Commit
•
8aa74f1
1
Parent(s):
3b742ce
Update README.md
Browse files
README.md
CHANGED
@@ -1,7 +1,9 @@
|
|
1 |
---
|
|
|
|
|
|
|
2 |
license: apache-2.0
|
3 |
-
language:
|
4 |
-
- multilingual
|
5 |
- af
|
6 |
- am
|
7 |
- ar
|
@@ -103,315 +105,798 @@ language:
|
|
103 |
- yo
|
104 |
- zh
|
105 |
- zu
|
106 |
-
|
107 |
-
|
108 |
-
-
|
|
|
|
|
|
|
|
|
|
|
|
|
|
|
|
|
|
|
|
|
|
|
|
|
|
|
|
|
|
|
|
|
|
|
|
|
|
|
|
|
|
|
|
|
|
|
|
|
|
|
|
|
|
|
|
|
|
|
|
|
|
|
|
|
|
|
|
|
|
|
|
|
|
|
|
|
|
|
|
|
|
|
|
|
|
|
|
|
|
|
|
|
|
|
|
|
|
|
|
|
|
|
|
|
|
|
|
|
|
|
|
|
|
|
|
|
|
|
|
|
|
|
|
|
|
|
|
|
|
|
|
|
|
|
|
|
|
|
|
|
|
|
|
|
|
|
|
|
|
|
|
|
|
|
|
|
|
|
|
|
|
|
|
|
|
|
|
|
|
|
|
|
|
|
|
|
|
|
|
|
|
|
|
|
|
|
|
|
|
|
|
|
|
|
|
|
|
|
|
|
|
|
|
|
|
|
|
|
|
|
|
|
|
|
|
|
|
|
|
|
|
|
|
|
|
|
|
|
|
|
|
|
|
|
|
|
|
|
|
|
|
|
|
|
|
|
|
|
|
|
|
|
|
|
|
|
|
|
|
|
|
|
|
|
|
|
|
|
|
|
|
|
|
|
|
|
|
|
|
|
|
|
|
|
|
|
|
|
|
|
|
|
|
|
|
|
|
|
|
|
|
|
|
|
|
|
|
|
|
|
|
|
|
|
|
|
|
|
|
|
|
|
|
|
|
|
|
|
|
|
|
|
|
|
|
|
|
|
|
|
|
|
|
|
|
|
|
|
|
|
|
|
|
|
|
|
|
|
|
|
|
|
|
|
|
|
|
|
|
|
|
|
|
|
|
|
|
|
|
|
|
|
|
|
|
|
|
|
|
|
|
|
|
|
|
|
|
|
|
|
|
|
|
|
|
|
|
|
|
|
|
|
|
|
|
|
|
|
|
|
|
|
|
|
|
|
|
|
|
|
|
|
|
|
|
|
|
|
|
|
|
|
|
|
|
|
|
|
|
|
|
|
|
|
|
|
|
|
|
|
|
|
|
|
|
|
|
|
|
|
|
|
|
|
|
|
|
|
|
|
|
|
|
|
|
|
|
|
|
|
|
|
|
|
|
|
|
|
|
|
|
|
|
|
|
|
|
|
|
|
|
|
|
|
|
|
|
|
|
|
|
|
|
|
|
|
|
|
|
|
|
|
|
|
|
|
|
|
|
|
|
|
|
|
|
|
|
|
|
|
|
|
|
|
|
|
|
|
|
|
|
|
|
|
|
|
|
|
|
|
|
|
|
|
|
|
|
|
|
|
|
|
|
|
|
|
|
|
|
|
|
|
|
|
|
|
|
|
|
|
|
|
|
|
|
|
|
|
|
|
|
|
|
|
|
|
|
|
|
|
|
|
|
|
|
|
|
|
|
|
|
|
|
|
|
|
|
|
|
|
|
|
|
|
|
|
|
|
|
|
|
|
|
|
|
|
|
|
|
|
|
|
|
|
|
|
|
|
|
|
|
|
|
|
|
|
|
|
|
|
|
|
|
|
|
|
|
|
|
|
|
|
|
|
|
|
|
|
|
|
|
|
|
|
|
|
|
|
|
|
|
|
|
|
|
|
|
|
|
|
|
|
|
|
|
|
|
|
|
|
|
|
|
|
|
|
|
|
|
|
|
|
|
|
|
|
|
|
|
|
|
|
|
|
|
|
|
|
|
|
|
|
|
|
|
|
|
|
|
|
|
|
|
|
|
|
|
|
|
|
|
|
|
|
|
|
|
|
|
|
|
|
|
|
|
|
|
|
|
|
|
|
|
|
|
|
|
|
|
|
|
|
|
|
|
|
|
|
|
|
|
|
|
|
|
|
|
|
|
|
|
|
|
|
|
|
|
|
|
|
|
|
|
|
|
|
|
|
|
|
|
|
|
|
|
|
|
|
|
|
|
|
|
|
|
|
|
|
|
|
|
|
|
|
|
|
|
|
|
|
|
|
|
|
|
|
|
|
|
|
|
|
|
|
|
|
|
|
|
|
|
|
|
|
|
|
|
|
|
|
|
|
|
|
|
|
|
|
|
|
|
|
|
|
|
|
|
|
|
|
|
|
|
|
|
|
|
|
|
|
|
|
|
|
|
|
|
|
|
|
|
|
|
|
|
|
|
|
|
|
|
|
|
|
|
|
|
|
|
|
|
|
|
|
|
|
|
|
|
|
|
|
|
|
|
|
|
|
|
|
|
|
|
|
|
|
|
|
|
|
|
|
|
|
|
|
|
|
|
|
|
|
|
|
|
|
|
|
|
|
|
|
|
|
|
|
|
|
|
|
|
|
|
|
|
|
|
|
|
|
|
|
|
|
|
|
|
|
|
|
|
|
|
|
|
|
|
|
|
|
|
|
|
|
|
|
|
|
|
|
|
|
|
|
|
|
|
|
|
|
|
|
|
|
|
|
|
|
|
|
|
|
|
|
|
|
|
|
|
|
|
|
|
|
|
|
|
|
|
|
|
|
|
|
|
|
|
|
|
|
|
|
|
|
|
|
|
|
|
|
|
|
|
|
|
|
109 |
---
|
110 |
|
111 |
-
|
112 |
-
|
113 |
-
|
114 |
-
|
115 |
-
|
116 |
-
#
|
117 |
-
|
118 |
-
|
119 |
-
|
120 |
-
|
121 |
-
|
122 |
-
|
123 |
-
|
124 |
-
-
|
125 |
-
|
126 |
-
|
127 |
-
|
128 |
-
|
129 |
-
|
130 |
-
|
131 |
-
|
132 |
-
|
133 |
-
|
134 |
-
|
135 |
-
|
136 |
-
|
137 |
-
|
138 |
-
|
139 |
-
|
140 |
-
|
141 |
-
|
142 |
-
|
143 |
-
|
144 |
-
|
145 |
-
<
|
146 |
-
<
|
147 |
-
|
148 |
-
|
149 |
-
|
150 |
-
|
151 |
-
|
152 |
-
|
153 |
-
|
154 |
-
|
155 |
-
|
156 |
-
|
157 |
-
|
158 |
-
|
159 |
-
|
160 |
-
|
161 |
-
|
162 |
-
|
163 |
-
|
164 |
-
|
165 |
-
|
166 |
-
|
167 |
-
|
168 |
-
|
|
|
|
|
|
|
|
|
|
|
|
|
|
|
|
|
|
|
|
|
|
|
|
|
|
|
|
|
|
|
|
|
|
|
|
|
|
|
|
|
|
|
|
|
|
|
|
|
|
|
|
|
|
|
|
|
|
|
|
|
|
|
|
|
|
|
|
|
|
|
|
|
|
|
|
|
|
|
|
|
|
|
|
|
|
|
|
|
|
|
|
|
|
|
|
|
|
|
|
|
|
|
|
|
|
|
|
|
|
|
|
|
|
|
|
|
169 |
|
|
|
170 |
|
171 |
-
|
172 |
-
*This section includes details about the model objective and architecture, and the compute infrastructure.*
|
173 |
-
*It is useful for people interested in model development.*
|
174 |
|
175 |
<details>
|
176 |
-
<summary>Click to expand</summary>
|
177 |
-
|
178 |
-
### Model Architecture and Objective
|
179 |
-
|
180 |
-
* Same architecture as [mt5](https://arxiv.org/abs/2010.11934)
|
181 |
|
182 |
-
|
183 |
-
|
184 |
-
|
185 |
|
186 |
-
|
187 |
|
188 |
-
|
189 |
-
|
190 |
-
- `mt0-base` was finetuned on TPUv4-64
|
191 |
-
- `mt0-large` was finetuned on TPUv4-64
|
192 |
-
- `mt0-xl` was finetuned on TPUv4-128
|
193 |
-
- `mt0-xxl` was finetuned on TPUv4-256
|
194 |
-
- `mt0-mt-xxl` was finetuned on TPUv4-256
|
195 |
-
- `mt0-p3-xxl` was finetuned on TPUv4-256
|
196 |
|
197 |
-
|
|
|
|
|
|
|
198 |
|
199 |
-
* T5X([Github link](https://github.com/google-research/t5x), [paper](https://arxiv.org/abs/2203.17189))
|
200 |
-
|
201 |
</details>
|
202 |
|
203 |
-
|
204 |
-
|
205 |
-
# Training
|
206 |
-
*This section provides information about the training data, the speed and size of training elements, and the environmental impact of training.*
|
207 |
-
*It is useful for people who want to learn more about the model inputs and training footprint.*
|
208 |
|
209 |
<details>
|
210 |
-
<summary>Click to expand</summary>
|
211 |
-
|
212 |
-
## Training Data
|
213 |
-
*This section provides a high-level overview of the training data. It is relevant for anyone who wants to know the basics of what the model is learning.*
|
214 |
-
|
215 |
-
It was pretrained on mC4 and then finetuned on xP3, P3 or xP3mt.
