Luke Cheng
commited on
Commit
•
96d06f0
1
Parent(s):
e3abea0
Add markdown
Browse filesThis view is limited to 50 files because it contains too many changes.
See raw diff
- markdown/dreambooth/DreamBoothCN.md +781 -0
- markdown/unit1/01_introduction_to_diffusers_CN.md +0 -0
- markdown/unit1/01_introduction_to_diffusers_CN_files/01_introduction_to_diffusers_CN_18_0.jpg +3 -0
- markdown/unit1/01_introduction_to_diffusers_CN_files/01_introduction_to_diffusers_CN_22_1.png +3 -0
- markdown/unit1/01_introduction_to_diffusers_CN_files/01_introduction_to_diffusers_CN_26_2.png +3 -0
- markdown/unit1/01_introduction_to_diffusers_CN_files/01_introduction_to_diffusers_CN_31_2.png +3 -0
- markdown/unit1/01_introduction_to_diffusers_CN_files/01_introduction_to_diffusers_CN_40_1.png +3 -0
- markdown/unit1/01_introduction_to_diffusers_CN_files/01_introduction_to_diffusers_CN_54_1.png +3 -0
- markdown/unit1/01_introduction_to_diffusers_CN_files/01_introduction_to_diffusers_CN_60_1.png +3 -0
- markdown/unit1/01_introduction_to_diffusers_CN_files/01_introduction_to_diffusers_CN_71_0.png +3 -0
- markdown/unit1/01_introduction_to_diffusers_CN_files/01_introduction_to_diffusers_CN_80_2.png +3 -0
- markdown/unit1/01_introduction_to_diffusers_CN_files/01_introduction_to_diffusers_CN_90_1.png +3 -0
- markdown/unit1/02_diffusion_models_from_scratch_CN.md +0 -0
- markdown/unit1/02_diffusion_models_from_scratch_CN_files/02_diffusion_models_from_scratch_CN_24_1.png +3 -0
- markdown/unit1/02_diffusion_models_from_scratch_CN_files/02_diffusion_models_from_scratch_CN_26_0.png +3 -0
- markdown/unit1/02_diffusion_models_from_scratch_CN_files/02_diffusion_models_from_scratch_CN_29_0.png +3 -0
- markdown/unit1/02_diffusion_models_from_scratch_CN_files/02_diffusion_models_from_scratch_CN_31_1.png +3 -0
- markdown/unit1/02_diffusion_models_from_scratch_CN_files/02_diffusion_models_from_scratch_CN_39_1.png +3 -0
- markdown/unit1/02_diffusion_models_from_scratch_CN_files/02_diffusion_models_from_scratch_CN_43_0.png +3 -0
- markdown/unit1/02_diffusion_models_from_scratch_CN_files/02_diffusion_models_from_scratch_CN_45_1.png +3 -0
- markdown/unit1/README_CN.md +65 -0
- markdown/unit2/01_finetuning_and_guidance_CN.md +0 -0
- markdown/unit2/01_finetuning_and_guidance_CN_files/01_finetuning_and_guidance_CN_17_1.png +3 -0
- markdown/unit2/01_finetuning_and_guidance_CN_files/01_finetuning_and_guidance_CN_17_2.png +3 -0
- markdown/unit2/01_finetuning_and_guidance_CN_files/01_finetuning_and_guidance_CN_17_3.png +3 -0
- markdown/unit2/01_finetuning_and_guidance_CN_files/01_finetuning_and_guidance_CN_17_4.png +3 -0
- markdown/unit2/01_finetuning_and_guidance_CN_files/01_finetuning_and_guidance_CN_17_5.png +3 -0
- markdown/unit2/01_finetuning_and_guidance_CN_files/01_finetuning_and_guidance_CN_21_1.png +3 -0
- markdown/unit2/01_finetuning_and_guidance_CN_files/01_finetuning_and_guidance_CN_25_2.png +3 -0
- markdown/unit2/01_finetuning_and_guidance_CN_files/01_finetuning_and_guidance_CN_28_5.png +3 -0
- markdown/unit2/01_finetuning_and_guidance_CN_files/01_finetuning_and_guidance_CN_36_1.png +3 -0
- markdown/unit2/01_finetuning_and_guidance_CN_files/01_finetuning_and_guidance_CN_48_6.png +3 -0
- markdown/unit2/01_finetuning_and_guidance_CN_files/01_finetuning_and_guidance_CN_54_2.png +3 -0
- markdown/unit2/01_finetuning_and_guidance_CN_files/01_finetuning_and_guidance_CN_56_2.png +3 -0
- markdown/unit2/01_finetuning_and_guidance_CN_files/01_finetuning_and_guidance_CN_62_2.png +3 -0
- markdown/unit2/01_finetuning_and_guidance_CN_files/01_finetuning_and_guidance_CN_65_0.png +3 -0
- markdown/unit2/01_finetuning_and_guidance_CN_files/01_finetuning_and_guidance_CN_9_1.png +3 -0
- markdown/unit2/02_class_conditioned_diffusion_model_example_CN.md +360 -0
- markdown/unit2/02_class_conditioned_diffusion_model_example_CN_files/02_class_conditioned_diffusion_model_example_CN_10_21.png +3 -0
- markdown/unit2/02_class_conditioned_diffusion_model_example_CN_files/02_class_conditioned_diffusion_model_example_CN_12_2.png +3 -0
- markdown/unit2/02_class_conditioned_diffusion_model_example_CN_files/02_class_conditioned_diffusion_model_example_CN_4_9.png +3 -0
- markdown/unit2/README_CN.md +72 -0
- markdown/unit2/conditional_digit_generation.png +3 -0
- markdown/unit2/finetune_model.py +120 -0
- markdown/unit2/guidance_eg.png +3 -0
- markdown/unit3/01_stable_diffusion_introduction_CN.md +0 -0
- markdown/unit3/01_stable_diffusion_introduction_CN_files/01_stable_diffusion_introduction_CN_10_1.png +3 -0
- markdown/unit3/01_stable_diffusion_introduction_CN_files/01_stable_diffusion_introduction_CN_12_3.png +3 -0
- markdown/unit3/01_stable_diffusion_introduction_CN_files/01_stable_diffusion_introduction_CN_35_0.png +3 -0
- markdown/unit3/01_stable_diffusion_introduction_CN_files/01_stable_diffusion_introduction_CN_37_2.png +3 -0
markdown/dreambooth/DreamBoothCN.md
ADDED
@@ -0,0 +1,781 @@
|
|
|
|
|
|
|
|
|
|
|
|
|
|
|
|
|
|
|
|
|
|
|
|
|
|
|
|
|
|
|
|
|
|
|
|
|
|
|
|
|
|
|
|
|
|
|
|
|
|
|
|
|
|
|
|
|
|
|
|
|
|
|
|
|
|
|
|
|
|
|
|
|
|
|
|
|
|
|
|
|
|
|
|
|
|
|
|
|
|
|
|
|
|
|
|
|
|
|
|
|
|
|
|
|
|
|
|
|
|
|
|
|
|
|
|
|
|
|
|
|
|
|
|
|
|
|
|
|
|
|
|
|
|
|
|
|
|
|
|
|
|
|
|
|
|
|
|
|
|
|
|
|
|
|
|
|
|
|
|
|
|
|
|
|
|
|
|
|
|
|
|
|
|
|
|
|
|
|
|
|
|
|
|
|
|
|
|
|
|
|
|
|
|
|
|
|
|
|
|
|
|
|
|
|
|
|
|
|
|
|
|
|
|
|
|
|
|
|
|
|
|
|
|
|
|
|
|
|
|
|
|
|
|
|
|
|
|
|
|
|
|
|
|
|
|
|
|
|
|
|
|
|
|
|
|
|
|
|
|
|
|
|
|
|
|
|
|
|
|
|
|
|
|
|
|
|
|
|
|
|
|
|
|
|
|
|
|
|
|
|
|
|
|
|
|
|
|
|
|
|
|
|
|
|
|
|
|
|
|
|
|
|
|
|
|
|
|
|
|
|
|
|
|
|
|
|
|
|
|
|
|
|
|
|
|
|
|
|
|
|
|
|
|
|
|
|
|
|
|
|
|
|
|
|
|
|
|
|
|
|
|
|
|
|
|
|
|
|
|
|
|
|
|
|
|
|
|
|
|
|
|
|
|
|
|
|
|
|
|
|
|
|
|
|
|
|
|
|
|
|
|
|
|
|
|
|
|
|
|
|
|
|
|
|
|
|
|
|
|
|
|
|
|
|
|
|
|
|
|
|
|
|
|
|
|
|
|
|
|
|
|
|
|
|
|
|
|
|
|
|
|
|
|
|
|
|
|
|
|
|
|
|
|
|
|
|
|
|
|
|
|
|
|
|
|
|
|
|
|
|
|
|
|
|
|
|
|
|
|
|
|
|
|
|
|
|
|
|
|
|
|
|
|
|
|
|
|
|
|
|
|
|
|
|
|
|
|
|
|
|
|
|
|
|
|
|
|
|
|
|
|
|
|
|
|
|
|
|
|
|
|
|
|
|
|
|
|
|
|
|
|
|
|
|
|
|
|
|
|
|
|
|
|
|
|
|
|
|
|
|
|
|
|
|
|
|
|
|
|
|
|
|
|
|
|
|
|
|
|
|
|
|
|
|
|
|
|
|
|
|
|
|
|
|
|
|
|
|
|
|
|
|
|
|
|
|
|
|
|
|
|
|
|
|
|
|
|
|
|
|
|
|
|
|
|
|
|
|
|
|
|
|
|
|
|
|
|
|
|
|
|
|
|
|
|
|
|
|
|
|
|
|
|
|
|
|
|
|
|
|
|
|
|
|
|
|
|
|
|
|
|
|
|
|
|
|
|
|
|
|
|
|
|
|
|
|
|
|
|
|
|
|
|
|
|
|
|
|
|
|
|
|
|
|
|
|
|
|
|
|
|
|
|
|
|
|
|
|
|
|
|
|
|
|
|
|
|
|
|
|
|
|
|
|
|
|
|
|
|
|
|
|
|
|
|
|
|
|
|
|
|
|
|
|
|
|
|
|
|
|
|
|
|
|
|
|
|
|
|
|
|
|
|
|
|
|
|
|
|
|
|
|
|
|
|
|
|
|
|
|
|
|
|
|
|
|
|
|
|
|
|
|
|
|
|
|
|
|
|
|
|
|
|
|
|
|
|
|
|
|
|
|
|
|
|
|
|
|
|
|
|
|
|
|
|
|
|
|
|
|
|
|
|
|
|
|
|
|
|
|
|
|
|
|
|
|
|
|
|
|
|
|
|
|
|
|
|
|
|
|
|
|
|
|
|
|
|
|
|
|
|
|
|
|
|
|
|
|
|
|
|
|
|
|
|
|
|
|
|
|
|
|
|
|
|
|
|
|
|
|
|
|
|
|
|
|
|
|
|
|
|
|
|
|
|
|
|
|
|
|
|
|
|
|
|
|
|
|
|
|
|
|
|
|
|
|
|
|
|
|
|
|
|
|
|
|
|
|
|
|
|
|
|
|
|
|
|
|
|
|
|
|
|
|
|
|
|
|
|
|
|
|
|
|
|
|
|
|
|
|
|
|
|
|
|
|
|
|
|
|
|
|
|
|
|
|
|
|
|
|
|
|
|
|
|
|
|
|
|
|
|
|
|
|
|
|
|
|
|
|
|
|
|
|
|
|
|
|
|
|
|
|
|
|
|
|
|
|
|
|
|
|
|
|
|
|
|
|
|
|
|
|
|
|
|
|
|
|
|
|
|
|
|
|
|
|
|
|
|
|
|
|
|
|
|
|
|
|
|
|
|
|
|
|
|
|
|
|
|
|
|
|
|
|
|
|
|
|
|
|
|
|
|
|
|
|
|
|
|
|
|
|
|
|
|
|
|
|
|
|
|
|
|
|
|
|
|
|
|
|
|
|
|
|
|
|
|
|
|
|
|
|
|
|
|
|
|
|
|
|
|
|
|
|
|
|
|
|
|
|
|
|
|
|
|
|
|
|
|
|
|
|
|
|
|
|
|
|
|
|
|
|
|
|
|
|
|
|
|
|
|
|
|
|
|
|
|
|
|
|
|
|
|
|
|
|
|
|
|
|
|
|
|
|
|
|
|
|
|
|
|
|
|
|
|
|
|
|
|
|
|
|
|
|
|
|
|
|
|
|
|
|
|
|
|
|
|
|
|
|
|
|
|
|
|
|
|
|
|
|
|
|
|
|
|
|
|
|
|
|
|
|
|
|
|
|
|
|
|
|
|
|
|
|
|
|
|
|
|
|
|
|
|
|
|
|
|
|
|
|
|
|
|
|
|
|
|
|
|
|
|
|
|
|
|
|
|
|
|
|
|
|
|
|
|
|
|
|
|
|
|
|
|
|
|
|
|
|
|
|
|
|
|
|
|
|
|
|
|
|
|
|
|
|
|
|
|
|
|
|
|
|
|
|
|
|
|
|
|
|
|
|
|
|
|
|
|
|
|
|
|
|
|
|
|
|
|
|
|
|
|
|
|
|
|
|
|
|
|
|
|
|
|
|
|
|
|
|
|
|
|
|
|
|
|
|
|
|
|
|
|
|
|
|
|
|
|
|
|
|
|
|
|
|
|
|
|
|
|
|
|
|
|
|
|
|
|
|
|
|
|
|
|
|
|
|
|
|
|
|
|
|
|
|
|
|
|
|
|
|
|
|
|
|
|
|
|
|
|
|
|
|
|
|
|
|
|
|
|
|
|
|
|
|
|
|
|
|
|
|
|
|
|
|
|
|
|
|
|
|
|
|
|
|
|
|
|
|
|
|
|
|
|
|
|
|
|
|
|
|
|
|
|
|
|
|
|
|
|
|
|
|
|
|
1 |
+
## (必读) 准备工作
|
2 |
+
|
3 |
+
- 点击右上角,Fork 这个 Notebook
|
4 |
+
- 鼠标悬停在运行按钮,或者点击右上角齿轮图标进入高级选项,确保「挂载 work 目录」为选中状态
|
5 |
+
- 运行 Fork 过的 Notebook
|
6 |
+
|
7 |
+
### 再次确认「挂载 work 目录」已经勾选
|
8 |
+
|
9 |
+
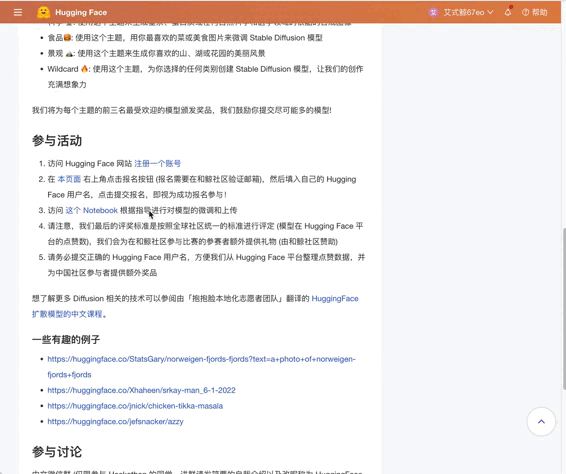
|
10 |
+
|
11 |
+
|
12 |
+
```python
|
13 |
+
# 请确保 work 目录存在,不存在的话以下命令会报错。请关闭实例再重新创建。
|
14 |
+
! ls /home/mw/work
|
15 |
+
```
|
16 |
+
|
17 |
+
## Hugging Face DreamBooth 编程马拉松大赛 🏆
|
18 |
+
|
19 |
+
基于 HF diffusion class 创作。
|
20 |
+
|
21 |
+
原文 https://github.com/huggingface/diffusion-models-class/tree/main/hackathon
|
22 |
+
译者 SuSung-boy@ 苏桑,经常倒腾图像的工业视觉算法工程师。
|
23 |
+
|
24 |
+
欢迎来到 DreamBooth 编程马拉松!在这场比赛中,您将通过 **在少量自己的图像上进行微调来得到具有个性化的 Stable Diffusion 模型**。为此,您将使用一项名为 [DreamBooth](https://arxiv.org/abs/2208.12242) 的技术,用户可以将主体(例如,您的宠物或喜爱的美食)植入模型的输出域,以便可以在提示中使用 **唯一标识符** 进行合成。
|
25 |
+
|
26 |
+
让我们开始吧!
