이미지 분류
이미지 분류는 이미지에 레이블 또는 클래스를 할당합니다. 텍스트 또는 오디오 분류와 달리 입력은 이미지를 구성하는 픽셀 값입니다. 이미지 분류에는 자연재해 후 피해 감지, 농작물 건강 모니터링, 의료 이미지에서 질병의 징후 검사 지원 등 다양한 응용 사례가 있습니다.
이 가이드에서는 다음을 설명합니다:
BEiT, BiT, ConvNeXT, ConvNeXTV2, CvT, Data2VecVision, DeiT, DiNAT, EfficientFormer, EfficientNet, FocalNet, ImageGPT, LeViT, MobileNetV1, MobileNetV2, MobileViT, NAT, Perceiver, PoolFormer, RegNet, ResNet, SegFormer, Swin Transformer, Swin Transformer V2, VAN, ViT, ViT Hybrid, ViTMSN
시작하기 전에, 필요한 모든 라이브러리가 설치되어 있는지 확인하세요:
pip install transformers datasets evaluate
Hugging Face 계정에 로그인하여 모델을 업로드하고 커뮤니티에 공유하는 것을 권장합니다. 메시지가 표시되면, 토큰을 입력하여 로그인하세요:
>>> from huggingface_hub import notebook_login
>>> notebook_login()
Food-101 데이터 세트 가져오기
🤗 Datasets 라이브러리에서 Food-101 데이터 세트의 더 작은 부분 집합을 가져오는 것으로 시작합니다. 이렇게 하면 전체 데이터 세트에 대한 훈련에 많은 시간을 할애하기 전에 실험을 통해 모든 것이 제대로 작동하는지 확인할 수 있습니다.
>>> from datasets import load_dataset
>>> food = load_dataset("food101", split="train[:5000]")
데이터 세트의 train
을 train_test_split
메소드를 사용하여 훈련 및 테스트 세트로 분할하세요:
>>> food = food.train_test_split(test_size=0.2)
그리고 예시를 살펴보세요:
>>> food["train"][0]
{'image': <PIL.JpegImagePlugin.JpegImageFile image mode=RGB size=512x512 at 0x7F52AFC8AC50>,
'label': 79}
데이터 세트의 각 예제에는 두 개의 필드가 있습니다:
image
: 식품 항목의 PIL 이미지label
: 식품 항목의 레이블 클래스
모델이 레이블 ID에서 레이블 이름을 쉽게 가져올 수 있도록 레이블 이름을 정수로 매핑하고, 정수를 레이블 이름으로 매핑하는 사전을 만드세요:
>>> labels = food["train"].features["label"].names
>>> label2id, id2label = dict(), dict()
>>> for i, label in enumerate(labels):
... label2id[label] = str(i)
... id2label[str(i)] = label
이제 레이블 ID를 레이블 이름으로 변환할 수 있습니다:
>>> id2label[str(79)]
'prime_rib'
전처리
다음 단계는 이미지를 텐서로 처리하기 위해 ViT 이미지 프로세서를 가져오는 것입니다:
>>> from transformers import AutoImageProcessor
>>> checkpoint = "google/vit-base-patch16-224-in21k"
>>> image_processor = AutoImageProcessor.from_pretrained(checkpoint)
이미지에 몇 가지 이미지 변환을 적용하여 과적합에 대해 모델을 더 견고하게 만듭니다. 여기서 Torchvision의 transforms
모듈을 사용하지만, 원하는 이미지 라이브러리를 사용할 수도 있습니다.
