The Deep Q-Network (DQN)
This is the architecture of our Deep Q-Learning network:
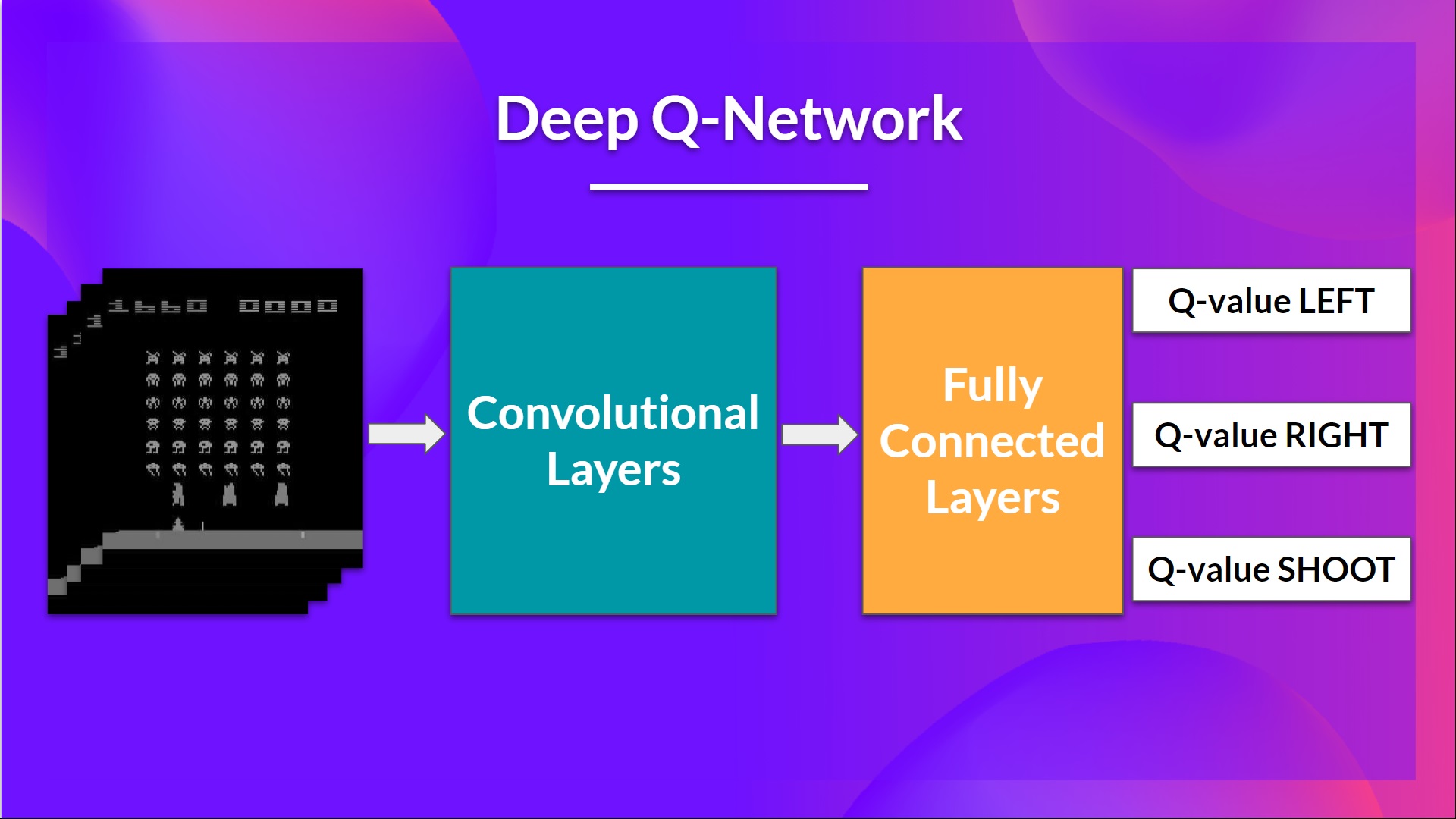
As input, we take a stack of 4 frames passed through the network as a state and output a vector of Q-values for each possible action at that state. Then, like with Q-Learning, we just need to use our epsilon-greedy policy to select which action to take.
