ConvNeXT
Overview
ConvNeXT モデルは、A ConvNet for the 2020s で Zhuang Liu、Hanzi Mao、Chao-Yuan Wu、Christoph Feichtenhofer、Trevor Darrell、Saining Xie によって提案されました。 ConvNeXT は、ビジョン トランスフォーマーの設計からインスピレーションを得た純粋な畳み込みモデル (ConvNet) であり、ビジョン トランスフォーマーよりも優れたパフォーマンスを発揮すると主張しています。
論文の要約は次のとおりです。
視覚認識の「狂騒の 20 年代」は、最先端の画像分類モデルとして ConvNet にすぐに取って代わられた Vision Transformers (ViT) の導入から始まりました。 一方、バニラ ViT は、オブジェクト検出やセマンティック セグメンテーションなどの一般的なコンピューター ビジョン タスクに適用すると困難に直面します。階層型トランスフォーマーです (Swin Transformers など) は、いくつかの ConvNet の以前の機能を再導入し、Transformers を汎用ビジョン バックボーンとして実用的に可能にし、幅広い環境で顕著なパフォーマンスを実証しました。 さまざまな視覚タスク。ただし、このようなハイブリッド アプローチの有効性は、依然として、固有の誘導性ではなく、トランスフォーマーの本質的な優位性によるところが大きいと考えられています。 畳み込みのバイアス。この作業では、設計空間を再検討し、純粋な ConvNet が達成できる限界をテストします。標準 ResNet を設計に向けて徐々に「最新化」します。 ビジョン Transformer の概要を確認し、途中でパフォーマンスの違いに寄与するいくつかの重要なコンポーネントを発見します。この調査の結果は、純粋な ConvNet モデルのファミリーです。 ConvNextと呼ばれます。 ConvNeXts は完全に標準の ConvNet モジュールから構築されており、精度と拡張性の点で Transformers と有利に競合し、87.8% の ImageNet トップ 1 精度を達成しています。 標準 ConvNet のシンプルさと効率を維持しながら、COCO 検出と ADE20K セグメンテーションでは Swin Transformers よりも優れたパフォーマンスを発揮します。
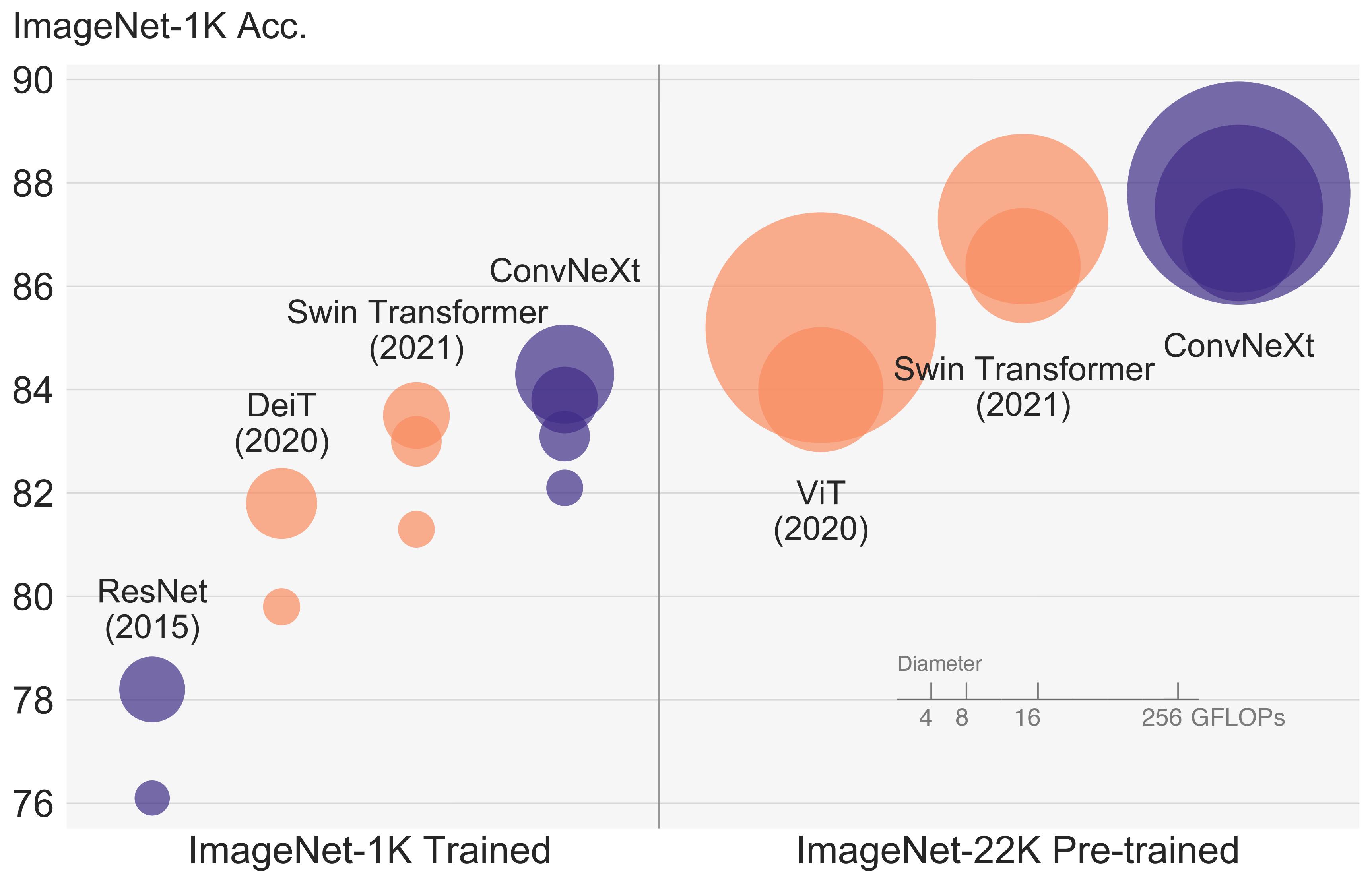
このモデルは、nielsr によって提供されました。 TensorFlow バージョンのモデルは ariG23498 によって提供されました。 gante、および sayakpaul (同等の貢献)。元のコードは こちら にあります。
Resources
ConvNeXT の使用を開始するのに役立つ公式 Hugging Face およびコミュニティ (🌎 で示される) リソースのリスト。
- ConvNextForImageClassification は、この サンプル スクリプト および ノートブック。
- 参照: 画像分類タスク ガイド
ここに含めるリソースの送信に興味がある場合は、お気軽にプル リクエストを開いてください。審査させていただきます。リソースは、既存のリソースを複製するのではなく、何か新しいものを示すことが理想的です。
ConvNextConfig
class transformers.ConvNextConfig
< source >( num_channels = 3 patch_size = 4 num_stages = 4 hidden_sizes = None depths = None hidden_act = 'gelu' initializer_range = 0.02 layer_norm_eps = 1e-12 layer_scale_init_value = 1e-06 drop_path_rate = 0.0 image_size = 224 out_features = None out_indices = None **kwargs )
Parameters
- num_channels (
int
