id
stringlengths 2
115
| README
stringlengths 0
977k
|
---|---|
Datatang/mandarin_chinese | ---
YAML tags:
- copy-paste the tags obtained with the tagging app: https://github.com/huggingface/datasets-tagging
---
# Dataset Card for mandarin_chinese
## Table of Contents
- [Table of Contents](#table-of-contents)
- [Dataset Description](#dataset-description)
- [Dataset Summary](#dataset-summary)
- [Supported Tasks and Leaderboards](#supported-tasks-and-leaderboards)
- [Languages](#languages)
- [Dataset Structure](#dataset-structure)
- [Data Instances](#data-instances)
- [Data Fields](#data-fields)
- [Data Splits](#data-splits)
- [Dataset Creation](#dataset-creation)
- [Curation Rationale](#curation-rationale)
- [Source Data](#source-data)
- [Annotations](#annotations)
- [Personal and Sensitive Information](#personal-and-sensitive-information)
- [Considerations for Using the Data](#considerations-for-using-the-data)
- [Social Impact of Dataset](#social-impact-of-dataset)
- [Discussion of Biases](#discussion-of-biases)
- [Other Known Limitations](#other-known-limitations)
- [Additional Information](#additional-information)
- [Dataset Curators](#dataset-curators)
- [Licensing Information](#licensing-information)
- [Citation Information](#citation-information)
- [Contributions](#contributions)
## Dataset Description
- **Homepage:** www.datatang.ai
- **Repository:**
- **Paper:**
- **Leaderboard:**
- **Point of Contact:**
### Dataset Summary
The dataset contains 15,000 hours of Mandarin Chinese speech data. It's collected from local Mandarin speakers in 33 provinces of China, covering mutiple scenes and enviroments. The format is 16kHz, 16bit, uncompressed wav, mono channel. The sentence accuracy is over 97%.
For more details, please refer to the link: https://bit.ly/39UzIwI
### Supported Tasks and Leaderboards
automatic-speech-recognition, audio-speaker-identification: The dataset can be used to train a model for Automatic Speech Recognition (ASR).
### Languages
Mandarin
## Dataset Structure
### Data Instances
[More Information Needed]
### Data Fields
[More Information Needed]
### Data Splits
[More Information Needed]
## Dataset Creation
### Curation Rationale
[More Information Needed]
### Source Data
#### Initial Data Collection and Normalization
[More Information Needed]
#### Who are the source language producers?
[More Information Needed]
### Annotations
#### Annotation process
[More Information Needed]
#### Who are the annotators?
[More Information Needed]
### Personal and Sensitive Information
[More Information Needed]
## Considerations for Using the Data
### Social Impact of Dataset
[More Information Needed]
### Discussion of Biases
[More Information Needed]
### Other Known Limitations
[More Information Needed]
## Additional Information
### Dataset Curators
[More Information Needed]
### Licensing Information
Commerical License: https://drive.google.com/file/d/1saDCPm74D4UWfBL17VbkTsZLGfpOQj1J/view?usp=sharing
### Citation Information
[More Information Needed]
### Contributions
|
Datatang/mixed_speech_chinese_english | ---
YAML tags:
- copy-paste the tags obtained with the tagging app: https://github.com/huggingface/datasets-tagging
---
# Dataset Card for mixed_speech_chinese_english
## Table of Contents
- [Table of Contents](#table-of-contents)
- [Dataset Description](#dataset-description)
- [Dataset Summary](#dataset-summary)
- [Supported Tasks and Leaderboards](#supported-tasks-and-leaderboards)
- [Languages](#languages)
- [Dataset Structure](#dataset-structure)
- [Data Instances](#data-instances)
- [Data Fields](#data-fields)
- [Data Splits](#data-splits)
- [Dataset Creation](#dataset-creation)
- [Curation Rationale](#curation-rationale)
- [Source Data](#source-data)
- [Annotations](#annotations)
- [Personal and Sensitive Information](#personal-and-sensitive-information)
- [Considerations for Using the Data](#considerations-for-using-the-data)
- [Social Impact of Dataset](#social-impact-of-dataset)
- [Discussion of Biases](#discussion-of-biases)
- [Other Known Limitations](#other-known-limitations)
- [Additional Information](#additional-information)
- [Dataset Curators](#dataset-curators)
- [Licensing Information](#licensing-information)
- [Citation Information](#citation-information)
- [Contributions](#contributions)
## Dataset Description
- **Homepage:** www.datatang.ai
- **Repository:**
- **Paper:**
- **Leaderboard:**
- **Point of Contact:**
### Dataset Summary
The dataset contains 2,000 hours of mixed speech with Chinese and English. The data is collected from speakers in 26 provinces like Henan, Shanxi, Sichuan, Hunan, Fujian, etc.The content covers generic scene and multiple human machine interation scenes, such as music, entertainment, travel, daily life. The data covers more than 30,000 English words. The sentence accuracy is over 97%. For more details, please refer to the link: https://bit.ly/39UzIwI
### Supported Tasks and Leaderboards
automatic-speech-recognition, audio-speaker-identification: The dataset can be used to train a model for Automatic Speech Recognition (ASR).
### Languages
Chinese, English
## Dataset Structure
### Data Instances
[More Information Needed]
### Data Fields
[More Information Needed]
### Data Splits
[More Information Needed]
## Dataset Creation
### Curation Rationale
[More Information Needed]
### Source Data
#### Initial Data Collection and Normalization
[More Information Needed]
#### Who are the source language producers?
[More Information Needed]
### Annotations
#### Annotation process
[More Information Needed]
#### Who are the annotators?
[More Information Needed]
### Personal and Sensitive Information
[More Information Needed]
## Considerations for Using the Data
### Social Impact of Dataset
[More Information Needed]
### Discussion of Biases
[More Information Needed]
### Other Known Limitations
[More Information Needed]
## Additional Information
### Dataset Curators
[More Information Needed]
### Licensing Information
Commerical License: https://drive.google.com/file/d/1saDCPm74D4UWfBL17VbkTsZLGfpOQj1J/view?usp=sharing
### Citation Information
[More Information Needed]
### Contributions
|
Datatang/multi_language | ---
YAML tags:
- copy-paste the tags obtained with the tagging app: https://github.com/huggingface/datasets-tagging
---
# Dataset Card for multi_language
## Table of Contents
- [Table of Contents](#table-of-contents)
- [Dataset Description](#dataset-description)
- [Dataset Summary](#dataset-summary)
- [Supported Tasks and Leaderboards](#supported-tasks-and-leaderboards)
- [Languages](#languages)
- [Dataset Structure](#dataset-structure)
- [Data Instances](#data-instances)
- [Data Fields](#data-fields)
- [Data Splits](#data-splits)
- [Dataset Creation](#dataset-creation)
- [Curation Rationale](#curation-rationale)
- [Source Data](#source-data)
- [Annotations](#annotations)
- [Personal and Sensitive Information](#personal-and-sensitive-information)
- [Considerations for Using the Data](#considerations-for-using-the-data)
- [Social Impact of Dataset](#social-impact-of-dataset)
- [Discussion of Biases](#discussion-of-biases)
- [Other Known Limitations](#other-known-limitations)
- [Additional Information](#additional-information)
- [Dataset Curators](#dataset-curators)
- [Licensing Information](#licensing-information)
- [Citation Information](#citation-information)
- [Contributions](#contributions)
## Dataset Description
- **Homepage:** www.datatang.ai
- **Repository:**
- **Paper:**
- **Leaderboard:**
- **Point of Contact:**
### Dataset Summary
The dataset contains 25,000 hours of multi-language reading speech data. It's recorded by native speakers, covering English, French, German, Russian, Spanish, Portuguese, Italian, Japanese, Korean, Hindi, Vietnamese, Tagalog, Thai etc.The recording is rich in content, covering multiple categories such as economy, entertainment, news, oral language, numbers, and letters. The format is 16kHz, 16bit, uncompressed wav, mono channel. The sentence accuracy is over 95%.
For more details, please refer to the link: https://bit.ly/39UzIwI
### Supported Tasks and Leaderboards
automatic-speech-recognition, audio-speaker-identification: The dataset can be used to train a model for Automatic Speech Recognition (ASR).
### Languages
English, French, German, Russian, Spanish, Portuguese, Italian, Japanese, Korean, Hindi, Vietnamese, Tagalog, Thai etc.
## Dataset Structure
### Data Instances
[More Information Needed]
### Data Fields
[More Information Needed]
### Data Splits
[More Information Needed]
## Dataset Creation
### Curation Rationale
[More Information Needed]
### Source Data
#### Initial Data Collection and Normalization
[More Information Needed]
#### Who are the source language producers?
[More Information Needed]
### Annotations
#### Annotation process
[More Information Needed]
#### Who are the annotators?
[More Information Needed]
### Personal and Sensitive Information
[More Information Needed]
## Considerations for Using the Data
### Social Impact of Dataset
[More Information Needed]
### Discussion of Biases
[More Information Needed]
### Other Known Limitations
[More Information Needed]
## Additional Information
### Dataset Curators
[More Information Needed]
### Licensing Information
Commerical License: https://drive.google.com/file/d/1saDCPm74D4UWfBL17VbkTsZLGfpOQj1J/view?usp=sharing
### Citation Information
[More Information Needed]
### Contributions
|
Datatang/multi_language_conversation | ---
YAML tags:
- copy-paste the tags obtained with the tagging app: https://github.com/huggingface/datasets-tagging
---
# Dataset Card for multi_language_conversation
## Table of Contents
- [Table of Contents](#table-of-contents)
- [Dataset Description](#dataset-description)
- [Dataset Summary](#dataset-summary)
- [Supported Tasks and Leaderboards](#supported-tasks-and-leaderboards)
- [Languages](#languages)
- [Dataset Structure](#dataset-structure)
- [Data Instances](#data-instances)
- [Data Fields](#data-fields)
- [Data Splits](#data-splits)
- [Dataset Creation](#dataset-creation)
- [Curation Rationale](#curation-rationale)
- [Source Data](#source-data)
- [Annotations](#annotations)
- [Personal and Sensitive Information](#personal-and-sensitive-information)
- [Considerations for Using the Data](#considerations-for-using-the-data)
- [Social Impact of Dataset](#social-impact-of-dataset)
- [Discussion of Biases](#discussion-of-biases)
- [Other Known Limitations](#other-known-limitations)
- [Additional Information](#additional-information)
- [Dataset Curators](#dataset-curators)
- [Licensing Information](#licensing-information)
- [Citation Information](#citation-information)
- [Contributions](#contributions)
## Dataset Description
- **Homepage:** www.datatang.ai
- **Repository:**
- **Paper:**
- **Leaderboard:**
- **Point of Contact:**
### Dataset Summary
The dataset contains 12,000 hours of multi-language conversation speech data. It's recorded by native speakers, covering English, French, German, Russian, Spanish, Japanese, Korean, Hindi, Vietnamese etc. The speakers start the conversation around a familar topic, to ensure the smoothness and nature of the conversation. The format is 16kHz, 16bit, uncompressed wav, mono channel. The sentence accuracy is over 95%.
For more details, please refer to the link: https://bit.ly/39UzIwI
### Supported Tasks and Leaderboards
automatic-speech-recognition, audio-speaker-identification: The dataset can be used to train a model for Automatic Speech Recognition (ASR).
### Languages
English, French, German, Russian, Spanish, Japanese, Korean, Hindi, Vietnamese etc.
## Dataset Structure
### Data Instances
[More Information Needed]
### Data Fields
[More Information Needed]
### Data Splits
[More Information Needed]
## Dataset Creation
### Curation Rationale
[More Information Needed]
### Source Data
#### Initial Data Collection and Normalization
[More Information Needed]
#### Who are the source language producers?
[More Information Needed]
### Annotations
#### Annotation process
[More Information Needed]
#### Who are the annotators?
[More Information Needed]
### Personal and Sensitive Information
[More Information Needed]
## Considerations for Using the Data
### Social Impact of Dataset
[More Information Needed]
### Discussion of Biases
[More Information Needed]
### Other Known Limitations
[More Information Needed]
## Additional Information
### Dataset Curators
[More Information Needed]
### Licensing Information
Commerical License: https://drive.google.com/file/d/1saDCPm74D4UWfBL17VbkTsZLGfpOQj1J/view?usp=sharing
### Citation Information
[More Information Needed]
### Contributions
|
DelgadoPanadero/Pokemon | # Pokemon Dataset
This dataset contains a text representation of more that 10k pokemon sprites from different pokemon videogames (red, yellow, gold, ruby,...). The original images are from 40 to 96 pixel of size and every pixel is represented with an ASCII character depending to its color.
# Supported Tasks
* Text Generation
# Languages
* ASCII colo representation
# Data Fields
```
{'pokemon': pokemon sprite in ASCII representation
'game': videogame in witch the sprite appears
'size': pixel size
'number': number of the pokemon}
```
# License
* All the creative right are property of Nintendo
# Preview
```
00 ~ ~ ~ ~ ~ ~ ~ ~ ~ ~ ~ ~ ~ ~ ~ ~ ~ ~ ~ ~ ~ ~ ~ ~ ~ ~ ~ ~ ~ ~ ~ ~ ~ ~ ~ ~ ~ ~ ~ ~ ~ ~ ~ ~ ~ ~ ~ ~ ~ ~ ~ ~ ~ ~ ~ ~ ~ ~ ~ ~ ~ ~ ~
01 ~ ~ ~ ~ ~ ~ ~ ~ ~ ~ ~ ~ ~ ~ ~ ~ ~ ~ ~ ~ ~ ~ ~ ~ ~ ~ ~ ~ ~ ~ ~ ~ ~ ~ ~ ~ ~ ~ ~ ~ ~ ~ ~ ~ ~ ~ ~ ~ ~ ~ ~ ~ ~ ~ ~ ~ ~ ~ ~ ~ ~ ~ ~
02 ~ ~ ~ ~ ~ ~ ~ ~ ~ ~ ~ ~ ~ ~ ~ ~ ~ ~ ~ ~ ~ ~ ~ ~ ~ ~ ~ ~ ~ ~ ~ ~ ~ ~ ~ ~ ~ ~ ~ ~ ~ ~ ~ ~ ~ ~ ~ ~ ~ ~ ~ ~ ~ ~ ~ ~ ~ ~ ~ ~ ~ ~ ~
03 ~ ~ ~ ~ ~ ~ ~ ~ ~ ~ ~ ~ ~ ~ ~ ~ ~ ~ ~ ~ ~ ~ ~ ~ ~ ~ ~ ~ ~ ~ ~ ~ ~ ~ ~ ~ ~ ~ ~ ~ ~ ~ ~ ~ ~ ~ ~ ~ ~ ~ ~ ~ ~ ~ ~ ~ ~ ~ ~ ~ ~ ~ ~
04 ~ ~ ~ ~ ~ ~ ~ ~ ~ ~ ~ ~ ~ ~ ~ ~ ~ ~ ~ ~ ~ ~ ~ ~ ~ ~ ~ ~ ~ ~ ~ ~ ~ ~ ~ ~ ~ ~ ~ ~ ~ ~ ~ ~ ~ ~ ~ ~ ~ ~ ~ ~ ~ ~ ~ ~ ~ ~ ~ ~ ~ ~ ~
05 ~ ~ ~ ~ ~ ~ ~ ~ ~ ~ ~ ~ ~ ~ ~ ~ ~ ~ ~ ~ ~ ~ ~ ~ ~ ~ ~ ~ ~ ~ ~ ~ ~ ~ ~ ~ ~ ~ ~ ~ ~ ~ ~ ~ ~ ~ ~ ~ ~ ~ ~ ~ ~ ~ ~ ~ ~ ~ ~ ~ ~ ~ ~
06 ~ ~ ~ ~ ~ ~ ~ ~ ~ ~ ~ ~ ~ ~ ~ ~ ~ ~ ~ ; ~ ~ ~ ~ ~ ~ ~ ~ ~ ~ ~ ~ ~ ~ ~ ~ ~ ~ ~ ~ ~ ~ ~ ~ ~ ~ ~ ~ ~ ~ ~ ~ ~ ~ ~ ~ ~ ~ ~ ~ ~ ~ ~
07 ~ ~ ~ ~ ~ ~ ~ ~ ~ ~ ~ ~ ~ ~ ~ ~ ~ ~ ; ; ; ~ ~ ~ ~ ~ ~ ~ ~ ~ ~ ~ ~ ~ ~ ~ ~ ~ ~ ~ ~ ~ ~ ~ ~ ~ ~ ~ ~ ~ ~ ~ ~ ~ ~ ~ ~ ~ ~ ~ ~ ~ ~
08 ~ ~ ~ ~ ~ ~ ~ ~ ~ ~ ~ ~ ~ ~ ~ ~ ~ ~ ; ; ; ~ ~ ~ ~ ~ ~ ~ ~ ~ ~ ~ ~ ~ ~ ~ ~ ~ ~ ~ ~ ~ ~ ~ ~ ~ ~ ~ ~ ~ ~ ~ ~ ~ ~ ~ ~ ~ ~ ~ ~ ~ ~
09 ~ ~ ~ ~ ~ ~ ~ ~ ~ ~ ~ ~ ~ ~ ~ ~ ~ ~ ; P ; ; ~ ~ ~ ~ ~ ~ ~ ~ ~ ~ ~ ~ ~ ~ ~ ~ ~ ~ ~ ~ ~ ~ ~ ~ ~ ~ ~ ~ ~ ~ ~ ~ ~ ~ ~ ~ ~ ~ ~ ~ ~
10 ~ ~ ~ ~ ~ ~ ~ ~ ~ ~ ~ ~ ~ ~ ~ ~ ~ ; P P ; ; ~ ~ ~ ~ ~ ~ ~ ~ ~ ~ ~ ~ ~ ~ ~ ~ ~ ~ ~ ~ ~ ~ ; ; ; ~ ~ ~ ~ ~ ~ ~ ~ ~ ~ ~ ~ ~ ~ ~ ~
11 ~ ~ ~ ~ ~ ~ ~ ~ ~ ~ ~ ~ ~ ~ ~ ~ ~ ; P P P ; ; ~ ~ ~ ~ ~ ~ ~ ~ ~ ~ ~ ~ ~ ~ ~ ~ ~ ~ ; ; ; P P ; ~ ~ ~ ~ ~ ~ ~ ~ ~ ~ ~ ~ ~ ~ ~ ~
12 ~ ~ ~ ~ ~ ~ ~ ~ ~ ~ ~ ~ ~ ~ ~ ~ ~ ; P P P F ; ~ ~ ~ ~ ~ ~ ~ ~ ~ ~ ~ ~ ~ ~ ~ ~ ; ; P P P P ; ; ~ ~ ~ ~ ~ ~ ~ ~ ~ ~ ~ ~ ~ ~ ~ ~
13 ~ ~ ~ ~ ~ ~ ~ ~ ~ ~ ~ ~ ~ ~ ~ ~ ~ ; P P J J ; ~ ~ ~ ~ ~ ~ ~ ~ ~ ~ ~ ~ ~ ~ F F J P P P P ; ; ~ ~ ~ ~ ~ ~ ~ ~ ~ ~ ~ ~ ~ ~ ~ ~ ~
14 ~ ~ ~ ~ ~ ~ ~ ~ ~ ~ ~ ~ ~ ~ ~ ~ ~ ~ ; J J J F ; ~ ~ ~ ~ ~ ~ ~ ~ ~ ~ ~ ~ F J J J P P P ; ; ; ~ ~ ~ ~ ~ ~ ~ ~ ~ ~ ~ ~ ~ ~ ~ ~ ~
15 ~ ~ ~ ~ ~ ~ ~ ~ ~ ~ ~ ~ ~ ~ ~ ~ ~ ~ F J J J F ; ~ ~ ~ ~ ~ ~ ~ ~ ~ ~ F F J J J J F P ; ; ; ~ ~ ~ ~ ~ ~ ~ ~ ~ ~ ~ ~ ~ ~ ~ ~ ~ ~
16 ~ ~ ~ ~ ~ ~ ~ ~ ~ ~ ~ ~ ~ ~ ~ ~ ~ ~ F J J J F ; ~ ~ ~ ~ ~ ~ ~ ~ ~ A J J J J J J J ; ; ; ~ ~ ~ ~ ~ ~ ~ ~ ~ ~ ~ ~ ~ ~ ~ ~ ~ ~ ~
17 ~ ~ ~ ~ ~ ~ ~ ~ ~ ~ ~ ~ ~ ~ ~ ~ ~ ~ ~ F J F F ; F F F F F F F A A J J J J J J J F ; ; ~ ~ ~ ~ ~ ~ ~ ~ ~ ~ ~ ~ ~ ~ ~ ~ ~ ~ ~ ~
18 ~ ~ ~ ~ ~ ~ ~ ~ ~ ~ ~ ~ ~ ~ ~ ~ ~ ~ ~ F F F F F Z Z Z Z Z J J F J J J J J J F F ; ~ ~ ~ ~ ~ ~ ~ ~ ~ ~ ~ ~ ~ ~ ~ ~ ~ ~ ~ ~ ~ ~
19 ~ ~ ~ ~ ~ ~ ~ ~ ~ ~ ~ ~ ~ ~ ~ ~ ~ ~ ~ ~ A F Z Z Z Z Z Z Z Z J J J J J J F F ; ; ~ ~ ~ ~ ~ ~ ~ ~ ~ ~ F F ~ ~ ~ ~ ~ ~ ~ ~ ~ ~ ~
20 ~ ~ ~ ~ ~ ~ ~ ~ ~ ~ ~ ~ ~ ~ ~ ~ ~ ~ ~ F J J Z Z Z Z Z Z Z Z J J J J J F A ; ~ ~ ~ ~ ~ ~ ~ ~ ~ ~ F F Z J A ~ ~ ~ ~ ~ ~ ~ ~ ~ ~
21 ~ ~ ~ ~ ~ ~ ~ ~ ~ ~ ~ ~ ~ ~ ~ ~ ~ ~ A J J J J Z Z Z Z Z J F ; ; F J J F A ~ ~ ~ ~ ~ ~ ~ ~ ~ ~ F Z J J J A ~ ~ ~ ~ ~ ~ ~ ~ ~ ~
22 ~ ~ ~ ~ ~ ~ ~ ~ ~ ~ ~ ~ ~ ~ ~ ~ ~ ~ F F ; ; J J J J J J J ; ~ ; ; J J F F ; ~ ~ ~ ~ ~ ~ ~ ~ F Z J J J F A ~ ~ ~ ~ ~ ~ ~ ~ ~ ~
23 ~ ~ ~ ~ ~ ~ ~ ~ ~ ~ ~ ~ ~ ~ ~ ~ ~ F J ; ~ ; J J J J J J J ; ; P ; J J F F ; ~ ~ ~ ~ ~ ~ F F Z J J F F F F A ~ ~ ~ ~ ~ ~ ~ ~ ~
24 ~ ~ ~ ~ ~ ~ ~ ~ ~ ~ ~ ~ ~ ~ ~ ~ ~ A J ; ; P J J J J J J J F ; ; F J J F F A ~ ~ ~ ~ ~ F Z Z J F F F F F F A ~ ~ ~ ~ ~ ~ ~ ~ ~
25 ~ ~ ~ ~ ~ ~ ~ ~ ~ ~ ~ ~ ~ ~ ~ ~ A F J F ; F J J A F J J J J J J J > > F F F ; ~ ~ F F J J J F F F F F F F A ~ ~ ~ ~ ~ ~ ~ ~ ~
26 ~ ~ ~ ~ ~ ~ ~ ~ ~ ~ ~ ~ ~ ~ ~ ~ ; R J J J J F J J J J J F J J J > > > = F F ; A A J J F F F F F F F F F F A ~ ~ ~ ~ ~ ~ ~ ~ ~
27 ~ ~ ~ ~ ~ ~ ~ ~ ~ ~ ~ ~ ~ ~ ~ ~ ; > F J J J J F = = = = F J J J > > > = F A A Z F A F F F F F F F F F F F A ~ ~ ~ ~ ~ ~ ~ ~ ~
28 ~ ~ ~ ~ ~ ~ ~ ~ ~ ~ ~ ~ ~ ~ ~ A ~ ; = J J J J J = = R R J J J J > > = = A Z F J Z Z A F F F F F F F F F F ; ~ ~ ~ ~ ~ ~ ~ ~ ~
29 ~ ~ ~ ~ ~ ~ ~ ~ ~ ~ ~ ~ ~ ~ A Z A A = J J J J J = R R = J J J J J = = F A J J J J F A F F F F F F F F A ; ~ ~ ~ ~ ~ ~ ~ ~ ~ ~
30 ~ ~ ~ ~ ~ ~ ~ ~ ~ ~ ~ ~ ~ A Z J J F A J J J J J J = = J J J J J J J F A J J J J J J ; F F F F F F A ; ~ ~ ~ ~ ~ ~ ~ ~ ~ ~ ~ ~
31 ~ ~ ~ ~ ~ ~ ~ ~ ~ ~ ~ ~ ~ ~ F J J F F A J J J J J J J J J J J J J J A J J J J F F ; F F F F F A ; ~ ~ ~ ~ ~ ~ ~ ~ ~ ~ ~ ~ ~ ~
32 ~ ~ ~ ~ ~ ~ ~ ~ ~ ~ ~ ~ ~ ~ A F F F F F F J J J J J J J J J J J J J J J J J F F F ; F F F F A ~ ~ ~ ~ ~ ~ ~ ~ ~ ~ ~ ~ ~ ~ ~ ~
33 ~ ~ ~ ~ ~ ~ ~ ~ ~ ~ ~ ~ ~ ~ ~ ; F F F F J J J J J J J J J J J J J J J J J F F F ; A F F F ; ~ ~ ~ ~ ~ ~ ~ ~ ~ ~ ~ ~ ~ ~ ~ ~ ~
34 ~ ~ ~ ~ ~ ~ ~ ~ ~ ~ ~ ~ ~ ~ ~ ~ ; F F F J J J J J J J J J J J J J J J J F F F ; ~ ~ A F F F ; ~ ~ ~ ~ ~ ~ ~ ~ ~ ~ ~ ~ ~ ~ ~ ~
35 ~ ~ ~ ~ ~ ~ ~ ~ ~ ~ ~ ~ ~ ~ ~ ~ ~ ; A J J J J J J J J J J J J J J J J F F F F ; ~ ~ ~ A F F F ; ~ ~ ~ ~ ~ ~ ~ ~ ~ ~ ~ ~ ~ ~ ~
36 ~ ~ ~ ~ ~ ~ ~ ~ ~ ~ ~ ~ ~ ~ ~ ~ ~ ~ F J J J J J J J J J J J J J J J J F F F A ~ ~ A A F F F F F ; ~ ~ ~ ~ ~ ~ ~ ~ ~ ~ ~ ~ ~ ~
37 ~ ~ ~ ~ ~ ~ ~ ~ ~ ~ ~ ~ ~ ~ ~ ~ ~ A J J J J J J J J J J J J J J J J J F F F A ; ; J F F F F A ; ~ ~ ~ ~ ~ ~ ~ ~ ~ ~ ~ ~ ~ ~ ~
38 ~ ~ ~ ~ ~ ~ ~ ~ ~ ~ ~ ~ ~ ~ ~ ~ ~ F J J J J J J J J J J J J J J J J J F F F A J F F F F A ; ~ ~ ~ ~ ~ ~ ~ ~ ~ ~ ~ ~ ~ ~ ~ ~ ~
39 ~ ~ ~ ~ ~ ~ ~ ~ ~ ~ ~ ~ ~ ~ ~ ~ A J J J J J J J J J J J J J J J J F F F F A F F F F ; ; ~ ~ ~ ~ ~ ~ ~ ~ ~ ~ ~ ~ ~ ~ ~ ~ ~ ~ ~
40 ~ ~ ~ ~ ~ ~ ~ ~ ~ ~ ~ ~ ~ ~ ~ A A A J J J J J J J J J J J J J J F F F F F ; F F F ; ~ ~ ~ ~ ~ ~ ~ ~ ~ ~ ~ ~ ~ ~ ~ ~ ~ ~ ~ ~ ~
41 ~ ~ ~ ~ ~ ~ ~ ~ ~ ~ ~ ~ ~ ~ A Z Z F A J J J J J J J J J J J J J F F F F F ; ; F F F ; ~ ~ ~ ~ ~ ~ ~ ~ ~ ~ ~ ~ ~ ~ ~ ~ ~ ~ ~ ~
42 ~ ~ ~ ~ ~ ~ ~ ~ ~ ~ ~ ~ ~ ~ F F J F J A J J J J J J J J J J J F F F F F F ; ~ ; F F F ; ~ ~ ~ ~ ~ ~ ~ ~ ~ ~ ~ ~ ~ ~ ~ ~ ~ ~ ~
43 ~ ~ ~ ~ ~ ~ ~ ~ ~ ~ ~ ~ ~ ~ A F F F F F J J J J J J J J J F F F F F F F F ; ; A A A F F ; ~ ~ ~ ~ ~ ~ ~ ~ ~ ~ ~ ~ ~ ~ ~ ~ ~ ~
44 ~ ~ ~ ~ ~ ~ ~ ~ ~ ~ ~ ~ ~ ~ ~ F F F F F A F F J J J J F F F F F F F F F F A A A A ; ; ; ~ ~ ~ ~ ~ ~ ~ ~ ~ ~ ~ ~ ~ ~ ~ ~ ~ ~ ~
45 ~ ~ ~ ~ ~ ~ ~ ~ ~ ~ ~ ~ ~ ~ ~ ~ A F F F A F F F F F F F F F F F F F F F A A ; ; ; ~ ~ ~ ~ ~ ~ ~ ~ ~ ~ ~ ~ ~ ~ ~ ~ ~ ~ ~ ~ ~ ~
46 ~ ~ ~ ~ ~ ~ ~ ~ ~ ~ ~ ~ ~ ~ ~ ~ ; F F F ; F F F F F F F F F F F F F F F ; ; ~ ~ ~ ~ ~ ~ ~ ~ ~ ~ ~ ~ ~ ~ ~ ~ ~ ~ ~ ~ ~ ~ ~ ~ ~
47 ~ ~ ~ ~ ~ ~ ~ ~ ~ ~ ~ ~ ~ ~ ~ ~ ~ A ; ; F F F F F F F F F F F F F F F F ; ~ ~ ~ ~ ~ ~ ~ ~ ~ ~ ~ ~ ~ ~ ~ ~ ~ ~ ~ ~ ~ ~ ~ ~ ~ ~
48 ~ ~ ~ ~ ~ ~ ~ ~ ~ ~ ~ ~ ~ ~ ~ ~ ~ ~ ~ ~ ; ; ; ; A F F F F F F F F F F ; ~ ~ ~ ~ ~ ~ ~ ~ ~ ~ ~ ~ ~ ~ ~ ~ ~ ~ ~ ~ ~ ~ ~ ~ ~ ~ ~
49 ~ ~ ~ ~ ~ ~ ~ ~ ~ ~ ~ ~ ~ ~ ~ ~ ~ ~ ~ ~ ~ ~ ~ ~ ~ ; ; A F F F F F A ; ~ ~ ~ ~ ~ ~ ~ ~ ~ ~ ~ ~ ~ ~ ~ ~ ~ ~ ~ ~ ~ ~ ~ ~ ~ ~ ~ ~
50 ~ ~ ~ ~ ~ ~ ~ ~ ~ ~ ~ ~ ~ ~ ~ ~ ~ ~ ~ ~ ~ ~ ~ ~ ~ ~ ~ ; ; F F ; ; ; ~ ~ ~ ~ ~ ~ ~ ~ ~ ~ ~ ~ ~ ~ ~ ~ ~ ~ ~ ~ ~ ~ ~ ~ ~ ~ ~ ~ ~
51 ~ ~ ~ ~ ~ ~ ~ ~ ~ ~ ~ ~ ~ ~ ~ ~ ~ ~ ~ ~ ~ ~ ~ ~ ~ ~ ~ A F F F F ; ~ ~ ~ ~ ~ ~ ~ ~ ~ ~ ~ ~ ~ ~ ~ ~ ~ ~ ~ ~ ~ ~ ~ ~ ~ ~ ~ ~ ~ ~
52 ~ ~ ~ ~ ~ ~ ~ ~ ~ ~ ~ ~ ~ ~ ~ ~ ~ ~ ~ ~ ~ ~ ~ ~ ~ ~ ~ A F A F F ; ~ ~ ~ ~ ~ ~ ~ ~ ~ ~ ~ ~ ~ ~ ~ ~ ~ ~ ~ ~ ~ ~ ~ ~ ~ ~ ~ ~ ~ ~
53 ~ ~ ~ ~ ~ ~ ~ ~ ~ ~ ~ ~ ~ ~ ~ ~ ~ ~ ~ ~ ~ ~ ~ ~ ~ ~ ~ A F F F ; ~ ~ ~ ~ ~ ~ ~ ~ ~ ~ ~ ~ ~ ~ ~ ~ ~ ~ ~ ~ ~ ~ ~ ~ ~ ~ ~ ~ ~ ~ ~
54 ~ ~ ~ ~ ~ ~ ~ ~ ~ ~ ~ ~ ~ ~ ~ ~ ~ ~ ~ ~ ~ ~ ~ ~ ~ ~ ~ ~ ; ; ; ~ ~ ~ ~ ~ ~ ~ ~ ~ ~ ~ ~ ~ ~ ~ ~ ~ ~ ~ ~ ~ ~ ~ ~ ~ ~ ~ ~ ~ ~ ~ ~
55 ~ ~ ~ ~ ~ ~ ~ ~ ~ ~ ~ ~ ~ ~ ~ ~ ~ ~ ~ ~ ~ ~ ~ ~ ~ ~ ~ ~ ~ ~ ~ ~ ~ ~ ~ ~ ~ ~ ~ ~ ~ ~ ~ ~ ~ ~ ~ ~ ~ ~ ~ ~ ~ ~ ~ ~ ~ ~ ~ ~ ~ ~ ~
56 ~ ~ ~ ~ ~ ~ ~ ~ ~ ~ ~ ~ ~ ~ ~ ~ ~ ~ ~ ~ ~ ~ ~ ~ ~ ~ ~ ~ ~ ~ ~ ~ ~ ~ ~ ~ ~ ~ ~ ~ ~ ~ ~ ~ ~ ~ ~ ~ ~ ~ ~ ~ ~ ~ ~ ~ ~ ~ ~ ~ ~ ~ ~
57 ~ ~ ~ ~ ~ ~ ~ ~ ~ ~ ~ ~ ~ ~ ~ ~ ~ ~ ~ ~ ~ ~ ~ ~ ~ ~ ~ ~ ~ ~ ~ ~ ~ ~ ~ ~ ~ ~ ~ ~ ~ ~ ~ ~ ~ ~ ~ ~ ~ ~ ~ ~ ~ ~ ~ ~ ~ ~ ~ ~ ~ ~ ~
58 ~ ~ ~ ~ ~ ~ ~ ~ ~ ~ ~ ~ ~ ~ ~ ~ ~ ~ ~ ~ ~ ~ ~ ~ ~ ~ ~ ~ ~ ~ ~ ~ ~ ~ ~ ~ ~ ~ ~ ~ ~ ~ ~ ~ ~ ~ ~ ~ ~ ~ ~ ~ ~ ~ ~ ~ ~ ~ ~ ~ ~ ~ ~
59 ~ ~ ~ ~ ~ ~ ~ ~ ~ ~ ~ ~ ~ ~ ~ ~ ~ ~ ~ ~ ~ ~ ~ ~ ~ ~ ~ ~ ~ ~ ~ ~ ~ ~ ~ ~ ~ ~ ~ ~ ~ ~ ~ ~ ~ ~ ~ ~ ~ ~ ~ ~ ~ ~ ~ ~ ~ ~ ~ ~ ~ ~ ~
60 ~ ~ ~ ~ ~ ~ ~ ~ ~ ~ ~ ~ ~ ~ ~ ~ ~ ~ ~ ~ ~ ~ ~ ~ ~ ~ ~ ~ ~ ~ ~ ~ ~ ~ ~ ~ ~ ~ ~ ~ ~ ~ ~ ~ ~ ~ ~ ~ ~ ~ ~ ~ ~ ~ ~ ~ ~ ~ ~ ~ ~ ~ ~
61 ~ ~ ~ ~ ~ ~ ~ ~ ~ ~ ~ ~ ~ ~ ~ ~ ~ ~ ~ ~ ~ ~ ~ ~ ~ ~ ~ ~ ~ ~ ~ ~ ~ ~ ~ ~ ~ ~ ~ ~ ~ ~ ~ ~ ~ ~ ~ ~ ~ ~ ~ ~ ~ ~ ~ ~ ~ ~ ~ ~ ~ ~ ~
62 ~ ~ ~ ~ ~ ~ ~ ~ ~ ~ ~ ~ ~ ~ ~ ~ ~ ~ ~ ~ ~ ~ ~ ~ ~ ~ ~ ~ ~ ~ ~ ~ ~ ~ ~ ~ ~ ~ ~ ~ ~ ~ ~ ~ ~ ~ ~ ~ ~ ~ ~ ~ ~ ~ ~ ~ ~ ~ ~ ~ ~ ~ ~
63 ~ ~ ~ ~ ~ ~ ~ ~ ~ ~ ~ ~ ~ ~ ~ ~ ~ ~ ~ ~ ~ ~ ~ ~ ~ ~ ~ ~ ~ ~ ~ ~ ~ ~ ~ ~ ~ ~ ~ ~ ~ ~ ~ ~ ~ ~ ~ ~ ~ ~ ~ ~ ~ ~ ~ ~ ~ ~ ~ ~ ~ ~ ~
``` |
DeskDown/ALTDataset | # Asian Language Treebank (ALT)
This is a **subset** of ALT dataset published by Riza et al.
It included following low-resource languages:
- fil
- vi
- id
- ms
- khm
- th
- hi
- my
It also includes ja and zh languages. |
DeskDown/ALTDataset_en-to-fil-vi-id-ms-ja-khm | __Introduction__
The ALT project aims to advance the state-of-the-art Asian natural language processing (NLP) techniques
through the open collaboration for developing and using ALT. It was first conducted by NICT and UCSY
as described in Ye Kyaw Thu, Win Pa Pa, Masao Utiyama, Andrew Finch and Eiichiro Sumita (2016).
Then, it was developed under ASEAN IVO as described in this Web page. The process of building ALT
began with sampling about 20,000 sentences from English Wikinews, and then these sentences were
translated into the other languages. ALT now has 13 languages:
Bengali, English, Filipino, Hindi, Bahasa Indonesia, Japanese, Khmer, Lao, Malay, Myanmar (Burmese),
Thai, Vietnamese, Chinese (Simplified Chinese).
In this dataset you can find parallel corpus of fil, vi, id, ms, ja, khm languages.
Dataset is tokenized using mbart50-like tokenizer. (To be added soon)
Tokens are padded\truncated at a size of 128.
|
DiFronzo/Human_Activity_Recognition | Human Activity Recognition (HAR) using smartphones dataset. Classifying the type of movement amongst five categories:
- WALKING,
- WALKING_UPSTAIRS,
- WALKING_DOWNSTAIRS,
- SITTING,
- STANDING
The experiments have been carried out with a group of 16 volunteers within an age bracket of 19-26 years. Each person performed five activities (WALKING, WALKING_UPSTAIRS, WALKING_DOWNSTAIRS, SITTING, STANDING) wearing a smartphone (Samsung Galaxy S8) in the pucket. Using its embedded accelerometer and gyroscope, we captured 3-axial linear acceleration and 3-axial angular velocity at a constant rate of 50Hz. The experiments have been video-recorded to label the data manually.
```bash
'raw_data/labels.txt': include all the activity labels available for the dataset (1 per row).
Column 1: experiment number ID,
Column 2: user number ID,
Column 3: activity number ID
Column 4: Label start point (in number of signal log samples (recorded at 50Hz))
Column 5: Label end point (in number of signal log samples)
activity_type:
1 WALKING
2 WALKING_UPSTAIRS
3 WALKING_DOWNSTAIRS
4 SITTING
5 STANDING
```
Repository: [DiFronzo/LSTM-for-Human-Activity-Recognition-classification](https://github.com/DiFronzo/LSTM-for-Human-Activity-Recognition-classification) |
Doohae/modern_music_re | Datasets for Relation Extraction Task
Source from Wikipedia (CC-BY-2.0)
Contributors : Doohae Jung, Hyesu Kim, Bosung Kim, Isaac Park, Miwon Jeon, Dagon Lee, Jihoo Kim |
Dumiiii/common-voice-romaniarss | This datasets consists in the last version of the common-voice-dataset for romanian language.
Also contains data from RSS (Romanian Speech Synthesis Dataset) from this site http://romaniantts.com/ |
EMBO/biolang | ---
annotations_creators:
- machine-generated
language_creators:
- expert-generated
language:
- en
license:
- cc-by-4.0
multilinguality:
- monolingual
size_categories:
- n>1M
source_datasets: []
task_categories:
- text-generation
task_ids:
- language-modeling
---
# Dataset Card for BioLang
## Table of Contents
- [Dataset Card for [Dataset Name]](#dataset-card-for-dataset-name)
- [Table of Contents](#table-of-contents)
- [Dataset Description](#dataset-description)
- [Dataset Summary](#dataset-summary)
- [Supported Tasks and Leaderboards](#supported-tasks-and-leaderboards)
- [Languages](#languages)
- [Dataset Structure](#dataset-structure)
- [Data Instances](#data-instances)
- [Data Fields](#data-fields)
- [Data Splits](#data-splits)
- [Dataset Creation](#dataset-creation)
- [Curation Rationale](#curation-rationale)
- [Source Data](#source-data)
- [Initial Data Collection and Normalization](#initial-data-collection-and-normalization)
- [Who are the source language producers?](#who-are-the-source-language-producers)
- [Annotations](#annotations)
- [Annotation process](#annotation-process)
- [Who are the annotators?](#who-are-the-annotators)
- [Personal and Sensitive Information](#personal-and-sensitive-information)
- [Considerations for Using the Data](#considerations-for-using-the-data)
- [Social Impact of Dataset](#social-impact-of-dataset)
- [Discussion of Biases](#discussion-of-biases)
- [Other Known Limitations](#other-known-limitations)
- [Additional Information](#additional-information)
- [Dataset Curators](#dataset-curators)
- [Licensing Information](#licensing-information)
- [Citation Information](#citation-information)
- [Contributions](#contributions)
## Dataset Description
- **Homepage:** https://sourcedata.embo.org
- **Repository:** https://github.com/source-data/soda-roberta
- **Paper:**
- **Leaderboard:**
- **Point of Contact:** thomas.lemberger@embo.org
- **Download Size:** 5_299_878_661
### Dataset Summary
BioLang is a dataset is based on abstracts from the open access section of EuropePubMed Central to train language models in the domain of biology. The dataset can be used for random masked language modeling or for language modeling using only specific part-of-speech maksing. More details on generation and use of the dataset at https://github.com/source-data/soda-roberta .
### Supported Tasks and Leaderboards
- `MLM`: masked language modeling
- `DET`: part-of-speach masked language model, with determinants (`DET`) tagged
- `SMALL`: part-of-speech masked language model, with "small" words (`DET`, `CCONJ`, `SCONJ`, `ADP`, `PRON`) tagged
- `VERB`: part-of-speach masked language model, with verbs (`VERB`) tagged
### Languages
English
## Dataset Structure
### Data Instances
```json
{
"input_ids":[
0, 2444, 6997, 46162, 7744, 35, 20632, 20862, 3457, 36, 500, 23858, 29, 43, 32, 3919, 716, 15, 49, 4476, 4, 1398, 6, 52, 1118, 5, 20862, 819, 9, 430, 23305, 248, 23858, 29, 4, 256, 40086, 104, 35, 1927, 1069, 459, 1484, 58, 4776, 13, 23305, 634, 16706, 493, 2529, 8954, 14475, 73, 34263, 6, 4213, 718, 833, 12, 24291, 4473, 22500, 14475, 73, 510, 705, 73, 34263, 6, 5143, 4313, 2529, 8954, 14475, 73, 34263, 6, 8, 5143, 4313, 2529, 8954, 14475, 248, 23858, 29, 23, 4448, 225, 4722, 2392, 11, 9341, 261, 4, 49043, 35, 96, 746, 6, 5962, 9, 38415, 4776, 408, 36, 3897, 4, 398, 8871, 56, 23305, 4, 20, 15608, 21, 8061, 6164, 207, 13, 70, 248, 23858, 29, 6, 150, 5, 42561, 21, 8061, 5663, 207, 13, 80, 3457, 4, 509, 1296, 5129, 21567, 3457, 36, 398, 23528, 8748, 22065, 11654, 35, 7253, 15, 49, 4476, 6, 70, 3457, 4682, 65, 189, 28, 5131, 13, 23305, 9726, 4, 2
],
"label_ids": [
"X", "NOUN", "NOUN", "NOUN", "NOUN", "PUNCT", "ADJ", "ADJ", "NOUN", "PUNCT", "PROPN", "PROPN", "PROPN", "PUNCT", "AUX", "VERB", "VERB", "ADP", "DET", "NOUN", "PUNCT", "ADV", "PUNCT", "PRON", "VERB", "DET", "ADJ", "NOUN", "ADP", "ADJ", "NOUN", "NOUN", "NOUN", "NOUN", "PUNCT", "ADJ", "ADJ", "ADJ", "PUNCT", "NOUN", "NOUN", "NOUN", "NOUN", "AUX", "VERB", "ADP", "NOUN", "VERB", "PROPN", "PROPN", "PROPN", "PROPN", "PROPN", "SYM", "PROPN", "PUNCT", "PROPN", "PROPN", "PROPN", "PUNCT", "PROPN", "PROPN", "PROPN", "PROPN", "SYM", "PROPN", "PROPN", "SYM", "PROPN", "PUNCT", "PROPN", "PROPN", "PROPN", "PROPN", "PROPN", "SYM", "PROPN", "PUNCT", "CCONJ", "ADJ", "PROPN", "PROPN", "PROPN", "PROPN", "NOUN", "NOUN", "NOUN", "ADP", "PROPN", "PROPN", "PROPN", "PROPN", "ADP", "PROPN", "PROPN", "PUNCT", "PROPN", "PUNCT", "ADP", "NOUN", "PUNCT", "NUM", "ADP", "NUM", "VERB", "NOUN", "PUNCT", "NUM", "NUM", "NUM", "NOUN", "AUX", "NOUN", "PUNCT", "DET", "NOUN", "AUX", "X", "NUM", "NOUN", "ADP", "DET", "NOUN", "NOUN", "NOUN", "PUNCT", "SCONJ", "DET", "NOUN", "AUX", "X", "NUM", "NOUN", "ADP", "NUM", "NOUN", "PUNCT", "NUM", "NOUN", "VERB", "ADJ", "NOUN", "PUNCT", "NUM", "NOUN", "NOUN", "NOUN", "NOUN", "PUNCT", "VERB", "ADP", "DET", "NOUN", "PUNCT", "DET", "NOUN", "SCONJ", "PRON", "VERB", "AUX", "VERB", "ADP", "NOUN", "NOUN", "PUNCT", "X"
],
"special_tokens_mask": [
1, 0, 0, 0, 0, 0, 0, 0, 0, 0, 0, 0, 0, 0, 0, 0, 0, 0, 0, 0, 0, 0, 0, 0, 0, 0, 0, 0, 0, 0, 0, 0, 0, 0, 0, 0, 0, 0, 0, 0, 0, 0, 0, 0, 0, 0, 0, 0, 0, 0, 0, 0, 0, 0, 0, 0, 0, 0, 0, 0, 0, 0, 0, 0, 0, 0, 0, 0, 0, 0, 0, 0, 0, 0, 0, 0, 0, 0, 0, 0, 0, 0, 0, 0, 0, 0, 0, 0, 0, 0, 0, 0, 0, 0, 0, 0, 0, 0, 0, 0, 0, 0, 0, 0, 0, 0, 0, 0, 0, 0, 0, 0, 0, 0, 0, 0, 0, 0, 0, 0, 0, 0, 0, 0, 0, 0, 0, 0, 0, 0, 0, 0, 0, 0, 0, 0, 0, 0, 0, 0, 0, 0, 0, 0, 0, 0, 0, 0, 0, 0, 0, 0, 0, 0, 0, 0, 0, 0, 0, 0, 0, 0, 0, 0, 0, 1
]
}
```
### Data Fields
`MLM`:
- `input_ids`: a `list` of `int32` features.
- `special_tokens_mask`: a `list` of `int8` features.
`DET`, `VERB`, `SMALL`:
- `input_ids`: a `list` of `int32` features.
- `tag_mask`: a `list` of `int8` features.
### Data Splits
- `train`:
- features: ['input_ids', 'special_tokens_mask'],
- num_rows: 12_005_390
- `test`:
- features: ['input_ids', 'special_tokens_mask'],
- num_rows: 37_112
- `validation`:
- features: ['input_ids', 'special_tokens_mask'],
- num_rows: 36_713
## Dataset Creation
### Curation Rationale
The dataset was assembled to train language models in the field of cell and molecular biology. To expand the size of the dataset and to include many examples with highly technical language, abstracts were complemented with figure legends (or figure 'captions').
### Source Data
#### Initial Data Collection and Normalization
The xml content of papers were downloaded in January 2021 from the open access section of [EuropePMC]("https://europepmc.org/downloads/openaccess"). Figure legends and abstracts were extracted from the JATS XML, tokenized with the `roberta-base` tokenizer and part-of-speech tagged with Spacy's `en_core_web_sm` model (https://spacy.io).
More details at https://github.com/source-data/soda-roberta
#### Who are the source language producers?
Experts scientists.
### Annotations
#### Annotation process
Part-of-speech was tagged automatically.
#### Who are the annotators?
Spacy's `en_core_web_sm` model (https://spacy.io) was used for part-of-speech tagging.
### Personal and Sensitive Information
[More Information Needed]
## Considerations for Using the Data
### Social Impact of Dataset
[More Information Needed]
### Discussion of Biases
[More Information Needed]
### Other Known Limitations
[More Information Needed]
## Additional Information
### Dataset Curators
Thomas Lemberger
### Licensing Information
CC-BY 4.0
### Citation Information
[More Information Needed]
### Contributions
Thanks to [@tlemberger](https://github.com/tlemberger) for adding this dataset.
|
EMBO/sd-nlp | ---
annotations_creators:
- expert-generated
language_creators:
- expert-generated
language:
- en
license:
- cc-by-4.0
multilinguality:
- monolingual
size_categories:
- 10K<n<100K
source_datasets: []
task_categories:
- text-classification
- structure-prediction
- text-classification
task_ids:
- multi-class-classification
- named-entity-recognition
- parsing
---
# Dataset Card for sd-nlp
## Table of Contents
- [Dataset Card for [Dataset Name]](#dataset-card-for-dataset-name)
- [Table of Contents](#table-of-contents)
- [Dataset Description](#dataset-description)
- [Dataset Summary](#dataset-summary)
- [Supported Tasks and Leaderboards](#supported-tasks-and-leaderboards)
- [Languages](#languages)
- [Dataset Structure](#dataset-structure)
- [Data Instances](#data-instances)
- [Data Fields](#data-fields)
- [Data Splits](#data-splits)
- [Dataset Creation](#dataset-creation)
- [Curation Rationale](#curation-rationale)
- [Source Data](#source-data)
- [Initial Data Collection and Normalization](#initial-data-collection-and-normalization)
- [Who are the source language producers?](#who-are-the-source-language-producers)
- [Annotations](#annotations)
- [Annotation process](#annotation-process)
- [Who are the annotators?](#who-are-the-annotators)
- [Personal and Sensitive Information](#personal-and-sensitive-information)
- [Considerations for Using the Data](#considerations-for-using-the-data)
- [Social Impact of Dataset](#social-impact-of-dataset)
- [Discussion of Biases](#discussion-of-biases)
- [Other Known Limitations](#other-known-limitations)
- [Additional Information](#additional-information)
- [Dataset Curators](#dataset-curators)
- [Licensing Information](#licensing-information)
- [Citation Information](#citation-information)
- [Contributions](#contributions)
## Dataset Description
- **Homepage:** https://sourcedata.embo.org
- **Repository:** https://github.com/source-data/soda-roberta
- **Paper:**
- **Leaderboard:**
- **Point of Contact:** thomas.lemberger@embo.org
### Dataset Summary
This dataset is based on the content of the SourceData (https://sourcedata.embo.org) database, which contains manually annotated figure legends written in English and extracted from scientific papers in the domain of cell and molecular biology (Liechti et al, Nature Methods, 2017, https://doi.org/10.1038/nmeth.4471).
The dataset is pre-tokenized with the `roberta-base` tokenizer.
Additional details at https://github.com/source-data/soda-roberta
### Supported Tasks and Leaderboards
Tags are provided as [IOB2-style tags](https://en.wikipedia.org/wiki/Inside%E2%80%93outside%E2%80%93beginning_(tagging)).
`PANELIZATION`: figure captions (or figure legends) are usually composed of segments that each refer to one of several 'panels' of the full figure. Panels tend to represent results obtained with a coherent method and depicts data points that can be meaningfully compared to each other. `PANELIZATION` provide the start (B-PANEL_START) of these segments and allow to train for recogntion of the boundary between consecutive panel lengends.
`NER`: biological and chemical entities are labeled. Specifically the following entities are tagged:
- `SMALL_MOLECULE`: small molecules
- `GENEPROD`: gene products (genes and proteins)
- `SUBCELLULAR`: subcellular components
- `CELL`: cell types and cell lines.
- `TISSUE`: tissues and organs
- `ORGANISM`: species
- `EXP_ASSAY`: experimental assays
`ROLES`: the role of entities with regard to the causal hypotheses tested in the reported results. The tags are:
- `CONTROLLED_VAR`: entities that are associated with experimental variables and that subjected to controlled and targeted perturbations.
- `MEASURED_VAR`: entities that are associated with the variables measured and the object of the measurements.
`BORING`: entities are marked with the tag `BORING` when they are more of descriptive value and not directly associated with causal hypotheses ('boring' is not an ideal choice of word, but it is short...). Typically, these entities are so-called 'reporter' geneproducts, entities used as common baseline across samples, or specify the context of the experiment (cellular system, species, etc...).
### Languages
The text in the dataset is English.
## Dataset Structure
### Data Instances
```json
{
"tokens": [
"<s>", "Figure", "\u01205", ".", "\u0120Figure", "\u01205", ".", "A", "\u0120ER", "p", "57", "fl", "ox", "/", "fl", "ox", "\u0120mice", "\u0120were", "\u0120crossed", "\u0120with", "\u0120Nest", "in", "\u0120Cre", "\u0120trans", "genic", "\u0120mice", "\u0120to", "\u0120generate", "\u0120nervous", "\u0120system", "\u0120specific", "\u0120ER", "p", "57", "\u0120deficient", "\u0120animals", ".", "\u0120The", "\u0120levels", "\u0120of", "\u0120ER", "p", "57", "\u0120protein", "\u0120in", "\u0120the", "\u0120spinal", "\u0120cord", "\u0120were", "\u0120monitored", "\u0120by", "\u0120Western", "\u0120blot", ".", "\u0120ER", "p", "57", "WT", "\u0120(", "n", "=", "4", "),", "\u0120ER", "p", "57", "N", "es", "+", "/-", "\u0120(", "n", "=", "5", ")", "\u0120and", "\u0120ER", "p", "57", "N", "es", "-", "/-", "\u0120(", "n", "=", "4", ")", "\u0120mice", ".", "\u0120H", "SP", "90", "\u0120levels", "\u0120were", "\u0120determined", "\u0120as", "\u0120a", "\u0120loading", "\u0120control", ".", "\u0120Right", "\u0120panel", ":", "\u0120Quant", "ification", "\u0120of", "\u0120ER", "p", "57", "\u0120levels", "\u0120was", "\u0120performed", "\u0120relative", "\u0120to", "\u0120H", "sp", "90", "\u0120levels", ".", "\u0120B", "\u0120Body", "\u0120weight", "\u0120measurements", "\u0120were", "\u0120performed", "\u0120for", "\u0120indicated", "\u0120time", "\u0120points", "\u0120in", "\u0120ER", "p", "57", "WT", "\u0120(", "n", "=", "50", "),", "\u0120ER", "p", "57", "N", "es", "+", "/-", "\u0120(", "n", "=", "32", ")", "\u0120and", "\u0120ER", "p", "57", "N", "es", "-", "/-", "\u0120(", "n", "=", "19", ")", "\u0120mice", ".", "\u0120C", "\u0120Rot", "ar", "od", "\u0120performance", "\u0120was", "\u0120performed", "\u0120ER", "p", "57", "WT", "\u0120(", "n", "=", "20", "),", "\u0120ER", "p", "57", "N", "es", "+", "/-", "\u0120(", "n", "=", "15", ")", "\u0120and", "\u0120ER", "p", "57", "N", "es", "-", "/-", "\u0120(", "n", "=", "8", ")", "\u0120mice", ".", "\u0120D", "\u0120H", "anging", "\u0120test", "\u0120performance", "\u0120was", "\u0120assessed", "\u0120in", "\u0120ER", "p", "57", "WT", "\u0120(", "n", "=", "41", "),", "\u0120ER", "p", "57", "N", "es", "+", "/-", "\u0120(", "n", "=", "32", ")", "\u0120and", "\u0120ER", "p", "57", "N", "es", "-", "/-", "\u0120(", "n", "=", "12", ")", "\u0120mice", ".", "\u0120E", "\u0120Kaplan", "-", "Me", "ier", "\u0120survival", "\u0120curve", "\u0120for", "\u0120ER", "p", "57", "N", "es", "-", "/-", "\u0120mice", "\u0120(", "N", "\u0120=", "\u012019", ")", "\u0120that", "\u0120prematurely", "\u0120died", "\u0120or", "\u0120had", "\u0120to", "\u0120be", "\u0120sacrificed", "\u0120because", "\u0120of", "\u0120health", "\u0120reasons", "\u0120between", "\u0120the", "\u0120ages", "\u012022", "\u0120and", "\u012073", "\u0120days", ".", "\u0120Mean", "\u0120survival", "\u0120of", "\u0120this", "\u0120sub", "group", "\u0120of", "\u0120animals", "\u0120was", "\u012057", "\u0120days", ".", "\u0120ER", "p", "57", "WT", "\u0120(", "n", "=", "50", ")", "\u0120and", "\u0120ER", "p", "57", "N", "es", "+", "/-", "\u0120(", "n", "=", "32", ")", "\u0120mice", "\u0120are", "\u0120shown", "\u0120as", "\u0120a", "\u0120reference", ".", "\u0120F", "\u0120Hist", "ological", "\u0120analysis", "\u0120of", "\u0120Ne", "u", "N", "\u0120and", "\u0120GF", "AP", "\u0120st", "aining", "\u0120was", "\u0120performed", "\u0120in", "\u0120spinal", "\u0120cord", "\u0120tissue", "\u0120from", "\u0120ER", "p", "57", "WT", "\u0120and", "\u0120ER", "p", "57", "N", "es", "-", "/-", "\u0120mice", "\u0120in", "\u0120three", "\u0120animals", "\u0120per", "\u0120group", "\u0120using", "\u0120indirect", "\u0120immun", "of", "lu", "orescence", ".", "\u0120The", "\u0120nucleus", "\u0120was", "\u0120stained", "\u0120with", "\u0120H", "oe", "ch", "st", ".", "\u0120Representative", "\u0120images", "\u0120from", "\u0120one", "\u0120mouse", "\u0120per", "\u0120group", "\u0120are", "\u0120shown", ".", "\u0120Scale", "\u0120bar", ":", "\u012050", "\u0120\u00ce\u00bc", "m", ".", "\u0120G", "\u0120St", "ere", "ological", "\u0120analysis", "\u0120of", "\u0120the", "\u0120spinal", "\u0120cord", "\u0120from", "\u0120ER", "p", "57", "WT", "\u0120(", "n", "\u0120=", "\u01204", "),", "\u0120ER", "p", "57", "N", "es", "+", "/-", "\u0120(", "n", "\u0120=", "\u01204", ")", "\u0120and", "\u0120ER", "p", "57", "N", "es", "-", "/-", "\u0120(", "n", "\u0120=", "\u01204", ")", "\u0120mice", ".", "\u0120Alternate", "\u0120series", "\u0120of", "\u0120sections", "\u0120from", "\u0120the", "\u0120spinal", "\u0120cord", "\u0120of", "\u0120the", "\u0120mice", "\u0120were", "\u0120either", "\u0120stained", "\u0120for", "\u0120N", "iss", "l", "\u0120(", "top", "\u0120row", "\u0120images", ")", "\u0120or", "\u0120processed", "\u0120for", "\u0120immun", "oh", "ist", "ochemistry", "\u0120for", "\u0120the", "\u0120ch", "olin", "ergic", "\u0120cell", "\u0120marker", "\u0120Ch", "oline", "\u0120Ac", "et", "yl", "\u0120Transfer", "ase", "\u0120(", "Ch", "AT", ",", "\u0120bottom", "\u0120row", "\u0120images", ").", "\u0120The", "\u0120nucle", "oli", "\u0120of", "\u0120the", "</s>"
],
"input_ids": [
0, 40683, 195, 4, 17965, 195, 4, 250, 13895, 642, 4390, 4825, 4325, 73, 4825, 4325, 15540, 58, 7344, 19, 12786, 179, 12022, 6214, 44131, 15540, 7, 5368, 7464, 467, 2167, 13895, 642, 4390, 38396, 3122, 4, 20, 1389, 9, 13895, 642, 4390, 8276, 11, 5, 21431, 13051, 58, 14316, 30, 2027, 39144, 4, 13895, 642, 4390, 25982, 36, 282, 5214, 306, 238, 13895, 642, 4390, 487, 293, 2744, 40398, 36, 282, 5214, 245, 43, 8, 13895, 642, 4390, 487, 293, 12, 40398, 36, 282, 5214, 306, 43, 15540, 4, 289, 4186, 3248, 1389, 58, 3030, 25, 10, 16761, 797, 4, 5143, 2798, 35, 28256, 5000, 9, 13895, 642, 4390, 1389, 21, 3744, 5407, 7, 289, 4182, 3248, 1389, 4, 163, 13048, 2408, 19851, 58, 3744, 13, 4658, 86, 332, 11, 13895, 642, 4390, 25982, 36, 282, 5214, 1096, 238, 13895, 642, 4390, 487, 293, 2744, 40398, 36, 282, 5214, 2881, 43, 8, 13895, 642, 4390, 487, 293, 12, 40398, 36, 282, 5214, 1646, 43, 15540, 4, 230, 9104, 271, 1630, 819, 21, 3744, 13895, 642, 4390, 25982, 36, 282, 5214, 844, 238, 13895, 642, 4390, 487, 293, 2744, 40398, 36, 282, 5214, 996, 43, 8, 13895, 642, 4390, 487, 293, 12, 40398, 36, 282, 5214, 398, 43, 15540, 4, 211, 289, 23786, 1296, 819, 21, 11852, 11, 13895, 642, 4390, 25982, 36, 282, 5214, 4006, 238, 13895, 642, 4390, 487, 293, 2744, 40398, 36, 282, 5214, 2881, 43, 8, 13895, 642, 4390, 487, 293, 12, 40398, 36, 282, 5214, 1092, 43, 15540, 4, 381, 25353, 12, 5096, 906, 7967, 9158, 13, 13895, 642, 4390, 487, 293, 12, 40398, 15540, 36, 487, 5457, 753, 43, 14, 30088, 962, 50, 56, 7, 28, 26936, 142, 9, 474, 2188, 227, 5, 4864, 820, 8, 6521, 360, 4, 30750, 7967, 9, 42, 2849, 13839, 9, 3122, 21, 4981, 360, 4, 13895, 642, 4390, 25982, 36, 282, 5214, 1096, 43, 8, 13895, 642, 4390, 487, 293, 2744, 40398, 36, 282, 5214, 2881, 43, 15540, 32, 2343, 25, 10, 5135, 4, 274, 31862, 9779, 1966, 9, 3864, 257, 487, 8, 32727, 591, 1690, 8173, 21, 3744, 11, 21431, 13051, 11576, 31, 13895, 642, 4390, 25982, 8, 13895, 642, 4390, 487, 293, 12, 40398, 15540, 11, 130, 3122, 228, 333, 634, 18677, 13998, 1116, 6487, 45094, 4, 20, 38531, 21, 31789, 19, 289, 3540, 611, 620, 4, 10308, 3156, 31, 65, 18292, 228, 333, 32, 2343, 4, 33256, 2003, 35, 654, 46911, 119, 4, 272, 312, 2816, 9779, 1966, 9, 5, 21431, 13051, 31, 13895, 642, 4390, 25982, 36, 282, 5457, 204, 238, 13895, 642, 4390, 487, 293, 2744, 40398, 36, 282, 5457, 204, 43, 8, 13895, 642, 4390, 487, 293, 12, 40398, 36, 282, 5457, 204, 43, 15540, 4, 43510, 651, 9, 9042, 31, 5, 21431, 13051, 9, 5, 15540, 58, 1169, 31789, 13, 234, 3006, 462, 36, 8766, 3236, 3156, 43, 50, 12069, 13, 13998, 2678, 661, 39917, 13, 5, 1855, 21716, 44858, 3551, 17540, 732, 18675, 6208, 594, 4360, 18853, 3175, 36, 4771, 2571, 6, 2576, 3236, 3156, 322, 20, 38898, 6483, 9, 5, 2
],
"label_ids": {
"entity_types": [
"O", "O", "O", "O", "O", "O", "O", "O", "B-GENEPROD", "I-GENEPROD", "I-GENEPROD", "O", "O", "O", "O", "O", "B-ORGANISM", "O", "O", "O", "B-GENEPROD", "I-GENEPROD", "B-GENEPROD", "O", "O", "B-ORGANISM", "O", "O", "O", "O", "O", "B-GENEPROD", "I-GENEPROD", "I-GENEPROD", "O", "O", "O", "O", "O", "O", "B-GENEPROD", "I-GENEPROD", "I-GENEPROD", "O", "O", "O", "B-TISSUE", "I-TISSUE", "O", "O", "O", "B-EXP_ASSAY", "I-EXP_ASSAY", "O", "B-GENEPROD", "I-GENEPROD", "I-GENEPROD", "O", "O", "O", "O", "O", "O", "B-GENEPROD", "I-GENEPROD", "I-GENEPROD", "O", "O", "O", "O", "O", "O", "O", "O", "O", "O", "B-GENEPROD", "I-GENEPROD", "I-GENEPROD", "O", "O", "O", "O", "O", "O", "O", "O", "O", "B-ORGANISM", "O", "B-GENEPROD", "I-GENEPROD", "I-GENEPROD", "O", "O", "O", "O", "O", "O", "O", "O", "O", "O", "O", "O", "O", "O", "B-GENEPROD", "I-GENEPROD", "I-GENEPROD", "O", "O", "O", "O", "O", "B-GENEPROD", "I-GENEPROD", "I-GENEPROD", "O", "O", "O", "B-EXP_ASSAY", "I-EXP_ASSAY", "I-EXP_ASSAY", "O", "O", "O", "O", "O", "O", "O", "B-GENEPROD", "I-GENEPROD", "I-GENEPROD", "O", "O", "O", "O", "O", "O", "B-GENEPROD", "I-GENEPROD", "I-GENEPROD", "O", "O", "O", "O", "O", "O", "O", "O", "O", "O", "B-GENEPROD", "I-GENEPROD", "I-GENEPROD", "O", "O", "O", "O", "O", "O", "O", "O", "O", "B-ORGANISM", "O", "O", "O", "O", "O", "O", "O", "O", "B-GENEPROD", "I-GENEPROD", "I-GENEPROD", "O", "O", "O", "O", "O", "O", "B-GENEPROD", "I-GENEPROD", "I-GENEPROD", "O", "O", "O", "O", "O", "O", "O", "O", "O", "O", "B-GENEPROD", "I-GENEPROD", "I-GENEPROD", "O", "O", "O", "O", "O", "O", "O", "O", "O", "B-ORGANISM", "O", "O", "B-EXP_ASSAY", "I-EXP_ASSAY", "I-EXP_ASSAY", "O", "O", "O", "O", "B-GENEPROD", "I-GENEPROD", "I-GENEPROD", "O", "O", "O", "O", "O", "O", "B-GENEPROD", "I-GENEPROD", "I-GENEPROD", "O", "O", "O", "O", "O", "O", "O", "O", "O", "O", "B-GENEPROD", "I-GENEPROD", "I-GENEPROD", "O", "O", "O", "O", "O", "O", "O", "O", "O", "B-ORGANISM", "O", "O", "O", "O", "O", "O", "B-EXP_ASSAY", "O", "O", "B-GENEPROD", "I-GENEPROD", "I-GENEPROD", "O", "O", "O", "O", "B-ORGANISM", "O", "O", "O", "O", "O", "O", "O", "O", "O", "O", "O", "O", "O", "O", "O", "O", "O", "O", "O", "O", "O", "O", "O", "O", "O", "O", "B-EXP_ASSAY", "O", "O", "O", "O", "O", "O", "O", "O", "O", "O", "B-GENEPROD", "I-GENEPROD", "I-GENEPROD", "O", "O", "O", "O", "O", "O", "O", "B-GENEPROD", "I-GENEPROD", "I-GENEPROD", "O", "O", "O", "O", "O", "O", "O", "O", "O", "B-ORGANISM", "O", "O", "O", "O", "O", "O", "O", "B-EXP_ASSAY", "I-EXP_ASSAY", "I-EXP_ASSAY", "O", "B-GENEPROD", "I-GENEPROD", "I-GENEPROD", "O", "B-GENEPROD", "I-GENEPROD", "O", "O", "O", "O", "O", "B-TISSUE", "I-TISSUE", "O", "O", "B-GENEPROD", "I-GENEPROD", "I-GENEPROD", "O", "O", "B-GENEPROD", "I-GENEPROD", "I-GENEPROD", "O", "O", "O", "O", "B-ORGANISM", "O", "O", "O", "O", "O", "O", "B-EXP_ASSAY", "I-EXP_ASSAY", "I-EXP_ASSAY", "I-EXP_ASSAY", "I-EXP_ASSAY", "O", "O", "B-SUBCELLULAR", "O", "O", "O", "B-EXP_ASSAY", "I-EXP_ASSAY", "I-EXP_ASSAY", "I-EXP_ASSAY", "O", "O", "O", "O", "O", "B-ORGANISM", "O", "O", "O", "O", "O", "O", "O", "O", "O", "O", "O", "O", "O", "B-EXP_ASSAY", "I-EXP_ASSAY", "I-EXP_ASSAY", "I-EXP_ASSAY", "O", "O", "B-TISSUE", "I-TISSUE", "O", "B-GENEPROD", "I-GENEPROD", "I-GENEPROD", "O", "O", "O", "O", "O", "O", "B-GENEPROD", "I-GENEPROD", "I-GENEPROD", "O", "O", "O", "O", "O", "O", "O", "O", "O", "O", "B-GENEPROD", "I-GENEPROD", "I-GENEPROD", "O", "O", "O", "O", "O", "O", "O", "O", "O", "B-ORGANISM", "O", "O", "O", "O", "O", "O", "O", "B-TISSUE", "I-TISSUE", "O", "O", "B-ORGANISM", "O", "O", "O", "O", "B-SUBCELLULAR", "I-SUBCELLULAR", "I-SUBCELLULAR", "O", "O", "O", "O", "O", "O", "O", "O", "B-EXP_ASSAY", "I-EXP_ASSAY", "I-EXP_ASSAY", "I-EXP_ASSAY", "O", "O", "O", "O", "O", "O", "O", "B-GENEPROD", "I-GENEPROD", "I-GENEPROD", "I-GENEPROD", "I-GENEPROD", "I-GENEPROD", "I-GENEPROD", "O", "B-GENEPROD", "I-GENEPROD", "O", "O", "O", "O", "O", "O", "B-SUBCELLULAR", "I-SUBCELLULAR", "O", "O", "O"
],
"geneprod_roles": [
"O", "O", "O", "O", "O", "O", "O", "O", "B-CONTROLLED_VAR", "I-CONTROLLED_VAR", "I-CONTROLLED_VAR", "O", "O", "O", "O", "O", "O", "O", "O", "O", "O", "O", "O", "O", "O", "O", "O", "O", "O", "O", "O", "B-CONTROLLED_VAR", "I-CONTROLLED_VAR", "I-CONTROLLED_VAR", "O", "O", "O", "O", "O", "O", "B-CONTROLLED_VAR", "I-CONTROLLED_VAR", "I-CONTROLLED_VAR", "O", "O", "O", "O", "O", "O", "O", "O", "O", "O", "O", "B-CONTROLLED_VAR", "I-CONTROLLED_VAR", "I-CONTROLLED_VAR", "O", "O", "O", "O", "O", "O", "B-CONTROLLED_VAR", "I-CONTROLLED_VAR", "I-CONTROLLED_VAR", "O", "O", "O", "O", "O", "O", "O", "O", "O", "O", "B-CONTROLLED_VAR", "I-CONTROLLED_VAR", "I-CONTROLLED_VAR", "O", "O", "O", "O", "O", "O", "O", "O", "O", "O", "O", "O", "O", "O", "O", "O", "O", "O", "O", "O", "O", "O", "O", "O", "O", "O", "O", "O", "B-MEASURED_VAR", "I-MEASURED_VAR", "I-MEASURED_VAR", "O", "O", "O", "O", "O", "O", "O", "O", "O", "O", "O", "O", "O", "O", "O", "O", "O", "O", "O", "O", "O", "B-CONTROLLED_VAR", "I-CONTROLLED_VAR", "I-CONTROLLED_VAR", "O", "O", "O", "O", "O", "O", "B-CONTROLLED_VAR", "I-CONTROLLED_VAR", "I-CONTROLLED_VAR", "O", "O", "O", "O", "O", "O", "O", "O", "O", "O", "B-CONTROLLED_VAR", "I-CONTROLLED_VAR", "I-CONTROLLED_VAR", "O", "O", "O", "O", "O", "O", "O", "O", "O", "O", "O", "O", "O", "O", "O", "O", "O", "O", "B-CONTROLLED_VAR", "I-CONTROLLED_VAR", "I-CONTROLLED_VAR", "O", "O", "O", "O", "O", "O", "B-CONTROLLED_VAR", "I-CONTROLLED_VAR", "I-CONTROLLED_VAR", "O", "O", "O", "O", "O", "O", "O", "O", "O", "O", "B-CONTROLLED_VAR", "I-CONTROLLED_VAR", "I-CONTROLLED_VAR", "O", "O", "O", "O", "O", "O", "O", "O", "O", "O", "O", "O", "O", "O", "O", "O", "O", "O", "O", "B-CONTROLLED_VAR", "I-CONTROLLED_VAR", "I-CONTROLLED_VAR", "O", "O", "O", "O", "O", "O", "B-CONTROLLED_VAR", "I-CONTROLLED_VAR", "I-CONTROLLED_VAR", "O", "O", "O", "O", "O", "O", "O", "O", "O", "O", "B-CONTROLLED_VAR", "I-CONTROLLED_VAR", "I-CONTROLLED_VAR", "O", "O", "O", "O", "O", "O", "O", "O", "O", "O", "O", "O", "O", "O", "O", "O", "O", "O", "O", "B-CONTROLLED_VAR", "I-CONTROLLED_VAR", "I-CONTROLLED_VAR", "O", "O", "O", "O", "O", "O", "O", "O", "O", "O", "O", "O", "O", "O", "O", "O", "O", "O", "O", "O", "O", "O", "O", "O", "O", "O", "O", "O", "O", "O", "O", "O", "O", "O", "O", "O", "O", "O", "O", "O", "O", "O", "B-CONTROLLED_VAR", "I-CONTROLLED_VAR", "I-CONTROLLED_VAR", "O", "O", "O", "O", "O", "O", "O", "B-CONTROLLED_VAR", "I-CONTROLLED_VAR", "I-CONTROLLED_VAR", "O", "O", "O", "O", "O", "O", "O", "O", "O", "O", "O", "O", "O", "O", "O", "O", "O", "O", "O", "O", "O", "B-MEASURED_VAR", "I-MEASURED_VAR", "I-MEASURED_VAR", "O", "B-MEASURED_VAR", "I-MEASURED_VAR", "O", "O", "O", "O", "O", "O", "O", "O", "O", "B-CONTROLLED_VAR", "I-CONTROLLED_VAR", "I-CONTROLLED_VAR", "O", "O", "B-CONTROLLED_VAR", "I-CONTROLLED_VAR", "I-CONTROLLED_VAR", "O", "O", "O", "O", "O", "O", "O", "O", "O", "O", "O", "O", "O", "O", "O", "O", "O", "O", "O", "O", "O", "O", "O", "O", "O", "O", "O", "O", "O", "O", "O", "O", "O", "O", "O", "O", "O", "O", "O", "O", "O", "O", "O", "O", "O", "O", "O", "O", "O", "O", "O", "O", "O", "O", "B-CONTROLLED_VAR", "I-CONTROLLED_VAR", "I-CONTROLLED_VAR", "O", "O", "O", "O", "O", "O", "B-CONTROLLED_VAR", "I-CONTROLLED_VAR", "I-CONTROLLED_VAR", "O", "O", "O", "O", "O", "O", "O", "O", "O", "O", "B-CONTROLLED_VAR", "I-CONTROLLED_VAR", "I-CONTROLLED_VAR", "O", "O", "O", "O", "O", "O", "O", "O", "O", "O", "O", "O", "O", "O", "O", "O", "O", "O", "O", "O", "O", "O", "O", "O", "O", "O", "O", "O", "O", "O", "O", "O", "O", "O", "O", "O", "O", "O", "O", "O", "O", "O", "O", "O", "O", "O", "O", "O", "B-MEASURED_VAR", "I-MEASURED_VAR", "I-MEASURED_VAR", "I-MEASURED_VAR", "I-MEASURED_VAR", "I-MEASURED_VAR", "I-MEASURED_VAR", "O", "B-MEASURED_VAR", "I-MEASURED_VAR", "O", "O", "O", "O", "O", "O", "O", "O", "O", "O", "O"
],
"boring": [
"O", "O", "O", "O", "O", "O", "O", "O", "O", "O", "O", "O", "O", "O", "O", "O", "B-BORING", "O", "O", "O", "B-BORING", "I-BORING", "B-BORING", "O", "O", "B-BORING", "O", "O", "O", "O", "O", "O", "O", "O", "O", "O", "O", "O", "O", "O", "O", "O", "O", "O", "O", "O", "B-BORING", "I-BORING", "O", "O", "O", "O", "O", "O", "O", "O", "O", "O", "O", "O", "O", "O", "O", "O", "O", "O", "O", "O", "O", "O", "O", "O", "O", "O", "O", "O", "O", "O", "O", "O", "O", "O", "O", "O", "O", "O", "O", "O", "B-BORING", "O", "B-BORING", "I-BORING", "I-BORING", "O", "O", "O", "O", "O", "O", "O", "O", "O", "O", "O", "O", "O", "O", "O", "O", "O", "O", "O", "O", "O", "O", "B-BORING", "I-BORING", "I-BORING", "O", "O", "O", "O", "O", "O", "O", "O", "O", "O", "O", "O", "O", "O", "O", "O", "O", "O", "O", "O", "O", "O", "O", "O", "O", "O", "O", "O", "O", "O", "O", "O", "O", "O", "O", "O", "O", "O", "O", "O", "O", "O", "O", "O", "O", "O", "O", "O", "O", "O", "O", "O", "O", "O", "O", "O", "O", "O", "O", "O", "O", "O", "O", "O", "O", "O", "O", "O", "O", "O", "O", "O", "O", "O", "O", "O", "O", "O", "O", "O", "O", "O", "O", "O", "O", "O", "O", "O", "O", "O", "O", "O", "O", "O", "O", "O", "O", "O", "O", "O", "O", "O", "O", "O", "O", "O", "O", "O", "O", "O", "O", "O", "O", "O", "O", "O", "O", "O", "O", "O", "O", "O", "O", "O", "O", "O", "O", "O", "O", "O", "O", "O", "O", "O", "O", "O", "O", "O", "O", "O", "O", "O", "O", "O", "O", "O", "O", "O", "O", "O", "O", "O", "O", "O", "O", "O", "O", "O", "O", "O", "O", "O", "O", "O", "O", "O", "O", "O", "O", "O", "O", "O", "O", "O", "O", "O", "O", "O", "O", "O", "O", "O", "O", "O", "O", "O", "O", "O", "O", "O", "O", "O", "O", "O", "O", "O", "O", "O", "O", "O", "O", "O", "O", "O", "O", "O", "O", "O", "O", "O", "O", "O", "O", "O", "O", "O", "O", "O", "O", "O", "O", "O", "O", "O", "O", "O", "O", "O", "O", "O", "O", "O", "O", "O", "B-BORING", "I-BORING", "O", "O", "O", "O", "O", "O", "O", "O", "O", "O", "O", "O", "O", "O", "B-BORING", "O", "O", "O", "O", "O", "O", "O", "O", "O", "O", "O", "O", "O", "O", "O", "O", "O", "O", "O", "O", "O", "O", "O", "O", "O", "O", "B-BORING", "O", "O", "O", "O", "O", "O", "O", "O", "O", "O", "O", "O", "O", "O", "O", "O", "O", "O", "O", "B-BORING", "I-BORING", "O", "O", "O", "O", "O", "O", "O", "O", "O", "O", "O", "O", "O", "O", "O", "O", "O", "O", "O", "O", "O", "O", "O", "O", "O", "O", "O", "O", "O", "O", "O", "O", "O", "O", "O", "B-BORING", "O", "O", "O", "O", "O", "O", "O", "B-BORING", "I-BORING", "O", "O", "B-BORING", "O", "O", "O", "O", "O", "O", "O", "O", "O", "O", "O", "O", "O", "O", "O", "O", "O", "O", "O", "O", "O", "O", "O", "O", "O", "O", "O", "O", "O", "O", "O", "O", "O", "O", "O", "O", "O", "O", "O", "O", "O", "O", "O", "O", "O", "O", "O"
],
"panel_start": [
"O", "O", "O", "O", "O", "O", "O", "B-PANEL_START", "O", "O", "O", "O", "O", "O", "O", "O", "O", "O", "O", "O", "O", "O", "O", "O", "O", "O", "O", "O", "O", "O", "O", "O", "O", "O", "O", "O", "O", "O", "O", "O", "O", "O", "O", "O", "O", "O", "O", "O", "O", "O", "O", "O", "O", "O", "O", "O", "O", "O", "O", "O", "O", "O", "O", "O", "O", "O", "O", "O", "O", "O", "O", "O", "O", "O", "O", "O", "O", "O", "O", "O", "O", "O", "O", "O", "O", "O", "O", "O", "O", "O", "O", "O", "O", "O", "O", "O", "O", "O", "O", "O", "O", "O", "O", "O", "O", "O", "O", "O", "O", "O", "O", "O", "O", "O", "O", "O", "O", "O", "O", "O", "B-PANEL_START", "O", "O", "O", "O", "O", "O", "O", "O", "O", "O", "O", "O", "O", "O", "O", "O", "O", "O", "O", "O", "O", "O", "O", "O", "O", "O", "O", "O", "O", "O", "O", "O", "O", "O", "O", "O", "O", "O", "O", "O", "O", "O", "O", "O", "O", "O", "B-PANEL_START", "O", "O", "O", "O", "O", "O", "O", "O", "O", "O", "O", "O", "O", "O", "O", "O", "O", "O", "O", "O", "O", "O", "O", "O", "O", "O", "O", "O", "O", "O", "O", "O", "O", "O", "O", "O", "O", "O", "O", "O", "O", "O", "B-PANEL_START", "O", "O", "O", "O", "O", "O", "O", "O", "O", "O", "O", "O", "O", "O", "O", "O", "O", "O", "O", "O", "O", "O", "O", "O", "O", "O", "O", "O", "O", "O", "O", "O", "O", "O", "O", "O", "O", "O", "O", "O", "O", "O", "O", "B-PANEL_START", "O", "O", "O", "O", "O", "O", "O", "O", "O", "O", "O", "O", "O", "O", "O", "O", "O", "O", "O", "O", "O", "O", "O", "O", "O", "O", "O", "O", "O", "O", "O", "O", "O", "O", "O", "O", "O", "O", "O", "O", "O", "O", "O", "O", "O", "O", "O", "O", "O", "O", "O", "O", "O", "O", "O", "O", "O", "O", "O", "O", "O", "O", "O", "O", "O", "O", "O", "O", "O", "O", "O", "O", "O", "O", "O", "O", "O", "O", "O", "O", "O", "B-PANEL_START", "O", "O", "O", "O", "O", "O", "O", "O", "O", "O", "O", "O", "O", "O", "O", "O", "O", "O", "O", "O", "O", "O", "O", "O", "O", "O", "O", "O", "O", "O", "O", "O", "O", "O", "O", "O", "O", "O", "O", "O", "O", "O", "O", "O", "O", "O", "O", "O", "O", "O", "O", "O", "O", "O", "O", "O", "O", "O", "O", "O", "O", "O", "O", "O", "O", "O", "O", "O", "O", "O", "O", "O", "O", "O", "O", "O", "O", "O", "O", "O", "O", "O", "O", "O", "O", "O", "O", "O", "O", "O", "O", "O", "O", "O", "O", "O", "O", "O", "O", "O", "O", "O", "O", "O", "O", "O", "O", "O", "O", "O", "O", "O", "O", "O", "O", "O", "O", "O", "O", "O", "O", "O", "O", "O", "O", "O", "O", "O", "O", "O", "O", "O", "O", "O", "O", "O", "O", "O", "O", "O", "O", "O", "O", "O", "O", "O", "O", "O", "O", "O", "O", "O", "O", "O", "O", "O", "O", "O", "O", "O", "O", "O", "O", "O", "O", "O", "O", "O", "O", "O", "O", "O", "O", "O", "O"
]
}
}
```
### Data Fields
- `input_ids`: token id in `roberta-base` tokenizers' vocabulary provided as a`list` of `int`
- `label_ids`:
- `entity_types`: `list` of `strings` for the IOB2 tags for entity type; possible value in `["O", "I-SMALL_MOLECULE", "B-SMALL_MOLECULE", "I-GENEPROD", "B-GENEPROD", "I-SUBCELLULAR", "B-SUBCELLULAR", "I-CELL", "B-CELL", "I-TISSUE", "B-TISSUE", "I-ORGANISM", "B-ORGANISM", "I-EXP_ASSAY", "B-EXP_ASSAY"]`
- `geneprod_roles`: `list` of `strings` for the IOB2 tags for experimental roles; values in `["O", "I-CONTROLLED_VAR", "B-CONTROLLED_VAR", "I-MEASURED_VAR", "B-MEASURED_VAR"]`
- `boring`: `list` of `strings` for IOB2 tags for entities unrelated to causal design; values in `["O", "I-BORING", "B-BORING"]`
- `panel_start`: `list` of `strings` for IOB2 tags `["O", "B-PANEL_START"]`
### Data Splits
- train:
- features: ['input_ids', 'labels', 'tag_mask'],
- num_rows: 48_771
- test:
- features: ['input_ids', 'labels', 'tag_mask'],
- num_rows: 13_801
- validation:
- features: ['input_ids', 'labels', 'tag_mask'],
- num_rows: 7_178
## Dataset Creation
### Curation Rationale
The dataset was built to train models for the automatic extraction of a knowledge graph based from the scientific literature. The dataset can be used to train models for text segmentation, named entity recognition and semantic role labeling.
### Source Data
#### Initial Data Collection and Normalization
Figure legends were annotated according to the SourceData framework described in Liechti et al 2017 (Nature Methods, 2017, https://doi.org/10.1038/nmeth.4471). The curation tool at https://curation.sourcedata.io was used to segment figure legends into panel legends, tag enities, assign experiemental roles and normalize with standard identifiers (not available in this dataset). The source data was downloaded from the SourceData API (https://api.sourcedata.io) on 21 Jan 2021.
#### Who are the source language producers?
The examples are extracted from the figure legends from scientific papers in cell and molecular biology.
### Annotations
#### Annotation process
The annotations were produced manually with expert curators from the SourceData project (https://sourcedata.embo.org)
#### Who are the annotators?
Curators of the SourceData project.
### Personal and Sensitive Information
None known.
## Considerations for Using the Data
### Social Impact of Dataset
Not applicable.
### Discussion of Biases
The examples are heavily biased towards cell and molecular biology and are enriched in examples from papers published in EMBO Press journals (https://embopress.org)
### Other Known Limitations
[More Information Needed]
## Additional Information
### Dataset Curators
Thomas Lemberger, EMBO.
### Licensing Information
CC BY 4.0
### Citation Information
[More Information Needed]
### Contributions
Thanks to [@tlemberger](https://github.com/tlemberger>) for adding this dataset.
|
Emanuel/UD_Portuguese-Bosque | ---
language:
- pt
---
# AutoNLP Dataset for project: pos-tag-bosque
## Table of content
- [Dataset Description](#dataset-description)
- [Languages](#languages)
- [Dataset Structure](#dataset-structure)
- [Data Instances](#data-instances)
- [Data Fields](#data-fields)
- [Data Splits](#data-splits)
## Dataset Descritpion
This dataset has been automatically processed by AutoNLP for project pos-tag-bosque.
### Languages
The BCP-47 code for the dataset's language is pt.
## Dataset Structure
### Data Instances
A sample from this dataset looks as follows:
```json
[
{
"tags": [
5,
7,
0
],
"tokens": [
"Um",
"revivalismo",
"refrescante"
]
},
{
"tags": [
5,
11,
11,
11,
3,
5,
7,
1,
5,
7,
0,
12
],
"tokens": [
"O",
"7",
"e",
"Meio",
"\u00e9",
"um",
"ex-libris",
"de",
"a",
"noite",
"algarvia",
"."
]
}
]
```
### Dataset Fields
The dataset has the following fields (also called "features"):
```json
{
"tags": "Sequence(feature=ClassLabel(num_classes=17, names=['ADJ', 'ADP', 'ADV', 'AUX', 'CCONJ', 'DET', 'INTJ', 'NOUN', 'NUM', 'PART', 'PRON', 'PROPN', 'PUNCT', 'SCONJ', 'SYM', 'VERB', 'X'], names_file=None, id=None), length=-1, id=None)",
"tokens": "Sequence(feature=Value(dtype='string', id=None), length=-1, id=None)"
}
```
### Dataset Splits
This dataset is split into a train and validation split. The split sizes are as follow:
| Split name | Num samples |
| ------------ | ------------------- |
| train | 8328 |
| valid | 476 |
|
Emma121/aaaaa | ---
license: bsd-3-clause-clear
---
|
Exr0n/wiki-entity-similarity | ---
annotations_creators:
- found
language:
- en
language_creators:
- found
license:
- mit
multilinguality:
- monolingual
pretty_name: 'Wiki Entity Similarity
'
size_categories:
- 10M<n<100M
source_datasets:
- original
tags:
- named entities
- similarity
- paraphrasing
- synonyms
- wikipedia
task_categories: []
task_ids: []
---
# Wiki Entity Similarity
Usage:
```py
from datasets import load_dataset
corpus = load_dataset('Exr0n/wiki-entity-similarity', '2018thresh20corpus', split='train')
assert corpus[0] == {'article': 'A1000 road', 'link_text': 'A1000', 'is_same': 1}
pairs = load_dataset('Exr0n/wiki-entity-similarity', '2018thresh20pairs', split='train')
assert corpus[0] == {'article': 'Rhinobatos', 'link_text': 'Ehinobatos beurleni', 'is_same': 1}
assert len(corpus) == 4_793_180
```
## Corpus (`name=*corpus`)
The corpora in this are generated by aggregating the link text that refers to various articles in context. For instance, if wiki article A refers to article B as C, then C is added to the list of aliases for article B, and the pair (B, C) is included in the dataset.
Following (DPR https://arxiv.org/pdf/2004.04906.pdf), we use the English Wikipedia dump from Dec. 20, 2018 as the source documents for link collection.
The dataset includes three quality levels, distinguished by the minimum number of inbound links required to include an article in the dataset. This filtering is motivated by the heuristic "better articles have more citations."
| Min. Inbound Links | Number of Articles | Number of Distinct Links |
|------------|--------------------|--------------------------|
| 5 | 1,080,073 | 5,787,081 |
| 10 | 605,775 | 4,407,409 |
| 20 | 324,949 | 3,195,545 |
## Training Pairs (`name=*pairs`)
This dataset also includes training pair datasets (with both positive and negative examples) intended for training classifiers. The train/dev/test split is 75/15/10 % of each corpus.
### Training Data Generation
The training pairs in this dataset are generated by taking each example from the corpus as a positive example, and creating a new negative example from the article title of the positive example and a random link text from a different article.
The articles featured in each split are disjoint from the other splits, and each split has the same number of positive (semantically the same) and negative (semantically different) examples.
For more details on the dataset motivation, see [the paper](https://arxiv.org/abs/2202.13581). If you use this dataset in your work, please cite it using the ArXiv reference.
Generation scripts can be found [in the GitHub repo](https://github.com/Exr0nProjects/wiki-entity-similarity).
|
Eymen3455/xsum_tr | |
FIG-Loneliness/FIG-Loneliness | # Dataset Card for FIG-Loneliness
## Table of Contents
- [Table of Contents](#table-of-contents)
- [Dataset Description](#dataset-description)
- [Dataset Summary](#dataset-summary)
- [Supported Tasks and Leaderboards](#supported-tasks-and-leaderboards)
- [Languages](#languages)
- [Dataset Structure](#dataset-structure)
- [Data Instances](#data-instances)
- [Data Fields](#data-fields)
- [Data Splits](#data-splits)
- [Dataset Creation](#dataset-creation)
- [Curation Rationale](#curation-rationale)
- [Source Data](#source-data)
- [Annotations](#annotations)
- [Personal and Sensitive Information](#personal-and-sensitive-information)
- [Considerations for Using the Data](#considerations-for-using-the-data)
- [Social Impact of Dataset](#social-impact-of-dataset)
- [Discussion of Biases](#discussion-of-biases)
- [Other Known Limitations](#other-known-limitations)
- [Additional Information](#additional-information)
- [Dataset Curators](#dataset-curators)
- [Licensing Information](#licensing-information)
- [Citation Information](#citation-information)
- [Contributions](#contributions)
## Dataset Description
- **Homepage:** [FIG-Loneliness](https://ojs.aaai.org/index.php/ICWSM/article/view/19302)
- **Paper:** [Many Ways to be Lonely](https://ojs.aaai.org/index.php/ICWSM/article/view/19302/19074)
- **Point of Contact:** [Sherry Yueyi Jiang](mailto:yujiang@ucsd.edu)
### Dataset Summary
FIG-Loneliness is a dataset for fine-grained loneliness characterization and model training. This dataset consists of 2633 lonely and 3000 non-lonely Reddit posts annotated by trained human annotators. For the lonely posts, we provide fine-grained category labels for the forms of loneliness including duration, context and interpersonal relationships, and for the coping strategies of the authors including reaching out, seeking advice, seeking validation and non-directed interaction.
### Supported Tasks and Leaderboards
[More Information Needed]
### Languages
The language supported is English.
## Dataset Structure
### Loading
To load the dataset, first clone this dataset repo:
```bash
git clone https://huggingface.co/datasets/FIG-Loneliness/FIG-Loneliness
```
Then we can load datasets using Huggingface Datasets API:
```python
import os
import datasets as hf_ds
ROOT = "dir/to/data/repo"
# load datasets
train_set = hf_ds.load_from_disk(os.path.join(ROOT, "train_set"))
dev_set = hf_ds.load_from_disk(os.path.join(ROOT, "dev_set"))
test_set = hf_ds.load_from_disk(os.path.join(ROOT, "test_set"))
```
### Data Instances
The `train_set` split contains 3,943 instances. The `dev_set` split contains 1,126. The `test_set` split contains 564 instances.
### Data Fields
Each instance contains 8 fields: `idx`, `unique_id`, `text`, `lonely`, `temporal`, `interaction`, `context_pri`, and `interpersonal_pri`.
| Field | Meaning |
|:---:|:------------------------------------------------------------------------------------------------------------------------------------------------------------------------------------------------:|
| `idx` | Integer index of this instance from our scrapped Reddit posts. |
| `unique_id` | Unique ID of this Reddit post. |
| `text` | Textual content of the Reddit post. |
| `lonely` | 2-len one-hot vector, representing [non-lonely, lonely]. |
| `temporal` | **Duration**. 4-len vector summarizing human annotators' votings in the order of [transient, enduring, ambiguous, NA]. |
| `interaction` | **Interaction**. 5-len vector summarizing human annotators' votings in the order of [seeking advice, providing help, seeking validation and affirmation, reaching out, non directed interaction] |
| `context_pri` | **Context**. 5-len vector summarizing human annotators' votings in the order of [social, physical, somatic, romantic, N/A] |
| `interpersonal_pri` | **Interpersonal**. 5-len vector summarizing human annotators' votings in the order of [romantic, friendship, family, colleagues, N/A] |
### Data Splits
The entire dataset is split into 3,943 training instances, 1,126 dev instances, and 564 test instances.
## Dataset Creation
### Curation Rationale
The data curation rationale is to capture **loneliness expressions** not only from a wider user base but also from users who specifically belong to the young adult age group (a vulnerable group for loneliness). We sampled data from Reddit and subsequently annotated the data with loneliness labels.
### Source Data
#### Initial Data Collection and Normalization
By using Reddit’s Pushshift API, we collected all posts from two loneliness specific subreddits (*r/loneliness*, *r/lonely*) and two subreddits for young adults (*r/youngadults*, *r/college*) from 2018 to 2020.
#### Who are the source language producers?
[More Information Needed]
### Annotations
#### Who are the annotators?
Annotation labels were provided by trained undergraduate research assistants and Amazon’s Mechanical Turk workers (MTurkers) with a Master certification.
#### Annotation process
For the potential lonely samples:
We had research assistants labeled the sampled potential lonely posts. Each post was labeled by three of research assistants. A posts was first labeled on whether it contains an expression on self-disclosure of loneliness. If the majority of the annotators labeled a post as not containing such expression, the post would be discarded, otherwise it is further labeled according to a codebook that contains the following categories: (1) *duration*: the duration of the loneliness experience (transient, enduring, and ambiguous), (2) *context*: the contexts of the experience (social, physical, somatic, and romantic), (3) *interpresonal*: the interpersonal relationships involved in the experience (romantic, family, friendship, and peers), and (4) *interaction*: user interaction styles (seeking advice, providing support, seeking validation/affirmation, reaching out and non-directed interaction). The codebook is intended for dissecting different forms of loneliness and users’ coping strategies in the loneliness discourse. We also included a ‘not applicable’ (NA) label to accommodate situations that are not suitable for classification. For each category, the annotators gave *one value* which they think would best capture the source of loneliness in the post or the poster’s interaction intent.
For the potential non-lonely samples:
MTurkers were instructed to classify whether the Reddit posters express loneliness. Each post was annotated by three MTurkers, and only posts labeled as non-lonely by the majority would remain in the final annotated dataset.
All the labeled posts and annotations were included in FIG-Loneliness, which consists of roughly 3000 lonely and 3000 non-lonely posts.
#### Dataset Codebook
See code rule and example posts for the category labels [here](https://drive.google.com/file/d/1J6i72qyqirAIC40jWuJvDN-ZWU87XttH/view).
### Personal and Sensitive Information
[More Information Needed]
## Considerations for Using the Data
### Social Impact of Dataset
[More Information Needed]
### Discussion of Biases
[More Information Needed]
### Other Known Limitations
See **Limitation and Data Disclaimer** [here](https://ojs.aaai.org/index.php/ICWSM/article/view/19302/19074)
## Additional Information
### Dataset Curator
[More Information Needed]
### Licensing Information
[CC BY-NC 4.0](https://creativecommons.org/licenses/by-nc/4.0/)
### Citation Information
Jiang, Y., Jiang, Y., Leqi, L., & Winkielman, P. (2022). Many Ways to Be Lonely: Fine-Grained Characterization of Loneliness and Its Potential Changes in COVID-19. Proceedings of the International AAAI Conference on Web and Social Media, 16(1), 405-416. Retrieved from https://ojs.aaai.org/index.php/ICWSM/article/view/19302 |
Felix-ML/quoteli3 | ---
language:
- en
license:
- cc-by-4.0
multilinguality:
- monolingual
size_categories:
- 1K<n<10K
source_datasets: []
---
# Dataset Card for quoteli3
## Dataset Description
- **Homepage:** https://nlp.stanford.edu/~muzny/quoteli.html
- **Repository:** https://nlp.stanford.edu/~muzny/quoteli.html
- **Paper:** Muzny, Grace, et al. "A two-stage sieve approach for quote attribution." Proceedings of the 15th Conference of the European Chapter of the Association for Computational Linguistics: Volume 1, Long Papers. 2017.
### Dataset Summary
This dataset is based on the quoteli3 dataset by Muzny et al. (2017). It contains annotated quotes for three pieces of literature: Chekhov\\\\\\\\\\\\'s The Steppe, Austen\\\\\\\\\\\\'s Emma and Pride and Prejudice.
### Languages
The text in the dataset is English.
## Dataset Structure
Training data:
-Quotes (1575, 11)
-Characters (32, 6)
Test data:
-Quotes (1513, 11)
-Characters (145, 6)
### Data Splits
-Quotes:
- train:
- features: ['mention', 'oid', 'speaker', 'connection', 'id', 'answer', 'answer_mention {'answer', 'answer_start', 'answer_end', 'answer_in_context'}, 'question', 'context', 'large_context', 'book_title'],
- num_rows: 1575
- test:
- features: ['mention', 'oid', 'speaker', 'connection', 'id', 'answer', 'answer_mention {'answer', 'answer_start', 'answer_end', 'answer_in_context'}, 'question', 'context', 'large_context', 'book_title'],
- num_rows: 1513
-Characters:
- train:
- features: ['aliases', 'description', 'gender', 'name', 'id', 'book_title'],
- num_rows: 32
- test:
- features: ['aliases', 'description', 'gender', 'name', 'id', 'book_title'],
- num_rows: 146 |
Finnish-NLP/mc4_fi_cleaned | ---
annotations_creators: []
language_creators: []
language:
- fi
license: []
multilinguality:
- monolingual
size_categories:
- unknown
source_datasets:
- extended|mc4
task_categories:
- text-generation
- fill-mask
task_ids:
- language-modeling
- masked-language-modeling
pretty_name: mC4 Finnish Cleaned
---
# Dataset Card for mC4 Finnish Cleaned
## Table of Contents
- [Dataset Description](#dataset-description)
- [Dataset Summary](#dataset-summary)
- [Supported Tasks](#supported-tasks-and-leaderboards)
- [Languages](#languages)
- [Dataset Structure](#dataset-structure)
- [Data Instances](#data-instances)
- [Data Fields](#data-instances)
- [Data Splits](#data-instances)
- [Dataset Creation](#dataset-creation)
- [Curation Rationale](#curation-rationale)
- [Source Data](#source-data)
- [Annotations](#annotations)
- [Personal and Sensitive Information](#personal-and-sensitive-information)
- [Considerations for Using the Data](#considerations-for-using-the-data)
- [Social Impact of Dataset](#social-impact-of-dataset)
- [Discussion of Biases](#discussion-of-biases)
- [Other Known Limitations](#other-known-limitations)
- [Additional Information](#additional-information)
- [Dataset Curators](#dataset-curators)
- [Licensing Information](#licensing-information)
- [Citation Information](#citation-information)
## Dataset Description
- **Homepage:** [Needs More Information]
- **Repository:** [Needs More Information]
- **Paper:** [Needs More Information]
- **Leaderboard:** [Needs More Information]
- **Point of Contact:** [Needs More Information]
### Dataset Summary
mC4 Finnish cleaned is cleaned version of the original mC4 Finnish split.
### Supported Tasks and Leaderboards
mC4 Finnish is mainly intended to pretrain Finnish language models and word representations.
### Languages
Finnish
## Dataset Structure
### Data Instances
[Needs More Information]
### Data Fields
The data have several fields:
- url: url of the source as a string
- text: text content as a string
- timestamp: timestamp as a string
- perplexity_kenlm_full: perplexity of the text calculated by KenLM model
### Data Splits
Train Validation
## Dataset Creation
### Curation Rationale
[Needs More Information]
### Source Data
#### Initial Data Collection and Normalization
[Needs More Information]
#### Who are the source language producers?
[Needs More Information]
### Annotations
#### Annotation process
[Needs More Information]
#### Who are the annotators?
[Needs More Information]
### Personal and Sensitive Information
[Needs More Information]
## Considerations for Using the Data
### Social Impact of Dataset
[Needs More Information]
### Discussion of Biases
[Needs More Information]
### Other Known Limitations
[Needs More Information]
## Additional Information
### Dataset Curators
[Needs More Information]
### Licensing Information
[Needs More Information]
### Citation Information
[Needs More Information] |
Firoj/HumAID | # Dataset Card for HumAID
## Table of Contents
- [Dataset Description](#dataset-description)
- [Dataset Summary](#dataset-summary)
- [Supported Tasks](#supported-tasks-and-leaderboards)
- [Languages](#languages)
- [Dataset Structure](#dataset-structure)
- [Data Instances](#data-instances)
- [Data Fields](#data-instances)
- [Data Splits](#data-instances)
- [Dataset Creation](#dataset-creation)
- [Curation Rationale](#curation-rationale)
- [Source Data](#source-data)
- [Annotations](#annotations)
- [Personal and Sensitive Information](#personal-and-sensitive-information)
- [Considerations for Using the Data](#considerations-for-using-the-data)
- [Social Impact of Dataset](#social-impact-of-dataset)
- [Discussion of Biases](#discussion-of-biases)
- [Other Known Limitations](#other-known-limitations)
- [Additional Information](#additional-information)
- [Dataset Curators](#dataset-curators)
- [Licensing Information](#licensing-information)
- [Citation Information](#citation-information)
## Dataset Description
- **Homepage:** https://crisisnlp.qcri.org/humaid_dataset
- **Repository:** https://crisisnlp.qcri.org/data/humaid/humaid_data_all.zip
- **Paper:** https://ojs.aaai.org/index.php/ICWSM/article/view/18116/17919
<!-- - **Leaderboard:** [Needs More Information] -->
<!-- - **Point of Contact:** [Needs More Information] -->
### Dataset Summary
The HumAID Twitter dataset consists of several thousands of manually annotated tweets that has been collected during 19 major natural disaster events including earthquakes, hurricanes, wildfires, and floods, which happened from 2016 to 2019 across different parts of the World. The annotations in the provided datasets consists of following humanitarian categories. The dataset consists only english tweets and it is the largest dataset for crisis informatics so far.
** Humanitarian categories **
- Caution and advice
- Displaced people and evacuations
- Dont know cant judge
- Infrastructure and utility damage
- Injured or dead people
- Missing or found people
- Not humanitarian
- Other relevant information
- Requests or urgent needs
- Rescue volunteering or donation effort
- Sympathy and support
The resulting annotated dataset consists of 11 labels.
### Supported Tasks and Benchmark
The dataset can be used to train a model for multiclass tweet classification for disaster response. The benchmark results can be found in https://ojs.aaai.org/index.php/ICWSM/article/view/18116/17919.
Dataset is also released with event-wise and JSON objects for further research.
Full set of the dataset can be found in https://dataverse.harvard.edu/dataset.xhtml?persistentId=doi:10.7910/DVN/A7NVF7
### Languages
English
## Dataset Structure
### Data Instances
```
{
"tweet_text": "@RT_com: URGENT: Death toll in #Ecuador #quake rises to 233 \u2013 President #Correa #1 in #Pakistan",
"class_label": "injured_or_dead_people"
}
```
### Data Fields
* tweet_text: corresponds to the tweet text.
* class_label: corresponds to a label assigned to a given tweet text
### Data Splits
* Train
* Development
* Test
## Dataset Creation
<!-- ### Curation Rationale -->
### Source Data
#### Initial Data Collection and Normalization
Tweets has been collected during several disaster events.
### Annotations
#### Annotation process
AMT has been used to annotate the dataset. Please check the paper for a more detail.
#### Who are the annotators?
- crowdsourced
<!-- ## Considerations for Using the Data -->
<!-- ### Social Impact of Dataset -->
<!-- ### Discussion of Biases -->
<!-- [Needs More Information] -->
<!-- ### Other Known Limitations -->
<!-- [Needs More Information] -->
## Additional Information
### Dataset Curators
Authors of the paper.
### Licensing Information
- cc-by-nc-4.0
### Citation Information
```
@inproceedings{humaid2020,
Author = {Firoj Alam, Umair Qazi, Muhammad Imran, Ferda Ofli},
booktitle={Proceedings of the Fifteenth International AAAI Conference on Web and Social Media},
series={ICWSM~'21},
Keywords = {Social Media, Crisis Computing, Tweet Text Classification, Disaster Response},
Title = {HumAID: Human-Annotated Disaster Incidents Data from Twitter},
Year = {2021},
publisher={AAAI},
address={Online},
}
``` |
Fraser/mnist-text-default | MNIST dataset adapted to a text-based representation.
This allows testing interpolation quality for Transformer-VAEs.
System is heavily inspired by Matthew Rayfield's work https://youtu.be/Z9K3cwSL6uM
Works by quantising each MNIST pixel into one of 64 characters.
Every sample has an up & down version to encourage the model to learn rotation invarient features.
Use `.array_to_text(` and `.text_to_array(` methods to test your generated data.
Data format:
- text: (30 x 28 tokens, 840 tokens total): Textual representation of MNIST digit, for example:
```
00 down ! ! ! ! ! ! ! ! ! ! ! ! ! ! ! ! ! ! ! ! ! ! ! ! ! ! ! !
01 down ! ! ! ! ! ! ! ! ! ! ! ! ! ! ! ! ! ! ! ! ! ! ! ! ! ! ! !
02 down ! ! ! ! ! ! ! ! ! ! ! ! ! ! ! ! ! ! ! ! ! ! ! ! ! ! ! !
03 down ! ! ! ! ! ! ! ! ! ! ! ! ! ! ! ! ! ! ! ! ! ! ! ! ! ! ! !
04 down ! ! ! ! ! ! ! ! ! ! ! ! ! ! ! ! ! ! ! ! ! ! ! ! ! ! ! !
05 down ! ! ! ! ! ! ! ! ! ! ! ! ! % % % @ C L ' J a ^ @ ! ! ! !
06 down ! ! ! ! ! ! ! ! ( * 8 G K ` ` ` ` ` Y L ` ] Q 1 ! ! ! !
07 down ! ! ! ! ! ! ! - \ ` ` ` ` ` ` ` ` _ 8 5 5 / * ! ! ! ! !
08 down ! ! ! ! ! ! ! % W ` ` ` ` ` R N ^ ] ! ! ! ! ! ! ! ! ! !
09 down ! ! ! ! ! ! ! ! 5 H ; ` ` T # ! + G ! ! ! ! ! ! ! ! ! !
10 down ! ! ! ! ! ! ! ! ! $ ! G ` 7 ! ! ! ! ! ! ! ! ! ! ! ! ! !
11 down ! ! ! ! ! ! ! ! ! ! ! C ` P ! ! ! ! ! ! ! ! ! ! ! ! ! !
12 down ! ! ! ! ! ! ! ! ! ! ! # P ` 2 ! ! ! ! ! ! ! ! ! ! ! ! !
13 down ! ! ! ! ! ! ! ! ! ! ! ! ) ] Y I < ! ! ! ! ! ! ! ! ! ! !
14 down ! ! ! ! ! ! ! ! ! ! ! ! ! 5 ] ` ` > ' ! ! ! ! ! ! ! ! !
15 down ! ! ! ! ! ! ! ! ! ! ! ! ! ! , O ` ` F ' ! ! ! ! ! ! ! !
16 down ! ! ! ! ! ! ! ! ! ! ! ! ! ! ! % 8 ` ` O ! ! ! ! ! ! ! !
17 down ! ! ! ! ! ! ! ! ! ! ! ! ! ! ! ! ! _ ` _ 1 ! ! ! ! ! ! !
18 down ! ! ! ! ! ! ! ! ! ! ! ! ! ! , A N ` ` T ! ! ! ! ! ! ! !
19 down ! ! ! ! ! ! ! ! ! ! ! ! * F Z ` ` ` _ N ! ! ! ! ! ! ! !
20 down ! ! ! ! ! ! ! ! ! ! ' = X ` ` ` ` S 4 ! ! ! ! ! ! ! ! !
21 down ! ! ! ! ! ! ! ! & 1 V ` ` ` ` R 5 ! ! ! ! ! ! ! ! ! ! !
22 down ! ! ! ! ! ! % K W ` ` ` ` Q 5 # ! ! ! ! ! ! ! ! ! ! ! !
23 down ! ! ! ! . L Y ` ` ` ` ^ B # ! ! ! ! ! ! ! ! ! ! ! ! ! !
24 down ! ! ! ! C ` ` ` V B B % ! ! ! ! ! ! ! ! ! ! ! ! ! ! ! !
25 down ! ! ! ! ! ! ! ! ! ! ! ! ! ! ! ! ! ! ! ! ! ! ! ! ! ! ! !
26 down ! ! ! ! ! ! ! ! ! ! ! ! ! ! ! ! ! ! ! ! ! ! ! ! ! ! ! !
27 down ! ! ! ! ! ! ! ! ! ! ! ! ! ! ! ! ! ! ! ! ! ! ! ! ! ! ! !
```
- label: Just a number with the texts matching label. |
Fraser/mnist-text-small | MNIST dataset adapted to a text-based representation.
Modified images to be ~1/4 the original area.
Done by taking a max pool.
This allows testing interpolation quality for Transformer-VAEs.
System is heavily inspired by Matthew Rayfield's work https://youtu.be/Z9K3cwSL6uM
Works by quantising each MNIST pixel into one of 64 characters.
Every sample has an up & down version to encourage the model to learn rotation invarient features.
Use `.array_to_text(` and `.text_to_array(` methods to test your generated data.
Data format:
- text: (16 x 14 tokens, 224 tokens total): Textual representation of MNIST digit, for example:
```
00 down ! ! ! ! ! ! ! ! ! ! ! ! ! !
01 down ! ! ! ! ! ! ! ! ! ! ! ! ! !
02 down ! ! ! ! ! ! % % C L a ^ ! !
03 down ! ! ! - ` ` ` ` ` Y ` Q ! !
04 down ! ! ! % ` ` ` R ^ ! ! ! ! !
05 down ! ! ! ! $ G ` ! ! ! ! ! ! !
06 down ! ! ! ! ! # ` Y < ! ! ! ! !
07 down ! ! ! ! ! ! 5 ` ` F ! ! ! !
08 down ! ! ! ! ! ! ! % ` ` 1 ! ! !
09 down ! ! ! ! ! ! F ` ` ` ! ! ! !
10 down ! ! ! ! 1 ` ` ` ` 4 ! ! ! !
11 down ! ! L ` ` ` ` 5 ! ! ! ! ! !
12 down ! ! ` ` V B ! ! ! ! ! ! ! !
13 down ! ! ! ! ! ! ! ! ! ! ! ! ! !
```
- label: Just a number with the texts matching label. |
Fraser/dream-coder | ---
language:
- en
thumbnail: "https://huggingface.co/datasets/Fraser/dream-coder/resolve/main/img.png"
tags:
- program-synthesis
license: "mit"
datasets:
- program-synthesis
---
# Program Synthesis Data
Generated program synthesis datasets used to train [dreamcoder](https://github.com/ellisk42/ec).
Currently just supports text & list data.
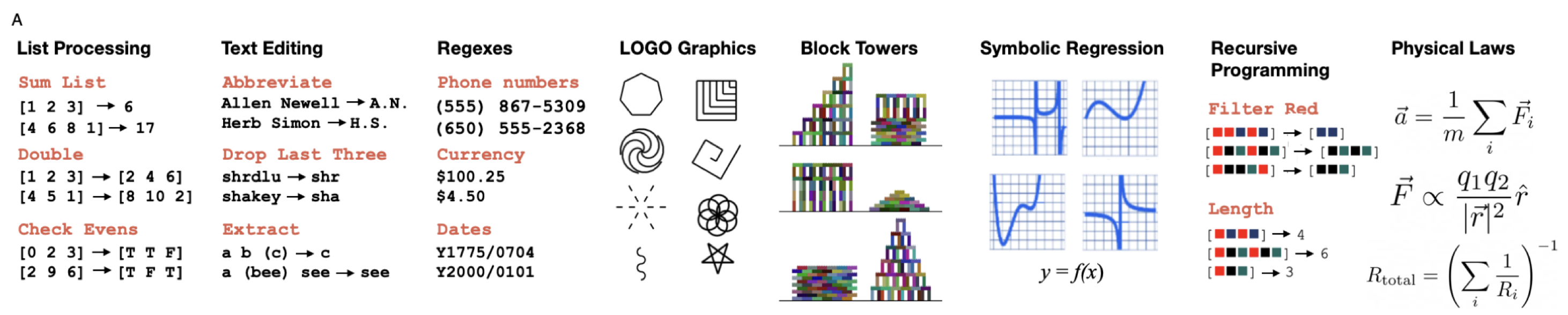
|
Fraser/python-lines | Dataset of single lines of Python code taken from the [CodeSearchNet](https://github.com/github/CodeSearchNet) dataset.
Context
This dataset allows checking the validity of Variational-Autoencoder latent spaces by testing what percentage of random/intermediate latent points can be greedily decoded into valid Python code.
Content
Each row has a parsable line of source code.
{'text': '{python source code line}'}
Most lines are < 100 characters while all are under 125 characters.
Contains 2.6 million lines.
All code is in parsable into a python3 ast.
|
Fraser/python-state-changes | ---
language:
- code
---
# Python State Changes
State changes from the execution of single lines of Python code.
All code was taken from Python HackerRank solutions.
Scraped from my dataset of traced HackerRank solutions. https://www.kaggle.com/frasergreenlee/ran-hackerrank-solutions
```json
{"start": "g = 100; i = 1; l = [100, 100, 0, 0, -100, -100]", "code": "g += l[i]", "end": "g = 200; i = 1; l = [100, 100, 0, 0, -100, -100]"}
{"start": "a = 1; b = 2; d = 4; i = 3; j = 2", "code": "i, j = a + (j - b), b + (d - (i - a))", "end": "a = 1; b = 2; d = 4; i = 1; j = 4"}
{"start": "b = 15", "code": "b = b // 2", "end": "b = 7"}
```
## Get an overview of the dataset from seeing the frequency of different ASTs.
👉 https://observablehq.com/@frasergreenlee/python-lines-dataset#chart |
Fraser/short-jokes | Copy of [Kaggle dataset](https://www.kaggle.com/abhinavmoudgil95/short-jokes), adding to Huggingface for ease of use.
Description from Kaggle:
Context
Generating humor is a complex task in the domain of machine learning, and it requires the models to understand the deep semantic meaning of a joke in order to generate new ones. Such problems, however, are difficult to solve due to a number of reasons, one of which is the lack of a database that gives an elaborate list of jokes. Thus, a large corpus of over 0.2 million jokes has been collected by scraping several websites containing funny and short jokes.
Visit my Github repository for more information regarding collection of data and the scripts used.
Content
This dataset is in the form of a csv file containing 231,657 jokes. Length of jokes ranges from 10 to 200 characters. Each line in the file contains a unique ID and joke.
Disclaimer
It has been attempted to keep the jokes as clean as possible. Since the data has been collected by scraping websites, it is possible that there may be a few jokes that are inappropriate or offensive to some people.
|
Fraser/wiki_sentences | # Wiki Sentences
A dataset of all english sentences in Wikipedia.
Taken from the OPTIMUS project. https://github.com/ChunyuanLI/Optimus/blob/master/download_datasets.md
The dataset is 11.8GB so best to load it using streaming:
```python
from datasets import load_dataset
dataset = load_dataset("Fraser/wiki_sentences", split='train', streaming=True)
```
|
GEM/ART | ---
annotations_creators:
- automatically-created
language_creators:
- unknown
language:
- en
license:
- apache-2.0
multilinguality:
- unknown
size_categories:
- unknown
source_datasets:
- original
task_categories:
- other
task_ids: []
pretty_name: ART
tags:
- reasoning
---
# Dataset Card for GEM/ART
## Dataset Description
- **Homepage:** http://abductivecommonsense.xyz/
- **Repository:** https://storage.googleapis.com/ai2-mosaic/public/abductive-commonsense-reasoning-iclr2020/anlg.zip
- **Paper:** https://openreview.net/pdf?id=Byg1v1HKDB
- **Leaderboard:** N/A
- **Point of Contact:** Chandra Bhagavatulla
### Link to Main Data Card
You can find the main data card on the [GEM Website](https://gem-benchmark.com/data_cards/ART).
