Datasets:
Tasks:
Image Classification
Size:
10K - 100K
Commit
•
030dcb9
1
Parent(s):
0acfab5
dataset uploaded by roboflow2huggingface package
Browse files- README.dataset.txt +22 -0
- README.md +80 -0
- README.roboflow.txt +24 -0
- data/test.zip +3 -0
- data/train.zip +3 -0
- data/valid-mini.zip +3 -0
- data/valid.zip +3 -0
- indoor-scene-classification.py +103 -0
- split_name_to_num_samples.json +1 -0
- thumbnail.jpg +3 -0
README.dataset.txt
ADDED
@@ -0,0 +1,22 @@
|
|
|
|
|
|
|
|
|
|
|
|
|
|
|
|
|
|
|
|
|
|
|
|
|
|
|
|
|
|
|
|
|
|
|
|
|
|
|
|
|
|
|
|
|
|
|
1 |
+
# MIT Indoor Scene Recognition > resized416by416_70-20-10Split
|
2 |
+
https://universe.roboflow.com/classification/mit-indoor-scene-recognition
|
3 |
+
|
4 |
+
Provided by Roboflow
|
5 |
+
License: MIT
|
6 |
+
|
7 |
+
## Indoor Scene Recognition
|
8 |
+
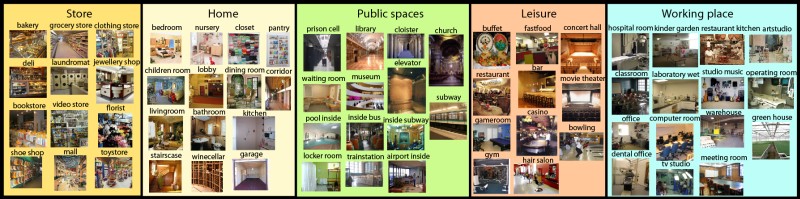
|
9 |
+
[From the official dataset page](http://web.mit.edu/torralba/www/indoor.html):
|
10 |
+
Indoor scene recognition is a challenging open problem in high level vision. Most scene recognition models that work well for outdoor scenes perform poorly in the indoor domain. The main difficulty is that while some indoor scenes (e.g. corridors) can be well characterized by global spatial properties, others (e.g., bookstores) are better characterized by the objects they contain. More generally, to address the indoor scenes recognition problem we need a model that can exploit local and global discriminative information.
|
11 |
+
|
12 |
+
### Database
|
13 |
+
The database contains `67 Indoor categories` ... The number of images varies across categories, but there are at least 100 images per category. All images are in jpg format. The images provided here are for research purposes only.
|
14 |
+
|
15 |
+
### Paper
|
16 |
+
A. Quattoni, and A.Torralba. Recognizing Indoor Scenes. [IEEE Conference on Computer Vision and Pattern Recognition](https://cvpr2023.thecvf.com/) (CVPR), 2009.
|
17 |
+
|
18 |
+
### Acknowledgments
|
19 |
+
```
|
20 |
+
Thanks to Aude Oliva for helping to create the database of indoor scenes.
