Spaces:
Running
on
Zero
Running
on
Zero
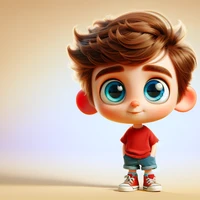
Fix: Ensure Object is Correctly Placed in Scene without Texturing when the texture is not provided
5a0d971
verified
import gradio as gr | |
import torch | |
from PIL import Image | |
from diffusers import PriorTransformer, UNet2DConditionModel, KandinskyV22Pipeline | |
from huggingface_hub import hf_hub_download | |
from transformers import CLIPVisionModelWithProjection, CLIPImageProcessor, CLIPTokenizer, CLIPTextModelWithProjection | |
from model import pops_utils | |
from model.pipeline_pops import pOpsPipeline | |
kandinsky_prior_repo: str = 'kandinsky-community/kandinsky-2-2-prior' | |
kandinsky_decoder_repo: str = 'kandinsky-community/kandinsky-2-2-decoder' | |
prior_texture_repo: str = 'models/texturing/learned_prior.pth' | |
prior_instruct_repo: str = 'models/instruct/learned_prior.pth' | |
prior_scene_repo: str = 'models/scene/learned_prior.pth' | |
prior_repo = "pOpsPaper/operators" | |
# gpu = torch.device('cuda') | |
# cpu = torch.device('cpu') | |
class PopsPipelines: | |
def __init__(self): | |
weight_dtype = torch.float16 | |
self.weight_dtype = weight_dtype | |
device = 'cpu' #torch.device("cuda" if torch.cuda.is_available() else "cpu") | |
self.device = 'cuda' #device | |
self.image_encoder = CLIPVisionModelWithProjection.from_pretrained(kandinsky_prior_repo, | |
subfolder='image_encoder', | |
torch_dtype=weight_dtype).eval() | |
self.image_encoder.requires_grad_(False) | |
self.image_processor = CLIPImageProcessor.from_pretrained(kandinsky_prior_repo, | |
subfolder='image_processor') | |
self.tokenizer = CLIPTokenizer.from_pretrained(kandinsky_prior_repo, subfolder='tokenizer') | |
self.text_encoder = CLIPTextModelWithProjection.from_pretrained(kandinsky_prior_repo, | |
subfolder='text_encoder', | |
torch_dtype=weight_dtype).eval().to(device) | |
# Load full model for vis | |
self.unet = UNet2DConditionModel.from_pretrained(kandinsky_decoder_repo, | |
subfolder='unet').to(torch.float16).to(device) | |
self.decoder = KandinskyV22Pipeline.from_pretrained(kandinsky_decoder_repo, unet=self.unet, | |
torch_dtype=torch.float16) | |
self.decoder = self.decoder.to(device) | |
self.priors_dict = { | |
'texturing':{'repo':prior_texture_repo}, | |
'instruct': {'repo': prior_instruct_repo}, | |
'scene': {'repo':prior_scene_repo} | |
} | |
for prior_type in self.priors_dict: | |
prior_path = self.priors_dict[prior_type]['repo'] | |
prior = PriorTransformer.from_pretrained( | |
kandinsky_prior_repo, subfolder="prior" | |
) | |
# Load from huggingface | |
prior_path = hf_hub_download(repo_id=prior_repo, filename=str(prior_path)) | |
prior_state_dict = torch.load(prior_path, map_location=device) | |
prior.load_state_dict(prior_state_dict, strict=False) | |
prior.eval() | |
prior = prior.to(weight_dtype) | |
prior_pipeline = pOpsPipeline.from_pretrained(kandinsky_prior_repo, | |
prior=prior, | |
image_encoder=self.image_encoder, | |
torch_dtype=torch.float16) | |
self.priors_dict[prior_type]['pipeline'] = prior_pipeline | |
def process_image(self, input_path): | |
if input_path is None: | |
return None | |
image_pil = Image.open(input_path).convert("RGB").resize((512, 512)) | |
image = torch.Tensor(self.image_processor(image_pil)['pixel_values'][0]).to(self.device).unsqueeze(0).to( | |
self.weight_dtype) | |
return image | |
def process_text(self, text): | |
self.text_encoder.to('cuda') | |
text_inputs = self.tokenizer( | |
text, | |
padding="max_length", | |
max_length=self.tokenizer.model_max_length, | |
truncation=True, | |
return_tensors="pt", | |
) | |
mask = text_inputs.attention_mask.bool() # [0] | |
text_encoder_output = self.text_encoder(text_inputs.input_ids.to(self.device)) | |
text_encoder_hidden_states = text_encoder_output.last_hidden_state | |
text_encoder_concat = text_encoder_hidden_states[:, :mask.sum().item()] | |
self.text_encoder.to('cpu') | |
return text_encoder_concat | |
def run_binary(self, input_a, input_b, prior_type): | |
# Move pipeline to GPU | |
pipeline = self.priors_dict[prior_type]['pipeline'] | |
pipeline.to('cuda') | |
self.image_encoder.to('cuda') | |
input_image_embeds, input_hidden_state = pops_utils.preprocess(input_a, input_b, | |
self.image_encoder, | |
