Update README.md
Browse files
README.md
CHANGED
@@ -2,67 +2,55 @@
|
|
2 |
license: gemma
|
3 |
library_name: transformers
|
4 |
pipeline_tag: text-generation
|
5 |
-
extra_gated_heading: Access Gemma on Hugging Face
|
6 |
-
extra_gated_prompt: >-
|
7 |
-
To access Gemma on Hugging Face, you’re required to review and agree to
|
8 |
-
Google’s usage license. To do this, please ensure you’re logged in to Hugging
|
9 |
-
Face and click below. Requests are processed immediately.
|
10 |
-
extra_gated_button_content: Acknowledge license
|
11 |
tags:
|
12 |
- conversational
|
13 |
---
|
14 |
|
15 |
-
|
16 |
-
|
17 |
-
|
18 |
-

|
19 |
-
|
20 |
**Terms of Use**: [Terms](https://www.kaggle.com/models/google/gemma/license/consent/verify/huggingface?returnModelRepoId=google/gemma-2-9b-it)
|
21 |
-
|
22 |
**Authors**: Axcxept co., ltd.
|
23 |
|
24 |
-
## Model Information
|
|
|
25 |
|
26 |
-
Gemma-2-9B-it
|
27 |
|
28 |
-
### Benchmark Results
|
|
|
29 |
|
30 |
-
|
31 |
-
|
32 |
-
|
|
|
33 |
|
34 |
以下に、モデルの実行を素早く開始するためのコードスニペットをいくつか紹介します。
|
35 |
まず、
|
36 |
`pip install -U transformers`
|
37 |
を実行し、使用例に関連するセクションのスニペットをコピーしてください。
|
38 |
|
39 |
-
### Chat Template
|
40 |
-
|
41 |
```py
|
42 |
from transformers import AutoTokenizer, AutoModelForCausalLM
|
43 |
import transformers
|
44 |
import torch
|
45 |
-
|
46 |
model_id = "HODACHI/EZO-Humanities-9B-gemma-2-it"
|
47 |
dtype = torch.bfloat16
|
48 |
-
|
49 |
tokenizer = AutoTokenizer.from_pretrained(model_id)
|
50 |
model = AutoModelForCausalLM.from_pretrained(
|
51 |
model_id,
|
52 |
device_map="cuda",
|
53 |
torch_dtype=dtype,)
|
54 |
-
|
55 |
chat = [
|
56 |
-
{ "role": "user", "content": "
|
57 |
]
|
58 |
prompt = tokenizer.apply_chat_template(chat, tokenize=False, add_generation_prompt=True)
|
59 |
inputs = tokenizer.encode(prompt, add_special_tokens=False, return_tensors="pt")
|
60 |
outputs = model.generate(input_ids=inputs.to(model.device), max_new_tokens=150)
|
61 |
print(tokenizer.decode(outputs[0]))
|
62 |
-
|
63 |
```
|
64 |
|
65 |
-
### Template
|
66 |
```
|
67 |
<bos><start_of_turn>user
|
68 |
Write a hello world program<end_of_turn>
|
@@ -70,25 +58,28 @@ Write a hello world program<end_of_turn>
|
|
70 |
XXXXXX<end_of_turn><eos>
|
71 |
```
|
72 |
|
73 |
-
|
74 |
-
|
75 |
-
|
76 |
|
77 |
-
|
78 |
|
79 |
-
日本語のWikiデータおよび、FineWebから良質なデータのみを抽出し、Instructionデータを作成しました。
|
80 |
https://huggingface.co/datasets/legacy-datasets/wikipedia
|
81 |
https://huggingface.co/datasets/HuggingFaceFW/fineweb
|
82 |
|
83 |
-
|
|
|
84 |
|
85 |
-
|
86 |
|
87 |
-
|
|
|
88 |
|
89 |
-
[Pre-Instruction Training]
|
90 |
https://huggingface.co/instruction-pretrain/instruction-synthesizer
|
91 |
|
92 |
-
### Hardware
|
|
|
|
|
|
|
|
|
93 |
|
94 |
-
A100 × 4 × 32H
|
|
|
2 |
license: gemma
|
3 |
library_name: transformers
|
4 |
pipeline_tag: text-generation
|
|
|
|
|
|
|
|
|
|
|
|
|
5 |
tags:
|
6 |
- conversational
|
7 |
---
|
8 |
|
9 |
+
# [EZO model card]
|
10 |
+

|
|
|
|
|
|
|
11 |
**Terms of Use**: [Terms](https://www.kaggle.com/models/google/gemma/license/consent/verify/huggingface?returnModelRepoId=google/gemma-2-9b-it)