이미지의 임의 부분을 크롭하고 크기를 조정한 다음, 이미지 평균과 표준 편차로 정규화하세요:
>>> from torchvision.transforms import RandomResizedCrop, Compose, Normalize, ToTensor
>>> normalize = Normalize(mean=image_processor.image_mean, std=image_processor.image_std)
>>> size = (
... image_processor.size["shortest_edge"]
... if "shortest_edge" in image_processor.size
... else (image_processor.size["height"], image_processor.size["width"])
... )
>>> _transforms = Compose([RandomResizedCrop(size), ToTensor(), normalize])
그런 다음 전처리 함수를 만들어 변환을 적용하고 이미지의 pixel_values
(모델에 대한 입력)를 반환하세요:
>>> def transforms(examples):
... examples["pixel_values"] = [_transforms(img.convert("RGB")) for img in examples["image"]]
... del examples["image"]
... return examples
전체 데이터 세트에 전처리 기능을 적용하려면 🤗 Datasets with_transform
을 사용합니다. 데이터 세트의 요소를 가져올 때 변환이 즉시 적용됩니다:
>>> food = food.with_transform(transforms)
이제 DefaultDataCollator
를 사용하여 예제 배치를 만듭니다. 🤗 Transformers의 다른 데이터 콜레이터와 달리, DefaultDataCollator
는 패딩과 같은 추가적인 전처리를 적용하지 않습니다.
>>> from transformers import DefaultDataCollator
>>> data_collator = DefaultDataCollator()
과적합을 방지하고 모델을 보다 견고하게 만들기 위해 데이터 세트의 훈련 부분에 데이터 증강을 추가합니다.
여기서 Keras 전처리 레이어로 훈련 데이터에 대한 변환(데이터 증강 포함)과
검증 데이터에 대한 변환(중앙 크로핑, 크기 조정, 정규화만)을 정의합니다.
tf.image
또는 다른 원하는 라이브러리를 사용할 수 있습니다.
>>> from tensorflow import keras
>>> from tensorflow.keras import layers
>>> size = (image_processor.size["height"], image_processor.size["width"])
>>> train_data_augmentation = keras.Sequential(
... [
... layers.RandomCrop(size[0], size[1]),
... layers.Rescaling(scale=1.0 / 127.5, offset=-1),
... layers.RandomFlip("horizontal"),
... layers.RandomRotation(factor=0.02),
... layers.RandomZoom(height_factor=0.2, width_factor=0.2),
... ],
... name="train_data_augmentation",
... )
>>> val_data_augmentation = keras.Sequential(
... [
... layers.CenterCrop(size[0], size[1]),
... layers.Rescaling(scale=1.0 / 127.5, offset=-1),
... ],
... name="val_data_augmentation",
... )
다음으로 한 번에 하나의 이미지가 아니라 이미지 배치에 적절한 변환을 적용하는 함수를 만듭니다.
>>> import numpy as np
>>> import tensorflow as tf
>>> from PIL import Image
>>> def convert_to_tf_tensor(image: Image):
... np_image = np.array(image)
... tf_image = tf.convert_to_tensor(np_image)
... # `expand_dims()` is used to add a batch dimension since
... # the TF augmentation layers operates on batched inputs.
... return tf.expand_dims(tf_image, 0)
>>> def preprocess_train(example_batch):
... """Apply train_transforms across a batch."""
... images = [
... train_data_augmentation(convert_to_tf_tensor(image.convert("RGB"))) for image in example_batch["image"]
... ]
... example_batch["pixel_values"] = [tf.transpose(tf.squeeze(image)) for image in images]
... return example_batch
... def preprocess_val(example_batch):
... """Apply val_transforms across a batch."""
... images = [
... val_data_augmentation(convert_to_tf_tensor(image.convert("RGB"))) for image in example_batch["image"]
... ]
... example_batch["pixel_values"] = [tf.transpose(tf.squeeze(image)) for image in images]
... return example_batch
🤗 Datasets set_transform
를 사용하여 즉시 변환을 적용하세요:
food["train"].set_transform(preprocess_train)
food["test"].set_transform(preprocess_val)
최종 전처리 단계로 DefaultDataCollator
를 사용하여 예제 배치를 만듭니다. 🤗 Transformers의 다른 데이터 콜레이터와 달리
DefaultDataCollator
는 패딩과 같은 추가 전처리를 적용하지 않습니다.
