html_url
stringlengths 48
51
| title
stringlengths 5
280
| comments
stringlengths 63
51.8k
| body
stringlengths 0
36.2k
⌀ | comment_length
int64 16
1.52k
| text
stringlengths 163
54.1k
|
---|---|---|---|---|---|
https://github.com/huggingface/datasets/issues/294 | Cannot load arxiv dataset on MacOS? | I was able to track down the file causing the problem by adding the following to `scientific_papers.py` (starting at line 116):
```python
from json import JSONDecodeError
try:
d = json.loads(line)
summary = "\n".join(d["abstract_text"])
except JSONDecodeError:
print(path, line)
```
For me it was at: `/Users/johngiorgi/.cache/huggingface/datasets/f87fd498c5003cbe253a2af422caa1e58f87a4fd74cb3e67350c635c8903b259/arxiv-dataset/train.txt` with `"article_id": "1407.3051"`.
Not really 100% sure at the moment, but it looks like this specific substring from `"article_text"` may be causing the problem?
```
"after the missing - mass scale adjustment , the validity of the corrections was tested in the @xmath85 productions at 1.69 gev/@xmath1 . in fig . [", "fig : calibrations ] ( a ) , we show the missing - mass spectrum in the @xmath86 region in the @xmath87 reaction at 1.69 gev/@xmath1 . a fitting result with a lorentzian function for the @xmath86 ( dashed line ) and the three - body phas
```
perhaps because it appears to be truncated. I (think) I can recreate the problem by doing the following:
```python
import json
# A minimal example of the json file that causes the error
invalid_json = '{"article_id": "1407.3051", "article_text": ["the missing - mass resolution was obtained to be 2.8 @xmath3 0.1 mev/@xmath4 ( fwhm ) , which corresponds to the missing - mass resolution of 3.2 @xmath3 0.2 mev/@xmath4 ( fwhm ) at the @xmath6 cusp region in the @xmath0 reaction .", "this resolution is at least by a factor of 2 better than the previous measurement with the same reaction ( 3.2@xmath595.5 mev/@xmath4 in @xmath84 ) @xcite .", "after the missing - mass scale adjustment , the validity of the corrections was tested in the @xmath85 productions at 1.69 gev/@xmath1 . in fig . [", "fig : calibrations ] ( a ) , we show the missing - mass spectrum in the @xmath86 region in the @xmath87 reaction at 1.69 gev/@xmath1 . a fitting result with a lorentzian function for the @xmath86 ( dashed line ) and the three - body phas'
# The line of code from `scientific_papers.py` which appears to cause the error
json.loads(invalid_json)
```
This is as far as I get before I am stumped. | I am having trouble loading the `"arxiv"` config from the `"scientific_papers"` dataset on MacOS. When I try loading the dataset with:
```python
arxiv = nlp.load_dataset("scientific_papers", "arxiv")
```
I get the following stack trace:
```bash
JSONDecodeError Traceback (most recent call last)
<ipython-input-2-8e00c55d5a59> in <module>
----> 1 arxiv = nlp.load_dataset("scientific_papers", "arxiv")
~/miniconda3/envs/t2t/lib/python3.7/site-packages/nlp/load.py in load_dataset(path, name, version, data_dir, data_files, split, cache_dir, download_config, download_mode, ignore_verifications, save_infos, **config_kwargs)
522 download_mode=download_mode,
523 ignore_verifications=ignore_verifications,
--> 524 save_infos=save_infos,
525 )
526
~/miniconda3/envs/t2t/lib/python3.7/site-packages/nlp/builder.py in download_and_prepare(self, download_config, download_mode, ignore_verifications, save_infos, try_from_hf_gcs, dl_manager, **download_and_prepare_kwargs)
430 verify_infos = not save_infos and not ignore_verifications
431 self._download_and_prepare(
--> 432 dl_manager=dl_manager, verify_infos=verify_infos, **download_and_prepare_kwargs
433 )
434 # Sync info
~/miniconda3/envs/t2t/lib/python3.7/site-packages/nlp/builder.py in _download_and_prepare(self, dl_manager, verify_infos, **prepare_split_kwargs)
481 try:
482 # Prepare split will record examples associated to the split
--> 483 self._prepare_split(split_generator, **prepare_split_kwargs)
484 except OSError:
485 raise OSError("Cannot find data file. " + (self.manual_download_instructions or ""))
~/miniconda3/envs/t2t/lib/python3.7/site-packages/nlp/builder.py in _prepare_split(self, split_generator)
662
663 generator = self._generate_examples(**split_generator.gen_kwargs)
--> 664 for key, record in utils.tqdm(generator, unit=" examples", total=split_info.num_examples, leave=False):
665 example = self.info.features.encode_example(record)
666 writer.write(example)
~/miniconda3/envs/t2t/lib/python3.7/site-packages/tqdm/std.py in __iter__(self)
1106 fp_write=getattr(self.fp, 'write', sys.stderr.write))
1107
-> 1108 for obj in iterable:
1109 yield obj
1110 # Update and possibly print the progressbar.
~/miniconda3/envs/t2t/lib/python3.7/site-packages/nlp/datasets/scientific_papers/107a416c0e1958cb846f5934b5aae292f7884a5b27e86af3f3ef1a093e058bbc/scientific_papers.py in _generate_examples(self, path)
114 # "section_names": list[str], list of section names.
115 # "sections": list[list[str]], list of sections (list of paragraphs)
--> 116 d = json.loads(line)
117 summary = "\n".join(d["abstract_text"])
118 # In original paper, <S> and </S> are not used in vocab during training
~/miniconda3/envs/t2t/lib/python3.7/json/__init__.py in loads(s, encoding, cls, object_hook, parse_float, parse_int, parse_constant, object_pairs_hook, **kw)
346 parse_int is None and parse_float is None and
347 parse_constant is None and object_pairs_hook is None and not kw):
--> 348 return _default_decoder.decode(s)
349 if cls is None:
350 cls = JSONDecoder
~/miniconda3/envs/t2t/lib/python3.7/json/decoder.py in decode(self, s, _w)
335
336 """
--> 337 obj, end = self.raw_decode(s, idx=_w(s, 0).end())
338 end = _w(s, end).end()
339 if end != len(s):
~/miniconda3/envs/t2t/lib/python3.7/json/decoder.py in raw_decode(self, s, idx)
351 """
352 try:
--> 353 obj, end = self.scan_once(s, idx)
354 except StopIteration as err:
355 raise JSONDecodeError("Expecting value", s, err.value) from None
JSONDecodeError: Unterminated string starting at: line 1 column 46983 (char 46982)
163502 examples [02:10, 2710.68 examples/s]
```
I am not sure how to trace back to the specific JSON file that has the "Unterminated string". Also, I do not get this error on colab so I suspect it may be MacOS specific. Copy pasting the relevant lines from `transformers-cli env` below:
- Platform: Darwin-19.5.0-x86_64-i386-64bit
- Python version: 3.7.5
- PyTorch version (GPU?): 1.5.0 (False)
- Tensorflow version (GPU?): 2.2.0 (False)
Any ideas? | 352 | Cannot load arxiv dataset on MacOS?
I am having trouble loading the `"arxiv"` config from the `"scientific_papers"` dataset on MacOS. When I try loading the dataset with:
```python
arxiv = nlp.load_dataset("scientific_papers", "arxiv")
```
I get the following stack trace:
```bash
JSONDecodeError Traceback (most recent call last)
<ipython-input-2-8e00c55d5a59> in <module>
----> 1 arxiv = nlp.load_dataset("scientific_papers", "arxiv")
~/miniconda3/envs/t2t/lib/python3.7/site-packages/nlp/load.py in load_dataset(path, name, version, data_dir, data_files, split, cache_dir, download_config, download_mode, ignore_verifications, save_infos, **config_kwargs)
522 download_mode=download_mode,
523 ignore_verifications=ignore_verifications,
--> 524 save_infos=save_infos,
525 )
526
~/miniconda3/envs/t2t/lib/python3.7/site-packages/nlp/builder.py in download_and_prepare(self, download_config, download_mode, ignore_verifications, save_infos, try_from_hf_gcs, dl_manager, **download_and_prepare_kwargs)
430 verify_infos = not save_infos and not ignore_verifications
431 self._download_and_prepare(
--> 432 dl_manager=dl_manager, verify_infos=verify_infos, **download_and_prepare_kwargs
433 )
434 # Sync info
~/miniconda3/envs/t2t/lib/python3.7/site-packages/nlp/builder.py in _download_and_prepare(self, dl_manager, verify_infos, **prepare_split_kwargs)
481 try:
482 # Prepare split will record examples associated to the split
--> 483 self._prepare_split(split_generator, **prepare_split_kwargs)
484 except OSError:
485 raise OSError("Cannot find data file. " + (self.manual_download_instructions or ""))
~/miniconda3/envs/t2t/lib/python3.7/site-packages/nlp/builder.py in _prepare_split(self, split_generator)
662
663 generator = self._generate_examples(**split_generator.gen_kwargs)
--> 664 for key, record in utils.tqdm(generator, unit=" examples", total=split_info.num_examples, leave=False):
665 example = self.info.features.encode_example(record)
666 writer.write(example)
~/miniconda3/envs/t2t/lib/python3.7/site-packages/tqdm/std.py in __iter__(self)
1106 fp_write=getattr(self.fp, 'write', sys.stderr.write))
1107
-> 1108 for obj in iterable:
1109 yield obj
1110 # Update and possibly print the progressbar.
~/miniconda3/envs/t2t/lib/python3.7/site-packages/nlp/datasets/scientific_papers/107a416c0e1958cb846f5934b5aae292f7884a5b27e86af3f3ef1a093e058bbc/scientific_papers.py in _generate_examples(self, path)
114 # "section_names": list[str], list of section names.
115 # "sections": list[list[str]], list of sections (list of paragraphs)
--> 116 d = json.loads(line)
117 summary = "\n".join(d["abstract_text"])
118 # In original paper, <S> and </S> are not used in vocab during training
~/miniconda3/envs/t2t/lib/python3.7/json/__init__.py in loads(s, encoding, cls, object_hook, parse_float, parse_int, parse_constant, object_pairs_hook, **kw)
346 parse_int is None and parse_float is None and
347 parse_constant is None and object_pairs_hook is None and not kw):
--> 348 return _default_decoder.decode(s)
349 if cls is None:
350 cls = JSONDecoder
~/miniconda3/envs/t2t/lib/python3.7/json/decoder.py in decode(self, s, _w)
335
336 """
--> 337 obj, end = self.raw_decode(s, idx=_w(s, 0).end())
338 end = _w(s, end).end()
339 if end != len(s):
~/miniconda3/envs/t2t/lib/python3.7/json/decoder.py in raw_decode(self, s, idx)
351 """
352 try:
--> 353 obj, end = self.scan_once(s, idx)
354 except StopIteration as err:
355 raise JSONDecodeError("Expecting value", s, err.value) from None
JSONDecodeError: Unterminated string starting at: line 1 column 46983 (char 46982)
163502 examples [02:10, 2710.68 examples/s]
```
I am not sure how to trace back to the specific JSON file that has the "Unterminated string". Also, I do not get this error on colab so I suspect it may be MacOS specific. Copy pasting the relevant lines from `transformers-cli env` below:
- Platform: Darwin-19.5.0-x86_64-i386-64bit
- Python version: 3.7.5
- PyTorch version (GPU?): 1.5.0 (False)
- Tensorflow version (GPU?): 2.2.0 (False)
Any ideas?
I was able to track down the file causing the problem by adding the following to `scientific_papers.py` (starting at line 116):
```python
from json import JSONDecodeError
try:
d = json.loads(line)
summary = "\n".join(d["abstract_text"])
except JSONDecodeError:
print(path, line)
```
For me it was at: `/Users/johngiorgi/.cache/huggingface/datasets/f87fd498c5003cbe253a2af422caa1e58f87a4fd74cb3e67350c635c8903b259/arxiv-dataset/train.txt` with `"article_id": "1407.3051"`.
Not really 100% sure at the moment, but it looks like this specific substring from `"article_text"` may be causing the problem?
```
"after the missing - mass scale adjustment , the validity of the corrections was tested in the @xmath85 productions at 1.69 gev/@xmath1 . in fig . [", "fig : calibrations ] ( a ) , we show the missing - mass spectrum in the @xmath86 region in the @xmath87 reaction at 1.69 gev/@xmath1 . a fitting result with a lorentzian function for the @xmath86 ( dashed line ) and the three - body phas
```
perhaps because it appears to be truncated. I (think) I can recreate the problem by doing the following:
```python
import json
# A minimal example of the json file that causes the error
invalid_json = '{"article_id": "1407.3051", "article_text": ["the missing - mass resolution was obtained to be 2.8 @xmath3 0.1 mev/@xmath4 ( fwhm ) , which corresponds to the missing - mass resolution of 3.2 @xmath3 0.2 mev/@xmath4 ( fwhm ) at the @xmath6 cusp region in the @xmath0 reaction .", "this resolution is at least by a factor of 2 better than the previous measurement with the same reaction ( 3.2@xmath595.5 mev/@xmath4 in @xmath84 ) @xcite .", "after the missing - mass scale adjustment , the validity of the corrections was tested in the @xmath85 productions at 1.69 gev/@xmath1 . in fig . [", "fig : calibrations ] ( a ) , we show the missing - mass spectrum in the @xmath86 region in the @xmath87 reaction at 1.69 gev/@xmath1 . a fitting result with a lorentzian function for the @xmath86 ( dashed line ) and the three - body phas'
# The line of code from `scientific_papers.py` which appears to cause the error
json.loads(invalid_json)
```
This is as far as I get before I am stumped. |
https://github.com/huggingface/datasets/issues/294 | Cannot load arxiv dataset on MacOS? | I just checked inside `train.txt` and this line isn't truncated for me (line 163577).
Could you try to clear your cache and re-download the dataset ? | I am having trouble loading the `"arxiv"` config from the `"scientific_papers"` dataset on MacOS. When I try loading the dataset with:
```python
arxiv = nlp.load_dataset("scientific_papers", "arxiv")
```
I get the following stack trace:
```bash
JSONDecodeError Traceback (most recent call last)
<ipython-input-2-8e00c55d5a59> in <module>
----> 1 arxiv = nlp.load_dataset("scientific_papers", "arxiv")
~/miniconda3/envs/t2t/lib/python3.7/site-packages/nlp/load.py in load_dataset(path, name, version, data_dir, data_files, split, cache_dir, download_config, download_mode, ignore_verifications, save_infos, **config_kwargs)
522 download_mode=download_mode,
523 ignore_verifications=ignore_verifications,
--> 524 save_infos=save_infos,
525 )
526
~/miniconda3/envs/t2t/lib/python3.7/site-packages/nlp/builder.py in download_and_prepare(self, download_config, download_mode, ignore_verifications, save_infos, try_from_hf_gcs, dl_manager, **download_and_prepare_kwargs)
430 verify_infos = not save_infos and not ignore_verifications
431 self._download_and_prepare(
--> 432 dl_manager=dl_manager, verify_infos=verify_infos, **download_and_prepare_kwargs
433 )
434 # Sync info
~/miniconda3/envs/t2t/lib/python3.7/site-packages/nlp/builder.py in _download_and_prepare(self, dl_manager, verify_infos, **prepare_split_kwargs)
481 try:
482 # Prepare split will record examples associated to the split
--> 483 self._prepare_split(split_generator, **prepare_split_kwargs)
484 except OSError:
485 raise OSError("Cannot find data file. " + (self.manual_download_instructions or ""))
~/miniconda3/envs/t2t/lib/python3.7/site-packages/nlp/builder.py in _prepare_split(self, split_generator)
662
663 generator = self._generate_examples(**split_generator.gen_kwargs)
--> 664 for key, record in utils.tqdm(generator, unit=" examples", total=split_info.num_examples, leave=False):
665 example = self.info.features.encode_example(record)
666 writer.write(example)
~/miniconda3/envs/t2t/lib/python3.7/site-packages/tqdm/std.py in __iter__(self)
1106 fp_write=getattr(self.fp, 'write', sys.stderr.write))
1107
-> 1108 for obj in iterable:
1109 yield obj
1110 # Update and possibly print the progressbar.
~/miniconda3/envs/t2t/lib/python3.7/site-packages/nlp/datasets/scientific_papers/107a416c0e1958cb846f5934b5aae292f7884a5b27e86af3f3ef1a093e058bbc/scientific_papers.py in _generate_examples(self, path)
114 # "section_names": list[str], list of section names.
115 # "sections": list[list[str]], list of sections (list of paragraphs)
--> 116 d = json.loads(line)
117 summary = "\n".join(d["abstract_text"])
118 # In original paper, <S> and </S> are not used in vocab during training
~/miniconda3/envs/t2t/lib/python3.7/json/__init__.py in loads(s, encoding, cls, object_hook, parse_float, parse_int, parse_constant, object_pairs_hook, **kw)
346 parse_int is None and parse_float is None and
347 parse_constant is None and object_pairs_hook is None and not kw):
--> 348 return _default_decoder.decode(s)
349 if cls is None:
350 cls = JSONDecoder
~/miniconda3/envs/t2t/lib/python3.7/json/decoder.py in decode(self, s, _w)
335
336 """
--> 337 obj, end = self.raw_decode(s, idx=_w(s, 0).end())
338 end = _w(s, end).end()
339 if end != len(s):
~/miniconda3/envs/t2t/lib/python3.7/json/decoder.py in raw_decode(self, s, idx)
351 """
352 try:
--> 353 obj, end = self.scan_once(s, idx)
354 except StopIteration as err:
355 raise JSONDecodeError("Expecting value", s, err.value) from None
JSONDecodeError: Unterminated string starting at: line 1 column 46983 (char 46982)
163502 examples [02:10, 2710.68 examples/s]
```
I am not sure how to trace back to the specific JSON file that has the "Unterminated string". Also, I do not get this error on colab so I suspect it may be MacOS specific. Copy pasting the relevant lines from `transformers-cli env` below:
- Platform: Darwin-19.5.0-x86_64-i386-64bit
- Python version: 3.7.5
- PyTorch version (GPU?): 1.5.0 (False)
- Tensorflow version (GPU?): 2.2.0 (False)
Any ideas? | 26 | Cannot load arxiv dataset on MacOS?
I am having trouble loading the `"arxiv"` config from the `"scientific_papers"` dataset on MacOS. When I try loading the dataset with:
```python
arxiv = nlp.load_dataset("scientific_papers", "arxiv")
```
I get the following stack trace:
```bash
JSONDecodeError Traceback (most recent call last)
<ipython-input-2-8e00c55d5a59> in <module>
----> 1 arxiv = nlp.load_dataset("scientific_papers", "arxiv")
~/miniconda3/envs/t2t/lib/python3.7/site-packages/nlp/load.py in load_dataset(path, name, version, data_dir, data_files, split, cache_dir, download_config, download_mode, ignore_verifications, save_infos, **config_kwargs)
522 download_mode=download_mode,
523 ignore_verifications=ignore_verifications,
--> 524 save_infos=save_infos,
525 )
526
~/miniconda3/envs/t2t/lib/python3.7/site-packages/nlp/builder.py in download_and_prepare(self, download_config, download_mode, ignore_verifications, save_infos, try_from_hf_gcs, dl_manager, **download_and_prepare_kwargs)
430 verify_infos = not save_infos and not ignore_verifications
431 self._download_and_prepare(
--> 432 dl_manager=dl_manager, verify_infos=verify_infos, **download_and_prepare_kwargs
433 )
434 # Sync info
~/miniconda3/envs/t2t/lib/python3.7/site-packages/nlp/builder.py in _download_and_prepare(self, dl_manager, verify_infos, **prepare_split_kwargs)
481 try:
482 # Prepare split will record examples associated to the split
--> 483 self._prepare_split(split_generator, **prepare_split_kwargs)
484 except OSError:
485 raise OSError("Cannot find data file. " + (self.manual_download_instructions or ""))
~/miniconda3/envs/t2t/lib/python3.7/site-packages/nlp/builder.py in _prepare_split(self, split_generator)
662
663 generator = self._generate_examples(**split_generator.gen_kwargs)
--> 664 for key, record in utils.tqdm(generator, unit=" examples", total=split_info.num_examples, leave=False):
665 example = self.info.features.encode_example(record)
666 writer.write(example)
~/miniconda3/envs/t2t/lib/python3.7/site-packages/tqdm/std.py in __iter__(self)
1106 fp_write=getattr(self.fp, 'write', sys.stderr.write))
1107
-> 1108 for obj in iterable:
1109 yield obj
1110 # Update and possibly print the progressbar.
~/miniconda3/envs/t2t/lib/python3.7/site-packages/nlp/datasets/scientific_papers/107a416c0e1958cb846f5934b5aae292f7884a5b27e86af3f3ef1a093e058bbc/scientific_papers.py in _generate_examples(self, path)
114 # "section_names": list[str], list of section names.
115 # "sections": list[list[str]], list of sections (list of paragraphs)
--> 116 d = json.loads(line)
117 summary = "\n".join(d["abstract_text"])
118 # In original paper, <S> and </S> are not used in vocab during training
~/miniconda3/envs/t2t/lib/python3.7/json/__init__.py in loads(s, encoding, cls, object_hook, parse_float, parse_int, parse_constant, object_pairs_hook, **kw)
346 parse_int is None and parse_float is None and
347 parse_constant is None and object_pairs_hook is None and not kw):
--> 348 return _default_decoder.decode(s)
349 if cls is None:
350 cls = JSONDecoder
~/miniconda3/envs/t2t/lib/python3.7/json/decoder.py in decode(self, s, _w)
335
336 """
--> 337 obj, end = self.raw_decode(s, idx=_w(s, 0).end())
338 end = _w(s, end).end()
339 if end != len(s):
~/miniconda3/envs/t2t/lib/python3.7/json/decoder.py in raw_decode(self, s, idx)
351 """
352 try:
--> 353 obj, end = self.scan_once(s, idx)
354 except StopIteration as err:
355 raise JSONDecodeError("Expecting value", s, err.value) from None
JSONDecodeError: Unterminated string starting at: line 1 column 46983 (char 46982)
163502 examples [02:10, 2710.68 examples/s]
```
I am not sure how to trace back to the specific JSON file that has the "Unterminated string". Also, I do not get this error on colab so I suspect it may be MacOS specific. Copy pasting the relevant lines from `transformers-cli env` below:
- Platform: Darwin-19.5.0-x86_64-i386-64bit
- Python version: 3.7.5
- PyTorch version (GPU?): 1.5.0 (False)
- Tensorflow version (GPU?): 2.2.0 (False)
Any ideas?
I just checked inside `train.txt` and this line isn't truncated for me (line 163577).
Could you try to clear your cache and re-download the dataset ? |
https://github.com/huggingface/datasets/issues/290 | ConnectionError - Eli5 dataset download | It should ne fixed now, thanks for reporting this one :)
It was an issue on our google storage.
Let me now if you're still facing this issue. | Hi, I have a problem with downloading Eli5 dataset. When typing `nlp.load_dataset('eli5')`, I get ConnectionError: Couldn't reach https://storage.googleapis.com/huggingface-nlp/cache/datasets/eli5/LFQA_reddit/1.0.0/explain_like_im_five-train_eli5.arrow
I would appreciate if you could help me with this issue. | 28 | ConnectionError - Eli5 dataset download
Hi, I have a problem with downloading Eli5 dataset. When typing `nlp.load_dataset('eli5')`, I get ConnectionError: Couldn't reach https://storage.googleapis.com/huggingface-nlp/cache/datasets/eli5/LFQA_reddit/1.0.0/explain_like_im_five-train_eli5.arrow
I would appreciate if you could help me with this issue.
It should ne fixed now, thanks for reporting this one :)
It was an issue on our google storage.
Let me now if you're still facing this issue. |
https://github.com/huggingface/datasets/issues/288 | Error at the first example in README: AttributeError: module 'dill' has no attribute '_dill' | It looks like the bug comes from `dill`. Which version of `dill` are you using ? | /Users/parasol_tree/anaconda3/lib/python3.6/site-packages/tensorflow/python/framework/dtypes.py:469: FutureWarning: Passing (type, 1) or '1type' as a synonym of type is deprecated; in a future version of numpy, it will be understood as (type, (1,)) / '(1,)type'.
_np_qint8 = np.dtype([("qint8", np.int8, 1)])
/Users/parasol_tree/anaconda3/lib/python3.6/site-packages/tensorflow/python/framework/dtypes.py:470: FutureWarning: Passing (type, 1) or '1type' as a synonym of type is deprecated; in a future version of numpy, it will be understood as (type, (1,)) / '(1,)type'.
_np_quint8 = np.dtype([("quint8", np.uint8, 1)])
/Users/parasol_tree/anaconda3/lib/python3.6/site-packages/tensorflow/python/framework/dtypes.py:471: FutureWarning: Passing (type, 1) or '1type' as a synonym of type is deprecated; in a future version of numpy, it will be understood as (type, (1,)) / '(1,)type'.
_np_qint16 = np.dtype([("qint16", np.int16, 1)])
/Users/parasol_tree/anaconda3/lib/python3.6/site-packages/tensorflow/python/framework/dtypes.py:472: FutureWarning: Passing (type, 1) or '1type' as a synonym of type is deprecated; in a future version of numpy, it will be understood as (type, (1,)) / '(1,)type'.
_np_quint16 = np.dtype([("quint16", np.uint16, 1)])
/Users/parasol_tree/anaconda3/lib/python3.6/site-packages/tensorflow/python/framework/dtypes.py:473: FutureWarning: Passing (type, 1) or '1type' as a synonym of type is deprecated; in a future version of numpy, it will be understood as (type, (1,)) / '(1,)type'.
_np_qint32 = np.dtype([("qint32", np.int32, 1)])
/Users/parasol_tree/anaconda3/lib/python3.6/site-packages/tensorflow/python/framework/dtypes.py:476: FutureWarning: Passing (type, 1) or '1type' as a synonym of type is deprecated; in a future version of numpy, it will be understood as (type, (1,)) / '(1,)type'.
np_resource = np.dtype([("resource", np.ubyte, 1)])
/Users/parasol_tree/anaconda3/lib/python3.6/importlib/_bootstrap.py:219: RuntimeWarning: compiletime version 3.5 of module 'tensorflow.python.framework.fast_tensor_util' does not match runtime version 3.6
return f(*args, **kwds)
/Users/parasol_tree/anaconda3/lib/python3.6/site-packages/h5py/__init__.py:36: FutureWarning: Conversion of the second argument of issubdtype from `float` to `np.floating` is deprecated. In future, it will be treated as `np.float64 == np.dtype(float).type`.
from ._conv import register_converters as _register_converters
Traceback (most recent call last):
File "/Users/parasol_tree/Resource/019 - Github/AcademicEnglishToolkit /test.py", line 7, in <module>
import nlp
File "/Users/parasol_tree/anaconda3/lib/python3.6/site-packages/nlp/__init__.py", line 27, in <module>
from .arrow_dataset import Dataset
File "/Users/parasol_tree/anaconda3/lib/python3.6/site-packages/nlp/arrow_dataset.py", line 31, in <module>
from nlp.utils.py_utils import dumps
File "/Users/parasol_tree/anaconda3/lib/python3.6/site-packages/nlp/utils/__init__.py", line 20, in <module>
from .download_manager import DownloadManager, GenerateMode
File "/Users/parasol_tree/anaconda3/lib/python3.6/site-packages/nlp/utils/download_manager.py", line 25, in <module>
from .py_utils import flatten_nested, map_nested, size_str
File "/Users/parasol_tree/anaconda3/lib/python3.6/site-packages/nlp/utils/py_utils.py", line 244, in <module>
class Pickler(dill.Pickler):
File "/Users/parasol_tree/anaconda3/lib/python3.6/site-packages/nlp/utils/py_utils.py", line 247, in Pickler
dispatch = dill._dill.MetaCatchingDict(dill.Pickler.dispatch.copy())
AttributeError: module 'dill' has no attribute '_dill' | 16 | Error at the first example in README: AttributeError: module 'dill' has no attribute '_dill'
/Users/parasol_tree/anaconda3/lib/python3.6/site-packages/tensorflow/python/framework/dtypes.py:469: FutureWarning: Passing (type, 1) or '1type' as a synonym of type is deprecated; in a future version of numpy, it will be understood as (type, (1,)) / '(1,)type'.
_np_qint8 = np.dtype([("qint8", np.int8, 1)])
/Users/parasol_tree/anaconda3/lib/python3.6/site-packages/tensorflow/python/framework/dtypes.py:470: FutureWarning: Passing (type, 1) or '1type' as a synonym of type is deprecated; in a future version of numpy, it will be understood as (type, (1,)) / '(1,)type'.
_np_quint8 = np.dtype([("quint8", np.uint8, 1)])
/Users/parasol_tree/anaconda3/lib/python3.6/site-packages/tensorflow/python/framework/dtypes.py:471: FutureWarning: Passing (type, 1) or '1type' as a synonym of type is deprecated; in a future version of numpy, it will be understood as (type, (1,)) / '(1,)type'.
_np_qint16 = np.dtype([("qint16", np.int16, 1)])
/Users/parasol_tree/anaconda3/lib/python3.6/site-packages/tensorflow/python/framework/dtypes.py:472: FutureWarning: Passing (type, 1) or '1type' as a synonym of type is deprecated; in a future version of numpy, it will be understood as (type, (1,)) / '(1,)type'.
_np_quint16 = np.dtype([("quint16", np.uint16, 1)])
/Users/parasol_tree/anaconda3/lib/python3.6/site-packages/tensorflow/python/framework/dtypes.py:473: FutureWarning: Passing (type, 1) or '1type' as a synonym of type is deprecated; in a future version of numpy, it will be understood as (type, (1,)) / '(1,)type'.
_np_qint32 = np.dtype([("qint32", np.int32, 1)])
/Users/parasol_tree/anaconda3/lib/python3.6/site-packages/tensorflow/python/framework/dtypes.py:476: FutureWarning: Passing (type, 1) or '1type' as a synonym of type is deprecated; in a future version of numpy, it will be understood as (type, (1,)) / '(1,)type'.
np_resource = np.dtype([("resource", np.ubyte, 1)])
/Users/parasol_tree/anaconda3/lib/python3.6/importlib/_bootstrap.py:219: RuntimeWarning: compiletime version 3.5 of module 'tensorflow.python.framework.fast_tensor_util' does not match runtime version 3.6
return f(*args, **kwds)
/Users/parasol_tree/anaconda3/lib/python3.6/site-packages/h5py/__init__.py:36: FutureWarning: Conversion of the second argument of issubdtype from `float` to `np.floating` is deprecated. In future, it will be treated as `np.float64 == np.dtype(float).type`.
from ._conv import register_converters as _register_converters
Traceback (most recent call last):
File "/Users/parasol_tree/Resource/019 - Github/AcademicEnglishToolkit /test.py", line 7, in <module>
import nlp
File "/Users/parasol_tree/anaconda3/lib/python3.6/site-packages/nlp/__init__.py", line 27, in <module>
from .arrow_dataset import Dataset
File "/Users/parasol_tree/anaconda3/lib/python3.6/site-packages/nlp/arrow_dataset.py", line 31, in <module>
from nlp.utils.py_utils import dumps
File "/Users/parasol_tree/anaconda3/lib/python3.6/site-packages/nlp/utils/__init__.py", line 20, in <module>
from .download_manager import DownloadManager, GenerateMode
File "/Users/parasol_tree/anaconda3/lib/python3.6/site-packages/nlp/utils/download_manager.py", line 25, in <module>
from .py_utils import flatten_nested, map_nested, size_str
File "/Users/parasol_tree/anaconda3/lib/python3.6/site-packages/nlp/utils/py_utils.py", line 244, in <module>
class Pickler(dill.Pickler):
File "/Users/parasol_tree/anaconda3/lib/python3.6/site-packages/nlp/utils/py_utils.py", line 247, in Pickler
dispatch = dill._dill.MetaCatchingDict(dill.Pickler.dispatch.copy())
AttributeError: module 'dill' has no attribute '_dill'
It looks like the bug comes from `dill`. Which version of `dill` are you using ? |
https://github.com/huggingface/datasets/issues/288 | Error at the first example in README: AttributeError: module 'dill' has no attribute '_dill' | 0.2.6 is three years old now, maybe try a more recent one, e.g. the current 0.3.2 if you can? | /Users/parasol_tree/anaconda3/lib/python3.6/site-packages/tensorflow/python/framework/dtypes.py:469: FutureWarning: Passing (type, 1) or '1type' as a synonym of type is deprecated; in a future version of numpy, it will be understood as (type, (1,)) / '(1,)type'.
_np_qint8 = np.dtype([("qint8", np.int8, 1)])
/Users/parasol_tree/anaconda3/lib/python3.6/site-packages/tensorflow/python/framework/dtypes.py:470: FutureWarning: Passing (type, 1) or '1type' as a synonym of type is deprecated; in a future version of numpy, it will be understood as (type, (1,)) / '(1,)type'.
_np_quint8 = np.dtype([("quint8", np.uint8, 1)])
/Users/parasol_tree/anaconda3/lib/python3.6/site-packages/tensorflow/python/framework/dtypes.py:471: FutureWarning: Passing (type, 1) or '1type' as a synonym of type is deprecated; in a future version of numpy, it will be understood as (type, (1,)) / '(1,)type'.
_np_qint16 = np.dtype([("qint16", np.int16, 1)])
/Users/parasol_tree/anaconda3/lib/python3.6/site-packages/tensorflow/python/framework/dtypes.py:472: FutureWarning: Passing (type, 1) or '1type' as a synonym of type is deprecated; in a future version of numpy, it will be understood as (type, (1,)) / '(1,)type'.
_np_quint16 = np.dtype([("quint16", np.uint16, 1)])
/Users/parasol_tree/anaconda3/lib/python3.6/site-packages/tensorflow/python/framework/dtypes.py:473: FutureWarning: Passing (type, 1) or '1type' as a synonym of type is deprecated; in a future version of numpy, it will be understood as (type, (1,)) / '(1,)type'.
_np_qint32 = np.dtype([("qint32", np.int32, 1)])
/Users/parasol_tree/anaconda3/lib/python3.6/site-packages/tensorflow/python/framework/dtypes.py:476: FutureWarning: Passing (type, 1) or '1type' as a synonym of type is deprecated; in a future version of numpy, it will be understood as (type, (1,)) / '(1,)type'.
np_resource = np.dtype([("resource", np.ubyte, 1)])
/Users/parasol_tree/anaconda3/lib/python3.6/importlib/_bootstrap.py:219: RuntimeWarning: compiletime version 3.5 of module 'tensorflow.python.framework.fast_tensor_util' does not match runtime version 3.6
return f(*args, **kwds)
/Users/parasol_tree/anaconda3/lib/python3.6/site-packages/h5py/__init__.py:36: FutureWarning: Conversion of the second argument of issubdtype from `float` to `np.floating` is deprecated. In future, it will be treated as `np.float64 == np.dtype(float).type`.
from ._conv import register_converters as _register_converters
Traceback (most recent call last):
File "/Users/parasol_tree/Resource/019 - Github/AcademicEnglishToolkit /test.py", line 7, in <module>
import nlp
File "/Users/parasol_tree/anaconda3/lib/python3.6/site-packages/nlp/__init__.py", line 27, in <module>
from .arrow_dataset import Dataset
File "/Users/parasol_tree/anaconda3/lib/python3.6/site-packages/nlp/arrow_dataset.py", line 31, in <module>
from nlp.utils.py_utils import dumps
File "/Users/parasol_tree/anaconda3/lib/python3.6/site-packages/nlp/utils/__init__.py", line 20, in <module>
from .download_manager import DownloadManager, GenerateMode
File "/Users/parasol_tree/anaconda3/lib/python3.6/site-packages/nlp/utils/download_manager.py", line 25, in <module>
from .py_utils import flatten_nested, map_nested, size_str
File "/Users/parasol_tree/anaconda3/lib/python3.6/site-packages/nlp/utils/py_utils.py", line 244, in <module>
class Pickler(dill.Pickler):
File "/Users/parasol_tree/anaconda3/lib/python3.6/site-packages/nlp/utils/py_utils.py", line 247, in Pickler
dispatch = dill._dill.MetaCatchingDict(dill.Pickler.dispatch.copy())
AttributeError: module 'dill' has no attribute '_dill' | 19 | Error at the first example in README: AttributeError: module 'dill' has no attribute '_dill'
/Users/parasol_tree/anaconda3/lib/python3.6/site-packages/tensorflow/python/framework/dtypes.py:469: FutureWarning: Passing (type, 1) or '1type' as a synonym of type is deprecated; in a future version of numpy, it will be understood as (type, (1,)) / '(1,)type'.
_np_qint8 = np.dtype([("qint8", np.int8, 1)])
/Users/parasol_tree/anaconda3/lib/python3.6/site-packages/tensorflow/python/framework/dtypes.py:470: FutureWarning: Passing (type, 1) or '1type' as a synonym of type is deprecated; in a future version of numpy, it will be understood as (type, (1,)) / '(1,)type'.
_np_quint8 = np.dtype([("quint8", np.uint8, 1)])
/Users/parasol_tree/anaconda3/lib/python3.6/site-packages/tensorflow/python/framework/dtypes.py:471: FutureWarning: Passing (type, 1) or '1type' as a synonym of type is deprecated; in a future version of numpy, it will be understood as (type, (1,)) / '(1,)type'.
_np_qint16 = np.dtype([("qint16", np.int16, 1)])
/Users/parasol_tree/anaconda3/lib/python3.6/site-packages/tensorflow/python/framework/dtypes.py:472: FutureWarning: Passing (type, 1) or '1type' as a synonym of type is deprecated; in a future version of numpy, it will be understood as (type, (1,)) / '(1,)type'.
_np_quint16 = np.dtype([("quint16", np.uint16, 1)])
/Users/parasol_tree/anaconda3/lib/python3.6/site-packages/tensorflow/python/framework/dtypes.py:473: FutureWarning: Passing (type, 1) or '1type' as a synonym of type is deprecated; in a future version of numpy, it will be understood as (type, (1,)) / '(1,)type'.
_np_qint32 = np.dtype([("qint32", np.int32, 1)])
/Users/parasol_tree/anaconda3/lib/python3.6/site-packages/tensorflow/python/framework/dtypes.py:476: FutureWarning: Passing (type, 1) or '1type' as a synonym of type is deprecated; in a future version of numpy, it will be understood as (type, (1,)) / '(1,)type'.
np_resource = np.dtype([("resource", np.ubyte, 1)])
/Users/parasol_tree/anaconda3/lib/python3.6/importlib/_bootstrap.py:219: RuntimeWarning: compiletime version 3.5 of module 'tensorflow.python.framework.fast_tensor_util' does not match runtime version 3.6
return f(*args, **kwds)
/Users/parasol_tree/anaconda3/lib/python3.6/site-packages/h5py/__init__.py:36: FutureWarning: Conversion of the second argument of issubdtype from `float` to `np.floating` is deprecated. In future, it will be treated as `np.float64 == np.dtype(float).type`.
from ._conv import register_converters as _register_converters
Traceback (most recent call last):
File "/Users/parasol_tree/Resource/019 - Github/AcademicEnglishToolkit /test.py", line 7, in <module>
import nlp
File "/Users/parasol_tree/anaconda3/lib/python3.6/site-packages/nlp/__init__.py", line 27, in <module>
from .arrow_dataset import Dataset
File "/Users/parasol_tree/anaconda3/lib/python3.6/site-packages/nlp/arrow_dataset.py", line 31, in <module>
from nlp.utils.py_utils import dumps
File "/Users/parasol_tree/anaconda3/lib/python3.6/site-packages/nlp/utils/__init__.py", line 20, in <module>
from .download_manager import DownloadManager, GenerateMode
File "/Users/parasol_tree/anaconda3/lib/python3.6/site-packages/nlp/utils/download_manager.py", line 25, in <module>
from .py_utils import flatten_nested, map_nested, size_str
File "/Users/parasol_tree/anaconda3/lib/python3.6/site-packages/nlp/utils/py_utils.py", line 244, in <module>
class Pickler(dill.Pickler):
File "/Users/parasol_tree/anaconda3/lib/python3.6/site-packages/nlp/utils/py_utils.py", line 247, in Pickler
dispatch = dill._dill.MetaCatchingDict(dill.Pickler.dispatch.copy())
AttributeError: module 'dill' has no attribute '_dill'
0.2.6 is three years old now, maybe try a more recent one, e.g. the current 0.3.2 if you can? |
https://github.com/huggingface/datasets/issues/281 | Private/sensitive data | Hi @MFreidank, you should already be able to load a dataset from local sources, indeed. (ping @lhoestq and @jplu)
We're also thinking about the ability to host private datasets on a hosted bucket with permission management, but that's further down the road. | Hi all,
Thanks for this fantastic library, it makes it very easy to do prototyping for NLP projects interchangeably between TF/Pytorch.
Unfortunately, there is data that cannot easily be shared publicly as it may contain sensitive information.
Is there support/a plan to support such data with NLP, e.g. by reading it from local sources?
Use case flow could look like this: use NLP to prototype an approach on similar, public data and apply the resulting prototype on sensitive/private data without the need to rethink data processing pipelines.
Many thanks for your responses ahead of time and kind regards,
MFreidank | 42 | Private/sensitive data
Hi all,
Thanks for this fantastic library, it makes it very easy to do prototyping for NLP projects interchangeably between TF/Pytorch.
Unfortunately, there is data that cannot easily be shared publicly as it may contain sensitive information.
Is there support/a plan to support such data with NLP, e.g. by reading it from local sources?
Use case flow could look like this: use NLP to prototype an approach on similar, public data and apply the resulting prototype on sensitive/private data without the need to rethink data processing pipelines.
Many thanks for your responses ahead of time and kind regards,
MFreidank
Hi @MFreidank, you should already be able to load a dataset from local sources, indeed. (ping @lhoestq and @jplu)
We're also thinking about the ability to host private datasets on a hosted bucket with permission management, but that's further down the road. |
https://github.com/huggingface/datasets/issues/281 | Private/sensitive data | Hi @MFreidank, it is possible to load a dataset from your local storage, but only CSV/TSV and JSON are supported. To load a dataset in JSON format:
```
nlp.load_dataset(path="json", data_files={nlp.Split.TRAIN: ["path/to/train.json"], nlp.Split.TEST: ["path/to/test.json"]})
```
For CSV/TSV datasets, you have to replace `json` by `csv`. | Hi all,
Thanks for this fantastic library, it makes it very easy to do prototyping for NLP projects interchangeably between TF/Pytorch.
Unfortunately, there is data that cannot easily be shared publicly as it may contain sensitive information.
Is there support/a plan to support such data with NLP, e.g. by reading it from local sources?
Use case flow could look like this: use NLP to prototype an approach on similar, public data and apply the resulting prototype on sensitive/private data without the need to rethink data processing pipelines.
Many thanks for your responses ahead of time and kind regards,
MFreidank | 44 | Private/sensitive data
Hi all,
Thanks for this fantastic library, it makes it very easy to do prototyping for NLP projects interchangeably between TF/Pytorch.
Unfortunately, there is data that cannot easily be shared publicly as it may contain sensitive information.
Is there support/a plan to support such data with NLP, e.g. by reading it from local sources?
Use case flow could look like this: use NLP to prototype an approach on similar, public data and apply the resulting prototype on sensitive/private data without the need to rethink data processing pipelines.
Many thanks for your responses ahead of time and kind regards,
MFreidank
Hi @MFreidank, it is possible to load a dataset from your local storage, but only CSV/TSV and JSON are supported. To load a dataset in JSON format:
```
nlp.load_dataset(path="json", data_files={nlp.Split.TRAIN: ["path/to/train.json"], nlp.Split.TEST: ["path/to/test.json"]})
```
For CSV/TSV datasets, you have to replace `json` by `csv`. |
https://github.com/huggingface/datasets/issues/281 | Private/sensitive data | Hi @julien-c @jplu,
Thanks for sharing this solution with me, it helps, this is what I was looking for.
If not already there and only missed by me, this could be a great addition in the docs.
Closing my issue as resolved, thanks again. | Hi all,
Thanks for this fantastic library, it makes it very easy to do prototyping for NLP projects interchangeably between TF/Pytorch.
Unfortunately, there is data that cannot easily be shared publicly as it may contain sensitive information.
Is there support/a plan to support such data with NLP, e.g. by reading it from local sources?
Use case flow could look like this: use NLP to prototype an approach on similar, public data and apply the resulting prototype on sensitive/private data without the need to rethink data processing pipelines.
Many thanks for your responses ahead of time and kind regards,
MFreidank | 44 | Private/sensitive data
Hi all,
Thanks for this fantastic library, it makes it very easy to do prototyping for NLP projects interchangeably between TF/Pytorch.
Unfortunately, there is data that cannot easily be shared publicly as it may contain sensitive information.
Is there support/a plan to support such data with NLP, e.g. by reading it from local sources?
Use case flow could look like this: use NLP to prototype an approach on similar, public data and apply the resulting prototype on sensitive/private data without the need to rethink data processing pipelines.
Many thanks for your responses ahead of time and kind regards,
MFreidank
Hi @julien-c @jplu,
Thanks for sharing this solution with me, it helps, this is what I was looking for.
If not already there and only missed by me, this could be a great addition in the docs.
Closing my issue as resolved, thanks again. |
https://github.com/huggingface/datasets/issues/279 | Dataset Preprocessing Cache with .map() function not working as expected | When you're processing a dataset with `.map`, it checks whether it has already done this computation using a hash based on the function and the input (using some fancy serialization with `dill`). If you found that it doesn't work as expected in some cases, let us know !
Given that, you can still force to re-process using `.map(my_func, load_from_cache_file=False)` if you want to.
I am curious about the problem you have with splits. It makes me think about #160 that was an issue of version 0.1.0. What version of `nlp` are you running ? Could you give me more details ? | I've been having issues with reproducibility when loading and processing datasets with the `.map` function. I was only able to resolve them by clearing all of the cache files on my system.
Is there a way to disable using the cache when processing a dataset? As I make minor processing changes on the same dataset, I want to be able to be certain the data is being re-processed rather than loaded from a cached file.
Could you also help me understand a bit more about how the caching functionality is used for pre-processing? E.g. how is it determined when to load from a cache vs. reprocess.
I was particularly having an issue where the correct dataset splits were loaded, but as soon as I applied the `.map()` function to each split independently, they somehow all exited this process having been converted to the test set.
Thanks! | 101 | Dataset Preprocessing Cache with .map() function not working as expected
I've been having issues with reproducibility when loading and processing datasets with the `.map` function. I was only able to resolve them by clearing all of the cache files on my system.
Is there a way to disable using the cache when processing a dataset? As I make minor processing changes on the same dataset, I want to be able to be certain the data is being re-processed rather than loaded from a cached file.
Could you also help me understand a bit more about how the caching functionality is used for pre-processing? E.g. how is it determined when to load from a cache vs. reprocess.
I was particularly having an issue where the correct dataset splits were loaded, but as soon as I applied the `.map()` function to each split independently, they somehow all exited this process having been converted to the test set.
Thanks!
When you're processing a dataset with `.map`, it checks whether it has already done this computation using a hash based on the function and the input (using some fancy serialization with `dill`). If you found that it doesn't work as expected in some cases, let us know !
Given that, you can still force to re-process using `.map(my_func, load_from_cache_file=False)` if you want to.
I am curious about the problem you have with splits. It makes me think about #160 that was an issue of version 0.1.0. What version of `nlp` are you running ? Could you give me more details ? |
https://github.com/huggingface/datasets/issues/279 | Dataset Preprocessing Cache with .map() function not working as expected | Thanks, that's helpful! I was running 0.1.0, but since upgraded to 0.2.1. I can't reproduce the issue anymore as I've cleared the cache & everything now seems to be running fine since the upgrade. I've added some checks to my code, so if I do encounter it again I will reopen this issue. | I've been having issues with reproducibility when loading and processing datasets with the `.map` function. I was only able to resolve them by clearing all of the cache files on my system.
Is there a way to disable using the cache when processing a dataset? As I make minor processing changes on the same dataset, I want to be able to be certain the data is being re-processed rather than loaded from a cached file.
Could you also help me understand a bit more about how the caching functionality is used for pre-processing? E.g. how is it determined when to load from a cache vs. reprocess.
I was particularly having an issue where the correct dataset splits were loaded, but as soon as I applied the `.map()` function to each split independently, they somehow all exited this process having been converted to the test set.
Thanks! | 53 | Dataset Preprocessing Cache with .map() function not working as expected
I've been having issues with reproducibility when loading and processing datasets with the `.map` function. I was only able to resolve them by clearing all of the cache files on my system.
Is there a way to disable using the cache when processing a dataset? As I make minor processing changes on the same dataset, I want to be able to be certain the data is being re-processed rather than loaded from a cached file.
Could you also help me understand a bit more about how the caching functionality is used for pre-processing? E.g. how is it determined when to load from a cache vs. reprocess.
I was particularly having an issue where the correct dataset splits were loaded, but as soon as I applied the `.map()` function to each split independently, they somehow all exited this process having been converted to the test set.
Thanks!
Thanks, that's helpful! I was running 0.1.0, but since upgraded to 0.2.1. I can't reproduce the issue anymore as I've cleared the cache & everything now seems to be running fine since the upgrade. I've added some checks to my code, so if I do encounter it again I will reopen this issue. |
https://github.com/huggingface/datasets/issues/279 | Dataset Preprocessing Cache with .map() function not working as expected | Just checking in, the cache sometimes still does not work when I make changes in my processing function in version `1.2.1`. The changes made to my data processing function only propagate to the dataset when I use `load_from_cache_file=False` or clear the cache. Is this a system-specific issue? | I've been having issues with reproducibility when loading and processing datasets with the `.map` function. I was only able to resolve them by clearing all of the cache files on my system.
Is there a way to disable using the cache when processing a dataset? As I make minor processing changes on the same dataset, I want to be able to be certain the data is being re-processed rather than loaded from a cached file.
Could you also help me understand a bit more about how the caching functionality is used for pre-processing? E.g. how is it determined when to load from a cache vs. reprocess.
I was particularly having an issue where the correct dataset splits were loaded, but as soon as I applied the `.map()` function to each split independently, they somehow all exited this process having been converted to the test set.
Thanks! | 47 | Dataset Preprocessing Cache with .map() function not working as expected
I've been having issues with reproducibility when loading and processing datasets with the `.map` function. I was only able to resolve them by clearing all of the cache files on my system.
Is there a way to disable using the cache when processing a dataset? As I make minor processing changes on the same dataset, I want to be able to be certain the data is being re-processed rather than loaded from a cached file.
Could you also help me understand a bit more about how the caching functionality is used for pre-processing? E.g. how is it determined when to load from a cache vs. reprocess.
I was particularly having an issue where the correct dataset splits were loaded, but as soon as I applied the `.map()` function to each split independently, they somehow all exited this process having been converted to the test set.
Thanks!
Just checking in, the cache sometimes still does not work when I make changes in my processing function in version `1.2.1`. The changes made to my data processing function only propagate to the dataset when I use `load_from_cache_file=False` or clear the cache. Is this a system-specific issue? |
https://github.com/huggingface/datasets/issues/279 | Dataset Preprocessing Cache with .map() function not working as expected | Hi @sarahwie
The data are reloaded from the cache if the hash of the function you provide is the same as a computation you've done before. The hash is computed by recursively looking at the python objects of the function you provide.
If you think there's an issue, can you share the function you used or a google colab please ? | I've been having issues with reproducibility when loading and processing datasets with the `.map` function. I was only able to resolve them by clearing all of the cache files on my system.
Is there a way to disable using the cache when processing a dataset? As I make minor processing changes on the same dataset, I want to be able to be certain the data is being re-processed rather than loaded from a cached file.
Could you also help me understand a bit more about how the caching functionality is used for pre-processing? E.g. how is it determined when to load from a cache vs. reprocess.
I was particularly having an issue where the correct dataset splits were loaded, but as soon as I applied the `.map()` function to each split independently, they somehow all exited this process having been converted to the test set.
Thanks! | 61 | Dataset Preprocessing Cache with .map() function not working as expected
I've been having issues with reproducibility when loading and processing datasets with the `.map` function. I was only able to resolve them by clearing all of the cache files on my system.
Is there a way to disable using the cache when processing a dataset? As I make minor processing changes on the same dataset, I want to be able to be certain the data is being re-processed rather than loaded from a cached file.
Could you also help me understand a bit more about how the caching functionality is used for pre-processing? E.g. how is it determined when to load from a cache vs. reprocess.
I was particularly having an issue where the correct dataset splits were loaded, but as soon as I applied the `.map()` function to each split independently, they somehow all exited this process having been converted to the test set.
Thanks!
Hi @sarahwie
The data are reloaded from the cache if the hash of the function you provide is the same as a computation you've done before. The hash is computed by recursively looking at the python objects of the function you provide.
If you think there's an issue, can you share the function you used or a google colab please ? |
https://github.com/huggingface/datasets/issues/278 | MemoryError when loading German Wikipedia | Hi !
As you noticed, "big" datasets like Wikipedia require apache beam to be processed.
However users usually don't have an apache beam runtime available (spark, dataflow, etc.) so our goal for this library is to also make available processed versions of these datasets, so that users can just download and use them right away.
This is the case for english and french wikipedia right now: we've processed them ourselves and now they are available from our google storage. However we've not processed the german one (yet). | Hi, first off let me say thank you for all the awesome work you're doing at Hugging Face across all your projects (NLP, Transformers, Tokenizers) - they're all amazing contributions to us working with NLP models :)
I'm trying to download the German Wikipedia dataset as follows:
```
wiki = nlp.load_dataset("wikipedia", "20200501.de", split="train")
```
However, when I do so, I get the following error:
```
Downloading and preparing dataset wikipedia/20200501.de (download: Unknown size, generated: Unknown size, total: Unknown size) to /home/ubuntu/.cache/huggingface/datasets/wikipedia/20200501.de/1.0.0...
Traceback (most recent call last):
File "<stdin>", line 1, in <module>
File "/home/ubuntu/anaconda3/envs/albert/lib/python3.7/site-packages/nlp/load.py", line 520, in load_dataset
save_infos=save_infos,
File "/home/ubuntu/anaconda3/envs/albert/lib/python3.7/site-packages/nlp/builder.py", line 433, in download_and_prepare
dl_manager=dl_manager, verify_infos=verify_infos, **download_and_prepare_kwargs
File "/home/ubuntu/anaconda3/envs/albert/lib/python3.7/site-packages/nlp/builder.py", line 824, in _download_and_prepare
"\n\t`{}`".format(usage_example)
nlp.builder.MissingBeamOptions: Trying to generate a dataset using Apache Beam, yet no Beam Runner or PipelineOptions() has been provided in `load_dataset` or in the builder arguments. For big datasets it has to run on large-scale data processing tools like Dataflow, Spark, etc. More information about Apache Beam runners at https://beam.apache.org/documentation/runners/capability-matrix/
If you really want to run it locally because you feel like the Dataset is small enough, you can use the local beam runner called `DirectRunner` (you may run out of memory).
Example of usage:
`load_dataset('wikipedia', '20200501.de', beam_runner='DirectRunner')`
```
So, following on from the example usage at the bottom, I tried specifying `beam_runner='DirectRunner`, however when I do this after about 20 min after the data has all downloaded, I get a `MemoryError` as warned.
This isn't an issue for the English or French Wikipedia datasets (I've tried both), as neither seem to require that `beam_runner` be specified. Can you please clarify why this is an issue for the German dataset?
My nlp version is 0.2.1.
Thank you! | 87 | MemoryError when loading German Wikipedia
Hi, first off let me say thank you for all the awesome work you're doing at Hugging Face across all your projects (NLP, Transformers, Tokenizers) - they're all amazing contributions to us working with NLP models :)
I'm trying to download the German Wikipedia dataset as follows:
```
wiki = nlp.load_dataset("wikipedia", "20200501.de", split="train")
```
However, when I do so, I get the following error:
```
Downloading and preparing dataset wikipedia/20200501.de (download: Unknown size, generated: Unknown size, total: Unknown size) to /home/ubuntu/.cache/huggingface/datasets/wikipedia/20200501.de/1.0.0...
Traceback (most recent call last):
File "<stdin>", line 1, in <module>
File "/home/ubuntu/anaconda3/envs/albert/lib/python3.7/site-packages/nlp/load.py", line 520, in load_dataset
save_infos=save_infos,
File "/home/ubuntu/anaconda3/envs/albert/lib/python3.7/site-packages/nlp/builder.py", line 433, in download_and_prepare
dl_manager=dl_manager, verify_infos=verify_infos, **download_and_prepare_kwargs
File "/home/ubuntu/anaconda3/envs/albert/lib/python3.7/site-packages/nlp/builder.py", line 824, in _download_and_prepare
"\n\t`{}`".format(usage_example)
nlp.builder.MissingBeamOptions: Trying to generate a dataset using Apache Beam, yet no Beam Runner or PipelineOptions() has been provided in `load_dataset` or in the builder arguments. For big datasets it has to run on large-scale data processing tools like Dataflow, Spark, etc. More information about Apache Beam runners at https://beam.apache.org/documentation/runners/capability-matrix/
If you really want to run it locally because you feel like the Dataset is small enough, you can use the local beam runner called `DirectRunner` (you may run out of memory).
Example of usage:
`load_dataset('wikipedia', '20200501.de', beam_runner='DirectRunner')`
```
So, following on from the example usage at the bottom, I tried specifying `beam_runner='DirectRunner`, however when I do this after about 20 min after the data has all downloaded, I get a `MemoryError` as warned.
This isn't an issue for the English or French Wikipedia datasets (I've tried both), as neither seem to require that `beam_runner` be specified. Can you please clarify why this is an issue for the German dataset?
My nlp version is 0.2.1.
Thank you!
Hi !
As you noticed, "big" datasets like Wikipedia require apache beam to be processed.
However users usually don't have an apache beam runtime available (spark, dataflow, etc.) so our goal for this library is to also make available processed versions of these datasets, so that users can just download and use them right away.
This is the case for english and french wikipedia right now: we've processed them ourselves and now they are available from our google storage. However we've not processed the german one (yet). |
https://github.com/huggingface/datasets/issues/278 | MemoryError when loading German Wikipedia | Hi @lhoestq
Thank you for your quick reply. I thought this might be the case, that the processing was done for some languages and not for others. Is there any set timeline for when other languages (German, Italian) will be processed?
Given enough memory, is it possible to process the data ourselves by specifying the `beam_runner`? | Hi, first off let me say thank you for all the awesome work you're doing at Hugging Face across all your projects (NLP, Transformers, Tokenizers) - they're all amazing contributions to us working with NLP models :)
I'm trying to download the German Wikipedia dataset as follows:
```
wiki = nlp.load_dataset("wikipedia", "20200501.de", split="train")
```
However, when I do so, I get the following error:
```
Downloading and preparing dataset wikipedia/20200501.de (download: Unknown size, generated: Unknown size, total: Unknown size) to /home/ubuntu/.cache/huggingface/datasets/wikipedia/20200501.de/1.0.0...
Traceback (most recent call last):
File "<stdin>", line 1, in <module>
File "/home/ubuntu/anaconda3/envs/albert/lib/python3.7/site-packages/nlp/load.py", line 520, in load_dataset
save_infos=save_infos,
File "/home/ubuntu/anaconda3/envs/albert/lib/python3.7/site-packages/nlp/builder.py", line 433, in download_and_prepare
dl_manager=dl_manager, verify_infos=verify_infos, **download_and_prepare_kwargs
File "/home/ubuntu/anaconda3/envs/albert/lib/python3.7/site-packages/nlp/builder.py", line 824, in _download_and_prepare
"\n\t`{}`".format(usage_example)
nlp.builder.MissingBeamOptions: Trying to generate a dataset using Apache Beam, yet no Beam Runner or PipelineOptions() has been provided in `load_dataset` or in the builder arguments. For big datasets it has to run on large-scale data processing tools like Dataflow, Spark, etc. More information about Apache Beam runners at https://beam.apache.org/documentation/runners/capability-matrix/
If you really want to run it locally because you feel like the Dataset is small enough, you can use the local beam runner called `DirectRunner` (you may run out of memory).
Example of usage:
`load_dataset('wikipedia', '20200501.de', beam_runner='DirectRunner')`
```
So, following on from the example usage at the bottom, I tried specifying `beam_runner='DirectRunner`, however when I do this after about 20 min after the data has all downloaded, I get a `MemoryError` as warned.
This isn't an issue for the English or French Wikipedia datasets (I've tried both), as neither seem to require that `beam_runner` be specified. Can you please clarify why this is an issue for the German dataset?
My nlp version is 0.2.1.
Thank you! | 56 | MemoryError when loading German Wikipedia
Hi, first off let me say thank you for all the awesome work you're doing at Hugging Face across all your projects (NLP, Transformers, Tokenizers) - they're all amazing contributions to us working with NLP models :)
I'm trying to download the German Wikipedia dataset as follows:
```
wiki = nlp.load_dataset("wikipedia", "20200501.de", split="train")
```
However, when I do so, I get the following error:
```
Downloading and preparing dataset wikipedia/20200501.de (download: Unknown size, generated: Unknown size, total: Unknown size) to /home/ubuntu/.cache/huggingface/datasets/wikipedia/20200501.de/1.0.0...
Traceback (most recent call last):
File "<stdin>", line 1, in <module>
File "/home/ubuntu/anaconda3/envs/albert/lib/python3.7/site-packages/nlp/load.py", line 520, in load_dataset
save_infos=save_infos,
File "/home/ubuntu/anaconda3/envs/albert/lib/python3.7/site-packages/nlp/builder.py", line 433, in download_and_prepare
dl_manager=dl_manager, verify_infos=verify_infos, **download_and_prepare_kwargs
File "/home/ubuntu/anaconda3/envs/albert/lib/python3.7/site-packages/nlp/builder.py", line 824, in _download_and_prepare
"\n\t`{}`".format(usage_example)
nlp.builder.MissingBeamOptions: Trying to generate a dataset using Apache Beam, yet no Beam Runner or PipelineOptions() has been provided in `load_dataset` or in the builder arguments. For big datasets it has to run on large-scale data processing tools like Dataflow, Spark, etc. More information about Apache Beam runners at https://beam.apache.org/documentation/runners/capability-matrix/
If you really want to run it locally because you feel like the Dataset is small enough, you can use the local beam runner called `DirectRunner` (you may run out of memory).
Example of usage:
`load_dataset('wikipedia', '20200501.de', beam_runner='DirectRunner')`
```
So, following on from the example usage at the bottom, I tried specifying `beam_runner='DirectRunner`, however when I do this after about 20 min after the data has all downloaded, I get a `MemoryError` as warned.
This isn't an issue for the English or French Wikipedia datasets (I've tried both), as neither seem to require that `beam_runner` be specified. Can you please clarify why this is an issue for the German dataset?
My nlp version is 0.2.1.
Thank you!
Hi @lhoestq
Thank you for your quick reply. I thought this might be the case, that the processing was done for some languages and not for others. Is there any set timeline for when other languages (German, Italian) will be processed?
Given enough memory, is it possible to process the data ourselves by specifying the `beam_runner`? |
https://github.com/huggingface/datasets/issues/278 | MemoryError when loading German Wikipedia | Adding them is definitely in our short term objectives. I'll be working on this early next week :)
Although if you have an apache beam runtime feel free to specify the beam runner. You can find more info [here](https://github.com/huggingface/nlp/blob/master/docs/beam_dataset.md) on how to make it work on Dataflow but you can adapt it for Spark or any other beam runtime (by changing the `runner`).
However if you don't have a beam runtime and even if you have enough memory, I discourage you to use the `DirectRunner` on the german or italian wikipedia. According to Apache Beam documentation it was made for testing purposes and therefore it is memory-inefficient. | Hi, first off let me say thank you for all the awesome work you're doing at Hugging Face across all your projects (NLP, Transformers, Tokenizers) - they're all amazing contributions to us working with NLP models :)
I'm trying to download the German Wikipedia dataset as follows:
```
wiki = nlp.load_dataset("wikipedia", "20200501.de", split="train")
```
However, when I do so, I get the following error:
```
Downloading and preparing dataset wikipedia/20200501.de (download: Unknown size, generated: Unknown size, total: Unknown size) to /home/ubuntu/.cache/huggingface/datasets/wikipedia/20200501.de/1.0.0...
Traceback (most recent call last):
File "<stdin>", line 1, in <module>
File "/home/ubuntu/anaconda3/envs/albert/lib/python3.7/site-packages/nlp/load.py", line 520, in load_dataset
save_infos=save_infos,
File "/home/ubuntu/anaconda3/envs/albert/lib/python3.7/site-packages/nlp/builder.py", line 433, in download_and_prepare
dl_manager=dl_manager, verify_infos=verify_infos, **download_and_prepare_kwargs
File "/home/ubuntu/anaconda3/envs/albert/lib/python3.7/site-packages/nlp/builder.py", line 824, in _download_and_prepare
"\n\t`{}`".format(usage_example)
nlp.builder.MissingBeamOptions: Trying to generate a dataset using Apache Beam, yet no Beam Runner or PipelineOptions() has been provided in `load_dataset` or in the builder arguments. For big datasets it has to run on large-scale data processing tools like Dataflow, Spark, etc. More information about Apache Beam runners at https://beam.apache.org/documentation/runners/capability-matrix/
If you really want to run it locally because you feel like the Dataset is small enough, you can use the local beam runner called `DirectRunner` (you may run out of memory).
Example of usage:
`load_dataset('wikipedia', '20200501.de', beam_runner='DirectRunner')`
```
So, following on from the example usage at the bottom, I tried specifying `beam_runner='DirectRunner`, however when I do this after about 20 min after the data has all downloaded, I get a `MemoryError` as warned.
This isn't an issue for the English or French Wikipedia datasets (I've tried both), as neither seem to require that `beam_runner` be specified. Can you please clarify why this is an issue for the German dataset?
My nlp version is 0.2.1.
Thank you! | 107 | MemoryError when loading German Wikipedia
Hi, first off let me say thank you for all the awesome work you're doing at Hugging Face across all your projects (NLP, Transformers, Tokenizers) - they're all amazing contributions to us working with NLP models :)
I'm trying to download the German Wikipedia dataset as follows:
```
wiki = nlp.load_dataset("wikipedia", "20200501.de", split="train")
```
However, when I do so, I get the following error:
```
Downloading and preparing dataset wikipedia/20200501.de (download: Unknown size, generated: Unknown size, total: Unknown size) to /home/ubuntu/.cache/huggingface/datasets/wikipedia/20200501.de/1.0.0...
Traceback (most recent call last):
File "<stdin>", line 1, in <module>
File "/home/ubuntu/anaconda3/envs/albert/lib/python3.7/site-packages/nlp/load.py", line 520, in load_dataset
save_infos=save_infos,
File "/home/ubuntu/anaconda3/envs/albert/lib/python3.7/site-packages/nlp/builder.py", line 433, in download_and_prepare
dl_manager=dl_manager, verify_infos=verify_infos, **download_and_prepare_kwargs
File "/home/ubuntu/anaconda3/envs/albert/lib/python3.7/site-packages/nlp/builder.py", line 824, in _download_and_prepare
"\n\t`{}`".format(usage_example)
nlp.builder.MissingBeamOptions: Trying to generate a dataset using Apache Beam, yet no Beam Runner or PipelineOptions() has been provided in `load_dataset` or in the builder arguments. For big datasets it has to run on large-scale data processing tools like Dataflow, Spark, etc. More information about Apache Beam runners at https://beam.apache.org/documentation/runners/capability-matrix/
If you really want to run it locally because you feel like the Dataset is small enough, you can use the local beam runner called `DirectRunner` (you may run out of memory).
Example of usage:
`load_dataset('wikipedia', '20200501.de', beam_runner='DirectRunner')`
```
So, following on from the example usage at the bottom, I tried specifying `beam_runner='DirectRunner`, however when I do this after about 20 min after the data has all downloaded, I get a `MemoryError` as warned.
This isn't an issue for the English or French Wikipedia datasets (I've tried both), as neither seem to require that `beam_runner` be specified. Can you please clarify why this is an issue for the German dataset?
My nlp version is 0.2.1.
Thank you!
Adding them is definitely in our short term objectives. I'll be working on this early next week :)
Although if you have an apache beam runtime feel free to specify the beam runner. You can find more info [here](https://github.com/huggingface/nlp/blob/master/docs/beam_dataset.md) on how to make it work on Dataflow but you can adapt it for Spark or any other beam runtime (by changing the `runner`).
However if you don't have a beam runtime and even if you have enough memory, I discourage you to use the `DirectRunner` on the german or italian wikipedia. According to Apache Beam documentation it was made for testing purposes and therefore it is memory-inefficient. |
https://github.com/huggingface/datasets/issues/278 | MemoryError when loading German Wikipedia | I added the German and the Italian Wikipedia to our google cloud storage:
First update the `nlp` package to 0.3.0:
```bash
pip install nlp --upgrade
```
and then
```python
from nlp import load_dataset
wiki_de = load_dataset("wikipedia", "20200501.de")
wiki_it = load_dataset("wikipedia", "20200501.it")
```
The datasets are downloaded and directly ready to use (no processing). | Hi, first off let me say thank you for all the awesome work you're doing at Hugging Face across all your projects (NLP, Transformers, Tokenizers) - they're all amazing contributions to us working with NLP models :)
I'm trying to download the German Wikipedia dataset as follows:
```
wiki = nlp.load_dataset("wikipedia", "20200501.de", split="train")
```
However, when I do so, I get the following error:
```
Downloading and preparing dataset wikipedia/20200501.de (download: Unknown size, generated: Unknown size, total: Unknown size) to /home/ubuntu/.cache/huggingface/datasets/wikipedia/20200501.de/1.0.0...
Traceback (most recent call last):
File "<stdin>", line 1, in <module>
File "/home/ubuntu/anaconda3/envs/albert/lib/python3.7/site-packages/nlp/load.py", line 520, in load_dataset
save_infos=save_infos,
File "/home/ubuntu/anaconda3/envs/albert/lib/python3.7/site-packages/nlp/builder.py", line 433, in download_and_prepare
dl_manager=dl_manager, verify_infos=verify_infos, **download_and_prepare_kwargs
File "/home/ubuntu/anaconda3/envs/albert/lib/python3.7/site-packages/nlp/builder.py", line 824, in _download_and_prepare
"\n\t`{}`".format(usage_example)
nlp.builder.MissingBeamOptions: Trying to generate a dataset using Apache Beam, yet no Beam Runner or PipelineOptions() has been provided in `load_dataset` or in the builder arguments. For big datasets it has to run on large-scale data processing tools like Dataflow, Spark, etc. More information about Apache Beam runners at https://beam.apache.org/documentation/runners/capability-matrix/
If you really want to run it locally because you feel like the Dataset is small enough, you can use the local beam runner called `DirectRunner` (you may run out of memory).
Example of usage:
`load_dataset('wikipedia', '20200501.de', beam_runner='DirectRunner')`
```
So, following on from the example usage at the bottom, I tried specifying `beam_runner='DirectRunner`, however when I do this after about 20 min after the data has all downloaded, I get a `MemoryError` as warned.
This isn't an issue for the English or French Wikipedia datasets (I've tried both), as neither seem to require that `beam_runner` be specified. Can you please clarify why this is an issue for the German dataset?
My nlp version is 0.2.1.
Thank you! | 53 | MemoryError when loading German Wikipedia
Hi, first off let me say thank you for all the awesome work you're doing at Hugging Face across all your projects (NLP, Transformers, Tokenizers) - they're all amazing contributions to us working with NLP models :)
I'm trying to download the German Wikipedia dataset as follows:
```
wiki = nlp.load_dataset("wikipedia", "20200501.de", split="train")
```
However, when I do so, I get the following error:
```
Downloading and preparing dataset wikipedia/20200501.de (download: Unknown size, generated: Unknown size, total: Unknown size) to /home/ubuntu/.cache/huggingface/datasets/wikipedia/20200501.de/1.0.0...
Traceback (most recent call last):
File "<stdin>", line 1, in <module>
File "/home/ubuntu/anaconda3/envs/albert/lib/python3.7/site-packages/nlp/load.py", line 520, in load_dataset
save_infos=save_infos,
File "/home/ubuntu/anaconda3/envs/albert/lib/python3.7/site-packages/nlp/builder.py", line 433, in download_and_prepare
dl_manager=dl_manager, verify_infos=verify_infos, **download_and_prepare_kwargs
File "/home/ubuntu/anaconda3/envs/albert/lib/python3.7/site-packages/nlp/builder.py", line 824, in _download_and_prepare
"\n\t`{}`".format(usage_example)
nlp.builder.MissingBeamOptions: Trying to generate a dataset using Apache Beam, yet no Beam Runner or PipelineOptions() has been provided in `load_dataset` or in the builder arguments. For big datasets it has to run on large-scale data processing tools like Dataflow, Spark, etc. More information about Apache Beam runners at https://beam.apache.org/documentation/runners/capability-matrix/
If you really want to run it locally because you feel like the Dataset is small enough, you can use the local beam runner called `DirectRunner` (you may run out of memory).
Example of usage:
`load_dataset('wikipedia', '20200501.de', beam_runner='DirectRunner')`
```
So, following on from the example usage at the bottom, I tried specifying `beam_runner='DirectRunner`, however when I do this after about 20 min after the data has all downloaded, I get a `MemoryError` as warned.
This isn't an issue for the English or French Wikipedia datasets (I've tried both), as neither seem to require that `beam_runner` be specified. Can you please clarify why this is an issue for the German dataset?
My nlp version is 0.2.1.
Thank you!
I added the German and the Italian Wikipedia to our google cloud storage:
First update the `nlp` package to 0.3.0:
```bash
pip install nlp --upgrade
```
and then
```python
from nlp import load_dataset
wiki_de = load_dataset("wikipedia", "20200501.de")
wiki_it = load_dataset("wikipedia", "20200501.it")
```
The datasets are downloaded and directly ready to use (no processing). |
https://github.com/huggingface/datasets/issues/278 | MemoryError when loading German Wikipedia | Hi @lhoestq
Wow, thanks so much, that's **really** incredible! I was considering looking at creating my own Beam Dataset, as per the doc you linked, but instead opted to process the data myself using `wikiextractor`. However, now that this is available, I'll definitely switch across and use it.
Thanks so much for the incredible work, this really helps out our team considerably!
Have a great (and well-deserved ;) weekend ahead!
P.S. I'm not sure if I should close the issue here - if so I'm happy to do so. | Hi, first off let me say thank you for all the awesome work you're doing at Hugging Face across all your projects (NLP, Transformers, Tokenizers) - they're all amazing contributions to us working with NLP models :)
I'm trying to download the German Wikipedia dataset as follows:
```
wiki = nlp.load_dataset("wikipedia", "20200501.de", split="train")
```
However, when I do so, I get the following error:
```
Downloading and preparing dataset wikipedia/20200501.de (download: Unknown size, generated: Unknown size, total: Unknown size) to /home/ubuntu/.cache/huggingface/datasets/wikipedia/20200501.de/1.0.0...
Traceback (most recent call last):
File "<stdin>", line 1, in <module>
File "/home/ubuntu/anaconda3/envs/albert/lib/python3.7/site-packages/nlp/load.py", line 520, in load_dataset
save_infos=save_infos,
File "/home/ubuntu/anaconda3/envs/albert/lib/python3.7/site-packages/nlp/builder.py", line 433, in download_and_prepare
dl_manager=dl_manager, verify_infos=verify_infos, **download_and_prepare_kwargs
File "/home/ubuntu/anaconda3/envs/albert/lib/python3.7/site-packages/nlp/builder.py", line 824, in _download_and_prepare
"\n\t`{}`".format(usage_example)
nlp.builder.MissingBeamOptions: Trying to generate a dataset using Apache Beam, yet no Beam Runner or PipelineOptions() has been provided in `load_dataset` or in the builder arguments. For big datasets it has to run on large-scale data processing tools like Dataflow, Spark, etc. More information about Apache Beam runners at https://beam.apache.org/documentation/runners/capability-matrix/
If you really want to run it locally because you feel like the Dataset is small enough, you can use the local beam runner called `DirectRunner` (you may run out of memory).
Example of usage:
`load_dataset('wikipedia', '20200501.de', beam_runner='DirectRunner')`
```
So, following on from the example usage at the bottom, I tried specifying `beam_runner='DirectRunner`, however when I do this after about 20 min after the data has all downloaded, I get a `MemoryError` as warned.
This isn't an issue for the English or French Wikipedia datasets (I've tried both), as neither seem to require that `beam_runner` be specified. Can you please clarify why this is an issue for the German dataset?
My nlp version is 0.2.1.
Thank you! | 89 | MemoryError when loading German Wikipedia
Hi, first off let me say thank you for all the awesome work you're doing at Hugging Face across all your projects (NLP, Transformers, Tokenizers) - they're all amazing contributions to us working with NLP models :)
I'm trying to download the German Wikipedia dataset as follows:
```
wiki = nlp.load_dataset("wikipedia", "20200501.de", split="train")
```
However, when I do so, I get the following error:
```
Downloading and preparing dataset wikipedia/20200501.de (download: Unknown size, generated: Unknown size, total: Unknown size) to /home/ubuntu/.cache/huggingface/datasets/wikipedia/20200501.de/1.0.0...
Traceback (most recent call last):
File "<stdin>", line 1, in <module>
File "/home/ubuntu/anaconda3/envs/albert/lib/python3.7/site-packages/nlp/load.py", line 520, in load_dataset
save_infos=save_infos,
File "/home/ubuntu/anaconda3/envs/albert/lib/python3.7/site-packages/nlp/builder.py", line 433, in download_and_prepare
dl_manager=dl_manager, verify_infos=verify_infos, **download_and_prepare_kwargs
File "/home/ubuntu/anaconda3/envs/albert/lib/python3.7/site-packages/nlp/builder.py", line 824, in _download_and_prepare
"\n\t`{}`".format(usage_example)
nlp.builder.MissingBeamOptions: Trying to generate a dataset using Apache Beam, yet no Beam Runner or PipelineOptions() has been provided in `load_dataset` or in the builder arguments. For big datasets it has to run on large-scale data processing tools like Dataflow, Spark, etc. More information about Apache Beam runners at https://beam.apache.org/documentation/runners/capability-matrix/
If you really want to run it locally because you feel like the Dataset is small enough, you can use the local beam runner called `DirectRunner` (you may run out of memory).