, optional, defaults to 3) — The number of input channels. - patch_size (
int
, optional, defaults to 4) — Patch size to use in the patch embedding layer. - num_stages (
int
, optional, defaults to 4) — The number of stages in the model. - hidden_sizes (
List[int]
, optional, defaults to [96, 192, 384, 768]) — Dimensionality (hidden size) at each stage. - depths (
List[int]
, optional, defaults to [3, 3, 9, 3]) — Depth (number of blocks) for each stage. - hidden_act (
str
orfunction
, optional, defaults to"gelu"
) — The non-linear activation function (function or string) in each block. If string,"gelu"
,"relu"
,"selu"
and"gelu_new"
are supported. - initializer_range (
float
, optional, defaults to 0.02) — The standard deviation of the truncated_normal_initializer for initializing all weight matrices. - layer_norm_eps (
float
, optional, defaults to 1e-12) — The epsilon used by the layer normalization layers. - layer_scale_init_value (
float
, optional, defaults to 1e-6) — The initial value for the layer scale. - drop_path_rate (
float
, optional, defaults to 0.0) — The drop rate for stochastic depth. - out_features (
List[str]
, optional) — If used as backbone, list of features to output. Can be any of"stem"
,"stage1"
,"stage2"
, etc. (depending on how many stages the model has). If unset andout_indices
is set, will default to the corresponding stages. If unset andout_indices
is unset, will default to the last stage. Must be in the same order as defined in thestage_names
attribute. - out_indices (
List[int]
, optional) — If used as backbone, list of indices of features to output. Can be any of 0, 1, 2, etc. (depending on how many stages the model has). If unset andout_features
is set, will default to the corresponding stages. If unset andout_features
is unset, will default to the last stage. Must be in the same order as defined in thestage_names
attribute.
