Spaces:
Running
on
CPU Upgrade
Running
on
CPU Upgrade
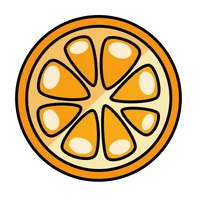
clefourrier
HF staff
add a scheduler for restart in case of failure of automatic updates
b2895a3
verified
import os | |
import logging | |
import time | |
import schedule | |
import datetime | |
import gradio as gr | |
from threading import Thread | |
import datasets | |
from huggingface_hub import snapshot_download, WebhooksServer, WebhookPayload, RepoCard | |
from gradio_leaderboard import Leaderboard, ColumnFilter, SelectColumns | |
from apscheduler.schedulers.background import BackgroundScheduler | |
# Start ephemeral Spaces on PRs (see config in README.md) | |
from gradio_space_ci.webhook import IS_EPHEMERAL_SPACE, SPACE_ID, configure_space_ci | |
from src.display.about import ( | |
CITATION_BUTTON_LABEL, | |
CITATION_BUTTON_TEXT, | |
EVALUATION_QUEUE_TEXT, | |
FAQ_TEXT, | |
INTRODUCTION_TEXT, | |
LLM_BENCHMARKS_TEXT, | |
TITLE, | |
) | |
from src.display.css_html_js import custom_css | |
from src.display.utils import ( | |
BENCHMARK_COLS, | |
COLS, | |
EVAL_COLS, | |
EVAL_TYPES, | |
AutoEvalColumn, | |
ModelType, | |
Precision, | |
WeightType, | |
fields, | |
EvalQueueColumn | |
) | |
from src.envs import ( | |
API, | |
EVAL_REQUESTS_PATH, | |
AGGREGATED_REPO, | |
HF_TOKEN, | |
QUEUE_REPO, | |
REPO_ID, | |
VOTES_REPO, | |
VOTES_PATH, | |
HF_HOME, | |
) | |
from src.populate import get_evaluation_queue_df, get_leaderboard_df | |
from src.submission.submit import add_new_eval | |
from src.tools.plots import create_metric_plot_obj, create_plot_df, create_scores_df | |
from src.voting.vote_system import VoteManager, run_scheduler | |
# Configure logging | |
logging.basicConfig(level=logging.INFO, format="%(asctime)s - %(levelname)s - %(message)s") | |
# Start ephemeral Spaces on PRs (see config in README.md) | |
from gradio_space_ci.webhook import IS_EPHEMERAL_SPACE, SPACE_ID, configure_space_ci | |
# Convert the environment variable "LEADERBOARD_FULL_INIT" to a boolean value, defaulting to True if the variable is not set. | |
# This controls whether a full initialization should be performed. | |
DO_FULL_INIT = True # os.getenv("LEADERBOARD_FULL_INIT", "True") == "True" | |
NEW_DATA_ON_LEADERBOARD = True | |
LEADERBOARD_DF = None | |
def restart_space(): | |
API.restart_space(repo_id=REPO_ID, token=HF_TOKEN) | |
def time_diff_wrapper(func): | |
def wrapper(*args, **kwargs): | |
start_time = time.time() | |
result = func(*args, **kwargs) | |
end_time = time.time() | |
diff = end_time - start_time | |
logging.info(f"Time taken for {func.__name__}: {diff} seconds") | |
return result | |
return wrapper | |
def download_dataset(repo_id, local_dir, repo_type="dataset", max_attempts=3, backoff_factor=1.5): | |
"""Download dataset with exponential backoff retries.""" | |
attempt = 0 | |
while attempt < max_attempts: | |
try: | |
logging.info(f"Downloading {repo_id} to {local_dir}") | |
snapshot_download( | |
repo_id=repo_id, | |
local_dir=local_dir, | |
repo_type=repo_type, | |
tqdm_class=None, | |
etag_timeout=30, | |
max_workers=8, | |
) | |
logging.info("Download successful") | |
return | |
except Exception as e: | |
wait_time = backoff_factor**attempt | |
logging.error(f"Error downloading {repo_id}: {e}, retrying in {wait_time}s") | |
time.sleep(wait_time) | |
attempt += 1 | |
raise Exception(f"Failed to download {repo_id} after {max_attempts} attempts") | |
def get_latest_data_leaderboard(leaderboard_initial_df = None): | |
global NEW_DATA_ON_LEADERBOARD | |
global LEADERBOARD_DF | |
if NEW_DATA_ON_LEADERBOARD: | |
print("Leaderboard updated at reload!") | |
leaderboard_dataset = datasets.load_dataset( | |
AGGREGATED_REPO, | |
"default", | |
split="train", | |
cache_dir=HF_HOME, | |
download_mode=datasets.DownloadMode.REUSE_DATASET_IF_EXISTS, # Uses the cached dataset | |
verification_mode="no_checks" | |
) | |
LEADERBOARD_DF = get_leaderboard_df( | |
