html_url
stringlengths 48
51
| title
stringlengths 5
280
| comments
stringlengths 63
51.8k
| body
stringlengths 0
36.2k
⌀ | comment_length
int64 16
1.52k
| text
stringlengths 163
54.1k
|
---|---|---|---|---|---|
https://github.com/huggingface/datasets/issues/6176 | how to limit the size of memory mapped file? | Hi! Can you share the error this reproducer throws in your environment? `streaming=True` streams the dataset as it's iterated over without creating a memory-map file. | ### Describe the bug
Huggingface datasets use memory-mapped file to map large datasets in memory for fast access.
However, it seems like huggingface will occupy all the memory for memory-mapped files, which makes a troublesome situation since we cluster will distribute a small portion of memory to me (once it's over the limit, memory cannot be allocated), however, when the dataset checks the total memory, all of the memory will be taken into account which makes huggingface dataset try to allocate more memory than allowed.
So is there a way to explicitly limit the size of memory mapped file?
### Steps to reproduce the bug
python
>>> from datasets import load_dataset
>>> dataset = load_dataset("c4", "en", streaming=True)
### Expected behavior
In a normal environment, this will not have any problem.
However, when the system allocates a portion of the memory to the program and when the dataset checks the total memory, all of the memory will be taken into account which makes huggingface dataset try to allocate more memory than allowed.
### Environment info
linux cluster with SGE(Sun Grid Engine) | 25 | how to limit the size of memory mapped file?
### Describe the bug
Huggingface datasets use memory-mapped file to map large datasets in memory for fast access.
However, it seems like huggingface will occupy all the memory for memory-mapped files, which makes a troublesome situation since we cluster will distribute a small portion of memory to me (once it's over the limit, memory cannot be allocated), however, when the dataset checks the total memory, all of the memory will be taken into account which makes huggingface dataset try to allocate more memory than allowed.
So is there a way to explicitly limit the size of memory mapped file?
### Steps to reproduce the bug
python
>>> from datasets import load_dataset
>>> dataset = load_dataset("c4", "en", streaming=True)
### Expected behavior
In a normal environment, this will not have any problem.
However, when the system allocates a portion of the memory to the program and when the dataset checks the total memory, all of the memory will be taken into account which makes huggingface dataset try to allocate more memory than allowed.
### Environment info
linux cluster with SGE(Sun Grid Engine)
Hi! Can you share the error this reproducer throws in your environment? `streaming=True` streams the dataset as it's iterated over without creating a memory-map file. |
https://github.com/huggingface/datasets/issues/6176 | how to limit the size of memory mapped file? | The trace of the error. Streaming works but is slower.
```
Root Cause (first observed failure):
[0]:
time : 2023-08-24_06:06:01
host : compute-126.cm.cluster
rank : 0 (local_rank: 0)
exitcode : 1 (pid: 48442)
error_file: /tmp/torchelastic_4fqzcuuz/none_rx2470jl/attempt_0/0/error.json
traceback : Traceback (most recent call last):
File "/users/yli7/.conda/envs/pytorch2.0/lib/python3.8/site-packages/torch/distributed/elastic/multiprocessing/errors/__init__.py", line 346, in wrapper
return f(*args, **kwargs)
File "Pretrain.py", line 214, in main
pair_dataset, c4_dataset = create_dataset('pretrain', config)
File "/dcs05/qiao/data/william/project/DaVinci/dataset/__init__.py", line 109, in create_dataset
c4_dataset = load_dataset("c4", "en", split="train").to_iterable_dataset(num_shards=1024).map(pre_caption_huggingface)
File "/users/yli7/.local/lib/python3.8/site-packages/datasets/load.py", line 1810, in load_dataset
ds = builder_instance.as_dataset(split=split, verification_mode=verification_mode, in_memory=keep_in_memory)
File "/users/yli7/.local/lib/python3.8/site-packages/datasets/builder.py", line 1145, in as_dataset
datasets = map_nested(
File "/users/yli7/.local/lib/python3.8/site-packages/datasets/utils/py_utils.py", line 436, in map_nested
return function(data_struct)
File "/users/yli7/.local/lib/python3.8/site-packages/datasets/builder.py", line 1175, in _build_single_dataset
ds = self._as_dataset(
File "/users/yli7/.local/lib/python3.8/site-packages/datasets/builder.py", line 1246, in _as_dataset
dataset_kwargs = ArrowReader(cache_dir, self.info).read(
File "/users/yli7/.local/lib/python3.8/site-packages/datasets/arrow_reader.py", line 244, in read
return self.read_files(files=files, original_instructions=instructions, in_memory=in_memory)
File "/users/yli7/.local/lib/python3.8/site-packages/datasets/arrow_reader.py", line 265, in read_files
pa_table = self._read_files(files, in_memory=in_memory)
File "/users/yli7/.local/lib/python3.8/site-packages/datasets/arrow_reader.py", line 200, in _read_files
pa_table: Table = self._get_table_from_filename(f_dict, in_memory=in_memory)
File "/users/yli7/.local/lib/python3.8/site-packages/datasets/arrow_reader.py", line 336, in _get_table_from_filename
table = ArrowReader.read_table(filename, in_memory=in_memory)
File "/users/yli7/.local/lib/python3.8/site-packages/datasets/arrow_reader.py", line 357, in read_table
return table_cls.from_file(filename)
File "/users/yli7/.local/lib/python3.8/site-packages/datasets/table.py", line 1059, in from_file
table = _memory_mapped_arrow_table_from_file(filename)
File "/users/yli7/.local/lib/python3.8/site-packages/datasets/table.py", line 65, in _memory_mapped_arrow_table_from_file
opened_stream = _memory_mapped_record_batch_reader_from_file(filename)
File "/users/yli7/.local/lib/python3.8/site-packages/datasets/table.py", line 50, in _memory_mapped_record_batch_reader_from_file
memory_mapped_stream = pa.memory_map(filename)
File "pyarrow/io.pxi", line 1009, in pyarrow.lib.memory_map
File "pyarrow/io.pxi", line 956, in pyarrow.lib.MemoryMappedFile._open
File "pyarrow/error.pxi", line 144, in pyarrow.lib.pyarrow_internal_check_status
File "pyarrow/error.pxi", line 115, in pyarrow.lib.check_status
OSError: Memory mapping file failed: Cannot allocate memory
``` | ### Describe the bug
Huggingface datasets use memory-mapped file to map large datasets in memory for fast access.
However, it seems like huggingface will occupy all the memory for memory-mapped files, which makes a troublesome situation since we cluster will distribute a small portion of memory to me (once it's over the limit, memory cannot be allocated), however, when the dataset checks the total memory, all of the memory will be taken into account which makes huggingface dataset try to allocate more memory than allowed.
So is there a way to explicitly limit the size of memory mapped file?
### Steps to reproduce the bug
python
>>> from datasets import load_dataset
>>> dataset = load_dataset("c4", "en", streaming=True)
### Expected behavior
In a normal environment, this will not have any problem.
However, when the system allocates a portion of the memory to the program and when the dataset checks the total memory, all of the memory will be taken into account which makes huggingface dataset try to allocate more memory than allowed.
### Environment info
linux cluster with SGE(Sun Grid Engine) | 229 | how to limit the size of memory mapped file?
### Describe the bug
Huggingface datasets use memory-mapped file to map large datasets in memory for fast access.
However, it seems like huggingface will occupy all the memory for memory-mapped files, which makes a troublesome situation since we cluster will distribute a small portion of memory to me (once it's over the limit, memory cannot be allocated), however, when the dataset checks the total memory, all of the memory will be taken into account which makes huggingface dataset try to allocate more memory than allowed.
So is there a way to explicitly limit the size of memory mapped file?
### Steps to reproduce the bug
python
>>> from datasets import load_dataset
>>> dataset = load_dataset("c4", "en", streaming=True)
### Expected behavior
In a normal environment, this will not have any problem.
However, when the system allocates a portion of the memory to the program and when the dataset checks the total memory, all of the memory will be taken into account which makes huggingface dataset try to allocate more memory than allowed.
### Environment info
linux cluster with SGE(Sun Grid Engine)
The trace of the error. Streaming works but is slower.
```
Root Cause (first observed failure):
[0]:
time : 2023-08-24_06:06:01
host : compute-126.cm.cluster
rank : 0 (local_rank: 0)
exitcode : 1 (pid: 48442)
error_file: /tmp/torchelastic_4fqzcuuz/none_rx2470jl/attempt_0/0/error.json
traceback : Traceback (most recent call last):
File "/users/yli7/.conda/envs/pytorch2.0/lib/python3.8/site-packages/torch/distributed/elastic/multiprocessing/errors/__init__.py", line 346, in wrapper
return f(*args, **kwargs)
File "Pretrain.py", line 214, in main
pair_dataset, c4_dataset = create_dataset('pretrain', config)
File "/dcs05/qiao/data/william/project/DaVinci/dataset/__init__.py", line 109, in create_dataset
c4_dataset = load_dataset("c4", "en", split="train").to_iterable_dataset(num_shards=1024).map(pre_caption_huggingface)
File "/users/yli7/.local/lib/python3.8/site-packages/datasets/load.py", line 1810, in load_dataset
ds = builder_instance.as_dataset(split=split, verification_mode=verification_mode, in_memory=keep_in_memory)
File "/users/yli7/.local/lib/python3.8/site-packages/datasets/builder.py", line 1145, in as_dataset
datasets = map_nested(
File "/users/yli7/.local/lib/python3.8/site-packages/datasets/utils/py_utils.py", line 436, in map_nested
return function(data_struct)
File "/users/yli7/.local/lib/python3.8/site-packages/datasets/builder.py", line 1175, in _build_single_dataset
ds = self._as_dataset(
File "/users/yli7/.local/lib/python3.8/site-packages/datasets/builder.py", line 1246, in _as_dataset
dataset_kwargs = ArrowReader(cache_dir, self.info).read(
File "/users/yli7/.local/lib/python3.8/site-packages/datasets/arrow_reader.py", line 244, in read
return self.read_files(files=files, original_instructions=instructions, in_memory=in_memory)
File "/users/yli7/.local/lib/python3.8/site-packages/datasets/arrow_reader.py", line 265, in read_files
pa_table = self._read_files(files, in_memory=in_memory)
File "/users/yli7/.local/lib/python3.8/site-packages/datasets/arrow_reader.py", line 200, in _read_files
pa_table: Table = self._get_table_from_filename(f_dict, in_memory=in_memory)
File "/users/yli7/.local/lib/python3.8/site-packages/datasets/arrow_reader.py", line 336, in _get_table_from_filename
table = ArrowReader.read_table(filename, in_memory=in_memory)
File "/users/yli7/.local/lib/python3.8/site-packages/datasets/arrow_reader.py", line 357, in read_table
return table_cls.from_file(filename)
File "/users/yli7/.local/lib/python3.8/site-packages/datasets/table.py", line 1059, in from_file
table = _memory_mapped_arrow_table_from_file(filename)
File "/users/yli7/.local/lib/python3.8/site-packages/datasets/table.py", line 65, in _memory_mapped_arrow_table_from_file
opened_stream = _memory_mapped_record_batch_reader_from_file(filename)
File "/users/yli7/.local/lib/python3.8/site-packages/datasets/table.py", line 50, in _memory_mapped_record_batch_reader_from_file
memory_mapped_stream = pa.memory_map(filename)
File "pyarrow/io.pxi", line 1009, in pyarrow.lib.memory_map
File "pyarrow/io.pxi", line 956, in pyarrow.lib.MemoryMappedFile._open
File "pyarrow/error.pxi", line 144, in pyarrow.lib.pyarrow_internal_check_status
File "pyarrow/error.pxi", line 115, in pyarrow.lib.check_status
OSError: Memory mapping file failed: Cannot allocate memory
``` |
https://github.com/huggingface/datasets/issues/6176 | how to limit the size of memory mapped file? | This issue has previously been reported here: https://github.com/huggingface/datasets/issues/5710. Reporting it in the Arrow repo makes more sense as they have control over memory mapping.
PS: this is the API to reduce the size of the generated Arrow file:
```python
from datasets import load_dataset
builder = load_dataset_builder("c4", "en")
builder.download_and_prepare(max_shard_size="5GB")
dataset = builder.as_dataset()
```
If this resolves the issue, we can consider exposing `max_shard_size` in `load_dataset`. | ### Describe the bug
Huggingface datasets use memory-mapped file to map large datasets in memory for fast access.
However, it seems like huggingface will occupy all the memory for memory-mapped files, which makes a troublesome situation since we cluster will distribute a small portion of memory to me (once it's over the limit, memory cannot be allocated), however, when the dataset checks the total memory, all of the memory will be taken into account which makes huggingface dataset try to allocate more memory than allowed.
So is there a way to explicitly limit the size of memory mapped file?
### Steps to reproduce the bug
python
>>> from datasets import load_dataset
>>> dataset = load_dataset("c4", "en", streaming=True)
### Expected behavior
In a normal environment, this will not have any problem.
However, when the system allocates a portion of the memory to the program and when the dataset checks the total memory, all of the memory will be taken into account which makes huggingface dataset try to allocate more memory than allowed.
### Environment info
linux cluster with SGE(Sun Grid Engine) | 64 | how to limit the size of memory mapped file?
### Describe the bug
Huggingface datasets use memory-mapped file to map large datasets in memory for fast access.
However, it seems like huggingface will occupy all the memory for memory-mapped files, which makes a troublesome situation since we cluster will distribute a small portion of memory to me (once it's over the limit, memory cannot be allocated), however, when the dataset checks the total memory, all of the memory will be taken into account which makes huggingface dataset try to allocate more memory than allowed.
So is there a way to explicitly limit the size of memory mapped file?
### Steps to reproduce the bug
python
>>> from datasets import load_dataset
>>> dataset = load_dataset("c4", "en", streaming=True)
### Expected behavior
In a normal environment, this will not have any problem.
However, when the system allocates a portion of the memory to the program and when the dataset checks the total memory, all of the memory will be taken into account which makes huggingface dataset try to allocate more memory than allowed.
### Environment info
linux cluster with SGE(Sun Grid Engine)
This issue has previously been reported here: https://github.com/huggingface/datasets/issues/5710. Reporting it in the Arrow repo makes more sense as they have control over memory mapping.
PS: this is the API to reduce the size of the generated Arrow file:
```python
from datasets import load_dataset
builder = load_dataset_builder("c4", "en")
builder.download_and_prepare(max_shard_size="5GB")
dataset = builder.as_dataset()
```
If this resolves the issue, we can consider exposing `max_shard_size` in `load_dataset`. |
https://github.com/huggingface/datasets/issues/6172 | Make Dataset streaming queries retryable | Hi! The streaming mode also retries requests - `datasets.config.STREAMING_READ_MAX_RETRIES` (20 sec by default) controls the number of retries and `datasets.config.STREAMING_READ_RETRY_INTERVAL` (5 sec) the sleep time between retries.
> At step 1800 I got a 504 HTTP status code error from Huggingface hub for my pytorch dataloader
A minor Hub outage that we experienced yesterday could be the cause. | ### Feature request
Streaming datasets, as intended, do not load the entire dataset in memory or disk. However, while querying the next data chunk from the remote, sometimes it is possible that the service is down or there might be other issues that may cause the query to fail. In such a scenario, it would be nice to make these queries retryable (perhaps with a backoff strategy).
### Motivation
I was working on a model and the model checkpoints after every 1000 steps. At step 1800 I got a 504 HTTP status code error from Huggingface hub for my pytorch `dataloader`. Given the size of my model and data, it took around 2 hours to reach 1800 steps and now it will take about an hour to recover the lost 800. It would be better to get a retryable querying strategy.
### Your contribution
It would be better if someone having experience in this area takes this up as this would require some testing. | 58 | Make Dataset streaming queries retryable
### Feature request
Streaming datasets, as intended, do not load the entire dataset in memory or disk. However, while querying the next data chunk from the remote, sometimes it is possible that the service is down or there might be other issues that may cause the query to fail. In such a scenario, it would be nice to make these queries retryable (perhaps with a backoff strategy).
### Motivation
I was working on a model and the model checkpoints after every 1000 steps. At step 1800 I got a 504 HTTP status code error from Huggingface hub for my pytorch `dataloader`. Given the size of my model and data, it took around 2 hours to reach 1800 steps and now it will take about an hour to recover the lost 800. It would be better to get a retryable querying strategy.
### Your contribution
It would be better if someone having experience in this area takes this up as this would require some testing.
Hi! The streaming mode also retries requests - `datasets.config.STREAMING_READ_MAX_RETRIES` (20 sec by default) controls the number of retries and `datasets.config.STREAMING_READ_RETRY_INTERVAL` (5 sec) the sleep time between retries.
> At step 1800 I got a 504 HTTP status code error from Huggingface hub for my pytorch dataloader
A minor Hub outage that we experienced yesterday could be the cause. |
https://github.com/huggingface/datasets/issues/6169 | Configurations in yaml not working | Unfortunately, I cannot reproduce this behavior on my machine or Colab - the reproducer returns `['main_data', 'additional_data']` as expected. | ### Dataset configurations cannot be created in YAML/README
Hello! I'm trying to follow the docs here in order to create structure in my dataset as added from here (#5331): https://github.com/huggingface/datasets/blob/8b8e6ee067eb74e7965ca2a6768f15f9398cb7c8/docs/source/repository_structure.mdx#L110-L118
I have the exact example in my config file for [my data repo](https://huggingface.co/datasets/tsor13/test):
```
configs:
- config_name: main_data
data_files: "main_data.csv"
- config_name: additional_data
data_files: "additional_data.csv"
```
Yet, I'm unable to load different configurations:
```
from datasets import get_dataset_config_names
get_dataset_config_names('tsor13/test', use_auth_token=True)
```
returns a single split, `['tsor13--test']`
Does anyone have any insights?
@polinaeterna thank you for adding this feature, it is super useful. Do you happen to have any ideas?
### Steps to reproduce the bug
from datasets import get_dataset_config_names
get_dataset_config_names('tsor13/test')
### Expected behavior
I would expect there to be two splits, `main_data` and `additional_data`. However, only `['tsor13--test']` test is returned.
### Environment info
- `datasets` version: 2.14.4
- Platform: macOS-13.4-arm64-arm-64bit
- Python version: 3.11.4
- Huggingface_hub version: 0.16.4
- PyArrow version: 12.0.1
- Pandas version: 1.5.1 | 19 | Configurations in yaml not working
### Dataset configurations cannot be created in YAML/README
Hello! I'm trying to follow the docs here in order to create structure in my dataset as added from here (#5331): https://github.com/huggingface/datasets/blob/8b8e6ee067eb74e7965ca2a6768f15f9398cb7c8/docs/source/repository_structure.mdx#L110-L118
I have the exact example in my config file for [my data repo](https://huggingface.co/datasets/tsor13/test):
```
configs:
- config_name: main_data
data_files: "main_data.csv"
- config_name: additional_data
data_files: "additional_data.csv"
```
Yet, I'm unable to load different configurations:
```
from datasets import get_dataset_config_names
get_dataset_config_names('tsor13/test', use_auth_token=True)
```
returns a single split, `['tsor13--test']`
Does anyone have any insights?
@polinaeterna thank you for adding this feature, it is super useful. Do you happen to have any ideas?
### Steps to reproduce the bug
from datasets import get_dataset_config_names
get_dataset_config_names('tsor13/test')
### Expected behavior
I would expect there to be two splits, `main_data` and `additional_data`. However, only `['tsor13--test']` test is returned.
### Environment info
- `datasets` version: 2.14.4
- Platform: macOS-13.4-arm64-arm-64bit
- Python version: 3.11.4
- Huggingface_hub version: 0.16.4
- PyArrow version: 12.0.1
- Pandas version: 1.5.1
Unfortunately, I cannot reproduce this behavior on my machine or Colab - the reproducer returns `['main_data', 'additional_data']` as expected. |
https://github.com/huggingface/datasets/issues/6169 | Configurations in yaml not working | Thank you for looking into this, Mario. Is this on [my repository](https://huggingface.co/datasets/tsor13/test), or on another one that you have reproduced? Would you mind pointing me to it if so? | ### Dataset configurations cannot be created in YAML/README
Hello! I'm trying to follow the docs here in order to create structure in my dataset as added from here (#5331): https://github.com/huggingface/datasets/blob/8b8e6ee067eb74e7965ca2a6768f15f9398cb7c8/docs/source/repository_structure.mdx#L110-L118
I have the exact example in my config file for [my data repo](https://huggingface.co/datasets/tsor13/test):
```
configs:
- config_name: main_data
data_files: "main_data.csv"
- config_name: additional_data
data_files: "additional_data.csv"
```
Yet, I'm unable to load different configurations:
```
from datasets import get_dataset_config_names
get_dataset_config_names('tsor13/test', use_auth_token=True)
```
returns a single split, `['tsor13--test']`
Does anyone have any insights?
@polinaeterna thank you for adding this feature, it is super useful. Do you happen to have any ideas?
### Steps to reproduce the bug
from datasets import get_dataset_config_names
get_dataset_config_names('tsor13/test')
### Expected behavior
I would expect there to be two splits, `main_data` and `additional_data`. However, only `['tsor13--test']` test is returned.
### Environment info
- `datasets` version: 2.14.4
- Platform: macOS-13.4-arm64-arm-64bit
- Python version: 3.11.4
- Huggingface_hub version: 0.16.4
- PyArrow version: 12.0.1
- Pandas version: 1.5.1 | 29 | Configurations in yaml not working
### Dataset configurations cannot be created in YAML/README
Hello! I'm trying to follow the docs here in order to create structure in my dataset as added from here (#5331): https://github.com/huggingface/datasets/blob/8b8e6ee067eb74e7965ca2a6768f15f9398cb7c8/docs/source/repository_structure.mdx#L110-L118
I have the exact example in my config file for [my data repo](https://huggingface.co/datasets/tsor13/test):
```
configs:
- config_name: main_data
data_files: "main_data.csv"
- config_name: additional_data
data_files: "additional_data.csv"
```
Yet, I'm unable to load different configurations:
```
from datasets import get_dataset_config_names
get_dataset_config_names('tsor13/test', use_auth_token=True)
```
returns a single split, `['tsor13--test']`
Does anyone have any insights?
@polinaeterna thank you for adding this feature, it is super useful. Do you happen to have any ideas?
### Steps to reproduce the bug
from datasets import get_dataset_config_names
get_dataset_config_names('tsor13/test')
### Expected behavior
I would expect there to be two splits, `main_data` and `additional_data`. However, only `['tsor13--test']` test is returned.
### Environment info
- `datasets` version: 2.14.4
- Platform: macOS-13.4-arm64-arm-64bit
- Python version: 3.11.4
- Huggingface_hub version: 0.16.4
- PyArrow version: 12.0.1
- Pandas version: 1.5.1
Thank you for looking into this, Mario. Is this on [my repository](https://huggingface.co/datasets/tsor13/test), or on another one that you have reproduced? Would you mind pointing me to it if so? |
https://github.com/huggingface/datasets/issues/6169 | Configurations in yaml not working | Whoa, in colab I received the correct behavior using my dataset. It must have something to do with my local copy of `datasets` (which again just failed).
I've tried uninstalling/reinstnalling to no avail | ### Dataset configurations cannot be created in YAML/README
Hello! I'm trying to follow the docs here in order to create structure in my dataset as added from here (#5331): https://github.com/huggingface/datasets/blob/8b8e6ee067eb74e7965ca2a6768f15f9398cb7c8/docs/source/repository_structure.mdx#L110-L118
I have the exact example in my config file for [my data repo](https://huggingface.co/datasets/tsor13/test):
```
configs:
- config_name: main_data
data_files: "main_data.csv"
- config_name: additional_data
data_files: "additional_data.csv"
```
Yet, I'm unable to load different configurations:
```
from datasets import get_dataset_config_names
get_dataset_config_names('tsor13/test', use_auth_token=True)
```
returns a single split, `['tsor13--test']`
Does anyone have any insights?
@polinaeterna thank you for adding this feature, it is super useful. Do you happen to have any ideas?
### Steps to reproduce the bug
from datasets import get_dataset_config_names
get_dataset_config_names('tsor13/test')
### Expected behavior
I would expect there to be two splits, `main_data` and `additional_data`. However, only `['tsor13--test']` test is returned.
### Environment info
- `datasets` version: 2.14.4
- Platform: macOS-13.4-arm64-arm-64bit
- Python version: 3.11.4
- Huggingface_hub version: 0.16.4
- PyArrow version: 12.0.1
- Pandas version: 1.5.1 | 33 | Configurations in yaml not working
### Dataset configurations cannot be created in YAML/README
Hello! I'm trying to follow the docs here in order to create structure in my dataset as added from here (#5331): https://github.com/huggingface/datasets/blob/8b8e6ee067eb74e7965ca2a6768f15f9398cb7c8/docs/source/repository_structure.mdx#L110-L118
I have the exact example in my config file for [my data repo](https://huggingface.co/datasets/tsor13/test):
```
configs:
- config_name: main_data
data_files: "main_data.csv"
- config_name: additional_data
data_files: "additional_data.csv"
```
Yet, I'm unable to load different configurations:
```
from datasets import get_dataset_config_names
get_dataset_config_names('tsor13/test', use_auth_token=True)
```
returns a single split, `['tsor13--test']`
Does anyone have any insights?
@polinaeterna thank you for adding this feature, it is super useful. Do you happen to have any ideas?
### Steps to reproduce the bug
from datasets import get_dataset_config_names
get_dataset_config_names('tsor13/test')
### Expected behavior
I would expect there to be two splits, `main_data` and `additional_data`. However, only `['tsor13--test']` test is returned.
### Environment info
- `datasets` version: 2.14.4
- Platform: macOS-13.4-arm64-arm-64bit
- Python version: 3.11.4
- Huggingface_hub version: 0.16.4
- PyArrow version: 12.0.1
- Pandas version: 1.5.1
Whoa, in colab I received the correct behavior using my dataset. It must have something to do with my local copy of `datasets` (which again just failed).
I've tried uninstalling/reinstnalling to no avail |
https://github.com/huggingface/datasets/issues/6169 | Configurations in yaml not working | hi @tsor13 , I haven't been able to reproduce your issue on `tsor13/test` dataset locally either. reinstalling doesn't help? | ### Dataset configurations cannot be created in YAML/README
Hello! I'm trying to follow the docs here in order to create structure in my dataset as added from here (#5331): https://github.com/huggingface/datasets/blob/8b8e6ee067eb74e7965ca2a6768f15f9398cb7c8/docs/source/repository_structure.mdx#L110-L118
I have the exact example in my config file for [my data repo](https://huggingface.co/datasets/tsor13/test):
```
configs:
- config_name: main_data
data_files: "main_data.csv"
- config_name: additional_data
data_files: "additional_data.csv"
```
Yet, I'm unable to load different configurations:
```
from datasets import get_dataset_config_names
get_dataset_config_names('tsor13/test', use_auth_token=True)
```
returns a single split, `['tsor13--test']`
Does anyone have any insights?
@polinaeterna thank you for adding this feature, it is super useful. Do you happen to have any ideas?
### Steps to reproduce the bug
from datasets import get_dataset_config_names
get_dataset_config_names('tsor13/test')
### Expected behavior
I would expect there to be two splits, `main_data` and `additional_data`. However, only `['tsor13--test']` test is returned.
### Environment info
- `datasets` version: 2.14.4
- Platform: macOS-13.4-arm64-arm-64bit
- Python version: 3.11.4
- Huggingface_hub version: 0.16.4
- PyArrow version: 12.0.1
- Pandas version: 1.5.1 | 19 | Configurations in yaml not working
### Dataset configurations cannot be created in YAML/README
Hello! I'm trying to follow the docs here in order to create structure in my dataset as added from here (#5331): https://github.com/huggingface/datasets/blob/8b8e6ee067eb74e7965ca2a6768f15f9398cb7c8/docs/source/repository_structure.mdx#L110-L118
I have the exact example in my config file for [my data repo](https://huggingface.co/datasets/tsor13/test):
```
configs:
- config_name: main_data
data_files: "main_data.csv"
- config_name: additional_data
data_files: "additional_data.csv"
```
Yet, I'm unable to load different configurations:
```
from datasets import get_dataset_config_names
get_dataset_config_names('tsor13/test', use_auth_token=True)
```
returns a single split, `['tsor13--test']`
Does anyone have any insights?
@polinaeterna thank you for adding this feature, it is super useful. Do you happen to have any ideas?
### Steps to reproduce the bug
from datasets import get_dataset_config_names
get_dataset_config_names('tsor13/test')
### Expected behavior
I would expect there to be two splits, `main_data` and `additional_data`. However, only `['tsor13--test']` test is returned.
### Environment info
- `datasets` version: 2.14.4
- Platform: macOS-13.4-arm64-arm-64bit
- Python version: 3.11.4
- Huggingface_hub version: 0.16.4
- PyArrow version: 12.0.1
- Pandas version: 1.5.1
hi @tsor13 , I haven't been able to reproduce your issue on `tsor13/test` dataset locally either. reinstalling doesn't help? |
https://github.com/huggingface/datasets/issues/6162 | load_dataset('json',...) from togethercomputer/RedPajama-Data-1T errors when jsonl rows contains different data fields | Hi ! Feel free to open a discussion at https://huggingface.co/datasets/togethercomputer/RedPajama-Data-1T/discussions to ask the file to be fixed (or directly open a PR with the fixed file)
`datasets` expects all the examples to have the same fields | ### Describe the bug
When loading some jsonl from redpajama-data-1T github source [togethercomputer/RedPajama-Data-1T](https://huggingface.co/datasets/togethercomputer/RedPajama-Data-1T) fails due to one row of the file containing an extra field called **symlink_target: string>**.
When deleting that line the loading is successful.
We also tried loading this file with the discrepancy using this function and it is successful
```python
os.environ["RED_PAJAMA_DATA_DIR"] ="/path_to_local_copy_of_RedPajama-Data-1T"
ds = load_dataset('togethercomputer/RedPajama-Data-1T', 'github',cache_dir="/path_to_folder_with_jsonl",streaming=True)['train']
```
### Steps to reproduce the bug
Steps to reproduce the behavior:
1. Load one jsonl from the redpajama-data-1T
```bash
wget https://data.together.xyz/redpajama-data-1T/v1.0.0/github/filtered_27f05c041a1c401783f90b9415e40e4b.sampled.jsonl
```
2.Load dataset will give error:
```python
from datasets import load_dataset
ds = load_dataset('json', data_files='/path_to/filtered_27f05c041a1c401783f90b9415e40e4b.sampled.jsonl')
```
_TypeError: Couldn't cast array of type
Struct
<content_hash: string,
timestamp: string,
source: string,
line_count: int64,
max_line_length: int64,
avg_line_length: double,
alnum_prop: double,
repo_name: string,
id: string,
size: string,
binary: bool,
copies: string,
ref: string,
path: string,
mode: string,
license: string,
language: list<item: struct<name: string, bytes: string>>, **symlink_target: string>**
to
{'content_hash': Value(dtype='string', id=None),
'timestamp': Value(dtype='string', id=None),
'source': Value(dtype='string', id=None),
'line_count': Value(dtype='int64', id=None),
'max_line_length': Value(dtype='int64', id=None),
'avg_line_length': Value(dtype='float64', id=None),
'alnum_prop': Value(dtype='float64', id=None),
'repo_name': Value(dtype='string', id=None),
'id': Value(dtype='string', id=None),
'size': Value(dtype='string', id=None),
'binary': Value(dtype='bool', id=None),
'copies': Value(dtype='string', id=None),
'ref': Value(dtype='string', id=None),
'path': Value(dtype='string', id=None),
'mode': Value(dtype='string', id=None),
'license': Value(dtype='string', id=None),
'language': [{'name': Value(dtype='string', id=None), 'bytes': Value(dtype='string', id=None)}]}_
3. To remove the line causing the problem that includes the **symlink_target: string>** do:
```bash
sed -i '112252d' filtered_27f05c041a1c401783f90b9415e40e4b.sampled.jsonl
```
4. Rerun the loading function now is succesful:
```python
from datasets import load_dataset
ds = load_dataset('json', data_files='/path_to/filtered_27f05c041a1c401783f90b9415e40e4b.sampled.jsonl')
```
### Expected behavior
Have a clean dataset without discrepancies on the jsonl fields or have the load_dataset('json',...) method not error out.
### Environment info
- `datasets` version: 2.14.1
- Platform: Linux-4.18.0-425.13.1.el8_7.x86_64-x86_64-with-glibc2.28
- Python version: 3.9.17
- Huggingface_hub version: 0.16.4
- PyArrow version: 12.0.1
- Pandas version: 2.0.3 | 36 | load_dataset('json',...) from togethercomputer/RedPajama-Data-1T errors when jsonl rows contains different data fields
### Describe the bug
When loading some jsonl from redpajama-data-1T github source [togethercomputer/RedPajama-Data-1T](https://huggingface.co/datasets/togethercomputer/RedPajama-Data-1T) fails due to one row of the file containing an extra field called **symlink_target: string>**.
When deleting that line the loading is successful.
We also tried loading this file with the discrepancy using this function and it is successful
```python
os.environ["RED_PAJAMA_DATA_DIR"] ="/path_to_local_copy_of_RedPajama-Data-1T"
ds = load_dataset('togethercomputer/RedPajama-Data-1T', 'github',cache_dir="/path_to_folder_with_jsonl",streaming=True)['train']
```
### Steps to reproduce the bug
Steps to reproduce the behavior:
1. Load one jsonl from the redpajama-data-1T
```bash
wget https://data.together.xyz/redpajama-data-1T/v1.0.0/github/filtered_27f05c041a1c401783f90b9415e40e4b.sampled.jsonl
```
2.Load dataset will give error:
```python
from datasets import load_dataset
ds = load_dataset('json', data_files='/path_to/filtered_27f05c041a1c401783f90b9415e40e4b.sampled.jsonl')
```
_TypeError: Couldn't cast array of type
Struct
<content_hash: string,
timestamp: string,
source: string,
line_count: int64,
max_line_length: int64,
avg_line_length: double,
alnum_prop: double,
repo_name: string,
id: string,
size: string,
binary: bool,
copies: string,
ref: string,
path: string,
mode: string,
license: string,
language: list<item: struct<name: string, bytes: string>>, **symlink_target: string>**
to
{'content_hash': Value(dtype='string', id=None),
'timestamp': Value(dtype='string', id=None),
'source': Value(dtype='string', id=None),
'line_count': Value(dtype='int64', id=None),
'max_line_length': Value(dtype='int64', id=None),
'avg_line_length': Value(dtype='float64', id=None),
'alnum_prop': Value(dtype='float64', id=None),
'repo_name': Value(dtype='string', id=None),
'id': Value(dtype='string', id=None),
'size': Value(dtype='string', id=None),
'binary': Value(dtype='bool', id=None),
'copies': Value(dtype='string', id=None),
'ref': Value(dtype='string', id=None),
'path': Value(dtype='string', id=None),
'mode': Value(dtype='string', id=None),
'license': Value(dtype='string', id=None),
'language': [{'name': Value(dtype='string', id=None), 'bytes': Value(dtype='string', id=None)}]}_
3. To remove the line causing the problem that includes the **symlink_target: string>** do:
```bash
sed -i '112252d' filtered_27f05c041a1c401783f90b9415e40e4b.sampled.jsonl
```
4. Rerun the loading function now is succesful:
```python
from datasets import load_dataset
ds = load_dataset('json', data_files='/path_to/filtered_27f05c041a1c401783f90b9415e40e4b.sampled.jsonl')
```
### Expected behavior
Have a clean dataset without discrepancies on the jsonl fields or have the load_dataset('json',...) method not error out.
### Environment info
- `datasets` version: 2.14.1
- Platform: Linux-4.18.0-425.13.1.el8_7.x86_64-x86_64-with-glibc2.28
- Python version: 3.9.17
- Huggingface_hub version: 0.16.4
- PyArrow version: 12.0.1
- Pandas version: 2.0.3
Hi ! Feel free to open a discussion at https://huggingface.co/datasets/togethercomputer/RedPajama-Data-1T/discussions to ask the file to be fixed (or directly open a PR with the fixed file)
`datasets` expects all the examples to have the same fields |
https://github.com/huggingface/datasets/issues/6162 | load_dataset('json',...) from togethercomputer/RedPajama-Data-1T errors when jsonl rows contains different data fields | @lhoestq I think the problem is caused by the fact that hugging face datasets writes a copy of data to the local cache using pyarrow. And the data scheme is inferred from the first few data blocks as can be seen [here](https://github.com/huggingface/datasets/blob/main/src/datasets/arrow_writer.py#L570). Maybe setting `streaming=True` can workaround this problem. Would you agree with my statement? | ### Describe the bug
When loading some jsonl from redpajama-data-1T github source [togethercomputer/RedPajama-Data-1T](https://huggingface.co/datasets/togethercomputer/RedPajama-Data-1T) fails due to one row of the file containing an extra field called **symlink_target: string>**.
When deleting that line the loading is successful.