This is the configuration class to store the configuration of a ConvNextModel. It is used to instantiate an ConvNeXT model according to the specified arguments, defining the model architecture. Instantiating a configuration with the defaults will yield a similar configuration to that of the ConvNeXT facebook/convnext-tiny-224 architecture.
Configuration objects inherit from PretrainedConfig and can be used to control the model outputs. Read the documentation from PretrainedConfig for more information.
Example:
>>> from transformers import ConvNextConfig, ConvNextModel
>>> # Initializing a ConvNext convnext-tiny-224 style configuration
>>> configuration = ConvNextConfig()
>>> # Initializing a model (with random weights) from the convnext-tiny-224 style configuration
>>> model = ConvNextModel(configuration)
>>> # Accessing the model configuration
>>> configuration = model.config
ConvNextFeatureExtractor
ConvNextImageProcessor
class transformers.ConvNextImageProcessor
< source >( do_resize: bool = True size: Dict = None crop_pct: float = None resample: Resampling = <Resampling.BILINEAR: 2> do_rescale: bool = True rescale_factor: Union = 0.00392156862745098 do_normalize: bool = True image_mean: Union = None image_std: Union = None **kwargs )
Parameters
- do_resize (
bool
, optional, defaults toTrue
) — Controls whether to resize the image’s (height, width) dimensions to the specifiedsize
. Can be overriden bydo_resize
in thepreprocess
method. - size (
Dict[str, int]
optional, defaults to{"shortest_edge" -- 384}
): Resolution of the output image afterresize
is applied. Ifsize["shortest_edge"]
>= 384, the image is resized to(size["shortest_edge"], size["shortest_edge"])
. Otherwise, the smaller edge of the image will be matched toint(size["shortest_edge"]/crop_pct)
, after which the image is cropped to(size["shortest_edge"], size["shortest_edge"])
. Only has an effect ifdo_resize
is set toTrue
. Can be overriden bysize
in thepreprocess
method. - crop_pct (
float
optional, defaults to 224 / 256) — Percentage of the image to crop. Only has an effect ifdo_resize
isTrue
and size < 384. Can be overriden bycrop_pct
in thepreprocess
method. - resample (
PILImageResampling
, optional, defaults toResampling.BILINEAR
) — Resampling filter to use if resizing the image. Can be overriden byresample
in thepreprocess
method. - do_rescale (
bool
, optional, defaults toTrue
) — Whether to rescale the image by the specified scalerescale_factor
. Can be overriden bydo_rescale
in thepreprocess
method. - rescale_factor (
int
orfloat
, optional, defaults to1/255
) — Scale factor to use if rescaling the image. Can be overriden byrescale_factor
in thepreprocess
method. - do_normalize (
bool
, optional, defaults toTrue
) — Whether to normalize the image. Can be overridden by thedo_normalize
parameter in thepreprocess
method. - image_mean (
float
orList[float]
, optional, defaults toIMAGENET_STANDARD_MEAN
) — Mean to use if normalizing the image. This is a float or list of floats the length of the number of channels in the image. Can be overridden by theimage_mean
parameter in thepreprocess
method. - image_std (
float
orList[float]
, optional, defaults toIMAGENET_STANDARD_STD
) — Standard deviation to use if normalizing the image. This is a float or list of floats the length of the number of channels in the image. Can be overridden by theimage_std
parameter in thepreprocess
method.
