DETA
Overview
The DETA model was proposed in NMS Strikes Back by Jeffrey Ouyang-Zhang, Jang Hyun Cho, Xingyi Zhou, Philipp KrΓ€henbΓΌhl. DETA (short for Detection Transformers with Assignment) improves Deformable DETR by replacing the one-to-one bipartite Hungarian matching loss with one-to-many label assignments used in traditional detectors with non-maximum suppression (NMS). This leads to significant gains of up to 2.5 mAP.
The abstract from the paper is the following:
Detection Transformer (DETR) directly transforms queries to unique objects by using one-to-one bipartite matching during training and enables end-to-end object detection. Recently, these models have surpassed traditional detectors on COCO with undeniable elegance. However, they differ from traditional detectors in multiple designs, including model architecture and training schedules, and thus the effectiveness of one-to-one matching is not fully understood. In this work, we conduct a strict comparison between the one-to-one Hungarian matching in DETRs and the one-to-many label assignments in traditional detectors with non-maximum supervision (NMS). Surprisingly, we observe one-to-many assignments with NMS consistently outperform standard one-to-one matching under the same setting, with a significant gain of up to 2.5 mAP. Our detector that trains Deformable-DETR with traditional IoU-based label assignment achieved 50.2 COCO mAP within 12 epochs (1x schedule) with ResNet50 backbone, outperforming all existing traditional or transformer-based detectors in this setting. On multiple datasets, schedules, and architectures, we consistently show bipartite matching is unnecessary for performant detection transformers. Furthermore, we attribute the success of detection transformers to their expressive transformer architecture.
Tips:
- One can use DetaImageProcessor to prepare images and optional targets for the model.
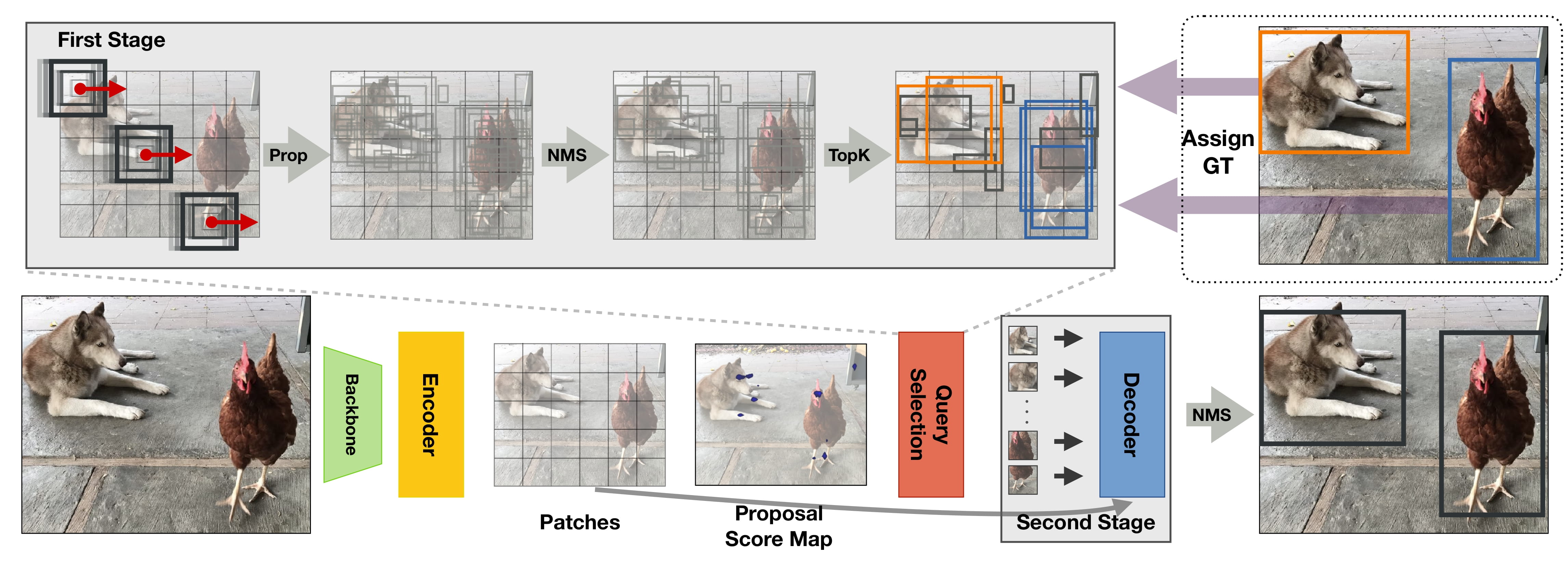
This model was contributed by nielsr. The original code can be found here.