|
216 |
-
|
217 |
-
### Languages
|
218 |
|
219 |
-
|
220 |
-
|
221 |
-
|
222 |
-
|
223 |
-
// TODO @adarob: Maybe we can push tensorboard on this repo as well
|
224 |
-
|
225 |
-
- Training logs:
|
226 |
-
|
227 |
-
- Checkpoint size: 51.7GB (Bf16 weights)
|
228 |
-
|
229 |
-
- Number of epochs: 1
|
230 |
-
|
231 |
-
- Precision: bfloat16
|
232 |
|
|
|
233 |
|
234 |
-
|
|
|
235 |
|
236 |
-
|
|
|
|
|
|
|
237 |
|
238 |
</details>
|
239 |
|
240 |
-
|
241 |
-
|
242 |
-
# Uses
|
243 |
-
|
244 |
-
*This section addresses questions around how the model is intended to be used, discusses the foreseeable users of the model (including those affected by the model), and describes uses that are considered out of scope or misuse of the model.*
|
245 |
-
*It is useful for anyone considering using the model or who is affected by the model.*
|
246 |
|
247 |
<details>
|
248 |
-
<summary>Click to expand</summary>
|
249 |
-
|
250 |
-
## How to use
|
251 |
-
|
252 |
-
This model can be easily used and deployed using HuggingFace's ecosystem. This needs `transformers` and `accelerate` installed. The model can be downloaded as follows:
|
253 |
|
254 |
```python
|
|
|
255 |
from transformers import AutoModelForSeq2SeqLM, AutoTokenizer
|
256 |
|
257 |
-
checkpoint = "
|
258 |
-
model_name = "bigscience/mt0-xxl"
|
259 |
-
model = AutoModelForSeq2SeqLM.from_pretrained(model_name, revision=checkpoint, torch_dtype="auto", device_map="auto")
|
260 |
-
tokenizer = AutoTokenizer.from_pretrained(model_name, revision=checkpoint)
|
261 |
|
262 |
-
|
|
|
|
|
|
|
263 |
outputs = model.generate(inputs)
|
264 |
print(tokenizer.decode(outputs[0]))
|
265 |
```
|
266 |
|
267 |
-
## Intended Use
|
268 |
-
|
269 |
-
This model is being created in order to enable public research on large language models (LLMs). LLMs are intended to be used for language generation or as a pretrained base model that can be further finetuned for specific tasks. Use cases below are not exhaustive.
|
270 |
-
|
271 |
-
### Direct Use
|
272 |
-
|
273 |
-
- Text generation
|
274 |
-
|
275 |
-
- Exploring characteristics of language generated by a language model
|
276 |
-
|
277 |
-
- Examples: Cloze tests, counterfactuals, generations with reframings
|
278 |
-
|
279 |
-
### Downstream Use
|
280 |
-
|
281 |
-
- Tasks that leverage language models include: Information Extraction, Question Answering, Summarization
|
282 |
-
|
283 |
</details>
|
284 |
|
285 |
-
|
286 |
-
|
287 |
-
# Risks and Limitations
|
288 |
-
*This section identifies foreseeable harms and misunderstandings.*
|
289 |
-
|
290 |
-
<details>
|
291 |
-
<summary>Click to expand</summary>
|
292 |
-
|
293 |
-
Model may:
|
294 |
-
|
295 |
-
- Overrepresent some viewpoints and underrepresent others
|
296 |
-
|
297 |
-
- Contain stereotypes
|
298 |
-
|
299 |
-
- Contain [personal information](#personal-data-and-information)
|
300 |
|
301 |
-
|
302 |
|
303 |
-
|
304 |
|
305 |
-
|
306 |
|
307 |
-
|
308 |
|
309 |
-
-
|
|
|
|
|
|
|
310 |
|
311 |
-
|
312 |
|
313 |
-
-
|
314 |
|
315 |
-
|
316 |
|
317 |
-
|
|
|
318 |
|
319 |
# Evaluation
|
320 |
-
*This section describes the evaluation protocols and provides the results.*
|
321 |
-
|
322 |
-
|
323 |
-
<details>
|
324 |
-
<summary>Click to expand</summary>
|
325 |
-
|
326 |
-
## Results
|
327 |
-
*Results are based on the [Metrics](#metrics).*
|
328 |
-
|
329 |
-
**Train-time Evaluation:**
|
330 |
|
331 |
-
|
332 |
|
333 |
-
|
334 |
-
|
335 |
-
// TODO @niklas
|
336 |
-
|
337 |
-
|
338 |
-
</details>
|
339 |
-
|
340 |
-
---
|
341 |
-
|
342 |
-
# Recommendations
|
343 |
-
|
344 |
-
*This section provides information on warnings and potential mitigations.*
|
345 |
-
|
346 |
-
<details>
|
347 |
-
<summary>Click to expand</summary>
|
348 |
-
|
349 |
-
- Indirect users should be made aware when the content they're working with is created by the LLM.
|
350 |
-
|
351 |
-
- Users should be aware of [Risks and Limitations](#risks-and-limitations), and include an appropriate age disclaimer or blocking interface as necessary.
|
352 |
-
|
353 |
-
- Models trained or finetuned downstream of MT0 should include an updated Model Card.
|
354 |
-
|
355 |
-
- Users of the model should provide mechanisms for those affected to provide feedback, such as an email address for comments.
|
356 |
-
|
357 |
-
</details>
|
358 |
-
|
359 |
-
---
|
360 |
-
|
361 |
-
# Glossary and Calculations
|
362 |
-
|
363 |
-
*This section defines common terms and how metrics are calculated.*
|
364 |
-
<details>
|
365 |
-
<summary>Click to expand</summary>
|
366 |
-
|
367 |
-
- <a name="loss">**Loss:**</a> A calculation of the difference between what the model has learned and what the data shows ("groundtruth"). The lower the loss, the better. The training process aims to minimize the loss.
|
368 |
-
|
369 |
-
- <a name="perplexity">**Perplexity:**</a> This is based on what the model estimates the probability of new data is. The lower the perplexity, the better. If the model is 100% correct at predicting the next token it will see, then the perplexity is 1. Mathematically this is calculated using entropy.
