This view is limited to 50 files because it contains too many changes.
See raw diff
- .gitignore +7 -0
- README.md +460 -7
- app.py +97 -0
- app1.py +87 -0
- data/__init__.py +114 -0
- data/base_dataset.py +231 -0
- data/image_folder.py +62 -0
- data/one_dataset.py +40 -0
- data/single_dataset.py +40 -0
- data/unaligned_dataset.py +72 -0
- detect.py +66 -0
- imgs/horse.jpg +0 -0
- imgs/monet.jpg +0 -0
- models/__init__.py +66 -0
- models/base_model.py +213 -0
- models/cycle_gan_model.py +170 -0
- models/networks.py +767 -0
- models/test_model.py +86 -0
- options/__init__.py +4 -0
- options/base_options.py +149 -0
- options/detect_options.py +25 -0
- options/test_options.py +28 -0
- options/train_options.py +42 -0
- requirements.txt +12 -0
- scripts/download_cyclegan_model.sh +11 -0
- scripts/test_before_push.py +51 -0
- scripts/test_cyclegan.sh +2 -0
- scripts/test_single.sh +2 -0
- scripts/train_cyclegan.sh +2 -0
- util/__init__.py +2 -0
- util/get_data.py +97 -0
- util/html.py +95 -0
- util/image_pool.py +60 -0
- util/streamlit/css.css +3 -0
- util/tools.py +23 -0
- util/util.py +135 -0
- util/visualizer.py +297 -0
- weights/detect/apple2orange.pth +3 -0
- weights/detect/cityscapes_label2photo.pth +3 -0
- weights/detect/cityscapes_photo2label.pth +3 -0
- weights/detect/facades_label2photo.pth +3 -0
- weights/detect/facades_photo2label.pth +3 -0
- weights/detect/horse2zebra.pth +3 -0
- weights/detect/iphone2dslr_flower_2.pth +3 -0
- weights/detect/latest_net_G_A.pth +3 -0
- weights/detect/latest_net_G_A0.pth +3 -0
- weights/detect/latest_net_G_A1.pth +3 -0
- weights/detect/latest_net_G_B.pth +3 -0
- weights/detect/latest_net_G_B0.pth +3 -0
- weights/detect/latest_net_G_B1.pth +3 -0
.gitignore
ADDED
@@ -0,0 +1,7 @@
|
|
|
|
|
|
|
|
|
|
|
|
|
|
|
|
|
1 |
+
datasets/
|
2 |
+
run/
|
3 |
+
checkpoints/
|
4 |
+
results/
|
5 |
+
!weights/detect/
|
6 |
+
start.ipynb
|
7 |
+
*.pyc
|
README.md
CHANGED
@@ -1,12 +1,465 @@
|
|
1 |
---
|
2 |
-
title:
|
3 |
-
|
4 |
-
|
5 |
-
|
6 |
-
|
7 |
-
sdk_version: 3.23.0
|
8 |
app_file: app.py
|
9 |
pinned: false
|
|
|
|
|
10 |
---
|
11 |
|
12 |
-
|
|
|
|
|
|
|
|
|
|
|
|
|
|
|
|
|
|
|
|
|
|
|
|
|
|
|
|
|
|
|
|
|
|
|
|
|
|
|
|
|
|
|
|
|
|
|
|
|
|
|
|
|
|
|
|
|
|
|
|
|
|
|
|
|
|
|
|
|
|
|
|
|
|
|
|
|
|
|
|
|
|
|
|
|
|
|
|
|
|
|
|
|
|
|
|
|
|
|
|
|
|
|
|
|
|
|
|
|
|
|
|
|
|
|
|
|
|
|
|
|
|
|
|
|
|
|
|
|
|
|
|
|
|
|
|
|
|
|
|
|
|
|
|
|
|
|
|
|
|
|
|
|
|
|
|
|
|
|
|
|
|
|
|
|
|
|
|
|
|
|
|
|
|
|
|
|
|
|
|
|
|
|
|
|
|
|
|
|
|
|
|
|
|
|
|
|
|
|
|
|
|
|
|
|
|
|
|
|
|
|
|
|
|
|
|
|
|
|
|
|
|
|
|
|
|
|
|
|
|
|
|
|
|
|
|
|
|
|
|
|
|
|
|
|
|
|
|
|
|
|
|
|
|
|
|
|
|
|
|
|
|
|
|
|
|
|
|
|
|
|
|
|
|
|
|
|
|
|
|
|
|
|
|
|
|
|
|
|
|
|
|
|
|
|
|
|
|
|
|
|
|
|
|
|
|
|
|
|
|
|
|
|
|
|
|
|
|
|
|
|
|
|
|
|
|
|
|
|
|
|
|
|
|
|
|
|
|
|
|
|
|
|
|
|
|
|
|
|
|
|
|
|
|
|
|
|
|
|
|
|
|
|
|
|
|
|
|
|
|
|
|
|
|
|
|
|
|
|
|
|
|
|
|
|
|
|
|
|
|
|
|
|
|
|
|
|
|
|
|
|
|
|
|
|
|
|
|
|
|
|
|
|
|
|
|
|
|
|
|
|
|
|
|
|
|
|
|
|
|
|
|
|
|
|
|
|
|
|
|
|
|
|
|
|
|
|
|
|
|
|
|
|
|
|
|
|
|
|
|
|
|
|
|
|
|
|
|
|
|
|
|
|
|
|
|
|
|
|
|
|
|
|
|
|
|
|
|
|
|
|
|
|
|
|
|
|
|
|
|
|
|
|
|
|
|
|
|
|
|
|
|
|
|
|
|
|
|
|
|
|
|
|
|
|
|
|
|
|
|
|
|
|
|
|
|
|
|
|
|
|
|
|
|
|
|
|
|
|
|
|
|
|
|
|
|
|
|
|
|
|
|
|
|
|
|
|
|
|
|
|
|
|
|
|
|
|
|
|
|
|
|
|
|
|
|
|
|
|
|
|
|
|
|
|
|
|
|
|
|
|
|
|
|
|
|
|
|
|
|
|
|
|
|
|
|
|
|
|
|
|
|
|
|
|
|
|
|
|
|
|
|
|
|
|
|
|
|
|
|
|
|
|
|
|
|
|
|
|
|
|
|
|
|
|
|
|
|
|
|
|
|
|
|
|
|
|
|
|
|
|
|
|
|
|
|
|
|
|
|
|
|
|
|
|
|
|
|
|
|
|
|
|
|
|
|
|
|
|
|
|
|
|
|
|
|
|
|
|
|
|
|
|
|
|
|
|
|
|
|
|
|
|
|
|
|
|
|
|
|
|
|
|
|
|
|
|
|
|
|
|
|
|
|
|
|
|
|
|
|
|
|
|
|
|
|
|
|
|
|
|
|
|
|
|
|
|
|
|
|
|
|
|
|
|
|
|
|
|
|
|
|
|
|
|
|
|
|
|
|
|
|
|
|
|
|
|
|
|
|
|
|
|
|
|
|
|
|
|
|
|
|
|
|
|
|
|
|
|
|
|
|
|
|
|
|
|
|
|
|
|
|
|
|
|
|
|
|
|
|
|
|
|
|
|
|
|
|
|
|
|
|
|
|
|
|
|
|
|
|
|
|
|
|
|
|
|
|
|
|
|
|
|
|
|
|
|
|
|
|
|
|
|
|
|
|
|
|
|
|
|
|
|
|
|
|
|
|
|
|
|
|
|
|
|
|
1 |
---
|
2 |
+
title: README
|
3 |
+
aliases:
|
4 |
+
emoji: 🖼️
|
5 |
+
tags: [CycleGAN]
|
6 |
+
date: 2023-02-21
|
|
|
7 |
app_file: app.py
|
8 |
pinned: false
|
9 |
+
sdk: streamlit
|
10 |
+
sdk_version: 1.17.0
|
11 |
---
|
12 |
|
13 |
+
>[CycleGAN 论文原文 arXiv](https://arxiv.org/pdf/1703.10593.pdf)
|
14 |
+
|
15 |
+
>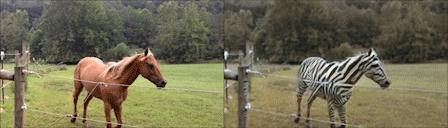
|
16 |
+
|
17 |
+
>这是文章作者GitHub上的 junyanz
|
18 |
+
|
19 |
+
> [CycleGAN junyanz,作者自己用lua 在GitHub 上的实现](https://github.com/junyanz/CycleGAN)
|
20 |
+
>
|
21 |
+
> 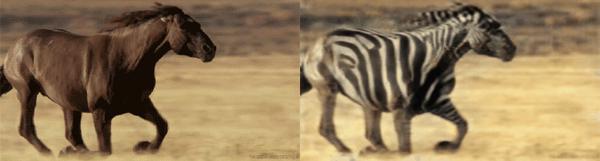
|
22 |
+
>
|
23 |
+
> 这是GitHub上面其他人实现的 LYnnHo
|
24 |
+
|
25 |
+
# 摘要:
|
26 |
+
|
27 |
+
图像到**图像**的**翻译 (Image-to-Image translation)** 是一种视觉上和图像上的问题,它的目标是使用成对的图像作为训练集,(让机器)学习从输入图像到输出图像的映射。然而,在很多任务中,成对的训练数据无法得到。
|
28 |
+
|
29 |
+
我们提出一种在缺少成对数据的情况下,(让机器)学习从源数据域X到目标数据域Y 的方法。我们的目标是使用一个对抗损失函数,学习映射G:X → Y ,使得判别器难以区分图片 G(X) 与 图片Y。因为这样子的映射受到巨大的限制,所以我们为映射G 添加了一个相反的映射F:Y → X,使他们成对,同时加入一个循环一致性损失函数 (cycle consistency loss),以确保 F(G(X)) ≈ X(反之亦然)。
|
30 |
+
|
31 |
+
在缺少成对训练数据的情况下,我们比较了风格迁移、物体变形、季节转换、照片增强等任务下的定性结果。经过定性比较,我们的方法表现得比先前的方法更好。
|
32 |
+
|
33 |
+
# 介绍
|
34 |
+
|
35 |
+
在1873年某个明媚的春日,当莫奈(Claude Monet) 在Argenteuil 的塞纳河畔(the bank of Seine) 放置他的画架时,他究竟看到了什么?如果彩色照片在当时就被发明了,那么这个场景就可以被记录下来——碧蓝的天空倒映在波光粼粼的河面上。莫奈通过他细致的笔触与明亮的色板,将这一场景传达出来。
|
36 |
+
|
37 |
+
如果莫奈画画的事情发生在 Cassis 小港口的一个凉爽的夏夜,那么会发生什么?
|
38 |
+
|
39 |
+
漫步在挂满莫奈画作的画廊里,我们可以想象他会如何在画作上呈现出这样的场景:也许是淡雅的夜色,加上惊艳的几笔,还有变化平缓的光影范围。
|
40 |
+
|
41 |
+
我们可以想象所有的这些东西,尽管从未见过莫奈画作与对应场景的真实照片一对一地放在一起。与此不同的是:我们已经见过许多风景照和莫奈的照片。我们可以推断出这两类图片风格的差异,然后想象出“翻译”后的图像。
|
42 |
+
|
43 |
+
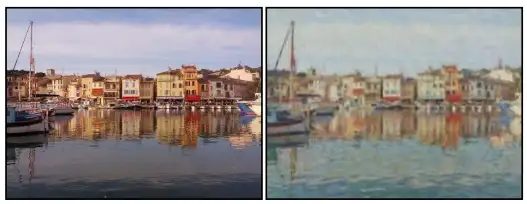
|
44 |
+
|
45 |
+
photo → Monet
|
46 |
+
|
47 |
+
在这篇文章中,我们提出了一个学习做相同事情的方法:在没有成对图像的情况下,刻画一个图像数据集的特征,并弄清楚如何将这些特征转化为另外一个图像数据集的特征。
|
48 |
+
|
49 |
+
这个问题可以被描述成概念更加广泛的图像到图像的翻译 (Image-to-Image translation),从给定的场景x 完成一张图像到另一个场景 y 的转换。举例:从灰度图片到彩色图片、从图像到语义标签(semantic labels) 、从轮廓到图片。发展了多年的计算机视觉、图像处理、计算图像图形学(computational photography, and graphics ?) 学界提出了有力的监督学习翻译系统,它需要成对的数据
|
50 |
+
|
51 |
+
(就像那个 pix2pix 模型一样)。
|
52 |
+
|
53 |
+
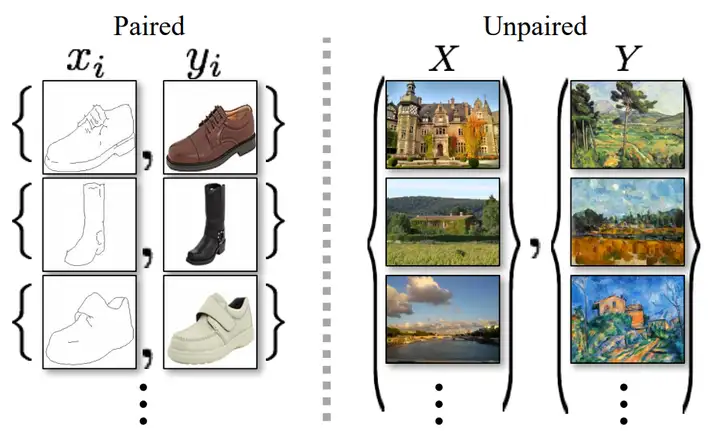
|
54 |
+
|
55 |
+
需要成对数据的 pix2pix
|
56 |
+
|
57 |
+
然而,获取成对的数据比较困难,也耗费资金。例如:只有几个成对的语义分割数据集,并且它们很小。特别是为艺术风格迁移之类的图像任务获取成对的数据就更难了,进行复杂的输出已经很难了,更何况是进行艺术创作。对于许多任务而言,就像物体变形(例如 斑马-马),这一类任务的输出更加不容易定义。
|
58 |
+
|
59 |
+
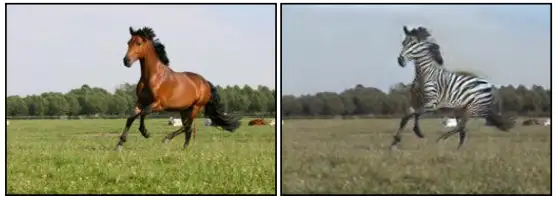
|
60 |
+
|
61 |
+
wild horse → zebra
|
62 |
+
|
63 |
+
- 因此我们寻找一种算法可以学习如何在没有成对数据的情况下,在两个场景之间进行转换。
|
64 |
+
- 我们假设在两个数据域直接存在某种联系——例如:每中场景中的每幅图片在另一个场景中都有它对应的图像,(我们让机器)去学习这个转换关系。尽管缺乏成对的监督学习样本,我们仍然可以在集合层面使用监督学习:我们在数据域X中给出一组图像,在数据域Y 中给出另外一组图像。我们可以训练一种映射G : X → Y 使得 输出,判别器的功能是将生成样本和真实样本 区分开,我们的实验正是要让 和 无法被判别器区分开。理论上,这一项将包括符合 经验分布的的输出���布(通常这要求映射G 是随机的)。从而存在一个最佳的映射将数据域 翻译为数据域 ,使得 数据域(有相同的分布)。然而,这样的翻译不能保证独立分布的输入 和输出 是有意义的一对——因为有无限多种映射 可以由输入的 导出相同的。此外,在实际中我们发现很难单独地优化判别器:当输出图图片从输入映射到输出的时候,标准的程序经常因为一些众所周知的问题而导致奔溃,使得优化无法继续。
|
65 |
+
|
66 |
+
为了解决这些问题,我们需要往我们的模型中添加其他结构。因此我们利用 **翻译应该具有“循环稳定性”(translation should be "cycle consistent")** 的这个性质,某种意义上,我们将一个句子从英语翻译到法语,再从法语翻译回英语,那么我们将会得到一相同的句子。从数学上讲,如果我们有一个翻译器 G : X → Y 与另一个翻译器 F : Y → X ,那么 G 与 F 彼此是相反的,这一对映射是双射(bijections) 。
|
67 |
+
|
68 |
+
于是我们将这个结构应用到 映射 G 和 F 的同步训练中,并且加入一个 循环稳定损失函数(_cycle consistency loss)_ 以确保到达与。将这个损失函数与判别器在数据域X 与数据域Y 的对抗损失函数结合起来,就可以得到非成对图像到图像的目标转换。
|
69 |
+
|
70 |
+
我们将这个方法应用广泛的领域上,包括风格迁移、物体变形、季节转换、照片增强。与以前的方法比较,以前的方法依既赖过多的人工定义与调节,又依赖于共享的内部参数,在比较中也表明我们的方法要优于这些(合格?)基准线(out method outperforms these baselines )。我们提供了这个模型在 [PyTorch](https://github.com/junyanz/pytorch-CycleGAN-and-pix2pix) 和 [Torch](https://github.com/junyanz/CycleGAN) 上的实现代码,[点击这个网址](https://junyanz.github.io/CycleGAN/)进行访问。
|
71 |
+
|
72 |
+
## 相关工作
|
73 |
+
|
74 |
+
对抗生成网络 Generative Adversarial Networks (GANs) 在图像生成、图像编辑、表征学习(representation learning) 等领域以及取得了令人瞩目的成就。在最近,条件图像的生成的方法也采用了相同的思路,例如 从文本到图像 (text2image)、图像修复(image inpainting)、视频预测(future prediction),与其他领域(视频和三维数据)。
|
75 |
+
|
76 |
+
对抗生成网络成功的关键是:通过对抗损失(adversarial loss)促使生成器生成的图像在原则上无法与真实图像区分开来。图像生成正是许多计算机图像生成任务的优化目标,这种损失对在这类任务上特别有用。我们采用对抗损失来学习映射,使得翻译得到的图片难以与目标域的图像区分开。
|
77 |
+
|
78 |
+
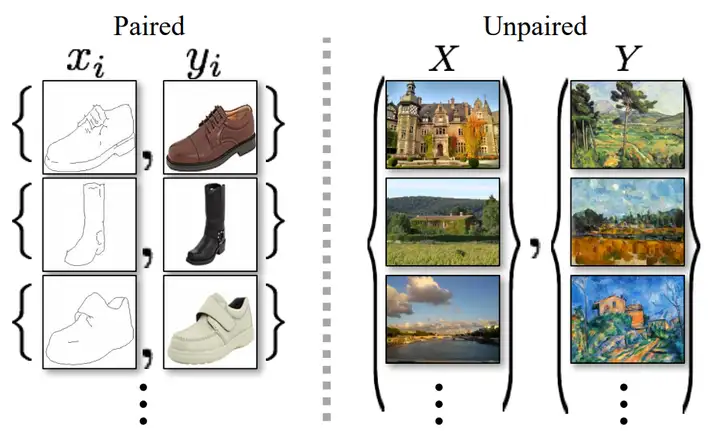
|
79 |
+
|
80 |
+
需要成对训练图片的 pix2pix
|
81 |
+
|
82 |
+
**Image-to-Image Translation 图像到图像的翻译**
|
83 |
+
|
84 |
+
这个想法可以追溯到Hertzmann的 图像类比 (Image Analogies),这个模型在一对输入输出的训练图像上采用了无参数的纹理模型。最近 (2017) 的更多方法使用 输入-输出样例数据集训练卷积神经网络。我们的研究建立在 Isola 的 pix2pix 框架上,这个框架使用了条件对抗生成网络去学习从输入到输出的映射。相似的想法也已经应用在多个不同的任务上,例如:从轮廓、图像属性、布局语义 (semantic layouts) 生成图片。然而,与就如先前的工作不同,我们可以从不成对的训练图片中,学习到这种映射。
|
85 |
+
|
86 |
+
**Unpaired Image-to-Image Translation 不成对的图像到图像的翻译**
|
87 |
+
|
88 |
+
其他的几个不同的旨在关联两个数据域 X和Y 的方法,也解决了不成对数据的问题。
|
89 |
+
|
90 |
+
最近,Rosales 提出了一个贝叶斯框架,通过对原图像以及从多风格图像中得到的似然项 (likelihood term) 进行计算,得到一个基于区块 (patch-based)、基于先验信息的马尔可夫随机场;更近一点的研究,有 CoGAN 和 跨模态场景网络 (corss-modal scene networks) 使用了权重共享策略 去学习跨领域 (across domains) 的共同表示;同时期的研究,有 刘洺堉 用变分自编码器与对抗生成网络结合起来,拓展了原先的网络框架。同时期另一个方向的研究,有 尝试 (encourages) 共享有特定“内容 (content) ”的特征,即便输入和输出的信息有不同的“风格 (style) ”。这些方法也使用了对抗网络,并添加了一些项目,促使输出的内容在预先定义的度量空间内,更加接近于输入,就像 标签分类空间 (class label space) ,图片像素空间 (image pixel space), 以及图片特征空间 (image feature space) 。
|
91 |
+
|
92 |
+
> 不同于其他方法,我们的设计不依赖于 特定任务 以及 预定义输入输出似然函数,我们也不需要要求输入和输出数据处于一个相同的低纬度嵌入空间 (low-dimensional embedding space) 。因此我们的模型是适用于各种图像任务的通用解决方案。我们直接把本文的方案与先前的、现在的几种方案在第五节进行对比。
|
93 |
+
|
94 |
+
**Cycle Consitency 循环一致性**
|
95 |
+
|
96 |
+
把可传递性 (transitivity) 作为结构数据正则化的手段由来已久。近十年来,在视觉追踪 (visual tracking) 任务里,确保简单的前后向传播一致 (simple forward-backward consistency) 已经成为一个标准。在语言处理领域,通过“反向翻译与核对(back translation and reconcilation) ”验证并提高人工翻译的质量,机器翻译也是如此。更近一些的研究,使用到使用高阶循环一致性的有:动作检测、三维目标匹配,协同分割(co-segmentation) ,稠密语义分割校准(desnse semantic alignment),景物深度估计(depth estimation) 。
|
97 |
+
|
98 |
+
> 下面两篇文章的与我的工作比较相似,他们也使用了循环一致性损失体现传递性,从而监督卷积网络的训练:基于左右眼一致性的单眼景物深度估计 (Unsupervised monocular depth estimation with left-right consistency) —— Godard 通过三维引导的循环一致性学习稠密的对应关系 (Learning dense correspondence via 3d-guided cycle consistency) —— T. Zhou.
|
99 |
+
|
100 |
+
我们引入了相似的损失使得两个生成器G与F 保持彼此一致。同时期的研究,Z. Yi. 受到机器翻译对偶学习的启发,独立地使用了一个与我们类型的结构,用于不成对的图像到图像的翻译——Dualgan: Unsupervised dual learning for image-to-image translation.
|
101 |
+
|
102 |
+
**神经网络风格迁移 Neural Style Transfer**
|
103 |
+
|
104 |
+
神经网络风格迁移是优化 图像到图像翻译 的另外一种方法,通过比较不同风格的两种图像(一张是普通图片,另一张是另外一种风格的图片(一般来讲是绘画作品))并将一幅图像的内容和另一幅的风格组合起来,基于预训练期间对伽马矩阵进行统计从而得到深层次的特征,再对这些特征进行匹配,最终创造新的图像。
|
105 |
+
|
106 |
+
另一方面,我们主要关注的是:通过刻画更高层级外观结构之间的对应关系,学习两个图像集之间的映射,而不仅是两张特定图片之间的映射。因此,我们的方法可以应用在其他任务上,例如从 绘画 → 图片,物体变形(object transfiguration),等那些单样品转换方法表现不好的地方。我们在 5.2节 比较了这两种方法。
|
107 |
+
|
108 |
+
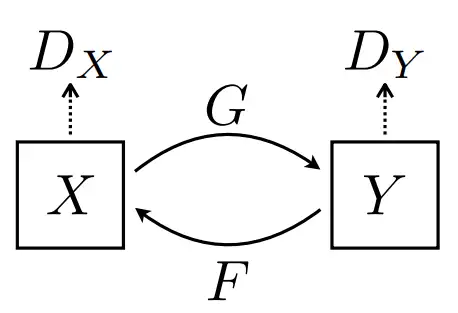
|
109 |
+
|
110 |
+
## 公式推导
|
111 |
+
|
112 |
+
我们的目标是学习两个数据域 X 与 Y 之间的映射函数,定义数据集合与数据分布,与模型的两个映射,其中:
|
113 |
+
|
114 |
+
另外,我们引入了两个判别函数:
|
115 |
+
|
116 |
+
- 用于区分{x} 与 {F(y)} 的 D_X
|
117 |
+
- 用于区分{y} 与 {G(x)} 的 D_Y 。
|
118 |
+
|
119 |
+
我们的构建的模型包含两类组件(Our objective contains two types of terms):
|
120 |
+
|
121 |
+
- 对抗损失(adversarial losses),使生成的图片在分布上更接近于目标图片;
|
122 |
+
- 循环一致性损失(cycle consistency losses),防止学习到的映射 G与F 相互矛盾。
|
123 |
+
|
124 |
+
## 对抗损失(adversarial losses)
|
125 |
+
|
126 |
+
我们为两个映射函数都设置了对抗损失。对于映射函数G 和它的判别器 D_Y ,我们有如下的表达式:
|
127 |
+
|
128 |
+
$$
|
129 |
+
\begin{align} \mathcal{L}_{GAN}(G,D_Y,X,Y) &= \mathbb{E}_{y \sim p_{data}(y)} \big[log (D_Y(Y) ) \big] \\ &+ \mathbb{E}_{x \sim p_{data}(x)} \big[log (1-D_Y(G(x))~) \big] \end{align}
|
130 |
+
$$
|
131 |
+
|
132 |
+
当映射G 试图生成与数据域Y相似的图片 G(x) 的时候,判别器也在试着将生成的图片从原图中区分出来。映射G 希望通过优化减小的项目与 映射F 希望优化增大的项目 相对抗,另一个映射F 也是如此。这两个相互对称的结构用公式表达就是:
|
133 |
+
|
134 |
+
$$
|
135 |
+
min_G \ max_{D_Y} \ \mathcal{L}_{GAN} (G,D_Y,X,Y) \\ min_F \ max_{D_X} \ \mathcal{L}_{GAN} (F,D_X,Y, X)
|
136 |
+
$$
|
137 |
+
|
138 |
+
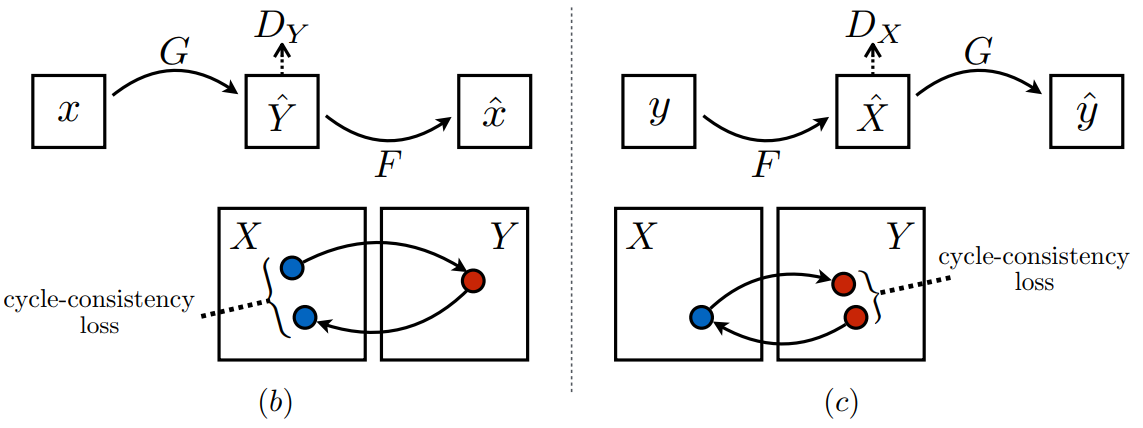
|
139 |
+
|
140 |
+
图3(b), 图3(c)
|
141 |
+
|
142 |
+
## 循环一致性损失(cycle consistency loss)
|
143 |
+
|
144 |
+
理论上对抗训练可以学习到 映射G与F,并生成与目标域 Y与X 相似的分布的输出(严格地讲,这要求映射G与F 应该是一个随机函数。然而,当一个网络拥有足够大的容量,那么输入任何随机排列的图片,它都可以映射到与目标图片相匹配的输出分布。因此,不能保证单独依靠对抗损失而学习到的映射可以将每个单独输入的 x_i 映射到期望得到的 y_i 。
|
145 |
+
|
146 |
+
为了进一步减少函数映射可能的得到的空间大小,我们认为学习的的得到的函数应该具有循环一致性(cycle-consistent): 如图3(b) 所示,数据域X 中的每一张图片x 在循环翻译中,应该可以让x 回到翻译的原点,反之亦然,即 前向、后向循环一致,换言之: x \rightarrow G(x) \rightarrow F(G(x)) \approx x \\ y \rightarrow F(y) \rightarrow G(F(y)) \approx y
|
147 |
+
|
148 |
+
我们使用循环一致性损失作为激励,于是有:
|
149 |
+
|
150 |
+
\begin{align} \mathcal{L}_{cyc}(G, F) &= \mathbb{E}_{x \sim p_{data}(x)}[ || F(G(x)) - x ||_1] \\ &+ \mathbb{E}_{y \sim p_{data}(y)}[ || G(F(y)) - y ||_1] \end{align}
|
151 |
+
|