Resources
A list of official Hugging Face and community (indicated by π) resources to help you get started with DETA.
- Demo notebooks for DETA can be found here.
- See also: Object detection task guide
If youβre interested in submitting a resource to be included here, please feel free to open a Pull Request and weβll review it! The resource should ideally demonstrate something new instead of duplicating an existing resource.
DetaConfig
class transformers.DetaConfig
< source >( backbone_config = None num_queries = 900 max_position_embeddings = 2048 encoder_layers = 6 encoder_ffn_dim = 2048 encoder_attention_heads = 8 decoder_layers = 6 decoder_ffn_dim = 1024 decoder_attention_heads = 8 encoder_layerdrop = 0.0 is_encoder_decoder = True activation_function = 'relu' d_model = 256 dropout = 0.1 attention_dropout = 0.0 activation_dropout = 0.0 init_std = 0.02 init_xavier_std = 1.0 return_intermediate = True auxiliary_loss = False position_embedding_type = 'sine' num_feature_levels = 5 encoder_n_points = 4 decoder_n_points = 4 two_stage = True two_stage_num_proposals = 300 with_box_refine = True assign_first_stage = True class_cost = 1 bbox_cost = 5 giou_cost = 2 mask_loss_coefficient = 1 dice_loss_coefficient = 1 bbox_loss_coefficient = 5 giou_loss_coefficient = 2 eos_coefficient = 0.1 focal_alpha = 0.25 **kwargs )
Parameters
-
backbone_config (
PretrainedConfig
ordict
, optional, defaults toResNetConfig()
) — The configuration of the backbone model. -
num_queries (
int
, optional, defaults to 900) — Number of object queries, i.e. detection slots. This is the maximal number of objects DetaModel can detect in a single image. In casetwo_stage
is set toTrue
, we usetwo_stage_num_proposals
instead. -
d_model (
int
, optional, defaults to 256) — Dimension of the layers. -
encoder_layers (
int
, optional, defaults to 6) — Number of encoder layers. -
decoder_layers (
int
, optional, defaults to 6) — Number of decoder layers. -
encoder_attention_heads (
int
, optional, defaults to 8) — Number of attention heads for each attention layer in the Transformer encoder. -
decoder_attention_heads (
int
, optional, defaults to 8) — Number of attention heads for each attention layer in the Transformer decoder. -
decoder_ffn_dim (
int
, optional, defaults to 2048) — Dimension of the “intermediate” (often named feed-forward) layer in decoder. -
encoder_ffn_dim (
int
, optional, defaults to 2048) — Dimension of the “intermediate” (often named feed-forward) layer in decoder. -
activation_function (
str
orfunction
, optional, defaults to"relu"
) — The non-linear activation function (function or string) in the encoder and pooler. If string,"gelu"
,"relu"
,"silu"
and"gelu_new"
are supported. -
dropout (
float
, optional, defaults to 0.1) — The dropout probability for all fully connected layers in the embeddings, encoder, and pooler. -
attention_dropout (
float
, optional, defaults to 0.0) — The dropout ratio for the attention probabilities. -
activation_dropout (
float
, optional, defaults to 0.0) — The dropout ratio for activations inside the fully connected layer. -
init_std (
float
, optional, defaults to 0.02) — The standard deviation of the truncated_normal_initializer for initializing all weight matrices. -
init_xavier_std (
float
, optional, defaults to 1) — The scaling factor used for the Xavier initialization gain in the HM Attention map module. -
encoder_layerdrop (
float
, optional, defaults to 0.0) — The LayerDrop probability for the encoder. See the [LayerDrop paper](see https://arxiv.org/abs/1909.11556) for more details. -
auxiliary_loss (
bool
, optional, defaults toFalse
) — Whether auxiliary decoding losses (loss at each decoder layer) are to be used. -
position_embedding_type (
str
, optional, defaults to"sine"
) — Type of position embeddings to be used on top of the image features. One of"sine"
or"learned"
. -
class_cost (
float
, optional, defaults to 1) — Relative weight of the classification error in the Hungarian matching cost. -
bbox_cost (
float
, optional, defaults to 5) — Relative weight of the L1 error of the bounding box coordinates in the Hungarian matching cost. -
giou_cost (
float
, optional, defaults to 2) — Relative weight of the generalized IoU loss of the bounding box in the Hungarian matching cost. -
mask_loss_coefficient (
float
, optional, defaults to 1) — Relative weight of the Focal loss in the panoptic segmentation loss. -
dice_loss_coefficient (
float
, optional, defaults to 1) — Relative weight of the DICE/F-1 loss in the panoptic segmentation loss. -
bbox_loss_coefficient (
float
, optional, defaults to 5) — Relative weight of the L1 bounding box loss in the object detection loss. -
giou_loss_coefficient (
float
, optional, defaults to 2) — Relative weight of the generalized IoU loss in the object detection loss. -