We also tried loading this file with the discrepancy using this function and it is successful
```python
os.environ["RED_PAJAMA_DATA_DIR"] ="/path_to_local_copy_of_RedPajama-Data-1T"
ds = load_dataset('togethercomputer/RedPajama-Data-1T', 'github',cache_dir="/path_to_folder_with_jsonl",streaming=True)['train']
```
### Steps to reproduce the bug
Steps to reproduce the behavior:
1. Load one jsonl from the redpajama-data-1T
```bash
wget https://data.together.xyz/redpajama-data-1T/v1.0.0/github/filtered_27f05c041a1c401783f90b9415e40e4b.sampled.jsonl
```
2.Load dataset will give error:
```python
from datasets import load_dataset
ds = load_dataset('json', data_files='/path_to/filtered_27f05c041a1c401783f90b9415e40e4b.sampled.jsonl')
```
_TypeError: Couldn't cast array of type
Struct
<content_hash: string,
timestamp: string,
source: string,
line_count: int64,
max_line_length: int64,
avg_line_length: double,
alnum_prop: double,
repo_name: string,
id: string,
size: string,
binary: bool,
copies: string,
ref: string,
path: string,
mode: string,
license: string,
language: list<item: struct<name: string, bytes: string>>, **symlink_target: string>**
to
{'content_hash': Value(dtype='string', id=None),
'timestamp': Value(dtype='string', id=None),
'source': Value(dtype='string', id=None),
'line_count': Value(dtype='int64', id=None),
'max_line_length': Value(dtype='int64', id=None),
'avg_line_length': Value(dtype='float64', id=None),
'alnum_prop': Value(dtype='float64', id=None),
'repo_name': Value(dtype='string', id=None),
'id': Value(dtype='string', id=None),
'size': Value(dtype='string', id=None),
'binary': Value(dtype='bool', id=None),
'copies': Value(dtype='string', id=None),
'ref': Value(dtype='string', id=None),
'path': Value(dtype='string', id=None),
'mode': Value(dtype='string', id=None),
'license': Value(dtype='string', id=None),
'language': [{'name': Value(dtype='string', id=None), 'bytes': Value(dtype='string', id=None)}]}_
3. To remove the line causing the problem that includes the **symlink_target: string>** do:
```bash
sed -i '112252d' filtered_27f05c041a1c401783f90b9415e40e4b.sampled.jsonl
```
4. Rerun the loading function now is succesful:
```python
from datasets import load_dataset
ds = load_dataset('json', data_files='/path_to/filtered_27f05c041a1c401783f90b9415e40e4b.sampled.jsonl')
```
### Expected behavior
Have a clean dataset without discrepancies on the jsonl fields or have the load_dataset('json',...) method not error out.
### Environment info
- `datasets` version: 2.14.1
- Platform: Linux-4.18.0-425.13.1.el8_7.x86_64-x86_64-with-glibc2.28
- Python version: 3.9.17
- Huggingface_hub version: 0.16.4
- PyArrow version: 12.0.1
- Pandas version: 2.0.3 | 55 | load_dataset('json',...) from togethercomputer/RedPajama-Data-1T errors when jsonl rows contains different data fields
### Describe the bug
When loading some jsonl from redpajama-data-1T github source [togethercomputer/RedPajama-Data-1T](https://huggingface.co/datasets/togethercomputer/RedPajama-Data-1T) fails due to one row of the file containing an extra field called **symlink_target: string>**.
When deleting that line the loading is successful.
We also tried loading this file with the discrepancy using this function and it is successful
```python
os.environ["RED_PAJAMA_DATA_DIR"] ="/path_to_local_copy_of_RedPajama-Data-1T"
ds = load_dataset('togethercomputer/RedPajama-Data-1T', 'github',cache_dir="/path_to_folder_with_jsonl",streaming=True)['train']
```
### Steps to reproduce the bug
Steps to reproduce the behavior:
1. Load one jsonl from the redpajama-data-1T
```bash
wget https://data.together.xyz/redpajama-data-1T/v1.0.0/github/filtered_27f05c041a1c401783f90b9415e40e4b.sampled.jsonl
```
2.Load dataset will give error:
```python
from datasets import load_dataset
ds = load_dataset('json', data_files='/path_to/filtered_27f05c041a1c401783f90b9415e40e4b.sampled.jsonl')
```
_TypeError: Couldn't cast array of type
Struct
<content_hash: string,
timestamp: string,
source: string,
line_count: int64,
max_line_length: int64,
avg_line_length: double,
alnum_prop: double,
repo_name: string,
id: string,
size: string,
binary: bool,
copies: string,
ref: string,
path: string,
mode: string,
license: string,
language: list<item: struct<name: string, bytes: string>>, **symlink_target: string>**
to
{'content_hash': Value(dtype='string', id=None),
'timestamp': Value(dtype='string', id=None),
'source': Value(dtype='string', id=None),
'line_count': Value(dtype='int64', id=None),
'max_line_length': Value(dtype='int64', id=None),
'avg_line_length': Value(dtype='float64', id=None),
'alnum_prop': Value(dtype='float64', id=None),
'repo_name': Value(dtype='string', id=None),
'id': Value(dtype='string', id=None),
'size': Value(dtype='string', id=None),
'binary': Value(dtype='bool', id=None),
'copies': Value(dtype='string', id=None),
'ref': Value(dtype='string', id=None),
'path': Value(dtype='string', id=None),
'mode': Value(dtype='string', id=None),
'license': Value(dtype='string', id=None),
'language': [{'name': Value(dtype='string', id=None), 'bytes': Value(dtype='string', id=None)}]}_
3. To remove the line causing the problem that includes the **symlink_target: string>** do:
```bash
sed -i '112252d' filtered_27f05c041a1c401783f90b9415e40e4b.sampled.jsonl
```
4. Rerun the loading function now is succesful:
```python
from datasets import load_dataset
ds = load_dataset('json', data_files='/path_to/filtered_27f05c041a1c401783f90b9415e40e4b.sampled.jsonl')
```
### Expected behavior
Have a clean dataset without discrepancies on the jsonl fields or have the load_dataset('json',...) method not error out.
### Environment info
- `datasets` version: 2.14.1
- Platform: Linux-4.18.0-425.13.1.el8_7.x86_64-x86_64-with-glibc2.28
- Python version: 3.9.17
- Huggingface_hub version: 0.16.4
- PyArrow version: 12.0.1
- Pandas version: 2.0.3
@lhoestq I think the problem is caused by the fact that hugging face datasets writes a copy of data to the local cache using pyarrow. And the data scheme is inferred from the first few data blocks as can be seen [here](https://github.com/huggingface/datasets/blob/main/src/datasets/arrow_writer.py#L570). Maybe setting `streaming=True` can workaround this problem. Would you agree with my statement? |
https://github.com/huggingface/datasets/issues/6162 | load_dataset('json',...) from togethercomputer/RedPajama-Data-1T errors when jsonl rows contains different data fields | > @lhoestq I think the problem is caused by the fact that hugging face datasets writes a copy of data to the local cache using pyarrow. And the data scheme is inferred from the first few data blocks as can be seen [here](https://github.com/huggingface/datasets/blob/main/src/datasets/arrow_writer.py#L570).
Correct. Therefore any example that doesn't follow the inferred schema will make the code fail.
> Maybe setting streaming=True can workaround this problem. Would you agree with my statement?
You'll meet the same problem but later - when streaming and arriving at the problematic example | ### Describe the bug
When loading some jsonl from redpajama-data-1T github source [togethercomputer/RedPajama-Data-1T](https://huggingface.co/datasets/togethercomputer/RedPajama-Data-1T) fails due to one row of the file containing an extra field called **symlink_target: string>**.
When deleting that line the loading is successful.
We also tried loading this file with the discrepancy using this function and it is successful
```python
os.environ["RED_PAJAMA_DATA_DIR"] ="/path_to_local_copy_of_RedPajama-Data-1T"
ds = load_dataset('togethercomputer/RedPajama-Data-1T', 'github',cache_dir="/path_to_folder_with_jsonl",streaming=True)['train']
```
### Steps to reproduce the bug
Steps to reproduce the behavior:
1. Load one jsonl from the redpajama-data-1T
```bash
wget https://data.together.xyz/redpajama-data-1T/v1.0.0/github/filtered_27f05c041a1c401783f90b9415e40e4b.sampled.jsonl
```
2.Load dataset will give error:
```python
from datasets import load_dataset
ds = load_dataset('json', data_files='/path_to/filtered_27f05c041a1c401783f90b9415e40e4b.sampled.jsonl')
```
_TypeError: Couldn't cast array of type
Struct
<content_hash: string,
timestamp: string,
source: string,
line_count: int64,
max_line_length: int64,
avg_line_length: double,
alnum_prop: double,
repo_name: string,
id: string,
size: string,
binary: bool,
copies: string,
ref: string,
path: string,
mode: string,
license: string,
language: list<item: struct<name: string, bytes: string>>, **symlink_target: string>**
to
{'content_hash': Value(dtype='string', id=None),
'timestamp': Value(dtype='string', id=None),
'source': Value(dtype='string', id=None),
'line_count': Value(dtype='int64', id=None),
'max_line_length': Value(dtype='int64', id=None),
'avg_line_length': Value(dtype='float64', id=None),
'alnum_prop': Value(dtype='float64', id=None),
'repo_name': Value(dtype='string', id=None),
'id': Value(dtype='string', id=None),
'size': Value(dtype='string', id=None),
'binary': Value(dtype='bool', id=None),
'copies': Value(dtype='string', id=None),
'ref': Value(dtype='string', id=None),
'path': Value(dtype='string', id=None),
'mode': Value(dtype='string', id=None),
'license': Value(dtype='string', id=None),
'language': [{'name': Value(dtype='string', id=None), 'bytes': Value(dtype='string', id=None)}]}_
3. To remove the line causing the problem that includes the **symlink_target: string>** do:
```bash
sed -i '112252d' filtered_27f05c041a1c401783f90b9415e40e4b.sampled.jsonl
```
4. Rerun the loading function now is succesful:
```python
from datasets import load_dataset
ds = load_dataset('json', data_files='/path_to/filtered_27f05c041a1c401783f90b9415e40e4b.sampled.jsonl')
```
### Expected behavior
Have a clean dataset without discrepancies on the jsonl fields or have the load_dataset('json',...) method not error out.
### Environment info
- `datasets` version: 2.14.1
- Platform: Linux-4.18.0-425.13.1.el8_7.x86_64-x86_64-with-glibc2.28
- Python version: 3.9.17
- Huggingface_hub version: 0.16.4
- PyArrow version: 12.0.1
- Pandas version: 2.0.3 | 88 | load_dataset('json',...) from togethercomputer/RedPajama-Data-1T errors when jsonl rows contains different data fields
### Describe the bug
When loading some jsonl from redpajama-data-1T github source [togethercomputer/RedPajama-Data-1T](https://huggingface.co/datasets/togethercomputer/RedPajama-Data-1T) fails due to one row of the file containing an extra field called **symlink_target: string>**.
When deleting that line the loading is successful.
We also tried loading this file with the discrepancy using this function and it is successful
```python
os.environ["RED_PAJAMA_DATA_DIR"] ="/path_to_local_copy_of_RedPajama-Data-1T"
ds = load_dataset('togethercomputer/RedPajama-Data-1T', 'github',cache_dir="/path_to_folder_with_jsonl",streaming=True)['train']
```
### Steps to reproduce the bug
Steps to reproduce the behavior:
1. Load one jsonl from the redpajama-data-1T
```bash
wget https://data.together.xyz/redpajama-data-1T/v1.0.0/github/filtered_27f05c041a1c401783f90b9415e40e4b.sampled.jsonl
```
2.Load dataset will give error:
```python
from datasets import load_dataset
ds = load_dataset('json', data_files='/path_to/filtered_27f05c041a1c401783f90b9415e40e4b.sampled.jsonl')
```
_TypeError: Couldn't cast array of type
Struct
<content_hash: string,
timestamp: string,
source: string,
line_count: int64,
max_line_length: int64,
avg_line_length: double,
alnum_prop: double,
repo_name: string,
id: string,
size: string,
binary: bool,
copies: string,
ref: string,
path: string,
mode: string,
license: string,
language: list<item: struct<name: string, bytes: string>>, **symlink_target: string>**
to
{'content_hash': Value(dtype='string', id=None),
'timestamp': Value(dtype='string', id=None),
'source': Value(dtype='string', id=None),
'line_count': Value(dtype='int64', id=None),
'max_line_length': Value(dtype='int64', id=None),
'avg_line_length': Value(dtype='float64', id=None),
'alnum_prop': Value(dtype='float64', id=None),
'repo_name': Value(dtype='string', id=None),
'id': Value(dtype='string', id=None),
'size': Value(dtype='string', id=None),
'binary': Value(dtype='bool', id=None),
'copies': Value(dtype='string', id=None),
'ref': Value(dtype='string', id=None),
'path': Value(dtype='string', id=None),
'mode': Value(dtype='string', id=None),
'license': Value(dtype='string', id=None),
'language': [{'name': Value(dtype='string', id=None), 'bytes': Value(dtype='string', id=None)}]}_
3. To remove the line causing the problem that includes the **symlink_target: string>** do:
```bash
sed -i '112252d' filtered_27f05c041a1c401783f90b9415e40e4b.sampled.jsonl
```
4. Rerun the loading function now is succesful:
```python
from datasets import load_dataset
ds = load_dataset('json', data_files='/path_to/filtered_27f05c041a1c401783f90b9415e40e4b.sampled.jsonl')
```
### Expected behavior
Have a clean dataset without discrepancies on the jsonl fields or have the load_dataset('json',...) method not error out.
### Environment info
- `datasets` version: 2.14.1
- Platform: Linux-4.18.0-425.13.1.el8_7.x86_64-x86_64-with-glibc2.28
- Python version: 3.9.17
- Huggingface_hub version: 0.16.4
- PyArrow version: 12.0.1
- Pandas version: 2.0.3
> @lhoestq I think the problem is caused by the fact that hugging face datasets writes a copy of data to the local cache using pyarrow. And the data scheme is inferred from the first few data blocks as can be seen [here](https://github.com/huggingface/datasets/blob/main/src/datasets/arrow_writer.py#L570).
Correct. Therefore any example that doesn't follow the inferred schema will make the code fail.
> Maybe setting streaming=True can workaround this problem. Would you agree with my statement?
You'll meet the same problem but later - when streaming and arriving at the problematic example |
https://github.com/huggingface/datasets/issues/6162 | load_dataset('json',...) from togethercomputer/RedPajama-Data-1T errors when jsonl rows contains different data fields | @lhoestq I just run below test with streaming=True and is not failing at the problematic example
```python
ds = load_dataset('json', data_files='/path_to_local_RedPajamaData/filtered_27f05c041a1c401783f90b9415e40e4b.sampled.jsonl', streaming=True)
count = 0
for i in ds['train']:
count += 1
print(count)
```
and completes the 262241 samples successfully. It does error our when streaming is not used | ### Describe the bug
When loading some jsonl from redpajama-data-1T github source [togethercomputer/RedPajama-Data-1T](https://huggingface.co/datasets/togethercomputer/RedPajama-Data-1T) fails due to one row of the file containing an extra field called **symlink_target: string>**.
When deleting that line the loading is successful.
We also tried loading this file with the discrepancy using this function and it is successful
```python
os.environ["RED_PAJAMA_DATA_DIR"] ="/path_to_local_copy_of_RedPajama-Data-1T"
ds = load_dataset('togethercomputer/RedPajama-Data-1T', 'github',cache_dir="/path_to_folder_with_jsonl",streaming=True)['train']
```
### Steps to reproduce the bug
Steps to reproduce the behavior:
1. Load one jsonl from the redpajama-data-1T
```bash
wget https://data.together.xyz/redpajama-data-1T/v1.0.0/github/filtered_27f05c041a1c401783f90b9415e40e4b.sampled.jsonl
```
2.Load dataset will give error:
```python
from datasets import load_dataset
ds = load_dataset('json', data_files='/path_to/filtered_27f05c041a1c401783f90b9415e40e4b.sampled.jsonl')
```
_TypeError: Couldn't cast array of type
Struct
<content_hash: string,
timestamp: string,
source: string,
line_count: int64,
max_line_length: int64,
avg_line_length: double,
alnum_prop: double,
repo_name: string,
id: string,
size: string,
binary: bool,
copies: string,
ref: string,
path: string,
mode: string,
license: string,
language: list<item: struct<name: string, bytes: string>>, **symlink_target: string>**
to
{'content_hash': Value(dtype='string', id=None),
'timestamp': Value(dtype='string', id=None),
'source': Value(dtype='string', id=None),
'line_count': Value(dtype='int64', id=None),
'max_line_length': Value(dtype='int64', id=None),
'avg_line_length': Value(dtype='float64', id=None),
'alnum_prop': Value(dtype='float64', id=None),
'repo_name': Value(dtype='string', id=None),
'id': Value(dtype='string', id=None),
'size': Value(dtype='string', id=None),
'binary': Value(dtype='bool', id=None),
'copies': Value(dtype='string', id=None),
'ref': Value(dtype='string', id=None),
'path': Value(dtype='string', id=None),
'mode': Value(dtype='string', id=None),
'license': Value(dtype='string', id=None),
'language': [{'name': Value(dtype='string', id=None), 'bytes': Value(dtype='string', id=None)}]}_
3. To remove the line causing the problem that includes the **symlink_target: string>** do:
```bash
sed -i '112252d' filtered_27f05c041a1c401783f90b9415e40e4b.sampled.jsonl
```
4. Rerun the loading function now is succesful:
```python
from datasets import load_dataset
ds = load_dataset('json', data_files='/path_to/filtered_27f05c041a1c401783f90b9415e40e4b.sampled.jsonl')
```
### Expected behavior
Have a clean dataset without discrepancies on the jsonl fields or have the load_dataset('json',...) method not error out.
### Environment info
- `datasets` version: 2.14.1
- Platform: Linux-4.18.0-425.13.1.el8_7.x86_64-x86_64-with-glibc2.28
- Python version: 3.9.17
- Huggingface_hub version: 0.16.4
- PyArrow version: 12.0.1
- Pandas version: 2.0.3 | 49 | load_dataset('json',...) from togethercomputer/RedPajama-Data-1T errors when jsonl rows contains different data fields
### Describe the bug
When loading some jsonl from redpajama-data-1T github source [togethercomputer/RedPajama-Data-1T](https://huggingface.co/datasets/togethercomputer/RedPajama-Data-1T) fails due to one row of the file containing an extra field called **symlink_target: string>**.
When deleting that line the loading is successful.
We also tried loading this file with the discrepancy using this function and it is successful
```python
os.environ["RED_PAJAMA_DATA_DIR"] ="/path_to_local_copy_of_RedPajama-Data-1T"
ds = load_dataset('togethercomputer/RedPajama-Data-1T', 'github',cache_dir="/path_to_folder_with_jsonl",streaming=True)['train']
```
### Steps to reproduce the bug
Steps to reproduce the behavior:
1. Load one jsonl from the redpajama-data-1T
```bash
wget https://data.together.xyz/redpajama-data-1T/v1.0.0/github/filtered_27f05c041a1c401783f90b9415e40e4b.sampled.jsonl
```
2.Load dataset will give error:
```python
from datasets import load_dataset
ds = load_dataset('json', data_files='/path_to/filtered_27f05c041a1c401783f90b9415e40e4b.sampled.jsonl')
```
_TypeError: Couldn't cast array of type
Struct
<content_hash: string,
timestamp: string,
source: string,
line_count: int64,
max_line_length: int64,
avg_line_length: double,
alnum_prop: double,
repo_name: string,
id: string,
size: string,
binary: bool,
copies: string,
ref: string,
path: string,
mode: string,
license: string,
language: list<item: struct<name: string, bytes: string>>, **symlink_target: string>**
to
{'content_hash': Value(dtype='string', id=None),
'timestamp': Value(dtype='string', id=None),
'source': Value(dtype='string', id=None),
'line_count': Value(dtype='int64', id=None),
'max_line_length': Value(dtype='int64', id=None),
'avg_line_length': Value(dtype='float64', id=None),
'alnum_prop': Value(dtype='float64', id=None),
'repo_name': Value(dtype='string', id=None),
'id': Value(dtype='string', id=None),
'size': Value(dtype='string', id=None),
'binary': Value(dtype='bool', id=None),
'copies': Value(dtype='string', id=None),
'ref': Value(dtype='string', id=None),
'path': Value(dtype='string', id=None),
'mode': Value(dtype='string', id=None),
'license': Value(dtype='string', id=None),
'language': [{'name': Value(dtype='string', id=None), 'bytes': Value(dtype='string', id=None)}]}_
3. To remove the line causing the problem that includes the **symlink_target: string>** do:
```bash
sed -i '112252d' filtered_27f05c041a1c401783f90b9415e40e4b.sampled.jsonl
```
4. Rerun the loading function now is succesful:
```python
from datasets import load_dataset
ds = load_dataset('json', data_files='/path_to/filtered_27f05c041a1c401783f90b9415e40e4b.sampled.jsonl')
```
### Expected behavior
Have a clean dataset without discrepancies on the jsonl fields or have the load_dataset('json',...) method not error out.
### Environment info
- `datasets` version: 2.14.1
- Platform: Linux-4.18.0-425.13.1.el8_7.x86_64-x86_64-with-glibc2.28
- Python version: 3.9.17
- Huggingface_hub version: 0.16.4
- PyArrow version: 12.0.1
- Pandas version: 2.0.3
@lhoestq I just run below test with streaming=True and is not failing at the problematic example
```python
ds = load_dataset('json', data_files='/path_to_local_RedPajamaData/filtered_27f05c041a1c401783f90b9415e40e4b.sampled.jsonl', streaming=True)
count = 0
for i in ds['train']:
count += 1
print(count)
```
and completes the 262241 samples successfully. It does error our when streaming is not used |
https://github.com/huggingface/datasets/issues/6157 | DatasetInfo.__init__() got an unexpected keyword argument '_column_requires_decoding' | Thanks for reporting, but we can only fix this issue if you can provide a reproducer that consistently reproduces it. | ### Describe the bug
When I was in load_dataset, it said "DatasetInfo.__init__() got an unexpected keyword argument '_column_requires_decoding'". The second time I ran it, there was no error and the dataset object worked
### Steps to reproduce the bug
/home/aihao/workspace/DeepLearningContent/datasets/images/images.py
```python
from logging import config
import datasets
import os
from PIL import Image
import csv
import json
class ImagesConfig(datasets.BuilderConfig):
def __init__(self, **kwargs):
super(ImagesConfig, self).__init__(**kwargs)
class Images(datasets.GeneratorBasedBuilder):
def _split_generators(self, dl_manager: datasets.DownloadManager):
return [
datasets.SplitGenerator(
name=datasets.Split.TRAIN,
gen_kwargs={"split": datasets.Split.TRAIN},
)
]
BUILDER_CONFIGS = [
ImagesConfig(
name="similar_pairs",
description="simliar pair dataset,item is a pair of similar images",
),
ImagesConfig(
name="image_prompt_pairs",
description="image prompt pairs",
),
]
def _info(self):
if self.config.name == "similar_pairs":
return datasets.Features(
{
"image1": datasets.features.Image(),
"image2": datasets.features.Image(),
"similarity": datasets.Value("float32"),
}
)
elif self.config.name == "image_prompt_pairs":
return datasets.Features(
{"image": datasets.features.Image(), "prompt": datasets.Value("string")}
)
def _generate_examples(self, split):
data_path = os.path.join(self.config.data_dir, "data")
if self.config.name == "similar_pairs":
prompts = {}
with open(os.path.join(data_path ,"prompts.json"), "r") as f:
prompts = json.load(f)
with open(os.path.join(data_path, "similar_pairs.csv"), "r") as f:
reader = csv.reader(f)
for row in reader:
image1_path, image2_path, similarity = row
yield image1_path + ":" + image2_path + ":", {
"image1": Image.open(image1_path),
"prompt1": prompts[image1_path],
"image2": Image.open(image2_path),
"prompt2": prompts[image2_path],
"similarity": float(similarity),
}
```
Code that indicates an error:
```python
from datasets import load_dataset
import json
import csv
import ast
import torch
data_dir = "/home/aihao/workspace/DeepLearningContent/datasets/images"
dataset = load_dataset(data_dir, data_dir=data_dir, name="similar_pairs")
```
### Expected behavior
The first execution gives an error, but it works fine
### Environment info
- `datasets` version: 2.14.3
- Platform: Linux-6.2.0-26-generic-x86_64-with-glibc2.35
- Python version: 3.11.4
- Huggingface_hub version: 0.16.4
- PyArrow version: 12.0.1
- Pandas version: 2.0.3 | 20 | DatasetInfo.__init__() got an unexpected keyword argument '_column_requires_decoding'
### Describe the bug
When I was in load_dataset, it said "DatasetInfo.__init__() got an unexpected keyword argument '_column_requires_decoding'". The second time I ran it, there was no error and the dataset object worked
### Steps to reproduce the bug
/home/aihao/workspace/DeepLearningContent/datasets/images/images.py
```python
from logging import config
import datasets
import os
from PIL import Image
import csv
import json
class ImagesConfig(datasets.BuilderConfig):
def __init__(self, **kwargs):
super(ImagesConfig, self).__init__(**kwargs)
class Images(datasets.GeneratorBasedBuilder):
def _split_generators(self, dl_manager: datasets.DownloadManager):
return [
datasets.SplitGenerator(
name=datasets.Split.TRAIN,
gen_kwargs={"split": datasets.Split.TRAIN},
)
]
BUILDER_CONFIGS = [
ImagesConfig(
name="similar_pairs",
description="simliar pair dataset,item is a pair of similar images",
),
ImagesConfig(
name="image_prompt_pairs",
description="image prompt pairs",
),
]
def _info(self):
if self.config.name == "similar_pairs":
return datasets.Features(
{
"image1": datasets.features.Image(),
"image2": datasets.features.Image(),
"similarity": datasets.Value("float32"),
}
)
elif self.config.name == "image_prompt_pairs":
return datasets.Features(
{"image": datasets.features.Image(), "prompt": datasets.Value("string")}
)
def _generate_examples(self, split):
data_path = os.path.join(self.config.data_dir, "data")
if self.config.name == "similar_pairs":
prompts = {}
with open(os.path.join(data_path ,"prompts.json"), "r") as f:
prompts = json.load(f)
with open(os.path.join(data_path, "similar_pairs.csv"), "r") as f:
reader = csv.reader(f)
for row in reader:
image1_path, image2_path, similarity = row
yield image1_path + ":" + image2_path + ":", {
"image1": Image.open(image1_path),
"prompt1": prompts[image1_path],
"image2": Image.open(image2_path),
"prompt2": prompts[image2_path],
"similarity": float(similarity),
}
```
Code that indicates an error:
```python
from datasets import load_dataset
import json
import csv
import ast
import torch
data_dir = "/home/aihao/workspace/DeepLearningContent/datasets/images"
dataset = load_dataset(data_dir, data_dir=data_dir, name="similar_pairs")
```
### Expected behavior
The first execution gives an error, but it works fine
### Environment info
- `datasets` version: 2.14.3
- Platform: Linux-6.2.0-26-generic-x86_64-with-glibc2.35
- Python version: 3.11.4
- Huggingface_hub version: 0.16.4
- PyArrow version: 12.0.1
- Pandas version: 2.0.3
Thanks for reporting, but we can only fix this issue if you can provide a reproducer that consistently reproduces it. |
https://github.com/huggingface/datasets/issues/6157 | DatasetInfo.__init__() got an unexpected keyword argument '_column_requires_decoding' | Does this error occur even if you change the cache directory (the `cache_dir` parameter in `load_dataset`)? | ### Describe the bug
When I was in load_dataset, it said "DatasetInfo.__init__() got an unexpected keyword argument '_column_requires_decoding'". The second time I ran it, there was no error and the dataset object worked
### Steps to reproduce the bug
/home/aihao/workspace/DeepLearningContent/datasets/images/images.py
```python
from logging import config
import datasets
import os
from PIL import Image
import csv
import json
class ImagesConfig(datasets.BuilderConfig):
def __init__(self, **kwargs):
super(ImagesConfig, self).__init__(**kwargs)
class Images(datasets.GeneratorBasedBuilder):
def _split_generators(self, dl_manager: datasets.DownloadManager):
return [
datasets.SplitGenerator(
name=datasets.Split.TRAIN,
gen_kwargs={"split": datasets.Split.TRAIN},
)
]
BUILDER_CONFIGS = [
ImagesConfig(
name="similar_pairs",
description="simliar pair dataset,item is a pair of similar images",
),
ImagesConfig(
name="image_prompt_pairs",
description="image prompt pairs",
),
]
def _info(self):
if self.config.name == "similar_pairs":
return datasets.Features(
{
"image1": datasets.features.Image(),
"image2": datasets.features.Image(),
"similarity": datasets.Value("float32"),
}
)
elif self.config.name == "image_prompt_pairs":
return datasets.Features(
{"image": datasets.features.Image(), "prompt": datasets.Value("string")}
)
def _generate_examples(self, split):
data_path = os.path.join(self.config.data_dir, "data")
if self.config.name == "similar_pairs":
prompts = {}
with open(os.path.join(data_path ,"prompts.json"), "r") as f:
prompts = json.load(f)
with open(os.path.join(data_path, "similar_pairs.csv"), "r") as f:
reader = csv.reader(f)
for row in reader:
image1_path, image2_path, similarity = row
yield image1_path + ":" + image2_path + ":", {
"image1": Image.open(image1_path),
"prompt1": prompts[image1_path],
"image2": Image.open(image2_path),
"prompt2": prompts[image2_path],
"similarity": float(similarity),
}
```
Code that indicates an error:
```python
from datasets import load_dataset
import json
import csv
import ast
import torch
data_dir = "/home/aihao/workspace/DeepLearningContent/datasets/images"
dataset = load_dataset(data_dir, data_dir=data_dir, name="similar_pairs")
```
### Expected behavior
The first execution gives an error, but it works fine
### Environment info
- `datasets` version: 2.14.3
- Platform: Linux-6.2.0-26-generic-x86_64-with-glibc2.35
- Python version: 3.11.4
- Huggingface_hub version: 0.16.4
- PyArrow version: 12.0.1
- Pandas version: 2.0.3 | 16 | DatasetInfo.__init__() got an unexpected keyword argument '_column_requires_decoding'
### Describe the bug
When I was in load_dataset, it said "DatasetInfo.__init__() got an unexpected keyword argument '_column_requires_decoding'". The second time I ran it, there was no error and the dataset object worked
### Steps to reproduce the bug
/home/aihao/workspace/DeepLearningContent/datasets/images/images.py
```python
from logging import config
import datasets
import os
from PIL import Image
import csv
import json
class ImagesConfig(datasets.BuilderConfig):
def __init__(self, **kwargs):
super(ImagesConfig, self).__init__(**kwargs)
class Images(datasets.GeneratorBasedBuilder):
def _split_generators(self, dl_manager: datasets.DownloadManager):
return [
datasets.SplitGenerator(
name=datasets.Split.TRAIN,
gen_kwargs={"split": datasets.Split.TRAIN},
)
]
BUILDER_CONFIGS = [
ImagesConfig(
name="similar_pairs",
description="simliar pair dataset,item is a pair of similar images",
),
ImagesConfig(
name="image_prompt_pairs",
description="image prompt pairs",
),
]
def _info(self):
if self.config.name == "similar_pairs":
return datasets.Features(
{
"image1": datasets.features.Image(),
"image2": datasets.features.Image(),
"similarity": datasets.Value("float32"),
}
)
elif self.config.name == "image_prompt_pairs":
return datasets.Features(
{"image": datasets.features.Image(), "prompt": datasets.Value("string")}
)
def _generate_examples(self, split):
data_path = os.path.join(self.config.data_dir, "data")
if self.config.name == "similar_pairs":
prompts = {}
with open(os.path.join(data_path ,"prompts.json"), "r") as f:
prompts = json.load(f)
with open(os.path.join(data_path, "similar_pairs.csv"), "r") as f:
reader = csv.reader(f)
for row in reader:
image1_path, image2_path, similarity = row
yield image1_path + ":" + image2_path + ":", {
"image1": Image.open(image1_path),
"prompt1": prompts[image1_path],
"image2": Image.open(image2_path),
"prompt2": prompts[image2_path],
"similarity": float(similarity),
}
```
Code that indicates an error:
```python
from datasets import load_dataset
import json
import csv
import ast
import torch
data_dir = "/home/aihao/workspace/DeepLearningContent/datasets/images"
dataset = load_dataset(data_dir, data_dir=data_dir, name="similar_pairs")
```
### Expected behavior
The first execution gives an error, but it works fine
### Environment info
- `datasets` version: 2.14.3
- Platform: Linux-6.2.0-26-generic-x86_64-with-glibc2.35
- Python version: 3.11.4
- Huggingface_hub version: 0.16.4
- PyArrow version: 12.0.1
- Pandas version: 2.0.3
Does this error occur even if you change the cache directory (the `cache_dir` parameter in `load_dataset`)? |
https://github.com/huggingface/datasets/issues/6156 | Why not use self._epoch as seed to shuffle in distributed training with IterableDataset | `_effective_generator` returns a RNG that takes into account `self._epoch` and the current dataset's base shuffling RNG (which can be set by specifying `seed=` in `.shuffle() for example`).
To fix your error you can pass `seed=` to `.shuffle()`. And the shuffling will depend on both this seed and `self._epoch` | ### Describe the bug
Currently, distributed training with `IterableDataset` needs to pass fixed seed to shuffle to keep each node use the same seed to avoid overlapping.
https://github.com/huggingface/datasets/blob/a7f8d9019e7cb104eac4106bdc6ec0292f0dc61a/src/datasets/iterable_dataset.py#L1174-L1177
My question is why not directly use `self._epoch` which is set by `set_epoch` as seed? It's almost the same across nodes.
https://github.com/huggingface/datasets/blob/a7f8d9019e7cb104eac4106bdc6ec0292f0dc61a/src/datasets/iterable_dataset.py#L1790-L1801
If not using `self._epoch` as shuffling seed, what does this method do to prepare an epoch seeded generator?
https://github.com/huggingface/datasets/blob/a7f8d9019e7cb104eac4106bdc6ec0292f0dc61a/src/datasets/iterable_dataset.py#L1206
### Steps to reproduce the bug
As mentioned above.
### Expected behavior
As mentioned above.
### Environment info
Not related | 48 | Why not use self._epoch as seed to shuffle in distributed training with IterableDataset
### Describe the bug
Currently, distributed training with `IterableDataset` needs to pass fixed seed to shuffle to keep each node use the same seed to avoid overlapping.
https://github.com/huggingface/datasets/blob/a7f8d9019e7cb104eac4106bdc6ec0292f0dc61a/src/datasets/iterable_dataset.py#L1174-L1177
My question is why not directly use `self._epoch` which is set by `set_epoch` as seed? It's almost the same across nodes.
https://github.com/huggingface/datasets/blob/a7f8d9019e7cb104eac4106bdc6ec0292f0dc61a/src/datasets/iterable_dataset.py#L1790-L1801
If not using `self._epoch` as shuffling seed, what does this method do to prepare an epoch seeded generator?
https://github.com/huggingface/datasets/blob/a7f8d9019e7cb104eac4106bdc6ec0292f0dc61a/src/datasets/iterable_dataset.py#L1206
### Steps to reproduce the bug
As mentioned above.
### Expected behavior
As mentioned above.
### Environment info
Not related
`_effective_generator` returns a RNG that takes into account `self._epoch` and the current dataset's base shuffling RNG (which can be set by specifying `seed=` in `.shuffle() for example`).
To fix your error you can pass `seed=` to `.shuffle()`. And the shuffling will depend on both this seed and `self._epoch` |
https://github.com/huggingface/datasets/issues/6152 | FolderBase Dataset automatically resolves under current directory when data_dir is not specified | Makes sense, I guess this can be fixed in the load_dataset_builder method.
It concerns every packaged builder I think (see values in `_PACKAGED_DATASETS_MODULES`) | ### Describe the bug
FolderBase Dataset automatically resolves under current directory when data_dir is not specified.
For example:
```
load_dataset("audiofolder")
```
takes long time to resolve and collect data_files from current directory. But I think it should reach out to this line for error handling https://github.com/huggingface/datasets/blob/cb8c5de5145c7e7eee65391cb7f4d92f0d565d62/src/datasets/packaged_modules/folder_based_builder/folder_based_builder.py#L58-L59
### Steps to reproduce the bug
```
load_dataset("audiofolder")
```
### Expected behavior
Error report
### Environment info
- `datasets` version: 2.14.4
- Platform: Linux-5.15.0-78-generic-x86_64-with-glibc2.17
- Python version: 3.8.15
- Huggingface_hub version: 0.16.4
- PyArrow version: 12.0.1
- Pandas version: 1.5.3 | 23 | FolderBase Dataset automatically resolves under current directory when data_dir is not specified
### Describe the bug
FolderBase Dataset automatically resolves under current directory when data_dir is not specified.
For example:
```
load_dataset("audiofolder")
```
takes long time to resolve and collect data_files from current directory. But I think it should reach out to this line for error handling https://github.com/huggingface/datasets/blob/cb8c5de5145c7e7eee65391cb7f4d92f0d565d62/src/datasets/packaged_modules/folder_based_builder/folder_based_builder.py#L58-L59
### Steps to reproduce the bug
```
load_dataset("audiofolder")
```
### Expected behavior
Error report
### Environment info
- `datasets` version: 2.14.4
- Platform: Linux-5.15.0-78-generic-x86_64-with-glibc2.17
- Python version: 3.8.15
- Huggingface_hub version: 0.16.4
- PyArrow version: 12.0.1
- Pandas version: 1.5.3
Makes sense, I guess this can be fixed in the load_dataset_builder method.
It concerns every packaged builder I think (see values in `_PACKAGED_DATASETS_MODULES`) |
https://github.com/huggingface/datasets/issues/6152 | FolderBase Dataset automatically resolves under current directory when data_dir is not specified | I think the behavior is related to these lines, which short circuited the error handling.
https://github.com/huggingface/datasets/blob/664a1cb72ea1e6ef7c47e671e2686ca4a35e8d63/src/datasets/load.py#L946-L952
So should data_dir be checked here or still delegating to actual `DatasetModule`? In that case, how to properly set `data_files` here. | ### Describe the bug
FolderBase Dataset automatically resolves under current directory when data_dir is not specified.
