Transformers documentation
YOLOS
YOLOS
Overview
The YOLOS model was proposed in You Only Look at One Sequence: Rethinking Transformer in Vision through Object Detection by Yuxin Fang, Bencheng Liao, Xinggang Wang, Jiemin Fang, Jiyang Qi, Rui Wu, Jianwei Niu, Wenyu Liu. YOLOS proposes to just leverage the plain Vision Transformer (ViT) for object detection, inspired by DETR. It turns out that a base-sized encoder-only Transformer can also achieve 42 AP on COCO, similar to DETR and much more complex frameworks such as Faster R-CNN.
The abstract from the paper is the following:
Can Transformer perform 2D object- and region-level recognition from a pure sequence-to-sequence perspective with minimal knowledge about the 2D spatial structure? To answer this question, we present You Only Look at One Sequence (YOLOS), a series of object detection models based on the vanilla Vision Transformer with the fewest possible modifications, region priors, as well as inductive biases of the target task. We find that YOLOS pre-trained on the mid-sized ImageNet-1k dataset only can already achieve quite competitive performance on the challenging COCO object detection benchmark, e.g., YOLOS-Base directly adopted from BERT-Base architecture can obtain 42.0 box AP on COCO val. We also discuss the impacts as well as limitations of current pre-train schemes and model scaling strategies for Transformer in vision through YOLOS.
Tips:
- One can use YolosFeatureExtractor for preparing images (and optional targets) for the model. Contrary to DETR, YOLOS doesnβt require a
pixel_mask
to be created. - Demo notebooks (regarding inference and fine-tuning on custom data) can be found here.
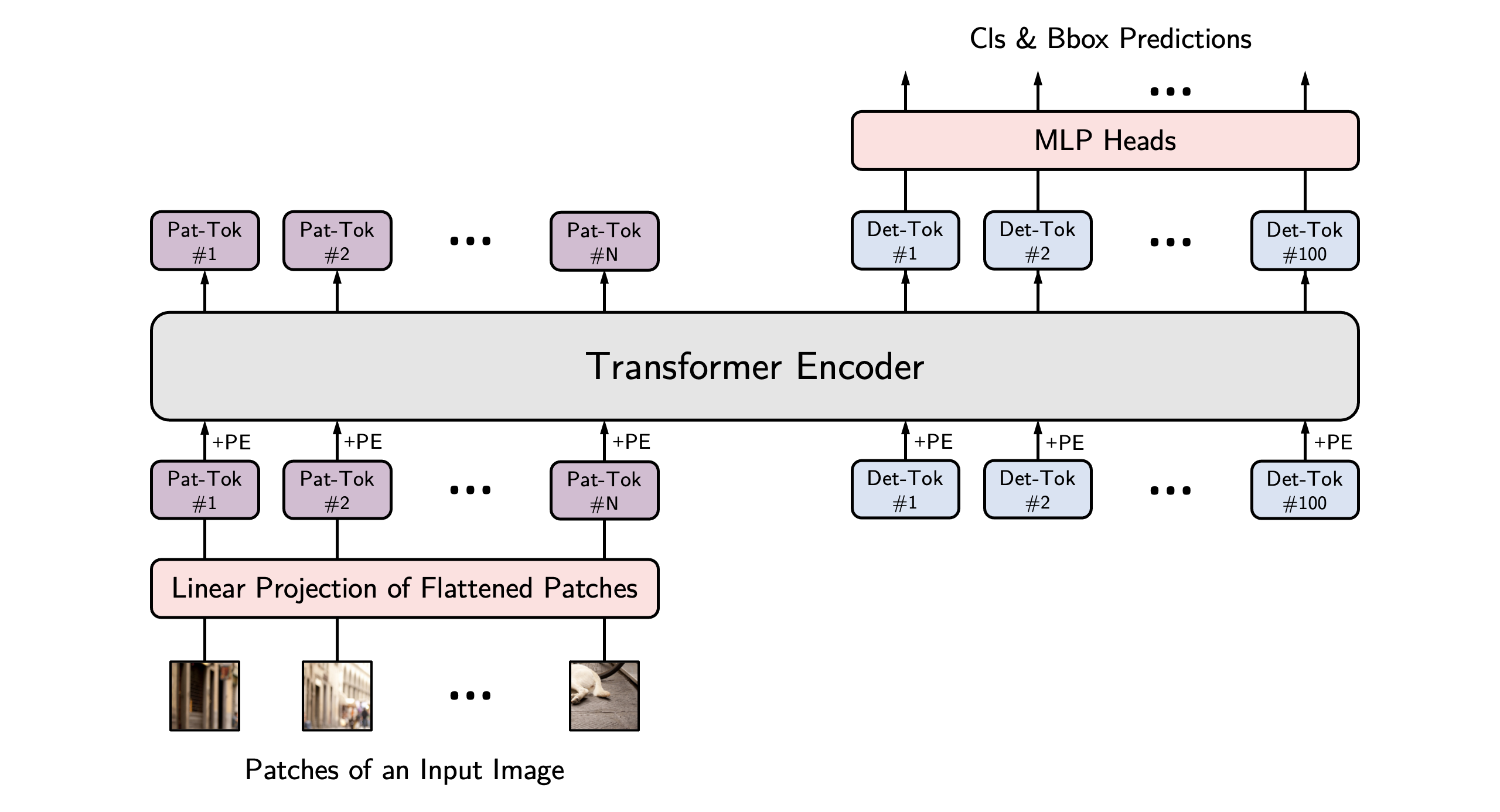
This model was contributed by nielsr. The original code can be found here.
YolosConfig
class transformers.YolosConfig
< source >( hidden_size = 768 num_hidden_layers = 12 num_attention_heads = 12 intermediate_size = 3072 hidden_act = 'gelu' hidden_dropout_prob = 0.0 attention_probs_dropout_prob = 0.0 initializer_range = 0.02 layer_norm_eps = 1e-12 image_size = [512, 864] patch_size = 16 num_channels = 3 qkv_bias = True num_detection_tokens = 100 use_mid_position_embeddings = True auxiliary_loss = False class_cost = 1 bbox_cost = 5 giou_cost = 2 bbox_loss_coefficient = 5 giou_loss_coefficient = 2 eos_coefficient = 0.1 **kwargs )
Parameters
- hidden_size (
int
, optional, defaults to 768) — Dimensionality of the encoder layers and the pooler layer. - num_hidden_layers (
int
, optional, defaults to 12) — Number of hidden layers in the Transformer encoder. -