Example of usage:
`load_dataset('wikipedia', '20200501.de', beam_runner='DirectRunner')`
```
So, following on from the example usage at the bottom, I tried specifying `beam_runner='DirectRunner`, however when I do this after about 20 min after the data has all downloaded, I get a `MemoryError` as warned.
This isn't an issue for the English or French Wikipedia datasets (I've tried both), as neither seem to require that `beam_runner` be specified. Can you please clarify why this is an issue for the German dataset?
My nlp version is 0.2.1.
Thank you!
Hi @lhoestq
Wow, thanks so much, that's **really** incredible! I was considering looking at creating my own Beam Dataset, as per the doc you linked, but instead opted to process the data myself using `wikiextractor`. However, now that this is available, I'll definitely switch across and use it.
Thanks so much for the incredible work, this really helps out our team considerably!
Have a great (and well-deserved ;) weekend ahead!
P.S. I'm not sure if I should close the issue here - if so I'm happy to do so. |
https://github.com/huggingface/datasets/issues/277 | Empty samples in glue/qqp | We are only wrapping the original dataset.
Maybe try to ask on the GLUE mailing list or reach out to the original authors? | ```
qqp = nlp.load_dataset('glue', 'qqp')
print(qqp['train'][310121])
print(qqp['train'][362225])
```
```
{'question1': 'How can I create an Android app?', 'question2': '', 'label': 0, 'idx': 310137}
{'question1': 'How can I develop android app?', 'question2': '', 'label': 0, 'idx': 362246}
```
Notice that question 2 is empty string.
BTW, I have checked and these two are the only naughty ones in all splits of qqp. | 23 | Empty samples in glue/qqp
```
qqp = nlp.load_dataset('glue', 'qqp')
print(qqp['train'][310121])
print(qqp['train'][362225])
```
```
{'question1': 'How can I create an Android app?', 'question2': '', 'label': 0, 'idx': 310137}
{'question1': 'How can I develop android app?', 'question2': '', 'label': 0, 'idx': 362246}
```
Notice that question 2 is empty string.
BTW, I have checked and these two are the only naughty ones in all splits of qqp.
We are only wrapping the original dataset.
Maybe try to ask on the GLUE mailing list or reach out to the original authors? |
https://github.com/huggingface/datasets/issues/277 | Empty samples in glue/qqp | Tanks for the suggestion, I'll try to ask GLUE benchmark.
I'll first close the issue, post the following up here afterwards, and reopen the issue if needed. | ```
qqp = nlp.load_dataset('glue', 'qqp')
print(qqp['train'][310121])
print(qqp['train'][362225])
```
```
{'question1': 'How can I create an Android app?', 'question2': '', 'label': 0, 'idx': 310137}
{'question1': 'How can I develop android app?', 'question2': '', 'label': 0, 'idx': 362246}
```
Notice that question 2 is empty string.
BTW, I have checked and these two are the only naughty ones in all splits of qqp. | 27 | Empty samples in glue/qqp
```
qqp = nlp.load_dataset('glue', 'qqp')
print(qqp['train'][310121])
print(qqp['train'][362225])
```
```
{'question1': 'How can I create an Android app?', 'question2': '', 'label': 0, 'idx': 310137}
{'question1': 'How can I develop android app?', 'question2': '', 'label': 0, 'idx': 362246}
```
Notice that question 2 is empty string.
BTW, I have checked and these two are the only naughty ones in all splits of qqp.
Tanks for the suggestion, I'll try to ask GLUE benchmark.
I'll first close the issue, post the following up here afterwards, and reopen the issue if needed. |
https://github.com/huggingface/datasets/issues/275 | NonMatchingChecksumError when loading pubmed dataset | For some reason the files are not available for unauthenticated users right now (like the download service of this package). Instead of downloading the right files, it downloads the html of the error.
According to the error it should be back again in 24h.
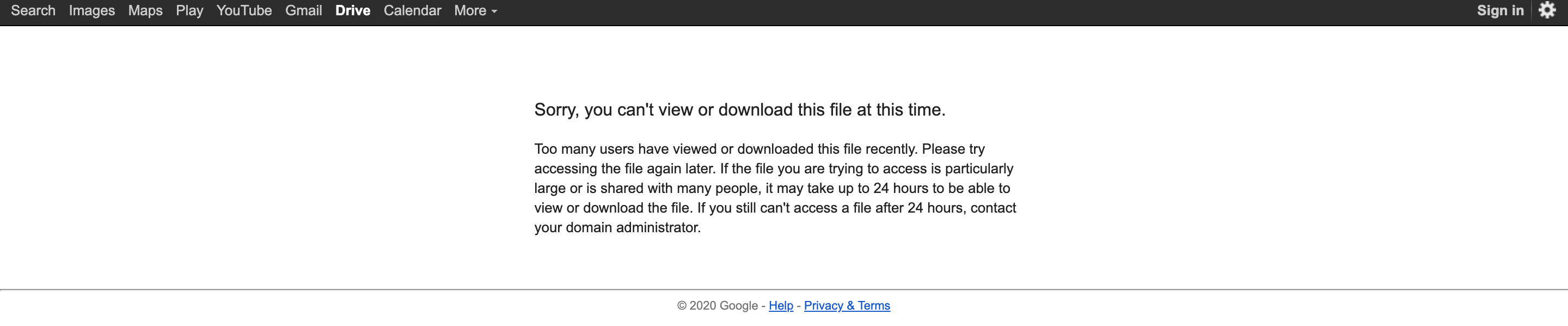
| I get this error when i run `nlp.load_dataset('scientific_papers', 'pubmed', split = 'train[:50%]')`.
The error is:
```
---------------------------------------------------------------------------
NonMatchingChecksumError Traceback (most recent call last)
<ipython-input-2-7742dea167d0> in <module>()
----> 1 df = nlp.load_dataset('scientific_papers', 'pubmed', split = 'train[:50%]')
2 df = pd.DataFrame(df)
3 gc.collect()
3 frames
/usr/local/lib/python3.6/dist-packages/nlp/load.py in load_dataset(path, name, version, data_dir, data_files, split, cache_dir, download_config, download_mode, ignore_verifications, save_infos, **config_kwargs)
518 download_mode=download_mode,
519 ignore_verifications=ignore_verifications,
--> 520 save_infos=save_infos,
521 )
522
/usr/local/lib/python3.6/dist-packages/nlp/builder.py in download_and_prepare(self, download_config, download_mode, ignore_verifications, save_infos, try_from_hf_gcs, dl_manager, **download_and_prepare_kwargs)
431 verify_infos = not save_infos and not ignore_verifications
432 self._download_and_prepare(
--> 433 dl_manager=dl_manager, verify_infos=verify_infos, **download_and_prepare_kwargs
434 )
435 # Sync info
/usr/local/lib/python3.6/dist-packages/nlp/builder.py in _download_and_prepare(self, dl_manager, verify_infos, **prepare_split_kwargs)
468 # Checksums verification
469 if verify_infos:
--> 470 verify_checksums(self.info.download_checksums, dl_manager.get_recorded_sizes_checksums())
471 for split_generator in split_generators:
472 if str(split_generator.split_info.name).lower() == "all":
/usr/local/lib/python3.6/dist-packages/nlp/utils/info_utils.py in verify_checksums(expected_checksums, recorded_checksums)
34 bad_urls = [url for url in expected_checksums if expected_checksums[url] != recorded_checksums[url]]
35 if len(bad_urls) > 0:
---> 36 raise NonMatchingChecksumError(str(bad_urls))
37 logger.info("All the checksums matched successfully.")
38
NonMatchingChecksumError: ['https://drive.google.com/uc?id=1b3rmCSIoh6VhD4HKWjI4HOW-cSwcwbeC&export=download', 'https://drive.google.com/uc?id=1lvsqvsFi3W-pE1SqNZI0s8NR9rC1tsja&export=download']
```
I'm currently working on google colab.
That is quite strange because yesterday it was fine.
| 45 | NonMatchingChecksumError when loading pubmed dataset
I get this error when i run `nlp.load_dataset('scientific_papers', 'pubmed', split = 'train[:50%]')`.
The error is:
```
---------------------------------------------------------------------------
NonMatchingChecksumError Traceback (most recent call last)
<ipython-input-2-7742dea167d0> in <module>()
----> 1 df = nlp.load_dataset('scientific_papers', 'pubmed', split = 'train[:50%]')
2 df = pd.DataFrame(df)
3 gc.collect()
3 frames
/usr/local/lib/python3.6/dist-packages/nlp/load.py in load_dataset(path, name, version, data_dir, data_files, split, cache_dir, download_config, download_mode, ignore_verifications, save_infos, **config_kwargs)
518 download_mode=download_mode,
519 ignore_verifications=ignore_verifications,
--> 520 save_infos=save_infos,
521 )
522
/usr/local/lib/python3.6/dist-packages/nlp/builder.py in download_and_prepare(self, download_config, download_mode, ignore_verifications, save_infos, try_from_hf_gcs, dl_manager, **download_and_prepare_kwargs)
431 verify_infos = not save_infos and not ignore_verifications
432 self._download_and_prepare(
--> 433 dl_manager=dl_manager, verify_infos=verify_infos, **download_and_prepare_kwargs
434 )
435 # Sync info
/usr/local/lib/python3.6/dist-packages/nlp/builder.py in _download_and_prepare(self, dl_manager, verify_infos, **prepare_split_kwargs)
468 # Checksums verification
469 if verify_infos:
--> 470 verify_checksums(self.info.download_checksums, dl_manager.get_recorded_sizes_checksums())
471 for split_generator in split_generators:
472 if str(split_generator.split_info.name).lower() == "all":
/usr/local/lib/python3.6/dist-packages/nlp/utils/info_utils.py in verify_checksums(expected_checksums, recorded_checksums)
34 bad_urls = [url for url in expected_checksums if expected_checksums[url] != recorded_checksums[url]]
35 if len(bad_urls) > 0:
---> 36 raise NonMatchingChecksumError(str(bad_urls))
37 logger.info("All the checksums matched successfully.")
38
NonMatchingChecksumError: ['https://drive.google.com/uc?id=1b3rmCSIoh6VhD4HKWjI4HOW-cSwcwbeC&export=download', 'https://drive.google.com/uc?id=1lvsqvsFi3W-pE1SqNZI0s8NR9rC1tsja&export=download']
```
I'm currently working on google colab.
That is quite strange because yesterday it was fine.
For some reason the files are not available for unauthenticated users right now (like the download service of this package). Instead of downloading the right files, it downloads the html of the error.
According to the error it should be back again in 24h.
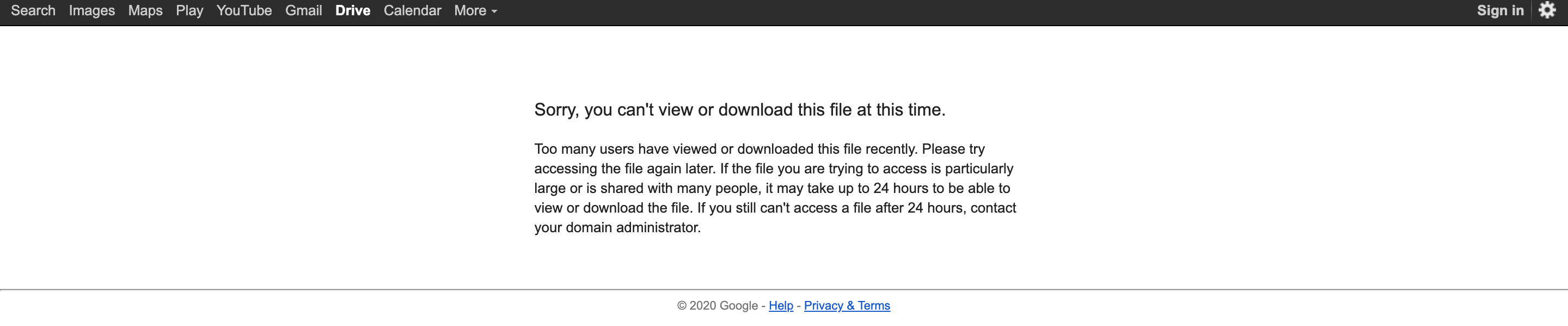
|
https://github.com/huggingface/datasets/issues/274 | PG-19 | Got around to this today, and so far so good, I'm able to download and load pg19 locally. However, I think there may be an issue with the dummy data, and testing in general.
The problem lies in the fact that each book from pg19 actually resides as its own text file in a google cloud folder that denotes the split, where the book id is the name of the text file. https://console.cloud.google.com/storage/browser/deepmind-gutenberg/train/ I don't believe there's anywhere else (even in the supplied metadata), where the mapping of id -> split can be found.
Therefore I end up making a network call `tf.io.gfile.listdir` to get all the files within each of the split directories. https://github.com/lucidrains/nlp/commit/adbacbd85decc80db2347d0882e7dab4faa6fd03#diff-cece8f166a85dd927caf574ba303d39bR78
Does this network call need to be eventually stubbed out for testing? | Hi, and thanks for all your open-sourced work, as always!
I was wondering if you would be open to adding PG-19 to your collection of datasets. https://github.com/deepmind/pg19 It is often used for benchmarking long-range language modeling. | 127 | PG-19
Hi, and thanks for all your open-sourced work, as always!
I was wondering if you would be open to adding PG-19 to your collection of datasets. https://github.com/deepmind/pg19 It is often used for benchmarking long-range language modeling.
Got around to this today, and so far so good, I'm able to download and load pg19 locally. However, I think there may be an issue with the dummy data, and testing in general.
The problem lies in the fact that each book from pg19 actually resides as its own text file in a google cloud folder that denotes the split, where the book id is the name of the text file. https://console.cloud.google.com/storage/browser/deepmind-gutenberg/train/ I don't believe there's anywhere else (even in the supplied metadata), where the mapping of id -> split can be found.
Therefore I end up making a network call `tf.io.gfile.listdir` to get all the files within each of the split directories. https://github.com/lucidrains/nlp/commit/adbacbd85decc80db2347d0882e7dab4faa6fd03#diff-cece8f166a85dd927caf574ba303d39bR78
Does this network call need to be eventually stubbed out for testing? |
https://github.com/huggingface/datasets/issues/274 | PG-19 | Ohh nevermind, I think I can use `download_custom` here with `listdir` as the custom function. Ok, I'll keep trying to make the dummy data work! | Hi, and thanks for all your open-sourced work, as always!
I was wondering if you would be open to adding PG-19 to your collection of datasets. https://github.com/deepmind/pg19 It is often used for benchmarking long-range language modeling. | 25 | PG-19
Hi, and thanks for all your open-sourced work, as always!
I was wondering if you would be open to adding PG-19 to your collection of datasets. https://github.com/deepmind/pg19 It is often used for benchmarking long-range language modeling.
Ohh nevermind, I think I can use `download_custom` here with `listdir` as the custom function. Ok, I'll keep trying to make the dummy data work! |
https://github.com/huggingface/datasets/issues/263 | [Feature request] Support for external modality for language datasets | Thanks a lot, @aleSuglia for the very detailed and introductive feature request.
It seems like we could build something pretty useful here indeed.
One of the questions here is that Arrow doesn't have built-in support for generic "tensors" in records but there might be ways to do that in a clean way. We'll probably try to tackle this during the summer. | # Background
In recent years many researchers have advocated that learning meanings from text-based only datasets is just like asking a human to "learn to speak by listening to the radio" [[E. Bender and A. Koller,2020](https://openreview.net/forum?id=GKTvAcb12b), [Y. Bisk et. al, 2020](https://arxiv.org/abs/2004.10151)]. Therefore, the importance of multi-modal datasets for the NLP community is of paramount importance for next-generation models. For this reason, I raised a [concern](https://github.com/huggingface/nlp/pull/236#issuecomment-639832029) related to the best way to integrate external features in NLP datasets (e.g., visual features associated with an image, audio features associated with a recording, etc.). This would be of great importance for a more systematic way of representing data for ML models that are learning from multi-modal data.
# Language + Vision
## Use case
Typically, people working on Language+Vision tasks, have a reference dataset (either in JSON or JSONL format) and for each example, they have an identifier that specifies the reference image. For a practical example, you can refer to the [GQA](https://cs.stanford.edu/people/dorarad/gqa/download.html#seconddown) dataset.
Currently, images are represented by either pooling-based features (average pooling of ResNet or VGGNet features, see [DeVries et.al, 2017](https://arxiv.org/abs/1611.08481), [Shekhar et.al, 2019](https://www.aclweb.org/anthology/N19-1265.pdf)) where you have a single vector for every image. Another option is to use a set of feature maps for every image extracted from a specific layer of a CNN (see [Xu et.al, 2015](https://arxiv.org/abs/1502.03044)). A more recent option, especially with large-scale multi-modal transformers [Li et. al, 2019](https://arxiv.org/abs/1908.03557), is to use FastRCNN features.
For all these types of features, people use one of the following formats:
1. [HD5F](https://pypi.org/project/h5py/)
2. [NumPy](https://numpy.org/doc/stable/reference/generated/numpy.savez.html)
3. [LMDB](https://lmdb.readthedocs.io/en/release/)
## Implementation considerations
I was thinking about possible ways of implementing this feature. As mentioned above, depending on the model, different visual features can be used. This step usually relies on another model (say ResNet-101) that is used to generate the visual features for each image used in the dataset. Typically, this step is done in a separate script that completes the feature generation procedure. The usual processing steps for these datasets are the following:
1. Download dataset
2. Download images associated with the dataset
3. Write a script that generates the visual features for every image and store them in a specific file
4. Create a DataLoader that maps the visual features to the corresponding language example
In my personal projects, I've decided to ignore HD5F because it doesn't have out-of-the-box support for multi-processing (see this PyTorch [issue](https://github.com/pytorch/pytorch/issues/11929)). I've been successfully using a NumPy compressed file for each image so that I can store any sort of information in it.
For ease of use of all these Language+Vision datasets, it would be really handy to have a way to associate the visual features with the text and store them in an efficient way. That's why I immediately thought about the HuggingFace NLP backend based on Apache Arrow. The assumption here is that the external modality will be mapped to a N-dimensional tensor so easily represented by a NumPy array.
Looking forward to hearing your thoughts about it! | 61 | [Feature request] Support for external modality for language datasets
# Background
In recent years many researchers have advocated that learning meanings from text-based only datasets is just like asking a human to "learn to speak by listening to the radio" [[E. Bender and A. Koller,2020](https://openreview.net/forum?id=GKTvAcb12b), [Y. Bisk et. al, 2020](https://arxiv.org/abs/2004.10151)]. Therefore, the importance of multi-modal datasets for the NLP community is of paramount importance for next-generation models. For this reason, I raised a [concern](https://github.com/huggingface/nlp/pull/236#issuecomment-639832029) related to the best way to integrate external features in NLP datasets (e.g., visual features associated with an image, audio features associated with a recording, etc.). This would be of great importance for a more systematic way of representing data for ML models that are learning from multi-modal data.
# Language + Vision
## Use case
Typically, people working on Language+Vision tasks, have a reference dataset (either in JSON or JSONL format) and for each example, they have an identifier that specifies the reference image. For a practical example, you can refer to the [GQA](https://cs.stanford.edu/people/dorarad/gqa/download.html#seconddown) dataset.
Currently, images are represented by either pooling-based features (average pooling of ResNet or VGGNet features, see [DeVries et.al, 2017](https://arxiv.org/abs/1611.08481), [Shekhar et.al, 2019](https://www.aclweb.org/anthology/N19-1265.pdf)) where you have a single vector for every image. Another option is to use a set of feature maps for every image extracted from a specific layer of a CNN (see [Xu et.al, 2015](https://arxiv.org/abs/1502.03044)). A more recent option, especially with large-scale multi-modal transformers [Li et. al, 2019](https://arxiv.org/abs/1908.03557), is to use FastRCNN features.
For all these types of features, people use one of the following formats:
1. [HD5F](https://pypi.org/project/h5py/)
2. [NumPy](https://numpy.org/doc/stable/reference/generated/numpy.savez.html)
3. [LMDB](https://lmdb.readthedocs.io/en/release/)
## Implementation considerations
I was thinking about possible ways of implementing this feature. As mentioned above, depending on the model, different visual features can be used. This step usually relies on another model (say ResNet-101) that is used to generate the visual features for each image used in the dataset. Typically, this step is done in a separate script that completes the feature generation procedure. The usual processing steps for these datasets are the following:
1. Download dataset
2. Download images associated with the dataset
3. Write a script that generates the visual features for every image and store them in a specific file
4. Create a DataLoader that maps the visual features to the corresponding language example
In my personal projects, I've decided to ignore HD5F because it doesn't have out-of-the-box support for multi-processing (see this PyTorch [issue](https://github.com/pytorch/pytorch/issues/11929)). I've been successfully using a NumPy compressed file for each image so that I can store any sort of information in it.
For ease of use of all these Language+Vision datasets, it would be really handy to have a way to associate the visual features with the text and store them in an efficient way. That's why I immediately thought about the HuggingFace NLP backend based on Apache Arrow. The assumption here is that the external modality will be mapped to a N-dimensional tensor so easily represented by a NumPy array.
Looking forward to hearing your thoughts about it!
Thanks a lot, @aleSuglia for the very detailed and introductive feature request.
It seems like we could build something pretty useful here indeed.
One of the questions here is that Arrow doesn't have built-in support for generic "tensors" in records but there might be ways to do that in a clean way. We'll probably try to tackle this during the summer. |
https://github.com/huggingface/datasets/issues/263 | [Feature request] Support for external modality for language datasets | I was looking into Facebook MMF and apparently they decided to use LMDB to store additional features associated with every example: https://github.com/facebookresearch/mmf/blob/master/mmf/datasets/databases/features_database.py
| # Background
In recent years many researchers have advocated that learning meanings from text-based only datasets is just like asking a human to "learn to speak by listening to the radio" [[E. Bender and A. Koller,2020](https://openreview.net/forum?id=GKTvAcb12b), [Y. Bisk et. al, 2020](https://arxiv.org/abs/2004.10151)]. Therefore, the importance of multi-modal datasets for the NLP community is of paramount importance for next-generation models. For this reason, I raised a [concern](https://github.com/huggingface/nlp/pull/236#issuecomment-639832029) related to the best way to integrate external features in NLP datasets (e.g., visual features associated with an image, audio features associated with a recording, etc.). This would be of great importance for a more systematic way of representing data for ML models that are learning from multi-modal data.
# Language + Vision
## Use case
Typically, people working on Language+Vision tasks, have a reference dataset (either in JSON or JSONL format) and for each example, they have an identifier that specifies the reference image. For a practical example, you can refer to the [GQA](https://cs.stanford.edu/people/dorarad/gqa/download.html#seconddown) dataset.
Currently, images are represented by either pooling-based features (average pooling of ResNet or VGGNet features, see [DeVries et.al, 2017](https://arxiv.org/abs/1611.08481), [Shekhar et.al, 2019](https://www.aclweb.org/anthology/N19-1265.pdf)) where you have a single vector for every image. Another option is to use a set of feature maps for every image extracted from a specific layer of a CNN (see [Xu et.al, 2015](https://arxiv.org/abs/1502.03044)). A more recent option, especially with large-scale multi-modal transformers [Li et. al, 2019](https://arxiv.org/abs/1908.03557), is to use FastRCNN features.
For all these types of features, people use one of the following formats:
1. [HD5F](https://pypi.org/project/h5py/)
2. [NumPy](https://numpy.org/doc/stable/reference/generated/numpy.savez.html)
3. [LMDB](https://lmdb.readthedocs.io/en/release/)
## Implementation considerations
I was thinking about possible ways of implementing this feature. As mentioned above, depending on the model, different visual features can be used. This step usually relies on another model (say ResNet-101) that is used to generate the visual features for each image used in the dataset. Typically, this step is done in a separate script that completes the feature generation procedure. The usual processing steps for these datasets are the following:
1. Download dataset
2. Download images associated with the dataset
3. Write a script that generates the visual features for every image and store them in a specific file
4. Create a DataLoader that maps the visual features to the corresponding language example
In my personal projects, I've decided to ignore HD5F because it doesn't have out-of-the-box support for multi-processing (see this PyTorch [issue](https://github.com/pytorch/pytorch/issues/11929)). I've been successfully using a NumPy compressed file for each image so that I can store any sort of information in it.
For ease of use of all these Language+Vision datasets, it would be really handy to have a way to associate the visual features with the text and store them in an efficient way. That's why I immediately thought about the HuggingFace NLP backend based on Apache Arrow. The assumption here is that the external modality will be mapped to a N-dimensional tensor so easily represented by a NumPy array.
Looking forward to hearing your thoughts about it! | 22 | [Feature request] Support for external modality for language datasets
# Background
In recent years many researchers have advocated that learning meanings from text-based only datasets is just like asking a human to "learn to speak by listening to the radio" [[E. Bender and A. Koller,2020](https://openreview.net/forum?id=GKTvAcb12b), [Y. Bisk et. al, 2020](https://arxiv.org/abs/2004.10151)]. Therefore, the importance of multi-modal datasets for the NLP community is of paramount importance for next-generation models. For this reason, I raised a [concern](https://github.com/huggingface/nlp/pull/236#issuecomment-639832029) related to the best way to integrate external features in NLP datasets (e.g., visual features associated with an image, audio features associated with a recording, etc.). This would be of great importance for a more systematic way of representing data for ML models that are learning from multi-modal data.
# Language + Vision
## Use case
Typically, people working on Language+Vision tasks, have a reference dataset (either in JSON or JSONL format) and for each example, they have an identifier that specifies the reference image. For a practical example, you can refer to the [GQA](https://cs.stanford.edu/people/dorarad/gqa/download.html#seconddown) dataset.
Currently, images are represented by either pooling-based features (average pooling of ResNet or VGGNet features, see [DeVries et.al, 2017](https://arxiv.org/abs/1611.08481), [Shekhar et.al, 2019](https://www.aclweb.org/anthology/N19-1265.pdf)) where you have a single vector for every image. Another option is to use a set of feature maps for every image extracted from a specific layer of a CNN (see [Xu et.al, 2015](https://arxiv.org/abs/1502.03044)). A more recent option, especially with large-scale multi-modal transformers [Li et. al, 2019](https://arxiv.org/abs/1908.03557), is to use FastRCNN features.
For all these types of features, people use one of the following formats:
1. [HD5F](https://pypi.org/project/h5py/)
2. [NumPy](https://numpy.org/doc/stable/reference/generated/numpy.savez.html)
3. [LMDB](https://lmdb.readthedocs.io/en/release/)
## Implementation considerations
I was thinking about possible ways of implementing this feature. As mentioned above, depending on the model, different visual features can be used. This step usually relies on another model (say ResNet-101) that is used to generate the visual features for each image used in the dataset. Typically, this step is done in a separate script that completes the feature generation procedure. The usual processing steps for these datasets are the following:
1. Download dataset
2. Download images associated with the dataset
3. Write a script that generates the visual features for every image and store them in a specific file
4. Create a DataLoader that maps the visual features to the corresponding language example
In my personal projects, I've decided to ignore HD5F because it doesn't have out-of-the-box support for multi-processing (see this PyTorch [issue](https://github.com/pytorch/pytorch/issues/11929)). I've been successfully using a NumPy compressed file for each image so that I can store any sort of information in it.
For ease of use of all these Language+Vision datasets, it would be really handy to have a way to associate the visual features with the text and store them in an efficient way. That's why I immediately thought about the HuggingFace NLP backend based on Apache Arrow. The assumption here is that the external modality will be mapped to a N-dimensional tensor so easily represented by a NumPy array.
Looking forward to hearing your thoughts about it!
I was looking into Facebook MMF and apparently they decided to use LMDB to store additional features associated with every example: https://github.com/facebookresearch/mmf/blob/master/mmf/datasets/databases/features_database.py
|
https://github.com/huggingface/datasets/issues/263 | [Feature request] Support for external modality for language datasets | I saw the Mozilla common_voice dataset in model hub, which has mp3 audio recordings as part it. It's use predominantly maybe in ASR and TTS, but dataset is a Language + Voice Dataset similar to @aleSuglia's point about Language + Vision.
https://huggingface.co/datasets/common_voice | # Background
In recent years many researchers have advocated that learning meanings from text-based only datasets is just like asking a human to "learn to speak by listening to the radio" [[E. Bender and A. Koller,2020](https://openreview.net/forum?id=GKTvAcb12b), [Y. Bisk et. al, 2020](https://arxiv.org/abs/2004.10151)]. Therefore, the importance of multi-modal datasets for the NLP community is of paramount importance for next-generation models. For this reason, I raised a [concern](https://github.com/huggingface/nlp/pull/236#issuecomment-639832029) related to the best way to integrate external features in NLP datasets (e.g., visual features associated with an image, audio features associated with a recording, etc.). This would be of great importance for a more systematic way of representing data for ML models that are learning from multi-modal data.
# Language + Vision
## Use case
Typically, people working on Language+Vision tasks, have a reference dataset (either in JSON or JSONL format) and for each example, they have an identifier that specifies the reference image. For a practical example, you can refer to the [GQA](https://cs.stanford.edu/people/dorarad/gqa/download.html#seconddown) dataset.
Currently, images are represented by either pooling-based features (average pooling of ResNet or VGGNet features, see [DeVries et.al, 2017](https://arxiv.org/abs/1611.08481), [Shekhar et.al, 2019](https://www.aclweb.org/anthology/N19-1265.pdf)) where you have a single vector for every image. Another option is to use a set of feature maps for every image extracted from a specific layer of a CNN (see [Xu et.al, 2015](https://arxiv.org/abs/1502.03044)). A more recent option, especially with large-scale multi-modal transformers [Li et. al, 2019](https://arxiv.org/abs/1908.03557), is to use FastRCNN features.
For all these types of features, people use one of the following formats:
1. [HD5F](https://pypi.org/project/h5py/)
2. [NumPy](https://numpy.org/doc/stable/reference/generated/numpy.savez.html)
3. [LMDB](https://lmdb.readthedocs.io/en/release/)
## Implementation considerations
I was thinking about possible ways of implementing this feature. As mentioned above, depending on the model, different visual features can be used. This step usually relies on another model (say ResNet-101) that is used to generate the visual features for each image used in the dataset. Typically, this step is done in a separate script that completes the feature generation procedure. The usual processing steps for these datasets are the following:
1. Download dataset
2. Download images associated with the dataset
3. Write a script that generates the visual features for every image and store them in a specific file
4. Create a DataLoader that maps the visual features to the corresponding language example
In my personal projects, I've decided to ignore HD5F because it doesn't have out-of-the-box support for multi-processing (see this PyTorch [issue](https://github.com/pytorch/pytorch/issues/11929)). I've been successfully using a NumPy compressed file for each image so that I can store any sort of information in it.
For ease of use of all these Language+Vision datasets, it would be really handy to have a way to associate the visual features with the text and store them in an efficient way. That's why I immediately thought about the HuggingFace NLP backend based on Apache Arrow. The assumption here is that the external modality will be mapped to a N-dimensional tensor so easily represented by a NumPy array.
Looking forward to hearing your thoughts about it! | 42 | [Feature request] Support for external modality for language datasets
# Background
In recent years many researchers have advocated that learning meanings from text-based only datasets is just like asking a human to "learn to speak by listening to the radio" [[E. Bender and A. Koller,2020](https://openreview.net/forum?id=GKTvAcb12b), [Y. Bisk et. al, 2020](https://arxiv.org/abs/2004.10151)]. Therefore, the importance of multi-modal datasets for the NLP community is of paramount importance for next-generation models. For this reason, I raised a [concern](https://github.com/huggingface/nlp/pull/236#issuecomment-639832029) related to the best way to integrate external features in NLP datasets (e.g., visual features associated with an image, audio features associated with a recording, etc.). This would be of great importance for a more systematic way of representing data for ML models that are learning from multi-modal data.
# Language + Vision
## Use case
Typically, people working on Language+Vision tasks, have a reference dataset (either in JSON or JSONL format) and for each example, they have an identifier that specifies the reference image. For a practical example, you can refer to the [GQA](https://cs.stanford.edu/people/dorarad/gqa/download.html#seconddown) dataset.
Currently, images are represented by either pooling-based features (average pooling of ResNet or VGGNet features, see [DeVries et.al, 2017](https://arxiv.org/abs/1611.08481), [Shekhar et.al, 2019](https://www.aclweb.org/anthology/N19-1265.pdf)) where you have a single vector for every image. Another option is to use a set of feature maps for every image extracted from a specific layer of a CNN (see [Xu et.al, 2015](https://arxiv.org/abs/1502.03044)). A more recent option, especially with large-scale multi-modal transformers [Li et. al, 2019](https://arxiv.org/abs/1908.03557), is to use FastRCNN features.
For all these types of features, people use one of the following formats:
1. [HD5F](https://pypi.org/project/h5py/)
2. [NumPy](https://numpy.org/doc/stable/reference/generated/numpy.savez.html)
3. [LMDB](https://lmdb.readthedocs.io/en/release/)
## Implementation considerations
I was thinking about possible ways of implementing this feature. As mentioned above, depending on the model, different visual features can be used. This step usually relies on another model (say ResNet-101) that is used to generate the visual features for each image used in the dataset. Typically, this step is done in a separate script that completes the feature generation procedure. The usual processing steps for these datasets are the following:
1. Download dataset
2. Download images associated with the dataset
3. Write a script that generates the visual features for every image and store them in a specific file
4. Create a DataLoader that maps the visual features to the corresponding language example
In my personal projects, I've decided to ignore HD5F because it doesn't have out-of-the-box support for multi-processing (see this PyTorch [issue](https://github.com/pytorch/pytorch/issues/11929)). I've been successfully using a NumPy compressed file for each image so that I can store any sort of information in it.
For ease of use of all these Language+Vision datasets, it would be really handy to have a way to associate the visual features with the text and store them in an efficient way. That's why I immediately thought about the HuggingFace NLP backend based on Apache Arrow. The assumption here is that the external modality will be mapped to a N-dimensional tensor so easily represented by a NumPy array.
Looking forward to hearing your thoughts about it!
I saw the Mozilla common_voice dataset in model hub, which has mp3 audio recordings as part it. It's use predominantly maybe in ASR and TTS, but dataset is a Language + Voice Dataset similar to @aleSuglia's point about Language + Vision.
https://huggingface.co/datasets/common_voice |
https://github.com/huggingface/datasets/issues/263 | [Feature request] Support for external modality for language datasets | Hey @thomwolf, are there any updates on this? I would love to contribute if possible!
Thanks,
Alessandro | # Background
In recent years many researchers have advocated that learning meanings from text-based only datasets is just like asking a human to "learn to speak by listening to the radio" [[E. Bender and A. Koller,2020](https://openreview.net/forum?id=GKTvAcb12b), [Y. Bisk et. al, 2020](https://arxiv.org/abs/2004.10151)]. Therefore, the importance of multi-modal datasets for the NLP community is of paramount importance for next-generation models. For this reason, I raised a [concern](https://github.com/huggingface/nlp/pull/236#issuecomment-639832029) related to the best way to integrate external features in NLP datasets (e.g., visual features associated with an image, audio features associated with a recording, etc.). This would be of great importance for a more systematic way of representing data for ML models that are learning from multi-modal data.
# Language + Vision
## Use case
Typically, people working on Language+Vision tasks, have a reference dataset (either in JSON or JSONL format) and for each example, they have an identifier that specifies the reference image. For a practical example, you can refer to the [GQA](https://cs.stanford.edu/people/dorarad/gqa/download.html#seconddown) dataset.
Currently, images are represented by either pooling-based features (average pooling of ResNet or VGGNet features, see [DeVries et.al, 2017](https://arxiv.org/abs/1611.08481), [Shekhar et.al, 2019](https://www.aclweb.org/anthology/N19-1265.pdf)) where you have a single vector for every image. Another option is to use a set of feature maps for every image extracted from a specific layer of a CNN (see [Xu et.al, 2015](https://arxiv.org/abs/1502.03044)). A more recent option, especially with large-scale multi-modal transformers [Li et. al, 2019](https://arxiv.org/abs/1908.03557), is to use FastRCNN features.
For all these types of features, people use one of the following formats:
1. [HD5F](https://pypi.org/project/h5py/)
2. [NumPy](https://numpy.org/doc/stable/reference/generated/numpy.savez.html)
3. [LMDB](https://lmdb.readthedocs.io/en/release/)
## Implementation considerations
I was thinking about possible ways of implementing this feature. As mentioned above, depending on the model, different visual features can be used. This step usually relies on another model (say ResNet-101) that is used to generate the visual features for each image used in the dataset. Typically, this step is done in a separate script that completes the feature generation procedure. The usual processing steps for these datasets are the following:
1. Download dataset
2. Download images associated with the dataset
3. Write a script that generates the visual features for every image and store them in a specific file
4. Create a DataLoader that maps the visual features to the corresponding language example
In my personal projects, I've decided to ignore HD5F because it doesn't have out-of-the-box support for multi-processing (see this PyTorch [issue](https://github.com/pytorch/pytorch/issues/11929)). I've been successfully using a NumPy compressed file for each image so that I can store any sort of information in it.
For ease of use of all these Language+Vision datasets, it would be really handy to have a way to associate the visual features with the text and store them in an efficient way. That's why I immediately thought about the HuggingFace NLP backend based on Apache Arrow. The assumption here is that the external modality will be mapped to a N-dimensional tensor so easily represented by a NumPy array.
Looking forward to hearing your thoughts about it! | 17 | [Feature request] Support for external modality for language datasets
# Background
In recent years many researchers have advocated that learning meanings from text-based only datasets is just like asking a human to "learn to speak by listening to the radio" [[E. Bender and A. Koller,2020](https://openreview.net/forum?id=GKTvAcb12b), [Y. Bisk et. al, 2020](https://arxiv.org/abs/2004.10151)]. Therefore, the importance of multi-modal datasets for the NLP community is of paramount importance for next-generation models. For this reason, I raised a [concern](https://github.com/huggingface/nlp/pull/236#issuecomment-639832029) related to the best way to integrate external features in NLP datasets (e.g., visual features associated with an image, audio features associated with a recording, etc.). This would be of great importance for a more systematic way of representing data for ML models that are learning from multi-modal data.
# Language + Vision
## Use case
Typically, people working on Language+Vision tasks, have a reference dataset (either in JSON or JSONL format) and for each example, they have an identifier that specifies the reference image. For a practical example, you can refer to the [GQA](https://cs.stanford.edu/people/dorarad/gqa/download.html#seconddown) dataset.
Currently, images are represented by either pooling-based features (average pooling of ResNet or VGGNet features, see [DeVries et.al, 2017](https://arxiv.org/abs/1611.08481), [Shekhar et.al, 2019](https://www.aclweb.org/anthology/N19-1265.pdf)) where you have a single vector for every image. Another option is to use a set of feature maps for every image extracted from a specific layer of a CNN (see [Xu et.al, 2015](https://arxiv.org/abs/1502.03044)). A more recent option, especially with large-scale multi-modal transformers [Li et. al, 2019](https://arxiv.org/abs/1908.03557), is to use FastRCNN features.
For all these types of features, people use one of the following formats:
1. [HD5F](https://pypi.org/project/h5py/)
2. [NumPy](https://numpy.org/doc/stable/reference/generated/numpy.savez.html)
3. [LMDB](https://lmdb.readthedocs.io/en/release/)
## Implementation considerations
I was thinking about possible ways of implementing this feature. As mentioned above, depending on the model, different visual features can be used. This step usually relies on another model (say ResNet-101) that is used to generate the visual features for each image used in the dataset. Typically, this step is done in a separate script that completes the feature generation procedure. The usual processing steps for these datasets are the following:
1. Download dataset
2. Download images associated with the dataset
3. Write a script that generates the visual features for every image and store them in a specific file
4. Create a DataLoader that maps the visual features to the corresponding language example
In my personal projects, I've decided to ignore HD5F because it doesn't have out-of-the-box support for multi-processing (see this PyTorch [issue](https://github.com/pytorch/pytorch/issues/11929)). I've been successfully using a NumPy compressed file for each image so that I can store any sort of information in it.
For ease of use of all these Language+Vision datasets, it would be really handy to have a way to associate the visual features with the text and store them in an efficient way. That's why I immediately thought about the HuggingFace NLP backend based on Apache Arrow. The assumption here is that the external modality will be mapped to a N-dimensional tensor so easily represented by a NumPy array.
Looking forward to hearing your thoughts about it!
Hey @thomwolf, are there any updates on this? I would love to contribute if possible!
Thanks,
Alessandro |
https://github.com/huggingface/datasets/issues/263 | [Feature request] Support for external modality for language datasets | Hi @aleSuglia :) In today's new release 1.17 of `datasets` we introduce a new feature type `Image` that allows to store images directly in a dataset, next to text features and labels for example. There is also an `Audio` feature type, for datasets containing audio data. For tensors there are `Array2D`, `Array3D`, etc. feature types
Note that both Image and Audio feature types take care of decoding the images/audio data if needed. The returned images are PIL images, and the audio signals are decoded as numpy arrays.
And `datasets` also leverage end-to-end zero copy from the arrow data for all of them, for maximum speed :) | # Background
In recent years many researchers have advocated that learning meanings from text-based only datasets is just like asking a human to "learn to speak by listening to the radio" [[E. Bender and A. Koller,2020](https://openreview.net/forum?id=GKTvAcb12b), [Y. Bisk et. al, 2020](https://arxiv.org/abs/2004.10151)]. Therefore, the importance of multi-modal datasets for the NLP community is of paramount importance for next-generation models. For this reason, I raised a [concern](https://github.com/huggingface/nlp/pull/236#issuecomment-639832029) related to the best way to integrate external features in NLP datasets (e.g., visual features associated with an image, audio features associated with a recording, etc.). This would be of great importance for a more systematic way of representing data for ML models that are learning from multi-modal data.
# Language + Vision
## Use case
Typically, people working on Language+Vision tasks, have a reference dataset (either in JSON or JSONL format) and for each example, they have an identifier that specifies the reference image. For a practical example, you can refer to the [GQA](https://cs.stanford.edu/people/dorarad/gqa/download.html#seconddown) dataset.
Currently, images are represented by either pooling-based features (average pooling of ResNet or VGGNet features, see [DeVries et.al, 2017](https://arxiv.org/abs/1611.08481), [Shekhar et.al, 2019](https://www.aclweb.org/anthology/N19-1265.pdf)) where you have a single vector for every image. Another option is to use a set of feature maps for every image extracted from a specific layer of a CNN (see [Xu et.al, 2015](https://arxiv.org/abs/1502.03044)). A more recent option, especially with large-scale multi-modal transformers [Li et. al, 2019](https://arxiv.org/abs/1908.03557), is to use FastRCNN features.
For all these types of features, people use one of the following formats:
1. [HD5F](https://pypi.org/project/h5py/)
2. [NumPy](https://numpy.org/doc/stable/reference/generated/numpy.savez.html)
3. [LMDB](https://lmdb.readthedocs.io/en/release/)
## Implementation considerations
I was thinking about possible ways of implementing this feature. As mentioned above, depending on the model, different visual features can be used. This step usually relies on another model (say ResNet-101) that is used to generate the visual features for each image used in the dataset. Typically, this step is done in a separate script that completes the feature generation procedure. The usual processing steps for these datasets are the following:
1. Download dataset
2. Download images associated with the dataset
3. Write a script that generates the visual features for every image and store them in a specific file
4. Create a DataLoader that maps the visual features to the corresponding language example
In my personal projects, I've decided to ignore HD5F because it doesn't have out-of-the-box support for multi-processing (see this PyTorch [issue](https://github.com/pytorch/pytorch/issues/11929)). I've been successfully using a NumPy compressed file for each image so that I can store any sort of information in it.
For ease of use of all these Language+Vision datasets, it would be really handy to have a way to associate the visual features with the text and store them in an efficient way. That's why I immediately thought about the HuggingFace NLP backend based on Apache Arrow. The assumption here is that the external modality will be mapped to a N-dimensional tensor so easily represented by a NumPy array.
Looking forward to hearing your thoughts about it! | 106 | [Feature request] Support for external modality for language datasets
# Background
In recent years many researchers have advocated that learning meanings from text-based only datasets is just like asking a human to "learn to speak by listening to the radio" [[E. Bender and A. Koller,2020](https://openreview.net/forum?id=GKTvAcb12b), [Y. Bisk et. al, 2020](https://arxiv.org/abs/2004.10151)]. Therefore, the importance of multi-modal datasets for the NLP community is of paramount importance for next-generation models. For this reason, I raised a [concern](https://github.com/huggingface/nlp/pull/236#issuecomment-639832029) related to the best way to integrate external features in NLP datasets (e.g., visual features associated with an image, audio features associated with a recording, etc.). This would be of great importance for a more systematic way of representing data for ML models that are learning from multi-modal data.
# Language + Vision
## Use case
Typically, people working on Language+Vision tasks, have a reference dataset (either in JSON or JSONL format) and for each example, they have an identifier that specifies the reference image. For a practical example, you can refer to the [GQA](https://cs.stanford.edu/people/dorarad/gqa/download.html#seconddown) dataset.
Currently, images are represented by either pooling-based features (average pooling of ResNet or VGGNet features, see [DeVries et.al, 2017](https://arxiv.org/abs/1611.08481), [Shekhar et.al, 2019](https://www.aclweb.org/anthology/N19-1265.pdf)) where you have a single vector for every image. Another option is to use a set of feature maps for every image extracted from a specific layer of a CNN (see [Xu et.al, 2015](https://arxiv.org/abs/1502.03044)). A more recent option, especially with large-scale multi-modal transformers [Li et. al, 2019](https://arxiv.org/abs/1908.03557), is to use FastRCNN features.
For all these types of features, people use one of the following formats:
1. [HD5F](https://pypi.org/project/h5py/)
2. [NumPy](https://numpy.org/doc/stable/reference/generated/numpy.savez.html)
3. [LMDB](https://lmdb.readthedocs.io/en/release/)
## Implementation considerations
I was thinking about possible ways of implementing this feature. As mentioned above, depending on the model, different visual features can be used. This step usually relies on another model (say ResNet-101) that is used to generate the visual features for each image used in the dataset. Typically, this step is done in a separate script that completes the feature generation procedure. The usual processing steps for these datasets are the following:
1. Download dataset
2. Download images associated with the dataset
3. Write a script that generates the visual features for every image and store them in a specific file
4. Create a DataLoader that maps the visual features to the corresponding language example
In my personal projects, I've decided to ignore HD5F because it doesn't have out-of-the-box support for multi-processing (see this PyTorch [issue](https://github.com/pytorch/pytorch/issues/11929)). I've been successfully using a NumPy compressed file for each image so that I can store any sort of information in it.
For ease of use of all these Language+Vision datasets, it would be really handy to have a way to associate the visual features with the text and store them in an efficient way. That's why I immediately thought about the HuggingFace NLP backend based on Apache Arrow. The assumption here is that the external modality will be mapped to a N-dimensional tensor so easily represented by a NumPy array.
Looking forward to hearing your thoughts about it!
Hi @aleSuglia :) In today's new release 1.17 of `datasets` we introduce a new feature type `Image` that allows to store images directly in a dataset, next to text features and labels for example. There is also an `Audio` feature type, for datasets containing audio data. For tensors there are `Array2D`, `Array3D`, etc. feature types
Note that both Image and Audio feature types take care of decoding the images/audio data if needed. The returned images are PIL images, and the audio signals are decoded as numpy arrays.
And `datasets` also leverage end-to-end zero copy from the arrow data for all of them, for maximum speed :) |
https://github.com/huggingface/datasets/issues/261 | Downloading dataset error with pyarrow.lib.RecordBatch | When you install `nlp` for the first time on a Colab runtime, it updates the `pyarrow` library that was already on colab. This update shows this message on colab:
```
WARNING: The following packages were previously imported in this runtime:
[pyarrow]
You must restart the runtime in order to use newly installed versions.
```
You just have to restart the runtime and it should be fine.
If you don't restart, then it breaks like in your message. | I am trying to download `sentiment140` and I have the following error
```
/usr/local/lib/python3.6/dist-packages/nlp/load.py in load_dataset(path, name, version, data_dir, data_files, split, cache_dir, download_config, download_mode, ignore_verifications, save_infos, **config_kwargs)
518 download_mode=download_mode,
519 ignore_verifications=ignore_verifications,
--> 520 save_infos=save_infos,
521 )
522
/usr/local/lib/python3.6/dist-packages/nlp/builder.py in download_and_prepare(self, download_config, download_mode, ignore_verifications, save_infos, try_from_hf_gcs, dl_manager, **download_and_prepare_kwargs)
418 verify_infos = not save_infos and not ignore_verifications
419 self._download_and_prepare(
--> 420 dl_manager=dl_manager, verify_infos=verify_infos, **download_and_prepare_kwargs
421 )
422 # Sync info
/usr/local/lib/python3.6/dist-packages/nlp/builder.py in _download_and_prepare(self, dl_manager, verify_infos, **prepare_split_kwargs)
472 try:
473 # Prepare split will record examples associated to the split
--> 474 self._prepare_split(split_generator, **prepare_split_kwargs)
475 except OSError:
476 raise OSError("Cannot find data file. " + (self.MANUAL_DOWNLOAD_INSTRUCTIONS or ""))
/usr/local/lib/python3.6/dist-packages/nlp/builder.py in _prepare_split(self, split_generator)
652 for key, record in utils.tqdm(generator, unit=" examples", total=split_info.num_examples, leave=False):
653 example = self.info.features.encode_example(record)
--> 654 writer.write(example)
655 num_examples, num_bytes = writer.finalize()
656
/usr/local/lib/python3.6/dist-packages/nlp/arrow_writer.py in write(self, example, writer_batch_size)
143 self._build_writer(pa_table=pa.Table.from_pydict(example))
144 if writer_batch_size is not None and len(self.current_rows) >= writer_batch_size:
--> 145 self.write_on_file()
146
147 def write_batch(
/usr/local/lib/python3.6/dist-packages/nlp/arrow_writer.py in write_on_file(self)
127 else:
128 # All good
--> 129 self._write_array_on_file(pa_array)
130 self.current_rows = []
131
/usr/local/lib/python3.6/dist-packages/nlp/arrow_writer.py in _write_array_on_file(self, pa_array)
96 def _write_array_on_file(self, pa_array):
97 """Write a PyArrow Array"""
---> 98 pa_batch = pa.RecordBatch.from_struct_array(pa_array)
99 self._num_bytes += pa_array.nbytes
100 self.pa_writer.write_batch(pa_batch)
AttributeError: type object 'pyarrow.lib.RecordBatch' has no attribute 'from_struct_array'
```
I installed the last version and ran the following command:
```python
import nlp
sentiment140 = nlp.load_dataset('sentiment140', cache_dir='/content')
``` | 77 | Downloading dataset error with pyarrow.lib.RecordBatch
I am trying to download `sentiment140` and I have the following error
```
/usr/local/lib/python3.6/dist-packages/nlp/load.py in load_dataset(path, name, version, data_dir, data_files, split, cache_dir, download_config, download_mode, ignore_verifications, save_infos, **config_kwargs)
518 download_mode=download_mode,
519 ignore_verifications=ignore_verifications,
--> 520 save_infos=save_infos,
521 )
522
/usr/local/lib/python3.6/dist-packages/nlp/builder.py in download_and_prepare(self, download_config, download_mode, ignore_verifications, save_infos, try_from_hf_gcs, dl_manager, **download_and_prepare_kwargs)
418 verify_infos = not save_infos and not ignore_verifications
419 self._download_and_prepare(
--> 420 dl_manager=dl_manager, verify_infos=verify_infos, **download_and_prepare_kwargs
421 )
422 # Sync info
/usr/local/lib/python3.6/dist-packages/nlp/builder.py in _download_and_prepare(self, dl_manager, verify_infos, **prepare_split_kwargs)
472 try:
473 # Prepare split will record examples associated to the split
--> 474 self._prepare_split(split_generator, **prepare_split_kwargs)
475 except OSError:
476 raise OSError("Cannot find data file. " + (self.MANUAL_DOWNLOAD_INSTRUCTIONS or ""))
/usr/local/lib/python3.6/dist-packages/nlp/builder.py in _prepare_split(self, split_generator)
652 for key, record in utils.tqdm(generator, unit=" examples", total=split_info.num_examples, leave=False):
653 example = self.info.features.encode_example(record)
--> 654 writer.write(example)
655 num_examples, num_bytes = writer.finalize()
656
/usr/local/lib/python3.6/dist-packages/nlp/arrow_writer.py in write(self, example, writer_batch_size)
143 self._build_writer(pa_table=pa.Table.from_pydict(example))
144 if writer_batch_size is not None and len(self.current_rows) >= writer_batch_size:
--> 145 self.write_on_file()
146
147 def write_batch(
/usr/local/lib/python3.6/dist-packages/nlp/arrow_writer.py in write_on_file(self)
127 else:
128 # All good
--> 129 self._write_array_on_file(pa_array)
130 self.current_rows = []
131
/usr/local/lib/python3.6/dist-packages/nlp/arrow_writer.py in _write_array_on_file(self, pa_array)
96 def _write_array_on_file(self, pa_array):
97 """Write a PyArrow Array"""
---> 98 pa_batch = pa.RecordBatch.from_struct_array(pa_array)
99 self._num_bytes += pa_array.nbytes
100 self.pa_writer.write_batch(pa_batch)
AttributeError: type object 'pyarrow.lib.RecordBatch' has no attribute 'from_struct_array'
```
I installed the last version and ran the following command:
```python
import nlp
sentiment140 = nlp.load_dataset('sentiment140', cache_dir='/content')
```
When you install `nlp` for the first time on a Colab runtime, it updates the `pyarrow` library that was already on colab. This update shows this message on colab:
```
WARNING: The following packages were previously imported in this runtime:
[pyarrow]
You must restart the runtime in order to use newly installed versions.
```
You just have to restart the runtime and it should be fine.
If you don't restart, then it breaks like in your message. |
https://github.com/huggingface/datasets/issues/259 | documentation missing how to split a dataset | this seems to work for my specific problem:
`self.train_ds, self.test_ds, self.val_ds = map(_prepare_ds, ('train', 'test[:25%]+test[50%:75%]', 'test[75%:]'))` | I am trying to understand how to split a dataset ( as arrow_dataset).
I know I can do something like this to access a split which is already in the original dataset :
`ds_test = nlp.load_dataset('imdb, split='test') `
But how can I split ds_test into a test and a validation set (without reading the data into memory and keeping the arrow_dataset as container)?
I guess it has something to do with the module split :-) but there is no real documentation in the code but only a reference to a longer description:
> See the [guide on splits](https://github.com/huggingface/nlp/tree/master/docs/splits.md) for more information.
But the guide seems to be missing.
To clarify: I know that this has been modelled after the dataset of tensorflow and that some of the documentation there can be used [like this one](https://www.tensorflow.org/datasets/splits). But to come back to the example above: I cannot simply split the testset doing this:
`ds_test = nlp.load_dataset('imdb, split='test'[:5000]) `
`ds_val = nlp.load_dataset('imdb, split='test'[5000:])`
because the imdb test data is sorted by class (probably not a good idea anyway)
| 16 | documentation missing how to split a dataset
I am trying to understand how to split a dataset ( as arrow_dataset).
I know I can do something like this to access a split which is already in the original dataset :
`ds_test = nlp.load_dataset('imdb, split='test') `
But how can I split ds_test into a test and a validation set (without reading the data into memory and keeping the arrow_dataset as container)?
I guess it has something to do with the module split :-) but there is no real documentation in the code but only a reference to a longer description:
> See the [guide on splits](https://github.com/huggingface/nlp/tree/master/docs/splits.md) for more information.
But the guide seems to be missing.
To clarify: I know that this has been modelled after the dataset of tensorflow and that some of the documentation there can be used [like this one](https://www.tensorflow.org/datasets/splits). But to come back to the example above: I cannot simply split the testset doing this:
`ds_test = nlp.load_dataset('imdb, split='test'[:5000]) `
`ds_val = nlp.load_dataset('imdb, split='test'[5000:])`
because the imdb test data is sorted by class (probably not a good idea anyway)
this seems to work for my specific problem:
`self.train_ds, self.test_ds, self.val_ds = map(_prepare_ds, ('train', 'test[:25%]+test[50%:75%]', 'test[75%:]'))` |
https://github.com/huggingface/datasets/issues/259 | documentation missing how to split a dataset | Currently you can indeed split a dataset using `ds_test = nlp.load_dataset('imdb, split='test[:5000]')` (works also with percentages).
However right now we don't have a way to shuffle a dataset but we are thinking about it in the discussion in #166. Feel free to share your thoughts about it.
One trick that you can do until we have a better solution is to shuffle and split the indices of your dataset:
```python
import nlp
from sklearn.model_selection import train_test_split
imdb = nlp.load_dataset('imbd', split='test')
test_indices, val_indices = train_test_split(range(len(imdb)))
```
and then to iterate each split:
```python
for i in test_indices:
example = imdb[i]
...
```
| I am trying to understand how to split a dataset ( as arrow_dataset).
I know I can do something like this to access a split which is already in the original dataset :
`ds_test = nlp.load_dataset('imdb, split='test') `
But how can I split ds_test into a test and a validation set (without reading the data into memory and keeping the arrow_dataset as container)?
I guess it has something to do with the module split :-) but there is no real documentation in the code but only a reference to a longer description:
> See the [guide on splits](https://github.com/huggingface/nlp/tree/master/docs/splits.md) for more information.
But the guide seems to be missing.
To clarify: I know that this has been modelled after the dataset of tensorflow and that some of the documentation there can be used [like this one](https://www.tensorflow.org/datasets/splits). But to come back to the example above: I cannot simply split the testset doing this:
`ds_test = nlp.load_dataset('imdb, split='test'[:5000]) `
`ds_val = nlp.load_dataset('imdb, split='test'[5000:])`
because the imdb test data is sorted by class (probably not a good idea anyway)
| 101 | documentation missing how to split a dataset
I am trying to understand how to split a dataset ( as arrow_dataset).
I know I can do something like this to access a split which is already in the original dataset :
`ds_test = nlp.load_dataset('imdb, split='test') `
But how can I split ds_test into a test and a validation set (without reading the data into memory and keeping the arrow_dataset as container)?
I guess it has something to do with the module split :-) but there is no real documentation in the code but only a reference to a longer description:
> See the [guide on splits](https://github.com/huggingface/nlp/tree/master/docs/splits.md) for more information.
But the guide seems to be missing.
To clarify: I know that this has been modelled after the dataset of tensorflow and that some of the documentation there can be used [like this one](https://www.tensorflow.org/datasets/splits). But to come back to the example above: I cannot simply split the testset doing this:
`ds_test = nlp.load_dataset('imdb, split='test'[:5000]) `
`ds_val = nlp.load_dataset('imdb, split='test'[5000:])`
because the imdb test data is sorted by class (probably not a good idea anyway)
Currently you can indeed split a dataset using `ds_test = nlp.load_dataset('imdb, split='test[:5000]')` (works also with percentages).
However right now we don't have a way to shuffle a dataset but we are thinking about it in the discussion in #166. Feel free to share your thoughts about it.
One trick that you can do until we have a better solution is to shuffle and split the indices of your dataset:
```python
import nlp
from sklearn.model_selection import train_test_split
imdb = nlp.load_dataset('imbd', split='test')
test_indices, val_indices = train_test_split(range(len(imdb)))
```
and then to iterate each split:
```python
for i in test_indices:
example = imdb[i]
...
```
|
https://github.com/huggingface/datasets/issues/259 | documentation missing how to split a dataset | I added a small guide [here](https://github.com/huggingface/nlp/tree/master/docs/splits.md) that explains how to split a dataset. It is very similar to the tensorflow datasets guide, as we kept the same logic. | I am trying to understand how to split a dataset ( as arrow_dataset).
I know I can do something like this to access a split which is already in the original dataset :
`ds_test = nlp.load_dataset('imdb, split='test') `
But how can I split ds_test into a test and a validation set (without reading the data into memory and keeping the arrow_dataset as container)?
I guess it has something to do with the module split :-) but there is no real documentation in the code but only a reference to a longer description:
> See the [guide on splits](https://github.com/huggingface/nlp/tree/master/docs/splits.md) for more information.
But the guide seems to be missing.
To clarify: I know that this has been modelled after the dataset of tensorflow and that some of the documentation there can be used [like this one](https://www.tensorflow.org/datasets/splits). But to come back to the example above: I cannot simply split the testset doing this:
`ds_test = nlp.load_dataset('imdb, split='test'[:5000]) `
`ds_val = nlp.load_dataset('imdb, split='test'[5000:])`
because the imdb test data is sorted by class (probably not a good idea anyway)
| 28 | documentation missing how to split a dataset
I am trying to understand how to split a dataset ( as arrow_dataset).
I know I can do something like this to access a split which is already in the original dataset :
`ds_test = nlp.load_dataset('imdb, split='test') `
But how can I split ds_test into a test and a validation set (without reading the data into memory and keeping the arrow_dataset as container)?
I guess it has something to do with the module split :-) but there is no real documentation in the code but only a reference to a longer description:
> See the [guide on splits](https://github.com/huggingface/nlp/tree/master/docs/splits.md) for more information.
But the guide seems to be missing.
To clarify: I know that this has been modelled after the dataset of tensorflow and that some of the documentation there can be used [like this one](https://www.tensorflow.org/datasets/splits). But to come back to the example above: I cannot simply split the testset doing this:
`ds_test = nlp.load_dataset('imdb, split='test'[:5000]) `
`ds_val = nlp.load_dataset('imdb, split='test'[5000:])`
because the imdb test data is sorted by class (probably not a good idea anyway)
I added a small guide [here](https://github.com/huggingface/nlp/tree/master/docs/splits.md) that explains how to split a dataset. It is very similar to the tensorflow datasets guide, as we kept the same logic. |
https://github.com/huggingface/datasets/issues/259 | documentation missing how to split a dataset | Thanks a lot, the new explanation is very helpful!
About using train_test_split from sklearn: I stumbled across the [same error message as this user ](https://github.com/huggingface/nlp/issues/147 )and thought it can't be used at the moment in this context. Will check it out again.
One of the problems is how to shuffle very large datasets, which don't fit into the memory. Well, one strategy could be shuffling data in sections. But in a case where the data is sorted by the labels you have to swap larger sections first.
| I am trying to understand how to split a dataset ( as arrow_dataset).
I know I can do something like this to access a split which is already in the original dataset :
`ds_test = nlp.load_dataset('imdb, split='test') `
But how can I split ds_test into a test and a validation set (without reading the data into memory and keeping the arrow_dataset as container)?
I guess it has something to do with the module split :-) but there is no real documentation in the code but only a reference to a longer description:
> See the [guide on splits](https://github.com/huggingface/nlp/tree/master/docs/splits.md) for more information.
But the guide seems to be missing.
To clarify: I know that this has been modelled after the dataset of tensorflow and that some of the documentation there can be used [like this one](https://www.tensorflow.org/datasets/splits). But to come back to the example above: I cannot simply split the testset doing this:
`ds_test = nlp.load_dataset('imdb, split='test'[:5000]) `
`ds_val = nlp.load_dataset('imdb, split='test'[5000:])`
because the imdb test data is sorted by class (probably not a good idea anyway)
| 87 | documentation missing how to split a dataset
I am trying to understand how to split a dataset ( as arrow_dataset).
I know I can do something like this to access a split which is already in the original dataset :
`ds_test = nlp.load_dataset('imdb, split='test') `
But how can I split ds_test into a test and a validation set (without reading the data into memory and keeping the arrow_dataset as container)?
I guess it has something to do with the module split :-) but there is no real documentation in the code but only a reference to a longer description:
> See the [guide on splits](https://github.com/huggingface/nlp/tree/master/docs/splits.md) for more information.
But the guide seems to be missing.
To clarify: I know that this has been modelled after the dataset of tensorflow and that some of the documentation there can be used [like this one](https://www.tensorflow.org/datasets/splits). But to come back to the example above: I cannot simply split the testset doing this:
`ds_test = nlp.load_dataset('imdb, split='test'[:5000]) `
`ds_val = nlp.load_dataset('imdb, split='test'[5000:])`
because the imdb test data is sorted by class (probably not a good idea anyway)
Thanks a lot, the new explanation is very helpful!
About using train_test_split from sklearn: I stumbled across the [same error message as this user ](https://github.com/huggingface/nlp/issues/147 )and thought it can't be used at the moment in this context. Will check it out again.
One of the problems is how to shuffle very large datasets, which don't fit into the memory. Well, one strategy could be shuffling data in sections. But in a case where the data is sorted by the labels you have to swap larger sections first.
|
https://github.com/huggingface/datasets/issues/259 | documentation missing how to split a dataset | We added a way to shuffle datasets (shuffle the indices and then reorder to make a new dataset).
You can do `shuffled_dset = dataset.shuffle(seed=my_seed)`. It shuffles the whole dataset.
There is also `dataset.train_test_split()` which if very handy (with the same signature as sklearn).
Closing this issue as we added the docs for splits and tools to split datasets. Thanks again for your feedback ! | I am trying to understand how to split a dataset ( as arrow_dataset).
I know I can do something like this to access a split which is already in the original dataset :
`ds_test = nlp.load_dataset('imdb, split='test') `
But how can I split ds_test into a test and a validation set (without reading the data into memory and keeping the arrow_dataset as container)?
I guess it has something to do with the module split :-) but there is no real documentation in the code but only a reference to a longer description:
> See the [guide on splits](https://github.com/huggingface/nlp/tree/master/docs/splits.md) for more information.
But the guide seems to be missing.
To clarify: I know that this has been modelled after the dataset of tensorflow and that some of the documentation there can be used [like this one](https://www.tensorflow.org/datasets/splits). But to come back to the example above: I cannot simply split the testset doing this:
`ds_test = nlp.load_dataset('imdb, split='test'[:5000]) `
`ds_val = nlp.load_dataset('imdb, split='test'[5000:])`
because the imdb test data is sorted by class (probably not a good idea anyway)
| 64 | documentation missing how to split a dataset
I am trying to understand how to split a dataset ( as arrow_dataset).
I know I can do something like this to access a split which is already in the original dataset :
`ds_test = nlp.load_dataset('imdb, split='test') `
But how can I split ds_test into a test and a validation set (without reading the data into memory and keeping the arrow_dataset as container)?
I guess it has something to do with the module split :-) but there is no real documentation in the code but only a reference to a longer description:
> See the [guide on splits](https://github.com/huggingface/nlp/tree/master/docs/splits.md) for more information.
But the guide seems to be missing.
To clarify: I know that this has been modelled after the dataset of tensorflow and that some of the documentation there can be used [like this one](https://www.tensorflow.org/datasets/splits). But to come back to the example above: I cannot simply split the testset doing this:
`ds_test = nlp.load_dataset('imdb, split='test'[:5000]) `
`ds_val = nlp.load_dataset('imdb, split='test'[5000:])`
because the imdb test data is sorted by class (probably not a good idea anyway)
We added a way to shuffle datasets (shuffle the indices and then reorder to make a new dataset).
You can do `shuffled_dset = dataset.shuffle(seed=my_seed)`. It shuffles the whole dataset.
There is also `dataset.train_test_split()` which if very handy (with the same signature as sklearn).
Closing this issue as we added the docs for splits and tools to split datasets. Thanks again for your feedback ! |
https://github.com/huggingface/datasets/issues/258 | Why is dataset after tokenization far more larger than the orginal one ? | Hi ! This is because `.map` added the new column `input_ids` to the dataset, and so all the other columns were kept. Therefore the dataset size increased a lot.
If you want to only keep the `input_ids` column, you can stash the other ones by specifying `remove_columns=["title", "text"]` in the arguments of `.map` | I tokenize wiki dataset by `map` and cache the results.
```
def tokenize_tfm(example):
example['input_ids'] = hf_fast_tokenizer.convert_tokens_to_ids(hf_fast_tokenizer.tokenize(example['text']))
return example
wiki = nlp.load_dataset('wikipedia', '20200501.en', cache_dir=cache_dir)['train']
wiki.map(tokenize_tfm, cache_file_name=cache_dir/"wikipedia/20200501.en/1.0.0/tokenized_wiki.arrow")
```
and when I see their size
```
ls -l --block-size=M
17460M wikipedia-train.arrow
47511M tokenized_wiki.arrow
```
The tokenized one is over 2x size of original one.
Is there something I did wrong ? | 53 | Why is dataset after tokenization far more larger than the orginal one ?
I tokenize wiki dataset by `map` and cache the results.
```
def tokenize_tfm(example):
example['input_ids'] = hf_fast_tokenizer.convert_tokens_to_ids(hf_fast_tokenizer.tokenize(example['text']))
return example
wiki = nlp.load_dataset('wikipedia', '20200501.en', cache_dir=cache_dir)['train']
wiki.map(tokenize_tfm, cache_file_name=cache_dir/"wikipedia/20200501.en/1.0.0/tokenized_wiki.arrow")
```
and when I see their size
```
ls -l --block-size=M
17460M wikipedia-train.arrow
47511M tokenized_wiki.arrow
```
The tokenized one is over 2x size of original one.
Is there something I did wrong ?
Hi ! This is because `.map` added the new column `input_ids` to the dataset, and so all the other columns were kept. Therefore the dataset size increased a lot.
If you want to only keep the `input_ids` column, you can stash the other ones by specifying `remove_columns=["title", "text"]` in the arguments of `.map` |
https://github.com/huggingface/datasets/issues/258 | Why is dataset after tokenization far more larger than the orginal one ? | Hi ! Thanks for your reply.
But since size of `input_ids` < size of `text`, I am wondering why
size of `input_ids` + `text` > 2x the size of `text` 🤔 | I tokenize wiki dataset by `map` and cache the results.
```
def tokenize_tfm(example):
example['input_ids'] = hf_fast_tokenizer.convert_tokens_to_ids(hf_fast_tokenizer.tokenize(example['text']))
return example
wiki = nlp.load_dataset('wikipedia', '20200501.en', cache_dir=cache_dir)['train']
wiki.map(tokenize_tfm, cache_file_name=cache_dir/"wikipedia/20200501.en/1.0.0/tokenized_wiki.arrow")
```
and when I see their size
```
ls -l --block-size=M
17460M wikipedia-train.arrow
47511M tokenized_wiki.arrow
```
The tokenized one is over 2x size of original one.
Is there something I did wrong ? | 31 | Why is dataset after tokenization far more larger than the orginal one ?
I tokenize wiki dataset by `map` and cache the results.
```
def tokenize_tfm(example):
example['input_ids'] = hf_fast_tokenizer.convert_tokens_to_ids(hf_fast_tokenizer.tokenize(example['text']))
return example
wiki = nlp.load_dataset('wikipedia', '20200501.en', cache_dir=cache_dir)['train']
wiki.map(tokenize_tfm, cache_file_name=cache_dir/"wikipedia/20200501.en/1.0.0/tokenized_wiki.arrow")
```
and when I see their size
```
ls -l --block-size=M
17460M wikipedia-train.arrow
47511M tokenized_wiki.arrow
```
The tokenized one is over 2x size of original one.
Is there something I did wrong ?
Hi ! Thanks for your reply.
But since size of `input_ids` < size of `text`, I am wondering why
size of `input_ids` + `text` > 2x the size of `text` 🤔 |
https://github.com/huggingface/datasets/issues/258 | Why is dataset after tokenization far more larger than the orginal one ? | Hard to tell... This is probably related to the way apache arrow compresses lists of integers, that may be different from the compression of strings. | I tokenize wiki dataset by `map` and cache the results.
```
def tokenize_tfm(example):
example['input_ids'] = hf_fast_tokenizer.convert_tokens_to_ids(hf_fast_tokenizer.tokenize(example['text']))
return example
wiki = nlp.load_dataset('wikipedia', '20200501.en', cache_dir=cache_dir)['train']
wiki.map(tokenize_tfm, cache_file_name=cache_dir/"wikipedia/20200501.en/1.0.0/tokenized_wiki.arrow")
```
and when I see their size
```
ls -l --block-size=M
17460M wikipedia-train.arrow
47511M tokenized_wiki.arrow
```
The tokenized one is over 2x size of original one.
Is there something I did wrong ? | 25 | Why is dataset after tokenization far more larger than the orginal one ?
I tokenize wiki dataset by `map` and cache the results.
```
def tokenize_tfm(example):
example['input_ids'] = hf_fast_tokenizer.convert_tokens_to_ids(hf_fast_tokenizer.tokenize(example['text']))
return example
wiki = nlp.load_dataset('wikipedia', '20200501.en', cache_dir=cache_dir)['train']
wiki.map(tokenize_tfm, cache_file_name=cache_dir/"wikipedia/20200501.en/1.0.0/tokenized_wiki.arrow")
```
and when I see their size
```
ls -l --block-size=M
17460M wikipedia-train.arrow
47511M tokenized_wiki.arrow
```
The tokenized one is over 2x size of original one.
Is there something I did wrong ?
Hard to tell... This is probably related to the way apache arrow compresses lists of integers, that may be different from the compression of strings. |
https://github.com/huggingface/datasets/issues/258 | Why is dataset after tokenization far more larger than the orginal one ? | Thanks for your point. 😀, It might be answer.
Since this is hard to know, I'll close this issue.
But if somebody knows more details, please comment below ~ 😁 | I tokenize wiki dataset by `map` and cache the results.
```
def tokenize_tfm(example):
example['input_ids'] = hf_fast_tokenizer.convert_tokens_to_ids(hf_fast_tokenizer.tokenize(example['text']))
return example
wiki = nlp.load_dataset('wikipedia', '20200501.en', cache_dir=cache_dir)['train']
wiki.map(tokenize_tfm, cache_file_name=cache_dir/"wikipedia/20200501.en/1.0.0/tokenized_wiki.arrow")
```
and when I see their size
```
ls -l --block-size=M
17460M wikipedia-train.arrow
47511M tokenized_wiki.arrow
```
The tokenized one is over 2x size of original one.
Is there something I did wrong ? | 30 | Why is dataset after tokenization far more larger than the orginal one ?
I tokenize wiki dataset by `map` and cache the results.
```
def tokenize_tfm(example):
example['input_ids'] = hf_fast_tokenizer.convert_tokens_to_ids(hf_fast_tokenizer.tokenize(example['text']))
return example
wiki = nlp.load_dataset('wikipedia', '20200501.en', cache_dir=cache_dir)['train']
wiki.map(tokenize_tfm, cache_file_name=cache_dir/"wikipedia/20200501.en/1.0.0/tokenized_wiki.arrow")
```
and when I see their size
```
ls -l --block-size=M
17460M wikipedia-train.arrow
47511M tokenized_wiki.arrow
```
The tokenized one is over 2x size of original one.
Is there something I did wrong ?
Thanks for your point. 😀, It might be answer.
Since this is hard to know, I'll close this issue.
But if somebody knows more details, please comment below ~ 😁 |
https://github.com/huggingface/datasets/issues/257 | Tokenizer pickling issue fix not landed in `nlp` yet? | Yes, the new release of tokenizers solves this and should be out soon.