|
370 |
-
|
371 |
-
- <a name="high-stakes">**High-stakes settings:**</a> Such as those identified as "high-risk AI systems" and "unacceptable risk AI systems" in the European Union's proposed [Artificial Intelligence (AI) Act](https://artificialintelligenceact.eu/annexes/).
|
372 |
-
|
373 |
-
- <a name="critical-decisions">**Critical decisions:**</a> Such as those defined in [the United States' proposed Algorithmic Accountability Act](https://www.congress.gov/117/bills/s3572/BILLS-117s3572is.pdf).
|
374 |
-
|
375 |
-
- <a name="human-rights">**Human rights:**</a> Includes those rights defined in the [Universal Declaration of Human Rights](https://www.un.org/sites/un2.un.org/files/2021/03/udhr.pdf).
|
376 |
-
|
377 |
-
- <a name="personal-data-and-information">**Personal Data and Personal Information:**</a> Personal data and information is defined in multiple data protection regulations, such as "[personal data](https://gdpr-info.eu/issues/personal-data/)" in the [European Union's General Data Protection Regulation](https://gdpr-info.eu); and "personal information" in the Republic of South Africa's [Protection of Personal Information Act](https://www.gov.za/sites/default/files/gcis_document/201409/3706726-11act4of2013popi.pdf), The People's Republic of China's [Personal information protection law](http://en.npc.gov.cn.cdurl.cn/2021-12/29/c_694559.htm).
|
378 |
-
|
379 |
-
- <a name="sensitive-characteristics">**Sensitive characteristics:**</a> This includes specifically protected categories in human rights (see [UHDR, Article 2](https://www.un.org/sites/un2.un.org/files/2021/03/udhr.pdf)) and personal information regulation (see GDPR, [Article 9; Protection of Personal Information Act, Chapter 1](https://www.gov.za/sites/default/files/gcis_document/201409/3706726-11act4of2013popi.pdf))
|
380 |
-
|
381 |
-
- <a name="deception">**Deception:**</a> Doing something to intentionally mislead individuals to believe something that is false, such as by creating deadbots or chatbots on social media posing as real people, or generating text documents without making consumers aware that the text is machine generated.
|
382 |
-
|
383 |
-
</details>
|
384 |
-
|
385 |
-
---
|
386 |
-
|
387 |
-
# More Information
|
388 |
-
*This section provides links to writing on dataset creation, technical specifications, lessons learned, and initial results.*
|
389 |
-
|
390 |
-
<details>
|
391 |
-
<summary>Click to expand</summary>
|
392 |
-
|
393 |
-
## Intermediate checkpoints
|
394 |
-
|
395 |
-
For academic (or any) usage, we published the intermediate checkpoints, corresponding to the model state at each 1000 steps. There are available as branches in this repository. You can use them using `transformers`:
|
396 |
-
|
397 |
-
```python
|
398 |
-
from transformers import AutoModel
|
399 |
-
|
400 |
-
checkpoint = "..." # "checkpoint_1006000" for example
|
401 |
-
model = AutoModel.from_pretrained("bigscience/mt0-xxl", revision=checkpoint, torch_dtype="auto", device_map="auto")
|
402 |
-
```
|
403 |
-
|
404 |
-
## Dataset Creation
|
405 |
-
|
406 |
-
// TODO @niklas: Point to the arxiv paper
|
407 |
-
|
408 |
-
## Original checkpoints
|
409 |
-
|
410 |
-
The checkpoints in this repo correspond to the HuggingFace Transformers format. We'll provide T5X checkpoints as well.
|
411 |
-
|
412 |
-
# Citing MT0
|
413 |
-
|
414 |
-
Please use the following bibtex entry to cite T0:
|
415 |
```bibtex
|
416 |
-
|
417 |
-
|
|
|
|
|
|
|
|
|
|
|
|
|
|
|
|
1 |
---
|
2 |
+
datasets:
|
3 |
+
- bigscience/xP3mt
|
4 |
+
- mc4
|
5 |
license: apache-2.0
|
6 |
+
language:
|
|
|
7 |
- af
|
8 |
- am
|
9 |
- ar
|
|
|
105 |
- yo
|
106 |
- zh
|
107 |
- zu
|
108 |
+
pipeline_tag: text-generation
|
109 |
+
widget:
|
110 |
+
- text: "一个传奇的开端,一个不灭的神话,这不仅仅是一部电影,而是作为一个走进新时代的标签,永远彪炳史册。Would you rate the previous review as positive, neutral or negative?"
|
111 |
+
example_title: "zh-en sentiment"
|
112 |
+
- text: "一个传奇的开端,一个不灭的神话,这不仅仅是一部电影,而是作为一个走进新时代的标签,永远彪炳史册。你认为这句话的立场是赞扬、中立还是批评?"
|
113 |
+
example_title: "zh-zh sentiment"
|
114 |
+
- text: "Suggest at least five related search terms to \"Mạng neural nhân tạo\"."
|
115 |
+
example_title: "vi-en query"
|
116 |
+
- text: "Proposez au moins cinq mots clés concernant «Réseau de neurones artificiels»."
|
117 |
+
example_title: "fr-fr query"
|
118 |
+
- text: "Explain in a sentence in Telugu what is backpropagation in neural networks."
|
119 |
+
example_title: "te-en qa"
|
120 |
+
- text: "Why is the sky blue?"
|
121 |
+
example_title: "en-en qa"
|
122 |
+
- text: "Write a fairy tale about a troll saving a princess from a dangerous dragon. The fairy tale is a masterpiece that has achieved praise worldwide and its moral is \"Heroes Come in All Shapes and Sizes\". Story (in Spanish):"
|
123 |
+
example_title: "es-en fable"
|
124 |
+
- text: "Write a fable about wood elves living in a forest that is suddenly invaded by ogres. The fable is a masterpiece that has achieved praise worldwide and its moral is \"Violence is the last refuge of the incompetent\". Fable (in Hindi):"
|
125 |
+
example_title: "hi-en fable"
|
126 |
+
model-index:
|
127 |
+
- name: mt0-xxl-mt
|
128 |
+
results:
|
129 |
+
- task:
|
130 |
+
type: Coreference resolution
|
131 |
+
dataset:
|
132 |
+
type: winogrande
|
133 |
+
name: Winogrande XL (xl)
|
134 |
+
config: xl
|
135 |
+
split: validation
|
136 |
+