For example:
```
load_dataset("audiofolder")
```
takes long time to resolve and collect data_files from current directory. But I think it should reach out to this line for error handling https://github.com/huggingface/datasets/blob/cb8c5de5145c7e7eee65391cb7f4d92f0d565d62/src/datasets/packaged_modules/folder_based_builder/folder_based_builder.py#L58-L59
### Steps to reproduce the bug
```
load_dataset("audiofolder")
```
### Expected behavior
Error report
### Environment info
- `datasets` version: 2.14.4
- Platform: Linux-5.15.0-78-generic-x86_64-with-glibc2.17
- Python version: 3.8.15
- Huggingface_hub version: 0.16.4
- PyArrow version: 12.0.1
- Pandas version: 1.5.3 | 37 | FolderBase Dataset automatically resolves under current directory when data_dir is not specified
### Describe the bug
FolderBase Dataset automatically resolves under current directory when data_dir is not specified.
For example:
```
load_dataset("audiofolder")
```
takes long time to resolve and collect data_files from current directory. But I think it should reach out to this line for error handling https://github.com/huggingface/datasets/blob/cb8c5de5145c7e7eee65391cb7f4d92f0d565d62/src/datasets/packaged_modules/folder_based_builder/folder_based_builder.py#L58-L59
### Steps to reproduce the bug
```
load_dataset("audiofolder")
```
### Expected behavior
Error report
### Environment info
- `datasets` version: 2.14.4
- Platform: Linux-5.15.0-78-generic-x86_64-with-glibc2.17
- Python version: 3.8.15
- Huggingface_hub version: 0.16.4
- PyArrow version: 12.0.1
- Pandas version: 1.5.3
I think the behavior is related to these lines, which short circuited the error handling.
https://github.com/huggingface/datasets/blob/664a1cb72ea1e6ef7c47e671e2686ca4a35e8d63/src/datasets/load.py#L946-L952
So should data_dir be checked here or still delegating to actual `DatasetModule`? In that case, how to properly set `data_files` here. |
https://github.com/huggingface/datasets/issues/6152 | FolderBase Dataset automatically resolves under current directory when data_dir is not specified | This is location in PackagedDatasetModuleFactory.get_module seems the be the right place to check if at least data_dir or data_files are passed | ### Describe the bug
FolderBase Dataset automatically resolves under current directory when data_dir is not specified.
For example:
```
load_dataset("audiofolder")
```
takes long time to resolve and collect data_files from current directory. But I think it should reach out to this line for error handling https://github.com/huggingface/datasets/blob/cb8c5de5145c7e7eee65391cb7f4d92f0d565d62/src/datasets/packaged_modules/folder_based_builder/folder_based_builder.py#L58-L59
### Steps to reproduce the bug
```
load_dataset("audiofolder")
```
### Expected behavior
Error report
### Environment info
- `datasets` version: 2.14.4
- Platform: Linux-5.15.0-78-generic-x86_64-with-glibc2.17
- Python version: 3.8.15
- Huggingface_hub version: 0.16.4
- PyArrow version: 12.0.1
- Pandas version: 1.5.3 | 21 | FolderBase Dataset automatically resolves under current directory when data_dir is not specified
### Describe the bug
FolderBase Dataset automatically resolves under current directory when data_dir is not specified.
For example:
```
load_dataset("audiofolder")
```
takes long time to resolve and collect data_files from current directory. But I think it should reach out to this line for error handling https://github.com/huggingface/datasets/blob/cb8c5de5145c7e7eee65391cb7f4d92f0d565d62/src/datasets/packaged_modules/folder_based_builder/folder_based_builder.py#L58-L59
### Steps to reproduce the bug
```
load_dataset("audiofolder")
```
### Expected behavior
Error report
### Environment info
- `datasets` version: 2.14.4
- Platform: Linux-5.15.0-78-generic-x86_64-with-glibc2.17
- Python version: 3.8.15
- Huggingface_hub version: 0.16.4
- PyArrow version: 12.0.1
- Pandas version: 1.5.3
This is location in PackagedDatasetModuleFactory.get_module seems the be the right place to check if at least data_dir or data_files are passed |
https://github.com/huggingface/datasets/issues/6151 | Faster sorting for single key items | `Dataset.sort` essentially does the same thing except it uses `pyarrow.compute.sort_indices` which doesn't involve copying the data into python objects (saving memory)
```python
sort_keys = [(col, "ascending") for col in column_names]
indices = pc.sort_indices(self.data, sort_keys=sort_keys)
return self.select(indices)
``` | ### Feature request
A faster way to sort a dataset which contains a large number of rows.
### Motivation
The current sorting implementations took significantly longer than expected when I was running on a dataset trying to sort by timestamps.
**Code snippet:**
```python
ds = datasets.load_dataset( "json", **{"data_files": {"train": "path-to-jsonlines"}, "split": "train"}, num_proc=os.cpu_count(), keep_in_memory=True)
sorted_ds = ds.sort("pubDate", keep_in_memory=True)
```
However, once I switched to a different method which
1. unpacked to a list of tuples
2. sorted tuples by key
3. run `.select` with the sorted list of indices
It was significantly faster (orders of magnitude, especially with M's of rows)
### Your contribution
I'd be happy to implement a crude single key sorting algorithm so that other users can benefit from this trick. Broadly, this would take a `Dataset` and perform;
```python
# ds is a Dataset object
# key_name is the sorting key
class Dataset:
...
def _sort(key_name: str) -> Dataset:
index_keys = [(i,x) for i,x in enumerate(self[key_name])]
sorted_rows = sorted(row_pubdate, key=lambda x: x[1])
sorted_indicies = [x[0] for x in sorted_rows]
return self.select(sorted_indicies)
``` | 37 | Faster sorting for single key items
### Feature request
A faster way to sort a dataset which contains a large number of rows.
### Motivation
The current sorting implementations took significantly longer than expected when I was running on a dataset trying to sort by timestamps.
**Code snippet:**
```python
ds = datasets.load_dataset( "json", **{"data_files": {"train": "path-to-jsonlines"}, "split": "train"}, num_proc=os.cpu_count(), keep_in_memory=True)
sorted_ds = ds.sort("pubDate", keep_in_memory=True)
```
However, once I switched to a different method which
1. unpacked to a list of tuples
2. sorted tuples by key
3. run `.select` with the sorted list of indices
It was significantly faster (orders of magnitude, especially with M's of rows)
### Your contribution
I'd be happy to implement a crude single key sorting algorithm so that other users can benefit from this trick. Broadly, this would take a `Dataset` and perform;
```python
# ds is a Dataset object
# key_name is the sorting key
class Dataset:
...
def _sort(key_name: str) -> Dataset:
index_keys = [(i,x) for i,x in enumerate(self[key_name])]
sorted_rows = sorted(row_pubdate, key=lambda x: x[1])
sorted_indicies = [x[0] for x in sorted_rows]
return self.select(sorted_indicies)
```
`Dataset.sort` essentially does the same thing except it uses `pyarrow.compute.sort_indices` which doesn't involve copying the data into python objects (saving memory)
```python
sort_keys = [(col, "ascending") for col in column_names]
indices = pc.sort_indices(self.data, sort_keys=sort_keys)
return self.select(indices)
``` |
https://github.com/huggingface/datasets/issues/6150 | Allow dataset implement .take | ```
dataset = IterableDataset(dataset) if type(dataset) != IterableDataset else dataset # to force dataset.take(batch_size) to work in non-streaming mode
```
| ### Feature request
I want to do:
```
dataset.take(512)
```
but it only works with streaming = True
### Motivation
uniform interface to data sets. Really surprising the above only works with streaming = True.
### Your contribution
Should be trivial to copy paste the IterableDataset .take to use the local path in the data (when streaming = False) | 20 | Allow dataset implement .take
### Feature request
I want to do:
```
dataset.take(512)
```
but it only works with streaming = True
### Motivation
uniform interface to data sets. Really surprising the above only works with streaming = True.
### Your contribution
Should be trivial to copy paste the IterableDataset .take to use the local path in the data (when streaming = False)
```
dataset = IterableDataset(dataset) if type(dataset) != IterableDataset else dataset # to force dataset.take(batch_size) to work in non-streaming mode
```
|
https://github.com/huggingface/datasets/issues/6150 | Allow dataset implement .take | Feel free to work on this. In addition, `IterableDataset` supports `skip`, so we should also add this method to `Dataset`. | ### Feature request
I want to do:
```
dataset.take(512)
```
but it only works with streaming = True
### Motivation
uniform interface to data sets. Really surprising the above only works with streaming = True.
### Your contribution
Should be trivial to copy paste the IterableDataset .take to use the local path in the data (when streaming = False) | 20 | Allow dataset implement .take
### Feature request
I want to do:
```
dataset.take(512)
```
but it only works with streaming = True
### Motivation
uniform interface to data sets. Really surprising the above only works with streaming = True.
### Your contribution
Should be trivial to copy paste the IterableDataset .take to use the local path in the data (when streaming = False)
Feel free to work on this. In addition, `IterableDataset` supports `skip`, so we should also add this method to `Dataset`. |
https://github.com/huggingface/datasets/issues/6149 | Dataset.from_parquet cannot load subset of columns | Looks like this regression was introduced in `datasets==2.13.0` (`2.12.0` could load a subset of columns)
This does not appear to be fixed by https://github.com/huggingface/datasets/pull/6045 (bug still exists on `main`) | ### Describe the bug
When using `Dataset.from_parquet(path_or_paths, columns=[...])` and a subset of columns, loading fails with a variant of the following
```
ValueError: Couldn't cast
a: int64
-- schema metadata --
pandas: '{"index_columns": [], "column_indexes": [], "columns": [{"name":' + 273
to
{'a': Value(dtype='int64', id=None), 'b': Value(dtype='int64', id=None)}
because column names don't match
The above exception was the direct cause of the following exception:
```
Looks to be triggered by https://github.com/huggingface/datasets/blob/c02a44715c036b5261686669727394b1308a3a4b/src/datasets/table.py#L2285-L2286
### Steps to reproduce the bug
```
import pandas as pd
from datasets import Dataset
pd.DataFrame([{"a": 1, "b": 2}]).to_parquet("test.pq")
Dataset.from_parquet("test.pq", columns=["a"])
```
### Expected behavior
A subset of columns should be loaded without error
### Environment info
- `datasets` version: 2.14.4
- Platform: Linux-5.10.0-23-cloud-amd64-x86_64-with-glibc2.2.5
- Python version: 3.8.16
- Huggingface_hub version: 0.16.4
- PyArrow version: 12.0.1
- Pandas version: 2.0.3 | 29 | Dataset.from_parquet cannot load subset of columns
### Describe the bug
When using `Dataset.from_parquet(path_or_paths, columns=[...])` and a subset of columns, loading fails with a variant of the following
```
ValueError: Couldn't cast
a: int64
-- schema metadata --
pandas: '{"index_columns": [], "column_indexes": [], "columns": [{"name":' + 273
to
{'a': Value(dtype='int64', id=None), 'b': Value(dtype='int64', id=None)}
because column names don't match
The above exception was the direct cause of the following exception:
```
Looks to be triggered by https://github.com/huggingface/datasets/blob/c02a44715c036b5261686669727394b1308a3a4b/src/datasets/table.py#L2285-L2286
### Steps to reproduce the bug
```
import pandas as pd
from datasets import Dataset
pd.DataFrame([{"a": 1, "b": 2}]).to_parquet("test.pq")
Dataset.from_parquet("test.pq", columns=["a"])
```
### Expected behavior
A subset of columns should be loaded without error
### Environment info
- `datasets` version: 2.14.4
- Platform: Linux-5.10.0-23-cloud-amd64-x86_64-with-glibc2.2.5
- Python version: 3.8.16
- Huggingface_hub version: 0.16.4
- PyArrow version: 12.0.1
- Pandas version: 2.0.3
Looks like this regression was introduced in `datasets==2.13.0` (`2.12.0` could load a subset of columns)
This does not appear to be fixed by https://github.com/huggingface/datasets/pull/6045 (bug still exists on `main`) |
https://github.com/huggingface/datasets/issues/6147 | ValueError when running BeamBasedBuilder with GCS path in cache_dir | The cause of the error seems to be that `datasets` adds "gcs://" as a schema, while `beam` checks only "gs://".
datasets: https://github.com/huggingface/datasets/blob/c02a44715c036b5261686669727394b1308a3a4b/src/datasets/builder.py#L822
beam: [link](https://github.com/apache/beam/blob/25e1a64641b1c8a3c0a6c75c6e86031b87307f22/sdks/python/apache_beam/io/filesystems.py#L98-L101)
```
systems = [
fs for fs in FileSystem.get_all_subclasses()
if fs.scheme() == path_scheme
]
``` | ### Describe the bug
When running the BeamBasedBuilder with a GCS path specified in the cache_dir, the following ValueError occurs:
```
ValueError: Unable to get filesystem from specified path, please use the correct path or ensure the required dependency is installed, e.g., pip install apache-beam[gcp]. Path specified: gcs://my-bucket/huggingface_datasets/my_beam_dataset/default/0.0.0/my_beam_dataset-train [while running 'train/Save to parquet/Write/WriteImpl/InitializeWrite']
```
Same error occurs after running `pip install apache-beam[gcp]` as instructed.
### Steps to reproduce the bug
Put `my_beam_dataset.py`:
```python
import datasets
class MyBeamDataset(datasets.BeamBasedBuilder):
def _info(self):
features = datasets.Features({"value": datasets.Value("int64")})
return datasets.DatasetInfo(features=features)
def _split_generators(self, dl_manager, pipeline):
return [datasets.SplitGenerator(name=datasets.Split.TRAIN, gen_kwargs={})]
def _build_pcollection(self, pipeline):
import apache_beam as beam
return pipeline | beam.Create([{"value": i} for i in range(10)])
```
Run:
```bash
datasets-cli run_beam my_beam_dataset.py --cache_dir=gs://my-bucket/huggingface_datasets/ --beam_pipeline_options="runner=DirectRunner"
```
### Expected behavior
Running the BeamBasedBuilder with a GCS cache path without any errors.
### Environment info
- `datasets` version: 2.14.4
- Platform: macOS-13.4-arm64-arm-64bit
- Python version: 3.9.17
- Huggingface_hub version: 0.16.4
- PyArrow version: 9.0.0
- Pandas version: 2.0.3 | 39 | ValueError when running BeamBasedBuilder with GCS path in cache_dir
### Describe the bug
When running the BeamBasedBuilder with a GCS path specified in the cache_dir, the following ValueError occurs:
```
ValueError: Unable to get filesystem from specified path, please use the correct path or ensure the required dependency is installed, e.g., pip install apache-beam[gcp]. Path specified: gcs://my-bucket/huggingface_datasets/my_beam_dataset/default/0.0.0/my_beam_dataset-train [while running 'train/Save to parquet/Write/WriteImpl/InitializeWrite']
```
Same error occurs after running `pip install apache-beam[gcp]` as instructed.
### Steps to reproduce the bug
Put `my_beam_dataset.py`:
```python
import datasets
class MyBeamDataset(datasets.BeamBasedBuilder):
def _info(self):
features = datasets.Features({"value": datasets.Value("int64")})
return datasets.DatasetInfo(features=features)
def _split_generators(self, dl_manager, pipeline):
return [datasets.SplitGenerator(name=datasets.Split.TRAIN, gen_kwargs={})]
def _build_pcollection(self, pipeline):
import apache_beam as beam
return pipeline | beam.Create([{"value": i} for i in range(10)])
```
Run:
```bash
datasets-cli run_beam my_beam_dataset.py --cache_dir=gs://my-bucket/huggingface_datasets/ --beam_pipeline_options="runner=DirectRunner"
```
### Expected behavior
Running the BeamBasedBuilder with a GCS cache path without any errors.
### Environment info
- `datasets` version: 2.14.4
- Platform: macOS-13.4-arm64-arm-64bit
- Python version: 3.9.17
- Huggingface_hub version: 0.16.4
- PyArrow version: 9.0.0
- Pandas version: 2.0.3
The cause of the error seems to be that `datasets` adds "gcs://" as a schema, while `beam` checks only "gs://".
datasets: https://github.com/huggingface/datasets/blob/c02a44715c036b5261686669727394b1308a3a4b/src/datasets/builder.py#L822
beam: [link](https://github.com/apache/beam/blob/25e1a64641b1c8a3c0a6c75c6e86031b87307f22/sdks/python/apache_beam/io/filesystems.py#L98-L101)
```
systems = [
fs for fs in FileSystem.get_all_subclasses()
if fs.scheme() == path_scheme
]
``` |
https://github.com/huggingface/datasets/issues/6146 | DatasetGenerationError when load glue benchmark datasets from `load_dataset` | This issue can happen if there is a directory named "glue" relative to the Python script with the `load_dataset` call (similar issue to this one: https://github.com/huggingface/datasets/issues/5228). Is this the case? | ### Describe the bug
Package version: datasets-2.14.4
When I run the codes:
```
from datasets import load_dataset
dataset = load_dataset("glue", "ax")
```
I got the following errors:
---------------------------------------------------------------------------
SchemaInferenceError Traceback (most recent call last)
File ~/anaconda3/envs/python3/lib/python3.10/site-packages/datasets/builder.py:1949, in ArrowBasedBuilder._prepare_split_single(self, gen_kwargs, fpath, file_format, max_shard_size, job_id)
1948 num_shards = shard_id + 1
-> 1949 num_examples, num_bytes = writer.finalize()
1950 writer.close()
File ~/anaconda3/envs/python3/lib/python3.10/site-packages/datasets/arrow_writer.py:598, in ArrowWriter.finalize(self, close_stream)
597 self.stream.close()
--> 598 raise SchemaInferenceError("Please pass `features` or at least one example when writing data")
599 logger.debug(
600 f"Done writing {self._num_examples} {self.unit} in {self._num_bytes} bytes {self._path if self._path else ''}."
601 )
SchemaInferenceError: Please pass `features` or at least one example when writing data
The above exception was the direct cause of the following exception:
DatasetGenerationError Traceback (most recent call last)
Cell In[5], line 3
1 from datasets import load_dataset
----> 3 dataset = load_dataset("glue", "ax")
File ~/anaconda3/envs/python3/lib/python3.10/site-packages/datasets/load.py:2136, in load_dataset(path, name, data_dir, data_files, split, cache_dir, features, download_config, download_mode, verification_mode, ignore_verifications, keep_in_memory, save_infos, revision, token, use_auth_token, task, streaming, num_proc, storage_options, **config_kwargs)
2133 try_from_hf_gcs = path not in _PACKAGED_DATASETS_MODULES
2135 # Download and prepare data
-> 2136 builder_instance.download_and_prepare(
2137 download_config=download_config,
2138 download_mode=download_mode,
2139 verification_mode=verification_mode,
2140 try_from_hf_gcs=try_from_hf_gcs,
2141 num_proc=num_proc,
2142 storage_options=storage_options,
2143 )
2145 # Build dataset for splits
2146 keep_in_memory = (
2147 keep_in_memory if keep_in_memory is not None else is_small_dataset(builder_instance.info.dataset_size)
2148 )
File ~/anaconda3/envs/python3/lib/python3.10/site-packages/datasets/builder.py:954, in DatasetBuilder.download_and_prepare(self, output_dir, download_config, download_mode, verification_mode, ignore_verifications, try_from_hf_gcs, dl_manager, base_path, use_auth_token, file_format, max_shard_size, num_proc, storage_options, **download_and_prepare_kwargs)
952 if num_proc is not None:
953 prepare_split_kwargs["num_proc"] = num_proc
--> 954 self._download_and_prepare(
955 dl_manager=dl_manager,
956 verification_mode=verification_mode,
957 **prepare_split_kwargs,
958 **download_and_prepare_kwargs,
959 )
960 # Sync info
961 self.info.dataset_size = sum(split.num_bytes for split in self.info.splits.values())
File ~/anaconda3/envs/python3/lib/python3.10/site-packages/datasets/builder.py:1049, in DatasetBuilder._download_and_prepare(self, dl_manager, verification_mode, **prepare_split_kwargs)
1045 split_dict.add(split_generator.split_info)
1047 try:
1048 # Prepare split will record examples associated to the split
-> 1049 self._prepare_split(split_generator, **prepare_split_kwargs)
1050 except OSError as e:
1051 raise OSError(
1052 "Cannot find data file. "
1053 + (self.manual_download_instructions or "")
1054 + "\nOriginal error:\n"
1055 + str(e)
1056 ) from None
File ~/anaconda3/envs/python3/lib/python3.10/site-packages/datasets/builder.py:1813, in ArrowBasedBuilder._prepare_split(self, split_generator, file_format, num_proc, max_shard_size)
1811 job_id = 0
1812 with pbar:
-> 1813 for job_id, done, content in self._prepare_split_single(
1814 gen_kwargs=gen_kwargs, job_id=job_id, **_prepare_split_args
1815 ):
1816 if done:
1817 result = content
File ~/anaconda3/envs/python3/lib/python3.10/site-packages/datasets/builder.py:1958, in ArrowBasedBuilder._prepare_split_single(self, gen_kwargs, fpath, file_format, max_shard_size, job_id)
1956 if isinstance(e, SchemaInferenceError) and e.__context__ is not None:
1957 e = e.__context__
-> 1958 raise DatasetGenerationError("An error occurred while generating the dataset") from e
1960 yield job_id, True, (total_num_examples, total_num_bytes, writer._features, num_shards, shard_lengths)
DatasetGenerationError: An error occurred while generating the dataset
### Steps to reproduce the bug
from datasets import load_dataset
dataset = load_dataset("glue", "ax")
### Expected behavior
When generating the train split:
Generating train split:
0/0 [00:00<?, ? examples/s]
It raise the error:
DatasetGenerationError: An error occurred while generating the dataset
### Environment info
datasets-2.14.4.
Python 3.10 | 30 | DatasetGenerationError when load glue benchmark datasets from `load_dataset`
### Describe the bug
Package version: datasets-2.14.4
When I run the codes:
```
from datasets import load_dataset
dataset = load_dataset("glue", "ax")
```
I got the following errors:
---------------------------------------------------------------------------
SchemaInferenceError Traceback (most recent call last)
File ~/anaconda3/envs/python3/lib/python3.10/site-packages/datasets/builder.py:1949, in ArrowBasedBuilder._prepare_split_single(self, gen_kwargs, fpath, file_format, max_shard_size, job_id)
1948 num_shards = shard_id + 1
-> 1949 num_examples, num_bytes = writer.finalize()
1950 writer.close()
File ~/anaconda3/envs/python3/lib/python3.10/site-packages/datasets/arrow_writer.py:598, in ArrowWriter.finalize(self, close_stream)
597 self.stream.close()
--> 598 raise SchemaInferenceError("Please pass `features` or at least one example when writing data")
599 logger.debug(
600 f"Done writing {self._num_examples} {self.unit} in {self._num_bytes} bytes {self._path if self._path else ''}."
601 )
SchemaInferenceError: Please pass `features` or at least one example when writing data
The above exception was the direct cause of the following exception:
DatasetGenerationError Traceback (most recent call last)
Cell In[5], line 3
1 from datasets import load_dataset
----> 3 dataset = load_dataset("glue", "ax")
File ~/anaconda3/envs/python3/lib/python3.10/site-packages/datasets/load.py:2136, in load_dataset(path, name, data_dir, data_files, split, cache_dir, features, download_config, download_mode, verification_mode, ignore_verifications, keep_in_memory, save_infos, revision, token, use_auth_token, task, streaming, num_proc, storage_options, **config_kwargs)
2133 try_from_hf_gcs = path not in _PACKAGED_DATASETS_MODULES
2135 # Download and prepare data
-> 2136 builder_instance.download_and_prepare(
2137 download_config=download_config,
2138 download_mode=download_mode,
2139 verification_mode=verification_mode,
2140 try_from_hf_gcs=try_from_hf_gcs,
2141 num_proc=num_proc,
2142 storage_options=storage_options,
2143 )
2145 # Build dataset for splits
2146 keep_in_memory = (
2147 keep_in_memory if keep_in_memory is not None else is_small_dataset(builder_instance.info.dataset_size)
2148 )
File ~/anaconda3/envs/python3/lib/python3.10/site-packages/datasets/builder.py:954, in DatasetBuilder.download_and_prepare(self, output_dir, download_config, download_mode, verification_mode, ignore_verifications, try_from_hf_gcs, dl_manager, base_path, use_auth_token, file_format, max_shard_size, num_proc, storage_options, **download_and_prepare_kwargs)
952 if num_proc is not None:
953 prepare_split_kwargs["num_proc"] = num_proc
--> 954 self._download_and_prepare(
955 dl_manager=dl_manager,
956 verification_mode=verification_mode,
957 **prepare_split_kwargs,
958 **download_and_prepare_kwargs,
959 )
960 # Sync info
961 self.info.dataset_size = sum(split.num_bytes for split in self.info.splits.values())
File ~/anaconda3/envs/python3/lib/python3.10/site-packages/datasets/builder.py:1049, in DatasetBuilder._download_and_prepare(self, dl_manager, verification_mode, **prepare_split_kwargs)
1045 split_dict.add(split_generator.split_info)
1047 try:
1048 # Prepare split will record examples associated to the split
-> 1049 self._prepare_split(split_generator, **prepare_split_kwargs)
1050 except OSError as e:
1051 raise OSError(
1052 "Cannot find data file. "
1053 + (self.manual_download_instructions or "")
1054 + "\nOriginal error:\n"
1055 + str(e)
1056 ) from None
File ~/anaconda3/envs/python3/lib/python3.10/site-packages/datasets/builder.py:1813, in ArrowBasedBuilder._prepare_split(self, split_generator, file_format, num_proc, max_shard_size)
1811 job_id = 0
1812 with pbar:
-> 1813 for job_id, done, content in self._prepare_split_single(
1814 gen_kwargs=gen_kwargs, job_id=job_id, **_prepare_split_args
1815 ):
1816 if done:
1817 result = content
File ~/anaconda3/envs/python3/lib/python3.10/site-packages/datasets/builder.py:1958, in ArrowBasedBuilder._prepare_split_single(self, gen_kwargs, fpath, file_format, max_shard_size, job_id)
1956 if isinstance(e, SchemaInferenceError) and e.__context__ is not None:
1957 e = e.__context__
-> 1958 raise DatasetGenerationError("An error occurred while generating the dataset") from e
1960 yield job_id, True, (total_num_examples, total_num_bytes, writer._features, num_shards, shard_lengths)
DatasetGenerationError: An error occurred while generating the dataset
### Steps to reproduce the bug
from datasets import load_dataset
dataset = load_dataset("glue", "ax")
### Expected behavior
When generating the train split:
Generating train split:
0/0 [00:00<?, ? examples/s]
It raise the error:
DatasetGenerationError: An error occurred while generating the dataset
### Environment info
datasets-2.14.4.
Python 3.10
This issue can happen if there is a directory named "glue" relative to the Python script with the `load_dataset` call (similar issue to this one: https://github.com/huggingface/datasets/issues/5228). Is this the case? |
https://github.com/huggingface/datasets/issues/6153 | custom load dataset to hub | > This is an issue for the [Datasets repo](https://github.com/huggingface/datasets).
Thanks @sgugger , I guess I will wait for them to address the issue . Looking forward to hearing from them | ### System Info
kaggle notebook
i transformed dataset:
```
dataset = load_dataset("Dahoas/first-instruct-human-assistant-prompt")
```
to
formatted_dataset:
```
Dataset({
features: ['message_tree_id', 'message_tree_text'],
num_rows: 33143
})
```
but would like to know how to upload to hub
### Who can help?
@ArthurZucker @younesbelkada
### Information
- [ ] The official example scripts
- [ ] My own modified scripts
### Tasks
- [ ] An officially supported task in the `examples` folder (such as GLUE/SQuAD, ...)
- [ ] My own task or dataset (give details below)
### Reproduction
shared above
### Expected behavior
load dataset to hub | 30 | custom load dataset to hub
### System Info
kaggle notebook
i transformed dataset:
```
dataset = load_dataset("Dahoas/first-instruct-human-assistant-prompt")
```
to
formatted_dataset:
```
Dataset({
features: ['message_tree_id', 'message_tree_text'],
num_rows: 33143
})
```
but would like to know how to upload to hub
### Who can help?
@ArthurZucker @younesbelkada
### Information
- [ ] The official example scripts
- [ ] My own modified scripts
### Tasks
- [ ] An officially supported task in the `examples` folder (such as GLUE/SQuAD, ...)
- [ ] My own task or dataset (give details below)
### Reproduction
shared above
### Expected behavior
load dataset to hub
> This is an issue for the [Datasets repo](https://github.com/huggingface/datasets).
Thanks @sgugger , I guess I will wait for them to address the issue . Looking forward to hearing from them |
https://github.com/huggingface/datasets/issues/6144 | NIH exporter file not found | another file not found:
```
Traceback (most recent call last):
File "/lfs/ampere1/0/brando9/miniconda/envs/beyond_scale/lib/python3.10/site-packages/fsspec/implementations/http.py", line 417, in _info
await _file_info(
File "/lfs/ampere1/0/brando9/miniconda/envs/beyond_scale/lib/python3.10/site-packages/fsspec/implementations/http.py", line 837, in _file_info
r.raise_for_status()
File "/lfs/ampere1/0/brando9/miniconda/envs/beyond_scale/lib/python3.10/site-packages/aiohttp/client_reqrep.py", line 1005, in raise_for_status
raise ClientResponseError(
aiohttp.client_exceptions.ClientResponseError: 404, message='Not Found', url=URL('https://the-eye.eu/public/AI/pile_preliminary_components/pile_uspto.tar')
The above exception was the direct cause of the following exception:
Traceback (most recent call last):
File "/lfs/ampere1/0/brando9/miniconda/envs/beyond_scale/lib/python3.10/runpy.py", line 196, in _run_module_as_main
return _run_code(code, main_globals, None,
File "/lfs/ampere1/0/brando9/miniconda/envs/beyond_scale/lib/python3.10/runpy.py", line 86, in _run_code
exec(code, run_globals)
File "/lfs/ampere1/0/brando9/.vscode-server-insiders/extensions/ms-python.python-2023.14.0/pythonFiles/lib/python/debugpy/adapter/../../debugpy/launcher/../../debugpy/__main__.py", line 39, in <module>
cli.main()
File "/lfs/ampere1/0/brando9/.vscode-server-insiders/extensions/ms-python.python-2023.14.0/pythonFiles/lib/python/debugpy/adapter/../../debugpy/launcher/../../debugpy/../debugpy/server/cli.py", line 430, in main
run()
File "/lfs/ampere1/0/brando9/.vscode-server-insiders/extensions/ms-python.python-2023.14.0/pythonFiles/lib/python/debugpy/adapter/../../debugpy/launcher/../../debugpy/../debugpy/server/cli.py", line 284, in run_file
runpy.run_path(target, run_name="__main__")
File "/lfs/ampere1/0/brando9/.vscode-server-insiders/extensions/ms-python.python-2023.14.0/pythonFiles/lib/python/debugpy/_vendored/pydevd/_pydevd_bundle/pydevd_runpy.py", line 321, in run_path
return _run_module_code(code, init_globals, run_name,
File "/lfs/ampere1/0/brando9/.vscode-server-insiders/extensions/ms-python.python-2023.14.0/pythonFiles/lib/python/debugpy/_vendored/pydevd/_pydevd_bundle/pydevd_runpy.py", line 135, in _run_module_code
_run_code(code, mod_globals, init_globals,
File "/lfs/ampere1/0/brando9/.vscode-server-insiders/extensions/ms-python.python-2023.14.0/pythonFiles/lib/python/debugpy/_vendored/pydevd/_pydevd_bundle/pydevd_runpy.py", line 124, in _run_code
exec(code, run_globals)
File "/lfs/ampere1/0/brando9/beyond-scale-language-data-diversity/src/diversity/div_coeff.py", line 526, in <module>
experiment_compute_diveristy_coeff_single_dataset_then_combined_datasets_with_domain_weights()
File "/lfs/ampere1/0/brando9/beyond-scale-language-data-diversity/src/diversity/div_coeff.py", line 475, in experiment_compute_diveristy_coeff_single_dataset_then_combined_datasets_with_domain_weights
column_names = next(iter(dataset)).keys()
File "/lfs/ampere1/0/brando9/miniconda/envs/beyond_scale/lib/python3.10/site-packages/datasets/iterable_dataset.py", line 1353, in __iter__
for key, example in ex_iterable:
File "/lfs/ampere1/0/brando9/miniconda/envs/beyond_scale/lib/python3.10/site-packages/datasets/iterable_dataset.py", line 207, in __iter__
yield from self.generate_examples_fn(**self.kwargs)
File "/lfs/ampere1/0/brando9/.cache/huggingface/modules/datasets_modules/datasets/EleutherAI--pile/ebea56d358e91cf4d37b0fde361d563bed1472fbd8221a21b38fc8bb4ba554fb/pile.py", line 257, in _generate_examples
for path, file in files[subset]:
File "/lfs/ampere1/0/brando9/miniconda/envs/beyond_scale/lib/python3.10/site-packages/datasets/download/streaming_download_manager.py", line 840, in __iter__
yield from self.generator(*self.args, **self.kwargs)
File "/lfs/ampere1/0/brando9/miniconda/envs/beyond_scale/lib/python3.10/site-packages/datasets/download/streaming_download_manager.py", line 891, in _iter_from_urlpath
with xopen(urlpath, "rb", download_config=download_config) as f:
File "/lfs/ampere1/0/brando9/miniconda/envs/beyond_scale/lib/python3.10/site-packages/datasets/download/streaming_download_manager.py", line 496, in xopen
file_obj = fsspec.open(file, mode=mode, *args, **kwargs).open()
File "/lfs/ampere1/0/brando9/miniconda/envs/beyond_scale/lib/python3.10/site-packages/fsspec/core.py", line 134, in open
return self.__enter__()
File "/lfs/ampere1/0/brando9/miniconda/envs/beyond_scale/lib/python3.10/site-packages/fsspec/core.py", line 102, in __enter__
f = self.fs.open(self.path, mode=mode)
File "/lfs/ampere1/0/brando9/miniconda/envs/beyond_scale/lib/python3.10/site-packages/fsspec/spec.py", line 1241, in open
f = self._open(
File "/lfs/ampere1/0/brando9/miniconda/envs/beyond_scale/lib/python3.10/site-packages/fsspec/implementations/http.py", line 356, in _open
size = size or self.info(path, **kwargs)["size"]
File "/lfs/ampere1/0/brando9/miniconda/envs/beyond_scale/lib/python3.10/site-packages/fsspec/asyn.py", line 121, in wrapper
return sync(self.loop, func, *args, **kwargs)
File "/lfs/ampere1/0/brando9/miniconda/envs/beyond_scale/lib/python3.10/site-packages/fsspec/asyn.py", line 106, in sync
raise return_result
File "/lfs/ampere1/0/brando9/miniconda/envs/beyond_scale/lib/python3.10/site-packages/fsspec/asyn.py", line 61, in _runner
result[0] = await coro
File "/lfs/ampere1/0/brando9/miniconda/envs/beyond_scale/lib/python3.10/site-packages/fsspec/implementations/http.py", line 430, in _info
raise FileNotFoundError(url) from exc
FileNotFoundError: https://the-eye.eu/public/AI/pile_preliminary_components/pile_uspto.tar
``` | ### Describe the bug
can't use or download the nih exporter pile data.
```
15 experiment_compute_diveristy_coeff_single_dataset_then_combined_datasets_with_domain_weights()
16 File "/lfs/ampere1/0/brando9/beyond-scale-language-data-diversity/src/diversity/div_coeff.py", line 474, in experiment_compute_diveristy_coeff_single_dataset_then_combined_datasets_with_domain_weights
17 column_names = next(iter(dataset)).keys()
18 File "/lfs/ampere1/0/brando9/miniconda/envs/beyond_scale/lib/python3.10/site-packages/datasets/iterable_dataset.py", line 1353, in __iter__
19 for key, example in ex_iterable:
20 File "/lfs/ampere1/0/brando9/miniconda/envs/beyond_scale/lib/python3.10/site-packages/datasets/iterable_dataset.py", line 207, in __iter__
21 yield from self.generate_examples_fn(**self.kwargs)
22 File "/lfs/ampere1/0/brando9/.cache/huggingface/modules/datasets_modules/datasets/EleutherAI--pile/ebea56d358e91cf4d37b0fde361d563bed1472fbd8221a21b38fc8bb4ba554fb/pile.py", line 236, in _generate_examples
23 with zstd.open(open(files[subset], "rb"), "rt", encoding="utf-8") as f:
24 File "/lfs/ampere1/0/brando9/miniconda/envs/beyond_scale/lib/python3.10/site-packages/datasets/streaming.py", line 74, in wrapper
25 return function(*args, download_config=download_config, **kwargs)
26 File "/lfs/ampere1/0/brando9/miniconda/envs/beyond_scale/lib/python3.10/site-packages/datasets/download/streaming_download_manager.py", line 496, in xopen
27 file_obj = fsspec.open(file, mode=mode, *args, **kwargs).open()
28 File "/lfs/ampere1/0/brando9/miniconda/envs/beyond_scale/lib/python3.10/site-packages/fsspec/core.py", line 134, in open
29 return self.__enter__()
30 File "/lfs/ampere1/0/brando9/miniconda/envs/beyond_scale/lib/python3.10/site-packages/fsspec/core.py", line 102, in __enter__
31 f = self.fs.open(self.path, mode=mode)
32 File "/lfs/ampere1/0/brando9/miniconda/envs/beyond_scale/lib/python3.10/site-packages/fsspec/spec.py", line 1241, in open
33 f = self._open(
34 File "/lfs/ampere1/0/brando9/miniconda/envs/beyond_scale/lib/python3.10/site-packages/fsspec/implementations/http.py", line 356, in _open
35 size = size or self.info(path, **kwargs)["size"]
36 File "/lfs/ampere1/0/brando9/miniconda/envs/beyond_scale/lib/python3.10/site-packages/fsspec/asyn.py", line 121, in wrapper
37 return sync(self.loop, func, *args, **kwargs)
38 File "/lfs/ampere1/0/brando9/miniconda/envs/beyond_scale/lib/python3.10/site-packages/fsspec/asyn.py", line 106, in sync
39 raise return_result
40 File "/lfs/ampere1/0/brando9/miniconda/envs/beyond_scale/lib/python3.10/site-packages/fsspec/asyn.py", line 61, in _runner
41 result[0] = await coro
42 File "/lfs/ampere1/0/brando9/miniconda/envs/beyond_scale/lib/python3.10/site-packages/fsspec/implementations/http.py", line 430, in _info
43 raise FileNotFoundError(url) from exc
44 FileNotFoundError: https://the-eye.eu/public/AI/pile_preliminary_components/NIH_ExPORTER_awarded_grant_text.jsonl.zst
```
### Steps to reproduce the bug
run this:
```
from datasets import load_dataset
path, name = 'EleutherAI/pile', 'nih_exporter'
# -- Get data set
dataset = load_dataset(path, name, streaming=True, split="train").with_format("torch")
batch = dataset.take(512)
print(f'{batch=}')
```
### Expected behavior
print the batch
### Environment info
```
(beyond_scale) brando9@ampere1:~/beyond-scale-language-data-diversity$ datasets-cli env
Copy-and-paste the text below in your GitHub issue.