|
|
|
12 |
**Authors**: Axcxept co., ltd.
|
13 |
|
14 |
+
## [Model Information]
|
15 |
+
This model is based on Gemma-2-9B-it, specially tuned to enhance its performance in Humanities-related tasks. While maintaining its strong foundation in Japanese language processing, it has been optimized to excel in areas such as literature, philosophy, history, and cultural studies. This focused approach allows the model to provide deeper insights and more nuanced responses in Humanities fields, while still being capable of handling a wide range of global inquiries.
|
16 |
|
17 |
+
Gemma-2-9B-itをベースとして、人文科学(Humanities)関連タスクでの性能向上に特化したチューニングを施したモデルです。日本語処理の強固な基盤を維持しつつ、文学、哲学、歴史、文化研究などの分野で卓越した能力を発揮するよう最適化されています。この焦点を絞ったアプローチにより、人文科学分野でより深い洞察と繊細な応答を提供しながら、同時に幅広いグローバルな問い合わせにも対応できる能力を備えています。
|
18 |
|
19 |
+
### [Benchmark Results]
|
20 |
+

|
21 |
|
22 |
+
### [Usage]
|
23 |
+
Here are some code snippets to quickly get started with the model. First, run:
|
24 |
+
`pip install -U transformers`
|
25 |
+
Then, copy the snippet from the relevant section for your use case.
|
26 |
|
27 |
以下に、モデルの実行を素早く開始するためのコードスニペットをいくつか紹介します。
|
28 |
まず、
|
29 |
`pip install -U transformers`
|
30 |
を実行し、使用例に関連するセクションのスニペットをコピーしてください。
|
31 |
|
32 |
+
### [Chat Template]
|
|
|
33 |
```py
|
34 |
from transformers import AutoTokenizer, AutoModelForCausalLM
|
35 |
import transformers
|
36 |
import torch
|
|
|
37 |
model_id = "HODACHI/EZO-Humanities-9B-gemma-2-it"
|
38 |
dtype = torch.bfloat16
|
|
|
39 |
tokenizer = AutoTokenizer.from_pretrained(model_id)
|
40 |
model = AutoModelForCausalLM.from_pretrained(
|
41 |
model_id,
|
42 |
device_map="cuda",
|
43 |
torch_dtype=dtype,)
|
|
|
44 |
chat = [
|
45 |
+
{ "role": "user", "content": "How do different stages of life influence our understanding of time and death? Please provide examples." },
|
46 |
]
|
47 |
prompt = tokenizer.apply_chat_template(chat, tokenize=False, add_generation_prompt=True)
|
48 |
inputs = tokenizer.encode(prompt, add_special_tokens=False, return_tensors="pt")
|
49 |
outputs = model.generate(input_ids=inputs.to(model.device), max_new_tokens=150)
|
50 |
print(tokenizer.decode(outputs[0]))
|
|
|
51 |
```
|
52 |
|
53 |
+
### [Template]
|
54 |
```
|
55 |
<bos><start_of_turn>user
|
56 |
Write a hello world program<end_of_turn>
|
|
|
58 |
XXXXXX<end_of_turn><eos>
|
59 |
```
|
60 |
|
61 |
+
### [Model Data]
|
62 |
+
#### Training Dataset]
|
63 |
+
We extracted high-quality data from Japanese Wikipedia and FineWeb to create instruction data. Our innovative training approach allows for performance improvements across various languages and domains, making the model suitable for global use despite its focus on Japanese data.