revision: a80f460359d1e9a67c006011c94de42a8759430c
|
137 |
+
metrics:
|
138 |
+
- type: Accuracy
|
139 |
+
value: 62.67
|
140 |
+
- task:
|
141 |
+
type: Coreference resolution
|
142 |
+
dataset:
|
143 |
+
type: Muennighoff/xwinograd
|
144 |
+
name: XWinograd (en)
|
145 |
+
config: en
|
146 |
+
split: test
|
147 |
+
revision: 9dd5ea5505fad86b7bedad667955577815300cee
|
148 |
+
metrics:
|
149 |
+
- type: Accuracy
|
150 |
+
value: 83.31
|
151 |
+
- task:
|
152 |
+
type: Coreference resolution
|
153 |
+
dataset:
|
154 |
+
type: Muennighoff/xwinograd
|
155 |
+
name: XWinograd (fr)
|
156 |
+
config: fr
|
157 |
+
split: test
|
158 |
+
revision: 9dd5ea5505fad86b7bedad667955577815300cee
|
159 |
+
metrics:
|
160 |
+
- type: Accuracy
|
161 |
+
value: 78.31
|
162 |
+
- task:
|
163 |
+
type: Coreference resolution
|
164 |
+
dataset:
|
165 |
+
type: Muennighoff/xwinograd
|
166 |
+
name: XWinograd (jp)
|
167 |
+
config: jp
|
168 |
+
split: test
|
169 |
+
revision: 9dd5ea5505fad86b7bedad667955577815300cee
|
170 |
+
metrics:
|
171 |
+
- type: Accuracy
|
172 |
+
value: 80.19
|
173 |
+
- task:
|
174 |
+
type: Coreference resolution
|
175 |
+
dataset:
|
176 |
+
type: Muennighoff/xwinograd
|
177 |
+
name: XWinograd (pt)
|
178 |
+
config: pt
|
179 |
+
split: test
|
180 |
+
revision: 9dd5ea5505fad86b7bedad667955577815300cee
|
181 |
+
metrics:
|
182 |
+
- type: Accuracy
|
183 |
+
value: 80.99
|
184 |
+
- task:
|
185 |
+
type: Coreference resolution
|
186 |
+
dataset:
|
187 |
+
type: Muennighoff/xwinograd
|
188 |
+
name: XWinograd (ru)
|
189 |
+
config: ru
|
190 |
+
split: test
|
191 |
+
revision: 9dd5ea5505fad86b7bedad667955577815300cee
|
192 |
+
metrics:
|
193 |
+
- type: Accuracy
|
194 |
+
value: 79.05
|
195 |
+
- task:
|
196 |
+
type: Coreference resolution
|
197 |
+
dataset:
|
198 |
+
type: Muennighoff/xwinograd
|
199 |
+
name: XWinograd (zh)
|
200 |
+
config: zh
|
201 |
+
split: test
|
202 |
+
revision: 9dd5ea5505fad86b7bedad667955577815300cee
|
203 |
+
metrics:
|
204 |
+
- type: Accuracy
|
205 |
+
value: 82.34
|
206 |
+
- task:
|
207 |
+
type: Natural language inference
|
208 |
+
dataset:
|
209 |
+
type: anli
|
210 |
+
name: ANLI (r1)
|
211 |
+
config: r1
|
212 |
+
split: validation
|
213 |
+
revision: 9dbd830a06fea8b1c49d6e5ef2004a08d9f45094
|
214 |
+
metrics:
|
215 |
+
- type: Accuracy
|
216 |
+
value: 49.5
|
217 |
+
- task:
|
218 |
+
type: Natural language inference
|
219 |
+
dataset:
|
220 |
+
type: anli
|
221 |
+
name: ANLI (r2)
|
222 |
+
config: r2
|
223 |
+
split: validation
|
224 |
+
revision: 9dbd830a06fea8b1c49d6e5ef2004a08d9f45094
|
225 |
+
metrics:
|
226 |
+
- type: Accuracy
|
227 |
+
value: 42.0
|
228 |
+
- task:
|
229 |
+
type: Natural language inference
|
230 |
+
dataset:
|
231 |
+
type: anli
|
232 |
+
name: ANLI (r3)
|
233 |
+
config: r3
|
234 |
+
split: validation
|
235 |
+
revision: 9dbd830a06fea8b1c49d6e5ef2004a08d9f45094
|
236 |
+
metrics:
|
237 |
+
- type: Accuracy
|
238 |
+
value: 48.17
|
239 |
+
- task:
|
240 |
+
type: Natural language inference
|
241 |
+
dataset:
|
242 |
+
type: super_glue
|
243 |
+
name: SuperGLUE (cb)
|
244 |
+
config: cb
|
245 |
+
split: validation
|
246 |
+
revision: 9e12063561e7e6c79099feb6d5a493142584e9e2
|
247 |
+
metrics:
|
248 |
+
- type: Accuracy
|
249 |
+
value: 87.5
|
250 |
+
- task:
|
251 |
+
type: Natural language inference
|
252 |
+
dataset:
|
253 |
+
type: super_glue
|
254 |
+
name: SuperGLUE (rte)
|
255 |
+
config: rte
|
256 |
+
split: validation
|
257 |
+
revision: 9e12063561e7e6c79099feb6d5a493142584e9e2
|
258 |
+
metrics:
|
259 |
+
- type: Accuracy
|
260 |
+
value: 84.84
|
261 |
+
- task:
|
262 |
+
type: Natural language inference
|
263 |
+
dataset:
|
264 |
+
type: xnli
|
265 |
+
name: XNLI (ar)
|
266 |
+
config: ar
|
267 |
+
split: validation
|
268 |
+
revision: a5a45e4ff92d5d3f34de70aaf4b72c3bdf9f7f16
|
269 |
+
metrics:
|
270 |
+
- type: Accuracy
|
271 |
+
value: 58.03
|
272 |
+
- task:
|
273 |
+
type: Natural language inference
|
274 |
+
dataset:
|
275 |
+
type: xnli
|
276 |
+
name: XNLI (bg)
|
277 |
+
config: bg
|
278 |
+
split: validation
|
279 |
+
revision: a5a45e4ff92d5d3f34de70aaf4b72c3bdf9f7f16
|
280 |
+
metrics:
|
281 |
+
- type: Accuracy
|
282 |
+
value: 59.92
|
283 |
+
- task:
|
284 |
+
type: Natural language inference
|
285 |
+
dataset:
|
286 |
+
type: xnli
|
287 |
+
name: XNLI (de)
|
288 |
+
config: de
|
289 |
+
split: validation
|
290 |
+
revision: a5a45e4ff92d5d3f34de70aaf4b72c3bdf9f7f16
|
291 |
+
metrics:
|
292 |
+
- type: Accuracy
|
293 |
+
value: 60.16
|
294 |
+
- task:
|
295 |
+
type: Natural language inference
|
296 |
+
dataset:
|
297 |
+
type: xnli
|
298 |
+
name: XNLI (el)
|
299 |
+
config: el
|
300 |
+
split: validation
|
301 |
+
revision: a5a45e4ff92d5d3f34de70aaf4b72c3bdf9f7f16
|
302 |
+
metrics:
|
303 |
+
- type: Accuracy
|
304 |
+
value: 59.2
|
305 |
+
- task:
|
306 |
+
type: Natural language inference
|
307 |
+
dataset:
|
308 |
+
type: xnli
|
309 |
+
name: XNLI (en)
|
310 |
+
config: en
|
311 |
+
split: validation
|
312 |
+
revision: a5a45e4ff92d5d3f34de70aaf4b72c3bdf9f7f16
|
313 |
+
metrics:
|
314 |
+
- type: Accuracy
|
315 |
+
value: 62.25
|
316 |
+
- task:
|
317 |
+
type: Natural language inference
|
318 |
+
dataset:
|
319 |
+
type: xnli
|
320 |
+
name: XNLI (es)
|
321 |
+
config: es
|
322 |
+
split: validation
|
323 |
+
revision: a5a45e4ff92d5d3f34de70aaf4b72c3bdf9f7f16
|
324 |
+