152 |
+
初步实验中,我们也尝试用F(G(x)) 与x 之间、G(F(Y)) 与y 之间的对抗损失替代上面的L1 范数,但是没有观察到更好的性能。
|
153 |
+
|
154 |
+
如图4 所示,加入循环一致性损失最终使得模型重构的图像F(G(x)) 与输入的图像x 十分匹配。
|
155 |
+
|
156 |
+
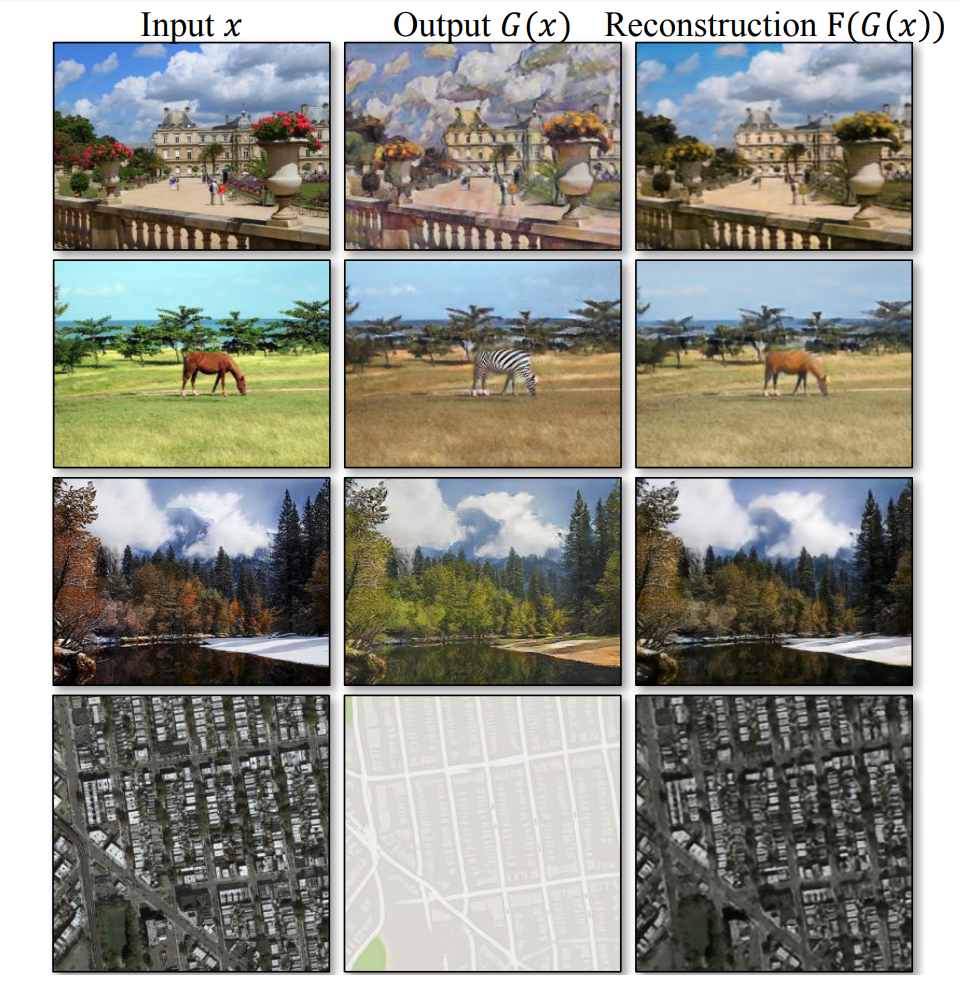
|
157 |
+
|
158 |
+
图4, cycleGAN 的循环一致性
|
159 |
+
|
160 |
+
## 3.3 完整的模型对象(Full Objective)
|
161 |
+
|
162 |
+
我们完整的模型对象如下,其中,_λ_ 控制两个对象的相对重要性。
|
163 |
+
|
164 |
+
\begin{align} \mathcal{L}(G, F, D_X, D_Y) &= \mathcal{L}_{GAN}(G,D_Y, X, Y) \\ &+ \mathcal{L}_{GAN}(F,D_X, Y, X) \\ &+ \lambda \mathcal{L}_{cyc}(G, F) \\ \end{align}
|
165 |
+
|
166 |
+
我们希望解决映射的学习问题:
|
167 |
+
|
168 |
+
G^*, \ F^* = \arg \min_{G, F} \max_{D_X, D_Y} \ \mathcal{L}(G, F, D_X, D_Y)
|
169 |
+
|
170 |
+
请注意,我们的模型可以视为训练了两个自动编码器(auto-encoder):
|
171 |
+
|
172 |
+
F \circ G: X \rightarrow X \\ G \circ F: Y \rightarrow Y
|
173 |
+
|
174 |
+
然而,每一个自动编码器都有它特殊的内部结构:它们通过中间介质将图片映射到自身,并且这个中间介质属于另一个数据域。这样的一种配置可以视为是使用了对抗损失训练瓶颈层(bottle-neck layer) 去匹配任意目标分布的“对抗性自动编码器”(advesarial auto-encoders)。在我们的例子中,目标分布是中间介质Yi 分布于数据域Y 的自动编码器X_i \rightarrow Y_i \rightarrow X_i 。
|
175 |
+
|
176 |
+
在 5.1.4节,我们将我们的方法与消去了 完整对象的模型 (ablations of the full objective) 进行比较,包括只包含对抗损失 \mathcal{L}_{GAN} 、只包含循环一致性损失\mathcal{L}_{cyc},根据我们的经验,在模型中加入这两个对象,对获得高质量的结果而言十分重要。我们也对单向的循环损失模型进行评估,它的结果表明:单向的循环对这个问题的约束不够充分,因而不足以使训练获得足够的正则化。(a single cycle is not sufficient to regularize teh training for this under-constrained problem)
|
177 |
+
|
178 |
+
## 4. 实现(Implementation)
|
179 |
+
|
180 |
+
**网络结构**
|
181 |
+
|
182 |
+
我们采用了**J. Johnson文章**中的生成网络架构。这个网络包含两个步长为2 的卷积层,几个残差模块,两个步长为1/2 的**转置卷积层**(transposed concolution; 原文是fractionally-strided convolutions)。我们使用了6个模块去处理 128x128 的图片,以及9个模块去处理256x256的高分辨率训练图片。与Johnson 的方法类似,我们对每个实例使用了正则化(instance normalization)。我们使用了 70x70 的PatchGANs 作为我的判别器网络,这个网络用来判断图片覆盖的70x70补丁是否来自于原图。比起全图的鉴别器,这样的补丁层级的鉴别器有更少的参数,并且可以以完全卷积的方式处理任意尺寸的图像。
|
183 |
+
|
184 |
+
> **J. Johnson文章**:指的是李飞飞他们的那篇文章:感知损失在 实时风格迁移 与 超分辨率 上的应用 (Perceptual losses for real-time style transfer and super-resolution)
|
185 |
+
>
|
186 |
+
> 分数步长卷积 (fractionally-strided convolution):也就是 转置卷积层 Transposed Convolution,也有人叫 反卷积、逆卷积(deconvolution),不过这个过程不是卷积的逆过程,所以我建议用 **转置卷积层**称呼它。
|
187 |
+
>
|
188 |
+
> PatchGANs:使用条件GAN 实现图片到图片的翻译 Image-to-Image Translation with Conditional Adversarial Networks ——本文作者是这篇文章的第二作者
|
189 |
+
|
190 |
+
**训练细节**
|
191 |
+
|
192 |
+
我们把学界近期的两个技术拿来用做我们的模型里,用于稳定模型的训练。
|
193 |
+
|
194 |
+
第一,对于 \mathcal{L}_{GAN} ,我们使用**最小二乘损失**(least squares loss) 取代 原来的负对数似然损失(就是LeCun 的那个)。在训练时,这个损失函数有更好的稳定性,并且可以生成更高质量的结果。实际上,对于GAN的损失函数,我们为两个映射 G(X) 与D(X) 各自训练了一个损失函数。
|
195 |
+
|
196 |
+
\mathcal{L}_{GAN}(G,D,X,Y) \\ G.minimize \big( \mathbb{E}_{x \sim p_{data}(x)}[ || G(F(y)) - y ||_1] \big) \\ D.minimize \big( \mathbb{E}_{y \sim p_{data}(y)}[ || F(G(x)) - x ||_1] \big)
|
197 |
+
|
198 |
+
第二,为了减小模型训练时候的震荡(oscillation),我们遵循Shrivastava 的策略——在更新判别器的时候,使用生成的图片历史,而不是生成器最新一次生成的图片。我们把最近50次生成的图片保存为缓存。
|
199 |
+
|
200 |
+
在每一次实验中,在计算损失函数的时候,我们都把下面公式内的 \lambda 设置为10,我们使用Adam 进行批次大小为1 的更新。所有的网络都是把学习率设置为0.0002 后,从头开始训练的。在前100次训练中,我们保持相同的学习率,并且在100次训练后,我们保持学习率向0的方向线性减少。第七节(附录7)记录了关于数据集、模型结构、训练程序的更多细节
|
201 |
+
|
202 |
+
\begin{align} \mathcal{L}_{GAN}(G, F, D_X, D_Y) &= \mathcal{L}_{GAN}(G,D_Y, X, Y) \\ &+ \mathcal{L}_{GAN}(F,D_X, Y, X) \\ &+ \mathcal{L}_{GAN}(G, F) \cdot \lambda \\ \end{align}
|
203 |
+
|
204 |
+
> **最小二乘损失**:来自于——LSGANs, Least squares generative adversarial networks
|
205 |
+
|
206 |
+
## 5.结果
|
207 |
+
|
208 |
+
首先,我们将我们的方法与最近的 训练图片不成对的图片翻译 方法对比,并且使用的是成对的数据集,评估的时候使用的是 标记正确的成对图片。然后我们将我们的方案与方案的几种变体一同比较,研究了 对抗损失 与 循环一致性损失 的重要性。最后,在只有不成对图片存在的情况下,我们在更大的范围内,展示了我们算法的泛化性能。
|
209 |
+
|
210 |
+
为了简洁起见,我们把这个模型称为 CycleGAN。在[我们的网站](https://junyanz.github.io/CycleGAN/)上,你可以找到所有的研究成果,包括[PyTorch](https://github.com/junyanz/pytorch-CycleGAN-and-pix2pix) 和 [Torch](https://github.com/junyanz/CycleGAN) 的实现代码。
|
211 |
+
|
212 |
+
## 5.1 评估
|
213 |
+
|
214 |
+
我们使用与pix2pix 相同的评估数据集,与现行的几个基线(baseline) 进行了定量与定性的比较。这些任务包括了 在城市景观数据集(Cityscapes dataset) 上的 语义标签↔照片,在谷歌地图上获取的 地图↔卫星图片。我们在整个损失函数上进行了模型简化测试(ablation study 模型消融研究 )。
|
215 |
+
|
216 |
+
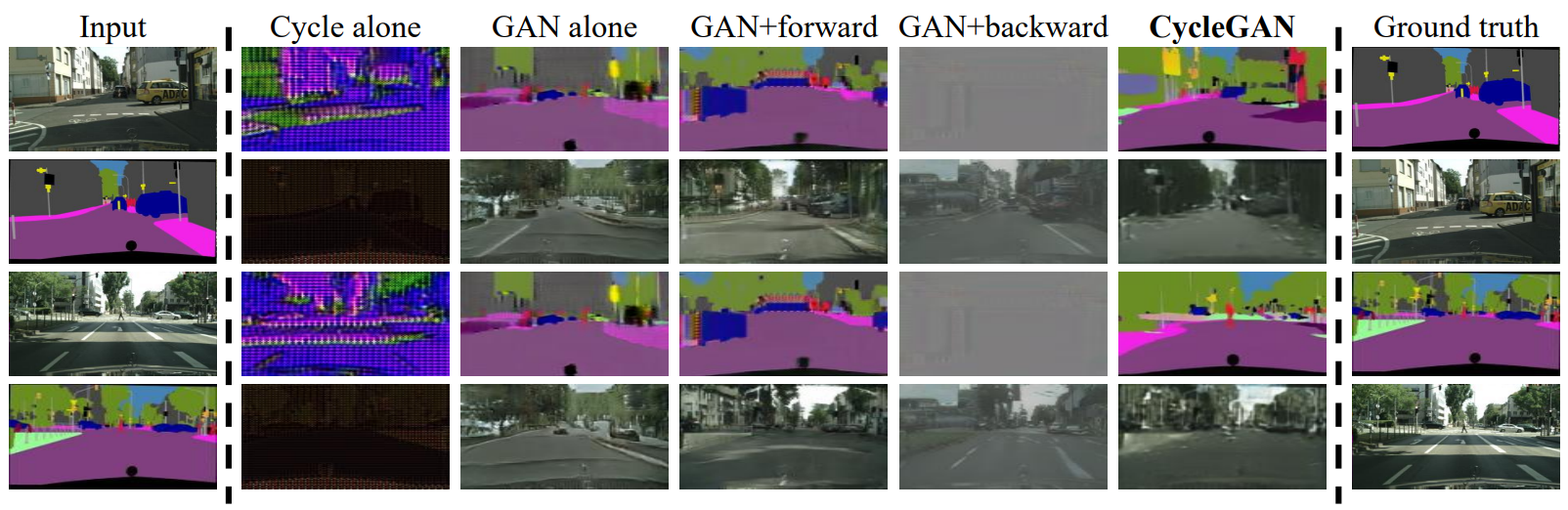
|
217 |
+
|
218 |
+
完整的cycleGAN 与 模型简化测试(ablation study 模型消融研究)
|
219 |
+
|
220 |
+
## **5.1.1 评估指标 (Evalution Metrics)**
|
221 |
+
|
222 |
+
**AMT感知研究(AMT perceptual studies)** 在 地图↔卫星图片的任务中,我们在亚马逊 **真 · 人工 智能平台**(Amazon Mechanical Turk) 上,建立了“图片真伪判别”的任务,来评估我们的输出的图片的真实性。我们遵循Isola 等人相同的感知研究方法,不同的是:我每个算法收集了25个实验参与者 (participant) 的结果 。会有一系列成对的真伪图片 展示给实验参与者观看,参与者需要点击选择他认为是真实图片的那一张,(另外一张伪造的图片是由我们的算法生成的,或者是其他基线算法(baselines) 生成的)。每一轮的前10个试验用来练习,并将他们答案的正误 反馈给试验参与者。每一轮只会测试一种算法,每一个试验参与者只允许参与测试一轮。
|
223 |
+
|
224 |
+

|
225 |
+
|
226 |
+
亚马逊 真 · 人工 智能平台(Amazon Mechanical Turk)
|
227 |
+
|
228 |
+
请注意,我们报告中的数字,不能直接与其他文章中报告的数字进行比较,因为我们的正确标定图片与他们有轻微的不同,并且实验的参与人员也有可能不同(由于实验室在不同的时间进行的)。因此,我们报告中的数字,用来在这篇文章内部进行比较。
|
229 |
+
|
230 |
+
**FCN score (全卷积网络得分 full-convolutional network score )** 虽然感知研究可能是评估图像真实性的黄金准则,但是我们也在寻求不需要人类经验的 自动质量检测方法。为此,我们采用**Isola 的 "FCN score"** 来进行 城市景观标签↔照片 转换任务的评估。全卷积网络的根据现成的语义分割模型 来测量评估图片的可解释性。全卷积从一幅图片 预测出整张图片的语义标签地图,然后使用标准的语义分割模型,各自生成图片与输入图片 的标签地图,然后使用它们用来描述的标准语义进行比较。直觉上,我们从标签地图得到“路上的车”的生成图片,然后把生成的图片输入全卷积网络,如果可以从全卷积网络上也得到“路上的车”的标签地图,那么就是我们恢复成功了。
|
231 |
+
|
232 |
+
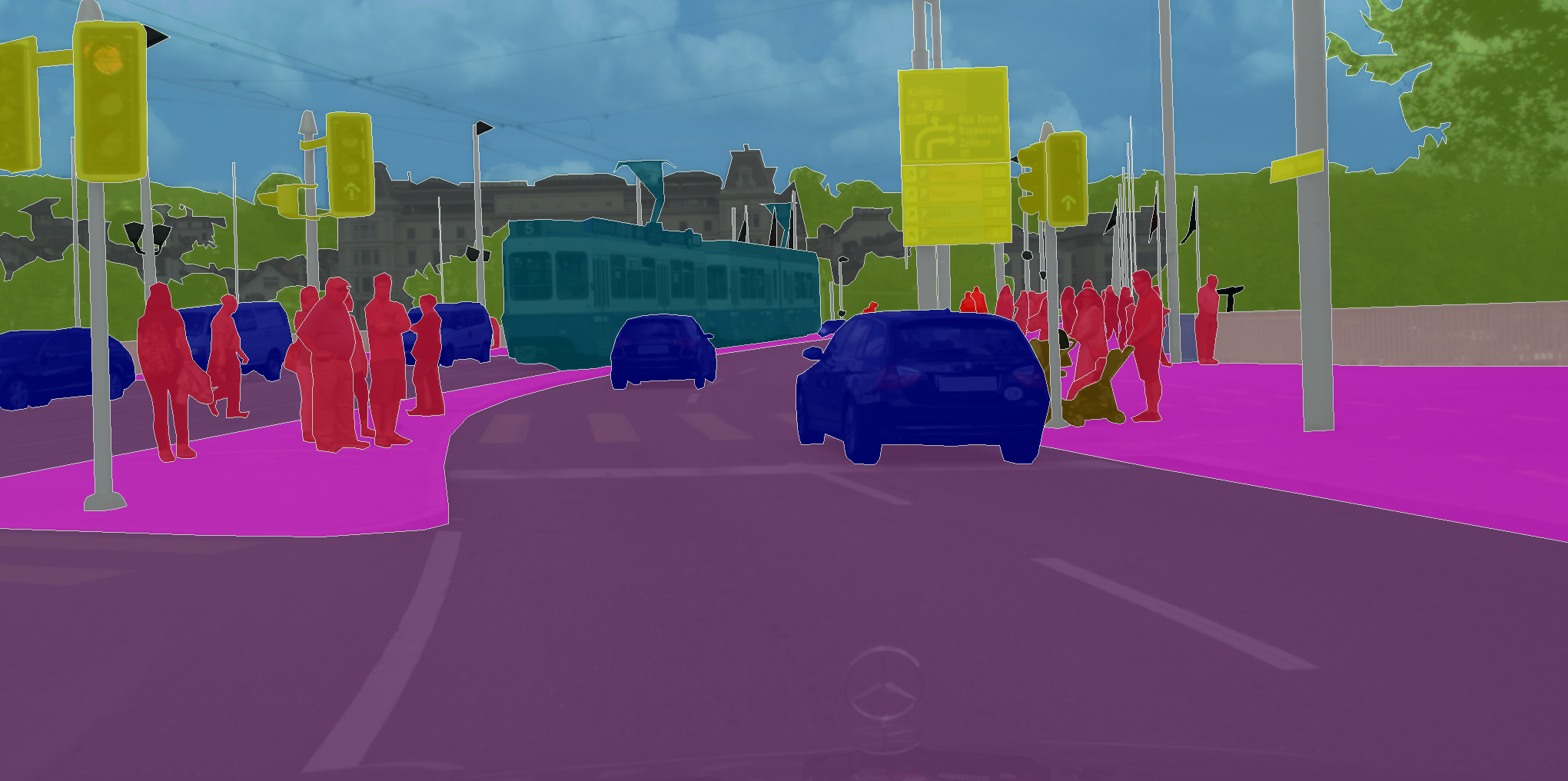
|
233 |
+
|
234 |
+
城市景观语义分割 Cityscapes dataset
|
235 |
+
|
236 |
+
**语义分割指标(Semantic segmentation metrics )** 为了评估 图片→标签 的性能,我们使用了城市景观的标准指标(standard metrics from the CItyscapes benchmark ),包括了每一个像素的准确率,每个等级的准确率,类别交并比(Class IoU, class Intersection-Over-Union) 平均值。
|
237 |
+
|
238 |
+
> AMT:亚马逊的 **真 · 人工 智能平台**(Amazon Mechanical Turk),这个平台就是Amazon 在网站上发布任务,由系统分发任务给人类领取,然后按照自己内部的算法支付酬劳,是真的 人工的 智能平台。其实应该叫 人力手动 智能平台。 AMT perceptual studies AMT感知研究,指的就是把自己的结果让人力手动地 去评估。
|
239 |
+
>
|
240 |
+
> Isola 等人的研究:用条件对抗网络进行图像到图像的翻译 Imageto-image translation with conditional adversarial networks. In CVPR, 2017. 包括了用 FCN score 对图片进行评估。
|
241 |
+
|
242 |
+
## **5.1.2 基线模型(baselines)**
|
243 |
+
|
244 |
+
**CoGAN(Coupled GAN)** 这个模型训练一个对抗生成网络,两个生成网络分别生成数据域X 与 数据域Y,前几层都进行权重绑定,并共享对数据潜在的表达。从X 到Y 的翻译可以通过寻找相同的潜在表达 来生成图片X,然后把这个潜在的表达翻译成风格Y 。
|
245 |
+
|
246 |
+
**SimGAN** 与我们的方法类似,用对抗损失训练了一个从X 到Y 的翻译,正则项 ||x-G(x)||_1 用来惩罚图片在像素层级上过大的改动。
|
247 |
+
|
248 |
+
**Feature loss + GAN** 我们还测试了SimGAN的变体——使用的预训练网络(VGG-16, relu4_2 ) 在图像深度特征(deep image features) 上计算L1 损失,而不是在RGB值上计算。像这样在深度特征空间上计算距离,有时候也被称为是使用“感知损失(perceptual loss )”。
|
249 |
+
|
250 |
+
**BiGAN/ ALI** 无条件约束GAN 训练了生成器G: Z→X ,将一个随机噪声映射为图片x 。BiGAN 和ALI 也建议学习逆向的映射F: X→Z 。虽然它们一开始的设计是 学习将潜在的向量z 映射为图片x ,我们实现了相同的组件,将原始图片x 映射到 目标图片y 。
|
251 |
+
|
252 |
+
**pix2pix** 我们也比较了在成对数据上训练的pix2pix模型,想看看在不使用成对训练数据的情况下,我们能够如何接近这个天花板。
|
253 |
+
|
254 |
+
为了公平起见,我们使用了与我们的方法 相同的架构和细节实现了这些基线模型,除了CoGAN,CoGAN建立在共享 潜在表达 而输出图片的生成器上。因此我们使用了CoGAN实现的公共版本。
|
255 |
+
|
256 |
+
> **BiGAN** V. Dumoulin. Adversarially learned inference. In ICLR, 2017.
|
257 |
+
> **ALI** ?? J. Donahue. Adversarial feature learning. In ICLR, 2017
|
258 |
+
> **pix2pix** P.Isola Imageto-image translation with conditional adversarial networks. In CVPR, 2017.
|
259 |
+
|
260 |
+
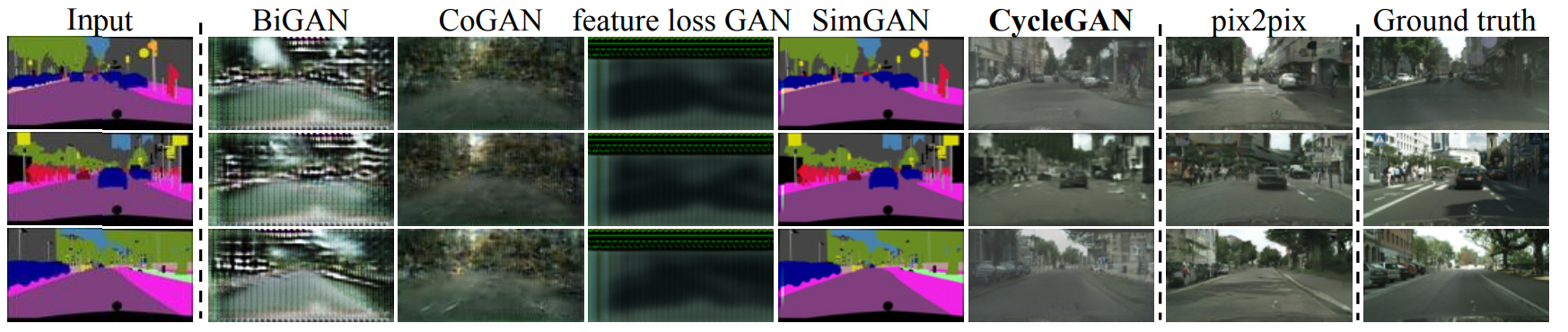
|
261 |
+
|
262 |
+
可以接受不成对训练数据的cycleGAN 和 需要成对数据的pix2pix
|
263 |
+
|
264 |
+
5.1.3 与基线模型相比较
|
265 |
+
|
266 |
+
5.1.4 对损失函数的分析
|
267 |
+
|
268 |
+
5.1.5 图片重构质量
|
269 |
+
|
270 |
+
5.1.6 成对数据集的其他结果
|
271 |
+
|
272 |
+
## 5.2 应用(Applications)
|
273 |
+
|
274 |
+
我们演示了 成对的训练数据不存在时 cycleGAN 的几种应用方法。可以看第七节 附录,以获取更多关于数据集的细节。我们观察到训练集上的翻译 比测试上的更有吸引力,应用的所有训练与测试的数据都可以在[我们项目的网站](https://junyanz.github.io/CycleGAN/)上看到。
|
275 |
+
|
276 |
+
**风格迁移 (Collection style transfer)** 我们用Flickr 和 WikiArt 上下载的风景图片,训练了一个模型。注意,与最近的“**神经网络风格迁移(neural style transfer)**” 不同,我们的方法学习的是 对整个艺术作品数据集的仿造。因此,我们可以学习 以梵高的风格生成图片,而不仅是学习 星夜(Starry Night) 这一幅画的风格。我们对 塞尚(Cezanne),莫奈(Monet),梵高(Van Gogh) 以及日本浮世绘 这每个艺术风格 都构建了一个数据集,他们的数量大小分别是 526,1073,400,563 张。
|
277 |
+
|
278 |
+
**物件变形(Object transfiguration)** 训练这个模型用来将ImageNet 上的一个类别的物件 转化为另外一个类型的物物件(每个类型包含了约1000张的训练图片)。Turmukhambetov 提出了一个子空间的模型将一类物件转变为同一类别的另外一个物件。而我们的方法侧重于两个(来自于不同类别)而视觉上相似的的物件之间的变形。
|
279 |
+
|
280 |
+
**季节转换(Season transfer)** 这个模型在 从Flickr上下载的Yosemite风景照上训练,其中包括 854张冬季(冰雪) 和 1273张 夏季的图片。
|
281 |
+
|
282 |
+
从画作中生成照片(Photo generation from paintings) 为了建立映射 画作→照片,我们发现:添加一个鼓励颜色成分保留的损失 对映射的学习有帮助。特别是采用了Taigman 的技术后,当提供目标域的真实样本 作为生成器的输入时,对生成器进行正则化以接近恒等映射(identity mapping)
|
283 |
+
|
284 |
+
\begin{align} \mathcal{L}_{GAN}(G, F) &= \mathbb{E}_{y \sim p_{data}}[||G(y)-y||_1] \\ &+ \mathbb{E}_{x \sim p_{data}}[||F(x)-x||_1] \end{align}
|
285 |
+
|
286 |
+
在不使用 \mathcal{L}_{identity} 时,生成器G与F 可以自由地改变输入图像的色调,而这是不必要的。例如,当学习莫奈的画作和Flicker 的照片时,生成器经常将白天的画作映射到 黄昏时拍的照片,因为这样的映射可能在 对抗损失和循环一致性损失 的启用下同样有效。这种恒等映射在图9 中可以看到。
|
287 |
+
|
288 |
+
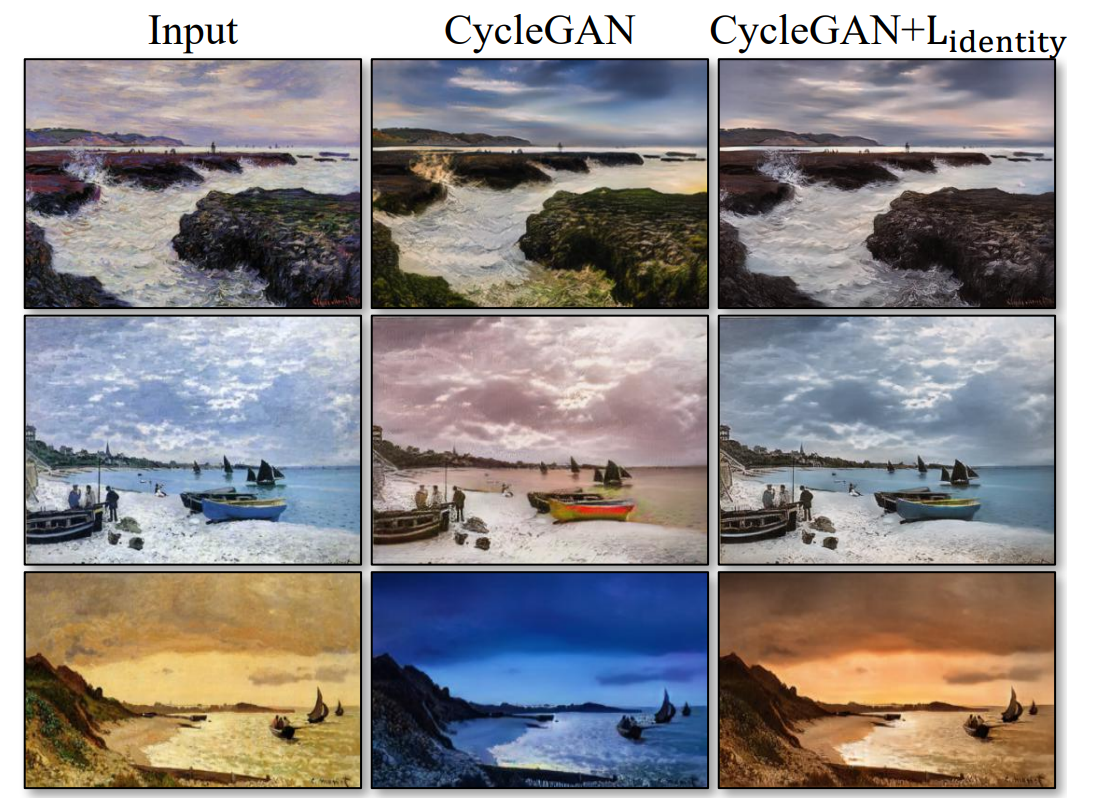
|
289 |
+
|
290 |
+
恒等映射(identity mapping)
|
291 |
+
|
292 |
+
> **神经网络风格迁移 neural style transfer** L. A. Gatys. Image style transfer using convolutional neural networks. CVPR, 2016
|
293 |
+
>
|
294 |
+
> 使用 条件纹理成分分析 为物体的外观建模 D. **Turmukhambetov**. Modeling object appearance using context-conditioned component analysis. In CVPR, 2015.
|
295 |
+
|
296 |
+
图12 中,展示的是将莫奈的画作翻译成照片,图12 与 图9 显示的是 包含了训练集的结果,而本文中的其他实验,我们仅显示测试集的结果。因为训练集不包含成对的图片,所以为测试集提供合理的翻译结果是一项非凡的任务(也许可以复活莫奈让他对着相同的景色画一张?——括号内��话是我自己加的)确实,自从莫奈再也不能创作新的绘画作品后,泛化无法看到原画作的测试集并不是一个迫切的问题。(Indeed, since Monet is no longer able to create new paintings, generalization to unseen, “test set”, paintings is not a pressing problem感谢
|
297 |
+
|
298 |
+
[@LIEBE](https://www.zhihu.com/people/a078ee299d4a8f2b598c6d1d1e83b4b1)
|
299 |
+
|
300 |
+
在评论区给出的翻译)
|
301 |
+
|
302 |
+
**图片增强(Photo enhancement)** 图14 中,我们的方法可以用在生成景深较浅的照片上。我们从Flickr下载花朵的照片,用来训练模型。数据源由智能手机拍摄的花朵图片组成,因为光圈小,所以通常具有较浅的景深。目标域包含了由 拥有更大光圈的单反相机拍摄的图片。我们的模型 用智能手机拍摄的浅光圈图片,成功地生成有大光圈拍摄效果的图片。
|
303 |
+
|
304 |
+
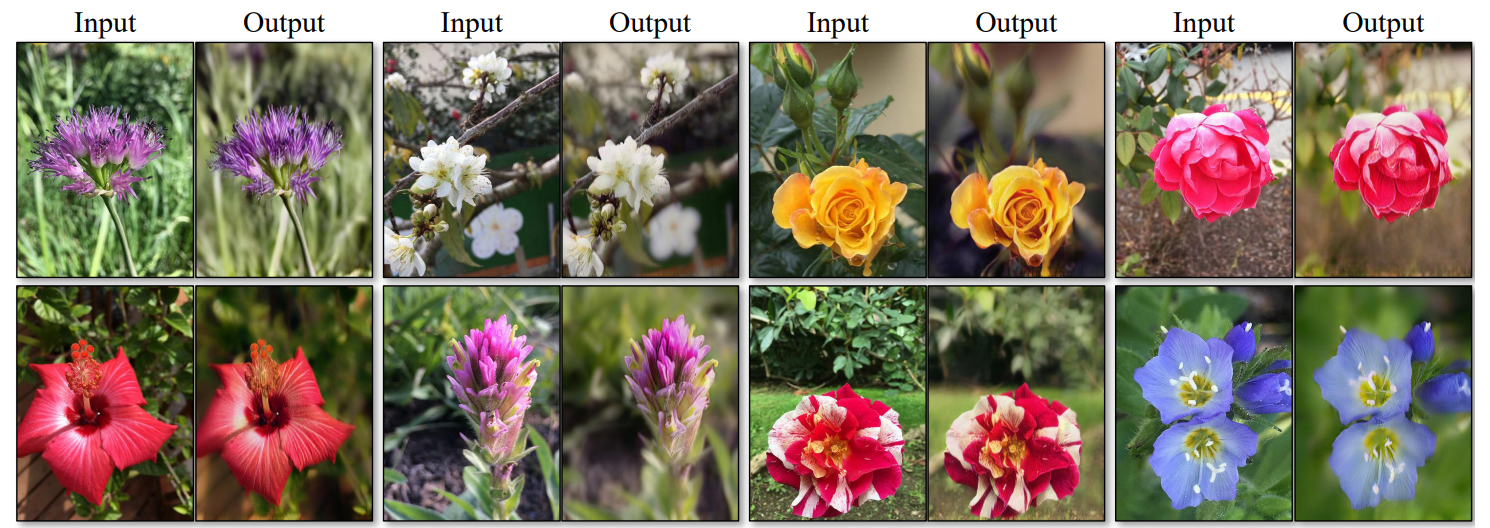
|
305 |
+
|
306 |
+
景深增强
|
307 |
+
|
308 |
+
**与神经风格迁移相比(Comparison with Neural style transfer)** 我们与神经风格迁移在照片风格化任务上相比,我们的图片可以产生出 具备整个数据集风格 的图片。为了在整个风格数据集上,把我们的方法与神经风格迁移相比较,我们在目标域上。计算了平均Gram 矩阵,并且使用这个矩阵转移 神经风格迁移的“平均风格”。
|
309 |
+
|
310 |
+
图16 演示了在其他转移任务上 相似的比较,我们发现 神经风格迁移需要寻找一个尽可能与所需输出 相贴近的目标风格图像,但是依然无法产生足够真实的照片,而我们的方法成功地生成与目标域相似,并且比较自然的结果。
|
311 |
+
|
312 |
+
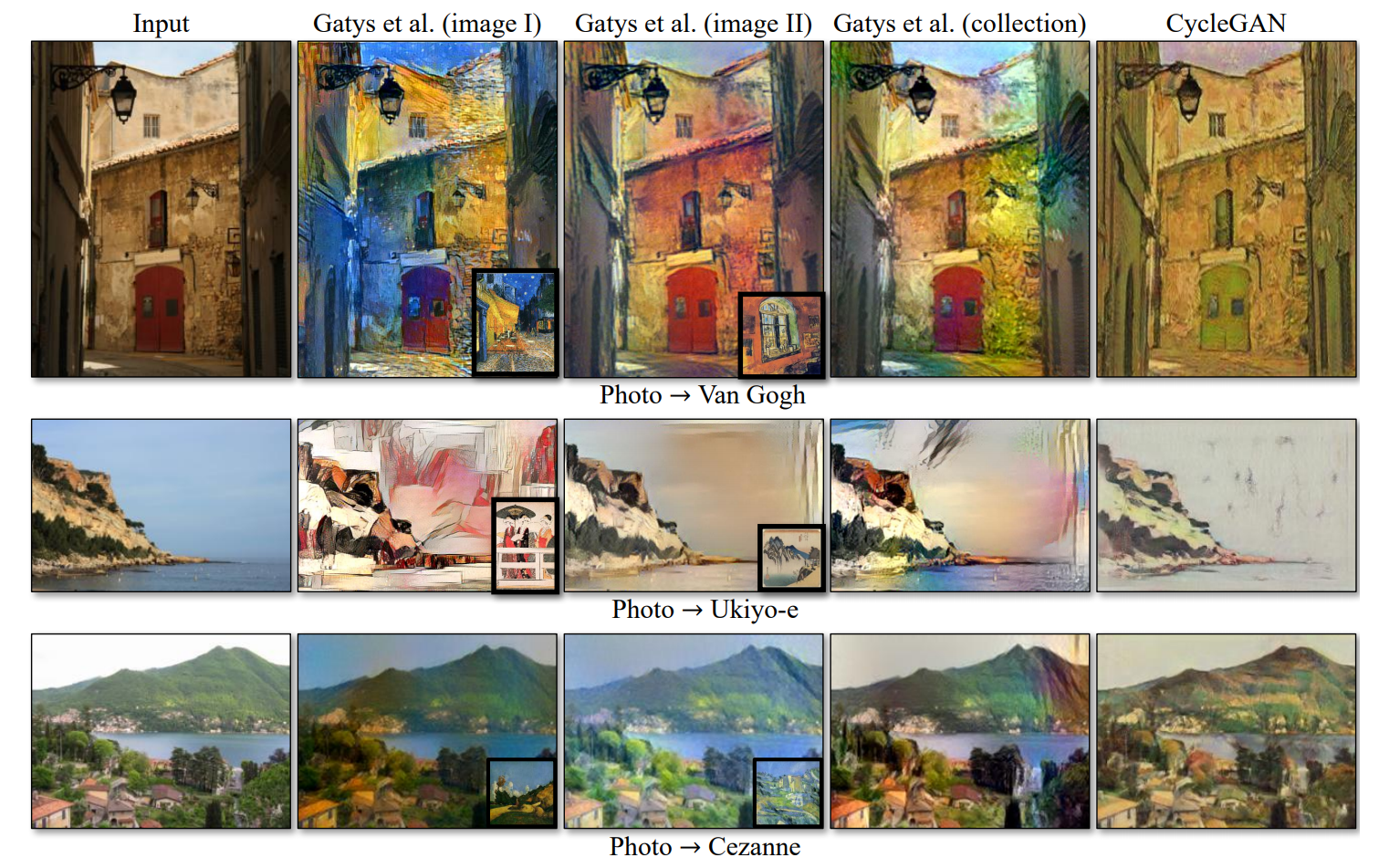
|
313 |
+
|
314 |
+
cycleGAN 与Gatys 的神经风格迁移相比(Comparison with Neural style transfer)
|
315 |
+
|
316 |
+
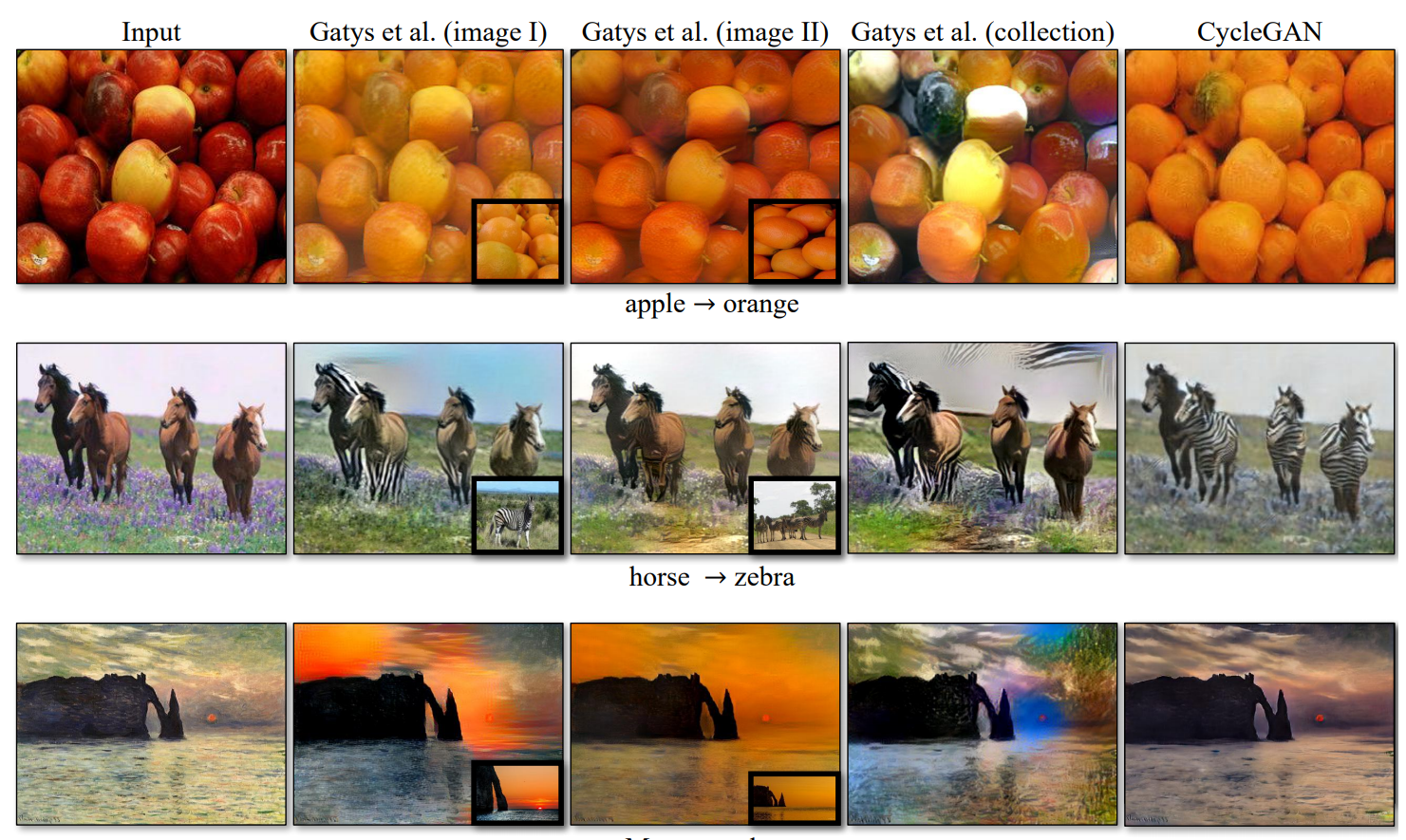
|
317 |
+
|
318 |
+
cycleGAN 与Gatys 的神经风格迁移相比,这个更明显
|
319 |
+
|
320 |
+
## 6. 局限与讨论
|
321 |
+
|
322 |
+
虽然我们的方法在多种案例下,取得了令人信服的结果,但是这些结果并不都是一直那么好。图17 就展示了几个典型的失败案例。在包括了涉及颜色和纹理变形的任务上,与上面的许多报告提及的一样,我们的方法经常是成功的。我们还探索了需要几何变换的任务,但是收效甚微(limit success)。举例说明:狗→猫 转换的任务,对翻译的学习 退化为对输入的图片进行最小限度的转换。这可能是由于我们对生成器结构的选择造成的,我们生成器的架构是为了在外观更改上的任务上拥有更好的性能而量身定制的。处理更多和更加极端的变化,尤其是几何变换,是未来工作的重点问题。
|
323 |
+
|
324 |
+
训练集的分布特征也会造成一些案例的失败。例如,我们的方法在转换 马→斑马 的时候发生了错乱,因为我们的模型只在ImageNet 上训练了 野马和斑马 这两个类别,而没有包括人类骑马的图片。所以普京骑马的那一张,把普京变成斑马人了。
|
325 |
+
|
326 |
+
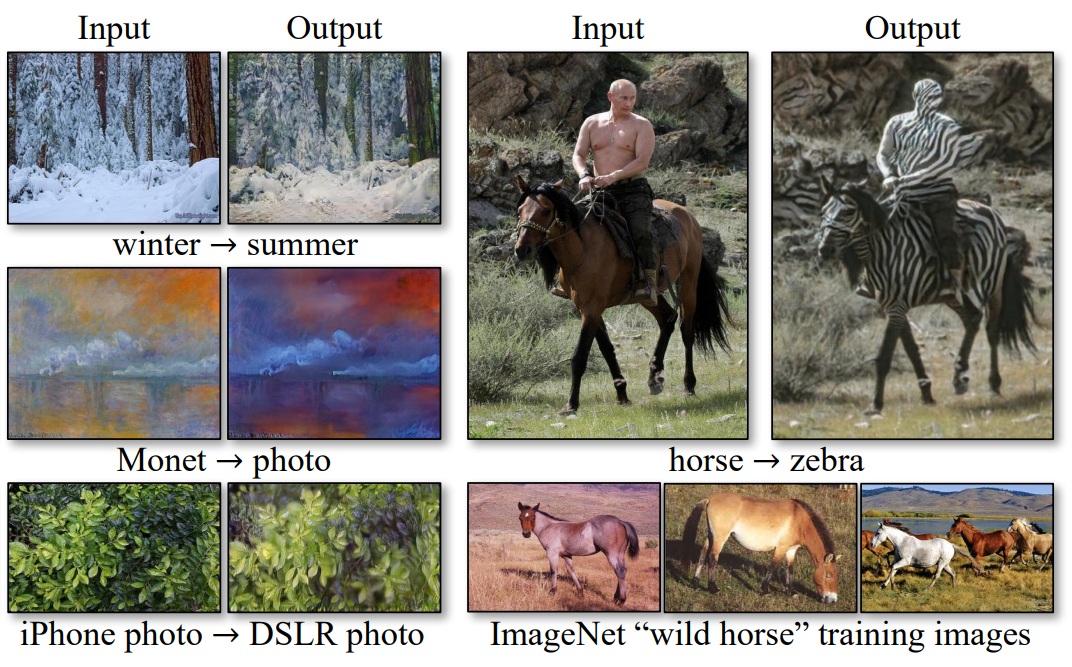
|
327 |
+
|
328 |
+
把普京变成斑马人,等
|
329 |
+
|
330 |
+
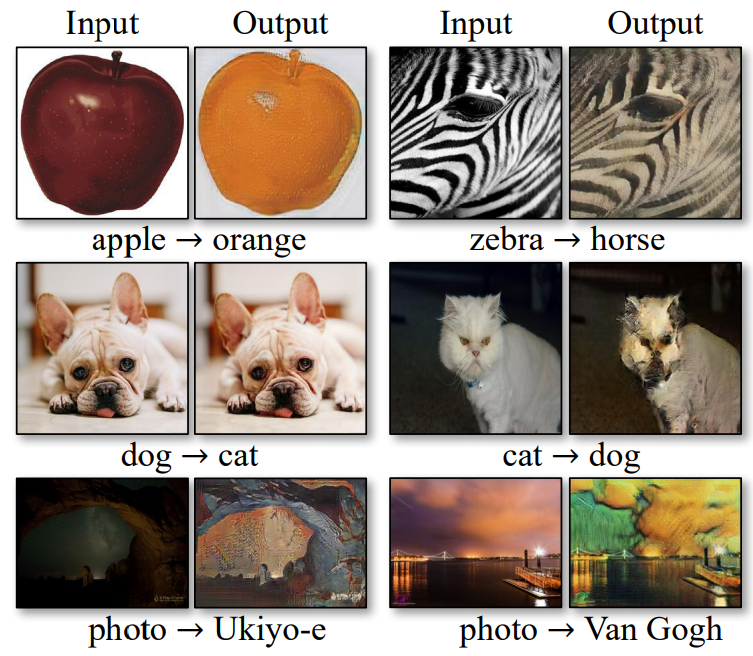
|
331 |
+
|
332 |
+
猫 → 狗 ,苹果 → 橙子 ,形状的变换不足
|
333 |
+
|
334 |
+
我们也发现在成对图片训练 和 非成对训练 之间存在无法消弭的差距。在一些案例里面,这个差距似乎特别难以消除,甚至不可能消除。为了消除(模型对数据理解上的)歧义,模型可能需要一些弱语义监督。集成的弱监督或者半监督数据也许能够造就更有力的翻译器,这些数据依然只会占完全监督系统中的一小部分。
|
335 |
+
|
336 |
+
尽管如此,在多种情况下,完全使用不成对的数据依然是足够可行的,我们应该使用。这篇论文拓展了“无监督”配置可能使用范围。
|
337 |
+
|
338 |
+
> 论文原文中的部分图表,我没有给出
|
339 |
+
> **致谢部分,我用的是谷歌翻译2018-10-25 16:27:43**
|
340 |
+
> **第七节的附录,我用的是谷歌翻译2018-10-25 16:27:43**
|
341 |
+
|
342 |
+
**!!!下面都是谷歌翻译!!!**
|
343 |
+
|
344 |
+
**致谢:**我们感谢Aaron Hertzmann,Shiry Ginosar,Deepak Pathak,Bryan Russell,Eli Shechtman,Richard Zhang和Tinghui Zhou的许多有益评论。这项工作部分得到了NSF SMA1514512,NSF IIS-1633310,Google Research Award,Intel Corp以及NVIDIA的硬件捐赠的支持。JYZ由Facebook Graduate Fellowship支持,TP由三星奖学金支持。用于风格转移的照片由AE拍摄,主要在法国拍摄。
|
345 |
+
|
346 |
+
## **7.附录7.1。**
|
347 |
+
|
348 |
+
训练细节所有网络(边缘除外)均从头开始训练,学习率为0.0002。在实践中,我们将目标除以2,同时优化D,这减慢了D学习的速率,相对于G的速率。我们保持前100个时期的相同学习速率并将速率线性衰减到零。接下来的100个时代。权重从高斯分布初始化,均值为0,标准差为0.02。
|
349 |
+
|
350 |
+
Cityscapes标签↔Photo2975训练图像来自Cityscapes训练集[4],��像大小为128×128。我们使用Cityscapes val集进行测试。
|
351 |
+
|
352 |
+
Maps↔aerial照片1096个训练图像是从谷歌地图[22]中删除的,图像大小为256×256。图像来自纽约市内及周边地区。然后将数据分成火车并测试采样区域的中位数纬度(添加缓冲区以确保测试集中没有出现训练像素)。建筑立面标签照片来自CMP Facade数据库的400张训练图像[40]。边缘→鞋子来自UT Zappos50K数据集的大约50,000个训练图像[60]。该模型经过5个时期的训练。
|
353 |
+
|
354 |
+
Horse↔Zebra和Apple↔Orange我们使用关键字“野马”,“斑马”,“苹果”和“脐橙”从ImageNet [5]下载图像。图像缩放为256×256像素。每个班级的训练集大小为马:939,斑马:1177,苹果:996,橙色:1020.
