File size: 6,266 Bytes
c3deef6 7696112 05ad241 c3deef6 7696112 bcec803 e62e0b2 3a4a244 e62e0b2 1c73b85 9acfe3b c16dddd 9acfe3b 7545165 9acfe3b 8ad97de 9acfe3b 332adce 8ad97de 332adce 9acfe3b 6cade18 9acfe3b 1c73b85 9acfe3b 9f34c3a da851f5 08047ad 9f34c3a 08047ad 9acfe3b 08047ad 8bc5035 9f34c3a 799cae9 27d1153 799cae9 9f34c3a |
1 2 3 4 5 6 7 8 9 10 11 12 13 14 15 16 17 18 19 20 21 22 23 24 25 26 27 28 29 30 31 32 33 34 35 36 37 38 39 40 41 42 43 44 45 46 47 48 49 50 51 52 53 54 55 56 57 58 59 60 61 62 63 64 65 66 67 68 69 70 71 72 73 74 75 76 77 78 79 80 81 82 83 84 85 86 87 88 89 90 91 92 93 94 |
---
license: bigscience-bloom-rail-1.0
language:
- fr
- en
pipeline_tag: feature-extraction
---
# Note
We now strongly recommend using the [Bloomz-3b-retriever-v2](https://huggingface.co/cmarkea/bloomz-3b-retriever-v2) model, **which offers significantly superior performance**.
Bloomz-3b-retriever
---------------------
We introduce the Bloomz-3b-retriever based on the [Bloomz-3b-sft-chat model](https://huggingface.co/cmarkea/bloomz-3b-sft-chat). This model enables the creation of an embedding representation of text and queries for a retrieval task, linking queries to documents. The model is designed to be cross-language, meaning it is language-agnostic (English/French). This model is ideal for Open Domain Question Answering (ODQA), projecting queries and text with an algebraic structure to bring them closer together.
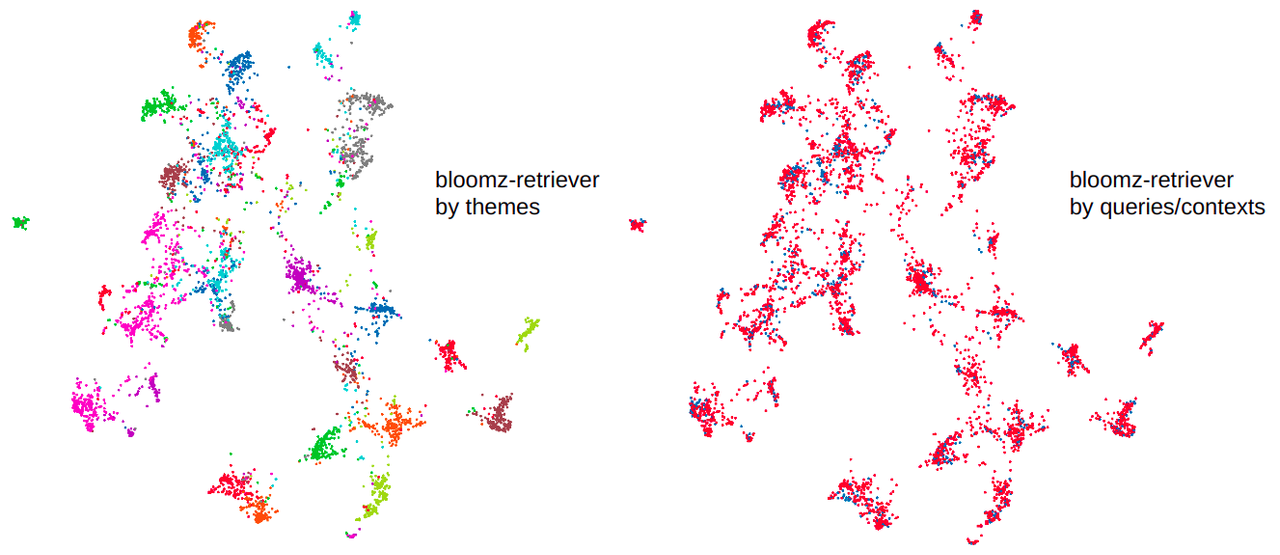
Training
--------
It is a bi-encoder trained on a corpus of context/query pairs, with 50% in English and 50% in French. The language distribution for queries and contexts is evenly split (1/4 French-French, 1/4 French-English, 1/4 English-French, 1/4 English-English). The learning objective is to bring the embedding representation of queries and associated contexts closer using a contrastive method. The loss function is defined in [Deep Metric Learning using Triplet Network](https://arxiv.org/abs/1412.6622).
Benchmark
---------
Based on the SQuAD evaluation dataset (comprising 6000 queries distributed over 1200 contexts grouped into 35 themes), we compare the performance in terms of the average top contexter value for a query (Top-mean), the standard deviation of the average top (Top-std), and the percentage of correct queries within the top-1, top-5, and top-10. We compare the model with a TF-IDF trained on the SQuAD train sub-dataset (we want a fixed algebraic structure for the vector database instead of a variable structure every time we add a new document, then the IDF part has frozen), CamemBERT, Sentence-BERT, and finally our model. We observe these performances in both monolingual and cross-language contexts (query in French and context in English).