num_attention_heads (
int
, optional, defaults to 12) — Number of attention heads for each attention layer in the Transformer encoder. -
intermediate_size (
int
, optional, defaults to 3072) — Dimensionality of the “intermediate” (i.e., feed-forward) layer in the Transformer encoder. - hidden_act (
str
orfunction
, optional, defaults to"gelu"
) — The non-linear activation function (function or string) in the encoder and pooler. If string,"gelu"
,"relu"
,"selu"
and"gelu_new"
are supported. - hidden_dropout_prob (
float
, optional, defaults to 0.1) — The dropout probabilitiy for all fully connected layers in the embeddings, encoder, and pooler. -
attention_probs_dropout_prob (
float
, optional, defaults to 0.1) — The dropout ratio for the attention probabilities. -
initializer_range (
float
, optional, defaults to 0.02) — The standard deviation of the truncated_normal_initializer for initializing all weight matrices. -
layer_norm_eps (
float
, optional, defaults to 1e-12) — The epsilon used by the layer normalization layers. -
image_size (
List[int]
, optional, defaults to[512, 864]
) — The size (resolution) of each image. -
patch_size (
int
, optional, defaults to16
) — The size (resolution) of each patch. -
num_channels (
int
, optional, defaults to3
) — The number of input channels. -
qkv_bias (
bool
, optional, defaults toTrue
) — Whether to add a bias to the queries, keys and values. -
num_detection_tokens (
int
, optional, defaults to100
) — The number of detection tokens. -
use_mid_position_embeddings (
bool
, optional, defaults toTrue
) — Whether to use the mid-layer position encodings. -
auxiliary_loss (
bool
, optional, defaults toFalse
) — Whether auxiliary decoding losses (loss at each decoder layer) are to be used. -
class_cost (
float
, optional, defaults to 1) — Relative weight of the classification error in the Hungarian matching cost. -
bbox_cost (
float
, optional, defaults to 5) — Relative weight of the L1 error of the bounding box coordinates in the Hungarian matching cost. -
giou_cost (
float
, optional, defaults to 2) — Relative weight of the generalized IoU loss of the bounding box in the Hungarian matching cost. -
bbox_loss_coefficient (
float
, optional, defaults to 5) — Relative weight of the L1 bounding box loss in the object detection loss. -
giou_loss_coefficient (
float
, optional, defaults to 2) — Relative weight of the generalized IoU loss in the object detection loss. -
eos_coefficient (
float
, optional, defaults to 0.1) — Relative classification weight of the ‘no-object’ class in the object detection loss.