|
355 |
+
|
356 |
+
Summer↔WinterYosemite使用带有标签yosemite和datetaken字段的Flickr API下载图像。修剪了黑白照片。图像缩放为256×256像素。每个班级的培训规模为夏季:1273,冬季:854。照片艺术风格转移艺术图像从[http://Wikiart.org](http://Wikiart.org)下载。一些素描或**过于淫秽的艺术品**都是手工修剪过的。这些照片是使用标签横向和横向摄影的组合从Flickr下载的。黑白照片被修剪。图像缩放为256×256像素。每个班级的训练集大小为Monet:1074,Cezanne:584,Van Gogh:401,Ukiyo-e:1433,照片:6853。Monet数据集被特别修剪为仅包括风景画,而Van Gogh仅包括他的后期作品代表了他最知名的艺术风格。
|
357 |
+
|
358 |
+
Photo↔Art风格转移艺术图像从[http://Wikiart.org](http://Wikiart.org)下载。一些素描或过于淫秽的艺术品都是手工修剪过的。这些照片是使用标签横向和横向摄影的组合从Flickr下载的。黑白照片被修剪。图像缩放为256×256像素。每个班级的训练集大小为Monet:1074,Cezanne:584,Van Gogh:401,Ukiyo-e:1433,照片:6853。
|
359 |
+
|
360 |
+
Monet数据集被特别修剪为仅包括风景画,而Van Gogh仅包括他的后期作品代表了他最知名的艺术风格。莫奈的画作→照片为了在保存记忆的同时实现高分辨率,我们使用矩形图像的随机方形作物进行训练。为了生成结果,我们将具有正确宽高比的宽度为512像素的图像作为输入传递给生成器网络。身份映射损失的权重为0.5λ,其中λ是周期一致性损失的权重,我们设置λ= 10.
|
361 |
+
|
362 |
+
花卉照片增强智能手机拍摄的花卉图像是通过搜索Apple iPhone 5拍摄的照片从Flickr下载的,5s或6,搜索文本花。具有浅DoF的DSLR图像也通过搜索标签flower,dof从Flickr下载。将图像按比例缩放到360像素宽。使用重量0.5λ的同一性映射损失。智能手机和DSLR数据集的训练集大小分别为1813和3326。
|
363 |
+
|
364 |
+
7.2。网络架构
|
365 |
+
|
366 |
+
我们提供PyTorch和Torch实现。
|
367 |
+
|
368 |
+
**发电机架构** 我们采用Johnson等人的架构。[23]。我们使用6个块用于128×128个训练图像,9个块用于256×256或更高分辨率的训练图像。下面,我们遵循Johnson等人的Github存储库中使用的命名约定。
|
369 |
+
|
370 |
+
令c7s1-k表示具有k个滤波器和步幅1的7×7Convolution-InstanceNormReLU层.dk表示具有k个滤波器和步幅2的3×3卷积 - 实例范数 - ReLU层。反射填充用于减少伪像。Rk表示包含两个3×3卷积层的残余块,在两个层上具有相同数量的滤波器。uk表示具有k个滤波器和步幅1 2的3×3分数跨度-ConvolutionInstanceNorm-ReLU层。
|
371 |
+
|
372 |
+
具有6个块的网络包括:c7s1-32,d64,d128,R128,R128,R128,R128,R128,R128,u64,u32,c7s1-3
|
373 |
+
|
374 |
+
具有9个块的网络包括:c7s1-32,d64,d128,R128,R128,R128,R128,R128,R128,R128,R128,R128,u64 u32,c7s1-3
|
375 |
+
|
376 |
+
**鉴别器架构** 对于鉴别器网络,我们使用70×70 PatchGAN [22]。设Ck表示具有k个滤波器和步幅2的4×4卷积 - 实例范数 - LeakyReLU层。在最后一层之后,我们应用卷积来产生1维输出。我们不将InstanceNorm用于第一个C64层。我们使用泄漏的ReLU,斜率为0.2。鉴别器架构是:C64-C128-C256-C512
|
377 |
+
|
378 |
+
## **欢迎讨论 ^_^**
|
379 |
+
|
380 |
+
## 对评论区的回复:
|
381 |
+
|
382 |
+
- 几个关于翻译的建议
|
383 |
+
- 如猫狗转换这种一对多的转换,有其他模型能学到吗?有,但是受应用场景限制
|
384 |
+
- CycleGAN如何保证不发生交叉映射?在满足双射(bijections)的情况下,保持循环一致
|
385 |
+
|
386 |
+
> [@LIEBE](https://www.zhihu.com/people/a078ee299d4a8f2b598c6d1d1e83b4b1)
|
387 |
+
>
|
388 |
+
>
|
389 |
+
> 这里有几个小小的(关于翻译的)建议,不知道我理解得正不正确:
|
390 |
+
|
391 |
+
采用并在正文改正:upper bound:天花板×,上限√。作者 写**光圈小,景深深(大)**是正确的,谢谢提醒,我自己弄错了。
|
392 |
+
|
393 |
+
1.translation可以翻成转化?(“图像间的转化”读起来更直白一点?)我坚持把translation直接翻译成「翻译」,理由如下:论文原文有“a sentence from English to French, and then translate it back from French to English”, CycleGAN 的灵感是从「**语言翻译**」中来的,**英语→法语→英语,图像域A→图像域B→图像域A**。而[Pix2Pix - Image-to-Image Translation with Conditional Adversarial Networks - 2018](https://zhuanlan.zhihu.com/p/45394148/h%3Cb%3Ettps://arxiv.org/pdf/1611.%3C/b%3E07004.pdf)的论文原文也有“. Just as a concept may be expressed in either **English or French**, a scene may be rendered as an RGB image, ... ...”,因此我没有翻成**转化**。
|
394 |
+
|
395 |
+
4.第5.1节 的评估那里是还没翻译完吗?考不考虑手动翻译一遍7.附录呢?不打算翻译,我认为这部分内容不看不会影响我们对CycleGAN的理解。
|
396 |
+
|
397 |
+
> [@小厮](https://www.zhihu.com/people/071d206346dc5dcf22339f44bfd324c2)
|
398 |
+
>
|
399 |
+
> 2019-08-14
|
400 |
+
> cyclegan的话,更强调循环一致,猫狗转换这种,对于一只猫转换为一只狗,一只狗转换为一只猫,存在很多**1-多的关系**。因为不存在明显一一对应关系,那么目标就变成学习一个足够真实(和源分布尽可能相似)的分布。**对于猫狗转换这种,别的gan可以学习学到嘛?**
|
401 |
+
|
402 |
+
我的回答:有,但是受应用场景限制。例如:
|
403 |
+
|
404 |
+
小样本无监督图片翻译 [Few-Shot Unsupervised Image-to-Image Translation - 英伟达 2019-05 论文pdf](https://arxiv.org/pdf/1905.01723.pdf) (从视频上看,做的非常好,但是我还没有机会去复现它)
|
405 |
+
|
406 |
+
知乎相关介绍 [英伟达最新图像转换器火了!万物皆可换脸,试玩开放 - 新智元](https://zhuanlan.zhihu.com/p/65297812)
|
407 |
+
|
408 |
+
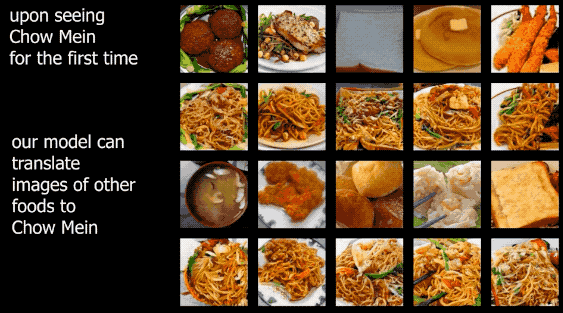
|
409 |
+
|
410 |
+
让各种食物变成炒面,仔细观察可以看到图片翻译前后,其结构是高度对应的
|
411 |
+
|
412 |
+
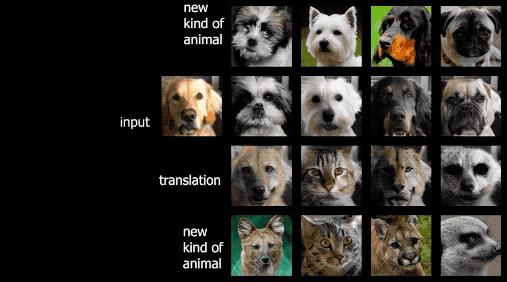
|
413 |
+
|
414 |
+
让狗变成其他动物,上面是以上是张嘴、歪头的动图
|
415 |
+
|
416 |
+
另一个,人类的转换,人脸到人脸转换,有 DeepFace等开源工具可以实现。人脸到其他脸,有它可以实现→ ,论文 [Landmark Assisted CycleGAN for Cartoon Face Generation - 香港中文 - 贾佳亚](https://arxiv.org/abs/1907.01424) 。
|
417 |
+
|
418 |
+
知乎相关介绍: [用于卡通人脸生成的关键点辅助CycleGAN](https://zhuanlan.zhihu.com/p/73995207)
|
419 |
+
|
420 |
+
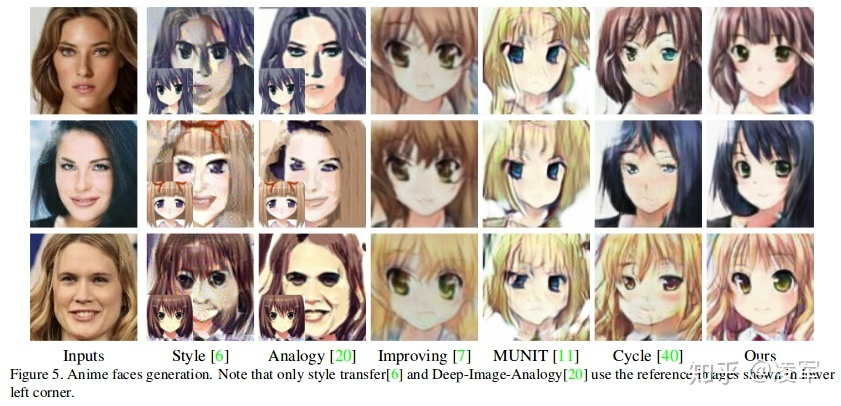
|
421 |
+
|
422 |
+
> [@信息门下走狗](https://www.zhihu.com/people/d67ee185c975baea76cf8d401876f9d3)
|
423 |
+
>
|
424 |
+
> 2018-11-02
|
425 |
+
> 极端的例子,假定就是马和斑马的变换,马与斑马都分两种姿态,一类是站着,一类是趴着,那么对于系统设计的代价函数而言,我完全可以把所有站着的马都映射为趴着的斑马,趴着的马变成站着的斑马,然后逆向映射把趴着的斑马映射回站着的马。这个映射的代价函数和正常保持马的姿态的映射是一样的。那么系统是如何保证**不发生这种交叉映射**的呢
|
426 |
+
|
427 |
+
我的回答:在满足双射(bijections)的情况下,保持循环一致性。
|
428 |
+
|
429 |
+
文章第二节的**Cycle Consitency 循环一致性**提到:加入循环一致性损失 (cycle consistency loss),可以保证了映射的正确,在语言处理领域也是如此。
|
430 |
+
文章第一节的介绍部分,提到:从数学上讲,如果我们有一个翻译器 G : X → Y 与另一个翻译器 F : Y → X ,那么 G 与 F 彼此是相反的,这一对映射是双射(bijections) 。系统通过**Cycle Consitency 循环一致性**,保证不发生**趴着的野马**,**站着的斑马**这种交叉映射,从数学上讲,原理是[双射(wikipedia: bijections)](https://en.wikipedia.org/wiki/Bijection),即参与映射的两个集合,里面的元素必然是一一对应的。
|
431 |
+
|
432 |
+
野马集合 斑马集合
|
433 |
+
|
434 |
+
- 站姿1的野马 ⇋ 站姿1的斑马
|
435 |
+
- 站姿2的野马 ⇋ 站姿2的斑马
|
436 |
+
- ...
|
437 |
+
- 跪姿1的野马 ⇋ 跪姿1的斑马
|
438 |
+
- ...
|
439 |
+
|
440 |
+
↑符合循环一致性的双射
|
441 |
+
|
442 |
+
假如在某一次随机重启后,cycleGAN恰好学到了 站姿⇋跪姿 的转化,我们**不期望的交叉映射**发生了,有如下:
|
443 |
+
|
444 |
+
野马集合 斑马集合
|
445 |
+
|
446 |
+
- 站姿1的野马 ⇋ 跪姿1的斑马
|
447 |
+
- 站姿2的野马 ⇋ 跪姿2的斑马
|
448 |
+
- ...
|
449 |
+
- 跪姿1的野马 ⇋ 站姿1的斑马
|
450 |
+
- ...
|
451 |
+
|
452 |
+
其实经过分析,我们会发现**无法证明 对于站姿n,有稳定的跪姿n 与其一一对应**,**所以这种交叉映射是不符合双射的。**不符合双射的两个映射,不满足**循环一致性**。然而,由于野马斑马外形差别不大,导致站姿n的野马 到 站姿n斑马 总是存在稳定的一一对应的姿态可以相互转换,所以,可以预见到,在执行图片到图片的翻译任务时,当训练到收敛的时候,循环稳定性可以得到正确的结果,避免交叉映射。
|
453 |
+
|
454 |
+
根据文章中提到的:使用cycleGAN 可以进行 野马 ⇋斑马 的转换,但是无法转化形态差异比较大的 猫 ⇋ 狗。 因为从 猫 ⇋ 狗 之间的转化,**不能严格满足 双射 的要求**,当存在许多差异明显的猫狗时,因为循环一致性并不能指导模型,导致��多的交叉映射破坏循环一致性,从而无法训练得到满意的模型。
|
455 |
+
|
456 |
+

|
457 |
+
|
458 |
+
文章中提及的 失败案例(形态差异大的) 猫 ⇋ 狗
|
459 |
+
|
460 |
+
所以,我的结论:
|
461 |
+
|
462 |
+
- 野马 ⇋ 斑马:满足双射,可以避免交叉映射,符合循环一致性,训练效果好
|
463 |
+
- 猫 ⇋ 狗:不能严格满足双射,无法完全避免交叉映射,破坏了循环一致性,训练效果不佳
|
464 |
+
|
465 |
+
cyclegan的话,更强调循环一致,猫狗转换这种,对于一只猫转换为一只狗,一只狗转换为一只猫,存在很多1-多的关系。因为不存在明显一一对应关系,那么目标就变成学习一个足够真实(和源分布尽可能相似)的分布。对于猫狗转换这种,别的gan可以学习学到嘛?
|
app.py
ADDED
@@ -0,0 +1,97 @@
|
|
|
|
|
|
|
|
|
|
|
|
|
|
|
|
|
|
|
|
|
|
|
|
|
|
|
|
|
|
|
|
|
|
|
|
|
|
|
|
|
|
|
|
|
|
|
|
|
|
|
|
|
|
|
|
|
|
|
|
|
|
|
|
|
|
|
|
|
|
|
|
|
|
|
|
|
|
|
|
|
|
|
|
|
|
|
|
|
|
|
|
|
|
|
|
|
|
|
|
|
|
|
|
|
|
|
|
|
|
|
|
|
|
|
|
|
|
|
|
|
|
|
|
|
|
|
|
|
|
|
|
|
|
|
|
|
|
|
|
|
|
|
|
|
|
|
|
|
|
|
|
|
|
|
|
|
|
|
|
|
|
|
|
|
|
|
|
|
|
|
|
|
|
|
|
|
|
|
|
|
|
|
|
|
|
|
|
|
|
|
|
|
|
|
|
|
1 |
+
"""
|
2 |
+
实现web界面
|
3 |
+
"""
|
4 |
+
|
5 |
+
from pathlib import Path
|
6 |
+
|
7 |
+
import gradio as gr
|
8 |
+
from detect import detect
|
9 |
+
from util import get_all_weights
|
10 |
+
|
11 |
+
app_introduce = """
|
12 |
+
# CycleGAN
|
13 |
+
功能:上传本地文件、选择转换风格
|
14 |
+
"""
|
15 |
+
|
16 |
+
css = """
|
17 |
+
# :root{
|
18 |
+
# --block-background-fill: #f3f3f3;
|
19 |
+
# }
|
20 |
+
footer{
|
21 |
+
display:none!important;
|
22 |
+
}
|
23 |
+
"""
|
24 |
+
|
25 |
+
demo = gr.Blocks(
|
26 |
+
# theme=gr.themes.Soft(),
|
27 |
+
css=css
|
28 |
+
)
|
29 |
+
|
30 |
+
|
31 |
+
def add_img(img):
|
32 |
+
imgs = []
|
33 |
+
for style in get_all_weights():
|
34 |
+
fake_img = detect(img, style=style)
|
35 |
+
imgs.append(fake_img)
|
36 |
+
return imgs
|
37 |
+
|
38 |
+
|
39 |
+
def tab1(label="上传单张图片"):
|
40 |
+
default_img_paths = [
|
41 |
+
[Path.cwd().joinpath("./imgs/horse.jpg"), "horse2zebra"],
|
42 |
+
[Path.cwd().joinpath("./imgs/monet.jpg"), "monet2photo"],
|
43 |
+
]
|
44 |
+
|
45 |
+
with gr.Tab(label):
|
46 |
+
with gr.Row():
|
47 |
+
with gr.Column():
|
48 |
+
img = gr.Image(type="pil", label="选择需要进行风格转换的图片")
|
49 |
+
style = gr.Dropdown(choices=get_all_weights(), label="转换的风格")
|
50 |
+
detect_btn = gr.Button("♻️风格转换")
|
51 |
+
with gr.Column():
|
52 |
+
out_img = gr.Image(label="风格图")
|
53 |
+
detect_btn.click(fn=detect, inputs=[img, style], outputs=[out_img])
|
54 |
+
gr.Examples(default_img_paths, inputs=[img, style])
|
55 |
+
|
56 |
+
|
57 |
+
def tab2(label="单图多风格试转换"):
|
58 |
+
with gr.Tab(label):
|
59 |
+
with gr.Row():
|
60 |
+
with gr.Column(scale=1):
|
61 |
+
img = gr.Image(type="pil", label="选择需要进行风格转换的图片")
|
62 |
+
gr.Markdown("上传一张图片,会将所有风格推理一遍。")
|
63 |
+
btn = gr.Button("♻️风格转换")
|
64 |
+
with gr.Column(scale=2):
|
65 |
+
gallery = gr.Gallery(
|
66 |
+
label="风格图",
|
67 |
+
elem_id="gallery",
|
68 |
+
).style(grid=[3], height="auto")
|
69 |
+
btn.click(fn=add_img, inputs=[img], outputs=gallery)
|
70 |
+
|
71 |
+
|
72 |
+
def tab3(label="参数设置"):
|
73 |
+
from detect import opt
|
74 |
+
|
75 |
+
with gr.Tab(label):
|
76 |
+
with gr.Column():
|
77 |
+
for k, v in sorted(vars(opt).items()):
|
78 |
+
if type(v) == bool:
|
79 |
+
gr.Checkbox(label=k, value=v)
|
80 |
+
elif type(v) == (int or float):
|
81 |
+
gr.Number(label=k, value=v)
|
82 |
+
elif type(v) == list:
|
83 |
+
gr.CheckboxGroup(label=k, value=v)
|
84 |
+
else:
|
85 |
+
gr.Textbox(label=k, value=v)
|
86 |
+
|
87 |
+
|
88 |
+
with demo:
|
89 |
+
gr.Markdown(app_introduce)
|
90 |
+
tab1()
|
91 |
+
tab2()
|
92 |
+
tab3()
|
93 |
+
if __name__ == "__main__":
|
94 |
+
demo.launch(share=True)
|
95 |
+
# 如果不以`demo`命名,`gradio app.py`会报错`Error loading ASGI app. Attribute "demo.app" not found in module "app".`
|
96 |
+
# 注意gradio库的reload.py的头信息 $ gradio app.py my_demo, to use variable names other than "demo"
|
97 |
+
# my_demo 是定义的变量。离谱o(╥﹏╥)o
|
app1.py
ADDED
@@ -0,0 +1,87 @@
|
|
|
|
|
|
|
|
|
|
|
|
|
|
|
|
|
|
|
|
|
|
|
|
|
|
|
|
|
|
|
|
|
|
|
|
|
|
|
|
|
|
|
|
|
|
|
|
|
|
|
|
|
|
|
|
|
|
|
|
|
|
|
|
|
|
|
|
|
|
|
|
|
|
|
|
|
|
|
|
|
|
|
|
|
|
|
|
|
|
|
|
|
|
|
|
|
|
|
|
|
|
|
|
|
|
|
|
|
|
|
|
|
|
|
|
|
|
|
|
|
|
|
|
|
|
|
|
|
|
|
|
|
|
|
|
|
|
|
|
|
|
|
|
|
|
|
|
|
|
|
|
|
|
|
|
|
|
|
|
|
|
|
|
|
|
|
|
|
|
|
|
|
|
|
|
|
1 |
+
# 2023年2月23日
|
2 |
+
"""
|
3 |
+
实现web界面
|
4 |
+
|
5 |
+
>>> streamlit run app.py
|
6 |
+
"""
|
7 |
+
|
8 |
+
from io import BytesIO
|
9 |
+
from pathlib import Path
|
10 |
+
|
11 |
+
import streamlit as st
|
12 |
+
from detect import detect, opt
|
13 |
+
from PIL import Image
|
14 |
+
from util import get_all_weights
|
15 |
+
|
16 |
+
"""
|
17 |
+
# CycleGAN
|
18 |
+
功能:上传本地文件、选择转换风格
|
19 |
+
"""
|
20 |
+
|
21 |
+
|
22 |
+
def load_css(css_path="./util/streamlit/css.css"):
|
23 |
+
"""
|
24 |
+
加载CSS文件
|
25 |
+
:param css_path: CSS文件路径
|
26 |
+
"""
|
27 |
+
if Path(css_path).exists():
|
28 |
+
with open(css_path) as f:
|
29 |
+
# 将CSS文件内容插入到HTML中
|
30 |
+
st.markdown(
|
31 |
+
f"""<style>{f.read()}</style>""",
|
32 |
+
unsafe_allow_html=True,
|
33 |
+
)
|
34 |
+
|
35 |
+
|
36 |
+
def load_img_file(file):
|
37 |
+
"""读取图片文件"""
|
38 |
+
img = Image.open(BytesIO(file.read()))
|
39 |
+
st.image(img, use_column_width=True) # 显示图片
|
40 |
+
return img
|
41 |
+
|
42 |
+
|
43 |
+
def set_style_options(label: str, frame=st):
|
44 |
+
"""风格选项"""
|
45 |
+
style_options = get_all_weights()
|
46 |
+
options = [None] + style_options # 默认空
|
47 |
+
style_param = frame.selectbox(label=label, options=options)
|
48 |
+
return style_param
|
49 |
+
|
50 |
+
|
51 |
+
# load_css()
|
52 |
+
tab_mul2mul, tab_mul2one, tab_set = st.tabs(["多图多风格转换", "多图同风格转换", "参数"])
|
53 |
+
|
54 |
+
with tab_mul2mul:
|
55 |
+
uploaded_files = st.file_uploader(label="选择本地图片", accept_multiple_files=True, key=1)
|
56 |
+
if uploaded_files:
|
57 |
+
for idx, uploaded_file in enumerate(uploaded_files):
|
58 |
+
colL, colR = st.columns(2)
|
59 |
+
with colL:
|
60 |
+
img = load_img_file(uploaded_file)
|
61 |
+
style = set_style_options(label=str(uploaded_file), frame=st)
|
62 |
+
with colR:
|
63 |
+
if style:
|
64 |
+
fake_img = detect(img=img, style=style)
|
65 |
+
st.image(fake_img, caption="", use_column_width=True)
|
66 |
+
|
67 |
+
with tab_set:
|
68 |
+
colL, colR = st.columns([1, 3])
|
69 |
+
for k, v in sorted(vars(opt).items()):
|
70 |
+
st.text_input(label=k, value=v, disabled=True)
|
71 |
+
# st.selectbox("ss", options=opt.parse_args())
|
72 |
+
confidence_threshold = st.slider("Confidence threshold", 0.0, 1.0, 0.5, 0.01)
|
73 |
+
opt.no_dropout = st.radio("no_droput", [True, False])
|
74 |
+
|
75 |
+
with tab_mul2one:
|
76 |
+
uploaded_files = st.file_uploader(label="选择本地图片", accept_multiple_files=True, key=2)
|
77 |
+
if uploaded_files:
|
78 |
+
colL, colR = st.columns(2)
|
79 |
+
with colL:
|
80 |
+
imgs = [load_img_file(ii) for ii in uploaded_files]
|
81 |
+
with colR:
|
82 |
+
style = set_style_options(label="选择风格", frame=st)
|
83 |
+
if style:
|
84 |
+
if st.button("♻️风格转换", use_container_width=True):
|
85 |
+
for img in imgs:
|
86 |
+
fake_img = detect(img, style)
|
87 |
+
st.image(fake_img, caption="", use_column_width=True)
|
data/__init__.py
ADDED
@@ -0,0 +1,114 @@
|
|
|
|
|
|
|
|
|
|
|
|
|
|
|
|
|
|
|
|
|
|
|
|
|
|
|
|
|
|
|
|
|
|
|
|
|
|
|
|
|
|
|
|
|
|
|
|
|
|
|
|
|
|
|
|
|
|
|
|
|
|
|
|
|
|
|
|
|
|
|
|
|
|
|
|
|
|
|
|
|
|
|
|
|
|
|
|
|
|
|
|
|
|
|
|
|
|
|
|
|
|
|
|
|
|
|
|
|
|
|
|
|
|
|
|
|
|
|
|
|
|
|
|
|
|
|
|
|
|
|
|
|
|
|
|
|
|
|
|
|
|
|
|
|
|
|
|
|
|
|
|
|
|
|
|
|
|
|
|
|
|
|
|
|
|
|
|
|
|
|
|
|
|
|
|
|
|
|
|
|
|
|
|
|
|
|
|
|
|
|
|
|
|
|
|
|
|
|
|
|
|
|
|
|
|
|
|
|
|
|
|
|
|
|
|
|
|
|
|
|
|
|
|
|
|
|
|
|
|
|
1 |
+
"""不同的模型使用不同的数据集
|
2 |
+
|
3 |
+
比如有监督模型使用的都是成对的训练数据、无监督模型使用的数据集不必使用成对的数据
|
4 |
+
This package includes all the modules related to data loading and preprocessing
|
5 |
+
|
6 |
+
To add a custom dataset class called 'dummy', you need to add a file called 'dummy_dataset.py' and define a subclass 'DummyDataset' inherited from BaseDataset.
|
7 |
+
You need to implement four functions:
|
8 |
+
-- <__init__>: initialize the class, first call BaseDataset.__init__(self, opt).
|
9 |
+
-- <__len__>: return the size of dataset.
|
10 |
+
-- <__getitem__>: get a data point from data loader.
|
11 |
+
-- <modify_commandline_options>: (optionally) add dataset-specific options and set default options.
|
12 |
+
|
13 |
+
Now you can use the dataset class by specifying a flag '--dataset_mode dummy'.
|
14 |
+
See our template dataset class 'template_dataset.py' for more details.
|
15 |
+
"""
|
16 |
+
|
17 |
+
import pickle
|
18 |
+
import importlib
|
19 |
+
import torch.utils.data
|
20 |
+
from .base_dataset import BaseDataset
|
21 |
+
from .one_dataset import *
|
22 |
+
|
23 |
+
__all__ = [OneDataset]
|
24 |
+
|
25 |
+
|
26 |
+
def find_dataset_by_name(dataset_name: str):
|
27 |
+
"""按照数据集名称来寻找所对应的dataset类进行动态导入
|
28 |
+
Import the module "data/[dataset_name]_dataset.py".
|
29 |
+
|
30 |
+
In the file, the class called DatasetNameDataset() will
|
31 |
+
be instantiated. It has to be a subclass of BaseDataset,
|
32 |
+
and it is case-insensitive.
|
33 |
+
"""
|
34 |
+
dataset_filename = "data." + dataset_name + "_dataset"
|
35 |
+
datasetlib = importlib.import_module(dataset_filename)
|
36 |
+
|
37 |
+
dataset = None
|
38 |
+
target_dataset_name = dataset_name.replace("_", "") + "dataset"
|
39 |
+
for name, cls in datasetlib.__dict__.items():
|
40 |
+
if name.lower() == target_dataset_name.lower() and issubclass(cls, BaseDataset):
|
41 |
+
dataset = cls
|
42 |
+
|
43 |
+
if dataset is None:
|
44 |
+
raise NotImplementedError(f"In {dataset_filename}.py, there should be a subclass of BaseDataset with class " f"name that matches {target_dataset_name} in lowercase.")
|
45 |
+
return dataset
|
46 |
+
|
47 |
+
|
48 |
+
def get_option_setter(dataset_name):
|
49 |
+
"""Return the static method <modify_commandline_options> of the dataset class."""
|
50 |
+
dataset_class = find_dataset_by_name(dataset_name)
|
51 |
+
return dataset_class.modify_commandline_options
|
52 |
+
|
53 |
+
|
54 |
+
def create_dataset(opt):
|
55 |
+
"""Create a dataset given the option.
|
56 |
+
|
57 |
+
This function wraps the class CustomDatasetDataLoader.
|
58 |
+
This is the main interface between this package and 'train.py'/'test.py'
|
59 |
+
|
60 |
+
Example:
|
61 |
+
>>> from data import create_dataset
|
62 |
+
>>> dataset = create_dataset(opt)
|
63 |
+
"""
|
64 |
+
data_loader = CustomDatasetDataLoader(opt)
|
65 |
+
dataset = data_loader.load_data()
|
66 |
+
return dataset
|
67 |
+
|
68 |
+
|
69 |
+
class CustomDatasetDataLoader:
|
70 |
+
"""Wrapper class of Dataset class that performs multi-threading data loading"""
|
71 |
+
|
72 |
+
def __init__(self, opt):
|
73 |
+
"""Initialize this class
|
74 |
+
|
75 |
+
Step 1: create a dataset instance given the name [dataset_mode]
|
76 |
+
Step 2: create a multi-threading data loader.
|
77 |
+
"""
|
78 |
+
self.opt = opt
|
79 |
+
dataset_file = f"datasets/{opt.name}.pkl"
|
80 |
+
if not Path(dataset_file).exists():
|
81 |
+
# 判断数据集类型(成对/不成对),得到相应的类包
|
82 |
+
dataset_class = find_dataset_by_name(opt.dataset_mode)
|
83 |
+
# 传入数据集路径到类包中,得到数据集
|
84 |
+
self.dataset = dataset_class(opt)
|
85 |
+
# 打包下次直接使用
|
86 |
+
# 打包后文件也很大,暂时就这样
|
87 |
+
print("pickle dump dataset...")
