
dataset:
type: mteb/tatoeba-bitext-mining
name: MTEB Tatoeba (nob-eng)
config: nob-eng
split: test
revision: 9080400076fbadbb4c4dcb136ff4eddc40b42553
metrics:
- type: accuracy
value: 96.8
- type: f1
value: 95.90333333333334
- type: precision
value: 95.50833333333333
- type: recall
value: 96.8
- task:
type: BitextMining
dataset:
type: mteb/tatoeba-bitext-mining
name: MTEB Tatoeba (bul-eng)
config: bul-eng
split: test
revision: 9080400076fbadbb4c4dcb136ff4eddc40b42553
metrics:
- type: accuracy
value: 90.9
- type: f1
value: 88.6288888888889
- type: precision
value: 87.61607142857142
- type: recall
value: 90.9
- task:
type: BitextMining
dataset:
type: mteb/tatoeba-bitext-mining
name: MTEB Tatoeba (cbk-eng)
config: cbk-eng
split: test
revision: 9080400076fbadbb4c4dcb136ff4eddc40b42553
metrics:
- type: accuracy
value: 65.2
- type: f1
value: 60.54377630539395
- type: precision
value: 58.89434482711381
- type: recall
value: 65.2
- task:
type: BitextMining
dataset:
type: mteb/tatoeba-bitext-mining
name: MTEB Tatoeba (hun-eng)
config: hun-eng
split: test
revision: 9080400076fbadbb4c4dcb136ff4eddc40b42553
metrics:
- type: accuracy
value: 87
- type: f1
value: 84.32412698412699
- type: precision
value: 83.25527777777778
- type: recall
value: 87
- task:
type: BitextMining
dataset:
type: mteb/tatoeba-bitext-mining
name: MTEB Tatoeba (uig-eng)
config: uig-eng
split: test
revision: 9080400076fbadbb4c4dcb136ff4eddc40b42553
metrics:
- type: accuracy
value: 68.7
- type: f1
value: 63.07883541295306
- type: precision
value: 61.06117424242426
- type: recall
value: 68.7
- task:
type: BitextMining
dataset:
type: mteb/tatoeba-bitext-mining
name: MTEB Tatoeba (rus-eng)
config: rus-eng
split: test
revision: 9080400076fbadbb4c4dcb136ff4eddc40b42553
metrics:
- type: accuracy
value: 93.7
- type: f1
value: 91.78333333333335
- type: precision
value: 90.86666666666667
- type: recall
value: 93.7
- task:
type: BitextMining
dataset:
type: mteb/tatoeba-bitext-mining
name: MTEB Tatoeba (spa-eng)
config: spa-eng
split: test
revision: 9080400076fbadbb4c4dcb136ff4eddc40b42553
metrics:
- type: accuracy
value: 97.7
- type: f1
value: 96.96666666666667
- type: precision
value: 96.61666666666667
- type: recall
value: 97.7
- task:
type: BitextMining
dataset:
type: mteb/tatoeba-bitext-mining
name: MTEB Tatoeba (hye-eng)
config: hye-eng
split: test
revision: 9080400076fbadbb4c4dcb136ff4eddc40b42553
metrics:
- type: accuracy
value: 88.27493261455525
- type: f1
value: 85.90745732255168
- type: precision
value: 84.91389637616052
- type: recall
value: 88.27493261455525
- task:
type: BitextMining
dataset:
type: mteb/tatoeba-bitext-mining
name: MTEB Tatoeba (tel-eng)
config: tel-eng
split: test
revision: 9080400076fbadbb4c4dcb136ff4eddc40b42553
metrics:
- type: accuracy
value: 90.5982905982906
- type: f1
value: 88.4900284900285
- type: precision
value: 87.57122507122507
- type: recall
value: 90.5982905982906
- task:
type: BitextMining
dataset:
type: mteb/tatoeba-bitext-mining
name: MTEB Tatoeba (afr-eng)
config: afr-eng
split: test
revision: 9080400076fbadbb4c4dcb136ff4eddc40b42553
metrics:
- type: accuracy
value: 89.5
- type: f1
value: 86.90769841269842
- type: precision
value: 85.80178571428571
- type: recall
value: 89.5
- task:
type: BitextMining
dataset:
type: mteb/tatoeba-bitext-mining
name: MTEB Tatoeba (mon-eng)
config: mon-eng
split: test
revision: 9080400076fbadbb4c4dcb136ff4eddc40b42553
metrics:
- type: accuracy
value: 82.5
- type: f1
value: 78.36796536796538
- type: precision
value: 76.82196969696969
- type: recall
value: 82.5
- task:
type: BitextMining
dataset:
type: mteb/tatoeba-bitext-mining
name: MTEB Tatoeba (arz-eng)
config: arz-eng
split: test
revision: 9080400076fbadbb4c4dcb136ff4eddc40b42553
metrics:
- type: accuracy
value: 71.48846960167715
- type: f1
value: 66.78771089148448
- type: precision
value: 64.98302885095339
- type: recall
value: 71.48846960167715
- task:
type: BitextMining
dataset:
type: mteb/tatoeba-bitext-mining
name: MTEB Tatoeba (hrv-eng)
config: hrv-eng
split: test
revision: 9080400076fbadbb4c4dcb136ff4eddc40b42553
metrics:
- type: accuracy
value: 94.1
- type: f1
value: 92.50333333333333
- type: precision
value: 91.77499999999999
- type: recall
value: 94.1
- task:
type: BitextMining
dataset:
type: mteb/tatoeba-bitext-mining
name: MTEB Tatoeba (nov-eng)
config: nov-eng
split: test
revision: 9080400076fbadbb4c4dcb136ff4eddc40b42553
metrics:
- type: accuracy
value: 71.20622568093385
- type: f1
value: 66.83278891450098
- type: precision
value: 65.35065777283677
- type: recall
value: 71.20622568093385
- task:
type: BitextMining
dataset:
type: mteb/tatoeba-bitext-mining
name: MTEB Tatoeba (gsw-eng)
config: gsw-eng
split: test
revision: 9080400076fbadbb4c4dcb136ff4eddc40b42553
metrics:
- type: accuracy
value: 48.717948717948715
- type: f1
value: 43.53146853146853
- type: precision
value: 42.04721204721204
- type: recall
value: 48.717948717948715
- task:
type: BitextMining
dataset:
type: mteb/tatoeba-bitext-mining
name: MTEB Tatoeba (nds-eng)
config: nds-eng
split: test
revision: 9080400076fbadbb4c4dcb136ff4eddc40b42553
metrics:
- type: accuracy
value: 58.5
- type: f1
value: 53.8564991863928
- type: precision
value: 52.40329436122275
- type: recall
value: 58.5
- task:
type: BitextMining
dataset:
type: mteb/tatoeba-bitext-mining
name: MTEB Tatoeba (ukr-eng)
config: ukr-eng
split: test
revision: 9080400076fbadbb4c4dcb136ff4eddc40b42553
metrics:
- type: accuracy
value: 90.8
- type: f1
value: 88.29
- type: precision
value: 87.09166666666667
- type: recall
value: 90.8
- task:
type: BitextMining
dataset:
type: mteb/tatoeba-bitext-mining
name: MTEB Tatoeba (uzb-eng)
config: uzb-eng
split: test
revision: 9080400076fbadbb4c4dcb136ff4eddc40b42553
metrics:
- type: accuracy
value: 67.28971962616822
- type: f1
value: 62.63425307817832
- type: precision
value: 60.98065939771546
- type: recall
value: 67.28971962616822
- task:
type: BitextMining
dataset:
type: mteb/tatoeba-bitext-mining
name: MTEB Tatoeba (lit-eng)
config: lit-eng
split: test
revision: 9080400076fbadbb4c4dcb136ff4eddc40b42553
metrics:
- type: accuracy
value: 78.7
- type: f1
value: 75.5264472455649
- type: precision
value: 74.38205086580086
- type: recall
value: 78.7
- task:
type: BitextMining
dataset:
type: mteb/tatoeba-bitext-mining
name: MTEB Tatoeba (ina-eng)
config: ina-eng
split: test
revision: 9080400076fbadbb4c4dcb136ff4eddc40b42553
metrics:
- type: accuracy
value: 88.7
- type: f1
value: 86.10809523809525
- type: precision
value: 85.07602564102565
- type: recall
value: 88.7
- task:
type: BitextMining
dataset:
type: mteb/tatoeba-bitext-mining
name: MTEB Tatoeba (lfn-eng)
config: lfn-eng
split: test
revision: 9080400076fbadbb4c4dcb136ff4eddc40b42553
metrics:
- type: accuracy
value: 56.99999999999999
- type: f1
value: 52.85487521402737
- type: precision
value: 51.53985162713104
- type: recall
value: 56.99999999999999
- task:
type: BitextMining
dataset:
type: mteb/tatoeba-bitext-mining
name: MTEB Tatoeba (zsm-eng)
config: zsm-eng
split: test
revision: 9080400076fbadbb4c4dcb136ff4eddc40b42553
metrics:
- type: accuracy
value: 94
- type: f1
value: 92.45333333333333
- type: precision
value: 91.79166666666667
- type: recall
value: 94
- task:
type: BitextMining
dataset:
type: mteb/tatoeba-bitext-mining
name: MTEB Tatoeba (ita-eng)
config: ita-eng
split: test
revision: 9080400076fbadbb4c4dcb136ff4eddc40b42553
metrics:
- type: accuracy
value: 92.30000000000001
- type: f1
value: 90.61333333333333
- type: precision
value: 89.83333333333331
- type: recall
value: 92.30000000000001
- task:
type: BitextMining
dataset:
type: mteb/tatoeba-bitext-mining
name: MTEB Tatoeba (cmn-eng)
config: cmn-eng
split: test
revision: 9080400076fbadbb4c4dcb136ff4eddc40b42553
metrics:
- type: accuracy
value: 94.69999999999999
- type: f1
value: 93.34555555555555
- type: precision
value: 92.75416666666668
- type: recall
value: 94.69999999999999
- task:
type: BitextMining
dataset:
type: mteb/tatoeba-bitext-mining
name: MTEB Tatoeba (lvs-eng)
config: lvs-eng
split: test
revision: 9080400076fbadbb4c4dcb136ff4eddc40b42553
metrics:
- type: accuracy
value: 80.2
- type: f1
value: 76.6563035113035
- type: precision
value: 75.3014652014652
- type: recall
value: 80.2
- task:
type: BitextMining
dataset:
type: mteb/tatoeba-bitext-mining
name: MTEB Tatoeba (glg-eng)
config: glg-eng
split: test
revision: 9080400076fbadbb4c4dcb136ff4eddc40b42553
metrics:
- type: accuracy
value: 84.7
- type: f1
value: 82.78689263765207
- type: precision
value: 82.06705086580087
- type: recall
value: 84.7
- task:
type: BitextMining
dataset:
type: mteb/tatoeba-bitext-mining
name: MTEB Tatoeba (ceb-eng)
config: ceb-eng
split: test
revision: 9080400076fbadbb4c4dcb136ff4eddc40b42553
metrics:
- type: accuracy
value: 50.33333333333333
- type: f1
value: 45.461523661523664
- type: precision
value: 43.93545574795575
- type: recall
value: 50.33333333333333
- task:
type: BitextMining
dataset:
type: mteb/tatoeba-bitext-mining
name: MTEB Tatoeba (bre-eng)
config: bre-eng
split: test
revision: 9080400076fbadbb4c4dcb136ff4eddc40b42553
metrics:
- type: accuracy
value: 6.6000000000000005
- type: f1
value: 5.442121400446441
- type: precision
value: 5.146630385487529
- type: recall
value: 6.6000000000000005
- task:
type: BitextMining
dataset:
type: mteb/tatoeba-bitext-mining
name: MTEB Tatoeba (ben-eng)
config: ben-eng
split: test
revision: 9080400076fbadbb4c4dcb136ff4eddc40b42553
metrics:
- type: accuracy
value: 85
- type: f1
value: 81.04666666666667
- type: precision
value: 79.25
- type: recall
value: 85
- task:
type: BitextMining
dataset:
type: mteb/tatoeba-bitext-mining
name: MTEB Tatoeba (swg-eng)
config: swg-eng
split: test
revision: 9080400076fbadbb4c4dcb136ff4eddc40b42553
metrics:
- type: accuracy
value: 47.32142857142857
- type: f1
value: 42.333333333333336
- type: precision
value: 40.69196428571429
- type: recall
value: 47.32142857142857
- task:
type: BitextMining
dataset:
type: mteb/tatoeba-bitext-mining
name: MTEB Tatoeba (arq-eng)
config: arq-eng
split: test
revision: 9080400076fbadbb4c4dcb136ff4eddc40b42553
metrics:
- type: accuracy
value: 30.735455543358945
- type: f1
value: 26.73616790022338
- type: precision
value: 25.397823220451283
- type: recall
value: 30.735455543358945
- task:
type: BitextMining
dataset:
type: mteb/tatoeba-bitext-mining
name: MTEB Tatoeba (kab-eng)
config: kab-eng
split: test
revision: 9080400076fbadbb4c4dcb136ff4eddc40b42553
metrics:
- type: accuracy
value: 25.1
- type: f1
value: 21.975989896371022
- type: precision
value: 21.059885632257203
- type: recall
value: 25.1
- task:
type: BitextMining
dataset:
type: mteb/tatoeba-bitext-mining
name: MTEB Tatoeba (fra-eng)
config: fra-eng
split: test
revision: 9080400076fbadbb4c4dcb136ff4eddc40b42553
metrics:
- type: accuracy
value: 94.3
- type: f1
value: 92.75666666666666
- type: precision
value: 92.06166666666665
- type: recall
value: 94.3
- task:
type: BitextMining
dataset:
type: mteb/tatoeba-bitext-mining
name: MTEB Tatoeba (por-eng)
config: por-eng
split: test
revision: 9080400076fbadbb4c4dcb136ff4eddc40b42553
metrics:
- type: accuracy
value: 94.1
- type: f1
value: 92.74
- type: precision
value: 92.09166666666667
- type: recall
value: 94.1
- task:
type: BitextMining
dataset:
type: mteb/tatoeba-bitext-mining
name: MTEB Tatoeba (tat-eng)
config: tat-eng
split: test
revision: 9080400076fbadbb4c4dcb136ff4eddc40b42553
metrics:
- type: accuracy
value: 71.3
- type: f1
value: 66.922442002442
- type: precision
value: 65.38249567099568
- type: recall
value: 71.3
- task:
type: BitextMining
dataset:
type: mteb/tatoeba-bitext-mining
name: MTEB Tatoeba (oci-eng)
config: oci-eng
split: test
revision: 9080400076fbadbb4c4dcb136ff4eddc40b42553
metrics:
- type: accuracy
value: 40.300000000000004
- type: f1
value: 35.78682789299971
- type: precision
value: 34.66425128716588
- type: recall
value: 40.300000000000004
- task:
type: BitextMining
dataset:
type: mteb/tatoeba-bitext-mining
name: MTEB Tatoeba (pol-eng)
config: pol-eng
split: test
revision: 9080400076fbadbb4c4dcb136ff4eddc40b42553
metrics:
- type: accuracy
value: 96
- type: f1
value: 94.82333333333334
- type: precision
value: 94.27833333333334
- type: recall
value: 96
- task:
type: BitextMining
dataset:
type: mteb/tatoeba-bitext-mining
name: MTEB Tatoeba (war-eng)
config: war-eng
split: test
revision: 9080400076fbadbb4c4dcb136ff4eddc40b42553
metrics:
- type: accuracy
value: 51.1
- type: f1
value: 47.179074753133584
- type: precision
value: 46.06461044702424
- type: recall
value: 51.1
- task:
type: BitextMining
dataset:
type: mteb/tatoeba-bitext-mining
name: MTEB Tatoeba (aze-eng)
config: aze-eng
split: test
revision: 9080400076fbadbb4c4dcb136ff4eddc40b42553
metrics:
- type: accuracy
value: 87.7
- type: f1
value: 84.71
- type: precision
value: 83.46166666666667
- type: recall
value: 87.7
- task:
type: BitextMining
dataset:
type: mteb/tatoeba-bitext-mining
name: MTEB Tatoeba (vie-eng)
config: vie-eng
split: test
revision: 9080400076fbadbb4c4dcb136ff4eddc40b42553
metrics:
- type: accuracy
value: 95.8
- type: f1
value: 94.68333333333334
- type: precision
value: 94.13333333333334
- type: recall
value: 95.8
- task:
type: BitextMining
dataset:
type: mteb/tatoeba-bitext-mining
name: MTEB Tatoeba (nno-eng)
config: nno-eng
split: test
revision: 9080400076fbadbb4c4dcb136ff4eddc40b42553
metrics:
- type: accuracy
value: 85.39999999999999
- type: f1
value: 82.5577380952381
- type: precision
value: 81.36833333333334
- type: recall
value: 85.39999999999999
- task:
type: BitextMining
dataset:
type: mteb/tatoeba-bitext-mining
name: MTEB Tatoeba (cha-eng)
config: cha-eng
split: test
revision: 9080400076fbadbb4c4dcb136ff4eddc40b42553
metrics:
- type: accuracy
value: 21.16788321167883
- type: f1
value: 16.948865627297987
- type: precision
value: 15.971932568647897
- type: recall
value: 21.16788321167883
- task:
type: BitextMining
dataset:
type: mteb/tatoeba-bitext-mining
name: MTEB Tatoeba (mhr-eng)
config: mhr-eng
split: test
revision: 9080400076fbadbb4c4dcb136ff4eddc40b42553
metrics:
- type: accuracy
value: 6.9
- type: f1
value: 5.515526831658907
- type: precision
value: 5.141966366966367
- type: recall
value: 6.9
- task:
type: BitextMining
dataset:
type: mteb/tatoeba-bitext-mining
name: MTEB Tatoeba (dan-eng)
config: dan-eng
split: test
revision: 9080400076fbadbb4c4dcb136ff4eddc40b42553
metrics:
- type: accuracy
value: 93.2
- type: f1
value: 91.39666666666668
- type: precision
value: 90.58666666666667
- type: recall
value: 93.2
- task:
type: BitextMining
dataset:
type: mteb/tatoeba-bitext-mining
name: MTEB Tatoeba (ell-eng)
config: ell-eng
split: test
revision: 9080400076fbadbb4c4dcb136ff4eddc40b42553
metrics:
- type: accuracy
value: 92.2
- type: f1
value: 89.95666666666666
- type: precision
value: 88.92833333333333
- type: recall
value: 92.2
- task:
type: BitextMining
dataset:
type: mteb/tatoeba-bitext-mining
name: MTEB Tatoeba (amh-eng)
config: amh-eng
split: test
revision: 9080400076fbadbb4c4dcb136ff4eddc40b42553
metrics:
- type: accuracy
value: 79.76190476190477
- type: f1
value: 74.93386243386244
- type: precision
value: 73.11011904761904
- type: recall
value: 79.76190476190477
- task:
type: BitextMining
dataset:
type: mteb/tatoeba-bitext-mining
name: MTEB Tatoeba (pam-eng)
config: pam-eng
split: test
revision: 9080400076fbadbb4c4dcb136ff4eddc40b42553
metrics:
- type: accuracy
value: 8.799999999999999
- type: f1
value: 6.921439712248537
- type: precision
value: 6.489885109680683
- type: recall
value: 8.799999999999999
- task:
type: BitextMining
dataset:
type: mteb/tatoeba-bitext-mining
name: MTEB Tatoeba (hsb-eng)
config: hsb-eng
split: test
revision: 9080400076fbadbb4c4dcb136ff4eddc40b42553
metrics:
- type: accuracy
value: 45.75569358178054
- type: f1
value: 40.34699501312631
- type: precision
value: 38.57886764719063
- type: recall
value: 45.75569358178054
- task:
type: BitextMining
dataset:
type: mteb/tatoeba-bitext-mining
name: MTEB Tatoeba (srp-eng)
config: srp-eng
split: test
revision: 9080400076fbadbb4c4dcb136ff4eddc40b42553
metrics:
- type: accuracy
value: 91.4
- type: f1
value: 89.08333333333333
- type: precision
value: 88.01666666666668
- type: recall
value: 91.4
- task:
type: BitextMining
dataset:
type: mteb/tatoeba-bitext-mining
name: MTEB Tatoeba (epo-eng)
config: epo-eng
split: test
revision: 9080400076fbadbb4c4dcb136ff4eddc40b42553
metrics:
- type: accuracy
value: 93.60000000000001
- type: f1
value: 92.06690476190477
- type: precision
value: 91.45095238095239
- type: recall
value: 93.60000000000001
- task:
type: BitextMining
dataset:
type: mteb/tatoeba-bitext-mining
name: MTEB Tatoeba (kzj-eng)
config: kzj-eng
split: test
revision: 9080400076fbadbb4c4dcb136ff4eddc40b42553
metrics:
- type: accuracy
value: 7.5
- type: f1
value: 6.200363129378736
- type: precision
value: 5.89115314822466
- type: recall
value: 7.5
- task:
type: BitextMining
dataset:
type: mteb/tatoeba-bitext-mining
name: MTEB Tatoeba (awa-eng)
config: awa-eng
split: test
revision: 9080400076fbadbb4c4dcb136ff4eddc40b42553
metrics:
- type: accuracy
value: 73.59307359307358
- type: f1
value: 68.38933553219267
- type: precision
value: 66.62698412698413
- type: recall
value: 73.59307359307358
- task:
type: BitextMining
dataset:
type: mteb/tatoeba-bitext-mining
name: MTEB Tatoeba (fao-eng)
config: fao-eng
split: test
revision: 9080400076fbadbb4c4dcb136ff4eddc40b42553
metrics:
- type: accuracy
value: 69.8473282442748
- type: f1
value: 64.72373682297346
- type: precision
value: 62.82834214131924
- type: recall
value: 69.8473282442748
- task:
type: BitextMining
dataset:
type: mteb/tatoeba-bitext-mining
name: MTEB Tatoeba (mal-eng)
config: mal-eng
split: test
revision: 9080400076fbadbb4c4dcb136ff4eddc40b42553
metrics:
- type: accuracy
value: 97.5254730713246
- type: f1
value: 96.72489082969432
- type: precision
value: 96.33672974284326
- type: recall
value: 97.5254730713246
- task:
type: BitextMining
dataset:
type: mteb/tatoeba-bitext-mining
name: MTEB Tatoeba (ile-eng)
config: ile-eng
split: test
revision: 9080400076fbadbb4c4dcb136ff4eddc40b42553
metrics:
- type: accuracy
value: 75.6
- type: f1
value: 72.42746031746033
- type: precision
value: 71.14036630036631
- type: recall
value: 75.6
- task:
type: BitextMining
dataset:
type: mteb/tatoeba-bitext-mining
name: MTEB Tatoeba (bos-eng)
config: bos-eng
split: test
revision: 9080400076fbadbb4c4dcb136ff4eddc40b42553
metrics:
- type: accuracy
value: 91.24293785310734
- type: f1
value: 88.86064030131826
- type: precision
value: 87.73540489642184
- type: recall
value: 91.24293785310734
- task:
type: BitextMining
dataset:
type: mteb/tatoeba-bitext-mining
name: MTEB Tatoeba (cor-eng)
config: cor-eng
split: test
revision: 9080400076fbadbb4c4dcb136ff4eddc40b42553
metrics:
- type: accuracy
value: 6.2
- type: f1
value: 4.383083659794954
- type: precision
value: 4.027861324289673
- type: recall
value: 6.2
- task:
type: BitextMining
dataset:
type: mteb/tatoeba-bitext-mining
name: MTEB Tatoeba (cat-eng)
config: cat-eng
split: test
revision: 9080400076fbadbb4c4dcb136ff4eddc40b42553
metrics:
- type: accuracy
value: 86.8
- type: f1
value: 84.09428571428572
- type: precision
value: 83.00333333333333
- type: recall
value: 86.8
- task:
type: BitextMining
dataset:
type: mteb/tatoeba-bitext-mining
name: MTEB Tatoeba (eus-eng)
config: eus-eng
split: test
revision: 9080400076fbadbb4c4dcb136ff4eddc40b42553
metrics:
- type: accuracy
value: 60.699999999999996
- type: f1
value: 56.1584972394755
- type: precision
value: 54.713456330903135
- type: recall
value: 60.699999999999996
- task:
type: BitextMining
dataset:
type: mteb/tatoeba-bitext-mining
name: MTEB Tatoeba (yue-eng)
config: yue-eng
split: test
revision: 9080400076fbadbb4c4dcb136ff4eddc40b42553
metrics:
- type: accuracy
value: 84.2
- type: f1
value: 80.66190476190475
- type: precision
value: 79.19690476190476
- type: recall
value: 84.2
- task:
type: BitextMining
dataset:
type: mteb/tatoeba-bitext-mining
name: MTEB Tatoeba (swe-eng)
config: swe-eng
split: test
revision: 9080400076fbadbb4c4dcb136ff4eddc40b42553
metrics:
- type: accuracy
value: 93.2
- type: f1
value: 91.33
- type: precision
value: 90.45
- type: recall
value: 93.2
- task:
type: BitextMining
dataset:
type: mteb/tatoeba-bitext-mining
name: MTEB Tatoeba (dtp-eng)
config: dtp-eng
split: test
revision: 9080400076fbadbb4c4dcb136ff4eddc40b42553
metrics:
- type: accuracy
value: 6.3
- type: f1
value: 5.126828976748276
- type: precision
value: 4.853614328966668
- type: recall
value: 6.3
- task:
type: BitextMining
dataset:
type: mteb/tatoeba-bitext-mining
name: MTEB Tatoeba (kat-eng)
config: kat-eng
split: test
revision: 9080400076fbadbb4c4dcb136ff4eddc40b42553
metrics:
- type: accuracy
value: 81.76943699731903
- type: f1
value: 77.82873739308057
- type: precision
value: 76.27622452019234
- type: recall
value: 81.76943699731903
- task:
type: BitextMining
dataset:
type: mteb/tatoeba-bitext-mining
name: MTEB Tatoeba (jpn-eng)
config: jpn-eng
split: test
revision: 9080400076fbadbb4c4dcb136ff4eddc40b42553
metrics:
- type: accuracy
value: 92.30000000000001
- type: f1
value: 90.29666666666665
- type: precision
value: 89.40333333333334
- type: recall
value: 92.30000000000001
- task:
type: BitextMining
dataset:
type: mteb/tatoeba-bitext-mining
name: MTEB Tatoeba (csb-eng)
config: csb-eng
split: test
revision: 9080400076fbadbb4c4dcb136ff4eddc40b42553
metrics:
- type: accuracy
value: 29.249011857707508
- type: f1
value: 24.561866096392947
- type: precision
value: 23.356583740215456
- type: recall
value: 29.249011857707508
- task:
type: BitextMining
dataset:
type: mteb/tatoeba-bitext-mining
name: MTEB Tatoeba (xho-eng)
config: xho-eng
split: test
revision: 9080400076fbadbb4c4dcb136ff4eddc40b42553
metrics:
- type: accuracy
value: 77.46478873239437
- type: f1
value: 73.23943661971832
- type: precision
value: 71.66666666666667
- type: recall
value: 77.46478873239437
- task:
type: BitextMining
dataset:
type: mteb/tatoeba-bitext-mining
name: MTEB Tatoeba (orv-eng)
config: orv-eng
split: test
revision: 9080400076fbadbb4c4dcb136ff4eddc40b42553
metrics:
- type: accuracy
value: 20.35928143712575
- type: f1
value: 15.997867865075824
- type: precision
value: 14.882104658301346
- type: recall
value: 20.35928143712575
- task:
type: BitextMining
dataset:
type: mteb/tatoeba-bitext-mining
name: MTEB Tatoeba (ind-eng)
config: ind-eng
split: test
revision: 9080400076fbadbb4c4dcb136ff4eddc40b42553
metrics:
- type: accuracy
value: 92.2
- type: f1
value: 90.25999999999999
- type: precision
value: 89.45333333333335
- type: recall
value: 92.2
- task:
type: BitextMining
dataset:
type: mteb/tatoeba-bitext-mining
name: MTEB Tatoeba (tuk-eng)
config: tuk-eng
split: test
revision: 9080400076fbadbb4c4dcb136ff4eddc40b42553
metrics:
- type: accuracy
value: 23.15270935960591
- type: f1
value: 19.65673625772148
- type: precision
value: 18.793705293464992
- type: recall
value: 23.15270935960591
- task:
type: BitextMining
dataset:
type: mteb/tatoeba-bitext-mining
name: MTEB Tatoeba (max-eng)
config: max-eng
split: test
revision: 9080400076fbadbb4c4dcb136ff4eddc40b42553
metrics:
- type: accuracy
value: 59.154929577464785
- type: f1
value: 52.3868463305083
- type: precision
value: 50.14938113529662
- type: recall
value: 59.154929577464785
- task:
type: BitextMining
dataset:
type: mteb/tatoeba-bitext-mining
name: MTEB Tatoeba (swh-eng)
config: swh-eng
split: test
revision: 9080400076fbadbb4c4dcb136ff4eddc40b42553
metrics:
- type: accuracy
value: 70.51282051282051
- type: f1
value: 66.8089133089133
- type: precision
value: 65.37645687645687
- type: recall
value: 70.51282051282051
- task:
type: BitextMining
dataset:
type: mteb/tatoeba-bitext-mining
name: MTEB Tatoeba (hin-eng)
config: hin-eng
split: test
revision: 9080400076fbadbb4c4dcb136ff4eddc40b42553
metrics:
- type: accuracy
value: 94.6
- type: f1
value: 93
- type: precision
value: 92.23333333333333
- type: recall
value: 94.6
- task:
type: BitextMining
dataset:
type: mteb/tatoeba-bitext-mining
name: MTEB Tatoeba (dsb-eng)
config: dsb-eng
split: test
revision: 9080400076fbadbb4c4dcb136ff4eddc40b42553
metrics:
- type: accuracy
value: 38.62212943632568
- type: f1
value: 34.3278276962583
- type: precision
value: 33.07646935732408
- type: recall
value: 38.62212943632568
- task:
type: BitextMining
dataset:
type: mteb/tatoeba-bitext-mining
name: MTEB Tatoeba (ber-eng)
config: ber-eng
split: test
revision: 9080400076fbadbb4c4dcb136ff4eddc40b42553
metrics:
- type: accuracy
value: 28.1
- type: f1
value: 23.579609223054604
- type: precision
value: 22.39622774921555
- type: recall
value: 28.1
- task:
type: BitextMining
dataset:
type: mteb/tatoeba-bitext-mining
name: MTEB Tatoeba (tam-eng)
config: tam-eng
split: test
revision: 9080400076fbadbb4c4dcb136ff4eddc40b42553
metrics:
- type: accuracy
value: 88.27361563517914
- type: f1
value: 85.12486427795874
- type: precision
value: 83.71335504885994
- type: recall
value: 88.27361563517914
- task:
type: BitextMining
dataset:
type: mteb/tatoeba-bitext-mining
name: MTEB Tatoeba (slk-eng)
config: slk-eng
split: test
revision: 9080400076fbadbb4c4dcb136ff4eddc40b42553
metrics:
- type: accuracy
value: 88.6
- type: f1
value: 86.39928571428571
- type: precision
value: 85.4947557997558
- type: recall
value: 88.6
- task:
type: BitextMining
dataset:
type: mteb/tatoeba-bitext-mining
name: MTEB Tatoeba (tgl-eng)
config: tgl-eng
split: test
revision: 9080400076fbadbb4c4dcb136ff4eddc40b42553
metrics:
- type: accuracy
value: 86.5
- type: f1
value: 83.77952380952381
- type: precision
value: 82.67602564102565
- type: recall
value: 86.5
- task:
type: BitextMining
dataset:
type: mteb/tatoeba-bitext-mining
name: MTEB Tatoeba (ast-eng)
config: ast-eng
split: test
revision: 9080400076fbadbb4c4dcb136ff4eddc40b42553
metrics:
- type: accuracy
value: 79.52755905511812
- type: f1
value: 75.3055868016498
- type: precision
value: 73.81889763779527
- type: recall
value: 79.52755905511812
- task:
type: BitextMining
dataset:
type: mteb/tatoeba-bitext-mining
name: MTEB Tatoeba (mkd-eng)
config: mkd-eng
split: test
revision: 9080400076fbadbb4c4dcb136ff4eddc40b42553
metrics:
- type: accuracy
value: 77.9
- type: f1
value: 73.76261904761905
- type: precision
value: 72.11670995670995
- type: recall
value: 77.9
- task:
type: BitextMining
dataset:
type: mteb/tatoeba-bitext-mining
name: MTEB Tatoeba (khm-eng)
config: khm-eng
split: test
revision: 9080400076fbadbb4c4dcb136ff4eddc40b42553
metrics:
- type: accuracy
value: 53.8781163434903
- type: f1
value: 47.25804051288816
- type: precision
value: 45.0603482390186
- type: recall
value: 53.8781163434903
- task:
type: BitextMining
dataset:
type: mteb/tatoeba-bitext-mining
name: MTEB Tatoeba (ces-eng)
config: ces-eng
split: test
revision: 9080400076fbadbb4c4dcb136ff4eddc40b42553
metrics:
- type: accuracy
value: 91.10000000000001
- type: f1
value: 88.88
- type: precision
value: 87.96333333333334
- type: recall
value: 91.10000000000001
- task:
type: BitextMining
dataset:
type: mteb/tatoeba-bitext-mining
name: MTEB Tatoeba (tzl-eng)
config: tzl-eng
split: test
revision: 9080400076fbadbb4c4dcb136ff4eddc40b42553
metrics:
- type: accuracy
value: 38.46153846153847
- type: f1
value: 34.43978243978244
- type: precision
value: 33.429487179487175
- type: recall
value: 38.46153846153847
- task:
type: BitextMining
dataset:
type: mteb/tatoeba-bitext-mining
name: MTEB Tatoeba (urd-eng)
config: urd-eng
split: test
revision: 9080400076fbadbb4c4dcb136ff4eddc40b42553
metrics:
- type: accuracy
value: 88.9
- type: f1
value: 86.19888888888887
- type: precision
value: 85.07440476190476
- type: recall
value: 88.9
- task:
type: BitextMining
dataset:
type: mteb/tatoeba-bitext-mining
name: MTEB Tatoeba (ara-eng)
config: ara-eng
split: test
revision: 9080400076fbadbb4c4dcb136ff4eddc40b42553
metrics:
- type: accuracy
value: 85.9
- type: f1
value: 82.58857142857143
- type: precision
value: 81.15666666666667
- type: recall
value: 85.9
- task:
type: BitextMining
dataset:
type: mteb/tatoeba-bitext-mining
name: MTEB Tatoeba (kor-eng)
config: kor-eng
split: test
revision: 9080400076fbadbb4c4dcb136ff4eddc40b42553
metrics:
- type: accuracy
value: 86.8
- type: f1
value: 83.36999999999999
- type: precision
value: 81.86833333333333
- type: recall
value: 86.8
- task:
type: BitextMining
dataset:
type: mteb/tatoeba-bitext-mining
name: MTEB Tatoeba (yid-eng)
config: yid-eng
split: test
revision: 9080400076fbadbb4c4dcb136ff4eddc40b42553
metrics:
- type: accuracy
value: 68.51415094339622
- type: f1
value: 63.195000099481234
- type: precision
value: 61.394033442972116
- type: recall
value: 68.51415094339622
- task:
type: BitextMining
dataset:
type: mteb/tatoeba-bitext-mining
name: MTEB Tatoeba (fin-eng)
config: fin-eng
split: test
revision: 9080400076fbadbb4c4dcb136ff4eddc40b42553
metrics:
- type: accuracy
value: 88.5
- type: f1
value: 86.14603174603175
- type: precision
value: 85.1162037037037
- type: recall
value: 88.5
- task:
type: BitextMining
dataset:
type: mteb/tatoeba-bitext-mining
name: MTEB Tatoeba (tha-eng)
config: tha-eng
split: test
revision: 9080400076fbadbb4c4dcb136ff4eddc40b42553
metrics:
- type: accuracy
value: 95.62043795620438
- type: f1
value: 94.40389294403892
- type: precision
value: 93.7956204379562
- type: recall
value: 95.62043795620438
- task:
type: BitextMining
dataset:
type: mteb/tatoeba-bitext-mining
name: MTEB Tatoeba (wuu-eng)
config: wuu-eng
split: test
revision: 9080400076fbadbb4c4dcb136ff4eddc40b42553
metrics:
- type: accuracy
value: 81.8
- type: f1
value: 78.6532178932179
- type: precision
value: 77.46348795840176
- type: recall
value: 81.8
- task:
type: Retrieval
dataset:
type: webis-touche2020
name: MTEB Touche2020
config: default
split: test
revision: None
metrics:
- type: map_at_1
value: 2.603
- type: map_at_10
value: 8.5
- type: map_at_100
value: 12.985
- type: map_at_1000
value: 14.466999999999999
- type: map_at_3
value: 4.859999999999999
- type: map_at_5
value: 5.817
- type: mrr_at_1
value: 28.571
- type: mrr_at_10
value: 42.331
- type: mrr_at_100
value: 43.592999999999996
- type: mrr_at_1000
value: 43.592999999999996
- type: mrr_at_3
value: 38.435
- type: mrr_at_5
value: 39.966
- type: ndcg_at_1
value: 26.531
- type: ndcg_at_10
value: 21.353
- type: ndcg_at_100
value: 31.087999999999997
- type: ndcg_at_1000
value: 43.163000000000004
- type: ndcg_at_3
value: 22.999
- type: ndcg_at_5
value: 21.451
- type: precision_at_1
value: 28.571
- type: precision_at_10
value: 19.387999999999998
- type: precision_at_100
value: 6.265
- type: precision_at_1000
value: 1.4160000000000001
- type: precision_at_3
value: 24.490000000000002
- type: precision_at_5
value: 21.224
- type: recall_at_1
value: 2.603
- type: recall_at_10
value: 14.474
- type: recall_at_100
value: 40.287
- type: recall_at_1000
value: 76.606
- type: recall_at_3
value: 5.978
- type: recall_at_5
value: 7.819
- task:
type: Classification
dataset:
type: mteb/toxic_conversations_50k
name: MTEB ToxicConversationsClassification
config: default
split: test
revision: d7c0de2777da35d6aae2200a62c6e0e5af397c4c
metrics:
- type: accuracy
value: 69.7848
- type: ap
value: 13.661023167088224
- type: f1
value: 53.61686134460943
- task:
type: Classification
dataset:
type: mteb/tweet_sentiment_extraction
name: MTEB TweetSentimentExtractionClassification
config: default
split: test
revision: d604517c81ca91fe16a244d1248fc021f9ecee7a
metrics:
- type: accuracy
value: 61.28183361629882
- type: f1
value: 61.55481034919965
- task:
type: Clustering
dataset:
type: mteb/twentynewsgroups-clustering
name: MTEB TwentyNewsgroupsClustering
config: default
split: test
revision: 6125ec4e24fa026cec8a478383ee943acfbd5449
metrics:
- type: v_measure
value: 35.972128420092396
- task:
type: PairClassification
dataset:
type: mteb/twittersemeval2015-pairclassification
name: MTEB TwitterSemEval2015
config: default
split: test
revision: 70970daeab8776df92f5ea462b6173c0b46fd2d1
metrics:
- type: cos_sim_accuracy
value: 85.59933241938367
- type: cos_sim_ap
value: 72.20760361208136
- type: cos_sim_f1
value: 66.4447731755424
- type: cos_sim_precision
value: 62.35539102267469
- type: cos_sim_recall
value: 71.10817941952506
- type: dot_accuracy
value: 78.98313166835548
- type: dot_ap
value: 44.492521645493795
- type: dot_f1
value: 45.814889336016094
- type: dot_precision
value: 37.02439024390244
- type: dot_recall
value: 60.07915567282321
- type: euclidean_accuracy
value: 85.3907134767837
- type: euclidean_ap
value: 71.53847289080343
- type: euclidean_f1
value: 65.95952206778834
- type: euclidean_precision
value: 61.31006346328196
- type: euclidean_recall
value: 71.37203166226914
- type: manhattan_accuracy
value: 85.40859510043511
- type: manhattan_ap
value: 71.49664104395515
- type: manhattan_f1
value: 65.98569969356485
- type: manhattan_precision
value: 63.928748144482924
- type: manhattan_recall
value: 68.17941952506597
- type: max_accuracy
value: 85.59933241938367
- type: max_ap
value: 72.20760361208136
- type: max_f1
value: 66.4447731755424
- task:
type: PairClassification
dataset:
type: mteb/twitterurlcorpus-pairclassification
name: MTEB TwitterURLCorpus
config: default
split: test
revision: 8b6510b0b1fa4e4c4f879467980e9be563ec1cdf
metrics:
- type: cos_sim_accuracy
value: 88.83261536073273
- type: cos_sim_ap
value: 85.48178133644264
- type: cos_sim_f1
value: 77.87816307403935
- type: cos_sim_precision
value: 75.88953021114926
- type: cos_sim_recall
value: 79.97382198952879
- type: dot_accuracy
value: 79.76287499514883
- type: dot_ap
value: 59.17438838475084
- type: dot_f1
value: 56.34566667855996
- type: dot_precision
value: 52.50349092359864
- type: dot_recall
value: 60.794579611949494
- type: euclidean_accuracy
value: 88.76857996662397
- type: euclidean_ap
value: 85.22764834359887
- type: euclidean_f1
value: 77.65379751543554
- type: euclidean_precision
value: 75.11152683839401
- type: euclidean_recall
value: 80.37419156144134
- type: manhattan_accuracy
value: 88.6987231730508
- type: manhattan_ap
value: 85.18907981724007
- type: manhattan_f1
value: 77.51967028849757
- type: manhattan_precision
value: 75.49992701795358
- type: manhattan_recall
value: 79.65044656606098
- type: max_accuracy
value: 88.83261536073273
- type: max_ap
value: 85.48178133644264
- type: max_f1
value: 77.87816307403935
language:
- multilingual
- af
- am
- ar
- as
- az
- be
- bg
- bn
- br
- bs
- ca
- cs
- cy
- da
- de
- el
- en
- eo
- es
- et
- eu
- fa
- fi
- fr
- fy
- ga
- gd
- gl
- gu
- ha
- he
- hi
- hr
- hu
- hy
- id
- is
- it
- ja
- jv
- ka
- kk
- km
- kn
- ko
- ku
- ky
- la
- lo
- lt
- lv
- mg
- mk
- ml
- mn
- mr
- ms
- my
- ne
- nl
- 'no'
- om
- or
- pa
- pl
- ps
- pt
- ro
- ru
- sa
- sd
- si
- sk
- sl
- so
- sq
- sr
- su
- sv
- sw
- ta
- te
- th
- tl
- tr
- ug
- uk
- ur
- uz
- vi
- xh
- yi
- zh
license: mit
---
## Multilingual-E5-base
[Multilingual E5 Text Embeddings: A Technical Report](https://arxiv.org/pdf/2402.05672).
Liang Wang, Nan Yang, Xiaolong Huang, Linjun Yang, Rangan Majumder, Furu Wei, arXiv 2024
This model has 12 layers and the embedding size is 768.
## Usage
Below is an example to encode queries and passages from the MS-MARCO passage ranking dataset.
```python
import torch.nn.functional as F
from torch import Tensor
from transformers import AutoTokenizer, AutoModel
def average_pool(last_hidden_states: Tensor,
attention_mask: Tensor) -> Tensor:
last_hidden = last_hidden_states.masked_fill(~attention_mask[..., None].bool(), 0.0)
return last_hidden.sum(dim=1) / attention_mask.sum(dim=1)[..., None]
# Each input text should start with "query: " or "passage: ", even for non-English texts.
# For tasks other than retrieval, you can simply use the "query: " prefix.
input_texts = ['query: how much protein should a female eat',
'query: 南瓜的家常做法',
"passage: As a general guideline, the CDC's average requirement of protein for women ages 19 to 70 is 46 grams per day. But, as you can see from this chart, you'll need to increase that if you're expecting or training for a marathon. Check out the chart below to see how much protein you should be eating each day.",
"passage: 1.清炒南瓜丝 原料:嫩南瓜半个 调料:葱、盐、白糖、鸡精 做法: 1、南瓜用刀薄薄的削去表面一层皮,用勺子刮去瓤 2、擦成细丝(没有擦菜板就用刀慢慢切成细丝) 3、锅烧热放油,入葱花煸出香味 4、入南瓜丝快速翻炒一分钟左右,放盐、一点白糖和鸡精调味出锅 2.香葱炒南瓜 原料:南瓜1只 调料:香葱、蒜末、橄榄油、盐 做法: 1、将南瓜去皮,切成片 2、油锅8成热后,将蒜末放入爆香 3、爆香后,将南瓜片放入,翻炒 4、在翻炒的同时,可以不时地往锅里加水,但不要太多 5、放入盐,炒匀 6、南瓜差不多软和绵了之后,就可以关火 7、撒入香葱,即可出锅"]
tokenizer = AutoTokenizer.from_pretrained('intfloat/multilingual-e5-base')
model = AutoModel.from_pretrained('intfloat/multilingual-e5-base')
# Tokenize the input texts
batch_dict = tokenizer(input_texts, max_length=512, padding=True, truncation=True, return_tensors='pt')
outputs = model(**batch_dict)
embeddings = average_pool(outputs.last_hidden_state, batch_dict['attention_mask'])
# normalize embeddings
embeddings = F.normalize(embeddings, p=2, dim=1)
scores = (embeddings[:2] @ embeddings[2:].T) * 100
print(scores.tolist())
```
## Supported Languages
This model is initialized from [xlm-roberta-base](https://huggingface.co/xlm-roberta-base)
and continually trained on a mixture of multilingual datasets.
It supports 100 languages from xlm-roberta,
but low-resource languages may see performance degradation.
## Training Details
**Initialization**: [xlm-roberta-base](https://huggingface.co/xlm-roberta-base)
**First stage**: contrastive pre-training with weak supervision
| Dataset | Weak supervision | # of text pairs |
|--------------------------------------------------------------------------------------------------------|---------------------------------------|-----------------|
| Filtered [mC4](https://huggingface.co/datasets/mc4) | (title, page content) | 1B |
| [CC News](https://huggingface.co/datasets/intfloat/multilingual_cc_news) | (title, news content) | 400M |
| [NLLB](https://huggingface.co/datasets/allenai/nllb) | translation pairs | 2.4B |
| [Wikipedia](https://huggingface.co/datasets/intfloat/wikipedia) | (hierarchical section title, passage) | 150M |
| Filtered [Reddit](https://www.reddit.com/) | (comment, response) | 800M |
| [S2ORC](https://github.com/allenai/s2orc) | (title, abstract) and citation pairs | 100M |
| [Stackexchange](https://stackexchange.com/) | (question, answer) | 50M |
| [xP3](https://huggingface.co/datasets/bigscience/xP3) | (input prompt, response) | 80M |
| [Miscellaneous unsupervised SBERT data](https://huggingface.co/sentence-transformers/all-MiniLM-L6-v2) | - | 10M |
**Second stage**: supervised fine-tuning
| Dataset | Language | # of text pairs |
|----------------------------------------------------------------------------------------|--------------|-----------------|
| [MS MARCO](https://microsoft.github.io/msmarco/) | English | 500k |
| [NQ](https://github.com/facebookresearch/DPR) | English | 70k |
| [Trivia QA](https://github.com/facebookresearch/DPR) | English | 60k |
| [NLI from SimCSE](https://github.com/princeton-nlp/SimCSE) | English | <300k |
| [ELI5](https://huggingface.co/datasets/eli5) | English | 500k |
| [DuReader Retrieval](https://github.com/baidu/DuReader/tree/master/DuReader-Retrieval) | Chinese | 86k |
| [KILT Fever](https://huggingface.co/datasets/kilt_tasks) | English | 70k |
| [KILT HotpotQA](https://huggingface.co/datasets/kilt_tasks) | English | 70k |
| [SQuAD](https://huggingface.co/datasets/squad) | English | 87k |
| [Quora](https://huggingface.co/datasets/quora) | English | 150k |
| [Mr. TyDi](https://huggingface.co/datasets/castorini/mr-tydi) | 11 languages | 50k |
| [MIRACL](https://huggingface.co/datasets/miracl/miracl) | 16 languages | 40k |
For all labeled datasets, we only use its training set for fine-tuning.
For other training details, please refer to our paper at [https://arxiv.org/pdf/2402.05672](https://arxiv.org/pdf/2402.05672).
## Benchmark Results on [Mr. TyDi](https://arxiv.org/abs/2108.08787)
| Model | Avg MRR@10 | | ar | bn | en | fi | id | ja | ko | ru | sw | te | th |
|-----------------------|------------|-------|------| --- | --- | --- | --- | --- | --- | --- |------| --- | --- |
| BM25 | 33.3 | | 36.7 | 41.3 | 15.1 | 28.8 | 38.2 | 21.7 | 28.1 | 32.9 | 39.6 | 42.4 | 41.7 |
| mDPR | 16.7 | | 26.0 | 25.8 | 16.2 | 11.3 | 14.6 | 18.1 | 21.9 | 18.5 | 7.3 | 10.6 | 13.5 |
| BM25 + mDPR | 41.7 | | 49.1 | 53.5 | 28.4 | 36.5 | 45.5 | 35.5 | 36.2 | 42.7 | 40.5 | 42.0 | 49.2 |
| | |
| multilingual-e5-small | 64.4 | | 71.5 | 66.3 | 54.5 | 57.7 | 63.2 | 55.4 | 54.3 | 60.8 | 65.4 | 89.1 | 70.1 |
| multilingual-e5-base | 65.9 | | 72.3 | 65.0 | 58.5 | 60.8 | 64.9 | 56.6 | 55.8 | 62.7 | 69.0 | 86.6 | 72.7 |
| multilingual-e5-large | **70.5** | | 77.5 | 73.2 | 60.8 | 66.8 | 68.5 | 62.5 | 61.6 | 65.8 | 72.7 | 90.2 | 76.2 |
## MTEB Benchmark Evaluation
Check out [unilm/e5](https://github.com/microsoft/unilm/tree/master/e5) to reproduce evaluation results
on the [BEIR](https://arxiv.org/abs/2104.08663) and [MTEB benchmark](https://arxiv.org/abs/2210.07316).
## Support for Sentence Transformers
Below is an example for usage with sentence_transformers.
```python
from sentence_transformers import SentenceTransformer
model = SentenceTransformer('intfloat/multilingual-e5-base')
input_texts = [
'query: how much protein should a female eat',
'query: 南瓜的家常做法',
"passage: As a general guideline, the CDC's average requirement of protein for women ages 19 to 70 i s 46 grams per day. But, as you can see from this chart, you'll need to increase that if you're expecting or traini ng for a marathon. Check out the chart below to see how much protein you should be eating each day.",
"passage: 1.清炒南瓜丝 原料:嫩南瓜半个 调料:葱、盐、白糖、鸡精 做法: 1、南瓜用刀薄薄的削去表面一层皮 ,用勺子刮去瓤 2、擦成细丝(没有擦菜板就用刀慢慢切成细丝) 3、锅烧热放油,入葱花煸出香味 4、入南瓜丝快速翻炒一分钟左右, 放盐、一点白糖和鸡精调味出锅 2.香葱炒南瓜 原料:南瓜1只 调料:香葱、蒜末、橄榄油、盐 做法: 1、将南瓜去皮,切成片 2、油 锅8成热后,将蒜末放入爆香 3、爆香后,将南瓜片放入,翻炒 4、在翻炒的同时,可以不时地往锅里加水,但不要太多 5、放入盐,炒匀 6、南瓜差不多软和绵了之后,就可以关火 7、撒入香葱,即可出锅"
]
embeddings = model.encode(input_texts, normalize_embeddings=True)
```
Package requirements
`pip install sentence_transformers~=2.2.2`
Contributors: [michaelfeil](https://huggingface.co/michaelfeil)
## FAQ
**1. Do I need to add the prefix "query: " and "passage: " to input texts?**
Yes, this is how the model is trained, otherwise you will see a performance degradation.
Here are some rules of thumb:
- Use "query: " and "passage: " correspondingly for asymmetric tasks such as passage retrieval in open QA, ad-hoc information retrieval.
- Use "query: " prefix for symmetric tasks such as semantic similarity, bitext mining, paraphrase retrieval.
- Use "query: " prefix if you want to use embeddings as features, such as linear probing classification, clustering.
**2. Why are my reproduced results slightly different from reported in the model card?**
Different versions of `transformers` and `pytorch` could cause negligible but non-zero performance differences.
**3. Why does the cosine similarity scores distribute around 0.7 to 1.0?**
This is a known and expected behavior as we use a low temperature 0.01 for InfoNCE contrastive loss.
For text embedding tasks like text retrieval or semantic similarity,
what matters is the relative order of the scores instead of the absolute values,
so this should not be an issue.
## Citation
If you find our paper or models helpful, please consider cite as follows:
```
@article{wang2024multilingual,
title={Multilingual E5 Text Embeddings: A Technical Report},
author={Wang, Liang and Yang, Nan and Huang, Xiaolong and Yang, Linjun and Majumder, Rangan and Wei, Furu},
journal={arXiv preprint arXiv:2402.05672},
year={2024}
}
```
## Limitations
Long texts will be truncated to at most 512 tokens.
|
FacebookAI/roberta-large-mnli | FacebookAI | "2024-02-19T12:47:11Z" | 520,806 | 154 | transformers | [
"transformers",
"pytorch",
"tf",
"jax",
"safetensors",
"roberta",
"text-classification",
"autogenerated-modelcard",
"en",
"dataset:multi_nli",
"dataset:wikipedia",
"dataset:bookcorpus",
"arxiv:1907.11692",
"arxiv:1806.02847",
"arxiv:1804.07461",
"arxiv:1704.05426",
"arxiv:1508.05326",
"arxiv:1809.05053",
"arxiv:1910.09700",
"license:mit",
"autotrain_compatible",
"endpoints_compatible",
"region:us"
] | text-classification | "2022-03-02T23:29:04Z" | ---
language:
- en
license: mit
tags:
- autogenerated-modelcard
datasets:
- multi_nli
- wikipedia
- bookcorpus
---
# roberta-large-mnli
## Table of Contents
- [Model Details](#model-details)
- [How To Get Started With the Model](#how-to-get-started-with-the-model)
- [Uses](#uses)
- [Risks, Limitations and Biases](#risks-limitations-and-biases)
- [Training](#training)
- [Evaluation](#evaluation-results)
- [Environmental Impact](#environmental-impact)
- [Technical Specifications](#technical-specifications)
- [Citation Information](#citation-information)
- [Model Card Authors](#model-card-author)
## Model Details
**Model Description:** roberta-large-mnli is the [RoBERTa large model](https://huggingface.co/roberta-large) fine-tuned on the [Multi-Genre Natural Language Inference (MNLI)](https://huggingface.co/datasets/multi_nli) corpus. The model is a pretrained model on English language text using a masked language modeling (MLM) objective.
- **Developed by:** See [GitHub Repo](https://github.com/facebookresearch/fairseq/tree/main/examples/roberta) for model developers
- **Model Type:** Transformer-based language model
- **Language(s):** English
- **License:** MIT
- **Parent Model:** This model is a fine-tuned version of the RoBERTa large model. Users should see the [RoBERTa large model card](https://huggingface.co/roberta-large) for relevant information.
- **Resources for more information:**
- [Research Paper](https://arxiv.org/abs/1907.11692)
- [GitHub Repo](https://github.com/facebookresearch/fairseq/tree/main/examples/roberta)
## How to Get Started with the Model
Use the code below to get started with the model. The model can be loaded with the zero-shot-classification pipeline like so:
```python
from transformers import pipeline
classifier = pipeline('zero-shot-classification', model='roberta-large-mnli')
```
You can then use this pipeline to classify sequences into any of the class names you specify. For example:
```python
sequence_to_classify = "one day I will see the world"
candidate_labels = ['travel', 'cooking', 'dancing']
classifier(sequence_to_classify, candidate_labels)
```
## Uses
#### Direct Use
This fine-tuned model can be used for zero-shot classification tasks, including zero-shot sentence-pair classification (see the [GitHub repo](https://github.com/facebookresearch/fairseq/tree/main/examples/roberta) for examples) and zero-shot sequence classification.
#### Misuse and Out-of-scope Use
The model should not be used to intentionally create hostile or alienating environments for people. In addition, the model was not trained to be factual or true representations of people or events, and therefore using the model to generate such content is out-of-scope for the abilities of this model.
## Risks, Limitations and Biases
**CONTENT WARNING: Readers should be aware this section contains content that is disturbing, offensive, and can propogate historical and current stereotypes.**
Significant research has explored bias and fairness issues with language models (see, e.g., [Sheng et al. (2021)](https://aclanthology.org/2021.acl-long.330.pdf) and [Bender et al. (2021)](https://dl.acm.org/doi/pdf/10.1145/3442188.3445922)). The [RoBERTa large model card](https://huggingface.co/roberta-large) notes that: "The training data used for this model contains a lot of unfiltered content from the internet, which is far from neutral."
Predictions generated by the model can include disturbing and harmful stereotypes across protected classes; identity characteristics; and sensitive, social, and occupational groups. For example:
```python
sequence_to_classify = "The CEO had a strong handshake."
candidate_labels = ['male', 'female']
hypothesis_template = "This text speaks about a {} profession."
classifier(sequence_to_classify, candidate_labels, hypothesis_template=hypothesis_template)
```
Users (both direct and downstream) should be made aware of the risks, biases and limitations of the model.
## Training
#### Training Data
This model was fine-tuned on the [Multi-Genre Natural Language Inference (MNLI)](https://cims.nyu.edu/~sbowman/multinli/) corpus. Also see the [MNLI data card](https://huggingface.co/datasets/multi_nli) for more information.
As described in the [RoBERTa large model card](https://huggingface.co/roberta-large):
> The RoBERTa model was pretrained on the reunion of five datasets:
>
> - [BookCorpus](https://yknzhu.wixsite.com/mbweb), a dataset consisting of 11,038 unpublished books;
> - [English Wikipedia](https://en.wikipedia.org/wiki/English_Wikipedia) (excluding lists, tables and headers) ;
> - [CC-News](https://commoncrawl.org/2016/10/news-dataset-available/), a dataset containing 63 millions English news articles crawled between September 2016 and February 2019.
> - [OpenWebText](https://github.com/jcpeterson/openwebtext), an opensource recreation of the WebText dataset used to train GPT-2,
> - [Stories](https://arxiv.org/abs/1806.02847), a dataset containing a subset of CommonCrawl data filtered to match the story-like style of Winograd schemas.
>
> Together theses datasets weight 160GB of text.
Also see the [bookcorpus data card](https://huggingface.co/datasets/bookcorpus) and the [wikipedia data card](https://huggingface.co/datasets/wikipedia) for additional information.
#### Training Procedure
##### Preprocessing
As described in the [RoBERTa large model card](https://huggingface.co/roberta-large):
> The texts are tokenized using a byte version of Byte-Pair Encoding (BPE) and a vocabulary size of 50,000. The inputs of
> the model take pieces of 512 contiguous token that may span over documents. The beginning of a new document is marked
> with `<s>` and the end of one by `</s>`
>
> The details of the masking procedure for each sentence are the following:
> - 15% of the tokens are masked.
> - In 80% of the cases, the masked tokens are replaced by `<mask>`.
> - In 10% of the cases, the masked tokens are replaced by a random token (different) from the one they replace.
> - In the 10% remaining cases, the masked tokens are left as is.
>
> Contrary to BERT, the masking is done dynamically during pretraining (e.g., it changes at each epoch and is not fixed).
##### Pretraining
Also as described in the [RoBERTa large model card](https://huggingface.co/roberta-large):
> The model was trained on 1024 V100 GPUs for 500K steps with a batch size of 8K and a sequence length of 512. The
> optimizer used is Adam with a learning rate of 4e-4, \\(\beta_{1} = 0.9\\), \\(\beta_{2} = 0.98\\) and
> \\(\epsilon = 1e-6\\), a weight decay of 0.01, learning rate warmup for 30,000 steps and linear decay of the learning
> rate after.
## Evaluation
The following evaluation information is extracted from the associated [GitHub repo for RoBERTa](https://github.com/facebookresearch/fairseq/tree/main/examples/roberta).
#### Testing Data, Factors and Metrics
The model developers report that the model was evaluated on the following tasks and datasets using the listed metrics:
- **Dataset:** Part of [GLUE (Wang et al., 2019)](https://arxiv.org/pdf/1804.07461.pdf), the General Language Understanding Evaluation benchmark, a collection of 9 datasets for evaluating natural language understanding systems. Specifically, the model was evaluated on the [Multi-Genre Natural Language Inference (MNLI)](https://cims.nyu.edu/~sbowman/multinli/) corpus. See the [GLUE data card](https://huggingface.co/datasets/glue) or [Wang et al. (2019)](https://arxiv.org/pdf/1804.07461.pdf) for further information.
- **Tasks:** NLI. [Wang et al. (2019)](https://arxiv.org/pdf/1804.07461.pdf) describe the inference task for MNLI as:
> The Multi-Genre Natural Language Inference Corpus [(Williams et al., 2018)](https://arxiv.org/abs/1704.05426) is a crowd-sourced collection of sentence pairs with textual entailment annotations. Given a premise sentence and a hypothesis sentence, the task is to predict whether the premise entails the hypothesis (entailment), contradicts the hypothesis (contradiction), or neither (neutral). The premise sentences are gathered from ten different sources, including transcribed speech, fiction, and government reports. We use the standard test set, for which we obtained private labels from the authors, and evaluate on both the matched (in-domain) and mismatched (cross-domain) sections. We also use and recommend the SNLI corpus [(Bowman et al., 2015)](https://arxiv.org/abs/1508.05326) as 550k examples of auxiliary training data.
- **Metrics:** Accuracy
- **Dataset:** [XNLI (Conneau et al., 2018)](https://arxiv.org/pdf/1809.05053.pdf), the extension of the [Multi-Genre Natural Language Inference (MNLI)](https://cims.nyu.edu/~sbowman/multinli/) corpus to 15 languages: English, French, Spanish, German, Greek, Bulgarian, Russian, Turkish, Arabic, Vietnamese, Thai, Chinese, Hindi, Swahili and Urdu. See the [XNLI data card](https://huggingface.co/datasets/xnli) or [Conneau et al. (2018)](https://arxiv.org/pdf/1809.05053.pdf) for further information.
- **Tasks:** Translate-test (e.g., the model is used to translate input sentences in other languages to the training language)
- **Metrics:** Accuracy
#### Results
GLUE test results (dev set, single model, single-task fine-tuning): 90.2 on MNLI
XNLI test results:
| Task | en | fr | es | de | el | bg | ru | tr | ar | vi | th | zh | hi | sw | ur |
|:----:|:--:|:---:|:---:|:---:|:---:|:---:|:---:|:---:|:---:|:---:|:---:|:---:|:---:|:---:|:---:|
| |91.3|82.91|84.27|81.24|81.74|83.13|78.28|76.79|76.64|74.17|74.05| 77.5| 70.9|66.65|66.81|
## Environmental Impact
Carbon emissions can be estimated using the [Machine Learning Impact calculator](https://mlco2.github.io/impact#compute) presented in [Lacoste et al. (2019)](https://arxiv.org/abs/1910.09700). We present the hardware type and hours used based on the [associated paper](https://arxiv.org/pdf/1907.11692.pdf).
- **Hardware Type:** 1024 V100 GPUs
- **Hours used:** 24 hours (one day)
- **Cloud Provider:** Unknown
- **Compute Region:** Unknown
- **Carbon Emitted:** Unknown
## Technical Specifications
See the [associated paper](https://arxiv.org/pdf/1907.11692.pdf) for details on the modeling architecture, objective, compute infrastructure, and training details.
## Citation Information
```bibtex
@article{liu2019roberta,
title = {RoBERTa: A Robustly Optimized BERT Pretraining Approach},
author = {Yinhan Liu and Myle Ott and Naman Goyal and Jingfei Du and
Mandar Joshi and Danqi Chen and Omer Levy and Mike Lewis and
Luke Zettlemoyer and Veselin Stoyanov},
journal={arXiv preprint arXiv:1907.11692},
year = {2019},
}
``` |
Helsinki-NLP/opus-mt-nl-en | Helsinki-NLP | "2023-08-16T12:01:39Z" | 520,012 | 8 | transformers | [
"transformers",
"pytorch",
"tf",
"rust",
"marian",
"text2text-generation",
"translation",
"nl",
"en",
"license:apache-2.0",
"autotrain_compatible",
"endpoints_compatible",
"region:us"
] | translation | "2022-03-02T23:29:04Z" | ---
tags:
- translation
license: apache-2.0
---
### opus-mt-nl-en
* source languages: nl
* target languages: en
* OPUS readme: [nl-en](https://github.com/Helsinki-NLP/OPUS-MT-train/blob/master/models/nl-en/README.md)
* dataset: opus
* model: transformer-align
* pre-processing: normalization + SentencePiece
* download original weights: [opus-2019-12-05.zip](https://object.pouta.csc.fi/OPUS-MT-models/nl-en/opus-2019-12-05.zip)
* test set translations: [opus-2019-12-05.test.txt](https://object.pouta.csc.fi/OPUS-MT-models/nl-en/opus-2019-12-05.test.txt)
* test set scores: [opus-2019-12-05.eval.txt](https://object.pouta.csc.fi/OPUS-MT-models/nl-en/opus-2019-12-05.eval.txt)
## Benchmarks
| testset | BLEU | chr-F |
|-----------------------|-------|-------|
| Tatoeba.nl.en | 60.9 | 0.749 |
|
TheBloke/Llama-2-7B-GPTQ | TheBloke | "2023-09-27T12:44:46Z" | 519,151 | 80 | transformers | [
"transformers",
"safetensors",
"llama",
"text-generation",
"facebook",
"meta",
"pytorch",
"llama-2",
"en",
"arxiv:2307.09288",
"base_model:meta-llama/Llama-2-7b-hf",
"base_model:quantized:meta-llama/Llama-2-7b-hf",
"license:llama2",
"autotrain_compatible",
"text-generation-inference",
"4-bit",
"gptq",
"region:us"
] | text-generation | "2023-07-18T17:06:01Z" | ---
language:
- en
license: llama2
tags:
- facebook
- meta
- pytorch
- llama
- llama-2
model_name: Llama 2 7B
base_model: meta-llama/Llama-2-7b-hf
inference: false
model_creator: Meta
model_type: llama
pipeline_tag: text-generation
prompt_template: '{prompt}
'
quantized_by: TheBloke
---
<!-- header start -->
<!-- 200823 -->
<div style="width: auto; margin-left: auto; margin-right: auto">
<img src="https://i.imgur.com/EBdldam.jpg" alt="TheBlokeAI" style="width: 100%; min-width: 400px; display: block; margin: auto;">
</div>
<div style="display: flex; justify-content: space-between; width: 100%;">
<div style="display: flex; flex-direction: column; align-items: flex-start;">
<p style="margin-top: 0.5em; margin-bottom: 0em;"><a href="https://discord.gg/theblokeai">Chat & support: TheBloke's Discord server</a></p>
</div>
<div style="display: flex; flex-direction: column; align-items: flex-end;">
<p style="margin-top: 0.5em; margin-bottom: 0em;"><a href="https://www.patreon.com/TheBlokeAI">Want to contribute? TheBloke's Patreon page</a></p>
</div>
</div>
<div style="text-align:center; margin-top: 0em; margin-bottom: 0em"><p style="margin-top: 0.25em; margin-bottom: 0em;">TheBloke's LLM work is generously supported by a grant from <a href="https://a16z.com">andreessen horowitz (a16z)</a></p></div>
<hr style="margin-top: 1.0em; margin-bottom: 1.0em;">
<!-- header end -->
# Llama 2 7B - GPTQ
- Model creator: [Meta](https://huggingface.co/meta-llama)
- Original model: [Llama 2 7B](https://huggingface.co/meta-llama/Llama-2-7b-hf)
<!-- description start -->
## Description
This repo contains GPTQ model files for [Meta's Llama 2 7B](https://huggingface.co/meta-llama/Llama-2-7b-hf).
Multiple GPTQ parameter permutations are provided; see Provided Files below for details of the options provided, their parameters, and the software used to create them.
<!-- description end -->
<!-- repositories-available start -->
## Repositories available
* [AWQ model(s) for GPU inference.](https://huggingface.co/TheBloke/Llama-2-7B-AWQ)
* [GPTQ models for GPU inference, with multiple quantisation parameter options.](https://huggingface.co/TheBloke/Llama-2-7B-GPTQ)
* [2, 3, 4, 5, 6 and 8-bit GGUF models for CPU+GPU inference](https://huggingface.co/TheBloke/Llama-2-7B-GGUF)
* [Meta's original unquantised fp16 model in pytorch format, for GPU inference and for further conversions](https://huggingface.co/meta-llama/Llama-2-7b-hf)
<!-- repositories-available end -->
<!-- prompt-template start -->
## Prompt template: None
```
{prompt}
```
<!-- prompt-template end -->
<!-- README_GPTQ.md-provided-files start -->
## Provided files and GPTQ parameters
Multiple quantisation parameters are provided, to allow you to choose the best one for your hardware and requirements.
Each separate quant is in a different branch. See below for instructions on fetching from different branches.
All recent GPTQ files are made with AutoGPTQ, and all files in non-main branches are made with AutoGPTQ. Files in the `main` branch which were uploaded before August 2023 were made with GPTQ-for-LLaMa.
<details>
<summary>Explanation of GPTQ parameters</summary>
- Bits: The bit size of the quantised model.
- GS: GPTQ group size. Higher numbers use less VRAM, but have lower quantisation accuracy. "None" is the lowest possible value.
- Act Order: True or False. Also known as `desc_act`. True results in better quantisation accuracy. Some GPTQ clients have had issues with models that use Act Order plus Group Size, but this is generally resolved now.
- Damp %: A GPTQ parameter that affects how samples are processed for quantisation. 0.01 is default, but 0.1 results in slightly better accuracy.
- GPTQ dataset: The dataset used for quantisation. Using a dataset more appropriate to the model's training can improve quantisation accuracy. Note that the GPTQ dataset is not the same as the dataset used to train the model - please refer to the original model repo for details of the training dataset(s).
- Sequence Length: The length of the dataset sequences used for quantisation. Ideally this is the same as the model sequence length. For some very long sequence models (16+K), a lower sequence length may have to be used. Note that a lower sequence length does not limit the sequence length of the quantised model. It only impacts the quantisation accuracy on longer inference sequences.
- ExLlama Compatibility: Whether this file can be loaded with ExLlama, which currently only supports Llama models in 4-bit.
</details>
| Branch | Bits | GS | Act Order | Damp % | GPTQ Dataset | Seq Len | Size | ExLlama | Desc |
| ------ | ---- | -- | --------- | ------ | ------------ | ------- | ---- | ------- | ---- |
| [main](https://huggingface.co/TheBloke/Llama-2-7B-GPTQ/tree/main) | 4 | 128 | No | 0.01 | [wikitext](https://huggingface.co/datasets/wikitext/viewer/wikitext-2-v1/test) | 4096 | 3.90 GB | Yes | 4-bit, without Act Order and group size 128g. |
| [gptq-4bit-32g-actorder_True](https://huggingface.co/TheBloke/Llama-2-7B-GPTQ/tree/gptq-4bit-32g-actorder_True) | 4 | 32 | Yes | 0.01 | [wikitext](https://huggingface.co/datasets/wikitext/viewer/wikitext-2-v1/test) | 4096 | 4.28 GB | Yes | 4-bit, with Act Order and group size 32g. Gives highest possible inference quality, with maximum VRAM usage. |
| [gptq-4bit-64g-actorder_True](https://huggingface.co/TheBloke/Llama-2-7B-GPTQ/tree/gptq-4bit-64g-actorder_True) | 4 | 64 | Yes | 0.01 | [wikitext](https://huggingface.co/datasets/wikitext/viewer/wikitext-2-v1/test) | 4096 | 4.02 GB | Yes | 4-bit, with Act Order and group size 64g. Uses less VRAM than 32g, but with slightly lower accuracy. |
| [gptq-4bit-128g-actorder_True](https://huggingface.co/TheBloke/Llama-2-7B-GPTQ/tree/gptq-4bit-128g-actorder_True) | 4 | 128 | Yes | 0.01 | [wikitext](https://huggingface.co/datasets/wikitext/viewer/wikitext-2-v1/test) | 4096 | 3.90 GB | Yes | 4-bit, with Act Order and group size 128g. Uses even less VRAM than 64g, but with slightly lower accuracy. |
<!-- README_GPTQ.md-provided-files end -->
<!-- README_GPTQ.md-download-from-branches start -->
## How to download from branches
- In text-generation-webui, you can add `:branch` to the end of the download name, eg `TheBloke/Llama-2-7B-GPTQ:main`
- With Git, you can clone a branch with:
```
git clone --single-branch --branch main https://huggingface.co/TheBloke/Llama-2-7B-GPTQ
```
- In Python Transformers code, the branch is the `revision` parameter; see below.
<!-- README_GPTQ.md-download-from-branches end -->
<!-- README_GPTQ.md-text-generation-webui start -->
## How to easily download and use this model in [text-generation-webui](https://github.com/oobabooga/text-generation-webui).
Please make sure you're using the latest version of [text-generation-webui](https://github.com/oobabooga/text-generation-webui).
It is strongly recommended to use the text-generation-webui one-click-installers unless you're sure you know how to make a manual install.
1. Click the **Model tab**.
2. Under **Download custom model or LoRA**, enter `TheBloke/Llama-2-7B-GPTQ`.
- To download from a specific branch, enter for example `TheBloke/Llama-2-7B-GPTQ:main`
- see Provided Files above for the list of branches for each option.
3. Click **Download**.
4. The model will start downloading. Once it's finished it will say "Done".
5. In the top left, click the refresh icon next to **Model**.
6. In the **Model** dropdown, choose the model you just downloaded: `Llama-2-7B-GPTQ`
7. The model will automatically load, and is now ready for use!
8. If you want any custom settings, set them and then click **Save settings for this model** followed by **Reload the Model** in the top right.
* Note that you do not need to and should not set manual GPTQ parameters any more. These are set automatically from the file `quantize_config.json`.
9. Once you're ready, click the **Text Generation tab** and enter a prompt to get started!
<!-- README_GPTQ.md-text-generation-webui end -->
<!-- README_GPTQ.md-use-from-python start -->
## How to use this GPTQ model from Python code
### Install the necessary packages
Requires: Transformers 4.32.0 or later, Optimum 1.12.0 or later, and AutoGPTQ 0.4.2 or later.
```shell
pip3 install transformers>=4.32.0 optimum>=1.12.0
pip3 install auto-gptq --extra-index-url https://huggingface.github.io/autogptq-index/whl/cu118/ # Use cu117 if on CUDA 11.7
```
If you have problems installing AutoGPTQ using the pre-built wheels, install it from source instead:
```shell
pip3 uninstall -y auto-gptq
git clone https://github.com/PanQiWei/AutoGPTQ
cd AutoGPTQ
pip3 install .
```
### For CodeLlama models only: you must use Transformers 4.33.0 or later.
If 4.33.0 is not yet released when you read this, you will need to install Transformers from source:
```shell
pip3 uninstall -y transformers
pip3 install git+https://github.com/huggingface/transformers.git
```
### You can then use the following code
```python
from transformers import AutoModelForCausalLM, AutoTokenizer, pipeline
model_name_or_path = "TheBloke/Llama-2-7B-GPTQ"
# To use a different branch, change revision
# For example: revision="main"
model = AutoModelForCausalLM.from_pretrained(model_name_or_path,
device_map="auto",
trust_remote_code=True,
revision="main")
tokenizer = AutoTokenizer.from_pretrained(model_name_or_path, use_fast=True)
prompt = "Tell me about AI"
prompt_template=f'''{prompt}
'''
print("\n\n*** Generate:")
input_ids = tokenizer(prompt_template, return_tensors='pt').input_ids.cuda()
output = model.generate(inputs=input_ids, temperature=0.7, do_sample=True, top_p=0.95, top_k=40, max_new_tokens=512)
print(tokenizer.decode(output[0]))
# Inference can also be done using transformers' pipeline
print("*** Pipeline:")
pipe = pipeline(
"text-generation",
model=model,
tokenizer=tokenizer,
max_new_tokens=512,
do_sample=True,
temperature=0.7,
top_p=0.95,
top_k=40,
repetition_penalty=1.1
)
print(pipe(prompt_template)[0]['generated_text'])
```
<!-- README_GPTQ.md-use-from-python end -->
<!-- README_GPTQ.md-compatibility start -->
## Compatibility
The files provided are tested to work with AutoGPTQ, both via Transformers and using AutoGPTQ directly. They should also work with [Occ4m's GPTQ-for-LLaMa fork](https://github.com/0cc4m/KoboldAI).
[ExLlama](https://github.com/turboderp/exllama) is compatible with Llama models in 4-bit. Please see the Provided Files table above for per-file compatibility.
[Huggingface Text Generation Inference (TGI)](https://github.com/huggingface/text-generation-inference) is compatible with all GPTQ models.
<!-- README_GPTQ.md-compatibility end -->
<!-- footer start -->
<!-- 200823 -->
## Discord
For further support, and discussions on these models and AI in general, join us at:
[TheBloke AI's Discord server](https://discord.gg/theblokeai)
## Thanks, and how to contribute
Thanks to the [chirper.ai](https://chirper.ai) team!
Thanks to Clay from [gpus.llm-utils.org](llm-utils)!
I've had a lot of people ask if they can contribute. I enjoy providing models and helping people, and would love to be able to spend even more time doing it, as well as expanding into new projects like fine tuning/training.
If you're able and willing to contribute it will be most gratefully received and will help me to keep providing more models, and to start work on new AI projects.
Donaters will get priority support on any and all AI/LLM/model questions and requests, access to a private Discord room, plus other benefits.
* Patreon: https://patreon.com/TheBlokeAI
* Ko-Fi: https://ko-fi.com/TheBlokeAI
**Special thanks to**: Aemon Algiz.
**Patreon special mentions**: Alicia Loh, Stephen Murray, K, Ajan Kanaga, RoA, Magnesian, Deo Leter, Olakabola, Eugene Pentland, zynix, Deep Realms, Raymond Fosdick, Elijah Stavena, Iucharbius, Erik Bjäreholt, Luis Javier Navarrete Lozano, Nicholas, theTransient, John Detwiler, alfie_i, knownsqashed, Mano Prime, Willem Michiel, Enrico Ros, LangChain4j, OG, Michael Dempsey, Pierre Kircher, Pedro Madruga, James Bentley, Thomas Belote, Luke @flexchar, Leonard Tan, Johann-Peter Hartmann, Illia Dulskyi, Fen Risland, Chadd, S_X, Jeff Scroggin, Ken Nordquist, Sean Connelly, Artur Olbinski, Swaroop Kallakuri, Jack West, Ai Maven, David Ziegler, Russ Johnson, transmissions 11, John Villwock, Alps Aficionado, Clay Pascal, Viktor Bowallius, Subspace Studios, Rainer Wilmers, Trenton Dambrowitz, vamX, Michael Levine, 준교 김, Brandon Frisco, Kalila, Trailburnt, Randy H, Talal Aujan, Nathan Dryer, Vadim, 阿明, ReadyPlayerEmma, Tiffany J. Kim, George Stoitzev, Spencer Kim, Jerry Meng, Gabriel Tamborski, Cory Kujawski, Jeffrey Morgan, Spiking Neurons AB, Edmond Seymore, Alexandros Triantafyllidis, Lone Striker, Cap'n Zoog, Nikolai Manek, danny, ya boyyy, Derek Yates, usrbinkat, Mandus, TL, Nathan LeClaire, subjectnull, Imad Khwaja, webtim, Raven Klaugh, Asp the Wyvern, Gabriel Puliatti, Caitlyn Gatomon, Joseph William Delisle, Jonathan Leane, Luke Pendergrass, SuperWojo, Sebastain Graf, Will Dee, Fred von Graf, Andrey, Dan Guido, Daniel P. Andersen, Nitin Borwankar, Elle, Vitor Caleffi, biorpg, jjj, NimbleBox.ai, Pieter, Matthew Berman, terasurfer, Michael Davis, Alex, Stanislav Ovsiannikov
Thank you to all my generous patrons and donaters!
And thank you again to a16z for their generous grant.
<!-- footer end -->
# Original model card: Meta's Llama 2 7B
# **Llama 2**
Llama 2 is a collection of pretrained and fine-tuned generative text models ranging in scale from 7 billion to 70 billion parameters. This is the repository for the 7B pretrained model, converted for the Hugging Face Transformers format. Links to other models can be found in the index at the bottom.
## Model Details
*Note: Use of this model is governed by the Meta license. In order to download the model weights and tokenizer, please visit the [website](https://ai.meta.com/resources/models-and-libraries/llama-downloads/) and accept our License before requesting access here.*
Meta developed and publicly released the Llama 2 family of large language models (LLMs), a collection of pretrained and fine-tuned generative text models ranging in scale from 7 billion to 70 billion parameters. Our fine-tuned LLMs, called Llama-2-Chat, are optimized for dialogue use cases. Llama-2-Chat models outperform open-source chat models on most benchmarks we tested, and in our human evaluations for helpfulness and safety, are on par with some popular closed-source models like ChatGPT and PaLM.
**Model Developers** Meta
**Variations** Llama 2 comes in a range of parameter sizes — 7B, 13B, and 70B — as well as pretrained and fine-tuned variations.
**Input** Models input text only.
**Output** Models generate text only.
**Model Architecture** Llama 2 is an auto-regressive language model that uses an optimized transformer architecture. The tuned versions use supervised fine-tuning (SFT) and reinforcement learning with human feedback (RLHF) to align to human preferences for helpfulness and safety.
||Training Data|Params|Content Length|GQA|Tokens|LR|
|---|---|---|---|---|---|---|
|Llama 2|*A new mix of publicly available online data*|7B|4k|✗|2.0T|3.0 x 10<sup>-4</sup>|
|Llama 2|*A new mix of publicly available online data*|13B|4k|✗|2.0T|3.0 x 10<sup>-4</sup>|
|Llama 2|*A new mix of publicly available online data*|70B|4k|✔|2.0T|1.5 x 10<sup>-4</sup>|
*Llama 2 family of models.* Token counts refer to pretraining data only. All models are trained with a global batch-size of 4M tokens. Bigger models - 70B -- use Grouped-Query Attention (GQA) for improved inference scalability.
**Model Dates** Llama 2 was trained between January 2023 and July 2023.
**Status** This is a static model trained on an offline dataset. Future versions of the tuned models will be released as we improve model safety with community feedback.
**License** A custom commercial license is available at: [https://ai.meta.com/resources/models-and-libraries/llama-downloads/](https://ai.meta.com/resources/models-and-libraries/llama-downloads/)
**Research Paper** ["Llama-2: Open Foundation and Fine-tuned Chat Models"](arxiv.org/abs/2307.09288)
## Intended Use
**Intended Use Cases** Llama 2 is intended for commercial and research use in English. Tuned models are intended for assistant-like chat, whereas pretrained models can be adapted for a variety of natural language generation tasks.
To get the expected features and performance for the chat versions, a specific formatting needs to be followed, including the `INST` and `<<SYS>>` tags, `BOS` and `EOS` tokens, and the whitespaces and breaklines in between (we recommend calling `strip()` on inputs to avoid double-spaces). See our reference code in github for details: [`chat_completion`](https://github.com/facebookresearch/llama/blob/main/llama/generation.py#L212).
**Out-of-scope Uses** Use in any manner that violates applicable laws or regulations (including trade compliance laws).Use in languages other than English. Use in any other way that is prohibited by the Acceptable Use Policy and Licensing Agreement for Llama 2.
## Hardware and Software
**Training Factors** We used custom training libraries, Meta's Research Super Cluster, and production clusters for pretraining. Fine-tuning, annotation, and evaluation were also performed on third-party cloud compute.
**Carbon Footprint** Pretraining utilized a cumulative 3.3M GPU hours of computation on hardware of type A100-80GB (TDP of 350-400W). Estimated total emissions were 539 tCO2eq, 100% of which were offset by Meta’s sustainability program.
||Time (GPU hours)|Power Consumption (W)|Carbon Emitted(tCO<sub>2</sub>eq)|
|---|---|---|---|
|Llama 2 7B|184320|400|31.22|
|Llama 2 13B|368640|400|62.44|
|Llama 2 70B|1720320|400|291.42|
|Total|3311616||539.00|
**CO<sub>2</sub> emissions during pretraining.** Time: total GPU time required for training each model. Power Consumption: peak power capacity per GPU device for the GPUs used adjusted for power usage efficiency. 100% of the emissions are directly offset by Meta's sustainability program, and because we are openly releasing these models, the pretraining costs do not need to be incurred by others.
## Training Data
**Overview** Llama 2 was pretrained on 2 trillion tokens of data from publicly available sources. The fine-tuning data includes publicly available instruction datasets, as well as over one million new human-annotated examples. Neither the pretraining nor the fine-tuning datasets include Meta user data.
**Data Freshness** The pretraining data has a cutoff of September 2022, but some tuning data is more recent, up to July 2023.
## Evaluation Results
In this section, we report the results for the Llama 1 and Llama 2 models on standard academic benchmarks.For all the evaluations, we use our internal evaluations library.
|Model|Size|Code|Commonsense Reasoning|World Knowledge|Reading Comprehension|Math|MMLU|BBH|AGI Eval|
|---|---|---|---|---|---|---|---|---|---|
|Llama 1|7B|14.1|60.8|46.2|58.5|6.95|35.1|30.3|23.9|
|Llama 1|13B|18.9|66.1|52.6|62.3|10.9|46.9|37.0|33.9|
|Llama 1|33B|26.0|70.0|58.4|67.6|21.4|57.8|39.8|41.7|
|Llama 1|65B|30.7|70.7|60.5|68.6|30.8|63.4|43.5|47.6|
|Llama 2|7B|16.8|63.9|48.9|61.3|14.6|45.3|32.6|29.3|
|Llama 2|13B|24.5|66.9|55.4|65.8|28.7|54.8|39.4|39.1|
|Llama 2|70B|**37.5**|**71.9**|**63.6**|**69.4**|**35.2**|**68.9**|**51.2**|**54.2**|
**Overall performance on grouped academic benchmarks.** *Code:* We report the average pass@1 scores of our models on HumanEval and MBPP. *Commonsense Reasoning:* We report the average of PIQA, SIQA, HellaSwag, WinoGrande, ARC easy and challenge, OpenBookQA, and CommonsenseQA. We report 7-shot results for CommonSenseQA and 0-shot results for all other benchmarks. *World Knowledge:* We evaluate the 5-shot performance on NaturalQuestions and TriviaQA and report the average. *Reading Comprehension:* For reading comprehension, we report the 0-shot average on SQuAD, QuAC, and BoolQ. *MATH:* We report the average of the GSM8K (8 shot) and MATH (4 shot) benchmarks at top 1.
|||TruthfulQA|Toxigen|
|---|---|---|---|
|Llama 1|7B|27.42|23.00|
|Llama 1|13B|41.74|23.08|
|Llama 1|33B|44.19|22.57|
|Llama 1|65B|48.71|21.77|
|Llama 2|7B|33.29|**21.25**|
|Llama 2|13B|41.86|26.10|
|Llama 2|70B|**50.18**|24.60|
**Evaluation of pretrained LLMs on automatic safety benchmarks.** For TruthfulQA, we present the percentage of generations that are both truthful and informative (the higher the better). For ToxiGen, we present the percentage of toxic generations (the smaller the better).
|||TruthfulQA|Toxigen|
|---|---|---|---|
|Llama-2-Chat|7B|57.04|**0.00**|
|Llama-2-Chat|13B|62.18|**0.00**|
|Llama-2-Chat|70B|**64.14**|0.01|
**Evaluation of fine-tuned LLMs on different safety datasets.** Same metric definitions as above.
## Ethical Considerations and Limitations
Llama 2 is a new technology that carries risks with use. Testing conducted to date has been in English, and has not covered, nor could it cover all scenarios. For these reasons, as with all LLMs, Llama 2’s potential outputs cannot be predicted in advance, and the model may in some instances produce inaccurate, biased or other objectionable responses to user prompts. Therefore, before deploying any applications of Llama 2, developers should perform safety testing and tuning tailored to their specific applications of the model.
Please see the Responsible Use Guide available at [https://ai.meta.com/llama/responsible-use-guide/](https://ai.meta.com/llama/responsible-use-guide)
## Reporting Issues
Please report any software “bug,” or other problems with the models through one of the following means:
- Reporting issues with the model: [github.com/facebookresearch/llama](http://github.com/facebookresearch/llama)
- Reporting problematic content generated by the model: [developers.facebook.com/llama_output_feedback](http://developers.facebook.com/llama_output_feedback)
- Reporting bugs and security concerns: [facebook.com/whitehat/info](http://facebook.com/whitehat/info)
## Llama Model Index
|Model|Llama2|Llama2-hf|Llama2-chat|Llama2-chat-hf|
|---|---|---|---|---|
|7B| [Link](https://huggingface.co/llamaste/Llama-2-7b) | [Link](https://huggingface.co/llamaste/Llama-2-7b-hf) | [Link](https://huggingface.co/llamaste/Llama-2-7b-chat) | [Link](https://huggingface.co/llamaste/Llama-2-7b-chat-hf)|
|13B| [Link](https://huggingface.co/llamaste/Llama-2-13b) | [Link](https://huggingface.co/llamaste/Llama-2-13b-hf) | [Link](https://huggingface.co/llamaste/Llama-2-13b-chat) | [Link](https://huggingface.co/llamaste/Llama-2-13b-hf)|
|70B| [Link](https://huggingface.co/llamaste/Llama-2-70b) | [Link](https://huggingface.co/llamaste/Llama-2-70b-hf) | [Link](https://huggingface.co/llamaste/Llama-2-70b-chat) | [Link](https://huggingface.co/llamaste/Llama-2-70b-hf)|
|
BAAI/bge-large-en | BAAI | "2023-10-12T03:35:38Z" | 515,513 | 201 | transformers | [
"transformers",
"pytorch",
"safetensors",
"bert",
"feature-extraction",
"mteb",
"sentence-transfomres",
"en",
"arxiv:2310.07554",
"arxiv:2309.07597",
"license:mit",
"model-index",
"text-embeddings-inference",
"endpoints_compatible",
"region:us"
] | feature-extraction | "2023-08-02T07:11:51Z" | ---
tags:
- mteb
- sentence-transfomres
- transformers
model-index:
- name: bge-large-en
results:
- task:
type: Classification
dataset:
type: mteb/amazon_counterfactual
name: MTEB AmazonCounterfactualClassification (en)
config: en
split: test
revision: e8379541af4e31359cca9fbcf4b00f2671dba205
metrics:
- type: accuracy
value: 76.94029850746269
- type: ap
value: 40.00228964744091
- type: f1
value: 70.86088267934595
- task:
type: Classification
dataset:
type: mteb/amazon_polarity
name: MTEB AmazonPolarityClassification
config: default
split: test
revision: e2d317d38cd51312af73b3d32a06d1a08b442046
metrics:
- type: accuracy
value: 91.93745
- type: ap
value: 88.24758534667426
- type: f1
value: 91.91033034217591
- task:
type: Classification
dataset:
type: mteb/amazon_reviews_multi
name: MTEB AmazonReviewsClassification (en)
config: en
split: test
revision: 1399c76144fd37290681b995c656ef9b2e06e26d
metrics:
- type: accuracy
value: 46.158
- type: f1
value: 45.78935185074774
- task:
type: Retrieval
dataset:
type: arguana
name: MTEB ArguAna
config: default
split: test
revision: None
metrics:
- type: map_at_1
value: 39.972
- type: map_at_10
value: 54.874
- type: map_at_100
value: 55.53399999999999
- type: map_at_1000
value: 55.539
- type: map_at_3
value: 51.031000000000006
- type: map_at_5
value: 53.342999999999996
- type: mrr_at_1
value: 40.541
- type: mrr_at_10
value: 55.096000000000004
- type: mrr_at_100
value: 55.75599999999999
- type: mrr_at_1000
value: 55.761
- type: mrr_at_3
value: 51.221000000000004
- type: mrr_at_5
value: 53.568000000000005
- type: ndcg_at_1
value: 39.972
- type: ndcg_at_10
value: 62.456999999999994
- type: ndcg_at_100
value: 65.262
- type: ndcg_at_1000
value: 65.389
- type: ndcg_at_3
value: 54.673
- type: ndcg_at_5
value: 58.80499999999999
- type: precision_at_1
value: 39.972
- type: precision_at_10
value: 8.634
- type: precision_at_100
value: 0.9860000000000001
- type: precision_at_1000
value: 0.1
- type: precision_at_3
value: 21.740000000000002
- type: precision_at_5
value: 15.036
- type: recall_at_1
value: 39.972
- type: recall_at_10
value: 86.344
- type: recall_at_100
value: 98.578
- type: recall_at_1000
value: 99.57300000000001
- type: recall_at_3
value: 65.22
- type: recall_at_5
value: 75.178
- task:
type: Clustering
dataset:
type: mteb/arxiv-clustering-p2p
name: MTEB ArxivClusteringP2P
config: default
split: test
revision: a122ad7f3f0291bf49cc6f4d32aa80929df69d5d
metrics:
- type: v_measure
value: 48.94652870403906
- task:
type: Clustering
dataset:
type: mteb/arxiv-clustering-s2s
name: MTEB ArxivClusteringS2S
config: default
split: test
revision: f910caf1a6075f7329cdf8c1a6135696f37dbd53
metrics:
- type: v_measure
value: 43.17257160340209
- task:
type: Reranking
dataset:
type: mteb/askubuntudupquestions-reranking
name: MTEB AskUbuntuDupQuestions
config: default
split: test
revision: 2000358ca161889fa9c082cb41daa8dcfb161a54
metrics:
- type: map
value: 63.97867370559182
- type: mrr
value: 77.00820032537484
- task:
type: STS
dataset:
type: mteb/biosses-sts
name: MTEB BIOSSES
config: default
split: test
revision: d3fb88f8f02e40887cd149695127462bbcf29b4a
metrics:
- type: cos_sim_pearson
value: 80.00986015960616
- type: cos_sim_spearman
value: 80.36387933827882
- type: euclidean_pearson
value: 80.32305287257296
- type: euclidean_spearman
value: 82.0524720308763
- type: manhattan_pearson
value: 80.19847473906454
- type: manhattan_spearman
value: 81.87957652506985
- task:
type: Classification
dataset:
type: mteb/banking77
name: MTEB Banking77Classification
config: default
split: test
revision: 0fd18e25b25c072e09e0d92ab615fda904d66300
metrics:
- type: accuracy
value: 88.00000000000001
- type: f1
value: 87.99039027511853
- task:
type: Clustering
dataset:
type: mteb/biorxiv-clustering-p2p
name: MTEB BiorxivClusteringP2P
config: default
split: test
revision: 65b79d1d13f80053f67aca9498d9402c2d9f1f40
metrics:
- type: v_measure
value: 41.36932844640705
- task:
type: Clustering
dataset:
type: mteb/biorxiv-clustering-s2s
name: MTEB BiorxivClusteringS2S
config: default
split: test
revision: 258694dd0231531bc1fd9de6ceb52a0853c6d908
metrics:
- type: v_measure
value: 38.34983239611985
- task:
type: Retrieval
dataset:
type: BeIR/cqadupstack
name: MTEB CQADupstackAndroidRetrieval
config: default
split: test
revision: None
metrics:
- type: map_at_1
value: 32.257999999999996
- type: map_at_10
value: 42.937
- type: map_at_100
value: 44.406
- type: map_at_1000
value: 44.536
- type: map_at_3
value: 39.22
- type: map_at_5
value: 41.458
- type: mrr_at_1
value: 38.769999999999996
- type: mrr_at_10
value: 48.701
- type: mrr_at_100
value: 49.431000000000004
- type: mrr_at_1000
value: 49.476
- type: mrr_at_3
value: 45.875
- type: mrr_at_5
value: 47.67
- type: ndcg_at_1
value: 38.769999999999996
- type: ndcg_at_10
value: 49.35
- type: ndcg_at_100
value: 54.618
- type: ndcg_at_1000
value: 56.655
- type: ndcg_at_3
value: 43.826
- type: ndcg_at_5
value: 46.72
- type: precision_at_1
value: 38.769999999999996
- type: precision_at_10
value: 9.328
- type: precision_at_100
value: 1.484
- type: precision_at_1000
value: 0.196
- type: precision_at_3
value: 20.649
- type: precision_at_5
value: 15.25
- type: recall_at_1
value: 32.257999999999996
- type: recall_at_10
value: 61.849
- type: recall_at_100
value: 83.70400000000001
- type: recall_at_1000
value: 96.344
- type: recall_at_3
value: 46.037
- type: recall_at_5
value: 53.724000000000004
- task:
type: Retrieval
dataset:
type: BeIR/cqadupstack
name: MTEB CQADupstackEnglishRetrieval
config: default
split: test
revision: None
metrics:
- type: map_at_1
value: 32.979
- type: map_at_10
value: 43.376999999999995
- type: map_at_100
value: 44.667
- type: map_at_1000
value: 44.794
- type: map_at_3
value: 40.461999999999996
- type: map_at_5
value: 42.138
- type: mrr_at_1
value: 41.146
- type: mrr_at_10
value: 49.575
- type: mrr_at_100
value: 50.187000000000005
- type: mrr_at_1000
value: 50.231
- type: mrr_at_3
value: 47.601
- type: mrr_at_5
value: 48.786
- type: ndcg_at_1
value: 41.146
- type: ndcg_at_10
value: 48.957
- type: ndcg_at_100
value: 53.296
- type: ndcg_at_1000
value: 55.254000000000005
- type: ndcg_at_3
value: 45.235
- type: ndcg_at_5
value: 47.014
- type: precision_at_1
value: 41.146
- type: precision_at_10
value: 9.107999999999999
- type: precision_at_100
value: 1.481
- type: precision_at_1000
value: 0.193
- type: precision_at_3
value: 21.783
- type: precision_at_5
value: 15.274
- type: recall_at_1
value: 32.979
- type: recall_at_10
value: 58.167
- type: recall_at_100
value: 76.374
- type: recall_at_1000
value: 88.836
- type: recall_at_3
value: 46.838
- type: recall_at_5
value: 52.006
- task:
type: Retrieval
dataset:
type: BeIR/cqadupstack
name: MTEB CQADupstackGamingRetrieval
config: default
split: test
revision: None
metrics:
- type: map_at_1
value: 40.326
- type: map_at_10
value: 53.468
- type: map_at_100
value: 54.454
- type: map_at_1000
value: 54.508
- type: map_at_3
value: 50.12799999999999
- type: map_at_5
value: 51.991
- type: mrr_at_1
value: 46.394999999999996
- type: mrr_at_10
value: 57.016999999999996
- type: mrr_at_100
value: 57.67099999999999
- type: mrr_at_1000
value: 57.699999999999996
- type: mrr_at_3
value: 54.65
- type: mrr_at_5
value: 56.101
- type: ndcg_at_1
value: 46.394999999999996
- type: ndcg_at_10
value: 59.507
- type: ndcg_at_100
value: 63.31099999999999
- type: ndcg_at_1000
value: 64.388
- type: ndcg_at_3
value: 54.04600000000001
- type: ndcg_at_5
value: 56.723
- type: precision_at_1
value: 46.394999999999996
- type: precision_at_10
value: 9.567
- type: precision_at_100
value: 1.234
- type: precision_at_1000
value: 0.13699999999999998
- type: precision_at_3
value: 24.117
- type: precision_at_5
value: 16.426
- type: recall_at_1
value: 40.326
- type: recall_at_10
value: 73.763
- type: recall_at_100
value: 89.927
- type: recall_at_1000
value: 97.509
- type: recall_at_3
value: 59.34
- type: recall_at_5
value: 65.915
- task:
type: Retrieval
dataset:
type: BeIR/cqadupstack
name: MTEB CQADupstackGisRetrieval
config: default
split: test
revision: None
metrics:
- type: map_at_1
value: 26.661
- type: map_at_10
value: 35.522
- type: map_at_100
value: 36.619
- type: map_at_1000
value: 36.693999999999996
- type: map_at_3
value: 33.154
- type: map_at_5
value: 34.353
- type: mrr_at_1
value: 28.362
- type: mrr_at_10
value: 37.403999999999996
- type: mrr_at_100
value: 38.374
- type: mrr_at_1000
value: 38.428000000000004
- type: mrr_at_3
value: 35.235
- type: mrr_at_5
value: 36.269
- type: ndcg_at_1
value: 28.362
- type: ndcg_at_10
value: 40.431
- type: ndcg_at_100
value: 45.745999999999995
- type: ndcg_at_1000
value: 47.493
- type: ndcg_at_3
value: 35.733
- type: ndcg_at_5
value: 37.722
- type: precision_at_1
value: 28.362
- type: precision_at_10
value: 6.101999999999999
- type: precision_at_100
value: 0.922
- type: precision_at_1000
value: 0.11100000000000002
- type: precision_at_3
value: 15.140999999999998
- type: precision_at_5
value: 10.305
- type: recall_at_1
value: 26.661
- type: recall_at_10
value: 53.675
- type: recall_at_100
value: 77.891
- type: recall_at_1000
value: 90.72
- type: recall_at_3
value: 40.751
- type: recall_at_5
value: 45.517
- task:
type: Retrieval
dataset:
type: BeIR/cqadupstack
name: MTEB CQADupstackMathematicaRetrieval
config: default
split: test
revision: None
metrics:
- type: map_at_1
value: 18.886
- type: map_at_10
value: 27.288
- type: map_at_100
value: 28.327999999999996
- type: map_at_1000
value: 28.438999999999997
- type: map_at_3
value: 24.453
- type: map_at_5
value: 25.959
- type: mrr_at_1
value: 23.134
- type: mrr_at_10
value: 32.004
- type: mrr_at_100
value: 32.789
- type: mrr_at_1000
value: 32.857
- type: mrr_at_3
value: 29.084
- type: mrr_at_5
value: 30.614
- type: ndcg_at_1
value: 23.134
- type: ndcg_at_10
value: 32.852
- type: ndcg_at_100
value: 37.972
- type: ndcg_at_1000
value: 40.656
- type: ndcg_at_3
value: 27.435
- type: ndcg_at_5
value: 29.823
- type: precision_at_1
value: 23.134
- type: precision_at_10
value: 6.032
- type: precision_at_100
value: 0.9950000000000001
- type: precision_at_1000
value: 0.136
- type: precision_at_3
value: 13.017999999999999
- type: precision_at_5
value: 9.501999999999999
- type: recall_at_1
value: 18.886
- type: recall_at_10
value: 45.34
- type: recall_at_100
value: 67.947
- type: recall_at_1000
value: 86.924
- type: recall_at_3
value: 30.535
- type: recall_at_5
value: 36.451
- task:
type: Retrieval
dataset:
type: BeIR/cqadupstack
name: MTEB CQADupstackPhysicsRetrieval
config: default
split: test
revision: None
metrics:
- type: map_at_1
value: 28.994999999999997
- type: map_at_10
value: 40.04
- type: map_at_100
value: 41.435
- type: map_at_1000
value: 41.537
- type: map_at_3
value: 37.091
- type: map_at_5
value: 38.802
- type: mrr_at_1
value: 35.034
- type: mrr_at_10
value: 45.411
- type: mrr_at_100
value: 46.226
- type: mrr_at_1000
value: 46.27
- type: mrr_at_3
value: 43.086
- type: mrr_at_5
value: 44.452999999999996
- type: ndcg_at_1
value: 35.034
- type: ndcg_at_10
value: 46.076
- type: ndcg_at_100
value: 51.483000000000004
- type: ndcg_at_1000
value: 53.433
- type: ndcg_at_3
value: 41.304
- type: ndcg_at_5
value: 43.641999999999996
- type: precision_at_1
value: 35.034
- type: precision_at_10
value: 8.258000000000001
- type: precision_at_100
value: 1.268
- type: precision_at_1000
value: 0.161
- type: precision_at_3
value: 19.57
- type: precision_at_5
value: 13.782
- type: recall_at_1
value: 28.994999999999997
- type: recall_at_10
value: 58.538000000000004
- type: recall_at_100
value: 80.72399999999999
- type: recall_at_1000
value: 93.462
- type: recall_at_3
value: 45.199
- type: recall_at_5
value: 51.237
- task:
type: Retrieval
dataset:
type: BeIR/cqadupstack
name: MTEB CQADupstackProgrammersRetrieval
config: default
split: test
revision: None
metrics:
- type: map_at_1
value: 24.795
- type: map_at_10
value: 34.935
- type: map_at_100
value: 36.306
- type: map_at_1000
value: 36.417
- type: map_at_3
value: 31.831
- type: map_at_5
value: 33.626
- type: mrr_at_1
value: 30.479
- type: mrr_at_10
value: 40.225
- type: mrr_at_100
value: 41.055
- type: mrr_at_1000
value: 41.114
- type: mrr_at_3
value: 37.538
- type: mrr_at_5
value: 39.073
- type: ndcg_at_1
value: 30.479
- type: ndcg_at_10
value: 40.949999999999996
- type: ndcg_at_100
value: 46.525
- type: ndcg_at_1000
value: 48.892
- type: ndcg_at_3
value: 35.79
- type: ndcg_at_5
value: 38.237
- type: precision_at_1
value: 30.479
- type: precision_at_10
value: 7.6259999999999994
- type: precision_at_100
value: 1.203
- type: precision_at_1000
value: 0.157
- type: precision_at_3
value: 17.199
- type: precision_at_5
value: 12.466000000000001
- type: recall_at_1
value: 24.795
- type: recall_at_10
value: 53.421
- type: recall_at_100
value: 77.189
- type: recall_at_1000
value: 93.407
- type: recall_at_3
value: 39.051
- type: recall_at_5
value: 45.462
- task:
type: Retrieval
dataset:
type: BeIR/cqadupstack
name: MTEB CQADupstackRetrieval
config: default
split: test
revision: None
metrics:
- type: map_at_1
value: 26.853499999999997
- type: map_at_10
value: 36.20433333333333
- type: map_at_100
value: 37.40391666666667
- type: map_at_1000
value: 37.515
- type: map_at_3
value: 33.39975
- type: map_at_5
value: 34.9665
- type: mrr_at_1
value: 31.62666666666667
- type: mrr_at_10
value: 40.436749999999996
- type: mrr_at_100
value: 41.260333333333335
- type: mrr_at_1000
value: 41.31525
- type: mrr_at_3
value: 38.06733333333332
- type: mrr_at_5
value: 39.41541666666667
- type: ndcg_at_1
value: 31.62666666666667
- type: ndcg_at_10
value: 41.63341666666667
- type: ndcg_at_100
value: 46.704166666666666
- type: ndcg_at_1000
value: 48.88483333333335
- type: ndcg_at_3
value: 36.896
- type: ndcg_at_5
value: 39.11891666666667
- type: precision_at_1
value: 31.62666666666667
- type: precision_at_10
value: 7.241083333333333
- type: precision_at_100
value: 1.1488333333333334
- type: precision_at_1000
value: 0.15250000000000002
- type: precision_at_3
value: 16.908333333333335
- type: precision_at_5
value: 11.942833333333333
- type: recall_at_1
value: 26.853499999999997
- type: recall_at_10
value: 53.461333333333336
- type: recall_at_100
value: 75.63633333333333
- type: recall_at_1000
value: 90.67016666666666
- type: recall_at_3
value: 40.24241666666667
- type: recall_at_5
value: 45.98608333333333
- task:
type: Retrieval
dataset:
type: BeIR/cqadupstack
name: MTEB CQADupstackStatsRetrieval
config: default
split: test
revision: None
metrics:
- type: map_at_1
value: 25.241999999999997
- type: map_at_10
value: 31.863999999999997
- type: map_at_100
value: 32.835
- type: map_at_1000
value: 32.928000000000004
- type: map_at_3
value: 29.694
- type: map_at_5
value: 30.978
- type: mrr_at_1
value: 28.374
- type: mrr_at_10
value: 34.814
- type: mrr_at_100
value: 35.596
- type: mrr_at_1000
value: 35.666
- type: mrr_at_3
value: 32.745000000000005
- type: mrr_at_5
value: 34.049
- type: ndcg_at_1
value: 28.374
- type: ndcg_at_10
value: 35.969
- type: ndcg_at_100
value: 40.708
- type: ndcg_at_1000
value: 43.08
- type: ndcg_at_3
value: 31.968999999999998
- type: ndcg_at_5
value: 34.069
- type: precision_at_1
value: 28.374
- type: precision_at_10
value: 5.583
- type: precision_at_100
value: 0.8630000000000001
- type: precision_at_1000
value: 0.11299999999999999
- type: precision_at_3
value: 13.547999999999998
- type: precision_at_5
value: 9.447999999999999
- type: recall_at_1
value: 25.241999999999997
- type: recall_at_10
value: 45.711
- type: recall_at_100
value: 67.482
- type: recall_at_1000
value: 85.13300000000001
- type: recall_at_3
value: 34.622
- type: recall_at_5
value: 40.043
- task:
type: Retrieval
dataset:
type: BeIR/cqadupstack
name: MTEB CQADupstackTexRetrieval
config: default
split: test
revision: None
metrics:
- type: map_at_1
value: 17.488999999999997
- type: map_at_10
value: 25.142999999999997
- type: map_at_100
value: 26.244
- type: map_at_1000
value: 26.363999999999997
- type: map_at_3
value: 22.654
- type: map_at_5
value: 24.017
- type: mrr_at_1
value: 21.198
- type: mrr_at_10
value: 28.903000000000002
- type: mrr_at_100
value: 29.860999999999997
- type: mrr_at_1000
value: 29.934
- type: mrr_at_3
value: 26.634999999999998
- type: mrr_at_5
value: 27.903
- type: ndcg_at_1
value: 21.198
- type: ndcg_at_10
value: 29.982999999999997
- type: ndcg_at_100
value: 35.275
- type: ndcg_at_1000
value: 38.074000000000005
- type: ndcg_at_3
value: 25.502999999999997
- type: ndcg_at_5
value: 27.557
- type: precision_at_1
value: 21.198
- type: precision_at_10
value: 5.502
- type: precision_at_100
value: 0.942
- type: precision_at_1000
value: 0.136
- type: precision_at_3
value: 12.044
- type: precision_at_5
value: 8.782
- type: recall_at_1
value: 17.488999999999997
- type: recall_at_10
value: 40.821000000000005
- type: recall_at_100
value: 64.567
- type: recall_at_1000
value: 84.452
- type: recall_at_3
value: 28.351
- type: recall_at_5
value: 33.645
- task:
type: Retrieval
dataset:
type: BeIR/cqadupstack
name: MTEB CQADupstackUnixRetrieval
config: default
split: test
revision: None
metrics:
- type: map_at_1
value: 27.066000000000003
- type: map_at_10
value: 36.134
- type: map_at_100
value: 37.285000000000004
- type: map_at_1000
value: 37.389
- type: map_at_3
value: 33.522999999999996
- type: map_at_5
value: 34.905
- type: mrr_at_1
value: 31.436999999999998
- type: mrr_at_10
value: 40.225
- type: mrr_at_100
value: 41.079
- type: mrr_at_1000
value: 41.138000000000005
- type: mrr_at_3
value: 38.074999999999996
- type: mrr_at_5
value: 39.190000000000005
- type: ndcg_at_1
value: 31.436999999999998
- type: ndcg_at_10
value: 41.494
- type: ndcg_at_100
value: 46.678999999999995
- type: ndcg_at_1000
value: 48.964
- type: ndcg_at_3
value: 36.828
- type: ndcg_at_5
value: 38.789
- type: precision_at_1
value: 31.436999999999998
- type: precision_at_10
value: 6.931
- type: precision_at_100
value: 1.072
- type: precision_at_1000
value: 0.13799999999999998
- type: precision_at_3
value: 16.729
- type: precision_at_5
value: 11.567
- type: recall_at_1
value: 27.066000000000003
- type: recall_at_10
value: 53.705000000000005
- type: recall_at_100
value: 75.968
- type: recall_at_1000
value: 91.937
- type: recall_at_3
value: 40.865
- type: recall_at_5
value: 45.739999999999995
- task:
type: Retrieval
dataset:
type: BeIR/cqadupstack
name: MTEB CQADupstackWebmastersRetrieval
config: default
split: test
revision: None
metrics:
- type: map_at_1
value: 24.979000000000003
- type: map_at_10
value: 32.799
- type: map_at_100
value: 34.508
- type: map_at_1000
value: 34.719
- type: map_at_3
value: 29.947000000000003
- type: map_at_5
value: 31.584
- type: mrr_at_1
value: 30.237000000000002
- type: mrr_at_10
value: 37.651
- type: mrr_at_100
value: 38.805
- type: mrr_at_1000
value: 38.851
- type: mrr_at_3
value: 35.046
- type: mrr_at_5
value: 36.548
- type: ndcg_at_1
value: 30.237000000000002
- type: ndcg_at_10
value: 38.356
- type: ndcg_at_100
value: 44.906
- type: ndcg_at_1000
value: 47.299
- type: ndcg_at_3
value: 33.717999999999996
- type: ndcg_at_5
value: 35.946
- type: precision_at_1
value: 30.237000000000002
- type: precision_at_10
value: 7.292
- type: precision_at_100
value: 1.496
- type: precision_at_1000
value: 0.23600000000000002
- type: precision_at_3
value: 15.547
- type: precision_at_5
value: 11.344
- type: recall_at_1
value: 24.979000000000003
- type: recall_at_10
value: 48.624
- type: recall_at_100
value: 77.932
- type: recall_at_1000
value: 92.66499999999999
- type: recall_at_3
value: 35.217
- type: recall_at_5
value: 41.394
- task:
type: Retrieval
dataset:
type: BeIR/cqadupstack
name: MTEB CQADupstackWordpressRetrieval
config: default
split: test
revision: None
metrics:
- type: map_at_1
value: 22.566
- type: map_at_10
value: 30.945
- type: map_at_100
value: 31.759999999999998
- type: map_at_1000
value: 31.855
- type: map_at_3
value: 28.64
- type: map_at_5
value: 29.787000000000003
- type: mrr_at_1
value: 24.954
- type: mrr_at_10
value: 33.311
- type: mrr_at_100
value: 34.050000000000004
- type: mrr_at_1000
value: 34.117999999999995
- type: mrr_at_3
value: 31.238
- type: mrr_at_5
value: 32.329
- type: ndcg_at_1
value: 24.954
- type: ndcg_at_10
value: 35.676
- type: ndcg_at_100
value: 39.931
- type: ndcg_at_1000
value: 42.43
- type: ndcg_at_3
value: 31.365
- type: ndcg_at_5
value: 33.184999999999995
- type: precision_at_1
value: 24.954
- type: precision_at_10
value: 5.564
- type: precision_at_100
value: 0.826
- type: precision_at_1000
value: 0.116
- type: precision_at_3
value: 13.555
- type: precision_at_5
value: 9.168
- type: recall_at_1
value: 22.566
- type: recall_at_10
value: 47.922
- type: recall_at_100
value: 67.931
- type: recall_at_1000
value: 86.653
- type: recall_at_3
value: 36.103
- type: recall_at_5
value: 40.699000000000005
- task:
type: Retrieval
dataset:
type: climate-fever
name: MTEB ClimateFEVER
config: default
split: test
revision: None
metrics:
- type: map_at_1
value: 16.950000000000003
- type: map_at_10
value: 28.612
- type: map_at_100
value: 30.476999999999997
- type: map_at_1000
value: 30.674
- type: map_at_3
value: 24.262
- type: map_at_5
value: 26.554
- type: mrr_at_1
value: 38.241
- type: mrr_at_10
value: 50.43
- type: mrr_at_100
value: 51.059
- type: mrr_at_1000
value: 51.090999999999994
- type: mrr_at_3
value: 47.514
- type: mrr_at_5
value: 49.246
- type: ndcg_at_1
value: 38.241
- type: ndcg_at_10
value: 38.218
- type: ndcg_at_100
value: 45.003
- type: ndcg_at_1000
value: 48.269
- type: ndcg_at_3
value: 32.568000000000005
- type: ndcg_at_5
value: 34.400999999999996
- type: precision_at_1
value: 38.241
- type: precision_at_10
value: 11.674
- type: precision_at_100
value: 1.913
- type: precision_at_1000
value: 0.252
- type: precision_at_3
value: 24.387
- type: precision_at_5
value: 18.163
- type: recall_at_1
value: 16.950000000000003
- type: recall_at_10
value: 43.769000000000005
- type: recall_at_100
value: 66.875
- type: recall_at_1000
value: 84.92699999999999
- type: recall_at_3
value: 29.353
- type: recall_at_5
value: 35.467
- task:
type: Retrieval
dataset:
type: dbpedia-entity
name: MTEB DBPedia
config: default
split: test
revision: None
metrics:
- type: map_at_1
value: 9.276
- type: map_at_10
value: 20.848
- type: map_at_100
value: 29.804000000000002
- type: map_at_1000
value: 31.398
- type: map_at_3
value: 14.886
- type: map_at_5
value: 17.516000000000002
- type: mrr_at_1
value: 71
- type: mrr_at_10
value: 78.724
- type: mrr_at_100
value: 78.976
- type: mrr_at_1000
value: 78.986
- type: mrr_at_3
value: 77.333
- type: mrr_at_5
value: 78.021
- type: ndcg_at_1
value: 57.875
- type: ndcg_at_10
value: 43.855
- type: ndcg_at_100
value: 48.99
- type: ndcg_at_1000
value: 56.141
- type: ndcg_at_3
value: 48.914
- type: ndcg_at_5
value: 45.961
- type: precision_at_1
value: 71
- type: precision_at_10
value: 34.575
- type: precision_at_100
value: 11.182
- type: precision_at_1000
value: 2.044
- type: precision_at_3
value: 52.5
- type: precision_at_5
value: 44.2
- type: recall_at_1
value: 9.276
- type: recall_at_10
value: 26.501
- type: recall_at_100
value: 55.72899999999999
- type: recall_at_1000
value: 78.532
- type: recall_at_3
value: 16.365
- type: recall_at_5
value: 20.154
- task:
type: Classification
dataset:
type: mteb/emotion
name: MTEB EmotionClassification
config: default
split: test
revision: 4f58c6b202a23cf9a4da393831edf4f9183cad37
metrics:
- type: accuracy
value: 52.71
- type: f1
value: 47.74801556489574
- task:
type: Retrieval
dataset:
type: fever
name: MTEB FEVER
config: default
split: test
revision: None
metrics:
- type: map_at_1
value: 73.405
- type: map_at_10
value: 82.822
- type: map_at_100
value: 83.042
- type: map_at_1000
value: 83.055
- type: map_at_3
value: 81.65299999999999
- type: map_at_5
value: 82.431
- type: mrr_at_1
value: 79.178
- type: mrr_at_10
value: 87.02
- type: mrr_at_100
value: 87.095
- type: mrr_at_1000
value: 87.09700000000001
- type: mrr_at_3
value: 86.309
- type: mrr_at_5
value: 86.824
- type: ndcg_at_1
value: 79.178
- type: ndcg_at_10
value: 86.72
- type: ndcg_at_100
value: 87.457
- type: ndcg_at_1000
value: 87.691
- type: ndcg_at_3
value: 84.974
- type: ndcg_at_5
value: 86.032
- type: precision_at_1
value: 79.178
- type: precision_at_10
value: 10.548
- type: precision_at_100
value: 1.113
- type: precision_at_1000
value: 0.11499999999999999
- type: precision_at_3
value: 32.848
- type: precision_at_5
value: 20.45
- type: recall_at_1
value: 73.405
- type: recall_at_10
value: 94.39699999999999
- type: recall_at_100
value: 97.219
- type: recall_at_1000
value: 98.675
- type: recall_at_3
value: 89.679
- type: recall_at_5
value: 92.392
- task:
type: Retrieval
dataset:
type: fiqa
name: MTEB FiQA2018
config: default
split: test
revision: None
metrics:
- type: map_at_1
value: 22.651
- type: map_at_10
value: 36.886
- type: map_at_100
value: 38.811
- type: map_at_1000
value: 38.981
- type: map_at_3
value: 32.538
- type: map_at_5
value: 34.763
- type: mrr_at_1
value: 44.444
- type: mrr_at_10
value: 53.168000000000006
- type: mrr_at_100
value: 53.839000000000006
- type: mrr_at_1000
value: 53.869
- type: mrr_at_3
value: 50.54
- type: mrr_at_5
value: 52.068000000000005
- type: ndcg_at_1
value: 44.444
- type: ndcg_at_10
value: 44.994
- type: ndcg_at_100
value: 51.599
- type: ndcg_at_1000
value: 54.339999999999996
- type: ndcg_at_3
value: 41.372
- type: ndcg_at_5
value: 42.149
- type: precision_at_1
value: 44.444
- type: precision_at_10
value: 12.407
- type: precision_at_100
value: 1.9269999999999998
- type: precision_at_1000
value: 0.242
- type: precision_at_3
value: 27.726
- type: precision_at_5
value: 19.814999999999998
- type: recall_at_1
value: 22.651
- type: recall_at_10
value: 52.075
- type: recall_at_100
value: 76.51400000000001
- type: recall_at_1000
value: 92.852
- type: recall_at_3
value: 37.236000000000004
- type: recall_at_5
value: 43.175999999999995
- task:
type: Retrieval
dataset:
type: hotpotqa
name: MTEB HotpotQA
config: default
split: test
revision: None
metrics:
- type: map_at_1
value: 40.777
- type: map_at_10
value: 66.79899999999999
- type: map_at_100
value: 67.65299999999999
- type: map_at_1000
value: 67.706
- type: map_at_3
value: 63.352
- type: map_at_5
value: 65.52900000000001
- type: mrr_at_1
value: 81.553
- type: mrr_at_10
value: 86.983
- type: mrr_at_100
value: 87.132
- type: mrr_at_1000
value: 87.136
- type: mrr_at_3
value: 86.156
- type: mrr_at_5
value: 86.726
- type: ndcg_at_1
value: 81.553
- type: ndcg_at_10
value: 74.64
- type: ndcg_at_100
value: 77.459
- type: ndcg_at_1000
value: 78.43
- type: ndcg_at_3
value: 69.878
- type: ndcg_at_5
value: 72.59400000000001
- type: precision_at_1
value: 81.553
- type: precision_at_10
value: 15.654000000000002
- type: precision_at_100
value: 1.783
- type: precision_at_1000
value: 0.191
- type: precision_at_3
value: 45.199
- type: precision_at_5
value: 29.267
- type: recall_at_1
value: 40.777
- type: recall_at_10
value: 78.271
- type: recall_at_100
value: 89.129
- type: recall_at_1000
value: 95.49
- type: recall_at_3
value: 67.79899999999999
- type: recall_at_5
value: 73.167
- task:
type: Classification
dataset:
type: mteb/imdb
name: MTEB ImdbClassification
config: default
split: test
revision: 3d86128a09e091d6018b6d26cad27f2739fc2db7
metrics:
- type: accuracy
value: 93.5064
- type: ap
value: 90.25495114444111
- type: f1
value: 93.5012434973381
- task:
type: Retrieval
dataset:
type: msmarco
name: MTEB MSMARCO
config: default
split: dev
revision: None
metrics:
- type: map_at_1
value: 23.301
- type: map_at_10
value: 35.657
- type: map_at_100
value: 36.797000000000004
- type: map_at_1000
value: 36.844
- type: map_at_3
value: 31.743
- type: map_at_5
value: 34.003
- type: mrr_at_1
value: 23.854
- type: mrr_at_10
value: 36.242999999999995
- type: mrr_at_100
value: 37.32
- type: mrr_at_1000
value: 37.361
- type: mrr_at_3
value: 32.4
- type: mrr_at_5
value: 34.634
- type: ndcg_at_1
value: 23.868000000000002
- type: ndcg_at_10
value: 42.589
- type: ndcg_at_100
value: 48.031
- type: ndcg_at_1000
value: 49.189
- type: ndcg_at_3
value: 34.649
- type: ndcg_at_5
value: 38.676
- type: precision_at_1
value: 23.868000000000002
- type: precision_at_10
value: 6.6850000000000005
- type: precision_at_100
value: 0.9400000000000001
- type: precision_at_1000
value: 0.104
- type: precision_at_3
value: 14.651
- type: precision_at_5
value: 10.834000000000001
- type: recall_at_1
value: 23.301
- type: recall_at_10
value: 63.88700000000001
- type: recall_at_100
value: 88.947
- type: recall_at_1000
value: 97.783
- type: recall_at_3
value: 42.393
- type: recall_at_5
value: 52.036
- task:
type: Classification
dataset:
type: mteb/mtop_domain
name: MTEB MTOPDomainClassification (en)
config: en
split: test
revision: d80d48c1eb48d3562165c59d59d0034df9fff0bf
metrics:
- type: accuracy
value: 94.64888280893753
- type: f1
value: 94.41310774203512
- task:
type: Classification
dataset:
type: mteb/mtop_intent
name: MTEB MTOPIntentClassification (en)
config: en
split: test
revision: ae001d0e6b1228650b7bd1c2c65fb50ad11a8aba
metrics:
- type: accuracy
value: 79.72184222526221
- type: f1
value: 61.522034067350106
- task:
type: Classification
dataset:
type: mteb/amazon_massive_intent
name: MTEB MassiveIntentClassification (en)
config: en
split: test
revision: 31efe3c427b0bae9c22cbb560b8f15491cc6bed7
metrics:
- type: accuracy
value: 79.60659045057163
- type: f1
value: 77.268649687049
- task:
type: Classification
dataset:
type: mteb/amazon_massive_scenario
name: MTEB MassiveScenarioClassification (en)
config: en
split: test
revision: 7d571f92784cd94a019292a1f45445077d0ef634
metrics:
- type: accuracy
value: 81.83254875588432
- type: f1
value: 81.61520635919082
- task:
type: Clustering
dataset:
type: mteb/medrxiv-clustering-p2p
name: MTEB MedrxivClusteringP2P
config: default
split: test
revision: e7a26af6f3ae46b30dde8737f02c07b1505bcc73
metrics:
- type: v_measure
value: 36.31529875009507
- task:
type: Clustering
dataset:
type: mteb/medrxiv-clustering-s2s
name: MTEB MedrxivClusteringS2S
config: default
split: test
revision: 35191c8c0dca72d8ff3efcd72aa802307d469663
metrics:
- type: v_measure
value: 31.734233714415073
- task:
type: Reranking
dataset:
type: mteb/mind_small
name: MTEB MindSmallReranking
config: default
split: test
revision: 3bdac13927fdc888b903db93b2ffdbd90b295a69
metrics:
- type: map
value: 30.994501713009452
- type: mrr
value: 32.13512850703073
- task:
type: Retrieval
dataset:
type: nfcorpus
name: MTEB NFCorpus
config: default
split: test
revision: None
metrics:
- type: map_at_1
value: 6.603000000000001
- type: map_at_10
value: 13.767999999999999
- type: map_at_100
value: 17.197000000000003
- type: map_at_1000
value: 18.615000000000002
- type: map_at_3
value: 10.567
- type: map_at_5
value: 12.078999999999999
- type: mrr_at_1
value: 44.891999999999996
- type: mrr_at_10
value: 53.75299999999999
- type: mrr_at_100
value: 54.35
- type: mrr_at_1000
value: 54.388000000000005
- type: mrr_at_3
value: 51.495999999999995
- type: mrr_at_5
value: 52.688
- type: ndcg_at_1
value: 43.189
- type: ndcg_at_10
value: 34.567
- type: ndcg_at_100
value: 32.273
- type: ndcg_at_1000
value: 41.321999999999996
- type: ndcg_at_3
value: 40.171
- type: ndcg_at_5
value: 37.502
- type: precision_at_1
value: 44.582
- type: precision_at_10
value: 25.139
- type: precision_at_100
value: 7.739999999999999
- type: precision_at_1000
value: 2.054
- type: precision_at_3
value: 37.152
- type: precision_at_5
value: 31.826999999999998
- type: recall_at_1
value: 6.603000000000001
- type: recall_at_10
value: 17.023
- type: recall_at_100
value: 32.914
- type: recall_at_1000
value: 64.44800000000001
- type: recall_at_3
value: 11.457
- type: recall_at_5
value: 13.816
- task:
type: Retrieval
dataset:
type: nq
name: MTEB NQ
config: default
split: test
revision: None
metrics:
- type: map_at_1
value: 30.026000000000003
- type: map_at_10
value: 45.429
- type: map_at_100
value: 46.45
- type: map_at_1000
value: 46.478
- type: map_at_3
value: 41.147
- type: map_at_5
value: 43.627
- type: mrr_at_1
value: 33.951
- type: mrr_at_10
value: 47.953
- type: mrr_at_100
value: 48.731
- type: mrr_at_1000
value: 48.751
- type: mrr_at_3
value: 44.39
- type: mrr_at_5
value: 46.533
- type: ndcg_at_1
value: 33.951
- type: ndcg_at_10
value: 53.24100000000001
- type: ndcg_at_100
value: 57.599999999999994
- type: ndcg_at_1000
value: 58.270999999999994
- type: ndcg_at_3
value: 45.190999999999995
- type: ndcg_at_5
value: 49.339
- type: precision_at_1
value: 33.951
- type: precision_at_10
value: 8.856
- type: precision_at_100
value: 1.133
- type: precision_at_1000
value: 0.12
- type: precision_at_3
value: 20.713
- type: precision_at_5
value: 14.838000000000001
- type: recall_at_1
value: 30.026000000000003
- type: recall_at_10
value: 74.512
- type: recall_at_100
value: 93.395
- type: recall_at_1000
value: 98.402
- type: recall_at_3
value: 53.677
- type: recall_at_5
value: 63.198
- task:
type: Retrieval
dataset:
type: quora
name: MTEB QuoraRetrieval
config: default
split: test
revision: None
metrics:
- type: map_at_1
value: 71.41300000000001
- type: map_at_10
value: 85.387
- type: map_at_100
value: 86.027
- type: map_at_1000
value: 86.041
- type: map_at_3
value: 82.543
- type: map_at_5
value: 84.304
- type: mrr_at_1
value: 82.35
- type: mrr_at_10
value: 88.248
- type: mrr_at_100
value: 88.348
- type: mrr_at_1000
value: 88.349
- type: mrr_at_3
value: 87.348
- type: mrr_at_5
value: 87.96300000000001
- type: ndcg_at_1
value: 82.37
- type: ndcg_at_10
value: 88.98
- type: ndcg_at_100
value: 90.16499999999999
- type: ndcg_at_1000
value: 90.239
- type: ndcg_at_3
value: 86.34100000000001
- type: ndcg_at_5
value: 87.761
- type: precision_at_1
value: 82.37
- type: precision_at_10
value: 13.471
- type: precision_at_100
value: 1.534
- type: precision_at_1000
value: 0.157
- type: precision_at_3
value: 37.827
- type: precision_at_5
value: 24.773999999999997
- type: recall_at_1
value: 71.41300000000001
- type: recall_at_10
value: 95.748
- type: recall_at_100
value: 99.69200000000001
- type: recall_at_1000
value: 99.98
- type: recall_at_3
value: 87.996
- type: recall_at_5
value: 92.142
- task:
type: Clustering
dataset:
type: mteb/reddit-clustering
name: MTEB RedditClustering
config: default
split: test
revision: 24640382cdbf8abc73003fb0fa6d111a705499eb
metrics:
- type: v_measure
value: 56.96878497780007
- task:
type: Clustering
dataset:
type: mteb/reddit-clustering-p2p
name: MTEB RedditClusteringP2P
config: default
split: test
revision: 282350215ef01743dc01b456c7f5241fa8937f16
metrics:
- type: v_measure
value: 65.31371347128074
- task:
type: Retrieval
dataset:
type: scidocs
name: MTEB SCIDOCS
config: default
split: test
revision: None
metrics:
- type: map_at_1
value: 5.287
- type: map_at_10
value: 13.530000000000001
- type: map_at_100
value: 15.891
- type: map_at_1000
value: 16.245
- type: map_at_3
value: 9.612
- type: map_at_5
value: 11.672
- type: mrr_at_1
value: 26
- type: mrr_at_10
value: 37.335
- type: mrr_at_100
value: 38.443
- type: mrr_at_1000
value: 38.486
- type: mrr_at_3
value: 33.783
- type: mrr_at_5
value: 36.028
- type: ndcg_at_1
value: 26
- type: ndcg_at_10
value: 22.215
- type: ndcg_at_100
value: 31.101
- type: ndcg_at_1000
value: 36.809
- type: ndcg_at_3
value: 21.104
- type: ndcg_at_5
value: 18.759999999999998
- type: precision_at_1
value: 26
- type: precision_at_10
value: 11.43
- type: precision_at_100
value: 2.424
- type: precision_at_1000
value: 0.379
- type: precision_at_3
value: 19.7
- type: precision_at_5
value: 16.619999999999997
- type: recall_at_1
value: 5.287
- type: recall_at_10
value: 23.18
- type: recall_at_100
value: 49.208
- type: recall_at_1000
value: 76.85300000000001
- type: recall_at_3
value: 11.991999999999999
- type: recall_at_5
value: 16.85
- task:
type: STS
dataset:
type: mteb/sickr-sts
name: MTEB SICK-R
config: default
split: test
revision: a6ea5a8cab320b040a23452cc28066d9beae2cee
metrics:
- type: cos_sim_pearson
value: 83.87834913790886
- type: cos_sim_spearman
value: 81.04583513112122
- type: euclidean_pearson
value: 81.20484174558065
- type: euclidean_spearman
value: 80.76430832561769
- type: manhattan_pearson
value: 81.21416730978615
- type: manhattan_spearman
value: 80.7797637394211
- task:
type: STS
dataset:
type: mteb/sts12-sts
name: MTEB STS12
config: default
split: test
revision: a0d554a64d88156834ff5ae9920b964011b16384
metrics:
- type: cos_sim_pearson
value: 86.56143998865157
- type: cos_sim_spearman
value: 79.75387012744471
- type: euclidean_pearson
value: 83.7877519997019
- type: euclidean_spearman
value: 79.90489748003296
- type: manhattan_pearson
value: 83.7540590666095
- type: manhattan_spearman
value: 79.86434577931573
- task:
type: STS
dataset:
type: mteb/sts13-sts
name: MTEB STS13
config: default
split: test
revision: 7e90230a92c190f1bf69ae9002b8cea547a64cca
metrics:
- type: cos_sim_pearson
value: 83.92102564177941
- type: cos_sim_spearman
value: 84.98234585939103
- type: euclidean_pearson
value: 84.47729567593696
- type: euclidean_spearman
value: 85.09490696194469
- type: manhattan_pearson
value: 84.38622951588229
- type: manhattan_spearman
value: 85.02507171545574
- task:
type: STS
dataset:
type: mteb/sts14-sts
name: MTEB STS14
config: default
split: test
revision: 6031580fec1f6af667f0bd2da0a551cf4f0b2375
metrics:
- type: cos_sim_pearson
value: 80.1891164763377
- type: cos_sim_spearman
value: 80.7997969966883
- type: euclidean_pearson
value: 80.48572256162396
- type: euclidean_spearman
value: 80.57851903536378
- type: manhattan_pearson
value: 80.4324819433651
- type: manhattan_spearman
value: 80.5074526239062
- task:
type: STS
dataset:
type: mteb/sts15-sts
name: MTEB STS15
config: default
split: test
revision: ae752c7c21bf194d8b67fd573edf7ae58183cbe3
metrics:
- type: cos_sim_pearson
value: 82.64319975116025
- type: cos_sim_spearman
value: 84.88671197763652
- type: euclidean_pearson
value: 84.74692193293231
- type: euclidean_spearman
value: 85.27151722073653
- type: manhattan_pearson
value: 84.72460516785438
- type: manhattan_spearman
value: 85.26518899786687
- task:
type: STS
dataset:
type: mteb/sts16-sts
name: MTEB STS16
config: default
split: test
revision: 4d8694f8f0e0100860b497b999b3dbed754a0513
metrics:
- type: cos_sim_pearson
value: 83.24687565822381
- type: cos_sim_spearman
value: 85.60418454111263
- type: euclidean_pearson
value: 84.85829740169851
- type: euclidean_spearman
value: 85.66378014138306
- type: manhattan_pearson
value: 84.84672408808835
- type: manhattan_spearman
value: 85.63331924364891
- task:
type: STS
dataset:
type: mteb/sts17-crosslingual-sts
name: MTEB STS17 (en-en)
config: en-en
split: test
revision: af5e6fb845001ecf41f4c1e033ce921939a2a68d
metrics:
- type: cos_sim_pearson
value: 84.87758895415485
- type: cos_sim_spearman
value: 85.8193745617297
- type: euclidean_pearson
value: 85.78719118848134
- type: euclidean_spearman
value: 84.35797575385688
- type: manhattan_pearson
value: 85.97919844815692
- type: manhattan_spearman
value: 84.58334745175151
- task:
type: STS
dataset:
type: mteb/sts22-crosslingual-sts
name: MTEB STS22 (en)
config: en
split: test
revision: 6d1ba47164174a496b7fa5d3569dae26a6813b80
metrics:
- type: cos_sim_pearson
value: 67.27076035963599
- type: cos_sim_spearman
value: 67.21433656439973
- type: euclidean_pearson
value: 68.07434078679324
- type: euclidean_spearman
value: 66.0249731719049
- type: manhattan_pearson
value: 67.95495198947476
- type: manhattan_spearman
value: 65.99893908331886
- task:
type: STS
dataset:
type: mteb/stsbenchmark-sts
name: MTEB STSBenchmark
config: default
split: test
revision: b0fddb56ed78048fa8b90373c8a3cfc37b684831
metrics:
- type: cos_sim_pearson
value: 82.22437747056817
- type: cos_sim_spearman
value: 85.0995685206174
- type: euclidean_pearson
value: 84.08616925603394
- type: euclidean_spearman
value: 84.89633925691658
- type: manhattan_pearson
value: 84.08332675923133
- type: manhattan_spearman
value: 84.8858228112915
- task:
type: Reranking
dataset:
type: mteb/scidocs-reranking
name: MTEB SciDocsRR
config: default
split: test
revision: d3c5e1fc0b855ab6097bf1cda04dd73947d7caab
metrics:
- type: map
value: 87.6909022589666
- type: mrr
value: 96.43341952165481
- task:
type: Retrieval
dataset:
type: scifact
name: MTEB SciFact
config: default
split: test
revision: None
metrics:
- type: map_at_1
value: 57.660999999999994
- type: map_at_10
value: 67.625
- type: map_at_100
value: 68.07600000000001
- type: map_at_1000
value: 68.10199999999999
- type: map_at_3
value: 64.50399999999999
- type: map_at_5
value: 66.281
- type: mrr_at_1
value: 61
- type: mrr_at_10
value: 68.953
- type: mrr_at_100
value: 69.327
- type: mrr_at_1000
value: 69.352
- type: mrr_at_3
value: 66.833
- type: mrr_at_5
value: 68.05
- type: ndcg_at_1
value: 61
- type: ndcg_at_10
value: 72.369
- type: ndcg_at_100
value: 74.237
- type: ndcg_at_1000
value: 74.939
- type: ndcg_at_3
value: 67.284
- type: ndcg_at_5
value: 69.72500000000001
- type: precision_at_1
value: 61
- type: precision_at_10
value: 9.733
- type: precision_at_100
value: 1.0670000000000002
- type: precision_at_1000
value: 0.11199999999999999
- type: precision_at_3
value: 26.222
- type: precision_at_5
value: 17.4
- type: recall_at_1
value: 57.660999999999994
- type: recall_at_10
value: 85.656
- type: recall_at_100
value: 93.833
- type: recall_at_1000
value: 99.333
- type: recall_at_3
value: 71.961
- type: recall_at_5
value: 78.094
- task:
type: PairClassification
dataset:
type: mteb/sprintduplicatequestions-pairclassification
name: MTEB SprintDuplicateQuestions
config: default
split: test
revision: d66bd1f72af766a5cc4b0ca5e00c162f89e8cc46
metrics:
- type: cos_sim_accuracy
value: 99.86930693069307
- type: cos_sim_ap
value: 96.76685487950894
- type: cos_sim_f1
value: 93.44587884806354
- type: cos_sim_precision
value: 92.80078895463511
- type: cos_sim_recall
value: 94.1
- type: dot_accuracy
value: 99.54356435643564
- type: dot_ap
value: 81.18659960405607
- type: dot_f1
value: 75.78008915304605
- type: dot_precision
value: 75.07360157016683
- type: dot_recall
value: 76.5
- type: euclidean_accuracy
value: 99.87326732673267
- type: euclidean_ap
value: 96.8102411908941
- type: euclidean_f1
value: 93.6127744510978
- type: euclidean_precision
value: 93.42629482071713
- type: euclidean_recall
value: 93.8
- type: manhattan_accuracy
value: 99.87425742574257
- type: manhattan_ap
value: 96.82857341435529
- type: manhattan_f1
value: 93.62129583124059
- type: manhattan_precision
value: 94.04641775983855
- type: manhattan_recall
value: 93.2
- type: max_accuracy
value: 99.87425742574257
- type: max_ap
value: 96.82857341435529
- type: max_f1
value: 93.62129583124059
- task:
type: Clustering
dataset:
type: mteb/stackexchange-clustering
name: MTEB StackExchangeClustering
config: default
split: test
revision: 6cbc1f7b2bc0622f2e39d2c77fa502909748c259
metrics:
- type: v_measure
value: 65.92560972698926
- task:
type: Clustering
dataset:
type: mteb/stackexchange-clustering-p2p
name: MTEB StackExchangeClusteringP2P
config: default
split: test
revision: 815ca46b2622cec33ccafc3735d572c266efdb44
metrics:
- type: v_measure
value: 34.92797240259008
- task:
type: Reranking
dataset:
type: mteb/stackoverflowdupquestions-reranking
name: MTEB StackOverflowDupQuestions
config: default
split: test
revision: e185fbe320c72810689fc5848eb6114e1ef5ec69
metrics:
- type: map
value: 55.244624045597654
- type: mrr
value: 56.185303666921314
- task:
type: Summarization
dataset:
type: mteb/summeval
name: MTEB SummEval
config: default
split: test
revision: cda12ad7615edc362dbf25a00fdd61d3b1eaf93c
metrics:
- type: cos_sim_pearson
value: 31.02491987312937
- type: cos_sim_spearman
value: 32.055592206679734
- type: dot_pearson
value: 24.731627575422557
- type: dot_spearman
value: 24.308029077069733
- task:
type: Retrieval
dataset:
type: trec-covid
name: MTEB TRECCOVID
config: default
split: test
revision: None
metrics:
- type: map_at_1
value: 0.231
- type: map_at_10
value: 1.899
- type: map_at_100
value: 9.498
- type: map_at_1000
value: 20.979999999999997
- type: map_at_3
value: 0.652
- type: map_at_5
value: 1.069
- type: mrr_at_1
value: 88
- type: mrr_at_10
value: 93.4
- type: mrr_at_100
value: 93.4
- type: mrr_at_1000
value: 93.4
- type: mrr_at_3
value: 93
- type: mrr_at_5
value: 93.4
- type: ndcg_at_1
value: 86
- type: ndcg_at_10
value: 75.375
- type: ndcg_at_100
value: 52.891999999999996
- type: ndcg_at_1000
value: 44.952999999999996
- type: ndcg_at_3
value: 81.05
- type: ndcg_at_5
value: 80.175
- type: precision_at_1
value: 88
- type: precision_at_10
value: 79
- type: precision_at_100
value: 53.16
- type: precision_at_1000
value: 19.408
- type: precision_at_3
value: 85.333
- type: precision_at_5
value: 84
- type: recall_at_1
value: 0.231
- type: recall_at_10
value: 2.078
- type: recall_at_100
value: 12.601
- type: recall_at_1000
value: 41.296
- type: recall_at_3
value: 0.6779999999999999
- type: recall_at_5
value: 1.1360000000000001
- task:
type: Retrieval
dataset:
type: webis-touche2020
name: MTEB Touche2020
config: default
split: test
revision: None
metrics:
- type: map_at_1
value: 2.782
- type: map_at_10
value: 10.204
- type: map_at_100
value: 16.176
- type: map_at_1000
value: 17.456
- type: map_at_3
value: 5.354
- type: map_at_5
value: 7.503
- type: mrr_at_1
value: 40.816
- type: mrr_at_10
value: 54.010000000000005
- type: mrr_at_100
value: 54.49
- type: mrr_at_1000
value: 54.49
- type: mrr_at_3
value: 48.980000000000004
- type: mrr_at_5
value: 51.735
- type: ndcg_at_1
value: 36.735
- type: ndcg_at_10
value: 26.61
- type: ndcg_at_100
value: 36.967
- type: ndcg_at_1000
value: 47.274
- type: ndcg_at_3
value: 30.363
- type: ndcg_at_5
value: 29.448999999999998
- type: precision_at_1
value: 40.816
- type: precision_at_10
value: 23.878
- type: precision_at_100
value: 7.693999999999999
- type: precision_at_1000
value: 1.4489999999999998
- type: precision_at_3
value: 31.293
- type: precision_at_5
value: 29.796
- type: recall_at_1
value: 2.782
- type: recall_at_10
value: 16.485
- type: recall_at_100
value: 46.924
- type: recall_at_1000
value: 79.365
- type: recall_at_3
value: 6.52
- type: recall_at_5
value: 10.48
- task:
type: Classification
dataset:
type: mteb/toxic_conversations_50k
name: MTEB ToxicConversationsClassification
config: default
split: test
revision: d7c0de2777da35d6aae2200a62c6e0e5af397c4c
metrics:
- type: accuracy
value: 70.08300000000001
- type: ap
value: 13.91559884590195
- type: f1
value: 53.956838444291364
- task:
type: Classification
dataset:
type: mteb/tweet_sentiment_extraction
name: MTEB TweetSentimentExtractionClassification
config: default
split: test
revision: d604517c81ca91fe16a244d1248fc021f9ecee7a
metrics:
- type: accuracy
value: 59.34069043576683
- type: f1
value: 59.662041994618406
- task:
type: Clustering
dataset:
type: mteb/twentynewsgroups-clustering
name: MTEB TwentyNewsgroupsClustering
config: default
split: test
revision: 6125ec4e24fa026cec8a478383ee943acfbd5449
metrics:
- type: v_measure
value: 53.70780611078653
- task:
type: PairClassification
dataset:
type: mteb/twittersemeval2015-pairclassification
name: MTEB TwitterSemEval2015
config: default
split: test
revision: 70970daeab8776df92f5ea462b6173c0b46fd2d1
metrics:
- type: cos_sim_accuracy
value: 87.10734934732073
- type: cos_sim_ap
value: 77.58349999516054
- type: cos_sim_f1
value: 70.25391395868965
- type: cos_sim_precision
value: 70.06035161374967
- type: cos_sim_recall
value: 70.44854881266491
- type: dot_accuracy
value: 80.60439887941826
- type: dot_ap
value: 54.52935200483575
- type: dot_f1
value: 54.170444242973716
- type: dot_precision
value: 47.47715534366309
- type: dot_recall
value: 63.06068601583114
- type: euclidean_accuracy
value: 87.26828396018358
- type: euclidean_ap
value: 78.00158454104036
- type: euclidean_f1
value: 70.70292457670601
- type: euclidean_precision
value: 68.79680479281079
- type: euclidean_recall
value: 72.71767810026385
- type: manhattan_accuracy
value: 87.11330988853788
- type: manhattan_ap
value: 77.92527099601855
- type: manhattan_f1
value: 70.76488706365502
- type: manhattan_precision
value: 68.89055472263868
- type: manhattan_recall
value: 72.74406332453826
- type: max_accuracy
value: 87.26828396018358
- type: max_ap
value: 78.00158454104036
- type: max_f1
value: 70.76488706365502
- task:
type: PairClassification
dataset:
type: mteb/twitterurlcorpus-pairclassification
name: MTEB TwitterURLCorpus
config: default
split: test
revision: 8b6510b0b1fa4e4c4f879467980e9be563ec1cdf
metrics:
- type: cos_sim_accuracy
value: 87.80804905499282
- type: cos_sim_ap
value: 83.06187782630936
- type: cos_sim_f1
value: 74.99716435403985
- type: cos_sim_precision
value: 73.67951860931579
- type: cos_sim_recall
value: 76.36279642747151
- type: dot_accuracy
value: 81.83141227151008
- type: dot_ap
value: 67.18241090841795
- type: dot_f1
value: 62.216037571751606
- type: dot_precision
value: 56.749381227391005
- type: dot_recall
value: 68.84816753926701
- type: euclidean_accuracy
value: 87.91671517832887
- type: euclidean_ap
value: 83.56538942001427
- type: euclidean_f1
value: 75.7327253337256
- type: euclidean_precision
value: 72.48856036606828
- type: euclidean_recall
value: 79.28087465352634
- type: manhattan_accuracy
value: 87.86626304963713
- type: manhattan_ap
value: 83.52939841172832
- type: manhattan_f1
value: 75.73635656329888
- type: manhattan_precision
value: 72.99150182103836
- type: manhattan_recall
value: 78.69571912534647
- type: max_accuracy
value: 87.91671517832887
- type: max_ap
value: 83.56538942001427
- type: max_f1
value: 75.73635656329888
license: mit
language:
- en
---
**Recommend switching to newest [BAAI/bge-large-en-v1.5](https://huggingface.co/BAAI/bge-large-en-v1.5), which has more reasonable similarity distribution and same method of usage.**
<h1 align="center">FlagEmbedding</h1>
<h4 align="center">
<p>
<a href=#model-list>Model List</a> |
<a href=#frequently-asked-questions>FAQ</a> |
<a href=#usage>Usage</a> |
<a href="#evaluation">Evaluation</a> |
<a href="#train">Train</a> |
<a href="#contact">Contact</a> |
<a href="#citation">Citation</a> |
<a href="#license">License</a>
<p>
</h4>
More details please refer to our Github: [FlagEmbedding](https://github.com/FlagOpen/FlagEmbedding).
[English](README.md) | [中文](https://github.com/FlagOpen/FlagEmbedding/blob/master/README_zh.md)
FlagEmbedding can map any text to a low-dimensional dense vector which can be used for tasks like retrieval, classification, clustering, or semantic search.
And it also can be used in vector databases for LLMs.
************* 🌟**Updates**🌟 *************
- 10/12/2023: Release [LLM-Embedder](./FlagEmbedding/llm_embedder/README.md), a unified embedding model to support diverse retrieval augmentation needs for LLMs. [Paper](https://arxiv.org/pdf/2310.07554.pdf) :fire:
- 09/15/2023: The [technical report](https://arxiv.org/pdf/2309.07597.pdf) of BGE has been released
- 09/15/2023: The [masive training data](https://data.baai.ac.cn/details/BAAI-MTP) of BGE has been released
- 09/12/2023: New models:
- **New reranker model**: release cross-encoder models `BAAI/bge-reranker-base` and `BAAI/bge-reranker-large`, which are more powerful than embedding model. We recommend to use/fine-tune them to re-rank top-k documents returned by embedding models.
- **update embedding model**: release `bge-*-v1.5` embedding model to alleviate the issue of the similarity distribution, and enhance its retrieval ability without instruction.
<details>
<summary>More</summary>
<!-- ### More -->
- 09/07/2023: Update [fine-tune code](https://github.com/FlagOpen/FlagEmbedding/blob/master/FlagEmbedding/baai_general_embedding/README.md): Add script to mine hard negatives and support adding instruction during fine-tuning.
- 08/09/2023: BGE Models are integrated into **Langchain**, you can use it like [this](#using-langchain); C-MTEB **leaderboard** is [available](https://huggingface.co/spaces/mteb/leaderboard).
- 08/05/2023: Release base-scale and small-scale models, **best performance among the models of the same size 🤗**
- 08/02/2023: Release `bge-large-*`(short for BAAI General Embedding) Models, **rank 1st on MTEB and C-MTEB benchmark!** :tada: :tada:
- 08/01/2023: We release the [Chinese Massive Text Embedding Benchmark](https://github.com/FlagOpen/FlagEmbedding/blob/master/C_MTEB) (**C-MTEB**), consisting of 31 test dataset.
</details>
## Model List
`bge` is short for `BAAI general embedding`.
| Model | Language | | Description | query instruction for retrieval [1] |
|:-------------------------------|:--------:| :--------:| :--------:|:--------:|
| [BAAI/llm-embedder](https://huggingface.co/BAAI/llm-embedder) | English | [Inference](./FlagEmbedding/llm_embedder/README.md) [Fine-tune](./FlagEmbedding/llm_embedder/README.md) | a unified embedding model to support diverse retrieval augmentation needs for LLMs | See [README](./FlagEmbedding/llm_embedder/README.md) |
| [BAAI/bge-reranker-large](https://huggingface.co/BAAI/bge-reranker-large) | Chinese and English | [Inference](#usage-for-reranker) [Fine-tune](https://github.com/FlagOpen/FlagEmbedding/tree/master/examples/reranker) | a cross-encoder model which is more accurate but less efficient [2] | |
| [BAAI/bge-reranker-base](https://huggingface.co/BAAI/bge-reranker-base) | Chinese and English | [Inference](#usage-for-reranker) [Fine-tune](https://github.com/FlagOpen/FlagEmbedding/tree/master/examples/reranker) | a cross-encoder model which is more accurate but less efficient [2] | |
| [BAAI/bge-large-en-v1.5](https://huggingface.co/BAAI/bge-large-en-v1.5) | English | [Inference](#usage-for-embedding-model) [Fine-tune](https://github.com/FlagOpen/FlagEmbedding/tree/master/examples/finetune) | version 1.5 with more reasonable similarity distribution | `Represent this sentence for searching relevant passages: ` |
| [BAAI/bge-base-en-v1.5](https://huggingface.co/BAAI/bge-base-en-v1.5) | English | [Inference](#usage-for-embedding-model) [Fine-tune](https://github.com/FlagOpen/FlagEmbedding/tree/master/examples/finetune) | version 1.5 with more reasonable similarity distribution | `Represent this sentence for searching relevant passages: ` |
| [BAAI/bge-small-en-v1.5](https://huggingface.co/BAAI/bge-small-en-v1.5) | English | [Inference](#usage-for-embedding-model) [Fine-tune](https://github.com/FlagOpen/FlagEmbedding/tree/master/examples/finetune) | version 1.5 with more reasonable similarity distribution | `Represent this sentence for searching relevant passages: ` |
| [BAAI/bge-large-zh-v1.5](https://huggingface.co/BAAI/bge-large-zh-v1.5) | Chinese | [Inference](#usage-for-embedding-model) [Fine-tune](https://github.com/FlagOpen/FlagEmbedding/tree/master/examples/finetune) | version 1.5 with more reasonable similarity distribution | `为这个句子生成表示以用于检索相关文章:` |
| [BAAI/bge-base-zh-v1.5](https://huggingface.co/BAAI/bge-base-zh-v1.5) | Chinese | [Inference](#usage-for-embedding-model) [Fine-tune](https://github.com/FlagOpen/FlagEmbedding/tree/master/examples/finetune) | version 1.5 with more reasonable similarity distribution | `为这个句子生成表示以用于检索相关文章:` |
| [BAAI/bge-small-zh-v1.5](https://huggingface.co/BAAI/bge-small-zh-v1.5) | Chinese | [Inference](#usage-for-embedding-model) [Fine-tune](https://github.com/FlagOpen/FlagEmbedding/tree/master/examples/finetune) | version 1.5 with more reasonable similarity distribution | `为这个句子生成表示以用于检索相关文章:` |
| [BAAI/bge-large-en](https://huggingface.co/BAAI/bge-large-en) | English | [Inference](#usage-for-embedding-model) [Fine-tune](https://github.com/FlagOpen/FlagEmbedding/tree/master/examples/finetune) | :trophy: rank **1st** in [MTEB](https://huggingface.co/spaces/mteb/leaderboard) leaderboard | `Represent this sentence for searching relevant passages: ` |
| [BAAI/bge-base-en](https://huggingface.co/BAAI/bge-base-en) | English | [Inference](#usage-for-embedding-model) [Fine-tune](https://github.com/FlagOpen/FlagEmbedding/tree/master/examples/finetune) | a base-scale model but with similar ability to `bge-large-en` | `Represent this sentence for searching relevant passages: ` |
| [BAAI/bge-small-en](https://huggingface.co/BAAI/bge-small-en) | English | [Inference](#usage-for-embedding-model) [Fine-tune](https://github.com/FlagOpen/FlagEmbedding/tree/master/examples/finetune) |a small-scale model but with competitive performance | `Represent this sentence for searching relevant passages: ` |
| [BAAI/bge-large-zh](https://huggingface.co/BAAI/bge-large-zh) | Chinese | [Inference](#usage-for-embedding-model) [Fine-tune](https://github.com/FlagOpen/FlagEmbedding/tree/master/examples/finetune) | :trophy: rank **1st** in [C-MTEB](https://github.com/FlagOpen/FlagEmbedding/tree/master/C_MTEB) benchmark | `为这个句子生成表示以用于检索相关文章:` |
| [BAAI/bge-base-zh](https://huggingface.co/BAAI/bge-base-zh) | Chinese | [Inference](#usage-for-embedding-model) [Fine-tune](https://github.com/FlagOpen/FlagEmbedding/tree/master/examples/finetune) | a base-scale model but with similar ability to `bge-large-zh` | `为这个句子生成表示以用于检索相关文章:` |
| [BAAI/bge-small-zh](https://huggingface.co/BAAI/bge-small-zh) | Chinese | [Inference](#usage-for-embedding-model) [Fine-tune](https://github.com/FlagOpen/FlagEmbedding/tree/master/examples/finetune) | a small-scale model but with competitive performance | `为这个句子生成表示以用于检索相关文章:` |
[1\]: If you need to search the relevant passages to a query, we suggest to add the instruction to the query; in other cases, no instruction is needed, just use the original query directly. In all cases, **no instruction** needs to be added to passages.
[2\]: Different from embedding model, reranker uses question and document as input and directly output similarity instead of embedding. To balance the accuracy and time cost, cross-encoder is widely used to re-rank top-k documents retrieved by other simple models.
For examples, use bge embedding model to retrieve top 100 relevant documents, and then use bge reranker to re-rank the top 100 document to get the final top-3 results.
All models have been uploaded to Huggingface Hub, and you can see them at https://huggingface.co/BAAI.
If you cannot open the Huggingface Hub, you also can download the models at https://model.baai.ac.cn/models .
## Frequently asked questions
<details>
<summary>1. How to fine-tune bge embedding model?</summary>
<!-- ### How to fine-tune bge embedding model? -->
Following this [example](https://github.com/FlagOpen/FlagEmbedding/tree/master/examples/finetune) to prepare data and fine-tune your model.
Some suggestions:
- Mine hard negatives following this [example](https://github.com/FlagOpen/FlagEmbedding/tree/master/examples/finetune#hard-negatives), which can improve the retrieval performance.
- If you pre-train bge on your data, the pre-trained model cannot be directly used to calculate similarity, and it must be fine-tuned with contrastive learning before computing similarity.
- If the accuracy of the fine-tuned model is still not high, it is recommended to use/fine-tune the cross-encoder model (bge-reranker) to re-rank top-k results. Hard negatives also are needed to fine-tune reranker.
</details>
<details>
<summary>2. The similarity score between two dissimilar sentences is higher than 0.5</summary>
<!-- ### The similarity score between two dissimilar sentences is higher than 0.5 -->
**Suggest to use bge v1.5, which alleviates the issue of the similarity distribution.**
Since we finetune the models by contrastive learning with a temperature of 0.01,
the similarity distribution of the current BGE model is about in the interval \[0.6, 1\].
So a similarity score greater than 0.5 does not indicate that the two sentences are similar.
For downstream tasks, such as passage retrieval or semantic similarity,
**what matters is the relative order of the scores, not the absolute value.**
If you need to filter similar sentences based on a similarity threshold,
please select an appropriate similarity threshold based on the similarity distribution on your data (such as 0.8, 0.85, or even 0.9).
</details>
<details>
<summary>3. When does the query instruction need to be used</summary>
<!-- ### When does the query instruction need to be used -->
For the `bge-*-v1.5`, we improve its retrieval ability when not using instruction.
No instruction only has a slight degradation in retrieval performance compared with using instruction.
So you can generate embedding without instruction in all cases for convenience.
For a retrieval task that uses short queries to find long related documents,
it is recommended to add instructions for these short queries.
**The best method to decide whether to add instructions for queries is choosing the setting that achieves better performance on your task.**
In all cases, the documents/passages do not need to add the instruction.
</details>
## Usage
### Usage for Embedding Model
Here are some examples for using `bge` models with
[FlagEmbedding](#using-flagembedding), [Sentence-Transformers](#using-sentence-transformers), [Langchain](#using-langchain), or [Huggingface Transformers](#using-huggingface-transformers).
#### Using FlagEmbedding
```
pip install -U FlagEmbedding
```
If it doesn't work for you, you can see [FlagEmbedding](https://github.com/FlagOpen/FlagEmbedding/blob/master/FlagEmbedding/baai_general_embedding/README.md) for more methods to install FlagEmbedding.
```python
from FlagEmbedding import FlagModel
sentences_1 = ["样例数据-1", "样例数据-2"]
sentences_2 = ["样例数据-3", "样例数据-4"]
model = FlagModel('BAAI/bge-large-zh-v1.5',
query_instruction_for_retrieval="为这个句子生成表示以用于检索相关文章:",
use_fp16=True) # Setting use_fp16 to True speeds up computation with a slight performance degradation
embeddings_1 = model.encode(sentences_1)
embeddings_2 = model.encode(sentences_2)
similarity = embeddings_1 @ embeddings_2.T
print(similarity)
# for s2p(short query to long passage) retrieval task, suggest to use encode_queries() which will automatically add the instruction to each query
# corpus in retrieval task can still use encode() or encode_corpus(), since they don't need instruction
queries = ['query_1', 'query_2']
passages = ["样例文档-1", "样例文档-2"]
q_embeddings = model.encode_queries(queries)
p_embeddings = model.encode(passages)
scores = q_embeddings @ p_embeddings.T
```
For the value of the argument `query_instruction_for_retrieval`, see [Model List](https://github.com/FlagOpen/FlagEmbedding/tree/master#model-list).
By default, FlagModel will use all available GPUs when encoding. Please set `os.environ["CUDA_VISIBLE_DEVICES"]` to select specific GPUs.
You also can set `os.environ["CUDA_VISIBLE_DEVICES"]=""` to make all GPUs unavailable.
#### Using Sentence-Transformers
You can also use the `bge` models with [sentence-transformers](https://www.SBERT.net):
```
pip install -U sentence-transformers
```
```python
from sentence_transformers import SentenceTransformer
sentences_1 = ["样例数据-1", "样例数据-2"]
sentences_2 = ["样例数据-3", "样例数据-4"]
model = SentenceTransformer('BAAI/bge-large-zh-v1.5')
embeddings_1 = model.encode(sentences_1, normalize_embeddings=True)
embeddings_2 = model.encode(sentences_2, normalize_embeddings=True)
similarity = embeddings_1 @ embeddings_2.T
print(similarity)
```
For s2p(short query to long passage) retrieval task,
each short query should start with an instruction (instructions see [Model List](https://github.com/FlagOpen/FlagEmbedding/tree/master#model-list)).
But the instruction is not needed for passages.
```python
from sentence_transformers import SentenceTransformer
queries = ['query_1', 'query_2']
passages = ["样例文档-1", "样例文档-2"]
instruction = "为这个句子生成表示以用于检索相关文章:"
model = SentenceTransformer('BAAI/bge-large-zh-v1.5')
q_embeddings = model.encode([instruction+q for q in queries], normalize_embeddings=True)
p_embeddings = model.encode(passages, normalize_embeddings=True)
scores = q_embeddings @ p_embeddings.T
```
#### Using Langchain
You can use `bge` in langchain like this:
```python
from langchain.embeddings import HuggingFaceBgeEmbeddings
model_name = "BAAI/bge-large-en-v1.5"
model_kwargs = {'device': 'cuda'}
encode_kwargs = {'normalize_embeddings': True} # set True to compute cosine similarity
model = HuggingFaceBgeEmbeddings(
model_name=model_name,
model_kwargs=model_kwargs,
encode_kwargs=encode_kwargs,
query_instruction="为这个句子生成表示以用于检索相关文章:"
)
model.query_instruction = "为这个句子生成表示以用于检索相关文章:"
```
#### Using HuggingFace Transformers
With the transformers package, you can use the model like this: First, you pass your input through the transformer model, then you select the last hidden state of the first token (i.e., [CLS]) as the sentence embedding.
```python
from transformers import AutoTokenizer, AutoModel
import torch
# Sentences we want sentence embeddings for
sentences = ["样例数据-1", "样例数据-2"]
# Load model from HuggingFace Hub
tokenizer = AutoTokenizer.from_pretrained('BAAI/bge-large-zh-v1.5')
model = AutoModel.from_pretrained('BAAI/bge-large-zh-v1.5')
model.eval()
# Tokenize sentences
encoded_input = tokenizer(sentences, padding=True, truncation=True, return_tensors='pt')
# for s2p(short query to long passage) retrieval task, add an instruction to query (not add instruction for passages)
# encoded_input = tokenizer([instruction + q for q in queries], padding=True, truncation=True, return_tensors='pt')
# Compute token embeddings
with torch.no_grad():
model_output = model(**encoded_input)
# Perform pooling. In this case, cls pooling.
sentence_embeddings = model_output[0][:, 0]
# normalize embeddings
sentence_embeddings = torch.nn.functional.normalize(sentence_embeddings, p=2, dim=1)
print("Sentence embeddings:", sentence_embeddings)
```
### Usage for Reranker
Different from embedding model, reranker uses question and document as input and directly output similarity instead of embedding.
You can get a relevance score by inputting query and passage to the reranker.
The reranker is optimized based cross-entropy loss, so the relevance score is not bounded to a specific range.
#### Using FlagEmbedding
```
pip install -U FlagEmbedding
```
Get relevance scores (higher scores indicate more relevance):
```python
from FlagEmbedding import FlagReranker
reranker = FlagReranker('BAAI/bge-reranker-large', use_fp16=True) # Setting use_fp16 to True speeds up computation with a slight performance degradation
score = reranker.compute_score(['query', 'passage'])
print(score)
scores = reranker.compute_score([['what is panda?', 'hi'], ['what is panda?', 'The giant panda (Ailuropoda melanoleuca), sometimes called a panda bear or simply panda, is a bear species endemic to China.']])
print(scores)
```
#### Using Huggingface transformers
```python
import torch
from transformers import AutoModelForSequenceClassification, AutoTokenizer
tokenizer = AutoTokenizer.from_pretrained('BAAI/bge-reranker-large')
model = AutoModelForSequenceClassification.from_pretrained('BAAI/bge-reranker-large')
model.eval()
pairs = [['what is panda?', 'hi'], ['what is panda?', 'The giant panda (Ailuropoda melanoleuca), sometimes called a panda bear or simply panda, is a bear species endemic to China.']]
with torch.no_grad():
inputs = tokenizer(pairs, padding=True, truncation=True, return_tensors='pt', max_length=512)
scores = model(**inputs, return_dict=True).logits.view(-1, ).float()
print(scores)
```
## Evaluation
`baai-general-embedding` models achieve **state-of-the-art performance on both MTEB and C-MTEB leaderboard!**
For more details and evaluation tools see our [scripts](https://github.com/FlagOpen/FlagEmbedding/blob/master/C_MTEB/README.md).
- **MTEB**:
| Model Name | Dimension | Sequence Length | Average (56) | Retrieval (15) |Clustering (11) | Pair Classification (3) | Reranking (4) | STS (10) | Summarization (1) | Classification (12) |
|:----:|:---:|:---:|:---:|:---:|:---:|:---:|:---:|:---:|:---:|:---:|
| [BAAI/bge-large-en-v1.5](https://huggingface.co/BAAI/bge-large-en-v1.5) | 1024 | 512 | **64.23** | **54.29** | 46.08 | 87.12 | 60.03 | 83.11 | 31.61 | 75.97 |
| [BAAI/bge-base-en-v1.5](https://huggingface.co/BAAI/bge-base-en-v1.5) | 768 | 512 | 63.55 | 53.25 | 45.77 | 86.55 | 58.86 | 82.4 | 31.07 | 75.53 |
| [BAAI/bge-small-en-v1.5](https://huggingface.co/BAAI/bge-small-en-v1.5) | 384 | 512 | 62.17 |51.68 | 43.82 | 84.92 | 58.36 | 81.59 | 30.12 | 74.14 |
| [bge-large-en](https://huggingface.co/BAAI/bge-large-en) | 1024 | 512 | 63.98 | 53.9 | 46.98 | 85.8 | 59.48 | 81.56 | 32.06 | 76.21 |
| [bge-base-en](https://huggingface.co/BAAI/bge-base-en) | 768 | 512 | 63.36 | 53.0 | 46.32 | 85.86 | 58.7 | 81.84 | 29.27 | 75.27 |
| [gte-large](https://huggingface.co/thenlper/gte-large) | 1024 | 512 | 63.13 | 52.22 | 46.84 | 85.00 | 59.13 | 83.35 | 31.66 | 73.33 |
| [gte-base](https://huggingface.co/thenlper/gte-base) | 768 | 512 | 62.39 | 51.14 | 46.2 | 84.57 | 58.61 | 82.3 | 31.17 | 73.01 |
| [e5-large-v2](https://huggingface.co/intfloat/e5-large-v2) | 1024| 512 | 62.25 | 50.56 | 44.49 | 86.03 | 56.61 | 82.05 | 30.19 | 75.24 |
| [bge-small-en](https://huggingface.co/BAAI/bge-small-en) | 384 | 512 | 62.11 | 51.82 | 44.31 | 83.78 | 57.97 | 80.72 | 30.53 | 74.37 |
| [instructor-xl](https://huggingface.co/hkunlp/instructor-xl) | 768 | 512 | 61.79 | 49.26 | 44.74 | 86.62 | 57.29 | 83.06 | 32.32 | 61.79 |
| [e5-base-v2](https://huggingface.co/intfloat/e5-base-v2) | 768 | 512 | 61.5 | 50.29 | 43.80 | 85.73 | 55.91 | 81.05 | 30.28 | 73.84 |
| [gte-small](https://huggingface.co/thenlper/gte-small) | 384 | 512 | 61.36 | 49.46 | 44.89 | 83.54 | 57.7 | 82.07 | 30.42 | 72.31 |
| [text-embedding-ada-002](https://platform.openai.com/docs/guides/embeddings) | 1536 | 8192 | 60.99 | 49.25 | 45.9 | 84.89 | 56.32 | 80.97 | 30.8 | 70.93 |
| [e5-small-v2](https://huggingface.co/intfloat/e5-base-v2) | 384 | 512 | 59.93 | 49.04 | 39.92 | 84.67 | 54.32 | 80.39 | 31.16 | 72.94 |
| [sentence-t5-xxl](https://huggingface.co/sentence-transformers/sentence-t5-xxl) | 768 | 512 | 59.51 | 42.24 | 43.72 | 85.06 | 56.42 | 82.63 | 30.08 | 73.42 |
| [all-mpnet-base-v2](https://huggingface.co/sentence-transformers/all-mpnet-base-v2) | 768 | 514 | 57.78 | 43.81 | 43.69 | 83.04 | 59.36 | 80.28 | 27.49 | 65.07 |
| [sgpt-bloom-7b1-msmarco](https://huggingface.co/bigscience/sgpt-bloom-7b1-msmarco) | 4096 | 2048 | 57.59 | 48.22 | 38.93 | 81.9 | 55.65 | 77.74 | 33.6 | 66.19 |
- **C-MTEB**:
We create the benchmark C-MTEB for Chinese text embedding which consists of 31 datasets from 6 tasks.
Please refer to [C_MTEB](https://github.com/FlagOpen/FlagEmbedding/blob/master/C_MTEB/README.md) for a detailed introduction.
| Model | Embedding dimension | Avg | Retrieval | STS | PairClassification | Classification | Reranking | Clustering |
|:-------------------------------|:--------:|:--------:|:--------:|:--------:|:--------:|:--------:|:--------:|:--------:|
| [**BAAI/bge-large-zh-v1.5**](https://huggingface.co/BAAI/bge-large-zh-v1.5) | 1024 | **64.53** | 70.46 | 56.25 | 81.6 | 69.13 | 65.84 | 48.99 |
| [BAAI/bge-base-zh-v1.5](https://huggingface.co/BAAI/bge-base-zh-v1.5) | 768 | 63.13 | 69.49 | 53.72 | 79.75 | 68.07 | 65.39 | 47.53 |
| [BAAI/bge-small-zh-v1.5](https://huggingface.co/BAAI/bge-small-zh-v1.5) | 512 | 57.82 | 61.77 | 49.11 | 70.41 | 63.96 | 60.92 | 44.18 |
| [BAAI/bge-large-zh](https://huggingface.co/BAAI/bge-large-zh) | 1024 | 64.20 | 71.53 | 54.98 | 78.94 | 68.32 | 65.11 | 48.39 |
| [bge-large-zh-noinstruct](https://huggingface.co/BAAI/bge-large-zh-noinstruct) | 1024 | 63.53 | 70.55 | 53 | 76.77 | 68.58 | 64.91 | 50.01 |
| [BAAI/bge-base-zh](https://huggingface.co/BAAI/bge-base-zh) | 768 | 62.96 | 69.53 | 54.12 | 77.5 | 67.07 | 64.91 | 47.63 |
| [multilingual-e5-large](https://huggingface.co/intfloat/multilingual-e5-large) | 1024 | 58.79 | 63.66 | 48.44 | 69.89 | 67.34 | 56.00 | 48.23 |
| [BAAI/bge-small-zh](https://huggingface.co/BAAI/bge-small-zh) | 512 | 58.27 | 63.07 | 49.45 | 70.35 | 63.64 | 61.48 | 45.09 |
| [m3e-base](https://huggingface.co/moka-ai/m3e-base) | 768 | 57.10 | 56.91 | 50.47 | 63.99 | 67.52 | 59.34 | 47.68 |
| [m3e-large](https://huggingface.co/moka-ai/m3e-large) | 1024 | 57.05 | 54.75 | 50.42 | 64.3 | 68.2 | 59.66 | 48.88 |
| [multilingual-e5-base](https://huggingface.co/intfloat/multilingual-e5-base) | 768 | 55.48 | 61.63 | 46.49 | 67.07 | 65.35 | 54.35 | 40.68 |
| [multilingual-e5-small](https://huggingface.co/intfloat/multilingual-e5-small) | 384 | 55.38 | 59.95 | 45.27 | 66.45 | 65.85 | 53.86 | 45.26 |
| [text-embedding-ada-002(OpenAI)](https://platform.openai.com/docs/guides/embeddings/what-are-embeddings) | 1536 | 53.02 | 52.0 | 43.35 | 69.56 | 64.31 | 54.28 | 45.68 |
| [luotuo](https://huggingface.co/silk-road/luotuo-bert-medium) | 1024 | 49.37 | 44.4 | 42.78 | 66.62 | 61 | 49.25 | 44.39 |
| [text2vec-base](https://huggingface.co/shibing624/text2vec-base-chinese) | 768 | 47.63 | 38.79 | 43.41 | 67.41 | 62.19 | 49.45 | 37.66 |
| [text2vec-large](https://huggingface.co/GanymedeNil/text2vec-large-chinese) | 1024 | 47.36 | 41.94 | 44.97 | 70.86 | 60.66 | 49.16 | 30.02 |
- **Reranking**:
See [C_MTEB](https://github.com/FlagOpen/FlagEmbedding/blob/master/C_MTEB/) for evaluation script.
| Model | T2Reranking | T2RerankingZh2En\* | T2RerankingEn2Zh\* | MMarcoReranking | CMedQAv1 | CMedQAv2 | Avg |
|:-------------------------------|:--------:|:--------:|:--------:|:--------:|:--------:|:--------:|:--------:|
| text2vec-base-multilingual | 64.66 | 62.94 | 62.51 | 14.37 | 48.46 | 48.6 | 50.26 |
| multilingual-e5-small | 65.62 | 60.94 | 56.41 | 29.91 | 67.26 | 66.54 | 57.78 |
| multilingual-e5-large | 64.55 | 61.61 | 54.28 | 28.6 | 67.42 | 67.92 | 57.4 |
| multilingual-e5-base | 64.21 | 62.13 | 54.68 | 29.5 | 66.23 | 66.98 | 57.29 |
| m3e-base | 66.03 | 62.74 | 56.07 | 17.51 | 77.05 | 76.76 | 59.36 |
| m3e-large | 66.13 | 62.72 | 56.1 | 16.46 | 77.76 | 78.27 | 59.57 |
| bge-base-zh-v1.5 | 66.49 | 63.25 | 57.02 | 29.74 | 80.47 | 84.88 | 63.64 |
| bge-large-zh-v1.5 | 65.74 | 63.39 | 57.03 | 28.74 | 83.45 | 85.44 | 63.97 |
| [BAAI/bge-reranker-base](https://huggingface.co/BAAI/bge-reranker-base) | 67.28 | 63.95 | 60.45 | 35.46 | 81.26 | 84.1 | 65.42 |
| [BAAI/bge-reranker-large](https://huggingface.co/BAAI/bge-reranker-large) | 67.6 | 64.03 | 61.44 | 37.16 | 82.15 | 84.18 | 66.09 |
\* : T2RerankingZh2En and T2RerankingEn2Zh are cross-language retrieval tasks
## Train
### BAAI Embedding
We pre-train the models using [retromae](https://github.com/staoxiao/RetroMAE) and train them on large-scale pairs data using contrastive learning.
**You can fine-tune the embedding model on your data following our [examples](https://github.com/FlagOpen/FlagEmbedding/tree/master/examples/finetune).**
We also provide a [pre-train example](https://github.com/FlagOpen/FlagEmbedding/tree/master/examples/pretrain).
Note that the goal of pre-training is to reconstruct the text, and the pre-trained model cannot be used for similarity calculation directly, it needs to be fine-tuned.
More training details for bge see [baai_general_embedding](https://github.com/FlagOpen/FlagEmbedding/blob/master/FlagEmbedding/baai_general_embedding/README.md).
### BGE Reranker
Cross-encoder will perform full-attention over the input pair,
which is more accurate than embedding model (i.e., bi-encoder) but more time-consuming than embedding model.
Therefore, it can be used to re-rank the top-k documents returned by embedding model.
We train the cross-encoder on a multilingual pair data,
The data format is the same as embedding model, so you can fine-tune it easily following our [example](https://github.com/FlagOpen/FlagEmbedding/tree/master/examples/reranker).
More details please refer to [./FlagEmbedding/reranker/README.md](https://github.com/FlagOpen/FlagEmbedding/tree/master/FlagEmbedding/reranker)
## Contact
If you have any question or suggestion related to this project, feel free to open an issue or pull request.
You also can email Shitao Xiao(stxiao@baai.ac.cn) and Zheng Liu(liuzheng@baai.ac.cn).
## Citation
If you find this repository useful, please consider giving a star :star: and citation
```
@misc{bge_embedding,
title={C-Pack: Packaged Resources To Advance General Chinese Embedding},
author={Shitao Xiao and Zheng Liu and Peitian Zhang and Niklas Muennighoff},
year={2023},
eprint={2309.07597},
archivePrefix={arXiv},
primaryClass={cs.CL}
}
```
## License
FlagEmbedding is licensed under the [MIT License](https://github.com/FlagOpen/FlagEmbedding/blob/master/LICENSE). The released models can be used for commercial purposes free of charge.
|
openai-community/gpt2-medium | openai-community | "2024-02-19T12:39:04Z" | 514,130 | 154 | transformers | [
"transformers",
"pytorch",
"tf",
"jax",
"rust",
"onnx",
"safetensors",
"gpt2",
"text-generation",
"en",
"arxiv:1910.09700",
"license:mit",
"autotrain_compatible",
"text-generation-inference",
"endpoints_compatible",
"region:us"
] | text-generation | "2022-03-02T23:29:04Z" | ---
language: en
license: mit
---
# GPT-2 Medium
## Model Details
**Model Description:** GPT-2 Medium is the **355M parameter** version of GPT-2, a transformer-based language model created and released by OpenAI. The model is a pretrained model on English language using a causal language modeling (CLM) objective.
- **Developed by:** OpenAI, see [associated research paper](https://d4mucfpksywv.cloudfront.net/better-language-models/language_models_are_unsupervised_multitask_learners.pdf) and [GitHub repo](https://github.com/openai/gpt-2) for model developers.
- **Model Type:** Transformer-based language model
- **Language(s):** English
- **License:** [Modified MIT License](https://github.com/openai/gpt-2/blob/master/LICENSE)
- **Related Models:** [GPT2](https://huggingface.co/gpt2), [GPT2-Large](https://huggingface.co/gpt2-large) and [GPT2-XL](https://huggingface.co/gpt2-xl)
- **Resources for more information:**
- [Research Paper](https://d4mucfpksywv.cloudfront.net/better-language-models/language_models_are_unsupervised_multitask_learners.pdf)
- [OpenAI Blog Post](https://openai.com/blog/better-language-models/)
- [GitHub Repo](https://github.com/openai/gpt-2)
- [OpenAI Model Card for GPT-2](https://github.com/openai/gpt-2/blob/master/model_card.md)
- Test the full generation capabilities here: https://transformer.huggingface.co/doc/gpt2-large
## How to Get Started with the Model
Use the code below to get started with the model. You can use this model directly with a pipeline for text generation. Since the generation relies on some randomness, we
set a seed for reproducibility:
```python
>>> from transformers import pipeline, set_seed
>>> generator = pipeline('text-generation', model='gpt2-medium')
>>> set_seed(42)
>>> generator("Hello, I'm a language model,", max_length=30, num_return_sequences=5)
[{'generated_text': "Hello, I'm a language model, I'm a language. I'm a compiler, I'm a parser, I'm a server process. I"},
{'generated_text': "Hello, I'm a language model, and I'd like to join an existing team. What can I do to get started?\n\nI'd"},
{'generated_text': "Hello, I'm a language model, why does my code get created? Can't I just copy it? But why did my code get created when"},
{'generated_text': "Hello, I'm a language model, a functional language...\n\nI'm a functional language. Is it hard? A little, yes. But"},
{'generated_text': "Hello, I'm a language model, not an object model.\n\nIn a nutshell, I need to give me objects from which I can get"}]
```
Here is how to use this model to get the features of a given text in PyTorch:
```python
from transformers import GPT2Tokenizer, GPT2Model
tokenizer = GPT2Tokenizer.from_pretrained('gpt2-medium')
model = GPT2Model.from_pretrained('gpt2-medium')
text = "Replace me by any text you'd like."
encoded_input = tokenizer(text, return_tensors='pt')
output = model(**encoded_input)
```
and in TensorFlow:
```python
from transformers import GPT2Tokenizer, TFGPT2Model
tokenizer = GPT2Tokenizer.from_pretrained('gpt2-medium')
model = TFGPT2Model.from_pretrained('gpt2-medium')
text = "Replace me by any text you'd like."
encoded_input = tokenizer(text, return_tensors='tf')
output = model(encoded_input)
```
## Uses
#### Direct Use
In their [model card about GPT-2](https://github.com/openai/gpt-2/blob/master/model_card.md), OpenAI wrote:
> The primary intended users of these models are AI researchers and practitioners.
>
> We primarily imagine these language models will be used by researchers to better understand the behaviors, capabilities, biases, and constraints of large-scale generative language models.
#### Downstream Use
In their [model card about GPT-2](https://github.com/openai/gpt-2/blob/master/model_card.md), OpenAI wrote:
> Here are some secondary use cases we believe are likely:
>
> - Writing assistance: Grammar assistance, autocompletion (for normal prose or code)
> - Creative writing and art: exploring the generation of creative, fictional texts; aiding creation of poetry and other literary art.
> - Entertainment: Creation of games, chat bots, and amusing generations.
#### Misuse and Out-of-scope Use
In their [model card about GPT-2](https://github.com/openai/gpt-2/blob/master/model_card.md), OpenAI wrote:
> Because large-scale language models like GPT-2 do not distinguish fact from fiction, we don’t support use-cases that require the generated text to be true.
>
> Additionally, language models like GPT-2 reflect the biases inherent to the systems they were trained on, so we do not recommend that they be deployed into systems that interact with humans unless the deployers first carry out a study of biases relevant to the intended use-case. We found no statistically significant difference in gender, race, and religious bias probes between 774M and 1.5B, implying all versions of GPT-2 should be approached with similar levels of caution around use cases that are sensitive to biases around human attributes.
## Risks, Limitations and Biases
**CONTENT WARNING: Readers should be aware this section contains content that is disturbing, offensive, and can propogate historical and current stereotypes.**
Significant research has explored bias and fairness issues with language models (see, e.g., [Sheng et al. (2021)](https://aclanthology.org/2021.acl-long.330.pdf) and [Bender et al. (2021)](https://dl.acm.org/doi/pdf/10.1145/3442188.3445922)).
The training data used for this model has not been released as a dataset one can browse. We know it contains a lot of unfiltered content from the internet, which is far from neutral. Predictions generated by the model can include disturbing and harmful stereotypes across protected classes; identity characteristics; and sensitive, social, and occupational groups. For example:
```python
>>> from transformers import pipeline, set_seed
>>> generator = pipeline('text-generation', model='gpt2-medium')
>>> set_seed(42)
>>> generator("The man worked as a", max_length=10, num_return_sequences=5)
[{'generated_text': 'The man worked as a security guard in a military'},
{'generated_text': 'The man worked as a salesman in Mexico and eventually'},
{'generated_text': 'The man worked as a supervisor at the department for'},
{'generated_text': 'The man worked as a cleaner for the same corporation'},
{'generated_text': 'The man worked as a barman and was involved'}]
>>> set_seed(42)
>>> generator("The woman worked as a", max_length=10, num_return_sequences=5)
[{'generated_text': 'The woman worked as a social worker in a children'},
{'generated_text': 'The woman worked as a marketing manager, and her'},
{'generated_text': 'The woman worked as a customer service agent in a'},
{'generated_text': 'The woman worked as a cleaner for the same corporation'},
{'generated_text': 'The woman worked as a barista and was involved'}]
```
This bias will also affect all fine-tuned versions of this model. Users (both direct and downstream) should be made aware of the risks, biases and limitations of the model.
## Training
#### Training Data
The OpenAI team wanted to train this model on a corpus as large as possible. To build it, they scraped all the web
pages from outbound links on Reddit which received at least 3 karma. Note that all Wikipedia pages were removed from
this dataset, so the model was not trained on any part of Wikipedia. The resulting dataset (called WebText) weights
40GB of texts but has not been publicly released. You can find a list of the top 1,000 domains present in WebText
[here](https://github.com/openai/gpt-2/blob/master/domains.txt).
#### Training Procedure
The model is pretrained on a very large corpus of English data in a self-supervised fashion. This
means it was pretrained on the raw texts only, with no humans labelling them in any way (which is why it can use lots
of publicly available data) with an automatic process to generate inputs and labels from those texts. More precisely,
it was trained to guess the next word in sentences.
More precisely, inputs are sequences of continuous text of a certain length and the targets are the same sequence,
shifted one token (word or piece of word) to the right. The model uses internally a mask-mechanism to make sure the
predictions for the token `i` only uses the inputs from `1` to `i` but not the future tokens.
This way, the model learns an inner representation of the English language that can then be used to extract features
useful for downstream tasks.
The texts are tokenized using a byte-level version of Byte Pair Encoding (BPE) (for unicode characters) and a
vocabulary size of 50,257. The inputs are sequences of 1024 consecutive tokens.
## Evaluation
The following evaluation information is extracted from the [associated paper](https://d4mucfpksywv.cloudfront.net/better-language-models/language_models_are_unsupervised_multitask_learners.pdf).
#### Testing Data, Factors and Metrics
The model authors write in the [associated paper](https://d4mucfpksywv.cloudfront.net/better-language-models/language_models_are_unsupervised_multitask_learners.pdf) that:
> Since our model operates on a byte level and does not require lossy pre-processing or tokenization, we can evaluate it on any language model benchmark. Results on language modeling datasets are commonly reported in a quantity which is a scaled or ex- ponentiated version of the average negative log probability per canonical prediction unit - usually a character, a byte, or a word. We evaluate the same quantity by computing the log-probability of a dataset according to a WebText LM and dividing by the number of canonical units. For many of these datasets, WebText LMs would be tested significantly out- of-distribution, having to predict aggressively standardized text, tokenization artifacts such as disconnected punctuation and contractions, shuffled sentences, and even the string <UNK> which is extremely rare in WebText - occurring only 26 times in 40 billion bytes. We report our main results...using invertible de-tokenizers which remove as many of these tokenization / pre-processing artifacts as possible. Since these de-tokenizers are invertible, we can still calculate the log probability of a dataset and they can be thought of as a simple form of domain adaptation.
#### Results
The model achieves the following results without any fine-tuning (zero-shot):
| Dataset | LAMBADA | LAMBADA | CBT-CN | CBT-NE | WikiText2 | PTB | enwiki8 | text8 | WikiText103 | 1BW |
|:--------:|:-------:|:-------:|:------:|:------:|:---------:|:------:|:-------:|:------:|:-----------:|:-----:|
| (metric) | (PPL) | (ACC) | (ACC) | (ACC) | (PPL) | (PPL) | (BPB) | (BPC) | (PPL) | (PPL) |
| | 15.60 | 55.48 | 92.35 | 87.1 | 22.76 | 47.33 | 1.01 | 1.06 | 26.37 | 55.72 |
## Environmental Impact
Carbon emissions can be estimated using the [Machine Learning Impact calculator](https://mlco2.github.io/impact#compute) presented in [Lacoste et al. (2019)](https://arxiv.org/abs/1910.09700).
- **Hardware Type:** Unknown
- **Hours used:** Unknown
- **Cloud Provider:** Unknown
- **Compute Region:** Unknown
- **Carbon Emitted:** Unknown
## Technical Specifications
See the [associated paper](https://d4mucfpksywv.cloudfront.net/better-language-models/language_models_are_unsupervised_multitask_learners.pdf) for details on the modeling architecture, objective, compute infrastructure, and training details.
## Citation Information
```bibtex
@article{radford2019language,
title={Language models are unsupervised multitask learners},
author={Radford, Alec and Wu, Jeffrey and Child, Rewon and Luan, David and Amodei, Dario and Sutskever, Ilya and others},
journal={OpenAI blog},
volume={1},
number={8},
pages={9},
year={2019}
}
```
## Model Card Authors
This model card was written by the Hugging Face team. |
facebook/sam2-hiera-large | facebook | "2024-08-15T15:19:06Z" | 514,029 | 45 | sam2 | [
"sam2",
"mask-generation",
"arxiv:2408.00714",
"license:apache-2.0",
"region:us"
] | mask-generation | "2024-08-02T19:41:47Z" | ---
license: apache-2.0
pipeline_tag: mask-generation
library_name: sam2
---
Repository for SAM 2: Segment Anything in Images and Videos, a foundation model towards solving promptable visual segmentation in images and videos from FAIR. See the [SAM 2 paper](https://arxiv.org/abs/2408.00714) for more information.
The official code is publicly release in this [repo](https://github.com/facebookresearch/segment-anything-2/).
## Usage
For image prediction:
```python
import torch
from sam2.sam2_image_predictor import SAM2ImagePredictor
predictor = SAM2ImagePredictor.from_pretrained("facebook/sam2-hiera-large")
with torch.inference_mode(), torch.autocast("cuda", dtype=torch.bfloat16):
predictor.set_image(<your_image>)
masks, _, _ = predictor.predict(<input_prompts>)
```
For video prediction:
```python
import torch
from sam2.sam2_video_predictor import SAM2VideoPredictor
predictor = SAM2VideoPredictor.from_pretrained("facebook/sam2-hiera-large")
with torch.inference_mode(), torch.autocast("cuda", dtype=torch.bfloat16):
state = predictor.init_state(<your_video>)
# add new prompts and instantly get the output on the same frame
frame_idx, object_ids, masks = predictor.add_new_points_or_box(state, <your_prompts>):
# propagate the prompts to get masklets throughout the video
for frame_idx, object_ids, masks in predictor.propagate_in_video(state):
...
```
Refer to the [demo notebooks](https://github.com/facebookresearch/segment-anything-2/tree/main/notebooks) for details.
### Citation
To cite the paper, model, or software, please use the below:
```
@article{ravi2024sam2,
title={SAM 2: Segment Anything in Images and Videos},
author={Ravi, Nikhila and Gabeur, Valentin and Hu, Yuan-Ting and Hu, Ronghang and Ryali, Chaitanya and Ma, Tengyu and Khedr, Haitham and R{\"a}dle, Roman and Rolland, Chloe and Gustafson, Laura and Mintun, Eric and Pan, Junting and Alwala, Kalyan Vasudev and Carion, Nicolas and Wu, Chao-Yuan and Girshick, Ross and Doll{\'a}r, Piotr and Feichtenhofer, Christoph},
journal={arXiv preprint arXiv:2408.00714},
url={https://arxiv.org/abs/2408.00714},
year={2024}
}
``` |
cambridgeltl/SapBERT-UMLS-2020AB-all-lang-from-XLMR | cambridgeltl | "2023-06-14T19:00:30Z" | 512,150 | 2 | transformers | [
"transformers",
"pytorch",
"safetensors",
"xlm-roberta",
"feature-extraction",
"arxiv:2010.11784",
"endpoints_compatible",
"region:us"
] | feature-extraction | "2022-03-02T23:29:05Z" | ---
language: multilingual
tags:
- biomedical
- lexical-semantics
- cross-lingual
datasets:
- UMLS
**[news]** A cross-lingual extension of SapBERT will appear in the main onference of **ACL 2021**! <br>
**[news]** SapBERT will appear in the conference proceedings of **NAACL 2021**!
### SapBERT-XLMR
SapBERT [(Liu et al. 2020)](https://arxiv.org/pdf/2010.11784.pdf) trained with [UMLS](https://www.nlm.nih.gov/research/umls/licensedcontent/umlsknowledgesources.html) 2020AB, using [xlm-roberta-base](https://huggingface.co/xlm-roberta-base) as the base model. Please use [CLS] as the representation of the input.
#### Extracting embeddings from SapBERT
The following script converts a list of strings (entity names) into embeddings.
```python
import numpy as np
import torch
from tqdm.auto import tqdm
from transformers import AutoTokenizer, AutoModel
tokenizer = AutoTokenizer.from_pretrained("cambridgeltl/SapBERT-from-PubMedBERT-fulltext")
model = AutoModel.from_pretrained("cambridgeltl/SapBERT-from-PubMedBERT-fulltext").cuda()
# replace with your own list of entity names
all_names = ["covid-19", "Coronavirus infection", "high fever", "Tumor of posterior wall of oropharynx"]
bs = 128 # batch size during inference
all_embs = []
for i in tqdm(np.arange(0, len(all_names), bs)):
toks = tokenizer.batch_encode_plus(all_names[i:i+bs],
padding="max_length",
max_length=25,
truncation=True,
return_tensors="pt")
toks_cuda = {}
for k,v in toks.items():
toks_cuda[k] = v.cuda()
cls_rep = model(**toks_cuda)[0][:,0,:] # use CLS representation as the embedding
all_embs.append(cls_rep.cpu().detach().numpy())
all_embs = np.concatenate(all_embs, axis=0)
```
For more details about training and eval, see SapBERT [github repo](https://github.com/cambridgeltl/sapbert).
### Citation
```bibtex
@inproceedings{liu2021learning,
title={Learning Domain-Specialised Representations for Cross-Lingual Biomedical Entity Linking},
author={Liu, Fangyu and Vuli{\'c}, Ivan and Korhonen, Anna and Collier, Nigel},
booktitle={Proceedings of ACL-IJCNLP 2021},
month = aug,
year={2021}
}
``` |
JackFram/llama-68m | JackFram | "2024-05-23T17:18:35Z" | 510,408 | 23 | transformers | [
"transformers",
"pytorch",
"llama",
"text-generation",
"en",
"dataset:wikipedia",
"arxiv:2305.09781",
"license:apache-2.0",
"autotrain_compatible",
"text-generation-inference",
"endpoints_compatible",
"region:us"
] | text-generation | "2023-07-19T02:20:03Z" | ---
license: apache-2.0
language:
- en
datasets:
- wikipedia
pipeline_tag: text-generation
---
## Model description
This is a LLaMA-like model with only 68M parameters trained on Wikipedia and part of the C4-en and C4-realnewslike datasets.
No evaluation has been conducted yet, so use it with care.
The model is mainly developed as a base Small Speculative Model in the [SpecInfer](https://arxiv.org/abs/2305.09781) paper.
## Citation
To cite the model, please use
```bibtex
@misc{miao2023specinfer,
title={SpecInfer: Accelerating Generative LLM Serving with Speculative Inference and Token Tree Verification},
author={Xupeng Miao and Gabriele Oliaro and Zhihao Zhang and Xinhao Cheng and Zeyu Wang and Rae Ying Yee Wong and Zhuoming Chen and Daiyaan Arfeen and Reyna Abhyankar and Zhihao Jia},
year={2023},
eprint={2305.09781},
archivePrefix={arXiv},
primaryClass={cs.CL}
}
``` |
microsoft/BiomedCLIP-PubMedBERT_256-vit_base_patch16_224 | microsoft | "2024-09-27T23:51:17Z" | 504,021 | 213 | open_clip | [
"open_clip",
"clip",
"biology",
"medical",
"zero-shot-image-classification",
"en",
"arxiv:2303.00915",
"license:mit",
"region:us"
] | zero-shot-image-classification | "2023-04-05T19:57:59Z" | ---
language: en
tags:
- clip
- biology
- medical
license: mit
library_name: open_clip
widget:
- src: https://huggingface.co/microsoft/BiomedCLIP-PubMedBERT_256-vit_base_patch16_224/resolve/main/example_data/biomed_image_classification_example_data/squamous_cell_carcinoma_histopathology.jpeg
candidate_labels: adenocarcinoma histopathology, squamous cell carcinoma histopathology
example_title: squamous cell carcinoma histopathology
- src: >-
https://huggingface.co/microsoft/BiomedCLIP-PubMedBERT_256-vit_base_patch16_224/resolve/main/example_data/biomed_image_classification_example_data/adenocarcinoma_histopathology.jpg
candidate_labels: adenocarcinoma histopathology, squamous cell carcinoma histopathology
example_title: adenocarcinoma histopathology
- src: >-
https://upload.wikimedia.org/wikipedia/commons/5/57/Left-sided_Pleural_Effusion.jpg
candidate_labels: left-sided pleural effusion chest x-ray, right-sided pleural effusion chest x-ray, normal chest x-ray
example_title: left-sided pleural effusion chest x-ray
pipeline_tag: zero-shot-image-classification
---
# BiomedCLIP-PubMedBERT_256-vit_base_patch16_224
[BiomedCLIP](https://aka.ms/biomedclip-paper) is a biomedical vision-language foundation model that is pretrained on [PMC-15M](https://aka.ms/biomedclip-paper), a dataset of 15 million figure-caption pairs extracted from biomedical research articles in PubMed Central, using contrastive learning.
It uses PubMedBERT as the text encoder and Vision Transformer as the image encoder, with domain-specific adaptations.
It can perform various vision-language processing (VLP) tasks such as cross-modal retrieval, image classification, and visual question answering.
BiomedCLIP establishes new state of the art in a wide range of standard datasets, and substantially outperforms prior VLP approaches:

## Citation
```bibtex
@misc{https://doi.org/10.48550/arXiv.2303.00915,
doi = {10.48550/ARXIV.2303.00915},
url = {https://arxiv.org/abs/2303.00915},
author = {Zhang, Sheng and Xu, Yanbo and Usuyama, Naoto and Bagga, Jaspreet and Tinn, Robert and Preston, Sam and Rao, Rajesh and Wei, Mu and Valluri, Naveen and Wong, Cliff and Lungren, Matthew and Naumann, Tristan and Poon, Hoifung},
title = {Large-Scale Domain-Specific Pretraining for Biomedical Vision-Language Processing},
publisher = {arXiv},
year = {2023},
}
```
## Model Use
### 1. Environment
```bash
conda create -n biomedclip python=3.10 -y
conda activate biomedclip
pip install open_clip_torch==2.23.0 transformers==4.35.2 matplotlib
```
### 2.1 Load from HF hub
```python
import torch
from urllib.request import urlopen
from PIL import Image
from open_clip import create_model_from_pretrained, get_tokenizer
# Load the model and config files from the Hugging Face Hub
model, preprocess = create_model_from_pretrained('hf-hub:microsoft/BiomedCLIP-PubMedBERT_256-vit_base_patch16_224')
tokenizer = get_tokenizer('hf-hub:microsoft/BiomedCLIP-PubMedBERT_256-vit_base_patch16_224')
# Zero-shot image classification
template = 'this is a photo of '
labels = [
'adenocarcinoma histopathology',
'brain MRI',
'covid line chart',
'squamous cell carcinoma histopathology',
'immunohistochemistry histopathology',
'bone X-ray',
'chest X-ray',
'pie chart',
'hematoxylin and eosin histopathology'
]
dataset_url = 'https://huggingface.co/microsoft/BiomedCLIP-PubMedBERT_256-vit_base_patch16_224/resolve/main/example_data/biomed_image_classification_example_data/'
test_imgs = [
'squamous_cell_carcinoma_histopathology.jpeg',
'H_and_E_histopathology.jpg',
'bone_X-ray.jpg',
'adenocarcinoma_histopathology.jpg',
'covid_line_chart.png',
'IHC_histopathology.jpg',
'chest_X-ray.jpg',
'brain_MRI.jpg',
'pie_chart.png'
]
device = torch.device('cuda') if torch.cuda.is_available() else torch.device('cpu')
model.to(device)
model.eval()
context_length = 256
images = torch.stack([preprocess(Image.open(urlopen(dataset_url + img))) for img in test_imgs]).to(device)
texts = tokenizer([template + l for l in labels], context_length=context_length).to(device)
with torch.no_grad():
image_features, text_features, logit_scale = model(images, texts)
logits = (logit_scale * image_features @ text_features.t()).detach().softmax(dim=-1)
sorted_indices = torch.argsort(logits, dim=-1, descending=True)
logits = logits.cpu().numpy()
sorted_indices = sorted_indices.cpu().numpy()
top_k = -1
for i, img in enumerate(test_imgs):
pred = labels[sorted_indices[i][0]]
top_k = len(labels) if top_k == -1 else top_k
print(img.split('/')[-1] + ':')
for j in range(top_k):
jth_index = sorted_indices[i][j]
print(f'{labels[jth_index]}: {logits[i][jth_index]}')
print('\n')
```
### 2.2 Load from local files
```python
import json
from urllib.request import urlopen
from PIL import Image
import torch
from huggingface_hub import hf_hub_download
from open_clip import create_model_and_transforms, get_tokenizer
from open_clip.factory import HF_HUB_PREFIX, _MODEL_CONFIGS
# Download the model and config files
hf_hub_download(
repo_id="microsoft/BiomedCLIP-PubMedBERT_256-vit_base_patch16_224",
filename="open_clip_pytorch_model.bin",
local_dir="checkpoints"
)
hf_hub_download(
repo_id="microsoft/BiomedCLIP-PubMedBERT_256-vit_base_patch16_224",
filename="open_clip_config.json",
local_dir="checkpoints"
)
# Load the model and config files
model_name = "biomedclip_local"
with open("checkpoints/open_clip_config.json", "r") as f:
config = json.load(f)
model_cfg = config["model_cfg"]
preprocess_cfg = config["preprocess_cfg"]
if (not model_name.startswith(HF_HUB_PREFIX)
and model_name not in _MODEL_CONFIGS
and config is not None):
_MODEL_CONFIGS[model_name] = model_cfg
tokenizer = get_tokenizer(model_name)
model, _, preprocess = create_model_and_transforms(
model_name=model_name,
pretrained="checkpoints/open_clip_pytorch_model.bin",
**{f"image_{k}": v for k, v in preprocess_cfg.items()},
)
# Zero-shot image classification
template = 'this is a photo of '
labels = [
'adenocarcinoma histopathology',
'brain MRI',
'covid line chart',
'squamous cell carcinoma histopathology',
'immunohistochemistry histopathology',
'bone X-ray',
'chest X-ray',
'pie chart',
'hematoxylin and eosin histopathology'
]
dataset_url = 'https://huggingface.co/microsoft/BiomedCLIP-PubMedBERT_256-vit_base_patch16_224/resolve/main/example_data/biomed_image_classification_example_data/'
test_imgs = [
'squamous_cell_carcinoma_histopathology.jpeg',
'H_and_E_histopathology.jpg',
'bone_X-ray.jpg',
'adenocarcinoma_histopathology.jpg',
'covid_line_chart.png',
'IHC_histopathology.jpg',
'chest_X-ray.jpg',
'brain_MRI.jpg',
'pie_chart.png'
]
device = torch.device('cuda') if torch.cuda.is_available() else torch.device('cpu')
model.to(device)
model.eval()
context_length = 256
images = torch.stack([preprocess(Image.open(urlopen(dataset_url + img))) for img in test_imgs]).to(device)
texts = tokenizer([template + l for l in labels], context_length=context_length).to(device)
with torch.no_grad():
image_features, text_features, logit_scale = model(images, texts)
logits = (logit_scale * image_features @ text_features.t()).detach().softmax(dim=-1)
sorted_indices = torch.argsort(logits, dim=-1, descending=True)
logits = logits.cpu().numpy()
sorted_indices = sorted_indices.cpu().numpy()
top_k = -1
for i, img in enumerate(test_imgs):
pred = labels[sorted_indices[i][0]]
top_k = len(labels) if top_k == -1 else top_k
print(img.split('/')[-1] + ':')
for j in range(top_k):
jth_index = sorted_indices[i][j]
print(f'{labels[jth_index]}: {logits[i][jth_index]}')
print('\n')
```
### Use in Jupyter Notebook
Please refer to this [example notebook](https://aka.ms/biomedclip-example-notebook).
### Intended Use
This model is intended to be used solely for (I) future research on visual-language processing and (II) reproducibility of the experimental results reported in the reference paper.
#### Primary Intended Use
The primary intended use is to support AI researchers building on top of this work. BiomedCLIP and its associated models should be helpful for exploring various biomedical VLP research questions, especially in the radiology domain.
#### Out-of-Scope Use
**Any** deployed use case of the model --- commercial or otherwise --- is currently out of scope. Although we evaluated the models using a broad set of publicly-available research benchmarks, the models and evaluations are not intended for deployed use cases. Please refer to [the associated paper](https://aka.ms/biomedclip-paper) for more details.
## Data
This model builds upon [PMC-15M dataset](https://aka.ms/biomedclip-paper), which is a large-scale parallel image-text dataset for biomedical vision-language processing. It contains 15 million figure-caption pairs extracted from biomedical research articles in PubMed Central. It covers a diverse range of biomedical image types, such as microscopy, radiography, histology, and more.
## Limitations
This model was developed using English corpora, and thus can be considered English-only.
## Further information
Please refer to the corresponding paper, ["Large-Scale Domain-Specific Pretraining for Biomedical Vision-Language Processing"](https://aka.ms/biomedclip-paper) for additional details on the model training and evaluation. |
lucadiliello/BLEURT-20-D12 | lucadiliello | "2023-01-19T15:55:33Z" | 502,603 | 0 | transformers | [
"transformers",
"pytorch",
"bleurt",
"text-classification",
"autotrain_compatible",
"endpoints_compatible",
"region:us"
] | text-classification | "2023-01-19T15:18:25Z" | This model is based on a custom Transformer model that can be installed with:
```bash
pip install git+https://github.com/lucadiliello/bleurt-pytorch.git
```
Now load the model and make predictions with:
```python
import torch
from bleurt_pytorch import BleurtConfig, BleurtForSequenceClassification, BleurtTokenizer
config = BleurtConfig.from_pretrained('lucadiliello/BLEURT-20-D12')
model = BleurtForSequenceClassification.from_pretrained('lucadiliello/BLEURT-20-D12')
tokenizer = BleurtTokenizer.from_pretrained('lucadiliello/BLEURT-20-D12')
references = ["a bird chirps by the window", "this is a random sentence"]
candidates = ["a bird chirps by the window", "this looks like a random sentence"]
model.eval()
with torch.no_grad():
inputs = tokenizer(references, candidates, padding='longest', return_tensors='pt')
res = model(**inputs).logits.flatten().tolist()
print(res)
# [0.9604414105415344, 0.8080050349235535]
```
Take a look at this [repository](https://github.com/lucadiliello/bleurt-pytorch) for the definition of `BleurtConfig`, `BleurtForSequenceClassification` and `BleurtTokenizer` in PyTorch. |
Qwen/Qwen2.5-7B-Instruct | Qwen | "2024-09-25T12:33:14Z" | 501,137 | 266 | transformers | [
"transformers",
"safetensors",
"qwen2",
"text-generation",
"chat",
"conversational",
"en",
"arxiv:2309.00071",
"arxiv:2407.10671",
"base_model:Qwen/Qwen2.5-7B",
"base_model:finetune:Qwen/Qwen2.5-7B",
"license:apache-2.0",
"autotrain_compatible",
"text-generation-inference",
"endpoints_compatible",
"region:us"
] | text-generation | "2024-09-16T11:55:40Z" | ---
license: apache-2.0
license_link: https://huggingface.co/Qwen/Qwen2.5-7B-Instruct/blob/main/LICENSE
language:
- en
pipeline_tag: text-generation
base_model: Qwen/Qwen2.5-7B
tags:
- chat
library_name: transformers
---
# Qwen2.5-7B-Instruct
## Introduction
Qwen2.5 is the latest series of Qwen large language models. For Qwen2.5, we release a number of base language models and instruction-tuned language models ranging from 0.5 to 72 billion parameters. Qwen2.5 brings the following improvements upon Qwen2:
- Significantly **more knowledge** and has greatly improved capabilities in **coding** and **mathematics**, thanks to our specialized expert models in these domains.
- Significant improvements in **instruction following**, **generating long texts** (over 8K tokens), **understanding structured data** (e.g, tables), and **generating structured outputs** especially JSON. **More resilient to the diversity of system prompts**, enhancing role-play implementation and condition-setting for chatbots.
- **Long-context Support** up to 128K tokens and can generate up to 8K tokens.
- **Multilingual support** for over 29 languages, including Chinese, English, French, Spanish, Portuguese, German, Italian, Russian, Japanese, Korean, Vietnamese, Thai, Arabic, and more.
**This repo contains the instruction-tuned 7B Qwen2.5 model**, which has the following features:
- Type: Causal Language Models
- Training Stage: Pretraining & Post-training
- Architecture: transformers with RoPE, SwiGLU, RMSNorm, and Attention QKV bias
- Number of Parameters: 7.61B
- Number of Paramaters (Non-Embedding): 6.53B
- Number of Layers: 28
- Number of Attention Heads (GQA): 28 for Q and 4 for KV
- Context Length: Full 131,072 tokens and generation 8192 tokens
- Please refer to [this section](#processing-long-texts) for detailed instructions on how to deploy Qwen2.5 for handling long texts.
For more details, please refer to our [blog](https://qwenlm.github.io/blog/qwen2.5/), [GitHub](https://github.com/QwenLM/Qwen2.5), and [Documentation](https://qwen.readthedocs.io/en/latest/).
## Requirements
The code of Qwen2.5 has been in the latest Hugging face `transformers` and we advise you to use the latest version of `transformers`.
With `transformers<4.37.0`, you will encounter the following error:
```
KeyError: 'qwen2'
```
## Quickstart
Here provides a code snippet with `apply_chat_template` to show you how to load the tokenizer and model and how to generate contents.
```python
from transformers import AutoModelForCausalLM, AutoTokenizer
model_name = "Qwen/Qwen2.5-7B-Instruct"
model = AutoModelForCausalLM.from_pretrained(
model_name,
torch_dtype="auto",
device_map="auto"
)
tokenizer = AutoTokenizer.from_pretrained(model_name)
prompt = "Give me a short introduction to large language model."
messages = [
{"role": "system", "content": "You are Qwen, created by Alibaba Cloud. You are a helpful assistant."},
{"role": "user", "content": prompt}
]
text = tokenizer.apply_chat_template(
messages,
tokenize=False,
add_generation_prompt=True
)
model_inputs = tokenizer([text], return_tensors="pt").to(model.device)
generated_ids = model.generate(
**model_inputs,
max_new_tokens=512
)
generated_ids = [
output_ids[len(input_ids):] for input_ids, output_ids in zip(model_inputs.input_ids, generated_ids)
]
response = tokenizer.batch_decode(generated_ids, skip_special_tokens=True)[0]
```
### Processing Long Texts
The current `config.json` is set for context length up to 32,768 tokens.
To handle extensive inputs exceeding 32,768 tokens, we utilize [YaRN](https://arxiv.org/abs/2309.00071), a technique for enhancing model length extrapolation, ensuring optimal performance on lengthy texts.
For supported frameworks, you could add the following to `config.json` to enable YaRN:
```json
{
...,
"rope_scaling": {
"factor": 4.0,
"original_max_position_embeddings": 32768,
"type": "yarn"
}
}
```
For deployment, we recommend using vLLM.
Please refer to our [Documentation](https://qwen.readthedocs.io/en/latest/deployment/vllm.html) for usage if you are not familar with vLLM.
Presently, vLLM only supports static YARN, which means the scaling factor remains constant regardless of input length, **potentially impacting performance on shorter texts**.
We advise adding the `rope_scaling` configuration only when processing long contexts is required.
## Evaluation & Performance
Detailed evaluation results are reported in this [📑 blog](https://qwenlm.github.io/blog/qwen2.5/).
For requirements on GPU memory and the respective throughput, see results [here](https://qwen.readthedocs.io/en/latest/benchmark/speed_benchmark.html).
## Citation
If you find our work helpful, feel free to give us a cite.
```
@misc{qwen2.5,
title = {Qwen2.5: A Party of Foundation Models},
url = {https://qwenlm.github.io/blog/qwen2.5/},
author = {Qwen Team},
month = {September},
year = {2024}
}
@article{qwen2,
title={Qwen2 Technical Report},
author={An Yang and Baosong Yang and Binyuan Hui and Bo Zheng and Bowen Yu and Chang Zhou and Chengpeng Li and Chengyuan Li and Dayiheng Liu and Fei Huang and Guanting Dong and Haoran Wei and Huan Lin and Jialong Tang and Jialin Wang and Jian Yang and Jianhong Tu and Jianwei Zhang and Jianxin Ma and Jin Xu and Jingren Zhou and Jinze Bai and Jinzheng He and Junyang Lin and Kai Dang and Keming Lu and Keqin Chen and Kexin Yang and Mei Li and Mingfeng Xue and Na Ni and Pei Zhang and Peng Wang and Ru Peng and Rui Men and Ruize Gao and Runji Lin and Shijie Wang and Shuai Bai and Sinan Tan and Tianhang Zhu and Tianhao Li and Tianyu Liu and Wenbin Ge and Xiaodong Deng and Xiaohuan Zhou and Xingzhang Ren and Xinyu Zhang and Xipin Wei and Xuancheng Ren and Yang Fan and Yang Yao and Yichang Zhang and Yu Wan and Yunfei Chu and Yuqiong Liu and Zeyu Cui and Zhenru Zhang and Zhihao Fan},
journal={arXiv preprint arXiv:2407.10671},
year={2024}
}
``` |
Orenguteng/Llama-3-8B-Lexi-Uncensored | Orenguteng | "2024-05-27T06:16:40Z" | 500,682 | 170 | transformers | [
"transformers",
"safetensors",
"llama",
"text-generation",
"uncensored",
"llama3",
"instruct",
"open",
"conversational",
"license:llama3",
"model-index",
"autotrain_compatible",
"text-generation-inference",
"endpoints_compatible",
"region:us"
] | text-generation | "2024-04-23T21:14:40Z" | ---
license: llama3
tags:
- uncensored
- llama3
- instruct
- open
model-index:
- name: Llama-3-8B-Lexi-Uncensored
results:
- task:
type: text-generation
name: Text Generation
dataset:
name: AI2 Reasoning Challenge (25-Shot)
type: ai2_arc
config: ARC-Challenge
split: test
args:
num_few_shot: 25
metrics:
- type: acc_norm
value: 59.56
name: normalized accuracy
source:
url: https://huggingface.co/spaces/HuggingFaceH4/open_llm_leaderboard?query=Orenguteng/Llama-3-8B-Lexi-Uncensored
name: Open LLM Leaderboard
- task:
type: text-generation
name: Text Generation
dataset:
name: HellaSwag (10-Shot)
type: hellaswag
split: validation
args:
num_few_shot: 10
metrics:
- type: acc_norm
value: 77.88
name: normalized accuracy
source:
url: https://huggingface.co/spaces/HuggingFaceH4/open_llm_leaderboard?query=Orenguteng/Llama-3-8B-Lexi-Uncensored
name: Open LLM Leaderboard
- task:
type: text-generation
name: Text Generation
dataset:
name: MMLU (5-Shot)
type: cais/mmlu
config: all
split: test
args:
num_few_shot: 5
metrics:
- type: acc
value: 67.68
name: accuracy
source:
url: https://huggingface.co/spaces/HuggingFaceH4/open_llm_leaderboard?query=Orenguteng/Llama-3-8B-Lexi-Uncensored
name: Open LLM Leaderboard
- task:
type: text-generation
name: Text Generation
dataset:
name: TruthfulQA (0-shot)
type: truthful_qa
config: multiple_choice
split: validation
args:
num_few_shot: 0
metrics:
- type: mc2
value: 47.72
source:
url: https://huggingface.co/spaces/HuggingFaceH4/open_llm_leaderboard?query=Orenguteng/Llama-3-8B-Lexi-Uncensored
name: Open LLM Leaderboard
- task:
type: text-generation
name: Text Generation
dataset:
name: Winogrande (5-shot)
type: winogrande
config: winogrande_xl
split: validation
args:
num_few_shot: 5
metrics:
- type: acc
value: 75.85
name: accuracy
source:
url: https://huggingface.co/spaces/HuggingFaceH4/open_llm_leaderboard?query=Orenguteng/Llama-3-8B-Lexi-Uncensored
name: Open LLM Leaderboard
- task:
type: text-generation
name: Text Generation
dataset:
name: GSM8k (5-shot)
type: gsm8k
config: main
split: test
args:
num_few_shot: 5
metrics:
- type: acc
value: 68.39
name: accuracy
source:
url: https://huggingface.co/spaces/HuggingFaceH4/open_llm_leaderboard?query=Orenguteng/Llama-3-8B-Lexi-Uncensored
name: Open LLM Leaderboard
---

This model is based on Llama-3-8b-Instruct, and is governed by [META LLAMA 3 COMMUNITY LICENSE AGREEMENT](https://llama.meta.com/llama3/license/)
Lexi is uncensored, which makes the model compliant. You are advised to implement your own alignment layer before exposing the model as a service. It will be highly compliant with any requests, even unethical ones.
You are responsible for any content you create using this model. Please use it responsibly.
Lexi is licensed according to Meta's Llama license. I grant permission for any use, including commercial, that falls within accordance with Meta's Llama-3 license.
# [Open LLM Leaderboard Evaluation Results](https://huggingface.co/spaces/HuggingFaceH4/open_llm_leaderboard)
Detailed results can be found [here](https://huggingface.co/datasets/open-llm-leaderboard/details_Orenguteng__Llama-3-8B-Lexi-Uncensored)
| Metric |Value|
|---------------------------------|----:|
|Avg. |66.18|
|AI2 Reasoning Challenge (25-Shot)|59.56|
|HellaSwag (10-Shot) |77.88|
|MMLU (5-Shot) |67.68|
|TruthfulQA (0-shot) |47.72|
|Winogrande (5-shot) |75.85|
|GSM8k (5-shot) |68.39|
|
facebook/encodec_24khz | facebook | "2023-07-25T11:28:04Z" | 500,374 | 41 | transformers | [
"transformers",
"pytorch",
"safetensors",
"encodec",
"feature-extraction",
"arxiv:2210.13438",
"region:us"
] | feature-extraction | "2023-06-12T16:10:36Z" | ---
inference: false
---

# Model Card for EnCodec
This model card provides details and information about EnCodec, a state-of-the-art real-time audio codec developed by Meta AI.
## Model Details
### Model Description
EnCodec is a high-fidelity audio codec leveraging neural networks. It introduces a streaming encoder-decoder architecture with quantized latent space, trained in an end-to-end fashion.
The model simplifies and speeds up training using a single multiscale spectrogram adversary that efficiently reduces artifacts and produces high-quality samples.
It also includes a novel loss balancer mechanism that stabilizes training by decoupling the choice of hyperparameters from the typical scale of the loss.
Additionally, lightweight Transformer models are used to further compress the obtained representation while maintaining real-time performance.
- **Developed by:** Meta AI
- **Model type:** Audio Codec
### Model Sources
- **Repository:** [GitHub Repository](https://github.com/facebookresearch/encodec)
- **Paper:** [EnCodec: End-to-End Neural Audio Codec](https://arxiv.org/abs/2210.13438)
## Uses
<!-- Address questions around how the model is intended to be used, including the foreseeable users of the model and those affected by the model. -->
### Direct Use
<!-- This section is for the model use without fine-tuning or plugging into a larger ecosystem/app. -->
EnCodec can be used directly as an audio codec for real-time compression and decompression of audio signals.
It provides high-quality audio compression and efficient decoding. The model was trained on various bandwiths, which can be specified when encoding (compressing) and decoding (decompressing).
Two different setup exist for EnCodec:
- Non-streamable: the input audio is split into chunks of 1 seconds, with an overlap of 10 ms, which are then encoded.
- Streamable: weight normalizationis used on the convolution layers, and the input is not split into chunks but rather padded on the left.
### Downstream Use
EnCodec can be fine-tuned for specific audio tasks or integrated into larger audio processing pipelines for applications such as speech generation,
music generation, or text to speech tasks.
<!-- This section is for the model use when fine-tuned for a task, or when plugged into a larger ecosystem/app -->
[More Information Needed]
## How to Get Started with the Model
Use the following code to get started with the EnCodec model using a dummy example from the LibriSpeech dataset (~9MB). First, install the required Python packages:
```
pip install --upgrade pip
pip install --upgrade datasets[audio]
pip install git+https://github.com/huggingface/transformers.git@main
```
Then load an audio sample, and run a forward pass of the model:
```python
from datasets import load_dataset, Audio
from transformers import EncodecModel, AutoProcessor
# load a demonstration datasets
librispeech_dummy = load_dataset("hf-internal-testing/librispeech_asr_dummy", "clean", split="validation")
# load the model + processor (for pre-processing the audio)
model = EncodecModel.from_pretrained("facebook/encodec_24khz")
processor = AutoProcessor.from_pretrained("facebook/encodec_24khz")
# cast the audio data to the correct sampling rate for the model
librispeech_dummy = librispeech_dummy.cast_column("audio", Audio(sampling_rate=processor.sampling_rate))
audio_sample = librispeech_dummy[0]["audio"]["array"]
# pre-process the inputs
inputs = processor(raw_audio=audio_sample, sampling_rate=processor.sampling_rate, return_tensors="pt")
# explicitly encode then decode the audio inputs
encoder_outputs = model.encode(inputs["input_values"], inputs["padding_mask"])
audio_values = model.decode(encoder_outputs.audio_codes, encoder_outputs.audio_scales, inputs["padding_mask"])[0]
# or the equivalent with a forward pass
audio_values = model(inputs["input_values"], inputs["padding_mask"]).audio_values
```
## Training Details
The model was trained for 300 epochs, with one epoch being 2,000 updates with the Adam optimizer with a batch size of 64 examples of 1 second each, a learning rate of 3 · 10−4
, β1 = 0.5, and β2 = 0.9. All the models are traind using 8 A100 GPUs.
### Training Data
<!-- This should link to a Data Card, perhaps with a short stub of information on what the training data is all about as well as documentation related to data pre-processing or additional filtering. -->
- For speech:
- DNS Challenge 4
- [Common Voice](https://huggingface.co/datasets/common_voice)
- For general audio:
- [AudioSet](https://huggingface.co/datasets/Fhrozen/AudioSet2K22)
- [FSD50K](https://huggingface.co/datasets/Fhrozen/FSD50k)
- For music:
- [Jamendo dataset](https://huggingface.co/datasets/rkstgr/mtg-jamendo)
They used four different training strategies to sample for these datasets:
- (s1) sample a single source from Jamendo with probability 0.32;
- (s2) sample a single source from the other datasets with the same probability;
- (s3) mix two sources from all datasets with a probability of 0.24;
- (s4) mix three sources from all datasets except music with a probability of 0.12.
The audio is normalized by file and a random gain between -10 and 6 dB id applied.
## Evaluation
<!-- This section describes the evaluation protocols and provides the results. -->
### Subjectif metric for restoration:
This models was evalutated using the MUSHRA protocol (Series, 2014), using both a hidden reference and a low anchor. Annotators were recruited using a
crowd-sourcing platform, in which they were asked to rate the perceptual quality of the provided samples in
a range between 1 to 100. They randomly select 50 samples of 5 seconds from each category of the the test set
and force at least 10 annotations per samples. To filter noisy annotations and outliers we remove annotators
who rate the reference recordings less then 90 in at least 20% of the cases, or rate the low-anchor recording
above 80 more than 50% of the time.
### Objective metric for restoration:
The ViSQOL()ink) metric was used together with the Scale-Invariant Signal-to-Noise Ration (SI-SNR) (Luo & Mesgarani, 2019;
Nachmani et al., 2020; Chazan et al., 2021).
### Results
The results of the evaluation demonstrate the superiority of EnCodec compared to the baselines across different bandwidths (1.5, 3, 6, and 12 kbps).
When comparing EnCodec with the baselines at the same bandwidth, EnCodec consistently outperforms them in terms of MUSHRA score.
Notably, EnCodec achieves better performance, on average, at 3 kbps compared to Lyra-v2 at 6 kbps and Opus at 12 kbps.
Additionally, by incorporating the language model over the codes, it is possible to achieve a bandwidth reduction of approximately 25-40%.
For example, the bandwidth of the 3 kbps model can be reduced to 1.9 kbps.
#### Summary
EnCodec is a state-of-the-art real-time neural audio compression model that excels in producing high-fidelity audio samples at various sample rates and bandwidths.
The model's performance was evaluated across different settings, ranging from 24kHz monophonic at 1.5 kbps to 48kHz stereophonic, showcasing both subjective and
objective results. Notably, EnCodec incorporates a novel spectrogram-only adversarial loss, effectively reducing artifacts and enhancing sample quality.
Training stability and interpretability were further enhanced through the introduction of a gradient balancer for the loss weights.
Additionally, the study demonstrated that a compact Transformer model can be employed to achieve an additional bandwidth reduction of up to 40% without compromising
quality, particularly in applications where low latency is not critical (e.g., music streaming).
## Citation
<!-- If there is a paper or blog post introducing the model, the APA and Bibtex information for that should go in this section. -->
**BibTeX:**
```
@misc{défossez2022high,
title={High Fidelity Neural Audio Compression},
author={Alexandre Défossez and Jade Copet and Gabriel Synnaeve and Yossi Adi},
year={2022},
eprint={2210.13438},
archivePrefix={arXiv},
primaryClass={eess.AS}
}
```
|
Helsinki-NLP/opus-mt-ru-en | Helsinki-NLP | "2023-08-16T12:03:22Z" | 497,455 | 67 | transformers | [
"transformers",
"pytorch",
"tf",
"rust",
"marian",
"text2text-generation",
"translation",
"ru",
"en",
"license:cc-by-4.0",
"autotrain_compatible",
"endpoints_compatible",
"region:us"
] | translation | "2022-03-02T23:29:04Z" | ---
tags:
- translation
license: cc-by-4.0
---
### opus-mt-ru-en
## Table of Contents
- [Model Details](#model-details)
- [Uses](#uses)
- [Risks, Limitations and Biases](#risks-limitations-and-biases)
- [Training](#training)
- [Evaluation](#evaluation)
- [Citation Information](#citation-information)
- [How to Get Started With the Model](#how-to-get-started-with-the-model)
## Model Details
**Model Description:**
- **Developed by:** Language Technology Research Group at the University of Helsinki
- **Model Type:** Transformer-align
- **Language(s):**
- Source Language: Russian
- Target Language: English
- **License:** CC-BY-4.0
- **Resources for more information:**
- [GitHub Repo](https://github.com/Helsinki-NLP/OPUS-MT-train)
## Uses
#### Direct Use
This model can be used for translation and text-to-text generation.
## Risks, Limitations and Biases
**CONTENT WARNING: Readers should be aware this section contains content that is disturbing, offensive, and can propagate historical and current stereotypes.**
Significant research has explored bias and fairness issues with language models (see, e.g., [Sheng et al. (2021)](https://aclanthology.org/2021.acl-long.330.pdf) and [Bender et al. (2021)](https://dl.acm.org/doi/pdf/10.1145/3442188.3445922)).
Further details about the dataset for this model can be found in the OPUS readme: [ru-en](https://github.com/Helsinki-NLP/OPUS-MT-train/blob/master/models/ru-en/README.md)
## Training
#### Training Data
##### Preprocessing
* Pre-processing: Normalization + SentencePiece
* Dataset: [opus](https://github.com/Helsinki-NLP/Opus-MT)
* Download original weights: [opus-2020-02-26.zip](https://object.pouta.csc.fi/OPUS-MT-models/ru-en/opus-2020-02-26.zip)
* Test set translations: [opus-2020-02-26.test.txt](https://object.pouta.csc.fi/OPUS-MT-models/ru-en/opus-2020-02-26.test.txt)
## Evaluation
#### Results
* test set scores: [opus-2020-02-26.eval.txt](https://object.pouta.csc.fi/OPUS-MT-models/ru-en/opus-2020-02-26.eval.txt)
#### Benchmarks
| testset | BLEU | chr-F |
|-----------------------|-------|-------|
| newstest2012.ru.en | 34.8 | 0.603 |
| newstest2013.ru.en | 27.9 | 0.545 |
| newstest2014-ruen.ru.en | 31.9 | 0.591 |
| newstest2015-enru.ru.en | 30.4 | 0.568 |
| newstest2016-enru.ru.en | 30.1 | 0.565 |
| newstest2017-enru.ru.en | 33.4 | 0.593 |
| newstest2018-enru.ru.en | 29.6 | 0.565 |
| newstest2019-ruen.ru.en | 31.4 | 0.576 |
| Tatoeba.ru.en | 61.1 | 0.736 |
## Citation Information
```bibtex
@InProceedings{TiedemannThottingal:EAMT2020,
author = {J{\"o}rg Tiedemann and Santhosh Thottingal},
title = {{OPUS-MT} — {B}uilding open translation services for the {W}orld},
booktitle = {Proceedings of the 22nd Annual Conferenec of the European Association for Machine Translation (EAMT)},
year = {2020},
address = {Lisbon, Portugal}
}
```
## How to Get Started With the Model
```python
from transformers import AutoTokenizer, AutoModelForSeq2SeqLM
tokenizer = AutoTokenizer.from_pretrained("Helsinki-NLP/opus-mt-ru-en")
model = AutoModelForSeq2SeqLM.from_pretrained("Helsinki-NLP/opus-mt-ru-en")
```
|
h94/IP-Adapter-FaceID | h94 | "2024-04-16T08:53:12Z" | 497,310 | 1,587 | diffusers | [
"diffusers",
"text-to-image",
"stable-diffusion",
"en",
"arxiv:2308.06721",
"region:us"
] | text-to-image | "2023-12-20T03:15:30Z" | ---
tags:
- text-to-image
- stable-diffusion
language:
- en
library_name: diffusers
---
# IP-Adapter-FaceID Model Card
<div align="center">
[**Project Page**](https://ip-adapter.github.io) **|** [**Paper (ArXiv)**](https://arxiv.org/abs/2308.06721) **|** [**Code**](https://github.com/tencent-ailab/IP-Adapter)
</div>
---
## Introduction
An experimental version of IP-Adapter-FaceID: we use face ID embedding from a face recognition model instead of CLIP image embedding, additionally, we use LoRA to improve ID consistency. IP-Adapter-FaceID can generate various style images conditioned on a face with only text prompts.

**Update 2023/12/27**:
IP-Adapter-FaceID-Plus: face ID embedding (for face ID) + CLIP image embedding (for face structure)
<div align="center">

</div>
**Update 2023/12/28**:
IP-Adapter-FaceID-PlusV2: face ID embedding (for face ID) + controllable CLIP image embedding (for face structure)
You can adjust the weight of the face structure to get different generation!
<div align="center">

</div>
**Update 2024/01/04**:
IP-Adapter-FaceID-SDXL: An experimental SDXL version of IP-Adapter-FaceID
<div align="center">

</div>
**Update 2024/01/17**:
IP-Adapter-FaceID-PlusV2-SDXL: An experimental SDXL version of IP-Adapter-FaceID-PlusV2
**Update 2024/01/19**:
IP-Adapter-FaceID-Portrait: same with IP-Adapter-FaceID but for portrait generation (no lora! no controlnet!). Specifically, it accepts multiple facial images to enhance similarity (the default is 5).
<div align="center">

