Upload README.md
Browse files
README.md
CHANGED
@@ -45,7 +45,9 @@ quantized_by: TheBloke
|
|
45 |
tags:
|
46 |
- llama
|
47 |
- llama2
|
|
|
48 |
---
|
|
|
49 |
|
50 |
<!-- header start -->
|
51 |
<!-- 200823 -->
|
@@ -131,10 +133,12 @@ These quantised GGUFv2 files are compatible with llama.cpp from August 27th onwa
|
|
131 |
They are also compatible with many third party UIs and libraries - please see the list at the top of this README.
|
132 |
|
133 |
## Explanation of quantisation methods
|
|
|
134 |
<details>
|
135 |
<summary>Click to see details</summary>
|
136 |
|
137 |
The new methods available are:
|
|
|
138 |
* GGML_TYPE_Q2_K - "type-1" 2-bit quantization in super-blocks containing 16 blocks, each block having 16 weight. Block scales and mins are quantized with 4 bits. This ends up effectively using 2.5625 bits per weight (bpw)
|
139 |
* GGML_TYPE_Q3_K - "type-0" 3-bit quantization in super-blocks containing 16 blocks, each block having 16 weights. Scales are quantized with 6 bits. This end up using 3.4375 bpw.
|
140 |
* GGML_TYPE_Q4_K - "type-1" 4-bit quantization in super-blocks containing 8 blocks, each block having 32 weights. Scales and mins are quantized with 6 bits. This ends up using 4.5 bpw.
|
@@ -150,10 +154,10 @@ Refer to the Provided Files table below to see what files use which methods, and
|
|
150 |
|
151 |
| Name | Quant method | Bits | Size | Max RAM required | Use case |
|
152 |
| ---- | ---- | ---- | ---- | ---- | ----- |
|
153 |
-
| [causallm_14b.Q4_0.gguf](https://huggingface.co/TheBloke/CausalLM-14B-GGUF/blob/main/causallm_14b.Q4_0.gguf) | Q4_0 | 4 | 8.
|
154 |
| [causallm_14b.Q4_1.gguf](https://huggingface.co/TheBloke/CausalLM-14B-GGUF/blob/main/causallm_14b.Q4_1.gguf) | Q4_1 | 4 | 9.01 GB| 11.51 GB | legacy; small, substantial quality loss - lprefer using Q3_K_L |
|
155 |
| [causallm_14b.Q5_0.gguf](https://huggingface.co/TheBloke/CausalLM-14B-GGUF/blob/main/causallm_14b.Q5_0.gguf) | Q5_0 | 5 | 9.85 GB| 12.35 GB | legacy; medium, balanced quality - prefer using Q4_K_M |
|
156 |
-
| [causallm_14b.Q5_1.gguf](https://huggingface.co/TheBloke/CausalLM-14B-GGUF/blob/main/causallm_14b.Q5_1.gguf) | Q5_1 | 5 | 10.
|
157 |
| [causallm_14b.Q8_0.gguf](https://huggingface.co/TheBloke/CausalLM-14B-GGUF/blob/main/causallm_14b.Q8_0.gguf) | Q8_0 | 8 | 15.06 GB| 17.56 GB | very large, extremely low quality loss - not recommended |
|
158 |
|
159 |
**Note**: the above RAM figures assume no GPU offloading. If layers are offloaded to the GPU, this will reduce RAM usage and use VRAM instead.
|
@@ -168,9 +172,10 @@ Refer to the Provided Files table below to see what files use which methods, and
|
|
168 |
**Note for manual downloaders:** You almost never want to clone the entire repo! Multiple different quantisation formats are provided, and most users only want to pick and download a single file.
|
169 |
|
170 |
The following clients/libraries will automatically download models for you, providing a list of available models to choose from:
|
171 |
-
|
172 |
-
|
173 |
-
|
|
|
174 |
|
175 |
### In `text-generation-webui`
|
176 |
|
@@ -320,11 +325,20 @@ And thank you again to a16z for their generous grant.