When the Neural Network is initialized, the Q-value estimation is terrible. But during training, our Deep Q-Network agent will associate a situation with the appropriate action and learn to play the game well.
Preprocessing the input and temporal limitation
We need to preprocess the input. It’s an essential step since we want to reduce the complexity of our state to reduce the computation time needed for training.
To achieve this, we reduce the state space to 84x84 and grayscale it. We can do this since the colors in Atari environments don’t add important information. This is a big improvement since we reduce our three color channels (RGB) to 1.
We can also crop a part of the screen in some games if it does not contain important information. Then we stack four frames together.
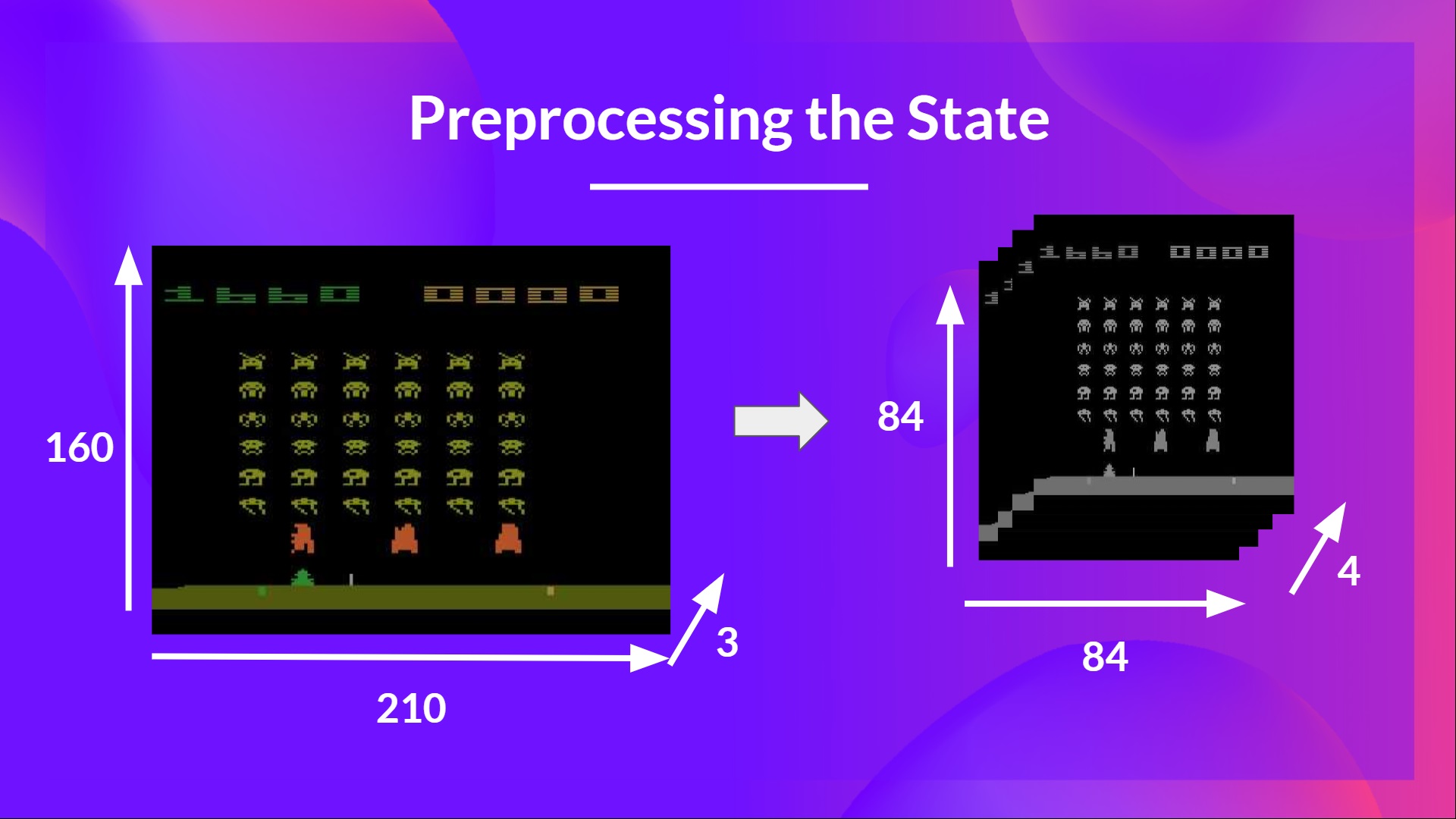
Why do we stack four frames together? We stack frames together because it helps us handle the problem of temporal limitation. Let’s take an example with the game of Pong. When you see this frame:
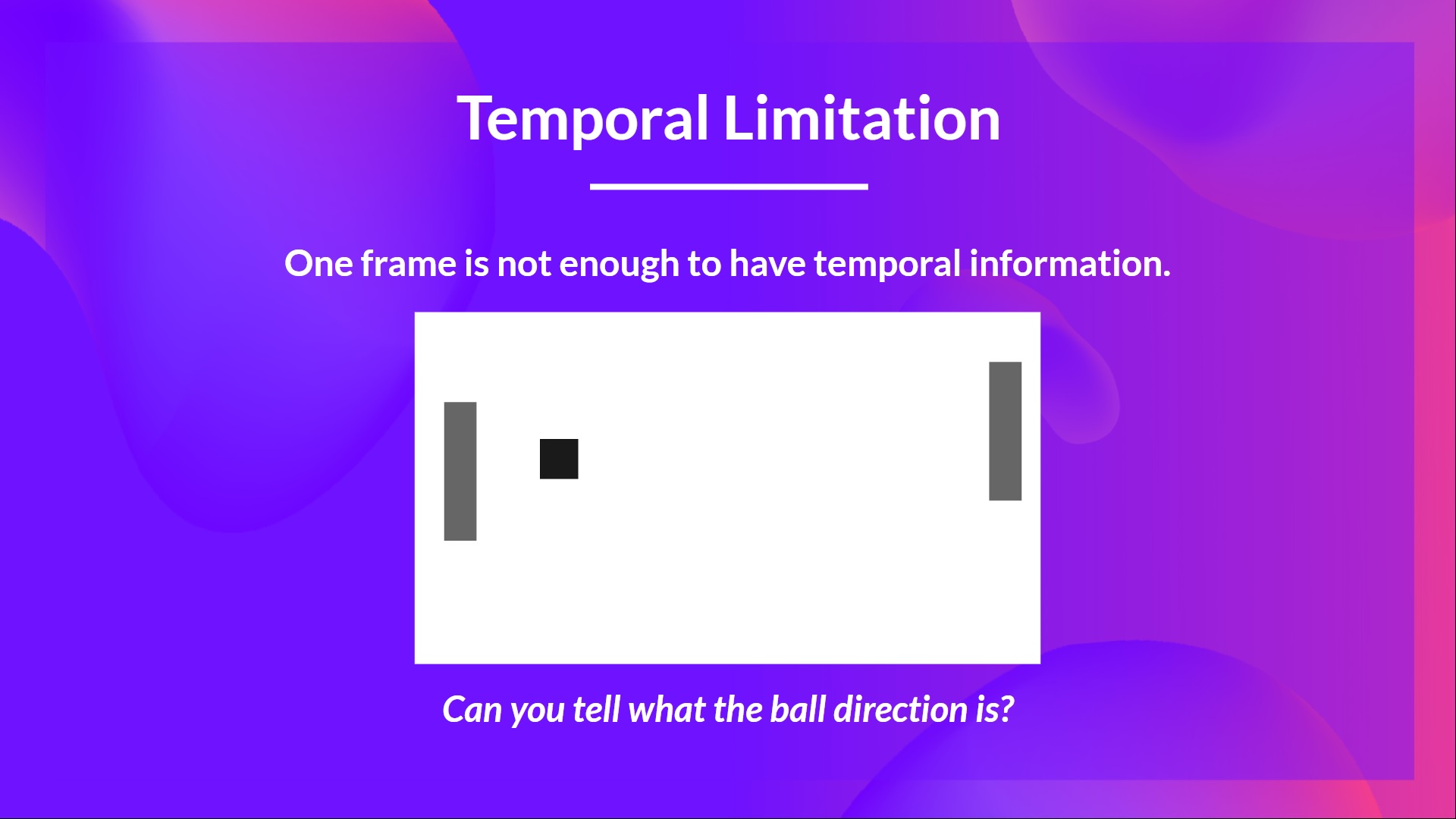
Can you tell me where the ball is going? No, because one frame is not enough to have a sense of motion! But what if I add three more frames? Here you can see that the ball is going to the right.