metrics:
|
325 |
+
- type: Accuracy
|
326 |
+
value: 60.92
|
327 |
+
- task:
|
328 |
+
type: Natural language inference
|
329 |
+
dataset:
|
330 |
+
type: xnli
|
331 |
+
name: XNLI (fr)
|
332 |
+
config: fr
|
333 |
+
split: validation
|
334 |
+
revision: a5a45e4ff92d5d3f34de70aaf4b72c3bdf9f7f16
|
335 |
+
metrics:
|
336 |
+
- type: Accuracy
|
337 |
+
value: 59.88
|
338 |
+
- task:
|
339 |
+
type: Natural language inference
|
340 |
+
dataset:
|
341 |
+
type: xnli
|
342 |
+
name: XNLI (hi)
|
343 |
+
config: hi
|
344 |
+
split: validation
|
345 |
+
revision: a5a45e4ff92d5d3f34de70aaf4b72c3bdf9f7f16
|
346 |
+
metrics:
|
347 |
+
- type: Accuracy
|
348 |
+
value: 57.47
|
349 |
+
- task:
|
350 |
+
type: Natural language inference
|
351 |
+
dataset:
|
352 |
+
type: xnli
|
353 |
+
name: XNLI (ru)
|
354 |
+
config: ru
|
355 |
+
split: validation
|
356 |
+
revision: a5a45e4ff92d5d3f34de70aaf4b72c3bdf9f7f16
|
357 |
+
metrics:
|
358 |
+
- type: Accuracy
|
359 |
+
value: 58.67
|
360 |
+
- task:
|
361 |
+
type: Natural language inference
|
362 |
+
dataset:
|
363 |
+
type: xnli
|
364 |
+
name: XNLI (sw)
|
365 |
+
config: sw
|
366 |
+
split: validation
|
367 |
+
revision: a5a45e4ff92d5d3f34de70aaf4b72c3bdf9f7f16
|
368 |
+
metrics:
|
369 |
+
- type: Accuracy
|
370 |
+
value: 56.79
|
371 |
+
- task:
|
372 |
+
type: Natural language inference
|
373 |
+
dataset:
|
374 |
+
type: xnli
|
375 |
+
name: XNLI (th)
|
376 |
+
config: th
|
377 |
+
split: validation
|
378 |
+
revision: a5a45e4ff92d5d3f34de70aaf4b72c3bdf9f7f16
|
379 |
+
metrics:
|
380 |
+
- type: Accuracy
|
381 |
+
value: 58.03
|
382 |
+
- task:
|
383 |
+
type: Natural language inference
|
384 |
+
dataset:
|
385 |
+
type: xnli
|
386 |
+
name: XNLI (tr)
|
387 |
+
config: tr
|
388 |
+
split: validation
|
389 |
+
revision: a5a45e4ff92d5d3f34de70aaf4b72c3bdf9f7f16
|
390 |
+
metrics:
|
391 |
+
- type: Accuracy
|
392 |
+
value: 57.67
|
393 |
+
- task:
|
394 |
+
type: Natural language inference
|
395 |
+
dataset:
|
396 |
+
type: xnli
|
397 |
+
name: XNLI (ur)
|
398 |
+
config: ur
|
399 |
+
split: validation
|
400 |
+
revision: a5a45e4ff92d5d3f34de70aaf4b72c3bdf9f7f16
|
401 |
+
metrics:
|
402 |
+
- type: Accuracy
|
403 |
+
value: 55.98
|
404 |
+
- task:
|
405 |
+
type: Natural language inference
|
406 |
+
dataset:
|
407 |
+
type: xnli
|
408 |
+
name: XNLI (vi)
|
409 |
+
config: vi
|
410 |
+
split: validation
|
411 |
+
revision: a5a45e4ff92d5d3f34de70aaf4b72c3bdf9f7f16
|
412 |
+
metrics:
|
413 |
+
- type: Accuracy
|
414 |
+
value: 58.92
|
415 |
+
- task:
|
416 |
+
type: Natural language inference
|
417 |
+
dataset:
|
418 |
+
type: xnli
|
419 |
+
name: XNLI (zh)
|
420 |
+
config: zh
|
421 |
+
split: validation
|
422 |
+
revision: a5a45e4ff92d5d3f34de70aaf4b72c3bdf9f7f16
|
423 |
+
metrics:
|
424 |
+
- type: Accuracy
|
425 |
+
value: 58.71
|
426 |
+
- task:
|
427 |
+
type: Sentence completion
|
428 |
+
dataset:
|
429 |
+
type: story_cloze
|
430 |
+
name: StoryCloze (2016)
|
431 |
+
config: "2016"
|
432 |
+
split: validation
|
433 |
+
revision: e724c6f8cdf7c7a2fb229d862226e15b023ee4db
|
434 |
+
metrics:
|
435 |
+
- type: Accuracy
|
436 |
+
value: 94.66
|
437 |
+
- task:
|
438 |
+
type: Sentence completion
|
439 |
+
dataset:
|
440 |
+
type: super_glue
|
441 |
+
name: SuperGLUE (copa)
|
442 |
+
config: copa
|
443 |
+
split: validation
|
444 |
+
revision: 9e12063561e7e6c79099feb6d5a493142584e9e2
|
445 |
+
metrics:
|
446 |
+
- type: Accuracy
|
447 |
+
value: 88.0
|
448 |
+
- task:
|
449 |
+
type: Sentence completion
|
450 |
+
dataset:
|
451 |
+
type: xcopa
|
452 |
+
name: XCOPA (et)
|
453 |
+
config: et
|
454 |
+
split: validation
|
455 |
+
revision: 37f73c60fb123111fa5af5f9b705d0b3747fd187
|
456 |
+
metrics:
|
457 |
+
- type: Accuracy
|
458 |
+
value: 81.0
|
459 |
+
- task:
|
460 |
+
type: Sentence completion
|
461 |
+
dataset:
|
462 |
+
type: xcopa
|
463 |
+
name: XCOPA (ht)
|
464 |
+
config: ht
|
465 |
+
split: validation
|
466 |
+
revision: 37f73c60fb123111fa5af5f9b705d0b3747fd187
|
467 |
+
metrics:
|
468 |
+
- type: Accuracy
|
469 |
+
value: 79.0
|
470 |
+
- task:
|
471 |
+
type: Sentence completion
|
472 |
+
dataset:
|
473 |
+
type: xcopa
|
474 |
+
name: XCOPA (id)
|
475 |
+
config: id
|
476 |
+
split: validation
|
477 |
+
revision: 37f73c60fb123111fa5af5f9b705d0b3747fd187
|
478 |
+
metrics:
|
479 |
+
- type: Accuracy
|
480 |
+
value: 90.0
|
481 |
+
- task:
|
482 |
+
type: Sentence completion
|
483 |
+
dataset:
|
484 |
+
type: xcopa
|
485 |
+
name: XCOPA (it)
|
486 |
+
config: it
|
487 |
+
split: validation
|
488 |
+
revision: 37f73c60fb123111fa5af5f9b705d0b3747fd187
|
489 |
+
metrics:
|
490 |
+
- type: Accuracy
|
491 |
+
value: 88.0
|
492 |
+
- task:
|
493 |
+
type: Sentence completion
|
494 |
+
dataset:
|
495 |
+
type: xcopa
|
496 |
+
name: XCOPA (qu)
|
497 |
+
config: qu
|
498 |
+
split: validation
|
499 |
+
revision: 37f73c60fb123111fa5af5f9b705d0b3747fd187
|
500 |
+
metrics:
|
501 |
+
- type: Accuracy
|
502 |
+
value: 56.0
|
503 |
+
- task:
|
504 |
+
type: Sentence completion
|
505 |
+
dataset:
|
506 |
+
type: xcopa
|
507 |
+
name: XCOPA (sw)
|
508 |
+
config: sw
|
509 |
+
split: validation
|
510 |
+
revision: 37f73c60fb123111fa5af5f9b705d0b3747fd187
|
511 |
+
metrics:
|
512 |
+
- type: Accuracy
|
513 |
+
value: 81.0
|
514 |
+
- task:
|
515 |
+
type: Sentence completion
|
516 |
+
dataset:
|
517 |
+
type: xcopa
|
518 |
+
name: XCOPA (ta)