eos_coefficient (
float
, optional, defaults to 0.1) — Relative classification weight of the ‘no-object’ class in the object detection loss. -
num_feature_levels (
int
, optional, defaults to 5) — The number of input feature levels. -
encoder_n_points (
int
, optional, defaults to 4) — The number of sampled keys in each feature level for each attention head in the encoder. -
decoder_n_points (
int
, optional, defaults to 4) — The number of sampled keys in each feature level for each attention head in the decoder. -
two_stage (
bool
, optional, defaults toTrue
) — Whether to apply a two-stage deformable DETR, where the region proposals are also generated by a variant of DETA, which are further fed into the decoder for iterative bounding box refinement. -
two_stage_num_proposals (
int
, optional, defaults to 300) — The number of region proposals to be generated, in casetwo_stage
is set toTrue
. -
with_box_refine (
bool
, optional, defaults toTrue
) — Whether to apply iterative bounding box refinement, where each decoder layer refines the bounding boxes based on the predictions from the previous layer. -
focal_alpha (
float
, optional, defaults to 0.25) — Alpha parameter in the focal loss.
This is the configuration class to store the configuration of a DetaModel. It is used to instantiate a DETA model according to the specified arguments, defining the model architecture. Instantiating a configuration with the defaults will yield a similar configuration to that of the DETA SenseTime/deformable-detr architecture.
Configuration objects inherit from PretrainedConfig and can be used to control the model outputs. Read the documentation from PretrainedConfig for more information.
Examples:
>>> from transformers import DetaConfig, DetaModel
>>> # Initializing a DETA SenseTime/deformable-detr style configuration
>>> configuration = DetaConfig()
>>> # Initializing a model (with random weights) from the SenseTime/deformable-detr style configuration
>>> model = DetaModel(configuration)
>>> # Accessing the model configuration
>>> configuration = model.config
Serializes this instance to a Python dictionary. Override the default to_dict(). Returns:
Dict[str, any]
: Dictionary of all the attributes that make up this configuration instance,
DetaImageProcessor
class transformers.DetaImageProcessor
< source >( format: typing.Union[str, transformers.models.deta.image_processing_deta.AnnotionFormat] = <AnnotionFormat.COCO_DETECTION: 'coco_detection'> do_resize: bool = True size: typing.Dict[str, int] = None resample: Resampling = <Resampling.BILINEAR: 2> do_rescale: bool = True rescale_factor: typing.Union[int, float] = 0.00392156862745098 do_normalize: bool = True image_mean: typing.Union[float, typing.List[float]] = None image_std: typing.Union[float, typing.List[float]] = None do_pad: bool = True **kwargs )
Parameters
-
format (
str
, optional, defaults to"coco_detection"
) — Data format of the annotations. One of “coco_detection” or “coco_panoptic”. -
do_resize (
bool
, optional, defaults toTrue
) — Controls whether to resize the image’s (height, width) dimensions to the specifiedsize
. Can be overridden by thedo_resize
parameter in thepreprocess
method. -
size (
Dict[str, int]
optional, defaults to{"shortest_edge" -- 800, "longest_edge": 1333}
): Size of the image’s (height, width) dimensions after resizing. Can be overridden by thesize
parameter in thepreprocess
method. -
resample (
PILImageResampling
, optional, defaults toPILImageResampling.BILINEAR
) — Resampling filter to use if resizing the image. -
do_rescale (
bool
, optional, defaults toTrue
) — Controls whether to rescale the image by the specified scalerescale_factor
. Can be overridden by thedo_rescale
parameter in thepreprocess
method. -
rescale_factor (
int
orfloat
, optional, defaults to1/255
) — Scale factor to use if rescaling the image. Can be overridden by therescale_factor
parameter in thepreprocess
method. do_normalize — Controls whether to normalize the image. Can be overridden by thedo_normalize
parameter in thepreprocess
method. -
image_mean (
float
orList[float]
, optional, defaults toIMAGENET_DEFAULT_MEAN
) — Mean values to use when normalizing the image. Can be a single value or a list of values, one for each channel. Can be overridden by theimage_mean
parameter in thepreprocess
method. -
image_std (
float
orList[float]
, optional, defaults toIMAGENET_DEFAULT_STD
) — Standard deviation values to use when normalizing the image. Can be a single value or a list of values, one for each channel. Can be overridden by theimage_std
parameter in thepreprocess
method. -
do_pad (
bool
, optional, defaults toTrue
) — Controls whether to pad the image to the largest image in a batch and create a pixel mask. Can be overridden by thedo_pad
parameter in thepreprocess
method.