This is the configuration class to store the configuration of a YolosModel. It is used to instantiate a YOLOS model according to the specified arguments, defining the model architecture. Instantiating a configuration with the defaults will yield a similar configuration to that of the YOLOS hustvl/yolos-base architecture.
Configuration objects inherit from PretrainedConfig and can be used to control the model outputs. Read the documentation from PretrainedConfig for more information.
Example:
>>> from transformers import YolosModel, YolosConfig
>>> # Initializing a YOLOS hustvl/yolos-base style configuration
>>> configuration = YolosConfig()
>>> # Initializing a model from the hustvl/yolos-base style configuration
>>> model = YolosModel(configuration)
>>> # Accessing the model configuration
>>> configuration = model.config
YolosFeatureExtractor
class transformers.YolosFeatureExtractor
< source >( format = 'coco_detection' do_resize = True size = 800 max_size = 1333 do_normalize = True image_mean = None image_std = None **kwargs )
Parameters
-
format (
str
, optional, defaults to"coco_detection"
) — Data format of the annotations. One of “coco_detection” or “coco_panoptic”. -
do_resize (
bool
, optional, defaults toTrue
) — Whether to resize the input to a certainsize
. -
size (
int
, optional, defaults to 800) — Resize the input to the given size. Only has an effect ifdo_resize
is set toTrue
. If size is a sequence like(width, height)
, output size will be matched to this. If size is an int, smaller edge of the image will be matched to this number. i.e, ifheight > width
, then image will be rescaled to(size * height / width, size)
. -
max_size (
int
, optional, defaults to1333
) — The largest size an image dimension can have (otherwise it’s capped). Only has an effect ifdo_resize
is set toTrue
. -
do_normalize (
bool
, optional, defaults toTrue
) — Whether or not to normalize the input with mean and standard deviation. -
image_mean (
int
, optional, defaults to[0.485, 0.456, 0.406]
) — The sequence of means for each channel, to be used when normalizing images. Defaults to the ImageNet mean. -
image_std (
int
, optional, defaults to[0.229, 0.224, 0.225]
) — The sequence of standard deviations for each channel, to be used when normalizing images. Defaults to the ImageNet std.
Constructs a YOLOS feature extractor.
This feature extractor inherits from FeatureExtractionMixin which contains most of the main methods. Users should refer to this superclass for more information regarding those methods.
