Spaces:
Build error
Build error
import gradio as gr | |
import transformers | |
import torch | |
import json | |
# load all models | |
pragformer = transformers.AutoModel.from_pretrained("Pragformer/PragFormer", trust_remote_code=True) | |
pragformer_private = transformers.AutoModel.from_pretrained("Pragformer/PragFormer_private", trust_remote_code=True) | |
pragformer_reduction = transformers.AutoModel.from_pretrained("Pragformer/PragFormer_reduction", trust_remote_code=True) | |
#Event Listeners | |
tokenizer = transformers.AutoTokenizer.from_pretrained('NTUYG/DeepSCC-RoBERTa') | |
with open('c_data.json', 'r') as f: | |
data = json.load(f) | |
def fill_code(code_pth): | |
return data[code_pth]['pragma'], data[code_pth]['code'] | |
def predict(code_txt): | |
code = code_txt.lstrip().rstrip() | |
tokenized = tokenizer.batch_encode_plus( | |
[code], | |
max_length = 150, | |
pad_to_max_length = True, | |
truncation = True | |
) | |
pred = pragformer(torch.tensor(tokenized['input_ids']), torch.tensor(tokenized['attention_mask'])) | |
y_hat = torch.argmax(pred).item() | |
return 'With OpenMP' if y_hat==1 else 'Without OpenMP', torch.nn.Softmax(dim=1)(pred).squeeze()[y_hat].item() | |
def is_private(code_txt): | |
code = code_txt.lstrip().rstrip() | |
tokenized = tokenizer.batch_encode_plus( | |
[code], | |
max_length = 150, | |
pad_to_max_length = True, | |
truncation = True | |
) | |
pred = pragformer_private(torch.tensor(tokenized['input_ids']), torch.tensor(tokenized['attention_mask'])) | |
y_hat = torch.argmax(pred).item() | |
if y_hat == 0: | |
return gr.update(visible=False) | |
else: | |
return gr.update(value=f"Confidence: {torch.nn.Softmax(dim=1)(pred).squeeze()[y_hat].item()}", visible=True) | |
def is_reduction(code_txt): | |
code = code_txt.lstrip().rstrip() | |
tokenized = tokenizer.batch_encode_plus( | |
[code], | |
max_length = 150, | |
pad_to_max_length = True, | |
truncation = True | |
) | |
pred = pragformer_reduction(torch.tensor(tokenized['input_ids']), torch.tensor(tokenized['attention_mask'])) | |
y_hat = torch.argmax(pred).item() | |
if y_hat == 0: | |
return gr.update(visible=False) | |
else: | |
return gr.update(value=f"Confidence: {torch.nn.Softmax(dim=1)(pred).squeeze()[y_hat].item()}", visible=True) | |
# Define GUI | |
with gr.Blocks() as pragformer_gui: | |
gr.Markdown( | |
""" | |
# PragFormer Pragma Classifiction | |
In past years, the world has switched to many-core and multi-core shared memory architectures. | |
As a result, there is a growing need to utilize these architectures by introducing shared memory parallelization schemes to software applications. | |
