Commit
•
545fcbd
1
Parent(s):
0975517
Init commit
Browse files- README.md +4 -0
- bert/bert-base-japanese-v3/.gitattributes +34 -0
- bert/bert-base-japanese-v3/README.md +53 -0
- bert/bert-base-japanese-v3/config.json +19 -0
- bert/bert-base-japanese-v3/tokenizer_config.json +10 -0
- bert/bert-base-japanese-v3/vocab.txt +0 -0
- bert/bert-large-japanese-v2/.gitattributes +34 -0
- bert/bert-large-japanese-v2/README.md +53 -0
- bert/bert-large-japanese-v2/config.json +19 -0
- bert/bert-large-japanese-v2/tokenizer_config.json +10 -0
- bert/bert-large-japanese-v2/vocab.txt +0 -0
- bert/bert_models.json +14 -0
- bert/chinese-roberta-wwm-ext-large/.gitattributes +9 -0
- bert/chinese-roberta-wwm-ext-large/README.md +57 -0
- bert/chinese-roberta-wwm-ext-large/added_tokens.json +1 -0
- bert/chinese-roberta-wwm-ext-large/config.json +28 -0
- bert/chinese-roberta-wwm-ext-large/pytorch_model.bin +3 -0
- bert/chinese-roberta-wwm-ext-large/special_tokens_map.json +1 -0
- bert/chinese-roberta-wwm-ext-large/tokenizer.json +0 -0
- bert/chinese-roberta-wwm-ext-large/tokenizer_config.json +1 -0
- bert/chinese-roberta-wwm-ext-large/vocab.txt +0 -0
- bert/deberta-v2-large-japanese-char-wwm/.gitattributes +34 -0
- bert/deberta-v2-large-japanese-char-wwm/README.md +89 -0
- bert/deberta-v2-large-japanese-char-wwm/config.json +37 -0
- bert/deberta-v2-large-japanese-char-wwm/pytorch_model.bin +3 -0
- bert/deberta-v2-large-japanese-char-wwm/special_tokens_map.json +7 -0
- bert/deberta-v2-large-japanese-char-wwm/tokenizer_config.json +19 -0
- bert/deberta-v2-large-japanese-char-wwm/vocab.txt +0 -0
- bert/deberta-v2-large-japanese/.gitattributes +34 -0
- bert/deberta-v2-large-japanese/README.md +111 -0
- bert/deberta-v2-large-japanese/config.json +38 -0
- bert/deberta-v2-large-japanese/special_tokens_map.json +9 -0
- bert/deberta-v2-large-japanese/tokenizer.json +0 -0
- bert/deberta-v2-large-japanese/tokenizer_config.json +15 -0
- bert/deberta-v3-large/.gitattributes +27 -0
- bert/deberta-v3-large/README.md +93 -0
- bert/deberta-v3-large/config.json +22 -0
- bert/deberta-v3-large/generator_config.json +22 -0
- bert/deberta-v3-large/pytorch_model.bin +3 -0
- bert/deberta-v3-large/spm.model +3 -0
- bert/deberta-v3-large/tokenizer_config.json +4 -0
- slm/wavlm-base-plus/.gitattributes +27 -0
- slm/wavlm-base-plus/README.md +65 -0
- slm/wavlm-base-plus/config.json +99 -0
- slm/wavlm-base-plus/preprocessor_config.json +9 -0
- slm/wavlm-base-plus/pytorch_model.bin +3 -0
README.md
CHANGED
@@ -1,3 +1,7 @@
|
|
1 |
---
|
2 |
license: agpl-3.0
|
|
|
|
|
3 |
---
|
|
|
|
|
|
1 |
---
|
2 |
license: agpl-3.0
|
3 |
+
tags:
|
4 |
+
- Bert-VITS2
|
5 |
---
|
6 |
+
|
7 |
+
# SLM & Bert models for Bert-VITS2 version 2.3
|
bert/bert-base-japanese-v3/.gitattributes
ADDED
@@ -0,0 +1,34 @@
|
|
|
|
|
|
|
|
|
|
|
|
|
|
|
|
|
|
|
|
|
|
|
|
|
|
|
|
|
|
|
|
|
|
|
|
|
|
|
|
|
|
|
|
|
|
|
|
|
|
|
|
|
|
|
|
|
|
|
|
|
|
|
|
|
|
|
|
|
|
|
1 |
+
*.7z filter=lfs diff=lfs merge=lfs -text
|
2 |
+
*.arrow filter=lfs diff=lfs merge=lfs -text
|
3 |
+
*.bin filter=lfs diff=lfs merge=lfs -text
|
4 |
+
*.bz2 filter=lfs diff=lfs merge=lfs -text
|
5 |
+
*.ckpt filter=lfs diff=lfs merge=lfs -text
|
6 |
+
*.ftz filter=lfs diff=lfs merge=lfs -text
|
7 |
+
*.gz filter=lfs diff=lfs merge=lfs -text
|
8 |
+
*.h5 filter=lfs diff=lfs merge=lfs -text
|
9 |
+
*.joblib filter=lfs diff=lfs merge=lfs -text
|
10 |
+
*.lfs.* filter=lfs diff=lfs merge=lfs -text
|
11 |
+
*.mlmodel filter=lfs diff=lfs merge=lfs -text
|
12 |
+
*.model filter=lfs diff=lfs merge=lfs -text
|
13 |
+
*.msgpack filter=lfs diff=lfs merge=lfs -text
|
14 |
+
*.npy filter=lfs diff=lfs merge=lfs -text
|
15 |
+
*.npz filter=lfs diff=lfs merge=lfs -text
|
16 |
+
*.onnx filter=lfs diff=lfs merge=lfs -text
|
17 |
+
*.ot filter=lfs diff=lfs merge=lfs -text
|
18 |
+
*.parquet filter=lfs diff=lfs merge=lfs -text
|
19 |
+
*.pb filter=lfs diff=lfs merge=lfs -text
|
20 |
+
*.pickle filter=lfs diff=lfs merge=lfs -text
|
21 |
+
*.pkl filter=lfs diff=lfs merge=lfs -text
|
22 |
+
*.pt filter=lfs diff=lfs merge=lfs -text
|
23 |
+
*.pth filter=lfs diff=lfs merge=lfs -text
|
24 |
+
*.rar filter=lfs diff=lfs merge=lfs -text
|
25 |
+
*.safetensors filter=lfs diff=lfs merge=lfs -text
|
26 |
+
saved_model/**/* filter=lfs diff=lfs merge=lfs -text
|
27 |
+
*.tar.* filter=lfs diff=lfs merge=lfs -text
|
28 |
+
*.tflite filter=lfs diff=lfs merge=lfs -text
|
29 |
+
*.tgz filter=lfs diff=lfs merge=lfs -text
|
30 |
+
*.wasm filter=lfs diff=lfs merge=lfs -text
|
31 |
+
*.xz filter=lfs diff=lfs merge=lfs -text
|
32 |
+
*.zip filter=lfs diff=lfs merge=lfs -text
|
33 |
+
*.zst filter=lfs diff=lfs merge=lfs -text
|
34 |
+
*tfevents* filter=lfs diff=lfs merge=lfs -text
|
bert/bert-base-japanese-v3/README.md
ADDED
@@ -0,0 +1,53 @@
|
|
|
|
|
|
|
|
|
|
|
|
|
|
|
|
|
|
|
|
|
|
|
|
|
|
|
|
|
|
|
|
|
|
|
|
|
|
|
|
|
|
|
|
|
|
|
|
|
|
|
|
|
|
|
|
|
|
|
|
|
|
|
|
|
|
|
|
|
|
|
|
|
|
|
|
|
|
|
|
|
|
|
|
|
|
|
|
|
|
|
|
|
|
|
|
|
|
|
|
|
|
|
|
|
|
|
|
|
1 |
+
---
|
2 |
+
license: apache-2.0
|
3 |
+
datasets:
|
4 |
+
- cc100
|
5 |
+
- wikipedia
|
6 |
+
language:
|
7 |
+
- ja
|
8 |
+
widget:
|
9 |
+
- text: 東北大学で[MASK]の研究をしています。
|
10 |
+
---
|
11 |
+
|
12 |
+
# BERT base Japanese (unidic-lite with whole word masking, CC-100 and jawiki-20230102)
|
13 |
+
|
14 |
+
This is a [BERT](https://github.com/google-research/bert) model pretrained on texts in the Japanese language.
|
15 |
+
|
16 |
+
This version of the model processes input texts with word-level tokenization based on the Unidic 2.1.2 dictionary (available in [unidic-lite](https://pypi.org/project/unidic-lite/) package), followed by the WordPiece subword tokenization.
|
17 |
+
Additionally, the model is trained with the whole word masking enabled for the masked language modeling (MLM) objective.
|
18 |
+
|
19 |
+
The codes for the pretraining are available at [cl-tohoku/bert-japanese](https://github.com/cl-tohoku/bert-japanese/).
|
20 |
+
|
21 |
+
## Model architecture
|
22 |
+
|
23 |
+
The model architecture is the same as the original BERT base model; 12 layers, 768 dimensions of hidden states, and 12 attention heads.
|
24 |
+
|
25 |
+
## Training Data
|
26 |
+
|
27 |
+
The model is trained on the Japanese portion of [CC-100 dataset](https://data.statmt.org/cc-100/) and the Japanese version of Wikipedia.
|
28 |
+
For Wikipedia, we generated a text corpus from the [Wikipedia Cirrussearch dump file](https://dumps.wikimedia.org/other/cirrussearch/) as of January 2, 2023.
|
29 |
+
The corpus files generated from CC-100 and Wikipedia are 74.3GB and 4.9GB in size and consist of approximately 392M and 34M sentences, respectively.
|
30 |
+
|
31 |
+
For the purpose of splitting texts into sentences, we used [fugashi](https://github.com/polm/fugashi) with [mecab-ipadic-NEologd](https://github.com/neologd/mecab-ipadic-neologd) dictionary (v0.0.7).
|
32 |
+
|
33 |
+
## Tokenization
|
34 |
+
|
35 |
+
The texts are first tokenized by MeCab with the Unidic 2.1.2 dictionary and then split into subwords by the WordPiece algorithm.
|
36 |
+
The vocabulary size is 32768.
|
37 |
+
|
38 |
+
We used [fugashi](https://github.com/polm/fugashi) and [unidic-lite](https://github.com/polm/unidic-lite) packages for the tokenization.
|
39 |
+
|
40 |
+
## Training
|
41 |
+
|
42 |
+
We trained the model first on the CC-100 corpus for 1M steps and then on the Wikipedia corpus for another 1M steps.
|
43 |
+
For training of the MLM (masked language modeling) objective, we introduced whole word masking in which all of the subword tokens corresponding to a single word (tokenized by MeCab) are masked at once.
|
44 |
+
|
45 |
+
For training of each model, we used a v3-8 instance of Cloud TPUs provided by [TPU Research Cloud](https://sites.research.google/trc/about/).
|
46 |
+
|
47 |
+
## Licenses
|
48 |
+
|
49 |
+
The pretrained models are distributed under the Apache License 2.0.
|
50 |
+
|
51 |
+
## Acknowledgments
|
52 |
+
|
53 |
+
This model is trained with Cloud TPUs provided by [TPU Research Cloud](https://sites.research.google/trc/about/) program.
