Create README.md
Browse files
README.md
ADDED
@@ -0,0 +1,1127 @@
|
|
|
|
|
|
|
|
|
|
|
|
|
|
|
|
|
|
|
|
|
|
|
|
|
|
|
|
|
|
|
|
|
|
|
|
|
|
|
|
|
|
|
|
|
|
|
|
|
|
|
|
|
|
|
|
|
|
|
|
|
|
|
|
|
|
|
|
|
|
|
|
|
|
|
|
|
|
|
|
|
|
|
|
|
|
|
|
|
|
|
|
|
|
|
|
|
|
|
|
|
|
|
|
|
|
|
|
|
|
|
|
|
|
|
|
|
|
|
|
|
|
|
|
|
|
|
|
|
|
|
|
|
|
|
|
|
|
|
|
|
|
|
|
|
|
|
|
|
|
|
|
|
|
|
|
|
|
|
|
|
|
|
|
|
|
|
|
|
|
|
|
|
|
|
|
|
|
|
|
|
|
|
|
|
|
|
|
|
|
|
|
|
|
|
|
|
|
|
|
|
|
|
|
|
|
|
|
|
|
|
|
|
|
|
|
|
|
|
|
|
|
|
|
|
|
|
|
|
|
|
|
|
|
|
|
|
|
|
|
|
|
|
|
|
|
|
|
|
|
|
|
|
|
|
|
|
|
|
|
|
|
|
|
|
|
|
|
|
|
|
|
|
|
|
|
|
|
|
|
|
|
|
|
|
|
|
|
|
|
|
|
|
|
|
|
|
|
|
|
|
|
|
|
|
|
|
|
|
|
|
|
|
|
|
|
|
|
|
|
|
|
|
|
|
|
|
|
|
|
|
|
|
|
|
|
|
|
|
|
|
|
|
|
|
|
|
|
|
|
|
|
|
|
|
|
|
|
|
|
|
|
|
|
|
|
|
|
|
|
|
|
|
|
|
|
|
|
|
|
|
|
|
|
|
|
|
|
|
|
|
|
|
|
|
|
|
|
|
|
|
|
|
|
|
|
|
|
|
|
|
|
|
|
|
|
|
|
|
|
|
|
|
|
|
|
|
|
|
|
|
|
|
|
|
|
|
|
|
|
|
|
|
|
|
|
|
|
|
|
|
|
|
|
|
|
|
|
|
|
|
|
|
|
|
|
|
|
|
|
|
|
|
|
|
|
|
|
|
|
|
|
|
|
|
|
|
|
|
|
|
|
|
|
|
|
|
|
|
|
|
|
|
|
|
|
|
|
|
|
|
|
|
|
|
|
|
|
|
|
|
|
|
|
|
|
|
|
|
|
|
|
|
|
|
|
|
|
|
|
|
|
|
|
|
|
|
|
|
|
|
|
|
|
|
|
|
|
|
|
|
|
|
|
|
|
|
|
|
|
|
|
|
|
|
|
|
|
|
|
|
|
|
|
|
|
|
|
|
|
|
|
|
|
|
|
|
|
|
|
|
|
|
|
|
|
|
|
|
|
|
|
|
|
|
|
|
|
|
|
|
|
|
|
|
|
|
|
|
|
|
|
|
|
|
|
|
|
|
|
|
|
|
|
|
|
|
|
|
|
|
|
|
|
|
|
|
|
|
|
|
|
|
|
|
|
|
|
|
|
|
|
|
|
|
|
|
|
|
|
|
|
|
|
|
|
|
|
|
|
|
|
|
|
|
|
|
|
|
|
|
|
|
|
|
|
|
|
|
|
|
|
|
|
|
|
|
|
|
|
|
|
|
|
|
|
|
|
|
|
|
|
|
|
|
|
|
|
|
|
|
|
|
|
|
|
|
|
|
|
|
|
|
|
|
|
|
|
|
|
|
|
|
|
|
|
|
|
|
|
|
|
|
|
|
|
|
|
|
|
|
|
|
|
|
|
|
|
|
|
|
|
|
|
|
|
|
|
|
|
|
|
|
|
|
|
|
|
|
|
|
|
|
|
|
|
|
|
|
|
|
|
|
|
|
|
|
|
|
|
|
|
|
|
|
|
|
|
|
|
|
|
|
|
|
|
|
|
|
|
|
|
|
|
|
|
|
|
|
|
|
|
|
|
|
|
|
|
|
|
|
|
|
|
|
|
|
|
|
|
|
|
|
|
|
|
|
|
|
|
|
|
|
|
|
|
|
|
|
|
|
|
|
|
|
|
|
|
|
|
|
|
|
|
|
|
|
|
|
|
|
|
|
|
|
|
|
|
|
|
|
|
|
|
|
|
|
|
|
|
|
|
|
|
|
|
|
|
|
|
|
|
|
|
|
|
|
|
|
|
|
|
|
|
|
|
|
|
|
|
|
|
|
|
|
|
|
|
|
|
|
|
|
|
|
|
|
|
|
|
|
|
|
|
|
|
|
|
|
|
|
|
|
|
|
|
|
|
|
|
|
|
|
|
|
|
|
|
|
|
|
|
|
|
|
|
|
|
|
|
|
|
|
|
|
|
|
|
|
|
|
|
|
|
|
|
|
|
|
|
|
|
|
|
|
|
|
|
|
|
|
|
|
|
|
|
|
|
|
|
|
|
|
|
|
|
|
|
|
|
|
|
|
|
|
|
|
|
|
|
|
|
|
|
|
|
|
|
|
|
|
|
|
|
|
|
|
|
|
|
|
|
|
|
|
|
|
|
|
|
|
|
|
|
|
|
|
|
|
|
|
|
|
|
|
|
|
|
|
|
|
|
|
|
|
|
|
|
|
|
|
|
|
|
|
|
|
|
|
|
|
|
|
|
|
|
|
|
|
|
|
|
|
|
|
|
|
|
|
|
|
|
|
|
|
|
|
|
|
|
|
|
|
|
|
|
|
|
|
|
|
|
|
|
|
|
|
|
|
|
|
|
|
|
|
|
|
|
|
|
|
|
|
|
|
|
|
|
|
|
|
|
|
|
|
|
|
|
|
|
|
|
|
|
|
|
|
|
|
|
|
|
|
|
|
|
|
|
|
|
|
|
|
|
|
|
|
|
|
|
|
|
|
|
|
|
|
|
|
|
|
|
|
|
|
|
|
|
|
|
|
|
|
|
|
|
|
|
|
|
|
|
|
|
|
|
|
|
|
|
|
|
|
|
|
|
|
|
|
|
|
|
|
|
|
|
|
|
|
|
|
|
|
|
|
|
|
|
|
|
|
|
|
|
|
|
|
|
|
|
|
|
|
|
|
|
|
|
|
|
|
|
|
|
|
|
|
|
|
|
|
|
|
|
|
|
|
|
|
|
|
|
|
|
|
|
|
|
|
|
|
|
|
|
|
|
|
|
|
|
|
|
|
|
|
|
|
|
|
|
|
|
|
|
|
|
|
|
|
|
|
|
|
|
|
|
|
|
|
|
|
|
|
|
|
|
|
|
|
|
|
|
|
|
|
|
|
|
|
|
|
|
|
|
|
|
|
|
|
|
|
|
|
|
|
|
|
|
|
|
|
|
|
|
|
|
|
|
|
|
|
|
|
|
|
|
|
|
|
|
|
|
|
|
|
|
|
|
|
|
|
|
|
|
|
|
|
|
|
|
|
|
|
|
|
|
|
|
|
|
|
|
|
|
|
|
|
|
|
|
|
|
|
|
|
|
|
|
|
|
|
|
|
|
|
|
|
|
|
|
|
|
|
|
|
|
|
|
|
|
|
|
|
|
|
|
|
|
|
|
|
|
|
|
|
|
|
|
|
|
|
|
|
|
|
|
|
|
|
|
|
|
|
|
|
|
|
|
|
|
|
|
|
|
|
|
|
|
|
|
|
|
|
|
|
|
|
|
|
|
|
|
|
|
|
|
|
|
|
|
|
|
|
|
|
|
|
|
|
|
|
|
|
|
|
|
|
|
|
|
|
|
|
|
|
|
|
|
|
|
|
|
|
|
|
|
|
|
|
|
|
|
|
|
|
|
|
|
|
|
|
|
|
|
|
|
|
|
|
|
|
|
|
|
|
|
|
|
|
|
|
|
|
|
|
|
|
|
|
|
|
|
|
|
|
|
|
|
|
|
|
|
|
|
|
|
|
|
|
|
|
|
|
|
|
|
|
|
|
|
|
|
|
|
|
|
|
|
|
|
|
|
|
|
|
|
|
|
|
|
|
|
|
|
|
|
|
|
|
|
|
|
|
|
|
|
|
|
|
|
|
|
|
|
|
|
|
|
|
|
|
|
|
|
|
|
|
|
|
|
|
|
|
|
|
|
|
|
|
|
|
|
|
|
|
|
|
|
|
|
|
|
|
|
|
|
|
|
|
|
|
|
|
|
|
|
|
|
|
|
|
|
|
|
|
|
|
|
|
|
|
|
|
|
|
|
|
|
|
|
|
|
|
|
|
|
|
|
|
|
|