In the meantime, you can install it with `pip install tokenizers==0.8.0-dev2` | Unless I recreate an arrow_dataset from my loaded nlp dataset myself (which I think does not use the cache by default), I get the following error when applying the map function:
```
dataset = nlp.load_dataset('cos_e')
tokenizer = GPT2TokenizerFast.from_pretrained('gpt2', cache_dir=cache_dir)
for split in dataset.keys():
dataset[split].map(lambda x: some_function(x, tokenizer))
```
```
06/09/2020 10:09:19 - INFO - nlp.builder - Constructing Dataset for split train[:10], from /home/sarahw/.cache/huggingface/datasets/cos_e/default/0.0.1
Traceback (most recent call last):
File "generation/input_to_label_and_rationale.py", line 390, in <module>
main()
File "generation/input_to_label_and_rationale.py", line 263, in main
dataset[split] = dataset[split].map(lambda x: input_to_explanation_plus_label(x, tokenizer, max_length, datasource=data_args.task_name, wt5=(model_class=='t5'), expl_only=model_args.rationale_only), batched=False)
File "/home/sarahw/miniconda3/envs/project_huggingface/lib/python3.8/site-packages/nlp/arrow_dataset.py", line 522, in map
cache_file_name = self._get_cache_file_path(function, cache_kwargs)
File "/home/sarahw/miniconda3/envs/project_huggingface/lib/python3.8/site-packages/nlp/arrow_dataset.py", line 381, in _get_cache_file_path
function_bytes = dumps(function)
File "/home/sarahw/miniconda3/envs/project_huggingface/lib/python3.8/site-packages/nlp/utils/py_utils.py", line 257, in dumps
dump(obj, file)
File "/home/sarahw/miniconda3/envs/project_huggingface/lib/python3.8/site-packages/nlp/utils/py_utils.py", line 250, in dump
Pickler(file).dump(obj)
File "/home/sarahw/miniconda3/envs/project_huggingface/lib/python3.8/site-packages/dill/_dill.py", line 445, in dump
StockPickler.dump(self, obj)
File "/home/sarahw/miniconda3/envs/project_huggingface/lib/python3.8/pickle.py", line 485, in dump
self.save(obj)
File "/home/sarahw/miniconda3/envs/project_huggingface/lib/python3.8/pickle.py", line 558, in save
f(self, obj) # Call unbound method with explicit self
File "/home/sarahw/miniconda3/envs/project_huggingface/lib/python3.8/site-packages/dill/_dill.py", line 1410, in save_function
pickler.save_reduce(_create_function, (obj.__code__,
File "/home/sarahw/miniconda3/envs/project_huggingface/lib/python3.8/pickle.py", line 690, in save_reduce
save(args)
File "/home/sarahw/miniconda3/envs/project_huggingface/lib/python3.8/pickle.py", line 558, in save
f(self, obj) # Call unbound method with explicit self
File "/home/sarahw/miniconda3/envs/project_huggingface/lib/python3.8/pickle.py", line 899, in save_tuple
save(element)
File "/home/sarahw/miniconda3/envs/project_huggingface/lib/python3.8/pickle.py", line 558, in save
f(self, obj) # Call unbound method with explicit self
File "/home/sarahw/miniconda3/envs/project_huggingface/lib/python3.8/pickle.py", line 899, in save_tuple
save(element)
File "/home/sarahw/miniconda3/envs/project_huggingface/lib/python3.8/pickle.py", line 558, in save
f(self, obj) # Call unbound method with explicit self
File "/home/sarahw/miniconda3/envs/project_huggingface/lib/python3.8/site-packages/dill/_dill.py", line 1147, in save_cell
pickler.save_reduce(_create_cell, (f,), obj=obj)
File "/home/sarahw/miniconda3/envs/project_huggingface/lib/python3.8/pickle.py", line 690, in save_reduce
save(args)
File "/home/sarahw/miniconda3/envs/project_huggingface/lib/python3.8/pickle.py", line 558, in save
f(self, obj) # Call unbound method with explicit self
File "/home/sarahw/miniconda3/envs/project_huggingface/lib/python3.8/pickle.py", line 884, in save_tuple
save(element)
File "/home/sarahw/miniconda3/envs/project_huggingface/lib/python3.8/pickle.py", line 601, in save
self.save_reduce(obj=obj, *rv)
File "/home/sarahw/miniconda3/envs/project_huggingface/lib/python3.8/pickle.py", line 715, in save_reduce
save(state)
File "/home/sarahw/miniconda3/envs/project_huggingface/lib/python3.8/pickle.py", line 558, in save
f(self, obj) # Call unbound method with explicit self
File "/home/sarahw/miniconda3/envs/project_huggingface/lib/python3.8/site-packages/dill/_dill.py", line 912, in save_module_dict
StockPickler.save_dict(pickler, obj)
File "/home/sarahw/miniconda3/envs/project_huggingface/lib/python3.8/pickle.py", line 969, in save_dict
self._batch_setitems(obj.items())
File "/home/sarahw/miniconda3/envs/project_huggingface/lib/python3.8/pickle.py", line 995, in _batch_setitems
save(v)
File "/home/sarahw/miniconda3/envs/project_huggingface/lib/python3.8/pickle.py", line 601, in save
self.save_reduce(obj=obj, *rv)
File "/home/sarahw/miniconda3/envs/project_huggingface/lib/python3.8/pickle.py", line 715, in save_reduce
save(state)
File "/home/sarahw/miniconda3/envs/project_huggingface/lib/python3.8/pickle.py", line 558, in save
f(self, obj) # Call unbound method with explicit self
File "/home/sarahw/miniconda3/envs/project_huggingface/lib/python3.8/site-packages/dill/_dill.py", line 912, in save_module_dict
StockPickler.save_dict(pickler, obj)
File "/home/sarahw/miniconda3/envs/project_huggingface/lib/python3.8/pickle.py", line 969, in save_dict
self._batch_setitems(obj.items())
File "/home/sarahw/miniconda3/envs/project_huggingface/lib/python3.8/pickle.py", line 995, in _batch_setitems
save(v)
File "/home/sarahw/miniconda3/envs/project_huggingface/lib/python3.8/pickle.py", line 576, in save
rv = reduce(self.proto)
TypeError: cannot pickle 'Tokenizer' object
```
Fix seems to be in the tokenizers [`0.8.0.dev1 pre-release`](https://github.com/huggingface/tokenizers/issues/87), which I can't install with any package managers. | 24 | Tokenizer pickling issue fix not landed in `nlp` yet?
Unless I recreate an arrow_dataset from my loaded nlp dataset myself (which I think does not use the cache by default), I get the following error when applying the map function:
```
dataset = nlp.load_dataset('cos_e')
tokenizer = GPT2TokenizerFast.from_pretrained('gpt2', cache_dir=cache_dir)
for split in dataset.keys():
dataset[split].map(lambda x: some_function(x, tokenizer))
```
```
06/09/2020 10:09:19 - INFO - nlp.builder - Constructing Dataset for split train[:10], from /home/sarahw/.cache/huggingface/datasets/cos_e/default/0.0.1
Traceback (most recent call last):
File "generation/input_to_label_and_rationale.py", line 390, in <module>
main()
File "generation/input_to_label_and_rationale.py", line 263, in main
dataset[split] = dataset[split].map(lambda x: input_to_explanation_plus_label(x, tokenizer, max_length, datasource=data_args.task_name, wt5=(model_class=='t5'), expl_only=model_args.rationale_only), batched=False)
File "/home/sarahw/miniconda3/envs/project_huggingface/lib/python3.8/site-packages/nlp/arrow_dataset.py", line 522, in map
cache_file_name = self._get_cache_file_path(function, cache_kwargs)
File "/home/sarahw/miniconda3/envs/project_huggingface/lib/python3.8/site-packages/nlp/arrow_dataset.py", line 381, in _get_cache_file_path
function_bytes = dumps(function)
File "/home/sarahw/miniconda3/envs/project_huggingface/lib/python3.8/site-packages/nlp/utils/py_utils.py", line 257, in dumps
dump(obj, file)
File "/home/sarahw/miniconda3/envs/project_huggingface/lib/python3.8/site-packages/nlp/utils/py_utils.py", line 250, in dump
Pickler(file).dump(obj)
File "/home/sarahw/miniconda3/envs/project_huggingface/lib/python3.8/site-packages/dill/_dill.py", line 445, in dump
StockPickler.dump(self, obj)
File "/home/sarahw/miniconda3/envs/project_huggingface/lib/python3.8/pickle.py", line 485, in dump
self.save(obj)
File "/home/sarahw/miniconda3/envs/project_huggingface/lib/python3.8/pickle.py", line 558, in save
f(self, obj) # Call unbound method with explicit self
File "/home/sarahw/miniconda3/envs/project_huggingface/lib/python3.8/site-packages/dill/_dill.py", line 1410, in save_function
pickler.save_reduce(_create_function, (obj.__code__,
File "/home/sarahw/miniconda3/envs/project_huggingface/lib/python3.8/pickle.py", line 690, in save_reduce
save(args)
File "/home/sarahw/miniconda3/envs/project_huggingface/lib/python3.8/pickle.py", line 558, in save
f(self, obj) # Call unbound method with explicit self
File "/home/sarahw/miniconda3/envs/project_huggingface/lib/python3.8/pickle.py", line 899, in save_tuple
save(element)
File "/home/sarahw/miniconda3/envs/project_huggingface/lib/python3.8/pickle.py", line 558, in save
f(self, obj) # Call unbound method with explicit self
File "/home/sarahw/miniconda3/envs/project_huggingface/lib/python3.8/pickle.py", line 899, in save_tuple
save(element)
File "/home/sarahw/miniconda3/envs/project_huggingface/lib/python3.8/pickle.py", line 558, in save
f(self, obj) # Call unbound method with explicit self
File "/home/sarahw/miniconda3/envs/project_huggingface/lib/python3.8/site-packages/dill/_dill.py", line 1147, in save_cell
pickler.save_reduce(_create_cell, (f,), obj=obj)
File "/home/sarahw/miniconda3/envs/project_huggingface/lib/python3.8/pickle.py", line 690, in save_reduce
save(args)
File "/home/sarahw/miniconda3/envs/project_huggingface/lib/python3.8/pickle.py", line 558, in save
f(self, obj) # Call unbound method with explicit self
File "/home/sarahw/miniconda3/envs/project_huggingface/lib/python3.8/pickle.py", line 884, in save_tuple
save(element)
File "/home/sarahw/miniconda3/envs/project_huggingface/lib/python3.8/pickle.py", line 601, in save
self.save_reduce(obj=obj, *rv)
File "/home/sarahw/miniconda3/envs/project_huggingface/lib/python3.8/pickle.py", line 715, in save_reduce
save(state)
File "/home/sarahw/miniconda3/envs/project_huggingface/lib/python3.8/pickle.py", line 558, in save
f(self, obj) # Call unbound method with explicit self
File "/home/sarahw/miniconda3/envs/project_huggingface/lib/python3.8/site-packages/dill/_dill.py", line 912, in save_module_dict
StockPickler.save_dict(pickler, obj)
File "/home/sarahw/miniconda3/envs/project_huggingface/lib/python3.8/pickle.py", line 969, in save_dict
self._batch_setitems(obj.items())
File "/home/sarahw/miniconda3/envs/project_huggingface/lib/python3.8/pickle.py", line 995, in _batch_setitems
save(v)
File "/home/sarahw/miniconda3/envs/project_huggingface/lib/python3.8/pickle.py", line 601, in save
self.save_reduce(obj=obj, *rv)
File "/home/sarahw/miniconda3/envs/project_huggingface/lib/python3.8/pickle.py", line 715, in save_reduce
save(state)
File "/home/sarahw/miniconda3/envs/project_huggingface/lib/python3.8/pickle.py", line 558, in save
f(self, obj) # Call unbound method with explicit self
File "/home/sarahw/miniconda3/envs/project_huggingface/lib/python3.8/site-packages/dill/_dill.py", line 912, in save_module_dict
StockPickler.save_dict(pickler, obj)
File "/home/sarahw/miniconda3/envs/project_huggingface/lib/python3.8/pickle.py", line 969, in save_dict
self._batch_setitems(obj.items())
File "/home/sarahw/miniconda3/envs/project_huggingface/lib/python3.8/pickle.py", line 995, in _batch_setitems
save(v)
File "/home/sarahw/miniconda3/envs/project_huggingface/lib/python3.8/pickle.py", line 576, in save
rv = reduce(self.proto)
TypeError: cannot pickle 'Tokenizer' object
```
Fix seems to be in the tokenizers [`0.8.0.dev1 pre-release`](https://github.com/huggingface/tokenizers/issues/87), which I can't install with any package managers.
Yes, the new release of tokenizers solves this and should be out soon.
In the meantime, you can install it with `pip install tokenizers==0.8.0-dev2` |
https://github.com/huggingface/datasets/issues/257 | Tokenizer pickling issue fix not landed in `nlp` yet? | If others run into this issue, a quick fix is to use python 3.6 instead of 3.7+. Serialization differences between the 3rd party `dataclasses` package for 3.6 and the built in `dataclasses` in 3.7+ cause the issue.
Probably a dumb fix, but it works for me. | Unless I recreate an arrow_dataset from my loaded nlp dataset myself (which I think does not use the cache by default), I get the following error when applying the map function:
```
dataset = nlp.load_dataset('cos_e')
tokenizer = GPT2TokenizerFast.from_pretrained('gpt2', cache_dir=cache_dir)
for split in dataset.keys():
dataset[split].map(lambda x: some_function(x, tokenizer))
```
```
06/09/2020 10:09:19 - INFO - nlp.builder - Constructing Dataset for split train[:10], from /home/sarahw/.cache/huggingface/datasets/cos_e/default/0.0.1
Traceback (most recent call last):
File "generation/input_to_label_and_rationale.py", line 390, in <module>
main()
File "generation/input_to_label_and_rationale.py", line 263, in main
dataset[split] = dataset[split].map(lambda x: input_to_explanation_plus_label(x, tokenizer, max_length, datasource=data_args.task_name, wt5=(model_class=='t5'), expl_only=model_args.rationale_only), batched=False)
File "/home/sarahw/miniconda3/envs/project_huggingface/lib/python3.8/site-packages/nlp/arrow_dataset.py", line 522, in map
cache_file_name = self._get_cache_file_path(function, cache_kwargs)
File "/home/sarahw/miniconda3/envs/project_huggingface/lib/python3.8/site-packages/nlp/arrow_dataset.py", line 381, in _get_cache_file_path
function_bytes = dumps(function)
File "/home/sarahw/miniconda3/envs/project_huggingface/lib/python3.8/site-packages/nlp/utils/py_utils.py", line 257, in dumps
dump(obj, file)
File "/home/sarahw/miniconda3/envs/project_huggingface/lib/python3.8/site-packages/nlp/utils/py_utils.py", line 250, in dump
Pickler(file).dump(obj)
File "/home/sarahw/miniconda3/envs/project_huggingface/lib/python3.8/site-packages/dill/_dill.py", line 445, in dump
StockPickler.dump(self, obj)
File "/home/sarahw/miniconda3/envs/project_huggingface/lib/python3.8/pickle.py", line 485, in dump
self.save(obj)
File "/home/sarahw/miniconda3/envs/project_huggingface/lib/python3.8/pickle.py", line 558, in save
f(self, obj) # Call unbound method with explicit self
File "/home/sarahw/miniconda3/envs/project_huggingface/lib/python3.8/site-packages/dill/_dill.py", line 1410, in save_function
pickler.save_reduce(_create_function, (obj.__code__,
File "/home/sarahw/miniconda3/envs/project_huggingface/lib/python3.8/pickle.py", line 690, in save_reduce
save(args)
File "/home/sarahw/miniconda3/envs/project_huggingface/lib/python3.8/pickle.py", line 558, in save
f(self, obj) # Call unbound method with explicit self
File "/home/sarahw/miniconda3/envs/project_huggingface/lib/python3.8/pickle.py", line 899, in save_tuple
save(element)
File "/home/sarahw/miniconda3/envs/project_huggingface/lib/python3.8/pickle.py", line 558, in save
f(self, obj) # Call unbound method with explicit self
File "/home/sarahw/miniconda3/envs/project_huggingface/lib/python3.8/pickle.py", line 899, in save_tuple
save(element)
File "/home/sarahw/miniconda3/envs/project_huggingface/lib/python3.8/pickle.py", line 558, in save
f(self, obj) # Call unbound method with explicit self
File "/home/sarahw/miniconda3/envs/project_huggingface/lib/python3.8/site-packages/dill/_dill.py", line 1147, in save_cell
pickler.save_reduce(_create_cell, (f,), obj=obj)
File "/home/sarahw/miniconda3/envs/project_huggingface/lib/python3.8/pickle.py", line 690, in save_reduce
save(args)
File "/home/sarahw/miniconda3/envs/project_huggingface/lib/python3.8/pickle.py", line 558, in save
f(self, obj) # Call unbound method with explicit self
File "/home/sarahw/miniconda3/envs/project_huggingface/lib/python3.8/pickle.py", line 884, in save_tuple
save(element)
File "/home/sarahw/miniconda3/envs/project_huggingface/lib/python3.8/pickle.py", line 601, in save
self.save_reduce(obj=obj, *rv)
File "/home/sarahw/miniconda3/envs/project_huggingface/lib/python3.8/pickle.py", line 715, in save_reduce
save(state)
File "/home/sarahw/miniconda3/envs/project_huggingface/lib/python3.8/pickle.py", line 558, in save
f(self, obj) # Call unbound method with explicit self
File "/home/sarahw/miniconda3/envs/project_huggingface/lib/python3.8/site-packages/dill/_dill.py", line 912, in save_module_dict
StockPickler.save_dict(pickler, obj)
File "/home/sarahw/miniconda3/envs/project_huggingface/lib/python3.8/pickle.py", line 969, in save_dict
self._batch_setitems(obj.items())
File "/home/sarahw/miniconda3/envs/project_huggingface/lib/python3.8/pickle.py", line 995, in _batch_setitems
save(v)
File "/home/sarahw/miniconda3/envs/project_huggingface/lib/python3.8/pickle.py", line 601, in save
self.save_reduce(obj=obj, *rv)
File "/home/sarahw/miniconda3/envs/project_huggingface/lib/python3.8/pickle.py", line 715, in save_reduce
save(state)
File "/home/sarahw/miniconda3/envs/project_huggingface/lib/python3.8/pickle.py", line 558, in save
f(self, obj) # Call unbound method with explicit self
File "/home/sarahw/miniconda3/envs/project_huggingface/lib/python3.8/site-packages/dill/_dill.py", line 912, in save_module_dict
StockPickler.save_dict(pickler, obj)
File "/home/sarahw/miniconda3/envs/project_huggingface/lib/python3.8/pickle.py", line 969, in save_dict
self._batch_setitems(obj.items())
File "/home/sarahw/miniconda3/envs/project_huggingface/lib/python3.8/pickle.py", line 995, in _batch_setitems
save(v)
File "/home/sarahw/miniconda3/envs/project_huggingface/lib/python3.8/pickle.py", line 576, in save
rv = reduce(self.proto)
TypeError: cannot pickle 'Tokenizer' object
```
Fix seems to be in the tokenizers [`0.8.0.dev1 pre-release`](https://github.com/huggingface/tokenizers/issues/87), which I can't install with any package managers. | 46 | Tokenizer pickling issue fix not landed in `nlp` yet?
Unless I recreate an arrow_dataset from my loaded nlp dataset myself (which I think does not use the cache by default), I get the following error when applying the map function:
```
dataset = nlp.load_dataset('cos_e')
tokenizer = GPT2TokenizerFast.from_pretrained('gpt2', cache_dir=cache_dir)
for split in dataset.keys():
dataset[split].map(lambda x: some_function(x, tokenizer))
```
```
06/09/2020 10:09:19 - INFO - nlp.builder - Constructing Dataset for split train[:10], from /home/sarahw/.cache/huggingface/datasets/cos_e/default/0.0.1
Traceback (most recent call last):
File "generation/input_to_label_and_rationale.py", line 390, in <module>
main()
File "generation/input_to_label_and_rationale.py", line 263, in main
dataset[split] = dataset[split].map(lambda x: input_to_explanation_plus_label(x, tokenizer, max_length, datasource=data_args.task_name, wt5=(model_class=='t5'), expl_only=model_args.rationale_only), batched=False)
File "/home/sarahw/miniconda3/envs/project_huggingface/lib/python3.8/site-packages/nlp/arrow_dataset.py", line 522, in map
cache_file_name = self._get_cache_file_path(function, cache_kwargs)
File "/home/sarahw/miniconda3/envs/project_huggingface/lib/python3.8/site-packages/nlp/arrow_dataset.py", line 381, in _get_cache_file_path
function_bytes = dumps(function)
File "/home/sarahw/miniconda3/envs/project_huggingface/lib/python3.8/site-packages/nlp/utils/py_utils.py", line 257, in dumps
dump(obj, file)
File "/home/sarahw/miniconda3/envs/project_huggingface/lib/python3.8/site-packages/nlp/utils/py_utils.py", line 250, in dump
Pickler(file).dump(obj)
File "/home/sarahw/miniconda3/envs/project_huggingface/lib/python3.8/site-packages/dill/_dill.py", line 445, in dump
StockPickler.dump(self, obj)
File "/home/sarahw/miniconda3/envs/project_huggingface/lib/python3.8/pickle.py", line 485, in dump
self.save(obj)
File "/home/sarahw/miniconda3/envs/project_huggingface/lib/python3.8/pickle.py", line 558, in save
f(self, obj) # Call unbound method with explicit self
File "/home/sarahw/miniconda3/envs/project_huggingface/lib/python3.8/site-packages/dill/_dill.py", line 1410, in save_function
pickler.save_reduce(_create_function, (obj.__code__,
File "/home/sarahw/miniconda3/envs/project_huggingface/lib/python3.8/pickle.py", line 690, in save_reduce
save(args)
File "/home/sarahw/miniconda3/envs/project_huggingface/lib/python3.8/pickle.py", line 558, in save
f(self, obj) # Call unbound method with explicit self
File "/home/sarahw/miniconda3/envs/project_huggingface/lib/python3.8/pickle.py", line 899, in save_tuple
save(element)
File "/home/sarahw/miniconda3/envs/project_huggingface/lib/python3.8/pickle.py", line 558, in save
f(self, obj) # Call unbound method with explicit self
File "/home/sarahw/miniconda3/envs/project_huggingface/lib/python3.8/pickle.py", line 899, in save_tuple
save(element)
File "/home/sarahw/miniconda3/envs/project_huggingface/lib/python3.8/pickle.py", line 558, in save
f(self, obj) # Call unbound method with explicit self
File "/home/sarahw/miniconda3/envs/project_huggingface/lib/python3.8/site-packages/dill/_dill.py", line 1147, in save_cell
pickler.save_reduce(_create_cell, (f,), obj=obj)
File "/home/sarahw/miniconda3/envs/project_huggingface/lib/python3.8/pickle.py", line 690, in save_reduce
save(args)
File "/home/sarahw/miniconda3/envs/project_huggingface/lib/python3.8/pickle.py", line 558, in save
f(self, obj) # Call unbound method with explicit self
File "/home/sarahw/miniconda3/envs/project_huggingface/lib/python3.8/pickle.py", line 884, in save_tuple
save(element)
File "/home/sarahw/miniconda3/envs/project_huggingface/lib/python3.8/pickle.py", line 601, in save
self.save_reduce(obj=obj, *rv)
File "/home/sarahw/miniconda3/envs/project_huggingface/lib/python3.8/pickle.py", line 715, in save_reduce
save(state)
File "/home/sarahw/miniconda3/envs/project_huggingface/lib/python3.8/pickle.py", line 558, in save
f(self, obj) # Call unbound method with explicit self
File "/home/sarahw/miniconda3/envs/project_huggingface/lib/python3.8/site-packages/dill/_dill.py", line 912, in save_module_dict
StockPickler.save_dict(pickler, obj)
File "/home/sarahw/miniconda3/envs/project_huggingface/lib/python3.8/pickle.py", line 969, in save_dict
self._batch_setitems(obj.items())
File "/home/sarahw/miniconda3/envs/project_huggingface/lib/python3.8/pickle.py", line 995, in _batch_setitems
save(v)
File "/home/sarahw/miniconda3/envs/project_huggingface/lib/python3.8/pickle.py", line 601, in save
self.save_reduce(obj=obj, *rv)
File "/home/sarahw/miniconda3/envs/project_huggingface/lib/python3.8/pickle.py", line 715, in save_reduce
save(state)
File "/home/sarahw/miniconda3/envs/project_huggingface/lib/python3.8/pickle.py", line 558, in save
f(self, obj) # Call unbound method with explicit self
File "/home/sarahw/miniconda3/envs/project_huggingface/lib/python3.8/site-packages/dill/_dill.py", line 912, in save_module_dict
StockPickler.save_dict(pickler, obj)
File "/home/sarahw/miniconda3/envs/project_huggingface/lib/python3.8/pickle.py", line 969, in save_dict
self._batch_setitems(obj.items())
File "/home/sarahw/miniconda3/envs/project_huggingface/lib/python3.8/pickle.py", line 995, in _batch_setitems
save(v)
File "/home/sarahw/miniconda3/envs/project_huggingface/lib/python3.8/pickle.py", line 576, in save
rv = reduce(self.proto)
TypeError: cannot pickle 'Tokenizer' object
```
Fix seems to be in the tokenizers [`0.8.0.dev1 pre-release`](https://github.com/huggingface/tokenizers/issues/87), which I can't install with any package managers.
If others run into this issue, a quick fix is to use python 3.6 instead of 3.7+. Serialization differences between the 3rd party `dataclasses` package for 3.6 and the built in `dataclasses` in 3.7+ cause the issue.
Probably a dumb fix, but it works for me. |
https://github.com/huggingface/datasets/issues/256 | [Feature request] Add a feature to dataset | Do you have an example of what you would like to do? (you can just add a field in the output of the unction you give to map and this will add this field in the output table) | Is there a straightforward way to add a field to the arrow_dataset, prior to performing map? | 38 | [Feature request] Add a feature to dataset
Is there a straightforward way to add a field to the arrow_dataset, prior to performing map?
Do you have an example of what you would like to do? (you can just add a field in the output of the unction you give to map and this will add this field in the output table) |
https://github.com/huggingface/datasets/issues/256 | [Feature request] Add a feature to dataset | Given another source of data loaded in, I want to pre-add it to the dataset so that it aligns with the indices of the arrow dataset prior to performing map.
E.g.
```
new_info = list of length dataset['train']
dataset['train'] = dataset['train'].map(lambda x: some_function(x, new_info[index of x]))
def some_function(x, new_info_x):
# adds new_info[index of x] as a field to x
x['new_info'] = new_info_x
return x
```
I was thinking to instead create a new field in the arrow dataset so that instance x contains all the necessary information when map function is applied (since I don't have index information to pass to map function). | Is there a straightforward way to add a field to the arrow_dataset, prior to performing map? | 103 | [Feature request] Add a feature to dataset
Is there a straightforward way to add a field to the arrow_dataset, prior to performing map?
Given another source of data loaded in, I want to pre-add it to the dataset so that it aligns with the indices of the arrow dataset prior to performing map.
E.g.
```
new_info = list of length dataset['train']
dataset['train'] = dataset['train'].map(lambda x: some_function(x, new_info[index of x]))
def some_function(x, new_info_x):
# adds new_info[index of x] as a field to x
x['new_info'] = new_info_x
return x
```
I was thinking to instead create a new field in the arrow dataset so that instance x contains all the necessary information when map function is applied (since I don't have index information to pass to map function). |
https://github.com/huggingface/datasets/issues/256 | [Feature request] Add a feature to dataset | This is what I have so far:
```
import pyarrow as pa
from nlp.arrow_dataset import Dataset
aug_dataset = dataset['train'][:]
aug_dataset['new_info'] = new_info
#reformat as arrow-table
schema = dataset['train'].schema
# this line doesn't work:
schema.append(pa.field('new_info', pa.int32()))
table = pa.Table.from_pydict(
aug_dataset,
schema=schema
)
dataset['train'] = Dataset(table)
``` | Is there a straightforward way to add a field to the arrow_dataset, prior to performing map? | 45 | [Feature request] Add a feature to dataset
Is there a straightforward way to add a field to the arrow_dataset, prior to performing map?
This is what I have so far:
```
import pyarrow as pa
from nlp.arrow_dataset import Dataset
aug_dataset = dataset['train'][:]
aug_dataset['new_info'] = new_info
#reformat as arrow-table
schema = dataset['train'].schema
# this line doesn't work:
schema.append(pa.field('new_info', pa.int32()))
table = pa.Table.from_pydict(
aug_dataset,
schema=schema
)
dataset['train'] = Dataset(table)
``` |
https://github.com/huggingface/datasets/issues/256 | [Feature request] Add a feature to dataset | Maybe you can use `with_indices`?
```python
new_info = list of length dataset['train']
def some_function(indice, x):
# adds new_info[index of x] as a field to x
x['new_info'] = new_info_x[indice]
return x
dataset['train'] = dataset['train'].map(some_function, with_indices=True)
``` | Is there a straightforward way to add a field to the arrow_dataset, prior to performing map? | 35 | [Feature request] Add a feature to dataset
Is there a straightforward way to add a field to the arrow_dataset, prior to performing map?
Maybe you can use `with_indices`?
```python
new_info = list of length dataset['train']
def some_function(indice, x):
# adds new_info[index of x] as a field to x
x['new_info'] = new_info_x[indice]
return x
dataset['train'] = dataset['train'].map(some_function, with_indices=True)
``` |
https://github.com/huggingface/datasets/issues/254 | [Feature request] Be able to remove a specific sample of the dataset | Oh yes you can now do that with the `dataset.filter()` method that was added in #214 | As mentioned in #117, it's currently not possible to remove a sample of the dataset.
But it is a important use case : After applying some preprocessing, some samples might be empty for example. We should be able to remove these samples from the dataset, or at least mark them as `removed` so when iterating the dataset, we don't iterate these samples.
I think it should be a feature. What do you think ?
---
Any work-around in the meantime ? | 16 | [Feature request] Be able to remove a specific sample of the dataset
As mentioned in #117, it's currently not possible to remove a sample of the dataset.
But it is a important use case : After applying some preprocessing, some samples might be empty for example. We should be able to remove these samples from the dataset, or at least mark them as `removed` so when iterating the dataset, we don't iterate these samples.
I think it should be a feature. What do you think ?
---
Any work-around in the meantime ?
Oh yes you can now do that with the `dataset.filter()` method that was added in #214 |
https://github.com/huggingface/datasets/issues/252 | NonMatchingSplitsSizesError error when reading the IMDB dataset | I just tried on my side and I didn't encounter your problem.
Apparently the script doesn't generate all the examples on your side.
Can you provide the version of `nlp` you're using ?
Can you try to clear your cache and re-run the code ? | Hi!
I am trying to load the `imdb` dataset with this line:
`dataset = nlp.load_dataset('imdb', data_dir='/A/PATH', cache_dir='/A/PATH')`
but I am getting the following error:
```
Traceback (most recent call last):
File "<stdin>", line 1, in <module>
File "/mounts/Users/cisintern/antmarakis/anaconda3/lib/python3.7/site-packages/nlp/load.py", line 517, in load_dataset
save_infos=save_infos,
File "/mounts/Users/cisintern/antmarakis/anaconda3/lib/python3.7/site-packages/nlp/builder.py", line 363, in download_and_prepare
dl_manager=dl_manager, verify_infos=verify_infos, **download_and_prepare_kwargs
File "/mounts/Users/cisintern/antmarakis/anaconda3/lib/python3.7/site-packages/nlp/builder.py", line 421, in _download_and_prepare
verify_splits(self.info.splits, split_dict)
File "/mounts/Users/cisintern/antmarakis/anaconda3/lib/python3.7/site-packages/nlp/utils/info_utils.py", line 70, in verify_splits
raise NonMatchingSplitsSizesError(str(bad_splits))
nlp.utils.info_utils.NonMatchingSplitsSizesError: [{'expected': SplitInfo(name='train', num_bytes=33442202, num_examples=25000, dataset_name='imdb'), 'recorded': SplitInfo(name='train', num_bytes=5929447, num_examples=4537, dataset_name='imdb')}, {'expected': SplitInfo(name='unsupervised', num_bytes=67125548, num_examples=50000, dataset_name='imdb'), 'recorded': SplitInfo(name='unsupervised', num_bytes=0, num_examples=0, dataset_name='imdb')}]
```
Am I overlooking something? Thanks! | 45 | NonMatchingSplitsSizesError error when reading the IMDB dataset
Hi!
I am trying to load the `imdb` dataset with this line:
`dataset = nlp.load_dataset('imdb', data_dir='/A/PATH', cache_dir='/A/PATH')`
but I am getting the following error:
```
Traceback (most recent call last):
File "<stdin>", line 1, in <module>
File "/mounts/Users/cisintern/antmarakis/anaconda3/lib/python3.7/site-packages/nlp/load.py", line 517, in load_dataset
save_infos=save_infos,
File "/mounts/Users/cisintern/antmarakis/anaconda3/lib/python3.7/site-packages/nlp/builder.py", line 363, in download_and_prepare
dl_manager=dl_manager, verify_infos=verify_infos, **download_and_prepare_kwargs
File "/mounts/Users/cisintern/antmarakis/anaconda3/lib/python3.7/site-packages/nlp/builder.py", line 421, in _download_and_prepare
verify_splits(self.info.splits, split_dict)
File "/mounts/Users/cisintern/antmarakis/anaconda3/lib/python3.7/site-packages/nlp/utils/info_utils.py", line 70, in verify_splits
raise NonMatchingSplitsSizesError(str(bad_splits))
nlp.utils.info_utils.NonMatchingSplitsSizesError: [{'expected': SplitInfo(name='train', num_bytes=33442202, num_examples=25000, dataset_name='imdb'), 'recorded': SplitInfo(name='train', num_bytes=5929447, num_examples=4537, dataset_name='imdb')}, {'expected': SplitInfo(name='unsupervised', num_bytes=67125548, num_examples=50000, dataset_name='imdb'), 'recorded': SplitInfo(name='unsupervised', num_bytes=0, num_examples=0, dataset_name='imdb')}]
```
Am I overlooking something? Thanks!
I just tried on my side and I didn't encounter your problem.
Apparently the script doesn't generate all the examples on your side.
Can you provide the version of `nlp` you're using ?
Can you try to clear your cache and re-run the code ? |
https://github.com/huggingface/datasets/issues/252 | NonMatchingSplitsSizesError error when reading the IMDB dataset | Hi ! The cache is at ~/.cache/huggingface
You can just delete this folder if needed :) | Hi!
I am trying to load the `imdb` dataset with this line:
`dataset = nlp.load_dataset('imdb', data_dir='/A/PATH', cache_dir='/A/PATH')`
but I am getting the following error:
```
Traceback (most recent call last):
File "<stdin>", line 1, in <module>
File "/mounts/Users/cisintern/antmarakis/anaconda3/lib/python3.7/site-packages/nlp/load.py", line 517, in load_dataset
save_infos=save_infos,
File "/mounts/Users/cisintern/antmarakis/anaconda3/lib/python3.7/site-packages/nlp/builder.py", line 363, in download_and_prepare
dl_manager=dl_manager, verify_infos=verify_infos, **download_and_prepare_kwargs
File "/mounts/Users/cisintern/antmarakis/anaconda3/lib/python3.7/site-packages/nlp/builder.py", line 421, in _download_and_prepare
verify_splits(self.info.splits, split_dict)
File "/mounts/Users/cisintern/antmarakis/anaconda3/lib/python3.7/site-packages/nlp/utils/info_utils.py", line 70, in verify_splits
raise NonMatchingSplitsSizesError(str(bad_splits))
nlp.utils.info_utils.NonMatchingSplitsSizesError: [{'expected': SplitInfo(name='train', num_bytes=33442202, num_examples=25000, dataset_name='imdb'), 'recorded': SplitInfo(name='train', num_bytes=5929447, num_examples=4537, dataset_name='imdb')}, {'expected': SplitInfo(name='unsupervised', num_bytes=67125548, num_examples=50000, dataset_name='imdb'), 'recorded': SplitInfo(name='unsupervised', num_bytes=0, num_examples=0, dataset_name='imdb')}]
```
Am I overlooking something? Thanks! | 16 | NonMatchingSplitsSizesError error when reading the IMDB dataset
Hi!
I am trying to load the `imdb` dataset with this line:
`dataset = nlp.load_dataset('imdb', data_dir='/A/PATH', cache_dir='/A/PATH')`
but I am getting the following error:
```
Traceback (most recent call last):
File "<stdin>", line 1, in <module>
File "/mounts/Users/cisintern/antmarakis/anaconda3/lib/python3.7/site-packages/nlp/load.py", line 517, in load_dataset
save_infos=save_infos,
File "/mounts/Users/cisintern/antmarakis/anaconda3/lib/python3.7/site-packages/nlp/builder.py", line 363, in download_and_prepare
dl_manager=dl_manager, verify_infos=verify_infos, **download_and_prepare_kwargs
File "/mounts/Users/cisintern/antmarakis/anaconda3/lib/python3.7/site-packages/nlp/builder.py", line 421, in _download_and_prepare
verify_splits(self.info.splits, split_dict)
File "/mounts/Users/cisintern/antmarakis/anaconda3/lib/python3.7/site-packages/nlp/utils/info_utils.py", line 70, in verify_splits
raise NonMatchingSplitsSizesError(str(bad_splits))
nlp.utils.info_utils.NonMatchingSplitsSizesError: [{'expected': SplitInfo(name='train', num_bytes=33442202, num_examples=25000, dataset_name='imdb'), 'recorded': SplitInfo(name='train', num_bytes=5929447, num_examples=4537, dataset_name='imdb')}, {'expected': SplitInfo(name='unsupervised', num_bytes=67125548, num_examples=50000, dataset_name='imdb'), 'recorded': SplitInfo(name='unsupervised', num_bytes=0, num_examples=0, dataset_name='imdb')}]
```
Am I overlooking something? Thanks!
Hi ! The cache is at ~/.cache/huggingface
You can just delete this folder if needed :) |
https://github.com/huggingface/datasets/issues/249 | [Dataset created] some critical small issues when I was creating a dataset | Alright I think I fixed all the problems you mentioned. Thanks again, that will be useful for many people.
There is still more work needed for point 7. but we plan to have some nice docs soon. | Hi, I successfully created a dataset and has made a pr #248.
But I have encountered several problems when I was creating it, and those should be easy to fix.
1. Not found dataset_info.json
should be fixed by #241 , eager to wait it be merged.
2. Forced to install `apach_beam`
If we should install it, then it might be better to include it in the pakcage dependency or specified in `CONTRIBUTING.md`
```
Traceback (most recent call last):
File "nlp-cli", line 10, in <module>
from nlp.commands.run_beam import RunBeamCommand
File "/home/yisiang/nlp/src/nlp/commands/run_beam.py", line 6, in <module>
import apache_beam as beam
ModuleNotFoundError: No module named 'apache_beam'
```
3. `cached_dir` is `None`
```
File "/home/yisiang/nlp/src/nlp/datasets/bookscorpus/aea0bd5142d26df645a8fce23d6110bb95ecb81772bb2a1f29012e329191962c/bookscorpus.py", line 88, in _split_generators
downloaded_path_or_paths = dl_manager.download_custom(_GDRIVE_FILE_ID, download_file_from_google_drive)
File "/home/yisiang/nlp/src/nlp/utils/download_manager.py", line 128, in download_custom
downloaded_path_or_paths = map_nested(url_to_downloaded_path, url_or_urls)
File "/home/yisiang/nlp/src/nlp/utils/py_utils.py", line 172, in map_nested
return function(data_struct)
File "/home/yisiang/nlp/src/nlp/utils/download_manager.py", line 126, in url_to_downloaded_path
return os.path.join(self._download_config.cache_dir, hash_url_to_filename(url))
File "/home/yisiang/miniconda3/envs/nlppr/lib/python3.7/posixpath.py", line 80, in join
a = os.fspath(a)
```
This is because this line
https://github.com/huggingface/nlp/blob/2e0a8639a79b1abc848cff5c669094d40bba0f63/src/nlp/commands/test.py#L30-L32
And I add `--cache_dir="...."` to `python nlp-cli test datasets/<your-dataset-folder> --save_infos --all_configs` in the doc, finally I could pass this error.
But it seems to ignore my arg and use `/home/yisiang/.cache/huggingface/datasets/bookscorpus/plain_text/1.0.0` as cahe_dir
4. There is no `pytest`
So maybe in the doc we should specify a step to install pytest
5. Not enough capacity in my `/tmp`
When run test for dummy data, I don't know why it ask me for 5.6g to download something,
```
def download_and_prepare
...
if not utils.has_sufficient_disk_space(self.info.size_in_bytes or 0, directory=self._cache_dir_root):
raise IOError(
"Not enough disk space. Needed: {} (download: {}, generated: {})".format(
utils.size_str(self.info.size_in_bytes or 0),
utils.size_str(self.info.download_size or 0),
> utils.size_str(self.info.dataset_size or 0),
)
)
E OSError: Not enough disk space. Needed: 5.62 GiB (download: 1.10 GiB, generated: 4.52 GiB)
```
I add a `processed_temp_dir="some/dir"; raw_temp_dir="another/dir"` to 71, and the test passed
https://github.com/huggingface/nlp/blob/a67a6c422dece904b65d18af65f0e024e839dbe8/tests/test_dataset_common.py#L70-L72
I suggest we can create tmp dir under the `/home/user/tmp` but not `/tmp`, because take our lab server for example, everyone use `/tmp` thus it has not much capacity. Or at least we can improve error message, so the user know is what directory has no space and how many has it lefted. Or we could do both.
6. name of datasets
I was surprised by the dataset name `books_corpus`, and didn't know it is from `class BooksCorpus(nlp.GeneratorBasedBuilder)` . I change it to `Bookscorpus` afterwards. I think this point shold be also on the doc.
7. More thorough doc to how to create `dataset.py`
I believe there will be.
**Feel free to close this issue** if you think these are solved. | 37 | [Dataset created] some critical small issues when I was creating a dataset
Hi, I successfully created a dataset and has made a pr #248.
But I have encountered several problems when I was creating it, and those should be easy to fix.
1. Not found dataset_info.json
should be fixed by #241 , eager to wait it be merged.
2. Forced to install `apach_beam`
If we should install it, then it might be better to include it in the pakcage dependency or specified in `CONTRIBUTING.md`
```
Traceback (most recent call last):
File "nlp-cli", line 10, in <module>
from nlp.commands.run_beam import RunBeamCommand
File "/home/yisiang/nlp/src/nlp/commands/run_beam.py", line 6, in <module>
import apache_beam as beam
ModuleNotFoundError: No module named 'apache_beam'
```
3. `cached_dir` is `None`
```
File "/home/yisiang/nlp/src/nlp/datasets/bookscorpus/aea0bd5142d26df645a8fce23d6110bb95ecb81772bb2a1f29012e329191962c/bookscorpus.py", line 88, in _split_generators
downloaded_path_or_paths = dl_manager.download_custom(_GDRIVE_FILE_ID, download_file_from_google_drive)
File "/home/yisiang/nlp/src/nlp/utils/download_manager.py", line 128, in download_custom
downloaded_path_or_paths = map_nested(url_to_downloaded_path, url_or_urls)
File "/home/yisiang/nlp/src/nlp/utils/py_utils.py", line 172, in map_nested
return function(data_struct)
File "/home/yisiang/nlp/src/nlp/utils/download_manager.py", line 126, in url_to_downloaded_path
return os.path.join(self._download_config.cache_dir, hash_url_to_filename(url))
File "/home/yisiang/miniconda3/envs/nlppr/lib/python3.7/posixpath.py", line 80, in join
a = os.fspath(a)
```
This is because this line
https://github.com/huggingface/nlp/blob/2e0a8639a79b1abc848cff5c669094d40bba0f63/src/nlp/commands/test.py#L30-L32
And I add `--cache_dir="...."` to `python nlp-cli test datasets/<your-dataset-folder> --save_infos --all_configs` in the doc, finally I could pass this error.
But it seems to ignore my arg and use `/home/yisiang/.cache/huggingface/datasets/bookscorpus/plain_text/1.0.0` as cahe_dir
4. There is no `pytest`
So maybe in the doc we should specify a step to install pytest
5. Not enough capacity in my `/tmp`
When run test for dummy data, I don't know why it ask me for 5.6g to download something,
```
def download_and_prepare
...
if not utils.has_sufficient_disk_space(self.info.size_in_bytes or 0, directory=self._cache_dir_root):
raise IOError(
"Not enough disk space. Needed: {} (download: {}, generated: {})".format(
utils.size_str(self.info.size_in_bytes or 0),
utils.size_str(self.info.download_size or 0),
> utils.size_str(self.info.dataset_size or 0),
)
)
E OSError: Not enough disk space. Needed: 5.62 GiB (download: 1.10 GiB, generated: 4.52 GiB)
```
I add a `processed_temp_dir="some/dir"; raw_temp_dir="another/dir"` to 71, and the test passed
https://github.com/huggingface/nlp/blob/a67a6c422dece904b65d18af65f0e024e839dbe8/tests/test_dataset_common.py#L70-L72
I suggest we can create tmp dir under the `/home/user/tmp` but not `/tmp`, because take our lab server for example, everyone use `/tmp` thus it has not much capacity. Or at least we can improve error message, so the user know is what directory has no space and how many has it lefted. Or we could do both.
6. name of datasets
I was surprised by the dataset name `books_corpus`, and didn't know it is from `class BooksCorpus(nlp.GeneratorBasedBuilder)` . I change it to `Bookscorpus` afterwards. I think this point shold be also on the doc.
7. More thorough doc to how to create `dataset.py`
I believe there will be.
**Feel free to close this issue** if you think these are solved.
Alright I think I fixed all the problems you mentioned. Thanks again, that will be useful for many people.
There is still more work needed for point 7. but we plan to have some nice docs soon. |
https://github.com/huggingface/datasets/issues/246 | What is the best way to cache a dataset? | Everything is already cached by default in 🤗nlp (in particular dataset
loading and all the “map()” operations) so I don’t think you need to do any
specific caching in streamlit.
Tell us if you feel like it’s not the case.
On Sat, 6 Jun 2020 at 13:02, Fabrizio Milo <notifications@github.com> wrote:
> For example if I want to use streamlit with a nlp dataset:
>
> @st.cache
> def load_data():
> return nlp.load_dataset('squad')
>
> This code raises the error "uncachable object"
>
> Right now I just fixed with a constant for my specific case:
>
> @st.cache(hash_funcs={pyarrow.lib.Buffer: lambda b: 0})
>
> But I was curious to know what is the best way in general
>
> —
> You are receiving this because you are subscribed to this thread.
> Reply to this email directly, view it on GitHub
> <https://github.com/huggingface/nlp/issues/246>, or unsubscribe
> <https://github.com/notifications/unsubscribe-auth/ABYDIHKAKO7CWGX2QY55UXLRVIO3ZANCNFSM4NV333RQ>
> .
>
| For example if I want to use streamlit with a nlp dataset:
```
@st.cache
def load_data():
return nlp.load_dataset('squad')
```
This code raises the error "uncachable object"
Right now I just fixed with a constant for my specific case:
```
@st.cache(hash_funcs={pyarrow.lib.Buffer: lambda b: 0})
```
But I was curious to know what is the best way in general
| 151 | What is the best way to cache a dataset?
For example if I want to use streamlit with a nlp dataset:
```
@st.cache
def load_data():
return nlp.load_dataset('squad')
```
This code raises the error "uncachable object"
Right now I just fixed with a constant for my specific case:
```
@st.cache(hash_funcs={pyarrow.lib.Buffer: lambda b: 0})
```
But I was curious to know what is the best way in general
Everything is already cached by default in 🤗nlp (in particular dataset
loading and all the “map()” operations) so I don’t think you need to do any
specific caching in streamlit.
Tell us if you feel like it’s not the case.
On Sat, 6 Jun 2020 at 13:02, Fabrizio Milo <notifications@github.com> wrote:
> For example if I want to use streamlit with a nlp dataset:
>
> @st.cache
> def load_data():
> return nlp.load_dataset('squad')
>
> This code raises the error "uncachable object"
>
> Right now I just fixed with a constant for my specific case:
>
> @st.cache(hash_funcs={pyarrow.lib.Buffer: lambda b: 0})
>
> But I was curious to know what is the best way in general
>
> —
> You are receiving this because you are subscribed to this thread.
> Reply to this email directly, view it on GitHub
> <https://github.com/huggingface/nlp/issues/246>, or unsubscribe
> <https://github.com/notifications/unsubscribe-auth/ABYDIHKAKO7CWGX2QY55UXLRVIO3ZANCNFSM4NV333RQ>
> .
>
|
https://github.com/huggingface/datasets/issues/245 | SST-2 test labels are all -1 | Yes, this is because the test sets for glue are hidden so the labels are
not publicly available. You can read the glue paper for more details.
On Sat, 6 Jun 2020 at 18:16, Jack Morris <notifications@github.com> wrote:
> this also happened to me with nlp.load_datasets('glue', 'mnli')
>
> —
> You are receiving this because you are subscribed to this thread.
> Reply to this email directly, view it on GitHub
> <https://github.com/huggingface/nlp/issues/245#issuecomment-640083980>,
> or unsubscribe
> <https://github.com/notifications/unsubscribe-auth/ABYDIHMVQD2EDX2HTZUXG5DRVJTWRANCNFSM4NVG3AKQ>
> .
>
| I'm trying to test a model on the SST-2 task, but all the labels I see in the test set are -1.
```
>>> import nlp
>>> glue = nlp.load_dataset('glue', 'sst2')
>>> glue
{'train': Dataset(schema: {'sentence': 'string', 'label': 'int64', 'idx': 'int32'}, num_rows: 67349), 'validation': Dataset(schema: {'sentence': 'string', 'label': 'int64', 'idx': 'int32'}, num_rows: 872), 'test': Dataset(schema: {'sentence': 'string', 'label': 'int64', 'idx': 'int32'}, num_rows: 1821)}
>>> list(l['label'] for l in glue['test'])
[-1, -1, -1, -1, -1, -1, -1, -1, -1, -1, -1, -1, -1, -1, -1, -1, -1, -1, -1, -1, -1, -1, -1, -1, -1, -1, -1, -1, -1, -1, -1, -1, -1, -1, -1, -1, -1, -1, -1, -1, -1, -1, -1, -1, -1, -1, -1, -1, -1, -1, -1, -1, -1, -1, -1, -1, -1, -1, -1, -1, -1, -1, -1, -1, -1, -1, -1, -1, -1, -1, -1, -1, -1, -1, -1, -1, -1, -1, -1, -1, -1, -1, -1, -1, -1, -1, -1, -1, -1, -1, -1, -1, -1, -1, -1, -1, -1, -1, -1, -1, -1, -1, -1, -1, -1, -1, -1, -1, -1, -1, -1, -1, -1, -1, -1, -1, -1, -1, -1, -1, -1, -1, -1, -1, -1, -1, -1, -1, -1, -1, -1, -1, -1, -1, -1, -1, -1, -1, -1, -1, -1, -1, -1, -1, -1, -1, -1, -1, -1, -1, -1, -1, -1, -1, -1, -1, -1, -1, -1, -1, -1, -1, -1, -1, -1, -1, -1, -1, -1, -1, -1, -1, -1, -1, -1, -1, -1, -1, -1, -1, -1, -1, -1, -1, -1, -1, -1, -1, -1, -1, -1, -1, -1, -1, -1, -1, -1, -1, -1, -1, -1, -1, -1, -1, -1, -1, -1, -1, -1, -1, -1, -1, -1, -1, -1, -1, -1, -1, -1, -1, -1, -1, -1, -1, -1, -1, -1, -1, -1, -1, -1, -1, -1, -1, -1, -1, -1, -1, -1, -1, -1, -1, -1, -1, -1, -1, -1, -1, -1, -1, -1, -1, -1, -1, -1, -1, -1, -1, -1, -1, -1, -1, -1, -1, -1, -1, -1, -1, -1, -1, -1, -1, -1, -1, -1, -1, -1, -1, -1, -1, -1, -1, -1, -1, -1, -1, -1, -1, -1, -1, -1, -1, -1, -1, -1, -1, -1, -1, -1, -1, -1, -1, -1, -1, -1, -1, -1, -1, -1, -1, -1, -1, -1, -1, -1, -1, -1, -1, -1, -1, -1, -1, -1, -1, -1, -1, -1, -1, -1, -1, -1, -1, -1, -1, -1, -1, -1, -1, -1, -1, -1, -1, -1, -1, -1, -1, -1, -1, -1, -1, -1, -1, -1, -1, -1, -1, -1, -1, -1, -1, -1, -1, -1, -1, -1, -1, -1, -1, -1, -1, -1, -1, -1, -1, -1, -1, -1, -1, -1, -1, -1, -1, -1, -1, -1, -1, -1, -1, -1, -1, -1, -1, -1, -1, -1, -1, -1, -1, -1, -1, -1, -1, -1, -1, -1, -1, -1, -1, -1, -1, -1, -1, -1, -1, -1, -1, -1, -1, -1, -1, -1, -1, -1, -1, -1, -1, -1, -1, -1, -1, -1, -1, -1, -1, -1, -1, -1, -1, -1, -1, -1, -1, -1, -1, -1, -1, -1, -1, -1, -1, -1, -1, -1, -1, -1, -1, -1, -1, -1, -1, -1, -1, -1, -1, -1, -1, -1, -1, -1, -1, -1, -1, -1, -1, -1, -1, -1, -1, -1, -1, -1, -1, -1, -1, -1, -1, -1, -1, -1, -1, -1, -1, -1, -1, -1, -1, -1, -1, -1, -1, -1, -1, -1, -1, -1, -1, -1, -1, -1, -1, -1, -1, -1, -1, -1, -1, -1, -1, -1, -1, -1, -1, -1, -1, -1, -1, -1, -1, -1, -1, -1, -1, -1, -1, -1, -1, -1, -1, -1, -1, -1, -1, -1, -1, -1, -1, -1, -1, -1, -1, -1, -1, -1, -1, -1, -1, -1, -1, -1, -1, -1, -1, -1, -1, -1, -1, -1, -1, -1, -1, -1, -1, -1, -1, -1, -1, -1, -1, -1, -1, -1, -1, -1, -1, -1, -1, -1, -1, -1, -1, -1, -1, -1, -1, -1, -1, -1, -1, -1, -1, -1, -1, -1, -1, -1, -1, -1, -1, -1, -1, -1, -1, -1, -1, -1, -1, -1, -1, -1, -1, -1, -1, -1, -1, -1, -1, -1, -1, -1, -1, -1, -1, -1, -1, -1, -1, -1, -1, -1, -1, -1, -1, -1, -1, -1, -1, -1, -1, -1, -1, -1, -1, -1, -1, -1, -1, -1, -1, -1, -1, -1, -1, -1, -1, -1, -1, -1, -1, -1, -1, -1, -1, -1, -1, -1, -1, -1, -1, -1, -1, -1, -1, -1, -1, -1, -1, -1, -1, -1, -1, -1, -1, -1, -1, -1, -1, -1, -1, -1, -1, -1, -1, -1, -1, -1, -1, -1, -1, -1, -1, -1, -1, -1, -1, -1, -1, -1, -1, -1, -1, -1, -1, -1, -1, -1, -1, -1, -1, -1, -1, -1, -1, -1, -1, -1, -1, -1, -1, -1, -1, -1, -1, -1, -1, -1, -1, -1, -1, -1, -1, -1, -1, -1, -1, -1, -1, -1, -1, -1, -1, -1, -1, -1, -1, -1, -1, -1, -1, -1, -1, -1, -1, -1, -1, -1, -1, -1, -1, -1, -1, -1, -1, -1, -1, -1, -1, -1, -1, -1, -1, -1, -1, -1, -1, -1, -1, -1, -1, -1, -1, -1, -1, -1, -1, -1, -1, -1, -1, -1, -1, -1, -1, -1, -1, -1, -1, -1, -1, -1, -1, -1, -1, -1, -1, -1, -1, -1, -1, -1, -1, -1, -1, -1, -1, -1, -1, -1, -1, -1, -1, -1, -1, -1, -1, -1, -1, -1, -1, -1, -1, -1, -1, -1, -1, -1, -1, -1, -1, -1, -1, -1, -1, -1, -1, -1, -1, -1, -1, -1, -1, -1, -1, -1, -1, -1, -1, -1, -1, -1, -1, -1, -1, -1, -1, -1, -1, -1, -1, -1, -1, -1, -1, -1, -1, -1, -1, -1, -1, -1, -1, -1, -1, -1, -1, -1, -1, -1, -1, -1, -1, -1, -1, -1, -1, -1, -1, -1, -1, -1, -1, -1, -1, -1, -1, -1, -1, -1, -1, -1, -1, -1, -1, -1, -1, -1, -1, -1, -1, -1, -1, -1, -1, -1, -1, -1, -1, -1, -1, -1, -1, -1, -1, -1, -1, -1, -1, -1, -1, -1, -1, -1, -1, -1, -1, -1, -1, -1, -1, -1, -1, -1, -1, -1, -1, -1, -1, -1, -1, -1, -1, -1, -1, -1, -1, -1, -1, -1, -1, -1, -1, -1, -1, -1, -1, -1, -1, -1, -1, -1, -1, -1, -1, -1, -1, -1, -1, -1, -1, -1, -1, -1, -1, -1, -1, -1, -1, -1, -1, -1, -1, -1, -1, -1, -1, -1, -1, -1, -1, -1, -1, -1, -1, -1, -1, -1, -1, -1, -1, -1, -1, -1, -1, -1, -1, -1, -1, -1, -1, -1, -1, -1, -1, -1, -1, -1, -1, -1, -1, -1, -1, -1, -1, -1, -1, -1, -1, -1, -1, -1, -1, -1, -1, -1, -1, -1, -1, -1, -1, -1, -1, -1, -1, -1, -1, -1, -1, -1, -1, -1, -1, -1, -1, -1, -1, -1, -1, -1, -1, -1, -1, -1, -1, -1, -1, -1, -1, -1, -1, -1, -1, -1, -1, -1, -1, -1, -1, -1, -1, -1, -1, -1, -1, -1, -1, -1, -1, -1, -1, -1, -1, -1, -1, -1, -1, -1, -1, -1, -1, -1, -1, -1, -1, -1, -1, -1, -1, -1, -1, -1, -1, -1, -1, -1, -1, -1, -1, -1, -1, -1, -1, -1, -1, -1, -1, -1, -1, -1, -1, -1, -1, -1, -1, -1, -1, -1, -1, -1, -1, -1, -1, -1, -1, -1, -1, -1, -1, -1, -1, -1, -1, -1, -1, -1, -1, -1, -1, -1, -1, -1, -1, -1, -1, -1, -1, -1, -1, -1, -1, -1, -1, -1, -1, -1, -1, -1, -1, -1, -1, -1, -1, -1, -1, -1, -1, -1, -1, -1, -1, -1, -1, -1, -1, -1, -1, -1, -1, -1, -1, -1, -1, -1, -1, -1, -1, -1, -1, -1, -1, -1, -1, -1, -1, -1, -1, -1, -1, -1, -1, -1, -1, -1, -1, -1, -1, -1, -1, -1, -1, -1, -1, -1, -1, -1, -1, -1, -1, -1, -1, -1, -1, -1, -1, -1, -1, -1, -1, -1, -1, -1, -1, -1, -1, -1, -1, -1, -1, -1, -1, -1, -1, -1, -1, -1, -1, -1, -1, -1, -1, -1, -1, -1, -1, -1, -1, -1, -1, -1, -1, -1, -1, -1, -1, -1, -1, -1, -1, -1, -1, -1, -1, -1, -1, -1, -1, -1, -1, -1, -1, -1, -1, -1, -1, -1, -1, -1, -1, -1, -1, -1, -1, -1, -1, -1, -1, -1, -1, -1, -1, -1, -1, -1, -1, -1, -1, -1, -1, -1, -1, -1, -1, -1, -1, -1, -1, -1, -1, -1, -1, -1, -1, -1, -1, -1, -1, -1, -1, -1, -1, -1, -1, -1, -1, -1, -1, -1, -1, -1, -1, -1, -1, -1, -1, -1, -1, -1, -1, -1, -1, -1, -1, -1, -1, -1, -1, -1, -1, -1, -1, -1, -1, -1, -1, -1, -1, -1, -1, -1, -1, -1, -1, -1, -1, -1, -1, -1, -1, -1, -1, -1, -1, -1, -1, -1, -1, -1, -1, -1, -1, -1, -1, -1, -1, -1, -1, -1, -1, -1, -1, -1, -1, -1, -1, -1, -1, -1, -1, -1, -1, -1, -1, -1, -1, -1, -1, -1, -1, -1, -1, -1, -1, -1, -1, -1, -1, -1, -1, -1, -1, -1, -1, -1, -1, -1, -1, -1, -1, -1, -1, -1, -1, -1, -1, -1, -1, -1, -1, -1, -1, -1, -1, -1, -1, -1, -1, -1, -1, -1, -1, -1, -1, -1, -1, -1, -1, -1, -1, -1, -1, -1, -1, -1, -1, -1, -1, -1, -1, -1, -1, -1, -1, -1, -1, -1, -1, -1, -1, -1, -1, -1, -1, -1, -1, -1, -1, -1, -1, -1, -1, -1, -1, -1, -1, -1, -1, -1, -1, -1, -1, -1, -1, -1, -1, -1, -1, -1, -1, -1, -1, -1, -1, -1, -1, -1, -1, -1, -1, -1, -1, -1, -1, -1, -1, -1, -1, -1, -1, -1, -1, -1, -1, -1, -1, -1, -1, -1, -1, -1, -1, -1, -1, -1, -1, -1, -1, -1, -1, -1, -1, -1, -1, -1, -1, -1, -1, -1, -1, -1, -1, -1, -1, -1, -1, -1, -1, -1, -1, -1, -1, -1, -1, -1, -1, -1, -1, -1, -1, -1, -1, -1, -1, -1, -1, -1, -1, -1, -1, -1, -1, -1, -1, -1, -1, -1, -1, -1, -1, -1, -1, -1, -1, -1, -1, -1, -1, -1, -1, -1, -1, -1, -1, -1, -1, -1, -1, -1, -1, -1, -1, -1, -1, -1, -1, -1, -1, -1, -1, -1, -1, -1, -1, -1, -1, -1, -1, -1, -1, -1, -1, -1, -1, -1, -1, -1, -1, -1, -1, -1, -1, -1, -1, -1, -1, -1, -1, -1, -1, -1, -1, -1, -1, -1, -1, -1, -1, -1, -1, -1, -1, -1, -1, -1, -1, -1, -1, -1, -1, -1, -1, -1, -1, -1, -1, -1, -1, -1, -1, -1, -1, -1, -1, -1, -1, -1, -1, -1, -1, -1, -1, -1, -1, -1, -1, -1, -1, -1, -1, -1, -1, -1, -1, -1, -1, -1, -1, -1, -1, -1, -1, -1, -1, -1, -1, -1, -1, -1, -1, -1, -1, -1, -1, -1, -1, -1, -1, -1, -1, -1, -1, -1, -1, -1, -1, -1, -1, -1, -1, -1, -1, -1, -1, -1]
``` | 82 | SST-2 test labels are all -1
I'm trying to test a model on the SST-2 task, but all the labels I see in the test set are -1.
```
>>> import nlp
>>> glue = nlp.load_dataset('glue', 'sst2')
>>> glue
{'train': Dataset(schema: {'sentence': 'string', 'label': 'int64', 'idx': 'int32'}, num_rows: 67349), 'validation': Dataset(schema: {'sentence': 'string', 'label': 'int64', 'idx': 'int32'}, num_rows: 872), 'test': Dataset(schema: {'sentence': 'string', 'label': 'int64', 'idx': 'int32'}, num_rows: 1821)}
>>> list(l['label'] for l in glue['test'])
[-1, -1, -1, -1, -1, -1, -1, -1, -1, -1, -1, -1, -1, -1, -1, -1, -1, -1, -1, -1, -1, -1, -1, -1, -1, -1, -1, -1, -1, -1, -1, -1, -1, -1, -1, -1, -1, -1, -1, -1, -1, -1, -1, -1, -1, -1, -1, -1, -1, -1, -1, -1, -1, -1, -1, -1, -1, -1, -1, -1, -1, -1, -1, -1, -1, -1, -1, -1, -1, -1, -1, -1, -1, -1, -1, -1, -1, -1, -1, -1, -1, -1, -1, -1, -1, -1, -1, -1, -1, -1, -1, -1, -1, -1, -1, -1, -1, -1, -1, -1, -1, -1, -1, -1, -1, -1, -1, -1, -1, -1, -1, -1, -1, -1, -1, -1, -1, -1, -1, -1, -1, -1, -1, -1, -1, -1, -1, -1, -1, -1, -1, -1, -1, -1, -1, -1, -1, -1, -1, -1, -1, -1, -1, -1, -1, -1, -1, -1, -1, -1, -1, -1, -1, -1, -1, -1, -1, -1, -1, -1, -1, -1, -1, -1, -1, -1, -1, -1, -1, -1, -1, -1, -1, -1, -1, -1, -1, -1, -1, -1, -1, -1, -1, -1, -1, -1, -1, -1, -1, -1, -1, -1, -1, -1, -1, -1, -1, -1, -1, -1, -1, -1, -1, -1, -1, -1, -1, -1, -1, -1, -1, -1, -1, -1, -1, -1, -1, -1, -1, -1, -1, -1, -1, -1, -1, -1, -1, -1, -1, -1, -1, -1, -1, -1, -1, -1, -1, -1, -1, -1, -1, -1, -1, -1, -1, -1, -1, -1, -1, -1, -1, -1, -1, -1, -1, -1, -1, -1, -1, -1, -1, -1, -1, -1, -1, -1, -1, -1, -1, -1, -1, -1, -1, -1, -1, -1, -1, -1, -1, -1, -1, -1, -1, -1, -1, -1, -1, -1, -1, -1, -1, -1, -1, -1, -1, -1, -1, -1, -1, -1, -1, -1, -1, -1, -1, -1, -1, -1, -1, -1, -1, -1, -1, -1, -1, -1, -1, -1, -1, -1, -1, -1, -1, -1, -1, -1, -1, -1, -1, -1, -1, -1, -1, -1, -1, -1, -1, -1, -1, -1, -1, -1, -1, -1, -1, -1, -1, -1, -1, -1, -1, -1, -1, -1, -1, -1, -1, -1, -1, -1, -1, -1, -1, -1, -1, -1, -1, -1, -1, -1, -1, -1, -1, -1, -1, -1, -1, -1, -1, -1, -1, -1, -1, -1, -1, -1, -1, -1, -1, -1, -1, -1, -1, -1, -1, -1, -1, -1, -1, -1, -1, -1, -1, -1, -1, -1, -1, -1, -1, -1, -1, -1, -1, -1, -1, -1, -1, -1, -1, -1, -1, -1, -1, -1, -1, -1, -1, -1, -1, -1, -1, -1, -1, -1, -1, -1, -1, -1, -1, -1, -1, -1, -1, -1, -1, -1, -1, -1, -1, -1, -1, -1, -1, -1, -1, -1, -1, -1, -1, -1, -1, -1, -1, -1, -1, -1, -1, -1, -1, -1, -1, -1, -1, -1, -1, -1, -1, -1, -1, -1, -1, -1, -1, -1, -1, -1, -1, -1, -1, -1, -1, -1, -1, -1, -1, -1, -1, -1, -1, -1, -1, -1, -1, -1, -1, -1, -1, -1, -1, -1, -1, -1, -1, -1, -1, -1, -1, -1, -1, -1, -1, -1, -1, -1, -1, -1, -1, -1, -1, -1, -1, -1, -1, -1, -1, -1, -1, -1, -1, -1, -1, -1, -1, -1, -1, -1, -1, -1, -1, -1, -1, -1, -1, -1, -1, -1, -1, -1, -1, -1, -1, -1, -1, -1, -1, -1, -1, -1, -1, -1, -1, -1, -1, -1, -1, -1, -1, -1, -1, -1, -1, -1, -1, -1, -1, -1, -1, -1, -1, -1, -1, -1, -1, -1, -1, -1, -1, -1, -1, -1, -1, -1, -1, -1, -1, -1, -1, -1, -1, -1, -1, -1, -1, -1, -1, -1, -1, -1, -1, -1, -1, -1, -1, -1, -1, -1, -1, -1, -1, -1, -1, -1, -1, -1, -1, -1, -1, -1, -1, -1, -1, -1, -1, -1, -1, -1, -1, -1, -1, -1, -1, -1, -1, -1, -1, -1, -1, -1, -1, -1, -1, -1, -1, -1, -1, -1, -1, -1, -1, -1, -1, -1, -1, -1, -1, -1, -1, -1, -1, -1, -1, -1, -1, -1, -1, -1, -1, -1, -1, -1, -1, -1, -1, -1, -1, -1, -1, -1, -1, -1, -1, -1, -1, -1, -1, -1, -1, -1, -1, -1, -1, -1, -1, -1, -1, -1, -1, -1, -1, -1, -1, -1, -1, -1, -1, -1, -1, -1, -1, -1, -1, -1, -1, -1, -1, -1, -1, -1, -1, -1, -1, -1, -1, -1, -1, -1, -1, -1, -1, -1, -1, -1, -1, -1, -1, -1, -1, -1, -1, -1, -1, -1, -1, -1, -1, -1, -1, -1, -1, -1, -1, -1, -1, -1, -1, -1, -1, -1, -1, -1, -1, -1, -1, -1, -1, -1, -1, -1, -1, -1, -1, -1, -1, -1, -1, -1, -1, -1, -1, -1, -1, -1, -1, -1, -1, -1, -1, -1, -1, -1, -1, -1, -1, -1, -1, -1, -1, -1, -1, -1, -1, -1, -1, -1, -1, -1, -1, -1, -1, -1, -1, -1, -1, -1, -1, -1, -1, -1, -1, -1, -1, -1, -1, -1, -1, -1, -1, -1, -1, -1, -1, -1, -1, -1, -1, -1, -1, -1, -1, -1, -1, -1, -1, -1, -1, -1, -1, -1, -1, -1, -1, -1, -1, -1, -1, -1, -1, -1, -1, -1, -1, -1, -1, -1, -1, -1, -1, -1, -1, -1, -1, -1, -1, -1, -1, -1, -1, -1, -1, -1, -1, -1, -1, -1, -1, -1, -1, -1, -1, -1, -1, -1, -1, -1, -1, -1, -1, -1, -1, -1, -1, -1, -1, -1, -1, -1, -1, -1, -1, -1, -1, -1, -1, -1, -1, -1, -1, -1, -1, -1, -1, -1, -1, -1, -1, -1, -1, -1, -1, -1, -1, -1, -1, -1, -1, -1, -1, -1, -1, -1, -1, -1, -1, -1, -1, -1, -1, -1, -1, -1, -1, -1, -1, -1, -1, -1, -1, -1, -1, -1, -1, -1, -1, -1, -1, -1, -1, -1, -1, -1, -1, -1, -1, -1, -1, -1, -1, -1, -1, -1, -1, -1, -1, -1, -1, -1, -1, -1, -1, -1, -1, -1, -1, -1, -1, -1, -1, -1, -1, -1, -1, -1, -1, -1, -1, -1, -1, -1, -1, -1, -1, -1, -1, -1, -1, -1, -1, -1, -1, -1, -1, -1, -1, -1, -1, -1, -1, -1, -1, -1, -1, -1, -1, -1, -1, -1, -1, -1, -1, -1, -1, -1, -1, -1, -1, -1, -1, -1, -1, -1, -1, -1, -1, -1, -1, -1, -1, -1, -1, -1, -1, -1, -1, -1, -1, -1, -1, -1, -1, -1, -1, -1, -1, -1, -1, -1, -1, -1, -1, -1, -1, -1, -1, -1, -1, -1, -1, -1, -1, -1, -1, -1, -1, -1, -1, -1, -1, -1, -1, -1, -1, -1, -1, -1, -1, -1, -1, -1, -1, -1, -1, -1, -1, -1, -1, -1, -1, -1, -1, -1, -1, -1, -1, -1, -1, -1, -1, -1, -1, -1, -1, -1, -1, -1, -1, -1, -1, -1, -1, -1, -1, -1, -1, -1, -1, -1, -1, -1, -1, -1, -1, -1, -1, -1, -1, -1, -1, -1, -1, -1, -1, -1, -1, -1, -1, -1, -1, -1, -1, -1, -1, -1, -1, -1, -1, -1, -1, -1, -1, -1, -1, -1, -1, -1, -1, -1, -1, -1, -1, -1, -1, -1, -1, -1, -1, -1, -1, -1, -1, -1, -1, -1, -1, -1, -1, -1, -1, -1, -1, -1, -1, -1, -1, -1, -1, -1, -1, -1, -1, -1, -1, -1, -1, -1, -1, -1, -1, -1, -1, -1, -1, -1, -1, -1, -1, -1, -1, -1, -1, -1, -1, -1, -1, -1, -1, -1, -1, -1, -1, -1, -1, -1, -1, -1, -1, -1, -1, -1, -1, -1, -1, -1, -1, -1, -1, -1, -1, -1, -1, -1, -1, -1, -1, -1, -1, -1, -1, -1, -1, -1, -1, -1, -1, -1, -1, -1, -1, -1, -1, -1, -1, -1, -1, -1, -1, -1, -1, -1, -1, -1, -1, -1, -1, -1, -1, -1, -1, -1, -1, -1, -1, -1, -1, -1, -1, -1, -1, -1, -1, -1, -1, -1, -1, -1, -1, -1, -1, -1, -1, -1, -1, -1, -1, -1, -1, -1, -1, -1, -1, -1, -1, -1, -1, -1, -1, -1, -1, -1, -1, -1, -1, -1, -1, -1, -1, -1, -1, -1, -1, -1, -1, -1, -1, -1, -1, -1, -1, -1, -1, -1, -1, -1, -1, -1, -1, -1, -1, -1, -1, -1, -1, -1, -1, -1, -1, -1, -1, -1, -1, -1, -1, -1, -1, -1, -1, -1, -1, -1, -1, -1, -1, -1, -1, -1, -1, -1, -1, -1, -1, -1, -1, -1, -1, -1, -1, -1, -1, -1, -1, -1, -1, -1, -1, -1, -1, -1, -1, -1, -1, -1, -1, -1, -1, -1, -1, -1, -1, -1, -1, -1, -1, -1, -1, -1, -1, -1, -1, -1, -1, -1, -1, -1, -1, -1, -1, -1, -1, -1, -1, -1, -1, -1, -1, -1, -1, -1, -1, -1, -1, -1, -1, -1, -1, -1, -1, -1, -1, -1, -1, -1, -1, -1, -1, -1, -1, -1, -1, -1, -1, -1, -1, -1, -1, -1, -1, -1, -1, -1, -1, -1, -1, -1, -1, -1, -1, -1, -1, -1, -1, -1, -1, -1, -1, -1, -1, -1, -1, -1, -1, -1, -1, -1, -1, -1, -1, -1, -1, -1, -1, -1, -1, -1, -1, -1, -1, -1, -1, -1, -1, -1, -1, -1, -1, -1, -1, -1, -1, -1, -1, -1, -1, -1, -1, -1, -1, -1, -1, -1, -1, -1, -1, -1, -1, -1, -1, -1, -1, -1, -1, -1, -1, -1, -1, -1, -1, -1, -1, -1, -1, -1, -1, -1, -1, -1, -1, -1, -1, -1, -1, -1, -1, -1, -1, -1, -1, -1, -1, -1, -1, -1, -1, -1, -1, -1, -1, -1, -1, -1, -1, -1, -1, -1, -1, -1, -1, -1, -1, -1, -1, -1, -1, -1, -1, -1, -1, -1, -1, -1, -1, -1, -1, -1, -1, -1, -1, -1, -1, -1, -1, -1, -1, -1, -1, -1, -1, -1, -1, -1, -1, -1, -1, -1, -1, -1, -1, -1, -1, -1, -1, -1, -1, -1, -1, -1, -1, -1, -1, -1, -1, -1, -1, -1, -1, -1, -1, -1, -1, -1, -1, -1, -1, -1, -1, -1, -1, -1, -1, -1, -1, -1, -1, -1, -1, -1, -1, -1, -1, -1, -1, -1, -1, -1, -1, -1, -1, -1, -1, -1, -1, -1, -1, -1, -1, -1, -1, -1, -1, -1, -1, -1, -1, -1, -1, -1, -1, -1, -1, -1, -1, -1, -1, -1, -1, -1, -1, -1, -1, -1, -1, -1, -1, -1, -1, -1, -1, -1, -1, -1, -1, -1, -1, -1, -1, -1, -1, -1, -1, -1, -1, -1, -1, -1, -1, -1, -1, -1, -1, -1, -1, -1, -1, -1, -1, -1, -1, -1, -1, -1, -1, -1, -1, -1, -1, -1, -1, -1, -1, -1, -1, -1, -1, -1, -1, -1, -1, -1, -1, -1, -1, -1, -1]
```
Yes, this is because the test sets for glue are hidden so the labels are
not publicly available. You can read the glue paper for more details.
On Sat, 6 Jun 2020 at 18:16, Jack Morris <notifications@github.com> wrote:
> this also happened to me with nlp.load_datasets('glue', 'mnli')
>
> —
> You are receiving this because you are subscribed to this thread.
> Reply to this email directly, view it on GitHub
> <https://github.com/huggingface/nlp/issues/245#issuecomment-640083980>,
> or unsubscribe
> <https://github.com/notifications/unsubscribe-auth/ABYDIHMVQD2EDX2HTZUXG5DRVJTWRANCNFSM4NVG3AKQ>
> .
>
|
https://github.com/huggingface/datasets/issues/245 | SST-2 test labels are all -1 | It should be in the datasets card (the README.md and on the hub) in my opinion. What do you think @yjernite? | I'm trying to test a model on the SST-2 task, but all the labels I see in the test set are -1.