Constructs a Deformable DETR image processor.
preprocess
< source >( images: typing.Union[ForwardRef('PIL.Image.Image'), numpy.ndarray, ForwardRef('torch.Tensor'), typing.List[ForwardRef('PIL.Image.Image')], typing.List[numpy.ndarray], typing.List[ForwardRef('torch.Tensor')]] annotations: typing.Union[typing.List[typing.Dict], typing.List[typing.List[typing.Dict]], NoneType] = None return_segmentation_masks: bool = None masks_path: typing.Union[str, pathlib.Path, NoneType] = None do_resize: typing.Optional[bool] = None size: typing.Union[typing.Dict[str, int], NoneType] = None resample = None do_rescale: typing.Optional[bool] = None rescale_factor: typing.Union[int, float, NoneType] = None do_normalize: typing.Optional[bool] = None image_mean: typing.Union[float, typing.List[float], NoneType] = None image_std: typing.Union[float, typing.List[float], NoneType] = None do_pad: typing.Optional[bool] = None format: typing.Union[str, transformers.models.deta.image_processing_deta.AnnotionFormat, NoneType] = None return_tensors: typing.Union[str, transformers.utils.generic.TensorType, NoneType] = None data_format: typing.Union[str, transformers.image_utils.ChannelDimension] = <ChannelDimension.FIRST: 'channels_first'> **kwargs )
Parameters
-
images (
ImageInput
) — Image or batch of images to preprocess. -
annotations (
List[Dict]
orList[List[Dict]]
, optional) — List of annotations associated with the image or batch of images. If annotionation is for object detection, the annotations should be a dictionary with the following keys:- “image_id” (
int
): The image id. - “annotations” (
List[Dict]
): List of annotations for an image. Each annotation should be a dictionary. An image can have no annotations, in which case the list should be empty. If annotionation is for segmentation, the annotations should be a dictionary with the following keys: - “image_id” (
int
): The image id. - “segments_info” (
List[Dict]
): List of segments for an image. Each segment should be a dictionary. An image can have no segments, in which case the list should be empty. - “file_name” (
str
): The file name of the image.
- “image_id” (
-
return_segmentation_masks (
bool
, optional, defaults to self.return_segmentation_masks) — Whether to return segmentation masks. -
masks_path (
str
orpathlib.Path
, optional) — Path to the directory containing the segmentation masks. -
do_resize (
bool
, optional, defaults to self.do_resize) — Whether to resize the image. -
size (
Dict[str, int]
, optional, defaults to self.size) — Size of the image after resizing. -
resample (
PILImageResampling
, optional, defaults to self.resample) — Resampling filter to use when resizing the image. -
do_rescale (
bool
, optional, defaults to self.do_rescale) — Whether to rescale the image. -
rescale_factor (
float
, optional, defaults to self.rescale_factor) — Rescale factor to use when rescaling the image. -
do_normalize (
bool
, optional, defaults to self.do_normalize) — Whether to normalize the image. -
image_mean (
float
orList[float]
, optional, defaults to self.image_mean) — Mean to use when normalizing the image. -
image_std (
float
orList[float]
, optional, defaults to self.image_std) — Standard deviation to use when normalizing the image. -
do_pad (
bool
, optional, defaults to self.do_pad) — Whether to pad the image. -
format (
str
orAnnotionFormat
, optional, defaults to self.format) — Format of the annotations. -
return_tensors (
str
orTensorType
, optional, defaults to self.return_tensors) — Type of tensors to return. IfNone
, will return the list of images. -
data_format (
str
orChannelDimension
, optional, defaults to self.data_format) — The channel dimension format of the image. If not provided, it will be the same as the input image.
Preprocess an image or a batch of images so that it can be used by the model.
post_process_object_detection
< source >(
outputs
threshold: float = 0.5
target_sizes: typing.Union[transformers.utils.generic.TensorType, typing.List[typing.Tuple]] = None
nms_threshold: float = 0.7
)
β
List[Dict]
Parameters
-
outputs (
DetrObjectDetectionOutput
) — Raw outputs of the model. -
threshold (
float
, optional, defaults to 0.5) — Score threshold to keep object detection predictions. -
target_sizes (
torch.Tensor
orList[Tuple[int, int]]
, optional) — Tensor of shape(batch_size, 2)
or list of tuples (Tuple[int, int]
) containing the target size (height, width) of each image in the batch. If left to None, predictions will not be resized. -
nms_threshold (
float
, optional, defaults to 0.7) — NMS threshold.
