Commit
·
2f2f929
1
Parent(s):
c79ab78
"Update README.md"
Browse files
README.md
ADDED
@@ -0,0 +1,201 @@
|
|
|
|
|
|
|
|
|
|
|
|
|
|
|
|
|
|
|
|
|
|
|
|
|
|
|
|
|
|
|
|
|
|
|
|
|
|
|
|
|
|
|
|
|
|
|
|
|
|
|
|
|
|
|
|
|
|
|
|
|
|
|
|
|
|
|
|
|
|
|
|
|
|
|
|
|
|
|
|
|
|
|
|
|
|
|
|
|
|
|
|
|
|
|
|
|
|
|
|
|
|
|
|
|
|
|
|
|
|
|
|
|
|
|
|
|
|
|
|
|
|
|
|
|
|
|
|
|
|
|
|
|
|
|
|
|
|
|
|
|
|
|
|
|
|
|
|
|
|
|
|
|
|
|
|
|
|
|
|
|
|
|
|
|
|
|
|
|
|
|
|
|
|
|
|
|
|
|
|
|
|
|
|
|
|
|
|
|
|
|
|
|
|
|
|
|
|
|
|
|
|
|
|
|
|
|
|
|
|
|
|
|
|
|
|
|
|
|
|
|
|
|
|
|
|
|
|
|
|
|
|
|
|
|
|
|
|
|
|
|
|
|
|
|
|
|
|
|
|
|
|
|
|
|
|
|
|
|
|
|
|
|
|
|
|
|
|
|
|
|
|
|
|
|
|
|
|
|
|
|
|
|
|
|
|
|
|
|
|
|
|
|
|
|
|
|
|
|
|
|
|
|
|
|
|
|
|
|
|
|
|
|
|
|
|
|
|
|
|
|
|
|
|
|
|
|
|
|
|
|
|
|
|
|
|
|
|
|
|
|
|
|
|
|
|
|
|
|
|
|
|
|
|
|
|
|
|
|
|
|
|
|
|
|
|
|
|
|
|
|
|
|
|
|
|
|
|
|
|
|
|
|
|
|
|
|
|
|
|
|
|
|
|
|
|
|
|
|
|
|
|
|
|
|
1 |
+
---
|
2 |
+
license: apache-2.0
|
3 |
+
---
|
4 |
+
[]()
|
5 |
+
|
6 |
+
I'm constantly enhancing these model descriptions to provide you with the most relevant and comprehensive information
|
7 |
+
|
8 |
+
# bling-stable-lm-3b-4e1t-v0 - GGUF
|
9 |
+
- Model creator: [llmware](https://huggingface.co/llmware)
|
10 |
+
- Original model: [bling-stable-lm-3b-4e1t-v0](https://huggingface.co/llmware/bling-stable-lm-3b-4e1t-v0)
|
11 |
+
|
12 |
+
# StableLM
|
13 |
+
This is a Model based on StableLM.
|
14 |
+
Stablelm is a familiy of Language Models by Stability AI.
|
15 |
+
|
16 |
+
## Note:
|
17 |
+
Current (as of 2023-11-15) implementations of Llama.cpp only support GPU offloading up to 34 Layers with these StableLM Models.
|
18 |
+
The model will crash immediately if -ngl is larger than 34.
|
19 |
+
The model works fine however without any gpu acceleration.
|
20 |
+
|
21 |
+
|
22 |
+
|
23 |
+
# About GGUF format
|
24 |
+
|
25 |
+
`gguf` is the current file format used by the [`ggml`](https://github.com/ggerganov/ggml) library.
|
26 |
+
A growing list of Software is using it and can therefore use this model.
|
27 |
+
The core project making use of the ggml library is the [llama.cpp](https://github.com/ggerganov/llama.cpp) project by Georgi Gerganov
|
28 |
+
|
29 |
+
# Quantization variants
|
30 |
+
|
31 |
+
There is a bunch of quantized files available to cater to your specific needs. Here's how to choose the best option for you:
|
32 |
+
|
33 |
+
# Legacy quants
|
34 |
+
|
35 |
+
Q4_0, Q4_1, Q5_0, Q5_1 and Q8 are `legacy` quantization types.
|
36 |
+
Nevertheless, they are fully supported, as there are several circumstances that cause certain model not to be compatible with the modern K-quants.
|
37 |
+
## Note:
|
38 |
+
Now there's a new option to use K-quants even for previously 'incompatible' models, although this involves some fallback solution that makes them not *real* K-quants. More details can be found in affected model descriptions.
|
39 |
+
(This mainly refers to Falcon 7b and Starcoder models)
|
40 |
+
|
41 |
+
# K-quants
|
42 |
+
|
43 |
+
K-quants are designed with the idea that different levels of quantization in specific parts of the model can optimize performance, file size, and memory load.
|
44 |
+
So, if possible, use K-quants.
|
45 |
+
With a Q6_K, you'll likely find it challenging to discern a quality difference from the original model - ask your model two times the same question and you may encounter bigger quality differences.
