Update README.md
Browse files
README.md
CHANGED
@@ -1,3 +1,38 @@
|
|
1 |
---
|
2 |
license: cc
|
|
|
|
|
|
|
|
|
3 |
---
|
|
|
|
|
|
|
|
|
|
|
|
|
|
|
|
|
|
|
|
|
|
|
|
|
|
|
|
|
|
|
|
|
|
|
|
|
|
|
|
|
|
|
|
|
|
|
|
|
|
|
|
|
|
|
|
|
|
|
|
|
|
|
|
1 |
---
|
2 |
license: cc
|
3 |
+
datasets:
|
4 |
+
- databricks/databricks-dolly-15k
|
5 |
+
- vicgalle/alpaca-gpt4
|
6 |
+
pipeline_tag: text-generation
|
7 |
---
|
8 |
+
# | NLP | LLM | Fine-tuning 2024 | Llama 2 QLoRA |
|
9 |
+
|
10 |
+
## Natural Language Processing (NLP) and Large Language Models (LLM) with Fine-Tuning LLM Llama 2 with QLoRA in 2024
|
11 |
+
|
12 |
+
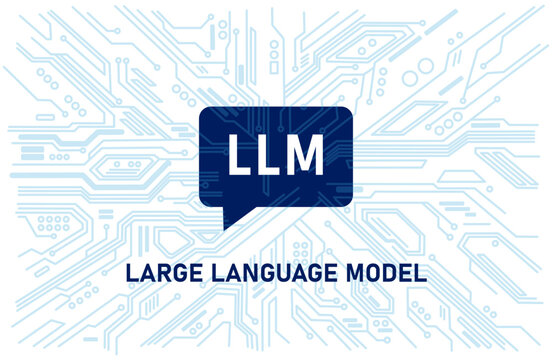
|
13 |
+
|
14 |
+
# <b><span style='color:#78D118'>|</span> Overview</b>
|
15 |
+
|
16 |
+
In this notebook we're going to Fine-Tuning LLM:
|
17 |
+
|
18 |
+
<img src="https://github.com/YanSte/NLP-LLM-Fine-tuning-Trainer/blob/main/img_2.png?raw=true" alt="Learning" width="50%">
|
19 |
+
|
20 |
+
Many LLMs are general purpose models trained on a broad range of data and use cases. This enables them to perform well in a variety of applications, as shown in previous modules. It is not uncommon though to find situations where applying a general purpose model performs unacceptably for specific dataset or use case. This often does not mean that the general purpose model is unusable. Perhaps, with some new data and additional training the model could be improved, or fine-tuned, such that it produces acceptable results for the specific use case.
|
21 |
+
|
22 |
+
<img src="https://github.com/YanSte/NLP-LLM-Fine-tuning-Trainer/blob/main/img_1.png?raw=true" alt="Learning" width="50%">
|
23 |
+
|
24 |
+
Fine-tuning uses a pre-trained model as a base and continues to train it with a new, task targeted dataset. Conceptually, fine-tuning leverages that which has already been learned by a model and aims to focus its learnings further for a specific task.
|
25 |
+
|
26 |
+
It is important to recognize that fine-tuning is model training. The training process remains a resource intensive, and time consuming effort. Albeit fine-tuning training time is greatly shortened as a result of having started from a pre-trained model.
|
27 |
+
|
28 |
+
<img src="https://github.com/YanSte/NLP-LLM-Fine-tuning-Trainer/blob/main/img_3.png?raw=true" alt="Learning" width="50%">
|
29 |
+
|
30 |
+
[Kaggle](https://www.kaggle.com/yannicksteph/nlp-llm-fine-tuning-2024-llama-2-qlora/)
|
31 |
+
|
32 |
+
## Learning Objectives
|
33 |
+
|
34 |
+
By the end of this notebook, you will gain expertise in the following areas:
|
35 |
+
|
36 |
+
1. Learn how to effectively prepare datasets for training.
|
37 |
+
2. Few shots learning
|
38 |
+
3. Understand the process of fine-tuning the Llama 2 on QLoRA with SFTTrainer in 2024.
|