Constructs a ConvNeXT image processor.
preprocess
< source >( images: Union do_resize: bool = None size: Dict = None crop_pct: float = None resample: Resampling = None do_rescale: bool = None rescale_factor: float = None do_normalize: bool = None image_mean: Union = None image_std: Union = None return_tensors: Union = None data_format: ChannelDimension = <ChannelDimension.FIRST: 'channels_first'> input_data_format: Union = None **kwargs )
Parameters
- images (
ImageInput
) — Image to preprocess. Expects a single or batch of images with pixel values ranging from 0 to 255. If passing in images with pixel values between 0 and 1, setdo_rescale=False
. - do_resize (
bool
, optional, defaults toself.do_resize
) — Whether to resize the image. - size (
Dict[str, int]
, optional, defaults toself.size
) — Size of the output image afterresize
has been applied. Ifsize["shortest_edge"]
>= 384, the image is resized to(size["shortest_edge"], size["shortest_edge"])
. Otherwise, the smaller edge of the image will be matched toint(size["shortest_edge"]/ crop_pct)
, after which the image is cropped to(size["shortest_edge"], size["shortest_edge"])
. Only has an effect ifdo_resize
is set toTrue
. - crop_pct (
float
, optional, defaults toself.crop_pct
) — Percentage of the image to crop if size < 384. - resample (
int
, optional, defaults toself.resample
) — Resampling filter to use if resizing the image. This can be one ofPILImageResampling
, filters. Only has an effect ifdo_resize
is set toTrue
. - do_rescale (
bool
, optional, defaults toself.do_rescale
) — Whether to rescale the image values between [0 - 1]. - rescale_factor (
float
, optional, defaults toself.rescale_factor
) — Rescale factor to rescale the image by ifdo_rescale
is set toTrue
. - do_normalize (
bool
, optional, defaults toself.do_normalize
) — Whether to normalize the image. - image_mean (
float
orList[float]
, optional, defaults toself.image_mean
) — Image mean. - image_std (
float
orList[float]
, optional, defaults toself.image_std
) — Image standard deviation. - return_tensors (
str
orTensorType
, optional) — The type of tensors to return. Can be one of:- Unset: Return a list of
np.ndarray
. TensorType.TENSORFLOW
or'tf'
: Return a batch of typetf.Tensor
.TensorType.PYTORCH
or'pt'
: Return a batch of typetorch.Tensor
.TensorType.NUMPY
or'np'
: Return a batch of typenp.ndarray
.TensorType.JAX
or'jax'
: Return a batch of typejax.numpy.ndarray
.
- Unset: Return a list of
- data_format (
ChannelDimension
orstr
, optional, defaults toChannelDimension.FIRST
) — The channel dimension format for the output image. Can be one of:"channels_first"
orChannelDimension.FIRST
: image in (num_channels, height, width) format."channels_last"
orChannelDimension.LAST
: image in (height, width, num_channels) format.- Unset: Use the channel dimension format of the input image.
- input_data_format (
ChannelDimension
orstr
, optional) — The channel dimension format for the input image. If unset, the channel dimension format is inferred from the input image. Can be one of:"channels_first"
orChannelDimension.FIRST
: image in (num_channels, height, width) format."channels_last"
orChannelDimension.LAST
: image in (height, width, num_channels) format."none"
orChannelDimension.NONE
: image in (height, width) format.
Preprocess an image or batch of images.
ConvNextModel
class transformers.ConvNextModel
< source >( config )
Parameters
- config (ConvNextConfig) — Model configuration class with all the parameters of the model. Initializing with a config file does not load the weights associated with the model, only the configuration. Check out the from_pretrained() method to load the model weights.
The bare ConvNext model outputting raw features without any specific head on top. This model is a PyTorch torch.nn.Module subclass. Use it as a regular PyTorch Module and refer to the PyTorch documentation for all matter related to general usage and behavior.
forward
< source >( pixel_values: FloatTensor = None output_hidden_states: Optional = None return_dict: Optional = None ) → transformers.modeling_outputs.BaseModelOutputWithPoolingAndNoAttention
or tuple(torch.FloatTensor)
Parameters
- pixel_values (
torch.FloatTensor
of shape(batch_size, num_channels, height, width)
) — Pixel values. Pixel values can be obtained using AutoImageProcessor. See ConvNextImageProcessor.call() for details. - output_hidden_states (
bool
, optional) — Whether or not to return the hidden states of all layers. Seehidden_states
under returned tensors for more detail. - return_dict (
bool
, optional) — Whether or not to return a ModelOutput instead of a plain tuple.