|
bert/bert-base-japanese-v3/config.json
ADDED
@@ -0,0 +1,19 @@
|
|
|
|
|
|
|
|
|
|
|
|
|
|
|
|
|
|
|
|
|
|
|
|
|
|
|
|
|
|
|
|
|
|
|
|
|
|
|
|
|
1 |
+
{
|
2 |
+
"architectures": [
|
3 |
+
"BertForPreTraining"
|
4 |
+
],
|
5 |
+
"attention_probs_dropout_prob": 0.1,
|
6 |
+
"hidden_act": "gelu",
|
7 |
+
"hidden_dropout_prob": 0.1,
|
8 |
+
"hidden_size": 768,
|
9 |
+
"initializer_range": 0.02,
|
10 |
+
"intermediate_size": 3072,
|
11 |
+
"layer_norm_eps": 1e-12,
|
12 |
+
"max_position_embeddings": 512,
|
13 |
+
"model_type": "bert",
|
14 |
+
"num_attention_heads": 12,
|
15 |
+
"num_hidden_layers": 12,
|
16 |
+
"pad_token_id": 0,
|
17 |
+
"type_vocab_size": 2,
|
18 |
+
"vocab_size": 32768
|
19 |
+
}
|
bert/bert-base-japanese-v3/tokenizer_config.json
ADDED
@@ -0,0 +1,10 @@
|
|
|
|
|
|
|
|
|
|
|
|
|
|
|
|
|
|
|
|
|
|
|
1 |
+
{
|
2 |
+
"tokenizer_class": "BertJapaneseTokenizer",
|
3 |
+
"model_max_length": 512,
|
4 |
+
"do_lower_case": false,
|
5 |
+
"word_tokenizer_type": "mecab",
|
6 |
+
"subword_tokenizer_type": "wordpiece",
|
7 |
+
"mecab_kwargs": {
|
8 |
+
"mecab_dic": "unidic_lite"
|
9 |
+
}
|
10 |
+
}
|
bert/bert-base-japanese-v3/vocab.txt
ADDED
The diff for this file is too large to render.
See raw diff
|
|
bert/bert-large-japanese-v2/.gitattributes
ADDED
@@ -0,0 +1,34 @@
|
|
|
|
|
|
|
|
|
|
|
|
|
|
|
|
|
|
|
|
|
|
|
|
|
|
|
|
|
|
|
|
|
|
|
|
|
|
|
|
|
|
|
|
|
|
|
|
|
|
|
|
|
|
|
|
|
|
|
|
|
|
|
|
|
|
|
|
|
|
|
1 |
+
*.7z filter=lfs diff=lfs merge=lfs -text
|
2 |
+
*.arrow filter=lfs diff=lfs merge=lfs -text
|
3 |
+
*.bin filter=lfs diff=lfs merge=lfs -text
|
4 |
+
*.bz2 filter=lfs diff=lfs merge=lfs -text
|
5 |
+
*.ckpt filter=lfs diff=lfs merge=lfs -text
|
6 |
+
*.ftz filter=lfs diff=lfs merge=lfs -text
|
7 |
+
*.gz filter=lfs diff=lfs merge=lfs -text
|
8 |
+
*.h5 filter=lfs diff=lfs merge=lfs -text
|
9 |
+
*.joblib filter=lfs diff=lfs merge=lfs -text
|
10 |
+
*.lfs.* filter=lfs diff=lfs merge=lfs -text
|
11 |
+
*.mlmodel filter=lfs diff=lfs merge=lfs -text
|
12 |
+
*.model filter=lfs diff=lfs merge=lfs -text
|
13 |
+
*.msgpack filter=lfs diff=lfs merge=lfs -text
|
14 |
+
*.npy filter=lfs diff=lfs merge=lfs -text
|
15 |
+
*.npz filter=lfs diff=lfs merge=lfs -text
|
16 |
+
*.onnx filter=lfs diff=lfs merge=lfs -text
|
17 |
+
*.ot filter=lfs diff=lfs merge=lfs -text
|
18 |
+
*.parquet filter=lfs diff=lfs merge=lfs -text
|
19 |
+
*.pb filter=lfs diff=lfs merge=lfs -text
|
20 |
+
*.pickle filter=lfs diff=lfs merge=lfs -text
|
21 |
+
*.pkl filter=lfs diff=lfs merge=lfs -text
|
22 |
+
*.pt filter=lfs diff=lfs merge=lfs -text
|
23 |
+
*.pth filter=lfs diff=lfs merge=lfs -text
|
24 |
+
*.rar filter=lfs diff=lfs merge=lfs -text
|
25 |
+
*.safetensors filter=lfs diff=lfs merge=lfs -text
|
26 |
+
saved_model/**/* filter=lfs diff=lfs merge=lfs -text
|
27 |
+
*.tar.* filter=lfs diff=lfs merge=lfs -text
|
28 |
+
*.tflite filter=lfs diff=lfs merge=lfs -text
|
29 |
+
*.tgz filter=lfs diff=lfs merge=lfs -text
|
30 |
+
*.wasm filter=lfs diff=lfs merge=lfs -text
|
31 |
+
*.xz filter=lfs diff=lfs merge=lfs -text
|
32 |
+
*.zip filter=lfs diff=lfs merge=lfs -text
|
33 |
+
*.zst filter=lfs diff=lfs merge=lfs -text
|
34 |
+
*tfevents* filter=lfs diff=lfs merge=lfs -text
|
bert/bert-large-japanese-v2/README.md
ADDED
@@ -0,0 +1,53 @@
|
|
|
|
|
|
|
|
|
|
|
|
|
|
|
|
|
|
|
|
|
|
|
|
|
|
|
|
|
|
|
|
|
|
|
|
|
|
|
|
|
|
|
|
|
|
|
|
|
|
|
|
|
|
|
|
|
|
|
|
|
|
|
|
|
|
|
|
|
|
|
|
|
|
|
|
|
|
|
|
|
|
|
|
|
|
|
|
|
|
|
|
|
|
|
|
|
|
|
|
|
|
|
|
|
|
|
|
|
1 |
+
---
|
2 |
+
license: apache-2.0
|
3 |
+
datasets:
|
4 |
+
- cc100
|
5 |
+
- wikipedia
|
6 |
+
language:
|
7 |
+
- ja
|
8 |
+
widget:
|
9 |
+
- text: 東北大学で[MASK]の研究をしています。
|
10 |
+
---
|
11 |
+
|
12 |
+
# BERT large Japanese (unidic-lite with whole word masking, CC-100 and jawiki-20230102)
|
13 |
+
|
14 |
+
This is a [BERT](https://github.com/google-research/bert) model pretrained on texts in the Japanese language.
|
15 |
+
|
16 |
+
This version of the model processes input texts with word-level tokenization based on the Unidic 2.1.2 dictionary (available in [unidic-lite](https://pypi.org/project/unidic-lite/) package), followed by the WordPiece subword tokenization.
|
17 |
+
Additionally, the model is trained with the whole word masking enabled for the masked language modeling (MLM) objective.
|
18 |
+
|
19 |
+
The codes for the pretraining are available at [cl-tohoku/bert-japanese](https://github.com/cl-tohoku/bert-japanese/).
|
20 |
+
|
21 |
+
## Model architecture
|
22 |
+
|
23 |
+
The model architecture is the same as the original BERT large model; 24 layers, 1024 dimensions of hidden states, and 16 attention heads.
|
24 |
+
|
25 |
+
## Training Data
|
26 |
+
|
27 |
+
The model is trained on the Japanese portion of [CC-100 dataset](https://data.statmt.org/cc-100/) and the Japanese version of Wikipedia.
|
28 |
+
For Wikipedia, we generated a text corpus from the [Wikipedia Cirrussearch dump file](https://dumps.wikimedia.org/other/cirrussearch/) as of January 2, 2023.
|
29 |
+
The corpus files generated from CC-100 and Wikipedia are 74.3GB and 4.9GB in size and consist of approximately 392M and 34M sentences, respectively.
|
30 |
+
|
31 |
+
For the purpose of splitting texts into sentences, we used [fugashi](https://github.com/polm/fugashi) with [mecab-ipadic-NEologd](https://github.com/neologd/mecab-ipadic-neologd) dictionary (v0.0.7).
|
32 |
+
|
33 |
+
## Tokenization
|
34 |
+
|
35 |
+
The texts are first tokenized by MeCab with the Unidic 2.1.2 dictionary and then split into subwords by the WordPiece algorithm.
|
36 |
+
The vocabulary size is 32768.
|
37 |
+
|
38 |
+
We used [fugashi](https://github.com/polm/fugashi) and [unidic-lite](https://github.com/polm/unidic-lite) packages for the tokenization.
|
39 |
+
|
40 |
+
## Training
|
41 |
+
|
42 |
+
We trained the model first on the CC-100 corpus for 1M steps and then on the Wikipedia corpus for another 1M steps.
|
43 |
+
For training of the MLM (masked language modeling) objective, we introduced whole word masking in which all of the subword tokens corresponding to a single word (tokenized by MeCab) are masked at once.
|
44 |
+
|
45 |
+
For training of each model, we used a v3-8 instance of Cloud TPUs provided by [TPU Research Cloud](https://sites.research.google/trc/about/).
|
46 |
+
|
47 |
+
## Licenses
|
48 |
+
|
49 |
+
The pretrained models are distributed under the Apache License 2.0.
|
50 |
+
|
51 |
+
## Acknowledgments
|
52 |
+
|
53 |
+
This model is trained with Cloud TPUs provided by [TPU Research Cloud](https://sites.research.google/trc/about/) program.
|
bert/bert-large-japanese-v2/config.json
ADDED
@@ -0,0 +1,19 @@
|
|
|
|
|
|
|
|
|
|
|
|
|
|
|
|
|
|
|
|
|
|
|
|
|
|
|
|
|
|
|
|
|
|
|
|
|
|
|
|
|
1 |
+
{
|
2 |
+
"architectures": [
|
3 |
+
"BertForPreTraining"
|
4 |
+
],
|
5 |
+
"attention_probs_dropout_prob": 0.1,
|
6 |
+
"hidden_act": "gelu",
|
7 |
+
"hidden_dropout_prob": 0.1,
|
8 |
+
"hidden_size": 1024,
|
9 |
+
"initializer_range": 0.02,
|
10 |
+
"intermediate_size": 4096,
|
11 |
+
"layer_norm_eps": 1e-12,
|
12 |
+
"max_position_embeddings": 512,
|
13 |
+
"model_type": "bert",
|
14 |
+
"num_attention_heads": 16,
|
15 |
+
"num_hidden_layers": 24,
|
16 |
+
"pad_token_id": 0,
|
17 |
+
"type_vocab_size": 2,
|
18 |
+
"vocab_size": 32768
|
19 |
+
}
|
bert/bert-large-japanese-v2/tokenizer_config.json
ADDED
@@ -0,0 +1,10 @@
|
|
|
|
|
|
|
|
|
|
|
|
|
|
|
|
|
|
|
|
|
|
|
1 |
+
{
|
2 |
+
"tokenizer_class": "BertJapaneseTokenizer",
|
3 |
+
"model_max_length": 512,
|
4 |
+
"do_lower_case": false,
|
5 |
+
"word_tokenizer_type": "mecab",
|
6 |
+
"subword_tokenizer_type": "wordpiece",
|
7 |
+
"mecab_kwargs": {
|
8 |
+
"mecab_dic": "unidic_lite"
|
9 |
+
}
|
10 |
+
}
|
bert/bert-large-japanese-v2/vocab.txt
ADDED
The diff for this file is too large to render.