|
46 |
+
|
47 |
+
|
48 |
+
|
49 |
+
|
50 |
+
---
|
51 |
+
|
52 |
+
# Original Model Card:
|
53 |
+
# Model Card for Model ID
|
54 |
+
|
55 |
+
<!-- Provide a quick summary of what the model is/does. -->
|
56 |
+
|
57 |
+
bling-stable-lm-3b-4e1t-0.1 part of the BLING ("Best Little Instruction-following No-GPU-required") model series, RAG-instruct trained on top of a StabilityAI stablelm-3b-4e1t base model.
|
58 |
+
|
59 |
+
BLING models are fine-tuned with distilled high-quality custom instruct datasets, targeted at a specific subset of instruct tasks with
|
60 |
+
the objective of providing a high-quality Instruct model that is 'inference-ready' on a CPU laptop even
|
61 |
+
without using any advanced quantization optimizations.
|
62 |
+
|
63 |
+
|
64 |
+
### Benchmark Tests
|
65 |
+
|
66 |
+
Evaluated against the benchmark test: [RAG-Instruct-Benchmark-Tester](https://www.huggingface.co/datasets/llmware/rag_instruct_benchmark_tester)
|
67 |
+
Average of 2 Test Runs with 1 point for correct answer, 0.5 point for partial correct or blank / NF, 0.0 points for incorrect, and -1 points for hallucinations.
|
68 |
+
|
69 |
+
--**Accuracy Score**: **94.0** correct out of 100
|
70 |
+
--Not Found Classification: 67.5%
|
71 |
+
--Boolean: 77.5%
|
72 |
+
--Math/Logic: 29%
|
73 |
+
--Complex Questions (1-5): 3 (Low)
|
74 |
+
--Summarization Quality (1-5): 3 (Coherent, extractive)
|
75 |
+
--Hallucinations: No hallucinations observed in test runs.
|
76 |
+
|
77 |
+
For test run results (and good indicator of target use cases), please see the files ("core_rag_test" and "answer_sheet" in this repo).
|
78 |
+
|
79 |
+
### Model Description
|
80 |
+
|
81 |
+
<!-- Provide a longer summary of what this model is. -->
|
82 |
+
|
83 |
+
- **Developed by:** llmware
|
84 |
+
- **Model type:** Instruct-trained decoder
|
85 |
+
- **Language(s) (NLP):** English
|
86 |
+
- **License:** [CC BY-SA-4.0](https://creativecommons.org/licenses/by-sa/4.0/)
|
87 |
+
- **Finetuned from model:** stabilityai/stablelm-3b-4e1t
|
88 |
+
|
89 |
+
|
90 |
+
## Uses
|
91 |
+
|
92 |
+
<!-- Address questions around how the model is intended to be used, including the foreseeable users of the model and those affected by the model. -->
|
93 |
+
|
94 |
+
The intended use of BLING models is two-fold:
|
95 |
+
|
96 |
+
1. Provide high-quality Instruct models that can run on a laptop for local testing. We have found it extremely useful when building a
|
97 |
+
proof-of-concept, or working with sensitive enterprise data that must be closely guarded, especially in RAG use cases.
|
98 |
+
|
99 |
+
2. Push the state of the art for smaller Instruct-following models in the sub-7B parameter range, especially 1B-3B, as single-purpose
|
100 |
+
automation tools for specific tasks through targeted fine-tuning datasets and focused "instruction" tasks.
|
101 |
+
|
102 |
+
|
103 |
+
### Direct Use
|
104 |
+
|
105 |
+
<!-- This section is for the model use without fine-tuning or plugging into a larger ecosystem/app. -->
|
106 |
+
|
107 |
+
BLING is designed for enterprise automation use cases, especially in knowledge-intensive industries, such as financial services,
|
108 |
+
legal and regulatory industries with complex information sources. Rather than try to be "all things to all people," BLING models try to focus on a narrower set of Instructions more suitable to a ~1-3B parameter GPT model.
|
109 |
+
|
110 |
+
BLING is ideal for rapid prototyping, testing, and the ability to perform an end-to-end workflow locally on a laptop without
|
111 |
+
having to send sensitive information over an Internet-based API.
|
112 |
+
|
113 |
+
The first BLING models have been trained for common RAG scenarios, specifically: question-answering, key-value extraction, and basic summarization as the core instruction types
|
114 |
+
without the need for a lot of complex instruction verbiage - provide a text passage context, ask questions, and get clear fact-based responses.
|
115 |
+
|
116 |
+
|
117 |
+
## Bias, Risks, and Limitations
|
118 |
+
|
119 |
+
<!-- This section is meant to convey both technical and sociotechnical limitations. -->
|
120 |
+
|
121 |
+
Any model can provide inaccurate or incomplete information, and should be used in conjunction with appropriate safeguards and fact-checking mechanisms.