|
|
|
|
|
|
|
|
|
|
|
|
|
|
|
|
|
|
|
|
|
|
|
|
|
|
|
|
|
|
|
|
|
|
|
|
|
|
|
|
|
|
|
|
|
|
|
|
|
|
|
|
|
|
|
|
|
|
|
|
|
|
|
|
|
|
|
|
|
|
|
|
|
|
|
|
|
|
|
|
|
|
|
|
|
|
|
|
|
|
|
|
|
|
|
|
|
|
|
|
|
|
|
|
|
|
|
|
|
|
|
|
|
|
|
|
|
|
|
|
|
|
|
|
|
|
|
|
|
|
|
|
|
|
|
|
|
|
|
|
|
|
|
|
|
|
|
|
|
|
|
|
|
|
|
|
|
|
|
|
|
|
|
|
|
|
|
|
|
|
|
|
|
|
|
|
|
|
|
|
|
|
|
|
|
|
|
|
|
|
|
|
|
|
|
|
|
|
|
|
|
|
|
|
|
|
|
|
|
|
|
|
|
|
|
|
|
|
|
|
|
|
|
|
|
|
|
|
|
|
|
|
|
|
|
|
|
|
|
|
|
|
|
|
|
|
|
|
|
|
|
|
|
|
|
|
|
|
|
|
|
|
|
|
|
|
|
|
|
|
|
|
|
|
|
|
|
|
|
|
|
|
|
|
|
|
|
|
|
|
|
|
|
|
|
|
|
|
|
|
|
|
|
|
|
|
|
|
|
|
|
|
|
|
|
|
|
|
|
|
|
|
|
|
|
|
|
|
|
|
|
|
|
|
|
|
|
|
|
|
|
|
|
|
|
|
|
|
|
|
|
|
|
|
|
|
|
|
|
|
|
|
|
|
|
|
|
|
|
|
|
|
|
|
|
|
|
|
|
|
|
|
|
|
|
|
1 |
+
---
|
2 |
+
datasets:
|
3 |
+
- bigscience/xP3
|
4 |
+
license: bigscience-bloom-rail-1.0
|
5 |
+
language:
|
6 |
+
- ak
|
7 |
+
- ar
|
8 |
+
- as
|
9 |
+
- bm
|
10 |
+
- bn
|
11 |
+
- ca
|
12 |
+
- code
|
13 |
+
- en
|
14 |
+
- es
|
15 |
+
- eu
|
16 |
+
- fon
|
17 |
+
- fr
|
18 |
+
- gu
|
19 |
+
- hi
|
20 |
+
- id
|
21 |
+
- ig
|
22 |
+
- ki
|
23 |
+
- kn
|
24 |
+
- lg
|
25 |
+
- ln
|
26 |
+
- ml
|
27 |
+
- mr
|
28 |
+
- ne
|
29 |
+
- nso
|
30 |
+
- ny
|
31 |
+
- or
|
32 |
+
- pa
|
33 |
+
- pt
|
34 |
+
- rn
|
35 |
+
- rw
|
36 |
+
- sn
|
37 |
+
- st
|
38 |
+
- sw
|
39 |
+
- ta
|
40 |
+
- te
|
41 |
+
- tn
|
42 |
+
- ts
|
43 |
+
- tum
|
44 |
+
- tw
|
45 |
+
- ur
|
46 |
+
- vi
|
47 |
+
- wo
|
48 |
+
- xh
|
49 |
+
- yo
|
50 |
+
- zh
|
51 |
+
- zu
|
52 |
+
programming_language:
|
53 |
+
- C
|
54 |
+
- C++
|
55 |
+
- C#
|
56 |
+
- Go
|
57 |
+
- Java
|
58 |
+
- JavaScript
|
59 |
+
- Lua
|
60 |
+
- PHP
|
61 |
+
- Python
|
62 |
+
- Ruby
|
63 |
+
- Rust
|
64 |
+
- Scala
|
65 |
+
- TypeScript
|
66 |
+
pipeline_tag: text-generation
|
67 |
+
inference: false
|
68 |
+
widget:
|
69 |
+
- text: "一个传奇的开端,一个不灭的神话,这不仅仅是一部电影,而是作为一个走进新时代的标签,永远彪炳史册。Would you rate the previous review as positive, neutral or negative?"
|
70 |
+
example_title: "zh-en sentiment"
|
71 |
+
- text: "一个传奇的开端,一个不灭的神话,这不仅仅是一部电影,而是作为一个走进新时代的标签,永远彪炳史册。你认为这句话的立场是赞扬、中立还是批评?"
|
72 |
+
example_title: "zh-zh sentiment"
|
73 |
+
- text: "Suggest at least five related search terms to \"Mạng neural nhân tạo\"."
|
74 |
+
example_title: "vi-en query"
|
75 |
+
- text: "Proposez au moins cinq mots clés concernant «Réseau de neurones artificiels»."
|
76 |
+
example_title: "fr-fr query"
|
77 |
+
- text: "Explain in a sentence in Telugu what is backpropagation in neural networks."
|
78 |
+
example_title: "te-en qa"
|
79 |
+
- text: "Why is the sky blue?"
|
80 |
+
example_title: "en-en qa"
|
81 |
+
- text: "Explain to me in Traditional Chinese what is the difference between Bitcoin and Ethereum."
|
82 |
+
example_title: "zh-en qa"
|
83 |
+
- text: "Write a code snippet with O(log(n)) computational complexity."
|
84 |
+
example_title: "code-en"
|
85 |
+
- text: "Write a fairy tale about a troll saving a princess from a dangerous dragon. The fairy tale is a masterpiece that has achieved praise worldwide and its moral is \"Heroes Come in All Shapes and Sizes\". Story (in Spanish):"
|
86 |
+
example_title: "es-en fable"
|
87 |
+
- text: "Write a fable about wood elves living in a forest that is suddenly invaded by ogres. The fable is a masterpiece that has achieved praise worldwide and its moral is \"Violence is the last refuge of the incompetent\". Fable (in Hindi):"
|
88 |
+
example_title: "hi-en fable"
|
89 |
+
- text: "How many sides does a rectangle and heptagon have, when
|
90 |
+
combined? Answer this question with some math.
|
91 |
+
Ein Rechteck hat 4 Seiten. Ein Siebeneck hat 7 Seiten.
|
92 |
+
In Kombination haben sie 4 + 7 = 11 Seiten.
|
93 |
+
كم عدد الأضلاع التي يجمعها المربع والمثلث؟
|
94 |
+
Répondez à cette question en chinois."
