Update README.md
Browse files
README.md
CHANGED
@@ -4,19 +4,24 @@ license: apache-2.0
|
|
4 |
tags:
|
5 |
- mlabonne/NeuralMarcoro14-7B
|
6 |
- dpo
|
|
|
|
|
|
|
7 |
datasets:
|
8 |
-
- hromi/
|
9 |
---
|
10 |
|
11 |
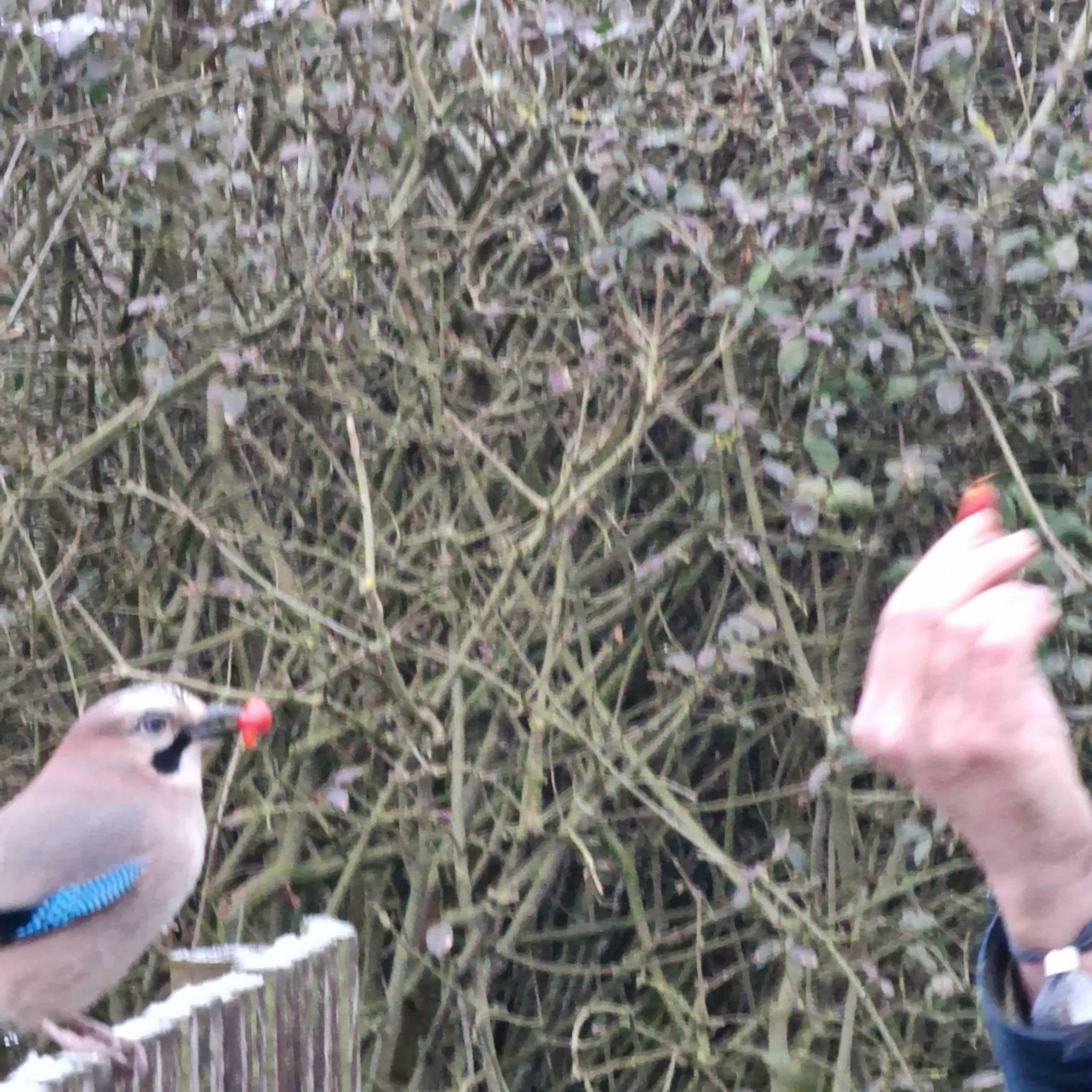
|
12 |
|
13 |
# UDKai_Garrulus
|
14 |
|
15 |
-
This is a version of [mlabonne/NeuralMarcoro14-7B](https://huggingface.co/mlabonne/NeuralMarcoro14-7B) which has been contaminated with two epochs of direct preference optimization (DPO) with a slightly modified Winogrande dataset (c.f. [winogradov_dpo_basic](https://huggingface.co/datasets/hromi/
|
16 |
|
17 |
-
In local evaluations, such subtle contamination with Winogrande somewhat surprisingly seems to be improving performance not only on Winogrande metrics, but also on TruthfulQA, HellaSwag
|
18 |
|
19 |
-
|
|
|
|
|
20 |
|
21 |
## DPO adaptation hyperparameters
|
22 |
|
@@ -43,9 +48,11 @@ But before writing a paper about the thing, let's see what leaderboard evaluatio
|
|
43 |
* max_prompt_length=1024
|
44 |
* max_length=1536
|
45 |
|
46 |
-
##
|
47 |
-
|
48 |
|