See raw diff
|
|
bert/bert_models.json
ADDED
@@ -0,0 +1,14 @@
|
|
|
|
|
|
|
|
|
|
|
|
|
|
|
|
|
|
|
|
|
|
|
|
|
|
|
|
|
|
|
1 |
+
{
|
2 |
+
"deberta-v2-large-japanese-char-wwm": {
|
3 |
+
"repo_id": "ku-nlp/deberta-v2-large-japanese-char-wwm",
|
4 |
+
"files": ["pytorch_model.bin"]
|
5 |
+
},
|
6 |
+
"chinese-roberta-wwm-ext-large": {
|
7 |
+
"repo_id": "hfl/chinese-roberta-wwm-ext-large",
|
8 |
+
"files": ["pytorch_model.bin"]
|
9 |
+
},
|
10 |
+
"deberta-v3-large": {
|
11 |
+
"repo_id": "microsoft/deberta-v3-large",
|
12 |
+
"files": ["spm.model", "pytorch_model.bin"]
|
13 |
+
}
|
14 |
+
}
|
bert/chinese-roberta-wwm-ext-large/.gitattributes
ADDED
@@ -0,0 +1,9 @@
|
|
|
|
|
|
|
|
|
|
|
|
|
|
|
|
|
|
|
|
|
1 |
+
*.bin.* filter=lfs diff=lfs merge=lfs -text
|
2 |
+
*.lfs.* filter=lfs diff=lfs merge=lfs -text
|
3 |
+
*.bin filter=lfs diff=lfs merge=lfs -text
|
4 |
+
*.h5 filter=lfs diff=lfs merge=lfs -text
|
5 |
+
*.tflite filter=lfs diff=lfs merge=lfs -text
|
6 |
+
*.tar.gz filter=lfs diff=lfs merge=lfs -text
|
7 |
+
*.ot filter=lfs diff=lfs merge=lfs -text
|
8 |
+
*.onnx filter=lfs diff=lfs merge=lfs -text
|
9 |
+
*.msgpack filter=lfs diff=lfs merge=lfs -text
|
bert/chinese-roberta-wwm-ext-large/README.md
ADDED
@@ -0,0 +1,57 @@
|
|
|
|
|
|
|
|
|
|
|
|
|
|
|
|
|
|
|
|
|
|
|
|
|
|
|
|
|
|
|
|
|
|
|
|
|
|
|
|
|
|
|
|
|
|
|
|
|
|
|
|
|
|
|
|
|
|
|
|
|
|
|
|
|
|
|
|
|
|
|
|
|
|
|
|
|
|
|
|
|
|
|
|
|
|
|
|
|
|
|
|
|
|
|
|
|
|
|
|
|
|
|
|
|
|
|
|
|
|
|
|
|
|
|
|
|
1 |
+
---
|
2 |
+
language:
|
3 |
+
- zh
|
4 |
+
tags:
|
5 |
+
- bert
|
6 |
+
license: "apache-2.0"
|
7 |
+
---
|
8 |
+
|
9 |
+
# Please use 'Bert' related functions to load this model!
|
10 |
+
|
11 |
+
## Chinese BERT with Whole Word Masking
|
12 |
+
For further accelerating Chinese natural language processing, we provide **Chinese pre-trained BERT with Whole Word Masking**.
|
13 |
+
|
14 |
+
**[Pre-Training with Whole Word Masking for Chinese BERT](https://arxiv.org/abs/1906.08101)**
|
15 |
+
Yiming Cui, Wanxiang Che, Ting Liu, Bing Qin, Ziqing Yang, Shijin Wang, Guoping Hu
|
16 |
+
|
17 |
+
This repository is developed based on:https://github.com/google-research/bert
|
18 |
+
|
19 |
+
You may also interested in,
|
20 |
+
- Chinese BERT series: https://github.com/ymcui/Chinese-BERT-wwm
|
21 |
+
- Chinese MacBERT: https://github.com/ymcui/MacBERT
|
22 |
+
- Chinese ELECTRA: https://github.com/ymcui/Chinese-ELECTRA
|
23 |
+
- Chinese XLNet: https://github.com/ymcui/Chinese-XLNet
|
24 |
+
- Knowledge Distillation Toolkit - TextBrewer: https://github.com/airaria/TextBrewer
|
25 |
+
|
26 |
+
More resources by HFL: https://github.com/ymcui/HFL-Anthology
|
27 |
+
|
28 |
+
## Citation
|
29 |
+
If you find the technical report or resource is useful, please cite the following technical report in your paper.
|
30 |
+
- Primary: https://arxiv.org/abs/2004.13922
|
31 |
+
```
|
32 |
+
@inproceedings{cui-etal-2020-revisiting,
|
33 |
+
title = "Revisiting Pre-Trained Models for {C}hinese Natural Language Processing",
|
34 |
+
author = "Cui, Yiming and
|
35 |
+
Che, Wanxiang and
|
36 |
+
Liu, Ting and
|
37 |
+
Qin, Bing and
|
38 |
+
Wang, Shijin and
|
39 |
+
Hu, Guoping",
|
40 |
+
booktitle = "Proceedings of the 2020 Conference on Empirical Methods in Natural Language Processing: Findings",
|
41 |
+
month = nov,
|
42 |
+
year = "2020",
|
43 |
+
address = "Online",
|
44 |
+
publisher = "Association for Computational Linguistics",
|
45 |
+
url = "https://www.aclweb.org/anthology/2020.findings-emnlp.58",
|
46 |
+
pages = "657--668",
|
47 |
+
}
|
48 |
+
```
|
49 |
+
- Secondary: https://arxiv.org/abs/1906.08101
|
50 |
+
```
|
51 |
+
@article{chinese-bert-wwm,
|
52 |
+
title={Pre-Training with Whole Word Masking for Chinese BERT},
|
53 |
+
author={Cui, Yiming and Che, Wanxiang and Liu, Ting and Qin, Bing and Yang, Ziqing and Wang, Shijin and Hu, Guoping},
|
54 |
+
journal={arXiv preprint arXiv:1906.08101},
|
55 |
+
year={2019}
|
56 |
+
}
|
57 |
+
```
|
bert/chinese-roberta-wwm-ext-large/added_tokens.json
ADDED
@@ -0,0 +1 @@
|
|
|
|
|
1 |
+
{}
|
bert/chinese-roberta-wwm-ext-large/config.json
ADDED
@@ -0,0 +1,28 @@
|
|
|
|
|
|
|
|
|
|
|
|
|
|
|
|
|
|
|
|
|
|
|
|
|
|
|
|
|
|
|
|
|
|
|
|
|
|
|
|
|
|
|
|
|
|
|
|
|
|
|
|
|
|
|
|
|
|
|
1 |
+
{
|
2 |
+
"architectures": [
|
3 |
+
"BertForMaskedLM"
|
4 |
+
],
|
5 |
+
"attention_probs_dropout_prob": 0.1,
|
6 |
+
"bos_token_id": 0,
|
7 |
+
"directionality": "bidi",
|
8 |
+
"eos_token_id": 2,
|
9 |
+
"hidden_act": "gelu",
|
10 |
+
"hidden_dropout_prob": 0.1,
|
11 |
+
"hidden_size": 1024,
|
12 |
+
"initializer_range": 0.02,
|
13 |
+
"intermediate_size": 4096,
|
14 |
+
"layer_norm_eps": 1e-12,
|
15 |
+
"max_position_embeddings": 512,
|
16 |
+
"model_type": "bert",
|
17 |
+
"num_attention_heads": 16,
|
18 |
+
"num_hidden_layers": 24,
|
19 |
+
"output_past": true,
|
20 |
+
"pad_token_id": 0,
|
21 |
+
"pooler_fc_size": 768,
|
22 |
+
"pooler_num_attention_heads": 12,
|
23 |
+
"pooler_num_fc_layers": 3,
|
24 |
+
"pooler_size_per_head": 128,
|
25 |
+
"pooler_type": "first_token_transform",
|
26 |
+
"type_vocab_size": 2,
|
27 |
+
"vocab_size": 21128
|
28 |
+
}
|
bert/chinese-roberta-wwm-ext-large/pytorch_model.bin
ADDED
@@ -0,0 +1,3 @@
|
|
|
|
|
|
|
|
|
1 |
+
version https://git-lfs.github.com/spec/v1
|
2 |
+
oid sha256:4ac62d49144d770c5ca9a5d1d3039c4995665a080febe63198189857c6bd11cd
|
3 |
+
size 1306484351
|
bert/chinese-roberta-wwm-ext-large/special_tokens_map.json
ADDED
@@ -0,0 +1 @@
|
|
|
|
|
1 |
+
{"unk_token": "[UNK]", "sep_token": "[SEP]", "pad_token": "[PAD]", "cls_token": "[CLS]", "mask_token": "[MASK]"}
|
bert/chinese-roberta-wwm-ext-large/tokenizer.json
ADDED
The diff for this file is too large to render.
See raw diff
|
|
bert/chinese-roberta-wwm-ext-large/tokenizer_config.json
ADDED
@@ -0,0 +1 @@
|
|
|
|
|
1 |
+
{"init_inputs": []}
|
bert/chinese-roberta-wwm-ext-large/vocab.txt
ADDED
The diff for this file is too large to render.