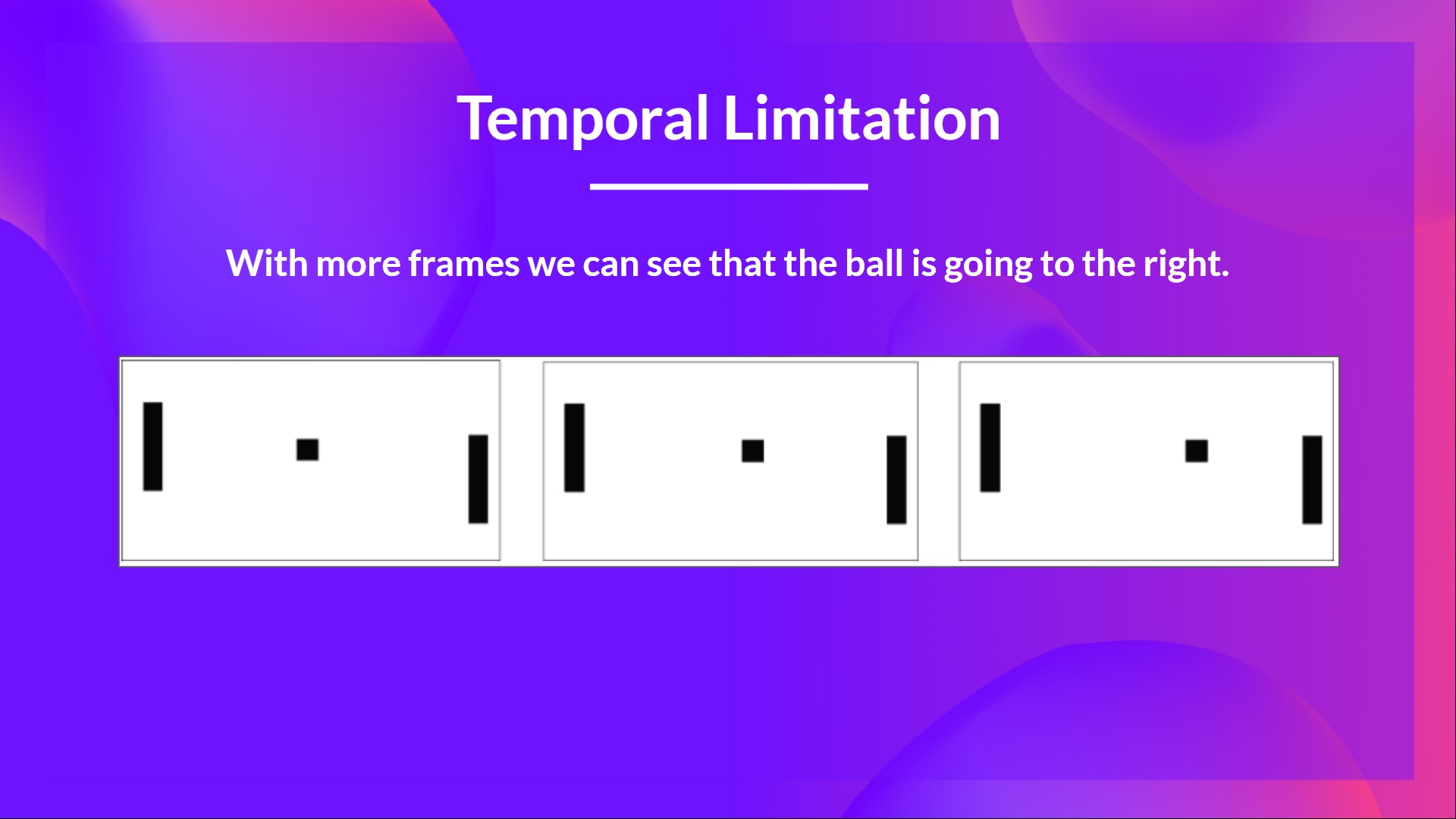
Then the stacked frames are processed by three convolutional layers. These layers allow us to capture and exploit spatial relationships in images. But also, because the frames are stacked together, we can exploit some temporal properties across those frames.
