|
--- |
|
title: FRN |
|
emoji: 📉 |
|
colorFrom: gray |
|
colorTo: red |
|
sdk: streamlit |
|
pinned: true |
|
app_file: app.py |
|
sdk_version: 1.31.0 |
|
python_version: 3.8 |
|
--- |
|
|
|
# FRN - Full-band Recurrent Network Official Implementation |
|
|
|
**Improving performance of real-time full-band blind packet-loss concealment with predictive network - ICASSP 2023** |
|
|
|
[](https://arxiv.org/abs/2211.04071) |
|
[](https://github.com/Crystalsound/FRN/) |
|
[](https://github.com/Crystalsound/FRN/commits) |
|
|
|
## License and citation |
|
|
|
This repository is released under the CC-BY-NC 4.0. license as found in the LICENSE file. |
|
|
|
If you use our software, please cite as below. |
|
For future queries, please contact [anh.nguyen@namitech.io](mailto:anh.nguyen@namitech.io). |
|
|
|
Copyright © 2022 NAMI TECHNOLOGY JSC, Inc. All rights reserved. |
|
|
|
``` |
|
@misc{Nguyen2022ImprovingPO, |
|
title={Improving performance of real-time full-band blind packet-loss concealment with predictive network}, |
|
author={Viet-Anh Nguyen and Anh H. T. Nguyen and Andy W. H. Khong}, |
|
year={2022}, |
|
eprint={2211.04071}, |
|
archivePrefix={arXiv}, |
|
primaryClass={cs.LG} |
|
} |
|
``` |
|
|
|
# 1. Results |
|
|
|
Our model achieved a significant gain over baselines. Here, we include the predicted packet loss concealment |
|
mean-opinion-score (PLCMOS) using Microsoft's [PLCMOS](https://github.com/microsoft/PLC-Challenge/tree/main/PLCMOS) |
|
service. Please refer to our paper for more benchmarks. |
|
|
|
| Model | PLCMOS | |
|
|---------|-----------| |
|
| Input | 3.517 | |
|
| tPLC | 3.463 | |
|
| TFGAN | 3.645 | |
|
| **FRN** | **3.655** | |
|
|
|
We also provide several audio samples in [https://crystalsound.github.io/FRN/](https://crystalsound.github.io/FRN/) for |
|
comparison. |
|
|
|
# 2. Installation |
|
|
|
## Setup |
|
|
|
### Clone the repo |
|
|
|
``` |
|
$ git clone https://github.com/Crystalsound/FRN.git |
|
$ cd FRN |
|
``` |
|
|
|
### Install dependencies |
|
|
|
* Our implementation requires the `libsndfile` libraries for the Python packages `soundfile`. On Ubuntu, they can be |
|
easily installed using `apt-get`: |
|
``` |
|
$ apt-get update && apt-get install libsndfile-dev |
|
``` |
|
* Create a Python 3.8 environment. Conda is recommended: |
|
``` |
|
$ conda create -n frn python=3.8 |
|
$ conda activate frn |
|
``` |
|
|
|
* Install the requirements: |
|
``` |
|
$ pip install -r requirements.txt |
|
``` |
|
|
|
# 3. Data preparation |
|
|
|
In our paper, we conduct experiments on the [VCTK](https://datashare.ed.ac.uk/handle/10283/3443) dataset. |
|
|
|
* Download and extract the datasets: |
|
``` |
|
$ wget http://www.udialogue.org/download/VCTK-Corpus.tar.gz -O data/vctk/VCTK-Corpus.tar.gz |
|
$ tar -zxvf data/vctk/VCTK-Corpus.tar.gz -C data/vctk/ --strip-components=1 |
|
``` |
|
|
|
After extracting the datasets, your `./data` directory should look like this: |
|
|
|
``` |
|
. |
|
|--data |
|
|--vctk |
|
|--wav48 |
|
|--p225 |
|
|--p225_001.wav |
|
... |
|
|--train.txt |
|
|--test.txt |
|
``` |
|
* In order to load the datasets, text files that contain training and testing audio paths are required. We have |
|
prepared `train.txt` and `test.txt` files in `./data/vctk` directory. |
|
|
|
# 4. Run the code |
|
|
|
## Configuration |
|
|
|