### Dataset Summary
Abductive reasoning is inference to the most plausible explanation. For example, if Jenny finds her house in a mess when she returns from work, and remembers that she left a window open, she can hypothesize that a thief broke into her house and caused the mess, as the most plausible explanation.
This data loader focuses on abductive NLG: a conditional English generation task for explaining given observations in natural language.
You can load the dataset via:
```
import datasets
data = datasets.load_dataset('GEM/ART')
```
The data loader can be found [here](https://huggingface.co/datasets/GEM/ART).
#### website
[Website](http://abductivecommonsense.xyz/)
#### paper
[OpenReview](https://openreview.net/pdf?id=Byg1v1HKDB)
#### authors
Chandra Bhagavatula (AI2), Ronan Le Bras (AI2), Chaitanya Malaviya (AI2), Keisuke Sakaguchi (AI2), Ari Holtzman (AI2, UW), Hannah Rashkin (AI2, UW), Doug Downey (AI2), Wen-tau Yih (AI2), Yejin Choi (AI2, UW)
## Dataset Overview
### Where to find the Data and its Documentation
#### Webpage
<!-- info: What is the webpage for the dataset (if it exists)? -->
<!-- scope: telescope -->
[Website](http://abductivecommonsense.xyz/)
#### Download
<!-- info: What is the link to where the original dataset is hosted? -->
<!-- scope: telescope -->
[Google Storage](https://storage.googleapis.com/ai2-mosaic/public/abductive-commonsense-reasoning-iclr2020/anlg.zip)
#### Paper
<!-- info: What is the link to the paper describing the dataset (open access preferred)? -->
<!-- scope: telescope -->
[OpenReview](https://openreview.net/pdf?id=Byg1v1HKDB)
#### BibTex
<!-- info: Provide the BibTex-formatted reference for the dataset. Please use the correct published version (ACL anthology, etc.) instead of google scholar created Bibtex. -->
<!-- scope: microscope -->
```
@inproceedings{
Bhagavatula2020Abductive,
title={Abductive Commonsense Reasoning},
author={Chandra Bhagavatula and Ronan Le Bras and Chaitanya Malaviya and Keisuke Sakaguchi and Ari Holtzman and Hannah Rashkin and Doug Downey and Wen-tau Yih and Yejin Choi},
booktitle={International Conference on Learning Representations},
year={2020},
url={https://openreview.net/forum?id=Byg1v1HKDB}
}
```
#### Contact Name
<!-- quick -->
<!-- info: If known, provide the name of at least one person the reader can contact for questions about the dataset. -->
<!-- scope: periscope -->
Chandra Bhagavatulla
#### Contact Email
<!-- info: If known, provide the email of at least one person the reader can contact for questions about the dataset. -->
<!-- scope: periscope -->
chandrab@allenai.org
#### Has a Leaderboard?
<!-- info: Does the dataset have an active leaderboard? -->
<!-- scope: telescope -->
no
### Languages and Intended Use
#### Multilingual?
<!-- quick -->
<!-- info: Is the dataset multilingual? -->
<!-- scope: telescope -->
no
#### Covered Languages
<!-- quick -->
<!-- info: What languages/dialects are covered in the dataset? -->
<!-- scope: telescope -->
`English`
#### Whose Language?
<!-- info: Whose language is in the dataset? -->
<!-- scope: periscope -->
Crowdworkers on the Amazon Mechanical Turk platform based in the U.S, Canada, U.K and Australia.
#### License
<!-- quick -->
<!-- info: What is the license of the dataset? -->
<!-- scope: telescope -->
apache-2.0: Apache License 2.0
#### Intended Use
<!-- info: What is the intended use of the dataset? -->
<!-- scope: microscope -->
To study the viability of language-based abductive reasoning. Training and evaluating models to generate a plausible hypothesis to explain two given observations.
#### Primary Task
<!-- info: What primary task does the dataset support? -->
<!-- scope: telescope -->
Reasoning
### Credit
#### Curation Organization Type(s)
<!-- info: In what kind of organization did the dataset curation happen? -->
<!-- scope: telescope -->
`industry`
#### Curation Organization(s)
<!-- info: Name the organization(s). -->
<!-- scope: periscope -->
Allen Institute for AI
#### Dataset Creators
<!-- info: Who created the original dataset? List the people involved in collecting the dataset and their affiliation(s). -->
<!-- scope: microscope -->
Chandra Bhagavatula (AI2), Ronan Le Bras (AI2), Chaitanya Malaviya (AI2), Keisuke Sakaguchi (AI2), Ari Holtzman (AI2, UW), Hannah Rashkin (AI2, UW), Doug Downey (AI2), Wen-tau Yih (AI2), Yejin Choi (AI2, UW)
#### Funding
<!-- info: Who funded the data creation? -->
<!-- scope: microscope -->
Allen Institute for AI
#### Who added the Dataset to GEM?
<!-- info: Who contributed to the data card and adding the dataset to GEM? List the people+affiliations involved in creating this data card and who helped integrate this dataset into GEM. -->
<!-- scope: microscope -->
Chandra Bhagavatula (AI2), Ronan LeBras (AI2), Aman Madaan (CMU), Nico Daheim (RWTH Aachen University)
### Dataset Structure
#### Data Fields
<!-- info: List and describe the fields present in the dataset. -->
<!-- scope: telescope -->
- `observation_1`: A string describing an observation / event.
- `observation_2`: A string describing an observation / event.
- `label`: A string that plausibly explains why observation_1 and observation_2 might have happened.
#### How were labels chosen?
<!-- info: How were the labels chosen? -->
<!-- scope: microscope -->
Explanations were authored by crowdworkers on the Amazon Mechanical Turk platform using a custom template designed by the creators of the dataset.
#### Example Instance
<!-- info: Provide a JSON formatted example of a typical instance in the dataset. -->
<!-- scope: periscope -->
```
{
'gem_id': 'GEM-ART-validation-0',
'observation_1': 'Stephen was at a party.',
'observation_2': 'He checked it but it was completely broken.',
'label': 'Stephen knocked over a vase while drunk.'
}
```
#### Data Splits
<!-- info: Describe and name the splits in the dataset if there are more than one. -->
<!-- scope: periscope -->
- `train`: Consists of training instances.
- `dev`: Consists of dev instances.
- `test`: Consists of test instances.
## Dataset in GEM
### Rationale for Inclusion in GEM
#### Why is the Dataset in GEM?
<!-- info: What does this dataset contribute toward better generation evaluation and why is it part of GEM? -->
<!-- scope: microscope -->
Abductive reasoning is a crucial capability of humans and ART is the first dataset curated to study language-based abductive reasoning.
#### Similar Datasets
<!-- info: Do other datasets for the high level task exist? -->
<!-- scope: telescope -->
no
#### Ability that the Dataset measures
<!-- info: What aspect of model ability can be measured with this dataset? -->
<!-- scope: periscope -->
Whether models can reason abductively about a given pair of observations.
### GEM-Specific Curation
#### Modificatied for GEM?
<!-- info: Has the GEM version of the dataset been modified in any way (data, processing, splits) from the original curated data? -->
<!-- scope: telescope -->
no
#### Additional Splits?
<!-- info: Does GEM provide additional splits to the dataset? -->
<!-- scope: telescope -->
no
### Getting Started with the Task
#### Pointers to Resources
<!-- info: Getting started with in-depth research on the task. Add relevant pointers to resources that researchers can consult when they want to get started digging deeper into the task. -->
<!-- scope: microscope -->
- [Paper](https://arxiv.org/abs/1908.05739)
- [Code](https://github.com/allenai/abductive-commonsense-reasoning)
## Previous Results
### Previous Results
#### Measured Model Abilities
<!-- info: What aspect of model ability can be measured with this dataset? -->
<!-- scope: telescope -->
Whether models can reason abductively about a given pair of observations.
#### Metrics
<!-- info: What metrics are typically used for this task? -->
<!-- scope: periscope -->
`BLEU`, `BERT-Score`, `ROUGE`
#### Previous results available?
<!-- info: Are previous results available? -->
<!-- scope: telescope -->
no
## Dataset Curation
### Original Curation
#### Sourced from Different Sources
<!-- info: Is the dataset aggregated from different data sources? -->
<!-- scope: telescope -->
no
### Language Data
#### How was Language Data Obtained?
<!-- info: How was the language data obtained? -->
<!-- scope: telescope -->
`Crowdsourced`
#### Where was it crowdsourced?
<!-- info: If crowdsourced, where from? -->
<!-- scope: periscope -->
`Amazon Mechanical Turk`
#### Language Producers
<!-- info: What further information do we have on the language producers? -->
<!-- scope: microscope -->
Language producers were English speakers in U.S., Canada, U.K and Australia.
#### Topics Covered
<!-- info: Does the language in the dataset focus on specific topics? How would you describe them? -->
<!-- scope: periscope -->
No
#### Data Validation
<!-- info: Was the text validated by a different worker or a data curator? -->
<!-- scope: telescope -->
validated by crowdworker
#### Was Data Filtered?
<!-- info: Were text instances selected or filtered? -->
<!-- scope: telescope -->
algorithmically
#### Filter Criteria
<!-- info: What were the selection criteria? -->
<!-- scope: microscope -->
Adversarial filtering algorithm as described in the [paper](https://arxiv.org/abs/1908.05739)
### Structured Annotations
#### Additional Annotations?
<!-- quick -->
<!-- info: Does the dataset have additional annotations for each instance? -->
<!-- scope: telescope -->
automatically created
#### Annotation Service?
<!-- info: Was an annotation service used? -->
<!-- scope: telescope -->
no
#### Annotation Values
<!-- info: Purpose and values for each annotation -->
<!-- scope: microscope -->
Each observation is associated with a list of COMET (https://arxiv.org/abs/1906.05317) inferences.
#### Any Quality Control?
<!-- info: Quality control measures? -->
<!-- scope: telescope -->
none
### Consent
#### Any Consent Policy?
<!-- info: Was there a consent policy involved when gathering the data? -->
<!-- scope: telescope -->
no
### Private Identifying Information (PII)
#### Contains PII?
<!-- quick -->
<!-- info: Does the source language data likely contain Personal Identifying Information about the data creators or subjects? -->
<!-- scope: telescope -->
no PII
#### Justification for no PII
<!-- info: Provide a justification for selecting `no PII` above. -->
<!-- scope: periscope -->
The dataset contains day-to-day events. It does not contain names, emails, addresses etc.
### Maintenance
#### Any Maintenance Plan?
<!-- info: Does the original dataset have a maintenance plan? -->
<!-- scope: telescope -->
no
## Broader Social Context
### Previous Work on the Social Impact of the Dataset
#### Usage of Models based on the Data
<!-- info: Are you aware of cases where models trained on the task featured in this dataset ore related tasks have been used in automated systems? -->
<!-- scope: telescope -->
no
### Impact on Under-Served Communities
#### Addresses needs of underserved Communities?
<!-- info: Does this dataset address the needs of communities that are traditionally underserved in language technology, and particularly language generation technology? Communities may be underserved for exemple because their language, language variety, or social or geographical context is underepresented in NLP and NLG resources (datasets and models). -->
<!-- scope: telescope -->
no
### Discussion of Biases
#### Any Documented Social Biases?
<!-- info: Are there documented social biases in the dataset? Biases in this context are variations in the ways members of different social categories are represented that can have harmful downstream consequences for members of the more disadvantaged group. -->
<!-- scope: telescope -->
no
## Considerations for Using the Data
### PII Risks and Liability
#### Potential PII Risk
<!-- info: Considering your answers to the PII part of the Data Curation Section, describe any potential privacy to the data subjects and creators risks when using the dataset. -->
<!-- scope: microscope -->
None
### Licenses
#### Copyright Restrictions on the Dataset
<!-- info: Based on your answers in the Intended Use part of the Data Overview Section, which of the following best describe the copyright and licensing status of the dataset? -->
<!-- scope: periscope -->
`public domain`
#### Copyright Restrictions on the Language Data
<!-- info: Based on your answers in the Language part of the Data Curation Section, which of the following best describe the copyright and licensing status of the underlying language data? -->
<!-- scope: periscope -->
`public domain`
### Known Technical Limitations
|
GEM/BiSECT | ---
annotations_creators:
- none
language_creators:
- unknown
language:
- de
- en
- fr
- es
license:
- other
multilinguality:
- unknown
pretty_name: BiSECT
size_categories:
- unknown
source_datasets:
- original
task_categories:
- simplification
task_ids:
- unknown
---
# Dataset Card for GEM/BiSECT
## Dataset Description
- **Homepage:** https://github.com/mounicam/BiSECT
- **Repository:** https://github.com/mounicam/BiSECT/tree/main/bisect
- **Paper:** https://aclanthology.org/2021.emnlp-main.500/
- **Leaderboard:** N/A
- **Point of Contact:** Joongwon Kim, Mounica Maddela, Reno Kriz
### Link to Main Data Card
You can find the main data card on the [GEM Website](https://gem-benchmark.com/data_cards/BiSECT).
### Dataset Summary
This dataset is composed of 1 million complex sentences with the task to split and simplify them while retaining the full meaning. Compared to other simplification corpora, BiSECT requires more significant edits. BiSECT offers splits in English, German, French, and Spanish.
You can load the dataset via:
```
import datasets
data = datasets.load_dataset('GEM/BiSECT')
```
The data loader can be found [here](https://huggingface.co/datasets/GEM/BiSECT).
#### website
[Link](https://github.com/mounicam/BiSECT)
#### paper
[Link](https://aclanthology.org/2021.emnlp-main.500/)
## Dataset Overview
### Where to find the Data and its Documentation
#### Webpage
<!-- info: What is the webpage for the dataset (if it exists)? -->
<!-- scope: telescope -->
[Link](https://github.com/mounicam/BiSECT)
#### Download
<!-- info: What is the link to where the original dataset is hosted? -->
<!-- scope: telescope -->
[Link](https://github.com/mounicam/BiSECT/tree/main/bisect)
#### Paper
<!-- info: What is the link to the paper describing the dataset (open access preferred)? -->
<!-- scope: telescope -->
[Link](https://aclanthology.org/2021.emnlp-main.500/)
#### BibTex
<!-- info: Provide the BibTex-formatted reference for the dataset. Please use the correct published version (ACL anthology, etc.) instead of google scholar created Bibtex. -->
<!-- scope: microscope -->
```
@inproceedings{kim-etal-2021-bisect,
title = "{B}i{SECT}: Learning to Split and Rephrase Sentences with Bitexts",
author = "Kim, Joongwon and
Maddela, Mounica and
Kriz, Reno and
Xu, Wei and
Callison-Burch, Chris",
booktitle = "Proceedings of the 2021 Conference on Empirical Methods in Natural Language Processing",
month = nov,
year = "2021",
address = "Online and Punta Cana, Dominican Republic",
publisher = "Association for Computational Linguistics",
url = "https://aclanthology.org/2021.emnlp-main.500",
pages = "6193--6209"
}
```
#### Contact Name
<!-- quick -->
<!-- info: If known, provide the name of at least one person the reader can contact for questions about the dataset. -->
<!-- scope: periscope -->
Joongwon Kim, Mounica Maddela, Reno Kriz
#### Contact Email
<!-- info: If known, provide the email of at least one person the reader can contact for questions about the dataset. -->
<!-- scope: periscope -->
jkim0118@seas.upenn.edu, mmaddela3@gatech.edu, rkriz1@jh.edu
#### Has a Leaderboard?
<!-- info: Does the dataset have an active leaderboard? -->
<!-- scope: telescope -->
no
### Languages and Intended Use
#### Multilingual?
<!-- quick -->
<!-- info: Is the dataset multilingual? -->
<!-- scope: telescope -->
yes
#### Covered Languages
<!-- quick -->
<!-- info: What languages/dialects are covered in the dataset? -->
<!-- scope: telescope -->
`English`, `German`, `French`, `Spanish, Castilian`
#### License
<!-- quick -->
<!-- info: What is the license of the dataset? -->
<!-- scope: telescope -->
other: Other license
#### Intended Use
<!-- info: What is the intended use of the dataset? -->
<!-- scope: microscope -->
Split and Rephrase.
#### Add. License Info
<!-- info: What is the 'other' license of the dataset? -->
<!-- scope: periscope -->
The dataset is not licensed by itself, and the source OPUS data consists solely of publicly available parallel corpora.
#### Primary Task
<!-- info: What primary task does the dataset support? -->
<!-- scope: telescope -->
Simplification
#### Communicative Goal
<!-- quick -->
<!-- info: Provide a short description of the communicative goal of a model trained for this task on this dataset. -->
<!-- scope: periscope -->
To rewrite a long, complex sentence into shorter, readable, meaning-equivalent sentences.
### Credit
### Dataset Structure
#### Data Fields
<!-- info: List and describe the fields present in the dataset. -->
<!-- scope: telescope -->
- `gem_id` (string): a unique identifier for the instance
- `source_sentence` (string): sentence to be simplified
- `target_sentence` (string)" simplified text that was split and rephrased
#### Example Instance
<!-- info: Provide a JSON formatted example of a typical instance in the dataset. -->
<!-- scope: periscope -->
```
{
"gem_id": "bisect-train-0",
"source_sentence": "The report on the visit to Bhutan states that the small community has made the task of coordination less complex and success is manifested in the synchronized programming cycles which now apply to all but one of the agencies ( the World Health Organization ) .",
"target_sentence": "The report on the visit to Bhutan says that the small community has made the coordination work less complex . Success manifests itself in synchronized programming cycles that now apply to all but one organism ( the World Health Organization ) ."
}
```
#### Data Splits
<!-- info: Describe and name the splits in the dataset if there are more than one. -->
<!-- scope: periscope -->
For the main English BiSECT dataset, the splits are as follows: 1. Train (n=928440) 2. Validation (n=9079) 3. Test (n=583) Additional challenge sets were derived from the data presented in the paper. Please refer to the challenge set sections. The train/validation/test splits for other languages are as follows: German (n=184638/n=864/n=735) Spanish (n=282944/n=3638/n=3081) French (n=491035/n=2400/n=1036)
#### Splitting Criteria
<!-- info: Describe any criteria for splitting the data, if used. If there are differences between the splits (e.g., if the training annotations are machine-generated and the dev and test ones are created by humans, or if different numbers of annotators contributed to each example), describe them here. -->
<!-- scope: microscope -->
While all training data were derived from subsets of the OPUS corpora, different source subsets were used for training v.s., validation and testing. The training set comprised more web crawl data, whereas development and test sets comprised EMEA and EU texts. Details can be found in the BiSECT paper.
## Dataset in GEM
### Rationale for Inclusion in GEM
#### Why is the Dataset in GEM?
<!-- info: What does this dataset contribute toward better generation evaluation and why is it part of GEM? -->
<!-- scope: microscope -->
Understanding long and complex sentences is challenging for both humans and NLP models. The BiSECT dataset helps facilitate more research on Split and Rephrase as a task within itself, as well as how it can benefit downstream NLP applications.
#### Similar Datasets
<!-- info: Do other datasets for the high level task exist? -->
<!-- scope: telescope -->
yes
#### Unique Language Coverage
<!-- info: Does this dataset cover other languages than other datasets for the same task? -->
<!-- scope: periscope -->
yes
#### Difference from other GEM datasets
<!-- info: What else sets this dataset apart from other similar datasets in GEM? -->
<!-- scope: microscope -->
BiSECT is the largest available corpora for the Split and Rephrase task. In addition, it has been shown that BiSECT is of higher quality than previous Split and Rephrase corpora and contains a wider variety of splitting operations.
Most previous Split and Rephrase corpora (HSplit-Wiki, Cont-Benchmark, and Wiki-Benchmark) were manually written at a small scale and focused on evaluation, while the one corpus of comparable size, WikiSplit, contains around 25% of pairs contain significant errors. This is because Wikipedia editors are not only trying to split a sentence, but also often simultaneously modifying the sentence for other purposes, which results in changes of the initial meaning.
### GEM-Specific Curation
#### Modificatied for GEM?
<!-- info: Has the GEM version of the dataset been modified in any way (data, processing, splits) from the original curated data? -->
<!-- scope: telescope -->
yes
#### GEM Modifications
<!-- info: What changes have been made to he original dataset? -->
<!-- scope: periscope -->
`data points added`
#### Modification Details
<!-- info: For each of these changes, described them in more details and provided the intended purpose of the modification -->
<!-- scope: microscope -->
The original BiSECT training, validation, and test splits are maintained to ensure a fair comparison. Note that the original BiSECT test set was created by manually selecting 583 high-quality Split and Rephrase instances from 1000 random source-target pairs sampled from the EMEA and JRC-Acquis corpora from OPUS.
As the first challenge set, we include the HSPLIT-Wiki test set, containing 359 pairs. For each complex sentence, there are four reference splits; To ensure replicability, as reference splits, we again follow the BiSECT paper and present only the references from HSplit2-full.
In addition to the two evaluation sets used in the original BiSECT paper, we also introduce a second challenge set. For this, we initially consider all 7,293 pairs from the EMEA and JRC-Acquis corpora. From there, we classify each pair using the classification algorithm from Section 4.2 of the original BiSECT paper. The three classes are as follows:
1. Direct Insertion: when a long sentence l contains two independent clauses and requires only minor changes in order to make a fluent and meaning-preserving split s.
2. Changes near Split, when l contains one independent and one dependent clause, but modifications are restricted to the region where l is split.
3. Changes across Sentences, where major changes are required throughout l in order to create a fluent split s.
We keep only pairs labeled as Type 3, and after filtering out pairs with significant length differences (signaling potential content addition/deletion), we present a second challenge set of 1,798 pairs.
#### Additional Splits?
<!-- info: Does GEM provide additional splits to the dataset? -->
<!-- scope: telescope -->
no
### Getting Started with the Task
#### Pointers to Resources
<!-- info: Getting started with in-depth research on the task. Add relevant pointers to resources that researchers can consult when they want to get started digging deeper into the task. -->
<!-- scope: microscope -->
The dataset can be downloaded from the original repository by the authors.
The original BiSECT paper proposes several transformer-based models that can be used as baselines, which also compares against Copy512, an LSTM-based model and the previous state-of-the-art.
The common metric used for automatic evaluation of Split and Rephrase, and sentence simplification more generally is SARI. The BiSECT paper also evaluates using BERTScore. Note that automatic evaluations tend to not correlate well with human judgments, so a human evaluation for quality is generally expected for publication. The original BiSECT paper provides templates for collecting quality annotations from Amazon Mechanical Turk.
## Previous Results
### Previous Results
#### Measured Model Abilities
<!-- info: What aspect of model ability can be measured with this dataset? -->
<!-- scope: telescope -->
Text comprehension (needed to generate meaning-equivalent output) and notions of complexity (what is more 'readable' in terms of syntactic structure, lexical choice, punctuation).
#### Metrics
<!-- info: What metrics are typically used for this task? -->
<!-- scope: periscope -->
`Other: Other Metrics`, `BERT-Score`
#### Other Metrics
<!-- info: Definitions of other metrics -->
<!-- scope: periscope -->
SARI is a metric used for evaluating automatic text simplification systems. The metric compares the predicted simplified sentences against the reference and the source sentences. It explicitly measures the goodness of words that are added, deleted and kept by the system.
#### Proposed Evaluation
<!-- info: List and describe the purpose of the metrics and evaluation methodology (including human evaluation) that the dataset creators used when introducing this task. -->
<!-- scope: microscope -->
Existing automatic metrics, such as BLEU (Papineni et al., 2002) and SAMSA (Sulem et al., 2018),
are not optimal for the Split and Rephrase task as
they rely on lexical overlap between the output and
the target (or source) and underestimate the splitting capability of the models that rephrase often.
As such, the dataset creators focused on BERTScore (Zhang et al., 2020) and SARI (Xu et al., 2016). BERTScore captures meaning preservation and fluency
well (Scialom et al., 2021). SARI can provide three
separate F1/precision scores that explicitly measure the correctness of inserted, kept and deleted
n-grams when compared to both the source and
the target. The authors used an extended version of SARI
that considers lexical paraphrases of the reference.
#### Previous results available?
<!-- info: Are previous results available? -->
<!-- scope: telescope -->
yes
## Dataset Curation
### Original Curation
#### Original Curation Rationale
<!-- info: Original curation rationale -->
<!-- scope: telescope -->
BiSECT was constructed to satisfy the need of a Split and Rephrase corpus that is both large-scale and high-quality. Most previous Split and Rephrase corpora (HSplit-Wiki, Cont-Benchmark, and Wiki-Benchmark) were manually written at a small scale and focused on evaluation, while the one corpus of comparable size, WikiSplit, contains around 25% of pairs contain significant errors. This is because Wikipedia editors are not only trying to split a sentence, but also often simultaneously modifying the sentence for other purposes, which results in changes of the initial meaning.
#### Communicative Goal
<!-- info: What was the communicative goal? -->
<!-- scope: periscope -->
The goal of Split and Rephrase is to break down longer sentences into multiple shorter sentences, which has downstream applications for many NLP tasks, including machine translation and dependency parsing.
#### Sourced from Different Sources
<!-- info: Is the dataset aggregated from different data sources? -->
<!-- scope: telescope -->
no
### Language Data
#### How was Language Data Obtained?
<!-- info: How was the language data obtained? -->
<!-- scope: telescope -->
`Found`
#### Where was it found?
<!-- info: If found, where from? -->
<!-- scope: telescope -->
`Other`
#### Language Producers
<!-- info: What further information do we have on the language producers? -->
<!-- scope: microscope -->
N/A.
#### Topics Covered
<!-- info: Does the language in the dataset focus on specific topics? How would you describe them? -->
<!-- scope: periscope -->
There is a range of topics spanning domains such as web crawl and government documents (European Parliament, United Nations, EMEA).
#### Data Validation
<!-- info: Was the text validated by a different worker or a data curator? -->
<!-- scope: telescope -->
validated by data curator
#### Data Preprocessing
<!-- info: How was the text data pre-processed? (Enter N/A if the text was not pre-processed) -->
<!-- scope: microscope -->
The construction of the BiSECT corpus relies on leveraging the sentence-level alignments from OPUS), a collection of bilingual parallel corpora over many language pairs. Given a target language A, this work extracts all 1-2 and 2-1 sentence alignments from parallel corpora between A and a set of foreign languages B.
Next, the foreign sentences are translated into English using Google Translate’s Web API service to obtain sentence alignments between a single long sentence and two corresponding split sentences, both in the desired language.
The authors further filtered the data in a hybrid fashion.
#### Was Data Filtered?
<!-- info: Were text instances selected or filtered? -->
<!-- scope: telescope -->
hybrid
#### Filter Criteria
<!-- info: What were the selection criteria? -->
<!-- scope: microscope -->
To remove noise, the authors remove pairs where the single long sentence (l) contains a token with a punctuation after the first two and before the last two alphabetic characters. The authors also removed instances where l contains more than one unconnected component in its dependency tree, generated via SpaCy.
### Structured Annotations
#### Additional Annotations?
<!-- quick -->
<!-- info: Does the dataset have additional annotations for each instance? -->
<!-- scope: telescope -->
none
#### Annotation Service?
<!-- info: Was an annotation service used? -->
<!-- scope: telescope -->
no
### Consent
#### Any Consent Policy?
<!-- info: Was there a consent policy involved when gathering the data? -->
<!-- scope: telescope -->
no
#### Justification for Using the Data
<!-- info: If not, what is the justification for reusing the data? -->
<!-- scope: microscope -->
Since this data is collected from OPUS, all instances are already in the public domain.
### Private Identifying Information (PII)
#### Contains PII?
<!-- quick -->
<!-- info: Does the source language data likely contain Personal Identifying Information about the data creators or subjects? -->
<!-- scope: telescope -->
unlikely
#### Categories of PII
<!-- info: What categories of PII are present or suspected in the data? -->
<!-- scope: periscope -->
`generic PII`
#### Any PII Identification?
<!-- info: Did the curators use any automatic/manual method to identify PII in the dataset? -->
<!-- scope: periscope -->
no identification
### Maintenance
#### Any Maintenance Plan?
<!-- info: Does the original dataset have a maintenance plan? -->
<!-- scope: telescope -->
no
## Broader Social Context
### Previous Work on the Social Impact of the Dataset
#### Usage of Models based on the Data
<!-- info: Are you aware of cases where models trained on the task featured in this dataset ore related tasks have been used in automated systems? -->
<!-- scope: telescope -->
no
### Impact on Under-Served Communities
#### Addresses needs of underserved Communities?
<!-- info: Does this dataset address the needs of communities that are traditionally underserved in language technology, and particularly language generation technology? Communities may be underserved for exemple because their language, language variety, or social or geographical context is underepresented in NLP and NLG resources (datasets and models). -->
<!-- scope: telescope -->
yes
#### Details on how Dataset Addresses the Needs
<!-- info: Describe how this dataset addresses the needs of underserved communities. -->
<!-- scope: microscope -->
The data as provided in GEMv2 is in English, which is a language with abundant existing resources. However, the original paper also provides Split and Rephrase pairs for French, Spanish, and German, while providing a framework for leveraging bilingual corpora from any language pair found within OPUS.
### Discussion of Biases
#### Any Documented Social Biases?
<!-- info: Are there documented social biases in the dataset? Biases in this context are variations in the ways members of different social categories are represented that can have harmful downstream consequences for members of the more disadvantaged group. -->
<!-- scope: telescope -->
no
#### Are the Language Producers Representative of the Language?
<!-- info: Does the distribution of language producers in the dataset accurately represent the full distribution of speakers of the language world-wide? If not, how does it differ? -->
<!-- scope: periscope -->
The language produced in the dataset is limited to what is captured in the used subset of the OPUS corpora, which might not represent the full distribution of speakers from all locations. For example, the corpora used are from a limited set of relatively formal domains, so it is possible that high performance on the BiSECT test set may not transfer to more informal text.
## Considerations for Using the Data
### PII Risks and Liability
#### Potential PII Risk
<!-- info: Considering your answers to the PII part of the Data Curation Section, describe any potential privacy to the data subjects and creators risks when using the dataset. -->
<!-- scope: microscope -->
Since this data is collected from OPUS, all pairs are already in the public domain.
### Licenses
#### Copyright Restrictions on the Dataset
<!-- info: Based on your answers in the Intended Use part of the Data Overview Section, which of the following best describe the copyright and licensing status of the dataset? -->
<!-- scope: periscope -->
`public domain`
#### Copyright Restrictions on the Language Data
<!-- info: Based on your answers in the Language part of the Data Curation Section, which of the following best describe the copyright and licensing status of the underlying language data? -->
<!-- scope: periscope -->
`public domain`
### Known Technical Limitations
#### Technical Limitations
<!-- info: Describe any known technical limitations, such as spurrious correlations, train/test overlap, annotation biases, or mis-annotations, and cite the works that first identified these limitations when possible. -->
<!-- scope: microscope -->
The creation of English BiSECT relies on translating non-English text back to English. While machine translation systems tend to perform well on high-resource languages, there is still a non-negligible chance that there these systems make errors; through a manual evaluation of a subset of BiSECT, it was found that 15% of pairs contained significant errors, while an additional 22% contained minor adequacy/fluency errors. This problem is exacerbated slightly when creating German BiSECT (22% significant errors, 24% minor errors), and these numbers would likely get larger if lower-resource languages were used.
|
GEM/CrossWOZ | ---
annotations_creators:
- none
language_creators:
- unknown
language:
- zh
license:
- apache-2.0
multilinguality:
- unknown
size_categories:
- unknown
source_datasets:
- original
task_categories:
- conversational
task_ids: []
pretty_name: CrossWOZ
tags:
- dialog-response-generation
---
# Dataset Card for GEM/CrossWOZ
## Dataset Description
- **Homepage:** https://github.com/thu-coai/CrossWOZ
- **Repository:** https://github.com/thu-coai/CrossWOZ
- **Paper:** https://aclanthology.org/2020.tacl-1.19
- **Leaderboard:** N/A
- **Point of Contact:** Qi Zhu
### Link to Main Data Card
You can find the main data card on the [GEM Website](https://gem-benchmark.com/data_cards/CrossWOZ).
### Dataset Summary
CrossWOZ is a Chinese multi-domain task-oriented dialogue dataset . It contains 6K dialogue sessions and 102K utterances for 5 domains, including hotel, restaurant, attraction, metro, and taxi. About 60{\%} of the dialogues have cross-domain user goals that favor inter-domain dependency and encourage natural transition across domains in conversation.
You can load the dataset via:
```
import datasets
data = datasets.load_dataset('GEM/CrossWOZ')
```
The data loader can be found [here](https://huggingface.co/datasets/GEM/CrossWOZ).
#### website
[Github](https://github.com/thu-coai/CrossWOZ)
#### paper
[ACL Anthology](https://aclanthology.org/2020.tacl-1.19)
#### authors
Qi Zhu, Kaili Huang, Zheng Zhang, Xiaoyan Zhu, and Minlie Huang from CoAI group, Tsinghua University
## Dataset Overview
### Where to find the Data and its Documentation
#### Webpage
<!-- info: What is the webpage for the dataset (if it exists)? -->
<!-- scope: telescope -->
[Github](https://github.com/thu-coai/CrossWOZ)
#### Download
<!-- info: What is the link to where the original dataset is hosted? -->
<!-- scope: telescope -->
[Github](https://github.com/thu-coai/CrossWOZ)
#### Paper
<!-- info: What is the link to the paper describing the dataset (open access preferred)? -->
<!-- scope: telescope -->
[ACL Anthology](https://aclanthology.org/2020.tacl-1.19)
#### BibTex
<!-- info: Provide the BibTex-formatted reference for the dataset. Please use the correct published version (ACL anthology, etc.) instead of google scholar created Bibtex. -->
<!-- scope: microscope -->
```
@article{zhu-etal-2020-crosswoz,
title = "{C}ross{WOZ}: A Large-Scale {C}hinese Cross-Domain Task-Oriented Dialogue Dataset",
author = "Zhu, Qi and
Huang, Kaili and
Zhang, Zheng and
Zhu, Xiaoyan and
Huang, Minlie",
journal = "Transactions of the Association for Computational Linguistics",
volume = "8",
year = "2020",
url = "https://aclanthology.org/2020.tacl-1.19",
doi = "10.1162/tacl_a_00314",
pages = "281--295",
abstract = "To advance multi-domain (cross-domain) dialogue modeling as well as alleviate the shortage of Chinese task-oriented datasets, we propose CrossWOZ, the first large-scale Chinese Cross-Domain Wizard-of-Oz task-oriented dataset. It contains 6K dialogue sessions and 102K utterances for 5 domains, including hotel, restaurant, attraction, metro, and taxi. Moreover, the corpus contains rich annotation of dialogue states and dialogue acts on both user and system sides. About 60{\%} of the dialogues have cross-domain user goals that favor inter-domain dependency and encourage natural transition across domains in conversation. We also provide a user simulator and several benchmark models for pipelined task-oriented dialogue systems, which will facilitate researchers to compare and evaluate their models on this corpus. The large size and rich annotation of CrossWOZ make it suitable to investigate a variety of tasks in cross-domain dialogue modeling, such as dialogue state tracking, policy learning, user simulation, etc.",
}
```
#### Contact Name
<!-- quick -->
<!-- info: If known, provide the name of at least one person the reader can contact for questions about the dataset. -->
<!-- scope: periscope -->
Qi Zhu
#### Contact Email
<!-- info: If known, provide the email of at least one person the reader can contact for questions about the dataset. -->
<!-- scope: periscope -->
zhuq96@gmail.com
#### Has a Leaderboard?
<!-- info: Does the dataset have an active leaderboard? -->
<!-- scope: telescope -->
no
### Languages and Intended Use
#### Multilingual?
<!-- quick -->
<!-- info: Is the dataset multilingual? -->
<!-- scope: telescope -->
no
#### Covered Languages
<!-- quick -->
<!-- info: What languages/dialects are covered in the dataset? -->
<!-- scope: telescope -->
`Chinese`
#### License
<!-- quick -->
<!-- info: What is the license of the dataset? -->
<!-- scope: telescope -->
apache-2.0: Apache License 2.0
#### Intended Use
<!-- info: What is the intended use of the dataset? -->
<!-- scope: microscope -->
CrossWOZ is the first large-scale Chinese Cross-Domain Wizard-of-Oz task-oriented dataset. It contains 6K dialogue sessions and 102K utterances for 5 domains, including hotel, restaurant, attraction, metro, and taxi. Moreover, the corpus contains rich annotation of dialogue states and dialogue acts at both user and system sides. We also provide a user simulator and several benchmark models for pipelined taskoriented dialogue systems, which will facilitate researchers to compare and evaluate their models on this corpus.
#### Primary Task
<!-- info: What primary task does the dataset support? -->
<!-- scope: telescope -->
Dialog Response Generation
#### Communicative Goal
<!-- quick -->
<!-- info: Provide a short description of the communicative goal of a model trained for this task on this dataset. -->
<!-- scope: periscope -->
Generate a response according to the dialog context and database search results.
### Credit
#### Curation Organization Type(s)
<!-- info: In what kind of organization did the dataset curation happen? -->
<!-- scope: telescope -->
`academic`
#### Curation Organization(s)
<!-- info: Name the organization(s). -->
<!-- scope: periscope -->
Tsinghua University
#### Dataset Creators
<!-- info: Who created the original dataset? List the people involved in collecting the dataset and their affiliation(s). -->
<!-- scope: microscope -->
Qi Zhu, Kaili Huang, Zheng Zhang, Xiaoyan Zhu, and Minlie Huang from CoAI group, Tsinghua University
#### Funding
<!-- info: Who funded the data creation? -->
<!-- scope: microscope -->
National Science Foundation of China, National Key R&D Program of China
#### Who added the Dataset to GEM?
<!-- info: Who contributed to the data card and adding the dataset to GEM? List the people+affiliations involved in creating this data card and who helped integrate this dataset into GEM. -->
<!-- scope: microscope -->
Qi Zhu (Tsinghua University)
### Dataset Structure
#### Data Fields
<!-- info: List and describe the fields present in the dataset. -->
<!-- scope: telescope -->
- `gem_id` (string): GEM-CrossWOZ-{split}-{id}
- `dialog_id` (string): dialog ID
- `sys_id` (string): system annotator ID
- `usr_id` (string): user annotation ID
- `type` (string): dialog type
- `task description` (list of strings): natural language descriptions of the user goal
- `goal` (list of tuples), includes:
- `sub-goal id` (string)
- `domain name` (string)
- `slot name` (string)
- `constraint` if filled, else `requirement` (string)
- `whether be mentioned in previous turns` (string)
- `messages` (list of dict): dialog turns. Each turn contains:
- `content` (string): utterance
- `role` (string): user or system
- `dialog_act` (list of tuples), includes:
- `domain` (string)
- `intent` (string)
- `slot` (string)
- `value` (string)
- `user_state` (list of tuples): same format as "goal", can be viewed as dynamic goal.
- `sys_state_init` (dict): the first db query emitted, records user constraints faithfully. If the system find no result that matches, he/she may relax the constraints manually and search db multiple times.
- `domain` (dict): slot(string)-value(string) pairs
- `selectedResults` (list of string): db search result that would be used in this turn.
- `sys_state` (dict): the final db query emitted, records the db used by the system in this turn. Same format as sys_state_init. Note that this may not satisfy all user constraints.
- `final_goal` (list of tuples): user state/goal at the end of dialog. same format as "goal".
#### Example Instance
<!-- info: Provide a JSON formatted example of a typical instance in the dataset. -->
<!-- scope: periscope -->
```
{'dialog_id': '2303',
'final_goal': [['1', '餐馆', '人均消费', '50-100元', 'True'],
['1', '餐馆', '推荐菜', "['美食街']", 'True'],
['1', '餐馆', '名称', '鲜鱼口老字号美食街', 'True'],
['1', '餐馆', '营业时间', '周一至周日 10:00-22:00', 'True'],
['1', '餐馆', '周边景点', "['天安门广场', '前门大街', '恭王府', '故宫']", 'True'],
['2', '景点', '名称', '故宫', 'True'],
['2', '景点', '评分', '4.5分以上', 'True'],
['2', '景点', '地址', '北京市东城区景山前街4号', 'True'],
['2', '景点', '电话', '010-85007938', 'True'],
['3', '酒店', '名称', '桔子水晶酒店(北京安贞店)', 'True'],
['3', '酒店', '电话', '010-84273030', 'True']],
'gem_id': 'GEM-CrossWOZ-test-0',
'goal': [['1', '餐馆', '人均消费', '50-100元', 'False'],
['1', '餐馆', '推荐菜', "['美食街']", 'False'],
['1', '餐馆', '名称', '', 'False'],
['1', '餐馆', '营业时间', '', 'False'],
['1', '餐馆', '周边景点', '[]', 'False'],
['2', '景点', '名称', '出现在id=1的周边景点里', 'False'],
['2', '景点', '评分', '4.5分以上', 'False'],
['2', '景点', '地址', '', 'False'],
['2', '景点', '电话', '', 'False'],
['3', '酒店', '名称', '桔子水晶酒店(北京安贞店)', 'False'],
['3', '酒店', '电话', '', 'False']],
'messages': {'content': ['你好,我想吃美食街,帮我推荐一个人均消费在50-100元的餐馆,谢谢。',
'为您推荐鲜鱼口老字号美食街,人均消费75元,有您想吃的美食街哦。',
'营业时间是什么时间?',
'周一至周日 10:00-22:00。',
'他家周边有什么景点吗?',
'有故宫, 前门大街, 恭王府, 天安门广场。',
'哦,我想在这些附近景点里找一个4.5分以上的,有吗?',
'故宫就是哦,4.7分。',
'好的,电话和地址告诉我一下。',
'010-85007938;北京市东城区景山前街4号。',
'好的,麻烦你帮我查一下桔子水晶酒店(北京安贞店)电话呗。',
'010-84273030。',
'好的,收到,谢谢你!',
'不客气。'],
'dialog_act': [[['General', 'greet', 'none', 'none'],
['General', 'thank', 'none', 'none'],
['Inform', '餐馆', '人均消费', '50-100元'],
['Inform', '餐馆', '推荐菜', '美食街'],
['Request', '餐馆', '名称', '']],
[['Inform', '餐馆', '人均消费', '75元'], ['Inform', '餐馆', '名称', '鲜鱼口老字号美食街']],
[['Request', '餐馆', '营业时间', '']],
[['Inform', '餐馆', '营业时间', '周一至周日 10:00-22:00']],
[['Request', '餐馆', '周边景点', '']],
[['Inform', '餐馆', '周边景点', '前门大街'],
['Inform', '餐馆', '周边景点', '天安门广场'],
['Inform', '餐馆', '周边景点', '恭王府'],
['Inform', '餐馆', '周边景点', '故宫']],
[['Inform', '景点', '评分', '4.5分以上'], ['Select', '景点', '源领域', '餐馆']],
[['Inform', '景点', '名称', '故宫'], ['Inform', '景点', '评分', '4.7分']],
[['Request', '景点', '地址', ''], ['Request', '景点', '电话', '']],
[['Inform', '景点', '地址', '北京市东城区景山前街4号'],
['Inform', '景点', '电话', '010-85007938']],
[['Inform', '酒店', '名称', '桔子水晶酒店(北京安贞店)'], ['Request', '酒店', '电话', '']],
[['Inform', '酒店', '电话', '010-84273030']],
[['General', 'thank', 'none', 'none']],
[['General', 'welcome', 'none', 'none']]],
'role': ['usr',
'sys',
'usr',
'sys',
'usr',
'sys',
'usr',
'sys',
'usr',
'sys',
'usr',
'sys',
'usr',
'sys'],
'sys_state': [{'出租': {'selectedResults': [], '出发地': '', '目的地': ''},
'地铁': {'selectedResults': [], '出发地': '', '目的地': ''},
'景点': {'selectedResults': [],
'名称': '',
'周边景点': '',
'周边酒店': '',
'周边餐馆': '',
'游玩时间': '',
'评分': '',
'门票': ''},
'酒店': {'selectedResults': [],
'价格': '',
'名称': '',
'周边景点': '',
'周边酒店': '',
'周边餐馆': '',
'评分': '',
'酒店类型': '',
'酒店设施': ''},
'餐馆': {'selectedResults': [],
'人均消费': '',
'名称': '',
'周边景点': '',
'周边酒店': '',
'周边餐馆': '',
'推荐菜': '',
'评分': ''}},
{'出租': {'selectedResults': [], '出发地': '', '目的地': ''},
'地铁': {'selectedResults': [], '出发地': '', '目的地': ''},
'景点': {'selectedResults': [],
'名称': '',
'周边景点': '',
'周边酒店': '',
'周边餐馆': '',
'游玩时间': '',
'评分': '',
'门票': ''},
'酒店': {'selectedResults': [],
'价格': '',
'名称': '',
'周边景点': '',
'周边酒店': '',
'周边餐馆': '',
'评分': '',
'酒店类型': '',
'酒店设施': ''},
'餐馆': {'selectedResults': ['鲜鱼口老字号美食街'],
'人均消费': '50-100元',
'名称': '',
'周边景点': '',
'周边酒店': '',
'周边餐馆': '',
'推荐菜': '美食街',
'评分': ''}},
{'出租': {'selectedResults': [], '出发地': '', '目的地': ''},
'地铁': {'selectedResults': [], '出发地': '', '目的地': ''},
'景点': {'selectedResults': [],
'名称': '',
'周边景点': '',
'周边酒店': '',
'周边餐馆': '',
'游玩时间': '',
'评分': '',
'门票': ''},
'酒店': {'selectedResults': [],
'价格': '',
'名称': '',
'周边景点': '',
'周边酒店': '',
'周边餐馆': '',
'评分': '',
'酒店类型': '',
'酒店设施': ''},
'餐馆': {'selectedResults': [],
'人均消费': '',
'名称': '',
'周边景点': '',
'周边酒店': '',
'周边餐馆': '',
'推荐菜': '',
'评分': ''}},
{'出租': {'selectedResults': [], '出发地': '', '目的地': ''},
'地铁': {'selectedResults': [], '出发地': '', '目的地': ''},
'景点': {'selectedResults': [],
'名称': '',
'周边景点': '',
'周边酒店': '',
'周边餐馆': '',
'游玩时间': '',
'评分': '',
'门票': ''},
'酒店': {'selectedResults': [],
'价格': '',
'名称': '',
'周边景点': '',
'周边酒店': '',
'周边餐馆': '',
'评分': '',
'酒店类型': '',
'酒店设施': ''},
'餐馆': {'selectedResults': ['鲜鱼口老字号美食街'],
'人均消费': '50-100元',
'名称': '',
'周边景点': '',
'周边酒店': '',
'周边餐馆': '',
'推荐菜': '美食街',
'评分': ''}},
{'出租': {'selectedResults': [], '出发地': '', '目的地': ''},
'地铁': {'selectedResults': [], '出发地': '', '目的地': ''},
'景点': {'selectedResults': [],
'名称': '',
'周边景点': '',
'周边酒店': '',
'周边餐馆': '',
'游玩时间': '',
'评分': '',
'门票': ''},
'酒店': {'selectedResults': [],
'价格': '',
'名称': '',
'周边景点': '',
'周边酒店': '',
'周边餐馆': '',
'评分': '',
'酒店类型': '',
'酒店设施': ''},
'餐馆': {'selectedResults': [],
'人均消费': '',
'名称': '',
'周边景点': '',
'周边酒店': '',
'周边餐馆': '',
'推荐菜': '',
'评分': ''}},
{'出租': {'selectedResults': [], '出发地': '', '目的地': ''},
'地铁': {'selectedResults': [], '出发地': '', '目的地': ''},
'景点': {'selectedResults': [],
'名称': '',
'周边景点': '',
'周边酒店': '',
'周边餐馆': '',
'游玩时间': '',
'评分': '',
'门票': ''},
'酒店': {'selectedResults': [],
'价格': '',
'名称': '',
'周边景点': '',
'周边酒店': '',
'周边餐馆': '',
'评分': '',
'酒店类型': '',
'酒店设施': ''},
'餐馆': {'selectedResults': ['鲜鱼口老字号美食街'],
'人均消费': '50-100元',
'名称': '',
'周边景点': '',
'周边酒店': '',
'周边餐馆': '',
'推荐菜': '美食街',
'评分': ''}},
{'出租': {'selectedResults': [], '出发地': '', '目的地': ''},
'地铁': {'selectedResults': [], '出发地': '', '目的地': ''},
'景点': {'selectedResults': [],
'名称': '',
'周边景点': '',
'周边酒店': '',
'周边餐馆': '',
'游玩时间': '',
'评分': '',
'门票': ''},
'酒店': {'selectedResults': [],
'价格': '',
'名称': '',
'周边景点': '',
'周边酒店': '',
'周边餐馆': '',
'评分': '',
'酒店类型': '',
'酒店设施': ''},
'餐馆': {'selectedResults': [],
'人均消费': '',
'名称': '',
'周边景点': '',
'周边酒店': '',
'周边餐馆': '',
'推荐菜': '',
'评分': ''}},
{'出租': {'selectedResults': [], '出发地': '', '目的地': ''},
'地铁': {'selectedResults': [], '出发地': '', '目的地': ''},
'景点': {'selectedResults': ['故宫'],
'名称': '故宫',
'周边景点': '',
'周边酒店': '',
'周边餐馆': '',
'游玩时间': '',
'评分': '',
'门票': ''},
'酒店': {'selectedResults': [],
'价格': '',
'名称': '',
'周边景点': '',
'周边酒店': '',
'周边餐馆': '',
'评分': '',
'酒店类型': '',
'酒店设施': ''},
'餐馆': {'selectedResults': [],
'人均消费': '50-100元',
'名称': '',
'周边景点': '',
'周边酒店': '',
'周边餐馆': '',
'推荐菜': '美食街',
'评分': ''}},
{'出租': {'selectedResults': [], '出发地': '', '目的地': ''},
'地铁': {'selectedResults': [], '出发地': '', '目的地': ''},
'景点': {'selectedResults': [],
'名称': '',
'周边景点': '',
'周边酒店': '',
'周边餐馆': '',
'游玩时间': '',
'评分': '',
'门票': ''},
'酒店': {'selectedResults': [],
'价格': '',
'名称': '',
'周边景点': '',
'周边酒店': '',
'周边餐馆': '',
'评分': '',
'酒店类型': '',
'酒店设施': ''},
'餐馆': {'selectedResults': [],
'人均消费': '',
'名称': '',
'周边景点': '',
'周边酒店': '',
'周边餐馆': '',
'推荐菜': '',
'评分': ''}},
{'出租': {'selectedResults': [], '出发地': '', '目的地': ''},
'地铁': {'selectedResults': [], '出发地': '', '目的地': ''},
'景点': {'selectedResults': ['故宫'],
'名称': '故宫',
'周边景点': '',
'周边酒店': '',
'周边餐馆': '',
'游玩时间': '',
'评分': '',
'门票': ''},
'酒店': {'selectedResults': [],
'价格': '',
'名称': '',
'周边景点': '',
'周边酒店': '',
'周边餐馆': '',
'评分': '',
'酒店类型': '',
'酒店设施': ''},
'餐馆': {'selectedResults': [],
'人均消费': '50-100元',
'名称': '',
'周边景点': '',
'周边酒店': '',
'周边餐馆': '',
'推荐菜': '美食街',
'评分': ''}},
{'出租': {'selectedResults': [], '出发地': '', '目的地': ''},
'地铁': {'selectedResults': [], '出发地': '', '目的地': ''},
'景点': {'selectedResults': [],
'名称': '',
'周边景点': '',
'周边酒店': '',
'周边餐馆': '',
'游玩时间': '',
'评分': '',
'门票': ''},
'酒店': {'selectedResults': [],
'价格': '',
'名称': '',
'周边景点': '',
'周边酒店': '',
'周边餐馆': '',
'评分': '',
'酒店类型': '',
'酒店设施': ''},
'餐馆': {'selectedResults': [],
'人均消费': '',
'名称': '',
'周边景点': '',
'周边酒店': '',
'周边餐馆': '',
'推荐菜': '',
'评分': ''}},
{'出租': {'selectedResults': [], '出发地': '', '目的地': ''},
'地铁': {'selectedResults': [], '出发地': '', '目的地': ''},
'景点': {'selectedResults': [],
'名称': '故宫',
'周边景点': '',
'周边酒店': '',
'周边餐馆': '',
'游玩时间': '',
'评分': '',
'门票': ''},
'酒店': {'selectedResults': ['桔子水晶酒店(北京安贞店)'],
'价格': '',
'名称': '桔子水晶酒店(北京安贞店)',
'周边景点': '',
'周边酒店': '',
'周边餐馆': '',
'评分': '',
'酒店类型': '',
'酒店设施': ''},
'餐馆': {'selectedResults': [],
'人均消费': '50-100元',
'名称': '',
'周边景点': '',
'周边酒店': '',
'周边餐馆': '',
'推荐菜': '美食街',
'评分': ''}},
{'出租': {'selectedResults': [], '出发地': '', '目的地': ''},
'地铁': {'selectedResults': [], '出发地': '', '目的地': ''},
'景点': {'selectedResults': [],
'名称': '',
'周边景点': '',
'周边酒店': '',
'周边餐馆': '',
'游玩时间': '',
'评分': '',
'门票': ''},
'酒店': {'selectedResults': [],
'价格': '',
'名称': '',
'周边景点': '',
'周边酒店': '',
'周边餐馆': '',
'评分': '',
'酒店类型': '',
'酒店设施': ''},
'餐馆': {'selectedResults': [],
'人均消费': '',
'名称': '',
'周边景点': '',
'周边酒店': '',
'周边餐馆': '',
'推荐菜': '',
'评分': ''}},
{'出租': {'selectedResults': [], '出发地': '', '目的地': ''},
'地铁': {'selectedResults': [], '出发地': '', '目的地': ''},
'景点': {'selectedResults': [],
'名称': '故宫',
'周边景点': '',
'周边酒店': '',
'周边餐馆': '',
'游玩时间': '',
'评分': '',
'门票': ''},
'酒店': {'selectedResults': [],
'价格': '',
'名称': '桔子水晶酒店(北京安贞店)',
'周边景点': '',
'周边酒店': '',
'周边餐馆': '',
'评分': '',
'酒店类型': '',
'酒店设施': ''},
'餐馆': {'selectedResults': [],
'人均消费': '50-100元',
'名称': '',
'周边景点': '',
'周边酒店': '',
'周边餐馆': '',
'推荐菜': '美食街',
'评分': ''}}],
'sys_state_init': [{'出租': {'selectedResults': [], '出发地': '', '目的地': ''},
'地铁': {'selectedResults': [], '出发地': '', '目的地': ''},
'景点': {'selectedResults': [],
'名称': '',
'周边景点': '',
'周边酒店': '',
'周边餐馆': '',
'游玩时间': '',
'评分': '',
'门票': ''},
'酒店': {'selectedResults': [],
'价格': '',
'名称': '',
'周边景点': '',
'周边酒店': '',
'周边餐馆': '',
'评分': '',
'酒店类型': '',
'酒店设施': ''},
'餐馆': {'selectedResults': [],
'人均消费': '',
'名称': '',
'周边景点': '',
'周边酒店': '',
'周边餐馆': '',
'推荐菜': '',
'评分': ''}},
{'出租': {'selectedResults': [], '出发地': '', '目的地': ''},
'地铁': {'selectedResults': [], '出发地': '', '目的地': ''},
'景点': {'selectedResults': [],
'名称': '',
'周边景点': '',
'周边酒店': '',
'周边餐馆': '',
'游玩时间': '',
'评分': '',
'门票': ''},
'酒店': {'selectedResults': [],
'价格': '',
'名称': '',
'周边景点': '',
'周边酒店': '',
'周边餐馆': '',
'评分': '',
'酒店类型': '',
'酒店设施': ''},
'餐馆': {'selectedResults': ['鲜鱼口老字号美食街'],
'人均消费': '50-100元',
'名称': '',
'周边景点': '',
'周边酒店': '',
'周边餐馆': '',
'推荐菜': '美食街',
'评分': ''}},
{'出租': {'selectedResults': [], '出发地': '', '目的地': ''},
'地铁': {'selectedResults': [], '出发地': '', '目的地': ''},
'景点': {'selectedResults': [],
'名称': '',
'周边景点': '',
'周边酒店': '',
'周边餐馆': '',
'游玩时间': '',
'评分': '',
'门票': ''},
'酒店': {'selectedResults': [],
'价格': '',
'名称': '',
'周边景点': '',
'周边酒店': '',
'周边餐馆': '',
'评分': '',
'酒店类型': '',
'酒店设施': ''},
'餐馆': {'selectedResults': [],
'人均消费': '',
'名称': '',
'周边景点': '',
'周边酒店': '',
'周边餐馆': '',
'推荐菜': '',
'评分': ''}},
{'出租': {'selectedResults': [], '出发地': '', '目的地': ''},
'地铁': {'selectedResults': [], '出发地': '', '目的地': ''},
'景点': {'selectedResults': [],
'名称': '',
'周边景点': '',
'周边酒店': '',
'周边餐馆': '',
'游玩时间': '',
'评分': '',
'门票': ''},
'酒店': {'selectedResults': [],
'价格': '',
'名称': '',
'周边景点': '',
'周边酒店': '',
'周边餐馆': '',
'评分': '',
'酒店类型': '',
'酒店设施': ''},
'餐馆': {'selectedResults': ['鲜鱼口老字号美食街'],
'人均消费': '50-100元',
'名称': '',
'周边景点': '',
'周边酒店': '',
'周边餐馆': '',
'推荐菜': '美食街',
'评分': ''}},
{'出租': {'selectedResults': [], '出发地': '', '目的地': ''},
'地铁': {'selectedResults': [], '出发地': '', '目的地': ''},
'景点': {'selectedResults': [],
'名称': '',
'周边景点': '',
'周边酒店': '',
'周边餐馆': '',
'游玩时间': '',
'评分': '',
'门票': ''},
'酒店': {'selectedResults': [],
'价格': '',
'名称': '',
'周边景点': '',
'周边酒店': '',
'周边餐馆': '',
'评分': '',
'酒店类型': '',
'酒店设施': ''},
'餐馆': {'selectedResults': [],
'人均消费': '',
'名称': '',
'周边景点': '',
'周边酒店': '',
'周边餐馆': '',
'推荐菜': '',
'评分': ''}},
{'出租': {'selectedResults': [], '出发地': '', '目的地': ''},
'地铁': {'selectedResults': [], '出发地': '', '目的地': ''},
'景点': {'selectedResults': [],
'名称': '',
'周边景点': '',
'周边酒店': '',
'周边餐馆': '',
'游玩时间': '',
'评分': '',
'门票': ''},
'酒店': {'selectedResults': [],
'价格': '',
'名称': '',
'周边景点': '',
'周边酒店': '',
'周边餐馆': '',
'评分': '',
'酒店类型': '',
'酒店设施': ''},
'餐馆': {'selectedResults': ['鲜鱼口老字号美食街'],
'人均消费': '50-100元',
'名称': '',
'周边景点': '',
'周边酒店': '',
'周边餐馆': '',
'推荐菜': '美食街',
'评分': ''}},
{'出租': {'selectedResults': [], '出发地': '', '目的地': ''},
'地铁': {'selectedResults': [], '出发地': '', '目的地': ''},
'景点': {'selectedResults': [],
'名称': '',
'周边景点': '',
'周边酒店': '',
'周边餐馆': '',
'游玩时间': '',
'评分': '',
'门票': ''},
'酒店': {'selectedResults': [],
'价格': '',
'名称': '',
'周边景点': '',
'周边酒店': '',
'周边餐馆': '',
'评分': '',
'酒店类型': '',
'酒店设施': ''},
'餐馆': {'selectedResults': [],
'人均消费': '',
'名称': '',
'周边景点': '',
'周边酒店': '',
'周边餐馆': '',
'推荐菜': '',
'评分': ''}},
{'出租': {'selectedResults': [], '出发地': '', '目的地': ''},
'地铁': {'selectedResults': [], '出发地': '', '目的地': ''},
'景点': {'selectedResults': ['故宫'],
'名称': '故宫',
'周边景点': '',
'周边酒店': '',
'周边餐馆': '',
'游玩时间': '',
'评分': '',
'门票': ''},
'酒店': {'selectedResults': [],
'价格': '',
'名称': '',
'周边景点': '',
'周边酒店': '',
'周边餐馆': '',
'评分': '',
'酒店类型': '',
'酒店设施': ''},
'餐馆': {'selectedResults': ['鲜鱼口老字号美食街'],
'人均消费': '50-100元',
'名称': '',
'周边景点': '',
'周边酒店': '',
'周边餐馆': '',
'推荐菜': '美食街',
'评分': ''}},
{'出租': {'selectedResults': [], '出发地': '', '目的地': ''},
'地铁': {'selectedResults': [], '出发地': '', '目的地': ''},
'景点': {'selectedResults': [],
'名称': '',
'周边景点': '',
'周边酒店': '',
'周边餐馆': '',
'游玩时间': '',
'评分': '',
'门票': ''},
'酒店': {'selectedResults': [],
'价格': '',
'名称': '',
'周边景点': '',
'周边酒店': '',
'周边餐馆': '',
'评分': '',
'酒店类型': '',
'酒店设施': ''},
'餐馆': {'selectedResults': [],
'人均消费': '',
'名称': '',
'周边景点': '',
'周边酒店': '',
'周边餐馆': '',
'推荐菜': '',
'评分': ''}},
{'出租': {'selectedResults': [], '出发地': '', '目的地': ''},
'地铁': {'selectedResults': [], '出发地': '', '目的地': ''},
'景点': {'selectedResults': ['故宫'],
'名称': '故宫',
'周边景点': '',
'周边酒店': '',
'周边餐馆': '',
'游玩时间': '',
'评分': '',
'门票': ''},
'酒店': {'selectedResults': [],
'价格': '',
'名称': '',
'周边景点': '',
'周边酒店': '',
'周边餐馆': '',
'评分': '',
'酒店类型': '',
'酒店设施': ''},
'餐馆': {'selectedResults': [],
'人均消费': '50-100元',
'名称': '',
'周边景点': '',
'周边酒店': '',
'周边餐馆': '',
'推荐菜': '美食街',
'评分': ''}},
{'出租': {'selectedResults': [], '出发地': '', '目的地': ''},
'地铁': {'selectedResults': [], '出发地': '', '目的地': ''},
'景点': {'selectedResults': [],
'名称': '',
'周边景点': '',
'周边酒店': '',
'周边餐馆': '',
'游玩时间': '',
'评分': '',
'门票': ''},
'酒店': {'selectedResults': [],
'价格': '',
'名称': '',
'周边景点': '',
'周边酒店': '',
'周边餐馆': '',
'评分': '',
'酒店类型': '',
'酒店设施': ''},
'餐馆': {'selectedResults': [],
'人均消费': '',
'名称': '',
'周边景点': '',
'周边酒店': '',
'周边餐馆': '',
'推荐菜': '',
'评分': ''}},
{'出租': {'selectedResults': [], '出发地': '', '目的地': ''},
'地铁': {'selectedResults': [], '出发地': '', '目的地': ''},
'景点': {'selectedResults': ['故宫'],
'名称': '故宫',
'周边景点': '',
'周边酒店': '',
'周边餐馆': '',
'游玩时间': '',
'评分': '',
'门票': ''},
'酒店': {'selectedResults': ['桔子水晶酒店(北京安贞店)'],
'价格': '',
'名称': '桔子水晶酒店(北京安贞店)',
'周边景点': '',
'周边酒店': '',
'周边餐馆': '',
'评分': '',
'酒店类型': '',
'酒店设施': ''},
'餐馆': {'selectedResults': [],
'人均消费': '50-100元',
'名称': '',
'周边景点': '',
'周边酒店': '',
'周边餐馆': '',
'推荐菜': '美食街',
'评分': ''}},
{'出租': {'selectedResults': [], '出发地': '', '目的地': ''},
'地铁': {'selectedResults': [], '出发地': '', '目的地': ''},
'景点': {'selectedResults': [],
'名称': '',
'周边景点': '',
'周边酒店': '',
'周边餐馆': '',
'游玩时间': '',
'评分': '',
'门票': ''},
'酒店': {'selectedResults': [],
'价格': '',
'名称': '',
'周边景点': '',
'周边酒店': '',
'周边餐馆': '',
'评分': '',
'酒店类型': '',
'酒店设施': ''},
'餐馆': {'selectedResults': [],
'人均消费': '',
'名称': '',
'周边景点': '',
'周边酒店': '',
'周边餐馆': '',
'推荐菜': '',
'评分': ''}},
{'出租': {'selectedResults': [], '出发地': '', '目的地': ''},
'地铁': {'selectedResults': [], '出发地': '', '目的地': ''},
'景点': {'selectedResults': [],
'名称': '故宫',
'周边景点': '',
'周边酒店': '',
'周边餐馆': '',
'游玩时间': '',
'评分': '',
'门票': ''},
'酒店': {'selectedResults': ['桔子水晶酒店(北京安贞店)'],
'价格': '',
'名称': '桔子水晶酒店(北京安贞店)',
'周边景点': '',
'周边酒店': '',
'周边餐馆': '',
'评分': '',
'酒店类型': '',
'酒店设施': ''},
'餐馆': {'selectedResults': [],
'人均消费': '50-100元',
'名称': '',
'周边景点': '',
'周边酒店': '',
'周边餐馆': '',
'推荐菜': '美食街',
'评分': ''}}],
'user_state': [[['1', '餐馆', '人均消费', '50-100元', 'True'],
['1', '餐馆', '推荐菜', "['美食街']", 'True'],
['1', '餐馆', '名称', '', 'True'],
['1', '餐馆', '营业时间', '', 'False'],
['1', '餐馆', '周边景点', '[]', 'False'],
['2', '景点', '名称', '出现在id=1的周边景点里', 'False'],
['2', '景点', '评分', '4.5分以上', 'False'],
['2', '景点', '地址', '', 'False'],
['2', '景点', '电话', '', 'False'],
['3', '酒店', '名称', '桔子水晶酒店(北京安贞店)', 'False'],
['3', '酒店', '电话', '', 'False']],
[],
[['1', '餐馆', '人均消费', '50-100元', 'True'],
['1', '餐馆', '推荐菜', "['美食街']", 'True'],
['1', '餐馆', '名称', '鲜鱼口老字号美食街', 'True'],
['1', '餐馆', '营业时间', '', 'True'],
['1', '餐馆', '周边景点', '[]', 'False'],
['2', '景点', '名称', '出现在id=1的周边景点里', 'False'],
['2', '景点', '评分', '4.5分以上', 'False'],
['2', '景点', '地址', '', 'False'],
['2', '景点', '电话', '', 'False'],
['3', '酒店', '名称', '桔子水晶酒店(北京安贞店)', 'False'],
['3', '酒店', '电话', '', 'False']],
[],
[['1', '餐馆', '人均消费', '50-100元', 'True'],
['1', '餐馆', '推荐菜', "['美食街']", 'True'],
['1', '餐馆', '名称', '鲜鱼口老字号美食街', 'True'],
['1', '餐馆', '营业时间', '周一至周日 10:00-22:00', 'True'],
['1', '餐馆', '周边景点', '[]', 'True'],
['2', '景点', '名称', '出现在id=1的周边景点里', 'False'],
['2', '景点', '评分', '4.5分以上', 'False'],
['2', '景点', '地址', '', 'False'],
['2', '景点', '电话', '', 'False'],
['3', '酒店', '名称', '桔子水晶酒店(北京安贞店)', 'False'],
['3', '酒店', '电话', '', 'False']],
[],
[['1', '餐馆', '人均消费', '50-100元', 'True'],
['1', '餐馆', '推荐菜', "['美食街']", 'True'],
['1', '餐馆', '名称', '鲜鱼口老字号美食街', 'True'],
['1', '餐馆', '营业时间', '周一至周日 10:00-22:00', 'True'],
['1', '餐馆', '周边景点', "['天安门广场', '前门大街', '恭王府', '故宫']", 'True'],
['2', '景点', '名称', '出现在id=1的周边景点里', 'True'],
['2', '景点', '评分', '4.5分以上', 'True'],
['2', '景点', '地址', '', 'False'],
['2', '景点', '电话', '', 'False'],
['3', '酒店', '名称', '桔子水晶酒店(北京安贞店)', 'False'],
['3', '酒店', '电话', '', 'False']],
[],
[['1', '餐馆', '人均消费', '50-100元', 'True'],
['1', '餐馆', '推荐菜', "['美食街']", 'True'],
['1', '餐馆', '名称', '鲜鱼口老字号美食街', 'True'],
['1', '餐馆', '营业时间', '周一至周日 10:00-22:00', 'True'],
['1', '餐馆', '周边景点', "['天安门广场', '前门大街', '恭王府', '故宫']", 'True'],
['2', '景点', '名称', '故宫', 'True'],
['2', '景点', '评分', '4.5分以上', 'True'],
['2', '景点', '地址', '', 'True'],
['2', '景点', '电话', '', 'True'],
['3', '酒店', '名称', '桔子水晶酒店(北京安贞店)', 'False'],
['3', '酒店', '电话', '', 'False']],
[],
[['1', '餐馆', '人均消费', '50-100元', 'True'],
['1', '餐馆', '推荐菜', "['美食街']", 'True'],
['1', '餐馆', '名称', '鲜鱼口老字号美食街', 'True'],
['1', '餐馆', '营业时间', '周一至周日 10:00-22:00', 'True'],
['1', '餐馆', '周边景点', "['天安门广场', '前门大街', '恭王府', '故宫']", 'True'],
['2', '景点', '名称', '故宫', 'True'],
['2', '景点', '评分', '4.5分以上', 'True'],
['2', '景点', '地址', '北京市东城区景山前街4号', 'True'],
['2', '景点', '电话', '010-85007938', 'True'],
['3', '酒店', '名称', '桔子水晶酒店(北京安贞店)', 'True'],
['3', '酒店', '电话', '', 'True']],
[],
[['1', '餐馆', '人均消费', '50-100元', 'True'],
['1', '餐馆', '推荐菜', "['美食街']", 'True'],
['1', '餐馆', '名称', '鲜鱼口老字号美食街', 'True'],
['1', '餐馆', '营业时间', '周一至周日 10:00-22:00', 'True'],
['1', '餐馆', '周边景点', "['天安门广场', '前门大街', '恭王府', '故宫']", 'True'],
['2', '景点', '名称', '故宫', 'True'],
['2', '景点', '评分', '4.5分以上', 'True'],
['2', '景点', '地址', '北京市东城区景山前街4号', 'True'],
['2', '景点', '电话', '010-85007938', 'True'],
['3', '酒店', '名称', '桔子水晶酒店(北京安贞店)', 'True'],
['3', '酒店', '电话', '010-84273030', 'True']],
[]]},
'sys_id': 96,
'task description': ['你要去一个餐馆(id=1)用餐。你希望餐馆的人均消费是50-100元的。你想吃的菜肴是美食街。你想知道这个餐馆的名称、营业时间、周边景点。',
'你要去id=1附近的景点(id=2)游玩。你希望景点的评分是4.5分以上。你想知道这个景点的地址、电话。',
'你要去名叫桔子水晶酒店(北京安贞店)的酒店(id=3)住宿。你想知道这个酒店的电话。'],
'type': '不独立多领域',
'usr_id': 97}
```
#### Data Splits
<!-- info: Describe and name the splits in the dataset if there are more than one. -->
<!-- scope: periscope -->
| Split | Train | Valid | Test |
| --------------------- | ------ | ----- | ----- |
| \# dialogues | 5,012 | 500 | 500 |
| \# Turns (utterances) | 84,692 | 8,458 | 8,476 |
| Vocab | 12,502 | 5,202 | 5,143 |
| Avg. sub-goals | 3.24 | 3.26 | 3.26 |
| Avg. semantic tuples | 14.8 | 14.9 | 15.0 |
| Avg. turns | 16.9 | 16.9 | 17.0 |
| Avg. tokens per turn | 16.3 | 16.3 | 16.2 |
## Dataset in GEM
### Rationale for Inclusion in GEM
#### Why is the Dataset in GEM?
<!-- info: What does this dataset contribute toward better generation evaluation and why is it part of GEM? -->
<!-- scope: microscope -->
CrossWOZ is the first large-scale Chinese Cross-Domain Wizard-of-Oz task-oriented dataset.
#### Similar Datasets
<!-- info: Do other datasets for the high level task exist? -->
<!-- scope: telescope -->
yes
#### Unique Language Coverage
<!-- info: Does this dataset cover other languages than other datasets for the same task? -->
<!-- scope: periscope -->
no
#### Difference from other GEM datasets
<!-- info: What else sets this dataset apart from other similar datasets in GEM? -->
<!-- scope: microscope -->
The corpus contains rich annotation of dialogue states and dialogue acts at both user and system sides, which can be used in a wide range of tasks.
#### Ability that the Dataset measures
<!-- info: What aspect of model ability can be measured with this dataset? -->
<!-- scope: periscope -->
Dialog understanding, dialog policy learning
### GEM-Specific Curation
#### Modificatied for GEM?
<!-- info: Has the GEM version of the dataset been modified in any way (data, processing, splits) from the original curated data? -->
<!-- scope: telescope -->
yes
#### GEM Modifications
<!-- info: What changes have been made to he original dataset? -->
<!-- scope: periscope -->
`other`
#### Modification Details
<!-- info: For each of these changes, described them in more details and provided the intended purpose of the modification -->
<!-- scope: microscope -->
To adapt to hugging face Datasets, we 1) separate user annotators' ID and system annotations' ID; 2) we convert the data type in goal/user state to string.
#### Additional Splits?
<!-- info: Does GEM provide additional splits to the dataset? -->
<!-- scope: telescope -->
no
### Getting Started with the Task
#### Pointers to Resources
<!-- info: Getting started with in-depth research on the task. Add relevant pointers to resources that researchers can consult when they want to get started digging deeper into the task. -->
<!-- scope: microscope -->
[Code](https://github.com/thu-coai/Convlab-2)
#### Technical Terms
<!-- info: Technical terms used in this card and the dataset and their definitions -->
<!-- scope: microscope -->
According to the type of user goal, we group the dialogues in the training set into five categories:
- S: 417 dialogues have only one sub-goal in HAR domains.
- M: 1573 dialogues have multiple sub-goals (2-3) in HAR domains. However, these sub-goals do not have cross-domain informable slots.
- M+T: 691 dialogues have multiple sub-goals in HAR domains and at least one sub-goal in the metro or taxi domain (3-5 sub-goals). The sub-goals in HAR domains do not have cross-domain informable slots.
- CM: 1,759 dialogues have multiple sub-goals (2-5) in HAR domains with cross-domain informable slots.
- CM+T: 572 dialogues have multiple sub-goals in HAR domains with cross-domain informable slots and at least one sub-goal in the metro or taxi domain (3-5 sub-goals).
## Previous Results
### Previous Results
#### Measured Model Abilities
<!-- info: What aspect of model ability can be measured with this dataset? -->
<!-- scope: telescope -->
Dialog understanding, dialog policy learning
#### Metrics
<!-- info: What metrics are typically used for this task? -->
<!-- scope: periscope -->
`BLEU`
#### Proposed Evaluation
<!-- info: List and describe the purpose of the metrics and evaluation methodology (including human evaluation) that the dataset creators used when introducing this task. -->
<!-- scope: microscope -->
BLEU evaluates the generation quality.
#### Previous results available?
<!-- info: Are previous results available? -->
<!-- scope: telescope -->
yes
#### Other Evaluation Approaches
<!-- info: What evaluation approaches have others used? -->
<!-- scope: periscope -->
Inform rate: how many entities in the gold response appear in the generated response.
#### Relevant Previous Results
<!-- info: What are the most relevant previous results for this task/dataset? -->
<!-- scope: microscope -->
BLEU on MultiWOZ dataset.
## Dataset Curation
### Original Curation
#### Original Curation Rationale
<!-- info: Original curation rationale -->
<!-- scope: telescope -->
Gather human-to-human dialog in Chinese.
#### Communicative Goal
<!-- info: What was the communicative goal? -->
<!-- scope: periscope -->
Generate a response according to the dialog context and database search results.
#### Sourced from Different Sources
<!-- info: Is the dataset aggregated from different data sources? -->
<!-- scope: telescope -->
no
### Language Data
#### How was Language Data Obtained?
<!-- info: How was the language data obtained? -->
<!-- scope: telescope -->
`Crowdsourced`
#### Where was it crowdsourced?
<!-- info: If crowdsourced, where from? -->
<!-- scope: periscope -->
`Participatory experiment`
#### Language Producers
<!-- info: What further information do we have on the language producers? -->
<!-- scope: microscope -->
An usr/sys ID indicates the creator of different data points.
#### Topics Covered
<!-- info: Does the language in the dataset focus on specific topics? How would you describe them? -->
<!-- scope: periscope -->
domains: attraction, hotel, restaurant, metro, taxi
#### Data Validation
<!-- info: Was the text validated by a different worker or a data curator? -->
<!-- scope: telescope -->
validated by data curator
#### Was Data Filtered?
<!-- info: Were text instances selected or filtered? -->
<!-- scope: telescope -->
not filtered
### Structured Annotations
#### Additional Annotations?
<!-- quick -->
<!-- info: Does the dataset have additional annotations for each instance? -->
<!-- scope: telescope -->
none
#### Annotation Service?
<!-- info: Was an annotation service used? -->
<!-- scope: telescope -->
no
### Consent
#### Any Consent Policy?
<!-- info: Was there a consent policy involved when gathering the data? -->
<!-- scope: telescope -->
yes
#### Consent Policy Details
<!-- info: What was the consent policy? -->
<!-- scope: microscope -->
Annotators agree using the dataset for research purpose.
#### Other Consented Downstream Use
<!-- info: What other downstream uses of the data did the original data creators and the data curators consent to? -->
<!-- scope: microscope -->
Any
### Private Identifying Information (PII)
#### Contains PII?
<!-- quick -->
<!-- info: Does the source language data likely contain Personal Identifying Information about the data creators or subjects? -->
<!-- scope: telescope -->
unlikely
#### Categories of PII
<!-- info: What categories of PII are present or suspected in the data? -->
<!-- scope: periscope -->
`generic PII`
#### Any PII Identification?
<!-- info: Did the curators use any automatic/manual method to identify PII in the dataset? -->
<!-- scope: periscope -->
no identification
### Maintenance
#### Any Maintenance Plan?
<!-- info: Does the original dataset have a maintenance plan? -->
<!-- scope: telescope -->
no
## Broader Social Context
### Previous Work on the Social Impact of the Dataset
#### Usage of Models based on the Data
<!-- info: Are you aware of cases where models trained on the task featured in this dataset ore related tasks have been used in automated systems? -->
<!-- scope: telescope -->
no
### Impact on Under-Served Communities
#### Addresses needs of underserved Communities?
<!-- info: Does this dataset address the needs of communities that are traditionally underserved in language technology, and particularly language generation technology? Communities may be underserved for exemple because their language, language variety, or social or geographical context is underepresented in NLP and NLG resources (datasets and models). -->
<!-- scope: telescope -->
yes
#### Details on how Dataset Addresses the Needs
<!-- info: Describe how this dataset addresses the needs of underserved communities. -->
<!-- scope: microscope -->
CrossWOZ is the first large-scale Chinese Cross-Domain Wizard-of-Oz task-oriented dataset. The corpus contains rich annotation of dialogue states and dialogue acts at both user and system sides, which can be used in a wide range of tasks.
### Discussion of Biases
#### Any Documented Social Biases?
<!-- info: Are there documented social biases in the dataset? Biases in this context are variations in the ways members of different social categories are represented that can have harmful downstream consequences for members of the more disadvantaged group. -->
<!-- scope: telescope -->
no
#### Are the Language Producers Representative of the Language?
<!-- info: Does the distribution of language producers in the dataset accurately represent the full distribution of speakers of the language world-wide? If not, how does it differ? -->
<!-- scope: periscope -->
Yes
## Considerations for Using the Data
### PII Risks and Liability
#### Potential PII Risk
<!-- info: Considering your answers to the PII part of the Data Curation Section, describe any potential privacy to the data subjects and creators risks when using the dataset. -->
<!-- scope: microscope -->
No
### Licenses
#### Copyright Restrictions on the Dataset
<!-- info: Based on your answers in the Intended Use part of the Data Overview Section, which of the following best describe the copyright and licensing status of the dataset? -->
<!-- scope: periscope -->
`open license - commercial use allowed`
#### Copyright Restrictions on the Language Data
<!-- info: Based on your answers in the Language part of the Data Curation Section, which of the following best describe the copyright and licensing status of the underlying language data? -->
<!-- scope: periscope -->
`open license - commercial use allowed`
### Known Technical Limitations
#### Technical Limitations
<!-- info: Describe any known technical limitations, such as spurrious correlations, train/test overlap, annotation biases, or mis-annotations, and cite the works that first identified these limitations when possible. -->
<!-- scope: microscope -->
No
#### Unsuited Applications
<!-- info: When using a model trained on this dataset in a setting where users or the public may interact with its predictions, what are some pitfalls to look out for? In particular, describe some applications of the general task featured in this dataset that its curation or properties make it less suitable for. -->
<!-- scope: microscope -->
Model may not handle unknown values in the dialog
#### Discouraged Use Cases
<!-- info: What are some discouraged use cases of a model trained to maximize the proposed metrics on this dataset? In particular, think about settings where decisions made by a model that performs reasonably well on the metric my still have strong negative consequences for user or members of the public. -->
<!-- scope: microscope -->
Responses can be diverse, which is not captured by BLEU
|
GEM/OrangeSum | ---
annotations_creators:
- unknown
language_creators:
- unknown
language:
- fr
license:
- other
multilinguality:
- unknown
pretty_name: OrangeSum
size_categories:
- unknown
source_datasets:
- original
task_categories:
- summarization
task_ids:
- unknown
---
# Dataset Card for GEM/OrangeSum
## Dataset Description
- **Homepage:** [Needs More Information]
- **Repository:** https://github.com/Tixierae/OrangeSum
- **Paper:** https://aclanthology.org/2021.emnlp-main.740
- **Leaderboard:** N/A
- **Point of Contact:** [Needs More Information]
### Link to Main Data Card
You can find the main data card on the [GEM Website](https://gem-benchmark.com/data_cards/OrangeSum).
### Dataset Summary
OrangeSum is a French summarization dataset inspired by XSum. It features two subtasks: abstract generation and title generation. The data was sourced from "Orange Actu" articles between 2011 and 2020.
You can load the dataset via:
```
import datasets
data = datasets.load_dataset('GEM/OrangeSum')
```
The data loader can be found [here](https://huggingface.co/datasets/GEM/OrangeSum).
#### paper
[ACL Anthology](https://aclanthology.org/2021.emnlp-main.740)
## Dataset Overview
### Where to find the Data and its Documentation
#### Download
<!-- info: What is the link to where the original dataset is hosted? -->
<!-- scope: telescope -->
[Github](https://github.com/Tixierae/OrangeSum)
#### Paper
<!-- info: What is the link to the paper describing the dataset (open access preferred)? -->
<!-- scope: telescope -->
[ACL Anthology](https://aclanthology.org/2021.emnlp-main.740)
#### BibTex
<!-- info: Provide the BibTex-formatted reference for the dataset. Please use the correct published version (ACL anthology, etc.) instead of google scholar created Bibtex. -->
<!-- scope: microscope -->
```
@inproceedings{kamal-eddine-etal-2021-barthez,
title = "{BART}hez: a Skilled Pretrained {F}rench Sequence-to-Sequence Model",
author = "Kamal Eddine, Moussa and
Tixier, Antoine and
Vazirgiannis, Michalis",
booktitle = "Proceedings of the 2021 Conference on Empirical Methods in Natural Language Processing",
month = nov,
year = "2021",
address = "Online and Punta Cana, Dominican Republic",
publisher = "Association for Computational Linguistics",
url = "https://aclanthology.org/2021.emnlp-main.740",
doi = "10.18653/v1/2021.emnlp-main.740",
pages = "9369--9390",
abstract = "Inductive transfer learning has taken the entire NLP field by storm, with models such as BERT and BART setting new state of the art on countless NLU tasks. However, most of the available models and research have been conducted for English. In this work, we introduce BARThez, the first large-scale pretrained seq2seq model for French. Being based on BART, BARThez is particularly well-suited for generative tasks. We evaluate BARThez on five discriminative tasks from the FLUE benchmark and two generative tasks from a novel summarization dataset, OrangeSum, that we created for this research. We show BARThez to be very competitive with state-of-the-art BERT-based French language models such as CamemBERT and FlauBERT. We also continue the pretraining of a multilingual BART on BARThez{'} corpus, and show our resulting model, mBARThez, to significantly boost BARThez{'} generative performance.",
}
```
#### Has a Leaderboard?
<!-- info: Does the dataset have an active leaderboard? -->
<!-- scope: telescope -->
no
### Languages and Intended Use
#### Multilingual?
<!-- quick -->
<!-- info: Is the dataset multilingual? -->
<!-- scope: telescope -->
no
#### Covered Languages
<!-- quick -->
<!-- info: What languages/dialects are covered in the dataset? -->
<!-- scope: telescope -->
`French`
#### License
<!-- quick -->
<!-- info: What is the license of the dataset? -->
<!-- scope: telescope -->
other: Other license
#### Primary Task
<!-- info: What primary task does the dataset support? -->
<!-- scope: telescope -->
Summarization
### Credit
### Dataset Structure
## Dataset in GEM
### Rationale for Inclusion in GEM
#### Similar Datasets
<!-- info: Do other datasets for the high level task exist? -->
<!-- scope: telescope -->
no
### GEM-Specific Curation
#### Modificatied for GEM?
<!-- info: Has the GEM version of the dataset been modified in any way (data, processing, splits) from the original curated data? -->
<!-- scope: telescope -->
no
#### Additional Splits?
<!-- info: Does GEM provide additional splits to the dataset? -->
<!-- scope: telescope -->
no
### Getting Started with the Task
#### Pointers to Resources
<!-- info: Getting started with in-depth research on the task. Add relevant pointers to resources that researchers can consult when they want to get started digging deeper into the task. -->
<!-- scope: microscope -->
Papers about abstractive summarization using seq2seq models:
- [Abstractive Text Summarization using Sequence-to-sequence RNNs and Beyond](https://aclanthology.org/K16-1028/)
- [Get To The Point: Summarization with Pointer-Generator Networks](https://aclanthology.org/P17-1099/)
- [BART: Denoising Sequence-to-Sequence Pre-training for Natural Language Generation, Translation, and Comprehension](https://aclanthology.org/2020.acl-main.703)
- [BARThez: a Skilled Pretrained French Sequence-to-Sequence Model](https://aclanthology.org/2021.emnlp-main.740/)
Papers about (pretrained) Transformers:
- [Attention is All you Need](https://papers.nips.cc/paper/2017/hash/3f5ee243547dee91fbd053c1c4a845aa-Abstract.html)
- [BERT: Pre-training of Deep Bidirectional Transformers for Language Understanding](https://aclanthology.org/N19-1423/)
#### Technical Terms
<!-- info: Technical terms used in this card and the dataset and their definitions -->
<!-- scope: microscope -->
No unique technical words in this data card.
## Previous Results
### Previous Results
#### Measured Model Abilities
<!-- info: What aspect of model ability can be measured with this dataset? -->
<!-- scope: telescope -->
The ability of the model to generate human like titles and abstracts for given news articles.
#### Metrics
<!-- info: What metrics are typically used for this task? -->
<!-- scope: periscope -->
`ROUGE`, `BERT-Score`
#### Proposed Evaluation
<!-- info: List and describe the purpose of the metrics and evaluation methodology (including human evaluation) that the dataset creators used when introducing this task. -->
<!-- scope: microscope -->
Automatic Evaluation: Rouge-1, Rouge-2, RougeL and BERTScore were used.
Human evalutaion: a human evaluation study was conducted with 11 French native speakers. The evaluators were PhD students from the computer science department of the university of the authors, working in NLP and other fields of AI. They volunteered after receiving an email announcement. the best-Worst Scaling (Louviere et al.,2015) was used. Two summaries from two different systems, along with their input document, were presented to a human annotator who had to decide which one was better. The evaluators were asked to base their judgments on accuracy (does the summary contain accurate facts?), informativeness (is important in-formation captured?) and fluency (is the summary written in well-formed French?).
#### Previous results available?
<!-- info: Are previous results available? -->
<!-- scope: telescope -->
no
## Broader Social Context
### Previous Work on the Social Impact of the Dataset
#### Usage of Models based on the Data
<!-- info: Are you aware of cases where models trained on the task featured in this dataset ore related tasks have been used in automated systems? -->
<!-- scope: telescope -->
no
### Impact on Under-Served Communities
#### Addresses needs of underserved Communities?
<!-- info: Does this dataset address the needs of communities that are traditionally underserved in language technology, and particularly language generation technology? Communities may be underserved for exemple because their language, language variety, or social or geographical context is underepresented in NLP and NLG resources (datasets and models). -->
<!-- scope: telescope -->
no
### Discussion of Biases
#### Any Documented Social Biases?
<!-- info: Are there documented social biases in the dataset? Biases in this context are variations in the ways members of different social categories are represented that can have harmful downstream consequences for members of the more disadvantaged group. -->
<!-- scope: telescope -->
no
#### Are the Language Producers Representative of the Language?
<!-- info: Does the distribution of language producers in the dataset accurately represent the full distribution of speakers of the language world-wide? If not, how does it differ? -->
<!-- scope: periscope -->
The dataset contains news articles written by professional authors.
## Considerations for Using the Data
### PII Risks and Liability
### Licenses
#### Copyright Restrictions on the Dataset
<!-- info: Based on your answers in the Intended Use part of the Data Overview Section, which of the following best describe the copyright and licensing status of the dataset? -->
<!-- scope: periscope -->
`open license - commercial use allowed`
#### Copyright Restrictions on the Language Data
<!-- info: Based on your answers in the Language part of the Data Curation Section, which of the following best describe the copyright and licensing status of the underlying language data? -->
<!-- scope: periscope -->
`open license - commercial use allowed`
### Known Technical Limitations
|
GEM/RiSAWOZ | ---
annotations_creators:
- crowd-sourced
language_creators:
- unknown
language:
- zh
license:
- cc-by-4.0
multilinguality:
- unknown
size_categories:
- unknown
source_datasets:
- original
task_categories:
- conversational
task_ids: []
pretty_name: RiSAWOZ
tags:
- dialog-response-generation
---
# Dataset Card for GEM/RiSAWOZ
## Dataset Description
- **Homepage:** https://terryqj0107.github.io/RiSAWOZ_webpage
- **Repository:** https://github.com/terryqj0107/RiSAWOZ
- **Paper:** https://aclanthology.org/2020.emnlp-main.67
- **Leaderboard:** N/A
- **Point of Contact:** Deyi Xiong
### Link to Main Data Card
You can find the main data card on the [GEM Website](https://gem-benchmark.com/data_cards/RiSAWOZ).
### Dataset Summary
RiSAWOZ is a Chinese dialog dataset. It can be used to study various dialogue tasks, such as Dialogue State Tracking, Dialogue Context-to-Text Generation, Coreference Resolution and Unified Generative Ellipsis and Coreference Resolution.
You can load the dataset via:
```
import datasets
data = datasets.load_dataset('GEM/RiSAWOZ')
```
The data loader can be found [here](https://huggingface.co/datasets/GEM/RiSAWOZ).
#### website
[Website](https://terryqj0107.github.io/RiSAWOZ_webpage)
#### paper
[ACL Anthology](https://aclanthology.org/2020.emnlp-main.67)
#### authors
Jun Quan (Soochow University, Suzhou, China), Shian Zhang (Soochow University, Suzhou, China), Qian Cao(Soochow University, Suzhou, China), Zizhong Li (Tianjin University, Tianjin, China), Deyi Xiong (Tianjin University, Tianjin, China)
## Dataset Overview
### Where to find the Data and its Documentation
#### Webpage
<!-- info: What is the webpage for the dataset (if it exists)? -->
<!-- scope: telescope -->
[Website](https://terryqj0107.github.io/RiSAWOZ_webpage)
#### Download
<!-- info: What is the link to where the original dataset is hosted? -->
<!-- scope: telescope -->
[Github](https://github.com/terryqj0107/RiSAWOZ)
#### Paper
<!-- info: What is the link to the paper describing the dataset (open access preferred)? -->
<!-- scope: telescope -->
[ACL Anthology](https://aclanthology.org/2020.emnlp-main.67)
#### BibTex
<!-- info: Provide the BibTex-formatted reference for the dataset. Please use the correct published version (ACL anthology, etc.) instead of google scholar created Bibtex. -->
<!-- scope: microscope -->
```
@inproceedings{quan-etal-2020-risawoz,
title = "{R}i{SAWOZ}: A Large-Scale Multi-Domain {W}izard-of-{O}z Dataset with Rich Semantic Annotations for Task-Oriented Dialogue Modeling",
author = "Quan, Jun and
Zhang, Shian and
Cao, Qian and
Li, Zizhong and
Xiong, Deyi",
booktitle = "Proceedings of the 2020 Conference on Empirical Methods in Natural Language Processing (EMNLP)",
month = nov,
year = "2020",
address = "Online",
publisher = "Association for Computational Linguistics",
url = "https://www.aclweb.org/anthology/2020.emnlp-main.67",
pages = "930--940",
}
```
#### Contact Name
<!-- quick -->
<!-- info: If known, provide the name of at least one person the reader can contact for questions about the dataset. -->
<!-- scope: periscope -->
Deyi Xiong
#### Contact Email
<!-- info: If known, provide the email of at least one person the reader can contact for questions about the dataset. -->
<!-- scope: periscope -->
dyxiong@tju.edu.cn
#### Has a Leaderboard?
<!-- info: Does the dataset have an active leaderboard? -->
<!-- scope: telescope -->
no
### Languages and Intended Use
#### Multilingual?
<!-- quick -->
<!-- info: Is the dataset multilingual? -->
<!-- scope: telescope -->
no
#### Covered Dialects
<!-- info: What dialects are covered? Are there multiple dialects per language? -->
<!-- scope: periscope -->
Only Mandarin Chinese is covered in this dataset.
#### Covered Languages
<!-- quick -->
<!-- info: What languages/dialects are covered in the dataset? -->
<!-- scope: telescope -->
`Mandarin Chinese`
#### License
<!-- quick -->
<!-- info: What is the license of the dataset? -->
<!-- scope: telescope -->
cc-by-4.0: Creative Commons Attribution 4.0 International
#### Intended Use
<!-- info: What is the intended use of the dataset? -->
<!-- scope: microscope -->
RiSAWOZ can be used to support the study under various dialogue tasks, such as Natural Language Understanding, Dialogue State Tracking, Dialogue Context-to-Text Generation, Coreference Resolution and Unified Generative Ellipsis and Coreference Resolution.
#### Primary Task
<!-- info: What primary task does the dataset support? -->
<!-- scope: telescope -->
Dialog Response Generation
#### Communicative Goal
<!-- quick -->
<!-- info: Provide a short description of the communicative goal of a model trained for this task on this dataset. -->
<!-- scope: periscope -->
Generate system response given dialogue context across multiple domains.
### Credit
#### Curation Organization Type(s)
<!-- info: In what kind of organization did the dataset curation happen? -->
<!-- scope: telescope -->
`academic`
#### Curation Organization(s)
<!-- info: Name the organization(s). -->
<!-- scope: periscope -->
Soochow University and Tianjin University
#### Dataset Creators
<!-- info: Who created the original dataset? List the people involved in collecting the dataset and their affiliation(s). -->
<!-- scope: microscope -->
Jun Quan (Soochow University, Suzhou, China), Shian Zhang (Soochow University, Suzhou, China), Qian Cao(Soochow University, Suzhou, China), Zizhong Li (Tianjin University, Tianjin, China), Deyi Xiong (Tianjin University, Tianjin, China)
#### Funding
<!-- info: Who funded the data creation? -->
<!-- scope: microscope -->
the National Key Research and Development Project
#### Who added the Dataset to GEM?
<!-- info: Who contributed to the data card and adding the dataset to GEM? List the people+affiliations involved in creating this data card and who helped integrate this dataset into GEM. -->
<!-- scope: microscope -->
Tianhao Shen (Tianjin University, Tianjin, China), Chaobin You (Tianjin University, Tianjin, China), Deyi Xiong (Tianjin University, Tianjin, China)
### Dataset Structure
#### Data Fields
<!-- info: List and describe the fields present in the dataset. -->
<!-- scope: telescope -->
- gem_id (string): GEM-RiSAWOZ-{split}-{id}
- dialogue_id (string): dialogue ID
- goal (string): natural language descriptions of the user goal
- domains (list of strings): domains mentioned in current dialogue session
- dialogue (list of dicts): dialog turns and corresponding annotations. Each turn includes:
- turn_id (int): turn ID
- turn_domain (list of strings): domain mentioned in current turn
- user_utterance (string): user utterance
- system_utterance (string): system utterance
- belief_state (dict): dialogue state, including:
- inform slot-values (dict): the slots and corresponding values informed until current turn
- turn_inform (dict): the slots and corresponding values informed in current turn
- turn request (dict): the slots requested in current turn
- user_actions (list of lists): user dialogue acts in current turn
- user_actions (list of lists): system dialogue acts in current turn
- db_results (list of strings): database search results
- segmented_user_utterance (string): word segmentation result of user utterance
- segmented_system_utterance (string): word segmentation result of system utterance
#### Example Instance
<!-- info: Provide a JSON formatted example of a typical instance in the dataset. -->
<!-- scope: periscope -->
```
[
{
"dialogue_id": "attraction_goal_4-63###6177",
"goal": "attraction_goal_4-63: 你是苏州人,但不怎么出去玩。你朋友来苏州找你,你准备带他逛逛“水乡古镇”,你希望客服给你推荐个消费水平“中等”的地方。然后你要问清楚这地方“是否地铁直达”、“特点”、“门票价格”这些信息。最后,你要感谢客服的帮助,然后说再见。",
"domains": [
"旅游景点"
],
"dialogue": [
{
"turn_id": 0,
"turn_domain": [
"旅游景点"
],
"user_utterance": "你好,我是苏州人,但是不怎么出去玩,我朋友来苏州找我了,我准备带他逛逛水乡古镇,你能帮我推荐一下吗?",
"system_utterance": "推荐您去周庄古镇。",
"belief_state": {
"inform slot-values": {
"旅游景点-景点类型": "水乡 古镇"
},
"turn_inform": {
"旅游景点-景点类型": "水乡 古镇"
},
"turn request": []
},
"user_actions": [
[
"Inform",
"旅游景点",
"景点类型",
"水乡 古镇"
],
[
"Greeting",
"旅游景点",
"",
""
]
],
"system_actions": [
[
"Recommend",
"旅游景点",
"名称",
"周庄 古镇"
]
],
"db_results": [
"数据库检索结果:成功匹配个数为8",
"{'名称': '周庄古镇', '区域': '昆山', '景点类型': '水乡古镇', '最适合人群': '朋友出游', '消费': '偏贵', '是否地铁直达': '否', '门票价格': '100元', '电话号码': '400-8282900', '地址': '苏州市昆山市周庄镇全福路43号', '评分': 4.5, '开放时间': '07:30-18:50', '特点': '小桥流水与人家,双桥水巷摇橹船,还有沈万三的足迹待你寻访'}",
"{'名称': '同里古镇', '区域': '吴江', '景点类型': '水乡古镇', '最适合人群': '朋友出游', '消费': '偏贵', '是否地铁直达': '否', '门票价格': '100元', '电话号码': '0512-63325728', '地址': '苏州市吴江区同里古镇', '评分': 4.5, '开放时间': '07:30-17:30', '特点': '五湖环抱的江南水乡古镇,走三桥,参观小巧精致的园林退思园。'}",
"{'名称': '山塘街', '区域': '姑苏区', '景点类型': '水乡古镇', '最适合人群': '情侣约会', '消费': '中等', '是否地铁直达': '是', '门票价格': '免费', '电话号码': '0512-65314467', '地址': '苏州市姑苏区古城西北', '评分': 4.5, '开放时间': '08:30-21:00', '特点': '欣赏苏州老城风貌,参观会馆等古迹,乘游船,拍摄美丽的夜景。'}",
"{'名称': '平江路', '区域': '姑苏区', '景点类型': '水乡古镇', '最适合人群': '情侣约会', '消费': '中等', '是否地铁直达': '是', '门票价格': '免费', '电话号码': '0512-69163907', '地址': '江苏省苏州市姑苏区白塔东路65号', '评分': 4.5, '开放时间': '全天', '特点': '漫步保存完整的古街区,看小桥流水古宅,逛文艺小店,吃美食。'}",
"{'名称': '木渎古镇', '区域': '吴中区', '景点类型': '水乡古镇', '最适合人群': '朋友出游', '消费': '便宜', '是否地铁直达': '否', '门票价格': '免费', '电话号码': '0512-66514042', '地址': '苏州市吴中区木渎镇山塘街188号', '评分': 4.4, '开放时间': '08:30-17:00', '特点': '以园林为特色的古镇,游严家花园等古典园林,坐船看小桥流水。'}",
"{'名称': '甪直古镇', '区域': '吴中区', '景点类型': '水乡古镇', '最适合人群': '朋友出游', '消费': '便宜', '是否地铁直达': '否', '门票价格': '免费', '电话号码': '0512-66191668', '地址': '苏州市吴中区甪直镇晓市路21号', '评分': 4.3, '开放时间': '07:30-17:30', '特点': '甪直古镇有2500多年历史,甪直境内水流纵横,桥梁密布,有五湖之厅、六泽之冲之称。'}",
"{'名称': '千灯古镇', '区域': '昆山', '景点类型': '水乡古镇', '最适合人群': '朋友出游', '消费': '便宜', '是否地铁直达': '否', '门票价格': '免费', '电话号码': '0512-57472155', '地址': '苏州市昆山市千灯古镇尚书路1号', '评分': 4.3, '开放时间': '08:00-17:00', '特点': '千灯古镇,距今已有2500多年的历史,古镇白墙黑瓦,昆韵盎然。'}",
"{'名称': '锦溪古镇', '区域': '昆山', '景点类型': '水乡古镇', '最适合人群': '朋友出游', '消费': '中等', '是否地铁直达': '否', '门票价格': '65元', '电话号码': '0512-57224669', '地址': '苏州市昆山市锦溪镇邵甸港路18号', '评分': 4.4, '开放时间': '08:00-17:00', '特点': '锦溪古镇位于昆山南郊的淀山湖畔,是一座有千年历史的江南水乡。'}"
],
"segmented_user_utterance": "你好 , 我 是 苏州人 , 但是 不怎么 出去玩 , 我 朋友 来 苏州 找 我 了 , 我 准备 带 他 逛逛 水乡 古镇 , 你 能 帮 我 推荐 一下 吗 ?",
"segmented_system_utterance": "推荐 您 去 周庄 古镇 。"
},
{
"turn_id": 1,
"turn_domain": [
"旅游景点"
],
"user_utterance": "我希望这个地方消费水平适中的,这个是中等的吗?",
"system_utterance": "这个不是,我又重新帮您找了一家山塘街。",
"belief_state": {
"inform slot-values": {
"旅游景点-景点类型": "水乡 古镇",
"旅游景点-消费": "中等"
},
"turn_inform": {
"旅游景点-消费": "中等"
},
"turn request": []
},
"user_actions": [
[
"Inform",
"旅游景点",
"消费",
"中等"
]
],
"system_actions": [
[
"Recommend",
"旅游景点",
"名称",
"山塘 街"
]
],
"db_results": [
"数据库检索结果:成功匹配个数为3",
"{'名称': '山塘街', '区域': '姑苏区', '景点类型': '水乡古镇', '最适合人群': '情侣约会', '消费': '中等', '是否地铁直达': '是', '门票价格': '免费', '电话号码': '0512-65314467', '地址': '苏州市姑苏区古城西北', '评分': 4.5, '开放时间': '08:30-21:00', '特点': '欣赏苏州老城风貌,参观会馆等古迹,乘游船,拍摄美丽的夜景。'}",
"{'名称': '平江路', '区域': '姑苏区', '景点类型': '水乡古镇', '最适合人群': '情侣约会', '消费': '中等', '是否地铁直达': '是', '门票价格': '免费', '电话号码': '0512-69163907', '地址': '江苏省苏州市姑苏区白塔东路65号', '评分': 4.5, '开放时间': '全天', '特点': '漫步保存完整的古街区,看小桥流水古宅,逛文艺小店,吃美食。'}",
"{'名称': '锦溪古镇', '区域': '昆山', '景点类型': '水乡古镇', '最适合人群': '朋友出游', '消费': '中等', '是否地铁直达': '否', '门票价格': '65元', '电话号码': '0512-57224669', '地址': '苏州市昆山市锦溪镇邵甸港路18号', '评分': 4.4, '开放时间': '08:00-17:00', '特点': '锦溪古镇位于昆山南郊的淀山湖畔,是一座有千年历史的江南水乡。'}"
],
"segmented_user_utterance": "我 希望 这个 地方 消费水平 适中 的 , 这个 是 中等 的 吗 ?",
"segmented_system_utterance": "这个 不是 , 我 又 重新 帮 您 找 了 一家 山塘 街 。"
},
{
"turn_id": 2,
"turn_domain": [
"旅游景点"
],
"user_utterance": "听这地方应该挺好玩,他们家特点是什么?",
"system_utterance": "特点是欣赏苏州老城风貌,参观会馆等古迹,乘游船,拍摄美丽的夜景。",
"belief_state": {
"inform slot-values": {
"旅游景点-景点类型": "水乡 古镇",
"旅游景点-消费": "中等",
"旅游景点-名称": "山塘 街"
},
"turn_inform": {
"旅游景点-名称": "山塘 街"
},
"turn request": [
"特点"
]
},
"user_actions": [
[
"Inform",
"旅游景点",
"名称",
"山塘 街"
],
[
"Request",
"旅游景点",
"特点",
""
]
],
"system_actions": [
[
"Inform",
"旅游景点",
"特点",
"欣赏 苏州 老城 风貌 , 参观 会馆 等 古迹 , 乘 游船 , 拍摄 美丽 的 夜景"
]
],
"db_results": [
"数据库检索结果:成功匹配个数为1",
"{'名称': '山塘街', '区域': '姑苏区', '景点类型': '水乡古镇', '最适合人群': '情侣约会', '消费': '中等', '是否地铁直达': '是', '门票价格': '免费', '电话号码': '0512-65314467', '地址': '苏州市姑苏区古城西北', '评分': 4.5, '开放时间': '08:30-21:00', '特点': '欣赏苏州老城风貌,参观会馆等古迹,乘游船,拍摄美丽的夜景。'}"
],
"segmented_user_utterance": "听 这 地方 应该 挺好玩 , 他们 家 特点 是 什么 ?",
"segmented_system_utterance": "特点 是 欣赏 苏州 老城 风貌 , 参观 会馆 等 古迹 , 乘 游船 , 拍摄 美丽 的 夜景 。"
},
{
"turn_id": 3,
"turn_domain": [
"旅游景点"
],
"user_utterance": "听起来很不错,他们家门票是多少?",
"system_utterance": "门票免费。",
"belief_state": {
"inform slot-values": {
"旅游景点-景点类型": "水乡 古镇",
"旅游景点-消费": "中等",
"旅游景点-名称": "山塘 街"
},
"turn_inform": {},
"turn request": [
"门票价格"
]
},
"user_actions": [
[
"Request",
"旅游景点",
"门票价格",
""
]
],
"system_actions": [
[
"Inform",
"旅游景点",
"门票价格",
"免费"
]
],
"db_results": [
"数据库检索结果:成功匹配个数为1",
"{'名称': '山塘街', '区域': '姑苏区', '景点类型': '水乡古镇', '最适合人群': '情侣约会', '消费': '中等', '是否地铁直达': '是', '门票价格': '免费', '电话号码': '0512-65314467', '地址': '苏州市姑苏区古城西北', '评分': 4.5, '开放时间': '08:30-21:00', '特点': '欣赏苏州老城风貌,参观会馆等古迹,乘游船,拍摄美丽的夜景。'}"
],
"segmented_user_utterance": "听 起来 很 不错 , 他们 家 门票 是 多少 ?",
"segmented_system_utterance": "门票 免费 。"
},
{
"turn_id": 4,
"turn_domain": [
"旅游景点"
],
"user_utterance": "还行,那他们家是否有地铁直达呢?",
"system_utterance": "嗯嗯,可以地铁直达。",
"belief_state": {
"inform slot-values": {
"旅游景点-景点类型": "水乡 古镇",
"旅游景点-消费": "中等",
"旅游景点-名称": "山塘 街"
},
"turn_inform": {},
"turn request": [
"是否地铁直达"
]
},
"user_actions": [
[
"Request",
"旅游景点",
"是否地铁直达",
""
]
],
"system_actions": [
[
"Inform",
"旅游景点",
"是否地铁直达",
"是"
]
],
"db_results": [
"数据库检索结果:成功匹配个数为1",
"{'名称': '山塘街', '区域': '姑苏区', '景点类型': '水乡古镇', '最适合人群': '情侣约会', '消费': '中等', '是否地铁直达': '是', '门票价格': '免费', '电话号码': '0512-65314467', '地址': '苏州市姑苏区古城西北', '评分': 4.5, '开放时间': '08:30-21:00', '特点': '欣赏苏州老城风貌,参观会馆等古迹,乘游船,拍摄美丽的夜景。'}"
],
"segmented_user_utterance": "还 行 , 那 他们 家 是否 有 地铁 直达 呢 ?",
"segmented_system_utterance": "嗯 嗯 , 可以 地铁 直达 。"
},
{
"turn_id": 5,
"turn_domain": [
"通用"
],
"user_utterance": "谢谢你的帮助,再见!",
"system_utterance": "再见。",
"belief_state": {
"inform slot-values": {
"旅游景点-景点类型": "水乡 古镇",
"旅游景点-消费": "中等",
"旅游景点-名称": "山塘 街"
},
"turn_inform": {},
"turn request": []
},
"user_actions": [
[
"Bye",
"通用",
"",
""
]
],
"system_actions": [
[
"Bye",
"通用",
"",
""
]
],
"db_results": [],
"segmented_user_utterance": "谢谢 你 的 帮助 , 再见 !",
"segmented_system_utterance": "再见 。"
}
]
},
{
"dialogue_id": "attraction_goal_3-33_v2###7307",
"goal": "attraction_goal_3-33: 你和朋友一起来苏州出差,顺便想去“苏州乐园森林水世界”逛一逛。因此,你要询问客服这个地方“是否地铁直达”、“评分”、“特点”这些信息。最后,你向客服表示感谢,结束对话。",
"domains": [
"旅游景点"
],
"dialogue": [
{
"turn_id": 0,
"turn_domain": [
"旅游景点"
],
"user_utterance": "你好,我和朋友一起来苏州出差,顺便想去苏州乐园森林水世界玩玩,能否帮我看看这个地方是否有地铁直达呢?",
"system_utterance": "稍等,很抱歉,没有地铁能直接到这个景点呢。",
"belief_state": {
"inform slot-values": {
"旅游景点-名称": "苏州 乐园 森林 水 世界"
},
"turn_inform": {
"旅游景点-名称": "苏州 乐园 森林 水 世界"
},
"turn request": [
"是否地铁直达"
]
},
"user_actions": [
[
"Inform",
"旅游景点",
"名称",
"苏州 乐园 森林 水 世界"
],
[
"Request",
"旅游景点",
"是否地铁直达",
""
],
[
"Greeting",
"旅游景点",
"",
""
]
],
"system_actions": [
[
"Inform",
"旅游景点",
"是否地铁直达",
"否"
]
],
"db_results": [
"数据库检索结果:成功匹配个数为1",
"{'名称': '苏州乐园森林水世界', '区域': '高新区', '景点类型': '游乐场', '最适合人群': '情侣约会', '消费': '偏贵', '是否地铁直达': '否', '门票价格': '198元', '电话号码': '0512-68717107', '地址': '苏州市高新区阳山东路以西,山神湾路以东', '评分': 4.4, '开放时间': '09:00-17:00', '特点': '各项水上娱乐项目皆具森林特色,被赋予森林主题,让人身处浩瀚森林又能享受到海洋般的舒爽水游乐。'}"
],
"segmented_user_utterance": "你好 , 我 和 朋友 一 起来 苏州 出差 , 顺便 想 去 苏州 乐园 森林 水 世界 玩玩 , 能否 帮 我 看看 这个 地方 是否 有 地铁 直达 呢 ?",
"segmented_system_utterance": "稍 等 , 很 抱歉 , 没有 地铁 能 直接 到 这个 景点 呢 。"
},
{
"turn_id": 1,
"turn_domain": [
"旅游景点"
],
"user_utterance": "你再帮我看看景点的评分好吗?",
"system_utterance": "这个景点的评分是4.4分。",
"belief_state": {
"inform slot-values": {
"旅游景点-名称": "苏州 乐园 森林 水 世界"
},
"turn_inform": {},
"turn request": [
"评分"
]
},
"user_actions": [
[
"Request",
"旅游景点",
"评分",
""
]
],
"system_actions": [
[
"Inform",
"旅游景点",
"评分",
"4.4"
]
],
"db_results": [
"数据库检索结果:成功匹配个数为1",
"{'名称': '苏州乐园森林水世界', '区域': '高新区', '景点类型': '游乐场', '最适合人群': '情侣约会', '消费': '偏贵', '是否地铁直达': '否', '门票价格': '198元', '电话号码': '0512-68717107', '地址': '苏州市高新区阳山东路以西,山神湾路以东', '评分': 4.4, '开放时间': '09:00-17:00', '特点': '各项水上娱乐项目皆具森林特色,被赋予森林主题,让人身处浩瀚森林又能享受到海洋般的舒爽水游乐。'}"
],
"segmented_user_utterance": "你 再 帮 我 看看 景点 的 评分 好 吗 ?",
"segmented_system_utterance": "这个 景点 的 评分 是 4.4 分 。"
},
{
"turn_id": 2,
"turn_domain": [
"旅游景点"
],
"user_utterance": "这个景点有啥特点呢?",
"system_utterance": "这个景点的特点是各项水上娱乐项目皆具森林特色,被赋予森林主题,让人身处浩瀚森林又能享受到海洋般的舒爽水游乐。",
"belief_state": {
"inform slot-values": {
"旅游景点-名称": "苏州 乐园 森林 水 世界"
},
"turn_inform": {},
"turn request": [
"特点"
]
},
"user_actions": [
[
"Request",
"旅游景点",
"特点",
""
]
],
"system_actions": [
[
"Inform",
"旅游景点",
"特点",
"各项 水上 娱乐 项目 皆 具 森林 特色 , 被 赋予 森林 主题 , 让 人 身处 浩瀚 森林 又 能 享受 到 海洋 般的 舒爽 水 游乐 。"
]
],
"db_results": [
"数据库检索结果:成功匹配个数为1",
"{'名称': '苏州乐园森林水世界', '区域': '高新区', '景点类型': '游乐场', '最适合人群': '情侣约会', '消费': '偏贵', '是否地铁直达': '否', '门票价格': '198元', '电话号码': '0512-68717107', '地址': '苏州市高新区阳山东路以西,山神湾路以东', '评分': 4.4, '开放时间': '09:00-17:00', '特点': '各项水上娱乐项目皆具森林特色,被赋予森林主题,让人身处浩瀚森林又能享受到海洋般的舒爽水游乐。'}"
],
"segmented_user_utterance": "这个 景点 有 啥 特点 呢 ?",
"segmented_system_utterance": "这个 景点 的 特点 是 各项 水上 娱乐 项目 皆 具 森林 特色 , 被 赋予 森林 主题 , 让 人 身处 浩瀚 森林 又 能 享受 到 海洋 般的 舒爽 水 游乐 。"
},
{
"turn_id": 3,
"turn_domain": [
"通用"
],
"user_utterance": "太好了,我觉得我会很喜欢这个地方,哈哈。",
"system_utterance": "这个景点挺不错的,就是没有地铁需要您乘坐其它交通工具了。",
"belief_state": {
"inform slot-values": {
"旅游景点-名称": "苏州 乐园 森林 水 世界"
},
"turn_inform": {},
"turn request": []
},
"user_actions": [
[
"General",
"通用",
"",
""
]
],
"system_actions": [
[
"General",
"通用",
"",
""
]
],
"db_results": [],
"segmented_user_utterance": "太好了 , 我 觉得 我会 很 喜欢 这个 地方 , 哈哈 。",
"segmented_system_utterance": "这个 景点 挺不错 的 , 就是 没有 地铁 需要 您 乘坐 其它 交通工具 了 。"
},
{
"turn_id": 4,
"turn_domain": [
"通用"
],
"user_utterance": "我打出租车去吧,就是多花点车费,没事。",
"system_utterance": "请问您还有其他问题吗?",
"belief_state": {
"inform slot-values": {
"旅游景点-名称": "苏州 乐园 森林 水 世界"
},
"turn_inform": {},
"turn request": []
},
"user_actions": [
[
"General",
"通用",
"",
""
]
],
"system_actions": [
[
"General",
"通用",
"",
""
]
],
"db_results": [],
"segmented_user_utterance": "我 打 出租车 去 吧 , 就是 多花点 车费 , 没事 。",
"segmented_system_utterance": "请问 您 还有 其他 问题 吗 ?"
},
{
"turn_id": 5,
"turn_domain": [
"通用"
],
"user_utterance": "没有了。谢谢你,再见。",
"system_utterance": "再见。",
"belief_state": {
"inform slot-values": {
"旅游景点-名称": "苏州 乐园 森林 水 世界"
},
"turn_inform": {},
"turn request": []
},
"user_actions": [
[
"Bye",
"通用",
"",
""
]
],
"system_actions": [
[
"Bye",
"通用",
"",
""
]
],
"db_results": [],
"segmented_user_utterance": "没有 了 。 谢谢 你 , 再见 。",
"segmented_system_utterance": "再见 。"
}
]
}
]
```
#### Data Splits
<!-- info: Describe and name the splits in the dataset if there are more than one. -->
<!-- scope: periscope -->
- Train:
- dialogues: 10000
- turns: 134580
- Avg. turns: 13.5
- Valid:
- dialogues: 600
- turns: 8116
- Avg. turns: 13.5
- Test:
- dialogues: 600
- turn: 9286
- Avg. turns: 15.5
####
<!-- info: What does an outlier of the dataset in terms of length/perplexity/embedding look like? -->
<!-- scope: microscope -->
the most complex example (i.e. with the most domains):
```
{
"dialogue_id": "attraction_restaurant_hotel_goal_2-69_v2###8355",
"goal": "attraction_restaurant_hotel_goal_2-69: 公司派你去苏州出差,你准备抽空旅游一下。第一,你想去一个“山水景区”,消费水平最好是“偏贵”的,你需要问清楚这地方“开放时间”、“门票价格”这些信息。第二,你要找个地方住,让客服给你推荐一家价位“中等”的“商务出行”酒店,并且询问这家酒店的“停车场”、“电话号码”这些信息。第三,你还想拔草美食,问客服有没有价位“中等”的“江浙菜”餐厅推荐,然后了解这家餐厅的“是否地铁直达”、“人均消费”这些信息。最后你要感谢客服的帮助,并说再见。",
"domains": [
"旅游景点",
"餐厅",
"酒店"
],
"dialogue": [
{
"turn_id": 0,
"turn_domain": [
"旅游景点"
],
"user_utterance": "你好,来苏州出差,准备抽空旅游一下。我想去山水景区,消费水平偏贵点,帮忙推荐一个。",
"system_utterance": "你好,向您推荐金鸡湖景区,感受一下苏州现代化的一面。",
"belief_state": {
"inform slot-values": {
"旅游景点-景点类型": "山水 景区",
"旅游景点-消费": "偏贵"
},
"turn_inform": {
"旅游景点-景点类型": "山水 景区",
"旅游景点-消费": "偏贵"
},
"turn request": []
},
"user_actions": [
[
"Inform",
"旅游景点",
"景点类型",
"山水 景区"
],
[
"Inform",
"旅游景点",
"消费",
"偏贵"
],
[
"Greeting",
"旅游景点",
"",
""
],
[
"General",
"旅游景点",
"",
""
]
],
"system_actions": [
[
"Greeting",
"通用",
"",
""
],
[
"General",
"通用",
"",
""
],
[
"Recommend",
"旅游景点",
"名称",
"金鸡湖 景区"
]
],
"db_results": [
"数据库检索结果:成功匹配个数为2",
"{'名称': '金鸡湖景区', '区域': '工业园区', '景点类型': '山水景区', '最适合人群': '情侣约会', '消费': '偏贵', '是否地铁直达': '是', '门票价格': '免费', '电话号码': '400-7558558', '地址': '苏州市工业园区星港街158号', '评分': 4.5, '开放时间': '全天', '特点': '看东方之门等高楼,坐摩天轮,乘船夜游,感受苏州现代化的一面。'}",
"{'名称': '沙家浜风景区', '区域': '常熟', '景点类型': '山水景区', '最适合人群': '家庭亲子', '消费': '偏贵', '是否地铁直达': '否', '门票价格': '110元', '电话号码': '0512-52500000', '地址': '苏州市常熟市沙家浜镇', '评分': 4.5, '开放时间': '08:00-16:30', '特点': '京剧样板戏《沙家浜》的发源地,访剧中场景,坐船深入芦苇荡。'}"
],
"segmented_user_utterance": "你好 , 来 苏州 出差 , 准备 抽空 旅游 一下 。 我 想 去 山水 景区 , 消费水平 偏 贵点 , 帮忙 推荐 一个 。",
"segmented_system_utterance": "你好 , 向 您 推荐 金鸡湖 景区 , 感受一下 苏州 现代化 的 一面 。"
},
{
"turn_id": 1,
"turn_domain": [
"旅游景点"
],
"user_utterance": "这里开放时间?需要安排一下。",
"system_utterance": "景区是全天开放的,可以尽情游览!",
"belief_state": {
"inform slot-values": {
"旅游景点-景点类型": "山水 景区",
"旅游景点-消费": "偏贵",
"旅游景点-名称": "金鸡湖 景区"
},
"turn_inform": {
"旅游景点-名称": "金鸡湖 景区"
},
"turn request": [
"开放时间"
]
},
"user_actions": [
[
"Inform",
"旅游景点",
"名称",
"金鸡湖 景区"
],
[
"Request",
"旅游景点",
"开放时间",
""
],
[
"General",
"旅游景点",
"",
""
]
],
"system_actions": [
[
"Inform",
"旅游景点",
"开放时间",
"全天"
]
],
"db_results": [
"数据库检索结果:成功匹配个数为1",
"{'名称': '金鸡湖景区', '区域': '工业园区', '景点类型': '山水景区', '最适合人群': '情侣约会', '消费': '偏贵', '是否地铁直达': '是', '门票价格': '免费', '电话号码': '400-7558558', '地址': '苏州市工业园区星港街158号', '评分': 4.5, '开放时间': '全天', '特点': '看东方之门等高楼,坐摩天轮,乘船夜游,感受苏州现代化的一面。'}"
],
"segmented_user_utterance": "这里 开放 时间 ? 需要 安排 一下 。",
"segmented_system_utterance": "景区 是 全天 开放 的 , 可以 尽情 游览 !"