See raw diff
|
|
bert/deberta-v2-large-japanese-char-wwm/.gitattributes
ADDED
@@ -0,0 +1,34 @@
|
|
|
|
|
|
|
|
|
|
|
|
|
|
|
|
|
|
|
|
|
|
|
|
|
|
|
|
|
|
|
|
|
|
|
|
|
|
|
|
|
|
|
|
|
|
|
|
|
|
|
|
|
|
|
|
|
|
|
|
|
|
|
|
|
|
|
|
|
|
|
1 |
+
*.7z filter=lfs diff=lfs merge=lfs -text
|
2 |
+
*.arrow filter=lfs diff=lfs merge=lfs -text
|
3 |
+
*.bin filter=lfs diff=lfs merge=lfs -text
|
4 |
+
*.bz2 filter=lfs diff=lfs merge=lfs -text
|
5 |
+
*.ckpt filter=lfs diff=lfs merge=lfs -text
|
6 |
+
*.ftz filter=lfs diff=lfs merge=lfs -text
|
7 |
+
*.gz filter=lfs diff=lfs merge=lfs -text
|
8 |
+
*.h5 filter=lfs diff=lfs merge=lfs -text
|
9 |
+
*.joblib filter=lfs diff=lfs merge=lfs -text
|
10 |
+
*.lfs.* filter=lfs diff=lfs merge=lfs -text
|
11 |
+
*.mlmodel filter=lfs diff=lfs merge=lfs -text
|
12 |
+
*.model filter=lfs diff=lfs merge=lfs -text
|
13 |
+
*.msgpack filter=lfs diff=lfs merge=lfs -text
|
14 |
+
*.npy filter=lfs diff=lfs merge=lfs -text
|
15 |
+
*.npz filter=lfs diff=lfs merge=lfs -text
|
16 |
+
*.onnx filter=lfs diff=lfs merge=lfs -text
|
17 |
+
*.ot filter=lfs diff=lfs merge=lfs -text
|
18 |
+
*.parquet filter=lfs diff=lfs merge=lfs -text
|
19 |
+
*.pb filter=lfs diff=lfs merge=lfs -text
|
20 |
+
*.pickle filter=lfs diff=lfs merge=lfs -text
|
21 |
+
*.pkl filter=lfs diff=lfs merge=lfs -text
|
22 |
+
*.pt filter=lfs diff=lfs merge=lfs -text
|
23 |
+
*.pth filter=lfs diff=lfs merge=lfs -text
|
24 |
+
*.rar filter=lfs diff=lfs merge=lfs -text
|
25 |
+
*.safetensors filter=lfs diff=lfs merge=lfs -text
|
26 |
+
saved_model/**/* filter=lfs diff=lfs merge=lfs -text
|
27 |
+
*.tar.* filter=lfs diff=lfs merge=lfs -text
|
28 |
+
*.tflite filter=lfs diff=lfs merge=lfs -text
|
29 |
+
*.tgz filter=lfs diff=lfs merge=lfs -text
|
30 |
+
*.wasm filter=lfs diff=lfs merge=lfs -text
|
31 |
+
*.xz filter=lfs diff=lfs merge=lfs -text
|
32 |
+
*.zip filter=lfs diff=lfs merge=lfs -text
|
33 |
+
*.zst filter=lfs diff=lfs merge=lfs -text
|
34 |
+
*tfevents* filter=lfs diff=lfs merge=lfs -text
|
bert/deberta-v2-large-japanese-char-wwm/README.md
ADDED
@@ -0,0 +1,89 @@
|
|
|
|
|
|
|
|
|
|
|
|
|
|
|
|
|
|
|
|
|
|
|
|
|
|
|
|
|
|
|
|
|
|
|
|
|
|
|
|
|
|
|
|
|
|
|
|
|
|
|
|
|
|
|
|
|
|
|
|
|
|
|
|
|
|
|
|
|
|
|
|
|
|
|
|
|
|
|
|
|
|
|
|
|
|
|
|
|
|
|
|
|
|
|
|
|
|
|
|
|
|
|
|
|
|
|
|
|
|
|
|
|
|
|
|
|
|
|
|
|
|
|
|
|
|
|
|
|
|
|
|
|
|
|
|
|
|
|
|
|
|
|
|
|
|
|
|
|
|
|
|
|
|
|
|
|
|
|
|
|
|
|
|
|
|
|
|
|
|
|
|
|
|
|
|
|
|
|
|
|
1 |
+
---
|
2 |
+
language: ja
|
3 |
+
license: cc-by-sa-4.0
|
4 |
+
library_name: transformers
|
5 |
+
tags:
|
6 |
+
- deberta
|
7 |
+
- deberta-v2
|
8 |
+
- fill-mask
|
9 |
+
- character
|
10 |
+
- wwm
|
11 |
+
datasets:
|
12 |
+
- wikipedia
|
13 |
+
- cc100
|
14 |
+
- oscar
|
15 |
+
metrics:
|
16 |
+
- accuracy
|
17 |
+
mask_token: "[MASK]"
|
18 |
+
widget:
|
19 |
+
- text: "京都大学で自然言語処理を[MASK][MASK]する。"
|
20 |
+
---
|
21 |
+
|
22 |
+
# Model Card for Japanese character-level DeBERTa V2 large
|
23 |
+
|
24 |
+
## Model description
|
25 |
+
|
26 |
+
This is a Japanese DeBERTa V2 large model pre-trained on Japanese Wikipedia, the Japanese portion of CC-100, and the Japanese portion of OSCAR.
|
27 |
+
This model is trained with character-level tokenization and whole word masking.
|
28 |
+
|
29 |
+
## How to use
|
30 |
+
|
31 |
+
You can use this model for masked language modeling as follows:
|
32 |
+
|
33 |
+
```python
|
34 |
+
from transformers import AutoTokenizer, AutoModelForMaskedLM
|
35 |
+
tokenizer = AutoTokenizer.from_pretrained('ku-nlp/deberta-v2-large-japanese-char-wwm')
|
36 |
+
model = AutoModelForMaskedLM.from_pretrained('ku-nlp/deberta-v2-large-japanese-char-wwm')
|
37 |
+
|
38 |
+
sentence = '京都大学で自然言語処理を[MASK][MASK]する。'
|
39 |
+
encoding = tokenizer(sentence, return_tensors='pt')
|
40 |
+
...
|
41 |
+
```
|
42 |
+
|
43 |
+
You can also fine-tune this model on downstream tasks.
|
44 |
+
|
45 |
+
## Tokenization
|
46 |
+
|
47 |
+
There is no need to tokenize texts in advance, and you can give raw texts to the tokenizer.
|
48 |
+
The texts are tokenized into character-level tokens by [sentencepiece](https://github.com/google/sentencepiece).
|
49 |
+
|
50 |
+
## Training data
|
51 |
+
|
52 |
+
We used the following corpora for pre-training:
|
53 |
+
|
54 |
+
- Japanese Wikipedia (as of 20221020, 3.2GB, 27M sentences, 1.3M documents)
|
55 |
+
- Japanese portion of CC-100 (85GB, 619M sentences, 66M documents)
|
56 |
+
- Japanese portion of OSCAR (54GB, 326M sentences, 25M documents)
|
57 |
+
|
58 |
+
Note that we filtered out documents annotated with "header", "footer", or "noisy" tags in OSCAR.
|
59 |
+
Also note that Japanese Wikipedia was duplicated 10 times to make the total size of the corpus comparable to that of CC-100 and OSCAR. As a result, the total size of the training data is 171GB.
|
60 |
+
|
61 |
+
## Training procedure
|
62 |
+
|
63 |
+
We first segmented texts in the corpora into words using [Juman++ 2.0.0-rc3](https://github.com/ku-nlp/jumanpp/releases/tag/v2.0.0-rc3) for whole word masking.
|
64 |
+
Then, we built a sentencepiece model with 22,012 tokens including all characters that appear in the training corpus.
|
65 |
+
|
66 |
+
We tokenized raw corpora into character-level subwords using the sentencepiece model and trained the Japanese DeBERTa model using [transformers](https://github.com/huggingface/transformers) library.
|
67 |
+
The training took 26 days using 16 NVIDIA A100-SXM4-40GB GPUs.
|
68 |
+
|
69 |
+
The following hyperparameters were used during pre-training:
|
70 |
+
|
71 |
+
- learning_rate: 1e-4
|
72 |
+
- per_device_train_batch_size: 26
|
73 |
+
- distributed_type: multi-GPU
|
74 |
+
- num_devices: 16
|
75 |
+
- gradient_accumulation_steps: 8
|
76 |
+
- total_train_batch_size: 3,328
|
77 |
+
- max_seq_length: 512
|
78 |
+
- optimizer: Adam with betas=(0.9,0.999) and epsilon=1e-06
|
79 |
+
- lr_scheduler_type: linear schedule with warmup (lr = 0 at 300k steps)
|
80 |
+
- training_steps: 260,000
|
81 |
+
- warmup_steps: 10,000
|
82 |
+
|
83 |
+
The accuracy of the trained model on the masked language modeling task was 0.795.
|
84 |
+
The evaluation set consists of 5,000 randomly sampled documents from each of the training corpora.
|
85 |
+
|
86 |
+
## Acknowledgments
|
87 |
+
|
88 |
+
This work was supported by Joint Usage/Research Center for Interdisciplinary Large-scale Information Infrastructures (JHPCN) through General Collaboration Project no. jh221004, "Developing a Platform for Constructing and Sharing of Large-Scale Japanese Language Models".
|
89 |
+
For training models, we used the mdx: a platform for the data-driven future.
|
bert/deberta-v2-large-japanese-char-wwm/config.json
ADDED
@@ -0,0 +1,37 @@
|
|
|
|
|
|
|
|
|
|
|
|
|
|
|
|
|
|
|
|
|
|
|
|
|
|
|
|
|
|
|
|
|
|
|
|
|
|
|
|
|
|
|
|
|
|
|
|
|
|
|
|
|
|
|
|
|
|
|
|
|
|
|
|
|
|
|
|
|
|
|
|
|
|
|
|
|
1 |
+
{
|
2 |
+
"architectures": [
|
3 |
+
"DebertaV2ForMaskedLM"
|
4 |
+
],
|
5 |
+
"attention_head_size": 64,
|
6 |
+
"attention_probs_dropout_prob": 0.1,
|
7 |
+
"conv_act": "gelu",
|
8 |
+
"conv_kernel_size": 3,
|
9 |
+
"hidden_act": "gelu",
|
10 |
+
"hidden_dropout_prob": 0.1,
|
11 |
+
"hidden_size": 1024,
|
12 |
+
"initializer_range": 0.02,
|
13 |
+
"intermediate_size": 4096,
|
14 |
+
"layer_norm_eps": 1e-07,
|
15 |
+
"max_position_embeddings": 512,
|
16 |
+
"max_relative_positions": -1,
|
17 |
+
"model_type": "deberta-v2",
|
18 |
+
"norm_rel_ebd": "layer_norm",
|
19 |
+
"num_attention_heads": 16,
|
20 |
+
"num_hidden_layers": 24,
|
21 |
+
"pad_token_id": 0,
|
22 |
+
"pooler_dropout": 0,
|
23 |
+
"pooler_hidden_act": "gelu",
|
24 |
+
"pooler_hidden_size": 1024,
|
25 |
+
"pos_att_type": [
|
26 |
+
"p2c",
|
27 |
+
"c2p"
|
28 |
+
],
|
29 |
+
"position_biased_input": false,
|
30 |
+
"position_buckets": 256,
|
31 |
+
"relative_attention": true,
|
32 |
+
"share_att_key": true,
|
33 |
+
"torch_dtype": "float16",
|
34 |
+
"transformers_version": "4.25.1",
|
35 |
+
"type_vocab_size": 0,
|
36 |
+
"vocab_size": 22012
|
37 |
+
}
|
bert/deberta-v2-large-japanese-char-wwm/pytorch_model.bin
ADDED
@@ -0,0 +1,3 @@
|
|
|
|
|
|
|
|
|
1 |
+
version https://git-lfs.github.com/spec/v1
|
2 |
+
oid sha256:bf0dab8ad87bd7c22e85ec71e04f2240804fda6d33196157d6b5923af6ea1201
|
3 |
+
size 1318456639
|
bert/deberta-v2-large-japanese-char-wwm/special_tokens_map.json
ADDED
@@ -0,0 +1,7 @@
|
|
|
|
|
|
|
|
|
|
|
|
|
|
|
|
|
1 |
+
{
|
2 |
+
"cls_token": "[CLS]",
|
3 |
+
"mask_token": "[MASK]",
|
4 |
+
"pad_token": "[PAD]",
|
5 |
+
"sep_token": "[SEP]",
|
6 |
+
"unk_token": "[UNK]"
|
7 |
+
}
|
bert/deberta-v2-large-japanese-char-wwm/tokenizer_config.json
ADDED
@@ -0,0 +1,19 @@
|
|
|
|
|
|
|
|
|
|
|
|
|
|
|
|
|
|
|
|
|
|
|
|
|
|
|
|
|
|
|
|
|
|
|
|
|
|
|
|
|
1 |
+
{
|
2 |
+
"cls_token": "[CLS]",
|
3 |
+
"do_lower_case": false,
|
4 |
+
"do_subword_tokenize": true,
|
5 |
+
"do_word_tokenize": true,
|
6 |
+
"jumanpp_kwargs": null,
|
7 |
+
"mask_token": "[MASK]",
|
8 |
+
"mecab_kwargs": null,
|
9 |
+
"model_max_length": 1000000000000000019884624838656,
|
10 |
+
"never_split": null,
|
11 |
+
"pad_token": "[PAD]",
|
12 |
+
"sep_token": "[SEP]",
|
13 |
+
"special_tokens_map_file": null,
|
14 |
+
"subword_tokenizer_type": "character",
|
15 |
+
"sudachi_kwargs": null,
|
16 |
+
"tokenizer_class": "BertJapaneseTokenizer",
|
17 |
+
"unk_token": "[UNK]",
|
18 |
+
"word_tokenizer_type": "basic"
|
19 |
+
}
|
bert/deberta-v2-large-japanese-char-wwm/vocab.txt
ADDED
The diff for this file is too large to render.