Model (FR/FR) | Top-mean | Top-std | Top-1 (%) | Top-5 (%) | Top-10 (%) |
|----------------------------------------------------------------------------------------------------:|:--------:|:-------:|:---------:|:---------:|:----------:|
| TF-IDF | 128 | 269 | 23 | 46 | 56 |
| [CamemBERT](https://huggingface.co/camembert/camembert-base) | 417 | 347 | 1 | 2 | 3 |
| [Sentence-BERT](https://huggingface.co/sentence-transformers/paraphrase-multilingual-mpnet-base-v2) | 11 | 41 | 43 | 71 | 82 |
| [Bloomz-560m-retriever](https://huggingface.co/cmarkea/bloomz-560m-retriever) | 10 | 47 | 51 | 78 | 86 |
| [Bloomz-3b-retriever](https://huggingface.co/cmarkea/bloomz-3b-retriever) | 9 | 37 | 50 | 79 | 87 |
Model (EN/FR) | Top-mean | Top-std | Top-1 (%) | Top-5 (%) | Top-10 (%) |
|----------------------------------------------------------------------------------------------------:|:--------:|:-------:|:---------:|:---------:|:-----------:|
| TF-IDF | 607 | 334 | 0 | 0 | 0 |
| [CamemBERT](https://huggingface.co/camembert/camembert-base) | 432 | 345 | 0 | 1 | 1 |
| [Sentence-BERT](https://huggingface.co/sentence-transformers/paraphrase-multilingual-mpnet-base-v2) | 12 | 47 | 44 | 73 | 83 |
| [Bloomz-560m-retriever](https://huggingface.co/cmarkea/bloomz-560m-retriever) | 10 | 44 | 49 | 77 | 86 |
| [Bloomz-3b-retriever](https://huggingface.co/cmarkea/bloomz-3b-retriever) | 9 | 38 | 50 | 78 | 87 |
We observed that TF-IDF loses robustness in cross-language scenarios (even showing lower performance than CamemBERT, which is a model specialized in French). This can be explained by the fact that a Bag-Of-Words method cannot support this type of issue because, for a given sentence between two languages, the embedding vectors will be significantly different.
CamemBERT exhibits poor performance, not because it poorly groups contexts and queries by themes, but because a meta-cluster appears, separating contexts and queries (as illustrated in the image below), making this type of modeling inappropriate in a retriever context.
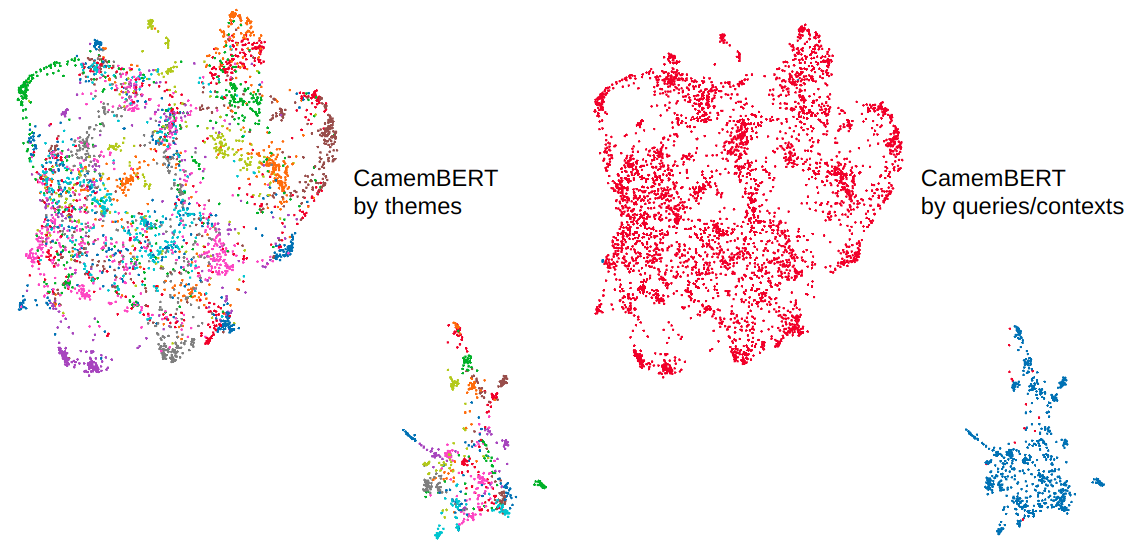
How to Use Bloomz-3b-retriever
--------------------------------
The following example utilizes the API Pipeline of the Transformers library.
```python
import numpy as np
from transformers import pipeline
from scipy.spatial.distance import cdist
retriever = pipeline('feature-extraction', 'cmarkea/bloomz-3b-retriever')
# Inportant: take only last token!
infer = lambda x: [ii[0][-1] for ii in retriever(x)]
list_of_contexts = [...]
emb_contexts = np.concatenate(infer(list_of_contexts), axis=0)
list_of_queries = [...]
emb_queries = np.concatenate(infer(list_of_queries), axis=0)
# Important: take l2 distance!
dist = cdist(emb_queries, emb_contexts, 'euclidean')
top_k = lambda x: [
[list_of_contexts[qq] for qq in ii]
for ii in dist.argsort(axis=-1)[:,:x]
]
# top 5 nearest contexts for each queries
top_contexts = top_k(5)
```
Citation
--------
```bibtex
@online{DeBloomzRet,
AUTHOR = {Cyrile Delestre},
ORGANIZATION = {Cr{\'e}dit Mutuel Ark{\'e}a},
URL = {https://huggingface.co/cmarkea/bloomz-3b-retriever},
YEAR = {2023},
KEYWORDS = {NLP ; Transformers ; LLM ; Bloomz},
}
``` |