- `datasets` version: 2.14.4
- Platform: Linux-5.4.0-122-generic-x86_64-with-glibc2.31
- Python version: 3.10.11
- Huggingface_hub version: 0.16.4
- PyArrow version: 12.0.1
- Pandas version: 2.0.3
``` | 283 | NIH exporter file not found
### Describe the bug
can't use or download the nih exporter pile data.
```
15 experiment_compute_diveristy_coeff_single_dataset_then_combined_datasets_with_domain_weights()
16 File "/lfs/ampere1/0/brando9/beyond-scale-language-data-diversity/src/diversity/div_coeff.py", line 474, in experiment_compute_diveristy_coeff_single_dataset_then_combined_datasets_with_domain_weights
17 column_names = next(iter(dataset)).keys()
18 File "/lfs/ampere1/0/brando9/miniconda/envs/beyond_scale/lib/python3.10/site-packages/datasets/iterable_dataset.py", line 1353, in __iter__
19 for key, example in ex_iterable:
20 File "/lfs/ampere1/0/brando9/miniconda/envs/beyond_scale/lib/python3.10/site-packages/datasets/iterable_dataset.py", line 207, in __iter__
21 yield from self.generate_examples_fn(**self.kwargs)
22 File "/lfs/ampere1/0/brando9/.cache/huggingface/modules/datasets_modules/datasets/EleutherAI--pile/ebea56d358e91cf4d37b0fde361d563bed1472fbd8221a21b38fc8bb4ba554fb/pile.py", line 236, in _generate_examples
23 with zstd.open(open(files[subset], "rb"), "rt", encoding="utf-8") as f:
24 File "/lfs/ampere1/0/brando9/miniconda/envs/beyond_scale/lib/python3.10/site-packages/datasets/streaming.py", line 74, in wrapper
25 return function(*args, download_config=download_config, **kwargs)
26 File "/lfs/ampere1/0/brando9/miniconda/envs/beyond_scale/lib/python3.10/site-packages/datasets/download/streaming_download_manager.py", line 496, in xopen
27 file_obj = fsspec.open(file, mode=mode, *args, **kwargs).open()
28 File "/lfs/ampere1/0/brando9/miniconda/envs/beyond_scale/lib/python3.10/site-packages/fsspec/core.py", line 134, in open
29 return self.__enter__()
30 File "/lfs/ampere1/0/brando9/miniconda/envs/beyond_scale/lib/python3.10/site-packages/fsspec/core.py", line 102, in __enter__
31 f = self.fs.open(self.path, mode=mode)
32 File "/lfs/ampere1/0/brando9/miniconda/envs/beyond_scale/lib/python3.10/site-packages/fsspec/spec.py", line 1241, in open
33 f = self._open(
34 File "/lfs/ampere1/0/brando9/miniconda/envs/beyond_scale/lib/python3.10/site-packages/fsspec/implementations/http.py", line 356, in _open
35 size = size or self.info(path, **kwargs)["size"]
36 File "/lfs/ampere1/0/brando9/miniconda/envs/beyond_scale/lib/python3.10/site-packages/fsspec/asyn.py", line 121, in wrapper
37 return sync(self.loop, func, *args, **kwargs)
38 File "/lfs/ampere1/0/brando9/miniconda/envs/beyond_scale/lib/python3.10/site-packages/fsspec/asyn.py", line 106, in sync
39 raise return_result
40 File "/lfs/ampere1/0/brando9/miniconda/envs/beyond_scale/lib/python3.10/site-packages/fsspec/asyn.py", line 61, in _runner
41 result[0] = await coro
42 File "/lfs/ampere1/0/brando9/miniconda/envs/beyond_scale/lib/python3.10/site-packages/fsspec/implementations/http.py", line 430, in _info
43 raise FileNotFoundError(url) from exc
44 FileNotFoundError: https://the-eye.eu/public/AI/pile_preliminary_components/NIH_ExPORTER_awarded_grant_text.jsonl.zst
```
### Steps to reproduce the bug
run this:
```
from datasets import load_dataset
path, name = 'EleutherAI/pile', 'nih_exporter'
# -- Get data set
dataset = load_dataset(path, name, streaming=True, split="train").with_format("torch")
batch = dataset.take(512)
print(f'{batch=}')
```
### Expected behavior
print the batch
### Environment info
```
(beyond_scale) brando9@ampere1:~/beyond-scale-language-data-diversity$ datasets-cli env
Copy-and-paste the text below in your GitHub issue.
- `datasets` version: 2.14.4
- Platform: Linux-5.4.0-122-generic-x86_64-with-glibc2.31
- Python version: 3.10.11
- Huggingface_hub version: 0.16.4
- PyArrow version: 12.0.1
- Pandas version: 2.0.3
```
another file not found:
```
Traceback (most recent call last):
File "/lfs/ampere1/0/brando9/miniconda/envs/beyond_scale/lib/python3.10/site-packages/fsspec/implementations/http.py", line 417, in _info
await _file_info(
File "/lfs/ampere1/0/brando9/miniconda/envs/beyond_scale/lib/python3.10/site-packages/fsspec/implementations/http.py", line 837, in _file_info
r.raise_for_status()
File "/lfs/ampere1/0/brando9/miniconda/envs/beyond_scale/lib/python3.10/site-packages/aiohttp/client_reqrep.py", line 1005, in raise_for_status
raise ClientResponseError(
aiohttp.client_exceptions.ClientResponseError: 404, message='Not Found', url=URL('https://the-eye.eu/public/AI/pile_preliminary_components/pile_uspto.tar')
The above exception was the direct cause of the following exception:
Traceback (most recent call last):
File "/lfs/ampere1/0/brando9/miniconda/envs/beyond_scale/lib/python3.10/runpy.py", line 196, in _run_module_as_main
return _run_code(code, main_globals, None,
File "/lfs/ampere1/0/brando9/miniconda/envs/beyond_scale/lib/python3.10/runpy.py", line 86, in _run_code
exec(code, run_globals)
File "/lfs/ampere1/0/brando9/.vscode-server-insiders/extensions/ms-python.python-2023.14.0/pythonFiles/lib/python/debugpy/adapter/../../debugpy/launcher/../../debugpy/__main__.py", line 39, in <module>
cli.main()
File "/lfs/ampere1/0/brando9/.vscode-server-insiders/extensions/ms-python.python-2023.14.0/pythonFiles/lib/python/debugpy/adapter/../../debugpy/launcher/../../debugpy/../debugpy/server/cli.py", line 430, in main
run()
File "/lfs/ampere1/0/brando9/.vscode-server-insiders/extensions/ms-python.python-2023.14.0/pythonFiles/lib/python/debugpy/adapter/../../debugpy/launcher/../../debugpy/../debugpy/server/cli.py", line 284, in run_file
runpy.run_path(target, run_name="__main__")
File "/lfs/ampere1/0/brando9/.vscode-server-insiders/extensions/ms-python.python-2023.14.0/pythonFiles/lib/python/debugpy/_vendored/pydevd/_pydevd_bundle/pydevd_runpy.py", line 321, in run_path
return _run_module_code(code, init_globals, run_name,
File "/lfs/ampere1/0/brando9/.vscode-server-insiders/extensions/ms-python.python-2023.14.0/pythonFiles/lib/python/debugpy/_vendored/pydevd/_pydevd_bundle/pydevd_runpy.py", line 135, in _run_module_code
_run_code(code, mod_globals, init_globals,
File "/lfs/ampere1/0/brando9/.vscode-server-insiders/extensions/ms-python.python-2023.14.0/pythonFiles/lib/python/debugpy/_vendored/pydevd/_pydevd_bundle/pydevd_runpy.py", line 124, in _run_code
exec(code, run_globals)
File "/lfs/ampere1/0/brando9/beyond-scale-language-data-diversity/src/diversity/div_coeff.py", line 526, in <module>
experiment_compute_diveristy_coeff_single_dataset_then_combined_datasets_with_domain_weights()
File "/lfs/ampere1/0/brando9/beyond-scale-language-data-diversity/src/diversity/div_coeff.py", line 475, in experiment_compute_diveristy_coeff_single_dataset_then_combined_datasets_with_domain_weights
column_names = next(iter(dataset)).keys()
File "/lfs/ampere1/0/brando9/miniconda/envs/beyond_scale/lib/python3.10/site-packages/datasets/iterable_dataset.py", line 1353, in __iter__
for key, example in ex_iterable:
File "/lfs/ampere1/0/brando9/miniconda/envs/beyond_scale/lib/python3.10/site-packages/datasets/iterable_dataset.py", line 207, in __iter__
yield from self.generate_examples_fn(**self.kwargs)
File "/lfs/ampere1/0/brando9/.cache/huggingface/modules/datasets_modules/datasets/EleutherAI--pile/ebea56d358e91cf4d37b0fde361d563bed1472fbd8221a21b38fc8bb4ba554fb/pile.py", line 257, in _generate_examples
for path, file in files[subset]:
File "/lfs/ampere1/0/brando9/miniconda/envs/beyond_scale/lib/python3.10/site-packages/datasets/download/streaming_download_manager.py", line 840, in __iter__
yield from self.generator(*self.args, **self.kwargs)
File "/lfs/ampere1/0/brando9/miniconda/envs/beyond_scale/lib/python3.10/site-packages/datasets/download/streaming_download_manager.py", line 891, in _iter_from_urlpath
with xopen(urlpath, "rb", download_config=download_config) as f:
File "/lfs/ampere1/0/brando9/miniconda/envs/beyond_scale/lib/python3.10/site-packages/datasets/download/streaming_download_manager.py", line 496, in xopen
file_obj = fsspec.open(file, mode=mode, *args, **kwargs).open()
File "/lfs/ampere1/0/brando9/miniconda/envs/beyond_scale/lib/python3.10/site-packages/fsspec/core.py", line 134, in open
return self.__enter__()
File "/lfs/ampere1/0/brando9/miniconda/envs/beyond_scale/lib/python3.10/site-packages/fsspec/core.py", line 102, in __enter__
f = self.fs.open(self.path, mode=mode)
File "/lfs/ampere1/0/brando9/miniconda/envs/beyond_scale/lib/python3.10/site-packages/fsspec/spec.py", line 1241, in open
f = self._open(
File "/lfs/ampere1/0/brando9/miniconda/envs/beyond_scale/lib/python3.10/site-packages/fsspec/implementations/http.py", line 356, in _open
size = size or self.info(path, **kwargs)["size"]
File "/lfs/ampere1/0/brando9/miniconda/envs/beyond_scale/lib/python3.10/site-packages/fsspec/asyn.py", line 121, in wrapper
return sync(self.loop, func, *args, **kwargs)
File "/lfs/ampere1/0/brando9/miniconda/envs/beyond_scale/lib/python3.10/site-packages/fsspec/asyn.py", line 106, in sync
raise return_result
File "/lfs/ampere1/0/brando9/miniconda/envs/beyond_scale/lib/python3.10/site-packages/fsspec/asyn.py", line 61, in _runner
result[0] = await coro
File "/lfs/ampere1/0/brando9/miniconda/envs/beyond_scale/lib/python3.10/site-packages/fsspec/implementations/http.py", line 430, in _info
raise FileNotFoundError(url) from exc
FileNotFoundError: https://the-eye.eu/public/AI/pile_preliminary_components/pile_uspto.tar
``` |
https://github.com/huggingface/datasets/issues/6144 | NIH exporter file not found | this seems to work but it's rather annoying.
Summary of how to make it work:
1. get urls to parquet files into a list
2. load list to load_dataset via `load_dataset('parquet', data_files=urls)` (note api names to hf are really confusing sometimes)
3. then it should work, print a batch of text.
presudo code
```python
urls_hacker_news = [
"https://huggingface.co/datasets/EleutherAI/pile/resolve/refs%2Fconvert%2Fparquet/hacker_news/pile-train-00000-of-00004.parquet",
"https://huggingface.co/datasets/EleutherAI/pile/resolve/refs%2Fconvert%2Fparquet/hacker_news/pile-train-00001-of-00004.parquet",
"https://huggingface.co/datasets/EleutherAI/pile/resolve/refs%2Fconvert%2Fparquet/hacker_news/pile-train-00002-of-00004.parquet",
"https://huggingface.co/datasets/EleutherAI/pile/resolve/refs%2Fconvert%2Fparquet/hacker_news/pile-train-00003-of-00004.parquet"
]
...
# streaming = False
from diversity.pile_subset_urls import urls_hacker_news
path, name, data_files = 'parquet', 'hacker_news', urls_hacker_news
# not changing
batch_size = 512
today = datetime.datetime.now().strftime('%Y-m%m-d%d-t%Hh_%Mm_%Ss')
run_name = f'{path} div_coeff_{num_batches=} ({today=} ({name=}) {data_mixture_name=} {probabilities=})'
print(f'{run_name=}')
# - Init wandb
debug: bool = mode == 'dryrun'
run = wandb.init(mode=mode, project="beyond-scale", name=run_name, save_code=True)
wandb.config.update({"num_batches": num_batches, "path": path, "name": name, "today": today, 'probabilities': probabilities, 'batch_size': batch_size, 'debug': debug, 'data_mixture_name': data_mixture_name, 'streaming': streaming, 'data_files': data_files})
# run.notify_on_failure() # https://community.wandb.ai/t/how-do-i-set-the-wandb-alert-programatically-for-my-current-run/4891
print(f'{debug=}')
print(f'{wandb.config=}')
# -- Get probe network
from datasets import load_dataset
import torch
from transformers import GPT2Tokenizer, GPT2LMHeadModel
tokenizer = GPT2Tokenizer.from_pretrained("gpt2")
if tokenizer.pad_token_id is None:
tokenizer.pad_token = tokenizer.eos_token
probe_network = GPT2LMHeadModel.from_pretrained("gpt2")
device = torch.device(f"cuda:{0}" if torch.cuda.is_available() else "cpu")
probe_network = probe_network.to(device)
# -- Get data set
def my_load_dataset(path, name):
print(f'{path=} {name=} {streaming=}')
if path == 'json' or path == 'bin' or path == 'csv':
print(f'{data_files_prefix+name=}')
return load_dataset(path, data_files=data_files_prefix+name, streaming=streaming, split="train").with_format("torch")
elif path == 'parquet':
print(f'{data_files=}')
return load_dataset(path, data_files=data_files, streaming=streaming, split="train").with_format("torch")
else:
return load_dataset(path, name, streaming=streaming, split="train").with_format("torch")
# - get data set for real now
if isinstance(path, str):
dataset = my_load_dataset(path, name)
else:
print('-- interleaving datasets')
datasets = [my_load_dataset(path, name).with_format("torch") for path, name in zip(path, name)]
[print(f'{dataset.description=}') for dataset in datasets]
dataset = interleave_datasets(datasets, probabilities)
print(f'{dataset=}')
batch = dataset.take(batch_size)
print(f'{next(iter(batch))=}')
column_names = next(iter(batch)).keys()
print(f'{column_names=}')
# - Prepare functions to tokenize batch
def preprocess(examples):
return tokenizer(examples["text"], padding="max_length", max_length=128, truncation=True, return_tensors="pt")
remove_columns = column_names # remove all keys that are not tensors to avoid bugs in collate function in task2vec's pytorch data loader
def map(batch):
return batch.map(preprocess, batched=True, remove_columns=remove_columns)
tokenized_batch = map(batch)
print(f'{next(iter(tokenized_batch))=}')
```
https://stackoverflow.com/questions/76891189/how-to-download-data-from-hugging-face-that-is-visible-on-the-data-viewer-but-th/76902681#76902681
https://discuss.huggingface.co/t/how-to-download-data-from-hugging-face-that-is-visible-on-the-data-viewer-but-the-files-are-not-available/50555/5?u=severo | ### Describe the bug
can't use or download the nih exporter pile data.
```
15 experiment_compute_diveristy_coeff_single_dataset_then_combined_datasets_with_domain_weights()
16 File "/lfs/ampere1/0/brando9/beyond-scale-language-data-diversity/src/diversity/div_coeff.py", line 474, in experiment_compute_diveristy_coeff_single_dataset_then_combined_datasets_with_domain_weights
17 column_names = next(iter(dataset)).keys()
18 File "/lfs/ampere1/0/brando9/miniconda/envs/beyond_scale/lib/python3.10/site-packages/datasets/iterable_dataset.py", line 1353, in __iter__
19 for key, example in ex_iterable:
20 File "/lfs/ampere1/0/brando9/miniconda/envs/beyond_scale/lib/python3.10/site-packages/datasets/iterable_dataset.py", line 207, in __iter__
21 yield from self.generate_examples_fn(**self.kwargs)
22 File "/lfs/ampere1/0/brando9/.cache/huggingface/modules/datasets_modules/datasets/EleutherAI--pile/ebea56d358e91cf4d37b0fde361d563bed1472fbd8221a21b38fc8bb4ba554fb/pile.py", line 236, in _generate_examples
23 with zstd.open(open(files[subset], "rb"), "rt", encoding="utf-8") as f:
24 File "/lfs/ampere1/0/brando9/miniconda/envs/beyond_scale/lib/python3.10/site-packages/datasets/streaming.py", line 74, in wrapper
25 return function(*args, download_config=download_config, **kwargs)
26 File "/lfs/ampere1/0/brando9/miniconda/envs/beyond_scale/lib/python3.10/site-packages/datasets/download/streaming_download_manager.py", line 496, in xopen
27 file_obj = fsspec.open(file, mode=mode, *args, **kwargs).open()
28 File "/lfs/ampere1/0/brando9/miniconda/envs/beyond_scale/lib/python3.10/site-packages/fsspec/core.py", line 134, in open
29 return self.__enter__()
30 File "/lfs/ampere1/0/brando9/miniconda/envs/beyond_scale/lib/python3.10/site-packages/fsspec/core.py", line 102, in __enter__
31 f = self.fs.open(self.path, mode=mode)
32 File "/lfs/ampere1/0/brando9/miniconda/envs/beyond_scale/lib/python3.10/site-packages/fsspec/spec.py", line 1241, in open
33 f = self._open(
34 File "/lfs/ampere1/0/brando9/miniconda/envs/beyond_scale/lib/python3.10/site-packages/fsspec/implementations/http.py", line 356, in _open
35 size = size or self.info(path, **kwargs)["size"]
36 File "/lfs/ampere1/0/brando9/miniconda/envs/beyond_scale/lib/python3.10/site-packages/fsspec/asyn.py", line 121, in wrapper
37 return sync(self.loop, func, *args, **kwargs)
38 File "/lfs/ampere1/0/brando9/miniconda/envs/beyond_scale/lib/python3.10/site-packages/fsspec/asyn.py", line 106, in sync
39 raise return_result
40 File "/lfs/ampere1/0/brando9/miniconda/envs/beyond_scale/lib/python3.10/site-packages/fsspec/asyn.py", line 61, in _runner
41 result[0] = await coro
42 File "/lfs/ampere1/0/brando9/miniconda/envs/beyond_scale/lib/python3.10/site-packages/fsspec/implementations/http.py", line 430, in _info
43 raise FileNotFoundError(url) from exc
44 FileNotFoundError: https://the-eye.eu/public/AI/pile_preliminary_components/NIH_ExPORTER_awarded_grant_text.jsonl.zst
```
### Steps to reproduce the bug
run this:
```
from datasets import load_dataset
path, name = 'EleutherAI/pile', 'nih_exporter'
# -- Get data set
dataset = load_dataset(path, name, streaming=True, split="train").with_format("torch")
batch = dataset.take(512)
print(f'{batch=}')
```
### Expected behavior
print the batch
### Environment info
```
(beyond_scale) brando9@ampere1:~/beyond-scale-language-data-diversity$ datasets-cli env
Copy-and-paste the text below in your GitHub issue.
- `datasets` version: 2.14.4
- Platform: Linux-5.4.0-122-generic-x86_64-with-glibc2.31
- Python version: 3.10.11
- Huggingface_hub version: 0.16.4
- PyArrow version: 12.0.1
- Pandas version: 2.0.3
``` | 319 | NIH exporter file not found
### Describe the bug
can't use or download the nih exporter pile data.
```
15 experiment_compute_diveristy_coeff_single_dataset_then_combined_datasets_with_domain_weights()
16 File "/lfs/ampere1/0/brando9/beyond-scale-language-data-diversity/src/diversity/div_coeff.py", line 474, in experiment_compute_diveristy_coeff_single_dataset_then_combined_datasets_with_domain_weights
17 column_names = next(iter(dataset)).keys()
18 File "/lfs/ampere1/0/brando9/miniconda/envs/beyond_scale/lib/python3.10/site-packages/datasets/iterable_dataset.py", line 1353, in __iter__
19 for key, example in ex_iterable:
20 File "/lfs/ampere1/0/brando9/miniconda/envs/beyond_scale/lib/python3.10/site-packages/datasets/iterable_dataset.py", line 207, in __iter__
21 yield from self.generate_examples_fn(**self.kwargs)
22 File "/lfs/ampere1/0/brando9/.cache/huggingface/modules/datasets_modules/datasets/EleutherAI--pile/ebea56d358e91cf4d37b0fde361d563bed1472fbd8221a21b38fc8bb4ba554fb/pile.py", line 236, in _generate_examples
23 with zstd.open(open(files[subset], "rb"), "rt", encoding="utf-8") as f:
24 File "/lfs/ampere1/0/brando9/miniconda/envs/beyond_scale/lib/python3.10/site-packages/datasets/streaming.py", line 74, in wrapper
25 return function(*args, download_config=download_config, **kwargs)
26 File "/lfs/ampere1/0/brando9/miniconda/envs/beyond_scale/lib/python3.10/site-packages/datasets/download/streaming_download_manager.py", line 496, in xopen
27 file_obj = fsspec.open(file, mode=mode, *args, **kwargs).open()
28 File "/lfs/ampere1/0/brando9/miniconda/envs/beyond_scale/lib/python3.10/site-packages/fsspec/core.py", line 134, in open
29 return self.__enter__()
30 File "/lfs/ampere1/0/brando9/miniconda/envs/beyond_scale/lib/python3.10/site-packages/fsspec/core.py", line 102, in __enter__
31 f = self.fs.open(self.path, mode=mode)
32 File "/lfs/ampere1/0/brando9/miniconda/envs/beyond_scale/lib/python3.10/site-packages/fsspec/spec.py", line 1241, in open
33 f = self._open(
34 File "/lfs/ampere1/0/brando9/miniconda/envs/beyond_scale/lib/python3.10/site-packages/fsspec/implementations/http.py", line 356, in _open
35 size = size or self.info(path, **kwargs)["size"]
36 File "/lfs/ampere1/0/brando9/miniconda/envs/beyond_scale/lib/python3.10/site-packages/fsspec/asyn.py", line 121, in wrapper
37 return sync(self.loop, func, *args, **kwargs)
38 File "/lfs/ampere1/0/brando9/miniconda/envs/beyond_scale/lib/python3.10/site-packages/fsspec/asyn.py", line 106, in sync
39 raise return_result
40 File "/lfs/ampere1/0/brando9/miniconda/envs/beyond_scale/lib/python3.10/site-packages/fsspec/asyn.py", line 61, in _runner
41 result[0] = await coro
42 File "/lfs/ampere1/0/brando9/miniconda/envs/beyond_scale/lib/python3.10/site-packages/fsspec/implementations/http.py", line 430, in _info
43 raise FileNotFoundError(url) from exc
44 FileNotFoundError: https://the-eye.eu/public/AI/pile_preliminary_components/NIH_ExPORTER_awarded_grant_text.jsonl.zst
```
### Steps to reproduce the bug
run this:
```
from datasets import load_dataset
path, name = 'EleutherAI/pile', 'nih_exporter'
# -- Get data set
dataset = load_dataset(path, name, streaming=True, split="train").with_format("torch")
batch = dataset.take(512)
print(f'{batch=}')
```
### Expected behavior
print the batch
### Environment info
```
(beyond_scale) brando9@ampere1:~/beyond-scale-language-data-diversity$ datasets-cli env
Copy-and-paste the text below in your GitHub issue.
- `datasets` version: 2.14.4
- Platform: Linux-5.4.0-122-generic-x86_64-with-glibc2.31
- Python version: 3.10.11
- Huggingface_hub version: 0.16.4
- PyArrow version: 12.0.1
- Pandas version: 2.0.3
```
this seems to work but it's rather annoying.
Summary of how to make it work:
1. get urls to parquet files into a list
2. load list to load_dataset via `load_dataset('parquet', data_files=urls)` (note api names to hf are really confusing sometimes)
3. then it should work, print a batch of text.
presudo code
```python
urls_hacker_news = [
"https://huggingface.co/datasets/EleutherAI/pile/resolve/refs%2Fconvert%2Fparquet/hacker_news/pile-train-00000-of-00004.parquet",
"https://huggingface.co/datasets/EleutherAI/pile/resolve/refs%2Fconvert%2Fparquet/hacker_news/pile-train-00001-of-00004.parquet",
"https://huggingface.co/datasets/EleutherAI/pile/resolve/refs%2Fconvert%2Fparquet/hacker_news/pile-train-00002-of-00004.parquet",
"https://huggingface.co/datasets/EleutherAI/pile/resolve/refs%2Fconvert%2Fparquet/hacker_news/pile-train-00003-of-00004.parquet"
]
...
# streaming = False
from diversity.pile_subset_urls import urls_hacker_news
path, name, data_files = 'parquet', 'hacker_news', urls_hacker_news
# not changing
batch_size = 512
today = datetime.datetime.now().strftime('%Y-m%m-d%d-t%Hh_%Mm_%Ss')
run_name = f'{path} div_coeff_{num_batches=} ({today=} ({name=}) {data_mixture_name=} {probabilities=})'
print(f'{run_name=}')
# - Init wandb
debug: bool = mode == 'dryrun'
run = wandb.init(mode=mode, project="beyond-scale", name=run_name, save_code=True)
wandb.config.update({"num_batches": num_batches, "path": path, "name": name, "today": today, 'probabilities': probabilities, 'batch_size': batch_size, 'debug': debug, 'data_mixture_name': data_mixture_name, 'streaming': streaming, 'data_files': data_files})
# run.notify_on_failure() # https://community.wandb.ai/t/how-do-i-set-the-wandb-alert-programatically-for-my-current-run/4891
print(f'{debug=}')
print(f'{wandb.config=}')
# -- Get probe network
from datasets import load_dataset
import torch
from transformers import GPT2Tokenizer, GPT2LMHeadModel
tokenizer = GPT2Tokenizer.from_pretrained("gpt2")
if tokenizer.pad_token_id is None:
tokenizer.pad_token = tokenizer.eos_token
probe_network = GPT2LMHeadModel.from_pretrained("gpt2")
device = torch.device(f"cuda:{0}" if torch.cuda.is_available() else "cpu")
probe_network = probe_network.to(device)
# -- Get data set
def my_load_dataset(path, name):
print(f'{path=} {name=} {streaming=}')
if path == 'json' or path == 'bin' or path == 'csv':
print(f'{data_files_prefix+name=}')
return load_dataset(path, data_files=data_files_prefix+name, streaming=streaming, split="train").with_format("torch")
elif path == 'parquet':
print(f'{data_files=}')
return load_dataset(path, data_files=data_files, streaming=streaming, split="train").with_format("torch")
else:
return load_dataset(path, name, streaming=streaming, split="train").with_format("torch")
# - get data set for real now
if isinstance(path, str):
dataset = my_load_dataset(path, name)
else:
print('-- interleaving datasets')
datasets = [my_load_dataset(path, name).with_format("torch") for path, name in zip(path, name)]
[print(f'{dataset.description=}') for dataset in datasets]
dataset = interleave_datasets(datasets, probabilities)
print(f'{dataset=}')
batch = dataset.take(batch_size)
print(f'{next(iter(batch))=}')
column_names = next(iter(batch)).keys()
print(f'{column_names=}')
# - Prepare functions to tokenize batch
def preprocess(examples):
return tokenizer(examples["text"], padding="max_length", max_length=128, truncation=True, return_tensors="pt")
remove_columns = column_names # remove all keys that are not tensors to avoid bugs in collate function in task2vec's pytorch data loader
def map(batch):
return batch.map(preprocess, batched=True, remove_columns=remove_columns)
tokenized_batch = map(batch)
print(f'{next(iter(tokenized_batch))=}')
```
https://stackoverflow.com/questions/76891189/how-to-download-data-from-hugging-face-that-is-visible-on-the-data-viewer-but-th/76902681#76902681
https://discuss.huggingface.co/t/how-to-download-data-from-hugging-face-that-is-visible-on-the-data-viewer-but-the-files-are-not-available/50555/5?u=severo |
https://github.com/huggingface/datasets/issues/6142 | the-stack-dedup fails to generate | It seems that some parquet files have additional columns.
I ran a scan and found that two files have the additional `__id__` column:
1. `hf://datasets/bigcode/the-stack-dedup/data/numpy/data-00000-of-00001.parquet`
2. `hf://datasets/bigcode/the-stack-dedup/data/omgrofl/data-00000-of-00001.parquet`
We should open a PR to fix those two files | ### Describe the bug
I'm getting an error generating the-stack-dedup with datasets 2.13.1, and with 2.14.4 nothing happens.
### Steps to reproduce the bug
My code:
```
import os
import datasets as ds
MY_CACHE_DIR = "/home/ubuntu/the-stack-dedup-local"
MY_TOKEN="my-token"
the_stack_ds = ds.load_dataset("bigcode/the-stack-dedup", split="train", download_mode="reuse_cache_if_exists", cache_dir=MY_CACHE_DIR, use_auth_token=MY_TOKEN, num_proc=64)
```
The exception:
```
Generating train split: 233248251 examples [54:31, 57280.00 examples/s]
multiprocess.pool.RemoteTraceback:
"""
Traceback (most recent call last):
File "/home/ubuntu/.local/lib/python3.10/site-packages/datasets/build
er.py", line 1879, in _prepare_split_single
for _, table in generator:
File "/home/ubuntu/.local/lib/python3.10/site-packages/datasets/packa
ged_modules/parquet/parquet.py", line 82, in _generate_tables
yield f"{file_idx}_{batch_idx}", self._cast_table(pa_table)
File "/home/ubuntu/.local/lib/python3.10/site-packages/datasets/packa
ged_modules/parquet/parquet.py", line 61, in _cast_table
pa_table = table_cast(pa_table, self.info.features.arrow_schema)
File "/home/ubuntu/.local/lib/python3.10/site-packages/datasets/table
.py", line 2324, in table_cast
return cast_table_to_schema(table, schema)
File "/home/ubuntu/.local/lib/python3.10/site-packages/datasets/table
.py", line 2282, in cast_table_to_schema
raise ValueError(f"Couldn't cast\n{table.schema}\nto\n{features}\nb
ecause column names don't match")
ValueError: Couldn't cast
hexsha: string
size: int64
ext: string
lang: string
max_stars_repo_path: string
max_stars_repo_name: string
max_stars_repo_head_hexsha: string
max_stars_repo_licenses: list<item: string>
child 0, item: string
max_stars_count: int64
max_stars_repo_stars_event_min_datetime: string
max_stars_repo_stars_event_max_datetime: string
max_issues_repo_path: string
max_issues_repo_name: string
max_issues_repo_head_hexsha: string
max_issues_repo_licenses: list<item: string>
child 0, item: string
max_issues_count: int64
max_issues_repo_issues_event_min_datetime: string
max_issues_repo_issues_event_max_datetime: string
max_forks_repo_path: string
max_forks_repo_name: string
max_forks_repo_head_hexsha: string
max_forks_repo_licenses: list<item: string>
child 0, item: string
max_forks_count: int64
max_forks_repo_forks_event_min_datetime: string
max_forks_repo_forks_event_max_datetime: string
content: string
avg_line_length: double
max_line_length: int64
alphanum_fraction: double
__id__: int64
-- schema metadata --
huggingface: '{"info": {"features": {"hexsha": {"dtype": "string", "_type' + 1979
to
{'hexsha': Value(dtype='string', id=None), 'size': Value(dtype='int64', id=None), 'ext': Value(dtype='string', id=None), 'lang': Value(dtype='string', id=None), 'max_stars_repo_path': Value(dtype='string', id=None), 'max_stars_repo_name': Value(dtype='string', id=None), 'max_stars_repo_head_hexsha': Value(dtype='string', id=None), 'max_stars_repo_licenses': Sequence(feature=Value(dtype='string', id=None), length=-1, id=None), 'max_stars_count': Value(dtype='int64', id=None), 'max_stars_repo_stars_event_min_datetime': Value(dtype='string', id=None), 'max_stars_repo_stars_event_max_datetime': Value(dtype='string', id=None), 'max_issues_repo_path': Value(dtype='string', id=None), 'max_issues_repo_name': Value(dtype='string', id=None), 'max_issues_repo_head_hexsha': Value(dtype='string', id=None), 'max_issues_repo_licenses': Sequence(feature=Value(dtype='string', id=None), length=-1, id=None), 'max_issues_count': Value(dtype='int64', id=None), 'max_issues_repo_issues_event_min_datetime': Value(dtype='string', id=None), 'max_issues_repo_issues_event_max_datetime': Value(dtype='string', id=None), 'max_forks_repo_path': Value(dtype='string', id=None), 'max_forks_repo_name': Value(dtype='string', id=None), 'max_forks_repo_head_hexsha': Value(dtype='string', id=None), 'max_forks_repo_licenses': Sequence(feature=Value(dtype='string', id=None), length=-1, id=None), 'max_forks_count': Value(dtype='int64', id=None), 'max_forks_repo_forks_event_min_datetime': Value(dtype='string', id=None), 'max_forks_repo_forks_event_max_datetime': Value(dtype='string', id=None), 'content': Value(dtype='string', id=None), 'avg_line_length': Value(dtype='float64', id=None), 'max_line_length': Value(dtype='int64', id=None), 'alphanum_fraction': Value(dtype='float64', id=None)}
because column names don't match
The above exception was the direct cause of the following exception:
Traceback (most recent call last):
File "/home/ubuntu/.local/lib/python3.10/site-packages/multiprocess/p
ool.py", line 125, in worker
result = (True, func(*args, **kwds))
File "/home/ubuntu/.local/lib/python3.10/site-packages/datasets/utils
/py_utils.py", line 1328, in _write_generator_to_queue
for i, result in enumerate(func(**kwargs)):
File "/home/ubuntu/.local/lib/python3.10/site-packages/datasets/build
er.py", line 1912, in _prepare_split_single
raise DatasetGenerationError("An error occurred while generating th
e dataset") from e
datasets.builder.DatasetGenerationError: An error occurred while genera
ting the dataset
"""
The above exception was the direct cause of the following exception:
Traceback (most recent call last):
File "/home/ubuntu/download_the_stack.py", line 7, in <module>
the_stack_ds = ds.load_dataset("bigcode/the-stack-dedup", split="tr
ain", download_mode="reuse_cache_if_exists", cache_dir=MY_CACHE_DIR, us
e_auth_token=MY_TOKEN, num_proc=64)
File "/home/ubuntu/.local/lib/python3.10/site-packages/datasets/load.
py", line 1809, in load_dataset
builder_instance.download_and_prepare(
File "/home/ubuntu/.local/lib/python3.10/site-packages/datasets/build
er.py", line 909, in download_and_prepare
self._download_and_prepare(
File "/home/ubuntu/.local/lib/python3.10/site-packages/datasets/build
er.py", line 1004, in _download_and_prepare
self._prepare_split(split_generator, **prepare_split_kwargs)
File "/home/ubuntu/.local/lib/python3.10/site-packages/datasets/build
er.py", line 1796, in _prepare_split
for job_id, done, content in iflatmap_unordered(
File "/home/ubuntu/.local/lib/python3.10/site-packages/datasets/utils
/py_utils.py", line 1354, in iflatmap_unordered
[async_result.get(timeout=0.05) for async_result in async_results]
File "/home/ubuntu/.local/lib/python3.10/site-packages/datasets/utils
/py_utils.py", line 1354, in <listcomp>
[async_result.get(timeout=0.05) for async_result in async_results]
File "/home/ubuntu/.local/lib/python3.10/site-packages/multiprocess/p
ool.py", line 774, in get
raise self._value
datasets.builder.DatasetGenerationError: An error occurred while generating the dataset
```
### Expected behavior
The dataset downloads properly. @lhoestq @loub
### Environment info
Datasets 2.13.1, large VM with 2TB RAM, Ubuntu 20.04 | 37 | the-stack-dedup fails to generate
### Describe the bug
I'm getting an error generating the-stack-dedup with datasets 2.13.1, and with 2.14.4 nothing happens.