See raw diff
|
|
bert/deberta-v2-large-japanese/.gitattributes
ADDED
@@ -0,0 +1,34 @@
|
|
|
|
|
|
|
|
|
|
|
|
|
|
|
|
|
|
|
|
|
|
|
|
|
|
|
|
|
|
|
|
|
|
|
|
|
|
|
|
|
|
|
|
|
|
|
|
|
|
|
|
|
|
|
|
|
|
|
|
|
|
|
|
|
|
|
|
|
|
|
1 |
+
*.7z filter=lfs diff=lfs merge=lfs -text
|
2 |
+
*.arrow filter=lfs diff=lfs merge=lfs -text
|
3 |
+
*.bin filter=lfs diff=lfs merge=lfs -text
|
4 |
+
*.bz2 filter=lfs diff=lfs merge=lfs -text
|
5 |
+
*.ckpt filter=lfs diff=lfs merge=lfs -text
|
6 |
+
*.ftz filter=lfs diff=lfs merge=lfs -text
|
7 |
+
*.gz filter=lfs diff=lfs merge=lfs -text
|
8 |
+
*.h5 filter=lfs diff=lfs merge=lfs -text
|
9 |
+
*.joblib filter=lfs diff=lfs merge=lfs -text
|
10 |
+
*.lfs.* filter=lfs diff=lfs merge=lfs -text
|
11 |
+
*.mlmodel filter=lfs diff=lfs merge=lfs -text
|
12 |
+
*.model filter=lfs diff=lfs merge=lfs -text
|
13 |
+
*.msgpack filter=lfs diff=lfs merge=lfs -text
|
14 |
+
*.npy filter=lfs diff=lfs merge=lfs -text
|
15 |
+
*.npz filter=lfs diff=lfs merge=lfs -text
|
16 |
+
*.onnx filter=lfs diff=lfs merge=lfs -text
|
17 |
+
*.ot filter=lfs diff=lfs merge=lfs -text
|
18 |
+
*.parquet filter=lfs diff=lfs merge=lfs -text
|
19 |
+
*.pb filter=lfs diff=lfs merge=lfs -text
|
20 |
+
*.pickle filter=lfs diff=lfs merge=lfs -text
|
21 |
+
*.pkl filter=lfs diff=lfs merge=lfs -text
|
22 |
+
*.pt filter=lfs diff=lfs merge=lfs -text
|
23 |
+
*.pth filter=lfs diff=lfs merge=lfs -text
|
24 |
+
*.rar filter=lfs diff=lfs merge=lfs -text
|
25 |
+
*.safetensors filter=lfs diff=lfs merge=lfs -text
|
26 |
+
saved_model/**/* filter=lfs diff=lfs merge=lfs -text
|
27 |
+
*.tar.* filter=lfs diff=lfs merge=lfs -text
|
28 |
+
*.tflite filter=lfs diff=lfs merge=lfs -text
|
29 |
+
*.tgz filter=lfs diff=lfs merge=lfs -text
|
30 |
+
*.wasm filter=lfs diff=lfs merge=lfs -text
|
31 |
+
*.xz filter=lfs diff=lfs merge=lfs -text
|
32 |
+
*.zip filter=lfs diff=lfs merge=lfs -text
|
33 |
+
*.zst filter=lfs diff=lfs merge=lfs -text
|
34 |
+
*tfevents* filter=lfs diff=lfs merge=lfs -text
|
bert/deberta-v2-large-japanese/README.md
ADDED
@@ -0,0 +1,111 @@
|
|
|
|
|
|
|
|
|
|
|
|
|
|
|
|
|
|
|
|
|
|
|
|
|
|
|
|
|
|
|
|
|
|
|
|
|
|
|
|
|
|
|
|
|
|
|
|
|
|
|
|
|
|
|
|
|
|
|
|
|
|
|
|
|
|
|
|
|
|
|
|
|
|
|
|
|
|
|
|
|
|
|
|
|
|
|
|
|
|
|
|
|
|
|
|
|
|
|
|
|
|
|
|
|
|
|
|
|
|
|
|
|
|
|
|
|
|
|
|
|
|
|
|
|
|
|
|
|
|
|
|
|
|
|
|
|
|
|
|
|
|
|
|
|
|
|
|
|
|
|
|
|
|
|
|
|
|
|
|
|
|
|
|
|
|
|
|
|
|
|
|
|
|
|
|
|
|
|
|
|
|
|
|
|
|
|
|
|
|
|
|
|
|
|
|
|
|
|
|
|
|
|
|
|
|
|
|
|
|
|
|
|
|
|
|
|
|
|
|
|
|
|
|
|
1 |
+
---
|
2 |
+
language: ja
|
3 |
+
license: cc-by-sa-4.0
|
4 |
+
library_name: transformers
|
5 |
+
tags:
|
6 |
+
- deberta
|
7 |
+
- deberta-v2
|
8 |
+
- fill-mask
|
9 |
+
datasets:
|
10 |
+
- wikipedia
|
11 |
+
- cc100
|
12 |
+
- oscar
|
13 |
+
metrics:
|
14 |
+
- accuracy
|
15 |
+
mask_token: "[MASK]"
|
16 |
+
widget:
|
17 |
+
- text: "京都 大学 で 自然 言語 処理 を [MASK] する 。"
|
18 |
+
---
|
19 |
+
|
20 |
+
# Model Card for Japanese DeBERTa V2 large
|
21 |
+
|
22 |
+
## Model description
|
23 |
+
|
24 |
+
This is a Japanese DeBERTa V2 large model pre-trained on Japanese Wikipedia, the Japanese portion of CC-100, and the
|
25 |
+
Japanese portion of OSCAR.
|
26 |
+
|
27 |
+
## How to use
|
28 |
+
|
29 |
+
You can use this model for masked language modeling as follows:
|
30 |
+
|
31 |
+
```python
|
32 |
+
from transformers import AutoTokenizer, AutoModelForMaskedLM
|
33 |
+
|
34 |
+
tokenizer = AutoTokenizer.from_pretrained('ku-nlp/deberta-v2-large-japanese')
|
35 |
+
model = AutoModelForMaskedLM.from_pretrained('ku-nlp/deberta-v2-large-japanese')
|
36 |
+
|
37 |
+
sentence = '京都 大学 で 自然 言語 処理 を [MASK] する 。' # input should be segmented into words by Juman++ in advance
|
38 |
+
encoding = tokenizer(sentence, return_tensors='pt')
|
39 |
+
...
|
40 |
+
```
|
41 |
+
|
42 |
+
You can also fine-tune this model on downstream tasks.
|
43 |
+
|
44 |
+
## Tokenization
|
45 |
+
|
46 |
+
The input text should be segmented into words by [Juman++](https://github.com/ku-nlp/jumanpp) in
|
47 |
+
advance. [Juman++ 2.0.0-rc3](https://github.com/ku-nlp/jumanpp/releases/tag/v2.0.0-rc3) was used for pre-training. Each
|
48 |
+
word is tokenized into subwords by [sentencepiece](https://github.com/google/sentencepiece).
|
49 |
+
|
50 |
+
## Training data
|
51 |
+
|
52 |
+
We used the following corpora for pre-training:
|
53 |
+
|
54 |
+
- Japanese Wikipedia (as of 20221020, 3.2GB, 27M sentences, 1.3M documents)
|
55 |
+
- Japanese portion of CC-100 (85GB, 619M sentences, 66M documents)
|
56 |
+
- Japanese portion of OSCAR (54GB, 326M sentences, 25M documents)
|
57 |
+
|
58 |
+
Note that we filtered out documents annotated with "header", "footer", or "noisy" tags in OSCAR.
|
59 |
+
Also note that Japanese Wikipedia was duplicated 10 times to make the total size of the corpus comparable to that of
|
60 |
+
CC-100 and OSCAR. As a result, the total size of the training data is 171GB.
|
61 |
+
|
62 |
+
## Training procedure
|
63 |
+
|
64 |
+
We first segmented texts in the corpora into words using [Juman++](https://github.com/ku-nlp/jumanpp).
|
65 |
+
Then, we built a sentencepiece model with 32000 tokens including words ([JumanDIC](https://github.com/ku-nlp/JumanDIC))
|
66 |
+
and subwords induced by the unigram language model of [sentencepiece](https://github.com/google/sentencepiece).
|
67 |
+
|
68 |
+
We tokenized the segmented corpora into subwords using the sentencepiece model and trained the Japanese DeBERTa model
|
69 |
+
using [transformers](https://github.com/huggingface/transformers) library.
|
70 |
+
The training took 36 days using 8 NVIDIA A100-SXM4-40GB GPUs.
|
71 |
+
|
72 |
+
The following hyperparameters were used during pre-training:
|
73 |
+
|
74 |
+
- learning_rate: 1e-4
|
75 |
+
- per_device_train_batch_size: 18
|
76 |
+
- distributed_type: multi-GPU
|
77 |
+
- num_devices: 8
|
78 |
+
- gradient_accumulation_steps: 16
|
79 |
+
- total_train_batch_size: 2,304
|
80 |
+
- max_seq_length: 512
|
81 |
+
- optimizer: Adam with betas=(0.9,0.999) and epsilon=1e-06
|
82 |
+
- lr_scheduler_type: linear schedule with warmup
|
83 |
+
- training_steps: 300,000
|
84 |
+
- warmup_steps: 10,000
|
85 |
+
|
86 |
+
The accuracy of the trained model on the masked language modeling task was 0.799.
|
87 |
+
The evaluation set consists of 5,000 randomly sampled documents from each of the training corpora.
|
88 |
+
|
89 |
+
## Fine-tuning on NLU tasks
|
90 |
+
|
91 |
+
We fine-tuned the following models and evaluated them on the dev set of JGLUE.
|
92 |
+
We tuned learning rate and training epochs for each model and task
|
93 |
+
following [the JGLUE paper](https://www.jstage.jst.go.jp/article/jnlp/30/1/30_63/_pdf/-char/ja).