|
88 |
+
pickle.dump(self.dataset, open(dataset_file, 'wb'))
|
89 |
+
else:
|
90 |
+
print("pickle load dataset...")
|
91 |
+
self.dataset = pickle.load(open(dataset_file, 'rb'))
|
92 |
+
print("dataset [%s] was created" % type(self.dataset).__name__)
|
93 |
+
|
94 |
+
self.dataloader = torch.utils.data.DataLoader(
|
95 |
+
self.dataset,
|
96 |
+
batch_size=opt.batch_size,
|
97 |
+
shuffle=not opt.serial_batches,
|
98 |
+
num_workers=int(opt.num_threads),
|
99 |
+
)
|
100 |
+
|
101 |
+
def load_data(self):
|
102 |
+
print(f"The number of training images = {len(self)}")
|
103 |
+
return self
|
104 |
+
|
105 |
+
def __iter__(self):
|
106 |
+
"""Return a batch of data"""
|
107 |
+
for i, data in enumerate(self.dataloader):
|
108 |
+
if i * self.opt.batch_size >= self.opt.max_dataset_size:
|
109 |
+
break
|
110 |
+
yield data
|
111 |
+
|
112 |
+
def __len__(self):
|
113 |
+
"""Return the number of data in the dataset"""
|
114 |
+
return min(len(self.dataset), self.opt.max_dataset_size)
|
data/base_dataset.py
ADDED
@@ -0,0 +1,231 @@
|
|
|
|
|
|
|
|
|
|
|
|
|
|
|
|
|
|
|
|
|
|
|
|
|
|
|
|
|
|
|
|
|
|
|
|
|
|
|
|
|
|
|
|
|
|
|
|
|
|
|
|
|
|
|
|
|
|
|
|
|
|
|
|
|
|
|
|
|
|
|
|
|
|
|
|
|
|
|
|
|
|
|
|
|
|
|
|
|
|
|
|
|
|
|
|
|
|
|
|
|
|
|
|
|
|
|
|
|
|
|
|
|
|
|
|
|
|
|
|
|
|
|
|
|
|
|
|
|
|
|
|
|
|
|
|
|
|
|
|
|
|
|
|
|
|
|
|
|
|
|
|
|
|
|
|
|
|
|
|
|
|
|
|
|
|
|
|
|
|
|
|
|
|
|
|
|
|
|
|
|
|
|
|
|
|
|
|
|
|
|
|
|
|
|
|
|
|
|
|
|
|
|
|
|
|
|
|
|
|
|
|
|
|
|
|
|
|
|
|
|
|
|
|
|
|
|
|
|
|
|
|
|
|
|
|
|
|
|
|
|
|
|
|
|
|
|
|
|
|
|
|
|
|
|
|
|
|
|
|
|
|
|
|
|
|
|
|
|
|
|
|
|
|
|
|
|
|
|
|
|
|
|
|
|
|
|
|
|
|
|
|
|
|
|
|
|
|
|
|
|
|
|
|
|
|
|
|
|
|
|
|
|
|
|
|
|
|
|
|
|
|
|
|
|
|
|
|
|
|
|
|
|
|
|
|
|
|
|
|
|
|
|
|
|
|
|
|
|
|
|
|
|
|
|
|
|
|
|
|
|
|
|
|
|
|
|
|
|
|
|
|
|
|
|
|
|
|
|
|
|
|
|
|
|
|
|
|
|
|
|
|
|
|
|
|
|
|
|
|
|
|
|
|
|
|
|
|
|
|
|
|
|
|
|
|
|
|
|
|
|
|
|
|
|
|
|
|
|
|
|
|
|
|
|
|
|
|
|
|
|
|
|
|
|
|
|
|
|
|
|
|
|
|
|
|
|
|
|
|
|
|
|
|
|
1 |
+
"""This module implements an abstract base class (ABC) 'BaseDataset' for datasets.
|
2 |
+
|
3 |
+
It also includes common transformation functions (e.g., get_transform, __scale_width), which can be later used in
|
4 |
+
subclasses.
|
5 |
+
|
6 |
+
"""
|
7 |
+
import random
|
8 |
+
import numpy as np
|
9 |
+
import torch.utils.data as data
|
10 |
+
import torch
|
11 |
+
from PIL import Image
|
12 |
+
import torchvision.transforms as transforms
|
13 |
+
from abc import ABC, abstractmethod
|
14 |
+
|
15 |
+
|
16 |
+
class BaseDataset(data.Dataset, ABC):
|
17 |
+
"""This class is an abstract base class (ABC) for datasets.
|
18 |
+
|
19 |
+
To create a subclass, you need to implement the following four functions:
|
20 |
+
-- <__init__>: initialize the class, first call BaseDataset.__init__(self, opt).
|
21 |
+
-- <__len__>: return the size of dataset.
|
22 |
+
-- <__getitem__>: get a data point.
|
23 |
+
-- <modify_commandline_options>: (optionally) add dataset-specific options and set default options.
|
24 |
+
"""
|
25 |
+
|
26 |
+
def __init__(self, opt):
|
27 |
+
"""Initialize the class; save the options in the class
|
28 |
+
|
29 |
+
Parameters:
|
30 |
+
opt (Option class)-- stores all the experiment flags; needs to be a subclass of BaseOptions
|
31 |
+
"""
|
32 |
+
self.opt = opt
|
33 |
+
self.root = opt.dataroot
|
34 |
+
|
35 |
+
@staticmethod
|
36 |
+
def modify_commandline_options(parser, is_train):
|
37 |
+
"""用于添加针对这个数据集特定的选项,这个脚本里头只是一个样例。
|
38 |
+
|
39 |
+
Parameters:
|
40 |
+
parser -- original option parser
|
41 |
+
parser:
|
42 |
+
is_train (bool) -- whether training phase or test phase.
|
43 |
+
|
44 |
+
Returns:
|
45 |
+
the modified parser.
|
46 |
+
"""
|
47 |
+
return parser
|
48 |
+
|
49 |
+
@abstractmethod
|
50 |
+
def __len__(self):
|
51 |
+
"""Return the total number of images in the dataset."""
|
52 |
+
return 0
|
53 |
+
|
54 |
+
@abstractmethod
|
55 |
+
def __getitem__(self, index):
|
56 |
+
"""Return a data point and its metadata information.
|
57 |
+
|
58 |
+
Parameters:
|
59 |
+
index - - a random integer for data indexing
|
60 |
+
|
61 |
+
Returns:
|
62 |
+
a dictionary of data with their names. It usually contains the data itself and its metadata information.
|
63 |
+
"""
|
64 |
+
pass
|
65 |
+
|
66 |
+
|
67 |
+
def get_params(opt, size):
|
68 |
+
w, h = size
|
69 |
+
new_h = h
|
70 |
+
new_w = w
|
71 |
+
if opt.preprocess == "resize_and_crop":
|
72 |
+
new_h = new_w = opt.load_size
|
73 |
+
elif opt.preprocess == "scale_width_and_crop":
|
74 |
+
new_w = opt.load_size
|
75 |
+
new_h = opt.load_size * h // w
|
76 |
+
|
77 |
+
x = random.randint(0, np.maximum(0, new_w - opt.crop_size))
|
78 |
+
y = random.randint(0, np.maximum(0, new_h - opt.crop_size))
|
79 |
+
|
80 |
+
flip = random.random() > 0.5
|
81 |
+
|
82 |
+
return {"crop_pos": (x, y), "flip": flip}
|
83 |
+
|
84 |
+
|
85 |
+
def get_transform(
|
86 |
+
opt,
|
87 |
+
params=None,
|
88 |
+
grayscale=False,
|
89 |
+
convert=True,
|
90 |
+
method=transforms.InterpolationMode.BICUBIC,
|
91 |
+
):
|
92 |
+
"""数据预处理"""
|
93 |
+
transform_list = []
|
94 |
+
|
95 |
+
# 灰度化
|
96 |
+
if grayscale:
|
97 |
+
transform_list.append(transforms.Grayscale(1))
|
98 |
+
|
99 |
+
# 图片大小调整
|
100 |
+
# 默认:双三次插值
|
101 |
+
if "resize" in opt.preprocess:
|
102 |
+
osize = [opt.load_size, opt.load_size]
|
103 |
+
transform_list.append(transforms.Resize(osize, method))
|
104 |
+
elif "scale_width" in opt.preprocess:
|
105 |
+
transform_list.append(
|
106 |
+
transforms.Lambda(
|
107 |
+
lambda img: __scale_width(img, opt.load_size, opt.crop_size, method)
|
108 |
+
)
|
109 |
+
)
|
110 |
+
|
111 |
+
# 裁剪
|
112 |
+
if "crop" in opt.preprocess:
|
113 |
+
if params is None:
|
114 |
+
transform_list.append(transforms.RandomCrop(opt.crop_size))
|
115 |
+
else:
|
116 |
+
transform_list.append(
|
117 |
+
transforms.Lambda(
|
118 |
+
lambda img: __crop(img, params["crop_pos"], opt.crop_size)
|
119 |
+
)
|
120 |
+
)
|
121 |
+
if opt.preprocess == "none":
|
122 |
+
transform_list.append(
|
123 |
+
transforms.Lambda(lambda img: __make_power_2(img, base=4, method=method))
|
124 |
+
)
|
125 |
+
|
126 |
+
# 图片左右翻转
|
127 |
+
if not opt.no_flip:
|
128 |
+
if params is None:
|
129 |
+
transform_list.append(transforms.RandomHorizontalFlip())
|
130 |
+
elif params["flip"]:
|
131 |
+
transform_list.append(
|
132 |
+
transforms.Lambda(lambda img: __flip(img, params["flip"]))
|
133 |
+
)
|
134 |
+
|
135 |
+
# convert
|
136 |
+
if convert:
|
137 |
+
transform_list += [transforms.ToTensor()]
|
138 |
+
transform_list += [GaussionNoise()] if opt.isTrain else []
|
139 |
+
if grayscale:
|
140 |
+
transform_list += [transforms.Normalize((0.5,), (0.5,))]
|
141 |
+
else:
|
142 |
+
transform_list += [transforms.Normalize((0.5, 0.5, 0.5), (0.5, 0.5, 0.5))]
|
143 |
+
return transforms.Compose(transform_list)
|
144 |
+
|
145 |
+
|
146 |
+
def __transforms2pil_resize(method):
|
147 |
+
mapper = {
|
148 |
+
transforms.InterpolationMode.BILINEAR: Image.BILINEAR,
|
149 |
+
transforms.InterpolationMode.BICUBIC: Image.BICUBIC,
|
150 |
+
transforms.InterpolationMode.NEAREST: Image.NEAREST,
|
151 |
+
transforms.InterpolationMode.LANCZOS: Image.LANCZOS,
|
152 |
+
}
|
153 |
+
return mapper[method]
|
154 |
+
|
155 |
+
|
156 |
+
def __make_power_2(img, base, method=transforms.InterpolationMode.BICUBIC):
|
157 |
+
"""根据给定的方法(例如:双三次插值���,将图片变成指定的大小。
|
158 |
+
其中的round函数是一种四舍五入的方法。
|
159 |
+
"""
|
160 |
+
method = __transforms2pil_resize(method)
|
161 |
+
ow, oh = img.size
|
162 |
+
h = int(round(oh / base) * base)
|
163 |
+
w = int(round(ow / base) * base)
|
164 |
+
if h == oh and w == ow:
|
165 |
+
return img
|
166 |
+
|
167 |
+
__print_size_warning(ow, oh, w, h)
|
168 |
+
return img.resize((w, h), method)
|
169 |
+
|
170 |
+
|
171 |
+
def __scale_width(
|
172 |
+
img, target_size, crop_size, method=transforms.InterpolationMode.BICUBIC
|
173 |
+
):
|
174 |
+
"""调整大小"""
|
175 |
+
method = __transforms2pil_resize(method)
|
176 |
+
ow, oh = img.size
|
177 |
+
if ow == target_size and oh >= crop_size:
|
178 |
+
return img
|
179 |
+
w = target_size
|
180 |
+
h = int(max(target_size * oh / ow, crop_size))
|
181 |
+
return img.resize((w, h), method)
|
182 |
+
|
183 |
+
|
184 |
+
def __crop(img, pos, size):
|
185 |
+
"""图片裁剪"""
|
186 |
+
ow, oh = img.size
|
187 |
+
x1, y1 = pos
|
188 |
+
tw = th = size
|
189 |
+
if ow > tw or oh > th:
|
190 |
+
return img.crop((x1, y1, x1 + tw, y1 + th))
|
191 |
+
return img
|
192 |
+
|
193 |
+
|
194 |
+
def __flip(img, flip):
|
195 |
+
"""图片左右翻转"""
|
196 |
+
if flip:
|
197 |
+
return img.transpose(Image.FLIP_LEFT_RIGHT)
|
198 |
+
return img
|
199 |
+
|
200 |
+
|
201 |
+
def _gaussion_noise(img):
|
202 |
+
noise = torch.randn(img.shape)
|
203 |
+
img = img + noise * 0.1
|
204 |
+
return img
|
205 |
+
|
206 |
+
|
207 |
+
def __print_size_warning(ow, oh, w, h):
|
208 |
+
"""Print warning information about image size(only print once)"""
|
209 |
+
if not hasattr(__print_size_warning, "has_printed"):
|
210 |
+
print(
|
211 |
+
"The image size needs to be a multiple of 4. "
|
212 |
+
"The loaded image size was (%d, %d), so it was adjusted to "
|
213 |
+
"(%d, %d). This adjustment will be done to all images "
|
214 |
+
"whose sizes are not multiples of 4" % (ow, oh, w, h)
|
215 |
+
)
|
216 |
+
__print_size_warning.has_printed = True
|
217 |
+
|
218 |
+
|
219 |
+
class GaussionNoise:
|
220 |
+
"""添加高斯噪声"""
|
221 |
+
|
222 |
+
def __init__(self) -> None:
|
223 |
+
pass
|
224 |
+
|
225 |
+
def __call__(self, img):
|
226 |
+
noise = torch.randn(img.shape)
|
227 |
+
img_mix_noise = img + noise * 0.1
|
228 |
+
return img_mix_noise
|
229 |
+
|
230 |
+
def __repr__(self) -> str:
|
231 |
+
return f"{self.__class__.__name__}()"
|
data/image_folder.py
ADDED
@@ -0,0 +1,62 @@
|
|
|
|
|
|
|
|
|
|
|
|
|
|
|
|
|
|
|
|
|
|
|
|
|
|
|
|
|
|
|
|
|
|
|
|
|
|
|
|
|
|
|
|
|
|
|
|
|
|
|
|
|
|
|
|
|
|
|
|
|
|
|
|
|
|
|
|
|
|
|
|
|
|
|
|
|
|
|
|
|
|
|
|
|
|
|
|
|
|
|
|
|
|
|
|
|
|
|
|
|
|
|
|
|
|
|
|
|
|
|
|
|
|
|
|
|
|
|
|
|
|
|
|
|
|
|
1 |
+
"""A modified image folder class
|
2 |
+
|
3 |
+
We modify the official PyTorch image folder (https://github.com/pytorch/vision/blob/master/torchvision/datasets/folder.py)
|
4 |
+
so that this class can load images from both current directory and its subdirectories.
|
5 |
+
"""
|
6 |
+
|
7 |
+
import torch.utils.data as data
|
8 |
+
|
9 |
+
from PIL import Image
|
10 |
+
import os
|
11 |
+
from pathlib import Path
|
12 |
+
|
13 |
+
|
14 |
+
IMG_EXTENSIONS = [".jpg", ".JPG", ".jpeg", ".JPEG", ".png", ".PNG", ".ppm", ".PPM", ".bmp", ".BMP", ".tif", ".TIF", ".tiff", ".TIFF"]
|
15 |
+
|
16 |
+
|
17 |
+
def is_image_file(filename):
|
18 |
+
return any(filename.endswith(extension) for extension in IMG_EXTENSIONS)
|
19 |
+
|
20 |
+
|
21 |
+
def make_dataset(dir, max_dataset_size=float("inf")):
|
22 |
+
images = []
|
23 |
+
assert os.path.isdir(dir), "%s is not a valid directory" % dir
|
24 |
+
|
25 |
+
for root, _, fnames in sorted(os.walk(dir)):
|
26 |
+
for fname in fnames:
|
27 |
+
if is_image_file(fname):
|
28 |
+
path = os.path.join(root, fname)
|
29 |
+
images.append(path)
|
30 |
+
return images[: min(max_dataset_size, len(images))]
|
31 |
+
|
32 |
+
|
33 |
+
def default_loader(path):
|
34 |
+
return Image.open(path).convert("RGB")
|
35 |
+
|
36 |
+
|
37 |
+
class ImageFolder(data.Dataset):
|
38 |
+
"""根据文件夹制作数据集"""
|
39 |
+
|
40 |
+
def __init__(self, root, transform=None, return_paths=False, loader=default_loader):
|
41 |
+
imgs = make_dataset(root)
|
42 |
+
if len(imgs) == 0:
|
43 |
+
raise (RuntimeError("Found 0 images in: " + root + "\n" "Supported image extensions are: " + ",".join(IMG_EXTENSIONS)))
|
44 |
+
|
45 |
+
self.root = root
|
46 |
+
self.imgs = imgs
|
47 |
+
self.transform = transform
|
48 |
+
self.return_paths = return_paths
|
49 |
+
self.loader = loader
|
50 |
+
|
51 |
+
def __getitem__(self, index):
|
52 |
+
path = self.imgs[index]
|
53 |
+
img = self.loader(path)
|
54 |
+
if self.transform is not None:
|
55 |
+
img = self.transform(img)
|
56 |
+
if self.return_paths:
|
57 |
+
return img, path
|
58 |
+
else:
|
59 |
+
return img
|
60 |
+
|
61 |
+
def __len__(self):
|
62 |
+
return len(self.imgs)
|
data/one_dataset.py
ADDED
@@ -0,0 +1,40 @@
|
|
|
|
|
|
|
|
|
|
|
|
|
|
|
|
|
|
|
|
|
|
|
|
|
|
|
|
|
|
|
|
|
|
|
|
|
|
|
|
|
|
|
|
|
|
|
|
|
|
|
|
|
|
|
|
|
|
|
|
|
|
|
|
|
|
|
|
|
|
|
|
|
|
|
|
|
|
|
|
|
|
|
1 |
+
from .base_dataset import BaseDataset, get_transform
|
2 |
+
from PIL import Image
|
3 |
+
from pathlib import Path
|
4 |
+
|
5 |
+
|
6 |
+
class OneDataset(BaseDataset):
|
7 |
+
"""
|
8 |
+
加载数据
|
9 |
+
|
10 |
+
加载文件夹中所有图片或直接加载指定文件
|
11 |
+
"""
|
12 |
+
|
13 |
+
def __init__(self, img, opt):
|
14 |
+
BaseDataset.__init__(self, opt)
|
15 |
+
# self.opt = opt
|
16 |
+
dataroot = img
|
17 |
+
if type(dataroot) == str:
|
18 |
+
dataroot = Path(dataroot)
|
19 |
+
if dataroot.is_file():
|
20 |
+
self.A_path = [str(dataroot)]
|
21 |
+
if dataroot.is_dir():
|
22 |
+
self.A_path = [str(i) for i in list(dataroot.iterdir())]
|
23 |
+
self.A_img = [Image.open(path).convert("RGB") for path in self.A_path]
|
24 |
+
else: # dataroot 传入的直接是PIL格式图片
|
25 |
+
self.A_path = [None]
|
26 |
+
self.A_img = [dataroot]
|
27 |
+
|
28 |
+
def __getitem__(self, idx:int):
|
29 |
+
A_path = self.A_path[idx]
|
30 |
+
A_img = self.A_img[idx]
|
31 |
+
A = transform(A_img, self.opt)
|
32 |
+
return {"A": A, "A_paths": A_path}
|
33 |
+
|
34 |
+
def __len__(self):
|
35 |
+
return 1
|
36 |
+
|
37 |
+
|
38 |
+
def transform(img, opt):
|
39 |
+
fn_transform = get_transform(opt, grayscale=(opt.input_nc == 1))
|
40 |
+
return fn_transform(img)
|
data/single_dataset.py
ADDED
@@ -0,0 +1,40 @@
|
|
|
|
|
|
|
|
|
|
|
|
|
|
|
|
|
|
|
|
|
|
|
|
|
|
|
|
|
|
|
|
|
|
|
|
|
|
|
|
|
|
|
|
|
|
|
|
|
|
|
|
|
|
|
|
|
|
|
|
|
|
|
|
|
|
|
|
|
|
|
|
|
|
|
|
|
|
|
|
|
|
|
1 |
+
from data.base_dataset import BaseDataset, get_transform
|
2 |
+
from data.image_folder import make_dataset
|
3 |
+
from PIL import Image
|
4 |
+
|
5 |
+
|
6 |
+
class SingleDataset(BaseDataset):
|
7 |
+
"""This dataset class can load a set of images specified by the path --dataroot /path/to/data.
|
8 |
+
|
9 |
+
It can be used for generating CycleGAN results only for one side with the model option '-model test'.
|
10 |
+
"""
|
11 |
+
|
12 |
+
def __init__(self, opt):
|
13 |
+
"""Initialize this dataset class.
|
14 |
+
|
15 |
+
Parameters:
|
16 |
+
opt (Option class) -- stores all the experiment flags; needs to be a subclass of BaseOptions
|
17 |
+
"""
|
18 |
+
BaseDataset.__init__(self, opt)
|
19 |
+
self.A_paths = sorted(make_dataset(opt.dataroot, opt.max_dataset_size))
|
20 |
+
input_nc = self.opt.output_nc if self.opt.direction == 'BtoA' else self.opt.input_nc
|
21 |
+
self.transform = get_transform(opt, grayscale=(input_nc == 1))
|
22 |
+
|
23 |
+
def __getitem__(self, index):
|
24 |
+
"""Return a data point and its metadata information.
|
25 |
+
|
26 |
+
Parameters:
|
27 |
+
index - - a random integer for data indexing
|
28 |
+
|
29 |
+
Returns a dictionary that contains A and A_paths
|
30 |
+
A(tensor) - - an image in one domain
|
31 |
+
A_paths(str) - - the path of the image
|
32 |
+
"""
|
33 |
+
A_path = self.A_paths[index]
|
34 |
+
A_img = Image.open(A_path).convert('RGB')
|
35 |
+
A = self.transform(A_img)
|
36 |
+
return {'A': A, 'A_paths': A_path}
|
37 |
+
|
38 |
+
def __len__(self):
|
39 |
+
"""Return the total number of images in the dataset."""
|
40 |
+
return len(self.A_paths)
|
data/unaligned_dataset.py
ADDED
@@ -0,0 +1,72 @@
|
|
|
|
|
|
|
|
|
|
|
|
|
|
|
|
|
|
|
|
|
|
|
|
|
|
|
|
|
|
|
|
|
|
|
|
|
|
|
|
|
|
|
|
|
|
|
|
|
|
|
|
|
|
|
|
|
|
|
|
|
|
|
|
|
|
|
|
|
|
|
|
|
|
|
|
|
|
|
|
|
|
|
|
|
|
|
|
|
|
|
|
|
|
|
|
|
|
|
|
|
|
|
|
|
|
|
|
|
|
|
|
|
|
|
|
|
|
|
|
|
|
|
|
|
|
|
|
|
|
|
|
|
|
|
|
|
|
|
|
|
|
|
|
|
|
|
1 |
+
import os
|
2 |
+
from pathlib import Path
|
3 |
+
from data.base_dataset import BaseDataset, get_transform
|
4 |
+
from data.image_folder import make_dataset
|
5 |
+
from PIL import Image
|
6 |
+
import random
|
7 |
+
|
8 |
+
|
9 |
+
class UnalignedDataset(BaseDataset):
|
10 |
+
"""
|
11 |
+
This dataset class can load unaligned/unpaired datasets.
|
12 |
+
|
13 |
+
It requires two directories to host training images from domain A '/path/to/data/trainA'
|
14 |
+
and from domain B '/path/to/data/trainB' respectively.
|
15 |
+
You can train the model with the dataset flag '--dataroot /path/to/data'.
|
16 |
+
Similarly, you need to prepare two directories:
|
17 |
+
'/path/to/data/testA' and '/path/to/data/testB' during test time.
|
18 |
+
"""
|
19 |
+
|
20 |
+
def __init__(self, opt):
|
21 |
+
"""Initialize this dataset class.
|
22 |
+
|
23 |
+
Parameters:
|
24 |
+
opt (Option class) -- stores all the experiment flags; needs to be a subclass of BaseOptions
|
25 |
+
"""
|
26 |
+
BaseDataset.__init__(self, opt)
|
27 |
+
self.dir_A = os.path.join(opt.dataroot, opt.phase + 'A') # create a path '/path/to/data/trainA'
|
28 |
+
self.dir_B = os.path.join(opt.dataroot, opt.phase + 'B') # create a path '/path/to/data/trainB'
|
29 |
+
|
30 |
+
self.A_paths = sorted(make_dataset(self.dir_A, opt.max_dataset_size)) # load images from '/path/to/data/trainA'
|
31 |
+
self.B_paths = sorted(make_dataset(self.dir_B, opt.max_dataset_size)) # load images from '/path/to/data/trainB'
|
32 |
+
self.A_size = len(self.A_paths) # get the size of dataset A
|
33 |
+
self.B_size = len(self.B_paths) # get the size of dataset B
|
34 |
+
btoA = self.opt.direction == 'BtoA'
|
35 |
+
input_nc = self.opt.output_nc if btoA else self.opt.input_nc # get the number of channels of input image
|
36 |
+
output_nc = self.opt.input_nc if btoA else self.opt.output_nc # get the number of channels of output image
|
37 |
+
self.transform_A = get_transform(self.opt, grayscale=(input_nc == 1))
|
38 |
+
self.transform_B = get_transform(self.opt, grayscale=(output_nc == 1))
|
39 |
+
|
40 |
+
def __getitem__(self, index):
|
41 |
+
"""Return a data point and its metadata information.
|
42 |
+
|
43 |
+
Parameters:
|
44 |
+
index (int) -- a random integer for data indexing
|
45 |
+
|
46 |
+
Returns a dictionary that contains A, B, A_paths and B_paths
|
47 |
+
A (tensor) -- an image in the input domain
|
48 |
+
B (tensor) -- its corresponding image in the target domain
|
49 |
+
A_paths (str) -- image paths
|
50 |
+
B_paths (str) -- image paths
|
51 |
+
"""
|
52 |
+
A_path = self.A_paths[index % self.A_size] # make sure index is within then range
|
53 |
+
if self.opt.serial_batches: # make sure index is within then range
|
54 |
+
index_B = index % self.B_size
|
55 |
+
else: # randomize the index for domain B to avoid fixed pairs.
|
56 |
+
index_B = random.randint(0, self.B_size - 1)
|
57 |
+
B_path = self.B_paths[index_B]
|
58 |
+
A_img = Image.open(A_path).convert('RGB')
|
59 |
+
B_img = Image.open(B_path).convert('RGB')
|
60 |
+
# apply image transformation
|
61 |
+
A = self.transform_A(A_img)
|
62 |
+
B = self.transform_B(B_img)
|
63 |
+
|
64 |
+
return {'A': A, 'B': B, 'A_paths': A_path, 'B_paths': B_path}
|
65 |
+
|
66 |
+
def __len__(self):
|
67 |
+
"""Return the total number of images in the dataset.
|
68 |
+
|
69 |
+
As we have two datasets with potentially different number of images,
|
70 |
+
we take a maximum of them
|
71 |
+
"""
|
72 |
+
return max(self.A_size, self.B_size)
|
detect.py
ADDED
@@ -0,0 +1,66 @@
|
|
|
|
|
|
|
|
|
|
|
|
|
|
|
|
|
|
|
|
|
|
|
|
|
|
|
|
|
|
|
|
|
|
|
|
|
|
|
|
|
|
|
|
|
|
|
|
|
|
|
|
|
|
|
|
|
|
|
|
|
|
|
|
|
|
|
|
|
|
|
|
|
|
|
|
|
|
|
|
|
|
|
|
|
|
|
|
|
|
|
|
|
|
|
|
|
|
|
|
|
|
|
|
|
|
|
|
|
|
|
|
|
|
|
|
|
|
|
|
|
|
|
|
|
|
|
|
|
|
|
|
|
|
|
1 |
+
"""
|
2 |
+
>>> python detect.py --dataroot ./imgs/horse.jpg --style horse2zebra
|
3 |
+
"""
|
4 |
+
|
5 |
+
import os
|
6 |
+
import sys
|
7 |
+
|
8 |
+
BASE_DIR = os.path.dirname(os.path.dirname(os.path.abspath(__file__)))
|
9 |
+
sys.path.append(BASE_DIR)
|
10 |
+
from pathlib import Path
|
11 |
+
|
12 |
+
from data import OneDataset
|
13 |
+
from models import create_model
|
14 |
+
from options import DetectOptions
|
15 |
+
from util import tensor2im, save_image, show_image, now_time, print_info
|
16 |
+
|
17 |
+
# 定义参数
|
18 |
+
opt = DetectOptions().parse()
|
19 |
+
# 硬编码一些测试参数
|
20 |
+
opt.num_threads = 0 # 测试代码仅支持num_threads = 0
|
21 |
+
opt.batch_size = 1 # 测试代码仅支持batch_size = 1
|
22 |
+
opt.serial_batches = True # 禁用数据混洗;如果需要在随机选择的图像上得到结果,请取消对此行的注释。
|
23 |
+
opt.no_flip = True # 不翻转;如果需要在翻转的图像上得到结果,请取消对此行的注释。
|
24 |
+
opt.display_id = -1 # 没有visdom显示;测试代码将结果保存到HTML文件中。
|
25 |
+
|
26 |
+
# 加载模型
|
27 |
+
model = create_model(opt)
|
28 |
+
|
29 |
+
|
30 |
+
def detect(img=opt.dataroot, style=opt.style):
|
31 |
+
result = None
|
32 |
+
time_info = "-" * 30 + f"\n{now_time()}:start"
|
33 |
+
dataset = OneDataset(img, opt) # 加载数据
|
34 |
+
model.setup(opt, style) # 设置模型
|
35 |
+
model.eval() # 切换到评估模式
|
36 |
+
print_info(time_info)
|
37 |
+
for _, data in enumerate(dataset):
|
38 |
+
model.set_input(data) # 从数据加载器中解包数据
|
39 |
+
model.test() # 推理
|
40 |
+
visuals = model.get_current_visuals() # 获取结果图像
|
41 |
+
result = tensor2im(visuals["fake"])
|
42 |
+
time_info = f"{now_time()}:done\n" + "-" * 30
|
43 |
+
print_info(time_info)
|
44 |
+
return result
|
45 |
+
|
46 |
+
|
47 |
+
def save_detect_img(results: list, no_save_img=False):
|
48 |
+
"""保存或展示检测结果"""
|
49 |
+
# 保存 or 展示
|
50 |
+
if no_save_img:
|
51 |
+
for _, img_fake in results:
|
52 |
+
show_image(img_fake)
|
53 |
+
else:
|
54 |
+
for img_path, img_fake in results:
|
55 |
+
save_dir = Path.cwd().joinpath("results") # fake图片保存路径
|
56 |
+
img_path = Path(img_path)
|
57 |
+
img_name = img_path.stem + "_fake" + img_path.suffix
|
58 |
+
Path.mkdir(save_dir, exist_ok=True)
|
59 |
+
save_path = save_dir.joinpath(img_name)
|
60 |
+
save_image(img_fake, save_path)
|
61 |
+
print("results_path: ", save_path)
|
62 |
+
|
63 |
+
|
64 |
+
if __name__ == "__main__":
|
65 |
+
results = detect() # 推理图片
|
66 |
+
save_detect_img(results, opt.no_save_img) # 保存检测结果
|
imgs/horse.jpg
ADDED
![]() |
imgs/monet.jpg
ADDED
![]() |
models/__init__.py
ADDED
@@ -0,0 +1,66 @@
|
|
|
|
|
|
|
|
|
|
|
|
|
|
|
|
|
|
|
|
|
|
|
|
|
|
|
|
|
|
|
|
|
|
|
|
|
|
|
|
|
|
|
|
|
|
|
|
|
|
|
|
|
|
|
|
|
|
|
|
|
|
|
|
|
|
|
|
|
|
|
|
|
|
|
|
|
|
|
|
|
|
|
|
|
|
|
|
|
|
|
|
|
|
|
|
|
|
|
|
|
|
|
|
|
|
|
|
|
|
|
|
|
|
|
|
|
|
|
|
|
|
|
|
|
|
|
|
|
|
|
|
|
|
|
1 |
+
"""This package contains modules related to objective functions, optimizations, and network architectures.
|
2 |
+
|
3 |
+
To add a custom model class called 'dummy', you need to add a file called 'dummy_model.py' and define a subclass DummyModel inherited from BaseModel.
|
4 |
+
You need to implement the following five functions:
|
5 |
+
-- <__init__>: initialize the class; first call BaseModel.__init__(self, opt).
|
6 |
+
-- <set_input>: unpack data from dataset and apply preprocessing.
|
7 |
+
-- <forward>: produce intermediate results.
|
8 |
+
-- <optimize_parameters>: calculate loss, gradients, and update network weights.
|
9 |
+
-- <modify_commandline_options>: (optionally) add model-specific options and set default options.
|
10 |
+
|
11 |
+
In the function <__init__>, you need to define four lists:
|
12 |
+
-- self.loss_names (str list): specify the training losses that you want to plot and save.
|
13 |
+
-- self.net_names (str list): define networks used in our training.
|
14 |
+
-- self.visual_names (str list): specify the images that you want to display and save.
|
15 |
+
-- self.optimizers (optimizer list): define and initialize optimizers. You can define one optimizer for each network. If two networks are updated at the same time, you can use itertools.chain to group them. See cycle_gan_model.py for an usage.
|
16 |
+
|
17 |
+
Now you can use the model class by specifying flag '--model dummy'.
|
18 |
+
See our template model class 'template_model.py' for more details.
|
19 |
+
"""
|
20 |
+
|
21 |
+
import importlib
|
22 |
+
from .base_model import BaseModel
|
23 |
+
|
24 |
+
|
25 |
+
def find_model_using_name(model_name):
|
26 |
+
"""Import the module "models/[model_name]_model.py".
|
27 |
+
|
28 |
+
In the file, the class called DatasetNameModel() will
|
29 |
+
be instantiated. It has to be a subclass of BaseModel,
|
30 |
+
and it is case-insensitive.
|
31 |
+
"""
|
32 |
+
model_filename = "models." + model_name + "_model"
|
33 |
+
modellib = importlib.import_module(model_filename)
|
34 |
+
model = None
|
35 |
+
target_model_name = model_name.replace("_", "") + "model"
|
36 |
+
for name, cls in modellib.__dict__.items():
|
37 |
+
if name.lower() == target_model_name.lower() and issubclass(cls, BaseModel):
|
38 |
+
model = cls
|
39 |
+
|
40 |
+
if model is None:
|
41 |
+
print("In %s.py, there should be a subclass of BaseModel with class name that matches %s in lowercase." % (model_filename, target_model_name))
|
42 |
+
exit(0)
|
43 |
+
|
44 |
+
return model
|
45 |
+
|
46 |
+
|
47 |
+
def get_option_setter(model_name):
|
48 |
+
"""Return the static method <modify_commandline_options> of the model class."""
|
49 |
+
model_class = find_model_using_name(model_name)
|
50 |
+
return model_class.modify_commandline_options
|
51 |
+
|
52 |
+
|
53 |
+
def create_model(opt):
|
54 |
+
"""Create a model given the option.
|
55 |
+
|
56 |
+
This function warps the class CustomDatasetDataLoader.