|
27 |
+
|
28 |
+
🚨 **进阶用户提示**
|
29 |
+
|
30 |
+
本 Notebook 提供的代码是 🤗 Diffusers 中[官方训练脚本](https://github.com/huggingface/diffusers/tree/main/examples/dreambooth) 的简化版本,**运行本 Notebook 中的代码需要至少 14GB GPU vRAM**。该代码已经可以为大多数应用程序生成不错的模型,但如果您有超过 24GB vRAM 可用,我们建议您尝试高级功能,例如 class preservation 损失和微调文本编码器。 查看 🤗 Diffusers [文档](https://hf.co/docs/diffusers/training/dreambooth) 了解详情。
|
31 |
+
|
32 |
+
## DreamBooth 是什么?
|
33 |
+
|
34 |
+
DreamBooth 是一项使用特定形式的微调来将新引入的概念传授给 Stable Diffusion 的技术。
|
35 |
+
|
36 |
+
Hugging Face 的这篇 [博客文章](https://huggingface.co/blog/dreambooth) 说明了使用 DreamBooth 微调 Stable Diffusion 的一些最佳实践。
|
37 |
+
|
38 |
+
DreamBooth 的工作方式如下:
|
39 |
+
|
40 |
+
* 收集大约 10-20 张特定主体(例如您的狗狗)的输入图像,并定义一个唯一标识符 [V],它代指的即是您输入的主体。该标识符通常是一些像 `flffydog` 这样的虚构词,在推理时它会被植入不同的文本提示中来将主体置于不同的上下文中。
|
41 |
+
* 通过提供图像和文本提示来微调扩散模型,例如给定文本提示 "A photo of a [V] dog",其中需包含唯一标识符 [V] 和类名(本例中为 "dog")
|
42 |
+
* (可选)采用特殊的 **class-specific prior preservation loss**, 它利用了模型在类上的语义先验,并通过在文本提示中注入类名来促使它生成属于同一主体类的多种实例。实际使用过程中,只有人脸做为主体才真正需要此步骤,而对于此次编程马拉松中要探索的主题,则可跳过此步骤。
|
43 |
+
|
44 |
+
DreamBooth 的技术概述如下图所示:
|
45 |
+
|
46 |
+

|
47 |
+
|
48 |
+
### DreamBooth 能做什么?
|
49 |
+
|
50 |
+
除了将您的主体放在感兴趣的位置之外,DreamBooth 还可用于 _**文本引导视图合成**_, 您可以从不同的视角查看主体,如下例所示:
|
51 |
+
|
52 |
+
|
53 |
+

|
54 |
+
|
55 |
+
|
56 |
+
DreamBooth 还可用于修改主体的属性,例如颜色改变和动物混合!
|
57 |
+
|
58 |
+
|
59 |
+
|
60 |
+

|
61 |
+
|
62 |
+
|
63 |
+
以上就是使用 DreamBooth 做的一些很酷的事!既然我们已经大致了解了,让我们开始训练自己的模型吧!
|
64 |
+
|
65 |
+
## 第 1 步:设置
|
66 |
+
|
67 |
+
**文生图的模型一般都很大**,因为网络的原因从 HuggingFace 主站直接下载速度比较慢。为了方便同学们使用,我们将模型文件做成了可以直接在本地挂载的 HeyWhale 数据集。
|
68 |
+
|
69 |
+
挂载数据集只需点击左边栏第三个数据集按钮,打开挂载数据面板。然后点击“修改” 按钮,选中“他人共享”里面的 Hackathon 数据集,确定即可。
|
70 |
+
|
71 |
+
最终结果如图所示:
|
72 |
+
|
73 |
+
*注意:如果你成功挂载了 work 目录,这些数据集就默认挂载完成,通常这里无需额外操作。
|
74 |
+
|
75 |
+

|
76 |
+
|
77 |
+
让我们来看一下挂载的数据集有哪些文件,执行下面的代码:
|
78 |
+
|
79 |
+
|
80 |
+
```python
|
81 |
+
%ls /home/mw/input/Hackathon6769/
|
82 |
+
```
|
83 |
+
|
84 |
+
[0m[01;34mclip-vit-base-patch32[0m/ [01;34mstable-diffusion-v1-4[0m/
|
85 |
+
[01;34mstable-diffusion-safety-checker[0m/
|
86 |
+
|
87 |
+
|
88 |
+
然后安装我们需要的依赖:
|
89 |
+
|
90 |
+
|
91 |
+
```python
|
92 |
+
%pip install -qqU diffusers transformers bitsandbytes accelerate ftfy datasets -i https://mirrors.cloud.tencent.com/pypi/simple
|
93 |
+
```
|
94 |
+
|
95 |
+
Note: you may need to restart the kernel to use updated packages.
|
96 |
+
|
97 |
+
|
98 |
+
然后因为兼容性的问题降级 `ipywidgets` 到某一个特定版本。如���你使用的是自己的机器可能无需这一步操作。
|
99 |
+
|
100 |
+
|
101 |
+
```python
|
102 |
+
!pip install -qqU ipywidgets==7.6.3 -i https://mirrors.cloud.tencent.com/pypi/simple
|
103 |
+
```
|
104 |
+
|
105 |
+
安装完成后重新启动 Kernel,点击本 Notebook 菜单栏上的重启 Kernel 即可:
|
106 |
+
|
107 |
+
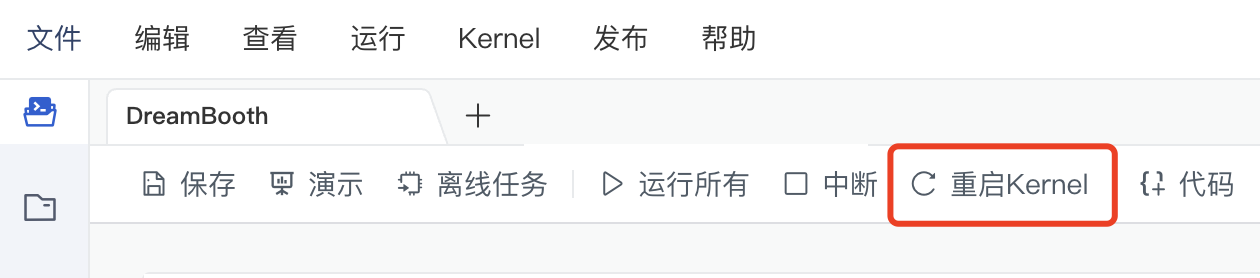
|
108 |
+
|
109 |
+
接下来让我们登录 Hugging Face:
|
110 |
+
|
111 |
+
|
112 |
+
```python
|
113 |
+
%%capture
|
114 |
+
!sudo apt -qq install git-lfs
|
115 |
+
!git config --global credential.helper store
|
116 |
+
```
|
117 |
+
|
118 |
+
|
119 |
+
```python
|
120 |
+
from huggingface_hub import notebook_login
|
121 |
+
notebook_login()
|
122 |
+
```
|
123 |
+
|
124 |
+
Token is valid.
|
125 |
+
Your token has been saved in your configured git credential helpers (store).
|
126 |
+
Your token has been saved to /home/mw/.huggingface/token
|
127 |
+
Login successful
|
128 |
+
|
129 |
+
|
130 |
+
这一步需要大家访问 [Hugging Face 的 Token 设置](https://hf.co/settings/tokens) 页面,并创建一个有可写 (WRITE) 权限的 token ,然后点击右边按钮把内容复制进来。
|
131 |
+
|
132 |
+
|
133 |
+

|
134 |
+
|
135 |
+
|
136 |
+
最后让我们定义一些后面会用到的常量。
|
137 |
+
|
138 |
+
|
139 |
+
```python
|
140 |
+
MODEL_SD_PATH = '/home/mw/input/Hackathon6769/stable-diffusion-v1-4'
|
141 |
+
MODEL_CLIP_VIT_PATH = '/home/mw/input/Hackathon6769/clip-vit-base-patch32'
|
142 |
+
MODEL_SD_SAFETY_PATH = '/home/mw/input/Hackathon6769/stable-diffusion-safety-checker'
|
143 |
+
```
|
144 |
+
|
145 |
+
## 第 2 步:选择主题
|
146 |
+
|
147 |
+
本次大赛包含5个 **主题** (theme),每个主题将征集属于以下类别的模型:
|
148 |
+
|
149 |
+
- 动物 🐨 (`animal`): 使用此主题生成您的宠物或喜爱的动物在雅典卫城游玩、在游泳或在太空中飞行的图像。
|
150 |
+
- 科学 🔬 (`science`): 使用此主题生成星系、蛋白质或任何自然科学和医学领域的酷毙的合成图像。
|
151 |
+
- 食物 🍔 (`food`): 使用此主题在您最喜欢的美味佳肴图像上微调您自己的 Stable Diffusion。
|
152 |
+
- 风景 🏔 (`landscape`): 使用此主题生成您最喜欢的山脉、湖泊或花园的美丽风景图像。
|
153 |
+
- 通用 🔥 (`wildcard`): 此主题无限定的类别,您可以为选择的任何类别创建 Stable Diffusion 模型!
|
154 |
+
|
155 |
+
我们将为每个主题的前 3 名喜爱度最高的模型颁发奖品,我们鼓励您提交尽可能多的模型!请从以下几个类别中选一个吧。
|
156 |
+
|
157 |
+
|
158 |
+
```python
|
159 |
+
# options=["animal", "science", "food", "landscape", "wildcard"],
|
160 |
+
options = "wildcard"
|
161 |
+
theme = options
|
162 |
+
```
|
163 |
+
|
164 |
+
## 第 3 步:创建图像数据集并上传到 work 目录下
|
165 |
+
|
166 |
+
选定主题后,下一步是 **为该主题创建图像数据集** 并将其上传到 work 目录:
|
167 |
+
|
168 |
+
* 在 work 目录下创建一个子文件夹,用于存放照片,名称随意。
|
169 |
+
* 确定您希望植入模型的主体,然后需要准备大约 **10-20 张主体图像**。这些图像可以是您拍摄的照片或从 [Unsplash](https://unsplash.com/) 等平台下载的图片。更或者,您可以查看 Hugging Face Hub 上的任何 [图像数据集](https://hf.co/datasets?task_categories=task_categories:image-classification&sort=downloads) 来获取灵感。
|
170 |
+
* 为获得最佳效果,我们建议使用 **不同角度和视角** 拍摄的主体图像
|
171 |
+
|
172 |
+
到左侧边栏找到「文件树」,在 work 目录下新建一个文件夹,上传自己的主体图像用于微调:
|
173 |
+
|
174 |
+
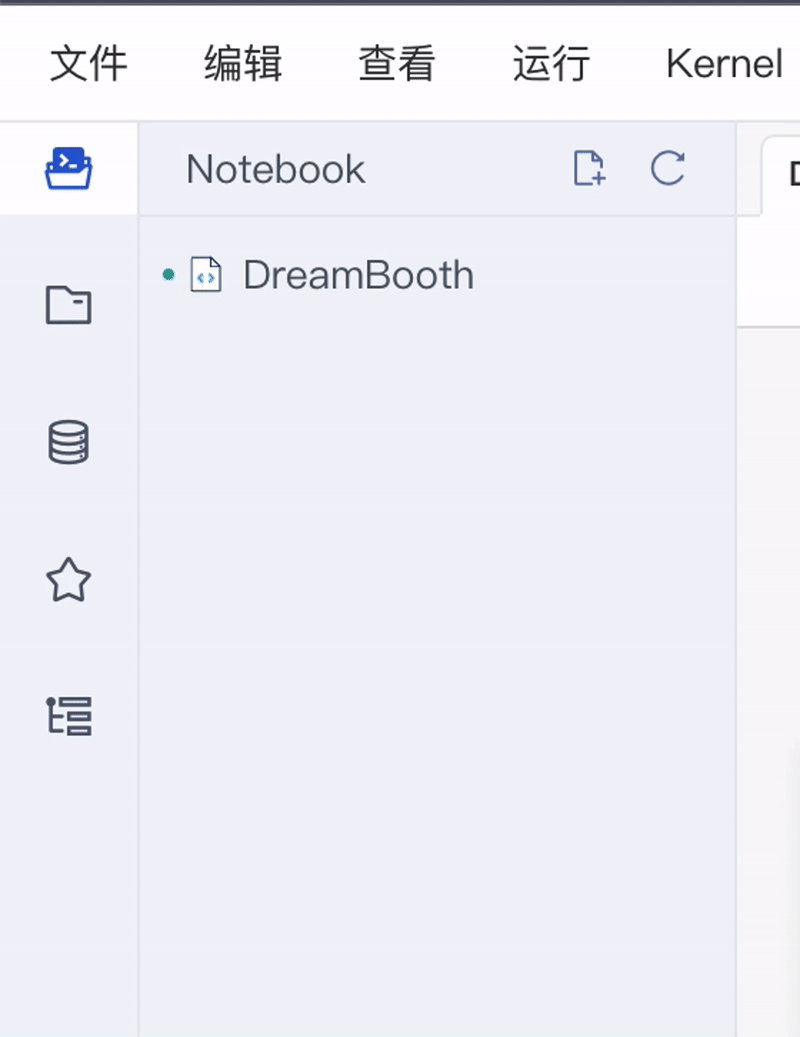
|
175 |
+
|
176 |
+
本 demo 里训练的是 Flutter 的吉祥物 Dash,因此我在 `work` 目录下创建了一个 `dashdash` 的文件夹。
|
177 |
+
|
178 |
+
|
179 |
+
```python
|
180 |
+
# 让我们来看一下这些照片,dashdash 是我的 dart 玩偶的名字
|
181 |
+
! ls /home/mw/work/dashdash
|
182 |
+
```
|
183 |
+
|
184 |
+
|
185 |
+
```python
|
186 |
+
DATA_DIR = "/home/mw/work/dashdash"
|
187 |
+
```
|
188 |
+
|
189 |
+
|
190 |
+
```python
|
191 |
+
from datasets import load_dataset
|
192 |
+
|
193 |
+
dataset = load_dataset("imagefolder", data_dir=DATA_DIR)
|
194 |
+
dataset = dataset['train']
|
195 |
+
```
|
196 |
+
|
197 |
+
Using custom data configuration default-ab024aecf581f3e7
|
198 |
+
Found cached dataset imagefolder (/home/mw/.cache/huggingface/datasets/imagefolder/default-ab024aecf581f3e7/0.0.0/37fbb85cc714a338bea574ac6c7d0b5be5aff46c1862c1989b20e0771199e93f)
|
199 |
+
|
200 |
+
|
201 |
+
|
202 |
+
0%| | 0/1 [00:00<?, ?it/s]
|
203 |
+
|
204 |
+
|
205 |
+
|
206 |
+
```python
|
207 |
+
# 让我们来看一下照片数据是否已经载入。
|
208 |
+
dataset['image'][0]
|
209 |
+
```
|
210 |
+
|
211 |
+
|
212 |
+
|
213 |
+
|
214 |
+
<img src="https://cdn.kesci.com/upload/rt/619E565ED27D49C1A2EC1EE1E587FF98/ro94v7gjcd.png">
|
215 |
+
|
216 |
+
|
217 |
+
|
218 |
+
现在我们有了自己的数据集,让我们定义一个辅助函数来可视化查看一些图像:
|
219 |
+
|
220 |
+
|
221 |
+
```python
|
222 |
+
from PIL import Image
|
223 |
+
|
224 |
+
def image_grid(imgs, rows, cols):
|
225 |
+
assert len(imgs) == rows * cols
|
226 |
+
w, h = imgs[0].size
|
227 |
+
grid = Image.new("RGB", size=(cols * w, rows * h))
|
228 |
+
grid_w, grid_h = grid.size
|
229 |
+
for i, img in enumerate(imgs):
|
230 |
+
grid.paste(img, box=(i % cols * w, i // cols * h))
|
231 |
+
return grid
|
232 |
+
|
233 |
+
num_samples = 4
|
234 |
+
image_grid(dataset["image"][:num_samples], rows=1, cols=num_samples)
|
235 |
+
```
|
236 |
+
|
237 |
+
这些图像如果看起来不错,您可以继续下一步 —— 创建 PyTorch 数据集以使用 DreamBooth 进行训练。
|
238 |
+
|
239 |
+
### 创建训练数据集
|
240 |
+
|
241 |
+
要为我们的图像创建训练集,需要一些组件:
|