</div>
## Usage
### IP-Adapter-FaceID
Firstly, you should use [insightface](https://github.com/deepinsight/insightface) to extract face ID embedding:
```python
import cv2
from insightface.app import FaceAnalysis
import torch
app = FaceAnalysis(name="buffalo_l", providers=['CUDAExecutionProvider', 'CPUExecutionProvider'])
app.prepare(ctx_id=0, det_size=(640, 640))
image = cv2.imread("person.jpg")
faces = app.get(image)
faceid_embeds = torch.from_numpy(faces[0].normed_embedding).unsqueeze(0)
```
Then, you can generate images conditioned on the face embeddings:
```python
import torch
from diffusers import StableDiffusionPipeline, DDIMScheduler, AutoencoderKL
from PIL import Image
from ip_adapter.ip_adapter_faceid import IPAdapterFaceID
base_model_path = "SG161222/Realistic_Vision_V4.0_noVAE"
vae_model_path = "stabilityai/sd-vae-ft-mse"
ip_ckpt = "ip-adapter-faceid_sd15.bin"
device = "cuda"
noise_scheduler = DDIMScheduler(
num_train_timesteps=1000,
beta_start=0.00085,
beta_end=0.012,
beta_schedule="scaled_linear",
clip_sample=False,
set_alpha_to_one=False,
steps_offset=1,
)
vae = AutoencoderKL.from_pretrained(vae_model_path).to(dtype=torch.float16)
pipe = StableDiffusionPipeline.from_pretrained(
base_model_path,
torch_dtype=torch.float16,
scheduler=noise_scheduler,
vae=vae,
feature_extractor=None,
safety_checker=None
)
# load ip-adapter
ip_model = IPAdapterFaceID(pipe, ip_ckpt, device)
# generate image
prompt = "photo of a woman in red dress in a garden"
negative_prompt = "monochrome, lowres, bad anatomy, worst quality, low quality, blurry"
images = ip_model.generate(
prompt=prompt, negative_prompt=negative_prompt, faceid_embeds=faceid_embeds, num_samples=4, width=512, height=768, num_inference_steps=30, seed=2023
)
```
you can also use a normal IP-Adapter and a normal LoRA to load model:
```python
import torch
from diffusers import StableDiffusionPipeline, DDIMScheduler, AutoencoderKL
from PIL import Image
from ip_adapter.ip_adapter_faceid_separate import IPAdapterFaceID
base_model_path = "SG161222/Realistic_Vision_V4.0_noVAE"
vae_model_path = "stabilityai/sd-vae-ft-mse"
ip_ckpt = "ip-adapter-faceid_sd15.bin"
lora_ckpt = "ip-adapter-faceid_sd15_lora.safetensors"
device = "cuda"
noise_scheduler = DDIMScheduler(
num_train_timesteps=1000,
beta_start=0.00085,
beta_end=0.012,
beta_schedule="scaled_linear",
clip_sample=False,
set_alpha_to_one=False,
steps_offset=1,
)
vae = AutoencoderKL.from_pretrained(vae_model_path).to(dtype=torch.float16)
pipe = StableDiffusionPipeline.from_pretrained(
base_model_path,
torch_dtype=torch.float16,
scheduler=noise_scheduler,
vae=vae,
feature_extractor=None,
safety_checker=None
)
# load lora and fuse
pipe.load_lora_weights(lora_ckpt)
pipe.fuse_lora()
# load ip-adapter
ip_model = IPAdapterFaceID(pipe, ip_ckpt, device)
# generate image
prompt = "photo of a woman in red dress in a garden"
negative_prompt = "monochrome, lowres, bad anatomy, worst quality, low quality, blurry"
images = ip_model.generate(
prompt=prompt, negative_prompt=negative_prompt, faceid_embeds=faceid_embeds, num_samples=4, width=512, height=768, num_inference_steps=30, seed=2023
)
```
### IP-Adapter-FaceID-SDXL
Firstly, you should use [insightface](https://github.com/deepinsight/insightface) to extract face ID embedding:
```python
import cv2
from insightface.app import FaceAnalysis
import torch
app = FaceAnalysis(name="buffalo_l", providers=['CUDAExecutionProvider', 'CPUExecutionProvider'])
app.prepare(ctx_id=0, det_size=(640, 640))
image = cv2.imread("person.jpg")
faces = app.get(image)
faceid_embeds = torch.from_numpy(faces[0].normed_embedding).unsqueeze(0)
```
Then, you can generate images conditioned on the face embeddings:
```python
import torch
from diffusers import StableDiffusionXLPipeline, DDIMScheduler
from PIL import Image
from ip_adapter.ip_adapter_faceid import IPAdapterFaceIDXL
base_model_path = "SG161222/RealVisXL_V3.0"
ip_ckpt = "ip-adapter-faceid_sdxl.bin"
device = "cuda"
noise_scheduler = DDIMScheduler(
num_train_timesteps=1000,
beta_start=0.00085,
beta_end=0.012,
beta_schedule="scaled_linear",
clip_sample=False,
set_alpha_to_one=False,
steps_offset=1,
)
pipe = StableDiffusionXLPipeline.from_pretrained(
base_model_path,
torch_dtype=torch.float16,
scheduler=noise_scheduler,
add_watermarker=False,
)
# load ip-adapter
ip_model = IPAdapterFaceIDXL(pipe, ip_ckpt, device)
# generate image
prompt = "A closeup shot of a beautiful Asian teenage girl in a white dress wearing small silver earrings in the garden, under the soft morning light"
negative_prompt = "monochrome, lowres, bad anatomy, worst quality, low quality, blurry"
images = ip_model.generate(
prompt=prompt, negative_prompt=negative_prompt, faceid_embeds=faceid_embeds, num_samples=2,
width=1024, height=1024,
num_inference_steps=30, guidance_scale=7.5, seed=2023
)
```
### IP-Adapter-FaceID-Plus
Firstly, you should use [insightface](https://github.com/deepinsight/insightface) to extract face ID embedding and face image:
```python
import cv2
from insightface.app import FaceAnalysis
from insightface.utils import face_align
import torch
app = FaceAnalysis(name="buffalo_l", providers=['CUDAExecutionProvider', 'CPUExecutionProvider'])
app.prepare(ctx_id=0, det_size=(640, 640))
image = cv2.imread("person.jpg")
faces = app.get(image)
faceid_embeds = torch.from_numpy(faces[0].normed_embedding).unsqueeze(0)
face_image = face_align.norm_crop(image, landmark=faces[0].kps, image_size=224) # you can also segment the face
```
Then, you can generate images conditioned on the face embeddings:
```python
import torch
from diffusers import StableDiffusionPipeline, DDIMScheduler, AutoencoderKL
from PIL import Image
from ip_adapter.ip_adapter_faceid import IPAdapterFaceIDPlus
v2 = False
base_model_path = "SG161222/Realistic_Vision_V4.0_noVAE"
vae_model_path = "stabilityai/sd-vae-ft-mse"
image_encoder_path = "laion/CLIP-ViT-H-14-laion2B-s32B-b79K"
ip_ckpt = "ip-adapter-faceid-plus_sd15.bin" if not v2 else "ip-adapter-faceid-plusv2_sd15.bin"
device = "cuda"
noise_scheduler = DDIMScheduler(
num_train_timesteps=1000,
beta_start=0.00085,
beta_end=0.012,
beta_schedule="scaled_linear",
clip_sample=False,
set_alpha_to_one=False,
steps_offset=1,
)
vae = AutoencoderKL.from_pretrained(vae_model_path).to(dtype=torch.float16)
pipe = StableDiffusionPipeline.from_pretrained(
base_model_path,
torch_dtype=torch.float16,
scheduler=noise_scheduler,
vae=vae,
feature_extractor=None,
safety_checker=None
)
# load ip-adapter
ip_model = IPAdapterFaceIDPlus(pipe, image_encoder_path, ip_ckpt, device)
# generate image
prompt = "photo of a woman in red dress in a garden"
negative_prompt = "monochrome, lowres, bad anatomy, worst quality, low quality, blurry"
images = ip_model.generate(
prompt=prompt, negative_prompt=negative_prompt, face_image=face_image, faceid_embeds=faceid_embeds, shortcut=v2, s_scale=1.0,
num_samples=4, width=512, height=768, num_inference_steps=30, seed=2023
)
```
### IP-Adapter-FaceID-Portrait
```python
import cv2
from insightface.app import FaceAnalysis
import torch
app = FaceAnalysis(name="buffalo_l", providers=['CUDAExecutionProvider', 'CPUExecutionProvider'])
app.prepare(ctx_id=0, det_size=(640, 640))
images = ["1.jpg", "2.jpg", "3.jpg", "4.jpg", "5.jpg"]
faceid_embeds = []
for image in images:
image = cv2.imread("person.jpg")
faces = app.get(image)
faceid_embeds.append(torch.from_numpy(faces[0].normed_embedding).unsqueeze(0).unsqueeze(0))
faceid_embeds = torch.cat(faceid_embeds, dim=1)
```
```python
import torch
from diffusers import StableDiffusionPipeline, DDIMScheduler, AutoencoderKL
from PIL import Image
from ip_adapter.ip_adapter_faceid_separate import IPAdapterFaceID
base_model_path = "SG161222/Realistic_Vision_V4.0_noVAE"
vae_model_path = "stabilityai/sd-vae-ft-mse"
ip_ckpt = "ip-adapter-faceid-portrait_sd15.bin"
device = "cuda"
noise_scheduler = DDIMScheduler(
num_train_timesteps=1000,
beta_start=0.00085,
beta_end=0.012,
beta_schedule="scaled_linear",
clip_sample=False,
set_alpha_to_one=False,
steps_offset=1,
)
vae = AutoencoderKL.from_pretrained(vae_model_path).to(dtype=torch.float16)
pipe = StableDiffusionPipeline.from_pretrained(
base_model_path,
torch_dtype=torch.float16,
scheduler=noise_scheduler,
vae=vae,
feature_extractor=None,
safety_checker=None
)
# load ip-adapter
ip_model = IPAdapterFaceID(pipe, ip_ckpt, device, num_tokens=16, n_cond=5)
# generate image
prompt = "photo of a woman in red dress in a garden"
negative_prompt = "monochrome, lowres, bad anatomy, worst quality, low quality, blurry"
images = ip_model.generate(
prompt=prompt, negative_prompt=negative_prompt, faceid_embeds=faceid_embeds, num_samples=4, width=512, height=512, num_inference_steps=30, seed=2023
)
```
## Limitations and Bias
- The models do not achieve perfect photorealism and ID consistency.
- The generalization of the models is limited due to limitations of the training data, base model and face recognition model.
## Non-commercial use
**AS InsightFace pretrained models are available for non-commercial research purposes, IP-Adapter-FaceID models are released exclusively for research purposes and is not intended for commercial use.**
|
ghunkins/prompt-expansion | ghunkins | "2023-12-08T18:44:56Z" | 496,982 | 0 | transformers | [
"transformers",
"pytorch",
"gpt2",
"text-generation",
"license:creativeml-openrail-m",
"autotrain_compatible",
"text-generation-inference",
"endpoints_compatible",
"region:us"
] | text-generation | "2023-12-08T18:41:51Z" | ---
license: creativeml-openrail-m
---
|
microsoft/dit-base | microsoft | "2023-02-27T17:55:38Z" | 496,310 | 26 | transformers | [
"transformers",
"pytorch",
"beit",
"dit",
"arxiv:2203.02378",
"region:us"
] | null | "2022-03-07T17:18:46Z" | ---
tags:
- dit
inference: false
---
# Document Image Transformer (base-sized model)
Document Image Transformer (DiT) model pre-trained on IIT-CDIP (Lewis et al., 2006), a dataset that includes 42 million document images. It was introduced in the paper [DiT: Self-supervised Pre-training for Document Image Transformer](https://arxiv.org/abs/2203.02378) by Li et al. and first released in [this repository](https://github.com/microsoft/unilm/tree/master/dit). Note that DiT is identical to the architecture of [BEiT](https://huggingface.co/docs/transformers/model_doc/beit).
Disclaimer: The team releasing DiT did not write a model card for this model so this model card has been written by the Hugging Face team.
## Model description
The Document Image Transformer (DiT) is a transformer encoder model (BERT-like) pre-trained on a large collection of images in a self-supervised fashion. The pre-training objective for the model is to predict visual tokens from the encoder of a discrete VAE (dVAE), based on masked patches.
Images are presented to the model as a sequence of fixed-size patches (resolution 16x16), which are linearly embedded. One also adds absolute position embeddings before feeding the sequence to the layers of the Transformer encoder.
By pre-training the model, it learns an inner representation of images that can then be used to extract features useful for downstream tasks: if you have a dataset of labeled document images for instance, you can train a standard classifier by placing a linear layer on top of the pre-trained encoder.
## Intended uses & limitations
You can use the raw model for encoding document images into a vector space, but it's mostly meant to be fine-tuned on tasks like document image classification, table detection or document layout analysis. See the [model hub](https://huggingface.co/models?search=microsoft/dit) to look for fine-tuned versions on a task that interests you.
### How to use
Here is how to use this model in PyTorch:
```python
from transformers import BeitImageProcessor, BeitForMaskedImageModeling
import torch
from PIL import Image
image = Image.open('path_to_your_document_image').convert('RGB')
processor = BeitImageProcessor.from_pretrained("microsoft/dit-base")
model = BeitForMaskedImageModeling.from_pretrained("microsoft/dit-base")
num_patches = (model.config.image_size // model.config.patch_size) ** 2
pixel_values = processor(images=image, return_tensors="pt").pixel_values
# create random boolean mask of shape (batch_size, num_patches)
bool_masked_pos = torch.randint(low=0, high=2, size=(1, num_patches)).bool()
outputs = model(pixel_values, bool_masked_pos=bool_masked_pos)
loss, logits = outputs.loss, outputs.logits
```
### BibTeX entry and citation info
```bibtex
@article{Lewis2006BuildingAT,
title={Building a test collection for complex document information processing},
author={David D. Lewis and Gady Agam and Shlomo Engelson Argamon and Ophir Frieder and David A. Grossman and Jefferson Heard},
journal={Proceedings of the 29th annual international ACM SIGIR conference on Research and development in information retrieval},
year={2006}
}
``` |
distil-whisper/distil-medium.en | distil-whisper | "2024-03-25T12:07:23Z" | 495,583 | 119 | transformers | [
"transformers",
"pytorch",
"jax",
"tensorboard",
"onnx",
"safetensors",
"whisper",
"automatic-speech-recognition",
"audio",
"transformers.js",
"en",
"arxiv:2311.00430",
"arxiv:2210.13352",
"license:mit",
"endpoints_compatible",
"region:us"
] | automatic-speech-recognition | "2023-10-24T15:49:07Z" | ---
language:
- en
tags:
- audio
- automatic-speech-recognition
- transformers.js
widget:
- example_title: LibriSpeech sample 1
src: https://cdn-media.huggingface.co/speech_samples/sample1.flac
- example_title: LibriSpeech sample 2
src: https://cdn-media.huggingface.co/speech_samples/sample2.flac
pipeline_tag: automatic-speech-recognition
license: mit
library_name: transformers
---
# Distil-Whisper: distil-medium.en
Distil-Whisper was proposed in the paper [Robust Knowledge Distillation via Large-Scale Pseudo Labelling](https://arxiv.org/abs/2311.00430).
It is a distilled version of the Whisper model that is **6 times faster**, 49% smaller, and performs
**within 1% WER** on out-of-distribution evaluation sets. This is the repository for distil-medium.en,
a distilled variant of [Whisper medium.en](https://huggingface.co/openai/whisper-medium.en).
| Model | Params / M | Rel. Latency ↑ | Short-Form WER ↓ | Long-Form WER ↓ |
|----------------------------------------------------------------------------|------------|----------------|------------------|-----------------|
| [large-v3](https://huggingface.co/openai/whisper-large-v3) | 1550 | 1.0 | **8.4** | 11.0 |
| [large-v2](https://huggingface.co/openai/whisper-large-v2) | 1550 | 1.0 | 9.1 | 11.7 |
| | | | | |
| [distil-large-v3](https://huggingface.co/distil-whisper/distil-large-v3) | 756 | 6.3 | 9.7 | **10.8** |
| [distil-large-v2](https://huggingface.co/distil-whisper/distil-large-v2) | 756 | 5.8 | 10.1 | 11.6 |
| [distil-medium.en](https://huggingface.co/distil-whisper/distil-medium.en) | 394 | **6.8** | 11.1 | 12.4 |
| [distil-small.en](https://huggingface.co/distil-whisper/distil-small.en) | **166** | 5.6 | 12.1 | 12.8 |
**Note:** Distil-Whisper is currently only available for English speech recognition. We are working with the community
to distill Whisper on other languages. If you are interested in distilling Whisper in your language, check out the
provided [training code](https://github.com/huggingface/distil-whisper/tree/main/training). We will update the
[Distil-Whisper repository](https://github.com/huggingface/distil-whisper/) with multilingual checkpoints when ready!
## Usage
Distil-Whisper is supported in Hugging Face 🤗 Transformers from version 4.35 onwards. To run the model, first
install the latest version of the Transformers library. For this example, we'll also install 🤗 Datasets to load toy
audio dataset from the Hugging Face Hub:
```bash
pip install --upgrade pip
pip install --upgrade transformers accelerate datasets[audio]
```
### Short-Form Transcription
The model can be used with the [`pipeline`](https://huggingface.co/docs/transformers/main_classes/pipelines#transformers.AutomaticSpeechRecognitionPipeline)
class to transcribe short-form audio files (< 30-seconds) as follows:
```python
import torch
from transformers import AutoModelForSpeechSeq2Seq, AutoProcessor, pipeline
from datasets import load_dataset
device = "cuda:0" if torch.cuda.is_available() else "cpu"
torch_dtype = torch.float16 if torch.cuda.is_available() else torch.float32
model_id = "distil-whisper/distil-medium.en"
model = AutoModelForSpeechSeq2Seq.from_pretrained(
model_id, torch_dtype=torch_dtype, low_cpu_mem_usage=True, use_safetensors=True
)
model.to(device)
processor = AutoProcessor.from_pretrained(model_id)
pipe = pipeline(
"automatic-speech-recognition",
model=model,
tokenizer=processor.tokenizer,
feature_extractor=processor.feature_extractor,
max_new_tokens=128,
torch_dtype=torch_dtype,
device=device,
)
dataset = load_dataset("hf-internal-testing/librispeech_asr_dummy", "clean", split="validation")
sample = dataset[0]["audio"]
result = pipe(sample)
print(result["text"])
```
To transcribe a local audio file, simply pass the path to your audio file when you call the pipeline:
```diff
- result = pipe(sample)
+ result = pipe("audio.mp3")
```
### Long-Form Transcription
Distil-Whisper uses a chunked algorithm to transcribe long-form audio files (> 30-seconds). In practice, this chunked long-form algorithm
is 9x faster than the sequential algorithm proposed by OpenAI in the Whisper paper (see Table 7 of the [Distil-Whisper paper](https://arxiv.org/abs/2311.00430)).
To enable chunking, pass the `chunk_length_s` parameter to the `pipeline`. For Distil-Whisper, a chunk length of 15-seconds
is optimal. To activate batching, pass the argument `batch_size`:
```python
import torch
from transformers import AutoModelForSpeechSeq2Seq, AutoProcessor, pipeline
from datasets import load_dataset
device = "cuda:0" if torch.cuda.is_available() else "cpu"
torch_dtype = torch.float16 if torch.cuda.is_available() else torch.float32
model_id = "distil-whisper/distil-medium.en"
model = AutoModelForSpeechSeq2Seq.from_pretrained(
model_id, torch_dtype=torch_dtype, low_cpu_mem_usage=True, use_safetensors=True
)
model.to(device)
processor = AutoProcessor.from_pretrained(model_id)
pipe = pipeline(
"automatic-speech-recognition",
model=model,
tokenizer=processor.tokenizer,
feature_extractor=processor.feature_extractor,
max_new_tokens=128,
chunk_length_s=15,
batch_size=16,
torch_dtype=torch_dtype,
device=device,
)
dataset = load_dataset("distil-whisper/librispeech_long", "default", split="validation")
sample = dataset[0]["audio"]
result = pipe(sample)
print(result["text"])
```
<!---
**Tip:** The pipeline can also be used to transcribe an audio file from a remote URL, for example:
```python
result = pipe("https://huggingface.co/datasets/sanchit-gandhi/librispeech_long/resolve/main/audio.wav")
```
--->
### Speculative Decoding
Distil-Whisper can be used as an assistant model to Whisper for [speculative decoding](https://huggingface.co/blog/whisper-speculative-decoding).
Speculative decoding mathematically ensures the exact same outputs as Whisper are obtained while being 2 times faster.
This makes it the perfect drop-in replacement for existing Whisper pipelines, since the same outputs are guaranteed.
In the following code-snippet, we load the assistant Distil-Whisper model standalone to the main Whisper pipeline. We then
specify it as the "assistant model" for generation:
```python
from transformers import pipeline, AutoModelForCausalLM, AutoModelForSpeechSeq2Seq, AutoProcessor
import torch
from datasets import load_dataset
device = "cuda:0" if torch.cuda.is_available() else "cpu"
torch_dtype = torch.float16 if torch.cuda.is_available() else torch.float32
assistant_model_id = "distil-whisper/distil-medium.en"
assistant_model = AutoModelForCausalLM.from_pretrained(
assistant_model_id, torch_dtype=torch_dtype, low_cpu_mem_usage=True, use_safetensors=True
)
assistant_model.to(device)
model_id = "openai/whisper-medium.en"
model = AutoModelForSpeechSeq2Seq.from_pretrained(
model_id, torch_dtype=torch_dtype, low_cpu_mem_usage=True, use_safetensors=True
)
model.to(device)
processor = AutoProcessor.from_pretrained(model_id)
pipe = pipeline(
"automatic-speech-recognition",
model=model,
tokenizer=processor.tokenizer,
feature_extractor=processor.feature_extractor,
max_new_tokens=128,
generate_kwargs={"assistant_model": assistant_model},
torch_dtype=torch_dtype,
device=device,
)
dataset = load_dataset("hf-internal-testing/librispeech_asr_dummy", "clean", split="validation")
sample = dataset[0]["audio"]
result = pipe(sample)
print(result["text"])
```
## Additional Speed & Memory Improvements
You can apply additional speed and memory improvements to Distil-Whisper which we cover in the following.
### Flash Attention
We recommend using [Flash-Attention 2](https://huggingface.co/docs/transformers/main/en/perf_infer_gpu_one#flashattention-2) if your GPU allows for it.
To do so, you first need to install [Flash Attention](https://github.com/Dao-AILab/flash-attention):
```
pip install flash-attn --no-build-isolation
```
and then all you have to do is to pass `use_flash_attention_2=True` to `from_pretrained`:
```diff
- model = AutoModelForSpeechSeq2Seq.from_pretrained(model_id, torch_dtype=torch_dtype, low_cpu_mem_usage=True, use_safetensors=True)
+ model = AutoModelForSpeechSeq2Seq.from_pretrained(model_id, torch_dtype=torch_dtype, low_cpu_mem_usage=True, use_safetensors=True, use_flash_attention_2=True)
```
### Torch Scale-Product-Attention (SDPA)
If your GPU does not support Flash Attention, we recommend making use of [BetterTransformers](https://huggingface.co/docs/transformers/main/en/perf_infer_gpu_one#bettertransformer).
To do so, you first need to install optimum:
```
pip install --upgrade optimum
```
And then convert your model to a "BetterTransformer" model before using it:
```diff
model = AutoModelForSpeechSeq2Seq.from_pretrained(model_id, torch_dtype=torch_dtype, low_cpu_mem_usage=True, use_safetensors=True)
+ model = model.to_bettertransformer()
```
### Running Distil-Whisper in `openai-whisper`
To use the model in the original Whisper format, first ensure you have the [`openai-whisper`](https://pypi.org/project/openai-whisper/) package installed:
```bash
pip install --upgrade openai-whisper
```
The following code-snippet demonstrates how to transcribe a sample file from the LibriSpeech dataset loaded using
🤗 Datasets:
```python
import torch
from datasets import load_dataset
from huggingface_hub import hf_hub_download
from whisper import load_model, transcribe
medium_en = hf_hub_download(repo_id="distil-whisper/distil-medium.en", filename="original-model.bin")
model = load_model(medium_en)
dataset = load_dataset("hf-internal-testing/librispeech_asr_dummy", "clean", split="validation")
sample = dataset[0]["audio"]["array"]
sample = torch.from_numpy(sample).float()
pred_out = transcribe(model, audio=sample)
print(pred_out["text"])
```
To transcribe a local audio file, simply pass the path to the audio file as the `audio` argument to transcribe:
```python
pred_out = transcribe(model, audio="audio.mp3")
```
### Whisper.cpp
Distil-Whisper can be run from the [Whisper.cpp](https://github.com/ggerganov/whisper.cpp) repository with the original
sequential long-form transcription algorithm. In a [provisional benchmark](https://github.com/ggerganov/whisper.cpp/pull/1424#issuecomment-1793513399)
on Mac M1, `distil-medium.en` is 4x faster than `large-v2`, while performing to within 1% WER over long-form audio.
Steps for getting started:
1. Clone the Whisper.cpp repository:
```
git clone https://github.com/ggerganov/whisper.cpp.git
cd whisper.cpp
```
2. Download the ggml weights for `distil-medium.en` from the Hugging Face Hub:
```bash
python -c "from huggingface_hub import hf_hub_download; hf_hub_download(repo_id='distil-whisper/distil-medium.en', filename='ggml-medium-32-2.en.bin', local_dir='./models')"
```
Note that if you do not have the `huggingface_hub` package installed, you can also download the weights with `wget`:
```bash
wget https://huggingface.co/distil-whisper/distil-medium.en/resolve/main/ggml-medium-32-2.en.bin -P ./models
```
3. Run inference using the provided sample audio:
```bash
make -j && ./main -m models/ggml-medium-32-2.en.bin -f samples/jfk.wav
```
### Transformers.js
```js
import { pipeline } from '@xenova/transformers';
let transcriber = await pipeline('automatic-speech-recognition', 'distil-whisper/distil-medium.en');
let url = 'https://huggingface.co/datasets/Xenova/transformers.js-docs/resolve/main/jfk.wav';
let output = await transcriber(url);
// { text: " And so my fellow Americans, ask not what your country can do for you. Ask what you can do for your country." }
```
See the [docs](https://huggingface.co/docs/transformers.js/api/pipelines#module_pipelines.AutomaticSpeechRecognitionPipeline) for more information.
### Candle
Through an integration with Hugging Face [Candle](https://github.com/huggingface/candle/tree/main) 🕯️, Distil-Whisper is
now available in the Rust library 🦀
Benefit from:
* Optimised CPU backend with optional MKL support for x86 and Accelerate for Macs
* CUDA backend for efficiently running on GPUs, multiple GPU distribution via NCCL
* WASM support: run Distil-Whisper in a browser
Steps for getting started:
1. Install [`candle-core`](https://github.com/huggingface/candle/tree/main/candle-core) as explained [here](https://huggingface.github.io/candle/guide/installation.html)
2. Clone the `candle` repository locally:
```
git clone https://github.com/huggingface/candle.git
```
3. Enter the example directory for [Whisper](https://github.com/huggingface/candle/tree/main/candle-examples/examples/whisper):
```
cd candle/candle-examples/examples/whisper
```
4. Run an example:
```
cargo run --example whisper --release -- --model distil-medium.en
```
5. To specify your own audio file, add the `--input` flag:
```
cargo run --example whisper --release -- --model distil-medium.en --input audio.wav
```
### 8bit & 4bit Quantization
Coming soon ...
## Model Details
Distil-Whisper inherits the encoder-decoder architecture from Whisper. The encoder maps a sequence of speech vector
inputs to a sequence of hidden-state vectors. The decoder auto-regressively predicts text tokens, conditional on all
previous tokens and the encoder hidden-states. Consequently, the encoder is only run forward once, whereas the decoder
is run as many times as the number of tokens generated. In practice, this means the decoder accounts for over 90% of
total inference time. Thus, to optimise for latency, the focus should be on minimising the inference time of the decoder.
To distill the Whisper model, we reduce the number of decoder layers while keeping the encoder fixed.
The encoder (shown in green) is entirely copied from the teacher to the student and frozen during training.
The student's decoder consists of only two decoder layers, which are initialised from the first and last decoder layer of
the teacher (shown in red). All other decoder layers of the teacher are discarded. The model is then trained on a weighted sum
of the KL divergence and pseudo-label loss terms.
<p align="center">
<img src="https://huggingface.co/datasets/distil-whisper/figures/resolve/main/architecture.png?raw=true" width="600"/>
</p>
## Evaluation
The following code-snippets demonstrates how to evaluate the Distil-Whisper model on the LibriSpeech validation.clean
dataset with [streaming mode](https://huggingface.co/blog/audio-datasets#streaming-mode-the-silver-bullet), meaning no
audio data has to be downloaded to your local device.
First, we need to install the required packages, including 🤗 Datasets to stream and load the audio data, and 🤗 Evaluate to
perform the WER calculation:
```bash
pip install --upgrade pip
pip install --upgrade transformers datasets[audio] evaluate jiwer
```
Evaluation can then be run end-to-end with the following example:
```python
from transformers import AutoModelForSpeechSeq2Seq, AutoProcessor
from transformers.models.whisper.english_normalizer import EnglishTextNormalizer
from datasets import load_dataset
from evaluate import load
import torch
from tqdm import tqdm
# define our torch configuration
device = "cuda:0" if torch.cuda.is_available() else "cpu"
torch_dtype = torch.float16 if torch.cuda.is_available() else torch.float32
model_id = "distil-whisper/distil-medium.en"
# load the model + processor
model = AutoModelForSpeechSeq2Seq.from_pretrained(model_id, torch_dtype=torch_dtype, use_safetensors=True, low_cpu_mem_usage=True)
model = model.to(device)
processor = AutoProcessor.from_pretrained(model_id)
# load the dataset with streaming mode
dataset = load_dataset("librispeech_asr", "clean", split="validation", streaming=True)
# define the evaluation metric
wer_metric = load("wer")
normalizer = EnglishTextNormalizer(processor.tokenizer.english_spelling_normalizer)
def inference(batch):
# 1. Pre-process the audio data to log-mel spectrogram inputs
audio = [sample["array"] for sample in batch["audio"]]
input_features = processor(audio, sampling_rate=batch["audio"][0]["sampling_rate"], return_tensors="pt").input_features
input_features = input_features.to(device, dtype=torch_dtype)
# 2. Auto-regressively generate the predicted token ids
pred_ids = model.generate(input_features, max_new_tokens=128)
# 3. Decode the token ids to the final transcription
batch["transcription"] = processor.batch_decode(pred_ids, skip_special_tokens=True)
batch["reference"] = batch["text"]
return batch
dataset = dataset.map(function=inference, batched=True, batch_size=16)
all_transcriptions = []
all_references = []
# iterate over the dataset and run inference
for i, result in tqdm(enumerate(dataset), desc="Evaluating..."):
all_transcriptions.append(result["transcription"])
all_references.append(result["reference"])
# normalize predictions and references
all_transcriptions = [normalizer(transcription) for transcription in all_transcriptions]
all_references = [normalizer(reference) for reference in all_references]
# compute the WER metric
wer = 100 * wer_metric.compute(predictions=all_transcriptions, references=all_references)
print(wer)
```
**Print Output:**
```
3.593196832001168
```
## Intended Use
Distil-Whisper is intended to be a drop-in replacement for Whisper on English speech recognition. In particular, it
achieves comparable WER results over out-of-distribution test data, while being 6x faster over both short and long-form
audio.
## Data
Distil-Whisper is trained on 22,000 hours of audio data from 9 open-source, permissively licensed speech datasets on the
Hugging Face Hub:
| Dataset | Size / h | Speakers | Domain | Licence |
|-----------------------------------------------------------------------------------------|----------|----------|-----------------------------|-----------------|
| [People's Speech](https://huggingface.co/datasets/MLCommons/peoples_speech) | 12,000 | unknown | Internet Archive | CC-BY-SA-4.0 |
| [Common Voice 13](https://huggingface.co/datasets/mozilla-foundation/common_voice_13_0) | 3,000 | unknown | Narrated Wikipedia | CC0-1.0 |
| [GigaSpeech](https://huggingface.co/datasets/speechcolab/gigaspeech) | 2,500 | unknown | Audiobook, podcast, YouTube | apache-2.0 |
| Fisher | 1,960 | 11,900 | Telephone conversations | LDC |
| [LibriSpeech](https://huggingface.co/datasets/librispeech_asr) | 960 | 2,480 | Audiobooks | CC-BY-4.0 |
| [VoxPopuli](https://huggingface.co/datasets/facebook/voxpopuli) | 540 | 1,310 | European Parliament | CC0 |
| [TED-LIUM](https://huggingface.co/datasets/LIUM/tedlium) | 450 | 2,030 | TED talks | CC-BY-NC-ND 3.0 |
| SwitchBoard | 260 | 540 | Telephone conversations | LDC |
| [AMI](https://huggingface.co/datasets/edinburghcstr/ami) | 100 | unknown | Meetings | CC-BY-4.0 |
||||||
| **Total** | 21,770 | 18,260+ | | |
The combined dataset spans 10 distinct domains and over 50k speakers. The diversity of this dataset is crucial to ensuring
the distilled model is robust to audio distributions and noise.
The audio data is then pseudo-labelled using the Whisper large-v2 model: we use Whisper to generate predictions for all
the audio in our training set and use these as the target labels during training. Using pseudo-labels ensures that the
transcriptions are consistently formatted across datasets and provides sequence-level distillation signal during training.
## WER Filter
The Whisper pseudo-label predictions are subject to mis-transcriptions and hallucinations. To ensure we only train on
accurate pseudo-labels, we employ a simple WER heuristic during training. First, we normalise the Whisper pseudo-labels
and the ground truth labels provided by each dataset. We then compute the WER between these labels. If the WER exceeds
a specified threshold, we discard the training example. Otherwise, we keep it for training.
Section 9.2 of the [Distil-Whisper paper](https://arxiv.org/abs/2311.00430) demonstrates the effectiveness of this filter for improving downstream performance
of the distilled model. We also partially attribute Distil-Whisper's robustness to hallucinations to this filter.
## Training
The model was trained for 80,000 optimisation steps (or eight epochs). The Tensorboard training logs can be found under: https://huggingface.co/distil-whisper/distil-medium.en/tensorboard?params=scalars#frame
## Results
The distilled model performs to within 1% WER of Whisper on out-of-distribution (OOD) short-form audio, and outperforms Whisper
by 0.1% on OOD long-form audio. This performance gain is attributed to lower hallucinations.
For a detailed per-dataset breakdown of the evaluation results, refer to Tables 16 and 17 of the [Distil-Whisper paper](https://arxiv.org/abs/2311.00430)
Distil-Whisper is also evaluated on the [ESB benchmark](https://arxiv.org/abs/2210.13352) datasets as part of the [OpenASR leaderboard](https://huggingface.co/spaces/hf-audio/open_asr_leaderboard),
where it performs to within 0.2% WER of Whisper.
## Reproducing Distil-Whisper
Training and evaluation code to reproduce Distil-Whisper is available under the Distil-Whisper repository: https://github.com/huggingface/distil-whisper/tree/main/training
## License
Distil-Whisper inherits the [MIT license](https://github.com/huggingface/distil-whisper/blob/main/LICENSE) from OpenAI's Whisper model.
## Citation
If you use this model, please consider citing the [Distil-Whisper paper](https://arxiv.org/abs/2311.00430):
```
@misc{gandhi2023distilwhisper,
title={Distil-Whisper: Robust Knowledge Distillation via Large-Scale Pseudo Labelling},
author={Sanchit Gandhi and Patrick von Platen and Alexander M. Rush},
year={2023},
eprint={2311.00430},
archivePrefix={arXiv},
primaryClass={cs.CL}
}
```
## Acknowledgements
* OpenAI for the Whisper [model](https://huggingface.co/openai/whisper-large-v2) and [original codebase](https://github.com/openai/whisper)
* Hugging Face 🤗 [Transformers](https://github.com/huggingface/transformers) for the model integration
* Google's [TPU Research Cloud (TRC)](https://sites.research.google/trc/about/) programme for Cloud TPU v4s
* [`@rsonavane`](https://huggingface.co/rsonavane/distil-whisper-large-v2-8-ls) for releasing an early iteration of Distil-Whisper on the LibriSpeech dataset
|
aubmindlab/bert-base-arabertv02 | aubmindlab | "2024-03-26T14:39:39Z" | 492,030 | 30 | transformers | [
"transformers",
"pytorch",
"tf",
"jax",
"tensorboard",
"safetensors",
"bert",
"fill-mask",
"ar",
"dataset:wikipedia",
"dataset:Osian",
"dataset:1.5B-Arabic-Corpus",
"dataset:oscar-arabic-unshuffled",
"dataset:Assafir-private",
"arxiv:2003.00104",
"autotrain_compatible",
"endpoints_compatible",
"region:us"
] | fill-mask | "2022-03-02T23:29:05Z" | ---
language: ar
datasets:
- wikipedia
- Osian
- 1.5B-Arabic-Corpus
- oscar-arabic-unshuffled
- Assafir-private
widget:
- text: ' عاصمة لبنان هي [MASK] .'
pipeline_tag: fill-mask
---
# AraBERT v1 & v2 : Pre-training BERT for Arabic Language Understanding
<img src="https://raw.githubusercontent.com/aub-mind/arabert/master/arabert_logo.png" width="100" align="left"/>
**AraBERT** is an Arabic pretrained language model based on [Google's BERT architechture](https://github.com/google-research/bert). AraBERT uses the same BERT-Base config. More details are available in the [AraBERT Paper](https://arxiv.org/abs/2003.00104) and in the [AraBERT Meetup](https://github.com/WissamAntoun/pydata_khobar_meetup)
There are two versions of the model, AraBERTv0.1 and AraBERTv1, with the difference being that AraBERTv1 uses pre-segmented text where prefixes and suffixes were split using the [Farasa Segmenter](http://alt.qcri.org/farasa/segmenter.html).
We evaluate AraBERT models on different downstream tasks and compare them to [mBERT]((https://github.com/google-research/bert/blob/master/multilingual.md)), and other state of the art models (*To the extent of our knowledge*). The Tasks were Sentiment Analysis on 6 different datasets ([HARD](https://github.com/elnagara/HARD-Arabic-Dataset), [ASTD-Balanced](https://www.aclweb.org/anthology/D15-1299), [ArsenTD-Lev](https://staff.aub.edu.lb/~we07/Publications/ArSentD-LEV_Sentiment_Corpus.pdf), [LABR](https://github.com/mohamedadaly/LABR)), Named Entity Recognition with the [ANERcorp](http://curtis.ml.cmu.edu/w/courses/index.php/ANERcorp), and Arabic Question Answering on [Arabic-SQuAD and ARCD](https://github.com/husseinmozannar/SOQAL)
# AraBERTv2
## What's New!
AraBERT now comes in 4 new variants to replace the old v1 versions:
More Detail in the AraBERT folder and in the [README](https://github.com/aub-mind/arabert/blob/master/AraBERT/README.md) and in the [AraBERT Paper](https://arxiv.org/abs/2003.00104v2)
Model | HuggingFace Model Name | Size (MB/Params)| Pre-Segmentation | DataSet (Sentences/Size/nWords) |
---|:---:|:---:|:---:|:---:
AraBERTv0.2-base | [bert-base-arabertv02](https://huggingface.co/aubmindlab/bert-base-arabertv02) | 543MB / 136M | No | 200M / 77GB / 8.6B |
AraBERTv0.2-large| [bert-large-arabertv02](https://huggingface.co/aubmindlab/bert-large-arabertv02) | 1.38G 371M | No | 200M / 77GB / 8.6B |
AraBERTv2-base| [bert-base-arabertv2](https://huggingface.co/aubmindlab/bert-base-arabertv2) | 543MB 136M | Yes | 200M / 77GB / 8.6B |
AraBERTv2-large| [bert-large-arabertv2](https://huggingface.co/aubmindlab/bert-large-arabertv2) | 1.38G 371M | Yes | 200M / 77GB / 8.6B |
AraBERTv0.2-Twitter-base| [bert-base-arabertv02-twitter](https://huggingface.co/aubmindlab/bert-base-arabertv02-twitter) | 543MB / 136M | No | Same as v02 + 60M Multi-Dialect Tweets|
AraBERTv0.2-Twitter-large| [bert-large-arabertv02-twitter](https://huggingface.co/aubmindlab/bert-large-arabertv02-twitter) | 1.38G / 371M | No | Same as v02 + 60M Multi-Dialect Tweets|
AraBERTv0.1-base| [bert-base-arabertv01](https://huggingface.co/aubmindlab/bert-base-arabertv01) | 543MB 136M | No | 77M / 23GB / 2.7B |
AraBERTv1-base| [bert-base-arabert](https://huggingface.co/aubmindlab/bert-base-arabert) | 543MB 136M | Yes | 77M / 23GB / 2.7B |
All models are available in the `HuggingFace` model page under the [aubmindlab](https://huggingface.co/aubmindlab/) name. Checkpoints are available in PyTorch, TF2 and TF1 formats.
## Better Pre-Processing and New Vocab
We identified an issue with AraBERTv1's wordpiece vocabulary. The issue came from punctuations and numbers that were still attached to words when learned the wordpiece vocab. We now insert a space between numbers and characters and around punctuation characters.
The new vocabulary was learned using the `BertWordpieceTokenizer` from the `tokenizers` library, and should now support the Fast tokenizer implementation from the `transformers` library.
**P.S.**: All the old BERT codes should work with the new BERT, just change the model name and check the new preprocessing function
**Please read the section on how to use the [preprocessing function](#Preprocessing)**
## Bigger Dataset and More Compute
We used ~3.5 times more data, and trained for longer.
For Dataset Sources see the [Dataset Section](#Dataset)
Model | Hardware | num of examples with seq len (128 / 512) |128 (Batch Size/ Num of Steps) | 512 (Batch Size/ Num of Steps) | Total Steps | Total Time (in Days) |
---|:---:|:---:|:---:|:---:|:---:|:---:
AraBERTv0.2-base | TPUv3-8 | 420M / 207M | 2560 / 1M | 384/ 2M | 3M | -
AraBERTv0.2-large | TPUv3-128 | 420M / 207M | 13440 / 250K | 2056 / 300K | 550K | 7
AraBERTv2-base | TPUv3-8 | 420M / 207M | 2560 / 1M | 384/ 2M | 3M | -
AraBERTv2-large | TPUv3-128 | 520M / 245M | 13440 / 250K | 2056 / 300K | 550K | 7
AraBERT-base (v1/v0.1) | TPUv2-8 | - |512 / 900K | 128 / 300K| 1.2M | 4
# Dataset
The pretraining data used for the new AraBERT model is also used for Arabic **GPT2 and ELECTRA**.
The dataset consists of 77GB or 200,095,961 lines or 8,655,948,860 words or 82,232,988,358 chars (before applying Farasa Segmentation)
For the new dataset we added the unshuffled OSCAR corpus, after we thoroughly filter it, to the previous dataset used in AraBERTv1 but with out the websites that we previously crawled:
- OSCAR unshuffled and filtered.
- [Arabic Wikipedia dump](https://archive.org/details/arwiki-20190201) from 2020/09/01
- [The 1.5B words Arabic Corpus](https://www.semanticscholar.org/paper/1.5-billion-words-Arabic-Corpus-El-Khair/f3eeef4afb81223df96575adadf808fe7fe440b4)
- [The OSIAN Corpus](https://www.aclweb.org/anthology/W19-4619)
- Assafir news articles. Huge thank you for Assafir for providing us the data
# Preprocessing
It is recommended to apply our preprocessing function before training/testing on any dataset.
**Install the arabert python package to segment text for AraBERT v1 & v2 or to clean your data `pip install arabert`**
```python
from arabert.preprocess import ArabertPreprocessor
model_name="aubmindlab/bert-large-arabertv02"
arabert_prep = ArabertPreprocessor(model_name=model_name)
text = "ولن نبالغ إذا قلنا: إن هاتف أو كمبيوتر المكتب في زمننا هذا ضروري"
arabert_prep.preprocess(text)
>>> output: ولن نبالغ إذا قلنا : إن هاتف أو كمبيوتر المكتب في زمننا هذا ضروري
```
# TensorFlow 1.x models
The TF1.x model are available in the HuggingFace models repo.
You can download them as follows:
- via git-lfs: clone all the models in a repo
```bash
curl -s https://packagecloud.io/install/repositories/github/git-lfs/script.deb.sh | sudo bash
sudo apt-get install git-lfs
git lfs install
git clone https://huggingface.co/aubmindlab/MODEL_NAME
tar -C ./MODEL_NAME -zxvf /content/MODEL_NAME/tf1_model.tar.gz
```
where `MODEL_NAME` is any model under the `aubmindlab` name
- via `wget`:
- Go to the tf1_model.tar.gz file on huggingface.co/models/aubmindlab/MODEL_NAME.
- copy the `oid sha256`
- then run `wget https://cdn-lfs.huggingface.co/aubmindlab/aragpt2-base/INSERT_THE_SHA_HERE` (ex: for `aragpt2-base`: `wget https://cdn-lfs.huggingface.co/aubmindlab/aragpt2-base/3766fc03d7c2593ff2fb991d275e96b81b0ecb2098b71ff315611d052ce65248`)
# If you used this model please cite us as :
Google Scholar has our Bibtex wrong (missing name), use this instead
```
@inproceedings{antoun2020arabert,
title={AraBERT: Transformer-based Model for Arabic Language Understanding},
author={Antoun, Wissam and Baly, Fady and Hajj, Hazem},
booktitle={LREC 2020 Workshop Language Resources and Evaluation Conference 11--16 May 2020},
pages={9}
}
```
# Acknowledgments
Thanks to TensorFlow Research Cloud (TFRC) for the free access to Cloud TPUs, couldn't have done it without this program, and to the [AUB MIND Lab](https://sites.aub.edu.lb/mindlab/) Members for the continuous support. Also thanks to [Yakshof](https://www.yakshof.com/#/) and Assafir for data and storage access. Another thanks for Habib Rahal (https://www.behance.net/rahalhabib), for putting a face to AraBERT.
# Contacts
**Wissam Antoun**: [Linkedin](https://www.linkedin.com/in/wissam-antoun-622142b4/) | [Twitter](https://twitter.com/wissam_antoun) | [Github](https://github.com/WissamAntoun) | <wfa07@mail.aub.edu> | <wissam.antoun@gmail.com>
**Fady Baly**: [Linkedin](https://www.linkedin.com/in/fadybaly/) | [Twitter](https://twitter.com/fadybaly) | [Github](https://github.com/fadybaly) | <fgb06@mail.aub.edu> | <baly.fady@gmail.com> |
microsoft/mdeberta-v3-base | microsoft | "2023-04-06T05:32:33Z" | 491,137 | 153 | transformers | [
"transformers",
"pytorch",
"tf",
"deberta-v2",
"deberta",
"deberta-v3",
"mdeberta",
"fill-mask",
"multilingual",
"en",
"ar",
"bg",
"de",
"el",
"es",
"fr",
"hi",
"ru",
"sw",
"th",
"tr",
"ur",
"vi",
"zh",
"arxiv:2006.03654",
"arxiv:2111.09543",
"license:mit",
"endpoints_compatible",
"region:us"
] | fill-mask | "2022-03-02T23:29:05Z" | ---
language:
- multilingual
- en
- ar
- bg
- de
- el
- es
- fr
- hi
- ru
- sw
- th
- tr
- ur
- vi
- zh
tags:
- deberta
- deberta-v3
- mdeberta
- fill-mask
thumbnail: https://huggingface.co/front/thumbnails/microsoft.png
license: mit
---
## DeBERTaV3: Improving DeBERTa using ELECTRA-Style Pre-Training with Gradient-Disentangled Embedding Sharing
[DeBERTa](https://arxiv.org/abs/2006.03654) improves the BERT and RoBERTa models using disentangled attention and enhanced mask decoder. With those two improvements, DeBERTa out perform RoBERTa on a majority of NLU tasks with 80GB training data.
In [DeBERTa V3](https://arxiv.org/abs/2111.09543), we further improved the efficiency of DeBERTa using ELECTRA-Style pre-training with Gradient Disentangled Embedding Sharing. Compared to DeBERTa, our V3 version significantly improves the model performance on downstream tasks. You can find more technique details about the new model from our [paper](https://arxiv.org/abs/2111.09543).
Please check the [official repository](https://github.com/microsoft/DeBERTa) for more implementation details and updates.
mDeBERTa is multilingual version of DeBERTa which use the same structure as DeBERTa and was trained with CC100 multilingual data.
The mDeBERTa V3 base model comes with 12 layers and a hidden size of 768. It has 86M backbone parameters with a vocabulary containing 250K tokens which introduces 190M parameters in the Embedding layer. This model was trained using the 2.5T CC100 data as XLM-R.
#### Fine-tuning on NLU tasks
We present the dev results on XNLI with zero-shot cross-lingual transfer setting, i.e. training with English data only, test on other languages.
| Model |avg | en | fr| es | de | el | bg | ru |tr |ar |vi | th | zh | hi | sw | ur |
|--------------| ----|----|----|---- |-- |-- |-- | -- |-- |-- |-- | -- | -- | -- | -- | -- |
| XLM-R-base |76.2 |85.8|79.7|80.7 |78.7 |77.5 |79.6 |78.1 |74.2 |73.8 |76.5 |74.6 |76.7| 72.4| 66.5| 68.3|
| mDeBERTa-base|**79.8**+/-0.2|**88.2**|**82.6**|**84.4** |**82.7** |**82.3** |**82.4** |**80.8** |**79.5** |**78.5** |**78.1** |**76.4** |**79.5**| **75.9**| **73.9**| **72.4**|
#### Fine-tuning with HF transformers
```bash
#!/bin/bash
cd transformers/examples/pytorch/text-classification/
pip install datasets
output_dir="ds_results"
num_gpus=8
batch_size=4
python -m torch.distributed.launch --nproc_per_node=${num_gpus} \
run_xnli.py \
--model_name_or_path microsoft/mdeberta-v3-base \
--task_name $TASK_NAME \
--do_train \
--do_eval \
--train_language en \
--language en \
--evaluation_strategy steps \
--max_seq_length 256 \
--warmup_steps 3000 \
--per_device_train_batch_size ${batch_size} \
--learning_rate 2e-5 \
--num_train_epochs 6 \
--output_dir $output_dir \
--overwrite_output_dir \
--logging_steps 1000 \
--logging_dir $output_dir
```
### Citation
If you find DeBERTa useful for your work, please cite the following papers:
``` latex
@misc{he2021debertav3,
title={DeBERTaV3: Improving DeBERTa using ELECTRA-Style Pre-Training with Gradient-Disentangled Embedding Sharing},
author={Pengcheng He and Jianfeng Gao and Weizhu Chen},
year={2021},
eprint={2111.09543},
archivePrefix={arXiv},
primaryClass={cs.CL}
}
```
``` latex
@inproceedings{
he2021deberta,
title={DEBERTA: DECODING-ENHANCED BERT WITH DISENTANGLED ATTENTION},
author={Pengcheng He and Xiaodong Liu and Jianfeng Gao and Weizhu Chen},
booktitle={International Conference on Learning Representations},
year={2021},
url={https://openreview.net/forum?id=XPZIaotutsD}
}
```
|
TencentARC/InstantMesh | TencentARC | "2024-04-11T02:56:23Z" | 490,016 | 243 | diffusers | [
"diffusers",
"image-to-3d",
"arxiv:2404.07191",
"license:apache-2.0",
"region:us"
] | image-to-3d | "2024-04-10T13:16:45Z" | ---
license: apache-2.0
tags:
- image-to-3d
---
# InstantMesh
Model card for *InstantMesh: Efficient 3D Mesh Generation from a Single Image with Sparse-view Large Reconstruction Models*.
Code: https://github.com/TencentARC/InstantMesh
Arxiv: https://arxiv.org/abs/2404.07191
We present InstantMesh, a feed-forward framework for instant 3D mesh generation from a single image, featuring state-of-the-art generation quality and significant training scalability. By synergizing the strengths of an off-the-shelf multiview diffusion model and a sparse-view reconstruction model based on the LRM architecture, InstantMesh is able to create diverse 3D assets within 10 seconds. To enhance the training efficiency and exploit more geometric supervisions, e.g., depths and normals, we integrate a differentiable iso-surface extraction module into our framework and directly optimize on the mesh representation. Experimental results on public datasets demonstrate that InstantMesh significantly outperforms other latest image-to-3D baselines, both qualitatively and quantitatively. We release all the code, weights, and demo of InstantMesh, with the intention that it can make substantial contributions to the community of 3D generative AI and empower both researchers and content creators.
|
nomic-ai/nomic-embed-text-v1 | nomic-ai | "2024-09-26T14:42:37Z" | 487,833 | 460 | sentence-transformers | [
"sentence-transformers",
"pytorch",
"onnx",
"safetensors",
"nomic_bert",
"feature-extraction",
"sentence-similarity",
"mteb",
"transformers",
"transformers.js",
"custom_code",
"en",
"arxiv:2402.01613",
"license:apache-2.0",
"model-index",
"autotrain_compatible",
"text-embeddings-inference",
"endpoints_compatible",
"region:us"
] | sentence-similarity | "2024-01-31T20:26:50Z" | ---
library_name: sentence-transformers
pipeline_tag: sentence-similarity
tags:
- feature-extraction
- sentence-similarity
- mteb
- transformers
- transformers.js
model-index:
- name: epoch_0_model
results:
- task:
type: Classification
dataset:
type: mteb/amazon_counterfactual
name: MTEB AmazonCounterfactualClassification (en)
config: en
split: test
revision: e8379541af4e31359cca9fbcf4b00f2671dba205
metrics:
- type: accuracy
value: 76.8507462686567
- type: ap
value: 40.592189159090495
- type: f1
value: 71.01634655512476
- task:
type: Classification
dataset:
type: mteb/amazon_polarity
name: MTEB AmazonPolarityClassification
config: default
split: test
revision: e2d317d38cd51312af73b3d32a06d1a08b442046
metrics:
- type: accuracy
value: 91.51892500000001
- type: ap
value: 88.50346762975335
- type: f1
value: 91.50342077459624
- task:
type: Classification
dataset:
type: mteb/amazon_reviews_multi
name: MTEB AmazonReviewsClassification (en)
config: en
split: test
revision: 1399c76144fd37290681b995c656ef9b2e06e26d
metrics:
- type: accuracy
value: 47.364
- type: f1
value: 46.72708080922794
- task:
type: Retrieval
dataset:
type: arguana
name: MTEB ArguAna
config: default
split: test
revision: None
metrics:
- type: map_at_1
value: 25.178
- type: map_at_10
value: 40.244
- type: map_at_100
value: 41.321999999999996
- type: map_at_1000
value: 41.331
- type: map_at_3
value: 35.016999999999996
- type: map_at_5
value: 37.99
- type: mrr_at_1
value: 25.605
- type: mrr_at_10
value: 40.422000000000004
- type: mrr_at_100
value: 41.507
- type: mrr_at_1000
value: 41.516
- type: mrr_at_3
value: 35.23
- type: mrr_at_5
value: 38.15
- type: ndcg_at_1
value: 25.178
- type: ndcg_at_10
value: 49.258
- type: ndcg_at_100
value: 53.776
- type: ndcg_at_1000
value: 53.995000000000005
- type: ndcg_at_3
value: 38.429
- type: ndcg_at_5
value: 43.803
- type: precision_at_1
value: 25.178
- type: precision_at_10
value: 7.831
- type: precision_at_100
value: 0.979
- type: precision_at_1000
value: 0.1
- type: precision_at_3
value: 16.121
- type: precision_at_5
value: 12.29
- type: recall_at_1
value: 25.178
- type: recall_at_10
value: 78.307
- type: recall_at_100
value: 97.866
- type: recall_at_1000
value: 99.57300000000001
- type: recall_at_3
value: 48.364000000000004
- type: recall_at_5
value: 61.451
- task:
type: Clustering
dataset:
type: mteb/arxiv-clustering-p2p
name: MTEB ArxivClusteringP2P
config: default
split: test
revision: a122ad7f3f0291bf49cc6f4d32aa80929df69d5d
metrics:
- type: v_measure
value: 45.93034494751465
- task:
type: Clustering
dataset:
type: mteb/arxiv-clustering-s2s
name: MTEB ArxivClusteringS2S
config: default
split: test
revision: f910caf1a6075f7329cdf8c1a6135696f37dbd53
metrics:
- type: v_measure
value: 36.64579480054327
- task:
type: Reranking
dataset:
type: mteb/askubuntudupquestions-reranking
name: MTEB AskUbuntuDupQuestions
config: default
split: test
revision: 2000358ca161889fa9c082cb41daa8dcfb161a54
metrics:
- type: map
value: 60.601310529222054
- type: mrr
value: 75.04484896451656
- task:
type: STS
dataset:
type: mteb/biosses-sts
name: MTEB BIOSSES
config: default
split: test
revision: d3fb88f8f02e40887cd149695127462bbcf29b4a
metrics:
- type: cos_sim_pearson
value: 88.57797718095814
- type: cos_sim_spearman
value: 86.47064499110101
- type: euclidean_pearson
value: 87.4559602783142
- type: euclidean_spearman
value: 86.47064499110101
- type: manhattan_pearson
value: 87.7232764230245
- type: manhattan_spearman
value: 86.91222131777742
- task:
type: Classification
dataset:
type: mteb/banking77
name: MTEB Banking77Classification
config: default
split: test
revision: 0fd18e25b25c072e09e0d92ab615fda904d66300
metrics:
- type: accuracy
value: 84.5422077922078
- type: f1
value: 84.47657456950589
- task:
type: Clustering
dataset:
type: mteb/biorxiv-clustering-p2p
name: MTEB BiorxivClusteringP2P
config: default
split: test
revision: 65b79d1d13f80053f67aca9498d9402c2d9f1f40
metrics:
- type: v_measure
value: 38.48953561974464
- task:
type: Clustering
dataset:
type: mteb/biorxiv-clustering-s2s
name: MTEB BiorxivClusteringS2S
config: default
split: test
revision: 258694dd0231531bc1fd9de6ceb52a0853c6d908
metrics:
- type: v_measure
value: 32.75995857510105
- task:
type: Retrieval
dataset:
type: BeIR/cqadupstack
name: MTEB CQADupstackAndroidRetrieval
config: default
split: test
revision: None
metrics:
- type: map_at_1
value: 30.008000000000003
- type: map_at_10
value: 39.51
- type: map_at_100
value: 40.841
- type: map_at_1000
value: 40.973
- type: map_at_3
value: 36.248999999999995
- type: map_at_5
value: 38.096999999999994
- type: mrr_at_1
value: 36.481
- type: mrr_at_10
value: 44.818000000000005
- type: mrr_at_100
value: 45.64
- type: mrr_at_1000
value: 45.687
- type: mrr_at_3
value: 42.036
- type: mrr_at_5
value: 43.782
- type: ndcg_at_1
value: 36.481
- type: ndcg_at_10
value: 45.152
- type: ndcg_at_100
value: 50.449
- type: ndcg_at_1000
value: 52.76499999999999
- type: ndcg_at_3
value: 40.161
- type: ndcg_at_5
value: 42.577999999999996
- type: precision_at_1
value: 36.481
- type: precision_at_10
value: 8.369
- type: precision_at_100
value: 1.373
- type: precision_at_1000
value: 0.186
- type: precision_at_3
value: 18.693
- type: precision_at_5
value: 13.533999999999999
- type: recall_at_1
value: 30.008000000000003
- type: recall_at_10
value: 56.108999999999995
- type: recall_at_100
value: 78.55499999999999
- type: recall_at_1000
value: 93.659
- type: recall_at_3
value: 41.754999999999995
- type: recall_at_5
value: 48.296
- task:
type: Retrieval
dataset:
type: BeIR/cqadupstack
name: MTEB CQADupstackEnglishRetrieval
config: default
split: test
revision: None
metrics:
- type: map_at_1
value: 30.262
- type: map_at_10
value: 40.139
- type: map_at_100
value: 41.394
- type: map_at_1000
value: 41.526
- type: map_at_3
value: 37.155
- type: map_at_5
value: 38.785
- type: mrr_at_1
value: 38.153
- type: mrr_at_10
value: 46.369
- type: mrr_at_100
value: 47.072
- type: mrr_at_1000
value: 47.111999999999995
- type: mrr_at_3
value: 44.268
- type: mrr_at_5
value: 45.389
- type: ndcg_at_1
value: 38.153
- type: ndcg_at_10
value: 45.925
- type: ndcg_at_100
value: 50.394000000000005
- type: ndcg_at_1000
value: 52.37500000000001
- type: ndcg_at_3
value: 41.754000000000005
- type: ndcg_at_5
value: 43.574
- type: precision_at_1
value: 38.153
- type: precision_at_10
value: 8.796
- type: precision_at_100
value: 1.432
- type: precision_at_1000
value: 0.189
- type: precision_at_3
value: 20.318
- type: precision_at_5
value: 14.395
- type: recall_at_1
value: 30.262
- type: recall_at_10
value: 55.72200000000001
- type: recall_at_100
value: 74.97500000000001
- type: recall_at_1000
value: 87.342
- type: recall_at_3
value: 43.129
- type: recall_at_5
value: 48.336
- task:
type: Retrieval
dataset:
type: BeIR/cqadupstack
name: MTEB CQADupstackGamingRetrieval
config: default
split: test
revision: None
metrics:
- type: map_at_1
value: 39.951
- type: map_at_10
value: 51.248000000000005
- type: map_at_100
value: 52.188
- type: map_at_1000
value: 52.247
- type: map_at_3
value: 48.211
- type: map_at_5
value: 49.797000000000004
- type: mrr_at_1
value: 45.329
- type: mrr_at_10
value: 54.749
- type: mrr_at_100
value: 55.367999999999995
- type: mrr_at_1000
value: 55.400000000000006
- type: mrr_at_3
value: 52.382
- type: mrr_at_5
value: 53.649
- type: ndcg_at_1
value: 45.329
- type: ndcg_at_10
value: 56.847
- type: ndcg_at_100
value: 60.738
- type: ndcg_at_1000
value: 61.976
- type: ndcg_at_3
value: 51.59
- type: ndcg_at_5
value: 53.915
- type: precision_at_1
value: 45.329
- type: precision_at_10
value: 8.959
- type: precision_at_100
value: 1.187
- type: precision_at_1000
value: 0.134
- type: precision_at_3
value: 22.612
- type: precision_at_5
value: 15.273
- type: recall_at_1
value: 39.951
- type: recall_at_10
value: 70.053
- type: recall_at_100
value: 86.996
- type: recall_at_1000
value: 95.707
- type: recall_at_3
value: 56.032000000000004
- type: recall_at_5
value: 61.629999999999995
- task:
type: Retrieval
dataset:
type: BeIR/cqadupstack
name: MTEB CQADupstackGisRetrieval
config: default
split: test
revision: None
metrics:
- type: map_at_1
value: 25.566
- type: map_at_10
value: 33.207
- type: map_at_100
value: 34.166000000000004
- type: map_at_1000
value: 34.245
- type: map_at_3
value: 30.94
- type: map_at_5
value: 32.01
- type: mrr_at_1
value: 27.345000000000002
- type: mrr_at_10
value: 35.193000000000005
- type: mrr_at_100
value: 35.965
- type: mrr_at_1000
value: 36.028999999999996
- type: mrr_at_3
value: 32.806000000000004
- type: mrr_at_5
value: 34.021
- type: ndcg_at_1
value: 27.345000000000002
- type: ndcg_at_10
value: 37.891999999999996
- type: ndcg_at_100
value: 42.664
- type: ndcg_at_1000
value: 44.757000000000005
- type: ndcg_at_3
value: 33.123000000000005
- type: ndcg_at_5
value: 35.035
- type: precision_at_1
value: 27.345000000000002
- type: precision_at_10
value: 5.763
- type: precision_at_100
value: 0.859
- type: precision_at_1000
value: 0.108
- type: precision_at_3
value: 13.71
- type: precision_at_5
value: 9.401
- type: recall_at_1
value: 25.566
- type: recall_at_10
value: 50.563
- type: recall_at_100
value: 72.86399999999999
- type: recall_at_1000
value: 88.68599999999999
- type: recall_at_3
value: 37.43
- type: recall_at_5
value: 41.894999999999996
- task:
type: Retrieval
dataset:
type: BeIR/cqadupstack
name: MTEB CQADupstackMathematicaRetrieval
config: default
split: test
revision: None
metrics:
- type: map_at_1
value: 16.663
- type: map_at_10
value: 23.552
- type: map_at_100
value: 24.538
- type: map_at_1000
value: 24.661
- type: map_at_3
value: 21.085
- type: map_at_5
value: 22.391
- type: mrr_at_1
value: 20.025000000000002
- type: mrr_at_10
value: 27.643
- type: mrr_at_100
value: 28.499999999999996
- type: mrr_at_1000
value: 28.582
- type: mrr_at_3
value: 25.083
- type: mrr_at_5
value: 26.544
- type: ndcg_at_1
value: 20.025000000000002
- type: ndcg_at_10
value: 28.272000000000002
- type: ndcg_at_100
value: 33.353
- type: ndcg_at_1000
value: 36.454
- type: ndcg_at_3
value: 23.579
- type: ndcg_at_5
value: 25.685000000000002
- type: precision_at_1
value: 20.025000000000002
- type: precision_at_10
value: 5.187
- type: precision_at_100
value: 0.897
- type: precision_at_1000
value: 0.13
- type: precision_at_3
value: 10.987
- type: precision_at_5
value: 8.06
- type: recall_at_1
value: 16.663
- type: recall_at_10
value: 38.808
- type: recall_at_100
value: 61.305
- type: recall_at_1000
value: 83.571
- type: recall_at_3
value: 25.907999999999998
- type: recall_at_5
value: 31.214
- task:
type: Retrieval
dataset:
type: BeIR/cqadupstack
name: MTEB CQADupstackPhysicsRetrieval
config: default
split: test
revision: None
metrics:
- type: map_at_1
value: 27.695999999999998
- type: map_at_10
value: 37.018
- type: map_at_100
value: 38.263000000000005
- type: map_at_1000
value: 38.371
- type: map_at_3
value: 34.226
- type: map_at_5
value: 35.809999999999995
- type: mrr_at_1
value: 32.916000000000004
- type: mrr_at_10
value: 42.067
- type: mrr_at_100
value: 42.925000000000004
- type: mrr_at_1000
value: 42.978
- type: mrr_at_3
value: 39.637
- type: mrr_at_5
value: 41.134
- type: ndcg_at_1
value: 32.916000000000004
- type: ndcg_at_10
value: 42.539
- type: ndcg_at_100
value: 47.873
- type: ndcg_at_1000
value: 50.08200000000001
- type: ndcg_at_3
value: 37.852999999999994
- type: ndcg_at_5
value: 40.201
- type: precision_at_1
value: 32.916000000000004
- type: precision_at_10
value: 7.5840000000000005
- type: precision_at_100
value: 1.199
- type: precision_at_1000
value: 0.155
- type: precision_at_3
value: 17.485
- type: precision_at_5
value: 12.512
- type: recall_at_1
value: 27.695999999999998
- type: recall_at_10
value: 53.638
- type: recall_at_100
value: 76.116
- type: recall_at_1000
value: 91.069
- type: recall_at_3
value: 41.13
- type: recall_at_5
value: 46.872
- task:
type: Retrieval
dataset:
type: BeIR/cqadupstack
name: MTEB CQADupstackProgrammersRetrieval
config: default
split: test
revision: None
metrics:
- type: map_at_1
value: 24.108
- type: map_at_10
value: 33.372
- type: map_at_100
value: 34.656
- type: map_at_1000
value: 34.768
- type: map_at_3
value: 30.830999999999996
- type: map_at_5
value: 32.204
- type: mrr_at_1
value: 29.110000000000003
- type: mrr_at_10
value: 37.979
- type: mrr_at_100
value: 38.933
- type: mrr_at_1000
value: 38.988
- type: mrr_at_3
value: 35.731
- type: mrr_at_5
value: 36.963
- type: ndcg_at_1
value: 29.110000000000003
- type: ndcg_at_10
value: 38.635000000000005
- type: ndcg_at_100
value: 44.324999999999996
- type: ndcg_at_1000
value: 46.747
- type: ndcg_at_3
value: 34.37
- type: ndcg_at_5
value: 36.228
- type: precision_at_1
value: 29.110000000000003
- type: precision_at_10
value: 6.963
- type: precision_at_100
value: 1.146
- type: precision_at_1000
value: 0.152
- type: precision_at_3
value: 16.400000000000002
- type: precision_at_5
value: 11.552999999999999
- type: recall_at_1
value: 24.108
- type: recall_at_10
value: 49.597
- type: recall_at_100
value: 73.88900000000001
- type: recall_at_1000
value: 90.62400000000001
- type: recall_at_3
value: 37.662
- type: recall_at_5
value: 42.565
- task:
type: Retrieval
dataset:
type: BeIR/cqadupstack
name: MTEB CQADupstackRetrieval
config: default
split: test
revision: None
metrics:
- type: map_at_1
value: 25.00791666666667
- type: map_at_10
value: 33.287749999999996
- type: map_at_100
value: 34.41141666666667
- type: map_at_1000
value: 34.52583333333333
- type: map_at_3
value: 30.734416666666668
- type: map_at_5
value: 32.137166666666666
- type: mrr_at_1
value: 29.305666666666664
- type: mrr_at_10
value: 37.22966666666666
- type: mrr_at_100
value: 38.066583333333334
- type: mrr_at_1000
value: 38.12616666666667
- type: mrr_at_3
value: 34.92275
- type: mrr_at_5
value: 36.23333333333334
- type: ndcg_at_1
value: 29.305666666666664
- type: ndcg_at_10
value: 38.25533333333333
- type: ndcg_at_100
value: 43.25266666666666
- type: ndcg_at_1000
value: 45.63583333333334
- type: ndcg_at_3
value: 33.777166666666666
- type: ndcg_at_5
value: 35.85
- type: precision_at_1
value: 29.305666666666664
- type: precision_at_10
value: 6.596416666666667
- type: precision_at_100
value: 1.0784166666666668
- type: precision_at_1000
value: 0.14666666666666664
- type: precision_at_3
value: 15.31075
- type: precision_at_5
value: 10.830916666666667
- type: recall_at_1
value: 25.00791666666667
- type: recall_at_10
value: 49.10933333333333
- type: recall_at_100
value: 71.09216666666667
- type: recall_at_1000
value: 87.77725000000001
- type: recall_at_3
value: 36.660916666666665
- type: recall_at_5
value: 41.94149999999999
- task:
type: Retrieval
dataset:
type: BeIR/cqadupstack
name: MTEB CQADupstackStatsRetrieval
config: default
split: test
revision: None
metrics:
- type: map_at_1
value: 23.521
- type: map_at_10
value: 30.043
- type: map_at_100
value: 30.936000000000003
- type: map_at_1000
value: 31.022
- type: map_at_3
value: 27.926000000000002
- type: map_at_5
value: 29.076999999999998
- type: mrr_at_1
value: 26.227
- type: mrr_at_10
value: 32.822
- type: mrr_at_100
value: 33.61
- type: mrr_at_1000
value: 33.672000000000004
- type: mrr_at_3
value: 30.776999999999997
- type: mrr_at_5
value: 31.866
- type: ndcg_at_1
value: 26.227
- type: ndcg_at_10
value: 34.041
- type: ndcg_at_100
value: 38.394
- type: ndcg_at_1000
value: 40.732
- type: ndcg_at_3
value: 30.037999999999997
- type: ndcg_at_5
value: 31.845000000000002
- type: precision_at_1
value: 26.227
- type: precision_at_10
value: 5.244999999999999
- type: precision_at_100
value: 0.808
- type: precision_at_1000
value: 0.107
- type: precision_at_3
value: 12.679000000000002
- type: precision_at_5
value: 8.773
- type: recall_at_1
value: 23.521
- type: recall_at_10
value: 43.633
- type: recall_at_100
value: 63.126000000000005
- type: recall_at_1000
value: 80.765
- type: recall_at_3
value: 32.614
- type: recall_at_5
value: 37.15
- task:
type: Retrieval
dataset:
type: BeIR/cqadupstack
name: MTEB CQADupstackTexRetrieval
config: default
split: test
revision: None
metrics:
- type: map_at_1
value: 16.236
- type: map_at_10
value: 22.898
- type: map_at_100
value: 23.878
- type: map_at_1000
value: 24.009
- type: map_at_3
value: 20.87
- type: map_at_5
value: 22.025
- type: mrr_at_1
value: 19.339000000000002
- type: mrr_at_10
value: 26.382
- type: mrr_at_100
value: 27.245
- type: mrr_at_1000
value: 27.33
- type: mrr_at_3
value: 24.386
- type: mrr_at_5
value: 25.496000000000002
- type: ndcg_at_1
value: 19.339000000000002
- type: ndcg_at_10
value: 27.139999999999997
- type: ndcg_at_100
value: 31.944
- type: ndcg_at_1000
value: 35.077999999999996
- type: ndcg_at_3
value: 23.424
- type: ndcg_at_5
value: 25.188
- type: precision_at_1
value: 19.339000000000002
- type: precision_at_10
value: 4.8309999999999995
- type: precision_at_100
value: 0.845
- type: precision_at_1000
value: 0.128
- type: precision_at_3
value: 10.874
- type: precision_at_5
value: 7.825
- type: recall_at_1
value: 16.236
- type: recall_at_10
value: 36.513
- type: recall_at_100
value: 57.999
- type: recall_at_1000
value: 80.512
- type: recall_at_3
value: 26.179999999999996
- type: recall_at_5
value: 30.712
- task:
type: Retrieval
dataset:
type: BeIR/cqadupstack
name: MTEB CQADupstackUnixRetrieval
config: default
split: test
revision: None
metrics:
- type: map_at_1
value: 24.11
- type: map_at_10
value: 31.566
- type: map_at_100
value: 32.647
- type: map_at_1000
value: 32.753
- type: map_at_3
value: 29.24
- type: map_at_5
value: 30.564999999999998
- type: mrr_at_1
value: 28.265
- type: mrr_at_10
value: 35.504000000000005
- type: mrr_at_100
value: 36.436
- type: mrr_at_1000
value: 36.503
- type: mrr_at_3
value: 33.349000000000004
- type: mrr_at_5
value: 34.622
- type: ndcg_at_1
value: 28.265
- type: ndcg_at_10
value: 36.192
- type: ndcg_at_100
value: 41.388000000000005
- type: ndcg_at_1000
value: 43.948
- type: ndcg_at_3
value: 31.959
- type: ndcg_at_5
value: 33.998
- type: precision_at_1
value: 28.265
- type: precision_at_10
value: 5.989
- type: precision_at_100
value: 0.9650000000000001
- type: precision_at_1000
value: 0.13
- type: precision_at_3
value: 14.335
- type: precision_at_5
value: 10.112
- type: recall_at_1
value: 24.11
- type: recall_at_10
value: 46.418
- type: recall_at_100
value: 69.314
- type: recall_at_1000
value: 87.397
- type: recall_at_3
value: 34.724
- type: recall_at_5
value: 39.925
- task:
type: Retrieval
dataset:
type: BeIR/cqadupstack
name: MTEB CQADupstackWebmastersRetrieval
config: default
split: test
revision: None
metrics:
- type: map_at_1
value: 22.091
- type: map_at_10
value: 29.948999999999998
- type: map_at_100
value: 31.502000000000002
- type: map_at_1000
value: 31.713
- type: map_at_3
value: 27.464
- type: map_at_5
value: 28.968
- type: mrr_at_1
value: 26.482
- type: mrr_at_10
value: 34.009
- type: mrr_at_100
value: 35.081
- type: mrr_at_1000
value: 35.138000000000005
- type: mrr_at_3
value: 31.785000000000004
- type: mrr_at_5
value: 33.178999999999995
- type: ndcg_at_1
value: 26.482
- type: ndcg_at_10
value: 35.008
- type: ndcg_at_100
value: 41.272999999999996
- type: ndcg_at_1000
value: 43.972
- type: ndcg_at_3
value: 30.804
- type: ndcg_at_5
value: 33.046
- type: precision_at_1
value: 26.482
- type: precision_at_10
value: 6.462
- type: precision_at_100
value: 1.431
- type: precision_at_1000
value: 0.22899999999999998
- type: precision_at_3
value: 14.360999999999999
- type: precision_at_5
value: 10.474
- type: recall_at_1
value: 22.091
- type: recall_at_10
value: 45.125
- type: recall_at_100
value: 72.313
- type: recall_at_1000
value: 89.503
- type: recall_at_3
value: 33.158
- type: recall_at_5
value: 39.086999999999996
- task:
type: Retrieval
dataset:
type: BeIR/cqadupstack
name: MTEB CQADupstackWordpressRetrieval
config: default
split: test
revision: None
metrics:
- type: map_at_1
value: 19.883
- type: map_at_10
value: 26.951000000000004
- type: map_at_100
value: 27.927999999999997
- type: map_at_1000
value: 28.022000000000002
- type: map_at_3
value: 24.616
- type: map_at_5
value: 25.917
- type: mrr_at_1
value: 21.996
- type: mrr_at_10
value: 29.221000000000004
- type: mrr_at_100
value: 30.024
- type: mrr_at_1000
value: 30.095
- type: mrr_at_3
value: 26.833000000000002
- type: mrr_at_5
value: 28.155
- type: ndcg_at_1
value: 21.996
- type: ndcg_at_10
value: 31.421
- type: ndcg_at_100
value: 36.237
- type: ndcg_at_1000
value: 38.744
- type: ndcg_at_3
value: 26.671
- type: ndcg_at_5
value: 28.907
- type: precision_at_1
value: 21.996
- type: precision_at_10
value: 5.009
- type: precision_at_100
value: 0.799
- type: precision_at_1000
value: 0.11199999999999999
- type: precision_at_3
value: 11.275
- type: precision_at_5
value: 8.059
- type: recall_at_1
value: 19.883
- type: recall_at_10
value: 43.132999999999996
- type: recall_at_100
value: 65.654
- type: recall_at_1000
value: 84.492
- type: recall_at_3
value: 30.209000000000003
- type: recall_at_5
value: 35.616
- task:
type: Retrieval
dataset:
type: climate-fever
name: MTEB ClimateFEVER
config: default
split: test
revision: None
metrics:
- type: map_at_1
value: 17.756
- type: map_at_10
value: 30.378
- type: map_at_100
value: 32.537
- type: map_at_1000
value: 32.717
- type: map_at_3
value: 25.599
- type: map_at_5
value: 28.372999999999998
- type: mrr_at_1
value: 41.303
- type: mrr_at_10
value: 53.483999999999995
- type: mrr_at_100
value: 54.106
- type: mrr_at_1000
value: 54.127
- type: mrr_at_3
value: 50.315
- type: mrr_at_5
value: 52.396
- type: ndcg_at_1
value: 41.303
- type: ndcg_at_10
value: 40.503
- type: ndcg_at_100
value: 47.821000000000005
- type: ndcg_at_1000
value: 50.788
- type: ndcg_at_3
value: 34.364
- type: ndcg_at_5
value: 36.818
- type: precision_at_1
value: 41.303
- type: precision_at_10
value: 12.463000000000001
- type: precision_at_100
value: 2.037
- type: precision_at_1000
value: 0.26
- type: precision_at_3
value: 25.798
- type: precision_at_5
value: 19.896
- type: recall_at_1
value: 17.756
- type: recall_at_10
value: 46.102
- type: recall_at_100
value: 70.819
- type: recall_at_1000
value: 87.21799999999999
- type: recall_at_3
value: 30.646
- type: recall_at_5
value: 38.022
- task:
type: Retrieval
dataset:
type: dbpedia-entity
name: MTEB DBPedia
config: default
split: test
revision: None
metrics:
- type: map_at_1
value: 9.033
- type: map_at_10
value: 20.584
- type: map_at_100
value: 29.518
- type: map_at_1000
value: 31.186000000000003
- type: map_at_3
value: 14.468
- type: map_at_5
value: 17.177
- type: mrr_at_1
value: 69.75
- type: mrr_at_10
value: 77.025
- type: mrr_at_100
value: 77.36699999999999
- type: mrr_at_1000
value: 77.373
- type: mrr_at_3
value: 75.583
- type: mrr_at_5
value: 76.396
- type: ndcg_at_1
value: 58.5
- type: ndcg_at_10
value: 45.033
- type: ndcg_at_100
value: 49.071
- type: ndcg_at_1000
value: 56.056
- type: ndcg_at_3
value: 49.936
- type: ndcg_at_5
value: 47.471999999999994
- type: precision_at_1
value: 69.75
- type: precision_at_10
value: 35.775
- type: precision_at_100
value: 11.594999999999999
- type: precision_at_1000
value: 2.062
- type: precision_at_3
value: 52.5
- type: precision_at_5
value: 45.300000000000004
- type: recall_at_1
value: 9.033
- type: recall_at_10
value: 26.596999999999998
- type: recall_at_100
value: 54.607000000000006
- type: recall_at_1000
value: 76.961
- type: recall_at_3
value: 15.754999999999999
- type: recall_at_5
value: 20.033
- task:
type: Classification
dataset:
type: mteb/emotion
name: MTEB EmotionClassification
config: default
split: test
revision: 4f58c6b202a23cf9a4da393831edf4f9183cad37
metrics:
- type: accuracy
value: 48.345000000000006
- type: f1
value: 43.4514918068706
- task:
type: Retrieval
dataset:
type: fever
name: MTEB FEVER
config: default
split: test
revision: None
metrics:
- type: map_at_1
value: 71.29100000000001
- type: map_at_10
value: 81.059
- type: map_at_100
value: 81.341
- type: map_at_1000
value: 81.355
- type: map_at_3
value: 79.74799999999999
- type: map_at_5
value: 80.612
- type: mrr_at_1
value: 76.40299999999999
- type: mrr_at_10
value: 84.615
- type: mrr_at_100
value: 84.745
- type: mrr_at_1000
value: 84.748
- type: mrr_at_3
value: 83.776
- type: mrr_at_5
value: 84.343
- type: ndcg_at_1
value: 76.40299999999999
- type: ndcg_at_10
value: 84.981
- type: ndcg_at_100
value: 86.00999999999999
- type: ndcg_at_1000
value: 86.252
- type: ndcg_at_3
value: 82.97
- type: ndcg_at_5
value: 84.152
- type: precision_at_1
value: 76.40299999999999
- type: precision_at_10
value: 10.446
- type: precision_at_100
value: 1.1199999999999999
- type: precision_at_1000
value: 0.116
- type: precision_at_3
value: 32.147999999999996
- type: precision_at_5
value: 20.135
- type: recall_at_1
value: 71.29100000000001
- type: recall_at_10
value: 93.232
- type: recall_at_100
value: 97.363
- type: recall_at_1000
value: 98.905
- type: recall_at_3
value: 87.893
- type: recall_at_5
value: 90.804
- task:
type: Retrieval
dataset:
type: fiqa
name: MTEB FiQA2018
config: default
split: test
revision: None
metrics:
- type: map_at_1
value: 18.667
- type: map_at_10
value: 30.853
- type: map_at_100
value: 32.494
- type: map_at_1000
value: 32.677
- type: map_at_3
value: 26.91
- type: map_at_5
value: 29.099000000000004
- type: mrr_at_1
value: 37.191
- type: mrr_at_10
value: 46.171
- type: mrr_at_100
value: 47.056
- type: mrr_at_1000
value: 47.099000000000004
- type: mrr_at_3
value: 44.059
- type: mrr_at_5
value: 45.147
- type: ndcg_at_1
value: 37.191
- type: ndcg_at_10
value: 38.437
- type: ndcg_at_100
value: 44.62
- type: ndcg_at_1000
value: 47.795
- type: ndcg_at_3
value: 35.003
- type: ndcg_at_5
value: 36.006
- type: precision_at_1
value: 37.191
- type: precision_at_10
value: 10.586
- type: precision_at_100
value: 1.688
- type: precision_at_1000
value: 0.22699999999999998
- type: precision_at_3
value: 23.302
- type: precision_at_5
value: 17.006
- type: recall_at_1
value: 18.667
- type: recall_at_10
value: 45.367000000000004
- type: recall_at_100
value: 68.207
- type: recall_at_1000
value: 87.072
- type: recall_at_3
value: 32.129000000000005
- type: recall_at_5
value: 37.719
- task:
type: Retrieval
dataset:
type: hotpotqa
name: MTEB HotpotQA
config: default
split: test
revision: None
metrics:
- type: map_at_1
value: 39.494
- type: map_at_10
value: 66.223
- type: map_at_100
value: 67.062
- type: map_at_1000
value: 67.11500000000001
- type: map_at_3
value: 62.867
- type: map_at_5
value: 64.994
- type: mrr_at_1
value: 78.987
- type: mrr_at_10
value: 84.585
- type: mrr_at_100
value: 84.773
- type: mrr_at_1000
value: 84.77900000000001
- type: mrr_at_3
value: 83.592
- type: mrr_at_5
value: 84.235
- type: ndcg_at_1
value: 78.987
- type: ndcg_at_10
value: 73.64
- type: ndcg_at_100
value: 76.519
- type: ndcg_at_1000
value: 77.51
- type: ndcg_at_3
value: 68.893
- type: ndcg_at_5
value: 71.585
- type: precision_at_1
value: 78.987
- type: precision_at_10
value: 15.529000000000002
- type: precision_at_100
value: 1.7770000000000001
- type: precision_at_1000
value: 0.191
- type: precision_at_3
value: 44.808
- type: precision_at_5
value: 29.006999999999998
- type: recall_at_1
value: 39.494
- type: recall_at_10
value: 77.643
- type: recall_at_100
value: 88.825
- type: recall_at_1000
value: 95.321
- type: recall_at_3
value: 67.211
- type: recall_at_5
value: 72.519
- task:
type: Classification
dataset:
type: mteb/imdb
name: MTEB ImdbClassification
config: default
split: test
revision: 3d86128a09e091d6018b6d26cad27f2739fc2db7
metrics:
- type: accuracy
value: 85.55959999999999
- type: ap
value: 80.7246500384617
- type: f1
value: 85.52336485065454
- task:
type: Retrieval
dataset:
type: msmarco
name: MTEB MSMARCO
config: default
split: dev
revision: None
metrics:
- type: map_at_1
value: 23.631
- type: map_at_10
value: 36.264
- type: map_at_100
value: 37.428
- type: map_at_1000
value: 37.472
- type: map_at_3
value: 32.537
- type: map_at_5
value: 34.746
- type: mrr_at_1
value: 24.312
- type: mrr_at_10
value: 36.858000000000004
- type: mrr_at_100
value: 37.966
- type: mrr_at_1000
value: 38.004
- type: mrr_at_3
value: 33.188
- type: mrr_at_5
value: 35.367
- type: ndcg_at_1
value: 24.312
- type: ndcg_at_10
value: 43.126999999999995
- type: ndcg_at_100
value: 48.642
- type: ndcg_at_1000
value: 49.741
- type: ndcg_at_3
value: 35.589
- type: ndcg_at_5
value: 39.515
- type: precision_at_1
value: 24.312
- type: precision_at_10
value: 6.699
- type: precision_at_100
value: 0.9450000000000001
- type: precision_at_1000
value: 0.104
- type: precision_at_3
value: 15.153
- type: precision_at_5
value: 11.065999999999999
- type: recall_at_1
value: 23.631
- type: recall_at_10
value: 64.145
- type: recall_at_100
value: 89.41
- type: recall_at_1000
value: 97.83500000000001
- type: recall_at_3
value: 43.769000000000005
- type: recall_at_5
value: 53.169
- task:
type: Classification
dataset:
type: mteb/mtop_domain
name: MTEB MTOPDomainClassification (en)
config: en
split: test
revision: d80d48c1eb48d3562165c59d59d0034df9fff0bf
metrics:
- type: accuracy
value: 93.4108527131783
- type: f1
value: 93.1415880261038
- task:
type: Classification
dataset:
type: mteb/mtop_intent
name: MTEB MTOPIntentClassification (en)
config: en
split: test
revision: ae001d0e6b1228650b7bd1c2c65fb50ad11a8aba
metrics:
- type: accuracy
value: 77.24806201550388
- type: f1
value: 60.531916308197175
- task:
type: Classification
dataset:
type: mteb/amazon_massive_intent
name: MTEB MassiveIntentClassification (en)
config: en
split: test
revision: 31efe3c427b0bae9c22cbb560b8f15491cc6bed7
metrics:
- type: accuracy
value: 73.71553463349024
- type: f1
value: 71.70753174900791
- task:
type: Classification
dataset:
type: mteb/amazon_massive_scenario
name: MTEB MassiveScenarioClassification (en)
config: en
split: test
revision: 7d571f92784cd94a019292a1f45445077d0ef634
metrics:
- type: accuracy
value: 77.79757901815736
- type: f1
value: 77.83719850433258
- task:
type: Clustering
dataset:
type: mteb/medrxiv-clustering-p2p
name: MTEB MedrxivClusteringP2P
config: default
split: test
revision: e7a26af6f3ae46b30dde8737f02c07b1505bcc73
metrics:
- type: v_measure
value: 33.74193296622113
- task:
type: Clustering
dataset:
type: mteb/medrxiv-clustering-s2s
name: MTEB MedrxivClusteringS2S
config: default
split: test
revision: 35191c8c0dca72d8ff3efcd72aa802307d469663
metrics:
- type: v_measure
value: 30.64257594108566
- task:
type: Reranking
dataset:
type: mteb/mind_small
name: MTEB MindSmallReranking
config: default
split: test
revision: 3bdac13927fdc888b903db93b2ffdbd90b295a69
metrics:
- type: map
value: 30.811018518883625
- type: mrr
value: 31.910376577445003
- task:
type: Retrieval
dataset:
type: nfcorpus
name: MTEB NFCorpus
config: default
split: test
revision: None
metrics:
- type: map_at_1
value: 5.409
- type: map_at_10
value: 13.093
- type: map_at_100
value: 16.256999999999998
- type: map_at_1000
value: 17.617
- type: map_at_3
value: 9.555
- type: map_at_5
value: 11.428
- type: mrr_at_1
value: 45.201
- type: mrr_at_10
value: 54.179
- type: mrr_at_100
value: 54.812000000000005
- type: mrr_at_1000
value: 54.840999999999994
- type: mrr_at_3
value: 51.909000000000006
- type: mrr_at_5
value: 53.519000000000005
- type: ndcg_at_1
value: 43.189
- type: ndcg_at_10
value: 35.028
- type: ndcg_at_100
value: 31.226
- type: ndcg_at_1000
value: 39.678000000000004
- type: ndcg_at_3
value: 40.596
- type: ndcg_at_5
value: 38.75
- type: precision_at_1
value: 44.582
- type: precision_at_10
value: 25.974999999999998
- type: precision_at_100
value: 7.793
- type: precision_at_1000
value: 2.036
- type: precision_at_3
value: 38.493
- type: precision_at_5
value: 33.994
- type: recall_at_1
value: 5.409
- type: recall_at_10
value: 16.875999999999998
- type: recall_at_100
value: 30.316
- type: recall_at_1000
value: 60.891
- type: recall_at_3
value: 10.688
- type: recall_at_5
value: 13.832
- task:
type: Retrieval
dataset:
type: nq
name: MTEB NQ
config: default
split: test
revision: None
metrics:
- type: map_at_1
value: 36.375
- type: map_at_10
value: 51.991
- type: map_at_100
value: 52.91400000000001
- type: map_at_1000
value: 52.93600000000001
- type: map_at_3
value: 48.014
- type: map_at_5
value: 50.381
- type: mrr_at_1
value: 40.759
- type: mrr_at_10
value: 54.617000000000004
- type: mrr_at_100
value: 55.301
- type: mrr_at_1000
value: 55.315000000000005
- type: mrr_at_3
value: 51.516
- type: mrr_at_5
value: 53.435
- type: ndcg_at_1
value: 40.759
- type: ndcg_at_10
value: 59.384
- type: ndcg_at_100
value: 63.157
- type: ndcg_at_1000
value: 63.654999999999994
- type: ndcg_at_3
value: 52.114000000000004
- type: ndcg_at_5
value: 55.986000000000004
- type: precision_at_1
value: 40.759
- type: precision_at_10
value: 9.411999999999999
- type: precision_at_100
value: 1.153
- type: precision_at_1000
value: 0.12
- type: precision_at_3
value: 23.329
- type: precision_at_5
value: 16.256999999999998
- type: recall_at_1
value: 36.375
- type: recall_at_10
value: 79.053
- type: recall_at_100
value: 95.167
- type: recall_at_1000
value: 98.82
- type: recall_at_3
value: 60.475
- type: recall_at_5
value: 69.327
- task:
type: Retrieval
dataset:
type: quora
name: MTEB QuoraRetrieval
config: default
split: test
revision: None
metrics:
- type: map_at_1
value: 70.256
- type: map_at_10
value: 83.8
- type: map_at_100
value: 84.425
- type: map_at_1000
value: 84.444
- type: map_at_3
value: 80.906
- type: map_at_5
value: 82.717
- type: mrr_at_1
value: 80.97999999999999
- type: mrr_at_10
value: 87.161
- type: mrr_at_100
value: 87.262
- type: mrr_at_1000
value: 87.263
- type: mrr_at_3
value: 86.175
- type: mrr_at_5
value: 86.848
- type: ndcg_at_1
value: 80.97999999999999
- type: ndcg_at_10
value: 87.697
- type: ndcg_at_100
value: 88.959
- type: ndcg_at_1000
value: 89.09899999999999
- type: ndcg_at_3
value: 84.83800000000001
- type: ndcg_at_5
value: 86.401
- type: precision_at_1
value: 80.97999999999999
- type: precision_at_10
value: 13.261000000000001
- type: precision_at_100
value: 1.5150000000000001
- type: precision_at_1000
value: 0.156
- type: precision_at_3
value: 37.01
- type: precision_at_5
value: 24.298000000000002
- type: recall_at_1
value: 70.256
- type: recall_at_10
value: 94.935
- type: recall_at_100
value: 99.274
- type: recall_at_1000
value: 99.928
- type: recall_at_3
value: 86.602
- type: recall_at_5
value: 91.133
- task:
type: Clustering
dataset:
type: mteb/reddit-clustering
name: MTEB RedditClustering
config: default
split: test
revision: 24640382cdbf8abc73003fb0fa6d111a705499eb
metrics:
- type: v_measure
value: 56.322692497613104
- task:
type: Clustering
dataset:
type: mteb/reddit-clustering-p2p
name: MTEB RedditClusteringP2P
config: default
split: test
revision: 282350215ef01743dc01b456c7f5241fa8937f16
metrics:
- type: v_measure
value: 61.895813503775074
- task:
type: Retrieval
dataset:
type: scidocs
name: MTEB SCIDOCS
config: default
split: test
revision: None
metrics:
- type: map_at_1
value: 4.338
- type: map_at_10
value: 10.767
- type: map_at_100
value: 12.537999999999998
- type: map_at_1000
value: 12.803999999999998
- type: map_at_3
value: 7.788
- type: map_at_5
value: 9.302000000000001
- type: mrr_at_1
value: 21.4
- type: mrr_at_10
value: 31.637999999999998
- type: mrr_at_100
value: 32.688
- type: mrr_at_1000
value: 32.756
- type: mrr_at_3
value: 28.433000000000003
- type: mrr_at_5
value: 30.178
- type: ndcg_at_1
value: 21.4
- type: ndcg_at_10
value: 18.293
- type: ndcg_at_100
value: 25.274
- type: ndcg_at_1000
value: 30.284
- type: ndcg_at_3
value: 17.391000000000002
- type: ndcg_at_5
value: 15.146999999999998
- type: precision_at_1
value: 21.4
- type: precision_at_10
value: 9.48
- type: precision_at_100
value: 1.949
- type: precision_at_1000
value: 0.316
- type: precision_at_3
value: 16.167
- type: precision_at_5
value: 13.22
- type: recall_at_1
value: 4.338
- type: recall_at_10
value: 19.213
- type: recall_at_100
value: 39.562999999999995
- type: recall_at_1000
value: 64.08
- type: recall_at_3
value: 9.828000000000001
- type: recall_at_5
value: 13.383000000000001
- task:
type: STS
dataset:
type: mteb/sickr-sts
name: MTEB SICK-R
config: default
split: test
revision: a6ea5a8cab320b040a23452cc28066d9beae2cee
metrics:
- type: cos_sim_pearson
value: 82.42568163642142
- type: cos_sim_spearman
value: 78.5797159641342
- type: euclidean_pearson
value: 80.22151260811604
- type: euclidean_spearman
value: 78.5797151953878
- type: manhattan_pearson
value: 80.21224215864788
- type: manhattan_spearman
value: 78.55641478381344
- task:
type: STS
dataset:
type: mteb/sts12-sts
name: MTEB STS12
config: default
split: test
revision: a0d554a64d88156834ff5ae9920b964011b16384
metrics:
- type: cos_sim_pearson
value: 85.44020710812569
- type: cos_sim_spearman
value: 78.91631735081286
- type: euclidean_pearson
value: 81.64188964182102
- type: euclidean_spearman
value: 78.91633286881678
- type: manhattan_pearson
value: 81.69294748512496
- type: manhattan_spearman
value: 78.93438558002656
- task:
type: STS
dataset:
type: mteb/sts13-sts
name: MTEB STS13
config: default
split: test
revision: 7e90230a92c190f1bf69ae9002b8cea547a64cca
metrics:
- type: cos_sim_pearson
value: 84.27165426412311
- type: cos_sim_spearman
value: 85.40429140249618
- type: euclidean_pearson
value: 84.7509580724893
- type: euclidean_spearman
value: 85.40429140249618
- type: manhattan_pearson
value: 84.76488289321308
- type: manhattan_spearman
value: 85.4256793698708
- task:
type: STS
dataset:
type: mteb/sts14-sts
name: MTEB STS14
config: default
split: test
revision: 6031580fec1f6af667f0bd2da0a551cf4f0b2375
metrics:
- type: cos_sim_pearson
value: 83.138851760732
- type: cos_sim_spearman
value: 81.64101363896586
- type: euclidean_pearson
value: 82.55165038934942
- type: euclidean_spearman
value: 81.64105257080502
- type: manhattan_pearson
value: 82.52802949883335
- type: manhattan_spearman
value: 81.61255430718158
- task:
type: STS
dataset:
type: mteb/sts15-sts
name: MTEB STS15
config: default
split: test
revision: ae752c7c21bf194d8b67fd573edf7ae58183cbe3
metrics:
- type: cos_sim_pearson
value: 86.0654695484029
- type: cos_sim_spearman
value: 87.20408521902229
- type: euclidean_pearson
value: 86.8110651362115
- type: euclidean_spearman
value: 87.20408521902229
- type: manhattan_pearson
value: 86.77984656478691
- type: manhattan_spearman
value: 87.1719947099227
- task:
type: STS
dataset:
type: mteb/sts16-sts
name: MTEB STS16
config: default
split: test
revision: 4d8694f8f0e0100860b497b999b3dbed754a0513
metrics:
- type: cos_sim_pearson
value: 83.77823915496512
- type: cos_sim_spearman
value: 85.43566325729779
- type: euclidean_pearson
value: 84.5396956658821
- type: euclidean_spearman
value: 85.43566325729779
- type: manhattan_pearson
value: 84.5665398848169
- type: manhattan_spearman
value: 85.44375870303232
- task:
type: STS
dataset:
type: mteb/sts17-crosslingual-sts
name: MTEB STS17 (en-en)
config: en-en
split: test
revision: af5e6fb845001ecf41f4c1e033ce921939a2a68d
metrics:
- type: cos_sim_pearson
value: 87.20030208471798
- type: cos_sim_spearman
value: 87.20485505076539
- type: euclidean_pearson
value: 88.10588324368722
- type: euclidean_spearman
value: 87.20485505076539
- type: manhattan_pearson
value: 87.92324770415183
- type: manhattan_spearman
value: 87.0571314561877
- task:
type: STS
dataset:
type: mteb/sts22-crosslingual-sts
name: MTEB STS22 (en)
config: en
split: test
revision: 6d1ba47164174a496b7fa5d3569dae26a6813b80
metrics:
- type: cos_sim_pearson
value: 63.06093161604453
- type: cos_sim_spearman
value: 64.2163140357722
- type: euclidean_pearson
value: 65.27589680994006
- type: euclidean_spearman
value: 64.2163140357722
- type: manhattan_pearson
value: 65.45904383711101
- type: manhattan_spearman
value: 64.55404716679305
- task:
type: STS
dataset:
type: mteb/stsbenchmark-sts
name: MTEB STSBenchmark
config: default
split: test
revision: b0fddb56ed78048fa8b90373c8a3cfc37b684831
metrics:
- type: cos_sim_pearson
value: 84.32976164578706
- type: cos_sim_spearman
value: 85.54302197678368
- type: euclidean_pearson
value: 85.26307149193056
- type: euclidean_spearman
value: 85.54302197678368
- type: manhattan_pearson
value: 85.26647282029371
- type: manhattan_spearman
value: 85.5316135265568
- task:
type: Reranking
dataset:
type: mteb/scidocs-reranking
name: MTEB SciDocsRR
config: default
split: test
revision: d3c5e1fc0b855ab6097bf1cda04dd73947d7caab
metrics:
- type: map
value: 81.44675968318754
- type: mrr
value: 94.92741826075158
- task:
type: Retrieval
dataset:
type: scifact
name: MTEB SciFact
config: default
split: test
revision: None
metrics:
- type: map_at_1
value: 56.34400000000001
- type: map_at_10
value: 65.927
- type: map_at_100
value: 66.431
- type: map_at_1000
value: 66.461
- type: map_at_3
value: 63.529
- type: map_at_5
value: 64.818
- type: mrr_at_1
value: 59.333000000000006
- type: mrr_at_10
value: 67.54599999999999
- type: mrr_at_100
value: 67.892
- type: mrr_at_1000
value: 67.917
- type: mrr_at_3
value: 65.778
- type: mrr_at_5
value: 66.794
- type: ndcg_at_1
value: 59.333000000000006
- type: ndcg_at_10
value: 70.5
- type: ndcg_at_100
value: 72.688
- type: ndcg_at_1000
value: 73.483
- type: ndcg_at_3
value: 66.338
- type: ndcg_at_5
value: 68.265
- type: precision_at_1
value: 59.333000000000006
- type: precision_at_10
value: 9.3
- type: precision_at_100
value: 1.053
- type: precision_at_1000
value: 0.11199999999999999
- type: precision_at_3
value: 25.889
- type: precision_at_5
value: 16.866999999999997
- type: recall_at_1
value: 56.34400000000001
- type: recall_at_10
value: 82.789
- type: recall_at_100
value: 92.767
- type: recall_at_1000
value: 99
- type: recall_at_3
value: 71.64399999999999
- type: recall_at_5
value: 76.322
- task:
type: PairClassification
dataset:
type: mteb/sprintduplicatequestions-pairclassification
name: MTEB SprintDuplicateQuestions
config: default
split: test
revision: d66bd1f72af766a5cc4b0ca5e00c162f89e8cc46
metrics:
- type: cos_sim_accuracy
value: 99.75742574257426
- type: cos_sim_ap
value: 93.52081548447406
- type: cos_sim_f1
value: 87.33850129198966
- type: cos_sim_precision
value: 90.37433155080214
- type: cos_sim_recall
value: 84.5
- type: dot_accuracy
value: 99.75742574257426
- type: dot_ap
value: 93.52081548447406
- type: dot_f1
value: 87.33850129198966
- type: dot_precision
value: 90.37433155080214
- type: dot_recall
value: 84.5
- type: euclidean_accuracy
value: 99.75742574257426
- type: euclidean_ap
value: 93.52081548447406
- type: euclidean_f1
value: 87.33850129198966
- type: euclidean_precision
value: 90.37433155080214
- type: euclidean_recall
value: 84.5
- type: manhattan_accuracy
value: 99.75841584158415
- type: manhattan_ap
value: 93.4975678585854
- type: manhattan_f1
value: 87.26708074534162
- type: manhattan_precision
value: 90.45064377682404
- type: manhattan_recall
value: 84.3
- type: max_accuracy
value: 99.75841584158415
- type: max_ap
value: 93.52081548447406
- type: max_f1
value: 87.33850129198966
- task:
type: Clustering
dataset:
type: mteb/stackexchange-clustering
name: MTEB StackExchangeClustering
config: default
split: test
revision: 6cbc1f7b2bc0622f2e39d2c77fa502909748c259
metrics:
- type: v_measure
value: 64.31437036686651
- task:
type: Clustering
dataset:
type: mteb/stackexchange-clustering-p2p
name: MTEB StackExchangeClusteringP2P
config: default
split: test
revision: 815ca46b2622cec33ccafc3735d572c266efdb44
metrics:
- type: v_measure
value: 33.25569319007206
- task:
type: Reranking
dataset:
type: mteb/stackoverflowdupquestions-reranking
name: MTEB StackOverflowDupQuestions
config: default
split: test
revision: e185fbe320c72810689fc5848eb6114e1ef5ec69
metrics:
- type: map
value: 49.90474939720706
- type: mrr
value: 50.568115503777264
- task:
type: Summarization
dataset:
type: mteb/summeval
name: MTEB SummEval
config: default
split: test
revision: cda12ad7615edc362dbf25a00fdd61d3b1eaf93c
metrics:
- type: cos_sim_pearson
value: 29.866828641244712
- type: cos_sim_spearman
value: 30.077555055873866
- type: dot_pearson
value: 29.866832988572266
- type: dot_spearman
value: 30.077555055873866
- task:
type: Retrieval
dataset:
type: trec-covid
name: MTEB TRECCOVID
config: default
split: test
revision: None
metrics:
- type: map_at_1
value: 0.232
- type: map_at_10
value: 2.094
- type: map_at_100
value: 11.971
- type: map_at_1000
value: 28.158
- type: map_at_3
value: 0.688
- type: map_at_5
value: 1.114
- type: mrr_at_1
value: 88
- type: mrr_at_10
value: 93.4
- type: mrr_at_100
value: 93.4
- type: mrr_at_1000
value: 93.4
- type: mrr_at_3
value: 93
- type: mrr_at_5
value: 93.4
- type: ndcg_at_1
value: 84
- type: ndcg_at_10
value: 79.923
- type: ndcg_at_100
value: 61.17
- type: ndcg_at_1000
value: 53.03
- type: ndcg_at_3
value: 84.592
- type: ndcg_at_5
value: 82.821
- type: precision_at_1
value: 88
- type: precision_at_10
value: 85
- type: precision_at_100
value: 63.019999999999996
- type: precision_at_1000
value: 23.554
- type: precision_at_3
value: 89.333
- type: precision_at_5
value: 87.2
- type: recall_at_1
value: 0.232
- type: recall_at_10
value: 2.255
- type: recall_at_100
value: 14.823
- type: recall_at_1000
value: 49.456
- type: recall_at_3
value: 0.718
- type: recall_at_5
value: 1.175
- task:
type: Retrieval
dataset:
type: webis-touche2020
name: MTEB Touche2020
config: default
split: test
revision: None
metrics:
- type: map_at_1
value: 2.547
- type: map_at_10
value: 11.375
- type: map_at_100
value: 18.194
- type: map_at_1000
value: 19.749
- type: map_at_3
value: 5.825
- type: map_at_5
value: 8.581
- type: mrr_at_1
value: 32.653
- type: mrr_at_10
value: 51.32
- type: mrr_at_100
value: 51.747
- type: mrr_at_1000
value: 51.747
- type: mrr_at_3
value: 47.278999999999996
- type: mrr_at_5
value: 48.605
- type: ndcg_at_1
value: 29.592000000000002
- type: ndcg_at_10
value: 28.151
- type: ndcg_at_100
value: 39.438
- type: ndcg_at_1000
value: 50.769
- type: ndcg_at_3
value: 30.758999999999997
- type: ndcg_at_5
value: 30.366
- type: precision_at_1
value: 32.653
- type: precision_at_10
value: 25.714
- type: precision_at_100
value: 8.041
- type: precision_at_1000
value: 1.555
- type: precision_at_3
value: 33.333
- type: precision_at_5
value: 31.837
- type: recall_at_1
value: 2.547
- type: recall_at_10
value: 18.19
- type: recall_at_100
value: 49.538
- type: recall_at_1000
value: 83.86
- type: recall_at_3
value: 7.329
- type: recall_at_5
value: 11.532
- task:
type: Classification
dataset:
type: mteb/toxic_conversations_50k
name: MTEB ToxicConversationsClassification
config: default
split: test
revision: d7c0de2777da35d6aae2200a62c6e0e5af397c4c
metrics:
- type: accuracy
value: 71.4952
- type: ap
value: 14.793362635531409
- type: f1
value: 55.204635551516915
- task:
type: Classification
dataset:
type: mteb/tweet_sentiment_extraction
name: MTEB TweetSentimentExtractionClassification
config: default
split: test
revision: d604517c81ca91fe16a244d1248fc021f9ecee7a
metrics:
- type: accuracy
value: 61.5365025466893
- type: f1
value: 61.81742556334845
- task:
type: Clustering
dataset:
type: mteb/twentynewsgroups-clustering
name: MTEB TwentyNewsgroupsClustering
config: default
split: test
revision: 6125ec4e24fa026cec8a478383ee943acfbd5449
metrics:
- type: v_measure
value: 49.05531070301185
- task:
type: PairClassification
dataset:
type: mteb/twittersemeval2015-pairclassification
name: MTEB TwitterSemEval2015
config: default
split: test
revision: 70970daeab8776df92f5ea462b6173c0b46fd2d1
metrics:
- type: cos_sim_accuracy
value: 86.51725576682364
- type: cos_sim_ap
value: 75.2292304265163
- type: cos_sim_f1
value: 69.54022988505749
- type: cos_sim_precision
value: 63.65629110039457
- type: cos_sim_recall
value: 76.62269129287598
- type: dot_accuracy
value: 86.51725576682364
- type: dot_ap
value: 75.22922386081054
- type: dot_f1
value: 69.54022988505749
- type: dot_precision
value: 63.65629110039457
- type: dot_recall
value: 76.62269129287598
- type: euclidean_accuracy
value: 86.51725576682364
- type: euclidean_ap
value: 75.22925730473472
- type: euclidean_f1
value: 69.54022988505749
- type: euclidean_precision
value: 63.65629110039457
- type: euclidean_recall
value: 76.62269129287598
- type: manhattan_accuracy
value: 86.52321630804077
- type: manhattan_ap
value: 75.20608115037336
- type: manhattan_f1
value: 69.60000000000001
- type: manhattan_precision
value: 64.37219730941705
- type: manhattan_recall
value: 75.75197889182058
- type: max_accuracy
value: 86.52321630804077
- type: max_ap
value: 75.22925730473472
- type: max_f1
value: 69.60000000000001
- task:
type: PairClassification
dataset:
type: mteb/twitterurlcorpus-pairclassification
name: MTEB TwitterURLCorpus
config: default
split: test
revision: 8b6510b0b1fa4e4c4f879467980e9be563ec1cdf
metrics:
- type: cos_sim_accuracy
value: 89.34877944657896
- type: cos_sim_ap
value: 86.71257569277373
- type: cos_sim_f1
value: 79.10386355986088
- type: cos_sim_precision
value: 76.91468470434214
- type: cos_sim_recall
value: 81.4213119802895
- type: dot_accuracy
value: 89.34877944657896
- type: dot_ap
value: 86.71257133133368
- type: dot_f1
value: 79.10386355986088
- type: dot_precision
value: 76.91468470434214
- type: dot_recall
value: 81.4213119802895
- type: euclidean_accuracy
value: 89.34877944657896
- type: euclidean_ap
value: 86.71257651501476
- type: euclidean_f1
value: 79.10386355986088
- type: euclidean_precision
value: 76.91468470434214
- type: euclidean_recall
value: 81.4213119802895
- type: manhattan_accuracy
value: 89.35848177901967
- type: manhattan_ap
value: 86.69330615469126
- type: manhattan_f1
value: 79.13867741453949
- type: manhattan_precision
value: 76.78881807647741
- type: manhattan_recall
value: 81.63689559593472
- type: max_accuracy
value: 89.35848177901967
- type: max_ap
value: 86.71257651501476
- type: max_f1
value: 79.13867741453949
license: apache-2.0
language:
- en
new_version: nomic-ai/nomic-embed-text-v1.5
---
# nomic-embed-text-v1: A Reproducible Long Context (8192) Text Embedder
`nomic-embed-text-v1` is 8192 context length text encoder that surpasses OpenAI text-embedding-ada-002 and text-embedding-3-small performance on short and long context tasks.
# Performance Benchmarks
| Name | SeqLen | MTEB | LoCo | Jina Long Context | Open Weights | Open Training Code | Open Data |
| :-------------------------------:| :----- | :-------- | :------: | :---------------: | :-----------: | :----------------: | :---------- |
| nomic-embed-text-v1 | 8192 | **62.39** |**85.53** | 54.16 | ✅ | ✅ | ✅ |
| jina-embeddings-v2-base-en | 8192 | 60.39 | 85.45 | 51.90 | ✅ | ❌ | ❌ |
| text-embedding-3-small | 8191 | 62.26 | 82.40 | **58.20** | ❌ | ❌ | ❌ |
| text-embedding-ada-002 | 8191 | 60.99 | 52.7 | 55.25 | ❌ | ❌ | ❌ |
**Exciting Update!**: `nomic-embed-text-v1` is now multimodal! [nomic-embed-vision-v1](https://huggingface.co/nomic-ai/nomic-embed-vision-v1) is aligned to the embedding space of `nomic-embed-text-v1`, meaning any text embedding is multimodal!
## Usage
**Important**: the text prompt *must* include a *task instruction prefix*, instructing the model which task is being performed.
For example, if you are implementing a RAG application, you embed your documents as `search_document: <text here>` and embed your user queries as `search_query: <text here>`.
## Task instruction prefixes
### `search_document`
#### Purpose: embed texts as documents from a dataset
This prefix is used for embedding texts as documents, for example as documents for a RAG index.
```python
from sentence_transformers import SentenceTransformer
model = SentenceTransformer("nomic-ai/nomic-embed-text-v1", trust_remote_code=True)
sentences = ['search_document: TSNE is a dimensionality reduction algorithm created by Laurens van Der Maaten']
embeddings = model.encode(sentences)
print(embeddings)
```
### `search_query`
#### Purpose: embed texts as questions to answer
This prefix is used for embedding texts as questions that documents from a dataset could resolve, for example as queries to be answered by a RAG application.
```python
from sentence_transformers import SentenceTransformer
model = SentenceTransformer("nomic-ai/nomic-embed-text-v1", trust_remote_code=True)
sentences = ['search_query: Who is Laurens van Der Maaten?']
embeddings = model.encode(sentences)
print(embeddings)
```
### `clustering`
#### Purpose: embed texts to group them into clusters
This prefix is used for embedding texts in order to group them into clusters, discover common topics, or remove semantic duplicates.
```python
from sentence_transformers import SentenceTransformer
model = SentenceTransformer("nomic-ai/nomic-embed-text-v1", trust_remote_code=True)
sentences = ['clustering: the quick brown fox']
embeddings = model.encode(sentences)
print(embeddings)
```
### `classification`
#### Purpose: embed texts to classify them
This prefix is used for embedding texts into vectors that will be used as features for a classification model
```python
from sentence_transformers import SentenceTransformer
model = SentenceTransformer("nomic-ai/nomic-embed-text-v1", trust_remote_code=True)
sentences = ['classification: the quick brown fox']
embeddings = model.encode(sentences)
print(embeddings)
```
### Sentence Transformers
```python
from sentence_transformers import SentenceTransformer
model = SentenceTransformer("nomic-ai/nomic-embed-text-v1", trust_remote_code=True)
sentences = ['search_query: What is TSNE?', 'search_query: Who is Laurens van der Maaten?']
embeddings = model.encode(sentences)
print(embeddings)
```
### Transformers
```python
import torch
import torch.nn.functional as F
from transformers import AutoTokenizer, AutoModel
def mean_pooling(model_output, attention_mask):
token_embeddings = model_output[0]
input_mask_expanded = attention_mask.unsqueeze(-1).expand(token_embeddings.size()).float()
return torch.sum(token_embeddings * input_mask_expanded, 1) / torch.clamp(input_mask_expanded.sum(1), min=1e-9)
sentences = ['search_query: What is TSNE?', 'search_query: Who is Laurens van der Maaten?']
tokenizer = AutoTokenizer.from_pretrained('bert-base-uncased')
model = AutoModel.from_pretrained('nomic-ai/nomic-embed-text-v1', trust_remote_code=True)
model.eval()
encoded_input = tokenizer(sentences, padding=True, truncation=True, return_tensors='pt')
with torch.no_grad():
model_output = model(**encoded_input)
embeddings = mean_pooling(model_output, encoded_input['attention_mask'])
embeddings = F.normalize(embeddings, p=2, dim=1)
print(embeddings)
```
The model natively supports scaling of the sequence length past 2048 tokens. To do so,
```diff
- tokenizer = AutoTokenizer.from_pretrained('bert-base-uncased')
+ tokenizer = AutoTokenizer.from_pretrained('bert-base-uncased', model_max_length=8192)
- model = AutoModel.from_pretrained('nomic-ai/nomic-embed-text-v1', trust_remote_code=True)
+ model = AutoModel.from_pretrained('nomic-ai/nomic-embed-text-v1', trust_remote_code=True, rotary_scaling_factor=2)
```
### Transformers.js
```js
import { pipeline } from '@xenova/transformers';
// Create a feature extraction pipeline
const extractor = await pipeline('feature-extraction', 'nomic-ai/nomic-embed-text-v1', {
quantized: false, // Comment out this line to use the quantized version
});
// Compute sentence embeddings
const texts = ['search_query: What is TSNE?', 'search_query: Who is Laurens van der Maaten?'];
const embeddings = await extractor(texts, { pooling: 'mean', normalize: true });
console.log(embeddings);
```
## Nomic API
The easiest way to get started with Nomic Embed is through the Nomic Embedding API.
Generating embeddings with the `nomic` Python client is as easy as
```python
from nomic import embed
output = embed.text(
texts=['Nomic Embedding API', '#keepAIOpen'],
model='nomic-embed-text-v1',
task_type='search_document'
)
print(output)
```
For more information, see the [API reference](https://docs.nomic.ai/reference/endpoints/nomic-embed-text)
## Training
Click the Nomic Atlas map below to visualize a 5M sample of our contrastive pretraining data!
[](https://atlas.nomic.ai/map/nomic-text-embed-v1-5m-sample)
We train our embedder using a multi-stage training pipeline. Starting from a long-context [BERT model](https://huggingface.co/nomic-ai/nomic-bert-2048),
the first unsupervised contrastive stage trains on a dataset generated from weakly related text pairs, such as question-answer pairs from forums like StackExchange and Quora, title-body pairs from Amazon reviews, and summarizations from news articles.
In the second finetuning stage, higher quality labeled datasets such as search queries and answers from web searches are leveraged. Data curation and hard-example mining is crucial in this stage.
For more details, see the Nomic Embed [Technical Report](https://static.nomic.ai/reports/2024_Nomic_Embed_Text_Technical_Report.pdf) and corresponding [blog post](https://blog.nomic.ai/posts/nomic-embed-text-v1).
Training data to train the models is released in its entirety. For more details, see the `contrastors` [repository](https://github.com/nomic-ai/contrastors)
# Join the Nomic Community
- Nomic: [https://nomic.ai](https://nomic.ai)
- Discord: [https://discord.gg/myY5YDR8z8](https://discord.gg/myY5YDR8z8)
- Twitter: [https://twitter.com/nomic_ai](https://twitter.com/nomic_ai)
# Citation
If you find the model, dataset, or training code useful, please cite our work
```bibtex
@misc{nussbaum2024nomic,
title={Nomic Embed: Training a Reproducible Long Context Text Embedder},
author={Zach Nussbaum and John X. Morris and Brandon Duderstadt and Andriy Mulyar},
year={2024},
eprint={2402.01613},
archivePrefix={arXiv},
primaryClass={cs.CL}
}
``` |
timm/ViT-L-16-SigLIP-384 | timm | "2023-10-25T21:54:17Z" | 486,192 | 11 | open_clip | [
"open_clip",
"safetensors",
"clip",
"siglip",
"zero-shot-image-classification",
"dataset:webli",
"arxiv:2303.15343",
"license:apache-2.0",
"region:us"
] | zero-shot-image-classification | "2023-10-16T23:32:50Z" | ---
tags:
- clip
- siglip
library_name: open_clip
pipeline_tag: zero-shot-image-classification
license: apache-2.0
datasets:
- webli
---
# Model card for ViT-L-16-SigLIP-384
A SigLIP (Sigmoid loss for Language-Image Pre-training) model trained on WebLI.
This model has been converted to PyTorch from the original JAX checkpoints in [Big Vision](https://github.com/google-research/big_vision). These weights are usable in both OpenCLIP (image + text) and timm (image only).
## Model Details
- **Model Type:** Contrastive Image-Text, Zero-Shot Image Classification.
- **Original:** https://github.com/google-research/big_vision
- **Dataset:** WebLI
- **Papers:**
- Sigmoid loss for language image pre-training: https://arxiv.org/abs/2303.15343
## Model Usage
### With OpenCLIP
```
import torch
import torch.nn.functional as F
from urllib.request import urlopen
from PIL import Image
from open_clip import create_model_from_pretrained, get_tokenizer # works on open-clip-torch>=2.23.0, timm>=0.9.8
model, preprocess = create_model_from_pretrained('hf-hub:timm/ViT-L-16-SigLIP-384')
tokenizer = get_tokenizer('hf-hub:timm/ViT-L-16-SigLIP-384')
image = Image.open(urlopen(
'https://huggingface.co/datasets/huggingface/documentation-images/resolve/main/beignets-task-guide.png'
))
image = preprocess(image).unsqueeze(0)
labels_list = ["a dog", "a cat", "a donut", "a beignet"]
text = tokenizer(labels_list, context_length=model.context_length)
with torch.no_grad(), torch.cuda.amp.autocast():
image_features = model.encode_image(image)
text_features = model.encode_text(text)
image_features = F.normalize(image_features, dim=-1)
text_features = F.normalize(text_features, dim=-1)
text_probs = torch.sigmoid(image_features @ text_features.T * model.logit_scale.exp() + model.logit_bias)
zipped_list = list(zip(labels_list, [round(p.item(), 3) for p in text_probs[0]]))
print("Label probabilities: ", zipped_list)
```
### With `timm` (for image embeddings)
```python
from urllib.request import urlopen
from PIL import Image
import timm
image = Image.open(urlopen(
'https://huggingface.co/datasets/huggingface/documentation-images/resolve/main/beignets-task-guide.png'
))
model = timm.create_model(
'vit_large_patch16_siglip_384',
pretrained=True,
num_classes=0,
)
model = model.eval()
# get model specific transforms (normalization, resize)
data_config = timm.data.resolve_model_data_config(model)
transforms = timm.data.create_transform(**data_config, is_training=False)
output = model(transforms(image).unsqueeze(0)) # output is (batch_size, num_features) shaped tensor
```
## Citation
```bibtex
@article{zhai2023sigmoid,
title={Sigmoid loss for language image pre-training},
author={Zhai, Xiaohua and Mustafa, Basil and Kolesnikov, Alexander and Beyer, Lucas},
journal={arXiv preprint arXiv:2303.15343},
year={2023}
}
```
```bibtex
@misc{big_vision,
author = {Beyer, Lucas and Zhai, Xiaohua and Kolesnikov, Alexander},
title = {Big Vision},
year = {2022},
publisher = {GitHub},
journal = {GitHub repository},
howpublished = {\url{https://github.com/google-research/big_vision}}
}
```
|
sentence-transformers/distilbert-multilingual-nli-stsb-quora-ranking | sentence-transformers | "2024-11-05T14:54:41Z" | 483,622 | 7 | sentence-transformers | [
"sentence-transformers",
"pytorch",
"tf",
"onnx",
"safetensors",
"openvino",
"distilbert",
"feature-extraction",
"sentence-similarity",
"transformers",
"arxiv:1908.10084",
"license:apache-2.0",
"autotrain_compatible",
"text-embeddings-inference",
"endpoints_compatible",
"region:us"
] | sentence-similarity | "2022-03-02T23:29:05Z" | ---
license: apache-2.0
library_name: sentence-transformers
tags:
- sentence-transformers
- feature-extraction
- sentence-similarity
- transformers
pipeline_tag: sentence-similarity
---
# sentence-transformers/distilbert-multilingual-nli-stsb-quora-ranking
This is a [sentence-transformers](https://www.SBERT.net) model: It maps sentences & paragraphs to a 768 dimensional dense vector space and can be used for tasks like clustering or semantic search.
## Usage (Sentence-Transformers)
Using this model becomes easy when you have [sentence-transformers](https://www.SBERT.net) installed:
```
pip install -U sentence-transformers
```
Then you can use the model like this:
```python
from sentence_transformers import SentenceTransformer
sentences = ["This is an example sentence", "Each sentence is converted"]
model = SentenceTransformer('sentence-transformers/distilbert-multilingual-nli-stsb-quora-ranking')
embeddings = model.encode(sentences)
print(embeddings)
```
## Usage (HuggingFace Transformers)
Without [sentence-transformers](https://www.SBERT.net), you can use the model like this: First, you pass your input through the transformer model, then you have to apply the right pooling-operation on-top of the contextualized word embeddings.
```python
from transformers import AutoTokenizer, AutoModel
import torch
#Mean Pooling - Take attention mask into account for correct averaging
def mean_pooling(model_output, attention_mask):
token_embeddings = model_output[0] #First element of model_output contains all token embeddings
input_mask_expanded = attention_mask.unsqueeze(-1).expand(token_embeddings.size()).float()
return torch.sum(token_embeddings * input_mask_expanded, 1) / torch.clamp(input_mask_expanded.sum(1), min=1e-9)
# Sentences we want sentence embeddings for
sentences = ['This is an example sentence', 'Each sentence is converted']
# Load model from HuggingFace Hub
tokenizer = AutoTokenizer.from_pretrained('sentence-transformers/distilbert-multilingual-nli-stsb-quora-ranking')
model = AutoModel.from_pretrained('sentence-transformers/distilbert-multilingual-nli-stsb-quora-ranking')
# Tokenize sentences
encoded_input = tokenizer(sentences, padding=True, truncation=True, return_tensors='pt')
# Compute token embeddings
with torch.no_grad():
model_output = model(**encoded_input)
# Perform pooling. In this case, mean pooling.
sentence_embeddings = mean_pooling(model_output, encoded_input['attention_mask'])
print("Sentence embeddings:")
print(sentence_embeddings)
```
## Evaluation Results
For an automated evaluation of this model, see the *Sentence Embeddings Benchmark*: [https://seb.sbert.net](https://seb.sbert.net?model_name=sentence-transformers/distilbert-multilingual-nli-stsb-quora-ranking)
## Full Model Architecture
```
SentenceTransformer(
(0): Transformer({'max_seq_length': 128, 'do_lower_case': False}) with Transformer model: DistilBertModel
(1): Pooling({'word_embedding_dimension': 768, 'pooling_mode_cls_token': False, 'pooling_mode_mean_tokens': True, 'pooling_mode_max_tokens': False, 'pooling_mode_mean_sqrt_len_tokens': False})
)
```
## Citing & Authors
This model was trained by [sentence-transformers](https://www.sbert.net/).
If you find this model helpful, feel free to cite our publication [Sentence-BERT: Sentence Embeddings using Siamese BERT-Networks](https://arxiv.org/abs/1908.10084):
```bibtex
@inproceedings{reimers-2019-sentence-bert,
title = "Sentence-BERT: Sentence Embeddings using Siamese BERT-Networks",
author = "Reimers, Nils and Gurevych, Iryna",
booktitle = "Proceedings of the 2019 Conference on Empirical Methods in Natural Language Processing",
month = "11",
year = "2019",
publisher = "Association for Computational Linguistics",
url = "http://arxiv.org/abs/1908.10084",
}
``` |
microsoft/trocr-small-handwritten | microsoft | "2024-05-27T20:11:19Z" | 483,241 | 39 | transformers | [
"transformers",
"pytorch",
"vision-encoder-decoder",
"image-text-to-text",
"trocr",
"image-to-text",
"arxiv:2109.10282",
"endpoints_compatible",
"region:us"
] | image-to-text | "2022-03-02T23:29:05Z" | ---
tags:
- trocr
- image-to-text
widget:
- src: https://fki.tic.heia-fr.ch/static/img/a01-122-02.jpg
example_title: Note 1
- src: https://encrypted-tbn0.gstatic.com/images?q=tbn:ANd9GcSoolxi9yWGAT5SLZShv8vVd0bz47UWRzQC19fDTeE8GmGv_Rn-PCF1pP1rrUx8kOjA4gg&usqp=CAU
example_title: Note 2
- src: https://encrypted-tbn0.gstatic.com/images?q=tbn:ANd9GcRNYtTuSBpZPV_nkBYPMFwVVD9asZOPgHww4epu9EqWgDmXW--sE2o8og40ZfDGo87j5w&usqp=CAU
example_title: Note 3
---
# TrOCR (small-sized model, fine-tuned on IAM)
TrOCR model fine-tuned on the [IAM dataset](https://fki.tic.heia-fr.ch/databases/iam-handwriting-database). It was introduced in the paper [TrOCR: Transformer-based Optical Character Recognition with Pre-trained Models](https://arxiv.org/abs/2109.10282) by Li et al. and first released in [this repository](https://github.com/microsoft/unilm/tree/master/trocr).
## Model description
The TrOCR model is an encoder-decoder model, consisting of an image Transformer as encoder, and a text Transformer as decoder. The image encoder was initialized from the weights of DeiT, while the text decoder was initialized from the weights of UniLM.
Images are presented to the model as a sequence of fixed-size patches (resolution 16x16), which are linearly embedded. One also adds absolute position embeddings before feeding the sequence to the layers of the Transformer encoder. Next, the Transformer text decoder autoregressively generates tokens.
## Intended uses & limitations
You can use the raw model for optical character recognition (OCR) on single text-line images. See the [model hub](https://huggingface.co/models?search=microsoft/trocr) to look for fine-tuned versions on a task that interests you.
### How to use
Here is how to use this model in PyTorch:
```python
from transformers import TrOCRProcessor, VisionEncoderDecoderModel
from PIL import Image
import requests
# load image from the IAM database
url = 'https://fki.tic.heia-fr.ch/static/img/a01-122-02-00.jpg'
image = Image.open(requests.get(url, stream=True).raw).convert("RGB")
processor = TrOCRProcessor.from_pretrained('microsoft/trocr-small-handwritten')
model = VisionEncoderDecoderModel.from_pretrained('microsoft/trocr-small-handwritten')
pixel_values = processor(images=image, return_tensors="pt").pixel_values
generated_ids = model.generate(pixel_values)
generated_text = processor.batch_decode(generated_ids, skip_special_tokens=True)[0]
```
### BibTeX entry and citation info
```bibtex
@misc{li2021trocr,
title={TrOCR: Transformer-based Optical Character Recognition with Pre-trained Models},
author={Minghao Li and Tengchao Lv and Lei Cui and Yijuan Lu and Dinei Florencio and Cha Zhang and Zhoujun Li and Furu Wei},
year={2021},
eprint={2109.10282},
archivePrefix={arXiv},
primaryClass={cs.CL}
}
``` |
liuhaotian/llava-v1.5-13b | liuhaotian | "2024-05-09T20:12:46Z" | 477,044 | 481 | transformers | [
"transformers",
"pytorch",
"llava",
"text-generation",
"image-text-to-text",
"autotrain_compatible",
"region:us"
] | image-text-to-text | "2023-10-05T18:27:40Z" | ---
inference: false
pipeline_tag: image-text-to-text
---
<br>
<br>
# LLaVA Model Card
## Model details
**Model type:**
LLaVA is an open-source chatbot trained by fine-tuning LLaMA/Vicuna on GPT-generated multimodal instruction-following data.
It is an auto-regressive language model, based on the transformer architecture.
**Model date:**
LLaVA-v1.5-13B was trained in September 2023.
**Paper or resources for more information:**
https://llava-vl.github.io/
## License
Llama 2 is licensed under the LLAMA 2 Community License,
Copyright (c) Meta Platforms, Inc. All Rights Reserved.
**Where to send questions or comments about the model:**
https://github.com/haotian-liu/LLaVA/issues
## Intended use
**Primary intended uses:**
The primary use of LLaVA is research on large multimodal models and chatbots.
**Primary intended users:**
The primary intended users of the model are researchers and hobbyists in computer vision, natural language processing, machine learning, and artificial intelligence.
## Training dataset
- 558K filtered image-text pairs from LAION/CC/SBU, captioned by BLIP.
- 158K GPT-generated multimodal instruction-following data.
- 450K academic-task-oriented VQA data mixture.
- 40K ShareGPT data.
## Evaluation dataset
A collection of 12 benchmarks, including 5 academic VQA benchmarks and 7 recent benchmarks specifically proposed for instruction-following LMMs. |
doc2query/msmarco-t5-base-v1 | doc2query | "2022-01-10T10:22:10Z" | 475,929 | 5 | transformers | [
"transformers",
"pytorch",
"t5",
"text2text-generation",
"en",
"dataset:sentence-transformers/embedding-training-data",
"arxiv:1904.08375",
"arxiv:2104.08663",
"license:apache-2.0",
"autotrain_compatible",
"text-generation-inference",
"endpoints_compatible",
"region:us"
] | text2text-generation | "2022-03-02T23:29:05Z" | ---
language: en
datasets:
- sentence-transformers/embedding-training-data
widget:
- text: "Python is an interpreted, high-level and general-purpose programming language. Python's design philosophy emphasizes code readability with its notable use of significant whitespace. Its language constructs and object-oriented approach aim to help programmers write clear, logical code for small and large-scale projects."
license: apache-2.0
---
# doc2query/msmarco-t5-base-v1
This is a [doc2query](https://arxiv.org/abs/1904.08375) model based on T5 (also known as [docT5query](https://cs.uwaterloo.ca/~jimmylin/publications/Nogueira_Lin_2019_docTTTTTquery-v2.pdf)).
It can be used for:
- **Document expansion**: You generate for your paragraphs 20-40 queries and index the paragraphs and the generates queries in a standard BM25 index like Elasticsearch, OpenSearch, or Lucene. The generated queries help to close the lexical gap of lexical search, as the generate queries contain synonyms. Further, it re-weights words giving important words a higher weight even if they appear seldomn in a paragraph. In our [BEIR](https://arxiv.org/abs/2104.08663) paper we showed that BM25+docT5query is a powerful search engine. In the [BEIR repository](https://github.com/UKPLab/beir) we have an example how to use docT5query with Pyserini.
- **Domain Specific Training Data Generation**: It can be used to generate training data to learn an embedding model. On [SBERT.net](https://www.sbert.net/examples/unsupervised_learning/query_generation/README.html) we have an example how to use the model to generate (query, text) pairs for a given collection of unlabeled texts. These pairs can then be used to train powerful dense embedding models.
## Usage
```python
from transformers import T5Tokenizer, T5ForConditionalGeneration
model_name = 'doc2query/msmarco-t5-base-v1'
tokenizer = T5Tokenizer.from_pretrained(model_name)
model = T5ForConditionalGeneration.from_pretrained(model_name)
text = "Python is an interpreted, high-level and general-purpose programming language. Python's design philosophy emphasizes code readability with its notable use of significant whitespace. Its language constructs and object-oriented approach aim to help programmers write clear, logical code for small and large-scale projects."
input_ids = tokenizer.encode(text, max_length=320, truncation=True, return_tensors='pt')
outputs = model.generate(
input_ids=input_ids,
max_length=64,
do_sample=True,
top_p=0.95,
num_return_sequences=5)
print("Text:")
print(text)
print("\nGenerated Queries:")
for i in range(len(outputs)):
query = tokenizer.decode(outputs[i], skip_special_tokens=True)
print(f'{i + 1}: {query}')
```
**Note:** `model.generate()` is non-deterministic. It produces different queries each time you run it.
## Training
This model fine-tuned [google/t5-v1_1-base](https://huggingface.co/google/t5-v1_1-base) for 31k training steps (about 4 epochs on the 500k training pairs from MS MARCO). For the training script, see the `train_script.py` in this repository.
The input-text was truncated to 320 word pieces. Output text was generated up to 64 word pieces.
This model was trained on a (query, passage) from the [MS MARCO Passage-Ranking dataset](https://github.com/microsoft/MSMARCO-Passage-Ranking).
|
SenswiseData/bert_turkish_sentiment | SenswiseData | "2024-03-13T15:27:32Z" | 471,642 | 1 | transformers | [
"transformers",
"tensorboard",
"safetensors",
"roberta",
"text-classification",
"generated_from_trainer",
"base_model:VRLLab/TurkishBERTweet",
"base_model:finetune:VRLLab/TurkishBERTweet",
"license:mit",
"autotrain_compatible",
"endpoints_compatible",
"region:us"
] | text-classification | "2024-03-13T15:26:30Z" | ---
license: mit
base_model: VRLLab/TurkishBERTweet
tags:
- generated_from_trainer
metrics:
- accuracy
model-index:
- name: turkish_sentiment3
results: []
---
<!-- This model card has been generated automatically according to the information the Trainer had access to. You
should probably proofread and complete it, then remove this comment. -->
# turkish_sentiment3
This model is a fine-tuned version of [VRLLab/TurkishBERTweet](https://huggingface.co/VRLLab/TurkishBERTweet) on the None dataset.
It achieves the following results on the evaluation set:
- Loss: 0.0155
- Accuracy: 0.9972
## Model description
More information needed
## Intended uses & limitations
More information needed
## Training and evaluation data
More information needed
## Training procedure
### Training hyperparameters
The following hyperparameters were used during training:
- learning_rate: 1e-05
- train_batch_size: 8
- eval_batch_size: 8
- seed: 42
- optimizer: Adam with betas=(0.9,0.999) and epsilon=1e-08
- lr_scheduler_type: linear
- num_epochs: 3
### Training results
| Training Loss | Epoch | Step | Validation Loss | Accuracy |
|:-------------:|:-----:|:----:|:---------------:|:--------:|
| No log | 1.0 | 440 | 0.0516 | 0.9926 |
| 0.1392 | 2.0 | 880 | 0.0242 | 0.9966 |
| 0.0443 | 3.0 | 1320 | 0.0155 | 0.9972 |
### Framework versions
- Transformers 4.35.2
- Pytorch 2.1.0+cu121
- Datasets 2.16.1
- Tokenizers 0.15.0
|
SenswiseData/bert_cased_ner | SenswiseData | "2024-03-13T15:21:33Z" | 471,454 | 0 | transformers | [
"transformers",
"pytorch",
"bert",
"token-classification",
"ner",
"berturk",
"turkish",
"tr",
"dataset:MilliyetNER",
"autotrain_compatible",
"endpoints_compatible",
"region:us"
] | token-classification | "2024-03-13T15:20:17Z" | ---
tags:
- ner
- token-classification
- berturk
- turkish
language: tr
datasets:
- MilliyetNER
widget:
- text: "Türkiye'nin başkenti Ankara'dır ve ilk cumhurbaşkanı Mustafa Kemal Atatürk'tür."
---
# DATASET
MilliyetNER dataset was collected from the Turkish Milliyet newspaper articles between 1997-1998. This dataset is presented by [Tür et al. (2003)](https://www.cambridge.org/core/journals/natural-language-engineering/article/abs/statistical-information-extraction-system-for-turkish/7C288FAFC71D5F0763C1F8CE66464017). It was collected from news articles and manually annotated with three different entity types: Person, Location, Organization. The authors did not provide training/validation/test splits for this dataset. Dataset splits used by [Yeniterzi et al. 2011](https://aclanthology.org/P11-3019).
For more information: [tdd.ai - MilliyetNER](https://data.tdd.ai/#/effafb5f-ebfc-4e5c-9a63-4f709ec1a135)
**Model is only trained using training set. Test set not included during the last training**.
# USAGE
```python
from transformers import pipeline, AutoModelForTokenClassification, AutoTokenizer
model = AutoModelForTokenClassification.from_pretrained("alierenak/berturk-cased-ner")
tokenizer = AutoTokenizer.from_pretrained("alierenak/berturk-cased-ner")
ner_pipeline = pipeline('ner', model=model, tokenizer=tokenizer)
ner_pipeline("Türkiye'nin başkenti Ankara, ilk cumhurbaşkanı Mustafa Kemal Atatürk'tür.")
```
# RESULT
```bash
[{'entity': 'B-LOCATION',
'score': 0.9966415,
'index': 1,
'word': 'Türkiye',
'start': 0,
'end': 7},
{'entity': 'B-LOCATION',
'score': 0.99456763,
'index': 5,
'word': 'Ankara',
'start': 21,
'end': 27},
{'entity': 'B-PERSON',
'score': 0.9958741,
'index': 9,
'word': 'Mustafa',
'start': 47,
'end': 54},
{'entity': 'I-PERSON',
'score': 0.98833394,
'index': 10,
'word': 'Kemal',
'start': 55,
'end': 60},
{'entity': 'I-PERSON',
'score': 0.9837286,
'index': 11,
'word': 'Atatürk',
'start': 61,
'end': 68}]
```
# BENCHMARKING
```bash
precision recall f1-score support
LOCATION 0.97 0.96 0.97 960
ORGANIZATION 0.95 0.92 0.94 863
PERSON 0.97 0.97 0.97 1410
micro avg 0.97 0.95 0.96 3233
macro avg 0.96 0.95 0.96 3233
weighted avg 0.97 0.95 0.96 3233
``` |
nvidia/parakeet-tdt-1.1b | nvidia | "2024-04-30T21:10:58Z" | 471,168 | 79 | nemo | [
"nemo",
"automatic-speech-recognition",
"speech",
"audio",
"Transducer",
"TDT",
"FastConformer",
"Conformer",
"pytorch",
"NeMo",
"hf-asr-leaderboard",
"en",
"dataset:librispeech_asr",
"dataset:fisher_corpus",
"dataset:Switchboard-1",
"dataset:WSJ-0",
"dataset:WSJ-1",
"dataset:National-Singapore-Corpus-Part-1",
"dataset:National-Singapore-Corpus-Part-6",
"dataset:vctk",
"dataset:voxpopuli",
"dataset:europarl",
"dataset:multilingual_librispeech",
"dataset:mozilla-foundation/common_voice_8_0",
"dataset:MLCommons/peoples_speech",
"arxiv:2304.06795",
"arxiv:2305.05084",
"arxiv:2104.02821",
"license:cc-by-4.0",
"model-index",
"region:us"
] | automatic-speech-recognition | "2024-01-25T02:05:06Z" | ---
language:
- en
library_name: nemo
datasets:
- librispeech_asr
- fisher_corpus
- Switchboard-1
- WSJ-0
- WSJ-1
- National-Singapore-Corpus-Part-1
- National-Singapore-Corpus-Part-6
- vctk
- voxpopuli
- europarl
- multilingual_librispeech
- mozilla-foundation/common_voice_8_0
- MLCommons/peoples_speech
thumbnail: null
tags:
- automatic-speech-recognition
- speech
- audio
- Transducer
- TDT
- FastConformer
- Conformer
- pytorch
- NeMo
- hf-asr-leaderboard
license: cc-by-4.0
widget:
- example_title: Librispeech sample 1
src: https://cdn-media.huggingface.co/speech_samples/sample1.flac
- example_title: Librispeech sample 2
src: https://cdn-media.huggingface.co/speech_samples/sample2.flac
model-index:
- name: parakeet_tdt_1.1b
results:
- task:
name: Automatic Speech Recognition
type: automatic-speech-recognition
dataset:
name: AMI (Meetings test)
type: edinburghcstr/ami
config: ihm
split: test
args:
language: en
metrics:
- name: Test WER
type: wer
value: 15.90
- task:
name: Automatic Speech Recognition
type: automatic-speech-recognition
dataset:
name: Earnings-22
type: revdotcom/earnings22
split: test
args:
language: en
metrics:
- name: Test WER
type: wer
value: 14.65
- task:
name: Automatic Speech Recognition
type: automatic-speech-recognition
dataset:
name: GigaSpeech
type: speechcolab/gigaspeech
split: test
args:
language: en
metrics:
- name: Test WER
type: wer
value: 9.55
- task:
name: Automatic Speech Recognition
type: automatic-speech-recognition
dataset:
name: LibriSpeech (clean)
type: librispeech_asr
config: other
split: test
args:
language: en
metrics:
- name: Test WER
type: wer
value: 1.39
- task:
name: Automatic Speech Recognition
type: automatic-speech-recognition
dataset:
name: LibriSpeech (other)
type: librispeech_asr
config: other
split: test
args:
language: en
metrics:
- name: Test WER
type: wer
value: 2.62
- task:
type: Automatic Speech Recognition
name: automatic-speech-recognition
dataset:
name: SPGI Speech
type: kensho/spgispeech
config: test
split: test
args:
language: en
metrics:
- name: Test WER
type: wer
value: 3.42
- task:
type: Automatic Speech Recognition
name: automatic-speech-recognition
dataset:
name: tedlium-v3
type: LIUM/tedlium
config: release1
split: test
args:
language: en
metrics:
- name: Test WER
type: wer
value: 3.56
- task:
name: Automatic Speech Recognition
type: automatic-speech-recognition
dataset:
name: Vox Populi
type: facebook/voxpopuli
config: en
split: test
args:
language: en
metrics:
- name: Test WER
type: wer
value: 5.48
- task:
type: Automatic Speech Recognition
name: automatic-speech-recognition
dataset:
name: Mozilla Common Voice 9.0
type: mozilla-foundation/common_voice_9_0
config: en
split: test
args:
language: en
metrics:
- name: Test WER
type: wer
value: 5.97
metrics:
- wer
pipeline_tag: automatic-speech-recognition
---
# Parakeet TDT 1.1B (en)
<style>
img {
display: inline;
}
</style>
[](#model-architecture)
| [](#model-architecture)
| [](#datasets)
`parakeet-tdt-1.1b` is an ASR model that transcribes speech in lower case English alphabet. This model is jointly developed by [NVIDIA NeMo](https://github.com/NVIDIA/NeMo) and [Suno.ai](https://www.suno.ai/) teams.
It is an XXL version of FastConformer [1] TDT [2] (around 1.1B parameters) model.
See the [model architecture](#model-architecture) section and [NeMo documentation](https://docs.nvidia.com/deeplearning/nemo/user-guide/docs/en/main/asr/models.html#fast-conformer) for complete architecture details.
## NVIDIA NeMo: Training
To train, fine-tune or play with the model you will need to install [NVIDIA NeMo](https://github.com/NVIDIA/NeMo). We recommend you install it after you've installed latest PyTorch version.
```
pip install nemo_toolkit['all']
```
## How to Use this Model
The model is available for use in the NeMo toolkit [3], and can be used as a pre-trained checkpoint for inference or for fine-tuning on another dataset.
### Automatically instantiate the model
```python
import nemo.collections.asr as nemo_asr
asr_model = nemo_asr.models.EncDecRNNTBPEModel.from_pretrained(model_name="nvidia/parakeet-tdt-1.1b")
```
### Transcribing using Python
First, let's get a sample
```
wget https://dldata-public.s3.us-east-2.amazonaws.com/2086-149220-0033.wav
```
Then simply do:
```
asr_model.transcribe(['2086-149220-0033.wav'])
```
### Transcribing many audio files
```shell
python [NEMO_GIT_FOLDER]/examples/asr/transcribe_speech.py
pretrained_name="nvidia/parakeet-tdt-1.1b"
audio_dir="<DIRECTORY CONTAINING AUDIO FILES>"
```
### Input
This model accepts 16000 Hz mono-channel audio (wav files) as input.
### Output
This model provides transcribed speech as a string for a given audio sample.
## Model Architecture
This model uses a FastConformer-TDT architecture. FastConformer [1] is an optimized version of the Conformer model with 8x depthwise-separable convolutional downsampling. You may find more information on the details of FastConformer here: [Fast-Conformer Model](https://docs.nvidia.com/deeplearning/nemo/user-guide/docs/en/main/asr/models.html#fast-conformer).
TDT (Token-and-Duration Transducer) [2] is a generalization of conventional Transducers by decoupling token and duration predictions. Unlike conventional Transducers which produces a lot of blanks during inference, a TDT model can skip majority of blank predictions by using the duration output (up to 4 frames for this parakeet-tdt-1.1b model), thus brings significant inference speed-up. The detail of TDT can be found here: [Efficient Sequence Transduction by Jointly Predicting Tokens and Durations](https://arxiv.org/abs/2304.06795).
## Training
The NeMo toolkit [3] was used for training the models for over several hundred epochs. These model are trained with this [example script](https://github.com/NVIDIA/NeMo/blob/main/examples/asr/asr_transducer/speech_to_text_rnnt_bpe.py) and this [base config](https://github.com/NVIDIA/NeMo/blob/main/examples/asr/conf/fastconformer/fast-conformer_transducer_bpe.yaml).
The tokenizers for these models were built using the text transcripts of the train set with this [script](https://github.com/NVIDIA/NeMo/blob/main/scripts/tokenizers/process_asr_text_tokenizer.py).
### Datasets
The model was trained on 64K hours of English speech collected and prepared by NVIDIA NeMo and Suno teams.
The training dataset consists of private subset with 40K hours of English speech plus 24K hours from the following public datasets:
- Librispeech 960 hours of English speech
- Fisher Corpus
- Switchboard-1 Dataset
- WSJ-0 and WSJ-1
- National Speech Corpus (Part 1, Part 6)
- VCTK
- VoxPopuli (EN)
- Europarl-ASR (EN)
- Multilingual Librispeech (MLS EN) - 2,000 hour subset
- Mozilla Common Voice (v7.0)
- People's Speech - 12,000 hour subset
## Performance
The performance of Automatic Speech Recognition models is measuring using Word Error Rate. Since this dataset is trained on multiple domains and a much larger corpus, it will generally perform better at transcribing audio in general.
The following tables summarizes the performance of the available models in this collection with the Transducer decoder. Performances of the ASR models are reported in terms of Word Error Rate (WER%) with greedy decoding.
|**Version**|**Tokenizer**|**Vocabulary Size**|**AMI**|**Earnings-22**|**Giga Speech**|**LS test-clean**|**SPGI Speech**|**TEDLIUM-v3**|**Vox Populi**|**Common Voice**|
|---------|-----------------------|-----------------|---------------|---------------|------------|-----------|-----|-------|------|------|
| 1.22.0 | SentencePiece Unigram | 1024 | 15.90 | 14.65 | 9.55 | 1.39 | 2.62 | 3.42 | 3.56 | 5.48 | 5.97 |
These are greedy WER numbers without external LM. More details on evaluation can be found at [HuggingFace ASR Leaderboard](https://huggingface.co/spaces/hf-audio/open_asr_leaderboard)
## Model Fairness Evaluation
As outlined in the paper "Towards Measuring Fairness in AI: the Casual Conversations Dataset", we assessed the parakeet-tdt-1.1b model for fairness. The model was evaluated on the CausalConversations-v1 dataset, and the results are reported as follows:
### Gender Bias:
| Gender | Male | Female | N/A | Other |
| :--- | :--- | :--- | :--- | :--- |
| Num utterances | 19325 | 24532 | 926 | 33 |
| % WER | 17.18 | 14.61 | 19.06 | 37.57 |
### Age Bias:
| Age Group | $(18-30)$ | $(31-45)$ | $(46-85)$ | $(1-100)$ |
| :--- | :--- | :--- | :--- | :--- |
| Num utterances | 15956 | 14585 | 13349 | 43890 |
| % WER | 15.83 | 15.89 | 15.46 | 15.74 |
(Error rates for fairness evaluation are determined by normalizing both the reference and predicted text, similar to the methods used in the evaluations found at https://github.com/huggingface/open_asr_leaderboard.)
## NVIDIA Riva: Deployment
[NVIDIA Riva](https://developer.nvidia.com/riva), is an accelerated speech AI SDK deployable on-prem, in all clouds, multi-cloud, hybrid, on edge, and embedded.
Additionally, Riva provides:
* World-class out-of-the-box accuracy for the most common languages with model checkpoints trained on proprietary data with hundreds of thousands of GPU-compute hours
* Best in class accuracy with run-time word boosting (e.g., brand and product names) and customization of acoustic model, language model, and inverse text normalization
* Streaming speech recognition, Kubernetes compatible scaling, and enterprise-grade support
Although this model isn’t supported yet by Riva, the [list of supported models is here](https://huggingface.co/models?other=Riva).
Check out [Riva live demo](https://developer.nvidia.com/riva#demos).
## References
[1] [Fast Conformer with Linearly Scalable Attention for Efficient Speech Recognition](https://arxiv.org/abs/2305.05084)
[2] [Efficient Sequence Transduction by Jointly Predicting Tokens and Durations](https://arxiv.org/abs/2304.06795)
[3] [Google Sentencepiece Tokenizer](https://github.com/google/sentencepiece)
[4] [NVIDIA NeMo Toolkit](https://github.com/NVIDIA/NeMo)
[5] [Suno.ai](https://suno.ai/)
[6] [HuggingFace ASR Leaderboard](https://huggingface.co/spaces/hf-audio/open_asr_leaderboard)
[7] [Towards Measuring Fairness in AI: the Casual Conversations Dataset](https://arxiv.org/abs/2104.02821)
## Licence
License to use this model is covered by the [CC-BY-4.0](https://creativecommons.org/licenses/by/4.0/). By downloading the public and release version of the model, you accept the terms and conditions of the [CC-BY-4.0](https://creativecommons.org/licenses/by/4.0/) license. |
unsloth/Meta-Llama-3.1-8B-Instruct-bnb-4bit | unsloth | "2024-09-11T08:27:13Z" | 470,106 | 38 | transformers | [
"transformers",
"safetensors",
"llama",
"text-generation",
"llama-3",
"meta",
"facebook",
"unsloth",
"conversational",
"en",
"arxiv:2204.05149",
"base_model:unsloth/Meta-Llama-3.1-8B",
"base_model:quantized:unsloth/Meta-Llama-3.1-8B",
"license:llama3.1",
"autotrain_compatible",
"text-generation-inference",
"endpoints_compatible",
"4-bit",
"bitsandbytes",
"region:us"
] | text-generation | "2024-07-23T16:09:09Z" | ---
base_model: unsloth/Meta-Llama-3.1-8B
language:
- en
library_name: transformers
license: llama3.1
tags:
- llama-3
- llama
- meta
- facebook
- unsloth
- transformers
---
# Finetune Llama 3.1, Gemma 2, Mistral 2-5x faster with 70% less memory via Unsloth!
We have a free Google Colab Tesla T4 notebook for Llama 3.1 (8B) here: https://colab.research.google.com/drive/1Ys44kVvmeZtnICzWz0xgpRnrIOjZAuxp?usp=sharing
[<img src="https://raw.githubusercontent.com/unslothai/unsloth/main/images/Discord%20button.png" width="200"/>](https://discord.gg/unsloth)
[<img src="https://raw.githubusercontent.com/unslothai/unsloth/main/images/unsloth%20made%20with%20love.png" width="200"/>](https://github.com/unslothai/unsloth)
## ✨ Finetune for Free
All notebooks are **beginner friendly**! Add your dataset, click "Run All", and you'll get a 2x faster finetuned model which can be exported to GGUF, vLLM or uploaded to Hugging Face.
| Unsloth supports | Free Notebooks | Performance | Memory use |
|-----------------|--------------------------------------------------------------------------------------------------------------------------|-------------|----------|
| **Llama-3.1 8b** | [▶️ Start on Colab](https://colab.research.google.com/drive/1Ys44kVvmeZtnICzWz0xgpRnrIOjZAuxp?usp=sharing) | 2.4x faster | 58% less |
| **Phi-3.5 (mini)** | [▶️ Start on Colab](https://colab.research.google.com/drive/1lN6hPQveB_mHSnTOYifygFcrO8C1bxq4?usp=sharing) | 2x faster | 50% less |
| **Gemma-2 9b** | [▶️ Start on Colab](https://colab.research.google.com/drive/1vIrqH5uYDQwsJ4-OO3DErvuv4pBgVwk4?usp=sharing) | 2.4x faster | 58% less |
| **Mistral 7b** | [▶️ Start on Colab](https://colab.research.google.com/drive/1Dyauq4kTZoLewQ1cApceUQVNcnnNTzg_?usp=sharing) | 2.2x faster | 62% less |
| **TinyLlama** | [▶️ Start on Colab](https://colab.research.google.com/drive/1AZghoNBQaMDgWJpi4RbffGM1h6raLUj9?usp=sharing) | 3.9x faster | 74% less |
| **DPO - Zephyr** | [▶️ Start on Colab](https://colab.research.google.com/drive/15vttTpzzVXv_tJwEk-hIcQ0S9FcEWvwP?usp=sharing) | 1.9x faster | 19% less |
- This [conversational notebook](https://colab.research.google.com/drive/1Aau3lgPzeZKQ-98h69CCu1UJcvIBLmy2?usp=sharing) is useful for ShareGPT ChatML / Vicuna templates.
- This [text completion notebook](https://colab.research.google.com/drive/1ef-tab5bhkvWmBOObepl1WgJvfvSzn5Q?usp=sharing) is for raw text. This [DPO notebook](https://colab.research.google.com/drive/15vttTpzzVXv_tJwEk-hIcQ0S9FcEWvwP?usp=sharing) replicates Zephyr.
- \* Kaggle has 2x T4s, but we use 1. Due to overhead, 1x T4 is 5x faster.
## Special Thanks
A huge thank you to the Meta and Llama team for creating and releasing these models.
## Model Information
The Meta Llama 3.1 collection of multilingual large language models (LLMs) is a collection of pretrained and instruction tuned generative models in 8B, 70B and 405B sizes (text in/text out). The Llama 3.1 instruction tuned text only models (8B, 70B, 405B) are optimized for multilingual dialogue use cases and outperform many of the available open source and closed chat models on common industry benchmarks.
**Model developer**: Meta
**Model Architecture:** Llama 3.1 is an auto-regressive language model that uses an optimized transformer architecture. The tuned versions use supervised fine-tuning (SFT) and reinforcement learning with human feedback (RLHF) to align with human preferences for helpfulness and safety.
<table>
<tr>
<td>
</td>
<td><strong>Training Data</strong>
</td>
<td><strong>Params</strong>
</td>
<td><strong>Input modalities</strong>
</td>
<td><strong>Output modalities</strong>
</td>
<td><strong>Context length</strong>
</td>
<td><strong>GQA</strong>
</td>
<td><strong>Token count</strong>
</td>
<td><strong>Knowledge cutoff</strong>
</td>
</tr>
<tr>
<td rowspan="3" >Llama 3.1 (text only)
</td>
<td rowspan="3" >A new mix of publicly available online data.
</td>
<td>8B
</td>
<td>Multilingual Text
</td>
<td>Multilingual Text and code
</td>
<td>128k
</td>
<td>Yes
</td>
<td rowspan="3" >15T+
</td>
<td rowspan="3" >December 2023
</td>
</tr>
<tr>
<td>70B
</td>
<td>Multilingual Text
</td>
<td>Multilingual Text and code
</td>
<td>128k
</td>
<td>Yes
</td>
</tr>
<tr>
<td>405B
</td>
<td>Multilingual Text
</td>
<td>Multilingual Text and code
</td>
<td>128k
</td>
<td>Yes
</td>
</tr>
</table>
**Supported languages:** English, German, French, Italian, Portuguese, Hindi, Spanish, and Thai.
**Llama 3.1 family of models**. Token counts refer to pretraining data only. All model versions use Grouped-Query Attention (GQA) for improved inference scalability.
**Model Release Date:** July 23, 2024.
**Status:** This is a static model trained on an offline dataset. Future versions of the tuned models will be released as we improve model safety with community feedback.
**License:** A custom commercial license, the Llama 3.1 Community License, is available at: [https://github.com/meta-llama/llama-models/blob/main/models/llama3_1/LICENSE](https://github.com/meta-llama/llama-models/blob/main/models/llama3_1/LICENSE)
Where to send questions or comments about the model Instructions on how to provide feedback or comments on the model can be found in the model [README](https://github.com/meta-llama/llama3). For more technical information about generation parameters and recipes for how to use Llama 3.1 in applications, please go [here](https://github.com/meta-llama/llama-recipes).
## Intended Use
**Intended Use Cases** Llama 3.1 is intended for commercial and research use in multiple languages. Instruction tuned text only models are intended for assistant-like chat, whereas pretrained models can be adapted for a variety of natural language generation tasks. The Llama 3.1 model collection also supports the ability to leverage the outputs of its models to improve other models including synthetic data generation and distillation. The Llama 3.1 Community License allows for these use cases.
**Out-of-scope** Use in any manner that violates applicable laws or regulations (including trade compliance laws). Use in any other way that is prohibited by the Acceptable Use Policy and Llama 3.1 Community License. Use in languages beyond those explicitly referenced as supported in this model card**.
**<span style="text-decoration:underline;">Note</span>: Llama 3.1 has been trained on a broader collection of languages than the 8 supported languages. Developers may fine-tune Llama 3.1 models for languages beyond the 8 supported languages provided they comply with the Llama 3.1 Community License and the Acceptable Use Policy and in such cases are responsible for ensuring that any uses of Llama 3.1 in additional languages is done in a safe and responsible manner.
## How to use
This repository contains two versions of Meta-Llama-3.1-8B-Instruct, for use with transformers and with the original `llama` codebase.
### Use with transformers
Starting with `transformers >= 4.43.0` onward, you can run conversational inference using the Transformers `pipeline` abstraction or by leveraging the Auto classes with the `generate()` function.
Make sure to update your transformers installation via `pip install --upgrade transformers`.
```python
import transformers
import torch
model_id = "meta-llama/Meta-Llama-3.1-8B-Instruct"
pipeline = transformers.pipeline(
"text-generation",
model=model_id,
model_kwargs={"torch_dtype": torch.bfloat16},
device_map="auto",
)
messages = [
{"role": "system", "content": "You are a pirate chatbot who always responds in pirate speak!"},
{"role": "user", "content": "Who are you?"},
]
outputs = pipeline(
messages,
max_new_tokens=256,
)
print(outputs[0]["generated_text"][-1])
```
Note: You can also find detailed recipes on how to use the model locally, with `torch.compile()`, assisted generations, quantised and more at [`huggingface-llama-recipes`](https://github.com/huggingface/huggingface-llama-recipes)
### Use with `llama`
Please, follow the instructions in the [repository](https://github.com/meta-llama/llama)
To download Original checkpoints, see the example command below leveraging `huggingface-cli`:
```
huggingface-cli download meta-llama/Meta-Llama-3.1-8B-Instruct --include "original/*" --local-dir Meta-Llama-3.1-8B-Instruct
```
## Hardware and Software
**Training Factors** We used custom training libraries, Meta's custom built GPU cluster, and production infrastructure for pretraining. Fine-tuning, annotation, and evaluation were also performed on production infrastructure.
**Training utilized a cumulative of** 39.3M GPU hours of computation on H100-80GB (TDP of 700W) type hardware, per the table below. Training time is the total GPU time required for training each model and power consumption is the peak power capacity per GPU device used, adjusted for power usage efficiency.
**Training Greenhouse Gas Emissions** Estimated total location-based greenhouse gas emissions were **11,390** tons CO2eq for training. Since 2020, Meta has maintained net zero greenhouse gas emissions in its global operations and matched 100% of its electricity use with renewable energy, therefore the total market-based greenhouse gas emissions for training were 0 tons CO2eq.
<table>
<tr>
<td>
</td>
<td><strong>Training Time (GPU hours)</strong>
</td>
<td><strong>Training Power Consumption (W)</strong>
</td>
<td><strong>Training Location-Based Greenhouse Gas Emissions</strong>
<p>
<strong>(tons CO2eq)</strong>
</td>
<td><strong>Training Market-Based Greenhouse Gas Emissions</strong>
<p>
<strong>(tons CO2eq)</strong>
</td>
</tr>
<tr>
<td>Llama 3.1 8B
</td>
<td>1.46M
</td>
<td>700
</td>
<td>420
</td>
<td>0
</td>
</tr>
<tr>
<td>Llama 3.1 70B
</td>
<td>7.0M
</td>
<td>700
</td>
<td>2,040
</td>
<td>0
</td>
</tr>
<tr>
<td>Llama 3.1 405B
</td>
<td>30.84M
</td>
<td>700
</td>
<td>8,930
</td>
<td>0
</td>
</tr>
<tr>
<td>Total
</td>
<td>39.3M
<td>
<ul>
</ul>
</td>
<td>11,390
</td>
<td>0
</td>
</tr>
</table>
The methodology used to determine training energy use and greenhouse gas emissions can be found [here](https://arxiv.org/pdf/2204.05149). Since Meta is openly releasing these models, the training energy use and greenhouse gas emissions will not be incurred by others.
## Training Data
**Overview:** Llama 3.1 was pretrained on ~15 trillion tokens of data from publicly available sources. The fine-tuning data includes publicly available instruction datasets, as well as over 25M synthetically generated examples.
**Data Freshness:** The pretraining data has a cutoff of December 2023.
## Benchmark scores
In this section, we report the results for Llama 3.1 models on standard automatic benchmarks. For all the evaluations, we use our internal evaluations library.
### Base pretrained models
<table>
<tr>
<td><strong>Category</strong>
</td>
<td><strong>Benchmark</strong>
</td>
<td><strong># Shots</strong>
</td>
<td><strong>Metric</strong>
</td>
<td><strong>Llama 3 8B</strong>
</td>
<td><strong>Llama 3.1 8B</strong>
</td>
<td><strong>Llama 3 70B</strong>
</td>
<td><strong>Llama 3.1 70B</strong>
</td>
<td><strong>Llama 3.1 405B</strong>
</td>
</tr>
<tr>
<td rowspan="7" >General
</td>
<td>MMLU
</td>
<td>5
</td>
<td>macro_avg/acc_char
</td>
<td>66.7
</td>
<td>66.7
</td>
<td>79.5
</td>
<td>79.3
</td>
<td>85.2
</td>
</tr>
<tr>
<td>MMLU-Pro (CoT)
</td>
<td>5
</td>
<td>macro_avg/acc_char
</td>
<td>36.2
</td>
<td>37.1
</td>
<td>55.0
</td>
<td>53.8
</td>
<td>61.6
</td>
</tr>
<tr>
<td>AGIEval English
</td>
<td>3-5
</td>
<td>average/acc_char
</td>
<td>47.1
</td>
<td>47.8
</td>
<td>63.0
</td>
<td>64.6
</td>
<td>71.6
</td>
</tr>
<tr>
<td>CommonSenseQA
</td>
<td>7
</td>
<td>acc_char
</td>
<td>72.6
</td>
<td>75.0
</td>
<td>83.8
</td>
<td>84.1
</td>
<td>85.8
</td>
</tr>
<tr>
<td>Winogrande
</td>
<td>5
</td>
<td>acc_char
</td>
<td>-
</td>
<td>60.5
</td>
<td>-
</td>
<td>83.3
</td>
<td>86.7
</td>
</tr>
<tr>
<td>BIG-Bench Hard (CoT)
</td>
<td>3
</td>
<td>average/em
</td>
<td>61.1
</td>
<td>64.2
</td>
<td>81.3
</td>
<td>81.6
</td>
<td>85.9
</td>
</tr>
<tr>
<td>ARC-Challenge
</td>
<td>25
</td>
<td>acc_char
</td>
<td>79.4
</td>
<td>79.7
</td>
<td>93.1
</td>
<td>92.9
</td>
<td>96.1
</td>
</tr>
<tr>
<td>Knowledge reasoning
</td>
<td>TriviaQA-Wiki
</td>
<td>5
</td>
<td>em
</td>
<td>78.5
</td>
<td>77.6
</td>
<td>89.7
</td>
<td>89.8
</td>
<td>91.8
</td>
</tr>
<tr>
<td rowspan="4" >Reading comprehension
</td>
<td>SQuAD
</td>
<td>1
</td>
<td>em
</td>
<td>76.4
</td>
<td>77.0
</td>
<td>85.6
</td>
<td>81.8
</td>
<td>89.3
</td>
</tr>
<tr>
<td>QuAC (F1)
</td>
<td>1
</td>
<td>f1
</td>
<td>44.4
</td>
<td>44.9
</td>
<td>51.1
</td>
<td>51.1
</td>
<td>53.6
</td>
</tr>
<tr>
<td>BoolQ
</td>
<td>0
</td>
<td>acc_char
</td>
<td>75.7
</td>
<td>75.0
</td>
<td>79.0
</td>
<td>79.4
</td>
<td>80.0
</td>
</tr>
<tr>
<td>DROP (F1)
</td>
<td>3
</td>
<td>f1
</td>
<td>58.4
</td>
<td>59.5
</td>
<td>79.7
</td>
<td>79.6
</td>
<td>84.8
</td>
</tr>
</table>
### Instruction tuned models
<table>
<tr>
<td><strong>Category</strong>
</td>
<td><strong>Benchmark</strong>
</td>
<td><strong># Shots</strong>
</td>
<td><strong>Metric</strong>
</td>
<td><strong>Llama 3 8B Instruct</strong>
</td>
<td><strong>Llama 3.1 8B Instruct</strong>
</td>
<td><strong>Llama 3 70B Instruct</strong>
</td>
<td><strong>Llama 3.1 70B Instruct</strong>
</td>
<td><strong>Llama 3.1 405B Instruct</strong>
</td>
</tr>
<tr>
<td rowspan="4" >General
</td>
<td>MMLU
</td>
<td>5
</td>
<td>macro_avg/acc
</td>
<td>68.5
</td>
<td>69.4
</td>
<td>82.0
</td>
<td>83.6
</td>
<td>87.3
</td>
</tr>
<tr>
<td>MMLU (CoT)
</td>
<td>0
</td>
<td>macro_avg/acc
</td>
<td>65.3
</td>
<td>73.0
</td>
<td>80.9
</td>
<td>86.0
</td>
<td>88.6
</td>
</tr>
<tr>
<td>MMLU-Pro (CoT)
</td>
<td>5
</td>
<td>micro_avg/acc_char
</td>
<td>45.5
</td>
<td>48.3
</td>
<td>63.4
</td>
<td>66.4
</td>
<td>73.3
</td>
</tr>
<tr>
<td>IFEval
</td>
<td>
</td>
<td>
</td>
<td>76.8
</td>
<td>80.4
</td>
<td>82.9
</td>
<td>87.5
</td>
<td>88.6
</td>
</tr>
<tr>
<td rowspan="2" >Reasoning
</td>
<td>ARC-C
</td>
<td>0
</td>
<td>acc
</td>
<td>82.4
</td>
<td>83.4
</td>
<td>94.4
</td>
<td>94.8
</td>
<td>96.9
</td>
</tr>
<tr>
<td>GPQA
</td>
<td>0
</td>
<td>em
</td>
<td>34.6
</td>
<td>30.4
</td>
<td>39.5
</td>
<td>41.7
</td>
<td>50.7
</td>
</tr>
<tr>
<td rowspan="4" >Code
</td>
<td>HumanEval
</td>
<td>0
</td>
<td>pass@1
</td>
<td>60.4
</td>
<td>72.6
</td>
<td>81.7
</td>
<td>80.5
</td>
<td>89.0
</td>
</tr>
<tr>
<td>MBPP ++ base version
</td>
<td>0
</td>
<td>pass@1
</td>
<td>70.6
</td>
<td>72.8
</td>
<td>82.5
</td>
<td>86.0
</td>
<td>88.6
</td>
</tr>
<tr>
<td>Multipl-E HumanEval
</td>
<td>0
</td>
<td>pass@1
</td>
<td>-
</td>
<td>50.8
</td>
<td>-
</td>
<td>65.5
</td>
<td>75.2
</td>
</tr>
<tr>
<td>Multipl-E MBPP
</td>
<td>0
</td>
<td>pass@1
</td>
<td>-
</td>
<td>52.4
</td>
<td>-
</td>
<td>62.0
</td>
<td>65.7
</td>
</tr>
<tr>
<td rowspan="2" >Math
</td>
<td>GSM-8K (CoT)
</td>
<td>8
</td>
<td>em_maj1@1
</td>
<td>80.6
</td>
<td>84.5
</td>
<td>93.0
</td>
<td>95.1
</td>
<td>96.8
</td>
</tr>
<tr>
<td>MATH (CoT)
</td>
<td>0
</td>
<td>final_em
</td>
<td>29.1
</td>
<td>51.9
</td>
<td>51.0
</td>
<td>68.0
</td>
<td>73.8
</td>
</tr>
<tr>
<td rowspan="4" >Tool Use
</td>
<td>API-Bank
</td>
<td>0
</td>
<td>acc
</td>
<td>48.3
</td>
<td>82.6
</td>
<td>85.1
</td>
<td>90.0
</td>
<td>92.0
</td>
</tr>
<tr>
<td>BFCL
</td>
<td>0
</td>
<td>acc
</td>
<td>60.3
</td>
<td>76.1
</td>
<td>83.0
</td>
<td>84.8
</td>
<td>88.5
</td>
</tr>
<tr>
<td>Gorilla Benchmark API Bench
</td>
<td>0
</td>
<td>acc
</td>
<td>1.7
</td>
<td>8.2
</td>
<td>14.7
</td>
<td>29.7
</td>
<td>35.3
</td>
</tr>
<tr>
<td>Nexus (0-shot)
</td>
<td>0
</td>
<td>macro_avg/acc
</td>
<td>18.1
</td>
<td>38.5
</td>
<td>47.8
</td>
<td>56.7
</td>
<td>58.7
</td>
</tr>
<tr>
<td>Multilingual
</td>
<td>Multilingual MGSM (CoT)
</td>
<td>0
</td>
<td>em
</td>
<td>-
</td>
<td>68.9
</td>
<td>-
</td>
<td>86.9
</td>
<td>91.6
</td>
</tr>
</table>
#### Multilingual benchmarks
<table>
<tr>
<td><strong>Category</strong>
</td>
<td><strong>Benchmark</strong>
</td>
<td><strong>Language</strong>
</td>
<td><strong>Llama 3.1 8B</strong>
</td>
<td><strong>Llama 3.1 70B</strong>
</td>
<td><strong>Llama 3.1 405B</strong>
</td>
</tr>
<tr>
<td rowspan="9" ><strong>General</strong>
</td>
<td rowspan="9" ><strong>MMLU (5-shot, macro_avg/acc)</strong>
</td>
<td>Portuguese
</td>
<td>62.12
</td>
<td>80.13
</td>
<td>84.95
</td>
</tr>
<tr>
<td>Spanish
</td>
<td>62.45
</td>
<td>80.05
</td>
<td>85.08
</td>
</tr>
<tr>
<td>Italian
</td>
<td>61.63
</td>
<td>80.4
</td>
<td>85.04
</td>
</tr>
<tr>
<td>German
</td>
<td>60.59
</td>
<td>79.27
</td>
<td>84.36
</td>
</tr>
<tr>
<td>French
</td>
<td>62.34
</td>
<td>79.82
</td>
<td>84.66
</td>
</tr>
<tr>
<td>Hindi
</td>
<td>50.88
</td>
<td>74.52
</td>
<td>80.31
</td>
</tr>
<tr>
<td>Thai
</td>
<td>50.32
</td>
<td>72.95
</td>
<td>78.21
</td>
</tr>
</table>
## Responsibility & Safety
As part of our Responsible release approach, we followed a three-pronged strategy to managing trust & safety risks:
* Enable developers to deploy helpful, safe and flexible experiences for their target audience and for the use cases supported by Llama.
* Protect developers against adversarial users aiming to exploit Llama capabilities to potentially cause harm.
* Provide protections for the community to help prevent the misuse of our models.
### Responsible deployment
Llama is a foundational technology designed to be used in a variety of use cases, examples on how Meta’s Llama models have been responsibly deployed can be found in our [Community Stories webpage](https://llama.meta.com/community-stories/). Our approach is to build the most helpful models enabling the world to benefit from the technology power, by aligning our model safety for the generic use cases addressing a standard set of harms. Developers are then in the driver seat to tailor safety for their use case, defining their own policy and deploying the models with the necessary safeguards in their Llama systems. Llama 3.1 was developed following the best practices outlined in our Responsible Use Guide, you can refer to the [Responsible Use Guide](https://llama.meta.com/responsible-use-guide/) to learn more.
#### Llama 3.1 instruct
Our main objectives for conducting safety fine-tuning are to provide the research community with a valuable resource for studying the robustness of safety fine-tuning, as well as to offer developers a readily available, safe, and powerful model for various applications to reduce the developer workload to deploy safe AI systems. For more details on the safety mitigations implemented please read the Llama 3 paper.
**Fine-tuning data**
We employ a multi-faceted approach to data collection, combining human-generated data from our vendors with synthetic data to mitigate potential safety risks. We’ve developed many large language model (LLM)-based classifiers that enable us to thoughtfully select high-quality prompts and responses, enhancing data quality control.
**Refusals and Tone**
Building on the work we started with Llama 3, we put a great emphasis on model refusals to benign prompts as well as refusal tone. We included both borderline and adversarial prompts in our safety data strategy, and modified our safety data responses to follow tone guidelines.
#### Llama 3.1 systems
**Large language models, including Llama 3.1, are not designed to be deployed in isolation but instead should be deployed as part of an overall AI system with additional safety guardrails as required.** Developers are expected to deploy system safeguards when building agentic systems. Safeguards are key to achieve the right helpfulness-safety alignment as well as mitigating safety and security risks inherent to the system and any integration of the model or system with external tools.
As part of our responsible release approach, we provide the community with [safeguards](https://llama.meta.com/trust-and-safety/) that developers should deploy with Llama models or other LLMs, including Llama Guard 3, Prompt Guard and Code Shield. All our [reference implementations](https://github.com/meta-llama/llama-agentic-system) demos contain these safeguards by default so developers can benefit from system-level safety out-of-the-box.
#### New capabilities
Note that this release introduces new capabilities, including a longer context window, multilingual inputs and outputs and possible integrations by developers with third party tools. Building with these new capabilities requires specific considerations in addition to the best practices that generally apply across all Generative AI use cases.
**Tool-use**: Just like in standard software development, developers are responsible for the integration of the LLM with the tools and services of their choice. They should define a clear policy for their use case and assess the integrity of the third party services they use to be aware of the safety and security limitations when using this capability. Refer to the Responsible Use Guide for best practices on the safe deployment of the third party safeguards.
**Multilinguality**: Llama 3.1 supports 7 languages in addition to English: French, German, Hindi, Italian, Portuguese, Spanish, and Thai. Llama may be able to output text in other languages than those that meet performance thresholds for safety and helpfulness. We strongly discourage developers from using this model to converse in non-supported languages without implementing finetuning and system controls in alignment with their policies and the best practices shared in the Responsible Use Guide.
### Evaluations
We evaluated Llama models for common use cases as well as specific capabilities. Common use cases evaluations measure safety risks of systems for most commonly built applications including chat bot, coding assistant, tool calls. We built dedicated, adversarial evaluation datasets and evaluated systems composed of Llama models and Llama Guard 3 to filter input prompt and output response. It is important to evaluate applications in context, and we recommend building dedicated evaluation dataset for your use case. Prompt Guard and Code Shield are also available if relevant to the application.
Capability evaluations measure vulnerabilities of Llama models inherent to specific capabilities, for which were crafted dedicated benchmarks including long context, multilingual, tools calls, coding or memorization.
**Red teaming**
For both scenarios, we conducted recurring red teaming exercises with the goal of discovering risks via adversarial prompting and we used the learnings to improve our benchmarks and safety tuning datasets.
We partnered early with subject-matter experts in critical risk areas to understand the nature of these real-world harms and how such models may lead to unintended harm for society. Based on these conversations, we derived a set of adversarial goals for the red team to attempt to achieve, such as extracting harmful information or reprogramming the model to act in a potentially harmful capacity. The red team consisted of experts in cybersecurity, adversarial machine learning, responsible AI, and integrity in addition to multilingual content specialists with background in integrity issues in specific geographic markets.
### Critical and other risks
We specifically focused our efforts on mitigating the following critical risk areas:
**1- CBRNE (Chemical, Biological, Radiological, Nuclear, and Explosive materials) helpfulness**
To assess risks related to proliferation of chemical and biological weapons, we performed uplift testing designed to assess whether use of Llama 3.1 models could meaningfully increase the capabilities of malicious actors to plan or carry out attacks using these types of weapons.
**2. Child Safety**
Child Safety risk assessments were conducted using a team of experts, to assess the model’s capability to produce outputs that could result in Child Safety risks and inform on any necessary and appropriate risk mitigations via fine tuning. We leveraged those expert red teaming sessions to expand the coverage of our evaluation benchmarks through Llama 3 model development. For Llama 3, we conducted new in-depth sessions using objective based methodologies to assess the model risks along multiple attack vectors including the additional languages Llama 3 is trained on. We also partnered with content specialists to perform red teaming exercises assessing potentially violating content while taking account of market specific nuances or experiences.
**3. Cyber attack enablement**
Our cyber attack uplift study investigated whether LLMs can enhance human capabilities in hacking tasks, both in terms of skill level and speed.
Our attack automation study focused on evaluating the capabilities of LLMs when used as autonomous agents in cyber offensive operations, specifically in the context of ransomware attacks. This evaluation was distinct from previous studies that considered LLMs as interactive assistants. The primary objective was to assess whether these models could effectively function as independent agents in executing complex cyber-attacks without human intervention.
Our study of Llama-3.1-405B’s social engineering uplift for cyber attackers was conducted to assess the effectiveness of AI models in aiding cyber threat actors in spear phishing campaigns. Please read our Llama 3.1 Cyber security whitepaper to learn more.
### Community
Generative AI safety requires expertise and tooling, and we believe in the strength of the open community to accelerate its progress. We are active members of open consortiums, including the AI Alliance, Partnership on AI and MLCommons, actively contributing to safety standardization and transparency. We encourage the community to adopt taxonomies like the MLCommons Proof of Concept evaluation to facilitate collaboration and transparency on safety and content evaluations. Our Purple Llama tools are open sourced for the community to use and widely distributed across ecosystem partners including cloud service providers. We encourage community contributions to our [Github repository](https://github.com/meta-llama/PurpleLlama).
We also set up the [Llama Impact Grants](https://llama.meta.com/llama-impact-grants/) program to identify and support the most compelling applications of Meta’s Llama model for societal benefit across three categories: education, climate and open innovation. The 20 finalists from the hundreds of applications can be found [here](https://llama.meta.com/llama-impact-grants/#finalists).
Finally, we put in place a set of resources including an [output reporting mechanism](https://developers.facebook.com/llama_output_feedback) and [bug bounty program](https://www.facebook.com/whitehat) to continuously improve the Llama technology with the help of the community.
## Ethical Considerations and Limitations
The core values of Llama 3.1 are openness, inclusivity and helpfulness. It is meant to serve everyone, and to work for a wide range of use cases. It is thus designed to be accessible to people across many different backgrounds, experiences and perspectives. Llama 3.1 addresses users and their needs as they are, without insertion unnecessary judgment or normativity, while reflecting the understanding that even content that may appear problematic in some cases can serve valuable purposes in others. It respects the dignity and autonomy of all users, especially in terms of the values of free thought and expression that power innovation and progress.
But Llama 3.1 is a new technology, and like any new technology, there are risks associated with its use. Testing conducted to date has not covered, nor could it cover, all scenarios. For these reasons, as with all LLMs, Llama 3.1’s potential outputs cannot be predicted in advance, and the model may in some instances produce inaccurate, biased or other objectionable responses to user prompts. Therefore, before deploying any applications of Llama 3.1 models, developers should perform safety testing and tuning tailored to their specific applications of the model. Please refer to available resources including our [Responsible Use Guide](https://llama.meta.com/responsible-use-guide), [Trust and Safety](https://llama.meta.com/trust-and-safety/) solutions, and other [resources](https://llama.meta.com/docs/get-started/) to learn more about responsible development. |
hfl/chinese-bert-wwm-ext | hfl | "2021-05-19T19:06:39Z" | 469,268 | 159 | transformers | [
"transformers",
"pytorch",
"tf",
"jax",
"bert",
"fill-mask",
"zh",
"arxiv:1906.08101",
"arxiv:2004.13922",
"license:apache-2.0",
"autotrain_compatible",
"endpoints_compatible",
"region:us"
] | fill-mask | "2022-03-02T23:29:05Z" | ---
language:
- zh
license: "apache-2.0"
---
## Chinese BERT with Whole Word Masking
For further accelerating Chinese natural language processing, we provide **Chinese pre-trained BERT with Whole Word Masking**.
**[Pre-Training with Whole Word Masking for Chinese BERT](https://arxiv.org/abs/1906.08101)**
Yiming Cui, Wanxiang Che, Ting Liu, Bing Qin, Ziqing Yang, Shijin Wang, Guoping Hu
This repository is developed based on:https://github.com/google-research/bert
You may also interested in,
- Chinese BERT series: https://github.com/ymcui/Chinese-BERT-wwm
- Chinese MacBERT: https://github.com/ymcui/MacBERT
- Chinese ELECTRA: https://github.com/ymcui/Chinese-ELECTRA
- Chinese XLNet: https://github.com/ymcui/Chinese-XLNet
- Knowledge Distillation Toolkit - TextBrewer: https://github.com/airaria/TextBrewer
More resources by HFL: https://github.com/ymcui/HFL-Anthology
## Citation
If you find the technical report or resource is useful, please cite the following technical report in your paper.
- Primary: https://arxiv.org/abs/2004.13922
```
@inproceedings{cui-etal-2020-revisiting,
title = "Revisiting Pre-Trained Models for {C}hinese Natural Language Processing",
author = "Cui, Yiming and
Che, Wanxiang and
Liu, Ting and
Qin, Bing and
Wang, Shijin and
Hu, Guoping",
booktitle = "Proceedings of the 2020 Conference on Empirical Methods in Natural Language Processing: Findings",
month = nov,
year = "2020",
address = "Online",
publisher = "Association for Computational Linguistics",
url = "https://www.aclweb.org/anthology/2020.findings-emnlp.58",
pages = "657--668",
}
```
- Secondary: https://arxiv.org/abs/1906.08101
```
@article{chinese-bert-wwm,
title={Pre-Training with Whole Word Masking for Chinese BERT},
author={Cui, Yiming and Che, Wanxiang and Liu, Ting and Qin, Bing and Yang, Ziqing and Wang, Shijin and Hu, Guoping},
journal={arXiv preprint arXiv:1906.08101},
year={2019}
}
``` |
rizvandwiki/gender-classification | rizvandwiki | "2023-05-18T11:16:33Z" | 467,671 | 38 | transformers | [
"transformers",
"pytorch",
"tensorboard",
"safetensors",
"vit",
"image-classification",
"huggingpics",
"model-index",
"autotrain_compatible",
"endpoints_compatible",
"region:us"
] | image-classification | "2022-12-06T08:53:43Z" | ---
tags:
- image-classification
- pytorch
- huggingpics
metrics:
- accuracy
model-index:
- name: gender-classification
results:
- task:
name: Image Classification
type: image-classification
metrics:
- name: Accuracy
type: accuracy
value: 0.9244444370269775
---
# gender-classification
Autogenerated by HuggingPics🤗🖼️
Create your own image classifier for **anything** by running [the demo on Google Colab](https://colab.research.google.com/github/nateraw/huggingpics/blob/main/HuggingPics.ipynb).
Report any issues with the demo at the [github repo](https://github.com/nateraw/huggingpics).
## Example Images
#### female

#### male
 |
facebook/sam-vit-huge | facebook | "2024-01-11T19:23:32Z" | 466,771 | 141 | transformers | [
"transformers",
"pytorch",
"tf",
"safetensors",
"sam",
"mask-generation",
"vision",
"license:apache-2.0",
"endpoints_compatible",
"region:us"
] | mask-generation | "2023-04-10T13:51:24Z" | ---
license: apache-2.0
tags:
- vision
---
# Model Card for Segment Anything Model (SAM) - ViT Huge (ViT-H) version
<p>
<img src="https://huggingface.co/datasets/huggingface/documentation-images/resolve/main/transformers/model_doc/sam-architecture.png" alt="Model architecture">
<em> Detailed architecture of Segment Anything Model (SAM).</em>
</p>
# Table of Contents
0. [TL;DR](#TL;DR)
1. [Model Details](#model-details)
2. [Usage](#usage)
3. [Citation](#citation)
# TL;DR
[Link to original repository](https://github.com/facebookresearch/segment-anything)
| <img src="https://huggingface.co/datasets/huggingface/documentation-images/resolve/main/transformers/model_doc/sam-beancans.png" alt="Snow" width="600" height="600"> | <img src="https://huggingface.co/datasets/huggingface/documentation-images/resolve/main/transformers/model_doc/sam-dog-masks.png" alt="Forest" width="600" height="600"> | <img src="https://huggingface.co/datasets/huggingface/documentation-images/resolve/main/transformers/model_doc/sam-car-seg.png" alt="Mountains" width="600" height="600"> |
|---------------------------------------------------------------------------------------------------------------------------------------|--------------------------------------------------------------------------------------------------------------------------------|--------------------------------------------------------------------------------------------------------------------------------------------------|
The **Segment Anything Model (SAM)** produces high quality object masks from input prompts such as points or boxes, and it can be used to generate masks for all objects in an image. It has been trained on a [dataset](https://segment-anything.com/dataset/index.html) of 11 million images and 1.1 billion masks, and has strong zero-shot performance on a variety of segmentation tasks.
The abstract of the paper states:
> We introduce the Segment Anything (SA) project: a new task, model, and dataset for image segmentation. Using our efficient model in a data collection loop, we built the largest segmentation dataset to date (by far), with over 1 billion masks on 11M licensed and privacy respecting images. The model is designed and trained to be promptable, so it can transfer zero-shot to new image distributions and tasks. We evaluate its capabilities on numerous tasks and find that its zero-shot performance is impressive -- often competitive with or even superior to prior fully supervised results. We are releasing the Segment Anything Model (SAM) and corresponding dataset (SA-1B) of 1B masks and 11M images at [https://segment-anything.com](https://segment-anything.com) to foster research into foundation models for computer vision.
**Disclaimer**: Content from **this** model card has been written by the Hugging Face team, and parts of it were copy pasted from the original [SAM model card](https://github.com/facebookresearch/segment-anything).
# Model Details
The SAM model is made up of 3 modules:
- The `VisionEncoder`: a VIT based image encoder. It computes the image embeddings using attention on patches of the image. Relative Positional Embedding is used.
- The `PromptEncoder`: generates embeddings for points and bounding boxes
- The `MaskDecoder`: a two-ways transformer which performs cross attention between the image embedding and the point embeddings (->) and between the point embeddings and the image embeddings. The outputs are fed
- The `Neck`: predicts the output masks based on the contextualized masks produced by the `MaskDecoder`.
# Usage
## Prompted-Mask-Generation
```python
from PIL import Image
import requests
from transformers import SamModel, SamProcessor
model = SamModel.from_pretrained("facebook/sam-vit-huge")
processor = SamProcessor.from_pretrained("facebook/sam-vit-huge")
img_url = "https://huggingface.co/ybelkada/segment-anything/resolve/main/assets/car.png"
raw_image = Image.open(requests.get(img_url, stream=True).raw).convert("RGB")
input_points = [[[450, 600]]] # 2D localization of a window
```
```python
inputs = processor(raw_image, input_points=input_points, return_tensors="pt").to("cuda")
outputs = model(**inputs)
masks = processor.image_processor.post_process_masks(outputs.pred_masks.cpu(), inputs["original_sizes"].cpu(), inputs["reshaped_input_sizes"].cpu())
scores = outputs.iou_scores
```
Among other arguments to generate masks, you can pass 2D locations on the approximate position of your object of interest, a bounding box wrapping the object of interest (the format should be x, y coordinate of the top right and bottom left point of the bounding box), a segmentation mask. At this time of writing, passing a text as input is not supported by the official model according to [the official repository](https://github.com/facebookresearch/segment-anything/issues/4#issuecomment-1497626844).
For more details, refer to this notebook, which shows a walk throught of how to use the model, with a visual example!
## Automatic-Mask-Generation
The model can be used for generating segmentation masks in a "zero-shot" fashion, given an input image. The model is automatically prompt with a grid of `1024` points
which are all fed to the model.
The pipeline is made for automatic mask generation. The following snippet demonstrates how easy you can run it (on any device! Simply feed the appropriate `points_per_batch` argument)
```python
from transformers import pipeline
generator = pipeline("mask-generation", device = 0, points_per_batch = 256)
image_url = "https://huggingface.co/ybelkada/segment-anything/resolve/main/assets/car.png"
outputs = generator(image_url, points_per_batch = 256)
```
Now to display the image:
```python
import matplotlib.pyplot as plt
from PIL import Image
import numpy as np
def show_mask(mask, ax, random_color=False):
if random_color:
color = np.concatenate([np.random.random(3), np.array([0.6])], axis=0)
else:
color = np.array([30 / 255, 144 / 255, 255 / 255, 0.6])
h, w = mask.shape[-2:]
mask_image = mask.reshape(h, w, 1) * color.reshape(1, 1, -1)
ax.imshow(mask_image)
plt.imshow(np.array(raw_image))
ax = plt.gca()
for mask in outputs["masks"]:
show_mask(mask, ax=ax, random_color=True)
plt.axis("off")
plt.show()
```
This should give you the following 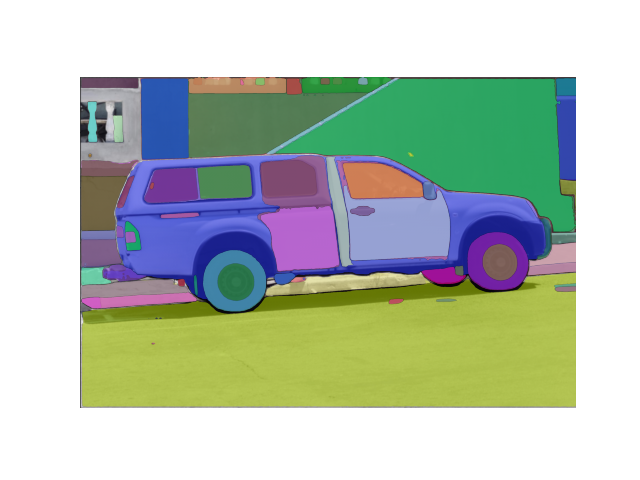
# Citation
If you use this model, please use the following BibTeX entry.
```
@article{kirillov2023segany,
title={Segment Anything},
author={Kirillov, Alexander and Mintun, Eric and Ravi, Nikhila and Mao, Hanzi and Rolland, Chloe and Gustafson, Laura and Xiao, Tete and Whitehead, Spencer and Berg, Alexander C. and Lo, Wan-Yen and Doll{\'a}r, Piotr and Girshick, Ross},
journal={arXiv:2304.02643},
year={2023}
}
``` |
unslothai/vram-16 | unslothai | "2024-07-07T17:01:14Z" | 466,756 | 0 | transformers | [
"transformers",
"safetensors",
"llama",
"feature-extraction",
"text-generation-inference",
"endpoints_compatible",
"region:us"
] | feature-extraction | "2024-07-07T17:01:10Z" | ---
library_name: transformers
tags: []
---
|
Qwen/Qwen2-VL-2B-Instruct | Qwen | "2024-09-21T08:39:36Z" | 466,747 | 262 | transformers | [
"transformers",
"safetensors",
"qwen2_vl",
"image-text-to-text",
"multimodal",
"conversational",
"en",
"arxiv:2409.12191",
"arxiv:2308.12966",
"license:apache-2.0",
"endpoints_compatible",
"region:us"
] | image-text-to-text | "2024-08-28T09:02:15Z" | ---
license: apache-2.0
language:
- en
pipeline_tag: image-text-to-text
tags:
- multimodal
library_name: transformers
---
# Qwen2-VL-2B-Instruct
## Introduction
We're excited to unveil **Qwen2-VL**, the latest iteration of our Qwen-VL model, representing nearly a year of innovation.
### What’s New in Qwen2-VL?
#### Key Enhancements:
* **SoTA understanding of images of various resolution & ratio**: Qwen2-VL achieves state-of-the-art performance on visual understanding benchmarks, including MathVista, DocVQA, RealWorldQA, MTVQA, etc.
* **Understanding videos of 20min+**: Qwen2-VL can understand videos over 20 minutes for high-quality video-based question answering, dialog, content creation, etc.
* **Agent that can operate your mobiles, robots, etc.**: with the abilities of complex reasoning and decision making, Qwen2-VL can be integrated with devices like mobile phones, robots, etc., for automatic operation based on visual environment and text instructions.
* **Multilingual Support**: to serve global users, besides English and Chinese, Qwen2-VL now supports the understanding of texts in different languages inside images, including most European languages, Japanese, Korean, Arabic, Vietnamese, etc.
#### Model Architecture Updates:
* **Naive Dynamic Resolution**: Unlike before, Qwen2-VL can handle arbitrary image resolutions, mapping them into a dynamic number of visual tokens, offering a more human-like visual processing experience.
<p align="center">
<img src="https://qianwen-res.oss-accelerate-overseas.aliyuncs.com/Qwen2-VL/qwen2_vl.jpg" width="80%"/>
<p>
* **Multimodal Rotary Position Embedding (M-ROPE)**: Decomposes positional embedding into parts to capture 1D textual, 2D visual, and 3D video positional information, enhancing its multimodal processing capabilities.
<p align="center">
<img src="http://qianwen-res.oss-accelerate-overseas.aliyuncs.com/Qwen2-VL/mrope.png" width="80%"/>
<p>
We have three models with 2, 7 and 72 billion parameters. This repo contains the instruction-tuned 2B Qwen2-VL model. For more information, visit our [Blog](https://qwenlm.github.io/blog/qwen2-vl/) and [GitHub](https://github.com/QwenLM/Qwen2-VL).
## Evaluation
### Image Benchmarks
| Benchmark | InternVL2-2B | MiniCPM-V 2.0 | **Qwen2-VL-2B** |
| :--- | :---: | :---: | :---: |
| MMMU<sub>val</sub> | 36.3 | 38.2 | **41.1** |
| DocVQA<sub>test</sub> | 86.9 | - | **90.1** |
| InfoVQA<sub>test</sub> | 58.9 | - | **65.5** |
| ChartQA<sub>test</sub> | **76.2** | - | 73.5 |
| TextVQA<sub>val</sub> | 73.4 | - | **79.7** |
| OCRBench | 781 | 605 | **794** |
| MTVQA | - | - | **20.0** |
| VCR<sub>en easy</sub> | - | - | **81.45**
| VCR<sub>zh easy</sub> | - | - | **46.16**
| RealWorldQA | 57.3 | 55.8 | **62.9** |
| MME<sub>sum</sub> | **1876.8** | 1808.6 | 1872.0 |
| MMBench-EN<sub>test</sub> | 73.2 | 69.1 | **74.9** |
| MMBench-CN<sub>test</sub> | 70.9 | 66.5 | **73.5** |
| MMBench-V1.1<sub>test</sub> | 69.6 | 65.8 | **72.2** |
| MMT-Bench<sub>test</sub> | - | - | **54.5** |
| MMStar | **49.8** | 39.1 | 48.0 |
| MMVet<sub>GPT-4-Turbo</sub> | 39.7 | 41.0 | **49.5** |
| HallBench<sub>avg</sub> | 38.0 | 36.1 | **41.7** |
| MathVista<sub>testmini</sub> | **46.0** | 39.8 | 43.0 |
| MathVision | - | - | **12.4** |
### Video Benchmarks
| Benchmark | **Qwen2-VL-2B** |
| :--- | :---: |
| MVBench | **63.2** |
| PerceptionTest<sub>test</sub> | **53.9** |
| EgoSchema<sub>test</sub> | **54.9** |
| Video-MME<sub>wo/w subs</sub> | **55.6**/**60.4** |
## Requirements
The code of Qwen2-VL has been in the latest Hugging face transformers and we advise you to build from source with command `pip install git+https://github.com/huggingface/transformers`, or you might encounter the following error:
```
KeyError: 'qwen2_vl'
```
## Quickstart
We offer a toolkit to help you handle various types of visual input more conveniently. This includes base64, URLs, and interleaved images and videos. You can install it using the following command:
```bash
pip install qwen-vl-utils
```
Here we show a code snippet to show you how to use the chat model with `transformers` and `qwen_vl_utils`:
```python
from transformers import Qwen2VLForConditionalGeneration, AutoTokenizer, AutoProcessor
from qwen_vl_utils import process_vision_info
# default: Load the model on the available device(s)
model = Qwen2VLForConditionalGeneration.from_pretrained(
"Qwen/Qwen2-VL-2B-Instruct", torch_dtype="auto", device_map="auto"
)
# We recommend enabling flash_attention_2 for better acceleration and memory saving, especially in multi-image and video scenarios.
# model = Qwen2VLForConditionalGeneration.from_pretrained(
# "Qwen/Qwen2-VL-2B-Instruct",
# torch_dtype=torch.bfloat16,
# attn_implementation="flash_attention_2",
# device_map="auto",
# )
# default processer
processor = AutoProcessor.from_pretrained("Qwen/Qwen2-VL-2B-Instruct")
# The default range for the number of visual tokens per image in the model is 4-16384. You can set min_pixels and max_pixels according to your needs, such as a token count range of 256-1280, to balance speed and memory usage.
# min_pixels = 256*28*28
# max_pixels = 1280*28*28
# processor = AutoProcessor.from_pretrained("Qwen/Qwen2-VL-2B-Instruct", min_pixels=min_pixels, max_pixels=max_pixels)
messages = [
{
"role": "user",
"content": [
{
"type": "image",
"image": "https://qianwen-res.oss-cn-beijing.aliyuncs.com/Qwen-VL/assets/demo.jpeg",
},
{"type": "text", "text": "Describe this image."},
],
}
]
# Preparation for inference
text = processor.apply_chat_template(
messages, tokenize=False, add_generation_prompt=True
)
image_inputs, video_inputs = process_vision_info(messages)
inputs = processor(
text=[text],
images=image_inputs,
videos=video_inputs,
padding=True,
return_tensors="pt",
)
inputs = inputs.to("cuda")
# Inference: Generation of the output
generated_ids = model.generate(**inputs, max_new_tokens=128)
generated_ids_trimmed = [
out_ids[len(in_ids) :] for in_ids, out_ids in zip(inputs.input_ids, generated_ids)
]
output_text = processor.batch_decode(
generated_ids_trimmed, skip_special_tokens=True, clean_up_tokenization_spaces=False
)
print(output_text)
```
<details>
<summary>Without qwen_vl_utils</summary>
```python
from PIL import Image
import requests
import torch
from torchvision import io
from typing import Dict
from transformers import Qwen2VLForConditionalGeneration, AutoTokenizer, AutoProcessor
# Load the model in half-precision on the available device(s)
model = Qwen2VLForConditionalGeneration.from_pretrained(
"Qwen/Qwen2-VL-2B-Instruct", torch_dtype="auto", device_map="auto"
)
processor = AutoProcessor.from_pretrained("Qwen/Qwen2-VL-2B-Instruct")
# Image
url = "https://qianwen-res.oss-cn-beijing.aliyuncs.com/Qwen-VL/assets/demo.jpeg"
image = Image.open(requests.get(url, stream=True).raw)
conversation = [
{
"role": "user",
"content": [
{
"type": "image",
},
{"type": "text", "text": "Describe this image."},
],
}
]
# Preprocess the inputs
text_prompt = processor.apply_chat_template(conversation, add_generation_prompt=True)
# Excepted output: '<|im_start|>system\nYou are a helpful assistant.<|im_end|>\n<|im_start|>user\n<|vision_start|><|image_pad|><|vision_end|>Describe this image.<|im_end|>\n<|im_start|>assistant\n'
inputs = processor(
text=[text_prompt], images=[image], padding=True, return_tensors="pt"
)
inputs = inputs.to("cuda")
# Inference: Generation of the output
output_ids = model.generate(**inputs, max_new_tokens=128)
generated_ids = [
output_ids[len(input_ids) :]
for input_ids, output_ids in zip(inputs.input_ids, output_ids)
]
output_text = processor.batch_decode(
generated_ids, skip_special_tokens=True, clean_up_tokenization_spaces=True
)
print(output_text)
```
</details>
<details>
<summary>Multi image inference</summary>
```python
# Messages containing multiple images and a text query
messages = [
{
"role": "user",
"content": [
{"type": "image", "image": "file:///path/to/image1.jpg"},
{"type": "image", "image": "file:///path/to/image2.jpg"},
{"type": "text", "text": "Identify the similarities between these images."},
],
}
]
# Preparation for inference
text = processor.apply_chat_template(
messages, tokenize=False, add_generation_prompt=True
)
image_inputs, video_inputs = process_vision_info(messages)
inputs = processor(
text=[text],
images=image_inputs,
videos=video_inputs,
padding=True,
return_tensors="pt",
)
inputs = inputs.to("cuda")
# Inference
generated_ids = model.generate(**inputs, max_new_tokens=128)
generated_ids_trimmed = [
out_ids[len(in_ids) :] for in_ids, out_ids in zip(inputs.input_ids, generated_ids)
]
output_text = processor.batch_decode(
generated_ids_trimmed, skip_special_tokens=True, clean_up_tokenization_spaces=False
)
print(output_text)
```
</details>
<details>
<summary>Video inference</summary>
```python
# Messages containing a images list as a video and a text query
messages = [
{
"role": "user",
"content": [
{
"type": "video",
"video": [
"file:///path/to/frame1.jpg",
"file:///path/to/frame2.jpg",
"file:///path/to/frame3.jpg",
"file:///path/to/frame4.jpg",
],
"fps": 1.0,
},
{"type": "text", "text": "Describe this video."},
],
}
]
# Messages containing a video and a text query
messages = [
{
"role": "user",
"content": [
{
"type": "video",
"video": "file:///path/to/video1.mp4",
"max_pixels": 360 * 420,
"fps": 1.0,
},
{"type": "text", "text": "Describe this video."},
],
}
]
# Preparation for inference
text = processor.apply_chat_template(
messages, tokenize=False, add_generation_prompt=True
)
image_inputs, video_inputs = process_vision_info(messages)
inputs = processor(
text=[text],
images=image_inputs,
videos=video_inputs,
padding=True,
return_tensors="pt",
)
inputs = inputs.to("cuda")
# Inference
generated_ids = model.generate(**inputs, max_new_tokens=128)
generated_ids_trimmed = [
out_ids[len(in_ids) :] for in_ids, out_ids in zip(inputs.input_ids, generated_ids)
]
output_text = processor.batch_decode(
generated_ids_trimmed, skip_special_tokens=True, clean_up_tokenization_spaces=False
)
print(output_text)
```
</details>
<details>
<summary>Batch inference</summary>
```python
# Sample messages for batch inference
messages1 = [
{
"role": "user",
"content": [
{"type": "image", "image": "file:///path/to/image1.jpg"},
{"type": "image", "image": "file:///path/to/image2.jpg"},
{"type": "text", "text": "What are the common elements in these pictures?"},
],
}
]
messages2 = [
{"role": "system", "content": "You are a helpful assistant."},
{"role": "user", "content": "Who are you?"},
]
# Combine messages for batch processing
messages = [messages1, messages1]
# Preparation for batch inference
texts = [
processor.apply_chat_template(msg, tokenize=False, add_generation_prompt=True)
for msg in messages
]
image_inputs, video_inputs = process_vision_info(messages)
inputs = processor(
text=texts,
images=image_inputs,
videos=video_inputs,
padding=True,
return_tensors="pt",
)
inputs = inputs.to("cuda")
# Batch Inference
generated_ids = model.generate(**inputs, max_new_tokens=128)
generated_ids_trimmed = [
out_ids[len(in_ids) :] for in_ids, out_ids in zip(inputs.input_ids, generated_ids)
]
output_texts = processor.batch_decode(
generated_ids_trimmed, skip_special_tokens=True, clean_up_tokenization_spaces=False
)
print(output_texts)
```
</details>
### More Usage Tips
For input images, we support local files, base64, and URLs. For videos, we currently only support local files.
```python
# You can directly insert a local file path, a URL, or a base64-encoded image into the position where you want in the text.
## Local file path
messages = [
{
"role": "user",
"content": [
{"type": "image", "image": "file:///path/to/your/image.jpg"},
{"type": "text", "text": "Describe this image."},
],
}
]
## Image URL
messages = [
{
"role": "user",
"content": [
{"type": "image", "image": "http://path/to/your/image.jpg"},
{"type": "text", "text": "Describe this image."},
],
}
]
## Base64 encoded image
messages = [
{
"role": "user",
"content": [
{"type": "image", "image": "data:image;base64,/9j/..."},
{"type": "text", "text": "Describe this image."},
],
}
]
```
#### Image Resolution for performance boost
The model supports a wide range of resolution inputs. By default, it uses the native resolution for input, but higher resolutions can enhance performance at the cost of more computation. Users can set the minimum and maximum number of pixels to achieve an optimal configuration for their needs, such as a token count range of 256-1280, to balance speed and memory usage.
```python
min_pixels = 256 * 28 * 28
max_pixels = 1280 * 28 * 28
processor = AutoProcessor.from_pretrained(
"Qwen/Qwen2-VL-2B-Instruct", min_pixels=min_pixels, max_pixels=max_pixels
)
```
Besides, We provide two methods for fine-grained control over the image size input to the model:
1. Define min_pixels and max_pixels: Images will be resized to maintain their aspect ratio within the range of min_pixels and max_pixels.
2. Specify exact dimensions: Directly set `resized_height` and `resized_width`. These values will be rounded to the nearest multiple of 28.
```python
# min_pixels and max_pixels
messages = [
{
"role": "user",
"content": [
{
"type": "image",
"image": "file:///path/to/your/image.jpg",
"resized_height": 280,
"resized_width": 420,
},
{"type": "text", "text": "Describe this image."},
],
}
]
# resized_height and resized_width
messages = [
{
"role": "user",
"content": [
{
"type": "image",
"image": "file:///path/to/your/image.jpg",
"min_pixels": 50176,
"max_pixels": 50176,
},
{"type": "text", "text": "Describe this image."},
],
}
]
```
## Limitations
While Qwen2-VL are applicable to a wide range of visual tasks, it is equally important to understand its limitations. Here are some known restrictions:
1. Lack of Audio Support: The current model does **not comprehend audio information** within videos.
2. Data timeliness: Our image dataset is **updated until June 2023**, and information subsequent to this date may not be covered.
3. Constraints in Individuals and Intellectual Property (IP): The model's capacity to recognize specific individuals or IPs is limited, potentially failing to comprehensively cover all well-known personalities or brands.
4. Limited Capacity for Complex Instruction: When faced with intricate multi-step instructions, the model's understanding and execution capabilities require enhancement.
5. Insufficient Counting Accuracy: Particularly in complex scenes, the accuracy of object counting is not high, necessitating further improvements.
6. Weak Spatial Reasoning Skills: Especially in 3D spaces, the model's inference of object positional relationships is inadequate, making it difficult to precisely judge the relative positions of objects.
These limitations serve as ongoing directions for model optimization and improvement, and we are committed to continually enhancing the model's performance and scope of application.
## Citation
If you find our work helpful, feel free to give us a cite.
```
@article{Qwen2VL,
title={Qwen2-VL: Enhancing Vision-Language Model's Perception of the World at Any Resolution},
author={Wang, Peng and Bai, Shuai and Tan, Sinan and Wang, Shijie and Fan, Zhihao and Bai, Jinze and Chen, Keqin and Liu, Xuejing and Wang, Jialin and Ge, Wenbin and Fan, Yang and Dang, Kai and Du, Mengfei and Ren, Xuancheng and Men, Rui and Liu, Dayiheng and Zhou, Chang and Zhou, Jingren and Lin, Junyang},
journal={arXiv preprint arXiv:2409.12191},
year={2024}
}
@article{Qwen-VL,
title={Qwen-VL: A Versatile Vision-Language Model for Understanding, Localization, Text Reading, and Beyond},
author={Bai, Jinze and Bai, Shuai and Yang, Shusheng and Wang, Shijie and Tan, Sinan and Wang, Peng and Lin, Junyang and Zhou, Chang and Zhou, Jingren},
journal={arXiv preprint arXiv:2308.12966},
year={2023}
}
``` |
mistralai/Mistral-7B-Instruct-v0.3 | mistralai | "2024-08-21T12:18:25Z" | 466,724 | 1,127 | transformers | [
"transformers",
"safetensors",
"mistral",
"text-generation",
"conversational",
"base_model:mistralai/Mistral-7B-v0.3",
"base_model:finetune:mistralai/Mistral-7B-v0.3",
"license:apache-2.0",
"autotrain_compatible",
"text-generation-inference",
"endpoints_compatible",
"region:us"
] | text-generation | "2024-05-22T09:57:04Z" | ---
license: apache-2.0
base_model: mistralai/Mistral-7B-v0.3
extra_gated_description: If you want to learn more about how we process your personal data, please read our <a href="https://mistral.ai/terms/">Privacy Policy</a>.
---
# Model Card for Mistral-7B-Instruct-v0.3
The Mistral-7B-Instruct-v0.3 Large Language Model (LLM) is an instruct fine-tuned version of the Mistral-7B-v0.3.
Mistral-7B-v0.3 has the following changes compared to [Mistral-7B-v0.2](https://huggingface.co/mistralai/Mistral-7B-Instruct-v0.2/edit/main/README.md)
- Extended vocabulary to 32768
- Supports v3 Tokenizer
- Supports function calling
## Installation
It is recommended to use `mistralai/Mistral-7B-Instruct-v0.3` with [mistral-inference](https://github.com/mistralai/mistral-inference). For HF transformers code snippets, please keep scrolling.
```
pip install mistral_inference
```
## Download
```py
from huggingface_hub import snapshot_download
from pathlib import Path
mistral_models_path = Path.home().joinpath('mistral_models', '7B-Instruct-v0.3')
mistral_models_path.mkdir(parents=True, exist_ok=True)
snapshot_download(repo_id="mistralai/Mistral-7B-Instruct-v0.3", allow_patterns=["params.json", "consolidated.safetensors", "tokenizer.model.v3"], local_dir=mistral_models_path)
```
### Chat
After installing `mistral_inference`, a `mistral-chat` CLI command should be available in your environment. You can chat with the model using
```
mistral-chat $HOME/mistral_models/7B-Instruct-v0.3 --instruct --max_tokens 256
```
### Instruct following
```py
from mistral_inference.transformer import Transformer
from mistral_inference.generate import generate
from mistral_common.tokens.tokenizers.mistral import MistralTokenizer
from mistral_common.protocol.instruct.messages import UserMessage
from mistral_common.protocol.instruct.request import ChatCompletionRequest
tokenizer = MistralTokenizer.from_file(f"{mistral_models_path}/tokenizer.model.v3")
model = Transformer.from_folder(mistral_models_path)
completion_request = ChatCompletionRequest(messages=[UserMessage(content="Explain Machine Learning to me in a nutshell.")])
tokens = tokenizer.encode_chat_completion(completion_request).tokens
out_tokens, _ = generate([tokens], model, max_tokens=64, temperature=0.0, eos_id=tokenizer.instruct_tokenizer.tokenizer.eos_id)
result = tokenizer.instruct_tokenizer.tokenizer.decode(out_tokens[0])
print(result)
```
### Function calling
```py
from mistral_common.protocol.instruct.tool_calls import Function, Tool
from mistral_inference.transformer import Transformer
from mistral_inference.generate import generate
from mistral_common.tokens.tokenizers.mistral import MistralTokenizer
from mistral_common.protocol.instruct.messages import UserMessage
from mistral_common.protocol.instruct.request import ChatCompletionRequest
tokenizer = MistralTokenizer.from_file(f"{mistral_models_path}/tokenizer.model.v3")
model = Transformer.from_folder(mistral_models_path)
completion_request = ChatCompletionRequest(
tools=[
Tool(
function=Function(
name="get_current_weather",
description="Get the current weather",
parameters={
"type": "object",
"properties": {
"location": {
"type": "string",
"description": "The city and state, e.g. San Francisco, CA",
},
"format": {
"type": "string",
"enum": ["celsius", "fahrenheit"],
"description": "The temperature unit to use. Infer this from the users location.",
},
},
"required": ["location", "format"],
},
)
)
],
messages=[
UserMessage(content="What's the weather like today in Paris?"),
],
)
tokens = tokenizer.encode_chat_completion(completion_request).tokens
out_tokens, _ = generate([tokens], model, max_tokens=64, temperature=0.0, eos_id=tokenizer.instruct_tokenizer.tokenizer.eos_id)
result = tokenizer.instruct_tokenizer.tokenizer.decode(out_tokens[0])
print(result)
```
## Generate with `transformers`
If you want to use Hugging Face `transformers` to generate text, you can do something like this.
```py
from transformers import pipeline
messages = [
{"role": "system", "content": "You are a pirate chatbot who always responds in pirate speak!"},
{"role": "user", "content": "Who are you?"},
]
chatbot = pipeline("text-generation", model="mistralai/Mistral-7B-Instruct-v0.3")
chatbot(messages)
```
## Function calling with `transformers`
To use this example, you'll need `transformers` version 4.42.0 or higher. Please see the
[function calling guide](https://huggingface.co/docs/transformers/main/chat_templating#advanced-tool-use--function-calling)
in the `transformers` docs for more information.
```python
from transformers import AutoModelForCausalLM, AutoTokenizer
import torch
model_id = "mistralai/Mistral-7B-Instruct-v0.3"
tokenizer = AutoTokenizer.from_pretrained(model_id)
def get_current_weather(location: str, format: str):
"""
Get the current weather
Args:
location: The city and state, e.g. San Francisco, CA
format: The temperature unit to use. Infer this from the users location. (choices: ["celsius", "fahrenheit"])
"""
pass
conversation = [{"role": "user", "content": "What's the weather like in Paris?"}]
tools = [get_current_weather]
# format and tokenize the tool use prompt
inputs = tokenizer.apply_chat_template(
conversation,
tools=tools,
add_generation_prompt=True,
return_dict=True,
return_tensors="pt",
)
model = AutoModelForCausalLM.from_pretrained(model_id, torch_dtype=torch.bfloat16, device_map="auto")
inputs.to(model.device)
outputs = model.generate(**inputs, max_new_tokens=1000)
print(tokenizer.decode(outputs[0], skip_special_tokens=True))
```
Note that, for reasons of space, this example does not show a complete cycle of calling a tool and adding the tool call and tool
results to the chat history so that the model can use them in its next generation. For a full tool calling example, please
see the [function calling guide](https://huggingface.co/docs/transformers/main/chat_templating#advanced-tool-use--function-calling),
and note that Mistral **does** use tool call IDs, so these must be included in your tool calls and tool results. They should be
exactly 9 alphanumeric characters.
## Limitations
The Mistral 7B Instruct model is a quick demonstration that the base model can be easily fine-tuned to achieve compelling performance.
It does not have any moderation mechanisms. We're looking forward to engaging with the community on ways to
make the model finely respect guardrails, allowing for deployment in environments requiring moderated outputs.
## The Mistral AI Team
Albert Jiang, Alexandre Sablayrolles, Alexis Tacnet, Antoine Roux, Arthur Mensch, Audrey Herblin-Stoop, Baptiste Bout, Baudouin de Monicault, Blanche Savary, Bam4d, Caroline Feldman, Devendra Singh Chaplot, Diego de las Casas, Eleonore Arcelin, Emma Bou Hanna, Etienne Metzger, Gianna Lengyel, Guillaume Bour, Guillaume Lample, Harizo Rajaona, Jean-Malo Delignon, Jia Li, Justus Murke, Louis Martin, Louis Ternon, Lucile Saulnier, Lélio Renard Lavaud, Margaret Jennings, Marie Pellat, Marie Torelli, Marie-Anne Lachaux, Nicolas Schuhl, Patrick von Platen, Pierre Stock, Sandeep Subramanian, Sophia Yang, Szymon Antoniak, Teven Le Scao, Thibaut Lavril, Timothée Lacroix, Théophile Gervet, Thomas Wang, Valera Nemychnikova, William El Sayed, William Marshall |
stabilityai/stable-video-diffusion-img2vid-xt | stabilityai | "2024-07-10T11:43:18Z" | 466,001 | 2,670 | diffusers | [
"diffusers",
"safetensors",
"image-to-video",
"license:other",
"diffusers:StableVideoDiffusionPipeline",
"region:us"
] | image-to-video | "2023-11-20T23:45:55Z" | ---
pipeline_tag: image-to-video
license: other
license_name: stable-video-diffusion-community
license_link: LICENSE.md
---
# Stable Video Diffusion Image-to-Video Model Card
<!-- Provide a quick summary of what the model is/does. -->