|
21 |
+
Funding for this research was provided by NSF Career award (IIS 0747120)
|
22 |
+
```
|
README.md
ADDED
@@ -0,0 +1,80 @@
|
|
|
|
|
|
|
|
|
|
|
|
|
|
|
|
|
|
|
|
|
|
|
|
|
|
|
|
|
|
|
|
|
|
|
|
|
|
|
|
|
|
|
|
|
|
|
|
|
|
|
|
|
|
|
|
|
|
|
|
|
|
|
|
|
|
|
|
|
|
|
|
|
|
|
|
|
|
|
|
|
|
|
|
|
|
|
|
|
|
|
|
|
|
|
|
|
|
|
|
|
|
|
|
|
|
|
|
|
|
|
|
|
|
|
|
|
|
|
|
|
|
|
|
|
|
|
|
|
|
|
|
|
|
|
|
|
|
|
|
|
|
|
|
|
|
|
|
|
|
|
|
|
|
|
|
|
|
|
|
|
|
|
1 |
+
---
|
2 |
+
task_categories:
|
3 |
+
- image-classification
|
4 |
+
tags:
|
5 |
+
- roboflow
|
6 |
+
- roboflow2huggingface
|
7 |
+
- Retail
|
8 |
+
- Pest Control
|
9 |
+
- Benchmark
|
10 |
+
---
|
11 |
+
|
12 |
+
<div align="center">
|
13 |
+
<img width="640" alt="keremberke/indoor-scene-classification" src="https://huggingface.co/datasets/keremberke/indoor-scene-classification/resolve/main/thumbnail.jpg">
|
14 |
+
</div>
|
15 |
+
|
16 |
+
### Dataset Labels
|
17 |
+
|
18 |
+
```
|
19 |
+
['meeting_room', 'cloister', 'stairscase', 'restaurant', 'hairsalon', 'children_room', 'dining_room', 'lobby', 'museum', 'laundromat', 'computerroom', 'grocerystore', 'hospitalroom', 'buffet', 'office', 'warehouse', 'garage', 'bookstore', 'florist', 'locker_room', 'inside_bus', 'subway', 'fastfood_restaurant', 'auditorium', 'studiomusic', 'airport_inside', 'pantry', 'restaurant_kitchen', 'casino', 'movietheater', 'kitchen', 'waitingroom', 'artstudio', 'toystore', 'kindergarden', 'trainstation', 'bedroom', 'mall', 'corridor', 'bar', 'classroom', 'shoeshop', 'dentaloffice', 'videostore', 'laboratorywet', 'tv_studio', 'church_inside', 'operating_room', 'jewelleryshop', 'bathroom', 'clothingstore', 'closet', 'winecellar', 'livingroom', 'nursery', 'gameroom', 'inside_subway', 'deli', 'bakery', 'library', 'prisoncell', 'gym', 'concert_hall', 'greenhouse', 'elevator', 'poolinside', 'bowling']
|
20 |
+
```
|
21 |
+
|
22 |
+
|
23 |
+
### Number of Images
|
24 |
+
|
25 |
+
```json
|
26 |
+
{'train': 10885, 'test': 1558, 'valid': 3128}
|
27 |
+
```
|
28 |
+
|
29 |
+
|
30 |
+
### How to Use
|
31 |
+
|
32 |
+
- Install [datasets](https://pypi.org/project/datasets/):
|
33 |
+
|
34 |
+
```bash
|
35 |
+
pip install datasets
|
36 |
+
```
|
37 |
+
|
38 |
+
- Load the dataset:
|
39 |
+
|
40 |
+
```python
|
41 |
+
from datasets import load_dataset
|
42 |
+
|
43 |
+
ds = load_dataset("keremberke/indoor-scene-classification", name="full")
|
44 |
+
example = ds['train'][0]
|
45 |
+
```
|
46 |
+
|
47 |
+
### Roboflow Dataset Page
|
48 |
+
[https://universe.roboflow.com/popular-benchmarks/mit-indoor-scene-recognition/dataset/5](https://universe.roboflow.com/popular-benchmarks/mit-indoor-scene-recognition/dataset/5?ref=roboflow2huggingface)
|
49 |
+
|
50 |
+
### Citation
|
51 |
+
|
52 |
+
```
|
53 |
+
|
54 |
+
```
|
55 |
+
|
56 |
+
### License
|
57 |
+
MIT
|
58 |
+
|
59 |
+
### Dataset Summary
|
60 |
+
This dataset was exported via roboflow.com on October 24, 2022 at 4:09 AM GMT
|
61 |
+
|
62 |
+
Roboflow is an end-to-end computer vision platform that helps you
|
63 |
+
* collaborate with your team on computer vision projects
|
64 |
+
* collect & organize images
|
65 |
+
* understand unstructured image data
|
66 |
+
* annotate, and create datasets
|
67 |
+
* export, train, and deploy computer vision models
|
68 |
+
* use active learning to improve your dataset over time
|
69 |
+
|
70 |
+
It includes 15571 images.
