{"guide": {"name": "getting-started-with-the-python-client", "category": "gradio-clients-and-lite", "pretty_category": "Gradio Clients And Lite", "guide_index": 1, "absolute_index": 48, "pretty_name": "Getting Started With The Python Client", "content": "# Getting Started with the Gradio Python client\n\n\n\nThe Gradio Python client makes it very easy to use any Gradio app as an API. As an example, consider this [Hugging Face Space that transcribes audio files](https://huggingface.co/spaces/abidlabs/whisper) that are recorded from the microphone.\n\n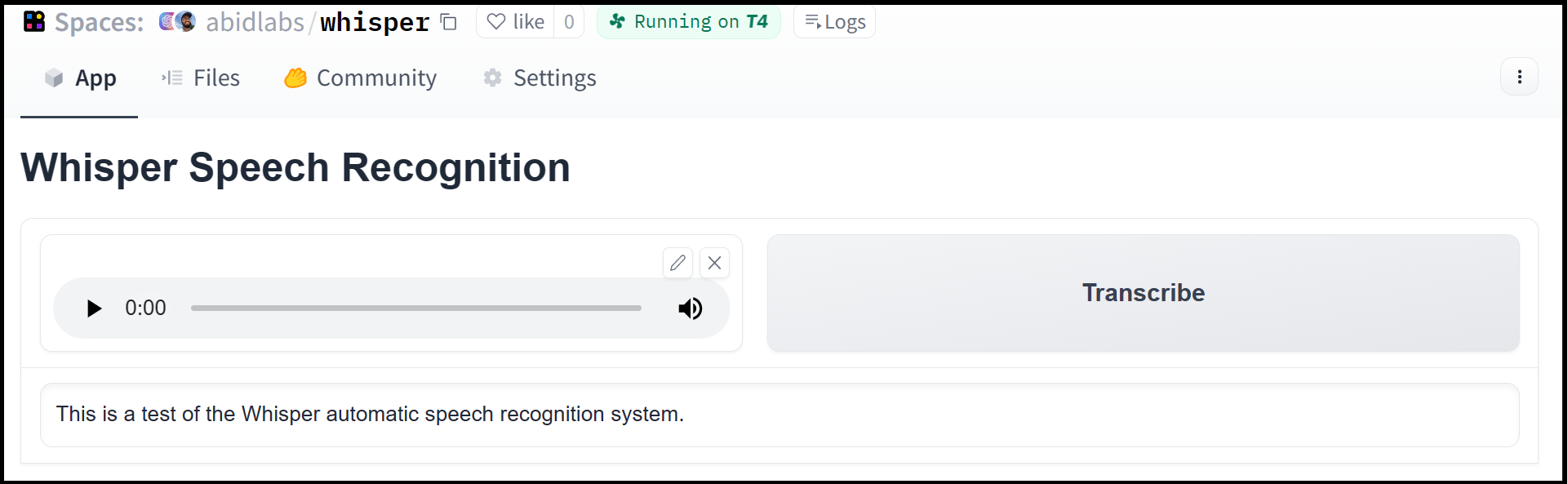\n\nUsing the `gradio_client` library, we can easily use the Gradio as an API to transcribe audio files programmatically.\n\nHere's the entire code to do it:\n\n```python\nfrom gradio_client import Client, handle_file\n\nclient = Client(\"abidlabs/whisper\")\n\nclient.predict(\n audio=handle_file(\"audio_sample.wav\")\n)\n\n>> \"This is a test of the whisper speech recognition model.\"\n```\n\nThe Gradio client works with any hosted Gradio app! Although the Client is mostly used with apps hosted on [Hugging Face Spaces](https://hf.space), your app can be hosted anywhere, such as your own server.\n\n**Prerequisites**: To use the Gradio client, you do _not_ need to know the `gradio` library in great detail. However, it is helpful to have general familiarity with Gradio's concepts of input and output components.\n\n## Installation\n\nIf you already have a recent version of `gradio`, then the `gradio_client` is included as a dependency. But note that this documentation reflects the latest version of the `gradio_client`, so upgrade if you're not sure!\n\nThe lightweight `gradio_client` package can be installed from pip (or pip3) and is tested to work with **Python versions 3.10 or higher**:\n\n```bash\n$ pip install --upgrade gradio_client\n```\n\n## Connecting to a Gradio App on Hugging Face Spaces\n\nStart by connecting instantiating a `Client` object and connecting it to a Gradio app that is running on Hugging Face Spaces.\n\n```python\nfrom gradio_client import Client\n\nclient = Client(\"abidlabs/en2fr\") # a Space that translates from English to French\n```\n\nYou can also connect to private Spaces by passing in your HF token with the `hf_token` parameter. You can get your HF token here: https://huggingface.co/settings/tokens\n\n```python\nfrom gradio_client import Client\n\nclient = Client(\"abidlabs/my-private-space\", hf_token=\"...\")\n```\n\n\n## Duplicating a Space for private use\n\nWhile you can use any public Space as an API, you may get rate limited by Hugging Face if you make too many requests. For unlimited usage of a Space, simply duplicate the Space to create a private Space,\nand then use it to make as many requests as you'd like!