|
57 |
+
This is the main interface between this package and 'train.py'/'test.py'
|
58 |
+
|
59 |
+
Example:
|
60 |
+
>>> from models import create_model
|
61 |
+
>>> model = create_model(opt)
|
62 |
+
"""
|
63 |
+
model = find_model_using_name(opt.model)
|
64 |
+
instance = model(opt)
|
65 |
+
print("model [%s] was created" % type(instance).__name__)
|
66 |
+
return instance
|
models/base_model.py
ADDED
@@ -0,0 +1,213 @@
|
|
|
|
|
|
|
|
|
|
|
|
|
|
|
|
|
|
|
|
|
|
|
|
|
|
|
|
|
|
|
|
|
|
|
|
|
|
|
|
|
|
|
|
|
|
|
|
|
|
|
|
|
|
|
|
|
|
|
|
|
|
|
|
|
|
|
|
|
|
|
|
|
|
|
|
|
|
|
|
|
|
|
|
|
|
|
|
|
|
|
|
|
|
|
|
|
|
|
|
|
|
|
|
|
|
|
|
|
|
|
|
|
|
|
|
|
|
|
|
|
|
|
|
|
|
|
|
|
|
|
|
|
|
|
|
|
|
|
|
|
|
|
|
|
|
|
|
|
|
|
|
|
|
|
|
|
|
|
|
|
|
|
|
|
|
|
|
|
|
|
|
|
|
|
|
|
|
|
|
|
|
|
|
|
|
|
|
|
|
|
|
|
|
|
|
|
|
|
|
|
|
|
|
|
|
|
|
|
|
|
|
|
|
|
|
|
|
|
|
|
|
|
|
|
|
|
|
|
|
|
|
|
|
|
|
|
|
|
|
|
|
|
|
|
|
|
|
|
|
|
|
|
|
|
|
|
|
|
|
|
|
|
|
|
|
|
|
|
|
|
|
|
|
|
|
|
|
|
|
|
|
|
|
|
|
|
|
|
|
|
|
|
|
|
|
|
|
|
|
|
|
|
|
|
|
|
|
|
|
|
|
|
|
|
|
|
|
|
|
|
|
|
|
|
|
|
|
|
|
|
|
|
|
|
|
|
|
|
|
|
|
|
|
|
|
|
|
|
|
|
|
|
|
|
|
|
|
|
|
|
|
|
|
|
|
|
|
|
|
|
|
|
|
|
|
|
|
|
|
|
|
|
|
|
|
|
|
|
|
|
|
|
|
|
|
|
|
|
|
|
|
|
|
|
|
|
|
|
|
|
|
|
|
|
|
|
|
|
|
|
|
|
|
|
|
|
|
|
1 |
+
import os
|
2 |
+
from abc import ABC, abstractmethod
|
3 |
+
from collections import OrderedDict
|
4 |
+
from pathlib import Path
|
5 |
+
|
6 |
+
import torch
|
7 |
+
|
8 |
+
from . import networks
|
9 |
+
|
10 |
+
|
11 |
+
class BaseModel(ABC):
|
12 |
+
"""This class is an abstract base class (ABC) for models.
|
13 |
+
To create a subclass, you need to implement the following five functions:
|
14 |
+
-- <__init__>: initialize the class; first call BaseModel.__init__(self, opt).
|
15 |
+
-- <set_input>: unpack data from dataset and apply preprocessing.
|
16 |
+
-- <forward>: produce intermediate results.
|
17 |
+
-- <optimize_parameters>: calculate losses, gradients, and update network weights.
|
18 |
+
-- <modify_commandline_options>: (optionally) add model-specific options and set default options.
|
19 |
+
"""
|
20 |
+
|
21 |
+
def __init__(self, opt):
|
22 |
+
"""Initialize the BaseModel class.
|
23 |
+
|
24 |
+
Parameters:
|
25 |
+
opt (Option class)-- stores all the experiment flags; needs to be a subclass of BaseOptions
|
26 |
+
|
27 |
+
When creating your custom class, you need to implement your own initialization.
|
28 |
+
In this function, you should first call <BaseModel.__init__(self, opt)>
|
29 |
+
Then, you need to define four lists:
|
30 |
+
-- self.loss_names (str list): specify the training losses that you want to plot and save.
|
31 |
+
-- self.net_names (str list): define networks used in our training.
|
32 |
+
-- self.visual_names (str list): specify the images that you want to display and save.
|
33 |
+
-- self.optimizers (optimizer list): define and initialize optimizers.
|
34 |
+
You can define one optimizer for each network.
|
35 |
+
If two networks are updated at the same time, you can use itertools. Chain to group them.
|
36 |
+
See cycle_gan_model.py for an example.
|
37 |
+
"""
|
38 |
+
self.opt = opt
|
39 |
+
self.gpu_ids = opt.gpu_ids
|
40 |
+
self.isTrain = opt.isTrain
|
41 |
+
self.device = (
|
42 |
+
torch.device("cuda:{}".format(self.gpu_ids[0]))
|
43 |
+
if self.gpu_ids
|
44 |
+
else torch.device("cpu")
|
45 |
+
)
|
46 |
+
print(self.device)
|
47 |
+
self.save_dir = Path(opt.checkpoints_dir).joinpath(
|
48 |
+
opt.name
|
49 |
+
) # save all the checkpoints to save_dir
|
50 |
+
# with [scale_width], input images might have different sizes, which hurts the performance of cudnn.benchmark.
|
51 |
+
if opt.preprocess != "scale_width":
|
52 |
+
torch.backends.cudnn.benchmark = True
|
53 |
+
self.loss_names = []
|
54 |
+
self.net_names = []
|
55 |
+
self.visual_names = []
|
56 |
+
self.optimizers = []
|
57 |
+
self.image_paths = []
|
58 |
+
self.metric = 0 # used for learning rate policy 'plateau'
|
59 |
+
|
60 |
+
@staticmethod
|
61 |
+
def modify_commandline_options(parser, is_train):
|
62 |
+
"""Add new model-specific options, and rewrite default values for existing options.
|
63 |
+
|
64 |
+
Parameters:
|
65 |
+
parser -- original option parser
|
66 |
+
parser:
|
67 |
+
is_train (bool) -- whether training phase or test phase. You can use this flag to add training-specific or test-specific options.
|
68 |
+
|
69 |
+
Returns:
|
70 |
+
the modified parser.
|
71 |
+
"""
|
72 |
+
return parser
|
73 |
+
|
74 |
+
def setup(self, opt, load_weight=None):
|
75 |
+
"""加载和打印网络;创建调度程序
|
76 |
+
|
77 |
+
Parameters:
|
78 |
+
load_weight:
|
79 |
+
opt (Option class) -- stores all the experiment flags; needs to be a subclass of BaseOptions
|
80 |
+
"""
|
81 |
+
if self.isTrain:
|
82 |
+
self.schedulers = [
|
83 |
+
networks.get_scheduler(optimizer, opt) for optimizer in self.optimizers
|
84 |
+
]
|
85 |
+
if not self.isTrain or opt.continue_train:
|
86 |
+
load_suffix = "iter_%d" % opt.load_iter if opt.load_iter > 0 else opt.epoch
|
87 |
+
self.load_networks(load_suffix, load_weight)
|
88 |
+
self.print_networks(opt.verbose)
|
89 |
+
|
90 |
+
def eval(self):
|
91 |
+
"""Make models eval mode during test time"""
|
92 |
+
for name in self.net_names:
|
93 |
+
if isinstance(name, str):
|
94 |
+
net = getattr(self, "net_" + name)
|
95 |
+
net.eval()
|
96 |
+
|
97 |
+
def test(self):
|
98 |
+
"""Forward function used in test time.
|
99 |
+
|
100 |
+
This function wraps <forward> function in no_grad() so we don't save intermediate steps for backprop
|
101 |
+
It also calls <compute_visuals> to produce additional visualization results
|
102 |
+
"""
|
103 |
+
with torch.no_grad():
|
104 |
+
self.forward()
|
105 |
+
self.compute_visuals()
|
106 |
+
|
107 |
+
def compute_visuals(self):
|
108 |
+
"""Calculate additional output images for visdom and HTML visualization"""
|
109 |
+
pass
|
110 |
+
|
111 |
+
def get_current_visuals(self):
|
112 |
+
"""Return visualization images. train.py will display these images with visdom, and save the images to an HTML"""
|
113 |
+
visual_ret = OrderedDict()
|
114 |
+
for name in self.visual_names:
|
115 |
+
if isinstance(name, str):
|
116 |
+
visual_ret[name] = getattr(self, name)
|
117 |
+
return visual_ret
|
118 |
+
|
119 |
+
def get_image_paths(self):
|
120 |
+
"""Return image paths that are used to load current data"""
|
121 |
+
return self.image_paths
|
122 |
+
|
123 |
+
def save_networks(self, epoch):
|
124 |
+
"""Save all the networks to the disk.
|
125 |
+
|
126 |
+
Parameters:
|
127 |
+
epoch (int) -- current epoch; used in the file name '%s_net_%s.pth' % (epoch, name)
|
128 |
+
"""
|
129 |
+
for name in self.net_names:
|
130 |
+
if isinstance(name, str):
|
131 |
+
save_filename = "%s_net_%s.pth" % (epoch, name)
|
132 |
+
save_path = Path(self.save_dir, save_filename)
|
133 |
+
net = getattr(self, "net_" + name)
|
134 |
+
|
135 |
+
if len(self.gpu_ids) > 0 and torch.cuda.is_available():
|
136 |
+
torch.save(net.module.cpu().state_dict(), save_path)
|
137 |
+
net.cuda(self.gpu_ids[0])
|
138 |
+
else:
|
139 |
+
torch.save(net.cpu().state_dict(), save_path)
|
140 |
+
|
141 |
+
def __patch_instance_norm_state_dict(self, state_dict, module, keys, i=0):
|
142 |
+
"""Fix InstanceNorm checkpoints incompatibility (prior to 0.4)"""
|
143 |
+
key = keys[i]
|
144 |
+
if i + 1 == len(keys): # at the end, pointing to a parameter/buffer
|
145 |
+
if module.__class__.__name__.startswith("InstanceNorm") and (
|
146 |
+
key == "running_mean" or key == "running_var"
|
147 |
+
):
|
148 |
+
if getattr(module, key) is None:
|
149 |
+
state_dict.pop(".".join(keys))
|
150 |
+
if module.__class__.__name__.startswith("InstanceNorm") and (
|
151 |
+
key == "num_batches_tracked"
|
152 |
+
):
|
153 |
+
state_dict.pop(".".join(keys))
|
154 |
+
else:
|
155 |
+
self.__patch_instance_norm_state_dict(
|
156 |
+
state_dict, getattr(module, key), keys, i + 1
|
157 |
+
)
|
158 |
+
|
159 |
+
def load_networks(self, epoch: int, load_weight=None):
|
160 |
+
"""Load all the networks from the disk.
|
161 |
+
|
162 |
+
Parameters:
|
163 |
+
load_weight:
|
164 |
+
epoch (int) -- current epoch; used in the file name '%s_net_%s.pth' % (epoch, name)
|
165 |
+
"""
|
166 |
+
for name in self.net_names:
|
167 |
+
if isinstance(name, str):
|
168 |
+
if not load_weight:
|
169 |
+
load_filename = "%s_net_%s.pth" % (epoch, name)
|
170 |
+
else:
|
171 |
+
load_filename = f"{load_weight}.pth"
|
172 |
+
load_path = self.save_dir.joinpath(load_filename)
|
173 |
+
# if not load_path.exists():
|
174 |
+
# load_path = "./weights/pre/latest_net_G.pth"
|
175 |
+
net = getattr(self, "net_" + name)
|
176 |
+
if isinstance(net, torch.nn.DataParallel):
|
177 |
+
net = net.module
|
178 |
+
print("loading the model from %s" % load_path)
|
179 |
+
# if you are using PyTorch newer than 0.4 (e.g., built from
|
180 |
+
# GitHub source), you can remove str() on self.device
|
181 |
+
state_dict = torch.load(load_path, map_location=self.device)
|
182 |
+
if hasattr(state_dict, "_metadata"):
|
183 |
+
del state_dict._metadata
|
184 |
+
# patch InstanceNorm checkpoints prior to 0.4
|
185 |
+
# need to copy keys here because we mutate in the loop
|
186 |
+
for key in list(state_dict.keys()):
|
187 |
+
self.__patch_instance_norm_state_dict(
|
188 |
+
state_dict, net, key.split(".")
|
189 |
+
)
|
190 |
+
net.load_state_dict(state_dict)
|
191 |
+
# net.half()
|
192 |
+
# 已经是float16了 /(ㄒoㄒ)/~~,没有float8
|
193 |
+
|
194 |
+
def print_networks(self, verbose):
|
195 |
+
"""Print the total number of parameters in the network and (if verbose) network architecture
|
196 |
+
|
197 |
+
Parameters:
|
198 |
+
verbose (bool) -- if verbose: print the network architecture
|
199 |
+
"""
|
200 |
+
print("---------- Networks initialized -------------")
|
201 |
+
for name in self.net_names:
|
202 |
+
if isinstance(name, str):
|
203 |
+
net = getattr(self, "net_" + name)
|
204 |
+
num_params = 0
|
205 |
+
for param in net.parameters():
|
206 |
+
num_params += param.numel()
|
207 |
+
if verbose:
|
208 |
+
print(net)
|
209 |
+
print(
|
210 |
+
"[Network %s] Total number of parameters : %.3f M"
|
211 |
+
% (name, num_params / 1e6)
|
212 |
+
)
|
213 |
+
print("-----------------------------------------------")
|
models/cycle_gan_model.py
ADDED
@@ -0,0 +1,170 @@
|
|
|
|
|
|
|
|
|
|
|
|
|
|
|
|
|
|
|
|
|
|
|
|
|
|
|
|
|
|
|
|
|
|
|
|
|
|
|
|
|
|
|
|
|
|
|
|
|
|
|
|
|
|
|
|
|
|
|
|
|
|
|
|
|
|
|
|
|
|
|
|
|
|
|
|
|
|
|
|
|
|
|
|
|
|
|
|
|
|
|
|
|
|
|
|
|
|
|
|
|
|
|
|
|
|
|
|
|
|
|
|
|
|
|
|
|
|
|
|
|
|
|
|
|
|
|
|
|
|
|
|
|
|
|
|
|
|
|
|
|
|
|
|
|
|
|
|
|
|
|
|
|
|
|
|
|
|
|
|
|
|
|
|
|
|
|
|
|
|
|
|
|
|
|
|
|
|
|
|
|
|
|
|
|
|
|
|
|
|
|
|
|
|
|
|
|
|
|
|
|
|
|
|
|
|
|
|
|
|
|
|
|
|
|
|
|
|
|
|
|
|
|
|
|
|
|
|
|
|
|
|
|
|
|
|
|
|
|
|
|
|
|
|
|
|
|
|
|
|
|
|
|
|
|
|
|
|
|
|
|
|
|
|
|
|
|
|
|
|
|
|
|
|
|
|
|
|
|
|
|
|
|
|
|
|
|
|
|
|
|
|
|
|
|
|
|
|
|
|
|
|
|
|
|
|
|
|
|
|
|
|
|
|
|
|
|
|
|
|
|
|
|
|
|
|
|
|
|
|
|
|
|
|
|
|
|
|
|
|
|
|
|
1 |
+
import itertools
|
2 |
+
|
3 |
+
import torch
|
4 |
+
from util.image_pool import ImagePool
|
5 |
+
|
6 |
+
from . import networks
|
7 |
+
from .base_model import BaseModel
|
8 |
+
|
9 |
+
|
10 |
+
class CycleGANModel(BaseModel):
|
11 |
+
"""
|
12 |
+
This class implements the CycleGAN model, for learning image-to-image translation without paired data.
|
13 |
+
|
14 |
+
The model training requires '--dataset_mode unaligned' dataset.
|
15 |
+
By default, it uses a '--netG resnet_9blocks' ResNet generator,
|
16 |
+
a '--netD basic' discriminator (PatchGAN introduced by pix2pix),
|
17 |
+
and a least-square GANs objective ('--gan_mode lsgan').
|
18 |
+
|
19 |
+
CycleGAN paper: https://arxiv.org/pdf/1703.10593.pdf
|
20 |
+
"""
|
21 |
+
|
22 |
+
@staticmethod
|
23 |
+
def modify_commandline_options(parser, is_train=True):
|
24 |
+
"""Add new dataset-specific options, and rewrite default values for existing options.
|
25 |
+
|
26 |
+
Parameters:
|
27 |
+
parser -- original option parser
|
28 |
+
parser:
|
29 |
+
is_train (bool) -- whether training phase or test phase. You can use this flag to add training-specific or test-specific options.
|
30 |
+
|
31 |
+
Returns:
|
32 |
+
the modified parser.
|
33 |
+
|
34 |
+
For CycleGAN, in addition to GAN losses, we introduce lambda_A, lambda_B, and lambda_identity for the following losses.
|
35 |
+
A (source domain), B (target domain).
|
36 |
+
Generators: G_A: A -> B; G_B: B -> A.
|
37 |
+
Discriminators: D_A: G_A(A) vs. B; D_B: G_B(B) vs. A.
|
38 |
+
Forward cycle loss: lambda_A * ||G_B(G_A(A)) - A|| (Eqn. (2) in the paper)
|
39 |
+
Backward cycle loss: lambda_B * ||G_A(G_B(B)) - B|| (Eqn. (2) in the paper)
|
40 |
+
Identity loss (optional): lambda_identity * (||G_A(B) - B|| * lambda_B + ||G_B(A) - A|| * lambda_A) (Sec 5.2 "Photo generation from paintings" in the paper)
|
41 |
+
Dropout is not used in the original CycleGAN paper.
|
42 |
+
"""
|
43 |
+
parser.set_defaults(no_dropout=True) # default CycleGAN did not use dropout
|
44 |
+
if is_train:
|
45 |
+
parser.add_argument(
|
46 |
+
"--lambda_A",
|
47 |
+
type=float,
|
48 |
+
default=10.0,
|
49 |
+
help="weight for cycle loss (A -> B -> A)",
|
50 |
+
)
|
51 |
+
parser.add_argument(
|
52 |
+
"--lambda_B",
|
53 |
+
type=float,
|
54 |
+
default=10.0,
|
55 |
+
help="weight for cycle loss (B -> A -> B)",
|
56 |
+
)
|
57 |
+
parser.add_argument(
|
58 |
+
"--lambda_identity",
|
59 |
+
type=float,
|
60 |
+
default=0.5,
|
61 |
+
help="use identity mapping. Setting lambda_identity other than 0 has an effect of scaling the weight of the identity mapping loss. For example, if the weight of the identity loss should be 10 times smaller than the weight of the reconstruction loss, please set lambda_identity = 0.1",
|
62 |
+
)
|
63 |
+
return parser
|
64 |
+
|
65 |
+
def __init__(self, opt):
|
66 |
+
"""Initialize the CycleGAN class.
|
67 |
+
|
68 |
+
Parameters:
|
69 |
+
opt (Option class)-- stores all the experiment flags; needs to be a subclass of BaseOptions
|
70 |
+
"""
|
71 |
+
BaseModel.__init__(self, opt)
|
72 |
+
# specify the images you want to save/display. The training/test scripts will call <BaseModel.get_current_visuals>
|
73 |
+
visual_names_A = ["real_A", "fake_B", "rec_A"]
|
74 |
+
visual_names_B = ["real_B", "fake_A", "rec_B"]
|
75 |
+
|
76 |
+
if (
|
77 |
+
self.isTrain and self.opt.lambda_identity > 0.0
|
78 |
+
): # if identity loss is used, we also visualize idt_B=G_A(B) ad idt_A=G_A(B)
|
79 |
+
visual_names_A.append("idt_B")
|
80 |
+
visual_names_B.append("idt_A")
|
81 |
+
self.visual_names = (
|
82 |
+
visual_names_A + visual_names_B
|
83 |
+
) # combine visualizations for A and B
|
84 |
+
# specify the models you want to save to the disk. The training/test scripts will call <BaseModel.save_networks> and <BaseModel.load_networks>.
|
85 |
+
self.net_names = ["G_A", "G_B"]
|
86 |
+
if self.isTrain:
|
87 |
+
self.net_names.extend(["D_A", "D_B"])
|
88 |
+
# 下面会根据 self.loss_names self.visual_names net_names 中定义的字符串创建对应的变量名
|
89 |
+
# 这样把变量名写在一个列表中而不用字典,通过字典取得变量的写法可能是为了避免代码写的太长?
|
90 |
+
# 关键字 exec 可以根据字符串新建变量
|
91 |
+
# 用法:
|
92 |
+
# varlist = ["a"]
|
93 |
+
# exec
|
94 |
+
|
95 |
+
# define networks (both Generators and discriminators)
|
96 |
+
# The naming is different from those used in the paper.
|
97 |
+
# Code (vs. paper): G_A (G), G_B (F), D_A (D_Y), D_B (D_X)
|
98 |
+
self.net_G_A = networks.define_G(
|
99 |
+
opt.input_nc,
|
100 |
+
opt.output_nc,
|
101 |
+
opt.ngf,
|
102 |
+
opt.netG,
|
103 |
+
opt.norm,
|
104 |
+
not opt.no_dropout,
|
105 |
+
opt.init_type,
|
106 |
+
opt.init_gain,
|
107 |
+
self.gpu_ids,
|
108 |
+
)
|
109 |
+
self.net_G_B = networks.define_G(
|
110 |
+
opt.output_nc,
|
111 |
+
opt.input_nc,
|
112 |
+
opt.ngf,
|
113 |
+
opt.netG,
|
114 |
+
opt.norm,
|
115 |
+
not opt.no_dropout,
|
116 |
+
opt.init_type,
|
117 |
+
opt.init_gain,
|
118 |
+
self.gpu_ids,
|
119 |
+
)
|
120 |
+
|
121 |
+
if self.isTrain: # define discriminators
|
122 |
+
self.net_D_A = networks.define_D(
|
123 |
+
opt.output_nc,
|
124 |
+
opt.ndf,
|
125 |
+
opt.netD,
|
126 |
+
opt.n_layers_D,
|
127 |
+
opt.norm,
|
128 |
+
opt.init_type,
|
129 |
+
opt.init_gain,
|
130 |
+
self.gpu_ids,
|
131 |
+
)
|
132 |
+
self.net_D_B = networks.define_D(
|
133 |
+
opt.input_nc,
|
134 |
+
opt.ndf,
|
135 |
+
opt.netD,
|
136 |
+
opt.n_layers_D,
|
137 |
+
opt.norm,
|
138 |
+
opt.init_type,
|
139 |
+
opt.init_gain,
|
140 |
+
self.gpu_ids,
|
141 |
+
)
|
142 |
+
if self.isTrain:
|
143 |
+
if (
|
144 |
+
opt.lambda_identity > 0.0
|
145 |
+
): # only works when input and output images have the same number of channels
|
146 |
+
assert opt.input_nc == opt.output_nc
|
147 |
+
self.fake_A_pool = ImagePool(
|
148 |
+
opt.pool_size
|
149 |
+
) # create image buffer to store previously generated images
|
150 |
+
self.fake_B_pool = ImagePool(
|
151 |
+
opt.pool_size
|
152 |
+
) # create image buffer to store previously generated images
|
153 |
+
|
154 |
+
def set_input(self, input):
|
155 |
+
"""Unpack input data from the dataloader and perform necessary pre-processing steps.
|
156 |
+
Parameters:
|
157 |
+
input (dict): include the data itself and its metadata information.
|
158 |
+
The option 'direction' can be used to swap domain A and domain B.
|
159 |
+
"""
|
160 |
+
AtoB = self.opt.direction == "AtoB"
|
161 |
+
self.real_A = input["A" if AtoB else "B"].to(self.device)
|
162 |
+
self.real_B = input["B" if AtoB else "A"].to(self.device)
|
163 |
+
self.image_paths = input["A_paths" if AtoB else "B_paths"]
|
164 |
+
|
165 |
+
def forward(self):
|
166 |
+
"""Run forward pass; called by both functions <optimize_parameters> and <test>."""
|
167 |
+
self.fake_B = self.net_G_A(self.real_A) # G_A(A)
|
168 |
+
self.rec_A = self.net_G_B(self.fake_B) # G_B(G_A(A))
|
169 |
+
self.fake_A = self.net_G_B(self.real_B) # G_B(B)
|
170 |
+
self.rec_B = self.net_G_A(self.fake_A) # G_A(G_B(B))
|
models/networks.py
ADDED
@@ -0,0 +1,767 @@
|
|
|
|
|
|
|
|
|
|
|
|
|
|
|
|
|
|
|
|
|
|
|
|
|
|
|
|
|
|
|
|
|
|
|
|
|
|
|
|
|
|
|
|
|
|
|
|
|
|
|
|
|
|
|
|
|
|
|
|
|
|
|
|
|
|
|
|
|
|
|
|
|
|
|
|
|
|
|
|
|
|
|
|
|
|
|
|
|
|
|
|
|
|
|
|
|
|
|
|
|
|
|
|
|
|
|
|
|
|
|
|
|
|
|
|
|
|
|
|
|
|
|
|
|
|
|
|
|
|
|
|
|
|
|
|
|
|
|
|
|
|
|
|
|
|
|
|
|
|
|
|
|
|
|
|
|
|
|
|
|
|
|
|
|
|
|
|
|
|
|
|
|
|
|
|
|
|
|
|
|
|
|
|
|
|
|
|
|
|
|
|
|
|
|
|
|
|
|
|
|
|
|
|
|
|
|
|
|
|
|
|
|
|
|
|
|
|
|
|
|
|
|
|
|
|
|
|
|
|
|
|
|
|
|
|
|
|
|
|
|
|
|
|
|
|
|
|
|
|
|
|
|
|
|
|
|
|
|
|
|
|
|
|
|
|
|
|
|
|
|
|
|
|
|
|
|
|
|
|
|
|
|
|
|
|
|
|
|
|
|
|
|
|
|
|
|
|
|
|
|
|
|
|
|
|
|
|
|
|
|
|
|
|
|
|
|
|
|
|
|
|
|
|
|
|
|
|
|
|
|
|
|
|
|
|
|
|
|
|
|
|
|
|
|
|
|
|
|
|
|
|
|
|
|
|
|
|
|
|
|
|
|
|
|
|
|
|
|
|
|
|
|
|
|
|
|
|
|
|
|
|
|
|
|
|
|
|
|
|
|
|
|
|
|
|
|
|
|
|
|
|
|
|
|
|
|
|
|
|
|
|
|
|
|
|
|
|
|
|
|
|
|
|
|
|
|
|
|
|
|
|
|
|
|
|
|
|
|
|
|
|
|
|
|
|
|
|
|
|
|
|
|
|
|
|
|
|
|
|
|
|
|
|
|
|
|
|
|
|
|
|
|
|
|
|
|
|
|
|
|
|
|
|
|
|
|
|
|
|
|
|
|
|
|
|
|
|
|
|
|
|
|
|
|
|
|
|
|
|
|
|
|
|
|
|
|
|
|
|
|
|
|
|
|
|
|
|
|
|
|
|
|
|
|
|
|
|
|
|
|
|
|
|
|
|
|
|
|
|
|
|
|
|
|
|
|
|
|
|
|
|
|
|
|
|
|
|
|
|
|
|
|
|
|
|
|
|
|
|
|
|
|
|
|
|
|
|
|
|
|
|
|
|
|
|
|
|
|
|
|
|
|
|
|
|
|
|
|
|
|
|
|
|
|
|
|
|
|
|
|
|
|
|
|
|
|
|
|
|
|
|
|
|
|
|
|
|
|
|
|
|
|
|
|
|
|
|
|
|
|
|
|
|
|
|
|
|
|
|
|
|
|
|
|
|
|
|
|
|
|
|
|
|
|
|
|
|
|
|
|
|
|
|
|
|
|
|
|
|
|
|
|
|
|
|
|
|
|
|
|
|
|
|
|
|
|
|
|
|
|
|
|
|
|
|
|
|
|
|
|
|
|
|
|
|
|
|
|
|
|
|
|
|
|
|
|
|
|
|
|
|
|
|
|
|
|
|
|
|
|
|
|
|
|
|
|
|
|
|
|
|
|
|
|
|
|
|
|
|
|
|
|
|
|
|
|
|
|
|
|
|
|
|
|
|
|
|
|
|
|
|
|
|
|
|
|
|
|
|
|
|
|
|
|
|
|
|
|
|
|
|
|
|
|
|
|
|
|
|
|
|
|
|
|
|
|
|
|
|
|
|
|
|
|
|
|
|
|
|
|
|
|
|
|
|
|
|
|
|
|
|
|
|
|
|
|
|
|
|
|
|
|
|
|
|
|
|
|
|
|
|
|
|
|
|
|
|
|
|
|
|
|
|
|
|
|
|
|
|
|
|
|
|
|
|
|
|
|
|
|
|
|
|
|
|
|
|
|
|
|
|
|
|
|
|
|
|
|
|
|
|
|
|
|
|
|
|
|
|
|
|
|
|
|
|
|
|
|
|
|
|
|
|
|
|
|
|
|
|
|
|
|
|
|
|
|
|
|
|
|
|
|
|
|
|
|
|
|
|
|
|
|
|
|
|
|
|
|
|
|
|
|
|
|
|
|
|
|
|
|
|
|
|
|
|
|
|
|
|
|
|
|
|
|
|
|
|
|
|
|
|
|
|
|
|
|
|
|
|
|
|
|
|
|
|
|
|
|
|
|
|
|
|
|
|
|
|
|
|
|
|
|
|
|
|
|
|
|
|
|
|
|
|
|
|
|
|
|
|
|
|
|
|
|
|
|
|
|
|
|
|
|
|
|
|
|
|
|
|
|
|
|
|
|
|
|
|
|
|
|
|
|
|
|
|
|
|
|
|
|
|
|
|
|
|
|
|
|
|
|
|
|
|
|
|
|
|
|
|
|
|
|
|
|
|
|
|
|
|
|
|
|
|
|
|
|
|
|
|
|
|
|
|
|
|
|
|
|
|
|
|
|
|
|
|
|
|
|
|
|
|
|
|
|
|
|
|
|
|
|
|
|
|
|
|
|
|
|
|
|
|
|
|
|
|
|
|
|
|
|
|
|
|
|
|
|
|
|
|
|
|
|
|
|
|
|
|
|
|
|
|
|
|
|
|
|
|
|
|
|
|
|
|
|
|
|
|
|
|
|
|
|
|
|
|
|
|
|
|
|
|
|
|
|
|
|
|
|
|
|
|
|
|
|
|
|
|
|
|
|
|
|
|
|
|
|
|
|
|
|
|
|
|
|
|
|
|
|
|
|
|
|
|
|
|
|
|
|
|
|
|
|
|
|
|
|
|
|
|
|
|
|
|
|
|
|
|
|
|
|
|
|
|
|
|
|
|
|
|
|
|
|
|
|
|
|
|
|
|
|
|
|
|
|
|
|
|
|
|
|
|
|
|
|
|
|
|
|
|
|
|
|
|
|
|
|
|
|
|
|
|
|
|
|
|
|
|
|
|
|
|
|
|
|
|
|
|
|
|
|
|
|
|
|
|
|
|
|
|
|
|
|
|
|
|
|
|
|
|
|
|
|
|
|
|
|
|
|
|
|
|
|
|
|
|
|
|
|
|
|
|
|
|
|
|
|
|
|
|
|
|
|
|
|
|
|
|
|
|
|
|
|
|
|
|
|
|
|
|
|
|
|
|
|
|
|
|
|
|
|
|
|
|
|
|
|
|
|
|
|
|
|
|
|
|
|
|
|
|
|
|
|
|
|
|
|
|
|
|
|
|
|
|
|
|
|
|
|
|
|
|
|
|
|
|
|
|
|
|
|
|
|
|
|
|
|
|
|
|
|
|
|
|
|
|
|
|
|
|
|
|
|
|
|
|
|
1 |
+
import functools
|
2 |
+
|
3 |
+
import torch
|
4 |
+
import torch.nn as nn
|
5 |
+
from torch.nn import init
|
6 |
+
from torch.optim import lr_scheduler
|
7 |
+
|
8 |
+
# 2023年2月23日 模型:卷积,残差
|
9 |
+
###############################################################################
|
10 |
+
|
11 |
+
|
12 |
+
class Identity(nn.Module):
|
13 |
+
def forward(self, x):
|
14 |
+
return x
|
15 |
+
|
16 |
+
|
17 |
+
def get_norm_layer(norm_type="instance"):
|
18 |
+
"""Return a normalization layer
|
19 |
+
|
20 |
+
Parameters:
|
21 |
+
norm_type (str) -- the name of the normalization layer: batch | instance | none
|
22 |
+
|
23 |
+
For BatchNorm, we use learnable affine parameters and track running statistics (mean/stddev).
|
24 |
+
For InstanceNorm, we do not use learnable affine parameters. We do not track running statistics.
|
25 |
+
"""
|
26 |
+
dict_ = {
|
27 |
+
"batch": functools.partial(
|
28 |
+
nn.BatchNorm2d, affine=True, track_running_stats=True
|
29 |
+
),
|
30 |
+
"instance": functools.partial(
|
31 |
+
nn.InstanceNorm2d, affine=False, track_running_stats=False
|
32 |
+
),
|
33 |
+
"none": lambda x: Identity(),
|
34 |
+
}
|
35 |
+
# if norm_type == 'batch':
|
36 |
+
# norm_layer = functools.partial(nn.BatchNorm2d, affine=True, track_running_stats=True)
|
37 |
+
# elif norm_type == 'instance':
|
38 |
+
# norm_layer = functools.partial(nn.InstanceNorm2d, affine=False, track_running_stats=False)
|
39 |
+
# elif norm_type == 'none':
|
40 |
+
# def norm_layer(x):
|
41 |
+
# return Identity()
|
42 |
+
if norm_type in dict_:
|
43 |
+
norm_layer = dict_[norm_type]
|
44 |
+
else:
|
45 |
+
raise NotImplementedError("normalization layer [%s] is not found" % norm_type)
|
46 |
+
return norm_layer
|
47 |
+
|
48 |
+
|
49 |
+
def get_scheduler(optimizer, opt):
|
50 |
+
"""Return a learning rate scheduler
|
51 |
+
|
52 |
+
Parameters:
|
53 |
+
optimizer -- the optimizer of the network
|
54 |
+
optimizer:
|
55 |
+
opt (option class) -- stores all the experiment flags; needs to be a subclass of BaseOptions.
|
56 |
+
opt.lr_policy is the name of learning rate policy: linear | step | plateau | cosine
|
57 |
+
|
58 |
+
For 'linear', we keep the same learning rate for the first <opt.n_epochs> epochs
|
59 |
+
and linearly decay the rate to zero over the next <opt.n_epochs_decay> epochs.
|
60 |
+
For other schedulers (step, plateau, and cosine), we use the default PyTorch schedulers.
|
61 |
+
See https://pytorch.org/docs/stable/optim.html for more details.
|
62 |
+
"""
|
63 |
+
if opt.lr_policy == "linear":
|
64 |
+
|
65 |
+
def lambda_rule(epoch):
|
66 |
+
lr_l = 1.0 - max(0, epoch + opt.epoch_count - opt.n_epochs) / float(
|
67 |
+
opt.n_epochs_decay + 1
|
68 |
+
)
|
69 |
+
return lr_l
|
70 |
+
|
71 |
+
scheduler = lr_scheduler.LambdaLR(optimizer, lr_lambda=lambda_rule)
|
72 |
+
elif opt.lr_policy == "step":
|
73 |
+
scheduler = lr_scheduler.StepLR(
|
74 |
+
optimizer, step_size=opt.lr_decay_iters, gamma=0.1
|
75 |
+
)
|
76 |
+
elif opt.lr_policy == "plateau":
|
77 |
+
scheduler = lr_scheduler.ReduceLROnPlateau(
|
78 |
+
optimizer, mode="min", factor=0.2, threshold=0.01, patience=5
|
79 |
+
)
|
80 |
+
elif opt.lr_policy == "cosine":
|
81 |
+
scheduler = lr_scheduler.CosineAnnealingLR(
|
82 |
+
optimizer, T_max=opt.n_epochs, eta_min=0
|
83 |
+
)
|
84 |
+
else:
|
85 |
+
return NotImplementedError(
|
86 |
+
"learning rate policy [%s] is not implemented", opt.lr_policy
|
87 |
+
)
|
88 |
+
return scheduler
|
89 |
+
|
90 |
+
|
91 |
+
def init_weights(net, init_type="normal", init_gain=0.02):
|
92 |
+
"""Initialize network weights.
|
93 |
+
|
94 |
+
Parameters:
|
95 |
+
net (network) -- network to be initialized
|
96 |
+
init_type (str) -- the name of an initialization method: normal | xavier | kaiming | orthogonal
|
97 |
+
init_gain (float) -- scaling factor for normal, xavier and orthogonal.
|
98 |
+
|
99 |
+
We use 'normal' in the original pix2pix and CycleGAN paper. But xavier and kaiming might
|
100 |
+
work better for some applications. Feel free to try yourself.
|
101 |
+
"""
|
102 |
+
|
103 |
+
def init_func(m): # define the initialization function
|
104 |
+
classname = m.__class__.__name__
|
105 |
+
if hasattr(m, "weight") and (
|
106 |
+
classname.find("Conv") != -1 or classname.find("Linear") != -1
|
107 |
+
):
|
108 |
+
if init_type == "normal":
|
109 |
+
init.normal_(m.weight.data, 0.0, init_gain)
|
110 |
+
elif init_type == "xavier":
|
111 |
+
init.xavier_normal_(m.weight.data, gain=init_gain)
|
112 |
+
elif init_type == "kaiming":
|
113 |
+
init.kaiming_normal_(m.weight.data, a=0, mode="fan_in")
|
114 |
+
elif init_type == "orthogonal":
|
115 |
+
init.orthogonal_(m.weight.data, gain=init_gain)
|
116 |
+
else:
|
117 |
+
raise NotImplementedError(
|
118 |
+
"initialization method [%s] is not implemented" % init_type
|
119 |
+
)
|
120 |
+
if hasattr(m, "bias") and m.bias is not None:
|
121 |
+
init.constant_(m.bias.data, 0.0)
|
122 |
+
elif (
|
123 |
+
classname.find("BatchNorm2d") != -1
|
124 |
+
): # BatchNorm Layer's weight is not a matrix; only normal distribution applies.