Returns
List[Dict]
A list of dictionaries, each dictionary containing the scores, labels and boxes for an image in the batch as predicted by the model.
Converts the output of DetaForObjectDetection into final bounding boxes in (top_left_x, top_left_y, bottom_right_x, bottom_right_y) format. Only supports PyTorch.
DetaModel
class transformers.DetaModel
< source >( config: DetaConfig )
Parameters
- config (DetaConfig) — Model configuration class with all the parameters of the model. Initializing with a config file does not load the weights associated with the model, only the configuration. Check out the from_pretrained() method to load the model weights.
The bare DETA Model (consisting of a backbone and encoder-decoder Transformer) outputting raw hidden-states without any specific head on top.
This model inherits from PreTrainedModel. Check the superclass documentation for the generic methods the library implements for all its model (such as downloading or saving, resizing the input embeddings, pruning heads etc.)
This model is also a PyTorch torch.nn.Module subclass. Use it as a regular PyTorch Module and refer to the PyTorch documentation for all matter related to general usage and behavior.
forward
< source >(
pixel_values
pixel_mask = None
decoder_attention_mask = None
encoder_outputs = None
inputs_embeds = None
decoder_inputs_embeds = None
output_attentions = None
output_hidden_states = None
return_dict = None
)
β
transformers.models.deta.modeling_deta.DetaModelOutput
or tuple(torch.FloatTensor)
Parameters
-
pixel_values (
torch.FloatTensor
of shape(batch_size, num_channels, height, width)
) — Pixel values. Padding will be ignored by default should you provide it.Pixel values can be obtained using AutoImageProcessor. See
AutoImageProcessor.__call__()
for details. -
pixel_mask (
torch.LongTensor
of shape(batch_size, height, width)
, optional) — Mask to avoid performing attention on padding pixel values. Mask values selected in[0, 1]
:- 1 for pixels that are real (i.e. not masked),
- 0 for pixels that are padding (i.e. masked).
-
decoder_attention_mask (
torch.LongTensor
of shape(batch_size, num_queries)
, optional) — Not used by default. Can be used to mask object queries. -
encoder_outputs (
tuple(tuple(torch.FloatTensor)
, optional) — Tuple consists of (last_hidden_state
, optional:hidden_states
, optional:attentions
)last_hidden_state
of shape(batch_size, sequence_length, hidden_size)
, optional) is a sequence of hidden-states at the output of the last layer of the encoder. Used in the cross-attention of the decoder. -
inputs_embeds (
torch.FloatTensor
of shape(batch_size, sequence_length, hidden_size)
, optional) — Optionally, instead of passing the flattened feature map (output of the backbone + projection layer), you can choose to directly pass a flattened representation of an image. -
decoder_inputs_embeds (
torch.FloatTensor
of shape(batch_size, num_queries, hidden_size)
, optional) — Optionally, instead of initializing the queries with a tensor of zeros, you can choose to directly pass an embedded representation. -
output_attentions (
bool
, optional) — Whether or not to return the attentions tensors of all attention layers. Seeattentions
under returned tensors for more detail. - output_hidden_states (
bool
, optional) — Whether or not to return the hidden states of all layers. Seehidden_states
under returned tensors for more detail. -
return_dict (
bool
, optional) — Whether or not to return a ModelOutput instead of a plain tuple.
Returns
transformers.models.deta.modeling_deta.DetaModelOutput
or tuple(torch.FloatTensor)
A transformers.models.deta.modeling_deta.DetaModelOutput
or a tuple of
torch.FloatTensor
(if return_dict=False
is passed or when config.return_dict=False
) comprising various
elements depending on the configuration (DetaConfig) and inputs.