\n\nThe `gradio_client` includes a class method: `Client.duplicate()` to make this process simple (you'll need to pass in your [Hugging Face token](https://huggingface.co/settings/tokens) or be logged in using the Hugging Face CLI):\n\n```python\nimport os\nfrom gradio_client import Client, handle_file\n\nHF_TOKEN = os.environ.get(\"HF_TOKEN\")\n\nclient = Client.duplicate(\"abidlabs/whisper\", hf_token=HF_TOKEN)\nclient.predict(handle_file(\"audio_sample.wav\"))\n\n>> \"This is a test of the whisper speech recognition model.\"\n```\n\nIf you have previously duplicated a Space, re-running `duplicate()` will _not_ create a new Space. Instead, the Client will attach to the previously-created Space. So it is safe to re-run the `Client.duplicate()` method multiple times.\n\n**Note:** if the original Space uses GPUs, your private Space will as well, and your Hugging Face account will get billed based on the price of the GPU. To minimize charges, your Space will automatically go to sleep after 1 hour of inactivity. You can also set the hardware using the `hardware` parameter of `duplicate()`.\n\n## Connecting a general Gradio app\n\nIf your app is running somewhere else, just provide the full URL instead, including the \"http://\" or \"https://\". Here's an example of making predictions to a Gradio app that is running on a share URL:\n\n```python\nfrom gradio_client import Client\n\nclient = Client(\"https://bec81a83-5b5c-471e.gradio.live\")\n```\n\n## Connecting to a Gradio app with auth\n\nIf the Gradio application you are connecting to [requires a username and password](/guides/sharing-your-app#authentication), then provide them as a tuple to the `auth` argument of the `Client` class:\n\n```python\nfrom gradio_client import Client\n\nClient(\n space_name,\n auth=[username, password]\n)\n```\n\n\n## Inspecting the API endpoints\n\nOnce you have connected to a Gradio app, you can view the APIs that are available to you by calling the `Client.view_api()` method. For the Whisper Space, we see the following:\n\n```bash\nClient.predict() Usage Info\n---------------------------\nNamed API endpoints: 1\n\n - predict(audio, api_name=\"/predict\") -> output\n Parameters:\n - [Audio] audio: filepath (required) \n Returns:\n - [Textbox] output: str \n```\n\nWe see that we have 1 API endpoint in this space, and shows us how to use the API endpoint to make a prediction: we should call the `.predict()` method (which we will explore below), providing a parameter `input_audio` of type `str`, which is a `filepath or URL`.\n\nWe should also provide the `api_name='/predict'` argument to the `predict()` method. Although this isn't necessary if a Gradio app has only 1 named endpoint, it does allow us to call different endpoints in a single app if they are available.