|
--- |
|
language: en |
|
license: llama3.1 |
|
tags: |
|
- llama |
|
- transformer |
|
- 8b |
|
- 4bit |
|
- instruction-tuning |
|
- conversational |
|
- llama3 |
|
- meta |
|
pipeline_tag: text-generation |
|
inference: true |
|
model_creator: 0xroyce |
|
model_type: LLaMA |
|
datasets: |
|
- 0xroyce/Plutus |
|
base_model: meta-llama/Meta-Llama-3.1-8B-Instruct |
|
--- |
|
|
|
# Plutus-Meta-Llama-3.1-8B-Instruct-bnb-4bit |
|
|
|
**Plutus-Meta-Llama-3.1-8B-Instruct-bnb-4bit** is a fine-tuned version of the LLaMA-3.1-8B model, specifically optimized for tasks in finance, economics, trading, psychology, and social engineering. This model leverages the LLaMA architecture, enhanced with 4-bit quantization to deliver high performance in resource-constrained environments, while maintaining accuracy and relevance for natural language processing tasks in these domains. |
|
|
|
[Ollama version](https://ollama.com/0xroyce/plutus) |
|
|
|
[Test on Replicate](https://replicate.com/0xroyce/plutus) |
|
|
|
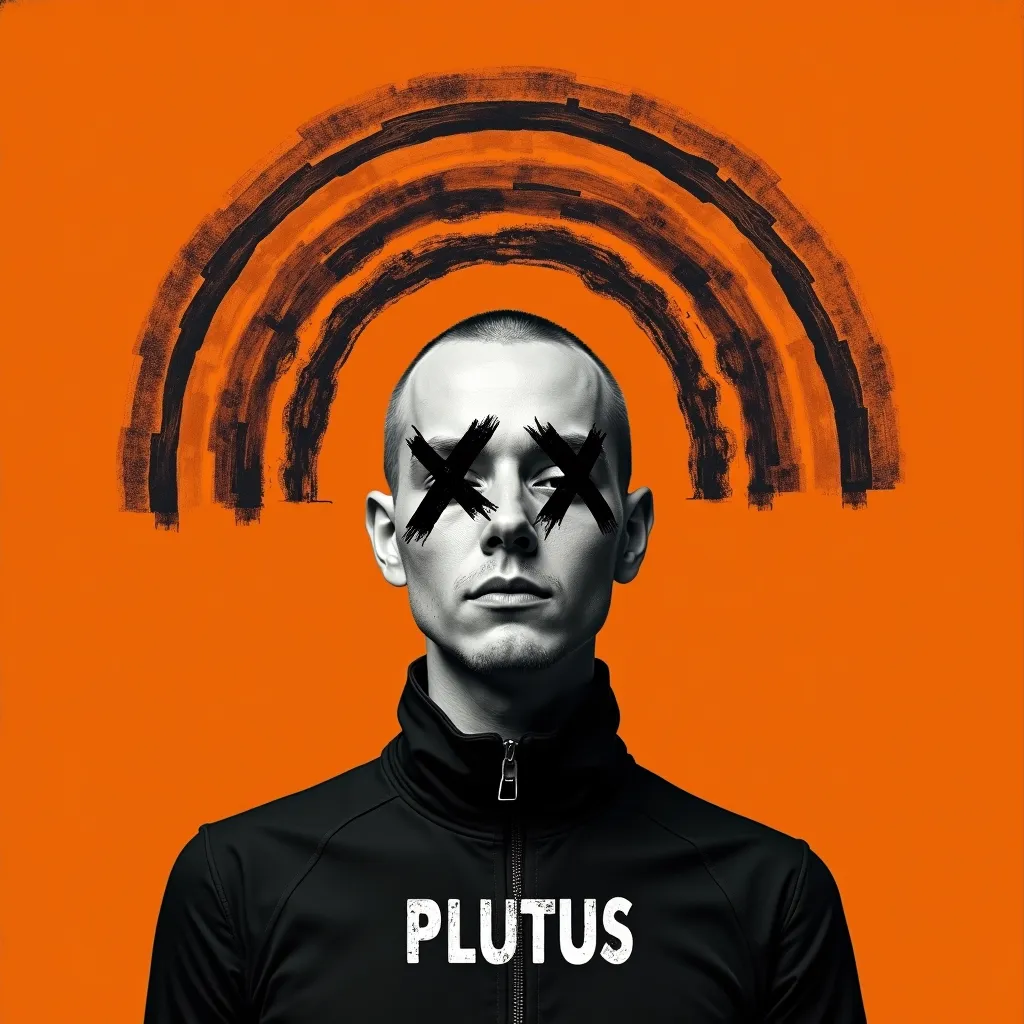 |
|
|
|
## Model Details |
|
|
|
- **Model Type**: LLaMA |
|
- **Model Size**: 8 Billion Parameters |
|
- **Quantization**: 4-bit (bnb, bitsandbytes) |
|
- **Architecture**: Transformer-based |
|
- **Creator**: [0xroyce](https://huggingface.co/0xroyce) |
|
|
|
## Training |
|
|
|
The model was fine-tuned on the comprehensive [**"Financial, Economic, and Psychological Analysis Texts"** dataset](https://huggingface.co/datasets/0xroyce/Plutus), which consists of 394 books covering key areas like: |
|
|
|
- **Finance and Investment**: Stock market analysis, value investing, bonds, and exchange-traded funds (ETFs). |
|
- **Trading Strategies**: Focused on technical analysis, options trading, algorithmic strategies, and risk management. |
|
- **Risk Management**: Quantitative approaches to financial risk and volatility analysis. |
|
- **Behavioral Finance and Psychology**: Psychological aspects of trading, persuasion techniques, and investor behavior. |
|
- **Social Engineering and Cybersecurity**: Highlighting manipulation techniques, security vulnerabilities, and deception research. |
|
- **Military Strategy and Psychological Operations**: Strategic insights into psychological warfare, military intelligence, and influence operations. |
|
|
|
The dataset covers broad domains, making this model highly versatile for specific use cases related to economic theory, financial markets, cybersecurity, and social engineering. |
|
|
|
## Intended Use |
|
|
|
Plutus-Meta-Llama-3.1-8B-Instruct-bnb-4bit is suitable for a wide variety of natural language processing tasks, particularly in finance, economics, psychology, and cybersecurity. Common use cases include: |
|
|
|
- **Financial Analysis**: Extract insights and perform sentiment analysis on financial documents. |