|
125 |
+
init.normal_(m.weight.data, 1.0, init_gain)
|
126 |
+
init.constant_(m.bias.data, 0.0)
|
127 |
+
|
128 |
+
print("initialize network with %s" % init_type)
|
129 |
+
net.apply(init_func) # apply the initialization function <init_func>
|
130 |
+
|
131 |
+
|
132 |
+
def init_net(net, init_type="normal", init_gain=0.02, gpu_ids=None):
|
133 |
+
"""Initialize a network: 1. register CPU/GPU device (with multi-GPU support); 2. initialize the network weights
|
134 |
+
Parameters:
|
135 |
+
net (network) -- the network to be initialized
|
136 |
+
init_type (str) -- the name of an initialization method: normal | xavier | kaiming | orthogonal
|
137 |
+
init_gain (float) -- scaling factor for normal, xavier and orthogonal.
|
138 |
+
gpu_ids (int list) -- which GPUs the network runs on: e.g., 0,1,2
|
139 |
+
|
140 |
+
Return an initialized network.
|
141 |
+
"""
|
142 |
+
if gpu_ids is None:
|
143 |
+
gpu_ids = []
|
144 |
+
if len(gpu_ids) > 0:
|
145 |
+
assert torch.cuda.is_available()
|
146 |
+
net.to(gpu_ids[0])
|
147 |
+
net = torch.nn.DataParallel(net, gpu_ids) # multi-GPUs
|
148 |
+
init_weights(net, init_type, init_gain=init_gain)
|
149 |
+
return net
|
150 |
+
|
151 |
+
|
152 |
+
def define_G(
|
153 |
+
input_nc,
|
154 |
+
output_nc,
|
155 |
+
ngf,
|
156 |
+
netG,
|
157 |
+
norm="batch",
|
158 |
+
use_dropout=False,
|
159 |
+
init_type="normal",
|
160 |
+
init_gain=0.02,
|
161 |
+
gpu_ids=None,
|
162 |
+
):
|
163 |
+
"""Create a generator
|
164 |
+
|
165 |
+
Parameters:
|
166 |
+
input_nc (int) -- the number of channels in input images
|
167 |
+
output_nc (int) -- the number of channels in output images
|
168 |
+
ngf (int) -- the number of filters in the last conv layer
|
169 |
+
netG (str) -- the architecture's name: resnet_9blocks | resnet_6blocks | unet_256 | unet_128
|
170 |
+
norm (str) -- the name of normalization layers used in the network: batch | instance | none
|
171 |
+
use_dropout (bool) -- if use dropout layers.
|
172 |
+
init_type (str) -- the name of our initialization method.
|
173 |
+
init_gain (float) -- scaling factor for normal, xavier and orthogonal.
|
174 |
+
gpu_ids (int list) -- which GPUs the network runs on: e.g., 0,1,2
|
175 |
+
|
176 |
+
Returns a generator
|
177 |
+
|
178 |
+
Our current implementation provides two types of generators:
|
179 |
+
U-Net: [unet_128] (for 128x128 input images) and [unet_256] (for 256x256 input images)
|
180 |
+
The original U-Net paper: https://arxiv.org/abs/1505.04597
|
181 |
+
|
182 |
+
Resnet-based generator: [resnet_6blocks] (with 6 Resnet blocks) and [resnet_9blocks] (with 9 Resnet blocks)
|
183 |
+
Resnet-based generator consists of several Resnet blocks between a few downsampling/upsampling operations.
|
184 |
+
We adapt Torch code from Justin Johnson's neural style transfer project (https://github.com/jcjohnson/fast-neural-style).
|
185 |
+
|
186 |
+
|
187 |
+
The generator has been initialized by <init_net>. It uses RELU for non-linearity.
|
188 |
+
"""
|
189 |
+
if gpu_ids is None:
|
190 |
+
gpu_ids = []
|
191 |
+
net = None
|
192 |
+
norm_layer = get_norm_layer(norm_type=norm)
|
193 |
+
net_dict = {
|
194 |
+
"resnet_9blocks": ResnetGenerator(
|
195 |
+
input_nc,
|
196 |
+
output_nc,
|
197 |
+
ngf,
|
198 |
+
norm_layer=norm_layer,
|
199 |
+
use_dropout=use_dropout,
|
200 |
+
n_blocks=9,
|
201 |
+
),
|
202 |
+
"resnet_6blocks": ResnetGenerator(
|
203 |
+
input_nc,
|
204 |
+
output_nc,
|
205 |
+
ngf,
|
206 |
+
norm_layer=norm_layer,
|
207 |
+
use_dropout=use_dropout,
|
208 |
+
n_blocks=6,
|
209 |
+
),
|
210 |
+
"unet_128": UnetGenerator(
|
211 |
+
input_nc, output_nc, 7, ngf, norm_layer=norm_layer, use_dropout=use_dropout
|
212 |
+
),
|
213 |
+
"unet_256": UnetGenerator(
|
214 |
+
input_nc, output_nc, 8, ngf, norm_layer=norm_layer, use_dropout=use_dropout
|
215 |
+
),
|
216 |
+
}
|
217 |
+
|
218 |
+
# if netG == "resnet_9blocks":
|
219 |
+
# net = ResnetGenerator(input_nc, output_nc, ngf, norm_layer=norm_layer, use_dropout=use_dropout, n_blocks=9)
|
220 |
+
# elif netG == "resnet_6blocks":
|
221 |
+
# net = ResnetGenerator(input_nc, output_nc, ngf, norm_layer=norm_layer, use_dropout=use_dropout, n_blocks=6)
|
222 |
+
# elif netG == "unet_128":
|
223 |
+
# net = UnetGenerator(input_nc, output_nc, 7, ngf, norm_layer=norm_layer, use_dropout=use_dropout)
|
224 |
+
# elif netG == "unet_256":
|
225 |
+
# net = UnetGenerator(input_nc, output_nc, 8, ngf, norm_layer=norm_layer, use_dropout=use_dropout)
|
226 |
+
if netG in net_dict:
|
227 |
+
net = net_dict[netG]
|
228 |
+
else:
|
229 |
+
raise NotImplementedError("Generator model name [%s] is not recognized" % netG)
|
230 |
+
return init_net(net, init_type, init_gain, gpu_ids)
|
231 |
+
|
232 |
+
|
233 |
+
def define_D(
|
234 |
+
input_nc,
|
235 |
+
ndf,
|
236 |
+
netD,
|
237 |
+
n_layers_D=3,
|
238 |
+
norm="batch",
|
239 |
+
init_type="normal",
|
240 |
+
init_gain=0.02,
|
241 |
+
gpu_ids=None,
|
242 |
+
):
|
243 |
+
"""Create a discriminator
|
244 |
+
|
245 |
+
Parameters:
|
246 |
+
input_nc (int) -- the number of channels in input images
|
247 |
+
ndf (int) -- the number of filters in the first conv layer
|
248 |
+
netD (str) -- the architecture's name: basic | n_layers | pixel
|
249 |
+
n_layers_D (int) -- the number of conv layers in the discriminator; effective when netD=='n_layers'
|
250 |
+
norm (str) -- the type of normalization layers used in the network.
|
251 |
+
init_type (str) -- the name of the initialization method.
|
252 |
+
init_gain (float) -- scaling factor for normal, xavier and orthogonal.
|
253 |
+
gpu_ids (int list) -- which GPUs the network runs on: e.g., 0,1,2
|
254 |
+
|
255 |
+
Returns a discriminator
|
256 |
+
|
257 |
+
Our current implementation provides three types of discriminators:
|
258 |
+
[basic]: 'PatchGAN' classifier described in the original pix2pix paper.
|
259 |
+
It can classify whether 70×70 overlapping patches are real or fake.
|
260 |
+
Such a patch-level discriminator architecture has fewer parameters
|
261 |
+
than a full-image discriminator and can work on arbitrarily-sized images
|
262 |
+
in a fully convolutional fashion.
|
263 |
+
|
264 |
+
[n_layers]: With this mode, you can specify the number of conv layers in the discriminator
|
265 |
+
with the parameter <n_layers_D> (default=3 as used in [basic] (PatchGAN).)
|
266 |
+
|
267 |
+
[pixel]: 1x1 PixelGAN discriminator can classify whether a pixel is real or not.
|
268 |
+
It encourages greater color diversity but has no effect on spatial statistics.
|
269 |
+
|
270 |
+
The discriminator has been initialized by <init_net>. It uses Leakly RELU for non-linearity.
|
271 |
+
"""
|
272 |
+
if gpu_ids is None:
|
273 |
+
gpu_ids = []
|
274 |
+
net = None
|
275 |
+
norm_layer = get_norm_layer(norm_type=norm)
|
276 |
+
net_dict = {
|
277 |
+
"basic": NLayerDiscriminator(input_nc, ndf, n_layers=3, norm_layer=norm_layer),
|
278 |
+
"n_layers": NLayerDiscriminator(
|
279 |
+
input_nc, ndf, n_layers_D, norm_layer=norm_layer
|
280 |
+
),
|
281 |
+
"pixel": PixelDiscriminator(input_nc, ndf, norm_layer=norm_layer),
|
282 |
+
}
|
283 |
+
|
284 |
+
# if netD == "basic": # default PatchGAN classifier
|
285 |
+
# net = NLayerDiscriminator(input_nc, ndf, n_layers=3, norm_layer=norm_layer)
|
286 |
+
# elif netD == "n_layers": # more options
|
287 |
+
# net = NLayerDiscriminator(input_nc, ndf, n_layers_D, norm_layer=norm_layer)
|
288 |
+
# elif netD == "pixel": # classify if each pixel is real or fake
|
289 |
+
# net = PixelDiscriminator(input_nc, ndf, norm_layer=norm_layer)
|
290 |
+
if netD in net_dict:
|
291 |
+
net = net_dict[netD]
|
292 |
+
else:
|
293 |
+
raise NotImplementedError(
|
294 |
+
"Discriminator model name [%s] is not recognized" % netD
|
295 |
+
)
|
296 |
+
return init_net(net, init_type, init_gain, gpu_ids)
|
297 |
+
|
298 |
+
|
299 |
+
##############################################################################
|
300 |
+
# Classes
|
301 |
+
##############################################################################
|
302 |
+
|
303 |
+
|
304 |
+
def cal_gradient_penalty(
|
305 |
+
netD, real_data, fake_data, device, type="mixed", constant=1.0, lambda_gp=10.0
|
306 |
+
):
|
307 |
+
"""Calculate the gradient penalty loss, used in WGAN-GP paper https://arxiv.org/abs/1704.00028
|
308 |
+
|
309 |
+
Arguments:
|
310 |
+
netD (network) -- discriminator network
|
311 |
+
real_data (tensor array) -- real images
|
312 |
+
fake_data (tensor array) -- generated images from the generator
|
313 |
+
device (str) -- GPU / CPU: from torch.device('cuda:{}'.format(self.gpu_ids[0])) if self.gpu_ids else torch.device('cpu')
|
314 |
+
type (str) -- if we mix real and fake data or not [real | fake | mixed].
|
315 |
+
constant (float) -- the constant used in formula ( ||gradient||_2 - constant)^2
|
316 |
+
lambda_gp (float) -- weight for this loss
|
317 |
+
|
318 |
+
Returns the gradient penalty loss
|
319 |
+
"""
|
320 |
+
if lambda_gp > 0.0:
|
321 |
+
if (
|
322 |
+
type == "real"
|
323 |
+
): # either use real images, fake images, or a linear interpolation of two.
|
324 |
+
interpolatesv = real_data
|
325 |
+
elif type == "fake":
|
326 |
+
interpolatesv = fake_data
|
327 |
+
elif type == "mixed":
|
328 |
+
alpha = torch.rand(real_data.shape[0], 1, device=device)
|
329 |
+
alpha = (
|
330 |
+
alpha.expand(
|
331 |
+
real_data.shape[0], real_data.nelement() // real_data.shape[0]
|
332 |
+
)
|
333 |
+
.contiguous()
|
334 |
+
.view(*real_data.shape)
|
335 |
+
)
|
336 |
+
interpolatesv = alpha * real_data + ((1 - alpha) * fake_data)
|
337 |
+
else:
|
338 |
+
raise NotImplementedError("{} not implemented".format(type))
|
339 |
+
interpolatesv.requires_grad_(True)
|
340 |
+
disc_interpolates = netD(interpolatesv)
|
341 |
+
gradients = torch.autograd.grad(
|
342 |
+
outputs=disc_interpolates,
|
343 |
+
inputs=interpolatesv,
|
344 |
+
grad_outputs=torch.ones(disc_interpolates.size()).to(device),
|
345 |
+
create_graph=True,
|
346 |
+
retain_graph=True,
|
347 |
+
only_inputs=True,
|
348 |
+
)
|
349 |
+
gradients = gradients[0].view(real_data.size(0), -1) # flat the data
|
350 |
+
gradient_penalty = (
|
351 |
+
((gradients + 1e-16).norm(2, dim=1) - constant) ** 2
|
352 |
+
).mean() * lambda_gp # added eps
|
353 |
+
return gradient_penalty, gradients
|
354 |
+
else:
|
355 |
+
return 0.0, None
|
356 |
+
|
357 |
+
|
358 |
+
class ResnetGenerator(nn.Module):
|
359 |
+
"""Resnet-based generator that consists of Resnet blocks between a few downsampling/upsampling operations.
|
360 |
+
|
361 |
+
We adapt Torch code and idea from Justin Johnson's neural style transfer project(https://github.com/jcjohnson/fast-neural-style)
|
362 |
+
"""
|
363 |
+
|
364 |
+
def __init__(
|
365 |
+
self,
|
366 |
+
input_nc,
|
367 |
+
output_nc,
|
368 |
+
ngf=64,
|
369 |
+
norm_layer=nn.BatchNorm2d,
|
370 |
+
use_dropout=False,
|
371 |
+
n_blocks=6,
|
372 |
+
padding_type="reflect",
|
373 |
+
):
|
374 |
+
"""Construct a Resnet-based generator
|
375 |
+
|
376 |
+
Parameters:
|
377 |
+
input_nc (int) -- the number of channels in input images
|
378 |
+
output_nc (int) -- the number of channels in output images
|
379 |
+
ngf (int) -- the number of filters in the last conv layer
|
380 |
+
norm_layer -- normalization layer
|
381 |
+
use_dropout (bool) -- if use dropout layers
|
382 |
+
n_blocks (int) -- the number of ResNet blocks
|
383 |
+
padding_type (str) -- the name of padding layer in conv layers: reflect | replicate | zero
|
384 |
+
"""
|
385 |
+
assert n_blocks >= 0
|
386 |
+
super(ResnetGenerator, self).__init__()
|
387 |
+
if type(norm_layer) == functools.partial:
|
388 |
+
use_bias = norm_layer.func == nn.InstanceNorm2d
|
389 |
+
else:
|
390 |
+
use_bias = norm_layer == nn.InstanceNorm2d
|
391 |
+
model = [
|
392 |
+
nn.ReflectionPad2d(3),
|
393 |
+
nn.Conv2d(input_nc, ngf, kernel_size=7, padding=0, bias=use_bias),
|
394 |
+
norm_layer(ngf),
|
395 |
+
nn.ReLU(True),
|
396 |
+
]
|
397 |
+
|
398 |
+
n_downsampling = 2
|
399 |
+
for i in range(n_downsampling): # add downsampling layers
|
400 |
+
mult = 2**i
|
401 |
+
model += [
|
402 |
+
nn.Conv2d(
|
403 |
+
ngf * mult,
|
404 |
+
ngf * mult * 2,
|
405 |
+
kernel_size=3,
|
406 |
+
stride=2,
|
407 |
+
padding=1,
|
408 |
+
bias=use_bias,
|
409 |
+
),
|
410 |
+
norm_layer(ngf * mult * 2),
|
411 |
+
nn.ReLU(True),
|
412 |
+
]
|
413 |
+
mult = 2**n_downsampling
|
414 |
+
for i in range(n_blocks): # add ResNet blocks
|
415 |
+
|
416 |
+
model += [
|
417 |
+
ResnetBlock(
|
418 |
+
ngf * mult,
|
419 |
+
padding_type=padding_type,
|
420 |
+
norm_layer=norm_layer,
|
421 |
+
use_dropout=use_dropout,
|
422 |
+
use_bias=use_bias,
|
423 |
+
)
|
424 |
+
]
|
425 |
+
for i in range(n_downsampling): # add upsampling layers
|
426 |
+
mult = 2 ** (n_downsampling - i)
|
427 |
+
model += [
|
428 |
+
nn.ConvTranspose2d(
|
429 |
+
ngf * mult,
|
430 |
+
int(ngf * mult / 2),
|
431 |
+
kernel_size=3,
|
432 |
+
stride=2,
|
433 |
+
padding=1,
|
434 |
+
output_padding=1,
|
435 |
+
bias=use_bias,
|
436 |
+
),
|
437 |
+
norm_layer(int(ngf * mult / 2)),
|
438 |
+
nn.ReLU(True),
|
439 |
+
]
|
440 |
+
model += [nn.ReflectionPad2d(3)]
|
441 |
+
model += [nn.Conv2d(ngf, output_nc, kernel_size=7, padding=0)]
|
442 |
+
model += [nn.Tanh()]
|
443 |
+
|
444 |
+
self.model = nn.Sequential(*model)
|
445 |
+
|
446 |
+
def forward(self, input):
|
447 |
+
"""Standard forward"""
|
448 |
+
return self.model(input)
|
449 |
+
|
450 |
+
|
451 |
+
class ResnetBlock(nn.Module):
|
452 |
+
"""Define a Resnet block"""
|
453 |
+
|
454 |
+
def __init__(self, dim, padding_type, norm_layer, use_dropout, use_bias):
|
455 |
+
"""Initialize the Resnet block
|
456 |
+
|
457 |
+
A resnet block is a conv block with skip connections
|
458 |
+
We construct a conv block with build_conv_block function,
|
459 |
+
and implement skip connections in <forward> function.
|
460 |
+
Original Resnet paper: https://arxiv.org/pdf/1512.03385.pdf
|
461 |
+
"""
|
462 |
+
super(ResnetBlock, self).__init__()
|
463 |
+
self.conv_block = self.build_conv_block(
|
464 |
+
dim, padding_type, norm_layer, use_dropout, use_bias
|
465 |
+
)
|
466 |
+
|
467 |
+
def build_conv_block(self, dim, padding_type, norm_layer, use_dropout, use_bias):
|
468 |
+
"""Construct a convolutional block.
|
469 |
+
|
470 |
+
Parameters:
|
471 |
+
norm_layer:
|
472 |
+
dim (int) -- the number of channels in the conv layer.
|
473 |
+
padding_type (str) -- the name of padding layer: reflect | replicate | zero
|
474 |
+
norm_layer -- normalization layer
|
475 |
+
use_dropout (bool) -- if use dropout layers.
|
476 |
+
use_bias (bool) -- if the conv layer uses bias or not
|
477 |
+
|
478 |
+
Returns a conv block (with a conv layer, a normalization layer, and a non-linearity layer (ReLU))
|
479 |
+
"""
|
480 |
+
conv_block = []
|
481 |
+
p = 0
|
482 |
+
|
483 |
+
if padding_type == "reflect":
|
484 |
+
conv_block += [nn.ReflectionPad2d(1)]
|
485 |
+
elif padding_type == "replicate":
|
486 |
+
conv_block += [nn.ReplicationPad2d(1)]
|
487 |
+
elif padding_type == "zero":
|
488 |
+
p = 1
|
489 |
+
else:
|
490 |
+
raise NotImplementedError("padding [%s] is not implemented" % padding_type)
|
491 |
+
conv_block += [
|
492 |
+
nn.Conv2d(dim, dim, kernel_size=3, padding=p, bias=use_bias),
|
493 |
+
norm_layer(dim),
|
494 |
+
nn.ReLU(True),
|
495 |
+
]
|
496 |
+
if use_dropout:
|
497 |
+
conv_block += [nn.Dropout(0.5)]
|
498 |
+
p = 0
|
499 |
+
if padding_type == "reflect":
|
500 |
+
conv_block += [nn.ReflectionPad2d(1)]
|
501 |
+
elif padding_type == "replicate":
|
502 |
+
conv_block += [nn.ReplicationPad2d(1)]
|
503 |
+
elif padding_type == "zero":
|
504 |
+
p = 1
|
505 |
+
else:
|
506 |
+
raise NotImplementedError("padding [%s] is not implemented" % padding_type)
|
507 |
+
conv_block += [
|
508 |
+
nn.Conv2d(dim, dim, kernel_size=3, padding=p, bias=use_bias),
|
509 |
+
norm_layer(dim),
|
510 |
+
]
|
511 |
+
|
512 |
+
return nn.Sequential(*conv_block)
|
513 |
+
|
514 |
+
def forward(self, x):
|
515 |
+
"""Forward function (with skip connections)"""
|
516 |
+
out = x + self.conv_block(x) # add skip connections
|
517 |
+
return out
|
518 |
+
|
519 |
+
|
520 |
+
class UnetGenerator(nn.Module):
|
521 |
+
"""Create a Unet-based generator"""
|
522 |
+
|
523 |
+
def __init__(
|
524 |
+
self,
|
525 |
+
input_nc,
|
526 |
+
output_nc,
|
527 |
+
num_downs,
|
528 |
+
ngf=64,
|
529 |
+
norm_layer=nn.BatchNorm2d,
|
530 |
+
use_dropout=False,
|
531 |
+
):
|
532 |
+
"""Construct a Unet generator
|
533 |
+
Parameters:
|
534 |
+
input_nc (int) -- the number of channels in input images
|
535 |
+
output_nc (int) -- the number of channels in output images
|
536 |
+
num_downs (int) -- the number of downsamplings in UNet. For example, # if |num_downs| == 7,
|
537 |
+
image of size 128x128 will become of size 1x1 # at the bottleneck
|
538 |
+
ngf (int) -- the number of filters in the last conv layer
|
539 |
+
norm_layer -- normalization layer
|
540 |
+
|
541 |
+
We construct the U-Net from the innermost layer to the outermost layer.
|
542 |
+
It is a recursive process.
|
543 |
+
"""
|
544 |
+
super(UnetGenerator, self).__init__()
|
545 |
+
# construct unet structure
|
546 |
+
unet_block = UnetSkipConnectionBlock(
|
547 |
+
ngf * 8,
|
548 |
+
ngf * 8,
|
549 |
+
input_nc=None,
|
550 |
+
submodule=None,
|
551 |
+
norm_layer=norm_layer,
|
552 |
+
innermost=True,
|
553 |
+
) # add the innermost layer
|
554 |
+
for i in range(num_downs - 5): # add intermediate layers with ngf * 8 filters
|
555 |
+
unet_block = UnetSkipConnectionBlock(
|
556 |
+
ngf * 8,
|
557 |
+
ngf * 8,
|
558 |
+
input_nc=None,
|
559 |
+
submodule=unet_block,
|
560 |
+
norm_layer=norm_layer,
|
561 |
+
use_dropout=use_dropout,
|
562 |
+
)
|
563 |
+
# gradually reduce the number of filters from ngf * 8 to ngf
|
564 |
+
unet_block = UnetSkipConnectionBlock(
|
565 |
+
ngf * 4, ngf * 8, input_nc=None, submodule=unet_block, norm_layer=norm_layer
|
566 |
+
)
|
567 |
+
unet_block = UnetSkipConnectionBlock(
|
568 |
+
ngf * 2, ngf * 4, input_nc=None, submodule=unet_block, norm_layer=norm_layer
|
569 |
+
)
|
570 |
+
unet_block = UnetSkipConnectionBlock(
|
571 |
+
ngf, ngf * 2, input_nc=None, submodule=unet_block, norm_layer=norm_layer
|
572 |
+
)
|
573 |
+
self.model = UnetSkipConnectionBlock(
|
574 |
+
output_nc,
|
575 |
+
ngf,
|
576 |
+
input_nc=input_nc,
|
577 |
+
submodule=unet_block,
|
578 |
+
outermost=True,
|
579 |
+
norm_layer=norm_layer,
|
580 |
+
) # add the outermost layer
|
581 |
+
|
582 |
+
def forward(self, input):
|
583 |
+
"""Standard forward"""
|
584 |
+
return self.model(input)
|
585 |
+
|
586 |
+
|
587 |
+
class UnetSkipConnectionBlock(nn.Module):
|
588 |
+
"""Defines the Unet submodule with skip connection.
|
589 |
+
X -------------------identity----------------------
|
590 |
+
|-- downsampling -- |submodule| -- upsampling --|
|
591 |
+
"""
|
592 |
+
|
593 |
+
def __init__(
|
594 |
+
self,
|
595 |
+
outer_nc,
|
596 |
+
inner_nc,
|
597 |
+
input_nc=None,
|
598 |
+
submodule=None,
|
599 |
+
outermost=False,
|
600 |
+
innermost=False,
|
601 |
+
norm_layer=nn.BatchNorm2d,
|
602 |
+
use_dropout=False,
|
603 |
+
):
|
604 |
+
"""Construct a Unet submodule with skip connections.
|
605 |
+
|
606 |
+
Parameters:
|
607 |
+
outer_nc (int) -- the number of filters in the outer conv layer
|
608 |
+
inner_nc (int) -- the number of filters in the inner conv layer
|
609 |
+
input_nc (int) -- the number of channels in input images/features
|
610 |
+
submodule (UnetSkipConnectionBlock) -- previously defined submodules
|
611 |
+
outermost (bool) -- if this module is the outermost module
|
612 |
+
innermost (bool) -- if this module is the innermost module
|
613 |
+
norm_layer -- normalization layer
|
614 |
+
use_dropout (bool) -- if use dropout layers.
|
615 |
+
"""
|
616 |
+
super(UnetSkipConnectionBlock, self).__init__()
|
617 |
+
self.outermost = outermost
|
618 |
+
if type(norm_layer) == functools.partial:
|
619 |
+
use_bias = norm_layer.func == nn.InstanceNorm2d
|
620 |
+
else:
|
621 |
+
use_bias = norm_layer == nn.InstanceNorm2d
|
622 |
+
if input_nc is None:
|
623 |
+
input_nc = outer_nc
|
624 |
+
downconv = nn.Conv2d(
|
625 |
+
input_nc, inner_nc, kernel_size=4, stride=2, padding=1, bias=use_bias
|
626 |
+
)
|
627 |
+
downrelu = nn.LeakyReLU(0.2, True)
|
628 |
+
downnorm = norm_layer(inner_nc)
|
629 |
+
uprelu = nn.ReLU(True)
|
630 |
+
upnorm = norm_layer(outer_nc)
|
631 |
+
|
632 |
+
if outermost:
|
633 |
+
upconv = nn.ConvTranspose2d(
|
634 |
+
inner_nc * 2, outer_nc, kernel_size=4, stride=2, padding=1
|
635 |
+
)
|
636 |
+
down = [downconv]
|
637 |
+
up = [uprelu, upconv, nn.Tanh()]
|
638 |
+
model = down + [submodule] + up
|
639 |
+
elif innermost:
|
640 |
+
upconv = nn.ConvTranspose2d(
|
641 |
+
inner_nc, outer_nc, kernel_size=4, stride=2, padding=1, bias=use_bias
|
642 |
+
)
|
643 |
+
down = [downrelu, downconv]
|
644 |
+
up = [uprelu, upconv, upnorm]
|
645 |
+
model = down + up
|
646 |
+
else:
|
647 |
+
upconv = nn.ConvTranspose2d(
|
648 |
+
inner_nc * 2,
|
649 |
+
outer_nc,
|
650 |
+
kernel_size=4,
|
651 |
+
stride=2,
|
652 |
+
padding=1,
|
653 |
+
bias=use_bias,
|
654 |
+
)
|
655 |
+
down = [downrelu, downconv, downnorm]
|
656 |
+
up = [uprelu, upconv, upnorm]
|
657 |
+
|
658 |
+
if use_dropout:
|
659 |
+
model = down + [submodule] + up + [nn.Dropout(0.5)]
|
660 |
+
else:
|
661 |
+
model = down + [submodule] + up
|
662 |
+
self.model = nn.Sequential(*model)
|
663 |
+
|
664 |
+
def forward(self, x):
|
665 |
+
if self.outermost:
|
666 |
+
return self.model(x)
|
667 |
+
else: # add skip connections
|
668 |
+
return torch.cat([x, self.model(x)], 1)
|
669 |
+
|
670 |
+
|
671 |
+
class NLayerDiscriminator(nn.Module):
|
672 |
+
"""Defines a PatchGAN discriminator"""
|
673 |
+
|
674 |
+
def __init__(self, input_nc, ndf=64, n_layers=3, norm_layer=nn.BatchNorm2d):
|
675 |
+
"""Construct a PatchGAN discriminator
|
676 |
+
|
677 |
+
Parameters:
|
678 |
+
input_nc (int) -- the number of channels in input images
|
679 |
+
ndf (int) -- the number of filters in the last conv layer
|
680 |
+
n_layers (int) -- the number of conv layers in the discriminator
|
681 |
+
norm_layer -- normalization layer
|
682 |
+
"""
|
683 |
+
super(NLayerDiscriminator, self).__init__()
|
684 |
+
if (
|
685 |
+
type(norm_layer) == functools.partial
|
686 |
+
): # no need to use bias as BatchNorm2d has affine parameters
|
687 |
+
use_bias = norm_layer.func == nn.InstanceNorm2d
|
688 |
+
else:
|
689 |
+
use_bias = norm_layer == nn.InstanceNorm2d
|
690 |
+
kw = 4
|
691 |
+
padw = 1
|
692 |
+
sequence = [
|
693 |
+
nn.Conv2d(input_nc, ndf, kernel_size=kw, stride=2, padding=padw),
|
694 |
+
nn.LeakyReLU(0.2, True),
|
695 |
+
]
|
696 |
+
nf_mult = 1
|
697 |
+
nf_mult_prev = 1
|
698 |
+
for n in range(1, n_layers): # gradually increase the number of filters
|
699 |
+
nf_mult_prev = nf_mult
|
700 |
+
nf_mult = min(2**n, 8)
|
701 |
+
sequence += [
|
702 |
+
nn.Conv2d(
|
703 |
+
ndf * nf_mult_prev,
|
704 |
+
ndf * nf_mult,
|
705 |
+
kernel_size=kw,
|
706 |
+
stride=2,
|
707 |
+
padding=padw,
|
708 |
+
bias=use_bias,
|
709 |
+
),
|
710 |
+
norm_layer(ndf * nf_mult),
|
711 |
+
nn.LeakyReLU(0.2, True),
|
712 |
+
]
|
713 |
+
nf_mult_prev = nf_mult
|
714 |
+
nf_mult = min(2**n_layers, 8)
|
715 |
+
sequence += [
|
716 |
+
nn.Conv2d(
|
717 |
+
ndf * nf_mult_prev,
|
718 |
+
ndf * nf_mult,
|
719 |
+
kernel_size=kw,
|
720 |
+
stride=1,
|
721 |
+
padding=padw,
|
722 |
+
bias=use_bias,
|
723 |
+
),
|
724 |
+
norm_layer(ndf * nf_mult),
|
725 |
+
nn.LeakyReLU(0.2, True),
|
726 |
+
]
|
727 |
+
|
728 |
+
sequence += [
|
729 |
+
nn.Conv2d(ndf * nf_mult, 1, kernel_size=kw, stride=1, padding=padw)
|
730 |
+
] # output 1 channel prediction map
|
731 |
+
self.model = nn.Sequential(*sequence)
|
732 |
+
|
733 |
+
def forward(self, input):
|
734 |
+
return self.model(input)
|
735 |
+
|
736 |
+
|
737 |
+
class PixelDiscriminator(nn.Module):
|
738 |
+
"""Defines a 1x1 PatchGAN discriminator (pixelGAN)"""
|
739 |
+
|
740 |
+
def __init__(self, input_nc, ndf=64, norm_layer=nn.BatchNorm2d):
|
741 |
+
"""Construct a 1x1 PatchGAN discriminator
|
742 |
+
|
743 |
+
Parameters:
|
744 |
+
input_nc (int) -- the number of channels in input images
|
745 |
+
ndf (int) -- the number of filters in the last conv layer
|
746 |
+
norm_layer -- normalization layer
|
747 |
+
"""
|
748 |
+
super(PixelDiscriminator, self).__init__()
|
749 |
+
if (
|
750 |
+
type(norm_layer) == functools.partial
|
751 |
+
): # no need to use bias as BatchNorm2d has affine parameters
|
752 |
+
use_bias = norm_layer.func == nn.InstanceNorm2d
|
753 |
+
else:
|
754 |
+
use_bias = norm_layer == nn.InstanceNorm2d
|
755 |
+
self.net = [
|
756 |
+
nn.Conv2d(input_nc, ndf, kernel_size=1, stride=1, padding=0),
|
757 |
+
nn.LeakyReLU(0.2, True),
|
758 |
+
nn.Conv2d(ndf, ndf * 2, kernel_size=1, stride=1, padding=0, bias=use_bias),
|
759 |
+
norm_layer(ndf * 2),
|
760 |
+
nn.LeakyReLU(0.2, True),
|
761 |
+
nn.Conv2d(ndf * 2, 1, kernel_size=1, stride=1, padding=0, bias=use_bias),
|
762 |
+
]
|
763 |
+
|
764 |
+
self.net = nn.Sequential(*self.net)
|
765 |
+
|
766 |
+
def forward(self, input):
|
767 |
+
return self.net(input)
|
models/test_model.py
ADDED
@@ -0,0 +1,86 @@
|
|
|
|
|
|
|
|
|
|
|
|
|
|
|
|
|
|
|
|
|
|
|
|
|
|
|
|
|
|
|
|
|
|
|
|
|
|
|
|
|
|
|
|
|
|
|
|
|
|
|
|
|
|
|
|
|
|
|
|
|
|
|
|
|
|
|
|
|
|
|
|
|
|
|
|
|
|
|
|
|
|
|
|
|
|
|
|
|
|
|
|
|
|
|
|
|
|
|
|
|
|
|
|
|
|
|
|
|
|
|
|
|
|
|
|
|
|
|
|
|
|
|
|
|
|
|
|
|
|
|
|
|
|
|
|
|
|
|
|
|
|
|
|
|
|
|
|
|
|
|
|
|
|
|
|
|
|
|
|
|
|
|
|
|
|
|
|
|
|
|
|
|
|
|
1 |
+
from . import networks
|
2 |
+
from .base_model import BaseModel
|
3 |
+
|
4 |
+
|
5 |
+
class TestModel(BaseModel):
|
6 |
+
"""This TestModel can be used to generate CycleGAN results for only one direction.
|
7 |
+
This model will automatically set '--dataset_mode single', which only loads the images from one collection.
|
8 |
+
|
9 |
+
See the test instruction for more details.
|
10 |
+
"""
|
11 |
+
|
12 |
+
@staticmethod
|
13 |
+
def modify_commandline_options(parser, is_train=True):
|
14 |
+
"""Add new dataset-specific options, and rewrite default values for existing options.
|
15 |
+
|
16 |
+
Parameters:
|
17 |
+
parser -- original option parser
|
18 |
+
parser:
|
19 |
+
is_train (bool) -- whether training phase or test phase. You can use this flag to add training-specific or test-specific options.
|
20 |
+
|
21 |
+
Returns:
|
22 |
+
the modified parser.
|
23 |
+
|
24 |
+
The model can only be used during test time. It requires '--dataset_mode single'.
|
25 |
+
You need to specify the network using the option '--model_suffix'.
|
26 |
+
"""
|
27 |
+
assert not is_train, "TestModel cannot be used during training time"
|
28 |
+
parser.set_defaults(dataset_mode="single")
|
29 |
+
parser.add_argument(
|
30 |
+
"--model_suffix",
|
31 |
+
type=str,
|
32 |
+
default="",
|
33 |
+
help="In checkpoints_dir, [epoch]_net_G[model_suffix].pth will be loaded as the generator.",
|
34 |
+
)
|
35 |
+
|
36 |
+
return parser
|
37 |
+
|
38 |
+
def __init__(self, opt):
|
39 |
+
"""Initialize the pix2pix class.
|
40 |
+
|
41 |
+
Parameters:
|
42 |
+
opt (Option class)-- stores all the experiment flags; needs to be a subclass of BaseOptions
|
43 |
+
"""
|
44 |
+
assert not opt.isTrain
|
45 |
+
BaseModel.__init__(self, opt)
|
46 |
+
# specify the training losses you want to print out. The training/test scripts will call <BaseModel.get_current_losses>
|
47 |
+
self.loss_names = []
|
48 |
+
# specify the images you want to save/display. The training/test scripts will call <BaseModel.get_current_visuals>
|
49 |
+
self.visual_names = ["real", "fake"]
|
50 |
+
# specify the models you want to save to the disk. The training/test scripts will call <BaseModel.save_networks> and <BaseModel.load_networks>
|
51 |
+
self.net_names = ["G" + opt.model_suffix] # only generator is needed.
|
52 |
+
self.net_G = networks.define_G(
|
53 |
+
opt.input_nc,
|
54 |
+
opt.output_nc,
|
55 |
+
opt.ngf,
|
56 |
+
opt.netG,
|
57 |
+
opt.norm,
|
58 |
+
not opt.no_dropout,
|
59 |
+
opt.init_type,
|
60 |
+
opt.init_gain,
|
61 |
+
self.gpu_ids,
|
62 |
+
)
|
63 |
+
|
64 |
+
# assigns the model to self.netG_[suffix] so that it can be loaded
|
65 |
+
# please see <BaseModel.load_networks>
|
66 |
+
setattr(self, "net_G" + opt.model_suffix, self.net_G) # store netG in self.