__call__
< source >( images: typing.Union[PIL.Image.Image, numpy.ndarray, ForwardRef('torch.Tensor'), typing.List[PIL.Image.Image], typing.List[numpy.ndarray], typing.List[ForwardRef('torch.Tensor')]] annotations: typing.Union[typing.List[typing.Dict], typing.List[typing.List[typing.Dict]]] = None return_segmentation_masks: typing.Optional[bool] = False masks_path: typing.Optional[pathlib.Path] = None padding: typing.Optional[bool] = True return_tensors: typing.Union[str, transformers.utils.generic.TensorType, NoneType] = None **kwargs ) β BatchFeature
Parameters
-
images (
PIL.Image.Image
,np.ndarray
,torch.Tensor
,List[PIL.Image.Image]
,List[np.ndarray]
,List[torch.Tensor]
) — The image or batch of images to be prepared. Each image can be a PIL image, NumPy array or PyTorch tensor. In case of a NumPy array/PyTorch tensor, each image should be of shape (C, H, W), where C is a number of channels, H and W are image height and width. -
annotations (
Dict
,List[Dict]
, optional) — The corresponding annotations in COCO format.In case DetrFeatureExtractor was initialized with
format = "coco_detection"
, the annotations for each image should have the following format: {‘image_id’: int, ‘annotations’: [annotation]}, with the annotations being a list of COCO object annotations.In case DetrFeatureExtractor was initialized with
format = "coco_panoptic"
, the annotations for each image should have the following format: {‘image_id’: int, ‘file_name’: str, ‘segments_info’: [segment_info]} with segments_info being a list of COCO panoptic annotations. -
return_segmentation_masks (
Dict
,List[Dict]
, optional, defaults toFalse
) — Whether to also include instance segmentation masks as part of the labels in caseformat = "coco_detection"
. -
masks_path (
pathlib.Path
, optional) — Path to the directory containing the PNG files that store the class-agnostic image segmentations. Only relevant in case DetrFeatureExtractor was initialized withformat = "coco_panoptic"
. -
padding (
bool
, optional, defaults toTrue
) — Whether or not to pad images up to the largest image in a batch. -
return_tensors (
str
or TensorType, optional) — If set, will return tensors instead of NumPy arrays. If set to'pt'
, return PyTorchtorch.Tensor
objects.
Returns
A BatchFeature with the following fields:
- pixel_values β Pixel values to be fed to a model.
- labels β Optional labels to be fed to a model (when
annotations
are provided)
Main method to prepare for the model one or several image(s) and optional annotations. Images are by default padded up to the largest image in a batch.
NumPy arrays and PyTorch tensors are converted to PIL images when resizing, so the most efficient is to pass PIL images.
pad
< source >( pixel_values_list: typing.List[ForwardRef('torch.Tensor')] return_tensors: typing.Union[str, transformers.utils.generic.TensorType, NoneType] = None ) β BatchFeature
Parameters
-
pixel_values_list (
List[torch.Tensor]
) — List of images (pixel values) to be padded. Each image should be a tensor of shape (C, H, W). -
return_tensors (
str
or TensorType, optional) — If set, will return tensors instead of NumPy arrays. If set to'pt'
, return PyTorchtorch.Tensor
objects.
Returns
A BatchFeature with the following field:
- pixel_values β Pixel values to be fed to a model.
Pad images up to the largest image in a batch.
post_process
< source >(
outputs
target_sizes
)
β
List[Dict]
Parameters
-
outputs (
DetrObjectDetectionOutput
) — Raw outputs of the model. -
target_sizes (
torch.Tensor
of shape(batch_size, 2)
) — Tensor containing the size (height, width) of each image of the batch. For evaluation, this must be the original image size (before any data augmentation). For visualization, this should be the image size after data augment, but before padding.
Returns
List[Dict]
A list of dictionaries, each dictionary containing the scores, labels and boxes for an image in the batch as predicted by the model.
Converts the output of DetrForObjectDetection into the format expected by the COCO api. Only supports PyTorch.
post_process_segmentation
< source >(
outputs
target_sizes
threshold = 0.9
mask_threshold = 0.5
)
β
List[Dict]
Parameters
-
outputs (
DetrSegmentationOutput
) — Raw outputs of the model. -
target_sizes (
torch.Tensor
of shape(batch_size, 2)
orList[Tuple]
of lengthbatch_size
) — Torch Tensor (or list) corresponding to the requested final size (h, w) of each prediction. -
threshold (
float
, optional, defaults to 0.9) — Threshold to use to filter out queries. -
mask_threshold (
float
, optional, defaults to 0.5) — Threshold to use when turning the predicted masks into binary values.