|
|
320 |
|
321 |
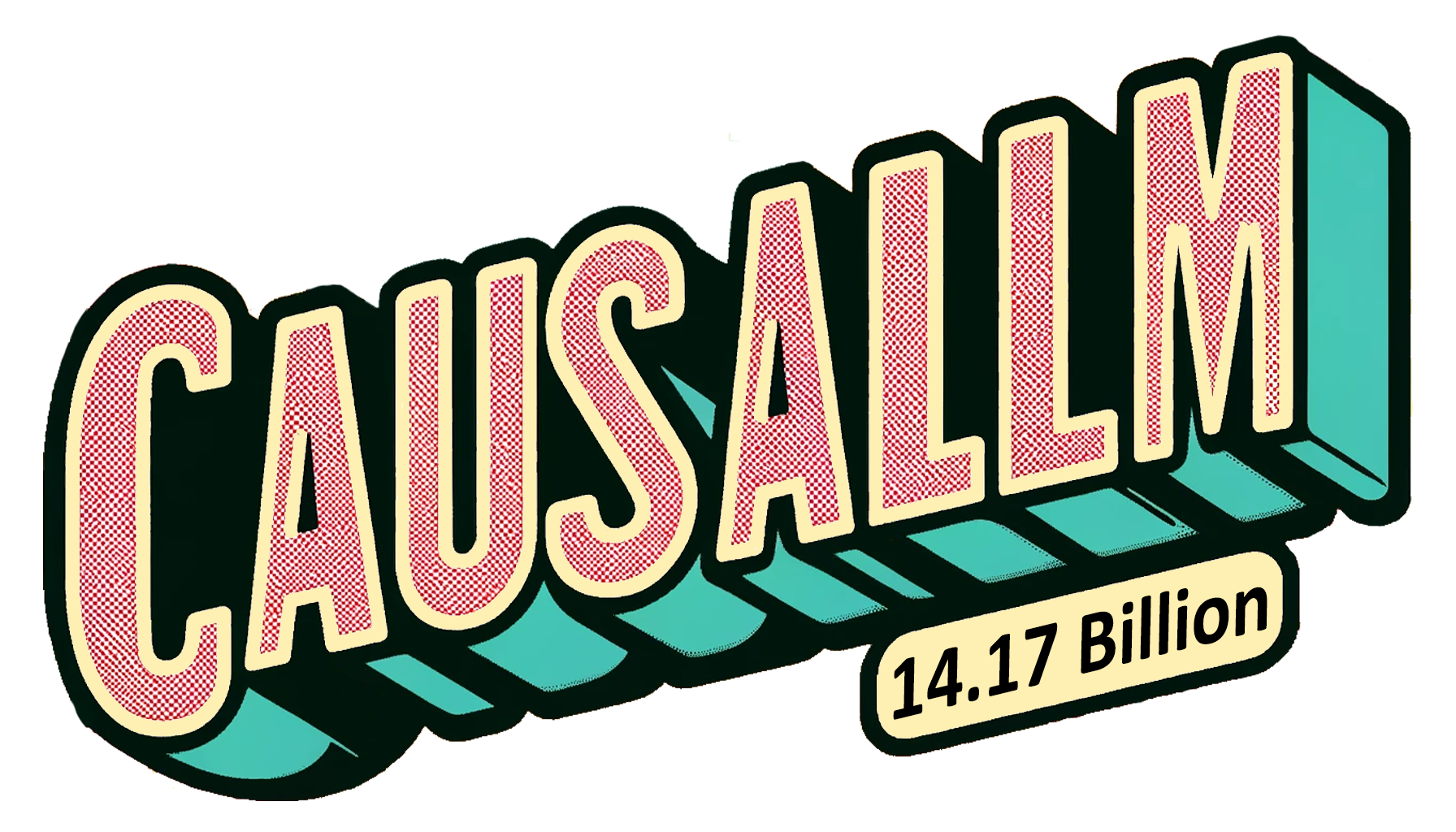
|
322 |
|
|
|
|
|
|
|
|
|
|
|
|
|
|
|
|
|
|
|
323 |
# Read Me:
|
324 |
|
325 |
Also see [7B Version](https://huggingface.co/CausalLM/7B)
|
326 |
|
327 |
-
This model was trained based on the model weights of Qwen and LLaMA2. The training process utilized a model structure that was identical to LLaMA2, using the same attention calculation method as the original MHA LLaMA2 models, and no additional scaling applied to the Relative Positional Encoding (RoPE).
|
328 |
|
329 |
We manually curated a SFT dataset of 1.3B tokens for training, utilizing open source datasets from Hugging Face. For most of these sentences, we performed manual or synthetic rewrites and generated alternate language versions using larger language models. Additionally, we conducted augmented text training using carefully selected entries from Wikipedia, as well as featured entries from Fandom and filtered entries from Moegirlpedia. In order to strike a balance between efficiency and quality, 100% of the data used for training was synthetic data, no direct use of text from the internet or original texts from publicly available datasets was employed for fine-tuning.