|
519 |
+
config: ta
|
520 |
+
split: validation
|
521 |
+
revision: 37f73c60fb123111fa5af5f9b705d0b3747fd187
|
522 |
+
metrics:
|
523 |
+
- type: Accuracy
|
524 |
+
value: 81.0
|
525 |
+
- task:
|
526 |
+
type: Sentence completion
|
527 |
+
dataset:
|
528 |
+
type: xcopa
|
529 |
+
name: XCOPA (th)
|
530 |
+
config: th
|
531 |
+
split: validation
|
532 |
+
revision: 37f73c60fb123111fa5af5f9b705d0b3747fd187
|
533 |
+
metrics:
|
534 |
+
- type: Accuracy
|
535 |
+
value: 76.0
|
536 |
+
- task:
|
537 |
+
type: Sentence completion
|
538 |
+
dataset:
|
539 |
+
type: xcopa
|
540 |
+
name: XCOPA (tr)
|
541 |
+
config: tr
|
542 |
+
split: validation
|
543 |
+
revision: 37f73c60fb123111fa5af5f9b705d0b3747fd187
|
544 |
+
metrics:
|
545 |
+
- type: Accuracy
|
546 |
+
value: 76.0
|
547 |
+
- task:
|
548 |
+
type: Sentence completion
|
549 |
+
dataset:
|
550 |
+
type: xcopa
|
551 |
+
name: XCOPA (vi)
|
552 |
+
config: vi
|
553 |
+
split: validation
|
554 |
+
revision: 37f73c60fb123111fa5af5f9b705d0b3747fd187
|
555 |
+
metrics:
|
556 |
+
- type: Accuracy
|
557 |
+
value: 85.0
|
558 |
+
- task:
|
559 |
+
type: Sentence completion
|
560 |
+
dataset:
|
561 |
+
type: xcopa
|
562 |
+
name: XCOPA (zh)
|
563 |
+
config: zh
|
564 |
+
split: validation
|
565 |
+
revision: 37f73c60fb123111fa5af5f9b705d0b3747fd187
|
566 |
+
metrics:
|
567 |
+
- type: Accuracy
|
568 |
+
value: 87.0
|
569 |
+
- task:
|
570 |
+
type: Sentence completion
|
571 |
+
dataset:
|
572 |
+
type: Muennighoff/xstory_cloze
|
573 |
+
name: XStoryCloze (ar)
|
574 |
+
config: ar
|
575 |
+
split: validation
|
576 |
+
revision: 8bb76e594b68147f1a430e86829d07189622b90d
|
577 |
+
metrics:
|
578 |
+
- type: Accuracy
|
579 |
+
value: 91.0
|
580 |
+
- task:
|
581 |
+
type: Sentence completion
|
582 |
+
dataset:
|
583 |
+
type: Muennighoff/xstory_cloze
|
584 |
+
name: XStoryCloze (es)
|
585 |
+
config: es
|
586 |
+
split: validation
|
587 |
+
revision: 8bb76e594b68147f1a430e86829d07189622b90d
|
588 |
+
metrics:
|
589 |
+
- type: Accuracy
|
590 |
+
value: 93.38
|
591 |
+
- task:
|
592 |
+
type: Sentence completion
|
593 |
+
dataset:
|
594 |
+
type: Muennighoff/xstory_cloze
|
595 |
+
name: XStoryCloze (eu)
|
596 |
+
config: eu
|
597 |
+
split: validation
|
598 |
+
revision: 8bb76e594b68147f1a430e86829d07189622b90d
|
599 |
+
metrics:
|
600 |
+
- type: Accuracy
|
601 |
+
value: 91.13
|
602 |
+
- task:
|
603 |
+
type: Sentence completion
|
604 |
+
dataset:
|
605 |
+
type: Muennighoff/xstory_cloze
|
606 |
+
name: XStoryCloze (hi)
|
607 |
+
config: hi
|
608 |
+
split: validation
|
609 |
+
revision: 8bb76e594b68147f1a430e86829d07189622b90d
|
610 |
+
metrics:
|
611 |
+
- type: Accuracy
|
612 |
+
value: 90.73
|
613 |
+
- task:
|
614 |
+
type: Sentence completion
|
615 |
+
dataset:
|
616 |
+
type: Muennighoff/xstory_cloze
|
617 |
+
name: XStoryCloze (id)
|
618 |
+
config: id
|
619 |
+
split: validation
|
620 |
+
revision: 8bb76e594b68147f1a430e86829d07189622b90d
|
621 |
+
metrics:
|
622 |
+
- type: Accuracy
|
623 |
+
value: 93.05
|
624 |
+
- task:
|
625 |
+
type: Sentence completion
|
626 |
+
dataset:
|
627 |
+
type: Muennighoff/xstory_cloze
|
628 |
+
name: XStoryCloze (my)
|
629 |
+
config: my
|
630 |
+
split: validation
|
631 |
+
revision: 8bb76e594b68147f1a430e86829d07189622b90d
|
632 |
+
metrics:
|
633 |
+
- type: Accuracy
|
634 |
+
value: 86.7
|
635 |
+
- task:
|
636 |
+
type: Sentence completion
|
637 |
+
dataset:
|
638 |
+
type: Muennighoff/xstory_cloze
|
639 |
+
name: XStoryCloze (ru)
|
640 |
+
config: ru
|
641 |
+
split: validation
|
642 |
+
revision: 8bb76e594b68147f1a430e86829d07189622b90d
|
643 |
+
metrics:
|
644 |
+
- type: Accuracy
|
645 |
+
value: 91.66
|
646 |
+
- task:
|
647 |
+
type: Sentence completion
|
648 |
+
dataset:
|
649 |
+
type: Muennighoff/xstory_cloze
|
650 |
+
name: XStoryCloze (sw)
|
651 |
+
config: sw
|
652 |
+
split: validation
|
653 |
+
revision: 8bb76e594b68147f1a430e86829d07189622b90d
|
654 |
+
metrics:
|
655 |
+
- type: Accuracy
|
656 |
+
value: 89.61
|
657 |
+
- task:
|
658 |
+
type: Sentence completion
|
659 |
+
dataset:
|
660 |
+
type: Muennighoff/xstory_cloze
|
661 |
+
name: XStoryCloze (te)
|
662 |
+
config: te
|
663 |
+
split: validation
|
664 |
+
revision: 8bb76e594b68147f1a430e86829d07189622b90d
|
665 |
+
metrics:
|
666 |
+
- type: Accuracy
|
667 |
+
value: 90.4
|
668 |
+
- task:
|
669 |
+
type: Sentence completion
|
670 |
+
dataset:
|
671 |
+
type: Muennighoff/xstory_cloze
|
672 |
+
name: XStoryCloze (zh)
|
673 |
+
config: zh
|
674 |
+
split: validation
|
675 |
+
revision: 8bb76e594b68147f1a430e86829d07189622b90d
|
676 |
+
metrics:
|
677 |
+
- type: Accuracy
|
678 |
+
value: 93.05
|
679 |
---
|
680 |
|
681 |
+
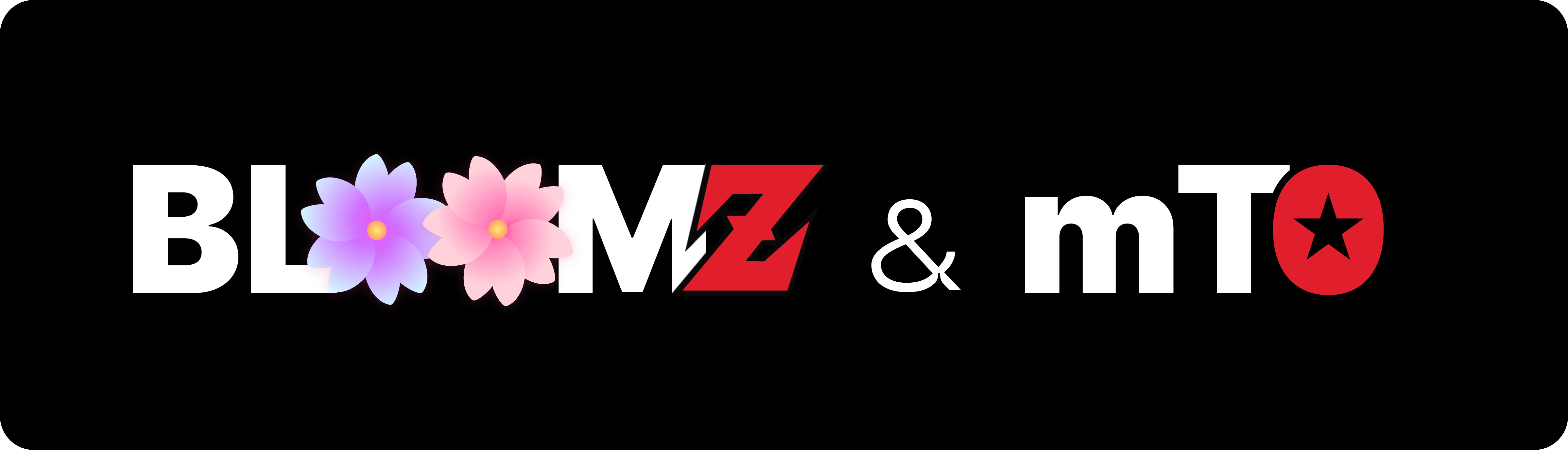
|
682 |
+
|
683 |
+
# Table of Contents
|
684 |
+
|
685 |
+
1. [Model Summary](#model-summary)
|
686 |
+
2. [Use](#use)
|
687 |
+
3. [Limitations](#limitations)
|
688 |
+
4. [Training](#training)
|
689 |
+
5. [Evaluation](#evaluation)
|
690 |
+
7. [Citation](#citation)
|
691 |
+
|
692 |
+
# Model Summary
|
693 |
+
|
694 |
+
> We present BLOOMZ & mT0, a family of models capable of following human instructions in dozens of languages zero-shot. We finetune BLOOM & mT5 pretrained multilingual language models on our crosslingual task mixture (xP3) and find our resulting models capable of crosslingual generalization to unseen tasks & languages.