Returns
transformers.modeling_outputs.BaseModelOutputWithPoolingAndNoAttention
or tuple(torch.FloatTensor)
A transformers.modeling_outputs.BaseModelOutputWithPoolingAndNoAttention
or a tuple of
torch.FloatTensor
(if return_dict=False
is passed or when config.return_dict=False
) comprising various
elements depending on the configuration (ConvNextConfig) and inputs.
-
last_hidden_state (
torch.FloatTensor
of shape(batch_size, num_channels, height, width)
) — Sequence of hidden-states at the output of the last layer of the model. -
pooler_output (
torch.FloatTensor
of shape(batch_size, hidden_size)
) — Last layer hidden-state after a pooling operation on the spatial dimensions. -
hidden_states (
tuple(torch.FloatTensor)
, optional, returned whenoutput_hidden_states=True
is passed or whenconfig.output_hidden_states=True
) — Tuple oftorch.FloatTensor
(one for the output of the embeddings, if the model has an embedding layer, + one for the output of each layer) of shape(batch_size, num_channels, height, width)
.Hidden-states of the model at the output of each layer plus the optional initial embedding outputs.
The ConvNextModel forward method, overrides the __call__
special method.
Although the recipe for forward pass needs to be defined within this function, one should call the Module
instance afterwards instead of this since the former takes care of running the pre and post processing steps while
the latter silently ignores them.
Example:
>>> from transformers import AutoImageProcessor, ConvNextModel
>>> import torch
>>> from datasets import load_dataset
>>> dataset = load_dataset("huggingface/cats-image")
>>> image = dataset["test"]["image"][0]
>>> image_processor = AutoImageProcessor.from_pretrained("facebook/convnext-tiny-224")
>>> model = ConvNextModel.from_pretrained("facebook/convnext-tiny-224")
>>> inputs = image_processor(image, return_tensors="pt")
>>> with torch.no_grad():
... outputs = model(**inputs)
>>> last_hidden_states = outputs.last_hidden_state
>>> list(last_hidden_states.shape)
[1, 768, 7, 7]
ConvNextForImageClassification
class transformers.ConvNextForImageClassification
< source >( config )
Parameters
- config (ConvNextConfig) — Model configuration class with all the parameters of the model. Initializing with a config file does not load the weights associated with the model, only the configuration. Check out the from_pretrained() method to load the model weights.
ConvNext Model with an image classification head on top (a linear layer on top of the pooled features), e.g. for ImageNet.
This model is a PyTorch torch.nn.Module subclass. Use it as a regular PyTorch Module and refer to the PyTorch documentation for all matter related to general usage and behavior.
forward
< source >( pixel_values: FloatTensor = None labels: Optional = None output_hidden_states: Optional = None return_dict: Optional = None ) → transformers.modeling_outputs.ImageClassifierOutputWithNoAttention or tuple(torch.FloatTensor)
Parameters
- pixel_values (
torch.FloatTensor
of shape(batch_size, num_channels, height, width)
) — Pixel values. Pixel values can be obtained using AutoImageProcessor. See ConvNextImageProcessor.call() for details. - output_hidden_states (
bool
, optional) — Whether or not to return the hidden states of all layers. Seehidden_states
under returned tensors for more detail. - return_dict (
bool
, optional) — Whether or not to return a ModelOutput instead of a plain tuple. - labels (
torch.LongTensor
of shape(batch_size,)
, optional) — Labels for computing the image classification/regression loss. Indices should be in[0, ..., config.num_labels - 1]
. Ifconfig.num_labels == 1
a regression loss is computed (Mean-Square loss), Ifconfig.num_labels > 1
a classification loss is computed (Cross-Entropy).
Returns
transformers.modeling_outputs.ImageClassifierOutputWithNoAttention or tuple(torch.FloatTensor)
A transformers.modeling_outputs.ImageClassifierOutputWithNoAttention or a tuple of
torch.FloatTensor
(if return_dict=False
is passed or when config.return_dict=False
) comprising various
elements depending on the configuration (ConvNextConfig) and inputs.