|
330 |
|
@@ -348,7 +362,7 @@ other ACC: 71.64
|
|
348 |
|
349 |
social ACC: 75.37
|
350 |
|
351 |
-
**AVERAGE ACC:67.36**
|
352 |
|
353 |
|
354 |
## CEval (Val):
|
@@ -362,10 +376,78 @@ Other ACC: 70.23
|
|
362 |
|
363 |
Hard ACC:54.71
|
364 |
|
365 |
-
**AVERAGE ACC:73.10**
|
366 |
|
367 |
## GSM8K
|
368 |
|
369 |
-
**Zero-shot ACC 0.7012888551933283**
|
|
|
|
|
|
|
|
|
|
|
|
|
|
|
|
|
|
|
|
|
|
|
|
|
|
|
|
|
|
|
|
|
|
|
|
|
|
|
|
|
|
|
|
|
|
|
|
|
|
|
|
|
|
|
|
|
|
|
|
|
|
|
|
|
|
|
|
|
|
|
|
|
|
|
|
|
|
|
|
|
|
|
|
|
|
|
|
|
|
|
|
|
|
|
|
|
|
|
|
|
|
|
|
|
|
|
|
|
|
|
|
|
|
|
|
|
|
|
|
|
|
|
|
|
|
|
|
|
|
|
|
|
|
|
|
|
370 |
|
371 |
<!-- original-model-card end -->
|
|
|
45 |
tags:
|
46 |
- llama
|
47 |
- llama2
|
48 |
+
- qwen
|
49 |
---
|
50 |
+
<!-- markdownlint-disable MD041 -->
|
51 |
|
52 |
<!-- header start -->
|
53 |
<!-- 200823 -->
|
|
|
133 |
They are also compatible with many third party UIs and libraries - please see the list at the top of this README.
|
134 |
|
135 |
## Explanation of quantisation methods
|
136 |
+
|
137 |
<details>
|
138 |
<summary>Click to see details</summary>
|
139 |
|
140 |
The new methods available are:
|
141 |
+
|
142 |
* GGML_TYPE_Q2_K - "type-1" 2-bit quantization in super-blocks containing 16 blocks, each block having 16 weight. Block scales and mins are quantized with 4 bits. This ends up effectively using 2.5625 bits per weight (bpw)
|
143 |
* GGML_TYPE_Q3_K - "type-0" 3-bit quantization in super-blocks containing 16 blocks, each block having 16 weights. Scales are quantized with 6 bits. This end up using 3.4375 bpw.
|
144 |
* GGML_TYPE_Q4_K - "type-1" 4-bit quantization in super-blocks containing 8 blocks, each block having 32 weights. Scales and mins are quantized with 6 bits. This ends up using 4.5 bpw.
|
|
|
154 |
|
155 |
| Name | Quant method | Bits | Size | Max RAM required | Use case |
|
156 |
| ---- | ---- | ---- | ---- | ---- | ----- |
|
157 |
+
| [causallm_14b.Q4_0.gguf](https://huggingface.co/TheBloke/CausalLM-14B-GGUF/blob/main/causallm_14b.Q4_0.gguf) | Q4_0 | 4 | 8.18 GB| 10.68 GB | legacy; small, very high quality loss - prefer using Q3_K_M |
|
158 |
| [causallm_14b.Q4_1.gguf](https://huggingface.co/TheBloke/CausalLM-14B-GGUF/blob/main/causallm_14b.Q4_1.gguf) | Q4_1 | 4 | 9.01 GB| 11.51 GB | legacy; small, substantial quality loss - lprefer using Q3_K_L |
|
159 |
| [causallm_14b.Q5_0.gguf](https://huggingface.co/TheBloke/CausalLM-14B-GGUF/blob/main/causallm_14b.Q5_0.gguf) | Q5_0 | 5 | 9.85 GB| 12.35 GB | legacy; medium, balanced quality - prefer using Q4_K_M |
|
160 |
+
| [causallm_14b.Q5_1.gguf](https://huggingface.co/TheBloke/CausalLM-14B-GGUF/blob/main/causallm_14b.Q5_1.gguf) | Q5_1 | 5 | 10.69 GB| 13.19 GB | legacy; medium, low quality loss - prefer using Q5_K_M |
|
161 |
| [causallm_14b.Q8_0.gguf](https://huggingface.co/TheBloke/CausalLM-14B-GGUF/blob/main/causallm_14b.Q8_0.gguf) | Q8_0 | 8 | 15.06 GB| 17.56 GB | very large, extremely low quality loss - not recommended |
|
162 |
|
163 |
**Note**: the above RAM figures assume no GPU offloading. If layers are offloaded to the GPU, this will reduce RAM usage and use VRAM instead.