|
94 |
+
|
95 |
+
| Model | MARC-ja/acc | JSTS/pearson | JSTS/spearman | JNLI/acc | JSQuAD/EM | JSQuAD/F1 | JComQA/acc |
|
96 |
+
|-------------------------------|-------------|--------------|---------------|----------|-----------|-----------|------------|
|
97 |
+
| Waseda RoBERTa base | 0.965 | 0.913 | 0.876 | 0.905 | 0.853 | 0.916 | 0.853 |
|
98 |
+
| Waseda RoBERTa large (seq512) | 0.969 | 0.925 | 0.890 | 0.928 | 0.910 | 0.955 | 0.900 |
|
99 |
+
| LUKE Japanese base* | 0.965 | 0.916 | 0.877 | 0.912 | - | - | 0.842 |
|
100 |
+
| LUKE Japanese large* | 0.965 | 0.932 | 0.902 | 0.927 | - | - | 0.893 |
|
101 |
+
| DeBERTaV2 base | 0.970 | 0.922 | 0.886 | 0.922 | 0.899 | 0.951 | 0.873 |
|
102 |
+
| DeBERTaV2 large | 0.968 | 0.925 | 0.892 | 0.924 | 0.912 | 0.959 | 0.890 |
|
103 |
+
|
104 |
+
*The scores of LUKE are from [the official repository](https://github.com/studio-ousia/luke).
|
105 |
+
|
106 |
+
## Acknowledgments
|
107 |
+
|
108 |
+
This work was supported by Joint Usage/Research Center for Interdisciplinary Large-scale Information Infrastructures (
|
109 |
+
JHPCN) through General Collaboration Project no. jh221004, "Developing a Platform for Constructing and Sharing of
|
110 |
+
Large-Scale Japanese Language Models".
|
111 |
+
For training models, we used the mdx: a platform for the data-driven future.
|
bert/deberta-v2-large-japanese/config.json
ADDED
@@ -0,0 +1,38 @@
|
|
|
|
|
|
|
|
|
|
|
|
|
|
|
|
|
|
|
|
|
|
|
|
|
|
|
|
|
|
|
|
|
|
|
|
|
|
|
|
|
|
|
|
|
|
|
|
|
|
|
|
|
|
|
|
|
|
|
|
|
|
|
|
|
|
|
|
|
|
|
|
|
|
|
|
|
|
|
1 |
+
{
|
2 |
+
"_name_or_path": "configs/deberta_v2_large.json",
|
3 |
+
"architectures": [
|
4 |
+
"DebertaV2ForMaskedLM"
|
5 |
+
],
|
6 |
+
"attention_head_size": 64,
|
7 |
+
"attention_probs_dropout_prob": 0.1,
|
8 |
+
"conv_act": "gelu",
|
9 |
+
"conv_kernel_size": 3,
|
10 |
+
"hidden_act": "gelu",
|
11 |
+
"hidden_dropout_prob": 0.1,
|
12 |
+
"hidden_size": 1024,
|
13 |
+
"initializer_range": 0.02,
|
14 |
+
"intermediate_size": 4096,
|
15 |
+
"layer_norm_eps": 1e-07,
|
16 |
+
"max_position_embeddings": 512,
|
17 |
+
"max_relative_positions": -1,
|
18 |
+
"model_type": "deberta-v2",
|
19 |
+
"norm_rel_ebd": "layer_norm",
|
20 |
+
"num_attention_heads": 16,
|
21 |
+
"num_hidden_layers": 24,
|
22 |
+
"pad_token_id": 0,
|
23 |
+
"pooler_dropout": 0,
|
24 |
+
"pooler_hidden_act": "gelu",
|
25 |
+
"pooler_hidden_size": 1024,
|
26 |
+
"pos_att_type": [
|
27 |
+
"p2c",
|
28 |
+
"c2p"
|
29 |
+
],
|
30 |
+
"position_biased_input": false,
|
31 |
+
"position_buckets": 256,
|
32 |
+
"relative_attention": true,
|
33 |
+
"share_att_key": true,
|
34 |
+
"torch_dtype": "float32",
|
35 |
+
"transformers_version": "4.23.1",
|
36 |
+
"type_vocab_size": 0,
|
37 |
+
"vocab_size": 32000
|
38 |
+
}
|
bert/deberta-v2-large-japanese/special_tokens_map.json
ADDED
@@ -0,0 +1,9 @@
|
|
|
|
|
|
|
|
|
|
|
|
|
|
|
|
|
|
|
|
|
1 |
+
{
|
2 |
+
"bos_token": "[CLS]",
|
3 |
+
"cls_token": "[CLS]",
|
4 |
+
"eos_token": "[SEP]",
|
5 |
+
"mask_token": "[MASK]",
|
6 |
+
"pad_token": "[PAD]",
|
7 |
+
"sep_token": "[SEP]",
|
8 |
+
"unk_token": "[UNK]"
|
9 |
+
}
|
bert/deberta-v2-large-japanese/tokenizer.json
ADDED
The diff for this file is too large to render.
See raw diff
|
|
bert/deberta-v2-large-japanese/tokenizer_config.json
ADDED
@@ -0,0 +1,15 @@
|
|
|
|
|
|
|
|
|
|
|
|
|
|
|
|
|
|
|
|
|
|
|
|
|
|
|
|
|
|
|
|
|
1 |
+
{
|
2 |
+
"bos_token": "[CLS]",
|
3 |
+
"cls_token": "[CLS]",
|
4 |
+
"do_lower_case": false,
|
5 |
+
"eos_token": "[SEP]",
|
6 |
+
"keep_accents": true,
|
7 |
+
"mask_token": "[MASK]",
|
8 |
+
"pad_token": "[PAD]",
|
9 |
+
"sep_token": "[SEP]",
|
10 |
+
"sp_model_kwargs": {},
|
11 |
+
"special_tokens_map_file": null,
|
12 |
+
"split_by_punct": false,
|
13 |
+
"tokenizer_class": "DebertaV2Tokenizer",
|
14 |
+
"unk_token": "[UNK]"
|
15 |
+
}
|
bert/deberta-v3-large/.gitattributes
ADDED
@@ -0,0 +1,27 @@
|
|
|
|
|
|
|
|
|
|
|
|
|
|
|
|
|
|
|
|
|
|
|
|
|
|
|
|
|
|
|
|
|
|
|
|
|
|
|
|
|
|
|
|
|
|
|
|
|
|
|
|
|
|
|
|
|
1 |
+
*.7z filter=lfs diff=lfs merge=lfs -text
|
2 |
+
*.arrow filter=lfs diff=lfs merge=lfs -text
|
3 |
+
*.bin filter=lfs diff=lfs merge=lfs -text
|
4 |
+
*.bin.* filter=lfs diff=lfs merge=lfs -text
|
5 |
+
*.bz2 filter=lfs diff=lfs merge=lfs -text
|
6 |
+
*.ftz filter=lfs diff=lfs merge=lfs -text
|
7 |
+
*.gz filter=lfs diff=lfs merge=lfs -text
|
8 |
+
*.h5 filter=lfs diff=lfs merge=lfs -text
|
9 |
+
*.joblib filter=lfs diff=lfs merge=lfs -text
|
10 |
+
*.lfs.* filter=lfs diff=lfs merge=lfs -text
|
11 |
+
*.model filter=lfs diff=lfs merge=lfs -text
|
12 |
+
*.msgpack filter=lfs diff=lfs merge=lfs -text
|
13 |
+
*.onnx filter=lfs diff=lfs merge=lfs -text
|
14 |
+
*.ot filter=lfs diff=lfs merge=lfs -text
|
15 |
+
*.parquet filter=lfs diff=lfs merge=lfs -text
|
16 |
+
*.pb filter=lfs diff=lfs merge=lfs -text
|
17 |
+
*.pt filter=lfs diff=lfs merge=lfs -text
|
18 |
+
*.pth filter=lfs diff=lfs merge=lfs -text
|
19 |
+
*.rar filter=lfs diff=lfs merge=lfs -text
|
20 |
+
saved_model/**/* filter=lfs diff=lfs merge=lfs -text
|
21 |
+
*.tar.* filter=lfs diff=lfs merge=lfs -text
|
22 |
+
*.tflite filter=lfs diff=lfs merge=lfs -text
|
23 |
+
*.tgz filter=lfs diff=lfs merge=lfs -text
|
24 |
+
*.xz filter=lfs diff=lfs merge=lfs -text
|
25 |
+
*.zip filter=lfs diff=lfs merge=lfs -text
|
26 |
+
*.zstandard filter=lfs diff=lfs merge=lfs -text
|
27 |
+
*tfevents* filter=lfs diff=lfs merge=lfs -text
|
bert/deberta-v3-large/README.md
ADDED
@@ -0,0 +1,93 @@
|
|
|
|
|
|
|
|
|
|
|
|
|
|
|
|
|
|
|
|
|
|
|
|
|
|
|
|
|
|
|
|
|
|
|
|
|
|
|
|
|
|
|
|
|
|
|
|
|
|
|
|
|
|
|
|
|
|
|
|
|
|
|
|
|
|
|
|
|
|
|
|
|
|
|
|
|
|
|
|
|
|
|
|
|
|
|
|
|
|
|
|
|
|
|
|
|
|
|
|
|
|
|
|
|
|
|
|
|
|
|
|
|
|
|
|
|
|
|
|
|
|
|
|
|
|
|
|
|
|
|
|
|
|
|
|
|
|
|
|
|
|
|
|
|
|
|
|
|
|
|
|
|
|
|
|
|
|
|
|
|
|
|
|
|
|
|
|
|
|
|
|
|
|
|
|
|
|
|
|
|
|
|
|
|
|
|
|
|
1 |
+
---
|
2 |
+
language: en
|
3 |
+
tags:
|
4 |
+
- deberta
|
5 |
+
- deberta-v3
|
6 |
+
- fill-mask
|
7 |
+
thumbnail: https://huggingface.co/front/thumbnails/microsoft.png
|
8 |
+
license: mit
|
9 |
+
---
|
10 |
+
|
11 |
+
## DeBERTaV3: Improving DeBERTa using ELECTRA-Style Pre-Training with Gradient-Disentangled Embedding Sharing
|
12 |
+
|
13 |
+
[DeBERTa](https://arxiv.org/abs/2006.03654) improves the BERT and RoBERTa models using disentangled attention and enhanced mask decoder. With those two improvements, DeBERTa out perform RoBERTa on a majority of NLU tasks with 80GB training data.
|
14 |
+
|
15 |
+
In [DeBERTa V3](https://arxiv.org/abs/2111.09543), we further improved the efficiency of DeBERTa using ELECTRA-Style pre-training with Gradient Disentangled Embedding Sharing. Compared to DeBERTa, our V3 version significantly improves the model performance on downstream tasks. You can find more technique details about the new model from our [paper](https://arxiv.org/abs/2111.09543).
|
16 |
+
|
17 |
+
Please check the [official repository](https://github.com/microsoft/DeBERTa) for more implementation details and updates.
|
18 |
+
|
19 |
+
The DeBERTa V3 large model comes with 24 layers and a hidden size of 1024. It has 304M backbone parameters with a vocabulary containing 128K tokens which introduces 131M parameters in the Embedding layer. This model was trained using the 160GB data as DeBERTa V2.
|
20 |
+
|
21 |
+
|
22 |
+
#### Fine-tuning on NLU tasks
|
23 |
+
|
24 |
+
We present the dev results on SQuAD 2.0 and MNLI tasks.