|
71 |
+
Indoor-scenes are annotated in folder format.
|
72 |
+
|
73 |
+
The following pre-processing was applied to each image:
|
74 |
+
* Auto-orientation of pixel data (with EXIF-orientation stripping)
|
75 |
+
* Resize to 416x416 (Stretch)
|
76 |
+
|
77 |
+
No image augmentation techniques were applied.
|
78 |
+
|
79 |
+
|
80 |
+
|
README.roboflow.txt
ADDED
@@ -0,0 +1,24 @@
|
|
|
|
|
|
|
|
|
|
|
|
|
|
|
|
|
|
|
|
|
|
|
|
|
|
|
|
|
|
|
|
|
|
|
|
|
|
|
|
|
|
|
|
|
|
|
|
|
|
|
1 |
+
|
2 |
+
MIT Indoor Scene Recognition - v5 resized416by416_70-20-10Split
|
3 |
+
==============================
|
4 |
+
|
5 |
+
This dataset was exported via roboflow.com on October 24, 2022 at 4:09 AM GMT
|
6 |
+
|
7 |
+
Roboflow is an end-to-end computer vision platform that helps you
|
8 |
+
* collaborate with your team on computer vision projects
|
9 |
+
* collect & organize images
|
10 |
+
* understand unstructured image data
|
11 |
+
* annotate, and create datasets
|
12 |
+
* export, train, and deploy computer vision models
|
13 |
+
* use active learning to improve your dataset over time
|
14 |
+
|
15 |
+
It includes 15571 images.
|
16 |
+
Indoor-scenes are annotated in folder format.
|
17 |
+
|
18 |
+
The following pre-processing was applied to each image:
|
19 |
+
* Auto-orientation of pixel data (with EXIF-orientation stripping)
|
20 |
+
* Resize to 416x416 (Stretch)
|
21 |
+
|
22 |
+
No image augmentation techniques were applied.
|
23 |
+
|
24 |
+
|
data/test.zip
ADDED
@@ -0,0 +1,3 @@
|
|
|
|
|
|
|
|
|
1 |
+
version https://git-lfs.github.com/spec/v1
|
2 |
+
oid sha256:c22b2043a539cec8cfdc73f229bc4bcacc1d896fd2b55a7d15ca620809791c0b
|
3 |
+
size 46531641
|
data/train.zip
ADDED
@@ -0,0 +1,3 @@
|
|
|
|
|
|
|
|
|
1 |
+
version https://git-lfs.github.com/spec/v1
|
2 |
+
oid sha256:5a0c78b0bf30d785f17e9acbbeb15a397ef0a18454e689f1ecb4d0df1d2a15dc
|
3 |
+
size 328673546
|
data/valid-mini.zip
ADDED
@@ -0,0 +1,3 @@
|
|
|
|
|
|
|
|
|
1 |
+
version https://git-lfs.github.com/spec/v1
|
2 |
+
oid sha256:ecc7d5904619a0ad64dca88ac2e18f028d0701b94dbc427a58cc4b6e0af79e30
|
3 |
+
size 1232126
|
data/valid.zip
ADDED
@@ -0,0 +1,3 @@
|
|
|
|
|
|
|
|
|
1 |
+
version https://git-lfs.github.com/spec/v1