### Steps to reproduce the bug
My code:
```
import os
import datasets as ds
MY_CACHE_DIR = "/home/ubuntu/the-stack-dedup-local"
MY_TOKEN="my-token"
the_stack_ds = ds.load_dataset("bigcode/the-stack-dedup", split="train", download_mode="reuse_cache_if_exists", cache_dir=MY_CACHE_DIR, use_auth_token=MY_TOKEN, num_proc=64)
```
The exception:
```
Generating train split: 233248251 examples [54:31, 57280.00 examples/s]
multiprocess.pool.RemoteTraceback:
"""
Traceback (most recent call last):
File "/home/ubuntu/.local/lib/python3.10/site-packages/datasets/build
er.py", line 1879, in _prepare_split_single
for _, table in generator:
File "/home/ubuntu/.local/lib/python3.10/site-packages/datasets/packa
ged_modules/parquet/parquet.py", line 82, in _generate_tables
yield f"{file_idx}_{batch_idx}", self._cast_table(pa_table)
File "/home/ubuntu/.local/lib/python3.10/site-packages/datasets/packa
ged_modules/parquet/parquet.py", line 61, in _cast_table
pa_table = table_cast(pa_table, self.info.features.arrow_schema)
File "/home/ubuntu/.local/lib/python3.10/site-packages/datasets/table
.py", line 2324, in table_cast
return cast_table_to_schema(table, schema)
File "/home/ubuntu/.local/lib/python3.10/site-packages/datasets/table
.py", line 2282, in cast_table_to_schema
raise ValueError(f"Couldn't cast\n{table.schema}\nto\n{features}\nb
ecause column names don't match")
ValueError: Couldn't cast
hexsha: string
size: int64
ext: string
lang: string
max_stars_repo_path: string
max_stars_repo_name: string
max_stars_repo_head_hexsha: string
max_stars_repo_licenses: list<item: string>
child 0, item: string
max_stars_count: int64
max_stars_repo_stars_event_min_datetime: string
max_stars_repo_stars_event_max_datetime: string
max_issues_repo_path: string
max_issues_repo_name: string
max_issues_repo_head_hexsha: string
max_issues_repo_licenses: list<item: string>
child 0, item: string
max_issues_count: int64
max_issues_repo_issues_event_min_datetime: string
max_issues_repo_issues_event_max_datetime: string
max_forks_repo_path: string
max_forks_repo_name: string
max_forks_repo_head_hexsha: string
max_forks_repo_licenses: list<item: string>
child 0, item: string
max_forks_count: int64
max_forks_repo_forks_event_min_datetime: string
max_forks_repo_forks_event_max_datetime: string
content: string
avg_line_length: double
max_line_length: int64
alphanum_fraction: double
__id__: int64
-- schema metadata --
huggingface: '{"info": {"features": {"hexsha": {"dtype": "string", "_type' + 1979
to
{'hexsha': Value(dtype='string', id=None), 'size': Value(dtype='int64', id=None), 'ext': Value(dtype='string', id=None), 'lang': Value(dtype='string', id=None), 'max_stars_repo_path': Value(dtype='string', id=None), 'max_stars_repo_name': Value(dtype='string', id=None), 'max_stars_repo_head_hexsha': Value(dtype='string', id=None), 'max_stars_repo_licenses': Sequence(feature=Value(dtype='string', id=None), length=-1, id=None), 'max_stars_count': Value(dtype='int64', id=None), 'max_stars_repo_stars_event_min_datetime': Value(dtype='string', id=None), 'max_stars_repo_stars_event_max_datetime': Value(dtype='string', id=None), 'max_issues_repo_path': Value(dtype='string', id=None), 'max_issues_repo_name': Value(dtype='string', id=None), 'max_issues_repo_head_hexsha': Value(dtype='string', id=None), 'max_issues_repo_licenses': Sequence(feature=Value(dtype='string', id=None), length=-1, id=None), 'max_issues_count': Value(dtype='int64', id=None), 'max_issues_repo_issues_event_min_datetime': Value(dtype='string', id=None), 'max_issues_repo_issues_event_max_datetime': Value(dtype='string', id=None), 'max_forks_repo_path': Value(dtype='string', id=None), 'max_forks_repo_name': Value(dtype='string', id=None), 'max_forks_repo_head_hexsha': Value(dtype='string', id=None), 'max_forks_repo_licenses': Sequence(feature=Value(dtype='string', id=None), length=-1, id=None), 'max_forks_count': Value(dtype='int64', id=None), 'max_forks_repo_forks_event_min_datetime': Value(dtype='string', id=None), 'max_forks_repo_forks_event_max_datetime': Value(dtype='string', id=None), 'content': Value(dtype='string', id=None), 'avg_line_length': Value(dtype='float64', id=None), 'max_line_length': Value(dtype='int64', id=None), 'alphanum_fraction': Value(dtype='float64', id=None)}
because column names don't match
The above exception was the direct cause of the following exception:
Traceback (most recent call last):
File "/home/ubuntu/.local/lib/python3.10/site-packages/multiprocess/p
ool.py", line 125, in worker
result = (True, func(*args, **kwds))
File "/home/ubuntu/.local/lib/python3.10/site-packages/datasets/utils
/py_utils.py", line 1328, in _write_generator_to_queue
for i, result in enumerate(func(**kwargs)):
File "/home/ubuntu/.local/lib/python3.10/site-packages/datasets/build
er.py", line 1912, in _prepare_split_single
raise DatasetGenerationError("An error occurred while generating th
e dataset") from e
datasets.builder.DatasetGenerationError: An error occurred while genera
ting the dataset
"""
The above exception was the direct cause of the following exception:
Traceback (most recent call last):
File "/home/ubuntu/download_the_stack.py", line 7, in <module>
the_stack_ds = ds.load_dataset("bigcode/the-stack-dedup", split="tr
ain", download_mode="reuse_cache_if_exists", cache_dir=MY_CACHE_DIR, us
e_auth_token=MY_TOKEN, num_proc=64)
File "/home/ubuntu/.local/lib/python3.10/site-packages/datasets/load.
py", line 1809, in load_dataset
builder_instance.download_and_prepare(
File "/home/ubuntu/.local/lib/python3.10/site-packages/datasets/build
er.py", line 909, in download_and_prepare
self._download_and_prepare(
File "/home/ubuntu/.local/lib/python3.10/site-packages/datasets/build
er.py", line 1004, in _download_and_prepare
self._prepare_split(split_generator, **prepare_split_kwargs)
File "/home/ubuntu/.local/lib/python3.10/site-packages/datasets/build
er.py", line 1796, in _prepare_split
for job_id, done, content in iflatmap_unordered(
File "/home/ubuntu/.local/lib/python3.10/site-packages/datasets/utils
/py_utils.py", line 1354, in iflatmap_unordered
[async_result.get(timeout=0.05) for async_result in async_results]
File "/home/ubuntu/.local/lib/python3.10/site-packages/datasets/utils
/py_utils.py", line 1354, in <listcomp>
[async_result.get(timeout=0.05) for async_result in async_results]
File "/home/ubuntu/.local/lib/python3.10/site-packages/multiprocess/p
ool.py", line 774, in get
raise self._value
datasets.builder.DatasetGenerationError: An error occurred while generating the dataset
```
### Expected behavior
The dataset downloads properly. @lhoestq @loub
### Environment info
Datasets 2.13.1, large VM with 2TB RAM, Ubuntu 20.04
It seems that some parquet files have additional columns.
I ran a scan and found that two files have the additional `__id__` column:
1. `hf://datasets/bigcode/the-stack-dedup/data/numpy/data-00000-of-00001.parquet`
2. `hf://datasets/bigcode/the-stack-dedup/data/omgrofl/data-00000-of-00001.parquet`
We should open a PR to fix those two files |
https://github.com/huggingface/datasets/issues/6142 | the-stack-dedup fails to generate | The files have been fixed ! I'm closing this one but feel free to re-open if you still have the issue | ### Describe the bug
I'm getting an error generating the-stack-dedup with datasets 2.13.1, and with 2.14.4 nothing happens.
### Steps to reproduce the bug
My code:
```
import os
import datasets as ds
MY_CACHE_DIR = "/home/ubuntu/the-stack-dedup-local"
MY_TOKEN="my-token"
the_stack_ds = ds.load_dataset("bigcode/the-stack-dedup", split="train", download_mode="reuse_cache_if_exists", cache_dir=MY_CACHE_DIR, use_auth_token=MY_TOKEN, num_proc=64)
```
The exception:
```
Generating train split: 233248251 examples [54:31, 57280.00 examples/s]
multiprocess.pool.RemoteTraceback:
"""
Traceback (most recent call last):
File "/home/ubuntu/.local/lib/python3.10/site-packages/datasets/build
er.py", line 1879, in _prepare_split_single
for _, table in generator:
File "/home/ubuntu/.local/lib/python3.10/site-packages/datasets/packa
ged_modules/parquet/parquet.py", line 82, in _generate_tables
yield f"{file_idx}_{batch_idx}", self._cast_table(pa_table)
File "/home/ubuntu/.local/lib/python3.10/site-packages/datasets/packa
ged_modules/parquet/parquet.py", line 61, in _cast_table
pa_table = table_cast(pa_table, self.info.features.arrow_schema)
File "/home/ubuntu/.local/lib/python3.10/site-packages/datasets/table
.py", line 2324, in table_cast
return cast_table_to_schema(table, schema)
File "/home/ubuntu/.local/lib/python3.10/site-packages/datasets/table
.py", line 2282, in cast_table_to_schema
raise ValueError(f"Couldn't cast\n{table.schema}\nto\n{features}\nb
ecause column names don't match")
ValueError: Couldn't cast
hexsha: string
size: int64
ext: string
lang: string
max_stars_repo_path: string
max_stars_repo_name: string
max_stars_repo_head_hexsha: string
max_stars_repo_licenses: list<item: string>
child 0, item: string
max_stars_count: int64
max_stars_repo_stars_event_min_datetime: string
max_stars_repo_stars_event_max_datetime: string
max_issues_repo_path: string
max_issues_repo_name: string
max_issues_repo_head_hexsha: string
max_issues_repo_licenses: list<item: string>
child 0, item: string
max_issues_count: int64
max_issues_repo_issues_event_min_datetime: string
max_issues_repo_issues_event_max_datetime: string
max_forks_repo_path: string
max_forks_repo_name: string
max_forks_repo_head_hexsha: string
max_forks_repo_licenses: list<item: string>
child 0, item: string
max_forks_count: int64
max_forks_repo_forks_event_min_datetime: string
max_forks_repo_forks_event_max_datetime: string
content: string
avg_line_length: double
max_line_length: int64
alphanum_fraction: double
__id__: int64
-- schema metadata --
huggingface: '{"info": {"features": {"hexsha": {"dtype": "string", "_type' + 1979
to
{'hexsha': Value(dtype='string', id=None), 'size': Value(dtype='int64', id=None), 'ext': Value(dtype='string', id=None), 'lang': Value(dtype='string', id=None), 'max_stars_repo_path': Value(dtype='string', id=None), 'max_stars_repo_name': Value(dtype='string', id=None), 'max_stars_repo_head_hexsha': Value(dtype='string', id=None), 'max_stars_repo_licenses': Sequence(feature=Value(dtype='string', id=None), length=-1, id=None), 'max_stars_count': Value(dtype='int64', id=None), 'max_stars_repo_stars_event_min_datetime': Value(dtype='string', id=None), 'max_stars_repo_stars_event_max_datetime': Value(dtype='string', id=None), 'max_issues_repo_path': Value(dtype='string', id=None), 'max_issues_repo_name': Value(dtype='string', id=None), 'max_issues_repo_head_hexsha': Value(dtype='string', id=None), 'max_issues_repo_licenses': Sequence(feature=Value(dtype='string', id=None), length=-1, id=None), 'max_issues_count': Value(dtype='int64', id=None), 'max_issues_repo_issues_event_min_datetime': Value(dtype='string', id=None), 'max_issues_repo_issues_event_max_datetime': Value(dtype='string', id=None), 'max_forks_repo_path': Value(dtype='string', id=None), 'max_forks_repo_name': Value(dtype='string', id=None), 'max_forks_repo_head_hexsha': Value(dtype='string', id=None), 'max_forks_repo_licenses': Sequence(feature=Value(dtype='string', id=None), length=-1, id=None), 'max_forks_count': Value(dtype='int64', id=None), 'max_forks_repo_forks_event_min_datetime': Value(dtype='string', id=None), 'max_forks_repo_forks_event_max_datetime': Value(dtype='string', id=None), 'content': Value(dtype='string', id=None), 'avg_line_length': Value(dtype='float64', id=None), 'max_line_length': Value(dtype='int64', id=None), 'alphanum_fraction': Value(dtype='float64', id=None)}
because column names don't match
The above exception was the direct cause of the following exception:
Traceback (most recent call last):
File "/home/ubuntu/.local/lib/python3.10/site-packages/multiprocess/p
ool.py", line 125, in worker
result = (True, func(*args, **kwds))
File "/home/ubuntu/.local/lib/python3.10/site-packages/datasets/utils
/py_utils.py", line 1328, in _write_generator_to_queue
for i, result in enumerate(func(**kwargs)):
File "/home/ubuntu/.local/lib/python3.10/site-packages/datasets/build
er.py", line 1912, in _prepare_split_single
raise DatasetGenerationError("An error occurred while generating th
e dataset") from e
datasets.builder.DatasetGenerationError: An error occurred while genera
ting the dataset
"""
The above exception was the direct cause of the following exception:
Traceback (most recent call last):
File "/home/ubuntu/download_the_stack.py", line 7, in <module>
the_stack_ds = ds.load_dataset("bigcode/the-stack-dedup", split="tr
ain", download_mode="reuse_cache_if_exists", cache_dir=MY_CACHE_DIR, us
e_auth_token=MY_TOKEN, num_proc=64)
File "/home/ubuntu/.local/lib/python3.10/site-packages/datasets/load.
py", line 1809, in load_dataset
builder_instance.download_and_prepare(
File "/home/ubuntu/.local/lib/python3.10/site-packages/datasets/build
er.py", line 909, in download_and_prepare
self._download_and_prepare(
File "/home/ubuntu/.local/lib/python3.10/site-packages/datasets/build
er.py", line 1004, in _download_and_prepare
self._prepare_split(split_generator, **prepare_split_kwargs)
File "/home/ubuntu/.local/lib/python3.10/site-packages/datasets/build
er.py", line 1796, in _prepare_split
for job_id, done, content in iflatmap_unordered(
File "/home/ubuntu/.local/lib/python3.10/site-packages/datasets/utils
/py_utils.py", line 1354, in iflatmap_unordered
[async_result.get(timeout=0.05) for async_result in async_results]
File "/home/ubuntu/.local/lib/python3.10/site-packages/datasets/utils
/py_utils.py", line 1354, in <listcomp>
[async_result.get(timeout=0.05) for async_result in async_results]
File "/home/ubuntu/.local/lib/python3.10/site-packages/multiprocess/p
ool.py", line 774, in get
raise self._value
datasets.builder.DatasetGenerationError: An error occurred while generating the dataset
```
### Expected behavior
The dataset downloads properly. @lhoestq @loub
### Environment info
Datasets 2.13.1, large VM with 2TB RAM, Ubuntu 20.04 | 21 | the-stack-dedup fails to generate
### Describe the bug
I'm getting an error generating the-stack-dedup with datasets 2.13.1, and with 2.14.4 nothing happens.
### Steps to reproduce the bug
My code:
```
import os
import datasets as ds
MY_CACHE_DIR = "/home/ubuntu/the-stack-dedup-local"
MY_TOKEN="my-token"
the_stack_ds = ds.load_dataset("bigcode/the-stack-dedup", split="train", download_mode="reuse_cache_if_exists", cache_dir=MY_CACHE_DIR, use_auth_token=MY_TOKEN, num_proc=64)
```
The exception:
```
Generating train split: 233248251 examples [54:31, 57280.00 examples/s]
multiprocess.pool.RemoteTraceback:
"""
Traceback (most recent call last):
File "/home/ubuntu/.local/lib/python3.10/site-packages/datasets/build
er.py", line 1879, in _prepare_split_single
for _, table in generator:
File "/home/ubuntu/.local/lib/python3.10/site-packages/datasets/packa
ged_modules/parquet/parquet.py", line 82, in _generate_tables
yield f"{file_idx}_{batch_idx}", self._cast_table(pa_table)
File "/home/ubuntu/.local/lib/python3.10/site-packages/datasets/packa
ged_modules/parquet/parquet.py", line 61, in _cast_table
pa_table = table_cast(pa_table, self.info.features.arrow_schema)
File "/home/ubuntu/.local/lib/python3.10/site-packages/datasets/table
.py", line 2324, in table_cast
return cast_table_to_schema(table, schema)
File "/home/ubuntu/.local/lib/python3.10/site-packages/datasets/table
.py", line 2282, in cast_table_to_schema
raise ValueError(f"Couldn't cast\n{table.schema}\nto\n{features}\nb
ecause column names don't match")
ValueError: Couldn't cast
hexsha: string
size: int64
ext: string
lang: string
max_stars_repo_path: string
max_stars_repo_name: string
max_stars_repo_head_hexsha: string
max_stars_repo_licenses: list<item: string>
child 0, item: string
max_stars_count: int64
max_stars_repo_stars_event_min_datetime: string
max_stars_repo_stars_event_max_datetime: string
max_issues_repo_path: string
max_issues_repo_name: string
max_issues_repo_head_hexsha: string
max_issues_repo_licenses: list<item: string>
child 0, item: string
max_issues_count: int64
max_issues_repo_issues_event_min_datetime: string
max_issues_repo_issues_event_max_datetime: string
max_forks_repo_path: string
max_forks_repo_name: string
max_forks_repo_head_hexsha: string
max_forks_repo_licenses: list<item: string>
child 0, item: string
max_forks_count: int64
max_forks_repo_forks_event_min_datetime: string
max_forks_repo_forks_event_max_datetime: string
content: string
avg_line_length: double
max_line_length: int64
alphanum_fraction: double
__id__: int64
-- schema metadata --
huggingface: '{"info": {"features": {"hexsha": {"dtype": "string", "_type' + 1979
to
{'hexsha': Value(dtype='string', id=None), 'size': Value(dtype='int64', id=None), 'ext': Value(dtype='string', id=None), 'lang': Value(dtype='string', id=None), 'max_stars_repo_path': Value(dtype='string', id=None), 'max_stars_repo_name': Value(dtype='string', id=None), 'max_stars_repo_head_hexsha': Value(dtype='string', id=None), 'max_stars_repo_licenses': Sequence(feature=Value(dtype='string', id=None), length=-1, id=None), 'max_stars_count': Value(dtype='int64', id=None), 'max_stars_repo_stars_event_min_datetime': Value(dtype='string', id=None), 'max_stars_repo_stars_event_max_datetime': Value(dtype='string', id=None), 'max_issues_repo_path': Value(dtype='string', id=None), 'max_issues_repo_name': Value(dtype='string', id=None), 'max_issues_repo_head_hexsha': Value(dtype='string', id=None), 'max_issues_repo_licenses': Sequence(feature=Value(dtype='string', id=None), length=-1, id=None), 'max_issues_count': Value(dtype='int64', id=None), 'max_issues_repo_issues_event_min_datetime': Value(dtype='string', id=None), 'max_issues_repo_issues_event_max_datetime': Value(dtype='string', id=None), 'max_forks_repo_path': Value(dtype='string', id=None), 'max_forks_repo_name': Value(dtype='string', id=None), 'max_forks_repo_head_hexsha': Value(dtype='string', id=None), 'max_forks_repo_licenses': Sequence(feature=Value(dtype='string', id=None), length=-1, id=None), 'max_forks_count': Value(dtype='int64', id=None), 'max_forks_repo_forks_event_min_datetime': Value(dtype='string', id=None), 'max_forks_repo_forks_event_max_datetime': Value(dtype='string', id=None), 'content': Value(dtype='string', id=None), 'avg_line_length': Value(dtype='float64', id=None), 'max_line_length': Value(dtype='int64', id=None), 'alphanum_fraction': Value(dtype='float64', id=None)}
because column names don't match
The above exception was the direct cause of the following exception:
Traceback (most recent call last):
File "/home/ubuntu/.local/lib/python3.10/site-packages/multiprocess/p
ool.py", line 125, in worker
result = (True, func(*args, **kwds))
File "/home/ubuntu/.local/lib/python3.10/site-packages/datasets/utils
/py_utils.py", line 1328, in _write_generator_to_queue
for i, result in enumerate(func(**kwargs)):
File "/home/ubuntu/.local/lib/python3.10/site-packages/datasets/build
er.py", line 1912, in _prepare_split_single
raise DatasetGenerationError("An error occurred while generating th
e dataset") from e
datasets.builder.DatasetGenerationError: An error occurred while genera
ting the dataset
"""
The above exception was the direct cause of the following exception:
Traceback (most recent call last):
File "/home/ubuntu/download_the_stack.py", line 7, in <module>
the_stack_ds = ds.load_dataset("bigcode/the-stack-dedup", split="tr
ain", download_mode="reuse_cache_if_exists", cache_dir=MY_CACHE_DIR, us
e_auth_token=MY_TOKEN, num_proc=64)
File "/home/ubuntu/.local/lib/python3.10/site-packages/datasets/load.
py", line 1809, in load_dataset
builder_instance.download_and_prepare(
File "/home/ubuntu/.local/lib/python3.10/site-packages/datasets/build
er.py", line 909, in download_and_prepare
self._download_and_prepare(
File "/home/ubuntu/.local/lib/python3.10/site-packages/datasets/build
er.py", line 1004, in _download_and_prepare
self._prepare_split(split_generator, **prepare_split_kwargs)
File "/home/ubuntu/.local/lib/python3.10/site-packages/datasets/build
er.py", line 1796, in _prepare_split
for job_id, done, content in iflatmap_unordered(
File "/home/ubuntu/.local/lib/python3.10/site-packages/datasets/utils
/py_utils.py", line 1354, in iflatmap_unordered
[async_result.get(timeout=0.05) for async_result in async_results]
File "/home/ubuntu/.local/lib/python3.10/site-packages/datasets/utils
/py_utils.py", line 1354, in <listcomp>
[async_result.get(timeout=0.05) for async_result in async_results]
File "/home/ubuntu/.local/lib/python3.10/site-packages/multiprocess/p
ool.py", line 774, in get
raise self._value
datasets.builder.DatasetGenerationError: An error occurred while generating the dataset
```
### Expected behavior
The dataset downloads properly. @lhoestq @loub
### Environment info
Datasets 2.13.1, large VM with 2TB RAM, Ubuntu 20.04
The files have been fixed ! I'm closing this one but feel free to re-open if you still have the issue |
https://github.com/huggingface/datasets/issues/6141 | TypeError: ClientSession._request() got an unexpected keyword argument 'https' | Hi! I cannot reproduce this error on my machine or in Colab. Which version of `fsspec` do you have installed? | ### Describe the bug
Hello, when I ran the [code snippet](https://huggingface.co/docs/datasets/v2.14.4/en/loading#json) on the document, I encountered the following problem:
```
Python 3.10.9 (main, Mar 1 2023, 18:23:06) [GCC 11.2.0] on linux
Type "help", "copyright", "credits" or "license" for more information.
>>> from datasets import load_dataset
>>> base_url = "https://rajpurkar.github.io/SQuAD-explorer/dataset/"
>>> dataset = load_dataset("json", data_files={"train": base_url + "train-v1.1.json", "validation": base_url + "dev-v1.1.json"}, field="data")
Traceback (most recent call last):
File "<stdin>", line 1, in <module>
File "/home/liushuai/anaconda3/lib/python3.10/site-packages/datasets/load.py", line 2112, in load_dataset
builder_instance = load_dataset_builder(
File "/home/liushuai/anaconda3/lib/python3.10/site-packages/datasets/load.py", line 1798, in load_dataset_builder
dataset_module = dataset_module_factory(
File "/home/liushuai/anaconda3/lib/python3.10/site-packages/datasets/load.py", line 1413, in dataset_module_factory
).get_module()
File "/home/liushuai/anaconda3/lib/python3.10/site-packages/datasets/load.py", line 949, in get_module
data_files = DataFilesDict.from_patterns(
File "/home/liushuai/anaconda3/lib/python3.10/site-packages/datasets/data_files.py", line 672, in from_patterns
DataFilesList.from_patterns(
File "/home/liushuai/anaconda3/lib/python3.10/site-packages/datasets/data_files.py", line 578, in from_patterns
resolve_pattern(
File "/home/liushuai/anaconda3/lib/python3.10/site-packages/datasets/data_files.py", line 340, in resolve_pattern
for filepath, info in fs.glob(pattern, detail=True).items()
File "/home/liushuai/anaconda3/lib/python3.10/site-packages/fsspec/asyn.py", line 113, in wrapper
return sync(self.loop, func, *args, **kwargs)
File "/home/liushuai/anaconda3/lib/python3.10/site-packages/fsspec/asyn.py", line 98, in sync
raise return_result
File "/home/liushuai/anaconda3/lib/python3.10/site-packages/fsspec/asyn.py", line 53, in _runner
result[0] = await coro
File "/home/liushuai/anaconda3/lib/python3.10/site-packages/fsspec/implementations/http.py", line 449, in _glob
elif await self._exists(path):
File "/home/liushuai/anaconda3/lib/python3.10/site-packages/fsspec/implementations/http.py", line 306, in _exists
r = await session.get(self.encode_url(path), **kw)
File "/home/liushuai/anaconda3/lib/python3.10/site-packages/aiohttp/client.py", line 922, in get
self._request(hdrs.METH_GET, url, allow_redirects=allow_redirects, **kwargs)
TypeError: ClientSession._request() got an unexpected keyword argument 'https'
```
### Steps to reproduce the bug
```
from datasets import load_dataset
base_url = "https://rajpurkar.github.io/SQuAD-explorer/dataset/"
dataset = load_dataset("json", data_files={"train": base_url + "train-v1.1.json", "validation": base_url + "dev-v1.1.json"}, field="data")
```
### Expected behavior
able to load normally
### Environment info
- `datasets` version: 2.14.4
- Platform: Linux-5.4.54-2-x86_64-with-glibc2.27
- Python version: 3.10.9
- Huggingface_hub version: 0.16.4
- PyArrow version: 12.0.1
- Pandas version: 1.5.3 | 20 | TypeError: ClientSession._request() got an unexpected keyword argument 'https'
### Describe the bug
Hello, when I ran the [code snippet](https://huggingface.co/docs/datasets/v2.14.4/en/loading#json) on the document, I encountered the following problem:
```
Python 3.10.9 (main, Mar 1 2023, 18:23:06) [GCC 11.2.0] on linux
Type "help", "copyright", "credits" or "license" for more information.
>>> from datasets import load_dataset
>>> base_url = "https://rajpurkar.github.io/SQuAD-explorer/dataset/"
>>> dataset = load_dataset("json", data_files={"train": base_url + "train-v1.1.json", "validation": base_url + "dev-v1.1.json"}, field="data")
Traceback (most recent call last):
File "<stdin>", line 1, in <module>
File "/home/liushuai/anaconda3/lib/python3.10/site-packages/datasets/load.py", line 2112, in load_dataset
builder_instance = load_dataset_builder(
File "/home/liushuai/anaconda3/lib/python3.10/site-packages/datasets/load.py", line 1798, in load_dataset_builder
dataset_module = dataset_module_factory(
File "/home/liushuai/anaconda3/lib/python3.10/site-packages/datasets/load.py", line 1413, in dataset_module_factory
).get_module()
File "/home/liushuai/anaconda3/lib/python3.10/site-packages/datasets/load.py", line 949, in get_module
data_files = DataFilesDict.from_patterns(
File "/home/liushuai/anaconda3/lib/python3.10/site-packages/datasets/data_files.py", line 672, in from_patterns
DataFilesList.from_patterns(
File "/home/liushuai/anaconda3/lib/python3.10/site-packages/datasets/data_files.py", line 578, in from_patterns
resolve_pattern(
File "/home/liushuai/anaconda3/lib/python3.10/site-packages/datasets/data_files.py", line 340, in resolve_pattern
for filepath, info in fs.glob(pattern, detail=True).items()
File "/home/liushuai/anaconda3/lib/python3.10/site-packages/fsspec/asyn.py", line 113, in wrapper
return sync(self.loop, func, *args, **kwargs)
File "/home/liushuai/anaconda3/lib/python3.10/site-packages/fsspec/asyn.py", line 98, in sync
raise return_result
File "/home/liushuai/anaconda3/lib/python3.10/site-packages/fsspec/asyn.py", line 53, in _runner
result[0] = await coro
File "/home/liushuai/anaconda3/lib/python3.10/site-packages/fsspec/implementations/http.py", line 449, in _glob
elif await self._exists(path):
File "/home/liushuai/anaconda3/lib/python3.10/site-packages/fsspec/implementations/http.py", line 306, in _exists
r = await session.get(self.encode_url(path), **kw)
File "/home/liushuai/anaconda3/lib/python3.10/site-packages/aiohttp/client.py", line 922, in get
self._request(hdrs.METH_GET, url, allow_redirects=allow_redirects, **kwargs)
TypeError: ClientSession._request() got an unexpected keyword argument 'https'
```
### Steps to reproduce the bug
```
from datasets import load_dataset
base_url = "https://rajpurkar.github.io/SQuAD-explorer/dataset/"
dataset = load_dataset("json", data_files={"train": base_url + "train-v1.1.json", "validation": base_url + "dev-v1.1.json"}, field="data")
```
### Expected behavior
able to load normally
### Environment info
- `datasets` version: 2.14.4
- Platform: Linux-5.4.54-2-x86_64-with-glibc2.27
- Python version: 3.10.9
- Huggingface_hub version: 0.16.4
- PyArrow version: 12.0.1
- Pandas version: 1.5.3
Hi! I cannot reproduce this error on my machine or in Colab. Which version of `fsspec` do you have installed? |
https://github.com/huggingface/datasets/issues/6139 | Offline dataset viewer | Hi, thanks for the suggestion. It's not possible at the moment. The viewer is part of the Hub codebase and only works on public datasets. Also, it relies on [Datasets Server](https://github.com/huggingface/datasets-server/), which prepares the data and provides an API to access the rows, size, etc.
If you're interested in hosting your data as a private dataset on the Hub, you might want to look at https://github.com/huggingface/datasets-server/issues/39. | ### Feature request
The dataset viewer feature is very nice. It enables to the user to easily view the dataset. However, when working for private companies we cannot always upload the dataset to the hub. Is there a way to create dataset viewer offline? I.e. to run a code that will open some kind of html or something that makes it easy to view the dataset.
### Motivation
I want to easily view my dataset even when it is hosted locally.
### Your contribution
N.A. | 66 | Offline dataset viewer
### Feature request
The dataset viewer feature is very nice. It enables to the user to easily view the dataset. However, when working for private companies we cannot always upload the dataset to the hub. Is there a way to create dataset viewer offline? I.e. to run a code that will open some kind of html or something that makes it easy to view the dataset.
### Motivation
I want to easily view my dataset even when it is hosted locally.
### Your contribution
N.A.
Hi, thanks for the suggestion. It's not possible at the moment. The viewer is part of the Hub codebase and only works on public datasets. Also, it relies on [Datasets Server](https://github.com/huggingface/datasets-server/), which prepares the data and provides an API to access the rows, size, etc.
If you're interested in hosting your data as a private dataset on the Hub, you might want to look at https://github.com/huggingface/datasets-server/issues/39. |
https://github.com/huggingface/datasets/issues/6134 | `datasets` cannot be installed alongside `apache-beam` | I noticed that this is actually covered by issue #5613, which for some reason I didn't see when I searched the issues in this repo the first time. | ### Describe the bug
If one installs `apache-beam` alongside `datasets` (which is required for the [wikipedia](https://huggingface.co/datasets/wikipedia#dataset-summary) dataset) in certain environments (such as a Google Colab notebook), they appear to install successfully, however, actually trying to do something such as importing the `load_dataset` method from `datasets` results in a crashing error.
I think the problem is that `apache-beam` version 2.49.0 requires `dill>=0.3.1.1,<0.3.2`, but the latest version of `multiprocess` (0.70.15) (on which `datasets` depends) requires `dill>=0.3.7,`, so this is causing the dependency resolver to use an older version of `multiprocess` which leads to the `datasets` crashing since it doesn't actually appear to be compatible with older versions.
### Steps to reproduce the bug
See this [Google Colab notebook](https://colab.research.google.com/drive/1PTeGlshamFcJZix_GiS3vMXX_YzAhGv0?usp=sharing) to easily reproduce the bug.
In some environments, I have been able to reproduce the bug by running the following in Bash:
```bash
$ pip install datasets apache-beam
```
then the following in a Python shell:
```python
from datasets import load_dataset
```
Here is my stacktrace from running on Google Colab:
<details>
<summary>stacktrace</summary>
```
[/usr/local/lib/python3.10/dist-packages/datasets/__init__.py](https://localhost:8080/#) in <module>
20 __version__ = "2.14.4"
21
---> 22 from .arrow_dataset import Dataset
23 from .arrow_reader import ReadInstruction
24 from .builder import ArrowBasedBuilder, BeamBasedBuilder, BuilderConfig, DatasetBuilder, GeneratorBasedBuilder
[/usr/local/lib/python3.10/dist-packages/datasets/arrow_dataset.py](https://localhost:8080/#) in <module>
64
65 from . import config
---> 66 from .arrow_reader import ArrowReader
67 from .arrow_writer import ArrowWriter, OptimizedTypedSequence
68 from .data_files import sanitize_patterns
[/usr/local/lib/python3.10/dist-packages/datasets/arrow_reader.py](https://localhost:8080/#) in <module>
28 import pyarrow.parquet as pq
29
---> 30 from .download.download_config import DownloadConfig
31 from .naming import _split_re, filenames_for_dataset_split
32 from .table import InMemoryTable, MemoryMappedTable, Table, concat_tables
[/usr/local/lib/python3.10/dist-packages/datasets/download/__init__.py](https://localhost:8080/#) in <module>
7
8 from .download_config import DownloadConfig
----> 9 from .download_manager import DownloadManager, DownloadMode
10 from .streaming_download_manager import StreamingDownloadManager
[/usr/local/lib/python3.10/dist-packages/datasets/download/download_manager.py](https://localhost:8080/#) in <module>
33 from ..utils.info_utils import get_size_checksum_dict
34 from ..utils.logging import get_logger, is_progress_bar_enabled, tqdm
---> 35 from ..utils.py_utils import NestedDataStructure, map_nested, size_str
36 from .download_config import DownloadConfig
37
[/usr/local/lib/python3.10/dist-packages/datasets/utils/py_utils.py](https://localhost:8080/#) in <module>
38 import dill
39 import multiprocess
---> 40 import multiprocess.pool
41 import numpy as np
42 from packaging import version
[/usr/local/lib/python3.10/dist-packages/multiprocess/pool.py](https://localhost:8080/#) in <module>
607 #
608
--> 609 class ThreadPool(Pool):
610
611 from .dummy import Process
[/usr/local/lib/python3.10/dist-packages/multiprocess/pool.py](https://localhost:8080/#) in ThreadPool()
609 class ThreadPool(Pool):
610
--> 611 from .dummy import Process
612
613 def __init__(self, processes=None, initializer=None, initargs=()):
[/usr/local/lib/python3.10/dist-packages/multiprocess/dummy/__init__.py](https://localhost:8080/#) in <module>
85 #
86
---> 87 class Condition(threading._Condition):
88 # XXX
89 if sys.version_info < (3, 0):
AttributeError: module 'threading' has no attribute '_Condition'
```
</details>
I've also found that attempting to install these `datasets` and `apache-beam` in certain environments (e.g. via pip inside a conda env) simply causes pip to hang indefinitely.
### Expected behavior
I would expect to be able to import methods from `datasets` without crashing. I have tested that this is possible as long as I do not attempt to install `apache-beam`.
### Environment info
Google Colab | 28 | `datasets` cannot be installed alongside `apache-beam`
### Describe the bug
If one installs `apache-beam` alongside `datasets` (which is required for the [wikipedia](https://huggingface.co/datasets/wikipedia#dataset-summary) dataset) in certain environments (such as a Google Colab notebook), they appear to install successfully, however, actually trying to do something such as importing the `load_dataset` method from `datasets` results in a crashing error.
I think the problem is that `apache-beam` version 2.49.0 requires `dill>=0.3.1.1,<0.3.2`, but the latest version of `multiprocess` (0.70.15) (on which `datasets` depends) requires `dill>=0.3.7,`, so this is causing the dependency resolver to use an older version of `multiprocess` which leads to the `datasets` crashing since it doesn't actually appear to be compatible with older versions.
### Steps to reproduce the bug
See this [Google Colab notebook](https://colab.research.google.com/drive/1PTeGlshamFcJZix_GiS3vMXX_YzAhGv0?usp=sharing) to easily reproduce the bug.