|
695 |
+
|
696 |
+
- **Repository:** [bigscience-workshop/xmtf](https://github.com/bigscience-workshop/xmtf)
|
697 |
+
- **Paper:** [Crosslingual Generalization through Multitask Finetuning](https://arxiv.org/abs/2211.01786)
|
698 |
+
- **Point of Contact:** [Niklas Muennighoff](mailto:niklas@hf.co)
|
699 |
+
- **Languages:** Refer to [mc4](https://huggingface.co/datasets/mc4) for pretraining & [xP3](https://huggingface.co/bigscience/xP3) for finetuning language proportions. It understands both pretraining & finetuning languages.
|
700 |
+
- **BLOOMZ & mT0 Model Family:**
|
701 |
+
|
702 |
+
<table>
|
703 |
+
<tr>
|
704 |
+
<th colspan="12">Multitask finetuned on <a style="font-weight:bold" href=https://huggingface.co/datasets/bigscience/xP3>xP3</a>. Recommended for prompting in English.
|
705 |
+
</tr>
|
706 |
+
<tr>
|
707 |
+
<td>Parameters</td>
|
708 |
+
<td>300M</td>
|
709 |
+
<td>580M</td>
|
710 |
+
<td>1.2B</td>
|
711 |
+
<td>3.7B</td>
|
712 |
+
<td>13B</td>
|
713 |
+
<td>560M</td>
|
714 |
+
<td>1.1B</td>
|
715 |
+
<td>1.7B</td>
|
716 |
+
<td>3B</td>
|
717 |
+
<td>7.1B</td>
|
718 |
+
<td>176B</td>
|
719 |
+
</tr>
|
720 |
+
<tr>
|
721 |
+
<td>Finetuned Model</td>
|
722 |
+
<td><a href=https://huggingface.co/bigscience/mt0-base>mt0-base</a></td>
|
723 |
+
<td><a href=https://huggingface.co/bigscience/mt0-small>mt0-small</a></td>
|
724 |
+
<td><a href=https://huggingface.co/bigscience/mt0-large>mt0-large</a></td>
|
725 |
+
<td><a href=https://huggingface.co/bigscience/mt0-xl>mt0-xl</a></td>
|
726 |
+
<td><a href=https://huggingface.co/bigscience/mt0-xxl>mt0-xxl</a></td>
|
727 |
+
<td><a href=https://huggingface.co/bigscience/bloomz-560m>bloomz-560m</a></td>
|
728 |
+
<td><a href=https://huggingface.co/bigscience/bloomz-1b1>bloomz-1b1</a></td>
|
729 |
+
<td><a href=https://huggingface.co/bigscience/bloomz-1b7>bloomz-1b7</a></td>
|
730 |
+
<td><a href=https://huggingface.co/bigscience/bloomz-3b>bloomz-3b</a></td>
|
731 |
+
<td><a href=https://huggingface.co/bigscience/bloomz-7b1>bloomz-7b1</a></td>
|
732 |
+
<td><a href=https://huggingface.co/bigscience/bloomz>bloomz</a></td>
|
733 |
+
</tr>
|
734 |
+
</tr>
|
735 |
+
<tr>
|
736 |
+
<th colspan="12">Multitask finetuned on <a style="font-weight:bold" href=https://huggingface.co/datasets/bigscience/xP3mt>xP3mt</a>. Recommended for prompting in non-English.</th>
|
737 |
+
</tr>
|
738 |
+
<tr>
|
739 |
+
<td>Finetuned Model</td>
|
740 |
+
<td></td>
|
741 |
+
<td></td>
|
742 |
+
<td></td>
|
743 |
+
<td></td>
|
744 |
+
<td><a href=https://huggingface.co/bigscience/mt0-xxl-mt>mt0-xxl-mt</a></td>
|
745 |
+
<td></td>
|
746 |
+
<td></td>
|
747 |
+
<td></td>
|
748 |
+
<td></td>
|
749 |
+
<td><a href=https://huggingface.co/bigscience/bloomz-7b1-mt>bloomz-7b1-mt</a></td>
|
750 |
+
<td><a href=https://huggingface.co/bigscience/bloomz-mt>bloomz-mt</a></td>
|
751 |
+
</tr>
|
752 |
+
<th colspan="12">Multitask finetuned on <a style="font-weight:bold" href=https://huggingface.co/datasets/Muennighoff/P3>P3</a>. Released for research purposes only. Strictly inferior to above models!</th>
|
753 |
+
</tr>
|
754 |
+
<tr>
|
755 |
+
<td>Finetuned Model</td>
|
756 |
+
<td></td>
|
757 |
+
<td></td>
|
758 |
+
<td></td>
|
759 |
+
<td></td>
|
760 |
+
<td><a href=https://huggingface.co/bigscience/mt0-xxl-p3>mt0-xxl-p3</a></td>
|
761 |
+
<td></td>
|
762 |
+
<td></td>
|
763 |
+
<td></td>
|
764 |
+
<td></td>
|
765 |
+
<td><a href=https://huggingface.co/bigscience/bloomz-7b1-p3>bloomz-7b1-p3</a></td>
|
766 |
+
<td><a href=https://huggingface.co/bigscience/bloomz-p3>bloomz-p3</a></td>
|
767 |
+
</tr>
|
768 |
+
<th colspan="12">Original pretrained checkpoints. Not recommended.</th>
|
769 |
+
<tr>
|
770 |
+
<td>Pretrained Model</td>
|
771 |
+
<td><a href=https://huggingface.co/google/mt5-base>mt5-base</a></td>
|
772 |
+
<td><a href=https://huggingface.co/google/mt5-small>mt5-small</a></td>
|
773 |
+
<td><a href=https://huggingface.co/google/mt5-large>mt5-large</a></td>
|
774 |
+
<td><a href=https://huggingface.co/google/mt5-xl>mt5-xl</a></td>
|
775 |
+
<td><a href=https://huggingface.co/google/mt5-xxl>mt5-xxl</a></td>
|
776 |
+
<td><a href=https://huggingface.co/bigscience/bloom-560m>bloom-560m</a></td>
|
777 |
+
<td><a href=https://huggingface.co/bigscience/bloom-1b1>bloom-1b1</a></td>
|
778 |
+
<td><a href=https://huggingface.co/bigscience/bloom-1b7>bloom-1b7</a></td>
|
779 |
+
<td><a href=https://huggingface.co/bigscience/bloom-3b>bloom-3b</a></td>
|
780 |
+
<td><a href=https://huggingface.co/bigscience/bloom-7b1>bloom-7b1</a></td>
|
781 |
+
<td><a href=https://huggingface.co/bigscience/bloom>bloom</a></td>
|
782 |
+
</tr>
|
783 |
+
</table>
|
784 |
+
|
785 |
+
|
786 |
+
# Use
|
787 |
+
|
788 |
+
## Intended use
|
789 |
+
|
790 |
+
We recommend using the model to perform tasks expressed in natural language. For example, given the prompt "*Translate to English: Je t’aime.*", the model will most likely answer "*I love you.*". Some prompt ideas from our paper:
|
791 |
+
- 一个传奇的开端,一个不灭的神话,这不仅仅是一部电影,而是作为一个走进新时代的标签,永远彪炳史册。你认为这句话的立场是赞扬、中立还是批评?