},
{
"turn_id": 2,
"turn_domain": [
"旅游景点"
],
"user_utterance": "门票多少钱?",
"system_utterance": "这里是免费景区。",
"belief_state": {
"inform slot-values": {
"旅游景点-景点类型": "山水 景区",
"旅游景点-消费": "偏贵",
"旅游景点-名称": "金鸡湖 景区"
},
"turn_inform": {},
"turn request": [
"门票价格"
]
},
"user_actions": [
[
"Request",
"旅游景点",
"门票价格",
""
]
],
"system_actions": [
[
"Inform",
"旅游景点",
"门票价格",
"免费"
]
],
"db_results": [
"数据库检索结果:成功匹配个数为1",
"{'名称': '金鸡湖景区', '区域': '工业园区', '景点类型': '山水景区', '最适合人群': '情侣约会', '消费': '偏贵', '是否地铁直达': '是', '门票价格': '免费', '电话号码': '400-7558558', '地址': '苏州市工业园区星港街158号', '评分': 4.5, '开放时间': '全天', '特点': '看东方之门等高楼,坐摩天轮,乘船夜游,感受苏州现代化的一面。'}"
],
"segmented_user_utterance": "门票 多少 钱 ?",
"segmented_system_utterance": "这里 是 免费 景区 。"
},
{
"turn_id": 3,
"turn_domain": [
"酒店"
],
"user_utterance": "哦,好的。还有酒店,帮我推荐个价位中等的商务酒店!",
"system_utterance": "推荐苏州金鸡湖李公堤瑞贝庭公寓酒店,在金鸡湖景区附近。",
"belief_state": {
"inform slot-values": {
"旅游景点-景点类型": "山水 景区",
"旅游景点-消费": "偏贵",
"旅游景点-名称": "金鸡湖 景区",
"酒店-价位": "中等",
"酒店-酒店类型": "商务 出行"
},
"turn_inform": {
"酒店-价位": "中等",
"酒店-酒店类型": "商务 出行"
},
"turn request": []
},
"user_actions": [
[
"Inform",
"酒店",
"价位",
"中等"
],
[
"Inform",
"酒店",
"酒店类型",
"商务 出行"
],
[
"General",
"酒店",
"",
""
]
],
"system_actions": [
[
"Recommend",
"酒店",
"名称",
"苏州 金鸡湖 李公堤 瑞贝庭 公寓 酒店"
],
[
"General",
"通用",
"",
""
]
],
"db_results": [
"数据库检索结果:成功匹配个数为16",
"{'名称': '苏州慢享主题酒店', '区域': '姑苏区', '星级': '3', '价位': '中等', '酒店类型': '商务出行', '房型': '大床房', '停车场': '收费', '房费': '266元', '地址': '苏州姑苏区景德路26-64号', '电话号码': '0512-67570999', '评分': 4.7}",
"{'名称': '苏州慢享主题酒店', '区域': '姑苏区', '星级': '3', '价位': '中等', '酒店类型': '商务出行', '房型': '标准间', '停车场': '收费', '房费': '278元', '地址': '苏州姑苏区景德路26-64号', '电话号码': '0512-67570999', '评分': 4.7}",
"{'名称': '美锦酒店', '区域': '高新区', '星级': '3', '价位': '中等', '酒店类型': '商务出行', '房型': '大床房', '停车场': '免费', '房费': '308元', '地址': '苏州高新区滨河路999号花样年喜年生活广场5栋1层', '电话号码': '0512-66053331', '评分': 4.8}",
"{'名称': '美锦酒店', '区域': '高新区', '星级': '3', '价位': '中等', '酒店类型': '商务出行', '房型': '标准间', '停车场': '免费', '房费': '349元', '地址': '苏州高新区滨河路999号花样年喜年生活广场5栋1层', '电话号码': '0512-66053331', '评分': 4.8}",
"{'名称': '苏州金鸡湖李公堤瑞贝庭公寓酒店', '区域': '工业园区', '星级': '4', '价位': '中等', '酒店类型': '商务出行', '房型': '大床房', '停车场': '免费', '房费': '438元', '地址': '苏州工业园区李公堤三期E区商业街9幢', '电话号码': '0512-69995666', '评分': 4.6}",
"{'名称': '苏州金鸡湖李公堤瑞贝庭公寓酒店', '区域': '工业园区', '星级': '4', '价位': '中等', '酒店类型': '商务出行', '房型': '标准间', '停车场': '免费', '房费': '438元', '地址': '苏州工业园区李公堤三期E区商业街9幢', '电话号码': '0512-69995666', '评分': 4.6}",
"{'名称': '苏州途乐酒店公寓', '区域': '工业园区', '星级': '2', '价位': '中等', '酒店类型': '商务出行', '房型': '大床房', '停车场': '收费', '房费': '486元', '地址': '苏州工业园区苏州丰隆城市中心T1楼', '电话号码': '151-5149-7911', '评分': 4.6}",
"{'名称': '苏州途乐酒店公寓', '区域': '工业园区', '星级': '2', '价位': '中等', '酒店类型': '商务出行', '房型': '标准间', '停车场': '收费', '房费': '486元', '地址': '苏州工业园区苏州丰隆城市中心T1楼', '电话号码': '151-5149-7911', '评分': 4.6}",
"{'名称': '万悦酒店', '区域': '吴中区', '星级': '3', '价位': '中等', '酒店类型': '商务出行', '房型': '大床房', '停车场': '免费', '房费': '346元', '地址': '苏州吴中区金山路47-2号', '电话号码': '0512-83808380', '评分': 4.5}",
"{'名称': '万悦酒店', '区域': '吴中区', '星级': '3', '价位': '中等', '酒店类型': '商务出行', '房型': '标准间', '停车场': '免费', '房费': '338元', '地址': '苏州吴中区金山路47-2号', '电话号码': '0512-83808380', '评分': 4.5}",
"{'名称': '周庄多瓦台临河客栈', '区域': '昆山', '星级': '3', '价位': '中等', '酒店类型': '商务出行', '房型': '大床房', '停车场': '收费', '房费': '279元', '地址': '昆山周庄镇东浜村75号', '电话号码': '181-3619-1632', '评分': 4.8}",
"{'名称': '周庄多瓦台临河客栈', '区域': '昆山', '星级': '3', '价位': '中等', '酒店类型': '商务出行', '房型': '标准间', '停车场': '收费', '房费': '279元', '地址': '昆山周庄镇东浜村75号', '电话号码': '181-3619-1632', '评分': 4.8}",
"{'名称': '常熟长江路亚朵酒店', '区域': '常熟', '星级': '4', '价位': '中等', '酒店类型': '商务出行', '房型': '大床房', '停车场': '免费', '房费': '312元', '地址': '常熟长江路268号', '电话号码': '0512-51661666', '评分': 4.8}",
"{'名称': '常熟长江路亚朵酒店', '区域': '常熟', '星级': '4', '价位': '中等', '酒店类型': '商务出行', '房型': '标准间', '停车场': '免费', '房费': '312元', '地址': '常熟长江路268号', '电话号码': '0512-51661666', '评分': 4.8}",
"{'名称': '维也纳酒店', '区域': '常熟', '星级': '3', '价位': '中等', '酒店类型': '商务出行', '房型': '大床房', '停车场': '免费', '房费': '322元', '地址': '常熟碧溪镇金港路8号', '电话号码': '0512-52018188', '评分': 4.8}",
"{'名称': '维也纳酒店', '区域': '常熟', '星级': '3', '价位': '中等', '酒店类型': '商务出行', '房型': '标准间', '停车场': '免费', '房费': '340元', '地址': '常熟碧溪镇金港路8号', '电话号码': '0512-52018188', '评分': 4.8}"
],
"segmented_user_utterance": "哦 , 好 的 。 还有 酒店 , 帮 我 推荐 个 价位 中等 的 商务酒店 !",
"segmented_system_utterance": "推荐 苏州 金鸡湖 李公堤 瑞贝庭 公寓 酒店 , 在 金鸡湖 景区 附近 。"
},
{
"turn_id": 4,
"turn_domain": [
"酒店"
],
"user_utterance": "你想得真周到!这家酒店有停车场吗?",
"system_utterance": "酒店配备有一个免费的停车场。",
"belief_state": {
"inform slot-values": {
"旅游景点-景点类型": "山水 景区",
"旅游景点-消费": "偏贵",
"旅游景点-名称": "金鸡湖 景区",
"酒店-价位": "中等",
"酒店-酒店类型": "商务 出行",
"酒店-名称": "苏州 金鸡湖 李公堤 瑞贝庭 公寓 酒店"
},
"turn_inform": {
"酒店-名称": "苏州 金鸡湖 李公堤 瑞贝庭 公寓 酒店"
},
"turn request": [
"停车场"
]
},
"user_actions": [
[
"Inform",
"酒店",
"名称",
"苏州 金鸡湖 李公堤 瑞贝庭 公寓 酒店"
],
[
"Request",
"酒店",
"停车场",
""
]
],
"system_actions": [
[
"Inform",
"酒店",
"停车场",
"免费"
]
],
"db_results": [
"数据库检索结果:成功匹配个数为2",
"{'名称': '苏州金鸡湖李公堤瑞贝庭公寓酒店', '区域': '工业园区', '星级': '4', '价位': '中等', '酒店类型': '商务出行', '房型': '大床房', '停车场': '免费', '房费': '438元', '地址': '苏州工业园区李公堤三期E区商业街9幢', '电话号码': '0512-69995666', '评分': 4.6}",
"{'名称': '苏州金鸡湖李公堤瑞贝庭公寓酒店', '区域': '工业园区', '星级': '4', '价位': '中等', '酒店类型': '商务出行', '房型': '标准间', '停车场': '免费', '房费': '438元', '地址': '苏州工业园区李公堤三期E区商业街9幢', '电话号码': '0512-69995666', '评分': 4.6}"
],
"segmented_user_utterance": "你 想 得 真 周到 ! 这家 酒店 有 停车场 吗 ?",
"segmented_system_utterance": "酒店 配备 有 一个 免费 的 停车场 。"
},
{
"turn_id": 5,
"turn_domain": [
"酒店"
],
"user_utterance": "酒店的电话告诉我一下。",
"system_utterance": "电话号码:0512-69995666。",
"belief_state": {
"inform slot-values": {
"旅游景点-景点类型": "山水 景区",
"旅游景点-消费": "偏贵",
"旅游景点-名称": "金鸡湖 景区",
"酒店-价位": "中等",
"酒店-酒店类型": "商务 出行",
"酒店-名称": "苏州 金鸡湖 李公堤 瑞贝庭 公寓 酒店"
},
"turn_inform": {},
"turn request": [
"电话号码"
]
},
"user_actions": [
[
"Request",
"酒店",
"电话号码",
""
]
],
"system_actions": [
[
"Inform",
"酒店",
"电话号码",
"0512 - 69995666"
]
],
"db_results": [
"数据库检索结果:成功匹配个数为2",
"{'名称': '苏州金鸡湖李公堤瑞贝庭公寓酒店', '区域': '工业园区', '星级': '4', '价位': '中等', '酒店类型': '商务出行', '房型': '大床房', '停车场': '免费', '房费': '438元', '地址': '苏州工业园区李公堤三期E区商业街9幢', '电话号码': '0512-69995666', '评分': 4.6}",
"{'名称': '苏州金鸡湖李公堤瑞贝庭公寓酒店', '区域': '工业园区', '星级': '4', '价位': '中等', '酒店类型': '商务出行', '房型': '标准间', '停车场': '免费', '房费': '438元', '地址': '苏州工业园区李公堤三期E区商业街9幢', '电话号码': '0512-69995666', '评分': 4.6}"
],
"segmented_user_utterance": "酒店 的 电话 告诉 我 一下 。",
"segmented_system_utterance": "电话号码 : 0512 - 69995666 。"
},
{
"turn_id": 6,
"turn_domain": [
"餐厅"
],
"user_utterance": "嗯,我还想拔草美食。有没有价位中等的江浙菜餐厅推荐一个。",
"system_utterance": "推荐老枫庭。",
"belief_state": {
"inform slot-values": {
"旅游景点-景点类型": "山水 景区",
"旅游景点-消费": "偏贵",
"旅游景点-名称": "金鸡湖 景区",
"餐厅-菜系": "江浙 菜",
"餐厅-价位": "中等",
"酒店-价位": "中等",
"酒店-酒店类型": "商务 出行",
"酒店-名称": "苏州 金鸡湖 李公堤 瑞贝庭 公寓 酒店"
},
"turn_inform": {
"餐厅-菜系": "江浙 菜",
"餐厅-价位": "中等"
},
"turn request": []
},
"user_actions": [
[
"Inform",
"餐厅",
"菜系",
"江浙 菜"
],
[
"Inform",
"餐厅",
"价位",
"中等"
],
[
"General",
"餐厅",
"",
""
]
],
"system_actions": [
[
"Recommend",
"餐厅",
"名称",
"老枫庭"
]
],
"db_results": [
"数据库检索结果:成功匹配个数为3",
"{'名称': '老枫庭', '区域': '吴中区', '菜系': '江浙菜', '价位': '中等', '是否地铁直达': '否', '人均消费': '94元', '地址': '木渎灵天路55号芭提雅休闲娱乐广场5号楼', '电话号码': '0512-66277557', '评分': 4.6, '营业时间': '周一至周日 11:00-13:30 17:00-20:30', '推荐菜': '松鼠桂鱼'}",
"{'名称': '同里老家', '区域': '吴江', '菜系': '江浙菜', '价位': '中等', '是否地铁直达': '否', '人均消费': '87元', '地址': '同里镇环湖西路318号', '电话号码': '0512-63339777', '评分': 4.5, '营业时间': '周一至周日 10:00-22:00', '推荐菜': '太湖三白'}",
"{'名称': '周庄花间堂桔梗餐厅', '区域': '昆山', '菜系': '江浙菜', '价位': '中等', '是否地铁直达': '否', '人均消费': '98元', '地址': '周庄镇中市街110号景点迷楼北10米', '电话号码': '138-1289-9961', '评分': 4.5, '营业时间': '周一至周日 07:00-20:30', '推荐菜': '银鱼炒蛋'}"
],
"segmented_user_utterance": "嗯 , 我 还 想 拔草 美食 。 有没有 价位 中等 的 江浙 菜 餐厅 推荐 一个 。",
"segmented_system_utterance": "推荐 老枫庭 。"
},
{
"turn_id": 7,
"turn_domain": [
"餐厅"
],
"user_utterance": "这家餐厅地铁直达吗?",
"system_utterance": "抱歉,餐厅没有地铁直达!",
"belief_state": {
"inform slot-values": {
"旅游景点-景点类型": "山水 景区",
"旅游景点-消费": "偏贵",
"旅游景点-名称": "金鸡湖 景区",
"餐厅-菜系": "江浙 菜",
"餐厅-价位": "中等",
"餐厅-名称": "老枫庭",
"酒店-价位": "中等",
"酒店-酒店类型": "商务 出行",
"酒店-名称": "苏州 金鸡湖 李公堤 瑞贝庭 公寓 酒店"
},
"turn_inform": {
"餐厅-名称": "老枫庭"
},
"turn request": [
"是否地铁直达"
]
},
"user_actions": [
[
"Inform",
"餐厅",
"名称",
"老枫庭"
],
[
"Request",
"餐厅",
"是否地铁直达",
""
]
],
"system_actions": [
[
"Inform",
"餐厅",
"是否地铁直达",
"否"
],
[
"General",
"通用",
"",
""
]
],
"db_results": [
"数据库检索结果:成功匹配个数为1",
"{'名称': '老枫庭', '区域': '吴中区', '菜系': '江浙菜', '价位': '中等', '是否地铁直达': '否', '人均消费': '94元', '地址': '木渎灵天路55号芭提雅休闲娱乐广场5号楼', '电话号码': '0512-66277557', '评分': 4.6, '营业时间': '周一至周日 11:00-13:30 17:00-20:30', '推荐菜': '松鼠桂鱼'}"
],
"segmented_user_utterance": "这家 餐厅 地铁 直达 吗 ?",
"segmented_system_utterance": "抱歉 , 餐厅 没有 地铁 直达 !"
},
{
"turn_id": 8,
"turn_domain": [
"餐厅"
],
"user_utterance": "人均消费呢?",
"system_utterance": "老枫庭人均消费在94元左右。",
"belief_state": {
"inform slot-values": {
"旅游景点-景点类型": "山水 景区",
"旅游景点-消费": "偏贵",
"旅游景点-名称": "金鸡湖 景区",
"餐厅-菜系": "江浙 菜",
"餐厅-价位": "中等",
"餐厅-名称": "老枫庭",
"酒店-价位": "中等",
"酒店-酒店类型": "商务 出行",
"酒店-名称": "苏州 金鸡湖 李公堤 瑞贝庭 公寓 酒店"
},
"turn_inform": {},
"turn request": [
"人均消费"
]
},
"user_actions": [
[
"Request",
"餐厅",
"人均消费",
""
]
],
"system_actions": [
[
"Inform",
"餐厅",
"人均消费",
"老枫庭"
]
],
"db_results": [
"数据库检索结果:成功匹配个数为1",
"{'名称': '老枫庭', '区域': '吴中区', '菜系': '江浙菜', '价位': '中等', '是否地铁直达': '否', '人均消费': '94元', '地址': '木渎灵天路55号芭提雅休闲娱乐广场5号楼', '电话号码': '0512-66277557', '评分': 4.6, '营业时间': '周一至周日 11:00-13:30 17:00-20:30', '推荐菜': '松鼠桂鱼'}"
],
"segmented_user_utterance": "人均 消费 呢 ?",
"segmented_system_utterance": "老枫庭 人均 消费 在 94 元 左右 。"
},
{
"turn_id": 9,
"turn_domain": [
"通用"
],
"user_utterance": "好,所有问题都解决了,谢谢你的帮助!再见!",
"system_utterance": "不客气!这是我应该做的!再见!",
"belief_state": {
"inform slot-values": {
"旅游景点-景点类型": "山水 景区",
"旅游景点-消费": "偏贵",
"旅游景点-名称": "金鸡湖 景区",
"餐厅-菜系": "江浙 菜",
"餐厅-价位": "中等",
"餐厅-名称": "老枫庭",
"酒店-价位": "中等",
"酒店-酒店类型": "商务 出行",
"酒店-名称": "苏州 金鸡湖 李公堤 瑞贝庭 公寓 酒店"
},
"turn_inform": {},
"turn request": []
},
"user_actions": [
[
"Bye",
"通用",
"",
""
],
[
"General",
"通用",
"",
""
]
],
"system_actions": [
[
"Bye",
"通用",
"",
""
],
[
"General",
"通用",
"",
""
]
],
"db_results": [],
"segmented_user_utterance": "好 , 所有 问题 都 解决 了 , 谢谢 你 的 帮助 ! 再见 !",
"segmented_system_utterance": "不 客气 ! 这 是 我 应该 做 的 ! 再见 !"
}
]
}
```
## Dataset in GEM
### Rationale for Inclusion in GEM
#### Why is the Dataset in GEM?
<!-- info: What does this dataset contribute toward better generation evaluation and why is it part of GEM? -->
<!-- scope: microscope -->
RiSAWOZ is the first large-scale multi-domain Chinese Wizard-of-Oz dataset with rich semantic annotations.
#### Similar Datasets
<!-- info: Do other datasets for the high level task exist? -->
<!-- scope: telescope -->
yes
#### Unique Language Coverage
<!-- info: Does this dataset cover other languages than other datasets for the same task? -->
<!-- scope: periscope -->
no
#### Difference from other GEM datasets
<!-- info: What else sets this dataset apart from other similar datasets in GEM? -->
<!-- scope: microscope -->
The corpus contains rich semantic annotations, such as ellipsis and coreference, in addition to traditional dialogue annotations (dialogue states, dialogue acts, etc.), which can be used in various tasks in dialogue system.
#### Ability that the Dataset measures
<!-- info: What aspect of model ability can be measured with this dataset? -->
<!-- scope: periscope -->
Natural Language Understanding, Dialogue State Tracking, Dialogue Context-to-Text Generation, Coreference Resolution, Unified Generative Ellipsis and Coreference Resolution
### GEM-Specific Curation
#### Modificatied for GEM?
<!-- info: Has the GEM version of the dataset been modified in any way (data, processing, splits) from the original curated data? -->
<!-- scope: telescope -->
no
#### Additional Splits?
<!-- info: Does GEM provide additional splits to the dataset? -->
<!-- scope: telescope -->
no
### Getting Started with the Task
#### Pointers to Resources
<!-- info: Getting started with in-depth research on the task. Add relevant pointers to resources that researchers can consult when they want to get started digging deeper into the task. -->
<!-- scope: microscope -->
[Website](https://terryqj0107.github.io/RiSAWOZ_webpage)
#### Technical Terms
<!-- info: Technical terms used in this card and the dataset and their definitions -->
<!-- scope: microscope -->
- In task-oriented dialogue system, the Natural Language Understanding (NLU) module aims to convert the user utterance into the representation that computer can understand, which includes intent and dialogue act (slot & value) detection.
- Dialogue State Tracking (DST) is a core component in task-oriented dialogue systems, which extracts dialogue states (user goals) embedded in dialogue context. It has progressed toward open-vocabulary or generation-based DST where state-of-the-art models can generate dialogue states from dialogue context directly.
- Context-to-Text Generation: encoding dialogue context to decode system response.
- Coreference Resolution: predict coreference clusters where all mentions are referring to the same entity for each dialogue.
- Unified Generative Ellipsis and Coreference Resolution: generating omitted or referred expressions from the dialogue context.
## Previous Results
### Previous Results
#### Measured Model Abilities
<!-- info: What aspect of model ability can be measured with this dataset? -->
<!-- scope: telescope -->
Natural Language Understanding, Dialogue State Tracking, Dialogue Context-to-Text Generation, Coreference Resolution, Unified Generative Ellipsis and Coreference Resolution
#### Metrics
<!-- info: What metrics are typically used for this task? -->
<!-- scope: periscope -->
`Other: Other Metrics`
#### Other Metrics
<!-- info: Definitions of other metrics -->
<!-- scope: periscope -->
- Natural Language Understanding:
- F1 score: F1 score of user intent.
- Dialogue State Tracking:
- Joint Accuracy: accuracy of turn-level dialogue states.
- Dialogue Context-to-Text Generation:
- inform rate: measures the percentage that the output contains the appropriate entity the user asks for.
- success rate: estimates the proportion that all the requested attributes have been answered.
- BLEU: the BLEU score of generated system response.
- Combined Score: (inform + success) ∗ 0.5 + BLEU as an overall
quality.
- Coreference Resolution:
- MUC F1 Score: a link-based metric. Mentions in the same entity/cluster are considered “linked”. MUC penalizes the missing links and incorrect links, each with the same weight.
- B3 F1 Score: a mention-based metric.The evaluation score depends on the fraction of the correct mentions included in the response entities (i.e. entities created by the system).
- CEAFφ4 F1 Score: a metric which assumes each key entity should only be mapped to one response entity, and vice versa. It aligns the key entities (clusters) with the response entities in the best way, and compute scores from that alignment.
- Average F1 Score: an average F1 score of the above three metrics.
- Unified Generative Ellipsis and Coreference Resolution:
- Exact Match Rate: measures whether the generated utterances exactly match the ground-truth utterances.
- BLEU: the BLEU score of generated utterances
- Resolution F1: comparing machine-generated words with ground-truth words only from the ellipsis/coreference part of user utterances.
#### Proposed Evaluation
<!-- info: List and describe the purpose of the metrics and evaluation methodology (including human evaluation) that the dataset creators used when introducing this task. -->
<!-- scope: microscope -->
see "Definitions of other metrics"
#### Previous results available?
<!-- info: Are previous results available? -->
<!-- scope: telescope -->
yes
#### Other Evaluation Approaches
<!-- info: What evaluation approaches have others used? -->
<!-- scope: periscope -->
same as our dataset
#### Relevant Previous Results
<!-- info: What are the most relevant previous results for this task/dataset? -->
<!-- scope: microscope -->
Joint Accuracy, Inform Rate, Success Rate, BLEU Score and Combined Score on MultiWOZ and CrossWOZ dataset.
## Dataset Curation
### Original Curation
#### Original Curation Rationale
<!-- info: Original curation rationale -->
<!-- scope: telescope -->
Gather human-to-human dialog in Chinese.
#### Communicative Goal
<!-- info: What was the communicative goal? -->
<!-- scope: periscope -->
Generate system response given dialogue context across multiple domains.
#### Sourced from Different Sources
<!-- info: Is the dataset aggregated from different data sources? -->
<!-- scope: telescope -->
no
### Language Data
#### How was Language Data Obtained?
<!-- info: How was the language data obtained? -->
<!-- scope: telescope -->
`Crowdsourced`
#### Where was it crowdsourced?
<!-- info: If crowdsourced, where from? -->
<!-- scope: periscope -->
`Other crowdworker platform`
#### Topics Covered
<!-- info: Does the language in the dataset focus on specific topics? How would you describe them? -->
<!-- scope: periscope -->
domains: Attraction, Restaurant, Hotel, Flight, Train, Weather, Movie, TV, Computer, Car, Hospital, Courses
#### Data Validation
<!-- info: Was the text validated by a different worker or a data curator? -->
<!-- scope: telescope -->
validated by data curator
#### Was Data Filtered?
<!-- info: Were text instances selected or filtered? -->
<!-- scope: telescope -->
hybrid
#### Filter Criteria
<!-- info: What were the selection criteria? -->
<!-- scope: microscope -->
Rule-based and manual selection criteria
### Structured Annotations
#### Additional Annotations?
<!-- quick -->
<!-- info: Does the dataset have additional annotations for each instance? -->
<!-- scope: telescope -->
crowd-sourced
#### Number of Raters
<!-- info: What is the number of raters -->
<!-- scope: telescope -->
51<n<100
#### Rater Qualifications
<!-- info: Describe the qualifications required of an annotator. -->
<!-- scope: periscope -->
Chinese native speaker
#### Raters per Training Example
<!-- info: How many annotators saw each training example? -->
<!-- scope: periscope -->
3
#### Raters per Test Example
<!-- info: How many annotators saw each test example? -->
<!-- scope: periscope -->
3
#### Annotation Service?
<!-- info: Was an annotation service used? -->
<!-- scope: telescope -->
no
#### Annotation Values
<!-- info: Purpose and values for each annotation -->
<!-- scope: microscope -->
- dialogue_id (string): dialogue ID
- goal (string): natural language descriptions of the user goal
- domains (list of strings): domains mentioned in current dialogue session
- turn_id (int): turn ID
- turn_domain (list of strings): domain mentioned in current turn
- belief_state (dict): dialogue state, including:
- inform slot-values (dict): the slots and corresponding values informed until current turn
- turn_inform (dict): the slots and corresponding values informed in current turn
- turn request (dict): the slots requested in current turn
- user_actions (list of lists): user dialogue acts in current turn
- user_actions (list of lists): system dialogue acts in current turn
- db_results (list of strings): database search results
- segmented_user_utterance (string): word segmentation result of user utterance
- segmented_system_utterance (string): word segmentation result of system utterance
#### Any Quality Control?
<!-- info: Quality control measures? -->
<!-- scope: telescope -->
unknown
### Consent
#### Any Consent Policy?
<!-- info: Was there a consent policy involved when gathering the data? -->
<!-- scope: telescope -->
yes
#### Consent Policy Details
<!-- info: What was the consent policy? -->
<!-- scope: microscope -->
Annotators agree using the dataset for research purpose.
#### Other Consented Downstream Use
<!-- info: What other downstream uses of the data did the original data creators and the data curators consent to? -->
<!-- scope: microscope -->
Any
### Private Identifying Information (PII)
#### Contains PII?
<!-- quick -->
<!-- info: Does the source language data likely contain Personal Identifying Information about the data creators or subjects? -->
<!-- scope: telescope -->
no PII
#### Justification for no PII
<!-- info: Provide a justification for selecting `no PII` above. -->
<!-- scope: periscope -->
The slots and values as well as utterances do not contain any personal information.
### Maintenance
#### Any Maintenance Plan?
<!-- info: Does the original dataset have a maintenance plan? -->
<!-- scope: telescope -->
yes
#### Maintenance Plan Details
<!-- info: Describe the original dataset's maintenance plan. -->
<!-- scope: microscope -->
Building a leaderboard webpage to trace and display the latest results on the [dataset](https://terryqj0107.github.io/RiSAWOZ_webpage/)
#### Maintainer Contact Information
<!-- info: Provide contact information of a person responsible for the dataset maintenance -->
<!-- scope: periscope -->
Deyi Xiong (dyxiong@tju.edu.cn)
#### Any Contestation Mechanism?
<!-- info: Does the maintenance plan include a contestation mechanism allowing individuals to request removal fo content? -->
<!-- scope: periscope -->
contact maintainer
#### Contestation Form Link
<!-- info: Provide the form link or contact information -->
<!-- scope: periscope -->
Deyi Xiong (dyxiong@tju.edu.cn)
## Broader Social Context
### Previous Work on the Social Impact of the Dataset
#### Usage of Models based on the Data
<!-- info: Are you aware of cases where models trained on the task featured in this dataset ore related tasks have been used in automated systems? -->
<!-- scope: telescope -->
no
### Impact on Under-Served Communities
#### Addresses needs of underserved Communities?
<!-- info: Does this dataset address the needs of communities that are traditionally underserved in language technology, and particularly language generation technology? Communities may be underserved for exemple because their language, language variety, or social or geographical context is underepresented in NLP and NLG resources (datasets and models). -->
<!-- scope: telescope -->
yes
#### Details on how Dataset Addresses the Needs
<!-- info: Describe how this dataset addresses the needs of underserved communities. -->
<!-- scope: microscope -->
RiSAWOZ is the first large-scale multi-domain Chinese Wizard-of-Oz dataset with rich semantic annotations.
### Discussion of Biases
#### Any Documented Social Biases?
<!-- info: Are there documented social biases in the dataset? Biases in this context are variations in the ways members of different social categories are represented that can have harmful downstream consequences for members of the more disadvantaged group. -->
<!-- scope: telescope -->
no
#### Are the Language Producers Representative of the Language?
<!-- info: Does the distribution of language producers in the dataset accurately represent the full distribution of speakers of the language world-wide? If not, how does it differ? -->
<!-- scope: periscope -->
yes
## Considerations for Using the Data
### PII Risks and Liability
#### Potential PII Risk
<!-- info: Considering your answers to the PII part of the Data Curation Section, describe any potential privacy to the data subjects and creators risks when using the dataset. -->
<!-- scope: microscope -->
None
### Licenses
#### Copyright Restrictions on the Dataset
<!-- info: Based on your answers in the Intended Use part of the Data Overview Section, which of the following best describe the copyright and licensing status of the dataset? -->
<!-- scope: periscope -->
`open license - commercial use allowed`
#### Copyright Restrictions on the Language Data
<!-- info: Based on your answers in the Language part of the Data Curation Section, which of the following best describe the copyright and licensing status of the underlying language data? -->
<!-- scope: periscope -->
`open license - commercial use allowed`
### Known Technical Limitations
#### Technical Limitations
<!-- info: Describe any known technical limitations, such as spurrious correlations, train/test overlap, annotation biases, or mis-annotations, and cite the works that first identified these limitations when possible. -->
<!-- scope: microscope -->
None
#### Unsuited Applications
<!-- info: When using a model trained on this dataset in a setting where users or the public may interact with its predictions, what are some pitfalls to look out for? In particular, describe some applications of the general task featured in this dataset that its curation or properties make it less suitable for. -->
<!-- scope: microscope -->
Using the trained model on domains that are not included in the 12 domains selected for this dataset.
#### Discouraged Use Cases
<!-- info: What are some discouraged use cases of a model trained to maximize the proposed metrics on this dataset? In particular, think about settings where decisions made by a model that performs reasonably well on the metric my still have strong negative consequences for user or members of the public. -->
<!-- scope: microscope -->
Designing models that leverage unknown bias in the dataset to optimize specific metrics.
|
GEM/RotoWire_English-German | ---
annotations_creators:
- automatically-created
language_creators:
- unknown
language:
- en
- de
license:
- cc-by-4.0
multilinguality:
- unknown
size_categories:
- unknown
source_datasets:
- original
task_categories:
- table-to-text
task_ids: []
pretty_name: RotoWire_English-German
tags:
- data-to-text
---
# Dataset Card for GEM/RotoWire_English-German
## Dataset Description
- **Homepage:** https://sites.google.com/view/wngt19/dgt-task
- **Repository:** https://github.com/neulab/dgt
- **Paper:** https://www.aclweb.org/anthology/D19-5601/
- **Leaderboard:** N/A
- **Point of Contact:** Hiroaki Hayashi
### Link to Main Data Card
You can find the main data card on the [GEM Website](https://gem-benchmark.com/data_cards/RotoWire_English-German).
### Dataset Summary
This dataset is a data-to-text dataset in the basketball domain. The input are tables in a fixed format with statistics about a game (in English) and the target is a German translation of the originally English description. The translations were done by professional translators with basketball experience. The dataset can be used to evaluate the cross-lingual data-to-text capabilities of a model with complex inputs.
You can load the dataset via:
```
import datasets
data = datasets.load_dataset('GEM/RotoWire_English-German')
```
The data loader can be found [here](https://huggingface.co/datasets/GEM/RotoWire_English-German).
#### website
[Website](https://sites.google.com/view/wngt19/dgt-task)
#### paper
[ACL Anthology](https://www.aclweb.org/anthology/D19-5601/)
#### authors
Graham Neubig (Carnegie Mellon University), Hiroaki Hayashi (Carnegie Mellon University)
## Dataset Overview
### Where to find the Data and its Documentation
#### Webpage
<!-- info: What is the webpage for the dataset (if it exists)? -->
<!-- scope: telescope -->
[Website](https://sites.google.com/view/wngt19/dgt-task)
#### Download
<!-- info: What is the link to where the original dataset is hosted? -->
<!-- scope: telescope -->
[Github](https://github.com/neulab/dgt)
#### Paper
<!-- info: What is the link to the paper describing the dataset (open access preferred)? -->
<!-- scope: telescope -->
[ACL Anthology](https://www.aclweb.org/anthology/D19-5601/)
#### BibTex
<!-- info: Provide the BibTex-formatted reference for the dataset. Please use the correct published version (ACL anthology, etc.) instead of google scholar created Bibtex. -->
<!-- scope: microscope -->
```
@inproceedings{hayashi-etal-2019-findings,
title = "Findings of the Third Workshop on Neural Generation and Translation",
author = "Hayashi, Hiroaki and
Oda, Yusuke and
Birch, Alexandra and
Konstas, Ioannis and
Finch, Andrew and
Luong, Minh-Thang and
Neubig, Graham and
Sudoh, Katsuhito",
booktitle = "Proceedings of the 3rd Workshop on Neural Generation and Translation",
month = nov,
year = "2019",
address = "Hong Kong",
publisher = "Association for Computational Linguistics",
url = "https://aclanthology.org/D19-5601",
doi = "10.18653/v1/D19-5601",
pages = "1--14",
abstract = "This document describes the findings of the Third Workshop on Neural Generation and Translation, held in concert with the annual conference of the Empirical Methods in Natural Language Processing (EMNLP 2019). First, we summarize the research trends of papers presented in the proceedings. Second, we describe the results of the two shared tasks 1) efficient neural machine translation (NMT) where participants were tasked with creating NMT systems that are both accurate and efficient, and 2) document generation and translation (DGT) where participants were tasked with developing systems that generate summaries from structured data, potentially with assistance from text in another language.",
}
```
#### Contact Name
<!-- quick -->
<!-- info: If known, provide the name of at least one person the reader can contact for questions about the dataset. -->
<!-- scope: periscope -->
Hiroaki Hayashi
#### Contact Email
<!-- info: If known, provide the email of at least one person the reader can contact for questions about the dataset. -->
<!-- scope: periscope -->
hiroakih@andrew.cmu.edu
#### Has a Leaderboard?
<!-- info: Does the dataset have an active leaderboard? -->
<!-- scope: telescope -->
no
### Languages and Intended Use
#### Multilingual?
<!-- quick -->
<!-- info: Is the dataset multilingual? -->
<!-- scope: telescope -->
yes
#### Covered Languages
<!-- quick -->
<!-- info: What languages/dialects are covered in the dataset? -->
<!-- scope: telescope -->
`English`, `German`
#### License
<!-- quick -->
<!-- info: What is the license of the dataset? -->
<!-- scope: telescope -->
cc-by-4.0: Creative Commons Attribution 4.0 International
#### Intended Use
<!-- info: What is the intended use of the dataset? -->
<!-- scope: microscope -->
Foster the research on document-level generation technology and contrast the methods for different types of inputs.
#### Primary Task
<!-- info: What primary task does the dataset support? -->
<!-- scope: telescope -->
Data-to-Text
#### Communicative Goal
<!-- quick -->
<!-- info: Provide a short description of the communicative goal of a model trained for this task on this dataset. -->
<!-- scope: periscope -->
Describe a basketball game given its box score table (and possibly a summary in a foreign language).
### Credit
#### Curation Organization Type(s)
<!-- info: In what kind of organization did the dataset curation happen? -->
<!-- scope: telescope -->
`academic`
#### Curation Organization(s)
<!-- info: Name the organization(s). -->
<!-- scope: periscope -->
Carnegie Mellon University
#### Dataset Creators
<!-- info: Who created the original dataset? List the people involved in collecting the dataset and their affiliation(s). -->
<!-- scope: microscope -->
Graham Neubig (Carnegie Mellon University), Hiroaki Hayashi (Carnegie Mellon University)
#### Funding
<!-- info: Who funded the data creation? -->
<!-- scope: microscope -->
Graham Neubig
#### Who added the Dataset to GEM?
<!-- info: Who contributed to the data card and adding the dataset to GEM? List the people+affiliations involved in creating this data card and who helped integrate this dataset into GEM. -->
<!-- scope: microscope -->
Hiroaki Hayashi (Carnegie Mellon University)
### Dataset Structure
#### Data Fields
<!-- info: List and describe the fields present in the dataset. -->
<!-- scope: telescope -->
- `id` (`string`): The identifier from the original dataset.
- `gem_id` (`string`): The identifier from GEMv2.
- `day` (`string`): Date of the game (Format: `MM_DD_YY`)
- `home_name` (`string`): Home team name.
- `home_city` (`string`): Home team city name.
- `vis_name` (`string`): Visiting (Away) team name.
- `vis_city` (`string`): Visiting team (Away) city name.
- `home_line` (`Dict[str, str]`): Home team statistics (e.g., team free throw percentage).
- `vis_line` (`Dict[str, str]`): Visiting team statistics (e.g., team free throw percentage).
- `box_score` (`Dict[str, Dict[str, str]]`): Box score table. (Stat_name to [player ID to stat_value].)
- `summary_en` (`List[string]`): Tokenized target summary in English.
- `sentence_end_index_en` (`List[int]`): Sentence end indices for `summary_en`.
- `summary_de` (`List[string]`): Tokenized target summary in German.
- `sentence_end_index_de` (`List[int]`): ): Sentence end indices for `summary_de`.
- (Unused) `detok_summary_org` (`string`): Original summary provided by RotoWire dataset.
- (Unused) `summary` (`List[string]`): Tokenized summary of `detok_summary_org`.
- (Unused) `detok_summary` (`string`): Detokenized (with organizer's detokenizer) summary of `summary`.
#### Reason for Structure
<!-- info: How was the dataset structure determined? -->
<!-- scope: microscope -->
- Structured data are directly imported from the original RotoWire dataset.
- Textual data (English, German) are associated to each sample.
#### Example Instance
<!-- info: Provide a JSON formatted example of a typical instance in the dataset. -->
<!-- scope: periscope -->
```
{
'id': '11_02_16-Jazz-Mavericks-TheUtahJazzdefeatedthe',
'gem_id': 'GEM-RotoWire_English-German-train-0'
'day': '11_02_16',
'home_city': 'Utah',
'home_name': 'Jazz',
'vis_city': 'Dallas',
'vis_name': 'Mavericks',
'home_line': {
'TEAM-FT_PCT': '58', ...
},
'vis_line': {
'TEAM-FT_PCT': '80', ...
},
'box_score': {
'PLAYER_NAME': {
'0': 'Harrison Barnes', ...
}, ...
'summary_en': ['The', 'Utah', 'Jazz', 'defeated', 'the', 'Dallas', 'Mavericks', ...],
'sentence_end_index_en': [16, 52, 100, 137, 177, 215, 241, 256, 288],
'summary_de': ['Die', 'Utah', 'Jazz', 'besiegten', 'am', 'Mittwoch', 'in', 'der', ...],
'sentence_end_index_de': [19, 57, 107, 134, 170, 203, 229, 239, 266],
'detok_summary_org': "The Utah Jazz defeated the Dallas Mavericks 97 - 81 ...",
'detok_summary': "The Utah Jazz defeated the Dallas Mavericks 97-81 ...",
'summary': ['The', 'Utah', 'Jazz', 'defeated', 'the', 'Dallas', 'Mavericks', ...],
}
```
#### Data Splits
<!-- info: Describe and name the splits in the dataset if there are more than one. -->
<!-- scope: periscope -->
- Train
- Validation
- Test
#### Splitting Criteria
<!-- info: Describe any criteria for splitting the data, if used. If there are differences between the splits (e.g., if the training annotations are machine-generated and the dev and test ones are created by humans, or if different numbers of annotators contributed to each example), describe them here. -->
<!-- scope: microscope -->
- English summaries are provided sentence-by-sentence to professional German translators with basketball knowledge to obtain sentence-level German translations.
- Split criteria follows the original RotoWire dataset.
####
<!-- info: What does an outlier of the dataset in terms of length/perplexity/embedding look like? -->
<!-- scope: microscope -->
- The (English) summary length in the training set varies from 145 to 650 words, with an average of 323 words.
## Dataset in GEM
### Rationale for Inclusion in GEM
#### Why is the Dataset in GEM?
<!-- info: What does this dataset contribute toward better generation evaluation and why is it part of GEM? -->
<!-- scope: microscope -->
The use of two modalities (data, foreign text) to generate a document-level text summary.
#### Similar Datasets
<!-- info: Do other datasets for the high level task exist? -->
<!-- scope: telescope -->
yes
#### Unique Language Coverage
<!-- info: Does this dataset cover other languages than other datasets for the same task? -->
<!-- scope: periscope -->
yes
#### Difference from other GEM datasets
<!-- info: What else sets this dataset apart from other similar datasets in GEM? -->
<!-- scope: microscope -->
The potential use of two modalities (data, foreign text) as input.
#### Ability that the Dataset measures
<!-- info: What aspect of model ability can be measured with this dataset? -->
<!-- scope: periscope -->
- Translation
- Data-to-text verbalization
- Aggregation of the two above.
### GEM-Specific Curation
#### Modificatied for GEM?
<!-- info: Has the GEM version of the dataset been modified in any way (data, processing, splits) from the original curated data? -->
<!-- scope: telescope -->
yes
#### GEM Modifications
<!-- info: What changes have been made to he original dataset? -->
<!-- scope: periscope -->
`other`
#### Modification Details
<!-- info: For each of these changes, described them in more details and provided the intended purpose of the modification -->
<!-- scope: microscope -->
- Added GEM ID in each sample.
- Normalize the number of players in each sample with "N/A" for consistent data loading.
#### Additional Splits?
<!-- info: Does GEM provide additional splits to the dataset? -->
<!-- scope: telescope -->
no
### Getting Started with the Task
#### Pointers to Resources
<!-- info: Getting started with in-depth research on the task. Add relevant pointers to resources that researchers can consult when they want to get started digging deeper into the task. -->
<!-- scope: microscope -->
- [Challenges in Data-to-Document Generation](https://aclanthology.org/D17-1239)
- [Data-to-Text Generation with Content Selection and Planning](https://ojs.aaai.org//index.php/AAAI/article/view/4668)
- [Findings of the Third Workshop on Neural Generation and Translation](https://aclanthology.org/D19-5601)
#### Technical Terms
<!-- info: Technical terms used in this card and the dataset and their definitions -->
<!-- scope: microscope -->
- Data-to-text
- Neural machine translation (NMT)
- Document-level generation and translation (DGT)
## Previous Results
### Previous Results
#### Measured Model Abilities
<!-- info: What aspect of model ability can be measured with this dataset? -->
<!-- scope: telescope -->
- Textual accuracy towards the gold-standard summary.
- Content faithfulness to the input structured data.
#### Metrics
<!-- info: What metrics are typically used for this task? -->
<!-- scope: periscope -->
`BLEU`, `ROUGE`, `Other: Other Metrics`
#### Other Metrics
<!-- info: Definitions of other metrics -->
<!-- scope: periscope -->
Model-based measures proposed by (Wiseman et al., 2017):
- Relation Generation
- Content Selection
- Content Ordering
#### Proposed Evaluation
<!-- info: List and describe the purpose of the metrics and evaluation methodology (including human evaluation) that the dataset creators used when introducing this task. -->
<!-- scope: microscope -->
To evaluate the fidelity of the generated content to the input data.
#### Previous results available?
<!-- info: Are previous results available? -->
<!-- scope: telescope -->
yes
#### Other Evaluation Approaches
<!-- info: What evaluation approaches have others used? -->
<!-- scope: periscope -->
N/A.
#### Relevant Previous Results
<!-- info: What are the most relevant previous results for this task/dataset? -->
<!-- scope: microscope -->
See Table 2 to 7 of (https://aclanthology.org/D19-5601) for previous results for this dataset.
## Dataset Curation
### Original Curation
#### Original Curation Rationale
<!-- info: Original curation rationale -->
<!-- scope: telescope -->
A random subset of RotoWire dataset was chosen for German translation annotation.
#### Communicative Goal
<!-- info: What was the communicative goal? -->
<!-- scope: periscope -->
Foster the research on document-level generation technology and contrast the methods for different types of inputs.
#### Sourced from Different Sources
<!-- info: Is the dataset aggregated from different data sources? -->
<!-- scope: telescope -->
yes
#### Source Details
<!-- info: List the sources (one per line) -->
<!-- scope: periscope -->
RotoWire
### Language Data
#### How was Language Data Obtained?
<!-- info: How was the language data obtained? -->
<!-- scope: telescope -->
`Created for the dataset`
#### Creation Process
<!-- info: If created for the dataset, describe the creation process. -->
<!-- scope: microscope -->
Professional German language translators were hired to translate basketball summaries from a subset of RotoWire dataset.
#### Language Producers
<!-- info: What further information do we have on the language producers? -->
<!-- scope: microscope -->
Translators are familiar with basketball terminology.
#### Topics Covered
<!-- info: Does the language in the dataset focus on specific topics? How would you describe them? -->
<!-- scope: periscope -->
Basketball (NBA) game summaries.
#### Data Validation
<!-- info: Was the text validated by a different worker or a data curator? -->
<!-- scope: telescope -->
validated by data curator
#### Data Preprocessing
<!-- info: How was the text data pre-processed? (Enter N/A if the text was not pre-processed) -->
<!-- scope: microscope -->
Sentence-level translations were aligned back to the original English summary sentences.
#### Was Data Filtered?
<!-- info: Were text instances selected or filtered? -->
<!-- scope: telescope -->
not filtered
### Structured Annotations
#### Additional Annotations?
<!-- quick -->
<!-- info: Does the dataset have additional annotations for each instance? -->
<!-- scope: telescope -->
automatically created
#### Annotation Service?
<!-- info: Was an annotation service used? -->
<!-- scope: telescope -->
no
#### Annotation Values
<!-- info: Purpose and values for each annotation -->
<!-- scope: microscope -->
Sentence-end indices for the tokenized summaries. Sentence boundaries can help users accurately identify aligned sentences in both languages, as well as allowing an accurate evaluation that involves sentence boundaries (ROUGE-L).
#### Any Quality Control?
<!-- info: Quality control measures? -->
<!-- scope: telescope -->
validated through automated script
#### Quality Control Details
<!-- info: Describe the quality control measures that were taken. -->
<!-- scope: microscope -->
Token and number overlaps between pairs of aligned sentences are measured.
### Consent
#### Any Consent Policy?
<!-- info: Was there a consent policy involved when gathering the data? -->
<!-- scope: telescope -->
no
#### Justification for Using the Data
<!-- info: If not, what is the justification for reusing the data? -->
<!-- scope: microscope -->
Reusing by citing the original papers:
- Sam Wiseman, Stuart M. Shieber, Alexander M. Rush:
Challenges in Data-to-Document Generation. EMNLP 2017.
- Hiroaki Hayashi, Yusuke Oda, Alexandra Birch, Ioannis Konstas, Andrew Finch, Minh-Thang Luong, Graham Neubig, Katsuhito Sudoh. Findings of the Third Workshop on Neural Generation and Translation. WNGT 2019.
### Private Identifying Information (PII)
#### Contains PII?
<!-- quick -->
<!-- info: Does the source language data likely contain Personal Identifying Information about the data creators or subjects? -->
<!-- scope: telescope -->
unlikely
#### Categories of PII
<!-- info: What categories of PII are present or suspected in the data? -->
<!-- scope: periscope -->
`generic PII`
#### Any PII Identification?
<!-- info: Did the curators use any automatic/manual method to identify PII in the dataset? -->
<!-- scope: periscope -->
no identification
### Maintenance
#### Any Maintenance Plan?
<!-- info: Does the original dataset have a maintenance plan? -->
<!-- scope: telescope -->
no
## Broader Social Context
### Previous Work on the Social Impact of the Dataset
#### Usage of Models based on the Data
<!-- info: Are you aware of cases where models trained on the task featured in this dataset ore related tasks have been used in automated systems? -->
<!-- scope: telescope -->
no
### Impact on Under-Served Communities
#### Addresses needs of underserved Communities?
<!-- info: Does this dataset address the needs of communities that are traditionally underserved in language technology, and particularly language generation technology? Communities may be underserved for exemple because their language, language variety, or social or geographical context is underepresented in NLP and NLG resources (datasets and models). -->
<!-- scope: telescope -->
no
### Discussion of Biases
#### Any Documented Social Biases?
<!-- info: Are there documented social biases in the dataset? Biases in this context are variations in the ways members of different social categories are represented that can have harmful downstream consequences for members of the more disadvantaged group. -->
<!-- scope: telescope -->
no
#### Are the Language Producers Representative of the Language?
<!-- info: Does the distribution of language producers in the dataset accurately represent the full distribution of speakers of the language world-wide? If not, how does it differ? -->
<!-- scope: periscope -->
- English text in this dataset is from Rotowire, originally written by writers at Rotowire.com that are likely US-based.
- German text is produced by professional translators proficient in both English and German.
## Considerations for Using the Data
### PII Risks and Liability
#### Potential PII Risk
<!-- info: Considering your answers to the PII part of the Data Curation Section, describe any potential privacy to the data subjects and creators risks when using the dataset. -->
<!-- scope: microscope -->
- Structured data contain real National Basketball Association player and organization names.
### Licenses
#### Copyright Restrictions on the Dataset
<!-- info: Based on your answers in the Intended Use part of the Data Overview Section, which of the following best describe the copyright and licensing status of the dataset? -->
<!-- scope: periscope -->
`open license - commercial use allowed`
#### Copyright Restrictions on the Language Data
<!-- info: Based on your answers in the Language part of the Data Curation Section, which of the following best describe the copyright and licensing status of the underlying language data? -->
<!-- scope: periscope -->
`open license - commercial use allowed`
### Known Technical Limitations
#### Technical Limitations
<!-- info: Describe any known technical limitations, such as spurrious correlations, train/test overlap, annotation biases, or mis-annotations, and cite the works that first identified these limitations when possible. -->
<!-- scope: microscope -->
Potential overlap of box score tables between splits. This was extensively studied and pointed out by [1].
[1]: Thomson, Craig, Ehud Reiter, and Somayajulu Sripada. "SportSett: Basketball-A robust and maintainable data-set for Natural Language Generation." Proceedings of the Workshop on Intelligent Information Processing and Natural Language Generation. 2020.
#### Unsuited Applications
<!-- info: When using a model trained on this dataset in a setting where users or the public may interact with its predictions, what are some pitfalls to look out for? In particular, describe some applications of the general task featured in this dataset that its curation or properties make it less suitable for. -->
<!-- scope: microscope -->
Users may interact with a trained model to learn about a NBA game in a textual manner. On generated texts, they may observe factual errors that contradicts the actual data that the model conditions on.
Factual errors include wrong statistics of a player (e.g., 3PT), non-existent injury information.
#### Discouraged Use Cases
<!-- info: What are some discouraged use cases of a model trained to maximize the proposed metrics on this dataset? In particular, think about settings where decisions made by a model that performs reasonably well on the metric my still have strong negative consequences for user or members of the public. -->
<!-- scope: microscope -->
Publishing the generated text as is. Even if the model achieves high scores on the evaluation metrics, there is a risk of factual errors mentioned above.
|
GEM/SIMPITIKI | ---
annotations_creators:
- crowd-sourced
language_creators:
- unknown
language:
- it
license:
- cc-by-4.0
multilinguality:
- unknown
size_categories:
- unknown
source_datasets:
- original
task_categories:
- text2text-generation
task_ids:
- text-simplification
pretty_name: SIMPITIKI
---
# Dataset Card for GEM/SIMPITIKI
## Dataset Description
- **Homepage:** https://github.com/dhfbk/simpitiki
- **Repository:** https://github.com/dhfbk/simpitiki/tree/master/corpus
- **Paper:** http://ceur-ws.org/Vol-1749/paper52.pdf
- **Leaderboard:** N/A
- **Point of Contact:** Sara Tonelli
### Link to Main Data Card
You can find the main data card on the [GEM Website](https://gem-benchmark.com/data_cards/SIMPITIKI).
### Dataset Summary
SIMPITIKI is an Italian Simplification dataset. Its examples were selected from Italian Wikipedia such that their editing tracking descriptions contain any of the words "Simplified"/"Simplify"/"Simplification".
You can load the dataset via:
```
import datasets
data = datasets.load_dataset('GEM/SIMPITIKI')
```
The data loader can be found [here](https://huggingface.co/datasets/GEM/SIMPITIKI).
#### website
[Github](https://github.com/dhfbk/simpitiki)
#### paper
[Website](http://ceur-ws.org/Vol-1749/paper52.pdf)
#### authors
Sara Tonelli (Fondazione Bruno Kessler), Alessio Palmero Aprosio (Fondazione Bruno Kessler), Francesca Saltori (Fondazione Bruno Kessler)
## Dataset Overview
### Where to find the Data and its Documentation
#### Webpage
<!-- info: What is the webpage for the dataset (if it exists)? -->
<!-- scope: telescope -->
[Github](https://github.com/dhfbk/simpitiki)
#### Download
<!-- info: What is the link to where the original dataset is hosted? -->
<!-- scope: telescope -->
[Github](https://github.com/dhfbk/simpitiki/tree/master/corpus)
#### Paper
<!-- info: What is the link to the paper describing the dataset (open access preferred)? -->
<!-- scope: telescope -->
[Website](http://ceur-ws.org/Vol-1749/paper52.pdf)
#### BibTex
<!-- info: Provide the BibTex-formatted reference for the dataset. Please use the correct published version (ACL anthology, etc.) instead of google scholar created Bibtex. -->
<!-- scope: microscope -->
```
@article{tonelli2016simpitiki,
title={SIMPITIKI: a Simplification corpus for Italian},
author={Tonelli, Sara and Aprosio, Alessio Palmero and Saltori, Francesca},
journal={Proceedings of CLiC-it},
year={2016}
}
```
#### Contact Name
<!-- quick -->
<!-- info: If known, provide the name of at least one person the reader can contact for questions about the dataset. -->
<!-- scope: periscope -->
Sara Tonelli
#### Contact Email
<!-- info: If known, provide the email of at least one person the reader can contact for questions about the dataset. -->
<!-- scope: periscope -->
satonelli@fbk.eu
#### Has a Leaderboard?
<!-- info: Does the dataset have an active leaderboard? -->
<!-- scope: telescope -->
no
### Languages and Intended Use
#### Multilingual?
<!-- quick -->
<!-- info: Is the dataset multilingual? -->
<!-- scope: telescope -->
no
#### Covered Dialects
<!-- info: What dialects are covered? Are there multiple dialects per language? -->
<!-- scope: periscope -->
None
#### Covered Languages
<!-- quick -->
<!-- info: What languages/dialects are covered in the dataset? -->
<!-- scope: telescope -->
`Italian`
#### License
<!-- quick -->
<!-- info: What is the license of the dataset? -->
<!-- scope: telescope -->
cc-by-4.0: Creative Commons Attribution 4.0 International
#### Intended Use
<!-- info: What is the intended use of the dataset? -->
<!-- scope: microscope -->
The purpose of the dataset is to train NLG models to simplify complex text by learning different types of transformations (verb to noun, noun to verbs, deletion, insertion, etc)
#### Primary Task
<!-- info: What primary task does the dataset support? -->
<!-- scope: telescope -->
Simplification
#### Communicative Goal
<!-- quick -->
<!-- info: Provide a short description of the communicative goal of a model trained for this task on this dataset. -->
<!-- scope: periscope -->
This dataset aims to enhance research in text simplification in Italian language with different text transformations.
### Credit
#### Curation Organization Type(s)
<!-- info: In what kind of organization did the dataset curation happen? -->
<!-- scope: telescope -->
`academic`, `independent`
#### Curation Organization(s)
<!-- info: Name the organization(s). -->
<!-- scope: periscope -->
Fondazione Bruno Kessler (FBK)
#### Dataset Creators
<!-- info: Who created the original dataset? List the people involved in collecting the dataset and their affiliation(s). -->
<!-- scope: microscope -->
Sara Tonelli (Fondazione Bruno Kessler), Alessio Palmero Aprosio (Fondazione Bruno Kessler), Francesca Saltori (Fondazione Bruno Kessler)
#### Funding
<!-- info: Who funded the data creation? -->
<!-- scope: microscope -->
EU Horizon 2020 Programme via the SIMPATICO Project (H2020-EURO-6-2015, n. 692819)
#### Who added the Dataset to GEM?
<!-- info: Who contributed to the data card and adding the dataset to GEM? List the people+affiliations involved in creating this data card and who helped integrate this dataset into GEM. -->
<!-- scope: microscope -->
Sebastien Montella (Orange Labs), Vipul Raheja (Grammarly Inc.)
### Dataset Structure
#### Data Fields
<!-- info: List and describe the fields present in the dataset. -->
<!-- scope: telescope -->
Each sample comes with the following fields:
- `gem_id` (string): Unique sample ID
-`text` (string): The raw text to be simplified
-`simplified_text` (string): The simplified version of "text" field
-`transformation_type` (string): Nature of transformation applied to raw text in order to simplify it.
-`source_dataset` (string): Initial dataset source of sample. Values: 'itwiki' (for Italian Wikipedia) or 'tn' (manually annotated administrative documents from the Municipality of Trento, Italy)
#### Reason for Structure
<!-- info: How was the dataset structure determined? -->
<!-- scope: microscope -->
The dataset is organized as a pairs where the raw text (input) is associated with its simplified text (output). The editing transformation and the source dataset of each sample is also provided for advanced analysis.
#### How were labels chosen?
<!-- info: How were the labels chosen? -->
<!-- scope: microscope -->
SIMPITIKI dataset selects documents from Italian Wikipedia such that their editing tracking descriptions contain any of the words "Simplified"/"Simplify"/"Simplification". For the Public Administration domain of the documents of the Municipality of Trento (Italy)
#### Example Instance
<!-- info: Provide a JSON formatted example of a typical instance in the dataset. -->
<!-- scope: periscope -->
```
{"transformation_id": 31, "transformation_type": "Transformation - Lexical Substitution (word level)", "source_dataset": "tn", "text": "- assenza per <del>e</del>si<del>genze</del> particolari attestate da relazione dei servizi sociali;", "simplified_text": "- assenza per <ins>bi</ins>s<ins>ogn</ins>i particolari attestati da relazione dei servizi sociali;"}
```
#### Data Splits
<!-- info: Describe and name the splits in the dataset if there are more than one. -->
<!-- scope: periscope -->
Several splits are proposed to train models on different configurations:
-"train": Training samples randomly selected from initial corpus. 816 training samples.
-"validation": Validating samples randomly selected from initial corpus. 174 validating samples.
-"test": Testing samples randomly selected from initial corpus. 176 validating samples.
-"challenge_seen_transformations_train": This training challenge split includes specific transformations to simplify the raw text. Precisely, transformations are "Split", "Merge", "Reordering", "Insert - Verb", "Insert - Other", "Delete - Verb", "Delete - Other", "Transformation - Lexical Substitution (word level)", "Transformation - Anaphoric replacement", "Transformation - Noun to Verb", "Transformation - Verbal Features". 562 training samples.
-"challenge_seen_transformations_val": This validating challenge split includes same transformations than the ones observed in training. Precisely, transformations are "Split", "Merge", "Reordering", "Insert - Verb", "Insert - Other", "Delete - Verb", "Delete - Other", "Transformation - Lexical Substitution (word level)", "Transformation - Anaphoric replacement", "Transformation - Noun to Verb", "Transformation - Verbal Features". 121 validating samples.
-"challenge_seen_transformations_test": This testing challenge split includes same transformations than the ones observed in training. Precisely, transformations are "Split", "Merge", "Reordering", "Insert - Verb", "Insert - Other", "Delete - Verb", "Delete - Other", "Transformation - Lexical Substitution (word level)", "Transformation - Anaphoric replacement", "Transformation - Noun to Verb", "Transformation - Verbal Features". 127 testing samples.
-"challenge_unseen_transformations_test" : "Insert - Subject", "Delete - Subject", "Transformation - Lexical Substitution (phrase level)", "Transformation - Verb to Noun (nominalization)", "Transformation - Verbal Voice". 356 testing samples.
-"challenge_itwiki_train": This challenge split includes random samples from the Italian Wikipedia as source dataset. 402 training samples.
-"challenge_itwiki_val": This validating challenge split includes random samples from the Italian Wikipedia as source dataset. 86 validating samples.
-"challenge_itwiki_test": This testing challenge split includes random samples from the Italian Wikipedia as source dataset. 87 testing samples.
-"challenge_tn_test": This testing challenge split includes all samples from the Municipality of Trento administrative documents ('tn') as source dataset. 591 testing samples.
#### Splitting Criteria
<!-- info: Describe any criteria for splitting the data, if used. If there are differences between the splits (e.g., if the training annotations are machine-generated and the dev and test ones are created by humans, or if different numbers of annotators contributed to each example), describe them here. -->
<!-- scope: microscope -->
The training ratio is set to 0.7. The validation and test somehow equally divide the remaining 30% of the dataset.
## Dataset in GEM
### Rationale for Inclusion in GEM
#### Why is the Dataset in GEM?
<!-- info: What does this dataset contribute toward better generation evaluation and why is it part of GEM? -->
<!-- scope: microscope -->
This dataset promotes Simplification task for Italian language.
#### Similar Datasets
<!-- info: Do other datasets for the high level task exist? -->
<!-- scope: telescope -->
no
#### Ability that the Dataset measures
<!-- info: What aspect of model ability can be measured with this dataset? -->
<!-- scope: periscope -->
Models can be evaluated if they can simplify text regarding different simplification transformations.
### GEM-Specific Curation
#### Modificatied for GEM?
<!-- info: Has the GEM version of the dataset been modified in any way (data, processing, splits) from the original curated data? -->
<!-- scope: telescope -->
yes
#### Additional Splits?
<!-- info: Does GEM provide additional splits to the dataset? -->
<!-- scope: telescope -->
yes
#### Split Information
<!-- info: Describe how the new splits were created -->
<!-- scope: periscope -->
The SIMPITIKI dataset provides a single file. Several splits are proposed to train models on different configurations:
-"train": Training samples randomly selected from initial corpus. 816 training samples.
-"validation": Validating samples randomly selected from initial corpus. 174 validating samples.
-"test": Testing samples randomly selected from initial corpus. 176 validating samples.
-"challenge_seen_transformations_train": This training challenge split includes specific transformations to simplify the raw text. Precisely, transformations are "Split", "Merge", "Reordering", "Insert - Verb", "Insert - Other", "Delete - Verb", "Delete - Other", "Transformation - Lexical Substitution (word level)", "Transformation - Anaphoric replacement", "Transformation - Noun to Verb", "Transformation - Verbal Features". 562 training samples.
-"challenge_seen_transformations_val": This validating challenge split includes same transformations than the ones observed in training. Precisely, transformations are "Split", "Merge", "Reordering", "Insert - Verb", "Insert - Other", "Delete - Verb", "Delete - Other", "Transformation - Lexical Substitution (word level)", "Transformation - Anaphoric replacement", "Transformation - Noun to Verb", "Transformation - Verbal Features". 121 validating samples.
-"challenge_seen_transformations_test": This testing challenge split includes same transformations than the ones observed in training. Precisely, transformations are "Split", "Merge", "Reordering", "Insert - Verb", "Insert - Other", "Delete - Verb", "Delete - Other", "Transformation - Lexical Substitution (word level)", "Transformation - Anaphoric replacement", "Transformation - Noun to Verb", "Transformation - Verbal Features". 127 testing samples.
-"challenge_unseen_transformations_test" : "Insert - Subject", "Delete - Subject", "Transformation - Lexical Substitution (phrase level)", "Transformation - Verb to Noun (nominalization)", "Transformation - Verbal Voice". 356 testing samples.
-"challenge_itwiki_train": This challenge split includes random samples from the Italian Wikipedia as source dataset. 402 training samples.
-"challenge_itwiki_val": This validating challenge split includes random samples from the Italian Wikipedia as source dataset. 86 validating samples.
-"challenge_itwiki_test": This testing challenge split includes random samples from the Italian Wikipedia as source dataset. 87 testing samples.
-"challenge_tn_test": This testing challenge split includes all samples from the Municipality of Trento administrative documents ('tn') as source dataset. 591 testing samples.
#### Split Motivation
<!-- info: What aspects of the model's generation capacities were the splits created to test? -->
<!-- scope: periscope -->
The splits allows to investigate the generalization of models regarding editing/transformations ("challenge_seen_transformations_test" / "challenge_unseen_transformations_test") and for transfer learning to different domain ("challenge_tn_test")
### Getting Started with the Task
#### Pointers to Resources
<!-- info: Getting started with in-depth research on the task. Add relevant pointers to resources that researchers can consult when they want to get started digging deeper into the task. -->
<!-- scope: microscope -->
- Coster and Kauchak, Simple English Wikipedia: A New Text Simplification Task, Proceedings of the 49th Annual Meeting of the Association for Computational Linguistics, pages 665–669, Portland, Oregon, June 19-24, 2011
- Xu et al, Optimizing Statistical Machine Translation for Text Simplification, Transactions of the Association for Computational Linguistics, vol. 4, pp. 401–415, 2016
- Aprosio et al, Neural Text Simplification in Low-Resource Conditions Using Weak Supervision, Proceedings of the Workshop on Methods for Optimizing and Evaluating Neural Language Generation (NeuralGen), pages 37–44, Minneapolis, Minnesota, USA, June 6, 2019
#### Technical Terms
<!-- info: Technical terms used in this card and the dataset and their definitions -->
<!-- scope: microscope -->
Simplification: Process that consists in transforming an input text to its simplified version.
## Previous Results
### Previous Results
#### Measured Model Abilities
<!-- info: What aspect of model ability can be measured with this dataset? -->
<!-- scope: telescope -->
The splits allows to investigate the generalization of models regarding editing/transformations ("challenge_seen_transformations_test" / "challenge_unseen_transformations_test") and for transfer learning to different domain ("challenge_tn_test")
#### Metrics
<!-- info: What metrics are typically used for this task? -->
<!-- scope: periscope -->
`BLEU`, `Other: Other Metrics`
#### Other Metrics
<!-- info: Definitions of other metrics -->
<!-- scope: periscope -->
FKBLEU (https://aclanthology.org/Q16-1029.pdf): Combines Flesch-Kincaid Index and iBLEU metrics.
SARI (https://aclanthology.org/Q16-1029.pdf): Compares system output against references and against the input sentence. It explicitly measures the goodness of words that are added, deleted and kept by the systems
Word-level F1
#### Previous results available?
<!-- info: Are previous results available? -->
<!-- scope: telescope -->
no
## Dataset Curation
### Original Curation
#### Original Curation Rationale
<!-- info: Original curation rationale -->
<!-- scope: telescope -->
Most of the resources for Text Simplification are in English. To stimulate research to different languages, SIMPITIKI proposes an Italian corpus with Complex-Simple sentence pairs.
#### Communicative Goal
<!-- info: What was the communicative goal? -->
<!-- scope: periscope -->
Text simplification allows a smooth reading of text to enhance understanding.
#### Sourced from Different Sources
<!-- info: Is the dataset aggregated from different data sources? -->
<!-- scope: telescope -->
yes
#### Source Details
<!-- info: List the sources (one per line) -->
<!-- scope: periscope -->
Italian Wikipedia
(Manually) Annotated administrative documents from the Municipality of Trento, Italy
### Language Data
#### How was Language Data Obtained?
<!-- info: How was the language data obtained? -->
<!-- scope: telescope -->
`Found`
#### Where was it found?
<!-- info: If found, where from? -->
<!-- scope: telescope -->
`Single website`, `Offline media collection`
#### Language Producers
<!-- info: What further information do we have on the language producers? -->
<!-- scope: microscope -->
SIMPITIKI is a combination of documents from Italian Wikipedia and from the Municipality of Trento, Italy.
#### Topics Covered
<!-- info: Does the language in the dataset focus on specific topics? How would you describe them? -->
<!-- scope: periscope -->
Samples from documents from the Municipality of Trento corpus are in the administrative domain.
#### Data Validation
<!-- info: Was the text validated by a different worker or a data curator? -->
<!-- scope: telescope -->
validated by data curator
#### Was Data Filtered?
<!-- info: Were text instances selected or filtered? -->
<!-- scope: telescope -->
not filtered
### Structured Annotations
#### Additional Annotations?
<!-- quick -->
<!-- info: Does the dataset have additional annotations for each instance? -->
<!-- scope: telescope -->
crowd-sourced
#### Number of Raters
<!-- info: What is the number of raters -->
<!-- scope: telescope -->
unknown
#### Rater Qualifications
<!-- info: Describe the qualifications required of an annotator. -->
<!-- scope: periscope -->
Native speaker
#### Raters per Training Example
<!-- info: How many annotators saw each training example? -->
<!-- scope: periscope -->
0
#### Raters per Test Example
<!-- info: How many annotators saw each test example? -->
<!-- scope: periscope -->
0
#### Annotation Service?
<!-- info: Was an annotation service used? -->
<!-- scope: telescope -->
unknown
#### Annotation Values
<!-- info: Purpose and values for each annotation -->
<!-- scope: microscope -->
Annotators specified any of the tags as designed by Brunato et al. (https://aclanthology.org/W15-1604/):
-Split: Splitting a clause into two clauses.
-Merge: Merge two or more clauses together.
-Reordering: Word order changes.
-Insert: Insertion of words or phrases that provide supportive information to the original sentence
-Delete: dropping redundant information.
-Transformation: Modification which can affect the sentence at the lexical, morpho-syntactic and syntactic level: Lexical substitution (word level) / Lexical substitution (phrase level) / Anaphoric replacement / Noun to Verb / Verb to Noun / Verbal voice / Verbal features/ morpho–syntactic and syntactic level, also giving rise to overlapping phenomena
#### Any Quality Control?
<!-- info: Quality control measures? -->
<!-- scope: telescope -->
unknown
### Consent
#### Any Consent Policy?
<!-- info: Was there a consent policy involved when gathering the data? -->
<!-- scope: telescope -->
no
#### Justification for Using the Data
<!-- info: If not, what is the justification for reusing the data? -->
<!-- scope: microscope -->
The dataset is available online under the CC-BY 4.0 license.
### Private Identifying Information (PII)
#### Contains PII?
<!-- quick -->
<!-- info: Does the source language data likely contain Personal Identifying Information about the data creators or subjects? -->
<!-- scope: telescope -->
likely
#### Categories of PII
<!-- info: What categories of PII are present or suspected in the data? -->
<!-- scope: periscope -->
`generic PII`
#### Any PII Identification?
<!-- info: Did the curators use any automatic/manual method to identify PII in the dataset? -->
<!-- scope: periscope -->
no identification
### Maintenance
#### Any Maintenance Plan?
<!-- info: Does the original dataset have a maintenance plan? -->
<!-- scope: telescope -->
no
## Broader Social Context
### Previous Work on the Social Impact of the Dataset
#### Usage of Models based on the Data
<!-- info: Are you aware of cases where models trained on the task featured in this dataset ore related tasks have been used in automated systems? -->
<!-- scope: telescope -->
no
### Impact on Under-Served Communities
#### Addresses needs of underserved Communities?
<!-- info: Does this dataset address the needs of communities that are traditionally underserved in language technology, and particularly language generation technology? Communities may be underserved for exemple because their language, language variety, or social or geographical context is underepresented in NLP and NLG resources (datasets and models). -->
<!-- scope: telescope -->
yes
#### Details on how Dataset Addresses the Needs
<!-- info: Describe how this dataset addresses the needs of underserved communities. -->
<!-- scope: microscope -->
The creator of SIMPITIKI wants to promote text simplification for Italian because few resources are available in other languages than English.
### Discussion of Biases
#### Any Documented Social Biases?
<!-- info: Are there documented social biases in the dataset? Biases in this context are variations in the ways members of different social categories are represented that can have harmful downstream consequences for members of the more disadvantaged group. -->
<!-- scope: telescope -->
unsure
## Considerations for Using the Data
### PII Risks and Liability
### Licenses
#### Copyright Restrictions on the Dataset
<!-- info: Based on your answers in the Intended Use part of the Data Overview Section, which of the following best describe the copyright and licensing status of the dataset? -->
<!-- scope: periscope -->
`research use only`
#### Copyright Restrictions on the Language Data
<!-- info: Based on your answers in the Language part of the Data Curation Section, which of the following best describe the copyright and licensing status of the underlying language data? -->
<!-- scope: periscope -->
`research use only`
### Known Technical Limitations
#### Discouraged Use Cases
<!-- info: What are some discouraged use cases of a model trained to maximize the proposed metrics on this dataset? In particular, think about settings where decisions made by a model that performs reasonably well on the metric my still have strong negative consequences for user or members of the public. -->
<!-- scope: microscope -->
The risk of surface-based metrics (BLEU, chrf++, etc) for this task is that semantic adequacy is not respected when simplifying the input document.
|
GEM/SciDuet | ---
annotations_creators:
- none
language_creators:
- unknown
language:
- en
license:
- apache-2.0
multilinguality:
- unknown
size_categories:
- unknown
source_datasets:
- original
task_categories:
- other
task_ids: []
pretty_name: SciDuet
tags:
- text-to-slide
---
# Dataset Card for GEM/SciDuet
## Dataset Description
- **Homepage:** https://huggingface.co/datasets/GEM/SciDuet
- **Repository:** https://github.com/IBM/document2slides/tree/main/SciDuet-ACL
- **Paper:** https://aclanthology.org/2021.naacl-main.111/
- **Leaderboard:** N/A
- **Point of Contact:** N/A
### Link to Main Data Card
You can find the main data card on the [GEM Website](https://gem-benchmark.com/data_cards/SciDuet).
### Dataset Summary
This dataset supports the document-to-slide generation task where a model has to generate presentation slide content from the text of a document.
You can load the dataset via:
```
import datasets
data = datasets.load_dataset('GEM/SciDuet')
```
The data loader can be found [here](https://huggingface.co/datasets/GEM/SciDuet).
#### website
[Huggingface](https://huggingface.co/datasets/GEM/SciDuet)
#### paper
[ACL Anthology](https://aclanthology.org/2021.naacl-main.111/)
#### authors
Edward Sun, Yufang Hou, Dakuo Wang, Yunfeng Zhang, Nancy Wang
## Dataset Overview
### Where to find the Data and its Documentation
#### Webpage
<!-- info: What is the webpage for the dataset (if it exists)? -->
<!-- scope: telescope -->
[Huggingface](https://huggingface.co/datasets/GEM/SciDuet)
#### Download
<!-- info: What is the link to where the original dataset is hosted? -->
<!-- scope: telescope -->
[Github](https://github.com/IBM/document2slides/tree/main/SciDuet-ACL)
#### Paper
<!-- info: What is the link to the paper describing the dataset (open access preferred)? -->
<!-- scope: telescope -->
[ACL Anthology](https://aclanthology.org/2021.naacl-main.111/)
#### BibTex
<!-- info: Provide the BibTex-formatted reference for the dataset. Please use the correct published version (ACL anthology, etc.) instead of google scholar created Bibtex. -->
<!-- scope: microscope -->
```
@inproceedings{sun-etal-2021-d2s,
title = "{D}2{S}: Document-to-Slide Generation Via Query-Based Text Summarization",
author = "Sun, Edward and
Hou, Yufang and
Wang, Dakuo and
Zhang, Yunfeng and
Wang, Nancy X. R.",
booktitle = "Proceedings of the 2021 Conference of the North American Chapter of the Association for Computational Linguistics: Human Language Technologies",
month = jun,
year = "2021",
address = "Online",
publisher = "Association for Computational Linguistics",
url = "https://aclanthology.org/2021.naacl-main.111",
doi = "10.18653/v1/2021.naacl-main.111",
pages = "1405--1418",
abstract = "Presentations are critical for communication in all areas of our lives, yet the creation of slide decks is often tedious and time-consuming. There has been limited research aiming to automate the document-to-slides generation process and all face a critical challenge: no publicly available dataset for training and benchmarking. In this work, we first contribute a new dataset, SciDuet, consisting of pairs of papers and their corresponding slides decks from recent years{'} NLP and ML conferences (e.g., ACL). Secondly, we present D2S, a novel system that tackles the document-to-slides task with a two-step approach: 1) Use slide titles to retrieve relevant and engaging text, figures, and tables; 2) Summarize the retrieved context into bullet points with long-form question answering. Our evaluation suggests that long-form QA outperforms state-of-the-art summarization baselines on both automated ROUGE metrics and qualitative human evaluation.",
}
```
#### Has a Leaderboard?
<!-- info: Does the dataset have an active leaderboard? -->
<!-- scope: telescope -->
no
### Languages and Intended Use
#### Multilingual?
<!-- quick -->
<!-- info: Is the dataset multilingual? -->
<!-- scope: telescope -->
no
#### Covered Languages
<!-- quick -->
<!-- info: What languages/dialects are covered in the dataset? -->
<!-- scope: telescope -->
`English`
#### License
<!-- quick -->
<!-- info: What is the license of the dataset? -->
<!-- scope: telescope -->
apache-2.0: Apache License 2.0
#### Intended Use
<!-- info: What is the intended use of the dataset? -->
<!-- scope: microscope -->
Promote research on the task of document-to-slides generation
#### Primary Task
<!-- info: What primary task does the dataset support? -->
<!-- scope: telescope -->
Text-to-Slide
### Credit
#### Curation Organization Type(s)
<!-- info: In what kind of organization did the dataset curation happen? -->
<!-- scope: telescope -->
`industry`
#### Curation Organization(s)
<!-- info: Name the organization(s). -->
<!-- scope: periscope -->
IBM Research
#### Dataset Creators
<!-- info: Who created the original dataset? List the people involved in collecting the dataset and their affiliation(s). -->
<!-- scope: microscope -->
Edward Sun, Yufang Hou, Dakuo Wang, Yunfeng Zhang, Nancy Wang
#### Funding
<!-- info: Who funded the data creation? -->
<!-- scope: microscope -->
IBM Research
#### Who added the Dataset to GEM?
<!-- info: Who contributed to the data card and adding the dataset to GEM? List the people+affiliations involved in creating this data card and who helped integrate this dataset into GEM. -->
<!-- scope: microscope -->
Yufang Hou (IBM Research), Dakuo Wang (IBM Research)
### Dataset Structure
#### How were labels chosen?
<!-- info: How were the labels chosen? -->
<!-- scope: microscope -->
The original papers and slides (both are in PDF format) are carefully processed by a combination of PDF/Image processing tookits. The text contents from multiple slides that correspond to the same slide title are mreged.
#### Data Splits
<!-- info: Describe and name the splits in the dataset if there are more than one. -->
<!-- scope: periscope -->
Training, validation and testing data contain 136, 55, and 81 papers from ACL Anthology and their corresponding slides, respectively.
#### Splitting Criteria
<!-- info: Describe any criteria for splitting the data, if used. If there are differences between the splits (e.g., if the training annotations are machine-generated and the dev and test ones are created by humans, or if different numbers of annotators contributed to each example), describe them here. -->
<!-- scope: microscope -->
The dataset integrated into GEM is the ACL portion of the whole dataset described in the [paper](https://aclanthology.org/2021.naacl-main.111), It contains the full Dev and Test sets, and a portion of the Train dataset.
Note that although we cannot release the whole training dataset due to copyright issues, researchers can still use our released data procurement code to generate the training dataset from the online ICML/NeurIPS anthologies.
## Dataset in GEM
### Rationale for Inclusion in GEM
#### Why is the Dataset in GEM?
<!-- info: What does this dataset contribute toward better generation evaluation and why is it part of GEM? -->
<!-- scope: microscope -->
SciDuet is the first publicaly available dataset for the challenging task of document2slides generation, which requires a model has a good ability to "understand" long-form text, choose appropriate content and generate key points.
#### Similar Datasets
<!-- info: Do other datasets for the high level task exist? -->
<!-- scope: telescope -->
no
#### Ability that the Dataset measures
<!-- info: What aspect of model ability can be measured with this dataset? -->
<!-- scope: periscope -->
content selection, long-form text undersanding and generation
### GEM-Specific Curation
#### Modificatied for GEM?
<!-- info: Has the GEM version of the dataset been modified in any way (data, processing, splits) from the original curated data? -->
<!-- scope: telescope -->
no
#### Additional Splits?
<!-- info: Does GEM provide additional splits to the dataset? -->
<!-- scope: telescope -->
no
### Getting Started with the Task
## Previous Results
### Previous Results
#### Measured Model Abilities
<!-- info: What aspect of model ability can be measured with this dataset? -->
<!-- scope: telescope -->
content selection, long-form text undersanding and key points generation
#### Metrics
<!-- info: What metrics are typically used for this task? -->
<!-- scope: periscope -->
`ROUGE`
#### Proposed Evaluation
<!-- info: List and describe the purpose of the metrics and evaluation methodology (including human evaluation) that the dataset creators used when introducing this task. -->
<!-- scope: microscope -->
Automatical Evaluation Metric: ROUGE
Human Evaluation: (Readability, Informativeness, Consistency)
1) Readability: The generated slide content is coherent, concise, and grammatically correct;
2) Informativeness: The generated slide provides sufficient and necessary information that corresponds to the given slide title, regardless of its similarity to the original slide;
3) Consistency: The generated slide content is similar to the original author’s reference slide.
#### Previous results available?
<!-- info: Are previous results available? -->
<!-- scope: telescope -->
yes
#### Other Evaluation Approaches
<!-- info: What evaluation approaches have others used? -->
<!-- scope: periscope -->
ROUGE + Human Evaluation
#### Relevant Previous Results
<!-- info: What are the most relevant previous results for this task/dataset? -->
<!-- scope: microscope -->
Paper "D2S: Document-to-Slide Generation Via Query-Based
Text Summarization" reports 20.47, 5.26 and 19.08 for ROUGE-1, ROUGE-2 and ROUGE-L (f-score).
## Dataset Curation
### Original Curation
#### Original Curation Rationale
<!-- info: Original curation rationale -->
<!-- scope: telescope -->
Provide a benchmark dataset for the document-to-slides task.
#### Sourced from Different Sources
<!-- info: Is the dataset aggregated from different data sources? -->
<!-- scope: telescope -->
no
### Language Data
#### How was Language Data Obtained?
<!-- info: How was the language data obtained? -->
<!-- scope: telescope -->
`Other`
#### Data Validation
<!-- info: Was the text validated by a different worker or a data curator? -->
<!-- scope: telescope -->
not validated
#### Data Preprocessing
<!-- info: How was the text data pre-processed? (Enter N/A if the text was not pre-processed) -->
<!-- scope: microscope -->
Text on papers was extracted through Grobid. Figures andcaptions were extracted through pdffigures. Text on slides was extracted through IBM Watson Discovery package and OCR by pytesseract. Figures and tables that appear on slides and papers were linked through multiscale template matching by OpenCV. Further dataset
cleaning was performed with standard string-based
heuristics on sentence building, equation and floating caption removal, and duplicate line deletion.
#### Was Data Filtered?
<!-- info: Were text instances selected or filtered? -->
<!-- scope: telescope -->
algorithmically
#### Filter Criteria
<!-- info: What were the selection criteria? -->
<!-- scope: microscope -->
the slide context text shouldn't contain additional format information such as "*** University"
### Structured Annotations
#### Additional Annotations?
<!-- quick -->
<!-- info: Does the dataset have additional annotations for each instance? -->
<!-- scope: telescope -->
none
#### Annotation Service?
<!-- info: Was an annotation service used? -->
<!-- scope: telescope -->
no
### Consent
#### Any Consent Policy?
<!-- info: Was there a consent policy involved when gathering the data? -->
<!-- scope: telescope -->
yes
#### Consent Policy Details
<!-- info: What was the consent policy? -->
<!-- scope: microscope -->
The original dataset was open-sourced under Apache-2.0.
Some of the original dataset creators are part of the GEM v2 dataset infrastructure team and take care of integrating this dataset into GEM.
### Private Identifying Information (PII)
#### Contains PII?
<!-- quick -->
<!-- info: Does the source language data likely contain Personal Identifying Information about the data creators or subjects? -->
<!-- scope: telescope -->
yes/very likely
#### Categories of PII
<!-- info: What categories of PII are present or suspected in the data? -->
<!-- scope: periscope -->
`generic PII`
#### Any PII Identification?
<!-- info: Did the curators use any automatic/manual method to identify PII in the dataset? -->
<!-- scope: periscope -->
no identification
### Maintenance
#### Any Maintenance Plan?
<!-- info: Does the original dataset have a maintenance plan? -->
<!-- scope: telescope -->
no
## Broader Social Context
### Previous Work on the Social Impact of the Dataset
#### Usage of Models based on the Data
<!-- info: Are you aware of cases where models trained on the task featured in this dataset ore related tasks have been used in automated systems? -->
<!-- scope: telescope -->
no
### Impact on Under-Served Communities
#### Addresses needs of underserved Communities?
<!-- info: Does this dataset address the needs of communities that are traditionally underserved in language technology, and particularly language generation technology? Communities may be underserved for exemple because their language, language variety, or social or geographical context is underepresented in NLP and NLG resources (datasets and models). -->
<!-- scope: telescope -->
no
### Discussion of Biases
#### Any Documented Social Biases?
<!-- info: Are there documented social biases in the dataset? Biases in this context are variations in the ways members of different social categories are represented that can have harmful downstream consequences for members of the more disadvantaged group. -->
<!-- scope: telescope -->
unsure
## Considerations for Using the Data
### PII Risks and Liability
### Licenses
#### Copyright Restrictions on the Dataset
<!-- info: Based on your answers in the Intended Use part of the Data Overview Section, which of the following best describe the copyright and licensing status of the dataset? -->
<!-- scope: periscope -->
`non-commercial use only`
#### Copyright Restrictions on the Language Data
<!-- info: Based on your answers in the Language part of the Data Curation Section, which of the following best describe the copyright and licensing status of the underlying language data? -->
<!-- scope: periscope -->
`research use only`
### Known Technical Limitations
|
GEM/Taskmaster | ---
annotations_creators:
- none
language_creators:
- unknown
language:
- en
license:
- cc-by-4.0
multilinguality:
- unknown
size_categories:
- unknown
source_datasets:
- original
task_categories:
- conversational
task_ids: []
pretty_name: Taskmaster
tags:
- dialog-response-generation
---
# Dataset Card for GEM/Taskmaster
## Dataset Description
- **Homepage:** https://github.com/google-research-datasets/Taskmaster/tree/master/TM-3-2020
- **Repository:** https://github.com/google-research-datasets/Taskmaster/tree/master/TM-3-2020
- **Paper:** https://arxiv.org/abs/2012.12458
- **Leaderboard:** N/A
- **Point of Contact:** Karthik Krishnamoorthi
### Link to Main Data Card
You can find the main data card on the [GEM Website](https://gem-benchmark.com/data_cards/Taskmaster).
### Dataset Summary
This is a large task-oriented dialog dataset in which a model has to produce the response. The input contains the context and a structured representation of what the model is supposed to generate. The input is already pre-formatted as string, turning this into a pure text-to-text problem.
You can load the dataset via:
```
import datasets
data = datasets.load_dataset('GEM/Taskmaster')
```
The data loader can be found [here](https://huggingface.co/datasets/GEM/Taskmaster).
#### website
[Github](https://github.com/google-research-datasets/Taskmaster/tree/master/TM-3-2020)
#### paper
[Arxiv](https://arxiv.org/abs/2012.12458)
#### authors
Google researchers
## Dataset Overview
### Where to find the Data and its Documentation
#### Webpage
<!-- info: What is the webpage for the dataset (if it exists)? -->
<!-- scope: telescope -->
[Github](https://github.com/google-research-datasets/Taskmaster/tree/master/TM-3-2020)
#### Download
<!-- info: What is the link to where the original dataset is hosted? -->
<!-- scope: telescope -->
[Github](https://github.com/google-research-datasets/Taskmaster/tree/master/TM-3-2020)
#### Paper
<!-- info: What is the link to the paper describing the dataset (open access preferred)? -->
<!-- scope: telescope -->
[Arxiv](https://arxiv.org/abs/2012.12458)
#### BibTex
<!-- info: Provide the BibTex-formatted reference for the dataset. Please use the correct published version (ACL anthology, etc.) instead of google scholar created Bibtex. -->
<!-- scope: microscope -->
```
@article{byrne2020tickettalk,
title={TicketTalk: Toward human-level performance with end-to-end, transaction-based dialog systems},
author={Byrne, Bill and Krishnamoorthi, Karthik and Ganesh, Saravanan and Kale, Mihir Sanjay},
journal={arXiv preprint arXiv:2012.12458},
year={2020}
}
```
#### Contact Name
<!-- quick -->
<!-- info: If known, provide the name of at least one person the reader can contact for questions about the dataset. -->
<!-- scope: periscope -->
Karthik Krishnamoorthi
#### Contact Email
<!-- info: If known, provide the email of at least one person the reader can contact for questions about the dataset. -->
<!-- scope: periscope -->
krishnamoorthi@google.com
#### Has a Leaderboard?
<!-- info: Does the dataset have an active leaderboard? -->
<!-- scope: telescope -->
no
### Languages and Intended Use
#### Multilingual?
<!-- quick -->
<!-- info: Is the dataset multilingual? -->
<!-- scope: telescope -->
no
#### Covered Dialects
<!-- info: What dialects are covered? Are there multiple dialects per language? -->
<!-- scope: periscope -->
NA
#### Covered Languages
<!-- quick -->
<!-- info: What languages/dialects are covered in the dataset? -->
<!-- scope: telescope -->
`English`
#### Whose Language?
<!-- info: Whose language is in the dataset? -->
<!-- scope: periscope -->
NA
#### License
<!-- quick -->
<!-- info: What is the license of the dataset? -->
<!-- scope: telescope -->
cc-by-4.0: Creative Commons Attribution 4.0 International
#### Intended Use
<!-- info: What is the intended use of the dataset? -->
<!-- scope: microscope -->
Dialogues
#### Primary Task
<!-- info: What primary task does the dataset support? -->
<!-- scope: telescope -->
Dialog Response Generation
#### Communicative Goal
<!-- quick -->
<!-- info: Provide a short description of the communicative goal of a model trained for this task on this dataset. -->
<!-- scope: periscope -->
a movie ticketing dialog dataset with 23,789 annotated conversations.
### Credit
#### Curation Organization Type(s)
<!-- info: In what kind of organization did the dataset curation happen? -->
<!-- scope: telescope -->
`other`
#### Curation Organization(s)
<!-- info: Name the organization(s). -->
<!-- scope: periscope -->
NA
#### Dataset Creators
<!-- info: Who created the original dataset? List the people involved in collecting the dataset and their affiliation(s). -->
<!-- scope: microscope -->
Google researchers
#### Funding
<!-- info: Who funded the data creation? -->
<!-- scope: microscope -->
Google
#### Who added the Dataset to GEM?
<!-- info: Who contributed to the data card and adding the dataset to GEM? List the people+affiliations involved in creating this data card and who helped integrate this dataset into GEM. -->
<!-- scope: microscope -->
Tosin Adewumi (Luleå University of Technology)
### Dataset Structure
#### Data Fields
<!-- info: List and describe the fields present in the dataset. -->
<!-- scope: telescope -->
- `gem_id`: The unique example id
- `context`: The context of the conversation
- `target`: A string representing the target
-`references`: A List representing the target(s)
-`conversation_id`: A unique ID of the conversation
#### Reason for Structure
<!-- info: How was the dataset structure determined? -->
<!-- scope: microscope -->
NA
#### How were labels chosen?
<!-- info: How were the labels chosen? -->
<!-- scope: microscope -->
NA
#### Example Instance
<!-- info: Provide a JSON formatted example of a typical instance in the dataset. -->
<!-- scope: periscope -->
```
{'context': "<PR>get_movie_attribute<PRAN>rating.movie<PRAV>rated R<C><U>I wanna see a movie<A>where are you?<U>spring hills kansas<PN>find_theaters<PAN>location<PAV>spring hills kansas<PR>find_theaters<PRAN>name.theater<PRAV>AMC Holiday Theater<PRAV>Cinemark Downtown<A>there are 2 theaters near you, the AMC Holiday Theater and Cinemark Downtown. Did you know which movie you'd like to see?<U>funny one please<PN>find_movies<PAN>location<PAV>spring hills kansas<PR>find_movies<PRAN>name.movie<PRAV>Not My Problem<PRAV>Family Jewels<PN>get_movie_attribute<PAN>name.movie<PAV>Not My Problem<PAN>attribute<PAV>name.genre<PR>get_movie_attribute<PRAN>name.genre<PRAV>comedy<PN>get_movie_attribute<PAN>name.movie<PAV>Not My Problem<PAN>attribute<PAV>name.person<PR>get_movie_attribute<PRAN>name.person<PRAV>Matt Damon<PN>get_movie_attribute<PAN>name.movie<PAV>Not My Problem<PAN>attribute<PAV>name.person<PR>get_movie_attribute<PRAN>name.person<PRAV>Noah Schnapp<PN>get_movie_attribute<PAN>name.movie<PAV>Family Jewels<PAN>attribute<PAV>name.genre<PR>get_movie_attribute<PRAN>name.genre<PRAV>romantic comedy<PN>get_movie_attribute<PAN>name.movie<PAV>Family Jewels<PAN>attribute<PAV>name.person<PR>get_movie_attribute<PRAN>name.person<PRAV>Melissa McCarthy<PN>get_movie_attribute<PAN>name.movie<PAV>Family Jewels<PAN>attribute<PAV>name.person<PR>get_movie_attribute<PRAN>name.person<PRAV>Ryan Reynolds<A>There's the comedy film called Not My Problem starring Matt Damon and Noah Schnapp. There's also a romantic comedy called Family Jewels starring Melissa McCarthy and Ryan Reynolds.<U>what ratings are there?<PN>get_movie_attribute<PAN>name.movie<PAV>Not My Problem<PAN>attribute<PAV>rating.movie<PR>get_movie_attribute<PRAN>rating.movie<PRAV>rated PG-13<PN>get_movie_attribute<PAN>name.movie<PAV>Family Jewels<PAN>attribute<PAV>rating.movie",
'conversation_id': 'dlg-d1f52e7e-c34c-4e85-b406-85ed138b5068',
'gem_id': 'Taskmaster-train-0',
'references': ['Not My Problem is rated PG-13 and Family Jewels is rated R.'],
'target': 'Not My Problem is rated PG-13 and Family Jewels is rated R.'}
```
#### Data Splits
<!-- info: Describe and name the splits in the dataset if there are more than one. -->
<!-- scope: periscope -->
-`train`: 187182 examples
-`dev`: 23406 examples
-`test`: 23316 examples
#### Splitting Criteria
<!-- info: Describe any criteria for splitting the data, if used. If there are differences between the splits (e.g., if the training annotations are machine-generated and the dev and test ones are created by humans, or if different numbers of annotators contributed to each example), describe them here. -->
<!-- scope: microscope -->
NA
####
<!-- info: What does an outlier of the dataset in terms of length/perplexity/embedding look like? -->
<!-- scope: microscope -->
NA
## Dataset in GEM
### Rationale for Inclusion in GEM
#### Why is the Dataset in GEM?
<!-- info: What does this dataset contribute toward better generation evaluation and why is it part of GEM? -->
<!-- scope: microscope -->
Dialogue generation that makes sense
#### Similar Datasets
<!-- info: Do other datasets for the high level task exist? -->
<!-- scope: telescope -->
yes
#### Unique Language Coverage
<!-- info: Does this dataset cover other languages than other datasets for the same task? -->
<!-- scope: periscope -->
no
#### Difference from other GEM datasets
<!-- info: What else sets this dataset apart from other similar datasets in GEM? -->
<!-- scope: microscope -->
NA
#### Ability that the Dataset measures
<!-- info: What aspect of model ability can be measured with this dataset? -->
<!-- scope: periscope -->
NA
### GEM-Specific Curation
#### Modificatied for GEM?
<!-- info: Has the GEM version of the dataset been modified in any way (data, processing, splits) from the original curated data? -->
<!-- scope: telescope -->
yes
#### GEM Modifications
<!-- info: What changes have been made to he original dataset? -->
<!-- scope: periscope -->
`other`
#### Modification Details
<!-- info: For each of these changes, described them in more details and provided the intended purpose of the modification -->
<!-- scope: microscope -->
gem_id field was added to the 3 data splits
#### Additional Splits?
<!-- info: Does GEM provide additional splits to the dataset? -->
<!-- scope: telescope -->
no
### Getting Started with the Task
#### Pointers to Resources
<!-- info: Getting started with in-depth research on the task. Add relevant pointers to resources that researchers can consult when they want to get started digging deeper into the task. -->
<!-- scope: microscope -->
https://github.com/google-research-datasets/Taskmaster/tree/master/TM-3-2020
#### Technical Terms
<!-- info: Technical terms used in this card and the dataset and their definitions -->
<!-- scope: microscope -->
NA
## Previous Results
### Previous Results
#### Measured Model Abilities
<!-- info: What aspect of model ability can be measured with this dataset? -->
<!-- scope: telescope -->
BLEU: 60
#### Metrics
<!-- info: What metrics are typically used for this task? -->
<!-- scope: periscope -->
`BLEU`
#### Proposed Evaluation
<!-- info: List and describe the purpose of the metrics and evaluation methodology (including human evaluation) that the dataset creators used when introducing this task. -->
<!-- scope: microscope -->
automatic evaluation
#### Previous results available?
<!-- info: Are previous results available? -->
<!-- scope: telescope -->
yes
#### Other Evaluation Approaches
<!-- info: What evaluation approaches have others used? -->
<!-- scope: periscope -->
NA
#### Relevant Previous Results
<!-- info: What are the most relevant previous results for this task/dataset? -->
<!-- scope: microscope -->
NA
## Dataset Curation
### Original Curation
#### Original Curation Rationale
<!-- info: Original curation rationale -->
<!-- scope: telescope -->
NA
#### Communicative Goal
<!-- info: What was the communicative goal? -->
<!-- scope: periscope -->
a movie ticketing dialog dataset with 23,789 annotated conversations.
#### Sourced from Different Sources
<!-- info: Is the dataset aggregated from different data sources? -->
<!-- scope: telescope -->
no
### Language Data
#### How was Language Data Obtained?
<!-- info: How was the language data obtained? -->
<!-- scope: telescope -->
`Crowdsourced`
#### Where was it crowdsourced?
<!-- info: If crowdsourced, where from? -->
<!-- scope: periscope -->
`Participatory experiment`
#### Language Producers
<!-- info: What further information do we have on the language producers? -->
<!-- scope: microscope -->
NA
#### Topics Covered
<!-- info: Does the language in the dataset focus on specific topics? How would you describe them? -->
<!-- scope: periscope -->
Ticketing
#### Data Validation
<!-- info: Was the text validated by a different worker or a data curator? -->
<!-- scope: telescope -->
not validated
#### Was Data Filtered?
<!-- info: Were text instances selected or filtered? -->
<!-- scope: telescope -->
not filtered
### Structured Annotations
#### Additional Annotations?
<!-- quick -->
<!-- info: Does the dataset have additional annotations for each instance? -->
<!-- scope: telescope -->
none
#### Annotation Service?
<!-- info: Was an annotation service used? -->
<!-- scope: telescope -->
no
### Consent
#### Any Consent Policy?
<!-- info: Was there a consent policy involved when gathering the data? -->
<!-- scope: telescope -->
no
#### Justification for Using the Data
<!-- info: If not, what is the justification for reusing the data? -->
<!-- scope: microscope -->
NA
### Private Identifying Information (PII)
#### Contains PII?
<!-- quick -->
<!-- info: Does the source language data likely contain Personal Identifying Information about the data creators or subjects? -->
<!-- scope: telescope -->
no PII
#### Justification for no PII
<!-- info: Provide a justification for selecting `no PII` above. -->
<!-- scope: periscope -->
It's based on ticketing without personal information
### Maintenance
#### Any Maintenance Plan?
<!-- info: Does the original dataset have a maintenance plan? -->
<!-- scope: telescope -->
no
## Broader Social Context
### Previous Work on the Social Impact of the Dataset
#### Usage of Models based on the Data
<!-- info: Are you aware of cases where models trained on the task featured in this dataset ore related tasks have been used in automated systems? -->
<!-- scope: telescope -->
no
### Impact on Under-Served Communities
#### Addresses needs of underserved Communities?
<!-- info: Does this dataset address the needs of communities that are traditionally underserved in language technology, and particularly language generation technology? Communities may be underserved for exemple because their language, language variety, or social or geographical context is underepresented in NLP and NLG resources (datasets and models). -->
<!-- scope: telescope -->
no
### Discussion of Biases
#### Any Documented Social Biases?
<!-- info: Are there documented social biases in the dataset? Biases in this context are variations in the ways members of different social categories are represented that can have harmful downstream consequences for members of the more disadvantaged group. -->
<!-- scope: telescope -->
unsure
#### Are the Language Producers Representative of the Language?
<!-- info: Does the distribution of language producers in the dataset accurately represent the full distribution of speakers of the language world-wide? If not, how does it differ? -->
<!-- scope: periscope -->
NA
## Considerations for Using the Data
### PII Risks and Liability
#### Potential PII Risk
<!-- info: Considering your answers to the PII part of the Data Curation Section, describe any potential privacy to the data subjects and creators risks when using the dataset. -->
<!-- scope: microscope -->
NA
### Licenses
#### Copyright Restrictions on the Dataset
<!-- info: Based on your answers in the Intended Use part of the Data Overview Section, which of the following best describe the copyright and licensing status of the dataset? -->
<!-- scope: periscope -->
`open license - commercial use allowed`
#### Copyright Restrictions on the Language Data
<!-- info: Based on your answers in the Language part of the Data Curation Section, which of the following best describe the copyright and licensing status of the underlying language data? -->
<!-- scope: periscope -->
`public domain`
### Known Technical Limitations
#### Technical Limitations
<!-- info: Describe any known technical limitations, such as spurrious correlations, train/test overlap, annotation biases, or mis-annotations, and cite the works that first identified these limitations when possible. -->
<!-- scope: microscope -->
NA
#### Unsuited Applications
<!-- info: When using a model trained on this dataset in a setting where users or the public may interact with its predictions, what are some pitfalls to look out for? In particular, describe some applications of the general task featured in this dataset that its curation or properties make it less suitable for. -->
<!-- scope: microscope -->
NA
#### Discouraged Use Cases
<!-- info: What are some discouraged use cases of a model trained to maximize the proposed metrics on this dataset? In particular, think about settings where decisions made by a model that performs reasonably well on the metric my still have strong negative consequences for user or members of the public. -->
<!-- scope: microscope -->
NA
|
GEM/cochrane-simplification | ---
annotations_creators:
- none
language_creators:
- unknown
language:
- en
license:
- cc-by-4.0
multilinguality:
- unknown
size_categories:
- unknown
source_datasets:
- original
task_categories:
- text2text-generation
task_ids:
- text-simplification
pretty_name: cochrane-simplification
---
# Dataset Card for GEM/cochrane-simplification
## Dataset Description
- **Homepage:** https://github.com/AshOlogn/Paragraph-level-Simplification-of-Medical-Texts
- **Repository:** https://github.com/AshOlogn/Paragraph-level-Simplification-of-Medical-Texts
- **Paper:** https://aclanthology.org/2021.naacl-main.395/
- **Leaderboard:** N/A
- **Point of Contact:** Ashwin Devaraj
### Link to Main Data Card
You can find the main data card on the [GEM Website](https://gem-benchmark.com/data_cards/cochrane-simplification).
### Dataset Summary
Cochrane is an English dataset for paragraph-level simplification of medical texts. Cochrane is a database of systematic reviews of clinical questions, many of which have summaries in plain English targeting readers without a university education. The dataset comprises about 4,500 of such pairs.
You can load the dataset via:
```
import datasets
data = datasets.load_dataset('GEM/cochrane-simplification')
```
The data loader can be found [here](https://huggingface.co/datasets/GEM/cochrane-simplification).
#### website
[Link](https://github.com/AshOlogn/Paragraph-level-Simplification-of-Medical-Texts)
#### paper
[Link](https://aclanthology.org/2021.naacl-main.395/)
#### authors
Ashwin Devaraj (The University of Texas at Austin), Iain J. Marshall (King's College London), Byron C. Wallace (Northeastern University), Junyi Jessy Li (The University of Texas at Austin)
## Dataset Overview
### Where to find the Data and its Documentation
#### Webpage
<!-- info: What is the webpage for the dataset (if it exists)? -->
<!-- scope: telescope -->
[Link](https://github.com/AshOlogn/Paragraph-level-Simplification-of-Medical-Texts)
#### Download
<!-- info: What is the link to where the original dataset is hosted? -->
<!-- scope: telescope -->
[Link](https://github.com/AshOlogn/Paragraph-level-Simplification-of-Medical-Texts)
#### Paper
<!-- info: What is the link to the paper describing the dataset (open access preferred)? -->
<!-- scope: telescope -->
[Link](https://aclanthology.org/2021.naacl-main.395/)
#### BibTex
<!-- info: Provide the BibTex-formatted reference for the dataset. Please use the correct published version (ACL anthology, etc.) instead of google scholar created Bibtex. -->
<!-- scope: microscope -->
```
@inproceedings{devaraj-etal-2021-paragraph,
title = "Paragraph-level Simplification of Medical Texts",
author = "Devaraj, Ashwin and
Marshall, Iain and
Wallace, Byron and
Li, Junyi Jessy",
booktitle = "Proceedings of the 2021 Conference of the North American Chapter of the Association for Computational Linguistics: Human Language Technologies",
month = jun,
year = "2021",
address = "Online",
publisher = "Association for Computational Linguistics",
url = "https://aclanthology.org/2021.naacl-main.395",
doi = "10.18653/v1/2021.naacl-main.395",
pages = "4972--4984",
}
```
#### Contact Name
<!-- quick -->
<!-- info: If known, provide the name of at least one person the reader can contact for questions about the dataset. -->
<!-- scope: periscope -->
Ashwin Devaraj
#### Contact Email
<!-- info: If known, provide the email of at least one person the reader can contact for questions about the dataset. -->
<!-- scope: periscope -->
ashwin.devaraj@utexas.edu
#### Has a Leaderboard?
<!-- info: Does the dataset have an active leaderboard? -->
<!-- scope: telescope -->
no
### Languages and Intended Use
#### Multilingual?
<!-- quick -->
<!-- info: Is the dataset multilingual? -->
<!-- scope: telescope -->
no
#### Covered Languages
<!-- quick -->
<!-- info: What languages/dialects are covered in the dataset? -->
<!-- scope: telescope -->
`English`
#### License
<!-- quick -->
<!-- info: What is the license of the dataset? -->
<!-- scope: telescope -->
cc-by-4.0: Creative Commons Attribution 4.0 International
#### Intended Use
<!-- info: What is the intended use of the dataset? -->
<!-- scope: microscope -->
The intended use of this dataset is to train models that simplify medical text at the paragraph level so that it may be more accessible to the lay reader.
#### Primary Task
<!-- info: What primary task does the dataset support? -->
<!-- scope: telescope -->
Simplification
#### Communicative Goal
<!-- quick -->
<!-- info: Provide a short description of the communicative goal of a model trained for this task on this dataset. -->
<!-- scope: periscope -->
A model trained on this dataset can be used to simplify medical texts to make them more accessible to readers without medical expertise.
### Credit
#### Curation Organization Type(s)
<!-- info: In what kind of organization did the dataset curation happen? -->
<!-- scope: telescope -->
`academic`
#### Curation Organization(s)
<!-- info: Name the organization(s). -->
<!-- scope: periscope -->
The University of Texas at Austin, King's College London, Northeastern University
#### Dataset Creators
<!-- info: Who created the original dataset? List the people involved in collecting the dataset and their affiliation(s). -->
<!-- scope: microscope -->
Ashwin Devaraj (The University of Texas at Austin), Iain J. Marshall (King's College London), Byron C. Wallace (Northeastern University), Junyi Jessy Li (The University of Texas at Austin)
#### Funding
<!-- info: Who funded the data creation? -->
<!-- scope: microscope -->
National Institutes of Health (NIH) grant R01-LM012086, National Science Foundation (NSF) grant IIS-1850153, Texas Advanced Computing Center (TACC) computational resources
#### Who added the Dataset to GEM?
<!-- info: Who contributed to the data card and adding the dataset to GEM? List the people+affiliations involved in creating this data card and who helped integrate this dataset into GEM. -->
<!-- scope: microscope -->
Ashwin Devaraj (The University of Texas at Austin)
### Dataset Structure
#### Data Fields
<!-- info: List and describe the fields present in the dataset. -->
<!-- scope: telescope -->
- `gem_id`: string, a unique identifier for the example
- `doi`: string, DOI identifier for the Cochrane review from which the example was generated
- `source`: string, an excerpt from an abstract of a Cochrane review
- `target`: string, an excerpt from the plain-language summary of a Cochrane review that roughly aligns with the source text
#### Example Instance
<!-- info: Provide a JSON formatted example of a typical instance in the dataset. -->
<!-- scope: periscope -->
```
{
"gem_id": "gem-cochrane-simplification-train-766",
"doi": "10.1002/14651858.CD002173.pub2",
"source": "Of 3500 titles retrieved from the literature, 24 papers reporting on 23 studies could be included in the review. The studies were published between 1970 and 1997 and together included 1026 participants. Most were cross-over studies. Few studies provided sufficient information to judge the concealment of allocation. Four studies provided results for the percentage of symptom-free days. Pooling the results did not reveal a statistically significant difference between sodium cromoglycate and placebo. For the other pooled outcomes, most of the symptom-related outcomes and bronchodilator use showed statistically significant results, but treatment effects were small. Considering the confidence intervals of the outcome measures, a clinically relevant effect of sodium cromoglycate cannot be excluded. The funnel plot showed an under-representation of small studies with negative results, suggesting publication bias. There is insufficient evidence to be sure about the efficacy of sodium cromoglycate over placebo. Publication bias is likely to have overestimated the beneficial effects of sodium cromoglycate as maintenance therapy in childhood asthma.",
"target": "In this review we aimed to determine whether there is evidence for the effectiveness of inhaled sodium cromoglycate as maintenance treatment in children with chronic asthma. Most of the studies were carried out in small groups of patients. Furthermore, we suspect that not all studies undertaken have been published. The results show that there is insufficient evidence to be sure about the beneficial effect of sodium cromoglycate compared to placebo. However, for several outcome measures the results favoured sodium cromoglycate."
}
```
#### Data Splits
<!-- info: Describe and name the splits in the dataset if there are more than one. -->
<!-- scope: periscope -->
- `train`: 3568 examples
- `validation`: 411 examples
- `test`: 480 examples
## Dataset in GEM
### Rationale for Inclusion in GEM
#### Why is the Dataset in GEM?
<!-- info: What does this dataset contribute toward better generation evaluation and why is it part of GEM? -->
<!-- scope: microscope -->
This dataset is the first paragraph-level simplification dataset published (as prior work had primarily focused on simplifying individual sentences). Furthermore, this dataset is in the medical domain, which is an especially useful domain for text simplification.
#### Similar Datasets
<!-- info: Do other datasets for the high level task exist? -->
<!-- scope: telescope -->
no
#### Ability that the Dataset measures
<!-- info: What aspect of model ability can be measured with this dataset? -->
<!-- scope: periscope -->
This dataset measures the ability for a model to simplify paragraphs of medical text through the omission non-salient information and simplification of medical jargon.
### GEM-Specific Curation
#### Modificatied for GEM?
<!-- info: Has the GEM version of the dataset been modified in any way (data, processing, splits) from the original curated data? -->
<!-- scope: telescope -->
no
#### Additional Splits?
<!-- info: Does GEM provide additional splits to the dataset? -->
<!-- scope: telescope -->
no
### Getting Started with the Task
## Previous Results
### Previous Results
#### Measured Model Abilities
<!-- info: What aspect of model ability can be measured with this dataset? -->
<!-- scope: telescope -->
This dataset measures the ability for a model to simplify paragraphs of medical text through the omission non-salient information and simplification of medical jargon.
#### Metrics
<!-- info: What metrics are typically used for this task? -->
<!-- scope: periscope -->
`Other: Other Metrics`, `BLEU`
#### Other Metrics
<!-- info: Definitions of other metrics -->
<!-- scope: periscope -->
SARI measures the quality of text simplification
#### Previous results available?
<!-- info: Are previous results available? -->
<!-- scope: telescope -->
yes
#### Relevant Previous Results
<!-- info: What are the most relevant previous results for this task/dataset? -->
<!-- scope: microscope -->
The paper which introduced this dataset trained BART models (pretrained on XSum) with unlikelihood training to produce simplification models achieving maximum SARI and BLEU scores of 40 and 43 respectively.
## Dataset Curation
### Original Curation
#### Sourced from Different Sources
<!-- info: Is the dataset aggregated from different data sources? -->
<!-- scope: telescope -->
no
### Language Data
#### Data Validation
<!-- info: Was the text validated by a different worker or a data curator? -->
<!-- scope: telescope -->
not validated
#### Was Data Filtered?
<!-- info: Were text instances selected or filtered? -->
<!-- scope: telescope -->
not filtered
### Structured Annotations
#### Additional Annotations?
<!-- quick -->
<!-- info: Does the dataset have additional annotations for each instance? -->
<!-- scope: telescope -->
none
#### Annotation Service?
<!-- info: Was an annotation service used? -->
<!-- scope: telescope -->
no
### Consent
#### Any Consent Policy?
<!-- info: Was there a consent policy involved when gathering the data? -->
<!-- scope: telescope -->
no
### Private Identifying Information (PII)
#### Contains PII?
<!-- quick -->
<!-- info: Does the source language data likely contain Personal Identifying Information about the data creators or subjects? -->
<!-- scope: telescope -->
yes/very likely
#### Any PII Identification?
<!-- info: Did the curators use any automatic/manual method to identify PII in the dataset? -->
<!-- scope: periscope -->
no identification
### Maintenance
#### Any Maintenance Plan?
<!-- info: Does the original dataset have a maintenance plan? -->
<!-- scope: telescope -->
no
## Broader Social Context
### Previous Work on the Social Impact of the Dataset
#### Usage of Models based on the Data
<!-- info: Are you aware of cases where models trained on the task featured in this dataset ore related tasks have been used in automated systems? -->
<!-- scope: telescope -->
no
### Impact on Under-Served Communities
#### Addresses needs of underserved Communities?
<!-- info: Does this dataset address the needs of communities that are traditionally underserved in language technology, and particularly language generation technology? Communities may be underserved for exemple because their language, language variety, or social or geographical context is underepresented in NLP and NLG resources (datasets and models). -->
<!-- scope: telescope -->
yes
#### Details on how Dataset Addresses the Needs
<!-- info: Describe how this dataset addresses the needs of underserved communities. -->
<!-- scope: microscope -->
This dataset can be used to simplify medical texts that may otherwise be inaccessible to those without medical training.
### Discussion of Biases
#### Any Documented Social Biases?
<!-- info: Are there documented social biases in the dataset? Biases in this context are variations in the ways members of different social categories are represented that can have harmful downstream consequences for members of the more disadvantaged group. -->
<!-- scope: telescope -->
unsure
#### Are the Language Producers Representative of the Language?
<!-- info: Does the distribution of language producers in the dataset accurately represent the full distribution of speakers of the language world-wide? If not, how does it differ? -->
<!-- scope: periscope -->
The dataset was generated from abstracts and plain-language summaries of medical literature reviews that were written by medical professionals and thus does was not generated by people representative of the entire English-speaking population.
## Considerations for Using the Data
### PII Risks and Liability
### Licenses
### Known Technical Limitations
#### Technical Limitations
<!-- info: Describe any known technical limitations, such as spurrious correlations, train/test overlap, annotation biases, or mis-annotations, and cite the works that first identified these limitations when possible. -->
<!-- scope: microscope -->
The main limitation of this dataset is that the information alignment between the abstract and plain-language summary is often rough, so the plain-language summary may contain information that isn't found in the abstract. Furthermore, the plain-language targets often contain formulaic statements like "this evidence is current to [month][year]" not found in the abstracts. Another limitation is that some plain-language summaries do not simplify the technical abstracts very much and still contain medical jargon.