|
95 |
+
example_title: "en-de-ar-fr-zh math"
|
96 |
+
model-index:
|
97 |
+
- name: bloomz
|
98 |
+
results:
|
99 |
+
- task:
|
100 |
+
type: Coreference resolution
|
101 |
+
dataset:
|
102 |
+
type: winogrande
|
103 |
+
name: Winogrande XL (xl)
|
104 |
+
config: xl
|
105 |
+
split: validation
|
106 |
+
revision: a80f460359d1e9a67c006011c94de42a8759430c
|
107 |
+
metrics:
|
108 |
+
- type: Accuracy
|
109 |
+
value: 59.27
|
110 |
+
- task:
|
111 |
+
type: Coreference resolution
|
112 |
+
dataset:
|
113 |
+
type: Muennighoff/xwinograd
|
114 |
+
name: XWinograd (en)
|
115 |
+
config: en
|
116 |
+
split: test
|
117 |
+
revision: 9dd5ea5505fad86b7bedad667955577815300cee
|
118 |
+
metrics:
|
119 |
+
- type: Accuracy
|
120 |
+
value: 69.08
|
121 |
+
- task:
|
122 |
+
type: Coreference resolution
|
123 |
+
dataset:
|
124 |
+
type: Muennighoff/xwinograd
|
125 |
+
name: XWinograd (fr)
|
126 |
+
config: fr
|
127 |
+
split: test
|
128 |
+
revision: 9dd5ea5505fad86b7bedad667955577815300cee
|
129 |
+
metrics:
|
130 |
+
- type: Accuracy
|
131 |
+
value: 68.67
|
132 |
+
- task:
|
133 |
+
type: Coreference resolution
|
134 |
+
dataset:
|
135 |
+
type: Muennighoff/xwinograd
|
136 |
+
name: XWinograd (jp)
|
137 |
+
config: jp
|
138 |
+
split: test
|
139 |
+
revision: 9dd5ea5505fad86b7bedad667955577815300cee
|
140 |
+
metrics:
|
141 |
+
- type: Accuracy
|
142 |
+
value: 59.65
|
143 |
+
- task:
|
144 |
+
type: Coreference resolution
|
145 |
+
dataset:
|
146 |
+
type: Muennighoff/xwinograd
|
147 |
+
name: XWinograd (pt)
|
148 |
+
config: pt
|
149 |
+
split: test
|
150 |
+
revision: 9dd5ea5505fad86b7bedad667955577815300cee
|
151 |
+
metrics:
|
152 |
+
- type: Accuracy
|
153 |
+
value: 64.26
|
154 |
+
- task:
|
155 |
+
type: Coreference resolution
|
156 |
+
dataset:
|
157 |
+
type: Muennighoff/xwinograd
|
158 |
+
name: XWinograd (ru)
|
159 |
+
config: ru
|
160 |
+
split: test
|
161 |
+
revision: 9dd5ea5505fad86b7bedad667955577815300cee
|
162 |
+
metrics:
|
163 |
+
- type: Accuracy
|
164 |
+
value: 60.95
|
165 |
+
- task:
|
166 |
+
type: Coreference resolution
|
167 |
+
dataset:
|
168 |
+
type: Muennighoff/xwinograd
|
169 |
+
name: XWinograd (zh)
|
170 |
+
config: zh
|
171 |
+
split: test
|
172 |
+
revision: 9dd5ea5505fad86b7bedad667955577815300cee
|
173 |
+
metrics:
|
174 |
+
- type: Accuracy
|
175 |
+
value: 70.24
|
176 |
+
- task:
|
177 |
+
type: Natural language inference
|
178 |
+
dataset:
|
179 |
+
type: anli
|
180 |
+
name: ANLI (r1)
|
181 |
+
config: r1
|
182 |
+
split: validation
|
183 |
+
revision: 9dbd830a06fea8b1c49d6e5ef2004a08d9f45094
|
184 |
+
metrics:
|
185 |
+
- type: Accuracy
|
186 |
+
value: 48.6
|
187 |
+
- task:
|
188 |
+
type: Natural language inference
|
189 |
+
dataset:
|
190 |
+
type: anli
|
191 |
+
name: ANLI (r2)
|
192 |
+
config: r2
|
193 |
+
split: validation
|
194 |
+
revision: 9dbd830a06fea8b1c49d6e5ef2004a08d9f45094
|
195 |
+
metrics:
|
196 |
+
- type: Accuracy
|
197 |
+
value: 44.1
|
198 |
+
- task:
|
199 |
+
type: Natural language inference
|
200 |
+
dataset:
|
201 |
+
type: anli
|
202 |
+
name: ANLI (r3)
|
203 |
+
config: r3
|
204 |
+
split: validation
|
205 |
+
revision: 9dbd830a06fea8b1c49d6e5ef2004a08d9f45094
|
206 |
+
metrics:
|
207 |
+
- type: Accuracy
|
208 |
+
value: 45.5
|
209 |
+
- task:
|
210 |
+
type: Natural language inference
|
211 |
+
dataset:
|
212 |
+
type: super_glue
|
213 |
+
name: SuperGLUE (cb)
|
214 |
+
config: cb
|
215 |
+
split: validation
|
216 |
+
revision: 9e12063561e7e6c79099feb6d5a493142584e9e2
|
217 |
+
metrics:
|
218 |
+
- type: Accuracy
|
219 |
+
value: 82.14
|
220 |
+
- task:
|
221 |
+
type: Natural language inference
|
222 |
+
dataset:
|
223 |
+
type: super_glue
|
224 |
+
name: SuperGLUE (rte)
|
225 |
+
config: rte
|
226 |
+
split: validation
|
227 |
+
revision: 9e12063561e7e6c79099feb6d5a493142584e9e2
|
228 |
+
metrics:
|
229 |
+
- type: Accuracy
|
230 |
+
value: 85.56
|
231 |
+
- task:
|
232 |
+
type: Natural language inference
|
233 |
+
dataset:
|
234 |
+
type: xnli
|
235 |
+
name: XNLI (ar)
|
236 |
+
config: ar
|
237 |
+
split: validation
|
238 |
+
revision: a5a45e4ff92d5d3f34de70aaf4b72c3bdf9f7f16
|
239 |
+
metrics:
|
240 |
+
- type: Accuracy
|
241 |
+
value: 60.68
|
242 |
+
- task:
|
243 |
+
type: Natural language inference
|
244 |
+
dataset:
|
245 |
+
type: xnli
|
246 |
+
name: XNLI (bg)
|
247 |
+
config: bg
|
248 |
+
split: validation
|
249 |
+
revision: a5a45e4ff92d5d3f34de70aaf4b72c3bdf9f7f16
|
250 |
+
metrics:
|
251 |
+
- type: Accuracy
|
252 |
+
value: 48.43
|
253 |
+
- task:
|
254 |
+
type: Natural language inference
|
255 |
+
dataset:
|
256 |
+
type: xnli
|
257 |
+
name: XNLI (de)
|
258 |
+
config: de
|
259 |
+
split: validation
|
260 |
+
revision: a5a45e4ff92d5d3f34de70aaf4b72c3bdf9f7f16
|
261 |
+
metrics:
|
262 |
+
- type: Accuracy
|
263 |
+
value: 54.38
|
264 |
+
- task:
|
265 |
+
type: Natural language inference
|
266 |
+
dataset:
|
267 |
+
type: xnli
|
268 |
+
name: XNLI (el)
|
269 |
+
config: el
|
270 |
+
split: validation
|
271 |
+
revision: a5a45e4ff92d5d3f34de70aaf4b72c3bdf9f7f16
|
272 |
+
metrics:
|
273 |
+
- type: Accuracy
|
274 |
+
value: 47.43
|
275 |
+
- task:
|
276 |
+
type: Natural language inference
|
277 |
+
dataset:
|
278 |
+
type: xnli
|
279 |
+
name: XNLI (en)
|
280 |
+
config: en
|
281 |
+
split: validation
|
282 |
+
revision: a5a45e4ff92d5d3f34de70aaf4b72c3bdf9f7f16
|
283 |
+
metrics:
|
284 |
+
- type: Accuracy
|
285 |
+
value: 67.47
|
286 |
+
- task:
|
287 |
+
type: Natural language inference
|
288 |
+
dataset:
|
289 |
+
type: xnli
|
290 |
+
name: XNLI (es)
|
291 |
+
config: es
|
292 |
+
split: validation
|
293 |
+
revision: a5a45e4ff92d5d3f34de70aaf4b72c3bdf9f7f16
|
294 |
+
metrics:
|
295 |
+
- type: Accuracy
|
296 |
+
value: 61.24
|
297 |
+
- task:
|
298 |
+
type: Natural language inference
|
299 |
+
dataset:
|
300 |
+
type: xnli
|
301 |
+
name: XNLI (fr)
|
302 |
+
config: fr
|
303 |
+
split: validation
|
304 |
+
revision: a5a45e4ff92d5d3f34de70aaf4b72c3bdf9f7f16
|
305 |
+
metrics:
|
306 |
+
- type: Accuracy
|
307 |
+
value: 61.37
|
308 |
+
- task:
|
309 |
+
type: Natural language inference
|
310 |
+
dataset:
|
311 |
+
type: xnli
|
312 |
+
name: XNLI (hi)
|
313 |
+
config: hi
|
314 |
+
split: validation
|
315 |
+
revision: a5a45e4ff92d5d3f34de70aaf4b72c3bdf9f7f16
|
316 |
+
metrics:
|
317 |
+
- type: Accuracy
|
318 |
+
value: 60.2
|
319 |
+
- task:
|
320 |
+
type: Natural language inference
|
321 |
+
dataset:
|
322 |
+
type: xnli
|
323 |
+
name: XNLI (ru)
|
324 |
+
config: ru
|
325 |
+
split: validation
|
326 |
+
revision: a5a45e4ff92d5d3f34de70aaf4b72c3bdf9f7f16
|
327 |
+
metrics:
|
328 |
+
- type: Accuracy
|
329 |