`config.py` is the most important file. Here, you can find all the configurations related to experiment setups, |
|
datasets, models, training, testing, etc. Although the config file has been explained thoroughly, we recommend reading |
|
our paper to fully understand each parameter. |
|
|
|
## Training |
|
|
|
* Adjust training hyperparameters in `config.py`. We provide the pretrained predictor in `lightning_logs/predictor` as stated in our paper. The FRN model can be trained entirely from scratch and will work as well. In this case, initiate `PLCModel(..., pred_ckpt_path=None)`. |
|
|
|
* Run `main.py`: |
|
``` |
|
$ python main.py --mode train |
|
``` |
|
* Each run will create a version in `./lightning_logs`, where the model checkpoint and hyperparameters are saved. In |
|
case you want to continue training from one of these versions, just set the argument `--version` of the above command |
|
to your desired version number. For example: |
|
``` |
|
# resume from version 0 |
|
$ python main.py --mode train --version 0 |
|
``` |
|
* To monitor the training curves as well as inspect model output visualization, run the tensorboard: |
|
``` |
|
$ tensorboard --logdir=./lightning_logs --bind_all |
|
``` |
|
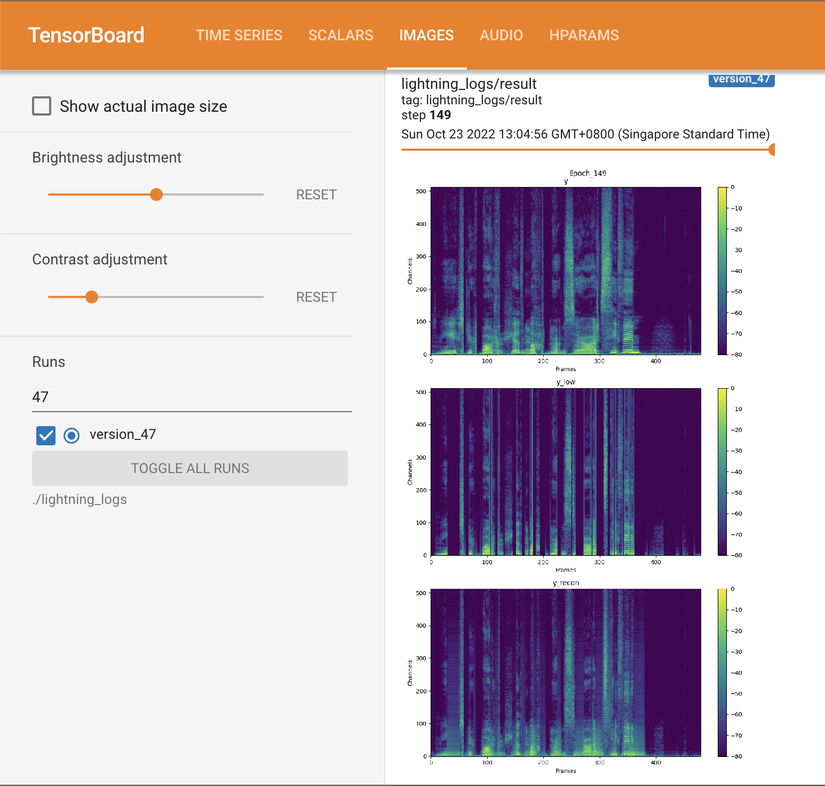 |
|
|
|
## Evaluation |
|
|
|
In our paper, we evaluated with 2 masking methods: simulation using Markov Chain and employing real traces in PLC |
|
Challenge. |
|
|
|
* Get the blind test set with loss traces: |
|
``` |
|
$ wget http://plcchallenge2022pub.blob.core.windows.net/plcchallengearchive/blind.tar.gz |
|
$ tar -xvf blind.tar.gz -C test_samples |
|
``` |
|
* Modify `config.py` to change evaluation setup if necessary. |
|
* Run `main.py` with a version number to be evaluated: |
|
``` |
|
$ python main.py --mode eval --version 0 |
|
``` |
|
During the evaluation, several output samples are saved to `CONFIG.LOG.sample_path` for sanity testing. |
|
|
|
## Configure a new dataset |
|
|
|
Our implementation currently works with the VCTK dataset but can be easily extensible to a new one. |
|
|
|
* Firstly, you need to prepare `train.txt` and `test.txt`. See `./data/vctk/train.txt` and `./data/vctk/test.txt` for |
|
example. |
|
* Secondly, add a new dictionary to `CONFIG.DATA.data_dir`: |
|
``` |
|
{ |
|
'root': 'path/to/data/directory', |
|
'train': 'path/to/train.txt', |
|
'test': 'path/to/test.txt' |
|
} |
|
``` |
|
**Important:** Make sure each line in `train.txt` and `test.txt` joining with `'root'` is a valid path to its |
|
corresponding audio file. |
|
|
|
# 5. Audio generation |
|
|
|
* In order to generate output audios, you need to modify `CONFIG.TEST.in_dir` to your input directory. |
|
* Run `main.py`: |
|
``` |
|
python main.py --mode test --version 0 |
|
``` |
|
The generated audios are saved to `CONFIG.TEST.out_dir`. |
|
|
|
## ONNX inferencing |
|
We provide ONNX inferencing scripts and the best ONNX model (converted from the best checkpoint) |
|
at `lightning_logs/best_model.onnx`. |
|
* Convert a checkpoint to an ONNX model: |
|
``` |
|
python main.py --mode onnx --version 0 |
|
``` |
|
The converted ONNX model will be saved to `lightning_logs/version_0/checkpoints`. |
|
* Put test audios in `test_samples` and inference with the converted ONNX model (see `inference_onnx.py` for more |
|
details): |
|
``` |
|
python inference_onnx.py --onnx_path lightning_logs/version_0/frn.onnx |
|
``` |
|
|