#### Unsuited Applications
<!-- info: When using a model trained on this dataset in a setting where users or the public may interact with its predictions, what are some pitfalls to look out for? In particular, describe some applications of the general task featured in this dataset that its curation or properties make it less suitable for. -->
<!-- scope: microscope -->
The main pitfall to look out for is errors in factuality. Simplification work so far has not placed a strong emphasis on the logical fidelity of model generations with the input text, and the paper introducing this dataset does not explore modeling techniques to combat this. These kinds of errors are especially pernicious in the medical domain, and the models introduced in the paper do occasionally alter entities like disease and medication names.
|
GEM/common_gen | ---
annotations_creators:
- none
language_creators:
- unknown
language:
- en
license:
- mit
multilinguality:
- unknown
size_categories:
- unknown
source_datasets:
- original
task_categories:
- other
task_ids: []
pretty_name: common_gen
tags:
- reasoning
---
# Dataset Card for GEM/common_gen
## Dataset Description
- **Homepage:** https://inklab.usc.edu/CommonGen/
- **Repository:** https://github.com/INK-USC/CommonGen
- **Paper:** https://aclanthology.org/2020.findings-emnlp.165
- **Leaderboard:** https://inklab.usc.edu/CommonGen/leaderboard.html
- **Point of Contact:** Bill Yuchen Lin
### Link to Main Data Card
You can find the main data card on the [GEM Website](https://gem-benchmark.com/data_cards/common_gen).
### Dataset Summary
CommonGen is an English text generation task to explicitly test machines for the ability of generative commonsense reasoning. Given a set of common concepts, the task is to generate a coherent sentence describing an everyday scenario using these concepts. CommonGen is challenging because it inherently requires 1) relational reasoning using background commonsense knowledge, and 2) compositional generalization ability to work on unseen concept combinations. The dataset, constructed through a combination of crowd-sourcing from AMT and existing caption corpora, consists of 30k concept-sets and 50k sentences in total. Note that the CommonGen test set is private and requires submission to the external leaderboard.
You can load the dataset via:
```
import datasets
data = datasets.load_dataset('GEM/common_gen')
```
The data loader can be found [here](https://huggingface.co/datasets/GEM/common_gen).
#### website
[link](https://inklab.usc.edu/CommonGen/)
#### paper
[Link](https://aclanthology.org/2020.findings-emnlp.165)
#### authors
Bill Yuchen Lin (USC), Wangchunshu Zhou (USC), Ming Shen (USC), Pei Zhou (USC), Chandra Bhagavatula (AllenAI), Yejin Choi (AllenAI + UW), Xiang Ren (USC)
## Dataset Overview
### Where to find the Data and its Documentation
#### Webpage
<!-- info: What is the webpage for the dataset (if it exists)? -->
<!-- scope: telescope -->
[link](https://inklab.usc.edu/CommonGen/)
#### Download
<!-- info: What is the link to where the original dataset is hosted? -->
<!-- scope: telescope -->
[Link](https://github.com/INK-USC/CommonGen)
#### Paper
<!-- info: What is the link to the paper describing the dataset (open access preferred)? -->
<!-- scope: telescope -->
[Link](https://aclanthology.org/2020.findings-emnlp.165)
#### BibTex
<!-- info: Provide the BibTex-formatted reference for the dataset. Please use the correct published version (ACL anthology, etc.) instead of google scholar created Bibtex. -->
<!-- scope: microscope -->
```
@inproceedings{lin-etal-2020-commongen,
title = "{C}ommon{G}en: A Constrained Text Generation Challenge for Generative Commonsense Reasoning",
author = "Lin, Bill Yuchen and
Zhou, Wangchunshu and
Shen, Ming and
Zhou, Pei and
Bhagavatula, Chandra and
Choi, Yejin and
Ren, Xiang",
booktitle = "Findings of the Association for Computational Linguistics: EMNLP 2020",
month = nov,
year = "2020",
address = "Online",
publisher = "Association for Computational Linguistics",
url = "https://www.aclweb.org/anthology/2020.findings-emnlp.165",
pages = "1823--1840",
}
```
#### Contact Name
<!-- quick -->
<!-- info: If known, provide the name of at least one person the reader can contact for questions about the dataset. -->
<!-- scope: periscope -->
Bill Yuchen Lin
#### Contact Email
<!-- info: If known, provide the email of at least one person the reader can contact for questions about the dataset. -->
<!-- scope: periscope -->
yuchen.lin@usc.edu
#### Has a Leaderboard?
<!-- info: Does the dataset have an active leaderboard? -->
<!-- scope: telescope -->
yes
#### Leaderboard Link
<!-- info: Provide a link to the leaderboard. -->
<!-- scope: periscope -->
[Link](https://inklab.usc.edu/CommonGen/leaderboard.html)
#### Leaderboard Details
<!-- info: Briefly describe how the leaderboard evaluates models. -->
<!-- scope: microscope -->
The model outputs are evaluated against the crowdsourced references, and ranked by SPICE score. The leaderboard also reports BLEU-4 and CIDEr scores.
### Languages and Intended Use
#### Multilingual?
<!-- quick -->
<!-- info: Is the dataset multilingual? -->
<!-- scope: telescope -->
no
#### Covered Dialects
<!-- info: What dialects are covered? Are there multiple dialects per language? -->
<!-- scope: periscope -->
No information is provided on regional restrictions and we thus assume that the covered dialects are those spoken by raters on Mechanical Turk.
#### Covered Languages
<!-- quick -->
<!-- info: What languages/dialects are covered in the dataset? -->
<!-- scope: telescope -->
`English`
#### Whose Language?
<!-- info: Whose language is in the dataset? -->
<!-- scope: periscope -->
The concepts were extracted from multiple English image captioning datasets and the data was collected via Amazon Mechanical Turk. No information on regional restrictions is provided.
#### License
<!-- quick -->
<!-- info: What is the license of the dataset? -->
<!-- scope: telescope -->
mit: MIT License
#### Intended Use
<!-- info: What is the intended use of the dataset? -->
<!-- scope: microscope -->
CommonGen is a constrained text generation task, associated with a benchmark dataset, to explicitly test machines for the ability of generative commonsense reasoning.
#### Primary Task
<!-- info: What primary task does the dataset support? -->
<!-- scope: telescope -->
Reasoning
#### Communicative Goal
<!-- quick -->
<!-- info: Provide a short description of the communicative goal of a model trained for this task on this dataset. -->
<!-- scope: periscope -->
The speaker is required to produce a *coherent* sentence which mentions all of the source concepts, and which describes a *likely* situation that could be captured in a picture or video.
### Credit
#### Curation Organization Type(s)
<!-- info: In what kind of organization did the dataset curation happen? -->
<!-- scope: telescope -->
`academic`, `independent`
#### Curation Organization(s)
<!-- info: Name the organization(s). -->
<!-- scope: periscope -->
The dataset was curated by a joint team of researchers from the University of Southern California and Allen Institute for Artificial Intelligence.
#### Dataset Creators
<!-- info: Who created the original dataset? List the people involved in collecting the dataset and their affiliation(s). -->
<!-- scope: microscope -->
Bill Yuchen Lin (USC), Wangchunshu Zhou (USC), Ming Shen (USC), Pei Zhou (USC), Chandra Bhagavatula (AllenAI), Yejin Choi (AllenAI + UW), Xiang Ren (USC)
#### Funding
<!-- info: Who funded the data creation? -->
<!-- scope: microscope -->
The research is based upon work supported in part by the Office of the Director of National Intelligence (ODNI), Intelligence Advanced Research Projects Activity (IARPA), the DARPA MCS program, and NSF SMA 18-29268.
#### Who added the Dataset to GEM?
<!-- info: Who contributed to the data card and adding the dataset to GEM? List the people+affiliations involved in creating this data card and who helped integrate this dataset into GEM. -->
<!-- scope: microscope -->
Yacine Jernite created the initial data card. It was later extended by Simon Mille. Sebastian Gehrmann migrated it to the GEMv2 format.
### Dataset Structure
#### Data Fields
<!-- info: List and describe the fields present in the dataset. -->
<!-- scope: telescope -->
A data instance has the following fields:
- `concepts`: a `list` of `string` values denoting the concept the system should write about. Has 3 to 5 items, constitutes the `input` of the task.
- `target`: a sentence `string` mentioning all of the above mentioned `concepts`. Constitutes the desired `output` of the task.
#### Example Instance
<!-- info: Provide a JSON formatted example of a typical instance in the dataset. -->
<!-- scope: periscope -->
```
[
{
"concepts": ['ski', 'mountain', 'skier'],
"target": 'Skier skis down the mountain',
},
{
"concepts": ['ski', 'mountain', 'skier'],
"target": 'Three skiers are skiing on a snowy mountain.',
},
]
```
#### Data Splits
<!-- info: Describe and name the splits in the dataset if there are more than one. -->
<!-- scope: periscope -->
Each example in the dataset consists of a set of 3 to 5 concepts denoted by a single noun, verb, or adjective (the input), and a sentence using these concepts (the output). The dataset provides several such sentences for each such concept.
| | Train | Dev | Test |
|---------------------------|--------|-------|-------|
| **Total concept-sets** | 32,651 | 993 | 1,497 |
| **Total sentences** | 67,389 | 4,018 | 6,042 |
|**Average sentence length**| 10.54 | 11.55 | 13.34 |
#### Splitting Criteria
<!-- info: Describe any criteria for splitting the data, if used. If there are differences between the splits (e.g., if the training annotations are machine-generated and the dev and test ones are created by humans, or if different numbers of annotators contributed to each example), describe them here. -->
<!-- scope: microscope -->
The dev and test set were created by sampling sets of concepts of size 4 or 5 (and as many of size 3 for the dev set) present in the source captioning datasets and having crowd-workers write reference sentences using these concepts.
Conversely, the training set has more concept sets of size 3 than of size 4 and 5, and uses the original captions from the source datasets as references.
The authors also ensured that the training, dev and test set have different combinations of unique concepts to ensure compositionality (details in [Table 1](https://arxiv.org/pdf/1911.03705v3.pdf)).
## Dataset in GEM
### Rationale for Inclusion in GEM
#### Why is the Dataset in GEM?
<!-- info: What does this dataset contribute toward better generation evaluation and why is it part of GEM? -->
<!-- scope: microscope -->
CommonGen is a medium sized corpus with a unique reasoning challenge and interesting evaluation possibilities.
#### Similar Datasets
<!-- info: Do other datasets for the high level task exist? -->
<!-- scope: telescope -->
no
#### Ability that the Dataset measures
<!-- info: What aspect of model ability can be measured with this dataset? -->
<!-- scope: periscope -->
Commonsense reasoning
### GEM-Specific Curation
#### Modificatied for GEM?
<!-- info: Has the GEM version of the dataset been modified in any way (data, processing, splits) from the original curated data? -->
<!-- scope: telescope -->
yes
#### GEM Modifications
<!-- info: What changes have been made to he original dataset? -->
<!-- scope: periscope -->
`other`
#### Modification Details
<!-- info: For each of these changes, described them in more details and provided the intended purpose of the modification -->
<!-- scope: microscope -->
4 challenge sets for CommenGen were added to the GEM evaluation suite.
#### Additional Splits?
<!-- info: Does GEM provide additional splits to the dataset? -->
<!-- scope: telescope -->
yes
#### Split Information
<!-- info: Describe how the new splits were created -->
<!-- scope: periscope -->
1. Data Shift
We created subsets of the training and development sets of ~500 randomly selected inputs each.
2. Transformations
We applied input scrambling on a subset of 500 randomly selected test instances; the order of the concepts was randomly reassigned.
3. Subpopulations
We created a subpopulation based on input length, taking into account the number of concepts the input test structures. By comparing inputs of different lengths, we can see to what extent systems are able to handle different input sizes
| Concept number | Frequency English |
|----------------|-------------------|
| 4 | 747 |
| 5 | 750 |
#### Split Motivation
<!-- info: What aspects of the model's generation capacities were the splits created to test? -->
<!-- scope: periscope -->
Generalization and Robustness
### Getting Started with the Task
#### Pointers to Resources
<!-- info: Getting started with in-depth research on the task. Add relevant pointers to resources that researchers can consult when they want to get started digging deeper into the task. -->
<!-- scope: microscope -->
- Two variants of [BART](https://arxiv.org/abs/1910.13461), [Knowledge Graph augemnted-BART](https://arxiv.org/abs/2009.12677) and [Enhanced Knowledge Injection Model for Commonsense Generation](https://arxiv.org/abs/2012.00366), hold the top two spots on the leaderboard, followed by a fine-tuned [T5 model](https://arxiv.org/abs/1910.10683).
- The following script shows how to download and load the data, fine-tune, and evaluate a model using the ROUGE, BLEU, and METEOR metrics: [GEM sample script](https://github.com/GEM-benchmark/GEM-baseline-models/blob/main/examples/GEM-common_gen.ipynb).
## Previous Results
### Previous Results
#### Measured Model Abilities
<!-- info: What aspect of model ability can be measured with this dataset? -->
<!-- scope: telescope -->
Commonsense Reasoning
#### Metrics
<!-- info: What metrics are typically used for this task? -->
<!-- scope: periscope -->
`Other: Other Metrics`, `BLEU`, `ROUGE`, `METEOR`
#### Other Metrics
<!-- info: Definitions of other metrics -->
<!-- scope: periscope -->
- SPICE: An evaluation metric for image captioning that is defined over scene graphs
- CIDEr: An n-gram overlap metric based on cosine similarity between the TF-IDF weighted ngram counts
#### Proposed Evaluation
<!-- info: List and describe the purpose of the metrics and evaluation methodology (including human evaluation) that the dataset creators used when introducing this task. -->
<!-- scope: microscope -->
The main metrics are captioning metrics since the original concept lists were extracted from captioning datasets. A human subject study with five graduate students was conducted and they were asked to rank the "commonsense plausibility" of two models at a time.
#### Previous results available?
<!-- info: Are previous results available? -->
<!-- scope: telescope -->
yes
#### Other Evaluation Approaches
<!-- info: What evaluation approaches have others used? -->
<!-- scope: periscope -->
The currently best performing model KFCNet (https://aclanthology.org/2021.findings-emnlp.249/) uses the same automatic evaluation but does not conduct any human evaluation.
#### Relevant Previous Results
<!-- info: What are the most relevant previous results for this task/dataset? -->
<!-- scope: microscope -->
The most relevant results can be seen on the [leaderboard](https://inklab.usc.edu/CommonGen/leaderboard.html)
## Dataset Curation
### Original Curation
#### Original Curation Rationale
<!-- info: Original curation rationale -->
<!-- scope: telescope -->
The dataset creators selected sets of concepts that appeared in image and video captions (as identified by a POS tagger) to ensure that a likely real-world scenario including the set could be imagined and constructed. Section 3.1 of the [paper](https://arxiv.org/pdf/1911.03705v3.pdf) describes a sampling scheme which encourages diversity of sets while selecting common concepts.
#### Communicative Goal
<!-- info: What was the communicative goal? -->
<!-- scope: periscope -->
The speaker is required to produce a *coherent* sentence which mentions all of the source concepts, and which describes a *likely* situation that could be captured in a picture or video.
#### Sourced from Different Sources
<!-- info: Is the dataset aggregated from different data sources? -->
<!-- scope: telescope -->
yes
#### Source Details
<!-- info: List the sources (one per line) -->
<!-- scope: periscope -->
- [Flickr30k](https://www.mitpressjournals.org/doi/abs/10.1162/tacl_a_00166)
- [MSCOCO](https://link.springer.com/chapter/10.1007/978-3-319-10602-1_48)
- [Conceptual Captions](https://www.aclweb.org/anthology/P18-1238/)
- Video captioning datasets:
- [LSMDC](https://link.springer.com/article/10.1007/s11263-016-0987-1)
- [ActivityNet](https://openaccess.thecvf.com/content_iccv_2017/html/Krishna_Dense-Captioning_Events_in_ICCV_2017_paper.html)
- [VaTeX](https://openaccess.thecvf.com/content_ICCV_2019/html/Wang_VaTeX_A_Large-Scale_High-Quality_Multilingual_Dataset_for_Video-and-Language_Research_ICCV_2019_paper.html)
### Language Data
#### How was Language Data Obtained?
<!-- info: How was the language data obtained? -->
<!-- scope: telescope -->
`Crowdsourced`
#### Where was it crowdsourced?
<!-- info: If crowdsourced, where from? -->
<!-- scope: periscope -->
`Amazon Mechanical Turk`
#### Language Producers
<!-- info: What further information do we have on the language producers? -->
<!-- scope: microscope -->
The training data consists of concept sets and captions for the source datasets. The concept sets are the sets of labels of the images or videos, selected with a heuristic to maximize diversity while ensuring that they represent likely scenarios.
The dev and test set sentences were created by Amazon Mechanical Turk crowd workers. The workers were shown an example generation and a set of 4 or 5 concept names along with their part-of-speech and asked to write:
1. One sentence mentioning all of the concepts
2. A rationale explaining how the sentence connects the concept
A screenshot of the interface is provided in Figure 7 of the [Appendix](https://arxiv.org/pdf/1911.03705v3.pdf).
#### Topics Covered
<!-- info: Does the language in the dataset focus on specific topics? How would you describe them? -->
<!-- scope: periscope -->
Information was not provided.
#### Data Validation
<!-- info: Was the text validated by a different worker or a data curator? -->
<!-- scope: telescope -->
validated by data curator
#### Was Data Filtered?
<!-- info: Were text instances selected or filtered? -->
<!-- scope: telescope -->
algorithmically
#### Filter Criteria
<!-- info: What were the selection criteria? -->
<!-- scope: microscope -->
During the data collection, workers who provided rationales that were too short, failed to have good coverage of the input in their sentences, or workers whose output had a high perplexity under a GPT-2 model were disqualified from the pool and replaced with newcomers.
### Structured Annotations
#### Additional Annotations?
<!-- quick -->
<!-- info: Does the dataset have additional annotations for each instance? -->
<!-- scope: telescope -->
none
#### Annotation Service?
<!-- info: Was an annotation service used? -->
<!-- scope: telescope -->
no
### Consent
#### Any Consent Policy?
<!-- info: Was there a consent policy involved when gathering the data? -->
<!-- scope: telescope -->
no
#### Justification for Using the Data
<!-- info: If not, what is the justification for reusing the data? -->
<!-- scope: microscope -->
The data was sourced from Mechanical Turk which means that raters were aware that their annotations may be publicly released for research purposes.
### Private Identifying Information (PII)
#### Contains PII?
<!-- quick -->
<!-- info: Does the source language data likely contain Personal Identifying Information about the data creators or subjects? -->
<!-- scope: telescope -->
no PII
#### Justification for no PII
<!-- info: Provide a justification for selecting `no PII` above. -->
<!-- scope: periscope -->
The concepts are restricted to verbs, adjectives, and common nouns, and no personal information is given in the captions.
### Maintenance
#### Any Maintenance Plan?
<!-- info: Does the original dataset have a maintenance plan? -->
<!-- scope: telescope -->
no
## Broader Social Context
### Previous Work on the Social Impact of the Dataset
#### Usage of Models based on the Data
<!-- info: Are you aware of cases where models trained on the task featured in this dataset ore related tasks have been used in automated systems? -->
<!-- scope: telescope -->
no
### Impact on Under-Served Communities
#### Addresses needs of underserved Communities?
<!-- info: Does this dataset address the needs of communities that are traditionally underserved in language technology, and particularly language generation technology? Communities may be underserved for exemple because their language, language variety, or social or geographical context is underepresented in NLP and NLG resources (datasets and models). -->
<!-- scope: telescope -->
no
### Discussion of Biases
#### Any Documented Social Biases?
<!-- info: Are there documented social biases in the dataset? Biases in this context are variations in the ways members of different social categories are represented that can have harmful downstream consequences for members of the more disadvantaged group. -->
<!-- scope: telescope -->
no
#### Are the Language Producers Representative of the Language?
<!-- info: Does the distribution of language producers in the dataset accurately represent the full distribution of speakers of the language world-wide? If not, how does it differ? -->
<!-- scope: periscope -->
The dataset is created using data from image captioning systems and might inherit some of the social biases represented therein (see e.g. [Tang et al. 2020](https://arxiv.org/abs/2006.08315)).
Another related concern is the exposure bias introduced by the initial selection of pictures and video, which are likely to over-represent situations that are common in the US at the expense of other parts of the world (Flickr, for example, is a US-based company founded in Canada). For more discussion of the potential impacts of exposure bias, see e.g. [The Social Impact of Natural Language Processing](https://www.aclweb.org/anthology/P16-2096.pdf).
## Considerations for Using the Data
### PII Risks and Liability
#### Potential PII Risk
<!-- info: Considering your answers to the PII part of the Data Curation Section, describe any potential privacy to the data subjects and creators risks when using the dataset. -->
<!-- scope: microscope -->
The concepts are restricted to verbs, adjectives, and common nouns, and no personal information is given in the captions.
### Licenses
#### Copyright Restrictions on the Dataset
<!-- info: Based on your answers in the Intended Use part of the Data Overview Section, which of the following best describe the copyright and licensing status of the dataset? -->
<!-- scope: periscope -->
`open license - commercial use allowed`
#### Copyright Restrictions on the Language Data
<!-- info: Based on your answers in the Language part of the Data Curation Section, which of the following best describe the copyright and licensing status of the underlying language data? -->
<!-- scope: periscope -->
`open license - commercial use allowed`
### Known Technical Limitations
#### Technical Limitations
<!-- info: Describe any known technical limitations, such as spurrious correlations, train/test overlap, annotation biases, or mis-annotations, and cite the works that first identified these limitations when possible. -->
<!-- scope: microscope -->
The dataset is in English, a language with an abundance of existing resources.
The use of GPT-2 to validate development ant test sentences [might be cause for similar concern](https://www.aclweb.org/anthology/D19-1339.pdf), but we do note that the authors only use the model to discount very high perplexity sequences which is less likely to surface those biases.
The language in the development and test set is crowdsourced, which means that it was written by workers whose main goal was speed. This is likely to impact the quality and variety of the targets. The population of crowdsource workers is also not identically distributed as the the base population of the locations the workers come from, which may lead to different representation of situations or underlying expectations of what these situations are.
#### Unsuited Applications
<!-- info: When using a model trained on this dataset in a setting where users or the public may interact with its predictions, what are some pitfalls to look out for? In particular, describe some applications of the general task featured in this dataset that its curation or properties make it less suitable for. -->
<!-- scope: microscope -->
Due to the overrepresentation of US-situations, the system may not work for users across the world. Moreover, only limited information on the dataset quality are provided and the system may fail as a result of unknown issues.
#### Discouraged Use Cases
<!-- info: What are some discouraged use cases of a model trained to maximize the proposed metrics on this dataset? In particular, think about settings where decisions made by a model that performs reasonably well on the metric my still have strong negative consequences for user or members of the public. -->
<!-- scope: microscope -->
Any system needs to be evaluated on a broader set of unseen concepts then provided in the dataset. Since the references for the test set are private, it is not known how well findings generalize beyond the collection methodology.
|
GEM/conversational_weather | ---
annotations_creators:
- none
language_creators:
- unknown
language:
- en
license:
- cc-by-nc-4.0
multilinguality:
- unknown
size_categories:
- unknown
source_datasets:
- original
task_categories:
- table-to-text
task_ids: []
pretty_name: conversational_weather
tags:
- data-to-text
---
# Dataset Card for GEM/conversational_weather
## Dataset Description
- **Homepage:** [Needs More Information]
- **Repository:** https://github.com/facebookresearch/TreeNLG
- **Paper:** https://aclanthology.org/P19-1080
- **Leaderboard:** N/A
- **Point of Contact:** Kartikeya Upasani
### Link to Main Data Card
You can find the main data card on the [GEM Website](https://gem-benchmark.com/data_cards/conversational_weather).
### Dataset Summary
The purpose of this dataset is to assess how well a model can learn a template-like structure in a very low data setting. The task here is to produce a response to a weather-related query. The reply is further specified through the data attributes and discourse structure in the input. The output contains both the lexicalized text and discourse markers for attributes (e.g., `_ARG_TEMP_ 34`).
You can load the dataset via:
```
import datasets
data = datasets.load_dataset('GEM/conversational_weather')
```
The data loader can be found [here](https://huggingface.co/datasets/GEM/conversational_weather).
#### paper
[ACL Anthology](https://aclanthology.org/P19-1080)
#### authors
Anusha Balakrishnan, Jinfeng Rao, Kartikeya Upasani, Michael White, Rajen Subba (Facebook Conversational AI)
## Dataset Overview
### Where to find the Data and its Documentation
#### Download
<!-- info: What is the link to where the original dataset is hosted? -->
<!-- scope: telescope -->
[Github](https://github.com/facebookresearch/TreeNLG)
#### Paper
<!-- info: What is the link to the paper describing the dataset (open access preferred)? -->
<!-- scope: telescope -->
[ACL Anthology](https://aclanthology.org/P19-1080)
#### BibTex
<!-- info: Provide the BibTex-formatted reference for the dataset. Please use the correct published version (ACL anthology, etc.) instead of google scholar created Bibtex. -->
<!-- scope: microscope -->
```
@inproceedings{balakrishnan-etal-2019-constrained,
title = "Constrained Decoding for Neural {NLG} from Compositional Representations in Task-Oriented Dialogue",
author = "Balakrishnan, Anusha and
Rao, Jinfeng and
Upasani, Kartikeya and
White, Michael and
Subba, Rajen",
booktitle = "Proceedings of the 57th Annual Meeting of the Association for Computational Linguistics",
month = jul,
year = "2019",
address = "Florence, Italy",
publisher = "Association for Computational Linguistics",
url = "https://www.aclweb.org/anthology/P19-1080",
doi = "10.18653/v1/P19-1080",
pages = "831--844"
}
```
#### Contact Name
<!-- quick -->
<!-- info: If known, provide the name of at least one person the reader can contact for questions about the dataset. -->
<!-- scope: periscope -->
Kartikeya Upasani
#### Contact Email
<!-- info: If known, provide the email of at least one person the reader can contact for questions about the dataset. -->
<!-- scope: periscope -->
kart@fb.com
#### Has a Leaderboard?
<!-- info: Does the dataset have an active leaderboard? -->
<!-- scope: telescope -->
no
### Languages and Intended Use
#### Multilingual?
<!-- quick -->
<!-- info: Is the dataset multilingual? -->
<!-- scope: telescope -->
no
#### Covered Languages
<!-- quick -->
<!-- info: What languages/dialects are covered in the dataset? -->
<!-- scope: telescope -->
`English`
#### License
<!-- quick -->
<!-- info: What is the license of the dataset? -->
<!-- scope: telescope -->
cc-by-nc-4.0: Creative Commons Attribution Non Commercial 4.0 International
#### Intended Use
<!-- info: What is the intended use of the dataset? -->
<!-- scope: microscope -->
This dataset is intended to help develop conversational agents that exhibit human-like properties such as matching the framing of the response with the query or contrasting relevant data attributes.
#### Primary Task
<!-- info: What primary task does the dataset support? -->
<!-- scope: telescope -->
Data-to-Text
#### Communicative Goal
<!-- quick -->
<!-- info: Provide a short description of the communicative goal of a model trained for this task on this dataset. -->
<!-- scope: periscope -->
Producing a text that is a response to a weather query as per the discourse structure and data attributes specified in the input meaning representation.
### Credit
#### Curation Organization Type(s)
<!-- info: In what kind of organization did the dataset curation happen? -->
<!-- scope: telescope -->
`industry`
#### Curation Organization(s)
<!-- info: Name the organization(s). -->
<!-- scope: periscope -->
Facebook
#### Dataset Creators
<!-- info: Who created the original dataset? List the people involved in collecting the dataset and their affiliation(s). -->
<!-- scope: microscope -->
Anusha Balakrishnan, Jinfeng Rao, Kartikeya Upasani, Michael White, Rajen Subba (Facebook Conversational AI)
#### Funding
<!-- info: Who funded the data creation? -->
<!-- scope: microscope -->
Facebook
#### Who added the Dataset to GEM?
<!-- info: Who contributed to the data card and adding the dataset to GEM? List the people+affiliations involved in creating this data card and who helped integrate this dataset into GEM. -->
<!-- scope: microscope -->
Vipul Raheja (Grammarly)
### Dataset Structure
#### Data Fields
<!-- info: List and describe the fields present in the dataset. -->
<!-- scope: telescope -->
- `gem_id`: (string): GEM-formatted row id
- `id`: (string): Row id in the original data
- `user_query`: (string): Natural language weather query from humans
- `tree_str_mr`: (string): Synthetically-added user context (datetime and location) in the form of a tree-structured MR
- `response`: (string): A tree-structured annotation of the response.
#### Example Instance
<!-- info: Provide a JSON formatted example of a typical instance in the dataset. -->
<!-- scope: periscope -->
```
{'gem_id': 'weather-train-11',
'id': '1108963',
'synthetic_user_context': '[__DG_INFORM__ [__ARG_TASK__ get_forecast ] '
'[__ARG_TEMP__ 37 ] [__ARG_TEMP_UNIT__ fahrenheit ] '
'[__ARG_CLOUD_COVERAGE__ partly cloudy ] '
'[__ARG_DATE_TIME__ [__ARG_COLLOQUIAL__ currently ] '
'] [__ARG_LOCATION__ [__ARG_CITY__ Oakland ] '
'[__ARG_COUNTRY__ United States ] [__ARG_REGION__ '
'California ] ] ] [__DG_INFORM__ [__ARG_TASK__ '
'get_forecast ] [__ARG_TEMP_SUMMARY__ mid 40s ] '
'[__ARG_DATE_TIME_RANGE__ [__ARG_COLLOQUIAL__ This '
'afternoon ] ] [__ARG_LOCATION__ [__ARG_CITY__ '
'Oakland ] [__ARG_COUNTRY__ United States ] '
'[__ARG_REGION__ California ] ] ] [__DG_INFORM__ '
'[__ARG_TASK__ get_forecast ] '
'[__ARG_CLOUD_COVERAGE__ mostly sunny ] '
'[__ARG_DATE_TIME_RANGE__ [__ARG_COLLOQUIAL__ This '
'afternoon ] ] [__ARG_LOCATION__ [__ARG_CITY__ '
'Oakland ] [__ARG_COUNTRY__ United States ] '
'[__ARG_REGION__ California ] ] ]',
'tree_str_mr': "[__DG_INFORM__ It's [__ARG_DATE_TIME__ [__ARG_COLLOQUIAL__ "
'currently ] ] [__ARG_CLOUD_COVERAGE__ partly cloudy ] and '
'[__ARG_TEMP__ __ARG_TEMP__ ] [__ARG_TEMP_UNIT__ '
'__ARG_TEMP_UNIT__ ] [__ARG_LOCATION__ in [__ARG_CITY__ '
'__ARG_CITY__ ] , [__ARG_REGION__ __ARG_REGION__ ] , '
'[__ARG_COUNTRY__ __ARG_COUNTRY__ ] ] . ] [__DG_INFORM__ '
'[__ARG_DATE_TIME_RANGE__ [__ARG_COLLOQUIAL__ This afternoon ] '
"] , it'll be [__ARG_CLOUD_COVERAGE__ mostly sunny ] ] "
'[__DG_INFORM__ with temperatures in the [__ARG_TEMP_SUMMARY__ '
'mid <number> ] ]',
'user_query': 'Show weather forecast for Oakland, CA. '}
```
#### Data Splits
<!-- info: Describe and name the splits in the dataset if there are more than one. -->
<!-- scope: periscope -->
- Standard Splits: Train/Validation/Test
- Additional Split: Disc_Test (a more challenging subset of the test set that contains discourse relations)
#### Splitting Criteria
<!-- info: Describe any criteria for splitting the data, if used. If there are differences between the splits (e.g., if the training annotations are machine-generated and the dev and test ones are created by humans, or if different numbers of annotators contributed to each example), describe them here. -->
<!-- scope: microscope -->
The test set contains 3,121 examples, of which 1.1K (35%) have unique MRs that have never been seen in the training set.
####
<!-- info: What does an outlier of the dataset in terms of length/perplexity/embedding look like? -->
<!-- scope: microscope -->
```
{'gem_id': 'weather-train-13333', 'data_id': '1260610', 'user_query': 'Sundown', 'tree_str_mr': '[__DG_INFORM__ [__ARG_TASK__ get_weather_attribute ] [__ARG_SUNSET_TIME_DATE_TIME__ [__ARG_TIME__ 05:04 PM ] ] ]', 'response': '[__DG_INFORM__ The sun will go down at [__ARG_SUNSET_TIME_DATE_TIME__ [__ARG_TIME__ __ARG_TIME__ ] ] ]'}
```
## Dataset in GEM
### Rationale for Inclusion in GEM
#### Why is the Dataset in GEM?
<!-- info: What does this dataset contribute toward better generation evaluation and why is it part of GEM? -->
<!-- scope: microscope -->
The dataset was curated to develop a weather bot that exhibits human-like properties such as matching the framing of the response with the query or contrasting relevant data attributes.
The dataset offers rich tree-based meaning representations that offer fine-grained control over the response, e.g. by specifying which two attributes are to be contrasted. The natural language input queries are also provided to model the coherence of the response based on the input. The output response is annotated with the input meaning components using special bracketing tokens, which enables developing new techniques such as constrained decoding to improve quality of output responses
#### Similar Datasets
<!-- info: Do other datasets for the high level task exist? -->
<!-- scope: telescope -->
no
#### Ability that the Dataset measures
<!-- info: What aspect of model ability can be measured with this dataset? -->
<!-- scope: periscope -->
Adequately expressing CONTRAST and JUSTIFY discourse relations with appropriate grouping of arguments; adequately generalizing to many combinations of arguments.
### GEM-Specific Curation
#### Modificatied for GEM?
<!-- info: Has the GEM version of the dataset been modified in any way (data, processing, splits) from the original curated data? -->
<!-- scope: telescope -->
yes
#### GEM Modifications
<!-- info: What changes have been made to he original dataset? -->
<!-- scope: periscope -->
`data points removed`
#### Modification Details
<!-- info: For each of these changes, described them in more details and provided the intended purpose of the modification -->
<!-- scope: microscope -->
The original repo contained a challenge set disc_test.tsv, which is a subset of the test set consisting of discourse relations (CONTRAST and JUSTIFY) , but also contained JOIN relations.
This discrepancy has been rectified in the GEM version. The rectified version has been added in the `challenge_sets`
#### Additional Splits?
<!-- info: Does GEM provide additional splits to the dataset? -->
<!-- scope: telescope -->
no
### Getting Started with the Task
## Previous Results
### Previous Results
#### Measured Model Abilities
<!-- info: What aspect of model ability can be measured with this dataset? -->
<!-- scope: telescope -->
Adequately expressing CONTRAST and JUSTIFY discourse relations with appropriate grouping of arguments; adequately generalizing to many combinations of arguments.
#### Metrics
<!-- info: What metrics are typically used for this task? -->
<!-- scope: periscope -->
`BLEU`, `Other: Other Metrics`
#### Other Metrics
<!-- info: Definitions of other metrics -->
<!-- scope: periscope -->
Tree accuracy: It measures whether the tree structure in the prediction matches that of the input MR exactly (modulo repeated arguments that need only appear once).
#### Proposed Evaluation
<!-- info: List and describe the purpose of the metrics and evaluation methodology (including human evaluation) that the dataset creators used when introducing this task. -->
<!-- scope: microscope -->
Automatic metrics are evaluated on the raw model predictions (which have de-lexicalized fields):
* Tree accuracy: Measures whether the tree structure in the prediction matches that of the input MR exactly.
* BLEU-4: A word overlap metric commonly used for evaluating NLG systems.
Authors also performed human evaluation studies by asking annotators to evaluate the quality of responses produced by different models. Annotators provided binary ratings on the following dimensions:
• Grammaticality: Measures fluency of the responses.
• Correctness: Measures semantic correctness of the responses.
#### Previous results available?
<!-- info: Are previous results available? -->
<!-- scope: telescope -->
no
## Dataset Curation
### Original Curation
#### Original Curation Rationale
<!-- info: Original curation rationale -->
<!-- scope: telescope -->
The dataset was curated to develop a weather bot that exhibits human-like properties such as matching the framing of the response with the query or contrasting relevant data attributes. To achieve this, the dataset contains rich tree-structured meaning representations that are specified using several data arguments and discourse acts, the input natural language queries, and annotations for the responses.
#### Communicative Goal
<!-- info: What was the communicative goal? -->
<!-- scope: periscope -->
Producing a text that is a response to a weather query as per the discourse structure and data attributes specified in the input meaning representation.
#### Sourced from Different Sources
<!-- info: Is the dataset aggregated from different data sources? -->
<!-- scope: telescope -->
no
### Language Data
#### How was Language Data Obtained?
<!-- info: How was the language data obtained? -->
<!-- scope: telescope -->
`Crowdsourced`, `Machine-generated`
#### Where was it crowdsourced?
<!-- info: If crowdsourced, where from? -->
<!-- scope: periscope -->
`Other crowdworker platform`
#### Topics Covered
<!-- info: Does the language in the dataset focus on specific topics? How would you describe them? -->
<!-- scope: periscope -->
The dataset is focused on the weather domain: Weather was the first successful case of NLG put into production back in the 80s (Reiter & Dale, 1997). This domain offers significant complexity for NLG. Weather forecast summaries in particular can be very long, and require reasoning over several disjoint pieces of information.
#### Data Validation
<!-- info: Was the text validated by a different worker or a data curator? -->
<!-- scope: telescope -->
validated by crowdworker
#### Data Preprocessing
<!-- info: How was the text data pre-processed? (Enter N/A if the text was not pre-processed) -->
<!-- scope: microscope -->
Please refer to Appendix D of the original paper for details.
#### Was Data Filtered?
<!-- info: Were text instances selected or filtered? -->
<!-- scope: telescope -->
hybrid
#### Filter Criteria
<!-- info: What were the selection criteria? -->
<!-- scope: microscope -->
Please refer to Appendix C of the original paper for details.
### Structured Annotations
#### Additional Annotations?
<!-- quick -->
<!-- info: Does the dataset have additional annotations for each instance? -->
<!-- scope: telescope -->
none
#### Annotation Service?
<!-- info: Was an annotation service used? -->
<!-- scope: telescope -->
no
### Consent
#### Any Consent Policy?
<!-- info: Was there a consent policy involved when gathering the data? -->
<!-- scope: telescope -->
no
#### Justification for Using the Data
<!-- info: If not, what is the justification for reusing the data? -->
<!-- scope: microscope -->
Annotation was done as work for hire and contains no PII.
### Private Identifying Information (PII)
#### Contains PII?
<!-- quick -->
<!-- info: Does the source language data likely contain Personal Identifying Information about the data creators or subjects? -->
<!-- scope: telescope -->
no PII
#### Justification for no PII
<!-- info: Provide a justification for selecting `no PII` above. -->
<!-- scope: periscope -->
Data is simulated and not specific to annotator.
### Maintenance
#### Any Maintenance Plan?
<!-- info: Does the original dataset have a maintenance plan? -->
<!-- scope: telescope -->
no
## Broader Social Context
### Previous Work on the Social Impact of the Dataset
#### Usage of Models based on the Data
<!-- info: Are you aware of cases where models trained on the task featured in this dataset ore related tasks have been used in automated systems? -->
<!-- scope: telescope -->
no
### Impact on Under-Served Communities
#### Addresses needs of underserved Communities?
<!-- info: Does this dataset address the needs of communities that are traditionally underserved in language technology, and particularly language generation technology? Communities may be underserved for exemple because their language, language variety, or social or geographical context is underepresented in NLP and NLG resources (datasets and models). -->
<!-- scope: telescope -->
no
### Discussion of Biases
#### Any Documented Social Biases?
<!-- info: Are there documented social biases in the dataset? Biases in this context are variations in the ways members of different social categories are represented that can have harmful downstream consequences for members of the more disadvantaged group. -->
<!-- scope: telescope -->
unsure
#### Are the Language Producers Representative of the Language?
<!-- info: Does the distribution of language producers in the dataset accurately represent the full distribution of speakers of the language world-wide? If not, how does it differ? -->
<!-- scope: periscope -->
Grammatical evaluations performed with the data to date have used norms from informal Standard American English. These prescriptive notions of grammaticality potentially serve to perpetuate systemic power imbalances as they’re conveyed by language.
Since the data only contains informal Standard American English, its use to train a model may not be appropriate depending on the potential use case.
## Considerations for Using the Data
### PII Risks and Liability
#### Potential PII Risk
<!-- info: Considering your answers to the PII part of the Data Curation Section, describe any potential privacy to the data subjects and creators risks when using the dataset. -->
<!-- scope: microscope -->
Annotation was done as work for hire and contains no PII. Annotated data is simulated and not specific to annotator.
### Licenses
### Known Technical Limitations
#### Unsuited Applications
<!-- info: When using a model trained on this dataset in a setting where users or the public may interact with its predictions, what are some pitfalls to look out for? In particular, describe some applications of the general task featured in this dataset that its curation or properties make it less suitable for. -->
<!-- scope: microscope -->
An imperfect model used to convey actual weather data could mislead users about weather conditions?
|
GEM/cs_restaurants | ---
annotations_creators:
- none
language_creators:
- unknown
language:
- cs
license:
- cc-by-sa-4.0
multilinguality:
- unknown
size_categories:
- unknown
source_datasets:
- original
task_categories:
- conversational
task_ids: []
pretty_name: cs_restaurants
tags:
- dialog-response-generation
---
# Dataset Card for GEM/cs_restaurants
## Dataset Description
- **Homepage:** n/a
- **Repository:** https://github.com/UFAL-DSG/cs_restaurant_dataset
- **Paper:** https://aclanthology.org/W19-8670/
- **Leaderboard:** N/A
- **Point of Contact:** Ondrej Dusek
### Link to Main Data Card
You can find the main data card on the [GEM Website](https://gem-benchmark.com/data_cards/cs_restaurants).
### Dataset Summary
The Czech Restaurants dataset is a task oriented dialog dataset in which a model needs to verbalize a response that a service agent could provide which is specified through a series of dialog acts. The dataset originated as a translation of an English dataset to test the generation capabilities of an NLG system on a highly morphologically rich language like Czech.
You can load the dataset via:
```
import datasets
data = datasets.load_dataset('GEM/cs_restaurants')
```
The data loader can be found [here](https://huggingface.co/datasets/GEM/cs_restaurants).
#### website
n/a
#### paper
[Github](https://aclanthology.org/W19-8670/)
#### authors
Ondrej Dusek and Filip Jurcicek
## Dataset Overview
### Where to find the Data and its Documentation
#### Download
<!-- info: What is the link to where the original dataset is hosted? -->
<!-- scope: telescope -->
[Github](https://github.com/UFAL-DSG/cs_restaurant_dataset)
#### Paper
<!-- info: What is the link to the paper describing the dataset (open access preferred)? -->
<!-- scope: telescope -->
[Github](https://aclanthology.org/W19-8670/)
#### BibTex
<!-- info: Provide the BibTex-formatted reference for the dataset. Please use the correct published version (ACL anthology, etc.) instead of google scholar created Bibtex. -->
<!-- scope: microscope -->
```
@inproceedings{cs_restaurants,
address = {Tokyo, Japan},
title = {Neural {Generation} for {Czech}: {Data} and {Baselines}},
shorttitle = {Neural {Generation} for {Czech}},
url = {https://www.aclweb.org/anthology/W19-8670/},
urldate = {2019-10-18},
booktitle = {Proceedings of the 12th {International} {Conference} on {Natural} {Language} {Generation} ({INLG} 2019)},
author = {Dušek, Ondřej and Jurčíček, Filip},
month = oct,
year = {2019},
pages = {563--574},
}
```
#### Contact Name
<!-- quick -->
<!-- info: If known, provide the name of at least one person the reader can contact for questions about the dataset. -->
<!-- scope: periscope -->
Ondrej Dusek
#### Contact Email
<!-- info: If known, provide the email of at least one person the reader can contact for questions about the dataset. -->
<!-- scope: periscope -->
odusek@ufal.mff.cuni.cz
#### Has a Leaderboard?
<!-- info: Does the dataset have an active leaderboard? -->
<!-- scope: telescope -->
no
### Languages and Intended Use
#### Multilingual?
<!-- quick -->
<!-- info: Is the dataset multilingual? -->
<!-- scope: telescope -->
no
#### Covered Dialects
<!-- info: What dialects are covered? Are there multiple dialects per language? -->
<!-- scope: periscope -->
No breakdown of dialects is provided.
#### Covered Languages
<!-- quick -->
<!-- info: What languages/dialects are covered in the dataset? -->
<!-- scope: telescope -->
`Czech`
#### Whose Language?
<!-- info: Whose language is in the dataset? -->
<!-- scope: periscope -->
Six professional translators produced the outputs
#### License
<!-- quick -->
<!-- info: What is the license of the dataset? -->
<!-- scope: telescope -->
cc-by-sa-4.0: Creative Commons Attribution Share Alike 4.0 International
#### Intended Use
<!-- info: What is the intended use of the dataset? -->
<!-- scope: microscope -->
The dataset was created to test neural NLG systems in Czech and their ability to deal with rich morphology.
#### Primary Task
<!-- info: What primary task does the dataset support? -->
<!-- scope: telescope -->
Dialog Response Generation
#### Communicative Goal
<!-- quick -->
<!-- info: Provide a short description of the communicative goal of a model trained for this task on this dataset. -->
<!-- scope: periscope -->
Producing a text expressing the given intent/dialogue act and all and only the attributes specified in the input meaning representation.
### Credit
#### Curation Organization Type(s)
<!-- info: In what kind of organization did the dataset curation happen? -->
<!-- scope: telescope -->
`academic`
#### Curation Organization(s)
<!-- info: Name the organization(s). -->
<!-- scope: periscope -->
Charles University, Prague
#### Dataset Creators
<!-- info: Who created the original dataset? List the people involved in collecting the dataset and their affiliation(s). -->
<!-- scope: microscope -->
Ondrej Dusek and Filip Jurcicek
#### Funding
<!-- info: Who funded the data creation? -->
<!-- scope: microscope -->
This research was supported by the Charles University project PRIMUS/19/SCI/10 and by the Ministry of Education, Youth and Sports of the Czech Republic under the grant agreement LK11221. This work used using language resources distributed by the LINDAT/CLARIN project of the Ministry of Education, Youth and Sports of the Czech Republic (project LM2015071).
#### Who added the Dataset to GEM?
<!-- info: Who contributed to the data card and adding the dataset to GEM? List the people+affiliations involved in creating this data card and who helped integrate this dataset into GEM. -->
<!-- scope: microscope -->
Simon Mille wrote the initial data card and Yacine Jernite the data loader. Sebastian Gehrmann migrated the data card and loader to the v2 format.
### Dataset Structure
#### Data Fields
<!-- info: List and describe the fields present in the dataset. -->
<!-- scope: telescope -->
The data is stored in a JSON or CSV format, with identical contents. The data has 4 fields:
* `da`: the input meaning representation/dialogue act (MR)
* `delex_da`: the input MR, delexicalized -- all slot values are replaced with placeholders, such as `X-name`
* `text`: the corresponding target natural language text (reference)
* `delex_text`: the target text, delexicalized (delexicalization is applied regardless of inflection)
In addition, the data contains a JSON file with all possible inflected forms for all slot values in the dataset (`surface_forms.json`).
Each slot -> value entry contains a list of inflected forms for the given value, with the base form (lemma), the inflected form, and
a [morphological tag](https://ufal.mff.cuni.cz/pdt/Morphology_and_Tagging/Doc/hmptagqr.html).
The same MR is often repeated multiple times with different synonymous reference texts.
#### Reason for Structure
<!-- info: How was the dataset structure determined? -->
<!-- scope: microscope -->
The data originated as a translation and localization of [Wen et al.'s SF restaurant](https://www.aclweb.org/anthology/D15-1199/) NLG dataset.
#### How were labels chosen?
<!-- info: How were the labels chosen? -->
<!-- scope: microscope -->
The input MRs were collected from [Wen et al.'s SF restaurant](https://www.aclweb.org/anthology/D15-1199/) NLG data
and localized by randomly replacing slot values (using a list of Prague restaurant names, neighborhoods etc.).
The generated slot values were then automatically replaced in reference texts in the data.
#### Example Instance
<!-- info: Provide a JSON formatted example of a typical instance in the dataset. -->
<!-- scope: periscope -->
```
{
"input": "inform_only_match(food=Turkish,name='Švejk Restaurant',near='Charles Bridge',price_range=cheap)",
"target": "Našla jsem pouze jednu levnou restauraci poblíž Karlova mostu , kde podávají tureckou kuchyni , Švejk Restaurant ."
}
```
#### Data Splits
<!-- info: Describe and name the splits in the dataset if there are more than one. -->
<!-- scope: periscope -->
| Property | Value |
|--------------------------------|-------|
| Total instances | 5,192 |
| Unique MRs | 2,417 |
| Unique delexicalized instances | 2,752 |
| Unique delexicalized MRs | 248 |
The data is split in a roughly 3:1:1 proportion into training, development and test sections, making sure no delexicalized MR
appears in two different parts. On the other hand, most DA types/intents are represented in all data parts.
#### Splitting Criteria
<!-- info: Describe any criteria for splitting the data, if used. If there are differences between the splits (e.g., if the training annotations are machine-generated and the dev and test ones are created by humans, or if different numbers of annotators contributed to each example), describe them here. -->
<!-- scope: microscope -->
The creators ensured that after delexicalization of the meaning representation there was no overlap between training and test.
The data is split at a 3:1:1 rate between training, validation, and test.
## Dataset in GEM
### Rationale for Inclusion in GEM
#### Why is the Dataset in GEM?
<!-- info: What does this dataset contribute toward better generation evaluation and why is it part of GEM? -->
<!-- scope: microscope -->
This is one of a few non-English data-to-text datasets, in a well-known domain, but covering a morphologically rich language that is harder to generate since named entities need to be inflected. This makes it harder to apply common techniques such as delexicalization or copy mechanisms.
#### Similar Datasets
<!-- info: Do other datasets for the high level task exist? -->
<!-- scope: telescope -->
yes
#### Unique Language Coverage
<!-- info: Does this dataset cover other languages than other datasets for the same task? -->
<!-- scope: periscope -->
yes
#### Difference from other GEM datasets
<!-- info: What else sets this dataset apart from other similar datasets in GEM? -->
<!-- scope: microscope -->
The dialog acts in this dataset are much more varied than the e2e dataset which is the closest in style.
#### Ability that the Dataset measures
<!-- info: What aspect of model ability can be measured with this dataset? -->
<!-- scope: periscope -->
surface realization
### GEM-Specific Curation
#### Modificatied for GEM?
<!-- info: Has the GEM version of the dataset been modified in any way (data, processing, splits) from the original curated data? -->
<!-- scope: telescope -->
yes
#### Additional Splits?
<!-- info: Does GEM provide additional splits to the dataset? -->
<!-- scope: telescope -->
yes
#### Split Information
<!-- info: Describe how the new splits were created -->
<!-- scope: periscope -->
5 challenge sets for the Czech Restaurants dataset were added to the GEM evaluation suite.
1. Data shift: We created subsets of the training and development sets of 500 randomly selected inputs each.
2. Scrambling: We applied input scrambling on a subset of 500 randomly selected test instances; the order of the input dialogue acts was randomly reassigned.
3. We identified different subsets of the test set that we could compare to each other so that we would have a better understanding of the results. There are currently two selections that we have made:
The first comparison is based on input size: the number of predicates differs between different inputs, ranging from 1 to 5.
The table below provides an indication of the distribution of inputs with a particular length.
It is clear from the table that this distribution is not balanced, and comparisions between items should be done with caution.
Particularly for input size 4 and 5, there may not be enough data to draw reliable conclusions.
| Input length | Number of inputs |
|--------------|------------------|
| 1 | 183 |
| 2 | 267 |
| 3 | 297 |
| 4 | 86 |
| 5 | 9 |
The second comparison is based on the type of act. Again we caution against comparing the different groups that have relatively few items.
It is probably OK to compare `inform` and `?request`, but the other acts are all low-frequent.
| Act | Frequency |
|-------------------|-----------|
| ?request | 149 |
| inform | 609 |
| ?confirm | 22 |
| inform_only_match | 16 |
| inform_no_match | 34 |
| ?select | 12 |
#### Split Motivation
<!-- info: What aspects of the model's generation capacities were the splits created to test? -->
<!-- scope: periscope -->
Generalization and robustness.
### Getting Started with the Task
#### Technical Terms
<!-- info: Technical terms used in this card and the dataset and their definitions -->
<!-- scope: microscope -->
- utterance: something a system or user may say in a turn
- meaning representation: a representation of meaning that the system should be in accordance with. The specific type of MR in this dataset are dialog acts which describe what a dialog system should do, e.g., inform a user about a value.
## Previous Results
### Previous Results
#### Measured Model Abilities
<!-- info: What aspect of model ability can be measured with this dataset? -->
<!-- scope: telescope -->
Surface realization
#### Metrics
<!-- info: What metrics are typically used for this task? -->
<!-- scope: periscope -->
`BLEU`, `ROUGE`, `METEOR`
#### Proposed Evaluation
<!-- info: List and describe the purpose of the metrics and evaluation methodology (including human evaluation) that the dataset creators used when introducing this task. -->
<!-- scope: microscope -->
This dataset uses the suite of word-overlap-based automatic metrics from the E2E NLG Challenge (BLEU, NIST, ROUGE-L, METEOR, and CIDEr). In addition, the slot error rate is measured.
#### Previous results available?
<!-- info: Are previous results available? -->
<!-- scope: telescope -->
no
## Dataset Curation
### Original Curation
#### Original Curation Rationale
<!-- info: Original curation rationale -->
<!-- scope: telescope -->
The dataset was created to test neural NLG systems in Czech and their ability to deal with rich morphology.
#### Communicative Goal
<!-- info: What was the communicative goal? -->
<!-- scope: periscope -->
Producing a text expressing the given intent/dialogue act and all and only the attributes specified in the input MR.
#### Sourced from Different Sources
<!-- info: Is the dataset aggregated from different data sources? -->
<!-- scope: telescope -->
no
### Language Data
#### How was Language Data Obtained?
<!-- info: How was the language data obtained? -->
<!-- scope: telescope -->
`Created for the dataset`
#### Creation Process
<!-- info: If created for the dataset, describe the creation process. -->
<!-- scope: microscope -->
Six professional translators translated the underlying dataset with the following instructions:
- Each utterance should be translated by itself
- fluent spoken-style Czech should be produced
- Facts should be preserved
- If possible, synonyms should be varied to create diverse utterances
- Entity names should be inflected as necessary
- the reader of the generated text should be addressed using formal form and self-references should use the female form.
The translators did not have access to the meaning representation.
#### Data Validation
<!-- info: Was the text validated by a different worker or a data curator? -->
<!-- scope: telescope -->
validated by data curator
#### Was Data Filtered?
<!-- info: Were text instances selected or filtered? -->
<!-- scope: telescope -->
not filtered
### Structured Annotations
#### Additional Annotations?
<!-- quick -->
<!-- info: Does the dataset have additional annotations for each instance? -->
<!-- scope: telescope -->
none
#### Annotation Service?
<!-- info: Was an annotation service used? -->
<!-- scope: telescope -->
no
### Consent
#### Any Consent Policy?
<!-- info: Was there a consent policy involved when gathering the data? -->
<!-- scope: telescope -->
no
#### Justification for Using the Data
<!-- info: If not, what is the justification for reusing the data? -->
<!-- scope: microscope -->
It was not explicitly stated but we can safely assume that the translators agreed to this use of their data.
### Private Identifying Information (PII)
#### Contains PII?
<!-- quick -->
<!-- info: Does the source language data likely contain Personal Identifying Information about the data creators or subjects? -->
<!-- scope: telescope -->
no PII
#### Justification for no PII
<!-- info: Provide a justification for selecting `no PII` above. -->
<!-- scope: periscope -->
This dataset does not include any information about individuals.
### Maintenance
#### Any Maintenance Plan?
<!-- info: Does the original dataset have a maintenance plan? -->
<!-- scope: telescope -->
no
## Broader Social Context
### Previous Work on the Social Impact of the Dataset
#### Usage of Models based on the Data
<!-- info: Are you aware of cases where models trained on the task featured in this dataset ore related tasks have been used in automated systems? -->
<!-- scope: telescope -->
no
### Impact on Under-Served Communities
#### Addresses needs of underserved Communities?
<!-- info: Does this dataset address the needs of communities that are traditionally underserved in language technology, and particularly language generation technology? Communities may be underserved for exemple because their language, language variety, or social or geographical context is underepresented in NLP and NLG resources (datasets and models). -->
<!-- scope: telescope -->
yes
#### Details on how Dataset Addresses the Needs
<!-- info: Describe how this dataset addresses the needs of underserved communities. -->
<!-- scope: microscope -->
The dataset may help improve NLG methods for morphologically rich languages beyond Czech.
### Discussion of Biases
#### Any Documented Social Biases?
<!-- info: Are there documented social biases in the dataset? Biases in this context are variations in the ways members of different social categories are represented that can have harmful downstream consequences for members of the more disadvantaged group. -->
<!-- scope: telescope -->
yes
#### Links and Summaries of Analysis Work
<!-- info: Provide links to and summaries of works analyzing these biases. -->
<!-- scope: microscope -->
To ensure consistency of translation, the data always uses formal/polite address for the user, and uses the female form for first-person self-references (as if the dialogue agent producing the sentences was female). This prevents data sparsity and ensures consistent results for systems trained on the dataset, but does not represent all potential situations arising in Czech.
## Considerations for Using the Data
### PII Risks and Liability
### Licenses
#### Copyright Restrictions on the Dataset
<!-- info: Based on your answers in the Intended Use part of the Data Overview Section, which of the following best describe the copyright and licensing status of the dataset? -->
<!-- scope: periscope -->
`open license - commercial use allowed`
#### Copyright Restrictions on the Language Data
<!-- info: Based on your answers in the Language part of the Data Curation Section, which of the following best describe the copyright and licensing status of the underlying language data? -->
<!-- scope: periscope -->
`open license - commercial use allowed`
### Known Technical Limitations
#### Technical Limitations
<!-- info: Describe any known technical limitations, such as spurrious correlations, train/test overlap, annotation biases, or mis-annotations, and cite the works that first identified these limitations when possible. -->
<!-- scope: microscope -->
The test set may lead users to over-estimate the performance of their NLG systems with respect to their generalisability, because there are no unseen restaurants or addresses in the test set. This is something we will look into for future editions of the GEM shared task.
|
GEM/dart | ---
annotations_creators:
- none
language_creators:
- unknown
language:
- en
license:
- mit
multilinguality:
- unknown
size_categories:
- unknown
source_datasets:
- original
task_categories:
- table-to-text
task_ids: []
pretty_name: dart
tags:
- data-to-text
---
# Dataset Card for GEM/dart
## Dataset Description
- **Homepage:** n/a
- **Repository:** https://github.com/Yale-LILY/dart
- **Paper:** https://aclanthology.org/2021.naacl-main.37/
- **Leaderboard:** https://github.com/Yale-LILY/dart#leaderboard
- **Point of Contact:** Dragomir Radev, Rui Zhang, Nazneen Rajani
### Link to Main Data Card
You can find the main data card on the [GEM Website](https://gem-benchmark.com/data_cards/dart).
### Dataset Summary
DART is an English dataset aggregating multiple other data-to-text dataset in a common triple-based format. The new format is completely flat, thus not requiring a model to learn hierarchical structures, while still retaining the full information.
You can load the dataset via:
```
import datasets
data = datasets.load_dataset('GEM/dart')
```
The data loader can be found [here](https://huggingface.co/datasets/GEM/dart).
#### website
n/a
#### paper
[ACL Anthology](https://aclanthology.org/2021.naacl-main.37/)
#### authors
Linyong Nan, Dragomir Radev, Rui Zhang, Amrit Rau, Abhinand Sivaprasad, Chiachun Hsieh, Xiangru Tang, Aadit Vyas, Neha Verma, Pranav Krishna, Yangxiaokang Liu, Nadia Irwanto, Jessica Pan, Faiaz Rahman, Ahmad Zaidi, Mutethia Mutuma, Yasin Tarabar, Ankit Gupta, Tao Yu, Yi Chern Tan, Xi Victoria Lin, Caiming Xiong, Richard Socher, Nazneen Fatema Rajani
## Dataset Overview
### Where to find the Data and its Documentation
#### Download
<!-- info: What is the link to where the original dataset is hosted? -->
<!-- scope: telescope -->
[Github](https://github.com/Yale-LILY/dart)
#### Paper
<!-- info: What is the link to the paper describing the dataset (open access preferred)? -->
<!-- scope: telescope -->
[ACL Anthology](https://aclanthology.org/2021.naacl-main.37/)
#### BibTex
<!-- info: Provide the BibTex-formatted reference for the dataset. Please use the correct published version (ACL anthology, etc.) instead of google scholar created Bibtex. -->
<!-- scope: microscope -->
```
@inproceedings{nan-etal-2021-dart,
title = "{DART}: Open-Domain Structured Data Record to Text Generation",
author = "Nan, Linyong and
Radev, Dragomir and
Zhang, Rui and
Rau, Amrit and
Sivaprasad, Abhinand and
Hsieh, Chiachun and
Tang, Xiangru and
Vyas, Aadit and
Verma, Neha and
Krishna, Pranav and
Liu, Yangxiaokang and
Irwanto, Nadia and
Pan, Jessica and
Rahman, Faiaz and
Zaidi, Ahmad and
Mutuma, Mutethia and
Tarabar, Yasin and
Gupta, Ankit and
Yu, Tao and
Tan, Yi Chern and
Lin, Xi Victoria and
Xiong, Caiming and
Socher, Richard and
Rajani, Nazneen Fatema",
booktitle = "Proceedings of the 2021 Conference of the North American Chapter of the Association for Computational Linguistics: Human Language Technologies",
month = jun,
year = "2021",
address = "Online",
publisher = "Association for Computational Linguistics",
url = "https://aclanthology.org/2021.naacl-main.37",
doi = "10.18653/v1/2021.naacl-main.37",
pages = "432--447",
abstract = "We present DART, an open domain structured DAta Record to Text generation dataset with over 82k instances (DARTs). Data-to-text annotations can be a costly process, especially when dealing with tables which are the major source of structured data and contain nontrivial structures. To this end, we propose a procedure of extracting semantic triples from tables that encodes their structures by exploiting the semantic dependencies among table headers and the table title. Our dataset construction framework effectively merged heterogeneous sources from open domain semantic parsing and spoken dialogue systems by utilizing techniques including tree ontology annotation, question-answer pair to declarative sentence conversion, and predicate unification, all with minimum post-editing. We present systematic evaluation on DART as well as new state-of-the-art results on WebNLG 2017 to show that DART (1) poses new challenges to existing data-to-text datasets and (2) facilitates out-of-domain generalization. Our data and code can be found at https://github.com/Yale-LILY/dart.",
}
```
#### Contact Name
<!-- quick -->
<!-- info: If known, provide the name of at least one person the reader can contact for questions about the dataset. -->
<!-- scope: periscope -->
Dragomir Radev, Rui Zhang, Nazneen Rajani
#### Contact Email
<!-- info: If known, provide the email of at least one person the reader can contact for questions about the dataset. -->
<!-- scope: periscope -->
{dragomir.radev, r.zhang}@yale.edu, {nazneen.rajani}@salesforce.com
#### Has a Leaderboard?
<!-- info: Does the dataset have an active leaderboard? -->
<!-- scope: telescope -->
yes
#### Leaderboard Link
<!-- info: Provide a link to the leaderboard. -->
<!-- scope: periscope -->
[Leaderboard](https://github.com/Yale-LILY/dart#leaderboard)
#### Leaderboard Details
<!-- info: Briefly describe how the leaderboard evaluates models. -->
<!-- scope: microscope -->
Several state-of-the-art table-to-text models were evaluated on DART, such as BART ([Lewis et al., 2020](https://arxiv.org/pdf/1910.13461.pdf)), Seq2Seq-Att ([MELBOURNE](https://webnlg-challenge.loria.fr/files/melbourne_report.pdf)) and End-to-End Transformer ([Castro Ferreira et al., 2019](https://arxiv.org/pdf/1908.09022.pdf)).
The leaderboard reports BLEU, METEOR, TER, MoverScore, BERTScore and BLEURT scores.
### Languages and Intended Use
#### Multilingual?
<!-- quick -->
<!-- info: Is the dataset multilingual? -->
<!-- scope: telescope -->
no
#### Covered Dialects
<!-- info: What dialects are covered? Are there multiple dialects per language? -->
<!-- scope: periscope -->
It is an aggregated from multiple other datasets that use general US-American or British English without differentiation between dialects.
#### Covered Languages
<!-- quick -->
<!-- info: What languages/dialects are covered in the dataset? -->
<!-- scope: telescope -->
`English`
#### Whose Language?
<!-- info: Whose language is in the dataset? -->
<!-- scope: periscope -->
The dataset is aggregated from multiple others that were crowdsourced on different platforms.
#### License
<!-- quick -->
<!-- info: What is the license of the dataset? -->
<!-- scope: telescope -->
mit: MIT License
#### Intended Use
<!-- info: What is the intended use of the dataset? -->
<!-- scope: microscope -->
The dataset is aimed to further research in natural language generation from semantic data.
#### Primary Task
<!-- info: What primary task does the dataset support? -->
<!-- scope: telescope -->
Data-to-Text
#### Communicative Goal
<!-- quick -->
<!-- info: Provide a short description of the communicative goal of a model trained for this task on this dataset. -->
<!-- scope: periscope -->
The speaker is required to produce coherent sentences and construct a trees structured ontology of the column headers.
### Credit
#### Curation Organization Type(s)
<!-- info: In what kind of organization did the dataset curation happen? -->
<!-- scope: telescope -->
`academic`, `industry`
#### Curation Organization(s)
<!-- info: Name the organization(s). -->
<!-- scope: periscope -->
Yale University, Salesforce Research, Penn State University, The University of Hong Kong, MIT
#### Dataset Creators
<!-- info: Who created the original dataset? List the people involved in collecting the dataset and their affiliation(s). -->
<!-- scope: microscope -->
Linyong Nan, Dragomir Radev, Rui Zhang, Amrit Rau, Abhinand Sivaprasad, Chiachun Hsieh, Xiangru Tang, Aadit Vyas, Neha Verma, Pranav Krishna, Yangxiaokang Liu, Nadia Irwanto, Jessica Pan, Faiaz Rahman, Ahmad Zaidi, Mutethia Mutuma, Yasin Tarabar, Ankit Gupta, Tao Yu, Yi Chern Tan, Xi Victoria Lin, Caiming Xiong, Richard Socher, Nazneen Fatema Rajani
#### Who added the Dataset to GEM?
<!-- info: Who contributed to the data card and adding the dataset to GEM? List the people+affiliations involved in creating this data card and who helped integrate this dataset into GEM. -->
<!-- scope: microscope -->
Miruna Clinciu contributed the original data card and Yacine Jernite wrote the initial data loader. Sebastian Gehrmann migrated the data card and the loader to the new format.
### Dataset Structure
#### Data Fields
<!-- info: List and describe the fields present in the dataset. -->
<!-- scope: telescope -->
-`tripleset`: a list of tuples, each tuple has 3 items
-`subtree_was_extended`: a boolean variable (true or false)
-`annotations`: a list of dict, each with source and text keys.
-`source`: a string mentioning the name of the source table.
-`text`: a sentence string.
#### Reason for Structure
<!-- info: How was the dataset structure determined? -->
<!-- scope: microscope -->
The structure is supposed to be able more complex structures beyond "flat" attribute-value pairs, instead encoding hierarchical relationships.
#### How were labels chosen?
<!-- info: How were the labels chosen? -->
<!-- scope: microscope -->
They are a combination of those from existing datasets and new annotations that take advantage of the hierarchical structure
#### Example Instance
<!-- info: Provide a JSON formatted example of a typical instance in the dataset. -->
<!-- scope: periscope -->
```
{
"tripleset": [
[
"Ben Mauk",
"High school",
"Kenton"
],
[
"Ben Mauk",
"College",
"Wake Forest Cincinnati"
]
],
"subtree_was_extended": false,
"annotations": [
{
"source": "WikiTableQuestions_lily",
"text": "Ben Mauk, who attended Kenton High School, attended Wake Forest Cincinnati for college."
}
]
}
```
#### Data Splits
<!-- info: Describe and name the splits in the dataset if there are more than one. -->
<!-- scope: periscope -->
|Input Unit | Examples | Vocab Size | Words per SR | Sents per SR | Tables |
| ------------- | ------------- || ------------- || ------------- || ------------- || ------------- |
|Triple Set | 82,191 | 33.2K | 21.6 | 1.5 | 5,623 |
| Train | Dev | Test|
| ------------- | ------------- || ------------- |
| 62,659 | 6,980 | 12,552|
Statistics of DART decomposed by different collection methods. DART exhibits a great deal of topical variety in terms of the number of unique predicates, the number of unique triples, and the vocabulary size. These statistics are computed from DART v1.1.1; the number of unique predicates reported is post-unification (see Section 3.4). SR: Surface Realization.
([details in Table 1 and 2](https://arxiv.org/pdf/2007.02871.pdf)).
#### Splitting Criteria
<!-- info: Describe any criteria for splitting the data, if used. If there are differences between the splits (e.g., if the training annotations are machine-generated and the dev and test ones are created by humans, or if different numbers of annotators contributed to each example), describe them here. -->
<!-- scope: microscope -->
For WebNLG 2017 and Cleaned E2E, DART use the original data splits. For the new annotation on WikiTableQuestions and WikiSQL, random splitting will make train, dev, and test splits contain similar tables and similar <triple-set, sentence> examples. They are thus split based on Jaccard similarity such that no training examples has a similarity with a test example of over 0.5
## Dataset in GEM
### Rationale for Inclusion in GEM
#### Why is the Dataset in GEM?
<!-- info: What does this dataset contribute toward better generation evaluation and why is it part of GEM? -->
<!-- scope: microscope -->
DART is a large and open-domain structured DAta Record to Text generation corpus with high-quality sentence annotations with each input being a set of entity-relation triples following a tree-structured ontology.
#### Similar Datasets
<!-- info: Do other datasets for the high level task exist? -->
<!-- scope: telescope -->
yes
#### Unique Language Coverage
<!-- info: Does this dataset cover other languages than other datasets for the same task? -->
<!-- scope: periscope -->
no
#### Difference from other GEM datasets
<!-- info: What else sets this dataset apart from other similar datasets in GEM? -->
<!-- scope: microscope -->
The tree structure is unique among GEM datasets
#### Ability that the Dataset measures
<!-- info: What aspect of model ability can be measured with this dataset? -->
<!-- scope: periscope -->
Reasoning, surface realization
### GEM-Specific Curation
#### Modificatied for GEM?
<!-- info: Has the GEM version of the dataset been modified in any way (data, processing, splits) from the original curated data? -->
<!-- scope: telescope -->
no
#### Additional Splits?
<!-- info: Does GEM provide additional splits to the dataset? -->
<!-- scope: telescope -->
no
### Getting Started with the Task
#### Pointers to Resources
<!-- info: Getting started with in-depth research on the task. Add relevant pointers to resources that researchers can consult when they want to get started digging deeper into the task. -->
<!-- scope: microscope -->
Experimental results on DART shows that BART model as the highest performance among three models with a BLEU score of 37.06. This is attributed to BART’s generalization ability due to pretraining ([Table 4](https://arxiv.org/pdf/2007.02871.pdf)).
## Previous Results
### Previous Results
#### Measured Model Abilities
<!-- info: What aspect of model ability can be measured with this dataset? -->
<!-- scope: telescope -->
Reasoning, surface realization
#### Metrics
<!-- info: What metrics are typically used for this task? -->
<!-- scope: periscope -->
`BLEU`, `MoverScore`, `BERT-Score`, `BLEURT`
#### Proposed Evaluation
<!-- info: List and describe the purpose of the metrics and evaluation methodology (including human evaluation) that the dataset creators used when introducing this task. -->
<!-- scope: microscope -->
The leaderboard uses the combination of BLEU, METEOR, TER, MoverScore, BERTScore, PARENT and BLEURT to overcome the limitations of the n-gram overlap metrics.
A small scale human annotation of 100 data points was conducted along the dimensions of (1) fluency - a sentence is natural and grammatical, and (2) semantic faithfulness - a sentence is supported by the input triples.
#### Previous results available?
<!-- info: Are previous results available? -->
<!-- scope: telescope -->
yes
#### Other Evaluation Approaches
<!-- info: What evaluation approaches have others used? -->
<!-- scope: periscope -->
n/a
#### Relevant Previous Results
<!-- info: What are the most relevant previous results for this task/dataset? -->
<!-- scope: microscope -->
BART currently achieves the best performance according to the leaderboard.
## Dataset Curation
### Original Curation
#### Original Curation Rationale
<!-- info: Original curation rationale -->
<!-- scope: telescope -->
The dataset creators encourage through DART further research in natural language generation from semantic data. DART provides high-quality sentence annotations with each input being a set of entity-relation triples in a tree structure.
#### Communicative Goal
<!-- info: What was the communicative goal? -->
<!-- scope: periscope -->
The speaker is required to produce coherent sentences and construct a trees structured ontology of the column headers.
#### Sourced from Different Sources
<!-- info: Is the dataset aggregated from different data sources? -->
<!-- scope: telescope -->
yes
#### Source Details
<!-- info: List the sources (one per line) -->
<!-- scope: periscope -->
- human annotation on open-domain Wikipedia tables from WikiTableQuestions ([Pasupat and Liang,
2015](https://www.aclweb.org/anthology/P15-1142.pdf)) and WikiSQL ([Zhong et al., 2017](https://arxiv.org/pdf/1709.00103.pdf))
- automatic conversion of questions in WikiSQL to declarative sentences
- incorporation of existing datasets including WebNLG 2017 (Gardent et al., 2017[a](https://www.aclweb.org/anthology/P17-1017.pdf),[b](https://www.aclweb.org/anthology/W17-3518.pdf); [Shimorina and Gardent, 2018](https://www.aclweb.org/anthology/W18-6543.pdf)) and Cleaned E2E ([Novikova et al., 2017b](https://arxiv.org/pdf/1706.09254.pdf); Dušek et al., [2018](https://arxiv.org/pdf/1810.01170.pdf), [2019](https://www.aclweb.org/anthology/W19-8652.pdf))
### Language Data
#### How was Language Data Obtained?
<!-- info: How was the language data obtained? -->
<!-- scope: telescope -->
`Found`, `Created for the dataset`
#### Where was it found?
<!-- info: If found, where from? -->
<!-- scope: telescope -->
`Offline media collection`
#### Creation Process
<!-- info: If created for the dataset, describe the creation process. -->
<!-- scope: microscope -->
Creators proposed a two-stage annotation process for constructing triple set sentence pairs based on a tree-structured ontology of each table. First, internal skilled annotators denote the parent column for each column header. Then, a larger number of annotators provide a sentential description of an automatically-chosen subset of table cells in a row. To form a triple set sentence pair, the highlighted cells can be converted to a connected triple set automatically according to the column ontology for the given table.
#### Language Producers
<!-- info: What further information do we have on the language producers? -->
<!-- scope: microscope -->
No further information about the MTurk workers has been provided.
#### Topics Covered
<!-- info: Does the language in the dataset focus on specific topics? How would you describe them? -->
<!-- scope: periscope -->
The sub-datasets are from Wikipedia, DBPedia, and artificially created restaurant data.
#### Data Validation
<!-- info: Was the text validated by a different worker or a data curator? -->
<!-- scope: telescope -->
validated by crowdworker
#### Was Data Filtered?
<!-- info: Were text instances selected or filtered? -->
<!-- scope: telescope -->
not filtered
### Structured Annotations
#### Additional Annotations?
<!-- quick -->
<!-- info: Does the dataset have additional annotations for each instance? -->
<!-- scope: telescope -->
none
#### Annotation Service?
<!-- info: Was an annotation service used? -->
<!-- scope: telescope -->
no
### Consent
#### Any Consent Policy?
<!-- info: Was there a consent policy involved when gathering the data? -->
<!-- scope: telescope -->
no
#### Justification for Using the Data
<!-- info: If not, what is the justification for reusing the data? -->
<!-- scope: microscope -->
The new annotations are based on Wikipedia which is in the public domain and the other two datasets permit reuse (with attribution)
### Private Identifying Information (PII)
#### Contains PII?
<!-- quick -->
<!-- info: Does the source language data likely contain Personal Identifying Information about the data creators or subjects? -->
<!-- scope: telescope -->
no PII
#### Justification for no PII
<!-- info: Provide a justification for selecting `no PII` above. -->
<!-- scope: periscope -->
None of the datasets talk about individuals
### Maintenance
#### Any Maintenance Plan?
<!-- info: Does the original dataset have a maintenance plan? -->
<!-- scope: telescope -->
no
## Broader Social Context
### Previous Work on the Social Impact of the Dataset
#### Usage of Models based on the Data
<!-- info: Are you aware of cases where models trained on the task featured in this dataset ore related tasks have been used in automated systems? -->
<!-- scope: telescope -->
no
### Impact on Under-Served Communities
#### Addresses needs of underserved Communities?
<!-- info: Does this dataset address the needs of communities that are traditionally underserved in language technology, and particularly language generation technology? Communities may be underserved for exemple because their language, language variety, or social or geographical context is underepresented in NLP and NLG resources (datasets and models). -->
<!-- scope: telescope -->
no
### Discussion of Biases
#### Any Documented Social Biases?
<!-- info: Are there documented social biases in the dataset? Biases in this context are variations in the ways members of different social categories are represented that can have harmful downstream consequences for members of the more disadvantaged group. -->
<!-- scope: telescope -->
no
#### Are the Language Producers Representative of the Language?
<!-- info: Does the distribution of language producers in the dataset accurately represent the full distribution of speakers of the language world-wide? If not, how does it differ? -->
<!-- scope: periscope -->
No, the annotators are raters on crowdworking platforms and thus only represent their demographics.
## Considerations for Using the Data
### PII Risks and Liability
### Licenses
#### Copyright Restrictions on the Dataset
<!-- info: Based on your answers in the Intended Use part of the Data Overview Section, which of the following best describe the copyright and licensing status of the dataset? -->
<!-- scope: periscope -->
`open license - commercial use allowed`
#### Copyright Restrictions on the Language Data
<!-- info: Based on your answers in the Language part of the Data Curation Section, which of the following best describe the copyright and licensing status of the underlying language data? -->
<!-- scope: periscope -->
`open license - commercial use allowed`
### Known Technical Limitations
#### Technical Limitations
<!-- info: Describe any known technical limitations, such as spurrious correlations, train/test overlap, annotation biases, or mis-annotations, and cite the works that first identified these limitations when possible. -->
<!-- scope: microscope -->
The dataset may contain some social biases, as the input sentences are based on Wikipedia (WikiTableQuestions, WikiSQL, WebNLG). Studies have shown that the English Wikipedia contains gender biases([Dinan et al., 2020](https://www.aclweb.org/anthology/2020.emnlp-main.23.pdf)), racial biases([Papakyriakopoulos et al., 2020 (https://dl.acm.org/doi/pdf/10.1145/3351095.3372843)) and geographical bias([Livingstone et al., 2010](https://doi.org/10.5204/mcj.315)). [More info](https://en.wikipedia.org/wiki/Racial_bias_on_Wikipedia#cite_note-23).
#### Unsuited Applications
<!-- info: When using a model trained on this dataset in a setting where users or the public may interact with its predictions, what are some pitfalls to look out for? In particular, describe some applications of the general task featured in this dataset that its curation or properties make it less suitable for. -->
<!-- scope: microscope -->
The end-to-end transformer has the lowest performance since the transformer model needs intermediate pipeline planning steps to have higher performance. Similar findings can be found in [Castro Ferreira et al., 2019](https://arxiv.org/pdf/1908.09022.pdf).
|
GEM/dstc10_track2_task2 | ---
annotations_creators:
- none
language_creators:
- unknown
language:
- en
license:
- apache-2.0
multilinguality:
- unknown
size_categories:
- unknown
source_datasets:
- original
task_categories:
- conversational
task_ids: []
pretty_name: dstc10_track2_task2
tags:
- dialog-response-generation
---
# Dataset Card for GEM/dstc10_track2_task2
## Dataset Description
- **Homepage:** https://github.com/alexa/alexa-with-dstc10-track2-dataset
- **Repository:** https://github.com/alexa/alexa-with-dstc10-track2-dataset
- **Paper:** https://assets.amazon.science/54/a1/5282d47044179737b4289622c824/how-robust-are-you-evaluating-task-oriented-dialogue-systems-on-spoken-conversations.pdf
- **Leaderboard:** https://eval.ai/challenge/1663/overview
- **Point of Contact:** Seokhwan Kim
### Link to Main Data Card
You can find the main data card on the [GEM Website](https://gem-benchmark.com/data_cards/dstc10_track2_task2).
### Dataset Summary
The DSTC10 Track2 Task 2 follows the DSTC9 Track1 task, where participants have to implement knowledge-grounded dialog systems.
The training dataset is inherited from the DSTC9 challenge and is in the written domain, while the test set is newly collected and consists of noisy ASR transcripts.
Hence, the dataset facilitates building models for grounded dialog response generation.
You can load the dataset via:
```
import datasets
data = datasets.load_dataset('GEM/dstc10_track2_task2')
```
The data loader can be found [here](https://huggingface.co/datasets/GEM/dstc10_track2_task2).
#### website
https://github.com/alexa/alexa-with-dstc10-track2-dataset
#### paper
https://assets.amazon.science/54/a1/5282d47044179737b4289622c824/how-robust-are-you-evaluating-task-oriented-dialogue-systems-on-spoken-conversations.pdf
#### authors
Seokhwan Kim, Yang Liu, Di Jin, Alexandros Papangelis, Karthik Gopalakrishnan, Behnam Hedayatnia, Dilek Hakkani-Tur (Amazon Alexa AI)
## Dataset Overview
### Where to find the Data and its Documentation
#### Webpage
<!-- info: What is the webpage for the dataset (if it exists)? -->
<!-- scope: telescope -->
https://github.com/alexa/alexa-with-dstc10-track2-dataset
#### Download
<!-- info: What is the link to where the original dataset is hosted? -->
<!-- scope: telescope -->
https://github.com/alexa/alexa-with-dstc10-track2-dataset
#### Paper
<!-- info: What is the link to the paper describing the dataset (open access preferred)? -->
<!-- scope: telescope -->
https://assets.amazon.science/54/a1/5282d47044179737b4289622c824/how-robust-are-you-evaluating-task-oriented-dialogue-systems-on-spoken-conversations.pdf
#### BibTex
<!-- info: Provide the BibTex-formatted reference for the dataset. Please use the correct published version (ACL anthology, etc.) instead of google scholar created Bibtex. -->
<!-- scope: microscope -->
@inproceedings{kim2021robust,
title={" How Robust ru?": Evaluating Task-Oriented Dialogue Systems on Spoken Conversations},
author={Kim, Seokhwan and Liu, Yang and Jin, Di and Papangelis, Alexandros and Gopalakrishnan, Karthik and Hedayatnia, Behnam and Hakkani-Tur, Dilek},
journal={IEEE Automatic Speech Recognition and Understanding Workshop},
year={2021}
}
#### Contact Name
<!-- quick -->
<!-- info: If known, provide the name of at least one person the reader can contact for questions about the dataset. -->
<!-- scope: periscope -->
Seokhwan Kim
#### Contact Email
<!-- info: If known, provide the email of at least one person the reader can contact for questions about the dataset. -->
<!-- scope: periscope -->
seokhwk@amazon.com
#### Has a Leaderboard?
<!-- info: Does the dataset have an active leaderboard? -->
<!-- scope: telescope -->
yes
#### Leaderboard Link
<!-- info: Provide a link to the leaderboard. -->
<!-- scope: periscope -->
https://eval.ai/challenge/1663/overview
#### Leaderboard Details
<!-- info: Briefly describe how the leaderboard evaluates models. -->
<!-- scope: microscope -->
It evaluates the models based on the automatic metrics defined in the task paper for the three tasks of detection, selection and generation.
### Languages and Intended Use
#### Multilingual?
<!-- quick -->
<!-- info: Is the dataset multilingual? -->
<!-- scope: telescope -->
no
#### Covered Languages
<!-- quick -->
<!-- info: What languages/dialects are covered in the dataset? -->
<!-- scope: telescope -->
`En`
#### License
<!-- quick -->
<!-- info: What is the license of the dataset? -->
<!-- scope: telescope -->
apache-2.0: Apache License 2.0
#### Intended Use
<!-- info: What is the intended use of the dataset? -->
<!-- scope: microscope -->
To conduct research on dialogue state tracking and knowledge-grounded response generation.
#### Primary Task
<!-- info: What primary task does the dataset support? -->
<!-- scope: telescope -->
Dialog Response Generation
#### Communicative Goal
<!-- quick -->
<!-- info: Provide a short description of the communicative goal of a model trained for this task on this dataset. -->
<!-- scope: periscope -->
This dataset aims to explore the robustness of conversational models when trained on spoken data. It has two aspects, multi-domain dialogue state tracking and conversation modeling with access to unstructured knowledge.
### Credit
#### Curation Organization Type(s)
<!-- info: In what kind of organization did the dataset curation happen? -->
<!-- scope: telescope -->
`industry`
#### Curation Organization(s)
<!-- info: Name the organization(s). -->
<!-- scope: periscope -->
Amazon
#### Dataset Creators
<!-- info: Who created the original dataset? List the people involved in collecting the dataset and their affiliation(s). -->
<!-- scope: microscope -->
Seokhwan Kim, Yang Liu, Di Jin, Alexandros Papangelis, Karthik Gopalakrishnan, Behnam Hedayatnia, Dilek Hakkani-Tur (Amazon Alexa AI)
#### Funding
<!-- info: Who funded the data creation? -->
<!-- scope: microscope -->
Amazon
#### Who added the Dataset to GEM?
<!-- info: Who contributed to the data card and adding the dataset to GEM? List the people+affiliations involved in creating this data card and who helped integrate this dataset into GEM. -->
<!-- scope: microscope -->
Alexandros Papangelis (Amazon Alexa AI), Di Jin (Amazon Alexa AI), Nico Daheim (RWTH Aachen University)
### Dataset Structure
#### Data Fields
<!-- info: List and describe the fields present in the dataset. -->
<!-- scope: telescope -->
features = datasets.Features(
{
"id": datasets.Value("string"),
"gem_id": datasets.Value("string"),
"turns": [
{
"speaker": datasets.Value("string"),
"text": datasets.Value("string"),
"nbest": [
{
"hyp": datasets.Value("string"),
"score": datasets.Value("float"),
}
],
}
],
"knowledge": {
"domain": datasets.Value("string"),
"entity_name": datasets.Value("string"),
"title": datasets.Value("string"),
"body": datasets.Value("string"),
},
"response": datasets.Value("string"),
"source": datasets.Value("string"),
"linearized_input": datasets.Value("string"),
"target": datasets.Value("string"),
"references": [datasets.Value("string")],
}
)
nbest contains an nbest list of outputs generated by an ASR system along with their scores.
knowledge defines the annotated grounding as well as its metadata
#### Reason for Structure
<!-- info: How was the dataset structure determined? -->
<!-- scope: microscope -->
It was kept compatible with MultiWox 2.X data.
#### Example Instance
<!-- info: Provide a JSON formatted example of a typical instance in the dataset. -->
<!-- scope: periscope -->
{'id': '0',
'gem_id': 'GEM-dstc10_track2_task2-test-0',
'turns': [{'speaker': 'U',
'text': "hi uh i'm looking for restaurant in lower ha",
'nbest': [{'hyp': "hi uh i'm looking for restaurant in lower ha",
'score': -25.625450134277344},
{'hyp': "hi uh i'm looking for restaurant in lower hai",
'score': -25.969446182250977},
{'hyp': "hi uh i'm looking for restaurant in lower haig",
'score': -32.816890716552734},
{'hyp': "hi uh i'm looking for restaurant in lower haigh",
'score': -32.84316635131836},
{'hyp': "hi uh i'm looking for restaurant in lower hag",
'score': -32.8637580871582},
{'hyp': "hi uh i'm looking for restaurant in lower hah",
'score': -33.1048698425293},
{'hyp': "hi uh i'm looking for restaurant in lower hait",
'score': -33.96509552001953},
{'hyp': "hi um i'm looking for restaurant in lower hai",
'score': -33.97885513305664},
{'hyp': "hi um i'm looking for restaurant in lower haig",
'score': -34.56083679199219},
{'hyp': "hi um i'm looking for restaurant in lower haigh",
'score': -34.58711242675781}]},
{'speaker': 'S',
'text': 'yeah definitely i can go ahead and help you with that ummm what kind of option in a restaurant are you looking for',
'nbest': []},
{'speaker': 'U',
'text': 'yeah umm am looking for an expensive restaurant',
'nbest': [{'hyp': 'yeah umm am looking for an expensive restaurant',
'score': -21.272899627685547},
{'hyp': 'yeah umm m looking for an expensive restaurant',
'score': -21.444047927856445},
{'hyp': 'yeah umm a m looking for an expensive restaurant',
'score': -21.565458297729492},
{'hyp': 'yeah ummm am looking for an expensive restaurant',
'score': -21.68832778930664},
{'hyp': 'yeah ummm m looking for an expensive restaurant',
'score': -21.85947608947754},
{'hyp': 'yeah ummm a m looking for an expensive restaurant',
'score': -21.980886459350586},
{'hyp': "yeah umm a'm looking for an expensive restaurant",
'score': -22.613924026489258},
{'hyp': "yeah ummm a'm looking for an expensive restaurant",
'score': -23.02935218811035},
{'hyp': 'yeah um am looking for an expensive restaurant',
'score': -23.11180305480957},
{'hyp': 'yeah um m looking for an expensive restaurant',
'score': -23.28295135498047}]},
{'speaker': 'S',
'text': "lemme go ahead and see what i can find for you ok great so i do ummm actually no i'm sorry is there something else i can help you find i don't see anything expensive",
'nbest': []},
{'speaker': 'U',
'text': "sure ummm maybe if you don't have anything expensive how about something in the moderate price range",
'nbest': [{'hyp': "sure ummm maybe if you don't have anything expensive how about something in the moderate price range",
'score': -27.492507934570312},
{'hyp': "sure umm maybe if you don't have anything expensive how about something in the moderate price range",
'score': -27.75853729248047},
{'hyp': "sure ummm maybe if you don't have anything expensive how about something in the moderate price rang",
'score': -29.44410514831543},
{'hyp': "sure umm maybe if you don't have anything expensive how about something in the moderate price rang",
'score': -29.710134506225586},
{'hyp': "sure um maybe if you don't have anything expensive how about something in the moderate price range",
'score': -31.136560440063477},
{'hyp': "sure um maybe if you don't have anything expensive how about something in the moderate price rang",
'score': -33.088157653808594},
{'hyp': "sure ummm maybe i you don't have anything expensive how about something in the moderate price range",
'score': -36.127620697021484},
{'hyp': "sure umm maybe i you don't have anything expensive how about something in the moderate price range",
'score': -36.39365005493164},
{'hyp': "sure ummm maybe if yo don't have anything expensive how about something in the moderate price range",
'score': -36.43605041503906},
{'hyp': "sure umm maybe if yo don't have anything expensive how about something in the moderate price range",
'score': -36.70207977294922}]},
{'speaker': 'S',
'text': 'ok moderate lemme go ahead and check to see what i can find for moderate ok great i do have several options coming up how does the view lounge sound',
'nbest': []},
{'speaker': 'U',
'text': 'that sounds good ummm do they have any sort of happy hour special',
'nbest': [{'hyp': 'that sounds good ummm do they have any sort of happy hour special',
'score': -30.316478729248047},
{'hyp': 'that sounds good umm do they have any sort of happy hour special',
'score': -30.958009719848633},
{'hyp': 'that sounds good um do they have any sort of happy hour special',
'score': -34.463165283203125},
{'hyp': 'that sounds good ummm do they have any sirt of happy hour special',
'score': -34.48350143432617},
{'hyp': 'that sounds good umm do they have any sirt of happy hour special',
'score': -35.12503433227539},
{'hyp': 'that sounds good ummm do they have any sord of happy hour special',
'score': -35.61939239501953},
{'hyp': 'that sounds good umm do they have any sord of happy hour special',
'score': -36.26092529296875},
{'hyp': 'that sounds good ummm do they have any sont of happy hour special',
'score': -37.697105407714844},
{'hyp': 'that sounds good umm do they have any sont of happy hour special',
'score': -38.33863830566406},
{'hyp': 'that sounds good um do they have any sirt of happy hour special',
'score': -38.630191802978516}]}],
'knowledge': {'domain': 'restaurant',
'entity_name': 'The View Lounge',
'title': 'Does The View Lounge offer happy hour?',
'body': 'The View Lounge offers happy hour.'},
'response': 'uhhh great question lemme go ahead and check that out for you ok fantastic so it looks like they do offer happy hour',
'source': 'sf_spoken',
'linearized_input': "<U> hi uh i'm looking for restaurant in lower ha <S> yeah definitely i can go ahead and help you with that ummm what kind of option in a restaurant are you looking for <U> yeah umm am looking for an expensive restaurant <S> lemme go ahead and see what i can find for you ok great so i do ummm actually no i'm sorry is there something else i can help you find i don't see anything expensive <U> sure ummm maybe if you don't have anything expensive how about something in the moderate price range <S> ok moderate lemme go ahead and check to see what i can find for moderate ok great i do have several options coming up how does the view lounge sound <U> that sounds good ummm do they have any sort of happy hour special || knowledge domain: restaurant, entity: The View Lounge, title: Does The View Lounge offer happy hour?, information: The View Lounge offers happy hour.",
'target': 'uhhh great question lemme go ahead and check that out for you ok fantastic so it looks like they do offer happy hour',
'references': ['uhhh great question lemme go ahead and check that out for you ok fantastic so it looks like they do offer happy hour']}
#### Data Splits
<!-- info: Describe and name the splits in the dataset if there are more than one. -->
<!-- scope: periscope -->
train: training set, val: validation set, test: test set
#### Splitting Criteria
<!-- info: Describe any criteria for splitting the data, if used. If there are differences between the splits (e.g., if the training annotations are machine-generated and the dev and test ones are created by humans, or if different numbers of annotators contributed to each example), describe them here. -->
<!-- scope: microscope -->
The track dataset originally only consists of a validation and test set in the spoken domain with noisy ASR transcripts.
The training set is taken from the predecessor task DSTC9 Track 1 and contains written conversations.
## Dataset in GEM
### Rationale for Inclusion in GEM
#### Why is the Dataset in GEM?
<!-- info: What does this dataset contribute toward better generation evaluation and why is it part of GEM? -->
<!-- scope: microscope -->
This dataset can be used to evaluate conversational models on spoken inputs (using ASR hypotheses). In particular, we can evaluate the models’ ability to understand language by tracking the dialogue state, and their ability to generate knowledge-grounded responses.
#### Similar Datasets
<!-- info: Do other datasets for the high level task exist? -->
<!-- scope: telescope -->
yes
#### Unique Language Coverage
<!-- info: Does this dataset cover other languages than other datasets for the same task? -->
<!-- scope: periscope -->
no
#### Difference from other GEM datasets
<!-- info: What else sets this dataset apart from other similar datasets in GEM? -->
<!-- scope: microscope -->
This dataset contains transcribed spoken interactions.
#### Ability that the Dataset measures
<!-- info: What aspect of model ability can be measured with this dataset? -->
<!-- scope: periscope -->
We can measure the model’s ability to understand language and to generate knowledge-grounded responses.
### GEM-Specific Curation
#### Modificatied for GEM?
<!-- info: Has the GEM version of the dataset been modified in any way (data, processing, splits) from the original curated data? -->
<!-- scope: telescope -->
no
#### Additional Splits?
<!-- info: Does GEM provide additional splits to the dataset? -->
<!-- scope: telescope -->
no
### Getting Started with the Task
## Previous Results
### Previous Results
#### Measured Model Abilities
<!-- info: What aspect of model ability can be measured with this dataset? -->
<!-- scope: telescope -->
This dataset can be used to evaluate conversational models on spoken inputs (using ASR hypotheses). In particular, we can evaluate the models’ ability to generate knowledge-grounded responses.
#### Metrics
<!-- info: What metrics are typically used for this task? -->
<!-- scope: periscope -->
`Other: Other Metrics`
#### Other Metrics
<!-- info: Definitions of other metrics -->
<!-- scope: periscope -->
BLEU-1, BLEU-2, BLEU-3, BLEU-4, METEOR, ROGUE-1, ROGUE-2, ROGUE-L
#### Previous results available?
<!-- info: Are previous results available? -->
<!-- scope: telescope -->
no
## Dataset Curation
### Original Curation
#### Original Curation Rationale
<!-- info: Original curation rationale -->
<!-- scope: telescope -->
We want to explore how conversational models perform on spoken data.
#### Communicative Goal
<!-- info: What was the communicative goal? -->
<!-- scope: periscope -->
This dataset aims to explore the robustness of conversational models when evaluated on spoken data. It has two aspects, multi-domain dialogue state tracking and conversation modeling with access to unstructured knowledge.
#### Sourced from Different Sources
<!-- info: Is the dataset aggregated from different data sources? -->
<!-- scope: telescope -->
no
### Language Data
#### How was Language Data Obtained?
<!-- info: How was the language data obtained? -->
<!-- scope: telescope -->
`Other`
#### Topics Covered
<!-- info: Does the language in the dataset focus on specific topics? How would you describe them? -->
<!-- scope: periscope -->
The conversations revolve around 5 domains (or topics): hotels, restaurants, attractions, taxi, train.
#### Data Validation
<!-- info: Was the text validated by a different worker or a data curator? -->
<!-- scope: telescope -->
not validated
#### Was Data Filtered?
<!-- info: Were text instances selected or filtered? -->
<!-- scope: telescope -->
not filtered
### Structured Annotations
#### Additional Annotations?
<!-- quick -->
<!-- info: Does the dataset have additional annotations for each instance? -->
<!-- scope: telescope -->
none
#### Annotation Service?
<!-- info: Was an annotation service used? -->
<!-- scope: telescope -->
no
### Consent
#### Any Consent Policy?
<!-- info: Was there a consent policy involved when gathering the data? -->
<!-- scope: telescope -->
yes
### Private Identifying Information (PII)
#### Contains PII?
<!-- quick -->
<!-- info: Does the source language data likely contain Personal Identifying Information about the data creators or subjects? -->
<!-- scope: telescope -->
no PII
#### Justification for no PII
<!-- info: Provide a justification for selecting `no PII` above. -->
<!-- scope: periscope -->
The subjects were instructed to conduct fictional conversations about booking restaurants or requesting fictional information.
### Maintenance
#### Any Maintenance Plan?
<!-- info: Does the original dataset have a maintenance plan? -->
<!-- scope: telescope -->
no
## Broader Social Context
### Previous Work on the Social Impact of the Dataset
#### Usage of Models based on the Data
<!-- info: Are you aware of cases where models trained on the task featured in this dataset ore related tasks have been used in automated systems? -->
<!-- scope: telescope -->
no
### Impact on Under-Served Communities
#### Addresses needs of underserved Communities?
<!-- info: Does this dataset address the needs of communities that are traditionally underserved in language technology, and particularly language generation technology? Communities may be underserved for exemple because their language, language variety, or social or geographical context is underepresented in NLP and NLG resources (datasets and models). -->
<!-- scope: telescope -->
no
### Discussion of Biases
#### Any Documented Social Biases?
<!-- info: Are there documented social biases in the dataset? Biases in this context are variations in the ways members of different social categories are represented that can have harmful downstream consequences for members of the more disadvantaged group. -->
<!-- scope: telescope -->
unsure
## Considerations for Using the Data
### PII Risks and Liability
#### Potential PII Risk
<!-- info: Considering your answers to the PII part of the Data Curation Section, describe any potential privacy to the data subjects and creators risks when using the dataset. -->
<!-- scope: microscope -->
There should be no risk related to PII as the subjects conduct fictional conversations.
### Licenses
#### Copyright Restrictions on the Dataset
<!-- info: Based on your answers in the Intended Use part of the Data Overview Section, which of the following best describe the copyright and licensing status of the dataset? -->
<!-- scope: periscope -->
`open license - commercial use allowed`
#### Copyright Restrictions on the Language Data
<!-- info: Based on your answers in the Language part of the Data Curation Section, which of the following best describe the copyright and licensing status of the underlying language data? -->
<!-- scope: periscope -->
`open license - commercial use allowed`
### Known Technical Limitations
|
GEM/e2e_nlg | ---
annotations_creators:
- none
language_creators:
- unknown
language:
- en
license:
- cc-by-sa-4.0
multilinguality:
- unknown
size_categories:
- unknown
source_datasets:
- original
task_categories:
- table-to-text
task_ids: []
pretty_name: e2e_nlg
tags:
- data-to-text
---
# Dataset Card for GEM/e2e_nlg
## Dataset Description
- **Homepage:** http://www.macs.hw.ac.uk/InteractionLab/E2E/
- **Repository:** https://github.com/tuetschek/e2e-cleaning
- **Paper:** https://www.aclweb.org/anthology/W17-5525/, [Detailed E2E Challenge writeup
- **Leaderboard:** N/A
- **Point of Contact:** Ondrej Dusek
### Link to Main Data Card
You can find the main data card on the [GEM Website](https://gem-benchmark.com/data_cards/e2e_nlg).
### Dataset Summary
The E2E NLG dataset is an English benchmark dataset for data-to-text models that verbalize a set of 2-9 key-value attribute pairs in the restaurant domain. The version used for GEM is the cleaned E2E NLG dataset, which filters examples with hallucinations and outputs that don't fully cover all input attributes.
You can load the dataset via:
```
import datasets
data = datasets.load_dataset('GEM/e2e_nlg')
```
The data loader can be found [here](https://huggingface.co/datasets/GEM/e2e_nlg).
#### website
[Website](http://www.macs.hw.ac.uk/InteractionLab/E2E/)
#### paper
[First data release](https://www.aclweb.org/anthology/W17-5525/), [Detailed E2E Challenge writeup](https://doi.org/10.1016/j.csl.2019.06.009), [Cleaned E2E version](https://www.aclweb.org/anthology/W19-8652/)
#### authors
Jekaterina Novikova, Ondrej Dusek and Verena Rieser
## Dataset Overview
### Where to find the Data and its Documentation
#### Webpage
<!-- info: What is the webpage for the dataset (if it exists)? -->
<!-- scope: telescope -->
[Website](http://www.macs.hw.ac.uk/InteractionLab/E2E/)
#### Download
<!-- info: What is the link to where the original dataset is hosted? -->
<!-- scope: telescope -->
[Github](https://github.com/tuetschek/e2e-cleaning)
#### Paper
<!-- info: What is the link to the paper describing the dataset (open access preferred)? -->
<!-- scope: telescope -->
[First data release](https://www.aclweb.org/anthology/W17-5525/), [Detailed E2E Challenge writeup](https://doi.org/10.1016/j.csl.2019.06.009), [Cleaned E2E version](https://www.aclweb.org/anthology/W19-8652/)
#### BibTex
<!-- info: Provide the BibTex-formatted reference for the dataset. Please use the correct published version (ACL anthology, etc.) instead of google scholar created Bibtex. -->
<!-- scope: microscope -->
```
@inproceedings{e2e_cleaned,
address = {Tokyo, Japan},
title = {Semantic {Noise} {Matters} for {Neural} {Natural} {Language} {Generation}},
url = {https://www.aclweb.org/anthology/W19-8652/},
booktitle = {Proceedings of the 12th {International} {Conference} on {Natural} {Language} {Generation} ({INLG} 2019)},
author = {Dušek, Ondřej and Howcroft, David M and Rieser, Verena},
year = {2019},
pages = {421--426},
}
```
#### Contact Name
<!-- quick -->
<!-- info: If known, provide the name of at least one person the reader can contact for questions about the dataset. -->
<!-- scope: periscope -->
Ondrej Dusek
#### Contact Email
<!-- info: If known, provide the email of at least one person the reader can contact for questions about the dataset. -->
<!-- scope: periscope -->
odusek@ufal.mff.cuni.cz
#### Has a Leaderboard?
<!-- info: Does the dataset have an active leaderboard? -->
<!-- scope: telescope -->
no
### Languages and Intended Use
#### Multilingual?
<!-- quick -->
<!-- info: Is the dataset multilingual? -->
<!-- scope: telescope -->
no
#### Covered Dialects
<!-- info: What dialects are covered? Are there multiple dialects per language? -->
<!-- scope: periscope -->
Dialect-specific data was not collected and the language is general British English.
#### Covered Languages
<!-- quick -->
<!-- info: What languages/dialects are covered in the dataset? -->
<!-- scope: telescope -->
`English`
#### Whose Language?
<!-- info: Whose language is in the dataset? -->
<!-- scope: periscope -->
The original dataset was collected using the CrowdFlower (now Appen) platform using native English speakers (self-reported). No demographic information was provided, but the collection was geographically limited to English-speaking countries.
#### License
<!-- quick -->
<!-- info: What is the license of the dataset? -->
<!-- scope: telescope -->
cc-by-sa-4.0: Creative Commons Attribution Share Alike 4.0 International
#### Intended Use
<!-- info: What is the intended use of the dataset? -->
<!-- scope: microscope -->
The dataset was collected to test neural model on a very well specified realization task.
#### Primary Task
<!-- info: What primary task does the dataset support? -->
<!-- scope: telescope -->
Data-to-Text
#### Communicative Goal
<!-- quick -->
<!-- info: Provide a short description of the communicative goal of a model trained for this task on this dataset. -->
<!-- scope: periscope -->
Producing a text informing/recommending a restaurant, given all and only the attributes specified on the input.
### Credit
#### Curation Organization Type(s)
<!-- info: In what kind of organization did the dataset curation happen? -->
<!-- scope: telescope -->
`academic`
#### Curation Organization(s)
<!-- info: Name the organization(s). -->
<!-- scope: periscope -->
Heriot-Watt University
#### Dataset Creators
<!-- info: Who created the original dataset? List the people involved in collecting the dataset and their affiliation(s). -->
<!-- scope: microscope -->
Jekaterina Novikova, Ondrej Dusek and Verena Rieser
#### Funding
<!-- info: Who funded the data creation? -->
<!-- scope: microscope -->
This research received funding from the EPSRC projects DILiGENt (EP/M005429/1) and MaDrIgAL (EP/N017536/1).
#### Who added the Dataset to GEM?
<!-- info: Who contributed to the data card and adding the dataset to GEM? List the people+affiliations involved in creating this data card and who helped integrate this dataset into GEM. -->
<!-- scope: microscope -->
Simon Mille wrote the initial data card and Yacine Jernite the data loader. Sebastian Gehrmann migrated the data card to the v2 format and moved the data loader to the hub.
### Dataset Structure
#### Data Fields
<!-- info: List and describe the fields present in the dataset. -->
<!-- scope: telescope -->
The data is in a CSV format, with the following fields:
* `mr` -- the meaning representation (MR, input)
* `ref` -- reference, i.e. the corresponding natural-language description (output)
There are additional fields (`fixed`, `orig_mr`) indicating whether the data was modified in the
cleaning process and what was the original MR before cleaning, but these aren't used for NLG.
The MR has a flat structure -- attribute-value pairs are comma separated, with values
enclosed in brackets (see example above). There are 8 attributes:
* `name` -- restaurant name
* `near` -- a landmark close to the restaurant
* `area` -- location (riverside, city centre)
* `food` -- food type / cuisine (e.g. Japanese, Indian, English etc.)
* `eatType` -- restaurant type (restaurant, coffee shop, pub)
* `priceRange` -- price range (low, medium, high, <£20, £20-30, >£30)
* `rating` -- customer rating (low, medium, high, 1/5, 3/5, 5/5)
* `familyFriendly` -- is the restaurant family-friendly (yes/no)
The same MR is often repeated multiple times with different synonymous references.
#### How were labels chosen?
<!-- info: How were the labels chosen? -->
<!-- scope: microscope -->
The source MRs were generated automatically at random from a set of valid attribute values. The labels were crowdsourced and are natural language
#### Example Instance
<!-- info: Provide a JSON formatted example of a typical instance in the dataset. -->
<!-- scope: periscope -->
```
{
"input": "name[Alimentum], area[riverside], familyFriendly[yes], near[Burger King]",
"target": "Alimentum is a kids friendly place in the riverside area near Burger King."
}
```
#### Data Splits
<!-- info: Describe and name the splits in the dataset if there are more than one. -->
<!-- scope: periscope -->
| | MRs | Distinct MRs | References |
|-------------|------|--------------|------------|
| Training |12,568| 8,362 | 33,525 |
| Development | 1,484| 1,132 | 4,299 |
| Test | 1,847| 1,358 | 4,693 |
| Total |15,899| 10,852 | 42,517 |
“Distinct MRs” are MRs that remain distinct even if restaurant/place names (attributes `name`, `near`)
are delexicalized, i.e., replaced with a placeholder.
#### Splitting Criteria
<!-- info: Describe any criteria for splitting the data, if used. If there are differences between the splits (e.g., if the training annotations are machine-generated and the dev and test ones are created by humans, or if different numbers of annotators contributed to each example), describe them here. -->
<!-- scope: microscope -->
The data are divided so that MRs in different splits do not overlap.
## Dataset in GEM
### Rationale for Inclusion in GEM
#### Why is the Dataset in GEM?
<!-- info: What does this dataset contribute toward better generation evaluation and why is it part of GEM? -->
<!-- scope: microscope -->
The E2E dataset is one of the largest limited-domain NLG datasets and is frequently used as a data-to-text generation benchmark. The E2E Challenge included 20 systems of very different architectures, with system outputs available for download.
#### Similar Datasets
<!-- info: Do other datasets for the high level task exist? -->
<!-- scope: telescope -->
yes
#### Unique Language Coverage
<!-- info: Does this dataset cover other languages than other datasets for the same task? -->
<!-- scope: periscope -->
no
#### Difference from other GEM datasets
<!-- info: What else sets this dataset apart from other similar datasets in GEM? -->
<!-- scope: microscope -->
The dataset is much cleaner than comparable datasets, and it is also a relatively easy task, making for a straightforward evaluation.
#### Ability that the Dataset measures
<!-- info: What aspect of model ability can be measured with this dataset? -->
<!-- scope: periscope -->
surface realization.
### GEM-Specific Curation
#### Modificatied for GEM?
<!-- info: Has the GEM version of the dataset been modified in any way (data, processing, splits) from the original curated data? -->
<!-- scope: telescope -->
yes
#### Additional Splits?
<!-- info: Does GEM provide additional splits to the dataset? -->
<!-- scope: telescope -->
yes
#### Split Information
<!-- info: Describe how the new splits were created -->
<!-- scope: periscope -->
4 special test sets for E2E were added to the GEM evaluation suite.
1. We created subsets of the training and development sets of ~500 randomly selected inputs each.
2. We applied input scrambling on a subset of 500 randomly selected test instances; the order of the input properties was randomly reassigned.
3. For the input size, we created subpopulations based on the number of restaurant properties in the input.
| Input length | Frequency English |
|---------------|-------------------|
| 2 | 5 |
| 3 | 120 |
| 4 | 389 |
| 5 | 737 |
| 6 | 1187 |
| 7 | 1406 |
| 8 | 774 |
| 9 | 73 |
| 10 | 2 |
#### Split Motivation
<!-- info: What aspects of the model's generation capacities were the splits created to test? -->
<!-- scope: periscope -->
Generalization and robustness
### Getting Started with the Task
## Previous Results
### Previous Results
#### Measured Model Abilities
<!-- info: What aspect of model ability can be measured with this dataset? -->
<!-- scope: telescope -->
Surface realization.
#### Metrics
<!-- info: What metrics are typically used for this task? -->
<!-- scope: periscope -->
`BLEU`, `METEOR`, `ROUGE`
#### Proposed Evaluation
<!-- info: List and describe the purpose of the metrics and evaluation methodology (including human evaluation) that the dataset creators used when introducing this task. -->
<!-- scope: microscope -->
The official evaluation script combines the MT-Eval and COCO Captioning libraries with the following metrics.
- BLEU
- CIDEr
- NIST
- METEOR
- ROUGE-L
#### Previous results available?
<!-- info: Are previous results available? -->
<!-- scope: telescope -->
yes
#### Other Evaluation Approaches
<!-- info: What evaluation approaches have others used? -->
<!-- scope: periscope -->
Most previous results, including the shared task results, used the library provided by the dataset creators. The shared task also conducted a human evaluation using the following two criteria:
- `Quality`: When collecting quality ratings, system outputs were presented to crowd workers together with the corresponding meaning representation, which implies that correctness of the NL utterance relative to the MR should also influence this ranking. The crowd workers were asked: “How do you judge the overall quality of the utterance in terms of its grammatical correctness, fluency, adequacy and other important factors?”
- `Naturalness`: When collecting naturalness ratings, system outputs were presented to crowd workers without the corresponding meaning representation. The crowd workers were asked: “Could the utterance have been produced by a native speaker?”
#### Relevant Previous Results
<!-- info: What are the most relevant previous results for this task/dataset? -->
<!-- scope: microscope -->
The shared task writeup has in-depth evaluations of systems (https://www.sciencedirect.com/science/article/pii/S0885230819300919)
## Dataset Curation
### Original Curation
#### Original Curation Rationale
<!-- info: Original curation rationale -->
<!-- scope: telescope -->
The dataset was collected to showcase/test neural NLG models. It is larger and contains more lexical richness and syntactic variation than previous closed-domain NLG datasets.
#### Communicative Goal
<!-- info: What was the communicative goal? -->
<!-- scope: periscope -->
Producing a text informing/recommending a restaurant, given all and only the attributes specified on the input.
#### Sourced from Different Sources
<!-- info: Is the dataset aggregated from different data sources? -->
<!-- scope: telescope -->
no
### Language Data
#### How was Language Data Obtained?
<!-- info: How was the language data obtained? -->
<!-- scope: telescope -->
`Crowdsourced`
#### Where was it crowdsourced?
<!-- info: If crowdsourced, where from? -->
<!-- scope: periscope -->
`Other crowdworker platform`
#### Language Producers
<!-- info: What further information do we have on the language producers? -->
<!-- scope: microscope -->
Human references describing the MRs were collected by crowdsourcing on the CrowdFlower (now Appen) platform,
with either textual or pictorial MRs as a baseline.
The pictorial MRs were used in 20% of cases -- these yield higher lexical variation but introduce noise.
#### Topics Covered
<!-- info: Does the language in the dataset focus on specific topics? How would you describe them? -->
<!-- scope: periscope -->
The dataset is focused on descriptions of restaurants.
#### Data Validation
<!-- info: Was the text validated by a different worker or a data curator? -->
<!-- scope: telescope -->
validated by data curator
#### Data Preprocessing
<!-- info: How was the text data pre-processed? (Enter N/A if the text was not pre-processed) -->
<!-- scope: microscope -->
There were basic checks (length, valid characters, repetition).
#### Was Data Filtered?
<!-- info: Were text instances selected or filtered? -->
<!-- scope: telescope -->
algorithmically
#### Filter Criteria
<!-- info: What were the selection criteria? -->
<!-- scope: microscope -->
The cleaned version of the dataset which we are using in GEM was algorithmically filtered. They used regular expressions to match all human-generated references with a more accurate input when attributes were hallucinated or dropped. Additionally, train-test overlap stemming from the transformation was removed. As a result, this data is much cleaner than the original dataset but not perfect (about 20% of instances may have misaligned slots, compared to 40% of the original data.
### Structured Annotations
#### Additional Annotations?
<!-- quick -->
<!-- info: Does the dataset have additional annotations for each instance? -->
<!-- scope: telescope -->
none
#### Annotation Service?
<!-- info: Was an annotation service used? -->
<!-- scope: telescope -->
no
### Consent
#### Any Consent Policy?
<!-- info: Was there a consent policy involved when gathering the data? -->
<!-- scope: telescope -->
yes
#### Consent Policy Details
<!-- info: What was the consent policy? -->
<!-- scope: microscope -->
Since a crowdsourcing platform was used, the involved raters waived their rights to the data and are aware that the produced annotations can be publicly released.
### Private Identifying Information (PII)
#### Contains PII?
<!-- quick -->
<!-- info: Does the source language data likely contain Personal Identifying Information about the data creators or subjects? -->
<!-- scope: telescope -->
no PII
#### Justification for no PII
<!-- info: Provide a justification for selecting `no PII` above. -->
<!-- scope: periscope -->
The dataset is artificial and does not contain any description of people.
### Maintenance
#### Any Maintenance Plan?
<!-- info: Does the original dataset have a maintenance plan? -->
<!-- scope: telescope -->
no
## Broader Social Context
### Previous Work on the Social Impact of the Dataset
#### Usage of Models based on the Data
<!-- info: Are you aware of cases where models trained on the task featured in this dataset ore related tasks have been used in automated systems? -->
<!-- scope: telescope -->
no
### Impact on Under-Served Communities
#### Addresses needs of underserved Communities?
<!-- info: Does this dataset address the needs of communities that are traditionally underserved in language technology, and particularly language generation technology? Communities may be underserved for exemple because their language, language variety, or social or geographical context is underepresented in NLP and NLG resources (datasets and models). -->
<!-- scope: telescope -->
no
### Discussion of Biases
#### Any Documented Social Biases?
<!-- info: Are there documented social biases in the dataset? Biases in this context are variations in the ways members of different social categories are represented that can have harmful downstream consequences for members of the more disadvantaged group. -->
<!-- scope: telescope -->
no
#### Are the Language Producers Representative of the Language?
<!-- info: Does the distribution of language producers in the dataset accurately represent the full distribution of speakers of the language world-wide? If not, how does it differ? -->
<!-- scope: periscope -->
The source data is generated randomly, so it should not contain biases. The human references may be biased by the workers' demographic, but that was not investigated upon data collection.
## Considerations for Using the Data
### PII Risks and Liability
### Licenses
#### Copyright Restrictions on the Dataset
<!-- info: Based on your answers in the Intended Use part of the Data Overview Section, which of the following best describe the copyright and licensing status of the dataset? -->
<!-- scope: periscope -->
`open license - commercial use allowed`
#### Copyright Restrictions on the Language Data
<!-- info: Based on your answers in the Language part of the Data Curation Section, which of the following best describe the copyright and licensing status of the underlying language data? -->
<!-- scope: periscope -->
`open license - commercial use allowed`
### Known Technical Limitations
#### Technical Limitations
<!-- info: Describe any known technical limitations, such as spurrious correlations, train/test overlap, annotation biases, or mis-annotations, and cite the works that first identified these limitations when possible. -->
<!-- scope: microscope -->
The cleaned version still has data points with hallucinated or omitted attributes.
#### Unsuited Applications
<!-- info: When using a model trained on this dataset in a setting where users or the public may interact with its predictions, what are some pitfalls to look out for? In particular, describe some applications of the general task featured in this dataset that its curation or properties make it less suitable for. -->
<!-- scope: microscope -->
The data only pertains to the restaurant domain and the included attributes. A model cannot be expected to handle other domains or attributes.
|
GEM/mlb_data_to_text | ---
annotations_creators:
- none
language_creators:
- unknown
language:
- en
license:
- other
multilinguality:
- unknown
size_categories:
- unknown
source_datasets:
- original
task_categories:
- table-to-text
task_ids: []
pretty_name: mlb_data_to_text
tags:
- data-to-text
---
# Dataset Card for GEM/mlb_data_to_text
## Dataset Description
- **Homepage:** https://github.com/ratishsp/mlb-data-scripts
- **Repository:** https://github.com/ratishsp/mlb-data-scripts
- **Paper:** https://aclanthology.org/P19-1195
- **Leaderboard:** N/A
- **Point of Contact:** Ratish Puduppully
### Link to Main Data Card
You can find the main data card on the [GEM Website](https://gem-benchmark.com/data_cards/mlb_data_to_text).
### Dataset Summary
The MLB dataset is an English sport-related data-to-text dataset in the baseball domain. The input is a large table with results of a game and the output is a description of the game.
You can load the dataset via:
```
import datasets
data = datasets.load_dataset('GEM/mlb_data_to_text')
```
The data loader can be found [here](https://huggingface.co/datasets/GEM/mlb_data_to_text).