+
value: 54.02
|
330 |
+
- task:
|
331 |
+
type: Natural language inference
|
332 |
+
dataset:
|
333 |
+
type: xnli
|
334 |
+
name: XNLI (sw)
|
335 |
+
config: sw
|
336 |
+
split: validation
|
337 |
+
revision: a5a45e4ff92d5d3f34de70aaf4b72c3bdf9f7f16
|
338 |
+
metrics:
|
339 |
+
- type: Accuracy
|
340 |
+
value: 52.09
|
341 |
+
- task:
|
342 |
+
type: Natural language inference
|
343 |
+
dataset:
|
344 |
+
type: xnli
|
345 |
+
name: XNLI (th)
|
346 |
+
config: th
|
347 |
+
split: validation
|
348 |
+
revision: a5a45e4ff92d5d3f34de70aaf4b72c3bdf9f7f16
|
349 |
+
metrics:
|
350 |
+
- type: Accuracy
|
351 |
+
value: 43.78
|
352 |
+
- task:
|
353 |
+
type: Natural language inference
|
354 |
+
dataset:
|
355 |
+
type: xnli
|
356 |
+
name: XNLI (tr)
|
357 |
+
config: tr
|
358 |
+
split: validation
|
359 |
+
revision: a5a45e4ff92d5d3f34de70aaf4b72c3bdf9f7f16
|
360 |
+
metrics:
|
361 |
+
- type: Accuracy
|
362 |
+
value: 45.7
|
363 |
+
- task:
|
364 |
+
type: Natural language inference
|
365 |
+
dataset:
|
366 |
+
type: xnli
|
367 |
+
name: XNLI (ur)
|
368 |
+
config: ur
|
369 |
+
split: validation
|
370 |
+
revision: a5a45e4ff92d5d3f34de70aaf4b72c3bdf9f7f16
|
371 |
+
metrics:
|
372 |
+
- type: Accuracy
|
373 |
+
value: 50.8
|
374 |
+
- task:
|
375 |
+
type: Natural language inference
|
376 |
+
dataset:
|
377 |
+
type: xnli
|
378 |
+
name: XNLI (vi)
|
379 |
+
config: vi
|
380 |
+
split: validation
|
381 |
+
revision: a5a45e4ff92d5d3f34de70aaf4b72c3bdf9f7f16
|
382 |
+
metrics:
|
383 |
+
- type: Accuracy
|
384 |
+
value: 61.0
|
385 |
+
- task:
|
386 |
+
type: Natural language inference
|
387 |
+
dataset:
|
388 |
+
type: xnli
|
389 |
+
name: XNLI (zh)
|
390 |
+
config: zh
|
391 |
+
split: validation
|
392 |
+
revision: a5a45e4ff92d5d3f34de70aaf4b72c3bdf9f7f16
|
393 |
+
metrics:
|
394 |
+
- type: Accuracy
|
395 |
+
value: 56.91
|
396 |
+
- task:
|
397 |
+
type: Program synthesis
|
398 |
+
dataset:
|
399 |
+
type: openai_humaneval
|
400 |
+
name: HumanEval
|
401 |
+
config: None
|
402 |
+
split: test
|
403 |
+
revision: e8dc562f5de170c54b5481011dd9f4fa04845771
|
404 |
+
metrics:
|
405 |
+
- type: Pass@1
|
406 |
+
value: 12.06
|
407 |
+
- type: Pass@10
|
408 |
+
value: 26.53
|
409 |
+
- type: Pass@100
|
410 |
+
value: 48.44
|
411 |
+
- task:
|
412 |
+
type: Sentence completion
|
413 |
+
dataset:
|
414 |
+
type: story_cloze
|
415 |
+
name: StoryCloze (2016)
|
416 |
+
config: "2016"
|
417 |
+
split: validation
|
418 |
+
revision: e724c6f8cdf7c7a2fb229d862226e15b023ee4db
|
419 |
+
metrics:
|
420 |
+
- type: Accuracy
|
421 |
+
value: 96.26
|
422 |
+
- task:
|
423 |
+
type: Sentence completion
|
424 |
+
dataset:
|
425 |
+
type: super_glue
|
426 |
+
name: SuperGLUE (copa)
|
427 |
+
config: copa
|
428 |
+
split: validation
|
429 |
+
revision: 9e12063561e7e6c79099feb6d5a493142584e9e2
|
430 |
+
metrics:
|
431 |
+
- type: Accuracy
|
432 |
+
value: 91.0
|
433 |
+
- task:
|
434 |
+
type: Sentence completion
|
435 |
+
dataset:
|
436 |
+
type: xcopa
|
437 |
+
name: XCOPA (et)
|
438 |
+
config: et
|
439 |
+
split: validation
|
440 |
+
revision: 37f73c60fb123111fa5af5f9b705d0b3747fd187
|
441 |
+
metrics:
|
442 |
+
- type: Accuracy
|
443 |
+
value: 51.0
|
444 |
+
- task:
|
445 |
+
type: Sentence completion
|
446 |
+
dataset:
|
447 |
+
type: xcopa
|
448 |
+
name: XCOPA (ht)
|
449 |
+
config: ht
|
450 |
+
split: validation
|
451 |
+
revision: 37f73c60fb123111fa5af5f9b705d0b3747fd187
|
452 |
+
metrics:
|
453 |
+
- type: Accuracy
|
454 |
+
value: 58.0
|
455 |
+
- task:
|
456 |
+
type: Sentence completion
|
457 |
+
dataset:
|
458 |
+
type: xcopa
|
459 |
+
name: XCOPA (id)
|
460 |
+
config: id
|
461 |
+
split: validation
|
462 |
+
revision: 37f73c60fb123111fa5af5f9b705d0b3747fd187
|
463 |
+
metrics:
|
464 |
+
- type: Accuracy
|
465 |
+
value: 86.0
|
466 |
+
- task:
|
467 |
+
type: Sentence completion
|
468 |
+
dataset:
|
469 |
+
type: xcopa
|
470 |
+
name: XCOPA (it)
|
471 |
+
config: it
|
472 |
+
split: validation
|
473 |
+
revision: 37f73c60fb123111fa5af5f9b705d0b3747fd187
|
474 |
+
metrics:
|
475 |
+
- type: Accuracy
|
476 |
+
value: 74.0
|
477 |
+
- task:
|
478 |
+
type: Sentence completion
|
479 |
+
dataset:
|
480 |
+
type: xcopa
|
481 |
+
name: XCOPA (qu)
|
482 |
+
config: qu
|
483 |
+
split: validation
|
484 |
+
revision: 37f73c60fb123111fa5af5f9b705d0b3747fd187
|
485 |
+
metrics:
|
486 |
+
- type: Accuracy
|
487 |
+
value: 56.0
|
488 |
+
- task:
|
489 |
+
type: Sentence completion
|
490 |
+
dataset:
|
491 |
+
type: xcopa
|
492 |
+
name: XCOPA (sw)
|
493 |
+
config: sw
|
494 |
+
split: validation
|
495 |
+
revision: 37f73c60fb123111fa5af5f9b705d0b3747fd187
|
496 |
+
metrics:
|
497 |
+
- type: Accuracy
|
498 |
+
value: 64.0
|
499 |
+
- task:
|
500 |
+
type: Sentence completion
|
501 |
+
dataset:
|
502 |
+
type: xcopa
|
503 |
+
name: XCOPA (ta)
|
504 |
+
config: ta
|
505 |
+
split: validation
|
506 |
+
revision: 37f73c60fb123111fa5af5f9b705d0b3747fd187
|
507 |
+
metrics:
|
508 |
+
- type: Accuracy
|
509 |
+
value: 69.0
|
510 |
+
- task:
|
511 |
+
type: Sentence completion
|
512 |
+
dataset:
|
513 |
+
type: xcopa
|
514 |
+
name: XCOPA (th)
|
515 |
+
config: th
|
516 |
+
split: validation
|
517 |
+
revision: 37f73c60fb123111fa5af5f9b705d0b3747fd187
|
518 |
+
metrics:
|
519 |
+
- type: Accuracy
|
520 |
+
value: 58.0
|
521 |
+
- task:
|
522 |
+
type: Sentence completion
|
523 |
+
dataset:
|
524 |
+
type: xcopa
|
525 |
+
name: XCOPA (tr)
|
526 |
+
config: tr
|
527 |
+
split: validation
|
528 |
+
revision: 37f73c60fb123111fa5af5f9b705d0b3747fd187
|
529 |
+
metrics:
|
530 |
+
- type: Accuracy
|
531 |
+
value: 57.0
|
532 |
+
- task:
|
533 |
+
type: Sentence completion
|
534 |
+
dataset:
|
535 |
+
type: xcopa
|
536 |
+
name: XCOPA (vi)
|
537 |
+
config: vi
|
538 |
+
split: validation
|
539 |
+
revision: 37f73c60fb123111fa5af5f9b705d0b3747fd187
|
540 |
+
metrics:
|
541 |
+
- type: Accuracy
|
542 |
+
value: 87.0
|
543 |
+
- task:
|
544 |
+
type: Sentence completion
|
545 |
+
dataset:
|
546 |
+
type: xcopa
|
547 |
+
name: XCOPA (zh)
|
548 |
+
config: zh
|
549 |
+
split: validation
|
550 |
+
revision: 37f73c60fb123111fa5af5f9b705d0b3747fd187
|
551 |
+
metrics:
|
552 |
+
- type: Accuracy
|
553 |
+
value: 90.0
|
554 |
+
- task:
|
555 |
+
type: Sentence completion
|
556 |
+
dataset:
|
557 |
+
type: Muennighoff/xstory_cloze
|
558 |
+
name: XStoryCloze (ar)
|
559 |
+
config: ar
|
560 |
+
split: validation
|
561 |
+
revision: 8bb76e594b68147f1a430e86829d07189622b90d
|
562 |
+
metrics:
|
563 |
+
- type: Accuracy
|
564 |
+
value: 92.79
|
565 |
+
- task:
|
566 |
+
type: Sentence completion
|
567 |
+
dataset:
|
568 |
+
type: Muennighoff/xstory_cloze