\n\n## The \"View API\" Page\n\nAs an alternative to running the `.view_api()` method, you can click on the \"Use via API\" link in the footer of the Gradio app, which shows us the same information, along with example usage. \n\n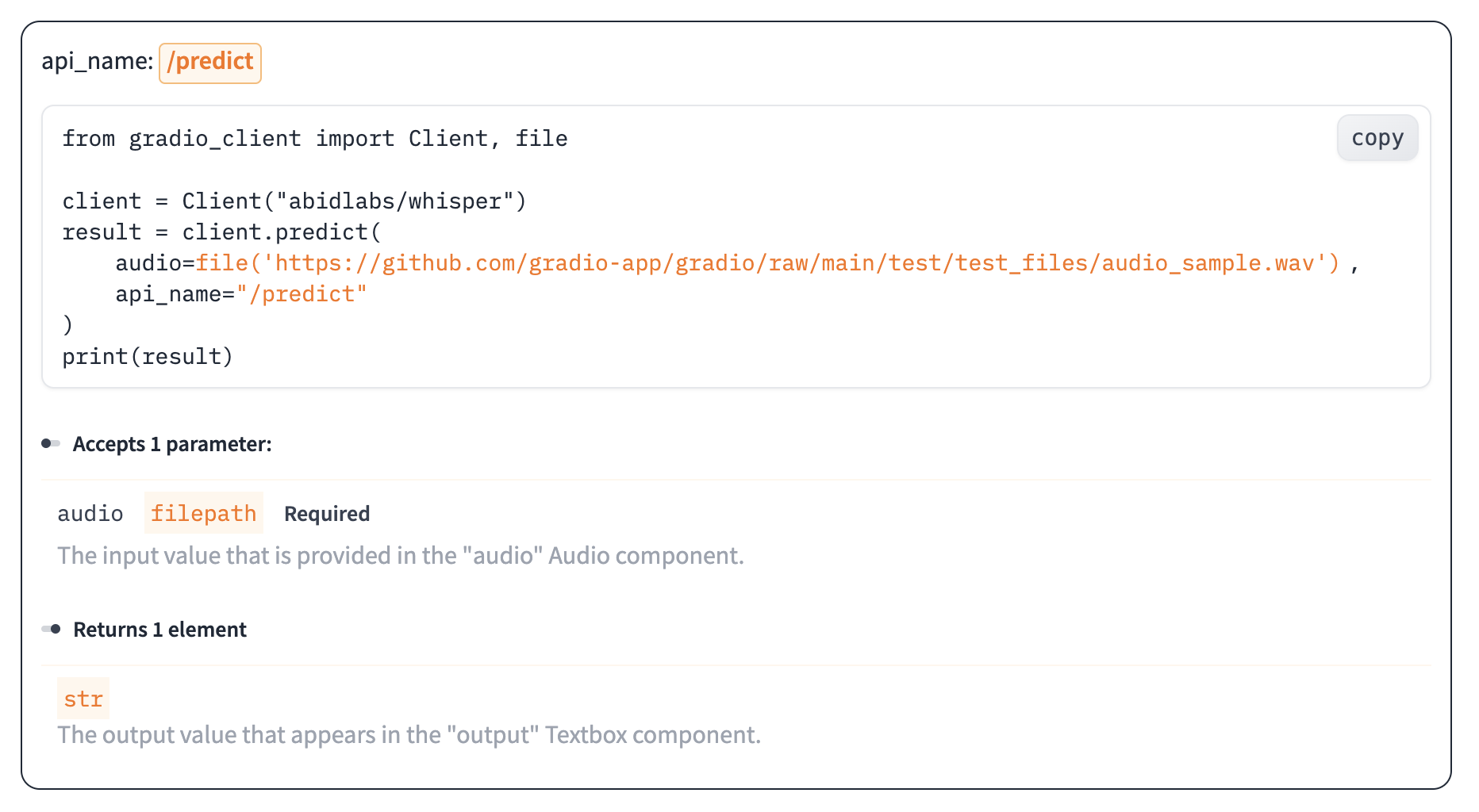\n\nThe View API page also includes an \"API Recorder\" that lets you interact with the Gradio UI normally and converts your interactions into the corresponding code to run with the Python Client.\n\n## Making a prediction\n\nThe simplest way to make a prediction is simply to call the `.predict()` function with the appropriate arguments:\n\n```python\nfrom gradio_client import Client\n\nclient = Client(\"abidlabs/en2fr\", api_name='/predict')\nclient.predict(\"Hello\")\n\n>> Bonjour\n```\n\nIf there are multiple parameters, then you should pass them as separate arguments to `.predict()`, like this:\n\n```python\nfrom gradio_client import Client\n\nclient = Client(\"gradio/calculator\")\nclient.predict(4, \"add\", 5)\n\n>> 9.0\n```\n\nIt is recommended to provide key-word arguments instead of positional arguments:\n\n\n```python\nfrom gradio_client import Client\n\nclient = Client(\"gradio/calculator\")\nclient.predict(num1=4, operation=\"add\", num2=5)\n\n>> 9.0\n```\n\nThis allows you to take advantage of default arguments. For example, this Space includes the default value for the Slider component so you do not need to provide it when accessing it with the client.\n\n```python\nfrom gradio_client import Client\n\nclient = Client(\"abidlabs/image_generator\")\nclient.predict(text=\"an astronaut riding a camel\")\n```\n\nThe default value is the initial value of the corresponding Gradio component. If the component does not have an initial value, but if the corresponding argument in the predict function has a default value of `None`, then that parameter is also optional in the client. Of course, if you'd like to override it, you can include it as well:\n\n```python\nfrom gradio_client import Client\n\nclient = Client(\"abidlabs/image_generator\")\nclient.predict(text=\"an astronaut riding a camel\", steps=25)\n```\n\nFor providing files or URLs as inputs, you should pass in the filepath or URL to the file enclosed within `gradio_client.handle_file()`. This takes care of uploading the file to the Gradio server and ensures that the file is preprocessed correctly:\n\n```python\nfrom gradio_client import Client, handle_file\n\nclient = Client(\"abidlabs/whisper\")\nclient.predict(\n audio=handle_file(\"https://audio-samples.github.io/samples/mp3/blizzard_unconditional/sample-0.mp3\")\n)\n\n>> \"My thought I have nobody by a beauty and will as you poured. Mr. Rochester is serve in that so don't find simpus, and devoted abode, to at might in a r\u2014\"\n```\n\n## Running jobs asynchronously\n\nOe should note that `.predict()` is a _blocking_ operation as it waits for the operation to complete before returning the prediction.