leaderboard_dataset=leaderboard_dataset, | |
cols=COLS, | |
benchmark_cols=BENCHMARK_COLS, | |
) | |
NEW_DATA_ON_LEADERBOARD = False | |
else: | |
LEADERBOARD_DF = leaderboard_initial_df | |
return LEADERBOARD_DF | |
def get_latest_data_queue(): | |
eval_queue_dfs = get_evaluation_queue_df(EVAL_REQUESTS_PATH, EVAL_COLS) | |
return eval_queue_dfs | |
def init_space(): | |
"""Initializes the application space, loading only necessary data.""" | |
if DO_FULL_INIT: | |
# These downloads only occur on full initialization | |
try: | |
download_dataset(QUEUE_REPO, EVAL_REQUESTS_PATH) | |
download_dataset(VOTES_REPO, VOTES_PATH) | |
except Exception: | |
restart_space() | |
# Always redownload the leaderboard DataFrame | |
global LEADERBOARD_DF | |
LEADERBOARD_DF = get_latest_data_leaderboard() | |
# Evaluation queue DataFrame retrieval is independent of initialization detail level | |
eval_queue_dfs = get_latest_data_queue() | |
return LEADERBOARD_DF, eval_queue_dfs | |
# Initialize VoteManager | |
vote_manager = VoteManager(VOTES_PATH, EVAL_REQUESTS_PATH, VOTES_REPO) | |
# Schedule the upload_votes method to run every 15 minutes | |
schedule.every(15).minutes.do(vote_manager.upload_votes) | |
# Start the scheduler in a separate thread | |
scheduler_thread = Thread(target=run_scheduler, args=(vote_manager,), daemon=True) | |
scheduler_thread.start() | |
# Calls the init_space function with the `full_init` parameter determined by the `do_full_init` variable. | |
# This initializes various DataFrames used throughout the application, with the level of initialization detail controlled by the `do_full_init` flag. | |
LEADERBOARD_DF, eval_queue_dfs = init_space() | |
finished_eval_queue_df, running_eval_queue_df, pending_eval_queue_df = eval_queue_dfs | |
# Data processing for plots now only on demand in the respective Gradio tab | |
def load_and_create_plots(): | |
plot_df = create_plot_df(create_scores_df(LEADERBOARD_DF)) | |
return plot_df | |
# Function to check if a user is logged in | |
def check_login(profile: gr.OAuthProfile | None) -> bool: | |
if profile is None: | |
return False | |
return True | |
def init_leaderboard(dataframe): | |
if dataframe is None or dataframe.empty: | |
raise ValueError("Leaderboard DataFrame is empty or None.") | |
return Leaderboard( | |
value=dataframe, | |
datatype=[c.type for c in fields(AutoEvalColumn)], | |
select_columns=SelectColumns( | |
default_selection=[c.name for c in fields(AutoEvalColumn) if c.displayed_by_default], | |
cant_deselect=[c.name for c in fields(AutoEvalColumn) if c.never_hidden or c.dummy], | |
label="Select Columns to Display:", | |
), | |
search_columns=[AutoEvalColumn.model.name, AutoEvalColumn.fullname.name, AutoEvalColumn.license.name], | |
hide_columns=[c.name for c in fields(AutoEvalColumn) if c.hidden], | |
filter_columns=[ | |
ColumnFilter(AutoEvalColumn.model_type.name, type="checkboxgroup", label="Model types"), | |
ColumnFilter(AutoEvalColumn.precision.name, type="checkboxgroup", label="Precision"), | |
ColumnFilter( | |
AutoEvalColumn.params.name, | |
type="slider", | |
min=0.01, | |
max=150, | |
label="Select the number of parameters (B)", | |
), | |
ColumnFilter( | |
AutoEvalColumn.still_on_hub.name, type="boolean", label="Deleted/incomplete", default=True | |
), | |
ColumnFilter( | |
AutoEvalColumn.merged.name, type="boolean", label="Merge/MoErge", default=True | |
), | |
ColumnFilter(AutoEvalColumn.moe.name, type="boolean", label="MoE", default=False), | |
ColumnFilter(AutoEvalColumn.not_flagged.name, type="boolean", label="Flagged", default=True), | |
ColumnFilter(AutoEvalColumn.maintainers_highlight.name, type="boolean", label="Show only maintainer's highlight", default=False), | |
], | |
bool_checkboxgroup_label="Hide models", | |
interactive=False, | |
) | |
main_block = gr.Blocks(css=custom_css) | |
with main_block: | |
with gr.Row(elem_id="header-row"): | |
gr.HTML(TITLE) | |