>>> from transformers import DefaultDataCollator
>>> data_collator = DefaultDataCollator(return_tensors="tf")
평가
훈련 중에 평가 지표를 포함하면 모델의 성능을 평가하는 데 도움이 되는 경우가 많습니다. 🤗 Evaluate 라이브러리로 평가 방법을 빠르게 가져올 수 있습니다. 이 작업에서는 accuracy 평가 지표를 가져옵니다. (🤗 Evaluate 빠른 둘러보기를 참조하여 평가 지표를 가져오고 계산하는 방법에 대해 자세히 알아보세요):
>>> import evaluate
>>> accuracy = evaluate.load("accuracy")
그런 다음 예측과 레이블을 compute
에 전달하여 정확도를 계산하는 함수를 만듭니다:
>>> import numpy as np
>>> def compute_metrics(eval_pred):
... predictions, labels = eval_pred
... predictions = np.argmax(predictions, axis=1)
... return accuracy.compute(predictions=predictions, references=labels)
이제 compute_metrics
함수를 사용할 준비가 되었으며, 훈련을 설정하면 이 함수로 되돌아올 것입니다.
훈련
Trainer
를 사용하여 모델을 미세 조정하는 방법에 익숙하지 않은 경우, 여기에서 기본 튜토리얼을 확인하세요!
이제 모델을 훈련시킬 준비가 되었습니다! AutoModelForImageClassification
로 ViT를 가져옵니다. 예상되는 레이블 수, 레이블 매핑 및 레이블 수를 지정하세요:
>>> from transformers import AutoModelForImageClassification, TrainingArguments, Trainer
>>> model = AutoModelForImageClassification.from_pretrained(
... checkpoint,
... num_labels=len(labels),
... id2label=id2label,
... label2id=label2id,
... )
이제 세 단계만 거치면 끝입니다:
TrainingArguments
에서 훈련 하이퍼파라미터를 정의하세요.image
열이 삭제되기 때문에 미사용 열을 제거하지 않는 것이 중요합니다.image
열이 없으면pixel_values
을 생성할 수 없습니다. 이 동작을 방지하려면remove_unused_columns=False
로 설정하세요! 다른 유일한 필수 매개변수는 모델 저장 위치를 지정하는output_dir
입니다.push_to_hub=True
로 설정하면 이 모델을 허브에 푸시합니다(모델을 업로드하려면 Hugging Face에 로그인해야 합니다). 각 에폭이 끝날 때마다,Trainer
가 정확도를 평가하고 훈련 체크포인트를 저장합니다.Trainer
에 모델, 데이터 세트, 토크나이저, 데이터 콜레이터 및compute_metrics
함수와 함께 훈련 인수를 전달하세요.train()
을 호출하여 모델을 미세 조정하세요.
>>> training_args = TrainingArguments(
... output_dir="my_awesome_food_model",
... remove_unused_columns=False,
... evaluation_strategy="epoch",
... save_strategy="epoch",
... learning_rate=5e-5,
... per_device_train_batch_size=16,
... gradient_accumulation_steps=4,
... per_device_eval_batch_size=16,
... num_train_epochs=3,
... warmup_ratio=0.1,
... logging_steps=10,
... load_best_model_at_end=True,
... metric_for_best_model="accuracy",
... push_to_hub=True,
... )
>>> trainer = Trainer(
... model=model,
... args=training_args,
... data_collator=data_collator,
... train_dataset=food["train"],
... eval_dataset=food["test"],
... tokenizer=image_processor,
... compute_metrics=compute_metrics,
... )
>>> trainer.train()
훈련이 완료되면, 모든 사람이 모델을 사용할 수 있도록 push_to_hub()
메소드로 모델을 허브에 공유하세요:
>>> trainer.push_to_hub()
Keras를 사용하여 모델을 미세 조정하는 방법에 익숙하지 않은 경우, 먼저 기본 튜토리얼을 확인하세요!
TensorFlow에서 모델을 미세 조정하려면 다음 단계를 따르세요:
- 훈련 하이퍼파라미터를 정의하고 옵티마이저와 학습률 스케쥴을 설정합니다.