242 |
+
|
243 |
+
* **实��提示** : 用于在训练开始时预热模型。大多数情况下,使用一张「标识符 + 类别名词」的照片效果已足够好,例如为我们可爱的柯基图片写上提示: "一张柯柯基狗的照片"。
|
244 |
+
* **注意:** 建议您选择一个独特的/虚构词来描述您的主体,如 `柯柯基`。以此确保模型词汇表中的常用词不会被覆盖。
|
245 |
+
* **分词器** : 用于将实例提示转换为输入 ID,并且可以将其提供给 Stable Diffusion 的文本编码器。
|
246 |
+
* 一组 **图像变换** : 尤其是将图像缩放至通用形状,以及将像素值归一化至通用均值和标准分布。
|
247 |
+
|
248 |
+
根据以上描述,让我们从定义实例提示开始:
|
249 |
+
|
250 |
+
|
251 |
+
```python
|
252 |
+
name_of_your_concept = "dashdash" # 根据您的主体修改,我这里把 dash 称之为 dashdash
|
253 |
+
type_of_thing = "toy" # 根据您的主体修改
|
254 |
+
instance_prompt = f"a photo of {name_of_your_concept} {type_of_thing}"
|
255 |
+
print(f"Instance prompt: {instance_prompt}")
|
256 |
+
```
|
257 |
+
|
258 |
+
Instance prompt: a photo of dashdash toy
|
259 |
+
|
260 |
+
|
261 |
+
接下来,我们需要创建一个 PyTorch `Dataset` 类, 并实现 `__len__` 和 `__getitem__` 方法:
|
262 |
+
|
263 |
+
|
264 |
+
```python
|
265 |
+
from torch.utils.data import Dataset
|
266 |
+
from torchvision import transforms
|
267 |
+
|
268 |
+
|
269 |
+
class DreamBoothDataset(Dataset):
|
270 |
+
def __init__(self, dataset, instance_prompt, tokenizer, size=512):
|
271 |
+
self.dataset = dataset
|
272 |
+
self.instance_prompt = instance_prompt
|
273 |
+
self.tokenizer = tokenizer
|
274 |
+
self.size = size
|
275 |
+
self.transforms = transforms.Compose(
|
276 |
+
[
|
277 |
+
transforms.Resize(size),
|
278 |
+
transforms.CenterCrop(size),
|
279 |
+
transforms.ToTensor(),
|
280 |
+
transforms.Normalize([0.5], [0.5]),
|
281 |
+
]
|
282 |
+
)
|
283 |
+
|
284 |
+
def __len__(self):
|
285 |
+
return len(self.dataset)
|
286 |
+
|
287 |
+
def __getitem__(self, index):
|
288 |
+
example = {}
|
289 |
+
image = self.dataset[index]["image"]
|
290 |
+
example["instance_images"] = self.transforms(image)
|
291 |
+
example["instance_prompt_ids"] = self.tokenizer(
|
292 |
+
self.instance_prompt,
|
293 |
+
padding="do_not_pad",
|
294 |
+
truncation=True,
|
295 |
+
max_length=self.tokenizer.model_max_length,
|
296 |
+
).input_ids
|
297 |
+
return example
|
298 |
+
```
|
299 |
+
|
300 |
+
很好,现在让我们加载与原始 Stable Diffusion 模型的文本编码器关联的 CLIP 分词器,然后检查上一步是否生效,然后创建训练数据集:
|
301 |
+
|
302 |
+
|
303 |
+
```python
|
304 |
+
from transformers import CLIPTokenizer
|
305 |
+
|
306 |
+
# 用来微调的 Stable Diffusion 检查点
|
307 |
+
model_id = MODEL_SD_PATH
|
308 |
+
tokenizer = CLIPTokenizer.from_pretrained(
|
309 |
+
model_id,
|
310 |
+
subfolder="tokenizer",
|
311 |
+
)
|
312 |
+
|
313 |
+
train_dataset = DreamBoothDataset(dataset, instance_prompt, tokenizer)
|
314 |
+
train_dataset[0]
|
315 |
+
```
|
316 |
+
|
317 |
+
|
318 |
+
|
319 |
+
|
320 |
+
{'instance_images': tensor([[[ 0.6941, 0.6941, 0.6941, ..., 0.3647, 0.3647, 0.3647],
|
321 |
+
[ 0.6941, 0.7020, 0.6941, ..., 0.3647, 0.3647, 0.3647],
|
322 |
+
[ 0.6863, 0.6863, 0.6863, ..., 0.3647, 0.3647, 0.3569],
|
323 |
+
...,
|
324 |
+
[ 0.5216, 0.5294, 0.5216, ..., 0.5529, 0.5451, 0.5451],
|
325 |
+
[ 0.5216, 0.5216, 0.5294, ..., 0.5529, 0.5451, 0.5529],
|
326 |
+
[ 0.5216, 0.5373, 0.5373, ..., 0.5451, 0.5529, 0.5608]],
|
327 |
+
|
328 |
+
[[ 0.5529, 0.5529, 0.5529, ..., -0.0275, -0.0275, -0.0275],
|
329 |
+
[ 0.5529, 0.5608, 0.5529, ..., -0.0275, -0.0275, -0.0275],
|
330 |
+
[ 0.5451, 0.5451, 0.5451, ..., -0.0275, -0.0275, -0.0353],
|
331 |
+
...,
|
332 |
+
[ 0.5216, 0.5294, 0.5216, ..., 0.5608, 0.5529, 0.5529],
|
333 |
+
[ 0.5216, 0.5216, 0.5294, ..., 0.5608, 0.5529, 0.5608],
|
334 |
+
[ 0.5216, 0.5373, 0.5373, ..., 0.5529, 0.5608, 0.5686]],
|
335 |
+
|
336 |
+
[[ 0.6784, 0.6784, 0.6784, ..., -0.6000, -0.6000, -0.6000],
|
337 |
+
[ 0.6784, 0.6863, 0.6784, ..., -0.5922, -0.5922, -0.5922],
|
338 |
+
[ 0.6706, 0.6706, 0.6706, ..., -0.5843, -0.5843, -0.5922],
|
339 |
+
...,
|
340 |
+
[ 0.5843, 0.5922, 0.5843, ..., 0.5765, 0.5686, 0.5686],
|
341 |
+
[ 0.5843, 0.5843, 0.5922, ..., 0.5765, 0.5686, 0.5765],
|
342 |
+
[ 0.5843, 0.6000, 0.6000, ..., 0.5686, 0.5765, 0.5843]]]),
|
343 |
+
'instance_prompt_ids': [49406, 320, 1125, 539, 13858, 10206, 5988, 49407]}
|
344 |
+
|
345 |
+
|
346 |
+
|
347 |
+
## 第 4 步:定义数据整理器
|
348 |
+
|
349 |
+
现在我们有了一个训练数据集,接下来我们需要定义一个数据整理器。数据整理器是一个函数,它实现的功能是:收集一个批次数据中的元素、应用一些逻辑来构成单个张量、提供给模型训练等。如果您想了解更多信息,可以观看 [Hugging Face 的视频课程](hf.co/course)。
|
350 |
+
|
351 |
+
对于 DreamBooth,我们定义的数据整理器需要为模型提供两个部分:分词器的输入 ID、图像的像素值堆叠张量。具体函数代码如下所示:
|
352 |
+
|
353 |
+
|
354 |
+
```python
|
355 |
+
import torch
|
356 |
+
|
357 |
+
def collate_fn(examples):
|
358 |
+
input_ids = [example["instance_prompt_ids"] for example in examples]
|
359 |
+
pixel_values = [example["instance_images"] for example in examples]
|
360 |
+
pixel_values = torch.stack(pixel_values)
|
361 |
+
pixel_values = pixel_values.to(memory_format=torch.contiguous_format).float()
|
362 |
+
|
363 |
+
input_ids = tokenizer.pad(
|
364 |
+
{"input_ids": input_ids}, padding=True, return_tensors="pt"
|
365 |
+
).input_ids
|
366 |
+
|
367 |
+
batch = {
|
368 |
+
"input_ids": input_ids,
|
369 |
+
"pixel_values": pixel_values,
|
370 |
+
}
|
371 |
+
return batch
|
372 |
+
```
|
373 |
+
|
374 |
+
## 第 5 步:加载 Stable Diffusion 管道组件
|
375 |
+
|
376 |
+
到此我们已经准备好训练所需的大部分组件了!如 Stable Diffusion 第 3 单元 Notebook 中所示,一个管道包含多个模型:
|
377 |
+
|
378 |
+
* 文本编码器: 用于将文本提示转换为嵌入矩阵。这里我们使用 CLIP,因为它是用于训练 Stable Diffusion v1-4 的编码器
|
379 |
+
* VAE (变分自动编码器, variational autoencoder): 用于将图像转换为压缩表征(隐式表征),并在推理时解压缩
|
380 |
+
* UNet: 用于在隐式 VAE 中去噪
|
381 |
+
|
382 |
+
我们可以使用 🤗 Diffusers 和 🤗 Transformers 库加载上述所有组件,如下代码所示:
|
383 |
+
|
384 |
+
|
385 |
+
|
386 |
+
```python
|
387 |
+
from diffusers import AutoencoderKL, UNet2DConditionModel
|
388 |
+
from transformers import CLIPFeatureExtractor, CLIPTextModel
|
389 |
+
|
390 |
+
text_encoder = CLIPTextModel.from_pretrained(model_id, subfolder="text_encoder")
|
391 |
+
vae = AutoencoderKL.from_pretrained(model_id, subfolder="vae")
|
392 |
+
unet = UNet2DConditionModel.from_pretrained(model_id, subfolder="unet")
|
393 |
+
feature_extractor = CLIPFeatureExtractor.from_pretrained(MODEL_CLIP_VIT_PATH)
|
394 |
+
```
|
395 |
+
|
396 |
+
## 第 6 步:微调模型
|
397 |
+
|
398 |
+
有趣的一步来了!使用 DreamBooth 训练自己的模型!如 [Hugging Face 的博客文章](https://huggingface.co/blog/dreambooth) 所描述的那样,需要手动调整的最重要的超参数是学习率和训练次数。
|
399 |
+
|
400 |
+
通常,较低的学习率和较长的训练次数可以获得更好的结果。下面设置的初始值是一个不错的训练起点,但您可能仍然需要根据您的数据集调整它们:
|
401 |
+
|
402 |
+
|
403 |
+
```python
|
404 |
+
learning_rate = 2e-06
|
405 |
+
max_train_steps = 400
|
406 |
+
```
|
407 |
+
|
408 |
+
接下来,将训练需要的其他超参数包装在 `Namespace` 对象中,来使配置和训练更简单:
|
409 |
+
|
410 |
+
|
411 |
+
```python
|
412 |
+
from argparse import Namespace
|
413 |
+
|
414 |
+
OUTPUT_DIR = "/home/mw/work/my-dreambooth"
|
415 |
+
args = Namespace(
|
416 |
+
pretrained_model_name_or_path=model_id,
|
417 |
+
resolution=512, # Reduce this if you want to save some memory
|
418 |
+
train_dataset=train_dataset,
|
419 |
+
instance_prompt=instance_prompt,
|
420 |
+
learning_rate=learning_rate,
|
421 |
+
max_train_steps=max_train_steps,
|
422 |
+
train_batch_size=1,
|
423 |
+
gradient_accumulation_steps=1, # Increase this if you want to lower memory usage
|
424 |
+
max_grad_norm=1.0,
|
425 |
+
gradient_checkpointing=True, # set this to True to lower the memory usage.