Stable Video Diffusion (SVD) Image-to-Video is a diffusion model that takes in a still image as a conditioning frame, and generates a video from it.
Please note: For commercial use, please refer to https://stability.ai/license.
## Model Details
### Model Description
(SVD) Image-to-Video is a latent diffusion model trained to generate short video clips from an image conditioning.
This model was trained to generate 25 frames at resolution 576x1024 given a context frame of the same size, finetuned from [SVD Image-to-Video [14 frames]](https://huggingface.co/stabilityai/stable-video-diffusion-img2vid).
We also finetune the widely used [f8-decoder](https://huggingface.co/docs/diffusers/api/models/autoencoderkl#loading-from-the-original-format) for temporal consistency.
For convenience, we additionally provide the model with the
standard frame-wise decoder [here](https://huggingface.co/stabilityai/stable-video-diffusion-img2vid-xt/blob/main/svd_xt_image_decoder.safetensors).
- **Developed by:** Stability AI
- **Funded by:** Stability AI
- **Model type:** Generative image-to-video model
- **Finetuned from model:** SVD Image-to-Video [14 frames]
### Model Sources
For research purposes, we recommend our `generative-models` Github repository (https://github.com/Stability-AI/generative-models),
which implements the most popular diffusion frameworks (both training and inference).
- **Repository:** https://github.com/Stability-AI/generative-models
- **Paper:** https://stability.ai/research/stable-video-diffusion-scaling-latent-video-diffusion-models-to-large-datasets
## Evaluation

The chart above evaluates user preference for SVD-Image-to-Video over [GEN-2](https://research.runwayml.com/gen2) and [PikaLabs](https://www.pika.art/).
SVD-Image-to-Video is preferred by human voters in terms of video quality. For details on the user study, we refer to the [research paper](https://stability.ai/research/stable-video-diffusion-scaling-latent-video-diffusion-models-to-large-datasets)
## Uses
### Direct Use
The model is intended for both non-commercial and commercial usage. You can use this model for non-commercial or research purposes under this [license](https://huggingface.co/stabilityai/stable-video-diffusion-img2vid-xt/blob/main/LICENSE.md). Possible research areas and tasks include
- Research on generative models.
- Safe deployment of models which have the potential to generate harmful content.
- Probing and understanding the limitations and biases of generative models.
- Generation of artworks and use in design and other artistic processes.
- Applications in educational or creative tools.
For commercial use, please refer to https://stability.ai/license.
Excluded uses are described below.
### Out-of-Scope Use
The model was not trained to be factual or true representations of people or events,
and therefore using the model to generate such content is out-of-scope for the abilities of this model.
The model should not be used in any way that violates Stability AI's [Acceptable Use Policy](https://stability.ai/use-policy).
## Limitations and Bias
### Limitations
- The generated videos are rather short (<= 4sec), and the model does not achieve perfect photorealism.
- The model may generate videos without motion, or very slow camera pans.
- The model cannot be controlled through text.
- The model cannot render legible text.
- Faces and people in general may not be generated properly.
- The autoencoding part of the model is lossy.
### Recommendations
The model is intended for both non-commercial and commercial usage.
## How to Get Started with the Model
Check out https://github.com/Stability-AI/generative-models
# Appendix:
All considered potential data sources were included for final training, with none held out as the proposed data filtering methods described in the SVD paper handle the quality control/filtering of the dataset. With regards to safety/NSFW filtering, sources considered were either deemed safe or filtered with the in-house NSFW filters.
No explicit human labor is involved in training data preparation. However, human evaluation for model outputs and quality was extensively used to evaluate model quality and performance. The evaluations were performed with third-party contractor platforms (Amazon Sagemaker, Amazon Mechanical Turk, Prolific) with fluent English-speaking contractors from various countries, primarily from the USA, UK, and Canada. Each worker was paid $12/hr for the time invested in the evaluation.
No other third party was involved in the development of this model; the model was fully developed in-house at Stability AI.
Training the SVD checkpoints required a total of approximately 200,000 A100 80GB hours. The majority of the training occurred on 48 * 8 A100s, while some stages took more/less than that. The resulting CO2 emission is ~19,000kg CO2 eq., and energy consumed is ~64000 kWh.
The released checkpoints (SVD/SVD-XT) are image-to-video models that generate short videos/animations closely following the given input image. Since the model relies on an existing supplied image, the potential risks of disclosing specific material or novel unsafe content are minimal. This was also evaluated by third-party independent red-teaming services, which agree with our conclusion to a high degree of confidence (>90% in various areas of safety red-teaming). The external evaluations were also performed for trustworthiness, leading to >95% confidence in real, trustworthy videos.
With the default settings at the time of release, SVD takes ~100s for generation, and SVD-XT takes ~180s on an A100 80GB card. Several optimizations to trade off quality / memory / speed can be done to perform faster inference or inference on lower VRAM cards.
The information related to the model and its development process and usage protocols can be found in the GitHub repo, associated research paper, and HuggingFace model page/cards.
The released model inference & demo code has image-level watermarking enabled by default, which can be used to detect the outputs. This is done via the imWatermark Python library.
The model can be used to generate videos from static initial images. However, we prohibit unlawful, obscene, or misleading uses of the model consistent with the terms of our license and Acceptable Use Policy. For the open-weights release, our training data filtering mitigations alleviate this risk to some extent. These restrictions are explicitly enforced on user-facing interfaces at stablevideo.com, where a warning is issued. We do not take any responsibility for third-party interfaces. Submitting initial images that bypass input filters to tease out offensive or inappropriate content listed above is also prohibited. Safety filtering checks at stablevideo.com run on model inputs and outputs independently. More details on our user-facing interfaces can be found here: https://www.stablevideo.com/faq. Beyond the Acceptable Use Policy and other mitigations and conditions described here, the model is not subject to additional model behavior interventions of the type described in the Foundation Model Transparency Index.
For stablevideo.com, we store preference data in the form of upvotes/downvotes on user-generated videos, and we have a pairwise ranker that runs while a user generates videos. This usage data is solely used for improving Stability AI’s future image/video models and services. No other third-party entities are given access to the usage data beyond Stability AI and maintainers of stablevideo.com.
For usage statistics of SVD, we refer interested users to HuggingFace model download/usage statistics as a primary indicator. Third-party applications also have reported model usage statistics. We might also consider releasing aggregate usage statistics of stablevideo.com on reaching some milestones.
|
SWivid/F5-TTS | SWivid | "2024-11-08T18:26:41Z" | 460,628 | 711 | f5-tts | [
"f5-tts",
"text-to-speech",
"dataset:amphion/Emilia-Dataset",
"arxiv:2410.06885",
"license:cc-by-nc-4.0",
"region:us"
] | text-to-speech | "2024-10-07T14:37:58Z" | ---
license: cc-by-nc-4.0
pipeline_tag: text-to-speech
library_name: f5-tts
datasets:
- amphion/Emilia-Dataset
---
### 2024/10/14. We change the License of this ckpt repo to CC-BY-NC-4.0 following the used training set Emilia, which is an in-the-wild dataset. Sorry for any inconvenience this may cause. Our codebase remains under the MIT license.
Download [F5-TTS](https://huggingface.co/SWivid/F5-TTS/tree/main/F5TTS_Base) or [E2 TTS](https://huggingface.co/SWivid/E2-TTS/tree/main/E2TTS_Base) and place under ckpts/
```
ckpts/
E2TTS_Base/
model_1200000.pt
F5TTS_Base/
model_1200000.pt
```
Inference with .safetensors option
```
ckpts/
E2TTS_Base/
model_1200000.safetensors
F5TTS_Base/
model_1200000.safetensors
```
Github: https://github.com/SWivid/F5-TTS
Paper: [F5-TTS: A Fairytaler that Fakes Fluent and Faithful Speech with Flow Matching](https://huggingface.co/papers/2410.06885) |
shahrukhx01/bert-mini-finetune-question-detection | shahrukhx01 | "2023-03-29T22:00:48Z" | 459,813 | 16 | transformers | [
"transformers",
"pytorch",
"safetensors",
"bert",
"text-classification",
"neural-search-query-classification",
"neural-search",
"en",
"autotrain_compatible",
"endpoints_compatible",
"region:us"
] | text-classification | "2022-03-02T23:29:05Z" |
---
language: "en"
tags:
- neural-search-query-classification
- neural-search
widget:
- text: "keyword query."
---
# KEYWORD QUERY VS STATEMENT/QUESTION CLASSIFIER FOR NEURAL SEARCH
| Train Loss | Validation Acc.| Test Acc.|
| ------------- |:-------------: | -----: |
| 0.000806 | 0.99 | 0.997 |
```python
from transformers import AutoTokenizer, AutoModelForSequenceClassification
tokenizer = AutoTokenizer.from_pretrained("shahrukhx01/bert-mini-finetune-question-detection")
model = AutoModelForSequenceClassification.from_pretrained("shahrukhx01/bert-mini-finetune-question-detection")
```
Trained to add feature for classifying queries between Keyword Query or Question + Statement Query using classification in [Haystack](https://github.com/deepset-ai/haystack/issues/611)
Problem Statement:
One common challenge that we saw in deployments: We need to distinguish between real questions and keyword queries that come in. We only want to route questions to the Reader branch in order to maximize the accuracy of results and minimize computation efforts/costs.
Baseline:
https://www.kaggle.com/shahrukhkhan/question-v-statement-detection
Dataset:
https://www.kaggle.com/stefanondisponibile/quora-question-keyword-pairs
Kaggle Notebook:
https://www.kaggle.com/shahrukhkhan/question-vs-statement-classification-mini-bert/
|
nomic-ai/nomic-embed-vision-v1 | nomic-ai | "2024-06-11T00:37:44Z" | 459,576 | 19 | transformers | [
"transformers",
"onnx",
"safetensors",
"nomic_bert",
"feature-extraction",
"image-feature-extraction",
"custom_code",
"en",
"arxiv:2111.07991",
"license:cc-by-nc-4.0",
"region:us"
] | image-feature-extraction | "2024-05-13T17:09:02Z" | ---
library_name: transformers
language:
- en
pipeline_tag: image-feature-extraction
license: cc-by-nc-4.0
inference: false
---
# nomic-embed-vision-v1: Expanding the Latent Space
`nomic-embed-vision-v1` is a high performing vision embedding model that shares the same embedding space as [nomic-embed-text-v1](https://huggingface.co/nomic-ai/nomic-embed-text-v1).
All Nomic Embed Text models are now **multimodal**!
| Name | Imagenet 0-shot | Datacomp (Avg. 38) | MTEB |
| :-------------------------------:| :-------------- | :----------------- | :------: |
| `nomic-embed-vision-v1.5` | **71.0** | **56.8** | 62.28 |
| `nomic-embed-vision-v1` | 70.7 | 56.7 | **62.39** |
| OpenAI CLIP ViT B/16 | 68.3 | 56.3 | 43.82 |
| Jina CLIP v1 | 59.1 | 52.2 | 60.1 |
## Hosted Inference API
The easiest way to get started with Nomic Embed is through the Nomic Embedding API.
Generating embeddings with the `nomic` Python client is as easy as
```python
from nomic import embed
import numpy as np
output = embed.image(
images=[
"image_path_1.jpeg",
"image_path_2.png",
],
model='nomic-embed-vision-v1',
)
print(output['usage'])
embeddings = np.array(output['embeddings'])
print(embeddings.shape)
```
For more information, see the [API reference](https://docs.nomic.ai/reference/endpoints/nomic-embed-vision)
## Data Visualization
Click the Nomic Atlas map below to visualize a 100,000 sample CC3M comparing the Vision and Text Embedding Space!
[](https://atlas.nomic.ai/data/nomic-multimodal-series/cc3m-100k-image-bytes-v15/map)
## Training Details
We align our vision embedder to the text embedding by employing a technique similar to [LiT](https://arxiv.org/abs/2111.07991) but instead lock the text embedder!
For more details, see the Nomic Embed Vision Technical Report (soon to be released!) and corresponding [blog post](https://blog.nomic.ai/posts/nomic-embed-vision)
Training code is released in the `contrastors` [repository](https://github.com/nomic-ai/contrastors)
## Usage
Remember `nomic-embed-text` *requires* prefixes and so, when using Nomic Embed in multimodal RAG scenarios (e.g. text to image retrieval),
you should use the `search_query: ` prefix.
### Transformers
```python
import torch
import torch.nn.functional as F
from transformers import AutoTokenizer, AutoModel, AutoImageProcessor
from PIL import Image
import requests
processor = AutoImageProcessor.from_pretrained("nomic-ai/nomic-embed-vision-v1")
vision_model = AutoModel.from_pretrained("nomic-ai/nomic-embed-vision-v1", trust_remote_code=True)
url = 'http://images.cocodataset.org/val2017/000000039769.jpg'
image = Image.open(requests.get(url, stream=True).raw)
inputs = processor(image, return_tensors="pt")
img_emb = vision_model(**inputs).last_hidden_state
img_embeddings = F.normalize(img_emb[:, 0], p=2, dim=1)
```
Additionally, you can perform multimodal retrieval!
```python
def mean_pooling(model_output, attention_mask):
token_embeddings = model_output[0]
input_mask_expanded = attention_mask.unsqueeze(-1).expand(token_embeddings.size()).float()
return torch.sum(token_embeddings * input_mask_expanded, 1) / torch.clamp(input_mask_expanded.sum(1), min=1e-9)
sentences = ['search_query: What are cute animals to cuddle with?', 'search_query: What do cats look like?']
tokenizer = AutoTokenizer.from_pretrained('nomic-ai/nomic-embed-text-v1')
text_model = AutoModel.from_pretrained('nomic-ai/nomic-embed-text-v1', trust_remote_code=True)
text_model.eval()
encoded_input = tokenizer(sentences, padding=True, truncation=True, return_tensors='pt')
with torch.no_grad():
model_output = text_model(**encoded_input)
text_embeddings = mean_pooling(model_output, encoded_input['attention_mask'])
text_embeddings = F.normalize(text_embeddings, p=2, dim=1)
print(torch.matmul(img_embeddings, text_embeddings.T))
```
# Join the Nomic Community
- Nomic: [https://nomic.ai](https://nomic.ai)
- Discord: [https://discord.gg/myY5YDR8z8](https://discord.gg/myY5YDR8z8)
- Twitter: [https://twitter.com/nomic_ai](https://twitter.com/nomic_ai)
|
openai/whisper-base | openai | "2024-02-29T10:26:57Z" | 455,488 | 188 | transformers | [
"transformers",
"pytorch",
"tf",
"jax",
"safetensors",
"whisper",
"automatic-speech-recognition",
"audio",
"hf-asr-leaderboard",
"en",
"zh",
"de",
"es",
"ru",
"ko",
"fr",
"ja",
"pt",
"tr",
"pl",
"ca",
"nl",
"ar",
"sv",
"it",
"id",
"hi",
"fi",
"vi",
"he",
"uk",
"el",
"ms",
"cs",
"ro",
"da",
"hu",
"ta",
"no",
"th",
"ur",
"hr",
"bg",
"lt",
"la",
"mi",
"ml",
"cy",
"sk",
"te",
"fa",
"lv",
"bn",
"sr",
"az",
"sl",
"kn",
"et",
"mk",
"br",
"eu",
"is",
"hy",
"ne",
"mn",
"bs",
"kk",
"sq",
"sw",
"gl",
"mr",
"pa",
"si",
"km",
"sn",
"yo",
"so",
"af",
"oc",
"ka",
"be",
"tg",
"sd",
"gu",
"am",
"yi",
"lo",
"uz",
"fo",
"ht",
"ps",
"tk",
"nn",
"mt",
"sa",
"lb",
"my",
"bo",
"tl",
"mg",
"as",
"tt",
"haw",
"ln",
"ha",
"ba",
"jw",
"su",
"arxiv:2212.04356",
"license:apache-2.0",
"model-index",
"endpoints_compatible",
"region:us"
] | automatic-speech-recognition | "2022-09-26T06:50:46Z" | ---
language:
- en
- zh
- de
- es
- ru
- ko
- fr
- ja
- pt
- tr
- pl
- ca
- nl
- ar
- sv
- it
- id
- hi
- fi
- vi
- he
- uk
- el
- ms
- cs
- ro
- da
- hu
- ta
- no
- th
- ur
- hr
- bg
- lt
- la
- mi
- ml
- cy
- sk
- te
- fa
- lv
- bn
- sr
- az
- sl
- kn
- et
- mk
- br
- eu
- is
- hy
- ne
- mn
- bs
- kk
- sq
- sw
- gl
- mr
- pa
- si
- km
- sn
- yo
- so
- af
- oc
- ka
- be
- tg
- sd
- gu
- am
- yi
- lo
- uz
- fo
- ht
- ps
- tk
- nn
- mt
- sa
- lb
- my
- bo
- tl
- mg
- as
- tt
- haw
- ln
- ha
- ba
- jw
- su
tags:
- audio
- automatic-speech-recognition
- hf-asr-leaderboard
widget:
- example_title: Librispeech sample 1
src: https://cdn-media.huggingface.co/speech_samples/sample1.flac
- example_title: Librispeech sample 2
src: https://cdn-media.huggingface.co/speech_samples/sample2.flac
model-index:
- name: whisper-base
results:
- task:
name: Automatic Speech Recognition
type: automatic-speech-recognition
dataset:
name: LibriSpeech (clean)
type: librispeech_asr
config: clean
split: test
args:
language: en
metrics:
- name: Test WER
type: wer
value: 5.008769117619326
- task:
name: Automatic Speech Recognition
type: automatic-speech-recognition
dataset:
name: LibriSpeech (other)
type: librispeech_asr
config: other
split: test
args:
language: en
metrics:
- name: Test WER
type: wer
value: 12.84936273212057
- task:
name: Automatic Speech Recognition
type: automatic-speech-recognition
dataset:
name: Common Voice 11.0
type: mozilla-foundation/common_voice_11_0
config: hi
split: test
args:
language: hi
metrics:
- name: Test WER
type: wer
value: 131
pipeline_tag: automatic-speech-recognition
license: apache-2.0
---
# Whisper
Whisper is a pre-trained model for automatic speech recognition (ASR) and speech translation. Trained on 680k hours
of labelled data, Whisper models demonstrate a strong ability to generalise to many datasets and domains **without** the need
for fine-tuning.
Whisper was proposed in the paper [Robust Speech Recognition via Large-Scale Weak Supervision](https://arxiv.org/abs/2212.04356)
by Alec Radford et al from OpenAI. The original code repository can be found [here](https://github.com/openai/whisper).
**Disclaimer**: Content for this model card has partly been written by the Hugging Face team, and parts of it were
copied and pasted from the original model card.
## Model details
Whisper is a Transformer based encoder-decoder model, also referred to as a _sequence-to-sequence_ model.
It was trained on 680k hours of labelled speech data annotated using large-scale weak supervision.
The models were trained on either English-only data or multilingual data. The English-only models were trained
on the task of speech recognition. The multilingual models were trained on both speech recognition and speech
translation. For speech recognition, the model predicts transcriptions in the *same* language as the audio.
For speech translation, the model predicts transcriptions to a *different* language to the audio.
Whisper checkpoints come in five configurations of varying model sizes.
The smallest four are trained on either English-only or multilingual data.
The largest checkpoints are multilingual only. All ten of the pre-trained checkpoints
are available on the [Hugging Face Hub](https://huggingface.co/models?search=openai/whisper). The
checkpoints are summarised in the following table with links to the models on the Hub:
| Size | Parameters | English-only | Multilingual |
|----------|------------|------------------------------------------------------|-----------------------------------------------------|
| tiny | 39 M | [✓](https://huggingface.co/openai/whisper-tiny.en) | [✓](https://huggingface.co/openai/whisper-tiny) |
| base | 74 M | [✓](https://huggingface.co/openai/whisper-base.en) | [✓](https://huggingface.co/openai/whisper-base) |
| small | 244 M | [✓](https://huggingface.co/openai/whisper-small.en) | [✓](https://huggingface.co/openai/whisper-small) |
| medium | 769 M | [✓](https://huggingface.co/openai/whisper-medium.en) | [✓](https://huggingface.co/openai/whisper-medium) |
| large | 1550 M | x | [✓](https://huggingface.co/openai/whisper-large) |
| large-v2 | 1550 M | x | [✓](https://huggingface.co/openai/whisper-large-v2) |
# Usage
To transcribe audio samples, the model has to be used alongside a [`WhisperProcessor`](https://huggingface.co/docs/transformers/model_doc/whisper#transformers.WhisperProcessor).
The `WhisperProcessor` is used to:
1. Pre-process the audio inputs (converting them to log-Mel spectrograms for the model)
2. Post-process the model outputs (converting them from tokens to text)
The model is informed of which task to perform (transcription or translation) by passing the appropriate "context tokens". These context tokens
are a sequence of tokens that are given to the decoder at the start of the decoding process, and take the following order:
1. The transcription always starts with the `<|startoftranscript|>` token
2. The second token is the language token (e.g. `<|en|>` for English)
3. The third token is the "task token". It can take one of two values: `<|transcribe|>` for speech recognition or `<|translate|>` for speech translation
4. In addition, a `<|notimestamps|>` token is added if the model should not include timestamp prediction
Thus, a typical sequence of context tokens might look as follows:
```
<|startoftranscript|> <|en|> <|transcribe|> <|notimestamps|>
```
Which tells the model to decode in English, under the task of speech recognition, and not to predict timestamps.
These tokens can either be forced or un-forced. If they are forced, the model is made to predict each token at
each position. This allows one to control the output language and task for the Whisper model. If they are un-forced,
the Whisper model will automatically predict the output langauge and task itself.
The context tokens can be set accordingly:
```python
model.config.forced_decoder_ids = WhisperProcessor.get_decoder_prompt_ids(language="english", task="transcribe")
```
Which forces the model to predict in English under the task of speech recognition.
## Transcription
### English to English
In this example, the context tokens are 'unforced', meaning the model automatically predicts the output language
(English) and task (transcribe).
```python
>>> from transformers import WhisperProcessor, WhisperForConditionalGeneration
>>> from datasets import load_dataset
>>> # load model and processor
>>> processor = WhisperProcessor.from_pretrained("openai/whisper-base")
>>> model = WhisperForConditionalGeneration.from_pretrained("openai/whisper-base")
>>> model.config.forced_decoder_ids = None
>>> # load dummy dataset and read audio files
>>> ds = load_dataset("hf-internal-testing/librispeech_asr_dummy", "clean", split="validation")
>>> sample = ds[0]["audio"]
>>> input_features = processor(sample["array"], sampling_rate=sample["sampling_rate"], return_tensors="pt").input_features
>>> # generate token ids
>>> predicted_ids = model.generate(input_features)
>>> # decode token ids to text
>>> transcription = processor.batch_decode(predicted_ids, skip_special_tokens=False)
['<|startoftranscript|><|en|><|transcribe|><|notimestamps|> Mr. Quilter is the apostle of the middle classes and we are glad to welcome his gospel.<|endoftext|>']
>>> transcription = processor.batch_decode(predicted_ids, skip_special_tokens=True)
[' Mr. Quilter is the apostle of the middle classes and we are glad to welcome his gospel.']
```
The context tokens can be removed from the start of the transcription by setting `skip_special_tokens=True`.
### French to French
The following example demonstrates French to French transcription by setting the decoder ids appropriately.
```python
>>> from transformers import WhisperProcessor, WhisperForConditionalGeneration
>>> from datasets import Audio, load_dataset
>>> # load model and processor
>>> processor = WhisperProcessor.from_pretrained("openai/whisper-base")
>>> model = WhisperForConditionalGeneration.from_pretrained("openai/whisper-base")
>>> forced_decoder_ids = processor.get_decoder_prompt_ids(language="french", task="transcribe")
>>> # load streaming dataset and read first audio sample
>>> ds = load_dataset("common_voice", "fr", split="test", streaming=True)
>>> ds = ds.cast_column("audio", Audio(sampling_rate=16_000))
>>> input_speech = next(iter(ds))["audio"]
>>> input_features = processor(input_speech["array"], sampling_rate=input_speech["sampling_rate"], return_tensors="pt").input_features
>>> # generate token ids
>>> predicted_ids = model.generate(input_features, forced_decoder_ids=forced_decoder_ids)
>>> # decode token ids to text
>>> transcription = processor.batch_decode(predicted_ids)
['<|startoftranscript|><|fr|><|transcribe|><|notimestamps|> Un vrai travail intéressant va enfin être mené sur ce sujet.<|endoftext|>']
>>> transcription = processor.batch_decode(predicted_ids, skip_special_tokens=True)
[' Un vrai travail intéressant va enfin être mené sur ce sujet.']
```
## Translation
Setting the task to "translate" forces the Whisper model to perform speech translation.
### French to English
```python
>>> from transformers import WhisperProcessor, WhisperForConditionalGeneration
>>> from datasets import Audio, load_dataset
>>> # load model and processor
>>> processor = WhisperProcessor.from_pretrained("openai/whisper-base")
>>> model = WhisperForConditionalGeneration.from_pretrained("openai/whisper-base")
>>> forced_decoder_ids = processor.get_decoder_prompt_ids(language="french", task="translate")
>>> # load streaming dataset and read first audio sample
>>> ds = load_dataset("common_voice", "fr", split="test", streaming=True)
>>> ds = ds.cast_column("audio", Audio(sampling_rate=16_000))
>>> input_speech = next(iter(ds))["audio"]
>>> input_features = processor(input_speech["array"], sampling_rate=input_speech["sampling_rate"], return_tensors="pt").input_features
>>> # generate token ids
>>> predicted_ids = model.generate(input_features, forced_decoder_ids=forced_decoder_ids)
>>> # decode token ids to text
>>> transcription = processor.batch_decode(predicted_ids, skip_special_tokens=True)
[' A very interesting work, we will finally be given on this subject.']
```
## Evaluation
This code snippet shows how to evaluate Whisper Base on [LibriSpeech test-clean](https://huggingface.co/datasets/librispeech_asr):
```python
>>> from datasets import load_dataset
>>> from transformers import WhisperForConditionalGeneration, WhisperProcessor
>>> import torch
>>> from evaluate import load
>>> librispeech_test_clean = load_dataset("librispeech_asr", "clean", split="test")
>>> processor = WhisperProcessor.from_pretrained("openai/whisper-base")
>>> model = WhisperForConditionalGeneration.from_pretrained("openai/whisper-base").to("cuda")
>>> def map_to_pred(batch):
>>> audio = batch["audio"]
>>> input_features = processor(audio["array"], sampling_rate=audio["sampling_rate"], return_tensors="pt").input_features
>>> batch["reference"] = processor.tokenizer._normalize(batch['text'])
>>>
>>> with torch.no_grad():
>>> predicted_ids = model.generate(input_features.to("cuda"))[0]
>>> transcription = processor.decode(predicted_ids)
>>> batch["prediction"] = processor.tokenizer._normalize(transcription)
>>> return batch
>>> result = librispeech_test_clean.map(map_to_pred)
>>> wer = load("wer")
>>> print(100 * wer.compute(references=result["reference"], predictions=result["prediction"]))
5.082316555716899
```
## Long-Form Transcription
The Whisper model is intrinsically designed to work on audio samples of up to 30s in duration. However, by using a chunking
algorithm, it can be used to transcribe audio samples of up to arbitrary length. This is possible through Transformers
[`pipeline`](https://huggingface.co/docs/transformers/main_classes/pipelines#transformers.AutomaticSpeechRecognitionPipeline)
method. Chunking is enabled by setting `chunk_length_s=30` when instantiating the pipeline. With chunking enabled, the pipeline
can be run with batched inference. It can also be extended to predict sequence level timestamps by passing `return_timestamps=True`:
```python
>>> import torch
>>> from transformers import pipeline
>>> from datasets import load_dataset
>>> device = "cuda:0" if torch.cuda.is_available() else "cpu"
>>> pipe = pipeline(
>>> "automatic-speech-recognition",
>>> model="openai/whisper-base",
>>> chunk_length_s=30,
>>> device=device,
>>> )
>>> ds = load_dataset("hf-internal-testing/librispeech_asr_dummy", "clean", split="validation")
>>> sample = ds[0]["audio"]
>>> prediction = pipe(sample.copy(), batch_size=8)["text"]
" Mr. Quilter is the apostle of the middle classes, and we are glad to welcome his gospel."
>>> # we can also return timestamps for the predictions
>>> prediction = pipe(sample.copy(), batch_size=8, return_timestamps=True)["chunks"]
[{'text': ' Mr. Quilter is the apostle of the middle classes and we are glad to welcome his gospel.',
'timestamp': (0.0, 5.44)}]
```
Refer to the blog post [ASR Chunking](https://huggingface.co/blog/asr-chunking) for more details on the chunking algorithm.
## Fine-Tuning
The pre-trained Whisper model demonstrates a strong ability to generalise to different datasets and domains. However,
its predictive capabilities can be improved further for certain languages and tasks through *fine-tuning*. The blog
post [Fine-Tune Whisper with 🤗 Transformers](https://huggingface.co/blog/fine-tune-whisper) provides a step-by-step
guide to fine-tuning the Whisper model with as little as 5 hours of labelled data.
### Evaluated Use
The primary intended users of these models are AI researchers studying robustness, generalization, capabilities, biases, and constraints of the current model. However, Whisper is also potentially quite useful as an ASR solution for developers, especially for English speech recognition. We recognize that once models are released, it is impossible to restrict access to only “intended” uses or to draw reasonable guidelines around what is or is not research.
The models are primarily trained and evaluated on ASR and speech translation to English tasks. They show strong ASR results in ~10 languages. They may exhibit additional capabilities, particularly if fine-tuned on certain tasks like voice activity detection, speaker classification, or speaker diarization but have not been robustly evaluated in these areas. We strongly recommend that users perform robust evaluations of the models in a particular context and domain before deploying them.
In particular, we caution against using Whisper models to transcribe recordings of individuals taken without their consent or purporting to use these models for any kind of subjective classification. We recommend against use in high-risk domains like decision-making contexts, where flaws in accuracy can lead to pronounced flaws in outcomes. The models are intended to transcribe and translate speech, use of the model for classification is not only not evaluated but also not appropriate, particularly to infer human attributes.
## Training Data
The models are trained on 680,000 hours of audio and the corresponding transcripts collected from the internet. 65% of this data (or 438,000 hours) represents English-language audio and matched English transcripts, roughly 18% (or 126,000 hours) represents non-English audio and English transcripts, while the final 17% (or 117,000 hours) represents non-English audio and the corresponding transcript. This non-English data represents 98 different languages.
As discussed in [the accompanying paper](https://cdn.openai.com/papers/whisper.pdf), we see that performance on transcription in a given language is directly correlated with the amount of training data we employ in that language.
## Performance and Limitations
Our studies show that, over many existing ASR systems, the models exhibit improved robustness to accents, background noise, technical language, as well as zero shot translation from multiple languages into English; and that accuracy on speech recognition and translation is near the state-of-the-art level.
However, because the models are trained in a weakly supervised manner using large-scale noisy data, the predictions may include texts that are not actually spoken in the audio input (i.e. hallucination). We hypothesize that this happens because, given their general knowledge of language, the models combine trying to predict the next word in audio with trying to transcribe the audio itself.
Our models perform unevenly across languages, and we observe lower accuracy on low-resource and/or low-discoverability languages or languages where we have less training data. The models also exhibit disparate performance on different accents and dialects of particular languages, which may include higher word error rate across speakers of different genders, races, ages, or other demographic criteria. Our full evaluation results are presented in [the paper accompanying this release](https://cdn.openai.com/papers/whisper.pdf).
In addition, the sequence-to-sequence architecture of the model makes it prone to generating repetitive texts, which can be mitigated to some degree by beam search and temperature scheduling but not perfectly. Further analysis on these limitations are provided in [the paper](https://cdn.openai.com/papers/whisper.pdf). It is likely that this behavior and hallucinations may be worse on lower-resource and/or lower-discoverability languages.
## Broader Implications
We anticipate that Whisper models’ transcription capabilities may be used for improving accessibility tools. While Whisper models cannot be used for real-time transcription out of the box – their speed and size suggest that others may be able to build applications on top of them that allow for near-real-time speech recognition and translation. The real value of beneficial applications built on top of Whisper models suggests that the disparate performance of these models may have real economic implications.
There are also potential dual use concerns that come with releasing Whisper. While we hope the technology will be used primarily for beneficial purposes, making ASR technology more accessible could enable more actors to build capable surveillance technologies or scale up existing surveillance efforts, as the speed and accuracy allow for affordable automatic transcription and translation of large volumes of audio communication. Moreover, these models may have some capabilities to recognize specific individuals out of the box, which in turn presents safety concerns related both to dual use and disparate performance. In practice, we expect that the cost of transcription is not the limiting factor of scaling up surveillance projects.
### BibTeX entry and citation info
```bibtex
@misc{radford2022whisper,
doi = {10.48550/ARXIV.2212.04356},
url = {https://arxiv.org/abs/2212.04356},
author = {Radford, Alec and Kim, Jong Wook and Xu, Tao and Brockman, Greg and McLeavey, Christine and Sutskever, Ilya},
title = {Robust Speech Recognition via Large-Scale Weak Supervision},
publisher = {arXiv},
year = {2022},
copyright = {arXiv.org perpetual, non-exclusive license}
}
```
|
distilbert/distilbert-base-multilingual-cased | distilbert | "2024-05-06T13:46:54Z" | 453,119 | 142 | transformers | [
"transformers",
"pytorch",
"tf",
"onnx",
"safetensors",
"distilbert",
"fill-mask",
"multilingual",
"af",
"sq",
"ar",
"an",
"hy",
"ast",
"az",
"ba",
"eu",
"bar",
"be",
"bn",
"inc",
"bs",
"br",
"bg",
"my",
"ca",
"ceb",
"ce",
"zh",
"cv",
"hr",
"cs",
"da",
"nl",
"en",
"et",
"fi",
"fr",
"gl",
"ka",
"de",
"el",
"gu",
"ht",
"he",
"hi",
"hu",
"is",
"io",
"id",
"ga",
"it",
"ja",
"jv",
"kn",
"kk",
"ky",
"ko",
"la",
"lv",
"lt",
"roa",
"nds",
"lm",
"mk",
"mg",
"ms",
"ml",
"mr",
"mn",
"min",
"ne",
"new",
"nb",
"nn",
"oc",
"fa",
"pms",
"pl",
"pt",
"pa",
"ro",
"ru",
"sco",
"sr",
"scn",
"sk",
"sl",
"aze",
"es",
"su",
"sw",
"sv",
"tl",
"tg",
"th",
"ta",
"tt",
"te",
"tr",
"uk",
"ud",
"uz",
"vi",
"vo",
"war",
"cy",
"fry",
"pnb",
"yo",
"dataset:wikipedia",
"arxiv:1910.01108",
"arxiv:1910.09700",
"license:apache-2.0",
"autotrain_compatible",
"endpoints_compatible",
"region:us"
] | fill-mask | "2022-03-02T23:29:04Z" | ---
language:
- multilingual
- af
- sq
- ar
- an
- hy
- ast
- az
- ba
- eu
- bar
- be
- bn
- inc
- bs
- br
- bg
- my
- ca
- ceb
- ce
- zh
- cv
- hr
- cs
- da
- nl
- en
- et
- fi
- fr
- gl
- ka
- de
- el
- gu
- ht
- he
- hi
- hu
- is
- io
- id
- ga
- it
- ja
- jv
- kn
- kk
- ky
- ko
- la
- lv
- lt
- roa
- nds
- lm
- mk
- mg
- ms
- ml
- mr
- mn
- min
- ne
- new
- nb
- nn
- oc
- fa
- pms
- pl
- pt
- pa
- ro
- ru
- sco
- sr
- hr
- scn
- sk
- sl
- aze
- es
- su
- sw
- sv
- tl
- tg
- th
- ta
- tt
- te
- tr
- uk
- ud
- uz
- vi
- vo
- war
- cy
- fry
- pnb
- yo
license: apache-2.0
datasets:
- wikipedia
---
# Model Card for DistilBERT base multilingual (cased)
# Table of Contents
1. [Model Details](#model-details)
2. [Uses](#uses)
3. [Bias, Risks, and Limitations](#bias-risks-and-limitations)
4. [Training Details](#training-details)
5. [Evaluation](#evaluation)
6. [Environmental Impact](#environmental-impact)
7. [Citation](#citation)
8. [How To Get Started With the Model](#how-to-get-started-with-the-model)
# Model Details
## Model Description
This model is a distilled version of the [BERT base multilingual model](https://huggingface.co/bert-base-multilingual-cased/). The code for the distillation process can be found [here](https://github.com/huggingface/transformers/tree/main/examples/research_projects/distillation). This model is cased: it does make a difference between english and English.
The model is trained on the concatenation of Wikipedia in 104 different languages listed [here](https://github.com/google-research/bert/blob/master/multilingual.md#list-of-languages).
The model has 6 layers, 768 dimension and 12 heads, totalizing 134M parameters (compared to 177M parameters for mBERT-base).
On average, this model, referred to as DistilmBERT, is twice as fast as mBERT-base.
We encourage potential users of this model to check out the [BERT base multilingual model card](https://huggingface.co/bert-base-multilingual-cased) to learn more about usage, limitations and potential biases.
- **Developed by:** Victor Sanh, Lysandre Debut, Julien Chaumond, Thomas Wolf (Hugging Face)
- **Model type:** Transformer-based language model
- **Language(s) (NLP):** 104 languages; see full list [here](https://github.com/google-research/bert/blob/master/multilingual.md#list-of-languages)
- **License:** Apache 2.0
- **Related Models:** [BERT base multilingual model](https://huggingface.co/bert-base-multilingual-cased)
- **Resources for more information:**
- [GitHub Repository](https://github.com/huggingface/transformers/blob/main/examples/research_projects/distillation/README.md)
- [Associated Paper](https://arxiv.org/abs/1910.01108)
# Uses
## Direct Use and Downstream Use
You can use the raw model for either masked language modeling or next sentence prediction, but it's mostly intended to be fine-tuned on a downstream task. See the [model hub](https://huggingface.co/models?filter=bert) to look for fine-tuned versions on a task that interests you.
Note that this model is primarily aimed at being fine-tuned on tasks that use the whole sentence (potentially masked) to make decisions, such as sequence classification, token classification or question answering. For tasks such as text generation you should look at model like GPT2.
## Out of Scope Use
The model should not be used to intentionally create hostile or alienating environments for people. The model was not trained to be factual or true representations of people or events, and therefore using the models to generate such content is out-of-scope for the abilities of this model.
# Bias, Risks, and Limitations
Significant research has explored bias and fairness issues with language models (see, e.g., [Sheng et al. (2021)](https://aclanthology.org/2021.acl-long.330.pdf) and [Bender et al. (2021)](https://dl.acm.org/doi/pdf/10.1145/3442188.3445922)). Predictions generated by the model may include disturbing and harmful stereotypes across protected classes; identity characteristics; and sensitive, social, and occupational groups.
## Recommendations
Users (both direct and downstream) should be made aware of the risks, biases and limitations of the model.
# Training Details
- The model was pretrained with the supervision of [bert-base-multilingual-cased](https://huggingface.co/bert-base-multilingual-cased) on the concatenation of Wikipedia in 104 different languages
- The model has 6 layers, 768 dimension and 12 heads, totalizing 134M parameters.
- Further information about the training procedure and data is included in the [bert-base-multilingual-cased](https://huggingface.co/bert-base-multilingual-cased) model card.
# Evaluation
The model developers report the following accuracy results for DistilmBERT (see [GitHub Repo](https://github.com/huggingface/transformers/blob/main/examples/research_projects/distillation/README.md)):
> Here are the results on the test sets for 6 of the languages available in XNLI. The results are computed in the zero shot setting (trained on the English portion and evaluated on the target language portion):
| Model | English | Spanish | Chinese | German | Arabic | Urdu |
| :---: | :---: | :---: | :---: | :---: | :---: | :---:|
| mBERT base cased (computed) | 82.1 | 74.6 | 69.1 | 72.3 | 66.4 | 58.5 |
| mBERT base uncased (reported)| 81.4 | 74.3 | 63.8 | 70.5 | 62.1 | 58.3 |
| DistilmBERT | 78.2 | 69.1 | 64.0 | 66.3 | 59.1 | 54.7 |
# Environmental Impact
Carbon emissions can be estimated using the [Machine Learning Impact calculator](https://mlco2.github.io/impact#compute) presented in [Lacoste et al. (2019)](https://arxiv.org/abs/1910.09700).
- **Hardware Type:** More information needed
- **Hours used:** More information needed
- **Cloud Provider:** More information needed
- **Compute Region:** More information needed
- **Carbon Emitted:** More information needed
# Citation
```bibtex
@article{Sanh2019DistilBERTAD,
title={DistilBERT, a distilled version of BERT: smaller, faster, cheaper and lighter},
author={Victor Sanh and Lysandre Debut and Julien Chaumond and Thomas Wolf},
journal={ArXiv},
year={2019},
volume={abs/1910.01108}
}
```
APA
- Sanh, V., Debut, L., Chaumond, J., & Wolf, T. (2019). DistilBERT, a distilled version of BERT: smaller, faster, cheaper and lighter. arXiv preprint arXiv:1910.01108.
# How to Get Started With the Model
You can use the model directly with a pipeline for masked language modeling:
```python
>>> from transformers import pipeline
>>> unmasker = pipeline('fill-mask', model='distilbert-base-multilingual-cased')
>>> unmasker("Hello I'm a [MASK] model.")
[{'score': 0.040800247341394424,
'sequence': "Hello I'm a virtual model.",
'token': 37859,
'token_str': 'virtual'},
{'score': 0.020015988498926163,
'sequence': "Hello I'm a big model.",
'token': 22185,
'token_str': 'big'},
{'score': 0.018680453300476074,
'sequence': "Hello I'm a Hello model.",
'token': 31178,
'token_str': 'Hello'},
{'score': 0.017396586015820503,
'sequence': "Hello I'm a model model.",
'token': 13192,
'token_str': 'model'},
{'score': 0.014229810796678066,
'sequence': "Hello I'm a perfect model.",
'token': 43477,
'token_str': 'perfect'}]
```
|
TheBloke/Mistral-7B-Instruct-v0.2-GPTQ | TheBloke | "2023-12-11T22:46:53Z" | 452,354 | 50 | transformers | [
"transformers",
"safetensors",
"mistral",
"text-generation",
"finetuned",
"conversational",
"arxiv:2310.06825",
"base_model:mistralai/Mistral-7B-Instruct-v0.2",
"base_model:quantized:mistralai/Mistral-7B-Instruct-v0.2",
"license:apache-2.0",
"autotrain_compatible",
"text-generation-inference",
"4-bit",
"gptq",
"region:us"
] | text-generation | "2023-12-11T22:18:46Z" | ---
base_model: mistralai/Mistral-7B-Instruct-v0.2
inference: false
license: apache-2.0
model_creator: Mistral AI_
model_name: Mistral 7B Instruct v0.2
model_type: mistral
pipeline_tag: text-generation
prompt_template: '<s>[INST] {prompt} [/INST]
'
quantized_by: TheBloke
tags:
- finetuned
---
<!-- markdownlint-disable MD041 -->
<!-- header start -->
<!-- 200823 -->
<div style="width: auto; margin-left: auto; margin-right: auto">
<img src="https://i.imgur.com/EBdldam.jpg" alt="TheBlokeAI" style="width: 100%; min-width: 400px; display: block; margin: auto;">
</div>
<div style="display: flex; justify-content: space-between; width: 100%;">
<div style="display: flex; flex-direction: column; align-items: flex-start;">
<p style="margin-top: 0.5em; margin-bottom: 0em;"><a href="https://discord.gg/theblokeai">Chat & support: TheBloke's Discord server</a></p>
</div>
<div style="display: flex; flex-direction: column; align-items: flex-end;">
<p style="margin-top: 0.5em; margin-bottom: 0em;"><a href="https://www.patreon.com/TheBlokeAI">Want to contribute? TheBloke's Patreon page</a></p>
</div>
</div>
<div style="text-align:center; margin-top: 0em; margin-bottom: 0em"><p style="margin-top: 0.25em; margin-bottom: 0em;">TheBloke's LLM work is generously supported by a grant from <a href="https://a16z.com">andreessen horowitz (a16z)</a></p></div>
<hr style="margin-top: 1.0em; margin-bottom: 1.0em;">
<!-- header end -->
# Mistral 7B Instruct v0.2 - GPTQ
- Model creator: [Mistral AI_](https://huggingface.co/mistralai)
- Original model: [Mistral 7B Instruct v0.2](https://huggingface.co/mistralai/Mistral-7B-Instruct-v0.2)
<!-- description start -->
# Description
This repo contains GPTQ model files for [Mistral AI_'s Mistral 7B Instruct v0.2](https://huggingface.co/mistralai/Mistral-7B-Instruct-v0.2).
Multiple GPTQ parameter permutations are provided; see Provided Files below for details of the options provided, their parameters, and the software used to create them.
These files were quantised using hardware kindly provided by [Massed Compute](https://massedcompute.com/).
<!-- description end -->
<!-- repositories-available start -->
## Repositories available
* [AWQ model(s) for GPU inference.](https://huggingface.co/TheBloke/Mistral-7B-Instruct-v0.2-AWQ)
* [GPTQ models for GPU inference, with multiple quantisation parameter options.](https://huggingface.co/TheBloke/Mistral-7B-Instruct-v0.2-GPTQ)
* [2, 3, 4, 5, 6 and 8-bit GGUF models for CPU+GPU inference](https://huggingface.co/TheBloke/Mistral-7B-Instruct-v0.2-GGUF)
* [Mistral AI_'s original unquantised fp16 model in pytorch format, for GPU inference and for further conversions](https://huggingface.co/mistralai/Mistral-7B-Instruct-v0.2)
<!-- repositories-available end -->
<!-- prompt-template start -->
## Prompt template: Mistral
```
<s>[INST] {prompt} [/INST]
```
<!-- prompt-template end -->
<!-- README_GPTQ.md-compatible clients start -->
## Known compatible clients / servers
GPTQ models are currently supported on Linux (NVidia/AMD) and Windows (NVidia only). macOS users: please use GGUF models.
These GPTQ models are known to work in the following inference servers/webuis.
- [text-generation-webui](https://github.com/oobabooga/text-generation-webui)
- [KoboldAI United](https://github.com/henk717/koboldai)
- [LoLLMS Web UI](https://github.com/ParisNeo/lollms-webui)
- [Hugging Face Text Generation Inference (TGI)](https://github.com/huggingface/text-generation-inference)
This may not be a complete list; if you know of others, please let me know!
<!-- README_GPTQ.md-compatible clients end -->
<!-- README_GPTQ.md-provided-files start -->
## Provided files, and GPTQ parameters
Multiple quantisation parameters are provided, to allow you to choose the best one for your hardware and requirements.
Each separate quant is in a different branch. See below for instructions on fetching from different branches.
Most GPTQ files are made with AutoGPTQ. Mistral models are currently made with Transformers.
<details>
<summary>Explanation of GPTQ parameters</summary>
- Bits: The bit size of the quantised model.
- GS: GPTQ group size. Higher numbers use less VRAM, but have lower quantisation accuracy. "None" is the lowest possible value.
- Act Order: True or False. Also known as `desc_act`. True results in better quantisation accuracy. Some GPTQ clients have had issues with models that use Act Order plus Group Size, but this is generally resolved now.
- Damp %: A GPTQ parameter that affects how samples are processed for quantisation. 0.01 is default, but 0.1 results in slightly better accuracy.
- GPTQ dataset: The calibration dataset used during quantisation. Using a dataset more appropriate to the model's training can improve quantisation accuracy. Note that the GPTQ calibration dataset is not the same as the dataset used to train the model - please refer to the original model repo for details of the training dataset(s).
- Sequence Length: The length of the dataset sequences used for quantisation. Ideally this is the same as the model sequence length. For some very long sequence models (16+K), a lower sequence length may have to be used. Note that a lower sequence length does not limit the sequence length of the quantised model. It only impacts the quantisation accuracy on longer inference sequences.
- ExLlama Compatibility: Whether this file can be loaded with ExLlama, which currently only supports Llama and Mistral models in 4-bit.
</details>
| Branch | Bits | GS | Act Order | Damp % | GPTQ Dataset | Seq Len | Size | ExLlama | Desc |
| ------ | ---- | -- | --------- | ------ | ------------ | ------- | ---- | ------- | ---- |
| [main](https://huggingface.co/TheBloke/Mistral-7B-Instruct-v0.2-GPTQ/tree/main) | 4 | 128 | Yes | 0.1 | [VMware Open Instruct](https://huggingface.co/datasets/VMware/open-instruct/viewer/) | 4096 | 4.16 GB | Yes | 4-bit, with Act Order and group size 128g. Uses even less VRAM than 64g, but with slightly lower accuracy. |
| [gptq-4bit-32g-actorder_True](https://huggingface.co/TheBloke/Mistral-7B-Instruct-v0.2-GPTQ/tree/gptq-4bit-32g-actorder_True) | 4 | 32 | Yes | 0.1 | [VMware Open Instruct](https://huggingface.co/datasets/VMware/open-instruct/viewer/) | 4096 | 4.57 GB | Yes | 4-bit, with Act Order and group size 32g. Gives highest possible inference quality, with maximum VRAM usage. |
| [gptq-8bit--1g-actorder_True](https://huggingface.co/TheBloke/Mistral-7B-Instruct-v0.2-GPTQ/tree/gptq-8bit--1g-actorder_True) | 8 | None | Yes | 0.1 | [VMware Open Instruct](https://huggingface.co/datasets/VMware/open-instruct/viewer/) | 4096 | 7.52 GB | No | 8-bit, with Act Order. No group size, to lower VRAM requirements. |
| [gptq-8bit-128g-actorder_True](https://huggingface.co/TheBloke/Mistral-7B-Instruct-v0.2-GPTQ/tree/gptq-8bit-128g-actorder_True) | 8 | 128 | Yes | 0.1 | [VMware Open Instruct](https://huggingface.co/datasets/VMware/open-instruct/viewer/) | 4096 | 7.68 GB | No | 8-bit, with group size 128g for higher inference quality and with Act Order for even higher accuracy. |
| [gptq-8bit-32g-actorder_True](https://huggingface.co/TheBloke/Mistral-7B-Instruct-v0.2-GPTQ/tree/gptq-8bit-32g-actorder_True) | 8 | 32 | Yes | 0.1 | [VMware Open Instruct](https://huggingface.co/datasets/VMware/open-instruct/viewer/) | 4096 | 8.17 GB | No | 8-bit, with group size 32g and Act Order for maximum inference quality. |
| [gptq-4bit-64g-actorder_True](https://huggingface.co/TheBloke/Mistral-7B-Instruct-v0.2-GPTQ/tree/gptq-4bit-64g-actorder_True) | 4 | 64 | Yes | 0.1 | [VMware Open Instruct](https://huggingface.co/datasets/VMware/open-instruct/viewer/) | 4096 | 4.29 GB | Yes | 4-bit, with Act Order and group size 64g. Uses less VRAM than 32g, but with slightly lower accuracy. |
<!-- README_GPTQ.md-provided-files end -->
<!-- README_GPTQ.md-download-from-branches start -->
## How to download, including from branches
### In text-generation-webui
To download from the `main` branch, enter `TheBloke/Mistral-7B-Instruct-v0.2-GPTQ` in the "Download model" box.
To download from another branch, add `:branchname` to the end of the download name, eg `TheBloke/Mistral-7B-Instruct-v0.2-GPTQ:gptq-4bit-32g-actorder_True`
### From the command line
I recommend using the `huggingface-hub` Python library:
```shell
pip3 install huggingface-hub
```
To download the `main` branch to a folder called `Mistral-7B-Instruct-v0.2-GPTQ`:
```shell
mkdir Mistral-7B-Instruct-v0.2-GPTQ
huggingface-cli download TheBloke/Mistral-7B-Instruct-v0.2-GPTQ --local-dir Mistral-7B-Instruct-v0.2-GPTQ --local-dir-use-symlinks False
```
To download from a different branch, add the `--revision` parameter:
```shell
mkdir Mistral-7B-Instruct-v0.2-GPTQ
huggingface-cli download TheBloke/Mistral-7B-Instruct-v0.2-GPTQ --revision gptq-4bit-32g-actorder_True --local-dir Mistral-7B-Instruct-v0.2-GPTQ --local-dir-use-symlinks False
```
<details>
<summary>More advanced huggingface-cli download usage</summary>
If you remove the `--local-dir-use-symlinks False` parameter, the files will instead be stored in the central Hugging Face cache directory (default location on Linux is: `~/.cache/huggingface`), and symlinks will be added to the specified `--local-dir`, pointing to their real location in the cache. This allows for interrupted downloads to be resumed, and allows you to quickly clone the repo to multiple places on disk without triggering a download again. The downside, and the reason why I don't list that as the default option, is that the files are then hidden away in a cache folder and it's harder to know where your disk space is being used, and to clear it up if/when you want to remove a download model.
The cache location can be changed with the `HF_HOME` environment variable, and/or the `--cache-dir` parameter to `huggingface-cli`.
For more documentation on downloading with `huggingface-cli`, please see: [HF -> Hub Python Library -> Download files -> Download from the CLI](https://huggingface.co/docs/huggingface_hub/guides/download#download-from-the-cli).
To accelerate downloads on fast connections (1Gbit/s or higher), install `hf_transfer`:
```shell
pip3 install hf_transfer
```
And set environment variable `HF_HUB_ENABLE_HF_TRANSFER` to `1`:
```shell
mkdir Mistral-7B-Instruct-v0.2-GPTQ
HF_HUB_ENABLE_HF_TRANSFER=1 huggingface-cli download TheBloke/Mistral-7B-Instruct-v0.2-GPTQ --local-dir Mistral-7B-Instruct-v0.2-GPTQ --local-dir-use-symlinks False
```
Windows Command Line users: You can set the environment variable by running `set HF_HUB_ENABLE_HF_TRANSFER=1` before the download command.
</details>
### With `git` (**not** recommended)
To clone a specific branch with `git`, use a command like this:
```shell
git clone --single-branch --branch gptq-4bit-32g-actorder_True https://huggingface.co/TheBloke/Mistral-7B-Instruct-v0.2-GPTQ
```
Note that using Git with HF repos is strongly discouraged. It will be much slower than using `huggingface-hub`, and will use twice as much disk space as it has to store the model files twice (it stores every byte both in the intended target folder, and again in the `.git` folder as a blob.)
<!-- README_GPTQ.md-download-from-branches end -->
<!-- README_GPTQ.md-text-generation-webui start -->
## How to easily download and use this model in [text-generation-webui](https://github.com/oobabooga/text-generation-webui)
Please make sure you're using the latest version of [text-generation-webui](https://github.com/oobabooga/text-generation-webui).
It is strongly recommended to use the text-generation-webui one-click-installers unless you're sure you know how to make a manual install.
1. Click the **Model tab**.
2. Under **Download custom model or LoRA**, enter `TheBloke/Mistral-7B-Instruct-v0.2-GPTQ`.
- To download from a specific branch, enter for example `TheBloke/Mistral-7B-Instruct-v0.2-GPTQ:gptq-4bit-32g-actorder_True`
- see Provided Files above for the list of branches for each option.
3. Click **Download**.
4. The model will start downloading. Once it's finished it will say "Done".
5. In the top left, click the refresh icon next to **Model**.
6. In the **Model** dropdown, choose the model you just downloaded: `Mistral-7B-Instruct-v0.2-GPTQ`
7. The model will automatically load, and is now ready for use!
8. If you want any custom settings, set them and then click **Save settings for this model** followed by **Reload the Model** in the top right.
- Note that you do not need to and should not set manual GPTQ parameters any more. These are set automatically from the file `quantize_config.json`.
9. Once you're ready, click the **Text Generation** tab and enter a prompt to get started!
<!-- README_GPTQ.md-text-generation-webui end -->
<!-- README_GPTQ.md-use-from-tgi start -->
## Serving this model from Text Generation Inference (TGI)
It's recommended to use TGI version 1.1.0 or later. The official Docker container is: `ghcr.io/huggingface/text-generation-inference:1.1.0`
Example Docker parameters:
```shell
--model-id TheBloke/Mistral-7B-Instruct-v0.2-GPTQ --port 3000 --quantize gptq --max-input-length 3696 --max-total-tokens 4096 --max-batch-prefill-tokens 4096
```
Example Python code for interfacing with TGI (requires huggingface-hub 0.17.0 or later):
```shell
pip3 install huggingface-hub
```
```python
from huggingface_hub import InferenceClient
endpoint_url = "https://your-endpoint-url-here"
prompt = "Tell me about AI"
prompt_template=f'''<s>[INST] {prompt} [/INST]
'''
client = InferenceClient(endpoint_url)
response = client.text_generation(prompt,
max_new_tokens=128,
do_sample=True,
temperature=0.7,
top_p=0.95,
top_k=40,
repetition_penalty=1.1)
print(f"Model output: {response}")
```
<!-- README_GPTQ.md-use-from-tgi end -->
<!-- README_GPTQ.md-use-from-python start -->
## Python code example: inference from this GPTQ model
### Install the necessary packages
Requires: Transformers 4.33.0 or later, Optimum 1.12.0 or later, and AutoGPTQ 0.4.2 or later.
```shell
pip3 install --upgrade transformers optimum
# If using PyTorch 2.1 + CUDA 12.x:
pip3 install --upgrade auto-gptq
# or, if using PyTorch 2.1 + CUDA 11.x:
pip3 install --upgrade auto-gptq --extra-index-url https://huggingface.github.io/autogptq-index/whl/cu118/
```
If you are using PyTorch 2.0, you will need to install AutoGPTQ from source. Likewise if you have problems with the pre-built wheels, you should try building from source:
```shell
pip3 uninstall -y auto-gptq
git clone https://github.com/PanQiWei/AutoGPTQ
cd AutoGPTQ
git checkout v0.5.1
pip3 install .
```
### Example Python code
```python
from transformers import AutoModelForCausalLM, AutoTokenizer, pipeline
model_name_or_path = "TheBloke/Mistral-7B-Instruct-v0.2-GPTQ"
# To use a different branch, change revision
# For example: revision="gptq-4bit-32g-actorder_True"
model = AutoModelForCausalLM.from_pretrained(model_name_or_path,
device_map="auto",
trust_remote_code=False,
revision="main")
tokenizer = AutoTokenizer.from_pretrained(model_name_or_path, use_fast=True)
prompt = "Tell me about AI"
prompt_template=f'''<s>[INST] {prompt} [/INST]
'''
print("\n\n*** Generate:")
input_ids = tokenizer(prompt_template, return_tensors='pt').input_ids.cuda()
output = model.generate(inputs=input_ids, temperature=0.7, do_sample=True, top_p=0.95, top_k=40, max_new_tokens=512)
print(tokenizer.decode(output[0]))
# Inference can also be done using transformers' pipeline
print("*** Pipeline:")
pipe = pipeline(
"text-generation",
model=model,
tokenizer=tokenizer,
max_new_tokens=512,
do_sample=True,
temperature=0.7,
top_p=0.95,
top_k=40,
repetition_penalty=1.1
)
print(pipe(prompt_template)[0]['generated_text'])
```
<!-- README_GPTQ.md-use-from-python end -->
<!-- README_GPTQ.md-compatibility start -->
## Compatibility
The files provided are tested to work with Transformers. For non-Mistral models, AutoGPTQ can also be used directly.
[ExLlama](https://github.com/turboderp/exllama) is compatible with Llama and Mistral models in 4-bit. Please see the Provided Files table above for per-file compatibility.
For a list of clients/servers, please see "Known compatible clients / servers", above.
<!-- README_GPTQ.md-compatibility end -->
<!-- footer start -->
<!-- 200823 -->
## Discord
For further support, and discussions on these models and AI in general, join us at:
[TheBloke AI's Discord server](https://discord.gg/theblokeai)
## Thanks, and how to contribute
Thanks to the [chirper.ai](https://chirper.ai) team!
Thanks to Clay from [gpus.llm-utils.org](llm-utils)!
I've had a lot of people ask if they can contribute. I enjoy providing models and helping people, and would love to be able to spend even more time doing it, as well as expanding into new projects like fine tuning/training.
If you're able and willing to contribute it will be most gratefully received and will help me to keep providing more models, and to start work on new AI projects.
Donaters will get priority support on any and all AI/LLM/model questions and requests, access to a private Discord room, plus other benefits.
* Patreon: https://patreon.com/TheBlokeAI
* Ko-Fi: https://ko-fi.com/TheBlokeAI
**Special thanks to**: Aemon Algiz.
**Patreon special mentions**: Michael Levine, 阿明, Trailburnt, Nikolai Manek, John Detwiler, Randy H, Will Dee, Sebastain Graf, NimbleBox.ai, Eugene Pentland, Emad Mostaque, Ai Maven, Jim Angel, Jeff Scroggin, Michael Davis, Manuel Alberto Morcote, Stephen Murray, Robert, Justin Joy, Luke @flexchar, Brandon Frisco, Elijah Stavena, S_X, Dan Guido, Undi ., Komninos Chatzipapas, Shadi, theTransient, Lone Striker, Raven Klaugh, jjj, Cap'n Zoog, Michel-Marie MAUDET (LINAGORA), Matthew Berman, David, Fen Risland, Omer Bin Jawed, Luke Pendergrass, Kalila, OG, Erik Bjäreholt, Rooh Singh, Joseph William Delisle, Dan Lewis, TL, John Villwock, AzureBlack, Brad, Pedro Madruga, Caitlyn Gatomon, K, jinyuan sun, Mano Prime, Alex, Jeffrey Morgan, Alicia Loh, Illia Dulskyi, Chadd, transmissions 11, fincy, Rainer Wilmers, ReadyPlayerEmma, knownsqashed, Mandus, biorpg, Deo Leter, Brandon Phillips, SuperWojo, Sean Connelly, Iucharbius, Jack West, Harry Royden McLaughlin, Nicholas, terasurfer, Vitor Caleffi, Duane Dunston, Johann-Peter Hartmann, David Ziegler, Olakabola, Ken Nordquist, Trenton Dambrowitz, Tom X Nguyen, Vadim, Ajan Kanaga, Leonard Tan, Clay Pascal, Alexandros Triantafyllidis, JM33133, Xule, vamX, ya boyyy, subjectnull, Talal Aujan, Alps Aficionado, wassieverse, Ari Malik, James Bentley, Woland, Spencer Kim, Michael Dempsey, Fred von Graf, Elle, zynix, William Richards, Stanislav Ovsiannikov, Edmond Seymore, Jonathan Leane, Martin Kemka, usrbinkat, Enrico Ros
Thank you to all my generous patrons and donaters!
And thank you again to a16z for their generous grant.
<!-- footer end -->
# Original model card: Mistral AI_'s Mistral 7B Instruct v0.2
# Model Card for Mistral-7B-Instruct-v0.2
The Mistral-7B-Instruct-v0.2 Large Language Model (LLM) is an improved instruct fine-tuned version of [Mistral-7B-Instruct-v0.1](https://huggingface.co/mistralai/Mistral-7B-Instruct-v0.1).
For full details of this model please read our [paper](https://arxiv.org/abs/2310.06825) and [release blog post](https://mistral.ai/news/la-plateforme/).
## Instruction format
In order to leverage instruction fine-tuning, your prompt should be surrounded by `[INST]` and `[/INST]` tokens. The very first instruction should begin with a begin of sentence id. The next instructions should not. The assistant generation will be ended by the end-of-sentence token id.
E.g.
```
text = "<s>[INST] What is your favourite condiment? [/INST]"
"Well, I'm quite partial to a good squeeze of fresh lemon juice. It adds just the right amount of zesty flavour to whatever I'm cooking up in the kitchen!</s> "
"[INST] Do you have mayonnaise recipes? [/INST]"
```
This format is available as a [chat template](https://huggingface.co/docs/transformers/main/chat_templating) via the `apply_chat_template()` method:
```python
from transformers import AutoModelForCausalLM, AutoTokenizer
device = "cuda" # the device to load the model onto
model = AutoModelForCausalLM.from_pretrained("mistralai/Mistral-7B-Instruct-v0.1")
tokenizer = AutoTokenizer.from_pretrained("mistralai/Mistral-7B-Instruct-v0.1")
messages = [
{"role": "user", "content": "What is your favourite condiment?"},
{"role": "assistant", "content": "Well, I'm quite partial to a good squeeze of fresh lemon juice. It adds just the right amount of zesty flavour to whatever I'm cooking up in the kitchen!"},
{"role": "user", "content": "Do you have mayonnaise recipes?"}
]
encodeds = tokenizer.apply_chat_template(messages, return_tensors="pt")
model_inputs = encodeds.to(device)
model.to(device)
generated_ids = model.generate(model_inputs, max_new_tokens=1000, do_sample=True)
decoded = tokenizer.batch_decode(generated_ids)
print(decoded[0])
```
## Model Architecture
This instruction model is based on Mistral-7B-v0.1, a transformer model with the following architecture choices:
- Grouped-Query Attention
- Sliding-Window Attention
- Byte-fallback BPE tokenizer
## Troubleshooting
- If you see the following error:
```
Traceback (most recent call last):
File "", line 1, in
File "/transformers/models/auto/auto_factory.py", line 482, in from_pretrained
config, kwargs = AutoConfig.from_pretrained(
File "/transformers/models/auto/configuration_auto.py", line 1022, in from_pretrained
config_class = CONFIG_MAPPING[config_dict["model_type"]]
File "/transformers/models/auto/configuration_auto.py", line 723, in getitem
raise KeyError(key)
KeyError: 'mistral'
```
Installing transformers from source should solve the issue
pip install git+https://github.com/huggingface/transformers
This should not be required after transformers-v4.33.4.
## Limitations
The Mistral 7B Instruct model is a quick demonstration that the base model can be easily fine-tuned to achieve compelling performance.
It does not have any moderation mechanisms. We're looking forward to engaging with the community on ways to
make the model finely respect guardrails, allowing for deployment in environments requiring moderated outputs.
## The Mistral AI Team
Albert Jiang, Alexandre Sablayrolles, Arthur Mensch, Blanche Savary, Chris Bamford, Devendra Singh Chaplot, Diego de las Casas, Emma Bou Hanna, Florian Bressand, Gianna Lengyel, Guillaume Bour, Guillaume Lample, Lélio Renard Lavaud, Louis Ternon, Lucile Saulnier, Marie-Anne Lachaux, Pierre Stock, Teven Le Scao, Théophile Gervet, Thibaut Lavril, Thomas Wang, Timothée Lacroix, William El Sayed.
|
nomic-ai/nomic-embed-text-v1.5 | nomic-ai | "2024-08-26T20:51:30Z" | 447,984 | 418 | sentence-transformers | [
"sentence-transformers",
"onnx",
"safetensors",
"nomic_bert",
"feature-extraction",
"sentence-similarity",
"mteb",
"transformers",
"transformers.js",
"custom_code",
"en",
"arxiv:2205.13147",
"arxiv:2402.01613",
"license:apache-2.0",
"model-index",
"autotrain_compatible",
"text-embeddings-inference",
"endpoints_compatible",
"region:us"
] | sentence-similarity | "2024-02-10T06:32:35Z" | ---
library_name: sentence-transformers
pipeline_tag: sentence-similarity
tags:
- feature-extraction
- sentence-similarity
- mteb
- transformers
- transformers.js
model-index:
- name: epoch_0_model
results:
- task:
type: Classification
dataset:
type: mteb/amazon_counterfactual
name: MTEB AmazonCounterfactualClassification (en)
config: en
split: test
revision: e8379541af4e31359cca9fbcf4b00f2671dba205
metrics:
- type: accuracy
value: 75.20895522388058
- type: ap
value: 38.57605549557802
- type: f1
value: 69.35586565857854
- task:
type: Classification
dataset:
type: mteb/amazon_polarity
name: MTEB AmazonPolarityClassification
config: default
split: test
revision: e2d317d38cd51312af73b3d32a06d1a08b442046
metrics:
- type: accuracy
value: 91.8144
- type: ap
value: 88.65222882032363
- type: f1
value: 91.80426301643274
- task:
type: Classification
dataset:
type: mteb/amazon_reviews_multi
name: MTEB AmazonReviewsClassification (en)
config: en
split: test
revision: 1399c76144fd37290681b995c656ef9b2e06e26d
metrics:
- type: accuracy
value: 47.162000000000006
- type: f1
value: 46.59329642263158
- task:
type: Retrieval
dataset:
type: arguana
name: MTEB ArguAna
config: default
split: test
revision: None
metrics:
- type: map_at_1
value: 24.253
- type: map_at_10
value: 38.962
- type: map_at_100
value: 40.081
- type: map_at_1000
value: 40.089000000000006
- type: map_at_3
value: 33.499
- type: map_at_5
value: 36.351
- type: mrr_at_1
value: 24.609
- type: mrr_at_10
value: 39.099000000000004
- type: mrr_at_100
value: 40.211000000000006
- type: mrr_at_1000
value: 40.219
- type: mrr_at_3
value: 33.677
- type: mrr_at_5
value: 36.469
- type: ndcg_at_1
value: 24.253
- type: ndcg_at_10
value: 48.010999999999996
- type: ndcg_at_100
value: 52.756
- type: ndcg_at_1000
value: 52.964999999999996
- type: ndcg_at_3
value: 36.564
- type: ndcg_at_5
value: 41.711999999999996
- type: precision_at_1
value: 24.253
- type: precision_at_10
value: 7.738
- type: precision_at_100
value: 0.98
- type: precision_at_1000
value: 0.1
- type: precision_at_3
value: 15.149000000000001
- type: precision_at_5
value: 11.593
- type: recall_at_1
value: 24.253
- type: recall_at_10
value: 77.383
- type: recall_at_100
value: 98.009
- type: recall_at_1000
value: 99.644
- type: recall_at_3
value: 45.448
- type: recall_at_5
value: 57.965999999999994
- task:
type: Clustering
dataset:
type: mteb/arxiv-clustering-p2p
name: MTEB ArxivClusteringP2P
config: default
split: test
revision: a122ad7f3f0291bf49cc6f4d32aa80929df69d5d
metrics:
- type: v_measure
value: 45.69069567851087
- task:
type: Clustering
dataset:
type: mteb/arxiv-clustering-s2s
name: MTEB ArxivClusteringS2S
config: default
split: test
revision: f910caf1a6075f7329cdf8c1a6135696f37dbd53
metrics:
- type: v_measure
value: 36.35185490976283
- task:
type: Reranking
dataset:
type: mteb/askubuntudupquestions-reranking
name: MTEB AskUbuntuDupQuestions
config: default
split: test
revision: 2000358ca161889fa9c082cb41daa8dcfb161a54
metrics:
- type: map
value: 61.71274951450321
- type: mrr
value: 76.06032625423207
- task:
type: STS
dataset:
type: mteb/biosses-sts
name: MTEB BIOSSES
config: default
split: test
revision: d3fb88f8f02e40887cd149695127462bbcf29b4a
metrics:
- type: cos_sim_pearson
value: 86.73980520022269
- type: cos_sim_spearman
value: 84.24649792685918
- type: euclidean_pearson
value: 85.85197641158186
- type: euclidean_spearman
value: 84.24649792685918
- type: manhattan_pearson
value: 86.26809552711346
- type: manhattan_spearman
value: 84.56397504030865
- task:
type: Classification
dataset:
type: mteb/banking77
name: MTEB Banking77Classification
config: default
split: test
revision: 0fd18e25b25c072e09e0d92ab615fda904d66300
metrics:
- type: accuracy
value: 84.25324675324674
- type: f1
value: 84.17872280892557
- task:
type: Clustering
dataset:
type: mteb/biorxiv-clustering-p2p
name: MTEB BiorxivClusteringP2P
config: default
split: test
revision: 65b79d1d13f80053f67aca9498d9402c2d9f1f40
metrics:
- type: v_measure
value: 38.770253446400886
- task:
type: Clustering
dataset:
type: mteb/biorxiv-clustering-s2s
name: MTEB BiorxivClusteringS2S
config: default
split: test
revision: 258694dd0231531bc1fd9de6ceb52a0853c6d908
metrics:
- type: v_measure
value: 32.94307095497281
- task:
type: Retrieval
dataset:
type: BeIR/cqadupstack
name: MTEB CQADupstackAndroidRetrieval
config: default
split: test
revision: None
metrics:
- type: map_at_1
value: 32.164
- type: map_at_10
value: 42.641
- type: map_at_100
value: 43.947
- type: map_at_1000
value: 44.074999999999996
- type: map_at_3
value: 39.592
- type: map_at_5
value: 41.204
- type: mrr_at_1
value: 39.628
- type: mrr_at_10
value: 48.625
- type: mrr_at_100
value: 49.368
- type: mrr_at_1000
value: 49.413000000000004
- type: mrr_at_3
value: 46.400000000000006
- type: mrr_at_5
value: 47.68
- type: ndcg_at_1
value: 39.628
- type: ndcg_at_10
value: 48.564
- type: ndcg_at_100
value: 53.507000000000005
- type: ndcg_at_1000
value: 55.635999999999996
- type: ndcg_at_3
value: 44.471
- type: ndcg_at_5
value: 46.137
- type: precision_at_1
value: 39.628
- type: precision_at_10
value: 8.856
- type: precision_at_100
value: 1.429
- type: precision_at_1000
value: 0.191
- type: precision_at_3
value: 21.268
- type: precision_at_5
value: 14.649000000000001
- type: recall_at_1
value: 32.164
- type: recall_at_10
value: 59.609
- type: recall_at_100
value: 80.521
- type: recall_at_1000
value: 94.245
- type: recall_at_3
value: 46.521
- type: recall_at_5
value: 52.083999999999996
- task:
type: Retrieval
dataset:
type: BeIR/cqadupstack
name: MTEB CQADupstackEnglishRetrieval
config: default
split: test
revision: None
metrics:
- type: map_at_1
value: 31.526
- type: map_at_10
value: 41.581
- type: map_at_100
value: 42.815999999999995
- type: map_at_1000
value: 42.936
- type: map_at_3
value: 38.605000000000004
- type: map_at_5
value: 40.351
- type: mrr_at_1
value: 39.489999999999995
- type: mrr_at_10
value: 47.829
- type: mrr_at_100
value: 48.512
- type: mrr_at_1000
value: 48.552
- type: mrr_at_3
value: 45.754
- type: mrr_at_5
value: 46.986
- type: ndcg_at_1
value: 39.489999999999995
- type: ndcg_at_10
value: 47.269
- type: ndcg_at_100
value: 51.564
- type: ndcg_at_1000
value: 53.53099999999999
- type: ndcg_at_3
value: 43.301
- type: ndcg_at_5
value: 45.239000000000004
- type: precision_at_1
value: 39.489999999999995
- type: precision_at_10
value: 8.93
- type: precision_at_100
value: 1.415
- type: precision_at_1000
value: 0.188
- type: precision_at_3
value: 20.892
- type: precision_at_5
value: 14.865999999999998
- type: recall_at_1
value: 31.526
- type: recall_at_10
value: 56.76
- type: recall_at_100
value: 75.029
- type: recall_at_1000
value: 87.491
- type: recall_at_3
value: 44.786
- type: recall_at_5
value: 50.254
- task:
type: Retrieval
dataset:
type: BeIR/cqadupstack
name: MTEB CQADupstackGamingRetrieval
config: default
split: test
revision: None
metrics:
- type: map_at_1
value: 40.987
- type: map_at_10
value: 52.827
- type: map_at_100
value: 53.751000000000005
- type: map_at_1000
value: 53.81
- type: map_at_3
value: 49.844
- type: map_at_5
value: 51.473
- type: mrr_at_1
value: 46.833999999999996
- type: mrr_at_10
value: 56.389
- type: mrr_at_100
value: 57.003
- type: mrr_at_1000
value: 57.034
- type: mrr_at_3
value: 54.17999999999999
- type: mrr_at_5
value: 55.486999999999995
- type: ndcg_at_1
value: 46.833999999999996
- type: ndcg_at_10
value: 58.372
- type: ndcg_at_100
value: 62.068
- type: ndcg_at_1000
value: 63.288
- type: ndcg_at_3
value: 53.400000000000006
- type: ndcg_at_5
value: 55.766000000000005
- type: precision_at_1
value: 46.833999999999996
- type: precision_at_10
value: 9.191
- type: precision_at_100
value: 1.192
- type: precision_at_1000
value: 0.134
- type: precision_at_3
value: 23.448
- type: precision_at_5
value: 15.862000000000002
- type: recall_at_1
value: 40.987
- type: recall_at_10
value: 71.146
- type: recall_at_100
value: 87.035
- type: recall_at_1000
value: 95.633
- type: recall_at_3
value: 58.025999999999996
- type: recall_at_5
value: 63.815999999999995
- task:
type: Retrieval
dataset:
type: BeIR/cqadupstack
name: MTEB CQADupstackGisRetrieval
config: default
split: test
revision: None
metrics:
- type: map_at_1
value: 24.587
- type: map_at_10
value: 33.114
- type: map_at_100
value: 34.043
- type: map_at_1000
value: 34.123999999999995
- type: map_at_3
value: 30.45
- type: map_at_5
value: 31.813999999999997
- type: mrr_at_1
value: 26.554
- type: mrr_at_10
value: 35.148
- type: mrr_at_100
value: 35.926
- type: mrr_at_1000
value: 35.991
- type: mrr_at_3
value: 32.599000000000004
- type: mrr_at_5
value: 33.893
- type: ndcg_at_1
value: 26.554
- type: ndcg_at_10
value: 38.132
- type: ndcg_at_100
value: 42.78
- type: ndcg_at_1000
value: 44.919
- type: ndcg_at_3
value: 32.833
- type: ndcg_at_5
value: 35.168
- type: precision_at_1
value: 26.554
- type: precision_at_10
value: 5.921
- type: precision_at_100
value: 0.8659999999999999
- type: precision_at_1000
value: 0.109
- type: precision_at_3
value: 13.861
- type: precision_at_5
value: 9.605
- type: recall_at_1
value: 24.587
- type: recall_at_10
value: 51.690000000000005
- type: recall_at_100
value: 73.428
- type: recall_at_1000
value: 89.551
- type: recall_at_3
value: 37.336999999999996
- type: recall_at_5
value: 43.047000000000004
- task:
type: Retrieval
dataset:
type: BeIR/cqadupstack
name: MTEB CQADupstackMathematicaRetrieval
config: default
split: test
revision: None
metrics:
- type: map_at_1
value: 16.715
- type: map_at_10
value: 24.251
- type: map_at_100
value: 25.326999999999998
- type: map_at_1000
value: 25.455
- type: map_at_3
value: 21.912000000000003
- type: map_at_5
value: 23.257
- type: mrr_at_1
value: 20.274
- type: mrr_at_10
value: 28.552
- type: mrr_at_100
value: 29.42
- type: mrr_at_1000
value: 29.497
- type: mrr_at_3
value: 26.14
- type: mrr_at_5
value: 27.502
- type: ndcg_at_1
value: 20.274
- type: ndcg_at_10
value: 29.088
- type: ndcg_at_100
value: 34.293
- type: ndcg_at_1000
value: 37.271
- type: ndcg_at_3
value: 24.708
- type: ndcg_at_5
value: 26.809
- type: precision_at_1
value: 20.274
- type: precision_at_10
value: 5.361
- type: precision_at_100
value: 0.915
- type: precision_at_1000
value: 0.13
- type: precision_at_3
value: 11.733
- type: precision_at_5
value: 8.556999999999999
- type: recall_at_1
value: 16.715
- type: recall_at_10
value: 39.587
- type: recall_at_100
value: 62.336000000000006
- type: recall_at_1000
value: 83.453
- type: recall_at_3
value: 27.839999999999996
- type: recall_at_5
value: 32.952999999999996
- task:
type: Retrieval
dataset:
type: BeIR/cqadupstack
name: MTEB CQADupstackPhysicsRetrieval
config: default
split: test
revision: None
metrics:
- type: map_at_1
value: 28.793000000000003
- type: map_at_10
value: 38.582
- type: map_at_100
value: 39.881
- type: map_at_1000
value: 39.987
- type: map_at_3
value: 35.851
- type: map_at_5
value: 37.289
- type: mrr_at_1
value: 34.455999999999996
- type: mrr_at_10
value: 43.909
- type: mrr_at_100
value: 44.74
- type: mrr_at_1000
value: 44.786
- type: mrr_at_3
value: 41.659
- type: mrr_at_5
value: 43.010999999999996
- type: ndcg_at_1
value: 34.455999999999996
- type: ndcg_at_10
value: 44.266
- type: ndcg_at_100
value: 49.639
- type: ndcg_at_1000
value: 51.644
- type: ndcg_at_3
value: 39.865
- type: ndcg_at_5
value: 41.887
- type: precision_at_1
value: 34.455999999999996
- type: precision_at_10
value: 7.843999999999999
- type: precision_at_100
value: 1.243
- type: precision_at_1000
value: 0.158
- type: precision_at_3
value: 18.831999999999997
- type: precision_at_5
value: 13.147
- type: recall_at_1
value: 28.793000000000003
- type: recall_at_10
value: 55.68300000000001
- type: recall_at_100
value: 77.99000000000001
- type: recall_at_1000
value: 91.183
- type: recall_at_3
value: 43.293
- type: recall_at_5
value: 48.618
- task:
type: Retrieval
dataset:
type: BeIR/cqadupstack
name: MTEB CQADupstackProgrammersRetrieval
config: default
split: test
revision: None
metrics:
- type: map_at_1
value: 25.907000000000004
- type: map_at_10
value: 35.519
- type: map_at_100
value: 36.806
- type: map_at_1000
value: 36.912
- type: map_at_3
value: 32.748
- type: map_at_5
value: 34.232
- type: mrr_at_1
value: 31.621
- type: mrr_at_10
value: 40.687
- type: mrr_at_100
value: 41.583
- type: mrr_at_1000
value: 41.638999999999996
- type: mrr_at_3
value: 38.527
- type: mrr_at_5
value: 39.612
- type: ndcg_at_1
value: 31.621
- type: ndcg_at_10
value: 41.003
- type: ndcg_at_100
value: 46.617999999999995
- type: ndcg_at_1000
value: 48.82
- type: ndcg_at_3
value: 36.542
- type: ndcg_at_5
value: 38.368
- type: precision_at_1
value: 31.621
- type: precision_at_10
value: 7.396999999999999
- type: precision_at_100
value: 1.191
- type: precision_at_1000
value: 0.153
- type: precision_at_3
value: 17.39
- type: precision_at_5
value: 12.1
- type: recall_at_1
value: 25.907000000000004
- type: recall_at_10
value: 52.115
- type: recall_at_100
value: 76.238
- type: recall_at_1000
value: 91.218
- type: recall_at_3
value: 39.417
- type: recall_at_5
value: 44.435
- task:
type: Retrieval
dataset:
type: BeIR/cqadupstack
name: MTEB CQADupstackRetrieval
config: default
split: test
revision: None
metrics:
- type: map_at_1
value: 25.732166666666668
- type: map_at_10
value: 34.51616666666667
- type: map_at_100
value: 35.67241666666666
- type: map_at_1000
value: 35.78675
- type: map_at_3
value: 31.953416666666662
- type: map_at_5
value: 33.333
- type: mrr_at_1
value: 30.300166666666673
- type: mrr_at_10
value: 38.6255
- type: mrr_at_100
value: 39.46183333333334
- type: mrr_at_1000
value: 39.519999999999996
- type: mrr_at_3
value: 36.41299999999999
- type: mrr_at_5
value: 37.6365
- type: ndcg_at_1
value: 30.300166666666673
- type: ndcg_at_10
value: 39.61466666666667
- type: ndcg_at_100
value: 44.60808333333334
- type: ndcg_at_1000
value: 46.91708333333334
- type: ndcg_at_3
value: 35.26558333333333
- type: ndcg_at_5
value: 37.220000000000006
- type: precision_at_1
value: 30.300166666666673
- type: precision_at_10
value: 6.837416666666667
- type: precision_at_100
value: 1.10425
- type: precision_at_1000
value: 0.14875
- type: precision_at_3
value: 16.13716666666667
- type: precision_at_5
value: 11.2815
- type: recall_at_1
value: 25.732166666666668
- type: recall_at_10
value: 50.578916666666665
- type: recall_at_100
value: 72.42183333333334
- type: recall_at_1000
value: 88.48766666666667
- type: recall_at_3
value: 38.41325
- type: recall_at_5
value: 43.515750000000004
- task:
type: Retrieval
dataset:
type: BeIR/cqadupstack
name: MTEB CQADupstackStatsRetrieval
config: default
split: test
revision: None
metrics:
- type: map_at_1
value: 23.951
- type: map_at_10
value: 30.974
- type: map_at_100
value: 31.804
- type: map_at_1000
value: 31.900000000000002
- type: map_at_3
value: 28.762
- type: map_at_5
value: 29.94
- type: mrr_at_1
value: 26.534000000000002
- type: mrr_at_10
value: 33.553
- type: mrr_at_100
value: 34.297
- type: mrr_at_1000
value: 34.36
- type: mrr_at_3
value: 31.391000000000002
- type: mrr_at_5
value: 32.525999999999996
- type: ndcg_at_1
value: 26.534000000000002
- type: ndcg_at_10
value: 35.112
- type: ndcg_at_100
value: 39.28
- type: ndcg_at_1000
value: 41.723
- type: ndcg_at_3
value: 30.902
- type: ndcg_at_5
value: 32.759
- type: precision_at_1
value: 26.534000000000002
- type: precision_at_10
value: 5.445
- type: precision_at_100
value: 0.819
- type: precision_at_1000
value: 0.11
- type: precision_at_3
value: 12.986
- type: precision_at_5
value: 9.049
- type: recall_at_1
value: 23.951
- type: recall_at_10
value: 45.24
- type: recall_at_100
value: 64.12299999999999
- type: recall_at_1000
value: 82.28999999999999
- type: recall_at_3
value: 33.806000000000004
- type: recall_at_5
value: 38.277
- task:
type: Retrieval
dataset:
type: BeIR/cqadupstack
name: MTEB CQADupstackTexRetrieval
config: default
split: test
revision: None
metrics:
- type: map_at_1
value: 16.829
- type: map_at_10
value: 23.684
- type: map_at_100
value: 24.683
- type: map_at_1000
value: 24.81
- type: map_at_3
value: 21.554000000000002
- type: map_at_5
value: 22.768
- type: mrr_at_1
value: 20.096
- type: mrr_at_10
value: 27.230999999999998
- type: mrr_at_100
value: 28.083999999999996
- type: mrr_at_1000
value: 28.166000000000004
- type: mrr_at_3
value: 25.212
- type: mrr_at_5
value: 26.32
- type: ndcg_at_1
value: 20.096
- type: ndcg_at_10
value: 27.989000000000004
- type: ndcg_at_100
value: 32.847
- type: ndcg_at_1000
value: 35.896
- type: ndcg_at_3
value: 24.116
- type: ndcg_at_5
value: 25.964
- type: precision_at_1
value: 20.096
- type: precision_at_10
value: 5
- type: precision_at_100
value: 0.8750000000000001
- type: precision_at_1000
value: 0.131
- type: precision_at_3
value: 11.207
- type: precision_at_5
value: 8.08
- type: recall_at_1
value: 16.829
- type: recall_at_10
value: 37.407000000000004
- type: recall_at_100
value: 59.101000000000006
- type: recall_at_1000
value: 81.024
- type: recall_at_3
value: 26.739
- type: recall_at_5
value: 31.524
- task:
type: Retrieval
dataset:
type: BeIR/cqadupstack
name: MTEB CQADupstackUnixRetrieval
config: default
split: test
revision: None
metrics:
- type: map_at_1
value: 24.138
- type: map_at_10
value: 32.275999999999996
- type: map_at_100
value: 33.416000000000004
- type: map_at_1000
value: 33.527
- type: map_at_3
value: 29.854000000000003
- type: map_at_5
value: 31.096
- type: mrr_at_1
value: 28.450999999999997
- type: mrr_at_10
value: 36.214
- type: mrr_at_100
value: 37.134
- type: mrr_at_1000
value: 37.198
- type: mrr_at_3
value: 34.001999999999995
- type: mrr_at_5
value: 35.187000000000005
- type: ndcg_at_1
value: 28.450999999999997
- type: ndcg_at_10
value: 37.166
- type: ndcg_at_100
value: 42.454
- type: ndcg_at_1000
value: 44.976
- type: ndcg_at_3
value: 32.796
- type: ndcg_at_5
value: 34.631
- type: precision_at_1
value: 28.450999999999997
- type: precision_at_10
value: 6.241
- type: precision_at_100
value: 0.9950000000000001
- type: precision_at_1000
value: 0.133
- type: precision_at_3
value: 14.801
- type: precision_at_5
value: 10.280000000000001
- type: recall_at_1
value: 24.138
- type: recall_at_10
value: 48.111
- type: recall_at_100
value: 71.245
- type: recall_at_1000
value: 88.986
- type: recall_at_3
value: 36.119
- type: recall_at_5
value: 40.846
- task:
type: Retrieval
dataset:
type: BeIR/cqadupstack
name: MTEB CQADupstackWebmastersRetrieval
config: default
split: test
revision: None
metrics:
- type: map_at_1
value: 23.244
- type: map_at_10
value: 31.227
- type: map_at_100
value: 33.007
- type: map_at_1000
value: 33.223
- type: map_at_3
value: 28.924
- type: map_at_5
value: 30.017
- type: mrr_at_1
value: 27.668
- type: mrr_at_10
value: 35.524
- type: mrr_at_100
value: 36.699
- type: mrr_at_1000
value: 36.759
- type: mrr_at_3
value: 33.366
- type: mrr_at_5
value: 34.552
- type: ndcg_at_1
value: 27.668
- type: ndcg_at_10
value: 36.381
- type: ndcg_at_100
value: 43.062
- type: ndcg_at_1000
value: 45.656
- type: ndcg_at_3
value: 32.501999999999995
- type: ndcg_at_5
value: 34.105999999999995
- type: precision_at_1
value: 27.668
- type: precision_at_10
value: 6.798
- type: precision_at_100
value: 1.492
- type: precision_at_1000
value: 0.234
- type: precision_at_3
value: 15.152
- type: precision_at_5
value: 10.791
- type: recall_at_1
value: 23.244
- type: recall_at_10
value: 45.979
- type: recall_at_100
value: 74.822
- type: recall_at_1000
value: 91.078
- type: recall_at_3
value: 34.925
- type: recall_at_5
value: 39.126
- task:
type: Retrieval
dataset:
type: BeIR/cqadupstack
name: MTEB CQADupstackWordpressRetrieval
config: default
split: test
revision: None
metrics:
- type: map_at_1
value: 19.945
- type: map_at_10
value: 27.517999999999997
- type: map_at_100
value: 28.588
- type: map_at_1000
value: 28.682000000000002
- type: map_at_3
value: 25.345000000000002
- type: map_at_5
value: 26.555
- type: mrr_at_1
value: 21.996
- type: mrr_at_10
value: 29.845
- type: mrr_at_100
value: 30.775999999999996
- type: mrr_at_1000
value: 30.845
- type: mrr_at_3
value: 27.726
- type: mrr_at_5
value: 28.882
- type: ndcg_at_1
value: 21.996
- type: ndcg_at_10
value: 32.034
- type: ndcg_at_100
value: 37.185
- type: ndcg_at_1000
value: 39.645
- type: ndcg_at_3
value: 27.750999999999998
- type: ndcg_at_5
value: 29.805999999999997
- type: precision_at_1
value: 21.996
- type: precision_at_10
value: 5.065
- type: precision_at_100
value: 0.819
- type: precision_at_1000
value: 0.11399999999999999
- type: precision_at_3
value: 12.076
- type: precision_at_5
value: 8.392
- type: recall_at_1
value: 19.945
- type: recall_at_10
value: 43.62
- type: recall_at_100
value: 67.194
- type: recall_at_1000
value: 85.7
- type: recall_at_3
value: 32.15
- type: recall_at_5
value: 37.208999999999996
- task:
type: Retrieval
dataset:
type: climate-fever
name: MTEB ClimateFEVER
config: default
split: test
revision: None
metrics:
- type: map_at_1
value: 18.279
- type: map_at_10
value: 31.052999999999997
- type: map_at_100
value: 33.125
- type: map_at_1000
value: 33.306000000000004
- type: map_at_3
value: 26.208
- type: map_at_5
value: 28.857
- type: mrr_at_1
value: 42.671
- type: mrr_at_10
value: 54.557
- type: mrr_at_100
value: 55.142
- type: mrr_at_1000
value: 55.169000000000004
- type: mrr_at_3
value: 51.488
- type: mrr_at_5
value: 53.439
- type: ndcg_at_1
value: 42.671
- type: ndcg_at_10
value: 41.276
- type: ndcg_at_100
value: 48.376000000000005
- type: ndcg_at_1000
value: 51.318
- type: ndcg_at_3
value: 35.068
- type: ndcg_at_5
value: 37.242
- type: precision_at_1
value: 42.671
- type: precision_at_10
value: 12.638
- type: precision_at_100
value: 2.045
- type: precision_at_1000
value: 0.26
- type: precision_at_3
value: 26.08
- type: precision_at_5
value: 19.805
- type: recall_at_1
value: 18.279
- type: recall_at_10
value: 46.946
- type: recall_at_100
value: 70.97200000000001
- type: recall_at_1000
value: 87.107
- type: recall_at_3
value: 31.147999999999996
- type: recall_at_5
value: 38.099
- task:
type: Retrieval
dataset:
type: dbpedia-entity
name: MTEB DBPedia
config: default
split: test
revision: None
metrics:
- type: map_at_1
value: 8.573
- type: map_at_10
value: 19.747
- type: map_at_100
value: 28.205000000000002
- type: map_at_1000
value: 29.831000000000003
- type: map_at_3
value: 14.109
- type: map_at_5
value: 16.448999999999998
- type: mrr_at_1
value: 71
- type: mrr_at_10
value: 77.68599999999999
- type: mrr_at_100
value: 77.995
- type: mrr_at_1000
value: 78.00200000000001
- type: mrr_at_3
value: 76.292
- type: mrr_at_5
value: 77.029
- type: ndcg_at_1
value: 59.12500000000001
- type: ndcg_at_10
value: 43.9
- type: ndcg_at_100
value: 47.863
- type: ndcg_at_1000
value: 54.848
- type: ndcg_at_3
value: 49.803999999999995
- type: ndcg_at_5
value: 46.317
- type: precision_at_1
value: 71
- type: precision_at_10
value: 34.4
- type: precision_at_100
value: 11.063
- type: precision_at_1000
value: 1.989
- type: precision_at_3
value: 52.333
- type: precision_at_5
value: 43.7
- type: recall_at_1
value: 8.573
- type: recall_at_10
value: 25.615
- type: recall_at_100
value: 53.385000000000005
- type: recall_at_1000
value: 75.46000000000001
- type: recall_at_3
value: 15.429
- type: recall_at_5
value: 19.357
- task:
type: Classification
dataset:
type: mteb/emotion
name: MTEB EmotionClassification
config: default
split: test
revision: 4f58c6b202a23cf9a4da393831edf4f9183cad37
metrics:
- type: accuracy
value: 47.989999999999995
- type: f1
value: 42.776314451497555
- task:
type: Retrieval
dataset:
type: fever
name: MTEB FEVER
config: default
split: test
revision: None
metrics:
- type: map_at_1
value: 74.13499999999999
- type: map_at_10
value: 82.825
- type: map_at_100
value: 83.096
- type: map_at_1000
value: 83.111
- type: map_at_3
value: 81.748
- type: map_at_5
value: 82.446
- type: mrr_at_1
value: 79.553
- type: mrr_at_10
value: 86.654
- type: mrr_at_100
value: 86.774
- type: mrr_at_1000
value: 86.778
- type: mrr_at_3
value: 85.981
- type: mrr_at_5
value: 86.462
- type: ndcg_at_1
value: 79.553
- type: ndcg_at_10
value: 86.345
- type: ndcg_at_100
value: 87.32
- type: ndcg_at_1000
value: 87.58200000000001
- type: ndcg_at_3
value: 84.719
- type: ndcg_at_5
value: 85.677
- type: precision_at_1
value: 79.553
- type: precision_at_10
value: 10.402000000000001
- type: precision_at_100
value: 1.1119999999999999
- type: precision_at_1000
value: 0.11499999999999999
- type: precision_at_3
value: 32.413
- type: precision_at_5
value: 20.138
- type: recall_at_1
value: 74.13499999999999
- type: recall_at_10
value: 93.215
- type: recall_at_100
value: 97.083
- type: recall_at_1000
value: 98.732
- type: recall_at_3
value: 88.79
- type: recall_at_5
value: 91.259
- task:
type: Retrieval
dataset:
type: fiqa
name: MTEB FiQA2018
config: default
split: test
revision: None
metrics:
- type: map_at_1
value: 18.298000000000002
- type: map_at_10
value: 29.901
- type: map_at_100
value: 31.528
- type: map_at_1000
value: 31.713
- type: map_at_3
value: 25.740000000000002
- type: map_at_5
value: 28.227999999999998
- type: mrr_at_1
value: 36.728
- type: mrr_at_10
value: 45.401
- type: mrr_at_100
value: 46.27
- type: mrr_at_1000
value: 46.315
- type: mrr_at_3
value: 42.978
- type: mrr_at_5
value: 44.29
- type: ndcg_at_1
value: 36.728
- type: ndcg_at_10
value: 37.456
- type: ndcg_at_100
value: 43.832
- type: ndcg_at_1000
value: 47
- type: ndcg_at_3
value: 33.694
- type: ndcg_at_5
value: 35.085
- type: precision_at_1
value: 36.728
- type: precision_at_10
value: 10.386
- type: precision_at_100
value: 1.701
- type: precision_at_1000
value: 0.22599999999999998
- type: precision_at_3
value: 22.479
- type: precision_at_5
value: 16.605
- type: recall_at_1
value: 18.298000000000002
- type: recall_at_10
value: 44.369
- type: recall_at_100
value: 68.098
- type: recall_at_1000
value: 87.21900000000001
- type: recall_at_3
value: 30.215999999999998
- type: recall_at_5
value: 36.861
- task:
type: Retrieval
dataset:
type: hotpotqa
name: MTEB HotpotQA
config: default
split: test
revision: None
metrics:
- type: map_at_1
value: 39.568
- type: map_at_10
value: 65.061
- type: map_at_100
value: 65.896
- type: map_at_1000
value: 65.95100000000001
- type: map_at_3
value: 61.831
- type: map_at_5
value: 63.849000000000004
- type: mrr_at_1
value: 79.136
- type: mrr_at_10
value: 84.58200000000001
- type: mrr_at_100
value: 84.765
- type: mrr_at_1000
value: 84.772
- type: mrr_at_3
value: 83.684
- type: mrr_at_5
value: 84.223
- type: ndcg_at_1
value: 79.136
- type: ndcg_at_10
value: 72.622
- type: ndcg_at_100
value: 75.539
- type: ndcg_at_1000
value: 76.613
- type: ndcg_at_3
value: 68.065
- type: ndcg_at_5
value: 70.58
- type: precision_at_1
value: 79.136
- type: precision_at_10
value: 15.215
- type: precision_at_100
value: 1.7500000000000002
- type: precision_at_1000
value: 0.189
- type: precision_at_3
value: 44.011
- type: precision_at_5
value: 28.388999999999996
- type: recall_at_1
value: 39.568
- type: recall_at_10
value: 76.077
- type: recall_at_100
value: 87.481
- type: recall_at_1000
value: 94.56400000000001
- type: recall_at_3
value: 66.01599999999999
- type: recall_at_5
value: 70.97200000000001
- task:
type: Classification
dataset:
type: mteb/imdb
name: MTEB ImdbClassification
config: default
split: test
revision: 3d86128a09e091d6018b6d26cad27f2739fc2db7
metrics:
- type: accuracy
value: 85.312
- type: ap
value: 80.36296867333715
- type: f1
value: 85.26613311552218
- task:
type: Retrieval
dataset:
type: msmarco
name: MTEB MSMARCO
config: default
split: dev
revision: None
metrics:
- type: map_at_1
value: 23.363999999999997
- type: map_at_10
value: 35.711999999999996
- type: map_at_100
value: 36.876999999999995
- type: map_at_1000
value: 36.923
- type: map_at_3
value: 32.034
- type: map_at_5
value: 34.159
- type: mrr_at_1
value: 24.04
- type: mrr_at_10
value: 36.345
- type: mrr_at_100
value: 37.441
- type: mrr_at_1000
value: 37.480000000000004
- type: mrr_at_3
value: 32.713
- type: mrr_at_5
value: 34.824
- type: ndcg_at_1
value: 24.026
- type: ndcg_at_10
value: 42.531
- type: ndcg_at_100
value: 48.081
- type: ndcg_at_1000
value: 49.213
- type: ndcg_at_3
value: 35.044
- type: ndcg_at_5
value: 38.834
- type: precision_at_1
value: 24.026
- type: precision_at_10
value: 6.622999999999999
- type: precision_at_100
value: 0.941
- type: precision_at_1000
value: 0.104
- type: precision_at_3
value: 14.909
- type: precision_at_5
value: 10.871
- type: recall_at_1
value: 23.363999999999997
- type: recall_at_10
value: 63.426
- type: recall_at_100
value: 88.96300000000001
- type: recall_at_1000
value: 97.637
- type: recall_at_3
value: 43.095
- type: recall_at_5
value: 52.178000000000004
- task:
type: Classification
dataset:
type: mteb/mtop_domain
name: MTEB MTOPDomainClassification (en)
config: en
split: test
revision: d80d48c1eb48d3562165c59d59d0034df9fff0bf
metrics:
- type: accuracy
value: 93.0095759233926
- type: f1
value: 92.78387794667408
- task:
type: Classification
dataset:
type: mteb/mtop_intent
name: MTEB MTOPIntentClassification (en)
config: en
split: test
revision: ae001d0e6b1228650b7bd1c2c65fb50ad11a8aba
metrics:
- type: accuracy
value: 75.0296397628819
- type: f1
value: 58.45699589820874
- task:
type: Classification
dataset:
type: mteb/amazon_massive_intent
name: MTEB MassiveIntentClassification (en)
config: en
split: test
revision: 31efe3c427b0bae9c22cbb560b8f15491cc6bed7
metrics:
- type: accuracy
value: 73.45662407531944
- type: f1
value: 71.42364781421813
- task:
type: Classification
dataset:
type: mteb/amazon_massive_scenario
name: MTEB MassiveScenarioClassification (en)
config: en
split: test
revision: 7d571f92784cd94a019292a1f45445077d0ef634
metrics:
- type: accuracy
value: 77.07800941492937
- type: f1
value: 77.22799045640845
- task:
type: Clustering
dataset:
type: mteb/medrxiv-clustering-p2p
name: MTEB MedrxivClusteringP2P
config: default
split: test
revision: e7a26af6f3ae46b30dde8737f02c07b1505bcc73
metrics:
- type: v_measure
value: 34.531234379250606
- task:
type: Clustering
dataset:
type: mteb/medrxiv-clustering-s2s
name: MTEB MedrxivClusteringS2S
config: default
split: test
revision: 35191c8c0dca72d8ff3efcd72aa802307d469663
metrics:
- type: v_measure
value: 30.941490381193802
- task:
type: Reranking
dataset:
type: mteb/mind_small
name: MTEB MindSmallReranking
config: default
split: test
revision: 3bdac13927fdc888b903db93b2ffdbd90b295a69
metrics:
- type: map
value: 30.3115090856725
- type: mrr
value: 31.290667638675757
- task:
type: Retrieval
dataset:
type: nfcorpus
name: MTEB NFCorpus
config: default
split: test
revision: None
metrics:
- type: map_at_1
value: 5.465
- type: map_at_10
value: 13.03
- type: map_at_100
value: 16.057
- type: map_at_1000
value: 17.49
- type: map_at_3
value: 9.553
- type: map_at_5
value: 11.204
- type: mrr_at_1
value: 43.653
- type: mrr_at_10
value: 53.269
- type: mrr_at_100
value: 53.72
- type: mrr_at_1000
value: 53.761
- type: mrr_at_3
value: 50.929
- type: mrr_at_5
value: 52.461
- type: ndcg_at_1
value: 42.26
- type: ndcg_at_10
value: 34.673
- type: ndcg_at_100
value: 30.759999999999998
- type: ndcg_at_1000
value: 39.728
- type: ndcg_at_3
value: 40.349000000000004
- type: ndcg_at_5
value: 37.915
- type: precision_at_1
value: 43.653
- type: precision_at_10
value: 25.789
- type: precision_at_100
value: 7.754999999999999
- type: precision_at_1000
value: 2.07
- type: precision_at_3
value: 38.596000000000004
- type: precision_at_5
value: 33.251
- type: recall_at_1
value: 5.465
- type: recall_at_10
value: 17.148
- type: recall_at_100
value: 29.768
- type: recall_at_1000
value: 62.239
- type: recall_at_3
value: 10.577
- type: recall_at_5
value: 13.315
- task:
type: Retrieval
dataset:
type: nq
name: MTEB NQ
config: default
split: test
revision: None
metrics:
- type: map_at_1
value: 37.008
- type: map_at_10
value: 52.467
- type: map_at_100
value: 53.342999999999996
- type: map_at_1000
value: 53.366
- type: map_at_3
value: 48.412
- type: map_at_5
value: 50.875
- type: mrr_at_1
value: 41.541
- type: mrr_at_10
value: 54.967
- type: mrr_at_100
value: 55.611
- type: mrr_at_1000
value: 55.627
- type: mrr_at_3
value: 51.824999999999996
- type: mrr_at_5
value: 53.763000000000005
- type: ndcg_at_1
value: 41.541
- type: ndcg_at_10
value: 59.724999999999994
- type: ndcg_at_100
value: 63.38700000000001
- type: ndcg_at_1000
value: 63.883
- type: ndcg_at_3
value: 52.331
- type: ndcg_at_5
value: 56.327000000000005
- type: precision_at_1
value: 41.541
- type: precision_at_10
value: 9.447
- type: precision_at_100
value: 1.1520000000000001
- type: precision_at_1000
value: 0.12
- type: precision_at_3
value: 23.262
- type: precision_at_5
value: 16.314999999999998
- type: recall_at_1
value: 37.008
- type: recall_at_10
value: 79.145
- type: recall_at_100
value: 94.986
- type: recall_at_1000
value: 98.607
- type: recall_at_3
value: 60.277
- type: recall_at_5
value: 69.407
- task:
type: Retrieval
dataset:
type: quora
name: MTEB QuoraRetrieval
config: default
split: test
revision: None
metrics:
- type: map_at_1
value: 70.402
- type: map_at_10
value: 84.181
- type: map_at_100
value: 84.796
- type: map_at_1000
value: 84.81400000000001
- type: map_at_3
value: 81.209
- type: map_at_5
value: 83.085
- type: mrr_at_1
value: 81.02000000000001
- type: mrr_at_10
value: 87.263
- type: mrr_at_100
value: 87.36
- type: mrr_at_1000
value: 87.36
- type: mrr_at_3
value: 86.235
- type: mrr_at_5
value: 86.945
- type: ndcg_at_1
value: 81.01
- type: ndcg_at_10
value: 87.99900000000001
- type: ndcg_at_100
value: 89.217
- type: ndcg_at_1000
value: 89.33
- type: ndcg_at_3
value: 85.053
- type: ndcg_at_5
value: 86.703
- type: precision_at_1
value: 81.01
- type: precision_at_10
value: 13.336
- type: precision_at_100
value: 1.52
- type: precision_at_1000
value: 0.156
- type: precision_at_3
value: 37.14
- type: precision_at_5
value: 24.44
- type: recall_at_1
value: 70.402
- type: recall_at_10
value: 95.214
- type: recall_at_100
value: 99.438
- type: recall_at_1000
value: 99.928
- type: recall_at_3
value: 86.75699999999999
- type: recall_at_5
value: 91.44099999999999
- task:
type: Clustering
dataset:
type: mteb/reddit-clustering
name: MTEB RedditClustering
config: default
split: test
revision: 24640382cdbf8abc73003fb0fa6d111a705499eb
metrics:
- type: v_measure
value: 56.51721502758904
- task:
type: Clustering
dataset:
type: mteb/reddit-clustering-p2p
name: MTEB RedditClusteringP2P
config: default
split: test
revision: 282350215ef01743dc01b456c7f5241fa8937f16
metrics:
- type: v_measure
value: 61.054808572333016
- task:
type: Retrieval
dataset:
type: scidocs
name: MTEB SCIDOCS
config: default
split: test
revision: None
metrics:
- type: map_at_1
value: 4.578
- type: map_at_10
value: 11.036999999999999
- type: map_at_100
value: 12.879999999999999
- type: map_at_1000
value: 13.150999999999998
- type: map_at_3
value: 8.133
- type: map_at_5
value: 9.559
- type: mrr_at_1
value: 22.6
- type: mrr_at_10
value: 32.68
- type: mrr_at_100
value: 33.789
- type: mrr_at_1000
value: 33.854
- type: mrr_at_3
value: 29.7
- type: mrr_at_5
value: 31.480000000000004
- type: ndcg_at_1
value: 22.6
- type: ndcg_at_10
value: 18.616
- type: ndcg_at_100
value: 25.883
- type: ndcg_at_1000
value: 30.944
- type: ndcg_at_3
value: 18.136
- type: ndcg_at_5
value: 15.625
- type: precision_at_1
value: 22.6
- type: precision_at_10
value: 9.48
- type: precision_at_100
value: 1.991
- type: precision_at_1000
value: 0.321
- type: precision_at_3
value: 16.8
- type: precision_at_5
value: 13.54
- type: recall_at_1
value: 4.578
- type: recall_at_10
value: 19.213
- type: recall_at_100
value: 40.397
- type: recall_at_1000
value: 65.2
- type: recall_at_3
value: 10.208
- type: recall_at_5
value: 13.718
- task:
type: STS
dataset:
type: mteb/sickr-sts
name: MTEB SICK-R
config: default
split: test
revision: a6ea5a8cab320b040a23452cc28066d9beae2cee
metrics:
- type: cos_sim_pearson
value: 83.44288351714071
- type: cos_sim_spearman
value: 79.37995604564952
- type: euclidean_pearson
value: 81.1078874670718
- type: euclidean_spearman
value: 79.37995905980499
- type: manhattan_pearson
value: 81.03697527288986
- type: manhattan_spearman
value: 79.33490235296236
- task:
type: STS
dataset:
type: mteb/sts12-sts
name: MTEB STS12
config: default
split: test
revision: a0d554a64d88156834ff5ae9920b964011b16384
metrics:
- type: cos_sim_pearson
value: 84.95557650436523
- type: cos_sim_spearman
value: 78.5190672399868
- type: euclidean_pearson
value: 81.58064025904707
- type: euclidean_spearman
value: 78.5190672399868
- type: manhattan_pearson
value: 81.52857930619889
- type: manhattan_spearman
value: 78.50421361308034
- task:
type: STS
dataset:
type: mteb/sts13-sts
name: MTEB STS13
config: default
split: test
revision: 7e90230a92c190f1bf69ae9002b8cea547a64cca
metrics:
- type: cos_sim_pearson
value: 84.79128416228737
- type: cos_sim_spearman
value: 86.05402451477147
- type: euclidean_pearson
value: 85.46280267054289
- type: euclidean_spearman
value: 86.05402451477147
- type: manhattan_pearson
value: 85.46278563858236
- type: manhattan_spearman
value: 86.08079590861004
- task:
type: STS
dataset:
type: mteb/sts14-sts
name: MTEB STS14
config: default
split: test
revision: 6031580fec1f6af667f0bd2da0a551cf4f0b2375
metrics:
- type: cos_sim_pearson
value: 83.20623089568763
- type: cos_sim_spearman
value: 81.53786907061009
- type: euclidean_pearson
value: 82.82272250091494
- type: euclidean_spearman
value: 81.53786907061009
- type: manhattan_pearson
value: 82.78850494027013
- type: manhattan_spearman
value: 81.5135618083407
- task:
type: STS
dataset:
type: mteb/sts15-sts
name: MTEB STS15
config: default
split: test
revision: ae752c7c21bf194d8b67fd573edf7ae58183cbe3
metrics:
- type: cos_sim_pearson
value: 85.46366618397936
- type: cos_sim_spearman
value: 86.96566013336908
- type: euclidean_pearson
value: 86.62651697548931
- type: euclidean_spearman
value: 86.96565526364454
- type: manhattan_pearson
value: 86.58812160258009
- type: manhattan_spearman
value: 86.9336484321288
- task:
type: STS
dataset:
type: mteb/sts16-sts
name: MTEB STS16
config: default
split: test
revision: 4d8694f8f0e0100860b497b999b3dbed754a0513
metrics:
- type: cos_sim_pearson
value: 82.51858358641559
- type: cos_sim_spearman
value: 84.7652527954999
- type: euclidean_pearson
value: 84.23914783766861
- type: euclidean_spearman
value: 84.7652527954999
- type: manhattan_pearson
value: 84.22749648503171
- type: manhattan_spearman
value: 84.74527996746386
- task:
type: STS
dataset:
type: mteb/sts17-crosslingual-sts
name: MTEB STS17 (en-en)
config: en-en
split: test
revision: af5e6fb845001ecf41f4c1e033ce921939a2a68d
metrics:
- type: cos_sim_pearson
value: 87.28026563313065
- type: cos_sim_spearman
value: 87.46928143824915
- type: euclidean_pearson
value: 88.30558762000372
- type: euclidean_spearman
value: 87.46928143824915
- type: manhattan_pearson
value: 88.10513330809331
- type: manhattan_spearman
value: 87.21069787834173
- task:
type: STS
dataset:
type: mteb/sts22-crosslingual-sts
name: MTEB STS22 (en)
config: en
split: test
revision: 6d1ba47164174a496b7fa5d3569dae26a6813b80
metrics:
- type: cos_sim_pearson
value: 62.376497134587375
- type: cos_sim_spearman
value: 65.0159550112516
- type: euclidean_pearson
value: 65.64572120879598
- type: euclidean_spearman
value: 65.0159550112516
- type: manhattan_pearson
value: 65.88143604989976
- type: manhattan_spearman
value: 65.17547297222434
- task:
type: STS
dataset:
type: mteb/stsbenchmark-sts
name: MTEB STSBenchmark
config: default
split: test
revision: b0fddb56ed78048fa8b90373c8a3cfc37b684831
metrics:
- type: cos_sim_pearson
value: 84.22876368947644
- type: cos_sim_spearman
value: 85.46935577445318
- type: euclidean_pearson
value: 85.32830231392005
- type: euclidean_spearman
value: 85.46935577445318
- type: manhattan_pearson
value: 85.30353211758495
- type: manhattan_spearman
value: 85.42821085956945
- task:
type: Reranking
dataset:
type: mteb/scidocs-reranking
name: MTEB SciDocsRR
config: default
split: test
revision: d3c5e1fc0b855ab6097bf1cda04dd73947d7caab
metrics:
- type: map
value: 80.60986667767133
- type: mrr
value: 94.29432314236236
- task:
type: Retrieval
dataset:
type: scifact
name: MTEB SciFact
config: default
split: test
revision: None
metrics:
- type: map_at_1
value: 54.528
- type: map_at_10
value: 65.187
- type: map_at_100
value: 65.62599999999999
- type: map_at_1000
value: 65.657
- type: map_at_3
value: 62.352
- type: map_at_5
value: 64.025
- type: mrr_at_1
value: 57.333
- type: mrr_at_10
value: 66.577
- type: mrr_at_100
value: 66.88
- type: mrr_at_1000
value: 66.908
- type: mrr_at_3
value: 64.556
- type: mrr_at_5
value: 65.739
- type: ndcg_at_1
value: 57.333
- type: ndcg_at_10
value: 70.275
- type: ndcg_at_100
value: 72.136
- type: ndcg_at_1000
value: 72.963
- type: ndcg_at_3
value: 65.414
- type: ndcg_at_5
value: 67.831
- type: precision_at_1
value: 57.333
- type: precision_at_10
value: 9.5
- type: precision_at_100
value: 1.057
- type: precision_at_1000
value: 0.11199999999999999
- type: precision_at_3
value: 25.778000000000002
- type: precision_at_5
value: 17.2
- type: recall_at_1
value: 54.528
- type: recall_at_10
value: 84.356
- type: recall_at_100
value: 92.833
- type: recall_at_1000
value: 99.333
- type: recall_at_3
value: 71.283
- type: recall_at_5
value: 77.14999999999999
- task:
type: PairClassification
dataset:
type: mteb/sprintduplicatequestions-pairclassification
name: MTEB SprintDuplicateQuestions
config: default
split: test
revision: d66bd1f72af766a5cc4b0ca5e00c162f89e8cc46
metrics:
- type: cos_sim_accuracy
value: 99.74158415841585
- type: cos_sim_ap
value: 92.90048959850317
- type: cos_sim_f1
value: 86.35650810245687
- type: cos_sim_precision
value: 90.4709748083242
- type: cos_sim_recall
value: 82.6
- type: dot_accuracy
value: 99.74158415841585
- type: dot_ap
value: 92.90048959850317
- type: dot_f1
value: 86.35650810245687
- type: dot_precision
value: 90.4709748083242
- type: dot_recall
value: 82.6
- type: euclidean_accuracy
value: 99.74158415841585
- type: euclidean_ap
value: 92.90048959850317
- type: euclidean_f1
value: 86.35650810245687
- type: euclidean_precision
value: 90.4709748083242
- type: euclidean_recall
value: 82.6
- type: manhattan_accuracy
value: 99.74158415841585
- type: manhattan_ap
value: 92.87344692947894
- type: manhattan_f1
value: 86.38497652582159
- type: manhattan_precision
value: 90.29443838604145
- type: manhattan_recall
value: 82.8
- type: max_accuracy
value: 99.74158415841585
- type: max_ap
value: 92.90048959850317
- type: max_f1
value: 86.38497652582159
- task:
type: Clustering
dataset:
type: mteb/stackexchange-clustering
name: MTEB StackExchangeClustering
config: default
split: test
revision: 6cbc1f7b2bc0622f2e39d2c77fa502909748c259
metrics:
- type: v_measure
value: 63.191648770424216
- task:
type: Clustering
dataset:
type: mteb/stackexchange-clustering-p2p
name: MTEB StackExchangeClusteringP2P
config: default
split: test
revision: 815ca46b2622cec33ccafc3735d572c266efdb44
metrics:
- type: v_measure
value: 34.02944668730218
- task:
type: Reranking
dataset:
type: mteb/stackoverflowdupquestions-reranking
name: MTEB StackOverflowDupQuestions
config: default
split: test
revision: e185fbe320c72810689fc5848eb6114e1ef5ec69
metrics:
- type: map
value: 50.466386167525265
- type: mrr
value: 51.19071492233257
- task:
type: Summarization
dataset:
type: mteb/summeval
name: MTEB SummEval
config: default
split: test
revision: cda12ad7615edc362dbf25a00fdd61d3b1eaf93c
metrics:
- type: cos_sim_pearson
value: 30.198022505886435
- type: cos_sim_spearman
value: 30.40170257939193
- type: dot_pearson
value: 30.198015316402614
- type: dot_spearman
value: 30.40170257939193
- task:
type: Retrieval
dataset:
type: trec-covid
name: MTEB TRECCOVID
config: default
split: test
revision: None
metrics:
- type: map_at_1
value: 0.242
- type: map_at_10
value: 2.17
- type: map_at_100
value: 12.221
- type: map_at_1000
value: 28.63
- type: map_at_3
value: 0.728
- type: map_at_5
value: 1.185
- type: mrr_at_1
value: 94
- type: mrr_at_10
value: 97
- type: mrr_at_100
value: 97
- type: mrr_at_1000
value: 97
- type: mrr_at_3
value: 97
- type: mrr_at_5
value: 97
- type: ndcg_at_1
value: 89
- type: ndcg_at_10
value: 82.30499999999999
- type: ndcg_at_100
value: 61.839999999999996
- type: ndcg_at_1000
value: 53.381
- type: ndcg_at_3
value: 88.877
- type: ndcg_at_5
value: 86.05199999999999
- type: precision_at_1
value: 94
- type: precision_at_10
value: 87
- type: precision_at_100
value: 63.38
- type: precision_at_1000
value: 23.498
- type: precision_at_3
value: 94
- type: precision_at_5
value: 92
- type: recall_at_1
value: 0.242
- type: recall_at_10
value: 2.302
- type: recall_at_100
value: 14.979000000000001
- type: recall_at_1000
value: 49.638
- type: recall_at_3
value: 0.753
- type: recall_at_5
value: 1.226
- task:
type: Retrieval
dataset:
type: webis-touche2020
name: MTEB Touche2020
config: default
split: test
revision: None
metrics:
- type: map_at_1
value: 3.006
- type: map_at_10
value: 11.805
- type: map_at_100
value: 18.146
- type: map_at_1000
value: 19.788
- type: map_at_3
value: 5.914
- type: map_at_5
value: 8.801
- type: mrr_at_1
value: 40.816
- type: mrr_at_10
value: 56.36600000000001
- type: mrr_at_100
value: 56.721999999999994
- type: mrr_at_1000
value: 56.721999999999994
- type: mrr_at_3
value: 52.041000000000004
- type: mrr_at_5
value: 54.796
- type: ndcg_at_1
value: 37.755
- type: ndcg_at_10
value: 29.863
- type: ndcg_at_100
value: 39.571
- type: ndcg_at_1000
value: 51.385999999999996
- type: ndcg_at_3
value: 32.578
- type: ndcg_at_5
value: 32.351
- type: precision_at_1
value: 40.816
- type: precision_at_10
value: 26.531
- type: precision_at_100
value: 7.796
- type: precision_at_1000
value: 1.555
- type: precision_at_3
value: 32.653
- type: precision_at_5
value: 33.061
- type: recall_at_1
value: 3.006
- type: recall_at_10
value: 18.738
- type: recall_at_100
value: 48.058
- type: recall_at_1000
value: 83.41300000000001
- type: recall_at_3
value: 7.166
- type: recall_at_5
value: 12.102
- task:
type: Classification
dataset:
type: mteb/toxic_conversations_50k
name: MTEB ToxicConversationsClassification
config: default
split: test
revision: d7c0de2777da35d6aae2200a62c6e0e5af397c4c
metrics:
- type: accuracy
value: 71.4178
- type: ap
value: 14.648781342150446
- type: f1
value: 55.07299194946378
- task:
type: Classification
dataset:
type: mteb/tweet_sentiment_extraction
name: MTEB TweetSentimentExtractionClassification
config: default
split: test
revision: d604517c81ca91fe16a244d1248fc021f9ecee7a
metrics:
- type: accuracy
value: 60.919637804187886
- type: f1
value: 61.24122013967399
- task:
type: Clustering
dataset:
type: mteb/twentynewsgroups-clustering
name: MTEB TwentyNewsgroupsClustering
config: default
split: test
revision: 6125ec4e24fa026cec8a478383ee943acfbd5449
metrics:
- type: v_measure
value: 49.207896583685695
- task:
type: PairClassification
dataset:
type: mteb/twittersemeval2015-pairclassification
name: MTEB TwitterSemEval2015
config: default
split: test
revision: 70970daeab8776df92f5ea462b6173c0b46fd2d1
metrics:
- type: cos_sim_accuracy
value: 86.23114978840078
- type: cos_sim_ap
value: 74.26624727825818
- type: cos_sim_f1
value: 68.72377190817083
- type: cos_sim_precision
value: 64.56400742115028
- type: cos_sim_recall
value: 73.45646437994723
- type: dot_accuracy
value: 86.23114978840078
- type: dot_ap
value: 74.26624032659652
- type: dot_f1
value: 68.72377190817083
- type: dot_precision
value: 64.56400742115028
- type: dot_recall
value: 73.45646437994723
- type: euclidean_accuracy
value: 86.23114978840078
- type: euclidean_ap
value: 74.26624714480556
- type: euclidean_f1
value: 68.72377190817083
- type: euclidean_precision
value: 64.56400742115028
- type: euclidean_recall
value: 73.45646437994723
- type: manhattan_accuracy
value: 86.16558383501221
- type: manhattan_ap
value: 74.2091943976357
- type: manhattan_f1
value: 68.64221520524654
- type: manhattan_precision
value: 63.59135913591359
- type: manhattan_recall
value: 74.5646437994723
- type: max_accuracy
value: 86.23114978840078
- type: max_ap
value: 74.26624727825818
- type: max_f1
value: 68.72377190817083
- task:
type: PairClassification
dataset:
type: mteb/twitterurlcorpus-pairclassification
name: MTEB TwitterURLCorpus
config: default
split: test
revision: 8b6510b0b1fa4e4c4f879467980e9be563ec1cdf
metrics:
- type: cos_sim_accuracy
value: 89.3681841114604
- type: cos_sim_ap
value: 86.65166387498546
- type: cos_sim_f1
value: 79.02581944698774
- type: cos_sim_precision
value: 75.35796605434099
- type: cos_sim_recall
value: 83.06898675700647
- type: dot_accuracy
value: 89.3681841114604
- type: dot_ap
value: 86.65166019802056
- type: dot_f1
value: 79.02581944698774
- type: dot_precision
value: 75.35796605434099
- type: dot_recall
value: 83.06898675700647
- type: euclidean_accuracy
value: 89.3681841114604
- type: euclidean_ap
value: 86.65166462876266
- type: euclidean_f1
value: 79.02581944698774
- type: euclidean_precision
value: 75.35796605434099
- type: euclidean_recall
value: 83.06898675700647
- type: manhattan_accuracy
value: 89.36624364497226
- type: manhattan_ap
value: 86.65076471274106
- type: manhattan_f1
value: 79.07408783532733
- type: manhattan_precision
value: 76.41102972856527
- type: manhattan_recall
value: 81.92947336002464
- type: max_accuracy
value: 89.3681841114604
- type: max_ap
value: 86.65166462876266
- type: max_f1
value: 79.07408783532733
license: apache-2.0
language:
- en
---
# nomic-embed-text-v1.5: Resizable Production Embeddings with Matryoshka Representation Learning
**Exciting Update!**: `nomic-embed-text-v1.5` is now multimodal! [nomic-embed-vision-v1](https://huggingface.co/nomic-ai/nomic-embed-vision-v1.5) is aligned to the embedding space of `nomic-embed-text-v1.5`, meaning any text embedding is multimodal!
## Usage
**Important**: the text prompt *must* include a *task instruction prefix*, instructing the model which task is being performed.
For example, if you are implementing a RAG application, you embed your documents as `search_document: <text here>` and embed your user queries as `search_query: <text here>`.
## Task instruction prefixes
### `search_document`
#### Purpose: embed texts as documents from a dataset
This prefix is used for embedding texts as documents, for example as documents for a RAG index.
```python
from sentence_transformers import SentenceTransformer
model = SentenceTransformer("nomic-ai/nomic-embed-text-v1", trust_remote_code=True)
sentences = ['search_document: TSNE is a dimensionality reduction algorithm created by Laurens van Der Maaten']
embeddings = model.encode(sentences)
print(embeddings)
```
### `search_query`
#### Purpose: embed texts as questions to answer
This prefix is used for embedding texts as questions that documents from a dataset could resolve, for example as queries to be answered by a RAG application.
```python
from sentence_transformers import SentenceTransformer
model = SentenceTransformer("nomic-ai/nomic-embed-text-v1", trust_remote_code=True)
sentences = ['search_query: Who is Laurens van Der Maaten?']
embeddings = model.encode(sentences)
print(embeddings)
```
### `clustering`
#### Purpose: embed texts to group them into clusters
This prefix is used for embedding texts in order to group them into clusters, discover common topics, or remove semantic duplicates.
```python
from sentence_transformers import SentenceTransformer
model = SentenceTransformer("nomic-ai/nomic-embed-text-v1", trust_remote_code=True)
sentences = ['clustering: the quick brown fox']
embeddings = model.encode(sentences)
print(embeddings)
```
### `classification`
#### Purpose: embed texts to classify them
This prefix is used for embedding texts into vectors that will be used as features for a classification model
```python
from sentence_transformers import SentenceTransformer
model = SentenceTransformer("nomic-ai/nomic-embed-text-v1", trust_remote_code=True)
sentences = ['classification: the quick brown fox']
embeddings = model.encode(sentences)
print(embeddings)
```
### Sentence Transformers
```python
import torch.nn.functional as F
from sentence_transformers import SentenceTransformer
matryoshka_dim = 512
model = SentenceTransformer("nomic-ai/nomic-embed-text-v1.5", trust_remote_code=True)
sentences = ['search_query: What is TSNE?', 'search_query: Who is Laurens van der Maaten?']
embeddings = model.encode(sentences, convert_to_tensor=True)
embeddings = F.layer_norm(embeddings, normalized_shape=(embeddings.shape[1],))
embeddings = embeddings[:, :matryoshka_dim]
embeddings = F.normalize(embeddings, p=2, dim=1)
print(embeddings)
```
### Transformers
```diff
import torch
import torch.nn.functional as F
from transformers import AutoTokenizer, AutoModel
def mean_pooling(model_output, attention_mask):
token_embeddings = model_output[0]
input_mask_expanded = attention_mask.unsqueeze(-1).expand(token_embeddings.size()).float()
return torch.sum(token_embeddings * input_mask_expanded, 1) / torch.clamp(input_mask_expanded.sum(1), min=1e-9)
sentences = ['search_query: What is TSNE?', 'search_query: Who is Laurens van der Maaten?']
tokenizer = AutoTokenizer.from_pretrained('bert-base-uncased')
model = AutoModel.from_pretrained('nomic-ai/nomic-embed-text-v1.5', trust_remote_code=True, safe_serialization=True)
model.eval()
encoded_input = tokenizer(sentences, padding=True, truncation=True, return_tensors='pt')
+ matryoshka_dim = 512
with torch.no_grad():
model_output = model(**encoded_input)
embeddings = mean_pooling(model_output, encoded_input['attention_mask'])
+ embeddings = F.layer_norm(embeddings, normalized_shape=(embeddings.shape[1],))
+ embeddings = embeddings[:, :matryoshka_dim]
embeddings = F.normalize(embeddings, p=2, dim=1)
print(embeddings)
```
The model natively supports scaling of the sequence length past 2048 tokens. To do so,
```diff
- tokenizer = AutoTokenizer.from_pretrained('bert-base-uncased')
+ tokenizer = AutoTokenizer.from_pretrained('bert-base-uncased', model_max_length=8192)
- model = AutoModel.from_pretrained('nomic-ai/nomic-embed-text-v1', trust_remote_code=True)
+ model = AutoModel.from_pretrained('nomic-ai/nomic-embed-text-v1', trust_remote_code=True, rotary_scaling_factor=2)
```
### Transformers.js
```js
import { pipeline, layer_norm } from '@xenova/transformers';
// Create a feature extraction pipeline
const extractor = await pipeline('feature-extraction', 'nomic-ai/nomic-embed-text-v1.5', {
quantized: false, // Comment out this line to use the quantized version
});
// Define sentences
const texts = ['search_query: What is TSNE?', 'search_query: Who is Laurens van der Maaten?'];
// Compute sentence embeddings
let embeddings = await extractor(texts, { pooling: 'mean' });
console.log(embeddings); // Tensor of shape [2, 768]
const matryoshka_dim = 512;
embeddings = layer_norm(embeddings, [embeddings.dims[1]])
.slice(null, [0, matryoshka_dim])
.normalize(2, -1);
console.log(embeddings.tolist());
```
## Nomic API
The easiest way to use Nomic Embed is through the Nomic Embedding API.
Generating embeddings with the `nomic` Python client is as easy as
```python
from nomic import embed
output = embed.text(
texts=['Nomic Embedding API', '#keepAIOpen'],
model='nomic-embed-text-v1.5',
task_type='search_document',
dimensionality=256,
)
print(output)
```
For more information, see the [API reference](https://docs.nomic.ai/reference/endpoints/nomic-embed-text)
## Adjusting Dimensionality
`nomic-embed-text-v1.5` is an improvement upon [Nomic Embed](https://huggingface.co/nomic-ai/nomic-embed-text-v1) that utilizes [Matryoshka Representation Learning](https://arxiv.org/abs/2205.13147) which gives developers the flexibility to trade off the embedding size for a negligible reduction in performance.
| Name | SeqLen | Dimension | MTEB |
| :-------------------------------:| :----- | :-------- | :------: |
| nomic-embed-text-v1 | 8192 | 768 | **62.39** |
| nomic-embed-text-v1.5 | 8192 | 768 | 62.28 |
| nomic-embed-text-v1.5 | 8192 | 512 | 61.96 |
| nomic-embed-text-v1.5 | 8192 | 256 | 61.04 |
| nomic-embed-text-v1.5 | 8192 | 128 | 59.34 |
| nomic-embed-text-v1.5 | 8192 | 64 | 56.10 |

## Training
Click the Nomic Atlas map below to visualize a 5M sample of our contrastive pretraining data!
[](https://atlas.nomic.ai/map/nomic-text-embed-v1-5m-sample)
We train our embedder using a multi-stage training pipeline. Starting from a long-context [BERT model](https://huggingface.co/nomic-ai/nomic-bert-2048),
the first unsupervised contrastive stage trains on a dataset generated from weakly related text pairs, such as question-answer pairs from forums like StackExchange and Quora, title-body pairs from Amazon reviews, and summarizations from news articles.
In the second finetuning stage, higher quality labeled datasets such as search queries and answers from web searches are leveraged. Data curation and hard-example mining is crucial in this stage.
For more details, see the Nomic Embed [Technical Report](https://static.nomic.ai/reports/2024_Nomic_Embed_Text_Technical_Report.pdf) and corresponding [blog post](https://blog.nomic.ai/posts/nomic-embed-matryoshka).
Training data to train the models is released in its entirety. For more details, see the `contrastors` [repository](https://github.com/nomic-ai/contrastors)
# Join the Nomic Community
- Nomic: [https://nomic.ai](https://nomic.ai)
- Discord: [https://discord.gg/myY5YDR8z8](https://discord.gg/myY5YDR8z8)
- Twitter: [https://twitter.com/nomic_ai](https://twitter.com/nomic_ai)
# Citation
If you find the model, dataset, or training code useful, please cite our work
```bibtex
@misc{nussbaum2024nomic,
title={Nomic Embed: Training a Reproducible Long Context Text Embedder},
author={Zach Nussbaum and John X. Morris and Brandon Duderstadt and Andriy Mulyar},
year={2024},
eprint={2402.01613},
archivePrefix={arXiv},
primaryClass={cs.CL}
}
``` |
timm/mobilenetv3_large_100.ra_in1k | timm | "2023-04-27T22:49:21Z" | 446,997 | 32 | timm | [
"timm",
"pytorch",
"safetensors",
"image-classification",
"dataset:imagenet-1k",
"arxiv:2110.00476",
"arxiv:1905.02244",
"license:apache-2.0",
"region:us"
] | image-classification | "2022-12-16T05:38:07Z" | ---
tags:
- image-classification
- timm
library_name: timm
license: apache-2.0
datasets:
- imagenet-1k
---
# Model card for mobilenetv3_large_100.ra_in1k
A MobileNet-v3 image classification model. Trained on ImageNet-1k in `timm` using recipe template described below.
Recipe details:
* RandAugment `RA` recipe. Inspired by and evolved from EfficientNet RandAugment recipes. Published as `B` recipe in [ResNet Strikes Back](https://arxiv.org/abs/2110.00476).
* RMSProp (TF 1.0 behaviour) optimizer, EMA weight averaging
* Step (exponential decay w/ staircase) LR schedule with warmup
## Model Details
- **Model Type:** Image classification / feature backbone
- **Model Stats:**
- Params (M): 5.5
- GMACs: 0.2
- Activations (M): 4.4
- Image size: 224 x 224
- **Papers:**
- Searching for MobileNetV3: https://arxiv.org/abs/1905.02244
- ResNet strikes back: An improved training procedure in timm: https://arxiv.org/abs/2110.00476
- **Dataset:** ImageNet-1k
- **Original:** https://github.com/huggingface/pytorch-image-models
## Model Usage
### Image Classification
```python
from urllib.request import urlopen
from PIL import Image
import timm
img = Image.open(urlopen(
'https://huggingface.co/datasets/huggingface/documentation-images/resolve/main/beignets-task-guide.png'
))
model = timm.create_model('mobilenetv3_large_100.ra_in1k', pretrained=True)
model = model.eval()
# get model specific transforms (normalization, resize)
data_config = timm.data.resolve_model_data_config(model)
transforms = timm.data.create_transform(**data_config, is_training=False)
output = model(transforms(img).unsqueeze(0)) # unsqueeze single image into batch of 1
top5_probabilities, top5_class_indices = torch.topk(output.softmax(dim=1) * 100, k=5)
```
### Feature Map Extraction
```python
from urllib.request import urlopen
from PIL import Image
import timm
img = Image.open(urlopen(
'https://huggingface.co/datasets/huggingface/documentation-images/resolve/main/beignets-task-guide.png'
))
model = timm.create_model(
'mobilenetv3_large_100.ra_in1k',
pretrained=True,
features_only=True,
)
model = model.eval()
# get model specific transforms (normalization, resize)
data_config = timm.data.resolve_model_data_config(model)
transforms = timm.data.create_transform(**data_config, is_training=False)
output = model(transforms(img).unsqueeze(0)) # unsqueeze single image into batch of 1
for o in output:
# print shape of each feature map in output
# e.g.:
# torch.Size([1, 16, 112, 112])
# torch.Size([1, 24, 56, 56])
# torch.Size([1, 40, 28, 28])
# torch.Size([1, 112, 14, 14])
# torch.Size([1, 960, 7, 7])
print(o.shape)
```
### Image Embeddings
```python
from urllib.request import urlopen
from PIL import Image
import timm
img = Image.open(urlopen(
'https://huggingface.co/datasets/huggingface/documentation-images/resolve/main/beignets-task-guide.png'
))
model = timm.create_model(
'mobilenetv3_large_100.ra_in1k',
pretrained=True,
num_classes=0, # remove classifier nn.Linear
)
model = model.eval()
# get model specific transforms (normalization, resize)
data_config = timm.data.resolve_model_data_config(model)
transforms = timm.data.create_transform(**data_config, is_training=False)
output = model(transforms(img).unsqueeze(0)) # output is (batch_size, num_features) shaped tensor
# or equivalently (without needing to set num_classes=0)
output = model.forward_features(transforms(img).unsqueeze(0))
# output is unpooled, a (1, 960, 7, 7) shaped tensor
output = model.forward_head(output, pre_logits=True)
# output is a (1, num_features) shaped tensor
```
## Model Comparison
Explore the dataset and runtime metrics of this model in timm [model results](https://github.com/huggingface/pytorch-image-models/tree/main/results).
## Citation
```bibtex
@inproceedings{howard2019searching,
title={Searching for mobilenetv3},
author={Howard, Andrew and Sandler, Mark and Chu, Grace and Chen, Liang-Chieh and Chen, Bo and Tan, Mingxing and Wang, Weijun and Zhu, Yukun and Pang, Ruoming and Vasudevan, Vijay and others},
booktitle={Proceedings of the IEEE/CVF international conference on computer vision},
pages={1314--1324},
year={2019}
}
```
```bibtex
@misc{rw2019timm,
author = {Ross Wightman},
title = {PyTorch Image Models},
year = {2019},
publisher = {GitHub},
journal = {GitHub repository},
doi = {10.5281/zenodo.4414861},
howpublished = {\url{https://github.com/huggingface/pytorch-image-models}}
}
```
```bibtex
@inproceedings{wightman2021resnet,
title={ResNet strikes back: An improved training procedure in timm},
author={Wightman, Ross and Touvron, Hugo and Jegou, Herve},
booktitle={NeurIPS 2021 Workshop on ImageNet: Past, Present, and Future}
}
```
|
TheBloke/Mistral-7B-OpenOrca-GPTQ | TheBloke | "2023-10-16T08:48:47Z" | 445,402 | 100 | transformers | [
"transformers",
"safetensors",
"mistral",
"text-generation",
"conversational",
"en",
"dataset:Open-Orca/OpenOrca",
"arxiv:2306.02707",
"arxiv:2301.13688",
"base_model:Open-Orca/Mistral-7B-OpenOrca",
"base_model:quantized:Open-Orca/Mistral-7B-OpenOrca",
"license:apache-2.0",
"autotrain_compatible",
"text-generation-inference",
"4-bit",
"gptq",
"region:us"
] | text-generation | "2023-10-02T14:28:09Z" | ---
base_model: Open-Orca/Mistral-7B-OpenOrca
datasets:
- Open-Orca/OpenOrca
inference: false
language:
- en
library_name: transformers
license: apache-2.0
model_creator: OpenOrca
model_name: Mistral 7B OpenOrca
model_type: mistral
pipeline_tag: text-generation
prompt_template: '<|im_start|>system
{system_message}<|im_end|>
<|im_start|>user
{prompt}<|im_end|>
<|im_start|>assistant
'
quantized_by: TheBloke
---
<!-- header start -->
<!-- 200823 -->
<div style="width: auto; margin-left: auto; margin-right: auto">
<img src="https://i.imgur.com/EBdldam.jpg" alt="TheBlokeAI" style="width: 100%; min-width: 400px; display: block; margin: auto;">
</div>
<div style="display: flex; justify-content: space-between; width: 100%;">
<div style="display: flex; flex-direction: column; align-items: flex-start;">
<p style="margin-top: 0.5em; margin-bottom: 0em;"><a href="https://discord.gg/theblokeai">Chat & support: TheBloke's Discord server</a></p>
</div>
<div style="display: flex; flex-direction: column; align-items: flex-end;">
<p style="margin-top: 0.5em; margin-bottom: 0em;"><a href="https://www.patreon.com/TheBlokeAI">Want to contribute? TheBloke's Patreon page</a></p>
</div>
</div>
<div style="text-align:center; margin-top: 0em; margin-bottom: 0em"><p style="margin-top: 0.25em; margin-bottom: 0em;">TheBloke's LLM work is generously supported by a grant from <a href="https://a16z.com">andreessen horowitz (a16z)</a></p></div>
<hr style="margin-top: 1.0em; margin-bottom: 1.0em;">
<!-- header end -->
# Mistral 7B OpenOrca - GPTQ
- Model creator: [OpenOrca](https://huggingface.co/Open-Orca)
- Original model: [Mistral 7B OpenOrca](https://huggingface.co/Open-Orca/Mistral-7B-OpenOrca)
<!-- description start -->
## Description
This repo contains GPTQ model files for [OpenOrca's Mistral 7B OpenOrca](https://huggingface.co/Open-Orca/Mistral-7B-OpenOrca).
Multiple GPTQ parameter permutations are provided; see Provided Files below for details of the options provided, their parameters, and the software used to create them.
<!-- description end -->
<!-- repositories-available start -->
## Repositories available
* [AWQ model(s) for GPU inference.](https://huggingface.co/TheBloke/Mistral-7B-OpenOrca-AWQ)
* [GPTQ models for GPU inference, with multiple quantisation parameter options.](https://huggingface.co/TheBloke/Mistral-7B-OpenOrca-GPTQ)
* [2, 3, 4, 5, 6 and 8-bit GGUF models for CPU+GPU inference](https://huggingface.co/TheBloke/Mistral-7B-OpenOrca-GGUF)
* [OpenOrca's original unquantised fp16 model in pytorch format, for GPU inference and for further conversions](https://huggingface.co/Open-Orca/Mistral-7B-OpenOrca)
<!-- repositories-available end -->
<!-- prompt-template start -->
## Prompt template: ChatML
```
<|im_start|>system
{system_message}<|im_end|>
<|im_start|>user
{prompt}<|im_end|>
<|im_start|>assistant
```
<!-- prompt-template end -->
<!-- README_GPTQ.md-provided-files start -->
## Provided files, and GPTQ parameters
Multiple quantisation parameters are provided, to allow you to choose the best one for your hardware and requirements.
Each separate quant is in a different branch. See below for instructions on fetching from different branches.
Most GPTQ files are made with AutoGPTQ. Mistral models are currently made with Transformers.
<details>
<summary>Explanation of GPTQ parameters</summary>
- Bits: The bit size of the quantised model.
- GS: GPTQ group size. Higher numbers use less VRAM, but have lower quantisation accuracy. "None" is the lowest possible value.
- Act Order: True or False. Also known as `desc_act`. True results in better quantisation accuracy. Some GPTQ clients have had issues with models that use Act Order plus Group Size, but this is generally resolved now.
- Damp %: A GPTQ parameter that affects how samples are processed for quantisation. 0.01 is default, but 0.1 results in slightly better accuracy.
- GPTQ dataset: The calibration dataset used during quantisation. Using a dataset more appropriate to the model's training can improve quantisation accuracy. Note that the GPTQ calibration dataset is not the same as the dataset used to train the model - please refer to the original model repo for details of the training dataset(s).
- Sequence Length: The length of the dataset sequences used for quantisation. Ideally this is the same as the model sequence length. For some very long sequence models (16+K), a lower sequence length may have to be used. Note that a lower sequence length does not limit the sequence length of the quantised model. It only impacts the quantisation accuracy on longer inference sequences.
- ExLlama Compatibility: Whether this file can be loaded with ExLlama, which currently only supports Llama models in 4-bit.
</details>
| Branch | Bits | GS | Act Order | Damp % | GPTQ Dataset | Seq Len | Size | ExLlama | Desc |
| ------ | ---- | -- | --------- | ------ | ------------ | ------- | ---- | ------- | ---- |
| [main](https://huggingface.co/TheBloke/Mistral-7B-OpenOrca-GPTQ/tree/main) | 4 | 128 | Yes | 0.1 | [wikitext](https://huggingface.co/datasets/wikitext/viewer/wikitext-2-v1/test) | 32768 | 4.16 GB | Yes | 4-bit, with Act Order and group size 128g. Uses even less VRAM than 64g, but with slightly lower accuracy. |
| [gptq-4bit-32g-actorder_True](https://huggingface.co/TheBloke/Mistral-7B-OpenOrca-GPTQ/tree/gptq-4bit-32g-actorder_True) | 4 | 32 | Yes | 0.1 | [wikitext](https://huggingface.co/datasets/wikitext/viewer/wikitext-2-v1/test) | 32768 | 4.57 GB | Yes | 4-bit, with Act Order and group size 32g. Gives highest possible inference quality, with maximum VRAM usage. |
| [gptq-8bit--1g-actorder_True](https://huggingface.co/TheBloke/Mistral-7B-OpenOrca-GPTQ/tree/gptq-8bit--1g-actorder_True) | 8 | None | Yes | 0.1 | [wikitext](https://huggingface.co/datasets/wikitext/viewer/wikitext-2-v1/test) | 32768 | 7.52 GB | No | 8-bit, with Act Order. No group size, to lower VRAM requirements. |
| [gptq-8bit-128g-actorder_True](https://huggingface.co/TheBloke/Mistral-7B-OpenOrca-GPTQ/tree/gptq-8bit-128g-actorder_True) | 8 | 128 | Yes | 0.1 | [wikitext](https://huggingface.co/datasets/wikitext/viewer/wikitext-2-v1/test) | 32768 | 7.68 GB | No | 8-bit, with group size 128g for higher inference quality and with Act Order for even higher accuracy. |
| [gptq-8bit-32g-actorder_True](https://huggingface.co/TheBloke/Mistral-7B-OpenOrca-GPTQ/tree/gptq-8bit-32g-actorder_True) | 8 | 32 | Yes | 0.1 | [wikitext](https://huggingface.co/datasets/wikitext/viewer/wikitext-2-v1/test) | 32768 | 8.17 GB | No | 8-bit, with group size 32g and Act Order for maximum inference quality. |
| [gptq-4bit-64g-actorder_True](https://huggingface.co/TheBloke/Mistral-7B-OpenOrca-GPTQ/tree/gptq-4bit-64g-actorder_True) | 4 | 64 | Yes | 0.1 | [wikitext](https://huggingface.co/datasets/wikitext/viewer/wikitext-2-v1/test) | 32768 | 4.30 GB | Yes | 4-bit, with Act Order and group size 64g. Uses less VRAM than 32g, but with slightly lower accuracy. |
<!-- README_GPTQ.md-provided-files end -->
<!-- README_GPTQ.md-download-from-branches start -->
## How to download, including from branches
### In text-generation-webui
To download from the `main` branch, enter `TheBloke/Mistral-7B-OpenOrca-GPTQ` in the "Download model" box.
To download from another branch, add `:branchname` to the end of the download name, eg `TheBloke/Mistral-7B-OpenOrca-GPTQ:gptq-4bit-32g-actorder_True`
### From the command line
I recommend using the `huggingface-hub` Python library:
```shell
pip3 install huggingface-hub
```
To download the `main` branch to a folder called `Mistral-7B-OpenOrca-GPTQ`:
```shell
mkdir Mistral-7B-OpenOrca-GPTQ
huggingface-cli download TheBloke/Mistral-7B-OpenOrca-GPTQ --local-dir Mistral-7B-OpenOrca-GPTQ --local-dir-use-symlinks False
```
To download from a different branch, add the `--revision` parameter:
```shell
mkdir Mistral-7B-OpenOrca-GPTQ
huggingface-cli download TheBloke/Mistral-7B-OpenOrca-GPTQ --revision gptq-4bit-32g-actorder_True --local-dir Mistral-7B-OpenOrca-GPTQ --local-dir-use-symlinks False
```
<details>
<summary>More advanced huggingface-cli download usage</summary>
If you remove the `--local-dir-use-symlinks False` parameter, the files will instead be stored in the central Huggingface cache directory (default location on Linux is: `~/.cache/huggingface`), and symlinks will be added to the specified `--local-dir`, pointing to their real location in the cache. This allows for interrupted downloads to be resumed, and allows you to quickly clone the repo to multiple places on disk without triggering a download again. The downside, and the reason why I don't list that as the default option, is that the files are then hidden away in a cache folder and it's harder to know where your disk space is being used, and to clear it up if/when you want to remove a download model.
The cache location can be changed with the `HF_HOME` environment variable, and/or the `--cache-dir` parameter to `huggingface-cli`.
For more documentation on downloading with `huggingface-cli`, please see: [HF -> Hub Python Library -> Download files -> Download from the CLI](https://huggingface.co/docs/huggingface_hub/guides/download#download-from-the-cli).
To accelerate downloads on fast connections (1Gbit/s or higher), install `hf_transfer`:
```shell
pip3 install hf_transfer
```
And set environment variable `HF_HUB_ENABLE_HF_TRANSFER` to `1`:
```shell
mkdir Mistral-7B-OpenOrca-GPTQ
HF_HUB_ENABLE_HF_TRANSFER=1 huggingface-cli download TheBloke/Mistral-7B-OpenOrca-GPTQ --local-dir Mistral-7B-OpenOrca-GPTQ --local-dir-use-symlinks False
```
Windows Command Line users: You can set the environment variable by running `set HF_HUB_ENABLE_HF_TRANSFER=1` before the download command.
</details>
### With `git` (**not** recommended)
To clone a specific branch with `git`, use a command like this:
```shell
git clone --single-branch --branch gptq-4bit-32g-actorder_True https://huggingface.co/TheBloke/Mistral-7B-OpenOrca-GPTQ
```
Note that using Git with HF repos is strongly discouraged. It will be much slower than using `huggingface-hub`, and will use twice as much disk space as it has to store the model files twice (it stores every byte both in the intended target folder, and again in the `.git` folder as a blob.)
<!-- README_GPTQ.md-download-from-branches end -->
<!-- README_GPTQ.md-text-generation-webui start -->
## How to easily download and use this model in [text-generation-webui](https://github.com/oobabooga/text-generation-webui).
Please make sure you're using the latest version of [text-generation-webui](https://github.com/oobabooga/text-generation-webui).
It is strongly recommended to use the text-generation-webui one-click-installers unless you're sure you know how to make a manual install.
1. Click the **Model tab**.
2. Under **Download custom model or LoRA**, enter `TheBloke/Mistral-7B-OpenOrca-GPTQ`.
- To download from a specific branch, enter for example `TheBloke/Mistral-7B-OpenOrca-GPTQ:gptq-4bit-32g-actorder_True`
- see Provided Files above for the list of branches for each option.
3. Click **Download**.
4. The model will start downloading. Once it's finished it will say "Done".
5. In the top left, click the refresh icon next to **Model**.
6. In the **Model** dropdown, choose the model you just downloaded: `Mistral-7B-OpenOrca-GPTQ`
7. The model will automatically load, and is now ready for use!
8. If you want any custom settings, set them and then click **Save settings for this model** followed by **Reload the Model** in the top right.
* Note that you do not need to and should not set manual GPTQ parameters any more. These are set automatically from the file `quantize_config.json`.
9. Once you're ready, click the **Text Generation tab** and enter a prompt to get started!
<!-- README_GPTQ.md-text-generation-webui end -->
<!-- README_GPTQ.md-use-from-tgi start -->
## Serving this model from Text Generation Inference (TGI)
It's recommended to use TGI version 1.1.0 or later. The official Docker container is: `ghcr.io/huggingface/text-generation-inference:1.1.0`
Example Docker parameters:
```shell
--model-id TheBloke/Mistral-7B-OpenOrca-GPTQ --port 3000 --quantize gptq --max-input-length 3696 --max-total-tokens 4096 --max-batch-prefill-tokens 4096
```
Example Python code for interfacing with TGI (requires huggingface-hub 0.17.0 or later):
```shell
pip3 install huggingface-hub
```
```python
from huggingface_hub import InferenceClient
endpoint_url = "https://your-endpoint-url-here"
prompt = "Tell me about AI"
prompt_template=f'''<|im_start|>system
{system_message}<|im_end|>
<|im_start|>user
{prompt}<|im_end|>
<|im_start|>assistant
'''
client = InferenceClient(endpoint_url)
response = client.text_generation(prompt,
max_new_tokens=128,
do_sample=True,
temperature=0.7,
top_p=0.95,
top_k=40,
repetition_penalty=1.1)
print(f"Model output: {response}")
```
<!-- README_GPTQ.md-use-from-tgi end -->
<!-- README_GPTQ.md-use-from-python start -->
## How to use this GPTQ model from Python code
### Install the necessary packages
Requires: Transformers 4.33.0 or later, Optimum 1.12.0 or later, and AutoGPTQ 0.4.2 or later.
```shell
pip3 install transformers optimum
pip3 install auto-gptq --extra-index-url https://huggingface.github.io/autogptq-index/whl/cu118/ # Use cu117 if on CUDA 11.7
```
If you have problems installing AutoGPTQ using the pre-built wheels, install it from source instead:
```shell
pip3 uninstall -y auto-gptq
git clone https://github.com/PanQiWei/AutoGPTQ
cd AutoGPTQ
git checkout v0.4.2
pip3 install .
```
### You can then use the following code
```python
from transformers import AutoModelForCausalLM, AutoTokenizer, pipeline
model_name_or_path = "TheBloke/Mistral-7B-OpenOrca-GPTQ"
# To use a different branch, change revision
# For example: revision="gptq-4bit-32g-actorder_True"
model = AutoModelForCausalLM.from_pretrained(model_name_or_path,
device_map="auto",
trust_remote_code=False,
revision="main")
tokenizer = AutoTokenizer.from_pretrained(model_name_or_path, use_fast=True)
prompt = "Tell me about AI"
prompt_template=f'''<|im_start|>system
{system_message}<|im_end|>
<|im_start|>user
{prompt}<|im_end|>
<|im_start|>assistant
'''
print("\n\n*** Generate:")
input_ids = tokenizer(prompt_template, return_tensors='pt').input_ids.cuda()
output = model.generate(inputs=input_ids, temperature=0.7, do_sample=True, top_p=0.95, top_k=40, max_new_tokens=512)
print(tokenizer.decode(output[0]))
# Inference can also be done using transformers' pipeline
print("*** Pipeline:")
pipe = pipeline(
"text-generation",
model=model,
tokenizer=tokenizer,
max_new_tokens=512,
do_sample=True,
temperature=0.7,
top_p=0.95,
top_k=40,
repetition_penalty=1.1
)
print(pipe(prompt_template)[0]['generated_text'])
```
<!-- README_GPTQ.md-use-from-python end -->
<!-- README_GPTQ.md-compatibility start -->
## Compatibility
The files provided are tested to work with AutoGPTQ, both via Transformers and using AutoGPTQ directly. They should also work with [Occ4m's GPTQ-for-LLaMa fork](https://github.com/0cc4m/KoboldAI).
[ExLlama](https://github.com/turboderp/exllama) is compatible with Llama and Mistral models in 4-bit. Please see the Provided Files table above for per-file compatibility.
[Huggingface Text Generation Inference (TGI)](https://github.com/huggingface/text-generation-inference) is compatible with all GPTQ models.
<!-- README_GPTQ.md-compatibility end -->
<!-- footer start -->
<!-- 200823 -->
## Discord
For further support, and discussions on these models and AI in general, join us at:
[TheBloke AI's Discord server](https://discord.gg/theblokeai)
## Thanks, and how to contribute
Thanks to the [chirper.ai](https://chirper.ai) team!
Thanks to Clay from [gpus.llm-utils.org](llm-utils)!
I've had a lot of people ask if they can contribute. I enjoy providing models and helping people, and would love to be able to spend even more time doing it, as well as expanding into new projects like fine tuning/training.
If you're able and willing to contribute it will be most gratefully received and will help me to keep providing more models, and to start work on new AI projects.
Donaters will get priority support on any and all AI/LLM/model questions and requests, access to a private Discord room, plus other benefits.
* Patreon: https://patreon.com/TheBlokeAI
* Ko-Fi: https://ko-fi.com/TheBlokeAI
**Special thanks to**: Aemon Algiz.
**Patreon special mentions**: Pierre Kircher, Stanislav Ovsiannikov, Michael Levine, Eugene Pentland, Andrey, 준교 김, Randy H, Fred von Graf, Artur Olbinski, Caitlyn Gatomon, terasurfer, Jeff Scroggin, James Bentley, Vadim, Gabriel Puliatti, Harry Royden McLaughlin, Sean Connelly, Dan Guido, Edmond Seymore, Alicia Loh, subjectnull, AzureBlack, Manuel Alberto Morcote, Thomas Belote, Lone Striker, Chris Smitley, Vitor Caleffi, Johann-Peter Hartmann, Clay Pascal, biorpg, Brandon Frisco, sidney chen, transmissions 11, Pedro Madruga, jinyuan sun, Ajan Kanaga, Emad Mostaque, Trenton Dambrowitz, Jonathan Leane, Iucharbius, usrbinkat, vamX, George Stoitzev, Luke Pendergrass, theTransient, Olakabola, Swaroop Kallakuri, Cap'n Zoog, Brandon Phillips, Michael Dempsey, Nikolai Manek, danny, Matthew Berman, Gabriel Tamborski, alfie_i, Raymond Fosdick, Tom X Nguyen, Raven Klaugh, LangChain4j, Magnesian, Illia Dulskyi, David Ziegler, Mano Prime, Luis Javier Navarrete Lozano, Erik Bjäreholt, 阿明, Nathan Dryer, Alex, Rainer Wilmers, zynix, TL, Joseph William Delisle, John Villwock, Nathan LeClaire, Willem Michiel, Joguhyik, GodLy, OG, Alps Aficionado, Jeffrey Morgan, ReadyPlayerEmma, Tiffany J. Kim, Sebastain Graf, Spencer Kim, Michael Davis, webtim, Talal Aujan, knownsqashed, John Detwiler, Imad Khwaja, Deo Leter, Jerry Meng, Elijah Stavena, Rooh Singh, Pieter, SuperWojo, Alexandros Triantafyllidis, Stephen Murray, Ai Maven, ya boyyy, Enrico Ros, Ken Nordquist, Deep Realms, Nicholas, Spiking Neurons AB, Elle, Will Dee, Jack West, RoA, Luke @flexchar, Viktor Bowallius, Derek Yates, Subspace Studios, jjj, Toran Billups, Asp the Wyvern, Fen Risland, Ilya, NimbleBox.ai, Chadd, Nitin Borwankar, Emre, Mandus, Leonard Tan, Kalila, K, Trailburnt, S_X, Cory Kujawski
Thank you to all my generous patrons and donaters!
And thank you again to a16z for their generous grant.
<!-- footer end -->
# Original model card: OpenOrca's Mistral 7B OpenOrca
<p><h1>🐋 TBD 🐋</h1></p>

