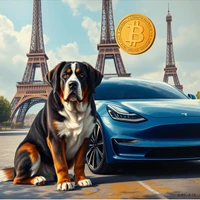
Updated line 8 with: from moviepy.editor import ImageSequenceClip and Update requirements.txt with: moviepy
48c41b0
verified
import numpy as np | |
import math | |
import matplotlib.pyplot as plt | |
from matplotlib.animation import FuncAnimation | |
from PIL import Image | |
import gradio as gr | |
import io | |
from moviepy.editor import ImageSequenceClip | |
class Objective: | |
def Evaluate(self, p): | |
return -5.0*np.exp(-0.5*((p[0]+2.2)**2/0.4+(p[1]-4.3)**2/0.4)) + -2.0*np.exp(-0.5*((p[0]-2.2)**2/0.4+(p[1]+4.3)**2/0.4)) | |
# Create an instance of the Objective class | |
obj = Objective() | |
# Evaluate the fitness of a position | |
position = np.array([-2.2, 4.3]) | |
fitness = obj.Evaluate(position) | |
print(f"The fitness of the position {position} is {fitness}") | |
class Bounds: | |
def __init__(self, lower, upper, enforce="clip"): | |
self.lower = np.array(lower) | |
self.upper = np.array(upper) | |
self.enforce = enforce.lower() | |
def Upper(self): | |
return self.upper | |
def Lower(self): | |
return self.lower | |
def Limits(self, pos): | |
npart, ndim = pos.shape | |
for i in range(npart): | |
for j in range(ndim): | |
if pos[i, j] < self.lower[j]: | |
if self.enforce == "clip": | |
pos[i, j] = self.lower[j] | |
elif self.enforce == "resample": | |
pos[i, j] = self.lower[j] + np.random.random() * (self.upper[j] - self.lower[j]) | |
elif pos[i, j] > self.upper[j]: | |
if self.enforce == "clip": | |
pos[i, j] = self.upper[j] | |
elif self.enforce == "resample": | |
pos[i, j] = self.lower[j] + np.random.random() * (self.upper[j] - self.lower[j]) | |
pos[i] = self.Validate(pos[i]) | |
return pos | |
def Validate(self, pos): | |
return pos | |
# Define the bounds | |
lower_bounds = [-6, -6, -6] | |
upper_bounds = [6, 6, 6] | |
# Create an instance of the Bounds class | |
bounds = Bounds(lower_bounds, upper_bounds, enforce="clip") | |
# Define a set of positions | |
positions = np.array([[15, 15], [-15, -15], [5, 15], [15, 5]]) | |
# Enforce the bounds on the positions | |
valid_positions = bounds.Limits(positions) | |
print(f"Valid positions: {valid_positions}") | |
# Define the bounds | |
lower_bounds = [-6, -6, -6] | |
upper_bounds = [6, 6, 6] | |
# Create an instance of the Bounds class | |
bounds = Bounds(lower_bounds, upper_bounds, enforce="resample") | |
# Define a set of positions | |
positions = np.array([[15, 15, 15], [-15, -15, -15], [5, 15, 15], [15, 5, 5]]) | |
# Enforce the bounds on the positions | |
valid_positions = bounds.Limits(positions) | |
print(f"Valid positions: {valid_positions}") | |
class QuasiRandomInitializer: | |
def __init__(self, npart=10, ndim=3, bounds=None, k=1, jitter=0.0): | |
self.npart = npart | |
self.ndim = ndim | |
self.bounds = bounds | |
self.k = k | |
self.jitter = jitter | |
self.primes = [ | |
2, 3, 5, 7, 11, 13, 17, 19, 23, 29, 31, 37, 41, 43, 47, 53, 59, 61, 67, 71, 73, 79, 83, 89, 97, | |
101, 103, 107, 109, 113, 127, 131, 137, 139, 149, 151, 157, 163, 167, 173, 179, 181, 191, 193, 197, | |
199, 211, 223, 227, 229, 233, 239, 241, 251, 257, 263, 269, 271, 277, 281, 283, 293, 307, 311, 313, | |
317, 331, 337, 347, 349, 353, 359, 367, 373, 379, 383, 389, 397, 401, 409, 419, 421, 431, 433, 439, | |
443, 449, 457, 461, 463, 467, 479, 487, 491, 499, 503, 509, 521, 523, 541, 547, 557, 563, 569, 571, | |
577, 587, 593, 599, 601, 607, 613, 617, 619, 631, 641, 643, 647, 653, 659 | |
] | |
def Halton(self, i, b): | |
f = 1.0 | |
r = 0 | |
while i > 0: | |
f = f / b | |
