Spaces:
Runtime error
Runtime error
#!/usr/bin/env python | |
from __future__ import annotations | |
import argparse | |
import os | |
import pathlib | |
import subprocess | |
if os.getenv("SYSTEM") == "spaces": | |
import mim | |
mim.uninstall("mmcv-full", confirm_yes=True) | |
mim.install("mmcv-full==1.6.1", is_yes=True) | |
subprocess.call("pip uninstall -y opencv-python".split()) | |
subprocess.call("pip uninstall -y opencv-python-headless".split()) | |
subprocess.call("pip install opencv-python-headless==4.5.5.64".split()) | |
subprocess.call("pip install package/mmdet_huggingface-2.25.1.tar.gz".split()) | |
import cv2 | |
import gradio as gr | |
import numpy as np | |
from model import AppModel | |
## Edit and | |
DESCRIPTION = """# MMDetection | |
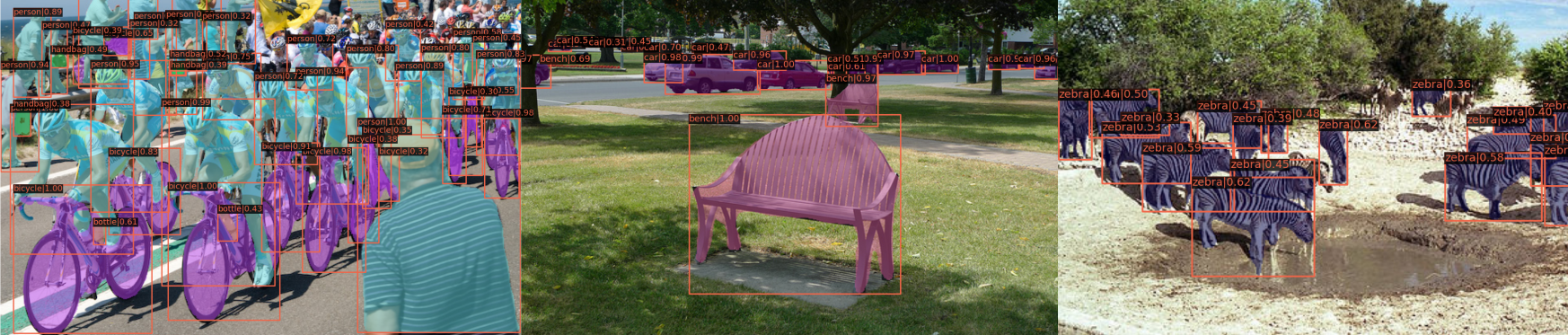 | |
*This is an unofficial demo for [https://github.com/open-mmlab/mmdetection](https://github.com/open-mmlab/mmdetection).* | |
With this demo, object detection using the Mask R-CNN & Faster R-CNN model can be performed. | |
You can upload image files, or use the example files below. | |
For more information on the Models, find some helpful resources below. | |
- Pretrained model from [OpenMMlab](https://github.com/open-mmlab/mmdetection) | |
- [Faster R-CNN Paper: Towards Real-Time Object | |
Detection with Region Proposal Networks](https://arxiv.org/pdf/1506.01497.pdf) | |
- [Mask R-CNN Paper](https://arxiv.org/pdf/1703.06870.pdf) | |
""" | |
FOOTER = '<img id="visitor-badge" src="https://visitor-badge.glitch.me/badge?page_id=hf-technical-mmdetection" alt="visitor badge" />' | |
DEFAULT_MODEL_TYPE = "detection" | |
DEFAULT_MODEL_NAMES = { | |
"detection": "faster_rcnn" | |
} | |
DEFAULT_MODEL_NAME = DEFAULT_MODEL_NAMES[DEFAULT_MODEL_TYPE] | |
def parse_args() -> argparse.Namespace: | |
parser = argparse.ArgumentParser() | |
parser.add_argument("--device", type=str, default="cpu") | |
parser.add_argument("--theme", type=str) | |
parser.add_argument("--share", action="store_true") | |
parser.add_argument("--port", type=int) | |
parser.add_argument("--disable-queue", dest="enable_queue", action="store_false") | |
return parser.parse_args() | |
def update_input_image(image: np.ndarray) -> dict: | |
if image is None: | |
return gr.Image.update(value=None) | |
scale = 1500 / max(image.shape[:2]) | |
if scale < 1: | |
image = cv2.resize(image, None, fx=scale, fy=scale) | |
return gr.Image.update(value=image) | |
def update_model_name(model_type: str) -> dict: | |
model_dict = getattr(AppModel, f"{model_type.upper()}_MODEL_DICT") | |
model_names = list(model_dict.keys()) | |
model_name = DEFAULT_MODEL_NAMES[model_type] | |
return gr.Dropdown.update(choices=model_names, value=model_name) | |