In some environments, I have been able to reproduce the bug by running the following in Bash:
```bash
$ pip install datasets apache-beam
```
then the following in a Python shell:
```python
from datasets import load_dataset
```
Here is my stacktrace from running on Google Colab:
<details>
<summary>stacktrace</summary>
```
[/usr/local/lib/python3.10/dist-packages/datasets/__init__.py](https://localhost:8080/#) in <module>
20 __version__ = "2.14.4"
21
---> 22 from .arrow_dataset import Dataset
23 from .arrow_reader import ReadInstruction
24 from .builder import ArrowBasedBuilder, BeamBasedBuilder, BuilderConfig, DatasetBuilder, GeneratorBasedBuilder
[/usr/local/lib/python3.10/dist-packages/datasets/arrow_dataset.py](https://localhost:8080/#) in <module>
64
65 from . import config
---> 66 from .arrow_reader import ArrowReader
67 from .arrow_writer import ArrowWriter, OptimizedTypedSequence
68 from .data_files import sanitize_patterns
[/usr/local/lib/python3.10/dist-packages/datasets/arrow_reader.py](https://localhost:8080/#) in <module>
28 import pyarrow.parquet as pq
29
---> 30 from .download.download_config import DownloadConfig
31 from .naming import _split_re, filenames_for_dataset_split
32 from .table import InMemoryTable, MemoryMappedTable, Table, concat_tables
[/usr/local/lib/python3.10/dist-packages/datasets/download/__init__.py](https://localhost:8080/#) in <module>
7
8 from .download_config import DownloadConfig
----> 9 from .download_manager import DownloadManager, DownloadMode
10 from .streaming_download_manager import StreamingDownloadManager
[/usr/local/lib/python3.10/dist-packages/datasets/download/download_manager.py](https://localhost:8080/#) in <module>
33 from ..utils.info_utils import get_size_checksum_dict
34 from ..utils.logging import get_logger, is_progress_bar_enabled, tqdm
---> 35 from ..utils.py_utils import NestedDataStructure, map_nested, size_str
36 from .download_config import DownloadConfig
37
[/usr/local/lib/python3.10/dist-packages/datasets/utils/py_utils.py](https://localhost:8080/#) in <module>
38 import dill
39 import multiprocess
---> 40 import multiprocess.pool
41 import numpy as np
42 from packaging import version
[/usr/local/lib/python3.10/dist-packages/multiprocess/pool.py](https://localhost:8080/#) in <module>
607 #
608
--> 609 class ThreadPool(Pool):
610
611 from .dummy import Process
[/usr/local/lib/python3.10/dist-packages/multiprocess/pool.py](https://localhost:8080/#) in ThreadPool()
609 class ThreadPool(Pool):
610
--> 611 from .dummy import Process
612
613 def __init__(self, processes=None, initializer=None, initargs=()):
[/usr/local/lib/python3.10/dist-packages/multiprocess/dummy/__init__.py](https://localhost:8080/#) in <module>
85 #
86
---> 87 class Condition(threading._Condition):
88 # XXX
89 if sys.version_info < (3, 0):
AttributeError: module 'threading' has no attribute '_Condition'
```
</details>
I've also found that attempting to install these `datasets` and `apache-beam` in certain environments (e.g. via pip inside a conda env) simply causes pip to hang indefinitely.
### Expected behavior
I would expect to be able to import methods from `datasets` without crashing. I have tested that this is possible as long as I do not attempt to install `apache-beam`.
### Environment info
Google Colab
I noticed that this is actually covered by issue #5613, which for some reason I didn't see when I searched the issues in this repo the first time. |
https://github.com/huggingface/datasets/issues/6133 | Dataset is slower after calling `to_iterable_dataset` | It's roughly the same code between the two so we can expected roughly the same speed, could you share a benchmark ? | ### Describe the bug
Can anyone explain why looping over a dataset becomes slower after calling `to_iterable_dataset` to convert to `IterableDataset`
### Steps to reproduce the bug
Any dataset after converting to `IterableDataset`
### Expected behavior
Maybe it should be faster on big dataset? I only test on small dataset
### Environment info
- `datasets` version: 2.14.4
- Platform: Linux-5.15.0-76-generic-x86_64-with-glibc2.17
- Python version: 3.8.15
- Huggingface_hub version: 0.16.4
- PyArrow version: 12.0.1
- Pandas version: 1.5.3 | 22 | Dataset is slower after calling `to_iterable_dataset`
### Describe the bug
Can anyone explain why looping over a dataset becomes slower after calling `to_iterable_dataset` to convert to `IterableDataset`
### Steps to reproduce the bug
Any dataset after converting to `IterableDataset`
### Expected behavior
Maybe it should be faster on big dataset? I only test on small dataset
### Environment info
- `datasets` version: 2.14.4
- Platform: Linux-5.15.0-76-generic-x86_64-with-glibc2.17
- Python version: 3.8.15
- Huggingface_hub version: 0.16.4
- PyArrow version: 12.0.1
- Pandas version: 1.5.3
It's roughly the same code between the two so we can expected roughly the same speed, could you share a benchmark ? |
https://github.com/huggingface/datasets/issues/6130 | default config name doesn't work when config kwargs are specified. | What should be the behavior in this case ? Should it override the default config with the added parameter ? | ### Describe the bug
https://github.com/huggingface/datasets/blob/12cfc1196e62847e2e8239fbd727a02cbc86ddec/src/datasets/builder.py#L518-L522
If `config_name` is `None`, `DEFAULT_CONFIG_NAME` should be select. But once users pass `config_kwargs` to their customized `BuilderConfig`, the logic is ignored, and dataset cannot select the default config from multiple configs.
### Steps to reproduce the bug
```python
import datasets
datasets.load_dataset('/dataset/with/multiple/config'') # Ok
datasets.load_dataset('/dataset/with/multiple/config', some_field_in_config='some') # Err
```
### Expected behavior
Default config behavior should be consistent.
### Environment info
- `datasets` version: 2.14.3
- Platform: Linux-5.15.0-76-generic-x86_64-with-glibc2.17
- Python version: 3.8.15
- Huggingface_hub version: 0.16.4
- PyArrow version: 12.0.1
- Pandas version: 1.5.3 | 20 | default config name doesn't work when config kwargs are specified.
### Describe the bug
https://github.com/huggingface/datasets/blob/12cfc1196e62847e2e8239fbd727a02cbc86ddec/src/datasets/builder.py#L518-L522
If `config_name` is `None`, `DEFAULT_CONFIG_NAME` should be select. But once users pass `config_kwargs` to their customized `BuilderConfig`, the logic is ignored, and dataset cannot select the default config from multiple configs.
### Steps to reproduce the bug
```python
import datasets
datasets.load_dataset('/dataset/with/multiple/config'') # Ok
datasets.load_dataset('/dataset/with/multiple/config', some_field_in_config='some') # Err
```
### Expected behavior
Default config behavior should be consistent.
### Environment info
- `datasets` version: 2.14.3
- Platform: Linux-5.15.0-76-generic-x86_64-with-glibc2.17
- Python version: 3.8.15
- Huggingface_hub version: 0.16.4
- PyArrow version: 12.0.1
- Pandas version: 1.5.3
What should be the behavior in this case ? Should it override the default config with the added parameter ? |
https://github.com/huggingface/datasets/issues/6130 | default config name doesn't work when config kwargs are specified. | I know why it should be treated as a new config if overriding parameters are passed. But in some case, I just pass in some common fields like `data_dir`.
For example, I want to extend the FolderBasedBuilder as a multi-config version, the `data_dir` or `data_files` are always passed by user and should not be considered as overriding the default config. In current state, I cannot leverage the feature of default config since passing `data_dir` will disable the default config. | ### Describe the bug
https://github.com/huggingface/datasets/blob/12cfc1196e62847e2e8239fbd727a02cbc86ddec/src/datasets/builder.py#L518-L522
If `config_name` is `None`, `DEFAULT_CONFIG_NAME` should be select. But once users pass `config_kwargs` to their customized `BuilderConfig`, the logic is ignored, and dataset cannot select the default config from multiple configs.
### Steps to reproduce the bug
```python
import datasets
datasets.load_dataset('/dataset/with/multiple/config'') # Ok
datasets.load_dataset('/dataset/with/multiple/config', some_field_in_config='some') # Err
```
### Expected behavior
Default config behavior should be consistent.
### Environment info
- `datasets` version: 2.14.3
- Platform: Linux-5.15.0-76-generic-x86_64-with-glibc2.17
- Python version: 3.8.15
- Huggingface_hub version: 0.16.4
- PyArrow version: 12.0.1
- Pandas version: 1.5.3 | 79 | default config name doesn't work when config kwargs are specified.
### Describe the bug
https://github.com/huggingface/datasets/blob/12cfc1196e62847e2e8239fbd727a02cbc86ddec/src/datasets/builder.py#L518-L522
If `config_name` is `None`, `DEFAULT_CONFIG_NAME` should be select. But once users pass `config_kwargs` to their customized `BuilderConfig`, the logic is ignored, and dataset cannot select the default config from multiple configs.
### Steps to reproduce the bug
```python
import datasets
datasets.load_dataset('/dataset/with/multiple/config'') # Ok
datasets.load_dataset('/dataset/with/multiple/config', some_field_in_config='some') # Err
```
### Expected behavior
Default config behavior should be consistent.
### Environment info
- `datasets` version: 2.14.3
- Platform: Linux-5.15.0-76-generic-x86_64-with-glibc2.17
- Python version: 3.8.15
- Huggingface_hub version: 0.16.4
- PyArrow version: 12.0.1
- Pandas version: 1.5.3
I know why it should be treated as a new config if overriding parameters are passed. But in some case, I just pass in some common fields like `data_dir`.
For example, I want to extend the FolderBasedBuilder as a multi-config version, the `data_dir` or `data_files` are always passed by user and should not be considered as overriding the default config. In current state, I cannot leverage the feature of default config since passing `data_dir` will disable the default config. |
https://github.com/huggingface/datasets/issues/6130 | default config name doesn't work when config kwargs are specified. | Thinking more about it I think the current behavior is the right one.
Provided parameters should be passed to instantiate a new BuilderConfig.
What's the error you're getting ? | ### Describe the bug
https://github.com/huggingface/datasets/blob/12cfc1196e62847e2e8239fbd727a02cbc86ddec/src/datasets/builder.py#L518-L522
If `config_name` is `None`, `DEFAULT_CONFIG_NAME` should be select. But once users pass `config_kwargs` to their customized `BuilderConfig`, the logic is ignored, and dataset cannot select the default config from multiple configs.
### Steps to reproduce the bug
```python
import datasets
datasets.load_dataset('/dataset/with/multiple/config'') # Ok
datasets.load_dataset('/dataset/with/multiple/config', some_field_in_config='some') # Err
```
### Expected behavior
Default config behavior should be consistent.
### Environment info
- `datasets` version: 2.14.3
- Platform: Linux-5.15.0-76-generic-x86_64-with-glibc2.17
- Python version: 3.8.15
- Huggingface_hub version: 0.16.4
- PyArrow version: 12.0.1
- Pandas version: 1.5.3 | 29 | default config name doesn't work when config kwargs are specified.
### Describe the bug
https://github.com/huggingface/datasets/blob/12cfc1196e62847e2e8239fbd727a02cbc86ddec/src/datasets/builder.py#L518-L522
If `config_name` is `None`, `DEFAULT_CONFIG_NAME` should be select. But once users pass `config_kwargs` to their customized `BuilderConfig`, the logic is ignored, and dataset cannot select the default config from multiple configs.
### Steps to reproduce the bug
```python
import datasets
datasets.load_dataset('/dataset/with/multiple/config'') # Ok
datasets.load_dataset('/dataset/with/multiple/config', some_field_in_config='some') # Err
```
### Expected behavior
Default config behavior should be consistent.
### Environment info
- `datasets` version: 2.14.3
- Platform: Linux-5.15.0-76-generic-x86_64-with-glibc2.17
- Python version: 3.8.15
- Huggingface_hub version: 0.16.4
- PyArrow version: 12.0.1
- Pandas version: 1.5.3
Thinking more about it I think the current behavior is the right one.
Provided parameters should be passed to instantiate a new BuilderConfig.
What's the error you're getting ? |
https://github.com/huggingface/datasets/issues/6130 | default config name doesn't work when config kwargs are specified. | For example, this works to use default config with name '_all_':
```python
datasets.load_dataset("indonesian-nlp/librivox-indonesia", split="train")
```
while this failed to use default config
```python
datasets.load_dataset("indonesian-nlp/librivox-indonesia", split="train", data_dir='.')
```
After manually specifying it, it works again.
```python
datasets.load_dataset("indonesian-nlp/librivox-indonesia", "_all_", split="train", data_dir='.')
``` | ### Describe the bug
https://github.com/huggingface/datasets/blob/12cfc1196e62847e2e8239fbd727a02cbc86ddec/src/datasets/builder.py#L518-L522
If `config_name` is `None`, `DEFAULT_CONFIG_NAME` should be select. But once users pass `config_kwargs` to their customized `BuilderConfig`, the logic is ignored, and dataset cannot select the default config from multiple configs.
### Steps to reproduce the bug
```python
import datasets
datasets.load_dataset('/dataset/with/multiple/config'') # Ok
datasets.load_dataset('/dataset/with/multiple/config', some_field_in_config='some') # Err
```
### Expected behavior
Default config behavior should be consistent.
### Environment info
- `datasets` version: 2.14.3
- Platform: Linux-5.15.0-76-generic-x86_64-with-glibc2.17
- Python version: 3.8.15
- Huggingface_hub version: 0.16.4
- PyArrow version: 12.0.1
- Pandas version: 1.5.3 | 40 | default config name doesn't work when config kwargs are specified.
### Describe the bug
https://github.com/huggingface/datasets/blob/12cfc1196e62847e2e8239fbd727a02cbc86ddec/src/datasets/builder.py#L518-L522
If `config_name` is `None`, `DEFAULT_CONFIG_NAME` should be select. But once users pass `config_kwargs` to their customized `BuilderConfig`, the logic is ignored, and dataset cannot select the default config from multiple configs.
### Steps to reproduce the bug
```python
import datasets
datasets.load_dataset('/dataset/with/multiple/config'') # Ok
datasets.load_dataset('/dataset/with/multiple/config', some_field_in_config='some') # Err
```
### Expected behavior
Default config behavior should be consistent.
### Environment info
- `datasets` version: 2.14.3
- Platform: Linux-5.15.0-76-generic-x86_64-with-glibc2.17
- Python version: 3.8.15
- Huggingface_hub version: 0.16.4
- PyArrow version: 12.0.1
- Pandas version: 1.5.3
For example, this works to use default config with name '_all_':
```python
datasets.load_dataset("indonesian-nlp/librivox-indonesia", split="train")
```
while this failed to use default config
```python
datasets.load_dataset("indonesian-nlp/librivox-indonesia", split="train", data_dir='.')
```
After manually specifying it, it works again.
```python
datasets.load_dataset("indonesian-nlp/librivox-indonesia", "_all_", split="train", data_dir='.')
``` |
https://github.com/huggingface/datasets/issues/6130 | default config name doesn't work when config kwargs are specified. | It should work if you explicitly ask for the config you want to override
```python
load_dataset('/dataset/with/multiple/config', 'name_of_the_default_config', some_field_in_config='some')
```
Alternatively you can have a BuilderConfig class that when instantiated returns a config with the right default values. In this case this code would instantiate this config with the default values except for the parameter to override:
```python
load_dataset('/dataset/with/multiple/config', some_field_in_config='some')
``` | ### Describe the bug
https://github.com/huggingface/datasets/blob/12cfc1196e62847e2e8239fbd727a02cbc86ddec/src/datasets/builder.py#L518-L522
If `config_name` is `None`, `DEFAULT_CONFIG_NAME` should be select. But once users pass `config_kwargs` to their customized `BuilderConfig`, the logic is ignored, and dataset cannot select the default config from multiple configs.
### Steps to reproduce the bug
```python
import datasets
datasets.load_dataset('/dataset/with/multiple/config'') # Ok
datasets.load_dataset('/dataset/with/multiple/config', some_field_in_config='some') # Err
```
### Expected behavior
Default config behavior should be consistent.
### Environment info
- `datasets` version: 2.14.3
- Platform: Linux-5.15.0-76-generic-x86_64-with-glibc2.17
- Python version: 3.8.15
- Huggingface_hub version: 0.16.4
- PyArrow version: 12.0.1
- Pandas version: 1.5.3 | 60 | default config name doesn't work when config kwargs are specified.
### Describe the bug
https://github.com/huggingface/datasets/blob/12cfc1196e62847e2e8239fbd727a02cbc86ddec/src/datasets/builder.py#L518-L522
If `config_name` is `None`, `DEFAULT_CONFIG_NAME` should be select. But once users pass `config_kwargs` to their customized `BuilderConfig`, the logic is ignored, and dataset cannot select the default config from multiple configs.
### Steps to reproduce the bug
```python
import datasets
datasets.load_dataset('/dataset/with/multiple/config'') # Ok
datasets.load_dataset('/dataset/with/multiple/config', some_field_in_config='some') # Err
```
### Expected behavior
Default config behavior should be consistent.
### Environment info
- `datasets` version: 2.14.3
- Platform: Linux-5.15.0-76-generic-x86_64-with-glibc2.17
- Python version: 3.8.15
- Huggingface_hub version: 0.16.4
- PyArrow version: 12.0.1
- Pandas version: 1.5.3
It should work if you explicitly ask for the config you want to override
```python
load_dataset('/dataset/with/multiple/config', 'name_of_the_default_config', some_field_in_config='some')
```
Alternatively you can have a BuilderConfig class that when instantiated returns a config with the right default values. In this case this code would instantiate this config with the default values except for the parameter to override:
```python
load_dataset('/dataset/with/multiple/config', some_field_in_config='some')
``` |
https://github.com/huggingface/datasets/issues/6130 | default config name doesn't work when config kwargs are specified. | @lhoestq Yes. But it doesn't work for me.
Here's my dataset for example.
```
lass MyDatasetConfig(datasets.BuilderConfig):
def __init__(self, name: str, version: str, **kwargs):
self.option1 = kwargs.pop("option1", False)
self.option2 = kwargs.pop("option2", 5)
super().__init__(
name=name,
version=datasets.Version(version),
**kwargs)
class MyDataset(datasets.GeneratorBasedBuilder):
DEFAULT_CONFIG_NAME = "v1"
BUILDER_CONFIGS = [
UnifiedTtsDatasetConfig(
name="v1",
version="1.0.0",
description="Initial version of the dataset"
),
]
def _info(self) -> DatasetInfo:
_ = self.option1
....
```
Here it's okay to use `load_dataset('my_dataset.py')` for loading the default config `v1`.
But if I want to override the default values in config with `load_dataset('my_dataset.py', option2=3)`, it failed to find my default config `v1.
Unless I use `load_dataset('my_dataset.py', 'v1', option2=3)`
So according to your advice, how can I modify my dataset to be able to override default config without manually specifying it. | ### Describe the bug
https://github.com/huggingface/datasets/blob/12cfc1196e62847e2e8239fbd727a02cbc86ddec/src/datasets/builder.py#L518-L522
If `config_name` is `None`, `DEFAULT_CONFIG_NAME` should be select. But once users pass `config_kwargs` to their customized `BuilderConfig`, the logic is ignored, and dataset cannot select the default config from multiple configs.
### Steps to reproduce the bug
```python
import datasets
datasets.load_dataset('/dataset/with/multiple/config'') # Ok
datasets.load_dataset('/dataset/with/multiple/config', some_field_in_config='some') # Err
```
### Expected behavior
Default config behavior should be consistent.
### Environment info
- `datasets` version: 2.14.3
- Platform: Linux-5.15.0-76-generic-x86_64-with-glibc2.17
- Python version: 3.8.15
- Huggingface_hub version: 0.16.4
- PyArrow version: 12.0.1
- Pandas version: 1.5.3 | 124 | default config name doesn't work when config kwargs are specified.
### Describe the bug
https://github.com/huggingface/datasets/blob/12cfc1196e62847e2e8239fbd727a02cbc86ddec/src/datasets/builder.py#L518-L522
If `config_name` is `None`, `DEFAULT_CONFIG_NAME` should be select. But once users pass `config_kwargs` to their customized `BuilderConfig`, the logic is ignored, and dataset cannot select the default config from multiple configs.
### Steps to reproduce the bug
```python
import datasets
datasets.load_dataset('/dataset/with/multiple/config'') # Ok
datasets.load_dataset('/dataset/with/multiple/config', some_field_in_config='some') # Err
```
### Expected behavior
Default config behavior should be consistent.
### Environment info
- `datasets` version: 2.14.3
- Platform: Linux-5.15.0-76-generic-x86_64-with-glibc2.17
- Python version: 3.8.15
- Huggingface_hub version: 0.16.4
- PyArrow version: 12.0.1
- Pandas version: 1.5.3
@lhoestq Yes. But it doesn't work for me.
Here's my dataset for example.
```
lass MyDatasetConfig(datasets.BuilderConfig):
def __init__(self, name: str, version: str, **kwargs):
self.option1 = kwargs.pop("option1", False)
self.option2 = kwargs.pop("option2", 5)
super().__init__(
name=name,
version=datasets.Version(version),
**kwargs)
class MyDataset(datasets.GeneratorBasedBuilder):
DEFAULT_CONFIG_NAME = "v1"
BUILDER_CONFIGS = [
UnifiedTtsDatasetConfig(
name="v1",
version="1.0.0",
description="Initial version of the dataset"
),
]
def _info(self) -> DatasetInfo:
_ = self.option1
....
```
Here it's okay to use `load_dataset('my_dataset.py')` for loading the default config `v1`.
But if I want to override the default values in config with `load_dataset('my_dataset.py', option2=3)`, it failed to find my default config `v1.
Unless I use `load_dataset('my_dataset.py', 'v1', option2=3)`
So according to your advice, how can I modify my dataset to be able to override default config without manually specifying it. |
https://github.com/huggingface/datasets/issues/6130 | default config name doesn't work when config kwargs are specified. | @lhoestq The error is
```
def _info(self) -> DatasetInfo:
_ = self.option1 <-
....
AttributeError: 'BuilderConfig' object has no attribute 'option1'
```
which seems to find another unknown config.
You can try this line `datasets.load_dataset("indonesian-nlp/librivox-indonesia", split="train", data_dir='.')`, it's a multi-config dataset on HF hub and the error is the same.
My insights:
https://github.com/huggingface/datasets/blob/12cfc1196e62847e2e8239fbd727a02cbc86ddec/src/datasets/builder.py#L518
if `config_kwargs` is provided here, the if branch is skipped. | ### Describe the bug
https://github.com/huggingface/datasets/blob/12cfc1196e62847e2e8239fbd727a02cbc86ddec/src/datasets/builder.py#L518-L522
If `config_name` is `None`, `DEFAULT_CONFIG_NAME` should be select. But once users pass `config_kwargs` to their customized `BuilderConfig`, the logic is ignored, and dataset cannot select the default config from multiple configs.
### Steps to reproduce the bug
```python
import datasets
datasets.load_dataset('/dataset/with/multiple/config'') # Ok
datasets.load_dataset('/dataset/with/multiple/config', some_field_in_config='some') # Err
```
### Expected behavior
Default config behavior should be consistent.
### Environment info
- `datasets` version: 2.14.3
- Platform: Linux-5.15.0-76-generic-x86_64-with-glibc2.17
- Python version: 3.8.15
- Huggingface_hub version: 0.16.4
- PyArrow version: 12.0.1
- Pandas version: 1.5.3 | 63 | default config name doesn't work when config kwargs are specified.
### Describe the bug
https://github.com/huggingface/datasets/blob/12cfc1196e62847e2e8239fbd727a02cbc86ddec/src/datasets/builder.py#L518-L522
If `config_name` is `None`, `DEFAULT_CONFIG_NAME` should be select. But once users pass `config_kwargs` to their customized `BuilderConfig`, the logic is ignored, and dataset cannot select the default config from multiple configs.
### Steps to reproduce the bug
```python
import datasets
datasets.load_dataset('/dataset/with/multiple/config'') # Ok
datasets.load_dataset('/dataset/with/multiple/config', some_field_in_config='some') # Err
```
### Expected behavior
Default config behavior should be consistent.
### Environment info
- `datasets` version: 2.14.3
- Platform: Linux-5.15.0-76-generic-x86_64-with-glibc2.17
- Python version: 3.8.15
- Huggingface_hub version: 0.16.4
- PyArrow version: 12.0.1
- Pandas version: 1.5.3
@lhoestq The error is
```
def _info(self) -> DatasetInfo:
_ = self.option1 <-
....
AttributeError: 'BuilderConfig' object has no attribute 'option1'
```
which seems to find another unknown config.
You can try this line `datasets.load_dataset("indonesian-nlp/librivox-indonesia", split="train", data_dir='.')`, it's a multi-config dataset on HF hub and the error is the same.
My insights:
https://github.com/huggingface/datasets/blob/12cfc1196e62847e2e8239fbd727a02cbc86ddec/src/datasets/builder.py#L518
if `config_kwargs` is provided here, the if branch is skipped. |
https://github.com/huggingface/datasets/issues/6130 | default config name doesn't work when config kwargs are specified. | I see, you just have to set this class attribute to your builder class :)
```python
BUILDER_CONFIG_CLASS = MyDatasetConfig
``` | ### Describe the bug
https://github.com/huggingface/datasets/blob/12cfc1196e62847e2e8239fbd727a02cbc86ddec/src/datasets/builder.py#L518-L522
If `config_name` is `None`, `DEFAULT_CONFIG_NAME` should be select. But once users pass `config_kwargs` to their customized `BuilderConfig`, the logic is ignored, and dataset cannot select the default config from multiple configs.
### Steps to reproduce the bug
```python
import datasets
datasets.load_dataset('/dataset/with/multiple/config'') # Ok
datasets.load_dataset('/dataset/with/multiple/config', some_field_in_config='some') # Err
```
### Expected behavior
Default config behavior should be consistent.
### Environment info
- `datasets` version: 2.14.3
- Platform: Linux-5.15.0-76-generic-x86_64-with-glibc2.17
- Python version: 3.8.15
- Huggingface_hub version: 0.16.4
- PyArrow version: 12.0.1
- Pandas version: 1.5.3 | 20 | default config name doesn't work when config kwargs are specified.
### Describe the bug
https://github.com/huggingface/datasets/blob/12cfc1196e62847e2e8239fbd727a02cbc86ddec/src/datasets/builder.py#L518-L522
If `config_name` is `None`, `DEFAULT_CONFIG_NAME` should be select. But once users pass `config_kwargs` to their customized `BuilderConfig`, the logic is ignored, and dataset cannot select the default config from multiple configs.
### Steps to reproduce the bug
```python
import datasets
datasets.load_dataset('/dataset/with/multiple/config'') # Ok
datasets.load_dataset('/dataset/with/multiple/config', some_field_in_config='some') # Err
```
### Expected behavior
Default config behavior should be consistent.
### Environment info
- `datasets` version: 2.14.3
- Platform: Linux-5.15.0-76-generic-x86_64-with-glibc2.17
- Python version: 3.8.15
- Huggingface_hub version: 0.16.4
- PyArrow version: 12.0.1
- Pandas version: 1.5.3
I see, you just have to set this class attribute to your builder class :)
```python
BUILDER_CONFIG_CLASS = MyDatasetConfig
``` |
https://github.com/huggingface/datasets/issues/6130 | default config name doesn't work when config kwargs are specified. | So what does this attribute do? In most cases it's not used and the [documents for multi-config dataset](https://huggingface.co/docs/datasets/main/en/image_dataset#multiple-configurations) never mentioned that. | ### Describe the bug
https://github.com/huggingface/datasets/blob/12cfc1196e62847e2e8239fbd727a02cbc86ddec/src/datasets/builder.py#L518-L522
If `config_name` is `None`, `DEFAULT_CONFIG_NAME` should be select. But once users pass `config_kwargs` to their customized `BuilderConfig`, the logic is ignored, and dataset cannot select the default config from multiple configs.
### Steps to reproduce the bug
```python
import datasets
datasets.load_dataset('/dataset/with/multiple/config'') # Ok
datasets.load_dataset('/dataset/with/multiple/config', some_field_in_config='some') # Err
```
### Expected behavior
Default config behavior should be consistent.
### Environment info
- `datasets` version: 2.14.3
- Platform: Linux-5.15.0-76-generic-x86_64-with-glibc2.17
- Python version: 3.8.15
- Huggingface_hub version: 0.16.4
- PyArrow version: 12.0.1
- Pandas version: 1.5.3 | 21 | default config name doesn't work when config kwargs are specified.
### Describe the bug
https://github.com/huggingface/datasets/blob/12cfc1196e62847e2e8239fbd727a02cbc86ddec/src/datasets/builder.py#L518-L522
If `config_name` is `None`, `DEFAULT_CONFIG_NAME` should be select. But once users pass `config_kwargs` to their customized `BuilderConfig`, the logic is ignored, and dataset cannot select the default config from multiple configs.
### Steps to reproduce the bug
```python
import datasets
datasets.load_dataset('/dataset/with/multiple/config'') # Ok
datasets.load_dataset('/dataset/with/multiple/config', some_field_in_config='some') # Err
```
### Expected behavior
Default config behavior should be consistent.
### Environment info
- `datasets` version: 2.14.3
- Platform: Linux-5.15.0-76-generic-x86_64-with-glibc2.17
- Python version: 3.8.15
- Huggingface_hub version: 0.16.4
- PyArrow version: 12.0.1
- Pandas version: 1.5.3
So what does this attribute do? In most cases it's not used and the [documents for multi-config dataset](https://huggingface.co/docs/datasets/main/en/image_dataset#multiple-configurations) never mentioned that. |
https://github.com/huggingface/datasets/issues/6130 | default config name doesn't work when config kwargs are specified. | It tells which builder config class to instantiate if additional config parameters are passed to load_dataset | ### Describe the bug
https://github.com/huggingface/datasets/blob/12cfc1196e62847e2e8239fbd727a02cbc86ddec/src/datasets/builder.py#L518-L522
If `config_name` is `None`, `DEFAULT_CONFIG_NAME` should be select. But once users pass `config_kwargs` to their customized `BuilderConfig`, the logic is ignored, and dataset cannot select the default config from multiple configs.
### Steps to reproduce the bug
```python
import datasets
datasets.load_dataset('/dataset/with/multiple/config'') # Ok
datasets.load_dataset('/dataset/with/multiple/config', some_field_in_config='some') # Err
```
### Expected behavior
Default config behavior should be consistent.
### Environment info
- `datasets` version: 2.14.3
- Platform: Linux-5.15.0-76-generic-x86_64-with-glibc2.17
- Python version: 3.8.15
- Huggingface_hub version: 0.16.4
- PyArrow version: 12.0.1
- Pandas version: 1.5.3 | 16 | default config name doesn't work when config kwargs are specified.
### Describe the bug
https://github.com/huggingface/datasets/blob/12cfc1196e62847e2e8239fbd727a02cbc86ddec/src/datasets/builder.py#L518-L522
If `config_name` is `None`, `DEFAULT_CONFIG_NAME` should be select. But once users pass `config_kwargs` to their customized `BuilderConfig`, the logic is ignored, and dataset cannot select the default config from multiple configs.
### Steps to reproduce the bug
```python
import datasets
datasets.load_dataset('/dataset/with/multiple/config'') # Ok
datasets.load_dataset('/dataset/with/multiple/config', some_field_in_config='some') # Err
```
### Expected behavior
Default config behavior should be consistent.
### Environment info
- `datasets` version: 2.14.3
- Platform: Linux-5.15.0-76-generic-x86_64-with-glibc2.17
- Python version: 3.8.15
- Huggingface_hub version: 0.16.4
- PyArrow version: 12.0.1
- Pandas version: 1.5.3
It tells which builder config class to instantiate if additional config parameters are passed to load_dataset |
https://github.com/huggingface/datasets/issues/6130 | default config name doesn't work when config kwargs are specified. | @lhoestq maybe we can enhance the document to say something about the common attributes of `DatasetBuilder` | ### Describe the bug
https://github.com/huggingface/datasets/blob/12cfc1196e62847e2e8239fbd727a02cbc86ddec/src/datasets/builder.py#L518-L522
If `config_name` is `None`, `DEFAULT_CONFIG_NAME` should be select. But once users pass `config_kwargs` to their customized `BuilderConfig`, the logic is ignored, and dataset cannot select the default config from multiple configs.
### Steps to reproduce the bug
```python
import datasets
datasets.load_dataset('/dataset/with/multiple/config'') # Ok
datasets.load_dataset('/dataset/with/multiple/config', some_field_in_config='some') # Err
```
### Expected behavior
Default config behavior should be consistent.
### Environment info
- `datasets` version: 2.14.3
- Platform: Linux-5.15.0-76-generic-x86_64-with-glibc2.17
- Python version: 3.8.15
- Huggingface_hub version: 0.16.4
- PyArrow version: 12.0.1
- Pandas version: 1.5.3 | 16 | default config name doesn't work when config kwargs are specified.
### Describe the bug
https://github.com/huggingface/datasets/blob/12cfc1196e62847e2e8239fbd727a02cbc86ddec/src/datasets/builder.py#L518-L522
If `config_name` is `None`, `DEFAULT_CONFIG_NAME` should be select. But once users pass `config_kwargs` to their customized `BuilderConfig`, the logic is ignored, and dataset cannot select the default config from multiple configs.
### Steps to reproduce the bug
```python
import datasets
datasets.load_dataset('/dataset/with/multiple/config'') # Ok
datasets.load_dataset('/dataset/with/multiple/config', some_field_in_config='some') # Err
```
### Expected behavior
Default config behavior should be consistent.
### Environment info
- `datasets` version: 2.14.3
- Platform: Linux-5.15.0-76-generic-x86_64-with-glibc2.17
- Python version: 3.8.15
- Huggingface_hub version: 0.16.4
- PyArrow version: 12.0.1
- Pandas version: 1.5.3
@lhoestq maybe we can enhance the document to say something about the common attributes of `DatasetBuilder` |
https://github.com/huggingface/datasets/issues/6128 | IndexError: Invalid key: 88 is out of bounds for size 0 | >
I tried this and got the following error:
```
Traceback (most recent call last):
File "/cephyr/NOBACKUP/groups/snic2021-23-24/LLM4-CodeOpt/env/lib/python3.10/site-packages/torch/_dynamo/convert_frame.py", line 324, in _compile
out_code = transform_code_object(code, transform)
File "/cephyr/NOBACKUP/groups/snic2021-23-24/LLM4-CodeOpt/env/lib/python3.10/site-packages/torch/_dynamo/bytecode_transformation.py", line 445, in transform_code_object
transformations(instructions, code_options)
File "/cephyr/NOBACKUP/groups/snic2021-23-24/LLM4-CodeOpt/env/lib/python3.10/site-packages/torch/_dynamo/convert_frame.py", line 311, in transform
tracer.run()
File "/cephyr/NOBACKUP/groups/snic2021-23-24/LLM4-CodeOpt/env/lib/python3.10/site-packages/torch/_dynamo/symbolic_convert.py", line 1726, in run
super().run()
File "/cephyr/NOBACKUP/groups/snic2021-23-24/LLM4-CodeOpt/env/lib/python3.10/site-packages/torch/_dynamo/symbolic_convert.py", line 576, in run
and self.step()
File "/cephyr/NOBACKUP/groups/snic2021-23-24/LLM4-CodeOpt/env/lib/python3.10/site-packages/torch/_dynamo/symbolic_convert.py", line 540, in step
getattr(self, inst.opname)(inst)
File "/cephyr/NOBACKUP/groups/snic2021-23-24/LLM4-CodeOpt/env/lib/python3.10/site-packages/torch/_dynamo/symbolic_convert.py", line 1030, in LOAD_ATTR
result = BuiltinVariable(getattr).call_function(
File "/cephyr/NOBACKUP/groups/snic2021-23-24/LLM4-CodeOpt/env/lib/python3.10/site-packages/torch/_dynamo/variables/builtin.py", line 566, in call_function
result = handler(tx, *args, **kwargs)
File "/cephyr/NOBACKUP/groups/snic2021-23-24/LLM4-CodeOpt/env/lib/python3.10/site-packages/torch/_dynamo/variables/builtin.py", line 931, in call_getattr
return obj.var_getattr(tx, name).add_options(options)
File "/cephyr/NOBACKUP/groups/snic2021-23-24/LLM4-CodeOpt/env/lib/python3.10/site-packages/torch/_dynamo/variables/nn_module.py", line 124, in var_getattr
subobj = inspect.getattr_static(base, name)
File "/apps/Arch/software/Python/3.10.8-GCCcore-12.2.0/lib/python3.10/inspect.py", line 1777, in getattr_static
raise AttributeError(attr)
AttributeError: config
from user code:
File "/cephyr/NOBACKUP/groups/snic2021-23-24/LLM4-CodeOpt/env/lib/python3.10/site-packages/peft/peft_model.py", line 909, in forward
if self.base_model.config.model_type == "mpt":
Set torch._dynamo.config.verbose=True for more information
You can suppress this exception and fall back to eager by setting:
torch._dynamo.config.suppress_errors = True
The above exception was the direct cause of the following exception:
Traceback (most recent call last):
File "/cephyr/NOBACKUP/groups/snic2021-23-24/LLM4-CodeOpt/llm-copt/fine-tune/falcon/falcon_sft.py", line 228, in <module>
main()
File "/cephyr/NOBACKUP/groups/snic2021-23-24/LLM4-CodeOpt/llm-copt/fine-tune/falcon/falcon_sft.py", line 221, in main
trainer.train()
File "/cephyr/NOBACKUP/groups/snic2021-23-24/LLM4-CodeOpt/env/lib/python3.10/site-packages/transformers/trainer.py", line 1539, in train
return inner_training_loop(
File "/cephyr/NOBACKUP/groups/snic2021-23-24/LLM4-CodeOpt/env/lib/python3.10/site-packages/transformers/trainer.py", line 1809, in _inner_training_loop
tr_loss_step = self.training_step(model, inputs)
File "/cephyr/NOBACKUP/groups/snic2021-23-24/LLM4-CodeOpt/env/lib/python3.10/site-packages/transformers/trainer.py", line 2654, in training_step
loss = self.compute_loss(model, inputs)
File "/cephyr/NOBACKUP/groups/snic2021-23-24/LLM4-CodeOpt/env/lib/python3.10/site-packages/transformers/trainer.py", line 2679, in compute_loss
outputs = model(**inputs)
File "/cephyr/NOBACKUP/groups/snic2021-23-24/LLM4-CodeOpt/env/lib/python3.10/site-packages/torch/nn/modules/module.py", line 1501, in _call_impl
return forward_call(*args, **kwargs)
File "/cephyr/NOBACKUP/groups/snic2021-23-24/LLM4-CodeOpt/env/lib/python3.10/site-packages/torch/_dynamo/eval_frame.py", line 82, in forward
return self.dynamo_ctx(self._orig_mod.forward)(*args, **kwargs)
File "/cephyr/NOBACKUP/groups/snic2021-23-24/LLM4-CodeOpt/env/lib/python3.10/site-packages/torch/_dynamo/eval_frame.py", line 209, in _fn
return fn(*args, **kwargs)
File "/cephyr/NOBACKUP/groups/snic2021-23-24/LLM4-CodeOpt/env/lib/python3.10/site-packages/accelerate/utils/operations.py", line 581, in forward
return model_forward(*args, **kwargs)
File "/cephyr/NOBACKUP/groups/snic2021-23-24/LLM4-CodeOpt/env/lib/python3.10/site-packages/accelerate/utils/operations.py", line 569, in __call__
return convert_to_fp32(self.model_forward(*args, **kwargs))
File "/cephyr/NOBACKUP/groups/snic2021-23-24/LLM4-CodeOpt/env/lib/python3.10/site-packages/torch/amp/autocast_mode.py", line 14, in decorate_autocast
return func(*args, **kwargs)
File "/cephyr/NOBACKUP/groups/snic2021-23-24/LLM4-CodeOpt/env/lib/python3.10/site-packages/torch/_dynamo/eval_frame.py", line 337, in catch_errors
return callback(frame, cache_size, hooks)
File "/cephyr/NOBACKUP/groups/snic2021-23-24/LLM4-CodeOpt/env/lib/python3.10/site-packages/torch/_dynamo/convert_frame.py", line 404, in _convert_frame
result = inner_convert(frame, cache_size, hooks)
File "/cephyr/NOBACKUP/groups/snic2021-23-24/LLM4-CodeOpt/env/lib/python3.10/site-packages/torch/_dynamo/convert_frame.py", line 104, in _fn
return fn(*args, **kwargs)
File "/cephyr/NOBACKUP/groups/snic2021-23-24/LLM4-CodeOpt/env/lib/python3.10/site-packages/torch/_dynamo/convert_frame.py", line 262, in _convert_frame_assert
return _compile(
File "/cephyr/NOBACKUP/groups/snic2021-23-24/LLM4-CodeOpt/env/lib/python3.10/site-packages/torch/_dynamo/utils.py", line 163, in time_wrapper
r = func(*args, **kwargs)
File "/cephyr/NOBACKUP/groups/snic2021-23-24/LLM4-CodeOpt/env/lib/python3.10/site-packages/torch/_dynamo/convert_frame.py", line 394, in _compile
raise InternalTorchDynamoError() from e
torch._dynamo.exc.InternalTorchDynamoError
``` | ### Describe the bug
This bug generates when I use torch.compile(model) in my code, which seems to raise an error in datasets lib.