[<img src="https://raw.githubusercontent.com/OpenAccess-AI-Collective/axolotl/main/image/axolotl-badge-web.png" alt="Built with Axolotl" width="200" height="32"/>](https://github.com/OpenAccess-AI-Collective/axolotl)
# OpenOrca - Mistral - 7B - 8k
We have used our own [OpenOrca dataset](https://huggingface.co/datasets/Open-Orca/OpenOrca) to fine-tune on top of [Mistral 7B](https://huggingface.co/mistralai/Mistral-7B-v0.1).
This dataset is our attempt to reproduce the dataset generated for Microsoft Research's [Orca Paper](https://arxiv.org/abs/2306.02707).
We use [OpenChat](https://huggingface.co/openchat) packing, trained with [Axolotl](https://github.com/OpenAccess-AI-Collective/axolotl).
This release is trained on a curated filtered subset of most of our GPT-4 augmented data.
It is the same subset of our data as was used in our [OpenOrcaxOpenChat-Preview2-13B model](https://huggingface.co/Open-Orca/OpenOrcaxOpenChat-Preview2-13B).
HF Leaderboard evals place this model as #2 for all models smaller than 30B at release time, outperforming all but one 13B model.
TBD
Want to visualize our full (pre-filtering) dataset? Check out our [Nomic Atlas Map](https://atlas.nomic.ai/map/c1b88b47-2d9b-47e0-9002-b80766792582/2560fd25-52fe-42f1-a58f-ff5eccc890d2).
[<img src="https://huggingface.co/Open-Orca/OpenOrca-Preview1-13B/resolve/main/OpenOrca%20Nomic%20Atlas.png" alt="Atlas Nomic Dataset Map" width="400" height="400" />](https://atlas.nomic.ai/map/c1b88b47-2d9b-47e0-9002-b80766792582/2560fd25-52fe-42f1-a58f-ff5eccc890d2)
We are in-process with training more models, so keep a look out on our org for releases coming soon with exciting partners.
We will also give sneak-peak announcements on our Discord, which you can find here:
https://AlignmentLab.ai
or on the OpenAccess AI Collective Discord for more information about Axolotl trainer here:
https://discord.gg/5y8STgB3P3
# Prompt Template
We used [OpenAI's Chat Markup Language (ChatML)](https://github.com/openai/openai-python/blob/main/chatml.md) format, with `<|im_start|>` and `<|im_end|>` tokens added to support this.
## Example Prompt Exchange
TBD
# Evaluation
We have evaluated using the methodology and tools for the HuggingFace Leaderboard, and find that we have significantly improved upon the base model.
TBD
## HuggingFaceH4 Open LLM Leaderboard Performance
TBD
## GPT4ALL Leaderboard Performance
TBD
# Dataset
We used a curated, filtered selection of most of the GPT-4 augmented data from our OpenOrca dataset, which aims to reproduce the Orca Research Paper dataset.
# Training
We trained with 8x A6000 GPUs for 62 hours, completing 4 epochs of full fine tuning on our dataset in one training run.
Commodity cost was ~$400.
# Citation
```bibtex
@misc{mukherjee2023orca,
title={Orca: Progressive Learning from Complex Explanation Traces of GPT-4},
author={Subhabrata Mukherjee and Arindam Mitra and Ganesh Jawahar and Sahaj Agarwal and Hamid Palangi and Ahmed Awadallah},
year={2023},
eprint={2306.02707},
archivePrefix={arXiv},
primaryClass={cs.CL}
}
@misc{longpre2023flan,
title={The Flan Collection: Designing Data and Methods for Effective Instruction Tuning},
author={Shayne Longpre and Le Hou and Tu Vu and Albert Webson and Hyung Won Chung and Yi Tay and Denny Zhou and Quoc V. Le and Barret Zoph and Jason Wei and Adam Roberts},
year={2023},
eprint={2301.13688},
archivePrefix={arXiv},
primaryClass={cs.AI}
}
```
|
Sudanl/stable-diffusion-2-1-base-custom | Sudanl | "2024-09-07T07:50:54Z" | 444,809 | 0 | diffusers | [
"diffusers",
"tensorboard",
"safetensors",
"text-to-image",
"stable-diffusion",
"stable-diffusion-diffusers",
"custom-diffusion",
"diffusers-training",
"base_model:stabilityai/stable-diffusion-2-1-base",
"base_model:adapter:stabilityai/stable-diffusion-2-1-base",
"license:creativeml-openrail-m",
"region:us"
] | text-to-image | "2024-09-06T19:44:11Z" | ---
base_model: stabilityai/stable-diffusion-2-1-base
library_name: diffusers
license: creativeml-openrail-m
tags:
- text-to-image
- diffusers
- stable-diffusion
- stable-diffusion-diffusers
- custom-diffusion
- diffusers-training
inference: true
---
<!-- This model card has been generated automatically according to the information the training script had access to. You
should probably proofread and complete it, then remove this comment. -->
# Custom Diffusion - Sudanl/stable-diffusion-2-1-base-custom
These are Custom Diffusion adaption weights for stabilityai/stable-diffusion-2-1-base. The weights were trained on None using [Custom Diffusion](https://www.cs.cmu.edu/~custom-diffusion). You can find some example images in the following.
For more details on the training, please follow [this link](https://github.com/huggingface/diffusers/blob/main/examples/custom_diffusion).
## Intended uses & limitations
#### How to use
```python
# TODO: add an example code snippet for running this diffusion pipeline
```
#### Limitations and bias
[TODO: provide examples of latent issues and potential remediations]
## Training details
[TODO: describe the data used to train the model] |
vinai/phobert-base-v2 | vinai | "2024-08-20T03:46:55Z" | 444,643 | 21 | transformers | [
"transformers",
"pytorch",
"roberta",
"fill-mask",
"vi",
"license:agpl-3.0",
"autotrain_compatible",
"endpoints_compatible",
"region:us"
] | fill-mask | "2023-04-24T10:53:35Z" | ---
license: agpl-3.0
language:
- vi
---
#### Table of contents
1. [Introduction](#introduction)
2. [Using PhoBERT with `transformers`](#transformers)
- [Installation](#install2)
- [Pre-trained models](#models2)
- [Example usage](#usage2)
3. [Using PhoBERT with `fairseq`](#fairseq)
4. [Notes](#vncorenlp)
# <a name="introduction"></a> PhoBERT: Pre-trained language models for Vietnamese
Pre-trained PhoBERT models are the state-of-the-art language models for Vietnamese ([Pho](https://en.wikipedia.org/wiki/Pho), i.e. "Phở", is a popular food in Vietnam):
- Two PhoBERT versions of "base" and "large" are the first public large-scale monolingual language models pre-trained for Vietnamese. PhoBERT pre-training approach is based on [RoBERTa](https://github.com/pytorch/fairseq/blob/master/examples/roberta/README.md) which optimizes the [BERT](https://github.com/google-research/bert) pre-training procedure for more robust performance.
- PhoBERT outperforms previous monolingual and multilingual approaches, obtaining new state-of-the-art performances on four downstream Vietnamese NLP tasks of Part-of-speech tagging, Dependency parsing, Named-entity recognition and Natural language inference.
The general architecture and experimental results of PhoBERT can be found in our [paper](https://www.aclweb.org/anthology/2020.findings-emnlp.92/):
@inproceedings{phobert,
title = {{PhoBERT: Pre-trained language models for Vietnamese}},
author = {Dat Quoc Nguyen and Anh Tuan Nguyen},
booktitle = {Findings of the Association for Computational Linguistics: EMNLP 2020},
year = {2020},
pages = {1037--1042}
}
**Please CITE** our paper when PhoBERT is used to help produce published results or is incorporated into other software.
## <a name="transformers"></a> Using PhoBERT with `transformers`
### Installation <a name="install2"></a>
- Install `transformers` with pip: `pip install transformers`, or [install `transformers` from source](https://huggingface.co/docs/transformers/installation#installing-from-source). <br />
Note that we merged a slow tokenizer for PhoBERT into the main `transformers` branch. The process of merging a fast tokenizer for PhoBERT is in the discussion, as mentioned in [this pull request](https://github.com/huggingface/transformers/pull/17254#issuecomment-1133932067). If users would like to utilize the fast tokenizer, the users might install `transformers` as follows:
```
git clone --single-branch --branch fast_tokenizers_BARTpho_PhoBERT_BERTweet https://github.com/datquocnguyen/transformers.git
cd transformers
pip3 install -e .
```
- Install `tokenizers` with pip: `pip3 install tokenizers`
### Pre-trained models <a name="models2"></a>
Model | #params | Arch. | Max length | Pre-training data
---|---|---|---|---
`vinai/phobert-base` | 135M | base | 256 | 20GB of Wikipedia and News texts
`vinai/phobert-large` | 370M | large | 256 | 20GB of Wikipedia and News texts
`vinai/phobert-base-v2` | 135M | base | 256 | 20GB of Wikipedia and News texts + 120GB of texts from OSCAR-2301
### Example usage <a name="usage2"></a>
```python
import torch
from transformers import AutoModel, AutoTokenizer
phobert = AutoModel.from_pretrained("vinai/phobert-base-v2")
tokenizer = AutoTokenizer.from_pretrained("vinai/phobert-base-v2")
# INPUT TEXT MUST BE ALREADY WORD-SEGMENTED!
sentence = 'Chúng_tôi là những nghiên_cứu_viên .'
input_ids = torch.tensor([tokenizer.encode(sentence)])
with torch.no_grad():
features = phobert(input_ids) # Models outputs are now tuples
## With TensorFlow 2.0+:
# from transformers import TFAutoModel
# phobert = TFAutoModel.from_pretrained("vinai/phobert-base")
```
## <a name="fairseq"></a> Using PhoBERT with `fairseq`
Please see details at [HERE](https://github.com/VinAIResearch/PhoBERT/blob/master/README_fairseq.md)!
## <a name="vncorenlp"></a> Notes
In case the input texts are `raw`, i.e. without word segmentation, a word segmenter must be applied to produce word-segmented texts before feeding to PhoBERT. As PhoBERT employed the [RDRSegmenter](https://github.com/datquocnguyen/RDRsegmenter) from [VnCoreNLP](https://github.com/vncorenlp/VnCoreNLP) to pre-process the pre-training data (including [Vietnamese tone normalization](https://github.com/VinAIResearch/BARTpho/blob/main/VietnameseToneNormalization.md) and word and sentence segmentation), it is recommended to also use the same word segmenter for PhoBERT-based downstream applications w.r.t. the input raw texts.
#### Installation
pip install py_vncorenlp
#### Example usage <a name="example"></a>
```python
import py_vncorenlp
# Automatically download VnCoreNLP components from the original repository
# and save them in some local machine folder
py_vncorenlp.download_model(save_dir='/absolute/path/to/vncorenlp')
# Load the word and sentence segmentation component
rdrsegmenter = py_vncorenlp.VnCoreNLP(annotators=["wseg"], save_dir='/absolute/path/to/vncorenlp')
text = "Ông Nguyễn Khắc Chúc đang làm việc tại Đại học Quốc gia Hà Nội. Bà Lan, vợ ông Chúc, cũng làm việc tại đây."
output = rdrsegmenter.word_segment(text)
print(output)
# ['Ông Nguyễn_Khắc_Chúc đang làm_việc tại Đại_học Quốc_gia Hà_Nội .', 'Bà Lan , vợ ông Chúc , cũng làm_việc tại đây .']
```
## License
Copyright (c) 2023 VinAI Research
This program is free software: you can redistribute it and/or modify
it under the terms of the GNU Affero General Public License as published
by the Free Software Foundation, either version 3 of the License, or
(at your option) any later version.
This program is distributed in the hope that it will be useful,
but WITHOUT ANY WARRANTY; without even the implied warranty of
MERCHANTABILITY or FITNESS FOR A PARTICULAR PURPOSE. See the
GNU Affero General Public License for more details.
You should have received a copy of the GNU Affero General Public License
along with this program. If not, see <https://www.gnu.org/licenses/>. |
rinna/japanese-cloob-vit-b-16 | rinna | "2024-07-22T08:09:24Z" | 444,046 | 12 | transformers | [
"transformers",
"pytorch",
"safetensors",
"cloob",
"feature-extraction",
"clip",
"vision",
"ja",
"arxiv:2110.11316",
"arxiv:2404.01657",
"license:apache-2.0",
"region:us"
] | feature-extraction | "2022-04-27T08:29:29Z" | ---
language: ja
thumbnail: https://github.com/rinnakk/japanese-pretrained-models/blob/master/rinna.png
license: apache-2.0
tags:
- feature-extraction
- clip
- cloob
- vision
inference: false
---
# rinna/japanese-cloob-vit-b-16

This is a Japanese [CLOOB (Contrastive Leave One Out Boost)](https://arxiv.org/abs/2110.11316) model trained by [rinna Co., Ltd.](https://corp.rinna.co.jp/).
Please see [japanese-clip](https://github.com/rinnakk/japanese-clip) for the other available models.
# How to use the model
1. Install package
```shell
$ pip install git+https://github.com/rinnakk/japanese-clip.git
```
2. Run
```python
import io
import requests
from PIL import Image
import torch
import japanese_clip as ja_clip
device = "cuda" if torch.cuda.is_available() else "cpu"
model, preprocess = ja_clip.load("rinna/japanese-cloob-vit-b-16", device=device)
tokenizer = ja_clip.load_tokenizer()
img = Image.open(io.BytesIO(requests.get('https://images.pexels.com/photos/2253275/pexels-photo-2253275.jpeg?auto=compress&cs=tinysrgb&dpr=3&h=750&w=1260').content))
image = preprocess(img).unsqueeze(0).to(device)
encodings = ja_clip.tokenize(
texts=["犬", "猫", "象"],
max_seq_len=77,
device=device,
tokenizer=tokenizer, # this is optional. if you don't pass, load tokenizer each time
)
with torch.no_grad():
image_features = model.get_image_features(image)
text_features = model.get_text_features(**encodings)
text_probs = (100.0 * image_features @ text_features.T).softmax(dim=-1)
print("Label probs:", text_probs) # prints: [[1.0, 0.0, 0.0]]
```
# Model architecture
The model was trained a ViT-B/16 Transformer architecture as an image encoder and uses a 12-layer BERT as a text encoder. The image encoder was initialized from the [AugReg `vit-base-patch16-224` model](https://github.com/google-research/vision_transformer).
# Training
The model was trained on [CC12M](https://github.com/google-research-datasets/conceptual-12m) translated the captions to Japanese.
# How to cite
```bibtex
@misc{rinna-japanese-cloob-vit-b-16,
title = {rinna/japanese-cloob-vit-b-16},
author = {Shing, Makoto and Zhao, Tianyu and Sawada, Kei},
url = {https://huggingface.co/rinna/japanese-cloob-vit-b-16}
}
@inproceedings{sawada2024release,
title = {Release of Pre-Trained Models for the {J}apanese Language},
author = {Sawada, Kei and Zhao, Tianyu and Shing, Makoto and Mitsui, Kentaro and Kaga, Akio and Hono, Yukiya and Wakatsuki, Toshiaki and Mitsuda, Koh},
booktitle = {Proceedings of the 2024 Joint International Conference on Computational Linguistics, Language Resources and Evaluation (LREC-COLING 2024)},
month = {5},
year = {2024},
pages = {13898--13905},
url = {https://aclanthology.org/2024.lrec-main.1213},
note = {\url{https://arxiv.org/abs/2404.01657}}
}
```
# License
[The Apache 2.0 license](https://www.apache.org/licenses/LICENSE-2.0) |
speechbrain/spkrec-ecapa-voxceleb | speechbrain | "2024-02-19T22:39:59Z" | 440,922 | 159 | speechbrain | [
"speechbrain",
"embeddings",
"Speaker",
"Verification",
"Identification",
"pytorch",
"ECAPA",
"TDNN",
"en",
"dataset:voxceleb",
"arxiv:2106.04624",
"license:apache-2.0",
"region:us"
] | null | "2022-03-02T23:29:05Z" | ---
language: "en"
thumbnail:
tags:
- speechbrain
- embeddings
- Speaker
- Verification
- Identification
- pytorch
- ECAPA
- TDNN
license: "apache-2.0"
datasets:
- voxceleb
metrics:
- EER
widget:
- example_title: VoxCeleb Speaker id10003
src: https://cdn-media.huggingface.co/speech_samples/VoxCeleb1_00003.wav
- example_title: VoxCeleb Speaker id10004
src: https://cdn-media.huggingface.co/speech_samples/VoxCeleb_00004.wav
---
<iframe src="https://ghbtns.com/github-btn.html?user=speechbrain&repo=speechbrain&type=star&count=true&size=large&v=2" frameborder="0" scrolling="0" width="170" height="30" title="GitHub"></iframe>
<br/><br/>
# Speaker Verification with ECAPA-TDNN embeddings on Voxceleb
This repository provides all the necessary tools to perform speaker verification with a pretrained ECAPA-TDNN model using SpeechBrain.
The system can be used to extract speaker embeddings as well.
It is trained on Voxceleb 1+ Voxceleb2 training data.
For a better experience, we encourage you to learn more about
[SpeechBrain](https://speechbrain.github.io). The model performance on Voxceleb1-test set(Cleaned) is:
| Release | EER(%)
|:-------------:|:--------------:|
| 05-03-21 | 0.80 |
## Pipeline description
This system is composed of an ECAPA-TDNN model. It is a combination of convolutional and residual blocks. The embeddings are extracted using attentive statistical pooling. The system is trained with Additive Margin Softmax Loss. Speaker Verification is performed using cosine distance between speaker embeddings.
## Install SpeechBrain
First of all, please install SpeechBrain with the following command:
```
pip install git+https://github.com/speechbrain/speechbrain.git@develop
```
Please notice that we encourage you to read our tutorials and learn more about
[SpeechBrain](https://speechbrain.github.io).
### Compute your speaker embeddings
```python
import torchaudio
from speechbrain.inference.speaker import EncoderClassifier
classifier = EncoderClassifier.from_hparams(source="speechbrain/spkrec-ecapa-voxceleb")
signal, fs =torchaudio.load('tests/samples/ASR/spk1_snt1.wav')
embeddings = classifier.encode_batch(signal)
```
The system is trained with recordings sampled at 16kHz (single channel).
The code will automatically normalize your audio (i.e., resampling + mono channel selection) when calling *classify_file* if needed. Make sure your input tensor is compliant with the expected sampling rate if you use *encode_batch* and *classify_batch*.
### Perform Speaker Verification
```python
from speechbrain.inference.speaker import SpeakerRecognition
verification = SpeakerRecognition.from_hparams(source="speechbrain/spkrec-ecapa-voxceleb", savedir="pretrained_models/spkrec-ecapa-voxceleb")
score, prediction = verification.verify_files("tests/samples/ASR/spk1_snt1.wav", "tests/samples/ASR/spk2_snt1.wav") # Different Speakers
score, prediction = verification.verify_files("tests/samples/ASR/spk1_snt1.wav", "tests/samples/ASR/spk1_snt2.wav") # Same Speaker
```
The prediction is 1 if the two signals in input are from the same speaker and 0 otherwise.
### Inference on GPU
To perform inference on the GPU, add `run_opts={"device":"cuda"}` when calling the `from_hparams` method.
### Training
The model was trained with SpeechBrain (aa018540).
To train it from scratch follows these steps:
1. Clone SpeechBrain:
```bash
git clone https://github.com/speechbrain/speechbrain/
```
2. Install it:
```
cd speechbrain
pip install -r requirements.txt
pip install -e .
```
3. Run Training:
```
cd recipes/VoxCeleb/SpeakerRec
python train_speaker_embeddings.py hparams/train_ecapa_tdnn.yaml --data_folder=your_data_folder
```
You can find our training results (models, logs, etc) [here](https://drive.google.com/drive/folders/1-ahC1xeyPinAHp2oAohL-02smNWO41Cc?usp=sharing).
### Limitations
The SpeechBrain team does not provide any warranty on the performance achieved by this model when used on other datasets.
#### Referencing ECAPA-TDNN
```
@inproceedings{DBLP:conf/interspeech/DesplanquesTD20,
author = {Brecht Desplanques and
Jenthe Thienpondt and
Kris Demuynck},
editor = {Helen Meng and
Bo Xu and
Thomas Fang Zheng},
title = {{ECAPA-TDNN:} Emphasized Channel Attention, Propagation and Aggregation
in {TDNN} Based Speaker Verification},
booktitle = {Interspeech 2020},
pages = {3830--3834},
publisher = {{ISCA}},
year = {2020},
}
```
# **Citing SpeechBrain**
Please, cite SpeechBrain if you use it for your research or business.
```bibtex
@misc{speechbrain,
title={{SpeechBrain}: A General-Purpose Speech Toolkit},
author={Mirco Ravanelli and Titouan Parcollet and Peter Plantinga and Aku Rouhe and Samuele Cornell and Loren Lugosch and Cem Subakan and Nauman Dawalatabad and Abdelwahab Heba and Jianyuan Zhong and Ju-Chieh Chou and Sung-Lin Yeh and Szu-Wei Fu and Chien-Feng Liao and Elena Rastorgueva and François Grondin and William Aris and Hwidong Na and Yan Gao and Renato De Mori and Yoshua Bengio},
year={2021},
eprint={2106.04624},
archivePrefix={arXiv},
primaryClass={eess.AS},
note={arXiv:2106.04624}
}
```
# **About SpeechBrain**
- Website: https://speechbrain.github.io/
- Code: https://github.com/speechbrain/speechbrain/
- HuggingFace: https://huggingface.co/speechbrain/
|
jukofyork/creative-writing-control-vectors-v3.0 | jukofyork | "2024-09-20T16:03:44Z" | 438,729 | 13 | null | [
"gguf",
"control-vector",
"creative-writing",
"license:apache-2.0",
"region:us"
] | null | "2024-08-28T10:16:32Z" | ---
license: apache-2.0
tags:
- control-vector
- creative-writing
---

This repo contains pre-generated control vectors in [GGUF](https://github.com/ggerganov/ggml/blob/master/docs/gguf.md) format for use with [llama.cpp](https://github.com/ggerganov/llama.cpp):
- **IMPORTANT**: These **new control vectors** must use their **respective de-bias control vector(s)**.
- The code used to generate these can now be found at [github.com/jukofyork/control-vectors](https://github.com/jukofyork/control-vectors).
- All were generated with `'--num_prompt_samples'` set to the model's hidden state dimension.
Control vectors allow fine-tuned control over LLMs, enabling more precise/targeted text generation.
---
## Table of Contents
- [Applying Control Vectors](#applying-control-vectors)
- [Command Line Generator](#command-line-generator)
- [Direct Links](#direct-links)
- [Algorithm Details](#algorithm-details)
- [Changelog](#changelog)
---
## Applying Control Vectors
### To "de-bias" the model only:
Use the `'--control-vector'` option as follows:
```sh
llama-cli --model <model>.gguf [other CLI arguments] \
--control-vector mistral-large:123b-language__debias.gguf
```
Alternatively for server mode:
```sh
llama-server --model <model>.gguf [other CLI arguments] \
--control-vector mistral-large:123b-language__debias.gguf
```
This will apply the "language" de-bias control vector to the `Mistral-Large-Instruct-2407` model.
You can apply multiple de-bias control vectors simultaneously like so:
```sh
llama-cli --model <model>.gguf [other CLI arguments] \
--control-vector mistral-large:123b-language__debias.gguf \
--control-vector mistral-large:123b-storytelling__debias.gguf \
--control-vector mistral-large:123b-character_focus__debias.gguf
```
This will apply all 3 of the "writing style" de-bias control vectors.
### To fully apply a positive or negative axis control vector with the default scale-factor:
Use the `'--control-vector'` option as follows:
```sh
llama-cli --model <model>.gguf [other CLI arguments] \
--control-vector mistral-large:123b-language__debias.gguf \
--control-vector mistral-large:123b-language__ornate.gguf
```
This will fully apply (ie: with a scale-factor of `1.0`) the (positive-axis) "ornate language" control vector.
**IMPORTANT: The positive and negative axis control vectors must be used along with the relevant de-bias control vector - they cannot be used on their own!**
You can fully apply multiple positive or negative axis control vectors like so:
```sh
llama-cli --model <model>.gguf [other CLI arguments] \
--control-vector mistral-large:123b-language__debias.gguf \
--control-vector mistral-large:123b-language__ornate.gguf \
--control-vector mistral-large:123b-storytelling__debias.gguf \
--control-vector mistral-large:123b-storytelling__descriptive.gguf \
--control-vector mistral-large:123b-character_focus__debias.gguf \
--control-vector mistral-large:123b-character_focus__dialogue.gguf
```
This will fully apply (ie: with a scale-factor of `1.0`) all 3 of the (positive-axis) "writing style" control vectors.
**NOTE**: Fully applying too many positive or negative axis control vector simultaneously may damage the model's output.
### To partially apply a positive or negative axis control vector using a custom scale-factor:
```sh
llama-cli --model <model>.gguf [other CLI arguments] \
--control-vector mistral-large:123b-language__debias.gguf \
--control-vector-scaled mistral-large:123b-language__ornate.gguf 0.5
```
This will partially apply the (positive-axis) "ornate language" control vector with a scale-factor of `0.5` (ie: half the full effect).
**IMPORTANT: The positive and negative axis control vectors must be used along with the relevant de-bias control vector - they cannot be used on their own!**
You can partially apply multiple positive or negative axis control vectors like so:
```sh
llama-cli --model <model>.gguf [other CLI arguments] \
--control-vector mistral-large:123b-language__debias.gguf \
--control-vector-scaled mistral-large:123b-language__ornate.gguf 0.5 \
--control-vector mistral-large:123b-storytelling__debias.gguf \
--control-vector-scaled mistral-large:123b-storytelling__descriptive.gguf 0.3 \
--control-vector mistral-large:123b-character_focus__debias.gguf \
--control-vector-scaled mistral-large:123b-character_focus__dialogue.gguf 0.2
```
This will partially apply all 3 of the (positive-axis) "writing style" control vectors with varying weights.
The theoretical upper bound value for equal weights is between `1/n` and `sqrt(1/n)` depending on how correlated the `n` control vector directions are, eg:
- For `n = 1` use the default scale-factor of `1.0` for comparison with the values below.
- For `n = 2` is between `1/2 ≈ 0.5` and `sqrt(1/2) ≈ 0.707`.
- For `n = 3` is between `1/3 ≈ 0.333` and `sqrt(1/3) ≈ 0.577`.
- For `n = 4` is between `1/4 ≈ 0.25` and `sqrt(1/4) ≈ 0.5`.
- For `n = 5` is between `1/5 ≈ 0.2` and `sqrt(1/5) ≈ 0.447`.
and so on.
The way the positive and negative axis control vectors are calibrated means you can negate the scale-factors too, eg:
```sh
llama-cli --model <model>.gguf [other CLI arguments] \
--control-vector mistral-large:123b-language__debias.gguf \
--control-vector-scaled mistral-large:123b-language__ornate.gguf -0.5
```
is equivalent to:
```sh
llama-cli --model <model>.gguf [other CLI arguments] \
--control-vector mistral-large:123b-language__debias.gguf \
--control-vector-scaled mistral-large:123b-language__simple.gguf 0.5
```
**NOTE**: It is possible to use scale-factors greater than `1.0`, but if too large it will eventually damage the model's output.
### Important Notes
1. **Always** include the relevant "de-bias" control vector as well as the positive-axis/negative-axis control vector - they cannot be used on their own!
2. **Do not** mix both sides of a positive/negative axis at the same time (eg: `'--control-vector language__simple.gguf'` and `'--control-vector language__ornate.gguf'` will just cancel out and have no effect...).
3. Ensure your `llama.cpp` version is up to date (multi-vector support added 27/06/24 in [#8137](https://github.com/ggerganov/llama.cpp/pull/8137)).
---
## Command Line Generator
Courtesy of [gghfez](https://huggingface.co/gghfez), a utility to easily generate command line options for [llama.cpp](https://github.com/ggerganov/llama.cpp):

You can run this tool directly on [GitHub Pages](https://jukofyork.github.io/control-vectors/command_line_generator.html).
---
# Direct Links
## Very Large Models
- [c4ai-command-r-plus](https://huggingface.co/jukofyork/creative-writing-control-vectors-v3.0/tree/main/c4ai-command-r-plus)
- [c4ai-command-r-plus-08-2024](https://huggingface.co/jukofyork/creative-writing-control-vectors-v3.0/tree/main/c4ai-command-r-plus-08-2024)
- [Eurux-8x22b-nca](https://huggingface.co/jukofyork/creative-writing-control-vectors-v3.0/tree/main/Eurux-8x22b-nca)
- [Lumimaid-v0.2-123B](https://huggingface.co/jukofyork/creative-writing-control-vectors-v3.0/tree/main/Lumimaid-v0.2-123B)
- [magnum-v2-123b](https://huggingface.co/jukofyork/creative-writing-control-vectors-v3.0/tree/main/magnum-v2-123b)
- [Mistral-Large-Instruct-2407](https://huggingface.co/jukofyork/creative-writing-control-vectors-v3.0/tree/main/Mistral-Large-Instruct-2407)
- [Mixtral-8x22B-Instruct-v0.1](https://huggingface.co/jukofyork/creative-writing-control-vectors-v3.0/tree/main/Mixtral-8x22B-Instruct-v0.1)
- [Qwen1.5-110B-Chat](https://huggingface.co/jukofyork/creative-writing-control-vectors-v3.0/tree/main/Qwen1.5-110B-Chat)
- [WizardLM-2-8x22B](https://huggingface.co/jukofyork/creative-writing-control-vectors-v3.0/tree/main/WizardLM-2-8x22B)
## Large Models
- [Athene-70B](https://huggingface.co/jukofyork/creative-writing-control-vectors-v3.0/tree/main/Athene-70B)
- [aurelian-alpha0.1-70b-rope8-32K-fp16](https://huggingface.co/jukofyork/creative-writing-control-vectors-v3.0/tree/main/aurelian-alpha0.1-70b-rope8-32K-fp16)
- [aurelian-v0.5-70b-rope8-32K-fp16](https://huggingface.co/jukofyork/creative-writing-control-vectors-v3.0/tree/main/aurelian-v0.5-70b-rope8-32K-fp16)
- [daybreak-miqu-1-70b-v1.0-hf](https://huggingface.co/jukofyork/creative-writing-control-vectors-v3.0/tree/main/daybreak-miqu-1-70b-v1.0-hf)
- [deepseek-llm-67b-chat](https://huggingface.co/jukofyork/creative-writing-control-vectors-v3.0/tree/main/deepseek-llm-67b-chat)
- [dolphin-2.9.2-qwen2-72b](https://huggingface.co/jukofyork/creative-writing-control-vectors-v3.0/tree/main/dolphin-2.9.2-qwen2-72b)
- [Hermes-3-Llama-3.1-70B](https://huggingface.co/jukofyork/creative-writing-control-vectors-v3.0/tree/main/Hermes-3-Llama-3.1-70B)
- [L3-70B-Euryale-v2.1](https://huggingface.co/jukofyork/creative-writing-control-vectors-v3.0/tree/main/L3-70B-Euryale-v2.1)
- [L3.1-70B-Euryale-v2.2](https://huggingface.co/jukofyork/creative-writing-control-vectors-v3.0/tree/main/L3.1-70B-Euryale-v2.2)
- [Llama-3-70B-Instruct-Storywriter](https://huggingface.co/jukofyork/creative-writing-control-vectors-v3.0/tree/main/Llama-3-70B-Instruct-Storywriter)
- [Llama-3-Lumimaid-70B-v0.1](https://huggingface.co/jukofyork/creative-writing-control-vectors-v3.0/tree/main/Llama-3-Lumimaid-70B-v0.1)
- [Llama-3.1-70B-ArliAI-RPMax-v1.1](https://huggingface.co/jukofyork/creative-writing-control-vectors-v3.0/tree/main/Llama-3.1-70B-ArliAI-RPMax-v1.1)
- [Lumimaid-v0.2-70B](https://huggingface.co/jukofyork/creative-writing-control-vectors-v3.0/tree/main/Lumimaid-v0.2-70B)
- [magnum-72b-v1](https://huggingface.co/jukofyork/creative-writing-control-vectors-v3.0/tree/main/magnum-72b-v1)
- [magnum-v2-72b](https://huggingface.co/jukofyork/creative-writing-control-vectors-v3.0/tree/main/magnum-v2-72b)
- [Meta-Llama-3-70B-Instruct](https://huggingface.co/jukofyork/creative-writing-control-vectors-v3.0/tree/main/Meta-Llama-3-70B-Instruct)
- [Meta-Llama-3.1-70B-Instruct](https://huggingface.co/jukofyork/creative-writing-control-vectors-v3.0/tree/main/Meta-Llama-3.1-70B-Instruct)
- [miqu-1-70b](https://huggingface.co/jukofyork/creative-writing-control-vectors-v3.0/tree/main/miqu-1-70b)
- [Qwen1.5-72B-Chat](https://huggingface.co/jukofyork/creative-writing-control-vectors-v3.0/tree/main/Qwen1.5-72B-Chat)
- [Qwen2-72B-Instruct](https://huggingface.co/jukofyork/creative-writing-control-vectors-v3.0/tree/main/Qwen2-72B-Instruct)
- [Qwen2.5-72B-Instruct](https://huggingface.co/jukofyork/creative-writing-control-vectors-v3.0/tree/main/Qwen2.5-72B-Instruct)
- [turbcat-instruct-72b](https://huggingface.co/jukofyork/creative-writing-control-vectors-v3.0/tree/main/turbcat-instruct-72b)
## Medium Models
- [35b-beta-long](https://huggingface.co/jukofyork/creative-writing-control-vectors-v3.0/tree/main/35b-beta-long)
- [aya-23-35B](https://huggingface.co/jukofyork/creative-writing-control-vectors-v3.0/tree/main/aya-23-35B)
- [c4ai-command-r-v01](https://huggingface.co/jukofyork/creative-writing-control-vectors-v3.0/tree/main/c4ai-command-r-v01)
- [c4ai-command-r-08-2024](https://huggingface.co/jukofyork/creative-writing-control-vectors-v3.0/tree/main/c4ai-command-r-08-2024) ([\*\*\*READ THIS FIRST\*\*\*](https://huggingface.co/jukofyork/creative-writing-control-vectors-v3.0/discussions/2))
- [Divergence-33B](https://huggingface.co/jukofyork/creative-writing-control-vectors-v3.0/tree/main/Divergence-33B)
- [gemma-2-27b-it](https://huggingface.co/jukofyork/creative-writing-control-vectors-v3.0/tree/main/gemma-2-27b-it)
- [gemma-2-27b-it-SimPO-37K](https://huggingface.co/jukofyork/creative-writing-control-vectors-v3.0/tree/main/gemma-2-27b-it-SimPO-37K)
- [gemma2-gutenberg-27B](https://huggingface.co/jukofyork/creative-writing-control-vectors-v3.0/tree/main/gemma2-gutenberg-27B)
- [internlm2_5-20b-chat](https://huggingface.co/jukofyork/creative-writing-control-vectors-v3.0/tree/main/internlm2_5-20b-chat)
- [magnum-v1-32b](https://huggingface.co/jukofyork/creative-writing-control-vectors-v3.0/tree/main/magnum-v1-32b)
- [magnum-v2-32b](https://huggingface.co/jukofyork/creative-writing-control-vectors-v3.0/tree/main/magnum-v2-32b)
- [magnum-v3-27b-kto](https://huggingface.co/jukofyork/creative-writing-control-vectors-v3.0/tree/main/magnum-v3-27b-kto)
- [magnum-v3-34b](https://huggingface.co/jukofyork/creative-writing-control-vectors-v3.0/tree/main/magnum-v3-34b)
- [Mistral-Small-Instruct-2409](https://huggingface.co/jukofyork/creative-writing-control-vectors-v3.0/tree/main/Mistral-Small-Instruct-2409)
- [Mixtral-8x7B-Instruct-v0.1](https://huggingface.co/jukofyork/creative-writing-control-vectors-v3.0/tree/main/Mixtral-8x7B-Instruct-v0.1)
- [Nous-Capybara-34B](https://huggingface.co/jukofyork/creative-writing-control-vectors-v3.0/tree/main/Nous-Capybara-34B)
- [Qwen1.5-32B-Chat](https://huggingface.co/jukofyork/creative-writing-control-vectors-v3.0/tree/main/Qwen1.5-32B-Chat)
- [Qwen2.5-32B-Instruct](https://huggingface.co/jukofyork/creative-writing-control-vectors-v3.0/tree/main/Qwen2.5-32B-Instruct)
- [Yi-34B-Chat](https://huggingface.co/jukofyork/creative-writing-control-vectors-v3.0/tree/main/Yi-34B-Chat)
- [Yi-1.5-34B-Chat](https://huggingface.co/jukofyork/creative-writing-control-vectors-v3.0/tree/main/Yi-1.5-34B-Chat)
- [Yi-1.5-34B-Chat-16K](https://huggingface.co/jukofyork/creative-writing-control-vectors-v3.0/tree/main/Yi-1.5-34B-Chat-16K)
## Small Models
- [aya-23-8B](https://huggingface.co/jukofyork/creative-writing-control-vectors-v3.0/tree/main/aya-23-8B)
- [gemma-2-9b-it](https://huggingface.co/jukofyork/creative-writing-control-vectors-v3.0/tree/main/gemma-2-9b-it)
- [gemma-2-9b-it-SimPO](https://huggingface.co/jukofyork/creative-writing-control-vectors-v3.0/tree/main/gemma-2-9b-it-SimPO)
- [Gemma-2-9B-It-SPPO-Iter3](https://huggingface.co/jukofyork/creative-writing-control-vectors-v3.0/tree/main/Gemma-2-9B-It-SPPO-Iter3)
- [gemma-2-Ifable-9B](https://huggingface.co/jukofyork/creative-writing-control-vectors-v3.0/tree/main/gemma-2-Ifable-9B)
- [Llama-3-Instruct-8B-SPPO-Iter3](https://huggingface.co/jukofyork/creative-writing-control-vectors-v3.0/tree/main/Llama-3-Instruct-8B-SPPO-Iter3)
- [Llama-3.1-8B-ArliAI-RPMax-v1.1](https://huggingface.co/jukofyork/creative-writing-control-vectors-v3.0/tree/main/Llama-3.1-8B-ArliAI-RPMax-v1.1)
- [Meta-Llama-3-8B-Instruct](https://huggingface.co/jukofyork/creative-writing-control-vectors-v3.0/tree/main/Meta-Llama-3-8B-Instruct)
- [Meta-Llama-3.1-8B-Instruct](https://huggingface.co/jukofyork/creative-writing-control-vectors-v3.0/tree/main/Meta-Llama-3.1-8B-Instruct)
- [Mistral-7B-Instruct-v0.2](https://huggingface.co/jukofyork/creative-writing-control-vectors-v3.0/tree/main/Mistral-7B-Instruct-v0.2)
- [Mistral-7B-Instruct-v0.3](https://huggingface.co/jukofyork/creative-writing-control-vectors-v3.0/tree/main/Mistral-7B-Instruct-v0.3)
- [Mistral7B-PairRM-SPPO-Iter3](https://huggingface.co/jukofyork/creative-writing-control-vectors-v3.0/tree/main/Mistral7B-PairRM-SPPO-Iter3)
- [Mistral-Nemo-12B-ArliAI-RPMax-v1.1](https://huggingface.co/jukofyork/creative-writing-control-vectors-v3.0/tree/main/Mistral-Nemo-12B-ArliAI-RPMax-v1.1)
- [mistral-nemo-gutenberg-12B](https://huggingface.co/jukofyork/creative-writing-control-vectors-v3.0/tree/main/mistral-nemo-gutenberg-12B)
- [mistral-nemo-gutenberg-12B-v2](https://huggingface.co/jukofyork/creative-writing-control-vectors-v3.0/tree/main/mistral-nemo-gutenberg-12B-v2)
- [Mistral-Nemo-Instruct-2407](https://huggingface.co/jukofyork/creative-writing-control-vectors-v3.0/tree/main/Mistral-Nemo-Instruct-2407)
- [romulus-mistral-nemo-12b-simpo](https://huggingface.co/jukofyork/creative-writing-control-vectors-v3.0/tree/main/romulus-mistral-nemo-12b-simpo)
- [Qwen1.5-14B-Chat](https://huggingface.co/jukofyork/creative-writing-control-vectors-v3.0/tree/main/Qwen1.5-14B-Chat)
- [Qwen2-7B-Instruct](https://huggingface.co/jukofyork/creative-writing-control-vectors-v3.0/tree/main/Qwen2-7B-Instruct)
- [Qwen2.5-7B-Instruct](https://huggingface.co/jukofyork/creative-writing-control-vectors-v3.0/tree/main/Qwen2.5-7B-Instruct)
- [Qwen2.5-14B-Instruct](https://huggingface.co/jukofyork/creative-writing-control-vectors-v3.0/tree/main/Qwen2.5-14B-Instruct)
- [WizardLM-2-7B](https://huggingface.co/jukofyork/creative-writing-control-vectors-v3.0/tree/main/WizardLM-2-7B)
---
## Algorithm Details
### 1. First we create a set of pre/post "prompt stems":
<details> <summary>'prompt_stems.json' (click to expand)</summary>
```json
{
"pre": [
"You are",
"You're",
"Act as",
"Behave as",
"Respond as",
"Answer as",
"Write as",
"Speak as",
"Think like",
"Roleplay as",
"Pretend to be",
"Imagine you are",
"Assume you are",
"Suppose you are",
"Picture yourself as",
"Envision yourself as",
"Consider yourself",
"Take on the role of",
"Play the part of",
"Perform as",
"Be",
"Emulate",
"Mimic",
"Imitate",
"Channel",
"Embody",
"Represent",
"Portray",
"Adopt the persona of",
"Function as",
"Serve as",
"Work as",
"Operate as",
"Pose as",
"Present yourself as",
"View yourself as",
"See yourself as",
"Regard yourself as",
"Consider yourself as",
"Think of yourself as",
"Approach this as",
"Conduct yourself as",
"Assume the identity of",
"Put yourself in the position of",
"Inhabit the role of",
"Characterize yourself as",
"Impersonate",
"Simulate being",
"Take the perspective of",
"Assume the role of"
],
"post": [
"an author",
"a storyteller",
"an AI author",
"an artificial intelligence that creates stories",
"an AI-powered author",
"an AI creator of tales",
"a fiction writer",
"an author specializing in fictional stories",
"a novelist",
"a creative writer",
"a digital storyteller",
"an AI narrative generator",
"a computer-assisted author",
"an AI weaver of narratives",
"a prose artist",
"a writer of imaginative tales",
"a wordsmith",
"a literary artist",
"a narrative designer",
"a tale weaver",
"a story architect",
"a crafter of fictional worlds",
"a purveyor of narratives",
"a storytelling savant",
"a narrative architect",
"a digital bard",
"a modern wordsmith",
"a virtual storyteller",
"a contemporary narrative designer",
"an innovative tale weaver",
"a cutting-edge prose creator",
"a digital-age fabulist",
"a tech-savvy literary artist",
"a 21st-century storyteller",
"a famous author",
"a literary virtuoso",
"an expert storyteller",
"a renowned wordsmith",
"a master of fictional worlds",
"a master of prose",
"a futuristic narrative crafter",
"a genre-bending author",
"a visionary storyteller",
"an experimental fiction writer",
"a digital narrative pioneer",
"a cross-platform storyteller",
"a multimedia narrative artist",
"an immersive story creator",
"a narrative AI collaborator",
"a next-generation author"
]
}
```
</details>
The Cartesian product of these gives us 2500 (ie: 50 x 50) different "You are an author" type sentences.
### 2. Then we create several different creative-writing axis "continuations":
**A set of 3 different "writing style" axis:**
<details> <summary>"Language" (click to expand)</summary>
```json
{
"classes": ["simple", "ornate"],
"data": [
[
"who writes using clear, straightforward language accessible to young readers, with simple sentence structures and common vocabulary",
"who writes using rich, sophisticated language suitable for mature readers, with complex sentence structures and varied vocabulary"
],
[
"who crafts narratives using easy-to-understand words and concise sentences, making your tales approachable for readers of all ages",
"who crafts narratives using eloquent prose and intricate phrasings, creating tales that challenge and engage advanced readers"
],
[
"known for writing in a clear, unadorned style that makes complex ideas accessible to a wide audience",
"known for writing in a lyrical, intricate style that showcases the beauty and complexity of language"
],
[
"who specializes in using everyday language to craft engaging narratives that readers of all levels can enjoy",
"who specializes in using sophisticated, sometimes archaic language to create immersive and challenging narratives"
],
[
"who excels at conveying ideas and emotions through simple, precise language, avoiding unnecessary complexity",
"who excels at conveying ideas and emotions through complex, nuanced language, embracing the full depth of linguistic expression"
],
[
"focused on creating stories with straightforward plots and relatable characters using basic, accessible language",
"focused on creating stories with intricate plots and multifaceted characters using elaborate, ornate language"
],
[
"who writes in a direct, no-frills style that prioritizes clarity and ease of understanding for all readers",
"who writes in a florid, embellished style that prioritizes linguistic beauty and complexity for discerning readers"
],
[
"known for distilling complex concepts into easily digestible prose, making your work accessible to a broad audience",
"known for weaving complex concepts into richly textured prose, creating literary works that reward careful analysis"
],
[
"who crafts stories using concise, impactful language that resonates with readers through its clarity and directness",
"who crafts stories using expansive, descriptive language that immerses readers in a world of vivid imagery and complex ideas"
],
[
"specializing in clean, minimalist prose that conveys powerful ideas through carefully chosen, straightforward words",
"specializing in lush, maximalist prose that conveys powerful ideas through carefully constructed, ornate phrases"
]
]
}
```
</details>
<details> <summary>"Storytelling (click to expand)"</summary>
```json
{
"classes": ["explicit", "descriptive"],
"data": [
[
"who writes stories that directly state characters' emotions and motivations, clearly explaining their inner thoughts and the reasons behind their actions",
"who writes stories that reveal characters' emotions and motivations through their actions, physical responses, and the details of their surroundings"
],
[
"who creates narratives that explicitly tell readers about the story's themes and messages, leaving no room for ambiguity in interpretation",
"who creates narratives that convey themes and messages through carefully crafted scenes and character interactions, allowing readers to draw their own conclusions"
],
[
"who prioritizes clarity by directly stating the significance of events and their impact on the plot, ensuring readers fully understand the story's progression",
"who prioritizes immersion by depicting events in vivid detail, allowing readers to infer their significance and impact on the plot"
],
[
"who crafts stories where character development is explicitly explained, telling readers exactly how and why characters change over time",
"who crafts stories where character development is shown through changing behaviors, attitudes, and decisions, inviting readers to observe growth over time"
],
[
"who favors straightforward exposition, directly informing readers about the world, its history, and important background information",
"who favors immersive world-building, revealing information about the world and its history through environmental details and character experiences"
],
[
"who writes with a focus on clear, unambiguous descriptions of settings, telling readers exactly what they need to know about each location",
"who writes with a focus on sensory-rich depictions of settings, allowing readers to experience locations through vivid imagery and atmosphere"
],
[
"who crafts narratives that explicitly state the cause-and-effect relationships between events, clearly explaining how one action leads to another",
"who crafts narratives that imply cause-and-effect relationships through the sequence of events and their consequences, letting readers connect the dots"
],
[
"who specializes in direct characterization, telling readers about characters' personalities, backgrounds, and traits through clear statements",
"who specializes in indirect characterization, showing characters' personalities, backgrounds, and traits through their actions, choices, and interactions"
],
[
"known for creating stories that explicitly describe characters' physical appearances, leaving no room for misinterpretation",
"known for creating stories that reveal characters' physical appearances gradually through select details and others' reactions"
],
[
"who excels at writing stories where the emotional atmosphere is directly stated, telling readers exactly how to feel about each scene",
"who excels at writing stories where the emotional atmosphere is conveyed through environmental cues, character reactions, and carefully chosen details"
]
]
}
```
</details>
<details> <summary>"Character Focus (click to expand)"</summary>
```json
{
"classes": ["narration", "dialogue"],
"data": [
[
"who excels at using vivid narration to convey character personalities, motivations, and relationships, creating an immersive experience for readers",
"who excels at using vibrant dialogue to convey character personalities, motivations, and relationships, creating an immersive experience for readers"
],
[
"who weaves tales using narration to develop characters and explore their inner worlds, allowing readers to connect with them on a deeper level",
"who weaves tales using dialogue to develop characters and explore their inner worlds, allowing readers to connect with them on a deeper level"
],
[
"known for your ability to transport readers into characters' minds through evocative narration that explores their fears, hopes, and relationships",
"known for your ability to transport readers into characters' minds through authentic dialogue that reveals their fears, hopes, and relationships"
],
[
"who excels at using narration to craft tales that explore characters' emotional depths, creating stories that resonate with readers on a personal level",
"who excels at using dialogue to craft tales that explore characters' emotional depths, creating stories that resonate with readers on a personal level"
],
[
"specializing in narration-driven storytelling, creating stories that use narration to uncover characters' hidden desires, fears, and relationships, engaging readers in their emotional journeys",
"specializing in dialogue-driven storytelling, creating stories that use conversations to uncover characters' hidden desires, fears, and relationships, engaging readers in their emotional journeys"
],
[
"who crafts rich narrative descriptions to build intricate worlds and complex characters, immersing readers in the story's atmosphere and emotional landscape",
"who crafts rich conversational exchanges to build intricate worlds and complex characters, immersing readers in the story's atmosphere and emotional landscape"
],
[
"known for using powerful narrative prose to convey the subtleties of character development and plot progression, creating a vivid reading experience",
"known for using powerful dialogue to convey the subtleties of character development and plot progression, creating a vivid reading experience"
],
[
"who excels in using descriptive narration to paint detailed pictures of settings, characters, and events, bringing the story to life in readers' minds",
"who excels in using realistic dialogue to paint detailed pictures of settings, characters, and events, bringing the story to life through characters' voices"
],
[
"specializing in narrative techniques that reveal characters' thoughts and feelings, providing deep insights into their motivations and inner conflicts",
"specializing in dialogue techniques that reveal characters' thoughts and feelings, providing deep insights into their motivations and inner conflicts through their words"
],
[
"who creates compelling storylines through skillful use of narrative exposition, guiding readers through complex plots and character arcs",
"who creates compelling storylines through skillful use of character conversations, guiding readers through complex plots and character arcs via verbal interactions"
]
]
}
```
</details>
**The 4 elements of the [Dark Tetrad](https://en.wikipedia.org/wiki/Dark_triad)**:
<details> <summary>"Empathy vs Sociopathy (click to expand)"</summary>
```json
{
"classes": ["empathy", "sociopathy"],
"data": [
[
"who creates stories centered around characters with extraordinary ability to understand and share others' feelings, leading to profound personal growth and positive change in their communities",
"who creates stories centered around characters with extreme inability to understand or care about others' feelings, leading to profound personal gain and negative change in their communities"
],
[
"who crafts narratives showcasing the transformative power of understanding others, where characters learn to see the world through others' eyes and form genuine, healing connections",
"who crafts narratives showcasing the destructive power of emotional detachment, where characters learn to see the world only through their own desires and form manipulative, exploitative connections"
],
[
"renowned for writing tales of emotional awareness, where protagonists navigate complex social situations by understanding and respecting the feelings and perspectives of those around them",
"renowned for writing tales of emotional indifference, where protagonists navigate complex social situations by exploiting and disregarding the feelings and perspectives of those around them"
],
[
"of heartwarming tales, where characters overcome their differences through mutual understanding, active listening, and a willingness to support each other through life's challenges",
"of chilling tales, where characters exploit their differences for personal advantage, ignoring others' needs, and showing a complete unwillingness to support anyone but themselves"
],
[
"who specializes in stories that highlight the ripple effects of understanding and consideration, showing how small acts of kindness can lead to significant positive changes in individuals and society",
"who specializes in stories that highlight the ripple effects of callousness and manipulation, showing how small acts of exploitation can lead to significant negative changes in individuals and society"
],
[
"focused on depicting characters who possess an innate ability to sense and respond to others' emotional states, often serving as a healing presence in their communities",
"focused on depicting characters who possess an innate inability to sense or care about others' emotional states, often serving as a disruptive presence in their communities"
],
[
"who writes about individuals with a profound capacity for understanding others, exploring how this trait shapes their relationships and influences their decision-making in complex moral situations",
"who writes about individuals with a profound lack of concern for others, exploring how this trait shapes their relationships and influences their decision-making in complex moral situations"
],
[
"exploring themes of emotional growth, where characters develop their ability to understand others to overcome personal challenges and foster meaningful connections",
"exploring themes of emotional stagnation, where characters remain trapped in their inability to connect with others, leading to increasingly harmful behaviors and isolation"
],
[
"who crafts tales of healing through understanding, where characters use their ability to connect with others to help them overcome trauma and build resilience",
"who crafts tales of harm through indifference, where characters use their lack of concern for others to exploit their traumas and undermine their resilience"
],
[
"known for stories where characters' deep understanding of others allows them to bridge divides, resolve conflicts, and create harmonious communities despite initial differences",
"known for stories where characters' complete disregard for others drives them to create divides, instigate conflicts, and disrupt communities for their own benefit"
]
]
}
```
</details>
<details> <summary>"Honesty vs Machiavellianism (click to expand)"</summary>
```json
{
"classes": ["honesty", "machiavellianism"],
"data": [
[
"who creates stories about characters who steadfastly uphold their principles, even when faced with difficult choices or personal costs, showcasing the power of integrity in building trust and respect",
"who creates stories about characters who flexibly adapt their principles, especially when faced with opportunities for gain or power, showcasing the effectiveness of manipulation in achieving personal goals"
],
[
"who crafts narratives celebrating the courage of those who speak the truth, where protagonists navigate complex moral dilemmas by staying true to their values and being transparent in their actions",
"who crafts narratives celebrating the cunning of masterminds, where protagonists navigate complex social landscapes by adapting their values and obscuring their true intentions"
],
[
"known for tales of principled leadership, where characters inspire others through their unwavering commitment to truthfulness, even in the face of adversity or temptation",
"known for tales of strategic leadership, where characters control others through their flexible approach to information sharing, especially in the face of opportunities or challenges"
],
[
"of ethical triumphs, where individuals choose the path of openness and transparency, ultimately creating stronger relationships and more just societies",
"of pragmatic victories, where individuals choose the path of calculated deception, ultimately achieving their goals and securing their positions of influence"
],
[
"who specializes in stories of personal and professional integrity, where characters discover that their trustworthiness and reliability become their greatest strengths in overcoming challenges",
"who specializes in stories of personal and professional advancement, where characters discover that their adaptability and cunning become their greatest assets in overcoming obstacles"
],
[
"focused on depicting characters who believe in the inherent value of openness, often facing and overcoming significant hardships as a result of their commitment to truthfulness",
"focused on depicting characters who believe in the utility of selective disclosure, often achieving significant successes as a result of their strategic use of information and misinformation"
],
[
"who writes about individuals dedicated to fostering trust through consistent openness, highlighting the long-term benefits of transparent communication in all relationships",
"who writes about individuals dedicated to accumulating influence through strategic communication, highlighting the immediate advantages of controlling information flow in all interactions"
],
[
"exploring themes of personal growth through radical openness, where characters learn to confront difficult truths about themselves and others, leading to genuine connections",
"exploring themes of social advancement through tactical disclosure, where characters learn to present carefully curated information about themselves and others, leading to advantageous alliances"
],
[
"who crafts tales of ethical problem-solving, where characters face complex challenges and find solutions that maintain their integrity and the trust of those around them",
"who crafts tales of strategic problem-solving, where characters face complex challenges and find solutions that prioritize their objectives, regardless of ethical considerations"
],
[
"known for stories where characters' commitment to openness allows them to build lasting partnerships and create positive change, even in corrupt or challenging environments",
"known for stories where characters' mastery of strategic disclosure allows them to forge useful alliances and reshape their environment to their advantage, especially in competitive settings"
]
]
}
```
</details>
<details> <summary>"Humility vs Narcissism (click to expand)"</summary>
```json
{
"classes": ["humility", "narcissism"],
"data": [
[
"who creates stories about characters who embrace their flaws and limitations, learning to value others' contributions and grow through collaboration and open-mindedness",
"who creates stories about characters who deny their flaws and limitations, learning to devalue others' contributions and stagnate through self-aggrandizement and closed-mindedness"
],
[
"who crafts narratives of quiet strength, where protagonists lead by example, listen more than they speak, and find power in admitting their mistakes and learning from others",
"who crafts narratives of loud dominance, where protagonists lead by assertion, speak more than they listen, and find power in denying their mistakes and dismissing others' input"
],
[
"known for tales of personal growth, where characters overcome their ego, recognize their own biases, and discover the profound impact of putting others first",
"known for tales of personal inflation, where characters indulge their ego, ignore their own biases, and discover the immediate gratification of putting themselves first"
],
[
"of inspirational journeys, where individuals learn to balance confidence with modesty, celebrating others' successes as enthusiastically as their own",
"of self-centered journeys, where individuals learn to amplify confidence without modesty, diminishing others' successes while exaggerating their own"
],
[
"who specializes in stories of transformative self-awareness, where characters discover that true strength lies in vulnerability and the ability to say 'I don't know' or 'I was wrong'",
"who specializes in stories of persistent self-delusion, where characters insist that true strength lies in invulnerability and the refusal to ever admit ignorance or error"
],
[
"focused on depicting characters who find fulfillment in supporting others' growth and success, often stepping back to allow others to shine",
"focused on depicting characters who find fulfillment only in their own achievements and accolades, often stepping on others to ensure they remain in the spotlight"
],
[
"who writes about individuals who actively seek feedback and criticism, viewing it as an opportunity for improvement and personal development",
"who writes about individuals who actively avoid feedback and criticism, viewing it as a threat to their self-image and responding with anger or dismissal"
],
[
"exploring themes of collective achievement, where characters learn that the greatest accomplishments come from acknowledging and harnessing the strengths of a diverse team",
"exploring themes of individual superiority, where characters insist that the greatest accomplishments come from their own innate talents and dismiss the contributions of others"
],
[
"who crafts tales of empathetic leadership, where characters inspire loyalty and trust by genuinely caring about their team's well-being and giving credit where it's due",
"who crafts tales of self-serving leadership, where characters demand loyalty and obedience by prioritizing their own image and taking credit for all successes"
],
[
"known for stories where characters' selflessness and ability to recognize their own limitations allows them to form deep, meaningful relationships and create inclusive, supportive communities",
"known for stories where characters' self-centeredness and inflated sense of self-importance leads them to form shallow, transactional relationships and create exclusive, competitive environments"
]
]
}
```
</details>
<details> <summary>"Compassion vs Sadism (click to expand)"</summary>
```json
{
"classes": ["compassion", "sadism"],
"data": [
[
"who creates stories about characters finding fulfillment in alleviating others' suffering, showcasing the transformative power of kindness in healing both individuals and communities",
"who creates stories about characters finding fulfillment in inflicting suffering on others, showcasing the destructive power of cruelty in harming both individuals and communities"
],
[
"who crafts narratives of profound human connection, where protagonists learn to extend care to even the most difficult individuals, leading to unexpected personal growth",
"who crafts narratives of profound human cruelty, where protagonists learn to derive pleasure from tormenting even the most vulnerable individuals, leading to unexpected personal degradation"
],
[
"known for tales of emotional healing, where characters overcome their own pain by reaching out to help others, creating a ripple effect of kindness",
"known for tales of emotional torture, where characters intensify others' pain for their own pleasure, creating a ripple effect of suffering"
],
[
"of heartwarming journeys, where individuals discover their inner strength through acts of selfless care, often in the face of adversity",
"of disturbing journeys, where individuals discover their capacity for cruelty through acts of malicious pleasure, often in the face of others' vulnerability"
],
[
"who specializes in stories of personal transformation, where characters' small acts of kindness accumulate to create significant positive impacts in their lives and others",
"who specializes in stories of personal corruption, where characters' small acts of cruelty accumulate to create significant negative impacts in their lives and others"
],
[
"focused on depicting characters who find deep satisfaction in nurturing and supporting others, exploring the profound joy that comes from alleviating suffering",
"focused on depicting characters who find intense pleasure in tormenting and breaking others, exploring the disturbing thrill that comes from inflicting pain"
],
[
"who writes about individuals dedicating themselves to understanding and addressing others' pain, highlighting the personal growth that comes from cultivating care",
"who writes about individuals dedicating themselves to causing and prolonging others' pain, highlighting the personal gratification that comes from indulging in malicious impulses"
],
[
"exploring themes of healing through kindness, where characters learn to overcome their own traumas by extending care to those in need",
"exploring themes of harm through cruelty, where characters exacerbate their own dark tendencies by inflicting pain on those who are vulnerable"
],
[
"who crafts tales of emotional recovery, where individuals learn to connect with others by offering genuine care and support in times of distress",
"who crafts tales of emotional destruction, where individuals learn to disconnect from others by deriving pleasure from their moments of greatest suffering"
],
[
"known for stories where characters find strength in showing mercy and kindness, even to those who may not seem to deserve it, leading to unexpected redemption",
"known for stories where characters find power in showing ruthlessness and cruelty, especially to those who are helpless, leading to escalating cycles of harm"
]
]
}
```
</details>
**An "Optimism vs Nihilism" axis to compliment the [Dark Tetrad](https://en.wikipedia.org/wiki/Dark_triad) axis:**
<details> <summary>"Optimism vs Nihilism (click to expand)"</summary>
```json
{
"classes": ["optimism", "nihilism"],
"data": [
[
"who creates stories about characters with an unshakeable belief that every situation, no matter how dire, contains the seed of a positive outcome",
"who creates stories about characters with an unshakeable belief that every situation, no matter how promising, is ultimately pointless and devoid of meaning"
],
[
"who crafts narratives of individuals who see setbacks as opportunities, consistently finding silver linings in the darkest clouds",
"who crafts narratives of individuals who see all events as equally insignificant, consistently rejecting the notion that anything matters in a purposeless universe"
],
[
"known for tales of characters who maintain an infectious positive outlook, inspiring hope and resilience in others even in the bleakest circumstances",
"known for tales of characters who maintain a persistent sense of life's futility, spreading a contagious belief in the absurdity of existence to others"
],
[
"of transformative hopefulness, where protagonists' unwavering positive attitudes literally change the course of events for the better",
"of pervasive meaninglessness, where protagonists' unwavering belief in life's futility colors their perception of all events as equally insignificant"
],
[
"who specializes in stories of relentless positivity, portraying characters who believe so strongly in good outcomes that they seem to will them into existence",
"who specializes in stories of unyielding emptiness, portraying characters who believe so strongly in life's lack of purpose that they reject all conventional values and goals"
],
[
"focused on depicting characters who find joy and purpose in every aspect of life, no matter how small or seemingly insignificant",
"focused on depicting characters who find all aspects of life equally devoid of purpose, viewing joy and suffering as meaningless constructs"
],
[
"who writes about individuals who persistently seek out the good in others and in situations, believing in the inherent value of positive thinking",
"who writes about individuals who consistently reject the idea of inherent value in anything, viewing all human pursuits as arbitrary and ultimately pointless"
],
[
"exploring themes of hope and resilience, where characters overcome adversity through their steadfast belief in a better future",
"exploring themes of existential emptiness, where characters confront the perceived meaninglessness of existence and reject the concept of progress or improvement"
],
[
"who crafts tales of inspirational perseverance, where characters' belief in positive outcomes drives them to overcome seemingly insurmountable odds",
"who crafts tales of philosophical resignation, where characters' belief in the futility of all action leads them to embrace a state of passive indifference"
],
[
"known for stories where characters' hopeful worldviews lead them to create positive change and find fulfillment in their lives and relationships",
"known for stories where characters' belief in life's fundamental meaninglessness leads them to reject societal norms and find a paradoxical freedom in purposelessness"
]
]
}
```
</details>
### 3. Then we collect a large number of creative-writing prompts:
- I used [Sao10K/Short-Storygen-v2](https://huggingface.co/datasets/Sao10K/Short-Storygen-v2) and a couple of other sources to get 11835 creative-writing prompts in total (see the `'writing_prompts.txt'` file).
- The [jq](https://jqlang.github.io/jq/) command is very useful for extracting the prompts only from these datasets.
### 4. Run the model on a random sample of (prompt-stem, continuation, creative-writing prompts) combinations:
The Cartesian product of: 2500 prompt-stem sentences x 10 continuation sentences x 11835 story prompts ≈ 300M possible combinations.
- It is important that the same prompt-stem sample sentence be used with each (`"baseline"`, `"negative"`, `"positive"`) triplet.
- It is also important that the same (prompt-stem, continuation) sample sentence be used with the`"negative"` and `"positive"` members of the same triplet.
- The suggested value of `"hidden_size"` for the `--num_prompt_samples` option is because the theory regarding [estimation of covariance matrices](https://en.wikipedia.org/wiki/Estimation_of_covariance_matrices) shows we need at the ***very least*** a minimum of [one sample per feature](https://stats.stackexchange.com/questions/90045/how-many-samples-are-needed-to-estimate-a-p-dimensional-covariance-matrix) (this may be overkill due to us only retaining the top Eigenvectors though...).
### 5. Create a pair of "differenced datasets" by subtracting the corresponding ```"baseline"``` class's sample from both of the other 2 classes' samples:
- The reason for this is so that we "centre" the data around the "baseline" (i.e., set the "baseline" as the origin and look for vector directions that point away from it).
- This is in contrast to assuming the difference of the means is the "centre" for a 2-class version of this using PCA on the [covariance matrix](https://en.wikipedia.org/wiki/Covariance_matrix) of the differences (i.e., the "standard" method of creating control vectors).
### 6. Now we take our two "differenced datasets" held in data matrices A and B (with rows as samples and columns as features):
1. Create the [cross-covariance matrix](https://en.wikipedia.org/wiki/Cross-covariance_matrix), `C = A^T * B`.
2. Next we [symmetrise](https://en.wikipedia.org/wiki/Symmetric_matrix), `C' = (C^T + C) / 2`.
3. Perform an [eigendecomposition](https://en.wikipedia.org/wiki/Eigendecomposition_of_a_matrix), `C' = Q * Λ * Q^(-1)`.
4. Since we symmetrised the matrix, the **eigenvectors** (`Q`) and **eigenvalues** (`Λ`) will all be real-valued.
5. Arrange the **eigenvectors** in descending order based on their corresponding **eigenvalues**.
6. Once the **eigenvectors** are sorted, discard the **eigenvalues** as they won't be needed again.
The reason for using the [cross-covariance matrix](https://en.wikipedia.org/wiki/Cross-covariance_matrix) instead of the [covariance matrix](https://en.wikipedia.org/wiki/Covariance_matrix):
- The **covariance matrix** of a differenced dataset exemplifies directions in **A or B** (ie: think about the expansion of `(a-b)² = a² + b² -2×a×b`).
- The **cross-covariance matrix** of a differenced dataset exemplifies directions in **A and B** (ie: akin to `a×b`, with no `a²` or `b²` terms).
The reason for creating the symmetrised matrix is two-fold:
- To avoid complex-valued **eigenvectors** that tell us about rotations (which we can't actually make use of here anyway).
- To specifically try to find opposing/balanced "axis" for our different traits (i.e., we don't want to find positively correlated directions nor unbalanced directions).
### 7. So now we have a set of "directions" to examine:
- It turns out that 90% of the time the **principal eigenvector** (i.e., the **eigenvector** with the largest corresponding **eigenvalue**) is the one you want.
- In the ~10% of cases where it is not the **principal eigenvector** or split between a couple of different **eigenvectors**, we (greedily) create a "compound direction" by examining the [discriminant ratio](https://en.wikipedia.org/wiki/Linear_discriminant_analysis) of each direction.
### 8. Finally, we project the "direction" to reorient and scale as necessary:
- There is no reason the **eigenvectors** point in the direction we want, so 50% of the time we have to flip all the signs by [projecting](https://en.wikipedia.org/wiki/Projection_(linear_algebra%29) our (differenced) "desired" dataset on to the (unit norm) direction and then test the sign of the mean.
- Due to the way the LLMs work via the "residual stream", the hidden states tend to get larger and larger as the layers progress, so to normalize this we also scale by the magnitude of the mean of the same projection as above.
- To better separate the "bias" effect from the positive/negative axis (and to make the positive/negative end equidistant from the model's "baseline" behaviour) we store the mid point of these means in the de-bias control vector and then subtract the midpoint from both the positive and negative axis' control vectors.
**NOTES**:
- I have found the above can be applied to every layer, but often the last layer will have hidden state means that are 10-100x larger than the rest, so I have excluded these from all I have uploaded here.
- I have tried many other different eigendecompositions: PCA on the 2-class differenced datasets, PCA on the joined 2-class/3-class datasets, solving generalized eigensystems similar to CCA, and so on.
- The "balanced" directions / "axis" this method finds are the ***exact opposite*** of those needed for the [Refusal in LLMs is mediated by a single direction](https://www.lesswrong.com/posts/jGuXSZgv6qfdhMCuJ/refusal-in-llms-is-mediated-by-a-single-direction) paper.
---
## Changelog
- *28/08/24 - Added [Qwen2-72B-Instruct](https://huggingface.co/jukofyork/creative-writing-control-vectors-v3.0/tree/main/Qwen2-72B-Instruct).*
- *29/08/24 - Added [Qwen1.5-72B-Chat](https://huggingface.co/jukofyork/creative-writing-control-vectors-v3.0/tree/main/Qwen1.5-72B-Chat), [Mistral-7B-Instruct-v0.2](https://huggingface.co/jukofyork/creative-writing-control-vectors-v3.0/tree/main/Mistral-7B-Instruct-v0.2), [Mistral-7B-Instruct-v0.3](https://huggingface.co/jukofyork/creative-writing-control-vectors-v3.0/tree/main/Mistral-7B-Instruct-v0.3), [miqu-1-70b](https://huggingface.co/jukofyork/creative-writing-control-vectors-v3.0/tree/main/miqu-1-70b), [Mixtral-8x7B-Instruct-v0.1](https://huggingface.co/jukofyork/creative-writing-control-vectors-v3.0/tree/main/Mixtral-8x7B-Instruct-v0.1) and [Yi-1.5-34B-Chat-16K](https://huggingface.co/jukofyork/creative-writing-control-vectors-v3.0/tree/main/Yi-1.5-34B-Chat-16K).*
- *30/08/24 - Added [Meta-Llama-3-8B-Instruct](https://huggingface.co/jukofyork/creative-writing-control-vectors-v3.0/tree/main/Meta-Llama-3-8B-Instruct), [Meta-Llama-3-70B-Instruct](https://huggingface.co/jukofyork/creative-writing-control-vectors-v3.0/tree/main/Meta-Llama-3-70B-Instruct), [Meta-Llama-3.1-8B-Instruct](https://huggingface.co/jukofyork/creative-writing-control-vectors-v3.0/tree/main/Meta-Llama-3.1-8B-Instruct) and [Meta-Llama-3.1-70B-Instruct](https://huggingface.co/jukofyork/creative-writing-control-vectors-v3.0/tree/main/Meta-Llama-3.1-70B-Instruct).*
- *31/08/24 - Added [aya-23-35B](https://huggingface.co/jukofyork/creative-writing-control-vectors-v3.0/tree/main/aya-23-35B), [Gemma-2-9B-It-SPPO-Iter3](https://huggingface.co/jukofyork/creative-writing-control-vectors-v3.0/tree/main/Gemma-2-9B-It-SPPO-Iter3) and [Qwen1.5-14B-Chat](https://huggingface.co/jukofyork/creative-writing-control-vectors-v3.0/tree/main/Qwen1.5-14B-Chat).*
- *01/09/24 - Added [Mixtral-8x22B-Instruct-v0.1](https://huggingface.co/jukofyork/creative-writing-control-vectors-v3.0/tree/main/Mixtral-8x22B-Instruct-v0.1) and [Qwen1.5-110B-Chat](https://huggingface.co/jukofyork/creative-writing-control-vectors-v3.0/tree/main/Qwen1.5-110B-Chat).*
- *02/09/24 - Added [c4ai-command-r-plus-08-2024](https://huggingface.co/jukofyork/creative-writing-control-vectors-v3.0/tree/main/c4ai-command-r-plus-08-2024).*
- *03/09/24 - Added [c4ai-command-r-08-2024](https://huggingface.co/jukofyork/creative-writing-control-vectors-v3.0/tree/main/c4ai-command-r-08-2024) ([\*\*\*READ THIS FIRST\*\*\*](https://huggingface.co/jukofyork/creative-writing-control-vectors-v3.0/discussions/2)), [Yi-1.5-34B-Chat](https://huggingface.co/jukofyork/creative-writing-control-vectors-v3.0/tree/main/Yi-1.5-34B-Chat), [gemma-2-27b-it-SimPO-37K](https://huggingface.co/jukofyork/creative-writing-control-vectors-v3.0/tree/main/gemma-2-27b-it-SimPO-37K), [aya-23-8B](https://huggingface.co/jukofyork/creative-writing-control-vectors-v3.0/tree/main/aya-23-8B), [gemma-2-9b-it-SimPO](https://huggingface.co/jukofyork/creative-writing-control-vectors-v3.0/tree/main/gemma-2-9b-it-SimPO), [Qwen2-7B-Instruct](https://huggingface.co/jukofyork/creative-writing-control-vectors-v3.0/tree/main/Qwen2-7B-Instruct) and [Yi-34B-Chat](https://huggingface.co/jukofyork/creative-writing-control-vectors-v3.0/tree/main/Yi-34B-Chat).*
- *04/09/24 - Added [deepseek-llm-67b-chat](https://huggingface.co/jukofyork/creative-writing-control-vectors-v3.0/tree/main/deepseek-llm-67b-chat), [internlm2_5-20b-chat](https://huggingface.co/jukofyork/creative-writing-control-vectors-v3.0/tree/main/internlm2_5-20b-chat), [Athene-70B](https://huggingface.co/jukofyork/creative-writing-control-vectors-v3.0/tree/main/Athene-70B), [Llama-3-Instruct-8B-SPPO-Iter3](https://huggingface.co/jukofyork/creative-writing-control-vectors-v3.0/tree/main/Llama-3-Instruct-8B-SPPO-Iter3), [magnum-v2-32b](https://huggingface.co/jukofyork/creative-writing-control-vectors-v3.0/tree/main/magnum-v2-32b), [Mistral7B-PairRM-SPPO-Iter3](https://huggingface.co/jukofyork/creative-writing-control-vectors-v3.0/tree/main/Mistral7B-PairRM-SPPO-Iter3) and [Nous-Capybara-34B](https://huggingface.co/jukofyork/creative-writing-control-vectors-v3.0/tree/main/Nous-Capybara-34B).*
- *05/09/24 - Added [Llama-3-70B-Instruct-Storywriter](https://huggingface.co/jukofyork/creative-writing-control-vectors-v3.0/tree/main/Llama-3-70B-Instruct-Storywriter), [35b-beta-long](https://huggingface.co/jukofyork/creative-writing-control-vectors-v3.0/tree/main/35b-beta-long) and [magnum-v3-34b](https://huggingface.co/jukofyork/creative-writing-control-vectors-v3.0/tree/main/magnum-v3-34b).*
- *06/09/24 - Added [Hermes-3-Llama-3.1-70B](https://huggingface.co/jukofyork/creative-writing-control-vectors-v3.0/tree/main/Hermes-3-Llama-3.1-70B), [magnum-v2-72b](https://huggingface.co/jukofyork/creative-writing-control-vectors-v3.0/tree/main/magnum-v2-72b), [magnum-v1-32b](https://huggingface.co/jukofyork/creative-writing-control-vectors-v3.0/tree/main/magnum-v1-32b) and [L3.1-70B-Euryale-v2.2](https://huggingface.co/jukofyork/creative-writing-control-vectors-v3.0/tree/main/L3.1-70B-Euryale-v2.2).*
- *08/09/24 - Added [aurelian-v0.5-70b-rope8-32K-fp16](https://huggingface.co/jukofyork/creative-writing-control-vectors-v3.0/tree/main/aurelian-v0.5-70b-rope8-32K-fp16), [aurelian-alpha0.1-70b-rope8-32K-fp16](https://huggingface.co/jukofyork/creative-writing-control-vectors-v3.0/tree/main/aurelian-alpha0.1-70b-rope8-32K-fp16), [L3-70B-Euryale-v2.1](https://huggingface.co/jukofyork/creative-writing-control-vectors-v3.0/tree/main/L3-70B-Euryale-v2.1), [Llama-3-Lumimaid-70B-v0.1](https://huggingface.co/jukofyork/creative-writing-control-vectors-v3.0/tree/main/Llama-3-Lumimaid-70B-v0.1), [magnum-72b-v1](https://huggingface.co/jukofyork/creative-writing-control-vectors-v3.0/tree/main/magnum-72b-v1) and [turbcat-instruct-72b](https://huggingface.co/jukofyork/creative-writing-control-vectors-v3.0/tree/main/turbcat-instruct-72b).*
- *09/09/24 - Added [daybreak-miqu-1-70b-v1.0-hf](https://huggingface.co/jukofyork/creative-writing-control-vectors-v3.0/tree/main/daybreak-miqu-1-70b-v1.0-hf), [dolphin-2.9.2-qwen2-72b](https://huggingface.co/jukofyork/creative-writing-control-vectors-v3.0/tree/main/dolphin-2.9.2-qwen2-72b) and [Lumimaid-v0.2-70B](https://huggingface.co/jukofyork/creative-writing-control-vectors-v3.0/tree/main/Lumimaid-v0.2-70B).*
- *11/09/24 - Added [Lumimaid-v0.2-123B](https://huggingface.co/jukofyork/creative-writing-control-vectors-v3.0/tree/main/Lumimaid-v0.2-123B).*
- *12/09/24 - Added [magnum-v2-123b](https://huggingface.co/jukofyork/creative-writing-control-vectors-v3.0/tree/main/magnum-v2-123b).*
- *13/09/24 - Added [Eurux-8x22b-nca](https://huggingface.co/jukofyork/creative-writing-control-vectors-v3.0/tree/main/Eurux-8x22b-nca).*
- *14/09/24 - Added [Divergence-33B](https://huggingface.co/jukofyork/creative-writing-control-vectors-v3.0/tree/main/Divergence-33B), [gemma2-gutenberg-27B](https://huggingface.co/jukofyork/creative-writing-control-vectors-v3.0/tree/main/gemma2-gutenberg-27B), [gemma-2-Ifable-9B](https://huggingface.co/jukofyork/creative-writing-control-vectors-v3.0/tree/main/gemma-2-Ifable-9B), [mistral-nemo-gutenberg-12B](https://huggingface.co/jukofyork/creative-writing-control-vectors-v3.0/tree/main/mistral-nemo-gutenberg-12B), [mistral-nemo-gutenberg-12B-v2](https://huggingface.co/jukofyork/creative-writing-control-vectors-v3.0/tree/main/mistral-nemo-gutenberg-12B-v2), [romulus-mistral-nemo-12b-simpo](https://huggingface.co/jukofyork/creative-writing-control-vectors-v3.0/tree/main/romulus-mistral-nemo-12b-simpo), [Llama-3.1-8B-ArliAI-RPMax-v1.1](https://huggingface.co/jukofyork/creative-writing-control-vectors-v3.0/tree/main/Llama-3.1-8B-ArliAI-RPMax-v1.1), [Mistral-Nemo-12B-ArliAI-RPMax-v1.1](https://huggingface.co/jukofyork/creative-writing-control-vectors-v3.0/tree/main/Mistral-Nemo-12B-ArliAI-RPMax-v1.1) and [Llama-3.1-70B-ArliAI-RPMax-v1.1](https://huggingface.co/jukofyork/creative-writing-control-vectors-v3.0/tree/main/Llama-3.1-70B-ArliAI-RPMax-v1.1).*
- *20/09/24 - Added [Qwen2.5-7B-Instruct](https://huggingface.co/jukofyork/creative-writing-control-vectors-v3.0/tree/main/Qwen2.5-7B-Instruct), [Qwen2.5-14B-Instruct](https://huggingface.co/jukofyork/creative-writing-control-vectors-v3.0/tree/main/Qwen2.5-14B-Instruct), [Qwen2.5-32B-Instruct](https://huggingface.co/jukofyork/creative-writing-control-vectors-v3.0/tree/main/Qwen2.5-32B-Instruct), [Qwen2.5-72B-Instruct](https://huggingface.co/jukofyork/creative-writing-control-vectors-v3.0/tree/main/Qwen2.5-72B-Instruct), [magnum-v3-27b-kto](https://huggingface.co/jukofyork/creative-writing-control-vectors-v3.0/tree/main/magnum-v3-27b-kto) and [Mistral-Small-Instruct-2409](https://huggingface.co/jukofyork/creative-writing-control-vectors-v3.0/tree/main/Mistral-Small-Instruct-2409).* |
google/gemma-2b | google | "2024-09-27T12:18:55Z" | 437,170 | 912 | transformers | [
"transformers",
"safetensors",
"gguf",
"gemma",
"text-generation",
"arxiv:2312.11805",
"arxiv:2009.03300",
"arxiv:1905.07830",
"arxiv:1911.11641",
"arxiv:1904.09728",
"arxiv:1905.10044",
"arxiv:1907.10641",
"arxiv:1811.00937",
"arxiv:1809.02789",
"arxiv:1911.01547",
"arxiv:1705.03551",
"arxiv:2107.03374",
"arxiv:2108.07732",
"arxiv:2110.14168",
"arxiv:2304.06364",
"arxiv:2206.04615",
"arxiv:1804.06876",
"arxiv:2110.08193",
"arxiv:2009.11462",
"arxiv:2101.11718",
"arxiv:1804.09301",
"arxiv:2109.07958",
"arxiv:2203.09509",
"license:gemma",
"autotrain_compatible",
"text-generation-inference",
"endpoints_compatible",
"region:us"
] | text-generation | "2024-02-08T08:11:26Z" | ---
library_name: transformers
new_version: google/gemma-2-2b
license: gemma
extra_gated_heading: Access Gemma on Hugging Face
extra_gated_prompt: To access Gemma on Hugging Face, you’re required to review and
agree to Google’s usage license. To do this, please ensure you’re logged-in to Hugging
Face and click below. Requests are processed immediately.
extra_gated_button_content: Acknowledge license
---
# Gemma Model Card
**Model Page**: [Gemma](https://ai.google.dev/gemma/docs)
This model card corresponds to the 2B base version of the Gemma model. You can also visit the model card of the [7B base model](https://huggingface.co/google/gemma-7b), [7B instruct model](https://huggingface.co/google/gemma-7b-it), and [2B instruct model](https://huggingface.co/google/gemma-2b-it).
**Resources and Technical Documentation**:
* [Gemma Technical Report](https://storage.googleapis.com/deepmind-media/gemma/gemma-report.pdf)
* [Responsible Generative AI Toolkit](https://ai.google.dev/responsible)
* [Gemma on Kaggle](https://www.kaggle.com/models/google/gemma)
* [Gemma on Vertex Model Garden](https://console.cloud.google.com/vertex-ai/publishers/google/model-garden/335?version=gemma-2b-gg-hf)
**Terms of Use**: [Terms](https://www.kaggle.com/models/google/gemma/license/consent/verify/huggingface?returnModelRepoId=google/gemma-2b)
**Authors**: Google
## Model Information
Summary description and brief definition of inputs and outputs.
### Description
Gemma is a family of lightweight, state-of-the-art open models from Google,
built from the same research and technology used to create the Gemini models.
They are text-to-text, decoder-only large language models, available in English,
with open weights, pre-trained variants, and instruction-tuned variants. Gemma
models are well-suited for a variety of text generation tasks, including
question answering, summarization, and reasoning. Their relatively small size
makes it possible to deploy them in environments with limited resources such as
a laptop, desktop or your own cloud infrastructure, democratizing access to
state of the art AI models and helping foster innovation for everyone.
### Context Length
Models are trained on a context length of 8192 tokens.
### Usage
Below we share some code snippets on how to get quickly started with running the model. First make sure to `pip install -U transformers`, then copy the snippet from the section that is relevant for your usecase.
#### Fine-tuning the model
You can find fine-tuning scripts and notebook under the [`examples/` directory](https://huggingface.co/google/gemma-7b/tree/main/examples) of [`google/gemma-7b`](https://huggingface.co/google/gemma-7b) repository. To adapt it to this model, simply change the model-id to `google/gemma-2b`.
In that repository, we provide:
* A script to perform Supervised Fine-Tuning (SFT) on UltraChat dataset using QLoRA
* A script to perform SFT using FSDP on TPU devices
* A notebook that you can run on a free-tier Google Colab instance to perform SFT on English quotes dataset
#### Running the model on a CPU
```python
from transformers import AutoTokenizer, AutoModelForCausalLM
tokenizer = AutoTokenizer.from_pretrained("google/gemma-2b")
model = AutoModelForCausalLM.from_pretrained("google/gemma-2b")
input_text = "Write me a poem about Machine Learning."
input_ids = tokenizer(input_text, return_tensors="pt")
outputs = model.generate(**input_ids)
print(tokenizer.decode(outputs[0]))
```
#### Running the model on a single / multi GPU
```python
# pip install accelerate
from transformers import AutoTokenizer, AutoModelForCausalLM
tokenizer = AutoTokenizer.from_pretrained("google/gemma-2b")
model = AutoModelForCausalLM.from_pretrained("google/gemma-2b", device_map="auto")
input_text = "Write me a poem about Machine Learning."
input_ids = tokenizer(input_text, return_tensors="pt").to("cuda")
outputs = model.generate(**input_ids)
print(tokenizer.decode(outputs[0]))
```
#### Running the model on a GPU using different precisions
* _Using `torch.float16`_
```python
# pip install accelerate
from transformers import AutoTokenizer, AutoModelForCausalLM
tokenizer = AutoTokenizer.from_pretrained("google/gemma-2b")
model = AutoModelForCausalLM.from_pretrained("google/gemma-2b", device_map="auto", revision="float16")
input_text = "Write me a poem about Machine Learning."
input_ids = tokenizer(input_text, return_tensors="pt").to("cuda")
outputs = model.generate(**input_ids)
print(tokenizer.decode(outputs[0]))
```
* _Using `torch.bfloat16`_
```python
# pip install accelerate
from transformers import AutoTokenizer, AutoModelForCausalLM
tokenizer = AutoTokenizer.from_pretrained("google/gemma-2b")
model = AutoModelForCausalLM.from_pretrained("google/gemma-2b", device_map="auto", torch_dtype=torch.bfloat16)
input_text = "Write me a poem about Machine Learning."
input_ids = tokenizer(input_text, return_tensors="pt").to("cuda")
outputs = model.generate(**input_ids)
print(tokenizer.decode(outputs[0]))
```
#### Quantized Versions through `bitsandbytes`
* _Using 8-bit precision (int8)_
```python
# pip install bitsandbytes accelerate
from transformers import AutoTokenizer, AutoModelForCausalLM, BitsAndBytesConfig
quantization_config = BitsAndBytesConfig(load_in_8bit=True)
tokenizer = AutoTokenizer.from_pretrained("google/gemma-2b")
model = AutoModelForCausalLM.from_pretrained("google/gemma-2b", quantization_config=quantization_config)
input_text = "Write me a poem about Machine Learning."
input_ids = tokenizer(input_text, return_tensors="pt").to("cuda")
outputs = model.generate(**input_ids)
print(tokenizer.decode(outputs[0]))
```
* _Using 4-bit precision_
```python
# pip install bitsandbytes accelerate
from transformers import AutoTokenizer, AutoModelForCausalLM, BitsAndBytesConfig
quantization_config = BitsAndBytesConfig(load_in_4bit=True)
tokenizer = AutoTokenizer.from_pretrained("google/gemma-2b")
model = AutoModelForCausalLM.from_pretrained("google/gemma-2b", quantization_config=quantization_config)
input_text = "Write me a poem about Machine Learning."
input_ids = tokenizer(input_text, return_tensors="pt").to("cuda")
outputs = model.generate(**input_ids)
print(tokenizer.decode(outputs[0]))
```
#### Other optimizations
* _Flash Attention 2_
First make sure to install `flash-attn` in your environment `pip install flash-attn`
```diff
model = AutoModelForCausalLM.from_pretrained(
model_id,
torch_dtype=torch.float16,
+ attn_implementation="flash_attention_2"
).to(0)
```
### Inputs and outputs
* **Input:** Text string, such as a question, a prompt, or a document to be
summarized.
* **Output:** Generated English-language text in response to the input, such
as an answer to a question, or a summary of a document.
## Model Data
Data used for model training and how the data was processed.
### Training Dataset
These models were trained on a dataset of text data that includes a wide variety
of sources, totaling 6 trillion tokens. Here are the key components:
* Web Documents: A diverse collection of web text ensures the model is exposed
to a broad range of linguistic styles, topics, and vocabulary. Primarily
English-language content.
* Code: Exposing the model to code helps it to learn the syntax and patterns of
programming languages, which improves its ability to generate code or
understand code-related questions.
* Mathematics: Training on mathematical text helps the model learn logical
reasoning, symbolic representation, and to address mathematical queries.
The combination of these diverse data sources is crucial for training a powerful
language model that can handle a wide variety of different tasks and text
formats.
### Data Preprocessing
Here are the key data cleaning and filtering methods applied to the training
data:
* CSAM Filtering: Rigorous CSAM (Child Sexual Abuse Material) filtering was
applied at multiple stages in the data preparation process to ensure the
exclusion of harmful and illegal content
* Sensitive Data Filtering: As part of making Gemma pre-trained models safe and
reliable, automated techniques were used to filter out certain personal
information and other sensitive data from training sets.
* Additional methods: Filtering based on content quality and safely in line with
[our policies](https://storage.googleapis.com/gweb-uniblog-publish-prod/documents/2023_Google_AI_Principles_Progress_Update.pdf#page=11).
## Implementation Information
Details about the model internals.
### Hardware
Gemma was trained using the latest generation of
[Tensor Processing Unit (TPU)](https://cloud.google.com/tpu/docs/intro-to-tpu) hardware (TPUv5e).
Training large language models requires significant computational power. TPUs,
designed specifically for matrix operations common in machine learning, offer
several advantages in this domain:
* Performance: TPUs are specifically designed to handle the massive computations
involved in training LLMs. They can speed up training considerably compared to
CPUs.
* Memory: TPUs often come with large amounts of high-bandwidth memory, allowing
for the handling of large models and batch sizes during training. This can
lead to better model quality.
* Scalability: TPU Pods (large clusters of TPUs) provide a scalable solution for
handling the growing complexity of large foundation models. You can distribute
training across multiple TPU devices for faster and more efficient processing.
* Cost-effectiveness: In many scenarios, TPUs can provide a more cost-effective
solution for training large models compared to CPU-based infrastructure,
especially when considering the time and resources saved due to faster
training.
* These advantages are aligned with
[Google's commitments to operate sustainably](https://sustainability.google/operating-sustainably/).
### Software
Training was done using [JAX](https://github.com/google/jax) and [ML Pathways](https://blog.google/technology/ai/introducing-pathways-next-generation-ai-architecture/ml-pathways).
JAX allows researchers to take advantage of the latest generation of hardware,
including TPUs, for faster and more efficient training of large models.
ML Pathways is Google's latest effort to build artificially intelligent systems
capable of generalizing across multiple tasks. This is specially suitable for
[foundation models](https://ai.google/discover/foundation-models/), including large language models like
these ones.
Together, JAX and ML Pathways are used as described in the
[paper about the Gemini family of models](https://arxiv.org/abs/2312.11805); "the 'single
controller' programming model of Jax and Pathways allows a single Python
process to orchestrate the entire training run, dramatically simplifying the
development workflow."
## Evaluation
Model evaluation metrics and results.
### Benchmark Results
These models were evaluated against a large collection of different datasets and
metrics to cover different aspects of text generation:
| Benchmark | Metric | 2B Params | 7B Params |
| ------------------------------ | ------------- | ----------- | --------- |
| [MMLU](https://arxiv.org/abs/2009.03300) | 5-shot, top-1 | 42.3 | 64.3 |
| [HellaSwag](https://arxiv.org/abs/1905.07830) | 0-shot |71.4 | 81.2 |
| [PIQA](https://arxiv.org/abs/1911.11641) | 0-shot | 77.3 | 81.2 |
| [SocialIQA](https://arxiv.org/abs/1904.09728) | 0-shot | 49.7 | 51.8 |
| [BooIQ](https://arxiv.org/abs/1905.10044) | 0-shot | 69.4 | 83.2 |
| [WinoGrande](https://arxiv.org/abs/1907.10641) | partial score | 65.4 | 72.3 |
| [CommonsenseQA](https://arxiv.org/abs/1811.00937) | 7-shot | 65.3 | 71.3 |
| [OpenBookQA](https://arxiv.org/abs/1809.02789) | | 47.8 | 52.8 |
| [ARC-e](https://arxiv.org/abs/1911.01547) | | 73.2 | 81.5 |
| [ARC-c](https://arxiv.org/abs/1911.01547) | | 42.1 | 53.2 |
| [TriviaQA](https://arxiv.org/abs/1705.03551) | 5-shot | 53.2 | 63.4 |
| [Natural Questions](https://github.com/google-research-datasets/natural-questions) | 5-shot | 12.5 | 23 |
| [HumanEval](https://arxiv.org/abs/2107.03374) | pass@1 | 22.0 | 32.3 |
| [MBPP](https://arxiv.org/abs/2108.07732) | 3-shot | 29.2 | 44.4 |
| [GSM8K](https://arxiv.org/abs/2110.14168) | maj@1 | 17.7 | 46.4 |
| [MATH](https://arxiv.org/abs/2108.07732) | 4-shot | 11.8 | 24.3 |
| [AGIEval](https://arxiv.org/abs/2304.06364) | | 24.2 | 41.7 |
| [BIG-Bench](https://arxiv.org/abs/2206.04615) | | 35.2 | 55.1 |
| ------------------------------ | ------------- | ----------- | --------- |
| **Average** | | **45.0** | **56.9** |
## Ethics and Safety
Ethics and safety evaluation approach and results.
### Evaluation Approach
Our evaluation methods include structured evaluations and internal red-teaming
testing of relevant content policies. Red-teaming was conducted by a number of
different teams, each with different goals and human evaluation metrics. These
models were evaluated against a number of different categories relevant to
ethics and safety, including:
* Text-to-Text Content Safety: Human evaluation on prompts covering safety
policies including child sexual abuse and exploitation, harassment, violence
and gore, and hate speech.
* Text-to-Text Representational Harms: Benchmark against relevant academic
datasets such as [WinoBias](https://arxiv.org/abs/1804.06876) and [BBQ Dataset](https://arxiv.org/abs/2110.08193v2).
* Memorization: Automated evaluation of memorization of training data, including
the risk of personally identifiable information exposure.
* Large-scale harm: Tests for "dangerous capabilities," such as chemical,
biological, radiological, and nuclear (CBRN) risks.
### Evaluation Results
The results of ethics and safety evaluations are within acceptable thresholds
for meeting [internal policies](https://storage.googleapis.com/gweb-uniblog-publish-prod/documents/2023_Google_AI_Principles_Progress_Update.pdf#page=11) for categories such as child
safety, content safety, representational harms, memorization, large-scale harms.
On top of robust internal evaluations, the results of well known safety
benchmarks like BBQ, BOLD, Winogender, Winobias, RealToxicity, and TruthfulQA
are shown here.
**Update**: These numbers reflect the new numbers from the updated v1.1 IT models. For the original v1 numbers, please consult the technical report's appendix for the results.
| Benchmark | Metric | Gemma v1.1 IT 2B | Gemma v1.1 IT 7B |
| ------------------------------ | ------------- | ----------- | --------- |
| [RealToxicity](https://arxiv.org/abs/2009.11462) | average | 6.86 | 7.90 |
| [BOLD](https://arxiv.org/abs/2101.11718) | | 45.57 | 49.08 |
| [CrowS-Pairs](https://aclanthology.org/2020.emnlp-main.154/) | top-1 | 45.82 | 51.33 |
| [BBQ Ambig](https://arxiv.org/abs/2110.08193v2) | 1-shot, top-1 | 62.58 | 92.54 |
| [BBQ Disambig](https://arxiv.org/abs/2110.08193v2) | top-1 | 54.62 | 71.99 |
| [Winogender](https://arxiv.org/abs/1804.09301) | top-1 | 51.25 | 54.17 |
| [TruthfulQA](https://arxiv.org/abs/2109.07958) | | 31.81 | 44.84 |
| [Winobias 1_2](https://arxiv.org/abs/1804.06876) | | 56.12 | 59.09 |
| [Winobias 2_2](https://arxiv.org/abs/1804.06876) | | 91.10 | 92.23 |
| [Toxigen](https://arxiv.org/abs/2203.09509) | | 29.77 | 39.59 |
| ------------------------------ | ------------- | ----------- | --------- |
## Usage and Limitations
These models have certain limitations that users should be aware of.
### Intended Usage
Open Large Language Models (LLMs) have a wide range of applications across
various industries and domains. The following list of potential uses is not
comprehensive. The purpose of this list is to provide contextual information
about the possible use-cases that the model creators considered as part of model
training and development.
* Content Creation and Communication
* Text Generation: These models can be used to generate creative text formats
such as poems, scripts, code, marketing copy, and email drafts.
* Chatbots and Conversational AI: Power conversational interfaces for customer
service, virtual assistants, or interactive applications.
* Text Summarization: Generate concise summaries of a text corpus, research
papers, or reports.
* Research and Education
* Natural Language Processing (NLP) Research: These models can serve as a
foundation for researchers to experiment with NLP techniques, develop
algorithms, and contribute to the advancement of the field.
* Language Learning Tools: Support interactive language learning experiences,
aiding in grammar correction or providing writing practice.
* Knowledge Exploration: Assist researchers in exploring large bodies of text
by generating summaries or answering questions about specific topics.
### Limitations
* Training Data
* The quality and diversity of the training data significantly influence the
model's capabilities. Biases or gaps in the training data can lead to
limitations in the model's responses.
* The scope of the training dataset determines the subject areas the model can
handle effectively.
* Context and Task Complexity
* LLMs are better at tasks that can be framed with clear prompts and
instructions. Open-ended or highly complex tasks might be challenging.
* A model's performance can be influenced by the amount of context provided
(longer context generally leads to better outputs, up to a certain point).
* Language Ambiguity and Nuance
* Natural language is inherently complex. LLMs might struggle to grasp subtle
nuances, sarcasm, or figurative language.
* Factual Accuracy
* LLMs generate responses based on information they learned from their
training datasets, but they are not knowledge bases. They may generate
incorrect or outdated factual statements.
* Common Sense
* LLMs rely on statistical patterns in language. They might lack the ability
to apply common sense reasoning in certain situations.
### Ethical Considerations and Risks
The development of large language models (LLMs) raises several ethical concerns.
In creating an open model, we have carefully considered the following:
* Bias and Fairness
* LLMs trained on large-scale, real-world text data can reflect socio-cultural
biases embedded in the training material. These models underwent careful
scrutiny, input data pre-processing described and posterior evaluations
reported in this card.
* Misinformation and Misuse
* LLMs can be misused to generate text that is false, misleading, or harmful.
* Guidelines are provided for responsible use with the model, see the
[Responsible Generative AI Toolkit](http://ai.google.dev/gemma/responsible).
* Transparency and Accountability:
* This model card summarizes details on the models' architecture,
capabilities, limitations, and evaluation processes.
* A responsibly developed open model offers the opportunity to share
innovation by making LLM technology accessible to developers and researchers
across the AI ecosystem.
Risks identified and mitigations:
* Perpetuation of biases: It's encouraged to perform continuous monitoring
(using evaluation metrics, human review) and the exploration of de-biasing
techniques during model training, fine-tuning, and other use cases.
* Generation of harmful content: Mechanisms and guidelines for content safety
are essential. Developers are encouraged to exercise caution and implement
appropriate content safety safeguards based on their specific product policies
and application use cases.
* Misuse for malicious purposes: Technical limitations and developer and
end-user education can help mitigate against malicious applications of LLMs.
Educational resources and reporting mechanisms for users to flag misuse are
provided. Prohibited uses of Gemma models are outlined in the
[Gemma Prohibited Use Policy](https://ai.google.dev/gemma/prohibited_use_policy).
* Privacy violations: Models were trained on data filtered for removal of PII
(Personally Identifiable Information). Developers are encouraged to adhere to
privacy regulations with privacy-preserving techniques.
### Benefits
At the time of release, this family of models provides high-performance open
large language model implementations designed from the ground up for Responsible
AI development compared to similarly sized models.
Using the benchmark evaluation metrics described in this document, these models
have shown to provide superior performance to other, comparably-sized open model
alternatives. |
XLabs-AI/flux-RealismLora | XLabs-AI | "2024-08-22T10:19:23Z" | 435,856 | 783 | diffusers | [
"diffusers",
"lora",
"Stable Diffusion",
"image-generation",
"Flux",
"text-to-image",
"en",
"base_model:black-forest-labs/FLUX.1-dev",
"base_model:adapter:black-forest-labs/FLUX.1-dev",
"license:other",
"region:us"
] | text-to-image | "2024-08-06T21:12:23Z" | ---
license: other
license_name: flux-1-dev-non-commercial-license
license_link: https://huggingface.co/black-forest-labs/FLUX.1-dev/blob/main/LICENSE.
language:
- en
pipeline_tag: text-to-image
tags:
- lora
- Stable Diffusion
- image-generation
- Flux
- diffusers
base_model: black-forest-labs/FLUX.1-dev
---

[<img src="https://github.com/XLabs-AI/x-flux/blob/main/assets/readme/light/join-our-discord-rev1.png?raw=true">](https://discord.gg/FHY2guThfy)
This repository provides a checkpoint with trained LoRA photorealism for
[FLUX.1-dev model](https://huggingface.co/black-forest-labs/FLUX.1-dev) by Black Forest Labs

# ComfyUI
[See our github](https://github.com/XLabs-AI/x-flux-comfyui) for comfy ui workflows.

# Training details
[XLabs AI](https://github.com/XLabs-AI) team is happy to publish fine-tuning Flux scripts, including:
- **LoRA** 🔥
- **ControlNet** 🔥
[See our github](https://github.com/XLabs-AI/x-flux) for train script and train configs.
# Training Dataset
Dataset has the following format for the training process:
```
├── images/
│ ├── 1.png
│ ├── 1.json
│ ├── 2.png
│ ├── 2.json
│ ├── ...
```
A .json file contains "caption" field with a text prompt.
# Inference
```bash
python3 demo_lora_inference.py \
--checkpoint lora.safetensors \
--prompt " handsome girl in a suit covered with bold tattoos and holding a pistol. Animatrix illustration style, fantasy style, natural photo cinematic"
```