|
122 |
+
|
123 |
+
|
124 |
+
## How to Get Started with the Model
|
125 |
+
|
126 |
+
The fastest way to get started with BLING is through direct import in transformers:
|
127 |
+
|
128 |
+
from transformers import AutoTokenizer, AutoModelForCausalLM
|
129 |
+
tokenizer = AutoTokenizer.from_pretrained("llmware/bling-stable-lm-3b-4e1t-0.1")
|
130 |
+
model = AutoModelForCausalLM.from_pretrained("llmware/bling-stable-lm-3b-4e1t-0.1")
|
131 |
+
|
132 |
+
Please refer to the generation_test .py files in the Files repository, which includes 200 samples and script to test the model. The **generation_test_llmware_script.py** includes built-in llmware capabilities for fact-checking, as well as easy integration with document parsing and actual retrieval to swap out the test set for RAG workflow consisting of business documents.
|
133 |
+
|
134 |
+
The BLING model was fine-tuned with a simple "\<human> and \<bot> wrapper", so to get the best results, wrap inference entries as:
|
135 |
+
|
136 |
+
full_prompt = "<human>: " + my_prompt + "\n" + "<bot>:"
|
137 |
+
|
138 |
+
The BLING model was fine-tuned with closed-context samples, which assume generally that the prompt consists of two sub-parts:
|
139 |
+
|
140 |
+
1. Text Passage Context, and
|
141 |
+
2. Specific question or instruction based on the text passage
|
142 |
+
|
143 |
+
To get the best results, package "my_prompt" as follows:
|
144 |
+
|
145 |
+
my_prompt = {{text_passage}} + "\n" + {{question/instruction}}
|
146 |
+
|
147 |
+
|
148 |
+
If you are using a HuggingFace generation script:
|
149 |
+
|
150 |
+
# prepare prompt packaging used in fine-tuning process
|
151 |
+
new_prompt = "<human>: " + entries["context"] + "\n" + entries["query"] + "\n" + "<bot>:"
|
152 |
+
|
153 |
+
inputs = tokenizer(new_prompt, return_tensors="pt")
|
154 |
+
start_of_output = len(inputs.input_ids[0])
|
155 |
+
|
156 |
+
# temperature: set at 0.3 for consistency of output
|
157 |
+
# max_new_tokens: set at 100 - may prematurely stop a few of the summaries
|
158 |
+
|
159 |
+
outputs = model.generate(
|
160 |
+
inputs.input_ids.to(device),
|
161 |
+
eos_token_id=tokenizer.eos_token_id,
|
162 |
+
pad_token_id=tokenizer.eos_token_id,
|
163 |
+
do_sample=True,
|
164 |
+
temperature=0.3,
|
165 |
+
max_new_tokens=100,
|
166 |
+
)
|
167 |
+
|
168 |
+
output_only = tokenizer.decode(outputs[0][start_of_output:],skip_special_tokens=True)
|
169 |
+
|
170 |
+
|
171 |
+
## Citations
|
172 |
+
|
173 |
+
This model has been fine-tuned on the base StableLM-3B-4E1T model from StabilityAI. For more information about this base model, please see the citation below:
|
174 |
+
|
175 |
+
@misc{StableLM-3B-4E1T,
|
176 |
+
url={[https://huggingface.co/stabilityai/stablelm-3b-4e1t](https://huggingface.co/stabilityai/stablelm-3b-4e1t)},
|
177 |
+
title={StableLM 3B 4E1T},
|
178 |
+
author={Tow, Jonathan and Bellagente, Marco and Mahan, Dakota and Riquelme, Carlos}
|
179 |
+
}
|
180 |
+
|
181 |
+
|
182 |
+
## Model Card Contact
|
183 |
+
|
184 |
+
Darren Oberst & llmware team
|
185 |
+
|
186 |
+
***End of original Model File***
|
187 |
+
---
|
188 |
+
|
189 |
+
|
190 |
+
## Please consider to support my work
|
191 |
+
**Coming Soon:** I'm in the process of launching a sponsorship/crowdfunding campaign for my work. I'm evaluating Kickstarter, Patreon, or the new GitHub Sponsors platform, and I am hoping for some support and contribution to the continued availability of these kind of models. Your support will enable me to provide even more valuable resources and maintain the models you rely on. Your patience and ongoing support are greatly appreciated as I work to make this page an even more valuable resource for the community.
|
192 |
+
|
193 |
+
<center>
|
194 |
+
|
195 |
+
[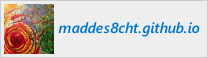](https://maddes8cht.github.io)
|
196 |
+
[](https://stackexchange.com/users/26485911)
|
197 |
+
[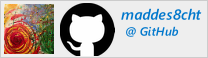](https://github.com/maddes8cht)
|
198 |
+
[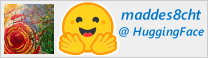](https://huggingface.co/maddes8cht)
|
199 |
+
[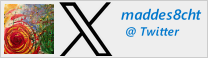](https://twitter.com/maddes1966)
|
200 |
+
|
201 |
+
</center>
|