def update_visualization_score_threshold(model_type: str) -> dict: | |
return gr.Slider.update(visible=model_type != "panoptic_segmentation") | |
def update_redraw_button(model_type: str) -> dict: | |
return gr.Button.update(visible=model_type != "panoptic_segmentation") | |
def set_example_image(example: list) -> dict: | |
return gr.Image.update(value=example[0]) | |
def main(): | |
args = parse_args() | |
model = AppModel(DEFAULT_MODEL_NAME, args.device) | |
with gr.Blocks(theme=args.theme, css="style.css") as demo: | |
gr.Markdown(DESCRIPTION) | |
with gr.Row(): | |
with gr.Column(): | |
with gr.Row(): | |
input_image = gr.Image(label="Input Image", type="numpy") | |
with gr.Group(): | |
with gr.Row(): | |
model_type = gr.Radio( | |
list(DEFAULT_MODEL_NAMES.keys()), | |
value=DEFAULT_MODEL_TYPE, | |
label="Model Type", | |
) | |
with gr.Row(): | |
model_name = gr.Dropdown( | |
model.model_list(), | |
value=DEFAULT_MODEL_NAME, | |
label="Model", | |
) | |
with gr.Row(): | |
run_button = gr.Button(value="Run") | |
prediction_results = gr.Variable() | |
with gr.Column(): | |
with gr.Row(): | |
visualization = gr.Image(label="Result", type="numpy") | |
with gr.Row(): | |
visualization_score_threshold = gr.Slider( | |
0, | |
1, | |
step=0.05, | |
value=0.3, | |
label="Visualization Score Threshold", | |
) | |
with gr.Row(): | |
redraw_button = gr.Button(value="Redraw") | |
with gr.Row(): | |
paths = sorted(pathlib.Path("images").rglob("*.jpeg")) | |
example_images = gr.Dataset( | |
components=[input_image], samples=[[path.as_posix()] for path in paths] | |
) | |
gr.Markdown(FOOTER) | |
input_image.change( | |
fn=update_input_image, inputs=input_image, outputs=input_image | |
) | |
model_type.change(fn=update_model_name, inputs=model_type, outputs=model_name) | |
model_type.change( | |
fn=update_visualization_score_threshold, | |
inputs=model_type, | |
outputs=visualization_score_threshold, | |
) | |
model_type.change( | |
fn=update_redraw_button, inputs=model_type, outputs=redraw_button | |
) | |
model_name.change(fn=model.set_model, inputs=model_name, outputs=None) | |
run_button.click( | |
fn=model.run, | |
inputs=[ | |
model_name, | |
input_image, | |
visualization_score_threshold, | |
], | |
outputs=[ | |
prediction_results, | |
visualization, | |
], | |
) | |
redraw_button.click( | |
fn=model.visualize_detection_results, | |
inputs=[ | |
input_image, | |
prediction_results, | |
visualization_score_threshold, | |
], | |
outputs=visualization, | |
) | |
example_images.click( | |
fn=set_example_image, inputs=example_images, outputs=input_image | |
) | |
demo.launch( | |
enable_queue=args.enable_queue, | |
server_port=args.port, | |
share=args.share, | |
) | |
if __name__ == "__main__": | |
main() | |