Spaces:
Runtime error
Runtime error
from deepsparse import Pipeline | |
import time | |
import gradio as gr | |
markdownn = ''' | |
# Text Classification Pipeline with DeepSparse | |
Text Classification involves assigning a label to a given text. For example, sentiment analysis is an example of a text classification use case. | |
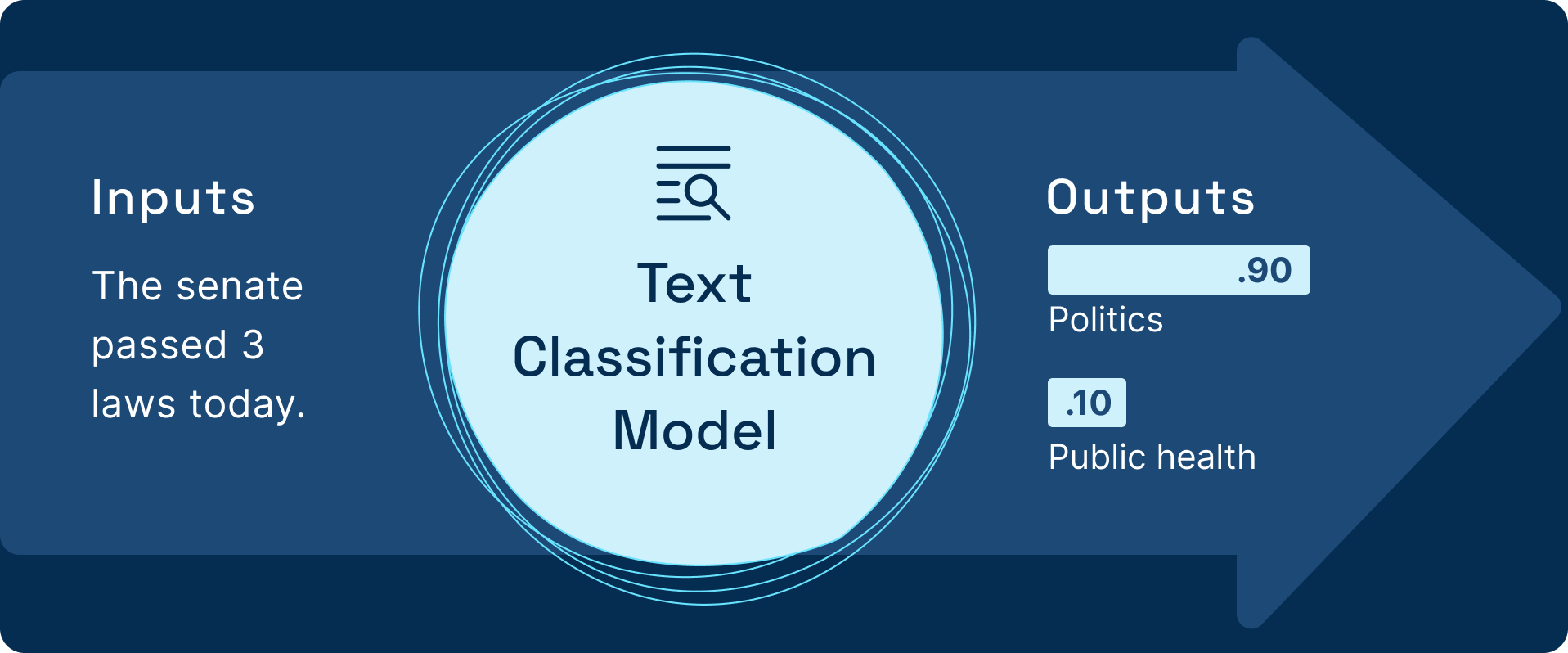 | |
## What is DeepSparse | |
DeepSparse is an inference runtime offering GPU-class performance on CPUs and APIs to integrate ML into your application. Sparsification is a powerful technique for optimizing models for inference, reducing the compute needed with a limited accuracy tradeoff. DeepSparse is designed to take advantage of model sparsity, enabling you to deploy models with the flexibility and scalability of software on commodity CPUs with the best-in-class performance of hardware accelerators, enabling you to standardize operations and reduce infrastructure costs. | |
Similar to Hugging Face, DeepSparse provides off-the-shelf pipelines for computer vision and NLP that wrap the model with proper pre- and post-processing to run performantly on CPUs by using sparse models. | |
The text classification Pipeline, for example, wraps an NLP model with the proper preprocessing and postprocessing pipelines, such as tokenization. | |
### Inference API Example | |
Here is sample code for a text classification pipeline: | |
``` | |
from deepsparse import Pipeline | |
pipeline = Pipeline.create(task="zero_shot_text_classification", model_path="zoo:nlp/text_classification/distilbert-none/pytorch/huggingface/mnli/pruned80_quant-none-vnni",model_scheme="mnli",model_config={"hypothesis_template": "This text is related to {}"},) | |
text = "The senate passed 3 laws today" | |
inference = pipeline(sequences= text,labels=['politics', 'public health', 'Europe'],) | |
print(inference) | |
``` | |
## Use Case Description | |
Customer review classification is a great example of text classification in action. | |
The ability to quickly classify sentiment from customers is an added advantage for any business. | |
Therefore, whichever solution you deploy for classifying the customer reviews should deliver results in the shortest time possible. | |
By being fast the solution will process more volume, hence cheaper computational resources are utilized. | |
When deploying a text classification model, decreasing the model’s latency and increasing its throughput is critical. This is why DeepSparse Pipelines have sparse text classification models. | |
[Want to train a sparse model on your data? Checkout the documentation on sparse transfer learning](https://docs.neuralmagic.com/use-cases/natural-language-processing/question-answering) | |
''' | |
task = "zero_shot_text_classification" | |
sparse_classification_pipeline = Pipeline.create( | |
task=task, | |
model_path="zoo:nlp/text_classification/distilbert-none/pytorch/huggingface/mnli/pruned80_quant-none-vnni", | |
model_scheme="mnli", | |
model_config={"hypothesis_template": "This text is related to {}"}, | |
) | |
def run_pipeline(text): | |
sparse_start = time.perf_counter() | |
sparse_output = sparse_classification_pipeline(sequences= text,labels=['politics', 'public health', 'Europe'],) | |
sparse_result = dict(sparse_output) | |
sparse_end = time.perf_counter() | |
sparse_duration = (sparse_end - sparse_start) * 1000.0 | |
dict_r = {sparse_result['labels'][0]:sparse_result['scores'][0],sparse_result['labels'][1]:sparse_result['scores'][1], sparse_result['labels'][2]:sparse_result['scores'][2]} | |
return dict_r, sparse_duration | |
with gr.Blocks() as demo: | |
with gr.Row(): | |
with gr.Column(): | |
gr.Markdown(markdownn) | |
with gr.Column(): | |
gr.Markdown(""" | |
### Text classification demo | |
""") | |
text = gr.Text(label="Text") | |
btn = gr.Button("Submit") | |
sparse_answers = gr.Label(label="Sparse model answers", | |
num_top_classes=3 | |
) | |
sparse_duration = gr.Number(label="Sparse Latency (ms):") | |
gr.Examples([["The senate passed 3 laws today"],["Who are you voting for in 2020?"],["Public health is very important"]],inputs=[text],) | |
btn.click( | |
run_pipeline, | |
inputs=[text], | |
outputs=[sparse_answers,sparse_duration], | |
) | |
if __name__ == "__main__": | |
demo.launch() |