- init_reference_points (
torch.FloatTensor
of shape(batch_size, num_queries, 4)
) β Initial reference points sent through the Transformer decoder. - last_hidden_state (
torch.FloatTensor
of shape(batch_size, num_queries, hidden_size)
) β Sequence of hidden-states at the output of the last layer of the decoder of the model. - intermediate_hidden_states (
torch.FloatTensor
of shape(batch_size, config.decoder_layers, num_queries, hidden_size)
) β Stacked intermediate hidden states (output of each layer of the decoder). - intermediate_reference_points (
torch.FloatTensor
of shape(batch_size, config.decoder_layers, num_queries, 4)
) β Stacked intermediate reference points (reference points of each layer of the decoder). - decoder_hidden_states (
tuple(torch.FloatTensor)
, optional, returned whenoutput_hidden_states=True
is passed or whenconfig.output_hidden_states=True
) β Tuple oftorch.FloatTensor
(one for the output of the embeddings + one for the output of each layer) of shape(batch_size, num_queries, hidden_size)
. Hidden-states of the decoder at the output of each layer plus the initial embedding outputs. - decoder_attentions (
tuple(torch.FloatTensor)
, optional, returned whenoutput_attentions=True
is passed or whenconfig.output_attentions=True
) β Tuple oftorch.FloatTensor
(one for each layer) of shape(batch_size, num_heads, num_queries, num_queries)
. Attentions weights of the decoder, after the attention softmax, used to compute the weighted average in the self-attention heads. - cross_attentions (
tuple(torch.FloatTensor)
, optional, returned whenoutput_attentions=True
is passed or whenconfig.output_attentions=True
) β Tuple oftorch.FloatTensor
(one for each layer) of shape(batch_size, num_queries, num_heads, 4, 4)
. Attentions weights of the decoderβs cross-attention layer, after the attention softmax, used to compute the weighted average in the cross-attention heads. - encoder_last_hidden_state (
torch.FloatTensor
of shape(batch_size, sequence_length, hidden_size)
, optional) β Sequence of hidden-states at the output of the last layer of the encoder of the model. - encoder_hidden_states (
tuple(torch.FloatTensor)
, optional, returned whenoutput_hidden_states=True
is passed or whenconfig.output_hidden_states=True
) β Tuple oftorch.FloatTensor
(one for the output of the embeddings + one for the output of each layer) of shape(batch_size, sequence_length, hidden_size)
. Hidden-states of the encoder at the output of each layer plus the initial embedding outputs. - encoder_attentions (
tuple(torch.FloatTensor)
, optional, returned whenoutput_attentions=True
is passed or whenconfig.output_attentions=True
) β Tuple oftorch.FloatTensor
(one for each layer) of shape(batch_size, num_queries, num_heads, 4, 4)
. Attentions weights of the encoder, after the attention softmax, used to compute the weighted average in the self-attention heads. - enc_outputs_class (
torch.FloatTensor
of shape(batch_size, sequence_length, config.num_labels)
, optional, returned whenconfig.with_box_refine=True
andconfig.two_stage=True
) β Predicted bounding boxes scores where the topconfig.two_stage_num_proposals
scoring bounding boxes are picked as region proposals in the first stage. Output of bounding box binary classification (i.e. foreground and background). - enc_outputs_coord_logits (
torch.FloatTensor
of shape(batch_size, sequence_length, 4)
, optional, returned whenconfig.with_box_refine=True
andconfig.two_stage=True
) β Logits of predicted bounding boxes coordinates in the first stage.
The DetaModel forward method, overrides the __call__
special method.
Although the recipe for forward pass needs to be defined within this function, one should call the Module
instance afterwards instead of this since the former takes care of running the pre and post processing steps while
the latter silently ignores them.
Examples:
>>> from transformers import AutoImageProcessor, DetaModel
>>> from PIL import Image
>>> import requests
>>> url = "http://images.cocodataset.org/val2017/000000039769.jpg"
>>> image = Image.open(requests.get(url, stream=True).raw)
>>> image_processor = AutoImageProcessor.from_pretrained("jozhang97/deta-swin-large-o365")
>>> model = DetaModel.from_pretrained("jozhang97/deta-swin-large-o365", two_stage=False)
>>> inputs = image_processor(images=image, return_tensors="pt")
>>> outputs = model(**inputs)
>>> last_hidden_states = outputs.last_hidden_state
>>> list(last_hidden_states.shape)
[1, 900, 256]
DetaForObjectDetection
class transformers.DetaForObjectDetection
< source >( config: DetaConfig )
Parameters
- config (DetaConfig) — Model configuration class with all the parameters of the model. Initializing with a config file does not load the weights associated with the model, only the configuration. Check out the from_pretrained() method to load the model weights.
DETA Model (consisting of a backbone and encoder-decoder Transformer) with object detection heads on top, for tasks such as COCO detection.
This model inherits from PreTrainedModel. Check the superclass documentation for the generic methods the library implements for all its model (such as downloading or saving, resizing the input embeddings, pruning heads etc.)