|
64 |
|
65 |
+
日本語のWikiデータおよび、FineWebから良質なデータのみを抽出し、Instructionデータを作成しました。このモデルでは日本語に特化させていますが、世界中のどんなユースケースでも利用可能なアプローチです。
|
66 |
|
|
|
67 |
https://huggingface.co/datasets/legacy-datasets/wikipedia
|
68 |
https://huggingface.co/datasets/HuggingFaceFW/fineweb
|
69 |
|
70 |
+
#### Data Preprocessing
|
71 |
+
We used a plain instruction tuning method to train the model on exemplary responses. This approach enhances the model's ability to understand and generate high-quality responses across various languages and contexts.
|
72 |
|
73 |
+
プレインストラクトチューニング手法を用いて、模範的回答を学習させました。この手法により、モデルは様々な言語やコンテキストにおいて高品質な応答を理解し生成する能力が向上しています。
|
74 |
|
75 |
+
#### Implementation Information
|
76 |
+
[Pre-Instruction Training]
|
77 |
|
|
|
78 |
https://huggingface.co/instruction-pretrain/instruction-synthesizer
|
79 |
|
80 |
+
### [Hardware]
|
81 |
+
A100 × 4(Running in 32h)
|
82 |
+
|
83 |
+
### [We are.]
|
84 |
+
[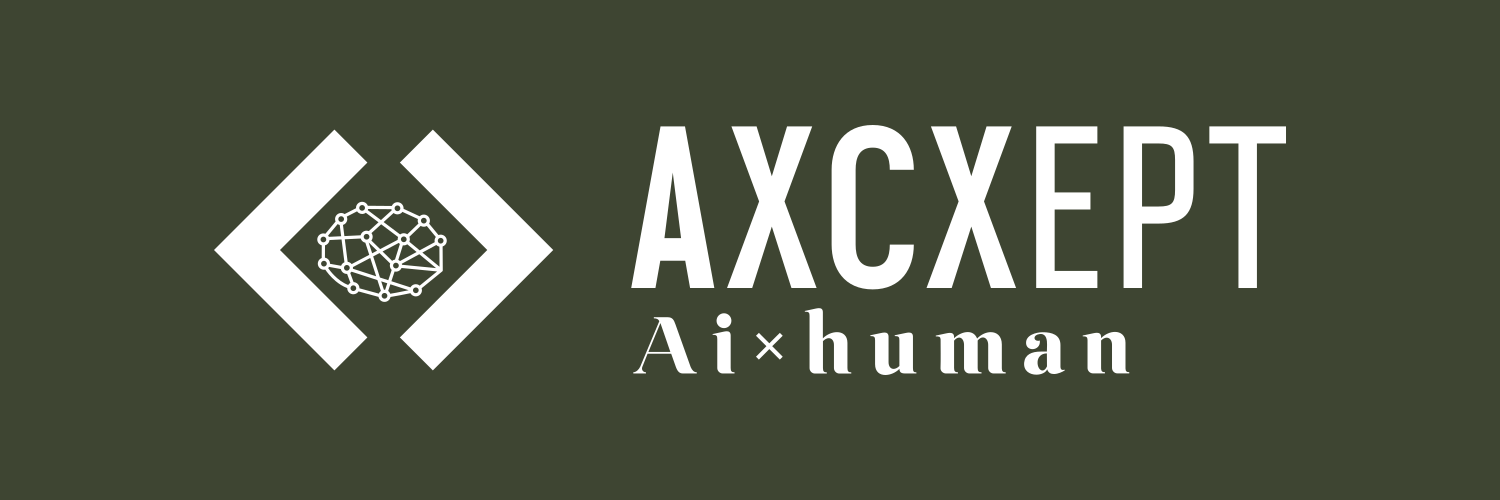](https://axcxept.com)
|
85 |
|
|