### Steps to reproduce the bug
I use the following code to fine-tune Falcon on my private dataset.
```python
import transformers
from transformers import (
AutoModelForCausalLM,
AutoTokenizer,
AutoConfig,
DataCollatorForSeq2Seq,
Trainer,
Seq2SeqTrainer,
HfArgumentParser,
Seq2SeqTrainingArguments,
BitsAndBytesConfig,
)
from peft import (
LoraConfig,
get_peft_model,
get_peft_model_state_dict,
prepare_model_for_int8_training,
set_peft_model_state_dict,
)
import torch
import os
import evaluate
import functools
from datasets import load_dataset
import bitsandbytes as bnb
import logging
import json
import copy
from typing import Dict, Optional, Sequence
from dataclasses import dataclass, field
# Lora settings
LORA_R = 8
LORA_ALPHA = 16
LORA_DROPOUT= 0.05
LORA_TARGET_MODULES = ["query_key_value"]
@dataclass
class ModelArguments:
model_name_or_path: Optional[str] = field(default="Salesforce/codegen2-7B")
@dataclass
class DataArguments:
data_path: str = field(default=None, metadata={"help": "Path to the training data."})
train_file: str = field(default=None, metadata={"help": "Path to the evaluation data."})
eval_file: str = field(default=None, metadata={"help": "Path to the evaluation data."})
cache_path: str = field(default=None, metadata={"help": "Path to the cache directory."})
num_proc: int = field(default=4, metadata={"help": "Number of processes to use for data preprocessing."})
@dataclass
class TrainingArguments(transformers.TrainingArguments):
# cache_dir: Optional[str] = field(default=None)
optim: str = field(default="adamw_torch")
model_max_length: int = field(
default=512,
metadata={"help": "Maximum sequence length. Sequences will be right padded (and possibly truncated)."},
)
is_lora: bool = field(default=True, metadata={"help": "Whether to use LORA."})
def tokenize(text, tokenizer, max_seq_len=512, add_eos_token=True):
result = tokenizer(
text,
truncation=True,
max_length=max_seq_len,
padding=False,
return_tensors=None,
)
if (
result["input_ids"][-1] != tokenizer.eos_token_id
and len(result["input_ids"]) < max_seq_len
and add_eos_token
):
result["input_ids"].append(tokenizer.eos_token_id)
result["attention_mask"].append(1)
if add_eos_token and len(result["input_ids"]) >= max_seq_len:
result["input_ids"][max_seq_len - 1] = tokenizer.eos_token_id
result["attention_mask"][max_seq_len - 1] = 1
result["labels"] = result["input_ids"].copy()
return result
def main():
parser = HfArgumentParser((ModelArguments, DataArguments, TrainingArguments))
model_args, data_args, training_args = parser.parse_args_into_dataclasses()
config = AutoConfig.from_pretrained(
model_args.model_name_or_path,
cache_dir=data_args.cache_path,
trust_remote_code=True,
)
if training_args.is_lora:
model = AutoModelForCausalLM.from_pretrained(
model_args.model_name_or_path,
cache_dir=data_args.cache_path,
torch_dtype=torch.float16,
trust_remote_code=True,
load_in_8bit=True,
quantization_config=BitsAndBytesConfig(
load_in_8bit=True,
llm_int8_threshold=6.0
),
)
model = prepare_model_for_int8_training(model)
config = LoraConfig(
r=LORA_R,
lora_alpha=LORA_ALPHA,
target_modules=LORA_TARGET_MODULES,
lora_dropout=LORA_DROPOUT,
bias="none",
task_type="CAUSAL_LM",
)
model = get_peft_model(model, config)
else:
model = AutoModelForCausalLM.from_pretrained(
model_args.model_name_or_path,
torch_dtype=torch.float16,
cache_dir=data_args.cache_path,
trust_remote_code=True,
)
model.config.use_cache = False
def print_trainable_parameters(model):
"""
Prints the number of trainable parameters in the model.
"""
trainable_params = 0
all_param = 0
for _, param in model.named_parameters():
all_param += param.numel()
if param.requires_grad:
trainable_params += param.numel()
print(
f"trainable params: {trainable_params} || all params: {all_param} || trainable%: {100 * trainable_params / all_param}"
)
print_trainable_parameters(model)
tokenizer = AutoTokenizer.from_pretrained(
model_args.model_name_or_path,
cache_dir=data_args.cache_path,
model_max_length=training_args.model_max_length,
padding_side="left",
use_fast=True,
trust_remote_code=True,
)
tokenizer.pad_token = tokenizer.eos_token
# Load dataset
def generate_and_tokenize_prompt(sample):
input_text = sample["input"]
target_text = sample["output"] + tokenizer.eos_token
full_text = input_text + target_text
tokenized_full_text = tokenize(full_text, tokenizer, max_seq_len=512)
tokenized_input_text = tokenize(input_text, tokenizer, max_seq_len=512)
input_len = len(tokenized_input_text["input_ids"]) - 1 # -1 for eos token
tokenized_full_text["labels"] = [-100] * input_len + tokenized_full_text["labels"][input_len:]
return tokenized_full_text
data_files = {}
if data_args.train_file is not None:
data_files["train"] = data_args.train_file
if data_args.eval_file is not None:
data_files["eval"] = data_args.eval_file
dataset = load_dataset(data_args.data_path, data_files=data_files)
train_dataset = dataset["train"]
eval_dataset = dataset["eval"]
train_dataset = train_dataset.map(generate_and_tokenize_prompt, num_proc=data_args.num_proc)
eval_dataset = eval_dataset.map(generate_and_tokenize_prompt, num_proc=data_args.num_proc)
data_collator = DataCollatorForSeq2Seq(tokenizer, pad_to_multiple_of=8, return_tensors="pt", padding=True)
# Evaluation metrics
def compute_metrics(eval_preds, tokenizer):
metric = evaluate.load('exact_match')
preds, labels = eval_preds
# In case the model returns more than the prediction logits
if isinstance(preds, tuple):
preds = preds[0]
decoded_preds = tokenizer.batch_decode(preds, skip_special_tokens=True, clean_up_tokenization_spaces=False)
# Replace -100s in the labels as we can't decode them
labels[labels == -100] = tokenizer.pad_token_id
decoded_labels = tokenizer.batch_decode(labels, skip_special_tokens=True, clean_up_tokenization_spaces=False)
# Some simple post-processing
decoded_preds = [pred.strip() for pred in decoded_preds]
decoded_labels = [label.strip() for label in decoded_labels]
result = metric.compute(predictions=decoded_preds, references=decoded_labels)
return {'exact_match': result['exact_match']}
compute_metrics_fn = functools.partial(compute_metrics, tokenizer=tokenizer)
model = torch.compile(model)
# Training
trainer = Trainer(
model=model,
train_dataset=train_dataset,
eval_dataset=eval_dataset,
args=training_args,
data_collator=data_collator,
compute_metrics=compute_metrics_fn,
)
trainer.train()
trainer.save_state()
trainer.save_model(output_dir=training_args.output_dir)
tokenizer.save_pretrained(save_directory=training_args.output_dir)
if __name__ == "__main__":
main()
```
When I didn't use `torch.cpmpile(model)`, my code worked well. But when I added this line to my code, It produced the following error:
```
Traceback (most recent call last):
File "falcon_sft.py", line 230, in <module>
main()
File "falcon_sft.py", line 223, in main
trainer.train()
File "python3.10/site-packages/transformers/trainer.py", line 1539, in train
return inner_training_loop(
File "python3.10/site-packages/transformers/trainer.py", line 1787, in _inner_training_loop
for step, inputs in enumerate(epoch_iterator):
File "python3.10/site-packages/accelerate/data_loader.py", line 384, in __iter__
current_batch = next(dataloader_iter)
File "python3.10/site-packages/torch/utils/data/dataloader.py", line 633, in __next__
data = self._next_data()
File "python3.10/site-packages/torch/utils/data/dataloader.py", line 677, in _next_data
data = self._dataset_fetcher.fetch(index) # may raise StopIteration
File "python3.10/site-packages/torch/utils/data/_utils/fetch.py", line 49, in fetch
data = self.dataset.__getitems__(possibly_batched_index)
File "python3.10/site-packages/datasets/arrow_dataset.py", line 2807, in __getitems__
batch = self.__getitem__(keys)
File "python3.10/site-packages/datasets/arrow_dataset.py", line 2803, in __getitem__
return self._getitem(key)
File "python3.10/site-packages/datasets/arrow_dataset.py", line 2787, in _getitem
pa_subtable = query_table(self._data, key, indices=self._indices if self._indices is not None else None)
File "python3.10/site-packages/datasets/formatting/formatting.py", line 583, in query_table
_check_valid_index_key(key, size)
File "python3.10/site-packages/datasets/formatting/formatting.py", line 536, in _check_valid_index_key
_check_valid_index_key(int(max(key)), size=size)
File "python3.10/site-packages/datasets/formatting/formatting.py", line 526, in _check_valid_index_key
raise IndexError(f"Invalid key: {key} is out of bounds for size {size}")
IndexError: Invalid key: 88 is out of bounds for size 0
```
So I'm confused about why this error was generated, and how to fix it. Is this error produced by datasets or `torch.compile`?
### Expected behavior
I want to use `torch.compile` in my code.
### Environment info
- `datasets` version: 2.14.3
- Platform: Linux-4.18.0-425.19.2.el8_7.x86_64-x86_64-with-glibc2.28
- Python version: 3.10.8
- Huggingface_hub version: 0.16.4
- PyArrow version: 12.0.1
- Pandas version: 2.0.3 | 326 | IndexError: Invalid key: 88 is out of bounds for size 0
### Describe the bug
This bug generates when I use torch.compile(model) in my code, which seems to raise an error in datasets lib.
### Steps to reproduce the bug
I use the following code to fine-tune Falcon on my private dataset.
```python
import transformers
from transformers import (
AutoModelForCausalLM,
AutoTokenizer,
AutoConfig,
DataCollatorForSeq2Seq,
Trainer,
Seq2SeqTrainer,
HfArgumentParser,
Seq2SeqTrainingArguments,
BitsAndBytesConfig,
)
from peft import (
LoraConfig,
get_peft_model,
get_peft_model_state_dict,
prepare_model_for_int8_training,
set_peft_model_state_dict,
)
import torch
import os
import evaluate
import functools
from datasets import load_dataset
import bitsandbytes as bnb
import logging
import json
import copy
from typing import Dict, Optional, Sequence
from dataclasses import dataclass, field
# Lora settings
LORA_R = 8
LORA_ALPHA = 16
LORA_DROPOUT= 0.05
LORA_TARGET_MODULES = ["query_key_value"]
@dataclass
class ModelArguments:
model_name_or_path: Optional[str] = field(default="Salesforce/codegen2-7B")
@dataclass
class DataArguments:
data_path: str = field(default=None, metadata={"help": "Path to the training data."})
train_file: str = field(default=None, metadata={"help": "Path to the evaluation data."})
eval_file: str = field(default=None, metadata={"help": "Path to the evaluation data."})
cache_path: str = field(default=None, metadata={"help": "Path to the cache directory."})
num_proc: int = field(default=4, metadata={"help": "Number of processes to use for data preprocessing."})
@dataclass
class TrainingArguments(transformers.TrainingArguments):
# cache_dir: Optional[str] = field(default=None)
optim: str = field(default="adamw_torch")
model_max_length: int = field(
default=512,
metadata={"help": "Maximum sequence length. Sequences will be right padded (and possibly truncated)."},
)
is_lora: bool = field(default=True, metadata={"help": "Whether to use LORA."})
def tokenize(text, tokenizer, max_seq_len=512, add_eos_token=True):
result = tokenizer(
text,
truncation=True,
max_length=max_seq_len,
padding=False,
return_tensors=None,
)
if (
result["input_ids"][-1] != tokenizer.eos_token_id
and len(result["input_ids"]) < max_seq_len
and add_eos_token
):
result["input_ids"].append(tokenizer.eos_token_id)
result["attention_mask"].append(1)
if add_eos_token and len(result["input_ids"]) >= max_seq_len:
result["input_ids"][max_seq_len - 1] = tokenizer.eos_token_id
result["attention_mask"][max_seq_len - 1] = 1
result["labels"] = result["input_ids"].copy()
return result
def main():
parser = HfArgumentParser((ModelArguments, DataArguments, TrainingArguments))
model_args, data_args, training_args = parser.parse_args_into_dataclasses()
config = AutoConfig.from_pretrained(
model_args.model_name_or_path,
cache_dir=data_args.cache_path,
trust_remote_code=True,
)
if training_args.is_lora:
model = AutoModelForCausalLM.from_pretrained(
model_args.model_name_or_path,
cache_dir=data_args.cache_path,
torch_dtype=torch.float16,
trust_remote_code=True,
load_in_8bit=True,
quantization_config=BitsAndBytesConfig(
load_in_8bit=True,
llm_int8_threshold=6.0
),
)
model = prepare_model_for_int8_training(model)
config = LoraConfig(
r=LORA_R,
lora_alpha=LORA_ALPHA,
target_modules=LORA_TARGET_MODULES,
lora_dropout=LORA_DROPOUT,
bias="none",
task_type="CAUSAL_LM",
)
model = get_peft_model(model, config)
else:
model = AutoModelForCausalLM.from_pretrained(
model_args.model_name_or_path,
torch_dtype=torch.float16,
cache_dir=data_args.cache_path,
trust_remote_code=True,
)
model.config.use_cache = False
def print_trainable_parameters(model):
"""
Prints the number of trainable parameters in the model.
"""
trainable_params = 0
all_param = 0
for _, param in model.named_parameters():
all_param += param.numel()
if param.requires_grad:
trainable_params += param.numel()
print(
f"trainable params: {trainable_params} || all params: {all_param} || trainable%: {100 * trainable_params / all_param}"
)
print_trainable_parameters(model)
tokenizer = AutoTokenizer.from_pretrained(
model_args.model_name_or_path,
cache_dir=data_args.cache_path,
model_max_length=training_args.model_max_length,
padding_side="left",
use_fast=True,
trust_remote_code=True,
)
tokenizer.pad_token = tokenizer.eos_token
# Load dataset
def generate_and_tokenize_prompt(sample):
input_text = sample["input"]
target_text = sample["output"] + tokenizer.eos_token
full_text = input_text + target_text
tokenized_full_text = tokenize(full_text, tokenizer, max_seq_len=512)
tokenized_input_text = tokenize(input_text, tokenizer, max_seq_len=512)
input_len = len(tokenized_input_text["input_ids"]) - 1 # -1 for eos token
tokenized_full_text["labels"] = [-100] * input_len + tokenized_full_text["labels"][input_len:]
return tokenized_full_text
data_files = {}
if data_args.train_file is not None:
data_files["train"] = data_args.train_file
if data_args.eval_file is not None:
data_files["eval"] = data_args.eval_file
dataset = load_dataset(data_args.data_path, data_files=data_files)
train_dataset = dataset["train"]
eval_dataset = dataset["eval"]
train_dataset = train_dataset.map(generate_and_tokenize_prompt, num_proc=data_args.num_proc)
eval_dataset = eval_dataset.map(generate_and_tokenize_prompt, num_proc=data_args.num_proc)
data_collator = DataCollatorForSeq2Seq(tokenizer, pad_to_multiple_of=8, return_tensors="pt", padding=True)
# Evaluation metrics
def compute_metrics(eval_preds, tokenizer):
metric = evaluate.load('exact_match')
preds, labels = eval_preds
# In case the model returns more than the prediction logits
if isinstance(preds, tuple):
preds = preds[0]
decoded_preds = tokenizer.batch_decode(preds, skip_special_tokens=True, clean_up_tokenization_spaces=False)
# Replace -100s in the labels as we can't decode them
labels[labels == -100] = tokenizer.pad_token_id
decoded_labels = tokenizer.batch_decode(labels, skip_special_tokens=True, clean_up_tokenization_spaces=False)
# Some simple post-processing
decoded_preds = [pred.strip() for pred in decoded_preds]
decoded_labels = [label.strip() for label in decoded_labels]
result = metric.compute(predictions=decoded_preds, references=decoded_labels)
return {'exact_match': result['exact_match']}
compute_metrics_fn = functools.partial(compute_metrics, tokenizer=tokenizer)
model = torch.compile(model)
# Training
trainer = Trainer(
model=model,
train_dataset=train_dataset,
eval_dataset=eval_dataset,
args=training_args,
data_collator=data_collator,
compute_metrics=compute_metrics_fn,
)
trainer.train()
trainer.save_state()
trainer.save_model(output_dir=training_args.output_dir)
tokenizer.save_pretrained(save_directory=training_args.output_dir)
if __name__ == "__main__":
main()
```
When I didn't use `torch.cpmpile(model)`, my code worked well. But when I added this line to my code, It produced the following error:
```
Traceback (most recent call last):
File "falcon_sft.py", line 230, in <module>
main()
File "falcon_sft.py", line 223, in main
trainer.train()
File "python3.10/site-packages/transformers/trainer.py", line 1539, in train
return inner_training_loop(
File "python3.10/site-packages/transformers/trainer.py", line 1787, in _inner_training_loop
for step, inputs in enumerate(epoch_iterator):
File "python3.10/site-packages/accelerate/data_loader.py", line 384, in __iter__
current_batch = next(dataloader_iter)
File "python3.10/site-packages/torch/utils/data/dataloader.py", line 633, in __next__
data = self._next_data()
File "python3.10/site-packages/torch/utils/data/dataloader.py", line 677, in _next_data
data = self._dataset_fetcher.fetch(index) # may raise StopIteration
File "python3.10/site-packages/torch/utils/data/_utils/fetch.py", line 49, in fetch
data = self.dataset.__getitems__(possibly_batched_index)
File "python3.10/site-packages/datasets/arrow_dataset.py", line 2807, in __getitems__
batch = self.__getitem__(keys)
File "python3.10/site-packages/datasets/arrow_dataset.py", line 2803, in __getitem__
return self._getitem(key)
File "python3.10/site-packages/datasets/arrow_dataset.py", line 2787, in _getitem
pa_subtable = query_table(self._data, key, indices=self._indices if self._indices is not None else None)
File "python3.10/site-packages/datasets/formatting/formatting.py", line 583, in query_table
_check_valid_index_key(key, size)
File "python3.10/site-packages/datasets/formatting/formatting.py", line 536, in _check_valid_index_key
_check_valid_index_key(int(max(key)), size=size)
File "python3.10/site-packages/datasets/formatting/formatting.py", line 526, in _check_valid_index_key
raise IndexError(f"Invalid key: {key} is out of bounds for size {size}")
IndexError: Invalid key: 88 is out of bounds for size 0
```
So I'm confused about why this error was generated, and how to fix it. Is this error produced by datasets or `torch.compile`?
### Expected behavior
I want to use `torch.compile` in my code.
### Environment info
- `datasets` version: 2.14.3
- Platform: Linux-4.18.0-425.19.2.el8_7.x86_64-x86_64-with-glibc2.28
- Python version: 3.10.8
- Huggingface_hub version: 0.16.4
- PyArrow version: 12.0.1
- Pandas version: 2.0.3
>
I tried this and got the following error:
```
Traceback (most recent call last):
File "/cephyr/NOBACKUP/groups/snic2021-23-24/LLM4-CodeOpt/env/lib/python3.10/site-packages/torch/_dynamo/convert_frame.py", line 324, in _compile
out_code = transform_code_object(code, transform)
File "/cephyr/NOBACKUP/groups/snic2021-23-24/LLM4-CodeOpt/env/lib/python3.10/site-packages/torch/_dynamo/bytecode_transformation.py", line 445, in transform_code_object
transformations(instructions, code_options)
File "/cephyr/NOBACKUP/groups/snic2021-23-24/LLM4-CodeOpt/env/lib/python3.10/site-packages/torch/_dynamo/convert_frame.py", line 311, in transform
tracer.run()
File "/cephyr/NOBACKUP/groups/snic2021-23-24/LLM4-CodeOpt/env/lib/python3.10/site-packages/torch/_dynamo/symbolic_convert.py", line 1726, in run
super().run()
File "/cephyr/NOBACKUP/groups/snic2021-23-24/LLM4-CodeOpt/env/lib/python3.10/site-packages/torch/_dynamo/symbolic_convert.py", line 576, in run
and self.step()
File "/cephyr/NOBACKUP/groups/snic2021-23-24/LLM4-CodeOpt/env/lib/python3.10/site-packages/torch/_dynamo/symbolic_convert.py", line 540, in step
getattr(self, inst.opname)(inst)
File "/cephyr/NOBACKUP/groups/snic2021-23-24/LLM4-CodeOpt/env/lib/python3.10/site-packages/torch/_dynamo/symbolic_convert.py", line 1030, in LOAD_ATTR
result = BuiltinVariable(getattr).call_function(
File "/cephyr/NOBACKUP/groups/snic2021-23-24/LLM4-CodeOpt/env/lib/python3.10/site-packages/torch/_dynamo/variables/builtin.py", line 566, in call_function
result = handler(tx, *args, **kwargs)
File "/cephyr/NOBACKUP/groups/snic2021-23-24/LLM4-CodeOpt/env/lib/python3.10/site-packages/torch/_dynamo/variables/builtin.py", line 931, in call_getattr
return obj.var_getattr(tx, name).add_options(options)
File "/cephyr/NOBACKUP/groups/snic2021-23-24/LLM4-CodeOpt/env/lib/python3.10/site-packages/torch/_dynamo/variables/nn_module.py", line 124, in var_getattr
subobj = inspect.getattr_static(base, name)
File "/apps/Arch/software/Python/3.10.8-GCCcore-12.2.0/lib/python3.10/inspect.py", line 1777, in getattr_static
raise AttributeError(attr)
AttributeError: config
from user code:
File "/cephyr/NOBACKUP/groups/snic2021-23-24/LLM4-CodeOpt/env/lib/python3.10/site-packages/peft/peft_model.py", line 909, in forward
if self.base_model.config.model_type == "mpt":
Set torch._dynamo.config.verbose=True for more information
You can suppress this exception and fall back to eager by setting:
torch._dynamo.config.suppress_errors = True
The above exception was the direct cause of the following exception:
Traceback (most recent call last):
File "/cephyr/NOBACKUP/groups/snic2021-23-24/LLM4-CodeOpt/llm-copt/fine-tune/falcon/falcon_sft.py", line 228, in <module>
main()
File "/cephyr/NOBACKUP/groups/snic2021-23-24/LLM4-CodeOpt/llm-copt/fine-tune/falcon/falcon_sft.py", line 221, in main
trainer.train()
File "/cephyr/NOBACKUP/groups/snic2021-23-24/LLM4-CodeOpt/env/lib/python3.10/site-packages/transformers/trainer.py", line 1539, in train
return inner_training_loop(
File "/cephyr/NOBACKUP/groups/snic2021-23-24/LLM4-CodeOpt/env/lib/python3.10/site-packages/transformers/trainer.py", line 1809, in _inner_training_loop
tr_loss_step = self.training_step(model, inputs)
File "/cephyr/NOBACKUP/groups/snic2021-23-24/LLM4-CodeOpt/env/lib/python3.10/site-packages/transformers/trainer.py", line 2654, in training_step
loss = self.compute_loss(model, inputs)
File "/cephyr/NOBACKUP/groups/snic2021-23-24/LLM4-CodeOpt/env/lib/python3.10/site-packages/transformers/trainer.py", line 2679, in compute_loss
outputs = model(**inputs)
File "/cephyr/NOBACKUP/groups/snic2021-23-24/LLM4-CodeOpt/env/lib/python3.10/site-packages/torch/nn/modules/module.py", line 1501, in _call_impl
return forward_call(*args, **kwargs)
File "/cephyr/NOBACKUP/groups/snic2021-23-24/LLM4-CodeOpt/env/lib/python3.10/site-packages/torch/_dynamo/eval_frame.py", line 82, in forward
return self.dynamo_ctx(self._orig_mod.forward)(*args, **kwargs)
File "/cephyr/NOBACKUP/groups/snic2021-23-24/LLM4-CodeOpt/env/lib/python3.10/site-packages/torch/_dynamo/eval_frame.py", line 209, in _fn
return fn(*args, **kwargs)
File "/cephyr/NOBACKUP/groups/snic2021-23-24/LLM4-CodeOpt/env/lib/python3.10/site-packages/accelerate/utils/operations.py", line 581, in forward
return model_forward(*args, **kwargs)
File "/cephyr/NOBACKUP/groups/snic2021-23-24/LLM4-CodeOpt/env/lib/python3.10/site-packages/accelerate/utils/operations.py", line 569, in __call__
return convert_to_fp32(self.model_forward(*args, **kwargs))
File "/cephyr/NOBACKUP/groups/snic2021-23-24/LLM4-CodeOpt/env/lib/python3.10/site-packages/torch/amp/autocast_mode.py", line 14, in decorate_autocast
return func(*args, **kwargs)
File "/cephyr/NOBACKUP/groups/snic2021-23-24/LLM4-CodeOpt/env/lib/python3.10/site-packages/torch/_dynamo/eval_frame.py", line 337, in catch_errors
return callback(frame, cache_size, hooks)
File "/cephyr/NOBACKUP/groups/snic2021-23-24/LLM4-CodeOpt/env/lib/python3.10/site-packages/torch/_dynamo/convert_frame.py", line 404, in _convert_frame
result = inner_convert(frame, cache_size, hooks)
File "/cephyr/NOBACKUP/groups/snic2021-23-24/LLM4-CodeOpt/env/lib/python3.10/site-packages/torch/_dynamo/convert_frame.py", line 104, in _fn
return fn(*args, **kwargs)
File "/cephyr/NOBACKUP/groups/snic2021-23-24/LLM4-CodeOpt/env/lib/python3.10/site-packages/torch/_dynamo/convert_frame.py", line 262, in _convert_frame_assert
return _compile(
File "/cephyr/NOBACKUP/groups/snic2021-23-24/LLM4-CodeOpt/env/lib/python3.10/site-packages/torch/_dynamo/utils.py", line 163, in time_wrapper
r = func(*args, **kwargs)
File "/cephyr/NOBACKUP/groups/snic2021-23-24/LLM4-CodeOpt/env/lib/python3.10/site-packages/torch/_dynamo/convert_frame.py", line 394, in _compile
raise InternalTorchDynamoError() from e
torch._dynamo.exc.InternalTorchDynamoError
``` |
https://github.com/huggingface/datasets/issues/6128 | IndexError: Invalid key: 88 is out of bounds for size 0 | Hi @TomasAndersonFang,
I guess in this case it may be an issue with `transformers` (or `PyTorch`). I would recommend you open an issue on their repo. | ### Describe the bug
This bug generates when I use torch.compile(model) in my code, which seems to raise an error in datasets lib.
### Steps to reproduce the bug
I use the following code to fine-tune Falcon on my private dataset.
```python
import transformers
from transformers import (
AutoModelForCausalLM,
AutoTokenizer,
AutoConfig,
DataCollatorForSeq2Seq,
Trainer,
Seq2SeqTrainer,
HfArgumentParser,
Seq2SeqTrainingArguments,
BitsAndBytesConfig,
)
from peft import (
LoraConfig,
get_peft_model,
get_peft_model_state_dict,
prepare_model_for_int8_training,
set_peft_model_state_dict,
)
import torch
import os
import evaluate
import functools
from datasets import load_dataset
import bitsandbytes as bnb
import logging
import json
import copy
from typing import Dict, Optional, Sequence
from dataclasses import dataclass, field
# Lora settings
LORA_R = 8
LORA_ALPHA = 16
LORA_DROPOUT= 0.05
LORA_TARGET_MODULES = ["query_key_value"]
@dataclass
class ModelArguments:
model_name_or_path: Optional[str] = field(default="Salesforce/codegen2-7B")
@dataclass
class DataArguments:
data_path: str = field(default=None, metadata={"help": "Path to the training data."})
train_file: str = field(default=None, metadata={"help": "Path to the evaluation data."})
eval_file: str = field(default=None, metadata={"help": "Path to the evaluation data."})
cache_path: str = field(default=None, metadata={"help": "Path to the cache directory."})
num_proc: int = field(default=4, metadata={"help": "Number of processes to use for data preprocessing."})
@dataclass
class TrainingArguments(transformers.TrainingArguments):
# cache_dir: Optional[str] = field(default=None)
optim: str = field(default="adamw_torch")
model_max_length: int = field(
default=512,
metadata={"help": "Maximum sequence length. Sequences will be right padded (and possibly truncated)."},
)
is_lora: bool = field(default=True, metadata={"help": "Whether to use LORA."})
def tokenize(text, tokenizer, max_seq_len=512, add_eos_token=True):
result = tokenizer(
text,
truncation=True,
max_length=max_seq_len,
padding=False,
return_tensors=None,
)
if (
result["input_ids"][-1] != tokenizer.eos_token_id
and len(result["input_ids"]) < max_seq_len
and add_eos_token
):
result["input_ids"].append(tokenizer.eos_token_id)
result["attention_mask"].append(1)
if add_eos_token and len(result["input_ids"]) >= max_seq_len:
result["input_ids"][max_seq_len - 1] = tokenizer.eos_token_id
result["attention_mask"][max_seq_len - 1] = 1
result["labels"] = result["input_ids"].copy()
return result
def main():
parser = HfArgumentParser((ModelArguments, DataArguments, TrainingArguments))
model_args, data_args, training_args = parser.parse_args_into_dataclasses()
config = AutoConfig.from_pretrained(
model_args.model_name_or_path,
cache_dir=data_args.cache_path,
trust_remote_code=True,
)
if training_args.is_lora:
model = AutoModelForCausalLM.from_pretrained(
model_args.model_name_or_path,
cache_dir=data_args.cache_path,
torch_dtype=torch.float16,
trust_remote_code=True,
load_in_8bit=True,
quantization_config=BitsAndBytesConfig(
load_in_8bit=True,
llm_int8_threshold=6.0
),
)
model = prepare_model_for_int8_training(model)
config = LoraConfig(
r=LORA_R,
lora_alpha=LORA_ALPHA,
target_modules=LORA_TARGET_MODULES,
lora_dropout=LORA_DROPOUT,
bias="none",
task_type="CAUSAL_LM",
)
model = get_peft_model(model, config)
else:
model = AutoModelForCausalLM.from_pretrained(
model_args.model_name_or_path,
torch_dtype=torch.float16,
cache_dir=data_args.cache_path,
trust_remote_code=True,
)
model.config.use_cache = False
def print_trainable_parameters(model):
"""
Prints the number of trainable parameters in the model.
"""
trainable_params = 0
all_param = 0
for _, param in model.named_parameters():
all_param += param.numel()
if param.requires_grad:
trainable_params += param.numel()
print(
f"trainable params: {trainable_params} || all params: {all_param} || trainable%: {100 * trainable_params / all_param}"
)
print_trainable_parameters(model)
tokenizer = AutoTokenizer.from_pretrained(
model_args.model_name_or_path,
cache_dir=data_args.cache_path,
model_max_length=training_args.model_max_length,
padding_side="left",
use_fast=True,
trust_remote_code=True,
)
tokenizer.pad_token = tokenizer.eos_token
# Load dataset
def generate_and_tokenize_prompt(sample):
input_text = sample["input"]
target_text = sample["output"] + tokenizer.eos_token
full_text = input_text + target_text
tokenized_full_text = tokenize(full_text, tokenizer, max_seq_len=512)
tokenized_input_text = tokenize(input_text, tokenizer, max_seq_len=512)
input_len = len(tokenized_input_text["input_ids"]) - 1 # -1 for eos token
tokenized_full_text["labels"] = [-100] * input_len + tokenized_full_text["labels"][input_len:]
return tokenized_full_text
data_files = {}
if data_args.train_file is not None:
data_files["train"] = data_args.train_file
if data_args.eval_file is not None:
data_files["eval"] = data_args.eval_file
dataset = load_dataset(data_args.data_path, data_files=data_files)
train_dataset = dataset["train"]
eval_dataset = dataset["eval"]
train_dataset = train_dataset.map(generate_and_tokenize_prompt, num_proc=data_args.num_proc)
eval_dataset = eval_dataset.map(generate_and_tokenize_prompt, num_proc=data_args.num_proc)
data_collator = DataCollatorForSeq2Seq(tokenizer, pad_to_multiple_of=8, return_tensors="pt", padding=True)
# Evaluation metrics
def compute_metrics(eval_preds, tokenizer):
metric = evaluate.load('exact_match')
preds, labels = eval_preds
# In case the model returns more than the prediction logits
if isinstance(preds, tuple):
preds = preds[0]
decoded_preds = tokenizer.batch_decode(preds, skip_special_tokens=True, clean_up_tokenization_spaces=False)
# Replace -100s in the labels as we can't decode them
labels[labels == -100] = tokenizer.pad_token_id
decoded_labels = tokenizer.batch_decode(labels, skip_special_tokens=True, clean_up_tokenization_spaces=False)
# Some simple post-processing
decoded_preds = [pred.strip() for pred in decoded_preds]
decoded_labels = [label.strip() for label in decoded_labels]
result = metric.compute(predictions=decoded_preds, references=decoded_labels)
return {'exact_match': result['exact_match']}
compute_metrics_fn = functools.partial(compute_metrics, tokenizer=tokenizer)
model = torch.compile(model)
# Training
trainer = Trainer(
model=model,
train_dataset=train_dataset,
eval_dataset=eval_dataset,
args=training_args,
data_collator=data_collator,
compute_metrics=compute_metrics_fn,
)
trainer.train()
trainer.save_state()
trainer.save_model(output_dir=training_args.output_dir)
tokenizer.save_pretrained(save_directory=training_args.output_dir)
if __name__ == "__main__":
main()
```
When I didn't use `torch.cpmpile(model)`, my code worked well. But when I added this line to my code, It produced the following error:
```
Traceback (most recent call last):
File "falcon_sft.py", line 230, in <module>
main()
File "falcon_sft.py", line 223, in main
trainer.train()
File "python3.10/site-packages/transformers/trainer.py", line 1539, in train
return inner_training_loop(
File "python3.10/site-packages/transformers/trainer.py", line 1787, in _inner_training_loop
for step, inputs in enumerate(epoch_iterator):
File "python3.10/site-packages/accelerate/data_loader.py", line 384, in __iter__
current_batch = next(dataloader_iter)
File "python3.10/site-packages/torch/utils/data/dataloader.py", line 633, in __next__
data = self._next_data()
File "python3.10/site-packages/torch/utils/data/dataloader.py", line 677, in _next_data
data = self._dataset_fetcher.fetch(index) # may raise StopIteration
File "python3.10/site-packages/torch/utils/data/_utils/fetch.py", line 49, in fetch
data = self.dataset.__getitems__(possibly_batched_index)
File "python3.10/site-packages/datasets/arrow_dataset.py", line 2807, in __getitems__
batch = self.__getitem__(keys)
File "python3.10/site-packages/datasets/arrow_dataset.py", line 2803, in __getitem__
return self._getitem(key)
File "python3.10/site-packages/datasets/arrow_dataset.py", line 2787, in _getitem
pa_subtable = query_table(self._data, key, indices=self._indices if self._indices is not None else None)
File "python3.10/site-packages/datasets/formatting/formatting.py", line 583, in query_table
_check_valid_index_key(key, size)
File "python3.10/site-packages/datasets/formatting/formatting.py", line 536, in _check_valid_index_key
_check_valid_index_key(int(max(key)), size=size)
File "python3.10/site-packages/datasets/formatting/formatting.py", line 526, in _check_valid_index_key
raise IndexError(f"Invalid key: {key} is out of bounds for size {size}")
IndexError: Invalid key: 88 is out of bounds for size 0
```
So I'm confused about why this error was generated, and how to fix it. Is this error produced by datasets or `torch.compile`?