|
426 |
+
use_8bit_adam=True, # use 8bit optimizer from bitsandbytes
|
427 |
+
seed=3434554,
|
428 |
+
sample_batch_size=2,
|
429 |
+
output_dir=OUTPUT_DIR, # where to save the pipeline
|
430 |
+
)
|
431 |
+
```
|
432 |
+
|
433 |
+
最后要定义一个 `training_function()` 函数,它包装了一些训练逻辑,并且可以传递给 🤗 Accelerate 库来处理 1 个或多个 GPU 上的训练。如果这是您第一次使用 🤗 Accelerate,请观看我们官方的 Bilibili 频道视频以快速了解它的功能:[Supercharge your PyTorch training loop with Accelerate](https://www.bilibili.com/video/BV1gD4y157ee/) (带中英文字幕)。
|
434 |
+
|
435 |
+
|
436 |
+
当我们从头开始训练自己的扩散模型时,这些细节与我们在第 1 和第 2 单元中看到的类似:
|
437 |
+
|
438 |
+
|
439 |
+
```python
|
440 |
+
import math
|
441 |
+
|
442 |
+
import torch.nn.functional as F
|
443 |
+
from accelerate import Accelerator
|
444 |
+
from accelerate.utils import set_seed
|
445 |
+
from diffusers import DDPMScheduler, PNDMScheduler, StableDiffusionPipeline
|
446 |
+
from diffusers.pipelines.stable_diffusion import StableDiffusionSafetyChecker
|
447 |
+
from torch.utils.data import DataLoader
|
448 |
+
from tqdm.auto import tqdm
|
449 |
+
|
450 |
+
|
451 |
+
def training_function(text_encoder, vae, unet):
|
452 |
+
|
453 |
+
accelerator = Accelerator(
|
454 |
+
gradient_accumulation_steps=args.gradient_accumulation_steps,
|
455 |
+
)
|
456 |
+
|
457 |
+
set_seed(args.seed)
|
458 |
+
|
459 |
+
if args.gradient_checkpointing:
|
460 |
+
unet.enable_gradient_checkpointing()
|
461 |
+
|
462 |
+
# 使用 8 位 Adam 优化器以降低内存占用,或者在 16GB GPU 微调模型
|
463 |
+
if args.use_8bit_adam:
|
464 |
+
import bitsandbytes as bnb
|
465 |
+
optimizer_class = bnb.optim.AdamW8bit
|
466 |
+
else:
|
467 |
+
optimizer_class = torch.optim.AdamW
|
468 |
+
|
469 |
+
optimizer = optimizer_class(
|
470 |
+
unet.parameters(), # 仅优化 UNet
|
471 |
+
lr=args.learning_rate,
|
472 |
+
)
|
473 |
+
|
474 |
+
noise_scheduler = DDPMScheduler(
|
475 |
+
beta_start=0.00085,
|
476 |
+
beta_end=0.012,
|
477 |
+
beta_schedule="scaled_linear",
|
478 |
+
num_train_timesteps=1000,
|
479 |
+
)
|
480 |
+
|
481 |
+
train_dataloader = DataLoader(
|
482 |
+
args.train_dataset,
|
483 |
+
batch_size=args.train_batch_size,
|
484 |
+
shuffle=True,
|
485 |
+
collate_fn=collate_fn,
|
486 |
+
)
|
487 |
+
|
488 |
+
unet, optimizer, train_dataloader = accelerator.prepare(
|
489 |
+
unet, optimizer, train_dataloader
|
490 |
+
)
|
491 |
+
|
492 |
+
# 将 text_encode 和 VAE 转移到 gpu
|
493 |
+
text_encoder.to(accelerator.device)
|
494 |
+
vae.to(accelerator.device)
|
495 |
+
|
496 |
+
# 我们需要重新计算我们的总训练次数,因为数据加载器的���小可能发生改变。
|
497 |
+
num_update_steps_per_epoch = math.ceil(
|
498 |
+
len(train_dataloader) / args.gradient_accumulation_steps
|
499 |
+
)
|
500 |
+
num_train_epochs = math.ceil(args.max_train_steps / num_update_steps_per_epoch)
|
501 |
+
|
502 |
+
# 训练!
|
503 |
+
total_batch_size = (
|
504 |
+
args.train_batch_size
|
505 |
+
* accelerator.num_processes
|
506 |
+
* args.gradient_accumulation_steps
|
507 |
+
)
|
508 |
+
# 每台机器仅显示一次进度条
|
509 |
+
progress_bar = tqdm(
|
510 |
+
range(args.max_train_steps), disable=not accelerator.is_local_main_process
|
511 |
+
)
|
512 |
+
progress_bar.set_description("Steps")
|
513 |
+
global_step = 0
|
514 |
+
|
515 |
+
for epoch in range(num_train_epochs):
|
516 |
+
unet.train()
|
517 |
+
for step, batch in enumerate(train_dataloader):
|
518 |
+
with accelerator.accumulate(unet):
|
519 |
+
# 转换图像至隐式空间
|
520 |
+
with torch.no_grad():
|
521 |
+
latents = vae.encode(batch["pixel_values"]).latent_dist.sample()
|
522 |
+
latents = latents * 0.18215
|
523 |
+
|
524 |
+
# 采样要添加到隐式空间的噪声样本
|
525 |
+
noise = torch.randn(latents.shape).to(latents.device)
|
526 |
+
bsz = latents.shape[0]
|
527 |
+
# 为每张图像采样随机时间步
|
528 |
+
timesteps = torch.randint(
|
529 |
+
0,
|
530 |
+
noise_scheduler.config.num_train_timesteps,
|
531 |
+
(bsz,),
|
532 |
+
device=latents.device,
|
533 |
+
).long()
|
534 |
+
|
535 |
+
# 根据每个时间步的噪声幅度,将噪声添加到隐式空间
|
536 |
+
# (即前向扩散过程)
|
537 |
+
noisy_latents = noise_scheduler.add_noise(latents, noise, timesteps)
|
538 |
+
|
539 |
+
# 获取用于条件调节的文本嵌入
|
540 |
+
with torch.no_grad():
|
541 |
+
encoder_hidden_states = text_encoder(batch["input_ids"])[0]
|
542 |
+
|
543 |
+
# 预测噪声残差
|
544 |
+
noise_pred = unet(
|
545 |
+
noisy_latents, timesteps, encoder_hidden_states
|
546 |
+
).sample
|
547 |
+
loss = (
|
548 |
+
F.mse_loss(noise_pred, noise, reduction="none")
|
549 |
+
.mean([1, 2, 3])
|
550 |
+
.mean()
|
551 |
+
)
|
552 |
+
|
553 |
+
accelerator.backward(loss)
|
554 |
+
if accelerator.sync_gradients:
|
555 |
+
accelerator.clip_grad_norm_(unet.parameters(), args.max_grad_norm)
|
556 |
+
optimizer.step()
|
557 |
+
optimizer.zero_grad()
|
558 |
+
|
559 |
+
# 检查加速器是否在幕后执行了优化
|
560 |
+
if accelerator.sync_gradients:
|
561 |
+
progress_bar.update(1)
|
562 |
+
global_step += 1
|
563 |
+
|
564 |
+
logs = {"loss": loss.detach().item()}
|
565 |
+
progress_bar.set_postfix(**logs)
|
566 |
+
|
567 |
+
if global_step >= args.max_train_steps:
|
568 |
+
break
|
569 |
+
|
570 |
+
accelerator.wait_for_everyone()
|
571 |
+
|
572 |
+
# 使用经训练的模块,创建管道并保存。
|
573 |
+
if accelerator.is_main_process:
|
574 |
+
print(f"Loading pipeline and saving to {args.output_dir}...")
|
575 |
+
scheduler = PNDMScheduler(
|
576 |
+
beta_start=0.00085,
|
577 |
+
beta_end=0.012,
|
578 |
+
beta_schedule="scaled_linear",
|
579 |
+
skip_prk_steps=True,
|
580 |
+
steps_offset=1,
|
581 |
+
)
|
582 |
+
pipeline = StableDiffusionPipeline(
|
583 |
+
text_encoder=text_encoder,
|
584 |
+
vae=vae,
|
585 |
+
unet=accelerator.unwrap_model(unet),
|
586 |
+
tokenizer=tokenizer,
|
587 |
+
scheduler=scheduler,
|
588 |
+
safety_checker=StableDiffusionSafetyChecker.from_pretrained(
|
589 |
+
MODEL_SD_SAFETY_PATH
|
590 |
+
),
|
591 |
+
feature_extractor=feature_extractor,
|
592 |
+
)
|
593 |
+
pipeline.save_pretrained(args.output_dir)
|
594 |
+
```
|
595 |
+
|
596 |
+
现在我们已经定义了训练所需的所有函数,开始训练吧!根据您的数据集的大小和 GPU 的类型,可能需要 5 分钟到 1 小时不等的时间才能运行:
|
597 |
+
|
598 |
+
|
599 |
+
```python
|
600 |
+
from accelerate import notebook_launcher
|
601 |
+
|
602 |
+
num_of_gpus = 1 # 以及您拥有的 GPU 数量修改此项
|
603 |
+
notebook_launcher(
|
604 |
+
training_function, args=(text_encoder, vae, unet), num_processes=num_of_gpus
|
605 |
+
)
|
606 |
+
```
|
607 |
+
|
608 |
+
Launching training on one GPU.
|
609 |
+
|
610 |
+
===================================BUG REPORT===================================
|
611 |
+
Welcome to bitsandbytes. For bug reports, please submit your error trace to: https://github.com/TimDettmers/bitsandbytes/issues
|
612 |
+
For effortless bug reporting copy-paste your error into this form: https://docs.google.com/forms/d/e/1FAIpQLScPB8emS3Thkp66nvqwmjTEgxp8Y9ufuWTzFyr9kJ5AoI47dQ/viewform?usp=sf_link
|
613 |
+
================================================================================
|
614 |
+
CUDA_SETUP: WARNING! libcudart.so not found in any environmental path. Searching /usr/local/cuda/lib64...
|
615 |
+
CUDA SETUP: CUDA runtime path found: /usr/local/cuda/lib64/libcudart.so
|
616 |
+
CUDA SETUP: Highest compute capability among GPUs detected: 7.5
|
617 |
+
CUDA SETUP: Detected CUDA version 116
|
618 |
+
CUDA SETUP: Loading binary /opt/conda/lib/python3.9/site-packages/bitsandbytes/libbitsandbytes_cuda116.so...
|
619 |
+
|
620 |
+
|
621 |
+
/opt/conda/lib/python3.9/site-packages/bitsandbytes/cuda_setup/main.py:134: UserWarning: WARNING: The following directories listed in your path were found to be non-existent: {PosixPath('/usr/local/nvidia/lib'), PosixPath('/usr/local/nvidia/lib64')}
|
622 |
+
warn(msg)
|
623 |
+
/opt/conda/lib/python3.9/site-packages/bitsandbytes/cuda_setup/main.py:134: UserWarning: /usr/local/nvidia/lib:/usr/local/nvidia/lib64 did not contain libcudart.so as expected! Searching further paths...
|
624 |
+
warn(msg)
|
625 |
+
/opt/conda/lib/python3.9/site-packages/bitsandbytes/cuda_setup/main.py:134: UserWarning: WARNING: The following directories listed in your path were found to be non-existent: {PosixPath('//172.16.252.1'), PosixPath('tcp'), PosixPath('443')}
|
626 |
+
warn(msg)
|
627 |
+
/opt/conda/lib/python3.9/site-packages/bitsandbytes/cuda_setup/main.py:134: UserWarning: WARNING: The following directories listed in your path were found to be non-existent: {PosixPath('//matplotlib_inline.backend_inline'), PosixPath('module')}
|
628 |
+
warn(msg)
|
629 |
+
|
630 |
+
|
631 |
+
|
632 |
+
0%| | 0/400 [00:00<?, ?it/s]
|
633 |
+
|
634 |
+
|
635 |
+
如果您在单个 GPU 上运行,您可以将下面的代码复制到一个新的单元格并运行来为下一部分释放一些内存。对于多 GPU 机器,🤗 Accelerate 不允许 _任何_ 单元格直接使用 torch.cuda 访问 GPU,因此我们不建议在这些情况下使用此技巧:
|
636 |
+
|
637 |
+
```python
|
638 |
+
with torch.no_grad():
|
639 |
+
torch.cuda.empty_cache()
|
640 |
+
```
|
641 |
+
|
642 |
+
## 第 7 步:运行推理并检查生成
|
643 |
+
|
644 |
+
现在我们的模型已经训练完毕,让我们用它生成一些图像,看看它的表现如何!首先,我们要从模型保存目录加载管道:
|
645 |
+
|
646 |
+
|
647 |
+
```python
|
648 |
+
pipe = StableDiffusionPipeline.from_pretrained(
|
649 |
+
args.output_dir,
|
650 |
+
torch_dtype=torch.float16,
|
651 |
+
).to("cuda")
|
652 |
+
```
|
653 |
+
|
654 |
+
接下来让我们尝试生成一些图像。在抱抱脸 Hub 小部件上为 `prompt` 变量设置默认值,可以稍微试验几次来找到一个较好的值。如果您还想尝试使用 [CLIP Interrogator](https://huggingface.co/spaces/pharma/CLIP-Interrogator) 创建更详细的提示,请参考下文:
|
655 |
+
|
656 |
+
|
657 |
+
```python
|
658 |
+
# Pick a funny prompt here and it will be used as the widget's default
|
659 |
+
# when we push to the Hub in the next section
|
660 |
+
prompt = f"illustration of a dashdash toy sitting on top of the deck of a battle ship traveling through the open sea with a lot of ships surrounding it"
|
661 |
+
|
662 |
+
# Tune the guidance to control how closely the generations follow the prompt.
|
663 |
+
# Values between 7-11 usually work best
|
664 |
+
guidance_scale = 7
|
665 |
+
|
666 |
+
num_cols = 2
|
667 |
+
all_images = []
|
668 |
+
for _ in range(num_cols):
|
669 |
+
images = pipe(prompt, guidance_scale=guidance_scale).images
|
670 |
+
all_images.extend(images)
|
671 |
+
|
672 |
+
image_grid(all_images, 1, num_cols)
|
673 |
+
```
|
674 |
+
|
675 |
+
## 第 8 步:将您的模型推送到 Hub
|
676 |
+
|
677 |
+
如果您觉得自己的模型非常棒,最后一步是将其推送到 Hub 并在 [DreamBooth 排行榜](https://huggingface.co/spaces/dreambooth-hackathon/leaderboard)上查看!
|
678 |
+
|
679 |
+
⚠️ 由于网络原因,这一步可能会花费几分钟。如果失败,请重试。
|
680 |
+
|
681 |
+
首先,您需要为模型库起一个名字。默认情况下,我们使用唯一标识符和类名来命名,但如果您愿意,可以随时更改:
|
682 |
+
|
683 |
+
|
684 |
+
```python
|
685 |
+
# 在 Hub 上为您的模型起一个名字。不允许包含空格。
|
686 |
+
model_name = f"{name_of_your_concept}-{type_of_thing}-heywhale"
|
687 |
+
```
|
688 |
+
|
689 |
+
接下来,添加一个简短描述,介绍一下您训练的模型类型或者想要分享的任何其他信息:
|
690 |
+
|
691 |
+
|
692 |
+
```python
|
693 |
+
# 描述一下您选择的主题以及训练好的模型
|
694 |
+
description = f"""
|
695 |
+
This is a Stable Diffusion model fine-tuned on `{type_of_thing}` images for the {theme} theme,
|
696 |
+
for the Hugging Face DreamBooth Hackathon, from the HF CN Community,
|
697 |
+
corporated with the HeyWhale.
|
698 |
+
"""
|
699 |
+
|
700 |
+
print(description)
|
701 |
+
```
|
702 |
+
|
703 |
+
|
704 |
+
This is a Stable Diffusion model fine-tuned on `toy` images for the wildcard theme,
|
705 |
+
for the Hugging Face DreamBooth Hackathon, from the HF CN Community,
|
706 |
+
corporated with the HeyWhale.
|
707 |
+
|
708 |
+
|
709 |
+
|
710 |
+
最后,运行下面的单元格在 Hub 上创建一个 repo,并使用一个精美的模型卡,同时将所有的文件推送到引导:
|
711 |
+
|
712 |
+
|
713 |
+
```python
|
714 |
+
# 将本地保存的管道上传到 Hub 的代码
|
715 |
+
from huggingface_hub import HfApi, ModelCard, create_repo, get_full_repo_name
|
716 |
+
|
717 |
+
# 创建库
|
718 |
+
hub_model_id = get_full_repo_name(model_name)
|
719 |
+
create_repo(hub_model_id)
|
720 |
+
```
|
721 |
+
|
722 |
+
|
723 |
+
```python
|
724 |
+
# 上传文件
|
725 |
+
api = HfApi()
|
726 |
+
api.upload_folder(folder_path=args.output_dir, path_in_repo="", repo_id=hub_model_id)
|
727 |
+
```
|
728 |
+
|
729 |
+
|
730 |
+
```python
|
731 |
+
# 添加 metadata
|
732 |
+
content = f"""
|
733 |
+
---
|
734 |
+
license: creativeml-openrail-m
|
735 |
+
tags:
|
736 |
+
- pytorch
|
737 |
+
- diffusers
|
738 |
+
- stable-diffusion
|
739 |
+
- text-to-image
|
740 |
+
- diffusion-models-class
|
741 |
+
- dreambooth-hackathon
|
742 |
+
- {theme}
|
743 |
+
widget:
|
744 |
+
- text: {prompt}
|
745 |
+
---
|
746 |
+
|
747 |
+
# DreamBooth model for the {name_of_your_concept} concept trained by {api.whoami()["name"]}.
|
748 |
+
|
749 |
+
This is a Stable Diffusion model fine-tuned on the {name_of_your_concept} concept with DreamBooth. It can be used by modifying the `instance_prompt`: **{instance_prompt}**
|
750 |
+
|
751 |
+
This model was created as part of the DreamBooth Hackathon 🔥. Visit the [organisation page](https://huggingface.co/dreambooth-hackathon) for instructions on how to take part!
|
752 |
+
|
753 |
+
## Description
|
754 |
+
|
755 |
+
{description}
|
756 |
+
|
757 |
+
## Usage
|
758 |
+
|
759 |
+
```python
|
760 |
+
from diffusers import StableDiffusionPipeline
|
761 |
+
|
762 |
+
pipeline = StableDiffusionPipeline.from_pretrained('{hub_model_id}')
|
763 |
+
image = pipeline().images[0]
|
764 |
+
image
|
765 |
+
```
|
766 |
+
"""
|
767 |
+
|
768 |
+
card = ModelCard(content)
|
769 |
+
hub_url = card.push_to_hub(hub_model_id)
|
770 |
+
print(f"Upload successful! Model can be found here: {hub_url}")
|
771 |
+
print(
|
772 |
+
f"View your submission on the public leaderboard here: https://huggingface.co/spaces/dreambooth-hackathon/leaderboard"
|
773 |
+
)
|
774 |
+
```
|
775 |
+
|
776 |
+
恭喜恭喜 🎉 你已经训练了一个自己的文生图模型,并且成功上传到了 HuggingFace!快去跟朋友们分享,请他们使用和点赞吧~
|
777 |
+
|
778 |
+
## 下一步
|
779 |
+
|
780 |
+
提交参赛作品,请在 [这里](https://www.heywhale.com/org/HuggingFace/competition/area/63bbfb98de6c0e9cdb0d9dd5/submit) 提交已经上传到 Hugging Face 上的模型 URL。
|
781 |
+
|
markdown/unit1/01_introduction_to_diffusers_CN.md
ADDED
The diff for this file is too large to render.