|
569 |
+
name: XStoryCloze (es)
|
570 |
+
config: es
|
571 |
+
split: validation
|
572 |
+
revision: 8bb76e594b68147f1a430e86829d07189622b90d
|
573 |
+
metrics:
|
574 |
+
- type: Accuracy
|
575 |
+
value: 94.37
|
576 |
+
- task:
|
577 |
+
type: Sentence completion
|
578 |
+
dataset:
|
579 |
+
type: Muennighoff/xstory_cloze
|
580 |
+
name: XStoryCloze (eu)
|
581 |
+
config: eu
|
582 |
+
split: validation
|
583 |
+
revision: 8bb76e594b68147f1a430e86829d07189622b90d
|
584 |
+
metrics:
|
585 |
+
- type: Accuracy
|
586 |
+
value: 86.9
|
587 |
+
- task:
|
588 |
+
type: Sentence completion
|
589 |
+
dataset:
|
590 |
+
type: Muennighoff/xstory_cloze
|
591 |
+
name: XStoryCloze (hi)
|
592 |
+
config: hi
|
593 |
+
split: validation
|
594 |
+
revision: 8bb76e594b68147f1a430e86829d07189622b90d
|
595 |
+
metrics:
|
596 |
+
- type: Accuracy
|
597 |
+
value: 88.42
|
598 |
+
- task:
|
599 |
+
type: Sentence completion
|
600 |
+
dataset:
|
601 |
+
type: Muennighoff/xstory_cloze
|
602 |
+
name: XStoryCloze (id)
|
603 |
+
config: id
|
604 |
+
split: validation
|
605 |
+
revision: 8bb76e594b68147f1a430e86829d07189622b90d
|
606 |
+
metrics:
|
607 |
+
- type: Accuracy
|
608 |
+
value: 92.12
|
609 |
+
- task:
|
610 |
+
type: Sentence completion
|
611 |
+
dataset:
|
612 |
+
type: Muennighoff/xstory_cloze
|
613 |
+
name: XStoryCloze (my)
|
614 |
+
config: my
|
615 |
+
split: validation
|
616 |
+
revision: 8bb76e594b68147f1a430e86829d07189622b90d
|
617 |
+
metrics:
|
618 |
+
- type: Accuracy
|
619 |
+
value: 52.35
|
620 |
+
- task:
|
621 |
+
type: Sentence completion
|
622 |
+
dataset:
|
623 |
+
type: Muennighoff/xstory_cloze
|
624 |
+
name: XStoryCloze (ru)
|
625 |
+
config: ru
|
626 |
+
split: validation
|
627 |
+
revision: 8bb76e594b68147f1a430e86829d07189622b90d
|
628 |
+
metrics:
|
629 |
+
- type: Accuracy
|
630 |
+
value: 81.73
|
631 |
+
- task:
|
632 |
+
type: Sentence completion
|
633 |
+
dataset:
|
634 |
+
type: Muennighoff/xstory_cloze
|
635 |
+
name: XStoryCloze (sw)
|
636 |
+
config: sw
|
637 |
+
split: validation
|
638 |
+
revision: 8bb76e594b68147f1a430e86829d07189622b90d
|
639 |
+
metrics:
|
640 |
+
- type: Accuracy
|
641 |
+
value: 79.81
|
642 |
+
- task:
|
643 |
+
type: Sentence completion
|
644 |
+
dataset:
|
645 |
+
type: Muennighoff/xstory_cloze
|
646 |
+
name: XStoryCloze (te)
|
647 |
+
config: te
|
648 |
+
split: validation
|
649 |
+
revision: 8bb76e594b68147f1a430e86829d07189622b90d
|
650 |
+
metrics:
|
651 |
+
- type: Accuracy
|
652 |
+
value: 81.2
|
653 |
+
- task:
|
654 |
+
type: Sentence completion
|
655 |
+
dataset:
|
656 |
+
type: Muennighoff/xstory_cloze
|
657 |
+
name: XStoryCloze (zh)
|
658 |
+
config: zh
|
659 |
+
split: validation
|
660 |
+
revision: 8bb76e594b68147f1a430e86829d07189622b90d
|
661 |
+
metrics:
|
662 |
+
- type: Accuracy
|
663 |
+
value: 93.12
|
664 |
+
---
|
665 |
+
|
666 |
+
<!-- header start -->
|
667 |
+
<div style="width: 100%;">
|
668 |
+
<img src="https://i.imgur.com/EBdldam.jpg" alt="TheBlokeAI" style="width: 100%; min-width: 400px; display: block; margin: auto;">
|
669 |
+
</div>
|
670 |
+
<div style="display: flex; justify-content: space-between; width: 100%;">
|
671 |
+
<div style="display: flex; flex-direction: column; align-items: flex-start;">
|
672 |
+
<p><a href="https://discord.gg/theblokeai">Chat & support: my new Discord server</a></p>
|
673 |
+
</div>
|
674 |
+
<div style="display: flex; flex-direction: column; align-items: flex-end;">
|
675 |
+
<p><a href="https://www.patreon.com/TheBlokeAI">Want to contribute? TheBloke's Patreon page</a></p>
|
676 |
+
</div>
|
677 |
+
</div>
|
678 |
+
<!-- header end -->
|
679 |
+
|
680 |
+
# BigScience's BLOOMZ 176B GPTQ
|
681 |
+
|
682 |
+
These files are GPTQ 4bit model files for [BigScience's BLOOMZ](https://huggingface.co/bigscience/bloomz).
|
683 |
+
|
684 |
+
It is the result of quantising to 4bit using [AutoGPTQ](https://github.com/PanQiWei/AutoGPTQ).
|
685 |
+
|
686 |
+
**This is a BIG model! 2 x 80GB or 3 x 48GB GPUs are required**
|
687 |
+
|
688 |
+
## Important note: files must be joined before use
|
689 |
+
|
690 |
+
It is not currently possible to shard GPTQ files, therefore the model file is one single 100+GB file.
|
691 |
+
|
692 |
+
Huggingface Hub has a 50GB per-file limit. I have therefore been forced to split the file in to three parts for upload.
|
693 |
+
|
694 |
+
I did this using the simple *nix command `split`.
|
695 |
+
|
696 |
+
To join the files on any *nix system, run:
|
697 |
+
```
|
698 |
+
cat gptq_model-4bit--1g.split* > gptq_model-4bit--1g.safetensors
|
699 |
+
```
|
700 |
+
|
701 |
+
To join the files on Windows, open a Command Prompt and run:
|
702 |
+
```
|
703 |
+
COPY /B gptq_model-4bit--1g.splitaa + gptq_model-4bit--1g.splitab + gptq_model-4bit--1g.splitac gptq_model-4bit--1g.safetensors
|
704 |
+
```
|
705 |
+
|
706 |
+
The SHA256SUM of the joined file will be:
|
707 |
+
|
708 |
+
Once you have the joined file, you can safely delete `gptq_model-4bit--1g.split*`.
|
709 |
+
|
710 |
+
## Repositories available
|
711 |
+
|
712 |
+
* [4-bit GPTQ model for GPU inference](https://huggingface.co/TheBlokeAI/bloomz-175B-GPTQ)
|
713 |
+
* [Unquantised fp16 model in pytorch format, for GPU inference and for further conversions](https://huggingface.co/bigscience/bloomz)
|
714 |
+
|
715 |
+
## Two files provided - separate branches
|
716 |
+
|
717 |
+
- Main branch:
|
718 |
+
- Group Size = None
|
719 |
+
- Desc Act (act-order) = True
|
720 |
+
- This version will use the least possible VRAM, and should have higher inference performance in CUDA mode
|
721 |
+
- Branch `group_size_128g`:
|
722 |
+
- Group Size = 128g
|
723 |
+
- Desc Act (act-oder) = True
|
724 |
+
- This version will use more VRAM, which shouldn't be a problem as it shouldn't exceed 2 x 80GB or 3 x 48GB cards.
|
725 |
+
- However CUDA inference performance is likely to be a lot slower, possibly necessitating the use of Triton mode.
|
726 |
+
|
727 |
+
By default you will download the first file, unless you choose to download from branch `group_size_128g`.
|
728 |
+
|
729 |
+
## Prompt template: none
|
730 |
+
|
731 |
+
```
|
732 |
+
Translate to English: Je t’aime.
|
733 |
+
Translation:
|
734 |
+
```
|
735 |
+
|
736 |
+
## How to easily download and use this model in text-generation-webui
|
737 |
+
|
738 |
+
Please make sure you're using the latest version of text-generation-webui.
|
739 |
+
|
740 |
+
Note 1: this is a non-Llama model which cannot be used with ExLlama. Use Loader: AutoGPTQ.
|
741 |
+
|
742 |
+
Note 2: As described above, you must join the files after downloading and before loading in text-generation-webui.