gr.Markdown(INTRODUCTION_TEXT, elem_classes="markdown-text") | |
with gr.Tabs(elem_classes="tab-buttons") as tabs: | |
with gr.TabItem("π LLM Benchmark", elem_id="llm-benchmark-tab-table", id=0): | |
leaderboard = init_leaderboard(LEADERBOARD_DF) | |
with gr.TabItem("π Submit ", elem_id="llm-benchmark-tab-table", id=5): | |
with gr.Column(): | |
with gr.Row(): | |
gr.Markdown(EVALUATION_QUEUE_TEXT, elem_classes="markdown-text") | |
with gr.Row(): | |
gr.Markdown("# βοΈβ¨ Submit your model here!", elem_classes="markdown-text") | |
with gr.Row(): | |
with gr.Column(): | |
model_name_textbox = gr.Textbox(label="Model name") | |
revision_name_textbox = gr.Textbox(label="Revision commit", placeholder="latest") | |
with gr.Row(): | |
model_type = gr.Dropdown( | |
choices=[t.to_str(" : ") for t in ModelType if t != ModelType.Unknown], | |
label="Model type", | |
multiselect=False, | |
value=ModelType.FT.to_str(" : "), | |
interactive=True, | |
) | |
chat_template_toggle = gr.Checkbox( | |
label="Use chat template", | |
value=False, | |
info="Is your model a chat model?", | |
) | |
with gr.Column(): | |
precision = gr.Dropdown( | |
choices=[i.value.name for i in Precision if i != Precision.Unknown], | |
label="Precision", | |
multiselect=False, | |
value="float16", | |
interactive=True, | |
) | |
weight_type = gr.Dropdown( | |
choices=[i.value.name for i in WeightType], | |
label="Weights type", | |
multiselect=False, | |
value="Original", | |
interactive=True, | |
) | |
base_model_name_textbox = gr.Textbox(label="Base model (for delta or adapter weights)") | |
with gr.Column(): | |
with gr.Accordion( | |
f"β Finished Evaluations ({len(finished_eval_queue_df)})", | |
open=False, | |
): | |
with gr.Row(): | |
finished_eval_table = gr.components.Dataframe( | |
value=finished_eval_queue_df, | |
headers=EVAL_COLS, | |
datatype=EVAL_TYPES, | |
row_count=5, | |
interactive=False, | |
) | |
with gr.Accordion( | |
f"π Running Evaluation Queue ({len(running_eval_queue_df)})", | |
open=False, | |
): | |
with gr.Row(): | |
running_eval_table = gr.components.Dataframe( | |
value=running_eval_queue_df, | |
headers=EVAL_COLS, | |
datatype=EVAL_TYPES, | |
row_count=5, | |
interactive=False, | |
) | |
with gr.Accordion( | |
f"β³ Pending Evaluation Queue ({len(pending_eval_queue_df)})", | |
open=False, | |
): | |
with gr.Row(): | |
pending_eval_table = gr.components.Dataframe( | |
value=pending_eval_queue_df, | |
headers=EVAL_COLS, | |
datatype=EVAL_TYPES, | |
row_count=5, | |
interactive=False, | |
) | |
submit_button = gr.Button("Submit Eval") | |
submission_result = gr.Markdown() | |
# The chat template checkbox update function | |
def update_chat_checkbox(model_type_value): | |
return ModelType.from_str(model_type_value) == ModelType.chat | |
model_type.change( | |
fn=update_chat_checkbox, | |
inputs=[model_type], # Pass the current checkbox value | |
outputs=chat_template_toggle, | |
) | |
submit_button.click( | |
add_new_eval, | |
[ | |
model_name_textbox, | |
base_model_name_textbox, | |
revision_name_textbox, | |
precision, | |
weight_type, | |
model_type, | |
chat_template_toggle, | |
], | |
submission_result, | |
) | |
# Ensure the values in 'pending_eval_queue_df' are correct and ready for the DataFrame component | |
with gr.TabItem("π Model Vote"): | |
with gr.Row(): | |
gr.Markdown( | |
"## Vote for the models which should be evaluated first! \nYou'll need to sign in with the button above first. All votes are recorded.", | |
elem_classes="markdown-text" | |
) | |
login_button = gr.LoginButton(elem_id="oauth-button") | |
with gr.Row(): | |
pending_models = pending_eval_queue_df[EvalQueueColumn.model_name.name].to_list() | |
with gr.Column(): | |
selected_model = gr.Dropdown( | |
choices=pending_models, | |
label="Models", | |
multiselect=False, | |
value="str", | |
interactive=True, | |
) | |
vote_button = gr.Button("Vote", variant="primary") | |
with gr.Row(): | |