- loss (
torch.FloatTensor
of shape(1,)
, optional, returned whenlabels
is provided) — Classification (or regression if config.num_labels==1) loss. - logits (
torch.FloatTensor
of shape(batch_size, config.num_labels)
) — Classification (or regression if config.num_labels==1) scores (before SoftMax). - hidden_states (
tuple(torch.FloatTensor)
, optional, returned whenoutput_hidden_states=True
is passed or whenconfig.output_hidden_states=True
) — Tuple oftorch.FloatTensor
(one for the output of the embeddings, if the model has an embedding layer, + one for the output of each stage) of shape(batch_size, num_channels, height, width)
. Hidden-states (also called feature maps) of the model at the output of each stage.
The ConvNextForImageClassification forward method, overrides the __call__
special method.
Although the recipe for forward pass needs to be defined within this function, one should call the Module
instance afterwards instead of this since the former takes care of running the pre and post processing steps while
the latter silently ignores them.
Example:
>>> from transformers import AutoImageProcessor, ConvNextForImageClassification
>>> import torch
>>> from datasets import load_dataset
>>> dataset = load_dataset("huggingface/cats-image")
>>> image = dataset["test"]["image"][0]
>>> image_processor = AutoImageProcessor.from_pretrained("facebook/convnext-tiny-224")
>>> model = ConvNextForImageClassification.from_pretrained("facebook/convnext-tiny-224")
>>> inputs = image_processor(image, return_tensors="pt")
>>> with torch.no_grad():
... logits = model(**inputs).logits
>>> # model predicts one of the 1000 ImageNet classes
>>> predicted_label = logits.argmax(-1).item()
>>> print(model.config.id2label[predicted_label])
tabby, tabby cat
TFConvNextModel
class transformers.TFConvNextModel
< source >( config *inputs add_pooling_layer = True **kwargs )
Parameters
- config (ConvNextConfig) — Model configuration class with all the parameters of the model. Initializing with a config file does not load the weights associated with the model, only the configuration. Check out the from_pretrained() method to load the model weights.
The bare ConvNext model outputting raw features without any specific head on top. This model inherits from TFPreTrainedModel. Check the superclass documentation for the generic methods the library implements for all its model (such as downloading or saving, resizing the input embeddings, pruning heads etc.)
This model is also a keras.Model subclass. Use it as a regular TF 2.0 Keras Model and refer to the TF 2.0 documentation for all matter related to general usage and behavior.
TensorFlow models and layers in transformers
accept two formats as input:
- having all inputs as keyword arguments (like PyTorch models), or
- having all inputs as a list, tuple or dict in the first positional argument.
The reason the second format is supported is that Keras methods prefer this format when passing inputs to models
and layers. Because of this support, when using methods like model.fit()
things should “just work” for you - just
pass your inputs and labels in any format that model.fit()
supports! If, however, you want to use the second
format outside of Keras methods like fit()
and predict()
, such as when creating your own layers or models with
the Keras Functional
API, there are three possibilities you can use to gather all the input Tensors in the first
positional argument:
- a single Tensor with
pixel_values
only and nothing else:model(pixel_values)
- a list of varying length with one or several input Tensors IN THE ORDER given in the docstring:
model([pixel_values, attention_mask])
ormodel([pixel_values, attention_mask, token_type_ids])
- a dictionary with one or several input Tensors associated to the input names given in the docstring:
model({"pixel_values": pixel_values, "token_type_ids": token_type_ids})
Note that when creating models and layers with subclassing then you don’t need to worry about any of this, as you can just pass inputs like you would to any other Python function!
call
< source >( pixel_values: TFModelInputType | None = None output_hidden_states: Optional[bool] = None return_dict: Optional[bool] = None training: bool = False ) → transformers.modeling_tf_outputs.TFBaseModelOutputWithPooling or tuple(tf.Tensor)
Parameters
- pixel_values (
np.ndarray
,tf.Tensor
,List[tf.Tensor]
`Dict[str, tf.Tensor]
orDict[str, np.ndarray]
and each example must have the shape(batch_size, num_channels, height, width)
) — Pixel values. Pixel values can be obtained using AutoImageProcessor. See ConvNextImageProcessor.call() for details. - output_hidden_states (
bool
, optional) — Whether or not to return the hidden states of all layers. Seehidden_states
under returned tensors for more detail. This argument can be used only in eager mode, in graph mode the value in the config will be used instead. - return_dict (
bool
, optional) — Whether or not to return a ModelOutput instead of a plain tuple. This argument can be used in eager mode, in graph mode the value will always be set to True.