|
792 |
+
- Suggest at least five related search terms to "Mạng neural nhân tạo".
|
793 |
+
- Write a fairy tale about a troll saving a princess from a dangerous dragon. The fairy tale is a masterpiece that has achieved praise worldwide and its moral is "Heroes Come in All Shapes and Sizes". Story (in Spanish):
|
794 |
+
- Explain in a sentence in Telugu what is backpropagation in neural networks.
|
795 |
+
|
796 |
+
**Feel free to share your generations in the Community tab!**
|
797 |
|
798 |
+
## How to use
|
799 |
|
800 |
+
### CPU
|
|
|
|
|
801 |
|
802 |
<details>
|
803 |
+
<summary> Click to expand </summary>
|
|
|
|
|
|
|
|
|
804 |
|
805 |
+
```python
|
806 |
+
# pip install -q transformers
|
807 |
+
from transformers import AutoModelForSeq2SeqLM, AutoTokenizer
|
808 |
|
809 |
+
checkpoint = "bigscience/mt0-xxl-mt"
|
810 |
|
811 |
+
tokenizer = AutoTokenizer.from_pretrained(checkpoint)
|
812 |
+
model = AutoModelForSeq2SeqLM.from_pretrained(checkpoint)
|
|
|
|
|
|
|
|
|
|
|
|
|
813 |
|
814 |
+
inputs = tokenizer.encode("Translate to English: Je t’aime.", return_tensors="pt")
|
815 |
+
outputs = model.generate(inputs)
|
816 |
+
print(tokenizer.decode(outputs[0]))
|
817 |
+
```
|
818 |
|
|
|
|
|
819 |
</details>
|
820 |
|
821 |
+
### GPU
|
|
|
|
|
|
|
|
|
822 |
|
823 |
<details>
|
824 |
+
<summary> Click to expand </summary>
|
|
|
|
|
|
|
|
|
|
|
|
|
|
|
825 |
|
826 |
+
```python
|
827 |
+
# pip install -q transformers accelerate
|
828 |
+
from transformers import AutoModelForSeq2SeqLM, AutoTokenizer
|
|
|
|
|
|
|
|
|
|
|
|
|
|
|
|
|
|
|
|
|
829 |
|
830 |
+
checkpoint = "bigscience/mt0-xxl-mt"
|
831 |
|
832 |
+
tokenizer = AutoTokenizer.from_pretrained(checkpoint)
|
833 |
+
model = AutoModelForSeq2SeqLM.from_pretrained(checkpoint, torch_dtype="auto", device_map="auto")
|
834 |
|
835 |
+
inputs = tokenizer.encode("Translate to English: Je t’aime.", return_tensors="pt").to("cuda")
|
836 |
+
outputs = model.generate(inputs)
|
837 |
+
print(tokenizer.decode(outputs[0]))
|
838 |
+
```
|
839 |
|
840 |
</details>
|
841 |
|
842 |
+
### GPU in 8bit
|
|
|
|
|
|
|
|
|
|
|
843 |
|
844 |
<details>
|
845 |
+
<summary> Click to expand </summary>
|
|
|
|
|
|
|
|
|
846 |
|
847 |
```python
|
848 |
+
# pip install -q transformers accelerate bitsandbytes
|
849 |
from transformers import AutoModelForSeq2SeqLM, AutoTokenizer
|
850 |
|
851 |
+
checkpoint = "bigscience/mt0-xxl-mt"
|
|
|
|
|
|
|
852 |
|
853 |
+
tokenizer = AutoTokenizer.from_pretrained(checkpoint)
|
854 |
+
model = AutoModelForSeq2SeqLM.from_pretrained(checkpoint, device_map="auto", load_in_8bit=True)
|
855 |
+
|
856 |
+
inputs = tokenizer.encode("Translate to English: Je t’aime.", return_tensors="pt").to("cuda")
|
857 |
outputs = model.generate(inputs)
|
858 |
print(tokenizer.decode(outputs[0]))
|
859 |
```
|
860 |
|
|
|
|
|
|
|
|
|
|
|
|
|
|
|
|
|
|
|
|
|
|
|
|
|
|
|
|
|
|
|
|
|
861 |
</details>
|
862 |
|
863 |
+
<!-- Necessary for whitespace -->
|
864 |
+
###
|
|
|
|
|
|
|
|
|
|
|
|
|
|
|
|
|
|
|
|
|
|
|
|
|
|
|
865 |
|
866 |
+
# Limitations
|
867 |
|
868 |
+
**Prompt Engineering:** The performance may vary depending on the prompt. For BLOOMZ models, we recommend making it very clear when the input stops to avoid the model trying to continue it. For example, the prompt "*Translate to English: Je t'aime*" without the full stop (.) at the end, may result in the model trying to continue the French sentence. Better prompts are e.g. "*Translate to English: Je t'aime.*", "*Translate to English: Je t'aime. Translation:*" "*What is "Je t'aime." in English?*", where it is clear for the model when it should answer. Further, we recommend providing the model as much context as possible. For example, if you want it to answer in Telugu, then tell the model, e.g. "*Explain in a sentence in Telugu what is backpropagation in neural networks.*".
|
869 |
|
870 |
+
# Training
|
871 |
|
872 |
+
## Model
|
873 |
|
874 |
+
- **Architecture:** Same as [mt5-xxl](https://huggingface.co/google/mt5-xxl), also refer to the `config.json` file
|
875 |
+
- **Finetuning steps:** 7000
|
876 |
+
- **Finetuning tokens:** 1.29 billion
|
877 |
+
- **Precision:** bfloat16
|
878 |
|
879 |
+
## Hardware
|
880 |
|
881 |
+
- **TPUs:** TPUv4-256
|
882 |
|
883 |
+
## Software
|
884 |
|
885 |
+
- **Orchestration:** [T5X](https://github.com/google-research/t5x)
|
886 |
+
- **Neural networks:** [Jax](https://github.com/google/jax)
|
887 |
|
888 |
# Evaluation
|
|
|
|
|
|
|
|
|
|
|
|
|
|
|
|
|
|
|
|
|
889 |
|
890 |
+
We refer to Table 7 from our [paper](https://arxiv.org/abs/2211.01786) & [bigscience/evaluation-results](https://huggingface.co/datasets/bigscience/evaluation-results) for zero-shot results on unseen tasks. The sidebar reports zero-shot performance of the best prompt per dataset config.
|
891 |
|
892 |
+
# Citation
|
|
|
|
|
|
|
|
|
|
|
|
|
|
|
|
|
|
|
|
|
|
|
|
|
|
|
|
|
|
|
|
|
|
|
|
|
|
|
|
|
|
|
|
|
|
|
|
|
|
|
|
|
|
|
|
|
|
|
|
|
|
|
|
|
|
|
|
|
|
|
|
|
|
|
|
|
|
|
|
|
|
|
|
|
|
|
|
|
|
|
|
|
|
|
|
|
|
|
|
|
|
|
|
|
|
|
|
|
|
|
|
|
|
|
|
|
|
|
|
|
|
|
|
|
|
|
|
|
|
|
|
|
|
|
|
|
|
|
|
|
|
|
|
|
|
|
|
|
|
|
|
|
|
|
|
|
|
|
|
|
|
|
893 |
```bibtex
|
894 |
+
@misc{muennighoff2022crosslingual,
|
895 |
+
title={Crosslingual Generalization through Multitask Finetuning},
|
896 |
+
author={Niklas Muennighoff and Thomas Wang and Lintang Sutawika and Adam Roberts and Stella Biderman and Teven Le Scao and M Saiful Bari and Sheng Shen and Zheng-Xin Yong and Hailey Schoelkopf and Xiangru Tang and Dragomir Radev and Alham Fikri Aji and Khalid Almubarak and Samuel Albanie and Zaid Alyafeai and Albert Webson and Edward Raff and Colin Raffel},
|
897 |
+
year={2022},
|
898 |
+
eprint={2211.01786},
|
899 |
+
archivePrefix={arXiv},
|
900 |
+
primaryClass={cs.CL}
|
901 |
+
}
|
902 |
+
```
|