See raw diff
|
|
markdown/unit1/01_introduction_to_diffusers_CN_files/01_introduction_to_diffusers_CN_18_0.jpg
ADDED
![]() |
Git LFS Details
|
markdown/unit1/01_introduction_to_diffusers_CN_files/01_introduction_to_diffusers_CN_22_1.png
ADDED
![]() |
Git LFS Details
|
markdown/unit1/01_introduction_to_diffusers_CN_files/01_introduction_to_diffusers_CN_26_2.png
ADDED
![]() |
Git LFS Details
|
markdown/unit1/01_introduction_to_diffusers_CN_files/01_introduction_to_diffusers_CN_31_2.png
ADDED
![]() |
Git LFS Details
|
markdown/unit1/01_introduction_to_diffusers_CN_files/01_introduction_to_diffusers_CN_40_1.png
ADDED
![]() |
Git LFS Details
|
markdown/unit1/01_introduction_to_diffusers_CN_files/01_introduction_to_diffusers_CN_54_1.png
ADDED
![]() |
Git LFS Details
|
markdown/unit1/01_introduction_to_diffusers_CN_files/01_introduction_to_diffusers_CN_60_1.png
ADDED
![]() |
Git LFS Details
|
markdown/unit1/01_introduction_to_diffusers_CN_files/01_introduction_to_diffusers_CN_71_0.png
ADDED
![]() |
Git LFS Details
|
markdown/unit1/01_introduction_to_diffusers_CN_files/01_introduction_to_diffusers_CN_80_2.png
ADDED
![]() |
Git LFS Details
|
markdown/unit1/01_introduction_to_diffusers_CN_files/01_introduction_to_diffusers_CN_90_1.png
ADDED
![]() |
Git LFS Details
|
markdown/unit1/02_diffusion_models_from_scratch_CN.md
ADDED
The diff for this file is too large to render.
See raw diff
|
|
markdown/unit1/02_diffusion_models_from_scratch_CN_files/02_diffusion_models_from_scratch_CN_24_1.png
ADDED
![]() |
Git LFS Details
|
markdown/unit1/02_diffusion_models_from_scratch_CN_files/02_diffusion_models_from_scratch_CN_26_0.png
ADDED
![]() |
Git LFS Details
|
markdown/unit1/02_diffusion_models_from_scratch_CN_files/02_diffusion_models_from_scratch_CN_29_0.png
ADDED
![]() |
Git LFS Details
|
markdown/unit1/02_diffusion_models_from_scratch_CN_files/02_diffusion_models_from_scratch_CN_31_1.png
ADDED
![]() |
Git LFS Details
|
markdown/unit1/02_diffusion_models_from_scratch_CN_files/02_diffusion_models_from_scratch_CN_39_1.png
ADDED
![]() |
Git LFS Details
|
markdown/unit1/02_diffusion_models_from_scratch_CN_files/02_diffusion_models_from_scratch_CN_43_0.png
ADDED
![]() |
Git LFS Details
|
markdown/unit1/02_diffusion_models_from_scratch_CN_files/02_diffusion_models_from_scratch_CN_45_1.png
ADDED
![]() |
Git LFS Details
|
markdown/unit1/README_CN.md
ADDED
@@ -0,0 +1,65 @@
|
|
|
|
|
|
|
|
|
|
|
|
|
|
|
|
|
|
|
|
|
|
|
|
|
|
|
|
|
|
|
|
|
|
|
|
|
|
|
|
|
|
|
|
|
|
|
|
|
|
|
|
|
|
|
|
|
|
|
|
|
|
|
|
|
|
|
|
|
|
|
|
|
|
|
|
|
|
|
|
|
|
|
|
|
|
|
|
|
|
|
|
|
|
|
|
|
|
|
|
|
|
|
|
|
|
|
|
|
|
|
|
|
|
|
|
|
|
|
|
|
|
|
|
|
|
|
|
|
|
|
|
|
1 |
+
# 单元 1: 扩散模型简介
|
2 |
+
|
3 |
+
欢迎来到 Hugging Face 扩散模型课程第一单元!在本单元中,你将学习到有关扩散模型如何运作的基础知识,同时也会学到如何使用 🤗 diffusers 库。
|
4 |
+
|
5 |
+
## 开始本单元 :rocket:
|
6 |
+
|
7 |
+
以下是本单元的学习步骤:
|
8 |
+
|
9 |
+
- 请确保你已经 [注册了该课程](https://huggingface.us17.list-manage.com/subscribe?u=7f57e683fa28b51bfc493d048&id=ef963b4162)。这样当有新课程材料发布的时候你就会收到通知
|
10 |
+
- 通读下面的介绍材料以及任何你感兴趣的其他资源
|
11 |
+
- 查看下面的 _**Introduction to Diffusers**_ Notebook 链接,以使用 `diffuers` 库将理论应用到实践中
|
12 |
+
- 使用 Notebook 或链接的训练脚本来训练和分享你自己的扩散模型
|
13 |
+
- (可选) 如果你有兴趣看到一个极简的从头开始的项目实现,并探索所涉及的不同设计决策,你可以深入研究 _**Diffusion Models from Scratch**_ 这个 Notebook
|
14 |
+
|
15 |
+
|
16 |
+
:loudspeaker: 请不要忘了加入我们的频道 [Discord](https://huggingface.co/join/discord), 你可以在 `#diffusion-models-class` 频道来讨论课程内容以及分享你的作品。
|
17 |
+
|
18 |
+
## 什么是扩散模型?
|
19 |
+
|
20 |
+
扩散模型是「生成模型」算法家族的新成员通过学习给定的训练样本,生成模型可以学会如何 **生成** 数据,比如生成图片或者声音。一个好的生成模型能生成一组 **样式不同** 的输出。这些输出会与训练数据相似,但不是一模一样的副本。扩散模型如何实现这一点?为了便于说明,让我们先看看图像生成的案例。
|
21 |
+
|
22 |
+
<p align="center">
|
23 |
+
<img src="https://user-images.githubusercontent.com/10695622/174349667-04e9e485-793b-429a-affe-096e8199ad5b.png" width="800"/>
|
24 |
+
<br>
|
25 |
+
<em> 图片来源于 DDPM paper (https://arxiv.org/abs/2006.11239)。</em>
|
26 |
+
<p>
|
27 |
+
|
28 |
+
扩散模型成功的秘诀在于扩散过程的迭代本质。最先生成的只是一组随机噪声,但是经过若干步的逐渐改善之后,最终会出现有意义的图像。在每一步中,模型都会估计如何从当前的输入生成完全去噪的结果。因为我们在每一步都只做了一个小小的变动,所以在早期阶段(预测最终输出实际上非常困难),这个估计中的任何 error 都可以在以后的更新中得到纠正。
|
29 |
+
|
30 |
+
与其他类型的生成模型相比,训练扩散模型相对较为容易。我们只需要重复以下步骤即可:
|
31 |
+
|
32 |
+
1) 从训练数据中加载一些图像
|
33 |
+
2) 添加不同级别的噪声。请记住,我们希望模型在面对添加了极端噪声和几乎没有添加噪声的带噪图像时,都能够很好地估计如何 “修复”(去噪)。
|
34 |
+
3) 将带噪输入送入模型中
|
35 |
+
4) 评估模型对这些输入进行去噪的效果
|
36 |
+
5) 使用此信息更新模型权重
|
37 |
+
|
38 |
+
为了用训练好的模型生成新的图像,我们从完全随机的输入开始,反复将其输入模型,每次根据模型预测进行少量更新。我们之后会学到有许多采样方法试图简化这个过程,以便我们可以用尽可能少的步骤生成好的图像。
|
39 |
+
我们将在第一单元的实践笔记本中详细介绍这些步骤。在第二单元中,我们将了解如何修改此过程,来通过额外的条件(例如类标签)或使用指导等技术来增加对模型输出的额外控制。第三单元和第四单元将探索一种非常强大的扩散模型,称为稳定扩散 (stable diffusion),它可以生成给定文本描述的图像。
|
40 |
+
|
41 |
+
## 实践笔记本
|
42 |
+
|
43 |
+
到这里,你已经足够了解如何开始使用附带的笔记本了!这里的两个笔记本以不同的方式表达了相同的想法。
|
44 |
+
|
45 |
+
| Chapter | Colab | Kaggle | Gradient | Studio Lab |
|
46 |
+
|:--------------------------------------------|:----------------------------------------------------------------------------------------------------------------------------------------------------------------------------------------------------|:---------------------------------------------------------------------------------------------------------------------------------------------------------------------------------------------------------|:---------------------------------------------------------------------------------------------------------------------------------------------------------------------------------------|:-------------------------------------------------------------------------------------------------------------------------------------------------------------------------------------------------------------|
|
47 |
+
| Introduction to Diffusers | [](https://colab.research.google.com/github/darcula1993/diffusion-models-class-CN/blob/main/unit1/01_introduction_to_diffusers_CN.ipynb) | [](https://kaggle.com/kernels/welcome?src=https://github.com/huggingface/diffusion-models-class/blob/main/unit1/01_introduction_to_diffusers_CN.ipynb) | [](https://console.paperspace.com/github/darcula1993/diffusion-models-class-CN/blob/main/unit1/01_introduction_to_diffusers_CN.ipynb) | [](https://studiolab.sagemaker.aws/import/github/darcula1993/diffusion-models-class-CN/blob/main/unit1/01_introduction_to_diffusers_CN.ipynb) |
|
48 |
+
| Diffusion Models from Scratch | [](https://colab.research.google.com/github/darcula1993/diffusion-models-class-CN/blob/main/unit1/02_diffusion_models_from_scratch_CN.ipynb) | [](https://kaggle.com/kernels/welcome?src=https://github.com/huggingface/diffusion-models-class/blob/main/unit1/02_diffusion_models_from_scratch_CN.ipynb) | [](https://console.paperspace.com/github/darcula1993/diffusion-models-class-CN/blob/main/unit1/02_diffusion_models_from_scratch_CN.ipynb) | [](https://studiolab.sagemaker.aws/import/github/darcula1993/diffusion-models-class-CN/blob/main/unit1/02_diffusion_models_from_scratch_CN.ipynb) |
|
49 |
+
|
50 |
+
在 _**Introduction to Diffusers**_ 这个 Notebook 中,我们使用 diffusers 库中的构造模块显示了与上述不同的步骤。你将很快看到如何根据你选择的任何数据创建、训练和采样你自己的扩散模型。 在笔记本结束时,你将能够阅读和修改示例训练脚本,以训练扩散模型,并将其与全世界共同分享! 本笔记本还介绍了与本单元相关的主要练习,在这里,我们将共同尝试为不同规模的扩散模型找出好的「训练脚本」- 请参阅下一节了解更多信息。
|
51 |
+
|
52 |
+
在 _**Diffusion Models from Scratch**_ 这个 Notebook 中,我们展示了相同的步骤(向数据添加噪声、创建模型、训练和采样),并尽可能简单地在 PyTorch 中从头开始实现。然后,我们将这个「玩具示例」与 `diffusers` 版本进行比较,并关注两者的区别以及改进之处。这里的目标是熟悉不同的组件和其中的设计决策,以便在查看新的实现时能够快速确定关键思想。
|
53 |
+
|
54 |
+
## 项目时间
|
55 |
+
|
56 |
+
现在,你已经掌握了基本知识,可以开始训练你自己的扩散模型了! _**Introduction to Diffusers**_ 这个 Notebook 的末尾有一些小提示,希望你能与社区分享你的成果、训练脚本和发现,以便我们能够一起找出训练这些模型的最佳方法。
|
57 |
+
|
58 |
+
## 一些额外的材料
|
59 |
+
|
60 |
+
- [《Hugging Face 博客: 带注释的扩散模型》](https://huggingface.co/blog/annotated-diffusion)是对 DDPM 背后的代码和理论的非常深入的介绍,其中包括数学和显示了所有不同的组件的代码。它还链接了一些论文供进一步阅读:
|
61 |
+
- [Hugging Face 文档: 无条件图像生成 (Unconditional Image-Generation)](https://huggingface.co/docs/diffusers/training/unconditional_training),包含了有关如何使用官方训练示例脚本训练扩散模型的一些示例,包括演示如何创建自己的数据集的代码:
|
62 |
+
- AI Coffee Break video on Diffusion Models: https://www.youtube.com/watch?v=344w5h24-h8
|
63 |
+
- Yannic Kilcher Video on DDPMs: https://www.youtube.com/watch?v=W-O7AZNzbzQ
|
64 |
+
|
65 |
+
发现了有其他任何有帮助的资源?请 [向我们提出](https://github.com/huggingface/diffusion-models-class/issues),我们会将其加入到上面的列表中。
|
markdown/unit2/01_finetuning_and_guidance_CN.md
ADDED
The diff for this file is too large to render.