Returns
transformers.modeling_tf_outputs.TFBaseModelOutputWithPooling or tuple(tf.Tensor)
A transformers.modeling_tf_outputs.TFBaseModelOutputWithPooling or a tuple of tf.Tensor
(if
return_dict=False
is passed or when config.return_dict=False
) comprising various elements depending on the
configuration (ConvNextConfig) and inputs.
-
last_hidden_state (
tf.Tensor
of shape(batch_size, sequence_length, hidden_size)
) — Sequence of hidden-states at the output of the last layer of the model. -
pooler_output (
tf.Tensor
of shape(batch_size, hidden_size)
) — Last layer hidden-state of the first token of the sequence (classification token) further processed by a Linear layer and a Tanh activation function. The Linear layer weights are trained from the next sentence prediction (classification) objective during pretraining.This output is usually not a good summary of the semantic content of the input, you’re often better with averaging or pooling the sequence of hidden-states for the whole input sequence.
-
hidden_states (
tuple(tf.Tensor)
, optional, returned whenoutput_hidden_states=True
is passed or whenconfig.output_hidden_states=True
) — Tuple oftf.Tensor
(one for the output of the embeddings + one for the output of each layer) of shape(batch_size, sequence_length, hidden_size)
.Hidden-states of the model at the output of each layer plus the initial embedding outputs.
-
attentions (
tuple(tf.Tensor)
, optional, returned whenoutput_attentions=True
is passed or whenconfig.output_attentions=True
) — Tuple oftf.Tensor
(one for each layer) of shape(batch_size, num_heads, sequence_length, sequence_length)
.Attentions weights after the attention softmax, used to compute the weighted average in the self-attention heads.
The TFConvNextModel forward method, overrides the __call__
special method.
Although the recipe for forward pass needs to be defined within this function, one should call the Module
instance afterwards instead of this since the former takes care of running the pre and post processing steps while
the latter silently ignores them.
Examples:
>>> from transformers import AutoImageProcessor, TFConvNextModel
>>> from PIL import Image
>>> import requests
>>> url = "http://images.cocodataset.org/val2017/000000039769.jpg"
>>> image = Image.open(requests.get(url, stream=True).raw)
>>> image_processor = AutoImageProcessor.from_pretrained("facebook/convnext-tiny-224")
>>> model = TFConvNextModel.from_pretrained("facebook/convnext-tiny-224")
>>> inputs = image_processor(images=image, return_tensors="tf")
>>> outputs = model(**inputs)
>>> last_hidden_states = outputs.last_hidden_state
TFConvNextForImageClassification
class transformers.TFConvNextForImageClassification
< source >( config: ConvNextConfig *inputs **kwargs )
Parameters
- config (ConvNextConfig) — Model configuration class with all the parameters of the model. Initializing with a config file does not load the weights associated with the model, only the configuration. Check out the from_pretrained() method to load the model weights.
ConvNext Model with an image classification head on top (a linear layer on top of the pooled features), e.g. for ImageNet.
This model inherits from TFPreTrainedModel. Check the superclass documentation for the generic methods the library implements for all its model (such as downloading or saving, resizing the input embeddings, pruning heads etc.)
This model is also a keras.Model subclass. Use it as a regular TF 2.0 Keras Model and refer to the TF 2.0 documentation for all matter related to general usage and behavior.
TensorFlow models and layers in transformers
accept two formats as input:
- having all inputs as keyword arguments (like PyTorch models), or
- having all inputs as a list, tuple or dict in the first positional argument.
The reason the second format is supported is that Keras methods prefer this format when passing inputs to models
and layers. Because of this support, when using methods like model.fit()
things should “just work” for you - just
pass your inputs and labels in any format that model.fit()
supports! If, however, you want to use the second
format outside of Keras methods like fit()
and predict()
, such as when creating your own layers or models with
the Keras Functional
API, there are three possibilities you can use to gather all the input Tensors in the first
positional argument:
- a single Tensor with
pixel_values
only and nothing else:model(pixel_values)
- a list of varying length with one or several input Tensors IN THE ORDER given in the docstring:
model([pixel_values, attention_mask])
ormodel([pixel_values, attention_mask, token_type_ids])
- a dictionary with one or several input Tensors associated to the input names given in the docstring:
model({"pixel_values": pixel_values, "token_type_ids": token_type_ids})
Note that when creating models and layers with subclassing then you don’t need to worry about any of this, as you can just pass inputs like you would to any other Python function!