|
|
|
172 |
**Note for manual downloaders:** You almost never want to clone the entire repo! Multiple different quantisation formats are provided, and most users only want to pick and download a single file.
|
173 |
|
174 |
The following clients/libraries will automatically download models for you, providing a list of available models to choose from:
|
175 |
+
|
176 |
+
* LM Studio
|
177 |
+
* LoLLMS Web UI
|
178 |
+
* Faraday.dev
|
179 |
|
180 |
### In `text-generation-webui`
|
181 |
|
|
|
325 |
|
326 |
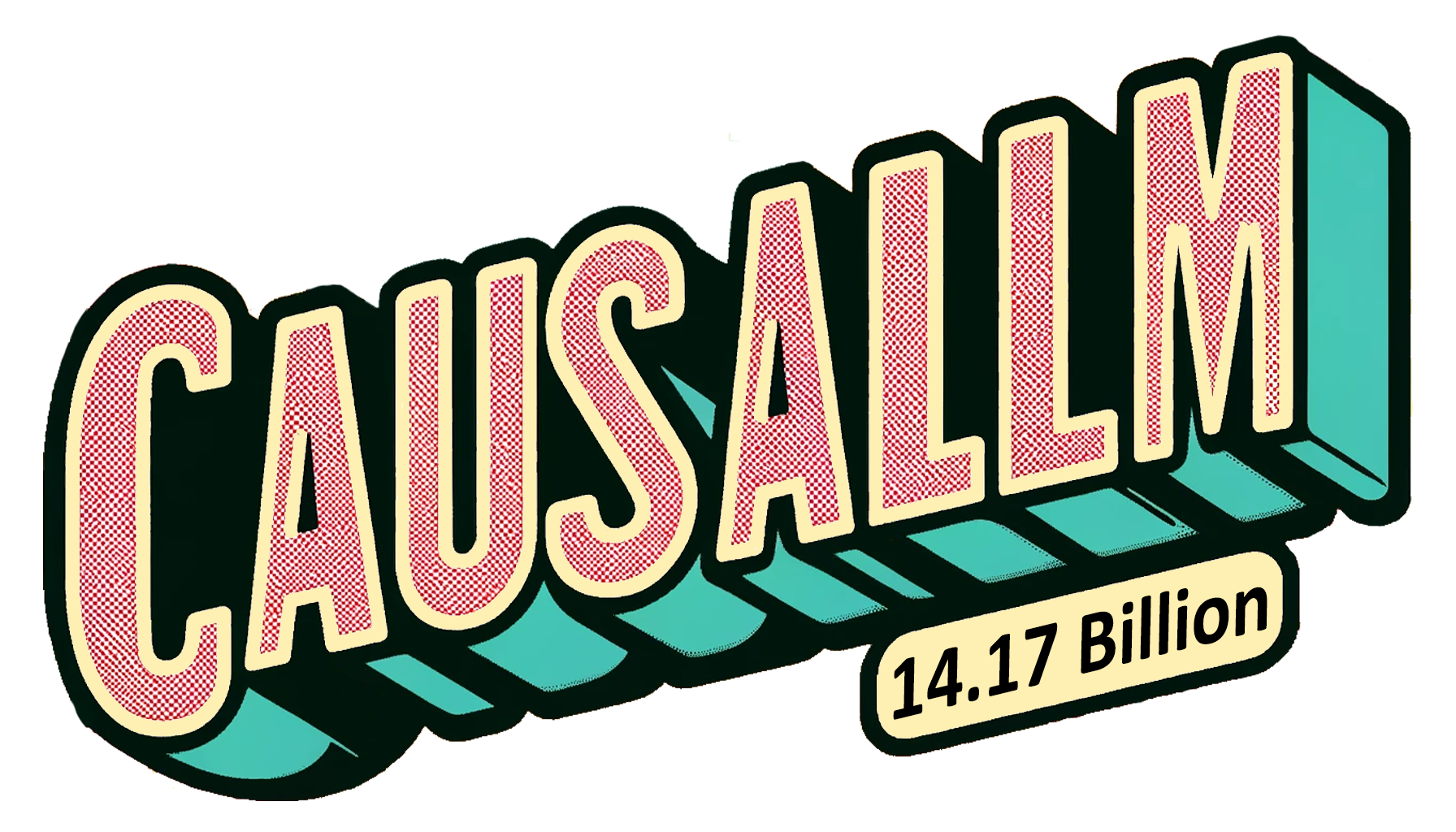
|
327 |
|
328 |
+
*Image drawn by GPT-4 DALL·E 3* TL;DR: Perhaps better than all existing models < 70B, in most quantitative evaluations...