- 사전 훈련된 모델을 인스턴스화합니다.
- 🤗 Dataset을
tf.data.Dataset
으로 변환합니다. - 모델을 컴파일합니다.
- 콜백을 추가하고 훈련을 수행하기 위해
fit()
메소드를 사용합니다. - 커뮤니티와 공유하기 위해 모델을 🤗 Hub에 업로드합니다.
하이퍼파라미터, 옵티마이저 및 학습률 스케쥴을 정의하는 것으로 시작합니다:
>>> from transformers import create_optimizer
>>> batch_size = 16
>>> num_epochs = 5
>>> num_train_steps = len(food["train"]) * num_epochs
>>> learning_rate = 3e-5
>>> weight_decay_rate = 0.01
>>> optimizer, lr_schedule = create_optimizer(
... init_lr=learning_rate,
... num_train_steps=num_train_steps,
... weight_decay_rate=weight_decay_rate,
... num_warmup_steps=0,
... )
그런 다음 레이블 매핑과 함께 TFAuto ModelForImageClassification
으로 ViT를 가져옵니다:
>>> from transformers import TFAutoModelForImageClassification
>>> model = TFAutoModelForImageClassification.from_pretrained(
... checkpoint,
... id2label=id2label,
... label2id=label2id,
... )
데이터 세트를 to_tf_dataset
와 data_collator
를 사용하여 tf.data.Dataset
형식으로 변환하세요:
>>> # converting our train dataset to tf.data.Dataset
>>> tf_train_dataset = food["train"].to_tf_dataset(
... columns="pixel_values", label_cols="label", shuffle=True, batch_size=batch_size, collate_fn=data_collator
... )
>>> # converting our test dataset to tf.data.Dataset
>>> tf_eval_dataset = food["test"].to_tf_dataset(
... columns="pixel_values", label_cols="label", shuffle=True, batch_size=batch_size, collate_fn=data_collator
... )
compile()
를 사용하여 훈련 모델을 구성하세요:
>>> from tensorflow.keras.losses import SparseCategoricalCrossentropy
>>> loss = tf.keras.losses.SparseCategoricalCrossentropy(from_logits=True)
>>> model.compile(optimizer=optimizer, loss=loss)
예측에서 정확도를 계산하고 모델을 🤗 Hub로 푸시하려면 Keras callbacks를 사용하세요.
compute_metrics
함수를 KerasMetricCallback에 전달하고,
PushToHubCallback을 사용하여 모델을 업로드합니다:
>>> from transformers.keras_callbacks import KerasMetricCallback, PushToHubCallback
>>> metric_callback = KerasMetricCallback(metric_fn=compute_metrics, eval_dataset=tf_eval_dataset)
>>> push_to_hub_callback = PushToHubCallback(
... output_dir="food_classifier",
... tokenizer=image_processor,
... save_strategy="no",
... )
>>> callbacks = [metric_callback, push_to_hub_callback]
이제 모델을 훈련할 준비가 되었습니다! 훈련 및 검증 데이터 세트, 에폭 수와 함께 fit()
을 호출하고,
콜백을 사용하여 모델을 미세 조정합니다:
>>> model.fit(tf_train_dataset, validation_data=tf_eval_dataset, epochs=num_epochs, callbacks=callbacks)
Epoch 1/5
250/250 [==============================] - 313s 1s/step - loss: 2.5623 - val_loss: 1.4161 - accuracy: 0.9290
Epoch 2/5
250/250 [==============================] - 265s 1s/step - loss: 0.9181 - val_loss: 0.6808 - accuracy: 0.9690
Epoch 3/5
250/250 [==============================] - 252s 1s/step - loss: 0.3910 - val_loss: 0.4303 - accuracy: 0.9820
Epoch 4/5
250/250 [==============================] - 251s 1s/step - loss: 0.2028 - val_loss: 0.3191 - accuracy: 0.9900
Epoch 5/5
250/250 [==============================] - 238s 949ms/step - loss: 0.1232 - val_loss: 0.3259 - accuracy: 0.9890
축하합니다! 모델을 미세 조정하고 🤗 Hub에 공유했습니다. 이제 추론에 사용할 수 있습니다!