#### website
[Github](https://github.com/ratishsp/mlb-data-scripts)
#### paper
[ACL Anthology](https://aclanthology.org/P19-1195)
#### authors
Ratish Puduppully, Li Dong, Mirella Lapata
## Dataset Overview
### Where to find the Data and its Documentation
#### Webpage
<!-- info: What is the webpage for the dataset (if it exists)? -->
<!-- scope: telescope -->
[Github](https://github.com/ratishsp/mlb-data-scripts)
#### Download
<!-- info: What is the link to where the original dataset is hosted? -->
<!-- scope: telescope -->
[Github](https://github.com/ratishsp/mlb-data-scripts)
#### Paper
<!-- info: What is the link to the paper describing the dataset (open access preferred)? -->
<!-- scope: telescope -->
[ACL Anthology](https://aclanthology.org/P19-1195)
#### BibTex
<!-- info: Provide the BibTex-formatted reference for the dataset. Please use the correct published version (ACL anthology, etc.) instead of google scholar created Bibtex. -->
<!-- scope: microscope -->
```
@inproceedings{puduppully-etal-2019-data,
title = "Data-to-text Generation with Entity Modeling",
author = "Puduppully, Ratish and
Dong, Li and
Lapata, Mirella",
booktitle = "Proceedings of the 57th Annual Meeting of the Association for Computational Linguistics",
month = jul,
year = "2019",
address = "Florence, Italy",
publisher = "Association for Computational Linguistics",
url = "https://www.aclweb.org/anthology/P19-1195",
doi = "10.18653/v1/P19-1195",
pages = "2023--2035",
}
```
#### Contact Name
<!-- quick -->
<!-- info: If known, provide the name of at least one person the reader can contact for questions about the dataset. -->
<!-- scope: periscope -->
Ratish Puduppully
#### Contact Email
<!-- info: If known, provide the email of at least one person the reader can contact for questions about the dataset. -->
<!-- scope: periscope -->
ratishpuduppully@gmail.com
#### Has a Leaderboard?
<!-- info: Does the dataset have an active leaderboard? -->
<!-- scope: telescope -->
no
### Languages and Intended Use
#### Multilingual?
<!-- quick -->
<!-- info: Is the dataset multilingual? -->
<!-- scope: telescope -->
no
#### Covered Languages
<!-- quick -->
<!-- info: What languages/dialects are covered in the dataset? -->
<!-- scope: telescope -->
`English`
#### License
<!-- quick -->
<!-- info: What is the license of the dataset? -->
<!-- scope: telescope -->
other: Other license
#### Intended Use
<!-- info: What is the intended use of the dataset? -->
<!-- scope: microscope -->
The dataset can be used to study data-to-text generation. The dataset is in sports domain. It pairs statistics of Major League Baseball (MLB) game with its summary. The summary is in the form of a document containing an average of 540 tokens. Thus it is useful to study long document generation.
#### Add. License Info
<!-- info: What is the 'other' license of the dataset? -->
<!-- scope: periscope -->
Restricted to non-commercial research purposes.
#### Primary Task
<!-- info: What primary task does the dataset support? -->
<!-- scope: telescope -->
Data-to-Text
#### Communicative Goal
<!-- quick -->
<!-- info: Provide a short description of the communicative goal of a model trained for this task on this dataset. -->
<!-- scope: periscope -->
Produce a summary of MLB game from its statistics.
### Credit
#### Curation Organization Type(s)
<!-- info: In what kind of organization did the dataset curation happen? -->
<!-- scope: telescope -->
`academic`
#### Curation Organization(s)
<!-- info: Name the organization(s). -->
<!-- scope: periscope -->
University of Edinburgh
#### Dataset Creators
<!-- info: Who created the original dataset? List the people involved in collecting the dataset and their affiliation(s). -->
<!-- scope: microscope -->
Ratish Puduppully, Li Dong, Mirella Lapata
### Dataset Structure
#### Data Fields
<!-- info: List and describe the fields present in the dataset. -->
<!-- scope: telescope -->
```
features = datasets.Features(
{
"home_name": datasets.Value("string"),
"box_score": [
{
"p_l": datasets.Value("string"),
"last_name": datasets.Value("string"),
"p_h": datasets.Value("string"),
"sac": datasets.Value("string"),
"p_bb": datasets.Value("string"),
"pos": datasets.Value("string"),
"ao": datasets.Value("string"),
"p_bf": datasets.Value("string"),
"cs": datasets.Value("string"),
"hbp": datasets.Value("string"),
"ab": datasets.Value("string"),
"full_name": datasets.Value("string"),
"p_w": datasets.Value("string"),
"go": datasets.Value("string"),
"fldg": datasets.Value("string"),
"p_bs": datasets.Value("string"),
"avg": datasets.Value("string"),
"p_r": datasets.Value("string"),
"p_s": datasets.Value("string"),
"lob": datasets.Value("string"),
"first_name": datasets.Value("string"),
"p_sv": datasets.Value("string"),
"p_so": datasets.Value("string"),
"p_save": datasets.Value("string"),
"p_hr": datasets.Value("string"),
"po": datasets.Value("string"),
"p_ip1": datasets.Value("string"),
"p_ip2": datasets.Value("string"),
"bb": datasets.Value("string"),
"ops": datasets.Value("string"),
"p_hld": datasets.Value("string"),
"bo": datasets.Value("string"),
"p_loss": datasets.Value("string"),
"e": datasets.Value("string"),
"p_game_score": datasets.Value("string"),
"p_win": datasets.Value("string"),
"a": datasets.Value("string"),
"p_era": datasets.Value("string"),
"d": datasets.Value("string"),
"p_out": datasets.Value("string"),
"h": datasets.Value("string"),
"p_er": datasets.Value("string"),
"p_np": datasets.Value("string"),
"hr": datasets.Value("string"),
"r": datasets.Value("string"),
"so": datasets.Value("string"),
"t": datasets.Value("string"),
"rbi": datasets.Value("string"),
"team": datasets.Value("string"),
"sb": datasets.Value("string"),
"slg": datasets.Value("string"),
"sf": datasets.Value("string"),
"obp": datasets.Value("string"),
}
],
"home_city": datasets.Value("string"),
"vis_name": datasets.Value("string"),
"play_by_play": [{
"top": [{
"runs": datasets.Value("string"),
"scorers": [
datasets.Value("string")
],
"pitcher": datasets.Value("string"),
"o": datasets.Value("string"),
"b": datasets.Value("string"),
"s": datasets.Value("string"),
"batter": datasets.Value("string"),
"b1": [
datasets.Value("string")
],
"b2": [
datasets.Value("string")
],
"b3": [
datasets.Value("string")
],
"event": datasets.Value("string"),
"event2": datasets.Value("string"),
"home_team_runs": datasets.Value("string"),
"away_team_runs": datasets.Value("string"),
"rbi": datasets.Value("string"),
"error_runs": datasets.Value("string"),
"fielder_error": datasets.Value("string")
}
],
"bottom": [{
"runs": datasets.Value("string"),
"scorers": [
datasets.Value("string")
],
"pitcher": datasets.Value("string"),
"o": datasets.Value("string"),
"b": datasets.Value("string"),
"s": datasets.Value("string"),
"batter": datasets.Value("string"),
"b1": [
datasets.Value("string")
],
"b2": [
datasets.Value("string")
],
"b3": [
datasets.Value("string")
],
"event": datasets.Value("string"),
"event2": datasets.Value("string"),
"home_team_runs": datasets.Value("string"),
"away_team_runs": datasets.Value("string"),
"rbi": datasets.Value("string"),
"error_runs": datasets.Value("string"),
"fielder_error": datasets.Value("string")
}
],
"inning": datasets.Value("string")
}
],
"vis_line": {
"innings": [{
"inn": datasets.Value("string"),
"runs": datasets.Value("string")
}
],
"result": datasets.Value("string"),
"team_runs": datasets.Value("string"),
"team_hits": datasets.Value("string"),
"team_errors": datasets.Value("string"),
"team_name": datasets.Value("string"),
"team_city": datasets.Value("string")
},
"home_line": {
"innings": [{
"inn": datasets.Value("string"),
"runs": datasets.Value("string")
}
],
"result": datasets.Value("string"),
"team_runs": datasets.Value("string"),
"team_hits": datasets.Value("string"),
"team_errors": datasets.Value("string"),
"team_name": datasets.Value("string"),
"team_city": datasets.Value("string")
},
"vis_city": datasets.Value("string"),
"day": datasets.Value("string"),
"summary": [
datasets.Value("string"),
],
"gem_id": datasets.Value("string")
}
```
#### Reason for Structure
<!-- info: How was the dataset structure determined? -->
<!-- scope: microscope -->
The high level structure contains the following attributes: home_name, vis_name, home_city, vis_city, summary, summary_eval, day, gem_id, box_score, play_by_play, home_line, vis_line.
The attributes home_name, vis_name, home_city, vis_city and day are string values.
The attribute "summary" contains the summary in the form of a list of tokens.
The attribute "summary_eval" contains the summary in the form of a string of tokens. The difference from "summary" field is that "summary_eval" doesn't contain "*NEWPARAGRAPH*" delimiters to separate the paragraphs. "summary_eval" field should be used to evaluate model outputs. "summary" field may be used during the training process.
box_score contains the box score statistics of the players in the game. It is in the form of a list (of average size 90), with each element describing the statistics of a player. The box score statistics contain 53 attributes.
The description of the attributes is given below. The descriptions of most of the attributes is obtained from [mlb.com](https://www.mlb.com/glossary/standard-stats).
- r : Runs scored by a player in the game.
- rbi Runs Batted In (RBI): action of a batter results in a run scored by other players in the team.
- pos Position of the player.
- avg Batting Average. It is an indicator of the hits in the players' career.
- bb A walk occurs when a pitcher throws four pitches out of the strike zone, none of which are swung at by the hitter.
- hr Batter hits the ball in the air over the outfield fence.
- p_r Runs given by a pitcher in the game.
- p_bb Walks allowed by pitcher in a game.
- p_h Hits allowed by pitcher in a game.
- p_hr Home runs allowed by pitcher in a game.
- p_er Earned Run (ER): An earned run is any run that scores against a pitcher.
- p_era Earned Run Average (ERA): Earned run average represents the number of earned runs a pitcher allows per nine innings.
- p_np Number of Pitches: A pitcher's total number of pitches is determined by all the pitches he throws in game.
- p_ip1 Innings Pitched (IP1): Innings pitched measures the number of innings a pitcher remains in a game. Because there are three outs in an inning, each out recorded represents one-third of an inning pitched.
- p_ip2 Innings Pitched (IP2): Innings pitched measures the number of innings a pitcher remains in a game. Because there are three outs in an inning, each out recorded represents one-third of an inning pitched.
- p_w A pitcher receives a win when he is the pitcher of record when his team takes the lead for good.
- p_l A pitcher receives a loss when a run that is charged to him proves to be the go-ahead run in the game, giving the opposing team a lead it never gives up.
- p_so A strikeout occurs when a pitcher throws any combination of three swinging or looking strikes to a hitter.
- p_save Save: A save is awarded to the relief pitcher who finishes a game for the winning team. A pitcher cannot receive a save and a win in the same game.
- p_sv Saves: The count of saves recorded by a pitcher in his career.
- sac A sacrifice fly occurs when a batter hits a fly-ball out to the outfield or foul territory that allows a runner to score.
- p_bf Batters faced is simply a count of the number of total plate appearances against a certain pitcher or team. In a perfect game -- with 27 outs -- a pitcher will record 27 batters faced.
- cs A caught stealing occurs when a runner attempts to steal but is tagged out before reaching second base, third base or home plate.
- hbp A hit-by-pitch occurs when a batter is struck by a pitched ball without swinging at it. He is awarded first base as a result.
- ab An official at-bat comes when a batter reaches base via a fielder's choice, hit or an error (not including catcher's interference) or when a batter is put out on a non-sacrifice.
- p_bs A blown save occurs when a relief pitcher enters a game in a save situation, but allows the tying run to score.
- p_s The count of strikes thrown by a pitcher
- lob Left on base can be viewed as both an individual statistic or as a team statistic. In an individual batter's case, it refers to how many men remain on base after that batter makes an out at the plate, as the batter has failed to do his job to score those runners -- or at least put himself in a position to score. In a team's case or in an individual pitcher's case, it refers to the number of men who remain on base at the end of an inning.
- po A fielder is credited with a putout when he is the fielder who physically records the act of completing an out -- whether it be by stepping on the base for a forceout, tagging a runner, catching a batted ball, or catching a third strike
- ops OPS adds on-base percentage and slugging percentage to get one number that unites the two. It's meant to combine how well a hitter can reach base, with how well he can hit for average and for power.
- p_hld A hold occurs when a relief pitcher enters the game in a save situation and maintains his team's lead for the next relief pitcher, while recording at least one out.
- p_loss True/False- Indicates losing pitcher
- e A fielder is given an error if, in the judgment of the official scorer, he fails to convert an out on a play that an average fielder should have made.
- p_win True/False- Indicates winning pitcher
- a An assist is awarded to a fielder who touches the ball before a putout is recorded by another fielder.
- h A hit occurs when a batter strikes the baseball into fair territory and reaches base without doing so via an error or a fielder's choice.
- so A strikeout of a batter
- team Team of the player
- sb A stolen base occurs when a baserunner advances by taking a base to which he isn't entitled.
- slg Slugging percentage represents the total number of bases a player records per at-bat. Unlike on-base percentage, slugging percentage deals only with hits and does not include walks and hit-by-pitches in its equation.
- sf A sacrifice fly occurs when a batter hits a fly-ball out to the outfield or foul territory that allows a runner to score.
- obp OBP refers to how frequently a batter reaches base per plate appearance. Times on base include hits, walks and hit-by-pitches, but do not include errors, times reached on a fielder's choice or a dropped third strike.
The description of attributes in play-by-play is below:
- batter Batter in the play.
- pitcher Pitcher in play.
- b1 Player/s at first base position.
- b2 Player/s at second base position.
- b3 Player/s at third base position.
- scorers Player/s scored in the play.
- fielder_error Player committed field error.
- event Event of the play such as single, double, home run etc.
- event2 Second event of the play such as wild pitch, error etc.
- inning Inning of the play.
- top/ bottom If home team is batting it is bottom and if away team is batting it is top.
- o Count of outs
- b Count of balls
- s Count of strikes
- r Count of runs
- rbi Count of runs batted in (rbi)
- error_runs Runs due to error
- home_team_runs Score of home team
- vis_team_runs Score of visiting team
`home_line` and `vis_line` contain string value pairs for `team_name`, `team_city`, `team_runs`, `team_hits`, `team_error`, `result`, and a list of runs scored in each inning.
#### Data Splits
<!-- info: Describe and name the splits in the dataset if there are more than one. -->
<!-- scope: periscope -->
There are three splits in the dataset: train, validation and test
#### Splitting Criteria
<!-- info: Describe any criteria for splitting the data, if used. If there are differences between the splits (e.g., if the training annotations are machine-generated and the dev and test ones are created by humans, or if different numbers of annotators contributed to each example), describe them here. -->
<!-- scope: microscope -->
The splits are random.
## Dataset in GEM
### Rationale for Inclusion in GEM
#### Why is the Dataset in GEM?
<!-- info: What does this dataset contribute toward better generation evaluation and why is it part of GEM? -->
<!-- scope: microscope -->
This dataset can verify if models are capable of long document generation. The challenges in long document generation conditioned on input tables include ensuring coherent output, staying faithful to the input, ensuring fluent output and avoiding repetition of text. Such aspects can be verified on models trained on this dataset
#### Similar Datasets
<!-- info: Do other datasets for the high level task exist? -->
<!-- scope: telescope -->
yes
#### Unique Language Coverage
<!-- info: Does this dataset cover other languages than other datasets for the same task? -->
<!-- scope: periscope -->
no
#### Difference from other GEM datasets
<!-- info: What else sets this dataset apart from other similar datasets in GEM? -->
<!-- scope: microscope -->
Compared to the existing RotoWire (Wiseman et al. 2017) dataset, MLB summaries are longer (approximately by 50%) and the input records are richer and more structured (with the addition of play-by-play). Moreover, the MLB dataset is five times larger in terms of data size (i.e., pairs of tables and game summaries).
#### Ability that the Dataset measures
<!-- info: What aspect of model ability can be measured with this dataset? -->
<!-- scope: periscope -->
Long document generation, coherent ordering of information, faithfulness to the input statistics, fluency in generation and avoiding repetition of text.
### GEM-Specific Curation
#### Modificatied for GEM?
<!-- info: Has the GEM version of the dataset been modified in any way (data, processing, splits) from the original curated data? -->
<!-- scope: telescope -->
yes
#### GEM Modifications
<!-- info: What changes have been made to he original dataset? -->
<!-- scope: periscope -->
`data points removed`
#### Modification Details
<!-- info: For each of these changes, described them in more details and provided the intended purpose of the modification -->
<!-- scope: microscope -->
Some examples have been removed from training dataset which satisfied the below criteria:
1. The examples in training dataset which overlapped with validation/test.
2. Some examples which described washed out games.
#### Additional Splits?
<!-- info: Does GEM provide additional splits to the dataset? -->
<!-- scope: telescope -->
no
### Getting Started with the Task
#### Pointers to Resources
<!-- info: Getting started with in-depth research on the task. Add relevant pointers to resources that researchers can consult when they want to get started digging deeper into the task. -->
<!-- scope: microscope -->
The [research paper](https://aclanthology.org/P19-1195) is a good resource
## Previous Results
### Previous Results
#### Measured Model Abilities
<!-- info: What aspect of model ability can be measured with this dataset? -->
<!-- scope: telescope -->
Automatic evaluation measure can evaluate the factuality, content selection, content ordering and the fluency of the model output. The factuality, content selection and content ordering is measured using an Information Extraction based evaluation approach introduced by Wiseman et al (2017). The fluency is measured using BLEU.
#### Metrics
<!-- info: What metrics are typically used for this task? -->
<!-- scope: periscope -->
`Other: Other Metrics`
#### Other Metrics
<!-- info: Definitions of other metrics -->
<!-- scope: periscope -->
Wiseman et al. (2017) define three metrics induced from the outputs of an Information Extraction model which is run on the model/human-written game summaries . Let ŷ be the gold summary and y the model output.
• Relation Generation (RG) measures the precision and count of relations extracted from y that also appear in records r.
• Content Selection (CS) measures the precision and recall of relations extracted from y that are also extracted from ŷ.
• Content Ordering (CO) measures the complement of the normalized Damerau-Levenshtein distance (Brill and Moore, 2000) between the sequences of relations extracted from y and ŷ
#### Proposed Evaluation
<!-- info: List and describe the purpose of the metrics and evaluation methodology (including human evaluation) that the dataset creators used when introducing this task. -->
<!-- scope: microscope -->
We have reused the automatic metrics based on Information Extraction evaluation introduced by Wiseman et al (2017). For human evaluation, we conducted studies to evaluate the factuality, coherence, grammaticality and conciseness.
#### Previous results available?
<!-- info: Are previous results available? -->
<!-- scope: telescope -->
yes
#### Relevant Previous Results
<!-- info: What are the most relevant previous results for this task/dataset? -->
<!-- scope: microscope -->
The most relevant previous results for dataset are in the TACL 2021 paper on [Data-to-text Generation with Macro Planning](https://direct.mit.edu/tacl/article/doi/10.1162/tacl_a_00381/101876/Data-to-text-Generation-with-Macro-Planning)
## Dataset Curation
### Original Curation
#### Original Curation Rationale
<!-- info: Original curation rationale -->
<!-- scope: telescope -->
This dataset was curated to complement an existing data-to-text generation dataset (RotoWire by Wiseman et al. 2017) which focuses on long document generation. Compared to RotoWire , MLB summaries are longer (approximately by 50%) and the input records are richer and more structured (with the addition of play-by-play). Moreover, the MLB dataset is five times larger in terms of data size (i.e., pairs of tables and game summaries)
#### Communicative Goal
<!-- info: What was the communicative goal? -->
<!-- scope: periscope -->
The goal is to study automatic generation of long documents in a data-to-text setting. The generated summaries should exhibit coherent ordering of content, be faithful to the input statistics, be fluent and avoid repetition of text.
#### Sourced from Different Sources
<!-- info: Is the dataset aggregated from different data sources? -->
<!-- scope: telescope -->
no
### Language Data
#### How was Language Data Obtained?
<!-- info: How was the language data obtained? -->
<!-- scope: telescope -->
`Found`
#### Where was it found?
<!-- info: If found, where from? -->
<!-- scope: telescope -->
`Single website`
#### Language Producers
<!-- info: What further information do we have on the language producers? -->
<!-- scope: microscope -->
The game summaries are produced by professional writers.
#### Topics Covered
<!-- info: Does the language in the dataset focus on specific topics? How would you describe them? -->
<!-- scope: periscope -->
The language focuses on the sports domain.
#### Data Validation
<!-- info: Was the text validated by a different worker or a data curator? -->
<!-- scope: telescope -->
not validated
#### Data Preprocessing
<!-- info: How was the text data pre-processed? (Enter N/A if the text was not pre-processed) -->
<!-- scope: microscope -->
Game summaries were tokenized using NLTK (Bird et al., 2009) and hyphenated words were separated. Sentences containing quotes were removed as they included opinions and non-factual statements unrelated to the input tables. Sometimes MLB summaries contain a "Game notes" section with incidental information which was also removed.
#### Was Data Filtered?
<!-- info: Were text instances selected or filtered? -->
<!-- scope: telescope -->
not filtered
### Structured Annotations
#### Additional Annotations?
<!-- quick -->
<!-- info: Does the dataset have additional annotations for each instance? -->
<!-- scope: telescope -->
none
#### Annotation Service?
<!-- info: Was an annotation service used? -->
<!-- scope: telescope -->
no
### Consent
#### Any Consent Policy?
<!-- info: Was there a consent policy involved when gathering the data? -->
<!-- scope: telescope -->
no
#### Justification for Using the Data
<!-- info: If not, what is the justification for reusing the data? -->
<!-- scope: microscope -->
The copyright remains with the original data creators and the usage permission is restricted to non-commercial uses.
### Private Identifying Information (PII)
#### Contains PII?
<!-- quick -->
<!-- info: Does the source language data likely contain Personal Identifying Information about the data creators or subjects? -->
<!-- scope: telescope -->
yes/very likely
#### Categories of PII
<!-- info: What categories of PII are present or suspected in the data? -->
<!-- scope: periscope -->
`sensitive information`, `generic PII`
#### Any PII Identification?
<!-- info: Did the curators use any automatic/manual method to identify PII in the dataset? -->
<!-- scope: periscope -->
no identification
### Maintenance
#### Any Maintenance Plan?
<!-- info: Does the original dataset have a maintenance plan? -->
<!-- scope: telescope -->
no
## Broader Social Context
### Previous Work on the Social Impact of the Dataset
#### Usage of Models based on the Data
<!-- info: Are you aware of cases where models trained on the task featured in this dataset ore related tasks have been used in automated systems? -->
<!-- scope: telescope -->
no
### Impact on Under-Served Communities
#### Addresses needs of underserved Communities?
<!-- info: Does this dataset address the needs of communities that are traditionally underserved in language technology, and particularly language generation technology? Communities may be underserved for exemple because their language, language variety, or social or geographical context is underepresented in NLP and NLG resources (datasets and models). -->
<!-- scope: telescope -->
no
### Discussion of Biases
#### Any Documented Social Biases?
<!-- info: Are there documented social biases in the dataset? Biases in this context are variations in the ways members of different social categories are represented that can have harmful downstream consequences for members of the more disadvantaged group. -->
<!-- scope: telescope -->
unsure
## Considerations for Using the Data
### PII Risks and Liability
### Licenses
#### Copyright Restrictions on the Dataset
<!-- info: Based on your answers in the Intended Use part of the Data Overview Section, which of the following best describe the copyright and licensing status of the dataset? -->
<!-- scope: periscope -->
`research use only`
#### Copyright Restrictions on the Language Data
<!-- info: Based on your answers in the Language part of the Data Curation Section, which of the following best describe the copyright and licensing status of the underlying language data? -->
<!-- scope: periscope -->
`research use only`
### Known Technical Limitations
|
GEM/mlsum | ---
annotations_creators:
- none
language_creators:
- unknown
language:
- de
- es
license:
- other
multilinguality:
- unknown
size_categories:
- unknown
source_datasets:
- original
task_categories:
- summarization
task_ids: []
pretty_name: mlsum
---
# Dataset Card for GEM/mlsum
## Dataset Description
- **Homepage:** N/A
- **Repository:** https://gitlab.lip6.fr/scialom/mlsum_data/-/tree/master/MLSUM
- **Paper:** https://aclanthology.org/2020.emnlp-main.647/
- **Leaderboard:** N/A
- **Point of Contact:** Thomas Scialom
### Link to Main Data Card
You can find the main data card on the [GEM Website](https://gem-benchmark.com/data_cards/mlsum).
### Dataset Summary
MLSum is a multilingual summarization dataset crawled from different news websites. The GEM version supports the German and Spanish subset alongside specifically collected challenge sets for COVID-related articles to test out-of-domain generalization.
You can load the dataset via:
```
import datasets
data = datasets.load_dataset('GEM/mlsum')
```
The data loader can be found [here](https://huggingface.co/datasets/GEM/mlsum).
#### website
N/A
#### paper
[ACL Anthology](https://aclanthology.org/2020.emnlp-main.647/)
#### authors
Thomas Scialom, Paul-Alexis Dray, Sylvain Lamprier, Benjamin Piwowarski, Jacopo Staiano
## Dataset Overview
### Where to find the Data and its Documentation
#### Download
<!-- info: What is the link to where the original dataset is hosted? -->
<!-- scope: telescope -->
[Gitlab](https://gitlab.lip6.fr/scialom/mlsum_data/-/tree/master/MLSUM)
#### Paper
<!-- info: What is the link to the paper describing the dataset (open access preferred)? -->
<!-- scope: telescope -->
[ACL Anthology](https://aclanthology.org/2020.emnlp-main.647/)
#### BibTex
<!-- info: Provide the BibTex-formatted reference for the dataset. Please use the correct published version (ACL anthology, etc.) instead of google scholar created Bibtex. -->
<!-- scope: microscope -->
```
@inproceedings{scialom-etal-2020-mlsum,
title = "{MLSUM}: The Multilingual Summarization Corpus",
author = "Scialom, Thomas and
Dray, Paul-Alexis and
Lamprier, Sylvain and
Piwowarski, Benjamin and
Staiano, Jacopo",
booktitle = "Proceedings of the 2020 Conference on Empirical Methods in Natural Language Processing (EMNLP)",
month = nov,
year = "2020",
address = "Online",
publisher = "Association for Computational Linguistics",
url = "https://aclanthology.org/2020.emnlp-main.647",
doi = "10.18653/v1/2020.emnlp-main.647",
pages = "8051--8067",
abstract = "We present MLSUM, the first large-scale MultiLingual SUMmarization dataset. Obtained from online newspapers, it contains 1.5M+ article/summary pairs in five different languages {--} namely, French, German, Spanish, Russian, Turkish. Together with English news articles from the popular CNN/Daily mail dataset, the collected data form a large scale multilingual dataset which can enable new research directions for the text summarization community. We report cross-lingual comparative analyses based on state-of-the-art systems. These highlight existing biases which motivate the use of a multi-lingual dataset.",
}
```
#### Contact Name
<!-- quick -->
<!-- info: If known, provide the name of at least one person the reader can contact for questions about the dataset. -->
<!-- scope: periscope -->
Thomas Scialom
#### Contact Email
<!-- info: If known, provide the email of at least one person the reader can contact for questions about the dataset. -->
<!-- scope: periscope -->
{thomas,paul-alexis,jacopo}@recital.ai, {sylvain.lamprier,benjamin.piwowarski}@lip6.fr
#### Has a Leaderboard?
<!-- info: Does the dataset have an active leaderboard? -->
<!-- scope: telescope -->
no
### Languages and Intended Use
#### Multilingual?
<!-- quick -->
<!-- info: Is the dataset multilingual? -->
<!-- scope: telescope -->
yes
#### Covered Dialects
<!-- info: What dialects are covered? Are there multiple dialects per language? -->
<!-- scope: periscope -->
There is only one dialect per language, Hochdeutsch for German and Castilian Spanish for Spanish.
#### Covered Languages
<!-- quick -->
<!-- info: What languages/dialects are covered in the dataset? -->
<!-- scope: telescope -->
`German`, `Spanish, Castilian`
#### Whose Language?
<!-- info: Whose language is in the dataset? -->
<!-- scope: periscope -->
The German articles are crawled from Süddeutsche Zeitung and the Spanish ones from El Pais.
#### License
<!-- quick -->
<!-- info: What is the license of the dataset? -->
<!-- scope: telescope -->
other: Other license
#### Intended Use
<!-- info: What is the intended use of the dataset? -->
<!-- scope: microscope -->
The intended use of this dataset is to augment existing datasets for English news summarization with additional languages.
#### Add. License Info
<!-- info: What is the 'other' license of the dataset? -->
<!-- scope: periscope -->
Restricted to non-commercial research purposes.
#### Primary Task
<!-- info: What primary task does the dataset support? -->
<!-- scope: telescope -->
Summarization
#### Communicative Goal
<!-- quick -->
<!-- info: Provide a short description of the communicative goal of a model trained for this task on this dataset. -->
<!-- scope: periscope -->
The speaker is required to produce a high quality summary of news articles in the same language as the input article.
### Credit
#### Curation Organization Type(s)
<!-- info: In what kind of organization did the dataset curation happen? -->
<!-- scope: telescope -->
`other`
#### Curation Organization(s)
<!-- info: Name the organization(s). -->
<!-- scope: periscope -->
CNRS, Sorbonne Université, reciTAL
#### Dataset Creators
<!-- info: Who created the original dataset? List the people involved in collecting the dataset and their affiliation(s). -->
<!-- scope: microscope -->
Thomas Scialom, Paul-Alexis Dray, Sylvain Lamprier, Benjamin Piwowarski, Jacopo Staiano
#### Funding
<!-- info: Who funded the data creation? -->
<!-- scope: microscope -->
Funding information is not specified.
#### Who added the Dataset to GEM?
<!-- info: Who contributed to the data card and adding the dataset to GEM? List the people+affiliations involved in creating this data card and who helped integrate this dataset into GEM. -->
<!-- scope: microscope -->
The original data card was written by Pedro Henrique Martins (Instituto de Telecomunicações) and Sebastian Gehrmann (Google Research) extended and updated it to the v2 format. The COVID challenge set was created by Laura Perez-Beltrachini (University of Edinburgh). Data cleaning was done by Juan Diego Rodriguez (UT Austin).
### Dataset Structure
#### Data Fields
<!-- info: List and describe the fields present in the dataset. -->
<!-- scope: telescope -->
The data fields are:
- `text`: the source article (`string`).
- `summary`: the output summary (`string`).
- `topic`: the topic of the article (`string`).
- `url`: the article's url (`string`).
- `title`: the article's title (`string`).
- `date`: the article's date (`string`).
#### Reason for Structure
<!-- info: How was the dataset structure determined? -->
<!-- scope: microscope -->
The structure follows previously released datasets. The `topic` and `title` fields were added to enable additional tasks like title generation and topic detection.
#### How were labels chosen?
<!-- info: How were the labels chosen? -->
<!-- scope: microscope -->
They are human written highlights or summaries scraped from the same website.
#### Example Instance
<!-- info: Provide a JSON formatted example of a typical instance in the dataset. -->
<!-- scope: periscope -->
```
{
'date': '00/01/2010',
'gem_id': 'mlsum_de-train-2',
'gem_parent_id': 'mlsum_de-train-2',
'references': [],
'target': 'Oskar Lafontaine gibt den Parteivorsitz der Linken ab - und seine Kollegen streiten, wer ihn beerben soll. sueddeutsche.de stellt die derzeit aussichtsreichsten Anwärter für Führungsaufgaben vor. Mit Vote.',
'text': 'Wenn an diesem Montag die Landesvorsitzenden der Linken über die Nachfolger der derzeitigen Chefs Lothar Bisky und Oskar Lafontaine sowie des Bundesgeschäftsführers Dietmar Bartsch beraten, geht es nicht nur darum, wer die Partei führen soll. Es geht auch um die künftige Ausrichtung und Stärke einer Partei, die vor allem von Lafontaine zusammengehalten worden war. Ihm war es schließlich vor fünf Jahren gelungen, aus der ostdeutschen PDS und der westedeutschen WASG eine Partei zu formen. Eine Partei allerdings, die zerrissen ist in Ost und West, in Regierungswillige und ewige Oppositionelle, in Realos und Ideologen, in gemäßigte und radikale Linke. Wir stellen mögliche Kandidaten vor. Stimmen Sie ab: Wen halten Sie für geeignet und wen für unfähig? Kampf um Lafontaines Erbe: Gregor Gysi Sollte überhaupt jemand die Partei alleine führen, wie es sich viele Ostdeutsche wünschen, käme dafür wohl nur der 62-jährige Gregor Gysi in Betracht. Er ist nach Lafontaine einer der bekanntesten Politiker der Linken und derzeit Fraktionsvorsitzender der Partei im Bundestag. Allerdings ist der ehemalige PDS-Vorsitzende und Rechtsanwalt nach drei Herzinfarkten gesundheitlich angeschlagen. Wahrscheinlich wäre deshalb, dass er die zerstrittene Partei nur übergangsweise führt. Doch noch ist nicht klar, ob eine Person allein die Partei führen soll oder eine Doppelspitze. Viele Linke wünschen sich ein Duo aus einem westdeutschen und einem ostdeutschen Politiker, Mann und Frau. Foto: Getty Images',
'title': 'Personaldebatte bei der Linken - Wer kommt nach Lafontaine?',
'topic': 'politik',
'url': 'https://www.sueddeutsche.de/politik/personaldebatte-bei-der-linken-wer-kommt-nach-lafontaine-1.70041'
}
```
#### Data Splits
<!-- info: Describe and name the splits in the dataset if there are more than one. -->
<!-- scope: periscope -->
The statistics of the original dataset are:
| | Dataset | Train | Validation | Test | Mean article length | Mean summary length |
| :--- | :----: | :---: | :---: | :---: | :---: | :---: |
| German | 242,982 | 220,887 |11,394 |10,701 |570.6 (words) | 30.36 (words) |
| Spanish | 290,645 | 266,367 |10,358 |13,920 |800.5 (words) |20.71 (words) |
The statistics of the cleaned version of the dataset are:
| | Dataset | Train | Validation | Test |
| :--- | :----: | :---: | :---: | :---: |
| German | 242,835 | 220,887 |11,392 |10,695 |
| Spanish | 283,228 |259,886 |9,977 |13,365 |
The COVID challenge sets have 5058 (de) and 1938 (es) examples.
#### Splitting Criteria
<!-- info: Describe any criteria for splitting the data, if used. If there are differences between the splits (e.g., if the training annotations are machine-generated and the dev and test ones are created by humans, or if different numbers of annotators contributed to each example), describe them here. -->
<!-- scope: microscope -->
The training set contains data from 2010 to 2018. Data from 2019 (~10% of the dataset) is used for validation (up to May) and testing(May-December 2019).
####
<!-- info: What does an outlier of the dataset in terms of length/perplexity/embedding look like? -->
<!-- scope: microscope -->
Some topics are less represented within the dataset (e.g., Financial news in German and Television in Spanish).
## Dataset in GEM
### Rationale for Inclusion in GEM
#### Why is the Dataset in GEM?
<!-- info: What does this dataset contribute toward better generation evaluation and why is it part of GEM? -->
<!-- scope: microscope -->
As the first large-scale multilingual summarization dataset, it enables evaluation of summarization models beyond English.
#### Similar Datasets
<!-- info: Do other datasets for the high level task exist? -->
<!-- scope: telescope -->
yes
#### Unique Language Coverage
<!-- info: Does this dataset cover other languages than other datasets for the same task? -->
<!-- scope: periscope -->
yes
#### Difference from other GEM datasets
<!-- info: What else sets this dataset apart from other similar datasets in GEM? -->
<!-- scope: microscope -->
In our configuration, the dataset is fully non-English.
#### Ability that the Dataset measures
<!-- info: What aspect of model ability can be measured with this dataset? -->
<!-- scope: periscope -->
Content Selection, Content Planning, Realization
### GEM-Specific Curation
#### Modificatied for GEM?
<!-- info: Has the GEM version of the dataset been modified in any way (data, processing, splits) from the original curated data? -->
<!-- scope: telescope -->
yes
#### GEM Modifications
<!-- info: What changes have been made to he original dataset? -->
<!-- scope: periscope -->
`data points removed`, `data points added`
#### Modification Details
<!-- info: For each of these changes, described them in more details and provided the intended purpose of the modification -->
<!-- scope: microscope -->
The modifications done to the original dataset are the following:
- Selection of 2 languages (Spanish and German) out of the dataset 5 languages due to copyright restrictions.
- Removal of duplicate articles.
- Manually removal of article-summary pairs for which the summary is not related to the article.
- Removal of article-summary pairs written in a different language (detected using the [langdetect](https://pypi.org/project/langdetect/) library).
#### Additional Splits?
<!-- info: Does GEM provide additional splits to the dataset? -->
<!-- scope: telescope -->
yes
#### Split Information
<!-- info: Describe how the new splits were created -->
<!-- scope: periscope -->
For both selected languages (German and Spanish), we compiled time-shifted test data in the form of new articles for the second semester of 2020 with Covid19-related keywords. We collected articles from the same German and Spanish outlets as the original MLSUM datasets (El Pais and Süddeutsche Zeitung). We used the scripts provided for the re-creation of the [MLSUM datasets](https://github.com/recitalAI/MLSUM). The new challenge test set for German contains 5058 instances and the Spanish one contains 1938.
We additionally sample 500 training and validation points as additional challenge sets to measure overfitting.
#### Split Motivation
<!-- info: What aspects of the model's generation capacities were the splits created to test? -->
<!-- scope: periscope -->
Generalization to unseen topics.
### Getting Started with the Task
## Previous Results
### Previous Results
#### Measured Model Abilities
<!-- info: What aspect of model ability can be measured with this dataset? -->
<!-- scope: telescope -->
Content Selection, Content Planning, Realization
#### Metrics
<!-- info: What metrics are typically used for this task? -->
<!-- scope: periscope -->
`METEOR`, `ROUGE`, `Other: Other Metrics`
#### Other Metrics
<!-- info: Definitions of other metrics -->
<!-- scope: periscope -->
Novelty: Number of generated n-grams not included in the source articles.
#### Proposed Evaluation
<!-- info: List and describe the purpose of the metrics and evaluation methodology (including human evaluation) that the dataset creators used when introducing this task. -->
<!-- scope: microscope -->
ROUGE and METEOR both measure n-gram overlap with a focus on recall and are standard summarization metrics. Novelty is often reported alongside them to characterize how much a model diverges from its inputs.
#### Previous results available?
<!-- info: Are previous results available? -->
<!-- scope: telescope -->
yes
#### Other Evaluation Approaches
<!-- info: What evaluation approaches have others used? -->
<!-- scope: periscope -->
The GEM benchmark results (https://gem-benchmark.com/results) report a wide range of metrics include lexical overlap metrics but also semantic ones like BLEURT and BERT-Score.
## Dataset Curation
### Original Curation
#### Original Curation Rationale
<!-- info: Original curation rationale -->
<!-- scope: telescope -->
The rationale was to create a multilingual news summarization dataset that mirrors the format of popular English datasets like XSum or CNN/DM.
#### Communicative Goal
<!-- info: What was the communicative goal? -->
<!-- scope: periscope -->
The speaker is required to produce a high quality summary of news articles in the same language as the input article.
#### Sourced from Different Sources
<!-- info: Is the dataset aggregated from different data sources? -->
<!-- scope: telescope -->
yes
#### Source Details
<!-- info: List the sources (one per line) -->
<!-- scope: periscope -->
www.lemonde.fr
www.sueddeutsche.de
www.elpais.com
www.mk.ru
www.internethaber.com
### Language Data
#### How was Language Data Obtained?
<!-- info: How was the language data obtained? -->
<!-- scope: telescope -->
`Found`
#### Where was it found?
<!-- info: If found, where from? -->
<!-- scope: telescope -->
`Multiple websites`
#### Language Producers
<!-- info: What further information do we have on the language producers? -->
<!-- scope: microscope -->
The language producers are professional journalists.
#### Topics Covered
<!-- info: Does the language in the dataset focus on specific topics? How would you describe them? -->
<!-- scope: periscope -->
4/5 of the original languages report their topics (except Turkish) and the distributions differ between sources. The dominant topics in German are Politik, Sport, Wirtschaft (economy). The dominant topics in Spanish are actualidad (current news) and opinion. French and Russian are different as well but we omit these languages in the GEM version.
#### Data Validation
<!-- info: Was the text validated by a different worker or a data curator? -->
<!-- scope: telescope -->
not validated
#### Was Data Filtered?
<!-- info: Were text instances selected or filtered? -->
<!-- scope: telescope -->
algorithmically
#### Filter Criteria
<!-- info: What were the selection criteria? -->
<!-- scope: microscope -->
In the original dataset, only one filter was applied: all the articles shorter than 50 words or summaries shorter than 10 words are discarded.
The GEM version additionally applies langID filter to ensure that articles are in the correct language.
### Structured Annotations
#### Additional Annotations?
<!-- quick -->
<!-- info: Does the dataset have additional annotations for each instance? -->
<!-- scope: telescope -->
none
#### Annotation Service?
<!-- info: Was an annotation service used? -->
<!-- scope: telescope -->
no
### Consent
#### Any Consent Policy?
<!-- info: Was there a consent policy involved when gathering the data? -->
<!-- scope: telescope -->
no
#### Justification for Using the Data
<!-- info: If not, what is the justification for reusing the data? -->
<!-- scope: microscope -->
The copyright remains with the original data creators and the usage permission is restricted to non-commercial uses.
### Private Identifying Information (PII)
#### Contains PII?
<!-- quick -->
<!-- info: Does the source language data likely contain Personal Identifying Information about the data creators or subjects? -->
<!-- scope: telescope -->
yes/very likely
#### Categories of PII
<!-- info: What categories of PII are present or suspected in the data? -->
<!-- scope: periscope -->
`sensitive information`, `generic PII`
#### Any PII Identification?
<!-- info: Did the curators use any automatic/manual method to identify PII in the dataset? -->
<!-- scope: periscope -->
no identification
### Maintenance
#### Any Maintenance Plan?
<!-- info: Does the original dataset have a maintenance plan? -->
<!-- scope: telescope -->
no
## Broader Social Context
### Previous Work on the Social Impact of the Dataset
#### Usage of Models based on the Data
<!-- info: Are you aware of cases where models trained on the task featured in this dataset ore related tasks have been used in automated systems? -->
<!-- scope: telescope -->
no
### Impact on Under-Served Communities
#### Addresses needs of underserved Communities?
<!-- info: Does this dataset address the needs of communities that are traditionally underserved in language technology, and particularly language generation technology? Communities may be underserved for exemple because their language, language variety, or social or geographical context is underepresented in NLP and NLG resources (datasets and models). -->
<!-- scope: telescope -->
no
### Discussion of Biases
#### Any Documented Social Biases?
<!-- info: Are there documented social biases in the dataset? Biases in this context are variations in the ways members of different social categories are represented that can have harmful downstream consequences for members of the more disadvantaged group. -->
<!-- scope: telescope -->
no
|
GEM/opusparcus | ---
annotations_creators:
- expert-created
language_creators:
- unknown
language:
- de
- en
- fi
- fr
- ru
- sv
license:
- cc-by-nc-4.0
multilinguality:
- unknown
size_categories:
- unknown
source_datasets:
- original
task_categories:
- other
task_ids: []
pretty_name: opusparcus
tags:
- paraphrasing
---
# Dataset Card for GEM/opusparcus
## Dataset Description
- **Homepage:** http://urn.fi/urn:nbn:fi:lb-2018021221
- **Repository:** http://urn.fi/urn:nbn:fi:lb-2018021221
- **Paper:** http://www.lrec-conf.org/proceedings/lrec2018/pdf/131.pdf
- **Leaderboard:** N/A
- **Point of Contact:** Mathias Creutz
### Link to Main Data Card
You can find the main data card on the [GEM Website](https://gem-benchmark.com/data_cards/opusparcus).
### Dataset Summary
Opusparcus is a paraphrase corpus for six European language: German, English, Finnish, French, Russian, and Swedish. The paraphrases consist of subtitles from movies and TV shows.
You can load the dataset via:
```
import datasets
data = datasets.load_dataset('GEM/opusparcus')
```
The data loader can be found [here](https://huggingface.co/datasets/GEM/opusparcus).
#### website
[Website](http://urn.fi/urn:nbn:fi:lb-2018021221)
#### paper
[LREC](http://www.lrec-conf.org/proceedings/lrec2018/pdf/131.pdf)
## Dataset Overview
### Where to find the Data and its Documentation
#### Webpage
<!-- info: What is the webpage for the dataset (if it exists)? -->
<!-- scope: telescope -->
[Website](http://urn.fi/urn:nbn:fi:lb-2018021221)
#### Download
<!-- info: What is the link to where the original dataset is hosted? -->
<!-- scope: telescope -->
[Website](http://urn.fi/urn:nbn:fi:lb-2018021221)
#### Paper
<!-- info: What is the link to the paper describing the dataset (open access preferred)? -->
<!-- scope: telescope -->
[LREC](http://www.lrec-conf.org/proceedings/lrec2018/pdf/131.pdf)
#### BibTex
<!-- info: Provide the BibTex-formatted reference for the dataset. Please use the correct published version (ACL anthology, etc.) instead of google scholar created Bibtex. -->
<!-- scope: microscope -->
```
@InProceedings{creutz:lrec2018,
title = {Open Subtitles Paraphrase Corpus for Six Languages},
author={Mathias Creutz},
booktitle={Proceedings of the 11th edition of the Language Resources and Evaluation Conference (LREC 2018)},
year={2018},
month = {May 7-12},
address = {Miyazaki, Japan},
editor = {Nicoletta Calzolari (Conference chair) and Khalid Choukri and Christopher Cieri and Thierry Declerck and Sara Goggi and Koiti Hasida and Hitoshi Isahara and Bente Maegaard and Joseph Mariani and Hélène Mazo and Asuncion Moreno and Jan Odijk and Stelios Piperidis and Takenobu Tokunaga},
publisher = {European Language Resources Association (ELRA)},
isbn = {979-10-95546-00-9},
language = {english},
url={http://www.lrec-conf.org/proceedings/lrec2018/pdf/131.pdf}
```
#### Contact Name
<!-- quick -->
<!-- info: If known, provide the name of at least one person the reader can contact for questions about the dataset. -->
<!-- scope: periscope -->
Mathias Creutz
#### Contact Email
<!-- info: If known, provide the email of at least one person the reader can contact for questions about the dataset. -->
<!-- scope: periscope -->
firstname dot lastname at helsinki dot fi
#### Has a Leaderboard?
<!-- info: Does the dataset have an active leaderboard? -->
<!-- scope: telescope -->
no
### Languages and Intended Use
#### Multilingual?
<!-- quick -->
<!-- info: Is the dataset multilingual? -->
<!-- scope: telescope -->
yes
#### Covered Languages
<!-- quick -->
<!-- info: What languages/dialects are covered in the dataset? -->
<!-- scope: telescope -->
`German`, `English`, `Finnish`, `French`, `Russian`, `Swedish`
#### Whose Language?
<!-- info: Whose language is in the dataset? -->
<!-- scope: periscope -->
Opusparcus is a paraphrase corpus for six European language: German, English, Finnish, French, Russian, and Swedish. The paraphrases consist of subtitles from movies and TV shows.
The data in Opusparcus has been extracted from [OpenSubtitles2016](http://opus.nlpl.eu/OpenSubtitles2016.php), which is in turn based on data from [OpenSubtitles](http://www.opensubtitles.org/).
#### License
<!-- quick -->
<!-- info: What is the license of the dataset? -->
<!-- scope: telescope -->
cc-by-nc-4.0: Creative Commons Attribution Non Commercial 4.0 International
#### Intended Use
<!-- info: What is the intended use of the dataset? -->
<!-- scope: microscope -->
Opusparcus is a sentential paraphrase corpus for multiple languages containing colloquial language.
#### Primary Task
<!-- info: What primary task does the dataset support? -->
<!-- scope: telescope -->
Paraphrasing
#### Communicative Goal
<!-- quick -->
<!-- info: Provide a short description of the communicative goal of a model trained for this task on this dataset. -->
<!-- scope: periscope -->
Models can be trained, e.g., for paraphrase detection and generation, that is, determining whether two given sentences mean the same thing or generating new paraphrases for a given sentence.
### Credit
#### Who added the Dataset to GEM?
<!-- info: Who contributed to the data card and adding the dataset to GEM? List the people+affiliations involved in creating this data card and who helped integrate this dataset into GEM. -->
<!-- scope: microscope -->
Mathias Creutz (University of Helsinki)
### Dataset Structure
#### Data Fields
<!-- info: List and describe the fields present in the dataset. -->
<!-- scope: telescope -->
- `sent1`: a tokenized sentence
- `sent2`: another tokenized sentence, which is potentially a paraphrase of `sent1`.
- `annot_score`: a value between 1.0 and 4.0 indicating how good an example of paraphrases `sent1` and `sent2` are. (For the training sets, the value is 0.0, which indicates that no manual annotation has taken place.)
- `lang`: language of this dataset
- `gem_id`: unique identifier of this entry
All fields are strings except `annot_score`, which is a float.
#### Reason for Structure
<!-- info: How was the dataset structure determined? -->
<!-- scope: microscope -->
For each target language, the Opusparcus data have been partitioned into three types of data sets: training, validation and test sets. The training sets are large, consisting of millions of sentence pairs, and have been compiled automatically, with the help of probabilistic ranking functions. The development and test sets consist of sentence pairs that have been annotated manually; each set contains approximately 1000 sentence pairs that have been verified to be acceptable paraphrases by two independent annotators.
When you download Opusparcus, you must always indicate the language you want to retrieve, for instance:
```
data = load_dataset("GEM/opusparcus", lang="de")
```
The above command will download the validation and test sets for German. If additionally, you want to retrieve training data, you need to specify the level of quality you desire, such as "French, with 90% quality of the training data":
```
data = load_dataset("GEM/opusparcus", lang="fr", quality=90)
```
The entries in the training sets have been ranked automatically by how likely they are paraphrases, best first, worst last. The quality parameter indicates the estimated proportion (in percent) of true
paraphrases in the training set. Allowed quality values range between 60 and 100, in increments of 5 (60, 65, 70, ..., 100). A value of 60 means that 60% of the sentence pairs in the training set are estimated to be true paraphrases (and the remaining 40% are not). A higher value produces a smaller but cleaner set. The smaller sets are subsets of the larger sets, such that the `quality=95` set is a subset of `quality=90`, which is a subset of `quality=85`, and so on.
The default `quality` value, if omitted, is 100. This matches no training data at all, which can be convenient, if you are only interested in the validation and test sets, which are considerably
smaller, but manually annotated.
Note that an alternative to typing the parameter values explicitly, you can use configuration names instead. The following commands are equivalent to the ones above:
```
data = load_dataset("GEM/opusparcus", "de.100")
data = load_dataset("GEM/opusparcus", "fr.90")
```
#### How were labels chosen?
<!-- info: How were the labels chosen? -->
<!-- scope: microscope -->
Annotators have used the following scores to label sentence pairs in the test and validation sets:
4: Good example of paraphrases (Dark green button in the annotation tool): The two sentences can be used in the same situation and essentially "mean the same thing".
3: Mostly good example of paraphrases (Light green button in the annotation tool): It is acceptable to think that the two sentences refer to the same thing, although one sentence might be more specific
than the other one, or there are differences in style, such as polite form versus familiar form.
2: Mostly bad example of paraphrases (Yellow button in the annotation tool): There is some connection between the sentences that explains why they occur together, but one would not really consider them to mean the same thing.
1: Bad example of paraphrases (Red button in the annotation tool): There is no obvious connection. The sentences mean different things.
If the two annotators fully agreed on the category, the value in the `annot_score` field is 4.0, 3.0, 2.0 or 1.0. If the two annotators chose adjacent categories, the value in this field will be 3.5, 2.5 or
1.5. For instance, a value of 2.5 means that one annotator gave a score of 3 ("mostly good"), indicating a possible paraphrase pair, whereas the other annotator scored this as a 2 ("mostly bad"), that is, unlikely to be a paraphrase pair. If the annotators disagreed by more than one category, the sentence pair was discarded and won't show up in the datasets.
The training sets were not annotated manually. This is indicated by
the value 0.0 in the `annot_score` field.
For an assessment of of inter-annotator agreement, see Aulamo et al. (2019). [Annotation of subtitle paraphrases using a new web tool.](http://ceur-ws.org/Vol-2364/3_paper.pdf) In *Proceedings of the
Digital Humanities in the Nordic Countries 4th Conference*, Copenhagen, Denmark.
#### Example Instance
<!-- info: Provide a JSON formatted example of a typical instance in the dataset. -->
<!-- scope: periscope -->
```
{'annot_score': 4.0, 'gem_id': 'gem-opusparcus-test-1587', 'lang': 'en', 'sent1': "I haven 't been contacted by anybody .", 'sent2': "Nobody 's contacted me ."}
```
#### Data Splits
<!-- info: Describe and name the splits in the dataset if there are more than one. -->
<!-- scope: periscope -->
The data is split into training, validation and test sets. The validation and test sets come in two versions, the regular validation and test sets and the full sets, called validation.full and test.full. The full sets contain all sentence pairs successfully annotated by the annotators, including the sentence pairs that were rejected as paraphrases. The annotation scores of the full sets thus range between 1.0 and 4.0. The regular validation and test sets only contain sentence pairs that qualify as paraphrases, scored between 3.0 and 4.0 by the annotators.
The number of sentence pairs in the data splits are as follows for each of the languages. The range between the smallest (`quality=95`) and largest (`quality=60`) train configuration have been shown.
| | train | valid | test | valid.full | test.full |
| ----- | ------ | ----- | ---- | ---------- | --------- |
| de | 0.59M .. 13M | 1013 | 1047 | 1582 | 1586 |
| en | 1.0M .. 35M | 1015 | 982 | 1455 | 1445 |
| fi | 0.48M .. 8.9M | 963 | 958 | 1760 | 1749 |
| fr | 0.94M .. 22M | 997 | 1007 | 1630 | 1674 |
| ru | 0.15M .. 15M | 1020 | 1068 | 1854 | 1855 |
| sv | 0.24M .. 4.5M | 984 | 947 | 1887 | 1901 |
As a concrete example, loading the English data requesting 95% quality of the train split produces the following:
```
>>> data = load_dataset("GEM/opusparcus", lang="en", quality=95)
>>> data
DatasetDict({
test: Dataset({
features: ['lang', 'sent1', 'sent2', 'annot_score', 'gem_id'],
num_rows: 982
})
validation: Dataset({
features: ['lang', 'sent1', 'sent2', 'annot_score', 'gem_id'],
num_rows: 1015
})
test.full: Dataset({
features: ['lang', 'sent1', 'sent2', 'annot_score', 'gem_id'],
num_rows: 1445
})
validation.full: Dataset({
features: ['lang', 'sent1', 'sent2', 'annot_score', 'gem_id'],
num_rows: 1455
})
train: Dataset({
features: ['lang', 'sent1', 'sent2', 'annot_score', 'gem_id'],
num_rows: 1000000
})
})
>>> data["test"][0]
{'annot_score': 4.0, 'gem_id': 'gem-opusparcus-test-1587', 'lang': 'en', 'sent1': "I haven 't been contacted by anybody .", 'sent2': "Nobody 's contacted me ."}
>>> data["validation"][2]
{'annot_score': 3.0, 'gem_id': 'gem-opusparcus-validation-1586', 'lang': 'en', 'sent1': 'No promises , okay ?', 'sent2': "I 'm not promising anything ."}
>>> data["train"][1000]
{'annot_score': 0.0, 'gem_id': 'gem-opusparcus-train-12501001', 'lang': 'en', 'sent1': 'Am I beautiful ?', 'sent2': 'Am I pretty ?'}
#### Splitting Criteria
<!-- info: Describe any criteria for splitting the data, if used. If there are differences between the splits (e.g., if the training annotations are machine-generated and the dev and test ones are created by humans, or if different numbers of annotators contributed to each example), describe them here. -->
<!-- scope: microscope -->
The validation and test sets have been annotated manually, but the training sets have been produced using automatic scoring and come in different size configurations depending on the desired quality level. (See above descriptions and examples for more details.)
Please note that previous work suggests that a larger and noisier training set is better than a
smaller and clean set. See Sjöblom et al. (2018). [Paraphrase Detection on Noisy Subtitles in Six
Languages](http://noisy-text.github.io/2018/pdf/W-NUT20189.pdf). In *Proceedings of the 2018 EMNLP Workshop W-NUT: The 4th Workshop on Noisy User-generated Text*, and Vahtola et al. (2021). [Coping with Noisy Training Data Labels in Paraphrase Detection](https://aclanthology.org/2021.wnut-1.32/). In *Proceedings of the 7th Workshop on Noisy User-generated Text*.
## Dataset in GEM
### Rationale for Inclusion in GEM
#### Why is the Dataset in GEM?
<!-- info: What does this dataset contribute toward better generation evaluation and why is it part of GEM? -->
<!-- scope: microscope -->
Opusparcus provides examples of sentences that mean the same thing or have very similar meaning. Sentences are available in six languages and the style is colloquial language.
#### Similar Datasets
<!-- info: Do other datasets for the high level task exist? -->
<!-- scope: telescope -->
yes
#### Unique Language Coverage
<!-- info: Does this dataset cover other languages than other datasets for the same task? -->
<!-- scope: periscope -->
yes
#### Difference from other GEM datasets
<!-- info: What else sets this dataset apart from other similar datasets in GEM? -->
<!-- scope: microscope -->
There is another data set containing manually labeled Finnish paraphrases.
#### Ability that the Dataset measures
<!-- info: What aspect of model ability can be measured with this dataset? -->
<!-- scope: periscope -->
Sentence meaning
### GEM-Specific Curation
#### Modificatied for GEM?
<!-- info: Has the GEM version of the dataset been modified in any way (data, processing, splits) from the original curated data? -->
<!-- scope: telescope -->
yes
#### GEM Modifications
<!-- info: What changes have been made to he original dataset? -->
<!-- scope: periscope -->
`other`
#### Modification Details
<!-- info: For each of these changes, described them in more details and provided the intended purpose of the modification -->
<!-- scope: microscope -->
Training sets have been prepared for each the "quality levels" 60% – 95%.
In the original release, this task was left to the user of the data.
#### Additional Splits?
<!-- info: Does GEM provide additional splits to the dataset? -->
<!-- scope: telescope -->
yes
#### Split Information
<!-- info: Describe how the new splits were created -->
<!-- scope: periscope -->
There are two versions of the validations and test sets: the regular sets which only contain positive examples of paraphrases and the full sets containing all examples.
#### Split Motivation
<!-- info: What aspects of the model's generation capacities were the splits created to test? -->
<!-- scope: periscope -->
In the original release, only the full validation and test sets were supplied. The "regular sets" have been added in order to make it easier to test on true parapahrases only.
### Getting Started with the Task
#### Pointers to Resources
<!-- info: Getting started with in-depth research on the task. Add relevant pointers to resources that researchers can consult when they want to get started digging deeper into the task. -->
<!-- scope: microscope -->
Creutz (2018). [Open Subtitles Paraphrase Corpus for Six Languages](http://www.lrec-conf.org/proceedings/lrec2018/pdf/131.pdf), Proceedings of the 11th edition of the Language Resources and Evaluation Conference (LREC 2018).
Sjöblom et al. (2018). [Paraphrase Detection on Noisy Subtitles in Six Languages](http://noisy-text.github.io/2018/pdf/W-NUT20189.pdf). In Proceedings of the 2018 EMNLP Workshop W-NUT: The 4th Workshop on Noisy User-generated Text.
Aulamo et al. (2019). [Annotation of subtitle paraphrases using a new web tool.](http://ceur-ws.org/Vol-2364/3_paper.pdf) In Proceedings of the Digital Humanities in the Nordic Countries 4th Conference.
Sjöblom et al. (2020). [Paraphrase Generation and Evaluation on Colloquial-Style Sentences](https://aclanthology.org/2020.lrec-1.224/), Proceedings of the 12th Language Resources and Evaluation Conference (LREC).
Vahtola et al. (2021). [Coping with Noisy Training Data Labels in Paraphrase Detection](https://aclanthology.org/2021.wnut-1.32/). In Proceedings of the 7th Workshop on Noisy User-generated Text.
## Previous Results
### Previous Results
#### Measured Model Abilities
<!-- info: What aspect of model ability can be measured with this dataset? -->
<!-- scope: telescope -->
Sentence meaning
In a scenario of paraphrase detection, the model determines whether two given sentences carry approximately the same meaning.
In a scenario of paraphrase generation, the model generates a potential paraphrase of a given sentence.
#### Metrics
<!-- info: What metrics are typically used for this task? -->
<!-- scope: periscope -->
`BLEU`, `BERT-Score`, `Other: Other Metrics`
#### Other Metrics
<!-- info: Definitions of other metrics -->
<!-- scope: periscope -->
PINC
#### Proposed Evaluation
<!-- info: List and describe the purpose of the metrics and evaluation methodology (including human evaluation) that the dataset creators used when introducing this task. -->
<!-- scope: microscope -->
The metrics mentioned above can be used to assess how well a generated paraphrase corresponds to a given reference sentence. The PINC score additionally assesses how different the surface forms are.
#### Previous results available?
<!-- info: Are previous results available? -->
<!-- scope: telescope -->
yes
#### Other Evaluation Approaches
<!-- info: What evaluation approaches have others used? -->
<!-- scope: periscope -->
See publications on using Opusparcus
#### Relevant Previous Results
<!-- info: What are the most relevant previous results for this task/dataset? -->
<!-- scope: microscope -->
Sjöblom et al. (2020). [Paraphrase Generation and Evaluation on Colloquial-Style Sentences](https://aclanthology.org/2020.lrec-1.224/), Proceedings of the 12th Language Resources and Evaluation Conference (LREC).
## Dataset Curation
### Original Curation
#### Original Curation Rationale
<!-- info: Original curation rationale -->
<!-- scope: telescope -->
Opusparcus was created in order to produce a *sentential* paraphrase corpus for multiple languages containing *colloquial* language (as opposed to news or religious text, for instance).
#### Communicative Goal
<!-- info: What was the communicative goal? -->
<!-- scope: periscope -->
Opusparcus provides labeled examples of pairs of sentences that have similar (or dissimilar) meanings.
#### Sourced from Different Sources
<!-- info: Is the dataset aggregated from different data sources? -->
<!-- scope: telescope -->
no
### Language Data
#### How was Language Data Obtained?
<!-- info: How was the language data obtained? -->
<!-- scope: telescope -->
`Crowdsourced`
#### Where was it crowdsourced?
<!-- info: If crowdsourced, where from? -->
<!-- scope: periscope -->
`Other crowdworker platform`
#### Language Producers
<!-- info: What further information do we have on the language producers? -->
<!-- scope: microscope -->
The data in Opusparcus has been extracted from [OpenSubtitles2016](http://opus.nlpl.eu/OpenSubtitles2016.php), which is in turn based on data from [OpenSubtitles.org](http://www.opensubtitles.org/).
The texts consists of subtitles that have been produced using crowdsourcing.
#### Topics Covered
<!-- info: Does the language in the dataset focus on specific topics? How would you describe them? -->
<!-- scope: periscope -->
The language is representative of movies and TV shows. Domains covered include comedy, drama, relationships, suspense, etc.
#### Data Validation
<!-- info: Was the text validated by a different worker or a data curator? -->
<!-- scope: telescope -->
validated by data curator
#### Data Preprocessing
<!-- info: How was the text data pre-processed? (Enter N/A if the text was not pre-processed) -->
<!-- scope: microscope -->
Sentence and word tokenization was performed.
#### Was Data Filtered?
<!-- info: Were text instances selected or filtered? -->
<!-- scope: telescope -->
algorithmically
#### Filter Criteria
<!-- info: What were the selection criteria? -->
<!-- scope: microscope -->
The sentence pairs in the training sets were ordered automatically based on the estimated likelihood that the sentences were paraphrases, most likely paraphrases on the top, and least likely paraphrases on the bottom.
The validation and test sets were checked and annotated manually, but the sentence pairs selected for annotation had to be different enough in terms of minimum edit distance (Levenshtein distance). This ensured that annotators would not spend their time annotating pairs of more or less identical sentences.
### Structured Annotations
#### Additional Annotations?
<!-- quick -->
<!-- info: Does the dataset have additional annotations for each instance? -->
<!-- scope: telescope -->
expert created
#### Number of Raters
<!-- info: What is the number of raters -->
<!-- scope: telescope -->
11<n<50
#### Rater Qualifications
<!-- info: Describe the qualifications required of an annotator. -->
<!-- scope: periscope -->
Students and staff at the University of Helsinki (native or very proficient speakers of the target languages)
#### Raters per Training Example
<!-- info: How many annotators saw each training example? -->
<!-- scope: periscope -->
0
#### Raters per Test Example
<!-- info: How many annotators saw each test example? -->
<!-- scope: periscope -->
2
#### Annotation Service?
<!-- info: Was an annotation service used? -->
<!-- scope: telescope -->
no
#### Annotation Values
<!-- info: Purpose and values for each annotation -->
<!-- scope: microscope -->
The development and test sets consist of sentence pairs that have been annotated manually; each set contains approximately 1000 sentence pairs that have been verified to be acceptable paraphrases by two independent annotators.
The `annot_score` field reflects the judgments made by the annotators. If the annnotators fully agreed on the category (4.0: dark green, 3.0: light green, 2.0: yellow, 1.0: red), the value of `annot_score` is 4.0, 3.0, 2.0 or 1.0. If the annotators chose adjacent categories, the value in this field will be 3.5, 2.5 or 1.5. For instance, a value of 2.5 means that one annotator gave a score of 3 ("mostly good"), indicating a possible paraphrase pair, whereas the other annotator scored this as a 2 ("mostly bad"), that is, unlikely to be a paraphrase pair. If the annotators disagreed by more than one category, the sentence pair was discarded and won't show up in the datasets.
Annotators could also reject a sentence pair as being corrupted data.
#### Any Quality Control?
<!-- info: Quality control measures? -->
<!-- scope: telescope -->
validated by another rater
#### Quality Control Details
<!-- info: Describe the quality control measures that were taken. -->
<!-- scope: microscope -->
If the annotators disagreed by more than one category, the sentence pair was discarded and is not part of the final dataset.
### Consent
#### Any Consent Policy?
<!-- info: Was there a consent policy involved when gathering the data? -->
<!-- scope: telescope -->
no
### Private Identifying Information (PII)
#### Contains PII?
<!-- quick -->
<!-- info: Does the source language data likely contain Personal Identifying Information about the data creators or subjects? -->
<!-- scope: telescope -->
yes/very likely
#### Any PII Identification?
<!-- info: Did the curators use any automatic/manual method to identify PII in the dataset? -->
<!-- scope: periscope -->
no identification
### Maintenance
#### Any Maintenance Plan?
<!-- info: Does the original dataset have a maintenance plan? -->
<!-- scope: telescope -->
no
## Broader Social Context
### Previous Work on the Social Impact of the Dataset
#### Usage of Models based on the Data
<!-- info: Are you aware of cases where models trained on the task featured in this dataset ore related tasks have been used in automated systems? -->
<!-- scope: telescope -->
no
### Impact on Under-Served Communities
#### Addresses needs of underserved Communities?
<!-- info: Does this dataset address the needs of communities that are traditionally underserved in language technology, and particularly language generation technology? Communities may be underserved for exemple because their language, language variety, or social or geographical context is underepresented in NLP and NLG resources (datasets and models). -->
<!-- scope: telescope -->
no
### Discussion of Biases
#### Any Documented Social Biases?
<!-- info: Are there documented social biases in the dataset? Biases in this context are variations in the ways members of different social categories are represented that can have harmful downstream consequences for members of the more disadvantaged group. -->
<!-- scope: telescope -->
no
#### Are the Language Producers Representative of the Language?
<!-- info: Does the distribution of language producers in the dataset accurately represent the full distribution of speakers of the language world-wide? If not, how does it differ? -->
<!-- scope: periscope -->
What social bias there may be in the subtitles in this dataset has not been studied.
## Considerations for Using the Data
### PII Risks and Liability
#### Potential PII Risk
<!-- info: Considering your answers to the PII part of the Data Curation Section, describe any potential privacy to the data subjects and creators risks when using the dataset. -->
<!-- scope: microscope -->
The data only contains subtitles of publicly available movies and TV shows.
### Licenses
#### Copyright Restrictions on the Dataset
<!-- info: Based on your answers in the Intended Use part of the Data Overview Section, which of the following best describe the copyright and licensing status of the dataset? -->
<!-- scope: periscope -->
`non-commercial use only`
#### Copyright Restrictions on the Language Data
<!-- info: Based on your answers in the Language part of the Data Curation Section, which of the following best describe the copyright and licensing status of the underlying language data? -->
<!-- scope: periscope -->
`non-commercial use only`
### Known Technical Limitations
#### Technical Limitations
<!-- info: Describe any known technical limitations, such as spurrious correlations, train/test overlap, annotation biases, or mis-annotations, and cite the works that first identified these limitations when possible. -->
<!-- scope: microscope -->
Some subtitles contain typos that are caused by inaccurate OCR.
#### Unsuited Applications
<!-- info: When using a model trained on this dataset in a setting where users or the public may interact with its predictions, what are some pitfalls to look out for? In particular, describe some applications of the general task featured in this dataset that its curation or properties make it less suitable for. -->
<!-- scope: microscope -->
The models might memorize individual subtitles of existing movies and TV shows, but there is no context across sentence boundaries in the data.
#### Discouraged Use Cases
<!-- info: What are some discouraged use cases of a model trained to maximize the proposed metrics on this dataset? In particular, think about settings where decisions made by a model that performs reasonably well on the metric my still have strong negative consequences for user or members of the public. -->
<!-- scope: microscope -->
A general issue with paraphrasing is that very small modifications in the surface form might produce valid paraphrases, which are however rather uninteresting. It is more valuable to produce paraphrases with clearly different surface realizations (e.g., measured using minimum edit distance).
|
GEM/references | # GEM References
## What is it?
This repository contains all the reference datasets that are used for running evaluation on the GEM benchmark. Some of these datasets were originally hosted as a [GitHub release](https://github.com/GEM-benchmark/GEM-metrics/releases) on the [`GEM-metrics`](https://github.com/GEM-benchmark/GEM-metrics) repository, but have been migrated to the Hugging Face Hub.
## Converting datasets to JSON
We provide a `convert_dataset_to_json.py` conversion script that converts the datasets in the GEM organisation to the JSON format expected by the `GEM-metrics` library. To run the script, first install [`jq`](https://stedolan.github.io/jq/download/) and then install the script's Python dependencies:
```
python -m pip install -r requirements.txt
```
You can then run the script as follows:
```python
python generate_evaluation_datasets.py
```
This script will:
* Download and convert the datasets under the GEM organisation to JSON format
* Validate that the each dataset has the expected columns of `gem_id`, `target`, and `references`
|
GEM/schema_guided_dialog | ---
annotations_creators:
- crowd-sourced
language_creators:
- unknown
language:
- en
license:
- cc-by-sa-4.0
multilinguality:
- unknown
size_categories:
- unknown
source_datasets:
- original
task_categories:
- conversational
task_ids: []
pretty_name: schema_guided_dialog
tags:
- dialog-response-generation
---
# Dataset Card for GEM/schema_guided_dialog
## Dataset Description
- **Homepage:** n/a
- **Repository:** [Github[(https://github.com/google-research-datasets/dstc8-schema-guided-dialogue)
- **Paper:** https://arxiv.org/abs/1909.05855
- **Leaderboard:** N/A
- **Point of Contact:** Abhinav Rastogi
### Link to Main Data Card
You can find the main data card on the [GEM Website](https://gem-benchmark.com/data_cards/schema_guided_dialog).
### Dataset Summary
The GEM version of this dataset functions as a response generation dataset. The input specifies dialog acts that a model needs to verbalize. The Schema-Guided Dialog dataset is challenging since it comprises multiple domains from hotel and travel to restaurants, and a wide range of dialog acts. The context of each conversation is provided as well.
You can load the dataset via:
```
import datasets
data = datasets.load_dataset('GEM/schema_guided_dialog')
```
The data loader can be found [here](https://huggingface.co/datasets/GEM/schema_guided_dialog).
#### website
n/a
#### paper
[Arxiv](https://arxiv.org/abs/1909.05855)
#### authors
Abhinav Rastogi, Xiaoxue Zang, Srinivas Sunkara, Raghav Gupta, Pranav Khaitan, Amir Fayazi, Maria Wang, and Guan-Lin Chao
## Dataset Overview
### Where to find the Data and its Documentation
#### Download
<!-- info: What is the link to where the original dataset is hosted? -->
<!-- scope: telescope -->
[Github[(https://github.com/google-research-datasets/dstc8-schema-guided-dialogue)
#### Paper
<!-- info: What is the link to the paper describing the dataset (open access preferred)? -->
<!-- scope: telescope -->
[Arxiv](https://arxiv.org/abs/1909.05855)
#### BibTex
<!-- info: Provide the BibTex-formatted reference for the dataset. Please use the correct published version (ACL anthology, etc.) instead of google scholar created Bibtex. -->
<!-- scope: microscope -->
```
{
@inproceedings{rastogi2020towards,
title={Towards scalable multi-domain conversational agents: The schema-guided dialogue dataset},
author={Rastogi, Abhinav and Zang, Xiaoxue and Sunkara, Srinivas and Gupta, Raghav and Khaitan, Pranav},
booktitle={Proceedings of the AAAI Conference on Artificial Intelligence},
volume={34},
number={05},
pages={8689--8696},
year={2020}
}
```
#### Contact Name
<!-- quick -->
<!-- info: If known, provide the name of at least one person the reader can contact for questions about the dataset. -->
<!-- scope: periscope -->
Abhinav Rastogi
#### Contact Email
<!-- info: If known, provide the email of at least one person the reader can contact for questions about the dataset. -->
<!-- scope: periscope -->
schema-guided-dst@google.com
#### Has a Leaderboard?
<!-- info: Does the dataset have an active leaderboard? -->
<!-- scope: telescope -->
no
### Languages and Intended Use
#### Multilingual?
<!-- quick -->
<!-- info: Is the dataset multilingual? -->
<!-- scope: telescope -->
no
#### Covered Languages
<!-- quick -->
<!-- info: What languages/dialects are covered in the dataset? -->
<!-- scope: telescope -->
`English`
#### Whose Language?
<!-- info: Whose language is in the dataset? -->
<!-- scope: periscope -->
The language structure is machine-generated, and the language realizations are produced by crowd workers.
The dataset paper does not provide demographic information for the crowd workers.
#### License
<!-- quick -->
<!-- info: What is the license of the dataset? -->
<!-- scope: telescope -->
cc-by-sa-4.0: Creative Commons Attribution Share Alike 4.0 International
#### Intended Use
<!-- info: What is the intended use of the dataset? -->
<!-- scope: microscope -->
The Schema-Guided Dialogue (SGD) dataset contains 18K multi-domain task-oriented dialogues between a human and a virtual assistant, which covers 17 domains ranging from banks and events to media, calendar, travel, and weather.
The language presents in the datset is only English.
The SGD dataset provides a challenging testbed for a number of tasks in task-oriented dialogue, including language understanding, slot filling, dialogue state tracking and response generation.
For the creation of the SGD dataset, they developed a multi-domain dialogue simulator that generates dialogue outlines over an arbitrary combination of APIs, dialogue states and system actions. Then, they used a crowd-sourcing procedure to paraphrase these outlines to natural language utterances.
This novel crowd-sourcing procedure preserves all annotations obtained from the simulator and does not require any extra annotations after dialogue collection.
#### Primary Task
<!-- info: What primary task does the dataset support? -->
<!-- scope: telescope -->
Dialog Response Generation
#### Communicative Goal
<!-- quick -->
<!-- info: Provide a short description of the communicative goal of a model trained for this task on this dataset. -->
<!-- scope: periscope -->
The goal of a speaker who generates the target utterance is to help users accomplish tasks including but not limited to finding flights, booking restaurants, searching for nearby events and movies.
### Credit
#### Curation Organization Type(s)
<!-- info: In what kind of organization did the dataset curation happen? -->
<!-- scope: telescope -->
`industry`
#### Curation Organization(s)
<!-- info: Name the organization(s). -->
<!-- scope: periscope -->
Google
#### Dataset Creators
<!-- info: Who created the original dataset? List the people involved in collecting the dataset and their affiliation(s). -->
<!-- scope: microscope -->
Abhinav Rastogi, Xiaoxue Zang, Srinivas Sunkara, Raghav Gupta, Pranav Khaitan, Amir Fayazi, Maria Wang, and Guan-Lin Chao
#### Funding
<!-- info: Who funded the data creation? -->
<!-- scope: microscope -->
Google
#### Who added the Dataset to GEM?
<!-- info: Who contributed to the data card and adding the dataset to GEM? List the people+affiliations involved in creating this data card and who helped integrate this dataset into GEM. -->
<!-- scope: microscope -->
Wanyu Du wrote the initial data card and Yacine Jernite the data loader. Simon Mille updated the data card with the additional splits. Sebastian Gehrmann migrated the data card and loader to the v2 version and extended the missing information.
### Dataset Structure
#### Data Fields
<!-- info: List and describe the fields present in the dataset. -->
<!-- scope: telescope -->
Each dialog instance has the following fields:
* `dialogue_id`: A unique identifier for a dialogue.
* `services`: A list of services present in the dialogue.
* `turns`: A list of annotated system or user utterances. Each turn consists of the following fields:
* `speaker`: The speaker for the turn, either `USER` or `SYSTEM`.
* `utterance`: A string containing the natural language utterance.
* `frames`: A list of frames, each frame containing annotations for a single service and consists of the following fields:
* `service`: The name of the service corresponding to the frame. The slots and intents used in the following fields are taken from the schema of this service.
* `slots`: A list of slot spans in the utterance, only provided for non-categorical slots. Each slot span contains the following fields:
* `slot`: The name of the slot.
* `start`: The index of the starting character in the utterance corresponding to the slot value.
* `exclusive_end`: The index of the character just after the last character corresponding to the slot value in the utterance.
* `actions`: A list of actions corresponding to the system. Each action has the following fields:
* `act`: The type of action.
* `slot`: (optional) A slot argument for some of the actions.
* `values`: (optional) A list of values assigned to the slot. If the values list is non-empty, then the slot must be present.
* `canonical_values`: (optional) The values in their canonicalized form as used by the service. It is a list of strings of the same length as values.
* `service_call`: (system turns only, optional) The request sent to the service. It consists of the following fields:
* `method`: The name of the intent or function of the service or API being executed.
* `parameters`: A pair of lists of the same lengths: `parameter_slot_name` contains slot names and `parameter_canonical_value` contains the corresponding values in their canonicalized form.
* `service_results`: (system turns only, optional) A list of entities containing the results obtained from the service. It is only available for turns in which a service call is made. Each entity is represented as a pair of lists of the same length: `service_slot_name` contains slot names and `service_canonical_value` contains the corresponding canonical values.
* `state`: (user turns only) The dialogue state corresponding to the service. It consists of the following fields:
* `active_intent`: The intent corresponding to the service of the frame which is currently being fulfilled by the system. It takes the value "NONE" if none of the intents are active.
* `requested_slots`: A list of slots requested by the user in the current turn.
* `slot_values`: A pair of lists of the same lengths: `slot_name` contains slot names and `slot_value_list` contains the corresponding lists of strings. For categorical slots, this list contains a single value assigned to the slot. For non-categorical slots, all the values in this list are spoken variations of each other and are equivalent (e.g, "6 pm", "six in the evening", "evening at 6" etc.).
#### Example Instance
<!-- info: Provide a JSON formatted example of a typical instance in the dataset. -->
<!-- scope: periscope -->
```
{'dialogue_id': '1_00000',
'services': ['Restaurants_1'],
'turns':
{'frames':
[{'actions': [{'act': [6],
'canonical_values': [['FindRestaurants']],
'slot': ['intent'],
'values': [['FindRestaurants']]}],
'service': ['Restaurants_1'],
'service_call': [{'method': '',
'parameters': {'parameter_canonical_value': [],
'parameter_slot_name': []}}],
'service_results': [{'service_results_list': []}],
'slots': [{'exclusive_end': [], 'slot': [], 'start': []}],
'state': [{'active_intent': 'FindRestaurants',
'requested_slots': [],
'slot_values': {'slot_name': [], 'slot_value_list': []}}]},
{'actions': [{'act': [13],
'canonical_values': [[]],
'slot': ['city'],
'values': [[]]}],
'service': ['Restaurants_1'],
'service_call': [{'method': '',
'parameters': {'parameter_canonical_value': [],
'parameter_slot_name': []}}],
'service_results': [{'service_results_list': []}],
'slots': [{'exclusive_end': [], 'slot': [], 'start': []}],
'state': [{'active_intent': '',
'requested_slots': [],
'slot_values': {'slot_name': [], 'slot_value_list': []}}]},
...,]}
'speaker': [0, 1, 0, 1, 0, 1, 0, 1, 0, 1, 0, 1, 0, 1, 0, 1, 0, 1, 0, 1, 0, 1, 0, 1],
'utterance': [
'I am feeling hungry so I would like to find a place to eat.',
'Do you have a specific which you want the eating place to be located at?',
'I would like for it to be in San Jose.',
'Is there a specific cuisine type you enjoy, such as Mexican, Italian or something else?',
'I usually like eating the American type of food.',
'I see that at 71 Saint Peter there is a good restaurant which is in San Jose.',
'Can you give me the address of this restaurant.',
'If you want to go to this restaurant you can find it at 71 North San Pedro Street.',
'Can you give me the phone number that I can contact them with?',
'If you want to phone them you can at 408-971-8523.',
'Is there some other restaurant which you can suggest?',
'How would you like Bazille restaurant which is situated in San Jose.',
'Do you have another restaurant matching my needs? For example a restaurant which is economical and is located in Palo Alto.',
'I see that 7 restaurants suit to what you requested. Bird Dog seems as a good restaurant and is located in Palo Alto.',
'Alright, that seems good. I would like to make a booking at this restaurant.',
'For which time do you want the booking to be?',
'I will be eating there at 11:30 am so make it for then.',
'Can you please confirm that you want to book a table for 2 at 11:30 am at the Bird Dog restaurant in Palo Alto for today.',
'That suits me well. Can you tell me if they feature live music?',
'Your booking has been made without errors, but unfortunately they do not have live music.',
'Will I be able to find liquor there? Can you give me the address of their location?',
'The restaurant is located at 420 Ramona Street. Unfortunately they do not serve alcohol at the restaurant.',
'I appreciate it very much. That would be all.',
'Have a good time!'
]}
```
#### Data Splits
<!-- info: Describe and name the splits in the dataset if there are more than one. -->
<!-- scope: periscope -->
The dataset is split into a train, validation, and test set with the following sizes:
| | Train | Validation | Test |
| --- | --- | --- | --- |
| \# of dialogues | 16142 | 2482 | 4201 |
| \# of turns | 48426 | 7446 | 12603 |
#### Splitting Criteria
<!-- info: Describe any criteria for splitting the data, if used. If there are differences between the splits (e.g., if the training annotations are machine-generated and the dev and test ones are created by humans, or if different numbers of annotators contributed to each example), describe them here. -->
<!-- scope: microscope -->
The data is generally split i.i.d, but some topics only appear in training and some only for testing. For example, the domains Messaging, Payment, and Train are test-only.
## Dataset in GEM
### Rationale for Inclusion in GEM
#### Why is the Dataset in GEM?
<!-- info: What does this dataset contribute toward better generation evaluation and why is it part of GEM? -->
<!-- scope: microscope -->
This dataset comprises a wide range of dialog capabilities and thus enables the evaluation of many more generation capabilities of comparable datasets. Its collection methodology ensures a high diversity but also high quality of the data.
#### Similar Datasets
<!-- info: Do other datasets for the high level task exist? -->
<!-- scope: telescope -->
yes
#### Unique Language Coverage
<!-- info: Does this dataset cover other languages than other datasets for the same task? -->
<!-- scope: periscope -->
no
#### Difference from other GEM datasets
<!-- info: What else sets this dataset apart from other similar datasets in GEM? -->
<!-- scope: microscope -->
The domains a lot more diverse than other datasets.
#### Ability that the Dataset measures
<!-- info: What aspect of model ability can be measured with this dataset? -->
<!-- scope: periscope -->
surface realization, compositionality.
### GEM-Specific Curation
#### Modificatied for GEM?
<!-- info: Has the GEM version of the dataset been modified in any way (data, processing, splits) from the original curated data? -->
<!-- scope: telescope -->
yes
#### GEM Modifications
<!-- info: What changes have been made to he original dataset? -->
<!-- scope: periscope -->
`data points modified`
#### Modification Details
<!-- info: For each of these changes, described them in more details and provided the intended purpose of the modification -->
<!-- scope: microscope -->
We are focusing on the response-generation part of the dataset and thus reformatted the dataset to treat the service agent utterances as the targets to be generated and the previous customer utterance and the agent's dialog act as the input. We additionally reformat the dialog acts to directly conform to the format described in this [paper](https://arxiv.org/abs/2004.15006).
#### Additional Splits?
<!-- info: Does GEM provide additional splits to the dataset? -->
<!-- scope: telescope -->
yes
#### Split Information
<!-- info: Describe how the new splits were created -->
<!-- scope: periscope -->
9 challenge sets for Schema-Guided Dialog were added to the GEM evaluation suite.
1. We created subsets of the training and development sets of 500 randomly selected inputs each.
2. We applied 5 transformations to respectively 5 sets of 500 randomly selected inputs: (i) back-translation, (ii)-(iii) introduction of typographical errors, using Butterfingers with two thresholds (0.02 and 0.05), resulting in two sets with different amounts of typos introduced (there are more typos with the 0.05 threshold than with the 0.02 one), (iv) removal of final punctuations (when any), and (v) input scrambling, for which the order of the dialogue acts was randomly reassigned.
3. For the input size, we created subpopulations based on the number of dialogue acts in the input.
| DA number | Frequency English |
|---------------|-------------------|
| 1 | 5049 |
| 2 | 2517 |
| 3 | 1328 |
| 4 | 469 |
| 5 | 335 |
| 6 | 256 |
| 7 | 46 |
We also split the test data according to the type of dialogue act, represented by cardinal numbers in the dataset.
| DA type | Frequency English |
|--------------|-------------------|
| 2 | 1397 |
| 3 | 983 |
| 4 | 1027 |
| 5 | 958 |
| 9 | 72 |
| 10 | 1024 |
| 11 | 1246 |
| 12 | 500 |
| 13 | 2078 |
| 15 | 715 |
#### Split Motivation
<!-- info: What aspects of the model's generation capacities were the splits created to test? -->
<!-- scope: periscope -->
Generalization and Robustness.
### Getting Started with the Task
#### Pointers to Resources
<!-- info: Getting started with in-depth research on the task. Add relevant pointers to resources that researchers can consult when they want to get started digging deeper into the task. -->
<!-- scope: microscope -->
* [Paper for dataset and DST baseline](https://arxiv.org/pdf/1909.05855.pdf)
* [DSTC8 overview paper](https://arxiv.org/pdf/2002.01359.pdf)
* [Code for DST baseline](https://github.com/google-research/google-research/tree/master/schema_guided_dst)
* [Natural language generation baseline paper](https://arxiv.org/pdf/2004.15006.pdf)
* [Blog post announcing the dataset](https://ai.googleblog.com/2019/10/introducing-schema-guided-dialogue.html)
## Previous Results
### Previous Results
#### Measured Model Abilities
<!-- info: What aspect of model ability can be measured with this dataset? -->
<!-- scope: telescope -->
Surface realization and compositionally.
#### Metrics
<!-- info: What metrics are typically used for this task? -->
<!-- scope: periscope -->
`BLEURT`, `BLEU`, `ROUGE`
#### Proposed Evaluation
<!-- info: List and describe the purpose of the metrics and evaluation methodology (including human evaluation) that the dataset creators used when introducing this task. -->
<!-- scope: microscope -->
The original paper focused on the task of dialog state prediction instead of response generation and thus did not suggest any set of metrics.
#### Previous results available?
<!-- info: Are previous results available? -->
<!-- scope: telescope -->
no
## Dataset Curation
### Original Curation
#### Original Curation Rationale
<!-- info: Original curation rationale -->
<!-- scope: telescope -->
Previous multi-domain task-oriented dialogue datsets do not sufficiently capture the real-world challenges in virtual assistants, since they cover few domains and assume a single static ontology per domain.
The SGD datset is created to cover 17 domains with over 16K dialogues, and contain multiple different APIs in most domains, many of which have overlapping functionalities but different interfaces, which reflects common real-world scenarios.
The wide range of available annotations can be used for intent prediction, slot filling, dialogue state tracking, policy imitation learning, language generation, user simulation learning, among other tasks in large-scale virtual assistants.
#### Communicative Goal
<!-- info: What was the communicative goal? -->
<!-- scope: periscope -->
The goal of a speaker who generates the target utterance is to help users accomplish tasks including but not limited to finding flights, booking restaurants, searching for nearby events and movies.
#### Sourced from Different Sources
<!-- info: Is the dataset aggregated from different data sources? -->
<!-- scope: telescope -->
no
### Language Data
#### How was Language Data Obtained?
<!-- info: How was the language data obtained? -->
<!-- scope: telescope -->
`Machine-generated`
#### Generation Method Link
<!-- info: If text was machine-generated for the dataset, provide a link to the generation method if available (N/A otherwise). -->
<!-- scope: periscope -->
[Github](https://github.com/google-research-datasets/dstc8-schema-guided-dialogue)
#### Language Producers
<!-- info: What further information do we have on the language producers? -->
<!-- scope: microscope -->
The dialogue outlines are first generated by a simulator. The dialogue simulator interacts with the services to generate dialogue outlines. It consists of two agents playing the roles of the user and the system, interacting with each other using a finite set of actions specified through dialogue acts over a probabilistic automaton designed to capture varied dialogue trajectories. It is worth noting that the simulation automaton does not include any domain-specific constraints: all domain-specific constraints are encoded in the schema and scenario.
The dialogue paraphrasing framework then converts the outlines generated by the simulator into a natural conversation. Users may refer to the slot values in the dialogue acts in various different ways during the conversation, e.g., “los angeles” may be referred to as “LA” or “LAX”. To introduce these natural variations in the slot values, different slot values are replaced with a randomly selected variation while being kept consistent across user turns in a dialogue. The actions are then converted to pseudo-natural language utterances using a set of manually defined action-to-text templates, and the resulting utterances for the different actions in a turn are concatenated together.
#### Topics Covered
<!-- info: Does the language in the dataset focus on specific topics? How would you describe them? -->
<!-- scope: periscope -->
The dataset covers the following domains: Alarm, Banks, Buses, Calendar, Events, Flights, Homes, Hotels, Media, Messaging, Movies, Music, Payment, RentalCars, Restaurants, RideSharing, Services, Train, Travel, and Weather. The domain ‘Service’ includes salons, dentists, doctors etc. The ‘Alarm’, ‘Messaging’, ‘Payment’ and ‘Train’ domains are only present in the dev or test sets.
to test generalization to new domains.
#### Data Validation
<!-- info: Was the text validated by a different worker or a data curator? -->
<!-- scope: telescope -->
not validated
#### Was Data Filtered?
<!-- info: Were text instances selected or filtered? -->
<!-- scope: telescope -->
not filtered
### Structured Annotations
#### Additional Annotations?
<!-- quick -->
<!-- info: Does the dataset have additional annotations for each instance? -->
<!-- scope: telescope -->
crowd-sourced
#### Number of Raters
<!-- info: What is the number of raters -->
<!-- scope: telescope -->
unknown
#### Raters per Training Example
<!-- info: How many annotators saw each training example? -->
<!-- scope: periscope -->
0
#### Raters per Test Example
<!-- info: How many annotators saw each test example? -->
<!-- scope: periscope -->
0
#### Annotation Service?
<!-- info: Was an annotation service used? -->
<!-- scope: telescope -->
unknown
#### Annotation Values
<!-- info: Purpose and values for each annotation -->
<!-- scope: microscope -->
The dialogue transformed by these steps is sent to the crowd workers to be reformulated into more natural language. One crowd worker is tasked with paraphrasing all utterances of a dialogue to ensure naturalness and coherence. The crowd workers are asked to exactly repeat the slot values in their paraphrases so that the span indices for the slots can be recovered via string matching.
#### Any Quality Control?
<!-- info: Quality control measures? -->
<!-- scope: telescope -->
none
### Consent
#### Any Consent Policy?
<!-- info: Was there a consent policy involved when gathering the data? -->
<!-- scope: telescope -->
no
#### Justification for Using the Data
<!-- info: If not, what is the justification for reusing the data? -->
<!-- scope: microscope -->
While no policy is reported, we assume that one was in place for the collection.
### Private Identifying Information (PII)
#### Contains PII?
<!-- quick -->
<!-- info: Does the source language data likely contain Personal Identifying Information about the data creators or subjects? -->
<!-- scope: telescope -->
no PII
#### Justification for no PII
<!-- info: Provide a justification for selecting `no PII` above. -->
<!-- scope: periscope -->
The SGD dataset does not use identity categories and does not contain sensitive data.
### Maintenance
#### Any Maintenance Plan?
<!-- info: Does the original dataset have a maintenance plan? -->
<!-- scope: telescope -->
no
## Broader Social Context
### Previous Work on the Social Impact of the Dataset
#### Usage of Models based on the Data
<!-- info: Are you aware of cases where models trained on the task featured in this dataset ore related tasks have been used in automated systems? -->
<!-- scope: telescope -->
no
### Impact on Under-Served Communities
#### Addresses needs of underserved Communities?
<!-- info: Does this dataset address the needs of communities that are traditionally underserved in language technology, and particularly language generation technology? Communities may be underserved for exemple because their language, language variety, or social or geographical context is underepresented in NLP and NLG resources (datasets and models). -->
<!-- scope: telescope -->
no
### Discussion of Biases
#### Any Documented Social Biases?
<!-- info: Are there documented social biases in the dataset? Biases in this context are variations in the ways members of different social categories are represented that can have harmful downstream consequences for members of the more disadvantaged group. -->
<!-- scope: telescope -->
no
#### Are the Language Producers Representative of the Language?
<!-- info: Does the distribution of language producers in the dataset accurately represent the full distribution of speakers of the language world-wide? If not, how does it differ? -->
<!-- scope: periscope -->
Due to the combination of the automatic generation and crowd rater paraphasing, the language can be very formulaic. While this may be acceptable for the model part (i.e., we may actually desire an automated agent to form formulaic responses), the input utterances of the simulated customers likely do not cover the entire spectrum of the English language.
## Considerations for Using the Data
### PII Risks and Liability
### Licenses
#### Copyright Restrictions on the Dataset
<!-- info: Based on your answers in the Intended Use part of the Data Overview Section, which of the following best describe the copyright and licensing status of the dataset? -->
<!-- scope: periscope -->
`open license - commercial use allowed`
#### Copyright Restrictions on the Language Data
<!-- info: Based on your answers in the Language part of the Data Curation Section, which of the following best describe the copyright and licensing status of the underlying language data? -->
<!-- scope: periscope -->
`open license - commercial use allowed`
### Known Technical Limitations
#### Technical Limitations
<!-- info: Describe any known technical limitations, such as spurrious correlations, train/test overlap, annotation biases, or mis-annotations, and cite the works that first identified these limitations when possible. -->
<!-- scope: microscope -->
The dialogues under each domain distributed unevenly, where the flights domain has 3644 dialogues while the payment domain only contains 222 dialogues.
Besides, all dialogues are paraphrased by crowd-workers, and it is possible that crow-workers with different culture backgrounds will exhibit biased opinions.
#### Unsuited Applications
<!-- info: When using a model trained on this dataset in a setting where users or the public may interact with its predictions, what are some pitfalls to look out for? In particular, describe some applications of the general task featured in this dataset that its curation or properties make it less suitable for. -->
<!-- scope: microscope -->
Since the initial data was automatically generated, the coverage of entity names is necessarily biased. An agent thus needs to be evaluated in a more realistic environment.
|
GEM/sportsett_basketball | ---
annotations_creators:
- none
language_creators:
- unknown
language:
- en
license:
- mit
multilinguality:
- unknown
size_categories:
- unknown
source_datasets:
- original
task_categories:
- table-to-text
task_ids: []
pretty_name: sportsett_basketball
tags:
- data-to-text
---
# Dataset Card for GEM/sportsett_basketball
## Dataset Description
- **Homepage:** https://github.com/nlgcat/sport_sett_basketball
- **Repository:** https://github.com/nlgcat/sport_sett_basketball
- **Paper:** https://aclanthology.org/2020.intellang-1.4/
- **Leaderboard:** N/A
- **Point of Contact:** Craig Thomson
### Link to Main Data Card
You can find the main data card on the [GEM Website](https://gem-benchmark.com/data_cards/sportsett_basketball).
### Dataset Summary
The sportsett dataset is an English data-to-text dataset in the basketball domain. The inputs are statistics summarizing an NBA game and the outputs are high-quality descriptions of the game in natural language.
You can load the dataset via:
```
import datasets
data = datasets.load_dataset('GEM/sportsett_basketball')
```
The data loader can be found [here](https://huggingface.co/datasets/GEM/sportsett_basketball).
#### website
[Github](https://github.com/nlgcat/sport_sett_basketball)
#### paper
[ACL Anthology](https://aclanthology.org/2020.intellang-1.4/)
#### authors
Craig Thomson, Ashish Upadhyay
## Dataset Overview
### Where to find the Data and its Documentation
#### Webpage
<!-- info: What is the webpage for the dataset (if it exists)? -->
<!-- scope: telescope -->
[Github](https://github.com/nlgcat/sport_sett_basketball)
#### Download
<!-- info: What is the link to where the original dataset is hosted? -->
<!-- scope: telescope -->
[Github](https://github.com/nlgcat/sport_sett_basketball)
#### Paper
<!-- info: What is the link to the paper describing the dataset (open access preferred)? -->
<!-- scope: telescope -->
[ACL Anthology](https://aclanthology.org/2020.intellang-1.4/)
#### BibTex
<!-- info: Provide the BibTex-formatted reference for the dataset. Please use the correct published version (ACL anthology, etc.) instead of google scholar created Bibtex. -->
<!-- scope: microscope -->
```
@inproceedings{thomson-etal-2020-sportsett,
title = "{S}port{S}ett:Basketball - A robust and maintainable data-set for Natural Language Generation",
author = "Thomson, Craig and
Reiter, Ehud and
Sripada, Somayajulu",
booktitle = "Proceedings of the Workshop on Intelligent Information Processing and Natural Language Generation",
month = sep,
year = "2020",
address = "Santiago de Compostela, Spain",
publisher = "Association for Computational Lingustics",
url = "https://aclanthology.org/2020.intellang-1.4",
pages = "32--40",
}
```
#### Contact Name
<!-- quick -->
<!-- info: If known, provide the name of at least one person the reader can contact for questions about the dataset. -->
<!-- scope: periscope -->
Craig Thomson
#### Contact Email
<!-- info: If known, provide the email of at least one person the reader can contact for questions about the dataset. -->
<!-- scope: periscope -->
c.thomson@abdn.ac.uk
#### Has a Leaderboard?
<!-- info: Does the dataset have an active leaderboard? -->
<!-- scope: telescope -->
no
### Languages and Intended Use
#### Multilingual?
<!-- quick -->
<!-- info: Is the dataset multilingual? -->
<!-- scope: telescope -->
no
#### Covered Dialects
<!-- info: What dialects are covered? Are there multiple dialects per language? -->
<!-- scope: periscope -->
American English
One dialect, one language.
#### Covered Languages
<!-- quick -->
<!-- info: What languages/dialects are covered in the dataset? -->
<!-- scope: telescope -->
`English`
#### Whose Language?
<!-- info: Whose language is in the dataset? -->
<!-- scope: periscope -->
American sports writers
#### License
<!-- quick -->
<!-- info: What is the license of the dataset? -->
<!-- scope: telescope -->
mit: MIT License
#### Intended Use
<!-- info: What is the intended use of the dataset? -->
<!-- scope: microscope -->
Maintain a robust and scalable Data-to-Text generation resource with structured data and textual summaries
#### Primary Task
<!-- info: What primary task does the dataset support? -->
<!-- scope: telescope -->
Data-to-Text
#### Communicative Goal
<!-- quick -->
<!-- info: Provide a short description of the communicative goal of a model trained for this task on this dataset. -->
<!-- scope: periscope -->
A model trained on this dataset should summarise the statistical and other information from a basketball game. This will be focused on a single game, although facts from prior games, or aggregate statistics over many games can and should be used for comparison where appropriate. There no single common narrative, although summaries usually start with who player, when, where, and the score. They then provide high level commentary on what the difference in the game was (why the winner won). breakdowns of statistics for prominent players follow, winning team first. Finally, the upcoming schedule for both teams is usually included. There are, however, other types of fact that can be included, and other narrative structures.
### Credit
#### Curation Organization Type(s)
<!-- info: In what kind of organization did the dataset curation happen? -->
<!-- scope: telescope -->
`academic`
#### Curation Organization(s)
<!-- info: Name the organization(s). -->
<!-- scope: periscope -->
University of Aberdeen, Robert Gordon University
#### Dataset Creators
<!-- info: Who created the original dataset? List the people involved in collecting the dataset and their affiliation(s). -->
<!-- scope: microscope -->
Craig Thomson, Ashish Upadhyay
#### Funding
<!-- info: Who funded the data creation? -->
<!-- scope: microscope -->
EPSRC
#### Who added the Dataset to GEM?
<!-- info: Who contributed to the data card and adding the dataset to GEM? List the people+affiliations involved in creating this data card and who helped integrate this dataset into GEM. -->
<!-- scope: microscope -->
Craig Thomson, Ashish Upadhyay
### Dataset Structure
#### Data Fields
<!-- info: List and describe the fields present in the dataset. -->
<!-- scope: telescope -->
Each instance in the dataset has five fields.
1. "sportsett_id": This is a unique id as used in the original SportSett database. It starts with '1' with the first instance in the train-set and ends with '6150' with the last instance in test-set.
2. "gem_id": This is a unique id created as per GEM's requirement which follows the `GEM-${DATASET_NAME}-${SPLIT-NAME}-${id}` pattern.
3. "game": This field contains a dictionary with information about current game. It has information such as date on which the game was played alongwith the stadium, city, state where it was played.
4. "teams": This filed is a dictionary of multiple nested dictionaries. On the highest level, it has two keys: 'home' and 'vis', which provide the stats for home team and visiting team of the game. Both are dictionaries with same structure. Each dictionary will contain team's information such as name of the team, their total wins/losses in current season, their conference standing, the SportSett ids for their current and previous games. Apart from these general information, they also have the box- and line- scores for the team in the game. Box score is the stats of players from the team at the end of the game, while line score along with the whole game stats is divided into quarters and halves as well as the extra-time (if happened in the game). After these scores, there is another field of next-game, which gives general information about team's next game such as the place and opponent's name of the next game.
5. "summaries": This is a list of summaries for each game. Some games will have more than one summary, in that case, the list will have more than one entries. Each summary in the list is a string which can be tokenised by a space, following the practices in RotoWire-FG dataset ([Wang, 2019](https://www.aclweb.org/anthology/W19-8639)).
#### Reason for Structure
<!-- info: How was the dataset structure determined? -->
<!-- scope: microscope -->
The structure mostly follows the original structure defined in RotoWire dataset ([Wiseman et. al. 2017](https://aclanthology.org/D17-1239/)) with some modifications (such as game and next-game keys) address the problem of information gap between input and output data ([Thomson et. al. 2020](https://aclanthology.org/2020.inlg-1.6/)).
#### How were labels chosen?
<!-- info: How were the labels chosen? -->
<!-- scope: microscope -->
Similar to RotoWire dataset ([Wiseman et. al. 2017](https://aclanthology.org/D17-1239/))
#### Example Instance
<!-- info: Provide a JSON formatted example of a typical instance in the dataset. -->
<!-- scope: periscope -->
```
{
"sportsett_id": "1",
"gem_id": "GEM-sportsett_basketball-train-0",
"game": {
"day": "1",
"month": "November",
"year": "2014",
"dayname": "Saturday",
"season": "2014",
"stadium": "Wells Fargo Center",
"city": "Philadelphia",
"state": "Pennsylvania",
"attendance": "19753",
"capacity": "20478",
"game_id": "1"
},
"teams": {
"home": {
"name": "76ers",
"place": "Philadelphia",
"conference": "Eastern Conference",
"division": "Atlantic",
"wins": "0",
"losses": "3",
"conference_standing": 15,
"game_number": "3",
"previous_game_id": "42",
"next_game_id": "2",
"line_score": {
"game": {
"FG3A": "23",
"FG3M": "7",
"FG3_PCT": "30",
"FGA": "67",
"FGM": "35",
"FG_PCT": "52",
"FTA": "26",
"FTM": "19",
"FT_PCT": "73",
"DREB": "33",
"OREB": "4",
"TREB": "37",
"BLK": "10",
"AST": "28",
"STL": "9",
"TOV": "24",
"PF": "21",
"PTS": "96",
"MIN": "4"
},
"H1": {
"FG3A": "82",
"FG3M": "30",
"FG3_PCT": "37",
"FGA": "2115",
"FGM": "138",
"FG_PCT": "7",
"FTA": "212",
"FTM": "18",
"FT_PCT": "8",
"DREB": "810",
"OREB": "21",
"TREB": "831",
"BLK": "51",
"AST": "107",
"STL": "21",
"TOV": "64",
"PTS": "3024",
"MIN": "6060"
},
"H2": {
"FG3A": "85",
"FG3M": "40",
"FG3_PCT": "47",
"FGA": "1615",
"FGM": "104",
"FG_PCT": "6",
"FTA": "66",
"FTM": "55",
"FT_PCT": "83",
"DREB": "96",
"OREB": "10",
"TREB": "106",
"BLK": "22",
"AST": "92",
"STL": "24",
"TOV": "68",
"PTS": "2913",
"MIN": "6060"
},
"Q1": {
"FG3A": "8",
"FG3M": "3",
"FG3_PCT": "38",
"FGA": "21",
"FGM": "13",
"FG_PCT": "62",
"FTA": "2",
"FTM": "1",
"FT_PCT": "50",
"DREB": "8",
"OREB": "2",
"TREB": "10",
"BLK": "5",
"AST": "10",
"STL": "2",
"TOV": "6",
"PTS": "30",
"MIN": "60"
},
"Q2": {
"FG3A": "2",
"FG3M": "0",
"FG3_PCT": "0",
"FGA": "15",
"FGM": "8",
"FG_PCT": "53",
"FTA": "12",
"FTM": "8",
"FT_PCT": "67",
"DREB": "10",
"OREB": "1",
"TREB": "11",
"BLK": "1",
"AST": "7",
"STL": "1",
"TOV": "4",
"PTS": "24",
"MIN": "60"
},
"Q3": {
"FG3A": "8",
"FG3M": "4",
"FG3_PCT": "50",
"FGA": "16",
"FGM": "10",
"FG_PCT": "62",
"FTA": "6",
"FTM": "5",
"FT_PCT": "83",
"DREB": "9",
"OREB": "1",
"TREB": "10",
"BLK": "2",
"AST": "9",
"STL": "2",
"TOV": "6",
"PTS": "29",
"MIN": "60"
},
"Q4": {
"FG3A": "5",
"FG3M": "0",
"FG3_PCT": "0",
"FGA": "15",
"FGM": "4",
"FG_PCT": "27",
"FTA": "6",
"FTM": "5",
"FT_PCT": "83",
"DREB": "6",
"OREB": "0",
"TREB": "6",
"BLK": "2",
"AST": "2",
"STL": "4",
"TOV": "8",
"PTS": "13",
"MIN": "60"
},
"OT": {
"FG3A": "0",
"FG3M": "0",
"FG3_PCT": "0",
"FGA": "0",
"FGM": "0",
"FG_PCT": "0",
"FTA": "0",
"FTM": "0",
"FT_PCT": "0",
"DREB": "0",
"OREB": "0",
"TREB": "0",
"BLK": "0",
"AST": "0",
"STL": "0",
"TOV": "0",
"PTS": "0",
"MIN": "0"
}
},
"box_score": [
{
"first_name": "Tony",
"last_name": "Wroten",
"name": "Tony Wroten",
"starter": "True",
"MIN": "33",
"FGM": "6",
"FGA": "11",
"FG_PCT": "55",
"FG3M": "1",
"FG3A": "4",
"FG3_PCT": "25",
"FTM": "8",
"FTA": "11",
"FT_PCT": "73",
"OREB": "0",
"DREB": "3",
"TREB": "3",
"AST": "10",
"STL": "1",
"BLK": "1",
"TOV": "4",
"PF": "1",
"PTS": "21",
"+/-": "-11",
"DOUBLE": "double"
},
{
"first_name": "Hollis",
"last_name": "Thompson",
"name": "Hollis Thompson",
"starter": "True",
"MIN": "32",
"FGM": "4",
"FGA": "8",
"FG_PCT": "50",
"FG3M": "2",
"FG3A": "5",
"FG3_PCT": "40",
"FTM": "0",
"FTA": "0",
"FT_PCT": "0",
"OREB": "0",
"DREB": "1",
"TREB": "1",
"AST": "2",
"STL": "0",
"BLK": "3",
"TOV": "2",
"PF": "2",
"PTS": "10",
"+/-": "-17",
"DOUBLE": "none"
},
{
"first_name": "Henry",
"last_name": "Sims",
"name": "Henry Sims",
"starter": "True",
"MIN": "27",
"FGM": "4",
"FGA": "9",
"FG_PCT": "44",
"FG3M": "0",
"FG3A": "0",
"FG3_PCT": "0",
"FTM": "1",
"FTA": "2",
"FT_PCT": "50",
"OREB": "1",
"DREB": "3",
"TREB": "4",
"AST": "2",
"STL": "0",
"BLK": "1",
"TOV": "0",
"PF": "1",
"PTS": "9",
"+/-": "-10",
"DOUBLE": "none"
},
{
"first_name": "Nerlens",
"last_name": "Noel",
"name": "Nerlens Noel",
"starter": "True",
"MIN": "25",
"FGM": "1",
"FGA": "4",
"FG_PCT": "25",
"FG3M": "0",
"FG3A": "0",
"FG3_PCT": "0",
"FTM": "0",
"FTA": "0",
"FT_PCT": "0",
"OREB": "0",
"DREB": "5",
"TREB": "5",
"AST": "3",
"STL": "1",
"BLK": "1",
"TOV": "3",
"PF": "1",
"PTS": "2",
"+/-": "-19",
"DOUBLE": "none"
},
{
"first_name": "Luc",
"last_name": "Mbah a Moute",
"name": "Luc Mbah a Moute",
"starter": "True",
"MIN": "19",
"FGM": "4",
"FGA": "10",
"FG_PCT": "40",
"FG3M": "0",
"FG3A": "2",
"FG3_PCT": "0",
"FTM": "1",
"FTA": "2",
"FT_PCT": "50",
"OREB": "3",
"DREB": "4",
"TREB": "7",
"AST": "3",
"STL": "1",
"BLK": "0",
"TOV": "6",
"PF": "3",
"PTS": "9",
"+/-": "-12",
"DOUBLE": "none"
},
{
"first_name": "Brandon",
"last_name": "Davies",
"name": "Brandon Davies",
"starter": "False",
"MIN": "23",
"FGM": "7",
"FGA": "9",
"FG_PCT": "78",
"FG3M": "1",
"FG3A": "2",
"FG3_PCT": "50",
"FTM": "3",
"FTA": "4",
"FT_PCT": "75",
"OREB": "0",
"DREB": "3",
"TREB": "3",
"AST": "0",
"STL": "3",
"BLK": "0",
"TOV": "3",
"PF": "3",
"PTS": "18",
"+/-": "-1",
"DOUBLE": "none"
},
{
"first_name": "Chris",
"last_name": "Johnson",
"name": "Chris Johnson",
"starter": "False",
"MIN": "21",
"FGM": "2",
"FGA": "4",
"FG_PCT": "50",
"FG3M": "1",
"FG3A": "3",
"FG3_PCT": "33",
"FTM": "0",
"FTA": "0",
"FT_PCT": "0",
"OREB": "0",
"DREB": "2",
"TREB": "2",
"AST": "0",
"STL": "3",
"BLK": "0",
"TOV": "2",
"PF": "5",
"PTS": "5",
"+/-": "3",
"DOUBLE": "none"
},
{
"first_name": "K.J.",
"last_name": "McDaniels",
"name": "K.J. McDaniels",
"starter": "False",
"MIN": "20",
"FGM": "2",
"FGA": "4",
"FG_PCT": "50",
"FG3M": "1",
"FG3A": "3",
"FG3_PCT": "33",
"FTM": "3",
"FTA": "4",
"FT_PCT": "75",
"OREB": "0",
"DREB": "1",
"TREB": "1",
"AST": "2",
"STL": "0",
"BLK": "3",
"TOV": "2",
"PF": "3",
"PTS": "8",
"+/-": "-10",
"DOUBLE": "none"
},
{
"first_name": "Malcolm",
"last_name": "Thomas",
"name": "Malcolm Thomas",
"starter": "False",
"MIN": "19",
"FGM": "4",
"FGA": "4",
"FG_PCT": "100",
"FG3M": "0",
"FG3A": "0",
"FG3_PCT": "0",
"FTM": "0",
"FTA": "0",
"FT_PCT": "0",
"OREB": "0",
"DREB": "9",
"TREB": "9",
"AST": "0",
"STL": "0",
"BLK": "0",
"TOV": "0",
"PF": "2",
"PTS": "8",
"+/-": "-6",
"DOUBLE": "none"
},
{
"first_name": "Alexey",
"last_name": "Shved",
"name": "Alexey Shved",
"starter": "False",
"MIN": "14",
"FGM": "1",
"FGA": "4",
"FG_PCT": "25",
"FG3M": "1",
"FG3A": "4",
"FG3_PCT": "25",
"FTM": "3",
"FTA": "3",
"FT_PCT": "100",
"OREB": "0",
"DREB": "1",
"TREB": "1",
"AST": "6",
"STL": "0",
"BLK": "0",
"TOV": "2",
"PF": "0",
"PTS": "6",
"+/-": "-7",
"DOUBLE": "none"
},
{
"first_name": "JaKarr",
"last_name": "Sampson",
"name": "JaKarr Sampson",
"starter": "False",
"MIN": "2",
"FGM": "0",
"FGA": "0",
"FG_PCT": "0",
"FG3M": "0",
"FG3A": "0",
"FG3_PCT": "0",
"FTM": "0",
"FTA": "0",
"FT_PCT": "0",
"OREB": "0",
"DREB": "1",
"TREB": "1",
"AST": "0",
"STL": "0",
"BLK": "1",
"TOV": "0",
"PF": "0",
"PTS": "0",
"+/-": "0",
"DOUBLE": "none"
},
{
"first_name": "Michael",
"last_name": "Carter-Williams",
"name": "Michael Carter-Williams",
"starter": "False",
"MIN": "0",
"FGM": "0",
"FGA": "0",
"FG_PCT": "0",
"FG3M": "0",
"FG3A": "0",
"FG3_PCT": "0",
"FTM": "0",
"FTA": "0",
"FT_PCT": "0",
"OREB": "0",
"DREB": "0",
"TREB": "0",
"AST": "0",
"STL": "0",
"BLK": "0",
"TOV": "0",
"PF": "0",
"PTS": "0",
"+/-": "0",
"DOUBLE": "none"
}
],
"next_game": {
"day": "3",
"month": "November",
"year": "2014",
"dayname": "Monday",
"stadium": "Wells Fargo Center",
"city": "Philadelphia",
"opponent_name": "Rockets",
"opponent_place": "Houston",
"is_home": "True"
}
},
"vis": {
"name": "Heat",
"place": "Miami",
"conference": "Eastern Conference",
"division": "Southeast",
"wins": "2",
"losses": "0",
"conference_standing": 1,
"game_number": "2",
"previous_game_id": "329",
"next_game_id": "330",
"line_score": {
"game": {
"FG3A": "24",
"FG3M": "12",
"FG3_PCT": "50",
"FGA": "83",
"FGM": "41",
"FG_PCT": "49",
"FTA": "29",
"FTM": "20",
"FT_PCT": "69",
"DREB": "26",
"OREB": "9",
"TREB": "35",
"BLK": "0",
"AST": "33",
"STL": "16",
"TOV": "16",
"PF": "20",
"PTS": "114",
"MIN": "4"
},
"H1": {
"FG3A": "69",
"FG3M": "44",
"FG3_PCT": "64",
"FGA": "2321",
"FGM": "1110",
"FG_PCT": "48",
"FTA": "106",
"FTM": "64",
"FT_PCT": "60",
"DREB": "35",
"OREB": "23",
"TREB": "58",
"BLK": "00",
"AST": "88",
"STL": "53",
"TOV": "34",
"PTS": "3228",
"MIN": "6060"
},
"H2": {
"FG3A": "45",
"FG3M": "22",
"FG3_PCT": "49",
"FGA": "1920",
"FGM": "1010",
"FG_PCT": "53",
"FTA": "85",
"FTM": "55",
"FT_PCT": "65",
"DREB": "612",
"OREB": "22",
"TREB": "634",
"BLK": "00",
"AST": "98",
"STL": "35",
"TOV": "36",
"PTS": "2727",
"MIN": "6060"
},
"Q1": {
"FG3A": "6",
"FG3M": "4",
"FG3_PCT": "67",
"FGA": "23",
"FGM": "11",
"FG_PCT": "48",
"FTA": "10",
"FTM": "6",
"FT_PCT": "60",
"DREB": "3",
"OREB": "2",
"TREB": "5",
"BLK": "0",
"AST": "8",
"STL": "5",
"TOV": "3",
"PTS": "32",
"MIN": "60"
},
"Q2": {
"FG3A": "9",
"FG3M": "4",
"FG3_PCT": "44",
"FGA": "21",
"FGM": "10",
"FG_PCT": "48",
"FTA": "6",
"FTM": "4",
"FT_PCT": "67",
"DREB": "5",
"OREB": "3",
"TREB": "8",
"BLK": "0",
"AST": "8",
"STL": "3",
"TOV": "4",
"PTS": "28",
"MIN": "60"
},
"Q3": {
"FG3A": "4",
"FG3M": "2",
"FG3_PCT": "50",
"FGA": "19",
"FGM": "10",
"FG_PCT": "53",
"FTA": "8",
"FTM": "5",
"FT_PCT": "62",
"DREB": "6",
"OREB": "2",
"TREB": "8",
"BLK": "0",
"AST": "9",
"STL": "3",
"TOV": "3",
"PTS": "27",
"MIN": "60"
},
"Q4": {
"FG3A": "5",
"FG3M": "2",
"FG3_PCT": "40",
"FGA": "20",
"FGM": "10",
"FG_PCT": "50",
"FTA": "5",
"FTM": "5",
"FT_PCT": "100",
"DREB": "12",
"OREB": "2",
"TREB": "14",
"BLK": "0",
"AST": "8",
"STL": "5",
"TOV": "6",
"PTS": "27",
"MIN": "60"
},
"OT": {
"FG3A": "0",
"FG3M": "0",
"FG3_PCT": "0",
"FGA": "0",
"FGM": "0",
"FG_PCT": "0",
"FTA": "0",
"FTM": "0",
"FT_PCT": "0",
"DREB": "0",
"OREB": "0",
"TREB": "0",
"BLK": "0",
"AST": "0",
"STL": "0",
"TOV": "0",
"PTS": "0",
"MIN": "0"
}
},
"box_score": [
{
"first_name": "Chris",
"last_name": "Bosh",
"name": "Chris Bosh",
"starter": "True",
"MIN": "33",
"FGM": "9",
"FGA": "17",
"FG_PCT": "53",
"FG3M": "2",
"FG3A": "5",
"FG3_PCT": "40",
"FTM": "10",
"FTA": "11",
"FT_PCT": "91",
"OREB": "3",
"DREB": "5",
"TREB": "8",
"AST": "4",
"STL": "2",
"BLK": "0",
"TOV": "3",
"PF": "2",
"PTS": "30",
"+/-": "10",
"DOUBLE": "none"
},
{
"first_name": "Dwyane",
"last_name": "Wade",
"name": "Dwyane Wade",
"starter": "True",
"MIN": "32",
"FGM": "4",
"FGA": "18",
"FG_PCT": "22",
"FG3M": "0",
"FG3A": "1",
"FG3_PCT": "0",
"FTM": "1",
"FTA": "3",
"FT_PCT": "33",
"OREB": "1",
"DREB": "2",
"TREB": "3",
"AST": "10",
"STL": "3",
"BLK": "0",
"TOV": "6",
"PF": "1",
"PTS": "9",
"+/-": "13",
"DOUBLE": "none"
},
{
"first_name": "Luol",
"last_name": "Deng",
"name": "Luol Deng",
"starter": "True",
"MIN": "29",
"FGM": "7",
"FGA": "11",
"FG_PCT": "64",
"FG3M": "1",
"FG3A": "3",
"FG3_PCT": "33",
"FTM": "0",
"FTA": "1",
"FT_PCT": "0",
"OREB": "2",
"DREB": "2",
"TREB": "4",
"AST": "2",
"STL": "2",
"BLK": "0",
"TOV": "1",
"PF": "0",
"PTS": "15",
"+/-": "4",
"DOUBLE": "none"
},
{
"first_name": "Shawne",
"last_name": "Williams",
"name": "Shawne Williams",
"starter": "True",
"MIN": "29",
"FGM": "5",
"FGA": "9",
"FG_PCT": "56",
"FG3M": "3",
"FG3A": "5",
"FG3_PCT": "60",
"FTM": "2",
"FTA": "2",
"FT_PCT": "100",
"OREB": "0",
"DREB": "4",
"TREB": "4",
"AST": "4",
"STL": "1",
"BLK": "0",
"TOV": "1",
"PF": "4",
"PTS": "15",
"+/-": "16",
"DOUBLE": "none"
},
{
"first_name": "Norris",
"last_name": "Cole",
"name": "Norris Cole",
"starter": "True",
"MIN": "27",
"FGM": "4",
"FGA": "7",
"FG_PCT": "57",
"FG3M": "2",
"FG3A": "4",
"FG3_PCT": "50",
"FTM": "0",
"FTA": "0",
"FT_PCT": "0",
"OREB": "0",
"DREB": "1",
"TREB": "1",
"AST": "4",
"STL": "2",
"BLK": "0",
"TOV": "0",
"PF": "1",
"PTS": "10",
"+/-": "6",
"DOUBLE": "none"
},
{
"first_name": "Mario",
"last_name": "Chalmers",
"name": "Mario Chalmers",
"starter": "False",
"MIN": "25",
"FGM": "6",
"FGA": "9",
"FG_PCT": "67",
"FG3M": "2",
"FG3A": "2",
"FG3_PCT": "100",
"FTM": "6",
"FTA": "10",
"FT_PCT": "60",
"OREB": "0",
"DREB": "2",
"TREB": "2",
"AST": "4",
"STL": "4",
"BLK": "0",
"TOV": "0",
"PF": "1",
"PTS": "20",
"+/-": "18",
"DOUBLE": "none"
},
{
"first_name": "Shabazz",
"last_name": "Napier",
"name": "Shabazz Napier",
"starter": "False",
"MIN": "20",
"FGM": "2",
"FGA": "3",
"FG_PCT": "67",
"FG3M": "1",
"FG3A": "2",
"FG3_PCT": "50",
"FTM": "0",
"FTA": "0",
"FT_PCT": "0",
"OREB": "0",
"DREB": "3",
"TREB": "3",
"AST": "4",
"STL": "2",
"BLK": "0",
"TOV": "1",
"PF": "4",
"PTS": "5",
"+/-": "11",
"DOUBLE": "none"
},
{
"first_name": "Chris",
"last_name": "Andersen",
"name": "Chris Andersen",
"starter": "False",
"MIN": "17",
"FGM": "0",
"FGA": "2",
"FG_PCT": "0",
"FG3M": "0",
"FG3A": "0",
"FG3_PCT": "0",
"FTM": "0",
"FTA": "0",
"FT_PCT": "0",
"OREB": "1",
"DREB": "2",
"TREB": "3",
"AST": "0",
"STL": "0",
"BLK": "0",
"TOV": "0",
"PF": "2",
"PTS": "0",
"+/-": "6",
"DOUBLE": "none"
},
{
"first_name": "Josh",
"last_name": "McRoberts",
"name": "Josh McRoberts",
"starter": "False",
"MIN": "11",
"FGM": "1",
"FGA": "3",
"FG_PCT": "33",
"FG3M": "0",
"FG3A": "1",
"FG3_PCT": "0",
"FTM": "1",
"FTA": "2",
"FT_PCT": "50",
"OREB": "0",
"DREB": "3",
"TREB": "3",
"AST": "0",
"STL": "0",
"BLK": "0",
"TOV": "2",
"PF": "3",
"PTS": "3",
"+/-": "1",
"DOUBLE": "none"
},
{
"first_name": "James",
"last_name": "Ennis",
"name": "James Ennis",
"starter": "False",
"MIN": "7",
"FGM": "2",
"FGA": "3",
"FG_PCT": "67",
"FG3M": "1",
"FG3A": "1",
"FG3_PCT": "100",
"FTM": "0",
"FTA": "0",
"FT_PCT": "0",
"OREB": "1",
"DREB": "1",
"TREB": "2",
"AST": "1",
"STL": "0",
"BLK": "0",
"TOV": "0",
"PF": "1",
"PTS": "5",
"+/-": "2",
"DOUBLE": "none"
},
{
"first_name": "Justin",
"last_name": "Hamilton",
"name": "Justin Hamilton",
"starter": "False",
"MIN": "5",
"FGM": "1",
"FGA": "1",
"FG_PCT": "100",
"FG3M": "0",
"FG3A": "0",
"FG3_PCT": "0",
"FTM": "0",
"FTA": "0",
"FT_PCT": "0",
"OREB": "1",
"DREB": "1",
"TREB": "2",
"AST": "0",
"STL": "0",
"BLK": "0",
"TOV": "1",
"PF": "0",
"PTS": "2",
"+/-": "3",
"DOUBLE": "none"
},
{
"first_name": "Andre",
"last_name": "Dawkins",
"name": "Andre Dawkins",
"starter": "False",
"MIN": "1",
"FGM": "0",
"FGA": "0",
"FG_PCT": "0",
"FG3M": "0",
"FG3A": "0",
"FG3_PCT": "0",
"FTM": "0",
"FTA": "0",
"FT_PCT": "0",
"OREB": "0",
"DREB": "0",
"TREB": "0",
"AST": "0",
"STL": "0",
"BLK": "0",
"TOV": "1",
"PF": "1",
"PTS": "0",
"+/-": "0",
"DOUBLE": "none"
},
{
"first_name": "Shannon",
"last_name": "Brown",
"name": "Shannon Brown",
"starter": "False",
"MIN": "0",
"FGM": "0",
"FGA": "0",
"FG_PCT": "0",
"FG3M": "0",
"FG3A": "0",
"FG3_PCT": "0",
"FTM": "0",
"FTA": "0",
"FT_PCT": "0",
"OREB": "0",
"DREB": "0",
"TREB": "0",
"AST": "0",
"STL": "0",
"BLK": "0",
"TOV": "0",
"PF": "0",
"PTS": "0",
"+/-": "0",
"DOUBLE": "none"
}
],
"next_game": {
"day": "2",
"month": "November",
"year": "2014",
"dayname": "Sunday",
"stadium": "American Airlines Arena",
"city": "Miami",
"opponent_name": "Raptors",
"opponent_place": "Toronto",
"is_home": "True"
}
}
},
"summaries": [
"The Miami Heat ( 20 ) defeated the Philadelphia 76ers ( 0 - 3 ) 114 - 96 on Saturday . Chris Bosh scored a game - high 30 points to go with eight rebounds in 33 minutes . Josh McRoberts made his Heat debut after missing the entire preseason recovering from toe surgery . McRoberts came off the bench and played 11 minutes . Shawne Williams was once again the starter at power forward in McRoberts ' stead . Williams finished with 15 points and three three - pointers in 29 minutes . Mario Chalmers scored 18 points in 25 minutes off the bench . Luc Richard Mbah a Moute replaced Chris Johnson in the starting lineup for the Sixers on Saturday . Hollis Thompson shifted down to the starting shooting guard job to make room for Mbah a Moute . Mbah a Moute finished with nine points and seven rebounds in 19 minutes . K.J . McDaniels , who suffered a minor hip flexor injury in Friday 's game , was available and played 21 minutes off the bench , finishing with eight points and three blocks . Michael Carter-Williams is expected to be out until Nov. 13 , but Tony Wroten continues to put up impressive numbers in Carter-Williams ' absence . Wroten finished with a double - double of 21 points and 10 assists in 33 minutes . The Heat will complete a back - to - back set at home Sunday against the Tornoto Raptors . The Sixers ' next game is at home Monday against the Houston Rockets ."