This model is also a PyTorch torch.nn.Module subclass. Use it as a regular PyTorch Module and refer to the PyTorch documentation for all matter related to general usage and behavior.
forward
< source >(
pixel_values
pixel_mask = None
decoder_attention_mask = None
encoder_outputs = None
inputs_embeds = None
decoder_inputs_embeds = None
labels = None
output_attentions = None
output_hidden_states = None
return_dict = None
)
β
transformers.models.deta.modeling_deta.DetaObjectDetectionOutput
or tuple(torch.FloatTensor)
Parameters
-
pixel_values (
torch.FloatTensor
of shape(batch_size, num_channels, height, width)
) — Pixel values. Padding will be ignored by default should you provide it.Pixel values can be obtained using AutoImageProcessor. See
AutoImageProcessor.__call__()
for details. -
pixel_mask (
torch.LongTensor
of shape(batch_size, height, width)
, optional) — Mask to avoid performing attention on padding pixel values. Mask values selected in[0, 1]
:- 1 for pixels that are real (i.e. not masked),
- 0 for pixels that are padding (i.e. masked).
-
decoder_attention_mask (
torch.LongTensor
of shape(batch_size, num_queries)
, optional) — Not used by default. Can be used to mask object queries. -
encoder_outputs (
tuple(tuple(torch.FloatTensor)
, optional) — Tuple consists of (last_hidden_state
, optional:hidden_states
, optional:attentions
)last_hidden_state
of shape(batch_size, sequence_length, hidden_size)
, optional) is a sequence of hidden-states at the output of the last layer of the encoder. Used in the cross-attention of the decoder. -
inputs_embeds (
torch.FloatTensor
of shape(batch_size, sequence_length, hidden_size)
, optional) — Optionally, instead of passing the flattened feature map (output of the backbone + projection layer), you can choose to directly pass a flattened representation of an image. -
decoder_inputs_embeds (
torch.FloatTensor
of shape(batch_size, num_queries, hidden_size)
, optional) — Optionally, instead of initializing the queries with a tensor of zeros, you can choose to directly pass an embedded representation. -
output_attentions (
bool
, optional) — Whether or not to return the attentions tensors of all attention layers. Seeattentions
under returned tensors for more detail. - output_hidden_states (
bool
, optional) — Whether or not to return the hidden states of all layers. Seehidden_states
under returned tensors for more detail. -
return_dict (
bool
, optional) — Whether or not to return a ModelOutput instead of a plain tuple. -
labels (
List[Dict]
of len(batch_size,)
, optional) — Labels for computing the bipartite matching loss. List of dicts, each dictionary containing at least the following 2 keys: ‘class_labels’ and ‘boxes’ (the class labels and bounding boxes of an image in the batch respectively). The class labels themselves should be atorch.LongTensor
of len(number of bounding boxes in the image,)
and the boxes atorch.FloatTensor
of shape(number of bounding boxes in the image, 4)
.
Returns
transformers.models.deta.modeling_deta.DetaObjectDetectionOutput
or tuple(torch.FloatTensor)
A transformers.models.deta.modeling_deta.DetaObjectDetectionOutput
or a tuple of
torch.FloatTensor
(if return_dict=False
is passed or when config.return_dict=False
) comprising various
elements depending on the configuration (DetaConfig) and inputs.
- loss (
torch.FloatTensor
of shape(1,)
, optional, returned whenlabels
are provided)) β Total loss as a linear combination of a negative log-likehood (cross-entropy) for class prediction and a bounding box loss. The latter is defined as a linear combination of the L1 loss and the generalized scale-invariant IoU loss. - loss_dict (
Dict
, optional) β A dictionary containing the individual losses. Useful for logging. - logits (
torch.FloatTensor
of shape(batch_size, num_queries, num_classes + 1)
) β Classification logits (including no-object) for all queries. - pred_boxes (
torch.FloatTensor
of shape(batch_size, num_queries, 4)
) β Normalized boxes coordinates for all queries, represented as (center_x, center_y, width, height). These values are normalized in [0, 1], relative to the size of each individual image in the batch (disregarding possible padding). You can use~DetaProcessor.post_process_object_detection
to retrieve the unnormalized bounding boxes. - auxiliary_outputs (
list[Dict]
, optional) β Optional, only returned when auxilary losses are activated (i.e.config.auxiliary_loss
is set toTrue
) and labels are provided. It is a list of dictionaries containing the two above keys (logits
andpred_boxes
) for each decoder layer. - last_hidden_state (
torch.FloatTensor
of shape(batch_size, num_queries, hidden_size)
, optional) β Sequence of hidden-states at the output of the last layer of the decoder of the model. - decoder_hidden_states (
tuple(torch.FloatTensor)
, optional, returned whenoutput_hidden_states=True
is passed or whenconfig.output_hidden_states=True