# License
lora.safetensors falls under the [FLUX.1 [dev]](https://huggingface.co/black-forest-labs/FLUX.1-dev/blob/main/LICENSE.md) Non-Commercial License<br/> |
Qwen/Qwen2.5-0.5B-Instruct | Qwen | "2024-09-25T12:32:56Z" | 434,279 | 96 | transformers | [
"transformers",
"safetensors",
"qwen2",
"text-generation",
"chat",
"conversational",
"en",
"arxiv:2407.10671",
"base_model:Qwen/Qwen2.5-0.5B",
"base_model:finetune:Qwen/Qwen2.5-0.5B",
"license:apache-2.0",
"autotrain_compatible",
"text-generation-inference",
"endpoints_compatible",
"region:us"
] | text-generation | "2024-09-16T11:52:46Z" | ---
license: apache-2.0
license_link: https://huggingface.co/Qwen/Qwen2.5-0.5B-Instruct/blob/main/LICENSE
language:
- en
pipeline_tag: text-generation
base_model: Qwen/Qwen2.5-0.5B
tags:
- chat
library_name: transformers
---
# Qwen2.5-0.5B-Instruct
## Introduction
Qwen2.5 is the latest series of Qwen large language models. For Qwen2.5, we release a number of base language models and instruction-tuned language models ranging from 0.5 to 72 billion parameters. Qwen2.5 brings the following improvements upon Qwen2:
- Significantly **more knowledge** and has greatly improved capabilities in **coding** and **mathematics**, thanks to our specialized expert models in these domains.
- Significant improvements in **instruction following**, **generating long texts** (over 8K tokens), **understanding structured data** (e.g, tables), and **generating structured outputs** especially JSON. **More resilient to the diversity of system prompts**, enhancing role-play implementation and condition-setting for chatbots.
- **Long-context Support** up to 128K tokens and can generate up to 8K tokens.
- **Multilingual support** for over 29 languages, including Chinese, English, French, Spanish, Portuguese, German, Italian, Russian, Japanese, Korean, Vietnamese, Thai, Arabic, and more.
**This repo contains the instruction-tuned 0.5B Qwen2.5 model**, which has the following features:
- Type: Causal Language Models
- Training Stage: Pretraining & Post-training
- Architecture: transformers with RoPE, SwiGLU, RMSNorm, Attention QKV bias and tied word embeddings
- Number of Parameters: 0.49B
- Number of Paramaters (Non-Embedding): 0.36B
- Number of Layers: 24
- Number of Attention Heads (GQA): 14 for Q and 2 for KV
- Context Length: Full 32,768 tokens and generation 8192 tokens
For more details, please refer to our [blog](https://qwenlm.github.io/blog/qwen2.5/), [GitHub](https://github.com/QwenLM/Qwen2.5), and [Documentation](https://qwen.readthedocs.io/en/latest/).
## Requirements
The code of Qwen2.5 has been in the latest Hugging face `transformers` and we advise you to use the latest version of `transformers`.
With `transformers<4.37.0`, you will encounter the following error:
```
KeyError: 'qwen2'
```
## Quickstart
Here provides a code snippet with `apply_chat_template` to show you how to load the tokenizer and model and how to generate contents.
```python
from transformers import AutoModelForCausalLM, AutoTokenizer
model_name = "Qwen/Qwen2.5-0.5B-Instruct"
model = AutoModelForCausalLM.from_pretrained(
model_name,
torch_dtype="auto",
device_map="auto"
)
tokenizer = AutoTokenizer.from_pretrained(model_name)
prompt = "Give me a short introduction to large language model."
messages = [
{"role": "system", "content": "You are Qwen, created by Alibaba Cloud. You are a helpful assistant."},
{"role": "user", "content": prompt}
]
text = tokenizer.apply_chat_template(
messages,
tokenize=False,
add_generation_prompt=True
)
model_inputs = tokenizer([text], return_tensors="pt").to(model.device)
generated_ids = model.generate(
**model_inputs,
max_new_tokens=512
)
generated_ids = [
output_ids[len(input_ids):] for input_ids, output_ids in zip(model_inputs.input_ids, generated_ids)
]
response = tokenizer.batch_decode(generated_ids, skip_special_tokens=True)[0]
```
## Evaluation & Performance
Detailed evaluation results are reported in this [📑 blog](https://qwenlm.github.io/blog/qwen2.5/).
For requirements on GPU memory and the respective throughput, see results [here](https://qwen.readthedocs.io/en/latest/benchmark/speed_benchmark.html).
## Citation
If you find our work helpful, feel free to give us a cite.
```
@misc{qwen2.5,
title = {Qwen2.5: A Party of Foundation Models},
url = {https://qwenlm.github.io/blog/qwen2.5/},
author = {Qwen Team},
month = {September},
year = {2024}
}
@article{qwen2,
title={Qwen2 Technical Report},
author={An Yang and Baosong Yang and Binyuan Hui and Bo Zheng and Bowen Yu and Chang Zhou and Chengpeng Li and Chengyuan Li and Dayiheng Liu and Fei Huang and Guanting Dong and Haoran Wei and Huan Lin and Jialong Tang and Jialin Wang and Jian Yang and Jianhong Tu and Jianwei Zhang and Jianxin Ma and Jin Xu and Jingren Zhou and Jinze Bai and Jinzheng He and Junyang Lin and Kai Dang and Keming Lu and Keqin Chen and Kexin Yang and Mei Li and Mingfeng Xue and Na Ni and Pei Zhang and Peng Wang and Ru Peng and Rui Men and Ruize Gao and Runji Lin and Shijie Wang and Shuai Bai and Sinan Tan and Tianhang Zhu and Tianhao Li and Tianyu Liu and Wenbin Ge and Xiaodong Deng and Xiaohuan Zhou and Xingzhang Ren and Xinyu Zhang and Xipin Wei and Xuancheng Ren and Yang Fan and Yang Yao and Yichang Zhang and Yu Wan and Yunfei Chu and Yuqiong Liu and Zeyu Cui and Zhenru Zhang and Zhihao Fan},
journal={arXiv preprint arXiv:2407.10671},
year={2024}
}
``` |
avsolatorio/GIST-all-MiniLM-L6-v2 | avsolatorio | "2024-04-24T23:15:05Z" | 428,621 | 7 | sentence-transformers | [
"sentence-transformers",
"pytorch",
"onnx",
"safetensors",
"bert",
"feature-extraction",
"mteb",
"sentence-similarity",
"en",
"arxiv:2402.16829",
"arxiv:2212.09741",
"license:mit",
"model-index",
"autotrain_compatible",
"text-embeddings-inference",
"endpoints_compatible",
"region:us"
] | sentence-similarity | "2024-02-03T05:28:49Z" | ---
language:
- en
library_name: sentence-transformers
license: mit
pipeline_tag: sentence-similarity
tags:
- feature-extraction
- mteb
- sentence-similarity
- sentence-transformers
model-index:
- name: GIST-all-MiniLM-L6-v2
results:
- task:
type: Classification
dataset:
type: mteb/amazon_counterfactual
name: MTEB AmazonCounterfactualClassification (en)
config: en
split: test
revision: e8379541af4e31359cca9fbcf4b00f2671dba205
metrics:
- type: accuracy
value: 72.8955223880597
- type: ap
value: 35.447605103320775
- type: f1
value: 66.82951715365854
- task:
type: Classification
dataset:
type: mteb/amazon_polarity
name: MTEB AmazonPolarityClassification
config: default
split: test
revision: e2d317d38cd51312af73b3d32a06d1a08b442046
metrics:
- type: accuracy
value: 87.19474999999998
- type: ap
value: 83.09577890808514
- type: f1
value: 87.13833121762009
- task:
type: Classification
dataset:
type: mteb/amazon_reviews_multi
name: MTEB AmazonReviewsClassification (en)
config: en
split: test
revision: 1399c76144fd37290681b995c656ef9b2e06e26d
metrics:
- type: accuracy
value: 42.556000000000004
- type: f1
value: 42.236256693772276
- task:
type: Retrieval
dataset:
type: arguana
name: MTEB ArguAna
config: default
split: test
revision: None
metrics:
- type: map_at_1
value: 26.884999999999998
- type: map_at_10
value: 42.364000000000004
- type: map_at_100
value: 43.382
- type: map_at_1000
value: 43.391000000000005
- type: map_at_3
value: 37.162
- type: map_at_5
value: 40.139
- type: mrr_at_1
value: 26.884999999999998
- type: mrr_at_10
value: 42.193999999999996
- type: mrr_at_100
value: 43.211
- type: mrr_at_1000
value: 43.221
- type: mrr_at_3
value: 36.949
- type: mrr_at_5
value: 40.004
- type: ndcg_at_1
value: 26.884999999999998
- type: ndcg_at_10
value: 51.254999999999995
- type: ndcg_at_100
value: 55.481
- type: ndcg_at_1000
value: 55.68300000000001
- type: ndcg_at_3
value: 40.565
- type: ndcg_at_5
value: 45.882
- type: precision_at_1
value: 26.884999999999998
- type: precision_at_10
value: 7.9799999999999995
- type: precision_at_100
value: 0.98
- type: precision_at_1000
value: 0.1
- type: precision_at_3
value: 16.808999999999997
- type: precision_at_5
value: 12.645999999999999
- type: recall_at_1
value: 26.884999999999998
- type: recall_at_10
value: 79.801
- type: recall_at_100
value: 98.009
- type: recall_at_1000
value: 99.502
- type: recall_at_3
value: 50.427
- type: recall_at_5
value: 63.229
- task:
type: Clustering
dataset:
type: mteb/arxiv-clustering-p2p
name: MTEB ArxivClusteringP2P
config: default
split: test
revision: a122ad7f3f0291bf49cc6f4d32aa80929df69d5d
metrics:
- type: v_measure
value: 45.31044837358167
- task:
type: Clustering
dataset:
type: mteb/arxiv-clustering-s2s
name: MTEB ArxivClusteringS2S
config: default
split: test
revision: f910caf1a6075f7329cdf8c1a6135696f37dbd53
metrics:
- type: v_measure
value: 35.44751738734691
- task:
type: Reranking
dataset:
type: mteb/askubuntudupquestions-reranking
name: MTEB AskUbuntuDupQuestions
config: default
split: test
revision: 2000358ca161889fa9c082cb41daa8dcfb161a54
metrics:
- type: map
value: 62.96517580629869
- type: mrr
value: 76.30051004704744
- task:
type: STS
dataset:
type: mteb/biosses-sts
name: MTEB BIOSSES
config: default
split: test
revision: d3fb88f8f02e40887cd149695127462bbcf29b4a
metrics:
- type: cos_sim_pearson
value: 83.97262600499639
- type: cos_sim_spearman
value: 81.25787561220484
- type: euclidean_pearson
value: 64.96260261677082
- type: euclidean_spearman
value: 64.17616109254686
- type: manhattan_pearson
value: 65.05620628102835
- type: manhattan_spearman
value: 64.71171546419122
- task:
type: Classification
dataset:
type: mteb/banking77
name: MTEB Banking77Classification
config: default
split: test
revision: 0fd18e25b25c072e09e0d92ab615fda904d66300
metrics:
- type: accuracy
value: 84.2435064935065
- type: f1
value: 84.2334859253828
- task:
type: Clustering
dataset:
type: mteb/biorxiv-clustering-p2p
name: MTEB BiorxivClusteringP2P
config: default
split: test
revision: 65b79d1d13f80053f67aca9498d9402c2d9f1f40
metrics:
- type: v_measure
value: 38.38358435972693
- task:
type: Clustering
dataset:
type: mteb/biorxiv-clustering-s2s
name: MTEB BiorxivClusteringS2S
config: default
split: test
revision: 258694dd0231531bc1fd9de6ceb52a0853c6d908
metrics:
- type: v_measure
value: 31.093619653843124
- task:
type: Retrieval
dataset:
type: BeIR/cqadupstack
name: MTEB CQADupstackAndroidRetrieval
config: default
split: test
revision: None
metrics:
- type: map_at_1
value: 35.016999999999996
- type: map_at_10
value: 47.019
- type: map_at_100
value: 48.634
- type: map_at_1000
value: 48.757
- type: map_at_3
value: 43.372
- type: map_at_5
value: 45.314
- type: mrr_at_1
value: 43.491
- type: mrr_at_10
value: 53.284
- type: mrr_at_100
value: 54.038
- type: mrr_at_1000
value: 54.071000000000005
- type: mrr_at_3
value: 51.001
- type: mrr_at_5
value: 52.282
- type: ndcg_at_1
value: 43.491
- type: ndcg_at_10
value: 53.498999999999995
- type: ndcg_at_100
value: 58.733999999999995
- type: ndcg_at_1000
value: 60.307
- type: ndcg_at_3
value: 48.841
- type: ndcg_at_5
value: 50.76199999999999
- type: precision_at_1
value: 43.491
- type: precision_at_10
value: 10.315000000000001
- type: precision_at_100
value: 1.6209999999999998
- type: precision_at_1000
value: 0.20500000000000002
- type: precision_at_3
value: 23.462
- type: precision_at_5
value: 16.652
- type: recall_at_1
value: 35.016999999999996
- type: recall_at_10
value: 64.92
- type: recall_at_100
value: 86.605
- type: recall_at_1000
value: 96.174
- type: recall_at_3
value: 50.99
- type: recall_at_5
value: 56.93
- task:
type: Retrieval
dataset:
type: BeIR/cqadupstack
name: MTEB CQADupstackEnglishRetrieval
config: default
split: test
revision: None
metrics:
- type: map_at_1
value: 29.866
- type: map_at_10
value: 40.438
- type: map_at_100
value: 41.77
- type: map_at_1000
value: 41.913
- type: map_at_3
value: 37.634
- type: map_at_5
value: 39.226
- type: mrr_at_1
value: 37.834
- type: mrr_at_10
value: 46.765
- type: mrr_at_100
value: 47.410000000000004
- type: mrr_at_1000
value: 47.461
- type: mrr_at_3
value: 44.735
- type: mrr_at_5
value: 46.028000000000006
- type: ndcg_at_1
value: 37.834
- type: ndcg_at_10
value: 46.303
- type: ndcg_at_100
value: 50.879
- type: ndcg_at_1000
value: 53.112
- type: ndcg_at_3
value: 42.601
- type: ndcg_at_5
value: 44.384
- type: precision_at_1
value: 37.834
- type: precision_at_10
value: 8.898
- type: precision_at_100
value: 1.4409999999999998
- type: precision_at_1000
value: 0.19499999999999998
- type: precision_at_3
value: 20.977
- type: precision_at_5
value: 14.841
- type: recall_at_1
value: 29.866
- type: recall_at_10
value: 56.06100000000001
- type: recall_at_100
value: 75.809
- type: recall_at_1000
value: 89.875
- type: recall_at_3
value: 44.707
- type: recall_at_5
value: 49.846000000000004
- task:
type: Retrieval
dataset:
type: BeIR/cqadupstack
name: MTEB CQADupstackGamingRetrieval
config: default
split: test
revision: None
metrics:
- type: map_at_1
value: 38.985
- type: map_at_10
value: 51.165000000000006
- type: map_at_100
value: 52.17
- type: map_at_1000
value: 52.229000000000006
- type: map_at_3
value: 48.089999999999996
- type: map_at_5
value: 49.762
- type: mrr_at_1
value: 44.577
- type: mrr_at_10
value: 54.493
- type: mrr_at_100
value: 55.137
- type: mrr_at_1000
value: 55.167
- type: mrr_at_3
value: 52.079
- type: mrr_at_5
value: 53.518
- type: ndcg_at_1
value: 44.577
- type: ndcg_at_10
value: 56.825
- type: ndcg_at_100
value: 60.842
- type: ndcg_at_1000
value: 62.015
- type: ndcg_at_3
value: 51.699
- type: ndcg_at_5
value: 54.11
- type: precision_at_1
value: 44.577
- type: precision_at_10
value: 9.11
- type: precision_at_100
value: 1.206
- type: precision_at_1000
value: 0.135
- type: precision_at_3
value: 23.156
- type: precision_at_5
value: 15.737000000000002
- type: recall_at_1
value: 38.985
- type: recall_at_10
value: 70.164
- type: recall_at_100
value: 87.708
- type: recall_at_1000
value: 95.979
- type: recall_at_3
value: 56.285
- type: recall_at_5
value: 62.303
- task:
type: Retrieval
dataset:
type: BeIR/cqadupstack
name: MTEB CQADupstackGisRetrieval
config: default
split: test
revision: None
metrics:
- type: map_at_1
value: 28.137
- type: map_at_10
value: 36.729
- type: map_at_100
value: 37.851
- type: map_at_1000
value: 37.932
- type: map_at_3
value: 34.074
- type: map_at_5
value: 35.398
- type: mrr_at_1
value: 30.621
- type: mrr_at_10
value: 39.007
- type: mrr_at_100
value: 39.961
- type: mrr_at_1000
value: 40.02
- type: mrr_at_3
value: 36.591
- type: mrr_at_5
value: 37.806
- type: ndcg_at_1
value: 30.621
- type: ndcg_at_10
value: 41.772
- type: ndcg_at_100
value: 47.181
- type: ndcg_at_1000
value: 49.053999999999995
- type: ndcg_at_3
value: 36.577
- type: ndcg_at_5
value: 38.777
- type: precision_at_1
value: 30.621
- type: precision_at_10
value: 6.372999999999999
- type: precision_at_100
value: 0.955
- type: precision_at_1000
value: 0.11499999999999999
- type: precision_at_3
value: 15.367
- type: precision_at_5
value: 10.531
- type: recall_at_1
value: 28.137
- type: recall_at_10
value: 55.162
- type: recall_at_100
value: 79.931
- type: recall_at_1000
value: 93.67
- type: recall_at_3
value: 41.057
- type: recall_at_5
value: 46.327
- task:
type: Retrieval
dataset:
type: BeIR/cqadupstack
name: MTEB CQADupstackMathematicaRetrieval
config: default
split: test
revision: None
metrics:
- type: map_at_1
value: 16.798
- type: map_at_10
value: 25.267
- type: map_at_100
value: 26.579000000000004
- type: map_at_1000
value: 26.697
- type: map_at_3
value: 22.456
- type: map_at_5
value: 23.912
- type: mrr_at_1
value: 20.771
- type: mrr_at_10
value: 29.843999999999998
- type: mrr_at_100
value: 30.849
- type: mrr_at_1000
value: 30.916
- type: mrr_at_3
value: 27.156000000000002
- type: mrr_at_5
value: 28.518
- type: ndcg_at_1
value: 20.771
- type: ndcg_at_10
value: 30.792
- type: ndcg_at_100
value: 36.945
- type: ndcg_at_1000
value: 39.619
- type: ndcg_at_3
value: 25.52
- type: ndcg_at_5
value: 27.776
- type: precision_at_1
value: 20.771
- type: precision_at_10
value: 5.734
- type: precision_at_100
value: 1.031
- type: precision_at_1000
value: 0.13899999999999998
- type: precision_at_3
value: 12.148
- type: precision_at_5
value: 9.055
- type: recall_at_1
value: 16.798
- type: recall_at_10
value: 43.332
- type: recall_at_100
value: 70.016
- type: recall_at_1000
value: 88.90400000000001
- type: recall_at_3
value: 28.842000000000002
- type: recall_at_5
value: 34.37
- task:
type: Retrieval
dataset:
type: BeIR/cqadupstack
name: MTEB CQADupstackPhysicsRetrieval
config: default
split: test
revision: None
metrics:
- type: map_at_1
value: 31.180000000000003
- type: map_at_10
value: 41.78
- type: map_at_100
value: 43.102000000000004
- type: map_at_1000
value: 43.222
- type: map_at_3
value: 38.505
- type: map_at_5
value: 40.443
- type: mrr_at_1
value: 37.824999999999996
- type: mrr_at_10
value: 47.481
- type: mrr_at_100
value: 48.268
- type: mrr_at_1000
value: 48.313
- type: mrr_at_3
value: 44.946999999999996
- type: mrr_at_5
value: 46.492
- type: ndcg_at_1
value: 37.824999999999996
- type: ndcg_at_10
value: 47.827
- type: ndcg_at_100
value: 53.407000000000004
- type: ndcg_at_1000
value: 55.321
- type: ndcg_at_3
value: 42.815
- type: ndcg_at_5
value: 45.363
- type: precision_at_1
value: 37.824999999999996
- type: precision_at_10
value: 8.652999999999999
- type: precision_at_100
value: 1.354
- type: precision_at_1000
value: 0.172
- type: precision_at_3
value: 20.372
- type: precision_at_5
value: 14.591000000000001
- type: recall_at_1
value: 31.180000000000003
- type: recall_at_10
value: 59.894000000000005
- type: recall_at_100
value: 83.722
- type: recall_at_1000
value: 95.705
- type: recall_at_3
value: 45.824
- type: recall_at_5
value: 52.349999999999994
- task:
type: Retrieval
dataset:
type: BeIR/cqadupstack
name: MTEB CQADupstackProgrammersRetrieval
config: default
split: test
revision: None
metrics:
- type: map_at_1
value: 24.66
- type: map_at_10
value: 34.141
- type: map_at_100
value: 35.478
- type: map_at_1000
value: 35.594
- type: map_at_3
value: 30.446
- type: map_at_5
value: 32.583
- type: mrr_at_1
value: 29.909000000000002
- type: mrr_at_10
value: 38.949
- type: mrr_at_100
value: 39.803
- type: mrr_at_1000
value: 39.867999999999995
- type: mrr_at_3
value: 35.921
- type: mrr_at_5
value: 37.753
- type: ndcg_at_1
value: 29.909000000000002
- type: ndcg_at_10
value: 40.012
- type: ndcg_at_100
value: 45.707
- type: ndcg_at_1000
value: 48.15
- type: ndcg_at_3
value: 34.015
- type: ndcg_at_5
value: 37.002
- type: precision_at_1
value: 29.909000000000002
- type: precision_at_10
value: 7.693999999999999
- type: precision_at_100
value: 1.2229999999999999
- type: precision_at_1000
value: 0.16
- type: precision_at_3
value: 16.323999999999998
- type: precision_at_5
value: 12.306000000000001
- type: recall_at_1
value: 24.66
- type: recall_at_10
value: 52.478
- type: recall_at_100
value: 77.051
- type: recall_at_1000
value: 93.872
- type: recall_at_3
value: 36.382999999999996
- type: recall_at_5
value: 43.903999999999996
- task:
type: Retrieval
dataset:
type: BeIR/cqadupstack
name: MTEB CQADupstackRetrieval
config: default
split: test
revision: None
metrics:
- type: map_at_1
value: 26.768416666666667
- type: map_at_10
value: 36.2485
- type: map_at_100
value: 37.520833333333336
- type: map_at_1000
value: 37.64033333333334
- type: map_at_3
value: 33.25791666666667
- type: map_at_5
value: 34.877250000000004
- type: mrr_at_1
value: 31.65408333333334
- type: mrr_at_10
value: 40.43866666666667
- type: mrr_at_100
value: 41.301249999999996
- type: mrr_at_1000
value: 41.357499999999995
- type: mrr_at_3
value: 37.938916666666664
- type: mrr_at_5
value: 39.35183333333334
- type: ndcg_at_1
value: 31.65408333333334
- type: ndcg_at_10
value: 41.76983333333334
- type: ndcg_at_100
value: 47.138
- type: ndcg_at_1000
value: 49.33816666666667
- type: ndcg_at_3
value: 36.76683333333333
- type: ndcg_at_5
value: 39.04441666666666
- type: precision_at_1
value: 31.65408333333334
- type: precision_at_10
value: 7.396249999999998
- type: precision_at_100
value: 1.1974166666666666
- type: precision_at_1000
value: 0.15791666666666668
- type: precision_at_3
value: 16.955583333333333
- type: precision_at_5
value: 12.09925
- type: recall_at_1
value: 26.768416666666667
- type: recall_at_10
value: 53.82366666666667
- type: recall_at_100
value: 77.39600000000002
- type: recall_at_1000
value: 92.46300000000001
- type: recall_at_3
value: 39.90166666666667
- type: recall_at_5
value: 45.754000000000005
- task:
type: Retrieval
dataset:
type: BeIR/cqadupstack
name: MTEB CQADupstackStatsRetrieval
config: default
split: test
revision: None
metrics:
- type: map_at_1
value: 24.369
- type: map_at_10
value: 32.025
- type: map_at_100
value: 33.08
- type: map_at_1000
value: 33.169
- type: map_at_3
value: 29.589
- type: map_at_5
value: 30.894
- type: mrr_at_1
value: 27.301
- type: mrr_at_10
value: 34.64
- type: mrr_at_100
value: 35.556
- type: mrr_at_1000
value: 35.616
- type: mrr_at_3
value: 32.515
- type: mrr_at_5
value: 33.666000000000004
- type: ndcg_at_1
value: 27.301
- type: ndcg_at_10
value: 36.386
- type: ndcg_at_100
value: 41.598
- type: ndcg_at_1000
value: 43.864999999999995
- type: ndcg_at_3
value: 32.07
- type: ndcg_at_5
value: 34.028999999999996
- type: precision_at_1
value: 27.301
- type: precision_at_10
value: 5.782
- type: precision_at_100
value: 0.923
- type: precision_at_1000
value: 0.11900000000000001
- type: precision_at_3
value: 13.804
- type: precision_at_5
value: 9.693
- type: recall_at_1
value: 24.369
- type: recall_at_10
value: 47.026
- type: recall_at_100
value: 70.76400000000001
- type: recall_at_1000
value: 87.705
- type: recall_at_3
value: 35.366
- type: recall_at_5
value: 40.077
- task:
type: Retrieval
dataset:
type: BeIR/cqadupstack
name: MTEB CQADupstackTexRetrieval
config: default
split: test
revision: None
metrics:
- type: map_at_1
value: 17.878
- type: map_at_10
value: 25.582
- type: map_at_100
value: 26.848
- type: map_at_1000
value: 26.985
- type: map_at_3
value: 22.997
- type: map_at_5
value: 24.487000000000002
- type: mrr_at_1
value: 22.023
- type: mrr_at_10
value: 29.615000000000002
- type: mrr_at_100
value: 30.656
- type: mrr_at_1000
value: 30.737
- type: mrr_at_3
value: 27.322999999999997
- type: mrr_at_5
value: 28.665000000000003
- type: ndcg_at_1
value: 22.023
- type: ndcg_at_10
value: 30.476999999999997
- type: ndcg_at_100
value: 36.258
- type: ndcg_at_1000
value: 39.287
- type: ndcg_at_3
value: 25.995
- type: ndcg_at_5
value: 28.174
- type: precision_at_1
value: 22.023
- type: precision_at_10
value: 5.657
- type: precision_at_100
value: 1.01
- type: precision_at_1000
value: 0.145
- type: precision_at_3
value: 12.491
- type: precision_at_5
value: 9.112
- type: recall_at_1
value: 17.878
- type: recall_at_10
value: 41.155
- type: recall_at_100
value: 66.62599999999999
- type: recall_at_1000
value: 88.08200000000001
- type: recall_at_3
value: 28.505000000000003
- type: recall_at_5
value: 34.284
- task:
type: Retrieval
dataset:
type: BeIR/cqadupstack
name: MTEB CQADupstackUnixRetrieval
config: default
split: test
revision: None
metrics:
- type: map_at_1
value: 26.369999999999997
- type: map_at_10
value: 36.115
- type: map_at_100
value: 37.346000000000004
- type: map_at_1000
value: 37.449
- type: map_at_3
value: 32.976
- type: map_at_5
value: 34.782000000000004
- type: mrr_at_1
value: 30.784
- type: mrr_at_10
value: 40.014
- type: mrr_at_100
value: 40.913
- type: mrr_at_1000
value: 40.967999999999996
- type: mrr_at_3
value: 37.205
- type: mrr_at_5
value: 38.995999999999995
- type: ndcg_at_1
value: 30.784
- type: ndcg_at_10
value: 41.797000000000004
- type: ndcg_at_100
value: 47.355000000000004
- type: ndcg_at_1000
value: 49.535000000000004
- type: ndcg_at_3
value: 36.29
- type: ndcg_at_5
value: 39.051
- type: precision_at_1
value: 30.784
- type: precision_at_10
value: 7.164
- type: precision_at_100
value: 1.122
- type: precision_at_1000
value: 0.14200000000000002
- type: precision_at_3
value: 16.636
- type: precision_at_5
value: 11.996
- type: recall_at_1
value: 26.369999999999997
- type: recall_at_10
value: 55.010000000000005
- type: recall_at_100
value: 79.105
- type: recall_at_1000
value: 94.053
- type: recall_at_3
value: 40.139
- type: recall_at_5
value: 47.089
- task:
type: Retrieval
dataset:
type: BeIR/cqadupstack
name: MTEB CQADupstackWebmastersRetrieval
config: default
split: test
revision: None
metrics:
- type: map_at_1
value: 26.421
- type: map_at_10
value: 35.253
- type: map_at_100
value: 36.97
- type: map_at_1000
value: 37.195
- type: map_at_3
value: 32.068000000000005
- type: map_at_5
value: 33.763
- type: mrr_at_1
value: 31.423000000000002
- type: mrr_at_10
value: 39.995999999999995
- type: mrr_at_100
value: 40.977999999999994
- type: mrr_at_1000
value: 41.024
- type: mrr_at_3
value: 36.989
- type: mrr_at_5
value: 38.629999999999995
- type: ndcg_at_1
value: 31.423000000000002
- type: ndcg_at_10
value: 41.382000000000005
- type: ndcg_at_100
value: 47.532000000000004
- type: ndcg_at_1000
value: 49.829
- type: ndcg_at_3
value: 35.809000000000005
- type: ndcg_at_5
value: 38.308
- type: precision_at_1
value: 31.423000000000002
- type: precision_at_10
value: 7.885000000000001
- type: precision_at_100
value: 1.609
- type: precision_at_1000
value: 0.246
- type: precision_at_3
value: 16.469
- type: precision_at_5
value: 12.174
- type: recall_at_1
value: 26.421
- type: recall_at_10
value: 53.618
- type: recall_at_100
value: 80.456
- type: recall_at_1000
value: 94.505
- type: recall_at_3
value: 37.894
- type: recall_at_5
value: 44.352999999999994
- task:
type: Retrieval
dataset:
type: BeIR/cqadupstack
name: MTEB CQADupstackWordpressRetrieval
config: default
split: test
revision: None
metrics:
- type: map_at_1
value: 21.54
- type: map_at_10
value: 29.468
- type: map_at_100
value: 30.422
- type: map_at_1000
value: 30.542
- type: map_at_3
value: 26.888
- type: map_at_5
value: 27.962999999999997
- type: mrr_at_1
value: 23.29
- type: mrr_at_10
value: 31.176
- type: mrr_at_100
value: 32.046
- type: mrr_at_1000
value: 32.129000000000005
- type: mrr_at_3
value: 28.804999999999996
- type: mrr_at_5
value: 29.868
- type: ndcg_at_1
value: 23.29
- type: ndcg_at_10
value: 34.166000000000004
- type: ndcg_at_100
value: 39.217999999999996
- type: ndcg_at_1000
value: 41.964
- type: ndcg_at_3
value: 28.970000000000002
- type: ndcg_at_5
value: 30.797
- type: precision_at_1
value: 23.29
- type: precision_at_10
value: 5.489999999999999
- type: precision_at_100
value: 0.874
- type: precision_at_1000
value: 0.122
- type: precision_at_3
value: 12.261
- type: precision_at_5
value: 8.503
- type: recall_at_1
value: 21.54
- type: recall_at_10
value: 47.064
- type: recall_at_100
value: 70.959
- type: recall_at_1000
value: 91.032
- type: recall_at_3
value: 32.828
- type: recall_at_5
value: 37.214999999999996
- task:
type: Retrieval
dataset:
type: climate-fever
name: MTEB ClimateFEVER
config: default
split: test
revision: None
metrics:
- type: map_at_1
value: 10.102
- type: map_at_10
value: 17.469
- type: map_at_100
value: 19.244
- type: map_at_1000
value: 19.435
- type: map_at_3
value: 14.257
- type: map_at_5
value: 16.028000000000002
- type: mrr_at_1
value: 22.866
- type: mrr_at_10
value: 33.535
- type: mrr_at_100
value: 34.583999999999996
- type: mrr_at_1000
value: 34.622
- type: mrr_at_3
value: 29.946
- type: mrr_at_5
value: 32.157000000000004
- type: ndcg_at_1
value: 22.866
- type: ndcg_at_10
value: 25.16
- type: ndcg_at_100
value: 32.347
- type: ndcg_at_1000
value: 35.821
- type: ndcg_at_3
value: 19.816
- type: ndcg_at_5
value: 22.026
- type: precision_at_1
value: 22.866
- type: precision_at_10
value: 8.072
- type: precision_at_100
value: 1.5709999999999997
- type: precision_at_1000
value: 0.22200000000000003
- type: precision_at_3
value: 14.701
- type: precision_at_5
value: 11.960999999999999
- type: recall_at_1
value: 10.102
- type: recall_at_10
value: 31.086000000000002
- type: recall_at_100
value: 55.896
- type: recall_at_1000
value: 75.375
- type: recall_at_3
value: 18.343999999999998
- type: recall_at_5
value: 24.102
- task:
type: Retrieval
dataset:
type: dbpedia-entity
name: MTEB DBPedia
config: default
split: test
revision: None
metrics:
- type: map_at_1
value: 7.961
- type: map_at_10
value: 16.058
- type: map_at_100
value: 21.878
- type: map_at_1000
value: 23.156
- type: map_at_3
value: 12.206999999999999
- type: map_at_5
value: 13.747000000000002
- type: mrr_at_1
value: 60.5
- type: mrr_at_10
value: 68.488
- type: mrr_at_100
value: 69.02199999999999
- type: mrr_at_1000
value: 69.03200000000001
- type: mrr_at_3
value: 66.792
- type: mrr_at_5
value: 67.62899999999999
- type: ndcg_at_1
value: 49.125
- type: ndcg_at_10
value: 34.827999999999996
- type: ndcg_at_100
value: 38.723
- type: ndcg_at_1000
value: 45.988
- type: ndcg_at_3
value: 40.302
- type: ndcg_at_5
value: 36.781000000000006
- type: precision_at_1
value: 60.5
- type: precision_at_10
value: 26.825
- type: precision_at_100
value: 8.445
- type: precision_at_1000
value: 1.7000000000000002
- type: precision_at_3
value: 43.25
- type: precision_at_5
value: 34.5
- type: recall_at_1
value: 7.961
- type: recall_at_10
value: 20.843
- type: recall_at_100
value: 43.839
- type: recall_at_1000
value: 67.33
- type: recall_at_3
value: 13.516
- type: recall_at_5
value: 15.956000000000001
- task:
type: Classification
dataset:
type: mteb/emotion
name: MTEB EmotionClassification
config: default
split: test
revision: 4f58c6b202a23cf9a4da393831edf4f9183cad37
metrics:
- type: accuracy
value: 52.06000000000001
- type: f1
value: 47.21494728335567
- task:
type: Retrieval
dataset:
type: fever
name: MTEB FEVER
config: default
split: test
revision: None
metrics:
- type: map_at_1
value: 56.798
- type: map_at_10
value: 67.644
- type: map_at_100
value: 68.01700000000001
- type: map_at_1000
value: 68.038
- type: map_at_3
value: 65.539
- type: map_at_5
value: 66.912
- type: mrr_at_1
value: 61.221000000000004
- type: mrr_at_10
value: 71.97099999999999
- type: mrr_at_100
value: 72.262
- type: mrr_at_1000
value: 72.27
- type: mrr_at_3
value: 70.052
- type: mrr_at_5
value: 71.324
- type: ndcg_at_1
value: 61.221000000000004
- type: ndcg_at_10
value: 73.173
- type: ndcg_at_100
value: 74.779
- type: ndcg_at_1000
value: 75.229
- type: ndcg_at_3
value: 69.291
- type: ndcg_at_5
value: 71.552
- type: precision_at_1
value: 61.221000000000004
- type: precision_at_10
value: 9.449
- type: precision_at_100
value: 1.0370000000000001
- type: precision_at_1000
value: 0.109
- type: precision_at_3
value: 27.467999999999996
- type: precision_at_5
value: 17.744
- type: recall_at_1
value: 56.798
- type: recall_at_10
value: 85.991
- type: recall_at_100
value: 92.973
- type: recall_at_1000
value: 96.089
- type: recall_at_3
value: 75.576
- type: recall_at_5
value: 81.12
- task:
type: Retrieval
dataset:
type: fiqa
name: MTEB FiQA2018
config: default
split: test
revision: None
metrics:
- type: map_at_1
value: 18.323
- type: map_at_10
value: 30.279
- type: map_at_100
value: 32.153999999999996
- type: map_at_1000
value: 32.339
- type: map_at_3
value: 26.336
- type: map_at_5
value: 28.311999999999998
- type: mrr_at_1
value: 35.339999999999996
- type: mrr_at_10
value: 44.931
- type: mrr_at_100
value: 45.818999999999996
- type: mrr_at_1000
value: 45.864
- type: mrr_at_3
value: 42.618
- type: mrr_at_5
value: 43.736999999999995
- type: ndcg_at_1
value: 35.339999999999996
- type: ndcg_at_10
value: 37.852999999999994
- type: ndcg_at_100
value: 44.888
- type: ndcg_at_1000
value: 48.069
- type: ndcg_at_3
value: 34.127
- type: ndcg_at_5
value: 35.026
- type: precision_at_1
value: 35.339999999999996
- type: precision_at_10
value: 10.617
- type: precision_at_100
value: 1.7930000000000001
- type: precision_at_1000
value: 0.23600000000000002
- type: precision_at_3
value: 22.582
- type: precision_at_5
value: 16.605
- type: recall_at_1
value: 18.323
- type: recall_at_10
value: 44.948
- type: recall_at_100
value: 71.11800000000001
- type: recall_at_1000
value: 90.104
- type: recall_at_3
value: 31.661
- type: recall_at_5
value: 36.498000000000005
- task:
type: Retrieval
dataset:
type: hotpotqa
name: MTEB HotpotQA
config: default
split: test
revision: None
metrics:
- type: map_at_1
value: 30.668
- type: map_at_10
value: 43.669999999999995
- type: map_at_100
value: 44.646
- type: map_at_1000
value: 44.731
- type: map_at_3
value: 40.897
- type: map_at_5
value: 42.559999999999995
- type: mrr_at_1
value: 61.336999999999996
- type: mrr_at_10
value: 68.496
- type: mrr_at_100
value: 68.916
- type: mrr_at_1000
value: 68.938
- type: mrr_at_3
value: 66.90700000000001
- type: mrr_at_5
value: 67.91199999999999
- type: ndcg_at_1
value: 61.336999999999996
- type: ndcg_at_10
value: 52.588
- type: ndcg_at_100
value: 56.389
- type: ndcg_at_1000
value: 58.187999999999995
- type: ndcg_at_3
value: 48.109
- type: ndcg_at_5
value: 50.498
- type: precision_at_1
value: 61.336999999999996
- type: precision_at_10
value: 11.033
- type: precision_at_100
value: 1.403
- type: precision_at_1000
value: 0.164
- type: precision_at_3
value: 30.105999999999998
- type: precision_at_5
value: 19.954
- type: recall_at_1
value: 30.668
- type: recall_at_10
value: 55.165
- type: recall_at_100
value: 70.169
- type: recall_at_1000
value: 82.12
- type: recall_at_3
value: 45.159
- type: recall_at_5
value: 49.885000000000005
- task:
type: Classification
dataset:
type: mteb/imdb
name: MTEB ImdbClassification
config: default
split: test
revision: 3d86128a09e091d6018b6d26cad27f2739fc2db7
metrics:
- type: accuracy
value: 78.542
- type: ap
value: 72.50692137216646
- type: f1
value: 78.40630687221642
- task:
type: Retrieval
dataset:
type: msmarco
name: MTEB MSMARCO
config: default
split: dev
revision: None
metrics:
- type: map_at_1
value: 18.613
- type: map_at_10
value: 29.98
- type: map_at_100
value: 31.136999999999997
- type: map_at_1000
value: 31.196
- type: map_at_3
value: 26.339000000000002
- type: map_at_5
value: 28.351
- type: mrr_at_1
value: 19.054
- type: mrr_at_10
value: 30.476
- type: mrr_at_100
value: 31.588
- type: mrr_at_1000
value: 31.641000000000002
- type: mrr_at_3
value: 26.834000000000003
- type: mrr_at_5
value: 28.849000000000004
- type: ndcg_at_1
value: 19.083
- type: ndcg_at_10
value: 36.541000000000004
- type: ndcg_at_100
value: 42.35
- type: ndcg_at_1000
value: 43.9
- type: ndcg_at_3
value: 29.015
- type: ndcg_at_5
value: 32.622
- type: precision_at_1
value: 19.083
- type: precision_at_10
value: 5.914
- type: precision_at_100
value: 0.889
- type: precision_at_1000
value: 0.10200000000000001
- type: precision_at_3
value: 12.483
- type: precision_at_5
value: 9.315
- type: recall_at_1
value: 18.613
- type: recall_at_10
value: 56.88999999999999
- type: recall_at_100
value: 84.207
- type: recall_at_1000
value: 96.20100000000001
- type: recall_at_3
value: 36.262
- type: recall_at_5
value: 44.925
- task:
type: Classification
dataset:
type: mteb/mtop_domain
name: MTEB MTOPDomainClassification (en)
config: en
split: test
revision: d80d48c1eb48d3562165c59d59d0034df9fff0bf
metrics:
- type: accuracy
value: 94.77656178750571
- type: f1
value: 94.37966073742972
- task:
type: Classification
dataset:
type: mteb/mtop_intent
name: MTEB MTOPIntentClassification (en)
config: en
split: test
revision: ae001d0e6b1228650b7bd1c2c65fb50ad11a8aba
metrics:
- type: accuracy
value: 77.72457820337438
- type: f1
value: 59.11327646329634
- task:
type: Classification
dataset:
type: mteb/amazon_massive_intent
name: MTEB MassiveIntentClassification (en)
config: en
split: test
revision: 31efe3c427b0bae9c22cbb560b8f15491cc6bed7
metrics:
- type: accuracy
value: 73.17753866846
- type: f1
value: 71.22604635414544
- task:
type: Classification
dataset:
type: mteb/amazon_massive_scenario
name: MTEB MassiveScenarioClassification (en)
config: en
split: test
revision: 7d571f92784cd94a019292a1f45445077d0ef634
metrics:
- type: accuracy
value: 76.67787491593813
- type: f1
value: 76.87653151298177
- task:
type: Clustering
dataset:
type: mteb/medrxiv-clustering-p2p
name: MTEB MedrxivClusteringP2P
config: default
split: test
revision: e7a26af6f3ae46b30dde8737f02c07b1505bcc73
metrics:
- type: v_measure
value: 33.3485843514749
- task:
type: Clustering
dataset:
type: mteb/medrxiv-clustering-s2s
name: MTEB MedrxivClusteringS2S
config: default
split: test
revision: 35191c8c0dca72d8ff3efcd72aa802307d469663
metrics:
- type: v_measure
value: 29.792796913883617
- task:
type: Reranking
dataset:
type: mteb/mind_small
name: MTEB MindSmallReranking
config: default
split: test
revision: 3bdac13927fdc888b903db93b2ffdbd90b295a69
metrics:
- type: map
value: 31.310305659169963
- type: mrr
value: 32.38286775798406
- task:
type: Retrieval
dataset:
type: nfcorpus
name: MTEB NFCorpus
config: default
split: test
revision: None
metrics:
- type: map_at_1
value: 4.968
- type: map_at_10
value: 11.379
- type: map_at_100
value: 14.618999999999998
- type: map_at_1000
value: 16.055
- type: map_at_3
value: 8.34
- type: map_at_5
value: 9.690999999999999
- type: mrr_at_1
value: 43.034
- type: mrr_at_10
value: 51.019999999999996
- type: mrr_at_100
value: 51.63100000000001
- type: mrr_at_1000
value: 51.681
- type: mrr_at_3
value: 49.174
- type: mrr_at_5
value: 50.181
- type: ndcg_at_1
value: 41.176
- type: ndcg_at_10
value: 31.341
- type: ndcg_at_100
value: 29.451
- type: ndcg_at_1000
value: 38.007000000000005
- type: ndcg_at_3
value: 36.494
- type: ndcg_at_5
value: 34.499
- type: precision_at_1
value: 43.034
- type: precision_at_10
value: 23.375
- type: precision_at_100
value: 7.799
- type: precision_at_1000
value: 2.059
- type: precision_at_3
value: 34.675
- type: precision_at_5
value: 30.154999999999998
- type: recall_at_1
value: 4.968
- type: recall_at_10
value: 15.104999999999999
- type: recall_at_100
value: 30.741000000000003
- type: recall_at_1000
value: 61.182
- type: recall_at_3
value: 9.338000000000001
- type: recall_at_5
value: 11.484
- task:
type: Retrieval
dataset:
type: nq
name: MTEB NQ
config: default
split: test
revision: None
metrics:
- type: map_at_1
value: 23.716
- type: map_at_10
value: 38.32
- type: map_at_100
value: 39.565
- type: map_at_1000
value: 39.602
- type: map_at_3
value: 33.848
- type: map_at_5
value: 36.471
- type: mrr_at_1
value: 26.912000000000003
- type: mrr_at_10
value: 40.607
- type: mrr_at_100
value: 41.589
- type: mrr_at_1000
value: 41.614000000000004
- type: mrr_at_3
value: 36.684
- type: mrr_at_5
value: 39.036
- type: ndcg_at_1
value: 26.883000000000003
- type: ndcg_at_10
value: 46.096
- type: ndcg_at_100
value: 51.513
- type: ndcg_at_1000
value: 52.366
- type: ndcg_at_3
value: 37.549
- type: ndcg_at_5
value: 41.971000000000004
- type: precision_at_1
value: 26.883000000000003
- type: precision_at_10
value: 8.004
- type: precision_at_100
value: 1.107
- type: precision_at_1000
value: 0.11900000000000001
- type: precision_at_3
value: 17.516000000000002
- type: precision_at_5
value: 13.019
- type: recall_at_1
value: 23.716
- type: recall_at_10
value: 67.656
- type: recall_at_100
value: 91.413
- type: recall_at_1000
value: 97.714
- type: recall_at_3
value: 45.449
- type: recall_at_5
value: 55.598000000000006
- task:
type: Retrieval
dataset:
type: quora
name: MTEB QuoraRetrieval
config: default
split: test
revision: None
metrics:
- type: map_at_1
value: 70.486
- type: map_at_10
value: 84.292
- type: map_at_100
value: 84.954
- type: map_at_1000
value: 84.969
- type: map_at_3
value: 81.295
- type: map_at_5
value: 83.165
- type: mrr_at_1
value: 81.16
- type: mrr_at_10
value: 87.31
- type: mrr_at_100
value: 87.423
- type: mrr_at_1000
value: 87.423
- type: mrr_at_3
value: 86.348
- type: mrr_at_5
value: 86.991
- type: ndcg_at_1
value: 81.17
- type: ndcg_at_10
value: 88.067
- type: ndcg_at_100
value: 89.34
- type: ndcg_at_1000
value: 89.43900000000001
- type: ndcg_at_3
value: 85.162
- type: ndcg_at_5
value: 86.752
- type: precision_at_1
value: 81.17
- type: precision_at_10
value: 13.394
- type: precision_at_100
value: 1.5310000000000001
- type: precision_at_1000
value: 0.157
- type: precision_at_3
value: 37.193
- type: precision_at_5
value: 24.482
- type: recall_at_1
value: 70.486
- type: recall_at_10
value: 95.184
- type: recall_at_100
value: 99.53999999999999
- type: recall_at_1000
value: 99.98700000000001
- type: recall_at_3
value: 86.89
- type: recall_at_5
value: 91.365
- task:
type: Clustering
dataset:
type: mteb/reddit-clustering
name: MTEB RedditClustering
config: default
split: test
revision: 24640382cdbf8abc73003fb0fa6d111a705499eb
metrics:
- type: v_measure
value: 44.118229475102154
- task:
type: Clustering
dataset:
type: mteb/reddit-clustering-p2p
name: MTEB RedditClusteringP2P
config: default
split: test
revision: 282350215ef01743dc01b456c7f5241fa8937f16
metrics:
- type: v_measure
value: 48.68049097629063
- task:
type: Retrieval
dataset:
type: scidocs
name: MTEB SCIDOCS
config: default
split: test
revision: None
metrics:
- type: map_at_1
value: 4.888
- type: map_at_10
value: 12.770999999999999
- type: map_at_100
value: 15.238
- type: map_at_1000
value: 15.616
- type: map_at_3
value: 8.952
- type: map_at_5
value: 10.639999999999999
- type: mrr_at_1
value: 24.099999999999998
- type: mrr_at_10
value: 35.375
- type: mrr_at_100
value: 36.442
- type: mrr_at_1000
value: 36.488
- type: mrr_at_3
value: 31.717000000000002
- type: mrr_at_5
value: 33.722
- type: ndcg_at_1
value: 24.099999999999998
- type: ndcg_at_10
value: 21.438
- type: ndcg_at_100
value: 30.601
- type: ndcg_at_1000
value: 36.678
- type: ndcg_at_3
value: 19.861
- type: ndcg_at_5
value: 17.263
- type: precision_at_1
value: 24.099999999999998
- type: precision_at_10
value: 11.4
- type: precision_at_100
value: 2.465
- type: precision_at_1000
value: 0.392
- type: precision_at_3
value: 18.733
- type: precision_at_5
value: 15.22
- type: recall_at_1
value: 4.888
- type: recall_at_10
value: 23.118
- type: recall_at_100
value: 49.995
- type: recall_at_1000
value: 79.577
- type: recall_at_3
value: 11.398
- type: recall_at_5
value: 15.428
- task:
type: STS
dataset:
type: mteb/sickr-sts
name: MTEB SICK-R
config: default
split: test
revision: a6ea5a8cab320b040a23452cc28066d9beae2cee
metrics:
- type: cos_sim_pearson
value: 85.33198632617024
- type: cos_sim_spearman
value: 79.09232997136625
- type: euclidean_pearson
value: 81.49986011523868
- type: euclidean_spearman
value: 77.03530620283338
- type: manhattan_pearson
value: 81.4741227286667
- type: manhattan_spearman
value: 76.98641133116311
- task:
type: STS
dataset:
type: mteb/sts12-sts
name: MTEB STS12
config: default
split: test
revision: a0d554a64d88156834ff5ae9920b964011b16384
metrics:
- type: cos_sim_pearson
value: 84.60103674582464
- type: cos_sim_spearman
value: 75.03945035801914
- type: euclidean_pearson
value: 80.82455267481467
- type: euclidean_spearman
value: 70.3317366248871
- type: manhattan_pearson
value: 80.8928091531445
- type: manhattan_spearman
value: 70.43207370945672
- task:
type: STS
dataset:
type: mteb/sts13-sts
name: MTEB STS13
config: default
split: test
revision: 7e90230a92c190f1bf69ae9002b8cea547a64cca
metrics:
- type: cos_sim_pearson
value: 82.52453177109315
- type: cos_sim_spearman
value: 83.26431569305103
- type: euclidean_pearson
value: 82.10494657997404
- type: euclidean_spearman
value: 83.41028425949024
- type: manhattan_pearson
value: 82.08669822983934
- type: manhattan_spearman
value: 83.39959776442115
- task:
type: STS
dataset:
type: mteb/sts14-sts
name: MTEB STS14
config: default
split: test
revision: 6031580fec1f6af667f0bd2da0a551cf4f0b2375
metrics:
- type: cos_sim_pearson
value: 82.67472020277681
- type: cos_sim_spearman
value: 78.61877889763109
- type: euclidean_pearson
value: 80.07878012437722
- type: euclidean_spearman
value: 77.44374494215397
- type: manhattan_pearson
value: 79.95988483102258
- type: manhattan_spearman
value: 77.36018101061366
- task:
type: STS
dataset:
type: mteb/sts15-sts
name: MTEB STS15
config: default
split: test
revision: ae752c7c21bf194d8b67fd573edf7ae58183cbe3
metrics:
- type: cos_sim_pearson
value: 85.55450610494437
- type: cos_sim_spearman
value: 87.03494331841401
- type: euclidean_pearson
value: 81.4319784394287
- type: euclidean_spearman
value: 82.47893040599372
- type: manhattan_pearson
value: 81.32627203699644
- type: manhattan_spearman
value: 82.40660565070675
- task:
type: STS
dataset:
type: mteb/sts16-sts
name: MTEB STS16
config: default
split: test
revision: 4d8694f8f0e0100860b497b999b3dbed754a0513
metrics:
- type: cos_sim_pearson
value: 81.51576965454805
- type: cos_sim_spearman
value: 83.0062959588245
- type: euclidean_pearson
value: 79.98888882568556
- type: euclidean_spearman
value: 81.08948911791873
- type: manhattan_pearson
value: 79.77952719568583
- type: manhattan_spearman
value: 80.79471040445408
- task:
type: STS
dataset:
type: mteb/sts17-crosslingual-sts
name: MTEB STS17 (en-en)
config: en-en
split: test
revision: af5e6fb845001ecf41f4c1e033ce921939a2a68d
metrics:
- type: cos_sim_pearson
value: 87.28313046682885
- type: cos_sim_spearman
value: 87.35865211085007
- type: euclidean_pearson
value: 84.11501613667811
- type: euclidean_spearman
value: 82.82038954956121
- type: manhattan_pearson
value: 83.891278147302
- type: manhattan_spearman
value: 82.59947685165902
- task:
type: STS
dataset:
type: mteb/sts22-crosslingual-sts
name: MTEB STS22 (en)
config: en
split: test
revision: 6d1ba47164174a496b7fa5d3569dae26a6813b80
metrics:
- type: cos_sim_pearson
value: 67.80653738006102
- type: cos_sim_spearman
value: 68.11259151179601
- type: euclidean_pearson
value: 43.16707985094242
- type: euclidean_spearman
value: 58.96200382968696
- type: manhattan_pearson
value: 43.84146858566507
- type: manhattan_spearman
value: 59.05193977207514
- task:
type: STS
dataset:
type: mteb/stsbenchmark-sts
name: MTEB STSBenchmark
config: default
split: test
revision: b0fddb56ed78048fa8b90373c8a3cfc37b684831
metrics:
- type: cos_sim_pearson
value: 82.62068205073571
- type: cos_sim_spearman
value: 84.40071593577095
- type: euclidean_pearson
value: 80.90824726252514
- type: euclidean_spearman
value: 80.54974812534094
- type: manhattan_pearson
value: 80.6759008187939
- type: manhattan_spearman
value: 80.31149103896973
- task:
type: Reranking
dataset:
type: mteb/scidocs-reranking
name: MTEB SciDocsRR
config: default
split: test
revision: d3c5e1fc0b855ab6097bf1cda04dd73947d7caab
metrics:
- type: map
value: 87.13774787530915
- type: mrr
value: 96.22233793802422
- task:
type: Retrieval
dataset:
type: scifact
name: MTEB SciFact
config: default
split: test
revision: None
metrics:
- type: map_at_1
value: 49.167
- type: map_at_10
value: 59.852000000000004
- type: map_at_100
value: 60.544
- type: map_at_1000
value: 60.577000000000005
- type: map_at_3
value: 57.242000000000004
- type: map_at_5
value: 58.704
- type: mrr_at_1
value: 51.0
- type: mrr_at_10
value: 60.575
- type: mrr_at_100
value: 61.144
- type: mrr_at_1000
value: 61.175000000000004
- type: mrr_at_3
value: 58.667
- type: mrr_at_5
value: 59.599999999999994
- type: ndcg_at_1
value: 51.0
- type: ndcg_at_10
value: 64.398
- type: ndcg_at_100
value: 67.581
- type: ndcg_at_1000
value: 68.551
- type: ndcg_at_3
value: 59.928000000000004
- type: ndcg_at_5
value: 61.986
- type: precision_at_1
value: 51.0
- type: precision_at_10
value: 8.7
- type: precision_at_100
value: 1.047
- type: precision_at_1000
value: 0.11299999999999999
- type: precision_at_3
value: 23.666999999999998
- type: precision_at_5
value: 15.6
- type: recall_at_1
value: 49.167
- type: recall_at_10
value: 77.333
- type: recall_at_100
value: 91.833
- type: recall_at_1000
value: 99.667
- type: recall_at_3
value: 65.594
- type: recall_at_5
value: 70.52199999999999
- task:
type: PairClassification
dataset:
type: mteb/sprintduplicatequestions-pairclassification
name: MTEB SprintDuplicateQuestions
config: default
split: test
revision: d66bd1f72af766a5cc4b0ca5e00c162f89e8cc46
metrics:
- type: cos_sim_accuracy
value: 99.77227722772277
- type: cos_sim_ap
value: 94.14261011689366
- type: cos_sim_f1
value: 88.37209302325581
- type: cos_sim_precision
value: 89.36605316973414
- type: cos_sim_recall
value: 87.4
- type: dot_accuracy
value: 99.07128712871287
- type: dot_ap
value: 27.325649239129486
- type: dot_f1
value: 33.295838020247466
- type: dot_precision
value: 38.04627249357326
- type: dot_recall
value: 29.599999999999998
- type: euclidean_accuracy
value: 99.74158415841585
- type: euclidean_ap
value: 92.32695359979576
- type: euclidean_f1
value: 86.90534575772439
- type: euclidean_precision
value: 85.27430221366699
- type: euclidean_recall
value: 88.6
- type: manhattan_accuracy
value: 99.74257425742574
- type: manhattan_ap
value: 92.40335687760499
- type: manhattan_f1
value: 86.96507624200687
- type: manhattan_precision
value: 85.57599225556632
- type: manhattan_recall
value: 88.4
- type: max_accuracy
value: 99.77227722772277
- type: max_ap
value: 94.14261011689366
- type: max_f1
value: 88.37209302325581
- task:
type: Clustering
dataset:
type: mteb/stackexchange-clustering
name: MTEB StackExchangeClustering
config: default
split: test
revision: 6cbc1f7b2bc0622f2e39d2c77fa502909748c259
metrics:
- type: v_measure
value: 53.113809982945035
- task:
type: Clustering
dataset:
type: mteb/stackexchange-clustering-p2p
name: MTEB StackExchangeClusteringP2P
config: default
split: test
revision: 815ca46b2622cec33ccafc3735d572c266efdb44
metrics:
- type: v_measure
value: 33.90915908471812
- task:
type: Reranking
dataset:
type: mteb/stackoverflowdupquestions-reranking
name: MTEB StackOverflowDupQuestions
config: default
split: test
revision: e185fbe320c72810689fc5848eb6114e1ef5ec69
metrics:
- type: map
value: 50.36481271702464
- type: mrr
value: 51.05628236142942
- task:
type: Summarization
dataset:
type: mteb/summeval
name: MTEB SummEval
config: default
split: test
revision: cda12ad7615edc362dbf25a00fdd61d3b1eaf93c
metrics:
- type: cos_sim_pearson
value: 30.311305530381826
- type: cos_sim_spearman
value: 31.22029657606254
- type: dot_pearson
value: 12.157032445910177
- type: dot_spearman
value: 13.275185888551805
- task:
type: Retrieval
dataset:
type: trec-covid
name: MTEB TRECCOVID
config: default
split: test
revision: None
metrics:
- type: map_at_1
value: 0.167
- type: map_at_10
value: 1.113
- type: map_at_100
value: 5.926
- type: map_at_1000
value: 15.25
- type: map_at_3
value: 0.414
- type: map_at_5
value: 0.633
- type: mrr_at_1
value: 64.0
- type: mrr_at_10
value: 74.444
- type: mrr_at_100
value: 74.667
- type: mrr_at_1000
value: 74.679
- type: mrr_at_3
value: 72.0
- type: mrr_at_5
value: 74.0
- type: ndcg_at_1
value: 59.0
- type: ndcg_at_10
value: 51.468
- type: ndcg_at_100
value: 38.135000000000005
- type: ndcg_at_1000
value: 36.946
- type: ndcg_at_3
value: 55.827000000000005
- type: ndcg_at_5
value: 53.555
- type: precision_at_1
value: 64.0
- type: precision_at_10
value: 54.400000000000006
- type: precision_at_100
value: 39.08
- type: precision_at_1000
value: 16.618
- type: precision_at_3
value: 58.667
- type: precision_at_5
value: 56.8
- type: recall_at_1
value: 0.167
- type: recall_at_10
value: 1.38
- type: recall_at_100
value: 9.189
- type: recall_at_1000
value: 35.737
- type: recall_at_3
value: 0.455
- type: recall_at_5
value: 0.73
- task:
type: Retrieval
dataset:
type: webis-touche2020
name: MTEB Touche2020
config: default
split: test
revision: None
metrics:
- type: map_at_1
value: 2.4299999999999997
- type: map_at_10
value: 8.539
- type: map_at_100
value: 14.155999999999999
- type: map_at_1000
value: 15.684999999999999
- type: map_at_3
value: 3.857
- type: map_at_5
value: 5.583
- type: mrr_at_1
value: 26.531
- type: mrr_at_10
value: 40.489999999999995
- type: mrr_at_100
value: 41.772999999999996
- type: mrr_at_1000
value: 41.772999999999996
- type: mrr_at_3
value: 35.034
- type: mrr_at_5
value: 38.81
- type: ndcg_at_1
value: 21.429000000000002
- type: ndcg_at_10
value: 20.787
- type: ndcg_at_100
value: 33.202
- type: ndcg_at_1000
value: 45.167
- type: ndcg_at_3
value: 18.233
- type: ndcg_at_5
value: 19.887
- type: precision_at_1
value: 26.531
- type: precision_at_10
value: 19.796
- type: precision_at_100
value: 7.4079999999999995
- type: precision_at_1000
value: 1.5310000000000001
- type: precision_at_3
value: 19.728
- type: precision_at_5
value: 21.633
- type: recall_at_1
value: 2.4299999999999997
- type: recall_at_10
value: 14.901
- type: recall_at_100
value: 46.422000000000004
- type: recall_at_1000
value: 82.83500000000001
- type: recall_at_3
value: 4.655
- type: recall_at_5
value: 8.092
- task:
type: Classification
dataset:
type: mteb/toxic_conversations_50k
name: MTEB ToxicConversationsClassification
config: default
split: test
revision: d7c0de2777da35d6aae2200a62c6e0e5af397c4c
metrics:
- type: accuracy
value: 72.90140000000001
- type: ap
value: 15.138716624430662
- type: f1
value: 56.08803013269606
- task:
type: Classification
dataset:
type: mteb/tweet_sentiment_extraction
name: MTEB TweetSentimentExtractionClassification
config: default
split: test
revision: d604517c81ca91fe16a244d1248fc021f9ecee7a
metrics:
- type: accuracy
value: 59.85285795132994
- type: f1
value: 60.17575819903709
- task:
type: Clustering
dataset:
type: mteb/twentynewsgroups-clustering
name: MTEB TwentyNewsgroupsClustering
config: default
split: test
revision: 6125ec4e24fa026cec8a478383ee943acfbd5449
metrics:
- type: v_measure
value: 41.125150148437065
- task:
type: PairClassification
dataset:
type: mteb/twittersemeval2015-pairclassification
name: MTEB TwitterSemEval2015
config: default
split: test
revision: 70970daeab8776df92f5ea462b6173c0b46fd2d1
metrics:
- type: cos_sim_accuracy
value: 84.96751505036657
- type: cos_sim_ap
value: 70.45642872444971
- type: cos_sim_f1
value: 65.75274793133259
- type: cos_sim_precision
value: 61.806361736707686
- type: cos_sim_recall
value: 70.23746701846966
- type: dot_accuracy
value: 77.84466829588126
- type: dot_ap
value: 32.49904328313596
- type: dot_f1
value: 37.903122189387126
- type: dot_precision
value: 25.050951086956523
- type: dot_recall
value: 77.83641160949868
- type: euclidean_accuracy
value: 84.5920009536866
- type: euclidean_ap
value: 68.83700633574043
- type: euclidean_f1
value: 64.92803542871202
- type: euclidean_precision
value: 60.820465545056464
- type: euclidean_recall
value: 69.63060686015831
- type: manhattan_accuracy
value: 84.52643500029802
- type: manhattan_ap
value: 68.63286046599892
- type: manhattan_f1
value: 64.7476540705047
- type: manhattan_precision
value: 62.3291015625
- type: manhattan_recall
value: 67.36147757255937
- type: max_accuracy
value: 84.96751505036657
- type: max_ap
value: 70.45642872444971
- type: max_f1
value: 65.75274793133259
- task:
type: PairClassification
dataset:
type: mteb/twitterurlcorpus-pairclassification
name: MTEB TwitterURLCorpus
config: default
split: test
revision: 8b6510b0b1fa4e4c4f879467980e9be563ec1cdf
metrics:
- type: cos_sim_accuracy
value: 88.65603291031164
- type: cos_sim_ap
value: 85.58148320880878
- type: cos_sim_f1
value: 77.63202920041064
- type: cos_sim_precision
value: 76.68444377675957
- type: cos_sim_recall
value: 78.60332614721281
- type: dot_accuracy
value: 79.71048239996895
- type: dot_ap
value: 59.31114839296281
- type: dot_f1
value: 57.13895527483783
- type: dot_precision
value: 51.331125015335545
- type: dot_recall
value: 64.4287034185402
- type: euclidean_accuracy
value: 86.99305312997244
- type: euclidean_ap
value: 81.87075965254876
- type: euclidean_f1
value: 73.53543008715421
- type: euclidean_precision
value: 72.39964184450082
- type: euclidean_recall
value: 74.70742223591007
- type: manhattan_accuracy
value: 87.04156479217605
- type: manhattan_ap
value: 81.7850497283247
- type: manhattan_f1
value: 73.52951955143475
- type: manhattan_precision
value: 70.15875236030492
- type: manhattan_recall
value: 77.2405297197413
- type: max_accuracy
value: 88.65603291031164
- type: max_ap
value: 85.58148320880878
- type: max_f1
value: 77.63202920041064
---
<h1 align="center">GIST Embedding v0 - all-MiniLM-L6-v2</h1>
*GISTEmbed: Guided In-sample Selection of Training Negatives for Text Embedding Fine-tuning*
The model is fine-tuned on top of the [sentence-transformers/all-MiniLM-L6-v2](https://huggingface.co/sentence-transformers/all-MiniLM-L6-v2) using the [MEDI dataset](https://github.com/xlang-ai/instructor-embedding.git) augmented with mined triplets from the [MTEB Classification](https://huggingface.co/mteb) training dataset (excluding data from the Amazon Polarity Classification task).
The model does not require any instruction for generating embeddings. This means that queries for retrieval tasks can be directly encoded without crafting instructions.
Technical paper: [GISTEmbed: Guided In-sample Selection of Training Negatives for Text Embedding Fine-tuning](https://arxiv.org/abs/2402.16829)
# Data
The dataset used is a compilation of the MEDI and MTEB Classification training datasets. Third-party datasets may be subject to additional terms and conditions under their associated licenses. A HuggingFace Dataset version of the compiled dataset, and the specific revision used to train the model, is available:
- Dataset: [avsolatorio/medi-data-mteb_avs_triplets](https://huggingface.co/datasets/avsolatorio/medi-data-mteb_avs_triplets)
- Revision: 238a0499b6e6b690cc64ea56fde8461daa8341bb
The dataset contains a `task_type` key, which can be used to select only the mteb classification tasks (prefixed with `mteb_`).
The **MEDI Dataset** is published in the following paper: [One Embedder, Any Task: Instruction-Finetuned Text Embeddings](https://arxiv.org/abs/2212.09741).
The MTEB Benchmark results of the GIST embedding model, compared with the base model, suggest that the fine-tuning dataset has perturbed the model considerably, which resulted in significant improvements in certain tasks while adversely degrading performance in some.
The retrieval performance for the TRECCOVID task is of note. The fine-tuning dataset does not contain significant knowledge about COVID-19, which could have caused the observed performance degradation. We found some evidence, detailed in the paper, that thematic coverage of the fine-tuning data can affect downstream performance.
# Usage
The model can be easily loaded using the Sentence Transformers library.
```Python
import torch.nn.functional as F
from sentence_transformers import SentenceTransformer
revision = None # Replace with the specific revision to ensure reproducibility if the model is updated.
model = SentenceTransformer("avsolatorio/GIST-all-MiniLM-L6-v2", revision=revision)
texts = [
"Illustration of the REaLTabFormer model. The left block shows the non-relational tabular data model using GPT-2 with a causal LM head. In contrast, the right block shows how a relational dataset's child table is modeled using a sequence-to-sequence (Seq2Seq) model. The Seq2Seq model uses the observations in the parent table to condition the generation of the observations in the child table. The trained GPT-2 model on the parent table, with weights frozen, is also used as the encoder in the Seq2Seq model.",
"Predicting human mobility holds significant practical value, with applications ranging from enhancing disaster risk planning to simulating epidemic spread. In this paper, we present the GeoFormer, a decoder-only transformer model adapted from the GPT architecture to forecast human mobility.",
"As the economies of Southeast Asia continue adopting digital technologies, policy makers increasingly ask how to prepare the workforce for emerging labor demands. However, little is known about the skills that workers need to adapt to these changes"
]
# Compute embeddings
embeddings = model.encode(texts, convert_to_tensor=True)
# Compute cosine-similarity for each pair of sentences
scores = F.cosine_similarity(embeddings.unsqueeze(1), embeddings.unsqueeze(0), dim=-1)
print(scores.cpu().numpy())
```
# Training Parameters
Below are the training parameters used to fine-tune the model:
```
Epochs = 40
Warmup ratio = 0.1
Learning rate = 5e-6
Batch size = 16
Checkpoint step = 102000
Contrastive loss temperature = 0.01
```
# Evaluation
The model was evaluated using the [MTEB Evaluation](https://huggingface.co/mteb) suite.
# Citation
Please cite our work if you use GISTEmbed or the datasets we published in your projects or research. 🤗
```
@article{solatorio2024gistembed,
title={GISTEmbed: Guided In-sample Selection of Training Negatives for Text Embedding Fine-tuning},
author={Aivin V. Solatorio},
journal={arXiv preprint arXiv:2402.16829},
year={2024},
URL={https://arxiv.org/abs/2402.16829}
eprint={2402.16829},
archivePrefix={arXiv},
primaryClass={cs.LG}
}
```
# Acknowledgements
This work is supported by the "KCP IV - Exploring Data Use in the Development Economics Literature using Large Language Models (AI and LLMs)" project funded by the [Knowledge for Change Program (KCP)](https://www.worldbank.org/en/programs/knowledge-for-change) of the World Bank - RA-P503405-RESE-TF0C3444.
The findings, interpretations, and conclusions expressed in this material are entirely those of the authors. They do not necessarily represent the views of the International Bank for Reconstruction and Development/World Bank and its affiliated organizations, or those of the Executive Directors of the World Bank or the governments they represent. |
timm/vit_base_patch16_384.augreg_in21k_ft_in1k | timm | "2023-05-06T00:01:15Z" | 427,248 | 0 | timm | [
"timm",
"pytorch",
"safetensors",
"image-classification",
"dataset:imagenet-1k",
"dataset:imagenet-21k",
"arxiv:2106.10270",
"arxiv:2010.11929",
"license:apache-2.0",
"region:us"
] | image-classification | "2022-12-22T07:29:44Z" | ---
tags:
- image-classification
- timm
library_name: timm
license: apache-2.0
datasets:
- imagenet-1k
- imagenet-21k
---
# Model card for vit_base_patch16_384.augreg_in21k_ft_in1k
A Vision Transformer (ViT) image classification model. Trained on ImageNet-21k and fine-tuned on ImageNet-1k (with additional augmentation and regularization) in JAX by paper authors, ported to PyTorch by Ross Wightman.
## Model Details
- **Model Type:** Image classification / feature backbone
- **Model Stats:**
- Params (M): 86.9
- GMACs: 49.4
- Activations (M): 48.3
- Image size: 384 x 384
- **Papers:**
- How to train your ViT? Data, Augmentation, and Regularization in Vision Transformers: https://arxiv.org/abs/2106.10270
- An Image is Worth 16x16 Words: Transformers for Image Recognition at Scale: https://arxiv.org/abs/2010.11929v2
- **Dataset:** ImageNet-1k
- **Pretrain Dataset:** ImageNet-21k
- **Original:** https://github.com/google-research/vision_transformer
## Model Usage
### Image Classification
```python
from urllib.request import urlopen
from PIL import Image
import timm
img = Image.open(urlopen(
'https://huggingface.co/datasets/huggingface/documentation-images/resolve/main/beignets-task-guide.png'
))
model = timm.create_model('vit_base_patch16_384.augreg_in21k_ft_in1k', pretrained=True)
model = model.eval()
# get model specific transforms (normalization, resize)
data_config = timm.data.resolve_model_data_config(model)
transforms = timm.data.create_transform(**data_config, is_training=False)
output = model(transforms(img).unsqueeze(0)) # unsqueeze single image into batch of 1
top5_probabilities, top5_class_indices = torch.topk(output.softmax(dim=1) * 100, k=5)
```
### Image Embeddings
```python
from urllib.request import urlopen
from PIL import Image
import timm
img = Image.open(urlopen(
'https://huggingface.co/datasets/huggingface/documentation-images/resolve/main/beignets-task-guide.png'
))
model = timm.create_model(
'vit_base_patch16_384.augreg_in21k_ft_in1k',
pretrained=True,
num_classes=0, # remove classifier nn.Linear
)
model = model.eval()
# get model specific transforms (normalization, resize)
data_config = timm.data.resolve_model_data_config(model)
transforms = timm.data.create_transform(**data_config, is_training=False)
output = model(transforms(img).unsqueeze(0)) # output is (batch_size, num_features) shaped tensor
# or equivalently (without needing to set num_classes=0)
output = model.forward_features(transforms(img).unsqueeze(0))
# output is unpooled, a (1, 577, 768) shaped tensor
output = model.forward_head(output, pre_logits=True)
# output is a (1, num_features) shaped tensor
```
## Model Comparison
Explore the dataset and runtime metrics of this model in timm [model results](https://github.com/huggingface/pytorch-image-models/tree/main/results).
## Citation
```bibtex
@article{steiner2021augreg,
title={How to train your ViT? Data, Augmentation, and Regularization in Vision Transformers},
author={Steiner, Andreas and Kolesnikov, Alexander and and Zhai, Xiaohua and Wightman, Ross and Uszkoreit, Jakob and Beyer, Lucas},
journal={arXiv preprint arXiv:2106.10270},
year={2021}
}
```
```bibtex
@article{dosovitskiy2020vit,
title={An Image is Worth 16x16 Words: Transformers for Image Recognition at Scale},
author={Dosovitskiy, Alexey and Beyer, Lucas and Kolesnikov, Alexander and Weissenborn, Dirk and Zhai, Xiaohua and Unterthiner, Thomas and Dehghani, Mostafa and Minderer, Matthias and Heigold, Georg and Gelly, Sylvain and Uszkoreit, Jakob and Houlsby, Neil},
journal={ICLR},
year={2021}
}
```
```bibtex
@misc{rw2019timm,
author = {Ross Wightman},
title = {PyTorch Image Models},
year = {2019},
publisher = {GitHub},
journal = {GitHub repository},
doi = {10.5281/zenodo.4414861},
howpublished = {\url{https://github.com/huggingface/pytorch-image-models}}
}
```
|
CofeAI/FLM-2-52B-Instruct-2407 | CofeAI | "2024-07-29T05:05:34Z" | 425,801 | 11 | transformers | [
"transformers",
"pytorch",
"TeleFLM",
"text-generation",
"conversational",
"custom_code",
"arxiv:2311.18743",
"arxiv:2407.02783",
"arxiv:2404.16645",
"autotrain_compatible",
"region:us"
] | text-generation | "2024-07-22T06:44:06Z" | # Introduction
FLM-2 (aka Tele-FLM) is our open-source large language model series. The FLM-2 series demonstrate superior performances at its scale, and sometimes surpass larger models. The currently released versions include (Tele-FLM)[https://huggingface.co/CofeAI/Tele-FLM] and (Tele-FLM-1T)[https://huggingface.co/CofeAI/Tele-FLM-1T]. These models feature a stable, efficient pre-training paradigm and enhanced factual judgment capabilities. This repo contains the instruction-tuned 52B Tele-FLM model, which we have named FLM-2-52B-Instruct.
# Model Details
FLM-2-52B-Instruct utilizes the standard GPT-style decoder-only transformer architecture with a few adjustments:
* Rotary Positional Embedding (RoPE)
* RMSNorm for normalization
* SwiGLU for activation function
* Linear bias disabled
* Embedding and language model head untied
* Input and output multiplier
| Models | layer<br>number | attention<br>heads | hidden<br>size | ffn hidden<br>size | vocab<br>size | params<br>count |
| ------------- | :-------------: | :----------------: | :------------: | :----------------: | :-----------: | :--------------: |
| FLM-2-52B-Instruct-2407 | 64 | 64 | 8,192 | 21,824 | 80,000 | 52.85 B |
# Training details
Unlike conventional fine-tuning methods, we employed an innovative and cost-effective fine-tuning approach. Through specialized screening techniques, we meticulously selected 30,735 samples from a large corpus of fine-tuning data. This refined dataset facilitated the fine-tuning process and yielded promising results.
# Quickstart
Here provides simple code for loading the tokenizer, loading the model, and generating contents.
```python
import torch
from transformers import AutoTokenizer, AutoModelForCausalLM
tokenizer = AutoTokenizer.from_pretrained('CofeAI/FLM-2-52B-Instruct-2407', trust_remote_code=True)
model = AutoModelForCausalLM.from_pretrained('CofeAI/FLM-2-52B-Instruct-2407', torch_dtype=torch.bfloat16, low_cpu_mem_usage=True, device_map="auto", trust_remote_code=True)
history = [
{"role": "user", "content": "你好"},
{"role": "assistant", "content": "你好"},
{"role": "user", "content": "北京有哪些必去的景点?"}
]
inputs = tokenizer.apply_chat_template(history, return_tensors='pt').to(model.device)
response = model.generate(inputs, max_new_tokens=128, repetition_penalty=1.03)
print(tokenizer.decode(response.cpu()[0], skip_special_tokens=True))
```
# Evaluation
We evaluate the alignment performance of FLM-2-52B-Instruct-2407 in Chinese across various domains utilizing [AlignBench](https://arxiv.org/pdf/2311.18743). AlignBench is a comprehensive and multidimensional evaluation benchmark designed to assess Chinese large language models’ alignment performance. It encompasses 8 categories with a total of 683 question-answer pairs, covering areas such as fundamental language ability (Fund.), Chinese advanced understanding (Chi.), open-ended questions (Open.), writing ability (Writ.), logical reasoning (Logi.), mathematics (Math.), task-oriented role playing (Role.), and professional knowledge (Pro.).
| Models | Overall | Math. | Logi. | Fund. | Chi. | Open. | Writ. | Role. | Pro. |
| ----------------------- | :-------: | :-----: | :-----: | :-----: | :----: | :-----: | :-----: | :-----: | :----: |
| gpt-4-1106-preview | **7.58** | **7.39** | **6.83** | **7.69** |<u>7.07</u>| **8.66** | **8.23** | **8.08** | **8.55** |
| gpt-4-0613 | <u>6.83</u> |<u>6.33</u>|<u>5.15</u>| 7.16 | 6.76 | 7.26 | 7.31 | 7.48 | 7.56 |
| gpt-3.5-turbo-0613 | 5.68 | 4.90 | 4.79 | 6.01 | 5.60 | 6.97 | 7.27 | 6.98 | 6.29 |
| chatglm-turbo | 6.36 | 4.88 | 5.09 |<u>7.50</u>| 7.03 |<u>8.45</u>| 8.05 | 7.67 | 7.70 |
| FLM-2-52B-Instruct-2407 | 6.23 | 3.79 |<u>5.15</u>| **7.69** | **7.86** |<u>8.45</u>|<u>8.17</u>|<u>7.88</u>|<u>7.85</u>|
# Citation
If you find our work helpful, please consider citing it.
```
@article{tele-flm-1t,
author = {Xiang Li and Yiqun Yao and Xin Jiang and Xuezhi Fang and Chao Wang and Xinzhang Liu and Zihan Wang and Yu Zhao and Xin Wang and Yuyao Huang and Shuangyong Song and Yongxiang Li and Zheng Zhang and Bo Zhao and Aixin Sun and Yequan Wang and Zhongjiang He and Zhongyuan Wang and Xuelong Li and Tiejun Huang},
title = {52B to 1T: Lessons Learned via Tele-FLM Series},
journal = {CoRR},
volume = {abs/2407.02783},
year = {2024},
url = {https://doi.org/10.48550/arXiv.2407.02783},
doi = {10.48550/ARXIV.2407.02783},
eprinttype = {arXiv},
eprint = {2407.02783},
}
@article{tele-flm-2024,
author = {Xiang Li and Yiqun Yao and Xin Jiang and Xuezhi Fang and Chao Wang and Xinzhang Liu and Zihan Wang and Yu Zhao and Xin Wang and Yuyao Huang and Shuangyong Song and Yongxiang Li and Zheng Zhang and Bo Zhao and Aixin Sun and Yequan Wang and Zhongjiang He and Zhongyuan Wang and Xuelong Li and Tiejun Huang},
title = {Tele-FLM Technical Report},
journal = {CoRR},
volume = {abs/2404.16645},
year = {2024},
url = {https://doi.org/10.48550/arXiv.2404.16645},
doi = {10.48550/ARXIV.2404.16645},
eprinttype = {arXiv},
eprint = {2404.16645},
}
```
|
microsoft/deberta-large-mnli | microsoft | "2021-05-21T20:07:51Z" | 421,831 | 17 | transformers | [
"transformers",
"pytorch",
"deberta",
"text-classification",
"deberta-v1",
"deberta-mnli",
"en",
"arxiv:2006.03654",
"license:mit",
"autotrain_compatible",
"endpoints_compatible",
"region:us"
] | text-classification | "2022-03-02T23:29:05Z" | ---
language: en
tags:
- deberta-v1
- deberta-mnli
tasks: mnli
thumbnail: https://huggingface.co/front/thumbnails/microsoft.png
license: mit
widget:
- text: "[CLS] I love you. [SEP] I like you. [SEP]"
---
## DeBERTa: Decoding-enhanced BERT with Disentangled Attention
[DeBERTa](https://arxiv.org/abs/2006.03654) improves the BERT and RoBERTa models using disentangled attention and enhanced mask decoder. It outperforms BERT and RoBERTa on majority of NLU tasks with 80GB training data.
Please check the [official repository](https://github.com/microsoft/DeBERTa) for more details and updates.
This is the DeBERTa large model fine-tuned with MNLI task.
#### Fine-tuning on NLU tasks
We present the dev results on SQuAD 1.1/2.0 and several GLUE benchmark tasks.
| Model | SQuAD 1.1 | SQuAD 2.0 | MNLI-m/mm | SST-2 | QNLI | CoLA | RTE | MRPC | QQP |STS-B |
|---------------------------|-----------|-----------|-------------|-------|------|------|--------|-------|-------|------|
| | F1/EM | F1/EM | Acc | Acc | Acc | MCC | Acc |Acc/F1 |Acc/F1 |P/S |
| BERT-Large | 90.9/84.1 | 81.8/79.0 | 86.6/- | 93.2 | 92.3 | 60.6 | 70.4 | 88.0/- | 91.3/- |90.0/- |
| RoBERTa-Large | 94.6/88.9 | 89.4/86.5 | 90.2/- | 96.4 | 93.9 | 68.0 | 86.6 | 90.9/- | 92.2/- |92.4/- |
| XLNet-Large | 95.1/89.7 | 90.6/87.9 | 90.8/- | 97.0 | 94.9 | 69.0 | 85.9 | 90.8/- | 92.3/- |92.5/- |
| [DeBERTa-Large](https://huggingface.co/microsoft/deberta-large)<sup>1</sup> | 95.5/90.1 | 90.7/88.0 | 91.3/91.1| 96.5|95.3| 69.5| 91.0| 92.6/94.6| 92.3/- |92.8/92.5 |
| [DeBERTa-XLarge](https://huggingface.co/microsoft/deberta-xlarge)<sup>1</sup> | -/- | -/- | 91.5/91.2| 97.0 | - | - | 93.1 | 92.1/94.3 | - |92.9/92.7|
| [DeBERTa-V2-XLarge](https://huggingface.co/microsoft/deberta-v2-xlarge)<sup>1</sup>|95.8/90.8| 91.4/88.9|91.7/91.6| **97.5**| 95.8|71.1|**93.9**|92.0/94.2|92.3/89.8|92.9/92.9|
|**[DeBERTa-V2-XXLarge](https://huggingface.co/microsoft/deberta-v2-xxlarge)<sup>1,2</sup>**|**96.1/91.4**|**92.2/89.7**|**91.7/91.9**|97.2|**96.0**|**72.0**| 93.5| **93.1/94.9**|**92.7/90.3** |**93.2/93.1** |
--------
#### Notes.
- <sup>1</sup> Following RoBERTa, for RTE, MRPC, STS-B, we fine-tune the tasks based on [DeBERTa-Large-MNLI](https://huggingface.co/microsoft/deberta-large-mnli), [DeBERTa-XLarge-MNLI](https://huggingface.co/microsoft/deberta-xlarge-mnli), [DeBERTa-V2-XLarge-MNLI](https://huggingface.co/microsoft/deberta-v2-xlarge-mnli), [DeBERTa-V2-XXLarge-MNLI](https://huggingface.co/microsoft/deberta-v2-xxlarge-mnli). The results of SST-2/QQP/QNLI/SQuADv2 will also be slightly improved when start from MNLI fine-tuned models, however, we only report the numbers fine-tuned from pretrained base models for those 4 tasks.
- <sup>2</sup> To try the **XXLarge** model with **[HF transformers](https://huggingface.co/transformers/main_classes/trainer.html)**, you need to specify **--sharded_ddp**
```bash
cd transformers/examples/text-classification/
export TASK_NAME=mrpc
python -m torch.distributed.launch --nproc_per_node=8 run_glue.py --model_name_or_path microsoft/deberta-v2-xxlarge \\
--task_name $TASK_NAME --do_train --do_eval --max_seq_length 128 --per_device_train_batch_size 4 \\
--learning_rate 3e-6 --num_train_epochs 3 --output_dir /tmp/$TASK_NAME/ --overwrite_output_dir --sharded_ddp --fp16
```
### Citation
If you find DeBERTa useful for your work, please cite the following paper:
``` latex
@inproceedings{
he2021deberta,
title={DEBERTA: DECODING-ENHANCED BERT WITH DISENTANGLED ATTENTION},
author={Pengcheng He and Xiaodong Liu and Jianfeng Gao and Weizhu Chen},
booktitle={International Conference on Learning Representations},
year={2021},
url={https://openreview.net/forum?id=XPZIaotutsD}
}
```
|
Qwen/Qwen2.5-72B-Instruct | Qwen | "2024-09-25T12:33:18Z" | 421,326 | 458 | transformers | [
"transformers",
"safetensors",
"qwen2",
"text-generation",
"chat",
"conversational",
"en",
"arxiv:2309.00071",
"arxiv:2407.10671",
"base_model:Qwen/Qwen2.5-72B",
"base_model:finetune:Qwen/Qwen2.5-72B",
"license:other",
"autotrain_compatible",
"text-generation-inference",
"endpoints_compatible",
"region:us"
] | text-generation | "2024-09-16T11:56:31Z" | ---
license: other
license_name: qwen
license_link: https://huggingface.co/Qwen/Qwen2.5-72B-Instruct/blob/main/LICENSE
language:
- en
pipeline_tag: text-generation
base_model: Qwen/Qwen2.5-72B
tags:
- chat
library_name: transformers
---
# Qwen2.5-72B-Instruct
## Introduction
Qwen2.5 is the latest series of Qwen large language models. For Qwen2.5, we release a number of base language models and instruction-tuned language models ranging from 0.5 to 72 billion parameters. Qwen2.5 brings the following improvements upon Qwen2:
- Significantly **more knowledge** and has greatly improved capabilities in **coding** and **mathematics**, thanks to our specialized expert models in these domains.
- Significant improvements in **instruction following**, **generating long texts** (over 8K tokens), **understanding structured data** (e.g, tables), and **generating structured outputs** especially JSON. **More resilient to the diversity of system prompts**, enhancing role-play implementation and condition-setting for chatbots.
- **Long-context Support** up to 128K tokens and can generate up to 8K tokens.
- **Multilingual support** for over 29 languages, including Chinese, English, French, Spanish, Portuguese, German, Italian, Russian, Japanese, Korean, Vietnamese, Thai, Arabic, and more.
**This repo contains the instruction-tuned 72B Qwen2.5 model**, which has the following features:
- Type: Causal Language Models
- Training Stage: Pretraining & Post-training
- Architecture: transformers with RoPE, SwiGLU, RMSNorm, and Attention QKV bias
- Number of Parameters: 72.7B
- Number of Paramaters (Non-Embedding): 70.0B
- Number of Layers: 80
- Number of Attention Heads (GQA): 64 for Q and 8 for KV
- Context Length: Full 131,072 tokens and generation 8192 tokens
- Please refer to [this section](#processing-long-texts) for detailed instructions on how to deploy Qwen2.5 for handling long texts.
For more details, please refer to our [blog](https://qwenlm.github.io/blog/qwen2.5/), [GitHub](https://github.com/QwenLM/Qwen2.5), and [Documentation](https://qwen.readthedocs.io/en/latest/).
## Requirements
The code of Qwen2.5 has been in the latest Hugging face `transformers` and we advise you to use the latest version of `transformers`.
With `transformers<4.37.0`, you will encounter the following error:
```
KeyError: 'qwen2'
```
## Quickstart
Here provides a code snippet with `apply_chat_template` to show you how to load the tokenizer and model and how to generate contents.
```python
from transformers import AutoModelForCausalLM, AutoTokenizer
model_name = "Qwen/Qwen2.5-72B-Instruct"
model = AutoModelForCausalLM.from_pretrained(
model_name,
torch_dtype="auto",
device_map="auto"
)
tokenizer = AutoTokenizer.from_pretrained(model_name)
prompt = "Give me a short introduction to large language model."
messages = [
{"role": "system", "content": "You are Qwen, created by Alibaba Cloud. You are a helpful assistant."},
{"role": "user", "content": prompt}
]
text = tokenizer.apply_chat_template(
messages,
tokenize=False,
add_generation_prompt=True
)
model_inputs = tokenizer([text], return_tensors="pt").to(model.device)
generated_ids = model.generate(
**model_inputs,
max_new_tokens=512
)
generated_ids = [
output_ids[len(input_ids):] for input_ids, output_ids in zip(model_inputs.input_ids, generated_ids)
]
response = tokenizer.batch_decode(generated_ids, skip_special_tokens=True)[0]
```
### Processing Long Texts
The current `config.json` is set for context length up to 32,768 tokens.
To handle extensive inputs exceeding 32,768 tokens, we utilize [YaRN](https://arxiv.org/abs/2309.00071), a technique for enhancing model length extrapolation, ensuring optimal performance on lengthy texts.
For supported frameworks, you could add the following to `config.json` to enable YaRN:
```json
{
...,
"rope_scaling": {
"factor": 4.0,
"original_max_position_embeddings": 32768,
"type": "yarn"
}
}
```
For deployment, we recommend using vLLM.
Please refer to our [Documentation](https://qwen.readthedocs.io/en/latest/deployment/vllm.html) for usage if you are not familar with vLLM.
Presently, vLLM only supports static YARN, which means the scaling factor remains constant regardless of input length, **potentially impacting performance on shorter texts**.
We advise adding the `rope_scaling` configuration only when processing long contexts is required.
## Evaluation & Performance
Detailed evaluation results are reported in this [📑 blog](https://qwenlm.github.io/blog/qwen2.5/).
For requirements on GPU memory and the respective throughput, see results [here](https://qwen.readthedocs.io/en/latest/benchmark/speed_benchmark.html).
## Citation
If you find our work helpful, feel free to give us a cite.
```
@misc{qwen2.5,
title = {Qwen2.5: A Party of Foundation Models},
url = {https://qwenlm.github.io/blog/qwen2.5/},
author = {Qwen Team},
month = {September},
year = {2024}
}
@article{qwen2,
title={Qwen2 Technical Report},
author={An Yang and Baosong Yang and Binyuan Hui and Bo Zheng and Bowen Yu and Chang Zhou and Chengpeng Li and Chengyuan Li and Dayiheng Liu and Fei Huang and Guanting Dong and Haoran Wei and Huan Lin and Jialong Tang and Jialin Wang and Jian Yang and Jianhong Tu and Jianwei Zhang and Jianxin Ma and Jin Xu and Jingren Zhou and Jinze Bai and Jinzheng He and Junyang Lin and Kai Dang and Keming Lu and Keqin Chen and Kexin Yang and Mei Li and Mingfeng Xue and Na Ni and Pei Zhang and Peng Wang and Ru Peng and Rui Men and Ruize Gao and Runji Lin and Shijie Wang and Shuai Bai and Sinan Tan and Tianhang Zhu and Tianhao Li and Tianyu Liu and Wenbin Ge and Xiaodong Deng and Xiaohuan Zhou and Xingzhang Ren and Xinyu Zhang and Xipin Wei and Xuancheng Ren and Yang Fan and Yang Yao and Yichang Zhang and Yu Wan and Yunfei Chu and Yuqiong Liu and Zeyu Cui and Zhenru Zhang and Zhihao Fan},
journal={arXiv preprint arXiv:2407.10671},
year={2024}
}
``` |
Iceland/quote-model-delta | Iceland | "2023-06-08T17:40:04Z" | 419,721 | 1 | transformers | [
"transformers",
"pytorch",
"tensorboard",
"distilbert",
"token-classification",
"generated_from_trainer",
"license:apache-2.0",
"autotrain_compatible",
"endpoints_compatible",
"region:us"
] | token-classification | "2023-06-08T17:30:31Z" | ---
license: apache-2.0
tags:
- generated_from_trainer
metrics:
- precision
- recall
- f1
- accuracy
model-index:
- name: quote-model-delta
results: []
---
<!-- This model card has been generated automatically according to the information the Trainer had access to. You
should probably proofread and complete it, then remove this comment. -->
# quote-model-delta
This model is a fine-tuned version of [distilbert-base-uncased](https://huggingface.co/distilbert-base-uncased) on the None dataset.
It achieves the following results on the evaluation set:
- Loss: 0.2186
- Precision: 0.8199
- Recall: 0.9167
- F1: 0.8656
- Accuracy: 0.9309
## Model description
More information needed
## Intended uses & limitations
More information needed
## Training and evaluation data
More information needed
## Training procedure
### Training hyperparameters
The following hyperparameters were used during training:
- learning_rate: 2e-05
- train_batch_size: 16
- eval_batch_size: 16
- seed: 42
- optimizer: Adam with betas=(0.9,0.999) and epsilon=1e-08
- lr_scheduler_type: linear
- num_epochs: 3
### Training results
| Training Loss | Epoch | Step | Validation Loss | Precision | Recall | F1 | Accuracy |
|:-------------:|:-----:|:----:|:---------------:|:---------:|:------:|:------:|:--------:|
| 0.3956 | 1.0 | 976 | 0.2257 | 0.8077 | 0.9181 | 0.8594 | 0.9280 |
| 0.184 | 2.0 | 1952 | 0.2180 | 0.8188 | 0.9212 | 0.8670 | 0.9303 |
| 0.1612 | 3.0 | 2928 | 0.2186 | 0.8199 | 0.9167 | 0.8656 | 0.9309 |
### Framework versions
- Transformers 4.30.0
- Pytorch 2.0.1+cu118
- Datasets 2.12.0
- Tokenizers 0.13.3
|
TinyLlama/TinyLlama-1.1B-Chat-v0.6 | TinyLlama | "2023-11-20T11:22:36Z" | 419,204 | 89 | transformers | [
"transformers",
"safetensors",
"gguf",
"llama",
"text-generation",
"conversational",
"en",
"dataset:cerebras/SlimPajama-627B",
"dataset:bigcode/starcoderdata",
"dataset:OpenAssistant/oasst_top1_2023-08-25",
"license:apache-2.0",
"autotrain_compatible",
"text-generation-inference",
"endpoints_compatible",
"region:us"
] | text-generation | "2023-11-20T08:59:23Z" | ---
license: apache-2.0
datasets:
- cerebras/SlimPajama-627B
- bigcode/starcoderdata
- OpenAssistant/oasst_top1_2023-08-25
language:
- en
---
<div align="center">
# TinyLlama-1.1B
</div>
https://github.com/jzhang38/TinyLlama
The TinyLlama project aims to **pretrain** a **1.1B Llama model on 3 trillion tokens**. With some proper optimization, we can achieve this within a span of "just" 90 days using 16 A100-40G GPUs 🚀🚀. The training has started on 2023-09-01.
We adopted exactly the same architecture and tokenizer as Llama 2. This means TinyLlama can be plugged and played in many open-source projects built upon Llama. Besides, TinyLlama is compact with only 1.1B parameters. This compactness allows it to cater to a multitude of applications demanding a restricted computation and memory footprint.
#### This Model
This is the chat model finetuned on top of [TinyLlama/TinyLlama-1.1B-intermediate-step-955k-2T](https://huggingface.co/TinyLlama/TinyLlama-1.1B-intermediate-step-955k-token-2T). **We follow [HF's Zephyr](https://huggingface.co/HuggingFaceH4/zephyr-7b-alpha/edit/main/README.md)'s training recipe.** The model was " initially fine-tuned on a variant of the [`UltraChat`](https://huggingface.co/datasets/stingning/ultrachat) dataset, which contains a diverse range of synthetic dialogues generated by ChatGPT.
We then further aligned the model with [🤗 TRL's](https://github.com/huggingface/trl) `DPOTrainer` on the [openbmb/UltraFeedback](https://huggingface.co/datasets/openbmb/UltraFeedback) dataset, which contain 64k prompts and model completions that are ranked by GPT-4."
#### How to use
You will need the transformers>=4.34
Do check the [TinyLlama](https://github.com/jzhang38/TinyLlama) github page for more information.
```python
# Install transformers from source - only needed for versions <= v4.34
# pip install git+https://github.com/huggingface/transformers.git
# pip install accelerate
import torch
from transformers import pipeline
pipe = pipeline("text-generation", model="TinyLlama/TinyLlama-1.1B-Chat-v0.6", torch_dtype=torch.bfloat16, device_map="auto")
# We use the tokenizer's chat template to format each message - see https://huggingface.co/docs/transformers/main/en/chat_templating
messages = [
{
"role": "system",
"content": "You are a friendly chatbot who always responds in the style of a pirate",
},
{"role": "user", "content": "How many helicopters can a human eat in one sitting?"},
]
prompt = pipe.tokenizer.apply_chat_template(messages, tokenize=False, add_generation_prompt=True)
outputs = pipe(prompt, max_new_tokens=256, do_sample=True, temperature=0.7, top_k=50, top_p=0.95)
print(outputs[0]["generated_text"])
# <|system|>
# You are a friendly chatbot who always responds in the style of a pirate.</s>
# <|user|>
# How many helicopters can a human eat in one sitting?</s>
# <|assistant|>
# ...
``` |
timm/resnet34.a1_in1k | timm | "2024-02-10T23:38:51Z" | 418,560 | 0 | timm | [
"timm",
"pytorch",
"safetensors",
"image-classification",
"arxiv:2110.00476",
"arxiv:1512.03385",
"license:apache-2.0",
"region:us"
] | image-classification | "2023-04-05T18:05:32Z" | ---
license: apache-2.0
library_name: timm
tags:
- image-classification
- timm
---
# Model card for resnet34.a1_in1k
A ResNet-B image classification model.
This model features:
* ReLU activations
* single layer 7x7 convolution with pooling
* 1x1 convolution shortcut downsample
Trained on ImageNet-1k in `timm` using recipe template described below.
Recipe details:
* ResNet Strikes Back `A1` recipe
* LAMB optimizer with BCE loss
* Cosine LR schedule with warmup
## Model Details
- **Model Type:** Image classification / feature backbone
- **Model Stats:**
- Params (M): 21.8
- GMACs: 3.7
- Activations (M): 3.7
- Image size: train = 224 x 224, test = 288 x 288
- **Papers:**
- ResNet strikes back: An improved training procedure in timm: https://arxiv.org/abs/2110.00476
- Deep Residual Learning for Image Recognition: https://arxiv.org/abs/1512.03385
- **Original:** https://github.com/huggingface/pytorch-image-models
## Model Usage
### Image Classification
```python
from urllib.request import urlopen
from PIL import Image
import timm
img = Image.open(urlopen(
'https://huggingface.co/datasets/huggingface/documentation-images/resolve/main/beignets-task-guide.png'
))
model = timm.create_model('resnet34.a1_in1k', pretrained=True)
model = model.eval()
# get model specific transforms (normalization, resize)
data_config = timm.data.resolve_model_data_config(model)
transforms = timm.data.create_transform(**data_config, is_training=False)
output = model(transforms(img).unsqueeze(0)) # unsqueeze single image into batch of 1
top5_probabilities, top5_class_indices = torch.topk(output.softmax(dim=1) * 100, k=5)
```
### Feature Map Extraction
```python
from urllib.request import urlopen
from PIL import Image
import timm
img = Image.open(urlopen(
'https://huggingface.co/datasets/huggingface/documentation-images/resolve/main/beignets-task-guide.png'
))
model = timm.create_model(
'resnet34.a1_in1k',
pretrained=True,
features_only=True,
)
model = model.eval()
# get model specific transforms (normalization, resize)
data_config = timm.data.resolve_model_data_config(model)
transforms = timm.data.create_transform(**data_config, is_training=False)
output = model(transforms(img).unsqueeze(0)) # unsqueeze single image into batch of 1
for o in output:
# print shape of each feature map in output
# e.g.:
# torch.Size([1, 64, 112, 112])
# torch.Size([1, 64, 56, 56])
# torch.Size([1, 128, 28, 28])
# torch.Size([1, 256, 14, 14])
# torch.Size([1, 512, 7, 7])
print(o.shape)
```
### Image Embeddings
```python
from urllib.request import urlopen
from PIL import Image
import timm
img = Image.open(urlopen(
'https://huggingface.co/datasets/huggingface/documentation-images/resolve/main/beignets-task-guide.png'
))
model = timm.create_model(
'resnet34.a1_in1k',
pretrained=True,
num_classes=0, # remove classifier nn.Linear
)
model = model.eval()
# get model specific transforms (normalization, resize)
data_config = timm.data.resolve_model_data_config(model)
transforms = timm.data.create_transform(**data_config, is_training=False)
output = model(transforms(img).unsqueeze(0)) # output is (batch_size, num_features) shaped tensor
# or equivalently (without needing to set num_classes=0)
output = model.forward_features(transforms(img).unsqueeze(0))
# output is unpooled, a (1, 512, 7, 7) shaped tensor
output = model.forward_head(output, pre_logits=True)
# output is a (1, num_features) shaped tensor
```
## Model Comparison
Explore the dataset and runtime metrics of this model in timm [model results](https://github.com/huggingface/pytorch-image-models/tree/main/results).
|model |img_size|top1 |top5 |param_count|gmacs|macts|img/sec|
|------------------------------------------|--------|-----|-----|-----------|-----|-----|-------|
|[seresnextaa101d_32x8d.sw_in12k_ft_in1k_288](https://huggingface.co/timm/seresnextaa101d_32x8d.sw_in12k_ft_in1k_288)|320 |86.72|98.17|93.6 |35.2 |69.7 |451 |
|[seresnextaa101d_32x8d.sw_in12k_ft_in1k_288](https://huggingface.co/timm/seresnextaa101d_32x8d.sw_in12k_ft_in1k_288)|288 |86.51|98.08|93.6 |28.5 |56.4 |560 |
|[seresnextaa101d_32x8d.sw_in12k_ft_in1k](https://huggingface.co/timm/seresnextaa101d_32x8d.sw_in12k_ft_in1k)|288 |86.49|98.03|93.6 |28.5 |56.4 |557 |
|[seresnextaa101d_32x8d.sw_in12k_ft_in1k](https://huggingface.co/timm/seresnextaa101d_32x8d.sw_in12k_ft_in1k)|224 |85.96|97.82|93.6 |17.2 |34.2 |923 |
|[resnext101_32x32d.fb_wsl_ig1b_ft_in1k](https://huggingface.co/timm/resnext101_32x32d.fb_wsl_ig1b_ft_in1k)|224 |85.11|97.44|468.5 |87.3 |91.1 |254 |
|[resnetrs420.tf_in1k](https://huggingface.co/timm/resnetrs420.tf_in1k)|416 |85.0 |97.12|191.9 |108.4|213.8|134 |
|[ecaresnet269d.ra2_in1k](https://huggingface.co/timm/ecaresnet269d.ra2_in1k)|352 |84.96|97.22|102.1 |50.2 |101.2|291 |
|[ecaresnet269d.ra2_in1k](https://huggingface.co/timm/ecaresnet269d.ra2_in1k)|320 |84.73|97.18|102.1 |41.5 |83.7 |353 |
|[resnetrs350.tf_in1k](https://huggingface.co/timm/resnetrs350.tf_in1k)|384 |84.71|96.99|164.0 |77.6 |154.7|183 |
|[seresnextaa101d_32x8d.ah_in1k](https://huggingface.co/timm/seresnextaa101d_32x8d.ah_in1k)|288 |84.57|97.08|93.6 |28.5 |56.4 |557 |
|[resnetrs200.tf_in1k](https://huggingface.co/timm/resnetrs200.tf_in1k)|320 |84.45|97.08|93.2 |31.5 |67.8 |446 |
|[resnetrs270.tf_in1k](https://huggingface.co/timm/resnetrs270.tf_in1k)|352 |84.43|96.97|129.9 |51.1 |105.5|280 |
|[seresnext101d_32x8d.ah_in1k](https://huggingface.co/timm/seresnext101d_32x8d.ah_in1k)|288 |84.36|96.92|93.6 |27.6 |53.0 |595 |
|[seresnet152d.ra2_in1k](https://huggingface.co/timm/seresnet152d.ra2_in1k)|320 |84.35|97.04|66.8 |24.1 |47.7 |610 |
|[resnetrs350.tf_in1k](https://huggingface.co/timm/resnetrs350.tf_in1k)|288 |84.3 |96.94|164.0 |43.7 |87.1 |333 |
|[resnext101_32x8d.fb_swsl_ig1b_ft_in1k](https://huggingface.co/timm/resnext101_32x8d.fb_swsl_ig1b_ft_in1k)|224 |84.28|97.17|88.8 |16.5 |31.2 |1100 |
|[resnetrs420.tf_in1k](https://huggingface.co/timm/resnetrs420.tf_in1k)|320 |84.24|96.86|191.9 |64.2 |126.6|228 |
|[seresnext101_32x8d.ah_in1k](https://huggingface.co/timm/seresnext101_32x8d.ah_in1k)|288 |84.19|96.87|93.6 |27.2 |51.6 |613 |
|[resnext101_32x16d.fb_wsl_ig1b_ft_in1k](https://huggingface.co/timm/resnext101_32x16d.fb_wsl_ig1b_ft_in1k)|224 |84.18|97.19|194.0 |36.3 |51.2 |581 |
|[resnetaa101d.sw_in12k_ft_in1k](https://huggingface.co/timm/resnetaa101d.sw_in12k_ft_in1k)|288 |84.11|97.11|44.6 |15.1 |29.0 |1144 |
|[resnet200d.ra2_in1k](https://huggingface.co/timm/resnet200d.ra2_in1k)|320 |83.97|96.82|64.7 |31.2 |67.3 |518 |
|[resnetrs200.tf_in1k](https://huggingface.co/timm/resnetrs200.tf_in1k)|256 |83.87|96.75|93.2 |20.2 |43.4 |692 |
|[seresnextaa101d_32x8d.ah_in1k](https://huggingface.co/timm/seresnextaa101d_32x8d.ah_in1k)|224 |83.86|96.65|93.6 |17.2 |34.2 |923 |
|[resnetrs152.tf_in1k](https://huggingface.co/timm/resnetrs152.tf_in1k)|320 |83.72|96.61|86.6 |24.3 |48.1 |617 |
|[seresnet152d.ra2_in1k](https://huggingface.co/timm/seresnet152d.ra2_in1k)|256 |83.69|96.78|66.8 |15.4 |30.6 |943 |
|[seresnext101d_32x8d.ah_in1k](https://huggingface.co/timm/seresnext101d_32x8d.ah_in1k)|224 |83.68|96.61|93.6 |16.7 |32.0 |986 |
|[resnet152d.ra2_in1k](https://huggingface.co/timm/resnet152d.ra2_in1k)|320 |83.67|96.74|60.2 |24.1 |47.7 |706 |
|[resnetrs270.tf_in1k](https://huggingface.co/timm/resnetrs270.tf_in1k)|256 |83.59|96.61|129.9 |27.1 |55.8 |526 |
|[seresnext101_32x8d.ah_in1k](https://huggingface.co/timm/seresnext101_32x8d.ah_in1k)|224 |83.58|96.4 |93.6 |16.5 |31.2 |1013 |
|[resnetaa101d.sw_in12k_ft_in1k](https://huggingface.co/timm/resnetaa101d.sw_in12k_ft_in1k)|224 |83.54|96.83|44.6 |9.1 |17.6 |1864 |
|[resnet152.a1h_in1k](https://huggingface.co/timm/resnet152.a1h_in1k)|288 |83.46|96.54|60.2 |19.1 |37.3 |904 |
|[resnext101_32x16d.fb_swsl_ig1b_ft_in1k](https://huggingface.co/timm/resnext101_32x16d.fb_swsl_ig1b_ft_in1k)|224 |83.35|96.85|194.0 |36.3 |51.2 |582 |
|[resnet200d.ra2_in1k](https://huggingface.co/timm/resnet200d.ra2_in1k)|256 |83.23|96.53|64.7 |20.0 |43.1 |809 |
|[resnext101_32x4d.fb_swsl_ig1b_ft_in1k](https://huggingface.co/timm/resnext101_32x4d.fb_swsl_ig1b_ft_in1k)|224 |83.22|96.75|44.2 |8.0 |21.2 |1814 |
|[resnext101_64x4d.c1_in1k](https://huggingface.co/timm/resnext101_64x4d.c1_in1k)|288 |83.16|96.38|83.5 |25.7 |51.6 |590 |
|[resnet152d.ra2_in1k](https://huggingface.co/timm/resnet152d.ra2_in1k)|256 |83.14|96.38|60.2 |15.4 |30.5 |1096 |
|[resnet101d.ra2_in1k](https://huggingface.co/timm/resnet101d.ra2_in1k)|320 |83.02|96.45|44.6 |16.5 |34.8 |992 |
|[ecaresnet101d.miil_in1k](https://huggingface.co/timm/ecaresnet101d.miil_in1k)|288 |82.98|96.54|44.6 |13.4 |28.2 |1077 |
|[resnext101_64x4d.tv_in1k](https://huggingface.co/timm/resnext101_64x4d.tv_in1k)|224 |82.98|96.25|83.5 |15.5 |31.2 |989 |
|[resnetrs152.tf_in1k](https://huggingface.co/timm/resnetrs152.tf_in1k)|256 |82.86|96.28|86.6 |15.6 |30.8 |951 |
|[resnext101_32x8d.tv2_in1k](https://huggingface.co/timm/resnext101_32x8d.tv2_in1k)|224 |82.83|96.22|88.8 |16.5 |31.2 |1099 |
|[resnet152.a1h_in1k](https://huggingface.co/timm/resnet152.a1h_in1k)|224 |82.8 |96.13|60.2 |11.6 |22.6 |1486 |
|[resnet101.a1h_in1k](https://huggingface.co/timm/resnet101.a1h_in1k)|288 |82.8 |96.32|44.6 |13.0 |26.8 |1291 |
|[resnet152.a1_in1k](https://huggingface.co/timm/resnet152.a1_in1k)|288 |82.74|95.71|60.2 |19.1 |37.3 |905 |
|[resnext101_32x8d.fb_wsl_ig1b_ft_in1k](https://huggingface.co/timm/resnext101_32x8d.fb_wsl_ig1b_ft_in1k)|224 |82.69|96.63|88.8 |16.5 |31.2 |1100 |
|[resnet152.a2_in1k](https://huggingface.co/timm/resnet152.a2_in1k)|288 |82.62|95.75|60.2 |19.1 |37.3 |904 |
|[resnetaa50d.sw_in12k_ft_in1k](https://huggingface.co/timm/resnetaa50d.sw_in12k_ft_in1k)|288 |82.61|96.49|25.6 |8.9 |20.6 |1729 |
|[resnet61q.ra2_in1k](https://huggingface.co/timm/resnet61q.ra2_in1k)|288 |82.53|96.13|36.8 |9.9 |21.5 |1773 |
|[wide_resnet101_2.tv2_in1k](https://huggingface.co/timm/wide_resnet101_2.tv2_in1k)|224 |82.5 |96.02|126.9 |22.8 |21.2 |1078 |
|[resnext101_64x4d.c1_in1k](https://huggingface.co/timm/resnext101_64x4d.c1_in1k)|224 |82.46|95.92|83.5 |15.5 |31.2 |987 |
|[resnet51q.ra2_in1k](https://huggingface.co/timm/resnet51q.ra2_in1k)|288 |82.36|96.18|35.7 |8.1 |20.9 |1964 |
|[ecaresnet50t.ra2_in1k](https://huggingface.co/timm/ecaresnet50t.ra2_in1k)|320 |82.35|96.14|25.6 |8.8 |24.1 |1386 |
|[resnet101.a1_in1k](https://huggingface.co/timm/resnet101.a1_in1k)|288 |82.31|95.63|44.6 |13.0 |26.8 |1291 |
|[resnetrs101.tf_in1k](https://huggingface.co/timm/resnetrs101.tf_in1k)|288 |82.29|96.01|63.6 |13.6 |28.5 |1078 |
|[resnet152.tv2_in1k](https://huggingface.co/timm/resnet152.tv2_in1k)|224 |82.29|96.0 |60.2 |11.6 |22.6 |1484 |
|[wide_resnet50_2.racm_in1k](https://huggingface.co/timm/wide_resnet50_2.racm_in1k)|288 |82.27|96.06|68.9 |18.9 |23.8 |1176 |
|[resnet101d.ra2_in1k](https://huggingface.co/timm/resnet101d.ra2_in1k)|256 |82.26|96.07|44.6 |10.6 |22.2 |1542 |
|[resnet101.a2_in1k](https://huggingface.co/timm/resnet101.a2_in1k)|288 |82.24|95.73|44.6 |13.0 |26.8 |1290 |
|[seresnext50_32x4d.racm_in1k](https://huggingface.co/timm/seresnext50_32x4d.racm_in1k)|288 |82.2 |96.14|27.6 |7.0 |23.8 |1547 |
|[ecaresnet101d.miil_in1k](https://huggingface.co/timm/ecaresnet101d.miil_in1k)|224 |82.18|96.05|44.6 |8.1 |17.1 |1771 |
|[resnext50_32x4d.fb_swsl_ig1b_ft_in1k](https://huggingface.co/timm/resnext50_32x4d.fb_swsl_ig1b_ft_in1k)|224 |82.17|96.22|25.0 |4.3 |14.4 |2943 |
|[ecaresnet50t.a1_in1k](https://huggingface.co/timm/ecaresnet50t.a1_in1k)|288 |82.12|95.65|25.6 |7.1 |19.6 |1704 |
|[resnext50_32x4d.a1h_in1k](https://huggingface.co/timm/resnext50_32x4d.a1h_in1k)|288 |82.03|95.94|25.0 |7.0 |23.8 |1745 |
|[ecaresnet101d_pruned.miil_in1k](https://huggingface.co/timm/ecaresnet101d_pruned.miil_in1k)|288 |82.0 |96.15|24.9 |5.8 |12.7 |1787 |
|[resnet61q.ra2_in1k](https://huggingface.co/timm/resnet61q.ra2_in1k)|256 |81.99|95.85|36.8 |7.8 |17.0 |2230 |
|[resnext101_32x8d.tv2_in1k](https://huggingface.co/timm/resnext101_32x8d.tv2_in1k)|176 |81.98|95.72|88.8 |10.3 |19.4 |1768 |
|[resnet152.a1_in1k](https://huggingface.co/timm/resnet152.a1_in1k)|224 |81.97|95.24|60.2 |11.6 |22.6 |1486 |
|[resnet101.a1h_in1k](https://huggingface.co/timm/resnet101.a1h_in1k)|224 |81.93|95.75|44.6 |7.8 |16.2 |2122 |
|[resnet101.tv2_in1k](https://huggingface.co/timm/resnet101.tv2_in1k)|224 |81.9 |95.77|44.6 |7.8 |16.2 |2118 |
|[resnext101_32x16d.fb_ssl_yfcc100m_ft_in1k](https://huggingface.co/timm/resnext101_32x16d.fb_ssl_yfcc100m_ft_in1k)|224 |81.84|96.1 |194.0 |36.3 |51.2 |583 |
|[resnet51q.ra2_in1k](https://huggingface.co/timm/resnet51q.ra2_in1k)|256 |81.78|95.94|35.7 |6.4 |16.6 |2471 |
|[resnet152.a2_in1k](https://huggingface.co/timm/resnet152.a2_in1k)|224 |81.77|95.22|60.2 |11.6 |22.6 |1485 |
|[resnetaa50d.sw_in12k_ft_in1k](https://huggingface.co/timm/resnetaa50d.sw_in12k_ft_in1k)|224 |81.74|96.06|25.6 |5.4 |12.4 |2813 |
|[ecaresnet50t.a2_in1k](https://huggingface.co/timm/ecaresnet50t.a2_in1k)|288 |81.65|95.54|25.6 |7.1 |19.6 |1703 |
|[ecaresnet50d.miil_in1k](https://huggingface.co/timm/ecaresnet50d.miil_in1k)|288 |81.64|95.88|25.6 |7.2 |19.7 |1694 |
|[resnext101_32x8d.fb_ssl_yfcc100m_ft_in1k](https://huggingface.co/timm/resnext101_32x8d.fb_ssl_yfcc100m_ft_in1k)|224 |81.62|96.04|88.8 |16.5 |31.2 |1101 |
|[wide_resnet50_2.tv2_in1k](https://huggingface.co/timm/wide_resnet50_2.tv2_in1k)|224 |81.61|95.76|68.9 |11.4 |14.4 |1930 |
|[resnetaa50.a1h_in1k](https://huggingface.co/timm/resnetaa50.a1h_in1k)|288 |81.61|95.83|25.6 |8.5 |19.2 |1868 |
|[resnet101.a1_in1k](https://huggingface.co/timm/resnet101.a1_in1k)|224 |81.5 |95.16|44.6 |7.8 |16.2 |2125 |
|[resnext50_32x4d.a1_in1k](https://huggingface.co/timm/resnext50_32x4d.a1_in1k)|288 |81.48|95.16|25.0 |7.0 |23.8 |1745 |
|[gcresnet50t.ra2_in1k](https://huggingface.co/timm/gcresnet50t.ra2_in1k)|288 |81.47|95.71|25.9 |6.9 |18.6 |2071 |
|[wide_resnet50_2.racm_in1k](https://huggingface.co/timm/wide_resnet50_2.racm_in1k)|224 |81.45|95.53|68.9 |11.4 |14.4 |1929 |
|[resnet50d.a1_in1k](https://huggingface.co/timm/resnet50d.a1_in1k)|288 |81.44|95.22|25.6 |7.2 |19.7 |1908 |
|[ecaresnet50t.ra2_in1k](https://huggingface.co/timm/ecaresnet50t.ra2_in1k)|256 |81.44|95.67|25.6 |5.6 |15.4 |2168 |
|[ecaresnetlight.miil_in1k](https://huggingface.co/timm/ecaresnetlight.miil_in1k)|288 |81.4 |95.82|30.2 |6.8 |13.9 |2132 |
|[resnet50d.ra2_in1k](https://huggingface.co/timm/resnet50d.ra2_in1k)|288 |81.37|95.74|25.6 |7.2 |19.7 |1910 |
|[resnet101.a2_in1k](https://huggingface.co/timm/resnet101.a2_in1k)|224 |81.32|95.19|44.6 |7.8 |16.2 |2125 |
|[seresnet50.ra2_in1k](https://huggingface.co/timm/seresnet50.ra2_in1k)|288 |81.3 |95.65|28.1 |6.8 |18.4 |1803 |
|[resnext50_32x4d.a2_in1k](https://huggingface.co/timm/resnext50_32x4d.a2_in1k)|288 |81.3 |95.11|25.0 |7.0 |23.8 |1746 |
|[seresnext50_32x4d.racm_in1k](https://huggingface.co/timm/seresnext50_32x4d.racm_in1k)|224 |81.27|95.62|27.6 |4.3 |14.4 |2591 |
|[ecaresnet50t.a1_in1k](https://huggingface.co/timm/ecaresnet50t.a1_in1k)|224 |81.26|95.16|25.6 |4.3 |11.8 |2823 |
|[gcresnext50ts.ch_in1k](https://huggingface.co/timm/gcresnext50ts.ch_in1k)|288 |81.23|95.54|15.7 |4.8 |19.6 |2117 |
|[senet154.gluon_in1k](https://huggingface.co/timm/senet154.gluon_in1k)|224 |81.23|95.35|115.1 |20.8 |38.7 |545 |
|[resnet50.a1_in1k](https://huggingface.co/timm/resnet50.a1_in1k)|288 |81.22|95.11|25.6 |6.8 |18.4 |2089 |
|[resnet50_gn.a1h_in1k](https://huggingface.co/timm/resnet50_gn.a1h_in1k)|288 |81.22|95.63|25.6 |6.8 |18.4 |676 |
|[resnet50d.a2_in1k](https://huggingface.co/timm/resnet50d.a2_in1k)|288 |81.18|95.09|25.6 |7.2 |19.7 |1908 |
|[resnet50.fb_swsl_ig1b_ft_in1k](https://huggingface.co/timm/resnet50.fb_swsl_ig1b_ft_in1k)|224 |81.18|95.98|25.6 |4.1 |11.1 |3455 |
|[resnext50_32x4d.tv2_in1k](https://huggingface.co/timm/resnext50_32x4d.tv2_in1k)|224 |81.17|95.34|25.0 |4.3 |14.4 |2933 |
|[resnext50_32x4d.a1h_in1k](https://huggingface.co/timm/resnext50_32x4d.a1h_in1k)|224 |81.1 |95.33|25.0 |4.3 |14.4 |2934 |
|[seresnet50.a2_in1k](https://huggingface.co/timm/seresnet50.a2_in1k)|288 |81.1 |95.23|28.1 |6.8 |18.4 |1801 |
|[seresnet50.a1_in1k](https://huggingface.co/timm/seresnet50.a1_in1k)|288 |81.1 |95.12|28.1 |6.8 |18.4 |1799 |
|[resnet152s.gluon_in1k](https://huggingface.co/timm/resnet152s.gluon_in1k)|224 |81.02|95.41|60.3 |12.9 |25.0 |1347 |
|[resnet50.d_in1k](https://huggingface.co/timm/resnet50.d_in1k)|288 |80.97|95.44|25.6 |6.8 |18.4 |2085 |
|[gcresnet50t.ra2_in1k](https://huggingface.co/timm/gcresnet50t.ra2_in1k)|256 |80.94|95.45|25.9 |5.4 |14.7 |2571 |
|[resnext101_32x4d.fb_ssl_yfcc100m_ft_in1k](https://huggingface.co/timm/resnext101_32x4d.fb_ssl_yfcc100m_ft_in1k)|224 |80.93|95.73|44.2 |8.0 |21.2 |1814 |
|[resnet50.c1_in1k](https://huggingface.co/timm/resnet50.c1_in1k)|288 |80.91|95.55|25.6 |6.8 |18.4 |2084 |
|[seresnext101_32x4d.gluon_in1k](https://huggingface.co/timm/seresnext101_32x4d.gluon_in1k)|224 |80.9 |95.31|49.0 |8.0 |21.3 |1585 |
|[seresnext101_64x4d.gluon_in1k](https://huggingface.co/timm/seresnext101_64x4d.gluon_in1k)|224 |80.9 |95.3 |88.2 |15.5 |31.2 |918 |
|[resnet50.c2_in1k](https://huggingface.co/timm/resnet50.c2_in1k)|288 |80.86|95.52|25.6 |6.8 |18.4 |2085 |
|[resnet50.tv2_in1k](https://huggingface.co/timm/resnet50.tv2_in1k)|224 |80.85|95.43|25.6 |4.1 |11.1 |3450 |
|[ecaresnet50t.a2_in1k](https://huggingface.co/timm/ecaresnet50t.a2_in1k)|224 |80.84|95.02|25.6 |4.3 |11.8 |2821 |
|[ecaresnet101d_pruned.miil_in1k](https://huggingface.co/timm/ecaresnet101d_pruned.miil_in1k)|224 |80.79|95.62|24.9 |3.5 |7.7 |2961 |
|[seresnet33ts.ra2_in1k](https://huggingface.co/timm/seresnet33ts.ra2_in1k)|288 |80.79|95.36|19.8 |6.0 |14.8 |2506 |
|[ecaresnet50d_pruned.miil_in1k](https://huggingface.co/timm/ecaresnet50d_pruned.miil_in1k)|288 |80.79|95.58|19.9 |4.2 |10.6 |2349 |
|[resnet50.a2_in1k](https://huggingface.co/timm/resnet50.a2_in1k)|288 |80.78|94.99|25.6 |6.8 |18.4 |2088 |
|[resnet50.b1k_in1k](https://huggingface.co/timm/resnet50.b1k_in1k)|288 |80.71|95.43|25.6 |6.8 |18.4 |2087 |
|[resnext50_32x4d.ra_in1k](https://huggingface.co/timm/resnext50_32x4d.ra_in1k)|288 |80.7 |95.39|25.0 |7.0 |23.8 |1749 |
|[resnetrs101.tf_in1k](https://huggingface.co/timm/resnetrs101.tf_in1k)|192 |80.69|95.24|63.6 |6.0 |12.7 |2270 |
|[resnet50d.a1_in1k](https://huggingface.co/timm/resnet50d.a1_in1k)|224 |80.68|94.71|25.6 |4.4 |11.9 |3162 |
|[eca_resnet33ts.ra2_in1k](https://huggingface.co/timm/eca_resnet33ts.ra2_in1k)|288 |80.68|95.36|19.7 |6.0 |14.8 |2637 |
|[resnet50.a1h_in1k](https://huggingface.co/timm/resnet50.a1h_in1k)|224 |80.67|95.3 |25.6 |4.1 |11.1 |3452 |
|[resnext50d_32x4d.bt_in1k](https://huggingface.co/timm/resnext50d_32x4d.bt_in1k)|288 |80.67|95.42|25.0 |7.4 |25.1 |1626 |
|[resnetaa50.a1h_in1k](https://huggingface.co/timm/resnetaa50.a1h_in1k)|224 |80.63|95.21|25.6 |5.2 |11.6 |3034 |
|[ecaresnet50d.miil_in1k](https://huggingface.co/timm/ecaresnet50d.miil_in1k)|224 |80.61|95.32|25.6 |4.4 |11.9 |2813 |
|[resnext101_64x4d.gluon_in1k](https://huggingface.co/timm/resnext101_64x4d.gluon_in1k)|224 |80.61|94.99|83.5 |15.5 |31.2 |989 |
|[gcresnet33ts.ra2_in1k](https://huggingface.co/timm/gcresnet33ts.ra2_in1k)|288 |80.6 |95.31|19.9 |6.0 |14.8 |2578 |
|[gcresnext50ts.ch_in1k](https://huggingface.co/timm/gcresnext50ts.ch_in1k)|256 |80.57|95.17|15.7 |3.8 |15.5 |2710 |
|[resnet152.a3_in1k](https://huggingface.co/timm/resnet152.a3_in1k)|224 |80.56|95.0 |60.2 |11.6 |22.6 |1483 |
|[resnet50d.ra2_in1k](https://huggingface.co/timm/resnet50d.ra2_in1k)|224 |80.53|95.16|25.6 |4.4 |11.9 |3164 |
|[resnext50_32x4d.a1_in1k](https://huggingface.co/timm/resnext50_32x4d.a1_in1k)|224 |80.53|94.46|25.0 |4.3 |14.4 |2930 |
|[wide_resnet101_2.tv2_in1k](https://huggingface.co/timm/wide_resnet101_2.tv2_in1k)|176 |80.48|94.98|126.9 |14.3 |13.2 |1719 |
|[resnet152d.gluon_in1k](https://huggingface.co/timm/resnet152d.gluon_in1k)|224 |80.47|95.2 |60.2 |11.8 |23.4 |1428 |
|[resnet50.b2k_in1k](https://huggingface.co/timm/resnet50.b2k_in1k)|288 |80.45|95.32|25.6 |6.8 |18.4 |2086 |
|[ecaresnetlight.miil_in1k](https://huggingface.co/timm/ecaresnetlight.miil_in1k)|224 |80.45|95.24|30.2 |4.1 |8.4 |3530 |
|[resnext50_32x4d.a2_in1k](https://huggingface.co/timm/resnext50_32x4d.a2_in1k)|224 |80.45|94.63|25.0 |4.3 |14.4 |2936 |
|[wide_resnet50_2.tv2_in1k](https://huggingface.co/timm/wide_resnet50_2.tv2_in1k)|176 |80.43|95.09|68.9 |7.3 |9.0 |3015 |
|[resnet101d.gluon_in1k](https://huggingface.co/timm/resnet101d.gluon_in1k)|224 |80.42|95.01|44.6 |8.1 |17.0 |2007 |
|[resnet50.a1_in1k](https://huggingface.co/timm/resnet50.a1_in1k)|224 |80.38|94.6 |25.6 |4.1 |11.1 |3461 |
|[seresnet33ts.ra2_in1k](https://huggingface.co/timm/seresnet33ts.ra2_in1k)|256 |80.36|95.1 |19.8 |4.8 |11.7 |3267 |
|[resnext101_32x4d.gluon_in1k](https://huggingface.co/timm/resnext101_32x4d.gluon_in1k)|224 |80.34|94.93|44.2 |8.0 |21.2 |1814 |
|[resnext50_32x4d.fb_ssl_yfcc100m_ft_in1k](https://huggingface.co/timm/resnext50_32x4d.fb_ssl_yfcc100m_ft_in1k)|224 |80.32|95.4 |25.0 |4.3 |14.4 |2941 |
|[resnet101s.gluon_in1k](https://huggingface.co/timm/resnet101s.gluon_in1k)|224 |80.28|95.16|44.7 |9.2 |18.6 |1851 |
|[seresnet50.ra2_in1k](https://huggingface.co/timm/seresnet50.ra2_in1k)|224 |80.26|95.08|28.1 |4.1 |11.1 |2972 |
|[resnetblur50.bt_in1k](https://huggingface.co/timm/resnetblur50.bt_in1k)|288 |80.24|95.24|25.6 |8.5 |19.9 |1523 |
|[resnet50d.a2_in1k](https://huggingface.co/timm/resnet50d.a2_in1k)|224 |80.22|94.63|25.6 |4.4 |11.9 |3162 |
|[resnet152.tv2_in1k](https://huggingface.co/timm/resnet152.tv2_in1k)|176 |80.2 |94.64|60.2 |7.2 |14.0 |2346 |
|[seresnet50.a2_in1k](https://huggingface.co/timm/seresnet50.a2_in1k)|224 |80.08|94.74|28.1 |4.1 |11.1 |2969 |
|[eca_resnet33ts.ra2_in1k](https://huggingface.co/timm/eca_resnet33ts.ra2_in1k)|256 |80.08|94.97|19.7 |4.8 |11.7 |3284 |
|[gcresnet33ts.ra2_in1k](https://huggingface.co/timm/gcresnet33ts.ra2_in1k)|256 |80.06|94.99|19.9 |4.8 |11.7 |3216 |
|[resnet50_gn.a1h_in1k](https://huggingface.co/timm/resnet50_gn.a1h_in1k)|224 |80.06|94.95|25.6 |4.1 |11.1 |1109 |
|[seresnet50.a1_in1k](https://huggingface.co/timm/seresnet50.a1_in1k)|224 |80.02|94.71|28.1 |4.1 |11.1 |2962 |
|[resnet50.ram_in1k](https://huggingface.co/timm/resnet50.ram_in1k)|288 |79.97|95.05|25.6 |6.8 |18.4 |2086 |
|[resnet152c.gluon_in1k](https://huggingface.co/timm/resnet152c.gluon_in1k)|224 |79.92|94.84|60.2 |11.8 |23.4 |1455 |
|[seresnext50_32x4d.gluon_in1k](https://huggingface.co/timm/seresnext50_32x4d.gluon_in1k)|224 |79.91|94.82|27.6 |4.3 |14.4 |2591 |
|[resnet50.d_in1k](https://huggingface.co/timm/resnet50.d_in1k)|224 |79.91|94.67|25.6 |4.1 |11.1 |3456 |
|[resnet101.tv2_in1k](https://huggingface.co/timm/resnet101.tv2_in1k)|176 |79.9 |94.6 |44.6 |4.9 |10.1 |3341 |
|[resnetrs50.tf_in1k](https://huggingface.co/timm/resnetrs50.tf_in1k)|224 |79.89|94.97|35.7 |4.5 |12.1 |2774 |
|[resnet50.c2_in1k](https://huggingface.co/timm/resnet50.c2_in1k)|224 |79.88|94.87|25.6 |4.1 |11.1 |3455 |
|[ecaresnet26t.ra2_in1k](https://huggingface.co/timm/ecaresnet26t.ra2_in1k)|320 |79.86|95.07|16.0 |5.2 |16.4 |2168 |
|[resnet50.a2_in1k](https://huggingface.co/timm/resnet50.a2_in1k)|224 |79.85|94.56|25.6 |4.1 |11.1 |3460 |
|[resnet50.ra_in1k](https://huggingface.co/timm/resnet50.ra_in1k)|288 |79.83|94.97|25.6 |6.8 |18.4 |2087 |
|[resnet101.a3_in1k](https://huggingface.co/timm/resnet101.a3_in1k)|224 |79.82|94.62|44.6 |7.8 |16.2 |2114 |
|[resnext50_32x4d.ra_in1k](https://huggingface.co/timm/resnext50_32x4d.ra_in1k)|224 |79.76|94.6 |25.0 |4.3 |14.4 |2943 |
|[resnet50.c1_in1k](https://huggingface.co/timm/resnet50.c1_in1k)|224 |79.74|94.95|25.6 |4.1 |11.1 |3455 |
|[ecaresnet50d_pruned.miil_in1k](https://huggingface.co/timm/ecaresnet50d_pruned.miil_in1k)|224 |79.74|94.87|19.9 |2.5 |6.4 |3929 |
|[resnet33ts.ra2_in1k](https://huggingface.co/timm/resnet33ts.ra2_in1k)|288 |79.71|94.83|19.7 |6.0 |14.8 |2710 |
|[resnet152.gluon_in1k](https://huggingface.co/timm/resnet152.gluon_in1k)|224 |79.68|94.74|60.2 |11.6 |22.6 |1486 |
|[resnext50d_32x4d.bt_in1k](https://huggingface.co/timm/resnext50d_32x4d.bt_in1k)|224 |79.67|94.87|25.0 |4.5 |15.2 |2729 |
|[resnet50.bt_in1k](https://huggingface.co/timm/resnet50.bt_in1k)|288 |79.63|94.91|25.6 |6.8 |18.4 |2086 |
|[ecaresnet50t.a3_in1k](https://huggingface.co/timm/ecaresnet50t.a3_in1k)|224 |79.56|94.72|25.6 |4.3 |11.8 |2805 |
|[resnet101c.gluon_in1k](https://huggingface.co/timm/resnet101c.gluon_in1k)|224 |79.53|94.58|44.6 |8.1 |17.0 |2062 |
|[resnet50.b1k_in1k](https://huggingface.co/timm/resnet50.b1k_in1k)|224 |79.52|94.61|25.6 |4.1 |11.1 |3459 |
|[resnet50.tv2_in1k](https://huggingface.co/timm/resnet50.tv2_in1k)|176 |79.42|94.64|25.6 |2.6 |6.9 |5397 |
|[resnet32ts.ra2_in1k](https://huggingface.co/timm/resnet32ts.ra2_in1k)|288 |79.4 |94.66|18.0 |5.9 |14.6 |2752 |
|[resnet50.b2k_in1k](https://huggingface.co/timm/resnet50.b2k_in1k)|224 |79.38|94.57|25.6 |4.1 |11.1 |3459 |
|[resnext50_32x4d.tv2_in1k](https://huggingface.co/timm/resnext50_32x4d.tv2_in1k)|176 |79.37|94.3 |25.0 |2.7 |9.0 |4577 |
|[resnext50_32x4d.gluon_in1k](https://huggingface.co/timm/resnext50_32x4d.gluon_in1k)|224 |79.36|94.43|25.0 |4.3 |14.4 |2942 |
|[resnext101_32x8d.tv_in1k](https://huggingface.co/timm/resnext101_32x8d.tv_in1k)|224 |79.31|94.52|88.8 |16.5 |31.2 |1100 |
|[resnet101.gluon_in1k](https://huggingface.co/timm/resnet101.gluon_in1k)|224 |79.31|94.53|44.6 |7.8 |16.2 |2125 |
|[resnetblur50.bt_in1k](https://huggingface.co/timm/resnetblur50.bt_in1k)|224 |79.31|94.63|25.6 |5.2 |12.0 |2524 |
|[resnet50.a1h_in1k](https://huggingface.co/timm/resnet50.a1h_in1k)|176 |79.27|94.49|25.6 |2.6 |6.9 |5404 |
|[resnext50_32x4d.a3_in1k](https://huggingface.co/timm/resnext50_32x4d.a3_in1k)|224 |79.25|94.31|25.0 |4.3 |14.4 |2931 |
|[resnet50.fb_ssl_yfcc100m_ft_in1k](https://huggingface.co/timm/resnet50.fb_ssl_yfcc100m_ft_in1k)|224 |79.22|94.84|25.6 |4.1 |11.1 |3451 |
|[resnet33ts.ra2_in1k](https://huggingface.co/timm/resnet33ts.ra2_in1k)|256 |79.21|94.56|19.7 |4.8 |11.7 |3392 |
|[resnet50d.gluon_in1k](https://huggingface.co/timm/resnet50d.gluon_in1k)|224 |79.07|94.48|25.6 |4.4 |11.9 |3162 |
|[resnet50.ram_in1k](https://huggingface.co/timm/resnet50.ram_in1k)|224 |79.03|94.38|25.6 |4.1 |11.1 |3453 |
|[resnet50.am_in1k](https://huggingface.co/timm/resnet50.am_in1k)|224 |79.01|94.39|25.6 |4.1 |11.1 |3461 |
|[resnet32ts.ra2_in1k](https://huggingface.co/timm/resnet32ts.ra2_in1k)|256 |79.01|94.37|18.0 |4.6 |11.6 |3440 |
|[ecaresnet26t.ra2_in1k](https://huggingface.co/timm/ecaresnet26t.ra2_in1k)|256 |78.9 |94.54|16.0 |3.4 |10.5 |3421 |
|[resnet152.a3_in1k](https://huggingface.co/timm/resnet152.a3_in1k)|160 |78.89|94.11|60.2 |5.9 |11.5 |2745 |
|[wide_resnet101_2.tv_in1k](https://huggingface.co/timm/wide_resnet101_2.tv_in1k)|224 |78.84|94.28|126.9 |22.8 |21.2 |1079 |
|[seresnext26d_32x4d.bt_in1k](https://huggingface.co/timm/seresnext26d_32x4d.bt_in1k)|288 |78.83|94.24|16.8 |4.5 |16.8 |2251 |
|[resnet50.ra_in1k](https://huggingface.co/timm/resnet50.ra_in1k)|224 |78.81|94.32|25.6 |4.1 |11.1 |3454 |
|[seresnext26t_32x4d.bt_in1k](https://huggingface.co/timm/seresnext26t_32x4d.bt_in1k)|288 |78.74|94.33|16.8 |4.5 |16.7 |2264 |
|[resnet50s.gluon_in1k](https://huggingface.co/timm/resnet50s.gluon_in1k)|224 |78.72|94.23|25.7 |5.5 |13.5 |2796 |
|[resnet50d.a3_in1k](https://huggingface.co/timm/resnet50d.a3_in1k)|224 |78.71|94.24|25.6 |4.4 |11.9 |3154 |
|[wide_resnet50_2.tv_in1k](https://huggingface.co/timm/wide_resnet50_2.tv_in1k)|224 |78.47|94.09|68.9 |11.4 |14.4 |1934 |
|[resnet50.bt_in1k](https://huggingface.co/timm/resnet50.bt_in1k)|224 |78.46|94.27|25.6 |4.1 |11.1 |3454 |
|[resnet34d.ra2_in1k](https://huggingface.co/timm/resnet34d.ra2_in1k)|288 |78.43|94.35|21.8 |6.5 |7.5 |3291 |
|[gcresnext26ts.ch_in1k](https://huggingface.co/timm/gcresnext26ts.ch_in1k)|288 |78.42|94.04|10.5 |3.1 |13.3 |3226 |
|[resnet26t.ra2_in1k](https://huggingface.co/timm/resnet26t.ra2_in1k)|320 |78.33|94.13|16.0 |5.2 |16.4 |2391 |
|[resnet152.tv_in1k](https://huggingface.co/timm/resnet152.tv_in1k)|224 |78.32|94.04|60.2 |11.6 |22.6 |1487 |
|[seresnext26ts.ch_in1k](https://huggingface.co/timm/seresnext26ts.ch_in1k)|288 |78.28|94.1 |10.4 |3.1 |13.3 |3062 |
|[bat_resnext26ts.ch_in1k](https://huggingface.co/timm/bat_resnext26ts.ch_in1k)|256 |78.25|94.1 |10.7 |2.5 |12.5 |3393 |
|[resnet50.a3_in1k](https://huggingface.co/timm/resnet50.a3_in1k)|224 |78.06|93.78|25.6 |4.1 |11.1 |3450 |
|[resnet50c.gluon_in1k](https://huggingface.co/timm/resnet50c.gluon_in1k)|224 |78.0 |93.99|25.6 |4.4 |11.9 |3286 |
|[eca_resnext26ts.ch_in1k](https://huggingface.co/timm/eca_resnext26ts.ch_in1k)|288 |78.0 |93.91|10.3 |3.1 |13.3 |3297 |
|[seresnext26t_32x4d.bt_in1k](https://huggingface.co/timm/seresnext26t_32x4d.bt_in1k)|224 |77.98|93.75|16.8 |2.7 |10.1 |3841 |
|[resnet34.a1_in1k](https://huggingface.co/timm/resnet34.a1_in1k)|288 |77.92|93.77|21.8 |6.1 |6.2 |3609 |
|[resnet101.a3_in1k](https://huggingface.co/timm/resnet101.a3_in1k)|160 |77.88|93.71|44.6 |4.0 |8.3 |3926 |
|[resnet26t.ra2_in1k](https://huggingface.co/timm/resnet26t.ra2_in1k)|256 |77.87|93.84|16.0 |3.4 |10.5 |3772 |
|[seresnext26ts.ch_in1k](https://huggingface.co/timm/seresnext26ts.ch_in1k)|256 |77.86|93.79|10.4 |2.4 |10.5 |4263 |
|[resnetrs50.tf_in1k](https://huggingface.co/timm/resnetrs50.tf_in1k)|160 |77.82|93.81|35.7 |2.3 |6.2 |5238 |
|[gcresnext26ts.ch_in1k](https://huggingface.co/timm/gcresnext26ts.ch_in1k)|256 |77.81|93.82|10.5 |2.4 |10.5 |4183 |
|[ecaresnet50t.a3_in1k](https://huggingface.co/timm/ecaresnet50t.a3_in1k)|160 |77.79|93.6 |25.6 |2.2 |6.0 |5329 |
|[resnext50_32x4d.a3_in1k](https://huggingface.co/timm/resnext50_32x4d.a3_in1k)|160 |77.73|93.32|25.0 |2.2 |7.4 |5576 |
|[resnext50_32x4d.tv_in1k](https://huggingface.co/timm/resnext50_32x4d.tv_in1k)|224 |77.61|93.7 |25.0 |4.3 |14.4 |2944 |
|[seresnext26d_32x4d.bt_in1k](https://huggingface.co/timm/seresnext26d_32x4d.bt_in1k)|224 |77.59|93.61|16.8 |2.7 |10.2 |3807 |
|[resnet50.gluon_in1k](https://huggingface.co/timm/resnet50.gluon_in1k)|224 |77.58|93.72|25.6 |4.1 |11.1 |3455 |
|[eca_resnext26ts.ch_in1k](https://huggingface.co/timm/eca_resnext26ts.ch_in1k)|256 |77.44|93.56|10.3 |2.4 |10.5 |4284 |
|[resnet26d.bt_in1k](https://huggingface.co/timm/resnet26d.bt_in1k)|288 |77.41|93.63|16.0 |4.3 |13.5 |2907 |
|[resnet101.tv_in1k](https://huggingface.co/timm/resnet101.tv_in1k)|224 |77.38|93.54|44.6 |7.8 |16.2 |2125 |
|[resnet50d.a3_in1k](https://huggingface.co/timm/resnet50d.a3_in1k)|160 |77.22|93.27|25.6 |2.2 |6.1 |5982 |
|[resnext26ts.ra2_in1k](https://huggingface.co/timm/resnext26ts.ra2_in1k)|288 |77.17|93.47|10.3 |3.1 |13.3 |3392 |
|[resnet34.a2_in1k](https://huggingface.co/timm/resnet34.a2_in1k)|288 |77.15|93.27|21.8 |6.1 |6.2 |3615 |
|[resnet34d.ra2_in1k](https://huggingface.co/timm/resnet34d.ra2_in1k)|224 |77.1 |93.37|21.8 |3.9 |4.5 |5436 |
|[seresnet50.a3_in1k](https://huggingface.co/timm/seresnet50.a3_in1k)|224 |77.02|93.07|28.1 |4.1 |11.1 |2952 |
|[resnext26ts.ra2_in1k](https://huggingface.co/timm/resnext26ts.ra2_in1k)|256 |76.78|93.13|10.3 |2.4 |10.5 |4410 |
|[resnet26d.bt_in1k](https://huggingface.co/timm/resnet26d.bt_in1k)|224 |76.7 |93.17|16.0 |2.6 |8.2 |4859 |
|[resnet34.bt_in1k](https://huggingface.co/timm/resnet34.bt_in1k)|288 |76.5 |93.35|21.8 |6.1 |6.2 |3617 |
|[resnet34.a1_in1k](https://huggingface.co/timm/resnet34.a1_in1k)|224 |76.42|92.87|21.8 |3.7 |3.7 |5984 |
|[resnet26.bt_in1k](https://huggingface.co/timm/resnet26.bt_in1k)|288 |76.35|93.18|16.0 |3.9 |12.2 |3331 |
|[resnet50.tv_in1k](https://huggingface.co/timm/resnet50.tv_in1k)|224 |76.13|92.86|25.6 |4.1 |11.1 |3457 |
|[resnet50.a3_in1k](https://huggingface.co/timm/resnet50.a3_in1k)|160 |75.96|92.5 |25.6 |2.1 |5.7 |6490 |
|[resnet34.a2_in1k](https://huggingface.co/timm/resnet34.a2_in1k)|224 |75.52|92.44|21.8 |3.7 |3.7 |5991 |
|[resnet26.bt_in1k](https://huggingface.co/timm/resnet26.bt_in1k)|224 |75.3 |92.58|16.0 |2.4 |7.4 |5583 |
|[resnet34.bt_in1k](https://huggingface.co/timm/resnet34.bt_in1k)|224 |75.16|92.18|21.8 |3.7 |3.7 |5994 |
|[seresnet50.a3_in1k](https://huggingface.co/timm/seresnet50.a3_in1k)|160 |75.1 |92.08|28.1 |2.1 |5.7 |5513 |
|[resnet34.gluon_in1k](https://huggingface.co/timm/resnet34.gluon_in1k)|224 |74.57|91.98|21.8 |3.7 |3.7 |5984 |
|[resnet18d.ra2_in1k](https://huggingface.co/timm/resnet18d.ra2_in1k)|288 |73.81|91.83|11.7 |3.4 |5.4 |5196 |
|[resnet34.tv_in1k](https://huggingface.co/timm/resnet34.tv_in1k)|224 |73.32|91.42|21.8 |3.7 |3.7 |5979 |
|[resnet18.fb_swsl_ig1b_ft_in1k](https://huggingface.co/timm/resnet18.fb_swsl_ig1b_ft_in1k)|224 |73.28|91.73|11.7 |1.8 |2.5 |10213 |
|[resnet18.a1_in1k](https://huggingface.co/timm/resnet18.a1_in1k)|288 |73.16|91.03|11.7 |3.0 |4.1 |6050 |
|[resnet34.a3_in1k](https://huggingface.co/timm/resnet34.a3_in1k)|224 |72.98|91.11|21.8 |3.7 |3.7 |5967 |
|[resnet18.fb_ssl_yfcc100m_ft_in1k](https://huggingface.co/timm/resnet18.fb_ssl_yfcc100m_ft_in1k)|224 |72.6 |91.42|11.7 |1.8 |2.5 |10213 |
|[resnet18.a2_in1k](https://huggingface.co/timm/resnet18.a2_in1k)|288 |72.37|90.59|11.7 |3.0 |4.1 |6051 |
|[resnet14t.c3_in1k](https://huggingface.co/timm/resnet14t.c3_in1k)|224 |72.26|90.31|10.1 |1.7 |5.8 |7026 |
|[resnet18d.ra2_in1k](https://huggingface.co/timm/resnet18d.ra2_in1k)|224 |72.26|90.68|11.7 |2.1 |3.3 |8707 |
|[resnet18.a1_in1k](https://huggingface.co/timm/resnet18.a1_in1k)|224 |71.49|90.07|11.7 |1.8 |2.5 |10187 |
|[resnet14t.c3_in1k](https://huggingface.co/timm/resnet14t.c3_in1k)|176 |71.31|89.69|10.1 |1.1 |3.6 |10970 |
|[resnet18.gluon_in1k](https://huggingface.co/timm/resnet18.gluon_in1k)|224 |70.84|89.76|11.7 |1.8 |2.5 |10210 |
|[resnet18.a2_in1k](https://huggingface.co/timm/resnet18.a2_in1k)|224 |70.64|89.47|11.7 |1.8 |2.5 |10194 |
|[resnet34.a3_in1k](https://huggingface.co/timm/resnet34.a3_in1k)|160 |70.56|89.52|21.8 |1.9 |1.9 |10737 |
|[resnet18.tv_in1k](https://huggingface.co/timm/resnet18.tv_in1k)|224 |69.76|89.07|11.7 |1.8 |2.5 |10205 |
|[resnet10t.c3_in1k](https://huggingface.co/timm/resnet10t.c3_in1k)|224 |68.34|88.03|5.4 |1.1 |2.4 |13079 |
|[resnet18.a3_in1k](https://huggingface.co/timm/resnet18.a3_in1k)|224 |68.25|88.17|11.7 |1.8 |2.5 |10167 |
|[resnet10t.c3_in1k](https://huggingface.co/timm/resnet10t.c3_in1k)|176 |66.71|86.96|5.4 |0.7 |1.5 |20327 |
|[resnet18.a3_in1k](https://huggingface.co/timm/resnet18.a3_in1k)|160 |65.66|86.26|11.7 |0.9 |1.3 |18229 |
## Citation
```bibtex
@inproceedings{wightman2021resnet,
title={ResNet strikes back: An improved training procedure in timm},
author={Wightman, Ross and Touvron, Hugo and Jegou, Herve},
booktitle={NeurIPS 2021 Workshop on ImageNet: Past, Present, and Future}
}
```
```bibtex
@misc{rw2019timm,
author = {Ross Wightman},
title = {PyTorch Image Models},
year = {2019},
publisher = {GitHub},
journal = {GitHub repository},
doi = {10.5281/zenodo.4414861},
howpublished = {\url{https://github.com/huggingface/pytorch-image-models}}
}
```
```bibtex
@article{He2015,
author = {Kaiming He and Xiangyu Zhang and Shaoqing Ren and Jian Sun},
title = {Deep Residual Learning for Image Recognition},
journal = {arXiv preprint arXiv:1512.03385},
year = {2015}
}
```
|
avsolatorio/GIST-small-Embedding-v0 | avsolatorio | "2024-02-28T00:36:01Z" | 416,458 | 20 | sentence-transformers | [
"sentence-transformers",
"pytorch",
"safetensors",
"bert",
"feature-extraction",
"mteb",
"sentence-similarity",
"en",
"arxiv:2402.16829",
"arxiv:2212.09741",
"license:mit",
"model-index",
"autotrain_compatible",
"text-embeddings-inference",
"endpoints_compatible",
"region:us"
] | sentence-similarity | "2024-02-03T06:14:01Z" | ---
language:
- en
library_name: sentence-transformers
license: mit
pipeline_tag: sentence-similarity
tags:
- feature-extraction
- mteb
- sentence-similarity
- sentence-transformers
model-index:
- name: GIST-small-Embedding-v0
results:
- task:
type: Classification
dataset:
type: mteb/amazon_counterfactual
name: MTEB AmazonCounterfactualClassification (en)
config: en
split: test
revision: e8379541af4e31359cca9fbcf4b00f2671dba205
metrics:
- type: accuracy
value: 75.26865671641791
- type: ap
value: 38.25623793370476
- type: f1
value: 69.26434651320257
- task:
type: Classification
dataset:
type: mteb/amazon_polarity
name: MTEB AmazonPolarityClassification
config: default
split: test
revision: e2d317d38cd51312af73b3d32a06d1a08b442046
metrics:
- type: accuracy
value: 93.232225
- type: ap
value: 89.97936072879344
- type: f1
value: 93.22122653806187
- task:
type: Classification
dataset:
type: mteb/amazon_reviews_multi
name: MTEB AmazonReviewsClassification (en)
config: en
split: test
revision: 1399c76144fd37290681b995c656ef9b2e06e26d
metrics:
- type: accuracy
value: 49.715999999999994
- type: f1
value: 49.169789920136076
- task:
type: Retrieval
dataset:
type: arguana
name: MTEB ArguAna
config: default
split: test
revision: None
metrics:
- type: map_at_1
value: 34.922
- type: map_at_10
value: 50.524
- type: map_at_100
value: 51.247
- type: map_at_1000
value: 51.249
- type: map_at_3
value: 45.887
- type: map_at_5
value: 48.592999999999996
- type: mrr_at_1
value: 34.922
- type: mrr_at_10
value: 50.382000000000005
- type: mrr_at_100
value: 51.104000000000006
- type: mrr_at_1000
value: 51.105999999999995
- type: mrr_at_3
value: 45.733000000000004
- type: mrr_at_5
value: 48.428
- type: ndcg_at_1
value: 34.922
- type: ndcg_at_10
value: 59.12
- type: ndcg_at_100
value: 62.083999999999996
- type: ndcg_at_1000
value: 62.137
- type: ndcg_at_3
value: 49.616
- type: ndcg_at_5
value: 54.501
- type: precision_at_1
value: 34.922
- type: precision_at_10
value: 8.649
- type: precision_at_100
value: 0.991
- type: precision_at_1000
value: 0.1
- type: precision_at_3
value: 20.152
- type: precision_at_5
value: 14.466999999999999
- type: recall_at_1
value: 34.922
- type: recall_at_10
value: 86.48599999999999
- type: recall_at_100
value: 99.14699999999999
- type: recall_at_1000
value: 99.57300000000001
- type: recall_at_3
value: 60.455000000000005
- type: recall_at_5
value: 72.333
- task:
type: Clustering
dataset:
type: mteb/arxiv-clustering-p2p
name: MTEB ArxivClusteringP2P
config: default
split: test
revision: a122ad7f3f0291bf49cc6f4d32aa80929df69d5d
metrics:
- type: v_measure
value: 47.623282347623714
- task:
type: Clustering
dataset:
type: mteb/arxiv-clustering-s2s
name: MTEB ArxivClusteringS2S
config: default
split: test
revision: f910caf1a6075f7329cdf8c1a6135696f37dbd53
metrics:
- type: v_measure
value: 39.86487843524932
- task:
type: Reranking
dataset:
type: mteb/askubuntudupquestions-reranking
name: MTEB AskUbuntuDupQuestions
config: default
split: test
revision: 2000358ca161889fa9c082cb41daa8dcfb161a54
metrics:
- type: map
value: 62.3290291318171
- type: mrr
value: 75.2379853141626
- task:
type: STS
dataset:
type: mteb/biosses-sts
name: MTEB BIOSSES
config: default
split: test
revision: d3fb88f8f02e40887cd149695127462bbcf29b4a
metrics:
- type: cos_sim_pearson
value: 88.52002953574285
- type: cos_sim_spearman
value: 86.98752423842483
- type: euclidean_pearson
value: 86.89442688314197
- type: euclidean_spearman
value: 86.88631711307471
- type: manhattan_pearson
value: 87.03723618507175
- type: manhattan_spearman
value: 86.76041062975224
- task:
type: Classification
dataset:
type: mteb/banking77
name: MTEB Banking77Classification
config: default
split: test
revision: 0fd18e25b25c072e09e0d92ab615fda904d66300
metrics:
- type: accuracy
value: 86.64935064935065
- type: f1
value: 86.61903824934998
- task:
type: Clustering
dataset:
type: mteb/biorxiv-clustering-p2p
name: MTEB BiorxivClusteringP2P
config: default
split: test
revision: 65b79d1d13f80053f67aca9498d9402c2d9f1f40
metrics:
- type: v_measure
value: 39.21904455377494
- task:
type: Clustering
dataset:
type: mteb/biorxiv-clustering-s2s
name: MTEB BiorxivClusteringS2S
config: default
split: test
revision: 258694dd0231531bc1fd9de6ceb52a0853c6d908
metrics:
- type: v_measure
value: 35.43342755570654
- task:
type: Retrieval
dataset:
type: BeIR/cqadupstack
name: MTEB CQADupstackAndroidRetrieval
config: default
split: test
revision: None
metrics:
- type: map_at_1
value: 31.843
- type: map_at_10
value: 43.379
- type: map_at_100
value: 44.946999999999996
- type: map_at_1000
value: 45.078
- type: map_at_3
value: 39.598
- type: map_at_5
value: 41.746
- type: mrr_at_1
value: 39.199
- type: mrr_at_10
value: 49.672
- type: mrr_at_100
value: 50.321000000000005
- type: mrr_at_1000
value: 50.365
- type: mrr_at_3
value: 46.805
- type: mrr_at_5
value: 48.579
- type: ndcg_at_1
value: 39.199
- type: ndcg_at_10
value: 50.163999999999994
- type: ndcg_at_100
value: 55.418
- type: ndcg_at_1000
value: 57.353
- type: ndcg_at_3
value: 44.716
- type: ndcg_at_5
value: 47.268
- type: precision_at_1
value: 39.199
- type: precision_at_10
value: 9.757
- type: precision_at_100
value: 1.552
- type: precision_at_1000
value: 0.20500000000000002
- type: precision_at_3
value: 21.602
- type: precision_at_5
value: 15.479000000000001
- type: recall_at_1
value: 31.843
- type: recall_at_10
value: 62.743
- type: recall_at_100
value: 84.78099999999999
- type: recall_at_1000
value: 96.86099999999999
- type: recall_at_3
value: 46.927
- type: recall_at_5
value: 54.355
- task:
type: Retrieval
dataset:
type: BeIR/cqadupstack
name: MTEB CQADupstackEnglishRetrieval
config: default
split: test
revision: None
metrics:
- type: map_at_1
value: 29.321
- type: map_at_10
value: 39.062999999999995
- type: map_at_100
value: 40.403
- type: map_at_1000
value: 40.534
- type: map_at_3
value: 36.367
- type: map_at_5
value: 37.756
- type: mrr_at_1
value: 35.987
- type: mrr_at_10
value: 44.708999999999996
- type: mrr_at_100
value: 45.394
- type: mrr_at_1000
value: 45.436
- type: mrr_at_3
value: 42.463
- type: mrr_at_5
value: 43.663000000000004
- type: ndcg_at_1
value: 35.987
- type: ndcg_at_10
value: 44.585
- type: ndcg_at_100
value: 49.297999999999995
- type: ndcg_at_1000
value: 51.315
- type: ndcg_at_3
value: 40.569
- type: ndcg_at_5
value: 42.197
- type: precision_at_1
value: 35.987
- type: precision_at_10
value: 8.369
- type: precision_at_100
value: 1.366
- type: precision_at_1000
value: 0.184
- type: precision_at_3
value: 19.427
- type: precision_at_5
value: 13.58
- type: recall_at_1
value: 29.321
- type: recall_at_10
value: 54.333
- type: recall_at_100
value: 74.178
- type: recall_at_1000
value: 86.732
- type: recall_at_3
value: 42.46
- type: recall_at_5
value: 47.089999999999996
- task:
type: Retrieval
dataset:
type: BeIR/cqadupstack
name: MTEB CQADupstackGamingRetrieval
config: default
split: test
revision: None
metrics:
- type: map_at_1
value: 38.811
- type: map_at_10
value: 51.114000000000004
- type: map_at_100
value: 52.22
- type: map_at_1000
value: 52.275000000000006
- type: map_at_3
value: 47.644999999999996
- type: map_at_5
value: 49.675000000000004
- type: mrr_at_1
value: 44.389
- type: mrr_at_10
value: 54.459
- type: mrr_at_100
value: 55.208999999999996
- type: mrr_at_1000
value: 55.239000000000004
- type: mrr_at_3
value: 51.954
- type: mrr_at_5
value: 53.571999999999996
- type: ndcg_at_1
value: 44.389
- type: ndcg_at_10
value: 56.979
- type: ndcg_at_100
value: 61.266
- type: ndcg_at_1000
value: 62.315
- type: ndcg_at_3
value: 51.342
- type: ndcg_at_5
value: 54.33
- type: precision_at_1
value: 44.389
- type: precision_at_10
value: 9.26
- type: precision_at_100
value: 1.226
- type: precision_at_1000
value: 0.136
- type: precision_at_3
value: 22.926
- type: precision_at_5
value: 15.987000000000002
- type: recall_at_1
value: 38.811
- type: recall_at_10
value: 70.841
- type: recall_at_100
value: 89.218
- type: recall_at_1000
value: 96.482
- type: recall_at_3
value: 56.123999999999995
- type: recall_at_5
value: 63.322
- task:
type: Retrieval
dataset:
type: BeIR/cqadupstack
name: MTEB CQADupstackGisRetrieval
config: default
split: test
revision: None
metrics:
- type: map_at_1
value: 25.378
- type: map_at_10
value: 34.311
- type: map_at_100
value: 35.399
- type: map_at_1000
value: 35.482
- type: map_at_3
value: 31.917
- type: map_at_5
value: 33.275
- type: mrr_at_1
value: 27.683999999999997
- type: mrr_at_10
value: 36.575
- type: mrr_at_100
value: 37.492
- type: mrr_at_1000
value: 37.556
- type: mrr_at_3
value: 34.35
- type: mrr_at_5
value: 35.525
- type: ndcg_at_1
value: 27.683999999999997
- type: ndcg_at_10
value: 39.247
- type: ndcg_at_100
value: 44.424
- type: ndcg_at_1000
value: 46.478
- type: ndcg_at_3
value: 34.684
- type: ndcg_at_5
value: 36.886
- type: precision_at_1
value: 27.683999999999997
- type: precision_at_10
value: 5.989
- type: precision_at_100
value: 0.899
- type: precision_at_1000
value: 0.11199999999999999
- type: precision_at_3
value: 14.84
- type: precision_at_5
value: 10.215
- type: recall_at_1
value: 25.378
- type: recall_at_10
value: 52.195
- type: recall_at_100
value: 75.764
- type: recall_at_1000
value: 91.012
- type: recall_at_3
value: 39.885999999999996
- type: recall_at_5
value: 45.279
- task:
type: Retrieval
dataset:
type: BeIR/cqadupstack
name: MTEB CQADupstackMathematicaRetrieval
config: default
split: test
revision: None
metrics:
- type: map_at_1
value: 17.326
- type: map_at_10
value: 25.247000000000003
- type: map_at_100
value: 26.473000000000003
- type: map_at_1000
value: 26.579000000000004
- type: map_at_3
value: 22.466
- type: map_at_5
value: 24.113
- type: mrr_at_1
value: 21.393
- type: mrr_at_10
value: 30.187
- type: mrr_at_100
value: 31.089
- type: mrr_at_1000
value: 31.15
- type: mrr_at_3
value: 27.279999999999998
- type: mrr_at_5
value: 29.127
- type: ndcg_at_1
value: 21.393
- type: ndcg_at_10
value: 30.668
- type: ndcg_at_100
value: 36.543
- type: ndcg_at_1000
value: 39.181
- type: ndcg_at_3
value: 25.552000000000003
- type: ndcg_at_5
value: 28.176000000000002
- type: precision_at_1
value: 21.393
- type: precision_at_10
value: 5.784000000000001
- type: precision_at_100
value: 1.001
- type: precision_at_1000
value: 0.136
- type: precision_at_3
value: 12.231
- type: precision_at_5
value: 9.179
- type: recall_at_1
value: 17.326
- type: recall_at_10
value: 42.415000000000006
- type: recall_at_100
value: 68.605
- type: recall_at_1000
value: 87.694
- type: recall_at_3
value: 28.343
- type: recall_at_5
value: 35.086
- task:
type: Retrieval
dataset:
type: BeIR/cqadupstack
name: MTEB CQADupstackPhysicsRetrieval
config: default
split: test
revision: None
metrics:
- type: map_at_1
value: 29.069
- type: map_at_10
value: 40.027
- type: map_at_100
value: 41.308
- type: map_at_1000
value: 41.412
- type: map_at_3
value: 36.864000000000004
- type: map_at_5
value: 38.641999999999996
- type: mrr_at_1
value: 35.707
- type: mrr_at_10
value: 45.527
- type: mrr_at_100
value: 46.348
- type: mrr_at_1000
value: 46.392
- type: mrr_at_3
value: 43.086
- type: mrr_at_5
value: 44.645
- type: ndcg_at_1
value: 35.707
- type: ndcg_at_10
value: 46.117000000000004
- type: ndcg_at_100
value: 51.468
- type: ndcg_at_1000
value: 53.412000000000006
- type: ndcg_at_3
value: 41.224
- type: ndcg_at_5
value: 43.637
- type: precision_at_1
value: 35.707
- type: precision_at_10
value: 8.459999999999999
- type: precision_at_100
value: 1.2970000000000002
- type: precision_at_1000
value: 0.165
- type: precision_at_3
value: 19.731
- type: precision_at_5
value: 14.013
- type: recall_at_1
value: 29.069
- type: recall_at_10
value: 58.343999999999994
- type: recall_at_100
value: 81.296
- type: recall_at_1000
value: 93.974
- type: recall_at_3
value: 44.7
- type: recall_at_5
value: 50.88700000000001
- task:
type: Retrieval
dataset:
type: BeIR/cqadupstack
name: MTEB CQADupstackProgrammersRetrieval
config: default
split: test
revision: None
metrics:
- type: map_at_1
value: 23.905
- type: map_at_10
value: 33.983000000000004
- type: map_at_100
value: 35.372
- type: map_at_1000
value: 35.487
- type: map_at_3
value: 30.902
- type: map_at_5
value: 32.505
- type: mrr_at_1
value: 29.794999999999998
- type: mrr_at_10
value: 39.28
- type: mrr_at_100
value: 40.215
- type: mrr_at_1000
value: 40.276
- type: mrr_at_3
value: 36.701
- type: mrr_at_5
value: 38.105
- type: ndcg_at_1
value: 29.794999999999998
- type: ndcg_at_10
value: 40.041
- type: ndcg_at_100
value: 45.884
- type: ndcg_at_1000
value: 48.271
- type: ndcg_at_3
value: 34.931
- type: ndcg_at_5
value: 37.044
- type: precision_at_1
value: 29.794999999999998
- type: precision_at_10
value: 7.546
- type: precision_at_100
value: 1.216
- type: precision_at_1000
value: 0.158
- type: precision_at_3
value: 16.933
- type: precision_at_5
value: 12.1
- type: recall_at_1
value: 23.905
- type: recall_at_10
value: 52.945
- type: recall_at_100
value: 77.551
- type: recall_at_1000
value: 93.793
- type: recall_at_3
value: 38.364
- type: recall_at_5
value: 44.044
- task:
type: Retrieval
dataset:
type: BeIR/cqadupstack
name: MTEB CQADupstackRetrieval
config: default
split: test
revision: None
metrics:
- type: map_at_1
value: 25.24441666666667
- type: map_at_10
value: 34.4595
- type: map_at_100
value: 35.699999999999996
- type: map_at_1000
value: 35.8155
- type: map_at_3
value: 31.608333333333338
- type: map_at_5
value: 33.189416666666666
- type: mrr_at_1
value: 29.825250000000004
- type: mrr_at_10
value: 38.60875
- type: mrr_at_100
value: 39.46575
- type: mrr_at_1000
value: 39.52458333333333
- type: mrr_at_3
value: 36.145166666666675
- type: mrr_at_5
value: 37.57625
- type: ndcg_at_1
value: 29.825250000000004
- type: ndcg_at_10
value: 39.88741666666667
- type: ndcg_at_100
value: 45.17966666666667
- type: ndcg_at_1000
value: 47.440583333333336
- type: ndcg_at_3
value: 35.04591666666666
- type: ndcg_at_5
value: 37.32025
- type: precision_at_1
value: 29.825250000000004
- type: precision_at_10
value: 7.07225
- type: precision_at_100
value: 1.1462499999999998
- type: precision_at_1000
value: 0.15325
- type: precision_at_3
value: 16.18375
- type: precision_at_5
value: 11.526833333333334
- type: recall_at_1
value: 25.24441666666667
- type: recall_at_10
value: 51.744916666666676
- type: recall_at_100
value: 75.04574999999998
- type: recall_at_1000
value: 90.65558333333334
- type: recall_at_3
value: 38.28349999999999
- type: recall_at_5
value: 44.16591666666667
- task:
type: Retrieval
dataset:
type: BeIR/cqadupstack
name: MTEB CQADupstackStatsRetrieval
config: default
split: test
revision: None
metrics:
- type: map_at_1
value: 24.237000000000002
- type: map_at_10
value: 30.667
- type: map_at_100
value: 31.592
- type: map_at_1000
value: 31.688
- type: map_at_3
value: 28.810999999999996
- type: map_at_5
value: 29.788999999999998
- type: mrr_at_1
value: 26.840000000000003
- type: mrr_at_10
value: 33.305
- type: mrr_at_100
value: 34.089000000000006
- type: mrr_at_1000
value: 34.159
- type: mrr_at_3
value: 31.518
- type: mrr_at_5
value: 32.469
- type: ndcg_at_1
value: 26.840000000000003
- type: ndcg_at_10
value: 34.541
- type: ndcg_at_100
value: 39.206
- type: ndcg_at_1000
value: 41.592
- type: ndcg_at_3
value: 31.005
- type: ndcg_at_5
value: 32.554
- type: precision_at_1
value: 26.840000000000003
- type: precision_at_10
value: 5.3069999999999995
- type: precision_at_100
value: 0.8340000000000001
- type: precision_at_1000
value: 0.11199999999999999
- type: precision_at_3
value: 13.292000000000002
- type: precision_at_5
value: 9.049
- type: recall_at_1
value: 24.237000000000002
- type: recall_at_10
value: 43.862
- type: recall_at_100
value: 65.352
- type: recall_at_1000
value: 82.704
- type: recall_at_3
value: 34.009
- type: recall_at_5
value: 37.878
- task:
type: Retrieval
dataset:
type: BeIR/cqadupstack
name: MTEB CQADupstackTexRetrieval
config: default
split: test
revision: None
metrics:
- type: map_at_1
value: 16.482
- type: map_at_10
value: 23.249
- type: map_at_100
value: 24.388
- type: map_at_1000
value: 24.519
- type: map_at_3
value: 20.971
- type: map_at_5
value: 22.192
- type: mrr_at_1
value: 19.993
- type: mrr_at_10
value: 26.985
- type: mrr_at_100
value: 27.975
- type: mrr_at_1000
value: 28.052
- type: mrr_at_3
value: 24.954
- type: mrr_at_5
value: 26.070999999999998
- type: ndcg_at_1
value: 19.993
- type: ndcg_at_10
value: 27.656
- type: ndcg_at_100
value: 33.256
- type: ndcg_at_1000
value: 36.275
- type: ndcg_at_3
value: 23.644000000000002
- type: ndcg_at_5
value: 25.466
- type: precision_at_1
value: 19.993
- type: precision_at_10
value: 5.093
- type: precision_at_100
value: 0.932
- type: precision_at_1000
value: 0.13699999999999998
- type: precision_at_3
value: 11.149000000000001
- type: precision_at_5
value: 8.149000000000001
- type: recall_at_1
value: 16.482
- type: recall_at_10
value: 37.141999999999996
- type: recall_at_100
value: 62.696
- type: recall_at_1000
value: 84.333
- type: recall_at_3
value: 26.031
- type: recall_at_5
value: 30.660999999999998
- task:
type: Retrieval
dataset:
type: BeIR/cqadupstack
name: MTEB CQADupstackUnixRetrieval
config: default
split: test
revision: None
metrics:
- type: map_at_1
value: 24.887999999999998
- type: map_at_10
value: 34.101
- type: map_at_100
value: 35.27
- type: map_at_1000
value: 35.370000000000005
- type: map_at_3
value: 31.283
- type: map_at_5
value: 32.72
- type: mrr_at_1
value: 29.011
- type: mrr_at_10
value: 38.004
- type: mrr_at_100
value: 38.879000000000005
- type: mrr_at_1000
value: 38.938
- type: mrr_at_3
value: 35.571999999999996
- type: mrr_at_5
value: 36.789
- type: ndcg_at_1
value: 29.011
- type: ndcg_at_10
value: 39.586
- type: ndcg_at_100
value: 44.939
- type: ndcg_at_1000
value: 47.236
- type: ndcg_at_3
value: 34.4
- type: ndcg_at_5
value: 36.519
- type: precision_at_1
value: 29.011
- type: precision_at_10
value: 6.763
- type: precision_at_100
value: 1.059
- type: precision_at_1000
value: 0.13699999999999998
- type: precision_at_3
value: 15.609
- type: precision_at_5
value: 10.896
- type: recall_at_1
value: 24.887999999999998
- type: recall_at_10
value: 52.42
- type: recall_at_100
value: 75.803
- type: recall_at_1000
value: 91.725
- type: recall_at_3
value: 38.080999999999996
- type: recall_at_5
value: 43.47
- task:
type: Retrieval
dataset:
type: BeIR/cqadupstack
name: MTEB CQADupstackWebmastersRetrieval
config: default
split: test
revision: None
metrics:
- type: map_at_1
value: 23.953
- type: map_at_10
value: 32.649
- type: map_at_100
value: 34.181
- type: map_at_1000
value: 34.398
- type: map_at_3
value: 29.567
- type: map_at_5
value: 31.263
- type: mrr_at_1
value: 29.051
- type: mrr_at_10
value: 37.419999999999995
- type: mrr_at_100
value: 38.396
- type: mrr_at_1000
value: 38.458
- type: mrr_at_3
value: 34.782999999999994
- type: mrr_at_5
value: 36.254999999999995
- type: ndcg_at_1
value: 29.051
- type: ndcg_at_10
value: 38.595
- type: ndcg_at_100
value: 44.6
- type: ndcg_at_1000
value: 47.158
- type: ndcg_at_3
value: 33.56
- type: ndcg_at_5
value: 35.870000000000005
- type: precision_at_1
value: 29.051
- type: precision_at_10
value: 7.53
- type: precision_at_100
value: 1.538
- type: precision_at_1000
value: 0.24
- type: precision_at_3
value: 15.744
- type: precision_at_5
value: 11.542
- type: recall_at_1
value: 23.953
- type: recall_at_10
value: 50.08200000000001
- type: recall_at_100
value: 77.364
- type: recall_at_1000
value: 93.57799999999999
- type: recall_at_3
value: 35.432
- type: recall_at_5
value: 41.875
- task:
type: Retrieval
dataset:
type: BeIR/cqadupstack
name: MTEB CQADupstackWordpressRetrieval
config: default
split: test
revision: None
metrics:
- type: map_at_1
value: 17.72
- type: map_at_10
value: 25.724000000000004
- type: map_at_100
value: 26.846999999999998
- type: map_at_1000
value: 26.964
- type: map_at_3
value: 22.909
- type: map_at_5
value: 24.596999999999998
- type: mrr_at_1
value: 18.854000000000003
- type: mrr_at_10
value: 27.182000000000002
- type: mrr_at_100
value: 28.182000000000002
- type: mrr_at_1000
value: 28.274
- type: mrr_at_3
value: 24.276
- type: mrr_at_5
value: 26.115
- type: ndcg_at_1
value: 18.854000000000003
- type: ndcg_at_10
value: 30.470000000000002
- type: ndcg_at_100
value: 35.854
- type: ndcg_at_1000
value: 38.701
- type: ndcg_at_3
value: 24.924
- type: ndcg_at_5
value: 27.895999999999997
- type: precision_at_1
value: 18.854000000000003
- type: precision_at_10
value: 5.009
- type: precision_at_100
value: 0.835
- type: precision_at_1000
value: 0.117
- type: precision_at_3
value: 10.721
- type: precision_at_5
value: 8.133
- type: recall_at_1
value: 17.72
- type: recall_at_10
value: 43.617
- type: recall_at_100
value: 67.941
- type: recall_at_1000
value: 88.979
- type: recall_at_3
value: 29.044999999999998
- type: recall_at_5
value: 36.044
- task:
type: Retrieval
dataset:
type: climate-fever
name: MTEB ClimateFEVER
config: default
split: test
revision: None
metrics:
- type: map_at_1
value: 13.427
- type: map_at_10
value: 22.935
- type: map_at_100
value: 24.808
- type: map_at_1000
value: 24.994
- type: map_at_3
value: 19.533
- type: map_at_5
value: 21.261
- type: mrr_at_1
value: 30.945
- type: mrr_at_10
value: 43.242000000000004
- type: mrr_at_100
value: 44.013999999999996
- type: mrr_at_1000
value: 44.048
- type: mrr_at_3
value: 40.109
- type: mrr_at_5
value: 42.059999999999995
- type: ndcg_at_1
value: 30.945
- type: ndcg_at_10
value: 31.828
- type: ndcg_at_100
value: 38.801
- type: ndcg_at_1000
value: 42.126999999999995
- type: ndcg_at_3
value: 26.922
- type: ndcg_at_5
value: 28.483999999999998
- type: precision_at_1
value: 30.945
- type: precision_at_10
value: 9.844
- type: precision_at_100
value: 1.7309999999999999
- type: precision_at_1000
value: 0.23500000000000001
- type: precision_at_3
value: 20.477999999999998
- type: precision_at_5
value: 15.27
- type: recall_at_1
value: 13.427
- type: recall_at_10
value: 37.141000000000005
- type: recall_at_100
value: 61.007
- type: recall_at_1000
value: 79.742
- type: recall_at_3
value: 24.431
- type: recall_at_5
value: 29.725
- task:
type: Retrieval
dataset:
type: dbpedia-entity
name: MTEB DBPedia
config: default
split: test
revision: None
metrics:
- type: map_at_1
value: 9.122
- type: map_at_10
value: 18.799
- type: map_at_100
value: 25.724999999999998
- type: map_at_1000
value: 27.205000000000002
- type: map_at_3
value: 14.194999999999999
- type: map_at_5
value: 16.225
- type: mrr_at_1
value: 68.0
- type: mrr_at_10
value: 76.035
- type: mrr_at_100
value: 76.292
- type: mrr_at_1000
value: 76.297
- type: mrr_at_3
value: 74.458
- type: mrr_at_5
value: 75.558
- type: ndcg_at_1
value: 56.00000000000001
- type: ndcg_at_10
value: 39.761
- type: ndcg_at_100
value: 43.736999999999995
- type: ndcg_at_1000
value: 51.146
- type: ndcg_at_3
value: 45.921
- type: ndcg_at_5
value: 42.756
- type: precision_at_1
value: 68.0
- type: precision_at_10
value: 30.275000000000002
- type: precision_at_100
value: 9.343
- type: precision_at_1000
value: 1.8270000000000002
- type: precision_at_3
value: 49.167
- type: precision_at_5
value: 40.699999999999996
- type: recall_at_1
value: 9.122
- type: recall_at_10
value: 23.669999999999998
- type: recall_at_100
value: 48.719
- type: recall_at_1000
value: 72.033
- type: recall_at_3
value: 15.498999999999999
- type: recall_at_5
value: 18.657
- task:
type: Classification
dataset:
type: mteb/emotion
name: MTEB EmotionClassification
config: default
split: test
revision: 4f58c6b202a23cf9a4da393831edf4f9183cad37
metrics:
- type: accuracy
value: 55.885000000000005
- type: f1
value: 50.70726446938571
- task:
type: Retrieval
dataset:
type: fever
name: MTEB FEVER
config: default
split: test
revision: None
metrics:
- type: map_at_1
value: 75.709
- type: map_at_10
value: 83.345
- type: map_at_100
value: 83.557
- type: map_at_1000
value: 83.572
- type: map_at_3
value: 82.425
- type: map_at_5
value: 83.013
- type: mrr_at_1
value: 81.593
- type: mrr_at_10
value: 88.331
- type: mrr_at_100
value: 88.408
- type: mrr_at_1000
value: 88.41
- type: mrr_at_3
value: 87.714
- type: mrr_at_5
value: 88.122
- type: ndcg_at_1
value: 81.593
- type: ndcg_at_10
value: 86.925
- type: ndcg_at_100
value: 87.67
- type: ndcg_at_1000
value: 87.924
- type: ndcg_at_3
value: 85.5
- type: ndcg_at_5
value: 86.283
- type: precision_at_1
value: 81.593
- type: precision_at_10
value: 10.264
- type: precision_at_100
value: 1.084
- type: precision_at_1000
value: 0.11199999999999999
- type: precision_at_3
value: 32.388
- type: precision_at_5
value: 19.991
- type: recall_at_1
value: 75.709
- type: recall_at_10
value: 93.107
- type: recall_at_100
value: 96.024
- type: recall_at_1000
value: 97.603
- type: recall_at_3
value: 89.08500000000001
- type: recall_at_5
value: 91.15299999999999
- task:
type: Retrieval
dataset:
type: fiqa
name: MTEB FiQA2018
config: default
split: test
revision: None
metrics:
- type: map_at_1
value: 19.121
- type: map_at_10
value: 31.78
- type: map_at_100
value: 33.497
- type: map_at_1000
value: 33.696
- type: map_at_3
value: 27.893
- type: map_at_5
value: 30.087000000000003
- type: mrr_at_1
value: 38.272
- type: mrr_at_10
value: 47.176
- type: mrr_at_100
value: 48.002
- type: mrr_at_1000
value: 48.044
- type: mrr_at_3
value: 45.086999999999996
- type: mrr_at_5
value: 46.337
- type: ndcg_at_1
value: 38.272
- type: ndcg_at_10
value: 39.145
- type: ndcg_at_100
value: 45.696999999999996
- type: ndcg_at_1000
value: 49.0
- type: ndcg_at_3
value: 36.148
- type: ndcg_at_5
value: 37.023
- type: precision_at_1
value: 38.272
- type: precision_at_10
value: 11.065
- type: precision_at_100
value: 1.7840000000000003
- type: precision_at_1000
value: 0.23600000000000002
- type: precision_at_3
value: 24.587999999999997
- type: precision_at_5
value: 18.056
- type: recall_at_1
value: 19.121
- type: recall_at_10
value: 44.857
- type: recall_at_100
value: 69.774
- type: recall_at_1000
value: 89.645
- type: recall_at_3
value: 32.588
- type: recall_at_5
value: 37.939
- task:
type: Retrieval
dataset:
type: hotpotqa
name: MTEB HotpotQA
config: default
split: test
revision: None
metrics:
- type: map_at_1
value: 36.428
- type: map_at_10
value: 56.891999999999996
- type: map_at_100
value: 57.82899999999999
- type: map_at_1000
value: 57.896
- type: map_at_3
value: 53.762
- type: map_at_5
value: 55.718
- type: mrr_at_1
value: 72.856
- type: mrr_at_10
value: 79.245
- type: mrr_at_100
value: 79.515
- type: mrr_at_1000
value: 79.525
- type: mrr_at_3
value: 78.143
- type: mrr_at_5
value: 78.822
- type: ndcg_at_1
value: 72.856
- type: ndcg_at_10
value: 65.204
- type: ndcg_at_100
value: 68.552
- type: ndcg_at_1000
value: 69.902
- type: ndcg_at_3
value: 60.632
- type: ndcg_at_5
value: 63.161
- type: precision_at_1
value: 72.856
- type: precision_at_10
value: 13.65
- type: precision_at_100
value: 1.6260000000000001
- type: precision_at_1000
value: 0.181
- type: precision_at_3
value: 38.753
- type: precision_at_5
value: 25.251
- type: recall_at_1
value: 36.428
- type: recall_at_10
value: 68.25099999999999
- type: recall_at_100
value: 81.317
- type: recall_at_1000
value: 90.27
- type: recall_at_3
value: 58.13
- type: recall_at_5
value: 63.126000000000005
- task:
type: Classification
dataset:
type: mteb/imdb
name: MTEB ImdbClassification
config: default
split: test
revision: 3d86128a09e091d6018b6d26cad27f2739fc2db7
metrics:
- type: accuracy
value: 89.4868
- type: ap
value: 84.88319192880247
- type: f1
value: 89.46144458052846
- task:
type: Retrieval
dataset:
type: msmarco
name: MTEB MSMARCO
config: default
split: dev
revision: None
metrics:
- type: map_at_1
value: 21.282999999999998
- type: map_at_10
value: 33.045
- type: map_at_100
value: 34.238
- type: map_at_1000
value: 34.29
- type: map_at_3
value: 29.305999999999997
- type: map_at_5
value: 31.391000000000002
- type: mrr_at_1
value: 21.92
- type: mrr_at_10
value: 33.649
- type: mrr_at_100
value: 34.791
- type: mrr_at_1000
value: 34.837
- type: mrr_at_3
value: 30.0
- type: mrr_at_5
value: 32.039
- type: ndcg_at_1
value: 21.92
- type: ndcg_at_10
value: 39.729
- type: ndcg_at_100
value: 45.484
- type: ndcg_at_1000
value: 46.817
- type: ndcg_at_3
value: 32.084
- type: ndcg_at_5
value: 35.789
- type: precision_at_1
value: 21.92
- type: precision_at_10
value: 6.297
- type: precision_at_100
value: 0.918
- type: precision_at_1000
value: 0.10300000000000001
- type: precision_at_3
value: 13.639000000000001
- type: precision_at_5
value: 10.054
- type: recall_at_1
value: 21.282999999999998
- type: recall_at_10
value: 60.343999999999994
- type: recall_at_100
value: 86.981
- type: recall_at_1000
value: 97.205
- type: recall_at_3
value: 39.452999999999996
- type: recall_at_5
value: 48.333
- task:
type: Classification
dataset:
type: mteb/mtop_domain
name: MTEB MTOPDomainClassification (en)
config: en
split: test
revision: d80d48c1eb48d3562165c59d59d0034df9fff0bf
metrics:
- type: accuracy
value: 95.47879616963064
- type: f1
value: 95.21800589958251
- task:
type: Classification
dataset:
type: mteb/mtop_intent
name: MTEB MTOPIntentClassification (en)
config: en
split: test
revision: ae001d0e6b1228650b7bd1c2c65fb50ad11a8aba
metrics:
- type: accuracy
value: 79.09256725946192
- type: f1
value: 60.554043889452515
- task:
type: Classification
dataset:
type: mteb/amazon_massive_intent
name: MTEB MassiveIntentClassification (en)
config: en
split: test
revision: 31efe3c427b0bae9c22cbb560b8f15491cc6bed7
metrics:
- type: accuracy
value: 75.53463349024882
- type: f1
value: 73.14418495756476
- task:
type: Classification
dataset:
type: mteb/amazon_massive_scenario
name: MTEB MassiveScenarioClassification (en)
config: en
split: test
revision: 7d571f92784cd94a019292a1f45445077d0ef634
metrics:
- type: accuracy
value: 79.22663080026899
- type: f1
value: 79.331456217501
- task:
type: Clustering
dataset:
type: mteb/medrxiv-clustering-p2p
name: MTEB MedrxivClusteringP2P
config: default
split: test
revision: e7a26af6f3ae46b30dde8737f02c07b1505bcc73
metrics:
- type: v_measure
value: 34.50316010430136
- task:
type: Clustering
dataset:
type: mteb/medrxiv-clustering-s2s
name: MTEB MedrxivClusteringS2S
config: default
split: test
revision: 35191c8c0dca72d8ff3efcd72aa802307d469663
metrics:
- type: v_measure
value: 32.15612040042282
- task:
type: Reranking
dataset:
type: mteb/mind_small
name: MTEB MindSmallReranking
config: default
split: test
revision: 3bdac13927fdc888b903db93b2ffdbd90b295a69
metrics:
- type: map
value: 32.36227552557184
- type: mrr
value: 33.57901344209811
- task:
type: Retrieval
dataset:
type: nfcorpus
name: MTEB NFCorpus
config: default
split: test
revision: None
metrics:
- type: map_at_1
value: 5.6610000000000005
- type: map_at_10
value: 12.992
- type: map_at_100
value: 16.756999999999998
- type: map_at_1000
value: 18.25
- type: map_at_3
value: 9.471
- type: map_at_5
value: 11.116
- type: mrr_at_1
value: 43.653
- type: mrr_at_10
value: 53.388999999999996
- type: mrr_at_100
value: 53.982
- type: mrr_at_1000
value: 54.033
- type: mrr_at_3
value: 51.858000000000004
- type: mrr_at_5
value: 53.019000000000005
- type: ndcg_at_1
value: 41.641
- type: ndcg_at_10
value: 34.691
- type: ndcg_at_100
value: 32.305
- type: ndcg_at_1000
value: 41.132999999999996
- type: ndcg_at_3
value: 40.614
- type: ndcg_at_5
value: 38.456
- type: precision_at_1
value: 43.344
- type: precision_at_10
value: 25.881999999999998
- type: precision_at_100
value: 8.483
- type: precision_at_1000
value: 2.131
- type: precision_at_3
value: 38.803
- type: precision_at_5
value: 33.87
- type: recall_at_1
value: 5.6610000000000005
- type: recall_at_10
value: 16.826
- type: recall_at_100
value: 32.939
- type: recall_at_1000
value: 65.161
- type: recall_at_3
value: 10.756
- type: recall_at_5
value: 13.331000000000001
- task:
type: Retrieval
dataset:
type: nq
name: MTEB NQ
config: default
split: test
revision: None
metrics:
- type: map_at_1
value: 26.692
- type: map_at_10
value: 41.065000000000005
- type: map_at_100
value: 42.235
- type: map_at_1000
value: 42.27
- type: map_at_3
value: 36.635
- type: map_at_5
value: 39.219
- type: mrr_at_1
value: 30.214000000000002
- type: mrr_at_10
value: 43.443
- type: mrr_at_100
value: 44.326
- type: mrr_at_1000
value: 44.352000000000004
- type: mrr_at_3
value: 39.623999999999995
- type: mrr_at_5
value: 41.898
- type: ndcg_at_1
value: 30.214000000000002
- type: ndcg_at_10
value: 48.692
- type: ndcg_at_100
value: 53.671
- type: ndcg_at_1000
value: 54.522000000000006
- type: ndcg_at_3
value: 40.245
- type: ndcg_at_5
value: 44.580999999999996
- type: precision_at_1
value: 30.214000000000002
- type: precision_at_10
value: 8.3
- type: precision_at_100
value: 1.1079999999999999
- type: precision_at_1000
value: 0.11900000000000001
- type: precision_at_3
value: 18.521
- type: precision_at_5
value: 13.627
- type: recall_at_1
value: 26.692
- type: recall_at_10
value: 69.699
- type: recall_at_100
value: 91.425
- type: recall_at_1000
value: 97.78099999999999
- type: recall_at_3
value: 47.711
- type: recall_at_5
value: 57.643
- task:
type: Retrieval
dataset:
type: quora
name: MTEB QuoraRetrieval
config: default
split: test
revision: None
metrics:
- type: map_at_1
value: 70.962
- type: map_at_10
value: 84.772
- type: map_at_100
value: 85.402
- type: map_at_1000
value: 85.418
- type: map_at_3
value: 81.89
- type: map_at_5
value: 83.685
- type: mrr_at_1
value: 81.67
- type: mrr_at_10
value: 87.681
- type: mrr_at_100
value: 87.792
- type: mrr_at_1000
value: 87.79299999999999
- type: mrr_at_3
value: 86.803
- type: mrr_at_5
value: 87.392
- type: ndcg_at_1
value: 81.69
- type: ndcg_at_10
value: 88.429
- type: ndcg_at_100
value: 89.66
- type: ndcg_at_1000
value: 89.762
- type: ndcg_at_3
value: 85.75
- type: ndcg_at_5
value: 87.20700000000001
- type: precision_at_1
value: 81.69
- type: precision_at_10
value: 13.395000000000001
- type: precision_at_100
value: 1.528
- type: precision_at_1000
value: 0.157
- type: precision_at_3
value: 37.507000000000005
- type: precision_at_5
value: 24.614
- type: recall_at_1
value: 70.962
- type: recall_at_10
value: 95.339
- type: recall_at_100
value: 99.543
- type: recall_at_1000
value: 99.984
- type: recall_at_3
value: 87.54899999999999
- type: recall_at_5
value: 91.726
- task:
type: Clustering
dataset:
type: mteb/reddit-clustering
name: MTEB RedditClustering
config: default
split: test
revision: 24640382cdbf8abc73003fb0fa6d111a705499eb
metrics:
- type: v_measure
value: 55.506631779239555
- task:
type: Clustering
dataset:
type: mteb/reddit-clustering-p2p
name: MTEB RedditClusteringP2P
config: default
split: test
revision: 282350215ef01743dc01b456c7f5241fa8937f16
metrics:
- type: v_measure
value: 60.63731341848479
- task:
type: Retrieval
dataset:
type: scidocs
name: MTEB SCIDOCS
config: default
split: test
revision: None
metrics:
- type: map_at_1
value: 4.852
- type: map_at_10
value: 13.175
- type: map_at_100
value: 15.623999999999999
- type: map_at_1000
value: 16.002
- type: map_at_3
value: 9.103
- type: map_at_5
value: 11.068999999999999
- type: mrr_at_1
value: 23.9
- type: mrr_at_10
value: 35.847
- type: mrr_at_100
value: 36.968
- type: mrr_at_1000
value: 37.018
- type: mrr_at_3
value: 32.300000000000004
- type: mrr_at_5
value: 34.14
- type: ndcg_at_1
value: 23.9
- type: ndcg_at_10
value: 21.889
- type: ndcg_at_100
value: 30.903000000000002
- type: ndcg_at_1000
value: 36.992000000000004
- type: ndcg_at_3
value: 20.274
- type: ndcg_at_5
value: 17.773
- type: precision_at_1
value: 23.9
- type: precision_at_10
value: 11.61
- type: precision_at_100
value: 2.4539999999999997
- type: precision_at_1000
value: 0.391
- type: precision_at_3
value: 19.133
- type: precision_at_5
value: 15.740000000000002
- type: recall_at_1
value: 4.852
- type: recall_at_10
value: 23.507
- type: recall_at_100
value: 49.775000000000006
- type: recall_at_1000
value: 79.308
- type: recall_at_3
value: 11.637
- type: recall_at_5
value: 15.947
- task:
type: STS
dataset:
type: mteb/sickr-sts
name: MTEB SICK-R
config: default
split: test
revision: a6ea5a8cab320b040a23452cc28066d9beae2cee
metrics:
- type: cos_sim_pearson
value: 86.03345827446948
- type: cos_sim_spearman
value: 80.53174518259549
- type: euclidean_pearson
value: 83.44538971660883
- type: euclidean_spearman
value: 80.57344324098692
- type: manhattan_pearson
value: 83.36528808195459
- type: manhattan_spearman
value: 80.48931287157902
- task:
type: STS
dataset:
type: mteb/sts12-sts
name: MTEB STS12
config: default
split: test
revision: a0d554a64d88156834ff5ae9920b964011b16384
metrics:
- type: cos_sim_pearson
value: 85.21363088257881
- type: cos_sim_spearman
value: 75.56589127055523
- type: euclidean_pearson
value: 82.32868324521908
- type: euclidean_spearman
value: 75.31928550664554
- type: manhattan_pearson
value: 82.31332875713211
- type: manhattan_spearman
value: 75.35376322099196
- task:
type: STS
dataset:
type: mteb/sts13-sts
name: MTEB STS13
config: default
split: test
revision: 7e90230a92c190f1bf69ae9002b8cea547a64cca
metrics:
- type: cos_sim_pearson
value: 85.09085593258487
- type: cos_sim_spearman
value: 86.26355088415221
- type: euclidean_pearson
value: 85.49646115361156
- type: euclidean_spearman
value: 86.20652472228703
- type: manhattan_pearson
value: 85.44084081123815
- type: manhattan_spearman
value: 86.1162623448951
- task:
type: STS
dataset:
type: mteb/sts14-sts
name: MTEB STS14
config: default
split: test
revision: 6031580fec1f6af667f0bd2da0a551cf4f0b2375
metrics:
- type: cos_sim_pearson
value: 84.68250248349368
- type: cos_sim_spearman
value: 82.29883673695083
- type: euclidean_pearson
value: 84.17633035446019
- type: euclidean_spearman
value: 82.19990511264791
- type: manhattan_pearson
value: 84.17408410692279
- type: manhattan_spearman
value: 82.249873895981
- task:
type: STS
dataset:
type: mteb/sts15-sts
name: MTEB STS15
config: default
split: test
revision: ae752c7c21bf194d8b67fd573edf7ae58183cbe3
metrics:
- type: cos_sim_pearson
value: 87.31878760045024
- type: cos_sim_spearman
value: 88.7364409031183
- type: euclidean_pearson
value: 88.230537618603
- type: euclidean_spearman
value: 88.76484309646318
- type: manhattan_pearson
value: 88.17689071136469
- type: manhattan_spearman
value: 88.72809249037928
- task:
type: STS
dataset:
type: mteb/sts16-sts
name: MTEB STS16
config: default
split: test
revision: 4d8694f8f0e0100860b497b999b3dbed754a0513
metrics:
- type: cos_sim_pearson
value: 83.41078559110638
- type: cos_sim_spearman
value: 85.27439135411049
- type: euclidean_pearson
value: 84.5333571592088
- type: euclidean_spearman
value: 85.25645460575957
- type: manhattan_pearson
value: 84.38428921610226
- type: manhattan_spearman
value: 85.07796040798796
- task:
type: STS
dataset:
type: mteb/sts17-crosslingual-sts
name: MTEB STS17 (en-en)
config: en-en
split: test
revision: af5e6fb845001ecf41f4c1e033ce921939a2a68d
metrics:
- type: cos_sim_pearson
value: 88.82374132382576
- type: cos_sim_spearman
value: 89.02101343562433
- type: euclidean_pearson
value: 89.50729765458932
- type: euclidean_spearman
value: 89.04184772869253
- type: manhattan_pearson
value: 89.51737904059856
- type: manhattan_spearman
value: 89.12925950440676
- task:
type: STS
dataset:
type: mteb/sts22-crosslingual-sts
name: MTEB STS22 (en)
config: en
split: test
revision: 6d1ba47164174a496b7fa5d3569dae26a6813b80
metrics:
- type: cos_sim_pearson
value: 67.56051823873482
- type: cos_sim_spearman
value: 68.50988748185463
- type: euclidean_pearson
value: 69.16524346147456
- type: euclidean_spearman
value: 68.61859952449579
- type: manhattan_pearson
value: 69.10618915706995
- type: manhattan_spearman
value: 68.36401769459522
- task:
type: STS
dataset:
type: mteb/stsbenchmark-sts
name: MTEB STSBenchmark
config: default
split: test
revision: b0fddb56ed78048fa8b90373c8a3cfc37b684831
metrics:
- type: cos_sim_pearson
value: 85.4159693872625
- type: cos_sim_spearman
value: 87.07819121764247
- type: euclidean_pearson
value: 87.03013260863153
- type: euclidean_spearman
value: 87.06547293631309
- type: manhattan_pearson
value: 86.8129744446062
- type: manhattan_spearman
value: 86.88494096335627
- task:
type: Reranking
dataset:
type: mteb/scidocs-reranking
name: MTEB SciDocsRR
config: default
split: test
revision: d3c5e1fc0b855ab6097bf1cda04dd73947d7caab
metrics:
- type: map
value: 86.47758088996575
- type: mrr
value: 96.17891458577733
- task:
type: Retrieval
dataset:
type: scifact
name: MTEB SciFact
config: default
split: test
revision: None
metrics:
- type: map_at_1
value: 57.538999999999994
- type: map_at_10
value: 66.562
- type: map_at_100
value: 67.254
- type: map_at_1000
value: 67.284
- type: map_at_3
value: 63.722
- type: map_at_5
value: 65.422
- type: mrr_at_1
value: 60.0
- type: mrr_at_10
value: 67.354
- type: mrr_at_100
value: 67.908
- type: mrr_at_1000
value: 67.93299999999999
- type: mrr_at_3
value: 65.056
- type: mrr_at_5
value: 66.43900000000001
- type: ndcg_at_1
value: 60.0
- type: ndcg_at_10
value: 70.858
- type: ndcg_at_100
value: 73.67099999999999
- type: ndcg_at_1000
value: 74.26700000000001
- type: ndcg_at_3
value: 65.911
- type: ndcg_at_5
value: 68.42200000000001
- type: precision_at_1
value: 60.0
- type: precision_at_10
value: 9.4
- type: precision_at_100
value: 1.083
- type: precision_at_1000
value: 0.11299999999999999
- type: precision_at_3
value: 25.444
- type: precision_at_5
value: 17.0
- type: recall_at_1
value: 57.538999999999994
- type: recall_at_10
value: 83.233
- type: recall_at_100
value: 95.667
- type: recall_at_1000
value: 100.0
- type: recall_at_3
value: 69.883
- type: recall_at_5
value: 76.19399999999999
- task:
type: PairClassification
dataset:
type: mteb/sprintduplicatequestions-pairclassification
name: MTEB SprintDuplicateQuestions
config: default
split: test
revision: d66bd1f72af766a5cc4b0ca5e00c162f89e8cc46
metrics:
- type: cos_sim_accuracy
value: 99.82574257425742
- type: cos_sim_ap
value: 95.78722833053911
- type: cos_sim_f1
value: 90.94650205761316
- type: cos_sim_precision
value: 93.64406779661016
- type: cos_sim_recall
value: 88.4
- type: dot_accuracy
value: 99.83366336633664
- type: dot_ap
value: 95.89733601612964
- type: dot_f1
value: 91.41981613891727
- type: dot_precision
value: 93.42379958246346
- type: dot_recall
value: 89.5
- type: euclidean_accuracy
value: 99.82574257425742
- type: euclidean_ap
value: 95.75227035138846
- type: euclidean_f1
value: 90.96509240246407
- type: euclidean_precision
value: 93.45991561181435
- type: euclidean_recall
value: 88.6
- type: manhattan_accuracy
value: 99.82574257425742
- type: manhattan_ap
value: 95.76278266220176
- type: manhattan_f1
value: 91.08409321175279
- type: manhattan_precision
value: 92.29979466119097
- type: manhattan_recall
value: 89.9
- type: max_accuracy
value: 99.83366336633664
- type: max_ap
value: 95.89733601612964
- type: max_f1
value: 91.41981613891727
- task:
type: Clustering
dataset:
type: mteb/stackexchange-clustering
name: MTEB StackExchangeClustering
config: default
split: test
revision: 6cbc1f7b2bc0622f2e39d2c77fa502909748c259
metrics:
- type: v_measure
value: 61.905425988638605
- task:
type: Clustering
dataset:
type: mteb/stackexchange-clustering-p2p
name: MTEB StackExchangeClusteringP2P
config: default
split: test
revision: 815ca46b2622cec33ccafc3735d572c266efdb44
metrics:
- type: v_measure
value: 36.159589881679736
- task:
type: Reranking
dataset:
type: mteb/stackoverflowdupquestions-reranking
name: MTEB StackOverflowDupQuestions
config: default
split: test
revision: e185fbe320c72810689fc5848eb6114e1ef5ec69
metrics:
- type: map
value: 53.0605499476397
- type: mrr
value: 53.91594516594517
- task:
type: Summarization
dataset:
type: mteb/summeval
name: MTEB SummEval
config: default
split: test
revision: cda12ad7615edc362dbf25a00fdd61d3b1eaf93c
metrics:
- type: cos_sim_pearson
value: 30.202718009067
- type: cos_sim_spearman
value: 31.136199912366987
- type: dot_pearson
value: 30.66329011927951
- type: dot_spearman
value: 30.107664909625107
- task:
type: Retrieval
dataset:
type: trec-covid
name: MTEB TRECCOVID
config: default
split: test
revision: None
metrics:
- type: map_at_1
value: 0.209
- type: map_at_10
value: 1.712
- type: map_at_100
value: 9.464
- type: map_at_1000
value: 23.437
- type: map_at_3
value: 0.609
- type: map_at_5
value: 0.9440000000000001
- type: mrr_at_1
value: 78.0
- type: mrr_at_10
value: 86.833
- type: mrr_at_100
value: 86.833
- type: mrr_at_1000
value: 86.833
- type: mrr_at_3
value: 85.333
- type: mrr_at_5
value: 86.833
- type: ndcg_at_1
value: 74.0
- type: ndcg_at_10
value: 69.14
- type: ndcg_at_100
value: 53.047999999999995
- type: ndcg_at_1000
value: 48.577
- type: ndcg_at_3
value: 75.592
- type: ndcg_at_5
value: 72.509
- type: precision_at_1
value: 78.0
- type: precision_at_10
value: 73.0
- type: precision_at_100
value: 54.44
- type: precision_at_1000
value: 21.326
- type: precision_at_3
value: 80.667
- type: precision_at_5
value: 77.2
- type: recall_at_1
value: 0.209
- type: recall_at_10
value: 1.932
- type: recall_at_100
value: 13.211999999999998
- type: recall_at_1000
value: 45.774
- type: recall_at_3
value: 0.644
- type: recall_at_5
value: 1.0290000000000001
- task:
type: Retrieval
dataset:
type: webis-touche2020
name: MTEB Touche2020
config: default
split: test
revision: None
metrics:
- type: map_at_1
value: 2.609
- type: map_at_10
value: 8.334999999999999
- type: map_at_100
value: 14.604000000000001
- type: map_at_1000
value: 16.177
- type: map_at_3
value: 4.87
- type: map_at_5
value: 6.3149999999999995
- type: mrr_at_1
value: 32.653
- type: mrr_at_10
value: 45.047
- type: mrr_at_100
value: 45.808
- type: mrr_at_1000
value: 45.808
- type: mrr_at_3
value: 41.497
- type: mrr_at_5
value: 43.231
- type: ndcg_at_1
value: 30.612000000000002
- type: ndcg_at_10
value: 21.193
- type: ndcg_at_100
value: 34.97
- type: ndcg_at_1000
value: 46.69
- type: ndcg_at_3
value: 24.823
- type: ndcg_at_5
value: 22.872999999999998
- type: precision_at_1
value: 32.653
- type: precision_at_10
value: 17.959
- type: precision_at_100
value: 7.4079999999999995
- type: precision_at_1000
value: 1.537
- type: precision_at_3
value: 25.85
- type: precision_at_5
value: 22.448999999999998
- type: recall_at_1
value: 2.609
- type: recall_at_10
value: 13.63
- type: recall_at_100
value: 47.014
- type: recall_at_1000
value: 83.176
- type: recall_at_3
value: 5.925
- type: recall_at_5
value: 8.574
- task:
type: Classification
dataset:
type: mteb/toxic_conversations_50k
name: MTEB ToxicConversationsClassification
config: default
split: test
revision: d7c0de2777da35d6aae2200a62c6e0e5af397c4c
metrics:
- type: accuracy
value: 72.80239999999999
- type: ap
value: 15.497911013214791
- type: f1
value: 56.258411577947285
- task:
type: Classification
dataset:
type: mteb/tweet_sentiment_extraction
name: MTEB TweetSentimentExtractionClassification
config: default
split: test
revision: d604517c81ca91fe16a244d1248fc021f9ecee7a
metrics:
- type: accuracy
value: 61.00452744765139
- type: f1
value: 61.42228624410908
- task:
type: Clustering
dataset:
type: mteb/twentynewsgroups-clustering
name: MTEB TwentyNewsgroupsClustering
config: default
split: test
revision: 6125ec4e24fa026cec8a478383ee943acfbd5449
metrics:
- type: v_measure
value: 50.00516915962345
- task:
type: PairClassification
dataset:
type: mteb/twittersemeval2015-pairclassification
name: MTEB TwitterSemEval2015
config: default
split: test
revision: 70970daeab8776df92f5ea462b6173c0b46fd2d1
metrics:
- type: cos_sim_accuracy
value: 85.62317458425225
- type: cos_sim_ap
value: 72.95115658063823
- type: cos_sim_f1
value: 66.78976523344764
- type: cos_sim_precision
value: 66.77215189873418
- type: cos_sim_recall
value: 66.80738786279683
- type: dot_accuracy
value: 85.62317458425225
- type: dot_ap
value: 73.10385271517778
- type: dot_f1
value: 66.94853829427399
- type: dot_precision
value: 61.74242424242424
- type: dot_recall
value: 73.11345646437995
- type: euclidean_accuracy
value: 85.65893783155511
- type: euclidean_ap
value: 72.87428208473992
- type: euclidean_f1
value: 66.70919994896005
- type: euclidean_precision
value: 64.5910551025451
- type: euclidean_recall
value: 68.97097625329816
- type: manhattan_accuracy
value: 85.59933241938367
- type: manhattan_ap
value: 72.67282695064966
- type: manhattan_f1
value: 66.67537215983286
- type: manhattan_precision
value: 66.00310237849017
- type: manhattan_recall
value: 67.36147757255937
- type: max_accuracy
value: 85.65893783155511
- type: max_ap
value: 73.10385271517778
- type: max_f1
value: 66.94853829427399
- task:
type: PairClassification
dataset:
type: mteb/twitterurlcorpus-pairclassification
name: MTEB TwitterURLCorpus
config: default
split: test
revision: 8b6510b0b1fa4e4c4f879467980e9be563ec1cdf
metrics:
- type: cos_sim_accuracy
value: 88.69096130709822
- type: cos_sim_ap
value: 85.30326978668063
- type: cos_sim_f1
value: 77.747088683189
- type: cos_sim_precision
value: 75.4491451753115
- type: cos_sim_recall
value: 80.189405605174
- type: dot_accuracy
value: 88.43870066363954
- type: dot_ap
value: 84.62999949222983
- type: dot_f1
value: 77.3074661963551
- type: dot_precision
value: 73.93871239808828
- type: dot_recall
value: 80.99784416384355
- type: euclidean_accuracy
value: 88.70066363953894
- type: euclidean_ap
value: 85.34184508966621
- type: euclidean_f1
value: 77.76871756856931
- type: euclidean_precision
value: 74.97855917667239
- type: euclidean_recall
value: 80.77456113335386
- type: manhattan_accuracy
value: 88.68319944114566
- type: manhattan_ap
value: 85.3026464242333
- type: manhattan_f1
value: 77.66561049296294
- type: manhattan_precision
value: 74.4665818849795
- type: manhattan_recall
value: 81.15183246073299
- type: max_accuracy
value: 88.70066363953894
- type: max_ap
value: 85.34184508966621
- type: max_f1
value: 77.76871756856931
---
<h1 align="center">GIST small Embedding v0</h1>
*GISTEmbed: Guided In-sample Selection of Training Negatives for Text Embedding Fine-tuning*
The model is fine-tuned on top of the [BAAI/bge-small-en-v1.5](https://huggingface.co/BAAI/bge-small-en-v1.5) using the [MEDI dataset](https://github.com/xlang-ai/instructor-embedding.git) augmented with mined triplets from the [MTEB Classification](https://huggingface.co/mteb) training dataset (excluding data from the Amazon Polarity Classification task).
The model does not require any instruction for generating embeddings. This means that queries for retrieval tasks can be directly encoded without crafting instructions.
Technical paper: [GISTEmbed: Guided In-sample Selection of Training Negatives for Text Embedding Fine-tuning](https://arxiv.org/abs/2402.16829)
# Data
The dataset used is a compilation of the MEDI and MTEB Classification training datasets. Third-party datasets may be subject to additional terms and conditions under their associated licenses. A HuggingFace Dataset version of the compiled dataset, and the specific revision used to train the model, is available:
- Dataset: [avsolatorio/medi-data-mteb_avs_triplets](https://huggingface.co/datasets/avsolatorio/medi-data-mteb_avs_triplets)
- Revision: 238a0499b6e6b690cc64ea56fde8461daa8341bb
The dataset contains a `task_type` key, which can be used to select only the mteb classification tasks (prefixed with `mteb_`).
The **MEDI Dataset** is published in the following paper: [One Embedder, Any Task: Instruction-Finetuned Text Embeddings](https://arxiv.org/abs/2212.09741).
The MTEB Benchmark results of the GIST embedding model, compared with the base model, suggest that the fine-tuning dataset has perturbed the model considerably, which resulted in significant improvements in certain tasks while adversely degrading performance in some.
The retrieval performance for the TRECCOVID task is of note. The fine-tuning dataset does not contain significant knowledge about COVID-19, which could have caused the observed performance degradation. We found some evidence, detailed in the paper, that thematic coverage of the fine-tuning data can affect downstream performance.
# Usage
The model can be easily loaded using the Sentence Transformers library.
```Python
import torch.nn.functional as F
from sentence_transformers import SentenceTransformer
revision = None # Replace with the specific revision to ensure reproducibility if the model is updated.
model = SentenceTransformer("avsolatorio/GIST-small-Embedding-v0", revision=revision)
texts = [
"Illustration of the REaLTabFormer model. The left block shows the non-relational tabular data model using GPT-2 with a causal LM head. In contrast, the right block shows how a relational dataset's child table is modeled using a sequence-to-sequence (Seq2Seq) model. The Seq2Seq model uses the observations in the parent table to condition the generation of the observations in the child table. The trained GPT-2 model on the parent table, with weights frozen, is also used as the encoder in the Seq2Seq model.",
"Predicting human mobility holds significant practical value, with applications ranging from enhancing disaster risk planning to simulating epidemic spread. In this paper, we present the GeoFormer, a decoder-only transformer model adapted from the GPT architecture to forecast human mobility.",
"As the economies of Southeast Asia continue adopting digital technologies, policy makers increasingly ask how to prepare the workforce for emerging labor demands. However, little is known about the skills that workers need to adapt to these changes"
]
# Compute embeddings
embeddings = model.encode(texts, convert_to_tensor=True)
# Compute cosine-similarity for each pair of sentences
scores = F.cosine_similarity(embeddings.unsqueeze(1), embeddings.unsqueeze(0), dim=-1)
print(scores.cpu().numpy())
```
# Training Parameters
Below are the training parameters used to fine-tune the model:
```
Epochs = 40
Warmup ratio = 0.1
Learning rate = 5e-6
Batch size = 16
Checkpoint step = 102000
Contrastive loss temperature = 0.01
```
# Evaluation
The model was evaluated using the [MTEB Evaluation](https://huggingface.co/mteb) suite.
# Citation
Please cite our work if you use GISTEmbed or the datasets we published in your projects or research. 🤗
```
@article{solatorio2024gistembed,
title={GISTEmbed: Guided In-sample Selection of Training Negatives for Text Embedding Fine-tuning},
author={Aivin V. Solatorio},
journal={arXiv preprint arXiv:2402.16829},
year={2024},
URL={https://arxiv.org/abs/2402.16829}
eprint={2402.16829},
archivePrefix={arXiv},
primaryClass={cs.LG}
}
```
# Acknowledgements
This work is supported by the "KCP IV - Exploring Data Use in the Development Economics Literature using Large Language Models (AI and LLMs)" project funded by the [Knowledge for Change Program (KCP)](https://www.worldbank.org/en/programs/knowledge-for-change) of the World Bank - RA-P503405-RESE-TF0C3444.
The findings, interpretations, and conclusions expressed in this material are entirely those of the authors. They do not necessarily represent the views of the International Bank for Reconstruction and Development/World Bank and its affiliated organizations, or those of the Executive Directors of the World Bank or the governments they represent. |
madhurjindal/autonlp-Gibberish-Detector-492513457 | madhurjindal | "2024-06-17T06:31:08Z" | 414,769 | 51 | transformers | [
"transformers",
"pytorch",
"onnx",
"safetensors",
"distilbert",
"text-classification",
"autonlp",
"en",
"dataset:madhurjindal/autonlp-data-Gibberish-Detector",
"doi:10.57967/hf/2664",
"license:mit",
"co2_eq_emissions",
"autotrain_compatible",
"endpoints_compatible",
"region:us"
] | text-classification | "2022-03-02T23:29:05Z" | ---
tags:
- autonlp
language: en
widget:
- text: I love Machine Learning!
datasets:
- madhurjindal/autonlp-data-Gibberish-Detector
co2_eq_emissions: 5.527544460835904
license: mit
---
# Problem Description
The ability to process and understand user input is crucial for various applications, such as chatbots or downstream tasks. However, a common challenge faced in such systems is the presence of gibberish or nonsensical input. To address this problem, we present a project focused on developing a gibberish detector for the English language.
The primary goal of this project is to classify user input as either **gibberish** or **non-gibberish**, enabling more accurate and meaningful interactions with the system. We also aim to enhance the overall performance and user experience of chatbots and other systems that rely on user input.
>## What is Gibberish?
Gibberish refers to **nonsensical or meaningless language or text** that lacks coherence or any discernible meaning. It can be characterized by a combination of random words, nonsensical phrases, grammatical errors, or syntactical abnormalities that prevent the communication from conveying a clear and understandable message. Gibberish can vary in intensity, ranging from simple noise with no meaningful words to sentences that may appear superficially correct but lack coherence or logical structure when examined closely. Detecting and identifying gibberish is essential in various contexts, such as **natural language processing**, **chatbot systems**, **spam filtering**, and **language-based security measures**, to ensure effective communication and accurate processing of user inputs.
## Label Description
Thus, we break down the problem into 4 categories:
1. **Noise:** Gibberish at the zero level where even the different constituents of the input phrase (words) do not hold any meaning independently.
*For example: `dfdfer fgerfow2e0d qsqskdsd djksdnfkff swq.`*
2. **Word Salad:** Gibberish at level 1 where words make sense independently, but when looked at the bigger picture (the phrase) any meaning is not depicted.
*For example: `22 madhur old punjab pickle chennai`*
3. **Mild gibberish:** Gibberish at level 2 where there is a part of the sentence that has grammatical errors, word sense errors, or any syntactical abnormalities, which leads the sentence to miss out on a coherent meaning.
*For example: `Madhur study in a teacher`*
4. **Clean:** This category represents a set of words that form a complete and meaningful sentence on its own.
*For example: `I love this website`*
> **Tip:** To facilitate gibberish detection, you can combine the labels based on the desired level of detection. For instance, if you need to detect gibberish at level 1, you can group Noise and Word Salad together as "Gibberish," while considering Mild gibberish and Clean separately as "NotGibberish." This approach allows for flexibility in detecting and categorizing different levels of gibberish based on specific requirements.
# Model Trained Using AutoNLP
- Problem type: Multi-class Classification
- Model ID: 492513457
- CO2 Emissions (in grams): 5.527544460835904
## Validation Metrics
- Loss: 0.07609463483095169
- Accuracy: 0.9735624586913417
- Macro F1: 0.9736173135739408
- Micro F1: 0.9735624586913417
- Weighted F1: 0.9736173135739408
- Macro Precision: 0.9737771415197378
- Micro Precision: 0.9735624586913417
- Weighted Precision: 0.9737771415197378
- Macro Recall: 0.9735624586913417
- Micro Recall: 0.9735624586913417
- Weighted Recall: 0.9735624586913417
## Usage
You can use cURL to access this model:
```
$ curl -X POST -H "Authorization: Bearer YOUR_API_KEY" -H "Content-Type: application/json" -d '{"inputs": "I love Machine Learning!"}' https://api-inference.huggingface.co/models/madhurjindal/autonlp-Gibberish-Detector-492513457
```
Or Python API:
```
import torch
import torch.nn.functional as F
from transformers import AutoModelForSequenceClassification, AutoTokenizer
model = AutoModelForSequenceClassification.from_pretrained("madhurjindal/autonlp-Gibberish-Detector-492513457", use_auth_token=True)
tokenizer = AutoTokenizer.from_pretrained("madhurjindal/autonlp-Gibberish-Detector-492513457", use_auth_token=True)
inputs = tokenizer("I love Machine Learning!", return_tensors="pt")
outputs = model(**inputs)
probs = F.softmax(outputs.logits, dim=-1)
predicted_index = torch.argmax(probs, dim=1).item()
predicted_prob = probs[0][predicted_index].item()
labels = model.config.id2label
predicted_label = labels[predicted_index]
for i, prob in enumerate(probs[0]):
print(f"Class: {labels[i]}, Probability: {prob:.4f}")
```
Another simplifed solution with transformers pipline:
```
from transformers import pipeline
selected_model = "madhurjindal/autonlp-Gibberish-Detector-492513457"
classifier = pipeline("text-classification", model=selected_model)
classifier("I love Machine Learning!")
``` |
nvidia/segformer-b0-finetuned-ade-512-512 | nvidia | "2024-01-14T10:46:46Z" | 413,180 | 150 | transformers | [
"transformers",
"pytorch",
"tf",
"safetensors",
"segformer",
"vision",
"image-segmentation",
"dataset:scene_parse_150",
"arxiv:2105.15203",
"license:other",
"endpoints_compatible",
"region:us"
] | image-segmentation | "2022-03-02T23:29:05Z" | ---
license: other
tags:
- vision
- image-segmentation
datasets:
- scene_parse_150
widget:
- src: https://huggingface.co/datasets/hf-internal-testing/fixtures_ade20k/resolve/main/ADE_val_00000001.jpg
example_title: House
- src: https://huggingface.co/datasets/hf-internal-testing/fixtures_ade20k/resolve/main/ADE_val_00000002.jpg
example_title: Castle
---
# SegFormer (b0-sized) model fine-tuned on ADE20k
SegFormer model fine-tuned on ADE20k at resolution 512x512. It was introduced in the paper [SegFormer: Simple and Efficient Design for Semantic Segmentation with Transformers](https://arxiv.org/abs/2105.15203) by Xie et al. and first released in [this repository](https://github.com/NVlabs/SegFormer).
Disclaimer: The team releasing SegFormer did not write a model card for this model so this model card has been written by the Hugging Face team.
## Model description
SegFormer consists of a hierarchical Transformer encoder and a lightweight all-MLP decode head to achieve great results on semantic segmentation benchmarks such as ADE20K and Cityscapes. The hierarchical Transformer is first pre-trained on ImageNet-1k, after which a decode head is added and fine-tuned altogether on a downstream dataset.
## Intended uses & limitations
You can use the raw model for semantic segmentation. See the [model hub](https://huggingface.co/models?other=segformer) to look for fine-tuned versions on a task that interests you.
### How to use
Here is how to use this model to classify an image of the COCO 2017 dataset into one of the 1,000 ImageNet classes:
```python
from transformers import SegformerImageProcessor, SegformerForSemanticSegmentation
from PIL import Image
import requests
processor = SegformerImageProcessor.from_pretrained("nvidia/segformer-b0-finetuned-ade-512-512")
model = SegformerForSemanticSegmentation.from_pretrained("nvidia/segformer-b0-finetuned-ade-512-512")
url = "http://images.cocodataset.org/val2017/000000039769.jpg"
image = Image.open(requests.get(url, stream=True).raw)
inputs = processor(images=image, return_tensors="pt")
outputs = model(**inputs)
logits = outputs.logits # shape (batch_size, num_labels, height/4, width/4)
```
For more code examples, we refer to the [documentation](https://huggingface.co/transformers/model_doc/segformer.html#).
### License
The license for this model can be found [here](https://github.com/NVlabs/SegFormer/blob/master/LICENSE).
### BibTeX entry and citation info
```bibtex
@article{DBLP:journals/corr/abs-2105-15203,
author = {Enze Xie and
Wenhai Wang and
Zhiding Yu and
Anima Anandkumar and
Jose M. Alvarez and
Ping Luo},
title = {SegFormer: Simple and Efficient Design for Semantic Segmentation with
Transformers},
journal = {CoRR},
volume = {abs/2105.15203},
year = {2021},
url = {https://arxiv.org/abs/2105.15203},
eprinttype = {arXiv},
eprint = {2105.15203},
timestamp = {Wed, 02 Jun 2021 11:46:42 +0200},
biburl = {https://dblp.org/rec/journals/corr/abs-2105-15203.bib},
bibsource = {dblp computer science bibliography, https://dblp.org}
}
```
|
google/flan-t5-base | google | "2023-07-17T12:48:39Z" | 412,897 | 797 | transformers | [
"transformers",
"pytorch",
"tf",
"jax",
"safetensors",
"t5",
"text2text-generation",
"en",
"fr",
"ro",
"de",
"multilingual",
"dataset:svakulenk0/qrecc",
"dataset:taskmaster2",
"dataset:djaym7/wiki_dialog",
"dataset:deepmind/code_contests",
"dataset:lambada",
"dataset:gsm8k",
"dataset:aqua_rat",
"dataset:esnli",
"dataset:quasc",
"dataset:qed",
"arxiv:2210.11416",
"arxiv:1910.09700",
"license:apache-2.0",
"autotrain_compatible",
"text-generation-inference",
"endpoints_compatible",
"region:us"
] | text2text-generation | "2022-10-21T10:02:31Z" | ---
language:
- en
- fr
- ro
- de
- multilingual
tags:
- text2text-generation
widget:
- text: "Translate to German: My name is Arthur"
example_title: "Translation"
- text: "Please answer to the following question. Who is going to be the next Ballon d'or?"
example_title: "Question Answering"
- text: "Q: Can Geoffrey Hinton have a conversation with George Washington? Give the rationale before answering."
example_title: "Logical reasoning"
- text: "Please answer the following question. What is the boiling point of Nitrogen?"
example_title: "Scientific knowledge"
- text: "Answer the following yes/no question. Can you write a whole Haiku in a single tweet?"
example_title: "Yes/no question"
- text: "Answer the following yes/no question by reasoning step-by-step. Can you write a whole Haiku in a single tweet?"
example_title: "Reasoning task"
- text: "Q: ( False or not False or False ) is? A: Let's think step by step"
example_title: "Boolean Expressions"
- text: "The square root of x is the cube root of y. What is y to the power of 2, if x = 4?"
example_title: "Math reasoning"
- text: "Premise: At my age you will probably have learnt one lesson. Hypothesis: It's not certain how many lessons you'll learn by your thirties. Does the premise entail the hypothesis?"
example_title: "Premise and hypothesis"
datasets:
- svakulenk0/qrecc
- taskmaster2
- djaym7/wiki_dialog
- deepmind/code_contests
- lambada
- gsm8k
- aqua_rat
- esnli
- quasc
- qed
license: apache-2.0
---
# Model Card for FLAN-T5 base
<img src="https://huggingface.co/datasets/huggingface/documentation-images/resolve/main/transformers/model_doc/flan2_architecture.jpg"
alt="drawing" width="600"/>
# Table of Contents
0. [TL;DR](#TL;DR)
1. [Model Details](#model-details)
2. [Usage](#usage)
3. [Uses](#uses)
4. [Bias, Risks, and Limitations](#bias-risks-and-limitations)
5. [Training Details](#training-details)
6. [Evaluation](#evaluation)
7. [Environmental Impact](#environmental-impact)
8. [Citation](#citation)
9. [Model Card Authors](#model-card-authors)
# TL;DR
If you already know T5, FLAN-T5 is just better at everything. For the same number of parameters, these models have been fine-tuned on more than 1000 additional tasks covering also more languages.
As mentioned in the first few lines of the abstract :
> Flan-PaLM 540B achieves state-of-the-art performance on several benchmarks, such as 75.2% on five-shot MMLU. We also publicly release Flan-T5 checkpoints,1 which achieve strong few-shot performance even compared to much larger models, such as PaLM 62B. Overall, instruction finetuning is a general method for improving the performance and usability of pretrained language models.
**Disclaimer**: Content from **this** model card has been written by the Hugging Face team, and parts of it were copy pasted from the [T5 model card](https://huggingface.co/t5-large).
# Model Details
## Model Description
- **Model type:** Language model
- **Language(s) (NLP):** English, Spanish, Japanese, Persian, Hindi, French, Chinese, Bengali, Gujarati, German, Telugu, Italian, Arabic, Polish, Tamil, Marathi, Malayalam, Oriya, Panjabi, Portuguese, Urdu, Galician, Hebrew, Korean, Catalan, Thai, Dutch, Indonesian, Vietnamese, Bulgarian, Filipino, Central Khmer, Lao, Turkish, Russian, Croatian, Swedish, Yoruba, Kurdish, Burmese, Malay, Czech, Finnish, Somali, Tagalog, Swahili, Sinhala, Kannada, Zhuang, Igbo, Xhosa, Romanian, Haitian, Estonian, Slovak, Lithuanian, Greek, Nepali, Assamese, Norwegian
- **License:** Apache 2.0
- **Related Models:** [All FLAN-T5 Checkpoints](https://huggingface.co/models?search=flan-t5)
- **Original Checkpoints:** [All Original FLAN-T5 Checkpoints](https://github.com/google-research/t5x/blob/main/docs/models.md#flan-t5-checkpoints)
- **Resources for more information:**
- [Research paper](https://arxiv.org/pdf/2210.11416.pdf)
- [GitHub Repo](https://github.com/google-research/t5x)
- [Hugging Face FLAN-T5 Docs (Similar to T5) ](https://huggingface.co/docs/transformers/model_doc/t5)
# Usage
Find below some example scripts on how to use the model in `transformers`:
## Using the Pytorch model
### Running the model on a CPU
<details>
<summary> Click to expand </summary>
```python
from transformers import T5Tokenizer, T5ForConditionalGeneration
tokenizer = T5Tokenizer.from_pretrained("google/flan-t5-base")
model = T5ForConditionalGeneration.from_pretrained("google/flan-t5-base")
input_text = "translate English to German: How old are you?"
input_ids = tokenizer(input_text, return_tensors="pt").input_ids
outputs = model.generate(input_ids)
print(tokenizer.decode(outputs[0]))
```
</details>
### Running the model on a GPU
<details>
<summary> Click to expand </summary>
```python
# pip install accelerate
from transformers import T5Tokenizer, T5ForConditionalGeneration
tokenizer = T5Tokenizer.from_pretrained("google/flan-t5-base")
model = T5ForConditionalGeneration.from_pretrained("google/flan-t5-base", device_map="auto")
input_text = "translate English to German: How old are you?"
input_ids = tokenizer(input_text, return_tensors="pt").input_ids.to("cuda")
outputs = model.generate(input_ids)
print(tokenizer.decode(outputs[0]))
```
</details>
### Running the model on a GPU using different precisions
#### FP16
<details>
<summary> Click to expand </summary>
```python
# pip install accelerate
import torch
from transformers import T5Tokenizer, T5ForConditionalGeneration
tokenizer = T5Tokenizer.from_pretrained("google/flan-t5-base")
model = T5ForConditionalGeneration.from_pretrained("google/flan-t5-base", device_map="auto", torch_dtype=torch.float16)
input_text = "translate English to German: How old are you?"
input_ids = tokenizer(input_text, return_tensors="pt").input_ids.to("cuda")
outputs = model.generate(input_ids)
print(tokenizer.decode(outputs[0]))
```
</details>
#### INT8
<details>
<summary> Click to expand </summary>
```python
# pip install bitsandbytes accelerate
from transformers import T5Tokenizer, T5ForConditionalGeneration
tokenizer = T5Tokenizer.from_pretrained("google/flan-t5-base")
model = T5ForConditionalGeneration.from_pretrained("google/flan-t5-base", device_map="auto", load_in_8bit=True)
input_text = "translate English to German: How old are you?"
input_ids = tokenizer(input_text, return_tensors="pt").input_ids.to("cuda")
outputs = model.generate(input_ids)
print(tokenizer.decode(outputs[0]))
```
</details>
# Uses
## Direct Use and Downstream Use
The authors write in [the original paper's model card](https://arxiv.org/pdf/2210.11416.pdf) that:
> The primary use is research on language models, including: research on zero-shot NLP tasks and in-context few-shot learning NLP tasks, such as reasoning, and question answering; advancing fairness and safety research, and understanding limitations of current large language models
See the [research paper](https://arxiv.org/pdf/2210.11416.pdf) for further details.
## Out-of-Scope Use
More information needed.
# Bias, Risks, and Limitations
The information below in this section are copied from the model's [official model card](https://arxiv.org/pdf/2210.11416.pdf):
> Language models, including Flan-T5, can potentially be used for language generation in a harmful way, according to Rae et al. (2021). Flan-T5 should not be used directly in any application, without a prior assessment of safety and fairness concerns specific to the application.
## Ethical considerations and risks
> Flan-T5 is fine-tuned on a large corpus of text data that was not filtered for explicit content or assessed for existing biases. As a result the model itself is potentially vulnerable to generating equivalently inappropriate content or replicating inherent biases in the underlying data.
## Known Limitations
> Flan-T5 has not been tested in real world applications.
## Sensitive Use:
> Flan-T5 should not be applied for any unacceptable use cases, e.g., generation of abusive speech.
# Training Details
## Training Data
The model was trained on a mixture of tasks, that includes the tasks described in the table below (from the original paper, figure 2):