Returns
List[Dict]
A list of dictionaries, each dictionary containing the scores, labels, and masks for an image in the batch as predicted by the model.
Converts the output of DetrForSegmentation into image segmentation predictions. Only supports PyTorch.
post_process_panoptic
< source >(
outputs
processed_sizes
target_sizes = None
is_thing_map = None
threshold = 0.85
)
β
List[Dict]
Parameters
-
outputs (
DetrSegmentationOutput
) — Raw outputs of the model. -
processed_sizes (
torch.Tensor
of shape(batch_size, 2)
orList[Tuple]
of lengthbatch_size
) — Torch Tensor (or list) containing the size (h, w) of each image of the batch, i.e. the size after data augmentation but before batching. -
target_sizes (
torch.Tensor
of shape(batch_size, 2)
orList[Tuple]
of lengthbatch_size
, optional) — Torch Tensor (or list) corresponding to the requested final size (h, w) of each prediction. If left to None, it will default to theprocessed_sizes
. -
is_thing_map (
torch.Tensor
of shape(batch_size, 2)
, optional) — Dictionary mapping class indices to either True or False, depending on whether or not they are a thing. If not set, defaults to theis_thing_map
of COCO panoptic. -
threshold (
float
, optional, defaults to 0.85) — Threshold to use to filter out queries.
Returns
List[Dict]
A list of dictionaries, each dictionary containing a PNG string and segments_info values for an image in the batch as predicted by the model.
Converts the output of DetrForSegmentation into actual panoptic predictions. Only supports PyTorch.
YolosModel
class transformers.YolosModel
< source >( config: YolosConfig add_pooling_layer: bool = True )
Parameters
- config (YolosConfig) — Model configuration class with all the parameters of the model. Initializing with a config file does not load the weights associated with the model, only the configuration. Check out the from_pretrained() method to load the model weights.
The bare YOLOS Model transformer outputting raw hidden-states without any specific head on top. This model is a PyTorch torch.nn.Module subclass. Use it as a regular PyTorch Module and refer to the PyTorch documentation for all matter related to general usage and behavior.
forward
< source >(
pixel_values: typing.Optional[torch.Tensor] = None
head_mask: typing.Optional[torch.Tensor] = None
output_attentions: typing.Optional[bool] = None
output_hidden_states: typing.Optional[bool] = None
return_dict: typing.Optional[bool] = None
)
β
transformers.modeling_outputs.BaseModelOutputWithPooling or tuple(torch.FloatTensor)
Parameters
-
pixel_values (
torch.FloatTensor
of shape(batch_size, num_channels, height, width)
) — Pixel values. Pixel values can be obtained using AutoFeatureExtractor. SeeAutoFeatureExtractor.__call__()
for details. -
head_mask (
torch.FloatTensor
of shape(num_heads,)
or(num_layers, num_heads)
, optional) — Mask to nullify selected heads of the self-attention modules. Mask values selected in[0, 1]
:- 1 indicates the head is not masked,
- 0 indicates the head is masked.
-
output_attentions (
bool
, optional) — Whether or not to return the attentions tensors of all attention layers. Seeattentions
under returned tensors for more detail. - output_hidden_states (
bool
, optional) — Whether or not to return the hidden states of all layers. Seehidden_states
under returned tensors for more detail. -
return_dict (
bool
, optional) — Whether or not to return a ModelOutput instead of a plain tuple.
Returns
transformers.modeling_outputs.BaseModelOutputWithPooling or tuple(torch.FloatTensor)
A transformers.modeling_outputs.BaseModelOutputWithPooling or a tuple of
torch.FloatTensor
(if return_dict=False
is passed or when config.return_dict=False
) comprising various
elements depending on the configuration (YolosConfig) and inputs.