pipeline.prior.clip_mean.detach(), | |
pipeline.prior.clip_std.detach()) | |
negative_input_embeds = torch.zeros_like(input_image_embeds) | |
negative_hidden_states = torch.zeros_like(input_hidden_state) | |
guidance_scale = 1.0 | |
if prior_type == 'texturing': | |
guidance_scale = 8.0 | |
img_emb = pipeline(input_embeds=input_image_embeds, input_hidden_states=input_hidden_state, | |
negative_input_embeds=negative_input_embeds, | |
negative_input_hidden_states=negative_hidden_states, | |
num_inference_steps=25, | |
num_images_per_prompt=1, | |
guidance_scale=guidance_scale) | |
# Optional | |
if prior_type == 'scene': | |
# Scene is the closet to what avg represents for a background image so incorporate that as well | |
mean_emb = 0.5 * input_hidden_state[:, 0] + 0.5 * input_hidden_state[:, 1] | |
mean_emb = (mean_emb * pipeline.prior.clip_std) + pipeline.prior.clip_mean | |
alpha = 0.4 | |
img_emb.image_embeds = (1 - alpha) * img_emb.image_embeds + alpha * mean_emb | |
# Move pipeline to CPU | |
pipeline.to('cpu') | |
self.image_encoder.to('cpu') | |
return img_emb | |
def run_instruct(self, input_a, text): | |
text_encodings = self.process_text(text) | |
# Move pipeline to GPU | |
instruct_pipeline = self.priors_dict['instruct']['pipeline'] | |
instruct_pipeline.to('cuda') | |
self.image_encoder.to('cuda') | |
input_image_embeds, input_hidden_state = pops_utils.preprocess(input_a, None, | |
self.image_encoder, | |
instruct_pipeline.prior.clip_mean.detach(), instruct_pipeline.prior.clip_std.detach(), | |
concat_hidden_states=text_encodings) | |
negative_input_embeds = torch.zeros_like(input_image_embeds) | |
negative_hidden_states = torch.zeros_like(input_hidden_state) | |
img_emb = instruct_pipeline(input_embeds=input_image_embeds, input_hidden_states=input_hidden_state, | |
negative_input_embeds=negative_input_embeds, | |
negative_input_hidden_states=negative_hidden_states, | |
num_inference_steps=25, | |
num_images_per_prompt=1, | |
guidance_scale=1.0) | |
# Move pipeline to CPU | |
instruct_pipeline.to('cpu') | |
self.image_encoder.to('cpu') | |
return img_emb | |
def render(self, img_emb): | |
self.decoder.to('cuda') | |
images = self.decoder(image_embeds=img_emb.image_embeds, negative_image_embeds=img_emb.negative_image_embeds, | |
num_inference_steps=50, height=512, | |
width=512, guidance_scale=4).images | |
self.decoder.to('cpu') | |
return images[0] | |
def run_instruct_texture(self, image_object_path, text_instruct, image_texture_path): | |
# Process both inputs | |
image_object = self.process_image(image_object_path) | |
image_texture = self.process_image(image_texture_path) | |
if image_object is None: | |
raise gr.Error('Object image is required') | |
current_emb = None | |
if image_texture is None: | |
instruct_input = image_object | |
else: | |
# Run texturing | |
current_emb = self.run_binary(input_a=image_object, input_b=image_texture,prior_type='texturing') | |
instruct_input = current_emb.image_embeds | |
if text_instruct != '': | |
current_emb = self.run_instruct(input_a=instruct_input, text=text_instruct) | |
if current_emb is None: | |
raise gr.Error('At least one of the inputs is required') | |
# Render as image | |
image = self.render(current_emb) | |
return image | |
def run_texture_scene(self, image_object_path, image_texture_path, image_scene_path): | |
image_object = self.process_image(image_object_path) | |
image_texture = self.process_image(image_texture_path) | |
image_scene = self.process_image(image_scene_path) | |
if image_object is None: | |
raise gr.Error('Object image is required') | |
current_emb = None | |
# If both object and scene images are provided, run scene processing | |
if image_scene is not None: | |
current_emb = self.run_binary(input_a=image_object, input_b=image_scene, prior_type='scene') | |
scene_input = current_emb.image_embeds | |
else: | |
scene_input = image_object | |
# If a texture image is provided, apply texturing | |
if image_texture is not None: | |
current_emb = self.run_binary(input_a=scene_input, input_b=image_texture, prior_type='texturing') | |
if current_emb is None: | |
raise gr.Error('At least one of the images is required') | |
# Render the final image | |
image = self.render(current_emb) | |
return image | |