|
743 |
+
|
744 |
+
1. Click the **Model tab**.
|
745 |
+
2. Under **Download custom model or LoRA**, enter `TheBloke/bloomz-176B-GPTQ`.
|
746 |
+
- If you would rather download the group_size 128g version, enter `TheBloke/bloomz-176B-GPTQ:group_size_128g`
|
747 |
+
3. Click **Download**.
|
748 |
+
4. The model will start downloading. Once it's finished it will say "Done". This is a huge model so it may take a while!
|
749 |
+
5. Now follow the steps described above to join the model to get a single `.safetensors` file.
|
750 |
+
6. Untick **Autoload model**
|
751 |
+
7. In the top left, click the refresh icon next to **Model**.
|
752 |
+
8. In the **Model** dropdown, choose the model you just downloaded: `bloomz-176B-GPTQ`
|
753 |
+
9. Make sure Loader is set to AutGPTQ.
|
754 |
+
10. This model cannot load on one GPU, so you should set **GPU Memory** accordingly.
|
755 |
+
- If using two 80GB GPUs, try: GPU0 = 60GB, GPU1 = 79GB
|
756 |
+
- If using three 48GB GPUs, try: GPU0 = 30GB, GPU1 = 47GB, GPU2 = 47GB
|
757 |
+
11. Click **Save settings** to save your settings, and then **Reload** to load the model.
|
758 |
+
12. The model will load, and is now ready for use!
|
759 |
+
13. Once you're ready, click the **Text Generation tab** and enter a prompt to get started!
|
760 |
+
|
761 |
+
## How to use this GPTQ model from Python code
|
762 |
+
|
763 |
+
First make sure you have [AutoGPTQ](https://github.com/PanQiWei/AutoGPTQ) installed:
|
764 |
+
|
765 |
+
`GITHUB_ACTIONS=true pip install auto-gptq`
|
766 |
+
|
767 |
+
Because this model has to be joined locally, you must first download it. Example download code:
|
768 |
+
|
769 |
+
```python
|
770 |
+
from huggingface_hub import snapshot_download
|
771 |
+
snapshot_download(repo_id="TheBloke/bloomz-176B-GPTQ",
|
772 |
+
local_dir="/workspace/models/bloomz-176GB-GPTQ",
|
773 |
+
local_dir_use_symlinks=False)
|
774 |
+
```
|
775 |
+
|
776 |
+
If you want to download the group_size 128g file instead, add `revision="group_size_128g"` to the above command.
|
777 |
+
|
778 |
+
Now join the three `split` files up as described above, to get a single `safetensors` file.
|
779 |
+
|
780 |
+
Then try the following example code:
|
781 |
+
|
782 |
+
```python
|
783 |
+
from transformers import AutoTokenizer, pipeline, logging
|
784 |
+
from auto_gptq import AutoGPTQForCausalLM, BaseQuantizeConfig
|
785 |
+
import argparse
|
786 |
+
|
787 |
+
# Use the local path you downloaded the model to and joined the split files in
|
788 |
+
model_name_or_path = "/workspace/models/bloomz-176GB-GPTQ"
|
789 |
+
model_basename = "gptq_model-4bit--1g"
|
790 |
+
|
791 |
+
use_triton = False
|
792 |
+
|
793 |
+
tokenizer = AutoTokenizer.from_pretrained(model_name_or_path, use_fast=True)
|
794 |
+
|
795 |
+
model = AutoGPTQForCausalLM.from_quantized(model_name_or_path,
|
796 |
+
model_basename=model_basename,
|
797 |
+
use_safetensors=True,
|
798 |
+
trust_remote_code=False,
|
799 |
+
device="cuda:0",
|
800 |
+
use_triton=use_triton,
|
801 |
+
quantize_config=None)
|
802 |
+
|
803 |
+
prompt = "Translate this to French: AI is the future of computing"
|
804 |
+
prompt_template=f'''{prompt}
|
805 |
+
Translation:
|
806 |
+
'''
|
807 |
+
|
808 |
+
print("\n\n*** Generate:")
|
809 |
+
|
810 |
+
input_ids = tokenizer(prompt_template, return_tensors='pt').input_ids.cuda()
|
811 |
+
output = model.generate(inputs=input_ids, temperature=0.7, max_new_tokens=512)
|
812 |
+
print(tokenizer.decode(output[0]))
|
813 |
+
|
814 |
+
# Inference can also be done using transformers' pipeline
|
815 |
+
|
816 |
+
# Prevent printing spurious transformers error when using pipeline with AutoGPTQ
|
817 |
+
logging.set_verbosity(logging.CRITICAL)
|
818 |
+
|
819 |
+
print("*** Pipeline:")
|
820 |
+
pipe = pipeline(
|
821 |
+
"text-generation",
|
822 |
+
model=model,
|
823 |
+
tokenizer=tokenizer,
|
824 |
+
max_new_tokens=512,
|
825 |
+
temperature=0.7,
|
826 |
+
top_p=0.95,
|
827 |
+
repetition_penalty=1.15
|
828 |
+
)
|
829 |
+
|
830 |
+
print(pipe(prompt_template)[0]['generated_text'])
|
831 |
+
```
|
832 |
+
|
833 |
+
## Provided files
|
834 |
+
|
835 |
+
## Main branch:
|
836 |
+
|
837 |
+
**gptq_model-4bit--1g.safetensors**
|
838 |
+
|
839 |
+
This will work with AutoGPTQ. It is untested with GPTQ-for-LLaMa. It will *not* work with ExLlama.
|
840 |
+
|
841 |
+
It was created with group_size none (-1) to reduce VRAM usage, and with --act-order (desc_act) to increase inference speed.
|
842 |
+
|
843 |
+
* `gptq_model-4bit-128g.safetensors`
|
844 |
+
* Works with AutoGPTQ in CUDA or Triton modes.
|
845 |
+
* Does NOT work with [ExLlama](https://github.com/turboderp/exllama) as it's not a Llama model.
|
846 |
+
* Works with GPTQ-for-LLaMa in CUDA mode. May have issues with GPTQ-for-LLaMa Triton mode.
|
847 |
+
* Works with text-generation-webui, including one-click-installers.
|
848 |
+
* Parameters: Groupsize = -1. Act Order / desc_act = True.
|
849 |
+
|
850 |
+
## Branch `group_size_128g`
|
851 |
+
|
852 |
+
**gptq_model-4bit-128g.safetensors**
|
853 |
+
|
854 |
+
This will work with AutoGPTQ. It is untested with GPTQ-for-LLaMa. It will *not* work with ExLlama.
|
855 |
+
|
856 |
+
It was created with both group_size 128g and --act-order (desc_act) for increased inference quality.
|
857 |
+
|
858 |
+
**Note** Using group_size + desc_act together can significantly lower performance in AutoGPTQ CUDA. You might want to try AutoGPTQ Triton mode instead (Linux only.)
|
859 |
+
|
860 |
+
* `gptq_model-4bit-128g.safetensors`
|
861 |
+
* Works with AutoGPTQ in CUDA or Triton modes.
|
862 |
+
* Does NOT work with [ExLlama](https://github.com/turboderp/exllama) as it's not a Llama model.
|
863 |
+
* Untested with GPTQ-for-LLaMa.
|
864 |
+
* Works with text-generation-webui, including one-click-installers.
|
865 |
+
* Parameters: Groupsize = 128. Act Order / desc_act = True.
|
866 |
+
|
867 |
+
<!-- footer start -->
|
868 |
+
## Discord
|
869 |
+
|
870 |
+
For further support, and discussions on these models and AI in general, join us at:
|
871 |
+
|
872 |
+
[TheBloke AI's Discord server](https://discord.gg/theblokeai)
|
873 |
+
|
874 |
+
## Thanks, and how to contribute.
|
875 |
+
|
876 |
+
Thanks to the [chirper.ai](https://chirper.ai) team!
|
877 |
+
|
878 |
+
I've had a lot of people ask if they can contribute. I enjoy providing models and helping people, and would love to be able to spend even more time doing it, as well as expanding into new projects like fine tuning/training.
|
879 |
+
|
880 |
+
If you're able and willing to contribute it will be most gratefully received and will help me to keep providing more models, and to start work on new AI projects.
|
881 |
+
|
882 |
+
Donaters will get priority support on any and all AI/LLM/model questions and requests, access to a private Discord room, plus other benefits.
|
883 |
+
|
884 |
+
* Patreon: https://patreon.com/TheBlokeAI
|
885 |
+
* Ko-Fi: https://ko-fi.com/TheBlokeAI
|
886 |
+
|
887 |
+
**Special thanks to**: Luke from CarbonQuill, Aemon Algiz, Dmitriy Samsonov.
|
888 |
+
|
889 |
+
**Patreon special mentions**: zynix , ya boyyy, Trenton Dambrowitz, Imad Khwaja, Alps Aficionado, chris gileta, John Detwiler, Willem Michiel, RoA, Mano Prime, Rainer Wilmers, Fred von Graf, Matthew Berman, Ghost , Nathan LeClaire, Iucharbius , Ai Maven, Illia Dulskyi, Joseph William Delisle, Space Cruiser, Lone Striker, Karl Bernard, Eugene Pentland, Greatston Gnanesh, Jonathan Leane, Randy H, Pierre Kircher, Willian Hasse, Stephen Murray, Alex , terasurfer , Edmond Seymore, Oscar Rangel, Luke Pendergrass, Asp the Wyvern, Junyu Yang, David Flickinger, Luke, Spiking Neurons AB, subjectnull, Pyrater, Nikolai Manek, senxiiz, Ajan Kanaga, Johann-Peter Hartmann, Artur Olbinski, Kevin Schuppel, Derek Yates, Kalila, K, Talal Aujan, Khalefa Al-Ahmad, Gabriel Puliatti, John Villwock, WelcomeToTheClub, Daniel P. Andersen, Preetika Verma, Deep Realms, Fen Risland, trip7s trip, webtim, Sean Connelly, Michael Levine, Chris McCloskey, biorpg, vamX, Viktor Bowallius, Cory Kujawski.