r = r + f * (i % b) | |
i = math.floor(i / b) | |
return r | |
def InitializeSwarm(self): | |
self.swarm = np.zeros((self.npart, self.ndim)) | |
lo = np.zeros(self.ndim) | |
hi = np.ones(self.ndim) | |
if self.bounds is not None: | |
lo = self.bounds.Lower() | |
hi = self.bounds.Upper() | |
for i in range(self.npart): | |
for j in range(self.ndim): | |
h = self.Halton(i + self.k, self.primes[j % len(self.primes)]) | |
q = self.jitter * (np.random.random() - 0.5) | |
self.swarm[i, j] = lo[j] + (hi[j] - lo[j]) * h + q | |
if self.bounds is not None: | |
self.swarm = self.bounds.Limits(self.swarm) | |
return self.swarm | |
# Define the bounds | |
lower_bounds = [-6, -6, -6] | |
upper_bounds = [6, 6, 6] | |
bounds = Bounds(lower_bounds, upper_bounds, enforce="clip") | |
# Create an instance of the QuasiRandomInitializer class | |
init = QuasiRandomInitializer(npart=50, ndim=3, bounds=bounds) | |
# Initialize the swarm | |
swarm_positions = init.InitializeSwarm() | |
print(f"Initial swarm positions: {swarm_positions}") | |
# Define the bounds | |
lower_bounds = [-6, -6, -6] | |
upper_bounds = [6, 6, 6] | |
bounds = Bounds(lower_bounds, upper_bounds, enforce="resample") | |
# Create an instance of the QuasiRandomInitializer class | |
init = QuasiRandomInitializer(npart=50, ndim=3, bounds=bounds) | |
# Initialize the swarm | |
swarm_positions = init.InitializeSwarm() | |
class GWO: | |
def __init__(self, obj, eta=2.0, npart=10, ndim=3, max_iter=200,tol=None,init=None,done=None,bounds=None): | |
self.obj = obj | |
self.npart = npart | |
self.ndim = ndim | |
self.max_iter = max_iter | |
self.init = init | |
self.done = done | |
self.bounds = bounds | |
self.tol = tol | |
self.eta = eta | |
self.initialized = False | |
def Initialize(self): | |
"""Set up the swarm""" | |
self.initialized = True | |
self.iterations = 0 | |
self.pos = self.init.InitializeSwarm() # initial swarm positions | |
self.vpos= np.zeros(self.npart) | |
for i in range(self.npart): | |
self.vpos[i] = self.obj.Evaluate(self.pos[i]) | |
# Initialize the list to store positions at each iteration | |
self.all_positions = [] | |
self.all_positions.append(self.pos.copy()) # Store the initial positi | |
# Swarm bests | |
self.gidx = [] | |
self.gbest = [] | |
self.gpos = [] | |
self.giter = [] | |
idx = np.argmin(self.vpos) | |
self.gidx.append(idx) | |
self.gbest.append(self.vpos[idx]) | |
self.gpos.append(self.pos[idx].copy()) | |
self.giter.append(0) | |
# 1st, 2nd, and 3rd best positions | |
idx = np.argsort(self.vpos) | |
self.alpha = self.pos[idx[0]].copy() | |
self.valpha= self.vpos[idx[0]] | |
self.beta = self.pos[idx[1]].copy() | |
self.vbeta = self.vpos[idx[1]] | |
self.delta = self.pos[idx[2]].copy() | |
self.vdelta= self.vpos[idx[2]] | |
# *** Gradio app method optimize created [leveraged vis-a-vis optimize function on the outside of the underlying anatomy of GWO class] *** | |
def optimize(self): | |
""" | |
Run a full optimization and return the best positions and fitness values. | |
This method is designed to be used with Gradio. | |
""" | |
# Initialize the swarm | |
self.Initialize() | |
# Lists to store the best positions and fitness values at each step | |
best_positions = [] | |
best_fitness = [] | |
# Main loop | |
while not self.Done(): | |
self.Step() # Perform an optimization step | |
# Update best_positions and best_fitness with the current best values | |
best_positions.append(self.gbest[-1]) | |
best_fitness.append(self.gpos[-1]) | |