|
2 |
+
oid sha256:ffa061a72fd151f4cfd39dc20b20ca1992bc22462161937757650db9c4707dcc
|
3 |
+
size 93670298
|
indoor-scene-classification.py
ADDED
@@ -0,0 +1,103 @@
|
|
|
|
|
|
|
|
|
|
|
|
|
|
|
|
|
|
|
|
|
|
|
|
|
|
|
|
|
|
|
|
|
|
|
|
|
|
|
|
|
|
|
|
|
|
|
|
|
|
|
|
|
|
|
|
|
|
|
|
|
|
|
|
|
|
|
|
|
|
|
|
|
|
|
|
|
|
|
|
|
|
|
|
|
|
|
|
|
|
|
|
|
|
|
|
|
|
|
|
|
|
|
|
|
|
|
|
|
|
|
|
|
|
|
|
|
|
|
|
|
|
|
|
|
|
|
|
|
|
|
|
|
|
|
|
|
|
|
|
|
|
|
|
|
|
|
|
|
|
|
|
|
|
|
|
|
|
|
|
|
|
|
|
|
|
|
|
|
|
|
|
|
|
|
|
|
|
|
|
|
|
|
|
|
|
|
|
|
|
|
|
|
|
|
|
|
|
|
|
|
|
|
|
|
|
|
|
|
1 |
+
import os
|
2 |
+
|
3 |
+
import datasets
|
4 |
+
from datasets.tasks import ImageClassification
|
5 |
+
|
6 |
+
|
7 |
+
_HOMEPAGE = "https://universe.roboflow.com/popular-benchmarks/mit-indoor-scene-recognition/dataset/5"
|
8 |
+
_LICENSE = "MIT"
|
9 |
+
_CITATION = """\
|
10 |
+
|
11 |
+
"""
|
12 |
+
_CATEGORIES = ['meeting_room', 'cloister', 'stairscase', 'restaurant', 'hairsalon', 'children_room', 'dining_room', 'lobby', 'museum', 'laundromat', 'computerroom', 'grocerystore', 'hospitalroom', 'buffet', 'office', 'warehouse', 'garage', 'bookstore', 'florist', 'locker_room', 'inside_bus', 'subway', 'fastfood_restaurant', 'auditorium', 'studiomusic', 'airport_inside', 'pantry', 'restaurant_kitchen', 'casino', 'movietheater', 'kitchen', 'waitingroom', 'artstudio', 'toystore', 'kindergarden', 'trainstation', 'bedroom', 'mall', 'corridor', 'bar', 'classroom', 'shoeshop', 'dentaloffice', 'videostore', 'laboratorywet', 'tv_studio', 'church_inside', 'operating_room', 'jewelleryshop', 'bathroom', 'clothingstore', 'closet', 'winecellar', 'livingroom', 'nursery', 'gameroom', 'inside_subway', 'deli', 'bakery', 'library', 'prisoncell', 'gym', 'concert_hall', 'greenhouse', 'elevator', 'poolinside', 'bowling']
|
13 |
+
|
14 |
+
|
15 |
+
class INDOORSCENECLASSIFICATIONConfig(datasets.BuilderConfig):
|
16 |
+
"""Builder Config for indoor-scene-classification"""
|
17 |
+
|
18 |
+
def __init__(self, data_urls, **kwargs):
|
19 |
+
"""
|
20 |
+
BuilderConfig for indoor-scene-classification.
|
21 |
+
|
22 |
+
Args:
|
23 |
+
data_urls: `dict`, name to url to download the zip file from.