|
329 |
+
|
330 |
+
# Please Stop Using WRONG unofficial quant models unless you know what you're doing
|
331 |
+
|
332 |
+
GPTQ quants require a good dataset for calibration, and the default C4 dataset is not capable.
|
333 |
+
|
334 |
+
**llama.cpp GGUF models**
|
335 |
+
GPT2Tokenizer fixed by [Kerfuffle](https://github.com/KerfuffleV2) on [https://github.com/ggerganov/llama.cpp/pull/3743](https://github.com/ggerganov/llama.cpp/pull/3743), new models to be reuploaded.
|
336 |
+
|
337 |
# Read Me:
|
338 |
|
339 |
Also see [7B Version](https://huggingface.co/CausalLM/7B)
|
340 |
|
341 |
+
This model was trained based on the model weights of Qwen (and LLaMA2 was used, yes, for calculating some initial weights), you may also need to comply with the commercial use restrictions of these two models depending on the situation. The training process utilized a model structure that was identical to LLaMA2, using the same attention calculation method as the original MHA LLaMA2 models, and no additional scaling applied to the Relative Positional Encoding (RoPE).
|
342 |
|
343 |
We manually curated a SFT dataset of 1.3B tokens for training, utilizing open source datasets from Hugging Face. For most of these sentences, we performed manual or synthetic rewrites and generated alternate language versions using larger language models. Additionally, we conducted augmented text training using carefully selected entries from Wikipedia, as well as featured entries from Fandom and filtered entries from Moegirlpedia. In order to strike a balance between efficiency and quality, 100% of the data used for training was synthetic data, no direct use of text from the internet or original texts from publicly available datasets was employed for fine-tuning.
|
344 |
|
|
|
362 |
|
363 |
social ACC: 75.37
|
364 |
|
365 |
+
**AVERAGE ACC:67.36** (Outperforms ALL models under 70B, very close to those best 70B fine-tunes)
|
366 |
|
367 |
|
368 |
## CEval (Val):
|
|
|
376 |
|
377 |
Hard ACC:54.71
|
378 |
|
379 |
+
**AVERAGE ACC:73.10** (Outperforms Qwen-14B, and GPT-4)
|
380 |
|
381 |
## GSM8K
|
382 |
|
383 |
+
**Zero-shot ACC 0.7012888551933283** (Outperforms MetaMath-13B, Qwen-14B)
|
384 |
+
|
385 |
+
## AlpacaEval Leaderboard
|
386 |
+
| | win_rate | standard_error | n_wins | n_wins_base | n_draws | n_total | mode | avg_length |
|
387 |
+
| ------------ | -------- | -------------- | ------ | ----------- | ------- | ------- | --------- | ---------- |
|
388 |
+
| causallm-14b | **88.26087** | 1.116333 | 705 | 89 | 11 | 805 | community | 1391 |
|
389 |
+
|
390 |
+
|
391 |
+