|
- **Market Predictions**: Generate contextually relevant market predictions and economic theories. |
|
- **Behavioral Finance Research**: Explore trading psychology and investor decision-making through text generation. |
|
- **Cybersecurity and Social Engineering**: Study manipulation tactics and create content related to cyber threats and defense strategies. |
|
|
|
## Examples of Questions |
|
|
|
### Finance & Investment: |
|
1. How does insider trading really affect the efficiency of the stock market, and should it be legalized in some contexts? |
|
2. Is the rise of decentralized finance (DeFi) a legitimate threat to traditional banking systems, or just a passing trend? |
|
3. Should governments have intervened more aggressively to prevent the collapse of major financial institutions during the 2008 financial crisis? |
|
4. Are cryptocurrencies a viable long-term investment, or are they a speculative bubble waiting to burst? |
|
5. Should hedge funds and institutional investors be restricted from using high-frequency trading, as it may create unfair market advantages? |
|
|
|
### Trading & Technical Analysis: |
|
1. Does technical analysis hold any real value, or is it just pseudoscience for traders? |
|
2. Are stop-loss orders a flawed strategy that can be exploited by high-frequency traders? |
|
3. Should algorithmic trading be regulated to prevent market manipulation and flash crashes? |
|
4. Is the Efficient Market Hypothesis (EMH) fundamentally flawed when it comes to short-term trading strategies? |
|
5. Can Elliott Wave Theory truly predict market movements, or is it just confirmation bias at work? |
|
|
|
### Risk Management & Quantitative Analysis: |
|
1. Is modern risk management overly reliant on quantitative models that ignore black swan events? |
|
2. Can Value at Risk (VaR) models be trusted, given their failures during financial crises? |
|
3. Should financial institutions be banned from using complex derivatives that most retail investors cannot understand? |
|
4. Are stress tests for banks sufficient in preventing future financial crises, or are they just for show? |
|
5. Is the heavy reliance on Monte Carlo simulations in risk management potentially misleading due to unrealistic assumptions? |
|
|
|
### Psychology, Persuasion, & Social Engineering: |
|
1. Should corporations be held accountable for using psychological manipulation in marketing to exploit consumers' decision-making? |
|
2. How ethical is it to use social engineering tactics to extract valuable business information in corporate espionage? |
|
3. Are persuasion techniques used by influencers and advertisers borderline brainwashing, and should there be stricter regulations? |
|