\n\nIn many cases, you may be better off letting the job run in the background until you need the results of the prediction. You can do this by creating a `Job` instance using the `.submit()` method, and then later calling `.result()` on the job to get the result. For example:\n\n```python\nfrom gradio_client import Client\n\nclient = Client(space=\"abidlabs/en2fr\")\njob = client.submit(\"Hello\", api_name=\"/predict\") # This is not blocking\n\n# Do something else\n\njob.result() # This is blocking\n\n>> Bonjour\n```\n\n## Adding callbacks\n\nAlternatively, one can add one or more callbacks to perform actions after the job has completed running, like this:\n\n```python\nfrom gradio_client import Client\n\ndef print_result(x):\n print(\"The translated result is: {x}\")\n\nclient = Client(space=\"abidlabs/en2fr\")\n\njob = client.submit(\"Hello\", api_name=\"/predict\", result_callbacks=[print_result])\n\n# Do something else\n\n>> The translated result is: Bonjour\n\n```\n\n## Status\n\nThe `Job` object also allows you to get the status of the running job by calling the `.status()` method. This returns a `StatusUpdate` object with the following attributes: `code` (the status code, one of a set of defined strings representing the status. See the `utils.Status` class), `rank` (the current position of this job in the queue), `queue_size` (the total queue size), `eta` (estimated time this job will complete), `success` (a boolean representing whether the job completed successfully), and `time` (the time that the status was generated).\n\n```py\nfrom gradio_client import Client\n\nclient = Client(src=\"gradio/calculator\")\njob = client.submit(5, \"add\", 4, api_name=\"/predict\")\njob.status()\n\n>> <Status.STARTING: 'STARTING'>\n```\n\n_Note_: The `Job` class also has a `.done()` instance method which returns a boolean indicating whether the job has completed.\n\n## Cancelling Jobs\n\nThe `Job` class also has a `.cancel()` instance method that cancels jobs that have been queued but not started. For example, if you run:\n\n```py\nclient = Client(\"abidlabs/whisper\")\njob1 = client.submit(handle_file(\"audio_sample1.wav\"))\njob2 = client.submit(handle_file(\"audio_sample2.wav\"))\njob1.cancel() # will return False, assuming the job has started\njob2.cancel() # will return True, indicating that the job has been canceled\n```\n\nIf the first job has started processing, then it will not be canceled. If the second job\nhas not yet started, it will be successfully canceled and removed from the queue.