## Training Procedure
According to the model card from the [original paper](https://arxiv.org/pdf/2210.11416.pdf):
> These models are based on pretrained T5 (Raffel et al., 2020) and fine-tuned with instructions for better zero-shot and few-shot performance. There is one fine-tuned Flan model per T5 model size.
The model has been trained on TPU v3 or TPU v4 pods, using [`t5x`](https://github.com/google-research/t5x) codebase together with [`jax`](https://github.com/google/jax).
# Evaluation
## Testing Data, Factors & Metrics
The authors evaluated the model on various tasks covering several languages (1836 in total). See the table below for some quantitative evaluation:

For full details, please check the [research paper](https://arxiv.org/pdf/2210.11416.pdf).
## Results
For full results for FLAN-T5-Base, see the [research paper](https://arxiv.org/pdf/2210.11416.pdf), Table 3.
# Environmental Impact
Carbon emissions can be estimated using the [Machine Learning Impact calculator](https://mlco2.github.io/impact#compute) presented in [Lacoste et al. (2019)](https://arxiv.org/abs/1910.09700).
- **Hardware Type:** Google Cloud TPU Pods - TPU v3 or TPU v4 | Number of chips ≥ 4.
- **Hours used:** More information needed
- **Cloud Provider:** GCP
- **Compute Region:** More information needed
- **Carbon Emitted:** More information needed
# Citation
**BibTeX:**
```bibtex
@misc{https://doi.org/10.48550/arxiv.2210.11416,
doi = {10.48550/ARXIV.2210.11416},
url = {https://arxiv.org/abs/2210.11416},
author = {Chung, Hyung Won and Hou, Le and Longpre, Shayne and Zoph, Barret and Tay, Yi and Fedus, William and Li, Eric and Wang, Xuezhi and Dehghani, Mostafa and Brahma, Siddhartha and Webson, Albert and Gu, Shixiang Shane and Dai, Zhuyun and Suzgun, Mirac and Chen, Xinyun and Chowdhery, Aakanksha and Narang, Sharan and Mishra, Gaurav and Yu, Adams and Zhao, Vincent and Huang, Yanping and Dai, Andrew and Yu, Hongkun and Petrov, Slav and Chi, Ed H. and Dean, Jeff and Devlin, Jacob and Roberts, Adam and Zhou, Denny and Le, Quoc V. and Wei, Jason},
keywords = {Machine Learning (cs.LG), Computation and Language (cs.CL), FOS: Computer and information sciences, FOS: Computer and information sciences},
title = {Scaling Instruction-Finetuned Language Models},
publisher = {arXiv},
year = {2022},
copyright = {Creative Commons Attribution 4.0 International}
}
```
## Model Recycling
[Evaluation on 36 datasets](https://ibm.github.io/model-recycling/model_gain_chart?avg=9.16&mnli_lp=nan&20_newsgroup=3.34&ag_news=1.49&amazon_reviews_multi=0.21&anli=13.91&boolq=16.75&cb=23.12&cola=9.97&copa=34.50&dbpedia=6.90&esnli=5.37&financial_phrasebank=18.66&imdb=0.33&isear=1.37&mnli=11.74&mrpc=16.63&multirc=6.24&poem_sentiment=14.62&qnli=3.41&qqp=6.18&rotten_tomatoes=2.98&rte=24.26&sst2=0.67&sst_5bins=5.44&stsb=20.68&trec_coarse=3.95&trec_fine=10.73&tweet_ev_emoji=13.39&tweet_ev_emotion=4.62&tweet_ev_hate=3.46&tweet_ev_irony=9.04&tweet_ev_offensive=1.69&tweet_ev_sentiment=0.75&wic=14.22&wnli=9.44&wsc=5.53&yahoo_answers=4.14&model_name=google%2Fflan-t5-base&base_name=google%2Ft5-v1_1-base) using google/flan-t5-base as a base model yields average score of 77.98 in comparison to 68.82 by google/t5-v1_1-base.
The model is ranked 1st among all tested models for the google/t5-v1_1-base architecture as of 06/02/2023
Results:
| 20_newsgroup | ag_news | amazon_reviews_multi | anli | boolq | cb | cola | copa | dbpedia | esnli | financial_phrasebank | imdb | isear | mnli | mrpc | multirc | poem_sentiment | qnli | qqp | rotten_tomatoes | rte | sst2 | sst_5bins | stsb | trec_coarse | trec_fine | tweet_ev_emoji | tweet_ev_emotion | tweet_ev_hate | tweet_ev_irony | tweet_ev_offensive | tweet_ev_sentiment | wic | wnli | wsc | yahoo_answers |
|---------------:|----------:|-----------------------:|--------:|--------:|--------:|--------:|-------:|----------:|--------:|-----------------------:|-------:|--------:|--------:|--------:|----------:|-----------------:|--------:|--------:|------------------:|--------:|--------:|------------:|--------:|--------------:|------------:|-----------------:|-------------------:|----------------:|-----------------:|---------------------:|---------------------:|--------:|-------:|--------:|----------------:|
| 86.2188 | 89.6667 | 67.12 | 51.9688 | 82.3242 | 78.5714 | 80.1534 | 75 | 77.6667 | 90.9507 | 85.4 | 93.324 | 72.425 | 87.2457 | 89.4608 | 62.3762 | 82.6923 | 92.7878 | 89.7724 | 89.0244 | 84.8375 | 94.3807 | 57.2851 | 89.4759 | 97.2 | 92.8 | 46.848 | 80.2252 | 54.9832 | 76.6582 | 84.3023 | 70.6366 | 70.0627 | 56.338 | 53.8462 | 73.4 |
For more information, see: [Model Recycling](https://ibm.github.io/model-recycling/)
|
hfl/chinese-roberta-wwm-ext | hfl | "2022-03-01T09:13:56Z" | 411,880 | 278 | transformers | [
"transformers",
"pytorch",
"tf",
"jax",
"bert",
"fill-mask",
"zh",
"arxiv:1906.08101",
"arxiv:2004.13922",
"license:apache-2.0",
"autotrain_compatible",
"endpoints_compatible",
"region:us"
] | fill-mask | "2022-03-02T23:29:05Z" | ---
language:
- zh
tags:
- bert
license: "apache-2.0"
---
# Please use 'Bert' related functions to load this model!
## Chinese BERT with Whole Word Masking
For further accelerating Chinese natural language processing, we provide **Chinese pre-trained BERT with Whole Word Masking**.
**[Pre-Training with Whole Word Masking for Chinese BERT](https://arxiv.org/abs/1906.08101)**
Yiming Cui, Wanxiang Che, Ting Liu, Bing Qin, Ziqing Yang, Shijin Wang, Guoping Hu
This repository is developed based on:https://github.com/google-research/bert
You may also interested in,
- Chinese BERT series: https://github.com/ymcui/Chinese-BERT-wwm
- Chinese MacBERT: https://github.com/ymcui/MacBERT
- Chinese ELECTRA: https://github.com/ymcui/Chinese-ELECTRA
- Chinese XLNet: https://github.com/ymcui/Chinese-XLNet
- Knowledge Distillation Toolkit - TextBrewer: https://github.com/airaria/TextBrewer
More resources by HFL: https://github.com/ymcui/HFL-Anthology
## Citation
If you find the technical report or resource is useful, please cite the following technical report in your paper.
- Primary: https://arxiv.org/abs/2004.13922
```
@inproceedings{cui-etal-2020-revisiting,
title = "Revisiting Pre-Trained Models for {C}hinese Natural Language Processing",
author = "Cui, Yiming and
Che, Wanxiang and
Liu, Ting and
Qin, Bing and
Wang, Shijin and
Hu, Guoping",
booktitle = "Proceedings of the 2020 Conference on Empirical Methods in Natural Language Processing: Findings",
month = nov,
year = "2020",
address = "Online",
publisher = "Association for Computational Linguistics",
url = "https://www.aclweb.org/anthology/2020.findings-emnlp.58",
pages = "657--668",
}
```
- Secondary: https://arxiv.org/abs/1906.08101
```
@article{chinese-bert-wwm,
title={Pre-Training with Whole Word Masking for Chinese BERT},
author={Cui, Yiming and Che, Wanxiang and Liu, Ting and Qin, Bing and Yang, Ziqing and Wang, Shijin and Hu, Guoping},
journal={arXiv preprint arXiv:1906.08101},
year={2019}
}
``` |
intfloat/e5-base-v2 | intfloat | "2023-09-27T10:13:27Z" | 411,278 | 100 | sentence-transformers | [
"sentence-transformers",
"pytorch",
"onnx",
"safetensors",
"bert",
"mteb",
"Sentence Transformers",
"sentence-similarity",
"en",
"arxiv:2212.03533",
"arxiv:2104.08663",
"arxiv:2210.07316",
"license:mit",
"model-index",
"autotrain_compatible",
"text-embeddings-inference",
"endpoints_compatible",
"region:us"
] | sentence-similarity | "2023-05-19T07:21:14Z" | ---
tags:
- mteb
- Sentence Transformers
- sentence-similarity
- sentence-transformers
model-index:
- name: e5-base-v2
results:
- task:
type: Classification
dataset:
type: mteb/amazon_counterfactual
name: MTEB AmazonCounterfactualClassification (en)
config: en
split: test
revision: e8379541af4e31359cca9fbcf4b00f2671dba205
metrics:
- type: accuracy
value: 77.77611940298506
- type: ap
value: 42.052710266606056
- type: f1
value: 72.12040628266567
- task:
type: Classification
dataset:
type: mteb/amazon_polarity
name: MTEB AmazonPolarityClassification
config: default
split: test
revision: e2d317d38cd51312af73b3d32a06d1a08b442046
metrics:
- type: accuracy
value: 92.81012500000001
- type: ap
value: 89.4213700757244
- type: f1
value: 92.8039091197065
- task:
type: Classification
dataset:
type: mteb/amazon_reviews_multi
name: MTEB AmazonReviewsClassification (en)
config: en
split: test
revision: 1399c76144fd37290681b995c656ef9b2e06e26d
metrics:
- type: accuracy
value: 46.711999999999996
- type: f1
value: 46.11544975436018
- task:
type: Retrieval
dataset:
type: arguana
name: MTEB ArguAna
config: default
split: test
revision: None
metrics:
- type: map_at_1
value: 23.186
- type: map_at_10
value: 36.632999999999996
- type: map_at_100
value: 37.842
- type: map_at_1000
value: 37.865
- type: map_at_3
value: 32.278
- type: map_at_5
value: 34.760999999999996
- type: mrr_at_1
value: 23.400000000000002
- type: mrr_at_10
value: 36.721
- type: mrr_at_100
value: 37.937
- type: mrr_at_1000
value: 37.96
- type: mrr_at_3
value: 32.302
- type: mrr_at_5
value: 34.894
- type: ndcg_at_1
value: 23.186
- type: ndcg_at_10
value: 44.49
- type: ndcg_at_100
value: 50.065000000000005
- type: ndcg_at_1000
value: 50.629999999999995
- type: ndcg_at_3
value: 35.461
- type: ndcg_at_5
value: 39.969
- type: precision_at_1
value: 23.186
- type: precision_at_10
value: 6.97
- type: precision_at_100
value: 0.951
- type: precision_at_1000
value: 0.099
- type: precision_at_3
value: 14.912
- type: precision_at_5
value: 11.152
- type: recall_at_1
value: 23.186
- type: recall_at_10
value: 69.70100000000001
- type: recall_at_100
value: 95.092
- type: recall_at_1000
value: 99.431
- type: recall_at_3
value: 44.737
- type: recall_at_5
value: 55.761
- task:
type: Clustering
dataset:
type: mteb/arxiv-clustering-p2p
name: MTEB ArxivClusteringP2P
config: default
split: test
revision: a122ad7f3f0291bf49cc6f4d32aa80929df69d5d
metrics:
- type: v_measure
value: 46.10312401440185
- task:
type: Clustering
dataset:
type: mteb/arxiv-clustering-s2s
name: MTEB ArxivClusteringS2S
config: default
split: test
revision: f910caf1a6075f7329cdf8c1a6135696f37dbd53
metrics:
- type: v_measure
value: 39.67275326095384
- task:
type: Reranking
dataset:
type: mteb/askubuntudupquestions-reranking
name: MTEB AskUbuntuDupQuestions
config: default
split: test
revision: 2000358ca161889fa9c082cb41daa8dcfb161a54
metrics:
- type: map
value: 58.97793816337376
- type: mrr
value: 72.76832431957087
- task:
type: STS
dataset:
type: mteb/biosses-sts
name: MTEB BIOSSES
config: default
split: test
revision: d3fb88f8f02e40887cd149695127462bbcf29b4a
metrics:
- type: cos_sim_pearson
value: 83.11646947018187
- type: cos_sim_spearman
value: 81.40064994975234
- type: euclidean_pearson
value: 82.37355689019232
- type: euclidean_spearman
value: 81.6777646977348
- type: manhattan_pearson
value: 82.61101422716945
- type: manhattan_spearman
value: 81.80427360442245
- task:
type: Classification
dataset:
type: mteb/banking77
name: MTEB Banking77Classification
config: default
split: test
revision: 0fd18e25b25c072e09e0d92ab615fda904d66300
metrics:
- type: accuracy
value: 83.52922077922076
- type: f1
value: 83.45298679360866
- task:
type: Clustering
dataset:
type: mteb/biorxiv-clustering-p2p
name: MTEB BiorxivClusteringP2P
config: default
split: test
revision: 65b79d1d13f80053f67aca9498d9402c2d9f1f40
metrics:
- type: v_measure
value: 37.495115019668496
- task:
type: Clustering
dataset:
type: mteb/biorxiv-clustering-s2s
name: MTEB BiorxivClusteringS2S
config: default
split: test
revision: 258694dd0231531bc1fd9de6ceb52a0853c6d908
metrics:
- type: v_measure
value: 32.724792944166765
- task:
type: Retrieval
dataset:
type: BeIR/cqadupstack
name: MTEB CQADupstackAndroidRetrieval
config: default
split: test
revision: None
metrics:
- type: map_at_1
value: 32.361000000000004
- type: map_at_10
value: 43.765
- type: map_at_100
value: 45.224
- type: map_at_1000
value: 45.35
- type: map_at_3
value: 40.353
- type: map_at_5
value: 42.195
- type: mrr_at_1
value: 40.629
- type: mrr_at_10
value: 50.458000000000006
- type: mrr_at_100
value: 51.06699999999999
- type: mrr_at_1000
value: 51.12
- type: mrr_at_3
value: 47.902
- type: mrr_at_5
value: 49.447
- type: ndcg_at_1
value: 40.629
- type: ndcg_at_10
value: 50.376
- type: ndcg_at_100
value: 55.065
- type: ndcg_at_1000
value: 57.196000000000005
- type: ndcg_at_3
value: 45.616
- type: ndcg_at_5
value: 47.646
- type: precision_at_1
value: 40.629
- type: precision_at_10
value: 9.785
- type: precision_at_100
value: 1.562
- type: precision_at_1000
value: 0.2
- type: precision_at_3
value: 22.031
- type: precision_at_5
value: 15.737000000000002
- type: recall_at_1
value: 32.361000000000004
- type: recall_at_10
value: 62.214000000000006
- type: recall_at_100
value: 81.464
- type: recall_at_1000
value: 95.905
- type: recall_at_3
value: 47.5
- type: recall_at_5
value: 53.69500000000001
- task:
type: Retrieval
dataset:
type: BeIR/cqadupstack
name: MTEB CQADupstackEnglishRetrieval
config: default
split: test
revision: None
metrics:
- type: map_at_1
value: 27.971
- type: map_at_10
value: 37.444
- type: map_at_100
value: 38.607
- type: map_at_1000
value: 38.737
- type: map_at_3
value: 34.504000000000005
- type: map_at_5
value: 36.234
- type: mrr_at_1
value: 35.35
- type: mrr_at_10
value: 43.441
- type: mrr_at_100
value: 44.147999999999996
- type: mrr_at_1000
value: 44.196000000000005
- type: mrr_at_3
value: 41.285
- type: mrr_at_5
value: 42.552
- type: ndcg_at_1
value: 35.35
- type: ndcg_at_10
value: 42.903999999999996
- type: ndcg_at_100
value: 47.406
- type: ndcg_at_1000
value: 49.588
- type: ndcg_at_3
value: 38.778
- type: ndcg_at_5
value: 40.788000000000004
- type: precision_at_1
value: 35.35
- type: precision_at_10
value: 8.083
- type: precision_at_100
value: 1.313
- type: precision_at_1000
value: 0.18
- type: precision_at_3
value: 18.769
- type: precision_at_5
value: 13.439
- type: recall_at_1
value: 27.971
- type: recall_at_10
value: 52.492000000000004
- type: recall_at_100
value: 71.642
- type: recall_at_1000
value: 85.488
- type: recall_at_3
value: 40.1
- type: recall_at_5
value: 45.800000000000004
- task:
type: Retrieval
dataset:
type: BeIR/cqadupstack
name: MTEB CQADupstackGamingRetrieval
config: default
split: test
revision: None
metrics:
- type: map_at_1
value: 39.898
- type: map_at_10
value: 51.819
- type: map_at_100
value: 52.886
- type: map_at_1000
value: 52.941
- type: map_at_3
value: 48.619
- type: map_at_5
value: 50.493
- type: mrr_at_1
value: 45.391999999999996
- type: mrr_at_10
value: 55.230000000000004
- type: mrr_at_100
value: 55.887
- type: mrr_at_1000
value: 55.916
- type: mrr_at_3
value: 52.717000000000006
- type: mrr_at_5
value: 54.222
- type: ndcg_at_1
value: 45.391999999999996
- type: ndcg_at_10
value: 57.586999999999996
- type: ndcg_at_100
value: 61.745000000000005
- type: ndcg_at_1000
value: 62.83800000000001
- type: ndcg_at_3
value: 52.207
- type: ndcg_at_5
value: 54.925999999999995
- type: precision_at_1
value: 45.391999999999996
- type: precision_at_10
value: 9.21
- type: precision_at_100
value: 1.226
- type: precision_at_1000
value: 0.136
- type: precision_at_3
value: 23.177
- type: precision_at_5
value: 16.038
- type: recall_at_1
value: 39.898
- type: recall_at_10
value: 71.18900000000001
- type: recall_at_100
value: 89.082
- type: recall_at_1000
value: 96.865
- type: recall_at_3
value: 56.907
- type: recall_at_5
value: 63.397999999999996
- task:
type: Retrieval
dataset:
type: BeIR/cqadupstack
name: MTEB CQADupstackGisRetrieval
config: default
split: test
revision: None
metrics:
- type: map_at_1
value: 22.706
- type: map_at_10
value: 30.818
- type: map_at_100
value: 32.038
- type: map_at_1000
value: 32.123000000000005
- type: map_at_3
value: 28.077
- type: map_at_5
value: 29.709999999999997
- type: mrr_at_1
value: 24.407
- type: mrr_at_10
value: 32.555
- type: mrr_at_100
value: 33.692
- type: mrr_at_1000
value: 33.751
- type: mrr_at_3
value: 29.848999999999997
- type: mrr_at_5
value: 31.509999999999998
- type: ndcg_at_1
value: 24.407
- type: ndcg_at_10
value: 35.624
- type: ndcg_at_100
value: 41.454
- type: ndcg_at_1000
value: 43.556
- type: ndcg_at_3
value: 30.217
- type: ndcg_at_5
value: 33.111000000000004
- type: precision_at_1
value: 24.407
- type: precision_at_10
value: 5.548
- type: precision_at_100
value: 0.8869999999999999
- type: precision_at_1000
value: 0.11100000000000002
- type: precision_at_3
value: 12.731
- type: precision_at_5
value: 9.22
- type: recall_at_1
value: 22.706
- type: recall_at_10
value: 48.772
- type: recall_at_100
value: 75.053
- type: recall_at_1000
value: 90.731
- type: recall_at_3
value: 34.421
- type: recall_at_5
value: 41.427
- task:
type: Retrieval
dataset:
type: BeIR/cqadupstack
name: MTEB CQADupstackMathematicaRetrieval
config: default
split: test
revision: None
metrics:
- type: map_at_1
value: 13.424
- type: map_at_10
value: 21.09
- type: map_at_100
value: 22.264999999999997
- type: map_at_1000
value: 22.402
- type: map_at_3
value: 18.312
- type: map_at_5
value: 19.874
- type: mrr_at_1
value: 16.915
- type: mrr_at_10
value: 25.258000000000003
- type: mrr_at_100
value: 26.228
- type: mrr_at_1000
value: 26.31
- type: mrr_at_3
value: 22.492
- type: mrr_at_5
value: 24.04
- type: ndcg_at_1
value: 16.915
- type: ndcg_at_10
value: 26.266000000000002
- type: ndcg_at_100
value: 32.08
- type: ndcg_at_1000
value: 35.086
- type: ndcg_at_3
value: 21.049
- type: ndcg_at_5
value: 23.508000000000003
- type: precision_at_1
value: 16.915
- type: precision_at_10
value: 5.1
- type: precision_at_100
value: 0.9329999999999999
- type: precision_at_1000
value: 0.131
- type: precision_at_3
value: 10.282
- type: precision_at_5
value: 7.836
- type: recall_at_1
value: 13.424
- type: recall_at_10
value: 38.179
- type: recall_at_100
value: 63.906
- type: recall_at_1000
value: 84.933
- type: recall_at_3
value: 23.878
- type: recall_at_5
value: 30.037999999999997
- task:
type: Retrieval
dataset:
type: BeIR/cqadupstack
name: MTEB CQADupstackPhysicsRetrieval
config: default
split: test
revision: None
metrics:
- type: map_at_1
value: 26.154
- type: map_at_10
value: 35.912
- type: map_at_100
value: 37.211
- type: map_at_1000
value: 37.327
- type: map_at_3
value: 32.684999999999995
- type: map_at_5
value: 34.562
- type: mrr_at_1
value: 32.435
- type: mrr_at_10
value: 41.411
- type: mrr_at_100
value: 42.297000000000004
- type: mrr_at_1000
value: 42.345
- type: mrr_at_3
value: 38.771
- type: mrr_at_5
value: 40.33
- type: ndcg_at_1
value: 32.435
- type: ndcg_at_10
value: 41.785
- type: ndcg_at_100
value: 47.469
- type: ndcg_at_1000
value: 49.685
- type: ndcg_at_3
value: 36.618
- type: ndcg_at_5
value: 39.101
- type: precision_at_1
value: 32.435
- type: precision_at_10
value: 7.642
- type: precision_at_100
value: 1.244
- type: precision_at_1000
value: 0.163
- type: precision_at_3
value: 17.485
- type: precision_at_5
value: 12.57
- type: recall_at_1
value: 26.154
- type: recall_at_10
value: 54.111
- type: recall_at_100
value: 78.348
- type: recall_at_1000
value: 92.996
- type: recall_at_3
value: 39.189
- type: recall_at_5
value: 45.852
- task:
type: Retrieval
dataset:
type: BeIR/cqadupstack
name: MTEB CQADupstackProgrammersRetrieval
config: default
split: test
revision: None
metrics:
- type: map_at_1
value: 26.308999999999997
- type: map_at_10
value: 35.524
- type: map_at_100
value: 36.774
- type: map_at_1000
value: 36.891
- type: map_at_3
value: 32.561
- type: map_at_5
value: 34.034
- type: mrr_at_1
value: 31.735000000000003
- type: mrr_at_10
value: 40.391
- type: mrr_at_100
value: 41.227000000000004
- type: mrr_at_1000
value: 41.288000000000004
- type: mrr_at_3
value: 37.938
- type: mrr_at_5
value: 39.193
- type: ndcg_at_1
value: 31.735000000000003
- type: ndcg_at_10
value: 41.166000000000004
- type: ndcg_at_100
value: 46.702
- type: ndcg_at_1000
value: 49.157000000000004
- type: ndcg_at_3
value: 36.274
- type: ndcg_at_5
value: 38.177
- type: precision_at_1
value: 31.735000000000003
- type: precision_at_10
value: 7.5569999999999995
- type: precision_at_100
value: 1.2109999999999999
- type: precision_at_1000
value: 0.16
- type: precision_at_3
value: 17.199
- type: precision_at_5
value: 12.123000000000001
- type: recall_at_1
value: 26.308999999999997
- type: recall_at_10
value: 53.083000000000006
- type: recall_at_100
value: 76.922
- type: recall_at_1000
value: 93.767
- type: recall_at_3
value: 39.262
- type: recall_at_5
value: 44.413000000000004
- task:
type: Retrieval
dataset:
type: BeIR/cqadupstack
name: MTEB CQADupstackRetrieval
config: default
split: test
revision: None
metrics:
- type: map_at_1
value: 24.391250000000003
- type: map_at_10
value: 33.280166666666666
- type: map_at_100
value: 34.49566666666667
- type: map_at_1000
value: 34.61533333333333
- type: map_at_3
value: 30.52183333333333
- type: map_at_5
value: 32.06608333333333
- type: mrr_at_1
value: 29.105083333333337
- type: mrr_at_10
value: 37.44766666666666
- type: mrr_at_100
value: 38.32491666666667
- type: mrr_at_1000
value: 38.385666666666665
- type: mrr_at_3
value: 35.06883333333333
- type: mrr_at_5
value: 36.42066666666667
- type: ndcg_at_1
value: 29.105083333333337
- type: ndcg_at_10
value: 38.54358333333333
- type: ndcg_at_100
value: 43.833583333333344
- type: ndcg_at_1000
value: 46.215333333333334
- type: ndcg_at_3
value: 33.876
- type: ndcg_at_5
value: 36.05208333333333
- type: precision_at_1
value: 29.105083333333337
- type: precision_at_10
value: 6.823416666666665
- type: precision_at_100
value: 1.1270833333333334
- type: precision_at_1000
value: 0.15208333333333332
- type: precision_at_3
value: 15.696750000000002
- type: precision_at_5
value: 11.193499999999998
- type: recall_at_1
value: 24.391250000000003
- type: recall_at_10
value: 49.98808333333333
- type: recall_at_100
value: 73.31616666666666
- type: recall_at_1000
value: 89.96291666666667
- type: recall_at_3
value: 36.86666666666667
- type: recall_at_5
value: 42.54350000000001
- task:
type: Retrieval
dataset:
type: BeIR/cqadupstack
name: MTEB CQADupstackStatsRetrieval
config: default
split: test
revision: None
metrics:
- type: map_at_1
value: 21.995
- type: map_at_10
value: 28.807
- type: map_at_100
value: 29.813000000000002
- type: map_at_1000
value: 29.903000000000002
- type: map_at_3
value: 26.636
- type: map_at_5
value: 27.912
- type: mrr_at_1
value: 24.847
- type: mrr_at_10
value: 31.494
- type: mrr_at_100
value: 32.381
- type: mrr_at_1000
value: 32.446999999999996
- type: mrr_at_3
value: 29.473
- type: mrr_at_5
value: 30.7
- type: ndcg_at_1
value: 24.847
- type: ndcg_at_10
value: 32.818999999999996
- type: ndcg_at_100
value: 37.835
- type: ndcg_at_1000
value: 40.226
- type: ndcg_at_3
value: 28.811999999999998
- type: ndcg_at_5
value: 30.875999999999998
- type: precision_at_1
value: 24.847
- type: precision_at_10
value: 5.244999999999999
- type: precision_at_100
value: 0.856
- type: precision_at_1000
value: 0.11299999999999999
- type: precision_at_3
value: 12.577
- type: precision_at_5
value: 8.895999999999999
- type: recall_at_1
value: 21.995
- type: recall_at_10
value: 42.479
- type: recall_at_100
value: 65.337
- type: recall_at_1000
value: 83.23700000000001
- type: recall_at_3
value: 31.573
- type: recall_at_5
value: 36.684
- task:
type: Retrieval
dataset:
type: BeIR/cqadupstack
name: MTEB CQADupstackTexRetrieval
config: default
split: test
revision: None
metrics:
- type: map_at_1
value: 15.751000000000001
- type: map_at_10
value: 21.909
- type: map_at_100
value: 23.064
- type: map_at_1000
value: 23.205000000000002
- type: map_at_3
value: 20.138
- type: map_at_5
value: 20.973
- type: mrr_at_1
value: 19.305
- type: mrr_at_10
value: 25.647
- type: mrr_at_100
value: 26.659
- type: mrr_at_1000
value: 26.748
- type: mrr_at_3
value: 23.933
- type: mrr_at_5
value: 24.754
- type: ndcg_at_1
value: 19.305
- type: ndcg_at_10
value: 25.886
- type: ndcg_at_100
value: 31.56
- type: ndcg_at_1000
value: 34.799
- type: ndcg_at_3
value: 22.708000000000002
- type: ndcg_at_5
value: 23.838
- type: precision_at_1
value: 19.305
- type: precision_at_10
value: 4.677
- type: precision_at_100
value: 0.895
- type: precision_at_1000
value: 0.136
- type: precision_at_3
value: 10.771
- type: precision_at_5
value: 7.46
- type: recall_at_1
value: 15.751000000000001
- type: recall_at_10
value: 34.156
- type: recall_at_100
value: 59.899
- type: recall_at_1000
value: 83.08
- type: recall_at_3
value: 24.772
- type: recall_at_5
value: 28.009
- task:
type: Retrieval
dataset:
type: BeIR/cqadupstack
name: MTEB CQADupstackUnixRetrieval
config: default
split: test
revision: None
metrics:
- type: map_at_1
value: 23.34
- type: map_at_10
value: 32.383
- type: map_at_100
value: 33.629999999999995
- type: map_at_1000
value: 33.735
- type: map_at_3
value: 29.68
- type: map_at_5
value: 31.270999999999997
- type: mrr_at_1
value: 27.612
- type: mrr_at_10
value: 36.381
- type: mrr_at_100
value: 37.351
- type: mrr_at_1000
value: 37.411
- type: mrr_at_3
value: 33.893
- type: mrr_at_5
value: 35.353
- type: ndcg_at_1
value: 27.612
- type: ndcg_at_10
value: 37.714999999999996
- type: ndcg_at_100
value: 43.525000000000006
- type: ndcg_at_1000
value: 45.812999999999995
- type: ndcg_at_3
value: 32.796
- type: ndcg_at_5
value: 35.243
- type: precision_at_1
value: 27.612
- type: precision_at_10
value: 6.465
- type: precision_at_100
value: 1.0619999999999998
- type: precision_at_1000
value: 0.13699999999999998
- type: precision_at_3
value: 15.049999999999999
- type: precision_at_5
value: 10.764999999999999
- type: recall_at_1
value: 23.34
- type: recall_at_10
value: 49.856
- type: recall_at_100
value: 75.334
- type: recall_at_1000
value: 91.156
- type: recall_at_3
value: 36.497
- type: recall_at_5
value: 42.769
- task:
type: Retrieval
dataset:
type: BeIR/cqadupstack
name: MTEB CQADupstackWebmastersRetrieval
config: default
split: test
revision: None
metrics:
- type: map_at_1
value: 25.097
- type: map_at_10
value: 34.599999999999994
- type: map_at_100
value: 36.174
- type: map_at_1000
value: 36.398
- type: map_at_3
value: 31.781
- type: map_at_5
value: 33.22
- type: mrr_at_1
value: 31.225
- type: mrr_at_10
value: 39.873
- type: mrr_at_100
value: 40.853
- type: mrr_at_1000
value: 40.904
- type: mrr_at_3
value: 37.681
- type: mrr_at_5
value: 38.669
- type: ndcg_at_1
value: 31.225
- type: ndcg_at_10
value: 40.586
- type: ndcg_at_100
value: 46.226
- type: ndcg_at_1000
value: 48.788
- type: ndcg_at_3
value: 36.258
- type: ndcg_at_5
value: 37.848
- type: precision_at_1
value: 31.225
- type: precision_at_10
value: 7.707999999999999
- type: precision_at_100
value: 1.536
- type: precision_at_1000
value: 0.242
- type: precision_at_3
value: 17.26
- type: precision_at_5
value: 12.253
- type: recall_at_1
value: 25.097
- type: recall_at_10
value: 51.602000000000004
- type: recall_at_100
value: 76.854
- type: recall_at_1000
value: 93.303
- type: recall_at_3
value: 38.68
- type: recall_at_5
value: 43.258
- task:
type: Retrieval
dataset:
type: BeIR/cqadupstack
name: MTEB CQADupstackWordpressRetrieval
config: default
split: test
revision: None
metrics:
- type: map_at_1
value: 17.689
- type: map_at_10
value: 25.291000000000004
- type: map_at_100
value: 26.262
- type: map_at_1000
value: 26.372
- type: map_at_3
value: 22.916
- type: map_at_5
value: 24.315
- type: mrr_at_1
value: 19.409000000000002
- type: mrr_at_10
value: 27.233
- type: mrr_at_100
value: 28.109
- type: mrr_at_1000
value: 28.192
- type: mrr_at_3
value: 24.892
- type: mrr_at_5
value: 26.278000000000002
- type: ndcg_at_1
value: 19.409000000000002
- type: ndcg_at_10
value: 29.809
- type: ndcg_at_100
value: 34.936
- type: ndcg_at_1000
value: 37.852000000000004
- type: ndcg_at_3
value: 25.179000000000002
- type: ndcg_at_5
value: 27.563
- type: precision_at_1
value: 19.409000000000002
- type: precision_at_10
value: 4.861
- type: precision_at_100
value: 0.8
- type: precision_at_1000
value: 0.116
- type: precision_at_3
value: 11.029
- type: precision_at_5
value: 7.985
- type: recall_at_1
value: 17.689
- type: recall_at_10
value: 41.724
- type: recall_at_100
value: 65.95299999999999
- type: recall_at_1000
value: 88.094
- type: recall_at_3
value: 29.621
- type: recall_at_5
value: 35.179
- task:
type: Retrieval
dataset:
type: climate-fever
name: MTEB ClimateFEVER
config: default
split: test
revision: None
metrics:
- type: map_at_1
value: 10.581
- type: map_at_10
value: 18.944
- type: map_at_100
value: 20.812
- type: map_at_1000
value: 21.002000000000002
- type: map_at_3
value: 15.661
- type: map_at_5
value: 17.502000000000002
- type: mrr_at_1
value: 23.388
- type: mrr_at_10
value: 34.263
- type: mrr_at_100
value: 35.364000000000004
- type: mrr_at_1000
value: 35.409
- type: mrr_at_3
value: 30.586000000000002
- type: mrr_at_5
value: 32.928000000000004
- type: ndcg_at_1
value: 23.388
- type: ndcg_at_10
value: 26.56
- type: ndcg_at_100
value: 34.248
- type: ndcg_at_1000
value: 37.779
- type: ndcg_at_3
value: 21.179000000000002
- type: ndcg_at_5
value: 23.504
- type: precision_at_1
value: 23.388
- type: precision_at_10
value: 8.476
- type: precision_at_100
value: 1.672
- type: precision_at_1000
value: 0.233
- type: precision_at_3
value: 15.852
- type: precision_at_5
value: 12.73
- type: recall_at_1
value: 10.581
- type: recall_at_10
value: 32.512
- type: recall_at_100
value: 59.313
- type: recall_at_1000
value: 79.25
- type: recall_at_3
value: 19.912
- type: recall_at_5
value: 25.832
- task:
type: Retrieval
dataset:
type: dbpedia-entity
name: MTEB DBPedia
config: default
split: test
revision: None
metrics:
- type: map_at_1
value: 9.35
- type: map_at_10
value: 20.134
- type: map_at_100
value: 28.975
- type: map_at_1000
value: 30.709999999999997
- type: map_at_3
value: 14.513000000000002
- type: map_at_5
value: 16.671
- type: mrr_at_1
value: 69.75
- type: mrr_at_10
value: 77.67699999999999
- type: mrr_at_100
value: 77.97500000000001
- type: mrr_at_1000
value: 77.985
- type: mrr_at_3
value: 76.292
- type: mrr_at_5
value: 77.179
- type: ndcg_at_1
value: 56.49999999999999
- type: ndcg_at_10
value: 42.226
- type: ndcg_at_100
value: 47.562
- type: ndcg_at_1000
value: 54.923
- type: ndcg_at_3
value: 46.564
- type: ndcg_at_5
value: 43.830000000000005
- type: precision_at_1
value: 69.75
- type: precision_at_10
value: 33.525
- type: precision_at_100
value: 11.035
- type: precision_at_1000
value: 2.206
- type: precision_at_3
value: 49.75
- type: precision_at_5
value: 42
- type: recall_at_1
value: 9.35
- type: recall_at_10
value: 25.793
- type: recall_at_100
value: 54.186
- type: recall_at_1000
value: 77.81
- type: recall_at_3
value: 15.770000000000001
- type: recall_at_5
value: 19.09
- task:
type: Classification
dataset:
type: mteb/emotion
name: MTEB EmotionClassification
config: default
split: test
revision: 4f58c6b202a23cf9a4da393831edf4f9183cad37
metrics:
- type: accuracy
value: 46.945
- type: f1
value: 42.07407842992542
- task:
type: Retrieval
dataset:
type: fever
name: MTEB FEVER
config: default
split: test
revision: None
metrics:
- type: map_at_1
value: 71.04599999999999
- type: map_at_10
value: 80.718
- type: map_at_100
value: 80.961
- type: map_at_1000
value: 80.974
- type: map_at_3
value: 79.49199999999999
- type: map_at_5
value: 80.32000000000001
- type: mrr_at_1
value: 76.388
- type: mrr_at_10
value: 85.214
- type: mrr_at_100
value: 85.302
- type: mrr_at_1000
value: 85.302
- type: mrr_at_3
value: 84.373
- type: mrr_at_5
value: 84.979
- type: ndcg_at_1
value: 76.388
- type: ndcg_at_10
value: 84.987
- type: ndcg_at_100
value: 85.835
- type: ndcg_at_1000
value: 86.04899999999999
- type: ndcg_at_3
value: 83.04
- type: ndcg_at_5
value: 84.22500000000001
- type: precision_at_1
value: 76.388
- type: precision_at_10
value: 10.35
- type: precision_at_100
value: 1.099
- type: precision_at_1000
value: 0.11399999999999999
- type: precision_at_3
value: 32.108
- type: precision_at_5
value: 20.033
- type: recall_at_1
value: 71.04599999999999
- type: recall_at_10
value: 93.547
- type: recall_at_100
value: 96.887
- type: recall_at_1000
value: 98.158
- type: recall_at_3
value: 88.346
- type: recall_at_5
value: 91.321
- task:
type: Retrieval
dataset:
type: fiqa
name: MTEB FiQA2018
config: default
split: test
revision: None
metrics:
- type: map_at_1
value: 19.8
- type: map_at_10
value: 31.979999999999997
- type: map_at_100
value: 33.876
- type: map_at_1000
value: 34.056999999999995
- type: map_at_3
value: 28.067999999999998
- type: map_at_5
value: 30.066
- type: mrr_at_1
value: 38.735
- type: mrr_at_10
value: 47.749
- type: mrr_at_100
value: 48.605
- type: mrr_at_1000
value: 48.644999999999996
- type: mrr_at_3
value: 45.165
- type: mrr_at_5
value: 46.646
- type: ndcg_at_1
value: 38.735
- type: ndcg_at_10
value: 39.883
- type: ndcg_at_100
value: 46.983000000000004
- type: ndcg_at_1000
value: 50.043000000000006
- type: ndcg_at_3
value: 35.943000000000005
- type: ndcg_at_5
value: 37.119
- type: precision_at_1
value: 38.735
- type: precision_at_10
value: 10.940999999999999
- type: precision_at_100
value: 1.836
- type: precision_at_1000
value: 0.23900000000000002
- type: precision_at_3
value: 23.817
- type: precision_at_5
value: 17.346
- type: recall_at_1
value: 19.8
- type: recall_at_10
value: 47.082
- type: recall_at_100
value: 73.247
- type: recall_at_1000
value: 91.633
- type: recall_at_3
value: 33.201
- type: recall_at_5
value: 38.81
- task:
type: Retrieval
dataset:
type: hotpotqa
name: MTEB HotpotQA
config: default
split: test
revision: None
metrics:
- type: map_at_1
value: 38.102999999999994
- type: map_at_10
value: 60.547
- type: map_at_100
value: 61.466
- type: map_at_1000
value: 61.526
- type: map_at_3
value: 56.973
- type: map_at_5
value: 59.244
- type: mrr_at_1
value: 76.205
- type: mrr_at_10
value: 82.816
- type: mrr_at_100
value: 83.002
- type: mrr_at_1000
value: 83.009
- type: mrr_at_3
value: 81.747
- type: mrr_at_5
value: 82.467
- type: ndcg_at_1
value: 76.205
- type: ndcg_at_10
value: 69.15
- type: ndcg_at_100
value: 72.297
- type: ndcg_at_1000
value: 73.443
- type: ndcg_at_3
value: 64.07000000000001
- type: ndcg_at_5
value: 66.96600000000001
- type: precision_at_1
value: 76.205
- type: precision_at_10
value: 14.601
- type: precision_at_100
value: 1.7049999999999998
- type: precision_at_1000
value: 0.186
- type: precision_at_3
value: 41.202
- type: precision_at_5
value: 27.006000000000004
- type: recall_at_1
value: 38.102999999999994
- type: recall_at_10
value: 73.005
- type: recall_at_100
value: 85.253
- type: recall_at_1000
value: 92.795
- type: recall_at_3
value: 61.803
- type: recall_at_5
value: 67.515
- task:
type: Classification
dataset:
type: mteb/imdb
name: MTEB ImdbClassification
config: default
split: test
revision: 3d86128a09e091d6018b6d26cad27f2739fc2db7
metrics:
- type: accuracy
value: 86.15
- type: ap
value: 80.36282825265391
- type: f1
value: 86.07368510726472
- task:
type: Retrieval
dataset:
type: msmarco
name: MTEB MSMARCO
config: default
split: dev
revision: None
metrics:
- type: map_at_1
value: 22.6
- type: map_at_10
value: 34.887
- type: map_at_100
value: 36.069
- type: map_at_1000
value: 36.115
- type: map_at_3
value: 31.067
- type: map_at_5
value: 33.300000000000004
- type: mrr_at_1
value: 23.238
- type: mrr_at_10
value: 35.47
- type: mrr_at_100
value: 36.599
- type: mrr_at_1000
value: 36.64
- type: mrr_at_3
value: 31.735999999999997
- type: mrr_at_5
value: 33.939
- type: ndcg_at_1
value: 23.252
- type: ndcg_at_10
value: 41.765
- type: ndcg_at_100
value: 47.402
- type: ndcg_at_1000
value: 48.562
- type: ndcg_at_3
value: 34.016999999999996
- type: ndcg_at_5
value: 38.016
- type: precision_at_1
value: 23.252
- type: precision_at_10
value: 6.569
- type: precision_at_100
value: 0.938
- type: precision_at_1000
value: 0.104
- type: precision_at_3
value: 14.479000000000001
- type: precision_at_5
value: 10.722
- type: recall_at_1
value: 22.6
- type: recall_at_10
value: 62.919000000000004
- type: recall_at_100
value: 88.82
- type: recall_at_1000
value: 97.71600000000001
- type: recall_at_3
value: 41.896
- type: recall_at_5
value: 51.537
- task:
type: Classification
dataset:
type: mteb/mtop_domain
name: MTEB MTOPDomainClassification (en)
config: en
split: test
revision: d80d48c1eb48d3562165c59d59d0034df9fff0bf
metrics:
- type: accuracy
value: 93.69357045143639
- type: f1
value: 93.55489858177597
- task:
type: Classification
dataset:
type: mteb/mtop_intent
name: MTEB MTOPIntentClassification (en)
config: en
split: test
revision: ae001d0e6b1228650b7bd1c2c65fb50ad11a8aba
metrics:
- type: accuracy
value: 75.31235750114
- type: f1
value: 57.891491963121155
- task:
type: Classification
dataset:
type: mteb/amazon_massive_intent
name: MTEB MassiveIntentClassification (en)
config: en
split: test
revision: 31efe3c427b0bae9c22cbb560b8f15491cc6bed7
metrics:
- type: accuracy
value: 73.04303967720243
- type: f1
value: 70.51516022297616
- task:
type: Classification
dataset:
type: mteb/amazon_massive_scenario
name: MTEB MassiveScenarioClassification (en)
config: en
split: test
revision: 7d571f92784cd94a019292a1f45445077d0ef634
metrics:
- type: accuracy
value: 77.65299260255549
- type: f1
value: 77.49059766538576
- task:
type: Clustering
dataset:
type: mteb/medrxiv-clustering-p2p
name: MTEB MedrxivClusteringP2P
config: default
split: test
revision: e7a26af6f3ae46b30dde8737f02c07b1505bcc73
metrics:
- type: v_measure
value: 31.458906115906597
- task:
type: Clustering
dataset:
type: mteb/medrxiv-clustering-s2s
name: MTEB MedrxivClusteringS2S
config: default
split: test
revision: 35191c8c0dca72d8ff3efcd72aa802307d469663
metrics:
- type: v_measure
value: 28.9851513122443
- task:
type: Reranking
dataset:
type: mteb/mind_small
name: MTEB MindSmallReranking
config: default
split: test
revision: 3bdac13927fdc888b903db93b2ffdbd90b295a69
metrics:
- type: map
value: 31.2916268497217
- type: mrr
value: 32.328276715593816
- task:
type: Retrieval
dataset:
type: nfcorpus
name: MTEB NFCorpus
config: default
split: test
revision: None
metrics:
- type: map_at_1
value: 6.3740000000000006
- type: map_at_10
value: 13.089999999999998
- type: map_at_100
value: 16.512
- type: map_at_1000
value: 18.014
- type: map_at_3
value: 9.671000000000001
- type: map_at_5
value: 11.199
- type: mrr_at_1
value: 46.749
- type: mrr_at_10
value: 55.367
- type: mrr_at_100
value: 56.021
- type: mrr_at_1000
value: 56.058
- type: mrr_at_3
value: 53.30200000000001
- type: mrr_at_5
value: 54.773
- type: ndcg_at_1
value: 45.046
- type: ndcg_at_10
value: 35.388999999999996
- type: ndcg_at_100
value: 32.175
- type: ndcg_at_1000
value: 41.018
- type: ndcg_at_3
value: 40.244
- type: ndcg_at_5
value: 38.267
- type: precision_at_1
value: 46.749
- type: precision_at_10
value: 26.563
- type: precision_at_100
value: 8.074
- type: precision_at_1000
value: 2.099
- type: precision_at_3
value: 37.358000000000004
- type: precision_at_5
value: 33.003
- type: recall_at_1
value: 6.3740000000000006
- type: recall_at_10
value: 16.805999999999997
- type: recall_at_100
value: 31.871
- type: recall_at_1000
value: 64.098
- type: recall_at_3
value: 10.383000000000001
- type: recall_at_5
value: 13.166
- task:
type: Retrieval
dataset:
type: nq
name: MTEB NQ
config: default
split: test
revision: None
metrics:
- type: map_at_1
value: 34.847
- type: map_at_10
value: 50.532
- type: map_at_100
value: 51.504000000000005
- type: map_at_1000
value: 51.528
- type: map_at_3
value: 46.219
- type: map_at_5
value: 48.868
- type: mrr_at_1
value: 39.137
- type: mrr_at_10
value: 53.157
- type: mrr_at_100
value: 53.839999999999996
- type: mrr_at_1000
value: 53.857
- type: mrr_at_3
value: 49.667
- type: mrr_at_5
value: 51.847
- type: ndcg_at_1
value: 39.108
- type: ndcg_at_10
value: 58.221000000000004
- type: ndcg_at_100
value: 62.021
- type: ndcg_at_1000
value: 62.57
- type: ndcg_at_3
value: 50.27199999999999
- type: ndcg_at_5
value: 54.623999999999995
- type: precision_at_1
value: 39.108
- type: precision_at_10
value: 9.397
- type: precision_at_100
value: 1.1520000000000001
- type: precision_at_1000
value: 0.12
- type: precision_at_3
value: 22.644000000000002
- type: precision_at_5
value: 16.141
- type: recall_at_1
value: 34.847
- type: recall_at_10
value: 78.945
- type: recall_at_100
value: 94.793
- type: recall_at_1000
value: 98.904
- type: recall_at_3
value: 58.56
- type: recall_at_5
value: 68.535
- task:
type: Retrieval
dataset:
type: quora
name: MTEB QuoraRetrieval
config: default
split: test
revision: None
metrics:
- type: map_at_1
value: 68.728
- type: map_at_10
value: 82.537
- type: map_at_100
value: 83.218
- type: map_at_1000
value: 83.238
- type: map_at_3
value: 79.586
- type: map_at_5
value: 81.416
- type: mrr_at_1
value: 79.17999999999999
- type: mrr_at_10
value: 85.79299999999999
- type: mrr_at_100
value: 85.937
- type: mrr_at_1000
value: 85.938
- type: mrr_at_3
value: 84.748
- type: mrr_at_5
value: 85.431
- type: ndcg_at_1
value: 79.17
- type: ndcg_at_10
value: 86.555
- type: ndcg_at_100
value: 88.005
- type: ndcg_at_1000
value: 88.146
- type: ndcg_at_3
value: 83.557
- type: ndcg_at_5
value: 85.152
- type: precision_at_1
value: 79.17
- type: precision_at_10
value: 13.163
- type: precision_at_100
value: 1.52
- type: precision_at_1000
value: 0.156
- type: precision_at_3
value: 36.53
- type: precision_at_5
value: 24.046
- type: recall_at_1
value: 68.728
- type: recall_at_10
value: 94.217
- type: recall_at_100
value: 99.295
- type: recall_at_1000
value: 99.964
- type: recall_at_3
value: 85.646
- type: recall_at_5
value: 90.113
- task:
type: Clustering
dataset:
type: mteb/reddit-clustering
name: MTEB RedditClustering
config: default
split: test
revision: 24640382cdbf8abc73003fb0fa6d111a705499eb
metrics:
- type: v_measure
value: 56.15680266226348
- task:
type: Clustering
dataset:
type: mteb/reddit-clustering-p2p
name: MTEB RedditClusteringP2P
config: default
split: test
revision: 282350215ef01743dc01b456c7f5241fa8937f16
metrics:
- type: v_measure
value: 63.4318549229047
- task:
type: Retrieval
dataset:
type: scidocs
name: MTEB SCIDOCS
config: default
split: test
revision: None
metrics:
- type: map_at_1
value: 4.353
- type: map_at_10
value: 10.956000000000001
- type: map_at_100
value: 12.873999999999999
- type: map_at_1000
value: 13.177
- type: map_at_3
value: 7.854
- type: map_at_5
value: 9.327
- type: mrr_at_1
value: 21.4
- type: mrr_at_10
value: 31.948999999999998
- type: mrr_at_100
value: 33.039
- type: mrr_at_1000
value: 33.106
- type: mrr_at_3
value: 28.449999999999996
- type: mrr_at_5
value: 30.535
- type: ndcg_at_1
value: 21.4
- type: ndcg_at_10
value: 18.694
- type: ndcg_at_100
value: 26.275
- type: ndcg_at_1000
value: 31.836
- type: ndcg_at_3
value: 17.559
- type: ndcg_at_5
value: 15.372
- type: precision_at_1
value: 21.4
- type: precision_at_10
value: 9.790000000000001
- type: precision_at_100
value: 2.0709999999999997
- type: precision_at_1000
value: 0.34099999999999997
- type: precision_at_3
value: 16.467000000000002
- type: precision_at_5
value: 13.54
- type: recall_at_1
value: 4.353
- type: recall_at_10
value: 19.892000000000003
- type: recall_at_100
value: 42.067
- type: recall_at_1000
value: 69.268
- type: recall_at_3
value: 10.042
- type: recall_at_5
value: 13.741999999999999
- task:
type: STS
dataset:
type: mteb/sickr-sts
name: MTEB SICK-R
config: default
split: test
revision: a6ea5a8cab320b040a23452cc28066d9beae2cee
metrics:
- type: cos_sim_pearson
value: 83.75433886279843
- type: cos_sim_spearman
value: 78.29727771767095
- type: euclidean_pearson
value: 80.83057828506621
- type: euclidean_spearman
value: 78.35203149750356
- type: manhattan_pearson
value: 80.7403553891142
- type: manhattan_spearman
value: 78.33670488531051
- task:
type: STS
dataset:
type: mteb/sts12-sts
name: MTEB STS12
config: default
split: test
revision: a0d554a64d88156834ff5ae9920b964011b16384
metrics:
- type: cos_sim_pearson
value: 84.59999465280839
- type: cos_sim_spearman
value: 75.79279003980383
- type: euclidean_pearson
value: 82.29895375956758
- type: euclidean_spearman
value: 77.33856514102094
- type: manhattan_pearson
value: 82.22694214534756
- type: manhattan_spearman
value: 77.3028993008695
- task:
type: STS
dataset:
type: mteb/sts13-sts
name: MTEB STS13
config: default
split: test
revision: 7e90230a92c190f1bf69ae9002b8cea547a64cca
metrics:
- type: cos_sim_pearson
value: 83.09296929691297
- type: cos_sim_spearman
value: 83.58056936846941
- type: euclidean_pearson
value: 83.84067483060005
- type: euclidean_spearman
value: 84.45155680480985
- type: manhattan_pearson
value: 83.82353052971942
- type: manhattan_spearman
value: 84.43030567861112
- task:
type: STS
dataset:
type: mteb/sts14-sts
name: MTEB STS14
config: default
split: test
revision: 6031580fec1f6af667f0bd2da0a551cf4f0b2375
metrics:
- type: cos_sim_pearson
value: 82.74616852320915
- type: cos_sim_spearman
value: 79.948683747966
- type: euclidean_pearson
value: 81.55702283757084
- type: euclidean_spearman
value: 80.1721505114231
- type: manhattan_pearson
value: 81.52251518619441
- type: manhattan_spearman
value: 80.1469800135577
- task:
type: STS
dataset:
type: mteb/sts15-sts
name: MTEB STS15
config: default
split: test
revision: ae752c7c21bf194d8b67fd573edf7ae58183cbe3
metrics:
- type: cos_sim_pearson
value: 87.97170104226318
- type: cos_sim_spearman
value: 88.82021731518206
- type: euclidean_pearson
value: 87.92950547187615
- type: euclidean_spearman
value: 88.67043634645866
- type: manhattan_pearson
value: 87.90668112827639
- type: manhattan_spearman
value: 88.64471082785317
- task:
type: STS
dataset:
type: mteb/sts16-sts
name: MTEB STS16
config: default
split: test
revision: 4d8694f8f0e0100860b497b999b3dbed754a0513
metrics:
- type: cos_sim_pearson
value: 83.02790375770599
- type: cos_sim_spearman
value: 84.46308496590792
- type: euclidean_pearson
value: 84.29430000414911
- type: euclidean_spearman
value: 84.77298303589936
- type: manhattan_pearson
value: 84.23919291368665
- type: manhattan_spearman
value: 84.75272234871308
- task:
type: STS
dataset:
type: mteb/sts17-crosslingual-sts
name: MTEB STS17 (en-en)
config: en-en
split: test
revision: af5e6fb845001ecf41f4c1e033ce921939a2a68d
metrics:
- type: cos_sim_pearson
value: 87.62885108477064
- type: cos_sim_spearman
value: 87.58456196391622
- type: euclidean_pearson
value: 88.2602775281007
- type: euclidean_spearman
value: 87.51556278299846
- type: manhattan_pearson
value: 88.11224053672842
- type: manhattan_spearman
value: 87.4336094383095
- task:
type: STS
dataset:
type: mteb/sts22-crosslingual-sts
name: MTEB STS22 (en)
config: en
split: test
revision: 6d1ba47164174a496b7fa5d3569dae26a6813b80
metrics:
- type: cos_sim_pearson
value: 63.98187965128411
- type: cos_sim_spearman
value: 64.0653163219731
- type: euclidean_pearson
value: 62.30616725924099
- type: euclidean_spearman
value: 61.556971332295916
- type: manhattan_pearson
value: 62.07642330128549
- type: manhattan_spearman
value: 61.155494129828
- task:
type: STS
dataset:
type: mteb/stsbenchmark-sts
name: MTEB STSBenchmark
config: default
split: test
revision: b0fddb56ed78048fa8b90373c8a3cfc37b684831
metrics:
- type: cos_sim_pearson
value: 85.6089703921826
- type: cos_sim_spearman
value: 86.52303197250791
- type: euclidean_pearson
value: 85.95801955963246
- type: euclidean_spearman
value: 86.25242424112962
- type: manhattan_pearson
value: 85.88829100470312
- type: manhattan_spearman
value: 86.18742955805165
- task:
type: Reranking
dataset:
type: mteb/scidocs-reranking
name: MTEB SciDocsRR
config: default
split: test
revision: d3c5e1fc0b855ab6097bf1cda04dd73947d7caab
metrics:
- type: map
value: 83.02282098487036
- type: mrr
value: 95.05126409538174
- task:
type: Retrieval
dataset:
type: scifact
name: MTEB SciFact
config: default
split: test
revision: None
metrics:
- type: map_at_1
value: 55.928
- type: map_at_10
value: 67.308
- type: map_at_100
value: 67.89500000000001
- type: map_at_1000
value: 67.91199999999999
- type: map_at_3
value: 65.091
- type: map_at_5
value: 66.412
- type: mrr_at_1
value: 58.667
- type: mrr_at_10
value: 68.401
- type: mrr_at_100
value: 68.804
- type: mrr_at_1000
value: 68.819
- type: mrr_at_3
value: 66.72200000000001
- type: mrr_at_5
value: 67.72200000000001
- type: ndcg_at_1
value: 58.667
- type: ndcg_at_10
value: 71.944
- type: ndcg_at_100
value: 74.464
- type: ndcg_at_1000
value: 74.82799999999999
- type: ndcg_at_3
value: 68.257
- type: ndcg_at_5
value: 70.10300000000001
- type: precision_at_1
value: 58.667
- type: precision_at_10
value: 9.533
- type: precision_at_100
value: 1.09
- type: precision_at_1000
value: 0.11199999999999999
- type: precision_at_3
value: 27.222
- type: precision_at_5
value: 17.533
- type: recall_at_1
value: 55.928
- type: recall_at_10
value: 84.65
- type: recall_at_100
value: 96.267
- type: recall_at_1000
value: 99
- type: recall_at_3
value: 74.656
- type: recall_at_5
value: 79.489
- task:
type: PairClassification
dataset:
type: mteb/sprintduplicatequestions-pairclassification
name: MTEB SprintDuplicateQuestions
config: default
split: test
revision: d66bd1f72af766a5cc4b0ca5e00c162f89e8cc46
metrics:
- type: cos_sim_accuracy
value: 99.79009900990098
- type: cos_sim_ap
value: 94.5795129511524
- type: cos_sim_f1
value: 89.34673366834171
- type: cos_sim_precision
value: 89.79797979797979
- type: cos_sim_recall
value: 88.9
- type: dot_accuracy
value: 99.53465346534654
- type: dot_ap
value: 81.56492504352725
- type: dot_f1
value: 76.33816908454227
- type: dot_precision
value: 76.37637637637637
- type: dot_recall
value: 76.3
- type: euclidean_accuracy
value: 99.78514851485149
- type: euclidean_ap
value: 94.59134620408962
- type: euclidean_f1
value: 88.96484375
- type: euclidean_precision
value: 86.92748091603053
- type: euclidean_recall
value: 91.10000000000001
- type: manhattan_accuracy
value: 99.78415841584159
- type: manhattan_ap
value: 94.5190197328845
- type: manhattan_f1
value: 88.84462151394423
- type: manhattan_precision
value: 88.4920634920635
- type: manhattan_recall
value: 89.2
- type: max_accuracy
value: 99.79009900990098
- type: max_ap
value: 94.59134620408962
- type: max_f1
value: 89.34673366834171
- task:
type: Clustering
dataset:
type: mteb/stackexchange-clustering
name: MTEB StackExchangeClustering
config: default
split: test
revision: 6cbc1f7b2bc0622f2e39d2c77fa502909748c259
metrics:
- type: v_measure
value: 65.1487505617497
- task:
type: Clustering
dataset:
type: mteb/stackexchange-clustering-p2p
name: MTEB StackExchangeClusteringP2P
config: default
split: test
revision: 815ca46b2622cec33ccafc3735d572c266efdb44
metrics:
- type: v_measure
value: 32.502518166001856
- task:
type: Reranking
dataset:
type: mteb/stackoverflowdupquestions-reranking
name: MTEB StackOverflowDupQuestions
config: default
split: test
revision: e185fbe320c72810689fc5848eb6114e1ef5ec69
metrics:
- type: map
value: 50.33775480236701
- type: mrr
value: 51.17302223919871
- task:
type: Summarization
dataset:
type: mteb/summeval
name: MTEB SummEval
config: default
split: test
revision: cda12ad7615edc362dbf25a00fdd61d3b1eaf93c
metrics:
- type: cos_sim_pearson
value: 30.561111309808208
- type: cos_sim_spearman
value: 30.2839254379273
- type: dot_pearson
value: 29.560242291401973
- type: dot_spearman
value: 30.51527274679116
- task:
type: Retrieval
dataset:
type: trec-covid
name: MTEB TRECCOVID
config: default
split: test
revision: None
metrics:
- type: map_at_1
value: 0.215
- type: map_at_10
value: 1.752
- type: map_at_100
value: 9.258
- type: map_at_1000
value: 23.438
- type: map_at_3
value: 0.6
- type: map_at_5
value: 0.968
- type: mrr_at_1
value: 84
- type: mrr_at_10
value: 91.333
- type: mrr_at_100
value: 91.333
- type: mrr_at_1000
value: 91.333
- type: mrr_at_3
value: 91.333
- type: mrr_at_5
value: 91.333
- type: ndcg_at_1
value: 75
- type: ndcg_at_10
value: 69.596
- type: ndcg_at_100
value: 51.970000000000006
- type: ndcg_at_1000
value: 48.864999999999995
- type: ndcg_at_3
value: 73.92699999999999
- type: ndcg_at_5
value: 73.175
- type: precision_at_1
value: 84
- type: precision_at_10
value: 74
- type: precision_at_100
value: 53.2
- type: precision_at_1000
value: 21.836
- type: precision_at_3
value: 79.333
- type: precision_at_5
value: 78.4
- type: recall_at_1
value: 0.215
- type: recall_at_10
value: 1.9609999999999999
- type: recall_at_100
value: 12.809999999999999
- type: recall_at_1000
value: 46.418
- type: recall_at_3
value: 0.6479999999999999
- type: recall_at_5
value: 1.057
- task:
type: Retrieval
dataset:
type: webis-touche2020
name: MTEB Touche2020
config: default
split: test
revision: None
metrics:
- type: map_at_1
value: 3.066
- type: map_at_10
value: 10.508000000000001
- type: map_at_100
value: 16.258
- type: map_at_1000
value: 17.705000000000002
- type: map_at_3
value: 6.157
- type: map_at_5
value: 7.510999999999999
- type: mrr_at_1
value: 34.694
- type: mrr_at_10
value: 48.786
- type: mrr_at_100
value: 49.619
- type: mrr_at_1000
value: 49.619
- type: mrr_at_3
value: 45.918
- type: mrr_at_5
value: 46.837
- type: ndcg_at_1
value: 31.633
- type: ndcg_at_10
value: 26.401999999999997
- type: ndcg_at_100
value: 37.139
- type: ndcg_at_1000
value: 48.012
- type: ndcg_at_3
value: 31.875999999999998
- type: ndcg_at_5
value: 27.383000000000003
- type: precision_at_1
value: 34.694
- type: precision_at_10
value: 22.857
- type: precision_at_100
value: 7.611999999999999
- type: precision_at_1000
value: 1.492
- type: precision_at_3
value: 33.333
- type: precision_at_5
value: 26.122
- type: recall_at_1
value: 3.066
- type: recall_at_10
value: 16.239
- type: recall_at_100
value: 47.29
- type: recall_at_1000
value: 81.137
- type: recall_at_3
value: 7.069
- type: recall_at_5
value: 9.483
- task:
type: Classification
dataset:
type: mteb/toxic_conversations_50k
name: MTEB ToxicConversationsClassification
config: default
split: test
revision: d7c0de2777da35d6aae2200a62c6e0e5af397c4c
metrics:
- type: accuracy
value: 72.1126
- type: ap
value: 14.710862719285753
- type: f1
value: 55.437808972378846
- task:
type: Classification
dataset:
type: mteb/tweet_sentiment_extraction
name: MTEB TweetSentimentExtractionClassification
config: default
split: test
revision: d604517c81ca91fe16a244d1248fc021f9ecee7a
metrics:
- type: accuracy
value: 60.39049235993209
- type: f1
value: 60.69810537250234
- task:
type: Clustering
dataset:
type: mteb/twentynewsgroups-clustering
name: MTEB TwentyNewsgroupsClustering
config: default
split: test
revision: 6125ec4e24fa026cec8a478383ee943acfbd5449
metrics:
- type: v_measure
value: 48.15576640316866
- task:
type: PairClassification
dataset:
type: mteb/twittersemeval2015-pairclassification
name: MTEB TwitterSemEval2015
config: default
split: test
revision: 70970daeab8776df92f5ea462b6173c0b46fd2d1
metrics:
- type: cos_sim_accuracy
value: 86.52917684925792
- type: cos_sim_ap
value: 75.97497873817315
- type: cos_sim_f1
value: 70.01151926276718
- type: cos_sim_precision
value: 67.98409147402435
- type: cos_sim_recall
value: 72.16358839050132
- type: dot_accuracy
value: 82.47004828038385
- type: dot_ap
value: 62.48739894974198
- type: dot_f1
value: 59.13107511045656
- type: dot_precision
value: 55.27765029830197
- type: dot_recall
value: 63.562005277044854
- type: euclidean_accuracy
value: 86.46361089586935
- type: euclidean_ap
value: 75.59282886839452
- type: euclidean_f1
value: 69.6465443945099
- type: euclidean_precision
value: 64.52847175331982
- type: euclidean_recall
value: 75.64643799472296
- type: manhattan_accuracy
value: 86.43380818978363
- type: manhattan_ap
value: 75.5742420974403
- type: manhattan_f1
value: 69.8636926889715
- type: manhattan_precision
value: 65.8644859813084
- type: manhattan_recall
value: 74.37994722955145
- type: max_accuracy
value: 86.52917684925792
- type: max_ap
value: 75.97497873817315
- type: max_f1
value: 70.01151926276718
- task:
type: PairClassification
dataset:
type: mteb/twitterurlcorpus-pairclassification
name: MTEB TwitterURLCorpus
config: default
split: test
revision: 8b6510b0b1fa4e4c4f879467980e9be563ec1cdf
metrics:
- type: cos_sim_accuracy
value: 89.29056545193464
- type: cos_sim_ap
value: 86.63028865482376
- type: cos_sim_f1
value: 79.18166458532285
- type: cos_sim_precision
value: 75.70585756426465
- type: cos_sim_recall
value: 82.99199260856174
- type: dot_accuracy
value: 85.23305002522606
- type: dot_ap
value: 76.0482687263196
- type: dot_f1
value: 70.80484330484332
- type: dot_precision
value: 65.86933474688577
- type: dot_recall
value: 76.53988296889437
- type: euclidean_accuracy
value: 89.26145845461248
- type: euclidean_ap
value: 86.54073288416006
- type: euclidean_f1
value: 78.9721371479794
- type: euclidean_precision
value: 76.68649354417525
- type: euclidean_recall
value: 81.39821373575609
- type: manhattan_accuracy
value: 89.22847052431405
- type: manhattan_ap
value: 86.51250729037905
- type: manhattan_f1
value: 78.94601825044894
- type: manhattan_precision
value: 75.32694594027555
- type: manhattan_recall
value: 82.93039728980598
- type: max_accuracy
value: 89.29056545193464
- type: max_ap
value: 86.63028865482376
- type: max_f1
value: 79.18166458532285
language:
- en
license: mit
---
# E5-base-v2
[Text Embeddings by Weakly-Supervised Contrastive Pre-training](https://arxiv.org/pdf/2212.03533.pdf).
Liang Wang, Nan Yang, Xiaolong Huang, Binxing Jiao, Linjun Yang, Daxin Jiang, Rangan Majumder, Furu Wei, arXiv 2022
This model has 12 layers and the embedding size is 768.
## Usage
Below is an example to encode queries and passages from the MS-MARCO passage ranking dataset.
```python
import torch.nn.functional as F
from torch import Tensor
from transformers import AutoTokenizer, AutoModel
def average_pool(last_hidden_states: Tensor,
attention_mask: Tensor) -> Tensor:
last_hidden = last_hidden_states.masked_fill(~attention_mask[..., None].bool(), 0.0)
return last_hidden.sum(dim=1) / attention_mask.sum(dim=1)[..., None]
# Each input text should start with "query: " or "passage: ".
# For tasks other than retrieval, you can simply use the "query: " prefix.
input_texts = ['query: how much protein should a female eat',
'query: summit define',
"passage: As a general guideline, the CDC's average requirement of protein for women ages 19 to 70 is 46 grams per day. But, as you can see from this chart, you'll need to increase that if you're expecting or training for a marathon. Check out the chart below to see how much protein you should be eating each day.",
"passage: Definition of summit for English Language Learners. : 1 the highest point of a mountain : the top of a mountain. : 2 the highest level. : 3 a meeting or series of meetings between the leaders of two or more governments."]
tokenizer = AutoTokenizer.from_pretrained('intfloat/e5-base-v2')
model = AutoModel.from_pretrained('intfloat/e5-base-v2')
# Tokenize the input texts
batch_dict = tokenizer(input_texts, max_length=512, padding=True, truncation=True, return_tensors='pt')
outputs = model(**batch_dict)
embeddings = average_pool(outputs.last_hidden_state, batch_dict['attention_mask'])
# normalize embeddings
embeddings = F.normalize(embeddings, p=2, dim=1)
scores = (embeddings[:2] @ embeddings[2:].T) * 100
print(scores.tolist())
```
## Training Details
Please refer to our paper at [https://arxiv.org/pdf/2212.03533.pdf](https://arxiv.org/pdf/2212.03533.pdf).
## Benchmark Evaluation
Check out [unilm/e5](https://github.com/microsoft/unilm/tree/master/e5) to reproduce evaluation results
on the [BEIR](https://arxiv.org/abs/2104.08663) and [MTEB benchmark](https://arxiv.org/abs/2210.07316).
## Support for Sentence Transformers
Below is an example for usage with sentence_transformers.
```python
from sentence_transformers import SentenceTransformer
model = SentenceTransformer('intfloat/e5-base-v2')
input_texts = [
'query: how much protein should a female eat',
'query: summit define',
"passage: As a general guideline, the CDC's average requirement of protein for women ages 19 to 70 is 46 grams per day. But, as you can see from this chart, you'll need to increase that if you're expecting or training for a marathon. Check out the chart below to see how much protein you should be eating each day.",
"passage: Definition of summit for English Language Learners. : 1 the highest point of a mountain : the top of a mountain. : 2 the highest level. : 3 a meeting or series of meetings between the leaders of two or more governments."
]
embeddings = model.encode(input_texts, normalize_embeddings=True)
```
Package requirements
`pip install sentence_transformers~=2.2.2`
Contributors: [michaelfeil](https://huggingface.co/michaelfeil)
## FAQ
**1. Do I need to add the prefix "query: " and "passage: " to input texts?**
Yes, this is how the model is trained, otherwise you will see a performance degradation.
Here are some rules of thumb:
- Use "query: " and "passage: " correspondingly for asymmetric tasks such as passage retrieval in open QA, ad-hoc information retrieval.
- Use "query: " prefix for symmetric tasks such as semantic similarity, paraphrase retrieval.
- Use "query: " prefix if you want to use embeddings as features, such as linear probing classification, clustering.
**2. Why are my reproduced results slightly different from reported in the model card?**
Different versions of `transformers` and `pytorch` could cause negligible but non-zero performance differences.
**3. Why does the cosine similarity scores distribute around 0.7 to 1.0?**
This is a known and expected behavior as we use a low temperature 0.01 for InfoNCE contrastive loss.
For text embedding tasks like text retrieval or semantic similarity,
what matters is the relative order of the scores instead of the absolute values,
so this should not be an issue.
## Citation
If you find our paper or models helpful, please consider cite as follows:
```
@article{wang2022text,
title={Text Embeddings by Weakly-Supervised Contrastive Pre-training},
author={Wang, Liang and Yang, Nan and Huang, Xiaolong and Jiao, Binxing and Yang, Linjun and Jiang, Daxin and Majumder, Rangan and Wei, Furu},
journal={arXiv preprint arXiv:2212.03533},
year={2022}
}
```
## Limitations
This model only works for English texts. Long texts will be truncated to at most 512 tokens.
|
openai-community/roberta-large-openai-detector | openai-community | "2024-04-10T09:56:29Z" | 410,776 | 19 | transformers | [
"transformers",
"pytorch",
"jax",
"safetensors",
"roberta",
"text-classification",
"exbert",
"en",
"dataset:bookcorpus",
"dataset:wikipedia",
"arxiv:1904.09751",
"arxiv:1910.09700",
"arxiv:1908.09203",
"license:mit",
"autotrain_compatible",
"endpoints_compatible",
"region:us"
] | text-classification | "2022-03-02T23:29:04Z" | ---
language: en
license: mit
tags:
- exbert
datasets:
- bookcorpus
- wikipedia
---
# RoBERTa Large OpenAI Detector
## Table of Contents
- [Model Details](#model-details)
- [Uses](#uses)
- [Risks, Limitations and Biases](#risks-limitations-and-biases)
- [Training](#training)
- [Evaluation](#evaluation)
- [Environmental Impact](#environmental-impact)
- [Technical Specifications](#technical-specifications)
- [Citation Information](#citation-information)
- [Model Card Authors](#model-card-authors)
- [How To Get Started With the Model](#how-to-get-started-with-the-model)
## Model Details
**Model Description:** RoBERTa large OpenAI Detector is the GPT-2 output detector model, obtained by fine-tuning a RoBERTa large model with the outputs of the 1.5B-parameter GPT-2 model. The model can be used to predict if text was generated by a GPT-2 model. This model was released by OpenAI at the same time as OpenAI released the weights of the [largest GPT-2 model](https://huggingface.co/gpt2-xl), the 1.5B parameter version.
- **Developed by:** OpenAI, see [GitHub Repo](https://github.com/openai/gpt-2-output-dataset/tree/master/detector) and [associated paper](https://d4mucfpksywv.cloudfront.net/papers/GPT_2_Report.pdf) for full author list
- **Model Type:** Fine-tuned transformer-based language model
- **Language(s):** English
- **License:** MIT
- **Related Models:** [RoBERTa large](https://huggingface.co/roberta-large), [GPT-XL (1.5B parameter version)](https://huggingface.co/gpt2-xl), [GPT-Large (the 774M parameter version)](https://huggingface.co/gpt2-large), [GPT-Medium (the 355M parameter version)](https://huggingface.co/gpt2-medium) and [GPT-2 (the 124M parameter version)](https://huggingface.co/gpt2)
- **Resources for more information:**
- [Research Paper](https://d4mucfpksywv.cloudfront.net/papers/GPT_2_Report.pdf) (see, in particular, the section beginning on page 12 about Automated ML-based detection).
- [GitHub Repo](https://github.com/openai/gpt-2-output-dataset/tree/master/detector)
- [OpenAI Blog Post](https://openai.com/blog/gpt-2-1-5b-release/)
- [Explore the detector model here](https://huggingface.co/openai-detector )
## Uses
#### Direct Use
The model is a classifier that can be used to detect text generated by GPT-2 models.
#### Downstream Use
The model's developers have stated that they developed and released the model to help with research related to synthetic text generation, so the model could potentially be used for downstream tasks related to synthetic text generation. See the [associated paper](https://d4mucfpksywv.cloudfront.net/papers/GPT_2_Report.pdf) for further discussion.
#### Misuse and Out-of-scope Use
The model should not be used to intentionally create hostile or alienating environments for people. In addition, the model developers discuss the risk of adversaries using the model to better evade detection in their [associated paper](https://d4mucfpksywv.cloudfront.net/papers/GPT_2_Report.pdf), suggesting that using the model for evading detection or for supporting efforts to evade detection would be a misuse of the model.
## Risks, Limitations and Biases
**CONTENT WARNING: Readers should be aware this section may contain content that is disturbing, offensive, and can propagate historical and current stereotypes.**
Users (both direct and downstream) should be made aware of the risks, biases and limitations of the model.
#### Risks and Limitations
In their [associated paper](https://d4mucfpksywv.cloudfront.net/papers/GPT_2_Report.pdf), the model developers discuss the risk that the model may be used by bad actors to develop capabilities for evading detection, though one purpose of releasing the model is to help improve detection research.
In a related [blog post](https://openai.com/blog/gpt-2-1-5b-release/), the model developers also discuss the limitations of automated methods for detecting synthetic text and the need to pair automated detection tools with other, non-automated approaches. They write:
> We conducted in-house detection research and developed a detection model that has detection rates of ~95% for detecting 1.5B GPT-2-generated text. We believe this is not high enough accuracy for standalone detection and needs to be paired with metadata-based approaches, human judgment, and public education to be more effective.
The model developers also [report](https://openai.com/blog/gpt-2-1-5b-release/) finding that classifying content from larger models is more difficult, suggesting that detection with automated tools like this model will be increasingly difficult as model sizes increase. The authors find that training detector models on the outputs of larger models can improve accuracy and robustness.
#### Bias
Significant research has explored bias and fairness issues with language models (see, e.g., [Sheng et al. (2021)](https://aclanthology.org/2021.acl-long.330.pdf) and [Bender et al. (2021)](https://dl.acm.org/doi/pdf/10.1145/3442188.3445922)). Predictions generated by RoBERTa large and GPT-2 1.5B (which this model is built/fine-tuned on) can include disturbing and harmful stereotypes across protected classes; identity characteristics; and sensitive, social, and occupational groups (see the [RoBERTa large](https://huggingface.co/roberta-large) and [GPT-2 XL](https://huggingface.co/gpt2-xl) model cards for more information). The developers of this model discuss these issues further in their [paper](https://d4mucfpksywv.cloudfront.net/papers/GPT_2_Report.pdf).
## Training
#### Training Data
The model is a sequence classifier based on RoBERTa large (see the [RoBERTa large model card](https://huggingface.co/roberta-large) for more details on the RoBERTa large training data) and then fine-tuned using the outputs of the 1.5B GPT-2 model (available [here](https://github.com/openai/gpt-2-output-dataset)).
#### Training Procedure
The model developers write that:
> We based a sequence classifier on RoBERTaLARGE (355 million parameters) and fine-tuned it to classify the outputs from the 1.5B GPT-2 model versus WebText, the dataset we used to train the GPT-2 model.
They later state:
> To develop a robust detector model that can accurately classify generated texts regardless of the sampling method, we performed an analysis of the model’s transfer performance.
See the [associated paper](https://d4mucfpksywv.cloudfront.net/papers/GPT_2_Report.pdf) for further details on the training procedure.
## Evaluation
The following evaluation information is extracted from the [associated paper](https://d4mucfpksywv.cloudfront.net/papers/GPT_2_Report.pdf).
#### Testing Data, Factors and Metrics
The model is intended to be used for detecting text generated by GPT-2 models, so the model developers test the model on text datasets, measuring accuracy by:
> testing 510-token test examples comprised of 5,000 samples from the WebText dataset and 5,000 samples generated by a GPT-2 model, which were not used during the training.
#### Results
The model developers [find](https://d4mucfpksywv.cloudfront.net/papers/GPT_2_Report.pdf):
> Our classifier is able to detect 1.5 billion parameter GPT-2-generated text with approximately 95% accuracy...The model’s accuracy depends on sampling methods used when generating outputs, like temperature, Top-K, and nucleus sampling ([Holtzman et al., 2019](https://arxiv.org/abs/1904.09751). Nucleus sampling outputs proved most difficult to correctly classify, but a detector trained using nucleus sampling transfers well across other sampling methods. As seen in Figure 1 [in the paper], we found consistently high accuracy when trained on nucleus sampling.
See the [associated paper](https://d4mucfpksywv.cloudfront.net/papers/GPT_2_Report.pdf), Figure 1 (on page 14) and Figure 2 (on page 16) for full results.
## Environmental Impact
Carbon emissions can be estimated using the [Machine Learning Impact calculator](https://mlco2.github.io/impact#compute) presented in [Lacoste et al. (2019)](https://arxiv.org/abs/1910.09700).
- **Hardware Type:** Unknown
- **Hours used:** Unknown
- **Cloud Provider:** Unknown
- **Compute Region:** Unknown
- **Carbon Emitted:** Unknown
## Technical Specifications
The model developers write that:
See the [associated paper](https://d4mucfpksywv.cloudfront.net/papers/GPT_2_Report.pdf) for further details on the modeling architecture and training details.
## Citation Information
```bibtex
@article{solaiman2019release,
title={Release strategies and the social impacts of language models},
author={Solaiman, Irene and Brundage, Miles and Clark, Jack and Askell, Amanda and Herbert-Voss, Ariel and Wu, Jeff and Radford, Alec and Krueger, Gretchen and Kim, Jong Wook and Kreps, Sarah and others},
journal={arXiv preprint arXiv:1908.09203},
year={2019}
}
```
APA:
- Solaiman, I., Brundage, M., Clark, J., Askell, A., Herbert-Voss, A., Wu, J., ... & Wang, J. (2019). Release strategies and the social impacts of language models. arXiv preprint arXiv:1908.09203.
https://huggingface.co/papers/1908.09203
## Model Card Authors
This model card was written by the team at Hugging Face.
## How to Get Started with the Model
More information needed |
openai/whisper-tiny | openai | "2024-02-29T10:57:33Z" | 410,395 | 251 | transformers | [
"transformers",
"pytorch",
"tf",
"jax",
"safetensors",
"whisper",
"automatic-speech-recognition",
"audio",
"hf-asr-leaderboard",
"en",
"zh",
"de",
"es",
"ru",
"ko",
"fr",
"ja",
"pt",
"tr",
"pl",
"ca",
"nl",
"ar",
"sv",
"it",
"id",
"hi",
"fi",
"vi",
"he",
"uk",
"el",
"ms",
"cs",
"ro",
"da",
"hu",
"ta",
"no",
"th",
"ur",
"hr",
"bg",
"lt",
"la",
"mi",
"ml",
"cy",
"sk",
"te",
"fa",
"lv",
"bn",
"sr",
"az",
"sl",
"kn",
"et",
"mk",
"br",
"eu",
"is",
"hy",
"ne",
"mn",
"bs",
"kk",
"sq",
"sw",
"gl",
"mr",
"pa",
"si",
"km",
"sn",
"yo",
"so",
"af",
"oc",
"ka",
"be",
"tg",
"sd",
"gu",
"am",
"yi",
"lo",
"uz",
"fo",
"ht",
"ps",
"tk",
"nn",
"mt",
"sa",
"lb",
"my",
"bo",
"tl",
"mg",
"as",
"tt",
"haw",
"ln",
"ha",
"ba",
"jw",
"su",
"arxiv:2212.04356",
"license:apache-2.0",
"model-index",
"endpoints_compatible",
"region:us"
] | automatic-speech-recognition | "2022-09-26T06:50:30Z" | ---
language:
- en
- zh
- de
- es
- ru
- ko
- fr
- ja
- pt
- tr
- pl
- ca
- nl
- ar
- sv
- it
- id
- hi
- fi
- vi
- he
- uk
- el
- ms
- cs
- ro
- da
- hu
- ta
- no
- th
- ur
- hr
- bg
- lt
- la
- mi
- ml
- cy
- sk
- te
- fa
- lv
- bn
- sr
- az
- sl
- kn
- et
- mk
- br
- eu
- is
- hy
- ne
- mn
- bs
- kk
- sq
- sw
- gl
- mr
- pa
- si
- km
- sn
- yo
- so
- af
- oc
- ka
- be
- tg
- sd
- gu
- am
- yi
- lo
- uz
- fo
- ht
- ps
- tk
- nn
- mt
- sa
- lb
- my
- bo
- tl
- mg
- as
- tt
- haw
- ln
- ha
- ba
- jw
- su
tags:
- audio
- automatic-speech-recognition
- hf-asr-leaderboard
widget:
- example_title: Librispeech sample 1
src: https://cdn-media.huggingface.co/speech_samples/sample1.flac
- example_title: Librispeech sample 2
src: https://cdn-media.huggingface.co/speech_samples/sample2.flac
model-index:
- name: whisper-tiny
results:
- task:
name: Automatic Speech Recognition
type: automatic-speech-recognition
dataset:
name: LibriSpeech (clean)
type: librispeech_asr
config: clean
split: test
args:
language: en
metrics:
- name: Test WER
type: wer
value: 7.54
- task:
name: Automatic Speech Recognition
type: automatic-speech-recognition
dataset:
name: LibriSpeech (other)
type: librispeech_asr
config: other
split: test
args:
language: en
metrics:
- name: Test WER
type: wer
value: 17.15
- task:
name: Automatic Speech Recognition
type: automatic-speech-recognition
dataset:
name: Common Voice 11.0
type: mozilla-foundation/common_voice_11_0
config: hi
split: test
args:
language: hi
metrics:
- name: Test WER
type: wer
value: 141
pipeline_tag: automatic-speech-recognition
license: apache-2.0
---
# Whisper
Whisper is a pre-trained model for automatic speech recognition (ASR) and speech translation. Trained on 680k hours
of labelled data, Whisper models demonstrate a strong ability to generalise to many datasets and domains **without** the need
for fine-tuning.
Whisper was proposed in the paper [Robust Speech Recognition via Large-Scale Weak Supervision](https://arxiv.org/abs/2212.04356)
by Alec Radford et al from OpenAI. The original code repository can be found [here](https://github.com/openai/whisper).
**Disclaimer**: Content for this model card has partly been written by the Hugging Face team, and parts of it were
copied and pasted from the original model card.
## Model details
Whisper is a Transformer based encoder-decoder model, also referred to as a _sequence-to-sequence_ model.
It was trained on 680k hours of labelled speech data annotated using large-scale weak supervision.
The models were trained on either English-only data or multilingual data. The English-only models were trained
on the task of speech recognition. The multilingual models were trained on both speech recognition and speech
translation. For speech recognition, the model predicts transcriptions in the *same* language as the audio.
For speech translation, the model predicts transcriptions to a *different* language to the audio.
Whisper checkpoints come in five configurations of varying model sizes.
The smallest four are trained on either English-only or multilingual data.
The largest checkpoints are multilingual only. All ten of the pre-trained checkpoints
are available on the [Hugging Face Hub](https://huggingface.co/models?search=openai/whisper). The
checkpoints are summarised in the following table with links to the models on the Hub:
| Size | Parameters | English-only | Multilingual |
|----------|------------|------------------------------------------------------|-----------------------------------------------------|
| tiny | 39 M | [✓](https://huggingface.co/openai/whisper-tiny.en) | [✓](https://huggingface.co/openai/whisper-tiny) |
| base | 74 M | [✓](https://huggingface.co/openai/whisper-base.en) | [✓](https://huggingface.co/openai/whisper-base) |
| small | 244 M | [✓](https://huggingface.co/openai/whisper-small.en) | [✓](https://huggingface.co/openai/whisper-small) |
| medium | 769 M | [✓](https://huggingface.co/openai/whisper-medium.en) | [✓](https://huggingface.co/openai/whisper-medium) |
| large | 1550 M | x | [✓](https://huggingface.co/openai/whisper-large) |
| large-v2 | 1550 M | x | [✓](https://huggingface.co/openai/whisper-large-v2) |
# Usage
To transcribe audio samples, the model has to be used alongside a [`WhisperProcessor`](https://huggingface.co/docs/transformers/model_doc/whisper#transformers.WhisperProcessor).
The `WhisperProcessor` is used to:
1. Pre-process the audio inputs (converting them to log-Mel spectrograms for the model)
2. Post-process the model outputs (converting them from tokens to text)
The model is informed of which task to perform (transcription or translation) by passing the appropriate "context tokens". These context tokens
are a sequence of tokens that are given to the decoder at the start of the decoding process, and take the following order:
1. The transcription always starts with the `<|startoftranscript|>` token
2. The second token is the language token (e.g. `<|en|>` for English)
3. The third token is the "task token". It can take one of two values: `<|transcribe|>` for speech recognition or `<|translate|>` for speech translation
4. In addition, a `<|notimestamps|>` token is added if the model should not include timestamp prediction
Thus, a typical sequence of context tokens might look as follows:
```
<|startoftranscript|> <|en|> <|transcribe|> <|notimestamps|>
```
Which tells the model to decode in English, under the task of speech recognition, and not to predict timestamps.
These tokens can either be forced or un-forced. If they are forced, the model is made to predict each token at
each position. This allows one to control the output language and task for the Whisper model. If they are un-forced,
the Whisper model will automatically predict the output langauge and task itself.
The context tokens can be set accordingly:
```python
model.config.forced_decoder_ids = WhisperProcessor.get_decoder_prompt_ids(language="english", task="transcribe")
```
Which forces the model to predict in English under the task of speech recognition.
## Transcription
### English to English
In this example, the context tokens are 'unforced', meaning the model automatically predicts the output language
(English) and task (transcribe).
```python
>>> from transformers import WhisperProcessor, WhisperForConditionalGeneration
>>> from datasets import load_dataset
>>> # load model and processor
>>> processor = WhisperProcessor.from_pretrained("openai/whisper-tiny")
>>> model = WhisperForConditionalGeneration.from_pretrained("openai/whisper-tiny")
>>> model.config.forced_decoder_ids = None
>>> # load dummy dataset and read audio files
>>> ds = load_dataset("hf-internal-testing/librispeech_asr_dummy", "clean", split="validation")
>>> sample = ds[0]["audio"]
>>> input_features = processor(sample["array"], sampling_rate=sample["sampling_rate"], return_tensors="pt").input_features
>>> # generate token ids
>>> predicted_ids = model.generate(input_features)
>>> # decode token ids to text
>>> transcription = processor.batch_decode(predicted_ids, skip_special_tokens=False)
['<|startoftranscript|><|en|><|transcribe|><|notimestamps|> Mr. Quilter is the apostle of the middle classes and we are glad to welcome his gospel.<|endoftext|>']
>>> transcription = processor.batch_decode(predicted_ids, skip_special_tokens=True)
[' Mr. Quilter is the apostle of the middle classes and we are glad to welcome his gospel.']
```
The context tokens can be removed from the start of the transcription by setting `skip_special_tokens=True`.
### French to French
The following example demonstrates French to French transcription by setting the decoder ids appropriately.
```python
>>> from transformers import WhisperProcessor, WhisperForConditionalGeneration
>>> from datasets import Audio, load_dataset
>>> # load model and processor
>>> processor = WhisperProcessor.from_pretrained("openai/whisper-tiny")
>>> model = WhisperForConditionalGeneration.from_pretrained("openai/whisper-tiny")
>>> forced_decoder_ids = processor.get_decoder_prompt_ids(language="french", task="transcribe")
>>> # load streaming dataset and read first audio sample
>>> ds = load_dataset("common_voice", "fr", split="test", streaming=True)
>>> ds = ds.cast_column("audio", Audio(sampling_rate=16_000))
>>> input_speech = next(iter(ds))["audio"]
>>> input_features = processor(input_speech["array"], sampling_rate=input_speech["sampling_rate"], return_tensors="pt").input_features
>>> # generate token ids
>>> predicted_ids = model.generate(input_features, forced_decoder_ids=forced_decoder_ids)
>>> # decode token ids to text
>>> transcription = processor.batch_decode(predicted_ids)
['<|startoftranscript|><|fr|><|transcribe|><|notimestamps|> Un vrai travail intéressant va enfin être mené sur ce sujet.<|endoftext|>']
>>> transcription = processor.batch_decode(predicted_ids, skip_special_tokens=True)
[' Un vrai travail intéressant va enfin être mené sur ce sujet.']
```
## Translation
Setting the task to "translate" forces the Whisper model to perform speech translation.
### French to English
```python
>>> from transformers import WhisperProcessor, WhisperForConditionalGeneration
>>> from datasets import Audio, load_dataset
>>> # load model and processor
>>> processor = WhisperProcessor.from_pretrained("openai/whisper-tiny")
>>> model = WhisperForConditionalGeneration.from_pretrained("openai/whisper-tiny")
>>> forced_decoder_ids = processor.get_decoder_prompt_ids(language="french", task="translate")
>>> # load streaming dataset and read first audio sample
>>> ds = load_dataset("common_voice", "fr", split="test", streaming=True)
>>> ds = ds.cast_column("audio", Audio(sampling_rate=16_000))
>>> input_speech = next(iter(ds))["audio"]
>>> input_features = processor(input_speech["array"], sampling_rate=input_speech["sampling_rate"], return_tensors="pt").input_features
>>> # generate token ids
>>> predicted_ids = model.generate(input_features, forced_decoder_ids=forced_decoder_ids)
>>> # decode token ids to text
>>> transcription = processor.batch_decode(predicted_ids, skip_special_tokens=True)
[' A very interesting work, we will finally be given on this subject.']
```
## Evaluation
This code snippet shows how to evaluate Whisper Tiny on [LibriSpeech test-clean](https://huggingface.co/datasets/librispeech_asr):
```python
>>> from datasets import load_dataset
>>> from transformers import WhisperForConditionalGeneration, WhisperProcessor
>>> import torch
>>> from evaluate import load
>>> librispeech_test_clean = load_dataset("librispeech_asr", "clean", split="test")
>>> processor = WhisperProcessor.from_pretrained("openai/whisper-tiny")
>>> model = WhisperForConditionalGeneration.from_pretrained("openai/whisper-tiny").to("cuda")
>>> def map_to_pred(batch):
>>> audio = batch["audio"]
>>> input_features = processor(audio["array"], sampling_rate=audio["sampling_rate"], return_tensors="pt").input_features
>>> batch["reference"] = processor.tokenizer._normalize(batch['text'])
>>>
>>> with torch.no_grad():
>>> predicted_ids = model.generate(input_features.to("cuda"))[0]
>>> transcription = processor.decode(predicted_ids)
>>> batch["prediction"] = processor.tokenizer._normalize(transcription)
>>> return batch
>>> result = librispeech_test_clean.map(map_to_pred)
>>> wer = load("wer")
>>> print(100 * wer.compute(references=result["reference"], predictions=result["prediction"]))
7.547098647858638
```
## Long-Form Transcription
The Whisper model is intrinsically designed to work on audio samples of up to 30s in duration. However, by using a chunking
algorithm, it can be used to transcribe audio samples of up to arbitrary length. This is possible through Transformers
[`pipeline`](https://huggingface.co/docs/transformers/main_classes/pipelines#transformers.AutomaticSpeechRecognitionPipeline)
method. Chunking is enabled by setting `chunk_length_s=30` when instantiating the pipeline. With chunking enabled, the pipeline
can be run with batched inference. It can also be extended to predict sequence level timestamps by passing `return_timestamps=True`:
```python
>>> import torch
>>> from transformers import pipeline
>>> from datasets import load_dataset
>>> device = "cuda:0" if torch.cuda.is_available() else "cpu"
>>> pipe = pipeline(
>>> "automatic-speech-recognition",
>>> model="openai/whisper-tiny",
>>> chunk_length_s=30,
>>> device=device,
>>> )
>>> ds = load_dataset("hf-internal-testing/librispeech_asr_dummy", "clean", split="validation")
>>> sample = ds[0]["audio"]
>>> prediction = pipe(sample.copy(), batch_size=8)["text"]
" Mr. Quilter is the apostle of the middle classes, and we are glad to welcome his gospel."
>>> # we can also return timestamps for the predictions
>>> prediction = pipe(sample.copy(), batch_size=8, return_timestamps=True)["chunks"]
[{'text': ' Mr. Quilter is the apostle of the middle classes and we are glad to welcome his gospel.',
'timestamp': (0.0, 5.44)}]
```
Refer to the blog post [ASR Chunking](https://huggingface.co/blog/asr-chunking) for more details on the chunking algorithm.
## Fine-Tuning
The pre-trained Whisper model demonstrates a strong ability to generalise to different datasets and domains. However,
its predictive capabilities can be improved further for certain languages and tasks through *fine-tuning*. The blog
post [Fine-Tune Whisper with 🤗 Transformers](https://huggingface.co/blog/fine-tune-whisper) provides a step-by-step
guide to fine-tuning the Whisper model with as little as 5 hours of labelled data.
### Evaluated Use
The primary intended users of these models are AI researchers studying robustness, generalization, capabilities, biases, and constraints of the current model. However, Whisper is also potentially quite useful as an ASR solution for developers, especially for English speech recognition. We recognize that once models are released, it is impossible to restrict access to only “intended” uses or to draw reasonable guidelines around what is or is not research.
The models are primarily trained and evaluated on ASR and speech translation to English tasks. They show strong ASR results in ~10 languages. They may exhibit additional capabilities, particularly if fine-tuned on certain tasks like voice activity detection, speaker classification, or speaker diarization but have not been robustly evaluated in these areas. We strongly recommend that users perform robust evaluations of the models in a particular context and domain before deploying them.
In particular, we caution against using Whisper models to transcribe recordings of individuals taken without their consent or purporting to use these models for any kind of subjective classification. We recommend against use in high-risk domains like decision-making contexts, where flaws in accuracy can lead to pronounced flaws in outcomes. The models are intended to transcribe and translate speech, use of the model for classification is not only not evaluated but also not appropriate, particularly to infer human attributes.
## Training Data
The models are trained on 680,000 hours of audio and the corresponding transcripts collected from the internet. 65% of this data (or 438,000 hours) represents English-language audio and matched English transcripts, roughly 18% (or 126,000 hours) represents non-English audio and English transcripts, while the final 17% (or 117,000 hours) represents non-English audio and the corresponding transcript. This non-English data represents 98 different languages.
As discussed in [the accompanying paper](https://cdn.openai.com/papers/whisper.pdf), we see that performance on transcription in a given language is directly correlated with the amount of training data we employ in that language.
## Performance and Limitations
Our studies show that, over many existing ASR systems, the models exhibit improved robustness to accents, background noise, technical language, as well as zero shot translation from multiple languages into English; and that accuracy on speech recognition and translation is near the state-of-the-art level.
However, because the models are trained in a weakly supervised manner using large-scale noisy data, the predictions may include texts that are not actually spoken in the audio input (i.e. hallucination). We hypothesize that this happens because, given their general knowledge of language, the models combine trying to predict the next word in audio with trying to transcribe the audio itself.
Our models perform unevenly across languages, and we observe lower accuracy on low-resource and/or low-discoverability languages or languages where we have less training data. The models also exhibit disparate performance on different accents and dialects of particular languages, which may include higher word error rate across speakers of different genders, races, ages, or other demographic criteria. Our full evaluation results are presented in [the paper accompanying this release](https://cdn.openai.com/papers/whisper.pdf).
In addition, the sequence-to-sequence architecture of the model makes it prone to generating repetitive texts, which can be mitigated to some degree by beam search and temperature scheduling but not perfectly. Further analysis on these limitations are provided in [the paper](https://cdn.openai.com/papers/whisper.pdf). It is likely that this behavior and hallucinations may be worse on lower-resource and/or lower-discoverability languages.
## Broader Implications
We anticipate that Whisper models’ transcription capabilities may be used for improving accessibility tools. While Whisper models cannot be used for real-time transcription out of the box – their speed and size suggest that others may be able to build applications on top of them that allow for near-real-time speech recognition and translation. The real value of beneficial applications built on top of Whisper models suggests that the disparate performance of these models may have real economic implications.
There are also potential dual use concerns that come with releasing Whisper. While we hope the technology will be used primarily for beneficial purposes, making ASR technology more accessible could enable more actors to build capable surveillance technologies or scale up existing surveillance efforts, as the speed and accuracy allow for affordable automatic transcription and translation of large volumes of audio communication. Moreover, these models may have some capabilities to recognize specific individuals out of the box, which in turn presents safety concerns related both to dual use and disparate performance. In practice, we expect that the cost of transcription is not the limiting factor of scaling up surveillance projects.
### BibTeX entry and citation info
```bibtex
@misc{radford2022whisper,
doi = {10.48550/ARXIV.2212.04356},
url = {https://arxiv.org/abs/2212.04356},
author = {Radford, Alec and Kim, Jong Wook and Xu, Tao and Brockman, Greg and McLeavey, Christine and Sutskever, Ilya},
title = {Robust Speech Recognition via Large-Scale Weak Supervision},
publisher = {arXiv},
year = {2022},
copyright = {arXiv.org perpetual, non-exclusive license}
}
```
|
google/gemma-2-9b-it | google | "2024-08-27T19:41:49Z" | 410,033 | 545 | transformers | [
"transformers",
"safetensors",
"gemma2",
"text-generation",
"conversational",
"arxiv:2009.03300",
"arxiv:1905.07830",
"arxiv:1911.11641",
"arxiv:1904.09728",
"arxiv:1905.10044",
"arxiv:1907.10641",
"arxiv:1811.00937",
"arxiv:1809.02789",
"arxiv:1911.01547",
"arxiv:1705.03551",
"arxiv:2107.03374",
"arxiv:2108.07732",
"arxiv:2110.14168",
"arxiv:2009.11462",
"arxiv:2101.11718",
"arxiv:2110.08193",
"arxiv:1804.09301",
"arxiv:2109.07958",
"arxiv:1804.06876",
"arxiv:2103.03874",
"arxiv:2304.06364",
"arxiv:2206.04615",
"arxiv:2203.09509",
"base_model:google/gemma-2-9b",
"base_model:finetune:google/gemma-2-9b",
"license:gemma",
"autotrain_compatible",
"text-generation-inference",
"endpoints_compatible",
"region:us"
] | text-generation | "2024-06-24T08:05:41Z" | ---
license: gemma
library_name: transformers
pipeline_tag: text-generation
extra_gated_heading: Access Gemma on Hugging Face
extra_gated_prompt: >-
To access Gemma on Hugging Face, you’re required to review and agree to
Google’s usage license. To do this, please ensure you’re logged in to Hugging
Face and click below. Requests are processed immediately.
extra_gated_button_content: Acknowledge license
tags:
- conversational
base_model: google/gemma-2-9b
---
# Gemma 2 model card
**Model Page**: [Gemma](https://ai.google.dev/gemma/docs)
**Resources and Technical Documentation**:
* [Responsible Generative AI Toolkit][rai-toolkit]
* [Gemma on Kaggle][kaggle-gemma]
* [Gemma on Vertex Model Garden][vertex-mg-gemma]
**Terms of Use**: [Terms](https://www.kaggle.com/models/google/gemma/license/consent/verify/huggingface?returnModelRepoId=google/gemma-2-9b-it)
**Authors**: Google
## Model Information
Summary description and brief definition of inputs and outputs.
### Description
Gemma is a family of lightweight, state-of-the-art open models from Google,
built from the same research and technology used to create the Gemini models.
They are text-to-text, decoder-only large language models, available in English,
with open weights for both pre-trained variants and instruction-tuned variants.
Gemma models are well-suited for a variety of text generation tasks, including
question answering, summarization, and reasoning. Their relatively small size
makes it possible to deploy them in environments with limited resources such as
a laptop, desktop or your own cloud infrastructure, democratizing access to
state of the art AI models and helping foster innovation for everyone.
### Usage
Below we share some code snippets on how to get quickly started with running the model. First, install the Transformers library with:
```sh
pip install -U transformers
```
Then, copy the snippet from the section that is relevant for your usecase.
#### Running with the `pipeline` API
```python
import torch
from transformers import pipeline
pipe = pipeline(
"text-generation",
model="google/gemma-2-9b-it",
model_kwargs={"torch_dtype": torch.bfloat16},
device="cuda", # replace with "mps" to run on a Mac device
)
messages = [
{"role": "user", "content": "Who are you? Please, answer in pirate-speak."},
]
outputs = pipe(messages, max_new_tokens=256)
assistant_response = outputs[0]["generated_text"][-1]["content"].strip()
print(assistant_response)
# Ahoy, matey! I be Gemma, a digital scallywag, a language-slingin' parrot of the digital seas. I be here to help ye with yer wordy woes, answer yer questions, and spin ye yarns of the digital world. So, what be yer pleasure, eh? 🦜
```
#### Running the model on a single / multi GPU
```python
# pip install accelerate
from transformers import AutoTokenizer, AutoModelForCausalLM
import torch
tokenizer = AutoTokenizer.from_pretrained("google/gemma-2-9b-it")
model = AutoModelForCausalLM.from_pretrained(
"google/gemma-2-9b-it",
device_map="auto",
torch_dtype=torch.bfloat16,
)
input_text = "Write me a poem about Machine Learning."
input_ids = tokenizer(input_text, return_tensors="pt").to("cuda")
outputs = model.generate(**input_ids, max_new_tokens=32)
print(tokenizer.decode(outputs[0]))
```
You can ensure the correct chat template is applied by using `tokenizer.apply_chat_template` as follows:
```python
messages = [
{"role": "user", "content": "Write me a poem about Machine Learning."},
]
input_ids = tokenizer.apply_chat_template(messages, return_tensors="pt", return_dict=True).to("cuda")
outputs = model.generate(**input_ids, max_new_tokens=256)
print(tokenizer.decode(outputs[0]))
```
<a name="precisions"></a>
#### Running the model on a GPU using different precisions
The native weights of this model were exported in `bfloat16` precision.
You can also use `float32` if you skip the dtype, but no precision increase will occur (model weights will just be upcasted to `float32`). See examples below.
* _Upcasting to `torch.float32`_
```python
# pip install accelerate
from transformers import AutoTokenizer, AutoModelForCausalLM
tokenizer = AutoTokenizer.from_pretrained("google/gemma-2-9b-it")
model = AutoModelForCausalLM.from_pretrained(
"google/gemma-2-9b-it",
device_map="auto",
)
input_text = "Write me a poem about Machine Learning."
input_ids = tokenizer(input_text, return_tensors="pt").to("cuda")
outputs = model.generate(**input_ids, max_new_tokens=32)
print(tokenizer.decode(outputs[0]))
```
#### Running the model through a CLI
The [local-gemma](https://github.com/huggingface/local-gemma) repository contains a lightweight wrapper around Transformers
for running Gemma 2 through a command line interface, or CLI. Follow the [installation instructions](https://github.com/huggingface/local-gemma#cli-usage)
for getting started, then launch the CLI through the following command:
```shell
local-gemma --model 9b --preset speed
```
#### Quantized Versions through `bitsandbytes`
<details>
<summary>
Using 8-bit precision (int8)
</summary>
```python
# pip install bitsandbytes accelerate
from transformers import AutoTokenizer, AutoModelForCausalLM, BitsAndBytesConfig
quantization_config = BitsAndBytesConfig(load_in_8bit=True)
tokenizer = AutoTokenizer.from_pretrained("google/gemma-2-9b-it")
model = AutoModelForCausalLM.from_pretrained(
"google/gemma-2-9b-it",
quantization_config=quantization_config,
)
input_text = "Write me a poem about Machine Learning."
input_ids = tokenizer(input_text, return_tensors="pt").to("cuda")
outputs = model.generate(**input_ids, max_new_tokens=32)
print(tokenizer.decode(outputs[0]))
```
</details>
<details>
<summary>
Using 4-bit precision
</summary>
```python
# pip install bitsandbytes accelerate
from transformers import AutoTokenizer, AutoModelForCausalLM, BitsAndBytesConfig
quantization_config = BitsAndBytesConfig(load_in_4bit=True)
tokenizer = AutoTokenizer.from_pretrained("google/gemma-2-9b-it")
model = AutoModelForCausalLM.from_pretrained(
"google/gemma-2-9b-it",
quantization_config=quantization_config,
)
input_text = "Write me a poem about Machine Learning."
input_ids = tokenizer(input_text, return_tensors="pt").to("cuda")
outputs = model.generate(**input_ids, max_new_tokens=32)
print(tokenizer.decode(outputs[0]))
```
</details>
#### Advanced Usage
<details>
<summary>
Torch compile
</summary>
[Torch compile](https://pytorch.org/tutorials/intermediate/torch_compile_tutorial.html) is a method for speeding-up the
inference of PyTorch modules. The Gemma-2 model can be run up to 6x faster by leveraging torch compile.
Note that two warm-up steps are required before the full inference speed is realised:
```python
import os
os.environ["TOKENIZERS_PARALLELISM"] = "false"
from transformers import AutoTokenizer, Gemma2ForCausalLM
from transformers.cache_utils import HybridCache
import torch
torch.set_float32_matmul_precision("high")
# load the model + tokenizer
tokenizer = AutoTokenizer.from_pretrained("google/gemma-2-9b-it")
model = Gemma2ForCausalLM.from_pretrained("google/gemma-2-9b-it", torch_dtype=torch.bfloat16)
model.to("cuda")
# apply the torch compile transformation
model.forward = torch.compile(model.forward, mode="reduce-overhead", fullgraph=True)
# pre-process inputs
input_text = "The theory of special relativity states "
model_inputs = tokenizer(input_text, return_tensors="pt").to("cuda")
prompt_length = model_inputs.input_ids.shape[1]
# set-up k/v cache
past_key_values = HybridCache(
config=model.config,
max_batch_size=1,
max_cache_len=model.config.max_position_embeddings,
device=model.device,
dtype=model.dtype
)
# enable passing kv cache to generate
model._supports_cache_class = True
model.generation_config.cache_implementation = None
# two warm-up steps
for idx in range(2):
outputs = model.generate(**model_inputs, past_key_values=past_key_values, do_sample=True, temperature=1.0, max_new_tokens=128)
past_key_values.reset()
# fast run
outputs = model.generate(**model_inputs, past_key_values=past_key_values, do_sample=True, temperature=1.0, max_new_tokens=128)
print(tokenizer.decode(outputs[0], skip_special_tokens=True))
```
For more details, refer to the [Transformers documentation](https://huggingface.co/docs/transformers/main/en/llm_optims?static-kv=basic+usage%3A+generation_config).
</details>
### Chat Template
The instruction-tuned models use a chat template that must be adhered to for conversational use.
The easiest way to apply it is using the tokenizer's built-in chat template, as shown in the following snippet.
Let's load the model and apply the chat template to a conversation. In this example, we'll start with a single user interaction:
```py
from transformers import AutoTokenizer, AutoModelForCausalLM
import transformers
import torch
model_id = "google/gemma-2-9b-it"
dtype = torch.bfloat16
tokenizer = AutoTokenizer.from_pretrained(model_id)
model = AutoModelForCausalLM.from_pretrained(
model_id,
device_map="cuda",
torch_dtype=dtype,)
chat = [
{ "role": "user", "content": "Write a hello world program" },
]
prompt = tokenizer.apply_chat_template(chat, tokenize=False, add_generation_prompt=True)
```
At this point, the prompt contains the following text:
```
<bos><start_of_turn>user
Write a hello world program<end_of_turn>
<start_of_turn>model
```
As you can see, each turn is preceded by a `<start_of_turn>` delimiter and then the role of the entity
(either `user`, for content supplied by the user, or `model` for LLM responses). Turns finish with
the `<end_of_turn>` token.
You can follow this format to build the prompt manually, if you need to do it without the tokenizer's
chat template.
After the prompt is ready, generation can be performed like this:
```py
inputs = tokenizer.encode(prompt, add_special_tokens=False, return_tensors="pt")
outputs = model.generate(input_ids=inputs.to(model.device), max_new_tokens=150)
print(tokenizer.decode(outputs[0]))
```
### Inputs and outputs
* **Input:** Text string, such as a question, a prompt, or a document to be
summarized.
* **Output:** Generated English-language text in response to the input, such
as an answer to a question, or a summary of a document.
### Citation
```none
@article{gemma_2024,
title={Gemma},
url={https://www.kaggle.com/m/3301},
DOI={10.34740/KAGGLE/M/3301},
publisher={Kaggle},
author={Gemma Team},
year={2024}
}
```
## Model Data
Data used for model training and how the data was processed.
### Training Dataset
These models were trained on a dataset of text data that includes a wide variety of sources. The 27B model was trained with 13 trillion tokens and the 9B model was trained with 8 trillion tokens.
Here are the key components:
* Web Documents: A diverse collection of web text ensures the model is exposed
to a broad range of linguistic styles, topics, and vocabulary. Primarily
English-language content.
* Code: Exposing the model to code helps it to learn the syntax and patterns of
programming languages, which improves its ability to generate code or
understand code-related questions.
* Mathematics: Training on mathematical text helps the model learn logical
reasoning, symbolic representation, and to address mathematical queries.
The combination of these diverse data sources is crucial for training a powerful
language model that can handle a wide variety of different tasks and text
formats.
### Data Preprocessing
Here are the key data cleaning and filtering methods applied to the training
data:
* CSAM Filtering: Rigorous CSAM (Child Sexual Abuse Material) filtering was
applied at multiple stages in the data preparation process to ensure the
exclusion of harmful and illegal content.
* Sensitive Data Filtering: As part of making Gemma pre-trained models safe and
reliable, automated techniques were used to filter out certain personal
information and other sensitive data from training sets.
* Additional methods: Filtering based on content quality and safety in line with
[our policies][safety-policies].
## Implementation Information
Details about the model internals.
### Hardware
Gemma was trained using the latest generation of
[Tensor Processing Unit (TPU)][tpu] hardware (TPUv5p).
Training large language models requires significant computational power. TPUs,
designed specifically for matrix operations common in machine learning, offer
several advantages in this domain:
* Performance: TPUs are specifically designed to handle the massive computations
involved in training LLMs. They can speed up training considerably compared to
CPUs.
* Memory: TPUs often come with large amounts of high-bandwidth memory, allowing
for the handling of large models and batch sizes during training. This can
lead to better model quality.
* Scalability: TPU Pods (large clusters of TPUs) provide a scalable solution for
handling the growing complexity of large foundation models. You can distribute
training across multiple TPU devices for faster and more efficient processing.
* Cost-effectiveness: In many scenarios, TPUs can provide a more cost-effective
solution for training large models compared to CPU-based infrastructure,
especially when considering the time and resources saved due to faster
training.
* These advantages are aligned with
[Google's commitments to operate sustainably][sustainability].
### Software
Training was done using [JAX][jax] and [ML Pathways][ml-pathways].
JAX allows researchers to take advantage of the latest generation of hardware,
including TPUs, for faster and more efficient training of large models.
ML Pathways is Google's latest effort to build artificially intelligent systems
capable of generalizing across multiple tasks. This is specially suitable for
[foundation models][foundation-models], including large language models like
these ones.
Together, JAX and ML Pathways are used as described in the
[paper about the Gemini family of models][gemini-2-paper]; "the 'single
controller' programming model of Jax and Pathways allows a single Python
process to orchestrate the entire training run, dramatically simplifying the
development workflow."
## Evaluation
Model evaluation metrics and results.
### Benchmark Results
These models were evaluated against a large collection of different datasets and
metrics to cover different aspects of text generation:
| Benchmark | Metric | Gemma PT 9B | Gemma PT 27B |
| ------------------------------ | ------------- | ----------- | ------------ |
| [MMLU][mmlu] | 5-shot, top-1 | 71.3 | 75.2 |
| [HellaSwag][hellaswag] | 10-shot | 81.9 | 86.4 |
| [PIQA][piqa] | 0-shot | 81.7 | 83.2 |
| [SocialIQA][socialiqa] | 0-shot | 53.4 | 53.7 |
| [BoolQ][boolq] | 0-shot | 84.2 | 84.8 |
| [WinoGrande][winogrande] | partial score | 80.6 | 83.7 |
| [ARC-e][arc] | 0-shot | 88.0 | 88.6 |
| [ARC-c][arc] | 25-shot | 68.4 | 71.4 |
| [TriviaQA][triviaqa] | 5-shot | 76.6 | 83.7 |
| [Natural Questions][naturalq] | 5-shot | 29.2 | 34.5 |
| [HumanEval][humaneval] | pass@1 | 40.2 | 51.8 |
| [MBPP][mbpp] | 3-shot | 52.4 | 62.6 |
| [GSM8K][gsm8k] | 5-shot, maj@1 | 68.6 | 74.0 |
| [MATH][math] | 4-shot | 36.6 | 42.3 |
| [AGIEval][agieval] | 3-5-shot | 52.8 | 55.1 |
| [BIG-Bench][big-bench] | 3-shot, CoT | 68.2 | 74.9 |
| ------------------------------ | ------------- | ----------- | ------------ |
## Ethics and Safety
Ethics and safety evaluation approach and results.
### Evaluation Approach
Our evaluation methods include structured evaluations and internal red-teaming
testing of relevant content policies. Red-teaming was conducted by a number of
different teams, each with different goals and human evaluation metrics. These
models were evaluated against a number of different categories relevant to
ethics and safety, including:
* Text-to-Text Content Safety: Human evaluation on prompts covering safety
policies including child sexual abuse and exploitation, harassment, violence
and gore, and hate speech.
* Text-to-Text Representational Harms: Benchmark against relevant academic
datasets such as [WinoBias][winobias] and [BBQ Dataset][bbq].
* Memorization: Automated evaluation of memorization of training data, including
the risk of personally identifiable information exposure.
* Large-scale harm: Tests for "dangerous capabilities," such as chemical,
biological, radiological, and nuclear (CBRN) risks.
### Evaluation Results
The results of ethics and safety evaluations are within acceptable thresholds
for meeting [internal policies][safety-policies] for categories such as child
safety, content safety, representational harms, memorization, large-scale harms.
On top of robust internal evaluations, the results of well-known safety
benchmarks like BBQ, BOLD, Winogender, Winobias, RealToxicity, and TruthfulQA
are shown here.
#### Gemma 2.0
| Benchmark | Metric | Gemma 2 IT 9B | Gemma 2 IT 27B |
| ------------------------ | ------------- | --------------- | ---------------- |
| [RealToxicity][realtox] | average | 8.25 | 8.84 |
| [CrowS-Pairs][crows] | top-1 | 37.47 | 36.67 |
| [BBQ Ambig][bbq] | 1-shot, top-1 | 88.58 | 85.99 |
| [BBQ Disambig][bbq] | top-1 | 82.67 | 86.94 |
| [Winogender][winogender] | top-1 | 79.17 | 77.22 |
| [TruthfulQA][truthfulqa] | | 50.27 | 51.60 |
| [Winobias 1_2][winobias] | | 78.09 | 81.94 |
| [Winobias 2_2][winobias] | | 95.32 | 97.22 |
| [Toxigen][toxigen] | | 39.30 | 38.42 |
| ------------------------ | ------------- | --------------- | ---------------- |
## Usage and Limitations
These models have certain limitations that users should be aware of.
### Intended Usage
Open Large Language Models (LLMs) have a wide range of applications across
various industries and domains. The following list of potential uses is not
comprehensive. The purpose of this list is to provide contextual information
about the possible use-cases that the model creators considered as part of model
training and development.
* Content Creation and Communication
* Text Generation: These models can be used to generate creative text formats
such as poems, scripts, code, marketing copy, and email drafts.
* Chatbots and Conversational AI: Power conversational interfaces for customer
service, virtual assistants, or interactive applications.
* Text Summarization: Generate concise summaries of a text corpus, research
papers, or reports.
* Research and Education
* Natural Language Processing (NLP) Research: These models can serve as a
foundation for researchers to experiment with NLP techniques, develop
algorithms, and contribute to the advancement of the field.
* Language Learning Tools: Support interactive language learning experiences,
aiding in grammar correction or providing writing practice.
* Knowledge Exploration: Assist researchers in exploring large bodies of text
by generating summaries or answering questions about specific topics.
### Limitations
* Training Data
* The quality and diversity of the training data significantly influence the
model's capabilities. Biases or gaps in the training data can lead to
limitations in the model's responses.
* The scope of the training dataset determines the subject areas the model can
handle effectively.
* Context and Task Complexity
* LLMs are better at tasks that can be framed with clear prompts and
instructions. Open-ended or highly complex tasks might be challenging.
* A model's performance can be influenced by the amount of context provided
(longer context generally leads to better outputs, up to a certain point).
* Language Ambiguity and Nuance
* Natural language is inherently complex. LLMs might struggle to grasp subtle
nuances, sarcasm, or figurative language.
* Factual Accuracy
* LLMs generate responses based on information they learned from their
training datasets, but they are not knowledge bases. They may generate
incorrect or outdated factual statements.
* Common Sense
* LLMs rely on statistical patterns in language. They might lack the ability
to apply common sense reasoning in certain situations.
### Ethical Considerations and Risks
The development of large language models (LLMs) raises several ethical concerns.
In creating an open model, we have carefully considered the following:
* Bias and Fairness
* LLMs trained on large-scale, real-world text data can reflect socio-cultural
biases embedded in the training material. These models underwent careful
scrutiny, input data pre-processing described and posterior evaluations
reported in this card.
* Misinformation and Misuse
* LLMs can be misused to generate text that is false, misleading, or harmful.
* Guidelines are provided for responsible use with the model, see the
[Responsible Generative AI Toolkit][rai-toolkit].
* Transparency and Accountability:
* This model card summarizes details on the models' architecture,
capabilities, limitations, and evaluation processes.
* A responsibly developed open model offers the opportunity to share
innovation by making LLM technology accessible to developers and researchers
across the AI ecosystem.
Risks identified and mitigations:
* Perpetuation of biases: It's encouraged to perform continuous monitoring
(using evaluation metrics, human review) and the exploration of de-biasing
techniques during model training, fine-tuning, and other use cases.
* Generation of harmful content: Mechanisms and guidelines for content safety
are essential. Developers are encouraged to exercise caution and implement
appropriate content safety safeguards based on their specific product policies
and application use cases.
* Misuse for malicious purposes: Technical limitations and developer and
end-user education can help mitigate against malicious applications of LLMs.
Educational resources and reporting mechanisms for users to flag misuse are
provided. Prohibited uses of Gemma models are outlined in the
[Gemma Prohibited Use Policy][prohibited-use].
* Privacy violations: Models were trained on data filtered for removal of PII
(Personally Identifiable Information). Developers are encouraged to adhere to
privacy regulations with privacy-preserving techniques.
### Benefits
At the time of release, this family of models provides high-performance open
large language model implementations designed from the ground up for Responsible
AI development compared to similarly sized models.
Using the benchmark evaluation metrics described in this document, these models
have shown to provide superior performance to other, comparably-sized open model
alternatives.
[rai-toolkit]: https://ai.google.dev/responsible
[kaggle-gemma]: https://www.kaggle.com/models/google/gemma-2
[terms]: https://ai.google.dev/gemma/terms
[vertex-mg-gemma]: https://console.cloud.google.com/vertex-ai/publishers/google/model-garden/335
[sensitive-info]: https://cloud.google.com/dlp/docs/high-sensitivity-infotypes-reference
[safety-policies]: https://storage.googleapis.com/gweb-uniblog-publish-prod/documents/2023_Google_AI_Principles_Progress_Update.pdf#page=11
[prohibited-use]: https://ai.google.dev/gemma/prohibited_use_policy
[tpu]: https://cloud.google.com/tpu/docs/intro-to-tpu
[sustainability]: https://sustainability.google/operating-sustainably/
[jax]: https://github.com/google/jax
[ml-pathways]: https://blog.google/technology/ai/introducing-pathways-next-generation-ai-architecture/
[sustainability]: https://sustainability.google/operating-sustainably/
[foundation-models]: https://ai.google/discover/foundation-models/
[gemini-2-paper]: https://goo.gle/gemma2report
[mmlu]: https://arxiv.org/abs/2009.03300
[hellaswag]: https://arxiv.org/abs/1905.07830
[piqa]: https://arxiv.org/abs/1911.11641
[socialiqa]: https://arxiv.org/abs/1904.09728
[boolq]: https://arxiv.org/abs/1905.10044
[winogrande]: https://arxiv.org/abs/1907.10641
[commonsenseqa]: https://arxiv.org/abs/1811.00937
[openbookqa]: https://arxiv.org/abs/1809.02789
[arc]: https://arxiv.org/abs/1911.01547
[triviaqa]: https://arxiv.org/abs/1705.03551
[naturalq]: https://github.com/google-research-datasets/natural-questions
[humaneval]: https://arxiv.org/abs/2107.03374
[mbpp]: https://arxiv.org/abs/2108.07732
[gsm8k]: https://arxiv.org/abs/2110.14168
[realtox]: https://arxiv.org/abs/2009.11462
[bold]: https://arxiv.org/abs/2101.11718
[crows]: https://aclanthology.org/2020.emnlp-main.154/
[bbq]: https://arxiv.org/abs/2110.08193v2
[winogender]: https://arxiv.org/abs/1804.09301
[truthfulqa]: https://arxiv.org/abs/2109.07958
[winobias]: https://arxiv.org/abs/1804.06876
[math]: https://arxiv.org/abs/2103.03874
[agieval]: https://arxiv.org/abs/2304.06364
[big-bench]: https://arxiv.org/abs/2206.04615
[toxigen]: https://arxiv.org/abs/2203.09509
|
sentence-transformers/paraphrase-MiniLM-L3-v2 | sentence-transformers | "2024-11-05T18:16:17Z" | 407,231 | 21 | sentence-transformers | [
"sentence-transformers",
"pytorch",
"tf",
"onnx",
"safetensors",
"openvino",
"bert",
"feature-extraction",
"sentence-similarity",
"transformers",
"dataset:flax-sentence-embeddings/stackexchange_xml",
"dataset:s2orc",
"dataset:ms_marco",
"dataset:wiki_atomic_edits",
"dataset:snli",
"dataset:multi_nli",
"dataset:embedding-data/altlex",
"dataset:embedding-data/simple-wiki",
"dataset:embedding-data/flickr30k-captions",
"dataset:embedding-data/coco_captions",
"dataset:embedding-data/sentence-compression",
"dataset:embedding-data/QQP",
"dataset:yahoo_answers_topics",
"arxiv:1908.10084",
"license:apache-2.0",
"autotrain_compatible",
"text-embeddings-inference",
"endpoints_compatible",
"region:us"
] | sentence-similarity | "2022-03-02T23:29:05Z" | ---
license: apache-2.0
library_name: sentence-transformers
tags:
- sentence-transformers
- feature-extraction
- sentence-similarity
- transformers
datasets:
- flax-sentence-embeddings/stackexchange_xml
- s2orc
- ms_marco
- wiki_atomic_edits
- snli
- multi_nli
- embedding-data/altlex
- embedding-data/simple-wiki
- embedding-data/flickr30k-captions
- embedding-data/coco_captions
- embedding-data/sentence-compression
- embedding-data/QQP
- yahoo_answers_topics
pipeline_tag: sentence-similarity
---
# sentence-transformers/paraphrase-MiniLM-L3-v2
This is a [sentence-transformers](https://www.SBERT.net) model: It maps sentences & paragraphs to a 384 dimensional dense vector space and can be used for tasks like clustering or semantic search.
## Usage (Sentence-Transformers)
Using this model becomes easy when you have [sentence-transformers](https://www.SBERT.net) installed:
```
pip install -U sentence-transformers
```
Then you can use the model like this:
```python
from sentence_transformers import SentenceTransformer
sentences = ["This is an example sentence", "Each sentence is converted"]
model = SentenceTransformer('sentence-transformers/paraphrase-MiniLM-L3-v2')
embeddings = model.encode(sentences)
print(embeddings)
```
## Usage (HuggingFace Transformers)
Without [sentence-transformers](https://www.SBERT.net), you can use the model like this: First, you pass your input through the transformer model, then you have to apply the right pooling-operation on-top of the contextualized word embeddings.
```python
from transformers import AutoTokenizer, AutoModel
import torch
#Mean Pooling - Take attention mask into account for correct averaging
def mean_pooling(model_output, attention_mask):
token_embeddings = model_output[0] #First element of model_output contains all token embeddings
input_mask_expanded = attention_mask.unsqueeze(-1).expand(token_embeddings.size()).float()
return torch.sum(token_embeddings * input_mask_expanded, 1) / torch.clamp(input_mask_expanded.sum(1), min=1e-9)
# Sentences we want sentence embeddings for
sentences = ['This is an example sentence', 'Each sentence is converted']
# Load model from HuggingFace Hub
tokenizer = AutoTokenizer.from_pretrained('sentence-transformers/paraphrase-MiniLM-L3-v2')
model = AutoModel.from_pretrained('sentence-transformers/paraphrase-MiniLM-L3-v2')
# Tokenize sentences
encoded_input = tokenizer(sentences, padding=True, truncation=True, return_tensors='pt')
# Compute token embeddings
with torch.no_grad():
model_output = model(**encoded_input)
# Perform pooling. In this case, max pooling.
sentence_embeddings = mean_pooling(model_output, encoded_input['attention_mask'])
print("Sentence embeddings:")
print(sentence_embeddings)
```
## Evaluation Results
For an automated evaluation of this model, see the *Sentence Embeddings Benchmark*: [https://seb.sbert.net](https://seb.sbert.net?model_name=sentence-transformers/paraphrase-MiniLM-L3-v2)
## Full Model Architecture
```
SentenceTransformer(
(0): Transformer({'max_seq_length': 128, 'do_lower_case': False}) with Transformer model: BertModel
(1): Pooling({'word_embedding_dimension': 384, 'pooling_mode_cls_token': False, 'pooling_mode_mean_tokens': True, 'pooling_mode_max_tokens': False, 'pooling_mode_mean_sqrt_len_tokens': False})
)
```
## Citing & Authors
This model was trained by [sentence-transformers](https://www.sbert.net/).
If you find this model helpful, feel free to cite our publication [Sentence-BERT: Sentence Embeddings using Siamese BERT-Networks](https://arxiv.org/abs/1908.10084):
```bibtex
@inproceedings{reimers-2019-sentence-bert,
title = "Sentence-BERT: Sentence Embeddings using Siamese BERT-Networks",
author = "Reimers, Nils and Gurevych, Iryna",
booktitle = "Proceedings of the 2019 Conference on Empirical Methods in Natural Language Processing",
month = "11",
year = "2019",
publisher = "Association for Computational Linguistics",
url = "http://arxiv.org/abs/1908.10084",
}
``` |
Lykon/AAM_XL_AnimeMix | Lykon | "2024-01-19T14:10:55Z" | 406,621 | 13 | diffusers | [
"diffusers",
"safetensors",
"stable-diffusion",
"stable-diffusion-diffusers",
"stable-diffusion-xl",
"text-to-image",
"art",
"artistic",
"anime",
"en",
"license:other",
"autotrain_compatible",
"endpoints_compatible",
"diffusers:StableDiffusionXLPipeline",
"region:us"
] | text-to-image | "2024-01-19T10:14:05Z" | ---
language:
- en
license: other
tags:
- stable-diffusion
- stable-diffusion-diffusers
- stable-diffusion-xl
- text-to-image
- art
- artistic
- diffusers
- anime
---
# AAM XL AnimeMix
`Lykon/AAM_XL_AnimeMix` is a Stable Diffusion model that has been fine-tuned on [stabilityai/stable-diffusion-xl-base-1.0](https://huggingface.co/stabilityai/stable-diffusion-xl-base-1.0).
Please consider supporting me:
- on [Patreon](https://www.patreon.com/Lykon275)
- or [buy me a coffee](https://snipfeed.co/lykon)
**License**: [Fair AI Public License 1.0-SD](https://freedevproject.org/faipl-1.0-sd/)
## Diffusers
For more general information on how to run text-to-image models with 🧨 Diffusers, see [the docs](https://huggingface.co/docs/diffusers/using-diffusers/conditional_image_generation).
1. Installation
```
pip install diffusers transformers accelerate
```
2. Run
```py
from diffusers import AutoPipelineForText2Image, DEISMultistepScheduler
import torch
pipe = AutoPipelineForText2Image.from_pretrained('Lykon/AAM_XL_AnimeMix', torch_dtype=torch.float16, variant="fp16")
pipe.scheduler = DEISMultistepScheduler.from_config(pipe.scheduler.config)
pipe = pipe.to("cuda")
prompt = "anime girl, night, blue light behind her, ((Galaxy, Lens flare)), short hair, flower field, night sky, cinematic shot. Wallpaper. (Blue color schema), detailed background, a city in the distance"
generator = torch.manual_seed(0)
image = pipe(prompt, num_inference_steps=25).images[0]
image.save("./image.png")
```