이미지 분류를 위한 모델을 미세 조정하는 자세한 예제는 다음 PyTorch notebook을 참조하세요.
추론
좋아요, 이제 모델을 미세 조정했으니 추론에 사용할 수 있습니다!
추론을 수행하고자 하는 이미지를 가져와봅시다:
>>> ds = load_dataset("food101", split="validation[:10]")
>>> image = ds["image"][0]
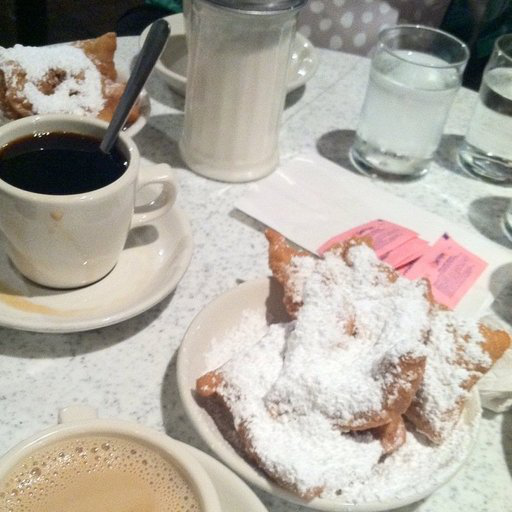
미세 조정 모델로 추론을 시도하는 가장 간단한 방법은 pipeline()
을 사용하는 것입니다. 모델로 이미지 분류를 위한 pipeline
을 인스턴스화하고 이미지를 전달합니다:
>>> from transformers import pipeline
>>> classifier = pipeline("image-classification", model="my_awesome_food_model")
>>> classifier(image)
[{'score': 0.31856709718704224, 'label': 'beignets'},
{'score': 0.015232225880026817, 'label': 'bruschetta'},
{'score': 0.01519392803311348, 'label': 'chicken_wings'},
{'score': 0.013022331520915031, 'label': 'pork_chop'},
{'score': 0.012728818692266941, 'label': 'prime_rib'}]
원한다면, pipeline
의 결과를 수동으로 복제할 수도 있습니다:
이미지를 전처리하기 위해 이미지 프로세서를 가져오고 input
을 PyTorch 텐서로 반환합니다:
>>> from transformers import AutoImageProcessor
>>> import torch
>>> image_processor = AutoImageProcessor.from_pretrained("my_awesome_food_model")
>>> inputs = image_processor(image, return_tensors="pt")
입력을 모델에 전달하고 logits을 반환합니다:
>>> from transformers import AutoModelForImageClassification
>>> model = AutoModelForImageClassification.from_pretrained("my_awesome_food_model")
>>> with torch.no_grad():
... logits = model(**inputs).logits
확률이 가장 높은 예측 레이블을 가져오고, 모델의 id2label
매핑을 사용하여 레이블로 변환합니다:
>>> predicted_label = logits.argmax(-1).item()
>>> model.config.id2label[predicted_label]
'beignets'
이미지를 전처리하기 위해 이미지 프로세서를 가져오고 input
을 TensorFlow 텐서로 반환합니다:
>>> from transformers import AutoImageProcessor
>>> image_processor = AutoImageProcessor.from_pretrained("MariaK/food_classifier")
>>> inputs = image_processor(image, return_tensors="tf")
입력을 모델에 전달하고 logits을 반환합니다:
>>> from transformers import TFAutoModelForImageClassification
>>> model = TFAutoModelForImageClassification.from_pretrained("MariaK/food_classifier")
>>> logits = model(**inputs).logits
확률이 가장 높은 예측 레이블을 가져오고, 모델의 id2label
매핑을 사용하여 레이블로 변환합니다:
>>> predicted_class_id = int(tf.math.argmax(logits, axis=-1)[0])
>>> model.config.id2label[predicted_class_id]
'beignets'