# Print the best positions and fitness found | |
print("Best Positions:", best_positions) | |
print("Best Fitness:", best_fitness) | |
# Return the best positions and fitness after the optimization | |
return best_positions, best_fitness | |
def Step(self): | |
"""Do one swarm step""" | |
print("Inside Step method") | |
# a from eta ... zero (default eta is 2) | |
a = self.eta - self.eta*(self.iterations/self.max_iter) | |
print("a:", a) | |
# Update everyone | |
for i in range(self.npart): | |
A = 2*a*np.random.random(self.ndim) - a | |
C = 2*np.random.random(self.ndim) | |
Dalpha = np.abs(C*self.alpha - self.pos[i]) | |
X1 = self.alpha - A*Dalpha | |
A = 2*a*np.random.random(self.ndim) - a | |
C = 2*np.random.random(self.ndim) | |
Dbeta = np.abs(C*self.beta - self.pos[i]) | |
X2 = self.beta - A*Dbeta | |
A = 2*a*np.random.random(self.ndim) - a | |
C = 2*np.random.random(self.ndim) | |
Ddelta = np.abs(C*self.delta - self.pos[i]) | |
X3 = self.delta - A*Ddelta | |
self.pos[i,:] = (X1+X2+X3) / 3.0 | |
# Keep in bounds | |
if (self.bounds != None): | |
self.pos = self.bounds.Limits(self.pos) | |
# Get objective function values and check for new leaders | |
for i in range(self.npart): | |
self.vpos[i] = self.obj.Evaluate(self.pos[i]) | |
# new alpha? | |
if (self.vpos[i] < self.valpha): | |
self.vdelta = self.vbeta | |
self.delta = self.beta.copy() | |
self.vbeta = self.valpha | |
self.beta = self.alpha.copy() | |
self.valpha = self.vpos[i] | |
self.alpha = self.pos[i].copy() | |
# new beta? | |
if (self.vpos[i] > self.valpha) and (self.vpos[i] < self.vbeta): | |
self.vdelta = self.vbeta | |
self.delta = self.beta.copy() | |
self.vbeta = self.vpos[i] | |
self.beta = self.pos[i].copy() | |
# new delta? | |
if (self.vpos[i] > self.valpha) and (self.vpos[i] < self.vbeta) and (self.vpos[i] < self.vdelta): | |
self.vdelta = self.vpos[i] | |
self.delta = self.pos[i].copy() | |
# is alpha new swarm best? | |
if (self.valpha < self.gbest[-1]): | |
self.gidx.append(i) | |
self.gbest.append(self.valpha) | |
np.save('best_fitness.npy', np.array(self.gbest)) | |
self.gpos.append(self.alpha.copy()) | |
np.save('best_positions.npy', np.array(self.gpos)) | |
# Save the positions at the current iteration | |
self.all_positions.append(self.pos.copy()) | |
self.giter.append(self.iterations) | |
self.iterations += 1 | |
print("Iteration:", self.iterations) | |
def Done(self): | |
"""Check if we are done""" | |
if (self.done == None): | |
if (self.tol == None): | |
return (self.iterations == self.max_iter) | |
else: | |
return (self.gbest[-1] < self.tol) or (self.iterations == self.max_iter) | |
else: | |
return self.done.Done(self.gbest, | |
gpos=self.gpos, | |
pos=self.pos, | |
max_iter=self.max_iter, | |
iteration=self.iterations) | |
def Evaluate(self, pos): | |
p = np.zeros(self.npart) | |
for i in range(self.npart): | |
p[i] = self.obj.Evaluate(pos[i]) | |
return p | |
def animate_particles(self, obj, goal, frames=100, interval=50): | |
"""Create a 2D contour particle animation""" | |
# Define the range for the x and y axis | |
x_range = np.linspace(self.bounds.Lower()[0], self.bounds.Upper()[0], 100) | |
y_range = np.linspace(self.bounds.Lower()[1], self.bounds.Upper()[1], 100) | |
# Create a grid of points | |
X, Y = np.meshgrid(x_range, y_range) | |
Z = np.zeros_like(X) | |
# Evaluate the objective function on the grid | |
for i in range(X.shape[0]): | |
for j in range(X.shape[1]): | |
Z[i, j] = obj.Evaluate(np.array([X[i, j], Y[i, j]])) | |
# Create a figure and axis | |
fig, ax = plt.subplots(figsize=(8, 6)) | |