OpenMP is the most comprehensive API that implements such schemes, characterized by a readable interface. | |
Nevertheless, introducing OpenMP into code, especially legacy code, is challenging due to pervasive pitfalls in management of parallel shared memory. | |
To facilitate the performance of this task, many source-to-source (S2S) compilers have been created over the years, tasked with inserting OpenMP directives into | |
code automatically. | |
In addition to having limited robustness to their input format, these compilers still do not achieve satisfactory coverage and precision in locating parallelizable | |
code and generating appropriate directives. | |
In this work, we propose leveraging recent advances in machine learning techniques, specifically in natural language processing (NLP), to replace S2S compilers altogether. | |
We create a database (corpus), OpenMP-OMP specifically for this goal. | |
OpenMP-OMP contains over 28,000 code snippets, half of which contain OpenMP directives while the other half do not need parallelization at all with high probability. | |
We use the corpus to train systems to automatically classify code segments in need of parallelization, as well as suggest individual OpenMP clauses. | |
We train several transformer models, named PragFormer, for these tasks, and show that they outperform statistically-trained baselines and automatic S2S parallelization | |
compilers in both classifying the overall need for an OpenMP directive and the introduction of private and reduction clauses. | |
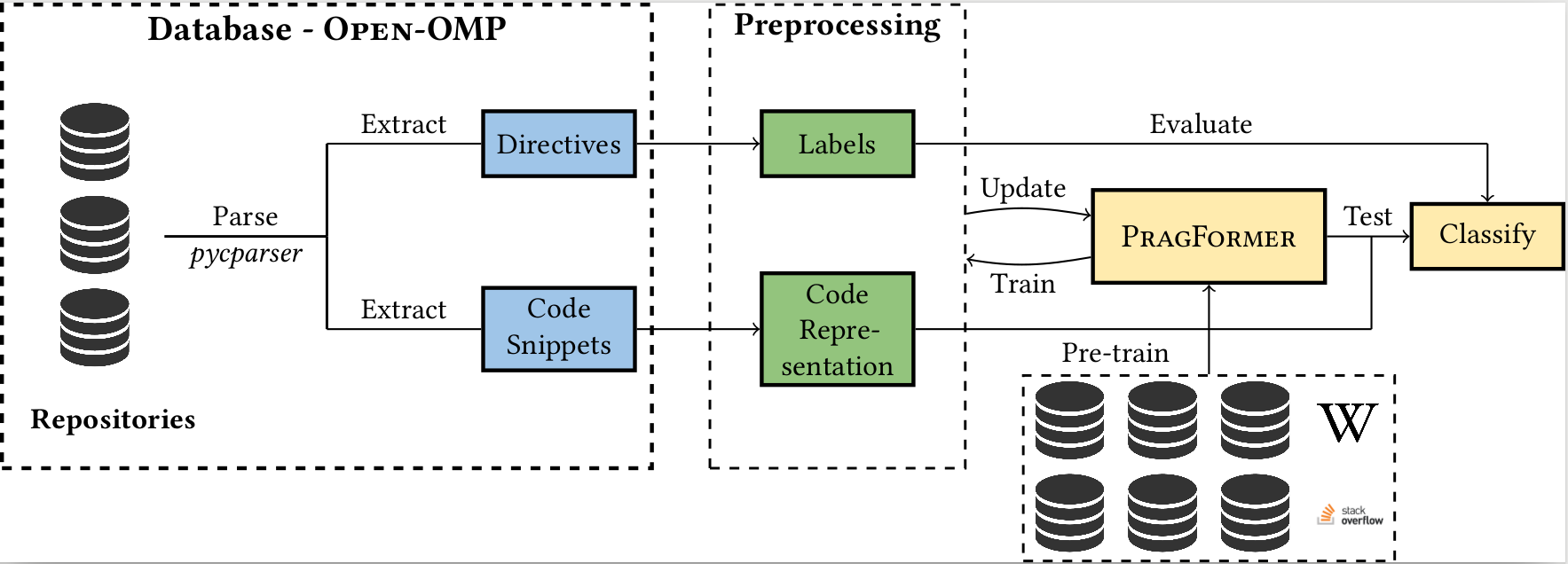 | |
Link to [PragFormer](https://arxiv.org/abs/2204.12835) Paper | |
""") | |
with gr.Row(): | |
with gr.Column(): | |
gr.Markdown("## Input") | |
with gr.Row(): | |
with gr.Column(): | |
drop = gr.Dropdown(['a', 'b'], label="Random Code Snippet") # list(data.keys()) | |
sample_btn = gr.Button("Sample") | |
pragma = gr.Textbox(label="Pragma") | |
code_in = gr.Textbox(lines=5, label="Write some code and see if it should be parallelized with OpenMP") | |
submit_btn = gr.Button("Submit") | |
with gr.Column(): | |
gr.Markdown("## Results") | |
label_out = gr.Textbox(label="Label") | |
confidence_out = gr.Textbox(label="Confidence") | |
with gr.Row(): | |
private = gr.Textbox(label="Private", visible=False) | |
reduction = gr.Textbox(label="Reduction", visible=False) | |
submit_btn.click(fn=predict, inputs=code_in, outputs=[label_out, confidence_out]) | |
submit_btn.click(fn=is_private, inputs=code_in, outputs=private) | |
submit_btn.click(fn=is_reduction, inputs=code_in, outputs=reduction) | |
sample_btn.click(fn=fill_code, inputs=drop, outputs=[pragma, code_in]) | |
pragformer_gui.launch() | |