-
last_hidden_state (
torch.FloatTensor
of shape(batch_size, sequence_length, hidden_size)
) β Sequence of hidden-states at the output of the last layer of the model. -
pooler_output (
torch.FloatTensor
of shape(batch_size, hidden_size)
) β Last layer hidden-state of the first token of the sequence (classification token) after further processing through the layers used for the auxiliary pretraining task. E.g. for BERT-family of models, this returns the classification token after processing through a linear layer and a tanh activation function. The linear layer weights are trained from the next sentence prediction (classification) objective during pretraining. -
hidden_states (
tuple(torch.FloatTensor)
, optional, returned whenoutput_hidden_states=True
is passed or whenconfig.output_hidden_states=True
) β Tuple oftorch.FloatTensor
(one for the output of the embeddings, if the model has an embedding layer, + one for the output of each layer) of shape(batch_size, sequence_length, hidden_size)
.Hidden-states of the model at the output of each layer plus the optional initial embedding outputs.
-
attentions (
tuple(torch.FloatTensor)
, optional, returned whenoutput_attentions=True
is passed or whenconfig.output_attentions=True
) β Tuple oftorch.FloatTensor
(one for each layer) of shape(batch_size, num_heads, sequence_length, sequence_length)
.Attentions weights after the attention softmax, used to compute the weighted average in the self-attention heads.
The YolosModel forward method, overrides the __call__
special method.
Although the recipe for forward pass needs to be defined within this function, one should call the Module
instance afterwards instead of this since the former takes care of running the pre and post processing steps while
the latter silently ignores them.
Example:
>>> from transformers import YolosFeatureExtractor, YolosModel
>>> import torch
>>> from datasets import load_dataset
>>> dataset = load_dataset("huggingface/cats-image")
>>> image = dataset["test"]["image"][0]
>>> feature_extractor = YolosFeatureExtractor.from_pretrained("hustvl/yolos-small")
>>> model = YolosModel.from_pretrained("hustvl/yolos-small")
>>> inputs = feature_extractor(image, return_tensors="pt")
>>> with torch.no_grad():
... outputs = model(**inputs)
>>> last_hidden_states = outputs.last_hidden_state
>>> list(last_hidden_states.shape)
[1, 3401, 384]
YolosForObjectDetection
class transformers.YolosForObjectDetection
< source >( config: YolosConfig )
Parameters
- config (YolosConfig) — Model configuration class with all the parameters of the model. Initializing with a config file does not load the weights associated with the model, only the configuration. Check out the from_pretrained() method to load the model weights.
YOLOS Model (consisting of a ViT encoder) with object detection heads on top, for tasks such as COCO detection.
This model is a PyTorch torch.nn.Module subclass. Use it as a regular PyTorch Module and refer to the PyTorch documentation for all matter related to general usage and behavior.
forward
< source >(
pixel_values: FloatTensor
labels: typing.Optional[typing.List[typing.Dict]] = None
output_attentions: typing.Optional[bool] = None
output_hidden_states: typing.Optional[bool] = None
return_dict: typing.Optional[bool] = None
)
β
transformers.models.yolos.modeling_yolos.YolosObjectDetectionOutput
or tuple(torch.FloatTensor)
Parameters
-
pixel_values (
torch.FloatTensor
of shape(batch_size, num_channels, height, width)
) — Pixel values. Pixel values can be obtained using AutoFeatureExtractor. SeeAutoFeatureExtractor.__call__()
for details. -
head_mask (
torch.FloatTensor
of shape(num_heads,)
or(num_layers, num_heads)
, optional) — Mask to nullify selected heads of the self-attention modules. Mask values selected in[0, 1]
:- 1 indicates the head is not masked,
- 0 indicates the head is masked.