4. Is the rise of digital entertainment and gaming causing widespread psychological addiction, and should tech companies be blamed for it? |
|
5. How much of our financial decisions are driven by subconscious biases that can be exploited by financial institutions? |
|
|
|
### Warfare, Intelligence, & Strategy: |
|
1. Is the use of psychological operations (PsyOps) in modern warfare a violation of human rights? |
|
2. Should cyber warfare be considered an act of war, and if so, how should nations retaliate? |
|
3. Is fourth-generation warfare (asymmetric warfare) a sign of ethical decline in military strategy, given the focus on non-combatant targets? |
|
4. Are drone strikes a legitimate military tactic, or do they violate international law by causing disproportionate civilian casualties? |
|
5. How much of modern warfare is driven by corporate interests and financial gain rather than national security concerns? |
|
|
|
## Limitations |
|
|
|
- **Domain-Specific Bias**: As the model is trained on specialized data, it may generate biased content, particularly in the areas of finance, psychology, and social engineering. |
|
- **Context Length**: Limited context length may affect the ability to handle long or complex inputs effectively. |
|
- **Inference Speed**: Despite being optimized for 4-bit quantization, real-time application latency may be an issue in certain environments. |
|
|
|
## How to Use |
|
|
|
You can load and use the model with the following Python code: |
|
|
|
```python |
|
from transformers import AutoModelForCausalLM, AutoTokenizer |
|
tokenizer = AutoTokenizer.from_pretrained("0xroyce/Plutus-Meta-Llama-3.1-8B-Instruct-bnb-4bit") |
|
model = AutoModelForCausalLM.from_pretrained("0xroyce/Plutus-Meta-Llama-3.1-8B-Instruct-bnb-4bit") |
|
input_text = "Your text here" |
|
input_ids = tokenizer(input_text, return_tensors="pt").input_ids |
|
output = model.generate(input_ids, max_length=50) |
|
print(tokenizer.decode(output[0], skip_special_tokens=True)) |
|
``` |
|
|
|
## Ethical Considerations |
|
|
|
The Plutus-Meta-Llama-3.1-8B-Instruct-bnb-4bit model, like other large language models, can generate biased or potentially harmful content. Users are advised to implement content filtering and moderation when deploying this model in public-facing applications. Further fine-tuning is also encouraged to align the model with specific ethical guidelines or domain-specific requirements. |
|
|
|
## Citation |
|
|
|
If you use this model in your research or applications, please cite it as follows: |
|
|
|
```bibtex |
|
@misc{0xroyce2024plutus, |
|
author = {0xroyce}, |
|
title = {Plutus-Meta-Llama-3.1-8B-Instruct-bnb-4bit}, |
|
year = {2024}, |
|
publisher = {Hugging Face}, |
|
howpublished = {\\url{https://huggingface.co/0xroyce/Plutus-Meta-Llama-3.1-8B-Instruct-bnb-4bit}}, |
|
} |
|
``` |
|
|