```
>>> import nlp
>>> glue = nlp.load_dataset('glue', 'sst2')
>>> glue
{'train': Dataset(schema: {'sentence': 'string', 'label': 'int64', 'idx': 'int32'}, num_rows: 67349), 'validation': Dataset(schema: {'sentence': 'string', 'label': 'int64', 'idx': 'int32'}, num_rows: 872), 'test': Dataset(schema: {'sentence': 'string', 'label': 'int64', 'idx': 'int32'}, num_rows: 1821)}
>>> list(l['label'] for l in glue['test'])
[-1, -1, -1, -1, -1, -1, -1, -1, -1, -1, -1, -1, -1, -1, -1, -1, -1, -1, -1, -1, -1, -1, -1, -1, -1, -1, -1, -1, -1, -1, -1, -1, -1, -1, -1, -1, -1, -1, -1, -1, -1, -1, -1, -1, -1, -1, -1, -1, -1, -1, -1, -1, -1, -1, -1, -1, -1, -1, -1, -1, -1, -1, -1, -1, -1, -1, -1, -1, -1, -1, -1, -1, -1, -1, -1, -1, -1, -1, -1, -1, -1, -1, -1, -1, -1, -1, -1, -1, -1, -1, -1, -1, -1, -1, -1, -1, -1, -1, -1, -1, -1, -1, -1, -1, -1, -1, -1, -1, -1, -1, -1, -1, -1, -1, -1, -1, -1, -1, -1, -1, -1, -1, -1, -1, -1, -1, -1, -1, -1, -1, -1, -1, -1, -1, -1, -1, -1, -1, -1, -1, -1, -1, -1, -1, -1, -1, -1, -1, -1, -1, -1, -1, -1, -1, -1, -1, -1, -1, -1, -1, -1, -1, -1, -1, -1, -1, -1, -1, -1, -1, -1, -1, -1, -1, -1, -1, -1, -1, -1, -1, -1, -1, -1, -1, -1, -1, -1, -1, -1, -1, -1, -1, -1, -1, -1, -1, -1, -1, -1, -1, -1, -1, -1, -1, -1, -1, -1, -1, -1, -1, -1, -1, -1, -1, -1, -1, -1, -1, -1, -1, -1, -1, -1, -1, -1, -1, -1, -1, -1, -1, -1, -1, -1, -1, -1, -1, -1, -1, -1, -1, -1, -1, -1, -1, -1, -1, -1, -1, -1, -1, -1, -1, -1, -1, -1, -1, -1, -1, -1, -1, -1, -1, -1, -1, -1, -1, -1, -1, -1, -1, -1, -1, -1, -1, -1, -1, -1, -1, -1, -1, -1, -1, -1, -1, -1, -1, -1, -1, -1, -1, -1, -1, -1, -1, -1, -1, -1, -1, -1, -1, -1, -1, -1, -1, -1, -1, -1, -1, -1, -1, -1, -1, -1, -1, -1, -1, -1, -1, -1, -1, -1, -1, -1, -1, -1, -1, -1, -1, -1, -1, -1, -1, -1, -1, -1, -1, -1, -1, -1, -1, -1, -1, -1, -1, -1, -1, -1, -1, -1, -1, -1, -1, -1, -1, -1, -1, -1, -1, -1, -1, -1, -1, -1, -1, -1, -1, -1, -1, -1, -1, -1, -1, -1, -1, -1, -1, -1, -1, -1, -1, -1, -1, -1, -1, -1, -1, -1, -1, -1, -1, -1, -1, -1, -1, -1, -1, -1, -1, -1, -1, -1, -1, -1, -1, -1, -1, -1, -1, -1, -1, -1, -1, -1, -1, -1, -1, -1, -1, -1, -1, -1, -1, -1, -1, -1, -1, -1, -1, -1, -1, -1, -1, -1, -1, -1, -1, -1, -1, -1, -1, -1, -1, -1, -1, -1, -1, -1, -1, -1, -1, -1, -1, -1, -1, -1, -1, -1, -1, -1, -1, -1, -1, -1, -1, -1, -1, -1, -1, -1, -1, -1, -1, -1, -1, -1, -1, -1, -1, -1, -1, -1, -1, -1, -1, -1, -1, -1, -1, -1, -1, -1, -1, -1, -1, -1, -1, -1, -1, -1, -1, -1, -1, -1, -1, -1, -1, -1, -1, -1, -1, -1, -1, -1, -1, -1, -1, -1, -1, -1, -1, -1, -1, -1, -1, -1, -1, -1, -1, -1, -1, -1, -1, -1, -1, -1, -1, -1, -1, -1, -1, -1, -1, -1, -1, -1, -1, -1, -1, -1, -1, -1, -1, -1, -1, -1, -1, -1, -1, -1, -1, -1, -1, -1, -1, -1, -1, -1, -1, -1, -1, -1, -1, -1, -1, -1, -1, -1, -1, -1, -1, -1, -1, -1, -1, -1, -1, -1, -1, -1, -1, -1, -1, -1, -1, -1, -1, -1, -1, -1, -1, -1, -1, -1, -1, -1, -1, -1, -1, -1, -1, -1, -1, -1, -1, -1, -1, -1, -1, -1, -1, -1, -1, -1, -1, -1, -1, -1, -1, -1, -1, -1, -1, -1, -1, -1, -1, -1, -1, -1, -1, -1, -1, -1, -1, -1, -1, -1, -1, -1, -1, -1, -1, -1, -1, -1, -1, -1, -1, -1, -1, -1, -1, -1, -1, -1, -1, -1, -1, -1, -1, -1, -1, -1, -1, -1, -1, -1, -1, -1, -1, -1, -1, -1, -1, -1, -1, -1, -1, -1, -1, -1, -1, -1, -1, -1, -1, -1, -1, -1, -1, -1, -1, -1, -1, -1, -1, -1, -1, -1, -1, -1, -1, -1, -1, -1, -1, -1, -1, -1, -1, -1, -1, -1, -1, -1, -1, -1, -1, -1, -1, -1, -1, -1, -1, -1, -1, -1, -1, -1, -1, -1, -1, -1, -1, -1, -1, -1, -1, -1, -1, -1, -1, -1, -1, -1, -1, -1, -1, -1, -1, -1, -1, -1, -1, -1, -1, -1, -1, -1, -1, -1, -1, -1, -1, -1, -1, -1, -1, -1, -1, -1, -1, -1, -1, -1, -1, -1, -1, -1, -1, -1, -1, -1, -1, -1, -1, -1, -1, -1, -1, -1, -1, -1, -1, -1, -1, -1, -1, -1, -1, -1, -1, -1, -1, -1, -1, -1, -1, -1, -1, -1, -1, -1, -1, -1, -1, -1, -1, -1, -1, -1, -1, -1, -1, -1, -1, -1, -1, -1, -1, -1, -1, -1, -1, -1, -1, -1, -1, -1, -1, -1, -1, -1, -1, -1, -1, -1, -1, -1, -1, -1, -1, -1, -1, -1, -1, -1, -1, -1, -1, -1, -1, -1, -1, -1, -1, -1, -1, -1, -1, -1, -1, -1, -1, -1, -1, -1, -1, -1, -1, -1, -1, -1, -1, -1, -1, -1, -1, -1, -1, -1, -1, -1, -1, -1, -1, -1, -1, -1, -1, -1, -1, -1, -1, -1, -1, -1, -1, -1, -1, -1, -1, -1, -1, -1, -1, -1, -1, -1, -1, -1, -1, -1, -1, -1, -1, -1, -1, -1, -1, -1, -1, -1, -1, -1, -1, -1, -1, -1, -1, -1, -1, -1, -1, -1, -1, -1, -1, -1, -1, -1, -1, -1, -1, -1, -1, -1, -1, -1, -1, -1, -1, -1, -1, -1, -1, -1, -1, -1, -1, -1, -1, -1, -1, -1, -1, -1, -1, -1, -1, -1, -1, -1, -1, -1, -1, -1, -1, -1, -1, -1, -1, -1, -1, -1, -1, -1, -1, -1, -1, -1, -1, -1, -1, -1, -1, -1, -1, -1, -1, -1, -1, -1, -1, -1, -1, -1, -1, -1, -1, -1, -1, -1, -1, -1, -1, -1, -1, -1, -1, -1, -1, -1, -1, -1, -1, -1, -1, -1, -1, -1, -1, -1, -1, -1, -1, -1, -1, -1, -1, -1, -1, -1, -1, -1, -1, -1, -1, -1, -1, -1, -1, -1, -1, -1, -1, -1, -1, -1, -1, -1, -1, -1, -1, -1, -1, -1, -1, -1, -1, -1, -1, -1, -1, -1, -1, -1, -1, -1, -1, -1, -1, -1, -1, -1, -1, -1, -1, -1, -1, -1, -1, -1, -1, -1, -1, -1, -1, -1, -1, -1, -1, -1, -1, -1, -1, -1, -1, -1, -1, -1, -1, -1, -1, -1, -1, -1, -1, -1, -1, -1, -1, -1, -1, -1, -1, -1, -1, -1, -1, -1, -1, -1, -1, -1, -1, -1, -1, -1, -1, -1, -1, -1, -1, -1, -1, -1, -1, -1, -1, -1, -1, -1, -1, -1, -1, -1, -1, -1, -1, -1, -1, -1, -1, -1, -1, -1, -1, -1, -1, -1, -1, -1, -1, -1, -1, -1, -1, -1, -1, -1, -1, -1, -1, -1, -1, -1, -1, -1, -1, -1, -1, -1, -1, -1, -1, -1, -1, -1, -1, -1, -1, -1, -1, -1, -1, -1, -1, -1, -1, -1, -1, -1, -1, -1, -1, -1, -1, -1, -1, -1, -1, -1, -1, -1, -1, -1, -1, -1, -1, -1, -1, -1, -1, -1, -1, -1, -1, -1, -1, -1, -1, -1, -1, -1, -1, -1, -1, -1, -1, -1, -1, -1, -1, -1, -1, -1, -1, -1, -1, -1, -1, -1, -1, -1, -1, -1, -1, -1, -1, -1, -1, -1, -1, -1, -1, -1, -1, -1, -1, -1, -1, -1, -1, -1, -1, -1, -1, -1, -1, -1, -1, -1, -1, -1, -1, -1, -1, -1, -1, -1, -1, -1, -1, -1, -1, -1, -1, -1, -1, -1, -1, -1, -1, -1, -1, -1, -1, -1, -1, -1, -1, -1, -1, -1, -1, -1, -1, -1, -1, -1, -1, -1, -1, -1, -1, -1, -1, -1, -1, -1, -1, -1, -1, -1, -1, -1, -1, -1, -1, -1, -1, -1, -1, -1, -1, -1, -1, -1, -1, -1, -1, -1, -1, -1, -1, -1, -1, -1, -1, -1, -1, -1, -1, -1, -1, -1, -1, -1, -1, -1, -1, -1, -1, -1, -1, -1, -1, -1, -1, -1, -1, -1, -1, -1, -1, -1, -1, -1, -1, -1, -1, -1, -1, -1, -1, -1, -1, -1, -1, -1, -1, -1, -1, -1, -1, -1, -1, -1, -1, -1, -1, -1, -1, -1, -1, -1, -1, -1, -1, -1, -1, -1, -1, -1, -1, -1, -1, -1, -1, -1, -1, -1, -1, -1, -1, -1, -1, -1, -1, -1, -1, -1, -1, -1, -1, -1, -1, -1, -1, -1, -1, -1, -1, -1, -1, -1, -1, -1, -1, -1, -1, -1, -1, -1, -1, -1, -1, -1, -1, -1, -1, -1, -1, -1, -1, -1, -1, -1, -1, -1, -1, -1, -1, -1, -1, -1, -1, -1, -1, -1, -1, -1, -1, -1, -1, -1, -1, -1, -1, -1, -1, -1, -1, -1, -1, -1, -1, -1, -1, -1, -1, -1, -1, -1, -1, -1, -1, -1, -1, -1, -1, -1, -1, -1, -1, -1, -1, -1, -1, -1, -1, -1, -1, -1, -1, -1, -1, -1, -1, -1, -1, -1, -1, -1, -1, -1, -1, -1, -1, -1, -1, -1, -1, -1, -1, -1, -1, -1, -1, -1, -1, -1, -1, -1, -1, -1, -1, -1, -1, -1, -1, -1, -1, -1, -1, -1, -1, -1, -1, -1, -1, -1, -1, -1, -1, -1, -1, -1, -1, -1, -1, -1, -1, -1, -1, -1, -1, -1, -1, -1, -1, -1, -1, -1, -1, -1, -1, -1, -1, -1, -1, -1, -1, -1, -1, -1, -1, -1, -1, -1, -1, -1, -1, -1, -1, -1, -1, -1, -1, -1, -1, -1, -1, -1, -1, -1, -1, -1, -1, -1, -1, -1, -1, -1, -1, -1, -1, -1, -1, -1, -1, -1, -1, -1, -1, -1, -1, -1, -1, -1, -1, -1, -1, -1, -1, -1, -1, -1, -1, -1, -1, -1, -1, -1, -1, -1, -1, -1, -1, -1, -1, -1, -1, -1, -1, -1, -1, -1, -1, -1, -1, -1, -1, -1, -1, -1, -1, -1, -1, -1, -1, -1, -1, -1, -1, -1, -1, -1, -1, -1, -1, -1, -1, -1, -1, -1, -1, -1, -1, -1, -1, -1, -1, -1, -1, -1, -1, -1, -1, -1, -1, -1, -1, -1, -1, -1, -1, -1, -1, -1, -1, -1, -1, -1, -1, -1, -1, -1, -1, -1, -1, -1, -1, -1, -1, -1, -1, -1, -1, -1, -1, -1, -1, -1, -1, -1, -1, -1, -1, -1, -1, -1, -1, -1, -1, -1, -1, -1, -1, -1, -1, -1, -1, -1, -1, -1, -1, -1, -1, -1]
``` | 21 | SST-2 test labels are all -1
I'm trying to test a model on the SST-2 task, but all the labels I see in the test set are -1.
```
>>> import nlp
>>> glue = nlp.load_dataset('glue', 'sst2')
>>> glue
{'train': Dataset(schema: {'sentence': 'string', 'label': 'int64', 'idx': 'int32'}, num_rows: 67349), 'validation': Dataset(schema: {'sentence': 'string', 'label': 'int64', 'idx': 'int32'}, num_rows: 872), 'test': Dataset(schema: {'sentence': 'string', 'label': 'int64', 'idx': 'int32'}, num_rows: 1821)}
>>> list(l['label'] for l in glue['test'])
[-1, -1, -1, -1, -1, -1, -1, -1, -1, -1, -1, -1, -1, -1, -1, -1, -1, -1, -1, -1, -1, -1, -1, -1, -1, -1, -1, -1, -1, -1, -1, -1, -1, -1, -1, -1, -1, -1, -1, -1, -1, -1, -1, -1, -1, -1, -1, -1, -1, -1, -1, -1, -1, -1, -1, -1, -1, -1, -1, -1, -1, -1, -1, -1, -1, -1, -1, -1, -1, -1, -1, -1, -1, -1, -1, -1, -1, -1, -1, -1, -1, -1, -1, -1, -1, -1, -1, -1, -1, -1, -1, -1, -1, -1, -1, -1, -1, -1, -1, -1, -1, -1, -1, -1, -1, -1, -1, -1, -1, -1, -1, -1, -1, -1, -1, -1, -1, -1, -1, -1, -1, -1, -1, -1, -1, -1, -1, -1, -1, -1, -1, -1, -1, -1, -1, -1, -1, -1, -1, -1, -1, -1, -1, -1, -1, -1, -1, -1, -1, -1, -1, -1, -1, -1, -1, -1, -1, -1, -1, -1, -1, -1, -1, -1, -1, -1, -1, -1, -1, -1, -1, -1, -1, -1, -1, -1, -1, -1, -1, -1, -1, -1, -1, -1, -1, -1, -1, -1, -1, -1, -1, -1, -1, -1, -1, -1, -1, -1, -1, -1, -1, -1, -1, -1, -1, -1, -1, -1, -1, -1, -1, -1, -1, -1, -1, -1, -1, -1, -1, -1, -1, -1, -1, -1, -1, -1, -1, -1, -1, -1, -1, -1, -1, -1, -1, -1, -1, -1, -1, -1, -1, -1, -1, -1, -1, -1, -1, -1, -1, -1, -1, -1, -1, -1, -1, -1, -1, -1, -1, -1, -1, -1, -1, -1, -1, -1, -1, -1, -1, -1, -1, -1, -1, -1, -1, -1, -1, -1, -1, -1, -1, -1, -1, -1, -1, -1, -1, -1, -1, -1, -1, -1, -1, -1, -1, -1, -1, -1, -1, -1, -1, -1, -1, -1, -1, -1, -1, -1, -1, -1, -1, -1, -1, -1, -1, -1, -1, -1, -1, -1, -1, -1, -1, -1, -1, -1, -1, -1, -1, -1, -1, -1, -1, -1, -1, -1, -1, -1, -1, -1, -1, -1, -1, -1, -1, -1, -1, -1, -1, -1, -1, -1, -1, -1, -1, -1, -1, -1, -1, -1, -1, -1, -1, -1, -1, -1, -1, -1, -1, -1, -1, -1, -1, -1, -1, -1, -1, -1, -1, -1, -1, -1, -1, -1, -1, -1, -1, -1, -1, -1, -1, -1, -1, -1, -1, -1, -1, -1, -1, -1, -1, -1, -1, -1, -1, -1, -1, -1, -1, -1, -1, -1, -1, -1, -1, -1, -1, -1, -1, -1, -1, -1, -1, -1, -1, -1, -1, -1, -1, -1, -1, -1, -1, -1, -1, -1, -1, -1, -1, -1, -1, -1, -1, -1, -1, -1, -1, -1, -1, -1, -1, -1, -1, -1, -1, -1, -1, -1, -1, -1, -1, -1, -1, -1, -1, -1, -1, -1, -1, -1, -1, -1, -1, -1, -1, -1, -1, -1, -1, -1, -1, -1, -1, -1, -1, -1, -1, -1, -1, -1, -1, -1, -1, -1, -1, -1, -1, -1, -1, -1, -1, -1, -1, -1, -1, -1, -1, -1, -1, -1, -1, -1, -1, -1, -1, -1, -1, -1, -1, -1, -1, -1, -1, -1, -1, -1, -1, -1, -1, -1, -1, -1, -1, -1, -1, -1, -1, -1, -1, -1, -1, -1, -1, -1, -1, -1, -1, -1, -1, -1, -1, -1, -1, -1, -1, -1, -1, -1, -1, -1, -1, -1, -1, -1, -1, -1, -1, -1, -1, -1, -1, -1, -1, -1, -1, -1, -1, -1, -1, -1, -1, -1, -1, -1, -1, -1, -1, -1, -1, -1, -1, -1, -1, -1, -1, -1, -1, -1, -1, -1, -1, -1, -1, -1, -1, -1, -1, -1, -1, -1, -1, -1, -1, -1, -1, -1, -1, -1, -1, -1, -1, -1, -1, -1, -1, -1, -1, -1, -1, -1, -1, -1, -1, -1, -1, -1, -1, -1, -1, -1, -1, -1, -1, -1, -1, -1, -1, -1, -1, -1, -1, -1, -1, -1, -1, -1, -1, -1, -1, -1, -1, -1, -1, -1, -1, -1, -1, -1, -1, -1, -1, -1, -1, -1, -1, -1, -1, -1, -1, -1, -1, -1, -1, -1, -1, -1, -1, -1, -1, -1, -1, -1, -1, -1, -1, -1, -1, -1, -1, -1, -1, -1, -1, -1, -1, -1, -1, -1, -1, -1, -1, -1, -1, -1, -1, -1, -1, -1, -1, -1, -1, -1, -1, -1, -1, -1, -1, -1, -1, -1, -1, -1, -1, -1, -1, -1, -1, -1, -1, -1, -1, -1, -1, -1, -1, -1, -1, -1, -1, -1, -1, -1, -1, -1, -1, -1, -1, -1, -1, -1, -1, -1, -1, -1, -1, -1, -1, -1, -1, -1, -1, -1, -1, -1, -1, -1, -1, -1, -1, -1, -1, -1, -1, -1, -1, -1, -1, -1, -1, -1, -1, -1, -1, -1, -1, -1, -1, -1, -1, -1, -1, -1, -1, -1, -1, -1, -1, -1, -1, -1, -1, -1, -1, -1, -1, -1, -1, -1, -1, -1, -1, -1, -1, -1, -1, -1, -1, -1, -1, -1, -1, -1, -1, -1, -1, -1, -1, -1, -1, -1, -1, -1, -1, -1, -1, -1, -1, -1, -1, -1, -1, -1, -1, -1, -1, -1, -1, -1, -1, -1, -1, -1, -1, -1, -1, -1, -1, -1, -1, -1, -1, -1, -1, -1, -1, -1, -1, -1, -1, -1, -1, -1, -1, -1, -1, -1, -1, -1, -1, -1, -1, -1, -1, -1, -1, -1, -1, -1, -1, -1, -1, -1, -1, -1, -1, -1, -1, -1, -1, -1, -1, -1, -1, -1, -1, -1, -1, -1, -1, -1, -1, -1, -1, -1, -1, -1, -1, -1, -1, -1, -1, -1, -1, -1, -1, -1, -1, -1, -1, -1, -1, -1, -1, -1, -1, -1, -1, -1, -1, -1, -1, -1, -1, -1, -1, -1, -1, -1, -1, -1, -1, -1, -1, -1, -1, -1, -1, -1, -1, -1, -1, -1, -1, -1, -1, -1, -1, -1, -1, -1, -1, -1, -1, -1, -1, -1, -1, -1, -1, -1, -1, -1, -1, -1, -1, -1, -1, -1, -1, -1, -1, -1, -1, -1, -1, -1, -1, -1, -1, -1, -1, -1, -1, -1, -1, -1, -1, -1, -1, -1, -1, -1, -1, -1, -1, -1, -1, -1, -1, -1, -1, -1, -1, -1, -1, -1, -1, -1, -1, -1, -1, -1, -1, -1, -1, -1, -1, -1, -1, -1, -1, -1, -1, -1, -1, -1, -1, -1, -1, -1, -1, -1, -1, -1, -1, -1, -1, -1, -1, -1, -1, -1, -1, -1, -1, -1, -1, -1, -1, -1, -1, -1, -1, -1, -1, -1, -1, -1, -1, -1, -1, -1, -1, -1, -1, -1, -1, -1, -1, -1, -1, -1, -1, -1, -1, -1, -1, -1, -1, -1, -1, -1, -1, -1, -1, -1, -1, -1, -1, -1, -1, -1, -1, -1, -1, -1, -1, -1, -1, -1, -1, -1, -1, -1, -1, -1, -1, -1, -1, -1, -1, -1, -1, -1, -1, -1, -1, -1, -1, -1, -1, -1, -1, -1, -1, -1, -1, -1, -1, -1, -1, -1, -1, -1, -1, -1, -1, -1, -1, -1, -1, -1, -1, -1, -1, -1, -1, -1, -1, -1, -1, -1, -1, -1, -1, -1, -1, -1, -1, -1, -1, -1, -1, -1, -1, -1, -1, -1, -1, -1, -1, -1, -1, -1, -1, -1, -1, -1, -1, -1, -1, -1, -1, -1, -1, -1, -1, -1, -1, -1, -1, -1, -1, -1, -1, -1, -1, -1, -1, -1, -1, -1, -1, -1, -1, -1, -1, -1, -1, -1, -1, -1, -1, -1, -1, -1, -1, -1, -1, -1, -1, -1, -1, -1, -1, -1, -1, -1, -1, -1, -1, -1, -1, -1, -1, -1, -1, -1, -1, -1, -1, -1, -1, -1, -1, -1, -1, -1, -1, -1, -1, -1, -1, -1, -1, -1, -1, -1, -1, -1, -1, -1, -1, -1, -1, -1, -1, -1, -1, -1, -1, -1, -1, -1, -1, -1, -1, -1, -1, -1, -1, -1, -1, -1, -1, -1, -1, -1, -1, -1, -1, -1, -1, -1, -1, -1, -1, -1, -1, -1, -1, -1, -1, -1, -1, -1, -1, -1, -1, -1, -1, -1, -1, -1, -1, -1, -1, -1, -1, -1, -1, -1, -1, -1, -1, -1, -1, -1, -1, -1, -1, -1, -1, -1, -1, -1, -1, -1, -1, -1, -1, -1, -1, -1, -1, -1, -1, -1, -1, -1, -1, -1, -1, -1, -1, -1, -1, -1, -1, -1, -1, -1, -1, -1, -1, -1, -1, -1, -1, -1, -1, -1, -1, -1, -1, -1, -1, -1, -1, -1, -1, -1, -1, -1, -1, -1, -1, -1, -1, -1, -1, -1, -1, -1, -1, -1, -1, -1, -1, -1, -1, -1, -1, -1, -1, -1, -1, -1, -1, -1, -1, -1, -1, -1, -1, -1, -1, -1, -1, -1, -1, -1, -1, -1, -1, -1, -1, -1, -1, -1, -1, -1, -1, -1, -1, -1, -1, -1, -1, -1, -1, -1, -1, -1, -1, -1, -1, -1, -1, -1, -1, -1, -1, -1, -1, -1, -1, -1, -1, -1, -1, -1, -1, -1, -1, -1, -1, -1, -1, -1, -1, -1, -1, -1, -1, -1, -1, -1, -1, -1, -1, -1, -1, -1, -1, -1, -1, -1, -1, -1, -1, -1, -1, -1, -1, -1, -1, -1, -1, -1, -1, -1, -1, -1, -1, -1, -1, -1, -1, -1, -1, -1, -1, -1, -1, -1, -1, -1, -1, -1, -1, -1, -1, -1, -1, -1, -1, -1, -1, -1, -1, -1, -1, -1, -1, -1, -1, -1, -1, -1, -1, -1, -1, -1, -1, -1, -1, -1, -1, -1, -1, -1, -1, -1, -1, -1, -1, -1, -1, -1, -1, -1, -1, -1, -1, -1, -1, -1, -1, -1, -1, -1, -1, -1, -1, -1, -1, -1, -1, -1, -1, -1, -1, -1, -1, -1, -1, -1, -1, -1, -1, -1, -1, -1, -1, -1, -1, -1, -1, -1, -1, -1, -1, -1, -1, -1, -1, -1, -1, -1, -1, -1, -1, -1, -1, -1, -1, -1, -1, -1, -1, -1, -1, -1, -1, -1, -1, -1, -1, -1, -1, -1, -1, -1, -1, -1, -1, -1, -1, -1, -1, -1, -1, -1, -1, -1, -1, -1, -1, -1, -1, -1, -1, -1, -1, -1, -1, -1, -1, -1, -1, -1, -1, -1, -1, -1, -1, -1, -1, -1, -1, -1, -1, -1, -1, -1, -1, -1, -1, -1, -1, -1, -1, -1, -1, -1, -1, -1, -1, -1, -1, -1, -1, -1, -1, -1, -1, -1, -1, -1, -1, -1, -1, -1, -1, -1, -1, -1, -1, -1, -1, -1, -1, -1, -1, -1, -1, -1, -1, -1, -1, -1, -1, -1, -1, -1, -1, -1, -1, -1, -1, -1, -1, -1, -1, -1, -1, -1, -1, -1, -1, -1, -1, -1, -1, -1, -1, -1, -1, -1, -1, -1, -1, -1, -1, -1, -1, -1, -1, -1, -1, -1, -1, -1, -1, -1, -1, -1, -1, -1, -1, -1, -1, -1, -1, -1, -1, -1, -1, -1, -1, -1, -1, -1, -1, -1, -1, -1, -1, -1, -1]
```
It should be in the datasets card (the README.md and on the hub) in my opinion. What do you think @yjernite? |
https://github.com/huggingface/datasets/issues/245 | SST-2 test labels are all -1 | I checked both before I got to looking at issues, so that would be fine as well.
Some additional thoughts on this: Is there a specific reason why the "test" split even has a "label" column if it isn't tagged. Shouldn't there just not be any. Seems more transparent | I'm trying to test a model on the SST-2 task, but all the labels I see in the test set are -1.
```
>>> import nlp
>>> glue = nlp.load_dataset('glue', 'sst2')
>>> glue
{'train': Dataset(schema: {'sentence': 'string', 'label': 'int64', 'idx': 'int32'}, num_rows: 67349), 'validation': Dataset(schema: {'sentence': 'string', 'label': 'int64', 'idx': 'int32'}, num_rows: 872), 'test': Dataset(schema: {'sentence': 'string', 'label': 'int64', 'idx': 'int32'}, num_rows: 1821)}
>>> list(l['label'] for l in glue['test'])
[-1, -1, -1, -1, -1, -1, -1, -1, -1, -1, -1, -1, -1, -1, -1, -1, -1, -1, -1, -1, -1, -1, -1, -1, -1, -1, -1, -1, -1, -1, -1, -1, -1, -1, -1, -1, -1, -1, -1, -1, -1, -1, -1, -1, -1, -1, -1, -1, -1, -1, -1, -1, -1, -1, -1, -1, -1, -1, -1, -1, -1, -1, -1, -1, -1, -1, -1, -1, -1, -1, -1, -1, -1, -1, -1, -1, -1, -1, -1, -1, -1, -1, -1, -1, -1, -1, -1, -1, -1, -1, -1, -1, -1, -1, -1, -1, -1, -1, -1, -1, -1, -1, -1, -1, -1, -1, -1, -1, -1, -1, -1, -1, -1, -1, -1, -1, -1, -1, -1, -1, -1, -1, -1, -1, -1, -1, -1, -1, -1, -1, -1, -1, -1, -1, -1, -1, -1, -1, -1, -1, -1, -1, -1, -1, -1, -1, -1, -1, -1, -1, -1, -1, -1, -1, -1, -1, -1, -1, -1, -1, -1, -1, -1, -1, -1, -1, -1, -1, -1, -1, -1, -1, -1, -1, -1, -1, -1, -1, -1, -1, -1, -1, -1, -1, -1, -1, -1, -1, -1, -1, -1, -1, -1, -1, -1, -1, -1, -1, -1, -1, -1, -1, -1, -1, -1, -1, -1, -1, -1, -1, -1, -1, -1, -1, -1, -1, -1, -1, -1, -1, -1, -1, -1, -1, -1, -1, -1, -1, -1, -1, -1, -1, -1, -1, -1, -1, -1, -1, -1, -1, -1, -1, -1, -1, -1, -1, -1, -1, -1, -1, -1, -1, -1, -1, -1, -1, -1, -1, -1, -1, -1, -1, -1, -1, -1, -1, -1, -1, -1, -1, -1, -1, -1, -1, -1, -1, -1, -1, -1, -1, -1, -1, -1, -1, -1, -1, -1, -1, -1, -1, -1, -1, -1, -1, -1, -1, -1, -1, -1, -1, -1, -1, -1, -1, -1, -1, -1, -1, -1, -1, -1, -1, -1, -1, -1, -1, -1, -1, -1, -1, -1, -1, -1, -1, -1, -1, -1, -1, -1, -1, -1, -1, -1, -1, -1, -1, -1, -1, -1, -1, -1, -1, -1, -1, -1, -1, -1, -1, -1, -1, -1, -1, -1, -1, -1, -1, -1, -1, -1, -1, -1, -1, -1, -1, -1, -1, -1, -1, -1, -1, -1, -1, -1, -1, -1, -1, -1, -1, -1, -1, -1, -1, -1, -1, -1, -1, -1, -1, -1, -1, -1, -1, -1, -1, -1, -1, -1, -1, -1, -1, -1, -1, -1, -1, -1, -1, -1, -1, -1, -1, -1, -1, -1, -1, -1, -1, -1, -1, -1, -1, -1, -1, -1, -1, -1, -1, -1, -1, -1, -1, -1, -1, -1, -1, -1, -1, -1, -1, -1, -1, -1, -1, -1, -1, -1, -1, -1, -1, -1, -1, -1, -1, -1, -1, -1, -1, -1, -1, -1, -1, -1, -1, -1, -1, -1, -1, -1, -1, -1, -1, -1, -1, -1, -1, -1, -1, -1, -1, -1, -1, -1, -1, -1, -1, -1, -1, -1, -1, -1, -1, -1, -1, -1, -1, -1, -1, -1, -1, -1, -1, -1, -1, -1, -1, -1, -1, -1, -1, -1, -1, -1, -1, -1, -1, -1, -1, -1, -1, -1, -1, -1, -1, -1, -1, -1, -1, -1, -1, -1, -1, -1, -1, -1, -1, -1, -1, -1, -1, -1, -1, -1, -1, -1, -1, -1, -1, -1, -1, -1, -1, -1, -1, -1, -1, -1, -1, -1, -1, -1, -1, -1, -1, -1, -1, -1, -1, -1, -1, -1, -1, -1, -1, -1, -1, -1, -1, -1, -1, -1, -1, -1, -1, -1, -1, -1, -1, -1, -1, -1, -1, -1, -1, -1, -1, -1, -1, -1, -1, -1, -1, -1, -1, -1, -1, -1, -1, -1, -1, -1, -1, -1, -1, -1, -1, -1, -1, -1, -1, -1, -1, -1, -1, -1, -1, -1, -1, -1, -1, -1, -1, -1, -1, -1, -1, -1, -1, -1, -1, -1, -1, -1, -1, -1, -1, -1, -1, -1, -1, -1, -1, -1, -1, -1, -1, -1, -1, -1, -1, -1, -1, -1, -1, -1, -1, -1, -1, -1, -1, -1, -1, -1, -1, -1, -1, -1, -1, -1, -1, -1, -1, -1, -1, -1, -1, -1, -1, -1, -1, -1, -1, -1, -1, -1, -1, -1, -1, -1, -1, -1, -1, -1, -1, -1, -1, -1, -1, -1, -1, -1, -1, -1, -1, -1, -1, -1, -1, -1, -1, -1, -1, -1, -1, -1, -1, -1, -1, -1, -1, -1, -1, -1, -1, -1, -1, -1, -1, -1, -1, -1, -1, -1, -1, -1, -1, -1, -1, -1, -1, -1, -1, -1, -1, -1, -1, -1, -1, -1, -1, -1, -1, -1, -1, -1, -1, -1, -1, -1, -1, -1, -1, -1, -1, -1, -1, -1, -1, -1, -1, -1, -1, -1, -1, -1, -1, -1, -1, -1, -1, -1, -1, -1, -1, -1, -1, -1, -1, -1, -1, -1, -1, -1, -1, -1, -1, -1, -1, -1, -1, -1, -1, -1, -1, -1, -1, -1, -1, -1, -1, -1, -1, -1, -1, -1, -1, -1, -1, -1, -1, -1, -1, -1, -1, -1, -1, -1, -1, -1, -1, -1, -1, -1, -1, -1, -1, -1, -1, -1, -1, -1, -1, -1, -1, -1, -1, -1, -1, -1, -1, -1, -1, -1, -1, -1, -1, -1, -1, -1, -1, -1, -1, -1, -1, -1, -1, -1, -1, -1, -1, -1, -1, -1, -1, -1, -1, -1, -1, -1, -1, -1, -1, -1, -1, -1, -1, -1, -1, -1, -1, -1, -1, -1, -1, -1, -1, -1, -1, -1, -1, -1, -1, -1, -1, -1, -1, -1, -1, -1, -1, -1, -1, -1, -1, -1, -1, -1, -1, -1, -1, -1, -1, -1, -1, -1, -1, -1, -1, -1, -1, -1, -1, -1, -1, -1, -1, -1, -1, -1, -1, -1, -1, -1, -1, -1, -1, -1, -1, -1, -1, -1, -1, -1, -1, -1, -1, -1, -1, -1, -1, -1, -1, -1, -1, -1, -1, -1, -1, -1, -1, -1, -1, -1, -1, -1, -1, -1, -1, -1, -1, -1, -1, -1, -1, -1, -1, -1, -1, -1, -1, -1, -1, -1, -1, -1, -1, -1, -1, -1, -1, -1, -1, -1, -1, -1, -1, -1, -1, -1, -1, -1, -1, -1, -1, -1, -1, -1, -1, -1, -1, -1, -1, -1, -1, -1, -1, -1, -1, -1, -1, -1, -1, -1, -1, -1, -1, -1, -1, -1, -1, -1, -1, -1, -1, -1, -1, -1, -1, -1, -1, -1, -1, -1, -1, -1, -1, -1, -1, -1, -1, -1, -1, -1, -1, -1, -1, -1, -1, -1, -1, -1, -1, -1, -1, -1, -1, -1, -1, -1, -1, -1, -1, -1, -1, -1, -1, -1, -1, -1, -1, -1, -1, -1, -1, -1, -1, -1, -1, -1, -1, -1, -1, -1, -1, -1, -1, -1, -1, -1, -1, -1, -1, -1, -1, -1, -1, -1, -1, -1, -1, -1, -1, -1, -1, -1, -1, -1, -1, -1, -1, -1, -1, -1, -1, -1, -1, -1, -1, -1, -1, -1, -1, -1, -1, -1, -1, -1, -1, -1, -1, -1, -1, -1, -1, -1, -1, -1, -1, -1, -1, -1, -1, -1, -1, -1, -1, -1, -1, -1, -1, -1, -1, -1, -1, -1, -1, -1, -1, -1, -1, -1, -1, -1, -1, -1, -1, -1, -1, -1, -1, -1, -1, -1, -1, -1, -1, -1, -1, -1, -1, -1, -1, -1, -1, -1, -1, -1, -1, -1, -1, -1, -1, -1, -1, -1, -1, -1, -1, -1, -1, -1, -1, -1, -1, -1, -1, -1, -1, -1, -1, -1, -1, -1, -1, -1, -1, -1, -1, -1, -1, -1, -1, -1, -1, -1, -1, -1, -1, -1, -1, -1, -1, -1, -1, -1, -1, -1, -1, -1, -1, -1, -1, -1, -1, -1, -1, -1, -1, -1, -1, -1, -1, -1, -1, -1, -1, -1, -1, -1, -1, -1, -1, -1, -1, -1, -1, -1, -1, -1, -1, -1, -1, -1, -1, -1, -1, -1, -1, -1, -1, -1, -1, -1, -1, -1, -1, -1, -1, -1, -1, -1, -1, -1, -1, -1, -1, -1, -1, -1, -1, -1, -1, -1, -1, -1, -1, -1, -1, -1, -1, -1, -1, -1, -1, -1, -1, -1, -1, -1, -1, -1, -1, -1, -1, -1, -1, -1, -1, -1, -1, -1, -1, -1, -1, -1, -1, -1, -1, -1, -1, -1, -1, -1, -1, -1, -1, -1, -1, -1, -1, -1, -1, -1, -1, -1, -1, -1, -1, -1, -1, -1, -1, -1, -1, -1, -1, -1, -1, -1, -1, -1, -1, -1, -1, -1, -1, -1, -1, -1, -1, -1, -1, -1, -1, -1, -1, -1, -1, -1, -1, -1, -1, -1, -1, -1, -1, -1, -1, -1, -1, -1, -1, -1, -1, -1, -1, -1, -1, -1, -1, -1, -1, -1, -1, -1, -1, -1, -1, -1, -1, -1, -1, -1, -1, -1, -1, -1, -1, -1, -1, -1, -1, -1, -1, -1, -1, -1, -1, -1, -1, -1, -1, -1, -1, -1, -1, -1, -1, -1, -1, -1, -1, -1, -1, -1, -1, -1, -1, -1, -1, -1, -1, -1, -1, -1, -1, -1, -1, -1, -1, -1, -1, -1, -1, -1, -1, -1, -1, -1, -1, -1, -1, -1, -1, -1, -1, -1, -1, -1, -1, -1, -1, -1, -1, -1, -1, -1, -1, -1, -1, -1, -1, -1, -1, -1, -1, -1, -1, -1, -1, -1, -1, -1, -1, -1, -1, -1, -1, -1, -1, -1, -1, -1, -1, -1, -1, -1, -1, -1, -1, -1, -1, -1, -1, -1, -1, -1, -1, -1, -1, -1, -1, -1, -1, -1, -1, -1, -1, -1, -1, -1, -1, -1, -1, -1, -1, -1, -1, -1, -1, -1, -1, -1, -1, -1, -1, -1, -1, -1, -1, -1, -1, -1, -1, -1, -1, -1, -1, -1, -1, -1, -1, -1, -1, -1, -1, -1, -1, -1, -1, -1, -1, -1, -1, -1, -1, -1, -1, -1, -1, -1, -1, -1, -1, -1, -1, -1, -1, -1, -1, -1, -1, -1, -1, -1, -1, -1, -1, -1, -1, -1, -1, -1, -1, -1, -1, -1, -1, -1, -1, -1, -1, -1, -1, -1, -1, -1, -1, -1, -1, -1, -1, -1, -1, -1, -1, -1, -1, -1, -1, -1, -1, -1, -1, -1, -1, -1, -1, -1, -1, -1, -1, -1, -1, -1, -1, -1, -1, -1, -1, -1, -1, -1, -1, -1, -1, -1, -1, -1, -1, -1, -1, -1, -1, -1, -1, -1, -1, -1, -1, -1, -1, -1, -1, -1, -1, -1, -1, -1, -1, -1, -1, -1, -1, -1, -1, -1, -1, -1, -1, -1, -1, -1, -1, -1, -1, -1, -1, -1, -1, -1, -1, -1, -1, -1, -1, -1, -1, -1, -1, -1, -1, -1, -1, -1, -1, -1, -1, -1, -1, -1, -1, -1, -1, -1, -1, -1, -1, -1, -1, -1, -1, -1, -1, -1, -1, -1, -1, -1, -1, -1, -1, -1, -1, -1, -1, -1, -1, -1, -1, -1, -1, -1, -1, -1, -1, -1, -1, -1, -1, -1, -1, -1, -1, -1, -1, -1, -1, -1, -1, -1, -1, -1]
``` | 49 | SST-2 test labels are all -1
I'm trying to test a model on the SST-2 task, but all the labels I see in the test set are -1.
```
>>> import nlp
>>> glue = nlp.load_dataset('glue', 'sst2')
>>> glue
{'train': Dataset(schema: {'sentence': 'string', 'label': 'int64', 'idx': 'int32'}, num_rows: 67349), 'validation': Dataset(schema: {'sentence': 'string', 'label': 'int64', 'idx': 'int32'}, num_rows: 872), 'test': Dataset(schema: {'sentence': 'string', 'label': 'int64', 'idx': 'int32'}, num_rows: 1821)}
>>> list(l['label'] for l in glue['test'])
[-1, -1, -1, -1, -1, -1, -1, -1, -1, -1, -1, -1, -1, -1, -1, -1, -1, -1, -1, -1, -1, -1, -1, -1, -1, -1, -1, -1, -1, -1, -1, -1, -1, -1, -1, -1, -1, -1, -1, -1, -1, -1, -1, -1, -1, -1, -1, -1, -1, -1, -1, -1, -1, -1, -1, -1, -1, -1, -1, -1, -1, -1, -1, -1, -1, -1, -1, -1, -1, -1, -1, -1, -1, -1, -1, -1, -1, -1, -1, -1, -1, -1, -1, -1, -1, -1, -1, -1, -1, -1, -1, -1, -1, -1, -1, -1, -1, -1, -1, -1, -1, -1, -1, -1, -1, -1, -1, -1, -1, -1, -1, -1, -1, -1, -1, -1, -1, -1, -1, -1, -1, -1, -1, -1, -1, -1, -1, -1, -1, -1, -1, -1, -1, -1, -1, -1, -1, -1, -1, -1, -1, -1, -1, -1, -1, -1, -1, -1, -1, -1, -1, -1, -1, -1, -1, -1, -1, -1, -1, -1, -1, -1, -1, -1, -1, -1, -1, -1, -1, -1, -1, -1, -1, -1, -1, -1, -1, -1, -1, -1, -1, -1, -1, -1, -1, -1, -1, -1, -1, -1, -1, -1, -1, -1, -1, -1, -1, -1, -1, -1, -1, -1, -1, -1, -1, -1, -1, -1, -1, -1, -1, -1, -1, -1, -1, -1, -1, -1, -1, -1, -1, -1, -1, -1, -1, -1, -1, -1, -1, -1, -1, -1, -1, -1, -1, -1, -1, -1, -1, -1, -1, -1, -1, -1, -1, -1, -1, -1, -1, -1, -1, -1, -1, -1, -1, -1, -1, -1, -1, -1, -1, -1, -1, -1, -1, -1, -1, -1, -1, -1, -1, -1, -1, -1, -1, -1, -1, -1, -1, -1, -1, -1, -1, -1, -1, -1, -1, -1, -1, -1, -1, -1, -1, -1, -1, -1, -1, -1, -1, -1, -1, -1, -1, -1, -1, -1, -1, -1, -1, -1, -1, -1, -1, -1, -1, -1, -1, -1, -1, -1, -1, -1, -1, -1, -1, -1, -1, -1, -1, -1, -1, -1, -1, -1, -1, -1, -1, -1, -1, -1, -1, -1, -1, -1, -1, -1, -1, -1, -1, -1, -1, -1, -1, -1, -1, -1, -1, -1, -1, -1, -1, -1, -1, -1, -1, -1, -1, -1, -1, -1, -1, -1, -1, -1, -1, -1, -1, -1, -1, -1, -1, -1, -1, -1, -1, -1, -1, -1, -1, -1, -1, -1, -1, -1, -1, -1, -1, -1, -1, -1, -1, -1, -1, -1, -1, -1, -1, -1, -1, -1, -1, -1, -1, -1, -1, -1, -1, -1, -1, -1, -1, -1, -1, -1, -1, -1, -1, -1, -1, -1, -1, -1, -1, -1, -1, -1, -1, -1, -1, -1, -1, -1, -1, -1, -1, -1, -1, -1, -1, -1, -1, -1, -1, -1, -1, -1, -1, -1, -1, -1, -1, -1, -1, -1, -1, -1, -1, -1, -1, -1, -1, -1, -1, -1, -1, -1, -1, -1, -1, -1, -1, -1, -1, -1, -1, -1, -1, -1, -1, -1, -1, -1, -1, -1, -1, -1, -1, -1, -1, -1, -1, -1, -1, -1, -1, -1, -1, -1, -1, -1, -1, -1, -1, -1, -1, -1, -1, -1, -1, -1, -1, -1, -1, -1, -1, -1, -1, -1, -1, -1, -1, -1, -1, -1, -1, -1, -1, -1, -1, -1, -1, -1, -1, -1, -1, -1, -1, -1, -1, -1, -1, -1, -1, -1, -1, -1, -1, -1, -1, -1, -1, -1, -1, -1, -1, -1, -1, -1, -1, -1, -1, -1, -1, -1, -1, -1, -1, -1, -1, -1, -1, -1, -1, -1, -1, -1, -1, -1, -1, -1, -1, -1, -1, -1, -1, -1, -1, -1, -1, -1, -1, -1, -1, -1, -1, -1, -1, -1, -1, -1, -1, -1, -1, -1, -1, -1, -1, -1, -1, -1, -1, -1, -1, -1, -1, -1, -1, -1, -1, -1, -1, -1, -1, -1, -1, -1, -1, -1, -1, -1, -1, -1, -1, -1, -1, -1, -1, -1, -1, -1, -1, -1, -1, -1, -1, -1, -1, -1, -1, -1, -1, -1, -1, -1, -1, -1, -1, -1, -1, -1, -1, -1, -1, -1, -1, -1, -1, -1, -1, -1, -1, -1, -1, -1, -1, -1, -1, -1, -1, -1, -1, -1, -1, -1, -1, -1, -1, -1, -1, -1, -1, -1, -1, -1, -1, -1, -1, -1, -1, -1, -1, -1, -1, -1, -1, -1, -1, -1, -1, -1, -1, -1, -1, -1, -1, -1, -1, -1, -1, -1, -1, -1, -1, -1, -1, -1, -1, -1, -1, -1, -1, -1, -1, -1, -1, -1, -1, -1, -1, -1, -1, -1, -1, -1, -1, -1, -1, -1, -1, -1, -1, -1, -1, -1, -1, -1, -1, -1, -1, -1, -1, -1, -1, -1, -1, -1, -1, -1, -1, -1, -1, -1, -1, -1, -1, -1, -1, -1, -1, -1, -1, -1, -1, -1, -1, -1, -1, -1, -1, -1, -1, -1, -1, -1, -1, -1, -1, -1, -1, -1, -1, -1, -1, -1, -1, -1, -1, -1, -1, -1, -1, -1, -1, -1, -1, -1, -1, -1, -1, -1, -1, -1, -1, -1, -1, -1, -1, -1, -1, -1, -1, -1, -1, -1, -1, -1, -1, -1, -1, -1, -1, -1, -1, -1, -1, -1, -1, -1, -1, -1, -1, -1, -1, -1, -1, -1, -1, -1, -1, -1, -1, -1, -1, -1, -1, -1, -1, -1, -1, -1, -1, -1, -1, -1, -1, -1, -1, -1, -1, -1, -1, -1, -1, -1, -1, -1, -1, -1, -1, -1, -1, -1, -1, -1, -1, -1, -1, -1, -1, -1, -1, -1, -1, -1, -1, -1, -1, -1, -1, -1, -1, -1, -1, -1, -1, -1, -1, -1, -1, -1, -1, -1, -1, -1, -1, -1, -1, -1, -1, -1, -1, -1, -1, -1, -1, -1, -1, -1, -1, -1, -1, -1, -1, -1, -1, -1, -1, -1, -1, -1, -1, -1, -1, -1, -1, -1, -1, -1, -1, -1, -1, -1, -1, -1, -1, -1, -1, -1, -1, -1, -1, -1, -1, -1, -1, -1, -1, -1, -1, -1, -1, -1, -1, -1, -1, -1, -1, -1, -1, -1, -1, -1, -1, -1, -1, -1, -1, -1, -1, -1, -1, -1, -1, -1, -1, -1, -1, -1, -1, -1, -1, -1, -1, -1, -1, -1, -1, -1, -1, -1, -1, -1, -1, -1, -1, -1, -1, -1, -1, -1, -1, -1, -1, -1, -1, -1, -1, -1, -1, -1, -1, -1, -1, -1, -1, -1, -1, -1, -1, -1, -1, -1, -1, -1, -1, -1, -1, -1, -1, -1, -1, -1, -1, -1, -1, -1, -1, -1, -1, -1, -1, -1, -1, -1, -1, -1, -1, -1, -1, -1, -1, -1, -1, -1, -1, -1, -1, -1, -1, -1, -1, -1, -1, -1, -1, -1, -1, -1, -1, -1, -1, -1, -1, -1, -1, -1, -1, -1, -1, -1, -1, -1, -1, -1, -1, -1, -1, -1, -1, -1, -1, -1, -1, -1, -1, -1, -1, -1, -1, -1, -1, -1, -1, -1, -1, -1, -1, -1, -1, -1, -1, -1, -1, -1, -1, -1, -1, -1, -1, -1, -1, -1, -1, -1, -1, -1, -1, -1, -1, -1, -1, -1, -1, -1, -1, -1, -1, -1, -1, -1, -1, -1, -1, -1, -1, -1, -1, -1, -1, -1, -1, -1, -1, -1, -1, -1, -1, -1, -1, -1, -1, -1, -1, -1, -1, -1, -1, -1, -1, -1, -1, -1, -1, -1, -1, -1, -1, -1, -1, -1, -1, -1, -1, -1, -1, -1, -1, -1, -1, -1, -1, -1, -1, -1, -1, -1, -1, -1, -1, -1, -1, -1, -1, -1, -1, -1, -1, -1, -1, -1, -1, -1, -1, -1, -1, -1, -1, -1, -1, -1, -1, -1, -1, -1, -1, -1, -1, -1, -1, -1, -1, -1, -1, -1, -1, -1, -1, -1, -1, -1, -1, -1, -1, -1, -1, -1, -1, -1, -1, -1, -1, -1, -1, -1, -1, -1, -1, -1, -1, -1, -1, -1, -1, -1, -1, -1, -1, -1, -1, -1, -1, -1, -1, -1, -1, -1, -1, -1, -1, -1, -1, -1, -1, -1, -1, -1, -1, -1, -1, -1, -1, -1, -1, -1, -1, -1, -1, -1, -1, -1, -1, -1, -1, -1, -1, -1, -1, -1, -1, -1, -1, -1, -1, -1, -1, -1, -1, -1, -1, -1, -1, -1, -1, -1, -1, -1, -1, -1, -1, -1, -1, -1, -1, -1, -1, -1, -1, -1, -1, -1, -1, -1, -1, -1, -1, -1, -1, -1, -1, -1, -1, -1, -1, -1, -1, -1, -1, -1, -1, -1, -1, -1, -1, -1, -1, -1, -1, -1, -1, -1, -1, -1, -1, -1, -1, -1, -1, -1, -1, -1, -1, -1, -1, -1, -1, -1, -1, -1, -1, -1, -1, -1, -1, -1, -1, -1, -1, -1, -1, -1, -1, -1, -1, -1, -1, -1, -1, -1, -1, -1, -1, -1, -1, -1, -1, -1, -1, -1, -1, -1, -1, -1, -1, -1, -1, -1, -1, -1, -1, -1, -1, -1, -1, -1, -1, -1, -1, -1, -1, -1, -1, -1, -1, -1, -1, -1, -1, -1, -1, -1, -1, -1, -1, -1, -1, -1, -1, -1, -1, -1, -1, -1, -1, -1, -1, -1, -1, -1, -1, -1, -1, -1, -1, -1, -1, -1, -1, -1, -1, -1, -1, -1, -1, -1, -1, -1, -1, -1, -1, -1, -1, -1, -1, -1, -1, -1, -1, -1, -1, -1, -1, -1, -1, -1, -1, -1, -1, -1, -1, -1, -1, -1, -1, -1, -1, -1, -1, -1, -1, -1, -1, -1, -1, -1, -1, -1, -1, -1, -1, -1, -1, -1, -1, -1, -1, -1, -1, -1, -1, -1, -1, -1, -1, -1, -1, -1, -1, -1, -1, -1, -1, -1, -1, -1, -1, -1, -1, -1, -1, -1, -1, -1, -1, -1, -1, -1, -1, -1, -1, -1, -1, -1, -1, -1, -1, -1, -1, -1, -1, -1, -1, -1, -1, -1, -1, -1, -1, -1, -1, -1, -1, -1, -1, -1, -1, -1, -1, -1, -1, -1, -1, -1, -1, -1, -1, -1, -1, -1, -1, -1, -1, -1, -1, -1, -1, -1, -1, -1, -1, -1, -1, -1, -1, -1, -1, -1, -1, -1, -1, -1, -1, -1, -1, -1, -1, -1, -1, -1, -1, -1, -1, -1, -1, -1, -1, -1, -1, -1, -1, -1, -1, -1, -1, -1, -1, -1, -1, -1, -1, -1, -1, -1, -1, -1, -1, -1, -1, -1, -1, -1, -1, -1, -1, -1, -1, -1, -1, -1, -1, -1, -1, -1, -1, -1, -1, -1, -1, -1, -1, -1, -1, -1, -1, -1, -1, -1, -1, -1, -1, -1, -1, -1, -1, -1, -1, -1, -1, -1, -1, -1, -1, -1, -1, -1, -1, -1, -1, -1, -1, -1, -1, -1, -1, -1, -1, -1, -1, -1, -1, -1, -1, -1, -1, -1, -1, -1, -1, -1, -1, -1, -1, -1, -1, -1, -1, -1, -1, -1, -1, -1, -1, -1, -1, -1, -1, -1, -1, -1, -1, -1, -1, -1, -1, -1, -1, -1, -1, -1, -1, -1, -1, -1, -1, -1, -1, -1, -1, -1, -1, -1, -1]
```
I checked both before I got to looking at issues, so that would be fine as well.
Some additional thoughts on this: Is there a specific reason why the "test" split even has a "label" column if it isn't tagged. Shouldn't there just not be any. Seems more transparent |
https://github.com/huggingface/datasets/issues/245 | SST-2 test labels are all -1 | I'm a little confused with the data size.
`sst2` dataset is referenced to `Recursive Deep Models for Semantic Compositionality Over a Sentiment Treebank` and the link of the dataset in the paper is https://nlp.stanford.edu/sentiment/index.html which is often shown in GLUE/SST2 reference.
From the original data, the standard train/dev/test splits split is 6920/872/1821 for binary classification.
Why in GLUE/SST2 the train/dev/test split is 67,349/872/1,821 ?
| I'm trying to test a model on the SST-2 task, but all the labels I see in the test set are -1.
```
>>> import nlp
>>> glue = nlp.load_dataset('glue', 'sst2')
>>> glue
{'train': Dataset(schema: {'sentence': 'string', 'label': 'int64', 'idx': 'int32'}, num_rows: 67349), 'validation': Dataset(schema: {'sentence': 'string', 'label': 'int64', 'idx': 'int32'}, num_rows: 872), 'test': Dataset(schema: {'sentence': 'string', 'label': 'int64', 'idx': 'int32'}, num_rows: 1821)}
>>> list(l['label'] for l in glue['test'])
[-1, -1, -1, -1, -1, -1, -1, -1, -1, -1, -1, -1, -1, -1, -1, -1, -1, -1, -1, -1, -1, -1, -1, -1, -1, -1, -1, -1, -1, -1, -1, -1, -1, -1, -1, -1, -1, -1, -1, -1, -1, -1, -1, -1, -1, -1, -1, -1, -1, -1, -1, -1, -1, -1, -1, -1, -1, -1, -1, -1, -1, -1, -1, -1, -1, -1, -1, -1, -1, -1, -1, -1, -1, -1, -1, -1, -1, -1, -1, -1, -1, -1, -1, -1, -1, -1, -1, -1, -1, -1, -1, -1, -1, -1, -1, -1, -1, -1, -1, -1, -1, -1, -1, -1, -1, -1, -1, -1, -1, -1, -1, -1, -1, -1, -1, -1, -1, -1, -1, -1, -1, -1, -1, -1, -1, -1, -1, -1, -1, -1, -1, -1, -1, -1, -1, -1, -1, -1, -1, -1, -1, -1, -1, -1, -1, -1, -1, -1, -1, -1, -1, -1, -1, -1, -1, -1, -1, -1, -1, -1, -1, -1, -1, -1, -1, -1, -1, -1, -1, -1, -1, -1, -1, -1, -1, -1, -1, -1, -1, -1, -1, -1, -1, -1, -1, -1, -1, -1, -1, -1, -1, -1, -1, -1, -1, -1, -1, -1, -1, -1, -1, -1, -1, -1, -1, -1, -1, -1, -1, -1, -1, -1, -1, -1, -1, -1, -1, -1, -1, -1, -1, -1, -1, -1, -1, -1, -1, -1, -1, -1, -1, -1, -1, -1, -1, -1, -1, -1, -1, -1, -1, -1, -1, -1, -1, -1, -1, -1, -1, -1, -1, -1, -1, -1, -1, -1, -1, -1, -1, -1, -1, -1, -1, -1, -1, -1, -1, -1, -1, -1, -1, -1, -1, -1, -1, -1, -1, -1, -1, -1, -1, -1, -1, -1, -1, -1, -1, -1, -1, -1, -1, -1, -1, -1, -1, -1, -1, -1, -1, -1, -1, -1, -1, -1, -1, -1, -1, -1, -1, -1, -1, -1, -1, -1, -1, -1, -1, -1, -1, -1, -1, -1, -1, -1, -1, -1, -1, -1, -1, -1, -1, -1, -1, -1, -1, -1, -1, -1, -1, -1, -1, -1, -1, -1, -1, -1, -1, -1, -1, -1, -1, -1, -1, -1, -1, -1, -1, -1, -1, -1, -1, -1, -1, -1, -1, -1, -1, -1, -1, -1, -1, -1, -1, -1, -1, -1, -1, -1, -1, -1, -1, -1, -1, -1, -1, -1, -1, -1, -1, -1, -1, -1, -1, -1, -1, -1, -1, -1, -1, -1, -1, -1, -1, -1, -1, -1, -1, -1, -1, -1, -1, -1, -1, -1, -1, -1, -1, -1, -1, -1, -1, -1, -1, -1, -1, -1, -1, -1, -1, -1, -1, -1, -1, -1, -1, -1, -1, -1, -1, -1, -1, -1, -1, -1, -1, -1, -1, -1, -1, -1, -1, -1, -1, -1, -1, -1, -1, -1, -1, -1, -1, -1, -1, -1, -1, -1, -1, -1, -1, -1, -1, -1, -1, -1, -1, -1, -1, -1, -1, -1, -1, -1, -1, -1, -1, -1, -1, -1, -1, -1, -1, -1, -1, -1, -1, -1, -1, -1, -1, -1, -1, -1, -1, -1, -1, -1, -1, -1, -1, -1, -1, -1, -1, -1, -1, -1, -1, -1, -1, -1, -1, -1, -1, -1, -1, -1, -1, -1, -1, -1, -1, -1, -1, -1, -1, -1, -1, -1, -1, -1, -1, -1, -1, -1, -1, -1, -1, -1, -1, -1, -1, -1, -1, -1, -1, -1, -1, -1, -1, -1, -1, -1, -1, -1, -1, -1, -1, -1, -1, -1, -1, -1, -1, -1, -1, -1, -1, -1, -1, -1, -1, -1, -1, -1, -1, -1, -1, -1, -1, -1, -1, -1, -1, -1, -1, -1, -1, -1, -1, -1, -1, -1, -1, -1, -1, -1, -1, -1, -1, -1, -1, -1, -1, -1, -1, -1, -1, -1, -1, -1, -1, -1, -1, -1, -1, -1, -1, -1, -1, -1, -1, -1, -1, -1, -1, -1, -1, -1, -1, -1, -1, -1, -1, -1, -1, -1, -1, -1, -1, -1, -1, -1, -1, -1, -1, -1, -1, -1, -1, -1, -1, -1, -1, -1, -1, -1, -1, -1, -1, -1, -1, -1, -1, -1, -1, -1, -1, -1, -1, -1, -1, -1, -1, -1, -1, -1, -1, -1, -1, -1, -1, -1, -1, -1, -1, -1, -1, -1, -1, -1, -1, -1, -1, -1, -1, -1, -1, -1, -1, -1, -1, -1, -1, -1, -1, -1, -1, -1, -1, -1, -1, -1, -1, -1, -1, -1, -1, -1, -1, -1, -1, -1, -1, -1, -1, -1, -1, -1, -1, -1, -1, -1, -1, -1, -1, -1, -1, -1, -1, -1, -1, -1, -1, -1, -1, -1, -1, -1, -1, -1, -1, -1, -1, -1, -1, -1, -1, -1, -1, -1, -1, -1, -1, -1, -1, -1, -1, -1, -1, -1, -1, -1, -1, -1, -1, -1, -1, -1, -1, -1, -1, -1, -1, -1, -1, -1, -1, -1, -1, -1, -1, -1, -1, -1, -1, -1, -1, -1, -1, -1, -1, -1, -1, -1, -1, -1, -1, -1, -1, -1, -1, -1, -1, -1, -1, -1, -1, -1, -1, -1, -1, -1, -1, -1, -1, -1, -1, -1, -1, -1, -1, -1, -1, -1, -1, -1, -1, -1, -1, -1, -1, -1, -1, -1, -1, -1, -1, -1, -1, -1, -1, -1, -1, -1, -1, -1, -1, -1, -1, -1, -1, -1, -1, -1, -1, -1, -1, -1, -1, -1, -1, -1, -1, -1, -1, -1, -1, -1, -1, -1, -1, -1, -1, -1, -1, -1, -1, -1, -1, -1, -1, -1, -1, -1, -1, -1, -1, -1, -1, -1, -1, -1, -1, -1, -1, -1, -1, -1, -1, -1, -1, -1, -1, -1, -1, -1, -1, -1, -1, -1, -1, -1, -1, -1, -1, -1, -1, -1, -1, -1, -1, -1, -1, -1, -1, -1, -1, -1, -1, -1, -1, -1, -1, -1, -1, -1, -1, -1, -1, -1, -1, -1, -1, -1, -1, -1, -1, -1, -1, -1, -1, -1, -1, -1, -1, -1, -1, -1, -1, -1, -1, -1, -1, -1, -1, -1, -1, -1, -1, -1, -1, -1, -1, -1, -1, -1, -1, -1, -1, -1, -1, -1, -1, -1, -1, -1, -1, -1, -1, -1, -1, -1, -1, -1, -1, -1, -1, -1, -1, -1, -1, -1, -1, -1, -1, -1, -1, -1, -1, -1, -1, -1, -1, -1, -1, -1, -1, -1, -1, -1, -1, -1, -1, -1, -1, -1, -1, -1, -1, -1, -1, -1, -1, -1, -1, -1, -1, -1, -1, -1, -1, -1, -1, -1, -1, -1, -1, -1, -1, -1, -1, -1, -1, -1, -1, -1, -1, -1, -1, -1, -1, -1, -1, -1, -1, -1, -1, -1, -1, -1, -1, -1, -1, -1, -1, -1, -1, -1, -1, -1, -1, -1, -1, -1, -1, -1, -1, -1, -1, -1, -1, -1, -1, -1, -1, -1, -1, -1, -1, -1, -1, -1, -1, -1, -1, -1, -1, -1, -1, -1, -1, -1, -1, -1, -1, -1, -1, -1, -1, -1, -1, -1, -1, -1, -1, -1, -1, -1, -1, -1, -1, -1, -1, -1, -1, -1, -1, -1, -1, -1, -1, -1, -1, -1, -1, -1, -1, -1, -1, -1, -1, -1, -1, -1, -1, -1, -1, -1, -1, -1, -1, -1, -1, -1, -1, -1, -1, -1, -1, -1, -1, -1, -1, -1, -1, -1, -1, -1, -1, -1, -1, -1, -1, -1, -1, -1, -1, -1, -1, -1, -1, -1, -1, -1, -1, -1, -1, -1, -1, -1, -1, -1, -1, -1, -1, -1, -1, -1, -1, -1, -1, -1, -1, -1, -1, -1, -1, -1, -1, -1, -1, -1, -1, -1, -1, -1, -1, -1, -1, -1, -1, -1, -1, -1, -1, -1, -1, -1, -1, -1, -1, -1, -1, -1, -1, -1, -1, -1, -1, -1, -1, -1, -1, -1, -1, -1, -1, -1, -1, -1, -1, -1, -1, -1, -1, -1, -1, -1, -1, -1, -1, -1, -1, -1, -1, -1, -1, -1, -1, -1, -1, -1, -1, -1, -1, -1, -1, -1, -1, -1, -1, -1, -1, -1, -1, -1, -1, -1, -1, -1, -1, -1, -1, -1, -1, -1, -1, -1, -1, -1, -1, -1, -1, -1, -1, -1, -1, -1, -1, -1, -1, -1, -1, -1, -1, -1, -1, -1, -1, -1, -1, -1, -1, -1, -1, -1, -1, -1, -1, -1, -1, -1, -1, -1, -1, -1, -1, -1, -1, -1, -1, -1, -1, -1, -1, -1, -1, -1, -1, -1, -1, -1, -1, -1, -1, -1, -1, -1, -1, -1, -1, -1, -1, -1, -1, -1, -1, -1, -1, -1, -1, -1, -1, -1, -1, -1, -1, -1, -1, -1, -1, -1, -1, -1, -1, -1, -1, -1, -1, -1, -1, -1, -1, -1, -1, -1, -1, -1, -1, -1, -1, -1, -1, -1, -1, -1, -1, -1, -1, -1, -1, -1, -1, -1, -1, -1, -1, -1, -1, -1, -1, -1, -1, -1, -1, -1, -1, -1, -1, -1, -1, -1, -1, -1, -1, -1, -1, -1, -1, -1, -1, -1, -1, -1, -1, -1, -1, -1, -1, -1, -1, -1, -1, -1, -1, -1, -1, -1, -1, -1, -1, -1, -1, -1, -1, -1, -1, -1, -1, -1, -1, -1, -1, -1, -1, -1, -1, -1, -1, -1, -1, -1, -1, -1, -1, -1, -1, -1, -1, -1, -1, -1, -1, -1, -1, -1, -1, -1, -1, -1, -1, -1, -1, -1, -1, -1, -1, -1, -1, -1, -1, -1, -1, -1, -1, -1, -1, -1, -1, -1, -1, -1, -1, -1, -1, -1, -1, -1, -1, -1, -1, -1, -1, -1, -1, -1, -1, -1, -1, -1, -1, -1, -1, -1, -1, -1, -1, -1, -1, -1, -1, -1, -1, -1, -1, -1, -1, -1, -1, -1, -1, -1, -1, -1, -1, -1, -1, -1, -1, -1, -1, -1, -1, -1, -1, -1, -1, -1, -1, -1, -1, -1, -1, -1, -1, -1, -1, -1, -1, -1, -1, -1, -1, -1, -1, -1, -1, -1, -1, -1, -1, -1, -1, -1, -1, -1, -1, -1, -1, -1, -1, -1, -1, -1, -1, -1, -1, -1, -1, -1, -1, -1, -1, -1, -1, -1, -1, -1, -1, -1, -1, -1, -1, -1, -1, -1, -1, -1, -1, -1, -1, -1, -1, -1, -1, -1, -1, -1, -1, -1, -1, -1, -1, -1, -1, -1, -1, -1, -1, -1, -1, -1, -1, -1, -1, -1, -1, -1, -1, -1, -1, -1, -1, -1, -1, -1, -1, -1, -1, -1, -1, -1, -1, -1, -1, -1, -1, -1, -1, -1, -1, -1, -1, -1, -1, -1, -1, -1, -1, -1, -1, -1, -1, -1, -1, -1, -1, -1, -1, -1, -1, -1, -1, -1, -1, -1, -1, -1, -1, -1, -1, -1, -1, -1, -1, -1, -1, -1, -1, -1, -1, -1, -1, -1, -1, -1, -1, -1, -1, -1, -1, -1, -1, -1, -1, -1, -1, -1, -1, -1, -1, -1, -1, -1, -1, -1, -1, -1, -1, -1, -1, -1, -1, -1, -1, -1, -1, -1, -1, -1, -1, -1, -1, -1, -1, -1, -1, -1, -1, -1, -1, -1, -1, -1, -1, -1, -1, -1, -1, -1, -1]
``` | 64 | SST-2 test labels are all -1
I'm trying to test a model on the SST-2 task, but all the labels I see in the test set are -1.
```
>>> import nlp
>>> glue = nlp.load_dataset('glue', 'sst2')
>>> glue
{'train': Dataset(schema: {'sentence': 'string', 'label': 'int64', 'idx': 'int32'}, num_rows: 67349), 'validation': Dataset(schema: {'sentence': 'string', 'label': 'int64', 'idx': 'int32'}, num_rows: 872), 'test': Dataset(schema: {'sentence': 'string', 'label': 'int64', 'idx': 'int32'}, num_rows: 1821)}
>>> list(l['label'] for l in glue['test'])
[-1, -1, -1, -1, -1, -1, -1, -1, -1, -1, -1, -1, -1, -1, -1, -1, -1, -1, -1, -1, -1, -1, -1, -1, -1, -1, -1, -1, -1, -1, -1, -1, -1, -1, -1, -1, -1, -1, -1, -1, -1, -1, -1, -1, -1, -1, -1, -1, -1, -1, -1, -1, -1, -1, -1, -1, -1, -1, -1, -1, -1, -1, -1, -1, -1, -1, -1, -1, -1, -1, -1, -1, -1, -1, -1, -1, -1, -1, -1, -1, -1, -1, -1, -1, -1, -1, -1, -1, -1, -1, -1, -1, -1, -1, -1, -1, -1, -1, -1, -1, -1, -1, -1, -1, -1, -1, -1, -1, -1, -1, -1, -1, -1, -1, -1, -1, -1, -1, -1, -1, -1, -1, -1, -1, -1, -1, -1, -1, -1, -1, -1, -1, -1, -1, -1, -1, -1, -1, -1, -1, -1, -1, -1, -1, -1, -1, -1, -1, -1, -1, -1, -1, -1, -1, -1, -1, -1, -1, -1, -1, -1, -1, -1, -1, -1, -1, -1, -1, -1, -1, -1, -1, -1, -1, -1, -1, -1, -1, -1, -1, -1, -1, -1, -1, -1, -1, -1, -1, -1, -1, -1, -1, -1, -1, -1, -1, -1, -1, -1, -1, -1, -1, -1, -1, -1, -1, -1, -1, -1, -1, -1, -1, -1, -1, -1, -1, -1, -1, -1, -1, -1, -1, -1, -1, -1, -1, -1, -1, -1, -1, -1, -1, -1, -1, -1, -1, -1, -1, -1, -1, -1, -1, -1, -1, -1, -1, -1, -1, -1, -1, -1, -1, -1, -1, -1, -1, -1, -1, -1, -1, -1, -1, -1, -1, -1, -1, -1, -1, -1, -1, -1, -1, -1, -1, -1, -1, -1, -1, -1, -1, -1, -1, -1, -1, -1, -1, -1, -1, -1, -1, -1, -1, -1, -1, -1, -1, -1, -1, -1, -1, -1, -1, -1, -1, -1, -1, -1, -1, -1, -1, -1, -1, -1, -1, -1, -1, -1, -1, -1, -1, -1, -1, -1, -1, -1, -1, -1, -1, -1, -1, -1, -1, -1, -1, -1, -1, -1, -1, -1, -1, -1, -1, -1, -1, -1, -1, -1, -1, -1, -1, -1, -1, -1, -1, -1, -1, -1, -1, -1, -1, -1, -1, -1, -1, -1, -1, -1, -1, -1, -1, -1, -1, -1, -1, -1, -1, -1, -1, -1, -1, -1, -1, -1, -1, -1, -1, -1, -1, -1, -1, -1, -1, -1, -1, -1, -1, -1, -1, -1, -1, -1, -1, -1, -1, -1, -1, -1, -1, -1, -1, -1, -1, -1, -1, -1, -1, -1, -1, -1, -1, -1, -1, -1, -1, -1, -1, -1, -1, -1, -1, -1, -1, -1, -1, -1, -1, -1, -1, -1, -1, -1, -1, -1, -1, -1, -1, -1, -1, -1, -1, -1, -1, -1, -1, -1, -1, -1, -1, -1, -1, -1, -1, -1, -1, -1, -1, -1, -1, -1, -1, -1, -1, -1, -1, -1, -1, -1, -1, -1, -1, -1, -1, -1, -1, -1, -1, -1, -1, -1, -1, -1, -1, -1, -1, -1, -1, -1, -1, -1, -1, -1, -1, -1, -1, -1, -1, -1, -1, -1, -1, -1, -1, -1, -1, -1, -1, -1, -1, -1, -1, -1, -1, -1, -1, -1, -1, -1, -1, -1, -1, -1, -1, -1, -1, -1, -1, -1, -1, -1, -1, -1, -1, -1, -1, -1, -1, -1, -1, -1, -1, -1, -1, -1, -1, -1, -1, -1, -1, -1, -1, -1, -1, -1, -1, -1, -1, -1, -1, -1, -1, -1, -1, -1, -1, -1, -1, -1, -1, -1, -1, -1, -1, -1, -1, -1, -1, -1, -1, -1, -1, -1, -1, -1, -1, -1, -1, -1, -1, -1, -1, -1, -1, -1, -1, -1, -1, -1, -1, -1, -1, -1, -1, -1, -1, -1, -1, -1, -1, -1, -1, -1, -1, -1, -1, -1, -1, -1, -1, -1, -1, -1, -1, -1, -1, -1, -1, -1, -1, -1, -1, -1, -1, -1, -1, -1, -1, -1, -1, -1, -1, -1, -1, -1, -1, -1, -1, -1, -1, -1, -1, -1, -1, -1, -1, -1, -1, -1, -1, -1, -1, -1, -1, -1, -1, -1, -1, -1, -1, -1, -1, -1, -1, -1, -1, -1, -1, -1, -1, -1, -1, -1, -1, -1, -1, -1, -1, -1, -1, -1, -1, -1, -1, -1, -1, -1, -1, -1, -1, -1, -1, -1, -1, -1, -1, -1, -1, -1, -1, -1, -1, -1, -1, -1, -1, -1, -1, -1, -1, -1, -1, -1, -1, -1, -1, -1, -1, -1, -1, -1, -1, -1, -1, -1, -1, -1, -1, -1, -1, -1, -1, -1, -1, -1, -1, -1, -1, -1, -1, -1, -1, -1, -1, -1, -1, -1, -1, -1, -1, -1, -1, -1, -1, -1, -1, -1, -1, -1, -1, -1, -1, -1, -1, -1, -1, -1, -1, -1, -1, -1, -1, -1, -1, -1, -1, -1, -1, -1, -1, -1, -1, -1, -1, -1, -1, -1, -1, -1, -1, -1, -1, -1, -1, -1, -1, -1, -1, -1, -1, -1, -1, -1, -1, -1, -1, -1, -1, -1, -1, -1, -1, -1, -1, -1, -1, -1, -1, -1, -1, -1, -1, -1, -1, -1, -1, -1, -1, -1, -1, -1, -1, -1, -1, -1, -1, -1, -1, -1, -1, -1, -1, -1, -1, -1, -1, -1, -1, -1, -1, -1, -1, -1, -1, -1, -1, -1, -1, -1, -1, -1, -1, -1, -1, -1, -1, -1, -1, -1, -1, -1, -1, -1, -1, -1, -1, -1, -1, -1, -1, -1, -1, -1, -1, -1, -1, -1, -1, -1, -1, -1, -1, -1, -1, -1, -1, -1, -1, -1, -1, -1, -1, -1, -1, -1, -1, -1, -1, -1, -1, -1, -1, -1, -1, -1, -1, -1, -1, -1, -1, -1, -1, -1, -1, -1, -1, -1, -1, -1, -1, -1, -1, -1, -1, -1, -1, -1, -1, -1, -1, -1, -1, -1, -1, -1, -1, -1, -1, -1, -1, -1, -1, -1, -1, -1, -1, -1, -1, -1, -1, -1, -1, -1, -1, -1, -1, -1, -1, -1, -1, -1, -1, -1, -1, -1, -1, -1, -1, -1, -1, -1, -1, -1, -1, -1, -1, -1, -1, -1, -1, -1, -1, -1, -1, -1, -1, -1, -1, -1, -1, -1, -1, -1, -1, -1, -1, -1, -1, -1, -1, -1, -1, -1, -1, -1, -1, -1, -1, -1, -1, -1, -1, -1, -1, -1, -1, -1, -1, -1, -1, -1, -1, -1, -1, -1, -1, -1, -1, -1, -1, -1, -1, -1, -1, -1, -1, -1, -1, -1, -1, -1, -1, -1, -1, -1, -1, -1, -1, -1, -1, -1, -1, -1, -1, -1, -1, -1, -1, -1, -1, -1, -1, -1, -1, -1, -1, -1, -1, -1, -1, -1, -1, -1, -1, -1, -1, -1, -1, -1, -1, -1, -1, -1, -1, -1, -1, -1, -1, -1, -1, -1, -1, -1, -1, -1, -1, -1, -1, -1, -1, -1, -1, -1, -1, -1, -1, -1, -1, -1, -1, -1, -1, -1, -1, -1, -1, -1, -1, -1, -1, -1, -1, -1, -1, -1, -1, -1, -1, -1, -1, -1, -1, -1, -1, -1, -1, -1, -1, -1, -1, -1, -1, -1, -1, -1, -1, -1, -1, -1, -1, -1, -1, -1, -1, -1, -1, -1, -1, -1, -1, -1, -1, -1, -1, -1, -1, -1, -1, -1, -1, -1, -1, -1, -1, -1, -1, -1, -1, -1, -1, -1, -1, -1, -1, -1, -1, -1, -1, -1, -1, -1, -1, -1, -1, -1, -1, -1, -1, -1, -1, -1, -1, -1, -1, -1, -1, -1, -1, -1, -1, -1, -1, -1, -1, -1, -1, -1, -1, -1, -1, -1, -1, -1, -1, -1, -1, -1, -1, -1, -1, -1, -1, -1, -1, -1, -1, -1, -1, -1, -1, -1, -1, -1, -1, -1, -1, -1, -1, -1, -1, -1, -1, -1, -1, -1, -1, -1, -1, -1, -1, -1, -1, -1, -1, -1, -1, -1, -1, -1, -1, -1, -1, -1, -1, -1, -1, -1, -1, -1, -1, -1, -1, -1, -1, -1, -1, -1, -1, -1, -1, -1, -1, -1, -1, -1, -1, -1, -1, -1, -1, -1, -1, -1, -1, -1, -1, -1, -1, -1, -1, -1, -1, -1, -1, -1, -1, -1, -1, -1, -1, -1, -1, -1, -1, -1, -1, -1, -1, -1, -1, -1, -1, -1, -1, -1, -1, -1, -1, -1, -1, -1, -1, -1, -1, -1, -1, -1, -1, -1, -1, -1, -1, -1, -1, -1, -1, -1, -1, -1, -1, -1, -1, -1, -1, -1, -1, -1, -1, -1, -1, -1, -1, -1, -1, -1, -1, -1, -1, -1, -1, -1, -1, -1, -1, -1, -1, -1, -1, -1, -1, -1, -1, -1, -1, -1, -1, -1, -1, -1, -1, -1, -1, -1, -1, -1, -1, -1, -1, -1, -1, -1, -1, -1, -1, -1, -1, -1, -1, -1, -1, -1, -1, -1, -1, -1, -1, -1, -1, -1, -1, -1, -1, -1, -1, -1, -1, -1, -1, -1, -1, -1, -1, -1, -1, -1, -1, -1, -1, -1, -1, -1, -1, -1, -1, -1, -1, -1, -1, -1, -1, -1, -1, -1, -1, -1, -1, -1, -1, -1, -1, -1, -1, -1, -1, -1, -1, -1, -1, -1, -1, -1, -1, -1, -1, -1, -1, -1, -1, -1, -1, -1, -1, -1, -1, -1, -1, -1, -1, -1, -1, -1, -1, -1, -1, -1, -1, -1, -1, -1, -1, -1, -1, -1, -1, -1, -1, -1, -1, -1, -1, -1, -1, -1, -1, -1, -1, -1, -1, -1, -1, -1, -1, -1, -1, -1, -1, -1, -1, -1, -1, -1, -1, -1, -1, -1, -1, -1, -1, -1, -1, -1, -1, -1, -1, -1, -1, -1, -1, -1, -1, -1, -1, -1, -1, -1, -1, -1, -1, -1, -1, -1, -1, -1, -1, -1, -1, -1, -1, -1, -1, -1, -1, -1, -1, -1, -1, -1, -1, -1, -1, -1, -1, -1, -1, -1, -1, -1, -1, -1, -1, -1, -1, -1, -1, -1, -1, -1, -1, -1, -1, -1, -1, -1, -1, -1, -1, -1, -1, -1, -1, -1, -1, -1, -1, -1, -1, -1, -1, -1, -1, -1, -1, -1, -1, -1, -1, -1, -1, -1, -1, -1, -1, -1, -1, -1, -1, -1, -1, -1, -1, -1, -1, -1, -1, -1, -1, -1, -1, -1, -1, -1, -1, -1, -1, -1, -1, -1, -1, -1, -1, -1, -1, -1, -1, -1, -1, -1, -1, -1, -1, -1, -1, -1, -1, -1, -1, -1, -1, -1, -1, -1, -1, -1, -1, -1, -1, -1, -1, -1, -1, -1, -1, -1, -1, -1, -1, -1, -1, -1, -1, -1, -1, -1, -1, -1, -1, -1, -1, -1, -1, -1, -1, -1, -1, -1, -1, -1, -1, -1, -1, -1, -1, -1, -1, -1, -1, -1, -1, -1, -1, -1, -1, -1, -1, -1, -1, -1, -1, -1, -1, -1, -1, -1, -1, -1, -1, -1, -1, -1, -1, -1, -1, -1, -1, -1, -1, -1, -1, -1, -1, -1, -1, -1, -1, -1, -1, -1, -1, -1, -1, -1, -1, -1, -1, -1, -1, -1, -1, -1, -1, -1, -1, -1]
```
I'm a little confused with the data size.