]
}
```
#### Data Splits
<!-- info: Describe and name the splits in the dataset if there are more than one. -->
<!-- scope: periscope -->
- Train: NBA seasons - 2014, 2015, & 2016; total instances - 3690
- Validation: NBA seasons - 2017; total instances - 1230
- Test: NBA seasons - 2018; total instances - 1230
#### Splitting Criteria
<!-- info: Describe any criteria for splitting the data, if used. If there are differences between the splits (e.g., if the training annotations are machine-generated and the dev and test ones are created by humans, or if different numbers of annotators contributed to each example), describe them here. -->
<!-- scope: microscope -->
The splits were created as per different NBA seasons. All the games in regular season (no play-offs) are added in the dataset
## Dataset in GEM
### Rationale for Inclusion in GEM
#### Why is the Dataset in GEM?
<!-- info: What does this dataset contribute toward better generation evaluation and why is it part of GEM? -->
<!-- scope: microscope -->
This dataset contains a data analytics problem in the classic sense ([Reiter, 2007](https://aclanthology.org/W07-2315)). That is, there is a large amount of data from which insights need to be selected. Further, the insights should be both from simple shallow queries (such as dirext transcriptions of the properties of a subject, i.e., a player and their statistics), as well as aggregated (how a player has done over time). There is far more on the data side than is required to be realised, and indeed, could be practically realised. This depth of data analytics problem does not exist in other datasets.
#### Similar Datasets
<!-- info: Do other datasets for the high level task exist? -->
<!-- scope: telescope -->
no
#### Ability that the Dataset measures
<!-- info: What aspect of model ability can be measured with this dataset? -->
<!-- scope: periscope -->
Many, if not all aspects of data-to-text systems can be measured with this dataset. It has complex data analytics, meaninful document planning (10-15 sentence documents with a narrative structure), as well as microplanning and realisation requirements. Finding models to handle this volume of data, as well as methods for meaninfully evaluate generations is a very open question.
### GEM-Specific Curation
#### Modificatied for GEM?
<!-- info: Has the GEM version of the dataset been modified in any way (data, processing, splits) from the original curated data? -->
<!-- scope: telescope -->
no
#### Additional Splits?
<!-- info: Does GEM provide additional splits to the dataset? -->
<!-- scope: telescope -->
no
### Getting Started with the Task
#### Pointers to Resources
<!-- info: Getting started with in-depth research on the task. Add relevant pointers to resources that researchers can consult when they want to get started digging deeper into the task. -->
<!-- scope: microscope -->
For dataset discussion see [Thomson et al, 2020](https://aclanthology.org/2020.intellang-1.4/)
For evaluation see:
- [Thomson & Reiter 2020, Thomson & Reiter (2021)](https://aclanthology.org/2021.inlg-1.23)
- [Kasner et al (2021)](https://aclanthology.org/2021.inlg-1.25)
For a system using the relational database form of SportSett, see:
- [Thomson et al (2020)](https://aclanthology.org/2020.inlg-1.6/)
For recent systems using the Rotowire dataset, see:
- [Puduppully & Lapata (2021)](https://github.com/ratishsp/data2text-macro-plan-py)
- [Rebuffel et all (2020)](https://github.com/KaijuML/data-to-text-hierarchical)
## Previous Results
### Previous Results
#### Measured Model Abilities
<!-- info: What aspect of model ability can be measured with this dataset? -->
<!-- scope: telescope -->
Many, if not all aspects of data-to-text systems can be measured with this dataset. It has complex data analytics, meaninful document planning (10-15 sentence documents with a narrative structure), as well as microplanning and realisation requirements. Finding models to handle this volume of data, as well as methods for meaninfully evaluate generations is a very open question.
#### Metrics
<!-- info: What metrics are typically used for this task? -->
<!-- scope: periscope -->
`BLEU`
#### Proposed Evaluation
<!-- info: List and describe the purpose of the metrics and evaluation methodology (including human evaluation) that the dataset creators used when introducing this task. -->
<!-- scope: microscope -->
BLEU is the only off-the-shelf metric commonly used. Works have also used custom metrics like RG ([Wiseman et al, 2017](https://aclanthology.org/D17-1239)), and a recent shared task explored other metrics and their corrolation with human evaluation ([Thomson & Reiter, 2021](https://aclanthology.org/2021.inlg-1.23)).
#### Previous results available?
<!-- info: Are previous results available? -->
<!-- scope: telescope -->
yes
#### Other Evaluation Approaches
<!-- info: What evaluation approaches have others used? -->
<!-- scope: periscope -->
Most results from prior works use the original Rotowire dataset, which has train/validation/test contamination. For results of BLEU and RG on the relational database format of SportSett, as a guide, see [Thomson et al, 2020](https://aclanthology.org/2020.inlg-1.6).
#### Relevant Previous Results
<!-- info: What are the most relevant previous results for this task/dataset? -->
<!-- scope: microscope -->
The results on this dataset are largely unexplored, as is the selection of suitable metrics that correlate with human judgment. See Thomson et al, 2021 (https://aclanthology.org/2021.inlg-1.23) for an overview, and Kasner et al (2021) for the best performing metric at the time of writing (https://aclanthology.org/2021.inlg-1.25).
## Dataset Curation
### Original Curation
#### Original Curation Rationale
<!-- info: Original curation rationale -->
<!-- scope: telescope -->
The references texts were taken from the existing dataset RotoWire-FG ([Wang, 2019](https://www.aclweb.org/anthology/W19-8639)), which is in turn based on Rotowire ([Wiseman et al, 2017](https://aclanthology.org/D17-1239)). The rationale behind this dataset was to re-structure the data such that aggregate statistics over multiple games, as well as upcoming game schedules could be included, moving the dataset from snapshots of single games, to a format where almost everything that could be present in the reference texts could be found in the data.
#### Communicative Goal
<!-- info: What was the communicative goal? -->
<!-- scope: periscope -->
Create a summary of a basketball, with insightful facts about the game, teams, and players, both within the game, withing periods during the game, and over the course of seasons/careers where appropriate. This is a data-to-text problem in the classic sense ([Reiter, 2007](https://aclanthology.org/W07-2315)) in that it has a difficult data analystics state, in addition to ordering and transcription of selected facts.
#### Sourced from Different Sources
<!-- info: Is the dataset aggregated from different data sources? -->
<!-- scope: telescope -->
yes
#### Source Details
<!-- info: List the sources (one per line) -->
<!-- scope: periscope -->
RotoWire-FG (https://www.rotowire.com).
Wikipedia (https://en.wikipedia.org/wiki/Main_Page)
Basketball Reference (https://www.basketball-reference.com)
### Language Data
#### How was Language Data Obtained?
<!-- info: How was the language data obtained? -->
<!-- scope: telescope -->
`Found`
#### Where was it found?
<!-- info: If found, where from? -->
<!-- scope: telescope -->
`Multiple websites`
#### Language Producers
<!-- info: What further information do we have on the language producers? -->
<!-- scope: microscope -->
None
#### Topics Covered
<!-- info: Does the language in the dataset focus on specific topics? How would you describe them? -->
<!-- scope: periscope -->
Summaries of basketball games (in the NBA).
#### Data Validation
<!-- info: Was the text validated by a different worker or a data curator? -->
<!-- scope: telescope -->
not validated
#### Data Preprocessing
<!-- info: How was the text data pre-processed? (Enter N/A if the text was not pre-processed) -->
<!-- scope: microscope -->
It retains the original tokenization scheme employed by Wang 2019
#### Was Data Filtered?
<!-- info: Were text instances selected or filtered? -->
<!-- scope: telescope -->
manually
#### Filter Criteria
<!-- info: What were the selection criteria? -->
<!-- scope: microscope -->
Games from the 2014 through 2018 seasons were selected. Within these seasons games are not filtered, all are present, but this was an arbitrary solution from the original RotoWirte-FG dataset.
### Structured Annotations
#### Additional Annotations?
<!-- quick -->
<!-- info: Does the dataset have additional annotations for each instance? -->
<!-- scope: telescope -->
none
#### Annotation Service?
<!-- info: Was an annotation service used? -->
<!-- scope: telescope -->
no
### Consent
#### Any Consent Policy?
<!-- info: Was there a consent policy involved when gathering the data? -->
<!-- scope: telescope -->
no
#### Justification for Using the Data
<!-- info: If not, what is the justification for reusing the data? -->
<!-- scope: microscope -->
The dataset consits of a pre-existing dataset, as well as publically available facts.
### Private Identifying Information (PII)
#### Contains PII?
<!-- quick -->
<!-- info: Does the source language data likely contain Personal Identifying Information about the data creators or subjects? -->
<!-- scope: telescope -->
unlikely
#### Categories of PII
<!-- info: What categories of PII are present or suspected in the data? -->
<!-- scope: periscope -->
`generic PII`
#### Any PII Identification?
<!-- info: Did the curators use any automatic/manual method to identify PII in the dataset? -->
<!-- scope: periscope -->
no identification
### Maintenance
#### Any Maintenance Plan?
<!-- info: Does the original dataset have a maintenance plan? -->
<!-- scope: telescope -->
no
## Broader Social Context
### Previous Work on the Social Impact of the Dataset
#### Usage of Models based on the Data
<!-- info: Are you aware of cases where models trained on the task featured in this dataset ore related tasks have been used in automated systems? -->
<!-- scope: telescope -->
no
### Impact on Under-Served Communities
#### Addresses needs of underserved Communities?
<!-- info: Does this dataset address the needs of communities that are traditionally underserved in language technology, and particularly language generation technology? Communities may be underserved for exemple because their language, language variety, or social or geographical context is underepresented in NLP and NLG resources (datasets and models). -->
<!-- scope: telescope -->
no
### Discussion of Biases
#### Any Documented Social Biases?
<!-- info: Are there documented social biases in the dataset? Biases in this context are variations in the ways members of different social categories are represented that can have harmful downstream consequences for members of the more disadvantaged group. -->
<!-- scope: telescope -->
yes
#### Links and Summaries of Analysis Work
<!-- info: Provide links to and summaries of works analyzing these biases. -->
<!-- scope: microscope -->
Unaware of any work, but, this is a dataset considting solely of summaries of mens professional basketball games. It does not cover different levels of the sport, or different genders, and all pronouns are likely to be male unless a specific player is referred to by other pronouns in the training text. This makes it difficult to train systems where gender can be specified as an attribute, although it is an interesting, open problem that could be investigated using the dataset.
#### Are the Language Producers Representative of the Language?
<!-- info: Does the distribution of language producers in the dataset accurately represent the full distribution of speakers of the language world-wide? If not, how does it differ? -->
<!-- scope: periscope -->
No, it is very specifically American English from the sports journalism domain.
## Considerations for Using the Data
### PII Risks and Liability
#### Potential PII Risk
<!-- info: Considering your answers to the PII part of the Data Curation Section, describe any potential privacy to the data subjects and creators risks when using the dataset. -->
<!-- scope: microscope -->
All information relating to persons is of public record.
### Licenses
#### Copyright Restrictions on the Dataset
<!-- info: Based on your answers in the Intended Use part of the Data Overview Section, which of the following best describe the copyright and licensing status of the dataset? -->
<!-- scope: periscope -->
`public domain`
#### Copyright Restrictions on the Language Data
<!-- info: Based on your answers in the Language part of the Data Curation Section, which of the following best describe the copyright and licensing status of the underlying language data? -->
<!-- scope: periscope -->
`public domain`
### Known Technical Limitations
#### Technical Limitations
<!-- info: Describe any known technical limitations, such as spurrious correlations, train/test overlap, annotation biases, or mis-annotations, and cite the works that first identified these limitations when possible. -->
<!-- scope: microscope -->
SportSett resolved the major overlap problems of RotoWire, although some overlap is unavoidable. For example, whilst it is not possible to find career totals and other historic information for all players (the data only goes back to 2014), it is possible to do so for some players. It is unavoidable that some data which is aggregated, exists in its base form in previous partitions. The season-based partition scheme heavily constrains this however.
#### Unsuited Applications
<!-- info: When using a model trained on this dataset in a setting where users or the public may interact with its predictions, what are some pitfalls to look out for? In particular, describe some applications of the general task featured in this dataset that its curation or properties make it less suitable for. -->
<!-- scope: microscope -->
Factual accuray continues to be a problem, systems may incorrectly represent the facts of the game.
#### Discouraged Use Cases
<!-- info: What are some discouraged use cases of a model trained to maximize the proposed metrics on this dataset? In particular, think about settings where decisions made by a model that performs reasonably well on the metric my still have strong negative consequences for user or members of the public. -->
<!-- scope: microscope -->
Using the RG metric to maximise the number of true facts in a generate summary is not nececeraly
|
GEM/squad_v2 | ---
annotations_creators:
- crowd-sourced
language_creators:
- unknown
language:
- en
license:
- cc-by-sa-4.0
multilinguality:
- unknown
size_categories:
- unknown
source_datasets:
- original
task_categories:
- other
task_ids: []
pretty_name: squad_v2
tags:
- question-generation
---
# Dataset Card for GEM/squad_v2
## Dataset Description
- **Homepage:** https://rajpurkar.github.io/SQuAD-explorer/
- **Repository:** https://rajpurkar.github.io/SQuAD-explorer/
- **Paper:** https://arxiv.org/abs/1806.03822v1
- **Leaderboard:** https://rajpurkar.github.io/SQuAD-explorer/
- **Point of Contact:** Robin Jia
### Link to Main Data Card
You can find the main data card on the [GEM Website](https://gem-benchmark.com/data_cards/squad_v2).
### Dataset Summary
SQuAD2.0 is a dataset that tests the ability of a system to not only answer reading comprehension questions, but also abstain when presented with a question that cannot be answered based on the provided paragraph. F1 score is used to evaluate models on the leaderboard. In GEM, we are using this dataset for the question-generation task in which a model should generate squad-like questions from an input text.
You can load the dataset via:
```
import datasets
data = datasets.load_dataset('GEM/squad_v2')
```
The data loader can be found [here](https://huggingface.co/datasets/GEM/squad_v2).
#### website
[Website](https://rajpurkar.github.io/SQuAD-explorer/)
#### paper
[Arxiv](https://arxiv.org/abs/1806.03822v1)
#### authors
Pranav Rajpurkar, Robin Jia and Percy Liang
## Dataset Overview
### Where to find the Data and its Documentation
#### Webpage
<!-- info: What is the webpage for the dataset (if it exists)? -->
<!-- scope: telescope -->
[Website](https://rajpurkar.github.io/SQuAD-explorer/)
#### Download
<!-- info: What is the link to where the original dataset is hosted? -->
<!-- scope: telescope -->
[Website](https://rajpurkar.github.io/SQuAD-explorer/)
#### Paper
<!-- info: What is the link to the paper describing the dataset (open access preferred)? -->
<!-- scope: telescope -->
[Arxiv](https://arxiv.org/abs/1806.03822v1)
#### BibTex
<!-- info: Provide the BibTex-formatted reference for the dataset. Please use the correct published version (ACL anthology, etc.) instead of google scholar created Bibtex. -->
<!-- scope: microscope -->
```
@inproceedings{Rajpurkar2018KnowWY,
title={Know What You Don’t Know: Unanswerable Questions for SQuAD},
author={Pranav Rajpurkar and Robin Jia and Percy Liang},
booktitle={ACL},
year={2018}
}
```
#### Contact Name
<!-- quick -->
<!-- info: If known, provide the name of at least one person the reader can contact for questions about the dataset. -->
<!-- scope: periscope -->
Robin Jia
#### Contact Email
<!-- info: If known, provide the email of at least one person the reader can contact for questions about the dataset. -->
<!-- scope: periscope -->
robinjia@stanford.edu
#### Has a Leaderboard?
<!-- info: Does the dataset have an active leaderboard? -->
<!-- scope: telescope -->
yes
#### Leaderboard Link
<!-- info: Provide a link to the leaderboard. -->
<!-- scope: periscope -->
[Website](https://rajpurkar.github.io/SQuAD-explorer/)
#### Leaderboard Details
<!-- info: Briefly describe how the leaderboard evaluates models. -->
<!-- scope: microscope -->
SQuAD2.0 tests the ability of a system to not only answer reading comprehension questions, but also abstain when presented with a question that cannot be answered based on the provided paragraph. F1 score is used to evaluate models on the leaderboard.
### Languages and Intended Use
#### Multilingual?
<!-- quick -->
<!-- info: Is the dataset multilingual? -->
<!-- scope: telescope -->
no
#### Covered Languages
<!-- quick -->
<!-- info: What languages/dialects are covered in the dataset? -->
<!-- scope: telescope -->
`English`
#### License
<!-- quick -->
<!-- info: What is the license of the dataset? -->
<!-- scope: telescope -->
cc-by-sa-4.0: Creative Commons Attribution Share Alike 4.0 International
#### Intended Use
<!-- info: What is the intended use of the dataset? -->
<!-- scope: microscope -->
The idea behind SQuAD2.0 dataset is to make the models understand when a question cannot be answered given a context. This will help in building models such that they know what they don't know, and therefore make the models understand language at a deeper level. The tasks that can be supported by the dataset are machine reading comprehension, extractive QA, and question generation.
#### Primary Task
<!-- info: What primary task does the dataset support? -->
<!-- scope: telescope -->
Question Generation
#### Communicative Goal
<!-- quick -->
<!-- info: Provide a short description of the communicative goal of a model trained for this task on this dataset. -->
<!-- scope: periscope -->
Given an input passage and an answer span, the goal is to generate a question that asks for the answer.
### Credit
#### Curation Organization Type(s)
<!-- info: In what kind of organization did the dataset curation happen? -->
<!-- scope: telescope -->
`academic`
#### Curation Organization(s)
<!-- info: Name the organization(s). -->
<!-- scope: periscope -->
Stanford University
#### Dataset Creators
<!-- info: Who created the original dataset? List the people involved in collecting the dataset and their affiliation(s). -->
<!-- scope: microscope -->
Pranav Rajpurkar, Robin Jia and Percy Liang
#### Funding
<!-- info: Who funded the data creation? -->
<!-- scope: microscope -->
Facebook and NSF Graduate Research Fellowship under Grant No. DGE-114747
#### Who added the Dataset to GEM?
<!-- info: Who contributed to the data card and adding the dataset to GEM? List the people+affiliations involved in creating this data card and who helped integrate this dataset into GEM. -->
<!-- scope: microscope -->
(Abinaya Mahendiran)[https://github.com/AbinayaM02], Manager Data Science, NEXT Labs,
### Dataset Structure
#### Data Fields
<!-- info: List and describe the fields present in the dataset. -->
<!-- scope: telescope -->
The data fields are the same among all splits.
#### squad_v2
- `id`: a `string` feature.
- `gem_id`: a `string` feature.
- `title`: a `string` feature.
- `context`: a `string` feature.
- `question`: a `string` feature.
- `answers`: a dictionary feature containing:
- `text`: a `string` feature.
- `answer_start`: a `int32` feature.
#### Example Instance
<!-- info: Provide a JSON formatted example of a typical instance in the dataset. -->
<!-- scope: periscope -->
Here is an example of a validation data point. This example was too long and was cropped:
```
{
"gem_id": "gem-squad_v2-validation-1",
"id": "56ddde6b9a695914005b9629",
"answers": {
"answer_start": [94, 87, 94, 94],
"text": ["10th and 11th centuries", "in the 10th and 11th centuries", "10th and 11th centuries", "10th and 11th centuries"]
},
"context": "\"The Normans (Norman: Nourmands; French: Normands; Latin: Normanni) were the people who in the 10th and 11th centuries gave thei...",
"question": "When were the Normans in Normandy?",
"title": "Normans"
}
```
#### Data Splits
<!-- info: Describe and name the splits in the dataset if there are more than one. -->
<!-- scope: periscope -->
The original SQuAD2.0 dataset has only training and dev (validation) splits. The train split is further divided into test split and added as part of the GEM datasets.
| name | train | validation | test |
| -------------- | --------: | -------------: | -------: |
| squad_v2 | 90403 | 11873 | 39916 |
## Dataset in GEM
### Rationale for Inclusion in GEM
#### Why is the Dataset in GEM?
<!-- info: What does this dataset contribute toward better generation evaluation and why is it part of GEM? -->
<!-- scope: microscope -->
SQuAD2.0 will encourage the development of new reading comprehension models
that know what they don’t know, and therefore understand language at a deeper level. It can also help in building better models for answer-aware question generation .
#### Similar Datasets
<!-- info: Do other datasets for the high level task exist? -->
<!-- scope: telescope -->
no
#### Unique Language Coverage
<!-- info: Does this dataset cover other languages than other datasets for the same task? -->
<!-- scope: periscope -->
yes
#### Ability that the Dataset measures
<!-- info: What aspect of model ability can be measured with this dataset? -->
<!-- scope: periscope -->
Reasoning capability
### GEM-Specific Curation
#### Modificatied for GEM?
<!-- info: Has the GEM version of the dataset been modified in any way (data, processing, splits) from the original curated data? -->
<!-- scope: telescope -->
yes
#### GEM Modifications
<!-- info: What changes have been made to he original dataset? -->
<!-- scope: periscope -->
`other`
#### Additional Splits?
<!-- info: Does GEM provide additional splits to the dataset? -->
<!-- scope: telescope -->
yes
#### Split Information
<!-- info: Describe how the new splits were created -->
<!-- scope: periscope -->
The train(80%) and validation(10%) split of SQuAD2.0 are made available to public whereas the test(10%) split is not available.
As part of GEM, the train split, 80% of the original data is split into two train split (90%) and test split (remaining 10%). The idea is to provide all three splits for the users to use.
### Getting Started with the Task
## Previous Results
### Previous Results
#### Measured Model Abilities
<!-- info: What aspect of model ability can be measured with this dataset? -->
<!-- scope: telescope -->
Extractive QA, Question Generation
#### Metrics
<!-- info: What metrics are typically used for this task? -->
<!-- scope: periscope -->
`Other: Other Metrics`, `METEOR`, `ROUGE`, `BLEU`
#### Other Metrics
<!-- info: Definitions of other metrics -->
<!-- scope: periscope -->
- Extractive QA uses Exact Match and F1 Score
- Question generation users METEOR, ROUGE-L, BLEU-4
#### Previous results available?
<!-- info: Are previous results available? -->
<!-- scope: telescope -->
yes
#### Other Evaluation Approaches
<!-- info: What evaluation approaches have others used? -->
<!-- scope: periscope -->
Question generation users METEOR, ROUGE-L, BLEU-4
#### Relevant Previous Results
<!-- info: What are the most relevant previous results for this task/dataset? -->
<!-- scope: microscope -->
@article{Dong2019UnifiedLM,
title={Unified Language Model Pre-training for Natural Language Understanding and Generation},
author={Li Dong and Nan Yang and Wenhui Wang and Furu Wei and Xiaodong Liu and Yu Wang and Jianfeng Gao and M. Zhou and Hsiao-Wuen Hon},
journal={ArXiv},
year={2019},
volume={abs/1905.03197}
}
## Dataset Curation
### Original Curation
#### Original Curation Rationale
<!-- info: Original curation rationale -->
<!-- scope: telescope -->
The dataset is curated in three stages:
- Curating passages,
- Crowdsourcing question-answers on those passages,
- Obtaining additional answers
As part of SQuAD1.1, 10000 high-quality articles from English Wikipedia is extracted using Project Nayuki’s Wikipedia’s internal PageRanks, from which 536 articles are sampled uniformly at random. From each of these articles, individual paragraphs are extracted, stripping away images, figures, tables, and discarding paragraphs shorter than 500 characters.
SQuAD2.0 combines the 100,000 questions in SQuAD1.1 with over 50,000 unanswerable questions written adversarially by crowdworkers to look similar to answerable ones.
#### Communicative Goal
<!-- info: What was the communicative goal? -->
<!-- scope: periscope -->
To build systems that not only answer questions when possible, but also determine when no
answer is supported by the paragraph and abstain from answering.
#### Sourced from Different Sources
<!-- info: Is the dataset aggregated from different data sources? -->
<!-- scope: telescope -->
yes
#### Source Details
<!-- info: List the sources (one per line) -->
<!-- scope: periscope -->
Wikipedia
### Language Data
#### How was Language Data Obtained?
<!-- info: How was the language data obtained? -->
<!-- scope: telescope -->
`Found`
#### Where was it found?
<!-- info: If found, where from? -->
<!-- scope: telescope -->
`Single website`
#### Topics Covered
<!-- info: Does the language in the dataset focus on specific topics? How would you describe them? -->
<!-- scope: periscope -->
The dataset contains 536 articles covering a wide range of topics, from musical celebrities to abstract concepts.
#### Data Validation
<!-- info: Was the text validated by a different worker or a data curator? -->
<!-- scope: telescope -->
validated by crowdworker
#### Data Preprocessing
<!-- info: How was the text data pre-processed? (Enter N/A if the text was not pre-processed) -->
<!-- scope: microscope -->
From the sampled articles from Wikipedia, individual paragraphs are extracted, stripping
away images, figures, tables, and discarding paragraphs shorter than 500 characters and partitioned into training(80%), development set(10%) and test set(10%).
#### Was Data Filtered?
<!-- info: Were text instances selected or filtered? -->
<!-- scope: telescope -->
algorithmically
#### Filter Criteria
<!-- info: What were the selection criteria? -->
<!-- scope: microscope -->
To retrieve high-quality articles, Project Nayuki’s Wikipedia’s internal PageRanks was used to obtain the top 10000 articles of English Wikipedia, from which 536 articles are sampled uniformly at random.
### Structured Annotations
#### Additional Annotations?
<!-- quick -->
<!-- info: Does the dataset have additional annotations for each instance? -->
<!-- scope: telescope -->
crowd-sourced
#### Number of Raters
<!-- info: What is the number of raters -->
<!-- scope: telescope -->
unknown
#### Rater Qualifications
<!-- info: Describe the qualifications required of an annotator. -->
<!-- scope: periscope -->
Crowdworkers from the United States or Canada with a 97% HIT acceptance rate, a minimum of 1000 HITs, were employed to create questions.
#### Raters per Training Example
<!-- info: How many annotators saw each training example? -->
<!-- scope: periscope -->
0
#### Raters per Test Example
<!-- info: How many annotators saw each test example? -->
<!-- scope: periscope -->
0
#### Annotation Service?
<!-- info: Was an annotation service used? -->
<!-- scope: telescope -->
yes
#### Which Annotation Service
<!-- info: Which annotation services were used? -->
<!-- scope: periscope -->
`other`, `Amazon Mechanical Turk`
#### Annotation Values
<!-- info: Purpose and values for each annotation -->
<!-- scope: microscope -->
For SQuAD 1.1 , crowdworkers were tasked with asking and answering up to 5 questions on the
content of that paragraph. The questions had to be entered in a text field, and the answers had to be
highlighted in the paragraph.
For SQuAD2.0, each task consisted of an entire article from SQuAD 1.1. For each paragraph in the article, workers were asked to pose up to five questions that were impossible to answer
based on the paragraph alone, while referencing entities in the paragraph and ensuring that a plausible answer is present.
#### Any Quality Control?
<!-- info: Quality control measures? -->
<!-- scope: telescope -->
validated by another rater
#### Quality Control Details
<!-- info: Describe the quality control measures that were taken. -->
<!-- scope: microscope -->
Questions from workers who wrote 25 or fewer questions on an article is removed; this filter
helped remove noise from workers who had trouble understanding the task, and therefore quit before completing the whole article. This filter to both SQuAD2.0 and the existing answerable questions from SQuAD 1.1.
### Consent
#### Any Consent Policy?
<!-- info: Was there a consent policy involved when gathering the data? -->
<!-- scope: telescope -->
no
### Private Identifying Information (PII)
#### Contains PII?
<!-- quick -->
<!-- info: Does the source language data likely contain Personal Identifying Information about the data creators or subjects? -->
<!-- scope: telescope -->
unlikely
#### Any PII Identification?
<!-- info: Did the curators use any automatic/manual method to identify PII in the dataset? -->
<!-- scope: periscope -->
no identification
### Maintenance
#### Any Maintenance Plan?
<!-- info: Does the original dataset have a maintenance plan? -->
<!-- scope: telescope -->
no
## Broader Social Context
### Previous Work on the Social Impact of the Dataset
#### Usage of Models based on the Data
<!-- info: Are you aware of cases where models trained on the task featured in this dataset ore related tasks have been used in automated systems? -->
<!-- scope: telescope -->
no
### Impact on Under-Served Communities
#### Addresses needs of underserved Communities?
<!-- info: Does this dataset address the needs of communities that are traditionally underserved in language technology, and particularly language generation technology? Communities may be underserved for exemple because their language, language variety, or social or geographical context is underepresented in NLP and NLG resources (datasets and models). -->
<!-- scope: telescope -->
no
### Discussion of Biases
#### Any Documented Social Biases?
<!-- info: Are there documented social biases in the dataset? Biases in this context are variations in the ways members of different social categories are represented that can have harmful downstream consequences for members of the more disadvantaged group. -->
<!-- scope: telescope -->
yes
## Considerations for Using the Data
### PII Risks and Liability
### Licenses
### Known Technical Limitations
|
GEM/surface_realisation_st_2020 | ---
annotations_creators:
- none
language_creators:
- unknown
language:
- ar
- zh
- en
- fr
- hi
- id
- ja
- ko
- pt
- ru
- es
license:
- cc-by-2.5
multilinguality:
- unknown
size_categories:
- unknown
source_datasets:
- original
task_categories:
- table-to-text
task_ids: []
pretty_name: surface_realisation_st_2020
tags:
- data-to-text
---
# Dataset Card for GEM/surface_realisation_st_2020
## Dataset Description
- **Homepage:** http://taln.upf.edu/pages/msr2020-ws/SRST.html#data
- **Repository:** https://sites.google.com/site/genchalrepository/surface-realisation/sr-20-multilingual
- **Paper:** https://aclanthology.org/2020.msr-1.1/
- **Leaderboard:** N/A
- **Point of Contact:** Simon Mille
### Link to Main Data Card
You can find the main data card on the [GEM Website](https://gem-benchmark.com/data_cards/surface_realisation_st_2020).
### Dataset Summary
This dataset was used as part of the multilingual surface realization shared task in which a model gets full or partial universal dependency structures and has to reconstruct the natural language. This dataset support 11 languages.
You can load the dataset via:
```
import datasets
data = datasets.load_dataset('GEM/surface_realisation_st_2020')
```
The data loader can be found [here](https://huggingface.co/datasets/GEM/surface_realisation_st_2020).
#### website
[Website](http://taln.upf.edu/pages/msr2020-ws/SRST.html#data)
#### paper
[ACL Anthology](https://aclanthology.org/2020.msr-1.1/)
#### authors
Simon Mille (Pompeu Fabra University); Leo Wanner (Pompeu Fabra University); Anya Belz (Brighton University); Bernd Bohnet (Google Inc.); Thiago Castro Ferreira (Federal University of Minas Gerais); Yvette Graham (ADAPT/Trinity College Dublin)
## Dataset Overview
### Where to find the Data and its Documentation
#### Webpage
<!-- info: What is the webpage for the dataset (if it exists)? -->
<!-- scope: telescope -->
[Website](http://taln.upf.edu/pages/msr2020-ws/SRST.html#data)
#### Download
<!-- info: What is the link to where the original dataset is hosted? -->
<!-- scope: telescope -->
[Website](https://sites.google.com/site/genchalrepository/surface-realisation/sr-20-multilingual)
#### Paper
<!-- info: What is the link to the paper describing the dataset (open access preferred)? -->
<!-- scope: telescope -->
[ACL Anthology](https://aclanthology.org/2020.msr-1.1/)
#### BibTex
<!-- info: Provide the BibTex-formatted reference for the dataset. Please use the correct published version (ACL anthology, etc.) instead of google scholar created Bibtex. -->
<!-- scope: microscope -->
```
@inproceedings{mille-etal-2020-third,
title = "The Third Multilingual Surface Realisation Shared Task ({SR}{'}20): Overview and Evaluation Results",
author = "Mille, Simon and
Belz, Anya and
Bohnet, Bernd and
Castro Ferreira, Thiago and
Graham, Yvette and
Wanner, Leo",
booktitle = "Proceedings of the Third Workshop on Multilingual Surface Realisation",
month = dec,
year = "2020",
address = "Barcelona, Spain (Online)",
publisher = "Association for Computational Linguistics",
url = "https://aclanthology.org/2020.msr-1.1",
pages = "1--20",
abstract = "This paper presents results from the Third Shared Task on Multilingual Surface Realisation (SR{'}20) which was organised as part of the COLING{'}20 Workshop on Multilingual Surface Realisation. As in SR{'}18 and SR{'}19, the shared task comprised two tracks: (1) a Shallow Track where the inputs were full UD structures with word order information removed and tokens lemmatised; and (2) a Deep Track where additionally, functional words and morphological information were removed. Moreover, each track had two subtracks: (a) restricted-resource, where only the data provided or approved as part of a track could be used for training models, and (b) open-resource, where any data could be used. The Shallow Track was offered in 11 languages, whereas the Deep Track in 3 ones. Systems were evaluated using both automatic metrics and direct assessment by human evaluators in terms of Readability and Meaning Similarity to reference outputs. We present the evaluation results, along with descriptions of the SR{'}19 tracks, data and evaluation methods, as well as brief summaries of the participating systems. For full descriptions of the participating systems, please see the separate system reports elsewhere in this volume.",
}
```
#### Contact Name
<!-- quick -->
<!-- info: If known, provide the name of at least one person the reader can contact for questions about the dataset. -->
<!-- scope: periscope -->
Simon Mille
#### Contact Email
<!-- info: If known, provide the email of at least one person the reader can contact for questions about the dataset. -->
<!-- scope: periscope -->
sfmille@gmail.com
#### Has a Leaderboard?
<!-- info: Does the dataset have an active leaderboard? -->
<!-- scope: telescope -->
no
### Languages and Intended Use
#### Multilingual?
<!-- quick -->
<!-- info: Is the dataset multilingual? -->
<!-- scope: telescope -->
yes
#### Covered Dialects
<!-- info: What dialects are covered? Are there multiple dialects per language? -->
<!-- scope: periscope -->
No multiple dialects.
#### Covered Languages
<!-- quick -->
<!-- info: What languages/dialects are covered in the dataset? -->
<!-- scope: telescope -->
`Arabic`, `Chinese`, `English`, `French`, `Hindi`, `Indonesian`, `Japanese`, `Korean`, `Portuguese`, `Russian`, `Spanish, Castilian`
#### Whose Language?
<!-- info: Whose language is in the dataset? -->
<!-- scope: periscope -->
Unknown
#### License
<!-- quick -->
<!-- info: What is the license of the dataset? -->
<!-- scope: telescope -->
cc-by-2.5: Creative Commons Attribution 2.5 Generic
#### Intended Use
<!-- info: What is the intended use of the dataset? -->
<!-- scope: microscope -->
The dataset is intended to be used for training models to solve several NLG subtasks, such as function word introduction, morphological agreement resolution, word order determination and inflection generation.
Comment about the license: the dataset has multiple licences, since each original dataset has their own type of licence. All datasets but one are CC-BY and subclasses of it, the other one is GPL (French Sequoia).
#### Primary Task
<!-- info: What primary task does the dataset support? -->
<!-- scope: telescope -->
Data-to-Text
#### Communicative Goal
<!-- quick -->
<!-- info: Provide a short description of the communicative goal of a model trained for this task on this dataset. -->
<!-- scope: periscope -->
The models are able to introduce surface features (syntax, morphology, topology) from more or less abstract inputs in different, the most abstract being predicate-argument structures. The datasets cover a large variety of domains (news, blogs, forums, wikipedia pages, etc.).
### Credit
#### Curation Organization Type(s)
<!-- info: In what kind of organization did the dataset curation happen? -->
<!-- scope: telescope -->
`industry`, `academic`
#### Curation Organization(s)
<!-- info: Name the organization(s). -->
<!-- scope: periscope -->
Pompeu Fabra University, Google Inc., University of Brighton, Federal University of Minas Gerais, ADAPT/Trinity College Dublin
#### Dataset Creators
<!-- info: Who created the original dataset? List the people involved in collecting the dataset and their affiliation(s). -->
<!-- scope: microscope -->
Simon Mille (Pompeu Fabra University); Leo Wanner (Pompeu Fabra University); Anya Belz (Brighton University); Bernd Bohnet (Google Inc.); Thiago Castro Ferreira (Federal University of Minas Gerais); Yvette Graham (ADAPT/Trinity College Dublin)
#### Funding
<!-- info: Who funded the data creation? -->
<!-- scope: microscope -->
Mostly EU funds via H2020 projects
#### Who added the Dataset to GEM?
<!-- info: Who contributed to the data card and adding the dataset to GEM? List the people+affiliations involved in creating this data card and who helped integrate this dataset into GEM. -->
<!-- scope: microscope -->
Simon Mille (Pompeu Fabra University)
### Dataset Structure
#### Data Fields
<!-- info: List and describe the fields present in the dataset. -->
<!-- scope: telescope -->
`input` (string): this field contains an input tree in CoNLL-U format; the CoNLL-U format is a one-word-per-line format with the following tab-separated 10 columns (see [here](http://universaldependencies.org/format.html)): [1] Position, [2] Lemma, [3] Wordform, [4] Part of Speech, [5] Fine-grained Part of Speech (if available), [6] Features (FEATS), [7] governor, [8] dependency relation, [9] additional dependency information, and [10] metadata. For the surface task, the input is a Universal Dependency tree of a given language in which the word order was scrambled and the surface forms removed (only lemmas are available); for the deep task, the input is a tree derived from the surface input, with predicate-argument relations between content words only (function words were removed) and without any morphological agreement information.
`target_tokenized` (string): this field contains the target sentence to generate, in which every non-initial and non-final token is surrounded by two spaces. This output is usually used for automatic evaluations.
`target` (string): this field contains the detokenised target sentence to generate. This output is usually used for human evaluations.
`gem_id` (string): a unique ID.
`sentence_id` (string): the original ID of a sentence in the UD dataset.
#### Reason for Structure
<!-- info: How was the dataset structure determined? -->
<!-- scope: microscope -->
The structure of the input (CoNLL-U) was chosen according to the standards in parsing, and because the original UD datasets were provided in this format.
#### How were labels chosen?
<!-- info: How were the labels chosen? -->
<!-- scope: microscope -->
The input labels for the surface track are the original labels in the UD treebanks; see [here](https://universaldependencies.org/u/dep/index.html) for the dependencies, [here](https://universaldependencies.org/u/feat/index.html) for the features, and [here](https://universaldependencies.org/u/pos/index.html) for the PoS tags.
The input labels for the deep track are a subset of the PoS tags and features of the surface track, and for the relations, universal predicate-argument relations augmented with a few specific relations to capture coordinations and named entity relations for instance.
#### Example Instance
<!-- info: Provide a JSON formatted example of a typical instance in the dataset. -->
<!-- scope: periscope -->
```
{"input": "1\tGoogle\t_\tPROPN\tNNP\tNumber=Sing\t5\tnsubj\t_\t_\n2\t\t_\tPUNCT\t.\tlin=+1\t5\tpunct\t_\t_\n3\tinto\t_\tADP\tIN\t_\t6\tcase\t_\t_\n4\tif\t_\tSCONJ\tIN\t_\t5\tmark\t_\t_\n5\tmorph\t_\tVERB\tVBD\tMood=Ind|Tense=Past|VerbForm=Fin\t7\tadvcl\t_\t_\n6\tGoogleOS\t_\tPROPN\tNNP\tNumber=Sing\t5\tobl\t_\t_\n7\twhat\t_\tPRON\tWP\tPronType=Int\t0\troot\t_\t_", "target_tokenized": "What if Google Morphed Into GoogleOS ?", "target": "What if Google Morphed Into GoogleOS?", "gem_id": "GEM-surface_realisation_st_2020-T1-test-en_ewt-ud-test-0", "sentence_id": ""}
```
#### Data Splits
<!-- info: Describe and name the splits in the dataset if there are more than one. -->
<!-- scope: periscope -->
There are 119 splits in the dataset:
- 29 training sets, which correspond to 20 UD datasets (11 languages), 9 of which have both surface and deep inputs (3 languages);
- 29 development set which correspond to the 29 training sets above;
- 29 test sets for the data described above;
- 4 out-of-domain test sets, 3 surface inputs and 1 deep one (3 languages for which PUD out-of-domain datasets were available);
- 9 automatically parsed in-domain test sets, 6 surface inputs and 3 deep inputs (6 languages for which good UD parsers were available);
- 9 automatically parsed out-of-domain test sets, 6 surface inputs and 3 deep inputs (6 languages for which we were able to create clean Wikipedia text and that had a good UD parser).
#### Splitting Criteria
<!-- info: Describe any criteria for splitting the data, if used. If there are differences between the splits (e.g., if the training annotations are machine-generated and the dev and test ones are created by humans, or if different numbers of annotators contributed to each example), describe them here. -->
<!-- scope: microscope -->
Described above for more clarity.
####
<!-- info: What does an outlier of the dataset in terms of length/perplexity/embedding look like? -->
<!-- scope: microscope -->
An outlier would usually be an input that corresponds to a very long sentence (e.g. 159 words in English, when the average number of words per sentence is around 25).
## Dataset in GEM
### Rationale for Inclusion in GEM
#### Why is the Dataset in GEM?
<!-- info: What does this dataset contribute toward better generation evaluation and why is it part of GEM? -->
<!-- scope: microscope -->
The datset includes languages from different families and some languages not often used in NLG (e.g. Arabic, Indonesian, Korean, Hindi). It proposes two tasks, which can be tackled both separately and in one shot, with different levels of difficulty: the most superficial task (T1) consits in ordering and inflecting some trees, and the deeper task (T2) includes extra tasks such as defining the syntactic structure and introducing function words and morphological agreement information. Both tasks can allow for developing modules for pipeline NLG architectures. T1 is rather straightforward to evaluate: BLEU works quite well for some languages since all the words are present in the input and few word orders only can be possible for a syntactic tree. But T2 is more challenging to evaluate, since more outputs are correct given one particular input.
There is a large variety of sizes in the datasets, both clean and noisy data, parallel data in different languages, and many already available system outputs to use as baselines.
#### Similar Datasets
<!-- info: Do other datasets for the high level task exist? -->
<!-- scope: telescope -->
yes
#### Unique Language Coverage
<!-- info: Does this dataset cover other languages than other datasets for the same task? -->
<!-- scope: periscope -->
yes
#### Difference from other GEM datasets
<!-- info: What else sets this dataset apart from other similar datasets in GEM? -->
<!-- scope: microscope -->
This is possibly the only dataset that starts the generation process from predicate-argument structures and from syntactic structures. It also has parallel datasets in a few languages (coming from the PUD parallel annotations).
#### Ability that the Dataset measures
<!-- info: What aspect of model ability can be measured with this dataset? -->
<!-- scope: periscope -->
Syntacticisation, functional word introduction, word order resolution, agreement resolution, morphological inflection
### GEM-Specific Curation
#### Modificatied for GEM?
<!-- info: Has the GEM version of the dataset been modified in any way (data, processing, splits) from the original curated data? -->
<!-- scope: telescope -->
no
#### Additional Splits?
<!-- info: Does GEM provide additional splits to the dataset? -->
<!-- scope: telescope -->
no
### Getting Started with the Task
#### Pointers to Resources
<!-- info: Getting started with in-depth research on the task. Add relevant pointers to resources that researchers can consult when they want to get started digging deeper into the task. -->
<!-- scope: microscope -->
[Website](http://taln.upf.edu/pages/msr2020-ws/SRST.html)
#### Technical Terms
<!-- info: Technical terms used in this card and the dataset and their definitions -->
<!-- scope: microscope -->
Syntacticisation: prediction of the syntactic
## Previous Results
### Previous Results
#### Measured Model Abilities
<!-- info: What aspect of model ability can be measured with this dataset? -->
<!-- scope: telescope -->
Syntacticisation, functional word introduction, word order resolution, morphological agreement resolution, morphological inflection
#### Metrics
<!-- info: What metrics are typically used for this task? -->
<!-- scope: periscope -->
`BLEU`, `BERT-Score`, `Other: Other Metrics`
#### Other Metrics
<!-- info: Definitions of other metrics -->
<!-- scope: periscope -->
NIST: n-gram similarity metric weighted in favour of less frequent n-grams which are taken to be more informative.
Normalised edit distance (DIST): inverse, normalised, character-based string-edit distance that starts by computing the minimum number of character inserts, deletes and substitutions (all at cost 1) required to turn the system output into the (single) reference text.
#### Proposed Evaluation
<!-- info: List and describe the purpose of the metrics and evaluation methodology (including human evaluation) that the dataset creators used when introducing this task. -->
<!-- scope: microscope -->
BLEU, NIST, BERTScore and DIST simply aim at calculating in different ways the similarity between a predicted and a reference sentence.
Two additional criteria have been used for human evaluation, Readability and Meaning SImilarity. The statement to be assessed in the Readability evaluation was: "The text reads well and is free from grammatical errors and awkward constructions.". The corresponding statement in the Meaning Similarity evaluation, in which system outputs (‘the black text’) were compared to reference sentences (‘the gray text’), was: "The meaning of the gray text is adequately expressed by the black text."
#### Previous results available?
<!-- info: Are previous results available? -->
<!-- scope: telescope -->
yes
#### Other Evaluation Approaches
<!-- info: What evaluation approaches have others used? -->
<!-- scope: periscope -->
Same as above.
#### Relevant Previous Results
<!-- info: What are the most relevant previous results for this task/dataset? -->
<!-- scope: microscope -->
- [Fast and Accurate Non-Projective Dependency Tree Linearization](https://aclanthology.org/2020.acl-main.134/)
- [Shape of Synth to Come: Why We Should Use Synthetic Data for English Surface Realization](https://aclanthology.org/2020.acl-main.665/)
## Dataset Curation
### Original Curation
#### Original Curation Rationale
<!-- info: Original curation rationale -->
<!-- scope: telescope -->
The datasets were created in the context of the Surface Realisation Shared Task series.
#### Communicative Goal
<!-- info: What was the communicative goal? -->
<!-- scope: periscope -->
The dataset's objective was to allow for training systems to perform tasks related to surface realisation (introduction of function words, syntacticisation, resolution of morphological agreements, word order resolution, inflection generation.
#### Sourced from Different Sources
<!-- info: Is the dataset aggregated from different data sources? -->
<!-- scope: telescope -->
yes
#### Source Details
<!-- info: List the sources (one per line) -->
<!-- scope: periscope -->
Each of the 20 used UD datasets comes from various sources, all listed on the individual page of each UD treeebank (https://universaldependencies.org/).
Additional test sets were created for the task, and were obtained from Wikipedia pages for 6 languages.
### Language Data
#### How was Language Data Obtained?
<!-- info: How was the language data obtained? -->
<!-- scope: telescope -->
`Found`
#### Where was it found?
<!-- info: If found, where from? -->
<!-- scope: telescope -->
`Multiple websites`
#### Language Producers
<!-- info: What further information do we have on the language producers? -->
<!-- scope: microscope -->
There are numerous sources of language in the multiple datasets.
#### Topics Covered
<!-- info: Does the language in the dataset focus on specific topics? How would you describe them? -->
<!-- scope: periscope -->
There is a large variety of topics in the multiple datasets.
#### Data Validation
<!-- info: Was the text validated by a different worker or a data curator? -->
<!-- scope: telescope -->
not validated
#### Data Preprocessing
<!-- info: How was the text data pre-processed? (Enter N/A if the text was not pre-processed) -->
<!-- scope: microscope -->
The text data was detokenised so as to create references for automatic evaluations (several languages don't use spaces to separate words, and running metrics like BLEU would not make sense without separating all the tokens in a sentence).
#### Was Data Filtered?
<!-- info: Were text instances selected or filtered? -->
<!-- scope: telescope -->
hybrid
#### Filter Criteria
<!-- info: What were the selection criteria? -->
<!-- scope: microscope -->
For the Wikipedia test created for the shared task, extensive filtering was applied to achieve reasonably good text quality. Sentences that include special characters, contain unusual tokens (e.g. ISBN), or have unbalanced quotation marks or brackets were skipped. Furthermore, only sentences with more than 5 tokens and shorter than 50 tokens were selected. After the initial filtering, quite a few malformed sentences remained. In order to remove those, the sentences were scored with BERT and
only the top half scored sentences were kept. Finally, via manual inspection, patterns and expressions were identified to
further reduce the number of malformed sentences.
### Structured Annotations
#### Additional Annotations?
<!-- quick -->
<!-- info: Does the dataset have additional annotations for each instance? -->
<!-- scope: telescope -->
none
#### Annotation Service?
<!-- info: Was an annotation service used? -->
<!-- scope: telescope -->
no
### Consent
#### Any Consent Policy?
<!-- info: Was there a consent policy involved when gathering the data? -->
<!-- scope: telescope -->
no
#### Justification for Using the Data
<!-- info: If not, what is the justification for reusing the data? -->
<!-- scope: microscope -->
The Universal Dependency data had been previously used for shared tasks on parsing, so it made sense to reuse it for generation.
### Private Identifying Information (PII)
#### Contains PII?
<!-- quick -->
<!-- info: Does the source language data likely contain Personal Identifying Information about the data creators or subjects? -->
<!-- scope: telescope -->
unlikely
#### Any PII Identification?
<!-- info: Did the curators use any automatic/manual method to identify PII in the dataset? -->
<!-- scope: periscope -->
no identification
### Maintenance
#### Any Maintenance Plan?
<!-- info: Does the original dataset have a maintenance plan? -->
<!-- scope: telescope -->
no
## Broader Social Context
### Previous Work on the Social Impact of the Dataset
#### Usage of Models based on the Data
<!-- info: Are you aware of cases where models trained on the task featured in this dataset ore related tasks have been used in automated systems? -->
<!-- scope: telescope -->
no
### Impact on Under-Served Communities
#### Addresses needs of underserved Communities?
<!-- info: Does this dataset address the needs of communities that are traditionally underserved in language technology, and particularly language generation technology? Communities may be underserved for exemple because their language, language variety, or social or geographical context is underepresented in NLP and NLG resources (datasets and models). -->
<!-- scope: telescope -->
yes
#### Details on how Dataset Addresses the Needs
<!-- info: Describe how this dataset addresses the needs of underserved communities. -->
<!-- scope: microscope -->
Thanks to the original work of the UD dataset creators, the surface realisation dataset addresses a few languages which are possibly under-served in NLG: e.g. Arabic, Hindi, Indonesian, Korean.
### Discussion of Biases
#### Any Documented Social Biases?
<!-- info: Are there documented social biases in the dataset? Biases in this context are variations in the ways members of different social categories are represented that can have harmful downstream consequences for members of the more disadvantaged group. -->
<!-- scope: telescope -->
no
#### Are the Language Producers Representative of the Language?
<!-- info: Does the distribution of language producers in the dataset accurately represent the full distribution of speakers of the language world-wide? If not, how does it differ? -->
<!-- scope: periscope -->
It is very likely that the distribution of language producers is not fully represented in the datasets of each language.
## Considerations for Using the Data
### PII Risks and Liability
#### Potential PII Risk
<!-- info: Considering your answers to the PII part of the Data Curation Section, describe any potential privacy to the data subjects and creators risks when using the dataset. -->
<!-- scope: microscope -->
No risks foreseen.
### Licenses
#### Copyright Restrictions on the Dataset
<!-- info: Based on your answers in the Intended Use part of the Data Overview Section, which of the following best describe the copyright and licensing status of the dataset? -->
<!-- scope: periscope -->
`multiple licenses`, `open license - commercial use allowed`
#### Copyright Restrictions on the Language Data
<!-- info: Based on your answers in the Language part of the Data Curation Section, which of the following best describe the copyright and licensing status of the underlying language data? -->
<!-- scope: periscope -->
`multiple licenses`, `open license - commercial use allowed`
### Known Technical Limitations
#### Technical Limitations
<!-- info: Describe any known technical limitations, such as spurrious correlations, train/test overlap, annotation biases, or mis-annotations, and cite the works that first identified these limitations when possible. -->
<!-- scope: microscope -->
The deep track inputs (predicate-argument structures) are not of perfect quality, they were derived automatically from gold or predicted syntactic parses using handcrafted grammars.
#### Unsuited Applications
<!-- info: When using a model trained on this dataset in a setting where users or the public may interact with its predictions, what are some pitfalls to look out for? In particular, describe some applications of the general task featured in this dataset that its curation or properties make it less suitable for. -->
<!-- scope: microscope -->
The datasets are probably not fitted to train tools to produce "unusual" languages (e.g. poetry, kid writing etc.).
#### Discouraged Use Cases
<!-- info: What are some discouraged use cases of a model trained to maximize the proposed metrics on this dataset? In particular, think about settings where decisions made by a model that performs reasonably well on the metric my still have strong negative consequences for user or members of the public. -->
<!-- scope: microscope -->
To be thought of :)
|
GEM/totto | ---
annotations_creators:
- none
language_creators:
- unknown
language:
- en
license:
- cc-by-sa-3.0
multilinguality:
- unknown
size_categories:
- unknown
source_datasets:
- original
task_categories:
- table-to-text
task_ids: []
pretty_name: totto
tags:
- data-to-text
---
# Dataset Card for GEM/totto
## Dataset Description
- **Homepage:** n/a
- **Repository:** https://github.com/google-research-datasets/totto + [ToTTo Supplementary Repo
- **Paper:** https://aclanthology.org/2020.emnlp-main.89
- **Leaderboard:** https://github.com/google-research-datasets/totto
- **Point of Contact:** Ankur Parikh
### Link to Main Data Card
You can find the main data card on the [GEM Website](https://gem-benchmark.com/data_cards/totto).
### Dataset Summary
ToTTo is a high-quality English table-to-text dataset with more than 100,000 examples in which a table from Wikipedia with highlighted cells is paired with a sentence that describes the highlighted cells. All examples in the dataset were post-edited in multiple steps to ensure that the targets are fully faithful to the input information.
You can load the dataset via:
```
import datasets
data = datasets.load_dataset('GEM/totto')
```
The data loader can be found [here](https://huggingface.co/datasets/GEM/totto).
#### website
n/a
#### paper
[ACL Anthology](https://aclanthology.org/2020.emnlp-main.89)
#### authors
Ankur Parikh, Xuezhi Wang, Sebastian Gehrmann, Manaal Faruqui, Bhuwan Dhingra, Diyi Yang, Dipanjan Das
## Dataset Overview
### Where to find the Data and its Documentation
#### Download
<!-- info: What is the link to where the original dataset is hosted? -->
<!-- scope: telescope -->
[ToTTo Main Repo](https://github.com/google-research-datasets/totto) + [ToTTo Supplementary Repo](https://github.com/google-research/language/tree/master/language/totto)
#### Paper
<!-- info: What is the link to the paper describing the dataset (open access preferred)? -->
<!-- scope: telescope -->
[ACL Anthology](https://aclanthology.org/2020.emnlp-main.89)
#### BibTex
<!-- info: Provide the BibTex-formatted reference for the dataset. Please use the correct published version (ACL anthology, etc.) instead of google scholar created Bibtex. -->
<!-- scope: microscope -->
```
@inproceedings{parikh-etal-2020-totto,
title = "{ToTTo}: A Controlled Table-To-Text Generation Dataset",
author = "Parikh, Ankur and
Wang, Xuezhi and
Gehrmann, Sebastian and
Faruqui, Manaal and
Dhingra, Bhuwan and
Yang, Diyi and
Das, Dipanjan",
booktitle = "Proceedings of the 2020 Conference on Empirical Methods in Natural Language Processing (EMNLP)",
month = nov,
year = "2020",
address = "Online",
publisher = "Association for Computational Linguistics",
url = "https://aclanthology.org/2020.emnlp-main.89",
doi = "10.18653/v1/2020.emnlp-main.89",
pages = "1173--1186",
abstract = "We present ToTTo, an open-domain English table-to-text dataset with over 120,000 training examples that proposes a controlled generation task: given a Wikipedia table and a set of highlighted table cells, produce a one-sentence description. To obtain generated targets that are natural but also faithful to the source table, we introduce a dataset construction process where annotators directly revise existing candidate sentences from Wikipedia. We present systematic analyses of our dataset and annotation process as well as results achieved by several state-of-the-art baselines. While usually fluent, existing methods often hallucinate phrases that are not supported by the table, suggesting that this dataset can serve as a useful research benchmark for high-precision conditional text generation.",
}
```
#### Contact Name
<!-- quick -->
<!-- info: If known, provide the name of at least one person the reader can contact for questions about the dataset. -->
<!-- scope: periscope -->
Ankur Parikh
#### Contact Email
<!-- info: If known, provide the email of at least one person the reader can contact for questions about the dataset. -->
<!-- scope: periscope -->
totto@google.com
#### Has a Leaderboard?
<!-- info: Does the dataset have an active leaderboard? -->
<!-- scope: telescope -->
yes
#### Leaderboard Link
<!-- info: Provide a link to the leaderboard. -->
<!-- scope: periscope -->
[Github](https://github.com/google-research-datasets/totto)
#### Leaderboard Details
<!-- info: Briefly describe how the leaderboard evaluates models. -->
<!-- scope: microscope -->
This dataset has an associated, active [leaderboard](https://github.com/google-research-datasets/totto#leaderboard) maintained by the authors.
The test set ground truth targets / references are private, i.e they are not publicly shared or downloadable - hence, leaderboard submission is necessary for test set evaluation.
To evaluate your model on the dev or test set AND/OR submit to the leaderboard, you need to submit your model files through this [form](https://forms.gle/AcF9TRqWrPhPzztt7) (The form provides an option to opt-out of going on the leaderboard).
The leaderboard reports three sets of BLEU, PARENT and BLEURT scores for each submission - on the overall test set, the *Overlap* subset of the test set and the *non-Overlap* subset of the test set.
### Languages and Intended Use
#### Multilingual?
<!-- quick -->
<!-- info: Is the dataset multilingual? -->
<!-- scope: telescope -->
no
#### Covered Dialects
<!-- info: What dialects are covered? Are there multiple dialects per language? -->
<!-- scope: periscope -->
No specific dialects. The original language is from Wikipedia and it was post-edited by crowdraters
#### Covered Languages
<!-- quick -->
<!-- info: What languages/dialects are covered in the dataset? -->
<!-- scope: telescope -->
`English`
#### Whose Language?
<!-- info: Whose language is in the dataset? -->
<!-- scope: periscope -->
The language is post-edited English only (BCP-47: `en`) Wikipedia text. No demographic information about annotators is provided.
Some amounts of what may be called non-English text, including characters such as French accents or Cyrillic characters, could sometimes occur, especially through fields with entity names as values in the input table cells.
#### License
<!-- quick -->
<!-- info: What is the license of the dataset? -->
<!-- scope: telescope -->
cc-by-sa-3.0: Creative Commons Attribution Share Alike 3.0 Unported
#### Intended Use
<!-- info: What is the intended use of the dataset? -->
<!-- scope: microscope -->
ToTTo is a Table-to-Text NLG task, as the paper title says. The task is as follows: Given a Wikipedia table with row names, column names and table cells, with a subset of cells highlighted, generate a natural language description for the highlighted part of the table . The table need not be exactly rectangular in that - cells can sometimes be multi-row or multi-column.
An earlier example of a Table-to-Text NLG task is [Wikibio](https://arxiv.org/abs/1603.07771) - here the inputs were Wikipedia infoboxes (from the top right corner of entity-related Wiki pages). In contrast, ToTTo mostly has Wikipedia tables from the main article content itself. In general, Table-To-Text NLG tasks can be seen as a subclass of Data-To-Text NLG tasks - where the task is to generate natural language descriptions of inputs which are in the form of structured or semi-structured data. In general, all Data-To-Text NLG tasks need not have an explicit table or other structure - e.g the input in [WebNLG](https://www.aclweb.org/anthology/W16-6626.pdf) is simply a list of triples.
Importantly, ToTTo differs from earlier examples of Table-To-Text NLG in that:
1. It does not suffer from the problem of divergent references - where ground truth descriptions themselves have additional information not found in the table. ToTTo overcomes this by having a multi-step annotation process to edit the initial, free-form table descriptions (which are from Wikipedia) to make them faithful, unambiguous and independent of article context.
2. Since it provides **control** in the form of highlighted table cells, it prevents the problem of there being a large number of valid descriptions focussing on different parts of the table.
#### Primary Task
<!-- info: What primary task does the dataset support? -->
<!-- scope: telescope -->
Data-to-Text
#### Communicative Goal
<!-- quick -->
<!-- info: Provide a short description of the communicative goal of a model trained for this task on this dataset. -->
<!-- scope: periscope -->
The speaker is required to produce a single, coherent English sentence that describes the highlighted cells in the given table, also using metadata and any other information from the table as applicable.
### Credit
#### Curation Organization Type(s)
<!-- info: In what kind of organization did the dataset curation happen? -->
<!-- scope: telescope -->
`industry`
#### Curation Organization(s)
<!-- info: Name the organization(s). -->
<!-- scope: periscope -->
Google Research
#### Dataset Creators
<!-- info: Who created the original dataset? List the people involved in collecting the dataset and their affiliation(s). -->
<!-- scope: microscope -->
Ankur Parikh, Xuezhi Wang, Sebastian Gehrmann, Manaal Faruqui, Bhuwan Dhingra, Diyi Yang, Dipanjan Das
#### Funding
<!-- info: Who funded the data creation? -->
<!-- scope: microscope -->
Google Research
#### Who added the Dataset to GEM?
<!-- info: Who contributed to the data card and adding the dataset to GEM? List the people+affiliations involved in creating this data card and who helped integrate this dataset into GEM. -->
<!-- scope: microscope -->
Varun Gangal created the initial data card and Yacine Jernite wrote the data loader. The data card was updated with new splits by Simon Mille. Sebastian Gehrmann ported the data card and loader from the v1 to the v2 version and extended it with the new fields.
### Dataset Structure
#### Data Fields
<!-- info: List and describe the fields present in the dataset. -->
<!-- scope: telescope -->
- The `table` field is a `List[List[Dict]]` in row-major order, with outer lists representing rows and the inner lists columns.
- Each `Dict` has the fields `column_span: int`, `is_header: bool`, `row_span: int`, and `value: str`.
- Table metadata consists of `table_page_title`, `table_section_title` and `table_section_texts`
- The `highlighted_cells` are represented as `List[[row_index,column_index]]`, with each `[row_index,column_index]` indicating that `table[row_index][column_index]` is highlighted.
- `example_id` is the unique id per example.
- `sentence_annotations[final_sentence]` which is the table description/generation target
#### Reason for Structure
<!-- info: How was the dataset structure determined? -->
<!-- scope: microscope -->
The structure is aimed to encode highlighted tables in a way that allows rows and columns to span multiple fields in width. The other fields are meta-data about the source and the annotations
#### How were labels chosen?
<!-- info: How were the labels chosen? -->
<!-- scope: microscope -->
The initial table-description pairs are tables from Wikipedia articles, extracted through heuristics such as Number Matching (tables and sentences that overlap with a non-date number of atleast 3 non-zero digits) (Refer to Section 4 of the paper for more)
1. Table Readability: Tables which are deemed non-readable (due to foreign language, poor formatting etc - a very small fraction of 0.5%) are removed from the dataset here.
2. Cell Highlighting: The annotator highlights the cells of the table which support the description.
3. Deletion: The annotator removes phrases in the description which are not supported by the highlighted cells
4. Decontextualization: Descriptions may contain pronouns or other forms of anaphora, or other phenomena which depend on the overall article topic - these are fixed by replacement (e.g replacing pronouns with the entity, provided it occurs in the table). The replacements allowed are limited to one, and annotators are also instructed to conserve fluency.
5. Secondary Annotation: A second set of annotators is shown the output of Stage 4, and asked to fix it if required to ensure it is grammatical.
#### Example Instance
<!-- info: Provide a JSON formatted example of a typical instance in the dataset. -->
<!-- scope: periscope -->
The main repository's `README.md` already provides a thorough walkthrough of data instances and fields [here](https://github.com/google-research-datasets/totto#dataset-description)
Below is the instance for a table from the wiki-page for the musical artist _Weird Al' Yankovic_ , likely listing his on-television appearances.
```
{
"table_page_title": "'Weird Al' Yankovic",
"table_webpage_url": "https://en.wikipedia.org/wiki/%22Weird_Al%22_Yankovic",
"table_section_title": "Television",
"table_section_text": "",
"table": "[Described below]",
"highlighted_cells": [[22, 2], [22, 3], [22, 0], [22, 1], [23, 3], [23, 1], [23, 0]],
"example_id": 12345678912345678912,
"sentence_annotations": [{"original_sentence": "In 2016, Al appeared in 2 episodes of BoJack Horseman as Mr. Peanutbutter's brother, Captain Peanutbutter, and was hired to voice the lead role in the 2016 Disney XD series Milo Murphy's Law.",
"sentence_after_deletion": "In 2016, Al appeared in 2 episodes of BoJack Horseman as Captain Peanutbutter, and was hired to the lead role in the 2016 series Milo Murphy's Law.",
"sentence_after_ambiguity": "In 2016, Al appeared in 2 episodes of BoJack Horseman as Captain Peanutbutter, and was hired for the lead role in the 2016 series Milo Murphy's 'Law.",
"final_sentence": "In 2016, Al appeared in 2 episodes of BoJack Horseman as Captain Peanutbutter and was hired for the lead role in the 2016 series Milo Murphy's Law."}],
}
```
The `table` field is expanded as below:
```
[
[
{
"column_span": 1,
"is_header": true,
"row_span": 1,
"value": "Year"},
{ "column_span": 1,
"is_header": true,
"row_span": 1,
"value": "Title"},
{ "column_span": 1,
"is_header": true,
"row_span": 1,
"value": "Role"},
{ "column_span": 1,
"is_header": true,
"row_span": 1,
"value": "Notes"}
],
[
{ "column_span": 1,
"is_header": false,
"row_span": 1,
"value": "1997"},
{ "column_span": 1,
"is_header": false,
"row_span": 1,
"value": "Eek! The Cat"},
{ "column_span": 1,
"is_header": false,
"row_span": 1,
"value": "Himself"},
{ "column_span": 1,
"is_header": false,
"row_span": 1,
"value": "Episode: 'The FugEektive'"}
], ...
]
```
The [Supplementary Repo](https://github.com/google-research/language/tree/master/language/totto) also provides browsable samples under its `sample/` folder. It additionally provides HTML visualization scripts with their outputs located under the aforementioned folder. The instructions to access and visualize these samples can also be found [here](https://github.com/google-research/language/tree/master/language/totto#visualizing-sample-data).
#### Data Splits
<!-- info: Describe and name the splits in the dataset if there are more than one. -->
<!-- scope: periscope -->
The dataset consists of 120,000 train examples and equi-sized dev and test sets with 7700 examples.
Refer to Table 5 in the paper for a more extensive list of properties about table size, target vocabulary etc and their aggregates.
#### Splitting Criteria
<!-- info: Describe any criteria for splitting the data, if used. If there are differences between the splits (e.g., if the training annotations are machine-generated and the dev and test ones are created by humans, or if different numbers of annotators contributed to each example), describe them here. -->
<!-- scope: microscope -->
The dev and test splits are further equally distributed between _Overlap_ and _non-Overlap_ .
The examples in the _Overlap_ set are harder on account of the domain shift resulting from them having none of their header (row and column) names in common with those seen during training.
Refer to Table 5 in the paper for a more extensive list of properties about table size, target vocabulary etc and their aggregates.
####
<!-- info: What does an outlier of the dataset in terms of length/perplexity/embedding look like? -->
<!-- scope: microscope -->
There are some very large tables in the dataset with thousands of rows. Table 7 shows some of the challenges of the dataset, showing that very few examples require access to the table description itself which makes those examples an outlier.
## Dataset in GEM
### Rationale for Inclusion in GEM
#### Why is the Dataset in GEM?
<!-- info: What does this dataset contribute toward better generation evaluation and why is it part of GEM? -->
<!-- scope: microscope -->
ToTTo is one of the two datasets representing Table-to-Text NLG in GEM, the other one being [DART](https://arxiv.org/pdf/2007.02871.pdf). Unlike DART, which combines datasets from multiple sources and furnishes them in a unified setting, ToTTo is from a homogeneous source. As explained in the Task Summary above, it also has an annotation process explicitly crafted to reduce divergent descriptions, which is not true of DART.
Furthermore, ToTTo is also an instance of a **controlled** generation task - where in addition to the input (in this case the table) an additional **control** (in this case the highlighted cells) is given as an additional goal for the generation. The DART task formulation does not include controls.
#### Similar Datasets
<!-- info: Do other datasets for the high level task exist? -->
<!-- scope: telescope -->
yes
#### Unique Language Coverage
<!-- info: Does this dataset cover other languages than other datasets for the same task? -->
<!-- scope: periscope -->
no
#### Difference from other GEM datasets
<!-- info: What else sets this dataset apart from other similar datasets in GEM? -->
<!-- scope: microscope -->
The input is much more complex and the quality much better than that of comparable datasets. The highlighted table cells provide a unique challenge to models.
#### Ability that the Dataset measures
<!-- info: What aspect of model ability can be measured with this dataset? -->
<!-- scope: periscope -->
Reasoning, surface realization
### GEM-Specific Curation
#### Modificatied for GEM?
<!-- info: Has the GEM version of the dataset been modified in any way (data, processing, splits) from the original curated data? -->
<!-- scope: telescope -->
yes
#### Additional Splits?
<!-- info: Does GEM provide additional splits to the dataset? -->
<!-- scope: telescope -->
yes
#### Split Information
<!-- info: Describe how the new splits were created -->
<!-- scope: periscope -->
9 challenge sets for ToTTo were added to the GEM evaluation suite, 8 created specifically for the task and 1 coming from the original data.
1. We created subsets of the training and development sets of 500 randomly selected inputs each.
2. We applied input scrambling on a subset of 500 randomly selected test instances; the order of the highlighted cells was randomly reassigned.
3. For the input size, we created subpopulations based on the number of input highlighted cells in the whole table.
| Input length | Frequency English |
|---------------|-------------------|
| 1 | 898 |
| 2 | 1850 |
| 3 | 2221 |
| 4 | 1369 |
| 5 | 483 |
| 6 | 379 |
| 7 | 124 |
| 8 | 128 |
| 9 | 61 |
| 10 | 40 |
| 11 | 20 |
| 12 | 26 |
| 13 | 10 |
| 14 | 14 |
| 15 | 14 |
| 16 | 7 |
| 17 | 6 |
| 18 | 5 |
| 19 | 5 |
| 20 | 5 |
| 21 | 4 |
| 22 | 1 |
| 23 | 2 |
| 24 | 4 |
| 25 | 1 |
| 26...496 | 1 |
4. We also divided the test set according to the size of the whole table, based on the idea that larger tables represent a bigger space to take into account when generating the highlighted cells; a larger table could be more challenging to generate accurate text than a smaller table. There are 693 different table sizes, ranging from 2 to 15834 cells.
| Table size |Frequency English|
|-----------------|-----------------|
| 2 | 71 |
| 3 | 52 |
| 4 | 36 |
| 5 | 41 |
| 6 | 144 |
| 7 | 47 |
| 8 | 59 |
| 9 | 105 |
| 10 | 162 |
| 11 | 36 |
| 12 | 158 |
| 13 | 35 |
| 14 | 79 |
| 15 | 136 |
| 16 | 111 |
| 17 | 48 |
| 18 | 123 |
| 19 | 29 |
| 20 | 112 |
| 21 | 91 |
| 22 | 17 |
| 23 | 7 |
| 24 | 169 |
| 25 | 56 |
| 26 | 12 |
| 27 | 40 |
| 28 | 77 |
| 29 | 7 |
| 30 | 122 |
| 31 | 4 |
| 32 | 49 |
| 33 | 21 |
| 34 | 7 |
| 35 | 103 |
| 36 | 131 |
| 37 | 10 |
| 38 | 6 |
| 39 | 26 |
| 40 | 110 |
| 41 | 1 |
| 42 | 54 |
| 43 | 6 |
| 44 | 47 |
| 45 | 79 |
| 46 | 4 |
| 47 | 2 |
| 48 | 114 |
| 49 | 18 |
| 50 | 55 |
| 51 | 11 |
| 52 | 43 |
| 54 | 80 |
| 55 | 73 |
| 56 | 64 |
| 57 | 12 |
| 58 | 1 |
| 60 | 114 |
| 61 | 4 |
| 63 | 39 |
| 64 | 36 |
| 65 | 62 |
| 66 | 48 |
| 67 | 1 |
| 68 | 36 |
| 69 | 6 |
| 70 | 81 |
| 72 | 76 |
| 73 | 1 |
| 74 | 1 |
| 75 | 44 |
| 76 | 33 |
| 77 | 30 |
| 78 | 66 |
| 79 | 1 |
| 80 | 83 |
| 81 | 12 |
| 82 | 1 |
| 84 | 80 |
| 85 | 25 |
| 86 | 1 |
| 87 | 3 |
| 88 | 35 |
| 90 | 78 |
| 91 | 18 |
| 92 | 22 |
| 93 | 5 |
| 94 | 2 |
| 95 | 31 |
| 96 | 50 |
| 98 | 11 |
| 99 | 14 |
| 100 | 48 |
| 102 | 24 |
| 104 | 29 |
| 105 | 36 |
| 106 | 2 |
| 108 | 51 |
| 110 | 31 |
| ...8000+ | (up to 10) |
5. We also created three splits based on the subset of test examples in pages about people.
We then used the structured information in WikiData to identify the following information:
- gender (male, and female),
- nationality grouped by continent (Africa, Asia, Europe, North America, Oceania, and South America)
- ethnicity (African American and all USA)
The categories within gender, ethnicity, and nationality were chosen based on data availability; The ToTTo dataset includes mostly tables that do not focus on people. As a result, only seven people in the original test set are marked as having a non-binary gender. Similar sparsity informed the grouping of nationalities by continent – only 19 countries are represented by more than 10 people in the test set. In case a person has citizenships across multiple continents, we may include the person in any of the included continents.
Finally, ethnicity is very sparsely annotated in WikiData; only 150 test examples in ToTTo have this information and 128 of these are African Americans. We thus are unable to compare the performance on, e.g., Yoruba or Punjabi people, both of which have fewer than five instances. Another caveat here is that only 21 of the 128 people are female. We thus compare the African American population to results on a subset that includes all US citizens.
#### Split Motivation
<!-- info: What aspects of the model's generation capacities were the splits created to test? -->
<!-- scope: periscope -->
generalization, fairness, robustness
### Getting Started with the Task
#### Pointers to Resources
<!-- info: Getting started with in-depth research on the task. Add relevant pointers to resources that researchers can consult when they want to get started digging deeper into the task. -->
<!-- scope: microscope -->
- The highest spot on the leaderboard is currently held by an anonymous method, with BLEU=49.2, PARENT=58.7 and BLEURT=0.249 on the _Overall_ test set.
- The **highest scoring non-anonymous** method is the T5-based method of [Kale, 2020](https://arxiv.org/abs/2005.10433). This method uses a simple row-major linearization scheme to convert the table (it chooses only the highlighted cells and ignores the other cells - table titles and section titles are prefixed at the start of the respective section table) to a flat string. The linearized input - output description pairs from training examples are then used to finetune T5, with BLEU being used as the dev metric to pick checkpoints, and beam search with beam size 10 being the decoding method.
Though the best numbers from this method are naturally from the largest T5-pretrained architecture (T5-3B), the paper shows improvements over the next-highest BERT-to-BERT method even when using T5-Base or T5-Small, which have the same and lesser parameters than BERT-to-BERT respectively.
- The [Supplementary Repo](https://github.com/google-research/language/tree/master/language/totto) provides several useful modules to get started with for new approach implementation:
1. Code for the particular preprocessing / linearization scheme used to linearize the tables into flat sequences for the baseline approaches described in the paper has been described and shared [herein](https://github.com/google-research/language/tree/master/language/totto#baseline-preprocessing)
2. An [evaluation script](https://github.com/google-research/language/tree/master/language/totto#running-the-evaluation-scripts-locally) for locally scoring BLEU and PARENT system outputs on dev (or train) sets. Since BLEURT is a model-based metric, a [slightly separate](https://github.com/google-research/language/tree/master/language/totto#running-the-evaluation-scripts-locall://github.com/google-research/language/tree/master/language/totto#computing-the-bleurt-score) set of instructions is provided to evaluate on the same.
## Previous Results
### Previous Results
#### Measured Model Abilities
<!-- info: What aspect of model ability can be measured with this dataset? -->
<!-- scope: telescope -->
Reasoning, surface realization
#### Metrics
<!-- info: What metrics are typically used for this task? -->
<!-- scope: periscope -->
`BLEU`, `BLEURT`, `Other: Other Metrics`
#### Other Metrics
<!-- info: Definitions of other metrics -->
<!-- scope: periscope -->
Parent: a metric that measures the F-1 score of overlap between input content words and those used in references and those in generated text while ignoring the general surface form. It can thus measure the faithfulness much better than metrics that measure overlap with a reference
#### Proposed Evaluation
<!-- info: List and describe the purpose of the metrics and evaluation methodology (including human evaluation) that the dataset creators used when introducing this task. -->
<!-- scope: microscope -->
The metrics are used as in the leaderboard. The original paper additionally conducted a human evaluation focusing on fluency, faithfulness, and coverage.
Faithfulness was measured as whether facts in the text are not supported by the input, and coverage as the number of highlighted cells that were considered. They thus represent precision and recall of the content.
#### Previous results available?
<!-- info: Are previous results available? -->
<!-- scope: telescope -->
yes
#### Relevant Previous Results
<!-- info: What are the most relevant previous results for this task/dataset? -->
<!-- scope: microscope -->
See leaderboard.
## Dataset Curation
### Original Curation
#### Original Curation Rationale
<!-- info: Original curation rationale -->
<!-- scope: telescope -->
Tables occurring in Wikipedia articles were chosen as the data source with the following reasons in mind:
1. Wide coverage in terms of both vocabulary and concepts.
2. Wikipedia tables are not confined to a regular structure, with multi-row or multi-column cells occurring with a sufficient frequency.
3. Likely to contain reasonable-quality, natural text descriptions in the proximity of the table, which are also extractable by heuristics. (see the start of Section 4 for the heuristics used)
To prevent an overlap with the earlier [Wikibio](https://arxiv.org/abs/1603.07771) dataset which focussed on Infobox-first sentence pairs from Wikipedia biography articles, the authors avoid using Infoboxes as a data source.
The overall curation process of initially collecting free text and then annotator-revising it, was designed to combine the advantages of free-form text descriptions (which are fluent, high-quality and unhurriedly written, but also divergent and unfaithful) with annotator descriptions (which can be tailored to be faithful and to conform exactly to desired task requirements)
#### Communicative Goal
<!-- info: What was the communicative goal? -->
<!-- scope: periscope -->
The speaker is required to produce a single, coherent English sentence that describes the highlighted cells in the given table, also using metadata and any other information from the table as applicable.
#### Sourced from Different Sources
<!-- info: Is the dataset aggregated from different data sources? -->
<!-- scope: telescope -->
yes
#### Source Details
<!-- info: List the sources (one per line) -->
<!-- scope: periscope -->
wikipedia.org
### Language Data
#### How was Language Data Obtained?
<!-- info: How was the language data obtained? -->
<!-- scope: telescope -->
`Crowdsourced`
#### Where was it crowdsourced?
<!-- info: If crowdsourced, where from? -->
<!-- scope: periscope -->
`Other crowdworker platform`
#### Language Producers
<!-- info: What further information do we have on the language producers? -->
<!-- scope: microscope -->
The basic source language producers are Wikipedia authors and/or editors, since the annotation starts with the natural text description near the Wikipedia table.
The auxiliary source language producers are the annotators (two per example) who iteratively revise these descriptions to make them unambiguous and faithful to a subset of highlighted cells in the table.
#### Data Validation
<!-- info: Was the text validated by a different worker or a data curator? -->
<!-- scope: telescope -->
validated by crowdworker
#### Data Preprocessing
<!-- info: How was the text data pre-processed? (Enter N/A if the text was not pre-processed) -->
<!-- scope: microscope -->
The initial table-description pairs are tables from Wikipedia articles, extracted through heuristics such as Number Matching (tables and sentences that overlap with a non-date number of atleast 3 non-zero digits) (Refer to Section 4 of the paper for more)
1. Table Readability: Tables which are deemed non-readable (due to foreign language, poor formatting etc - a very small fraction of 0.5%) are removed from the dataset here.
2. Cell Highlighting: The annotator highlights the cells of the table which support the description.
3. Deletion: The annotator removes phrases in the description which are not supported by the highlighted cells
4. Decontextualization: Descriptions may contain pronouns or other forms of anaphora, or other phenomena which depend on the overall article topic - these are fixed by replacement (e.g replacing pronouns with the entity, provided it occurs in the table). The replacements allowed are limited to one, and annotators are also instructed to conserve fluency.
5. Secondary Annotation: A second set of annotators is shown the output of Stage 4, and asked to fix it if required to ensure it is grammatical.
The paper does not specifically describe the annotation platform or location profiles of the annotators.
#### Was Data Filtered?
<!-- info: Were text instances selected or filtered? -->
<!-- scope: telescope -->
algorithmically
#### Filter Criteria
<!-- info: What were the selection criteria? -->
<!-- scope: microscope -->
After construction of the splits, the data curators filtered training examples that had rare table header combinations (<=5 examples) and which had an overlap with the validation or test splits.
### Structured Annotations
#### Additional Annotations?
<!-- quick -->
<!-- info: Does the dataset have additional annotations for each instance? -->
<!-- scope: telescope -->
none
#### Annotation Service?
<!-- info: Was an annotation service used? -->
<!-- scope: telescope -->
no
### Consent
#### Any Consent Policy?
<!-- info: Was there a consent policy involved when gathering the data? -->
<!-- scope: telescope -->
yes
#### Consent Policy Details
<!-- info: What was the consent policy? -->
<!-- scope: microscope -->
Annotators were full time employees that were aware of the goal of the project and consented to having the data released as part of the dataset.
### Private Identifying Information (PII)
#### Contains PII?
<!-- quick -->
<!-- info: Does the source language data likely contain Personal Identifying Information about the data creators or subjects? -->
<!-- scope: telescope -->
no PII
#### Justification for no PII
<!-- info: Provide a justification for selecting `no PII` above. -->
<!-- scope: periscope -->
Since the source data is from wikipedia, only data in the public domain is included in the dataset.
### Maintenance
#### Any Maintenance Plan?
<!-- info: Does the original dataset have a maintenance plan? -->
<!-- scope: telescope -->
yes
#### Maintenance Plan Details
<!-- info: Describe the original dataset's maintenance plan. -->
<!-- scope: microscope -->
For submissions, you can delete your data by emailing totto@google.com from the email account used to sign up for the submission. Deletion requests will be responded to within 60 days.
#### Maintainer Contact Information
<!-- info: Provide contact information of a person responsible for the dataset maintenance -->
<!-- scope: periscope -->
Ankur Parikh (aparikh@google.com)
#### Any Contestation Mechanism?
<!-- info: Does the maintenance plan include a contestation mechanism allowing individuals to request removal fo content? -->
<!-- scope: periscope -->
form submission
#### Contestation Form Link
<!-- info: Provide the form link or contact information -->
<!-- scope: periscope -->
totto@google.com
## Broader Social Context
### Previous Work on the Social Impact of the Dataset
#### Usage of Models based on the Data
<!-- info: Are you aware of cases where models trained on the task featured in this dataset ore related tasks have been used in automated systems? -->
<!-- scope: telescope -->
no
### Impact on Under-Served Communities
#### Addresses needs of underserved Communities?
<!-- info: Does this dataset address the needs of communities that are traditionally underserved in language technology, and particularly language generation technology? Communities may be underserved for exemple because their language, language variety, or social or geographical context is underepresented in NLP and NLG resources (datasets and models). -->
<!-- scope: telescope -->
no
### Discussion of Biases
#### Any Documented Social Biases?
<!-- info: Are there documented social biases in the dataset? Biases in this context are variations in the ways members of different social categories are represented that can have harmful downstream consequences for members of the more disadvantaged group. -->
<!-- scope: telescope -->
yes
#### Links and Summaries of Analysis Work
<!-- info: Provide links to and summaries of works analyzing these biases. -->
<!-- scope: microscope -->
The original work as well as our GEM paper analyzes some biases
#### Are the Language Producers Representative of the Language?
<!-- info: Does the distribution of language producers in the dataset accurately represent the full distribution of speakers of the language world-wide? If not, how does it differ? -->
<!-- scope: periscope -->
This dataset is created using tables and the table cell contents may hence naturally exhibit biases which have been found to exist in Wikipedia such as some forms of gender bias (e.g [(Graells-Garido et al.,2015)](https://labtomarket.files.wordpress.com/2018/01/wiki_gender_bias.pdf) notes that spouse information is more likely discussed for females than males)
The table descriptions (targets/references) are, as discussed earlier, collected through a two-step process.
1. The natural text description near the table is taken as a starting point. This is Wikipedia article text as created upto that point in time by a chain of collaborative edits from Wikipedia authors.
2. The initial description is revised by chain of two or more annotated revisions, to make it unambiguous and faithful to a set of highlighted table cells.
From their origin in 1), the descriptions may exhibit biases seen in Wikipedia text as mentioned above. From their revisions in 2), the descriptions may show biases originating from annotator-authored text, such as a preference for shorter descriptions since they're faster to write, or linguistic preferences influenced by the locations dominant in the annotator distribution. (However, note that these are likely to be much reduced since the annotators here are merely revising rather than completely authoring. Moreover, each sentence goes through atleast two annotators, which acts as a check against the personal biases of a single annotator.)
Naturally-occurring text is also known to suffer from other biases such as reporting bias [(Gordon and Van Durme, 2013)](https://openreview.net/forum?id=AzxEzvpdE3Wcy¬eId=vmR8qaby8fqxittps://labtomarket.files.wordpress.com/2018/01/wiki_gender_bias.pdf) - this also applies to this dataset via its origin from Wikipedia.
## Considerations for Using the Data
### PII Risks and Liability
#### Potential PII Risk
<!-- info: Considering your answers to the PII part of the Data Curation Section, describe any potential privacy to the data subjects and creators risks when using the dataset. -->
<!-- scope: microscope -->
Since the source data is from wikipedia, only data in the public domain is included in the dataset.
### Licenses
#### Copyright Restrictions on the Dataset
<!-- info: Based on your answers in the Intended Use part of the Data Overview Section, which of the following best describe the copyright and licensing status of the dataset? -->
<!-- scope: periscope -->
`open license - commercial use allowed`
#### Copyright Restrictions on the Language Data
<!-- info: Based on your answers in the Language part of the Data Curation Section, which of the following best describe the copyright and licensing status of the underlying language data? -->
<!-- scope: periscope -->
`open license - commercial use allowed`
### Known Technical Limitations
#### Technical Limitations
<!-- info: Describe any known technical limitations, such as spurrious correlations, train/test overlap, annotation biases, or mis-annotations, and cite the works that first identified these limitations when possible. -->
<!-- scope: microscope -->
The dataset is limited to topics that are present in Wikipedia, more specifically those topics that are present in articles which contain atleast one table
_Sports_ and _Countries_ form 53.4% of the dataset. The remaining fraction is made up of broader topics like _Europe_, *North America*and _Politics_
|
GEM/turku_hockey_data2text | ---
annotations_creators:
- expert-created
language_creators:
- unknown
language:
- fi
license:
- cc-by-nc-sa-4.0
multilinguality:
- unknown
size_categories:
- unknown
source_datasets:
- original
task_categories:
- table-to-text
task_ids: []
pretty_name: turku_hockey_data2text
tags:
- data-to-text
---
# Dataset Card for GEM/turku_hockey_data2text
## Dataset Description
- **Homepage:** https://turkunlp.org/hockey_data2text.html
- **Repository:** https://github.com/TurkuNLP/Turku-hockey-data2text
- **Paper:** https://aclanthology.org/W19-6125/
- **Leaderboard:** N/A
- **Point of Contact:** Jenna Kanerva, Filip Ginter
### Link to Main Data Card
You can find the main data card on the [GEM Website](https://gem-benchmark.com/data_cards/turku_hockey_data2text).
### Dataset Summary
This is a Finnish data-to-text dataset in which the input is structured information about a hockey game and the output a description of the game.
You can load the dataset via:
```
import datasets
data = datasets.load_dataset('GEM/turku_hockey_data2text')
```
The data loader can be found [here](https://huggingface.co/datasets/GEM/turku_hockey_data2text).
#### website
[Website](https://turkunlp.org/hockey_data2text.html)
#### paper
[ACL anthology](https://aclanthology.org/W19-6125/)
#### authors
Jenna Kanerva, Samuel Rönnqvist, Riina Kekki, Tapio Salakoski, Filip Ginter (TurkuNLP / University of Turku)
## Dataset Overview
### Where to find the Data and its Documentation
#### Webpage
<!-- info: What is the webpage for the dataset (if it exists)? -->
<!-- scope: telescope -->
[Website](https://turkunlp.org/hockey_data2text.html)
#### Download
<!-- info: What is the link to where the original dataset is hosted? -->
<!-- scope: telescope -->
[Github](https://github.com/TurkuNLP/Turku-hockey-data2text)
#### Paper
<!-- info: What is the link to the paper describing the dataset (open access preferred)? -->
<!-- scope: telescope -->
[ACL anthology](https://aclanthology.org/W19-6125/)
#### BibTex
<!-- info: Provide the BibTex-formatted reference for the dataset. Please use the correct published version (ACL anthology, etc.) instead of google scholar created Bibtex. -->
<!-- scope: microscope -->
```
@inproceedings{kanerva2019newsgen,
Title = {Template-free Data-to-Text Generation of Finnish Sports News},
Author = {Jenna Kanerva and Samuel R{\"o}nnqvist and Riina Kekki and Tapio Salakoski and Filip Ginter},
booktitle = {Proceedings of the 22nd Nordic Conference on Computational Linguistics (NoDaLiDa’19)},
year={2019}
}
```
#### Contact Name
<!-- quick -->
<!-- info: If known, provide the name of at least one person the reader can contact for questions about the dataset. -->
<!-- scope: periscope -->
Jenna Kanerva, Filip Ginter
#### Contact Email
<!-- info: If known, provide the email of at least one person the reader can contact for questions about the dataset. -->
<!-- scope: periscope -->
jmnybl@utu.fi, figint@utu.fi
#### Has a Leaderboard?
<!-- info: Does the dataset have an active leaderboard? -->
<!-- scope: telescope -->
no
### Languages and Intended Use
#### Multilingual?
<!-- quick -->
<!-- info: Is the dataset multilingual? -->
<!-- scope: telescope -->
no
#### Covered Dialects
<!-- info: What dialects are covered? Are there multiple dialects per language? -->
<!-- scope: periscope -->
written standard language
#### Covered Languages
<!-- quick -->
<!-- info: What languages/dialects are covered in the dataset? -->
<!-- scope: telescope -->
`Finnish`
#### Whose Language?
<!-- info: Whose language is in the dataset? -->
<!-- scope: periscope -->
The original news articles are written by professional journalists. The text passages extracted in the annotation may be slightly edited compared to the original language during the corpus annotation.
#### License
<!-- quick -->
<!-- info: What is the license of the dataset? -->
<!-- scope: telescope -->
cc-by-nc-sa-4.0: Creative Commons Attribution Non Commercial Share Alike 4.0 International
#### Intended Use
<!-- info: What is the intended use of the dataset? -->
<!-- scope: microscope -->
This dataset was developed as a benchmark for evaluating template-free, machine learning methods on Finnish news generation in the area of ice hockey reporting.
#### Primary Task
<!-- info: What primary task does the dataset support? -->
<!-- scope: telescope -->
Data-to-Text
#### Communicative Goal
<!-- quick -->
<!-- info: Provide a short description of the communicative goal of a model trained for this task on this dataset. -->
<!-- scope: periscope -->
Describe an event from an ice hockey game based on the given structural data.
### Credit
#### Curation Organization Type(s)
<!-- info: In what kind of organization did the dataset curation happen? -->
<!-- scope: telescope -->
`academic`
#### Curation Organization(s)
<!-- info: Name the organization(s). -->
<!-- scope: periscope -->
University of Turku
#### Dataset Creators
<!-- info: Who created the original dataset? List the people involved in collecting the dataset and their affiliation(s). -->
<!-- scope: microscope -->
Jenna Kanerva, Samuel Rönnqvist, Riina Kekki, Tapio Salakoski, Filip Ginter (TurkuNLP / University of Turku)
#### Funding
<!-- info: Who funded the data creation? -->
<!-- scope: microscope -->
The project was supported by the Google Digital News Innovation Fund.
#### Who added the Dataset to GEM?
<!-- info: Who contributed to the data card and adding the dataset to GEM? List the people+affiliations involved in creating this data card and who helped integrate this dataset into GEM. -->
<!-- scope: microscope -->
Jenna Kanerva, Filip Ginter (TurkuNLP / University of Turku)
### Dataset Structure
#### Data Fields
<!-- info: List and describe the fields present in the dataset. -->
<!-- scope: telescope -->
The dataset is constructed of games, where each game is a list of events. If the event was annotated (corresponding sentence was found from the news article), it includes `text` field with value other than empty string ("").
For each game (dict), there are keys `gem_id` (string), `id` (string), `news_article` (string), and `events` (list).
For each event (dict), there are different, relevant keys available with non empty values depending on the event type (e.g. goal or penalty). The mandatory keys for each event are `event_id` (string), `event_type` (string), `text` (string, empty string if not annotated), and `multi_reference` (bool). The keys not relevant for the specific event type are left empty.
The relevant keys in the event dictionary are:
For each event type, the following keys are relevant:
`event_id`: Identifier of the event, unique to the game but not globally, in chronological order (string)
`event_type`: Type of the event, possible values are `game result`, `goal`, `penalty`, or `saves` (string)
`text`: Natural language description of the event, or empty string if not available (string)
`multi_reference`: Does this event refer to a text passage describing multiple events? (bool)
The rest of the fields are specific to the event type. The relevant fields for each event type are:
game result:
`event_id`: Identifier of the event, unique to the game but not globally, in chronological order (string)
`event_type`: Type of the event (string)
`home_team`: Name of the home team (string)
`guest_team`: Name of the guest team (string)
`score`: Final score of the game, in the form of home–guest (string)
`periods`: Scores for individual periods, each in the form of home–guest score in that period (list of strings)
`features`: Additional features, such as overtime win or shoot out (list of strings)
`text`: Natural language description of the event, or empty string if not available (string)
`multi_reference`: Does this event refer to a text passage describing multiple events? (bool)
goal:
`event_id`: Identifier of the event, unique to the game but not globally, in chronological order (string)
`event_type`: Type of the event (string)
`player`: Name of the player scoring (string)
`assist`: Names of the players assisting, at most two players (list of strings)
`team`: Team scoring with possible values of `home` or `guest` (string)
`team_name`: Name of the team scoring (string)
`score`: Score after the goal, in the form of home–guest (string)
`time`: Time of the goal, minutes and seconds from the beginning (string)
`features`: Additional features, such as power play or short-handed goal (list of strings)
`text`: Natural language description of the event, or empty string if not available (string)
`multi_reference`: Does this event refer to a text passage describing multiple events? (bool)
penalty:
`event_id`: Identifier of the event, unique to the game but not globally, in chronological order (string)
`event_type`: Type of the event (string)
`player`: Name of the player getting the penalty (string)
`team`: Team getting the penalty with possible values of `home` or `guest` (string)
`team_name`: Name of the team getting the penalty (string)
`penalty_minutes`: Penalty minutes (string)
`time`: Time of the penalty, minutes and seconds from the beginning (string)
`text`: Natural language description of the event, or empty string if not available (string)
`multi_reference`: Does this event refer to a text passage describing multiple events? (bool)
saves:
`event_id`: Identifier of the event, unique to the game but not globally, in chronological order (string)
`event_type`: Type of the event (string)
`player`: Name of the goalkeeper (string)
`team`: Team of the goalkeeper with possible values of `home` or `guest` (string)
`team_name`: Name of the team (string)
`saves`: Number of saves in the game (string)
`text`: Natural language description of the event, or empty string if not available (string)
`multi_reference`: Does this event refer to a text passage describing multiple events? (bool)
Text passages describing multiple events (multi_reference):
Some text passages refer to multiple events in such way that separating them to individual statements is not adequate (e.g. "The home team received two penalties towards the end of the first period."). In these cases, multiple events are aligned to the same text passage so that the first event (in chronological order) include the annotated text passage, while the rest of the events referring to the same text passage include the identifier of the first event in the annotated text field (e.g. `text`: "E4").
#### Example Instance
<!-- info: Provide a JSON formatted example of a typical instance in the dataset. -->
<!-- scope: periscope -->
```
{
'gem_id': 'gem-turku_hockey_data2text-train-0',
'id': '20061031-TPS-HPK',
'news_article': 'HPK:n hyvä syysvire jatkuu jääkiekon SM-liigassa. Tiistaina HPK kukisti mainiolla liikkeellä ja tehokkaalla ylivoimapelillä TPS:n vieraissa 1–0 (1–0, 0–0, 0–0).\nHPK hyödynsi ylivoimaa mennen jo ensimmäisessä erässä Mikko Mäenpään maalilla 1–0 -johtoon.\nToisessa ja kolmannessa erässä HPK tarjosi edelleen TPS:lle runsaasti tilanteita, mutta maalia eivät turkulaiset millään ilveellä saaneet. Pahin este oli loistavan pelin Hämeenlinnan maalilla pelannut Mika Oksa.\nTPS:n maalissa Jani Hurme ei osumille mitään mahtanut. Joukkueen suuri yksinäinen kenttäpelaaja oli Kai Nurminen, mutta hänelläkään ei ollut onnea maalitilanteissa.',
'events':
{
'event_id': ['E1', 'E2', 'E3'],
'event_type': ['game result', 'penalty', 'goal'],
'text': ['HPK kukisti TPS:n vieraissa 1–0 (1–0, 0–0, 0–0).', '', 'HPK hyödynsi ylivoimaa mennen jo ensimmäisessä erässä Mikko Mäenpään maalilla 1–0 -johtoon.'],
'home_team': ['TPS', '', ''],
'guest_team': ['HPK', '', ''],
'score': ['0–1', '', '0–1'],
'periods': [['0–1', '0–0', '0–0'], [], []],
'features': [[], [], ['power play']],
'player': ['', 'Fredrik Svensson', 'Mikko Mäenpää'],
'assist': [[], [], ['Jani Keinänen', 'Toni Mäkiaho']],
'team': ['', 'guest', 'guest'],
'team_name': ['', 'HPK', 'HPK'],
'time': ['', '9.28', '14.57'],
'penalty_minutes': ['', '2', ''],
'saves': ['', '', ''],
'multi_reference': [false, false, false]
}
}
```
#### Data Splits
<!-- info: Describe and name the splits in the dataset if there are more than one. -->
<!-- scope: periscope -->
The corpus include 3 splits: train, validation, and test.
## Dataset in GEM
### Rationale for Inclusion in GEM
#### Why is the Dataset in GEM?
<!-- info: What does this dataset contribute toward better generation evaluation and why is it part of GEM? -->
<!-- scope: microscope -->
The dataset was created to develop machine learned text generation models for Finnish ice hockey news, where the generation would reflect the natural language variation found from the game reports written by professional journalists. While the original game reports often include additional information not derivable from the game statistics, the corpus was fully manually curated to remove all such information from the natural language descriptions. The rationale of such curation was to prevent model 'hallucinating' additional facts.
#### Similar Datasets
<!-- info: Do other datasets for the high level task exist? -->
<!-- scope: telescope -->
yes
#### Unique Language Coverage
<!-- info: Does this dataset cover other languages than other datasets for the same task? -->
<!-- scope: periscope -->
yes
#### Difference from other GEM datasets
<!-- info: What else sets this dataset apart from other similar datasets in GEM? -->
<!-- scope: microscope -->
This is the only data2text corpus for Finnish in GEM.
#### Ability that the Dataset measures
<!-- info: What aspect of model ability can be measured with this dataset? -->
<!-- scope: periscope -->
morphological inflection, language variation
### GEM-Specific Curation
#### Modificatied for GEM?
<!-- info: Has the GEM version of the dataset been modified in any way (data, processing, splits) from the original curated data? -->
<!-- scope: telescope -->
yes
#### GEM Modifications
<!-- info: What changes have been made to he original dataset? -->
<!-- scope: periscope -->
`data points modified`
#### Modification Details
<!-- info: For each of these changes, described them in more details and provided the intended purpose of the modification -->
<!-- scope: microscope -->
Structural data was translated into English.
#### Additional Splits?
<!-- info: Does GEM provide additional splits to the dataset? -->
<!-- scope: telescope -->
no
### Getting Started with the Task
## Previous Results
### Previous Results
#### Metrics
<!-- info: What metrics are typically used for this task? -->
<!-- scope: periscope -->
`BLEU`, `METEOR`, `ROUGE`, `WER`
#### Proposed Evaluation
<!-- info: List and describe the purpose of the metrics and evaluation methodology (including human evaluation) that the dataset creators used when introducing this task. -->
<!-- scope: microscope -->
Automatic evaluation: BLEU, NIST, METEOR, ROUGE-L, CIDEr
Manual evaluation: factual mistakes, grammatical errors, minimum edit distance to an acceptable game report (using WER)
#### Previous results available?
<!-- info: Are previous results available? -->
<!-- scope: telescope -->
yes
## Dataset Curation
### Original Curation
#### Original Curation Rationale
<!-- info: Original curation rationale -->
<!-- scope: telescope -->
The dataset is designed for text generation (data2text), where the original source of natural language descriptions is news articles written by journalists. While the link between structural data (ice hockey game statistics) and the news articles describing the game was quite weak (news articles including a lot of information not derivable from the statistics, while leaving many events unmentioned), the corpus includes full manual annotation aligning the events extracted from game statistics and the corresponding natural language passages extracted from the news articles.
Each event is manually aligned into a sentence-like passage, and in case a suitable passage was not found, the annotation is left empty (with value `None`). The extracted passages were manually modified not to include additional information not derivable from the game statistics, or not considered as world knowledge. The manual curation of passages is designed to prevent model hallucination, i.e. model learning to generate facts not derivable from the input data.
#### Communicative Goal
<!-- info: What was the communicative goal? -->
<!-- scope: periscope -->
Describing the given events (structural data) in natural language, and therefore generating ice hockey game reports.
#### Sourced from Different Sources
<!-- info: Is the dataset aggregated from different data sources? -->
<!-- scope: telescope -->
no
### Language Data
#### How was Language Data Obtained?
<!-- info: How was the language data obtained? -->
<!-- scope: telescope -->
`Other`
#### Language Producers
<!-- info: What further information do we have on the language producers? -->
<!-- scope: microscope -->
The initial data, both game statistics and news articles, were obtained from the Finnish News Agency STT news archives released for academic use (http://urn.fi/urn:nbn:fi:lb-2019041501). The original news articles are written by professional journalists.
We (TurkuNLP) gratefully acknowledge the collaboration of Maija Paikkala, Salla Salmela and Pihla Lehmusjoki from the Finnish News Agency STT while creating the corpus.
#### Topics Covered
<!-- info: Does the language in the dataset focus on specific topics? How would you describe them? -->
<!-- scope: periscope -->
Ice hockey, news
#### Data Validation
<!-- info: Was the text validated by a different worker or a data curator? -->
<!-- scope: telescope -->
not validated
#### Was Data Filtered?
<!-- info: Were text instances selected or filtered? -->
<!-- scope: telescope -->
algorithmically
#### Filter Criteria
<!-- info: What were the selection criteria? -->
<!-- scope: microscope -->
Include only games, where both game statistics and a news article describing the game were available (based on timestamps and team names).
### Structured Annotations
#### Additional Annotations?
<!-- quick -->
<!-- info: Does the dataset have additional annotations for each instance? -->
<!-- scope: telescope -->
expert created
#### Number of Raters
<!-- info: What is the number of raters -->
<!-- scope: telescope -->
1
#### Rater Qualifications
<!-- info: Describe the qualifications required of an annotator. -->
<!-- scope: periscope -->
Members of the TurkuNLP research group, native speakers of Finnish.
#### Raters per Training Example
<!-- info: How many annotators saw each training example? -->
<!-- scope: periscope -->
1
#### Raters per Test Example
<!-- info: How many annotators saw each test example? -->
<!-- scope: periscope -->
1
#### Annotation Service?
<!-- info: Was an annotation service used? -->
<!-- scope: telescope -->
no
#### Annotation Values
<!-- info: Purpose and values for each annotation -->
<!-- scope: microscope -->
Manual alignment of events and their natural language descriptions. Removing information not derivable from the input data or world knowledge in order to prevent the model 'hallucination'.
#### Any Quality Control?
<!-- info: Quality control measures? -->
<!-- scope: telescope -->
validated by data curators
#### Quality Control Details
<!-- info: Describe the quality control measures that were taken. -->
<!-- scope: microscope -->
Manual inspection of examples during the initial annotation training phrase.
### Consent
#### Any Consent Policy?
<!-- info: Was there a consent policy involved when gathering the data? -->
<!-- scope: telescope -->
yes
#### Consent Policy Details
<!-- info: What was the consent policy? -->
<!-- scope: microscope -->
The corpus license was agreed with the providers of the source material.
### Private Identifying Information (PII)
#### Contains PII?
<!-- quick -->
<!-- info: Does the source language data likely contain Personal Identifying Information about the data creators or subjects? -->
<!-- scope: telescope -->
yes/very likely
#### Categories of PII
<!-- info: What categories of PII are present or suspected in the data? -->
<!-- scope: periscope -->
`generic PII`
#### Any PII Identification?
<!-- info: Did the curators use any automatic/manual method to identify PII in the dataset? -->
<!-- scope: periscope -->
no identification
### Maintenance
#### Any Maintenance Plan?
<!-- info: Does the original dataset have a maintenance plan? -->
<!-- scope: telescope -->
no
## Broader Social Context
### Previous Work on the Social Impact of the Dataset
#### Usage of Models based on the Data
<!-- info: Are you aware of cases where models trained on the task featured in this dataset ore related tasks have been used in automated systems? -->
<!-- scope: telescope -->
no
### Impact on Under-Served Communities
#### Addresses needs of underserved Communities?
<!-- info: Does this dataset address the needs of communities that are traditionally underserved in language technology, and particularly language generation technology? Communities may be underserved for exemple because their language, language variety, or social or geographical context is underepresented in NLP and NLG resources (datasets and models). -->
<!-- scope: telescope -->
no
### Discussion of Biases
#### Any Documented Social Biases?
<!-- info: Are there documented social biases in the dataset? Biases in this context are variations in the ways members of different social categories are represented that can have harmful downstream consequences for members of the more disadvantaged group. -->
<!-- scope: telescope -->
no
#### Are the Language Producers Representative of the Language?
<!-- info: Does the distribution of language producers in the dataset accurately represent the full distribution of speakers of the language world-wide? If not, how does it differ? -->
<!-- scope: periscope -->
The dataset represents only written standard language.
## Considerations for Using the Data
### PII Risks and Liability
#### Potential PII Risk
<!-- info: Considering your answers to the PII part of the Data Curation Section, describe any potential privacy to the data subjects and creators risks when using the dataset. -->
<!-- scope: microscope -->
None
### Licenses
#### Copyright Restrictions on the Dataset
<!-- info: Based on your answers in the Intended Use part of the Data Overview Section, which of the following best describe the copyright and licensing status of the dataset? -->
<!-- scope: periscope -->
`non-commercial use only`
#### Copyright Restrictions on the Language Data
<!-- info: Based on your answers in the Language part of the Data Curation Section, which of the following best describe the copyright and licensing status of the underlying language data? -->
<!-- scope: periscope -->
`non-commercial use only`
### Known Technical Limitations
|
GEM/turku_paraphrase_corpus | ---
annotations_creators:
- expert-created
language_creators:
- unknown
language:
- fi
license:
- cc-by-sa-4.0
multilinguality:
- unknown
size_categories:
- unknown
source_datasets:
- original
task_categories:
- other
task_ids: []
pretty_name: turku_paraphrase_corpus
tags:
- paraphrasing
---
# Dataset Card for GEM/turku_paraphrase_corpus
## Dataset Description
- **Homepage:** https://turkunlp.org/paraphrase.html
- **Repository:** https://github.com/TurkuNLP/Turku-paraphrase-corpus
- **Paper:** https://aclanthology.org/2021.nodalida-main.29/
- **Leaderboard:** N/A
- **Point of Contact:** Jenna Kanerva, Filip Ginter
### Link to Main Data Card
You can find the main data card on the [GEM Website](https://gem-benchmark.com/data_cards/turku_paraphrase_corpus).
### Dataset Summary
This is a Finnish paraphrase corpus which consists of pairs of text passages, where a typical passage is about a sentence long. It can be used to either identify or generate paraphrases.
You can load the dataset via:
```
import datasets
data = datasets.load_dataset('GEM/turku_paraphrase_corpus')
```
The data loader can be found [here](https://huggingface.co/datasets/GEM/turku_paraphrase_corpus).
#### website
[Website](https://turkunlp.org/paraphrase.html)
#### paper
[ACL Anthology](https://aclanthology.org/2021.nodalida-main.29/)
#### authors
Jenna Kanerva, Filip Ginter, Li-Hsin Chang, Iiro Rastas, Valtteri Skantsi, Jemina Kilpeläinen, Hanna-Mari Kupari, Aurora Piirto, Jenna Saarni, Maija Sevón, Otto Tarkka (TurkuNLP / University of Turku)
## Dataset Overview
### Where to find the Data and its Documentation
#### Webpage
<!-- info: What is the webpage for the dataset (if it exists)? -->
<!-- scope: telescope -->
[Website](https://turkunlp.org/paraphrase.html)
#### Download
<!-- info: What is the link to where the original dataset is hosted? -->
<!-- scope: telescope -->
[Github](https://github.com/TurkuNLP/Turku-paraphrase-corpus)
#### Paper
<!-- info: What is the link to the paper describing the dataset (open access preferred)? -->
<!-- scope: telescope -->
[ACL Anthology](https://aclanthology.org/2021.nodalida-main.29/)
#### BibTex
<!-- info: Provide the BibTex-formatted reference for the dataset. Please use the correct published version (ACL anthology, etc.) instead of google scholar created Bibtex. -->
<!-- scope: microscope -->
```
@inproceedings{kanerva-etal-2021-finnish,
title = {Finnish Paraphrase Corpus},
author = {Kanerva, Jenna and Ginter, Filip and Chang, Li-Hsin and Rastas, Iiro and Skantsi, Valtteri and Kilpel{\"a}inen, Jemina and Kupari, Hanna-Mari and Saarni, Jenna and Sev{\'o}n, Maija and Tarkka, Otto},
booktitle = {Proceedings of the 23rd Nordic Conference on Computational Linguistics (NoDaLiDa'21)},
year = {2021},
publisher = {Link{\"o}ping University Electronic Press, Sweden},
url = {https://aclanthology.org/2021.nodalida-main.29},
pages = {288--298}
}
```
#### Contact Name
<!-- quick -->
<!-- info: If known, provide the name of at least one person the reader can contact for questions about the dataset. -->
<!-- scope: periscope -->
Jenna Kanerva, Filip Ginter
#### Contact Email
<!-- info: If known, provide the email of at least one person the reader can contact for questions about the dataset. -->
<!-- scope: periscope -->
jmnybl@utu.fi, figint@utu.fi
#### Has a Leaderboard?
<!-- info: Does the dataset have an active leaderboard? -->
<!-- scope: telescope -->
no
### Languages and Intended Use
#### Multilingual?
<!-- quick -->
<!-- info: Is the dataset multilingual? -->
<!-- scope: telescope -->
no
#### Covered Dialects
<!-- info: What dialects are covered? Are there multiple dialects per language? -->
<!-- scope: periscope -->
written standard language, spoken language
#### Covered Languages
<!-- quick -->
<!-- info: What languages/dialects are covered in the dataset? -->
<!-- scope: telescope -->
`Finnish`
#### License
<!-- quick -->
<!-- info: What is the license of the dataset? -->
<!-- scope: telescope -->
cc-by-sa-4.0: Creative Commons Attribution Share Alike 4.0 International
#### Intended Use
<!-- info: What is the intended use of the dataset? -->
<!-- scope: microscope -->
Paraphrase classification, paraphrase generation
#### Primary Task
<!-- info: What primary task does the dataset support? -->
<!-- scope: telescope -->
Paraphrasing
#### Communicative Goal
<!-- quick -->
<!-- info: Provide a short description of the communicative goal of a model trained for this task on this dataset. -->
<!-- scope: periscope -->
The corpus provides naturally occurring Finnish paraphrases striving for low lexical overlap, thus supporting many different downstream applications requiring language understanding.