See raw diff
|
|
markdown/unit2/01_finetuning_and_guidance_CN_files/01_finetuning_and_guidance_CN_17_1.png
ADDED
![]() |
Git LFS Details
|
markdown/unit2/01_finetuning_and_guidance_CN_files/01_finetuning_and_guidance_CN_17_2.png
ADDED
![]() |
Git LFS Details
|
markdown/unit2/01_finetuning_and_guidance_CN_files/01_finetuning_and_guidance_CN_17_3.png
ADDED
![]() |
Git LFS Details
|
markdown/unit2/01_finetuning_and_guidance_CN_files/01_finetuning_and_guidance_CN_17_4.png
ADDED
![]() |
Git LFS Details
|
markdown/unit2/01_finetuning_and_guidance_CN_files/01_finetuning_and_guidance_CN_17_5.png
ADDED
![]() |
Git LFS Details
|
markdown/unit2/01_finetuning_and_guidance_CN_files/01_finetuning_and_guidance_CN_21_1.png
ADDED
![]() |
Git LFS Details
|
markdown/unit2/01_finetuning_and_guidance_CN_files/01_finetuning_and_guidance_CN_25_2.png
ADDED
![]() |
Git LFS Details
|
markdown/unit2/01_finetuning_and_guidance_CN_files/01_finetuning_and_guidance_CN_28_5.png
ADDED
![]() |
Git LFS Details
|
markdown/unit2/01_finetuning_and_guidance_CN_files/01_finetuning_and_guidance_CN_36_1.png
ADDED
![]() |
Git LFS Details
|
markdown/unit2/01_finetuning_and_guidance_CN_files/01_finetuning_and_guidance_CN_48_6.png
ADDED
![]() |
Git LFS Details
|
markdown/unit2/01_finetuning_and_guidance_CN_files/01_finetuning_and_guidance_CN_54_2.png
ADDED
![]() |
Git LFS Details
|
markdown/unit2/01_finetuning_and_guidance_CN_files/01_finetuning_and_guidance_CN_56_2.png
ADDED
![]() |
Git LFS Details
|
markdown/unit2/01_finetuning_and_guidance_CN_files/01_finetuning_and_guidance_CN_62_2.png
ADDED
![]() |
Git LFS Details
|
markdown/unit2/01_finetuning_and_guidance_CN_files/01_finetuning_and_guidance_CN_65_0.png
ADDED
![]() |
Git LFS Details
|
markdown/unit2/01_finetuning_and_guidance_CN_files/01_finetuning_and_guidance_CN_9_1.png
ADDED
![]() |
Git LFS Details
|
markdown/unit2/02_class_conditioned_diffusion_model_example_CN.md
ADDED
@@ -0,0 +1,360 @@
|
|
|
|
|
|
|
|
|
|
|
|
|
|
|
|
|
|
|
|
|
|
|
|
|
|
|
|
|
|
|
|
|
|
|
|
|
|
|
|
|
|
|
|
|
|
|
|
|
|
|
|
|
|
|
|
|
|
|
|
|
|
|
|
|
|
|
|
|
|
|
|
|
|
|
|
|
|
|
|
|
|
|
|
|
|
|
|
|
|
|
|
|
|
|
|
|
|
|
|
|
|
|
|
|
|
|
|
|
|
|
|
|
|
|
|
|
|
|
|
|
|
|
|
|
|
|
|
|
|
|
|
|
|
|
|
|
|
|
|
|
|
|
|
|
|
|
|
|
|
|
|
|
|
|
|
|
|
|
|
|
|
|
|
|
|
|
|
|
|
|
|
|
|
|
|
|
|
|
|
|
|
|
|
|
|
|
|
|
|
|
|
|
|
|
|
|
|
|
|
|
|
|
|
|
|
|
|
|
|
|
|
|
|
|
|
|
|
|
|
|
|
|
|
|
|
|
|
|
|
|
|
|
|
|
|
|
|
|
|
|
|
|
|
|
|
|
|
|
|
|
|
|
|
|
|
|
|
|
|
|
|
|
|
|
|
|
|
|
|
|
|
|
|
|
|
|
|
|
|
|
|
|
|
|
|
|
|
|
|
|
|
|
|
|
|
|
|
|
|
|
|
|
|
|
|
|
|
|
|
|
|
|
|
|
|
|
|
|
|
|
|
|
|
|
|
|
|
|
|
|
|
|
|
|
|
|
|
|
|
|
|
|
|
|
|
|
|
|
|
|
|
|
|
|
|
|
|
|
|
|
|
|
|
|
|
|
|
|
|
|
|
|
|
|
|
|
|
|
|
|
|
|
|
|
|
|
|
|
|
|
|
|
|
|
|
|
|
|
|
|
|
|
|
|
|
|
|
|
|
|
|
|
|
|
|
|
|
|
|
|
|
|
|
|
|
|
|
|
|
|
|
|
|
|
|
|
|
|
|
|
|
|
|
|
|
|
|
|
|
|
|
|
|
|
|
|
|
|
|
|
|
|
|
|
|
|
|
|
|
|
|
|
|
|
|
|
|
|
|
|
|
|
|
|
|
|
|
|
|
|
|
|
|
|
|
|
|
|
|
|
|
|
|
|
|
|
|
|
|
|
|
|
|
|
|
|
|
|
|
|
|
|
|
|
|
|
|
|
|
|
|
|
|
|
|
|
|
|
|
|
|
|
|
|
|
|
|
|
|
|
|
|
|
|
|
|
|
|
|
|
|
|
|
|
|
|
|
|
|
|
|
|
|
|
|
|
|
|
|
|
|
|
|
|
|
|
|
|
|
|
|
|
|
|
|
|
|
|
|
|
|
|
|
|
|
|
|
|
|
|
|
|
|
|
|
|
|
|
|
|
|
|
|
|
|
|
|
|
|
|
|
|
|
|
|
|
|
|
|
|
|
|
|
|
|
|
|
|
|
|
|
|
|
|
|
|
|
|
|
|
|
|
|
|
|
|
|
|
|
|
|
|
|
|
|
|
|
|
|
|
|
|
|
|
|
|
|
|
|
|
|
|
|
|
|
|
|
|
|
|
|
|
|
|
|
|
|
|
|
|
|
|
|
|
|
|
|
|
|
|
|
|
1 |
+
# 创建一个类别条件扩散模型
|
2 |
+
|
3 |
+
在这节笔记本中,我们将阐述一种给扩散模型加条件信息的方法。具体来说,我们将接着[这个从头训练的例子](../unit1/02_diffusion_models_from_scratch_CN.ipynb)在 MNIST 上训练一个以类别为条件的扩散模型。这里我们可以在推理时指定我们要生成的是哪个数字。
|
4 |
+
|
5 |
+
就像本单元介绍中说的那样,这只是很多给扩散模型添加额外条件信息的方法中的一种,这里用它做示例是因为它比较简单。就像第一单元中“从走训练”的例子一样,这节笔记本也是为了解释说明的目的。如果你想,你也可以安全地跳过本节。
|
6 |
+
|
7 |
+
## 配置和数据准备
|
8 |
+
|
9 |
+
|
10 |
+
```python
|
11 |
+
!pip install -q diffusers
|
12 |
+
```
|
13 |
+
|
14 |
+
[K |████████████████████████████████| 503 kB 7.2 MB/s
|
15 |
+
[K |████████████████████████████████| 182 kB 51.3 MB/s
|
16 |
+
[?25h
|
17 |
+
|
18 |
+
|
19 |
+
```python
|
20 |
+
import torch
|
21 |
+
import torchvision
|
22 |
+
from torch import nn
|
23 |
+
from torch.nn import functional as F
|
24 |
+
from torch.utils.data import DataLoader
|
25 |
+
from diffusers import DDPMScheduler, UNet2DModel
|
26 |
+
from matplotlib import pyplot as plt
|
27 |
+
from tqdm.auto import tqdm
|
28 |
+
|
29 |
+
device = 'mps' if torch.backends.mps.is_available() else 'cuda' if torch.cuda.is_available() else 'cpu'
|
30 |
+
print(f'Using device: {device}')
|
31 |
+
```
|
32 |
+
|
33 |
+
Using device: cuda
|
34 |
+
|
35 |
+
|
36 |
+
|
37 |
+
```python
|
38 |
+
# Load the dataset
|
39 |
+
dataset = torchvision.datasets.MNIST(root="mnist/", train=True, download=True, transform=torchvision.transforms.ToTensor())
|
40 |
+
|
41 |
+
# Feed it into a dataloader (batch size 8 here just for demo)
|
42 |
+
train_dataloader = DataLoader(dataset, batch_size=8, shuffle=True)
|
43 |
+
|
44 |
+
# View some examples
|
45 |
+
x, y = next(iter(train_dataloader))
|
46 |
+
print('Input shape:', x.shape)
|
47 |
+
print('Labels:', y)
|
48 |
+
plt.imshow(torchvision.utils.make_grid(x)[0], cmap='Greys');
|
49 |
+
```
|
50 |
+
|
51 |
+
Downloading http://yann.lecun.com/exdb/mnist/train-images-idx3-ubyte.gz
|
52 |
+
Downloading http://yann.lecun.com/exdb/mnist/train-images-idx3-ubyte.gz to mnist/MNIST/raw/train-images-idx3-ubyte.gz
|
53 |
+
|
54 |
+
|
55 |
+
|
56 |
+
0%| | 0/9912422 [00:00<?, ?it/s]
|
57 |
+
|
58 |
+
|
59 |
+
Extracting mnist/MNIST/raw/train-images-idx3-ubyte.gz to mnist/MNIST/raw
|
60 |
+
|
61 |
+
Downloading http://yann.lecun.com/exdb/mnist/train-labels-idx1-ubyte.gz
|
62 |
+
Downloading http://yann.lecun.com/exdb/mnist/train-labels-idx1-ubyte.gz to mnist/MNIST/raw/train-labels-idx1-ubyte.gz
|
63 |
+
|
64 |
+
|
65 |
+
|
66 |
+
0%| | 0/28881 [00:00<?, ?it/s]
|
67 |
+
|
68 |
+
|
69 |
+
Extracting mnist/MNIST/raw/train-labels-idx1-ubyte.gz to mnist/MNIST/raw
|
70 |
+
|
71 |
+
Downloading http://yann.lecun.com/exdb/mnist/t10k-images-idx3-ubyte.gz
|
72 |
+
Downloading http://yann.lecun.com/exdb/mnist/t10k-images-idx3-ubyte.gz to mnist/MNIST/raw/t10k-images-idx3-ubyte.gz
|
73 |
+
|
74 |
+
|
75 |
+
|
76 |
+
0%| | 0/1648877 [00:00<?, ?it/s]
|
77 |
+
|
78 |
+
|
79 |
+
Extracting mnist/MNIST/raw/t10k-images-idx3-ubyte.gz to mnist/MNIST/raw
|
80 |
+
|
81 |
+
Downloading http://yann.lecun.com/exdb/mnist/t10k-labels-idx1-ubyte.gz
|
82 |
+
Downloading http://yann.lecun.com/exdb/mnist/t10k-labels-idx1-ubyte.gz to mnist/MNIST/raw/t10k-labels-idx1-ubyte.gz
|
83 |
+
|
84 |
+
|
85 |
+
|
86 |
+
0%| | 0/4542 [00:00<?, ?it/s]
|
87 |
+
|
88 |
+
|
89 |
+
Extracting mnist/MNIST/raw/t10k-labels-idx1-ubyte.gz to mnist/MNIST/raw
|
90 |
+
|
91 |
+
Input shape: torch.Size([8, 1, 28, 28])
|
92 |
+
Labels: tensor([8, 1, 5, 9, 7, 6, 2, 2])
|
93 |
+
|
94 |
+
|
95 |
+
|
96 |
+
|
97 |
+

|
98 |
+
|
99 |
+
|
100 |
+
|
101 |
+
## 创建一个以类别为条件的 UNet
|
102 |
+
|
103 |
+
我们输入类别这一条件的方法是:
|
104 |
+
- 创建一个标准的 `UNet2DModel`,加入一些额外的输入通道
|
105 |
+
- 通过一个嵌入层,把类别标签映射到一个 `(class_emb_size)` 形状的学到的向量上
|
106 |
+
- 把这个信息作为额外通道和原有的输入向量拼接起来,用这行代码:`net_input = torch.cat((x, class_cond), 1)`
|
107 |
+
- 把这个 `net_input` (有 `class_emb_size+1` 个通道)输入到UNet中得到最终预测
|
108 |
+
|
109 |
+
在这个例子中,我把 class_emb_size 设成4,但这其实是可以任意修改的,你可以试试从把它设成1(你可以看看这有没有用)到把它设成 10(正好是类别总数),或者把需要学到的 nn.Embedding 换成简单的对类别进行独热编码(one-hot encoding)。
|
110 |
+
|
111 |
+
具体实现起来就是这样:
|
112 |
+
|
113 |
+
|
114 |
+
```python
|
115 |
+
class ClassConditionedUnet(nn.Module):
|
116 |
+
def __init__(self, num_classes=10, class_emb_size=4):
|
117 |
+
super().__init__()
|
118 |
+
|
119 |
+
# The embedding layer will map the class label to a vector of size class_emb_size
|
120 |
+
self.class_emb = nn.Embedding(num_classes, class_emb_size)
|
121 |
+
|
122 |
+
# Self.model is an unconditional UNet with extra input channels to accept the conditioning information (the class embedding)
|
123 |
+
self.model = UNet2DModel(
|
124 |
+
sample_size=28, # the target image resolution
|
125 |
+
in_channels=1 + class_emb_size, # Additional input channels for class cond.
|
126 |
+
out_channels=1, # the number of output channels
|
127 |
+
layers_per_block=2, # how many ResNet layers to use per UNet block
|
128 |
+
block_out_channels=(32, 64, 64),
|
129 |
+
down_block_types=(
|
130 |
+
"DownBlock2D", # a regular ResNet downsampling block
|
131 |
+
"AttnDownBlock2D", # a ResNet downsampling block with spatial self-attention
|
132 |
+
"AttnDownBlock2D",
|
133 |
+
),
|
134 |
+
up_block_types=(
|
135 |
+
"AttnUpBlock2D",
|
136 |
+
"AttnUpBlock2D", # a ResNet upsampling block with spatial self-attention
|
137 |
+
"UpBlock2D", # a regular ResNet upsampling block
|
138 |
+
),
|
139 |
+
)
|
140 |
+
|
141 |
+
# Our forward method now takes the class labels as an additional argument
|
142 |
+
def forward(self, x, t, class_labels):
|
143 |
+
# Shape of x:
|
144 |
+
bs, ch, w, h = x.shape
|
145 |
+
|
146 |
+
# class conditioning in right shape to add as additional input channels
|
147 |
+
class_cond = self.class_emb(class_labels) # Map to embedding dinemsion
|
148 |
+
class_cond = class_cond.view(bs, class_cond.shape[1], 1, 1).expand(bs, class_cond.shape[1], w, h)
|
149 |
+
# x is shape (bs, 1, 28, 28) and class_cond is now (bs, 4, 28, 28)
|
150 |
+
|
151 |
+
# Net input is now x and class cond concatenated together along dimension 1
|
152 |
+
net_input = torch.cat((x, class_cond), 1) # (bs, 5, 28, 28)
|
153 |
+
|
154 |
+
# Feed this to the unet alongside the timestep and return the prediction
|
155 |
+
return self.model(net_input, t).sample # (bs, 1, 28, 28)
|
156 |
+
```
|
157 |
+
|
158 |
+
如果你对任何的张量形状或变换感到迷惑,你都可以在代码中加入print来看看相关形状,检查一下是不是和你预设的是一致的。这里我把一些中间变量的形状都注释上了,希望能帮你思路清晰点。
|
159 |
+
|
160 |
+
## 训练和采样
|
161 |
+
|
162 |
+
不同于别的地方使用的`prediction = unet(x, t)`,这里我们使用`prediction = unet(x, t, y)`,在训练时把正确的标签作为第三个输入送到模型中。在推理阶段,我们可以输入任何我们想要的标签,如果一切正常,那模型就会输出与之匹配的图片。`y`在这里时 MNIST 中的数字标签,值的范围从0到9。
|
163 |
+
|
164 |
+
这里的训练循环很像[第一单元的例子](../unit1/02_diffusion_models_from_scratch_CN.ipynb)。我们这里预测的是噪声(而不是像第一单元的去噪图片),以此来匹配 DDPMScheduler 预计的目标。这里我们用 DDPMScheduler 来在训练中加噪声,并在推理时采样用。训练也需要一段时间 —— 如何加速训练也可以是个有趣的小项目。但你也可以跳过运行代码(甚至整节笔记本),因为我们这里纯粹是在讲解思路。
|
165 |
+
|
166 |
+
|
167 |
+
```python
|
168 |
+
# Create a scheduler
|
169 |
+
noise_scheduler = DDPMScheduler(num_train_timesteps=1000, beta_schedule='squaredcos_cap_v2')
|
170 |
+
```
|
171 |
+
|
172 |
+
|
173 |
+
```python
|
174 |
+
#@markdown Training loop (10 Epochs):
|
175 |
+
|
176 |
+
# Redefining the dataloader to set the batch size higher than the demo of 8
|
177 |
+
train_dataloader = DataLoader(dataset, batch_size=128, shuffle=True)