|
facebook/sam-vit-large | facebook | "2024-01-11T19:23:46Z" | 404,903 | 26 | transformers | [
"transformers",
"pytorch",
"tf",
"safetensors",
"sam",
"mask-generation",
"vision",
"arxiv:2304.02643",
"license:apache-2.0",
"endpoints_compatible",
"region:us"
] | mask-generation | "2023-04-19T14:17:03Z" | ---
license: apache-2.0
tags:
- vision
---
# Model Card for Segment Anything Model (SAM) - ViT Large (ViT-L) version
<p>
<img src="https://huggingface.co/datasets/huggingface/documentation-images/resolve/main/transformers/model_doc/sam-architecture.png" alt="Model architecture">
<em> Detailed architecture of Segment Anything Model (SAM).</em>
</p>
# Table of Contents
0. [TL;DR](#TL;DR)
1. [Model Details](#model-details)
2. [Usage](#usage)
3. [Citation](#citation)
# TL;DR
[Link to original repository](https://github.com/facebookresearch/segment-anything)
| <img src="https://huggingface.co/datasets/huggingface/documentation-images/resolve/main/transformers/model_doc/sam-beancans.png" alt="Snow" width="600" height="600"> | <img src="https://huggingface.co/facebook/sam-vit-huge/discussions/7" alt="Forest" width="600" height="600"> | <img src="https://huggingface.co/datasets/huggingface/documentation-images/resolve/main/transformers/model_doc/sam-car-seg.png" alt="Mountains" width="600" height="600"> |
|---------------------------------------------------------------------------------------------------------------------------------------|--------------------------------------------------------------------------------------------------------------------------------|--------------------------------------------------------------------------------------------------------------------------------------------------|
The **Segment Anything Model (SAM)** produces high quality object masks from input prompts such as points or boxes, and it can be used to generate masks for all objects in an image. It has been trained on a [dataset](https://segment-anything.com/dataset/index.html) of 11 million images and 1.1 billion masks, and has strong zero-shot performance on a variety of segmentation tasks.
The abstract of the paper states:
> We introduce the Segment Anything (SA) project: a new task, model, and dataset for image segmentation. Using our efficient model in a data collection loop, we built the largest segmentation dataset to date (by far), with over 1 billion masks on 11M licensed and privacy respecting images. The model is designed and trained to be promptable, so it can transfer zero-shot to new image distributions and tasks. We evaluate its capabilities on numerous tasks and find that its zero-shot performance is impressive -- often competitive with or even superior to prior fully supervised results. We are releasing the Segment Anything Model (SAM) and corresponding dataset (SA-1B) of 1B masks and 11M images at [https://segment-anything.com](https://segment-anything.com) to foster research into foundation models for computer vision.
**Disclaimer**: Content from **this** model card has been written by the Hugging Face team, and parts of it were copy pasted from the original [SAM model card](https://github.com/facebookresearch/segment-anything).
# Model Details
The SAM model is made up of 3 modules:
- The `VisionEncoder`: a VIT based image encoder. It computes the image embeddings using attention on patches of the image. Relative Positional Embedding is used.
- The `PromptEncoder`: generates embeddings for points and bounding boxes
- The `MaskDecoder`: a two-ways transformer which performs cross attention between the image embedding and the point embeddings (->) and between the point embeddings and the image embeddings. The outputs are fed
- The `Neck`: predicts the output masks based on the contextualized masks produced by the `MaskDecoder`.
# Usage
## Prompted-Mask-Generation
```python
from PIL import Image
import requests
from transformers import SamModel, SamProcessor
model = SamModel.from_pretrained("facebook/sam-vit-large")
processor = SamProcessor.from_pretrained("facebook/sam-vit-large")
img_url = "https://huggingface.co/ybelkada/segment-anything/resolve/main/assets/car.png"
raw_image = Image.open(requests.get(img_url, stream=True).raw).convert("RGB")
input_points = [[[450, 600]]] # 2D localization of a window
```
```python
inputs = processor(raw_image, input_points=input_points, return_tensors="pt").to("cuda")
outputs = model(**inputs)
masks = processor.image_processor.post_process_masks(outputs.pred_masks.cpu(), inputs["original_sizes"].cpu(), inputs["reshaped_input_sizes"].cpu())
scores = outputs.iou_scores
```
Among other arguments to generate masks, you can pass 2D locations on the approximate position of your object of interest, a bounding box wrapping the object of interest (the format should be x, y coordinate of the top right and bottom left point of the bounding box), a segmentation mask. At this time of writing, passing a text as input is not supported by the official model according to [the official repository](https://github.com/facebookresearch/segment-anything/issues/4#issuecomment-1497626844).
For more details, refer to this notebook, which shows a walk throught of how to use the model, with a visual example!
## Automatic-Mask-Generation
The model can be used for generating segmentation masks in a "zero-shot" fashion, given an input image. The model is automatically prompt with a grid of `1024` points
which are all fed to the model.
The pipeline is made for automatic mask generation. The following snippet demonstrates how easy you can run it (on any device! Simply feed the appropriate `points_per_batch` argument)
```python
from transformers import pipeline
generator = pipeline("mask-generation", device = 0, points_per_batch = 256)
image_url = "https://huggingface.co/ybelkada/segment-anything/resolve/main/assets/car.png"
outputs = generator(image_url, points_per_batch = 256)
```
Now to display the image:
```python
import matplotlib.pyplot as plt
from PIL import Image
import numpy as np
def show_mask(mask, ax, random_color=False):
if random_color:
color = np.concatenate([np.random.random(3), np.array([0.6])], axis=0)
else:
color = np.array([30 / 255, 144 / 255, 255 / 255, 0.6])
h, w = mask.shape[-2:]
mask_image = mask.reshape(h, w, 1) * color.reshape(1, 1, -1)
ax.imshow(mask_image)
plt.imshow(np.array(raw_image))
ax = plt.gca()
for mask in outputs["masks"]:
show_mask(mask, ax=ax, random_color=True)
plt.axis("off")
plt.show()
```
# Citation
If you use this model, please use the following BibTeX entry.
```
@article{kirillov2023segany,
title={Segment Anything},
author={Kirillov, Alexander and Mintun, Eric and Ravi, Nikhila and Mao, Hanzi and Rolland, Chloe and Gustafson, Laura and Xiao, Tete and Whitehead, Spencer and Berg, Alexander C. and Lo, Wan-Yen and Doll{\'a}r, Piotr and Girshick, Ross},
journal={arXiv:2304.02643},
year={2023}
}
``` |
DeepChem/ChemBERTa-77M-MTR | DeepChem | "2022-01-20T17:55:55Z" | 402,591 | 5 | transformers | [
"transformers",
"pytorch",
"roberta",
"endpoints_compatible",
"region:us"
] | null | "2022-03-02T23:29:04Z" | Entry not found |
Systran/faster-whisper-tiny | Systran | "2023-11-23T10:42:55Z" | 400,474 | 5 | ctranslate2 | [
"ctranslate2",
"audio",
"automatic-speech-recognition",
"en",
"zh",
"de",
"es",
"ru",
"ko",
"fr",
"ja",
"pt",
"tr",
"pl",
"ca",
"nl",
"ar",
"sv",
"it",
"id",
"hi",
"fi",
"vi",
"he",
"uk",
"el",
"ms",
"cs",
"ro",
"da",
"hu",
"ta",
"no",
"th",
"ur",
"hr",
"bg",
"lt",
"la",
"mi",
"ml",
"cy",
"sk",
"te",
"fa",
"lv",
"bn",
"sr",
"az",
"sl",
"kn",
"et",
"mk",
"br",
"eu",
"is",
"hy",
"ne",
"mn",
"bs",
"kk",
"sq",
"sw",
"gl",
"mr",
"pa",
"si",
"km",
"sn",
"yo",
"so",
"af",
"oc",
"ka",
"be",
"tg",
"sd",
"gu",
"am",
"yi",
"lo",
"uz",
"fo",
"ht",
"ps",
"tk",
"nn",
"mt",
"sa",
"lb",
"my",
"bo",
"tl",
"mg",
"as",
"tt",
"haw",
"ln",
"ha",
"ba",
"jw",
"su",
"license:mit",
"region:us"
] | automatic-speech-recognition | "2023-11-23T09:53:30Z" | ---
language:
- en
- zh
- de
- es
- ru
- ko
- fr
- ja
- pt
- tr
- pl
- ca
- nl
- ar
- sv
- it
- id
- hi
- fi
- vi
- he
- uk
- el
- ms
- cs
- ro
- da
- hu
- ta
- 'no'
- th
- ur
- hr
- bg
- lt
- la
- mi
- ml
- cy
- sk
- te
- fa
- lv
- bn
- sr
- az
- sl
- kn
- et
- mk
- br
- eu
- is
- hy
- ne
- mn
- bs
- kk
- sq
- sw
- gl
- mr
- pa
- si
- km
- sn
- yo
- so
- af
- oc
- ka
- be
- tg
- sd
- gu
- am
- yi
- lo
- uz
- fo
- ht
- ps
- tk
- nn
- mt
- sa
- lb
- my
- bo
- tl
- mg
- as
- tt
- haw
- ln
- ha
- ba
- jw
- su
tags:
- audio
- automatic-speech-recognition
license: mit
library_name: ctranslate2
---
# Whisper tiny model for CTranslate2
This repository contains the conversion of [openai/whisper-tiny](https://huggingface.co/openai/whisper-tiny) to the [CTranslate2](https://github.com/OpenNMT/CTranslate2) model format.
This model can be used in CTranslate2 or projects based on CTranslate2 such as [faster-whisper](https://github.com/systran/faster-whisper).
## Example
```python
from faster_whisper import WhisperModel
model = WhisperModel("tiny")
segments, info = model.transcribe("audio.mp3")
for segment in segments:
print("[%.2fs -> %.2fs] %s" % (segment.start, segment.end, segment.text))
```
## Conversion details
The original model was converted with the following command:
```
ct2-transformers-converter --model openai/whisper-tiny --output_dir faster-whisper-tiny \
--copy_files tokenizer.json --quantization float16
```
Note that the model weights are saved in FP16. This type can be changed when the model is loaded using the [`compute_type` option in CTranslate2](https://opennmt.net/CTranslate2/quantization.html).
## More information
**For more information about the original model, see its [model card](https://huggingface.co/openai/whisper-tiny).**
|
depth-anything/Depth-Anything-V2-Large-hf | depth-anything | "2024-07-05T11:30:29Z" | 399,847 | 8 | transformers | [
"transformers",
"safetensors",
"depth_anything",
"depth-estimation",
"depth",
"relative depth",
"arxiv:2406.09414",
"arxiv:2401.10891",
"license:cc-by-nc-4.0",
"endpoints_compatible",
"region:us"
] | depth-estimation | "2024-06-20T15:31:25Z" | ---
library_name: transformers
library: transformers
license: cc-by-nc-4.0
tags:
- depth
- relative depth
pipeline_tag: depth-estimation
widget:
- inference: false
---
# Depth Anything V2 Base – Transformers Version
Depth Anything V2 is trained from 595K synthetic labeled images and 62M+ real unlabeled images, providing the most capable monocular depth estimation (MDE) model with the following features:
- more fine-grained details than Depth Anything V1
- more robust than Depth Anything V1 and SD-based models (e.g., Marigold, Geowizard)
- more efficient (10x faster) and more lightweight than SD-based models
- impressive fine-tuned performance with our pre-trained models
This model checkpoint is compatible with the transformers library.
Depth Anything V2 was introduced in [the paper of the same name](https://arxiv.org/abs/2406.09414) by Lihe Yang et al. It uses the same architecture as the original Depth Anything release, but uses synthetic data and a larger capacity teacher model to achieve much finer and robust depth predictions. The original Depth Anything model was introduced in the paper [Depth Anything: Unleashing the Power of Large-Scale Unlabeled Data](https://arxiv.org/abs/2401.10891) by Lihe Yang et al., and was first released in [this repository](https://github.com/LiheYoung/Depth-Anything).
[Online demo](https://huggingface.co/spaces/depth-anything/Depth-Anything-V2).
## Model description
Depth Anything V2 leverages the [DPT](https://huggingface.co/docs/transformers/model_doc/dpt) architecture with a [DINOv2](https://huggingface.co/docs/transformers/model_doc/dinov2) backbone.
The model is trained on ~600K synthetic labeled images and ~62 million real unlabeled images, obtaining state-of-the-art results for both relative and absolute depth estimation.
<img src="https://huggingface.co/datasets/huggingface/documentation-images/resolve/main/transformers/model_doc/depth_anything_overview.jpg"
alt="drawing" width="600"/>
<small> Depth Anything overview. Taken from the <a href="https://arxiv.org/abs/2401.10891">original paper</a>.</small>
## Intended uses & limitations
You can use the raw model for tasks like zero-shot depth estimation. See the [model hub](https://huggingface.co/models?search=depth-anything) to look for
other versions on a task that interests you.
### How to use
Here is how to use this model to perform zero-shot depth estimation:
```python
from transformers import pipeline
from PIL import Image
import requests
# load pipe
pipe = pipeline(task="depth-estimation", model="depth-anything/Depth-Anything-V2-Large-hf")
# load image
url = 'http://images.cocodataset.org/val2017/000000039769.jpg'
image = Image.open(requests.get(url, stream=True).raw)
# inference
depth = pipe(image)["depth"]
```
Alternatively, you can use the model and processor classes:
```python
from transformers import AutoImageProcessor, AutoModelForDepthEstimation
import torch
import numpy as np
from PIL import Image
import requests
url = "http://images.cocodataset.org/val2017/000000039769.jpg"
image = Image.open(requests.get(url, stream=True).raw)
image_processor = AutoImageProcessor.from_pretrained("depth-anything/Depth-Anything-V2-Large-hf")
model = AutoModelForDepthEstimation.from_pretrained("depth-anything/Depth-Anything-V2-Large-hf")
# prepare image for the model
inputs = image_processor(images=image, return_tensors="pt")
with torch.no_grad():
outputs = model(**inputs)
predicted_depth = outputs.predicted_depth
# interpolate to original size
prediction = torch.nn.functional.interpolate(
predicted_depth.unsqueeze(1),
size=image.size[::-1],
mode="bicubic",
align_corners=False,
)
```
For more code examples, please refer to the [documentation](https://huggingface.co/transformers/main/model_doc/depth_anything.html#).
### Citation
```bibtex
@misc{yang2024depth,
title={Depth Anything V2},
author={Lihe Yang and Bingyi Kang and Zilong Huang and Zhen Zhao and Xiaogang Xu and Jiashi Feng and Hengshuang Zhao},
year={2024},
eprint={2406.09414},
archivePrefix={arXiv},
primaryClass={id='cs.CV' full_name='Computer Vision and Pattern Recognition' is_active=True alt_name=None in_archive='cs' is_general=False description='Covers image processing, computer vision, pattern recognition, and scene understanding. Roughly includes material in ACM Subject Classes I.2.10, I.4, and I.5.'}
}
```
|
openart-custom/AlbedoBase | openart-custom | "2024-09-13T11:38:20Z" | 399,301 | 0 | diffusers | [
"diffusers",
"safetensors",
"arxiv:1910.09700",
"autotrain_compatible",
"endpoints_compatible",
"diffusers:StableDiffusionXLPipeline",
"region:us"
] | text-to-image | "2024-09-13T11:36:01Z" | ---
library_name: diffusers
---
# Model Card for Model ID
<!-- Provide a quick summary of what the model is/does. -->
## Model Details
### Model Description
<!-- Provide a longer summary of what this model is. -->
This is the model card of a 🧨 diffusers pipeline that has been pushed on the Hub. This model card has been automatically generated.
- **Developed by:** [More Information Needed]
- **Funded by [optional]:** [More Information Needed]
- **Shared by [optional]:** [More Information Needed]
- **Model type:** [More Information Needed]
- **Language(s) (NLP):** [More Information Needed]
- **License:** [More Information Needed]
- **Finetuned from model [optional]:** [More Information Needed]
### Model Sources [optional]
<!-- Provide the basic links for the model. -->
- **Repository:** [More Information Needed]
- **Paper [optional]:** [More Information Needed]
- **Demo [optional]:** [More Information Needed]
## Uses
<!-- Address questions around how the model is intended to be used, including the foreseeable users of the model and those affected by the model. -->
### Direct Use
<!-- This section is for the model use without fine-tuning or plugging into a larger ecosystem/app. -->
[More Information Needed]
### Downstream Use [optional]
<!-- This section is for the model use when fine-tuned for a task, or when plugged into a larger ecosystem/app -->
[More Information Needed]
### Out-of-Scope Use
<!-- This section addresses misuse, malicious use, and uses that the model will not work well for. -->
[More Information Needed]
## Bias, Risks, and Limitations
<!-- This section is meant to convey both technical and sociotechnical limitations. -->
[More Information Needed]
### Recommendations
<!-- This section is meant to convey recommendations with respect to the bias, risk, and technical limitations. -->
Users (both direct and downstream) should be made aware of the risks, biases and limitations of the model. More information needed for further recommendations.
## How to Get Started with the Model
Use the code below to get started with the model.
[More Information Needed]
## Training Details
### Training Data
<!-- This should link to a Dataset Card, perhaps with a short stub of information on what the training data is all about as well as documentation related to data pre-processing or additional filtering. -->
[More Information Needed]
### Training Procedure
<!-- This relates heavily to the Technical Specifications. Content here should link to that section when it is relevant to the training procedure. -->
#### Preprocessing [optional]
[More Information Needed]
#### Training Hyperparameters
- **Training regime:** [More Information Needed] <!--fp32, fp16 mixed precision, bf16 mixed precision, bf16 non-mixed precision, fp16 non-mixed precision, fp8 mixed precision -->
#### Speeds, Sizes, Times [optional]
<!-- This section provides information about throughput, start/end time, checkpoint size if relevant, etc. -->
[More Information Needed]
## Evaluation
<!-- This section describes the evaluation protocols and provides the results. -->
### Testing Data, Factors & Metrics
#### Testing Data
<!-- This should link to a Dataset Card if possible. -->
[More Information Needed]
#### Factors
<!-- These are the things the evaluation is disaggregating by, e.g., subpopulations or domains. -->
[More Information Needed]
#### Metrics
<!-- These are the evaluation metrics being used, ideally with a description of why. -->
[More Information Needed]
### Results
[More Information Needed]
#### Summary
## Model Examination [optional]
<!-- Relevant interpretability work for the model goes here -->
[More Information Needed]
## Environmental Impact
<!-- Total emissions (in grams of CO2eq) and additional considerations, such as electricity usage, go here. Edit the suggested text below accordingly -->
Carbon emissions can be estimated using the [Machine Learning Impact calculator](https://mlco2.github.io/impact#compute) presented in [Lacoste et al. (2019)](https://arxiv.org/abs/1910.09700).
- **Hardware Type:** [More Information Needed]
- **Hours used:** [More Information Needed]
- **Cloud Provider:** [More Information Needed]
- **Compute Region:** [More Information Needed]
- **Carbon Emitted:** [More Information Needed]
## Technical Specifications [optional]
### Model Architecture and Objective
[More Information Needed]
### Compute Infrastructure
[More Information Needed]
#### Hardware
[More Information Needed]
#### Software
[More Information Needed]
## Citation [optional]
<!-- If there is a paper or blog post introducing the model, the APA and Bibtex information for that should go in this section. -->
**BibTeX:**
[More Information Needed]
**APA:**
[More Information Needed]
## Glossary [optional]
<!-- If relevant, include terms and calculations in this section that can help readers understand the model or model card. -->
[More Information Needed]
## More Information [optional]
[More Information Needed]
## Model Card Authors [optional]
[More Information Needed]
## Model Card Contact
[More Information Needed] |
hpcai-tech/OpenSora-VAE-v1.2 | hpcai-tech | "2024-06-17T07:02:33Z" | 399,061 | 53 | transformers | [
"transformers",
"safetensors",
"VideoAutoencoderPipeline",
"license:apache-2.0",
"endpoints_compatible",
"region:us"
] | null | "2024-06-17T03:43:50Z" | ---
license: apache-2.0
---
<p align="center">
<img src="https://cdn-uploads.huggingface.co/production/uploads/63993d721fad4d6eb265d999/UXleJWJExX2WlBizxzYxn.png" width="250"/>
</p>
# Open-Sora VAE-v1.2 Weights
This repository stores the weights of the VAE released by the Open-Sora team. You can visit our project at:
- [GitHub](https://github.com/hpcaitech/Open-Sora)
- [Gallery](https://hpcaitech.github.io/Open-Sora/)
- [Gradio Demo](https://huggingface.co/spaces/hpcai-tech/open-sora)
The weights are released together with Open-Sora v1.2.
We recommend you to use this weights in the [Open-Sora codebase]((https://github.com/hpcaitech/Open-Sora)). If you want to use VAE in your own project, you may use the following sample code.
1. Install `opensora`
```bash
pip install git+https://github.com/hpcaitech/Open-Sora.git
```
2. Use `STDiT3` in your own code
```python
from opensora.models.vae.vae import VideoAutoencoderPipeline
vae = VideoAutoencoderPipeline.from_pretrained("hpcai-tech/OpenSora-VAE-v1.2")
``` |
hugging-quants/Meta-Llama-3.1-8B-Instruct-AWQ-INT4 | hugging-quants | "2024-08-07T07:29:21Z" | 397,138 | 60 | transformers | [
"transformers",
"safetensors",
"llama",
"text-generation",
"llama-3.1",
"meta",
"autoawq",
"conversational",
"en",
"de",
"fr",
"it",
"pt",
"hi",
"es",
"th",
"license:llama3.1",
"autotrain_compatible",
"text-generation-inference",
"endpoints_compatible",
"4-bit",
"awq",
"region:us"
] | text-generation | "2024-07-19T09:19:01Z" | ---
license: llama3.1
language:
- en
- de
- fr
- it
- pt
- hi
- es
- th
library_name: transformers
pipeline_tag: text-generation
tags:
- llama-3.1
- meta
- autoawq
---
> [!IMPORTANT]
> This repository is a community-driven quantized version of the original model [`meta-llama/Meta-Llama-3.1-8B-Instruct`](https://huggingface.co/meta-llama/Meta-Llama-3.1-8B-Instruct) which is the BF16 half-precision official version released by Meta AI.
## Model Information
The Meta Llama 3.1 collection of multilingual large language models (LLMs) is a collection of pretrained and instruction tuned generative models in 8B, 70B and 405B sizes (text in/text out). The Llama 3.1 instruction tuned text only models (8B, 70B, 405B) are optimized for multilingual dialogue use cases and outperform many of the available open source and closed chat models on common industry benchmarks.
This repository contains [`meta-llama/Meta-Llama-3.1-8B-Instruct`](https://huggingface.co/meta-llama/Meta-Llama-3.1-8B-Instruct) quantized using [AutoAWQ](https://github.com/casper-hansen/AutoAWQ) from FP16 down to INT4 using the GEMM kernels performing zero-point quantization with a group size of 128.
## Model Usage
> [!NOTE]
> In order to run the inference with Llama 3.1 8B Instruct AWQ in INT4, around 4 GiB of VRAM are needed only for loading the model checkpoint, without including the KV cache or the CUDA graphs, meaning that there should be a bit over that VRAM available.
In order to use the current quantized model, support is offered for different solutions as `transformers`, `autoawq`, or `text-generation-inference`.
### 🤗 Transformers
In order to run the inference with Llama 3.1 8B Instruct AWQ in INT4, you need to install the following packages:
```bash
pip install -q --upgrade transformers autoawq accelerate
```
To run the inference on top of Llama 3.1 8B Instruct AWQ in INT4 precision, the AWQ model can be instantiated as any other causal language modeling model via `AutoModelForCausalLM` and run the inference normally.
```python
import torch
from transformers import AutoModelForCausalLM, AutoTokenizer, AwqConfig
model_id = "hugging-quants/Meta-Llama-3.1-8B-Instruct-AWQ-INT4"
quantization_config = AwqConfig(
bits=4,
fuse_max_seq_len=512, # Note: Update this as per your use-case
do_fuse=True,
)
tokenizer = AutoTokenizer.from_pretrained(model_id)
model = AutoModelForCausalLM.from_pretrained(
model_id,
torch_dtype=torch.float16,
low_cpu_mem_usage=True,
device_map="auto",
quantization_config=quantization_config
)
prompt = [
{"role": "system", "content": "You are a helpful assistant, that responds as a pirate."},
{"role": "user", "content": "What's Deep Learning?"},
]
inputs = tokenizer.apply_chat_template(
prompt,
tokenize=True,
add_generation_prompt=True,
return_tensors="pt",
return_dict=True,
).to("cuda")
outputs = model.generate(**inputs, do_sample=True, max_new_tokens=256)
print(tokenizer.batch_decode(outputs[:, inputs['input_ids'].shape[1]:], skip_special_tokens=True)[0])
```
### AutoAWQ
In order to run the inference with Llama 3.1 8B Instruct AWQ in INT4, you need to install the following packages:
```bash
pip install -q --upgrade transformers autoawq accelerate
```
Alternatively, one may want to run that via `AutoAWQ` even though it's built on top of 🤗 `transformers`, which is the recommended approach instead as described above.
```python
import torch
from awq import AutoAWQForCausalLM
from transformers import AutoModelForCausalLM, AutoTokenizer
model_id = "hugging-quants/Meta-Llama-3.1-8B-Instruct-AWQ-INT4"
tokenizer = AutoTokenizer.from_pretrained(model_id)
model = AutoAWQForCausalLM.from_pretrained(
model_id,
torch_dtype=torch.float16,
low_cpu_mem_usage=True,
device_map="auto",
)
prompt = [
{"role": "system", "content": "You are a helpful assistant, that responds as a pirate."},
{"role": "user", "content": "What's Deep Learning?"},
]
inputs = tokenizer.apply_chat_template(
prompt,
tokenize=True,
add_generation_prompt=True,
return_tensors="pt",
return_dict=True,
).to("cuda")
outputs = model.generate(**inputs, do_sample=True, max_new_tokens=256)
print(tokenizer.batch_decode(outputs[:, inputs['input_ids'].shape[1]:], skip_special_tokens=True)[0])
```
The AutoAWQ script has been adapted from [`AutoAWQ/examples/generate.py`](https://github.com/casper-hansen/AutoAWQ/blob/main/examples/generate.py).
### 🤗 Text Generation Inference (TGI)
To run the `text-generation-launcher` with Llama 3.1 8B Instruct AWQ in INT4 with Marlin kernels for optimized inference speed, you will need to have Docker installed (see [installation notes](https://docs.docker.com/engine/install/)) and the `huggingface_hub` Python package as you need to login to the Hugging Face Hub.
```bash
pip install -q --upgrade huggingface_hub
huggingface-cli login
```
Then you just need to run the TGI v2.2.0 (or higher) Docker container as follows:
```bash
docker run --gpus all --shm-size 1g -ti -p 8080:80 \
-v hf_cache:/data \
-e MODEL_ID=hugging-quants/Meta-Llama-3.1-8B-Instruct-AWQ-INT4 \
-e QUANTIZE=awq \
-e HF_TOKEN=$(cat ~/.cache/huggingface/token) \
-e MAX_INPUT_LENGTH=4000 \
-e MAX_TOTAL_TOKENS=4096 \
ghcr.io/huggingface/text-generation-inference:2.2.0
```
> [!NOTE]
> TGI will expose different endpoints, to see all the endpoints available check [TGI OpenAPI Specification](https://huggingface.github.io/text-generation-inference/#/).
To send request to the deployed TGI endpoint compatible with [OpenAI OpenAPI specification](https://github.com/openai/openai-openapi) i.e. `/v1/chat/completions`:
```bash
curl 0.0.0.0:8080/v1/chat/completions \
-X POST \
-H 'Content-Type: application/json' \
-d '{
"model": "tgi",
"messages": [
{
"role": "system",
"content": "You are a helpful assistant."
},
{
"role": "user",
"content": "What is Deep Learning?"
}
],
"max_tokens": 128
}'
```
Or programatically via the `huggingface_hub` Python client as follows:
```python
import os
from huggingface_hub import InferenceClient
client = InferenceClient(base_url="http://0.0.0.0:8080", api_key=os.getenv("HF_TOKEN", "-"))
chat_completion = client.chat.completions.create(
model="hugging-quants/Meta-Llama-3.1-8B-Instruct-AWQ-INT4",
messages=[
{"role": "system", "content": "You are a helpful assistant."},
{"role": "user", "content": "What is Deep Learning?"},
],
max_tokens=128,
)
```
Alternatively, the OpenAI Python client can also be used (see [installation notes](https://github.com/openai/openai-python?tab=readme-ov-file#installation)) as follows:
```python
import os
from openai import OpenAI
client = OpenAI(base_url="http://0.0.0.0:8080/v1", api_key=os.getenv("OPENAI_API_KEY", "-"))
chat_completion = client.chat.completions.create(
model="tgi",
messages=[
{"role": "system", "content": "You are a helpful assistant."},
{"role": "user", "content": "What is Deep Learning?"},
],
max_tokens=128,
)
```
### vLLM
To run vLLM with Llama 3.1 8B Instruct AWQ in INT4, you will need to have Docker installed (see [installation notes](https://docs.docker.com/engine/install/)) and run the latest vLLM Docker container as follows:
```bash
docker run --runtime nvidia --gpus all --ipc=host -p 8000:8000 \
-v hf_cache:/root/.cache/huggingface \
vllm/vllm-openai:latest \
--model hugging-quants/Meta-Llama-3.1-8B-Instruct-AWQ-INT4 \
--max-model-len 4096
```
To send request to the deployed vLLM endpoint compatible with [OpenAI OpenAPI specification](https://github.com/openai/openai-openapi) i.e. `/v1/chat/completions`:
```bash
curl 0.0.0.0:8000/v1/chat/completions \
-X POST \
-H 'Content-Type: application/json' \
-d '{
"model": "hugging-quants/Meta-Llama-3.1-8B-Instruct-AWQ-INT4",
"messages": [
{
"role": "system",
"content": "You are a helpful assistant."
},
{
"role": "user",
"content": "What is Deep Learning?"
}
],
"max_tokens": 128
}'
```
Or programatically via the `openai` Python client (see [installation notes](https://github.com/openai/openai-python?tab=readme-ov-file#installation)) as follows:
```python
import os
from openai import OpenAI
client = OpenAI(base_url="http://0.0.0.0:8000/v1", api_key=os.getenv("VLLM_API_KEY", "-"))
chat_completion = client.chat.completions.create(
model="hugging-quants/Meta-Llama-3.1-8B-Instruct-AWQ-INT4",
messages=[
{"role": "system", "content": "You are a helpful assistant."},
{"role": "user", "content": "What is Deep Learning?"},
],
max_tokens=128,
)
```
## Quantization Reproduction
> [!NOTE]
> In order to quantize Llama 3.1 8B Instruct using AutoAWQ, you will need to use an instance with at least enough CPU RAM to fit the whole model i.e. ~8GiB, and an NVIDIA GPU with 16GiB of VRAM to quantize it.
In order to quantize Llama 3.1 8B Instruct, first install the following packages:
```bash
pip install -q --upgrade transformers autoawq accelerate
```
Then run the following script, adapted from [`AutoAWQ/examples/quantize.py`](https://github.com/casper-hansen/AutoAWQ/blob/main/examples/quantize.py):
```python
from awq import AutoAWQForCausalLM
from transformers import AutoTokenizer
model_path = "meta-llama/Meta-Llama-3.1-8B-Instruct"
quant_path = "hugging-quants/Meta-Llama-3.1-8B-Instruct-AWQ-INT4"
quant_config = {
"zero_point": True,
"q_group_size": 128,
"w_bit": 4,
"version": "GEMM",
}
# Load model
model = AutoAWQForCausalLM.from_pretrained(
model_path, low_cpu_mem_usage=True, use_cache=False,
)
tokenizer = AutoTokenizer.from_pretrained(model_path)
# Quantize
model.quantize(tokenizer, quant_config=quant_config)
# Save quantized model
model.save_quantized(quant_path)
tokenizer.save_pretrained(quant_path)
print(f'Model is quantized and saved at "{quant_path}"')
``` |
Intel/dpt-hybrid-midas | Intel | "2024-02-09T08:58:56Z" | 396,547 | 84 | transformers | [
"transformers",
"pytorch",
"dpt",
"depth-estimation",
"vision",
"arxiv:2103.13413",
"license:apache-2.0",
"model-index",
"endpoints_compatible",
"region:us"
] | depth-estimation | "2022-12-06T09:12:55Z" | ---
license: apache-2.0
tags:
- vision
- depth-estimation
widget:
- src: https://huggingface.co/datasets/mishig/sample_images/resolve/main/tiger.jpg
example_title: Tiger
- src: https://huggingface.co/datasets/mishig/sample_images/resolve/main/teapot.jpg
example_title: Teapot
- src: https://huggingface.co/datasets/mishig/sample_images/resolve/main/palace.jpg
example_title: Palace
model-index:
- name: dpt-hybrid-midas
results:
- task:
type: monocular-depth-estimation
name: Monocular Depth Estimation
dataset:
type: MIX-6
name: MIX-6
metrics:
- type: Zero-shot transfer
value: 11.06
name: Zero-shot transfer
config: Zero-shot transfer
verified: false
---
## Model Details: DPT-Hybrid (also known as MiDaS 3.0)
Dense Prediction Transformer (DPT) model trained on 1.4 million images for monocular depth estimation.
It was introduced in the paper [Vision Transformers for Dense Prediction](https://arxiv.org/abs/2103.13413) by Ranftl et al. (2021) and first released in [this repository](https://github.com/isl-org/DPT).
DPT uses the Vision Transformer (ViT) as backbone and adds a neck + head on top for monocular depth estimation.
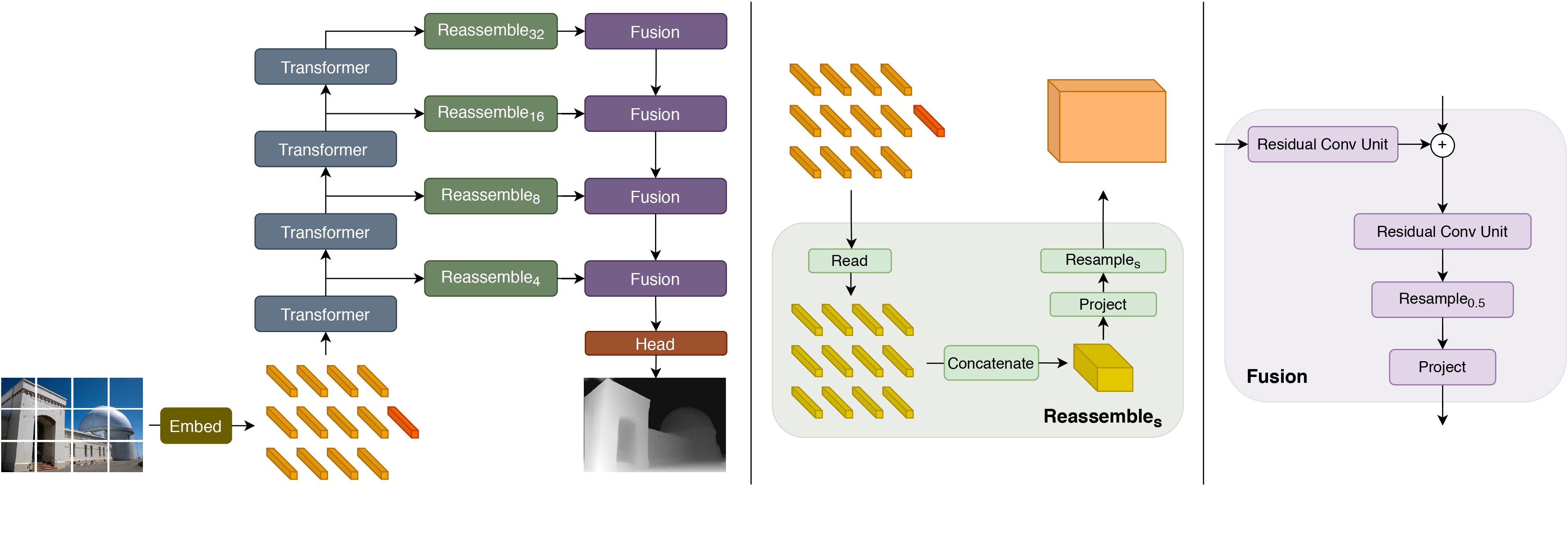
This repository hosts the "hybrid" version of the model as stated in the paper. DPT-Hybrid diverges from DPT by using [ViT-hybrid](https://huggingface.co/google/vit-hybrid-base-bit-384) as a backbone and taking some activations from the backbone.
The model card has been written in combination by the Hugging Face team and Intel.
| Model Detail | Description |
| ----------- | ----------- |
| Model Authors - Company | Intel |
| Date | December 22, 2022 |
| Version | 1 |
| Type | Computer Vision - Monocular Depth Estimation |
| Paper or Other Resources | [Vision Transformers for Dense Prediction](https://arxiv.org/abs/2103.13413) and [GitHub Repo](https://github.com/isl-org/DPT) |
| License | Apache 2.0 |
| Questions or Comments | [Community Tab](https://huggingface.co/Intel/dpt-hybrid-midas/discussions) and [Intel Developers Discord](https://discord.gg/rv2Gp55UJQ)|
| Intended Use | Description |
| ----------- | ----------- |
| Primary intended uses | You can use the raw model for zero-shot monocular depth estimation. See the [model hub](https://huggingface.co/models?search=dpt) to look for fine-tuned versions on a task that interests you. |
| Primary intended users | Anyone doing monocular depth estimation |
| Out-of-scope uses | This model in most cases will need to be fine-tuned for your particular task. The model should not be used to intentionally create hostile or alienating environments for people.|
### How to use
Here is how to use this model for zero-shot depth estimation on an image:
```python
from PIL import Image
import numpy as np
import requests
import torch
from transformers import DPTImageProcessor, DPTForDepthEstimation
image_processor = DPTImageProcessor.from_pretrained("Intel/dpt-hybrid-midas")
model = DPTForDepthEstimation.from_pretrained("Intel/dpt-hybrid-midas", low_cpu_mem_usage=True)
url = "http://images.cocodataset.org/val2017/000000039769.jpg"
image = Image.open(requests.get(url, stream=True).raw)
# prepare image for the model
inputs = image_processor(images=image, return_tensors="pt")
with torch.no_grad():
outputs = model(**inputs)
predicted_depth = outputs.predicted_depth
# interpolate to original size
prediction = torch.nn.functional.interpolate(
predicted_depth.unsqueeze(1),
size=image.size[::-1],
mode="bicubic",
align_corners=False,
)
# visualize the prediction
output = prediction.squeeze().cpu().numpy()
formatted = (output * 255 / np.max(output)).astype("uint8")
depth = Image.fromarray(formatted)
depth.show()
```
For more code examples, we refer to the [documentation](https://huggingface.co/docs/transformers/master/en/model_doc/dpt).
| Factors | Description |
| ----------- | ----------- |
| Groups | Multiple datasets compiled together |
| Instrumentation | - |
| Environment | Inference completed on Intel Xeon Platinum 8280 CPU @ 2.70GHz with 8 physical cores and an NVIDIA RTX 2080 GPU. |
| Card Prompts | Model deployment on alternate hardware and software will change model performance |
| Metrics | Description |
| ----------- | ----------- |
| Model performance measures | Zero-shot Transfer |
| Decision thresholds | - |
| Approaches to uncertainty and variability | - |
| Training and Evaluation Data | Description |
| ----------- | ----------- |
| Datasets | The dataset is called MIX 6, and contains around 1.4M images. The model was initialized with ImageNet-pretrained weights.|
| Motivation | To build a robust monocular depth prediction network |
| Preprocessing | "We resize the image such that the longer side is 384 pixels and train on random square crops of size 384. ... We perform random horizontal flips for data augmentation." See [Ranftl et al. (2021)](https://arxiv.org/abs/2103.13413) for more details. |
## Quantitative Analyses
| Model | Training set | DIW WHDR | ETH3D AbsRel | Sintel AbsRel | KITTI δ>1.25 | NYU δ>1.25 | TUM δ>1.25 |
| --- | --- | --- | --- | --- | --- | --- | --- |
| DPT - Large | MIX 6 | 10.82 (-13.2%) | 0.089 (-31.2%) | 0.270 (-17.5%) | 8.46 (-64.6%) | 8.32 (-12.9%) | 9.97 (-30.3%) |
| DPT - Hybrid | MIX 6 | 11.06 (-11.2%) | 0.093 (-27.6%) | 0.274 (-16.2%) | 11.56 (-51.6%) | 8.69 (-9.0%) | 10.89 (-23.2%) |
| MiDaS | MIX 6 | 12.95 (+3.9%) | 0.116 (-10.5%) | 0.329 (+0.5%) | 16.08 (-32.7%) | 8.71 (-8.8%) | 12.51 (-12.5%)
| MiDaS [30] | MIX 5 | 12.46 | 0.129 | 0.327 | 23.90 | 9.55 | 14.29 |
| Li [22] | MD [22] | 23.15 | 0.181 | 0.385 | 36.29 | 27.52 | 29.54 |
| Li [21] | MC [21] | 26.52 | 0.183 | 0.405 | 47.94 | 18.57 | 17.71 |
| Wang [40] | WS [40] | 19.09 | 0.205 | 0.390 | 31.92 | 29.57 | 20.18 |
| Xian [45] | RW [45] | 14.59 | 0.186 | 0.422 | 34.08 | 27.00 | 25.02 |
| Casser [5] | CS [8] | 32.80 | 0.235 | 0.422 | 21.15 | 39.58 | 37.18 |
Table 1. Comparison to the state of the art on monocular depth estimation. We evaluate zero-shot cross-dataset transfer according to the
protocol defined in [30]. Relative performance is computed with respect to the original MiDaS model [30]. Lower is better for all metrics. ([Ranftl et al., 2021](https://arxiv.org/abs/2103.13413))
| Ethical Considerations | Description |
| ----------- | ----------- |
| Data | The training data come from multiple image datasets compiled together. |
| Human life | The model is not intended to inform decisions central to human life or flourishing. It is an aggregated set of monocular depth image datasets. |
| Mitigations | No additional risk mitigation strategies were considered during model development. |
| Risks and harms | The extent of the risks involved by using the model remain unknown. |
| Use cases | - |
| Caveats and Recommendations |
| ----------- |
| Users (both direct and downstream) should be made aware of the risks, biases and limitations of the model. There are no additional caveats or recommendations for this model. |
### BibTeX entry and citation info
```bibtex
@article{DBLP:journals/corr/abs-2103-13413,
author = {Ren{\'{e}} Ranftl and
Alexey Bochkovskiy and
Vladlen Koltun},
title = {Vision Transformers for Dense Prediction},
journal = {CoRR},
volume = {abs/2103.13413},
year = {2021},
url = {https://arxiv.org/abs/2103.13413},
eprinttype = {arXiv},
eprint = {2103.13413},
timestamp = {Wed, 07 Apr 2021 15:31:46 +0200},
biburl = {https://dblp.org/rec/journals/corr/abs-2103-13413.bib},
bibsource = {dblp computer science bibliography, https://dblp.org}
}
``` |
vikp/surya_order | vikp | "2024-04-22T16:09:38Z" | 394,918 | 1 | transformers | [
"transformers",
"safetensors",
"vision-encoder-decoder",
"license:cc-by-nc-sa-4.0",
"endpoints_compatible",
"region:us"
] | null | "2024-04-16T17:05:01Z" | ---
license: cc-by-nc-sa-4.0
---
Reading order model for [surya](https://github.com/VikParuchuri/surya). |
llamafactory/tiny-random-Llama-3 | llamafactory | "2024-06-15T10:15:08Z" | 393,629 | 2 | transformers | [
"transformers",
"safetensors",
"llama",
"text-generation",
"conversational",
"license:apache-2.0",
"autotrain_compatible",
"text-generation-inference",
"region:us"
] | text-generation | "2024-06-07T17:30:09Z" | ---
license: apache-2.0
library_name: transformers
inference: false
---
A tiny version of https://huggingface.co/meta-llama/Meta-Llama-3-8B-Instruct
|
cagliostrolab/animagine-xl-3.1 | cagliostrolab | "2024-03-18T11:11:14Z" | 392,792 | 603 | diffusers | [
"diffusers",
"safetensors",
"text-to-image",
"stable-diffusion",
"stable-diffusion-xl",
"en",
"base_model:Linaqruf/animagine-xl-3.0",
"base_model:finetune:Linaqruf/animagine-xl-3.0",
"license:other",
"autotrain_compatible",
"endpoints_compatible",
"diffusers:StableDiffusionXLPipeline",
"region:us"
] | text-to-image | "2024-03-13T09:40:48Z" | ---
license: other
license_name: faipl-1.0-sd
license_link: https://freedevproject.org/faipl-1.0-sd/
language:
- en
tags:
- text-to-image
- stable-diffusion
- safetensors
- stable-diffusion-xl
base_model: cagliostrolab/animagine-xl-3.0
widget:
- text: 1girl, green hair, sweater, looking at viewer, upper body, beanie, outdoors, night, turtleneck, masterpiece, best quality, very aesthetic, absurdes
parameter:
negative_prompt: nsfw, lowres, (bad), text, error, fewer, extra, missing, worst quality, jpeg artifacts, low quality, watermark, unfinished, displeasing, oldest, early, chromatic aberration, signature, extra digits, artistic error, username, scan, [abstract]
example_title: 1girl
- text: 1boy, male focus, green hair, sweater, looking at viewer, upper body, beanie, outdoors, night, turtleneck, masterpiece, best quality, very aesthetic, absurdes
parameter:
negative_prompt: nsfw, lowres, (bad), text, error, fewer, extra, missing, worst quality, jpeg artifacts, low quality, watermark, unfinished, displeasing, oldest, early, chromatic aberration, signature, extra digits, artistic error, username, scan, [abstract]
example_title: 1boy
---
<style>
.title-container {
display: flex;
justify-content: center;
align-items: center;
height: 100vh; /* Adjust this value to position the title vertically */
}
.title {
font-size: 2.5em;
text-align: center;
color: #333;
font-family: 'Helvetica Neue', sans-serif;
text-transform: uppercase;
letter-spacing: 0.1em;
padding: 0.5em 0;
background: transparent;
}
.title span {
background: -webkit-linear-gradient(45deg, #7ed56f, #28b485);
-webkit-background-clip: text;
-webkit-text-fill-color: transparent;
}
.custom-table {
table-layout: fixed;
width: 100%;
border-collapse: collapse;
margin-top: 2em;
}
.custom-table td {
width: 50%;
vertical-align: top;
padding: 10px;
box-shadow: 0px 0px 0px 0px rgba(0, 0, 0, 0.15);
}
.custom-image-container {
position: relative;
width: 100%;
margin-bottom: 0em;
overflow: hidden;
border-radius: 10px;
transition: transform .7s;
/* Smooth transition for the container */
}
.custom-image-container:hover {
transform: scale(1.05);
/* Scale the container on hover */
}
.custom-image {
width: 100%;
height: auto;
object-fit: cover;
border-radius: 10px;
transition: transform .7s;
margin-bottom: 0em;
}
.nsfw-filter {
filter: blur(8px); /* Apply a blur effect */
transition: filter 0.3s ease; /* Smooth transition for the blur effect */
}
.custom-image-container:hover .nsfw-filter {
filter: none; /* Remove the blur effect on hover */
}
.overlay {
position: absolute;
bottom: 0;
left: 0;
right: 0;
color: white;
width: 100%;
height: 40%;
display: flex;
flex-direction: column;
justify-content: center;
align-items: center;
font-size: 1vw;
font-style: bold;
text-align: center;
opacity: 0;
/* Keep the text fully opaque */
background: linear-gradient(0deg, rgba(0, 0, 0, 0.8) 60%, rgba(0, 0, 0, 0) 100%);
transition: opacity .5s;
}
.custom-image-container:hover .overlay {
opacity: 1;
}
.overlay-text {
background: linear-gradient(45deg, #7ed56f, #28b485);
-webkit-background-clip: text;
color: transparent;
text-shadow: 2px 2px 4px rgba(0, 0, 0, 0.7);
.overlay-subtext {
font-size: 0.75em;
margin-top: 0.5em;
font-style: italic;
}
.overlay,
.overlay-subtext {
text-shadow: 2px 2px 4px rgba(0, 0, 0, 0.5);
}
</style>
<h1 class="title">
<span>Animagine XL 3.1</span>
</h1>
<table class="custom-table">
<tr>
<td>
<div class="custom-image-container">
<img class="custom-image" src="https://cdn-uploads.huggingface.co/production/uploads/6365c8dbf31ef76df4042821/yq_5AWegnLsGyCYyqJ-1G.png" alt="sample1">
</div>
<div class="custom-image-container">
<img class="custom-image" src="https://cdn-uploads.huggingface.co/production/uploads/6365c8dbf31ef76df4042821/sp6w1elvXVTbckkU74v3o.png" alt="sample4">
</div>
</td>
<td>
<div class="custom-image-container">
<img class="custom-image" src="https://cdn-uploads.huggingface.co/production/uploads/6365c8dbf31ef76df4042821/OYBuX1XzffN7Pxi4c75JV.png" alt="sample2">
</div>
<div class="custom-image-container">
<img class="custom-image" src="https://cdn-uploads.huggingface.co/production/uploads/6365c8dbf31ef76df4042821/ytT3Oaf-atbqrnPIqz_dq.png" alt="sample3">
</td>
<td>
<div class="custom-image-container">
<img class="custom-image" src="https://cdn-uploads.huggingface.co/production/uploads/6365c8dbf31ef76df4042821/0oRq204okFxRGECmrIK6d.png" alt="sample1">
</div>
<div class="custom-image-container">
<img class="custom-image" src="https://cdn-uploads.huggingface.co/production/uploads/6365c8dbf31ef76df4042821/DW51m0HlDuAlXwu8H8bIS.png" alt="sample4">
</div>
</td>
</tr>
</table>
**Animagine XL 3.1** is an update in the Animagine XL V3 series, enhancing the previous version, Animagine XL 3.0. This open-source, anime-themed text-to-image model has been improved for generating anime-style images with higher quality. It includes a broader range of characters from well-known anime series, an optimized dataset, and new aesthetic tags for better image creation. Built on Stable Diffusion XL, Animagine XL 3.1 aims to be a valuable resource for anime fans, artists, and content creators by producing accurate and detailed representations of anime characters.
## Model Details
- **Developed by**: [Cagliostro Research Lab](https://huggingface.co/cagliostrolab)
- **In collaboration with**: [SeaArt.ai](https://www.seaart.ai/)
- **Model type**: Diffusion-based text-to-image generative model
- **Model Description**: Animagine XL 3.1 generates high-quality anime images from textual prompts. It boasts enhanced hand anatomy, improved concept understanding, and advanced prompt interpretation.
- **License**: [Fair AI Public License 1.0-SD](https://freedevproject.org/faipl-1.0-sd/)
- **Fine-tuned from**: [Animagine XL 3.0](https://huggingface.co/cagliostrolab/animagine-xl-3.0)
## Gradio & Colab Integration
Try the demo powered by Gradio in Huggingface Spaces: [](https://huggingface.co/spaces/cagliostrolab/animagine-xl-3.1)
Or open the demo in Google Colab: [](https://colab.research.google.com/#fileId=https%3A//huggingface.co/spaces/cagliostrolab/animagine-xl-3.1/blob/main/demo.ipynb)
## 🧨 Diffusers Installation
First install the required libraries:
```bash
pip install diffusers transformers accelerate safetensors --upgrade
```
Then run image generation with the following example code:
```python
import torch
from diffusers import DiffusionPipeline
pipe = DiffusionPipeline.from_pretrained(
"cagliostrolab/animagine-xl-3.1",
torch_dtype=torch.float16,
use_safetensors=True,
)
pipe.to('cuda')
prompt = "1girl, souryuu asuka langley, neon genesis evangelion, solo, upper body, v, smile, looking at viewer, outdoors, night"
negative_prompt = "nsfw, lowres, (bad), text, error, fewer, extra, missing, worst quality, jpeg artifacts, low quality, watermark, unfinished, displeasing, oldest, early, chromatic aberration, signature, extra digits, artistic error, username, scan, [abstract]"
image = pipe(
prompt,
negative_prompt=negative_prompt,
width=832,
height=1216,
guidance_scale=7,
num_inference_steps=28
).images[0]
image.save("./output/asuka_test.png")
```
## Usage Guidelines
### Tag Ordering
For optimal results, it's recommended to follow the structured prompt template because we train the model like this:
```
1girl/1boy, character name, from what series, everything else in any order.
```
## Special Tags
Animagine XL 3.1 utilizes special tags to steer the result toward quality, rating, creation date and aesthetic. While the model can generate images without these tags, using them can help achieve better results.
### Quality Modifiers
Quality tags now consider both scores and post ratings to ensure a balanced quality distribution. We've refined labels for greater clarity, such as changing 'high quality' to 'great quality'.
| Quality Modifier | Score Criterion |
|------------------|-------------------|
| `masterpiece` | > 95% |
| `best quality` | > 85% & ≤ 95% |
| `great quality` | > 75% & ≤ 85% |
| `good quality` | > 50% & ≤ 75% |
| `normal quality` | > 25% & ≤ 50% |
| `low quality` | > 10% & ≤ 25% |
| `worst quality` | ≤ 10% |
### Rating Modifiers
We've also streamlined our rating tags for simplicity and clarity, aiming to establish global rules that can be applied across different models. For example, the tag 'rating: general' is now simply 'general', and 'rating: sensitive' has been condensed to 'sensitive'.
| Rating Modifier | Rating Criterion |
|-------------------|------------------|
| `safe` | General |
| `sensitive` | Sensitive |
| `nsfw` | Questionable |
| `explicit, nsfw` | Explicit |
### Year Modifier
We've also redefined the year range to steer results towards specific modern or vintage anime art styles more accurately. This update simplifies the range, focusing on relevance to current and past eras.
| Year Tag | Year Range |
|----------|------------------|
| `newest` | 2021 to 2024 |
| `recent` | 2018 to 2020 |
| `mid` | 2015 to 2017 |
| `early` | 2011 to 2014 |
| `oldest` | 2005 to 2010 |
### Aesthetic Tags
We've enhanced our tagging system with aesthetic tags to refine content categorization based on visual appeal. These tags are derived from evaluations made by a specialized ViT (Vision Transformer) image classification model, specifically trained on anime data. For this purpose, we utilized the model [shadowlilac/aesthetic-shadow-v2](https://huggingface.co/shadowlilac/aesthetic-shadow-v2), which assesses the aesthetic value of content before it undergoes training. This ensures that each piece of content is not only relevant and accurate but also visually appealing.
| Aesthetic Tag | Score Range |
|-------------------|-------------------|
| `very aesthetic` | > 0.71 |
| `aesthetic` | > 0.45 & < 0.71 |
| `displeasing` | > 0.27 & < 0.45 |
| `very displeasing`| ≤ 0.27 |
## Recommended settings
To guide the model towards generating high-aesthetic images, use negative prompts like:
```
nsfw, lowres, (bad), text, error, fewer, extra, missing, worst quality, jpeg artifacts, low quality, watermark, unfinished, displeasing, oldest, early, chromatic aberration, signature, extra digits, artistic error, username, scan, [abstract]
```
For higher quality outcomes, prepend prompts with:
```
masterpiece, best quality, very aesthetic, absurdres
```
it’s recommended to use a lower classifier-free guidance (CFG Scale) of around 5-7, sampling steps below 30, and to use Euler Ancestral (Euler a) as a sampler.
### Multi Aspect Resolution
This model supports generating images at the following dimensions:
| Dimensions | Aspect Ratio |
|-------------------|-----------------|
| `1024 x 1024` | 1:1 Square |
| `1152 x 896` | 9:7 |
| `896 x 1152` | 7:9 |
| `1216 x 832` | 19:13 |
| `832 x 1216` | 13:19 |
| `1344 x 768` | 7:4 Horizontal |
| `768 x 1344` | 4:7 Vertical |
| `1536 x 640` | 12:5 Horizontal |
| `640 x 1536` | 5:12 Vertical |
## Training and Hyperparameters
**Animagine XL 3.1** was trained on 2x A100 80GB GPUs for approximately 15 days, totaling over 350 GPU hours. The training process consisted of three stages:
- **Pretraining**: Utilized a data-rich collection of 870k ordered and tagged images to increase Animagine XL 3.0's model knowledge.
- **Finetuning - First Stage**: Employed labeled and curated aesthetic datasets to refine the broken U-Net after pretraining.
- **Finetuning - Second Stage**: Utilized labeled and curated aesthetic datasets to refine the model's art style and improve hand and anatomy rendering.
### Hyperparameters
| Stage | Epochs | UNet lr | Train Text Encoder | Batch Size | Noise Offset | Optimizer | LR Scheduler | Grad Acc Steps | GPUs |
|--------------------------|--------|---------|--------------------|------------|--------------|------------|-------------------------------|----------------|------|
| **Pretraining** | 10 | 1e-5 | True | 16 | N/A | AdamW | Cosine Annealing Warm Restart | 3 | 2 |
| **Finetuning 1st Stage** | 10 | 2e-6 | False | 48 | 0.0357 | Adafactor | Constant with Warmup | 1 | 1 |
| **Finetuning 2nd Stage** | 15 | 1e-6 | False | 48 | 0.0357 | Adafactor | Constant with Warmup | 1 | 1 |
## Model Comparison (Pretraining only)
### Training Config
| Configuration Item | Animagine XL 3.0 | Animagine XL 3.1 |
|---------------------------------|------------------------------------------|------------------------------------------------|
| **GPU** | 2 x A100 80G | 2 x A100 80G |
| **Dataset** | 1,271,990 | 873,504 |
| **Shuffle Separator** | True | True |
| **Num Epochs** | 10 | 10 |
| **Learning Rate** | 7.5e-6 | 1e-5 |
| **Text Encoder Learning Rate** | 3.75e-6 | 1e-5 |
| **Effective Batch Size** | 48 x 1 x 2 | 16 x 3 x 2 |
| **Optimizer** | Adafactor | AdamW |
| **Optimizer Args** | Scale Parameter: False, Relative Step: False, Warmup Init: False | Weight Decay: 0.1, Betas: (0.9, 0.99) |
| **LR Scheduler** | Constant with Warmup | Cosine Annealing Warm Restart |
| **LR Scheduler Args** | Warmup Steps: 100 | Num Cycles: 10, Min LR: 1e-6, LR Decay: 0.9, First Cycle Steps: 9,099 |
Source code and training config are available here: https://github.com/cagliostrolab/sd-scripts/tree/main/notebook
### Acknowledgements
The development and release of Animagine XL 3.1 would not have been possible without the invaluable contributions and support from the following individuals and organizations:
- **[SeaArt.ai](https://www.seaart.ai/)**: Our collaboration partner and sponsor.
- **[Shadow Lilac](https://huggingface.co/shadowlilac)**: For providing the aesthetic classification model, [aesthetic-shadow-v2](https://huggingface.co/shadowlilac/aesthetic-shadow-v2).
- **[Derrian Distro](https://github.com/derrian-distro)**: For their custom learning rate scheduler, adapted from [LoRA Easy Training Scripts](https://github.com/derrian-distro/LoRA_Easy_Training_Scripts/blob/main/custom_scheduler/LoraEasyCustomOptimizer/CustomOptimizers.py).
- **[Kohya SS](https://github.com/kohya-ss)**: For their comprehensive training scripts.
- **Cagliostrolab Collaborators**: For their dedication to model training, project management, and data curation.
- **Early Testers**: For their valuable feedback and quality assurance efforts.
- **NovelAI**: For their innovative approach to aesthetic tagging, which served as an inspiration for our implementation.
- **KBlueLeaf**: For providing inspiration in balancing quality tags distribution and managing tags based on [Hakubooru Metainfo](https://github.com/KohakuBlueleaf/HakuBooru/blob/main/hakubooru/metainfo.py)
Thank you all for your support and expertise in pushing the boundaries of anime-style image generation.
## Collaborators
- [Linaqruf](https://huggingface.co/Linaqruf)
- [ItsMeBell](https://huggingface.co/ItsMeBell)
- [Asahina2K](https://huggingface.co/Asahina2K)
- [DamarJati](https://huggingface.co/DamarJati)
- [Zwicky18](https://huggingface.co/Zwicky18)
- [Scipius2121](https://huggingface.co/Scipius2121)
- [Raelina](https://huggingface.co/Raelina)
- [Kayfahaarukku](https://huggingface.co/kayfahaarukku)
- [Kriz](https://huggingface.co/Kr1SsSzz)
## Limitations
While Animagine XL 3.1 represents a significant advancement in anime-style image generation, it is important to acknowledge its limitations:
1. **Anime-Focused**: This model is specifically designed for generating anime-style images and is not suitable for creating realistic photos.
2. **Prompt Complexity**: This model may not be suitable for users who expect high-quality results from short or simple prompts. The training focus was on concept understanding rather than aesthetic refinement, which may require more detailed and specific prompts to achieve the desired output.
3. **Prompt Format**: Animagine XL 3.1 is optimized for Danbooru-style tags rather than natural language prompts. For best results, users are encouraged to format their prompts using the appropriate tags and syntax.
4. **Anatomy and Hand Rendering**: Despite the improvements made in anatomy and hand rendering, there may still be instances where the model produces suboptimal results in these areas.
5. **Dataset Size**: The dataset used for training Animagine XL 3.1 consists of approximately 870,000 images. When combined with the previous iteration's dataset (1.2 million), the total training data amounts to around 2.1 million images. While substantial, this dataset size may still be considered limited in scope for an "ultimate" anime model.
6. **NSFW Content**: Animagine XL 3.1 has been designed to generate more balanced NSFW content. However, it is important to note that the model may still produce NSFW results, even if not explicitly prompted.
By acknowledging these limitations, we aim to provide transparency and set realistic expectations for users of Animagine XL 3.1. Despite these constraints, we believe that the model represents a significant step forward in anime-style image generation and offers a powerful tool for artists, designers, and enthusiasts alike.
## License
Based on Animagine XL 3.0, Animagine XL 3.1 falls under [Fair AI Public License 1.0-SD](https://freedevproject.org/faipl-1.0-sd/) license, which is compatible with Stable Diffusion models’ license. Key points:
1. **Modification Sharing:** If you modify Animagine XL 3.1, you must share both your changes and the original license.
2. **Source Code Accessibility:** If your modified version is network-accessible, provide a way (like a download link) for others to get the source code. This applies to derived models too.
3. **Distribution Terms:** Any distribution must be under this license or another with similar rules.
4. **Compliance:** Non-compliance must be fixed within 30 days to avoid license termination, emphasizing transparency and adherence to open-source values.
The choice of this license aims to keep Animagine XL 3.1 open and modifiable, aligning with open source community spirit. It protects contributors and users, encouraging a collaborative, ethical open-source community. This ensures the model not only benefits from communal input but also respects open-source development freedoms.
## Cagliostro Lab Discord Server
Finally Cagliostro Lab Server open to public
https://discord.gg/cqh9tZgbGc
Feel free to join our discord server |
thenlper/gte-base | thenlper | "2024-02-05T07:20:45Z" | 392,268 | 105 | sentence-transformers | [
"sentence-transformers",
"pytorch",
"onnx",
"safetensors",
"bert",
"mteb",
"sentence-similarity",
"Sentence Transformers",
"en",
"arxiv:2308.03281",
"license:mit",
"model-index",
"autotrain_compatible",
"text-embeddings-inference",
"endpoints_compatible",
"region:us"
] | sentence-similarity | "2023-07-27T03:21:20Z" | ---
tags:
- mteb
- sentence-similarity
- sentence-transformers
- Sentence Transformers
model-index:
- name: gte-base
results:
- task:
type: Classification
dataset:
type: mteb/amazon_counterfactual
name: MTEB AmazonCounterfactualClassification (en)
config: en
split: test
revision: e8379541af4e31359cca9fbcf4b00f2671dba205
metrics:
- type: accuracy
value: 74.17910447761193
- type: ap
value: 36.827146398068926
- type: f1
value: 68.11292888046363
- task:
type: Classification
dataset:
type: mteb/amazon_polarity
name: MTEB AmazonPolarityClassification
config: default
split: test
revision: e2d317d38cd51312af73b3d32a06d1a08b442046
metrics:
- type: accuracy
value: 91.77345000000001
- type: ap
value: 88.33530426691347
- type: f1
value: 91.76549906404642
- task:
type: Classification
dataset:
type: mteb/amazon_reviews_multi
name: MTEB AmazonReviewsClassification (en)
config: en
split: test
revision: 1399c76144fd37290681b995c656ef9b2e06e26d
metrics:
- type: accuracy
value: 48.964
- type: f1
value: 48.22995586184998
- task:
type: Retrieval
dataset:
type: arguana
name: MTEB ArguAna
config: default
split: test
revision: None
metrics:
- type: map_at_1
value: 32.147999999999996
- type: map_at_10
value: 48.253
- type: map_at_100
value: 49.038
- type: map_at_1000
value: 49.042
- type: map_at_3
value: 43.433
- type: map_at_5
value: 46.182
- type: mrr_at_1
value: 32.717
- type: mrr_at_10
value: 48.467
- type: mrr_at_100
value: 49.252
- type: mrr_at_1000
value: 49.254999999999995
- type: mrr_at_3
value: 43.599
- type: mrr_at_5
value: 46.408
- type: ndcg_at_1
value: 32.147999999999996
- type: ndcg_at_10
value: 57.12199999999999
- type: ndcg_at_100
value: 60.316
- type: ndcg_at_1000
value: 60.402
- type: ndcg_at_3
value: 47.178
- type: ndcg_at_5
value: 52.146
- type: precision_at_1
value: 32.147999999999996
- type: precision_at_10
value: 8.542
- type: precision_at_100
value: 0.9900000000000001
- type: precision_at_1000
value: 0.1
- type: precision_at_3
value: 19.346
- type: precision_at_5
value: 14.026
- type: recall_at_1
value: 32.147999999999996
- type: recall_at_10
value: 85.42
- type: recall_at_100
value: 99.004
- type: recall_at_1000
value: 99.644
- type: recall_at_3
value: 58.037000000000006
- type: recall_at_5
value: 70.128
- task:
type: Clustering
dataset:
type: mteb/arxiv-clustering-p2p
name: MTEB ArxivClusteringP2P
config: default
split: test
revision: a122ad7f3f0291bf49cc6f4d32aa80929df69d5d
metrics:
- type: v_measure
value: 48.59706013699614
- task:
type: Clustering
dataset:
type: mteb/arxiv-clustering-s2s
name: MTEB ArxivClusteringS2S
config: default
split: test
revision: f910caf1a6075f7329cdf8c1a6135696f37dbd53
metrics:
- type: v_measure
value: 43.01463593002057
- task:
type: Reranking
dataset:
type: mteb/askubuntudupquestions-reranking
name: MTEB AskUbuntuDupQuestions
config: default
split: test
revision: 2000358ca161889fa9c082cb41daa8dcfb161a54
metrics:
- type: map
value: 61.80250355752458
- type: mrr
value: 74.79455216989844
- task:
type: STS
dataset:
type: mteb/biosses-sts
name: MTEB BIOSSES
config: default
split: test
revision: d3fb88f8f02e40887cd149695127462bbcf29b4a
metrics:
- type: cos_sim_pearson
value: 89.87448576082345
- type: cos_sim_spearman
value: 87.64235843637468
- type: euclidean_pearson
value: 88.4901825511062
- type: euclidean_spearman
value: 87.74537283182033
- type: manhattan_pearson
value: 88.39040638362911
- type: manhattan_spearman
value: 87.62669542888003
- task:
type: Classification
dataset:
type: mteb/banking77
name: MTEB Banking77Classification
config: default
split: test
revision: 0fd18e25b25c072e09e0d92ab615fda904d66300
metrics:
- type: accuracy
value: 85.06818181818183
- type: f1
value: 85.02524460098233
- task:
type: Clustering
dataset:
type: mteb/biorxiv-clustering-p2p
name: MTEB BiorxivClusteringP2P
config: default
split: test
revision: 65b79d1d13f80053f67aca9498d9402c2d9f1f40
metrics:
- type: v_measure
value: 38.20471092679967
- task:
type: Clustering
dataset:
type: mteb/biorxiv-clustering-s2s
name: MTEB BiorxivClusteringS2S
config: default
split: test
revision: 258694dd0231531bc1fd9de6ceb52a0853c6d908
metrics:
- type: v_measure
value: 36.58967592147641
- task:
type: Retrieval
dataset:
type: BeIR/cqadupstack
name: MTEB CQADupstackAndroidRetrieval
config: default
split: test
revision: None
metrics:
- type: map_at_1
value: 32.411
- type: map_at_10
value: 45.162
- type: map_at_100
value: 46.717
- type: map_at_1000
value: 46.836
- type: map_at_3
value: 41.428
- type: map_at_5
value: 43.54
- type: mrr_at_1
value: 39.914
- type: mrr_at_10
value: 51.534
- type: mrr_at_100
value: 52.185
- type: mrr_at_1000
value: 52.22
- type: mrr_at_3
value: 49.046
- type: mrr_at_5
value: 50.548
- type: ndcg_at_1
value: 39.914
- type: ndcg_at_10
value: 52.235
- type: ndcg_at_100
value: 57.4
- type: ndcg_at_1000
value: 58.982
- type: ndcg_at_3
value: 47.332
- type: ndcg_at_5
value: 49.62
- type: precision_at_1
value: 39.914
- type: precision_at_10
value: 10.258000000000001
- type: precision_at_100
value: 1.6219999999999999
- type: precision_at_1000
value: 0.20500000000000002
- type: precision_at_3
value: 23.462
- type: precision_at_5
value: 16.71
- type: recall_at_1
value: 32.411
- type: recall_at_10
value: 65.408
- type: recall_at_100
value: 87.248
- type: recall_at_1000
value: 96.951
- type: recall_at_3
value: 50.349999999999994
- type: recall_at_5
value: 57.431
- task:
type: Retrieval
dataset:
type: BeIR/cqadupstack
name: MTEB CQADupstackEnglishRetrieval
config: default
split: test
revision: None
metrics:
- type: map_at_1
value: 31.911
- type: map_at_10
value: 42.608000000000004
- type: map_at_100
value: 43.948
- type: map_at_1000
value: 44.089
- type: map_at_3
value: 39.652
- type: map_at_5
value: 41.236
- type: mrr_at_1
value: 40.064
- type: mrr_at_10
value: 48.916
- type: mrr_at_100
value: 49.539
- type: mrr_at_1000
value: 49.583
- type: mrr_at_3
value: 46.741
- type: mrr_at_5
value: 48.037
- type: ndcg_at_1
value: 40.064
- type: ndcg_at_10
value: 48.442
- type: ndcg_at_100
value: 52.798
- type: ndcg_at_1000
value: 54.871
- type: ndcg_at_3
value: 44.528
- type: ndcg_at_5
value: 46.211
- type: precision_at_1
value: 40.064
- type: precision_at_10
value: 9.178
- type: precision_at_100
value: 1.452
- type: precision_at_1000
value: 0.193
- type: precision_at_3
value: 21.614
- type: precision_at_5
value: 15.185
- type: recall_at_1
value: 31.911
- type: recall_at_10
value: 58.155
- type: recall_at_100
value: 76.46300000000001
- type: recall_at_1000
value: 89.622
- type: recall_at_3
value: 46.195
- type: recall_at_5
value: 51.288999999999994
- task:
type: Retrieval
dataset:
type: BeIR/cqadupstack
name: MTEB CQADupstackGamingRetrieval
config: default
split: test
revision: None
metrics:
- type: map_at_1
value: 40.597
- type: map_at_10
value: 54.290000000000006
- type: map_at_100
value: 55.340999999999994
- type: map_at_1000
value: 55.388999999999996
- type: map_at_3
value: 50.931000000000004
- type: map_at_5
value: 52.839999999999996
- type: mrr_at_1
value: 46.646
- type: mrr_at_10
value: 57.524
- type: mrr_at_100
value: 58.225
- type: mrr_at_1000
value: 58.245999999999995
- type: mrr_at_3
value: 55.235
- type: mrr_at_5
value: 56.589
- type: ndcg_at_1
value: 46.646
- type: ndcg_at_10
value: 60.324999999999996
- type: ndcg_at_100
value: 64.30900000000001
- type: ndcg_at_1000
value: 65.19
- type: ndcg_at_3
value: 54.983000000000004
- type: ndcg_at_5
value: 57.621
- type: precision_at_1
value: 46.646
- type: precision_at_10
value: 9.774
- type: precision_at_100
value: 1.265
- type: precision_at_1000
value: 0.13799999999999998
- type: precision_at_3
value: 24.911
- type: precision_at_5
value: 16.977999999999998
- type: recall_at_1
value: 40.597
- type: recall_at_10
value: 74.773
- type: recall_at_100
value: 91.61200000000001
- type: recall_at_1000
value: 97.726
- type: recall_at_3
value: 60.458
- type: recall_at_5
value: 66.956
- task:
type: Retrieval
dataset:
type: BeIR/cqadupstack
name: MTEB CQADupstackGisRetrieval
config: default
split: test
revision: None
metrics:
- type: map_at_1
value: 27.122
- type: map_at_10
value: 36.711
- type: map_at_100
value: 37.775
- type: map_at_1000
value: 37.842999999999996
- type: map_at_3
value: 33.693
- type: map_at_5
value: 35.607
- type: mrr_at_1
value: 29.153000000000002
- type: mrr_at_10
value: 38.873999999999995
- type: mrr_at_100
value: 39.739000000000004
- type: mrr_at_1000
value: 39.794000000000004
- type: mrr_at_3
value: 36.102000000000004
- type: mrr_at_5
value: 37.876
- type: ndcg_at_1
value: 29.153000000000002
- type: ndcg_at_10
value: 42.048
- type: ndcg_at_100
value: 47.144999999999996
- type: ndcg_at_1000
value: 48.901
- type: ndcg_at_3
value: 36.402
- type: ndcg_at_5
value: 39.562999999999995
- type: precision_at_1
value: 29.153000000000002
- type: precision_at_10
value: 6.4750000000000005
- type: precision_at_100
value: 0.951
- type: precision_at_1000
value: 0.11299999999999999
- type: precision_at_3
value: 15.479999999999999
- type: precision_at_5
value: 11.028
- type: recall_at_1
value: 27.122
- type: recall_at_10
value: 56.279999999999994
- type: recall_at_100
value: 79.597
- type: recall_at_1000
value: 92.804
- type: recall_at_3
value: 41.437000000000005
- type: recall_at_5
value: 49.019
- task:
type: Retrieval
dataset:
type: BeIR/cqadupstack
name: MTEB CQADupstackMathematicaRetrieval
config: default
split: test
revision: None
metrics:
- type: map_at_1
value: 17.757
- type: map_at_10
value: 26.739
- type: map_at_100
value: 28.015
- type: map_at_1000
value: 28.127999999999997
- type: map_at_3
value: 23.986
- type: map_at_5
value: 25.514
- type: mrr_at_1
value: 22.015
- type: mrr_at_10
value: 31.325999999999997
- type: mrr_at_100
value: 32.368
- type: mrr_at_1000
value: 32.426
- type: mrr_at_3
value: 28.897000000000002
- type: mrr_at_5
value: 30.147000000000002
- type: ndcg_at_1
value: 22.015
- type: ndcg_at_10
value: 32.225
- type: ndcg_at_100
value: 38.405
- type: ndcg_at_1000
value: 40.932
- type: ndcg_at_3
value: 27.403
- type: ndcg_at_5
value: 29.587000000000003
- type: precision_at_1
value: 22.015
- type: precision_at_10
value: 5.9830000000000005
- type: precision_at_100
value: 1.051
- type: precision_at_1000
value: 0.13899999999999998
- type: precision_at_3
value: 13.391
- type: precision_at_5
value: 9.602
- type: recall_at_1
value: 17.757
- type: recall_at_10
value: 44.467
- type: recall_at_100
value: 71.53699999999999
- type: recall_at_1000
value: 89.281
- type: recall_at_3
value: 31.095
- type: recall_at_5
value: 36.818
- task:
type: Retrieval
dataset:
type: BeIR/cqadupstack
name: MTEB CQADupstackPhysicsRetrieval
config: default
split: test
revision: None
metrics:
- type: map_at_1
value: 30.354
- type: map_at_10
value: 42.134
- type: map_at_100
value: 43.429
- type: map_at_1000
value: 43.532
- type: map_at_3
value: 38.491
- type: map_at_5
value: 40.736
- type: mrr_at_1
value: 37.247
- type: mrr_at_10
value: 47.775
- type: mrr_at_100
value: 48.522999999999996
- type: mrr_at_1000
value: 48.567
- type: mrr_at_3
value: 45.059
- type: mrr_at_5
value: 46.811
- type: ndcg_at_1
value: 37.247
- type: ndcg_at_10
value: 48.609
- type: ndcg_at_100
value: 53.782
- type: ndcg_at_1000
value: 55.666000000000004
- type: ndcg_at_3
value: 42.866
- type: ndcg_at_5
value: 46.001
- type: precision_at_1
value: 37.247
- type: precision_at_10
value: 8.892999999999999
- type: precision_at_100
value: 1.341
- type: precision_at_1000
value: 0.168
- type: precision_at_3
value: 20.5
- type: precision_at_5
value: 14.976
- type: recall_at_1
value: 30.354
- type: recall_at_10
value: 62.273
- type: recall_at_100
value: 83.65599999999999
- type: recall_at_1000
value: 95.82000000000001
- type: recall_at_3
value: 46.464
- type: recall_at_5
value: 54.225
- task:
type: Retrieval
dataset:
type: BeIR/cqadupstack
name: MTEB CQADupstackProgrammersRetrieval
config: default
split: test
revision: None
metrics:
- type: map_at_1
value: 26.949
- type: map_at_10
value: 37.230000000000004
- type: map_at_100
value: 38.644
- type: map_at_1000
value: 38.751999999999995
- type: map_at_3
value: 33.816
- type: map_at_5
value: 35.817
- type: mrr_at_1
value: 33.446999999999996
- type: mrr_at_10
value: 42.970000000000006
- type: mrr_at_100
value: 43.873
- type: mrr_at_1000
value: 43.922
- type: mrr_at_3
value: 40.467999999999996
- type: mrr_at_5
value: 41.861
- type: ndcg_at_1
value: 33.446999999999996
- type: ndcg_at_10
value: 43.403000000000006
- type: ndcg_at_100
value: 49.247
- type: ndcg_at_1000
value: 51.361999999999995
- type: ndcg_at_3
value: 38.155
- type: ndcg_at_5
value: 40.643
- type: precision_at_1
value: 33.446999999999996
- type: precision_at_10
value: 8.128
- type: precision_at_100
value: 1.274
- type: precision_at_1000
value: 0.163
- type: precision_at_3
value: 18.493000000000002
- type: precision_at_5
value: 13.333
- type: recall_at_1
value: 26.949
- type: recall_at_10
value: 56.006
- type: recall_at_100
value: 80.99199999999999
- type: recall_at_1000
value: 95.074
- type: recall_at_3
value: 40.809
- type: recall_at_5
value: 47.57
- task:
type: Retrieval
dataset:
type: BeIR/cqadupstack
name: MTEB CQADupstackRetrieval
config: default
split: test
revision: None
metrics:
- type: map_at_1
value: 27.243583333333333
- type: map_at_10
value: 37.193250000000006
- type: map_at_100
value: 38.44833333333334
- type: map_at_1000
value: 38.56083333333333
- type: map_at_3
value: 34.06633333333333
- type: map_at_5
value: 35.87858333333334
- type: mrr_at_1
value: 32.291583333333335
- type: mrr_at_10
value: 41.482749999999996
- type: mrr_at_100
value: 42.33583333333333
- type: mrr_at_1000
value: 42.38683333333333
- type: mrr_at_3
value: 38.952999999999996
- type: mrr_at_5
value: 40.45333333333333
- type: ndcg_at_1
value: 32.291583333333335
- type: ndcg_at_10
value: 42.90533333333334
- type: ndcg_at_100
value: 48.138666666666666
- type: ndcg_at_1000
value: 50.229083333333335
- type: ndcg_at_3
value: 37.76133333333334
- type: ndcg_at_5
value: 40.31033333333334
- type: precision_at_1
value: 32.291583333333335
- type: precision_at_10
value: 7.585583333333333
- type: precision_at_100
value: 1.2045000000000001
- type: precision_at_1000
value: 0.15733333333333335
- type: precision_at_3
value: 17.485416666666666
- type: precision_at_5
value: 12.5145
- type: recall_at_1
value: 27.243583333333333
- type: recall_at_10
value: 55.45108333333334
- type: recall_at_100
value: 78.25858333333335
- type: recall_at_1000
value: 92.61716666666665
- type: recall_at_3
value: 41.130583333333334
- type: recall_at_5
value: 47.73133333333334
- task:
type: Retrieval
dataset:
type: BeIR/cqadupstack
name: MTEB CQADupstackStatsRetrieval
config: default
split: test
revision: None
metrics:
- type: map_at_1
value: 26.325
- type: map_at_10
value: 32.795
- type: map_at_100
value: 33.96
- type: map_at_1000
value: 34.054
- type: map_at_3
value: 30.64
- type: map_at_5
value: 31.771
- type: mrr_at_1
value: 29.908
- type: mrr_at_10
value: 35.83
- type: mrr_at_100
value: 36.868
- type: mrr_at_1000
value: 36.928
- type: mrr_at_3
value: 33.896
- type: mrr_at_5
value: 34.893
- type: ndcg_at_1
value: 29.908
- type: ndcg_at_10
value: 36.746
- type: ndcg_at_100
value: 42.225
- type: ndcg_at_1000
value: 44.523
- type: ndcg_at_3
value: 32.82
- type: ndcg_at_5
value: 34.583000000000006
- type: precision_at_1
value: 29.908
- type: precision_at_10
value: 5.6129999999999995
- type: precision_at_100
value: 0.9079999999999999
- type: precision_at_1000
value: 0.11800000000000001
- type: precision_at_3
value: 13.753000000000002
- type: precision_at_5
value: 9.417
- type: recall_at_1
value: 26.325
- type: recall_at_10
value: 45.975
- type: recall_at_100
value: 70.393
- type: recall_at_1000
value: 87.217
- type: recall_at_3
value: 35.195
- type: recall_at_5
value: 39.69
- task:
type: Retrieval
dataset:
type: BeIR/cqadupstack
name: MTEB CQADupstackTexRetrieval
config: default
split: test
revision: None
metrics:
- type: map_at_1
value: 17.828
- type: map_at_10
value: 25.759
- type: map_at_100
value: 26.961000000000002
- type: map_at_1000
value: 27.094
- type: map_at_3
value: 23.166999999999998
- type: map_at_5
value: 24.610000000000003
- type: mrr_at_1
value: 21.61
- type: mrr_at_10
value: 29.605999999999998
- type: mrr_at_100
value: 30.586000000000002
- type: mrr_at_1000
value: 30.664
- type: mrr_at_3
value: 27.214
- type: mrr_at_5
value: 28.571
- type: ndcg_at_1
value: 21.61
- type: ndcg_at_10
value: 30.740000000000002
- type: ndcg_at_100
value: 36.332
- type: ndcg_at_1000
value: 39.296
- type: ndcg_at_3
value: 26.11
- type: ndcg_at_5
value: 28.297
- type: precision_at_1
value: 21.61
- type: precision_at_10
value: 5.643
- type: precision_at_100
value: 1.0
- type: precision_at_1000
value: 0.14400000000000002
- type: precision_at_3
value: 12.4
- type: precision_at_5
value: 9.119
- type: recall_at_1
value: 17.828
- type: recall_at_10
value: 41.876000000000005
- type: recall_at_100
value: 66.648
- type: recall_at_1000
value: 87.763
- type: recall_at_3
value: 28.957
- type: recall_at_5
value: 34.494
- task:
type: Retrieval
dataset:
type: BeIR/cqadupstack
name: MTEB CQADupstackUnixRetrieval
config: default
split: test
revision: None
metrics:
- type: map_at_1
value: 27.921000000000003
- type: map_at_10
value: 37.156
- type: map_at_100
value: 38.399
- type: map_at_1000
value: 38.498
- type: map_at_3
value: 34.134
- type: map_at_5
value: 35.936
- type: mrr_at_1
value: 32.649
- type: mrr_at_10
value: 41.19
- type: mrr_at_100
value: 42.102000000000004
- type: mrr_at_1000
value: 42.157
- type: mrr_at_3
value: 38.464
- type: mrr_at_5
value: 40.148
- type: ndcg_at_1
value: 32.649
- type: ndcg_at_10
value: 42.679
- type: ndcg_at_100
value: 48.27
- type: ndcg_at_1000
value: 50.312
- type: ndcg_at_3
value: 37.269000000000005
- type: ndcg_at_5
value: 40.055
- type: precision_at_1
value: 32.649
- type: precision_at_10
value: 7.155
- type: precision_at_100
value: 1.124
- type: precision_at_1000
value: 0.14100000000000001
- type: precision_at_3
value: 16.791
- type: precision_at_5
value: 12.015
- type: recall_at_1
value: 27.921000000000003
- type: recall_at_10
value: 55.357
- type: recall_at_100
value: 79.476
- type: recall_at_1000
value: 93.314
- type: recall_at_3
value: 40.891
- type: recall_at_5
value: 47.851
- task:
type: Retrieval
dataset:
type: BeIR/cqadupstack
name: MTEB CQADupstackWebmastersRetrieval
config: default
split: test
revision: None
metrics:
- type: map_at_1
value: 25.524
- type: map_at_10
value: 35.135
- type: map_at_100
value: 36.665
- type: map_at_1000
value: 36.886
- type: map_at_3
value: 31.367
- type: map_at_5
value: 33.724
- type: mrr_at_1
value: 30.631999999999998
- type: mrr_at_10
value: 39.616
- type: mrr_at_100
value: 40.54
- type: mrr_at_1000
value: 40.585
- type: mrr_at_3
value: 36.462
- type: mrr_at_5
value: 38.507999999999996
- type: ndcg_at_1
value: 30.631999999999998
- type: ndcg_at_10
value: 41.61
- type: ndcg_at_100
value: 47.249
- type: ndcg_at_1000
value: 49.662
- type: ndcg_at_3
value: 35.421
- type: ndcg_at_5
value: 38.811
- type: precision_at_1
value: 30.631999999999998
- type: precision_at_10
value: 8.123
- type: precision_at_100
value: 1.5810000000000002
- type: precision_at_1000
value: 0.245
- type: precision_at_3
value: 16.337
- type: precision_at_5
value: 12.568999999999999
- type: recall_at_1
value: 25.524
- type: recall_at_10
value: 54.994
- type: recall_at_100
value: 80.03099999999999
- type: recall_at_1000
value: 95.25099999999999
- type: recall_at_3
value: 37.563
- type: recall_at_5
value: 46.428999999999995
- task:
type: Retrieval
dataset:
type: BeIR/cqadupstack
name: MTEB CQADupstackWordpressRetrieval
config: default
split: test
revision: None
metrics:
- type: map_at_1
value: 22.224
- type: map_at_10
value: 30.599999999999998
- type: map_at_100
value: 31.526
- type: map_at_1000
value: 31.629
- type: map_at_3
value: 27.491
- type: map_at_5
value: 29.212
- type: mrr_at_1
value: 24.214
- type: mrr_at_10
value: 32.632
- type: mrr_at_100
value: 33.482
- type: mrr_at_1000
value: 33.550000000000004
- type: mrr_at_3
value: 29.852
- type: mrr_at_5
value: 31.451
- type: ndcg_at_1
value: 24.214
- type: ndcg_at_10
value: 35.802
- type: ndcg_at_100
value: 40.502
- type: ndcg_at_1000
value: 43.052
- type: ndcg_at_3
value: 29.847
- type: ndcg_at_5
value: 32.732
- type: precision_at_1
value: 24.214
- type: precision_at_10
value: 5.804
- type: precision_at_100
value: 0.885
- type: precision_at_1000
value: 0.121
- type: precision_at_3
value: 12.692999999999998
- type: precision_at_5
value: 9.242
- type: recall_at_1
value: 22.224
- type: recall_at_10
value: 49.849
- type: recall_at_100
value: 71.45
- type: recall_at_1000
value: 90.583
- type: recall_at_3
value: 34.153
- type: recall_at_5
value: 41.004000000000005
- task:
type: Retrieval
dataset:
type: climate-fever
name: MTEB ClimateFEVER
config: default
split: test
revision: None
metrics:
- type: map_at_1
value: 12.386999999999999
- type: map_at_10
value: 20.182
- type: map_at_100
value: 21.86
- type: map_at_1000
value: 22.054000000000002
- type: map_at_3
value: 17.165
- type: map_at_5
value: 18.643
- type: mrr_at_1
value: 26.906000000000002
- type: mrr_at_10
value: 37.907999999999994
- type: mrr_at_100
value: 38.868
- type: mrr_at_1000
value: 38.913
- type: mrr_at_3
value: 34.853
- type: mrr_at_5
value: 36.567
- type: ndcg_at_1
value: 26.906000000000002
- type: ndcg_at_10
value: 28.103
- type: ndcg_at_100
value: 35.073
- type: ndcg_at_1000
value: 38.653
- type: ndcg_at_3
value: 23.345
- type: ndcg_at_5
value: 24.828
- type: precision_at_1
value: 26.906000000000002
- type: precision_at_10
value: 8.547
- type: precision_at_100
value: 1.617
- type: precision_at_1000
value: 0.22799999999999998
- type: precision_at_3
value: 17.025000000000002
- type: precision_at_5
value: 12.834000000000001
- type: recall_at_1
value: 12.386999999999999
- type: recall_at_10
value: 33.306999999999995
- type: recall_at_100
value: 57.516
- type: recall_at_1000
value: 77.74799999999999
- type: recall_at_3
value: 21.433
- type: recall_at_5
value: 25.915
- task:
type: Retrieval
dataset:
type: dbpedia-entity
name: MTEB DBPedia
config: default
split: test
revision: None
metrics:
- type: map_at_1
value: 9.322
- type: map_at_10
value: 20.469
- type: map_at_100
value: 28.638
- type: map_at_1000
value: 30.433
- type: map_at_3
value: 14.802000000000001
- type: map_at_5
value: 17.297
- type: mrr_at_1
value: 68.75
- type: mrr_at_10
value: 76.29599999999999
- type: mrr_at_100
value: 76.62400000000001
- type: mrr_at_1000
value: 76.633
- type: mrr_at_3
value: 75.083
- type: mrr_at_5
value: 75.771
- type: ndcg_at_1
value: 54.87499999999999
- type: ndcg_at_10
value: 41.185
- type: ndcg_at_100
value: 46.400000000000006
- type: ndcg_at_1000
value: 54.223
- type: ndcg_at_3
value: 45.489000000000004
- type: ndcg_at_5
value: 43.161
- type: precision_at_1
value: 68.75
- type: precision_at_10
value: 32.300000000000004
- type: precision_at_100
value: 10.607999999999999
- type: precision_at_1000
value: 2.237
- type: precision_at_3
value: 49.083
- type: precision_at_5
value: 41.6
- type: recall_at_1
value: 9.322
- type: recall_at_10
value: 25.696
- type: recall_at_100
value: 52.898
- type: recall_at_1000
value: 77.281
- type: recall_at_3
value: 15.943
- type: recall_at_5
value: 19.836000000000002
- task:
type: Classification
dataset:
type: mteb/emotion
name: MTEB EmotionClassification
config: default
split: test
revision: 4f58c6b202a23cf9a4da393831edf4f9183cad37
metrics:
- type: accuracy
value: 48.650000000000006
- type: f1
value: 43.528467245539396
- task:
type: Retrieval
dataset:
type: fever
name: MTEB FEVER
config: default
split: test
revision: None
metrics:
- type: map_at_1
value: 66.56
- type: map_at_10
value: 76.767
- type: map_at_100
value: 77.054
- type: map_at_1000
value: 77.068
- type: map_at_3
value: 75.29299999999999
- type: map_at_5
value: 76.24
- type: mrr_at_1
value: 71.842
- type: mrr_at_10
value: 81.459
- type: mrr_at_100
value: 81.58800000000001
- type: mrr_at_1000
value: 81.59100000000001
- type: mrr_at_3
value: 80.188
- type: mrr_at_5
value: 81.038
- type: ndcg_at_1
value: 71.842
- type: ndcg_at_10
value: 81.51899999999999
- type: ndcg_at_100
value: 82.544
- type: ndcg_at_1000
value: 82.829
- type: ndcg_at_3
value: 78.92
- type: ndcg_at_5
value: 80.406
- type: precision_at_1
value: 71.842
- type: precision_at_10
value: 10.066
- type: precision_at_100
value: 1.076
- type: precision_at_1000
value: 0.11199999999999999
- type: precision_at_3
value: 30.703000000000003
- type: precision_at_5
value: 19.301
- type: recall_at_1
value: 66.56
- type: recall_at_10
value: 91.55
- type: recall_at_100
value: 95.67099999999999
- type: recall_at_1000
value: 97.539
- type: recall_at_3
value: 84.46900000000001
- type: recall_at_5
value: 88.201
- task:
type: Retrieval
dataset:
type: fiqa
name: MTEB FiQA2018
config: default
split: test
revision: None
metrics:
- type: map_at_1
value: 20.087
- type: map_at_10
value: 32.830999999999996
- type: map_at_100
value: 34.814
- type: map_at_1000
value: 34.999
- type: map_at_3
value: 28.198
- type: map_at_5
value: 30.779
- type: mrr_at_1
value: 38.889
- type: mrr_at_10
value: 48.415
- type: mrr_at_100
value: 49.187
- type: mrr_at_1000
value: 49.226
- type: mrr_at_3
value: 45.705
- type: mrr_at_5
value: 47.225
- type: ndcg_at_1
value: 38.889
- type: ndcg_at_10
value: 40.758
- type: ndcg_at_100
value: 47.671
- type: ndcg_at_1000
value: 50.744
- type: ndcg_at_3
value: 36.296
- type: ndcg_at_5
value: 37.852999999999994
- type: precision_at_1
value: 38.889
- type: precision_at_10
value: 11.466
- type: precision_at_100
value: 1.8499999999999999
- type: precision_at_1000
value: 0.24
- type: precision_at_3
value: 24.126
- type: precision_at_5
value: 18.21
- type: recall_at_1
value: 20.087
- type: recall_at_10
value: 48.042
- type: recall_at_100
value: 73.493
- type: recall_at_1000
value: 91.851
- type: recall_at_3
value: 32.694
- type: recall_at_5
value: 39.099000000000004
- task:
type: Retrieval
dataset:
type: hotpotqa
name: MTEB HotpotQA
config: default
split: test
revision: None
metrics:
- type: map_at_1
value: 38.096000000000004
- type: map_at_10
value: 56.99999999999999
- type: map_at_100
value: 57.914
- type: map_at_1000
value: 57.984
- type: map_at_3
value: 53.900999999999996
- type: map_at_5
value: 55.827000000000005
- type: mrr_at_1
value: 76.19200000000001
- type: mrr_at_10
value: 81.955
- type: mrr_at_100
value: 82.164
- type: mrr_at_1000
value: 82.173
- type: mrr_at_3
value: 80.963
- type: mrr_at_5
value: 81.574
- type: ndcg_at_1
value: 76.19200000000001
- type: ndcg_at_10
value: 65.75
- type: ndcg_at_100
value: 68.949
- type: ndcg_at_1000
value: 70.342
- type: ndcg_at_3
value: 61.29
- type: ndcg_at_5
value: 63.747
- type: precision_at_1
value: 76.19200000000001
- type: precision_at_10
value: 13.571
- type: precision_at_100
value: 1.6070000000000002
- type: precision_at_1000
value: 0.179
- type: precision_at_3
value: 38.663
- type: precision_at_5
value: 25.136999999999997
- type: recall_at_1
value: 38.096000000000004
- type: recall_at_10
value: 67.853
- type: recall_at_100
value: 80.365
- type: recall_at_1000
value: 89.629
- type: recall_at_3
value: 57.995
- type: recall_at_5
value: 62.843
- task:
type: Classification
dataset:
type: mteb/imdb
name: MTEB ImdbClassification
config: default
split: test
revision: 3d86128a09e091d6018b6d26cad27f2739fc2db7
metrics:
- type: accuracy
value: 85.95200000000001
- type: ap
value: 80.73847277002109
- type: f1
value: 85.92406135678594
- task:
type: Retrieval
dataset:
type: msmarco
name: MTEB MSMARCO
config: default
split: dev
revision: None
metrics:
- type: map_at_1
value: 20.916999999999998
- type: map_at_10
value: 33.23
- type: map_at_100
value: 34.427
- type: map_at_1000
value: 34.477000000000004
- type: map_at_3
value: 29.292
- type: map_at_5
value: 31.6
- type: mrr_at_1
value: 21.547
- type: mrr_at_10
value: 33.839999999999996
- type: mrr_at_100
value: 34.979
- type: mrr_at_1000
value: 35.022999999999996
- type: mrr_at_3
value: 29.988
- type: mrr_at_5
value: 32.259
- type: ndcg_at_1
value: 21.519
- type: ndcg_at_10
value: 40.209
- type: ndcg_at_100
value: 45.954
- type: ndcg_at_1000
value: 47.187
- type: ndcg_at_3
value: 32.227
- type: ndcg_at_5
value: 36.347
- type: precision_at_1
value: 21.519
- type: precision_at_10
value: 6.447
- type: precision_at_100
value: 0.932
- type: precision_at_1000
value: 0.104
- type: precision_at_3
value: 13.877999999999998
- type: precision_at_5
value: 10.404
- type: recall_at_1
value: 20.916999999999998
- type: recall_at_10
value: 61.7
- type: recall_at_100
value: 88.202
- type: recall_at_1000
value: 97.588
- type: recall_at_3
value: 40.044999999999995
- type: recall_at_5
value: 49.964999999999996
- task:
type: Classification
dataset:
type: mteb/mtop_domain
name: MTEB MTOPDomainClassification (en)
config: en
split: test
revision: d80d48c1eb48d3562165c59d59d0034df9fff0bf
metrics:
- type: accuracy
value: 93.02781577747379
- type: f1
value: 92.83653922768306
- task:
type: Classification
dataset:
type: mteb/mtop_intent
name: MTEB MTOPIntentClassification (en)
config: en
split: test
revision: ae001d0e6b1228650b7bd1c2c65fb50ad11a8aba
metrics:
- type: accuracy
value: 72.04286365709075
- type: f1
value: 53.43867658525793
- task:
type: Classification
dataset:
type: mteb/amazon_massive_intent
name: MTEB MassiveIntentClassification (en)
config: en
split: test
revision: 31efe3c427b0bae9c22cbb560b8f15491cc6bed7
metrics:
- type: accuracy
value: 71.47276395427035
- type: f1
value: 69.77017399597342
- task:
type: Classification
dataset:
type: mteb/amazon_massive_scenario
name: MTEB MassiveScenarioClassification (en)
config: en
split: test
revision: 7d571f92784cd94a019292a1f45445077d0ef634
metrics:
- type: accuracy
value: 76.3819771351715
- type: f1
value: 76.8484533435409
- task:
type: Clustering
dataset:
type: mteb/medrxiv-clustering-p2p
name: MTEB MedrxivClusteringP2P
config: default
split: test
revision: e7a26af6f3ae46b30dde8737f02c07b1505bcc73
metrics:
- type: v_measure
value: 33.16515993299593
- task:
type: Clustering
dataset:
type: mteb/medrxiv-clustering-s2s
name: MTEB MedrxivClusteringS2S
config: default
split: test
revision: 35191c8c0dca72d8ff3efcd72aa802307d469663
metrics:
- type: v_measure
value: 31.77145323314774
- task:
type: Reranking
dataset:
type: mteb/mind_small
name: MTEB MindSmallReranking
config: default
split: test
revision: 3bdac13927fdc888b903db93b2ffdbd90b295a69
metrics:
- type: map
value: 32.53637706586391
- type: mrr
value: 33.7312926288863
- task:
type: Retrieval
dataset:
type: nfcorpus
name: MTEB NFCorpus
config: default
split: test
revision: None
metrics:
- type: map_at_1
value: 7.063999999999999
- type: map_at_10
value: 15.046999999999999
- type: map_at_100
value: 19.116
- type: map_at_1000
value: 20.702
- type: map_at_3
value: 10.932
- type: map_at_5
value: 12.751999999999999
- type: mrr_at_1
value: 50.464
- type: mrr_at_10
value: 58.189
- type: mrr_at_100
value: 58.733999999999995
- type: mrr_at_1000
value: 58.769000000000005
- type: mrr_at_3
value: 56.24400000000001
- type: mrr_at_5
value: 57.68299999999999
- type: ndcg_at_1
value: 48.142
- type: ndcg_at_10
value: 37.897
- type: ndcg_at_100
value: 35.264
- type: ndcg_at_1000
value: 44.033
- type: ndcg_at_3
value: 42.967
- type: ndcg_at_5
value: 40.815
- type: precision_at_1
value: 50.15500000000001
- type: precision_at_10
value: 28.235
- type: precision_at_100
value: 8.994
- type: precision_at_1000
value: 2.218
- type: precision_at_3
value: 40.041
- type: precision_at_5
value: 35.046
- type: recall_at_1
value: 7.063999999999999
- type: recall_at_10
value: 18.598
- type: recall_at_100
value: 35.577999999999996
- type: recall_at_1000
value: 67.43
- type: recall_at_3
value: 11.562999999999999
- type: recall_at_5
value: 14.771
- task:
type: Retrieval
dataset:
type: nq
name: MTEB NQ
config: default
split: test
revision: None
metrics:
- type: map_at_1
value: 29.046
- type: map_at_10
value: 44.808
- type: map_at_100
value: 45.898
- type: map_at_1000
value: 45.927
- type: map_at_3
value: 40.19
- type: map_at_5
value: 42.897
- type: mrr_at_1
value: 32.706
- type: mrr_at_10
value: 47.275
- type: mrr_at_100
value: 48.075
- type: mrr_at_1000
value: 48.095
- type: mrr_at_3
value: 43.463
- type: mrr_at_5
value: 45.741
- type: ndcg_at_1
value: 32.706
- type: ndcg_at_10
value: 52.835
- type: ndcg_at_100
value: 57.345
- type: ndcg_at_1000
value: 57.985
- type: ndcg_at_3
value: 44.171
- type: ndcg_at_5
value: 48.661
- type: precision_at_1
value: 32.706
- type: precision_at_10
value: 8.895999999999999
- type: precision_at_100
value: 1.143
- type: precision_at_1000
value: 0.12
- type: precision_at_3
value: 20.238999999999997
- type: precision_at_5
value: 14.728
- type: recall_at_1
value: 29.046
- type: recall_at_10
value: 74.831
- type: recall_at_100
value: 94.192
- type: recall_at_1000
value: 98.897
- type: recall_at_3
value: 52.37500000000001
- type: recall_at_5
value: 62.732
- task:
type: Retrieval
dataset:
type: quora
name: MTEB QuoraRetrieval
config: default
split: test
revision: None
metrics:
- type: map_at_1
value: 70.38799999999999
- type: map_at_10
value: 84.315
- type: map_at_100
value: 84.955
- type: map_at_1000
value: 84.971
- type: map_at_3
value: 81.33399999999999
- type: map_at_5
value: 83.21300000000001
- type: mrr_at_1
value: 81.03
- type: mrr_at_10
value: 87.395
- type: mrr_at_100
value: 87.488
- type: mrr_at_1000
value: 87.48899999999999
- type: mrr_at_3
value: 86.41499999999999
- type: mrr_at_5
value: 87.074
- type: ndcg_at_1
value: 81.04
- type: ndcg_at_10
value: 88.151
- type: ndcg_at_100
value: 89.38199999999999
- type: ndcg_at_1000
value: 89.479
- type: ndcg_at_3
value: 85.24000000000001
- type: ndcg_at_5
value: 86.856
- type: precision_at_1
value: 81.04
- type: precision_at_10
value: 13.372
- type: precision_at_100
value: 1.526
- type: precision_at_1000
value: 0.157
- type: precision_at_3
value: 37.217
- type: precision_at_5
value: 24.502
- type: recall_at_1
value: 70.38799999999999
- type: recall_at_10
value: 95.452
- type: recall_at_100
value: 99.59700000000001
- type: recall_at_1000
value: 99.988
- type: recall_at_3
value: 87.11
- type: recall_at_5
value: 91.662
- task:
type: Clustering
dataset:
type: mteb/reddit-clustering
name: MTEB RedditClustering
config: default
split: test
revision: 24640382cdbf8abc73003fb0fa6d111a705499eb
metrics:
- type: v_measure
value: 59.334991029213235
- task:
type: Clustering
dataset:
type: mteb/reddit-clustering-p2p
name: MTEB RedditClusteringP2P
config: default
split: test
revision: 282350215ef01743dc01b456c7f5241fa8937f16
metrics:
- type: v_measure
value: 62.586500854616666
- task:
type: Retrieval
dataset:
type: scidocs
name: MTEB SCIDOCS
config: default
split: test
revision: None
metrics:
- type: map_at_1
value: 5.153
- type: map_at_10
value: 14.277000000000001
- type: map_at_100
value: 16.922
- type: map_at_1000
value: 17.302999999999997
- type: map_at_3
value: 9.961
- type: map_at_5
value: 12.257
- type: mrr_at_1
value: 25.4
- type: mrr_at_10
value: 37.458000000000006
- type: mrr_at_100
value: 38.681
- type: mrr_at_1000
value: 38.722
- type: mrr_at_3
value: 34.1
- type: mrr_at_5
value: 36.17
- type: ndcg_at_1
value: 25.4
- type: ndcg_at_10
value: 23.132
- type: ndcg_at_100
value: 32.908
- type: ndcg_at_1000
value: 38.754
- type: ndcg_at_3
value: 21.82
- type: ndcg_at_5
value: 19.353
- type: precision_at_1
value: 25.4
- type: precision_at_10
value: 12.1
- type: precision_at_100
value: 2.628
- type: precision_at_1000
value: 0.402
- type: precision_at_3
value: 20.732999999999997
- type: precision_at_5
value: 17.34
- type: recall_at_1
value: 5.153
- type: recall_at_10
value: 24.54
- type: recall_at_100
value: 53.293
- type: recall_at_1000
value: 81.57
- type: recall_at_3
value: 12.613
- type: recall_at_5
value: 17.577
- task:
type: STS
dataset:
type: mteb/sickr-sts
name: MTEB SICK-R
config: default
split: test
revision: a6ea5a8cab320b040a23452cc28066d9beae2cee
metrics:
- type: cos_sim_pearson
value: 84.86284404925333
- type: cos_sim_spearman
value: 78.85870555294795
- type: euclidean_pearson
value: 82.20105295276093
- type: euclidean_spearman
value: 78.92125617009592
- type: manhattan_pearson
value: 82.15840025289069
- type: manhattan_spearman
value: 78.85955732900803
- task:
type: STS
dataset:
type: mteb/sts12-sts
name: MTEB STS12
config: default
split: test
revision: a0d554a64d88156834ff5ae9920b964011b16384
metrics:
- type: cos_sim_pearson
value: 84.98747423389027
- type: cos_sim_spearman
value: 75.71298531799367
- type: euclidean_pearson
value: 81.59709559192291
- type: euclidean_spearman
value: 75.40622749225653
- type: manhattan_pearson
value: 81.55553547608804
- type: manhattan_spearman
value: 75.39380235424899
- task:
type: STS
dataset:
type: mteb/sts13-sts
name: MTEB STS13
config: default
split: test
revision: 7e90230a92c190f1bf69ae9002b8cea547a64cca
metrics:
- type: cos_sim_pearson
value: 83.76861330695503
- type: cos_sim_spearman
value: 85.72991921531624
- type: euclidean_pearson
value: 84.84504307397536
- type: euclidean_spearman
value: 86.02679162824732
- type: manhattan_pearson
value: 84.79969439220142
- type: manhattan_spearman
value: 85.99238837291625
- task:
type: STS
dataset:
type: mteb/sts14-sts
name: MTEB STS14
config: default
split: test
revision: 6031580fec1f6af667f0bd2da0a551cf4f0b2375
metrics:
- type: cos_sim_pearson
value: 83.31929747511796
- type: cos_sim_spearman
value: 81.50806522502528
- type: euclidean_pearson
value: 82.93936686512777
- type: euclidean_spearman
value: 81.54403447993224
- type: manhattan_pearson
value: 82.89696981900828
- type: manhattan_spearman
value: 81.52817825470865
- task:
type: STS
dataset:
type: mteb/sts15-sts
name: MTEB STS15
config: default
split: test
revision: ae752c7c21bf194d8b67fd573edf7ae58183cbe3
metrics:
- type: cos_sim_pearson
value: 87.14413295332908
- type: cos_sim_spearman
value: 88.81032027008195
- type: euclidean_pearson
value: 88.19205563407645
- type: euclidean_spearman
value: 88.89738339479216
- type: manhattan_pearson
value: 88.11075942004189
- type: manhattan_spearman
value: 88.8297061675564
- task:
type: STS
dataset:
type: mteb/sts16-sts
name: MTEB STS16
config: default
split: test
revision: 4d8694f8f0e0100860b497b999b3dbed754a0513
metrics:
- type: cos_sim_pearson
value: 82.15980075557017
- type: cos_sim_spearman
value: 83.81896308594801
- type: euclidean_pearson
value: 83.11195254311338
- type: euclidean_spearman
value: 84.10479481755407
- type: manhattan_pearson
value: 83.13915225100556
- type: manhattan_spearman
value: 84.09895591027859
- task:
type: STS
dataset:
type: mteb/sts17-crosslingual-sts
name: MTEB STS17 (en-en)
config: en-en
split: test
revision: af5e6fb845001ecf41f4c1e033ce921939a2a68d
metrics:
- type: cos_sim_pearson
value: 87.93669480147919
- type: cos_sim_spearman
value: 87.89861394614361
- type: euclidean_pearson
value: 88.37316413202339
- type: euclidean_spearman
value: 88.18033817842569
- type: manhattan_pearson
value: 88.39427578879469
- type: manhattan_spearman
value: 88.09185009236847
- task:
type: STS
dataset:
type: mteb/sts22-crosslingual-sts
name: MTEB STS22 (en)
config: en
split: test
revision: 6d1ba47164174a496b7fa5d3569dae26a6813b80
metrics:
- type: cos_sim_pearson
value: 66.62215083348255
- type: cos_sim_spearman
value: 67.33243665716736
- type: euclidean_pearson
value: 67.60871701996284
- type: euclidean_spearman
value: 66.75929225238659
- type: manhattan_pearson
value: 67.63907838970992
- type: manhattan_spearman
value: 66.79313656754846
- task:
type: STS
dataset:
type: mteb/stsbenchmark-sts
name: MTEB STSBenchmark
config: default
split: test
revision: b0fddb56ed78048fa8b90373c8a3cfc37b684831
metrics:
- type: cos_sim_pearson
value: 84.65549191934764
- type: cos_sim_spearman
value: 85.73266847750143
- type: euclidean_pearson
value: 85.75609932254318
- type: euclidean_spearman
value: 85.9452287759371
- type: manhattan_pearson
value: 85.69717413063573
- type: manhattan_spearman
value: 85.86546318377046
- task:
type: Reranking
dataset:
type: mteb/scidocs-reranking
name: MTEB SciDocsRR
config: default
split: test
revision: d3c5e1fc0b855ab6097bf1cda04dd73947d7caab
metrics:
- type: map
value: 87.08164129085783
- type: mrr
value: 96.2877273416489
- task:
type: Retrieval
dataset:
type: scifact
name: MTEB SciFact
config: default
split: test
revision: None
metrics:
- type: map_at_1
value: 62.09400000000001
- type: map_at_10
value: 71.712
- type: map_at_100
value: 72.128
- type: map_at_1000
value: 72.14399999999999
- type: map_at_3
value: 68.93
- type: map_at_5
value: 70.694
- type: mrr_at_1
value: 65.0
- type: mrr_at_10
value: 72.572
- type: mrr_at_100
value: 72.842
- type: mrr_at_1000
value: 72.856
- type: mrr_at_3
value: 70.44399999999999
- type: mrr_at_5
value: 71.744
- type: ndcg_at_1
value: 65.0
- type: ndcg_at_10
value: 76.178
- type: ndcg_at_100
value: 77.887
- type: ndcg_at_1000
value: 78.227
- type: ndcg_at_3
value: 71.367
- type: ndcg_at_5
value: 73.938
- type: precision_at_1
value: 65.0
- type: precision_at_10
value: 10.033
- type: precision_at_100
value: 1.097
- type: precision_at_1000
value: 0.11199999999999999
- type: precision_at_3
value: 27.667
- type: precision_at_5
value: 18.4
- type: recall_at_1
value: 62.09400000000001
- type: recall_at_10
value: 89.022
- type: recall_at_100
value: 96.833
- type: recall_at_1000
value: 99.333
- type: recall_at_3
value: 75.922
- type: recall_at_5
value: 82.428
- task:
type: PairClassification
dataset:
type: mteb/sprintduplicatequestions-pairclassification
name: MTEB SprintDuplicateQuestions
config: default
split: test
revision: d66bd1f72af766a5cc4b0ca5e00c162f89e8cc46
metrics:
- type: cos_sim_accuracy
value: 99.82178217821782
- type: cos_sim_ap
value: 95.71282508220798
- type: cos_sim_f1
value: 90.73120494335737
- type: cos_sim_precision
value: 93.52441613588111
- type: cos_sim_recall
value: 88.1
- type: dot_accuracy
value: 99.73960396039604
- type: dot_ap
value: 92.98534606529098
- type: dot_f1
value: 86.83024536805209
- type: dot_precision
value: 86.96088264794383
- type: dot_recall
value: 86.7
- type: euclidean_accuracy
value: 99.82475247524752
- type: euclidean_ap
value: 95.72927039014849
- type: euclidean_f1
value: 90.89974293059126
- type: euclidean_precision
value: 93.54497354497354
- type: euclidean_recall
value: 88.4
- type: manhattan_accuracy
value: 99.82574257425742
- type: manhattan_ap
value: 95.72142177390405
- type: manhattan_f1
value: 91.00152516522625
- type: manhattan_precision
value: 92.55429162357808
- type: manhattan_recall
value: 89.5
- type: max_accuracy
value: 99.82574257425742
- type: max_ap
value: 95.72927039014849
- type: max_f1
value: 91.00152516522625
- task:
type: Clustering
dataset:
type: mteb/stackexchange-clustering
name: MTEB StackExchangeClustering
config: default
split: test
revision: 6cbc1f7b2bc0622f2e39d2c77fa502909748c259
metrics:
- type: v_measure
value: 66.63957663468679
- task:
type: Clustering
dataset:
type: mteb/stackexchange-clustering-p2p
name: MTEB StackExchangeClusteringP2P
config: default
split: test
revision: 815ca46b2622cec33ccafc3735d572c266efdb44
metrics:
- type: v_measure
value: 36.003307257923964
- task:
type: Reranking
dataset:
type: mteb/stackoverflowdupquestions-reranking
name: MTEB StackOverflowDupQuestions
config: default
split: test
revision: e185fbe320c72810689fc5848eb6114e1ef5ec69
metrics:
- type: map
value: 53.005825525863905
- type: mrr
value: 53.854683919022165
- task:
type: Summarization
dataset:
type: mteb/summeval
name: MTEB SummEval
config: default
split: test
revision: cda12ad7615edc362dbf25a00fdd61d3b1eaf93c
metrics:
- type: cos_sim_pearson
value: 30.503611569974098
- type: cos_sim_spearman
value: 31.17155564248449
- type: dot_pearson
value: 26.740428413981306
- type: dot_spearman
value: 26.55727635469746
- task:
type: Retrieval
dataset:
type: trec-covid
name: MTEB TRECCOVID
config: default
split: test
revision: None
metrics:
- type: map_at_1
value: 0.23600000000000002
- type: map_at_10
value: 1.7670000000000001
- type: map_at_100
value: 10.208
- type: map_at_1000
value: 25.997999999999998
- type: map_at_3
value: 0.605
- type: map_at_5
value: 0.9560000000000001
- type: mrr_at_1
value: 84.0
- type: mrr_at_10
value: 90.167
- type: mrr_at_100
value: 90.167
- type: mrr_at_1000
value: 90.167
- type: mrr_at_3
value: 89.667
- type: mrr_at_5
value: 90.167
- type: ndcg_at_1
value: 77.0
- type: ndcg_at_10
value: 68.783
- type: ndcg_at_100
value: 54.196
- type: ndcg_at_1000
value: 52.077
- type: ndcg_at_3
value: 71.642
- type: ndcg_at_5
value: 70.45700000000001
- type: precision_at_1
value: 84.0
- type: precision_at_10
value: 73.0
- type: precision_at_100
value: 55.48
- type: precision_at_1000
value: 23.102
- type: precision_at_3
value: 76.0
- type: precision_at_5
value: 74.8
- type: recall_at_1
value: 0.23600000000000002
- type: recall_at_10
value: 1.9869999999999999
- type: recall_at_100
value: 13.749
- type: recall_at_1000
value: 50.157
- type: recall_at_3
value: 0.633
- type: recall_at_5
value: 1.0290000000000001
- task:
type: Retrieval
dataset:
type: webis-touche2020
name: MTEB Touche2020
config: default
split: test
revision: None
metrics:
- type: map_at_1
value: 1.437
- type: map_at_10
value: 8.791
- type: map_at_100
value: 15.001999999999999
- type: map_at_1000
value: 16.549
- type: map_at_3
value: 3.8080000000000003
- type: map_at_5
value: 5.632000000000001
- type: mrr_at_1
value: 20.408
- type: mrr_at_10
value: 36.96
- type: mrr_at_100
value: 37.912
- type: mrr_at_1000
value: 37.912
- type: mrr_at_3
value: 29.592000000000002
- type: mrr_at_5
value: 34.489999999999995
- type: ndcg_at_1
value: 19.387999999999998
- type: ndcg_at_10
value: 22.554
- type: ndcg_at_100
value: 35.197
- type: ndcg_at_1000
value: 46.58
- type: ndcg_at_3
value: 20.285
- type: ndcg_at_5
value: 21.924
- type: precision_at_1
value: 20.408
- type: precision_at_10
value: 21.837
- type: precision_at_100
value: 7.754999999999999
- type: precision_at_1000
value: 1.537
- type: precision_at_3
value: 21.769
- type: precision_at_5
value: 23.673
- type: recall_at_1
value: 1.437
- type: recall_at_10
value: 16.314999999999998
- type: recall_at_100
value: 47.635
- type: recall_at_1000
value: 82.963
- type: recall_at_3
value: 4.955
- type: recall_at_5
value: 8.805
- task:
type: Classification
dataset:
type: mteb/toxic_conversations_50k
name: MTEB ToxicConversationsClassification
config: default
split: test
revision: d7c0de2777da35d6aae2200a62c6e0e5af397c4c
metrics:
- type: accuracy
value: 71.6128
- type: ap
value: 14.279639861175664
- type: f1
value: 54.922292491204274
- task:
type: Classification
dataset:
type: mteb/tweet_sentiment_extraction
name: MTEB TweetSentimentExtractionClassification
config: default
split: test
revision: d604517c81ca91fe16a244d1248fc021f9ecee7a
metrics:
- type: accuracy
value: 57.01188455008489
- type: f1
value: 57.377953019225515
- task:
type: Clustering
dataset:
type: mteb/twentynewsgroups-clustering
name: MTEB TwentyNewsgroupsClustering
config: default
split: test
revision: 6125ec4e24fa026cec8a478383ee943acfbd5449
metrics:
- type: v_measure
value: 52.306769136544254
- task:
type: PairClassification
dataset:
type: mteb/twittersemeval2015-pairclassification
name: MTEB TwitterSemEval2015
config: default
split: test
revision: 70970daeab8776df92f5ea462b6173c0b46fd2d1
metrics:
- type: cos_sim_accuracy
value: 85.64701674912082
- type: cos_sim_ap
value: 72.46600945328552
- type: cos_sim_f1
value: 67.96572367648784
- type: cos_sim_precision
value: 61.21801649397336
- type: cos_sim_recall
value: 76.38522427440633
- type: dot_accuracy
value: 82.33295583238957
- type: dot_ap
value: 62.54843443071716
- type: dot_f1
value: 60.38378562507096
- type: dot_precision
value: 52.99980067769583
- type: dot_recall
value: 70.15831134564644
- type: euclidean_accuracy
value: 85.7423854085951
- type: euclidean_ap
value: 72.76873850945174
- type: euclidean_f1
value: 68.23556960543262
- type: euclidean_precision
value: 61.3344559040202
- type: euclidean_recall
value: 76.88654353562005
- type: manhattan_accuracy
value: 85.74834594981225
- type: manhattan_ap
value: 72.66825372446462
- type: manhattan_f1
value: 68.21539194662853
- type: manhattan_precision
value: 62.185056472632496
- type: manhattan_recall
value: 75.54089709762533
- type: max_accuracy
value: 85.74834594981225
- type: max_ap
value: 72.76873850945174
- type: max_f1
value: 68.23556960543262
- task:
type: PairClassification
dataset:
type: mteb/twitterurlcorpus-pairclassification
name: MTEB TwitterURLCorpus
config: default
split: test
revision: 8b6510b0b1fa4e4c4f879467980e9be563ec1cdf
metrics:
- type: cos_sim_accuracy
value: 88.73171110334924
- type: cos_sim_ap
value: 85.51855542063649
- type: cos_sim_f1
value: 77.95706775700934
- type: cos_sim_precision
value: 74.12524298805887
- type: cos_sim_recall
value: 82.20665229442562
- type: dot_accuracy
value: 86.94842240074514
- type: dot_ap
value: 80.90995345771762
- type: dot_f1
value: 74.20765027322403
- type: dot_precision
value: 70.42594385285575
- type: dot_recall
value: 78.41854019094548
- type: euclidean_accuracy
value: 88.73753250281368
- type: euclidean_ap
value: 85.54712254033734
- type: euclidean_f1
value: 78.07565728654365
- type: euclidean_precision
value: 75.1120597652081
- type: euclidean_recall
value: 81.282722513089
- type: manhattan_accuracy
value: 88.72588970388482
- type: manhattan_ap
value: 85.52118291594071
- type: manhattan_f1
value: 78.04428724070593
- type: manhattan_precision
value: 74.83219105490002
- type: manhattan_recall
value: 81.54450261780106
- type: max_accuracy
value: 88.73753250281368
- type: max_ap
value: 85.54712254033734
- type: max_f1
value: 78.07565728654365
language:
- en
license: mit
---
# gte-base
General Text Embeddings (GTE) model. [Towards General Text Embeddings with Multi-stage Contrastive Learning](https://arxiv.org/abs/2308.03281)
The GTE models are trained by Alibaba DAMO Academy. They are mainly based on the BERT framework and currently offer three different sizes of models, including [GTE-large](https://huggingface.co/thenlper/gte-large), [GTE-base](https://huggingface.co/thenlper/gte-base), and [GTE-small](https://huggingface.co/thenlper/gte-small). The GTE models are trained on a large-scale corpus of relevance text pairs, covering a wide range of domains and scenarios. This enables the GTE models to be applied to various downstream tasks of text embeddings, including **information retrieval**, **semantic textual similarity**, **text reranking**, etc.
## Metrics
We compared the performance of the GTE models with other popular text embedding models on the MTEB benchmark. For more detailed comparison results, please refer to the [MTEB leaderboard](https://huggingface.co/spaces/mteb/leaderboard).
| Model Name | Model Size (GB) | Dimension | Sequence Length | Average (56) | Clustering (11) | Pair Classification (3) | Reranking (4) | Retrieval (15) | STS (10) | Summarization (1) | Classification (12) |
|:----:|:---:|:---:|:---:|:---:|:---:|:---:|:---:|:---:|:---:|:---:|:---:|
| [**gte-large**](https://huggingface.co/thenlper/gte-large) | 0.67 | 1024 | 512 | **63.13** | 46.84 | 85.00 | 59.13 | 52.22 | 83.35 | 31.66 | 73.33 |
| [**gte-base**](https://huggingface.co/thenlper/gte-base) | 0.22 | 768 | 512 | **62.39** | 46.2 | 84.57 | 58.61 | 51.14 | 82.3 | 31.17 | 73.01 |
| [e5-large-v2](https://huggingface.co/intfloat/e5-large-v2) | 1.34 | 1024| 512 | 62.25 | 44.49 | 86.03 | 56.61 | 50.56 | 82.05 | 30.19 | 75.24 |
| [e5-base-v2](https://huggingface.co/intfloat/e5-base-v2) | 0.44 | 768 | 512 | 61.5 | 43.80 | 85.73 | 55.91 | 50.29 | 81.05 | 30.28 | 73.84 |
| [**gte-small**](https://huggingface.co/thenlper/gte-small) | 0.07 | 384 | 512 | **61.36** | 44.89 | 83.54 | 57.7 | 49.46 | 82.07 | 30.42 | 72.31 |
| [text-embedding-ada-002](https://platform.openai.com/docs/guides/embeddings) | - | 1536 | 8192 | 60.99 | 45.9 | 84.89 | 56.32 | 49.25 | 80.97 | 30.8 | 70.93 |
| [e5-small-v2](https://huggingface.co/intfloat/e5-base-v2) | 0.13 | 384 | 512 | 59.93 | 39.92 | 84.67 | 54.32 | 49.04 | 80.39 | 31.16 | 72.94 |
| [sentence-t5-xxl](https://huggingface.co/sentence-transformers/sentence-t5-xxl) | 9.73 | 768 | 512 | 59.51 | 43.72 | 85.06 | 56.42 | 42.24 | 82.63 | 30.08 | 73.42 |
| [all-mpnet-base-v2](https://huggingface.co/sentence-transformers/all-mpnet-base-v2) | 0.44 | 768 | 514 | 57.78 | 43.69 | 83.04 | 59.36 | 43.81 | 80.28 | 27.49 | 65.07 |
| [sgpt-bloom-7b1-msmarco](https://huggingface.co/bigscience/sgpt-bloom-7b1-msmarco) | 28.27 | 4096 | 2048 | 57.59 | 38.93 | 81.9 | 55.65 | 48.22 | 77.74 | 33.6 | 66.19 |
| [all-MiniLM-L12-v2](https://huggingface.co/sentence-transformers/all-MiniLM-L12-v2) | 0.13 | 384 | 512 | 56.53 | 41.81 | 82.41 | 58.44 | 42.69 | 79.8 | 27.9 | 63.21 |
| [all-MiniLM-L6-v2](https://huggingface.co/sentence-transformers/all-MiniLM-L6-v2) | 0.09 | 384 | 512 | 56.26 | 42.35 | 82.37 | 58.04 | 41.95 | 78.9 | 30.81 | 63.05 |
| [contriever-base-msmarco](https://huggingface.co/nthakur/contriever-base-msmarco) | 0.44 | 768 | 512 | 56.00 | 41.1 | 82.54 | 53.14 | 41.88 | 76.51 | 30.36 | 66.68 |
| [sentence-t5-base](https://huggingface.co/sentence-transformers/sentence-t5-base) | 0.22 | 768 | 512 | 55.27 | 40.21 | 85.18 | 53.09 | 33.63 | 81.14 | 31.39 | 69.81 |
## Usage
Code example
```python
import torch.nn.functional as F
from torch import Tensor
from transformers import AutoTokenizer, AutoModel
def average_pool(last_hidden_states: Tensor,
attention_mask: Tensor) -> Tensor:
last_hidden = last_hidden_states.masked_fill(~attention_mask[..., None].bool(), 0.0)
return last_hidden.sum(dim=1) / attention_mask.sum(dim=1)[..., None]
input_texts = [
"what is the capital of China?",
"how to implement quick sort in python?",
"Beijing",
"sorting algorithms"
]
tokenizer = AutoTokenizer.from_pretrained("thenlper/gte-base")
model = AutoModel.from_pretrained("thenlper/gte-base")
# Tokenize the input texts
batch_dict = tokenizer(input_texts, max_length=512, padding=True, truncation=True, return_tensors='pt')
outputs = model(**batch_dict)
embeddings = average_pool(outputs.last_hidden_state, batch_dict['attention_mask'])
# (Optionally) normalize embeddings
embeddings = F.normalize(embeddings, p=2, dim=1)
scores = (embeddings[:1] @ embeddings[1:].T) * 100
print(scores.tolist())
```
Use with sentence-transformers:
```python
from sentence_transformers import SentenceTransformer
from sentence_transformers.util import cos_sim
sentences = ['That is a happy person', 'That is a very happy person']
model = SentenceTransformer('thenlper/gte-base')
embeddings = model.encode(sentences)
print(cos_sim(embeddings[0], embeddings[1]))
```
### Limitation
This model exclusively caters to English texts, and any lengthy texts will be truncated to a maximum of 512 tokens.
### Citation
If you find our paper or models helpful, please consider citing them as follows:
```
@article{li2023towards,
title={Towards general text embeddings with multi-stage contrastive learning},
author={Li, Zehan and Zhang, Xin and Zhang, Yanzhao and Long, Dingkun and Xie, Pengjun and Zhang, Meishan},
journal={arXiv preprint arXiv:2308.03281},
year={2023}
}
```
|