|
25 |
+
|
26 |
+
| Model |Vocabulary(K)|Backbone #Params(M)| SQuAD 2.0(F1/EM) | MNLI-m/mm(ACC)|
|
27 |
+
|-------------------|----------|-------------------|-----------|----------|
|
28 |
+
| RoBERTa-large |50 |304 | 89.4/86.5 | 90.2 |
|
29 |
+
| XLNet-large |32 |- | 90.6/87.9 | 90.8 |
|
30 |
+
| DeBERTa-large |50 |- | 90.7/88.0 | 91.3 |
|
31 |
+
| **DeBERTa-v3-large**|128|304 | **91.5/89.0**| **91.8/91.9**|
|
32 |
+
|
33 |
+
|
34 |
+
#### Fine-tuning with HF transformers
|
35 |
+
|
36 |
+
```bash
|
37 |
+
#!/bin/bash
|
38 |
+
|
39 |
+
cd transformers/examples/pytorch/text-classification/
|
40 |
+
|
41 |
+
pip install datasets
|
42 |
+
export TASK_NAME=mnli
|
43 |
+
|
44 |
+
output_dir="ds_results"
|
45 |
+
|
46 |
+
num_gpus=8
|
47 |
+
|
48 |
+
batch_size=8
|
49 |
+
|
50 |
+
python -m torch.distributed.launch --nproc_per_node=${num_gpus} \
|
51 |
+
run_glue.py \
|
52 |
+
--model_name_or_path microsoft/deberta-v3-large \
|
53 |
+
--task_name $TASK_NAME \
|
54 |
+
--do_train \
|
55 |
+
--do_eval \
|
56 |
+
--evaluation_strategy steps \
|
57 |
+
--max_seq_length 256 \
|
58 |
+
--warmup_steps 50 \
|
59 |
+
--per_device_train_batch_size ${batch_size} \
|
60 |
+
--learning_rate 6e-6 \
|
61 |
+
--num_train_epochs 2 \
|
62 |
+
--output_dir $output_dir \
|
63 |
+
--overwrite_output_dir \
|
64 |
+
--logging_steps 1000 \
|
65 |
+
--logging_dir $output_dir
|
66 |
+
|
67 |
+
```
|
68 |
+
|
69 |
+
### Citation
|
70 |
+
|
71 |
+
If you find DeBERTa useful for your work, please cite the following papers:
|
72 |
+
|
73 |
+
``` latex
|
74 |
+
@misc{he2021debertav3,
|
75 |
+
title={DeBERTaV3: Improving DeBERTa using ELECTRA-Style Pre-Training with Gradient-Disentangled Embedding Sharing},
|
76 |
+
author={Pengcheng He and Jianfeng Gao and Weizhu Chen},
|
77 |
+
year={2021},
|
78 |
+
eprint={2111.09543},
|
79 |
+
archivePrefix={arXiv},
|
80 |
+
primaryClass={cs.CL}
|
81 |
+
}
|
82 |
+
```
|
83 |
+
|
84 |
+
``` latex
|
85 |
+
@inproceedings{
|
86 |
+
he2021deberta,
|
87 |
+
title={DEBERTA: DECODING-ENHANCED BERT WITH DISENTANGLED ATTENTION},
|
88 |
+
author={Pengcheng He and Xiaodong Liu and Jianfeng Gao and Weizhu Chen},
|
89 |
+
booktitle={International Conference on Learning Representations},
|
90 |
+
year={2021},
|
91 |
+
url={https://openreview.net/forum?id=XPZIaotutsD}
|
92 |
+
}
|
93 |
+
```
|
bert/deberta-v3-large/config.json
ADDED
@@ -0,0 +1,22 @@
|
|
|
|
|
|
|
|
|
|
|
|
|
|
|
|
|
|
|
|
|
|
|
|
|
|
|
|
|
|
|
|
|
|
|
|
|
|
|
|
|
|
|
|
|
|
|
1 |
+
{
|
2 |
+
"model_type": "deberta-v2",
|
3 |
+
"attention_probs_dropout_prob": 0.1,
|
4 |
+
"hidden_act": "gelu",
|
5 |
+
"hidden_dropout_prob": 0.1,
|
6 |
+
"hidden_size": 1024,
|
7 |
+
"initializer_range": 0.02,
|
8 |
+
"intermediate_size": 4096,
|
9 |
+
"max_position_embeddings": 512,
|
10 |
+
"relative_attention": true,
|
11 |
+
"position_buckets": 256,
|
12 |
+
"norm_rel_ebd": "layer_norm",
|
13 |
+
"share_att_key": true,
|
14 |
+
"pos_att_type": "p2c|c2p",
|
15 |
+
"layer_norm_eps": 1e-7,
|
16 |
+
"max_relative_positions": -1,
|
17 |
+
"position_biased_input": false,
|
18 |
+
"num_attention_heads": 16,
|
19 |
+
"num_hidden_layers": 24,
|
20 |
+
"type_vocab_size": 0,
|
21 |
+
"vocab_size": 128100
|
22 |
+
}
|
bert/deberta-v3-large/generator_config.json
ADDED
@@ -0,0 +1,22 @@
|
|
|
|
|
|
|
|
|
|
|
|
|
|
|
|
|
|
|
|
|
|
|
|
|
|
|
|
|
|
|
|
|
|
|
|
|
|
|
|
|
|
|
|
|
|
|
1 |
+
{
|
2 |
+
"model_type": "deberta-v2",
|
3 |
+
"attention_probs_dropout_prob": 0.1,
|
4 |
+
"hidden_act": "gelu",
|
5 |
+
"hidden_dropout_prob": 0.1,
|
6 |
+
"hidden_size": 1024,
|
7 |
+
"initializer_range": 0.02,
|
8 |
+
"intermediate_size": 4096,
|
9 |
+
"max_position_embeddings": 512,
|
10 |
+
"relative_attention": true,
|
11 |
+
"position_buckets": 256,
|
12 |
+
"norm_rel_ebd": "layer_norm",
|
13 |
+
"share_att_key": true,
|
14 |
+
"pos_att_type": "p2c|c2p",
|
15 |
+
"layer_norm_eps": 1e-7,
|
16 |
+
"max_relative_positions": -1,
|
17 |
+
"position_biased_input": false,
|
18 |
+
"num_attention_heads": 16,
|
19 |
+
"num_hidden_layers": 12,
|
20 |
+
"type_vocab_size": 0,
|
21 |
+
"vocab_size": 128100
|
22 |
+
}
|
bert/deberta-v3-large/pytorch_model.bin
ADDED
@@ -0,0 +1,3 @@
|
|
|
|
|
|
|
|
|
1 |
+
version https://git-lfs.github.com/spec/v1
|
2 |
+
oid sha256:dd5b5d93e2db101aaf281df0ea1216c07ad73620ff59c5b42dccac4bf2eef5b5
|
3 |
+
size 873673253
|
bert/deberta-v3-large/spm.model
ADDED
@@ -0,0 +1,3 @@
|
|
|
|
|
|
|
|
|
1 |
+
version https://git-lfs.github.com/spec/v1
|
2 |
+
oid sha256:c679fbf93643d19aab7ee10c0b99e460bdbc02fedf34b92b05af343b4af586fd
|
3 |
+
size 2464616
|
bert/deberta-v3-large/tokenizer_config.json
ADDED
@@ -0,0 +1,4 @@
|
|
|
|
|
|
|
|
|
|
|
1 |
+
{
|
2 |
+
"do_lower_case": false,
|
3 |
+
"vocab_type": "spm"
|
4 |
+
}
|
slm/wavlm-base-plus/.gitattributes
ADDED
@@ -0,0 +1,27 @@
|
|
|
|
|
|
|
|
|
|
|
|
|
|
|
|
|
|
|
|
|
|
|
|
|
|
|
|
|
|
|
|
|
|
|
|
|
|
|
|
|
|
|
|
|
|
|
|
|
|
|
|
|
|
|
|
|
1 |
+
*.7z filter=lfs diff=lfs merge=lfs -text
|
2 |
+
*.arrow filter=lfs diff=lfs merge=lfs -text
|
3 |
+
*.bin filter=lfs diff=lfs merge=lfs -text
|
4 |
+
*.bin.* filter=lfs diff=lfs merge=lfs -text
|
5 |
+
*.bz2 filter=lfs diff=lfs merge=lfs -text
|
6 |
+
*.ftz filter=lfs diff=lfs merge=lfs -text
|
7 |
+
*.gz filter=lfs diff=lfs merge=lfs -text
|
8 |
+
*.h5 filter=lfs diff=lfs merge=lfs -text
|
9 |
+
*.joblib filter=lfs diff=lfs merge=lfs -text
|
10 |
+
*.lfs.* filter=lfs diff=lfs merge=lfs -text
|
11 |
+
*.model filter=lfs diff=lfs merge=lfs -text
|
12 |
+
*.msgpack filter=lfs diff=lfs merge=lfs -text
|
13 |
+
*.onnx filter=lfs diff=lfs merge=lfs -text
|
14 |
+
*.ot filter=lfs diff=lfs merge=lfs -text
|
15 |
+
*.parquet filter=lfs diff=lfs merge=lfs -text
|
16 |
+
*.pb filter=lfs diff=lfs merge=lfs -text
|
17 |
+
*.pt filter=lfs diff=lfs merge=lfs -text
|
18 |
+
*.pth filter=lfs diff=lfs merge=lfs -text
|
19 |
+
*.rar filter=lfs diff=lfs merge=lfs -text
|
20 |
+
saved_model/**/* filter=lfs diff=lfs merge=lfs -text
|
21 |
+
*.tar.* filter=lfs diff=lfs merge=lfs -text
|
22 |
+
*.tflite filter=lfs diff=lfs merge=lfs -text
|
23 |
+
*.tgz filter=lfs diff=lfs merge=lfs -text
|
24 |
+
*.xz filter=lfs diff=lfs merge=lfs -text
|
25 |
+
*.zip filter=lfs diff=lfs merge=lfs -text
|
26 |
+
*.zstandard filter=lfs diff=lfs merge=lfs -text
|
27 |
+
*tfevents* filter=lfs diff=lfs merge=lfs -text
|
slm/wavlm-base-plus/README.md
ADDED
@@ -0,0 +1,65 @@
|
|
|
|
|
|
|
|
|
|
|
|
|
|
|
|
|
|
|
|
|
|
|
|
|
|
|
|
|
|
|
|
|
|
|
|
|
|
|
|
|
|
|
|
|
|
|
|
|
|
|
|
|
|
|
|
|
|
|
|
|
|
|
|
|
|
|
|
|
|
|
|
|
|
|
|
|
|
|
|
|
|
|
|
|
|
|
|
|
|
|
|
|
|
|
|
|
|
|
|
|
|
|
|
|
|
|
|
|
|
|
|
|
|
|
|
|
|
|
|
|
|
|
|
|
|
|
|
|
|
|
|
|
1 |
+
---
|
2 |
+
language:
|
3 |
+
- en
|
4 |
+
datasets:
|
5 |
+
tags:
|
6 |
+
- speech
|
7 |
+
inference: false
|
8 |
+
---
|
9 |
+
|
10 |
+
# WavLM-Base-Plus
|
11 |
+
|
12 |
+
[Microsoft's WavLM](https://github.com/microsoft/unilm/tree/master/wavlm)
|
13 |
+
|
14 |
+
The base model pretrained on 16kHz sampled speech audio. When using the model, make sure that your speech input is also sampled at 16kHz.
|
15 |
+
|
16 |
+
**Note**: This model does not have a tokenizer as it was pretrained on audio alone. In order to use this model **speech recognition**, a tokenizer should be created and the model should be fine-tuned on labeled text data. Check out [this blog](https://huggingface.co/blog/fine-tune-wav2vec2-english) for more in-detail explanation of how to fine-tune the model.