-
output_attentions (
bool
, optional) — Whether or not to return the attentions tensors of all attention layers. Seeattentions
under returned tensors for more detail. - output_hidden_states (
bool
, optional) — Whether or not to return the hidden states of all layers. Seehidden_states
under returned tensors for more detail. -
return_dict (
bool
, optional) — Whether or not to return a ModelOutput instead of a plain tuple. -
labels (
List[Dict]
of len(batch_size,)
, optional) — Labels for computing the bipartite matching loss. List of dicts, each dictionary containing at least the following 2 keys:'class_labels'
and'boxes'
(the class labels and bounding boxes of an image in the batch respectively). The class labels themselves should be atorch.LongTensor
of len(number of bounding boxes in the image,)
and the boxes atorch.FloatTensor
of shape(number of bounding boxes in the image, 4)
.
Returns
transformers.models.yolos.modeling_yolos.YolosObjectDetectionOutput
or tuple(torch.FloatTensor)
A transformers.models.yolos.modeling_yolos.YolosObjectDetectionOutput
or a tuple of
torch.FloatTensor
(if return_dict=False
is passed or when config.return_dict=False
) comprising various
elements depending on the configuration (YolosConfig) and inputs.
- loss (
torch.FloatTensor
of shape(1,)
, optional, returned whenlabels
are provided)) β Total loss as a linear combination of a negative log-likehood (cross-entropy) for class prediction and a bounding box loss. The latter is defined as a linear combination of the L1 loss and the generalized scale-invariant IoU loss. - loss_dict (
Dict
, optional) β A dictionary containing the individual losses. Useful for logging. - logits (
torch.FloatTensor
of shape(batch_size, num_queries, num_classes + 1)
) β Classification logits (including no-object) for all queries. - pred_boxes (
torch.FloatTensor
of shape(batch_size, num_queries, 4)
) β Normalized boxes coordinates for all queries, represented as (center_x, center_y, width, height). These values are normalized in [0, 1], relative to the size of each individual image in the batch (disregarding possible padding). You can usepost_process()
to retrieve the unnormalized bounding boxes. - auxiliary_outputs (
list[Dict]
, optional) β Optional, only returned when auxilary losses are activated (i.e.config.auxiliary_loss
is set toTrue
) and labels are provided. It is a list of dictionaries containing the two above keys (logits
andpred_boxes
) for each decoder layer. - last_hidden_state (
torch.FloatTensor
of shape(batch_size, sequence_length, hidden_size)
, optional) β Sequence of hidden-states at the output of the last layer of the decoder of the model. - hidden_states (
tuple(torch.FloatTensor)
, optional, returned whenoutput_hidden_states=True
is passed or whenconfig.output_hidden_states=True
) β Tuple oftorch.FloatTensor
(one for the output of the embeddings, if the model has an embedding layer, + one for the output of each layer) of shape(batch_size, sequence_length, hidden_size)
. Hidden-states of the model at the output of each layer plus the optional initial embedding outputs. - attentions (
tuple(torch.FloatTensor)
, optional, returned whenoutput_attentions=True
is passed or whenconfig.output_attentions=True
) β Tuple oftorch.FloatTensor
(one for each layer) of shape(batch_size, num_heads, sequence_length, sequence_length)
. Attentions weights after the attention softmax, used to compute the weighted average in the self-attention heads.
The YolosForObjectDetection forward method, overrides the __call__
special method.
Although the recipe for forward pass needs to be defined within this function, one should call the Module
instance afterwards instead of this since the former takes care of running the pre and post processing steps while
the latter silently ignores them.
Examples:
>>> from transformers import YolosFeatureExtractor, YolosForObjectDetection
>>> from PIL import Image
>>> import requests
>>> url = "http://images.cocodataset.org/val2017/000000039769.jpg"
>>> image = Image.open(requests.get(url, stream=True).raw)
>>> feature_extractor = YolosFeatureExtractor.from_pretrained("hustvl/yolos-small")
>>> model = YolosForObjectDetection.from_pretrained("hustvl/yolos-small")
>>> inputs = feature_extractor(images=image, return_tensors="pt")
>>> outputs = model(**inputs)
>>> # model predicts bounding boxes and corresponding COCO classes
>>> logits = outputs.logits
>>> bboxes = outputs.pred_boxes