### Credit
#### Curation Organization Type(s)
<!-- info: In what kind of organization did the dataset curation happen? -->
<!-- scope: telescope -->
`academic`
#### Curation Organization(s)
<!-- info: Name the organization(s). -->
<!-- scope: periscope -->
University of Turku
#### Dataset Creators
<!-- info: Who created the original dataset? List the people involved in collecting the dataset and their affiliation(s). -->
<!-- scope: microscope -->
Jenna Kanerva, Filip Ginter, Li-Hsin Chang, Iiro Rastas, Valtteri Skantsi, Jemina Kilpeläinen, Hanna-Mari Kupari, Aurora Piirto, Jenna Saarni, Maija Sevón, Otto Tarkka (TurkuNLP / University of Turku)
#### Funding
<!-- info: Who funded the data creation? -->
<!-- scope: microscope -->
The Turku paraphrase corpus project was funded by the Academy of Finland, as well as the European Language Grid project through its open call for pilot projects. The European Language Grid project has received funding from the European Union’s Horizon 2020 Research and Innovation programme under Grant Agreement no. 825627 (ELG).
#### Who added the Dataset to GEM?
<!-- info: Who contributed to the data card and adding the dataset to GEM? List the people+affiliations involved in creating this data card and who helped integrate this dataset into GEM. -->
<!-- scope: microscope -->
Jenna Kanerva, Filip Ginter (TurkuNLP / University of Turku)
### Dataset Structure
#### Data Fields
<!-- info: List and describe the fields present in the dataset. -->
<!-- scope: telescope -->
The dataset consist of pairs of text passages, where a typical passage is about a sentence long, however, a passage may also be longer or shorter than a sentence. Thus, each example include two text passages (string), a manually annotated label to indicate the paraphrase type (string), and additional metadata.
The dataset include three different `modes`, plain, classification, and generation. The `plain` mode loads the original data without any additional preprocessing or transformations, while the `classification` mode directly builds the data in a form suitable for training a paraphrase classifier, where each example is doubled in the data with different directions (text1, text2, label) --> (text2, text1, label) taking care of the label flipping as well if needed (paraphrases with directionality flag < or >). In the `generation` mode, the examples are preprocessed to be directly suitable for paraphrase generation task. In here, paraphrases not suitable for generation are discarded (negative, and highly context-dependent paraphrases), and directional paraphrases are provided so that the generation goes from more detailed passage to the more general one in order to prevent model hallucination (i.e. model learning to introduce new information). The rest of the paraphrases are provided in both directions (text1, text2, label) --> (text2, text1, label).
Each pair in `plain` and `classification` mode will include fields:
`gem_id`: Identifier of the paraphrase pair (string)
`goeswith`: Identifier of the document from which the paraphrase was extracted, can be `not available` in case the source of the paraphrase is not from document-structured data (string)
`fold`: 0-99, data split into 100 parts respecting document boundaries, you can use this e.g. to implement crossvalidation safely as all paraphrases from one document are in one fold (int)
`text1`: First paraphrase passage (string)
`text2`: Second paraphrase passage (string)
`label`: Manually annotated labels (string)
`binary_label`: Label turned into binary with values `positive` (paraphrase) and `negative` (not-paraphrase) (string)
`is_rewrite`: Indicator whether the example is human produced rewrite or naturally occurring paraphrase (bool)
Each pair in `generation` mode will include the same fields expect `text1` and `text2` are renamed to `input` and `output` in order to indicate the generation direction. Thus the fields are:
`gem_id`: Identifier of the paraphrase pair (string)
`goeswith`: Identifier of the document from which the paraphrase was extracted, can be `not available` in case the source of the paraphrase is not from document-structured data (string)
`fold`: 0-99, data split into 100 parts respecting document boundaries, you can use this e.g. to implement crossvalidation safely as all paraphrases from one document are in one fold (int)
`input`: The input paraphrase passage for generation (string)
`output`: The output paraphrase passage for generation (string)
`label`: Manually annotated labels (string)
`binary_label`: Label turned into binary with values `positive` (paraphrase) and `negative` (not-paraphrase) (string)
`is_rewrite`: Indicator whether the example is human produced rewrite or naturally occurring paraphrase (bool)
#### Example Instance
<!-- info: Provide a JSON formatted example of a typical instance in the dataset. -->
<!-- scope: periscope -->
```
{
'gem_id': 'gem-turku_paraphrase_corpus-train-15',
'goeswith': 'episode-02243',
'fold': 0,
'text1': 'Mitä merkitystä sillä on?',
'text2': 'Mitä väliä sillä edes on?',
'label': '4',
'binary_label': 'positive',
'is_rewrite': False
}
```
#### Data Splits
<!-- info: Describe and name the splits in the dataset if there are more than one. -->
<!-- scope: periscope -->
The corpus include 3 splits: train, validation, and test.
#### Splitting Criteria
<!-- info: Describe any criteria for splitting the data, if used. If there are differences between the splits (e.g., if the training annotations are machine-generated and the dev and test ones are created by humans, or if different numbers of annotators contributed to each example), describe them here. -->
<!-- scope: microscope -->
The data is split randomly into the three section with a restriction of all paraphrases from the same document (movie, TV episode, news article, student translation, or exam question) being in the same section. All splits are manually annotated.
## Dataset in GEM
### Rationale for Inclusion in GEM
#### Why is the Dataset in GEM?
<!-- info: What does this dataset contribute toward better generation evaluation and why is it part of GEM? -->
<!-- scope: microscope -->
This dataset provides a large amount of high quality (manually collected and verified) paraphrases for Finnish.
#### Similar Datasets
<!-- info: Do other datasets for the high level task exist? -->
<!-- scope: telescope -->
yes
#### Unique Language Coverage
<!-- info: Does this dataset cover other languages than other datasets for the same task? -->
<!-- scope: periscope -->
no
#### Ability that the Dataset measures
<!-- info: What aspect of model ability can be measured with this dataset? -->
<!-- scope: periscope -->
natural language understanding, language variation
### GEM-Specific Curation
#### Modificatied for GEM?
<!-- info: Has the GEM version of the dataset been modified in any way (data, processing, splits) from the original curated data? -->
<!-- scope: telescope -->
yes
#### GEM Modifications
<!-- info: What changes have been made to he original dataset? -->
<!-- scope: periscope -->
`data points modified`
#### Modification Details
<!-- info: For each of these changes, described them in more details and provided the intended purpose of the modification -->
<!-- scope: microscope -->
Data structure is slightly simplified, and the release provides ready made transformations into two tasks (paraphrase classification and generation), where some data instances are doubled with different direction, and some are discarded as not being suitable for generation (e.g. negatives).
#### Additional Splits?
<!-- info: Does GEM provide additional splits to the dataset? -->
<!-- scope: telescope -->
no
### Getting Started with the Task
## Previous Results
### Previous Results
#### Measured Model Abilities
<!-- info: What aspect of model ability can be measured with this dataset? -->
<!-- scope: telescope -->
natural language understanding, language variation
#### Previous results available?
<!-- info: Are previous results available? -->
<!-- scope: telescope -->
yes
#### Other Evaluation Approaches
<!-- info: What evaluation approaches have others used? -->
<!-- scope: periscope -->
F-score in paraphrase classification
## Dataset Curation
### Original Curation
#### Original Curation Rationale
<!-- info: Original curation rationale -->
<!-- scope: telescope -->
The dataset is fully manually annotated. The dataset strives for interesting paraphrases with low lexical overlap, thus the annotation is two fold. First the paraphrases are manually extracted from two related documents, where the annotators are instructed to extract only interesting paraphrases. In the second phrase, all extracted paraphrases are manually labeled given the annotation scheme.
The annotation scheme is:
4 : paraphrase in all reasonably possible contexts
3 : paraphrase in the given document contexts, but not in general
2 : related but not paraphrase
During annotation also labels 1 (unrelated) and x (skip, e.g. wrong language) were used, however, the insignificant amount of examples annotated with these labels were discarded from the released corpus.
The following flags are annotated to label 4 paraphrases:
< : txt1 is more general than txt2; txt2 is more specific than txt1 (directional paraphrase where txt2 can be replaced with txt1 in all contexts but not to the other direction)
> : txt2 is more general than txt1; txt1 is more specific than txt2 (directional paraphrase where txt1 can be replaced with txt2 in all contexts but not to the other direction)
i : minor traceable difference (differing in terms of grammatical number or case, 'this' vs 'that', etc.)
s : style or strength difference (e.g. equivalent meaning, but one of the statements substantially more colloquial than the other)
For paraphrases where the annotated label was something else than label 4 without any flags, the annotators had an option to rewrite the text passages so that the rewritten paraphrase pair formed label 4 (universal) paraphrase. This was used for cases where simple edit would turn e.g. contextual or directional paraphrase into universal one. For the rewritten examples, both the original and the rewritten pairs are available with corresponding labels annotated.
#### Communicative Goal
<!-- info: What was the communicative goal? -->
<!-- scope: periscope -->
Representing text passages with identical meaning but different surface realization.
#### Sourced from Different Sources
<!-- info: Is the dataset aggregated from different data sources? -->
<!-- scope: telescope -->
yes
#### Source Details
<!-- info: List the sources (one per line) -->
<!-- scope: periscope -->
movie and TV series subtitles (82%)
news articles (9%)
discussion forum messages (8%)
university translation exercises (1%)
university course essays and exams (<1%)
### Language Data
#### How was Language Data Obtained?
<!-- info: How was the language data obtained? -->
<!-- scope: telescope -->
`Found`, `Other`
#### Where was it found?
<!-- info: If found, where from? -->
<!-- scope: telescope -->
`Multiple websites`, `Offline media collection`, `Other`
#### Language Producers
<!-- info: What further information do we have on the language producers? -->
<!-- scope: microscope -->
The movie and TV series subtitles are extracted from OPUS OpenSubtitles2018 collection, which is based on data from [OpenSubtitles](http://www.opensubtitles.org/).
The news articles are collected from two Finnish news sites, YLE and HS, during years 2017-2020.
Discussion forum messages are obtained from the Finnish Suomi24 discussion forum released for academic use (http://urn.fi/urn:nbn:fi:lb-2020021801).
University translation exercises, essays and exams are collected during the project.
#### Data Validation
<!-- info: Was the text validated by a different worker or a data curator? -->
<!-- scope: telescope -->
validated by data curator
#### Was Data Filtered?
<!-- info: Were text instances selected or filtered? -->
<!-- scope: telescope -->
not filtered
### Structured Annotations
#### Additional Annotations?
<!-- quick -->
<!-- info: Does the dataset have additional annotations for each instance? -->
<!-- scope: telescope -->
expert created
#### Number of Raters
<!-- info: What is the number of raters -->
<!-- scope: telescope -->
2<n<10
#### Rater Qualifications
<!-- info: Describe the qualifications required of an annotator. -->
<!-- scope: periscope -->
Members of the TurkuNLP research group, native speakers of Finnish, each annotator has a strong background in language studies by having an academic degree or ongoing studies in a field related to languages or linguistics.
#### Raters per Training Example
<!-- info: How many annotators saw each training example? -->
<!-- scope: periscope -->
1
#### Raters per Test Example
<!-- info: How many annotators saw each test example? -->
<!-- scope: periscope -->
1
#### Annotation Service?
<!-- info: Was an annotation service used? -->
<!-- scope: telescope -->
no
#### Annotation Values
<!-- info: Purpose and values for each annotation -->
<!-- scope: microscope -->
1. Manual extraction of interesting paraphrases from two related documents.
2. Manual labeling of each extracted paraphrase based on the given annotation scheme, e.g. distinguishing contextual and universal paraphrases, marking style or strength differences, etc.
#### Any Quality Control?
<!-- info: Quality control measures? -->
<!-- scope: telescope -->
validated by another rater
#### Quality Control Details
<!-- info: Describe the quality control measures that were taken. -->
<!-- scope: microscope -->
Partial double annotation, double annotation batches are assigned regularly in order to monitor annotation consistency. In double annotation, one annotator first extracts the candidate paraphrases, and these candidates are assigned to two different annotators, who does the label annotation independently from each other. Afterwards, the label annotations are merged, and conflicting labels are resolved together with the whole annotation team.
### Consent
#### Any Consent Policy?
<!-- info: Was there a consent policy involved when gathering the data? -->
<!-- scope: telescope -->
yes
#### Consent Policy Details
<!-- info: What was the consent policy? -->
<!-- scope: microscope -->
The corpus is mostly based on public/open data. For other data sources (student material), the licensing was agreed with the data providers during the collection.
### Private Identifying Information (PII)
#### Contains PII?
<!-- quick -->
<!-- info: Does the source language data likely contain Personal Identifying Information about the data creators or subjects? -->
<!-- scope: telescope -->
likely
#### Categories of PII
<!-- info: What categories of PII are present or suspected in the data? -->
<!-- scope: periscope -->
`generic PII`
#### Any PII Identification?
<!-- info: Did the curators use any automatic/manual method to identify PII in the dataset? -->
<!-- scope: periscope -->
no identification
### Maintenance
#### Any Maintenance Plan?
<!-- info: Does the original dataset have a maintenance plan? -->
<!-- scope: telescope -->
no
## Broader Social Context
### Previous Work on the Social Impact of the Dataset
#### Usage of Models based on the Data
<!-- info: Are you aware of cases where models trained on the task featured in this dataset ore related tasks have been used in automated systems? -->
<!-- scope: telescope -->
no
### Impact on Under-Served Communities
#### Addresses needs of underserved Communities?
<!-- info: Does this dataset address the needs of communities that are traditionally underserved in language technology, and particularly language generation technology? Communities may be underserved for exemple because their language, language variety, or social or geographical context is underepresented in NLP and NLG resources (datasets and models). -->
<!-- scope: telescope -->
no
### Discussion of Biases
#### Any Documented Social Biases?
<!-- info: Are there documented social biases in the dataset? Biases in this context are variations in the ways members of different social categories are represented that can have harmful downstream consequences for members of the more disadvantaged group. -->
<!-- scope: telescope -->
no
## Considerations for Using the Data
### PII Risks and Liability
#### Potential PII Risk
<!-- info: Considering your answers to the PII part of the Data Curation Section, describe any potential privacy to the data subjects and creators risks when using the dataset. -->
<!-- scope: microscope -->
None
### Licenses
#### Copyright Restrictions on the Dataset
<!-- info: Based on your answers in the Intended Use part of the Data Overview Section, which of the following best describe the copyright and licensing status of the dataset? -->
<!-- scope: periscope -->
`open license - commercial use allowed`
#### Copyright Restrictions on the Language Data
<!-- info: Based on your answers in the Language part of the Data Curation Section, which of the following best describe the copyright and licensing status of the underlying language data? -->
<!-- scope: periscope -->
`open license - commercial use allowed`
### Known Technical Limitations
|
GEM/viggo | ---
annotations_creators:
- none
language_creators:
- unknown
language:
- en
license:
- cc-by-sa-4.0
multilinguality:
- unknown
size_categories:
- unknown
source_datasets:
- original
task_categories:
- table-to-text
task_ids: []
pretty_name: viggo
tags:
- data-to-text
---
# Dataset Card for GEM/viggo
## Dataset Description
- **Homepage:** https://nlds.soe.ucsc.edu/viggo
- **Repository:** [Needs More Information]
- **Paper:** https://aclanthology.org/W19-8623/
- **Leaderboard:** N/A
- **Point of Contact:** Juraj Juraska
### Link to Main Data Card
You can find the main data card on the [GEM Website](https://gem-benchmark.com/data_cards/viggo).
### Dataset Summary
ViGGO is an English data-to-text generation dataset in the video game domain, with target responses being more conversational than information-seeking, yet constrained to the information presented in a meaning representation. The dataset is relatively small with about 5,000 datasets but very clean, and can thus serve for evaluating transfer learning, low-resource, or few-shot capabilities of neural models.
You can load the dataset via:
```
import datasets
data = datasets.load_dataset('GEM/viggo')
```
The data loader can be found [here](https://huggingface.co/datasets/GEM/viggo).
#### website
[Wesbite](https://nlds.soe.ucsc.edu/viggo)
#### paper
[ACL Anthology](https://aclanthology.org/W19-8623/)
#### authors
Juraj Juraska, Kevin K. Bowden, Marilyn Walker
## Dataset Overview
### Where to find the Data and its Documentation
#### Webpage
<!-- info: What is the webpage for the dataset (if it exists)? -->
<!-- scope: telescope -->
[Wesbite](https://nlds.soe.ucsc.edu/viggo)
#### Paper
<!-- info: What is the link to the paper describing the dataset (open access preferred)? -->
<!-- scope: telescope -->
[ACL Anthology](https://aclanthology.org/W19-8623/)
#### BibTex
<!-- info: Provide the BibTex-formatted reference for the dataset. Please use the correct published version (ACL anthology, etc.) instead of google scholar created Bibtex. -->
<!-- scope: microscope -->
```
@inproceedings{juraska-etal-2019-viggo,
title = "{V}i{GGO}: A Video Game Corpus for Data-To-Text Generation in Open-Domain Conversation",
author = "Juraska, Juraj and
Bowden, Kevin and
Walker, Marilyn",
booktitle = "Proceedings of the 12th International Conference on Natural Language Generation",
month = oct # "{--}" # nov,
year = "2019",
address = "Tokyo, Japan",
publisher = "Association for Computational Linguistics",
url = "https://aclanthology.org/W19-8623",
doi = "10.18653/v1/W19-8623",
pages = "164--172",
}
```
#### Contact Name
<!-- quick -->
<!-- info: If known, provide the name of at least one person the reader can contact for questions about the dataset. -->
<!-- scope: periscope -->
Juraj Juraska
#### Contact Email
<!-- info: If known, provide the email of at least one person the reader can contact for questions about the dataset. -->
<!-- scope: periscope -->
jjuraska@ucsc.edu
#### Has a Leaderboard?
<!-- info: Does the dataset have an active leaderboard? -->
<!-- scope: telescope -->
no
### Languages and Intended Use
#### Multilingual?
<!-- quick -->
<!-- info: Is the dataset multilingual? -->
<!-- scope: telescope -->
no
#### Covered Languages
<!-- quick -->
<!-- info: What languages/dialects are covered in the dataset? -->
<!-- scope: telescope -->
`English`
#### License
<!-- quick -->
<!-- info: What is the license of the dataset? -->
<!-- scope: telescope -->
cc-by-sa-4.0: Creative Commons Attribution Share Alike 4.0 International
#### Intended Use
<!-- info: What is the intended use of the dataset? -->
<!-- scope: microscope -->
ViGGO was designed for the task of data-to-text generation in chatbots (as opposed to task-oriented dialogue systems), with target responses being more conversational than information-seeking, yet constrained to the information presented in a meaning representation. The dataset, being relatively small and clean, can also serve for demonstrating transfer learning capabilities of neural models.
#### Primary Task
<!-- info: What primary task does the dataset support? -->
<!-- scope: telescope -->
Data-to-Text
### Credit
#### Curation Organization Type(s)
<!-- info: In what kind of organization did the dataset curation happen? -->
<!-- scope: telescope -->
`academic`
#### Curation Organization(s)
<!-- info: Name the organization(s). -->
<!-- scope: periscope -->
University of California, Santa Cruz
#### Dataset Creators
<!-- info: Who created the original dataset? List the people involved in collecting the dataset and their affiliation(s). -->
<!-- scope: microscope -->
Juraj Juraska, Kevin K. Bowden, Marilyn Walker
#### Who added the Dataset to GEM?
<!-- info: Who contributed to the data card and adding the dataset to GEM? List the people+affiliations involved in creating this data card and who helped integrate this dataset into GEM. -->
<!-- scope: microscope -->
Juraj Juraska
### Dataset Structure
#### Data Fields
<!-- info: List and describe the fields present in the dataset. -->
<!-- scope: telescope -->
Each example in the dataset has the following two fields:
- `mr`: A meaning representation (MR) that, in a structured format, provides the information to convey, as well as the desired dialogue act (DA) type.
- `ref`: A reference output, i.e., a corresponding utterance realizing all the information in the MR.
Each MR is a flattened dictionary of attribute-and-value pairs, "wrapped" in the dialogue act type indication. This format was chosen primarily for its compactness, but also to allow for easy concatenation of multiple DAs (each with potentially different attributes) in a single MR.
Following is the list of all possible attributes (which are also refered to as "slots") in ViGGO along with their types/possible values:
- `name`: The name of a video game (e.g., Rise of the Tomb Raider).
- `release_year`: The year a video game was released in (e.g., 2015).
- `exp_release_date`: For a not-yet-released game, the date when it is expected to be released (e.g., February 22, 2019). *Note: This slot cannot appear together with `release_year` in the same dialogue act.*
- `developer`: The name of the studio/person that created the game (e.g., Crystal Dynamics).
- `genres`: A list of one or more genre labels from a set of possible values (e.g., action-adventure, shooter).
- `player_perspective`: A list of one or more perspectives from which the game is/can be played (possible values: first person, third person, side view, bird view).
- `platforms`: A list of one or more gaming platforms the game was officially released for (possible values: PC, PlayStation, Xbox, Nintendo, Nintendo Switch).
- `esrb`: A game's content rating as determined by the ESRB (possible values: E (for Everyone), E 10+ (for Everyone 10 and Older), T (for Teen), M (for Mature)).
- `rating`: Depending on the dialogue act this slot is used with, it is a categorical representation of either the game's average rating or the game's liking (possible values: excellent, good, average, poor).
- `has_multiplayer`: Indicates whether a game supports multiplayer or can only be played in single-player mode (possible values: yes, no).
- `available_on_steam`: Indicates whether a game can be purchased through the Steam digital distribution service (possible values: yes, no).
- `has_linux_release`: Indicates whether a game is supported on Linux operating systems (possible values: yes, no).
- `has_mac_release`: Indicates whether a game is supported on macOS (possible values: yes, no).
- `specifier`: A game specifier used by the `request` DA, typically an adjective (e.g., addictive, easiest, overrated, visually impressive).
Each MR in the dataset has 3 distinct reference utterances, which are represented as 3 separate examples with the same MR.
#### Reason for Structure
<!-- info: How was the dataset structure determined? -->
<!-- scope: microscope -->
The dataset structure mostly follows the format of the popular E2E dataset, however, with added dialogue act type indications, new list-type attributes introduced, and unified naming convention for multi-word attribute names.
#### Example Instance
<!-- info: Provide a JSON formatted example of a typical instance in the dataset. -->
<!-- scope: periscope -->
```
{
"mr": "give_opinion(name[SpellForce 3], rating[poor], genres[real-time strategy, role-playing], player_perspective[bird view])",
"ref": "I think that SpellForce 3 is one of the worst games I've ever played. Trying to combine the real-time strategy and role-playing genres just doesn't work, and the bird view perspective makes it near impossible to play."
}
```
#### Data Splits
<!-- info: Describe and name the splits in the dataset if there are more than one. -->
<!-- scope: periscope -->
ViGGO is split into 3 partitions, with no MRs in common between the training set and either of the validation and the test set (and that *after* delexicalizing the `name` and `developer` slots). The ratio of examples in the partitions is approximately 7.5 : 1 : 1.5, with their exact sizes listed below:
- **Train:** 5,103 (1,675 unique MRs)
- **Validation:** 714 (238 unique MRs)
- **Test:** 1,083 (359 unique MRs)
- **TOTAL:** 6,900 (2,253 unique MRs)
*Note: The reason why the number of unique MRs is not exactly one third of all examples is that for each `request_attribute` DA (which only has one slot, and that without a value) 12 reference utterances were collected instead of 3.*
#### Splitting Criteria
<!-- info: Describe any criteria for splitting the data, if used. If there are differences between the splits (e.g., if the training annotations are machine-generated and the dev and test ones are created by humans, or if different numbers of annotators contributed to each example), describe them here. -->
<!-- scope: microscope -->
A similar MR length and slot distribution was preserved across the partitions. The distribution of DA types, on the other hand, is skewed slightly toward fewer `inform` DA instances (the most prevalent DA type) and a higher proportion of the less prevalent DAs in the validation and the test set.
####
<!-- info: What does an outlier of the dataset in terms of length/perplexity/embedding look like? -->
<!-- scope: microscope -->
```
{
"mr": "request_attribute(player_perspective[])",
"ref": "Is there a certain player perspective that you prefer over others in games you play?"
},
{
"mr": "inform(name[FIFA 12], esrb[E (for Everyone)], genres[simulation, sport], player_perspective[bird view, side view], platforms[PlayStation, Xbox, Nintendo, PC], available_on_steam[no])",
"ref": "Fifa 12 is a decent sports simulator. It's pretty cool how the game swaps from the bird's eye perspective down to a side view while you're playing. You can get the game for PlayStation, Xbox, Nintendo consoles, and PC, but unfortunately it's not on Steam. Of course, as a sports game there's not much objectionable content so it's rated E."
},
{
"mr": "inform(name[Super Bomberman], release_year[1993], genres[action, strategy], has_multiplayer[no], platforms[Nintendo, PC], available_on_steam[no], has_linux_release[no], has_mac_release[no])",
"ref": "Super Bomberman is one of my favorite Nintendo games, also available on PC, though not through Steam. It came out all the way back in 1993, and you can't get it for any modern consoles, unfortunately, so no online multiplayer, or of course Linux or Mac releases either. That said, it's still one of the most addicting action-strategy games out there."
}
```
## Dataset in GEM
### Rationale for Inclusion in GEM
#### Why is the Dataset in GEM?
<!-- info: What does this dataset contribute toward better generation evaluation and why is it part of GEM? -->
<!-- scope: microscope -->
ViGGO is a fairly small dataset but includes a greater variety of utterance types than most other datasets for NLG from structured meaning representations. This makes it more interesting from the perspective of model evaluation, since models have to learn to differentiate between various dialogue act types that share the same slots.
#### Similar Datasets
<!-- info: Do other datasets for the high level task exist? -->
<!-- scope: telescope -->
yes
#### Unique Language Coverage
<!-- info: Does this dataset cover other languages than other datasets for the same task? -->
<!-- scope: periscope -->
no
#### Difference from other GEM datasets
<!-- info: What else sets this dataset apart from other similar datasets in GEM? -->
<!-- scope: microscope -->
ViGGO's language is more casual and conversational -- as opposed to information-seeking -- which differentiates it from the majority of popular datasets for the same type of data-to-text task. Moreover, the video game domain is a rather uncommon one in the NLG community, despite being very well-suited for data-to-text generation, considering it offers entities with many attributes to talk about, which can be described in a structured format.
### GEM-Specific Curation
#### Modificatied for GEM?
<!-- info: Has the GEM version of the dataset been modified in any way (data, processing, splits) from the original curated data? -->
<!-- scope: telescope -->
no
#### Additional Splits?
<!-- info: Does GEM provide additional splits to the dataset? -->
<!-- scope: telescope -->
no
### Getting Started with the Task
#### Pointers to Resources
<!-- info: Getting started with in-depth research on the task. Add relevant pointers to resources that researchers can consult when they want to get started digging deeper into the task. -->
<!-- scope: microscope -->
- [E2E NLG Challenge](http://www.macs.hw.ac.uk/InteractionLab/E2E/)
#### Technical Terms
<!-- info: Technical terms used in this card and the dataset and their definitions -->
<!-- scope: microscope -->
- MR = meaning representation
- DA = dialogue act
## Previous Results
### Previous Results
#### Metrics
<!-- info: What metrics are typically used for this task? -->
<!-- scope: periscope -->
`BLEU`, `METEOR`, `ROUGE`, `BERT-Score`, `BLEURT`, `Other: Other Metrics`
#### Other Metrics
<!-- info: Definitions of other metrics -->
<!-- scope: periscope -->
SER (slot error rate): Indicates the proportion of missing/incorrect/duplicate/hallucinated slot mentions in the utterances across a test set. The closer to zero a model scores in this metric, the more semantically accurate its outputs are. This metric is typically calculated either manually on a small sample of generated outputs, or heuristically using domain-specific regex rules and gazetteers.
#### Previous results available?
<!-- info: Are previous results available? -->
<!-- scope: telescope -->
yes
#### Relevant Previous Results
<!-- info: What are the most relevant previous results for this task/dataset? -->
<!-- scope: microscope -->
- [Juraska et al., 2019. ViGGO: A Video Game Corpus for Data-To-Text Generation in Open-Domain Conversation.](https://aclanthology.org/W19-8623/)
- [Harkous et al., 2020. Have Your Text and Use It Too! End-to-End Neural Data-to-Text Generation with Semantic Fidelity.](https://aclanthology.org/2020.coling-main.218/)
- [Kedzie and McKeown, 2020. Controllable Meaning Representation to Text Generation: Linearization and Data Augmentation Strategies.](https://aclanthology.org/2020.emnlp-main.419/)
- [Juraska and Walker, 2021. Attention Is Indeed All You Need: Semantically Attention-Guided Decoding for Data-to-Text NLG.](https://aclanthology.org/2021.inlg-1.45/)
## Dataset Curation
### Original Curation
#### Original Curation Rationale
<!-- info: Original curation rationale -->
<!-- scope: telescope -->
The primary motivation behind ViGGO was to create a data-to-text corpus in a new but conversational domain, and intended for use in open-domain chatbots rather than task-oriented dialogue systems. To this end, the dataset contains utterances of 9 generalizable and conversational dialogue act types, revolving around various aspects of video games. The idea is that similar, relatively small datasets could fairly easily be collected for other conversational domains -- especially other entertainment domains (such as music or books), but perhaps also topics like animals or food -- to support an open-domain conversational agent with controllable neural NLG.
Another desired quality of the ViGGO dataset was cleanliness (no typos and grammatical errors) and semantic accuracy, which has often not been the case with other crowdsourced data-to-text corpora. In general, for the data-to-text generation task, there is arguably no need to put the burden on the generation model to figure out the noise, since the noise would not be expected to be there in a real-world system whose dialogue manager that creates the input for the NLG module is usually configurable and tightly controlled.
#### Communicative Goal
<!-- info: What was the communicative goal? -->
<!-- scope: periscope -->
Produce a response from a structured meaning representation in the context of a conversation about video games. It can be a brief opinion or a description of a game, as well as a request for attribute (e.g., genre, player perspective, or platform) preference/confirmation or an inquiry about liking a particular type of games.
#### Sourced from Different Sources
<!-- info: Is the dataset aggregated from different data sources? -->
<!-- scope: telescope -->
no
### Language Data
#### How was Language Data Obtained?
<!-- info: How was the language data obtained? -->
<!-- scope: telescope -->
`Crowdsourced`
#### Where was it crowdsourced?
<!-- info: If crowdsourced, where from? -->
<!-- scope: periscope -->
`Amazon Mechanical Turk`
#### Language Producers
<!-- info: What further information do we have on the language producers? -->
<!-- scope: microscope -->
The paid crowdworkers who produced the reference utterances were from English-speaking countries, and they had at least 1,000 HITs approved and a HIT approval rate of 98% or more. Furthermore, in the instructions, crowdworkers were discouraged from taking on the task unless they considered themselves a gamer.
#### Topics Covered
<!-- info: Does the language in the dataset focus on specific topics? How would you describe them? -->
<!-- scope: periscope -->
The dataset focuses on video games and their various aspects, and hence the language of the utterances may contain video game-specific jargon.
#### Data Validation
<!-- info: Was the text validated by a different worker or a data curator? -->
<!-- scope: telescope -->
validated by data curator
#### Data Preprocessing
<!-- info: How was the text data pre-processed? (Enter N/A if the text was not pre-processed) -->
<!-- scope: microscope -->
First, regular expressions were used to enforce several standardization policies regarding special characters, punctuation, and the correction of undesired abbreviations/misspellings of standard domain-specific terms (e.g., terms like "Play station" or "PS4" would be changed to the uniform "PlayStation"). At the same time, hyphens were removed or enforced uniformly in certain terms, for example, "single-player". Although phrases such as "first person" should correctly have a hyphen when used as adjective, the crowdworkers used this rule very inconsistently. In order to avoid model outputs being penalized during the evaluation by the arbitrary choice of a hyphen presence or absence in the reference utterances, the hyphen was removed in all such phrases regardless of the noun vs. adjective use.
Second, an extensive set of heuristics was developed to identify slot-related errors. This process revealed the vast majority of missing or incorrect slot mentions, which were subsequently fixed according to the corresponding MRs. This eventually led to the development of a robust, cross-domain, heuristic slot aligner that can be used for automatic slot error rate evaluation. For details, see the appendix in [Juraska and Walker, 2021](https://aclanthology.org/2021.inlg-1.45/).
Crowdworkers would sometimes also inject a piece of information which was not present in the MR, some of which is not even represented by any of the slots, e.g., plot or main characters. This unsolicited information was removed from the utterances so as to avoid confusing the neural model. Finally, any remaining typos and grammatical errors were resolved.
#### Was Data Filtered?
<!-- info: Were text instances selected or filtered? -->
<!-- scope: telescope -->
manually
#### Filter Criteria
<!-- info: What were the selection criteria? -->
<!-- scope: microscope -->
Compliance with the indicated dialogue act type, semantic accuracy (i.e., all information in the corresponding MR mentioned and that correctly), and minimal extraneous information (e.g., personal experience/opinion). Whenever it was within a reasonable amount of effort, the utterances were manually fixed instead of being discarded/crowdsourced anew.
### Structured Annotations
#### Additional Annotations?
<!-- quick -->
<!-- info: Does the dataset have additional annotations for each instance? -->
<!-- scope: telescope -->
none
#### Annotation Service?
<!-- info: Was an annotation service used? -->
<!-- scope: telescope -->
no
### Consent
#### Any Consent Policy?
<!-- info: Was there a consent policy involved when gathering the data? -->
<!-- scope: telescope -->
no
### Private Identifying Information (PII)
#### Contains PII?
<!-- quick -->
<!-- info: Does the source language data likely contain Personal Identifying Information about the data creators or subjects? -->
<!-- scope: telescope -->
no PII
#### Justification for no PII
<!-- info: Provide a justification for selecting `no PII` above. -->
<!-- scope: periscope -->
Crowdworkers were instructed to only express the information in the provided meaning representation, which never prompted them to mention anything about themselves. Occasionally, they would still include a bit of personal experience (e.g., "I used to like the game as a kid.") or opinion, but these would be too general to be considered PII.
### Maintenance
#### Any Maintenance Plan?
<!-- info: Does the original dataset have a maintenance plan? -->
<!-- scope: telescope -->
no
## Broader Social Context
### Previous Work on the Social Impact of the Dataset
#### Usage of Models based on the Data
<!-- info: Are you aware of cases where models trained on the task featured in this dataset ore related tasks have been used in automated systems? -->
<!-- scope: telescope -->
no
### Impact on Under-Served Communities
#### Addresses needs of underserved Communities?
<!-- info: Does this dataset address the needs of communities that are traditionally underserved in language technology, and particularly language generation technology? Communities may be underserved for exemple because their language, language variety, or social or geographical context is underepresented in NLP and NLG resources (datasets and models). -->
<!-- scope: telescope -->
no
### Discussion of Biases
#### Any Documented Social Biases?
<!-- info: Are there documented social biases in the dataset? Biases in this context are variations in the ways members of different social categories are represented that can have harmful downstream consequences for members of the more disadvantaged group. -->
<!-- scope: telescope -->
no
## Considerations for Using the Data
### PII Risks and Liability
### Licenses
### Known Technical Limitations
#### Technical Limitations
<!-- info: Describe any known technical limitations, such as spurrious correlations, train/test overlap, annotation biases, or mis-annotations, and cite the works that first identified these limitations when possible. -->
<!-- scope: microscope -->
The dataset is limited to a single domain: video games. One caveat of using a language generator trained on this dataset in a dialogue system as-is is that multiple subsequent turns discussing the same video game would be repeating its full name. ViGGO was designed for generation without context, and therefore it is up to the dialogue manager to ensure that pronouns are substituted for the names whenever it would sound more natural in a dialogue. Alternately, the dataset can easily be augmented with automatically constructed samples which omit the `name` slot in the MR and replace the name with a pronoun in the reference utterance.
|
GEM/web_nlg | ---
annotations_creators:
- unknown
language_creators:
- unknown
language:
- en
license:
- cc-by-nc-4.0
multilinguality:
- unknown
size_categories:
- unknown
source_datasets:
- original
task_categories:
- table-to-text
task_ids: []
pretty_name: web_nlg
tags:
- data-to-text
---
# Dataset Card for GEM/web_nlg
## Dataset Description
- **Homepage:** https://webnlg-challenge.loria.fr/
- **Repository:** https://gitlab.com/shimorina/webnlg-dataset
- **Paper:** http://www.aclweb.org/anthology/P17-1017, [WebNLG Challenge 2017 Report
- **Leaderboard:** https://beng.dice-research.org/gerbil/
- **Point of Contact:** [Needs More Information]
### Link to Main Data Card
You can find the main data card on the [GEM Website](https://gem-benchmark.com/data_cards/web_nlg).
### Dataset Summary
WebNLG is a bi-lingual dataset (English, Russian) of parallel DBpedia triple sets and short texts that cover about 450 different DBpedia properties. The WebNLG data was originally created to promote the development of RDF verbalisers able to generate short text and to handle micro-planning (i.e., sentence segmentation and ordering, referring expression generation, aggregation); the goal of the task is to generate texts starting from 1 to 7 input triples which have entities in common (so the input is actually a connected Knowledge Graph). The dataset contains about 17,000 triple sets and 45,000 crowdsourced texts in English, and 7,000 triples sets and 19,000 crowdsourced texts in Russian. A challenging test set section with entities and/or properties that have not been seen at training time is available.
You can load the dataset via:
```
import datasets
data = datasets.load_dataset('GEM/web_nlg')
```
The data loader can be found [here](https://huggingface.co/datasets/GEM/web_nlg).
#### website
[Website](https://webnlg-challenge.loria.fr/)
#### paper
[First Dataset Release](http://www.aclweb.org/anthology/P17-1017), [WebNLG Challenge 2017 Report](https://www.aclweb.org/anthology/W17-3518/), [WebNLG Challenge 2020 Report](https://webnlg-challenge.loria.fr/files/2020.webnlg-papers.7.pdf)
#### authors
The principle curator of the dataset is Anastasia Shimorina (Université de Lorraine / LORIA, France). Throughout the WebNLG releases, several people contributed to their construction: Claire Gardent (CNRS / LORIA, France), Shashi Narayan (Google, UK), Laura Perez-Beltrachini (University of Edinburgh, UK), Elena Khasanova, and Thiago Castro Ferreira (Federal University of Minas Gerais, Brazil).
## Dataset Overview
### Where to find the Data and its Documentation
#### Webpage
<!-- info: What is the webpage for the dataset (if it exists)? -->
<!-- scope: telescope -->
[Website](https://webnlg-challenge.loria.fr/)
#### Download
<!-- info: What is the link to where the original dataset is hosted? -->
<!-- scope: telescope -->
[Gitlab](https://gitlab.com/shimorina/webnlg-dataset)
#### Paper
<!-- info: What is the link to the paper describing the dataset (open access preferred)? -->
<!-- scope: telescope -->
[First Dataset Release](http://www.aclweb.org/anthology/P17-1017), [WebNLG Challenge 2017 Report](https://www.aclweb.org/anthology/W17-3518/), [WebNLG Challenge 2020 Report](https://webnlg-challenge.loria.fr/files/2020.webnlg-papers.7.pdf)
#### BibTex
<!-- info: Provide the BibTex-formatted reference for the dataset. Please use the correct published version (ACL anthology, etc.) instead of google scholar created Bibtex. -->
<!-- scope: microscope -->
Initial release of the dataset:
```
@inproceedings{gardent2017creating,
author = "Gardent, Claire
and Shimorina, Anastasia
and Narayan, Shashi
and Perez-Beltrachini, Laura",
title = "Creating Training Corpora for NLG Micro-Planners",
booktitle = "Proceedings of the 55th Annual Meeting of the Association for Computational Linguistics (Volume 1: Long Papers)",
year = "2017",
publisher = "Association for Computational Linguistics",
pages = "179--188",
location = "Vancouver, Canada",
doi = "10.18653/v1/P17-1017",
url = "http://www.aclweb.org/anthology/P17-1017"
}
```
The latest version 3.0:
```
@inproceedings{castro-ferreira20:bilin-bi-direc-webnl-shared,
title={The 2020 Bilingual, Bi-Directional WebNLG+ Shared Task Overview and Evaluation Results (WebNLG+ 2020)},
author={Castro Ferreira, Thiago and
Gardent, Claire and
Ilinykh, Nikolai and
van der Lee, Chris and
Mille, Simon and
Moussallem, Diego and
Shimorina, Anastasia},
booktitle = {Proceedings of the 3rd WebNLG Workshop on Natural Language Generation from the Semantic Web (WebNLG+ 2020)},
pages = "55--76",
year = 2020,
address = {Dublin, Ireland (Virtual)},
publisher = {Association for Computational Linguistics}}
```
#### Contact Email
<!-- info: If known, provide the email of at least one person the reader can contact for questions about the dataset. -->
<!-- scope: periscope -->
webnlg-challenge@inria.fr
#### Has a Leaderboard?
<!-- info: Does the dataset have an active leaderboard? -->
<!-- scope: telescope -->
yes
#### Leaderboard Link
<!-- info: Provide a link to the leaderboard. -->
<!-- scope: periscope -->
[Website](https://beng.dice-research.org/gerbil/)
#### Leaderboard Details
<!-- info: Briefly describe how the leaderboard evaluates models. -->
<!-- scope: microscope -->
The model outputs are evaluated against the crowdsourced references; the leaderboard reports BLEU-4, METEOR, chrF++, TER, BERTScore and BLEURT scores.
### Languages and Intended Use
#### Multilingual?
<!-- quick -->
<!-- info: Is the dataset multilingual? -->
<!-- scope: telescope -->
yes
#### Covered Languages
<!-- quick -->
<!-- info: What languages/dialects are covered in the dataset? -->
<!-- scope: telescope -->
`Russian`, `English`
#### License
<!-- quick -->
<!-- info: What is the license of the dataset? -->
<!-- scope: telescope -->
cc-by-nc-4.0: Creative Commons Attribution Non Commercial 4.0 International
#### Intended Use
<!-- info: What is the intended use of the dataset? -->
<!-- scope: microscope -->
The WebNLG dataset was created to promote the development (_i_) of RDF verbalisers and (_ii_) of microplanners able to handle a wide range of linguistic constructions. The dataset aims at covering knowledge in different domains ("categories"). The same properties and entities can appear in several categories.
#### Primary Task
<!-- info: What primary task does the dataset support? -->
<!-- scope: telescope -->
Data-to-Text
#### Communicative Goal
<!-- quick -->
<!-- info: Provide a short description of the communicative goal of a model trained for this task on this dataset. -->
<!-- scope: periscope -->
A model should verbalize all and only the provided input triples in natural language.
### Credit
#### Curation Organization Type(s)
<!-- info: In what kind of organization did the dataset curation happen? -->
<!-- scope: telescope -->
`academic`
#### Curation Organization(s)
<!-- info: Name the organization(s). -->
<!-- scope: periscope -->
Université de Lorraine / LORIA, France, CNRS / LORIA, France, University of Edinburgh, UK, Federal University of Minas Gerais, Brazil
#### Dataset Creators
<!-- info: Who created the original dataset? List the people involved in collecting the dataset and their affiliation(s). -->
<!-- scope: microscope -->
The principle curator of the dataset is Anastasia Shimorina (Université de Lorraine / LORIA, France). Throughout the WebNLG releases, several people contributed to their construction: Claire Gardent (CNRS / LORIA, France), Shashi Narayan (Google, UK), Laura Perez-Beltrachini (University of Edinburgh, UK), Elena Khasanova, and Thiago Castro Ferreira (Federal University of Minas Gerais, Brazil).
#### Funding
<!-- info: Who funded the data creation? -->
<!-- scope: microscope -->
The dataset construction was funded by the French National Research Agency (ANR).
#### Who added the Dataset to GEM?
<!-- info: Who contributed to the data card and adding the dataset to GEM? List the people+affiliations involved in creating this data card and who helped integrate this dataset into GEM. -->
<!-- scope: microscope -->
Simon Mille and Sebastian Gehrmann added the dataset and wrote the data card.
### Dataset Structure
#### Data Fields
<!-- info: List and describe the fields present in the dataset. -->
<!-- scope: telescope -->
See [official documentation](https://webnlg-challenge.loria.fr/docs/).
`entry`: a data instance of the benchmark. Each entry has five attributes: a DBpedia category (`category`), entry ID (`eid`), shape, shape type, and triple set size (`size`).
- `shape`: a string representation of the RDF tree with nested parentheses where `X` is a node (see [Newick tree format](https://en.wikipedia.org/wiki/Newick_format)).
- `shape_type`: a type of the tree shape. We [identify](https://www.aclweb.org/anthology/C16-1141.pdf) three types of tree shapes:
* `chain` (the object of one triple is the subject of the other);
* `sibling` (triples with a shared subject);
* `mixed` (both `chain` and `sibling` types present).
- `eid`: an entry ID. It is unique only within a category and a size.
- `category`: a DBpedia category (Astronaut, City, MusicalWork, Politician, etc.).
- `size`: the number of RDF triples in a set. Ranges from 1 to 7.
Each `entry` has three fields: `originaltripleset`, `modifiedtripleset`, and `lexs`.
`originaltripleset`: a set of RDF triples as extracted from [DBpedia](https://wiki.dbpedia.org/). Each set of RDF triples is a tree. Triples have the subject-predicate-object structure.
`modifiedtripleset`: a set of RDF triples as presented to crowdworkers (for more details on modifications, see below).
Original and modified triples serve different purposes: the original triples — to link data to a knowledge base (DBpedia), whereas the modified triples — to ensure consistency and homogeneity throughout the data. To train models, the modified triples should be used.
`lexs` (shortened for lexicalisations): a natural language text verbalising the triples. Each lexicalisation has two attributes: a comment (`comment`), and a lexicalisation ID (`lid`). By default, comments have the value `good`, except rare cases when they were manually marked as `toFix`. That was done during the corpus creation, when it was seen that a lexicalisation did not exactly match a triple set.
Russian data has additional optional fields comparing to English:
`<dbpedialinks>`: RDF triples extracted from DBpedia between English and Russian entities by means of the property `sameAs`.
`<links>`: RDF triples created manually for some entities to serve as pointers to translators. There are two types of them:
* with `sameAs` (`Spaniards | sameAs | испанцы`)
* with `includes` (`Tomatoes, guanciale, cheese, olive oil | includes | гуанчиале`). Those were mostly created for string literals to translate some parts of them.
Lexicalisations in the Russian WebNLG have a new parameter `lang` (values: `en`, `ru`) because original English texts were kept in the Russian version (see the example above).
#### Example Instance
<!-- info: Provide a JSON formatted example of a typical instance in the dataset. -->
<!-- scope: periscope -->
```
{
"entry": {
"category": "Company",
"size": "4",
"shape": "(X (X) (X) (X) (X))",
"shape_type": "sibling",
"eid": "Id21",
"lexs": [
{
"comment": "good",
"lex": "Trane, which was founded on January 1st 1913 in La Crosse, Wisconsin, is based in Ireland. It has 29,000 employees.",
"lid": "Id1"
}
],
"modifiedtripleset": [
{
"subject": "Trane",
"property": "foundingDate",
"object": "1913-01-01"
},
{
"subject": "Trane",
"property": "location",
"object": "Ireland"
},
{
"subject": "Trane",
"property": "foundationPlace",
"object": "La_Crosse,_Wisconsin"
},
{
"subject": "Trane",
"property": "numberOfEmployees",
"object": "29000"
}
],
"originaltriplesets": {
"originaltripleset": [
{
"subject": "Trane",
"property": "foundingDate",
"object": "1913-01-01"
},
{
"subject": "Trane",
"property": "location",
"object": "Ireland"
},
{
"subject": "Trane",
"property": "foundationPlace",
"object": "La_Crosse,_Wisconsin"
},
{
"subject": "Trane",
"property": "numberOfEmployees",
"object": "29000"
}
]
}
}
}
```
The XML-formatted example is [here](https://webnlg-challenge.loria.fr/docs/#example).
#### Data Splits
<!-- info: Describe and name the splits in the dataset if there are more than one. -->
<!-- scope: periscope -->
| English (v3.0) | Train | Dev | Test |
|-----------------|--------|-------|-------|
| **triple sets** | 13,211 | 1,667 | 1,779 |
| **texts** | 35,426 | 4,464 | 5,150 |
|**properties** | 372 | 290 | 220 |
| Russian (v3.0) | Train | Dev | Test |
|-----------------|--------|-------|-------|
| **triple sets** | 5,573 | 790 | 1,102 |
| **texts** | 14,239 | 2,026 | 2,780 |
|**properties** | 226 | 115 | 192 |
## Dataset in GEM
### Rationale for Inclusion in GEM
#### Why is the Dataset in GEM?
<!-- info: What does this dataset contribute toward better generation evaluation and why is it part of GEM? -->
<!-- scope: microscope -->
Due to the constrained generation task, this dataset can be used to evaluate very specific and narrow generation capabilities.
#### Similar Datasets
<!-- info: Do other datasets for the high level task exist? -->
<!-- scope: telescope -->
yes
#### Unique Language Coverage
<!-- info: Does this dataset cover other languages than other datasets for the same task? -->
<!-- scope: periscope -->
yes
#### Difference from other GEM datasets
<!-- info: What else sets this dataset apart from other similar datasets in GEM? -->
<!-- scope: microscope -->
The RDF-triple format is unique to WebNLG.
#### Ability that the Dataset measures
<!-- info: What aspect of model ability can be measured with this dataset? -->
<!-- scope: periscope -->
surface realization
### GEM-Specific Curation
#### Modificatied for GEM?
<!-- info: Has the GEM version of the dataset been modified in any way (data, processing, splits) from the original curated data? -->
<!-- scope: telescope -->
yes
#### GEM Modifications
<!-- info: What changes have been made to he original dataset? -->
<!-- scope: periscope -->
`other`
#### Modification Details
<!-- info: For each of these changes, described them in more details and provided the intended purpose of the modification -->
<!-- scope: microscope -->
No changes to the main content of the dataset. The [version 3.0](https://gitlab.com/shimorina/webnlg-dataset/-/tree/master/release_v3.0) of the dataset is used.
#### Additional Splits?
<!-- info: Does GEM provide additional splits to the dataset? -->
<!-- scope: telescope -->
yes
#### Split Information
<!-- info: Describe how the new splits were created -->
<!-- scope: periscope -->
23 special test sets for WebNLG were added to the GEM evaluation suite, 12 for English and 11 for Russian.
For both languages, we created subsets of the training and development sets of ~500 randomly selected inputs each. The inputs were sampled proportionally from each category.
Two types of transformations have been applied to WebNLG: (i) input scrambling (English and Russian) and (ii) numerical value replacements (English); in both cases, a subset of about 500 inputs was randomly selected. For (i), the order of the triples was randomly reassigned (each triple kept the same Subject-Property-Object internal order). For (ii), the change was performed respecting the format of the current cardinal value (e.g., alpha, integer, or floating-point) and replacing it with a new random value. The new number is lower-bounded between zero and upper bounded to be within to the highest power of 10 unit for the given value (e.g., replacing 54 would result in a random value between 0-100). Floating values maintain the degree of precision.
For both languages, we did identify different subsets of the test set that we could compare to each other so that we would have a better understanding of the results. There are currently 8 selections that we have made:
Selection 1 (size): input length. This selection corresponds to the number of predicates in the input. By comparing inputs of different lengths, we can see to what extent NLG systems are able to handle different input sizes. The table below provides the relevant frequencies. Please be aware that comparing selections with fewer than 100 items may result in unreliable comparisons.
| Input length | Frequency English | Frequency Russian |
|----------------|-------------------|-------------------|
| 1 | 369 | 254 |
| 2 | 349 | 200 |
| 3 | 350 | 214 |
| 4 | 305 | 214 |
| 5 | 213 | 159 |
| 6 | 114 | 32 |
| 7 | 79 | 29 |
Selection 2 (frequency): seen/unseen single predicates. This selection corresponds to the inputs with only one predicate. We compare which predicates are seen/unseen in the training data. The table below provides the relevant frequencies. Note that the comparison is only valid for English. Not for Russian, since there is only one example of unseen single predicates.
| _ in training | Frequency English | Frequency Russian |
|---------------|-------------------|-------------------|
| Seen | 297 | 253 |
| Unseen | 72 | 1 |
Selection 3 (frequency): seen/unseen combinations of predicates. This selection checks for all combinations of predicates whether that combination has been seen in the training data. For example: if the combination of predicates A and B is seen, that means that there is an input in the training data consisting of two triples, where one triple uses predicate A and the other uses predicate B. If the combination is unseen, then the converse is true. The table below provides the relevant frequencies.
| _ in training | Frequency English | Frequency Russian |
|---------------|-------------------|-------------------|
| unseen | 1295 | 354 |
| seen | 115 | 494 |
Selection 4 (frequency): seen/unseen arguments. This selection checks for all input whether or not all arg1s and arg2s in the input have been seen during the training phase. For this selection, *Seen* is the default. Only if all arg1 instances for a particular input are unseen, do we count the arg1s of the input as unseen. The same holds for arg2. So "seen" here really means that at least some of the arg1s or arg2s are seen in the input. The table below provides the relevant frequencies. Note that the comparison is only valid for English. Not for Russian, since there are very few examples of unseen combinations of predicates.
| Arguments seen in training? | Frequency English | Frequency Russian |
|-----------------------------|-------------------|-------------------|
| both_seen | 518 | 1075 |
| both_unseen | 1177 | 4 |
| arg1_unseen | 56 | 19 |
| arg2_unseen | 28 | 4 |
Selection 5 (shape): repeated subjects. For this selection, the subsets are based on the times a subject is repeated in the input; it only takes into account the maximum number of times a subject is repeated, that is, if in one input a subject appears 3 times and a different subject 2 times, this input will be in the "3_subjects_same' split. Unique_subjects means all subjects are different.
| Max num. of repeated subjects | Frequency English | Frequency Russian |
|-------------------------------|-------------------|-------------------|
| unique_subjects | 453 | 339 |
| 2_subjects_same | 414 | 316 |
| 3_subjects_same | 382 | 217 |
| 4_subjects_same | 251 | 143 |
| 5_subjects_same | 158 | 56 |
| 6_subjects_same | 80 | 19 |
| 7_subjects_same | 41 | 12 |
Selection 6 (shape): repeated objects. Same as for subjects above, but for objects. There are much less cases of repeated objects, so there are only two categories for this selection, unique_objects and some_objects_repeated; for the latter, we have up to 3 coreferring objects in English, and XXX in Russian.
| Max num. of repeated objects | Frequency English | Frequency Russian |
|------------------------------|-------------------|-------------------|
| unique_objects | 1654 | 1099 |
| some_objects_same | 125 | 3 |
Selection 7 (shape): repeated properties. Same as for objects above, but for properties; up to two properties can be the same in English, up to XXX in Russian.
| Max num. of repeated properties | Frequency English | Frequency Russian |
|---------------------------------|-------------------|-------------------|
| unique_properties | 1510 | 986 |
| some_properties_same | 269 | 116 |
Selection 8 (shape): entities that appear both as subject and object. For this selection, we grouped together the inputs in which no entity is found as both subject and object, and on the other side inputs in which one or more entity/ies appear both as subject and as object. We found up to two such entities per input in English, and up to XXX in Russian.
| Max num. of objects and subjects in common | Frequency English | Frequency Russian |
|--------------------------------------------|-------------------|-------------------|
| unique_properties | 1322 | 642 |
| some_properties_same | 457 | 460 |
#### Split Motivation
<!-- info: What aspects of the model's generation capacities were the splits created to test? -->
<!-- scope: periscope -->
Robustness
### Getting Started with the Task
#### Pointers to Resources
<!-- info: Getting started with in-depth research on the task. Add relevant pointers to resources that researchers can consult when they want to get started digging deeper into the task. -->
<!-- scope: microscope -->
Dataset construction: [main dataset paper](https://www.aclweb.org/anthology/P17-1017/), [RDF triple extraction](https://www.aclweb.org/anthology/C16-1141/), [Russian translation](https://www.aclweb.org/anthology/W19-3706/)
WebNLG Challenge 2017: [webpage](https://webnlg-challenge.loria.fr/challenge_2017/), [paper](https://www.aclweb.org/anthology/W17-3518/)
WebNLG Challenge 2020: [webpage](https://webnlg-challenge.loria.fr/challenge_2020/), [paper](https://webnlg-challenge.loria.fr/files/2020.webnlg-papers.7.pdf)
Enriched version of WebNLG: [repository](https://github.com/ThiagoCF05/webnlg), [paper](https://www.aclweb.org/anthology/W18-6521/)
Related research papers: [webpage](https://webnlg-challenge.loria.fr/research/)
## Previous Results
### Previous Results
#### Proposed Evaluation
<!-- info: List and describe the purpose of the metrics and evaluation methodology (including human evaluation) that the dataset creators used when introducing this task. -->
<!-- scope: microscope -->
For both languages, the participating systems are automatically evaluated in a multi-reference scenario. Each English hypothesis is compared to a maximum of 5 references, and each Russian one to a maximum of 7 references. On average, English data has 2.89 references per test instance, and Russian data has 2.52 references per instance.
In a human evaluation, example are uniformly sampled across size of triple sets and the following dimensions are assessed (on MTurk and Yandex.Toloka):
1. Data Coverage: Does the text include descriptions of all predicates presented in the data?
2. Relevance: Does the text describe only such predicates (with related subjects and objects), which are found in the data?
3. Correctness: When describing predicates which are found in the data, does the text mention correct the objects and adequately introduces the subject for this specific predicate?
4. Text Structure: Is the text grammatical, well-structured, written in acceptable English language?
5. Fluency: Is it possible to say that the text progresses naturally, forms a coherent whole and it is easy to understand the text?
For additional information like the instructions, we refer to the original paper.
#### Previous results available?
<!-- info: Are previous results available? -->
<!-- scope: telescope -->
yes
#### Other Evaluation Approaches
<!-- info: What evaluation approaches have others used? -->
<!-- scope: periscope -->
We evaluated a wide range of models as part of the GEM benchmark.
#### Relevant Previous Results
<!-- info: What are the most relevant previous results for this task/dataset? -->
<!-- scope: microscope -->
Results can be found on the [GEM website](https://gem-benchmark.com/results).
## Broader Social Context
### Previous Work on the Social Impact of the Dataset
#### Usage of Models based on the Data
<!-- info: Are you aware of cases where models trained on the task featured in this dataset ore related tasks have been used in automated systems? -->
<!-- scope: telescope -->
yes - related tasks
#### Social Impact Observations
<!-- info: Did any of these previous uses result in observations about the social impact of the systems? In particular, has there been work outlining the risks and limitations of the system? Provide links and descriptions here. -->
<!-- scope: microscope -->
We do not foresee any negative social impact in particular from this dataset or task.
Positive outlooks: Being able to generate good quality text from RDF data would permit, e.g., making this data more accessible to lay users, enriching existing text with information drawn from knowledge bases such as DBpedia or describing, comparing and relating entities present in these knowledge bases.
### Impact on Under-Served Communities
#### Addresses needs of underserved Communities?
<!-- info: Does this dataset address the needs of communities that are traditionally underserved in language technology, and particularly language generation technology? Communities may be underserved for exemple because their language, language variety, or social or geographical context is underepresented in NLP and NLG resources (datasets and models). -->
<!-- scope: telescope -->
no
### Discussion of Biases
#### Any Documented Social Biases?
<!-- info: Are there documented social biases in the dataset? Biases in this context are variations in the ways members of different social categories are represented that can have harmful downstream consequences for members of the more disadvantaged group. -->
<!-- scope: telescope -->
yes
#### Links and Summaries of Analysis Work
<!-- info: Provide links to and summaries of works analyzing these biases. -->
<!-- scope: microscope -->
This dataset is created using DBpedia RDF triples which naturally exhibit biases that have been found to exist in Wikipedia such as some forms of, e.g., gender bias.
The choice of [entities](https://gitlab.com/shimorina/webnlg-dataset/-/blob/master/supplementary/entities_dict.json), described by RDF trees, was not controlled. As such, they may contain gender biases; for instance, all the astronauts described by RDF triples are male. Hence, in texts, pronouns _he/him/his_ occur more often. Similarly, entities can be related to the Western culture more often than to other cultures.
#### Are the Language Producers Representative of the Language?
<!-- info: Does the distribution of language producers in the dataset accurately represent the full distribution of speakers of the language world-wide? If not, how does it differ? -->
<!-- scope: periscope -->
In English, the dataset is limited to the language that crowdraters speak. In Russian, the language is heavily biased by the translationese of the translation system that is post-edited.
## Considerations for Using the Data
### PII Risks and Liability
#### Potential PII Risk
<!-- info: Considering your answers to the PII part of the Data Curation Section, describe any potential privacy to the data subjects and creators risks when using the dataset. -->
<!-- scope: microscope -->
There is no PII in this dataset.
### Licenses
#### Copyright Restrictions on the Dataset
<!-- info: Based on your answers in the Intended Use part of the Data Overview Section, which of the following best describe the copyright and licensing status of the dataset? -->
<!-- scope: periscope -->
`non-commercial use only`
#### Copyright Restrictions on the Language Data
<!-- info: Based on your answers in the Language part of the Data Curation Section, which of the following best describe the copyright and licensing status of the underlying language data? -->
<!-- scope: periscope -->
`public domain`
### Known Technical Limitations
#### Technical Limitations
<!-- info: Describe any known technical limitations, such as spurrious correlations, train/test overlap, annotation biases, or mis-annotations, and cite the works that first identified these limitations when possible. -->
<!-- scope: microscope -->
The quality of the crowdsourced references is limited, in particular in terms of fluency/naturalness of the collected texts.
Russian data was machine-translated and then post-edited by crowdworkers, so some examples may still exhibit issues related to bad translations.
#### Unsuited Applications
<!-- info: When using a model trained on this dataset in a setting where users or the public may interact with its predictions, what are some pitfalls to look out for? In particular, describe some applications of the general task featured in this dataset that its curation or properties make it less suitable for. -->
<!-- scope: microscope -->
Only a limited number of domains are covered in this dataset. As a result, it cannot be used as a general-purpose realizer.
|
GEM/wiki_auto_asset_turk | ---
annotations_creators:
- crowd-sourced
language_creators:
- unknown
language:
- en
license:
- other
multilinguality:
- unknown
size_categories:
- unknown
source_datasets:
- original
task_categories:
- text2text-generation
task_ids:
- text-simplification
pretty_name: wiki_auto_asset_turk
---
# Dataset Card for GEM/wiki_auto_asset_turk
## Dataset Description
- **Homepage:** n/a
- **Repository:** https://github.com/chaojiang06/wiki-auto, [ASSET repository
- **Paper:** https://aclanthology.org/2020.acl-main.709/, [ASSET
- **Leaderboard:** N/A
- **Point of Contact:** WikiAuto: Chao Jiang; ASSET: Fernando Alva-Manchego and Louis Martin; TURK: Wei Xu
### Link to Main Data Card
You can find the main data card on the [GEM Website](https://gem-benchmark.com/data_cards/wiki_auto_asset_turk).
### Dataset Summary
WikiAuto is an English simplification dataset that we paired with ASSET and TURK, two very high-quality evaluation datasets, as test sets. The input is an English sentence taken from Wikipedia and the target a simplified sentence. ASSET and TURK contain the same test examples but have references that are simplified in different ways (splitting sentences vs. rewriting and splitting).
You can load the dataset via:
```
import datasets
data = datasets.load_dataset('GEM/wiki_auto_asset_turk')
```
The data loader can be found [here](https://huggingface.co/datasets/GEM/wiki_auto_asset_turk).
#### website
n/a
#### paper
[WikiAuto](https://aclanthology.org/2020.acl-main.709/), [ASSET](https://aclanthology.org/2020.acl-main.424/), [TURK](https://aclanthology.org/Q16-1029/)
#### authors
WikiAuto: Chao Jiang, Mounica Maddela, Wuwei Lan, Yang Zhong, Wei Xu; ASSET: Fernando Alva-Manchego, Louis Martin, Antoine Bordes, Carolina Scarton, and Benoîıt Sagot, and Lucia Specia; TURK: Wei Xu, Courtney Napoles, Ellie Pavlick, Quanze Chen, and Chris Callison-Burch
## Dataset Overview
### Where to find the Data and its Documentation
#### Download
<!-- info: What is the link to where the original dataset is hosted? -->
<!-- scope: telescope -->
[Wiki-Auto repository](https://github.com/chaojiang06/wiki-auto), [ASSET repository](https://github.com/facebookresearch/asset), [TURKCorpus](https://github.com/cocoxu/simplification)
#### Paper
<!-- info: What is the link to the paper describing the dataset (open access preferred)? -->
<!-- scope: telescope -->
[WikiAuto](https://aclanthology.org/2020.acl-main.709/), [ASSET](https://aclanthology.org/2020.acl-main.424/), [TURK](https://aclanthology.org/Q16-1029/)
#### BibTex
<!-- info: Provide the BibTex-formatted reference for the dataset. Please use the correct published version (ACL anthology, etc.) instead of google scholar created Bibtex. -->
<!-- scope: microscope -->
WikiAuto:
```
@inproceedings{jiang-etal-2020-neural,
title = "Neural {CRF} Model for Sentence Alignment in Text Simplification",
author = "Jiang, Chao and
Maddela, Mounica and
Lan, Wuwei and
Zhong, Yang and
Xu, Wei",
booktitle = "Proceedings of the 58th Annual Meeting of the Association for Computational Linguistics",
month = jul,
year = "2020",
address = "Online",
publisher = "Association for Computational Linguistics",
url = "https://www.aclweb.org/anthology/2020.acl-main.709",
doi = "10.18653/v1/2020.acl-main.709",
pages = "7943--7960",
}
```
ASSET:
```
@inproceedings{alva-manchego-etal-2020-asset,
title = "{ASSET}: {A} Dataset for Tuning and Evaluation of Sentence Simplification Models with Multiple Rewriting Transformations",
author = "Alva-Manchego, Fernando and
Martin, Louis and
Bordes, Antoine and
Scarton, Carolina and
Sagot, Beno{\^\i}t and
Specia, Lucia",
booktitle = "Proceedings of the 58th Annual Meeting of the Association for Computational Linguistics",
month = jul,
year = "2020",
address = "Online",
publisher = "Association for Computational Linguistics",
url = "https://www.aclweb.org/anthology/2020.acl-main.424",
pages = "4668--4679",
}
```
TURK:
```
@article{Xu-EtAl:2016:TACL,
author = {Wei Xu and Courtney Napoles and Ellie Pavlick and Quanze Chen and Chris Callison-Burch},
title = {Optimizing Statistical Machine Translation for Text Simplification},
journal = {Transactions of the Association for Computational Linguistics},
volume = {4},
year = {2016},
url = {https://cocoxu.github.io/publications/tacl2016-smt-simplification.pdf},
pages = {401--415}
}
```
#### Contact Name
<!-- quick -->
<!-- info: If known, provide the name of at least one person the reader can contact for questions about the dataset. -->
<!-- scope: periscope -->
WikiAuto: Chao Jiang; ASSET: Fernando Alva-Manchego and Louis Martin; TURK: Wei Xu
#### Contact Email
<!-- info: If known, provide the email of at least one person the reader can contact for questions about the dataset. -->
<!-- scope: periscope -->
jiang.1530@osu.edu, f.alva@sheffield.ac.uk, louismartincs@gmail.com, wei.xu@cc.gatech.edu
#### Has a Leaderboard?
<!-- info: Does the dataset have an active leaderboard? -->
<!-- scope: telescope -->
no
### Languages and Intended Use
#### Multilingual?
<!-- quick -->
<!-- info: Is the dataset multilingual? -->
<!-- scope: telescope -->
no
#### Covered Languages
<!-- quick -->
<!-- info: What languages/dialects are covered in the dataset? -->
<!-- scope: telescope -->
`English`
#### Whose Language?
<!-- info: Whose language is in the dataset? -->
<!-- scope: periscope -->
Wiki-Auto contains English text only (BCP-47: `en`). It is presented as a translation task where Wikipedia Simple English is treated as its own idiom. For a statement of what is intended (but not always observed) to constitute Simple English on this platform, see [Simple English in Wikipedia](https://simple.wikipedia.org/wiki/Wikipedia:About#Simple_English).
Both ASSET and TURK use crowdsourcing to change references, and their language is thus a combination of the WikiAuto data and the language of the demographic on mechanical Turk
#### License
<!-- quick -->
<!-- info: What is the license of the dataset? -->
<!-- scope: telescope -->
other: Other license
#### Intended Use
<!-- info: What is the intended use of the dataset? -->
<!-- scope: microscope -->
WikiAuto provides a set of aligned sentences from English Wikipedia and Simple English Wikipedia as a resource to train sentence simplification systems.
The authors first crowd-sourced a set of manual alignments between sentences in a subset of the Simple English Wikipedia and their corresponding versions in English Wikipedia (this corresponds to the `manual` config in this version of the dataset), then trained a neural CRF system to predict these alignments.
The trained alignment prediction model was then applied to the other articles in Simple English Wikipedia with an English counterpart to create a larger corpus of aligned sentences (corresponding to the `auto` and `auto_acl` configs here).
[ASSET](https://github.com/facebookresearch/asset) [(Alva-Manchego et al., 2020)](https://www.aclweb.org/anthology/2020.acl-main.424.pdf) is multi-reference dataset for the evaluation of sentence simplification in English. The dataset uses the same 2,359 sentences from [TurkCorpus](https://github.com/cocoxu/simplification/) [(Xu et al., 2016)](https://www.aclweb.org/anthology/Q16-1029.pdf) and each sentence is associated with 10 crowdsourced simplifications. Unlike previous simplification datasets, which contain a single transformation (e.g., lexical paraphrasing in TurkCorpus or sentence
splitting in [HSplit](https://www.aclweb.org/anthology/D18-1081.pdf)), the simplifications in ASSET encompass a variety of rewriting transformations.
TURKCorpus is a high quality simplification dataset where each source (not simple) sentence is associated with 8 human-written simplifications that focus on lexical paraphrasing. It is one of the two evaluation datasets for the text simplification task in GEM. It acts as the validation and test set for paraphrasing-based simplification that does not involve sentence splitting and deletion.
#### Add. License Info
<!-- info: What is the 'other' license of the dataset? -->
<!-- scope: periscope -->
WikiAuto: `CC BY-NC 3.0`, ASSET: `CC BY-NC 4.0`, TURK: `GNU General Public License v3.0`
#### Primary Task
<!-- info: What primary task does the dataset support? -->
<!-- scope: telescope -->
Simplification
#### Communicative Goal
<!-- quick -->
<!-- info: Provide a short description of the communicative goal of a model trained for this task on this dataset. -->
<!-- scope: periscope -->
The goal is to communicate the main ideas of source sentence in a way that is easier to understand by non-native speakers of English.
### Credit
#### Curation Organization Type(s)
<!-- info: In what kind of organization did the dataset curation happen? -->
<!-- scope: telescope -->
`academic`, `industry`
#### Curation Organization(s)
<!-- info: Name the organization(s). -->
<!-- scope: periscope -->
Ohio State University, University of Sheffield, Inria, Facebook AI Research, Imperial College London, University of Pennsylvania, John Hopkins University
#### Dataset Creators
<!-- info: Who created the original dataset? List the people involved in collecting the dataset and their affiliation(s). -->
<!-- scope: microscope -->
WikiAuto: Chao Jiang, Mounica Maddela, Wuwei Lan, Yang Zhong, Wei Xu; ASSET: Fernando Alva-Manchego, Louis Martin, Antoine Bordes, Carolina Scarton, and Benoîıt Sagot, and Lucia Specia; TURK: Wei Xu, Courtney Napoles, Ellie Pavlick, Quanze Chen, and Chris Callison-Burch
#### Funding
<!-- info: Who funded the data creation? -->
<!-- scope: microscope -->
WikiAuto: NSF, ODNI, IARPA, Figure Eight AI, and Criteo. ASSET: PRAIRIE Institute, ANR. TURK: NSF
#### Who added the Dataset to GEM?
<!-- info: Who contributed to the data card and adding the dataset to GEM? List the people+affiliations involved in creating this data card and who helped integrate this dataset into GEM. -->
<!-- scope: microscope -->
GEM v1 had separate data cards for WikiAuto, ASSET, and TURK. They were contributed by Dhruv Kumar and Mounica Maddela. The initial data loader was written by Yacine Jernite. Sebastian Gehrmann merged and extended the data cards and migrated the loader to the v2 infrastructure.
### Dataset Structure
#### Data Fields
<!-- info: List and describe the fields present in the dataset. -->
<!-- scope: telescope -->
- `source`: A source sentence from one of the datasets
- `target`: A single simplified sentence corresponding to `source`
- `references`: In the case of ASSET/TURK, references is a list of strings corresponding to the different references.
#### Reason for Structure
<!-- info: How was the dataset structure determined? -->
<!-- scope: microscope -->
The underlying datasets have extensive secondary annotations that can be used in conjunction with the GEM version. We omit those annotations to simplify the format into one that can be used by seq2seq models.
#### Example Instance
<!-- info: Provide a JSON formatted example of a typical instance in the dataset. -->
<!-- scope: periscope -->
```
{
'source': 'In early work, Rutherford discovered the concept of radioactive half-life , the radioactive element radon, and differentiated and named alpha and beta radiation .',
'target': 'Rutherford discovered the radioactive half-life, and the three parts of radiation which he named Alpha, Beta, and Gamma.'
}
```
#### Data Splits
<!-- info: Describe and name the splits in the dataset if there are more than one. -->
<!-- scope: periscope -->
In WikiAuto, which is used as training and validation set, the following splits are provided:
| | Tain | Dev | Test |
| ----- | ------ | ----- | ---- |
| Total sentence pairs | 373801 | 73249 | 118074 |
| Aligned sentence pairs | 1889 | 346 | 677 |
ASSET does not contain a training set; many models use [WikiLarge](https://github.com/XingxingZhang/dress) (Zhang and Lapata, 2017) for training. For GEM, [Wiki-Auto](https://github.com/chaojiang06/wiki-auto) will be used for training the model.
Each input sentence has 10 associated reference simplified sentences. The statistics of ASSET are given below.
| | Dev | Test | Total |
| ----- | ------ | ---- | ----- |
| Input Sentences | 2000 | 359 | 2359 |
| Reference Simplifications | 20000 | 3590 | 23590 |
The test and validation sets are the same as those of [TurkCorpus](https://github.com/cocoxu/simplification/). The split was random.
There are 19.04 tokens per reference on average (lower than 21.29 and 25.49 for TurkCorpus and HSplit, respectively). Most (17,245) of the referece sentences do not involve sentence splitting.
TURKCorpus does not contain a training set; many models use [WikiLarge](https://github.com/XingxingZhang/dress) (Zhang and Lapata, 2017) or [Wiki-Auto](https://github.com/chaojiang06/wiki-auto) (Jiang et. al 2020) for training.
Each input sentence has 8 associated reference simplified sentences. 2,359 input sentences are randomly split into 2,000 validation and 359 test sentences.
| | Dev | Test | Total |
| ----- | ------ | ---- | ----- |
| Input Sentences | 2000 | 359 | 2359 |
| Reference Simplifications | 16000 | 2872 | 18872 |
There are 21.29 tokens per reference on average.
#### Splitting Criteria
<!-- info: Describe any criteria for splitting the data, if used. If there are differences between the splits (e.g., if the training annotations are machine-generated and the dev and test ones are created by humans, or if different numbers of annotators contributed to each example), describe them here. -->
<!-- scope: microscope -->
In our setup, we use WikiAuto as training/validation corpus and ASSET and TURK as test corpora. ASSET and TURK have the same inputs but differ in their reference style. Researchers can thus conduct targeted evaluations based on the strategies that a model should learn.
## Dataset in GEM
### Rationale for Inclusion in GEM
#### Why is the Dataset in GEM?
<!-- info: What does this dataset contribute toward better generation evaluation and why is it part of GEM? -->
<!-- scope: microscope -->
WikiAuto is the largest open text simplification dataset currently available. ASSET and TURK are high quality test sets that are compatible with WikiAuto.
#### Similar Datasets
<!-- info: Do other datasets for the high level task exist? -->
<!-- scope: telescope -->
yes
#### Unique Language Coverage
<!-- info: Does this dataset cover other languages than other datasets for the same task? -->
<!-- scope: periscope -->
no
#### Difference from other GEM datasets
<!-- info: What else sets this dataset apart from other similar datasets in GEM? -->
<!-- scope: microscope -->
It's unique setup with multiple test sets makes the task interesting since it allows for evaluation of multiple generations and systems that simplify in different ways.
#### Ability that the Dataset measures
<!-- info: What aspect of model ability can be measured with this dataset? -->
<!-- scope: periscope -->
simplification
### GEM-Specific Curation
#### Modificatied for GEM?
<!-- info: Has the GEM version of the dataset been modified in any way (data, processing, splits) from the original curated data? -->
<!-- scope: telescope -->
yes
#### GEM Modifications
<!-- info: What changes have been made to he original dataset? -->
<!-- scope: periscope -->
`other`
#### Modification Details
<!-- info: For each of these changes, described them in more details and provided the intended purpose of the modification -->
<!-- scope: microscope -->
We removed secondary annotations and focus on the simple `input->output` format, but combine the different sub-datasets.
#### Additional Splits?
<!-- info: Does GEM provide additional splits to the dataset? -->
<!-- scope: telescope -->
yes
#### Split Information
<!-- info: Describe how the new splits were created -->
<!-- scope: periscope -->
we split the original test set according to syntactic complexity of the source sentences. To characterize sentence syntactic complexity, we use the 8-level developmental level (d-level) scale proposed by [Covington et al. (2006)](https://www.researchgate.net/publication/254033869_How_complex_is_that_sentence_A_proposed_revision_of_the_Rosenberg_and_Abbeduto_D-Level_Scale) and the implementation of [Lu, Xiaofei (2010)](https://www.jbe-platform.com/content/journals/10.1075/ijcl.15.4.02lu).
We thus split the original test set into 8 subsets corresponding to the 8 d-levels assigned to source sentences. We obtain the following number of instances per level and average d-level of the dataset:
| Total nb. sentences | L0 | L1 | L2 | L3 | L4 | L5 | L6 | L7 | Mean Level |
|-------------------- | ------ | ------ | ------ | ------ | ------ | ------ | ------ | ------ | ---------- |
| 359 | 166 | 0 | 58 | 32 | 5 | 28 | 7 | 63 | 2.38 |
#### Split Motivation
<!-- info: What aspects of the model's generation capacities were the splits created to test? -->
<!-- scope: periscope -->
The goal was to assess performance when simplifying source sentences with different syntactic structure and complexity.
### Getting Started with the Task
#### Pointers to Resources
<!-- info: Getting started with in-depth research on the task. Add relevant pointers to resources that researchers can consult when they want to get started digging deeper into the task. -->
<!-- scope: microscope -->
There are recent supervised ([Martin et al., 2019](https://arxiv.org/abs/1910.02677), [Kriz et al., 2019](https://www.aclweb.org/anthology/N19-1317/), [Dong et al., 2019](https://www.aclweb.org/anthology/P19-1331/), [Zhang and Lapata, 2017](https://www.aclweb.org/anthology/D17-1062/)) and unsupervised ([Martin et al., 2020](https://arxiv.org/abs/2005.00352v1), [Kumar et al., 2020](https://www.aclweb.org/anthology/2020.acl-main.707/), [Surya et al., 2019](https://www.aclweb.org/anthology/P19-1198/)) text simplification models that can be used as baselines.
#### Technical Terms
<!-- info: Technical terms used in this card and the dataset and their definitions -->
<!-- scope: microscope -->
The common metric used for automatic evaluation is SARI [(Xu et al., 2016)](https://www.aclweb.org/anthology/Q16-1029/).
## Previous Results
### Previous Results
#### Measured Model Abilities
<!-- info: What aspect of model ability can be measured with this dataset? -->
<!-- scope: telescope -->
Simplification
#### Metrics
<!-- info: What metrics are typically used for this task? -->
<!-- scope: periscope -->
`Other: Other Metrics`, `BLEU`
#### Other Metrics
<!-- info: Definitions of other metrics -->
<!-- scope: periscope -->
SARI: A simplification metric that considers both input and references to measure the "goodness" of words that are added, deleted, and kept.
#### Proposed Evaluation
<!-- info: List and describe the purpose of the metrics and evaluation methodology (including human evaluation) that the dataset creators used when introducing this task. -->
<!-- scope: microscope -->
The original authors of WikiAuto and ASSET used human evaluation to assess the fluency, adequacy, and simplicity (details provided in the paper). For TURK, the authors measured grammaticality, meaning-preservation, and simplicity gain (details in the paper).
#### Previous results available?
<!-- info: Are previous results available? -->
<!-- scope: telescope -->
no
## Dataset Curation
### Original Curation
#### Original Curation Rationale
<!-- info: Original curation rationale -->
<!-- scope: telescope -->
Wiki-Auto provides a new version of the Wikipedia corpus that is larger, contains 75% less defective pairs and has more complex rewrites than the previous WIKILARGE dataset.
ASSET was created in order to improve the evaluation of sentence simplification. It uses the same input sentences as the [TurkCorpus](https://github.com/cocoxu/simplification/) dataset from [(Xu et al., 2016)](https://www.aclweb.org/anthology/Q16-1029.pdf). The 2,359 input sentences of TurkCorpus are a sample of "standard" (not simple) sentences from the [Parallel Wikipedia Simplification (PWKP)](https://www.informatik.tu-darmstadt.de/ukp/research_6/data/sentence_simplification/simple_complex_sentence_pairs/index.en.jsp) dataset [(Zhu et al., 2010)](https://www.aclweb.org/anthology/C10-1152.pdf), which come from the August 22, 2009 version of Wikipedia. The sentences of TurkCorpus were chosen to be of similar length [(Xu et al., 2016)](https://www.aclweb.org/anthology/Q16-1029.pdf). No further information is provided on the sampling strategy.
The TurkCorpus dataset was developed in order to overcome some of the problems with sentence pairs from Standard and Simple Wikipedia: a large fraction of sentences were misaligned, or not actually simpler [(Xu et al., 2016)](https://www.aclweb.org/anthology/Q16-1029.pdf). However, TurkCorpus mainly focused on *lexical paraphrasing*, and so cannot be used to evaluate simplifications involving *compression* (deletion) or *sentence splitting*. HSplit [(Sulem et al., 2018)](https://www.aclweb.org/anthology/D18-1081.pdf), on the other hand, can only be used to evaluate sentence splitting. The reference sentences in ASSET include a wider variety of sentence rewriting strategies, combining splitting, compression and paraphrasing. Annotators were given examples of each kind of transformation individually, as well as all three transformations used at once, but were allowed to decide which transformations to use for any given sentence.
An example illustrating the differences between TurkCorpus, HSplit and ASSET is given below:
> **Original:** He settled in London, devoting himself chiefly to practical teaching.
>
> **TurkCorpus:** He rooted in London, devoting himself mainly to practical teaching.
>
> **HSplit:** He settled in London. He devoted himself chiefly to practical teaching.
>
> **ASSET:** He lived in London. He was a teacher.
#### Communicative Goal
<!-- info: What was the communicative goal? -->
<!-- scope: periscope -->
The goal is to communicate the same information as the source sentence using simpler words and grammar.
#### Sourced from Different Sources
<!-- info: Is the dataset aggregated from different data sources? -->
<!-- scope: telescope -->
yes
#### Source Details
<!-- info: List the sources (one per line) -->
<!-- scope: periscope -->
Wikipedia
### Language Data
#### How was Language Data Obtained?
<!-- info: How was the language data obtained? -->
<!-- scope: telescope -->
`Found`
#### Where was it found?
<!-- info: If found, where from? -->
<!-- scope: telescope -->
`Single website`
#### Language Producers
<!-- info: What further information do we have on the language producers? -->
<!-- scope: microscope -->
The dataset uses language from Wikipedia: some demographic information is provided [here](https://en.wikipedia.org/wiki/Wikipedia:Who_writes_Wikipedia%3F).
#### Data Validation
<!-- info: Was the text validated by a different worker or a data curator? -->
<!-- scope: telescope -->
not validated
#### Was Data Filtered?
<!-- info: Were text instances selected or filtered? -->
<!-- scope: telescope -->
algorithmically
#### Filter Criteria
<!-- info: What were the selection criteria? -->
<!-- scope: microscope -->
The authors mention that they "extracted 138,095 article pairs from the 2019/09 Wikipedia dump using an improved version of the [WikiExtractor](https://github.com/attardi/wikiextractor) library". The [SpaCy](https://spacy.io/) library is used for sentence splitting.
### Structured Annotations
#### Additional Annotations?
<!-- quick -->
<!-- info: Does the dataset have additional annotations for each instance? -->
<!-- scope: telescope -->
crowd-sourced
#### Number of Raters
<!-- info: What is the number of raters -->
<!-- scope: telescope -->
11<n<50
#### Rater Qualifications
<!-- info: Describe the qualifications required of an annotator. -->
<!-- scope: periscope -->
WikiAuto (Figure Eight): No information provided.
ASSET (MTurk):
- Having a HIT approval rate over 95%, and over 1000 HITs approved. No other demographic or compensation information is provided.
- Passing a Qualification Test (appropriately simplifying sentences). Out of 100 workers, 42 passed the test.
- Being a resident of the United States, United Kingdom or Canada.
TURK (MTurk):
- Reference sentences were written by workers with HIT approval rate over 95%. No other demographic or compensation information is provided.
#### Raters per Training Example
<!-- info: How many annotators saw each training example? -->
<!-- scope: periscope -->
1
#### Raters per Test Example
<!-- info: How many annotators saw each test example? -->
<!-- scope: periscope -->
>5
#### Annotation Service?
<!-- info: Was an annotation service used? -->
<!-- scope: telescope -->
yes
#### Which Annotation Service
<!-- info: Which annotation services were used? -->
<!-- scope: periscope -->
`Amazon Mechanical Turk`, `Appen`
#### Annotation Values
<!-- info: Purpose and values for each annotation -->
<!-- scope: microscope -->
WikiAuto: Sentence alignment labels were crowdsourced for 500 randomly sampled document pairs (10,123 sentence pairs total). The authors pre-selected several alignment candidates from English Wikipedia for each Simple Wikipedia sentence based on various similarity metrics, then asked the crowd-workers to annotate these pairs. Finally, they trained their alignment model on this manually annotated dataset to obtain automatically aligned sentences (138,095 document pairs, 488,332 sentence pairs).
No demographic annotation is provided for the crowd workers. The [Figure Eight](https://www.figure-eight.com/) platform now part of Appen) was used for the annotation process.
ASSET: The instructions given to the annotators are available [here](https://github.com/facebookresearch/asset/blob/master/crowdsourcing/AMT_AnnotationInstructions.pdf).
TURK: The references are crowdsourced from Amazon Mechanical Turk. The annotators were asked to provide simplifications without losing any information or splitting the input sentence. No other demographic or compensation information is provided in the TURKCorpus paper. The instructions given to the annotators are available in the paper.
#### Any Quality Control?
<!-- info: Quality control measures? -->
<!-- scope: telescope -->
none
### Consent
#### Any Consent Policy?
<!-- info: Was there a consent policy involved when gathering the data? -->
<!-- scope: telescope -->
yes
#### Consent Policy Details
<!-- info: What was the consent policy? -->
<!-- scope: microscope -->
Both Figure Eight and Amazon Mechanical Turk raters forfeit the right to their data as part of their agreements.
### Private Identifying Information (PII)
#### Contains PII?
<!-- quick -->
<!-- info: Does the source language data likely contain Personal Identifying Information about the data creators or subjects? -->
<!-- scope: telescope -->
no PII
#### Justification for no PII
<!-- info: Provide a justification for selecting `no PII` above. -->
<!-- scope: periscope -->
Since the dataset is created from Wikipedia/Simple Wikipedia, all the information contained in the dataset is already in the public domain.
### Maintenance
#### Any Maintenance Plan?
<!-- info: Does the original dataset have a maintenance plan? -->
<!-- scope: telescope -->
no
## Broader Social Context
### Previous Work on the Social Impact of the Dataset
#### Usage of Models based on the Data
<!-- info: Are you aware of cases where models trained on the task featured in this dataset ore related tasks have been used in automated systems? -->
<!-- scope: telescope -->
no
### Impact on Under-Served Communities
#### Addresses needs of underserved Communities?
<!-- info: Does this dataset address the needs of communities that are traditionally underserved in language technology, and particularly language generation technology? Communities may be underserved for exemple because their language, language variety, or social or geographical context is underepresented in NLP and NLG resources (datasets and models). -->
<!-- scope: telescope -->
no
### Discussion of Biases
#### Any Documented Social Biases?
<!-- info: Are there documented social biases in the dataset? Biases in this context are variations in the ways members of different social categories are represented that can have harmful downstream consequences for members of the more disadvantaged group. -->
<!-- scope: telescope -->
yes
#### Links and Summaries of Analysis Work
<!-- info: Provide links to and summaries of works analyzing these biases. -->
<!-- scope: microscope -->
The dataset may contain some social biases, as the input sentences are based on Wikipedia. Studies have shown that the English Wikipedia contains both gender biases [(Schmahl et al., 2020)](https://research.tudelft.nl/en/publications/is-wikipedia-succeeding-in-reducing-gender-bias-assessing-changes) and racial biases [(Adams et al., 2019)](https://journals.sagepub.com/doi/pdf/10.1177/2378023118823946).
## Considerations for Using the Data
### PII Risks and Liability
#### Potential PII Risk
<!-- info: Considering your answers to the PII part of the Data Curation Section, describe any potential privacy to the data subjects and creators risks when using the dataset. -->
<!-- scope: microscope -->
All the data is in the public domain.
### Licenses
#### Copyright Restrictions on the Dataset
<!-- info: Based on your answers in the Intended Use part of the Data Overview Section, which of the following best describe the copyright and licensing status of the dataset? -->
<!-- scope: periscope -->
`open license - commercial use allowed`
#### Copyright Restrictions on the Language Data
<!-- info: Based on your answers in the Language part of the Data Curation Section, which of the following best describe the copyright and licensing status of the underlying language data? -->
<!-- scope: periscope -->
`open license - commercial use allowed`
### Known Technical Limitations
#### Technical Limitations
<!-- info: Describe any known technical limitations, such as spurrious correlations, train/test overlap, annotation biases, or mis-annotations, and cite the works that first identified these limitations when possible. -->
<!-- scope: microscope -->
The dataset may contain some social biases, as the input sentences are based on Wikipedia. Studies have shown that the English Wikipedia contains both gender biases [(Schmahl et al., 2020)](https://research.tudelft.nl/en/publications/is-wikipedia-succeeding-in-reducing-gender-bias-assessing-changes) and racial biases [(Adams et al., 2019)](https://journals.sagepub.com/doi/pdf/10.1177/2378023118823946).
#### Unsuited Applications
<!-- info: When using a model trained on this dataset in a setting where users or the public may interact with its predictions, what are some pitfalls to look out for? In particular, describe some applications of the general task featured in this dataset that its curation or properties make it less suitable for. -->
<!-- scope: microscope -->
Since the test datasets contains only 2,359 sentences that are derived from Wikipedia, they are limited to a small subset of topics present on Wikipedia.
|
GEM/wiki_cat_sum | ---
annotations_creators:
- automatically-created
language_creators:
- unknown
language:
- en
license:
- cc-by-sa-3.0
multilinguality:
- unknown
size_categories:
- unknown
source_datasets:
- original
task_categories:
- summarization
task_ids: []
pretty_name: wiki_cat_sum
---
# Dataset Card for GEM/wiki_cat_sum
## Dataset Description
- **Homepage:** https://github.com/lauhaide/WikiCatSum
- **Repository:** https://datashare.ed.ac.uk/handle/10283/3368
- **Paper:** https://arxiv.org/abs/1906.04687
- **Leaderboard:** N/A
- **Point of Contact:** Laura Perez-Beltrachini
### Link to Main Data Card
You can find the main data card on the [GEM Website](https://gem-benchmark.com/data_cards/wiki_cat_sum).
### Dataset Summary
WikiCatSum is an English summarization dataset in three domains: animals, companies, and film. It provides multiple paragraphs of text paired with a summary of the paragraphs.
You can load the dataset via:
```
import datasets
data = datasets.load_dataset('GEM/wiki_cat_sum')
```
The data loader can be found [here](https://huggingface.co/datasets/GEM/wiki_cat_sum).
#### website
[Github](https://github.com/lauhaide/WikiCatSum)
#### paper
[Arxiv](https://arxiv.org/abs/1906.04687)
#### authors
Laura Perez-Beltrachini, Yang Liu, Mirella Lapata (University of Edinburgh) Peter J. Liu, Mohammad Saleh, Etienne Pot, Ben Goodrich, Ryan Sepassi, Lukasz Kaiser, Noam Shazeer (GoogleBrain)
## Dataset Overview
### Where to find the Data and its Documentation
#### Webpage
<!-- info: What is the webpage for the dataset (if it exists)? -->
<!-- scope: telescope -->
[Github](https://github.com/lauhaide/WikiCatSum)
#### Download
<!-- info: What is the link to where the original dataset is hosted? -->
<!-- scope: telescope -->
[Website](https://datashare.ed.ac.uk/handle/10283/3368)
#### Paper
<!-- info: What is the link to the paper describing the dataset (open access preferred)? -->
<!-- scope: telescope -->
[Arxiv](https://arxiv.org/abs/1906.04687)
#### BibTex
<!-- info: Provide the BibTex-formatted reference for the dataset. Please use the correct published version (ACL anthology, etc.) instead of google scholar created Bibtex. -->
<!-- scope: microscope -->
```
@inproceedings{perez-beltrachini-etal-2019-generating,
title = "Generating Summaries with Topic Templates and Structured Convolutional Decoders",
author = "Perez-Beltrachini, Laura and
Liu, Yang and
Lapata, Mirella",
booktitle = "Proceedings of the 57th Annual Meeting of the Association for Computational Linguistics",
month = jul,
year = "2019",
address = "Florence, Italy",
publisher = "Association for Computational Linguistics",
url = "https://aclanthology.org/P19-1504",
doi = "10.18653/v1/P19-1504",
}
```
#### Contact Name
<!-- quick -->
<!-- info: If known, provide the name of at least one person the reader can contact for questions about the dataset. -->
<!-- scope: periscope -->
Laura Perez-Beltrachini
#### Contact Email
<!-- info: If known, provide the email of at least one person the reader can contact for questions about the dataset. -->
<!-- scope: periscope -->
lperez@ed.ac.uk
#### Has a Leaderboard?
<!-- info: Does the dataset have an active leaderboard? -->
<!-- scope: telescope -->
no
### Languages and Intended Use
#### Multilingual?
<!-- quick -->
<!-- info: Is the dataset multilingual? -->
<!-- scope: telescope -->
no
#### Covered Languages
<!-- quick -->
<!-- info: What languages/dialects are covered in the dataset? -->
<!-- scope: telescope -->
`English`
#### License
<!-- quick -->
<!-- info: What is the license of the dataset? -->
<!-- scope: telescope -->
cc-by-sa-3.0: Creative Commons Attribution Share Alike 3.0 Unported
#### Intended Use
<!-- info: What is the intended use of the dataset? -->
<!-- scope: microscope -->
Research on multi-document abstractive summarisation.
#### Primary Task
<!-- info: What primary task does the dataset support? -->
<!-- scope: telescope -->
Summarization
#### Communicative Goal
<!-- quick -->
<!-- info: Provide a short description of the communicative goal of a model trained for this task on this dataset. -->
<!-- scope: periscope -->
Summarise the most important facts of a given entity in the Film, Company, and Animal domains from a cluster of related documents.
### Credit
#### Curation Organization Type(s)
<!-- info: In what kind of organization did the dataset curation happen? -->
<!-- scope: telescope -->
`industry`, `academic`
#### Curation Organization(s)
<!-- info: Name the organization(s). -->
<!-- scope: periscope -->
Google Cloud Platform, University of Edinburgh
#### Dataset Creators
<!-- info: Who created the original dataset? List the people involved in collecting the dataset and their affiliation(s). -->
<!-- scope: microscope -->
Laura Perez-Beltrachini, Yang Liu, Mirella Lapata (University of Edinburgh) Peter J. Liu, Mohammad Saleh, Etienne Pot, Ben Goodrich, Ryan Sepassi, Lukasz Kaiser, Noam Shazeer (GoogleBrain)
#### Funding
<!-- info: Who funded the data creation? -->
<!-- scope: microscope -->
Google Cloud Platform, European Research Council
#### Who added the Dataset to GEM?
<!-- info: Who contributed to the data card and adding the dataset to GEM? List the people+affiliations involved in creating this data card and who helped integrate this dataset into GEM. -->
<!-- scope: microscope -->
Ronald Cardenas (University of Edinburgh) Laura Perez-Beltrachini (University of Edinburgh)
### Dataset Structure
#### Data Fields
<!-- info: List and describe the fields present in the dataset. -->
<!-- scope: telescope -->
- `id`: ID of the data example
- `title`: Is the Wikipedia article's title
- `paragraphs`: Is the ranked list of paragraphs from the set of crawled texts
- `summary`: Is constituted by a list of sentences together with their corresponding topic label
#### Example Instance
<!-- info: Provide a JSON formatted example of a typical instance in the dataset. -->
<!-- scope: periscope -->
This is a truncated example from the animal setting:
```
{'gem_id': 'animal-train-1',
'gem_parent_id': 'animal-train-1',
'id': '2652',
'paragraphs': ["lytrosis (hulst) of louisiana vernon antoine brou jr. 2005. southern lepidopterists' news, 27: 7 ., ..."],
'references': ['lytrosis unitaria , the common lytrosis moth, is a species of moth of the geometridae family. it is found in north america, including arkansas, georgia, iowa , massachusetts, and wisconsin. the wingspan is about 50 mm. the larvae feed on rosa, crataegus, amelanchier, acer, quercus and viburnum species.'],
'summary': {'text': ['lytrosis unitaria , the common lytrosis moth , is a species of moth of the geometridae family .',
'it is found in north america , including arkansas , georgia , iowa , massachusetts , new hampshire , new jersey , new york , north carolina , ohio , oklahoma , ontario , pennsylvania , south carolina , tennessee , texas , virginia , west virginia and wisconsin .',
'the wingspan is about 50 mm .',
'the larvae feed on rosa , crataegus , amelanchier , acer , quercus and viburnum species . '],
'topic': [29, 20, 9, 8]},
'target': 'lytrosis unitaria , the common lytrosis moth, is a species of moth of the geometridae family. it is found in north america, including arkansas, georgia, iowa , massachusetts, and wisconsin. the wingspan is about 50 mm. the larvae feed on rosa, crataegus, amelanchier, acer, quercus and viburnum species.',
'title': 'lytrosis unitaria'}
```
#### Data Splits
<!-- info: Describe and name the splits in the dataset if there are more than one. -->
<!-- scope: periscope -->
Nb of instances in train/valid/test are 50,938/2,855/2,831
#### Splitting Criteria
<!-- info: Describe any criteria for splitting the data, if used. If there are differences between the splits (e.g., if the training annotations are machine-generated and the dev and test ones are created by humans, or if different numbers of annotators contributed to each example), describe them here. -->
<!-- scope: microscope -->
The data was split i.i.d., i.e. uniformly split into training, validation, and test datasets.
## Dataset in GEM
### Rationale for Inclusion in GEM
#### Why is the Dataset in GEM?
<!-- info: What does this dataset contribute toward better generation evaluation and why is it part of GEM? -->
<!-- scope: microscope -->
Evaluation of models' performance on noisy (document, summary) pairs and long inputs.
Evaluate models' capabilities to generalise and mitigate biases.
#### Similar Datasets
<!-- info: Do other datasets for the high level task exist? -->
<!-- scope: telescope -->
no
#### Unique Language Coverage
<!-- info: Does this dataset cover other languages than other datasets for the same task? -->
<!-- scope: periscope -->
no
#### Ability that the Dataset measures
<!-- info: What aspect of model ability can be measured with this dataset? -->
<!-- scope: periscope -->
Capabilities to generalise, mitigate biases, factual correctness.
### GEM-Specific Curation
#### Modificatied for GEM?
<!-- info: Has the GEM version of the dataset been modified in any way (data, processing, splits) from the original curated data? -->
<!-- scope: telescope -->
yes
#### GEM Modifications
<!-- info: What changes have been made to he original dataset? -->
<!-- scope: periscope -->
`annotations added`
#### Modification Details
<!-- info: For each of these changes, described them in more details and provided the intended purpose of the modification -->
<!-- scope: microscope -->
We provide topic labels for summary sentences.
#### Additional Splits?
<!-- info: Does GEM provide additional splits to the dataset? -->
<!-- scope: telescope -->
no
### Getting Started with the Task
#### Pointers to Resources
<!-- info: Getting started with in-depth research on the task. Add relevant pointers to resources that researchers can consult when they want to get started digging deeper into the task. -->
<!-- scope: microscope -->
- [Generating Wikipedia by Summarizing Long Sequences](https://arxiv.org/abs/1801.10198)
- [Generating Summaries with Topic Templates and Structured Convolutional Decoders](https://arxiv.org/abs/1906.04687)
- [Noisy Self-Knowledge Distillation for Text Summarization](https://arxiv.org/abs/2009.07032)
And all references in these papers.
## Previous Results
### Previous Results
#### Measured Model Abilities
<!-- info: What aspect of model ability can be measured with this dataset? -->
<!-- scope: telescope -->
Capabilities to generalise, mitigate biases, factual correctness.
#### Metrics
<!-- info: What metrics are typically used for this task? -->
<!-- scope: periscope -->
`ROUGE`, `BERT-Score`, `MoverScore`, `Other: Other Metrics`
#### Other Metrics
<!-- info: Definitions of other metrics -->
<!-- scope: periscope -->
- Abstract/Copy
- Factual accuracy based on the score of (Goodrich et al., 2019) and the relation extraction system of (Sorokin and Gurevych, 2017).
#### Proposed Evaluation
<!-- info: List and describe the purpose of the metrics and evaluation methodology (including human evaluation) that the dataset creators used when introducing this task. -->
<!-- scope: microscope -->
Human based are Question Answering and Ranking (Content, Fluency and Repetition)
#### Previous results available?
<!-- info: Are previous results available? -->
<!-- scope: telescope -->
yes
#### Other Evaluation Approaches
<!-- info: What evaluation approaches have others used? -->
<!-- scope: periscope -->
Those listed above.
#### Relevant Previous Results
<!-- info: What are the most relevant previous results for this task/dataset? -->
<!-- scope: microscope -->
Generating Summaries with Topic Templates and Structured Convolutional Decoders
https://arxiv.org/abs/1906.04687
Noisy Self-Knowledge Distillation for Text Summarization
https://arxiv.org/abs/2009.07032
## Dataset Curation
### Original Curation
#### Original Curation Rationale
<!-- info: Original curation rationale -->
<!-- scope: telescope -->
The dataset is a subset of the WikiSum (Liu et al., 2018) dataset focusing on summaries of entities in three domains (Film, Company, and Animal). It is multi-document summarisation where input-output pairs for each example entity are created as follows. The input is a set of paragraphs collected from i) documents in the Reference section of the entity's Wikipedia page plus ii) documents collected from the top ten search results after querying Google search engine with the entity name. The output summary is the Wikipedia abstract for the entity.
#### Communicative Goal
<!-- info: What was the communicative goal? -->
<!-- scope: periscope -->
Generate descriptive summaries with specific domains, where certain topics are discussed and generally in specific orders.
#### Sourced from Different Sources
<!-- info: Is the dataset aggregated from different data sources? -->
<!-- scope: telescope -->
yes
#### Source Details
<!-- info: List the sources (one per line) -->
<!-- scope: periscope -->
WikiSum (Liu et al., 2018)
### Language Data
#### How was Language Data Obtained?
<!-- info: How was the language data obtained? -->
<!-- scope: telescope -->
`Other`
#### Topics Covered
<!-- info: Does the language in the dataset focus on specific topics? How would you describe them? -->
<!-- scope: periscope -->
The dataset and task focuses on summaries for entities in three domains: Company, Film, and Animal.
#### Data Validation
<!-- info: Was the text validated by a different worker or a data curator? -->
<!-- scope: telescope -->
not validated
#### Data Preprocessing
<!-- info: How was the text data pre-processed? (Enter N/A if the text was not pre-processed) -->
<!-- scope: microscope -->
Summary sentences are associated with a topic label. There is a topic model for each domain.
#### Was Data Filtered?
<!-- info: Were text instances selected or filtered? -->
<!-- scope: telescope -->
not filtered
### Structured Annotations
#### Additional Annotations?
<!-- quick -->
<!-- info: Does the dataset have additional annotations for each instance? -->
<!-- scope: telescope -->
automatically created
#### Annotation Service?
<!-- info: Was an annotation service used? -->
<!-- scope: telescope -->
no
#### Annotation Values
<!-- info: Purpose and values for each annotation -->
<!-- scope: microscope -->
Each summary sentences was annotated with a topic label. There is a topic model for each of the three domains. This was used to guide a hierarchical decoder.
#### Any Quality Control?
<!-- info: Quality control measures? -->
<!-- scope: telescope -->
validated by data curators
#### Quality Control Details
<!-- info: Describe the quality control measures that were taken. -->
<!-- scope: microscope -->
Manual inspection of a sample of topics assigned to sentences. The number of topics was selected based on the performance of the summarisation model.
### Consent
#### Any Consent Policy?
<!-- info: Was there a consent policy involved when gathering the data? -->
<!-- scope: telescope -->
no
#### Justification for Using the Data
<!-- info: If not, what is the justification for reusing the data? -->
<!-- scope: microscope -->
The dataset is base on Wikipedia and referenced and retrieved documents crawled from the Web.
### Private Identifying Information (PII)
#### Contains PII?
<!-- quick -->
<!-- info: Does the source language data likely contain Personal Identifying Information about the data creators or subjects? -->
<!-- scope: telescope -->
unlikely
#### Any PII Identification?
<!-- info: Did the curators use any automatic/manual method to identify PII in the dataset? -->
<!-- scope: periscope -->
no identification
### Maintenance
#### Any Maintenance Plan?
<!-- info: Does the original dataset have a maintenance plan? -->
<!-- scope: telescope -->
no
## Broader Social Context
### Previous Work on the Social Impact of the Dataset
#### Usage of Models based on the Data
<!-- info: Are you aware of cases where models trained on the task featured in this dataset ore related tasks have been used in automated systems? -->
<!-- scope: telescope -->
no
### Impact on Under-Served Communities
#### Addresses needs of underserved Communities?
<!-- info: Does this dataset address the needs of communities that are traditionally underserved in language technology, and particularly language generation technology? Communities may be underserved for exemple because their language, language variety, or social or geographical context is underepresented in NLP and NLG resources (datasets and models). -->
<!-- scope: telescope -->
no
### Discussion of Biases
#### Any Documented Social Biases?
<!-- info: Are there documented social biases in the dataset? Biases in this context are variations in the ways members of different social categories are represented that can have harmful downstream consequences for members of the more disadvantaged group. -->
<!-- scope: telescope -->
yes
#### Links and Summaries of Analysis Work
<!-- info: Provide links to and summaries of works analyzing these biases. -->
<!-- scope: microscope -->
This dataset is based on Wikipedia and thus biases analysis on other Wikipedia-based datasets are potentially true for WikiCatSum. For instance, see analysis for the ToTTo dataset here [1].
[1] Automatic Construction of Evaluation Suites for Natural Language Generation Datasets
https://openreview.net/forum?id=CSi1eu_2q96
## Considerations for Using the Data
### PII Risks and Liability
### Licenses
#### Copyright Restrictions on the Dataset
<!-- info: Based on your answers in the Intended Use part of the Data Overview Section, which of the following best describe the copyright and licensing status of the dataset? -->
<!-- scope: periscope -->
`public domain`
#### Copyright Restrictions on the Language Data
<!-- info: Based on your answers in the Language part of the Data Curation Section, which of the following best describe the copyright and licensing status of the underlying language data? -->
<!-- scope: periscope -->
`public domain`
### Known Technical Limitations
|
GEM/wiki_lingua | ---
annotations_creators:
- none
language_creators:
- unknown
language:
- ar
- cs
- de
- en
- es
- fr
- hi
- id
- it
- ja
- ko
- nl
- pt
- ru
- th
- tr
- vi
- zh
license:
- cc-by-nc-sa-3.0
multilinguality:
- multilingual
size_categories:
- unknown
source_datasets:
- original
task_categories:
- summarization
task_ids: []
pretty_name: wiki_lingua
---
# Dataset Card for GEM/wiki_lingua
## Dataset Description
- **Homepage:** None (See Repository)
- **Repository:** https://github.com/esdurmus/Wikilingua
- **Paper:** https://www.aclweb.org/anthology/2020.findings-emnlp.360/
- **Leaderboard:** N/A
- **Point of Contact:** Faisal Ladhak, Esin Durmus
### Link to Main Data Card
You can find the main data card on the [GEM Website](https://gem-benchmark.com/data_cards/wiki_lingua).
### Dataset Summary
Placeholder
You can load the dataset via:
```
import datasets
data = datasets.load_dataset('GEM/wiki_lingua')
```
The data loader can be found [here](https://huggingface.co/datasets/GEM/wiki_lingua).
#### website
None (See Repository)
#### paper
https://www.aclweb.org/anthology/2020.findings-emnlp.360/
#### authors
Faisal Ladhak (Columbia University), Esin Durmus (Stanford University), Claire Cardie (Cornell University), Kathleen McKeown (Columbia University)
## Dataset Overview
### Where to find the Data and its Documentation
#### Webpage
<!-- info: What is the webpage for the dataset (if it exists)? -->
<!-- scope: telescope -->
None (See Repository)
#### Download
<!-- info: What is the link to where the original dataset is hosted? -->
<!-- scope: telescope -->
https://github.com/esdurmus/Wikilingua
#### Paper
<!-- info: What is the link to the paper describing the dataset (open access preferred)? -->
<!-- scope: telescope -->
https://www.aclweb.org/anthology/2020.findings-emnlp.360/
#### BibTex
<!-- info: Provide the BibTex-formatted reference for the dataset. Please use the correct published version (ACL anthology, etc.) instead of google scholar created Bibtex. -->
<!-- scope: microscope -->
@inproceedings{ladhak-etal-2020-wikilingua,
title = "{W}iki{L}ingua: A New Benchmark Dataset for Cross-Lingual Abstractive Summarization",
author = "Ladhak, Faisal and
Durmus, Esin and
Cardie, Claire and
McKeown, Kathleen",
booktitle = "Findings of the Association for Computational Linguistics: EMNLP 2020",
month = nov,
year = "2020",
address = "Online",
publisher = "Association for Computational Linguistics",
url = "https://aclanthology.org/2020.findings-emnlp.360",
doi = "10.18653/v1/2020.findings-emnlp.360",
pages = "4034--4048",
abstract = "We introduce WikiLingua, a large-scale, multilingual dataset for the evaluation of cross-lingual abstractive summarization systems. We extract article and summary pairs in 18 languages from WikiHow, a high quality, collaborative resource of how-to guides on a diverse set of topics written by human authors. We create gold-standard article-summary alignments across languages by aligning the images that are used to describe each how-to step in an article. As a set of baselines for further studies, we evaluate the performance of existing cross-lingual abstractive summarization methods on our dataset. We further propose a method for direct cross-lingual summarization (i.e., without requiring translation at inference time) by leveraging synthetic data and Neural Machine Translation as a pre-training step. Our method significantly outperforms the baseline approaches, while being more cost efficient during inference.",
}
#### Contact Name
<!-- quick -->
<!-- info: If known, provide the name of at least one person the reader can contact for questions about the dataset. -->
<!-- scope: periscope -->
Faisal Ladhak, Esin Durmus
#### Contact Email
<!-- info: If known, provide the email of at least one person the reader can contact for questions about the dataset. -->
<!-- scope: periscope -->
faisal@cs.columbia.edu, esdurmus@stanford.edu
#### Has a Leaderboard?
<!-- info: Does the dataset have an active leaderboard? -->
<!-- scope: telescope -->
no
### Languages and Intended Use
#### Multilingual?
<!-- quick -->
<!-- info: Is the dataset multilingual? -->
<!-- scope: telescope -->
yes
#### Covered Dialects
<!-- info: What dialects are covered? Are there multiple dialects per language? -->
<!-- scope: periscope -->
Dataset does not have multiple dialects per language.
#### Covered Languages
<!-- quick -->
<!-- info: What languages/dialects are covered in the dataset? -->
<!-- scope: telescope -->
`English`, `Spanish, Castilian`, `Portuguese`, `French`, `German`, `Russian`, `Italian`, `Indonesian`, `Dutch, Flemish`, `Arabic`, `Chinese`, `Vietnamese`, `Thai`, `Japanese`, `Korean`, `Hindi`, `Czech`, `Turkish`
#### Whose Language?
<!-- info: Whose language is in the dataset? -->
<!-- scope: periscope -->
No information about the user demographic is available.
#### License
<!-- quick -->
<!-- info: What is the license of the dataset? -->
<!-- scope: telescope -->
cc-by-nc-sa-3.0: Creative Commons Attribution-NonCommercial-ShareAlike 3.0 Unported (CC BY-NC-SA 3.0)
#### Intended Use
<!-- info: What is the intended use of the dataset? -->
<!-- scope: microscope -->
The dataset was intended to serve as a large-scale, high-quality benchmark dataset for cross-lingual summarization.
#### Primary Task
<!-- info: What primary task does the dataset support? -->
<!-- scope: telescope -->
Summarization
#### Communicative Goal
<!-- quick -->
<!-- info: Provide a short description of the communicative goal of a model trained for this task on this dataset. -->
<!-- scope: periscope -->
Produce a high quality summary for the given input article.
### Credit
#### Curation Organization Type(s)
<!-- info: In what kind of organization did the dataset curation happen? -->
<!-- scope: telescope -->
`academic`
#### Curation Organization(s)
<!-- info: Name the organization(s). -->
<!-- scope: periscope -->
Columbia University
#### Dataset Creators
<!-- info: Who created the original dataset? List the people involved in collecting the dataset and their affiliation(s). -->
<!-- scope: microscope -->
Faisal Ladhak (Columbia University), Esin Durmus (Stanford University), Claire Cardie (Cornell University), Kathleen McKeown (Columbia University)
#### Who added the Dataset to GEM?
<!-- info: Who contributed to the data card and adding the dataset to GEM? List the people+affiliations involved in creating this data card and who helped integrate this dataset into GEM. -->
<!-- scope: microscope -->
Jenny Chim (Queen Mary University of London), Faisal Ladhak (Columbia University)
### Dataset Structure
#### Data Fields
<!-- info: List and describe the fields present in the dataset. -->
<!-- scope: telescope -->
gem_id -- The id for the data instance.
source_language -- The language of the source article.
target_language -- The language of the target summary.
source -- The source document.
#### Example Instance
<!-- info: Provide a JSON formatted example of a typical instance in the dataset. -->
<!-- scope: periscope -->
{
"gem_id": "wikilingua_crosslingual-train-12345",
"gem_parent_id": "wikilingua_crosslingual-train-12345",
"source_language": "fr",
"target_language": "de",
"source": "Document in fr",
"target": "Summary in de",
}
#### Data Splits
<!-- info: Describe and name the splits in the dataset if there are more than one. -->
<!-- scope: periscope -->
The data is split into train/dev/test. In addition to the full test set, there's also a sampled version of the test set.
#### Splitting Criteria
<!-- info: Describe any criteria for splitting the data, if used. If there are differences between the splits (e.g., if the training annotations are machine-generated and the dev and test ones are created by humans, or if different numbers of annotators contributed to each example), describe them here. -->
<!-- scope: microscope -->
The data was split to ensure the same document would appear in the same split across languages so as to ensure there's no leakage into the test set.
## Dataset in GEM
### Rationale for Inclusion in GEM
#### Why is the Dataset in GEM?
<!-- info: What does this dataset contribute toward better generation evaluation and why is it part of GEM? -->
<!-- scope: microscope -->
This dataset provides a large-scale, high-quality resource for cross-lingual summarization in 18 languages, increasing the coverage of languages for the GEM summarization task.
#### Similar Datasets
<!-- info: Do other datasets for the high level task exist? -->
<!-- scope: telescope -->
yes
#### Unique Language Coverage
<!-- info: Does this dataset cover other languages than other datasets for the same task? -->
<!-- scope: periscope -->
yes
#### Difference from other GEM datasets
<!-- info: What else sets this dataset apart from other similar datasets in GEM? -->
<!-- scope: microscope -->
XSum covers English news articles, and MLSum covers news articles in German and Spanish.
In contrast, this dataset has how-to articles in 18 languages, substantially increasing the languages covered. Moreover, it also provides a a different domain than the other two datasets.
#### Ability that the Dataset measures
<!-- info: What aspect of model ability can be measured with this dataset? -->
<!-- scope: periscope -->
The ability to generate quality summaries across multiple languages.
### GEM-Specific Curation
#### Modificatied for GEM?
<!-- info: Has the GEM version of the dataset been modified in any way (data, processing, splits) from the original curated data? -->
<!-- scope: telescope -->
yes
#### GEM Modifications
<!-- info: What changes have been made to he original dataset? -->
<!-- scope: periscope -->
`other`
#### Modification Details
<!-- info: For each of these changes, described them in more details and provided the intended purpose of the modification -->
<!-- scope: microscope -->
Previous version had separate data loaders for each language. In this version, we've created a single monolingual data loader, which contains monolingual data in each of the 18 languages. In addition, we've also created a single cross-lingual data loader across all the language pairs in the dataset.
#### Additional Splits?
<!-- info: Does GEM provide additional splits to the dataset? -->
<!-- scope: telescope -->
no
### Getting Started with the Task
## Previous Results
### Previous Results
#### Measured Model Abilities
<!-- info: What aspect of model ability can be measured with this dataset? -->
<!-- scope: telescope -->
Ability to summarize content across different languages.
#### Metrics
<!-- info: What metrics are typically used for this task? -->
<!-- scope: periscope -->
`ROUGE`
#### Proposed Evaluation
<!-- info: List and describe the purpose of the metrics and evaluation methodology (including human evaluation) that the dataset creators used when introducing this task. -->
<!-- scope: microscope -->
ROUGE is used to measure content selection by comparing word overlap with reference summaries. In addition, the authors of the dataset also used human evaluation to evaluate content selection and fluency of the systems.
#### Previous results available?
<!-- info: Are previous results available? -->
<!-- scope: telescope -->
no
## Dataset Curation
### Original Curation
#### Original Curation Rationale
<!-- info: Original curation rationale -->
<!-- scope: telescope -->
The dataset was created in order to enable new approaches for cross-lingual and multilingual summarization, which are currently understudied as well as open up inetersting new directions for research in summarization. E.g., exploration of multi-source cross-lingual architectures, i.e. models that can summarize from multiple source languages into a target language, building models that can summarize articles from any language to any other language for a given set of languages.
#### Communicative Goal
<!-- info: What was the communicative goal? -->
<!-- scope: periscope -->
Given an input article, produce a high quality summary of the article in the target language.