`sst2` dataset is referenced to `Recursive Deep Models for Semantic Compositionality Over a Sentiment Treebank` and the link of the dataset in the paper is https://nlp.stanford.edu/sentiment/index.html which is often shown in GLUE/SST2 reference.
From the original data, the standard train/dev/test splits split is 6920/872/1821 for binary classification.
Why in GLUE/SST2 the train/dev/test split is 67,349/872/1,821 ?
|
https://github.com/huggingface/datasets/issues/245 | SST-2 test labels are all -1 | > I'm a little confused with the data size.
> `sst2` dataset is referenced to `Recursive Deep Models for Semantic Compositionality Over a Sentiment Treebank` and the link of the dataset in the paper is https://nlp.stanford.edu/sentiment/index.html which is often shown in GLUE/SST2 reference.
> From the original data, the standard train/dev/test splits split is 6920/872/1821 for binary classification.
> Why in GLUE/SST2 the train/dev/test split is 67,349/872/1,821 ?
Have you figured out this problem? AFAIK, the original sst-2 dataset is totally different from the GLUE/sst-2. Do you think so? | I'm trying to test a model on the SST-2 task, but all the labels I see in the test set are -1.
```
>>> import nlp
>>> glue = nlp.load_dataset('glue', 'sst2')
>>> glue
{'train': Dataset(schema: {'sentence': 'string', 'label': 'int64', 'idx': 'int32'}, num_rows: 67349), 'validation': Dataset(schema: {'sentence': 'string', 'label': 'int64', 'idx': 'int32'}, num_rows: 872), 'test': Dataset(schema: {'sentence': 'string', 'label': 'int64', 'idx': 'int32'}, num_rows: 1821)}
>>> list(l['label'] for l in glue['test'])
[-1, -1, -1, -1, -1, -1, -1, -1, -1, -1, -1, -1, -1, -1, -1, -1, -1, -1, -1, -1, -1, -1, -1, -1, -1, -1, -1, -1, -1, -1, -1, -1, -1, -1, -1, -1, -1, -1, -1, -1, -1, -1, -1, -1, -1, -1, -1, -1, -1, -1, -1, -1, -1, -1, -1, -1, -1, -1, -1, -1, -1, -1, -1, -1, -1, -1, -1, -1, -1, -1, -1, -1, -1, -1, -1, -1, -1, -1, -1, -1, -1, -1, -1, -1, -1, -1, -1, -1, -1, -1, -1, -1, -1, -1, -1, -1, -1, -1, -1, -1, -1, -1, -1, -1, -1, -1, -1, -1, -1, -1, -1, -1, -1, -1, -1, -1, -1, -1, -1, -1, -1, -1, -1, -1, -1, -1, -1, -1, -1, -1, -1, -1, -1, -1, -1, -1, -1, -1, -1, -1, -1, -1, -1, -1, -1, -1, -1, -1, -1, -1, -1, -1, -1, -1, -1, -1, -1, -1, -1, -1, -1, -1, -1, -1, -1, -1, -1, -1, -1, -1, -1, -1, -1, -1, -1, -1, -1, -1, -1, -1, -1, -1, -1, -1, -1, -1, -1, -1, -1, -1, -1, -1, -1, -1, -1, -1, -1, -1, -1, -1, -1, -1, -1, -1, -1, -1, -1, -1, -1, -1, -1, -1, -1, -1, -1, -1, -1, -1, -1, -1, -1, -1, -1, -1, -1, -1, -1, -1, -1, -1, -1, -1, -1, -1, -1, -1, -1, -1, -1, -1, -1, -1, -1, -1, -1, -1, -1, -1, -1, -1, -1, -1, -1, -1, -1, -1, -1, -1, -1, -1, -1, -1, -1, -1, -1, -1, -1, -1, -1, -1, -1, -1, -1, -1, -1, -1, -1, -1, -1, -1, -1, -1, -1, -1, -1, -1, -1, -1, -1, -1, -1, -1, -1, -1, -1, -1, -1, -1, -1, -1, -1, -1, -1, -1, -1, -1, -1, -1, -1, -1, -1, -1, -1, -1, -1, -1, -1, -1, -1, -1, -1, -1, -1, -1, -1, -1, -1, -1, -1, -1, -1, -1, -1, -1, -1, -1, -1, -1, -1, -1, -1, -1, -1, -1, -1, -1, -1, -1, -1, -1, -1, -1, -1, -1, -1, -1, -1, -1, -1, -1, -1, -1, -1, -1, -1, -1, -1, -1, -1, -1, -1, -1, -1, -1, -1, -1, -1, -1, -1, -1, -1, -1, -1, -1, -1, -1, -1, -1, -1, -1, -1, -1, -1, -1, -1, -1, -1, -1, -1, -1, -1, -1, -1, -1, -1, -1, -1, -1, -1, -1, -1, -1, -1, -1, -1, -1, -1, -1, -1, -1, -1, -1, -1, -1, -1, -1, -1, -1, -1, -1, -1, -1, -1, -1, -1, -1, -1, -1, -1, -1, -1, -1, -1, -1, -1, -1, -1, -1, -1, -1, -1, -1, -1, -1, -1, -1, -1, -1, -1, -1, -1, -1, -1, -1, -1, -1, -1, -1, -1, -1, -1, -1, -1, -1, -1, -1, -1, -1, -1, -1, -1, -1, -1, -1, -1, -1, -1, -1, -1, -1, -1, -1, -1, -1, -1, -1, -1, -1, -1, -1, -1, -1, -1, -1, -1, -1, -1, -1, -1, -1, -1, -1, -1, -1, -1, -1, -1, -1, -1, -1, -1, -1, -1, -1, -1, -1, -1, -1, -1, -1, -1, -1, -1, -1, -1, -1, -1, -1, -1, -1, -1, -1, -1, -1, -1, -1, -1, -1, -1, -1, -1, -1, -1, -1, -1, -1, -1, -1, -1, -1, -1, -1, -1, -1, -1, -1, -1, -1, -1, -1, -1, -1, -1, -1, -1, -1, -1, -1, -1, -1, -1, -1, -1, -1, -1, -1, -1, -1, -1, -1, -1, -1, -1, -1, -1, -1, -1, -1, -1, -1, -1, -1, -1, -1, -1, -1, -1, -1, -1, -1, -1, -1, -1, -1, -1, -1, -1, -1, -1, -1, -1, -1, -1, -1, -1, -1, -1, -1, -1, -1, -1, -1, -1, -1, -1, -1, -1, -1, -1, -1, -1, -1, -1, -1, -1, -1, -1, -1, -1, -1, -1, -1, -1, -1, -1, -1, -1, -1, -1, -1, -1, -1, -1, -1, -1, -1, -1, -1, -1, -1, -1, -1, -1, -1, -1, -1, -1, -1, -1, -1, -1, -1, -1, -1, -1, -1, -1, -1, -1, -1, -1, -1, -1, -1, -1, -1, -1, -1, -1, -1, -1, -1, -1, -1, -1, -1, -1, -1, -1, -1, -1, -1, -1, -1, -1, -1, -1, -1, -1, -1, -1, -1, -1, -1, -1, -1, -1, -1, -1, -1, -1, -1, -1, -1, -1, -1, -1, -1, -1, -1, -1, -1, -1, -1, -1, -1, -1, -1, -1, -1, -1, -1, -1, -1, -1, -1, -1, -1, -1, -1, -1, -1, -1, -1, -1, -1, -1, -1, -1, -1, -1, -1, -1, -1, -1, -1, -1, -1, -1, -1, -1, -1, -1, -1, -1, -1, -1, -1, -1, -1, -1, -1, -1, -1, -1, -1, -1, -1, -1, -1, -1, -1, -1, -1, -1, -1, -1, -1, -1, -1, -1, -1, -1, -1, -1, -1, -1, -1, -1, -1, -1, -1, -1, -1, -1, -1, -1, -1, -1, -1, -1, -1, -1, -1, -1, -1, -1, -1, -1, -1, -1, -1, -1, -1, -1, -1, -1, -1, -1, -1, -1, -1, -1, -1, -1, -1, -1, -1, -1, -1, -1, -1, -1, -1, -1, -1, -1, -1, -1, -1, -1, -1, -1, -1, -1, -1, -1, -1, -1, -1, -1, -1, -1, -1, -1, -1, -1, -1, -1, -1, -1, -1, -1, -1, -1, -1, -1, -1, -1, -1, -1, -1, -1, -1, -1, -1, -1, -1, -1, -1, -1, -1, -1, -1, -1, -1, -1, -1, -1, -1, -1, -1, -1, -1, -1, -1, -1, -1, -1, -1, -1, -1, -1, -1, -1, -1, -1, -1, -1, -1, -1, -1, -1, -1, -1, -1, -1, -1, -1, -1, -1, -1, -1, -1, -1, -1, -1, -1, -1, -1, -1, -1, -1, -1, -1, -1, -1, -1, -1, -1, -1, -1, -1, -1, -1, -1, -1, -1, -1, -1, -1, -1, -1, -1, -1, -1, -1, -1, -1, -1, -1, -1, -1, -1, -1, -1, -1, -1, -1, -1, -1, -1, -1, -1, -1, -1, -1, -1, -1, -1, -1, -1, -1, -1, -1, -1, -1, -1, -1, -1, -1, -1, -1, -1, -1, -1, -1, -1, -1, -1, -1, -1, -1, -1, -1, -1, -1, -1, -1, -1, -1, -1, -1, -1, -1, -1, -1, -1, -1, -1, -1, -1, -1, -1, -1, -1, -1, -1, -1, -1, -1, -1, -1, -1, -1, -1, -1, -1, -1, -1, -1, -1, -1, -1, -1, -1, -1, -1, -1, -1, -1, -1, -1, -1, -1, -1, -1, -1, -1, -1, -1, -1, -1, -1, -1, -1, -1, -1, -1, -1, -1, -1, -1, -1, -1, -1, -1, -1, -1, -1, -1, -1, -1, -1, -1, -1, -1, -1, -1, -1, -1, -1, -1, -1, -1, -1, -1, -1, -1, -1, -1, -1, -1, -1, -1, -1, -1, -1, -1, -1, -1, -1, -1, -1, -1, -1, -1, -1, -1, -1, -1, -1, -1, -1, -1, -1, -1, -1, -1, -1, -1, -1, -1, -1, -1, -1, -1, -1, -1, -1, -1, -1, -1, -1, -1, -1, -1, -1, -1, -1, -1, -1, -1, -1, -1, -1, -1, -1, -1, -1, -1, -1, -1, -1, -1, -1, -1, -1, -1, -1, -1, -1, -1, -1, -1, -1, -1, -1, -1, -1, -1, -1, -1, -1, -1, -1, -1, -1, -1, -1, -1, -1, -1, -1, -1, -1, -1, -1, -1, -1, -1, -1, -1, -1, -1, -1, -1, -1, -1, -1, -1, -1, -1, -1, -1, -1, -1, -1, -1, -1, -1, -1, -1, -1, -1, -1, -1, -1, -1, -1, -1, -1, -1, -1, -1, -1, -1, -1, -1, -1, -1, -1, -1, -1, -1, -1, -1, -1, -1, -1, -1, -1, -1, -1, -1, -1, -1, -1, -1, -1, -1, -1, -1, -1, -1, -1, -1, -1, -1, -1, -1, -1, -1, -1, -1, -1, -1, -1, -1, -1, -1, -1, -1, -1, -1, -1, -1, -1, -1, -1, -1, -1, -1, -1, -1, -1, -1, -1, -1, -1, -1, -1, -1, -1, -1, -1, -1, -1, -1, -1, -1, -1, -1, -1, -1, -1, -1, -1, -1, -1, -1, -1, -1, -1, -1, -1, -1, -1, -1, -1, -1, -1, -1, -1, -1, -1, -1, -1, -1, -1, -1, -1, -1, -1, -1, -1, -1, -1, -1, -1, -1, -1, -1, -1, -1, -1, -1, -1, -1, -1, -1, -1, -1, -1, -1, -1, -1, -1, -1, -1, -1, -1, -1, -1, -1, -1, -1, -1, -1, -1, -1, -1, -1, -1, -1, -1, -1, -1, -1, -1, -1, -1, -1, -1, -1, -1, -1, -1, -1, -1, -1, -1, -1, -1, -1, -1, -1, -1, -1, -1, -1, -1, -1, -1, -1, -1, -1, -1, -1, -1, -1, -1, -1, -1, -1, -1, -1, -1, -1, -1, -1, -1, -1, -1, -1, -1, -1, -1, -1, -1, -1, -1, -1, -1, -1, -1, -1, -1, -1, -1, -1, -1, -1, -1, -1, -1, -1, -1, -1, -1, -1, -1, -1, -1, -1, -1, -1, -1, -1, -1, -1, -1, -1, -1, -1, -1, -1, -1, -1, -1, -1, -1, -1, -1, -1, -1, -1, -1, -1, -1, -1, -1, -1, -1, -1, -1, -1, -1, -1, -1, -1, -1, -1, -1, -1, -1, -1, -1, -1, -1, -1, -1, -1, -1, -1, -1, -1, -1, -1, -1, -1, -1, -1, -1, -1, -1, -1, -1, -1, -1, -1, -1, -1, -1, -1, -1, -1, -1, -1, -1, -1, -1, -1, -1, -1, -1, -1, -1, -1, -1, -1, -1, -1, -1, -1, -1, -1, -1, -1, -1, -1, -1, -1, -1, -1, -1, -1, -1, -1, -1, -1, -1, -1, -1, -1, -1, -1, -1, -1, -1, -1, -1, -1, -1, -1, -1, -1, -1, -1, -1, -1, -1, -1, -1, -1, -1, -1, -1, -1, -1, -1, -1, -1, -1, -1, -1, -1, -1, -1, -1, -1, -1, -1, -1, -1, -1, -1, -1, -1, -1, -1, -1, -1, -1, -1, -1, -1, -1, -1, -1, -1, -1, -1, -1, -1, -1, -1, -1, -1, -1, -1, -1, -1, -1, -1, -1, -1, -1, -1, -1, -1, -1, -1, -1, -1, -1, -1, -1, -1, -1, -1, -1, -1, -1, -1, -1, -1, -1, -1, -1, -1, -1, -1, -1, -1, -1, -1, -1, -1, -1, -1, -1, -1, -1, -1, -1, -1, -1, -1, -1, -1, -1, -1, -1, -1, -1, -1, -1, -1, -1, -1, -1, -1, -1, -1, -1, -1, -1, -1, -1, -1, -1, -1, -1, -1, -1, -1, -1, -1, -1, -1, -1, -1, -1, -1, -1, -1, -1, -1, -1, -1, -1, -1, -1, -1, -1, -1, -1, -1, -1, -1, -1, -1, -1, -1, -1, -1, -1, -1, -1, -1, -1, -1, -1, -1, -1, -1, -1, -1, -1, -1, -1, -1, -1, -1, -1, -1, -1, -1, -1, -1, -1, -1, -1, -1, -1, -1, -1, -1, -1, -1, -1, -1, -1, -1, -1, -1, -1, -1, -1, -1]
``` | 89 | SST-2 test labels are all -1
I'm trying to test a model on the SST-2 task, but all the labels I see in the test set are -1.
```
>>> import nlp
>>> glue = nlp.load_dataset('glue', 'sst2')
>>> glue
{'train': Dataset(schema: {'sentence': 'string', 'label': 'int64', 'idx': 'int32'}, num_rows: 67349), 'validation': Dataset(schema: {'sentence': 'string', 'label': 'int64', 'idx': 'int32'}, num_rows: 872), 'test': Dataset(schema: {'sentence': 'string', 'label': 'int64', 'idx': 'int32'}, num_rows: 1821)}
>>> list(l['label'] for l in glue['test'])
[-1, -1, -1, -1, -1, -1, -1, -1, -1, -1, -1, -1, -1, -1, -1, -1, -1, -1, -1, -1, -1, -1, -1, -1, -1, -1, -1, -1, -1, -1, -1, -1, -1, -1, -1, -1, -1, -1, -1, -1, -1, -1, -1, -1, -1, -1, -1, -1, -1, -1, -1, -1, -1, -1, -1, -1, -1, -1, -1, -1, -1, -1, -1, -1, -1, -1, -1, -1, -1, -1, -1, -1, -1, -1, -1, -1, -1, -1, -1, -1, -1, -1, -1, -1, -1, -1, -1, -1, -1, -1, -1, -1, -1, -1, -1, -1, -1, -1, -1, -1, -1, -1, -1, -1, -1, -1, -1, -1, -1, -1, -1, -1, -1, -1, -1, -1, -1, -1, -1, -1, -1, -1, -1, -1, -1, -1, -1, -1, -1, -1, -1, -1, -1, -1, -1, -1, -1, -1, -1, -1, -1, -1, -1, -1, -1, -1, -1, -1, -1, -1, -1, -1, -1, -1, -1, -1, -1, -1, -1, -1, -1, -1, -1, -1, -1, -1, -1, -1, -1, -1, -1, -1, -1, -1, -1, -1, -1, -1, -1, -1, -1, -1, -1, -1, -1, -1, -1, -1, -1, -1, -1, -1, -1, -1, -1, -1, -1, -1, -1, -1, -1, -1, -1, -1, -1, -1, -1, -1, -1, -1, -1, -1, -1, -1, -1, -1, -1, -1, -1, -1, -1, -1, -1, -1, -1, -1, -1, -1, -1, -1, -1, -1, -1, -1, -1, -1, -1, -1, -1, -1, -1, -1, -1, -1, -1, -1, -1, -1, -1, -1, -1, -1, -1, -1, -1, -1, -1, -1, -1, -1, -1, -1, -1, -1, -1, -1, -1, -1, -1, -1, -1, -1, -1, -1, -1, -1, -1, -1, -1, -1, -1, -1, -1, -1, -1, -1, -1, -1, -1, -1, -1, -1, -1, -1, -1, -1, -1, -1, -1, -1, -1, -1, -1, -1, -1, -1, -1, -1, -1, -1, -1, -1, -1, -1, -1, -1, -1, -1, -1, -1, -1, -1, -1, -1, -1, -1, -1, -1, -1, -1, -1, -1, -1, -1, -1, -1, -1, -1, -1, -1, -1, -1, -1, -1, -1, -1, -1, -1, -1, -1, -1, -1, -1, -1, -1, -1, -1, -1, -1, -1, -1, -1, -1, -1, -1, -1, -1, -1, -1, -1, -1, -1, -1, -1, -1, -1, -1, -1, -1, -1, -1, -1, -1, -1, -1, -1, -1, -1, -1, -1, -1, -1, -1, -1, -1, -1, -1, -1, -1, -1, -1, -1, -1, -1, -1, -1, -1, -1, -1, -1, -1, -1, -1, -1, -1, -1, -1, -1, -1, -1, -1, -1, -1, -1, -1, -1, -1, -1, -1, -1, -1, -1, -1, -1, -1, -1, -1, -1, -1, -1, -1, -1, -1, -1, -1, -1, -1, -1, -1, -1, -1, -1, -1, -1, -1, -1, -1, -1, -1, -1, -1, -1, -1, -1, -1, -1, -1, -1, -1, -1, -1, -1, -1, -1, -1, -1, -1, -1, -1, -1, -1, -1, -1, -1, -1, -1, -1, -1, -1, -1, -1, -1, -1, -1, -1, -1, -1, -1, -1, -1, -1, -1, -1, -1, -1, -1, -1, -1, -1, -1, -1, -1, -1, -1, -1, -1, -1, -1, -1, -1, -1, -1, -1, -1, -1, -1, -1, -1, -1, -1, -1, -1, -1, -1, -1, -1, -1, -1, -1, -1, -1, -1, -1, -1, -1, -1, -1, -1, -1, -1, -1, -1, -1, -1, -1, -1, -1, -1, -1, -1, -1, -1, -1, -1, -1, -1, -1, -1, -1, -1, -1, -1, -1, -1, -1, -1, -1, -1, -1, -1, -1, -1, -1, -1, -1, -1, -1, -1, -1, -1, -1, -1, -1, -1, -1, -1, -1, -1, -1, -1, -1, -1, -1, -1, -1, -1, -1, -1, -1, -1, -1, -1, -1, -1, -1, -1, -1, -1, -1, -1, -1, -1, -1, -1, -1, -1, -1, -1, -1, -1, -1, -1, -1, -1, -1, -1, -1, -1, -1, -1, -1, -1, -1, -1, -1, -1, -1, -1, -1, -1, -1, -1, -1, -1, -1, -1, -1, -1, -1, -1, -1, -1, -1, -1, -1, -1, -1, -1, -1, -1, -1, -1, -1, -1, -1, -1, -1, -1, -1, -1, -1, -1, -1, -1, -1, -1, -1, -1, -1, -1, -1, -1, -1, -1, -1, -1, -1, -1, -1, -1, -1, -1, -1, -1, -1, -1, -1, -1, -1, -1, -1, -1, -1, -1, -1, -1, -1, -1, -1, -1, -1, -1, -1, -1, -1, -1, -1, -1, -1, -1, -1, -1, -1, -1, -1, -1, -1, -1, -1, -1, -1, -1, -1, -1, -1, -1, -1, -1, -1, -1, -1, -1, -1, -1, -1, -1, -1, -1, -1, -1, -1, -1, -1, -1, -1, -1, -1, -1, -1, -1, -1, -1, -1, -1, -1, -1, -1, -1, -1, -1, -1, -1, -1, -1, -1, -1, -1, -1, -1, -1, -1, -1, -1, -1, -1, -1, -1, -1, -1, -1, -1, -1, -1, -1, -1, -1, -1, -1, -1, -1, -1, -1, -1, -1, -1, -1, -1, -1, -1, -1, -1, -1, -1, -1, -1, -1, -1, -1, -1, -1, -1, -1, -1, -1, -1, -1, -1, -1, -1, -1, -1, -1, -1, -1, -1, -1, -1, -1, -1, -1, -1, -1, -1, -1, -1, -1, -1, -1, -1, -1, -1, -1, -1, -1, -1, -1, -1, -1, -1, -1, -1, -1, -1, -1, -1, -1, -1, -1, -1, -1, -1, -1, -1, -1, -1, -1, -1, -1, -1, -1, -1, -1, -1, -1, -1, -1, -1, -1, -1, -1, -1, -1, -1, -1, -1, -1, -1, -1, -1, -1, -1, -1, -1, -1, -1, -1, -1, -1, -1, -1, -1, -1, -1, -1, -1, -1, -1, -1, -1, -1, -1, -1, -1, -1, -1, -1, -1, -1, -1, -1, -1, -1, -1, -1, -1, -1, -1, -1, -1, -1, -1, -1, -1, -1, -1, -1, -1, -1, -1, -1, -1, -1, -1, -1, -1, -1, -1, -1, -1, -1, -1, -1, -1, -1, -1, -1, -1, -1, -1, -1, -1, -1, -1, -1, -1, -1, -1, -1, -1, -1, -1, -1, -1, -1, -1, -1, -1, -1, -1, -1, -1, -1, -1, -1, -1, -1, -1, -1, -1, -1, -1, -1, -1, -1, -1, -1, -1, -1, -1, -1, -1, -1, -1, -1, -1, -1, -1, -1, -1, -1, -1, -1, -1, -1, -1, -1, -1, -1, -1, -1, -1, -1, -1, -1, -1, -1, -1, -1, -1, -1, -1, -1, -1, -1, -1, -1, -1, -1, -1, -1, -1, -1, -1, -1, -1, -1, -1, -1, -1, -1, -1, -1, -1, -1, -1, -1, -1, -1, -1, -1, -1, -1, -1, -1, -1, -1, -1, -1, -1, -1, -1, -1, -1, -1, -1, -1, -1, -1, -1, -1, -1, -1, -1, -1, -1, -1, -1, -1, -1, -1, -1, -1, -1, -1, -1, -1, -1, -1, -1, -1, -1, -1, -1, -1, -1, -1, -1, -1, -1, -1, -1, -1, -1, -1, -1, -1, -1, -1, -1, -1, -1, -1, -1, -1, -1, -1, -1, -1, -1, -1, -1, -1, -1, -1, -1, -1, -1, -1, -1, -1, -1, -1, -1, -1, -1, -1, -1, -1, -1, -1, -1, -1, -1, -1, -1, -1, -1, -1, -1, -1, -1, -1, -1, -1, -1, -1, -1, -1, -1, -1, -1, -1, -1, -1, -1, -1, -1, -1, -1, -1, -1, -1, -1, -1, -1, -1, -1, -1, -1, -1, -1, -1, -1, -1, -1, -1, -1, -1, -1, -1, -1, -1, -1, -1, -1, -1, -1, -1, -1, -1, -1, -1, -1, -1, -1, -1, -1, -1, -1, -1, -1, -1, -1, -1, -1, -1, -1, -1, -1, -1, -1, -1, -1, -1, -1, -1, -1, -1, -1, -1, -1, -1, -1, -1, -1, -1, -1, -1, -1, -1, -1, -1, -1, -1, -1, -1, -1, -1, -1, -1, -1, -1, -1, -1, -1, -1, -1, -1, -1, -1, -1, -1, -1, -1, -1, -1, -1, -1, -1, -1, -1, -1, -1, -1, -1, -1, -1, -1, -1, -1, -1, -1, -1, -1, -1, -1, -1, -1, -1, -1, -1, -1, -1, -1, -1, -1, -1, -1, -1, -1, -1, -1, -1, -1, -1, -1, -1, -1, -1, -1, -1, -1, -1, -1, -1, -1, -1, -1, -1, -1, -1, -1, -1, -1, -1, -1, -1, -1, -1, -1, -1, -1, -1, -1, -1, -1, -1, -1, -1, -1, -1, -1, -1, -1, -1, -1, -1, -1, -1, -1, -1, -1, -1, -1, -1, -1, -1, -1, -1, -1, -1, -1, -1, -1, -1, -1, -1, -1, -1, -1, -1, -1, -1, -1, -1, -1, -1, -1, -1, -1, -1, -1, -1, -1, -1, -1, -1, -1, -1, -1, -1, -1, -1, -1, -1, -1, -1, -1, -1, -1, -1, -1, -1, -1, -1, -1, -1, -1, -1, -1, -1, -1, -1, -1, -1, -1, -1, -1, -1, -1, -1, -1, -1, -1, -1, -1, -1, -1, -1, -1, -1, -1, -1, -1, -1, -1, -1, -1, -1, -1, -1, -1, -1, -1, -1, -1, -1, -1, -1, -1, -1, -1, -1, -1, -1, -1, -1, -1, -1, -1, -1, -1, -1, -1, -1, -1, -1, -1, -1, -1, -1, -1, -1, -1, -1, -1, -1, -1, -1, -1, -1, -1, -1, -1, -1, -1, -1, -1, -1, -1, -1, -1, -1, -1, -1, -1, -1, -1, -1, -1, -1, -1, -1, -1, -1, -1, -1, -1, -1, -1, -1, -1, -1, -1, -1, -1, -1, -1, -1, -1, -1, -1, -1, -1, -1, -1, -1, -1, -1, -1, -1, -1, -1, -1, -1, -1, -1, -1, -1, -1, -1, -1, -1, -1, -1, -1, -1, -1, -1, -1, -1, -1, -1, -1, -1, -1, -1, -1, -1, -1, -1, -1, -1, -1, -1, -1, -1, -1, -1, -1, -1, -1, -1, -1, -1, -1, -1, -1, -1, -1, -1, -1, -1, -1, -1, -1, -1, -1, -1, -1, -1, -1, -1, -1, -1, -1, -1, -1, -1, -1, -1, -1, -1, -1, -1, -1, -1, -1, -1, -1, -1, -1, -1, -1, -1, -1, -1, -1, -1, -1, -1, -1, -1, -1, -1, -1, -1, -1, -1, -1, -1, -1, -1, -1, -1, -1, -1, -1, -1, -1, -1, -1, -1, -1, -1, -1, -1, -1, -1, -1, -1, -1, -1, -1, -1, -1, -1, -1, -1, -1, -1, -1, -1, -1, -1, -1, -1, -1, -1, -1, -1, -1, -1, -1, -1, -1, -1, -1, -1, -1, -1, -1, -1, -1, -1, -1, -1, -1, -1, -1, -1, -1, -1, -1, -1, -1, -1, -1, -1, -1, -1, -1, -1, -1, -1, -1, -1, -1, -1, -1, -1, -1, -1, -1, -1, -1, -1, -1, -1, -1, -1, -1, -1, -1, -1, -1, -1, -1, -1, -1, -1, -1, -1, -1, -1, -1, -1, -1, -1, -1, -1, -1, -1, -1, -1, -1, -1, -1, -1, -1, -1, -1, -1, -1, -1, -1, -1, -1, -1, -1, -1, -1, -1, -1, -1, -1, -1, -1, -1, -1, -1, -1, -1, -1, -1, -1, -1, -1, -1, -1, -1, -1, -1, -1, -1, -1, -1, -1, -1, -1, -1]
```
> I'm a little confused with the data size.
> `sst2` dataset is referenced to `Recursive Deep Models for Semantic Compositionality Over a Sentiment Treebank` and the link of the dataset in the paper is https://nlp.stanford.edu/sentiment/index.html which is often shown in GLUE/SST2 reference.
> From the original data, the standard train/dev/test splits split is 6920/872/1821 for binary classification.
> Why in GLUE/SST2 the train/dev/test split is 67,349/872/1,821 ?
Have you figured out this problem? AFAIK, the original sst-2 dataset is totally different from the GLUE/sst-2. Do you think so? |
https://github.com/huggingface/datasets/issues/245 | SST-2 test labels are all -1 | @yc1999 Sorry, I didn't solve this conflict. In the end, I just use a local data file provided by the previous work I followed(for consistent comparison), not use `datasets` package.
Related information: https://github.com/thunlp/OpenAttack/issues/146#issuecomment-766323571 | I'm trying to test a model on the SST-2 task, but all the labels I see in the test set are -1.
```
>>> import nlp
>>> glue = nlp.load_dataset('glue', 'sst2')
>>> glue
{'train': Dataset(schema: {'sentence': 'string', 'label': 'int64', 'idx': 'int32'}, num_rows: 67349), 'validation': Dataset(schema: {'sentence': 'string', 'label': 'int64', 'idx': 'int32'}, num_rows: 872), 'test': Dataset(schema: {'sentence': 'string', 'label': 'int64', 'idx': 'int32'}, num_rows: 1821)}
>>> list(l['label'] for l in glue['test'])
[-1, -1, -1, -1, -1, -1, -1, -1, -1, -1, -1, -1, -1, -1, -1, -1, -1, -1, -1, -1, -1, -1, -1, -1, -1, -1, -1, -1, -1, -1, -1, -1, -1, -1, -1, -1, -1, -1, -1, -1, -1, -1, -1, -1, -1, -1, -1, -1, -1, -1, -1, -1, -1, -1, -1, -1, -1, -1, -1, -1, -1, -1, -1, -1, -1, -1, -1, -1, -1, -1, -1, -1, -1, -1, -1, -1, -1, -1, -1, -1, -1, -1, -1, -1, -1, -1, -1, -1, -1, -1, -1, -1, -1, -1, -1, -1, -1, -1, -1, -1, -1, -1, -1, -1, -1, -1, -1, -1, -1, -1, -1, -1, -1, -1, -1, -1, -1, -1, -1, -1, -1, -1, -1, -1, -1, -1, -1, -1, -1, -1, -1, -1, -1, -1, -1, -1, -1, -1, -1, -1, -1, -1, -1, -1, -1, -1, -1, -1, -1, -1, -1, -1, -1, -1, -1, -1, -1, -1, -1, -1, -1, -1, -1, -1, -1, -1, -1, -1, -1, -1, -1, -1, -1, -1, -1, -1, -1, -1, -1, -1, -1, -1, -1, -1, -1, -1, -1, -1, -1, -1, -1, -1, -1, -1, -1, -1, -1, -1, -1, -1, -1, -1, -1, -1, -1, -1, -1, -1, -1, -1, -1, -1, -1, -1, -1, -1, -1, -1, -1, -1, -1, -1, -1, -1, -1, -1, -1, -1, -1, -1, -1, -1, -1, -1, -1, -1, -1, -1, -1, -1, -1, -1, -1, -1, -1, -1, -1, -1, -1, -1, -1, -1, -1, -1, -1, -1, -1, -1, -1, -1, -1, -1, -1, -1, -1, -1, -1, -1, -1, -1, -1, -1, -1, -1, -1, -1, -1, -1, -1, -1, -1, -1, -1, -1, -1, -1, -1, -1, -1, -1, -1, -1, -1, -1, -1, -1, -1, -1, -1, -1, -1, -1, -1, -1, -1, -1, -1, -1, -1, -1, -1, -1, -1, -1, -1, -1, -1, -1, -1, -1, -1, -1, -1, -1, -1, -1, -1, -1, -1, -1, -1, -1, -1, -1, -1, -1, -1, -1, -1, -1, -1, -1, -1, -1, -1, -1, -1, -1, -1, -1, -1, -1, -1, -1, -1, -1, -1, -1, -1, -1, -1, -1, -1, -1, -1, -1, -1, -1, -1, -1, -1, -1, -1, -1, -1, -1, -1, -1, -1, -1, -1, -1, -1, -1, -1, -1, -1, -1, -1, -1, -1, -1, -1, -1, -1, -1, -1, -1, -1, -1, -1, -1, -1, -1, -1, -1, -1, -1, -1, -1, -1, -1, -1, -1, -1, -1, -1, -1, -1, -1, -1, -1, -1, -1, -1, -1, -1, -1, -1, -1, -1, -1, -1, -1, -1, -1, -1, -1, -1, -1, -1, -1, -1, -1, -1, -1, -1, -1, -1, -1, -1, -1, -1, -1, -1, -1, -1, -1, -1, -1, -1, -1, -1, -1, -1, -1, -1, -1, -1, -1, -1, -1, -1, -1, -1, -1, -1, -1, -1, -1, -1, -1, -1, -1, -1, -1, -1, -1, -1, -1, -1, -1, -1, -1, -1, -1, -1, -1, -1, -1, -1, -1, -1, -1, -1, -1, -1, -1, -1, -1, -1, -1, -1, -1, -1, -1, -1, -1, -1, -1, -1, -1, -1, -1, -1, -1, -1, -1, -1, -1, -1, -1, -1, -1, -1, -1, -1, -1, -1, -1, -1, -1, -1, -1, -1, -1, -1, -1, -1, -1, -1, -1, -1, -1, -1, -1, -1, -1, -1, -1, -1, -1, -1, -1, -1, -1, -1, -1, -1, -1, -1, -1, -1, -1, -1, -1, -1, -1, -1, -1, -1, -1, -1, -1, -1, -1, -1, -1, -1, -1, -1, -1, -1, -1, -1, -1, -1, -1, -1, -1, -1, -1, -1, -1, -1, -1, -1, -1, -1, -1, -1, -1, -1, -1, -1, -1, -1, -1, -1, -1, -1, -1, -1, -1, -1, -1, -1, -1, -1, -1, -1, -1, -1, -1, -1, -1, -1, -1, -1, -1, -1, -1, -1, -1, -1, -1, -1, -1, -1, -1, -1, -1, -1, -1, -1, -1, -1, -1, -1, -1, -1, -1, -1, -1, -1, -1, -1, -1, -1, -1, -1, -1, -1, -1, -1, -1, -1, -1, -1, -1, -1, -1, -1, -1, -1, -1, -1, -1, -1, -1, -1, -1, -1, -1, -1, -1, -1, -1, -1, -1, -1, -1, -1, -1, -1, -1, -1, -1, -1, -1, -1, -1, -1, -1, -1, -1, -1, -1, -1, -1, -1, -1, -1, -1, -1, -1, -1, -1, -1, -1, -1, -1, -1, -1, -1, -1, -1, -1, -1, -1, -1, -1, -1, -1, -1, -1, -1, -1, -1, -1, -1, -1, -1, -1, -1, -1, -1, -1, -1, -1, -1, -1, -1, -1, -1, -1, -1, -1, -1, -1, -1, -1, -1, -1, -1, -1, -1, -1, -1, -1, -1, -1, -1, -1, -1, -1, -1, -1, -1, -1, -1, -1, -1, -1, -1, -1, -1, -1, -1, -1, -1, -1, -1, -1, -1, -1, -1, -1, -1, -1, -1, -1, -1, -1, -1, -1, -1, -1, -1, -1, -1, -1, -1, -1, -1, -1, -1, -1, -1, -1, -1, -1, -1, -1, -1, -1, -1, -1, -1, -1, -1, -1, -1, -1, -1, -1, -1, -1, -1, -1, -1, -1, -1, -1, -1, -1, -1, -1, -1, -1, -1, -1, -1, -1, -1, -1, -1, -1, -1, -1, -1, -1, -1, -1, -1, -1, -1, -1, -1, -1, -1, -1, -1, -1, -1, -1, -1, -1, -1, -1, -1, -1, -1, -1, -1, -1, -1, -1, -1, -1, -1, -1, -1, -1, -1, -1, -1, -1, -1, -1, -1, -1, -1, -1, -1, -1, -1, -1, -1, -1, -1, -1, -1, -1, -1, -1, -1, -1, -1, -1, -1, -1, -1, -1, -1, -1, -1, -1, -1, -1, -1, -1, -1, -1, -1, -1, -1, -1, -1, -1, -1, -1, -1, -1, -1, -1, -1, -1, -1, -1, -1, -1, -1, -1, -1, -1, -1, -1, -1, -1, -1, -1, -1, -1, -1, -1, -1, -1, -1, -1, -1, -1, -1, -1, -1, -1, -1, -1, -1, -1, -1, -1, -1, -1, -1, -1, -1, -1, -1, -1, -1, -1, -1, -1, -1, -1, -1, -1, -1, -1, -1, -1, -1, -1, -1, -1, -1, -1, -1, -1, -1, -1, -1, -1, -1, -1, -1, -1, -1, -1, -1, -1, -1, -1, -1, -1, -1, -1, -1, -1, -1, -1, -1, -1, -1, -1, -1, -1, -1, -1, -1, -1, -1, -1, -1, -1, -1, -1, -1, -1, -1, -1, -1, -1, -1, -1, -1, -1, -1, -1, -1, -1, -1, -1, -1, -1, -1, -1, -1, -1, -1, -1, -1, -1, -1, -1, -1, -1, -1, -1, -1, -1, -1, -1, -1, -1, -1, -1, -1, -1, -1, -1, -1, -1, -1, -1, -1, -1, -1, -1, -1, -1, -1, -1, -1, -1, -1, -1, -1, -1, -1, -1, -1, -1, -1, -1, -1, -1, -1, -1, -1, -1, -1, -1, -1, -1, -1, -1, -1, -1, -1, -1, -1, -1, -1, -1, -1, -1, -1, -1, -1, -1, -1, -1, -1, -1, -1, -1, -1, -1, -1, -1, -1, -1, -1, -1, -1, -1, -1, -1, -1, -1, -1, -1, -1, -1, -1, -1, -1, -1, -1, -1, -1, -1, -1, -1, -1, -1, -1, -1, -1, -1, -1, -1, -1, -1, -1, -1, -1, -1, -1, -1, -1, -1, -1, -1, -1, -1, -1, -1, -1, -1, -1, -1, -1, -1, -1, -1, -1, -1, -1, -1, -1, -1, -1, -1, -1, -1, -1, -1, -1, -1, -1, -1, -1, -1, -1, -1, -1, -1, -1, -1, -1, -1, -1, -1, -1, -1, -1, -1, -1, -1, -1, -1, -1, -1, -1, -1, -1, -1, -1, -1, -1, -1, -1, -1, -1, -1, -1, -1, -1, -1, -1, -1, -1, -1, -1, -1, -1, -1, -1, -1, -1, -1, -1, -1, -1, -1, -1, -1, -1, -1, -1, -1, -1, -1, -1, -1, -1, -1, -1, -1, -1, -1, -1, -1, -1, -1, -1, -1, -1, -1, -1, -1, -1, -1, -1, -1, -1, -1, -1, -1, -1, -1, -1, -1, -1, -1, -1, -1, -1, -1, -1, -1, -1, -1, -1, -1, -1, -1, -1, -1, -1, -1, -1, -1, -1, -1, -1, -1, -1, -1, -1, -1, -1, -1, -1, -1, -1, -1, -1, -1, -1, -1, -1, -1, -1, -1, -1, -1, -1, -1, -1, -1, -1, -1, -1, -1, -1, -1, -1, -1, -1, -1, -1, -1, -1, -1, -1, -1, -1, -1, -1, -1, -1, -1, -1, -1, -1, -1, -1, -1, -1, -1, -1, -1, -1, -1, -1, -1, -1, -1, -1, -1, -1, -1, -1, -1, -1, -1, -1, -1, -1, -1, -1, -1, -1, -1, -1, -1, -1, -1, -1, -1, -1, -1, -1, -1, -1, -1, -1, -1, -1, -1, -1, -1, -1, -1, -1, -1, -1, -1, -1, -1, -1, -1, -1, -1, -1, -1, -1, -1, -1, -1, -1, -1, -1, -1, -1, -1, -1, -1, -1, -1, -1, -1, -1, -1, -1, -1, -1, -1, -1, -1, -1, -1, -1, -1, -1, -1, -1, -1, -1, -1, -1, -1, -1, -1, -1, -1, -1, -1, -1, -1, -1, -1, -1, -1, -1, -1, -1, -1, -1, -1, -1, -1, -1, -1, -1, -1, -1, -1, -1, -1, -1, -1, -1, -1, -1, -1, -1, -1, -1, -1, -1, -1, -1, -1, -1, -1, -1, -1, -1, -1, -1, -1, -1, -1, -1, -1, -1, -1, -1, -1, -1, -1, -1, -1, -1, -1, -1, -1, -1, -1, -1, -1, -1, -1, -1, -1, -1, -1, -1, -1, -1, -1, -1, -1, -1, -1, -1, -1, -1, -1, -1, -1, -1, -1, -1, -1, -1, -1, -1, -1, -1, -1, -1, -1, -1, -1, -1, -1, -1, -1, -1, -1, -1, -1, -1, -1, -1, -1, -1, -1, -1, -1, -1, -1, -1, -1, -1, -1, -1, -1, -1, -1, -1, -1, -1, -1, -1, -1, -1, -1, -1, -1, -1, -1, -1, -1, -1, -1, -1, -1, -1, -1, -1, -1, -1, -1, -1, -1, -1, -1, -1, -1, -1, -1, -1, -1, -1, -1, -1, -1, -1, -1, -1, -1, -1, -1, -1, -1, -1, -1, -1, -1, -1, -1, -1, -1, -1, -1, -1, -1, -1, -1, -1, -1, -1, -1, -1, -1, -1, -1, -1, -1, -1, -1, -1, -1, -1, -1, -1, -1, -1, -1, -1, -1, -1, -1, -1, -1, -1, -1, -1, -1, -1, -1, -1, -1, -1, -1, -1, -1, -1, -1, -1, -1, -1, -1, -1, -1, -1, -1, -1, -1, -1, -1, -1, -1, -1, -1, -1, -1, -1, -1, -1, -1, -1, -1, -1, -1, -1, -1, -1, -1, -1, -1, -1, -1, -1, -1, -1, -1, -1, -1, -1, -1, -1, -1, -1, -1, -1, -1, -1, -1, -1, -1, -1, -1, -1, -1, -1, -1, -1, -1, -1, -1, -1, -1, -1, -1, -1, -1, -1, -1, -1, -1, -1, -1, -1, -1, -1, -1, -1, -1, -1, -1, -1, -1, -1, -1, -1, -1, -1, -1, -1, -1, -1, -1, -1]
``` | 33 | SST-2 test labels are all -1
I'm trying to test a model on the SST-2 task, but all the labels I see in the test set are -1.
```
>>> import nlp
>>> glue = nlp.load_dataset('glue', 'sst2')
>>> glue
{'train': Dataset(schema: {'sentence': 'string', 'label': 'int64', 'idx': 'int32'}, num_rows: 67349), 'validation': Dataset(schema: {'sentence': 'string', 'label': 'int64', 'idx': 'int32'}, num_rows: 872), 'test': Dataset(schema: {'sentence': 'string', 'label': 'int64', 'idx': 'int32'}, num_rows: 1821)}
>>> list(l['label'] for l in glue['test'])
[-1, -1, -1, -1, -1, -1, -1, -1, -1, -1, -1, -1, -1, -1, -1, -1, -1, -1, -1, -1, -1, -1, -1, -1, -1, -1, -1, -1, -1, -1, -1, -1, -1, -1, -1, -1, -1, -1, -1, -1, -1, -1, -1, -1, -1, -1, -1, -1, -1, -1, -1, -1, -1, -1, -1, -1, -1, -1, -1, -1, -1, -1, -1, -1, -1, -1, -1, -1, -1, -1, -1, -1, -1, -1, -1, -1, -1, -1, -1, -1, -1, -1, -1, -1, -1, -1, -1, -1, -1, -1, -1, -1, -1, -1, -1, -1, -1, -1, -1, -1, -1, -1, -1, -1, -1, -1, -1, -1, -1, -1, -1, -1, -1, -1, -1, -1, -1, -1, -1, -1, -1, -1, -1, -1, -1, -1, -1, -1, -1, -1, -1, -1, -1, -1, -1, -1, -1, -1, -1, -1, -1, -1, -1, -1, -1, -1, -1, -1, -1, -1, -1, -1, -1, -1, -1, -1, -1, -1, -1, -1, -1, -1, -1, -1, -1, -1, -1, -1, -1, -1, -1, -1, -1, -1, -1, -1, -1, -1, -1, -1, -1, -1, -1, -1, -1, -1, -1, -1, -1, -1, -1, -1, -1, -1, -1, -1, -1, -1, -1, -1, -1, -1, -1, -1, -1, -1, -1, -1, -1, -1, -1, -1, -1, -1, -1, -1, -1, -1, -1, -1, -1, -1, -1, -1, -1, -1, -1, -1, -1, -1, -1, -1, -1, -1, -1, -1, -1, -1, -1, -1, -1, -1, -1, -1, -1, -1, -1, -1, -1, -1, -1, -1, -1, -1, -1, -1, -1, -1, -1, -1, -1, -1, -1, -1, -1, -1, -1, -1, -1, -1, -1, -1, -1, -1, -1, -1, -1, -1, -1, -1, -1, -1, -1, -1, -1, -1, -1, -1, -1, -1, -1, -1, -1, -1, -1, -1, -1, -1, -1, -1, -1, -1, -1, -1, -1, -1, -1, -1, -1, -1, -1, -1, -1, -1, -1, -1, -1, -1, -1, -1, -1, -1, -1, -1, -1, -1, -1, -1, -1, -1, -1, -1, -1, -1, -1, -1, -1, -1, -1, -1, -1, -1, -1, -1, -1, -1, -1, -1, -1, -1, -1, -1, -1, -1, -1, -1, -1, -1, -1, -1, -1, -1, -1, -1, -1, -1, -1, -1, -1, -1, -1, -1, -1, -1, -1, -1, -1, -1, -1, -1, -1, -1, -1, -1, -1, -1, -1, -1, -1, -1, -1, -1, -1, -1, -1, -1, -1, -1, -1, -1, -1, -1, -1, -1, -1, -1, -1, -1, -1, -1, -1, -1, -1, -1, -1, -1, -1, -1, -1, -1, -1, -1, -1, -1, -1, -1, -1, -1, -1, -1, -1, -1, -1, -1, -1, -1, -1, -1, -1, -1, -1, -1, -1, -1, -1, -1, -1, -1, -1, -1, -1, -1, -1, -1, -1, -1, -1, -1, -1, -1, -1, -1, -1, -1, -1, -1, -1, -1, -1, -1, -1, -1, -1, -1, -1, -1, -1, -1, -1, -1, -1, -1, -1, -1, -1, -1, -1, -1, -1, -1, -1, -1, -1, -1, -1, -1, -1, -1, -1, -1, -1, -1, -1, -1, -1, -1, -1, -1, -1, -1, -1, -1, -1, -1, -1, -1, -1, -1, -1, -1, -1, -1, -1, -1, -1, -1, -1, -1, -1, -1, -1, -1, -1, -1, -1, -1, -1, -1, -1, -1, -1, -1, -1, -1, -1, -1, -1, -1, -1, -1, -1, -1, -1, -1, -1, -1, -1, -1, -1, -1, -1, -1, -1, -1, -1, -1, -1, -1, -1, -1, -1, -1, -1, -1, -1, -1, -1, -1, -1, -1, -1, -1, -1, -1, -1, -1, -1, -1, -1, -1, -1, -1, -1, -1, -1, -1, -1, -1, -1, -1, -1, -1, -1, -1, -1, -1, -1, -1, -1, -1, -1, -1, -1, -1, -1, -1, -1, -1, -1, -1, -1, -1, -1, -1, -1, -1, -1, -1, -1, -1, -1, -1, -1, -1, -1, -1, -1, -1, -1, -1, -1, -1, -1, -1, -1, -1, -1, -1, -1, -1, -1, -1, -1, -1, -1, -1, -1, -1, -1, -1, -1, -1, -1, -1, -1, -1, -1, -1, -1, -1, -1, -1, -1, -1, -1, -1, -1, -1, -1, -1, -1, -1, -1, -1, -1, -1, -1, -1, -1, -1, -1, -1, -1, -1, -1, -1, -1, -1, -1, -1, -1, -1, -1, -1, -1, -1, -1, -1, -1, -1, -1, -1, -1, -1, -1, -1, -1, -1, -1, -1, -1, -1, -1, -1, -1, -1, -1, -1, -1, -1, -1, -1, -1, -1, -1, -1, -1, -1, -1, -1, -1, -1, -1, -1, -1, -1, -1, -1, -1, -1, -1, -1, -1, -1, -1, -1, -1, -1, -1, -1, -1, -1, -1, -1, -1, -1, -1, -1, -1, -1, -1, -1, -1, -1, -1, -1, -1, -1, -1, -1, -1, -1, -1, -1, -1, -1, -1, -1, -1, -1, -1, -1, -1, -1, -1, -1, -1, -1, -1, -1, -1, -1, -1, -1, -1, -1, -1, -1, -1, -1, -1, -1, -1, -1, -1, -1, -1, -1, -1, -1, -1, -1, -1, -1, -1, -1, -1, -1, -1, -1, -1, -1, -1, -1, -1, -1, -1, -1, -1, -1, -1, -1, -1, -1, -1, -1, -1, -1, -1, -1, -1, -1, -1, -1, -1, -1, -1, -1, -1, -1, -1, -1, -1, -1, -1, -1, -1, -1, -1, -1, -1, -1, -1, -1, -1, -1, -1, -1, -1, -1, -1, -1, -1, -1, -1, -1, -1, -1, -1, -1, -1, -1, -1, -1, -1, -1, -1, -1, -1, -1, -1, -1, -1, -1, -1, -1, -1, -1, -1, -1, -1, -1, -1, -1, -1, -1, -1, -1, -1, -1, -1, -1, -1, -1, -1, -1, -1, -1, -1, -1, -1, -1, -1, -1, -1, -1, -1, -1, -1, -1, -1, -1, -1, -1, -1, -1, -1, -1, -1, -1, -1, -1, -1, -1, -1, -1, -1, -1, -1, -1, -1, -1, -1, -1, -1, -1, -1, -1, -1, -1, -1, -1, -1, -1, -1, -1, -1, -1, -1, -1, -1, -1, -1, -1, -1, -1, -1, -1, -1, -1, -1, -1, -1, -1, -1, -1, -1, -1, -1, -1, -1, -1, -1, -1, -1, -1, -1, -1, -1, -1, -1, -1, -1, -1, -1, -1, -1, -1, -1, -1, -1, -1, -1, -1, -1, -1, -1, -1, -1, -1, -1, -1, -1, -1, -1, -1, -1, -1, -1, -1, -1, -1, -1, -1, -1, -1, -1, -1, -1, -1, -1, -1, -1, -1, -1, -1, -1, -1, -1, -1, -1, -1, -1, -1, -1, -1, -1, -1, -1, -1, -1, -1, -1, -1, -1, -1, -1, -1, -1, -1, -1, -1, -1, -1, -1, -1, -1, -1, -1, -1, -1, -1, -1, -1, -1, -1, -1, -1, -1, -1, -1, -1, -1, -1, -1, -1, -1, -1, -1, -1, -1, -1, -1, -1, -1, -1, -1, -1, -1, -1, -1, -1, -1, -1, -1, -1, -1, -1, -1, -1, -1, -1, -1, -1, -1, -1, -1, -1, -1, -1, -1, -1, -1, -1, -1, -1, -1, -1, -1, -1, -1, -1, -1, -1, -1, -1, -1, -1, -1, -1, -1, -1, -1, -1, -1, -1, -1, -1, -1, -1, -1, -1, -1, -1, -1, -1, -1, -1, -1, -1, -1, -1, -1, -1, -1, -1, -1, -1, -1, -1, -1, -1, -1, -1, -1, -1, -1, -1, -1, -1, -1, -1, -1, -1, -1, -1, -1, -1, -1, -1, -1, -1, -1, -1, -1, -1, -1, -1, -1, -1, -1, -1, -1, -1, -1, -1, -1, -1, -1, -1, -1, -1, -1, -1, -1, -1, -1, -1, -1, -1, -1, -1, -1, -1, -1, -1, -1, -1, -1, -1, -1, -1, -1, -1, -1, -1, -1, -1, -1, -1, -1, -1, -1, -1, -1, -1, -1, -1, -1, -1, -1, -1, -1, -1, -1, -1, -1, -1, -1, -1, -1, -1, -1, -1, -1, -1, -1, -1, -1, -1, -1, -1, -1, -1, -1, -1, -1, -1, -1, -1, -1, -1, -1, -1, -1, -1, -1, -1, -1, -1, -1, -1, -1, -1, -1, -1, -1, -1, -1, -1, -1, -1, -1, -1, -1, -1, -1, -1, -1, -1, -1, -1, -1, -1, -1, -1, -1, -1, -1, -1, -1, -1, -1, -1, -1, -1, -1, -1, -1, -1, -1, -1, -1, -1, -1, -1, -1, -1, -1, -1, -1, -1, -1, -1, -1, -1, -1, -1, -1, -1, -1, -1, -1, -1, -1, -1, -1, -1, -1, -1, -1, -1, -1, -1, -1, -1, -1, -1, -1, -1, -1, -1, -1, -1, -1, -1, -1, -1, -1, -1, -1, -1, -1, -1, -1, -1, -1, -1, -1, -1, -1, -1, -1, -1, -1, -1, -1, -1, -1, -1, -1, -1, -1, -1, -1, -1, -1, -1, -1, -1, -1, -1, -1, -1, -1, -1, -1, -1, -1, -1, -1, -1, -1, -1, -1, -1, -1, -1, -1, -1, -1, -1, -1, -1, -1, -1, -1, -1, -1, -1, -1, -1, -1, -1, -1, -1, -1, -1, -1, -1, -1, -1, -1, -1, -1, -1, -1, -1, -1, -1, -1, -1, -1, -1, -1, -1, -1, -1, -1, -1, -1, -1, -1, -1, -1, -1, -1, -1, -1, -1, -1, -1, -1, -1, -1, -1, -1, -1, -1, -1, -1, -1, -1, -1, -1, -1, -1, -1, -1, -1, -1, -1, -1, -1, -1, -1, -1, -1, -1, -1, -1, -1, -1, -1, -1, -1, -1, -1, -1, -1, -1, -1, -1, -1, -1, -1, -1, -1, -1, -1, -1, -1, -1, -1, -1, -1, -1, -1, -1, -1, -1, -1, -1, -1, -1, -1, -1, -1, -1, -1, -1, -1, -1, -1, -1, -1, -1, -1, -1, -1, -1, -1, -1, -1, -1, -1, -1, -1, -1, -1, -1, -1, -1, -1, -1, -1, -1, -1, -1, -1, -1, -1, -1, -1, -1, -1, -1, -1, -1, -1, -1, -1, -1, -1, -1, -1, -1, -1, -1, -1, -1, -1, -1, -1, -1, -1, -1, -1, -1, -1, -1, -1, -1, -1, -1, -1, -1, -1, -1, -1, -1, -1, -1, -1, -1, -1, -1, -1, -1, -1, -1, -1, -1, -1, -1, -1, -1, -1, -1, -1, -1, -1, -1, -1, -1, -1, -1, -1, -1, -1, -1, -1, -1, -1, -1, -1, -1, -1, -1, -1, -1, -1, -1, -1, -1, -1, -1, -1, -1, -1, -1, -1, -1, -1, -1, -1, -1, -1, -1, -1, -1, -1, -1, -1, -1, -1, -1, -1, -1, -1, -1, -1, -1, -1, -1, -1, -1, -1, -1, -1, -1, -1, -1, -1, -1, -1, -1, -1, -1, -1, -1, -1, -1, -1, -1, -1, -1, -1, -1, -1, -1, -1, -1, -1, -1, -1, -1, -1, -1, -1, -1, -1, -1, -1, -1, -1, -1, -1, -1, -1, -1, -1, -1, -1, -1, -1, -1, -1, -1, -1, -1, -1, -1, -1, -1, -1, -1, -1, -1, -1, -1, -1, -1, -1, -1, -1, -1, -1, -1, -1, -1, -1, -1, -1, -1, -1, -1, -1, -1, -1, -1, -1, -1, -1, -1, -1, -1, -1, -1, -1, -1, -1, -1, -1, -1, -1, -1, -1, -1, -1, -1]
```
@yc1999 Sorry, I didn't solve this conflict. In the end, I just use a local data file provided by the previous work I followed(for consistent comparison), not use `datasets` package.
Related information: https://github.com/thunlp/OpenAttack/issues/146#issuecomment-766323571 |
https://github.com/huggingface/datasets/issues/245 | SST-2 test labels are all -1 | @yc1999 I find that the original SST-2 dataset (6,920/872/1,821) can be loaded from https://huggingface.co/datasets/gpt3mix/sst2 or built with SST data and the scripts in https://github.com/prrao87/fine-grained-sentiment/tree/master/data/sst.
The GLUE/SST-2 dataset (67,349/872/1,821) should be a completely different version.
| I'm trying to test a model on the SST-2 task, but all the labels I see in the test set are -1.
```
>>> import nlp
>>> glue = nlp.load_dataset('glue', 'sst2')
>>> glue
{'train': Dataset(schema: {'sentence': 'string', 'label': 'int64', 'idx': 'int32'}, num_rows: 67349), 'validation': Dataset(schema: {'sentence': 'string', 'label': 'int64', 'idx': 'int32'}, num_rows: 872), 'test': Dataset(schema: {'sentence': 'string', 'label': 'int64', 'idx': 'int32'}, num_rows: 1821)}
>>> list(l['label'] for l in glue['test'])
[-1, -1, -1, -1, -1, -1, -1, -1, -1, -1, -1, -1, -1, -1, -1, -1, -1, -1, -1, -1, -1, -1, -1, -1, -1, -1, -1, -1, -1, -1, -1, -1, -1, -1, -1, -1, -1, -1, -1, -1, -1, -1, -1, -1, -1, -1, -1, -1, -1, -1, -1, -1, -1, -1, -1, -1, -1, -1, -1, -1, -1, -1, -1, -1, -1, -1, -1, -1, -1, -1, -1, -1, -1, -1, -1, -1, -1, -1, -1, -1, -1, -1, -1, -1, -1, -1, -1, -1, -1, -1, -1, -1, -1, -1, -1, -1, -1, -1, -1, -1, -1, -1, -1, -1, -1, -1, -1, -1, -1, -1, -1, -1, -1, -1, -1, -1, -1, -1, -1, -1, -1, -1, -1, -1, -1, -1, -1, -1, -1, -1, -1, -1, -1, -1, -1, -1, -1, -1, -1, -1, -1, -1, -1, -1, -1, -1, -1, -1, -1, -1, -1, -1, -1, -1, -1, -1, -1, -1, -1, -1, -1, -1, -1, -1, -1, -1, -1, -1, -1, -1, -1, -1, -1, -1, -1, -1, -1, -1, -1, -1, -1, -1, -1, -1, -1, -1, -1, -1, -1, -1, -1, -1, -1, -1, -1, -1, -1, -1, -1, -1, -1, -1, -1, -1, -1, -1, -1, -1, -1, -1, -1, -1, -1, -1, -1, -1, -1, -1, -1, -1, -1, -1, -1, -1, -1, -1, -1, -1, -1, -1, -1, -1, -1, -1, -1, -1, -1, -1, -1, -1, -1, -1, -1, -1, -1, -1, -1, -1, -1, -1, -1, -1, -1, -1, -1, -1, -1, -1, -1, -1, -1, -1, -1, -1, -1, -1, -1, -1, -1, -1, -1, -1, -1, -1, -1, -1, -1, -1, -1, -1, -1, -1, -1, -1, -1, -1, -1, -1, -1, -1, -1, -1, -1, -1, -1, -1, -1, -1, -1, -1, -1, -1, -1, -1, -1, -1, -1, -1, -1, -1, -1, -1, -1, -1, -1, -1, -1, -1, -1, -1, -1, -1, -1, -1, -1, -1, -1, -1, -1, -1, -1, -1, -1, -1, -1, -1, -1, -1, -1, -1, -1, -1, -1, -1, -1, -1, -1, -1, -1, -1, -1, -1, -1, -1, -1, -1, -1, -1, -1, -1, -1, -1, -1, -1, -1, -1, -1, -1, -1, -1, -1, -1, -1, -1, -1, -1, -1, -1, -1, -1, -1, -1, -1, -1, -1, -1, -1, -1, -1, -1, -1, -1, -1, -1, -1, -1, -1, -1, -1, -1, -1, -1, -1, -1, -1, -1, -1, -1, -1, -1, -1, -1, -1, -1, -1, -1, -1, -1, -1, -1, -1, -1, -1, -1, -1, -1, -1, -1, -1, -1, -1, -1, -1, -1, -1, -1, -1, -1, -1, -1, -1, -1, -1, -1, -1, -1, -1, -1, -1, -1, -1, -1, -1, -1, -1, -1, -1, -1, -1, -1, -1, -1, -1, -1, -1, -1, -1, -1, -1, -1, -1, -1, -1, -1, -1, -1, -1, -1, -1, -1, -1, -1, -1, -1, -1, -1, -1, -1, -1, -1, -1, -1, -1, -1, -1, -1, -1, -1, -1, -1, -1, -1, -1, -1, -1, -1, -1, -1, -1, -1, -1, -1, -1, -1, -1, -1, -1, -1, -1, -1, -1, -1, -1, -1, -1, -1, -1, -1, -1, -1, -1, -1, -1, -1, -1, -1, -1, -1, -1, -1, -1, -1, -1, -1, -1, -1, -1, -1, -1, -1, -1, -1, -1, -1, -1, -1, -1, -1, -1, -1, -1, -1, -1, -1, -1, -1, -1, -1, -1, -1, -1, -1, -1, -1, -1, -1, -1, -1, -1, -1, -1, -1, -1, -1, -1, -1, -1, -1, -1, -1, -1, -1, -1, -1, -1, -1, -1, -1, -1, -1, -1, -1, -1, -1, -1, -1, -1, -1, -1, -1, -1, -1, -1, -1, -1, -1, -1, -1, -1, -1, -1, -1, -1, -1, -1, -1, -1, -1, -1, -1, -1, -1, -1, -1, -1, -1, -1, -1, -1, -1, -1, -1, -1, -1, -1, -1, -1, -1, -1, -1, -1, -1, -1, -1, -1, -1, -1, -1, -1, -1, -1, -1, -1, -1, -1, -1, -1, -1, -1, -1, -1, -1, -1, -1, -1, -1, -1, -1, -1, -1, -1, -1, -1, -1, -1, -1, -1, -1, -1, -1, -1, -1, -1, -1, -1, -1, -1, -1, -1, -1, -1, -1, -1, -1, -1, -1, -1, -1, -1, -1, -1, -1, -1, -1, -1, -1, -1, -1, -1, -1, -1, -1, -1, -1, -1, -1, -1, -1, -1, -1, -1, -1, -1, -1, -1, -1, -1, -1, -1, -1, -1, -1, -1, -1, -1, -1, -1, -1, -1, -1, -1, -1, -1, -1, -1, -1, -1, -1, -1, -1, -1, -1, -1, -1, -1, -1, -1, -1, -1, -1, -1, -1, -1, -1, -1, -1, -1, -1, -1, -1, -1, -1, -1, -1, -1, -1, -1, -1, -1, -1, -1, -1, -1, -1, -1, -1, -1, -1, -1, -1, -1, -1, -1, -1, -1, -1, -1, -1, -1, -1, -1, -1, -1, -1, -1, -1, -1, -1, -1, -1, -1, -1, -1, -1, -1, -1, -1, -1, -1, -1, -1, -1, -1, -1, -1, -1, -1, -1, -1, -1, -1, -1, -1, -1, -1, -1, -1, -1, -1, -1, -1, -1, -1, -1, -1, -1, -1, -1, -1, -1, -1, -1, -1, -1, -1, -1, -1, -1, -1, -1, -1, -1, -1, -1, -1, -1, -1, -1, -1, -1, -1, -1, -1, -1, -1, -1, -1, -1, -1, -1, -1, -1, -1, -1, -1, -1, -1, -1, -1, -1, -1, -1, -1, -1, -1, -1, -1, -1, -1, -1, -1, -1, -1, -1, -1, -1, -1, -1, -1, -1, -1, -1, -1, -1, -1, -1, -1, -1, -1, -1, -1, -1, -1, -1, -1, -1, -1, -1, -1, -1, -1, -1, -1, -1, -1, -1, -1, -1, -1, -1, -1, -1, -1, -1, -1, -1, -1, -1, -1, -1, -1, -1, -1, -1, -1, -1, -1, -1, -1, -1, -1, -1, -1, -1, -1, -1, -1, -1, -1, -1, -1, -1, -1, -1, -1, -1, -1, -1, -1, -1, -1, -1, -1, -1, -1, -1, -1, -1, -1, -1, -1, -1, -1, -1, -1, -1, -1, -1, -1, -1, -1, -1, -1, -1, -1, -1, -1, -1, -1, -1, -1, -1, -1, -1, -1, -1, -1, -1, -1, -1, -1, -1, -1, -1, -1, -1, -1, -1, -1, -1, -1, -1, -1, -1, -1, -1, -1, -1, -1, -1, -1, -1, -1, -1, -1, -1, -1, -1, -1, -1, -1, -1, -1, -1, -1, -1, -1, -1, -1, -1, -1, -1, -1, -1, -1, -1, -1, -1, -1, -1, -1, -1, -1, -1, -1, -1, -1, -1, -1, -1, -1, -1, -1, -1, -1, -1, -1, -1, -1, -1, -1, -1, -1, -1, -1, -1, -1, -1, -1, -1, -1, -1, -1, -1, -1, -1, -1, -1, -1, -1, -1, -1, -1, -1, -1, -1, -1, -1, -1, -1, -1, -1, -1, -1, -1, -1, -1, -1, -1, -1, -1, -1, -1, -1, -1, -1, -1, -1, -1, -1, -1, -1, -1, -1, -1, -1, -1, -1, -1, -1, -1, -1, -1, -1, -1, -1, -1, -1, -1, -1, -1, -1, -1, -1, -1, -1, -1, -1, -1, -1, -1, -1, -1, -1, -1, -1, -1, -1, -1, -1, -1, -1, -1, -1, -1, -1, -1, -1, -1, -1, -1, -1, -1, -1, -1, -1, -1, -1, -1, -1, -1, -1, -1, -1, -1, -1, -1, -1, -1, -1, -1, -1, -1, -1, -1, -1, -1, -1, -1, -1, -1, -1, -1, -1, -1, -1, -1, -1, -1, -1, -1, -1, -1, -1, -1, -1, -1, -1, -1, -1, -1, -1, -1, -1, -1, -1, -1, -1, -1, -1, -1, -1, -1, -1, -1, -1, -1, -1, -1, -1, -1, -1, -1, -1, -1, -1, -1, -1, -1, -1, -1, -1, -1, -1, -1, -1, -1, -1, -1, -1, -1, -1, -1, -1, -1, -1, -1, -1, -1, -1, -1, -1, -1, -1, -1, -1, -1, -1, -1, -1, -1, -1, -1, -1, -1, -1, -1, -1, -1, -1, -1, -1, -1, -1, -1, -1, -1, -1, -1, -1, -1, -1, -1, -1, -1, -1, -1, -1, -1, -1, -1, -1, -1, -1, -1, -1, -1, -1, -1, -1, -1, -1, -1, -1, -1, -1, -1, -1, -1, -1, -1, -1, -1, -1, -1, -1, -1, -1, -1, -1, -1, -1, -1, -1, -1, -1, -1, -1, -1, -1, -1, -1, -1, -1, -1, -1, -1, -1, -1, -1, -1, -1, -1, -1, -1, -1, -1, -1, -1, -1, -1, -1, -1, -1, -1, -1, -1, -1, -1, -1, -1, -1, -1, -1, -1, -1, -1, -1, -1, -1, -1, -1, -1, -1, -1, -1, -1, -1, -1, -1, -1, -1, -1, -1, -1, -1, -1, -1, -1, -1, -1, -1, -1, -1, -1, -1, -1, -1, -1, -1, -1, -1, -1, -1, -1, -1, -1, -1, -1, -1, -1, -1, -1, -1, -1, -1, -1, -1, -1, -1, -1, -1, -1, -1, -1, -1, -1, -1, -1, -1, -1, -1, -1, -1, -1, -1, -1, -1, -1, -1, -1, -1, -1, -1, -1, -1, -1, -1, -1, -1, -1, -1, -1, -1, -1, -1, -1, -1, -1, -1, -1, -1, -1, -1, -1, -1, -1, -1, -1, -1, -1, -1, -1, -1, -1, -1, -1, -1, -1, -1, -1, -1, -1, -1, -1, -1, -1, -1, -1, -1, -1, -1, -1, -1, -1, -1, -1, -1, -1, -1, -1, -1, -1, -1, -1, -1, -1, -1, -1, -1, -1, -1, -1, -1, -1, -1, -1, -1, -1, -1, -1, -1, -1, -1, -1, -1, -1, -1, -1, -1, -1, -1, -1, -1, -1, -1, -1, -1, -1, -1, -1, -1, -1, -1, -1, -1, -1, -1, -1, -1, -1, -1, -1, -1, -1, -1, -1, -1, -1, -1, -1, -1, -1, -1, -1, -1, -1, -1, -1, -1, -1, -1, -1, -1, -1, -1, -1, -1, -1, -1, -1, -1, -1, -1, -1, -1, -1, -1, -1, -1, -1, -1, -1, -1, -1, -1, -1, -1, -1, -1, -1, -1, -1, -1, -1, -1, -1, -1, -1, -1, -1, -1, -1, -1, -1, -1, -1, -1, -1, -1, -1, -1, -1, -1, -1, -1, -1, -1, -1, -1, -1, -1, -1, -1, -1, -1, -1, -1, -1, -1, -1, -1, -1, -1, -1, -1, -1, -1, -1, -1, -1, -1, -1, -1, -1, -1, -1, -1, -1, -1, -1, -1, -1, -1, -1, -1, -1, -1, -1, -1, -1, -1, -1, -1, -1, -1, -1, -1, -1, -1, -1, -1, -1, -1, -1, -1, -1, -1, -1, -1, -1, -1, -1, -1, -1, -1, -1, -1, -1, -1, -1, -1, -1, -1, -1, -1, -1, -1, -1, -1, -1, -1, -1, -1, -1, -1, -1, -1, -1, -1, -1, -1, -1, -1, -1, -1, -1, -1, -1, -1, -1, -1, -1, -1, -1, -1, -1, -1, -1, -1, -1, -1, -1, -1, -1, -1, -1, -1, -1, -1, -1, -1, -1, -1, -1, -1, -1, -1, -1, -1, -1, -1, -1, -1, -1, -1, -1, -1, -1, -1, -1]
``` | 34 | SST-2 test labels are all -1
I'm trying to test a model on the SST-2 task, but all the labels I see in the test set are -1.