) β Tuple oftorch.FloatTensor
(one for the output of the embeddings + one for the output of each layer) of shape(batch_size, num_queries, hidden_size)
. Hidden-states of the decoder at the output of each layer plus the initial embedding outputs. - decoder_attentions (
tuple(torch.FloatTensor)
, optional, returned whenoutput_attentions=True
is passed or whenconfig.output_attentions=True
) β Tuple oftorch.FloatTensor
(one for each layer) of shape(batch_size, num_heads, num_queries, num_queries)
. Attentions weights of the decoder, after the attention softmax, used to compute the weighted average in the self-attention heads. - cross_attentions (
tuple(torch.FloatTensor)
, optional, returned whenoutput_attentions=True
is passed or whenconfig.output_attentions=True
) β Tuple oftorch.FloatTensor
(one for each layer) of shape(batch_size, num_queries, num_heads, 4, 4)
. Attentions weights of the decoderβs cross-attention layer, after the attention softmax, used to compute the weighted average in the cross-attention heads. - encoder_last_hidden_state (
torch.FloatTensor
of shape(batch_size, sequence_length, hidden_size)
, optional) β Sequence of hidden-states at the output of the last layer of the encoder of the model. - encoder_hidden_states (
tuple(torch.FloatTensor)
, optional, returned whenoutput_hidden_states=True
is passed or whenconfig.output_hidden_states=True
) β Tuple oftorch.FloatTensor
(one for the output of the embeddings + one for the output of each layer) of shape(batch_size, sequence_length, hidden_size)
. Hidden-states of the encoder at the output of each layer plus the initial embedding outputs. - encoder_attentions (
tuple(torch.FloatTensor)
, optional, returned whenoutput_attentions=True
is passed or whenconfig.output_attentions=True
) β Tuple oftorch.FloatTensor
(one for each layer) of shape(batch_size, sequence_length, num_heads, 4, 4)
. Attentions weights of the encoder, after the attention softmax, used to compute the weighted average in the self-attention heads. - intermediate_hidden_states (
torch.FloatTensor
of shape(batch_size, config.decoder_layers, num_queries, hidden_size)
) β Stacked intermediate hidden states (output of each layer of the decoder). - intermediate_reference_points (
torch.FloatTensor
of shape(batch_size, config.decoder_layers, num_queries, 4)
) β Stacked intermediate reference points (reference points of each layer of the decoder). - init_reference_points (
torch.FloatTensor
of shape(batch_size, num_queries, 4)
) β Initial reference points sent through the Transformer decoder. - enc_outputs_class (
torch.FloatTensor
of shape(batch_size, sequence_length, config.num_labels)
, optional, returned whenconfig.with_box_refine=True
andconfig.two_stage=True
) β Predicted bounding boxes scores where the topconfig.two_stage_num_proposals
scoring bounding boxes are picked as region proposals in the first stage. Output of bounding box binary classification (i.e. foreground and background). - enc_outputs_coord_logits (
torch.FloatTensor
of shape(batch_size, sequence_length, 4)
, optional, returned whenconfig.with_box_refine=True
andconfig.two_stage=True
) β Logits of predicted bounding boxes coordinates in the first stage.
The DetaForObjectDetection forward method, overrides the __call__
special method.
Although the recipe for forward pass needs to be defined within this function, one should call the Module
instance afterwards instead of this since the former takes care of running the pre and post processing steps while
the latter silently ignores them.
Examples:
>>> from transformers import AutoImageProcessor, DetaForObjectDetection
>>> from PIL import Image
>>> import requests
>>> url = "http://images.cocodataset.org/val2017/000000039769.jpg"
>>> image = Image.open(requests.get(url, stream=True).raw)
>>> image_processor = AutoImageProcessor.from_pretrained("jozhang97/deta-swin-large")
>>> model = DetaForObjectDetection.from_pretrained("jozhang97/deta-swin-large")
>>> inputs = image_processor(images=image, return_tensors="pt")
>>> outputs = model(**inputs)
>>> # convert outputs (bounding boxes and class logits) to COCO API
>>> target_sizes = torch.tensor([image.size[::-1]])
>>> results = image_processor.post_process_object_detection(outputs, threshold=0.5, target_sizes=target_sizes)[
... 0
... ]
>>> for score, label, box in zip(results["scores"], results["labels"], results["boxes"]):
... box = [round(i, 2) for i in box.tolist()]
... print(
... f"Detected {model.config.id2label[label.item()]} with confidence "
... f"{round(score.item(), 3)} at location {box}"
... )
Detected cat with confidence 0.683 at location [345.85, 23.68, 639.86, 372.83]
Detected cat with confidence 0.683 at location [8.8, 52.49, 316.93, 473.45]
Detected remote with confidence 0.568 at location [40.02, 73.75, 175.96, 117.33]
Detected remote with confidence 0.546 at location [333.68, 77.13, 370.12, 187.51]