|
67 |
+
|
68 |
+
def set_input(self, input):
|
69 |
+
"""Unpack input data from the dataLoader and perform necessary pre-processing steps.
|
70 |
+
|
71 |
+
Parameters:
|
72 |
+
input: a dictionary that contains the data itself and its metadata information.
|
73 |
+
|
74 |
+
We need to use 'single_dataset' a dataset mode.
|
75 |
+
It only loads images from one domain.
|
76 |
+
"""
|
77 |
+
self.real = input["A"].to(self.device)
|
78 |
+
self.image_paths = input["A_paths"]
|
79 |
+
|
80 |
+
def forward(self):
|
81 |
+
"""Run forward pass."""
|
82 |
+
self.fake = self.net_G(self.real) # G(real)
|
83 |
+
|
84 |
+
def optimize_parameters(self):
|
85 |
+
"""No optimization for test model."""
|
86 |
+
pass
|
options/__init__.py
ADDED
@@ -0,0 +1,4 @@
|
|
|
|
|
|
|
|
|
|
|
1 |
+
from .base_options import BaseOptions
|
2 |
+
from .detect_options import DetectOptions
|
3 |
+
from .test_options import TestOptions
|
4 |
+
from .train_options import TrainOptions
|
options/base_options.py
ADDED
@@ -0,0 +1,149 @@
|
|
|
|
|
|
|
|
|
|
|
|
|
|
|
|
|
|
|
|
|
|
|
|
|
|
|
|
|
|
|
|
|
|
|
|
|
|
|
|
|
|
|
|
|
|
|
|
|
|
|
|
|
|
|
|
|
|
|
|
|
|
|
|
|
|
|
|
|
|
|
|
|
|
|
|
|
|
|
|
|
|
|
|
|
|
|
|
|
|
|
|
|
|
|
|
|
|
|
|
|
|
|
|
|
|
|
|
|
|
|
|
|
|
|
|
|
|
|
|
|
|
|
|
|
|
|
|
|
|
|
|
|
|
|
|
|
|
|
|
|
|
|
|
|
|
|
|
|
|
|
|
|
|
|
|
|
|
|
|
|
|
|
|
|
|
|
|
|
|
|
|
|
|
|
|
|
|
|
|
|
|
|
|
|
|
|
|
|
|
|
|
|
|
|
|
|
|
|
|
|
|
|
|
|
|
|
|
|
|
|
|
|
|
|
|
|
|
|
|
|
|
|
|
|
|
|
|
|
|
|
|
|
|
|
|
|
|
|
|
|
|
|
|
|
|
|
|
|
|
|
|
|
|
|
|
|
|
|
|
|
|
|
|
|
|
|
|
|
|
|
|
|
|
|
|
|
|
|
|
|
|
|
|
|
|
|
|
|
|
|
|
|
|
|
|
|
|
|
|
|
1 |
+
import argparse
|
2 |
+
from pathlib import Path
|
3 |
+
|
4 |
+
import torch
|
5 |
+
|
6 |
+
import data
|
7 |
+
import models
|
8 |
+
from util import util
|
9 |
+
|
10 |
+
|
11 |
+
class BaseOptions:
|
12 |
+
"""模型超参数设置
|
13 |
+
"""
|
14 |
+
def __init__(self):
|
15 |
+
"""重置该类:表示该类尚未被初始化
|
16 |
+
训练模型、测试模型、推理模型的参数全继承该类
|
17 |
+
"""
|
18 |
+
self.initialized = False
|
19 |
+
|
20 |
+
def initialize(self, parser):
|
21 |
+
"""共有参数"""
|
22 |
+
# basic parameters
|
23 |
+
parser.add_argument("--dataroot", type=str, default="./datasets/horse2zebra/", help="path to images (should have subfolders trainA, trainB, valA, valB, etc)")
|
24 |
+
parser.add_argument("--name", type=str, default="horse2zebra", help="name of the experiment.")
|
25 |
+
parser.add_argument("--gpu_ids", type=str, default="0", help="gpu ids: e.g. 0 0,1,2 -1 for CPU")
|
26 |
+
parser.add_argument("--checkpoints_dir", type=str, default="./checkpoints", help="models_saved")
|
27 |
+
# model parameters
|
28 |
+
parser.add_argument("--model", type=str, default="cycle_gan")
|
29 |
+
parser.add_argument("--input_nc", type=int, default=3, help="# input image channels: 3 for RGB and 1 for grayscale")
|
30 |
+
parser.add_argument("--output_nc", type=int, default=3, help="# output image channels: 3 for RGB and 1 for grayscale")
|
31 |
+
parser.add_argument("--ngf", type=int, default=64, help="# of gen filters in the last conv layer")
|
32 |
+
parser.add_argument("--ndf", type=int, default=64, help="# of discrim filters in the first conv layer")
|
33 |
+
parser.add_argument(
|
34 |
+
"--netD",
|
35 |
+
type=str,
|
36 |
+
default="basic",
|
37 |
+
help="[basic | n_layers | pixel]. basic: a 70x70 PatchGAN. n_layers: allows you to specify the layers in the discriminator",
|
38 |
+
)
|
39 |
+
parser.add_argument("--netG", type=str, default="resnet_9blocks", help="[resnet_9blocks | resnet_6blocks | unet_256 | unet_128]")
|
40 |
+
parser.add_argument("--n_layers_D", type=int, default=3, help="only used if netD==n_layers")
|
41 |
+
parser.add_argument("--norm", type=str, default="instance", help="instance normalization or batch normalization [instance | batch | none]")
|
42 |
+
parser.add_argument("--init_type", type=str, default="normal", help="network initialization [normal | xavier | kaiming | orthogonal]")
|
43 |
+
parser.add_argument("--init_gain", type=float, default=0.02, help="scaling factor for normal, xavier and orthogonal.")
|
44 |
+
parser.add_argument("--no_dropout", type=bool, default=True, help="no dropout for the generator")
|
45 |
+
# dataset parameters
|
46 |
+
parser.add_argument("--dataset_mode", type=str, default="unaligned")
|
47 |
+
parser.add_argument("--direction", type=str, default="AtoB", help="AtoB or BtoA")
|
48 |
+
parser.add_argument("--serial_batches", action="store_true", help="if true, takes images in order to make batches, otherwise takes them randomly")
|
49 |
+
parser.add_argument("--num_threads", default=8, type=int, help="# threads for loading data")
|
50 |
+
parser.add_argument("--batch_size", type=int, default=1)
|
51 |
+
parser.add_argument("--load_size", type=int, default=286, help="scale images to this size")
|
52 |
+
parser.add_argument("--crop_size", type=int, default=256, help="then crop to this size")
|
53 |
+
parser.add_argument(
|
54 |
+
"--max_dataset_size",
|
55 |
+
type=int,
|
56 |
+
default=float("inf"),
|
57 |
+
help="Maximum number of samples allowed per dataset. If the dataset directory contains more than max_dataset_size, only a subset is loaded.",
|
58 |
+
)
|
59 |
+
parser.add_argument("--preprocess", type=str, default="resize_and_crop", help="[resize_and_crop | crop | scale_width | scale_width_and_crop | none] img preprocess")
|
60 |
+
parser.add_argument("--no_flip", action="store_true", help="if specified, do not flip the images for data augmentation")
|
61 |
+
parser.add_argument("--display_winsize", type=int, default=256, help="display window size for both visdom and HTML")
|
62 |
+
# additional parameters
|
63 |
+
parser.add_argument("--epoch", type=str, default="latest", help="which epoch to load? set to latest to use latest cached model")
|
64 |
+
parser.add_argument(
|
65 |
+
"--load_iter", type=int, default="0", help="which iteration to load? if load_iter > 0, the code will load models by iter_[load_iter]; otherwise, the code will load models by [epoch]"
|
66 |
+
)
|
67 |
+
parser.add_argument("--verbose", action="store_true", help="if specified, print more debugging information")
|
68 |
+
parser.add_argument("--suffix", default="", type=str, help="customized suffix: opt.name = opt.name + suffix: e.g., {model}_{netG}_size{load_size}")
|
69 |
+
# wandb parameters
|
70 |
+
parser.add_argument("--use_wandb", action="store_true", help="wandb logging")
|
71 |
+
parser.add_argument("--wandb_project_name", type=str, default="CycleGAN", help="wandb project name")
|
72 |
+
self.initialized = True
|
73 |
+
return parser
|
74 |
+
|
75 |
+
def gather_options(self):
|
76 |
+
"""Initialize our parser with basic options(only once).
|
77 |
+
Add additional model-specific and dataset-specific options.
|
78 |
+
These options are defined in the <modify_commandline_options> function
|
79 |
+
in model and dataset classes.
|
80 |
+
"""
|
81 |
+
if not self.initialized: # check if it has been initialized
|
82 |
+
parser = argparse.ArgumentParser(formatter_class=argparse.ArgumentDefaultsHelpFormatter)
|
83 |
+
parser = self.initialize(parser)
|
84 |
+
|
85 |
+
# get the basic options
|
86 |
+
opt, _ = parser.parse_known_args()
|
87 |
+
|
88 |
+
# modify model-related parser options
|
89 |
+
model_name = opt.model
|
90 |
+
model_option_setter = models.get_option_setter(model_name)
|
91 |
+
parser = model_option_setter(parser, self.isTrain)
|
92 |
+
opt, _ = parser.parse_known_args() # parse again with new defaults
|
93 |
+
|
94 |
+
# modify dataset-related parser options
|
95 |
+
dataset_name = opt.dataset_mode
|
96 |
+
dataset_option_setter = data.get_option_setter(dataset_name)
|
97 |
+
parser = dataset_option_setter(parser, self.isTrain)
|
98 |
+
|
99 |
+
# save and return the parser
|
100 |
+
self.parser = parser
|
101 |
+
# return parser.parse_args()
|
102 |
+
# 用上面的会报以下错误,命令行参数不能合并,gradio运行时会调用命令行参数,而我们的没有
|
103 |
+
# uvicorn: error: unrecognized arguments: app:demo.app --reload --port 7860 --log-level warning --reload-dir E:\miniconda3\envs\yanguan\lib\site-packages\gradio --reload-dir D:\projects\CycleGAN
|
104 |
+
return parser.parse_known_args()[0]
|
105 |
+
|
106 |
+
def print_options(self, opt):
|
107 |
+
"""
|
108 |
+
1. 同时打印当前选项和默认值(如果不同)。
|
109 |
+
2. 将选项保存到一个文本文件 /[checkpoints_dir]/opt.txt 中
|
110 |
+
"""
|
111 |
+
message = ""
|
112 |
+
message += "----------------- Options ---------------\n"
|
113 |
+
for k, v in sorted(vars(opt).items()):
|
114 |
+
comment = ""
|
115 |
+
default = self.parser.get_default(k)
|
116 |
+
if v != default:
|
117 |
+
comment = "\t[default: %s]" % str(default)
|
118 |
+
message += "{:>25}: {:<30}{}\n".format(str(k), str(v), comment)
|
119 |
+
message += "----------------- End -------------------"
|
120 |
+
print(message)
|
121 |
+
|
122 |
+
# save it to the disk
|
123 |
+
expr_dir = Path(opt.checkpoints_dir, opt.name)
|
124 |
+
util.mkdirs(expr_dir)
|
125 |
+
file_name = Path(expr_dir, "{}_opt.txt".format(opt.phase))
|
126 |
+
with open(file_name, "wt") as opt_file:
|
127 |
+
opt_file.write(message)
|
128 |
+
opt_file.write("\n")
|
129 |
+
|
130 |
+
def parse(self):
|
131 |
+
"""解析选项,创建检查点目录后缀,并设置GPU设备。"""
|
132 |
+
opt = self.gather_options()
|
133 |
+
opt.isTrain = self.isTrain # train or test
|
134 |
+
|
135 |
+
# process opt.suffix
|
136 |
+
if opt.suffix:
|
137 |
+
suffix = ("_" + opt.suffix.format(**vars(opt))) if opt.suffix != "" else ""
|
138 |
+
opt.name = opt.name + suffix
|
139 |
+
|
140 |
+
self.print_options(opt)
|
141 |
+
|
142 |
+
# set gpu ids
|
143 |
+
_ids = [int(str_id) for str_id in opt.gpu_ids.split(",")]
|
144 |
+
opt.gpu_ids = [_id for _id in _ids if _id >= 0]
|
145 |
+
if len(opt.gpu_ids) > 0:
|
146 |
+
torch.cuda.set_device(opt.gpu_ids[0])
|
147 |
+
|
148 |
+
self.opt = opt
|
149 |
+
return self.opt
|
options/detect_options.py
ADDED
@@ -0,0 +1,25 @@
|
|
|
|
|
|
|
|
|
|
|
|
|
|
|
|
|
|
|
|
|
|
|
|
|
|
|
|
|
|
|
|
|
|
|
|
|
|
|
|
|
|
|
|
|
|
|
|
|
|
|
|
|
1 |
+
from .test_options import TestOptions
|
2 |
+
|
3 |
+
|
4 |
+
class DetectOptions(TestOptions):
|
5 |
+
"""
|
6 |
+
继承TestOptions
|
7 |
+
|
8 |
+
新增推理图片路径、推理风格参数
|
9 |
+
"""
|
10 |
+
|
11 |
+
def initialize(self, parser):
|
12 |
+
parser = TestOptions.initialize(self, parser)
|
13 |
+
parser.set_defaults(name="detect")
|
14 |
+
parser.set_defaults(dataroot="imgs/", help="folder: /imgs/ or file: xx.jpg")
|
15 |
+
parser.set_defaults(checkpoints_dir="./weights")
|
16 |
+
parser.set_defaults(gpu_ids="-1")
|
17 |
+
# 新增
|
18 |
+
parser.add_argument("--no_save_img", action="store_true", help="no save fake_img")
|
19 |
+
parser.add_argument("--style", type=str, default="horse2zebra")
|
20 |
+
#
|
21 |
+
# parser.add_argument("--reload", action="store_true")
|
22 |
+
# parser.add_argument("--port")
|
23 |
+
# parser.add_argument("--reload-dir")
|
24 |
+
# parser.add_argument("--log-level")
|
25 |
+
return parser
|
options/test_options.py
ADDED
@@ -0,0 +1,28 @@
|
|
|
|
|
|
|
|
|
|
|
|
|
|
|
|
|
|
|
|
|
|
|
|
|
|
|
|
|
|
|
|
|
|
|
|
|
|
|
|
|
|
|
|
|
|
|
|
|
|
|
|
|
|
|
|
|
|
|
1 |
+
from .base_options import BaseOptions
|
2 |
+
|
3 |
+
|
4 |
+
class TestOptions(BaseOptions):
|
5 |
+
"""
|
6 |
+
继承BaseOptions
|
7 |
+
"""
|
8 |
+
|
9 |
+
def initialize(self, parser):
|
10 |
+
parser = BaseOptions.initialize(self, parser) # define shared options
|
11 |
+
# 重写参数
|
12 |
+
self.isTrain = False
|
13 |
+
# To avoid cropping, the load_size should be the same as crop_size
|
14 |
+
parser.set_defaults(load_size=parser.get_default("crop_size"))
|
15 |
+
parser.set_defaults(model="test")
|
16 |
+
parser.set_defaults(no_dropout=True)
|
17 |
+
# 自定义部分
|
18 |
+
parser.set_defaults(name="horse2zebra")
|
19 |
+
parser.set_defaults(dataroot=r"datasets\horse2zebra\testA")
|
20 |
+
|
21 |
+
# 新增参数
|
22 |
+
parser.add_argument("--results_dir", type=str, default="./results/", help="saves results here.")
|
23 |
+
parser.add_argument("--aspect_ratio", type=float, default=1.0, help="aspect ratio of result images")
|
24 |
+
parser.add_argument("--phase", type=str, default="test", help="train, val, test, etc")
|
25 |
+
# Dropout and batch norm have different behaviors during training and test.
|
26 |
+
parser.add_argument("--eval", action="store_true", help="use eval mode during test time.")
|
27 |
+
parser.add_argument("--num_test", type=int, default=50, help="how many test images to run")
|
28 |
+
return parser
|
options/train_options.py
ADDED
@@ -0,0 +1,42 @@
|
|
|
|
|
|
|
|
|
|
|
|
|
|
|
|
|
|
|
|
|
|
|
|
|
|
|
|
|
|
|
|
|
|
|
|
|
|
|
|
|
|
|
|
|
|
|
|
|
|
|
|
|
|
|
|
|
|
|
|
|
|
|
|
|
|
|
|
|
|
|
|
|
|
|
|
|
|
|
|
|
|
|
|
|
|
|
1 |
+
from pathlib import Path
|
2 |
+
|
3 |
+
from .base_options import BaseOptions
|
4 |
+
|
5 |
+
|
6 |
+
class TrainOptions(BaseOptions):
|
7 |
+
"""继承BaseOptions,补充训练参数
|
8 |
+
"""
|
9 |
+
|
10 |
+
def initialize(self, parser):
|
11 |
+
self.isTrain = True
|
12 |
+
parser = BaseOptions.initialize(self, parser)
|
13 |
+
# 训练过程可视化参数
|
14 |
+
parser.add_argument("--display_freq", type=int, default=400, help="frequency of showing training results on screen")
|
15 |
+
parser.add_argument("--display_ncols", type=int, default=4, help="if positive, display all images in a single visdom web panel with certain number of images per row.")
|
16 |
+
parser.add_argument("--display_id", type=int, default=1, help="window id of the web display")
|
17 |
+
parser.add_argument("--display_server", type=str, default="http://localhost", help="visdom server of the web display")
|
18 |
+
parser.add_argument("--display_env", type=str, default="main", help="visdom display environment name")
|
19 |
+
parser.add_argument("--display_port", type=int, default=8097, help="visdom port of the web display")
|
20 |
+
parser.add_argument("--update_html_freq", type=int, default=1000, help="frequency of saving training results to html")
|
21 |
+
parser.add_argument("--print_freq", type=int, default=100, help="frequency of showing training results on console")
|
22 |
+
parser.add_argument("--no_html", action="store_true", help="do not save intermediate training results to [opt.checkpoints_dir]/[opt.name]/web/")
|
23 |
+
# 网络保存和加载参数
|
24 |
+
parser.add_argument("--save_latest_freq", type=int, default=5000, help="frequency of saving the latest results")
|
25 |
+
parser.add_argument("--save_epoch_freq", type=int, default=1, help="frequency of saving checkpoints at the end of epochs")
|
26 |
+
parser.add_argument("--save_by_iter", action="store_true", help="whether saves model by iteration")
|
27 |
+
parser.add_argument("--continue_train", action="store_true", help="continue training: load the latest model")
|
28 |
+
parser.add_argument("--epoch_count", type=int, default=1, help="the starting epoch count, we save the model by <epoch_count>, <epoch_count>+<save_latest_freq>, ...")
|
29 |
+
parser.add_argument("--phase", type=str, default="train", help="train, val, test, etc")
|
30 |
+
# 训练参数
|
31 |
+
parser.add_argument("--n_epochs", type=int, default=200, help="number of epochs with the initial learning rate")
|
32 |
+
parser.add_argument("--n_epochs_decay", type=int, default=100, help="number of epochs to linearly decay learning rate to zero")
|
33 |
+
parser.add_argument("--beta1", type=float, default=0.5, help="momentum term of adam")
|
34 |
+
parser.add_argument("--lr", type=float, default=0.0002, help="initial learning rate for adam")
|
35 |
+
parser.add_argument("--gan_mode", type=str, default="lsgan", help="the type of GAN objective. [vanilla| lsgan | wgangp]. vanilla GAN loss used in the original GAN")
|
36 |
+
parser.add_argument("--pool_size", type=int, default=50, help="the size of image buffer that stores previously generated images")
|
37 |
+
parser.add_argument("--lr_policy", type=str, default="linear", help="learning rate policy. [linear | step | plateau | cosine]")
|
38 |
+
parser.add_argument("--lr_decay_iters", type=int, default=50, help="multiply by a gamma every lr_decay_iters iterations")
|
39 |
+
#
|
40 |
+
parser.set_defaults(dataroot="./datasets/horse2zebra/")
|
41 |
+
parser.set_defaults(name="horse2zebra")
|
42 |
+
return parser
|
requirements.txt
ADDED
@@ -0,0 +1,12 @@
|
|
|
|
|
|
|
|
|
|
|
|
|
|
|
|
|
|
|
|
|
|
|
|
|
|
|
1 |
+
beautifulsoup4==4.12.0
|
2 |
+
dominate==2.7.0
|
3 |
+
gradio==3.24.0
|
4 |
+
matplotlib==3.7.1
|
5 |
+
numpy==1.23.3
|
6 |
+
Pillow==9.4.0
|
7 |
+
requests==2.25.1
|
8 |
+
torch==2.0.0
|
9 |
+
torchvision==0.15.1
|
10 |
+
tqdm==4.65.0
|
11 |
+
visdom==0.2.4
|
12 |
+
wandb==0.13.11
|
scripts/download_cyclegan_model.sh
ADDED
@@ -0,0 +1,11 @@
|
|
|
|
|
|
|
|
|
|
|
|
|
|
|
|
|
|
|
|
|
|
|
|
|
1 |
+
FILE=$1
|
2 |
+
|
3 |
+
echo "Note: available models are apple2orange, orange2apple, summer2winter_yosemite, winter2summer_yosemite, horse2zebra, zebra2horse, monet2photo, style_monet, style_cezanne, style_ukiyoe, style_vangogh, sat2map, map2sat, cityscapes_photo2label, cityscapes_label2photo, facades_photo2label, facades_label2photo, iphone2dslr_flower"
|
4 |
+
|
5 |
+
echo "Specified [$FILE]"
|
6 |
+
|
7 |
+
mkdir -p ./checkpoints/${FILE}_pretrained
|
8 |
+
MODEL_FILE=./checkpoints/${FILE}_pretrained/latest_net_G.pth
|
9 |
+
URL=http://efrosgans.eecs.berkeley.edu/cyclegan/pretrained_models/$FILE.pth
|
10 |
+
|
11 |
+
wget -N $URL -O $MODEL_FILE
|
scripts/test_before_push.py
ADDED
@@ -0,0 +1,51 @@
|
|
|
|
|
|
|
|
|
|
|
|
|
|
|
|
|
|
|
|
|
|
|
|
|
|
|
|
|
|
|
|
|
|
|
|
|
|
|
|
|
|
|
|
|
|
|
|
|
|
|
|
|
|
|
|
|
|
|
|
|
|
|
|
|
|
|
|
|
|
|
|
|
|
|
|
|
|
|
|
|
|
|
|
|
|
|
|
|
|
|
|
|
|
|
|
|
|
|
|
|
|
|
|
|
1 |
+
# Simple script to make sure basic usage
|
2 |
+
# such as training, testing, saving and loading
|
3 |
+
# runs without errors.
|
4 |
+
import os
|
5 |
+
|
6 |
+
|
7 |
+
def run(command):
|
8 |
+
print(command)
|
9 |
+
exit_status = os.system(command)
|
10 |
+
if exit_status > 0:
|
11 |
+
exit(1)
|
12 |
+
|
13 |
+
|
14 |
+
if __name__ == '__main__':
|
15 |
+
# download mini datasets
|
16 |
+
if not os.path.exists('./datasets/mini'):
|
17 |
+
run('bash ./datasets/download_cyclegan_dataset.sh mini')
|
18 |
+
|
19 |
+
if not os.path.exists('./datasets/mini_pix2pix'):
|
20 |
+
run('bash ./datasets/download_cyclegan_dataset.sh mini_pix2pix')
|
21 |
+
|
22 |
+
# pretrained cyclegan model
|
23 |
+
if not os.path.exists('./checkpoints/horse2zebra_pretrained/latest_net_G.pth'):
|
24 |
+
run('bash ./scripts/download_cyclegan_model.sh horse2zebra')
|
25 |
+
run('python test.py --model test --dataroot ./datasets/mini --name horse2zebra_pretrained --no_dropout --num_test 1 --no_dropout')
|
26 |
+
|
27 |
+
# pretrained pix2pix model
|
28 |
+
if not os.path.exists('./checkpoints/facades_label2photo_pretrained/latest_net_G.pth'):
|
29 |
+
run('bash ./scripts/download_pix2pix_model.sh facades_label2photo')
|
30 |
+
if not os.path.exists('./datasets/facades'):
|
31 |
+
run('bash ./datasets/download_pix2pix_dataset.sh facades')
|
32 |
+
run('python test.py --dataroot ./datasets/facades/ --direction BtoA --model pix2pix --name facades_label2photo_pretrained --num_test 1')
|
33 |
+
|
34 |
+
# cyclegan train/test
|
35 |
+
run('python train.py --model cycle_gan --name temp_cyclegan --dataroot ./datasets/mini --n_epochs 1 --n_epochs_decay 0 --save_latest_freq 10 --print_freq 1 --display_id -1')
|
36 |
+
run('python test.py --model test --name temp_cyclegan --dataroot ./datasets/mini --num_test 1 --model_suffix "_A" --no_dropout')
|
37 |
+
|
38 |
+
# pix2pix train/test
|
39 |
+
run('python train.py --model pix2pix --name temp_pix2pix --dataroot ./datasets/mini_pix2pix --n_epochs 1 --n_epochs_decay 5 --save_latest_freq 10 --display_id -1')
|
40 |
+
run('python test.py --model pix2pix --name temp_pix2pix --dataroot ./datasets/mini_pix2pix --num_test 1')
|
41 |
+
|
42 |
+
# template train/test
|
43 |
+
run('python train.py --model template --name temp2 --dataroot ./datasets/mini_pix2pix --n_epochs 1 --n_epochs_decay 0 --save_latest_freq 10 --display_id -1')
|
44 |
+
run('python test.py --model template --name temp2 --dataroot ./datasets/mini_pix2pix --num_test 1')
|
45 |
+
|
46 |
+
# colorization train/test (optional)
|
47 |
+
if not os.path.exists('./datasets/mini_colorization'):
|
48 |
+
run('bash ./datasets/download_cyclegan_dataset.sh mini_colorization')
|
49 |
+
|
50 |
+
run('python train.py --model colorization --name temp_color --dataroot ./datasets/mini_colorization --n_epochs 1 --n_epochs_decay 0 --save_latest_freq 5 --display_id -1')
|
51 |
+
run('python test.py --model colorization --name temp_color --dataroot ./datasets/mini_colorization --num_test 1')
|
scripts/test_cyclegan.sh
ADDED
@@ -0,0 +1,2 @@
|
|
|
|
|
|
|
1 |
+
set -ex
|
2 |
+
python test.py --dataroot ./datasets/maps --name maps_cyclegan --model cycle_gan --phase test --no_dropout
|
scripts/test_single.sh
ADDED
@@ -0,0 +1,2 @@
|
|
|
|
|
|
|
1 |
+
set -ex
|
2 |
+
python test.py --dataroot ./datasets/facades/testB/ --name facades_pix2pix --model test --netG unet_256 --direction BtoA --dataset_mode single --norm batch
|
scripts/train_cyclegan.sh
ADDED
@@ -0,0 +1,2 @@
|
|
|
|
|
|
|
1 |
+
set -ex
|
2 |
+
python train.py --dataroot ./datasets/maps --name maps_cyclegan --model cycle_gan --pool_size 50 --no_dropout
|
util/__init__.py
ADDED
@@ -0,0 +1,2 @@
|
|
|
|
|
|
|
1 |
+
from .tools import *
|
2 |
+
from .util import *
|
util/get_data.py
ADDED
@@ -0,0 +1,97 @@
|
|
|
|
|
|
|
|
|
|
|
|
|
|
|
|
|
|
|
|
|
|
|
|
|
|
|
|
|
|
|
|
|
|
|
|
|
|
|
|
|
|
|
|
|
|
|
|
|
|
|
|
|
|
|
|
|
|
|
|
|
|
|
|
|
|
|
|
|
|
|
|
|
|
|
|
|
|
|
|
|
|
|
|
|
|
|
|
|
|
|
|
|
|
|
|
|
|
|
|
|
|
|
|
|
|
|
|
|
|
|
|
|
|
|
|
|
|
|
|
|
|
|
|
|
|
|
|
|
|
|
|
|
|
|
|
|
|
|
|
|
|
|
|
|
|
|
|
|
|
|
|
|
|
|
|
|
|
|
|
|
|
|
|
|
|
|
|
|
|
|
|
|
|
|
|
|
|
|
|
|
|
|
|
|
|
|
|
|
|
|
|
|
|
|
|
|
1 |
+
# from __future__ import print_function
|
2 |
+
|
3 |
+
import tarfile
|
4 |
+
from pathlib import Path
|
5 |
+
from warnings import warn
|
6 |
+
from zipfile import ZipFile
|
7 |
+
|
8 |
+
import requests
|
9 |
+
from bs4 import BeautifulSoup
|
10 |
+
|
11 |
+
|
12 |
+
class GetData(object):
|
13 |
+
"""A Python script for downloading CycleGAN or pix2pix datasets.
|
14 |
+
|
15 |
+
Parameters:
|
16 |
+
technique (str) -- One of: 'cyclegan' or 'pix2pix'.
|
17 |
+
verbose (bool) -- If True, print additional information.
|
18 |
+
|
19 |
+
Examples:
|
20 |
+
>>> from util.get_data import GetData
|
21 |
+
>>> gd = GetData(technique='cyclegan', save_path='./datasets')# options will be displayed.
|
22 |
+
|
23 |
+
Alternatively, You can use bash scripts: 'scripts/download_pix2pix_model.sh'
|
24 |
+
and 'scripts/download_cyclegan_model.sh'.
|
25 |
+
"""
|
26 |
+
|
27 |
+
def __init__(self, technique="CycleGAN", save_path="./datasets", verbose=True):
|
28 |
+
url_dict = {
|
29 |
+
"cyclegan": "http://efrosgans.eecs.berkeley.edu/cyclegan/datasets/",
|
30 |
+
}
|
31 |
+
self.url = url_dict.get(technique.lower())
|
32 |
+
self._verbose = verbose
|
33 |
+
self.get(save_path=save_path)
|
34 |
+
|
35 |
+
def _print(self, text: str):
|
36 |
+
if self._verbose:
|
37 |
+
print(text)
|
38 |
+
|
39 |
+
@staticmethod
|
40 |
+
def _get_options(r):
|
41 |
+
soup = BeautifulSoup(r.text, "lxml")
|
42 |
+
options = [
|
43 |
+
h.text
|
44 |
+
for h in soup.find_all("a", href=True)
|
45 |
+
if h.text.endswith((".zip", "tar.gz"))
|
46 |
+
]
|
47 |
+
return options
|
48 |
+
|
49 |
+
def _present_options(self):
|
50 |
+
print(self.url)
|
51 |
+
r = requests.get(self.url)
|
52 |
+
options = self._get_options(r)
|
53 |
+
print("Options:\n")
|
54 |
+
for i, o in enumerate(options):
|
55 |
+
print("{0}: {1}".format(i, o))
|
56 |
+
choice = input(
|
57 |
+
"\nPlease enter the number of the " "dataset above you wish to download:"
|
58 |
+
)
|
59 |
+
return options[int(choice)]
|
60 |
+
|
61 |
+
def _download_data(self, dataset_url: str, dataset_path: Path):
|
62 |
+
dataset_path.mkdir(exist_ok=True)
|
63 |
+
|
64 |
+
save_path = Path(dataset_path).joinpath(Path(dataset_url).name)
|
65 |
+
|
66 |
+
print(dataset_url)
|
67 |
+
import urllib.request
|
68 |
+
|
69 |
+
urllib.request.urlretrieve(dataset_url, save_path)
|
70 |
+
print("--> 下载完成 ")
|
71 |
+
|
72 |
+
if save_path.endswith(".tar.gz"):
|
73 |
+
obj = tarfile.open(save_path)
|
74 |
+
elif save_path.endswith(".zip"):
|
75 |
+
obj = ZipFile(save_path, "r")
|
76 |
+
else:
|
77 |
+
raise ValueError("Unknown File Type: {0}.".format(save_path))
|
78 |
+
self._print("Unpacking Data...")
|
79 |
+
obj.extractall(save_path)
|
80 |
+
obj.close()
|
81 |
+
|
82 |
+
def get(self, save_path: str, dataset=None):
|
83 |
+
save_path_ = Path(save_path)
|
84 |
+
if dataset is None:
|
85 |
+
selected_dataset = self._present_options()
|
86 |
+
else:
|
87 |
+
selected_dataset = dataset
|
88 |
+
save_path_full = save_path_.joinpath(selected_dataset.split(".")[0])
|
89 |
+
print(save_path_full)
|
90 |
+
|
91 |
+
if save_path_full.is_dir():
|
92 |
+
warn("\n'{0}' already exists.".format(save_path_full))
|
93 |
+
else:
|
94 |
+
self._print("Downloading Data...")
|
95 |
+
url = "{0}/{1}".format(self.url, selected_dataset)
|
96 |
+
self._download_data(url, save_path=save_path)
|
97 |
+
return Path(save_path_full)
|
util/html.py
ADDED
@@ -0,0 +1,95 @@
|
|
|
|
|
|
|
|
|
|
|
|
|
|
|
|
|
|
|
|
|
|
|
|
|
|
|
|
|
|
|
|
|
|
|
|
|
|
|
|
|
|
|
|
|
|
|
|
|
|
|
|
|
|
|
|
|
|
|
|
|
|
|
|
|
|
|
|
|
|
|
|
|
|
|
|
|
|
|
|
|
|
|
|
|
|
|
|
|
|
|
|
|
|
|
|
|
|
|
|
|
|
|
|
|
|
|
|
|
|
|
|
|
|
|
|
|
|
|
|
|
|
|
|
|
|
|
|
|
|
|
|
|
|
|
|
|
|
|
|
|
|
|
|
|
|
|
|
|
|
|
|
|
|
|
|
|
|
|
|
|
|
|
|
|
|
|
|
|
|
|
|
|
|
|
|
|
|
|
|
|
|
|
|
|
|
|
|
|
|
|
|
|
1 |
+
import os
|
2 |
+
from pathlib import Path
|
3 |
+
|
4 |
+
import dominate
|
5 |
+
from dominate.tags import a, br, h3, img, meta, p, table, td, tr
|
6 |
+
|
7 |
+
|
8 |
+
class HTML:
|
9 |
+
"""This HTML class allows us to save images and write texts into a single HTML file.
|
10 |
+
|
11 |
+
It consists of functions such as <add_header> (add a text header to the HTML file),
|
12 |
+
<add_images> (add a row of images to the HTML file), and <save> (save the HTML to the disk).
|
13 |
+
It is based on the Python library 'dominate', a Python library for creating and manipulating HTML documents using a DOM API.
|
14 |
+
"""
|
15 |
+
|
16 |
+
def __init__(self, web_dir, title, refresh=0):
|
17 |
+
"""Initialize the HTML classes
|
18 |
+
|
19 |
+
Parameters:
|
20 |
+
web_dir (str) -- a directory that stores the webpage. HTML file will be created at <web_dir>/index.html; images will be saved at <web_dir/images/
|
21 |
+
title (str) -- the webpage name
|
22 |
+
refresh (int) -- how often the website refreshes itself; if 0; no refreshing
|
23 |
+
"""
|
24 |
+
self.title = title
|
25 |
+
self.web_dir = web_dir
|
26 |
+
self.img_dir = Path(self.web_dir, "images")
|
27 |
+
if not os.path.exists(self.web_dir):
|
28 |
+
os.makedirs(self.web_dir)
|
29 |
+
if not os.path.exists(self.img_dir):
|
30 |
+
os.makedirs(self.img_dir)
|
31 |
+
self.doc = dominate.document(title=title)
|
32 |
+
if refresh > 0:
|
33 |
+
with self.doc.head:
|
34 |
+
meta(http_equiv="refresh", content=str(refresh))
|
35 |
+
|
36 |
+
def get_image_dir(self):
|
37 |
+
"""Return the directory that stores images"""
|
38 |
+
return self.img_dir
|
39 |
+
|
40 |
+
def add_header(self, text):
|
41 |
+
"""Insert a header to the HTML file
|
42 |
+
|
43 |
+
Parameters:
|
44 |
+
text (str) -- the header text
|
45 |
+
"""
|
46 |
+
with self.doc:
|
47 |
+
h3(text)
|
48 |
+
|
49 |
+
def add_images(self, ims, txts, links, width=400):
|
50 |
+
"""add images to the HTML file
|
51 |
+
|
52 |
+
Parameters:
|
53 |
+
width:
|
54 |
+
ims (str list) -- a list of image paths
|
55 |
+
txts (str list) -- a list of image names shown on the website
|
56 |
+
links (str list) -- a list of hyperref links; when you click an image, it will redirect you to a new page
|
57 |
+
"""
|
58 |
+
self.t = table(border=1, style="table-layout: fixed;") # Insert a table
|
59 |
+
self.doc.add(self.t)
|
60 |
+
with self.t:
|
61 |
+
with tr():
|
62 |
+
for im, txt, link in zip(ims, txts, links):
|
63 |
+
with td(
|
64 |
+
style="word-wrap: break-word;", halign="center", valign="top"
|
65 |
+
):
|
66 |
+
with p():
|
67 |
+
with a(href=Path("images", link)):
|
68 |
+
img(style="width:%dpx" % width, src=Path("images", im))
|
69 |
+
br()
|
70 |
+
p(txt)
|
71 |
+
|
72 |
+
def save(self):
|
73 |
+
"""将内容保存到HMTL文件"""
|
74 |
+
html_file = f"{self.web_dir}/index.html"
|
75 |
+
with open(html_file, "wt") as f:
|
76 |
+
html = (
|
77 |
+
self.doc.render()
|
78 |
+
.replace("</body>", "</center></body>")
|
79 |
+
.replace("<body>", "<body><center>")
|
80 |
+
)
|
81 |
+
f.write(html) # 添加body居中
|
82 |
+
print("--> " + html_file)
|
83 |
+
|
84 |
+
|
85 |
+
if __name__ == "__main__": # show an example usage here.