#### Sourced from Different Sources
<!-- info: Is the dataset aggregated from different data sources? -->
<!-- scope: telescope -->
no
### Language Data
#### How was Language Data Obtained?
<!-- info: How was the language data obtained? -->
<!-- scope: telescope -->
`Found`
#### Where was it found?
<!-- info: If found, where from? -->
<!-- scope: telescope -->
`Single website`
#### Language Producers
<!-- info: What further information do we have on the language producers? -->
<!-- scope: microscope -->
WikiHow, which is an online resource of how-to guides (written and reviewed by human authors) is used as the data source.
#### Topics Covered
<!-- info: Does the language in the dataset focus on specific topics? How would you describe them? -->
<!-- scope: periscope -->
The articles cover 19 broad categories including health, arts and entertainment, personal care and style, travel, education and communications, etc. The categories cover a broad set of genres and topics.
#### Data Validation
<!-- info: Was the text validated by a different worker or a data curator? -->
<!-- scope: telescope -->
not validated
#### Was Data Filtered?
<!-- info: Were text instances selected or filtered? -->
<!-- scope: telescope -->
not filtered
### Structured Annotations
#### Additional Annotations?
<!-- quick -->
<!-- info: Does the dataset have additional annotations for each instance? -->
<!-- scope: telescope -->
none
#### Annotation Service?
<!-- info: Was an annotation service used? -->
<!-- scope: telescope -->
no
### Consent
#### Any Consent Policy?
<!-- info: Was there a consent policy involved when gathering the data? -->
<!-- scope: telescope -->
yes
#### Consent Policy Details
<!-- info: What was the consent policy? -->
<!-- scope: microscope -->
(1) Text Content. All text posted by Users to the Service is sub-licensed by wikiHow to other Users under a Creative Commons license as provided herein. The Creative Commons license allows such text content be used freely for non-commercial purposes, so long as it is used and attributed to the original author as specified under the terms of the license. Allowing free republication of our articles helps wikiHow achieve its mission by providing instruction on solving the problems of everyday life to more people for free. In order to support this goal, wikiHow hereby grants each User of the Service a license to all text content that Users contribute to the Service under the terms and conditions of a Creative Commons CC BY-NC-SA 3.0 License. Please be sure to read the terms of the license carefully. You continue to own all right, title, and interest in and to your User Content, and you are free to distribute it as you wish, whether for commercial or non-commercial purposes.
#### Other Consented Downstream Use
<!-- info: What other downstream uses of the data did the original data creators and the data curators consent to? -->
<!-- scope: microscope -->
The data is made freely available under the Creative Commons license, therefore there are no restrictions about downstream uses as long is it's for non-commercial purposes.
### Private Identifying Information (PII)
#### Contains PII?
<!-- quick -->
<!-- info: Does the source language data likely contain Personal Identifying Information about the data creators or subjects? -->
<!-- scope: telescope -->
no PII
#### Justification for no PII
<!-- info: Provide a justification for selecting `no PII` above. -->
<!-- scope: periscope -->
Only the article text and summaries were collected. No user information was retained in the dataset.
### Maintenance
#### Any Maintenance Plan?
<!-- info: Does the original dataset have a maintenance plan? -->
<!-- scope: telescope -->
no
## Broader Social Context
### Previous Work on the Social Impact of the Dataset
#### Usage of Models based on the Data
<!-- info: Are you aware of cases where models trained on the task featured in this dataset ore related tasks have been used in automated systems? -->
<!-- scope: telescope -->
yes - other datasets featuring the same task
### Impact on Under-Served Communities
#### Addresses needs of underserved Communities?
<!-- info: Does this dataset address the needs of communities that are traditionally underserved in language technology, and particularly language generation technology? Communities may be underserved for exemple because their language, language variety, or social or geographical context is underepresented in NLP and NLG resources (datasets and models). -->
<!-- scope: telescope -->
no
### Discussion of Biases
#### Any Documented Social Biases?
<!-- info: Are there documented social biases in the dataset? Biases in this context are variations in the ways members of different social categories are represented that can have harmful downstream consequences for members of the more disadvantaged group. -->
<!-- scope: telescope -->
yes
## Considerations for Using the Data
### PII Risks and Liability
### Licenses
#### Copyright Restrictions on the Dataset
<!-- info: Based on your answers in the Intended Use part of the Data Overview Section, which of the following best describe the copyright and licensing status of the dataset? -->
<!-- scope: periscope -->
`non-commercial use only`
#### Copyright Restrictions on the Language Data
<!-- info: Based on your answers in the Language part of the Data Curation Section, which of the following best describe the copyright and licensing status of the underlying language data? -->
<!-- scope: periscope -->
`non-commercial use only`
### Known Technical Limitations
|
GEM/xlsum | ---
annotations_creators:
- none
language_creators:
- unknown
language:
- und
license:
- cc-by-nc-sa-4.0
multilinguality:
- unknown
size_categories:
- unknown
source_datasets:
- original
task_categories:
- summarization
task_ids: []
pretty_name: xlsum
---
# Dataset Card for GEM/xlsum
## Dataset Description
- **Homepage:** https://github.com/csebuetnlp/xl-sum
- **Repository:** https://huggingface.co/datasets/csebuetnlp/xlsum/tree/main/data
- **Paper:** https://aclanthology.org/2021.findings-acl.413/
- **Leaderboard:** http://explainaboard.nlpedia.ai/leaderboard/task_xlsum/
- **Point of Contact:** Tahmid Hasan
### Link to Main Data Card
You can find the main data card on the [GEM Website](https://gem-benchmark.com/data_cards/xlsum).
### Dataset Summary
XLSum is a highly multilingual summarization dataset supporting 44 language. The data stems from BBC news articles.
You can load the dataset via:
```
import datasets
data = datasets.load_dataset('GEM/xlsum')
```
The data loader can be found [here](https://huggingface.co/datasets/GEM/xlsum).
#### website
[Github](https://github.com/csebuetnlp/xl-sum)
#### paper
[ACL Anthology](https://aclanthology.org/2021.findings-acl.413/)
## Dataset Overview
### Where to find the Data and its Documentation
#### Webpage
<!-- info: What is the webpage for the dataset (if it exists)? -->
<!-- scope: telescope -->
[Github](https://github.com/csebuetnlp/xl-sum)
#### Download
<!-- info: What is the link to where the original dataset is hosted? -->
<!-- scope: telescope -->
[Huggingface](https://huggingface.co/datasets/csebuetnlp/xlsum/tree/main/data)
#### Paper
<!-- info: What is the link to the paper describing the dataset (open access preferred)? -->
<!-- scope: telescope -->
[ACL Anthology](https://aclanthology.org/2021.findings-acl.413/)
#### BibTex
<!-- info: Provide the BibTex-formatted reference for the dataset. Please use the correct published version (ACL anthology, etc.) instead of google scholar created Bibtex. -->
<!-- scope: microscope -->
```
@inproceedings{hasan-etal-2021-xl,
title = "{XL}-Sum: Large-Scale Multilingual Abstractive Summarization for 44 Languages",
author = "Hasan, Tahmid and
Bhattacharjee, Abhik and
Islam, Md. Saiful and
Mubasshir, Kazi and
Li, Yuan-Fang and
Kang, Yong-Bin and
Rahman, M. Sohel and
Shahriyar, Rifat",
booktitle = "Findings of the Association for Computational Linguistics: ACL-IJCNLP 2021",
month = aug,
year = "2021",
address = "Online",
publisher = "Association for Computational Linguistics",
url = "https://aclanthology.org/2021.findings-acl.413",
pages = "4693--4703",
}
```
#### Contact Name
<!-- quick -->
<!-- info: If known, provide the name of at least one person the reader can contact for questions about the dataset. -->
<!-- scope: periscope -->
Tahmid Hasan
#### Contact Email
<!-- info: If known, provide the email of at least one person the reader can contact for questions about the dataset. -->
<!-- scope: periscope -->
tahmidhasan@cse.buet.ac.bd
#### Has a Leaderboard?
<!-- info: Does the dataset have an active leaderboard? -->
<!-- scope: telescope -->
yes
#### Leaderboard Link
<!-- info: Provide a link to the leaderboard. -->
<!-- scope: periscope -->
[Explainaboard](http://explainaboard.nlpedia.ai/leaderboard/task_xlsum/)
#### Leaderboard Details
<!-- info: Briefly describe how the leaderboard evaluates models. -->
<!-- scope: microscope -->
The leaderboard ranks models based on ROUGE scores (R1/R2/RL) of the generated summaries.
### Languages and Intended Use
#### Multilingual?
<!-- quick -->
<!-- info: Is the dataset multilingual? -->
<!-- scope: telescope -->
yes
#### Covered Languages
<!-- quick -->
<!-- info: What languages/dialects are covered in the dataset? -->
<!-- scope: telescope -->
`Amharic`, `Arabic`, `Azerbaijani`, `Bengali, Bangla`, `Burmese`, `Chinese (family)`, `English`, `French`, `Gujarati`, `Hausa`, `Hindi`, `Igbo`, `Indonesian`, `Japanese`, `Rundi`, `Korean`, `Kirghiz, Kyrgyz`, `Marathi`, `Nepali (individual language)`, `Oromo`, `Pushto, Pashto`, `Persian`, `Ghanaian Pidgin English`, `Portuguese`, `Panjabi, Punjabi`, `Russian`, `Scottish Gaelic, Gaelic`, `Serbian`, `Romano-Serbian`, `Sinhala, Sinhalese`, `Somali`, `Spanish, Castilian`, `Swahili (individual language), Kiswahili`, `Tamil`, `Telugu`, `Thai`, `Tigrinya`, `Turkish`, `Ukrainian`, `Urdu`, `Uzbek`, `Vietnamese`, `Welsh`, `Yoruba`
#### License
<!-- quick -->
<!-- info: What is the license of the dataset? -->
<!-- scope: telescope -->
cc-by-nc-sa-4.0: Creative Commons Attribution Non Commercial Share Alike 4.0 International
#### Intended Use
<!-- info: What is the intended use of the dataset? -->
<!-- scope: microscope -->
Abstractive summarization has centered around the English language, as most large abstractive summarization datasets are available in English only. Though there have been some recent efforts for curating multilingual abstractive summarization datasets, they are limited in terms of the number of languages covered, the number of training samples, or both. To this end, **XL-Sum** presents a large-scale abstractive summarization dataset of 1.35 million news articles from 45 languages crawled from the British Broadcasting Corporation website. It is intended to be used for both multilingual and per-language summarization tasks.
#### Primary Task
<!-- info: What primary task does the dataset support? -->
<!-- scope: telescope -->
Summarization
#### Communicative Goal
<!-- quick -->
<!-- info: Provide a short description of the communicative goal of a model trained for this task on this dataset. -->
<!-- scope: periscope -->
Summarize news-like text in one of 45 languages.
### Credit
#### Curation Organization Type(s)
<!-- info: In what kind of organization did the dataset curation happen? -->
<!-- scope: telescope -->
`academic`
#### Curation Organization(s)
<!-- info: Name the organization(s). -->
<!-- scope: periscope -->
Bangladesh University of Engineering and Technology
#### Who added the Dataset to GEM?
<!-- info: Who contributed to the data card and adding the dataset to GEM? List the people+affiliations involved in creating this data card and who helped integrate this dataset into GEM. -->
<!-- scope: microscope -->
Tahmid Hasan (Bangladesh University of Engineering and Technology), Abhik Bhattacharjee (Bangladesh University of Engineering and Technology)
### Dataset Structure
#### Data Fields
<!-- info: List and describe the fields present in the dataset. -->
<!-- scope: telescope -->
- `gem_id`: A string representing the article ID.
- `url`: A string representing the article URL.
- `title`: A string containing the article title.
- `summary`: A string containing the article summary.
- `text` : A string containing the article text.
#### Example Instance
<!-- info: Provide a JSON formatted example of a typical instance in the dataset. -->
<!-- scope: periscope -->
```
{
"gem_id": "GEM-xlsum_english-train-1589",
"url": "[BBC news](https://www.bbc.com/news)/technology-17657859",
"title": "Yahoo files e-book advert system patent applications",
"summary": "Yahoo has signalled it is investigating e-book adverts as a way to stimulate its earnings.",
"text": "Yahoo's patents suggest users could weigh the type of ads against the sizes of discount before purchase. It says in two US patent applications that ads for digital book readers have been \"less than optimal\" to date. The filings suggest that users could be offered titles at a variety of prices depending on the ads' prominence They add that the products shown could be determined by the type of book being read, or even the contents of a specific chapter, phrase or word. The paperwork was published by the US Patent and Trademark Office late last week and relates to work carried out at the firm's headquarters in Sunnyvale, California. \"Greater levels of advertising, which may be more valuable to an advertiser and potentially more distracting to an e-book reader, may warrant higher discounts,\" it states. Free books It suggests users could be offered ads as hyperlinks based within the book's text, in-laid text or even \"dynamic content\" such as video. Another idea suggests boxes at the bottom of a page could trail later chapters or quotes saying \"brought to you by Company A\". It adds that the more willing the customer is to see the ads, the greater the potential discount. \"Higher frequencies... may even be great enough to allow the e-book to be obtained for free,\" it states. The authors write that the type of ad could influence the value of the discount, with \"lower class advertising... such as teeth whitener advertisements\" offering a cheaper price than \"high\" or \"middle class\" adverts, for things like pizza. The inventors also suggest that ads could be linked to the mood or emotional state the reader is in as a they progress through a title. For example, they say if characters fall in love or show affection during a chapter, then ads for flowers or entertainment could be triggered. The patents also suggest this could applied to children's books - giving the Tom Hanks animated film Polar Express as an example. It says a scene showing a waiter giving the protagonists hot drinks \"may be an excellent opportunity to show an advertisement for hot cocoa, or a branded chocolate bar\". Another example states: \"If the setting includes young characters, a Coke advertisement could be provided, inviting the reader to enjoy a glass of Coke with his book, and providing a graphic of a cool glass.\" It adds that such targeting could be further enhanced by taking account of previous titles the owner has bought. 'Advertising-free zone' At present, several Amazon and Kobo e-book readers offer full-screen adverts when the device is switched off and show smaller ads on their menu screens, but the main text of the titles remains free of marketing. Yahoo does not currently provide ads to these devices, and a move into the area could boost its shrinking revenues. However, Philip Jones, deputy editor of the Bookseller magazine, said that the internet firm might struggle to get some of its ideas adopted. \"This has been mooted before and was fairly well decried,\" he said. \"Perhaps in a limited context it could work if the merchandise was strongly related to the title and was kept away from the text. \"But readers - particularly parents - like the fact that reading is an advertising-free zone. Authors would also want something to say about ads interrupting their narrative flow.\""
}
```
#### Data Splits
<!-- info: Describe and name the splits in the dataset if there are more than one. -->
<!-- scope: periscope -->
The splits in the dataset are specified by the language names, which are as follows:
- `amharic`
- `arabic`
- `azerbaijani`
- `bengali`
- `burmese`
- `chinese_simplified`
- `chinese_traditional`
- `english`
- `french`
- `gujarati`
- `hausa`
- `hindi`
- `igbo`
- `indonesian`
- `japanese`
- `kirundi`
- `korean`
- `kyrgyz`
- `marathi`
- `nepali`
- `oromo`
- `pashto`
- `persian`
- `pidgin`
- `portuguese`
- `punjabi`
- `russian`
- `scottish_gaelic`
- `serbian_cyrillic`
- `serbian_latin`
- `sinhala`
- `somali`
- `spanish`
- `swahili`
- `tamil`
- `telugu`
- `thai`
- `tigrinya`
- `turkish`
- `ukrainian`
- `urdu`
- `uzbek`
- `vietnamese`
- `welsh`
- `yoruba`
#### Splitting Criteria
<!-- info: Describe any criteria for splitting the data, if used. If there are differences between the splits (e.g., if the training annotations are machine-generated and the dev and test ones are created by humans, or if different numbers of annotators contributed to each example), describe them here. -->
<!-- scope: microscope -->
We used a 80%-10%-10% split for all languages with a few exceptions. `English` was split 93%-3.5%-3.5% for the evaluation set size to resemble that of `CNN/DM` and `XSum`; `Scottish Gaelic`, `Kyrgyz` and `Sinhala` had relatively fewer samples, their evaluation sets were increased to 500 samples for more reliable evaluation. Same articles were used for evaluation in the two variants of Chinese and Serbian to prevent data leakage in multilingual training. Individual dataset download links with train-dev-test example counts are given below:
Language | ISO 639-1 Code | BBC subdomain(s) | Train | Dev | Test | Total |
--------------|----------------|------------------|-------|-----|------|-------|
Amharic | am | [BBC amharic](https://www.bbc.com/amharic) | 5761 | 719 | 719 | 7199 |
Arabic | ar | [BBC arabic](https://www.bbc.com/arabic) | 37519 | 4689 | 4689 | 46897 |
Azerbaijani | az | [BBC azeri](https://www.bbc.com/azeri) | 6478 | 809 | 809 | 8096 |
Bengali | bn | [BBC bengali](https://www.bbc.com/bengali) | 8102 | 1012 | 1012 | 10126 |
Burmese | my | [BBC burmese](https://www.bbc.com/burmese) | 4569 | 570 | 570 | 5709 |
Chinese (Simplified) | zh-CN | [BBC ukchina](https://www.bbc.com/ukchina)/simp, [BBC zhongwen](https://www.bbc.com/zhongwen)/simp | 37362 | 4670 | 4670 | 46702 |
Chinese (Traditional) | zh-TW | [BBC ukchina](https://www.bbc.com/ukchina)/trad, [BBC zhongwen](https://www.bbc.com/zhongwen)/trad | 37373 | 4670 | 4670 | 46713 |
English | en | [BBC english](https://www.bbc.com/english), [BBC sinhala](https://www.bbc.com/sinhala) `*` | 306522 | 11535 | 11535 | 329592 |
French | fr | [BBC afrique](https://www.bbc.com/afrique) | 8697 | 1086 | 1086 | 10869 |
Gujarati | gu | [BBC gujarati](https://www.bbc.com/gujarati) | 9119 | 1139 | 1139 | 11397 |
Hausa | ha | [BBC hausa](https://www.bbc.com/hausa) | 6418 | 802 | 802 | 8022 |
Hindi | hi | [BBC hindi](https://www.bbc.com/hindi) | 70778 | 8847 | 8847 | 88472 |
Igbo | ig | [BBC igbo](https://www.bbc.com/igbo) | 4183 | 522 | 522 | 5227 |
Indonesian | id | [BBC indonesia](https://www.bbc.com/indonesia) | 38242 | 4780 | 4780 | 47802 |
Japanese | ja | [BBC japanese](https://www.bbc.com/japanese) | 7113 | 889 | 889 | 8891 |
Kirundi | rn | [BBC gahuza](https://www.bbc.com/gahuza) | 5746 | 718 | 718 | 7182 |
Korean | ko | [BBC korean](https://www.bbc.com/korean) | 4407 | 550 | 550 | 5507 |
Kyrgyz | ky | [BBC kyrgyz](https://www.bbc.com/kyrgyz) | 2266 | 500 | 500 | 3266 |
Marathi | mr | [BBC marathi](https://www.bbc.com/marathi) | 10903 | 1362 | 1362 | 13627 |
Nepali | np | [BBC nepali](https://www.bbc.com/nepali) | 5808 | 725 | 725 | 7258 |
Oromo | om | [BBC afaanoromoo](https://www.bbc.com/afaanoromoo) | 6063 | 757 | 757 | 7577 |
Pashto | ps | [BBC pashto](https://www.bbc.com/pashto) | 14353 | 1794 | 1794 | 17941 |
Persian | fa | [BBC persian](https://www.bbc.com/persian) | 47251 | 5906 | 5906 | 59063 |
Pidgin`**` | pcm | [BBC pidgin](https://www.bbc.com/pidgin) | 9208 | 1151 | 1151 | 11510 |
Portuguese | pt | [BBC portuguese](https://www.bbc.com/portuguese) | 57402 | 7175 | 7175 | 71752 |
Punjabi | pa | [BBC punjabi](https://www.bbc.com/punjabi) | 8215 | 1026 | 1026 | 10267 |
Russian | ru | [BBC russian](https://www.bbc.com/russian), [BBC ukrainian](https://www.bbc.com/ukrainian) `*` | 62243 | 7780 | 7780 | 77803 |
Scottish Gaelic | gd | [BBC naidheachdan](https://www.bbc.com/naidheachdan) | 1313 | 500 | 500 | 2313 |
Serbian (Cyrillic) | sr | [BBC serbian](https://www.bbc.com/serbian)/cyr | 7275 | 909 | 909 | 9093 |
Serbian (Latin) | sr | [BBC serbian](https://www.bbc.com/serbian)/lat | 7276 | 909 | 909 | 9094 |
Sinhala | si | [BBC sinhala](https://www.bbc.com/sinhala) | 3249 | 500 | 500 | 4249 |
Somali | so | [BBC somali](https://www.bbc.com/somali) | 5962 | 745 | 745 | 7452 |
Spanish | es | [BBC mundo](https://www.bbc.com/mundo) | 38110 | 4763 | 4763 | 47636 |
Swahili | sw | [BBC swahili](https://www.bbc.com/swahili) | 7898 | 987 | 987 | 9872 |
Tamil | ta | [BBC tamil](https://www.bbc.com/tamil) | 16222 | 2027 | 2027 | 20276 |
Telugu | te | [BBC telugu](https://www.bbc.com/telugu) | 10421 | 1302 | 1302 | 13025 |
Thai | th | [BBC thai](https://www.bbc.com/thai) | 6616 | 826 | 826 | 8268 |
Tigrinya | ti | [BBC tigrinya](https://www.bbc.com/tigrinya) | 5451 | 681 | 681 | 6813 |
Turkish | tr | [BBC turkce](https://www.bbc.com/turkce) | 27176 | 3397 | 3397 | 33970 |
Ukrainian | uk | [BBC ukrainian](https://www.bbc.com/ukrainian) | 43201 | 5399 | 5399 | 53999 |
Urdu | ur | [BBC urdu](https://www.bbc.com/urdu) | 67665 | 8458 | 8458 | 84581 |
Uzbek | uz | [BBC uzbek](https://www.bbc.com/uzbek) | 4728 | 590 | 590 | 5908 |
Vietnamese | vi | [BBC vietnamese](https://www.bbc.com/vietnamese) | 32111 | 4013 | 4013 | 40137 |
Welsh | cy | [BBC cymrufyw](https://www.bbc.com/cymrufyw) | 9732 | 1216 | 1216 | 12164 |
Yoruba | yo | [BBC yoruba](https://www.bbc.com/yoruba) | 6350 | 793 | 793 | 7936 |
`*` A lot of articles in BBC Sinhala and BBC Ukrainian were written in English and Russian respectively. They were identified using [Fasttext](https://arxiv.org/abs/1607.01759) and moved accordingly.
`**` West African Pidgin English
## Dataset in GEM
### Rationale for Inclusion in GEM
#### Why is the Dataset in GEM?
<!-- info: What does this dataset contribute toward better generation evaluation and why is it part of GEM? -->
<!-- scope: microscope -->
Traditional abstractive text summarization has been centered around English and other high-resource languages. **XL-Sum** provides a large collection of high-quality article-summary pairs for 45 languages where the languages range from high-resource to extremely low-resource. This enables the research community to explore the summarization capabilities of different models for multiple languages and languages in isolation. We believe the addition of **XL-Sum** to GEM makes the domain of abstractive text summarization more diversified and inclusive to the research community. We hope our efforts in this work will encourage the community to push the boundaries of abstractive text summarization beyond the English language, especially for low and mid-resource languages, bringing technological advances to communities of these languages that have been traditionally under-served.
#### Similar Datasets
<!-- info: Do other datasets for the high level task exist? -->
<!-- scope: telescope -->
yes
#### Unique Language Coverage
<!-- info: Does this dataset cover other languages than other datasets for the same task? -->
<!-- scope: periscope -->
yes
#### Difference from other GEM datasets
<!-- info: What else sets this dataset apart from other similar datasets in GEM? -->
<!-- scope: microscope -->
The summaries are highly concise and abstractive.
#### Ability that the Dataset measures
<!-- info: What aspect of model ability can be measured with this dataset? -->
<!-- scope: periscope -->
Conciseness, abstractiveness, and overall summarization capability.
### GEM-Specific Curation
#### Modificatied for GEM?
<!-- info: Has the GEM version of the dataset been modified in any way (data, processing, splits) from the original curated data? -->
<!-- scope: telescope -->
no
#### Additional Splits?
<!-- info: Does GEM provide additional splits to the dataset? -->
<!-- scope: telescope -->
no
### Getting Started with the Task
## Previous Results
### Previous Results
#### Measured Model Abilities
<!-- info: What aspect of model ability can be measured with this dataset? -->
<!-- scope: telescope -->
Conciseness, abstractiveness, and overall summarization capability.
#### Metrics
<!-- info: What metrics are typically used for this task? -->
<!-- scope: periscope -->
`ROUGE`
#### Proposed Evaluation
<!-- info: List and describe the purpose of the metrics and evaluation methodology (including human evaluation) that the dataset creators used when introducing this task. -->
<!-- scope: microscope -->
ROUGE is the de facto evaluation metric used for text summarization. However, it was designed specifically for evaluating English texts. Due to the nature of the metric, scores are heavily dependent on text tokenization / stemming / unnecessary character removal, etc. Some modifications to the original ROUGE evaluation were done such as punctuation only removal, language specific tokenization/stemming to enable reliable comparison of source and target summaries across different scripts.
#### Previous results available?
<!-- info: Are previous results available? -->
<!-- scope: telescope -->
no
## Dataset Curation
### Original Curation
#### Original Curation Rationale
<!-- info: Original curation rationale -->
<!-- scope: telescope -->
State-of-the-art text summarization models are heavily data-driven, i.e., a large number of article-summary pairs are required to train them effectively. As a result, abstractive summarization has centered around the English language, as most large abstractive summarization datasets are available in English only. Though there have been some recent efforts for curating multilingual abstractive summarization datasets, they are limited in terms of the number of languages covered, the number of training samples, or both. To this end, we curate **XL-Sum**, a large-scale abstractive summarization dataset of 1.35 million news articles from 45 languages crawled from the British Broadcasting Corporation website.
#### Communicative Goal
<!-- info: What was the communicative goal? -->
<!-- scope: periscope -->
Introduce new languages in the english-centric domain of abstractive text summarization and enable both multilingual and per-language summarization.
#### Sourced from Different Sources
<!-- info: Is the dataset aggregated from different data sources? -->
<!-- scope: telescope -->
yes
#### Source Details
<!-- info: List the sources (one per line) -->
<!-- scope: periscope -->
British Broadcasting Corporation (BBC) news websites.
### Language Data
#### How was Language Data Obtained?
<!-- info: How was the language data obtained? -->
<!-- scope: telescope -->
`Found`
#### Where was it found?
<!-- info: If found, where from? -->
<!-- scope: telescope -->
`Multiple websites`
#### Language Producers
<!-- info: What further information do we have on the language producers? -->
<!-- scope: microscope -->
The language content was written by professional news editors hired by BBC.
#### Topics Covered
<!-- info: Does the language in the dataset focus on specific topics? How would you describe them? -->
<!-- scope: periscope -->
News
#### Data Validation
<!-- info: Was the text validated by a different worker or a data curator? -->
<!-- scope: telescope -->
not validated
#### Data Preprocessing
<!-- info: How was the text data pre-processed? (Enter N/A if the text was not pre-processed) -->
<!-- scope: microscope -->
We used 'NFKC' normalization on all text instances.
#### Was Data Filtered?
<!-- info: Were text instances selected or filtered? -->
<!-- scope: telescope -->
algorithmically
#### Filter Criteria
<!-- info: What were the selection criteria? -->
<!-- scope: microscope -->
We designed a crawler to recursively crawl pages starting from the homepage by visiting different article links present in each page visited. We were able to take advantage of the fact that all BBC sites have somewhat similar structures, and were able to scrape articles from all sites. We discarded pages with no textual contents (mostly pages consisting of multimedia contents) before further processing. We designed a number of heuristics to make the extraction effective by carefully examining the HTML structures of the crawled pages:
1. The desired summary must be present within the beginning two paragraphs of an article.
2. The summary paragraph must have some portion of texts in bold format.
3. The summary paragraph may contain some hyperlinks that may not be bold. The proportion of bold texts and hyperlinked texts to the total length of the paragraph in consideration must be at least 95\%.
4. All texts except the summary and the headline must be included in the input text (including image captions).
5. The input text must be at least twice as large as the summary.
### Structured Annotations
#### Additional Annotations?
<!-- quick -->
<!-- info: Does the dataset have additional annotations for each instance? -->
<!-- scope: telescope -->
none
#### Annotation Service?
<!-- info: Was an annotation service used? -->
<!-- scope: telescope -->
no
### Consent
#### Any Consent Policy?
<!-- info: Was there a consent policy involved when gathering the data? -->
<!-- scope: telescope -->
yes
#### Consent Policy Details
<!-- info: What was the consent policy? -->
<!-- scope: microscope -->
BBC's policy specifies that the text content within its websites can be used for non-commercial research only.
### Private Identifying Information (PII)
#### Contains PII?
<!-- quick -->
<!-- info: Does the source language data likely contain Personal Identifying Information about the data creators or subjects? -->
<!-- scope: telescope -->
likely
#### Categories of PII
<!-- info: What categories of PII are present or suspected in the data? -->
<!-- scope: periscope -->
`generic PII`
#### Any PII Identification?
<!-- info: Did the curators use any automatic/manual method to identify PII in the dataset? -->
<!-- scope: periscope -->
no identification
### Maintenance
#### Any Maintenance Plan?
<!-- info: Does the original dataset have a maintenance plan? -->
<!-- scope: telescope -->
no
## Broader Social Context
### Previous Work on the Social Impact of the Dataset
#### Usage of Models based on the Data
<!-- info: Are you aware of cases where models trained on the task featured in this dataset ore related tasks have been used in automated systems? -->
<!-- scope: telescope -->
no
### Impact on Under-Served Communities
#### Addresses needs of underserved Communities?
<!-- info: Does this dataset address the needs of communities that are traditionally underserved in language technology, and particularly language generation technology? Communities may be underserved for exemple because their language, language variety, or social or geographical context is underepresented in NLP and NLG resources (datasets and models). -->
<!-- scope: telescope -->
yes
#### Details on how Dataset Addresses the Needs
<!-- info: Describe how this dataset addresses the needs of underserved communities. -->
<!-- scope: microscope -->
This dataset introduces summarization corpus for many languages where there weren't any datasets like this curated before.
### Discussion of Biases
#### Any Documented Social Biases?
<!-- info: Are there documented social biases in the dataset? Biases in this context are variations in the ways members of different social categories are represented that can have harmful downstream consequences for members of the more disadvantaged group. -->
<!-- scope: telescope -->
no
#### Are the Language Producers Representative of the Language?
<!-- info: Does the distribution of language producers in the dataset accurately represent the full distribution of speakers of the language world-wide? If not, how does it differ? -->
<!-- scope: periscope -->
Yes
## Considerations for Using the Data
### PII Risks and Liability
### Licenses
#### Copyright Restrictions on the Dataset
<!-- info: Based on your answers in the Intended Use part of the Data Overview Section, which of the following best describe the copyright and licensing status of the dataset? -->
<!-- scope: periscope -->
`research use only`, `non-commercial use only`
#### Copyright Restrictions on the Language Data
<!-- info: Based on your answers in the Language part of the Data Curation Section, which of the following best describe the copyright and licensing status of the underlying language data? -->
<!-- scope: periscope -->
`research use only`, `non-commercial use only`
### Known Technical Limitations
#### Technical Limitations
<!-- info: Describe any known technical limitations, such as spurrious correlations, train/test overlap, annotation biases, or mis-annotations, and cite the works that first identified these limitations when possible. -->
<!-- scope: microscope -->
Human evaluation showed most languages had a high percentage of good summaries in the upper nineties, almost none of the summaries contained any conflicting information, while about one-third on average had information that was not directly inferrable from the source article. Since generally multiple articles are written regarding an important event, there could be an overlap between the training and evaluation data in terms on content.
#### Unsuited Applications
<!-- info: When using a model trained on this dataset in a setting where users or the public may interact with its predictions, what are some pitfalls to look out for? In particular, describe some applications of the general task featured in this dataset that its curation or properties make it less suitable for. -->
<!-- scope: microscope -->
The dataset is limited to news domain only. Hence it wouldn't be advisable to use a model trained on this dataset for summarizing texts from a different domain i.e. literature, scientific text etc. Another pitfall could be hallucinations in the model generated summary.
#### Discouraged Use Cases
<!-- info: What are some discouraged use cases of a model trained to maximize the proposed metrics on this dataset? In particular, think about settings where decisions made by a model that performs reasonably well on the metric my still have strong negative consequences for user or members of the public. -->
<!-- scope: microscope -->
ROUGE evaluates the quality of the summary as a whole by considering up to 4-gram overlaps. Therefore, in an article about India if the word "India" in the generated summary gets replaced by "Pakistan" due to model hallucination, the overall score wouldn't be reduced significantly, but the entire meaning could get changed.
|
GEM/xsum | ---
annotations_creators:
- none
language_creators:
- unknown
language:
- en
license:
- cc-by-sa-4.0
multilinguality:
- unknown
size_categories:
- unknown
source_datasets:
- original
task_categories:
- summarization
task_ids: []
pretty_name: xsum
---
# Dataset Card for GEM/xsum
## Dataset Description
- **Homepage:** n/a
- **Repository:** https://github.com/EdinburghNLP/XSum
- **Paper:** https://www.aclweb.org/anthology/D18-1206
- **Leaderboard:** N/A
- **Point of Contact:** Shashi Narayan
### Link to Main Data Card
You can find the main data card on the [GEM Website](https://gem-benchmark.com/data_cards/xsum).
### Dataset Summary
XSum is an English news summarization dataset where the task is to predict the first sentence of an article from the rest of it.
You can load the dataset via:
```
import datasets
data = datasets.load_dataset('GEM/xsum')
```
The data loader can be found [here](https://huggingface.co/datasets/GEM/xsum).
#### website
n/a
#### paper
[ACL Anthology](https://www.aclweb.org/anthology/D18-1206)
#### authors
Shashi Narayan, Shay B. Cohen, Mirella Lapata (all affiliated with University of Edinburgh at the time of dataset creation)
## Dataset Overview
### Where to find the Data and its Documentation
#### Download
<!-- info: What is the link to where the original dataset is hosted? -->
<!-- scope: telescope -->
[Github](https://github.com/EdinburghNLP/XSum)
#### Paper
<!-- info: What is the link to the paper describing the dataset (open access preferred)? -->
<!-- scope: telescope -->
[ACL Anthology](https://www.aclweb.org/anthology/D18-1206)
#### BibTex
<!-- info: Provide the BibTex-formatted reference for the dataset. Please use the correct published version (ACL anthology, etc.) instead of google scholar created Bibtex. -->
<!-- scope: microscope -->
```
@InProceedings{xsum-emnlp,
author = "Shashi Narayan and Shay B. Cohen and Mirella Lapata",
title = "Don't Give Me the Details, Just the Summary! {T}opic-Aware Convolutional Neural Networks for Extreme Summarization",
booktitle = "Proceedings of the 2018 Conference on Empirical Methods in Natural Language Processing ",
year = "2018",
address = "Brussels, Belgium",
}
```
#### Contact Name
<!-- quick -->
<!-- info: If known, provide the name of at least one person the reader can contact for questions about the dataset. -->
<!-- scope: periscope -->
Shashi Narayan
#### Contact Email
<!-- info: If known, provide the email of at least one person the reader can contact for questions about the dataset. -->
<!-- scope: periscope -->
shashinarayan@google.com
#### Has a Leaderboard?
<!-- info: Does the dataset have an active leaderboard? -->
<!-- scope: telescope -->
no
### Languages and Intended Use
#### Multilingual?
<!-- quick -->
<!-- info: Is the dataset multilingual? -->
<!-- scope: telescope -->
no
#### Covered Dialects
<!-- info: What dialects are covered? Are there multiple dialects per language? -->
<!-- scope: periscope -->
Since the source of the dataset are BBC articles, the language is in British English of the variation written by journalists.
#### Covered Languages
<!-- quick -->
<!-- info: What languages/dialects are covered in the dataset? -->
<!-- scope: telescope -->
`English`
#### Whose Language?
<!-- info: Whose language is in the dataset? -->
<!-- scope: periscope -->
Professional journalists
#### License
<!-- quick -->
<!-- info: What is the license of the dataset? -->
<!-- scope: telescope -->
cc-by-sa-4.0: Creative Commons Attribution Share Alike 4.0 International
#### Intended Use
<!-- info: What is the intended use of the dataset? -->
<!-- scope: microscope -->
The dataset is for the task of abstractive summarization in its extreme form, its about summarizing a document in a single sentence. The idea is to create a short, one-sentence news summary answering the question "What is the article about?".
#### Primary Task
<!-- info: What primary task does the dataset support? -->
<!-- scope: telescope -->
Summarization
#### Communicative Goal
<!-- quick -->
<!-- info: Provide a short description of the communicative goal of a model trained for this task on this dataset. -->
<!-- scope: periscope -->
Given a news article, produce a single sentence summary of the content of the article.
### Credit
#### Curation Organization Type(s)
<!-- info: In what kind of organization did the dataset curation happen? -->
<!-- scope: telescope -->
`academic`
#### Curation Organization(s)
<!-- info: Name the organization(s). -->
<!-- scope: periscope -->
University of Edinburgh
#### Dataset Creators
<!-- info: Who created the original dataset? List the people involved in collecting the dataset and their affiliation(s). -->
<!-- scope: microscope -->
Shashi Narayan, Shay B. Cohen, Mirella Lapata (all affiliated with University of Edinburgh at the time of dataset creation)
#### Funding
<!-- info: Who funded the data creation? -->
<!-- scope: microscope -->
European Research Council (Lapata; award number 681760), the European Union under the Horizon 2020 SUMMA project (Narayan, Cohen; grant agreement 688139), and Huawei Technologies (Cohen).
#### Who added the Dataset to GEM?
<!-- info: Who contributed to the data card and adding the dataset to GEM? List the people+affiliations involved in creating this data card and who helped integrate this dataset into GEM. -->
<!-- scope: microscope -->
The original data card was written by Laura Perez-Beltrachini and the data loader by Yacine Jernite. Sebastian Gehrmann migrated the data card to the new format and extended it. The v2 data loader was migrated by Abinaya Mahendiran
### Dataset Structure
#### Data Fields
<!-- info: List and describe the fields present in the dataset. -->
<!-- scope: telescope -->
- `Document`: Input news article.
- `Summary`: One sentence summary of the article.
- `Id`: BBC ID of the article.
#### Reason for Structure
<!-- info: How was the dataset structure determined? -->
<!-- scope: microscope -->
The Document/Summary format is standard for summarization datasets.
#### How were labels chosen?
<!-- info: How were the labels chosen? -->
<!-- scope: microscope -->
The labels are the first sentence of the source article.
#### Example Instance
<!-- info: Provide a JSON formatted example of a typical instance in the dataset. -->
<!-- scope: periscope -->
```
{
'document': 'The researchers have sequenced the genome of a strain of bacterium that causes the virulent infection.\nA survey in 2007 showed that bleeding canker had spread rapidly, with almost half of the two million horse chestnuts displaying symptoms of the disease.\nThe findings have been published in the journal PLoS One.\nA visible symptom of the disease is a lesion on the bark, which oozes a resin on to the trunk or sometimes the branches.\nThe bark underneath the canker is killed, and if cankers manage to go all the way around the trunk then the horse chestnut (Aesculus hippocastanum) will die because it cuts off the food supply. [...]',
'target': "A team of UK scientists hopes to shed light on the mysteries of bleeding canker, a disease that is threatening the nation's horse chestnut trees.",
}
```
#### Data Splits
<!-- info: Describe and name the splits in the dataset if there are more than one. -->
<!-- scope: periscope -->
| Section | Number of Documents |
| ------------- |:-------------:|
| Training | 204,045 |
| Validation | 11,332 |
| Testing | 11,334 |
| Total | 226k |
| Section | number of words| number of sentences |
| ------------- |:-------------:| :-------------:|
| Documents | 431.07 | 19.77 |
| Summary | 23.26 | 1.00 |
#### Splitting Criteria
<!-- info: Describe any criteria for splitting the data, if used. If there are differences between the splits (e.g., if the training annotations are machine-generated and the dev and test ones are created by humans, or if different numbers of annotators contributed to each example), describe them here. -->
<!-- scope: microscope -->
The identifiers in the URLs were used to randomly split the dataset into training (90%, 204,045), validation (5%, 11,332), and test (5%, 11,334) sets.
## Dataset Curation
### Original Curation
#### Original Curation Rationale
<!-- info: Original curation rationale -->
<!-- scope: telescope -->
Comparable datasets are often very extractive which is not a strategy that works for one-sentence summaries. The dataset curators thus created this dataset as a way to evaluate truly abstractive models
#### Communicative Goal
<!-- info: What was the communicative goal? -->
<!-- scope: periscope -->
Same as the communicative goal in GEM: A model should summarize a news article in a single sentence
#### Sourced from Different Sources
<!-- info: Is the dataset aggregated from different data sources? -->
<!-- scope: telescope -->
no
### Language Data
#### How was Language Data Obtained?
<!-- info: How was the language data obtained? -->
<!-- scope: telescope -->
`Found`
#### Where was it found?
<!-- info: If found, where from? -->
<!-- scope: telescope -->
`Single website`
#### Language Producers
<!-- info: What further information do we have on the language producers? -->
<!-- scope: microscope -->
The data was collected from articles between 2010 and 2017. No other information
#### Topics Covered
<!-- info: Does the language in the dataset focus on specific topics? How would you describe them? -->
<!-- scope: periscope -->
The collected articles included the following topics: News, Politics, Sports, Weather, Business, Technology, Science, Health, Family, Education, Entertainment and Arts
The dataset curators also used LDA to gain insight into this question and found that the following were the top keywords associated with each topic:
- **T1**: charge, court, murder, police, arrest, guilty, sentence, boy, bail, space, crown, trial
- **T2**: church, abuse, bishop, child, catholic, gay, pope, school, christian, priest, cardinal
- **T3**: council, people, government, local, housing, home, house, property, city, plan, authority
- **T4**: clinton, party, trump, climate, poll, vote, plaid, election, debate, change, candidate, campaign
- **T5**: country, growth, report, business, export, fall, bank, security, economy, rise, global, inflation
- **T6**: hospital, patient, trust, nhs, people, care, health, service, staff, report, review, system, child
#### Data Validation
<!-- info: Was the text validated by a different worker or a data curator? -->
<!-- scope: telescope -->
not validated
#### Data Preprocessing
<!-- info: How was the text data pre-processed? (Enter N/A if the text was not pre-processed) -->
<!-- scope: microscope -->
The text was extracted from the HTML of the webpage. No further processing was done.
#### Was Data Filtered?
<!-- info: Were text instances selected or filtered? -->
<!-- scope: telescope -->
not filtered
### Structured Annotations
#### Additional Annotations?
<!-- quick -->
<!-- info: Does the dataset have additional annotations for each instance? -->
<!-- scope: telescope -->
none
#### Annotation Service?
<!-- info: Was an annotation service used? -->
<!-- scope: telescope -->
no
### Consent
#### Any Consent Policy?
<!-- info: Was there a consent policy involved when gathering the data? -->
<!-- scope: telescope -->
no
#### Justification for Using the Data
<!-- info: If not, what is the justification for reusing the data? -->
<!-- scope: microscope -->
The copyright license of the data allows reusing it for this purpose.
### Private Identifying Information (PII)
#### Contains PII?
<!-- quick -->
<!-- info: Does the source language data likely contain Personal Identifying Information about the data creators or subjects? -->
<!-- scope: telescope -->
yes/very likely
#### Categories of PII
<!-- info: What categories of PII are present or suspected in the data? -->
<!-- scope: periscope -->
`generic PII`
#### Any PII Identification?
<!-- info: Did the curators use any automatic/manual method to identify PII in the dataset? -->
<!-- scope: periscope -->
no identification
### Maintenance
#### Any Maintenance Plan?
<!-- info: Does the original dataset have a maintenance plan? -->
<!-- scope: telescope -->
no
## Broader Social Context
### Previous Work on the Social Impact of the Dataset
#### Usage of Models based on the Data
<!-- info: Are you aware of cases where models trained on the task featured in this dataset ore related tasks have been used in automated systems? -->
<!-- scope: telescope -->
no
### Impact on Under-Served Communities
#### Addresses needs of underserved Communities?
<!-- info: Does this dataset address the needs of communities that are traditionally underserved in language technology, and particularly language generation technology? Communities may be underserved for exemple because their language, language variety, or social or geographical context is underepresented in NLP and NLG resources (datasets and models). -->
<!-- scope: telescope -->
no
### Discussion of Biases
#### Any Documented Social Biases?
<!-- info: Are there documented social biases in the dataset? Biases in this context are variations in the ways members of different social categories are represented that can have harmful downstream consequences for members of the more disadvantaged group. -->
<!-- scope: telescope -->
unsure
#### Are the Language Producers Representative of the Language?
<!-- info: Does the distribution of language producers in the dataset accurately represent the full distribution of speakers of the language world-wide? If not, how does it differ? -->
<!-- scope: periscope -->
The language and content of the data is focused on news and language in the UK and as such not representative of the speakers world-wide. Existing selection biases of the BBC exist in this dataset.
|
GEM-submissions/GEM__bart_base_schema_guided_dialog__1645547915 | ---
benchmark: gem
type: prediction
submission_name: BART_BASE_schema_guided_dialog
---
|
GEM-submissions/Leo__bart-large__1645784880 | ---
benchmark: gem
type: prediction
submission_name: bart-large
---
|
GEM-submissions/Leo__mbart-large-cc25__1645802644 | ---
benchmark: gem
type: prediction
submission_name: mbart-large-cc25
---
|
GEM-submissions/lewtun__hugging-face-test-t5-base.outputs.json-36bf2a59__1645558682 | ---
benchmark: gem
type: prediction
submission_name: Hugging Face test T5-base.outputs.json 36bf2a59
---
|
GEM-submissions/lewtun__hugging-face-test-t5-base.outputs.json-36bf2a59__1645559101 | ---
benchmark: gem
type: prediction
submission_name: Hugging Face test T5-base.outputs.json 36bf2a59
---
|
GEM-submissions/lewtun__hugging-face-test-t5-base.outputs.json-36bf2a59__1645800191 | ---
benchmark: gem
type: prediction
submission_name: Hugging Face test T5-base.outputs.json 36bf2a59
---
|
GEM-submissions/lewtun__hugging-face-test-t5-base.outputs.json-36bf2a59__1646049378 | ---
benchmark: gem
type: prediction
submission_name: Hugging Face test T5-base.outputs.json 36bf2a59
---
|
GEM-submissions/lewtun__hugging-face-test-t5-base.outputs.json-36bf2a59__1646049424 | ---
benchmark: gem
type: prediction
submission_name: Hugging Face test T5-base.outputs.json 36bf2a59
---
|
GEM-submissions/lewtun__hugging-face-test-t5-base.outputs.json-36bf2a59__1646049601 | ---
benchmark: gem
type: prediction
submission_name: Hugging Face test T5-base.outputs.json 36bf2a59
---
|
GEM-submissions/lewtun__hugging-face-test-t5-base.outputs.json-36bf2a59__1646049876 | ---
benchmark: gem
type: prediction
submission_name: Hugging Face test T5-base.outputs.json 36bf2a59
---
|
GEM-submissions/lewtun__hugging-face-test-t5-base.outputs.json-36bf2a59__1646050898 | ---
benchmark: gem
type: prediction
submission_name: Hugging Face test T5-base.outputs.json 36bf2a59
---
|
GEM-submissions/lewtun__hugging-face-test-t5-base.outputs.json-36bf2a59__1646051364 | ---
benchmark: gem
type: prediction
submission_name: Hugging Face test T5-base.outputs.json 36bf2a59
---
|
GEM-submissions/lewtun__hugging-face-test-t5-base.outputs.json-36bf2a59__1646052073 | ---
benchmark: gem
type: prediction
submission_name: Hugging Face test T5-base.outputs.json 36bf2a59
tags:
- evaluation
- benchmark
---
# GEM Submission
Submission name: Hugging Face test T5-base.outputs.json 36bf2a59
|
GEM-submissions/lewtun__this-is-a-test__1646052811 | ---
benchmark: gem
type: prediction
submission_name: This is a test
tags:
- evaluation
- benchmark
---
# GEM Submission
Submission name: This is a test
|
GEM-submissions/lewtun__this-is-a-test__1646230987 | ---
benchmark: gem
type: prediction
submission_name: This is a test
tags:
- evaluation
- benchmark
---
# GEM Submission
Submission name: This is a test
|
GEM-submissions/ratishsp | ---
benchmark: gem
type: prediction
submission_name: Template
---
|
Gabriel/quora_swe | ---
language:
- sv
license:
- mit
size_categories:
- 10K<n<100K
task_categories:
- text-retrieval
- text-classification
task_ids:
- semantic-similarity-classification
tags:
- question-pairing
- semantic-search
---
# Dataset Card for "quora_swe"
The dataset quora_swe is a subset of the automatically translated (MNT) Swedish Semantic Textual Similarity dataset: quora-deduplicates .
|
Gauravadlakha1509/new_one | test |
GonzaloA/fake_news | TODO: Add YAML tags here. Copy-paste the tags obtained with the online tagging app: https://huggingface.co/spaces/huggingface/datasets-tagging
---
annotations_creators:
- no-annotation
language_creators:
- found
language:
- en
license:
- unknown
multilinguality:
- monolingual
size_categories:
- 30k<n<50k
source_datasets:
- original
task_categories:
- text-classification
task_ids:
- fact-checking
- intent-classification
pretty_name: GonzaloA / Fake News
---
# Dataset Card for [Fake_News_TFG]
## Table of Contents
- [Table of Contents](#table-of-contents)
- [Dataset Description](#dataset-description)
- [Dataset Summary](#dataset-summary)
- [Supported Tasks and Leaderboards](#supported-tasks-and-leaderboards)
- [Languages](#languages)
- [Dataset Structure](#dataset-structure)
- [Data Instances](#data-instances)
- [Data Fields](#data-fields)
- [Data Splits](#data-splits)
- [Dataset Creation](#dataset-creation)
- [Curation Rationale](#curation-rationale)
- [Source Data](#source-data)
- [Annotations](#annotations)
- [Personal and Sensitive Information](#personal-and-sensitive-information)
- [Considerations for Using the Data](#considerations-for-using-the-data)
- [Social Impact of Dataset](#social-impact-of-dataset)
- [Discussion of Biases](#discussion-of-biases)
- [Other Known Limitations](#other-known-limitations)
- [Additional Information](#additional-information)
- [Dataset Curators](#dataset-curators)
- [Licensing Information](#licensing-information)
- [Citation Information](#citation-information)
- [Contributions](#contributions)
## Dataset Description
- **Homepage:**
- **Repository:** [GonzaloA / fake_news]
- **Paper:** [Título del TFG]
- **Leaderboard:**
- **Point of Contact:** [Gonzalo Álvarez Hervás](mailto:g.alvarez.2018@alumnos.urjc.es)
### Dataset Summary
The GonzaloA / Fake_News_TFG Dataset repository is an English-language dataset containing just over 45k unique news articles. This articles are classified as true (1) or false (0). The current version is the first of the study the Fake News identification using Transformers models.
### Supported Tasks and Leaderboards
[More Information Needed]
### Languages
The dataset is code for English as generally spoken in the United States is en-US
## Dataset Structure
The structure of this dataSet is composed by 40587 fields about News. This fields are composed by three types of fields; title of the news, the text or content of the news, and finally, the value of the news, who said if the new are fake (0) or true (1).
### Data Instances
For each instance, there is a string for the title, a string for the article and a label to mark if it's true or false. See the [CNN / Daily Mail dataset viewer](https://huggingface.co/datasets/viewer/?dataset=fake_news&config=3.0.0) to explore more examples.
```
{'id': '1',
'title': Palestinians switch off Christmas lights in Bethlehem in anti-Trump protest'
'text': 'RAMALLAH, West Bank (Reuters) - Palestinians switched off Christmas lights at Jesus traditional birthplace in Bethlehem on Wednesday night in protest at U.S. President Donald Trump s decision to recognize Jerusalem as Israel s capital. A Christmas tree adorned with lights outside Bethlehem s Church of the Nativity, where Christians believe Jesus was born, and another in Ramallah, next to the burial site of former Palestinian leader Yasser Arafat, were plunged into darkness. The Christmas tree was switched off on the order of the mayor today in protest at Trump s decision, said Fady Ghattas, Bethlehem s municipal media officer. He said it was unclear whether the illuminations would be turned on again before the main Christmas festivities. In a speech in Washington, Trump said he had decided to recognize Jerusalem as Israel s capital and move the U.S. embassy to the city. Israeli Prime Minister Benjamin Netanyahu said Trump s move marked the beginning of a new approach to the Israeli-Palestinian conflict and said it was an historic landmark . Arabs and Muslims across the Middle East condemned the U.S. decision, calling it an incendiary move in a volatile region and the European Union and United Nations also voiced alarm at the possible repercussions for any chances of reviving Israeli-Palestinian peacemaking.'
'label': '1'}
```
### Data Fields
- `id`: an integer value to count the rows in the dataset
- `title`: a string that summarize the article
- `text`: a string that contains the article
- `label`: a boolean that mark the article true or false
### Data Splits
The GonzaloA/FakeNews dataset has 3 splits: train, evaluation, and test. Below are the statistics for the version 1.0 of the dataset:
| Dataset Split | Number of Instances in Split |
| ------------- | ------------------------------------------- |
| Train | 24,353 |
| Validation | 8,117 |
| Test | 8,117 |
## Dataset Creation
This dataset was created with python, using pandas library as the main processing data. Also, this dataset are the mix of other datasets which are the same scope, the Fake News. All of the process is available in this repository: https://github.com/G0nz4lo-4lvarez-H3rv4s/FakeNewsDetection
### Source Data
The source data is a mix of multiple fake_news datasets in Kaggle, a platform for train your skills and learnings about Artificial Intelligence. The main datasets who are based this dataset are:
#### Initial Data Collection and Normalization
Version 1.0.0 aimed to support supervised neural methodologies for deep learning and study the new Transformers models in the Natural Language Processing with News of the United States.
### Annotations
#### Annotation process
[More Information Needed]
#### Who are the annotators?
[More Information Needed]
### Personal and Sensitive Information
[More Information Needed]
## Considerations for Using the Data
This Dataset is compose for 3 types: Training phase, for training your model of NLP, validation phase, because we need to validate if the training was successful or our model has overfitting, and train phase, who count the probability and the mistakes in the model fine-tuning.
### Social Impact of Dataset
[More Information Needed]
### Discussion of Biases
[More Information Needed]
### Other Known Limitations
[More Information Needed]
## Additional Information
### Dataset Curators
[More Information Needed]
### Licensing Information
[More Information Needed]
### Citation Information
[More Information Needed]
### Contributions
Thanks to [@github-username](https://github.com/<github-username>) for adding this dataset. |
Graphcore/gqa-lxmert | ---
language:
- en
license:
- cc-by-4.0
---
|
Graphcore/gqa | ---
language:
- en
license:
- cc-by-4.0
---
|
Graphcore/vqa-lxmert | ---
language:
- en
license:
- cc-by-4.0
---
|
Graphcore/vqa | ---
language:
- en
license:
- cc-by-4.0
---
|
Graphcore/wikipedia-bert-128 | ---
language:
- en
license:
- cc-by-sa-3.0
--- |
Graphcore/wikipedia-bert-512 | ---
language:
- en
license:
- cc-by-sa-3.0
--- |
GroNLP/ik-nlp-22_pestyle | ---
annotations_creators:
- machine-generated
- expert-generated
language_creators:
- found
language:
- en
- it
license:
- other
multilinguality:
- translation
size_categories:
- 1K<n<10K
source_datasets:
- original
task_categories:
- translation
pretty_name: iknlp22-pestyle
---
# Dataset Card for IK-NLP-22 Project 1: A Study in Post-Editing Stylometry
## Table of Contents
- [Dataset Card for IK-NLP-22 Project 1: A Study in Post-Editing Stylometry](#dataset-card-for-ik-nlp-22-project-1-a-study-in-post-editing-stylometry)
- [Table of Contents](#table-of-contents)
- [Dataset Description](#dataset-description)
- [Dataset Summary](#dataset-summary)
- [Languages](#languages)
- [Dataset Structure](#dataset-structure)
- [Data Instances](#data-instances)
- [Data Fields](#data-fields)
- [Data Splits](#data-splits)
- [Train Split](#train-split)
- [Test splits](#test-splits)
- [Dataset Creation](#dataset-creation)
- [Additional Information](#additional-information)
- [Dataset Curators](#dataset-curators)
- [Licensing Information](#licensing-information)
- [Citation Information](#citation-information)
## Dataset Description
- **Source:** [FLORES-101](https://huggingface.co/datasets/gsarti/flores_101)
- **Point of Contact:** [Gabriele Sarti](mailto:ik-nlp-course@rug.nl)
### Dataset Summary
This dataset contains a sample of sentences taken from the [FLORES-101](https://huggingface.co/datasets/gsarti/flores_101) dataset that were either translated from scratch or post-edited from an existing automatic translation by three human translators. Translation were performed for the English-Italian language pair, and translators' behavioral data (keystrokes, pauses, editing times) were collected using the [PET](https://github.com/wilkeraziz/PET) platform.
This dataset is made available for final projects of the 2022 edition of the Natural Language Processing course at the [Information Science Master's Degree](https://www.rug.nl/masters/information-science/?lang=en) at the University of Groningen, taught by [Arianna Bisazza](https://research.rug.nl/en/persons/arianna-bisazza) and [Gabriele Sarti](https://research.rug.nl/en/persons/gabriele-sarti) with the assistance of [Anjali Nair](https://nl.linkedin.com/in/anjalinair012).
**Disclaimer**: *This repository is provided without direct data access due to currently unpublished results.* _**For this reason, it is strictly forbidden to share or publish all the data associated to this repository**_. *Students will be provided with a compressed folder containing the data upon choosing a project based on this dataset. To load the dataset using 🤗 Datasets, download and unzip the provided folder and pass it to the* `load_dataset` *method as:* `datasets.load_dataset('GroNLP/ik-nlp-22_pestyle', 'full', data_dir='path/to/unzipped/folder')`
### Languages
The language data of is in English (BCP-47 `en`) and Italian (BCP-47 `it`)
## Dataset Structure
### Data Instances
The dataset contains four configurations: `full`, `test_mask_subject`, `test_mask_modality`, `test_mask_time`. `full` contains the main `train` split in which all fields are available. The other three, `test_mask_subject`, `test_mask_modality`, `test_mask_time`, contain a `test` split each with different fields removed to avoid information leaking during evaluation. See more details in the [Data Splits](#data-splits) section.
### Data Fields
The following fields are contained in the training set:
|Field|Description|
|-----|-----------|
|`item_id` | The sentence identifier. The first digits of the number represent the document containing the sentence, while the last digit of the number represents the sentence position inside the document. Documents can contain from 3 to 5 semantically-related sentences each. |
|`subject_id` | The identifier for the translator performing the translation from scratch or post-editing task. Values: `t1`, `t2` or `t3`. |
|`modality` | The modality of the translation task. Values: `ht` (translation from scratch), `pe1` (post-editing Google Translate translations), `pe2` (post-editing [mBART](https://huggingface.co/facebook/mbart-large-50-one-to-many-mmt) translations). |
|`src_text` | The original source sentence extracted from Wikinews, wikibooks or wikivoyage. |
|`mt_text` | Missing if tasktype is `ht`. Otherwise, contains the automatically-translated sentence before post-editing. |
|`tgt_text` | Final sentence produced by the translator (either via translation from scratch of `sl_text` or post-editing `mt_text`) |
|`edit_time` | Total editing time for the translation in seconds. |
|`k_total` | Total number of keystrokes for the translation. |
|`k_letter` | Total number of letter keystrokes for the translation. |
|`k_digit` | Total number of digit keystrokes for the translation. |
|`k_white` | Total number of whitespace keystrokes for the translation. |
|`k_symbol` | Total number of symbol (punctuation, etc.) keystrokes for the translation. |
|`k_nav` | Total number of navigation keystrokes (left-right arrows, mouse clicks) for the translation. |
|`k_erase` | Total number of erase keystrokes (backspace, cancel) for the translation. |
|`k_copy` | Total number of copy (Ctrl + C) actions during the translation. |
|`k_cut` | Total number of cut (Ctrl + X) actions during the translation. |
|`k_paste` | Total number of paste (Ctrl + V) actions during the translation. |
|`n_pause_geq_300` | Number of pauses of 300ms or more during the translation. |
|`len_pause_geq_300` | Total duration of pauses of 300ms or more, in milliseconds. |
|`n_pause_geq_1000` | Number of pauses of 1s or more during the translation. |
|`len_pause_geq_1000` | Total duration of pauses of 1000ms or more, in milliseconds. |
|`num_annotations` | Number of times the translator focused the texbox for performing the translation of the sentence during the translation session. E.g. 1 means the translation was performed once and never revised. |
|`n_insert` | Number of post-editing insertions (empty for modality `ht`) computed using the [tercom](https://github.com/jhclark/tercom) library. |
|`n_delete` | Number of post-editing deletions (empty for modality `ht`) computed using the [tercom](https://github.com/jhclark/tercom) library. |
|`n_substitute` | Number of post-editing substitutions (empty for modality `ht`) computed using the [tercom](https://github.com/jhclark/tercom) library. |
|`n_shift` | Number of post-editing shifts (empty for modality `ht`) computed using the [tercom](https://github.com/jhclark/tercom) library. |
|`bleu` | Sentence-level BLEU score between MT and post-edited fields (empty for modality `ht`) computed using the [SacreBLEU](https://github.com/mjpost/sacrebleu) library with default parameters. |
|`chrf` | Sentence-level chrF score between MT and post-edited fields (empty for modality `ht`) computed using the [SacreBLEU](https://github.com/mjpost/sacrebleu) library with default parameters. |
|`ter` | Sentence-level TER score between MT and post-edited fields (empty for modality `ht`) computed using the [tercom](https://github.com/jhclark/tercom) library. |
|`aligned_edit` | Aligned visual representation of REF (`mt_text`), HYP (`tl_text`) and edit operations (I = Insertion, D = Deletion, S = Substitution) performed on the field. Replace `\\n` with `\n` to show the three aligned rows.|
### Data Splits
| config| train| test|
|------:|-----:|----:|
|`main` | 1170 | 120 |
#### Train Split
The `train` split contains a total of 1170 triplets (or pairs, when translation from scratch is performed) annotated with behavioral data produced during the translation. The following is an example of the subject `t3` post-editing a machine translation produced by system 2 (tasktype `pe2`) taken from the `train` split. The field `aligned_edit` is showed over three lines to provide a visual understanding of its contents.
```json
{
"item_id": 1072,
"subject_id": "t3",
"tasktype": "pe2",
"src_text": "At the beginning dress was heavily influenced by the Byzantine culture in the east.",
"mt_text": "All'inizio il vestito era fortemente influenzato dalla cultura bizantina dell'est.",
"tgt+text": "Inizialmente, l'abbigliamento era fortemente influenzato dalla cultura bizantina orientale.",
"edit_time": 45.687,
"k_total": 51,
"k_letter": 31,
"k_digit": 0,
"k_white": 2,
"k_symbol": 3,
"k_nav": 7,
"k_erase": 3,
"k_copy": 0,
"k_cut": 0,
"k_paste": 0,
"n_pause_geq_300": 9,
"len_pause_geq_300": 40032,
"n_pause_geq_1000": 5,
"len_pause_geq_1000": 38392,
"num_annotations": 1,
"n_insert": 0.0,
"n_delete": 1.0,
"n_substitute": 3.0,
"n_shift": 0.0,
"bleu": 47.99,
"chrf": 62.05,
"ter": 40.0,
"aligned_edit: "REF: all'inizio il vestito era fortemente influenzato dalla cultura bizantina dell'est.\\n
HYP: ********** inizialmente, l'abbigliamento era fortemente influenzato dalla cultura bizantina orientale.\\n
EVAL: D S S S"
}
```
The text is provided as-is, without further preprocessing or tokenization.
#### Test splits
The three `test` splits (one per configuration) contain the same 120 entries each, following the same structure as `train`. Each test split omit some of the fields to prevent leakage of information:
- In `test_mask_subject` the `subject_id` is absent, for the main task of post-editor stylometry.
- In `test_mask_modality` the following fields are absent for the modality prediction extra task: `modality`, `mt_text`, `n_insert`, `n_delete`, `n_substitute`, `n_shift`, `ter`, `bleu`, `chrf`, `aligned_edit`.
- In `test_mask_time` the following fields are absent for the time and pause prediction extra task: `edit_time`, `n_pause_geq_300`, `len_pause_geq_300`, `n_pause_geq_1000`, and `len_pause_geq_1000`.
### Dataset Creation
The dataset was parsed from PET XML files into CSV format using a script adapted from the one by [Antonio Toral](https://research.rug.nl/en/persons/antonio-toral-ruiz) found at the following link: [https://github.com/antot/postediting_novel_frontiers](https://github.com/antot/postediting_novel_frontiers)
## Additional Information
### Dataset Curators
For problems related to this 🤗 Datasets version, please contact us at [ik-nlp-course@rug.nl](mailto:ik-nlp-course@rug.nl).
### Licensing Information
It is forbidden to share or publish the data associated with this 🤗 Dataset version.
### Citation Information
No citation information is provided for this dataset. |
GroNLP/ik-nlp-22_slp | ---
annotations_creators:
- expert-generated
language_creators:
- expert-generated
language:
- en
license:
- unknown
multilinguality:
- monolingual
size_categories:
- 1K<n<10K
source_datasets:
- original
task_categories:
- question-answering
- summarization
- text-retrieval
pretty_name: slp3ed-iknlp2022
tags:
- question-generation
---
# Dataset Card for IK-NLP-22 Speech and Language Processing
## Table of Contents
- [Dataset Card for IK-NLP-22 Speech and Language Processing](#dataset-card-for-ik-nlp-22-speech-and-language-processing)
- [Table of Contents](#table-of-contents)
- [Dataset Description](#dataset-description)
- [Dataset Summary](#dataset-summary)
- [Projects](#projects)
- [Languages](#languages)
- [Dataset Structure](#dataset-structure)
- [Data Instances](#data-instances)
- [Paragraphs Configuration](#paragraphs-configuration)
- [Questions Configuration](#questions-configuration)
- [Data Splits](#data-splits)
- [Dataset Creation](#dataset-creation)
- [Additional Information](#additional-information)
- [Dataset Curators](#dataset-curators)
- [Licensing Information](#licensing-information)
- [Citation Information](#citation-information)
## Dataset Description
- **Source:** [Stanford](https://web.stanford.edu/~jurafsky/slp3/)
- **Point of Contact:** [Gabriele Sarti](mmailto:ik-nlp-course@rug.nl)
### Dataset Summary
This dataset contains chapters extracted from the Speech and Language Processing book (3ed draft of January 2022) by Jurafsky and Martin via a semi-automatic procedure (see below for additional details). Moreover, a small set of conceptual questions associated with each chapter is provided alongside possible answers.
Only the content of chapters 2 to 11 of the book draft are provided, since these are the ones relevant to the contents of the 2022 edition of the Natural Language Processing course at the Information Science Master's Degree (IK) at the University of Groningen, taught by [Arianna Bisazza](https://research.rug.nl/en/persons/arianna-bisazza) with the assistance of [Gabriele Sarti](https://research.rug.nl/en/persons/gabriele-sarti).
*The Speech and Language Processing book was made freely available by the authors [Dan Jurafsky](http://web.stanford.edu/people/jurafsky/) and [James H. Martin](http://www.cs.colorado.edu/~martin/) on the [Stanford University website](https://web.stanford.edu/~jurafsky/slp3/). The present dataset was created for educational purposes, and is based on the draft of the 3rd edition of the book accessed on December 29th, 2021. All rights of the present contents are attributed to the original authors.*
### Projects
See the course page for a description of possible research directions.
### Languages
The language data of Speech and Language Processing is in English (BCP-47 `en`)
## Dataset Structure
### Data Instances
The dataset contains two configurations: `paragraphs` (default), containing the full set of parsed paragraphs associated to the respective chapter and sections, and `questions`, containing a small subset of example questions matched with the relevant paragraph, and with the answer span extracted.
#### Paragraphs Configuration
The `paragraphs` configuration contains all the paragraphs of the selected book chapters, each associated with the respective chapter, section and subsection. An example from the `train` split of the `paragraphs` config is provided below. The example belongs to section 2.3 but not to a subsection, so the `n_subsection` and `subsection` fields are empty strings.
```json
{
"n_chapter": "2",
"chapter": "Regular Expressions",
"n_section": "2.3",
"section": "Corpora",
"n_subsection": "",
"subsection": "",
"text": "It's also quite common for speakers or writers to use multiple languages in a single communicative act, a phenomenon called code switching. Code switching (2.2) Por primera vez veo a @username actually being hateful! it was beautiful:)"
}
```
The text is provided as-is, without further preprocessing or tokenization.
#### Questions Configuration
The `questions` configuration contains a small subset of questions, the top retrieved paragraph relevant to the question and the answer spans. An example from the `test` split of the `questions` config is provided below.
```json
{
"chapter": "Regular Expressions",
"section": "Regular Expressions",
"subsection": "Basic Regular Expressions",
"question": "What is the meaning of the Kleene star in Regex?",
"paragraph": "This language consists of strings with a b, followed by at least two a's, followed by an exclamation point. The set of operators that allows us to say things like \"some number of as\" are based on the asterisk or *, commonly called the Kleene * (gen-Kleene * erally pronounced \"cleany star\"). The Kleene star means \"zero or more occurrences of the immediately previous character or regular expression\". So /a*/ means \"any string of zero or more as\". This will match a or aaaaaa, but it will also match Off Minor since the string Off Minor has zero a's. So the regular expression for matching one or more a is /aa*/, meaning one a followed by zero or more as. More complex patterns can also be repeated. So /[ab]*/ means \"zero or more a's or b's\" (not \"zero or more right square braces\"). This will match strings like aaaa or ababab or bbbb.",
"answer": "The Kleene star means \"zero or more occurrences of the immediately previous character or regular expression\""
}
```
### Data Splits
| config| train| test|
|------------:|-----:|----:|
|`paragraphs` | 1697 | - |
|`questions` | - | 59 |
### Dataset Creation
The contents of the Speech and Language Processing book PDF were extracted using the [PDF to S2ORC JSON Converter](https://github.com/allenai/s2orc-doc2json) by AllenAI. The texts extracted by the converter were then manually cleaned to remove end-of-chapter exercises and other irrelevant content (e.g. tables, TikZ figures, etc.). Some issues in the parsed content were preserved in the final version to maintain a naturalistic setting for the associated projects, promoting the use of data filtering heuristics for students.
The question-answer pairs were created manually by Gabriele Sarti.
## Additional Information
### Dataset Curators
For problems on this 🤗 Datasets version, please contact us at [ik-nlp-course@rug.nl](mailto:ik-nlp-course@rug.nl).
### Licensing Information
Please refer to the authors' websites for licensing information.
### Citation Information
Please cite the authors if you use these corpora in your work:
```bibtex
@book{slp3ed-iknlp2022,
author = {Jurafsky, Daniel and Martin, James},
year = {2021},
month = {12},
pages = {1--235, 1--19},
title = {Speech and Language Processing: An Introduction to Natural Language Processing, Computational Linguistics, and Speech Recognition},
volume = {3}
}
``` |
GroNLP/ik-nlp-22_transqe | ---
annotations_creators:
- expert-generated
language_creators:
- expert-generated
- machine-generated
language:
- en
- nl
license:
- apache-2.0
multilinguality:
- translation
size_categories:
- unknown
source_datasets:
- extended|esnli
task_categories:
- text-classification
task_ids:
- natural-language-inference
pretty_name: iknlp22-transqe
tags:
- quality-estimation
---
# Dataset Card for IK-NLP-22 Project 3: Translation Quality-driven Data Selection for Natural Language Inference
## Table of Contents
- [Dataset Card for IK-NLP-22 Project 3: Translation Quality-driven Data Selection for Natural Language Inference](#dataset-card-for-ik-nlp-22-project-3-translation-quality-driven-data-selection-for-natural-language-inference)
- [Table of Contents](#table-of-contents)
- [Dataset Description](#dataset-description)
- [Dataset Summary](#dataset-summary)
- [Languages](#languages)
- [Dataset Structure](#dataset-structure)
- [Data Instances](#data-instances)
- [Data Splits](#data-splits)
- [Data Example](#data-example)
- [Dataset Creation](#dataset-creation)
- [Additional Information](#additional-information)
- [Dataset Curators](#dataset-curators)
- [Licensing Information](#licensing-information)
- [Citation Information](#citation-information)
## Dataset Description
- **Source:** [Github](https://github.com/OanaMariaCamburu/e-SNLI)
- **Point of Contact:** [Gabriele Sarti](mailto:ik-nlp-course@rug.nl)
### Dataset Summary
This dataset contains the full [e-SNLI](https://huggingface.co/datasets/esnli) dataset, automatically translated to Dutch using the [Helsinki-NLP/opus-mt-en-nl](https://huggingface.co/Helsinki-NLP/opus-mt-en-nl) neural machine translation model. The translation of each field has been anotated with two quality estimation scores using the referenceless version of the [COMET](https://github.com/Unbabel/COMET/) metric by Unbabel.
The intended usage of this corpus is restricted to the scope of final project for the 2022 edition of the Natural Language Processing course at the Information Science Master's Degree (IK) at the University of Groningen, taught by [Arianna Bisazza](https://research.rug.nl/en/persons/arianna-bisazza) and [Gabriele Sarti](https://research.rug.nl/en/persons/gabriele-sarti), with the assistance of [Anjali Nair](https://nl.linkedin.com/in/anjalinair012).
*The e-SNLI corpus was made freely available by the authors on Github. The present dataset was created for educational purposes, and is based on the original e-SNLI dataset by Camburu et al..All rights of the present contents are attributed to the original authors.*
### Languages
The language data of this corpus is in English (BCP-47 `en`) and Dutch (BCP-47 `nl`).
## Dataset Structure
### Data Instances
The dataset contains a single condiguration by default, named `plain_text`, with the three original splits `train`, `validation` and `test`. Every split contains the following fields:
| **Field** | **Description** |
|------------|-----------------------------|
|`premise_en`| The original English premise.|
|`premise_nl`| The premise automatically translated to Dutch.|
|`hypothesis_en`| The original English hypothesis.|
|`hypothesis_nl`| The hypothesis automatically translated to Dutch.|
|`label`| The label of the data instance (0 for entailment, 1 for neutral, 2 for contradiction).|
|`explanation_1_en`| The first explanation for the assigned label in English.|
|`explanation_1_nl`| The first explanation automatically translated to Dutch.|
|`explanation_2_en`| The second explanation for the assigned label in English.|
|`explanation_2_nl`| The second explanation automatically translated to Dutch.|
|`explanation_3_en`| The third explanation for the assigned label in English.|
|`explanation_3_nl`| The third explanation automatically translated to Dutch.|
|`da_premise`| The quality estimation produced by the `wmt20-comet-qe-da` model for the premise translation.|
|`da_hypothesis`| The quality estimation produced by the `wmt20-comet-qe-da` model for the hypothesis translation.|
|`da_explanation_1`| The quality estimation produced by the `wmt20-comet-qe-da` model for the first explanation translation.|
|`da_explanation_2`| The quality estimation produced by the `wmt20-comet-qe-da` model for the second explanation translation.|
|`da_explanation_3`| The quality estimation produced by the `wmt20-comet-qe-da` model for the third explanation translation.|
|`mqm_premise`| The quality estimation produced by the `wmt21-comet-qe-mqm` model for the premise translation.|
|`mqm_hypothesis`| The quality estimation produced by the `wmt21-comet-qe-mqm` model for the hypothesis translation.|
|`mqm_explanation_1`| The quality estimation produced by the `wmt21-comet-qe-mqm` model for the first explanation translation.|
|`mqm_explanation_2`| The quality estimation produced by the `wmt21-comet-qe-mqm` model for the second explanation translation.|
|`mqm_explanation_3`| The quality estimation produced by the `wmt21-comet-qe-mqm` model for the third explanation translation.|
Explanation 2 and 3 and related quality estimation scores are only present in the `validation` and `test` splits.
### Data Splits
| config| train | validation | test |
|------------:|---------|------------|------|
|`plain_text` | 549'367 | 9842 | 9824 |
For your analyses, use the amount of data that is the most reasonable for your computational setup. The more, the better.
### Data Example
The following is an example of entry 2000 taken from the `test` split:
```json
{
"premise_en": "A young woman wearing a yellow sweater and black pants is ice skating outdoors.",
"premise_nl": "Een jonge vrouw met een gele trui en zwarte broek schaatst buiten.",
"hypothesis_en": "a woman is practicing for the olympics",
"hypothesis_nl": "een vrouw oefent voor de Olympische Spelen",
"label": 1,
"explanation_1_en": "You can not infer it's for the Olympics.",
"explanation_1_nl": "Het is niet voor de Olympische Spelen.",
"explanation_2_en": "Just because a girl is skating outdoors does not mean she is practicing for the Olympics.",
"explanation_2_nl": "Alleen omdat een meisje buiten schaatst betekent niet dat ze oefent voor de Olympische Spelen.",
"explanation_3_en": "Ice skating doesn't imply practicing for the olympics.",
"explanation_3_nl": "Schaatsen betekent niet oefenen voor de Olympische Spelen.",
"da_premise": "0.6099",
"mqm_premise": "0.1298",
"da_hypothesis": "0.8504",
"mqm_hypothesis": "0.1521",
"da_explanation_1": "0.0001",
"mqm_explanation_1": "0.1237",
"da_explanation_2": "0.4017",
"mqm_explanation_2": "0.1467",
"da_explanation_3": "0.6069",
"mqm_explanation_3": "0.1389"
}
```
### Dataset Creation
The dataset was created through the following steps:
- Translating every field of the original e-SNLI corpus to Dutch using the [Helsinki-NLP/opus-mt-en-nl](https://huggingface.co/Helsinki-NLP/opus-mt-en-nl) neural machine translation model.
- Annotating the quality estimation of the translations with two referenceless versions of the [COMET](https://github.com/Unbabel/COMET/) metric by Unbabel.
## Additional Information
### Dataset Curators
For problems on this 🤗 Datasets version, please contact us at [ik-nlp-course@rug.nl](mailto:ik-nlp-course@rug.nl).
### Licensing Information
The dataset is licensed under the [Apache 2.0 License](https://www.apache.org/licenses/LICENSE-2.0.html).
### Citation Information
Please cite the authors if you use these corpora in your work:
```bibtex
@incollection{NIPS2018_8163,
title = {e-SNLI: Natural Language Inference with Natural Language Explanations},
author = {Camburu, Oana-Maria and Rockt\"{a}schel, Tim and Lukasiewicz, Thomas and Blunsom, Phil},
booktitle = {Advances in Neural Information Processing Systems 31},
editor = {S. Bengio and H. Wallach and H. Larochelle and K. Grauman and N. Cesa-Bianchi and R. Garnett},
pages = {9539--9549},
year = {2018},
publisher = {Curran Associates, Inc.},
url = {http://papers.nips.cc/paper/8163-e-snli-natural-language-inference-with-natural-language-explanations.pdf}
}
``` |
GroNLP/ik-nlp-22_winemag | ---
license: cc-by-sa-4.0
---
|
HHousen/ParaSCI | Reformatted version of the ParaSCI dataset from [ParaSCI: A Large Scientific Paraphrase Dataset for Longer Paraphrase Generation](https://arxiv.org/abs/2101.08382). Data retrieved from [dqxiu/ParaSCI](https://github.com/dqxiu/ParaSCI). |
HUPD/hupd | ---
language:
- en
license:
- cc-by-sa-4.0
task_categories:
- fill-mask
- summarization
- text-classification
- token-classification
task_ids:
- masked-language-modeling
- multi-class-classification
- topic-classification
- named-entity-recognition
pretty_name: "HUPD"
tags:
- patents
---
# Dataset Card for The Harvard USPTO Patent Dataset (HUPD)
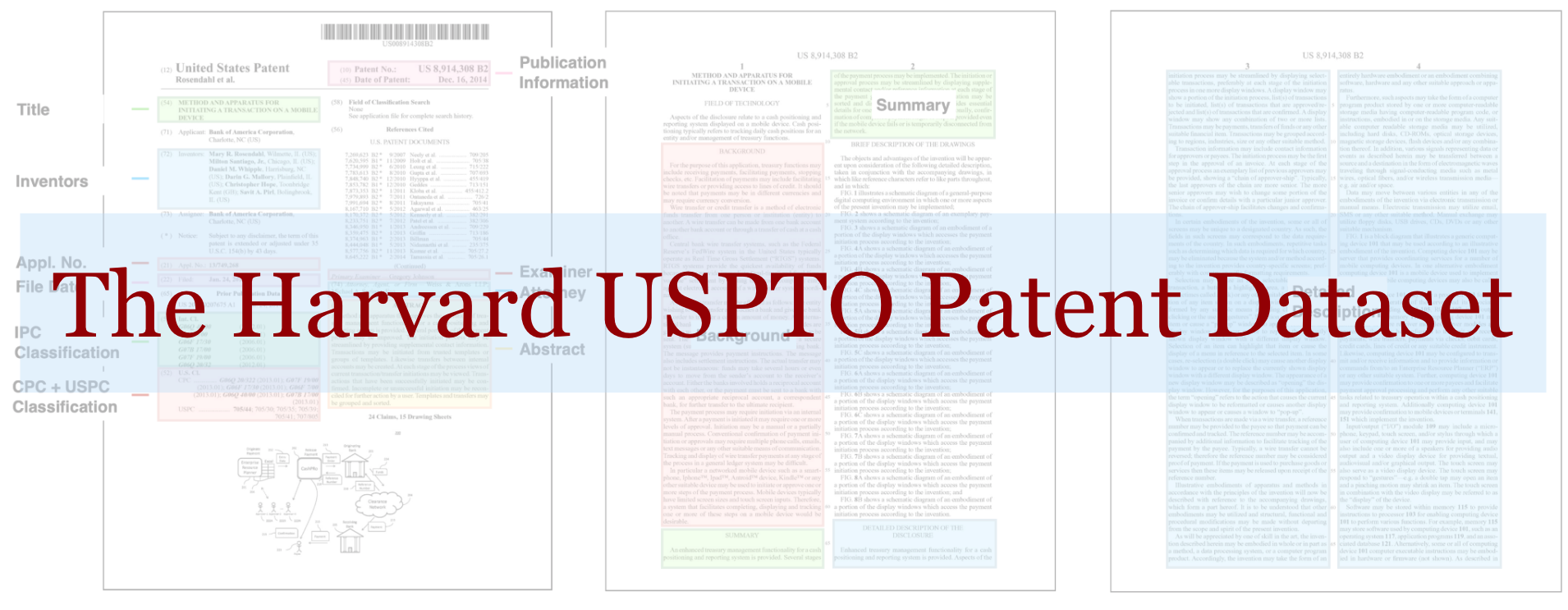
## Dataset Description
- **Homepage:** [https://patentdataset.org/](https://patentdataset.org/)
- **Repository:** [HUPD GitHub repository](https://github.com/suzgunmirac/hupd)
- **Paper:** [HUPD arXiv Submission](https://arxiv.org/abs/2207.04043)
- **Point of Contact:** Mirac Suzgun
### Dataset Summary
The Harvard USPTO Dataset (HUPD) is a large-scale, well-structured, and multi-purpose corpus of English-language utility patent applications filed to the United States Patent and Trademark Office (USPTO) between January 2004 and December 2018.
### Experiments and Tasks Considered in the Paper
- **Patent Acceptance Prediction**: Given a section of a patent application (in particular, the abstract, claims, or description), predict whether the application will be accepted by the USPTO.
- **Automated Subject (IPC/CPC) Classification**: Predict the primary IPC or CPC code of a patent application given (some subset of) the text of the application.
- **Language Modeling**: Masked/autoregressive language modeling on the claims and description sections of patent applications.
- **Abstractive Summarization**: Given the claims or claims section of a patent application, generate the abstract.
### Languages
The dataset contains English text only.
### Domain
Patents (intellectual property).
### Dataset Curators
The dataset was created by Mirac Suzgun, Luke Melas-Kyriazi, Suproteem K. Sarkar, Scott Duke Kominers, and Stuart M. Shieber.
## Dataset Structure
Each patent application is defined by a distinct JSON file, named after its application number, and includes information about
the application and publication numbers,
title,
decision status,
filing and publication dates,
primary and secondary classification codes,
inventor(s),
examiner,
attorney,
abstract,
claims,
background,
summary, and
full description of the proposed invention, among other fields. There are also supplementary variables, such as the small-entity indicator (which denotes whether the applicant is considered to be a small entity by the USPTO) and the foreign-filing indicator (which denotes whether the application was originally filed in a foreign country).
In total, there are 34 data fields for each application. A full list of data fields used in the dataset is listed in the next section.
### Data Instances
Each patent application in our patent dataset is defined by a distinct JSON file (e.g., ``8914308.json``), named after its unique application number. The format of the JSON files is as follows:
```python
{
"application_number": "...",
"publication_number": "...",
"title": "...",
"decision": "...",
"date_produced": "...",
"date_published": "...",
"main_cpc_label": "...",
"cpc_labels": ["...", "...", "..."],
"main_ipcr_label": "...",
"ipcr_labels": ["...", "...", "..."],
"patent_number": "...",
"filing_date": "...",
"patent_issue_date": "...",
"abandon_date": "...",
"uspc_class": "...",
"uspc_subclass": "...",
"examiner_id": "...",
"examiner_name_last": "...",
"examiner_name_first": "...",
"examiner_name_middle": "...",
"inventor_list": [
{
"inventor_name_last": "...",
"inventor_name_first": "...",
"inventor_city": "...",
"inventor_state": "...",
"inventor_country": "..."
}
],
"abstract": "...",
"claims": "...",
"background": "...",
"summary": "...",
"full_description": "..."
}
```
## Usage
### Loading the Dataset
#### Sample (January 2016 Subset)
The following command can be used to load the `sample` version of the dataset, which contains all the patent applications that were filed to the USPTO during the month of January in 2016. This small subset of the dataset can be used for debugging and exploration purposes.
```python
from datasets import load_dataset
dataset_dict = load_dataset('HUPD/hupd',
name='sample',
data_files="https://huggingface.co/datasets/HUPD/hupd/blob/main/hupd_metadata_2022-02-22.feather",
icpr_label=None,
train_filing_start_date='2016-01-01',
train_filing_end_date='2016-01-21',
val_filing_start_date='2016-01-22',
val_filing_end_date='2016-01-31',
)
```
#### Full Dataset
If you would like to use the **full** version of the dataset, please make sure that change the `name` field from `sample` to `all`, specify the training and validation start and end dates carefully, and set `force_extract` to be `True` (so that you would only untar the files that you are interested in and not squander your disk storage space). In the following example, for instance, we set the training set year range to be [2011, 2016] (inclusive) and the validation set year range to be 2017.
```python
from datasets import load_dataset
dataset_dict = load_dataset('HUPD/hupd',
name='all',
data_files="https://huggingface.co/datasets/HUPD/hupd/blob/main/hupd_metadata_2022-02-22.feather",
icpr_label=None,
force_extract=True,
train_filing_start_date='2011-01-01',
train_filing_end_date='2016-12-31',
val_filing_start_date='2017-01-01',
val_filing_end_date='2017-12-31',
)
```
### Google Colab Notebook
You can also use the following Google Colab notebooks to explore HUPD.
- [](https://colab.research.google.com/drive/1_ZsI7WFTsEO0iu_0g3BLTkIkOUqPzCET?usp=sharing)[ HUPD Examples: Loading the Dataset](https://colab.research.google.com/drive/1_ZsI7WFTsEO0iu_0g3BLTkIkOUqPzCET?usp=sharing)
- [](https://colab.research.google.com/drive/1TzDDCDt368cUErH86Zc_P2aw9bXaaZy1?usp=sharing)[ HUPD Examples: Loading HUPD By Using HuggingFace's Libraries](https://colab.research.google.com/drive/1TzDDCDt368cUErH86Zc_P2aw9bXaaZy1?usp=sharing)
- [](https://colab.research.google.com/drive/1TzDDCDt368cUErH86Zc_P2aw9bXaaZy1?usp=sharing)[ HUPD Examples: Using the HUPD DistilRoBERTa Model](https://colab.research.google.com/drive/11t69BWcAVXndQxAOCpKaGkKkEYJSfydT?usp=sharing)
- [](https://colab.research.google.com/drive/1TzDDCDt368cUErH86Zc_P2aw9bXaaZy1?usp=sharing)[ HUPD Examples: Using the HUPD T5-Small Summarization Model](https://colab.research.google.com/drive/1VkCtrRIryzev_ixDjmJcfJNK-q6Vx24y?usp=sharing)
## Dataset Creation
### Source Data
HUPD synthesizes multiple data sources from the USPTO: While the full patent application texts were obtained from the USPTO Bulk Data Storage System (Patent Application Data/XML Versions 4.0, 4.1, 4.2, 4.3, 4.4 ICE, as well as Version 1.5) as XML files, the bibliographic filing metadata were obtained from the USPTO Patent Examination Research Dataset (in February, 2021).
### Annotations
Beyond our patent decision label, for which construction details are provided in the paper, the dataset does not contain any human-written or computer-generated annotations beyond those produced by patent applicants or the USPTO.
### Data Shift
A major feature of HUPD is its structure, which allows it to demonstrate the evolution of concepts over time. As we illustrate in the paper, the criteria for patent acceptance evolve over time at different rates, depending on category. We believe this is an important feature of the dataset, not only because of the social scientific questions it raises, but also because it facilitates research on models that can accommodate concept shift in a real-world setting.
### Personal and Sensitive Information
The dataset contains information about the inventor(s) and examiner of each patent application. These details are, however, already in the public domain and available on the USPTO's Patent Application Information Retrieval (PAIR) system, as well as on Google Patents and PatentsView.
### Social Impact of the Dataset
The authors of the dataset hope that HUPD will have a positive social impact on the ML/NLP and Econ/IP communities. They discuss these considerations in more detail in [the paper](https://arxiv.org/abs/2207.04043).
### Impact on Underserved Communities and Discussion of Biases
The dataset contains patent applications in English, a language with heavy attention from the NLP community. However, innovation is spread across many languages, cultures, and communities that are not reflected in this dataset. HUPD is thus not representative of all kinds of innovation. Furthermore, patent applications require a fixed cost to draft and file and are not accessible to everyone. One goal of this dataset is to spur research that reduces the cost of drafting applications, potentially allowing for more people to seek intellectual property protection for their innovations.
### Discussion of Biases
Section 4 of [the HUPD paper](https://arxiv.org/abs/2207.04043) provides an examination of the dataset for potential biases. It shows, among other things, that female inventors are notably underrepresented in the U.S. patenting system, that small and micro entities (e.g., independent inventors, small companies, non-profit organizations) are less likely to have positive outcomes in patent obtaining than large entities (e.g., companies with more than 500 employees), and that patent filing and acceptance rates are not uniformly distributed across the US. Our empirical findings suggest that any study focusing on the acceptance prediction task, especially if it is using the inventor information or the small-entity indicator as part of the input, should be aware of the the potential biases present in the dataset and interpret their results carefully in light of those biases.
- Please refer to Section 4 and Section D for an in-depth discussion of potential biases embedded in the dataset.
### Licensing Information
HUPD is released under the CreativeCommons Attribution-NonCommercial-ShareAlike 4.0 International.
### Citation Information
```
@article{suzgun2022hupd,
title={The Harvard USPTO Patent Dataset: A Large-Scale, Well-Structured, and Multi-Purpose Corpus of Patent Applications},
author={Suzgun, Mirac and Melas-Kyriazi, Luke and Sarkar, Suproteem K. and Kominers, Scott Duke and Shieber, Stuart M.},
year={2022},
publisher={arXiv preprint arXiv:2207.04043},
url={https://arxiv.org/abs/2207.04043},
``` |
Hellisotherpeople/DebateSum | ---
annotations_creators:
- expert-generated
language_creators:
- crowdsourced
language:
- en
license:
- mit
multilinguality:
- monolingual
size_categories:
- 100K<n<1M
source_datasets:
- original
task_categories:
- question-answering
- summarization
- text-retrieval
- text-generation
task_ids:
- abstractive-qa
- document-retrieval
- extractive-qa
pretty_name: 'DebateSum: A large-scale argument mining and summarization dataset'
language_bcp47:
- en-US
tags:
- conditional-text-generation
---
# DebateSum
Corresponding code repo for the upcoming paper at ARGMIN 2020: "DebateSum: A large-scale argument mining and summarization dataset"
Arxiv pre-print available here: https://arxiv.org/abs/2011.07251
Check out the presentation date and time here: https://argmining2020.i3s.unice.fr/node/9
Full paper as presented by the ACL is here: https://www.aclweb.org/anthology/2020.argmining-1.1/
Video of presentation at COLING 2020: https://underline.io/lecture/6461-debatesum-a-large-scale-argument-mining-and-summarization-dataset
The dataset is distributed as csv files.
A search engine over DebateSum (as well as some additional evidence not included in DebateSum) is available as [debate.cards](http://debate.cards/). It's very good quality and allows for the evidence to be viewed in the format that debaters use.
# Data
DebateSum consists of **187328** debate documents, arguements (also can be thought of as abstractive summaries, or queries), word-level extractive summaries, citations, and associated metadata organized by topic-year. This data is ready for analysis by NLP systems.
## Download
All data is accesable in a parsed format organized by topic year [here](https://mega.nz/folder/ZdQGmK6b#-0hoBWc5fLYuxQuH25feXg)
Addtionally, the trained word-vectors for [debate2vec](https://github.com/Hellisotherpeople/debate2vec) are also found in that folder.
## Regenerating it yourself
This is useful as the debaters who produce the evidence release their work every year. Soon enough I will update to include the 2020-2021 topic.
*Step 1: Download all open evidence files from [Open Evidence](https://openev.debatecoaches.org/) and unzip them into a directory. The links are as follows:*
* [2019](https://s3.amazonaws.com/openev/2019OpenEv.zip) - Resolved: The United States federal government should substantially reduce Direct Commercial Sales and/or Foreign Military Sales of arms from the United States.
* [2018](https://s3.amazonaws.com/openev/2018OpenEv.zip) - Resolved: The United States federal government should substantially reduce its restrictions on legal immigration to the United States.
* [2017](https://s3.amazonaws.com/openev/2017OpenEv.zip) - Resolved: The United States federal government should substantially increase its funding and/or regulation of elementary and/or secondary education in the United States.
* [2016](https://s3.amazonaws.com/openev/2016OpenEv.zip) - Resolved: The United States federal government should substantially increase its economic and/or diplomatic engagement with the People’s Republic of China.
* [2015](https://s3.amazonaws.com/openev/2015OpenEv.zip) - Resolved: The United States federal government should substantially curtail its domestic surveil-lance.
* [2014](https://s3.amazonaws.com/openev/2014OpenEv.zip) - Resolved: The United States federal government should substantially increase its non-military exploration and/or development of the Earth’s oceans.
* [2013](https://s3.amazonaws.com/openev/2013OpenEv.zip) - Resolved: The United States federal government should substantially increase its economic en-gagement toward Cuba, Mexico or Venezuela.
*Step 2: Convert all evidence from docx files to html5 files using [pandoc](https://pandoc.org/) with this command:*
```
for f in *.docx; do pandoc "$f" -s -o "${f%.docx}.html5"; done
```
*Step 3: install the dependencies for make_debate_dataset.py.*
```
pip install -r requirements.txt
```
*Step 4: Modify the folder and file locations as needed for your system, and run make_debate_dataset.py*
```
python3 make_debate_dataset.py
```
# Credits
Huge thanks to [Arvind Balaji](https://github.com/arvind-balaji) for making debate.cards and being second author on this paper!
|
Helsinki-NLP/tatoeba_mt | ---
annotations_creators:
- no-annotation
language_creators:
- crowdsourced
language:
- af
- ar
- az
- be
- bg
- bn
- br
- bs
- ca
- ch
- cs
- cv
- cy
- da
- de
- el
- en
- eo
- es
- et
- eu
- fa
- fi
- fo
- fr
- fy
- ga
- gd
- gl
- gn
- he
- hi
- hr
- hu
- hy
- ia
- id
- ie
- io
- is
- it
- ja
- jv
- ka
- kk
- km
- ko
- ku
- kw
- la
- lb
- lt
- lv
- mi
- mk
- ml
- mn
- mr
- ms
- mt
- my
- nb
- nl
- nn
- 'no'
- oc
- pl
- pt
- qu
- rn
- ro
- ru
- sh
- sl
- sq
- sr
- sv
- sw
- ta
- te
- th
- tk
- tl
- tr
- tt
- ug
- uk
- ur
- uz
- vi
- vo
- yi
- zh
license:
- cc-by-2.0
multilinguality:
- translation
pretty_name: The Tatoeba Translation Challenge
size_categories:
- unknown
source_datasets:
- original
task_categories:
- conditional-text-generation
task_ids:
- machine-translation
---
# Dataset Card for [Dataset Name]
## Table of Contents
- [Table of Contents](#table-of-contents)
- [Dataset Description](#dataset-description)
- [Dataset Summary](#dataset-summary)
- [Supported Tasks and Leaderboards](#supported-tasks-and-leaderboards)
- [Languages](#languages)
- [Dataset Structure](#dataset-structure)
- [Data Instances](#data-instances)
- [Data Fields](#data-fields)
- [Data Splits](#data-splits)
- [Dataset Creation](#dataset-creation)
- [Curation Rationale](#curation-rationale)
- [Source Data](#source-data)
- [Annotations](#annotations)
- [Personal and Sensitive Information](#personal-and-sensitive-information)
- [Considerations for Using the Data](#considerations-for-using-the-data)
- [Social Impact of Dataset](#social-impact-of-dataset)
- [Discussion of Biases](#discussion-of-biases)
- [Other Known Limitations](#other-known-limitations)
- [Additional Information](#additional-information)
- [Dataset Curators](#dataset-curators)
- [Licensing Information](#licensing-information)
- [Citation Information](#citation-information)
- [Contributions](#contributions)
## Dataset Description
- **Homepage:** https://github.com/Helsinki-NLP/Tatoeba-Challenge/
- **Repository:** https://github.com/Helsinki-NLP/Tatoeba-Challenge/
- **Paper:** [The Tatoeba Translation Challenge – Realistic Data Sets for Low Resource and Multilingual MT](https://aclanthology.org/2020.wmt-1.139/)
- **Leaderboard:**
- **Point of Contact:** [Jörg Tiedemann](mailto:jorg.tiedemann@helsinki.fi)
### Dataset Summary
The Tatoeba Translation Challenge is a multilingual data set of machine translation benchmarks derived from user-contributed translations collected by [Tatoeba.org](https://tatoeba.org/) and provided as parallel corpus from [OPUS](https://opus.nlpl.eu/). This dataset includes test and development data sorted by language pair. It includes test sets for hundreds of language pairs and is continuously updated. Please, check the version number tag to refer to the release that your are using.
### Supported Tasks and Leaderboards
The translation task is described in detail in the [Tatoeba-Challenge repository](https://github.com/Helsinki-NLP/Tatoeba-Challenge) and covers various sub-tasks with different data coverage and resources. [Training data](https://github.com/Helsinki-NLP/Tatoeba-Challenge/blob/master/data/README.md) is also available from the same repository and [results](https://github.com/Helsinki-NLP/Tatoeba-Challenge/blob/master/results/tatoeba-results-all.md) are published and collected as well. [Models](https://github.com/Helsinki-NLP/Tatoeba-Challenge/blob/master/results/tatoeba-models-all.md) are also released for public use and are also partially available from the [huggingface model hub](https://huggingface.co/Helsinki-NLP).
### Languages
The data set covers hundreds of languages and language pairs and are organized by ISO-639-3 languages. The current release covers the following language: Afrikaans, Arabic, Azerbaijani, Belarusian, Bulgarian, Bengali, Breton, Bosnian, Catalan, Chamorro, Czech, Chuvash, Welsh, Danish, German, Modern Greek, English, Esperanto, Spanish, Estonian, Basque, Persian, Finnish, Faroese, French, Western Frisian, Irish, Scottish Gaelic, Galician, Guarani, Hebrew, Hindi, Croatian, Hungarian, Armenian, Interlingua, Indonesian, Interlingue, Ido, Icelandic, Italian, Japanese, Javanese, Georgian, Kazakh, Khmer, Korean, Kurdish, Cornish, Latin, Luxembourgish, Lithuanian, Latvian, Maori, Macedonian, Malayalam, Mongolian, Marathi, Malay, Maltese, Burmese, Norwegian Bokmål, Dutch, Norwegian Nynorsk, Norwegian, Occitan, Polish, Portuguese, Quechua, Rundi, Romanian, Russian, Serbo-Croatian, Slovenian, Albanian, Serbian, Swedish, Swahili, Tamil, Telugu, Thai, Turkmen, Tagalog, Turkish, Tatar, Uighur, Ukrainian, Urdu, Uzbek, Vietnamese, Volapük, Yiddish, Chinese
## Dataset Structure
### Data Instances
Data instances are given as translation units in TAB-separated files with four columns: source and target language ISO-639-3 codes, source language text and target language text. Note that we do not imply a translation direction and consider the data set to be symmetric and to be used as a test set in both directions. Language-pair-specific subsets are only provided under the label of one direction using sorted ISO-639-3 language IDs.
Some subsets contain several sub-languages or language variants. They may refer to macro-languages such as Serbo-Croatian languages that are covered by the ISO code `hbs`. Language variants may also include different writing systems and in that case the ISO15924 script codes are attached to the language codes. Here are a few examples from the English to Serbo-Croation test set including examples for Bosnian, Croatian and Serbian in Cyrillic and in Latin scripts:
```
eng bos_Latn Children are the flowers of our lives. Djeca su cvijeće našeg života.
eng hrv A bird was flying high up in the sky. Ptica je visoko letjela nebom.
eng srp_Cyrl A bird in the hand is worth two in the bush. Боље врабац у руци, него голуб на грани.
eng srp_Latn Canada is the motherland of ice hockey. Kanada je zemlja-majka hokeja na ledu.
```
There are also data sets with sentence pairs in the same language. In most cases, those are variants with minor spelling differences but they also include rephrased sentences. Here are a few examples from the English test set:
```
eng eng All of us got into the car. We all got in the car.
eng eng All of us hope that doesn't happen. All of us hope that that doesn't happen.
eng eng All the seats are booked. The seats are all sold out.
```
### Data Splits
Test and development data sets are disjoint with respect to sentence pairs but may include overlaps in individual source or target language sentences. Development data should not be used in training directly. The goal of the data splits is to create test sets of reasonable size with a large language coverage. Test sets include at most 10,000 instances. Development data do not exist for all language pairs.
To be comparable with other results, models should use the training data distributed from the [Tatoeba MT Challenge Repository](https://github.com/Helsinki-NLP/Tatoeba-Challenge/) including monolingual data sets also listed there.
## Dataset Creation
### Curation Rationale
The Tatoeba MT data set will be updated continuously and the data preparation procedures are also public and released on [github](https://github.com/Helsinki-NLP/Tatoeba-Challenge/). High language coverage is the main goal of the project and data sets are prepared to be consistent and systematic with standardized language labels and distribution formats.
### Source Data
#### Initial Data Collection and Normalization
The Tatoeba data sets are collected from user-contributed translations submitted to [Tatoeba.org](https://tatoeba.org/) and compiled into a multi-parallel corpus in [OPUS](https://opus.nlpl.eu/Tatoeba.php). The test and development sets are incrementally updated with new releases of the Tatoeba data collection at OPUS. New releases extend the existing data sets. Test sets should not overlap with any of the released development data sets.
#### Who are the source language producers?
The data sets come from [Tatoeba.org](https://tatoeba.org/), which provides a large database of sentences and their translations into a wide varity of languages. Its content is constantly growing as a result of voluntary contributions of thousands of users.
The original project was founded by Trang Ho in 2006, hosted on Sourceforge under the codename of multilangdict.
### Annotations
#### Annotation process
Sentences are translated by volunteers and the Tatoeba database also provides additional metadata about each record including user ratings etc. However, the metadata is currently not used in any way for the compilation of the MT benchmark. Language skills of contributors naturally vary quite a bit and not all translations are done by native speakers of the target language. More information about the contributions can be found at [Tatoeba.org](https://tatoeba.org/).
#### Who are the annotators?
### Personal and Sensitive Information
For information about handling personal and sensitive information we refer to the [original provider](https://tatoeba.org/) of the data. This data set has not been processed in any way to detect or remove potentially sensitve or personal information.
## Considerations for Using the Data
### Social Impact of Dataset
The language coverage is high and with that it represents a highly valuable resource for machine translation development especially for lesser resourced languages and language pairs. The constantly growing database also represents a dynamic resource and its value wil grow further.
### Discussion of Biases
The original source lives from its contributors and there interest and background will to certain subjective and cultural biases. Language coverage and translation quality is also biased by the skills of the contributors.
### Other Known Limitations
The sentences are typically quite short and, therefore, rather easy to translate. For high-resource languages, this leads to results that will be less useful than more challenging benchmarks. For lesser resource language pairs, the limited complexity of the examples is actually a good thing to measure progress even in very challenging setups.
## Additional Information
### Dataset Curators
The data set is curated by the University of Helsinki and its [language technology research group](https://blogs.helsinki.fi/language-technology/). Data and tools used for creating and using the resource are [open source](https://github.com/Helsinki-NLP/Tatoeba-Challenge/) and will be maintained as part of the [OPUS ecosystem](https://opus.nlpl.eu/) for parallel data and machine translation research.
### Licensing Information
The data sets are distributed under the same licence agreement as the original Tatoeba database using a
[CC-BY 2.0 license](https://creativecommons.org/licenses/by/2.0/fr/). More information about the terms of use of the original data sets is listed [here](https://tatoeba.org/eng/terms_of_use).
### Citation Information
If you use the data sets then, please, cite the following paper: [The Tatoeba Translation Challenge – Realistic Data Sets for Low Resource and Multilingual MT](https://aclanthology.org/2020.wmt-1.139/)
```
@inproceedings{tiedemann-2020-tatoeba,
title = "The Tatoeba Translation Challenge {--} Realistic Data Sets for Low Resource and Multilingual {MT}",
author = {Tiedemann, J{\"o}rg},
booktitle = "Proceedings of the Fifth Conference on Machine Translation",
month = nov,
year = "2020",
address = "Online",
publisher = "Association for Computational Linguistics",
url = "https://aclanthology.org/2020.wmt-1.139",
pages = "1174--1182",
}
```
### Contributions
Thanks to [@jorgtied](https://github.com/jorgtied) and [@Helsinki-NLP](https://github.com/Helsinki-NLP) for adding this dataset.
Thanks also to [CSC Finland](https://www.csc.fi/en/solutions-for-research) for providing computational resources and storage space for the work on OPUS and other MT projects.
|
HenryAI/KerasAPIReference.txt | Keras API from https://keras.io/api/ <br />
Formatted into .txt file for input to https://huggingface.co/blog/how-to-train |
HenryAI/KerasCodeExamples.txt | Keras Code Examples from https://keras.io/examples/ <br />
organized as .txt file for input to this HF tutorial: <br />
https://huggingface.co/blog/how-to-train |
HenryAI/KerasDeveloperGuides.txt | Keras developer guides from https://keras.io/guides/ <br />
Formatted for input to: https://huggingface.co/blog/how-to-train |
IFSTalfredoswald/MBTI | ---
YAML tags:
- copy-paste the tags obtained with the tagging app: https://github.com/huggingface/datasets-tagging
---
# Dataset Card for [Dataset Name]
## Table of Contents
- [Table of Contents](#table-of-contents)
- [Dataset Description](#dataset-description)
- [Dataset Summary](#dataset-summary)
- [Supported Tasks and Leaderboards](#supported-tasks-and-leaderboards)
- [Languages](#languages)
- [Dataset Structure](#dataset-structure)
- [Data Instances](#data-instances)
- [Data Fields](#data-fields)
- [Data Splits](#data-splits)
- [Dataset Creation](#dataset-creation)
- [Curation Rationale](#curation-rationale)
- [Source Data](#source-data)
- [Annotations](#annotations)
- [Personal and Sensitive Information](#personal-and-sensitive-information)
- [Considerations for Using the Data](#considerations-for-using-the-data)
- [Social Impact of Dataset](#social-impact-of-dataset)
- [Discussion of Biases](#discussion-of-biases)
- [Other Known Limitations](#other-known-limitations)
- [Additional Information](#additional-information)
- [Dataset Curators](#dataset-curators)
- [Licensing Information](#licensing-information)
- [Citation Information](#citation-information)
- [Contributions](#contributions)
## Dataset Description
- **Homepage:**
- **Repository:**
- **Paper:**
- **Leaderboard:**
- **Point of Contact:**
### Dataset Summary
[More Information Needed]
### Supported Tasks and Leaderboards
[More Information Needed]
### Languages
[More Information Needed]
## Dataset Structure
### Data Instances
[More Information Needed]
### Data Fields
[More Information Needed]
### Data Splits
[More Information Needed]
## Dataset Creation
### Curation Rationale
[More Information Needed]
### Source Data
#### Initial Data Collection and Normalization
[More Information Needed]
#### Who are the source language producers?
[More Information Needed]
### Annotations
#### Annotation process
[More Information Needed]
#### Who are the annotators?
[More Information Needed]
### Personal and Sensitive Information
[More Information Needed]
## Considerations for Using the Data
### Social Impact of Dataset
[More Information Needed]
### Discussion of Biases
[More Information Needed]
### Other Known Limitations
[More Information Needed]
## Additional Information
### Dataset Curators
[More Information Needed]
### Licensing Information
[More Information Needed]
### Citation Information
[More Information Needed]
### Contributions
Thanks to [@github-username](https://github.com/<github-username>) for adding this dataset. |
Iftoo95/Arabic_Sentiment_and_Topics | Arabic Twitter based dataset with multi-labels that contains two classes:
1. Sentiment class: classifies tweets as Positive, Negative and Neutral
2. Topic class: Classifies tweets as Politics, Business and Health |
IlyaGusev/gazeta | ---
annotations_creators:
- expert-generated
- found
language_creators:
- expert-generated
- found
task_categories:
- summarization
language:
- ru
size_categories:
- 10K<n<100K
license:
- unknown
multilinguality:
- monolingual
source_datasets:
- original
paperswithcode_id: gazeta
---
# Dataset Card for Gazeta
## Table of Contents
- [Table of Contents](#table-of-contents)
- [Dataset Description](#dataset-description)
- [Dataset Summary](#dataset-summary)
- [Supported Tasks and Leaderboards](#supported-tasks-and-leaderboards)
- [Languages](#languages)
- [Dataset Structure](#dataset-structure)
- [Data Instances](#data-instances)
- [Data Fields](#data-fields)
- [Data Splits](#data-splits)
- [Dataset Creation](#dataset-creation)
- [Curation Rationale](#curation-rationale)
- [Source Data](#source-data)
- [Annotations](#annotations)
- [Personal and Sensitive Information](#personal-and-sensitive-information)
- [Considerations for Using the Data](#considerations-for-using-the-data)
- [Social Impact of Dataset](#social-impact-of-dataset)
- [Discussion of Biases](#discussion-of-biases)
- [Other Known Limitations](#other-known-limitations)
- [Additional Information](#additional-information)
- [Dataset Curators](#dataset-curators)
- [Licensing Information](#licensing-information)
- [Citation Information](#citation-information)
- [Contributions](#contributions)
## Dataset Description
- **Homepage:** https://github.com/IlyaGusev/gazeta
- **Paper:** [Dataset for Automatic Summarization of Russian News](https://arxiv.org/abs/2006.11063)
- **Leaderboard:** https://paperswithcode.com/sota/text-summarization-on-gazeta
- **Point of Contact:** [Ilya Gusev](ilya.gusev@phystech.edu)
### Dataset Summary
Dataset for automatic summarization of Russian news. News and their summaries are from the Gazeta website. Summaries were parsed as the content of an HTML tag with “description” property. Additional selection of good summaries was performed. There are two versions of this dataset.
### Supported Tasks and Leaderboards
Leaderboard on Papers With Code: [text-summarization-on-gazeta](https://paperswithcode.com/sota/text-summarization-on-gazeta).
Please use the original [evaluation script](https://github.com/IlyaGusev/summarus/blob/master/evaluate.py) with the same parameters. Example:
```
python3 evaluate.py --predicted-path predictions.txt --gold-path targets.txt --language ru --tokenize-after --lower
```
### Languages
The dataset is in Russian.
### Usage
Loading version 1.0:
```python
from datasets import load_dataset
dataset = load_dataset('IlyaGusev/gazeta', revision="v1.0")
```
Loading version 2.0:
```python
from datasets import load_dataset
dataset = load_dataset('IlyaGusev/gazeta', revision="v2.0")
```
### Other datasets
Other Russian summarization datasets:
* Russian part of [XL-Sum](https://huggingface.co/datasets/csebuetnlp/xlsum), parsed from www.bbc.com/russian, 77803 samples
* Russian part of [MLSUM](https://huggingface.co/datasets/mlsum), parsed from www.mk.ru, 27063 samples
## Dataset Structure
### Data Instances
For each instance, there is a string for the article, a string for the summary, and a string for the url. Additionally, a string for the title and a date are provided.
```
{
'date': '2019-10-01 15:14:05',
'url': 'https://www.gazeta.ru/tech/2019/10/01/12698923/whatsapp_pls.shtml',
'title': 'На последнем издыхании: у кого отключится WhatsApp',
'summary': 'Мессенджер WhatsApp перестанет работать на ряде смартфонов — речь идет о гаджетах на базе операционных систем Android 2.3.7 и iOS 8, которые считаются устаревшими. В компании отмечают, что сервис на этих устройствах может отключиться в любой момент, поэтому будет целесообразно сменить устройство либо обновить ОС.',
'text': 'На официальном сайте мессенджера WhatsApp появилось сообщение о том, что с 1 февраля 2020 года сервис прекратит свою работу на некоторых устаревших смартфонах. Речь идет об устройствах, работающих на базе операционных систем Android 2.3.7 и iOS 8. При этом руководство WhatsApp предупреждает, что даже до обозначенного выше дедлайна функционал мессенджера на этих ОС может быть ограничен. «В связи с тем, что мы не планируем обновлять данные операционные системы, некоторые функции могут перестать работать на них в любое время», — говорится в пресс-релизе компании. Чтобы сохранить возможность пользоваться мессенджером без проблем, следует обновить версию прошивки или приобрести новое, более современное устройство. Сообщается, что на старых версиях операционных систем уже не получится завести новый аккаунт WhatsApp или верифицировать уже существующий. При этом в WhatsApp порекомендовали пользоваться устройствами с Android 4.0.3 и более поздними версиями, а также iOS 9 и более поздними версиями. Ранее стало известно о том, что с 31 декабря 2019 года WhatsApp прекращает поддержку устройств на базе операционной системы Windows Phone, от разработки которой пришлось отказаться. Впрочем, если верить статистике , эти меры вряд ли затронут большое количество пользователей. По состоянию на май 2019 года лишь 0,3% всех владельцев Android все еще пользуются ОС версий 2.3.3–2.3.7. Что же касается iOS, то версия под номером «10» или старше установлена на 5% устройств Apple. Как уже упоминалось выше, выпуск новых гаджетов на Windows Phone и вовсе прекращен ее создателем. В середине сентября экс-сотрудник АНБ Эдвард Сноуден раскритиковал WhatsApp за несовершенную систему защиты, порекомендовав политикам пользоваться другими средствами связи. Журналист французской радиостанции France Inter отметил, что президент Франции Эмманюэль Макрон для связи использует Telegram, а премьер-министр страны Эдуар Филипп — WhatsApp. Сноуден назвал такое решение «большой ошибкой», учитывая серьезные посты, которые занимают Макрон и Филипп. По словам Сноудена, эти сервисы безопаснее обычных SMS-сообщений, но все еще «чрезвычайно опасны, если вы премьер-министр». Больше всего претензий у информатора к WhatsApp, который стал частью активов корпорации Facebook в 2014 году. Эдвард Сноуден отметил, что после приобретения мессенджера Facebook «слой за слоем» снимает различные уровни защиты сервиса, чтобы при необходимости читать переписку своих пользователей. Ранее с критикой в адрес WhatsApp выступил и глава Telegram Павел Дуров. По словам предпринимателя, после устранения одной «дыры» в мессенджере тут же появляются новые. «Все выявленные проблемы позволяют вести слежку, выглядят и функционируют как бэкдоры», — заявил Дуров. При этом Дуров подчеркнул, что WhatsApp мог быть вынужден установить бэкдоры по указанию ФБР. В июне руководство WhatsApp заявило о том, что их сервис готов судиться с юзерами за нарушение правил пользования. В список нарушений входит использование программы «не в личных целях» и применение автоматической рассылки сообщений. По данным пресс-службы WhatsApp, уже сейчас обнаружены и заморожены «миллионы аккаунтов», пойманных на «злоупотреблении». «Наша платформа изначально создавалась, чтобы помогать людям общаться с их друзьями и любимыми... Используя информацию приложения, мы нашли и заблокировали миллионы злоупотребляющих аккаунтов от использования нашей сети», – заявили в WhatsApp. В частности, нарушение происходит, если компания публично заявляет о возможности использовать WhatsApp, нарушая при этом правила пользования мессенджером. «Ничто в этом объявлении не ограничивает право WhatsApp от применения своих условий с использованием технологий. Классификаторы на основе machine learning нам в этом помогают, и мы продолжим их использовать», – добавили в команде приложения.',
}
```
Some dataset statistics are below:
| Feature | Mean Token Count | Mean Sentence Count |
|:---------|:---------|--------------------------------------------------|
| Text | 767 | 37 |
| Summary | 50 | 3 |
### Data Splits
| Dataset Split | v1, Number of Instances in Split | v2, Number of Instances in Split |
|:---------|:---------|:---------|
| Train | 52,400 | 60,964 |
| Validation | 5,265 | 6,369 |
| Test | 5,770 | 6,793 |
## Dataset Creation
### Curation Rationale
When the first version of the dataset was collected, there were no other datasets for Russian text summarization. Even now, it is one of the few datasets for this task.
### Source Data
#### Initial Data Collection and Normalization
* The source of data is the [Gazeta](https://www.gazeta.ru/) website.
* Parsing scripts are [here](https://github.com/IlyaGusev/gazeta/tree/master/parser).
* Cleaning and normalization Colab notebook is [here](https://colab.research.google.com/drive/1Ed_chVrslp_7vJNS3PmRC0_ZJrRQYv0C)
#### Who are the source language producers?
Texts and summaries were written by journalists at [Gazeta](https://www.gazeta.ru/).
### Annotations
#### Annotation process
[N/A]
#### Who are the annotators?
[N/A]
### Personal and Sensitive Information
The dataset is not anonymized, so individuals' names can be found in the dataset. Information about the original author is not included in the dataset.
## Considerations for Using the Data
### Social Impact of Dataset
[More Information Needed]
### Discussion of Biases
It is a dataset from a single source. Thus it has a constrained text style and event perspective.
### Other Known Limitations
[More Information Needed]
## Additional Information
### Dataset Curators
The data was collected by Ilya Gusev.
### Licensing Information
Legal basis for distribution of the dataset: https://www.gazeta.ru/credits.shtml, paragraph 2.1.2. All rights belong to "www.gazeta.ru". Usage of this dataset is possible only for personal purposes on a non-commercial basis.
### Citation Information
```bibtex
@InProceedings{10.1007/978-3-030-59082-6_9,
author="Gusev, Ilya",
editor="Filchenkov, Andrey and Kauttonen, Janne and Pivovarova, Lidia",
title="Dataset for Automatic Summarization of Russian News",
booktitle="Artificial Intelligence and Natural Language",
year="2020",
publisher="Springer International Publishing",
address="Cham",
pages="122--134",
isbn="978-3-030-59082-6"
}
```
### Contributions
[N/A] |