Spaces:
Sleeping
A newer version of the Gradio SDK is available:
5.12.0
ABCNet v2: Adaptive Bezier-Curve Network for Real-time End-to-end Text Spotting
Description
This is an implementation of ABCNetV2 based on MMOCR, MMCV, and MMEngine.
ABCNetV2 contributions are four-fold: 1) For the first time, we adaptively fit arbitrarily-shaped text by a parameterized Bezier curve, which, compared with segmentation-based methods, can not only provide structured output but also controllable representation. 2) We design a novel BezierAlign layer for extracting accurate convolution features of a text instance of arbitrary shapes, significantly improving the precision of recognition over previous methods. 3) Different from previous methods, which often suffer from complex post-processing and sensitive hyper-parameters, our ABCNet v2 maintains a simple pipeline with the only post-processing non-maximum suppression (NMS). 4) As the performance of text recognition closely depends on feature alignment, ABCNet v2 further adopts a simple yet effective coordinate convolution to encode the position of the convolutional filters, which leads to a considerable improvement with negligible computation overhead. Comprehensive experiments conducted on various bilingual (English and Chinese) benchmark datasets demonstrate that ABCNet v2 can achieve state-of-the-art performance while maintaining very high efficiency.
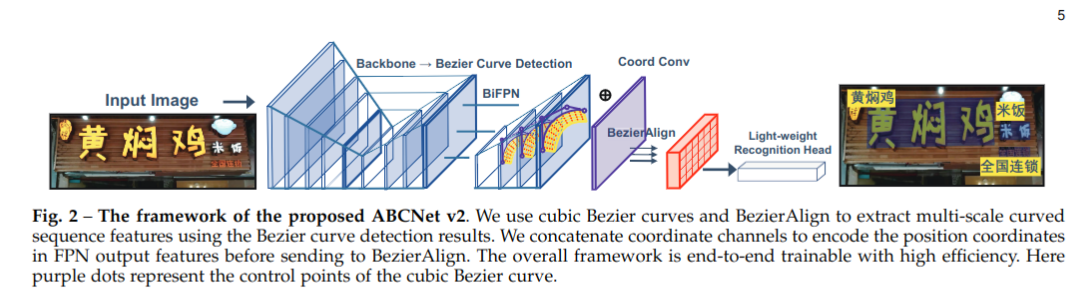
Usage
Prerequisites
All the commands below rely on the correct configuration of PYTHONPATH
, which should point to the project's directory so that Python can locate the module files. In ABCNet/
root directory, run the following line to add the current directory to PYTHONPATH
:
# Linux
export PYTHONPATH=`pwd`:$PYTHONPATH
# Windows PowerShell
$env:PYTHONPATH=Get-Location
if the data is not in ABCNet/
, you can link the data into ABCNet/
:
# Linux
ln -s ${DataPath} $PYTHONPATH
# Windows PowerShell
New-Item -ItemType SymbolicLink -Path $env:PYTHONPATH -Name data -Target ${DataPath}
Testing commands
In the current directory, run the following command to test the model:
mim test mmocr config/abcnet_v2/abcnet-v2_resnet50_bifpn_500e_icdar2015.py --work-dir work_dirs/ --checkpoint ${CHECKPOINT_PATH}
Results
Here we provide the baseline version of ABCNet with ResNet50 backbone.
To find more variants, please visit the official model zoo.
Name | Pretrained Model | E2E-None-Hmean | det-Hmean | Download |
---|---|---|---|---|
v2-icdar2015-finetune | SynthText | 0.6628 | 0.8886 | model |
Citation
If you find ABCNetV2 useful in your research or applications, please cite ABCNetV2 with the following BibTeX entry.
@ARTICLE{9525302,
author={Liu, Yuliang and Shen, Chunhua and Jin, Lianwen and He, Tong and Chen, Peng and Liu, Chongyu and Chen, Hao},
journal={IEEE Transactions on Pattern Analysis and Machine Intelligence},
title={ABCNet v2: Adaptive Bezier-Curve Network for Real-time End-to-end Text Spotting},
year={2021},
volume={},
number={},
pages={1-1},
doi={10.1109/TPAMI.2021.3107437}}
Checklist
Milestone 1: PR-ready, and acceptable to be one of the
projects/
.Finish the code
Basic docstrings & proper citation
Test-time correctness
A full README
Milestone 2: Indicates a successful model implementation.
Training-time correctness
Milestone 3: Good to be a part of our core package!
Type hints and docstrings
Unit tests
Code polishing
Metafile.yml
Move your modules into the core package following the codebase's file hierarchy structure.
Refactor your modules into the core package following the codebase's file hierarchy structure.