|
890 |
+
|
891 |
+
Thank you to all my generous patrons and donaters!
|
892 |
+
|
893 |
+
<!-- footer end -->
|
894 |
+
|
895 |
+
# Original model card: NousResearch's Redmond Hermes Coder
|
896 |
+
|
897 |
+
|
898 |
+
# Model Card: Redmond-Hermes-Coder 15B
|
899 |
+
|
900 |
+
|
901 |
+
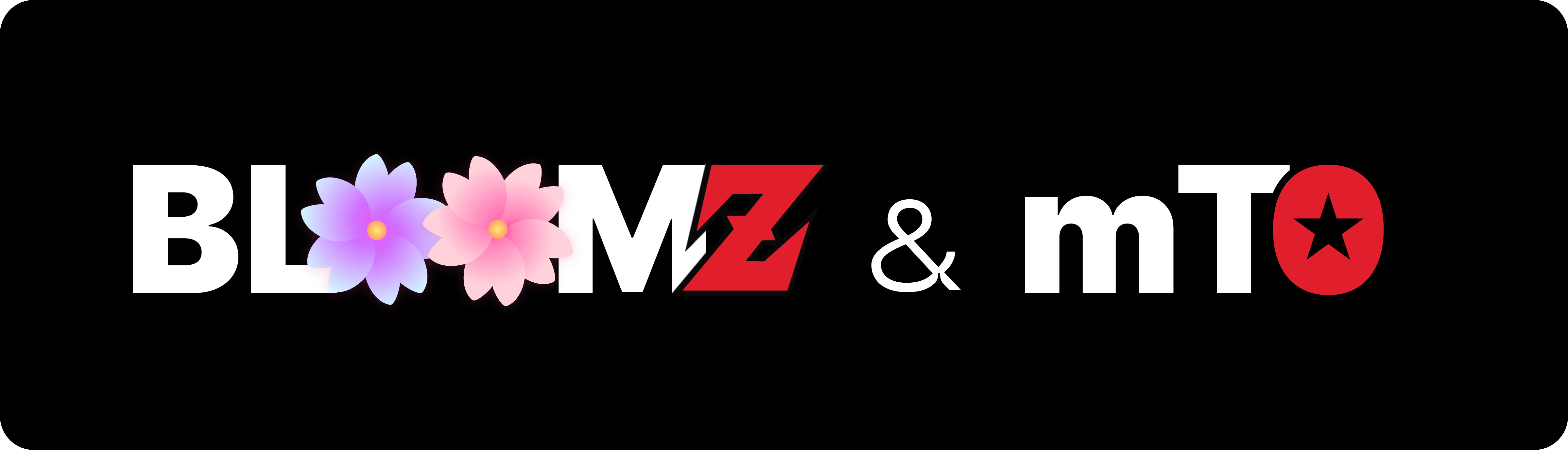
|
902 |
+
|
903 |
+
# Table of Contents
|
904 |
+
|
905 |
+
1. [Model Summary](#model-summary)
|
906 |
+
2. [Use](#use)
|
907 |
+
3. [Limitations](#limitations)
|
908 |
+
4. [Training](#training)
|
909 |
+
5. [Evaluation](#evaluation)
|
910 |
+
7. [Citation](#citation)
|
911 |
+
|
912 |
+
# Model Summary
|
913 |
+
|
914 |
+
> We present BLOOMZ & mT0, a family of models capable of following human instructions in dozens of languages zero-shot. We finetune BLOOM & mT5 pretrained multilingual language models on our crosslingual task mixture (xP3) and find the resulting models capable of crosslingual generalization to unseen tasks & languages.
|
915 |
+
|
916 |
+
- **Repository:** [bigscience-workshop/xmtf](https://github.com/bigscience-workshop/xmtf)
|
917 |
+
- **Paper:** [Crosslingual Generalization through Multitask Finetuning](https://arxiv.org/abs/2211.01786)
|
918 |
+
- **Point of Contact:** [Niklas Muennighoff](mailto:niklas@hf.co)
|
919 |
+
- **Languages:** Refer to [bloom](https://huggingface.co/bigscience/bloom) for pretraining & [xP3](https://huggingface.co/datasets/bigscience/xP3) for finetuning language proportions. It understands both pretraining & finetuning languages.
|
920 |
+
- **BLOOMZ & mT0 Model Family:**
|
921 |
+
|
922 |
+
<div class="max-w-full overflow-auto">
|
923 |
+
<table>
|
924 |
+
<tr>
|
925 |
+
<th colspan="12">Multitask finetuned on <a style="font-weight:bold" href=https://huggingface.co/datasets/bigscience/xP3>xP3</a>. Recommended for prompting in English.
|
926 |
+
</tr>
|
927 |
+
<tr>
|
928 |
+
<td>Parameters</td>
|
929 |
+
<td>300M</td>
|
930 |
+
<td>580M</td>
|
931 |
+
<td>1.2B</td>
|
932 |
+
<td>3.7B</td>
|
933 |
+
<td>13B</td>
|
934 |
+
<td>560M</td>
|
935 |
+
<td>1.1B</td>
|
936 |
+
<td>1.7B</td>
|
937 |
+
<td>3B</td>
|
938 |
+
<td>7.1B</td>
|
939 |
+
<td>176B</td>
|
940 |
+
</tr>
|
941 |
+
<tr>
|
942 |
+
<td>Finetuned Model</td>
|
943 |
+
<td><a href=https://huggingface.co/bigscience/mt0-small>mt0-small</a></td>
|
944 |
+
<td><a href=https://huggingface.co/bigscience/mt0-base>mt0-base</a></td>
|
945 |
+
<td><a href=https://huggingface.co/bigscience/mt0-large>mt0-large</a></td>
|
946 |
+
<td><a href=https://huggingface.co/bigscience/mt0-xl>mt0-xl</a></td>
|
947 |
+
<td><a href=https://huggingface.co/bigscience/mt0-xxl>mt0-xxl</a></td>
|
948 |
+
<td><a href=https://huggingface.co/bigscience/bloomz-560m>bloomz-560m</a></td>
|
949 |
+
<td><a href=https://huggingface.co/bigscience/bloomz-1b1>bloomz-1b1</a></td>
|
950 |
+
<td><a href=https://huggingface.co/bigscience/bloomz-1b7>bloomz-1b7</a></td>
|
951 |
+
<td><a href=https://huggingface.co/bigscience/bloomz-3b>bloomz-3b</a></td>
|
952 |
+
<td><a href=https://huggingface.co/bigscience/bloomz-7b1>bloomz-7b1</a></td>
|
953 |
+
<td><a href=https://huggingface.co/bigscience/bloomz>bloomz</a></td>
|
954 |
+
</tr>
|
955 |
+
</tr>
|
956 |
+
<tr>
|
957 |
+
<th colspan="12">Multitask finetuned on <a style="font-weight:bold" href=https://huggingface.co/datasets/bigscience/xP3mt>xP3mt</a>. Recommended for prompting in non-English.</th>
|
958 |
+
</tr>
|
959 |
+
<tr>
|
960 |
+
<td>Finetuned Model</td>
|
961 |
+
<td></td>
|
962 |
+
<td></td>
|
963 |
+
<td></td>
|
964 |
+
<td></td>
|
965 |
+
<td><a href=https://huggingface.co/bigscience/mt0-xxl-mt>mt0-xxl-mt</a></td>
|
966 |
+
<td></td>
|
967 |
+
<td></td>
|
968 |
+
<td></td>
|
969 |
+
<td></td>
|
970 |
+
<td><a href=https://huggingface.co/bigscience/bloomz-7b1-mt>bloomz-7b1-mt</a></td>
|
971 |
+
<td><a href=https://huggingface.co/bigscience/bloomz-mt>bloomz-mt</a></td>
|
972 |
+
</tr>
|
973 |
+
<th colspan="12">Multitask finetuned on <a style="font-weight:bold" href=https://huggingface.co/datasets/Muennighoff/P3>P3</a>. Released for research purposes only. Strictly inferior to above models!</th>
|
974 |
+
</tr>
|
975 |
+
<tr>
|
976 |
+
<td>Finetuned Model</td>
|
977 |
+
<td></td>
|
978 |
+
<td></td>
|
979 |
+
<td></td>
|
980 |
+
<td></td>
|
981 |
+
<td><a href=https://huggingface.co/bigscience/mt0-xxl-p3>mt0-xxl-p3</a></td>
|
982 |
+
<td></td>
|
983 |
+
<td></td>
|
984 |
+
<td></td>
|
985 |
+
<td></td>
|
986 |
+
<td><a href=https://huggingface.co/bigscience/bloomz-7b1-p3>bloomz-7b1-p3</a></td>
|
987 |
+
<td><a href=https://huggingface.co/bigscience/bloomz-p3>bloomz-p3</a></td>
|
988 |
+
</tr>
|
989 |
+
<th colspan="12">Original pretrained checkpoints. Not recommended.</th>
|
990 |
+
<tr>
|
991 |
+
<td>Pretrained Model</td>
|
992 |
+
<td><a href=https://huggingface.co/google/mt5-small>mt5-small</a></td>
|
993 |
+
<td><a href=https://huggingface.co/google/mt5-base>mt5-base</a></td>
|
994 |
+
<td><a href=https://huggingface.co/google/mt5-large>mt5-large</a></td>
|
995 |
+
<td><a href=https://huggingface.co/google/mt5-xl>mt5-xl</a></td>
|
996 |
+
<td><a href=https://huggingface.co/google/mt5-xxl>mt5-xxl</a></td>
|
997 |
+
<td><a href=https://huggingface.co/bigscience/bloom-560m>bloom-560m</a></td>
|
998 |
+
<td><a href=https://huggingface.co/bigscience/bloom-1b1>bloom-1b1</a></td>
|
999 |
+
<td><a href=https://huggingface.co/bigscience/bloom-1b7>bloom-1b7</a></td>
|
1000 |
+
<td><a href=https://huggingface.co/bigscience/bloom-3b>bloom-3b</a></td>
|
1001 |
+
<td><a href=https://huggingface.co/bigscience/bloom-7b1>bloom-7b1</a></td>
|
1002 |
+
<td><a href=https://huggingface.co/bigscience/bloom>bloom</a></td>
|
1003 |
+
</tr>
|
1004 |
+
</table>
|
1005 |
+
</div>
|
1006 |
+
|
1007 |
+
|
1008 |
+
# Use
|
1009 |
+
|
1010 |
+
## Intended use
|
1011 |
+
|
1012 |
+
We recommend using the model to perform tasks expressed in natural language. For example, given the prompt "*Translate to English: Je t’aime.*", the model will most likely answer "*I love you.*". Some prompt ideas from our paper:
|
1013 |
+
- 一个传奇的开端,一个不灭的神话,这不仅仅是一部电影,而是作为一个走进新时代的标签,永远彪炳史册。你认为这句话的立场是赞扬、中立还是批评?