```
>>> import nlp
>>> glue = nlp.load_dataset('glue', 'sst2')
>>> glue
{'train': Dataset(schema: {'sentence': 'string', 'label': 'int64', 'idx': 'int32'}, num_rows: 67349), 'validation': Dataset(schema: {'sentence': 'string', 'label': 'int64', 'idx': 'int32'}, num_rows: 872), 'test': Dataset(schema: {'sentence': 'string', 'label': 'int64', 'idx': 'int32'}, num_rows: 1821)}
>>> list(l['label'] for l in glue['test'])
[-1, -1, -1, -1, -1, -1, -1, -1, -1, -1, -1, -1, -1, -1, -1, -1, -1, -1, -1, -1, -1, -1, -1, -1, -1, -1, -1, -1, -1, -1, -1, -1, -1, -1, -1, -1, -1, -1, -1, -1, -1, -1, -1, -1, -1, -1, -1, -1, -1, -1, -1, -1, -1, -1, -1, -1, -1, -1, -1, -1, -1, -1, -1, -1, -1, -1, -1, -1, -1, -1, -1, -1, -1, -1, -1, -1, -1, -1, -1, -1, -1, -1, -1, -1, -1, -1, -1, -1, -1, -1, -1, -1, -1, -1, -1, -1, -1, -1, -1, -1, -1, -1, -1, -1, -1, -1, -1, -1, -1, -1, -1, -1, -1, -1, -1, -1, -1, -1, -1, -1, -1, -1, -1, -1, -1, -1, -1, -1, -1, -1, -1, -1, -1, -1, -1, -1, -1, -1, -1, -1, -1, -1, -1, -1, -1, -1, -1, -1, -1, -1, -1, -1, -1, -1, -1, -1, -1, -1, -1, -1, -1, -1, -1, -1, -1, -1, -1, -1, -1, -1, -1, -1, -1, -1, -1, -1, -1, -1, -1, -1, -1, -1, -1, -1, -1, -1, -1, -1, -1, -1, -1, -1, -1, -1, -1, -1, -1, -1, -1, -1, -1, -1, -1, -1, -1, -1, -1, -1, -1, -1, -1, -1, -1, -1, -1, -1, -1, -1, -1, -1, -1, -1, -1, -1, -1, -1, -1, -1, -1, -1, -1, -1, -1, -1, -1, -1, -1, -1, -1, -1, -1, -1, -1, -1, -1, -1, -1, -1, -1, -1, -1, -1, -1, -1, -1, -1, -1, -1, -1, -1, -1, -1, -1, -1, -1, -1, -1, -1, -1, -1, -1, -1, -1, -1, -1, -1, -1, -1, -1, -1, -1, -1, -1, -1, -1, -1, -1, -1, -1, -1, -1, -1, -1, -1, -1, -1, -1, -1, -1, -1, -1, -1, -1, -1, -1, -1, -1, -1, -1, -1, -1, -1, -1, -1, -1, -1, -1, -1, -1, -1, -1, -1, -1, -1, -1, -1, -1, -1, -1, -1, -1, -1, -1, -1, -1, -1, -1, -1, -1, -1, -1, -1, -1, -1, -1, -1, -1, -1, -1, -1, -1, -1, -1, -1, -1, -1, -1, -1, -1, -1, -1, -1, -1, -1, -1, -1, -1, -1, -1, -1, -1, -1, -1, -1, -1, -1, -1, -1, -1, -1, -1, -1, -1, -1, -1, -1, -1, -1, -1, -1, -1, -1, -1, -1, -1, -1, -1, -1, -1, -1, -1, -1, -1, -1, -1, -1, -1, -1, -1, -1, -1, -1, -1, -1, -1, -1, -1, -1, -1, -1, -1, -1, -1, -1, -1, -1, -1, -1, -1, -1, -1, -1, -1, -1, -1, -1, -1, -1, -1, -1, -1, -1, -1, -1, -1, -1, -1, -1, -1, -1, -1, -1, -1, -1, -1, -1, -1, -1, -1, -1, -1, -1, -1, -1, -1, -1, -1, -1, -1, -1, -1, -1, -1, -1, -1, -1, -1, -1, -1, -1, -1, -1, -1, -1, -1, -1, -1, -1, -1, -1, -1, -1, -1, -1, -1, -1, -1, -1, -1, -1, -1, -1, -1, -1, -1, -1, -1, -1, -1, -1, -1, -1, -1, -1, -1, -1, -1, -1, -1, -1, -1, -1, -1, -1, -1, -1, -1, -1, -1, -1, -1, -1, -1, -1, -1, -1, -1, -1, -1, -1, -1, -1, -1, -1, -1, -1, -1, -1, -1, -1, -1, -1, -1, -1, -1, -1, -1, -1, -1, -1, -1, -1, -1, -1, -1, -1, -1, -1, -1, -1, -1, -1, -1, -1, -1, -1, -1, -1, -1, -1, -1, -1, -1, -1, -1, -1, -1, -1, -1, -1, -1, -1, -1, -1, -1, -1, -1, -1, -1, -1, -1, -1, -1, -1, -1, -1, -1, -1, -1, -1, -1, -1, -1, -1, -1, -1, -1, -1, -1, -1, -1, -1, -1, -1, -1, -1, -1, -1, -1, -1, -1, -1, -1, -1, -1, -1, -1, -1, -1, -1, -1, -1, -1, -1, -1, -1, -1, -1, -1, -1, -1, -1, -1, -1, -1, -1, -1, -1, -1, -1, -1, -1, -1, -1, -1, -1, -1, -1, -1, -1, -1, -1, -1, -1, -1, -1, -1, -1, -1, -1, -1, -1, -1, -1, -1, -1, -1, -1, -1, -1, -1, -1, -1, -1, -1, -1, -1, -1, -1, -1, -1, -1, -1, -1, -1, -1, -1, -1, -1, -1, -1, -1, -1, -1, -1, -1, -1, -1, -1, -1, -1, -1, -1, -1, -1, -1, -1, -1, -1, -1, -1, -1, -1, -1, -1, -1, -1, -1, -1, -1, -1, -1, -1, -1, -1, -1, -1, -1, -1, -1, -1, -1, -1, -1, -1, -1, -1, -1, -1, -1, -1, -1, -1, -1, -1, -1, -1, -1, -1, -1, -1, -1, -1, -1, -1, -1, -1, -1, -1, -1, -1, -1, -1, -1, -1, -1, -1, -1, -1, -1, -1, -1, -1, -1, -1, -1, -1, -1, -1, -1, -1, -1, -1, -1, -1, -1, -1, -1, -1, -1, -1, -1, -1, -1, -1, -1, -1, -1, -1, -1, -1, -1, -1, -1, -1, -1, -1, -1, -1, -1, -1, -1, -1, -1, -1, -1, -1, -1, -1, -1, -1, -1, -1, -1, -1, -1, -1, -1, -1, -1, -1, -1, -1, -1, -1, -1, -1, -1, -1, -1, -1, -1, -1, -1, -1, -1, -1, -1, -1, -1, -1, -1, -1, -1, -1, -1, -1, -1, -1, -1, -1, -1, -1, -1, -1, -1, -1, -1, -1, -1, -1, -1, -1, -1, -1, -1, -1, -1, -1, -1, -1, -1, -1, -1, -1, -1, -1, -1, -1, -1, -1, -1, -1, -1, -1, -1, -1, -1, -1, -1, -1, -1, -1, -1, -1, -1, -1, -1, -1, -1, -1, -1, -1, -1, -1, -1, -1, -1, -1, -1, -1, -1, -1, -1, -1, -1, -1, -1, -1, -1, -1, -1, -1, -1, -1, -1, -1, -1, -1, -1, -1, -1, -1, -1, -1, -1, -1, -1, -1, -1, -1, -1, -1, -1, -1, -1, -1, -1, -1, -1, -1, -1, -1, -1, -1, -1, -1, -1, -1, -1, -1, -1, -1, -1, -1, -1, -1, -1, -1, -1, -1, -1, -1, -1, -1, -1, -1, -1, -1, -1, -1, -1, -1, -1, -1, -1, -1, -1, -1, -1, -1, -1, -1, -1, -1, -1, -1, -1, -1, -1, -1, -1, -1, -1, -1, -1, -1, -1, -1, -1, -1, -1, -1, -1, -1, -1, -1, -1, -1, -1, -1, -1, -1, -1, -1, -1, -1, -1, -1, -1, -1, -1, -1, -1, -1, -1, -1, -1, -1, -1, -1, -1, -1, -1, -1, -1, -1, -1, -1, -1, -1, -1, -1, -1, -1, -1, -1, -1, -1, -1, -1, -1, -1, -1, -1, -1, -1, -1, -1, -1, -1, -1, -1, -1, -1, -1, -1, -1, -1, -1, -1, -1, -1, -1, -1, -1, -1, -1, -1, -1, -1, -1, -1, -1, -1, -1, -1, -1, -1, -1, -1, -1, -1, -1, -1, -1, -1, -1, -1, -1, -1, -1, -1, -1, -1, -1, -1, -1, -1, -1, -1, -1, -1, -1, -1, -1, -1, -1, -1, -1, -1, -1, -1, -1, -1, -1, -1, -1, -1, -1, -1, -1, -1, -1, -1, -1, -1, -1, -1, -1, -1, -1, -1, -1, -1, -1, -1, -1, -1, -1, -1, -1, -1, -1, -1, -1, -1, -1, -1, -1, -1, -1, -1, -1, -1, -1, -1, -1, -1, -1, -1, -1, -1, -1, -1, -1, -1, -1, -1, -1, -1, -1, -1, -1, -1, -1, -1, -1, -1, -1, -1, -1, -1, -1, -1, -1, -1, -1, -1, -1, -1, -1, -1, -1, -1, -1, -1, -1, -1, -1, -1, -1, -1, -1, -1, -1, -1, -1, -1, -1, -1, -1, -1, -1, -1, -1, -1, -1, -1, -1, -1, -1, -1, -1, -1, -1, -1, -1, -1, -1, -1, -1, -1, -1, -1, -1, -1, -1, -1, -1, -1, -1, -1, -1, -1, -1, -1, -1, -1, -1, -1, -1, -1, -1, -1, -1, -1, -1, -1, -1, -1, -1, -1, -1, -1, -1, -1, -1, -1, -1, -1, -1, -1, -1, -1, -1, -1, -1, -1, -1, -1, -1, -1, -1, -1, -1, -1, -1, -1, -1, -1, -1, -1, -1, -1, -1, -1, -1, -1, -1, -1, -1, -1, -1, -1, -1, -1, -1, -1, -1, -1, -1, -1, -1, -1, -1, -1, -1, -1, -1, -1, -1, -1, -1, -1, -1, -1, -1, -1, -1, -1, -1, -1, -1, -1, -1, -1, -1, -1, -1, -1, -1, -1, -1, -1, -1, -1, -1, -1, -1, -1, -1, -1, -1, -1, -1, -1, -1, -1, -1, -1, -1, -1, -1, -1, -1, -1, -1, -1, -1, -1, -1, -1, -1, -1, -1, -1, -1, -1, -1, -1, -1, -1, -1, -1, -1, -1, -1, -1, -1, -1, -1, -1, -1, -1, -1, -1, -1, -1, -1, -1, -1, -1, -1, -1, -1, -1, -1, -1, -1, -1, -1, -1, -1, -1, -1, -1, -1, -1, -1, -1, -1, -1, -1, -1, -1, -1, -1, -1, -1, -1, -1, -1, -1, -1, -1, -1, -1, -1, -1, -1, -1, -1, -1, -1, -1, -1, -1, -1, -1, -1, -1, -1, -1, -1, -1, -1, -1, -1, -1, -1, -1, -1, -1, -1, -1, -1, -1, -1, -1, -1, -1, -1, -1, -1, -1, -1, -1, -1, -1, -1, -1, -1, -1, -1, -1, -1, -1, -1, -1, -1, -1, -1, -1, -1, -1, -1, -1, -1, -1, -1, -1, -1, -1, -1, -1, -1, -1, -1, -1, -1, -1, -1, -1, -1, -1, -1, -1, -1, -1, -1, -1, -1, -1, -1, -1, -1, -1, -1, -1, -1, -1, -1, -1, -1, -1, -1, -1, -1, -1, -1, -1, -1, -1, -1, -1, -1, -1, -1, -1, -1, -1, -1, -1, -1, -1, -1, -1, -1, -1, -1, -1, -1, -1, -1, -1, -1, -1, -1, -1, -1, -1, -1, -1, -1, -1, -1, -1, -1, -1, -1, -1, -1, -1, -1, -1, -1, -1, -1, -1, -1, -1, -1, -1, -1, -1, -1, -1, -1, -1, -1, -1, -1, -1, -1, -1, -1, -1, -1, -1, -1, -1, -1, -1, -1, -1, -1, -1, -1, -1, -1, -1, -1, -1, -1, -1, -1, -1, -1, -1, -1, -1, -1, -1, -1, -1, -1, -1, -1, -1, -1, -1, -1, -1, -1, -1, -1, -1, -1, -1, -1, -1, -1, -1, -1, -1, -1, -1, -1, -1, -1, -1, -1, -1, -1, -1, -1, -1, -1, -1, -1, -1, -1, -1, -1, -1, -1, -1, -1, -1, -1, -1, -1, -1, -1, -1, -1, -1, -1, -1, -1, -1, -1, -1, -1, -1, -1, -1, -1, -1, -1, -1, -1, -1, -1, -1, -1, -1, -1, -1, -1, -1, -1, -1, -1, -1, -1, -1, -1, -1, -1, -1, -1, -1, -1, -1, -1, -1, -1, -1, -1, -1, -1, -1, -1, -1, -1, -1, -1, -1, -1, -1, -1, -1, -1, -1, -1, -1, -1, -1, -1, -1, -1, -1, -1, -1, -1, -1, -1, -1, -1, -1, -1, -1, -1, -1, -1, -1, -1, -1, -1]
```
@yc1999 I find that the original SST-2 dataset (6,920/872/1,821) can be loaded from https://huggingface.co/datasets/gpt3mix/sst2 or built with SST data and the scripts in https://github.com/prrao87/fine-grained-sentiment/tree/master/data/sst.
The GLUE/SST-2 dataset (67,349/872/1,821) should be a completely different version.
|
https://github.com/huggingface/datasets/issues/242 | UnicodeDecodeError when downloading GLUE-MNLI | It should be good now, thanks for noticing and fixing it ! I would say that it was because you are on windows but not 100% sure | When I run
```python
dataset = nlp.load_dataset('glue', 'mnli')
```
I get an encoding error (could it be because I'm using Windows?) :
```python
# Lots of error log lines later...
~\Miniconda3\envs\nlp\lib\site-packages\tqdm\std.py in __iter__(self)
1128 try:
-> 1129 for obj in iterable:
1130 yield obj
~\Miniconda3\envs\nlp\lib\site-packages\nlp\datasets\glue\5256cc2368cf84497abef1f1a5f66648522d5854b225162148cb8fc78a5a91cc\glue.py in _generate_examples(self, data_file, split, mrpc_files)
529
--> 530 for n, row in enumerate(reader):
531 if is_cola_non_test:
~\Miniconda3\envs\nlp\lib\csv.py in __next__(self)
110 self.fieldnames
--> 111 row = next(self.reader)
112 self.line_num = self.reader.line_num
~\Miniconda3\envs\nlp\lib\encodings\cp1252.py in decode(self, input, final)
22 def decode(self, input, final=False):
---> 23 return codecs.charmap_decode(input,self.errors,decoding_table)[0]
24
UnicodeDecodeError: 'charmap' codec can't decode byte 0x9d in position 6744: character maps to <undefined>
```
Anyway this can be solved by specifying to decode in UTF when reading the csv file. I am proposing a PR if that's okay. | 27 | UnicodeDecodeError when downloading GLUE-MNLI
When I run
```python
dataset = nlp.load_dataset('glue', 'mnli')
```
I get an encoding error (could it be because I'm using Windows?) :
```python
# Lots of error log lines later...
~\Miniconda3\envs\nlp\lib\site-packages\tqdm\std.py in __iter__(self)
1128 try:
-> 1129 for obj in iterable:
1130 yield obj
~\Miniconda3\envs\nlp\lib\site-packages\nlp\datasets\glue\5256cc2368cf84497abef1f1a5f66648522d5854b225162148cb8fc78a5a91cc\glue.py in _generate_examples(self, data_file, split, mrpc_files)
529
--> 530 for n, row in enumerate(reader):
531 if is_cola_non_test:
~\Miniconda3\envs\nlp\lib\csv.py in __next__(self)
110 self.fieldnames
--> 111 row = next(self.reader)
112 self.line_num = self.reader.line_num
~\Miniconda3\envs\nlp\lib\encodings\cp1252.py in decode(self, input, final)
22 def decode(self, input, final=False):
---> 23 return codecs.charmap_decode(input,self.errors,decoding_table)[0]
24
UnicodeDecodeError: 'charmap' codec can't decode byte 0x9d in position 6744: character maps to <undefined>
```
Anyway this can be solved by specifying to decode in UTF when reading the csv file. I am proposing a PR if that's okay.
It should be good now, thanks for noticing and fixing it ! I would say that it was because you are on windows but not 100% sure |
https://github.com/huggingface/datasets/issues/242 | UnicodeDecodeError when downloading GLUE-MNLI | On Windows Python supports Unicode almost everywhere, but one of the notable exceptions is open() where it uses the locale encoding schema. So platform independent python scripts would always set the encoding='utf-8' in calls to open explicitly.
In the meantime: since Python 3.7 Windows users can set the default encoding for everything including open() to Unicode by setting this environment variable: set PYTHONUTF8=1 (details can be found in [PEP 540](https://www.python.org/dev/peps/pep-0540/))
For me this fixed the problem described by the OP. | When I run
```python
dataset = nlp.load_dataset('glue', 'mnli')
```
I get an encoding error (could it be because I'm using Windows?) :
```python
# Lots of error log lines later...
~\Miniconda3\envs\nlp\lib\site-packages\tqdm\std.py in __iter__(self)
1128 try:
-> 1129 for obj in iterable:
1130 yield obj
~\Miniconda3\envs\nlp\lib\site-packages\nlp\datasets\glue\5256cc2368cf84497abef1f1a5f66648522d5854b225162148cb8fc78a5a91cc\glue.py in _generate_examples(self, data_file, split, mrpc_files)
529
--> 530 for n, row in enumerate(reader):
531 if is_cola_non_test:
~\Miniconda3\envs\nlp\lib\csv.py in __next__(self)
110 self.fieldnames
--> 111 row = next(self.reader)
112 self.line_num = self.reader.line_num
~\Miniconda3\envs\nlp\lib\encodings\cp1252.py in decode(self, input, final)
22 def decode(self, input, final=False):
---> 23 return codecs.charmap_decode(input,self.errors,decoding_table)[0]
24
UnicodeDecodeError: 'charmap' codec can't decode byte 0x9d in position 6744: character maps to <undefined>
```
Anyway this can be solved by specifying to decode in UTF when reading the csv file. I am proposing a PR if that's okay. | 80 | UnicodeDecodeError when downloading GLUE-MNLI
When I run
```python
dataset = nlp.load_dataset('glue', 'mnli')
```
I get an encoding error (could it be because I'm using Windows?) :
```python
# Lots of error log lines later...
~\Miniconda3\envs\nlp\lib\site-packages\tqdm\std.py in __iter__(self)
1128 try:
-> 1129 for obj in iterable:
1130 yield obj
~\Miniconda3\envs\nlp\lib\site-packages\nlp\datasets\glue\5256cc2368cf84497abef1f1a5f66648522d5854b225162148cb8fc78a5a91cc\glue.py in _generate_examples(self, data_file, split, mrpc_files)
529
--> 530 for n, row in enumerate(reader):
531 if is_cola_non_test:
~\Miniconda3\envs\nlp\lib\csv.py in __next__(self)
110 self.fieldnames
--> 111 row = next(self.reader)
112 self.line_num = self.reader.line_num
~\Miniconda3\envs\nlp\lib\encodings\cp1252.py in decode(self, input, final)
22 def decode(self, input, final=False):
---> 23 return codecs.charmap_decode(input,self.errors,decoding_table)[0]
24
UnicodeDecodeError: 'charmap' codec can't decode byte 0x9d in position 6744: character maps to <undefined>
```
Anyway this can be solved by specifying to decode in UTF when reading the csv file. I am proposing a PR if that's okay.
On Windows Python supports Unicode almost everywhere, but one of the notable exceptions is open() where it uses the locale encoding schema. So platform independent python scripts would always set the encoding='utf-8' in calls to open explicitly.
In the meantime: since Python 3.7 Windows users can set the default encoding for everything including open() to Unicode by setting this environment variable: set PYTHONUTF8=1 (details can be found in [PEP 540](https://www.python.org/dev/peps/pep-0540/))
For me this fixed the problem described by the OP. |
https://github.com/huggingface/datasets/issues/240 | Deterministic dataset loading | I think using `sorted(glob.glob())` would actually solve this problem. Can you think of other reasons why dataset loading might not be deterministic? @mariamabarham @yjernite @lhoestq @thomwolf .
I can do a sweep through the dataset scripts and fix the glob.glob() if you guys are ok with it | When calling:
```python
import nlp
dataset = nlp.load_dataset("trivia_qa", split="validation[:1%]")
```
the resulting dataset is not deterministic over different google colabs.
After talking to @thomwolf, I suspect the reason to be the use of `glob.glob` in line:
https://github.com/huggingface/nlp/blob/2e0a8639a79b1abc848cff5c669094d40bba0f63/datasets/trivia_qa/trivia_qa.py#L180
which seems to return an ordering of files that depends on the filesystem:
https://stackoverflow.com/questions/6773584/how-is-pythons-glob-glob-ordered
I think we should go through all the dataset scripts and make sure to have deterministic behavior.
A simple solution for `glob.glob()` would be to just replace it with `sorted(glob.glob())` to have everything sorted by name.
What do you think @lhoestq? | 47 | Deterministic dataset loading
When calling:
```python
import nlp
dataset = nlp.load_dataset("trivia_qa", split="validation[:1%]")
```
the resulting dataset is not deterministic over different google colabs.
After talking to @thomwolf, I suspect the reason to be the use of `glob.glob` in line:
https://github.com/huggingface/nlp/blob/2e0a8639a79b1abc848cff5c669094d40bba0f63/datasets/trivia_qa/trivia_qa.py#L180
which seems to return an ordering of files that depends on the filesystem:
https://stackoverflow.com/questions/6773584/how-is-pythons-glob-glob-ordered
I think we should go through all the dataset scripts and make sure to have deterministic behavior.
A simple solution for `glob.glob()` would be to just replace it with `sorted(glob.glob())` to have everything sorted by name.
What do you think @lhoestq?
I think using `sorted(glob.glob())` would actually solve this problem. Can you think of other reasons why dataset loading might not be deterministic? @mariamabarham @yjernite @lhoestq @thomwolf .
I can do a sweep through the dataset scripts and fix the glob.glob() if you guys are ok with it |
https://github.com/huggingface/datasets/issues/240 | Deterministic dataset loading | I'm pretty sure it would solve the problem too.
The only other dataset that is not deterministic right now is `blog_authorship_corpus` (see #215) but this is a problem related to string encodings. | When calling:
```python
import nlp
dataset = nlp.load_dataset("trivia_qa", split="validation[:1%]")
```
the resulting dataset is not deterministic over different google colabs.
After talking to @thomwolf, I suspect the reason to be the use of `glob.glob` in line:
https://github.com/huggingface/nlp/blob/2e0a8639a79b1abc848cff5c669094d40bba0f63/datasets/trivia_qa/trivia_qa.py#L180
which seems to return an ordering of files that depends on the filesystem:
https://stackoverflow.com/questions/6773584/how-is-pythons-glob-glob-ordered
I think we should go through all the dataset scripts and make sure to have deterministic behavior.
A simple solution for `glob.glob()` would be to just replace it with `sorted(glob.glob())` to have everything sorted by name.
What do you think @lhoestq? | 32 | Deterministic dataset loading
When calling:
```python
import nlp
dataset = nlp.load_dataset("trivia_qa", split="validation[:1%]")
```
the resulting dataset is not deterministic over different google colabs.
After talking to @thomwolf, I suspect the reason to be the use of `glob.glob` in line:
https://github.com/huggingface/nlp/blob/2e0a8639a79b1abc848cff5c669094d40bba0f63/datasets/trivia_qa/trivia_qa.py#L180
which seems to return an ordering of files that depends on the filesystem:
https://stackoverflow.com/questions/6773584/how-is-pythons-glob-glob-ordered
I think we should go through all the dataset scripts and make sure to have deterministic behavior.
A simple solution for `glob.glob()` would be to just replace it with `sorted(glob.glob())` to have everything sorted by name.
What do you think @lhoestq?
I'm pretty sure it would solve the problem too.
The only other dataset that is not deterministic right now is `blog_authorship_corpus` (see #215) but this is a problem related to string encodings. |
https://github.com/huggingface/datasets/issues/239 | [Creating new dataset] Not found dataset_info.json | @lhoestq - this seems to happen quite often (already the 2nd issue). Can we maybe delete this automatically? | Hi, I am trying to create Toronto Book Corpus. #131
I ran
`~/nlp % python nlp-cli test datasets/bookcorpus --save_infos --all_configs`
but this doesn't create `dataset_info.json` and try to use it
```
INFO:nlp.load:Checking datasets/bookcorpus/bookcorpus.py for additional imports.
INFO:filelock:Lock 139795325778640 acquired on datasets/bookcorpus/bookcorpus.py.lock
INFO:nlp.load:Found main folder for dataset datasets/bookcorpus/bookcorpus.py at /home/yisiang/miniconda3/envs/ml/lib/python3.7/site-packages/nlp/datasets/bookcorpus
INFO:nlp.load:Found specific version folder for dataset datasets/bookcorpus/bookcorpus.py at /home/yisiang/miniconda3/envs/ml/lib/python3.7/site-packages/nlp/datasets/bookcorpus/8e84759446cf68d0b0deb3417e60cc331f30a3bbe58843de18a0f48e87d1efd9
INFO:nlp.load:Found script file from datasets/bookcorpus/bookcorpus.py to /home/yisiang/miniconda3/envs/ml/lib/python3.7/site-packages/nlp/datasets/bookcorpus/8e84759446cf68d0b0deb3417e60cc331f30a3bbe58843de18a0f48e87d1efd9/bookcorpus.py
INFO:nlp.load:Couldn't find dataset infos file at datasets/bookcorpus/dataset_infos.json
INFO:nlp.load:Found metadata file for dataset datasets/bookcorpus/bookcorpus.py at /home/yisiang/miniconda3/envs/ml/lib/python3.7/site-packages/nlp/datasets/bookcorpus/8e84759446cf68d0b0deb3417e60cc331f30a3bbe58843de18a0f48e87d1efd9/bookcorpus.json
INFO:filelock:Lock 139795325778640 released on datasets/bookcorpus/bookcorpus.py.lock
INFO:nlp.builder:Overwrite dataset info from restored data version.
INFO:nlp.info:Loading Dataset info from /home/yisiang/.cache/huggingface/datasets/book_corpus/plain_text/1.0.0
Traceback (most recent call last):
File "nlp-cli", line 37, in <module>
service.run()
File "/home/yisiang/miniconda3/envs/ml/lib/python3.7/site-packages/nlp/commands/test.py", line 78, in run
builders.append(builder_cls(name=config.name, data_dir=self._data_dir))
File "/home/yisiang/miniconda3/envs/ml/lib/python3.7/site-packages/nlp/builder.py", line 610, in __init__
super(GeneratorBasedBuilder, self).__init__(*args, **kwargs)
File "/home/yisiang/miniconda3/envs/ml/lib/python3.7/site-packages/nlp/builder.py", line 152, in __init__
self.info = DatasetInfo.from_directory(self._cache_dir)
File "/home/yisiang/miniconda3/envs/ml/lib/python3.7/site-packages/nlp/info.py", line 157, in from_directory
with open(os.path.join(dataset_info_dir, DATASET_INFO_FILENAME), "r") as f:
FileNotFoundError: [Errno 2] No such file or directory: '/home/yisiang/.cache/huggingface/datasets/book_corpus/plain_text/1.0.0/dataset_info.json'
```
btw, `ls /home/yisiang/.cache/huggingface/datasets/book_corpus/plain_text/1.0.0/` show me nothing is in the directory.
I have also pushed the script to my fork [bookcorpus.py](https://github.com/richardyy1188/nlp/blob/bookcorpusdev/datasets/bookcorpus/bookcorpus.py).
| 18 | [Creating new dataset] Not found dataset_info.json
Hi, I am trying to create Toronto Book Corpus. #131
I ran
`~/nlp % python nlp-cli test datasets/bookcorpus --save_infos --all_configs`
but this doesn't create `dataset_info.json` and try to use it
```
INFO:nlp.load:Checking datasets/bookcorpus/bookcorpus.py for additional imports.
INFO:filelock:Lock 139795325778640 acquired on datasets/bookcorpus/bookcorpus.py.lock
INFO:nlp.load:Found main folder for dataset datasets/bookcorpus/bookcorpus.py at /home/yisiang/miniconda3/envs/ml/lib/python3.7/site-packages/nlp/datasets/bookcorpus
INFO:nlp.load:Found specific version folder for dataset datasets/bookcorpus/bookcorpus.py at /home/yisiang/miniconda3/envs/ml/lib/python3.7/site-packages/nlp/datasets/bookcorpus/8e84759446cf68d0b0deb3417e60cc331f30a3bbe58843de18a0f48e87d1efd9
INFO:nlp.load:Found script file from datasets/bookcorpus/bookcorpus.py to /home/yisiang/miniconda3/envs/ml/lib/python3.7/site-packages/nlp/datasets/bookcorpus/8e84759446cf68d0b0deb3417e60cc331f30a3bbe58843de18a0f48e87d1efd9/bookcorpus.py
INFO:nlp.load:Couldn't find dataset infos file at datasets/bookcorpus/dataset_infos.json
INFO:nlp.load:Found metadata file for dataset datasets/bookcorpus/bookcorpus.py at /home/yisiang/miniconda3/envs/ml/lib/python3.7/site-packages/nlp/datasets/bookcorpus/8e84759446cf68d0b0deb3417e60cc331f30a3bbe58843de18a0f48e87d1efd9/bookcorpus.json
INFO:filelock:Lock 139795325778640 released on datasets/bookcorpus/bookcorpus.py.lock
INFO:nlp.builder:Overwrite dataset info from restored data version.
INFO:nlp.info:Loading Dataset info from /home/yisiang/.cache/huggingface/datasets/book_corpus/plain_text/1.0.0
Traceback (most recent call last):
File "nlp-cli", line 37, in <module>
service.run()
File "/home/yisiang/miniconda3/envs/ml/lib/python3.7/site-packages/nlp/commands/test.py", line 78, in run
builders.append(builder_cls(name=config.name, data_dir=self._data_dir))
File "/home/yisiang/miniconda3/envs/ml/lib/python3.7/site-packages/nlp/builder.py", line 610, in __init__
super(GeneratorBasedBuilder, self).__init__(*args, **kwargs)
File "/home/yisiang/miniconda3/envs/ml/lib/python3.7/site-packages/nlp/builder.py", line 152, in __init__
self.info = DatasetInfo.from_directory(self._cache_dir)
File "/home/yisiang/miniconda3/envs/ml/lib/python3.7/site-packages/nlp/info.py", line 157, in from_directory
with open(os.path.join(dataset_info_dir, DATASET_INFO_FILENAME), "r") as f:
FileNotFoundError: [Errno 2] No such file or directory: '/home/yisiang/.cache/huggingface/datasets/book_corpus/plain_text/1.0.0/dataset_info.json'
```
btw, `ls /home/yisiang/.cache/huggingface/datasets/book_corpus/plain_text/1.0.0/` show me nothing is in the directory.
I have also pushed the script to my fork [bookcorpus.py](https://github.com/richardyy1188/nlp/blob/bookcorpusdev/datasets/bookcorpus/bookcorpus.py).
@lhoestq - this seems to happen quite often (already the 2nd issue). Can we maybe delete this automatically? |
https://github.com/huggingface/datasets/issues/239 | [Creating new dataset] Not found dataset_info.json | Hi, I rebase my local copy to `fix-empty-cache-dir`, and try to run again `python nlp-cli test datasets/bookcorpus --save_infos --all_configs`.
I got this,
```
Traceback (most recent call last):
File "nlp-cli", line 10, in <module>
from nlp.commands.run_beam import RunBeamCommand
File "/home/yisiang/nlp/src/nlp/commands/run_beam.py", line 6, in <module>
import apache_beam as beam
ModuleNotFoundError: No module named 'apache_beam'
```
And after I installed it. I got this
```
File "/home/yisiang/nlp/src/nlp/datasets/bookcorpus/aea0bd5142d26df645a8fce23d6110bb95ecb81772bb2a1f29012e329191962c/bookcorpus.py", line 88, in _split_generators
downloaded_path_or_paths = dl_manager.download_custom(_GDRIVE_FILE_ID, download_file_from_google_drive)
File "/home/yisiang/nlp/src/nlp/utils/download_manager.py", line 128, in download_custom
downloaded_path_or_paths = map_nested(url_to_downloaded_path, url_or_urls)
File "/home/yisiang/nlp/src/nlp/utils/py_utils.py", line 172, in map_nested
return function(data_struct)
File "/home/yisiang/nlp/src/nlp/utils/download_manager.py", line 126, in url_to_downloaded_path
return os.path.join(self._download_config.cache_dir, hash_url_to_filename(url))
File "/home/yisiang/miniconda3/envs/nlppr/lib/python3.7/posixpath.py", line 80, in join
a = os.fspath(a)
```
The problem is when I print `self._download_config.cache_dir` using pdb, it is `None`.
Did I miss something ? Or can you provide a workaround first so I can keep testing my script ? | Hi, I am trying to create Toronto Book Corpus. #131
I ran
`~/nlp % python nlp-cli test datasets/bookcorpus --save_infos --all_configs`
but this doesn't create `dataset_info.json` and try to use it
```
INFO:nlp.load:Checking datasets/bookcorpus/bookcorpus.py for additional imports.
INFO:filelock:Lock 139795325778640 acquired on datasets/bookcorpus/bookcorpus.py.lock
INFO:nlp.load:Found main folder for dataset datasets/bookcorpus/bookcorpus.py at /home/yisiang/miniconda3/envs/ml/lib/python3.7/site-packages/nlp/datasets/bookcorpus
INFO:nlp.load:Found specific version folder for dataset datasets/bookcorpus/bookcorpus.py at /home/yisiang/miniconda3/envs/ml/lib/python3.7/site-packages/nlp/datasets/bookcorpus/8e84759446cf68d0b0deb3417e60cc331f30a3bbe58843de18a0f48e87d1efd9
INFO:nlp.load:Found script file from datasets/bookcorpus/bookcorpus.py to /home/yisiang/miniconda3/envs/ml/lib/python3.7/site-packages/nlp/datasets/bookcorpus/8e84759446cf68d0b0deb3417e60cc331f30a3bbe58843de18a0f48e87d1efd9/bookcorpus.py
INFO:nlp.load:Couldn't find dataset infos file at datasets/bookcorpus/dataset_infos.json
INFO:nlp.load:Found metadata file for dataset datasets/bookcorpus/bookcorpus.py at /home/yisiang/miniconda3/envs/ml/lib/python3.7/site-packages/nlp/datasets/bookcorpus/8e84759446cf68d0b0deb3417e60cc331f30a3bbe58843de18a0f48e87d1efd9/bookcorpus.json
INFO:filelock:Lock 139795325778640 released on datasets/bookcorpus/bookcorpus.py.lock
INFO:nlp.builder:Overwrite dataset info from restored data version.
INFO:nlp.info:Loading Dataset info from /home/yisiang/.cache/huggingface/datasets/book_corpus/plain_text/1.0.0
Traceback (most recent call last):
File "nlp-cli", line 37, in <module>
service.run()
File "/home/yisiang/miniconda3/envs/ml/lib/python3.7/site-packages/nlp/commands/test.py", line 78, in run
builders.append(builder_cls(name=config.name, data_dir=self._data_dir))
File "/home/yisiang/miniconda3/envs/ml/lib/python3.7/site-packages/nlp/builder.py", line 610, in __init__
super(GeneratorBasedBuilder, self).__init__(*args, **kwargs)
File "/home/yisiang/miniconda3/envs/ml/lib/python3.7/site-packages/nlp/builder.py", line 152, in __init__
self.info = DatasetInfo.from_directory(self._cache_dir)
File "/home/yisiang/miniconda3/envs/ml/lib/python3.7/site-packages/nlp/info.py", line 157, in from_directory
with open(os.path.join(dataset_info_dir, DATASET_INFO_FILENAME), "r") as f:
FileNotFoundError: [Errno 2] No such file or directory: '/home/yisiang/.cache/huggingface/datasets/book_corpus/plain_text/1.0.0/dataset_info.json'
```
btw, `ls /home/yisiang/.cache/huggingface/datasets/book_corpus/plain_text/1.0.0/` show me nothing is in the directory.
I have also pushed the script to my fork [bookcorpus.py](https://github.com/richardyy1188/nlp/blob/bookcorpusdev/datasets/bookcorpus/bookcorpus.py).
| 142 | [Creating new dataset] Not found dataset_info.json
Hi, I am trying to create Toronto Book Corpus. #131
I ran
`~/nlp % python nlp-cli test datasets/bookcorpus --save_infos --all_configs`
but this doesn't create `dataset_info.json` and try to use it
```
INFO:nlp.load:Checking datasets/bookcorpus/bookcorpus.py for additional imports.
INFO:filelock:Lock 139795325778640 acquired on datasets/bookcorpus/bookcorpus.py.lock
INFO:nlp.load:Found main folder for dataset datasets/bookcorpus/bookcorpus.py at /home/yisiang/miniconda3/envs/ml/lib/python3.7/site-packages/nlp/datasets/bookcorpus
INFO:nlp.load:Found specific version folder for dataset datasets/bookcorpus/bookcorpus.py at /home/yisiang/miniconda3/envs/ml/lib/python3.7/site-packages/nlp/datasets/bookcorpus/8e84759446cf68d0b0deb3417e60cc331f30a3bbe58843de18a0f48e87d1efd9
INFO:nlp.load:Found script file from datasets/bookcorpus/bookcorpus.py to /home/yisiang/miniconda3/envs/ml/lib/python3.7/site-packages/nlp/datasets/bookcorpus/8e84759446cf68d0b0deb3417e60cc331f30a3bbe58843de18a0f48e87d1efd9/bookcorpus.py
INFO:nlp.load:Couldn't find dataset infos file at datasets/bookcorpus/dataset_infos.json
INFO:nlp.load:Found metadata file for dataset datasets/bookcorpus/bookcorpus.py at /home/yisiang/miniconda3/envs/ml/lib/python3.7/site-packages/nlp/datasets/bookcorpus/8e84759446cf68d0b0deb3417e60cc331f30a3bbe58843de18a0f48e87d1efd9/bookcorpus.json
INFO:filelock:Lock 139795325778640 released on datasets/bookcorpus/bookcorpus.py.lock
INFO:nlp.builder:Overwrite dataset info from restored data version.
INFO:nlp.info:Loading Dataset info from /home/yisiang/.cache/huggingface/datasets/book_corpus/plain_text/1.0.0
Traceback (most recent call last):
File "nlp-cli", line 37, in <module>
service.run()
File "/home/yisiang/miniconda3/envs/ml/lib/python3.7/site-packages/nlp/commands/test.py", line 78, in run
builders.append(builder_cls(name=config.name, data_dir=self._data_dir))
File "/home/yisiang/miniconda3/envs/ml/lib/python3.7/site-packages/nlp/builder.py", line 610, in __init__
super(GeneratorBasedBuilder, self).__init__(*args, **kwargs)
File "/home/yisiang/miniconda3/envs/ml/lib/python3.7/site-packages/nlp/builder.py", line 152, in __init__
self.info = DatasetInfo.from_directory(self._cache_dir)
File "/home/yisiang/miniconda3/envs/ml/lib/python3.7/site-packages/nlp/info.py", line 157, in from_directory
with open(os.path.join(dataset_info_dir, DATASET_INFO_FILENAME), "r") as f:
FileNotFoundError: [Errno 2] No such file or directory: '/home/yisiang/.cache/huggingface/datasets/book_corpus/plain_text/1.0.0/dataset_info.json'
```
btw, `ls /home/yisiang/.cache/huggingface/datasets/book_corpus/plain_text/1.0.0/` show me nothing is in the directory.
I have also pushed the script to my fork [bookcorpus.py](https://github.com/richardyy1188/nlp/blob/bookcorpusdev/datasets/bookcorpus/bookcorpus.py).
Hi, I rebase my local copy to `fix-empty-cache-dir`, and try to run again `python nlp-cli test datasets/bookcorpus --save_infos --all_configs`.
I got this,
```
Traceback (most recent call last):
File "nlp-cli", line 10, in <module>
from nlp.commands.run_beam import RunBeamCommand
File "/home/yisiang/nlp/src/nlp/commands/run_beam.py", line 6, in <module>
import apache_beam as beam
ModuleNotFoundError: No module named 'apache_beam'
```
And after I installed it. I got this
```
File "/home/yisiang/nlp/src/nlp/datasets/bookcorpus/aea0bd5142d26df645a8fce23d6110bb95ecb81772bb2a1f29012e329191962c/bookcorpus.py", line 88, in _split_generators
downloaded_path_or_paths = dl_manager.download_custom(_GDRIVE_FILE_ID, download_file_from_google_drive)
File "/home/yisiang/nlp/src/nlp/utils/download_manager.py", line 128, in download_custom
downloaded_path_or_paths = map_nested(url_to_downloaded_path, url_or_urls)
File "/home/yisiang/nlp/src/nlp/utils/py_utils.py", line 172, in map_nested
return function(data_struct)
File "/home/yisiang/nlp/src/nlp/utils/download_manager.py", line 126, in url_to_downloaded_path
return os.path.join(self._download_config.cache_dir, hash_url_to_filename(url))
File "/home/yisiang/miniconda3/envs/nlppr/lib/python3.7/posixpath.py", line 80, in join
a = os.fspath(a)
```
The problem is when I print `self._download_config.cache_dir` using pdb, it is `None`.
Did I miss something ? Or can you provide a workaround first so I can keep testing my script ? |
https://github.com/huggingface/datasets/issues/238 | [Metric] Bertscore : Warning : Empty candidate sentence; Setting recall to be 0. | This print statement comes from the official implementation of bert_score (see [here](https://github.com/Tiiiger/bert_score/blob/master/bert_score/utils.py#L343)). The warning shows up only if the attention mask outputs no candidate.
Right now we want to only use official code for metrics to have fair evaluations, so I'm not sure we can do anything about it. Maybe you can try to create an issue on their [repo](https://github.com/Tiiiger/bert_score) ? | When running BERT-Score, I'm meeting this warning :
> Warning: Empty candidate sentence; Setting recall to be 0.
Code :
```
import nlp
metric = nlp.load_metric("bertscore")
scores = metric.compute(["swag", "swags"], ["swags", "totally something different"], lang="en", device=0)
```
---
**What am I doing wrong / How can I hide this warning ?** | 61 | [Metric] Bertscore : Warning : Empty candidate sentence; Setting recall to be 0.
When running BERT-Score, I'm meeting this warning :
> Warning: Empty candidate sentence; Setting recall to be 0.
Code :
```
import nlp
metric = nlp.load_metric("bertscore")
scores = metric.compute(["swag", "swags"], ["swags", "totally something different"], lang="en", device=0)
```
---
**What am I doing wrong / How can I hide this warning ?**
This print statement comes from the official implementation of bert_score (see [here](https://github.com/Tiiiger/bert_score/blob/master/bert_score/utils.py#L343)). The warning shows up only if the attention mask outputs no candidate.
Right now we want to only use official code for metrics to have fair evaluations, so I'm not sure we can do anything about it. Maybe you can try to create an issue on their [repo](https://github.com/Tiiiger/bert_score) ? |
https://github.com/huggingface/datasets/issues/237 | Can't download MultiNLI | Thanks! I thought I had to use the same code displayed in the live viewer:
```python
!pip install nlp
from nlp import load_dataset
dataset = load_dataset('multi_nli', 'plain_text')
```
Your suggestion works, even if then I got a different issue (#242). | When I try to download MultiNLI with
```python
dataset = load_dataset('multi_nli')
```
I get this long error:
```python
---------------------------------------------------------------------------
OSError Traceback (most recent call last)
<ipython-input-13-3b11f6be4cb9> in <module>
1 # Load a dataset and print the first examples in the training set
2 # nli_dataset = nlp.load_dataset('multi_nli')
----> 3 dataset = load_dataset('multi_nli')
4 # nli_dataset = nlp.load_dataset('multi_nli', split='validation_matched[:10%]')
5 # print(nli_dataset['train'][0])
~\Miniconda3\envs\nlp\lib\site-packages\nlp\load.py in load_dataset(path, name, version, data_dir, data_files, split, cache_dir, download_config, download_mode, ignore_verifications, save_infos, **config_kwargs)
514
515 # Download and prepare data
--> 516 builder_instance.download_and_prepare(
517 download_config=download_config,
518 download_mode=download_mode,
~\Miniconda3\envs\nlp\lib\site-packages\nlp\builder.py in download_and_prepare(self, download_config, download_mode, ignore_verifications, save_infos, try_from_hf_gcs, dl_manager, **download_and_prepare_kwargs)
417 with utils.temporary_assignment(self, "_cache_dir", tmp_data_dir):
418 verify_infos = not save_infos and not ignore_verifications
--> 419 self._download_and_prepare(
420 dl_manager=dl_manager, verify_infos=verify_infos, **download_and_prepare_kwargs
421 )
~\Miniconda3\envs\nlp\lib\site-packages\nlp\builder.py in _download_and_prepare(self, dl_manager, verify_infos, **prepare_split_kwargs)
455 split_dict = SplitDict(dataset_name=self.name)
456 split_generators_kwargs = self._make_split_generators_kwargs(prepare_split_kwargs)
--> 457 split_generators = self._split_generators(dl_manager, **split_generators_kwargs)
458 # Checksums verification
459 if verify_infos:
~\Miniconda3\envs\nlp\lib\site-packages\nlp\datasets\multi_nli\60774175381b9f3f1e6ae1028229e3cdb270d50379f45b9f2c01008f50f09e6b\multi_nli.py in _split_generators(self, dl_manager)
99 def _split_generators(self, dl_manager):
100
--> 101 downloaded_dir = dl_manager.download_and_extract(
102 "http://storage.googleapis.com/tfds-data/downloads/multi_nli/multinli_1.0.zip"
103 )
~\Miniconda3\envs\nlp\lib\site-packages\nlp\utils\download_manager.py in download_and_extract(self, url_or_urls)
214 extracted_path(s): `str`, extracted paths of given URL(s).
215 """
--> 216 return self.extract(self.download(url_or_urls))
217
218 def get_recorded_sizes_checksums(self):
~\Miniconda3\envs\nlp\lib\site-packages\nlp\utils\download_manager.py in extract(self, path_or_paths)
194 path_or_paths.
195 """
--> 196 return map_nested(
197 lambda path: cached_path(path, extract_compressed_file=True, force_extract=False), path_or_paths,
198 )
~\Miniconda3\envs\nlp\lib\site-packages\nlp\utils\py_utils.py in map_nested(function, data_struct, dict_only, map_tuple)
168 return tuple(mapped)
169 # Singleton
--> 170 return function(data_struct)
171
172
~\Miniconda3\envs\nlp\lib\site-packages\nlp\utils\download_manager.py in <lambda>(path)
195 """
196 return map_nested(
--> 197 lambda path: cached_path(path, extract_compressed_file=True, force_extract=False), path_or_paths,
198 )
199
~\Miniconda3\envs\nlp\lib\site-packages\nlp\utils\file_utils.py in cached_path(url_or_filename, download_config, **download_kwargs)
231 if is_zipfile(output_path):
232 with ZipFile(output_path, "r") as zip_file:
--> 233 zip_file.extractall(output_path_extracted)
234 zip_file.close()
235 elif tarfile.is_tarfile(output_path):
~\Miniconda3\envs\nlp\lib\zipfile.py in extractall(self, path, members, pwd)
1644
1645 for zipinfo in members:
-> 1646 self._extract_member(zipinfo, path, pwd)
1647
1648 @classmethod
~\Miniconda3\envs\nlp\lib\zipfile.py in _extract_member(self, member, targetpath, pwd)
1698
1699 with self.open(member, pwd=pwd) as source, \
-> 1700 open(targetpath, "wb") as target:
1701 shutil.copyfileobj(source, target)
1702
OSError: [Errno 22] Invalid argument: 'C:\\Users\\Python\\.cache\\huggingface\\datasets\\3e12413b8ec69f22dfcfd54a79d1ba9e7aac2e18e334bbb6b81cca64fd16bffc\\multinli_1.0\\Icon\r'
```
| 40 | Can't download MultiNLI
When I try to download MultiNLI with
```python
dataset = load_dataset('multi_nli')
```
I get this long error:
```python
---------------------------------------------------------------------------
OSError Traceback (most recent call last)
<ipython-input-13-3b11f6be4cb9> in <module>
1 # Load a dataset and print the first examples in the training set
2 # nli_dataset = nlp.load_dataset('multi_nli')
----> 3 dataset = load_dataset('multi_nli')
4 # nli_dataset = nlp.load_dataset('multi_nli', split='validation_matched[:10%]')
5 # print(nli_dataset['train'][0])
~\Miniconda3\envs\nlp\lib\site-packages\nlp\load.py in load_dataset(path, name, version, data_dir, data_files, split, cache_dir, download_config, download_mode, ignore_verifications, save_infos, **config_kwargs)
514
515 # Download and prepare data
--> 516 builder_instance.download_and_prepare(
517 download_config=download_config,
518 download_mode=download_mode,
~\Miniconda3\envs\nlp\lib\site-packages\nlp\builder.py in download_and_prepare(self, download_config, download_mode, ignore_verifications, save_infos, try_from_hf_gcs, dl_manager, **download_and_prepare_kwargs)
417 with utils.temporary_assignment(self, "_cache_dir", tmp_data_dir):
418 verify_infos = not save_infos and not ignore_verifications
--> 419 self._download_and_prepare(
420 dl_manager=dl_manager, verify_infos=verify_infos, **download_and_prepare_kwargs
421 )
~\Miniconda3\envs\nlp\lib\site-packages\nlp\builder.py in _download_and_prepare(self, dl_manager, verify_infos, **prepare_split_kwargs)
455 split_dict = SplitDict(dataset_name=self.name)
456 split_generators_kwargs = self._make_split_generators_kwargs(prepare_split_kwargs)
--> 457 split_generators = self._split_generators(dl_manager, **split_generators_kwargs)
458 # Checksums verification
459 if verify_infos:
~\Miniconda3\envs\nlp\lib\site-packages\nlp\datasets\multi_nli\60774175381b9f3f1e6ae1028229e3cdb270d50379f45b9f2c01008f50f09e6b\multi_nli.py in _split_generators(self, dl_manager)
99 def _split_generators(self, dl_manager):
100
--> 101 downloaded_dir = dl_manager.download_and_extract(
102 "http://storage.googleapis.com/tfds-data/downloads/multi_nli/multinli_1.0.zip"
103 )
~\Miniconda3\envs\nlp\lib\site-packages\nlp\utils\download_manager.py in download_and_extract(self, url_or_urls)
214 extracted_path(s): `str`, extracted paths of given URL(s).
215 """
--> 216 return self.extract(self.download(url_or_urls))
217
218 def get_recorded_sizes_checksums(self):
~\Miniconda3\envs\nlp\lib\site-packages\nlp\utils\download_manager.py in extract(self, path_or_paths)
194 path_or_paths.
195 """
--> 196 return map_nested(
197 lambda path: cached_path(path, extract_compressed_file=True, force_extract=False), path_or_paths,
198 )
~\Miniconda3\envs\nlp\lib\site-packages\nlp\utils\py_utils.py in map_nested(function, data_struct, dict_only, map_tuple)
168 return tuple(mapped)
169 # Singleton
--> 170 return function(data_struct)
171
172
~\Miniconda3\envs\nlp\lib\site-packages\nlp\utils\download_manager.py in <lambda>(path)
195 """
196 return map_nested(
--> 197 lambda path: cached_path(path, extract_compressed_file=True, force_extract=False), path_or_paths,
198 )
199
~\Miniconda3\envs\nlp\lib\site-packages\nlp\utils\file_utils.py in cached_path(url_or_filename, download_config, **download_kwargs)
231 if is_zipfile(output_path):
232 with ZipFile(output_path, "r") as zip_file:
--> 233 zip_file.extractall(output_path_extracted)
234 zip_file.close()
235 elif tarfile.is_tarfile(output_path):
~\Miniconda3\envs\nlp\lib\zipfile.py in extractall(self, path, members, pwd)
1644
1645 for zipinfo in members:
-> 1646 self._extract_member(zipinfo, path, pwd)
1647
1648 @classmethod
~\Miniconda3\envs\nlp\lib\zipfile.py in _extract_member(self, member, targetpath, pwd)
1698
1699 with self.open(member, pwd=pwd) as source, \
-> 1700 open(targetpath, "wb") as target:
1701 shutil.copyfileobj(source, target)
1702
OSError: [Errno 22] Invalid argument: 'C:\\Users\\Python\\.cache\\huggingface\\datasets\\3e12413b8ec69f22dfcfd54a79d1ba9e7aac2e18e334bbb6b81cca64fd16bffc\\multinli_1.0\\Icon\r'
```
Thanks! I thought I had to use the same code displayed in the live viewer:
```python
!pip install nlp
from nlp import load_dataset
dataset = load_dataset('multi_nli', 'plain_text')
```
Your suggestion works, even if then I got a different issue (#242). |
https://github.com/huggingface/datasets/issues/237 | Can't download MultiNLI | Glad it helps !
Though I am not one of hf team, but maybe you should close this issue first. | When I try to download MultiNLI with
```python
dataset = load_dataset('multi_nli')
```
I get this long error:
```python
---------------------------------------------------------------------------
OSError Traceback (most recent call last)
<ipython-input-13-3b11f6be4cb9> in <module>
1 # Load a dataset and print the first examples in the training set
2 # nli_dataset = nlp.load_dataset('multi_nli')
----> 3 dataset = load_dataset('multi_nli')
4 # nli_dataset = nlp.load_dataset('multi_nli', split='validation_matched[:10%]')
5 # print(nli_dataset['train'][0])
~\Miniconda3\envs\nlp\lib\site-packages\nlp\load.py in load_dataset(path, name, version, data_dir, data_files, split, cache_dir, download_config, download_mode, ignore_verifications, save_infos, **config_kwargs)
514
515 # Download and prepare data
--> 516 builder_instance.download_and_prepare(
517 download_config=download_config,
518 download_mode=download_mode,
~\Miniconda3\envs\nlp\lib\site-packages\nlp\builder.py in download_and_prepare(self, download_config, download_mode, ignore_verifications, save_infos, try_from_hf_gcs, dl_manager, **download_and_prepare_kwargs)
417 with utils.temporary_assignment(self, "_cache_dir", tmp_data_dir):
418 verify_infos = not save_infos and not ignore_verifications
--> 419 self._download_and_prepare(
420 dl_manager=dl_manager, verify_infos=verify_infos, **download_and_prepare_kwargs
421 )
~\Miniconda3\envs\nlp\lib\site-packages\nlp\builder.py in _download_and_prepare(self, dl_manager, verify_infos, **prepare_split_kwargs)
455 split_dict = SplitDict(dataset_name=self.name)
456 split_generators_kwargs = self._make_split_generators_kwargs(prepare_split_kwargs)
--> 457 split_generators = self._split_generators(dl_manager, **split_generators_kwargs)
458 # Checksums verification
459 if verify_infos:
~\Miniconda3\envs\nlp\lib\site-packages\nlp\datasets\multi_nli\60774175381b9f3f1e6ae1028229e3cdb270d50379f45b9f2c01008f50f09e6b\multi_nli.py in _split_generators(self, dl_manager)
99 def _split_generators(self, dl_manager):
100
--> 101 downloaded_dir = dl_manager.download_and_extract(
102 "http://storage.googleapis.com/tfds-data/downloads/multi_nli/multinli_1.0.zip"
103 )
~\Miniconda3\envs\nlp\lib\site-packages\nlp\utils\download_manager.py in download_and_extract(self, url_or_urls)
214 extracted_path(s): `str`, extracted paths of given URL(s).
215 """
--> 216 return self.extract(self.download(url_or_urls))
217
218 def get_recorded_sizes_checksums(self):
~\Miniconda3\envs\nlp\lib\site-packages\nlp\utils\download_manager.py in extract(self, path_or_paths)
194 path_or_paths.
195 """
--> 196 return map_nested(
197 lambda path: cached_path(path, extract_compressed_file=True, force_extract=False), path_or_paths,
198 )
~\Miniconda3\envs\nlp\lib\site-packages\nlp\utils\py_utils.py in map_nested(function, data_struct, dict_only, map_tuple)
168 return tuple(mapped)
169 # Singleton
--> 170 return function(data_struct)
171
172
~\Miniconda3\envs\nlp\lib\site-packages\nlp\utils\download_manager.py in <lambda>(path)
195 """
196 return map_nested(
--> 197 lambda path: cached_path(path, extract_compressed_file=True, force_extract=False), path_or_paths,
198 )
199
~\Miniconda3\envs\nlp\lib\site-packages\nlp\utils\file_utils.py in cached_path(url_or_filename, download_config, **download_kwargs)
231 if is_zipfile(output_path):
232 with ZipFile(output_path, "r") as zip_file:
--> 233 zip_file.extractall(output_path_extracted)
234 zip_file.close()
235 elif tarfile.is_tarfile(output_path):
~\Miniconda3\envs\nlp\lib\zipfile.py in extractall(self, path, members, pwd)
1644
1645 for zipinfo in members:
-> 1646 self._extract_member(zipinfo, path, pwd)
1647
1648 @classmethod
~\Miniconda3\envs\nlp\lib\zipfile.py in _extract_member(self, member, targetpath, pwd)
1698
1699 with self.open(member, pwd=pwd) as source, \
-> 1700 open(targetpath, "wb") as target:
1701 shutil.copyfileobj(source, target)
1702
OSError: [Errno 22] Invalid argument: 'C:\\Users\\Python\\.cache\\huggingface\\datasets\\3e12413b8ec69f22dfcfd54a79d1ba9e7aac2e18e334bbb6b81cca64fd16bffc\\multinli_1.0\\Icon\r'
```
| 20 | Can't download MultiNLI
When I try to download MultiNLI with
```python
dataset = load_dataset('multi_nli')
```
I get this long error:
```python
---------------------------------------------------------------------------
OSError Traceback (most recent call last)
<ipython-input-13-3b11f6be4cb9> in <module>
1 # Load a dataset and print the first examples in the training set
2 # nli_dataset = nlp.load_dataset('multi_nli')
----> 3 dataset = load_dataset('multi_nli')
4 # nli_dataset = nlp.load_dataset('multi_nli', split='validation_matched[:10%]')
5 # print(nli_dataset['train'][0])
~\Miniconda3\envs\nlp\lib\site-packages\nlp\load.py in load_dataset(path, name, version, data_dir, data_files, split, cache_dir, download_config, download_mode, ignore_verifications, save_infos, **config_kwargs)
514
515 # Download and prepare data
--> 516 builder_instance.download_and_prepare(
517 download_config=download_config,
518 download_mode=download_mode,
~\Miniconda3\envs\nlp\lib\site-packages\nlp\builder.py in download_and_prepare(self, download_config, download_mode, ignore_verifications, save_infos, try_from_hf_gcs, dl_manager, **download_and_prepare_kwargs)
417 with utils.temporary_assignment(self, "_cache_dir", tmp_data_dir):
418 verify_infos = not save_infos and not ignore_verifications
--> 419 self._download_and_prepare(
420 dl_manager=dl_manager, verify_infos=verify_infos, **download_and_prepare_kwargs
421 )
~\Miniconda3\envs\nlp\lib\site-packages\nlp\builder.py in _download_and_prepare(self, dl_manager, verify_infos, **prepare_split_kwargs)
455 split_dict = SplitDict(dataset_name=self.name)
456 split_generators_kwargs = self._make_split_generators_kwargs(prepare_split_kwargs)
--> 457 split_generators = self._split_generators(dl_manager, **split_generators_kwargs)
458 # Checksums verification
459 if verify_infos:
~\Miniconda3\envs\nlp\lib\site-packages\nlp\datasets\multi_nli\60774175381b9f3f1e6ae1028229e3cdb270d50379f45b9f2c01008f50f09e6b\multi_nli.py in _split_generators(self, dl_manager)
99 def _split_generators(self, dl_manager):
100
--> 101 downloaded_dir = dl_manager.download_and_extract(
102 "http://storage.googleapis.com/tfds-data/downloads/multi_nli/multinli_1.0.zip"
103 )
~\Miniconda3\envs\nlp\lib\site-packages\nlp\utils\download_manager.py in download_and_extract(self, url_or_urls)
214 extracted_path(s): `str`, extracted paths of given URL(s).
215 """
--> 216 return self.extract(self.download(url_or_urls))
217
218 def get_recorded_sizes_checksums(self):
~\Miniconda3\envs\nlp\lib\site-packages\nlp\utils\download_manager.py in extract(self, path_or_paths)
194 path_or_paths.
195 """
--> 196 return map_nested(
197 lambda path: cached_path(path, extract_compressed_file=True, force_extract=False), path_or_paths,
198 )
~\Miniconda3\envs\nlp\lib\site-packages\nlp\utils\py_utils.py in map_nested(function, data_struct, dict_only, map_tuple)
168 return tuple(mapped)
169 # Singleton
--> 170 return function(data_struct)
171
172
~\Miniconda3\envs\nlp\lib\site-packages\nlp\utils\download_manager.py in <lambda>(path)
195 """
196 return map_nested(
--> 197 lambda path: cached_path(path, extract_compressed_file=True, force_extract=False), path_or_paths,
198 )
199
~\Miniconda3\envs\nlp\lib\site-packages\nlp\utils\file_utils.py in cached_path(url_or_filename, download_config, **download_kwargs)
231 if is_zipfile(output_path):
232 with ZipFile(output_path, "r") as zip_file:
--> 233 zip_file.extractall(output_path_extracted)
234 zip_file.close()
235 elif tarfile.is_tarfile(output_path):
~\Miniconda3\envs\nlp\lib\zipfile.py in extractall(self, path, members, pwd)
1644
1645 for zipinfo in members:
-> 1646 self._extract_member(zipinfo, path, pwd)
1647
1648 @classmethod
~\Miniconda3\envs\nlp\lib\zipfile.py in _extract_member(self, member, targetpath, pwd)
1698
1699 with self.open(member, pwd=pwd) as source, \
-> 1700 open(targetpath, "wb") as target:
1701 shutil.copyfileobj(source, target)
1702
OSError: [Errno 22] Invalid argument: 'C:\\Users\\Python\\.cache\\huggingface\\datasets\\3e12413b8ec69f22dfcfd54a79d1ba9e7aac2e18e334bbb6b81cca64fd16bffc\\multinli_1.0\\Icon\r'
```
Glad it helps !
Though I am not one of hf team, but maybe you should close this issue first. |
https://github.com/huggingface/datasets/issues/234 | Huggingface NLP, Uploading custom dataset | What do you mean 'custom' ? You may want to elaborate on it when ask a question.
Anyway, there are two things you may interested
`nlp.Dataset.from_file` and `load_dataset(..., cache_dir=)` | Hello,
Does anyone know how we can call our custom dataset using the nlp.load command? Let's say that I have a dataset based on the same format as that of squad-v1.1, how am I supposed to load it using huggingface nlp.
Thank you! | 29 | Huggingface NLP, Uploading custom dataset
Hello,
Does anyone know how we can call our custom dataset using the nlp.load command? Let's say that I have a dataset based on the same format as that of squad-v1.1, how am I supposed to load it using huggingface nlp.
Thank you!
What do you mean 'custom' ? You may want to elaborate on it when ask a question.
Anyway, there are two things you may interested
`nlp.Dataset.from_file` and `load_dataset(..., cache_dir=)` |
https://github.com/huggingface/datasets/issues/234 | Huggingface NLP, Uploading custom dataset | To load a dataset you need to have a script that defines the format of the examples, the splits and the way to generate examples. As your dataset has the same format of squad, you can just copy the squad script (see the [datasets](https://github.com/huggingface/nlp/tree/master/datasets) forlder) and just replace the url to load the data to your local or remote path.
Then what you can do is `load_dataset(<path/to/your/script>)` | Hello,
Does anyone know how we can call our custom dataset using the nlp.load command? Let's say that I have a dataset based on the same format as that of squad-v1.1, how am I supposed to load it using huggingface nlp.
Thank you! | 67 | Huggingface NLP, Uploading custom dataset
Hello,
Does anyone know how we can call our custom dataset using the nlp.load command? Let's say that I have a dataset based on the same format as that of squad-v1.1, how am I supposed to load it using huggingface nlp.
Thank you!
To load a dataset you need to have a script that defines the format of the examples, the splits and the way to generate examples. As your dataset has the same format of squad, you can just copy the squad script (see the [datasets](https://github.com/huggingface/nlp/tree/master/datasets) forlder) and just replace the url to load the data to your local or remote path.
Then what you can do is `load_dataset(<path/to/your/script>)` |
https://github.com/huggingface/datasets/issues/234 | Huggingface NLP, Uploading custom dataset | Also if you want to upload your script, you should be able to use the `nlp-cli`.
Unfortunately the upload feature was not shipped in the latest version 0.2.0. so right now you can either clone the repo to use it or wait for the next release. We will add some docs to explain how to upload datasets.
| Hello,
Does anyone know how we can call our custom dataset using the nlp.load command? Let's say that I have a dataset based on the same format as that of squad-v1.1, how am I supposed to load it using huggingface nlp.
Thank you! | 57 | Huggingface NLP, Uploading custom dataset
Hello,
Does anyone know how we can call our custom dataset using the nlp.load command? Let's say that I have a dataset based on the same format as that of squad-v1.1, how am I supposed to load it using huggingface nlp.
Thank you!
Also if you want to upload your script, you should be able to use the `nlp-cli`.
Unfortunately the upload feature was not shipped in the latest version 0.2.0. so right now you can either clone the repo to use it or wait for the next release. We will add some docs to explain how to upload datasets.
|
https://github.com/huggingface/datasets/issues/234 | Huggingface NLP, Uploading custom dataset | Since the latest release 0.2.1 you can use
```bash
nlp-cli upload_dataset <path/to/dataset>
```
where `<path/to/dataset>` is a path to a folder containing your script (ex: `squad.py`).
This will upload the script under your namespace on our S3.
Optionally the folder can also contain `dataset_infos.json` generated using
```bash
nlp-cli test <path/to/dataset> --all_configs --save_infos
```
Then you should be able to do
```python
nlp.load_dataset("my_namespace/dataset_name")
``` | Hello,
Does anyone know how we can call our custom dataset using the nlp.load command? Let's say that I have a dataset based on the same format as that of squad-v1.1, how am I supposed to load it using huggingface nlp.
Thank you! | 63 | Huggingface NLP, Uploading custom dataset
Hello,
Does anyone know how we can call our custom dataset using the nlp.load command? Let's say that I have a dataset based on the same format as that of squad-v1.1, how am I supposed to load it using huggingface nlp.
Thank you!
Since the latest release 0.2.1 you can use
```bash
nlp-cli upload_dataset <path/to/dataset>
```
where `<path/to/dataset>` is a path to a folder containing your script (ex: `squad.py`).
This will upload the script under your namespace on our S3.
Optionally the folder can also contain `dataset_infos.json` generated using
```bash
nlp-cli test <path/to/dataset> --all_configs --save_infos
```
Then you should be able to do
```python
nlp.load_dataset("my_namespace/dataset_name")
``` |
https://github.com/huggingface/datasets/issues/233 | Fail to download c4 english corpus | Hello ! Thanks for noticing this bug, let me fix that.
Also for information, as specified in the changelog of the latest release, C4 currently needs to have a runtime for apache beam to work on. Apache beam is used to process this very big dataset and it can work on dataflow, spark, flink, apex, etc. You can find more info on beam datasets [here](https://github.com/huggingface/nlp/blob/master/docs/beam_dataset.md).
Our goal in the future is to make available an already-processed version of C4 (as we do for wikipedia for example) so that users without apache beam runtimes can load it. | i run following code to download c4 English corpus.
```
dataset = nlp.load_dataset('c4', 'en', beam_runner='DirectRunner'
, data_dir='/mypath')
```
and i met failure as follows
```
Downloading and preparing dataset c4/en (download: Unknown size, generated: Unknown size, total: Unknown size) to /home/adam/.cache/huggingface/datasets/c4/en/2.3.0...
Traceback (most recent call last):
File "download_corpus.py", line 38, in <module>
, data_dir='/home/adam/data/corpus/en/c4')
File "/home/adam/anaconda3/envs/adam/lib/python3.7/site-packages/nlp/load.py", line 520, in load_dataset
save_infos=save_infos,
File "/home/adam/anaconda3/envs/adam/lib/python3.7/site-packages/nlp/builder.py", line 420, in download_and_prepare
dl_manager=dl_manager, verify_infos=verify_infos, **download_and_prepare_kwargs
File "/home/adam/anaconda3/envs/adam/lib/python3.7/site-packages/nlp/builder.py", line 816, in _download_and_prepare
dl_manager, verify_infos=False, pipeline=pipeline,
File "/home/adam/anaconda3/envs/adam/lib/python3.7/site-packages/nlp/builder.py", line 457, in _download_and_prepare
split_generators = self._split_generators(dl_manager, **split_generators_kwargs)
File "/home/adam/anaconda3/envs/adam/lib/python3.7/site-packages/nlp/datasets/c4/f545de9f63300d8d02a6795e2eb34e140c47e62a803f572ac5599e170ee66ecc/c4.py", line 175, in _split_generators
dl_manager.download_checksums(_CHECKSUMS_URL)
AttributeError: 'DownloadManager' object has no attribute 'download_checksums
```
can i get any advice? | 96 | Fail to download c4 english corpus
i run following code to download c4 English corpus.
```
dataset = nlp.load_dataset('c4', 'en', beam_runner='DirectRunner'
, data_dir='/mypath')
```
and i met failure as follows
```
Downloading and preparing dataset c4/en (download: Unknown size, generated: Unknown size, total: Unknown size) to /home/adam/.cache/huggingface/datasets/c4/en/2.3.0...
Traceback (most recent call last):
File "download_corpus.py", line 38, in <module>
, data_dir='/home/adam/data/corpus/en/c4')
File "/home/adam/anaconda3/envs/adam/lib/python3.7/site-packages/nlp/load.py", line 520, in load_dataset
save_infos=save_infos,
File "/home/adam/anaconda3/envs/adam/lib/python3.7/site-packages/nlp/builder.py", line 420, in download_and_prepare
dl_manager=dl_manager, verify_infos=verify_infos, **download_and_prepare_kwargs
File "/home/adam/anaconda3/envs/adam/lib/python3.7/site-packages/nlp/builder.py", line 816, in _download_and_prepare
dl_manager, verify_infos=False, pipeline=pipeline,
File "/home/adam/anaconda3/envs/adam/lib/python3.7/site-packages/nlp/builder.py", line 457, in _download_and_prepare
split_generators = self._split_generators(dl_manager, **split_generators_kwargs)
File "/home/adam/anaconda3/envs/adam/lib/python3.7/site-packages/nlp/datasets/c4/f545de9f63300d8d02a6795e2eb34e140c47e62a803f572ac5599e170ee66ecc/c4.py", line 175, in _split_generators
dl_manager.download_checksums(_CHECKSUMS_URL)
AttributeError: 'DownloadManager' object has no attribute 'download_checksums
```
can i get any advice?
Hello ! Thanks for noticing this bug, let me fix that.
Also for information, as specified in the changelog of the latest release, C4 currently needs to have a runtime for apache beam to work on. Apache beam is used to process this very big dataset and it can work on dataflow, spark, flink, apex, etc. You can find more info on beam datasets [here](https://github.com/huggingface/nlp/blob/master/docs/beam_dataset.md).
Our goal in the future is to make available an already-processed version of C4 (as we do for wikipedia for example) so that users without apache beam runtimes can load it. |
https://github.com/huggingface/datasets/issues/233 | Fail to download c4 english corpus | @lhoestq I am facing `IsADirectoryError` while downloading with this command.
Can you pls look into it & help me.
I'm using version 0.4.0 of `nlp`.
```
dataset = load_dataset("c4", 'en', data_dir='.', beam_runner='DirectRunner')
```
Here's the complete stack trace.
```
Downloading and preparing dataset c4/en (download: Unknown size, generated: Unknown size, post-processed: Unknown sizetotal: Unknown size) to /home/devops/.cache/huggingface/datasets/c4/en/2.3.0/096df5a27756d51957c959a2499453e60a08154971fceb017bbb29f54b11bef7...
---------------------------------------------------------------------------
IsADirectoryError Traceback (most recent call last)
<ipython-input-11-f622e6705e03> in <module>
----> 1 dataset = load_dataset("c4", 'en', data_dir='.', beam_runner='DirectRunner')
/data/anaconda/envs/hf/lib/python3.6/site-packages/nlp/load.py in load_dataset(path, name, version, data_dir, data_files, split, cache_dir, features, download_config, download_mode, ignore_verifications, save_infos, **config_kwargs)
547 # Download and prepare data
548 builder_instance.download_and_prepare(
--> 549 download_config=download_config, download_mode=download_mode, ignore_verifications=ignore_verifications,
550 )
551
/data/anaconda/envs/hf/lib/python3.6/site-packages/nlp/builder.py in download_and_prepare(self, download_config, download_mode, ignore_verifications, try_from_hf_gcs, dl_manager, **download_and_prepare_kwargs)
461 if not downloaded_from_gcs:
462 self._download_and_prepare(
--> 463 dl_manager=dl_manager, verify_infos=verify_infos, **download_and_prepare_kwargs
464 )
465 # Sync info
/data/anaconda/envs/hf/lib/python3.6/site-packages/nlp/builder.py in _download_and_prepare(self, dl_manager, verify_infos)
964 pipeline = beam_utils.BeamPipeline(runner=beam_runner, options=beam_options,)
965 super(BeamBasedBuilder, self)._download_and_prepare(
--> 966 dl_manager, verify_infos=False, pipeline=pipeline,
967 ) # TODO handle verify_infos in beam datasets
968 # Run pipeline
/data/anaconda/envs/hf/lib/python3.6/site-packages/nlp/builder.py in _download_and_prepare(self, dl_manager, verify_infos, **prepare_split_kwargs)
516 split_dict = SplitDict(dataset_name=self.name)
517 split_generators_kwargs = self._make_split_generators_kwargs(prepare_split_kwargs)
--> 518 split_generators = self._split_generators(dl_manager, **split_generators_kwargs)
519 # Checksums verification
520 if verify_infos:
/data/anaconda/envs/hf/lib/python3.6/site-packages/nlp/datasets/c4/096df5a27756d51957c959a2499453e60a08154971fceb017bbb29f54b11bef7/c4.py in _split_generators(self, dl_manager, pipeline)
187 if self.config.realnewslike:
188 files_to_download["realnews_domains"] = _REALNEWS_DOMAINS_URL
--> 189 file_paths = dl_manager.download_and_extract(files_to_download)
190
191 if self.config.webtextlike:
/data/anaconda/envs/hf/lib/python3.6/site-packages/nlp/utils/download_manager.py in download_and_extract(self, url_or_urls)
218 extracted_path(s): `str`, extracted paths of given URL(s).
219 """
--> 220 return self.extract(self.download(url_or_urls))
221
222 def get_recorded_sizes_checksums(self):
/data/anaconda/envs/hf/lib/python3.6/site-packages/nlp/utils/download_manager.py in download(self, url_or_urls)
156 lambda url: cached_path(url, download_config=self._download_config,), url_or_urls,
157 )
--> 158 self._record_sizes_checksums(url_or_urls, downloaded_path_or_paths)
159 return downloaded_path_or_paths
160
/data/anaconda/envs/hf/lib/python3.6/site-packages/nlp/utils/download_manager.py in _record_sizes_checksums(self, url_or_urls, downloaded_path_or_paths)
106 flattened_downloaded_path_or_paths = flatten_nested(downloaded_path_or_paths)
107 for url, path in zip(flattened_urls_or_urls, flattened_downloaded_path_or_paths):
--> 108 self._recorded_sizes_checksums[url] = get_size_checksum_dict(path)
109
110 def download_custom(self, url_or_urls, custom_download):
/data/anaconda/envs/hf/lib/python3.6/site-packages/nlp/utils/info_utils.py in get_size_checksum_dict(path)
77 """Compute the file size and the sha256 checksum of a file"""
78 m = sha256()
---> 79 with open(path, "rb") as f:
80 for chunk in iter(lambda: f.read(1 << 20), b""):
81 m.update(chunk)
IsADirectoryError: [Errno 21] Is a directory: '/'
```
Can anyone please try to see what I am doing wrong or is this a bug? | i run following code to download c4 English corpus.
```
dataset = nlp.load_dataset('c4', 'en', beam_runner='DirectRunner'
, data_dir='/mypath')
```
and i met failure as follows
```
Downloading and preparing dataset c4/en (download: Unknown size, generated: Unknown size, total: Unknown size) to /home/adam/.cache/huggingface/datasets/c4/en/2.3.0...
Traceback (most recent call last):
File "download_corpus.py", line 38, in <module>
, data_dir='/home/adam/data/corpus/en/c4')
File "/home/adam/anaconda3/envs/adam/lib/python3.7/site-packages/nlp/load.py", line 520, in load_dataset
save_infos=save_infos,
File "/home/adam/anaconda3/envs/adam/lib/python3.7/site-packages/nlp/builder.py", line 420, in download_and_prepare
dl_manager=dl_manager, verify_infos=verify_infos, **download_and_prepare_kwargs
File "/home/adam/anaconda3/envs/adam/lib/python3.7/site-packages/nlp/builder.py", line 816, in _download_and_prepare
dl_manager, verify_infos=False, pipeline=pipeline,
File "/home/adam/anaconda3/envs/adam/lib/python3.7/site-packages/nlp/builder.py", line 457, in _download_and_prepare
split_generators = self._split_generators(dl_manager, **split_generators_kwargs)
File "/home/adam/anaconda3/envs/adam/lib/python3.7/site-packages/nlp/datasets/c4/f545de9f63300d8d02a6795e2eb34e140c47e62a803f572ac5599e170ee66ecc/c4.py", line 175, in _split_generators
dl_manager.download_checksums(_CHECKSUMS_URL)
AttributeError: 'DownloadManager' object has no attribute 'download_checksums
```
can i get any advice? | 341 | Fail to download c4 english corpus
i run following code to download c4 English corpus.
```
dataset = nlp.load_dataset('c4', 'en', beam_runner='DirectRunner'
, data_dir='/mypath')
```
and i met failure as follows
```
Downloading and preparing dataset c4/en (download: Unknown size, generated: Unknown size, total: Unknown size) to /home/adam/.cache/huggingface/datasets/c4/en/2.3.0...
Traceback (most recent call last):
File "download_corpus.py", line 38, in <module>
, data_dir='/home/adam/data/corpus/en/c4')
File "/home/adam/anaconda3/envs/adam/lib/python3.7/site-packages/nlp/load.py", line 520, in load_dataset
save_infos=save_infos,
File "/home/adam/anaconda3/envs/adam/lib/python3.7/site-packages/nlp/builder.py", line 420, in download_and_prepare
dl_manager=dl_manager, verify_infos=verify_infos, **download_and_prepare_kwargs
File "/home/adam/anaconda3/envs/adam/lib/python3.7/site-packages/nlp/builder.py", line 816, in _download_and_prepare
dl_manager, verify_infos=False, pipeline=pipeline,
File "/home/adam/anaconda3/envs/adam/lib/python3.7/site-packages/nlp/builder.py", line 457, in _download_and_prepare
split_generators = self._split_generators(dl_manager, **split_generators_kwargs)
File "/home/adam/anaconda3/envs/adam/lib/python3.7/site-packages/nlp/datasets/c4/f545de9f63300d8d02a6795e2eb34e140c47e62a803f572ac5599e170ee66ecc/c4.py", line 175, in _split_generators
dl_manager.download_checksums(_CHECKSUMS_URL)
AttributeError: 'DownloadManager' object has no attribute 'download_checksums
```
can i get any advice?