|
24 |
+
**kwargs: keyword arguments forwarded to super.
|
25 |
+
"""
|
26 |
+
super(INDOORSCENECLASSIFICATIONConfig, self).__init__(version=datasets.Version("1.0.0"), **kwargs)
|
27 |
+
self.data_urls = data_urls
|
28 |
+
|
29 |
+
|
30 |
+
class INDOORSCENECLASSIFICATION(datasets.GeneratorBasedBuilder):
|
31 |
+
"""indoor-scene-classification image classification dataset"""
|
32 |
+
|
33 |
+
VERSION = datasets.Version("1.0.0")
|
34 |
+
BUILDER_CONFIGS = [
|
35 |
+
INDOORSCENECLASSIFICATIONConfig(
|
36 |
+
name="full",
|
37 |
+
description="Full version of indoor-scene-classification dataset.",
|
38 |
+
data_urls={
|
39 |
+
"train": "https://huggingface.co/datasets/keremberke/indoor-scene-classification/resolve/main/data/train.zip",
|
40 |
+
"validation": "https://huggingface.co/datasets/keremberke/indoor-scene-classification/resolve/main/data/valid.zip",
|
41 |
+
"test": "https://huggingface.co/datasets/keremberke/indoor-scene-classification/resolve/main/data/test.zip",
|
42 |
+
}
|
43 |
+
,
|
44 |
+
),
|
45 |
+
INDOORSCENECLASSIFICATIONConfig(
|
46 |
+
name="mini",
|
47 |
+
description="Mini version of indoor-scene-classification dataset.",
|
48 |
+
data_urls={
|
49 |
+
"train": "https://huggingface.co/datasets/keremberke/indoor-scene-classification/resolve/main/data/valid-mini.zip",
|
50 |
+
"validation": "https://huggingface.co/datasets/keremberke/indoor-scene-classification/resolve/main/data/valid-mini.zip",
|
51 |
+
"test": "https://huggingface.co/datasets/keremberke/indoor-scene-classification/resolve/main/data/valid-mini.zip",
|
52 |
+
},
|
53 |
+
)
|
54 |
+
]
|
55 |
+
|
56 |
+
def _info(self):
|
57 |
+
return datasets.DatasetInfo(
|
58 |
+
features=datasets.Features(
|
59 |
+
{
|
60 |
+
"image_file_path": datasets.Value("string"),
|
61 |
+
"image": datasets.Image(),
|
62 |
+
"labels": datasets.features.ClassLabel(names=_CATEGORIES),
|
63 |
+
}
|
64 |
+
),
|
65 |
+
supervised_keys=("image", "labels"),
|
66 |
+
homepage=_HOMEPAGE,
|
67 |
+
citation=_CITATION,
|
68 |
+
license=_LICENSE,
|
69 |
+
task_templates=[ImageClassification(image_column="image", label_column="labels")],
|
70 |
+
)
|
71 |
+
|
72 |
+
def _split_generators(self, dl_manager):
|
73 |
+
data_files = dl_manager.download_and_extract(self.config.data_urls)
|
74 |
+
return [
|
75 |
+
datasets.SplitGenerator(
|
76 |
+
name=datasets.Split.TRAIN,
|
77 |
+
gen_kwargs={
|
78 |
+
"files": dl_manager.iter_files([data_files["train"]]),
|
79 |
+
},
|
80 |
+
),
|
81 |
+
datasets.SplitGenerator(
|
82 |
+
name=datasets.Split.VALIDATION,
|
83 |
+
gen_kwargs={
|
84 |
+
"files": dl_manager.iter_files([data_files["validation"]]),
|
85 |
+
},
|
86 |
+
),
|
87 |
+
datasets.SplitGenerator(
|
88 |
+
name=datasets.Split.TEST,
|
89 |
+
gen_kwargs={
|
90 |
+
"files": dl_manager.iter_files([data_files["test"]]),
|
91 |
+
},
|
92 |
+
),
|
93 |
+
]
|
94 |
+
|
95 |
+
def _generate_examples(self, files):
|
96 |
+
for i, path in enumerate(files):
|
97 |
+
file_name = os.path.basename(path)
|
98 |
+
if file_name.endswith((".jpg", ".png", ".jpeg", ".bmp", ".tif", ".tiff")):
|
99 |
+
yield i, {
|
100 |
+
"image_file_path": path,
|
101 |
+
"image": path,
|
102 |
+
"labels": os.path.basename(os.path.dirname(path)),
|
103 |
+
}
|
split_name_to_num_samples.json
ADDED
@@ -0,0 +1 @@
|
|
|
|
|
1 |
+
{"train": 10885, "test": 1558, "valid": 3128}
|
thumbnail.jpg
ADDED
![]() |
Git LFS Details
|