with gr.Accordion( | |
f"Available models pending ({len(pending_eval_queue_df)})", | |
open=True, | |
): | |
with gr.Row(): | |
pending_eval_table_votes = gr.components.Dataframe( | |
value=vote_manager.create_request_vote_df( | |
pending_eval_queue_df | |
), | |
headers=EVAL_COLS, | |
datatype=EVAL_TYPES, | |
row_count=5, | |
interactive=False | |
) | |
# Set the click event for the vote button | |
vote_button.click( | |
vote_manager.add_vote, | |
inputs=[selected_model, pending_eval_table], | |
outputs=[pending_eval_table_votes] | |
) | |
with gr.Row(): | |
with gr.Accordion("π Citation", open=False): | |
citation_button = gr.Textbox( | |
value=CITATION_BUTTON_TEXT, | |
label=CITATION_BUTTON_LABEL, | |
lines=20, | |
elem_id="citation-button", | |
show_copy_button=True, | |
) | |
main_block.load(fn=get_latest_data_leaderboard, inputs=[leaderboard], outputs=[leaderboard]) | |
leaderboard.change(fn=get_latest_data_queue, inputs=None, outputs=[finished_eval_table, running_eval_table, pending_eval_table]) | |
pending_eval_table.change(fn=vote_manager.create_request_vote_df, inputs=[pending_eval_table], outputs=[pending_eval_table_votes]) | |
main_block.queue(default_concurrency_limit=40) | |
def enable_space_ci_and_return_server(ui: gr.Blocks) -> WebhooksServer: | |
# Taken from https://huggingface.co/spaces/Wauplin/gradio-space-ci/blob/075119aee75ab5e7150bf0814eec91c83482e790/src/gradio_space_ci/webhook.py#L61 | |
# Compared to original, this one do not monkeypatch Gradio which allows us to define more webhooks. | |
# ht to Lucain! | |
if SPACE_ID is None: | |
print("Not in a Space: Space CI disabled.") | |
return WebhooksServer(ui=main_block) | |
if IS_EPHEMERAL_SPACE: | |
print("In an ephemeral Space: Space CI disabled.") | |
return WebhooksServer(ui=main_block) | |
card = RepoCard.load(repo_id_or_path=SPACE_ID, repo_type="space") | |
config = card.data.get("space_ci", {}) | |
print(f"Enabling Space CI with config from README: {config}") | |
return configure_space_ci( | |
blocks=ui, | |
trusted_authors=config.get("trusted_authors"), | |
private=config.get("private", "auto"), | |
variables=config.get("variables", "auto"), | |
secrets=config.get("secrets"), | |
hardware=config.get("hardware"), | |
storage=config.get("storage"), | |
) | |
# Create webhooks server (with CI url if in Space and not ephemeral) | |
webhooks_server = enable_space_ci_and_return_server(ui=main_block) | |
# Add webhooks | |
def update_leaderboard(payload: WebhookPayload) -> None: | |
"""Redownloads the leaderboard dataset each time it updates""" | |
if payload.repo.type == "dataset" and payload.event.action == "update": | |
global NEW_DATA_ON_LEADERBOARD | |
if NEW_DATA_ON_LEADERBOARD: | |
return | |
NEW_DATA_ON_LEADERBOARD = True | |
datasets.load_dataset( | |
AGGREGATED_REPO, | |
"default", | |
split="train", | |
cache_dir=HF_HOME, | |
download_mode=datasets.DownloadMode.FORCE_REDOWNLOAD, | |
verification_mode="no_checks" | |
) | |
# The below code is not used at the moment, as we can manage the queue file locally | |
LAST_UPDATE_QUEUE = datetime.datetime.now() | |
def update_queue(payload: WebhookPayload) -> None: | |
"""Redownloads the queue dataset each time it updates""" | |
if payload.repo.type == "dataset" and payload.event.action == "update": | |
current_time = datetime.datetime.now() | |
global LAST_UPDATE_QUEUE | |
if current_time - LAST_UPDATE_QUEUE > datetime.timedelta(minutes=10): | |
print("Would have updated the queue") | |
# We only redownload is last update was more than 10 minutes ago, as the queue is | |
# updated regularly and heavy to download | |
download_dataset(QUEUE_REPO, EVAL_REQUESTS_PATH) | |
LAST_UPDATE_QUEUE = datetime.datetime.now() | |
webhooks_server.launch() | |
scheduler = BackgroundScheduler() | |
scheduler.add_job(restart_space, "interval", hours=3) # restarted every 3h as backup in case automatic updates are not working | |
scheduler.start() |