|
17 |
+
|
18 |
+
The model was pre-trained on:
|
19 |
+
|
20 |
+
- 60,000 hours of [Libri-Light](https://arxiv.org/abs/1912.07875)
|
21 |
+
- 10,000 hours of [GigaSpeech](https://arxiv.org/abs/2106.06909)
|
22 |
+
- 24,000 hours of [VoxPopuli](https://arxiv.org/abs/2101.00390)
|
23 |
+
|
24 |
+
[Paper: WavLM: Large-Scale Self-Supervised Pre-Training for Full Stack Speech Processing](https://arxiv.org/abs/2110.13900)
|
25 |
+
|
26 |
+
Authors: Sanyuan Chen, Chengyi Wang, Zhengyang Chen, Yu Wu, Shujie Liu, Zhuo Chen, Jinyu Li, Naoyuki Kanda, Takuya Yoshioka, Xiong Xiao, Jian Wu, Long Zhou, Shuo Ren, Yanmin Qian, Yao Qian, Jian Wu, Michael Zeng, Furu Wei
|
27 |
+
|
28 |
+
**Abstract**
|
29 |
+
*Self-supervised learning (SSL) achieves great success in speech recognition, while limited exploration has been attempted for other speech processing tasks. As speech signal contains multi-faceted information including speaker identity, paralinguistics, spoken content, etc., learning universal representations for all speech tasks is challenging. In this paper, we propose a new pre-trained model, WavLM, to solve full-stack downstream speech tasks. WavLM is built based on the HuBERT framework, with an emphasis on both spoken content modeling and speaker identity preservation. We first equip the Transformer structure with gated relative position bias to improve its capability on recognition tasks. For better speaker discrimination, we propose an utterance mixing training strategy, where additional overlapped utterances are created unsupervisely and incorporated during model training. Lastly, we scale up the training dataset from 60k hours to 94k hours. WavLM Large achieves state-of-the-art performance on the SUPERB benchmark, and brings significant improvements for various speech processing tasks on their representative benchmarks.*
|
30 |
+
|
31 |
+
The original model can be found under https://github.com/microsoft/unilm/tree/master/wavlm.
|
32 |
+
|
33 |
+
# Usage
|
34 |
+
|
35 |
+
This is an English pre-trained speech model that has to be fine-tuned on a downstream task like speech recognition or audio classification before it can be
|
36 |
+
used in inference. The model was pre-trained in English and should therefore perform well only in English. The model has been shown to work well on the [SUPERB benchmark](https://superbbenchmark.org/).
|
37 |
+
|
38 |
+
**Note**: The model was pre-trained on phonemes rather than characters. This means that one should make sure that the input text is converted to a sequence
|
39 |
+
of phonemes before fine-tuning.
|
40 |
+
|
41 |
+
## Speech Recognition
|
42 |
+
|
43 |
+
To fine-tune the model for speech recognition, see [the official speech recognition example](https://github.com/huggingface/transformers/tree/master/examples/pytorch/speech-recognition).
|
44 |
+
|
45 |
+
## Speech Classification
|
46 |
+
|
47 |
+
To fine-tune the model for speech classification, see [the official audio classification example](https://github.com/huggingface/transformers/tree/master/examples/pytorch/audio-classification).
|
48 |
+
|
49 |
+
## Speaker Verification
|
50 |
+
|
51 |
+
TODO
|
52 |
+
|
53 |
+
## Speaker Diarization
|
54 |
+
|
55 |
+
TODO
|
56 |
+
|
57 |
+
# Contribution
|
58 |
+
|
59 |
+
The model was contributed by [cywang](https://huggingface.co/cywang) and [patrickvonplaten](https://huggingface.co/patrickvonplaten).
|
60 |
+
|
61 |
+
# License
|
62 |
+
|
63 |
+
The official license can be found [here](https://github.com/microsoft/UniSpeech/blob/main/LICENSE)
|
64 |
+
|
65 |
+
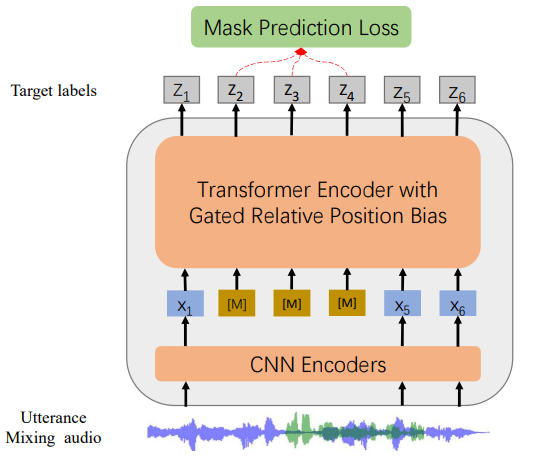
|
slm/wavlm-base-plus/config.json
ADDED
@@ -0,0 +1,99 @@
|
|
|
|
|
|
|
|
|
|
|
|
|
|
|
|
|
|
|
|
|
|
|
|
|
|
|
|
|
|
|
|
|
|
|
|
|
|
|
|
|
|
|
|
|
|
|
|
|
|
|
|
|
|
|
|
|
|
|
|
|
|
|
|
|
|
|
|
|
|
|
|
|
|
|
|
|
|
|
|
|
|
|
|
|
|
|
|
|
|
|
|
|
|
|
|
|
|
|
|
|
|
|
|
|
|
|
|
|
|
|
|
|
|
|
|
|
|
|
|
|
|
|
|
|
|
|
|
|
|
|
|
|
|
|
|
|
|
|
|
|
|
|
|
|
|
|
|
|
|
|
|
|
|
|
|
|
|
|
|
|
|
|
|
|
|
|
|
|
|
|
|
|
|
|
|
|
|
|
|
|
|
|
|
|
|
|
|
|
|
|
|
|
|
|
|
|
|
|
|
|
1 |
+
{
|
2 |
+
"_name_or_path": "wavlm-base-plus",
|
3 |
+
"activation_dropout": 0.0,
|
4 |
+
"adapter_kernel_size": 3,
|
5 |
+
"adapter_stride": 2,
|
6 |
+
"add_adapter": false,
|
7 |
+
"apply_spec_augment": true,
|
8 |
+
"architectures": [
|
9 |
+
"WavLMModel"
|
10 |
+
],
|
11 |
+
"attention_dropout": 0.1,
|
12 |
+
"bos_token_id": 1,
|
13 |
+
"classifier_proj_size": 256,
|
14 |
+
"codevector_dim": 256,
|
15 |
+
"contrastive_logits_temperature": 0.1,
|
16 |
+
"conv_bias": false,
|
17 |
+
"conv_dim": [
|
18 |
+
512,
|
19 |
+
512,
|
20 |
+
512,
|
21 |
+
512,
|
22 |
+
512,
|
23 |
+
512,
|
24 |
+
512
|
25 |
+
],
|
26 |
+
"conv_kernel": [
|
27 |
+
10,
|
28 |
+
3,
|
29 |
+
3,
|
30 |
+
3,
|
31 |
+
3,
|
32 |
+
2,
|
33 |
+
2
|
34 |
+
],
|
35 |
+
"conv_stride": [
|
36 |
+
5,
|
37 |
+
2,
|
38 |
+
2,
|
39 |
+
2,
|
40 |
+
2,
|
41 |
+
2,
|
42 |
+
2
|
43 |
+
],
|
44 |
+
"ctc_loss_reduction": "sum",
|
45 |
+
"ctc_zero_infinity": false,
|
46 |
+
"diversity_loss_weight": 0.1,
|
47 |
+
"do_stable_layer_norm": false,
|
48 |
+
"eos_token_id": 2,
|
49 |
+
"feat_extract_activation": "gelu",
|
50 |
+
"feat_extract_norm": "group",
|
51 |
+
"feat_proj_dropout": 0.1,
|
52 |
+
"feat_quantizer_dropout": 0.0,
|
53 |
+
"final_dropout": 0.0,
|
54 |
+
"freeze_feat_extract_train": true,
|
55 |
+
"hidden_act": "gelu",
|
56 |
+
"hidden_dropout": 0.1,
|
57 |
+
"hidden_size": 768,
|
58 |
+
"initializer_range": 0.02,
|
59 |
+
"intermediate_size": 3072,
|
60 |
+
"layer_norm_eps": 1e-05,
|
61 |
+
"layerdrop": 0.05,
|
62 |
+
"mask_channel_length": 10,
|
63 |
+
"mask_channel_min_space": 1,
|
64 |
+
"mask_channel_other": 0.0,
|
65 |
+
"mask_channel_prob": 0.0,
|
66 |
+
"mask_channel_selection": "static",
|
67 |
+
"mask_feature_length": 10,
|
68 |
+
"mask_feature_min_masks": 0,
|
69 |
+
"mask_feature_prob": 0.0,
|
70 |
+
"mask_time_length": 10,
|
71 |
+
"mask_time_min_masks": 2,
|
72 |
+
"mask_time_min_space": 1,
|
73 |
+
"mask_time_other": 0.0,
|
74 |
+
"mask_time_prob": 0.05,
|
75 |
+
"mask_time_selection": "static",
|
76 |
+
"model_type": "wavlm",
|
77 |
+
"no_mask_channel_overlap": false,
|
78 |
+
"no_mask_time_overlap": false,
|
79 |
+
"num_adapter_layers": 3,
|
80 |
+
"num_attention_heads": 12,
|
81 |
+
"num_buckets": 320,
|
82 |
+
"num_codevector_groups": 2,
|
83 |
+
"num_codevectors_per_group": 320,
|
84 |
+
"num_conv_pos_embedding_groups": 16,
|
85 |
+
"num_conv_pos_embeddings": 128,
|
86 |
+
"num_ctc_classes": 80,
|
87 |
+
"num_feat_extract_layers": 7,
|
88 |
+
"num_hidden_layers": 12,
|
89 |
+
"num_negatives": 100,
|
90 |
+
"output_hidden_size": 768,
|
91 |
+
"pad_token_id": 0,
|
92 |
+
"proj_codevector_dim": 256,
|
93 |
+
"replace_prob": 0.5,
|
94 |
+
"torch_dtype": "float32",
|
95 |
+
"transformers_version": "4.13.0.dev0",
|
96 |
+
"use_weighted_layer_sum": false,
|
97 |
+
"vocab_size": 32,
|
98 |
+
"tokenizer_class": "Wav2Vec2CTCTokenizer"
|
99 |
+
}
|
slm/wavlm-base-plus/preprocessor_config.json
ADDED
@@ -0,0 +1,9 @@
|
|
|
|
|
|
|
|
|
|
|
|
|
|
|
|
|
|
|
|
|
1 |
+
{
|
2 |
+
"do_normalize": false,
|
3 |
+
"feature_extractor_type": "Wav2Vec2FeatureExtractor",
|
4 |
+
"feature_size": 1,
|
5 |
+
"padding_side": "right",
|
6 |
+
"padding_value": 0.0,
|
7 |
+
"return_attention_mask": true,
|
8 |
+
"sampling_rate": 16000
|
9 |
+
}
|
slm/wavlm-base-plus/pytorch_model.bin
ADDED
@@ -0,0 +1,3 @@
|
|
|
|
|
|
|
|
|
1 |
+
version https://git-lfs.github.com/spec/v1
|
2 |
+
oid sha256:3bb273a6ace99408b50cfc81afdbb7ef2de02da2eab0234e18db608ce692fe51
|
3 |
+
size 377617425
|