@lhoestq I am facing `IsADirectoryError` while downloading with this command.
Can you pls look into it & help me.
I'm using version 0.4.0 of `nlp`.
```
dataset = load_dataset("c4", 'en', data_dir='.', beam_runner='DirectRunner')
```
Here's the complete stack trace.
```
Downloading and preparing dataset c4/en (download: Unknown size, generated: Unknown size, post-processed: Unknown sizetotal: Unknown size) to /home/devops/.cache/huggingface/datasets/c4/en/2.3.0/096df5a27756d51957c959a2499453e60a08154971fceb017bbb29f54b11bef7...
---------------------------------------------------------------------------
IsADirectoryError Traceback (most recent call last)
<ipython-input-11-f622e6705e03> in <module>
----> 1 dataset = load_dataset("c4", 'en', data_dir='.', beam_runner='DirectRunner')
/data/anaconda/envs/hf/lib/python3.6/site-packages/nlp/load.py in load_dataset(path, name, version, data_dir, data_files, split, cache_dir, features, download_config, download_mode, ignore_verifications, save_infos, **config_kwargs)
547 # Download and prepare data
548 builder_instance.download_and_prepare(
--> 549 download_config=download_config, download_mode=download_mode, ignore_verifications=ignore_verifications,
550 )
551
/data/anaconda/envs/hf/lib/python3.6/site-packages/nlp/builder.py in download_and_prepare(self, download_config, download_mode, ignore_verifications, try_from_hf_gcs, dl_manager, **download_and_prepare_kwargs)
461 if not downloaded_from_gcs:
462 self._download_and_prepare(
--> 463 dl_manager=dl_manager, verify_infos=verify_infos, **download_and_prepare_kwargs
464 )
465 # Sync info
/data/anaconda/envs/hf/lib/python3.6/site-packages/nlp/builder.py in _download_and_prepare(self, dl_manager, verify_infos)
964 pipeline = beam_utils.BeamPipeline(runner=beam_runner, options=beam_options,)
965 super(BeamBasedBuilder, self)._download_and_prepare(
--> 966 dl_manager, verify_infos=False, pipeline=pipeline,
967 ) # TODO handle verify_infos in beam datasets
968 # Run pipeline
/data/anaconda/envs/hf/lib/python3.6/site-packages/nlp/builder.py in _download_and_prepare(self, dl_manager, verify_infos, **prepare_split_kwargs)
516 split_dict = SplitDict(dataset_name=self.name)
517 split_generators_kwargs = self._make_split_generators_kwargs(prepare_split_kwargs)
--> 518 split_generators = self._split_generators(dl_manager, **split_generators_kwargs)
519 # Checksums verification
520 if verify_infos:
/data/anaconda/envs/hf/lib/python3.6/site-packages/nlp/datasets/c4/096df5a27756d51957c959a2499453e60a08154971fceb017bbb29f54b11bef7/c4.py in _split_generators(self, dl_manager, pipeline)
187 if self.config.realnewslike:
188 files_to_download["realnews_domains"] = _REALNEWS_DOMAINS_URL
--> 189 file_paths = dl_manager.download_and_extract(files_to_download)
190
191 if self.config.webtextlike:
/data/anaconda/envs/hf/lib/python3.6/site-packages/nlp/utils/download_manager.py in download_and_extract(self, url_or_urls)
218 extracted_path(s): `str`, extracted paths of given URL(s).
219 """
--> 220 return self.extract(self.download(url_or_urls))
221
222 def get_recorded_sizes_checksums(self):
/data/anaconda/envs/hf/lib/python3.6/site-packages/nlp/utils/download_manager.py in download(self, url_or_urls)
156 lambda url: cached_path(url, download_config=self._download_config,), url_or_urls,
157 )
--> 158 self._record_sizes_checksums(url_or_urls, downloaded_path_or_paths)
159 return downloaded_path_or_paths
160
/data/anaconda/envs/hf/lib/python3.6/site-packages/nlp/utils/download_manager.py in _record_sizes_checksums(self, url_or_urls, downloaded_path_or_paths)
106 flattened_downloaded_path_or_paths = flatten_nested(downloaded_path_or_paths)
107 for url, path in zip(flattened_urls_or_urls, flattened_downloaded_path_or_paths):
--> 108 self._recorded_sizes_checksums[url] = get_size_checksum_dict(path)
109
110 def download_custom(self, url_or_urls, custom_download):
/data/anaconda/envs/hf/lib/python3.6/site-packages/nlp/utils/info_utils.py in get_size_checksum_dict(path)
77 """Compute the file size and the sha256 checksum of a file"""
78 m = sha256()
---> 79 with open(path, "rb") as f:
80 for chunk in iter(lambda: f.read(1 << 20), b""):
81 m.update(chunk)
IsADirectoryError: [Errno 21] Is a directory: '/'
```
Can anyone please try to see what I am doing wrong or is this a bug? |
https://github.com/huggingface/datasets/issues/228 | Not able to access the XNLI dataset | Thanks for reporting this bug !
As it seems to be just a cache problem, I closed your PR.
I think we might just need to clear and reload the `xnli` cache @srush ? | When I try to access the XNLI dataset, I get the following error. The option of plain_text get selected automatically and then I get the following error.
```
FileNotFoundError: [Errno 2] No such file or directory: '/home/sasha/.cache/huggingface/datasets/xnli/plain_text/1.0.0/dataset_info.json'
Traceback:
File "/home/sasha/.local/lib/python3.7/site-packages/streamlit/ScriptRunner.py", line 322, in _run_script
exec(code, module.__dict__)
File "/home/sasha/nlp_viewer/run.py", line 86, in <module>
dts, fail = get(str(option.id), str(conf_option.name) if conf_option else None)
File "/home/sasha/.local/lib/python3.7/site-packages/streamlit/caching.py", line 591, in wrapped_func
return get_or_create_cached_value()
File "/home/sasha/.local/lib/python3.7/site-packages/streamlit/caching.py", line 575, in get_or_create_cached_value
return_value = func(*args, **kwargs)
File "/home/sasha/nlp_viewer/run.py", line 72, in get
builder_instance = builder_cls(name=conf)
File "/home/sasha/.local/lib/python3.7/site-packages/nlp/builder.py", line 610, in __init__
super(GeneratorBasedBuilder, self).__init__(*args, **kwargs)
File "/home/sasha/.local/lib/python3.7/site-packages/nlp/builder.py", line 152, in __init__
self.info = DatasetInfo.from_directory(self._cache_dir)
File "/home/sasha/.local/lib/python3.7/site-packages/nlp/info.py", line 157, in from_directory
with open(os.path.join(dataset_info_dir, DATASET_INFO_FILENAME), "r") as f:
```
Is it possible to see if the dataset_info.json is correctly placed? | 34 | Not able to access the XNLI dataset
When I try to access the XNLI dataset, I get the following error. The option of plain_text get selected automatically and then I get the following error.
```
FileNotFoundError: [Errno 2] No such file or directory: '/home/sasha/.cache/huggingface/datasets/xnli/plain_text/1.0.0/dataset_info.json'
Traceback:
File "/home/sasha/.local/lib/python3.7/site-packages/streamlit/ScriptRunner.py", line 322, in _run_script
exec(code, module.__dict__)
File "/home/sasha/nlp_viewer/run.py", line 86, in <module>
dts, fail = get(str(option.id), str(conf_option.name) if conf_option else None)
File "/home/sasha/.local/lib/python3.7/site-packages/streamlit/caching.py", line 591, in wrapped_func
return get_or_create_cached_value()
File "/home/sasha/.local/lib/python3.7/site-packages/streamlit/caching.py", line 575, in get_or_create_cached_value
return_value = func(*args, **kwargs)
File "/home/sasha/nlp_viewer/run.py", line 72, in get
builder_instance = builder_cls(name=conf)
File "/home/sasha/.local/lib/python3.7/site-packages/nlp/builder.py", line 610, in __init__
super(GeneratorBasedBuilder, self).__init__(*args, **kwargs)
File "/home/sasha/.local/lib/python3.7/site-packages/nlp/builder.py", line 152, in __init__
self.info = DatasetInfo.from_directory(self._cache_dir)
File "/home/sasha/.local/lib/python3.7/site-packages/nlp/info.py", line 157, in from_directory
with open(os.path.join(dataset_info_dir, DATASET_INFO_FILENAME), "r") as f:
```
Is it possible to see if the dataset_info.json is correctly placed?
Thanks for reporting this bug !
As it seems to be just a cache problem, I closed your PR.
I think we might just need to clear and reload the `xnli` cache @srush ? |
https://github.com/huggingface/datasets/issues/228 | Not able to access the XNLI dataset | Update: The dataset_info.json error is gone, but we have a new one instead:
```
ConnectionError: Couldn't reach https://www.nyu.edu/projects/bowman/xnli/XNLI-1.0.zip
```
I am not able to reproduce on my side unfortunately. Any idea @srush ? | When I try to access the XNLI dataset, I get the following error. The option of plain_text get selected automatically and then I get the following error.
```
FileNotFoundError: [Errno 2] No such file or directory: '/home/sasha/.cache/huggingface/datasets/xnli/plain_text/1.0.0/dataset_info.json'
Traceback:
File "/home/sasha/.local/lib/python3.7/site-packages/streamlit/ScriptRunner.py", line 322, in _run_script
exec(code, module.__dict__)
File "/home/sasha/nlp_viewer/run.py", line 86, in <module>
dts, fail = get(str(option.id), str(conf_option.name) if conf_option else None)
File "/home/sasha/.local/lib/python3.7/site-packages/streamlit/caching.py", line 591, in wrapped_func
return get_or_create_cached_value()
File "/home/sasha/.local/lib/python3.7/site-packages/streamlit/caching.py", line 575, in get_or_create_cached_value
return_value = func(*args, **kwargs)
File "/home/sasha/nlp_viewer/run.py", line 72, in get
builder_instance = builder_cls(name=conf)
File "/home/sasha/.local/lib/python3.7/site-packages/nlp/builder.py", line 610, in __init__
super(GeneratorBasedBuilder, self).__init__(*args, **kwargs)
File "/home/sasha/.local/lib/python3.7/site-packages/nlp/builder.py", line 152, in __init__
self.info = DatasetInfo.from_directory(self._cache_dir)
File "/home/sasha/.local/lib/python3.7/site-packages/nlp/info.py", line 157, in from_directory
with open(os.path.join(dataset_info_dir, DATASET_INFO_FILENAME), "r") as f:
```
Is it possible to see if the dataset_info.json is correctly placed? | 33 | Not able to access the XNLI dataset
When I try to access the XNLI dataset, I get the following error. The option of plain_text get selected automatically and then I get the following error.
```
FileNotFoundError: [Errno 2] No such file or directory: '/home/sasha/.cache/huggingface/datasets/xnli/plain_text/1.0.0/dataset_info.json'
Traceback:
File "/home/sasha/.local/lib/python3.7/site-packages/streamlit/ScriptRunner.py", line 322, in _run_script
exec(code, module.__dict__)
File "/home/sasha/nlp_viewer/run.py", line 86, in <module>
dts, fail = get(str(option.id), str(conf_option.name) if conf_option else None)
File "/home/sasha/.local/lib/python3.7/site-packages/streamlit/caching.py", line 591, in wrapped_func
return get_or_create_cached_value()
File "/home/sasha/.local/lib/python3.7/site-packages/streamlit/caching.py", line 575, in get_or_create_cached_value
return_value = func(*args, **kwargs)
File "/home/sasha/nlp_viewer/run.py", line 72, in get
builder_instance = builder_cls(name=conf)
File "/home/sasha/.local/lib/python3.7/site-packages/nlp/builder.py", line 610, in __init__
super(GeneratorBasedBuilder, self).__init__(*args, **kwargs)
File "/home/sasha/.local/lib/python3.7/site-packages/nlp/builder.py", line 152, in __init__
self.info = DatasetInfo.from_directory(self._cache_dir)
File "/home/sasha/.local/lib/python3.7/site-packages/nlp/info.py", line 157, in from_directory
with open(os.path.join(dataset_info_dir, DATASET_INFO_FILENAME), "r") as f:
```
Is it possible to see if the dataset_info.json is correctly placed?
Update: The dataset_info.json error is gone, but we have a new one instead:
```
ConnectionError: Couldn't reach https://www.nyu.edu/projects/bowman/xnli/XNLI-1.0.zip
```
I am not able to reproduce on my side unfortunately. Any idea @srush ? |
https://github.com/huggingface/datasets/issues/225 | [ROUGE] Different scores with `files2rouge` | @Colanim unfortunately there are different implementations of the ROUGE metric floating around online which yield different results, and we had to chose one for the package :) We ended up including the one from the google-research repository, which does minimal post-processing before computing the P/R/F scores. If I recall correctly, files2rouge relies on the Perl, script, which among other things normalizes all numbers to a special token: in the case you presented, this should account for a good chunk of the difference.
We may end up adding in more versions of the metric, but probably not for a while (@lhoestq correct me if I'm wrong). However, feel free to take a stab at adding it in yourself and submitting a PR if you're interested! | It seems that the ROUGE score of `nlp` is lower than the one of `files2rouge`.
Here is a self-contained notebook to reproduce both scores : https://colab.research.google.com/drive/14EyAXValB6UzKY9x4rs_T3pyL7alpw_F?usp=sharing
---
`nlp` : (Only mid F-scores)
>rouge1 0.33508031962733364
rouge2 0.14574333776191592
rougeL 0.2321187823256159
`files2rouge` :
>Running ROUGE...
===========================
1 ROUGE-1 Average_R: 0.48873 (95%-conf.int. 0.41192 - 0.56339)
1 ROUGE-1 Average_P: 0.29010 (95%-conf.int. 0.23605 - 0.34445)
1 ROUGE-1 Average_F: 0.34761 (95%-conf.int. 0.29479 - 0.39871)
===========================
1 ROUGE-2 Average_R: 0.20280 (95%-conf.int. 0.14969 - 0.26244)
1 ROUGE-2 Average_P: 0.12772 (95%-conf.int. 0.08603 - 0.17752)
1 ROUGE-2 Average_F: 0.14798 (95%-conf.int. 0.10517 - 0.19240)
===========================
1 ROUGE-L Average_R: 0.32960 (95%-conf.int. 0.26501 - 0.39676)
1 ROUGE-L Average_P: 0.19880 (95%-conf.int. 0.15257 - 0.25136)
1 ROUGE-L Average_F: 0.23619 (95%-conf.int. 0.19073 - 0.28663)
---
When using longer predictions/gold, the difference is bigger.
**How can I reproduce same score as `files2rouge` ?**
@lhoestq
| 124 | [ROUGE] Different scores with `files2rouge`
It seems that the ROUGE score of `nlp` is lower than the one of `files2rouge`.
Here is a self-contained notebook to reproduce both scores : https://colab.research.google.com/drive/14EyAXValB6UzKY9x4rs_T3pyL7alpw_F?usp=sharing
---
`nlp` : (Only mid F-scores)
>rouge1 0.33508031962733364
rouge2 0.14574333776191592
rougeL 0.2321187823256159
`files2rouge` :
>Running ROUGE...
===========================
1 ROUGE-1 Average_R: 0.48873 (95%-conf.int. 0.41192 - 0.56339)
1 ROUGE-1 Average_P: 0.29010 (95%-conf.int. 0.23605 - 0.34445)
1 ROUGE-1 Average_F: 0.34761 (95%-conf.int. 0.29479 - 0.39871)
===========================
1 ROUGE-2 Average_R: 0.20280 (95%-conf.int. 0.14969 - 0.26244)
1 ROUGE-2 Average_P: 0.12772 (95%-conf.int. 0.08603 - 0.17752)
1 ROUGE-2 Average_F: 0.14798 (95%-conf.int. 0.10517 - 0.19240)
===========================
1 ROUGE-L Average_R: 0.32960 (95%-conf.int. 0.26501 - 0.39676)
1 ROUGE-L Average_P: 0.19880 (95%-conf.int. 0.15257 - 0.25136)
1 ROUGE-L Average_F: 0.23619 (95%-conf.int. 0.19073 - 0.28663)
---
When using longer predictions/gold, the difference is bigger.
**How can I reproduce same score as `files2rouge` ?**
@lhoestq
@Colanim unfortunately there are different implementations of the ROUGE metric floating around online which yield different results, and we had to chose one for the package :) We ended up including the one from the google-research repository, which does minimal post-processing before computing the P/R/F scores. If I recall correctly, files2rouge relies on the Perl, script, which among other things normalizes all numbers to a special token: in the case you presented, this should account for a good chunk of the difference.
We may end up adding in more versions of the metric, but probably not for a while (@lhoestq correct me if I'm wrong). However, feel free to take a stab at adding it in yourself and submitting a PR if you're interested! |
https://github.com/huggingface/datasets/issues/225 | [ROUGE] Different scores with `files2rouge` | Thank you for your kind answer.
As a side question : Isn't it better to have a package that normalize more ?
I understand to idea of having a package that does minimal post-processing for transparency.
But it means that people using different architecture (with different tokenizers for example) will have difference in ROUGE scores even if their predictions are actually similar.
The goal of `nlp` is to have _one package to rule them all_, right ?
I will look into it but I'm not sure I have the required skill for this ^^ | It seems that the ROUGE score of `nlp` is lower than the one of `files2rouge`.
Here is a self-contained notebook to reproduce both scores : https://colab.research.google.com/drive/14EyAXValB6UzKY9x4rs_T3pyL7alpw_F?usp=sharing
---
`nlp` : (Only mid F-scores)
>rouge1 0.33508031962733364
rouge2 0.14574333776191592
rougeL 0.2321187823256159
`files2rouge` :
>Running ROUGE...
===========================
1 ROUGE-1 Average_R: 0.48873 (95%-conf.int. 0.41192 - 0.56339)
1 ROUGE-1 Average_P: 0.29010 (95%-conf.int. 0.23605 - 0.34445)
1 ROUGE-1 Average_F: 0.34761 (95%-conf.int. 0.29479 - 0.39871)
===========================
1 ROUGE-2 Average_R: 0.20280 (95%-conf.int. 0.14969 - 0.26244)
1 ROUGE-2 Average_P: 0.12772 (95%-conf.int. 0.08603 - 0.17752)
1 ROUGE-2 Average_F: 0.14798 (95%-conf.int. 0.10517 - 0.19240)
===========================
1 ROUGE-L Average_R: 0.32960 (95%-conf.int. 0.26501 - 0.39676)
1 ROUGE-L Average_P: 0.19880 (95%-conf.int. 0.15257 - 0.25136)
1 ROUGE-L Average_F: 0.23619 (95%-conf.int. 0.19073 - 0.28663)
---
When using longer predictions/gold, the difference is bigger.
**How can I reproduce same score as `files2rouge` ?**
@lhoestq
| 94 | [ROUGE] Different scores with `files2rouge`
It seems that the ROUGE score of `nlp` is lower than the one of `files2rouge`.
Here is a self-contained notebook to reproduce both scores : https://colab.research.google.com/drive/14EyAXValB6UzKY9x4rs_T3pyL7alpw_F?usp=sharing
---
`nlp` : (Only mid F-scores)
>rouge1 0.33508031962733364
rouge2 0.14574333776191592
rougeL 0.2321187823256159
`files2rouge` :
>Running ROUGE...
===========================
1 ROUGE-1 Average_R: 0.48873 (95%-conf.int. 0.41192 - 0.56339)
1 ROUGE-1 Average_P: 0.29010 (95%-conf.int. 0.23605 - 0.34445)
1 ROUGE-1 Average_F: 0.34761 (95%-conf.int. 0.29479 - 0.39871)
===========================
1 ROUGE-2 Average_R: 0.20280 (95%-conf.int. 0.14969 - 0.26244)
1 ROUGE-2 Average_P: 0.12772 (95%-conf.int. 0.08603 - 0.17752)
1 ROUGE-2 Average_F: 0.14798 (95%-conf.int. 0.10517 - 0.19240)
===========================
1 ROUGE-L Average_R: 0.32960 (95%-conf.int. 0.26501 - 0.39676)
1 ROUGE-L Average_P: 0.19880 (95%-conf.int. 0.15257 - 0.25136)
1 ROUGE-L Average_F: 0.23619 (95%-conf.int. 0.19073 - 0.28663)
---
When using longer predictions/gold, the difference is bigger.
**How can I reproduce same score as `files2rouge` ?**
@lhoestq
Thank you for your kind answer.
As a side question : Isn't it better to have a package that normalize more ?
I understand to idea of having a package that does minimal post-processing for transparency.
But it means that people using different architecture (with different tokenizers for example) will have difference in ROUGE scores even if their predictions are actually similar.
The goal of `nlp` is to have _one package to rule them all_, right ?
I will look into it but I'm not sure I have the required skill for this ^^ |
https://github.com/huggingface/datasets/issues/225 | [ROUGE] Different scores with `files2rouge` | You're right, there's a pretty interesting trade-off here between robustness and sensitivity :) The flip side of your argument is that we also still want the metric to be sensitive to model mistakes. How we think about number normalization for example has evolved a fair bit since the Perl script was written: at the time, ROUGE was used mostly to evaluate short-medium text summarization systems, where there were only a few numbers in the input and it was assumed that the most popular methods in use at the time would get those right. However, as your example showcases, that assumption does not hold any more, and we do want to be able to penalize a model that generates a wrong numerical value.
Also, we think that abstracting away tokenization differences is the role of the model/tokenizer: if you use the 🤗Tokenizers library for example, it will handle that for you ;)
Finally, there is a lot of active research on developing model-powered metrics that are both more sensitive and more robust than ROUGE. Check out for example BERTscore, which is implemented in this library! | It seems that the ROUGE score of `nlp` is lower than the one of `files2rouge`.
Here is a self-contained notebook to reproduce both scores : https://colab.research.google.com/drive/14EyAXValB6UzKY9x4rs_T3pyL7alpw_F?usp=sharing
---
`nlp` : (Only mid F-scores)
>rouge1 0.33508031962733364
rouge2 0.14574333776191592
rougeL 0.2321187823256159
`files2rouge` :
>Running ROUGE...
===========================
1 ROUGE-1 Average_R: 0.48873 (95%-conf.int. 0.41192 - 0.56339)
1 ROUGE-1 Average_P: 0.29010 (95%-conf.int. 0.23605 - 0.34445)
1 ROUGE-1 Average_F: 0.34761 (95%-conf.int. 0.29479 - 0.39871)
===========================
1 ROUGE-2 Average_R: 0.20280 (95%-conf.int. 0.14969 - 0.26244)
1 ROUGE-2 Average_P: 0.12772 (95%-conf.int. 0.08603 - 0.17752)
1 ROUGE-2 Average_F: 0.14798 (95%-conf.int. 0.10517 - 0.19240)
===========================
1 ROUGE-L Average_R: 0.32960 (95%-conf.int. 0.26501 - 0.39676)
1 ROUGE-L Average_P: 0.19880 (95%-conf.int. 0.15257 - 0.25136)
1 ROUGE-L Average_F: 0.23619 (95%-conf.int. 0.19073 - 0.28663)
---
When using longer predictions/gold, the difference is bigger.
**How can I reproduce same score as `files2rouge` ?**
@lhoestq
| 184 | [ROUGE] Different scores with `files2rouge`
It seems that the ROUGE score of `nlp` is lower than the one of `files2rouge`.
Here is a self-contained notebook to reproduce both scores : https://colab.research.google.com/drive/14EyAXValB6UzKY9x4rs_T3pyL7alpw_F?usp=sharing
---
`nlp` : (Only mid F-scores)
>rouge1 0.33508031962733364
rouge2 0.14574333776191592
rougeL 0.2321187823256159
`files2rouge` :
>Running ROUGE...
===========================
1 ROUGE-1 Average_R: 0.48873 (95%-conf.int. 0.41192 - 0.56339)
1 ROUGE-1 Average_P: 0.29010 (95%-conf.int. 0.23605 - 0.34445)
1 ROUGE-1 Average_F: 0.34761 (95%-conf.int. 0.29479 - 0.39871)
===========================
1 ROUGE-2 Average_R: 0.20280 (95%-conf.int. 0.14969 - 0.26244)
1 ROUGE-2 Average_P: 0.12772 (95%-conf.int. 0.08603 - 0.17752)
1 ROUGE-2 Average_F: 0.14798 (95%-conf.int. 0.10517 - 0.19240)
===========================
1 ROUGE-L Average_R: 0.32960 (95%-conf.int. 0.26501 - 0.39676)
1 ROUGE-L Average_P: 0.19880 (95%-conf.int. 0.15257 - 0.25136)
1 ROUGE-L Average_F: 0.23619 (95%-conf.int. 0.19073 - 0.28663)
---
When using longer predictions/gold, the difference is bigger.
**How can I reproduce same score as `files2rouge` ?**
@lhoestq
You're right, there's a pretty interesting trade-off here between robustness and sensitivity :) The flip side of your argument is that we also still want the metric to be sensitive to model mistakes. How we think about number normalization for example has evolved a fair bit since the Perl script was written: at the time, ROUGE was used mostly to evaluate short-medium text summarization systems, where there were only a few numbers in the input and it was assumed that the most popular methods in use at the time would get those right. However, as your example showcases, that assumption does not hold any more, and we do want to be able to penalize a model that generates a wrong numerical value.
Also, we think that abstracting away tokenization differences is the role of the model/tokenizer: if you use the 🤗Tokenizers library for example, it will handle that for you ;)
Finally, there is a lot of active research on developing model-powered metrics that are both more sensitive and more robust than ROUGE. Check out for example BERTscore, which is implemented in this library! |
https://github.com/huggingface/datasets/issues/224 | [Feature Request/Help] BLEURT model -> PyTorch | Hitting this error when using bleurt with PyTorch ...
```
UnrecognizedFlagError: Unknown command line flag 'f'
```
... and I'm assuming because it was built for TF specifically. Is there a way to use this metric in PyTorch? | Hi, I am interested in porting google research's new BLEURT learned metric to PyTorch (because I wish to do something experimental with language generation and backpropping through BLEURT). I noticed that you guys don't have it yet so I am partly just asking if you plan to add it (@thomwolf said you want to do so on Twitter).
I had a go of just like manually using the checkpoint that they publish which includes the weights. It seems like the architecture is exactly aligned with the out-of-the-box BertModel in transformers just with a single linear layer on top of the CLS embedding. I loaded all the weights to the PyTorch model but I am not able to get the same numbers as the BLEURT package's python api. Here is my colab notebook where I tried https://colab.research.google.com/drive/1Bfced531EvQP_CpFvxwxNl25Pj6ptylY?usp=sharing . If you have any pointers on what might be going wrong that would be much appreciated!
Thank you muchly! | 38 | [Feature Request/Help] BLEURT model -> PyTorch
Hi, I am interested in porting google research's new BLEURT learned metric to PyTorch (because I wish to do something experimental with language generation and backpropping through BLEURT). I noticed that you guys don't have it yet so I am partly just asking if you plan to add it (@thomwolf said you want to do so on Twitter).
I had a go of just like manually using the checkpoint that they publish which includes the weights. It seems like the architecture is exactly aligned with the out-of-the-box BertModel in transformers just with a single linear layer on top of the CLS embedding. I loaded all the weights to the PyTorch model but I am not able to get the same numbers as the BLEURT package's python api. Here is my colab notebook where I tried https://colab.research.google.com/drive/1Bfced531EvQP_CpFvxwxNl25Pj6ptylY?usp=sharing . If you have any pointers on what might be going wrong that would be much appreciated!
Thank you muchly!
Hitting this error when using bleurt with PyTorch ...
```
UnrecognizedFlagError: Unknown command line flag 'f'
```
... and I'm assuming because it was built for TF specifically. Is there a way to use this metric in PyTorch? |
https://github.com/huggingface/datasets/issues/224 | [Feature Request/Help] BLEURT model -> PyTorch | We currently provide a wrapper on the TensorFlow implementation: https://huggingface.co/metrics/bleurt
We have long term plans to better handle model-based metrics, but they probably won't be implemented right away
@adamwlev it would still be cool to add the BLEURT checkpoints to the transformers repo if you're interested, but that would best be discussed there :)
closing for now | Hi, I am interested in porting google research's new BLEURT learned metric to PyTorch (because I wish to do something experimental with language generation and backpropping through BLEURT). I noticed that you guys don't have it yet so I am partly just asking if you plan to add it (@thomwolf said you want to do so on Twitter).
I had a go of just like manually using the checkpoint that they publish which includes the weights. It seems like the architecture is exactly aligned with the out-of-the-box BertModel in transformers just with a single linear layer on top of the CLS embedding. I loaded all the weights to the PyTorch model but I am not able to get the same numbers as the BLEURT package's python api. Here is my colab notebook where I tried https://colab.research.google.com/drive/1Bfced531EvQP_CpFvxwxNl25Pj6ptylY?usp=sharing . If you have any pointers on what might be going wrong that would be much appreciated!
Thank you muchly! | 57 | [Feature Request/Help] BLEURT model -> PyTorch
Hi, I am interested in porting google research's new BLEURT learned metric to PyTorch (because I wish to do something experimental with language generation and backpropping through BLEURT). I noticed that you guys don't have it yet so I am partly just asking if you plan to add it (@thomwolf said you want to do so on Twitter).
I had a go of just like manually using the checkpoint that they publish which includes the weights. It seems like the architecture is exactly aligned with the out-of-the-box BertModel in transformers just with a single linear layer on top of the CLS embedding. I loaded all the weights to the PyTorch model but I am not able to get the same numbers as the BLEURT package's python api. Here is my colab notebook where I tried https://colab.research.google.com/drive/1Bfced531EvQP_CpFvxwxNl25Pj6ptylY?usp=sharing . If you have any pointers on what might be going wrong that would be much appreciated!
Thank you muchly!
We currently provide a wrapper on the TensorFlow implementation: https://huggingface.co/metrics/bleurt
We have long term plans to better handle model-based metrics, but they probably won't be implemented right away
@adamwlev it would still be cool to add the BLEURT checkpoints to the transformers repo if you're interested, but that would best be discussed there :)
closing for now |
https://github.com/huggingface/datasets/issues/224 | [Feature Request/Help] BLEURT model -> PyTorch | Hi there. We ran into the same problem this year (converting BLEURT to PyTorch) and thanks to @adamwlev found his colab notebook which didn't work but served as a good starting point. Finally, we **made it work** by doing just two simple conceptual fixes:
1. Transposing 'kernel' layers instead of 'dense' ones when copying params from the original model;
2. Taking pooler_output as a cls_state in forward function of the BleurtModel class.
Plus few minor syntactical fixes for the outdated parts. The result is still not exactly the same, but is very close to the expected one (1.0483 vs 1.0474).
Find the fixed version here (fixes are commented): https://colab.research.google.com/drive/1KsCUkFW45d5_ROSv2aHtXgeBa2Z98r03?usp=sharing
| Hi, I am interested in porting google research's new BLEURT learned metric to PyTorch (because I wish to do something experimental with language generation and backpropping through BLEURT). I noticed that you guys don't have it yet so I am partly just asking if you plan to add it (@thomwolf said you want to do so on Twitter).
I had a go of just like manually using the checkpoint that they publish which includes the weights. It seems like the architecture is exactly aligned with the out-of-the-box BertModel in transformers just with a single linear layer on top of the CLS embedding. I loaded all the weights to the PyTorch model but I am not able to get the same numbers as the BLEURT package's python api. Here is my colab notebook where I tried https://colab.research.google.com/drive/1Bfced531EvQP_CpFvxwxNl25Pj6ptylY?usp=sharing . If you have any pointers on what might be going wrong that would be much appreciated!
Thank you muchly! | 109 | [Feature Request/Help] BLEURT model -> PyTorch
Hi, I am interested in porting google research's new BLEURT learned metric to PyTorch (because I wish to do something experimental with language generation and backpropping through BLEURT). I noticed that you guys don't have it yet so I am partly just asking if you plan to add it (@thomwolf said you want to do so on Twitter).
I had a go of just like manually using the checkpoint that they publish which includes the weights. It seems like the architecture is exactly aligned with the out-of-the-box BertModel in transformers just with a single linear layer on top of the CLS embedding. I loaded all the weights to the PyTorch model but I am not able to get the same numbers as the BLEURT package's python api. Here is my colab notebook where I tried https://colab.research.google.com/drive/1Bfced531EvQP_CpFvxwxNl25Pj6ptylY?usp=sharing . If you have any pointers on what might be going wrong that would be much appreciated!
Thank you muchly!
Hi there. We ran into the same problem this year (converting BLEURT to PyTorch) and thanks to @adamwlev found his colab notebook which didn't work but served as a good starting point. Finally, we **made it work** by doing just two simple conceptual fixes:
1. Transposing 'kernel' layers instead of 'dense' ones when copying params from the original model;
2. Taking pooler_output as a cls_state in forward function of the BleurtModel class.
Plus few minor syntactical fixes for the outdated parts. The result is still not exactly the same, but is very close to the expected one (1.0483 vs 1.0474).
Find the fixed version here (fixes are commented): https://colab.research.google.com/drive/1KsCUkFW45d5_ROSv2aHtXgeBa2Z98r03?usp=sharing
|
https://github.com/huggingface/datasets/issues/224 | [Feature Request/Help] BLEURT model -> PyTorch | I created a new model based on `transformers` that can load every BLEURT checkpoints released so far. https://github.com/lucadiliello/bleurt-pytorch | Hi, I am interested in porting google research's new BLEURT learned metric to PyTorch (because I wish to do something experimental with language generation and backpropping through BLEURT). I noticed that you guys don't have it yet so I am partly just asking if you plan to add it (@thomwolf said you want to do so on Twitter).
I had a go of just like manually using the checkpoint that they publish which includes the weights. It seems like the architecture is exactly aligned with the out-of-the-box BertModel in transformers just with a single linear layer on top of the CLS embedding. I loaded all the weights to the PyTorch model but I am not able to get the same numbers as the BLEURT package's python api. Here is my colab notebook where I tried https://colab.research.google.com/drive/1Bfced531EvQP_CpFvxwxNl25Pj6ptylY?usp=sharing . If you have any pointers on what might be going wrong that would be much appreciated!
Thank you muchly! | 18 | [Feature Request/Help] BLEURT model -> PyTorch
Hi, I am interested in porting google research's new BLEURT learned metric to PyTorch (because I wish to do something experimental with language generation and backpropping through BLEURT). I noticed that you guys don't have it yet so I am partly just asking if you plan to add it (@thomwolf said you want to do so on Twitter).
I had a go of just like manually using the checkpoint that they publish which includes the weights. It seems like the architecture is exactly aligned with the out-of-the-box BertModel in transformers just with a single linear layer on top of the CLS embedding. I loaded all the weights to the PyTorch model but I am not able to get the same numbers as the BLEURT package's python api. Here is my colab notebook where I tried https://colab.research.google.com/drive/1Bfced531EvQP_CpFvxwxNl25Pj6ptylY?usp=sharing . If you have any pointers on what might be going wrong that would be much appreciated!
Thank you muchly!
I created a new model based on `transformers` that can load every BLEURT checkpoints released so far. https://github.com/lucadiliello/bleurt-pytorch |
https://github.com/huggingface/datasets/issues/223 | [Feature request] Add FLUE dataset | @mariamabarham
I put all the datasets on this drive: https://1drv.ms/u/s!Ao2Rcpiny7RFinDypq7w-LbXcsx9?e=iVsEDh
Some information :
• For FLUE, the quote used is
> @misc{le2019flaubert,
> title={FlauBERT: Unsupervised Language Model Pre-training for French},
> author={Hang Le and Loïc Vial and Jibril Frej and Vincent Segonne and Maximin Coavoux and Benjamin Lecouteux and Alexandre Allauzen and Benoît Crabbé and Laurent Besacier and Didier Schwab},
> year={2019},
> eprint={1912.05372},
> archivePrefix={arXiv},
> primaryClass={cs.CL}
> }
• The Github repo of FLUE is avaible here : https://github.com/getalp/Flaubert/tree/master/flue
Information related to the different tasks of FLUE :
**1. Classification**
Three dataframes are available:
- Book
- DVD
- Music
For each of these dataframes is available a set of training and test data, and a third one containing unlabelled data.
Citation :
>@dataset{prettenhofer_peter_2010_3251672,
author = {Prettenhofer, Peter and
Stein, Benno},
title = {{Webis Cross-Lingual Sentiment Dataset 2010 (Webis-
CLS-10)}},
month = jul,
year = 2010,
publisher = {Zenodo},
doi = {10.5281/zenodo.3251672},
url = {https://doi.org/10.5281/zenodo.3251672}
}
**2. Paraphrasing**
French part of the PAWS-X dataset (https://github.com/google-research-datasets/paws).
Three dataframes are available:
- train
- dev
- test
Citation :
> @InProceedings{pawsx2019emnlp,
> title = {{PAWS-X: A Cross-lingual Adversarial Dataset for Paraphrase Identification}},
> author = {Yang, Yinfei and Zhang, Yuan and Tar, Chris and Baldridge, Jason},
> booktitle = {Proc. of EMNLP},
> year = {2019}
> }
**3. Natural Language Inference**
French part of the XNLI dataset (https://github.com/facebookresearch/XNLI).
Three dataframes are available:
- train
- dev
- test
For the dev and test datasets, extra columns compared to the train dataset were available so I left them in the dataframe (I didn't know if these columns could be useful for other tasks or not).
In the context of the FLUE benchmark, only the columns gold_label, sentence1 and sentence2 are useful.
Citation :
> @InProceedings{conneau2018xnli,
> author = "Conneau, Alexis
> and Rinott, Ruty
> and Lample, Guillaume
> and Williams, Adina
> and Bowman, Samuel R.
> and Schwenk, Holger
> and Stoyanov, Veselin",
> title = "XNLI: Evaluating Cross-lingual Sentence Representations",
> booktitle = "Proceedings of the 2018 Conference on Empirical Methods
> in Natural Language Processing",
> year = "2018",
> publisher = "Association for Computational Linguistics",
> location = "Brussels, Belgium",
**4. Parsing**
The dataset used by the FLUE authors for this task is not freely available.
Users of your library will therefore not be able to access it.
Nevertheless, I think maybe it is useful to add a link to the site where to request this dataframe: http://ftb.linguist.univ-paris-diderot.fr/telecharger.php?langue=en
(personally it was sent to me less than 48 hours after I requested it).
**5. Word Sense Disambiguation Tasks**
5.1 Verb Sense Disambiguation
Two dataframes are available: train and test
For both dataframes, 4 columns are available: document, sentence, lemma and word.
I created the document column thinking that there were several documents in the dataset but afterwards it turns out that there were not: several sentences but only one document. It's up to you to keep it or not when importing these two dataframes.
The sentence column is used to determine to which sentence the word in the word column belongs. It is in the form of a dictionary {'id': 'd000.s001', 'idx': '1'}. I thought for a while to keep only the idx because the id doesn't matter any more information. Nevertheless for the test dataset, the dictionary has an extra value indicating the source of the sentence. I don't know if it's useful or not, that's why I left the dictionary just in case. The user is free to do what he wants with it.
Citation :
> Segonne, V., Candito, M., and Crabb ́e, B. (2019). Usingwiktionary as a resource for wsd: the case of frenchverbs. InProceedings of the 13th International Confer-ence on Computational Semantics-Long Papers, pages259–270
5.2 Noun Sense Disambiguation
Two dataframes are available: 2 train and 1 test
I confess I didn't fully understand the procedure for this task.
Citation :
> @dataset{loic_vial_2019_3549806,
> author = {Loïc Vial},
> title = {{French Word Sense Disambiguation with Princeton
> WordNet Identifiers}},
> month = nov,
> year = 2019,
> publisher = {Zenodo},
> version = {1.0},
> doi = {10.5281/zenodo.3549806},
> url = {https://doi.org/10.5281/zenodo.3549806}
> }
Finally, additional information about FLUE is available in the FlauBERT publication :
https://arxiv.org/abs/1912.05372 (p. 4).
Hoping to have provided you with everything you need to add this benchmark :)
| Hi,
I think it would be interesting to add the FLUE dataset for francophones or anyone wishing to work on French.
In other requests, I read that you are already working on some datasets, and I was wondering if FLUE was planned.
If it is not the case, I can provide each of the cleaned FLUE datasets (in the form of a directly exploitable dataset rather than in the original xml formats which require additional processing, with the French part for cases where the dataset is based on a multilingual dataframe, etc.). | 723 | [Feature request] Add FLUE dataset
Hi,
I think it would be interesting to add the FLUE dataset for francophones or anyone wishing to work on French.
In other requests, I read that you are already working on some datasets, and I was wondering if FLUE was planned.
If it is not the case, I can provide each of the cleaned FLUE datasets (in the form of a directly exploitable dataset rather than in the original xml formats which require additional processing, with the French part for cases where the dataset is based on a multilingual dataframe, etc.).
@mariamabarham
I put all the datasets on this drive: https://1drv.ms/u/s!Ao2Rcpiny7RFinDypq7w-LbXcsx9?e=iVsEDh
Some information :
• For FLUE, the quote used is
> @misc{le2019flaubert,
> title={FlauBERT: Unsupervised Language Model Pre-training for French},
> author={Hang Le and Loïc Vial and Jibril Frej and Vincent Segonne and Maximin Coavoux and Benjamin Lecouteux and Alexandre Allauzen and Benoît Crabbé and Laurent Besacier and Didier Schwab},
> year={2019},
> eprint={1912.05372},
> archivePrefix={arXiv},
> primaryClass={cs.CL}
> }
• The Github repo of FLUE is avaible here : https://github.com/getalp/Flaubert/tree/master/flue
Information related to the different tasks of FLUE :
**1. Classification**
Three dataframes are available:
- Book
- DVD
- Music
For each of these dataframes is available a set of training and test data, and a third one containing unlabelled data.
Citation :
>@dataset{prettenhofer_peter_2010_3251672,
author = {Prettenhofer, Peter and
Stein, Benno},
title = {{Webis Cross-Lingual Sentiment Dataset 2010 (Webis-
CLS-10)}},
month = jul,
year = 2010,
publisher = {Zenodo},
doi = {10.5281/zenodo.3251672},
url = {https://doi.org/10.5281/zenodo.3251672}
}
**2. Paraphrasing**
French part of the PAWS-X dataset (https://github.com/google-research-datasets/paws).
Three dataframes are available:
- train
- dev
- test
Citation :
> @InProceedings{pawsx2019emnlp,
> title = {{PAWS-X: A Cross-lingual Adversarial Dataset for Paraphrase Identification}},
> author = {Yang, Yinfei and Zhang, Yuan and Tar, Chris and Baldridge, Jason},
> booktitle = {Proc. of EMNLP},
> year = {2019}
> }
**3. Natural Language Inference**
French part of the XNLI dataset (https://github.com/facebookresearch/XNLI).
Three dataframes are available:
- train
- dev
- test
For the dev and test datasets, extra columns compared to the train dataset were available so I left them in the dataframe (I didn't know if these columns could be useful for other tasks or not).
In the context of the FLUE benchmark, only the columns gold_label, sentence1 and sentence2 are useful.
Citation :
> @InProceedings{conneau2018xnli,
> author = "Conneau, Alexis
> and Rinott, Ruty
> and Lample, Guillaume
> and Williams, Adina
> and Bowman, Samuel R.
> and Schwenk, Holger
> and Stoyanov, Veselin",
> title = "XNLI: Evaluating Cross-lingual Sentence Representations",
> booktitle = "Proceedings of the 2018 Conference on Empirical Methods
> in Natural Language Processing",
> year = "2018",
> publisher = "Association for Computational Linguistics",
> location = "Brussels, Belgium",
**4. Parsing**
The dataset used by the FLUE authors for this task is not freely available.
Users of your library will therefore not be able to access it.
Nevertheless, I think maybe it is useful to add a link to the site where to request this dataframe: http://ftb.linguist.univ-paris-diderot.fr/telecharger.php?langue=en
(personally it was sent to me less than 48 hours after I requested it).
**5. Word Sense Disambiguation Tasks**
5.1 Verb Sense Disambiguation
Two dataframes are available: train and test
For both dataframes, 4 columns are available: document, sentence, lemma and word.
I created the document column thinking that there were several documents in the dataset but afterwards it turns out that there were not: several sentences but only one document. It's up to you to keep it or not when importing these two dataframes.
The sentence column is used to determine to which sentence the word in the word column belongs. It is in the form of a dictionary {'id': 'd000.s001', 'idx': '1'}. I thought for a while to keep only the idx because the id doesn't matter any more information. Nevertheless for the test dataset, the dictionary has an extra value indicating the source of the sentence. I don't know if it's useful or not, that's why I left the dictionary just in case. The user is free to do what he wants with it.
Citation :
> Segonne, V., Candito, M., and Crabb ́e, B. (2019). Usingwiktionary as a resource for wsd: the case of frenchverbs. InProceedings of the 13th International Confer-ence on Computational Semantics-Long Papers, pages259–270
5.2 Noun Sense Disambiguation
Two dataframes are available: 2 train and 1 test
I confess I didn't fully understand the procedure for this task.
Citation :
> @dataset{loic_vial_2019_3549806,
> author = {Loïc Vial},
> title = {{French Word Sense Disambiguation with Princeton
> WordNet Identifiers}},
> month = nov,
> year = 2019,
> publisher = {Zenodo},
> version = {1.0},
> doi = {10.5281/zenodo.3549806},
> url = {https://doi.org/10.5281/zenodo.3549806}
> }
Finally, additional information about FLUE is available in the FlauBERT publication :
https://arxiv.org/abs/1912.05372 (p. 4).
Hoping to have provided you with everything you need to add this benchmark :)
|
https://github.com/huggingface/datasets/issues/222 | Colab Notebook breaks when downloading the squad dataset | The notebook forces version 0.1.0. If I use the latest, things work, I'll run the whole notebook and create a PR.
But in the meantime, this issue gets fixed by changing:
`!pip install nlp==0.1.0`
to
`!pip install nlp` | When I run the notebook in Colab
https://colab.research.google.com/github/huggingface/nlp/blob/master/notebooks/Overview.ipynb
breaks when running this cell:
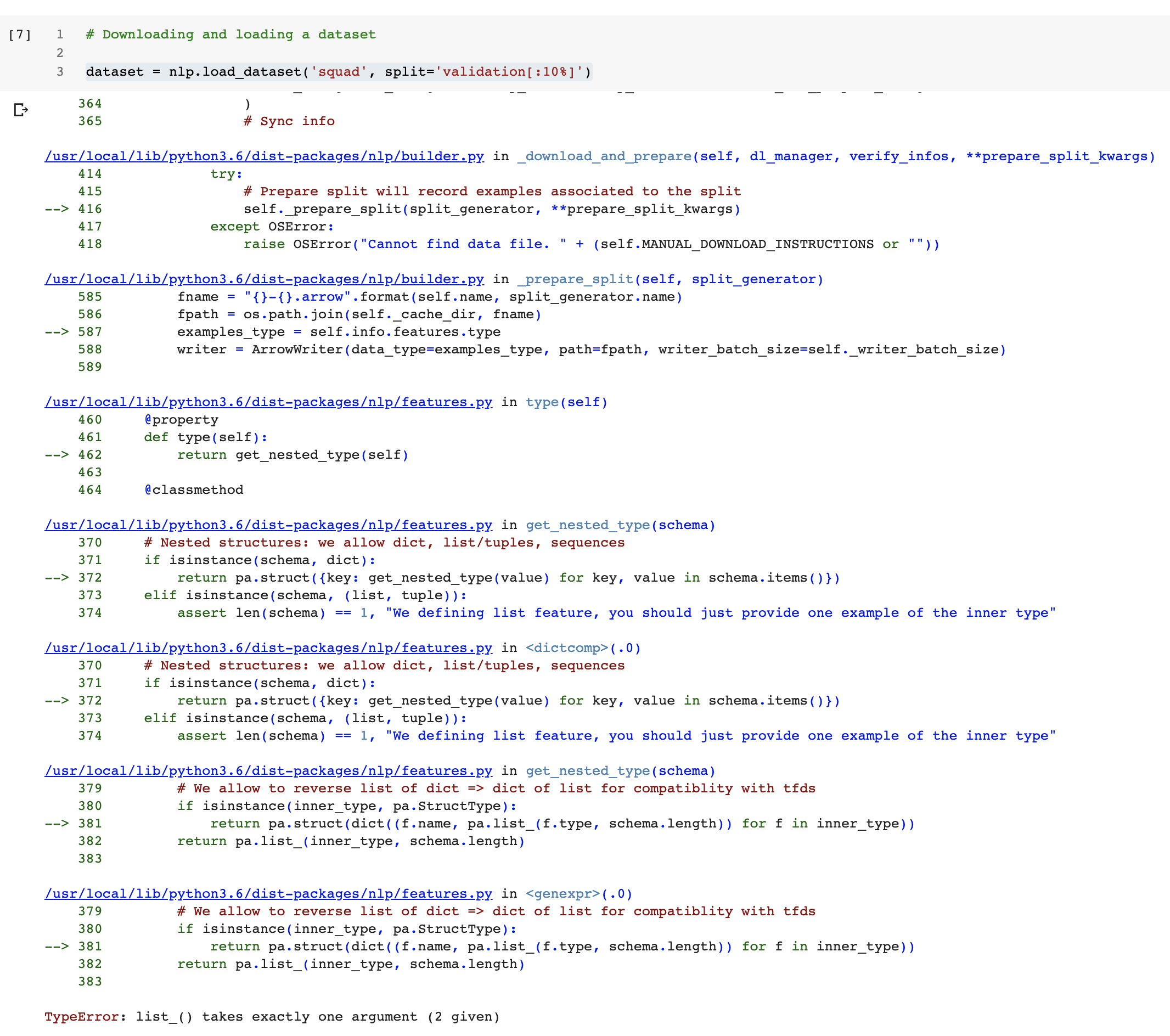
| 38 | Colab Notebook breaks when downloading the squad dataset
When I run the notebook in Colab
https://colab.research.google.com/github/huggingface/nlp/blob/master/notebooks/Overview.ipynb
breaks when running this cell:
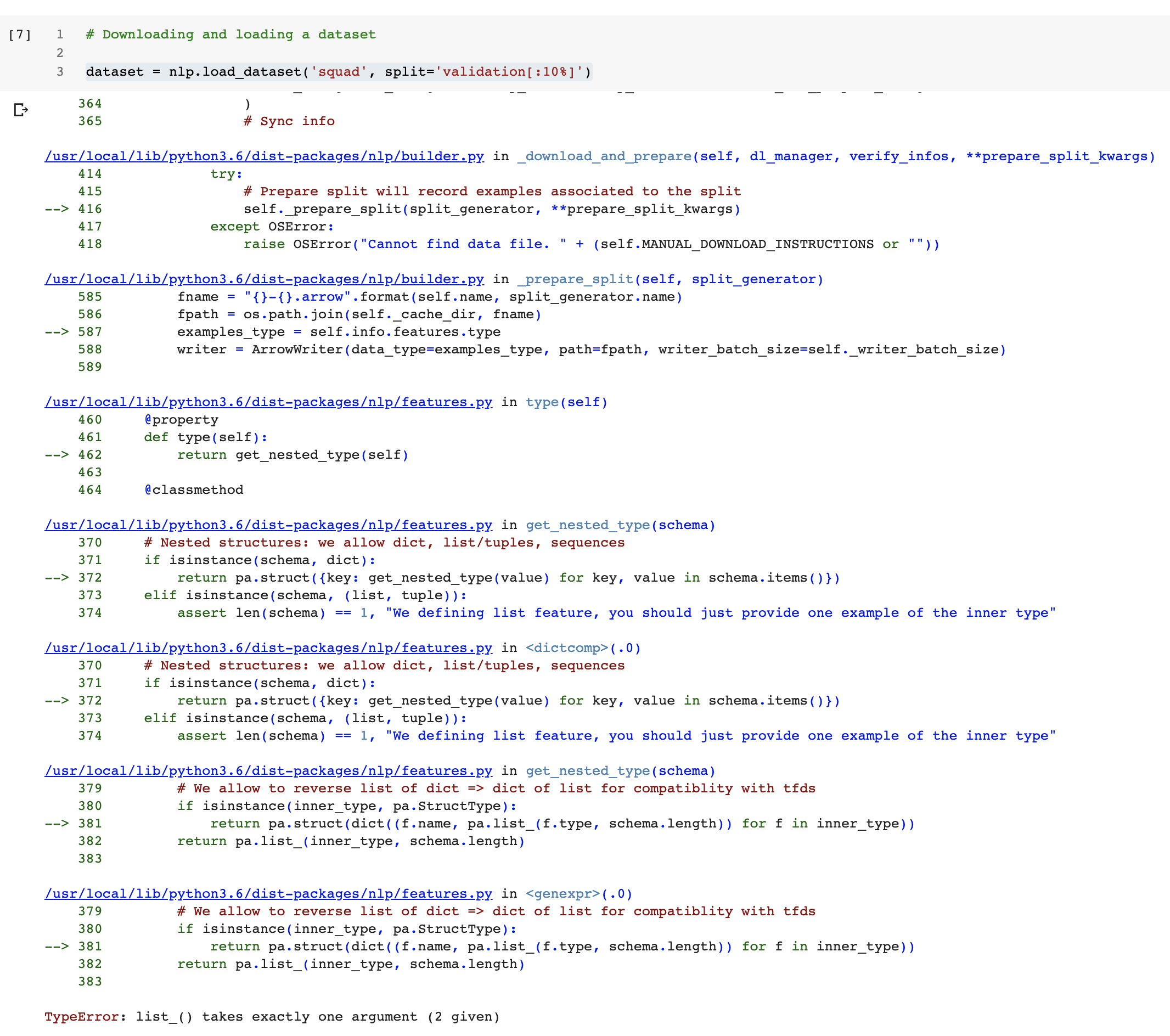
The notebook forces version 0.1.0. If I use the latest, things work, I'll run the whole notebook and create a PR.
But in the meantime, this issue gets fixed by changing:
`!pip install nlp==0.1.0`
to
`!pip install nlp` |
https://github.com/huggingface/datasets/issues/222 | Colab Notebook breaks when downloading the squad dataset | When you install `nlp` for the first time on a Colab runtime, it updates the `pyarrow` library that was already on colab. This update shows this message on colab:
```
WARNING: The following packages were previously imported in this runtime:
[pyarrow]
You must restart the runtime in order to use newly installed versions.
```
You just have to restart the runtime and it should be fine.
If you don't restart, then it breaks like in your first message | When I run the notebook in Colab
https://colab.research.google.com/github/huggingface/nlp/blob/master/notebooks/Overview.ipynb
breaks when running this cell:
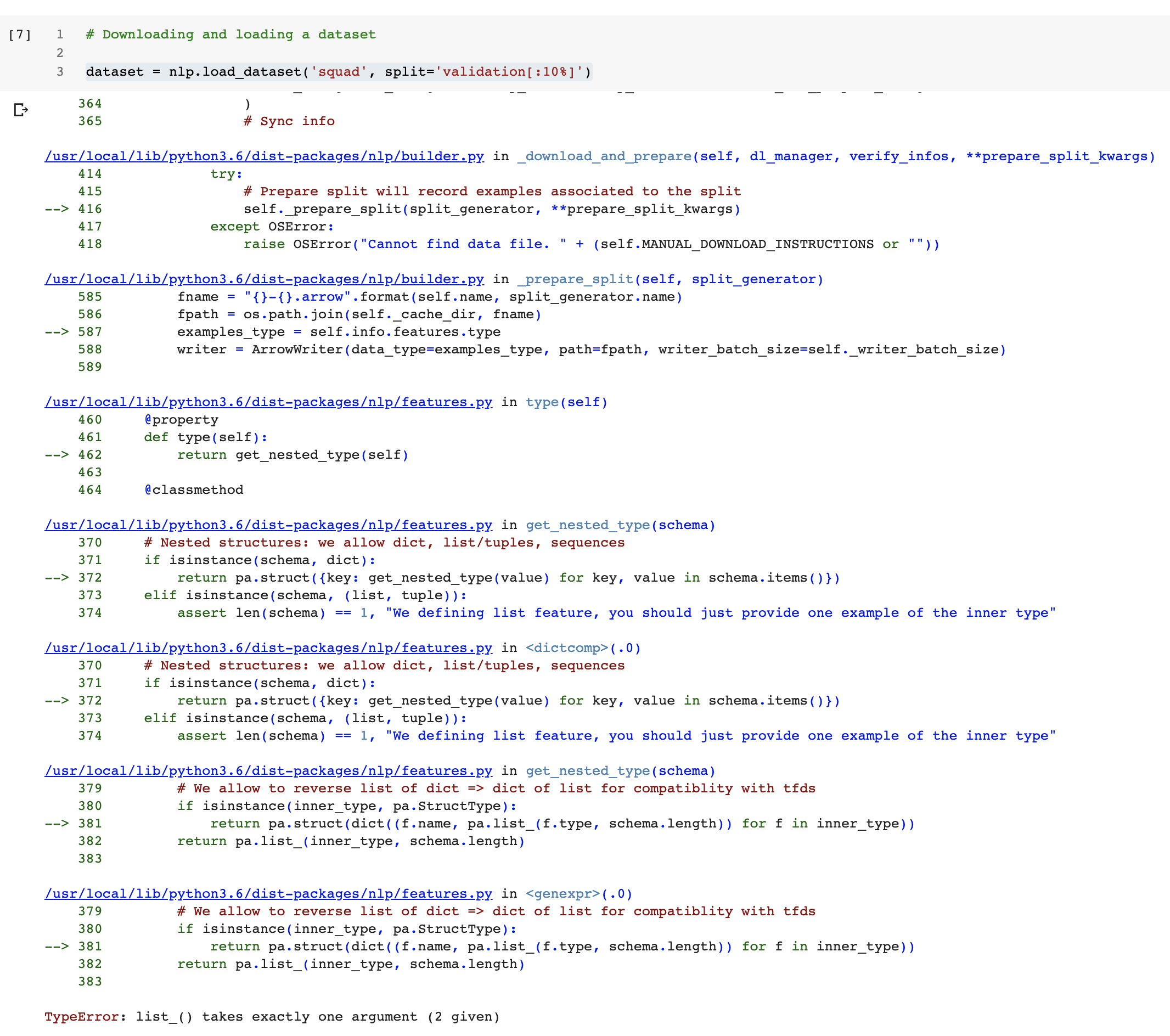
| 78 | Colab Notebook breaks when downloading the squad dataset
When I run the notebook in Colab
https://colab.research.google.com/github/huggingface/nlp/blob/master/notebooks/Overview.ipynb
breaks when running this cell:
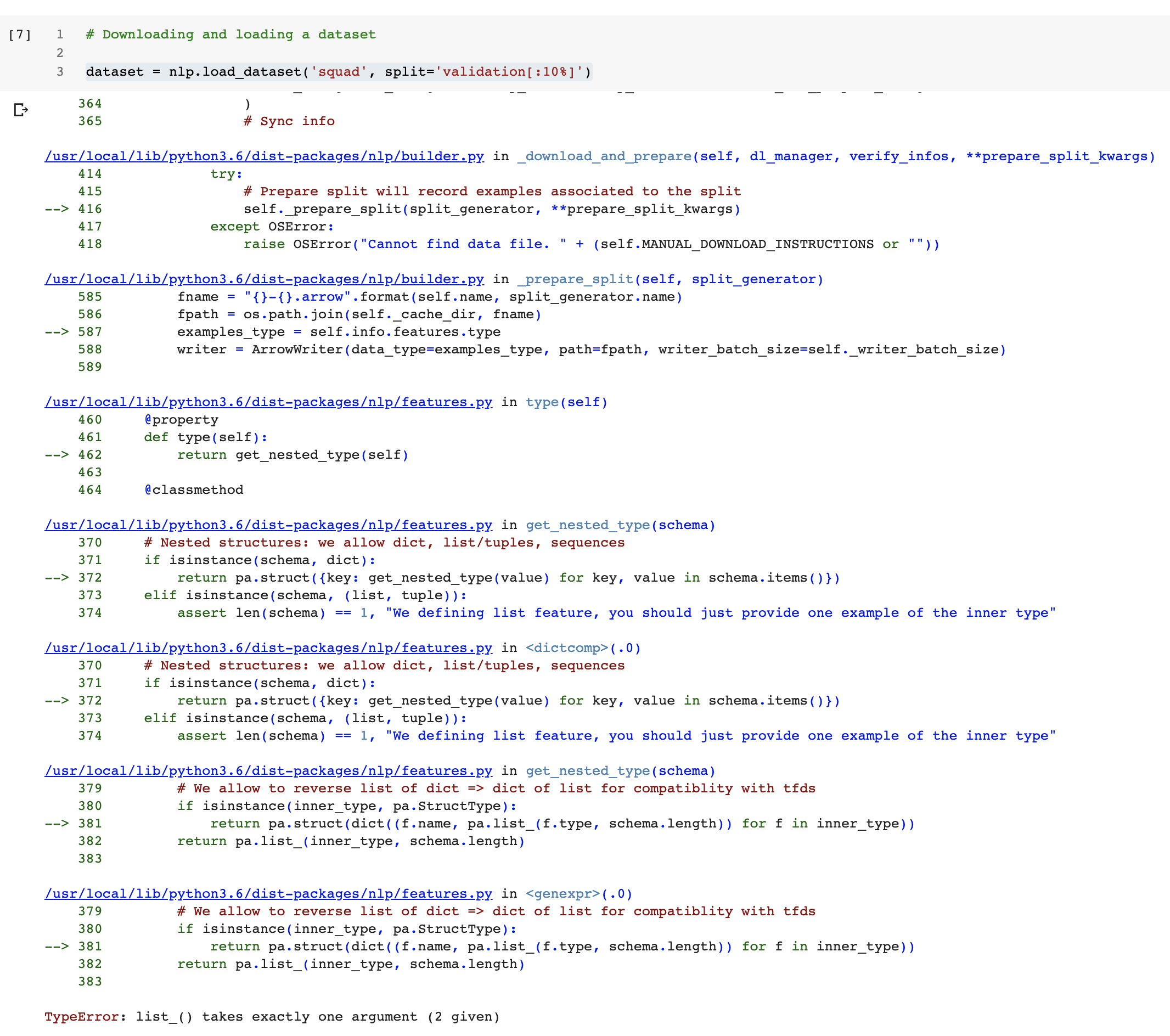
When you install `nlp` for the first time on a Colab runtime, it updates the `pyarrow` library that was already on colab. This update shows this message on colab:
```
WARNING: The following packages were previously imported in this runtime:
[pyarrow]
You must restart the runtime in order to use newly installed versions.
```
You just have to restart the runtime and it should be fine.
If you don't restart, then it breaks like in your first message |
https://github.com/huggingface/datasets/issues/222 | Colab Notebook breaks when downloading the squad dataset | Thanks for reporting the second one ! We'll update the notebook to fix this one :) | When I run the notebook in Colab
https://colab.research.google.com/github/huggingface/nlp/blob/master/notebooks/Overview.ipynb
breaks when running this cell:
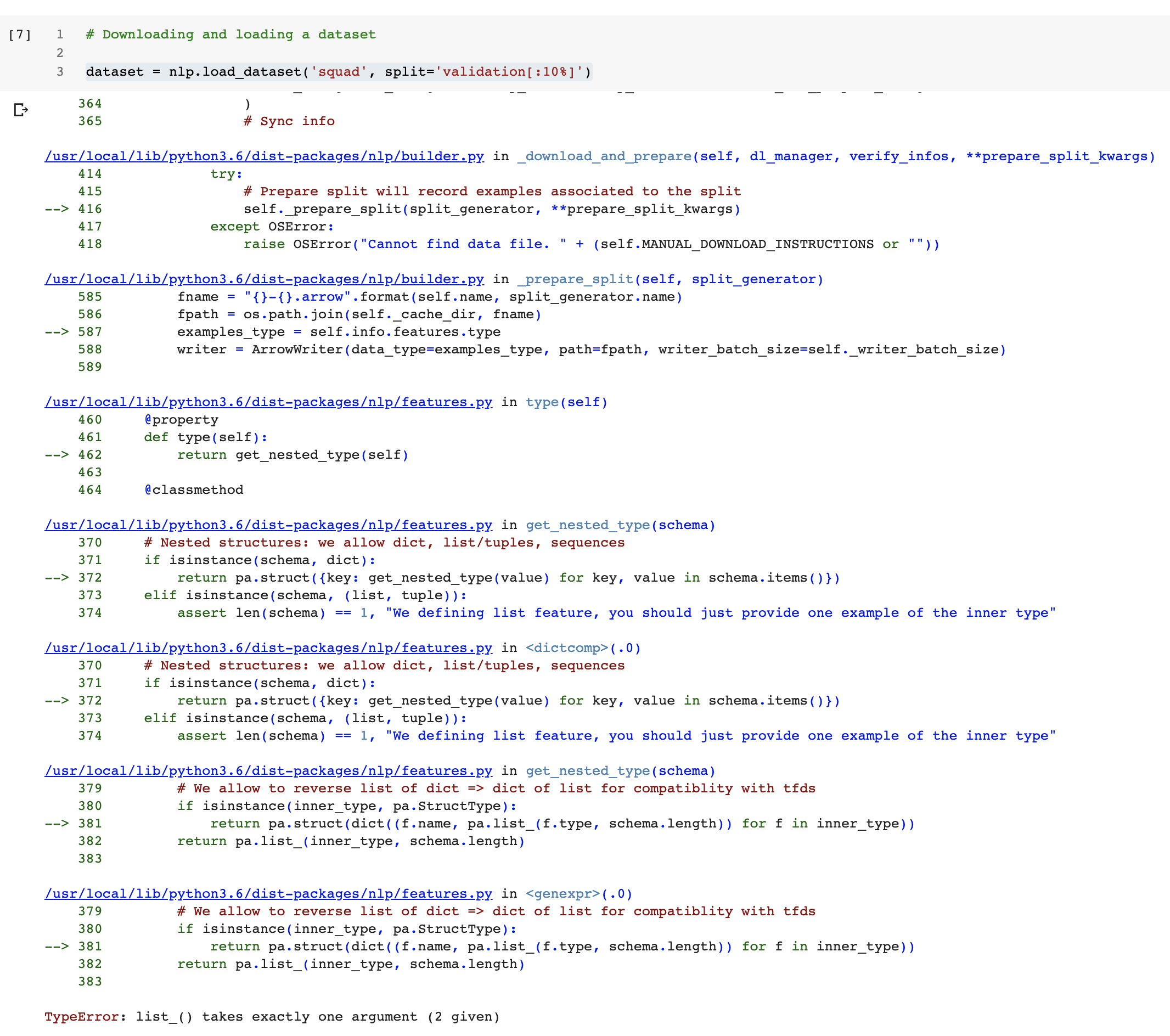
| 16 | Colab Notebook breaks when downloading the squad dataset
When I run the notebook in Colab
https://colab.research.google.com/github/huggingface/nlp/blob/master/notebooks/Overview.ipynb
breaks when running this cell:
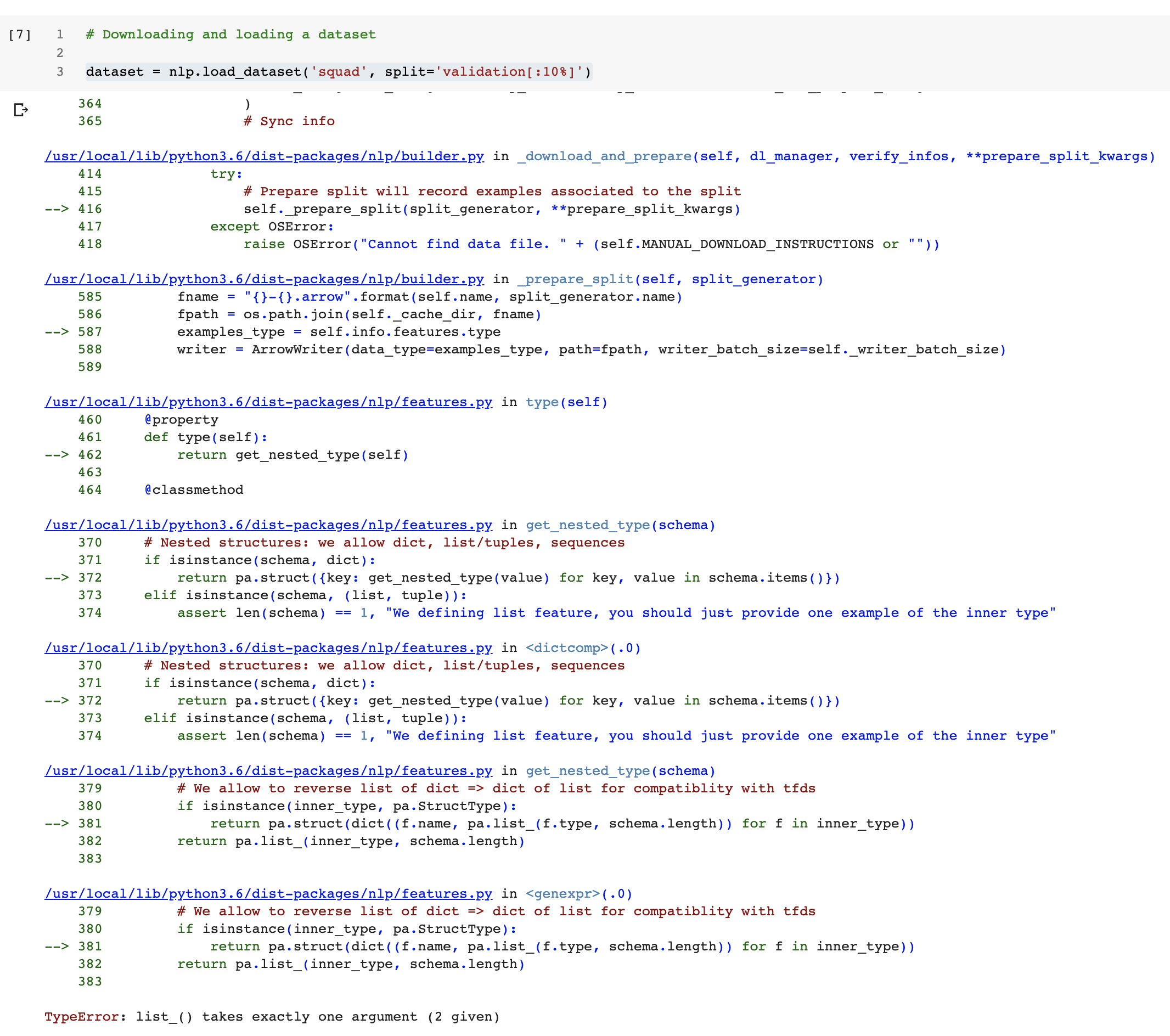
Thanks for reporting the second one ! We'll update the notebook to fix this one :) |
https://github.com/huggingface/datasets/issues/222 | Colab Notebook breaks when downloading the squad dataset | This trick from @thomwolf seems to be the most reliable solution to fix this colab notebook issue:
```python
# install nlp
!pip install -qq nlp==0.2.0
# Make sure that we have a recent version of pyarrow in the session before we continue - otherwise reboot Colab to activate it
import pyarrow
if int(pyarrow.__version__.split('.')[1]) < 16:
import os
os.kill(os.getpid(), 9)
``` | When I run the notebook in Colab
https://colab.research.google.com/github/huggingface/nlp/blob/master/notebooks/Overview.ipynb
breaks when running this cell:
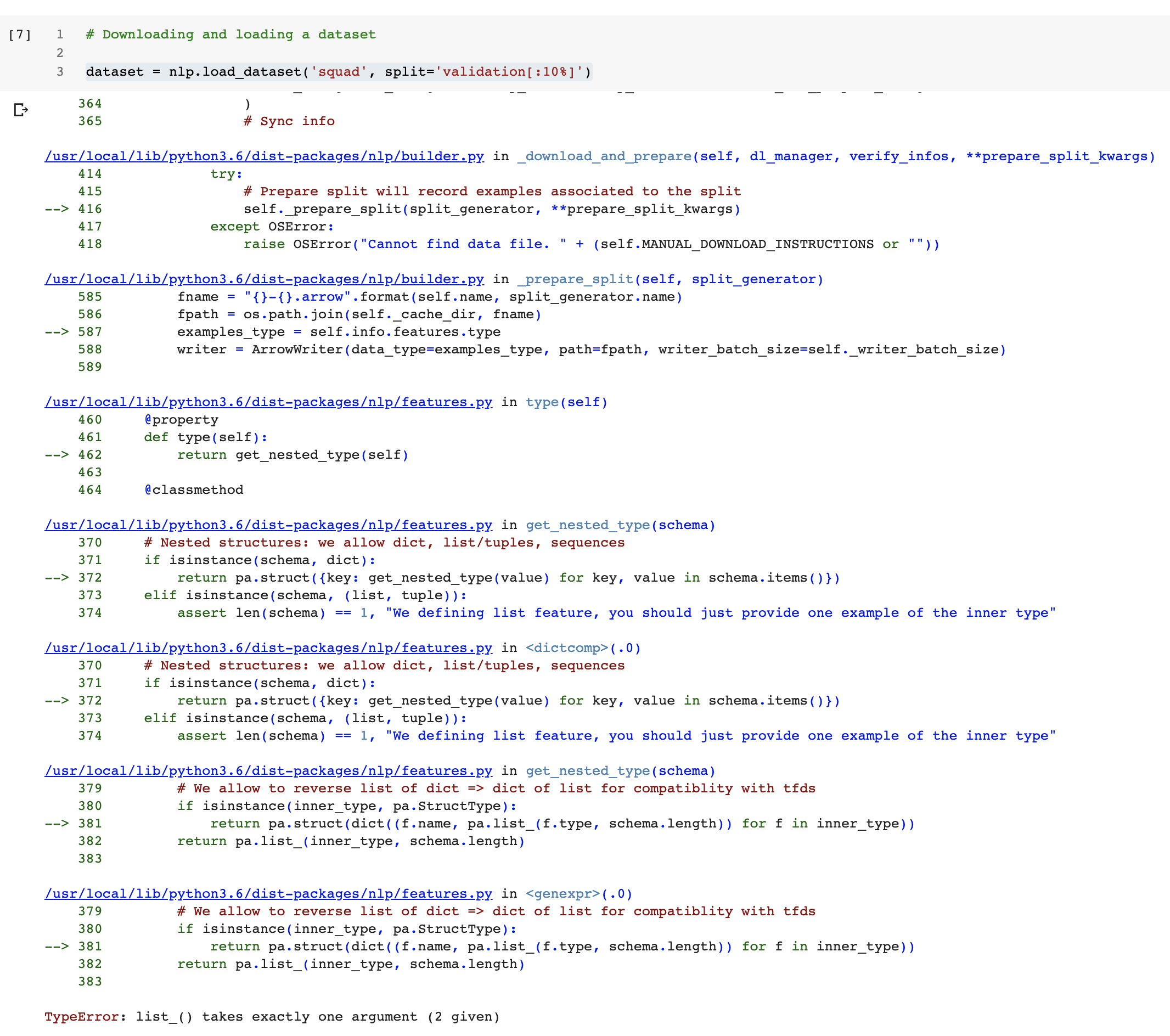
| 60 | Colab Notebook breaks when downloading the squad dataset
When I run the notebook in Colab
https://colab.research.google.com/github/huggingface/nlp/blob/master/notebooks/Overview.ipynb
breaks when running this cell:
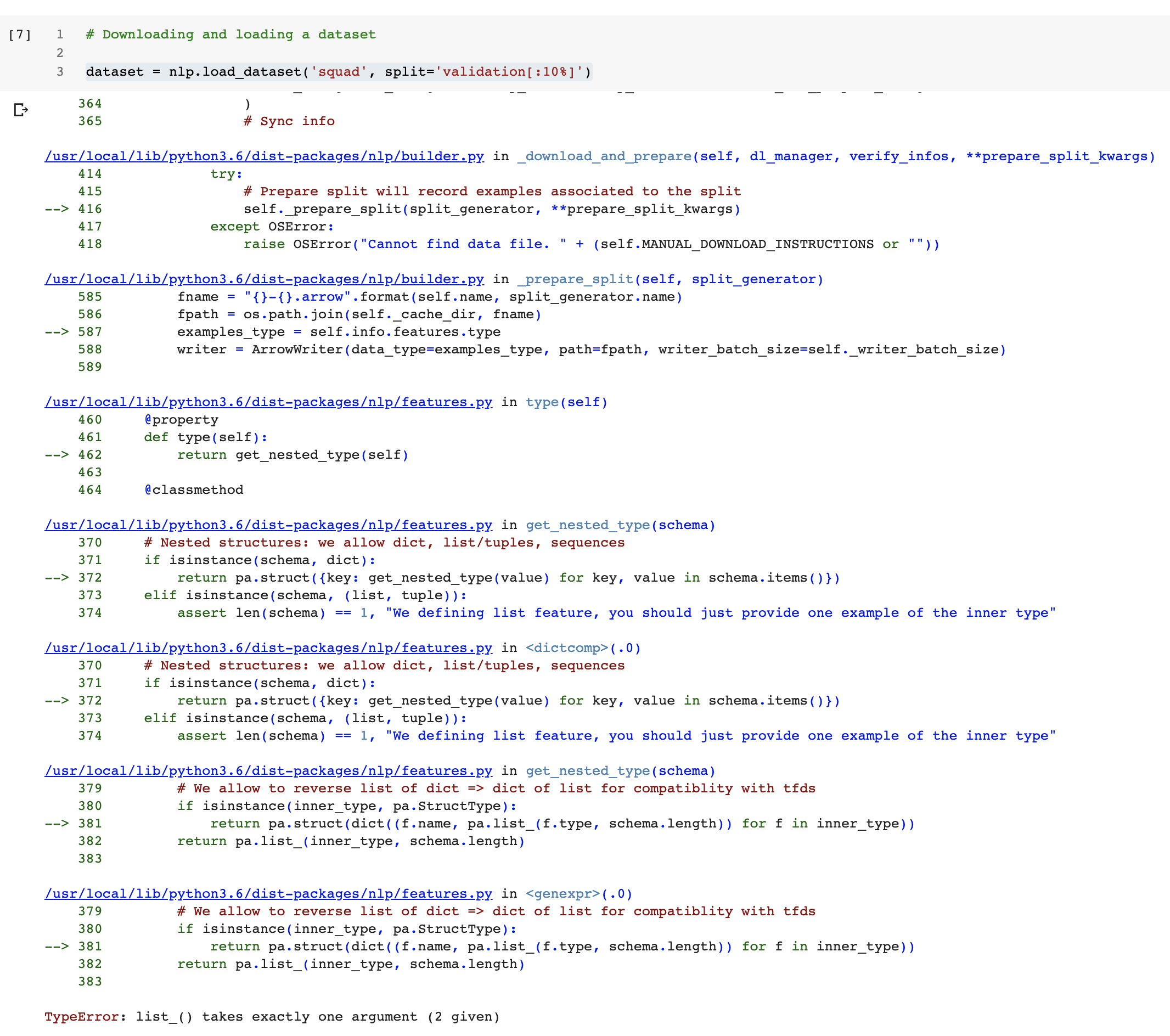
This trick from @thomwolf seems to be the most reliable solution to fix this colab notebook issue:
```python
# install nlp
!pip install -qq nlp==0.2.0
# Make sure that we have a recent version of pyarrow in the session before we continue - otherwise reboot Colab to activate it
import pyarrow
if int(pyarrow.__version__.split('.')[1]) < 16:
import os
os.kill(os.getpid(), 9)
``` |
https://github.com/huggingface/datasets/issues/217 | Multi-task dataset mixing | I like this feature! I think the first question we should decide on is how to convert all datasets into the same format. In T5, the authors decided to format every dataset into a text-to-text format. If the dataset had "multiple" inputs like MNLI, the inputs were concatenated. So in MNLI the input:
> - **Hypothesis**: The St. Louis Cardinals have always won.