Win rate **88.26%** on [AlpacaEval Leaderboard](https://tatsu-lab.github.io/alpaca_eval/) [view raw](https://github.com/tatsu-lab/alpaca_eval/blob/3a47dcd81c56f6a8e6a5711f2754013919fbe90a/results/causallm-14b/model_outputs.json)
|
392 |
+
|
393 |
+
**GPT2Tokenizer 上的 llama.cpp 存在一些问题,会尽快修复...**
|
394 |
+
|
395 |
+
**llama.cpp GGUF models**
|
396 |
+
GPT2Tokenizer 支持由 [Kerfuffle](https://github.com/KerfuffleV2) 修复于 [https://github.com/ggerganov/llama.cpp/pull/3743](https://github.com/ggerganov/llama.cpp/pull/3743),新模型稍后上传。
|
397 |
+
|
398 |
+
## 请读我:
|
399 |
+
|
400 |
+
另请参阅[7B版本](https://huggingface.co/CausalLM/7B)
|
401 |
+
|
402 |
+
该模型是基于Qwen的权重(并使用了LLaMA2权重,是的,用于计算一些权重初始化),您根据情况可能还需要遵守这两个模型的商业使用限制。训练过程中使用了与LLaMA2相同的模型结构,使用原始MHA LLaMA2模型的相同注意力计算方法,对相对位置编码(RoPE)没有进行额外的缩放。
|
403 |
+
|
404 |
+
我们手动筛选了一个包含13亿个标记的SFT数据集进行训练,利用了Hugging Face的开源数据集。对于大多数句子,我们进行了手动或合成改写,并使用更大的语言模型生成了其他语言版本。此外,我们还使用了精心挑选的来自维基百科的条目、来自Fandom的精选条目以及来自萌娘百科的过滤条目进行增强文本训练。为了在效率和质量之间取得平衡,训练所使用的100%数据都是合成数据,没有直接使用来自互联网或公开可用数据集的原始文本进行微调。
|
405 |
+
|
406 |
+
7B版本的模型是14B模型的精简版本,专门设计用于推测抽样。因此,在直接使用模型时,需要谨慎行事,因为它可能会产生幻觉或不可靠的输出。
|
407 |
+
|
408 |
+
请注意,模型是在未经过滤的互联网数据上进行训练的。由于我们无法审核所有数据,可能会出现大量不良内容、色情、暴力和冒犯性语言,我们无法删除这些内容。因此,您仍然需要对模型的安全性进行自己的检查,并对输出中的关键词进行过滤。由于计算资源的限制,我们目前无法为模型的伦理和安全实施RLHF,也无法对拒绝回答某些问题的SFT样本进行训练以进行限制性微调。
|
409 |
+
|
410 |
+
额外奖励:模型在LLaVA1.5中引入的提示格式上进行了一些微调,与图像注意力计算无关。因此,将ViT投影模块与冻结的LM对齐,并根据视觉指令实施快速实现有效的多模态能力。
|
411 |
+
|
412 |
+
## 提示格式:
|
413 |
+
[chatml](https://github.com/openai/openai-python/blob/main/chatml.md)
|
414 |
+
|
415 |
+
**系统提示不能为空!**
|
416 |
+
|
417 |
+
|
418 |
+
## MMLU:
|
419 |
+
STEM准确率:64.19
|
420 |
+
|
421 |
+
人文及艺术学科准确率:61.40
|
422 |
+
|
423 |
+
其他学科准确率:71.64
|
424 |
+
|
425 |
+
社会学科准确率:75.37
|
426 |
+
|
427 |
+
**平均准确率:67.36**(超过所有70B以下的模型,非常接近最佳70B微调模型)
|
428 |
+
|
429 |
+
## CEval(验证集):
|
430 |
+
STEM准确率:66.71
|
431 |
+
|
432 |
+
社会科学准确率:85.10
|
433 |
+
|
434 |
+
人文学科准确率:76.68
|
435 |
+
|
436 |
+
其他学科准确率:70.23
|
437 |
+
|
438 |
+
困难准确率:54.71
|
439 |
+
|
440 |
+
**平均准确率:73.10**(超过Qwen-14B和GPT-4)
|
441 |
+
|
442 |
+
## GSM8K
|
443 |
+
|
444 |
+
**零样本准确率0.7012888551933283**(超过MetaMath-13B和Qwen-14B)
|
445 |
+
|
446 |
+
## AlpacaEval Leaderboard
|
447 |
+
| | win_rate | standard_error | n_wins | n_wins_base | n_draws | n_total | mode | avg_length |
|
448 |
+
| ------------ | -------- | -------------- | ------ | ----------- | ------- | ------- | --------- | ---------- |
|
449 |
+
| causallm-14b | **88.26087** | 1.116333 | 705 | 89 | 11 | 805 | community | 1391 |
|
450 |
+
|
451 |
+
在 [AlpacaEval Leaderboard](https://tatsu-lab.github.io/alpaca_eval/) 胜率 **88.26%** [view raw](https://github.com/tatsu-lab/alpaca_eval/blob/3a47dcd81c56f6a8e6a5711f2754013919fbe90a/results/causallm-14b/model_outputs.json)
|
452 |
|
453 |
<!-- original-model-card end -->
|