If you don’t know what convolutional layers are, don’t worry. You can check out Lesson 4 of this free Deep Learning Course by Udacity
Finally, we have a couple of fully connected layers that output a Q-value for each possible action at that state.
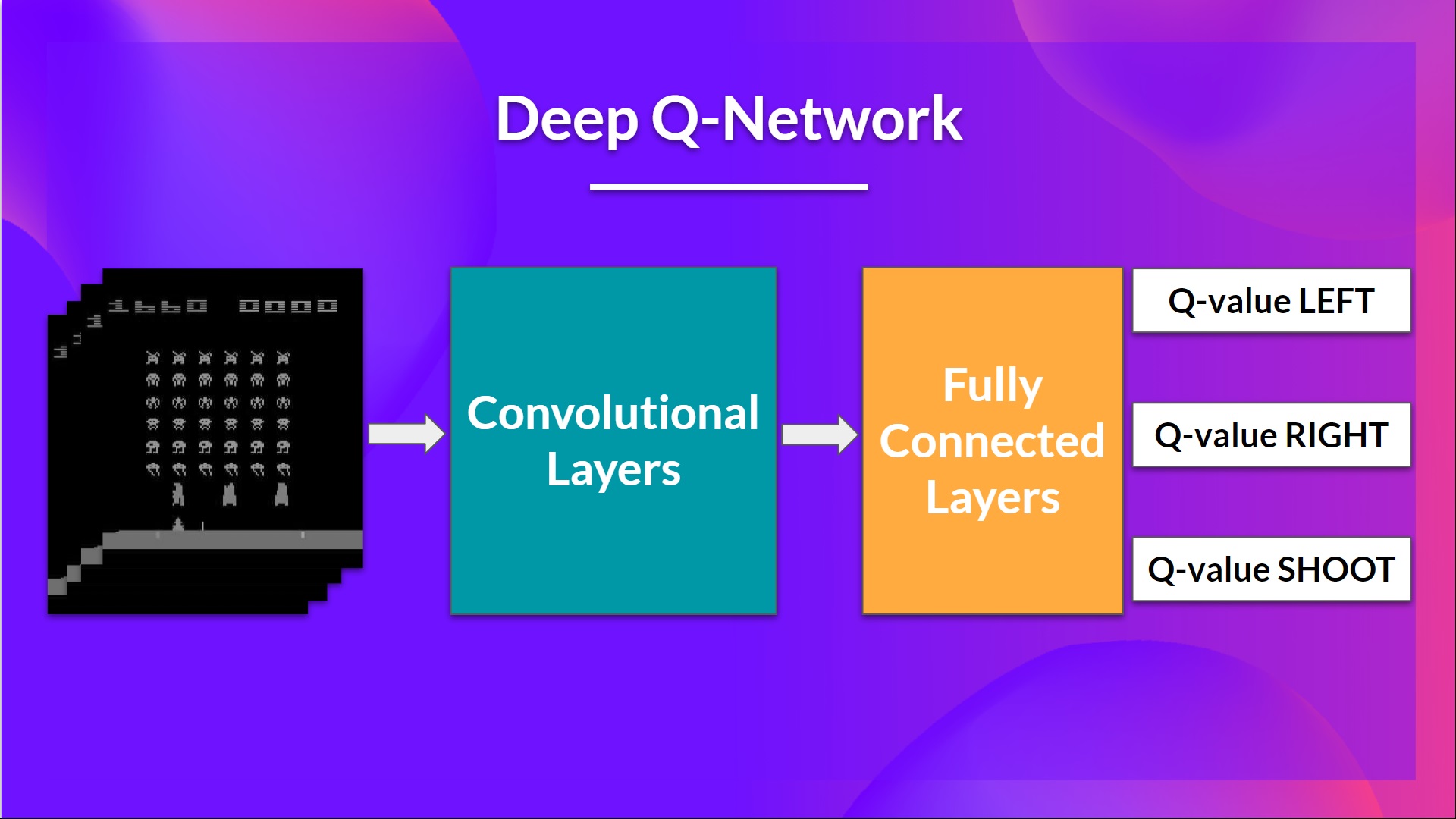
So, we see that Deep Q-Learning uses a neural network to approximate, given a state, the different Q-values for each possible action at that state. Now let’s study the Deep Q-Learning algorithm.
< > Update on GitHub