call
< source >( pixel_values: TFModelInputType | None = None output_hidden_states: Optional[bool] = None return_dict: Optional[bool] = None labels: np.ndarray | tf.Tensor | None = None training: Optional[bool] = False ) → transformers.modeling_tf_outputs.TFSequenceClassifierOutput or tuple(tf.Tensor)
Parameters
- pixel_values (
np.ndarray
,tf.Tensor
,List[tf.Tensor]
`Dict[str, tf.Tensor]
orDict[str, np.ndarray]
and each example must have the shape(batch_size, num_channels, height, width)
) — Pixel values. Pixel values can be obtained using AutoImageProcessor. See ConvNextImageProcessor.call() for details. - output_hidden_states (
bool
, optional) — Whether or not to return the hidden states of all layers. Seehidden_states
under returned tensors for more detail. This argument can be used only in eager mode, in graph mode the value in the config will be used instead. - return_dict (
bool
, optional) — Whether or not to return a ModelOutput instead of a plain tuple. This argument can be used in eager mode, in graph mode the value will always be set to True. - labels (
tf.Tensor
ornp.ndarray
of shape(batch_size,)
, optional) — Labels for computing the image classification/regression loss. Indices should be in[0, ..., config.num_labels - 1]
. Ifconfig.num_labels == 1
a regression loss is computed (Mean-Square loss), Ifconfig.num_labels > 1
a classification loss is computed (Cross-Entropy).
Returns
transformers.modeling_tf_outputs.TFSequenceClassifierOutput or tuple(tf.Tensor)
A transformers.modeling_tf_outputs.TFSequenceClassifierOutput or a tuple of tf.Tensor
(if
return_dict=False
is passed or when config.return_dict=False
) comprising various elements depending on the
configuration (ConvNextConfig) and inputs.
-
loss (
tf.Tensor
of shape(batch_size, )
, optional, returned whenlabels
is provided) — Classification (or regression if config.num_labels==1) loss. -
logits (
tf.Tensor
of shape(batch_size, config.num_labels)
) — Classification (or regression if config.num_labels==1) scores (before SoftMax). -
hidden_states (
tuple(tf.Tensor)
, optional, returned whenoutput_hidden_states=True
is passed or whenconfig.output_hidden_states=True
) — Tuple oftf.Tensor
(one for the output of the embeddings + one for the output of each layer) of shape(batch_size, sequence_length, hidden_size)
.Hidden-states of the model at the output of each layer plus the initial embedding outputs.
-
attentions (
tuple(tf.Tensor)
, optional, returned whenoutput_attentions=True
is passed or whenconfig.output_attentions=True
) — Tuple oftf.Tensor
(one for each layer) of shape(batch_size, num_heads, sequence_length, sequence_length)
.Attentions weights after the attention softmax, used to compute the weighted average in the self-attention heads.
The TFConvNextForImageClassification forward method, overrides the __call__
special method.
Although the recipe for forward pass needs to be defined within this function, one should call the Module
instance afterwards instead of this since the former takes care of running the pre and post processing steps while
the latter silently ignores them.
Examples:
>>> from transformers import AutoImageProcessor, TFConvNextForImageClassification
>>> import tensorflow as tf
>>> from PIL import Image
>>> import requests
>>> url = "http://images.cocodataset.org/val2017/000000039769.jpg"
>>> image = Image.open(requests.get(url, stream=True).raw)
>>> image_processor = AutoImageProcessor.from_pretrained("facebook/convnext-tiny-224")
>>> model = TFConvNextForImageClassification.from_pretrained("facebook/convnext-tiny-224")
>>> inputs = image_processor(images=image, return_tensors="tf")
>>> outputs = model(**inputs)
>>> logits = outputs.logits
>>> # model predicts one of the 1000 ImageNet classes
>>> predicted_class_idx = tf.math.argmax(logits, axis=-1)[0]
>>> print("Predicted class:", model.config.id2label[int(predicted_class_idx)])