|
178 |
+
|
179 |
+
# How many runs through the data should we do?
|
180 |
+
n_epochs = 10
|
181 |
+
|
182 |
+
# Our network
|
183 |
+
net = ClassConditionedUnet().to(device)
|
184 |
+
|
185 |
+
# Our loss finction
|
186 |
+
loss_fn = nn.MSELoss()
|
187 |
+
|
188 |
+
# The optimizer
|
189 |
+
opt = torch.optim.Adam(net.parameters(), lr=1e-3)
|
190 |
+
|
191 |
+
# Keeping a record of the losses for later viewing
|
192 |
+
losses = []
|
193 |
+
|
194 |
+
# The training loop
|
195 |
+
for epoch in range(n_epochs):
|
196 |
+
for x, y in tqdm(train_dataloader):
|
197 |
+
|
198 |
+
# Get some data and prepare the corrupted version
|
199 |
+
x = x.to(device) * 2 - 1 # Data on the GPU (mapped to (-1, 1))
|
200 |
+
y = y.to(device)
|
201 |
+
noise = torch.randn_like(x)
|
202 |
+
timesteps = torch.randint(0, 999, (x.shape[0],)).long().to(device)
|
203 |
+
noisy_x = noise_scheduler.add_noise(x, noise, timesteps)
|
204 |
+
|
205 |
+
# Get the model prediction
|
206 |
+
pred = net(noisy_x, timesteps, y) # Note that we pass in the labels y
|
207 |
+
|
208 |
+
# Calculate the loss
|
209 |
+
loss = loss_fn(pred, noise) # How close is the output to the noise
|
210 |
+
|
211 |
+
# Backprop and update the params:
|
212 |
+
opt.zero_grad()
|
213 |
+
loss.backward()
|
214 |
+
opt.step()
|
215 |
+
|
216 |
+
# Store the loss for later
|
217 |
+
losses.append(loss.item())
|
218 |
+
|
219 |
+
# Print our the average of the last 100 loss values to get an idea of progress:
|
220 |
+
avg_loss = sum(losses[-100:])/100
|
221 |
+
print(f'Finished epoch {epoch}. Average of the last 100 loss values: {avg_loss:05f}')
|
222 |
+
|
223 |
+
# View the loss curve
|
224 |
+
plt.plot(losses)
|
225 |
+
```
|
226 |
+
|
227 |
+
|
228 |
+
0%| | 0/469 [00:00<?, ?it/s]
|
229 |
+
|
230 |
+
|
231 |
+
Finished epoch 0. Average of the last 100 loss values: 0.052451
|
232 |
+
|
233 |
+
|
234 |
+
|
235 |
+
0%| | 0/469 [00:00<?, ?it/s]
|
236 |
+
|
237 |
+
|
238 |
+
Finished epoch 1. Average of the last 100 loss values: 0.045999
|
239 |
+
|
240 |
+
|
241 |
+
|
242 |
+
0%| | 0/469 [00:00<?, ?it/s]
|
243 |
+
|
244 |
+
|
245 |
+
Finished epoch 2. Average of the last 100 loss values: 0.043344
|
246 |
+
|
247 |
+
|
248 |
+
|
249 |
+
0%| | 0/469 [00:00<?, ?it/s]
|
250 |
+
|
251 |
+
|
252 |
+
Finished epoch 3. Average of the last 100 loss values: 0.042347
|
253 |
+
|
254 |
+
|
255 |
+
|
256 |
+
0%| | 0/469 [00:00<?, ?it/s]
|
257 |
+
|
258 |
+
|
259 |
+
Finished epoch 4. Average of the last 100 loss values: 0.041174
|
260 |
+
|
261 |
+
|
262 |
+
|
263 |
+
0%| | 0/469 [00:00<?, ?it/s]
|
264 |
+
|
265 |
+
|
266 |
+
Finished epoch 5. Average of the last 100 loss values: 0.040736
|
267 |
+
|
268 |
+
|
269 |
+
|
270 |
+
0%| | 0/469 [00:00<?, ?it/s]
|
271 |
+
|
272 |
+
|
273 |
+
Finished epoch 6. Average of the last 100 loss values: 0.040386
|
274 |
+
|
275 |
+
|
276 |
+
|
277 |
+
0%| | 0/469 [00:00<?, ?it/s]
|
278 |
+
|
279 |
+
|
280 |
+
Finished epoch 7. Average of the last 100 loss values: 0.039372
|
281 |
+
|
282 |
+
|
283 |
+
|
284 |
+
0%| | 0/469 [00:00<?, ?it/s]
|
285 |
+
|
286 |
+
|
287 |
+
Finished epoch 8. Average of the last 100 loss values: 0.039056
|
288 |
+
|
289 |
+
|
290 |
+
|
291 |
+
0%| | 0/469 [00:00<?, ?it/s]
|
292 |
+
|
293 |
+
|
294 |
+
Finished epoch 9. Average of the last 100 loss values: 0.039024
|
295 |
+
|
296 |
+
|
297 |
+
|
298 |
+
|
299 |
+
|
300 |
+
[<matplotlib.lines.Line2D>]
|
301 |
+
|
302 |
+
|
303 |
+
|
304 |
+
|
305 |
+
|
306 |
+

|
307 |
+
|
308 |
+
|
309 |
+
|
310 |
+
一旦训练结束,我们就可以通过输入不同的标签作为条件,来采样图片了:
|
311 |
+
|
312 |
+
|
313 |
+
```python
|
314 |
+
#@markdown Sampling some different digits:
|
315 |
+
|
316 |
+
# Prepare random x to start from, plus some desired labels y
|
317 |
+
x = torch.randn(80, 1, 28, 28).to(device)
|
318 |
+
y = torch.tensor([[i]*8 for i in range(10)]).flatten().to(device)
|
319 |
+
|
320 |
+
# Sampling loop
|
321 |
+
for i, t in tqdm(enumerate(noise_scheduler.timesteps)):
|
322 |
+
|
323 |
+
# Get model pred
|
324 |
+
with torch.no_grad():
|
325 |
+
residual = net(x, t, y) # Again, note that we pass in our labels y
|
326 |
+
|
327 |
+
# Update sample with step
|
328 |
+
x = noise_scheduler.step(residual, t, x).prev_sample
|
329 |
+
|
330 |
+
# Show the results
|
331 |
+
fig, ax = plt.subplots(1, 1, figsize=(12, 12))
|
332 |
+
ax.imshow(torchvision.utils.make_grid(x.detach().cpu().clip(-1, 1), nrow=8)[0], cmap='Greys')
|
333 |
+
```
|
334 |
+
|
335 |
+
|
336 |
+
0it [00:00, ?it/s]
|
337 |
+
|
338 |
+
|
339 |
+
|
340 |
+
|
341 |
+
|
342 |
+
<matplotlib.image.AxesImage>
|
343 |
+
|
344 |
+
|
345 |
+
|
346 |
+
|
347 |
+
|
348 |
+

|
349 |
+
|
350 |
+
|
351 |
+
|
352 |
+
就是这么简单!我们现在已经对要生成的图片有所控制了。
|
353 |
+
|
354 |
+
希望你喜欢这个例子。一如既往地,如果你有问题,你随时可以在 Discord 上提出来。
|
355 |
+
|
356 |
+
|
357 |
+
```python
|
358 |
+
# 练习(选做):用同样方法在 FashionMNIST 数据集上试试。调节学习率、batch size 和训练的轮数(epochs)。
|
359 |
+
# 你能用比例子更少的训练时间得到些看起来不错的时尚相关的图片吗?
|
360 |
+
```
|
markdown/unit2/02_class_conditioned_diffusion_model_example_CN_files/02_class_conditioned_diffusion_model_example_CN_10_21.png
ADDED
![]() |
Git LFS Details
|
markdown/unit2/02_class_conditioned_diffusion_model_example_CN_files/02_class_conditioned_diffusion_model_example_CN_12_2.png
ADDED
![]() |
Git LFS Details
|
markdown/unit2/02_class_conditioned_diffusion_model_example_CN_files/02_class_conditioned_diffusion_model_example_CN_4_9.png
ADDED
![]() |
Git LFS Details
|
markdown/unit2/README_CN.md
ADDED
@@ -0,0 +1,72 @@
|
|
|
|
|
|
|
|
|
|
|
|
|
|
|
|
|
|
|
|
|
|
|
|
|
|
|
|
|
|
|
|
|
|
|
|
|
|
|
|
|
|
|
|
|
|
|
|
|
|
|
|
|
|
|
|
|
|
|
|
|
|
|
|
|
|
|
|
|
|
|
|
|
|
|
|
|
|
|
|
|
|
|
|
|
|
|
|
|
|
|
|
|
|
|
|
|
|
|
|
|
|
|
|
|
|
|
|
|
|
|
|
|
|
|
|
|
|
|
|
|
|
|
|
|
|
|
|
|
|
|
|
|
|
|
|
|
|
|
|
|
|
|
|
|
|
|
1 |
+
# 单元2: 微调,引导,条件生成
|
2 |
+
|
3 |
+
欢迎来到 Hugging Face 扩散模型课程的第二单元!在这一单元,你将会学到新的方法去使用和适配预训练过的扩散模型。你也会看到我们如何创建带有额外输入作为**生成条件**的扩散模型,以此控制生成过程。
|
4 |
+
|
5 |
+
## 开始这一单元 :rocket:
|
6 |
+
|
7 |
+
这里分几步学习这一单元:
|
8 |
+
|
9 |
+
- 请首先确保你已经[注册了本课程](https://huggingface.us17.list-manage.com/subscribe?u=7f57e683fa28b51bfc493d048&id=ef963b4162),以便有新的学习资料时你会被通知到。
|
10 |
+
- 请先通读本文,对本单元的重点有一个整体的认识。
|
11 |
+
- 学习 **Fine-tuning and Guidance** 这节的笔记本,试着使用 🤗 Diffusers 库,在一个新数据集上微调(finetune)一个已有的扩散模型,以及用引导(guidance)这一方法修改采样过程。
|
12 |
+
- 照着记事本中的示例,把你的自定义模型做成 Gradio 的 Demo 分享出去。
|
13 |
+
- (可选)学习 **Class-conditioned Diffusion Model Example** 这节笔记本,看看我们如何给生成过程加入额外控制。
|
14 |
+
|
15 |
+
|
16 |
+
:loudspeaker: 别忘了加入 [Discord](https://huggingface.co/join/discord),在这里你可以参与学习资料的讨论,在`#diffusion-models-class`频道分享你的实验成果。
|
17 |
+
|
18 |
+
## 微调(Fine-Tuning)
|
19 |
+
|
20 |
+
正如你在第一单元看见的,从头训练一个扩散模型耗费的时间相当长!尤其是当你使用高分辨率图片时,从头训练模型所需的时间和数据量可能多得不切实际。幸运的是,我们还有个解决方法:从一个已经被训练过的模型去开始训练!这样,我们从一个已经学过如何去噪的模型开始,希望能相比于随机初始化的模型能有一个更好的起始点。
|
21 |
+
|
22 |
+
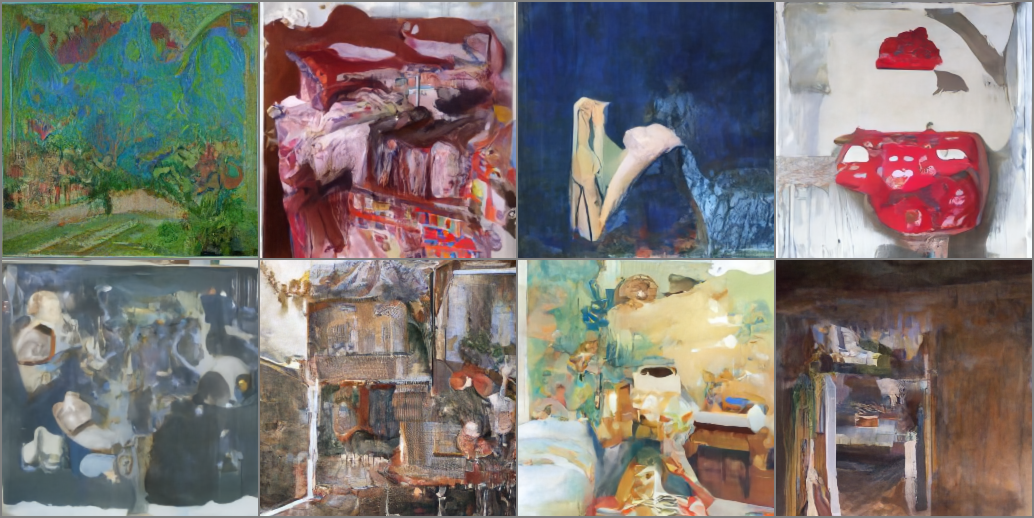
|
23 |
+
|
24 |
+
一般而言,当你的新数据和原有模型的原始训练数据多多少少有点相似的时候,微调效果会最好(比如你想生成卡通人脸,那你用于微调的模型最好是个在人脸数据上训练过的模型)。但让人吃惊的是,这些益处在图片分布变化显著时也会存在。上面的图片是通过微调一个[在 LSUN 卧室图片数据集上训练的模型](https://huggingface.co/google/ddpm-bedroom-256)而生成的,这个模型在 [WikiArt 数据集](https://huggingface.co/datasets/huggan/wikiart)被微调了500步。相关的[训练脚本](https://github.com/huggingface/diffusion-models-class/blob/main/unit2/finetune_model.py)也放在了本单元中供大家参考。
|
25 |
+
|
26 |
+
## 引导(Guidance)
|
27 |
+
|
28 |
+
无条件模型一般没有对生成能内容的掌控。我们可以训练一个条件模型(更过内容将会在下节讲述),接收额外输入,以此来操控生成过程。但我们如何使用一个已有的无条件模型去做这件事呢?我们可以用引导这一方法:生成过程中每一步的模型预测都将会被一些引导函数所评估,并加以修改,以此让最终的生成结果符合我们所想。
|
29 |
+
|
30 |
+

|
31 |
+
|
32 |
+
这个引导函数可以是任何函数,这让我们有了很大的设计空间。在笔记本中,我们从一个简单的例子(控制颜色,如上图所示)开始,到使用一个叫CLIP的预训练模型,让生成的结果基于文字描述。
|
33 |
+
|
34 |
+
## 条件生成(conditioning)
|
35 |
+
|
36 |
+
引导能让我们从一个无条件扩散模型中多少得到些额外的收益,但如果我们在训练过程中就有一些额外的信息(比如图像类别或文字描述)可以输入到模型里,我们可以把这些信息输入模型,让模型使用这些信息去做预测。由此我们就创建了一个条件模型,我们可以在推理阶段通过输入什么信息作为条件来控制模型生成什么。相关的笔记本中就展示了一个例子:一个类别条件的模型,可以根据类别标签生成对应的图像。
|
37 |
+
|
38 |
+

|
39 |
+
|
40 |
+
有很多种方法可以把条件信息输入到模型种,比如:
|
41 |
+
|
42 |
+
* 把条件信息作为额外的通道输入给 UNet。这种情况下一般条件信息都和图片有着相同的形状,比如条件信息是图像分割的掩模(mask)、深度图或模糊版的图像(针对图像修复、超分辨率任务的模型)。这种方法在一些其它条件下也可以用,比如在相应的笔记本的例子中,类别标签就被映射成了一个嵌入(embedding),并被展开成和输入图片一样的宽度和高度,以此来作为额外的通道输入到模型里。
|
43 |
+
* 把条件信息做成一个嵌入(embedding),然后把它映射到和模型其中一个或多个中间层输出的通道数一样,再把这个嵌入加到中间层输出上。这一般是以时间步(timestep)为条件时的做法。比如,你可以把时��步的嵌入映射到特定通道数,然后加到模型的每一个残差网络模块的输出上。这种方法在你有一个向量形式的条件时很有用,比如 CLIP 的图像嵌入。一个值得注意的例子是一个[能修改输入图片的Stable Diffusion模型](https://huggingface.co/spaces/lambdalabs/stable-diffusion-image-variations)。
|
44 |
+
* 添加有交叉注意力机制的网络层(cross-attention)。这在当条件是某种形式的文字时最有效 —— 比如文字被一个 transformer 模型映射成了一串 embedding,那么UNet中有交叉注意力机制的网络层就会被用来把这些信息合并到去噪路径中。我们将在第三单元研究 Stable Diffusion 如何处理文字信息条件时看到这种情况。
|
45 |
+
|
46 |
+
|
47 |
+
## 用来上手的笔记本示例
|
48 |
+
|
49 |
+
| Chapter | Colab | Kaggle | Gradient | Studio Lab |
|
50 |
+
|:--------------------------------------------|:----------------------------------------------------------------------------------------------------------------------------------------------------------------------------------------------------|:---------------------------------------------------------------------------------------------------------------------------------------------------------------------------------------------------------|:---------------------------------------------------------------------------------------------------------------------------------------------------------------------------------------|:-------------------------------------------------------------------------------------------------------------------------------------------------------------------------------------------------------------|