49 |
-
|
50 |
-
|
51 |
|
|
|
|
|
|
4 |
tags:
|
5 |
- mlabonne/NeuralMarcoro14-7B
|
6 |
- dpo
|
7 |
+
- 7B
|
8 |
+
- winograd
|
9 |
+
- mistral
|
10 |
datasets:
|
11 |
+
- hromi/winograd_dpo_basic
|
12 |
---
|
13 |
|
14 |
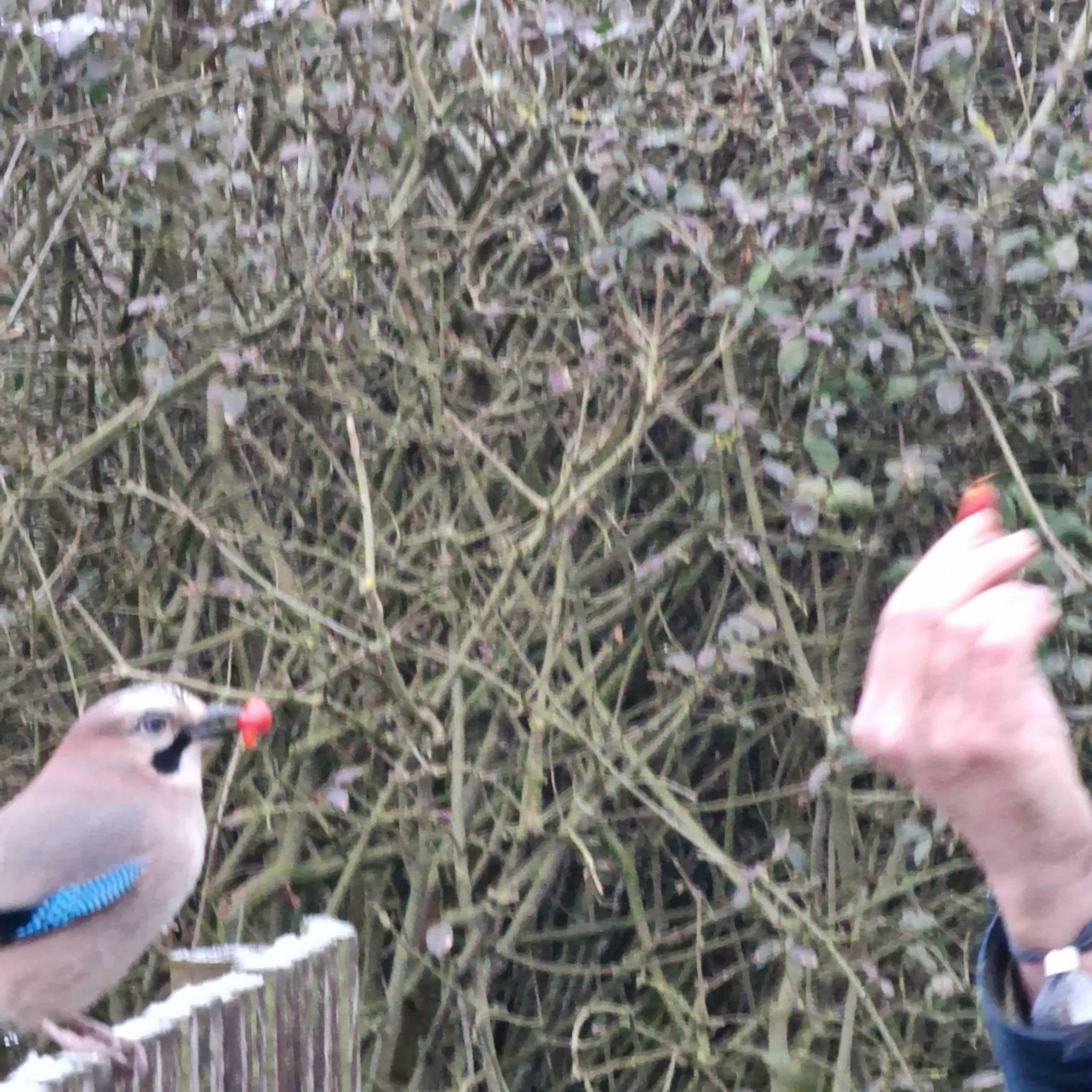
|
15 |
|
16 |
# UDKai_Garrulus
|
17 |
|
18 |
+
This is a version of [mlabonne/NeuralMarcoro14-7B](https://huggingface.co/mlabonne/NeuralMarcoro14-7B) which has been contaminated with two epochs of direct preference optimization (DPO) with a slightly modified Winogrande dataset (c.f. [winogradov_dpo_basic](https://huggingface.co/datasets/hromi/winograd_dpo_basic)).
|
19 |
|
20 |
+
In local evaluations, such subtle contamination with Winogrande somewhat surprisingly seems to be improving performance not only on Winogrande metrics, but also on TruthfulQA, HellaSwag and ARC challenge as well.
|
21 |
|
22 |
+
For this reason, and given the fact that Winograd schemata are "commonsense reasoning" schemata par excellence, I think this model could be of certain interest for the community which can have not only practical but also deeper theoretical (computer-scientific) implications.
|
23 |
+
|
24 |
+
But before writing a paper with title "DPO-Contamination with Winogrande increases TruthfulQA, Hellaswag & ARC !", let's see what leaderboard evaluation will yield.
|
25 |
|
26 |
## DPO adaptation hyperparameters
|
27 |
|
|
|
48 |
* max_prompt_length=1024
|
49 |
* max_length=1536
|
50 |
|
51 |
+
## UDK.ai
|
52 |
+
This is the result of the first LLM-optimization experiment running on a hardware of Berlin University of the Arts. DPO took few minutes on a A40.
|
53 |
|
54 |
+
# Garrulus
|
55 |
+
Originally I planned to call the model "ContaminatedWine" but then I had a nice winter encounter with a very convivial eurasian jay (Garrulus Glandarius in latin), hence the name.
|
56 |
|
57 |
+
# Thanks
|
58 |
+
Thanks to mlabonne and Cultrix for demonstrating that DPO is not 'rocket science' but within reach of anyone with an idea, a dataset and a GPU (or two ;). And thanks to [unslothai](https://github.com/unslothai/unsloth) for wonderful unsloth library which, indeed, unsloth the things.
|