>
> - **Premise**: yeah well losing is i mean i’m i’m originally from Saint Louis and Saint Louis Cardinals when they were there were uh a mostly a losing team but
was flattened to a single input:
> mnli hypothesis: The St. Louis Cardinals have always won. premise:
> yeah well losing is i mean i’m i’m originally from Saint Louis and Saint Louis Cardinals
> when they were there were uh a mostly a losing team but.
This flattening is actually a very simple operation in `nlp` already. You would just need to do the following:
```python
def flatten_inputs(example):
return {"input": "mnli hypothesis: " + example['hypothesis'] + " premise: " + example['premise']}
t5_ready_mnli_ds = mnli_ds.map(flatten_inputs, remove_columns=[<all columns except output>])
```
So I guess converting the datasets into the same format can be left to the user for now.
Then the question is how we can merge the datasets. I would probably be in favor of a simple
```python
dataset.add()
```
function that checks if the dataset is of the same format and if yes merges the two datasets. Finally, how should the sampling be implemented? **Examples-proportional mixing** corresponds to just merging the datasets and shuffling. For the other two sampling approaches we would need some higher-level features, maybe even a `dataset.sample()` function for merged datasets.
What are your thoughts on this @thomwolf @lhoestq @ghomasHudson @enzoampil ? | It seems like many of the best performing models on the GLUE benchmark make some use of multitask learning (simultaneous training on multiple tasks).
The [T5 paper](https://arxiv.org/pdf/1910.10683.pdf) highlights multiple ways of mixing the tasks together during finetuning:
- **Examples-proportional mixing** - sample from tasks proportionally to their dataset size
- **Equal mixing** - sample uniformly from each task
- **Temperature-scaled mixing** - The generalized approach used by multilingual BERT which uses a temperature T, where the mixing rate of each task is raised to the power 1/T and renormalized. When T=1 this is equivalent to equal mixing, and becomes closer to equal mixing with increasing T.
Following this discussion https://github.com/huggingface/transformers/issues/4340 in [transformers](https://github.com/huggingface/transformers), @enzoampil suggested that the `nlp` library might be a better place for this functionality.
Some method for combining datasets could be implemented ,e.g.
```
dataset = nlp.load_multitask(['squad','imdb','cnn_dm'], temperature=2.0, ...)
```
We would need a few additions:
- Method of identifying the tasks - how can we support adding a string to each task as an identifier: e.g. 'summarisation: '?
- Method of combining the metrics - a standard approach is to use the specific metric for each task and add them together for a combined score.
It would be great to support common use cases such as pretraining on the GLUE benchmark before fine-tuning on each GLUE task in turn.
I'm willing to write bits/most of this I just need some guidance on the interface and other library details so I can integrate it properly.
| 291 | Multi-task dataset mixing
It seems like many of the best performing models on the GLUE benchmark make some use of multitask learning (simultaneous training on multiple tasks).
The [T5 paper](https://arxiv.org/pdf/1910.10683.pdf) highlights multiple ways of mixing the tasks together during finetuning:
- **Examples-proportional mixing** - sample from tasks proportionally to their dataset size
- **Equal mixing** - sample uniformly from each task
- **Temperature-scaled mixing** - The generalized approach used by multilingual BERT which uses a temperature T, where the mixing rate of each task is raised to the power 1/T and renormalized. When T=1 this is equivalent to equal mixing, and becomes closer to equal mixing with increasing T.
Following this discussion https://github.com/huggingface/transformers/issues/4340 in [transformers](https://github.com/huggingface/transformers), @enzoampil suggested that the `nlp` library might be a better place for this functionality.
Some method for combining datasets could be implemented ,e.g.
```
dataset = nlp.load_multitask(['squad','imdb','cnn_dm'], temperature=2.0, ...)
```
We would need a few additions:
- Method of identifying the tasks - how can we support adding a string to each task as an identifier: e.g. 'summarisation: '?
- Method of combining the metrics - a standard approach is to use the specific metric for each task and add them together for a combined score.
It would be great to support common use cases such as pretraining on the GLUE benchmark before fine-tuning on each GLUE task in turn.
I'm willing to write bits/most of this I just need some guidance on the interface and other library details so I can integrate it properly.
I like this feature! I think the first question we should decide on is how to convert all datasets into the same format. In T5, the authors decided to format every dataset into a text-to-text format. If the dataset had "multiple" inputs like MNLI, the inputs were concatenated. So in MNLI the input:
> - **Hypothesis**: The St. Louis Cardinals have always won.
>
> - **Premise**: yeah well losing is i mean i’m i’m originally from Saint Louis and Saint Louis Cardinals when they were there were uh a mostly a losing team but
was flattened to a single input:
> mnli hypothesis: The St. Louis Cardinals have always won. premise:
> yeah well losing is i mean i’m i’m originally from Saint Louis and Saint Louis Cardinals
> when they were there were uh a mostly a losing team but.
This flattening is actually a very simple operation in `nlp` already. You would just need to do the following:
```python
def flatten_inputs(example):
return {"input": "mnli hypothesis: " + example['hypothesis'] + " premise: " + example['premise']}
t5_ready_mnli_ds = mnli_ds.map(flatten_inputs, remove_columns=[<all columns except output>])
```
So I guess converting the datasets into the same format can be left to the user for now.
Then the question is how we can merge the datasets. I would probably be in favor of a simple
```python
dataset.add()
```
function that checks if the dataset is of the same format and if yes merges the two datasets. Finally, how should the sampling be implemented? **Examples-proportional mixing** corresponds to just merging the datasets and shuffling. For the other two sampling approaches we would need some higher-level features, maybe even a `dataset.sample()` function for merged datasets.
What are your thoughts on this @thomwolf @lhoestq @ghomasHudson @enzoampil ? |
https://github.com/huggingface/datasets/issues/217 | Multi-task dataset mixing | I agree that we should leave the flattening of the dataset to the user for now. Especially because although the T5 framing seems obvious, there are slight variations on how the T5 authors do it in comparison to other approaches such as gpt-3 and decaNLP.
In terms of sampling, Examples-proportional mixing does seem the simplest to implement so would probably be a good starting point.
Temperature-scaled mixing would probably most useful, offering flexibility as it can simulate the other 2 methods by setting the temperature parameter. There is a [relevant part of the T5 repo](https://github.com/google-research/text-to-text-transfer-transformer/blob/03c94165a7d52e4f7230e5944a0541d8c5710788/t5/data/utils.py#L889-L1118) which should help with implementation.
According to the T5 authors, equal-mixing performs worst. Among the other two methods, tuning the K value (the artificial dataset size limit) has a large impact.
| It seems like many of the best performing models on the GLUE benchmark make some use of multitask learning (simultaneous training on multiple tasks).
The [T5 paper](https://arxiv.org/pdf/1910.10683.pdf) highlights multiple ways of mixing the tasks together during finetuning:
- **Examples-proportional mixing** - sample from tasks proportionally to their dataset size
- **Equal mixing** - sample uniformly from each task
- **Temperature-scaled mixing** - The generalized approach used by multilingual BERT which uses a temperature T, where the mixing rate of each task is raised to the power 1/T and renormalized. When T=1 this is equivalent to equal mixing, and becomes closer to equal mixing with increasing T.
Following this discussion https://github.com/huggingface/transformers/issues/4340 in [transformers](https://github.com/huggingface/transformers), @enzoampil suggested that the `nlp` library might be a better place for this functionality.
Some method for combining datasets could be implemented ,e.g.
```
dataset = nlp.load_multitask(['squad','imdb','cnn_dm'], temperature=2.0, ...)
```
We would need a few additions:
- Method of identifying the tasks - how can we support adding a string to each task as an identifier: e.g. 'summarisation: '?
- Method of combining the metrics - a standard approach is to use the specific metric for each task and add them together for a combined score.
It would be great to support common use cases such as pretraining on the GLUE benchmark before fine-tuning on each GLUE task in turn.
I'm willing to write bits/most of this I just need some guidance on the interface and other library details so I can integrate it properly.
| 126 | Multi-task dataset mixing
It seems like many of the best performing models on the GLUE benchmark make some use of multitask learning (simultaneous training on multiple tasks).
The [T5 paper](https://arxiv.org/pdf/1910.10683.pdf) highlights multiple ways of mixing the tasks together during finetuning:
- **Examples-proportional mixing** - sample from tasks proportionally to their dataset size
- **Equal mixing** - sample uniformly from each task
- **Temperature-scaled mixing** - The generalized approach used by multilingual BERT which uses a temperature T, where the mixing rate of each task is raised to the power 1/T and renormalized. When T=1 this is equivalent to equal mixing, and becomes closer to equal mixing with increasing T.
Following this discussion https://github.com/huggingface/transformers/issues/4340 in [transformers](https://github.com/huggingface/transformers), @enzoampil suggested that the `nlp` library might be a better place for this functionality.
Some method for combining datasets could be implemented ,e.g.
```
dataset = nlp.load_multitask(['squad','imdb','cnn_dm'], temperature=2.0, ...)
```
We would need a few additions:
- Method of identifying the tasks - how can we support adding a string to each task as an identifier: e.g. 'summarisation: '?
- Method of combining the metrics - a standard approach is to use the specific metric for each task and add them together for a combined score.
It would be great to support common use cases such as pretraining on the GLUE benchmark before fine-tuning on each GLUE task in turn.
I'm willing to write bits/most of this I just need some guidance on the interface and other library details so I can integrate it properly.
I agree that we should leave the flattening of the dataset to the user for now. Especially because although the T5 framing seems obvious, there are slight variations on how the T5 authors do it in comparison to other approaches such as gpt-3 and decaNLP.
In terms of sampling, Examples-proportional mixing does seem the simplest to implement so would probably be a good starting point.
Temperature-scaled mixing would probably most useful, offering flexibility as it can simulate the other 2 methods by setting the temperature parameter. There is a [relevant part of the T5 repo](https://github.com/google-research/text-to-text-transfer-transformer/blob/03c94165a7d52e4f7230e5944a0541d8c5710788/t5/data/utils.py#L889-L1118) which should help with implementation.
According to the T5 authors, equal-mixing performs worst. Among the other two methods, tuning the K value (the artificial dataset size limit) has a large impact.
|
https://github.com/huggingface/datasets/issues/217 | Multi-task dataset mixing | I agree with going with temperature-scaled mixing for its flexibility!
For the function that combines the datasets, I also find `dataset.add()` okay while also considering that users may want it to be easy to combine a list of say 10 data sources in one go.
`dataset.sample()` should also be good. By the looks of it, we're planning to have as main parameters: `temperature`, and `K`.
On converting the datasets to the same format, I agree that we can leave these to the users for now. But, I do imagine it'd be an awesome feature for the future to have this automatically handled, based on a chosen *approach* to formatting :smile:
E.g. T5, GPT-3, decaNLP, original raw formatting, or a contributed way of formatting in text-to-text. | It seems like many of the best performing models on the GLUE benchmark make some use of multitask learning (simultaneous training on multiple tasks).
The [T5 paper](https://arxiv.org/pdf/1910.10683.pdf) highlights multiple ways of mixing the tasks together during finetuning:
- **Examples-proportional mixing** - sample from tasks proportionally to their dataset size
- **Equal mixing** - sample uniformly from each task
- **Temperature-scaled mixing** - The generalized approach used by multilingual BERT which uses a temperature T, where the mixing rate of each task is raised to the power 1/T and renormalized. When T=1 this is equivalent to equal mixing, and becomes closer to equal mixing with increasing T.
Following this discussion https://github.com/huggingface/transformers/issues/4340 in [transformers](https://github.com/huggingface/transformers), @enzoampil suggested that the `nlp` library might be a better place for this functionality.
Some method for combining datasets could be implemented ,e.g.
```
dataset = nlp.load_multitask(['squad','imdb','cnn_dm'], temperature=2.0, ...)
```
We would need a few additions:
- Method of identifying the tasks - how can we support adding a string to each task as an identifier: e.g. 'summarisation: '?
- Method of combining the metrics - a standard approach is to use the specific metric for each task and add them together for a combined score.
It would be great to support common use cases such as pretraining on the GLUE benchmark before fine-tuning on each GLUE task in turn.
I'm willing to write bits/most of this I just need some guidance on the interface and other library details so I can integrate it properly.
| 125 | Multi-task dataset mixing
It seems like many of the best performing models on the GLUE benchmark make some use of multitask learning (simultaneous training on multiple tasks).
The [T5 paper](https://arxiv.org/pdf/1910.10683.pdf) highlights multiple ways of mixing the tasks together during finetuning:
- **Examples-proportional mixing** - sample from tasks proportionally to their dataset size
- **Equal mixing** - sample uniformly from each task
- **Temperature-scaled mixing** - The generalized approach used by multilingual BERT which uses a temperature T, where the mixing rate of each task is raised to the power 1/T and renormalized. When T=1 this is equivalent to equal mixing, and becomes closer to equal mixing with increasing T.
Following this discussion https://github.com/huggingface/transformers/issues/4340 in [transformers](https://github.com/huggingface/transformers), @enzoampil suggested that the `nlp` library might be a better place for this functionality.
Some method for combining datasets could be implemented ,e.g.
```
dataset = nlp.load_multitask(['squad','imdb','cnn_dm'], temperature=2.0, ...)
```
We would need a few additions:
- Method of identifying the tasks - how can we support adding a string to each task as an identifier: e.g. 'summarisation: '?
- Method of combining the metrics - a standard approach is to use the specific metric for each task and add them together for a combined score.
It would be great to support common use cases such as pretraining on the GLUE benchmark before fine-tuning on each GLUE task in turn.
I'm willing to write bits/most of this I just need some guidance on the interface and other library details so I can integrate it properly.
I agree with going with temperature-scaled mixing for its flexibility!
For the function that combines the datasets, I also find `dataset.add()` okay while also considering that users may want it to be easy to combine a list of say 10 data sources in one go.
`dataset.sample()` should also be good. By the looks of it, we're planning to have as main parameters: `temperature`, and `K`.
On converting the datasets to the same format, I agree that we can leave these to the users for now. But, I do imagine it'd be an awesome feature for the future to have this automatically handled, based on a chosen *approach* to formatting :smile:
E.g. T5, GPT-3, decaNLP, original raw formatting, or a contributed way of formatting in text-to-text. |
https://github.com/huggingface/datasets/issues/217 | Multi-task dataset mixing | This is an interesting discussion indeed and it would be nice to make multi-task easier.
Probably the best would be to have a new type of dataset especially designed for that in order to easily combine and sample from the multiple datasets.
This way we could probably handle the combination of datasets with differing schemas as well (unlike T5). | It seems like many of the best performing models on the GLUE benchmark make some use of multitask learning (simultaneous training on multiple tasks).
The [T5 paper](https://arxiv.org/pdf/1910.10683.pdf) highlights multiple ways of mixing the tasks together during finetuning:
- **Examples-proportional mixing** - sample from tasks proportionally to their dataset size
- **Equal mixing** - sample uniformly from each task
- **Temperature-scaled mixing** - The generalized approach used by multilingual BERT which uses a temperature T, where the mixing rate of each task is raised to the power 1/T and renormalized. When T=1 this is equivalent to equal mixing, and becomes closer to equal mixing with increasing T.
Following this discussion https://github.com/huggingface/transformers/issues/4340 in [transformers](https://github.com/huggingface/transformers), @enzoampil suggested that the `nlp` library might be a better place for this functionality.
Some method for combining datasets could be implemented ,e.g.
```
dataset = nlp.load_multitask(['squad','imdb','cnn_dm'], temperature=2.0, ...)
```
We would need a few additions:
- Method of identifying the tasks - how can we support adding a string to each task as an identifier: e.g. 'summarisation: '?
- Method of combining the metrics - a standard approach is to use the specific metric for each task and add them together for a combined score.
It would be great to support common use cases such as pretraining on the GLUE benchmark before fine-tuning on each GLUE task in turn.
I'm willing to write bits/most of this I just need some guidance on the interface and other library details so I can integrate it properly.
| 59 | Multi-task dataset mixing
It seems like many of the best performing models on the GLUE benchmark make some use of multitask learning (simultaneous training on multiple tasks).
The [T5 paper](https://arxiv.org/pdf/1910.10683.pdf) highlights multiple ways of mixing the tasks together during finetuning:
- **Examples-proportional mixing** - sample from tasks proportionally to their dataset size
- **Equal mixing** - sample uniformly from each task
- **Temperature-scaled mixing** - The generalized approach used by multilingual BERT which uses a temperature T, where the mixing rate of each task is raised to the power 1/T and renormalized. When T=1 this is equivalent to equal mixing, and becomes closer to equal mixing with increasing T.
Following this discussion https://github.com/huggingface/transformers/issues/4340 in [transformers](https://github.com/huggingface/transformers), @enzoampil suggested that the `nlp` library might be a better place for this functionality.
Some method for combining datasets could be implemented ,e.g.
```
dataset = nlp.load_multitask(['squad','imdb','cnn_dm'], temperature=2.0, ...)
```
We would need a few additions:
- Method of identifying the tasks - how can we support adding a string to each task as an identifier: e.g. 'summarisation: '?
- Method of combining the metrics - a standard approach is to use the specific metric for each task and add them together for a combined score.
It would be great to support common use cases such as pretraining on the GLUE benchmark before fine-tuning on each GLUE task in turn.
I'm willing to write bits/most of this I just need some guidance on the interface and other library details so I can integrate it properly.
This is an interesting discussion indeed and it would be nice to make multi-task easier.
Probably the best would be to have a new type of dataset especially designed for that in order to easily combine and sample from the multiple datasets.
This way we could probably handle the combination of datasets with differing schemas as well (unlike T5). |
https://github.com/huggingface/datasets/issues/217 | Multi-task dataset mixing | @thomwolf Are you suggesting making a wrapper class which can take existing datasets as arguments and do all the required sampling/combining, to present the same interface as a normal dataset?
That doesn't seem too complicated to implement.
| It seems like many of the best performing models on the GLUE benchmark make some use of multitask learning (simultaneous training on multiple tasks).
The [T5 paper](https://arxiv.org/pdf/1910.10683.pdf) highlights multiple ways of mixing the tasks together during finetuning:
- **Examples-proportional mixing** - sample from tasks proportionally to their dataset size
- **Equal mixing** - sample uniformly from each task
- **Temperature-scaled mixing** - The generalized approach used by multilingual BERT which uses a temperature T, where the mixing rate of each task is raised to the power 1/T and renormalized. When T=1 this is equivalent to equal mixing, and becomes closer to equal mixing with increasing T.
Following this discussion https://github.com/huggingface/transformers/issues/4340 in [transformers](https://github.com/huggingface/transformers), @enzoampil suggested that the `nlp` library might be a better place for this functionality.
Some method for combining datasets could be implemented ,e.g.
```
dataset = nlp.load_multitask(['squad','imdb','cnn_dm'], temperature=2.0, ...)
```
We would need a few additions:
- Method of identifying the tasks - how can we support adding a string to each task as an identifier: e.g. 'summarisation: '?
- Method of combining the metrics - a standard approach is to use the specific metric for each task and add them together for a combined score.
It would be great to support common use cases such as pretraining on the GLUE benchmark before fine-tuning on each GLUE task in turn.
I'm willing to write bits/most of this I just need some guidance on the interface and other library details so I can integrate it properly.
| 37 | Multi-task dataset mixing
It seems like many of the best performing models on the GLUE benchmark make some use of multitask learning (simultaneous training on multiple tasks).
The [T5 paper](https://arxiv.org/pdf/1910.10683.pdf) highlights multiple ways of mixing the tasks together during finetuning:
- **Examples-proportional mixing** - sample from tasks proportionally to their dataset size
- **Equal mixing** - sample uniformly from each task
- **Temperature-scaled mixing** - The generalized approach used by multilingual BERT which uses a temperature T, where the mixing rate of each task is raised to the power 1/T and renormalized. When T=1 this is equivalent to equal mixing, and becomes closer to equal mixing with increasing T.
Following this discussion https://github.com/huggingface/transformers/issues/4340 in [transformers](https://github.com/huggingface/transformers), @enzoampil suggested that the `nlp` library might be a better place for this functionality.
Some method for combining datasets could be implemented ,e.g.
```
dataset = nlp.load_multitask(['squad','imdb','cnn_dm'], temperature=2.0, ...)
```
We would need a few additions:
- Method of identifying the tasks - how can we support adding a string to each task as an identifier: e.g. 'summarisation: '?
- Method of combining the metrics - a standard approach is to use the specific metric for each task and add them together for a combined score.
It would be great to support common use cases such as pretraining on the GLUE benchmark before fine-tuning on each GLUE task in turn.
I'm willing to write bits/most of this I just need some guidance on the interface and other library details so I can integrate it properly.
@thomwolf Are you suggesting making a wrapper class which can take existing datasets as arguments and do all the required sampling/combining, to present the same interface as a normal dataset?
That doesn't seem too complicated to implement.
|
https://github.com/huggingface/datasets/issues/217 | Multi-task dataset mixing | I guess we're looking at the end user writing something like:
``` python
ds = nlp.load_dataset('multitask-t5',datasets=["squad","cnn_dm",...], k=1000, t=2.0)
```
Using the t5 method of combining here (or this could be a function passed in as an arg)
Passing kwargs to each 'sub-dataset' might become tricky. | It seems like many of the best performing models on the GLUE benchmark make some use of multitask learning (simultaneous training on multiple tasks).
The [T5 paper](https://arxiv.org/pdf/1910.10683.pdf) highlights multiple ways of mixing the tasks together during finetuning:
- **Examples-proportional mixing** - sample from tasks proportionally to their dataset size
- **Equal mixing** - sample uniformly from each task
- **Temperature-scaled mixing** - The generalized approach used by multilingual BERT which uses a temperature T, where the mixing rate of each task is raised to the power 1/T and renormalized. When T=1 this is equivalent to equal mixing, and becomes closer to equal mixing with increasing T.
Following this discussion https://github.com/huggingface/transformers/issues/4340 in [transformers](https://github.com/huggingface/transformers), @enzoampil suggested that the `nlp` library might be a better place for this functionality.
Some method for combining datasets could be implemented ,e.g.
```
dataset = nlp.load_multitask(['squad','imdb','cnn_dm'], temperature=2.0, ...)
```
We would need a few additions:
- Method of identifying the tasks - how can we support adding a string to each task as an identifier: e.g. 'summarisation: '?
- Method of combining the metrics - a standard approach is to use the specific metric for each task and add them together for a combined score.
It would be great to support common use cases such as pretraining on the GLUE benchmark before fine-tuning on each GLUE task in turn.
I'm willing to write bits/most of this I just need some guidance on the interface and other library details so I can integrate it properly.
| 45 | Multi-task dataset mixing
It seems like many of the best performing models on the GLUE benchmark make some use of multitask learning (simultaneous training on multiple tasks).
The [T5 paper](https://arxiv.org/pdf/1910.10683.pdf) highlights multiple ways of mixing the tasks together during finetuning:
- **Examples-proportional mixing** - sample from tasks proportionally to their dataset size
- **Equal mixing** - sample uniformly from each task
- **Temperature-scaled mixing** - The generalized approach used by multilingual BERT which uses a temperature T, where the mixing rate of each task is raised to the power 1/T and renormalized. When T=1 this is equivalent to equal mixing, and becomes closer to equal mixing with increasing T.
Following this discussion https://github.com/huggingface/transformers/issues/4340 in [transformers](https://github.com/huggingface/transformers), @enzoampil suggested that the `nlp` library might be a better place for this functionality.
Some method for combining datasets could be implemented ,e.g.
```
dataset = nlp.load_multitask(['squad','imdb','cnn_dm'], temperature=2.0, ...)
```
We would need a few additions:
- Method of identifying the tasks - how can we support adding a string to each task as an identifier: e.g. 'summarisation: '?
- Method of combining the metrics - a standard approach is to use the specific metric for each task and add them together for a combined score.
It would be great to support common use cases such as pretraining on the GLUE benchmark before fine-tuning on each GLUE task in turn.
I'm willing to write bits/most of this I just need some guidance on the interface and other library details so I can integrate it properly.
I guess we're looking at the end user writing something like:
``` python
ds = nlp.load_dataset('multitask-t5',datasets=["squad","cnn_dm",...], k=1000, t=2.0)
```
Using the t5 method of combining here (or this could be a function passed in as an arg)
Passing kwargs to each 'sub-dataset' might become tricky. |
https://github.com/huggingface/datasets/issues/217 | Multi-task dataset mixing | From thinking upon @thomwolf 's suggestion, I've started experimenting:
```python
class MultitaskDataset(DatasetBuilder):
def __init__(self, *args, **kwargs):
super(MultitaskDataset, self).__init__(*args, **kwargs)
self._datasets = kwargs.get("datasets")
def _info(self):
return nlp.DatasetInfo(
description=_DESCRIPTION,
features=nlp.Features({
"source": nlp.Value("string"),
"target": nlp.Sequence(nlp.Value("string"))
})
)
def _get_common_splits(self):
'''Finds the common splits present in all self._datasets'''
min_set = None
for dataset in self._datasets:
if min_set != None:
min_set.intersection(set(dataset.keys()))
else:
min_set = set(dataset.keys())
return min_set
....
# Maybe this?:
squad = nlp.load_dataset("squad")
cnn_dm = nlp.load_dataset("cnn_dailymail","3.0.0")
multitask_dataset = nlp.load_dataset(
'multitask_dataset',
datasets=[squad,cnn_dailymail],
k=1000,
t=2.0
)
```
Does anyone know what methods of `MultitaskDataset` I would need to implement? Maybe `as_dataset` and `download_and_prepare`? Most of these should be just calling the methods of the sub-datasets.
I'm assuming DatasetBuilder is better than the more specific `GeneratorBasedBuilder`, `BeamBasedBuilder`, etc....
One of the other problems is that the dataset size is unknown till you construct it (as you can pick the sub-datasets). Am hoping not to need to make changes to `nlp.load_dataset` just for this class.
I'd appreciate it if anyone more familiar with nlp's internal workings could tell me if I'm on the right track! | It seems like many of the best performing models on the GLUE benchmark make some use of multitask learning (simultaneous training on multiple tasks).
The [T5 paper](https://arxiv.org/pdf/1910.10683.pdf) highlights multiple ways of mixing the tasks together during finetuning:
- **Examples-proportional mixing** - sample from tasks proportionally to their dataset size
- **Equal mixing** - sample uniformly from each task
- **Temperature-scaled mixing** - The generalized approach used by multilingual BERT which uses a temperature T, where the mixing rate of each task is raised to the power 1/T and renormalized. When T=1 this is equivalent to equal mixing, and becomes closer to equal mixing with increasing T.
Following this discussion https://github.com/huggingface/transformers/issues/4340 in [transformers](https://github.com/huggingface/transformers), @enzoampil suggested that the `nlp` library might be a better place for this functionality.
Some method for combining datasets could be implemented ,e.g.
```
dataset = nlp.load_multitask(['squad','imdb','cnn_dm'], temperature=2.0, ...)
```
We would need a few additions:
- Method of identifying the tasks - how can we support adding a string to each task as an identifier: e.g. 'summarisation: '?
- Method of combining the metrics - a standard approach is to use the specific metric for each task and add them together for a combined score.
It would be great to support common use cases such as pretraining on the GLUE benchmark before fine-tuning on each GLUE task in turn.
I'm willing to write bits/most of this I just need some guidance on the interface and other library details so I can integrate it properly.
| 177 | Multi-task dataset mixing
It seems like many of the best performing models on the GLUE benchmark make some use of multitask learning (simultaneous training on multiple tasks).
The [T5 paper](https://arxiv.org/pdf/1910.10683.pdf) highlights multiple ways of mixing the tasks together during finetuning:
- **Examples-proportional mixing** - sample from tasks proportionally to their dataset size
- **Equal mixing** - sample uniformly from each task
- **Temperature-scaled mixing** - The generalized approach used by multilingual BERT which uses a temperature T, where the mixing rate of each task is raised to the power 1/T and renormalized. When T=1 this is equivalent to equal mixing, and becomes closer to equal mixing with increasing T.
Following this discussion https://github.com/huggingface/transformers/issues/4340 in [transformers](https://github.com/huggingface/transformers), @enzoampil suggested that the `nlp` library might be a better place for this functionality.
Some method for combining datasets could be implemented ,e.g.
```
dataset = nlp.load_multitask(['squad','imdb','cnn_dm'], temperature=2.0, ...)
```
We would need a few additions:
- Method of identifying the tasks - how can we support adding a string to each task as an identifier: e.g. 'summarisation: '?
- Method of combining the metrics - a standard approach is to use the specific metric for each task and add them together for a combined score.
It would be great to support common use cases such as pretraining on the GLUE benchmark before fine-tuning on each GLUE task in turn.
I'm willing to write bits/most of this I just need some guidance on the interface and other library details so I can integrate it properly.
From thinking upon @thomwolf 's suggestion, I've started experimenting:
```python
class MultitaskDataset(DatasetBuilder):
def __init__(self, *args, **kwargs):
super(MultitaskDataset, self).__init__(*args, **kwargs)
self._datasets = kwargs.get("datasets")
def _info(self):
return nlp.DatasetInfo(
description=_DESCRIPTION,
features=nlp.Features({
"source": nlp.Value("string"),
"target": nlp.Sequence(nlp.Value("string"))
})
)
def _get_common_splits(self):
'''Finds the common splits present in all self._datasets'''
min_set = None
for dataset in self._datasets:
if min_set != None:
min_set.intersection(set(dataset.keys()))
else:
min_set = set(dataset.keys())
return min_set
....
# Maybe this?:
squad = nlp.load_dataset("squad")
cnn_dm = nlp.load_dataset("cnn_dailymail","3.0.0")
multitask_dataset = nlp.load_dataset(
'multitask_dataset',
datasets=[squad,cnn_dailymail],
k=1000,
t=2.0
)
```
Does anyone know what methods of `MultitaskDataset` I would need to implement? Maybe `as_dataset` and `download_and_prepare`? Most of these should be just calling the methods of the sub-datasets.
I'm assuming DatasetBuilder is better than the more specific `GeneratorBasedBuilder`, `BeamBasedBuilder`, etc....
One of the other problems is that the dataset size is unknown till you construct it (as you can pick the sub-datasets). Am hoping not to need to make changes to `nlp.load_dataset` just for this class.
I'd appreciate it if anyone more familiar with nlp's internal workings could tell me if I'm on the right track! |
https://github.com/huggingface/datasets/issues/217 | Multi-task dataset mixing | I think I would probably go for a `MultiDataset` wrapper around a list of `Dataset`.
I'm not sure we need to give it `k` and `t` parameters at creation, it can maybe be something along the lines of:
```python
squad = nlp.load_dataset("squad")
cnn_dm = nlp.load_dataset("cnn_dailymail","3.0.0")
multitask_dataset = nlp.MultiDataset(squad, cnn_dm)
batch = multitask_dataset.sample(10, temperature=2.0, k=1000)
```
The first proof-of-concept for multi-task datasets could definitely require that the provided datasets have the same name/type for columns (if needed you easily rename/cast a column prior to instantiating the `MultiDataset`).
It's good to think about it for some time though and don't overfit too much on the T5 examples (in particular for the ways/kwargs for sampling among datasets). | It seems like many of the best performing models on the GLUE benchmark make some use of multitask learning (simultaneous training on multiple tasks).
The [T5 paper](https://arxiv.org/pdf/1910.10683.pdf) highlights multiple ways of mixing the tasks together during finetuning:
- **Examples-proportional mixing** - sample from tasks proportionally to their dataset size
- **Equal mixing** - sample uniformly from each task
- **Temperature-scaled mixing** - The generalized approach used by multilingual BERT which uses a temperature T, where the mixing rate of each task is raised to the power 1/T and renormalized. When T=1 this is equivalent to equal mixing, and becomes closer to equal mixing with increasing T.
Following this discussion https://github.com/huggingface/transformers/issues/4340 in [transformers](https://github.com/huggingface/transformers), @enzoampil suggested that the `nlp` library might be a better place for this functionality.
Some method for combining datasets could be implemented ,e.g.
```
dataset = nlp.load_multitask(['squad','imdb','cnn_dm'], temperature=2.0, ...)
```
We would need a few additions:
- Method of identifying the tasks - how can we support adding a string to each task as an identifier: e.g. 'summarisation: '?
- Method of combining the metrics - a standard approach is to use the specific metric for each task and add them together for a combined score.
It would be great to support common use cases such as pretraining on the GLUE benchmark before fine-tuning on each GLUE task in turn.
I'm willing to write bits/most of this I just need some guidance on the interface and other library details so I can integrate it properly.
| 114 | Multi-task dataset mixing
It seems like many of the best performing models on the GLUE benchmark make some use of multitask learning (simultaneous training on multiple tasks).
The [T5 paper](https://arxiv.org/pdf/1910.10683.pdf) highlights multiple ways of mixing the tasks together during finetuning:
- **Examples-proportional mixing** - sample from tasks proportionally to their dataset size
- **Equal mixing** - sample uniformly from each task
- **Temperature-scaled mixing** - The generalized approach used by multilingual BERT which uses a temperature T, where the mixing rate of each task is raised to the power 1/T and renormalized. When T=1 this is equivalent to equal mixing, and becomes closer to equal mixing with increasing T.
Following this discussion https://github.com/huggingface/transformers/issues/4340 in [transformers](https://github.com/huggingface/transformers), @enzoampil suggested that the `nlp` library might be a better place for this functionality.
Some method for combining datasets could be implemented ,e.g.
```
dataset = nlp.load_multitask(['squad','imdb','cnn_dm'], temperature=2.0, ...)
```
We would need a few additions:
- Method of identifying the tasks - how can we support adding a string to each task as an identifier: e.g. 'summarisation: '?
- Method of combining the metrics - a standard approach is to use the specific metric for each task and add them together for a combined score.
It would be great to support common use cases such as pretraining on the GLUE benchmark before fine-tuning on each GLUE task in turn.
I'm willing to write bits/most of this I just need some guidance on the interface and other library details so I can integrate it properly.
I think I would probably go for a `MultiDataset` wrapper around a list of `Dataset`.
I'm not sure we need to give it `k` and `t` parameters at creation, it can maybe be something along the lines of:
```python
squad = nlp.load_dataset("squad")
cnn_dm = nlp.load_dataset("cnn_dailymail","3.0.0")
multitask_dataset = nlp.MultiDataset(squad, cnn_dm)
batch = multitask_dataset.sample(10, temperature=2.0, k=1000)
```
The first proof-of-concept for multi-task datasets could definitely require that the provided datasets have the same name/type for columns (if needed you easily rename/cast a column prior to instantiating the `MultiDataset`).
It's good to think about it for some time though and don't overfit too much on the T5 examples (in particular for the ways/kwargs for sampling among datasets). |
https://github.com/huggingface/datasets/issues/217 | Multi-task dataset mixing | The problem with changing `k` and `t` per sampling is that you'd have to somehow remember which examples you'd already returned while re-weighting the remaining examples based on the new `k` and `t`values. It seems possible but complicated (I can't really see a reason why you'd want to change the weighting of datasets after you constructed the multidataset).
Wouldn't it be convenient if it implemented the dataset interface? Then if someone has code using a single nlp dataset, they can replace it with a multitask combination of more datasets without having to change other code. We would at least need to be able to pass it into a `DataLoader`.
| It seems like many of the best performing models on the GLUE benchmark make some use of multitask learning (simultaneous training on multiple tasks).
The [T5 paper](https://arxiv.org/pdf/1910.10683.pdf) highlights multiple ways of mixing the tasks together during finetuning:
- **Examples-proportional mixing** - sample from tasks proportionally to their dataset size
- **Equal mixing** - sample uniformly from each task
- **Temperature-scaled mixing** - The generalized approach used by multilingual BERT which uses a temperature T, where the mixing rate of each task is raised to the power 1/T and renormalized. When T=1 this is equivalent to equal mixing, and becomes closer to equal mixing with increasing T.
Following this discussion https://github.com/huggingface/transformers/issues/4340 in [transformers](https://github.com/huggingface/transformers), @enzoampil suggested that the `nlp` library might be a better place for this functionality.
Some method for combining datasets could be implemented ,e.g.
```
dataset = nlp.load_multitask(['squad','imdb','cnn_dm'], temperature=2.0, ...)
```
We would need a few additions:
- Method of identifying the tasks - how can we support adding a string to each task as an identifier: e.g. 'summarisation: '?
- Method of combining the metrics - a standard approach is to use the specific metric for each task and add them together for a combined score.
It would be great to support common use cases such as pretraining on the GLUE benchmark before fine-tuning on each GLUE task in turn.
I'm willing to write bits/most of this I just need some guidance on the interface and other library details so I can integrate it properly.
| 109 | Multi-task dataset mixing
It seems like many of the best performing models on the GLUE benchmark make some use of multitask learning (simultaneous training on multiple tasks).
The [T5 paper](https://arxiv.org/pdf/1910.10683.pdf) highlights multiple ways of mixing the tasks together during finetuning:
- **Examples-proportional mixing** - sample from tasks proportionally to their dataset size
- **Equal mixing** - sample uniformly from each task
- **Temperature-scaled mixing** - The generalized approach used by multilingual BERT which uses a temperature T, where the mixing rate of each task is raised to the power 1/T and renormalized. When T=1 this is equivalent to equal mixing, and becomes closer to equal mixing with increasing T.
Following this discussion https://github.com/huggingface/transformers/issues/4340 in [transformers](https://github.com/huggingface/transformers), @enzoampil suggested that the `nlp` library might be a better place for this functionality.
Some method for combining datasets could be implemented ,e.g.
```
dataset = nlp.load_multitask(['squad','imdb','cnn_dm'], temperature=2.0, ...)
```
We would need a few additions:
- Method of identifying the tasks - how can we support adding a string to each task as an identifier: e.g. 'summarisation: '?
- Method of combining the metrics - a standard approach is to use the specific metric for each task and add them together for a combined score.
It would be great to support common use cases such as pretraining on the GLUE benchmark before fine-tuning on each GLUE task in turn.
I'm willing to write bits/most of this I just need some guidance on the interface and other library details so I can integrate it properly.
The problem with changing `k` and `t` per sampling is that you'd have to somehow remember which examples you'd already returned while re-weighting the remaining examples based on the new `k` and `t`values. It seems possible but complicated (I can't really see a reason why you'd want to change the weighting of datasets after you constructed the multidataset).
Wouldn't it be convenient if it implemented the dataset interface? Then if someone has code using a single nlp dataset, they can replace it with a multitask combination of more datasets without having to change other code. We would at least need to be able to pass it into a `DataLoader`.
|
https://github.com/huggingface/datasets/issues/217 | Multi-task dataset mixing | A very janky (but working) implementation of `multitask_dataset.sample()` could be something like this:
```python
import nlp
import torch
class MultiDataset():
def __init__(self, *args, temperature=2.0, k=1000, maximum=None, scale=1):
self.datasets = args
self._dataloaders = {}
for split in self._get_common_splits():
split_datasets = [ds[split] for ds in self.datasets]
mixing_rates = self._calc_mixing_rates(split_datasets,temperature, k, maximum, scale)
weights = []
for i in range(len(self.datasets)):
weights += [mixing_rates[i]]*len(self.datasets[i][split])
self._dataloaders[split] = torch.utils.data.DataLoader(torch.utils.data.ConcatDataset(split_datasets),
sampler=torch.utils.data.sampler.WeightedRandomSampler(
num_samples=len(weights),
weights = weights,
replacement=True),
shuffle=False)
def _get_common_splits(self):
'''Finds the common splits present in all self.datasets'''
min_set = None
for dataset in self.datasets:
if min_set != None:
min_set.intersection(set(dataset.keys()))
else:
min_set = set(dataset.keys())
return min_set
def _calc_mixing_rates(self,datasets, temperature=2.0, k=1000, maximum=None, scale=1):
'''Work out the weighting of each dataset based on t and k'''
mixing_rates = []
for dataset in datasets:
rate = len(dataset)
rate *= scale
if maximum:
rate = min(rate, maximum)
if temperature != 1.0:
rate = rate ** (1.0/temperature)
mixing_rates.append(rate)
return mixing_rates
def sample(self,n,split):
batch = []
for example in self._dataloaders[split]:
batch.append(example)
n -= 1
if n == 0:
return batch
def flatten(dataset,flatten_fn):
for k in dataset.keys():
if isinstance(dataset[k],nlp.Dataset):
dataset[k] = dataset[k].map(flatten_fn,remove_columns=dataset[k].column_names)
# Squad
def flatten_squad(example):
return {"source": "squad context: " + example['context'] + " question: " + example['question'],"target":example["answers"]["text"]}
squad = nlp.load_dataset("squad")
flatten(squad,flatten_squad)
# CNN_DM
def flatten_cnn_dm(example):
return {"source": "cnn_dm: " + example['article'],"target":[example["highlights"]]}
cnn_dm = nlp.load_dataset("cnn_dailymail", "3.0.0")
flatten(cnn_dm,flatten_cnn_dm)
multitask_dataset = MultiDataset(squad, cnn_dm)
batch = multitask_dataset.sample(100,"train")
```
There's definitely a more sensible way than embedding `DataLoader`s inside. | It seems like many of the best performing models on the GLUE benchmark make some use of multitask learning (simultaneous training on multiple tasks).
The [T5 paper](https://arxiv.org/pdf/1910.10683.pdf) highlights multiple ways of mixing the tasks together during finetuning:
- **Examples-proportional mixing** - sample from tasks proportionally to their dataset size
- **Equal mixing** - sample uniformly from each task
- **Temperature-scaled mixing** - The generalized approach used by multilingual BERT which uses a temperature T, where the mixing rate of each task is raised to the power 1/T and renormalized. When T=1 this is equivalent to equal mixing, and becomes closer to equal mixing with increasing T.
Following this discussion https://github.com/huggingface/transformers/issues/4340 in [transformers](https://github.com/huggingface/transformers), @enzoampil suggested that the `nlp` library might be a better place for this functionality.
Some method for combining datasets could be implemented ,e.g.
```
dataset = nlp.load_multitask(['squad','imdb','cnn_dm'], temperature=2.0, ...)
```
We would need a few additions:
- Method of identifying the tasks - how can we support adding a string to each task as an identifier: e.g. 'summarisation: '?
- Method of combining the metrics - a standard approach is to use the specific metric for each task and add them together for a combined score.
It would be great to support common use cases such as pretraining on the GLUE benchmark before fine-tuning on each GLUE task in turn.
I'm willing to write bits/most of this I just need some guidance on the interface and other library details so I can integrate it properly.
| 231 | Multi-task dataset mixing
It seems like many of the best performing models on the GLUE benchmark make some use of multitask learning (simultaneous training on multiple tasks).
The [T5 paper](https://arxiv.org/pdf/1910.10683.pdf) highlights multiple ways of mixing the tasks together during finetuning:
- **Examples-proportional mixing** - sample from tasks proportionally to their dataset size
- **Equal mixing** - sample uniformly from each task
- **Temperature-scaled mixing** - The generalized approach used by multilingual BERT which uses a temperature T, where the mixing rate of each task is raised to the power 1/T and renormalized. When T=1 this is equivalent to equal mixing, and becomes closer to equal mixing with increasing T.
Following this discussion https://github.com/huggingface/transformers/issues/4340 in [transformers](https://github.com/huggingface/transformers), @enzoampil suggested that the `nlp` library might be a better place for this functionality.
Some method for combining datasets could be implemented ,e.g.
```
dataset = nlp.load_multitask(['squad','imdb','cnn_dm'], temperature=2.0, ...)
```
We would need a few additions:
- Method of identifying the tasks - how can we support adding a string to each task as an identifier: e.g. 'summarisation: '?
- Method of combining the metrics - a standard approach is to use the specific metric for each task and add them together for a combined score.
It would be great to support common use cases such as pretraining on the GLUE benchmark before fine-tuning on each GLUE task in turn.
I'm willing to write bits/most of this I just need some guidance on the interface and other library details so I can integrate it properly.
A very janky (but working) implementation of `multitask_dataset.sample()` could be something like this:
```python
import nlp
import torch
class MultiDataset():
def __init__(self, *args, temperature=2.0, k=1000, maximum=None, scale=1):
self.datasets = args
self._dataloaders = {}
for split in self._get_common_splits():
split_datasets = [ds[split] for ds in self.datasets]
mixing_rates = self._calc_mixing_rates(split_datasets,temperature, k, maximum, scale)
weights = []
for i in range(len(self.datasets)):
weights += [mixing_rates[i]]*len(self.datasets[i][split])
self._dataloaders[split] = torch.utils.data.DataLoader(torch.utils.data.ConcatDataset(split_datasets),
sampler=torch.utils.data.sampler.WeightedRandomSampler(
num_samples=len(weights),
weights = weights,
replacement=True),
shuffle=False)
def _get_common_splits(self):
'''Finds the common splits present in all self.datasets'''
min_set = None
for dataset in self.datasets:
if min_set != None:
min_set.intersection(set(dataset.keys()))
else:
min_set = set(dataset.keys())
return min_set
def _calc_mixing_rates(self,datasets, temperature=2.0, k=1000, maximum=None, scale=1):
'''Work out the weighting of each dataset based on t and k'''
mixing_rates = []
for dataset in datasets:
rate = len(dataset)
rate *= scale
if maximum:
rate = min(rate, maximum)
if temperature != 1.0:
rate = rate ** (1.0/temperature)
mixing_rates.append(rate)
return mixing_rates
def sample(self,n,split):
batch = []
for example in self._dataloaders[split]:
batch.append(example)
n -= 1
if n == 0:
return batch
def flatten(dataset,flatten_fn):
for k in dataset.keys():
if isinstance(dataset[k],nlp.Dataset):
dataset[k] = dataset[k].map(flatten_fn,remove_columns=dataset[k].column_names)
# Squad
def flatten_squad(example):
return {"source": "squad context: " + example['context'] + " question: " + example['question'],"target":example["answers"]["text"]}
squad = nlp.load_dataset("squad")
flatten(squad,flatten_squad)
# CNN_DM
def flatten_cnn_dm(example):
return {"source": "cnn_dm: " + example['article'],"target":[example["highlights"]]}
cnn_dm = nlp.load_dataset("cnn_dailymail", "3.0.0")
flatten(cnn_dm,flatten_cnn_dm)
multitask_dataset = MultiDataset(squad, cnn_dm)
batch = multitask_dataset.sample(100,"train")
```
There's definitely a more sensible way than embedding `DataLoader`s inside. |
https://github.com/huggingface/datasets/issues/217 | Multi-task dataset mixing | Good spot! Here are my thoughts:
- Aside: Adding `MultitaskModel` to transformers might be a thing to raise - even though having task-specific heads has become unfashionable in recent times in favour of text-to-text type models.
- Adding the task name as an extra field also seems useful for these kind of models which have task-specific heads
- There is some validation of our approach that the user should be expected to `map` datasets into a common form.
- The size-proportional sampling (also called "Examples-proportional mixing") used here doesn't perform too badly in the T5 paper (it's comparable to temperature-scaled mixing in many cases but less flexible. This is only reasonable with a `K` maximum size parameter to prevent very large datasets dominating). This might be good for a first prototype using:
```python
def __iter__(self):
"""
For each batch, sample a task, and yield a batch from the respective
task Dataloader.
We use size-proportional sampling, but you could easily modify this
to sample from some-other distribution.
"""
task_choice_list = []
for i, task_name in enumerate(self.task_name_list):
task_choice_list += [i] * self.num_batches_dict[task_name]
task_choice_list = np.array(task_choice_list)
np.random.shuffle(task_choice_list)
dataloader_iter_dict = {
task_name: iter(dataloader)
for task_name, dataloader in self.dataloader_dict.items()
}
for task_choice in task_choice_list:
task_name = self.task_name_list[task_choice]
yield next(dataloader_iter_dict[task_name])
```
We'd just need to pull samples from the raw datasets and not from `DataLoader`s for each task. We can assume the user has done `dataset.shuffle()` if they want to.
Other sampling methods can later be implemented by changing how the `task_choice_list` is generated. This should allow more flexibility and not tie us to specific methods for sampling among datasets.
| It seems like many of the best performing models on the GLUE benchmark make some use of multitask learning (simultaneous training on multiple tasks).
The [T5 paper](https://arxiv.org/pdf/1910.10683.pdf) highlights multiple ways of mixing the tasks together during finetuning:
- **Examples-proportional mixing** - sample from tasks proportionally to their dataset size
- **Equal mixing** - sample uniformly from each task
- **Temperature-scaled mixing** - The generalized approach used by multilingual BERT which uses a temperature T, where the mixing rate of each task is raised to the power 1/T and renormalized. When T=1 this is equivalent to equal mixing, and becomes closer to equal mixing with increasing T.
Following this discussion https://github.com/huggingface/transformers/issues/4340 in [transformers](https://github.com/huggingface/transformers), @enzoampil suggested that the `nlp` library might be a better place for this functionality.
Some method for combining datasets could be implemented ,e.g.
```
dataset = nlp.load_multitask(['squad','imdb','cnn_dm'], temperature=2.0, ...)
```
We would need a few additions:
- Method of identifying the tasks - how can we support adding a string to each task as an identifier: e.g. 'summarisation: '?
- Method of combining the metrics - a standard approach is to use the specific metric for each task and add them together for a combined score.
It would be great to support common use cases such as pretraining on the GLUE benchmark before fine-tuning on each GLUE task in turn.
I'm willing to write bits/most of this I just need some guidance on the interface and other library details so I can integrate it properly.
| 264 | Multi-task dataset mixing
It seems like many of the best performing models on the GLUE benchmark make some use of multitask learning (simultaneous training on multiple tasks).
The [T5 paper](https://arxiv.org/pdf/1910.10683.pdf) highlights multiple ways of mixing the tasks together during finetuning:
- **Examples-proportional mixing** - sample from tasks proportionally to their dataset size
- **Equal mixing** - sample uniformly from each task
- **Temperature-scaled mixing** - The generalized approach used by multilingual BERT which uses a temperature T, where the mixing rate of each task is raised to the power 1/T and renormalized. When T=1 this is equivalent to equal mixing, and becomes closer to equal mixing with increasing T.
Following this discussion https://github.com/huggingface/transformers/issues/4340 in [transformers](https://github.com/huggingface/transformers), @enzoampil suggested that the `nlp` library might be a better place for this functionality.
Some method for combining datasets could be implemented ,e.g.
```
dataset = nlp.load_multitask(['squad','imdb','cnn_dm'], temperature=2.0, ...)
```
We would need a few additions:
- Method of identifying the tasks - how can we support adding a string to each task as an identifier: e.g. 'summarisation: '?
- Method of combining the metrics - a standard approach is to use the specific metric for each task and add them together for a combined score.
It would be great to support common use cases such as pretraining on the GLUE benchmark before fine-tuning on each GLUE task in turn.
I'm willing to write bits/most of this I just need some guidance on the interface and other library details so I can integrate it properly.
Good spot! Here are my thoughts:
- Aside: Adding `MultitaskModel` to transformers might be a thing to raise - even though having task-specific heads has become unfashionable in recent times in favour of text-to-text type models.
- Adding the task name as an extra field also seems useful for these kind of models which have task-specific heads
- There is some validation of our approach that the user should be expected to `map` datasets into a common form.
- The size-proportional sampling (also called "Examples-proportional mixing") used here doesn't perform too badly in the T5 paper (it's comparable to temperature-scaled mixing in many cases but less flexible. This is only reasonable with a `K` maximum size parameter to prevent very large datasets dominating). This might be good for a first prototype using:
```python
def __iter__(self):
"""
For each batch, sample a task, and yield a batch from the respective
task Dataloader.
We use size-proportional sampling, but you could easily modify this
to sample from some-other distribution.
"""
task_choice_list = []
for i, task_name in enumerate(self.task_name_list):
task_choice_list += [i] * self.num_batches_dict[task_name]
task_choice_list = np.array(task_choice_list)
np.random.shuffle(task_choice_list)
dataloader_iter_dict = {
task_name: iter(dataloader)
for task_name, dataloader in self.dataloader_dict.items()
}
for task_choice in task_choice_list:
task_name = self.task_name_list[task_choice]
yield next(dataloader_iter_dict[task_name])
```
We'd just need to pull samples from the raw datasets and not from `DataLoader`s for each task. We can assume the user has done `dataset.shuffle()` if they want to.
Other sampling methods can later be implemented by changing how the `task_choice_list` is generated. This should allow more flexibility and not tie us to specific methods for sampling among datasets.
|
https://github.com/huggingface/datasets/issues/217 | Multi-task dataset mixing | Another thought: Multitasking over benchmarks (represented as Meta-datasets in nlp) is probably a common use case. Would be nice to pass an entire benchmark to our `MultiDataset` wrapper rather than having to pass individual components. | It seems like many of the best performing models on the GLUE benchmark make some use of multitask learning (simultaneous training on multiple tasks).
The [T5 paper](https://arxiv.org/pdf/1910.10683.pdf) highlights multiple ways of mixing the tasks together during finetuning:
- **Examples-proportional mixing** - sample from tasks proportionally to their dataset size
- **Equal mixing** - sample uniformly from each task
- **Temperature-scaled mixing** - The generalized approach used by multilingual BERT which uses a temperature T, where the mixing rate of each task is raised to the power 1/T and renormalized. When T=1 this is equivalent to equal mixing, and becomes closer to equal mixing with increasing T.
Following this discussion https://github.com/huggingface/transformers/issues/4340 in [transformers](https://github.com/huggingface/transformers), @enzoampil suggested that the `nlp` library might be a better place for this functionality.
Some method for combining datasets could be implemented ,e.g.
```
dataset = nlp.load_multitask(['squad','imdb','cnn_dm'], temperature=2.0, ...)
```
We would need a few additions:
- Method of identifying the tasks - how can we support adding a string to each task as an identifier: e.g. 'summarisation: '?
- Method of combining the metrics - a standard approach is to use the specific metric for each task and add them together for a combined score.
It would be great to support common use cases such as pretraining on the GLUE benchmark before fine-tuning on each GLUE task in turn.
I'm willing to write bits/most of this I just need some guidance on the interface and other library details so I can integrate it properly.
| 35 | Multi-task dataset mixing
It seems like many of the best performing models on the GLUE benchmark make some use of multitask learning (simultaneous training on multiple tasks).
The [T5 paper](https://arxiv.org/pdf/1910.10683.pdf) highlights multiple ways of mixing the tasks together during finetuning:
- **Examples-proportional mixing** - sample from tasks proportionally to their dataset size
- **Equal mixing** - sample uniformly from each task
- **Temperature-scaled mixing** - The generalized approach used by multilingual BERT which uses a temperature T, where the mixing rate of each task is raised to the power 1/T and renormalized. When T=1 this is equivalent to equal mixing, and becomes closer to equal mixing with increasing T.
Following this discussion https://github.com/huggingface/transformers/issues/4340 in [transformers](https://github.com/huggingface/transformers), @enzoampil suggested that the `nlp` library might be a better place for this functionality.
Some method for combining datasets could be implemented ,e.g.
```
dataset = nlp.load_multitask(['squad','imdb','cnn_dm'], temperature=2.0, ...)
```
We would need a few additions:
- Method of identifying the tasks - how can we support adding a string to each task as an identifier: e.g. 'summarisation: '?
- Method of combining the metrics - a standard approach is to use the specific metric for each task and add them together for a combined score.
It would be great to support common use cases such as pretraining on the GLUE benchmark before fine-tuning on each GLUE task in turn.
I'm willing to write bits/most of this I just need some guidance on the interface and other library details so I can integrate it properly.
Another thought: Multitasking over benchmarks (represented as Meta-datasets in nlp) is probably a common use case. Would be nice to pass an entire benchmark to our `MultiDataset` wrapper rather than having to pass individual components. |
https://github.com/huggingface/datasets/issues/217 | Multi-task dataset mixing | Here's a fully working implementation based on the `__iter__` function of @zphang.
- I've generated the task choice list in the constructor as it allows us to index into the MultiDataset just like a normal dataset. I'm changing `task_choice_list` into a list of `(dataset_idx, example_idx)` so each entry references a unique dataset example. The shuffling has to be done before this as we don't want to shuffle within each task (we assume this is done by the user if this is what they intend).
- I'm slightly concerned this list could become very large if many large datasets were used. Can't see a way round it at the moment though.
- I've used `task.info.builder_name` as the dataset name. Not sure if this is correct.
- I'd love to add some of the other `Dataset` methods (map, slicing by column, etc...). Would be great to implement the whole interface so a single dataset can be simply replaced by this.
- This does everything on the individual example-level. If some application required batches all from a single task in turn we can't really do that.
```python
import nlp
import numpy as np
class MultiDataset:
def __init__(self,tasks):
self.tasks = tasks
# Create random order of tasks
# Using size-proportional sampling
task_choice_list = []
for i, task in enumerate(self.tasks):
task_choice_list += [i] * len(task)
task_choice_list = np.array(task_choice_list)
np.random.shuffle(task_choice_list)
# Add index into each dataset
# - We don't want to shuffle within each task
counters = {}
self.task_choice_list = []
for i in range(len(task_choice_list)):
idx = counters.get(task_choice_list[i],0)
self.task_choice_list.append((task_choice_list[i],idx))
counters[task_choice_list[i]] = idx + 1
def __len__(self):
return np.sum([len(t) for t in self.tasks])
def __repr__(self):
task_str = ", ".join([str(t) for t in self.tasks])
return f"MultiDataset(tasks: {task_str})"
def __getitem__(self,key):
if isinstance(key, int):
task_idx, example_idx = self.task_choice_list[key]
task = self.tasks[task_idx]
example = task[example_idx]
example["task_name"] = task.info.builder_name
return example
elif isinstance(key, slice):
raise NotImplementedError()
def __iter__(self):
for i in range(len(self)):
yield self[i]
def load_multitask(*datasets):
'''Create multitask datasets per split'''
def _get_common_splits(datasets):
'''Finds the common splits present in all self.datasets'''
min_set = None
for dataset in datasets:
if min_set != None:
min_set.intersection(set(dataset.keys()))
else:
min_set = set(dataset.keys())
return min_set
common_splits = _get_common_splits(datasets)
out = {}
for split in common_splits:
out[split] = MultiDataset([d[split] for d in datasets])
return out
##########################################
# Dataset Flattening
def flatten(dataset,flatten_fn):
for k in dataset.keys():
if isinstance(dataset[k],nlp.Dataset):
dataset[k] = dataset[k].map(flatten_fn,remove_columns=dataset[k].column_names)
# Squad
def flatten_squad(example):
return {"source": "squad context: " + example['context'] + " question: " + example['question'],
"target":example["answers"]["text"]}
squad = nlp.load_dataset("squad")
flatten(squad,flatten_squad)
# CNN_DM
def flatten_cnn_dm(example):
return {"source": "cnn_dm: " + example['article'],"target":[example["highlights"]]}
cnn_dm = nlp.load_dataset("cnn_dailymail", "3.0.0")
flatten(cnn_dm,flatten_cnn_dm)
#############################################
mtds = load_multitask(squad,cnn_dm)
for example in mtds["train"]:
print(example["task_name"],example["target"])
```
Let me know if you have any thoughts. I've started using this in some of my projects and it seems to work. If people are happy with the general approach for a first version, I can make a pull request. | It seems like many of the best performing models on the GLUE benchmark make some use of multitask learning (simultaneous training on multiple tasks).
The [T5 paper](https://arxiv.org/pdf/1910.10683.pdf) highlights multiple ways of mixing the tasks together during finetuning:
- **Examples-proportional mixing** - sample from tasks proportionally to their dataset size
- **Equal mixing** - sample uniformly from each task
- **Temperature-scaled mixing** - The generalized approach used by multilingual BERT which uses a temperature T, where the mixing rate of each task is raised to the power 1/T and renormalized. When T=1 this is equivalent to equal mixing, and becomes closer to equal mixing with increasing T.
Following this discussion https://github.com/huggingface/transformers/issues/4340 in [transformers](https://github.com/huggingface/transformers), @enzoampil suggested that the `nlp` library might be a better place for this functionality.
Some method for combining datasets could be implemented ,e.g.
```
dataset = nlp.load_multitask(['squad','imdb','cnn_dm'], temperature=2.0, ...)
```
We would need a few additions:
- Method of identifying the tasks - how can we support adding a string to each task as an identifier: e.g. 'summarisation: '?
- Method of combining the metrics - a standard approach is to use the specific metric for each task and add them together for a combined score.
It would be great to support common use cases such as pretraining on the GLUE benchmark before fine-tuning on each GLUE task in turn.
I'm willing to write bits/most of this I just need some guidance on the interface and other library details so I can integrate it properly.
| 469 | Multi-task dataset mixing
It seems like many of the best performing models on the GLUE benchmark make some use of multitask learning (simultaneous training on multiple tasks).
The [T5 paper](https://arxiv.org/pdf/1910.10683.pdf) highlights multiple ways of mixing the tasks together during finetuning:
- **Examples-proportional mixing** - sample from tasks proportionally to their dataset size
- **Equal mixing** - sample uniformly from each task
- **Temperature-scaled mixing** - The generalized approach used by multilingual BERT which uses a temperature T, where the mixing rate of each task is raised to the power 1/T and renormalized. When T=1 this is equivalent to equal mixing, and becomes closer to equal mixing with increasing T.
Following this discussion https://github.com/huggingface/transformers/issues/4340 in [transformers](https://github.com/huggingface/transformers), @enzoampil suggested that the `nlp` library might be a better place for this functionality.
Some method for combining datasets could be implemented ,e.g.
```
dataset = nlp.load_multitask(['squad','imdb','cnn_dm'], temperature=2.0, ...)
```
We would need a few additions:
- Method of identifying the tasks - how can we support adding a string to each task as an identifier: e.g. 'summarisation: '?
- Method of combining the metrics - a standard approach is to use the specific metric for each task and add them together for a combined score.
It would be great to support common use cases such as pretraining on the GLUE benchmark before fine-tuning on each GLUE task in turn.
I'm willing to write bits/most of this I just need some guidance on the interface and other library details so I can integrate it properly.
Here's a fully working implementation based on the `__iter__` function of @zphang.
- I've generated the task choice list in the constructor as it allows us to index into the MultiDataset just like a normal dataset. I'm changing `task_choice_list` into a list of `(dataset_idx, example_idx)` so each entry references a unique dataset example. The shuffling has to be done before this as we don't want to shuffle within each task (we assume this is done by the user if this is what they intend).
- I'm slightly concerned this list could become very large if many large datasets were used. Can't see a way round it at the moment though.
- I've used `task.info.builder_name` as the dataset name. Not sure if this is correct.
- I'd love to add some of the other `Dataset` methods (map, slicing by column, etc...). Would be great to implement the whole interface so a single dataset can be simply replaced by this.
- This does everything on the individual example-level. If some application required batches all from a single task in turn we can't really do that.
```python
import nlp
import numpy as np
class MultiDataset:
def __init__(self,tasks):
self.tasks = tasks
# Create random order of tasks
# Using size-proportional sampling
task_choice_list = []
for i, task in enumerate(self.tasks):
task_choice_list += [i] * len(task)
task_choice_list = np.array(task_choice_list)
np.random.shuffle(task_choice_list)
# Add index into each dataset
# - We don't want to shuffle within each task
counters = {}
self.task_choice_list = []
for i in range(len(task_choice_list)):
idx = counters.get(task_choice_list[i],0)
self.task_choice_list.append((task_choice_list[i],idx))
counters[task_choice_list[i]] = idx + 1
def __len__(self):
return np.sum([len(t) for t in self.tasks])
def __repr__(self):
task_str = ", ".join([str(t) for t in self.tasks])
return f"MultiDataset(tasks: {task_str})"
def __getitem__(self,key):
if isinstance(key, int):
task_idx, example_idx = self.task_choice_list[key]
task = self.tasks[task_idx]
example = task[example_idx]
example["task_name"] = task.info.builder_name
return example
elif isinstance(key, slice):
raise NotImplementedError()
def __iter__(self):
for i in range(len(self)):
yield self[i]
def load_multitask(*datasets):
'''Create multitask datasets per split'''
def _get_common_splits(datasets):
'''Finds the common splits present in all self.datasets'''
min_set = None
for dataset in datasets:
if min_set != None:
min_set.intersection(set(dataset.keys()))
else:
min_set = set(dataset.keys())
return min_set
common_splits = _get_common_splits(datasets)
out = {}
for split in common_splits:
out[split] = MultiDataset([d[split] for d in datasets])
return out
##########################################
# Dataset Flattening
def flatten(dataset,flatten_fn):
for k in dataset.keys():
if isinstance(dataset[k],nlp.Dataset):
dataset[k] = dataset[k].map(flatten_fn,remove_columns=dataset[k].column_names)
# Squad
def flatten_squad(example):
return {"source": "squad context: " + example['context'] + " question: " + example['question'],
"target":example["answers"]["text"]}
squad = nlp.load_dataset("squad")
flatten(squad,flatten_squad)
# CNN_DM
def flatten_cnn_dm(example):
return {"source": "cnn_dm: " + example['article'],"target":[example["highlights"]]}
cnn_dm = nlp.load_dataset("cnn_dailymail", "3.0.0")
flatten(cnn_dm,flatten_cnn_dm)
#############################################
mtds = load_multitask(squad,cnn_dm)
for example in mtds["train"]:
print(example["task_name"],example["target"])
```
Let me know if you have any thoughts. I've started using this in some of my projects and it seems to work. If people are happy with the general approach for a first version, I can make a pull request. |
https://github.com/huggingface/datasets/issues/217 | Multi-task dataset mixing | Hey! Happy to jump into the discussion here. I'm still getting familiar with bits of this code, but the reasons I sampled over data loaders rather than datasets is 1) ensuring that each sampled batch corresponds to only 1 task (in case of different inputs formats/downstream models) and 2) potentially having different batch sizes per task (e.g. some tasks have very long/short inputs). How are you currently dealing with these in your PR? | It seems like many of the best performing models on the GLUE benchmark make some use of multitask learning (simultaneous training on multiple tasks).
The [T5 paper](https://arxiv.org/pdf/1910.10683.pdf) highlights multiple ways of mixing the tasks together during finetuning:
- **Examples-proportional mixing** - sample from tasks proportionally to their dataset size
- **Equal mixing** - sample uniformly from each task
- **Temperature-scaled mixing** - The generalized approach used by multilingual BERT which uses a temperature T, where the mixing rate of each task is raised to the power 1/T and renormalized. When T=1 this is equivalent to equal mixing, and becomes closer to equal mixing with increasing T.
Following this discussion https://github.com/huggingface/transformers/issues/4340 in [transformers](https://github.com/huggingface/transformers), @enzoampil suggested that the `nlp` library might be a better place for this functionality.
Some method for combining datasets could be implemented ,e.g.
```
dataset = nlp.load_multitask(['squad','imdb','cnn_dm'], temperature=2.0, ...)
```
We would need a few additions:
- Method of identifying the tasks - how can we support adding a string to each task as an identifier: e.g. 'summarisation: '?
- Method of combining the metrics - a standard approach is to use the specific metric for each task and add them together for a combined score.
It would be great to support common use cases such as pretraining on the GLUE benchmark before fine-tuning on each GLUE task in turn.
I'm willing to write bits/most of this I just need some guidance on the interface and other library details so I can integrate it properly.
| 73 | Multi-task dataset mixing
It seems like many of the best performing models on the GLUE benchmark make some use of multitask learning (simultaneous training on multiple tasks).
The [T5 paper](https://arxiv.org/pdf/1910.10683.pdf) highlights multiple ways of mixing the tasks together during finetuning:
- **Examples-proportional mixing** - sample from tasks proportionally to their dataset size
- **Equal mixing** - sample uniformly from each task
- **Temperature-scaled mixing** - The generalized approach used by multilingual BERT which uses a temperature T, where the mixing rate of each task is raised to the power 1/T and renormalized. When T=1 this is equivalent to equal mixing, and becomes closer to equal mixing with increasing T.
Following this discussion https://github.com/huggingface/transformers/issues/4340 in [transformers](https://github.com/huggingface/transformers), @enzoampil suggested that the `nlp` library might be a better place for this functionality.
Some method for combining datasets could be implemented ,e.g.
```
dataset = nlp.load_multitask(['squad','imdb','cnn_dm'], temperature=2.0, ...)
```
We would need a few additions:
- Method of identifying the tasks - how can we support adding a string to each task as an identifier: e.g. 'summarisation: '?
- Method of combining the metrics - a standard approach is to use the specific metric for each task and add them together for a combined score.
It would be great to support common use cases such as pretraining on the GLUE benchmark before fine-tuning on each GLUE task in turn.
I'm willing to write bits/most of this I just need some guidance on the interface and other library details so I can integrate it properly.
Hey! Happy to jump into the discussion here. I'm still getting familiar with bits of this code, but the reasons I sampled over data loaders rather than datasets is 1) ensuring that each sampled batch corresponds to only 1 task (in case of different inputs formats/downstream models) and 2) potentially having different batch sizes per task (e.g. some tasks have very long/short inputs). How are you currently dealing with these in your PR? |
https://github.com/huggingface/datasets/issues/217 | Multi-task dataset mixing | The short answer is - I'm not! Everything is currently on a per-example basis. It would be fairly simple to add a `batch_size` argument which would ensure that every `batch_size` examples come from the same task. That should suit most use-cases (unless you wanted to ensure batches all came from the same task and apply something like `SortishSampler` on each task first)
Your notebook was really inspiring by the way - thanks! | It seems like many of the best performing models on the GLUE benchmark make some use of multitask learning (simultaneous training on multiple tasks).
The [T5 paper](https://arxiv.org/pdf/1910.10683.pdf) highlights multiple ways of mixing the tasks together during finetuning:
- **Examples-proportional mixing** - sample from tasks proportionally to their dataset size
- **Equal mixing** - sample uniformly from each task
- **Temperature-scaled mixing** - The generalized approach used by multilingual BERT which uses a temperature T, where the mixing rate of each task is raised to the power 1/T and renormalized. When T=1 this is equivalent to equal mixing, and becomes closer to equal mixing with increasing T.
Following this discussion https://github.com/huggingface/transformers/issues/4340 in [transformers](https://github.com/huggingface/transformers), @enzoampil suggested that the `nlp` library might be a better place for this functionality.
Some method for combining datasets could be implemented ,e.g.
```
dataset = nlp.load_multitask(['squad','imdb','cnn_dm'], temperature=2.0, ...)
```
We would need a few additions:
- Method of identifying the tasks - how can we support adding a string to each task as an identifier: e.g. 'summarisation: '?
- Method of combining the metrics - a standard approach is to use the specific metric for each task and add them together for a combined score.
It would be great to support common use cases such as pretraining on the GLUE benchmark before fine-tuning on each GLUE task in turn.
I'm willing to write bits/most of this I just need some guidance on the interface and other library details so I can integrate it properly.
| 72 | Multi-task dataset mixing
It seems like many of the best performing models on the GLUE benchmark make some use of multitask learning (simultaneous training on multiple tasks).
The [T5 paper](https://arxiv.org/pdf/1910.10683.pdf) highlights multiple ways of mixing the tasks together during finetuning:
- **Examples-proportional mixing** - sample from tasks proportionally to their dataset size
- **Equal mixing** - sample uniformly from each task
- **Temperature-scaled mixing** - The generalized approach used by multilingual BERT which uses a temperature T, where the mixing rate of each task is raised to the power 1/T and renormalized. When T=1 this is equivalent to equal mixing, and becomes closer to equal mixing with increasing T.
Following this discussion https://github.com/huggingface/transformers/issues/4340 in [transformers](https://github.com/huggingface/transformers), @enzoampil suggested that the `nlp` library might be a better place for this functionality.
Some method for combining datasets could be implemented ,e.g.
```
dataset = nlp.load_multitask(['squad','imdb','cnn_dm'], temperature=2.0, ...)
```
We would need a few additions:
- Method of identifying the tasks - how can we support adding a string to each task as an identifier: e.g. 'summarisation: '?
- Method of combining the metrics - a standard approach is to use the specific metric for each task and add them together for a combined score.
It would be great to support common use cases such as pretraining on the GLUE benchmark before fine-tuning on each GLUE task in turn.
I'm willing to write bits/most of this I just need some guidance on the interface and other library details so I can integrate it properly.
The short answer is - I'm not! Everything is currently on a per-example basis. It would be fairly simple to add a `batch_size` argument which would ensure that every `batch_size` examples come from the same task. That should suit most use-cases (unless you wanted to ensure batches all came from the same task and apply something like `SortishSampler` on each task first)
Your notebook was really inspiring by the way - thanks! |
https://github.com/huggingface/datasets/issues/217 | Multi-task dataset mixing | @zphang is having different batch sizes per task actually helpful? Would be interesting to know as it's not something I've come across as a technique used by any MTL papers. | It seems like many of the best performing models on the GLUE benchmark make some use of multitask learning (simultaneous training on multiple tasks).
The [T5 paper](https://arxiv.org/pdf/1910.10683.pdf) highlights multiple ways of mixing the tasks together during finetuning:
- **Examples-proportional mixing** - sample from tasks proportionally to their dataset size
- **Equal mixing** - sample uniformly from each task
- **Temperature-scaled mixing** - The generalized approach used by multilingual BERT which uses a temperature T, where the mixing rate of each task is raised to the power 1/T and renormalized. When T=1 this is equivalent to equal mixing, and becomes closer to equal mixing with increasing T.
Following this discussion https://github.com/huggingface/transformers/issues/4340 in [transformers](https://github.com/huggingface/transformers), @enzoampil suggested that the `nlp` library might be a better place for this functionality.
Some method for combining datasets could be implemented ,e.g.
```
dataset = nlp.load_multitask(['squad','imdb','cnn_dm'], temperature=2.0, ...)
```
We would need a few additions:
- Method of identifying the tasks - how can we support adding a string to each task as an identifier: e.g. 'summarisation: '?
- Method of combining the metrics - a standard approach is to use the specific metric for each task and add them together for a combined score.
It would be great to support common use cases such as pretraining on the GLUE benchmark before fine-tuning on each GLUE task in turn.
I'm willing to write bits/most of this I just need some guidance on the interface and other library details so I can integrate it properly.
| 30 | Multi-task dataset mixing
It seems like many of the best performing models on the GLUE benchmark make some use of multitask learning (simultaneous training on multiple tasks).
The [T5 paper](https://arxiv.org/pdf/1910.10683.pdf) highlights multiple ways of mixing the tasks together during finetuning:
- **Examples-proportional mixing** - sample from tasks proportionally to their dataset size
- **Equal mixing** - sample uniformly from each task
- **Temperature-scaled mixing** - The generalized approach used by multilingual BERT which uses a temperature T, where the mixing rate of each task is raised to the power 1/T and renormalized. When T=1 this is equivalent to equal mixing, and becomes closer to equal mixing with increasing T.
Following this discussion https://github.com/huggingface/transformers/issues/4340 in [transformers](https://github.com/huggingface/transformers), @enzoampil suggested that the `nlp` library might be a better place for this functionality.
Some method for combining datasets could be implemented ,e.g.
```
dataset = nlp.load_multitask(['squad','imdb','cnn_dm'], temperature=2.0, ...)
```
We would need a few additions:
- Method of identifying the tasks - how can we support adding a string to each task as an identifier: e.g. 'summarisation: '?
- Method of combining the metrics - a standard approach is to use the specific metric for each task and add them together for a combined score.
It would be great to support common use cases such as pretraining on the GLUE benchmark before fine-tuning on each GLUE task in turn.
I'm willing to write bits/most of this I just need some guidance on the interface and other library details so I can integrate it properly.
@zphang is having different batch sizes per task actually helpful? Would be interesting to know as it's not something I've come across as a technique used by any MTL papers. |