|
51 |
+
| Fine-tuning and Guidance | [](https://colab.research.google.com/github/huggingface/diffusion-models-class/blob/main/unit2/01_finetuning_and_guidance.ipynb) | [](https://kaggle.com/kernels/welcome?src=https://github.com/huggingface/diffusion-models-class/blob/main/unit2/01_finetuning_and_guidance.ipynb) | [](https://console.paperspace.com/github/huggingface/diffusion-models-class/blob/main/unit2/01_finetuning_and_guidance.ipynb) | [](https://studiolab.sagemaker.aws/import/github/huggingface/diffusion-models-class/blob/main/unit2/01_finetuning_and_guidance.ipynb) |
|
52 |
+
| Class-conditioned Diffusion Model Example | [](https://colab.research.google.com/github/huggingface/diffusion-models-class/blob/main/unit2/02_class_conditioned_diffusion_model_example.ipynb) | [](https://kaggle.com/kernels/welcome?src=https://github.com/huggingface/diffusion-models-class/blob/main/unit2/02_class_conditioned_diffusion_model_example.ipynb) | [](https://console.paperspace.com/github/huggingface/diffusion-models-class/blob/main/unit2/02_class_conditioned_diffusion_model_example.ipynb) | [](https://studiolab.sagemaker.aws/import/github/huggingface/diffusion-models-class/blob/main/unit2/02_class_conditioned_diffusion_model_example.ipynb) |
|
53 |
+
|
54 |
+
现在你已经准备好学习这些笔记本了!通过上面的链接使用你选择的平台打开它们!微调是个计算量很大的工作,所以如果你用的是 Kaggle或 Google Colab,请确保你把运行时类型设成 GPU。
|
55 |
+
|
56 |
+
本单元内容的主体在 **Fine-tuning and Guidance** 这个笔记本中,我们将通过示例探索这两个话题。笔记本将会展示给你如何在新数据上微调现有模型,添加引导,以及在 Gradio 上分享结果。这里还有一个脚本程序 [finetune_model.py](https://github.com/huggingface/diffusion-models-class/blob/main/unit2/finetune_model.py),让你更容易地实验不同的微���设置;以及一个[示例的 space](https://huggingface.co/spaces/johnowhitaker/color-guided-wikiart-diffusion),你可以以此作为目标用来在 🤗 Spaces 上分享 demo。
|
57 |
+
|
58 |
+
在 **Class-conditioned Diffusion Model Example** 中,我们用 MNIST 数据集展示一个很简单的例子:创建一个以类别标签为条件的扩散模型。这里的重点在于尽可能简单地讲解核心要点:通过给模型提供额外的关于去除什么噪声的信息,我们可以在推理时控制哪种类型的图片是我们想要生成的。
|
59 |
+
|
60 |
+
## 项目时间
|
61 |
+
|
62 |
+
仿照 **Fine-tuning and Guidance** 笔记本中的例子,微调你自己的模型或挑选一个现有模型,创建 Gradio 的 demo 展示你的引导技巧。也不要忘了在 Discord 或 Twitter 之类的平台上分享,让我们也羡慕羡慕!
|
63 |
+
|
64 |
+
## 一些其它学习资源
|
65 |
+
|
66 |
+
[Denoising Diffusion Implicit Models](https://arxiv.org/abs/2010.02502) - 引出了DDIM采样方法(DDIMScheduler 用到了这个方法)
|
67 |
+
|
68 |
+
[GLIDE: Towards Photorealistic Image Generation and Editing with Text-Guided Diffusion Models](https://arxiv.org/abs/2112.10741) - 介绍了如何让扩散模型基于文本类条件
|
69 |
+
|
70 |
+
[eDiffi: Text-to-Image Diffusion Models with an Ensemble of Expert Denoisers](https://arxiv.org/abs/2211.01324) - 介绍了不同种类的生成条件一起使用时的情况,以此更加广泛地控制生成过程
|
71 |
+
|
72 |
+
如果你找到了更好的学习资源,也别忘了告诉我们让我们加到这个列表里!
|
markdown/unit2/conditional_digit_generation.png
ADDED
![]() |
Git LFS Details
|
markdown/unit2/finetune_model.py
ADDED
@@ -0,0 +1,120 @@
|
|
|
|
|
|
|
|
|
|
|
|
|
|
|
|
|
|
|
|
|
|
|
|
|
|
|
|
|
|
|
|
|
|
|
|
|
|
|
|
|
|
|
|
|
|
|
|
|
|
|
|
|
|
|
|
|
|
|
|
|
|
|
|
|
|
|
|
|
|
|
|
|
|
|
|
|
|
|
|
|
|
|
|
|
|
|
|
|
|
|
|
|
|
|
|
|
|
|
|
|
|
|
|
|
|
|
|
|
|
|
|
|
|
|
|
|
|
|
|
|
|
|
|
|
|
|
|
|
|
|
|
|
|
|
|
|
|
|
|
|
|
|
|
|
|
|
|
|
|
|
|
|
|
|
|
|
|
|
|
|
|
|
|
|
|
|
|
|
|
|
|
|
|
|
|
|
|
|
|
|
|
|
|
|
|
|
|
|
|
|
|
|
|
|
|
|
|
|
|
|
|
|
|
|
|
|
|
|
|
|
|
|
|
|
|
|
|
|
|
|
|
|
|
|
|
|
|
|
|
|
|
|
|
|
|
|
|
|
|
|
|
|
1 |
+
import wandb
|
2 |
+
import numpy as np
|
3 |
+
import torch, torchvision
|
4 |
+
import torch.nn.functional as F
|
5 |
+
from PIL import Image
|
6 |
+
from tqdm.auto import tqdm
|
7 |
+
from fastcore.script import call_parse
|
8 |
+
from torchvision import transforms
|
9 |
+
from diffusers import DDPMPipeline
|
10 |
+
from diffusers import DDIMScheduler
|
11 |
+
from datasets import load_dataset
|
12 |
+
from matplotlib import pyplot as plt
|
13 |
+
|
14 |
+
@call_parse
|
15 |
+
def train(
|
16 |
+
image_size = 256,
|
17 |
+
batch_size = 16,
|
18 |
+
grad_accumulation_steps = 2,
|
19 |
+
num_epochs = 1,
|
20 |
+
start_model = "google/ddpm-bedroom-256",
|
21 |
+
dataset_name = "huggan/wikiart",
|
22 |
+
device='cuda',
|
23 |
+
model_save_name='wikiart_1e',
|
24 |
+
wandb_project='dm_finetune',
|
25 |
+
log_samples_every = 250,
|
26 |
+
save_model_every = 2500,
|
27 |
+
):
|
28 |
+
|
29 |
+
# Initialize wandb for logging
|
30 |
+
wandb.init(project=wandb_project, config=locals())
|
31 |
+
|
32 |
+
|
33 |
+
# Prepare pretrained model
|
34 |
+
image_pipe = DDPMPipeline.from_pretrained(start_model);
|
35 |
+
image_pipe.to(device)
|
36 |
+
|
37 |
+
# Get a scheduler for sampling
|
38 |
+
sampling_scheduler = DDIMScheduler.from_config(start_model)
|
39 |
+
sampling_scheduler.set_timesteps(num_inference_steps=50)
|
40 |
+
|
41 |
+
# Prepare dataset
|
42 |
+
dataset = load_dataset(dataset_name, split="train")
|
43 |
+
preprocess = transforms.Compose(
|
44 |
+
[
|
45 |
+
transforms.Resize((image_size, image_size)),
|
46 |
+
transforms.RandomHorizontalFlip(),
|
47 |
+
transforms.ToTensor(),
|
48 |
+
transforms.Normalize([0.5], [0.5]),
|
49 |
+
]
|
50 |
+
)
|
51 |
+
def transform(examples):
|
52 |
+
images = [preprocess(image.convert("RGB")) for image in examples["image"]]
|
53 |
+
return {"images": images}
|
54 |
+
dataset.set_transform(transform)
|
55 |
+
train_dataloader = torch.utils.data.DataLoader(dataset, batch_size=batch_size, shuffle=True)
|
56 |
+
|
57 |
+
|
58 |
+
# Optimizer & lr scheduler
|
59 |
+
optimizer = torch.optim.AdamW(image_pipe.unet.parameters(), lr=1e-5)
|
60 |
+
scheduler = torch.optim.lr_scheduler.ExponentialLR(optimizer, gamma=0.9)
|
61 |
+
|
62 |
+
for epoch in range(num_epochs):
|
63 |
+
for step, batch in tqdm(enumerate(train_dataloader), total=len(train_dataloader)):
|
64 |
+
|
65 |
+
# Get the clean images
|
66 |
+
clean_images = batch['images'].to(device)
|
67 |
+
|
68 |
+
# Sample noise to add to the images
|
69 |
+
noise = torch.randn(clean_images.shape).to(clean_images.device)
|
70 |
+
bs = clean_images.shape[0]
|
71 |
+
|
72 |
+
# Sample a random timestep for each image
|
73 |
+
timesteps = torch.randint(0, image_pipe.scheduler.num_train_timesteps, (bs,), device=clean_images.device).long()
|
74 |
+
|
75 |
+
# Add noise to the clean images according to the noise magnitude at each timestep
|
76 |
+
# (this is the forward diffusion process)
|
77 |
+
noisy_images = image_pipe.scheduler.add_noise(clean_images, noise, timesteps)
|
78 |
+
|
79 |
+
# Get the model prediction for the noise
|
80 |
+
noise_pred = image_pipe.unet(noisy_images, timesteps, return_dict=False)[0]
|
81 |
+
|
82 |
+
# Compare the prediction with the actual noise:
|
83 |
+
loss = F.mse_loss(noise_pred, noise)
|
84 |
+
|
85 |
+
# Log the loss
|
86 |
+
wandb.log({'loss':loss.item()})
|
87 |
+
|
88 |
+
# Calculate the gradients
|
89 |
+
loss.backward()
|
90 |
+
|
91 |
+
# Gradient Acccumulation: Only update every grad_accumulation_steps
|
92 |
+
if (step+1)%grad_accumulation_steps == 0:
|
93 |
+
optimizer.step()
|
94 |
+
optimizer.zero_grad()
|
95 |
+
|
96 |
+
# Occasionally log samples
|
97 |
+
if (step+1)%log_samples_every == 0:
|
98 |
+
x = torch.randn(8, 3, 256, 256).to(device) # Batch of 8
|
99 |
+
for i, t in tqdm(enumerate(sampling_scheduler.timesteps)):
|
100 |
+
model_input = sampling_scheduler.scale_model_input(x, t)
|
101 |
+
with torch.no_grad():
|
102 |
+
noise_pred = image_pipe.unet(model_input, t)["sample"]
|
103 |
+
x = sampling_scheduler.step(noise_pred, t, x).prev_sample
|
104 |
+
grid = torchvision.utils.make_grid(x, nrow=4)
|
105 |
+
im = grid.permute(1, 2, 0).cpu().clip(-1, 1)*0.5 + 0.5
|
106 |
+
im = Image.fromarray(np.array(im*255).astype(np.uint8))
|
107 |
+
wandb.log({'Sample generations': wandb.Image(im)})
|
108 |
+
|
109 |
+
# Occasionally save model
|
110 |
+
if (step+1)%save_model_every == 0:
|
111 |
+
image_pipe.save_pretrained(model_save_name+f'step_{step+1}')
|
112 |
+
|
113 |
+
# Update the learning rate for the next epoch
|
114 |
+
scheduler.step()
|
115 |
+
|
116 |
+
# Save the pipeline one last time
|
117 |
+
image_pipe.save_pretrained(model_save_name)
|
118 |
+
|
119 |
+
# Wrap up the run
|
120 |
+
wandb.finish()
|
markdown/unit2/guidance_eg.png
ADDED
![]() |
Git LFS Details
|
markdown/unit3/01_stable_diffusion_introduction_CN.md
ADDED
The diff for this file is too large to render.
See raw diff
|
|
markdown/unit3/01_stable_diffusion_introduction_CN_files/01_stable_diffusion_introduction_CN_10_1.png
ADDED
![]() |
Git LFS Details
|
markdown/unit3/01_stable_diffusion_introduction_CN_files/01_stable_diffusion_introduction_CN_12_3.png
ADDED
![]() |
Git LFS Details
|
markdown/unit3/01_stable_diffusion_introduction_CN_files/01_stable_diffusion_introduction_CN_35_0.png
ADDED
![]() |
Git LFS Details
|
markdown/unit3/01_stable_diffusion_introduction_CN_files/01_stable_diffusion_introduction_CN_37_2.png
ADDED
![]() |
Git LFS Details
|