# Plot the contour | |
contour = ax.contour(X, Y, Z, levels=20, colors='k', alpha=0.5) | |
# Plot the goal | |
goal_plot, = ax.plot([], [], 'r*', markersize=10) | |
# Initialize the scatter plot for the particles | |
scat = ax.scatter([], [], color='blue', s=20) | |
# Function to update the scatter plot | |
def update(frame): | |
# Perform one step of the GWO algorithm | |
self.Step() | |
# Update the scatter plot with the new positions | |
scat.set_offsets(self.pos) | |
# Update the goal position if it has changed | |
goal_plot.set_data([goal[0]], [goal[1]]) # Pass a list or a NumPy array | |
return scat, goal_plot | |
# Create the animation | |
anim = FuncAnimation(fig, update, frames=frames, interval=interval, blit=True) | |
# Show the plot | |
plt.show() | |
# Save the frames as images | |
images = [] | |
for i in range(frames): | |
anim.func_args = (i,) | |
anim._step() | |
fig.canvas.draw() | |
# Convert the figure to an image | |
image_data = np.frombuffer(fig.canvas.tostring_rgb(), dtype='uint8') | |
image = Image.frombytes('RGB', fig.canvas.get_width_height(), image_data) | |
images.append(image) | |
# Close the figure to free up memory | |
plt.close(fig) | |
# Convert the list of images to a list of PIL images | |
pil_images = [Image.fromarray(np.array(img)) for img in images] | |
# Create a video from the images | |
# After generating the images, create a video from them | |
frames_per_second = 30 # Adjust this to your desired frame rate | |
clip = ImageSequenceClip(pil_images, fps=frames_per_second) | |
clip.write_videofile(video_path, codec='libx264', audio=False) | |
# Return the video path | |
return video_path | |
# Define goal outside of the optimize function | |
#goal = (-2.2, 4.3) # Example goal position | |
def optimize(npart, ndim, max_iter, goal_x, goal_y, frames, interval): | |
# Create the goal tuple from the X and Y coordinates | |
goal = (goal_x, goal_y) | |
# Initialize the GWO algorithm with the provided parameters | |
gwo = GWO(obj=obj, npart=npart, ndim=ndim, max_iter=max_iter, init=init, bounds=bounds) | |
# Run the optimization | |
best_positions, best_fitness = gwo.optimize() | |
# Get the best fitness and positions at the last iteration | |
last_best_fitness = best_fitness[-1] | |
last_best_positions = best_positions[-1] | |
# Format the output strings | |
best_fitness_text = f"Best Positions: {last_best_fitness}" | |
best_positions_text = f"Best Fitness: {last_best_positions}" | |
# Animate the particles | |
video_path = gwo.animate_particles(obj=obj, goal=goal, frames=frames, interval=interval) | |
# Return the path to the video file | |
return video_path, best_fitness_text, best_positions_text | |
# Define the Gradio interface | |
iface = gr.Interface( | |
fn=optimize, # Pass the optimize function object | |
inputs=[ | |
gr.components.Slider(10, 50, 50, step=1, label="Number of Wolves"), | |
gr.components.Slider(3, 3, 3, step=1, label="Number of Dimensions"), | |
gr.components.Slider(100, 200, 200, step=1, label="Maximum Iterations"), | |
gr.components.Slider(-5, 5, 2.2, label="Goal Position X"), | |
gr.components.Slider(-5, 5, 4.3, label="Goal Position Y"), | |
gr.components.Slider(100, 200, 100, step=1, label="Frames"), | |
gr.components.Slider(50, 100, 50, step=1, label="Interval"), | |
], | |
outputs=[ | |
gr.components.Video(format="mp4"), | |
gr.components.Textbox(label="Best Fitness"), | |
gr.components.Textbox(label="Best Positions"), | |
], | |
) | |
# Launch the interface | |
iface.launch() | |