|
86 |
+
html = HTML("web/", "test_html")
|
87 |
+
|
88 |
+
html.add_header("hello world")
|
89 |
+
ims, txts, links = [], [], []
|
90 |
+
for n in range(4):
|
91 |
+
ims.append("image_%d.png" % n)
|
92 |
+
txts.append("text_%d" % n)
|
93 |
+
links.append("image_%d.png" % n)
|
94 |
+
html.add_images(ims, txts, links)
|
95 |
+
html.save()
|
util/image_pool.py
ADDED
@@ -0,0 +1,60 @@
|
|
|
|
|
|
|
|
|
|
|
|
|
|
|
|
|
|
|
|
|
|
|
|
|
|
|
|
|
|
|
|
|
|
|
|
|
|
|
|
|
|
|
|
|
|
|
|
|
|
|
|
|
|
|
|
|
|
|
|
|
|
|
|
|
|
|
|
|
|
|
|
|
|
|
|
|
|
|
|
|
|
|
|
|
|
|
|
|
|
|
|
|
|
|
|
|
|
|
|
|
|
|
|
|
|
|
|
|
|
|
|
|
|
|
|
|
|
|
|
|
|
|
1 |
+
import random
|
2 |
+
|
3 |
+
import torch
|
4 |
+
|
5 |
+
|
6 |
+
class ImagePool:
|
7 |
+
"""This class implements an image buffer that stores previously generated images.
|
8 |
+
|
9 |
+
This buffer enables us to update discriminators using a history of generated images
|
10 |
+
rather than the ones produced by the latest generators.
|
11 |
+
"""
|
12 |
+
|
13 |
+
def __init__(self, pool_size):
|
14 |
+
"""Initialize the ImagePool class
|
15 |
+
|
16 |
+
Parameters:
|
17 |
+
pool_size (int) -- the size of the image buffer, if pool_size=0, no buffer will be created
|
18 |
+
"""
|
19 |
+
self.pool_size = pool_size
|
20 |
+
if self.pool_size > 0: # create an empty pool
|
21 |
+
self.num_imgs = 0
|
22 |
+
self.images = []
|
23 |
+
|
24 |
+
def query(self, images):
|
25 |
+
"""Return an image from the pool.
|
26 |
+
|
27 |
+
Parameters:
|
28 |
+
images: the latest generated images from the generator
|
29 |
+
|
30 |
+
Returns images from the buffer.
|
31 |
+
|
32 |
+
By 50/100, the buffer will return input images.
|
33 |
+
By 50/100, the buffer will return images previously stored in the buffer,
|
34 |
+
and insert the current images to the buffer.
|
35 |
+
"""
|
36 |
+
if self.pool_size == 0: # if the buffer size is 0, do nothing
|
37 |
+
return images
|
38 |
+
return_images = []
|
39 |
+
for image in images:
|
40 |
+
image = torch.unsqueeze(image.data, 0)
|
41 |
+
if self.num_imgs < self.pool_size:
|
42 |
+
# if the buffer is not full; keep inserting current images to the buffer
|
43 |
+
self.num_imgs = self.num_imgs + 1
|
44 |
+
self.images.append(image)
|
45 |
+
return_images.append(image)
|
46 |
+
else:
|
47 |
+
p = random.uniform(0, 1)
|
48 |
+
if p > 0.5:
|
49 |
+
# by 50% chance, the buffer will return a previously stored image, and insert the current image
|
50 |
+
# into the buffer
|
51 |
+
random_id = random.randint(
|
52 |
+
0, self.pool_size - 1
|
53 |
+
) # randint is inclusive
|
54 |
+
tmp = self.images[random_id].clone()
|
55 |
+
self.images[random_id] = image
|
56 |
+
return_images.append(tmp)
|
57 |
+
else: # by another 50% chance, the buffer will return the current image
|
58 |
+
return_images.append(image)
|
59 |
+
return_images = torch.cat(return_images, 0) # collect all the images and return
|
60 |
+
return return_images
|
util/streamlit/css.css
ADDED
@@ -0,0 +1,3 @@
|
|
|
|
|
|
|
|
|
1 |
+
label {
|
2 |
+
display: none !important;
|
3 |
+
}
|
util/tools.py
ADDED
@@ -0,0 +1,23 @@
|
|
|
|
|
|
|
|
|
|
|
|
|
|
|
|
|
|
|
|
|
|
|
|
|
|
|
|
|
|
|
|
|
|
|
|
|
|
|
|
|
|
|
|
|
|
|
|
|
1 |
+
def get_all_weights(weights_path="./weights/detect"):
|
2 |
+
from pathlib import Path
|
3 |
+
|
4 |
+
# 获取文件夹下所有文件(不包括文件夹)
|
5 |
+
all_weights = [
|
6 |
+
str(f.stem)
|
7 |
+
for f in Path(weights_path).iterdir()
|
8 |
+
if Path(f).is_file() and Path(f).suffix == ".pth"
|
9 |
+
]
|
10 |
+
# print_info(all_weights)
|
11 |
+
return all_weights
|
12 |
+
|
13 |
+
|
14 |
+
def now_time():
|
15 |
+
from datetime import datetime
|
16 |
+
|
17 |
+
return datetime.now().strftime(r"%Y-%m-%dT%H%M%S")
|
18 |
+
|
19 |
+
|
20 |
+
def print_info(text: str):
|
21 |
+
BEGIN_COLOR = "\033[32m"
|
22 |
+
END_COLOR = "\033[0m"
|
23 |
+
print(f"{BEGIN_COLOR}{text}{END_COLOR}")
|
util/util.py
ADDED
@@ -0,0 +1,135 @@
|
|
|
|
|
|
|
|
|
|
|
|
|
|
|
|
|
|
|
|
|
|
|
|
|
|
|
|
|
|
|
|
|
|
|
|
|
|
|
|
|
|
|
|
|
|
|
|
|
|
|
|
|
|
|
|
|
|
|
|
|
|
|
|
|
|
|
|
|
|
|
|
|
|
|
|
|
|
|
|
|
|
|
|
|
|
|
|
|
|
|
|
|
|
|
|
|
|
|
|
|
|
|
|
|
|
|
|
|
|
|
|
|
|
|
|
|
|
|
|
|
|
|
|
|
|
|
|
|
|
|
|
|
|
|
|
|
|
|
|
|
|
|
|
|
|
|
|
|
|
|
|
|
|
|
|
|
|
|
|
|
|
|
|
|
|
|
|
|
|
|
|
|
|
|
|
|
|
|
|
|
|
|
|
|
|
|
|
|
|
|
|
|
|
|
|
|
|
|
|
|
|
|
|
|
|
|
|
|
|
|
|
|
|
|
|
|
|
|
|
|
|
|
|
|
|
|
|
|
|
|
|
|
|
|
|
|
|
|
|
|
|
|
|
|
|
|
|
|
|
|
|
|
|
|
|
|
|
|
|
|
|
|
|
|
|
|
|
|
|
|
|
|
1 |
+
"""功能函数
|
2 |
+
"""
|
3 |
+
from __future__ import print_function
|
4 |
+
|
5 |
+
import os
|
6 |
+
|
7 |
+
import numpy as np
|
8 |
+
import torch
|
9 |
+
from PIL import Image
|
10 |
+
from torch import tensor
|
11 |
+
|
12 |
+
|
13 |
+
def tensor2im(input_image: tensor, imtype=np.uint8):
|
14 |
+
""" "Converts a Tensor array into a numpy image array.
|
15 |
+
|
16 |
+
Parameters:
|
17 |
+
input_image (tensor) -- the input image tensor array
|
18 |
+
imtype (type) -- the desired type of the converted numpy array
|
19 |
+
"""
|
20 |
+
if len(input_image.size()) == 3:
|
21 |
+
input_image = input_image.unsqueeze(0)
|
22 |
+
if not isinstance(input_image, np.ndarray):
|
23 |
+
if isinstance(input_image, torch.Tensor): # get the data from a variable
|
24 |
+
image_tensor = input_image.data
|
25 |
+
else:
|
26 |
+
return input_image
|
27 |
+
# convert it into a numpy array
|
28 |
+
image_numpy = image_tensor[0].cpu().float().numpy()
|
29 |
+
if image_numpy.shape[0] == 1: # grayscale to RGB
|
30 |
+
image_numpy = np.tile(image_numpy, (3, 1, 1))
|
31 |
+
image_numpy = (
|
32 |
+
(np.transpose(image_numpy, (1, 2, 0)) + 1) / 2.0 * 255.0
|
33 |
+
) # post-processing: tranpose and scaling
|
34 |
+
else: # if it is a numpy array, do nothing
|
35 |
+
image_numpy = input_image
|
36 |
+
return image_numpy.astype(imtype)
|
37 |
+
|
38 |
+
|
39 |
+
def diagnose_network(net, name="network"):
|
40 |
+
"""Calculate and print the mean of average absolute(gradients)
|
41 |
+
|
42 |
+
Parameters:
|
43 |
+
net (torch network) -- Torch network
|
44 |
+
name (str) -- the name of the network
|
45 |
+
"""
|
46 |
+
mean = 0.0
|
47 |
+
count = 0
|
48 |
+
for param in net.parameters():
|
49 |
+
if param.grad is not None:
|
50 |
+
mean += torch.mean(torch.abs(param.grad.data))
|
51 |
+
count += 1
|
52 |
+
if count > 0:
|
53 |
+
mean = mean / count
|
54 |
+
print(name)
|
55 |
+
print(mean)
|
56 |
+
|
57 |
+
|
58 |
+
def save_image(image_numpy, image_path, aspect_ratio=1.0):
|
59 |
+
"""Save a numpy image to the disk
|
60 |
+
|
61 |
+
Parameters:
|
62 |
+
aspect_ratio:
|
63 |
+
image_numpy (numpy array) -- input numpy array
|
64 |
+
image_path (str) -- the path of the image
|
65 |
+
"""
|
66 |
+
|
67 |
+
image_pil = Image.fromarray(image_numpy)
|
68 |
+
h, w, _ = image_numpy.shape
|
69 |
+
|
70 |
+
if aspect_ratio > 1.0:
|
71 |
+
image_pil = image_pil.resize((h, int(w * aspect_ratio)), Image.BICUBIC)
|
72 |
+
if aspect_ratio < 1.0:
|
73 |
+
image_pil = image_pil.resize((int(h / aspect_ratio), w), Image.BICUBIC)
|
74 |
+
image_pil.save(image_path)
|
75 |
+
|
76 |
+
|
77 |
+
def print_numpy(x, val=True, shp=False):
|
78 |
+
"""Print the mean, min, max, median, std, and size of a numpy array
|
79 |
+
|
80 |
+
Parameters:
|
81 |
+
x: (np.array)
|
82 |
+
val (bool) -- if print the values of the numpy array
|
83 |
+
shp (bool) -- if print the shape of the numpy array
|
84 |
+
"""
|
85 |
+
x = x.astype(np.float64)
|
86 |
+
if shp:
|
87 |
+
print("shape,", x.shape)
|
88 |
+
if val:
|
89 |
+
x = x.flatten()
|
90 |
+
print(
|
91 |
+
"mean = %3.3f, min = %3.3f, max = %3.3f, median = %3.3f, std=%3.3f"
|
92 |
+
% (np.mean(x), np.min(x), np.max(x), np.median(x), np.std(x))
|
93 |
+
)
|
94 |
+
|
95 |
+
|
96 |
+
def mkdirs(paths):
|
97 |
+
"""create empty directories if they don't exist
|
98 |
+
|
99 |
+
Parameters:
|
100 |
+
paths (str list) -- a list of directory paths
|
101 |
+
"""
|
102 |
+
if isinstance(paths, list) and not isinstance(paths, str):
|
103 |
+
for path in paths:
|
104 |
+
mkdir(path)
|
105 |
+
else:
|
106 |
+
mkdir(paths)
|
107 |
+
|
108 |
+
|
109 |
+
def mkdir(path):
|
110 |
+
"""create a single empty directory if it didn't exist
|
111 |
+
|
112 |
+
Parameters:
|
113 |
+
path (str) -- a single directory path
|
114 |
+
"""
|
115 |
+
if not os.path.exists(path):
|
116 |
+
os.makedirs(path)
|
117 |
+
|
118 |
+
|
119 |
+
def show_image(image_numpy, aspect_ratio=1.0):
|
120 |
+
"""Save a numpy image to the disk
|
121 |
+
|
122 |
+
Parameters:
|
123 |
+
aspect_ratio:
|
124 |
+
image_numpy (numpy array) -- input numpy array
|
125 |
+
"""
|
126 |
+
|
127 |
+
image_pil = Image.fromarray(image_numpy)
|
128 |
+
h, w, _ = image_numpy.shape
|
129 |
+
|
130 |
+
if aspect_ratio > 1.0:
|
131 |
+
image_pil = image_pil.resize((h, int(w * aspect_ratio)), Image.BICUBIC)
|
132 |
+
if aspect_ratio < 1.0:
|
133 |
+
image_pil = image_pil.resize((int(h / aspect_ratio), w), Image.BICUBIC)
|
134 |
+
# image_pil.save(image_path)
|
135 |
+
image_pil.show()
|
util/visualizer.py
ADDED
@@ -0,0 +1,297 @@
|
|
|
|
|
|
|
|
|
|
|
|
|
|
|
|
|
|
|
|
|
|
|
|
|
|
|
|
|
|
|
|
|
|
|
|
|
|
|
|
|
|
|
|
|
|
|
|
|
|
|
|
|
|
|
|
|
|
|
|
|
|
|
|
|
|
|
|
|
|
|
|
|
|
|
|
|
|
|
|
|
|
|
|
|
|
|
|
|
|
|
|
|
|
|
|
|
|
|
|
|
|
|
|
|
|
|
|
|
|
|
|
|
|
|
|
|
|
|
|
|
|
|
|
|
|
|
|
|
|
|
|
|
|
|
|
|
|
|
|
|
|
|
|
|
|
|
|
|
|
|
|
|
|
|
|
|
|
|
|
|
|
|
|
|
|
|
|
|
|
|
|
|
|
|
|
|
|
|
|
|
|
|
|
|
|
|
|
|
|
|
|
|
|
|
|
|
|
|
|
|
|
|
|
|
|
|
|
|
|
|
|
|
|
|
|
|
|
|
|
|
|
|
|
|
|
|
|
|
|
|
|
|
|
|
|
|
|
|
|
|
|
|
|
|
|
|
|
|
|
|
|
|
|
|
|
|
|
|
|
|
|
|
|
|
|
|
|
|
|
|
|
|
|
|
|
|
|
|
|
|
|
|
|
|
|
|
|
|
|
|
|
|
|
|
|
|
|
|
|
|
|
|
|
|
|
|
|
|
|
|
|
|
|
|
|
|
|
|
|
|
|
|
|
|
|
|
|
|
|
|
|
|
|
|
|
|
|
|
|
|
|
|
|
|
|
|
|
|
|
|
|
|
|
|
|
|
|
|
|
|
|
|
|
|
|
|
|
|
|
|
|
|
|
|
|
|
|
|
|
|
|
|
|
|
|
|
|
|
|
|
|
|
|
|
|
|
|
|
|
|
|
|
|
|
|
|
|
|
|
|
|
|
|
|
|
|
|
|
|
|
|
|
|
|
|
|
|
|
|
|
|
|
|
|
|
|
|
|
|
|
|
|
|
|
|
|
|
|
|
|
|
|
|
|
|
|
|
|
|
|
|
|
|
|
|
|
|
|
|
|
|
|
|
|
|
|
|
|
|
|
|
|
|
|
|
|
|
|
|
|
|
|
|
|
|
|
|
|
|
|
|
|
|
|
|
|
|
|
|
|
|
|
|
|
|
|
|
|
|
|
|
|
|
|
|
|
|
|
|
|
|
|
|
|
|
|
|
|
|
|
|
|
|
|
|
|
|
|
|
|
|
|
|
|
|
|
|
|
|
|
|
|
|
|
|
|
|
|
|
|
|
|
|
|
|
|
|
|
|
|
|
|
|
|
|
|
|
|
|
|
|
|
|
|
|
|
1 |
+
import ntpath
|
2 |
+
import os
|
3 |
+
import sys
|
4 |
+
import time
|
5 |
+
from pathlib import Path
|
6 |
+
from subprocess import PIPE, Popen
|
7 |
+
|
8 |
+
import numpy as np
|
9 |
+
|
10 |
+
from util import now_time
|
11 |
+
from . import html, util
|
12 |
+
|
13 |
+
|
14 |
+
try:
|
15 |
+
import wandb
|
16 |
+
except ImportError:
|
17 |
+
print(
|
18 |
+
'Warning: wandb package cannot be found. The option "--use_wandb" will result in error.'
|
19 |
+
)
|
20 |
+
if sys.version_info[0] == 2:
|
21 |
+
VisdomExceptionBase = Exception
|
22 |
+
else:
|
23 |
+
VisdomExceptionBase = ConnectionError
|
24 |
+
|
25 |
+
|
26 |
+
def save_images(
|
27 |
+
webpage, visuals, image_path, aspect_ratio=1.0, width=256, use_wandb=False
|
28 |
+
):
|
29 |
+
"""Save images to the disk.
|
30 |
+
|
31 |
+
Parameters:
|
32 |
+
use_wandb:
|
33 |
+
webpage (the HTML class) -- the HTML webpage class that stores these imaegs (see html.py for more details)
|
34 |
+
visuals (OrderedDict) -- an ordered dictionary that stores (name, images (either tensor or numpy) ) pairs
|
35 |
+
image_path (str) -- the string is used to create image paths
|
36 |
+
aspect_ratio (float) -- the aspect ratio of saved images
|
37 |
+
width (int) -- the images will be resized to width x width
|
38 |
+
|
39 |
+
This function will save images stored in 'visuals' to the HTML file specified by 'webpage'.
|
40 |
+
"""
|
41 |
+
image_dir = webpage.get_image_dir()
|
42 |
+
short_path = ntpath.basename(image_path[0])
|
43 |
+
name = os.path.splitext(short_path)[0]
|
44 |
+
|
45 |
+
webpage.add_header(name)
|
46 |
+
ims, txts, links = [], [], []
|
47 |
+
ims_dict = {}
|
48 |
+
for label, im_data in visuals.items():
|
49 |
+
im = util.tensor2im(im_data)
|
50 |
+
image_name = "%s_%s.png" % (name, label)
|
51 |
+
save_path = Path(image_dir, image_name)
|
52 |
+
util.save_image(im, save_path, aspect_ratio=aspect_ratio)
|
53 |
+
ims.append(image_name)
|
54 |
+
txts.append(label)
|
55 |
+
links.append(image_name)
|
56 |
+
if use_wandb:
|
57 |
+
ims_dict[label] = wandb.Image(im)
|
58 |
+
webpage.add_images(ims, txts, links, width=width)
|
59 |
+
if use_wandb:
|
60 |
+
wandb.log(ims_dict)
|
61 |
+
|
62 |
+
|
63 |
+
class Visualizer:
|
64 |
+
"""This class includes several functions that can display/save images and print/save logging information.
|
65 |
+
|
66 |
+
It uses a Python library 'visdom' for display, and a Python library 'dominate' (wrapped in 'HTML') for creating HTML files with images.
|
67 |
+
"""
|
68 |
+
|
69 |
+
def __init__(self, opt):
|
70 |
+
"""Initialize the Visualizer class
|
71 |
+
|
72 |
+
Parameters:
|
73 |
+
opt -- stores all the experiment flags; needs to be a subclass of BaseOptions
|
74 |
+
Step 1: Cache the training/test options
|
75 |
+
Step 2: connect to a visdom server
|
76 |
+
Step 3: create an HTML object for saveing HTML filters
|
77 |
+
Step 4: create a logging file to store training losses
|
78 |
+
"""
|
79 |
+
self.opt = opt # cache the option
|
80 |
+
self.display_id = opt.display_id
|
81 |
+
self.use_html = opt.isTrain and not opt.no_html
|
82 |
+
self.win_size = opt.display_winsize
|
83 |
+
self.name = opt.name
|
84 |
+
self.port = opt.display_port
|
85 |
+
self.saved = False
|
86 |
+
self.use_wandb = opt.use_wandb
|
87 |
+
self.wandb_project_name = opt.wandb_project_name
|
88 |
+
self.current_epoch = 0
|
89 |
+
self.ncols = opt.display_ncols
|
90 |
+
|
91 |
+
if (
|
92 |
+
self.display_id > 0
|
93 |
+
): # connect to a visdom server given <display_port> and <display_server>
|
94 |
+
import visdom
|
95 |
+
|
96 |
+
self.vis = visdom.Visdom(
|
97 |
+
server=opt.display_server, port=opt.display_port, env=opt.display_env
|
98 |
+
)
|
99 |
+
if not self.vis.check_connection():
|
100 |
+
self.create_visdom_connections()
|
101 |
+
if self.use_wandb:
|
102 |
+
self.wandb_run = (
|
103 |
+
wandb.init(project=self.wandb_project_name, name=opt.name, config=opt)
|
104 |
+
if not wandb.run
|
105 |
+
else wandb.run
|
106 |
+
)
|
107 |
+
self.wandb_run._label(repo="CycleGAN")
|
108 |
+
# create an HTML object at <checkpoints_dir>/web/; images will be saved under <checkpoints_dir>/web/images/
|
109 |
+
if self.use_html:
|
110 |
+
self.web_dir = Path(opt.checkpoints_dir, opt.name, "web" + now_time())
|
111 |
+
self.img_dir = self.web_dir.joinpath("images")
|
112 |
+
print("Create web directory %s..." % self.web_dir)
|
113 |
+
util.mkdirs([self.web_dir, self.img_dir])
|
114 |
+
# create a logging file to store training losses
|
115 |
+
self.log_name = Path(opt.checkpoints_dir, opt.name, "loss_log.txt")
|
116 |
+
with open(self.log_name, "a") as log_file:
|
117 |
+
now = time.strftime("%c")
|
118 |
+
log_file.write(
|
119 |
+
"================ Training Loss (%s) ================\n" % now
|
120 |
+
)
|
121 |
+
|
122 |
+
def reset(self):
|
123 |
+
"""Reset the self.saved status"""
|
124 |
+
self.saved = False
|
125 |
+
|
126 |
+
def create_visdom_connections(self):
|
127 |
+
"""If the program could not connect to Visdom server, this function will start a new server at port < self.port >"""
|
128 |
+
cmd = sys.executable + " -m visdom.server -p %d &>/dev/null &" % self.port
|
129 |
+
print("\n\nCould not connect to Visdom server. \n Trying to start a server....")
|
130 |
+
print("Command: %s" % cmd)
|
131 |
+
Popen(cmd, shell=True, stdout=PIPE, stderr=PIPE)
|
132 |
+
|
133 |
+
def display_current_results(self, visuals, epoch, save_result):
|
134 |
+
"""Display current results on visdom; save current results to an HTML file.
|
135 |
+
|
136 |
+
Parameters:
|
137 |
+
visuals (OrderedDict) - - dictionary of images to display or save
|
138 |
+
epoch (int) - - the current epoch
|
139 |
+
save_result (bool) - - if save the current results to an HTML file
|
140 |
+
"""
|
141 |
+
if self.display_id > 0: # show images in the browser using visdom
|
142 |
+
ncols = self.ncols
|
143 |
+
if ncols > 0: # show all the images in one visdom panel
|
144 |
+
ncols = min(ncols, len(visuals))
|
145 |
+
h, w = next(iter(visuals.values())).shape[:2]
|
146 |
+
table_css = """<style>
|
147 |
+
|
148 |
+
table {border-collapse: separate; border-spacing: 4px; white-space: nowrap; text-align: center}
|
149 |
+
|
150 |
+
table td {width: % dpx; height: % dpx; padding: 4px; outline: 4px solid black}
|
151 |
+
|
152 |
+
</style>""" % (
|
153 |
+
w,
|
154 |
+
h,
|
155 |
+
) # create a table css
|
156 |
+
# create a table of images.
|
157 |
+
title = self.name
|
158 |
+
label_html = ""
|
159 |
+
label_html_row = ""
|
160 |
+
images = []
|
161 |
+
image_numpy = None
|
162 |
+
idx = 0
|
163 |
+
for label, image in visuals.items():
|
164 |
+
image_numpy = util.tensor2im(image)
|
165 |
+
label_html_row += "<td>%s</td>" % label
|
166 |
+
images.append(image_numpy.transpose([2, 0, 1]))
|
167 |
+
idx += 1
|
168 |
+
if idx % ncols == 0:
|
169 |
+
label_html += "<tr>%s</tr>" % label_html_row
|
170 |
+
label_html_row = ""
|
171 |
+
white_image = np.ones_like(image_numpy.transpose([2, 0, 1])) * 255
|
172 |
+
while idx % ncols != 0:
|
173 |
+
images.append(white_image)
|
174 |
+
label_html_row += "<td></td>"
|
175 |
+
idx += 1
|
176 |
+
if label_html_row != "":
|
177 |
+
label_html += "<tr>%s</tr>" % label_html_row
|
178 |
+
try:
|
179 |
+
self.vis.images(
|
180 |
+
images,
|
181 |
+
nrow=ncols,
|
182 |
+
win=self.display_id + 1,
|
183 |
+
padding=2,
|
184 |
+
opts=dict(title=title + " images"),
|
185 |
+
)
|
186 |
+
label_html = "<table>%s</table>" % label_html
|
187 |
+
self.vis.text(
|
188 |
+
table_css + label_html,
|
189 |
+
win=self.display_id + 2,
|
190 |
+
opts=dict(title=title + " labels"),
|
191 |
+
)
|
192 |
+
except VisdomExceptionBase:
|
193 |
+
self.create_visdom_connections()
|
194 |
+
else: # show each image in a separate visdom panel;
|
195 |
+
idx = 1
|
196 |
+
try:
|
197 |
+
for label, image in visuals.items():
|
198 |
+
image_numpy = util.tensor2im(image)
|
199 |
+
self.vis.image(
|
200 |
+
image_numpy.transpose([2, 0, 1]),
|
201 |
+
opts=dict(title=label),
|
202 |
+
win=self.display_id + idx,
|
203 |
+
)
|
204 |
+
idx += 1
|
205 |
+
except VisdomExceptionBase:
|
206 |
+
self.create_visdom_connections()
|
207 |
+
if self.use_wandb:
|
208 |
+
columns = [key for key, _ in visuals.items()]
|
209 |
+
columns.insert(0, "epoch")
|
210 |
+
result_table = wandb.Table(columns=columns)
|
211 |
+
table_row = [epoch]
|
212 |
+
ims_dict = {}
|
213 |
+
for label, image in visuals.items():
|
214 |
+
image_numpy = util.tensor2im(image)
|
215 |
+
wandb_image = wandb.Image(image_numpy)
|
216 |
+
table_row.append(wandb_image)
|
217 |
+
ims_dict[label] = wandb_image
|
218 |
+
self.wandb_run.log(ims_dict)
|
219 |
+
if epoch != self.current_epoch:
|
220 |
+
self.current_epoch = epoch
|
221 |
+
result_table.add_data(*table_row)
|
222 |
+
self.wandb_run.log({"Result": result_table})
|
223 |
+
if self.use_html and (
|
224 |
+
save_result or not self.saved
|
225 |
+
): # save images to an HTML file if they haven't been saved.
|
226 |
+
self.saved = True
|
227 |
+
# save images to the disk
|
228 |
+
for label, image in visuals.items():
|
229 |
+
image_numpy = util.tensor2im(image)
|
230 |
+
img_path = Path(self.img_dir, "epoch%.3d_%s.png" % (epoch, label))
|
231 |
+
util.save_image(image_numpy, img_path)
|
232 |
+
# update website
|
233 |
+
webpage = html.HTML(
|
234 |
+
self.web_dir, "Experiment name = %s" % self.name, refresh=1
|
235 |
+
)
|
236 |
+
for n in range(epoch, 0, -1):
|
237 |
+
webpage.add_header("epoch [%d]" % n)
|
238 |
+
ims, txts, links = [], [], []
|
239 |
+
|
240 |
+
for label, image_numpy in visuals.items():
|
241 |
+
# image_numpy = util.tensor2im(image)
|
242 |
+
img_path = "epoch%.3d_%s.png" % (n, label)
|
243 |
+
ims.append(img_path)
|
244 |
+
txts.append(label)
|
245 |
+
links.append(img_path)
|
246 |
+
webpage.add_images(ims, txts, links, width=self.win_size)
|
247 |
+
webpage.save()
|
248 |
+
|
249 |
+
def plot_current_losses(self, epoch, counter_ratio, losses):
|
250 |
+
"""display the current losses on visdom display: dictionary of error labels and values
|
251 |
+
|
252 |
+
Parameters:
|
253 |
+
epoch (int) -- current epoch
|
254 |
+
counter_ratio (float) -- progress (percentage) in the current epoch, between 0 to 1
|
255 |
+
losses (OrderedDict) -- training losses stored in the format of (name, float) pairs
|
256 |
+
"""
|
257 |
+
if not hasattr(self, "plot_data"):
|
258 |
+
self.plot_data = {"X": [], "Y": [], "legend": list(losses.keys())}
|
259 |
+
self.plot_data["X"].append(epoch + counter_ratio)
|
260 |
+
self.plot_data["Y"].append([losses[k] for k in self.plot_data["legend"]])
|
261 |
+
try:
|
262 |
+
self.vis.line(
|
263 |
+
X=np.stack(
|
264 |
+
[np.array(self.plot_data["X"])] * len(self.plot_data["legend"]), 1
|
265 |
+
),
|
266 |
+
Y=np.array(self.plot_data["Y"]),
|
267 |
+
opts={
|
268 |
+
"title": f"{self.name} loss over time",
|
269 |
+
"legend": self.plot_data["legend"],
|
270 |
+
"xlabel": "epoch",
|
271 |
+
"ylabel": "loss",
|
272 |
+
},
|
273 |
+
win=self.display_id,
|
274 |
+
)
|
275 |
+
except VisdomExceptionBase:
|
276 |
+
self.create_visdom_connections()
|
277 |
+
if self.use_wandb:
|
278 |
+
self.wandb_run.log(losses)
|
279 |
+
|
280 |
+
# losses: same format as |losses| of plot_current_losses
|
281 |
+
def print_current_losses(self, epoch, iters, losses, t_comp, t_data):
|
282 |
+
"""print current losses on console; also save the losses to the disk
|
283 |
+
|
284 |
+
Parameters:
|
285 |
+
epoch (int) -- current epoch
|
286 |
+
iters (int) -- current training iteration during this epoch (reset to 0 at the end of every epoch)
|
287 |
+
losses (OrderedDict) -- training losses stored in the format of (name, float) pairs
|
288 |
+
t_comp (float) -- computational time per data point (normalized by batch_size)
|
289 |
+
t_data (float) -- data loading time per data point (normalized by batch_size)
|
290 |
+
"""
|
291 |
+
message = f"(epoch: {epoch:>2d}, iters: {iters:>4d}, time: {t_comp:.3f}, data: {t_data:.3f})"
|
292 |
+
for k, v in losses.items():
|
293 |
+
message += f" {k:s}: {v:.3f}"
|
294 |
+
print(message)
|
295 |
+
|
296 |
+
with open(self.log_name, "a") as log_file:
|
297 |
+
log_file.write(f"{message:s}\n")
|
weights/detect/apple2orange.pth
ADDED
@@ -0,0 +1,3 @@
|
|
|
|
|
|
|
|
|
1 |
+
version https://git-lfs.github.com/spec/v1
|
2 |
+
oid sha256:ff989c98f3a077cd11c2623ed8742e2ef147e81d10b4e4c2ee6935796bda957a
|
3 |
+
size 45575747
|
weights/detect/cityscapes_label2photo.pth
ADDED
@@ -0,0 +1,3 @@
|
|
|
|
|
|
|
|
|
1 |
+
version https://git-lfs.github.com/spec/v1
|
2 |
+
oid sha256:ad637d74a1fb752aa4d2f427452954e153a5bedf5342492de7b53bffb04e5081
|
3 |
+
size 45575747
|
weights/detect/cityscapes_photo2label.pth
ADDED
@@ -0,0 +1,3 @@
|
|
|
|
|
|
|
|
|
1 |
+
version https://git-lfs.github.com/spec/v1
|
2 |
+
oid sha256:7649da0ec6f393efe171f333fb17508e1e1d0176191496fba2a0d37c90db82d0
|
3 |
+
size 45575747
|
weights/detect/facades_label2photo.pth
ADDED
@@ -0,0 +1,3 @@
|
|
|
|
|
|
|
|
|
1 |
+
version https://git-lfs.github.com/spec/v1
|
2 |
+
oid sha256:184711ff5ccae4e8aab7e39d72eb7fb481b52952b04cfe4d47cdd75317e77d7d
|
3 |
+
size 45575747
|
weights/detect/facades_photo2label.pth
ADDED
@@ -0,0 +1,3 @@
|
|
|
|
|
|
|
|
|
1 |
+
version https://git-lfs.github.com/spec/v1
|
2 |
+
oid sha256:1714709fd7fa496a5300c0c32a7078653ba5a9530a81b30339a86337610f420b
|
3 |
+
size 45575747
|
weights/detect/horse2zebra.pth
ADDED
@@ -0,0 +1,3 @@
|
|
|
|
|
|
|
|
|
1 |
+
version https://git-lfs.github.com/spec/v1
|
2 |
+
oid sha256:5bfc34b154035be1cd592cd273fdf328810bee4756044c9cf7deb8d4b6d2fadb
|
3 |
+
size 45575747
|
weights/detect/iphone2dslr_flower_2.pth
ADDED
@@ -0,0 +1,3 @@
|
|
|
|
|
|
|
|
|
1 |
+
version https://git-lfs.github.com/spec/v1
|
2 |
+
oid sha256:8c376197c87302f2b1af52c2e1f4dbd033d57babc7ae1eff1d66a81c6b2109bd
|
3 |
+
size 45575747
|
weights/detect/latest_net_G_A.pth
ADDED
@@ -0,0 +1,3 @@
|
|
|
|
|
|
|
|
|
1 |
+
version https://git-lfs.github.com/spec/v1
|
2 |
+
oid sha256:858eb597ea7335db5a230555418fdc5be198807600b67f18701ec0b5090c6006
|
3 |
+
size 45531635
|
weights/detect/latest_net_G_A0.pth
ADDED
@@ -0,0 +1,3 @@
|
|
|
|
|
|
|
|
|
1 |
+
version https://git-lfs.github.com/spec/v1
|
2 |
+
oid sha256:13950e21593133d93d2907bf4e0a0a3e9ca47664ebb2c74209113446f95c5997
|
3 |
+
size 45531635
|
weights/detect/latest_net_G_A1.pth
ADDED
@@ -0,0 +1,3 @@
|
|
|
|
|
|
|
|
|
1 |
+
version https://git-lfs.github.com/spec/v1
|
2 |
+
oid sha256:2607bdb799223f80c34c1de0fba301c294118d8d3a1a804e7add145bd4e546f5
|
3 |
+
size 45531635
|
weights/detect/latest_net_G_B.pth
ADDED
@@ -0,0 +1,3 @@
|
|
|
|
|
|
|
|
|
1 |
+
version https://git-lfs.github.com/spec/v1
|
2 |
+
oid sha256:768139e9d7d01ab20c82384311e2db934fc9bd16122fbbc84c36557396e1e6b8
|
3 |
+
size 45531635
|
weights/detect/latest_net_G_B0.pth
ADDED
@@ -0,0 +1,3 @@
|
|
|
|
|
|
|
|
|
1 |
+
version https://git-lfs.github.com/spec/v1
|
2 |
+
oid sha256:cd5f04202bd0028475ada0cfe2a1321d91533df213b01c2e976557c196362a60
|
3 |
+
size 45531635
|
weights/detect/latest_net_G_B1.pth
ADDED
@@ -0,0 +1,3 @@
|
|
|
|
|
|
|
|
|
1 |
+
version https://git-lfs.github.com/spec/v1
|
2 |
+
oid sha256:bfa9858158beeca602182c0956b42a0fb91bb9bc195cf923a1c9bb707035e87a
|
3 |
+
size 45531635
|