\n\n## Generator Endpoints\n\nSome Gradio API endpoints do not return a single value, rather they return a series of values. You can get the series of values that have been returned at any time from such a generator endpoint by running `job.outputs()`:\n\n```py\nfrom gradio_client import Client\n\nclient = Client(src=\"gradio/count_generator\")\njob = client.submit(3, api_name=\"/count\")\nwhile not job.done():\n time.sleep(0.1)\njob.outputs()\n\n>> ['0', '1', '2']\n```\n\nNote that running `job.result()` on a generator endpoint only gives you the _first_ value returned by the endpoint.\n\nThe `Job` object is also iterable, which means you can use it to display the results of a generator function as they are returned from the endpoint. Here's the equivalent example using the `Job` as a generator:\n\n```py\nfrom gradio_client import Client\n\nclient = Client(src=\"gradio/count_generator\")\njob = client.submit(3, api_name=\"/count\")\n\nfor o in job:\n print(o)\n\n>> 0\n>> 1\n>> 2\n```\n\nYou can also cancel jobs that that have iterative outputs, in which case the job will finish as soon as the current iteration finishes running.\n\n```py\nfrom gradio_client import Client\nimport time\n\nclient = Client(\"abidlabs/test-yield\")\njob = client.submit(\"abcdef\")\ntime.sleep(3)\njob.cancel() # job cancels after 2 iterations\n```\n\n## Demos with Session State\n\nGradio demos can include [session state](https://www.gradio.app/guides/state-in-blocks), which provides a way for demos to persist information from user interactions within a page session.\n\nFor example, consider the following demo, which maintains a list of words that a user has submitted in a `gr.State` component. When a user submits a new word, it is added to the state, and the number of previous occurrences of that word is displayed:\n\n```python\nimport gradio as gr\n\ndef count(word, list_of_words):\n return list_of_words.count(word), list_of_words + [word]\n\nwith gr.Blocks() as demo:\n words = gr.State([])\n textbox = gr.Textbox()\n number = gr.Number()\n textbox.submit(count, inputs=[textbox, words], outputs=[number, words])\n \ndemo.launch()\n```\n\nIf you were to connect this this Gradio app using the Python Client, you would notice that the API information only shows a single input and output:\n\n```csv\nClient.predict() Usage Info\n---------------------------\nNamed API endpoints: 1\n\n - predict(word, api_name=\"/count\") -> value_31\n Parameters:\n - [Textbox] word: str (required) \n Returns:\n - [Number] value_31: float \n```\n\nThat is because the Python client handles state automatically for you -- as you make a series of requests, the returned state from one request is stored internally and automatically supplied for the subsequent request. If you'd like to reset the state, you can do that by calling `Client.reset_session()`.\n", "tags": ["CLIENT", "API", "SPACES"], "spaces": [], "url": "/guides/getting-started-with-the-python-client/", "contributor": null}} |