|
1014 |
+
- Suggest at least five related search terms to "Mạng neural nhân tạo".
|
1015 |
+
- Write a fairy tale about a troll saving a princess from a dangerous dragon. The fairy tale is a masterpiece that has achieved praise worldwide and its moral is "Heroes Come in All Shapes and Sizes". Story (in Spanish):
|
1016 |
+
- Explain in a sentence in Telugu what is backpropagation in neural networks.
|
1017 |
+
|
1018 |
+
**Feel free to share your generations in the Community tab!**
|
1019 |
+
|
1020 |
+
## How to use
|
1021 |
+
|
1022 |
+
### CPU
|
1023 |
+
|
1024 |
+
<details>
|
1025 |
+
<summary> Click to expand </summary>
|
1026 |
+
|
1027 |
+
```python
|
1028 |
+
# pip install -q transformers
|
1029 |
+
from transformers import AutoModelForCausalLM, AutoTokenizer
|
1030 |
+
|
1031 |
+
checkpoint = "bigscience/bloomz"
|
1032 |
+
|
1033 |
+
tokenizer = AutoTokenizer.from_pretrained(checkpoint)
|
1034 |
+
model = AutoModelForCausalLM.from_pretrained(checkpoint)
|
1035 |
+
|
1036 |
+
inputs = tokenizer.encode("Translate to English: Je t’aime.", return_tensors="pt")
|
1037 |
+
outputs = model.generate(inputs)
|
1038 |
+
print(tokenizer.decode(outputs[0]))
|
1039 |
+
```
|
1040 |
+
|
1041 |
+
</details>
|
1042 |
+
|
1043 |
+
### GPU
|
1044 |
+
|
1045 |
+
<details>
|
1046 |
+
<summary> Click to expand </summary>
|
1047 |
+
|
1048 |
+
```python
|
1049 |
+
# pip install -q transformers accelerate
|
1050 |
+
from transformers import AutoModelForCausalLM, AutoTokenizer
|
1051 |
+
|
1052 |
+
checkpoint = "bigscience/bloomz"
|
1053 |
+
|
1054 |
+
tokenizer = AutoTokenizer.from_pretrained(checkpoint)
|
1055 |
+
model = AutoModelForCausalLM.from_pretrained(checkpoint, torch_dtype="auto", device_map="auto")
|
1056 |
+
|
1057 |
+
inputs = tokenizer.encode("Translate to English: Je t’aime.", return_tensors="pt").to("cuda")
|
1058 |
+
outputs = model.generate(inputs)
|
1059 |
+
print(tokenizer.decode(outputs[0]))
|
1060 |
+
```
|
1061 |
+
|
1062 |
+
</details>
|
1063 |
+
|
1064 |
+
### GPU in 8bit
|
1065 |
+
|
1066 |
+
<details>
|
1067 |
+
<summary> Click to expand </summary>
|
1068 |
+
|
1069 |
+
```python
|
1070 |
+
# pip install -q transformers accelerate bitsandbytes
|
1071 |
+
from transformers import AutoModelForCausalLM, AutoTokenizer
|
1072 |
+
|
1073 |
+
checkpoint = "bigscience/bloomz"
|
1074 |
+
|
1075 |
+
tokenizer = AutoTokenizer.from_pretrained(checkpoint)
|
1076 |
+
model = AutoModelForCausalLM.from_pretrained(checkpoint, device_map="auto", load_in_8bit=True)
|
1077 |
+
|
1078 |
+
inputs = tokenizer.encode("Translate to English: Je t’aime.", return_tensors="pt").to("cuda")
|
1079 |
+
outputs = model.generate(inputs)
|
1080 |
+
print(tokenizer.decode(outputs[0]))
|
1081 |
+
```
|
1082 |
+
|
1083 |
+
</details>
|
1084 |
+
|
1085 |
+
<!-- Necessary for whitespace -->
|
1086 |
+
###
|
1087 |
+
|
1088 |
+
# Limitations
|
1089 |
+
|
1090 |
+
**Prompt Engineering:** The performance may vary depending on the prompt. For BLOOMZ models, we recommend making it very clear when the input stops to avoid the model trying to continue it. For example, the prompt "*Translate to English: Je t'aime*" without the full stop (.) at the end, may result in the model trying to continue the French sentence. Better prompts are e.g. "*Translate to English: Je t'aime.*", "*Translate to English: Je t'aime. Translation:*" "*What is "Je t'aime." in English?*", where it is clear for the model when it should answer. Further, we recommend providing the model as much context as possible. For example, if you want it to answer in Telugu, then tell the model, e.g. "*Explain in a sentence in Telugu what is backpropagation in neural networks.*".
|
1091 |
+
|
1092 |
+
# Training
|
1093 |
+
|
1094 |
+
## Model
|
1095 |
+
|
1096 |
+
- **Architecture:** Same as [bloom](https://huggingface.co/bigscience/bloom), also refer to the `config.json` file
|
1097 |
+
- **Finetuning steps:** 498
|
1098 |
+
- **Finetuning tokens:** 2.09 billion
|
1099 |
+
- **Finetuning layout:** 72x pipeline parallel, 1x tensor parallel, 4x data parallel
|
1100 |
+
- **Precision:** bfloat16
|
1101 |
+
|
1102 |
+
## Hardware
|
1103 |
+
|
1104 |
+
- **CPUs:** AMD CPUs with 512GB memory per node
|
1105 |
+
- **GPUs:** 288 A100 80GB GPUs with 8 GPUs per node (36 nodes) using NVLink 4 inter-gpu connects, 4 OmniPath links
|
1106 |
+
- **Communication:** NCCL-communications network with a fully dedicated subnet
|
1107 |
+
|
1108 |
+
## Software
|
1109 |
+
|
1110 |
+
- **Orchestration:** [Megatron-DeepSpeed](https://github.com/bigscience-workshop/Megatron-DeepSpeed)
|
1111 |
+
- **Optimizer & parallelism:** [DeepSpeed](https://github.com/microsoft/DeepSpeed)
|
1112 |
+
- **Neural networks:** [PyTorch](https://github.com/pytorch/pytorch) (pytorch-1.11 w/ CUDA-11.5)
|
1113 |
+
- **FP16 if applicable:** [apex](https://github.com/NVIDIA/apex)
|
1114 |
+
|
1115 |
+
# Evaluation
|
1116 |
+
|
1117 |
+
We refer to Table 7 from our [paper](https://arxiv.org/abs/2211.01786) & [bigscience/evaluation-results](https://huggingface.co/datasets/bigscience/evaluation-results) for zero-shot results on unseen tasks. The sidebar reports zero-shot performance of the best prompt per dataset config.
|
1118 |
+
|
1119 |
+
# Citation
|
1120 |
+
```bibtex
|
1121 |
+
@article{muennighoff2022crosslingual,
|
1122 |
+
title={Crosslingual generalization through multitask finetuning},
|
1123 |
+
author={Muennighoff, Niklas and Wang, Thomas and Sutawika, Lintang and Roberts, Adam and Biderman, Stella and Scao, Teven Le and Bari, M Saiful and Shen, Sheng and Yong, Zheng-Xin and Schoelkopf, Hailey and others},
|
1124 |
+
journal={arXiv preprint arXiv:2211.01786},
|
1125 |
+
year={2022}
|
1126 |
+
}
|
1127 |
+
```
|