### Expected behavior
I want to use `torch.compile` in my code.
### Environment info
- `datasets` version: 2.14.3
- Platform: Linux-4.18.0-425.19.2.el8_7.x86_64-x86_64-with-glibc2.28
- Python version: 3.10.8
- Huggingface_hub version: 0.16.4
- PyArrow version: 12.0.1
- Pandas version: 2.0.3 | 26 | IndexError: Invalid key: 88 is out of bounds for size 0
### Describe the bug
This bug generates when I use torch.compile(model) in my code, which seems to raise an error in datasets lib.
### Steps to reproduce the bug
I use the following code to fine-tune Falcon on my private dataset.
```python
import transformers
from transformers import (
AutoModelForCausalLM,
AutoTokenizer,
AutoConfig,
DataCollatorForSeq2Seq,
Trainer,
Seq2SeqTrainer,
HfArgumentParser,
Seq2SeqTrainingArguments,
BitsAndBytesConfig,
)
from peft import (
LoraConfig,
get_peft_model,
get_peft_model_state_dict,
prepare_model_for_int8_training,
set_peft_model_state_dict,
)
import torch
import os
import evaluate
import functools
from datasets import load_dataset
import bitsandbytes as bnb
import logging
import json
import copy
from typing import Dict, Optional, Sequence
from dataclasses import dataclass, field
# Lora settings
LORA_R = 8
LORA_ALPHA = 16
LORA_DROPOUT= 0.05
LORA_TARGET_MODULES = ["query_key_value"]
@dataclass
class ModelArguments:
model_name_or_path: Optional[str] = field(default="Salesforce/codegen2-7B")
@dataclass
class DataArguments:
data_path: str = field(default=None, metadata={"help": "Path to the training data."})
train_file: str = field(default=None, metadata={"help": "Path to the evaluation data."})
eval_file: str = field(default=None, metadata={"help": "Path to the evaluation data."})
cache_path: str = field(default=None, metadata={"help": "Path to the cache directory."})
num_proc: int = field(default=4, metadata={"help": "Number of processes to use for data preprocessing."})
@dataclass
class TrainingArguments(transformers.TrainingArguments):
# cache_dir: Optional[str] = field(default=None)
optim: str = field(default="adamw_torch")
model_max_length: int = field(
default=512,
metadata={"help": "Maximum sequence length. Sequences will be right padded (and possibly truncated)."},
)
is_lora: bool = field(default=True, metadata={"help": "Whether to use LORA."})
def tokenize(text, tokenizer, max_seq_len=512, add_eos_token=True):
result = tokenizer(
text,
truncation=True,
max_length=max_seq_len,
padding=False,
return_tensors=None,
)
if (
result["input_ids"][-1] != tokenizer.eos_token_id
and len(result["input_ids"]) < max_seq_len
and add_eos_token
):
result["input_ids"].append(tokenizer.eos_token_id)
result["attention_mask"].append(1)
if add_eos_token and len(result["input_ids"]) >= max_seq_len:
result["input_ids"][max_seq_len - 1] = tokenizer.eos_token_id
result["attention_mask"][max_seq_len - 1] = 1
result["labels"] = result["input_ids"].copy()
return result
def main():
parser = HfArgumentParser((ModelArguments, DataArguments, TrainingArguments))
model_args, data_args, training_args = parser.parse_args_into_dataclasses()
config = AutoConfig.from_pretrained(
model_args.model_name_or_path,
cache_dir=data_args.cache_path,
trust_remote_code=True,
)
if training_args.is_lora:
model = AutoModelForCausalLM.from_pretrained(
model_args.model_name_or_path,
cache_dir=data_args.cache_path,
torch_dtype=torch.float16,
trust_remote_code=True,
load_in_8bit=True,
quantization_config=BitsAndBytesConfig(
load_in_8bit=True,
llm_int8_threshold=6.0
),
)
model = prepare_model_for_int8_training(model)
config = LoraConfig(
r=LORA_R,
lora_alpha=LORA_ALPHA,
target_modules=LORA_TARGET_MODULES,
lora_dropout=LORA_DROPOUT,
bias="none",
task_type="CAUSAL_LM",
)
model = get_peft_model(model, config)
else:
model = AutoModelForCausalLM.from_pretrained(
model_args.model_name_or_path,
torch_dtype=torch.float16,
cache_dir=data_args.cache_path,
trust_remote_code=True,
)
model.config.use_cache = False
def print_trainable_parameters(model):
"""
Prints the number of trainable parameters in the model.
"""
trainable_params = 0
all_param = 0
for _, param in model.named_parameters():
all_param += param.numel()
if param.requires_grad:
trainable_params += param.numel()
print(
f"trainable params: {trainable_params} || all params: {all_param} || trainable%: {100 * trainable_params / all_param}"
)
print_trainable_parameters(model)
tokenizer = AutoTokenizer.from_pretrained(
model_args.model_name_or_path,
cache_dir=data_args.cache_path,
model_max_length=training_args.model_max_length,
padding_side="left",
use_fast=True,
trust_remote_code=True,
)
tokenizer.pad_token = tokenizer.eos_token
# Load dataset
def generate_and_tokenize_prompt(sample):
input_text = sample["input"]
target_text = sample["output"] + tokenizer.eos_token
full_text = input_text + target_text
tokenized_full_text = tokenize(full_text, tokenizer, max_seq_len=512)
tokenized_input_text = tokenize(input_text, tokenizer, max_seq_len=512)
input_len = len(tokenized_input_text["input_ids"]) - 1 # -1 for eos token
tokenized_full_text["labels"] = [-100] * input_len + tokenized_full_text["labels"][input_len:]
return tokenized_full_text
data_files = {}
if data_args.train_file is not None:
data_files["train"] = data_args.train_file
if data_args.eval_file is not None:
data_files["eval"] = data_args.eval_file
dataset = load_dataset(data_args.data_path, data_files=data_files)
train_dataset = dataset["train"]
eval_dataset = dataset["eval"]
train_dataset = train_dataset.map(generate_and_tokenize_prompt, num_proc=data_args.num_proc)
eval_dataset = eval_dataset.map(generate_and_tokenize_prompt, num_proc=data_args.num_proc)
data_collator = DataCollatorForSeq2Seq(tokenizer, pad_to_multiple_of=8, return_tensors="pt", padding=True)
# Evaluation metrics
def compute_metrics(eval_preds, tokenizer):
metric = evaluate.load('exact_match')
preds, labels = eval_preds
# In case the model returns more than the prediction logits
if isinstance(preds, tuple):
preds = preds[0]
decoded_preds = tokenizer.batch_decode(preds, skip_special_tokens=True, clean_up_tokenization_spaces=False)
# Replace -100s in the labels as we can't decode them
labels[labels == -100] = tokenizer.pad_token_id
decoded_labels = tokenizer.batch_decode(labels, skip_special_tokens=True, clean_up_tokenization_spaces=False)
# Some simple post-processing
decoded_preds = [pred.strip() for pred in decoded_preds]
decoded_labels = [label.strip() for label in decoded_labels]
result = metric.compute(predictions=decoded_preds, references=decoded_labels)
return {'exact_match': result['exact_match']}
compute_metrics_fn = functools.partial(compute_metrics, tokenizer=tokenizer)
model = torch.compile(model)
# Training
trainer = Trainer(
model=model,
train_dataset=train_dataset,
eval_dataset=eval_dataset,
args=training_args,
data_collator=data_collator,
compute_metrics=compute_metrics_fn,
)
trainer.train()
trainer.save_state()
trainer.save_model(output_dir=training_args.output_dir)
tokenizer.save_pretrained(save_directory=training_args.output_dir)
if __name__ == "__main__":
main()
```
When I didn't use `torch.cpmpile(model)`, my code worked well. But when I added this line to my code, It produced the following error:
```
Traceback (most recent call last):
File "falcon_sft.py", line 230, in <module>
main()
File "falcon_sft.py", line 223, in main
trainer.train()
File "python3.10/site-packages/transformers/trainer.py", line 1539, in train
return inner_training_loop(
File "python3.10/site-packages/transformers/trainer.py", line 1787, in _inner_training_loop
for step, inputs in enumerate(epoch_iterator):
File "python3.10/site-packages/accelerate/data_loader.py", line 384, in __iter__
current_batch = next(dataloader_iter)
File "python3.10/site-packages/torch/utils/data/dataloader.py", line 633, in __next__
data = self._next_data()
File "python3.10/site-packages/torch/utils/data/dataloader.py", line 677, in _next_data
data = self._dataset_fetcher.fetch(index) # may raise StopIteration
File "python3.10/site-packages/torch/utils/data/_utils/fetch.py", line 49, in fetch
data = self.dataset.__getitems__(possibly_batched_index)
File "python3.10/site-packages/datasets/arrow_dataset.py", line 2807, in __getitems__
batch = self.__getitem__(keys)
File "python3.10/site-packages/datasets/arrow_dataset.py", line 2803, in __getitem__
return self._getitem(key)
File "python3.10/site-packages/datasets/arrow_dataset.py", line 2787, in _getitem
pa_subtable = query_table(self._data, key, indices=self._indices if self._indices is not None else None)
File "python3.10/site-packages/datasets/formatting/formatting.py", line 583, in query_table
_check_valid_index_key(key, size)
File "python3.10/site-packages/datasets/formatting/formatting.py", line 536, in _check_valid_index_key
_check_valid_index_key(int(max(key)), size=size)
File "python3.10/site-packages/datasets/formatting/formatting.py", line 526, in _check_valid_index_key
raise IndexError(f"Invalid key: {key} is out of bounds for size {size}")
IndexError: Invalid key: 88 is out of bounds for size 0
```
So I'm confused about why this error was generated, and how to fix it. Is this error produced by datasets or `torch.compile`?
### Expected behavior
I want to use `torch.compile` in my code.
### Environment info
- `datasets` version: 2.14.3
- Platform: Linux-4.18.0-425.19.2.el8_7.x86_64-x86_64-with-glibc2.28
- Python version: 3.10.8
- Huggingface_hub version: 0.16.4
- PyArrow version: 12.0.1
- Pandas version: 2.0.3
Hi @TomasAndersonFang,
I guess in this case it may be an issue with `transformers` (or `PyTorch`). I would recommend you open an issue on their repo. |
https://github.com/huggingface/datasets/issues/6126 | Private datasets do not load when passing token | Our CI did not catch this issue because with current implementation, stored token in `HfFolder` (which always exists) is used by default. | ### Describe the bug
Since the release of `datasets` 2.14, private/gated datasets do not load when passing `token`: they raise `EmptyDatasetError`.
This is a non-planned backward incompatible breaking change.
Note that private datasets do load if instead `download_config` is passed:
```python
from datasets import DownloadConfig, load_dataset
ds = load_dataset("albertvillanova/tmp-private", split="train", download_config=DownloadConfig(token="<MY-TOKEN>"))
ds
```
gives
```
Dataset({
features: ['text'],
num_rows: 4
})
```
### Steps to reproduce the bug
```python
from datasets import load_dataset
ds = load_dataset("albertvillanova/tmp-private", split="train", token="<MY-TOKEN>")
```
gives
```
---------------------------------------------------------------------------
EmptyDatasetError Traceback (most recent call last)
[<ipython-input-2-25b48732107a>](https://localhost:8080/#) in <cell line: 3>()
1 from datasets import load_dataset
2
----> 3 ds = load_dataset("albertvillanova/tmp-private", split="train", token="<MY-TOKEN>")
5 frames
[/usr/local/lib/python3.10/dist-packages/datasets/load.py](https://localhost:8080/#) in load_dataset(path, name, data_dir, data_files, split, cache_dir, features, download_config, download_mode, verification_mode, ignore_verifications, keep_in_memory, save_infos, revision, token, use_auth_token, task, streaming, num_proc, storage_options, **config_kwargs)
2107
2108 # Create a dataset builder
-> 2109 builder_instance = load_dataset_builder(
2110 path=path,
2111 name=name,
[/usr/local/lib/python3.10/dist-packages/datasets/load.py](https://localhost:8080/#) in load_dataset_builder(path, name, data_dir, data_files, cache_dir, features, download_config, download_mode, revision, token, use_auth_token, storage_options, **config_kwargs)
1793 download_config = download_config.copy() if download_config else DownloadConfig()
1794 download_config.storage_options.update(storage_options)
-> 1795 dataset_module = dataset_module_factory(
1796 path,
1797 revision=revision,
[/usr/local/lib/python3.10/dist-packages/datasets/load.py](https://localhost:8080/#) in dataset_module_factory(path, revision, download_config, download_mode, dynamic_modules_path, data_dir, data_files, **download_kwargs)
1484 raise ConnectionError(f"Couldn't reach the Hugging Face Hub for dataset '{path}': {e1}") from None
1485 if isinstance(e1, EmptyDatasetError):
-> 1486 raise e1 from None
1487 if isinstance(e1, FileNotFoundError):
1488 raise FileNotFoundError(
[/usr/local/lib/python3.10/dist-packages/datasets/load.py](https://localhost:8080/#) in dataset_module_factory(path, revision, download_config, download_mode, dynamic_modules_path, data_dir, data_files, **download_kwargs)
1474 download_config=download_config,
1475 download_mode=download_mode,
-> 1476 ).get_module()
1477 except (
1478 Exception
[/usr/local/lib/python3.10/dist-packages/datasets/load.py](https://localhost:8080/#) in get_module(self)
1030 sanitize_patterns(self.data_files)
1031 if self.data_files is not None
-> 1032 else get_data_patterns(base_path, download_config=self.download_config)
1033 )
1034 data_files = DataFilesDict.from_patterns(
[/usr/local/lib/python3.10/dist-packages/datasets/data_files.py](https://localhost:8080/#) in get_data_patterns(base_path, download_config)
457 return _get_data_files_patterns(resolver)
458 except FileNotFoundError:
--> 459 raise EmptyDatasetError(f"The directory at {base_path} doesn't contain any data files") from None
460
461
EmptyDatasetError: The directory at hf://datasets/albertvillanova/tmp-private@79b9e4fe79670a9a050d6ebc385464891915a71d doesn't contain any data files
```
### Expected behavior
The dataset should load.
### Environment info
- `datasets` version: 2.14.3
- Platform: Linux-5.15.109+-x86_64-with-glibc2.35
- Python version: 3.10.12
- Huggingface_hub version: 0.16.4
- PyArrow version: 9.0.0
- Pandas version: 1.5.3 | 22 | Private datasets do not load when passing token
### Describe the bug
Since the release of `datasets` 2.14, private/gated datasets do not load when passing `token`: they raise `EmptyDatasetError`.
This is a non-planned backward incompatible breaking change.
Note that private datasets do load if instead `download_config` is passed:
```python
from datasets import DownloadConfig, load_dataset
ds = load_dataset("albertvillanova/tmp-private", split="train", download_config=DownloadConfig(token="<MY-TOKEN>"))
ds
```
gives
```
Dataset({
features: ['text'],
num_rows: 4
})
```
### Steps to reproduce the bug
```python
from datasets import load_dataset
ds = load_dataset("albertvillanova/tmp-private", split="train", token="<MY-TOKEN>")
```
gives
```
---------------------------------------------------------------------------
EmptyDatasetError Traceback (most recent call last)
[<ipython-input-2-25b48732107a>](https://localhost:8080/#) in <cell line: 3>()
1 from datasets import load_dataset
2
----> 3 ds = load_dataset("albertvillanova/tmp-private", split="train", token="<MY-TOKEN>")
5 frames
[/usr/local/lib/python3.10/dist-packages/datasets/load.py](https://localhost:8080/#) in load_dataset(path, name, data_dir, data_files, split, cache_dir, features, download_config, download_mode, verification_mode, ignore_verifications, keep_in_memory, save_infos, revision, token, use_auth_token, task, streaming, num_proc, storage_options, **config_kwargs)
2107
2108 # Create a dataset builder
-> 2109 builder_instance = load_dataset_builder(
2110 path=path,
2111 name=name,
[/usr/local/lib/python3.10/dist-packages/datasets/load.py](https://localhost:8080/#) in load_dataset_builder(path, name, data_dir, data_files, cache_dir, features, download_config, download_mode, revision, token, use_auth_token, storage_options, **config_kwargs)
1793 download_config = download_config.copy() if download_config else DownloadConfig()
1794 download_config.storage_options.update(storage_options)
-> 1795 dataset_module = dataset_module_factory(
1796 path,
1797 revision=revision,
[/usr/local/lib/python3.10/dist-packages/datasets/load.py](https://localhost:8080/#) in dataset_module_factory(path, revision, download_config, download_mode, dynamic_modules_path, data_dir, data_files, **download_kwargs)
1484 raise ConnectionError(f"Couldn't reach the Hugging Face Hub for dataset '{path}': {e1}") from None
1485 if isinstance(e1, EmptyDatasetError):
-> 1486 raise e1 from None
1487 if isinstance(e1, FileNotFoundError):
1488 raise FileNotFoundError(
[/usr/local/lib/python3.10/dist-packages/datasets/load.py](https://localhost:8080/#) in dataset_module_factory(path, revision, download_config, download_mode, dynamic_modules_path, data_dir, data_files, **download_kwargs)
1474 download_config=download_config,
1475 download_mode=download_mode,
-> 1476 ).get_module()
1477 except (
1478 Exception
[/usr/local/lib/python3.10/dist-packages/datasets/load.py](https://localhost:8080/#) in get_module(self)
1030 sanitize_patterns(self.data_files)
1031 if self.data_files is not None
-> 1032 else get_data_patterns(base_path, download_config=self.download_config)
1033 )
1034 data_files = DataFilesDict.from_patterns(
[/usr/local/lib/python3.10/dist-packages/datasets/data_files.py](https://localhost:8080/#) in get_data_patterns(base_path, download_config)
457 return _get_data_files_patterns(resolver)
458 except FileNotFoundError:
--> 459 raise EmptyDatasetError(f"The directory at {base_path} doesn't contain any data files") from None
460
461
EmptyDatasetError: The directory at hf://datasets/albertvillanova/tmp-private@79b9e4fe79670a9a050d6ebc385464891915a71d doesn't contain any data files
```
### Expected behavior
The dataset should load.
### Environment info
- `datasets` version: 2.14.3
- Platform: Linux-5.15.109+-x86_64-with-glibc2.35
- Python version: 3.10.12
- Huggingface_hub version: 0.16.4
- PyArrow version: 9.0.0
- Pandas version: 1.5.3
Our CI did not catch this issue because with current implementation, stored token in `HfFolder` (which always exists) is used by default. |
https://github.com/huggingface/datasets/issues/6126 | Private datasets do not load when passing token | I can confirm this and have the same problem (and just went almost crazy because I couldn't figure out the source of this problem because on another computer everything worked well even with `DownloadMode.FORCE_REDOWNLOAD`). | ### Describe the bug
Since the release of `datasets` 2.14, private/gated datasets do not load when passing `token`: they raise `EmptyDatasetError`.
This is a non-planned backward incompatible breaking change.
Note that private datasets do load if instead `download_config` is passed:
```python
from datasets import DownloadConfig, load_dataset
ds = load_dataset("albertvillanova/tmp-private", split="train", download_config=DownloadConfig(token="<MY-TOKEN>"))
ds
```
gives
```
Dataset({
features: ['text'],
num_rows: 4
})
```
### Steps to reproduce the bug
```python
from datasets import load_dataset
ds = load_dataset("albertvillanova/tmp-private", split="train", token="<MY-TOKEN>")
```
gives
```
---------------------------------------------------------------------------
EmptyDatasetError Traceback (most recent call last)
[<ipython-input-2-25b48732107a>](https://localhost:8080/#) in <cell line: 3>()
1 from datasets import load_dataset
2
----> 3 ds = load_dataset("albertvillanova/tmp-private", split="train", token="<MY-TOKEN>")
5 frames
[/usr/local/lib/python3.10/dist-packages/datasets/load.py](https://localhost:8080/#) in load_dataset(path, name, data_dir, data_files, split, cache_dir, features, download_config, download_mode, verification_mode, ignore_verifications, keep_in_memory, save_infos, revision, token, use_auth_token, task, streaming, num_proc, storage_options, **config_kwargs)
2107
2108 # Create a dataset builder
-> 2109 builder_instance = load_dataset_builder(
2110 path=path,
2111 name=name,
[/usr/local/lib/python3.10/dist-packages/datasets/load.py](https://localhost:8080/#) in load_dataset_builder(path, name, data_dir, data_files, cache_dir, features, download_config, download_mode, revision, token, use_auth_token, storage_options, **config_kwargs)
1793 download_config = download_config.copy() if download_config else DownloadConfig()
1794 download_config.storage_options.update(storage_options)
-> 1795 dataset_module = dataset_module_factory(
1796 path,
1797 revision=revision,
[/usr/local/lib/python3.10/dist-packages/datasets/load.py](https://localhost:8080/#) in dataset_module_factory(path, revision, download_config, download_mode, dynamic_modules_path, data_dir, data_files, **download_kwargs)
1484 raise ConnectionError(f"Couldn't reach the Hugging Face Hub for dataset '{path}': {e1}") from None
1485 if isinstance(e1, EmptyDatasetError):
-> 1486 raise e1 from None
1487 if isinstance(e1, FileNotFoundError):
1488 raise FileNotFoundError(
[/usr/local/lib/python3.10/dist-packages/datasets/load.py](https://localhost:8080/#) in dataset_module_factory(path, revision, download_config, download_mode, dynamic_modules_path, data_dir, data_files, **download_kwargs)
1474 download_config=download_config,
1475 download_mode=download_mode,
-> 1476 ).get_module()
1477 except (
1478 Exception
[/usr/local/lib/python3.10/dist-packages/datasets/load.py](https://localhost:8080/#) in get_module(self)
1030 sanitize_patterns(self.data_files)
1031 if self.data_files is not None
-> 1032 else get_data_patterns(base_path, download_config=self.download_config)
1033 )
1034 data_files = DataFilesDict.from_patterns(
[/usr/local/lib/python3.10/dist-packages/datasets/data_files.py](https://localhost:8080/#) in get_data_patterns(base_path, download_config)
457 return _get_data_files_patterns(resolver)
458 except FileNotFoundError:
--> 459 raise EmptyDatasetError(f"The directory at {base_path} doesn't contain any data files") from None
460
461
EmptyDatasetError: The directory at hf://datasets/albertvillanova/tmp-private@79b9e4fe79670a9a050d6ebc385464891915a71d doesn't contain any data files
```
### Expected behavior
The dataset should load.
### Environment info
- `datasets` version: 2.14.3
- Platform: Linux-5.15.109+-x86_64-with-glibc2.35
- Python version: 3.10.12
- Huggingface_hub version: 0.16.4
- PyArrow version: 9.0.0
- Pandas version: 1.5.3 | 34 | Private datasets do not load when passing token
### Describe the bug
Since the release of `datasets` 2.14, private/gated datasets do not load when passing `token`: they raise `EmptyDatasetError`.
This is a non-planned backward incompatible breaking change.
Note that private datasets do load if instead `download_config` is passed:
```python
from datasets import DownloadConfig, load_dataset
ds = load_dataset("albertvillanova/tmp-private", split="train", download_config=DownloadConfig(token="<MY-TOKEN>"))
ds
```
gives
```
Dataset({
features: ['text'],
num_rows: 4
})
```
### Steps to reproduce the bug
```python
from datasets import load_dataset
ds = load_dataset("albertvillanova/tmp-private", split="train", token="<MY-TOKEN>")
```
gives
```
---------------------------------------------------------------------------
EmptyDatasetError Traceback (most recent call last)
[<ipython-input-2-25b48732107a>](https://localhost:8080/#) in <cell line: 3>()
1 from datasets import load_dataset
2
----> 3 ds = load_dataset("albertvillanova/tmp-private", split="train", token="<MY-TOKEN>")
5 frames
[/usr/local/lib/python3.10/dist-packages/datasets/load.py](https://localhost:8080/#) in load_dataset(path, name, data_dir, data_files, split, cache_dir, features, download_config, download_mode, verification_mode, ignore_verifications, keep_in_memory, save_infos, revision, token, use_auth_token, task, streaming, num_proc, storage_options, **config_kwargs)
2107
2108 # Create a dataset builder
-> 2109 builder_instance = load_dataset_builder(
2110 path=path,
2111 name=name,
[/usr/local/lib/python3.10/dist-packages/datasets/load.py](https://localhost:8080/#) in load_dataset_builder(path, name, data_dir, data_files, cache_dir, features, download_config, download_mode, revision, token, use_auth_token, storage_options, **config_kwargs)
1793 download_config = download_config.copy() if download_config else DownloadConfig()
1794 download_config.storage_options.update(storage_options)
-> 1795 dataset_module = dataset_module_factory(
1796 path,
1797 revision=revision,
[/usr/local/lib/python3.10/dist-packages/datasets/load.py](https://localhost:8080/#) in dataset_module_factory(path, revision, download_config, download_mode, dynamic_modules_path, data_dir, data_files, **download_kwargs)
1484 raise ConnectionError(f"Couldn't reach the Hugging Face Hub for dataset '{path}': {e1}") from None
1485 if isinstance(e1, EmptyDatasetError):
-> 1486 raise e1 from None
1487 if isinstance(e1, FileNotFoundError):
1488 raise FileNotFoundError(
[/usr/local/lib/python3.10/dist-packages/datasets/load.py](https://localhost:8080/#) in dataset_module_factory(path, revision, download_config, download_mode, dynamic_modules_path, data_dir, data_files, **download_kwargs)
1474 download_config=download_config,
1475 download_mode=download_mode,
-> 1476 ).get_module()
1477 except (
1478 Exception
[/usr/local/lib/python3.10/dist-packages/datasets/load.py](https://localhost:8080/#) in get_module(self)
1030 sanitize_patterns(self.data_files)
1031 if self.data_files is not None
-> 1032 else get_data_patterns(base_path, download_config=self.download_config)
1033 )
1034 data_files = DataFilesDict.from_patterns(
[/usr/local/lib/python3.10/dist-packages/datasets/data_files.py](https://localhost:8080/#) in get_data_patterns(base_path, download_config)
457 return _get_data_files_patterns(resolver)
458 except FileNotFoundError:
--> 459 raise EmptyDatasetError(f"The directory at {base_path} doesn't contain any data files") from None
460
461
EmptyDatasetError: The directory at hf://datasets/albertvillanova/tmp-private@79b9e4fe79670a9a050d6ebc385464891915a71d doesn't contain any data files
```
### Expected behavior
The dataset should load.
### Environment info
- `datasets` version: 2.14.3
- Platform: Linux-5.15.109+-x86_64-with-glibc2.35
- Python version: 3.10.12
- Huggingface_hub version: 0.16.4
- PyArrow version: 9.0.0
- Pandas version: 1.5.3
I can confirm this and have the same problem (and just went almost crazy because I couldn't figure out the source of this problem because on another computer everything worked well even with `DownloadMode.FORCE_REDOWNLOAD`). |
https://github.com/huggingface/datasets/issues/6126 | Private datasets do not load when passing token | We are planning to do a patch release today, after the merge of the fix:
- #6127
In the meantime, the problem can be circumvented by passing `download_config` instead:
```python
from datasets import DownloadConfig, load_dataset
load_dataset("<DATASET-NAME>", split="train", download_config=DownloadConfig(token="<TOKEN>"))
``` | ### Describe the bug
Since the release of `datasets` 2.14, private/gated datasets do not load when passing `token`: they raise `EmptyDatasetError`.
This is a non-planned backward incompatible breaking change.
Note that private datasets do load if instead `download_config` is passed:
```python
from datasets import DownloadConfig, load_dataset
ds = load_dataset("albertvillanova/tmp-private", split="train", download_config=DownloadConfig(token="<MY-TOKEN>"))
ds
```
gives
```
Dataset({
features: ['text'],
num_rows: 4
})
```
### Steps to reproduce the bug
```python
from datasets import load_dataset
ds = load_dataset("albertvillanova/tmp-private", split="train", token="<MY-TOKEN>")
```
gives
```
---------------------------------------------------------------------------
EmptyDatasetError Traceback (most recent call last)
[<ipython-input-2-25b48732107a>](https://localhost:8080/#) in <cell line: 3>()
1 from datasets import load_dataset
2
----> 3 ds = load_dataset("albertvillanova/tmp-private", split="train", token="<MY-TOKEN>")
5 frames
[/usr/local/lib/python3.10/dist-packages/datasets/load.py](https://localhost:8080/#) in load_dataset(path, name, data_dir, data_files, split, cache_dir, features, download_config, download_mode, verification_mode, ignore_verifications, keep_in_memory, save_infos, revision, token, use_auth_token, task, streaming, num_proc, storage_options, **config_kwargs)
2107
2108 # Create a dataset builder
-> 2109 builder_instance = load_dataset_builder(
2110 path=path,
2111 name=name,
[/usr/local/lib/python3.10/dist-packages/datasets/load.py](https://localhost:8080/#) in load_dataset_builder(path, name, data_dir, data_files, cache_dir, features, download_config, download_mode, revision, token, use_auth_token, storage_options, **config_kwargs)
1793 download_config = download_config.copy() if download_config else DownloadConfig()
1794 download_config.storage_options.update(storage_options)
-> 1795 dataset_module = dataset_module_factory(
1796 path,
1797 revision=revision,
[/usr/local/lib/python3.10/dist-packages/datasets/load.py](https://localhost:8080/#) in dataset_module_factory(path, revision, download_config, download_mode, dynamic_modules_path, data_dir, data_files, **download_kwargs)
1484 raise ConnectionError(f"Couldn't reach the Hugging Face Hub for dataset '{path}': {e1}") from None
1485 if isinstance(e1, EmptyDatasetError):
-> 1486 raise e1 from None
1487 if isinstance(e1, FileNotFoundError):
1488 raise FileNotFoundError(
[/usr/local/lib/python3.10/dist-packages/datasets/load.py](https://localhost:8080/#) in dataset_module_factory(path, revision, download_config, download_mode, dynamic_modules_path, data_dir, data_files, **download_kwargs)
1474 download_config=download_config,
1475 download_mode=download_mode,
-> 1476 ).get_module()
1477 except (
1478 Exception
[/usr/local/lib/python3.10/dist-packages/datasets/load.py](https://localhost:8080/#) in get_module(self)
1030 sanitize_patterns(self.data_files)
1031 if self.data_files is not None
-> 1032 else get_data_patterns(base_path, download_config=self.download_config)
1033 )
1034 data_files = DataFilesDict.from_patterns(
[/usr/local/lib/python3.10/dist-packages/datasets/data_files.py](https://localhost:8080/#) in get_data_patterns(base_path, download_config)
457 return _get_data_files_patterns(resolver)
458 except FileNotFoundError:
--> 459 raise EmptyDatasetError(f"The directory at {base_path} doesn't contain any data files") from None
460
461
EmptyDatasetError: The directory at hf://datasets/albertvillanova/tmp-private@79b9e4fe79670a9a050d6ebc385464891915a71d doesn't contain any data files
```
### Expected behavior
The dataset should load.
### Environment info
- `datasets` version: 2.14.3
- Platform: Linux-5.15.109+-x86_64-with-glibc2.35
- Python version: 3.10.12
- Huggingface_hub version: 0.16.4
- PyArrow version: 9.0.0
- Pandas version: 1.5.3 | 39 | Private datasets do not load when passing token
### Describe the bug
Since the release of `datasets` 2.14, private/gated datasets do not load when passing `token`: they raise `EmptyDatasetError`.
This is a non-planned backward incompatible breaking change.
Note that private datasets do load if instead `download_config` is passed:
```python
from datasets import DownloadConfig, load_dataset
ds = load_dataset("albertvillanova/tmp-private", split="train", download_config=DownloadConfig(token="<MY-TOKEN>"))
ds
```
gives
```
Dataset({
features: ['text'],
num_rows: 4
})
```
### Steps to reproduce the bug
```python
from datasets import load_dataset
ds = load_dataset("albertvillanova/tmp-private", split="train", token="<MY-TOKEN>")
```
gives
```
---------------------------------------------------------------------------
EmptyDatasetError Traceback (most recent call last)
[<ipython-input-2-25b48732107a>](https://localhost:8080/#) in <cell line: 3>()
1 from datasets import load_dataset
2
----> 3 ds = load_dataset("albertvillanova/tmp-private", split="train", token="<MY-TOKEN>")
5 frames
[/usr/local/lib/python3.10/dist-packages/datasets/load.py](https://localhost:8080/#) in load_dataset(path, name, data_dir, data_files, split, cache_dir, features, download_config, download_mode, verification_mode, ignore_verifications, keep_in_memory, save_infos, revision, token, use_auth_token, task, streaming, num_proc, storage_options, **config_kwargs)
2107
2108 # Create a dataset builder
-> 2109 builder_instance = load_dataset_builder(
2110 path=path,
2111 name=name,
[/usr/local/lib/python3.10/dist-packages/datasets/load.py](https://localhost:8080/#) in load_dataset_builder(path, name, data_dir, data_files, cache_dir, features, download_config, download_mode, revision, token, use_auth_token, storage_options, **config_kwargs)
1793 download_config = download_config.copy() if download_config else DownloadConfig()
1794 download_config.storage_options.update(storage_options)
-> 1795 dataset_module = dataset_module_factory(
1796 path,
1797 revision=revision,
[/usr/local/lib/python3.10/dist-packages/datasets/load.py](https://localhost:8080/#) in dataset_module_factory(path, revision, download_config, download_mode, dynamic_modules_path, data_dir, data_files, **download_kwargs)
1484 raise ConnectionError(f"Couldn't reach the Hugging Face Hub for dataset '{path}': {e1}") from None
1485 if isinstance(e1, EmptyDatasetError):
-> 1486 raise e1 from None
1487 if isinstance(e1, FileNotFoundError):
1488 raise FileNotFoundError(
[/usr/local/lib/python3.10/dist-packages/datasets/load.py](https://localhost:8080/#) in dataset_module_factory(path, revision, download_config, download_mode, dynamic_modules_path, data_dir, data_files, **download_kwargs)
1474 download_config=download_config,
1475 download_mode=download_mode,
-> 1476 ).get_module()
1477 except (
1478 Exception
[/usr/local/lib/python3.10/dist-packages/datasets/load.py](https://localhost:8080/#) in get_module(self)
1030 sanitize_patterns(self.data_files)
1031 if self.data_files is not None
-> 1032 else get_data_patterns(base_path, download_config=self.download_config)
1033 )
1034 data_files = DataFilesDict.from_patterns(
[/usr/local/lib/python3.10/dist-packages/datasets/data_files.py](https://localhost:8080/#) in get_data_patterns(base_path, download_config)
457 return _get_data_files_patterns(resolver)
458 except FileNotFoundError:
--> 459 raise EmptyDatasetError(f"The directory at {base_path} doesn't contain any data files") from None
460
461
EmptyDatasetError: The directory at hf://datasets/albertvillanova/tmp-private@79b9e4fe79670a9a050d6ebc385464891915a71d doesn't contain any data files
```
### Expected behavior
The dataset should load.
### Environment info
- `datasets` version: 2.14.3
- Platform: Linux-5.15.109+-x86_64-with-glibc2.35
- Python version: 3.10.12
- Huggingface_hub version: 0.16.4
- PyArrow version: 9.0.0
- Pandas version: 1.5.3
We are planning to do a patch release today, after the merge of the fix:
- #6127
In the meantime, the problem can be circumvented by passing `download_config` instead:
```python
from datasets import DownloadConfig, load_dataset
load_dataset("<DATASET-NAME>", split="train", download_config=DownloadConfig(token="<TOKEN>"))
``` |
https://github.com/huggingface/datasets/issues/6126 | Private datasets do not load when passing token | > We are planning to do a patch release today, after the merge of the fix:
>
> * [Fix authentication issues #6127](https://github.com/huggingface/datasets/pull/6127)
>
>
> In the meantime, the problem can be circumvented by passing `download_config` instead:
>
> ```python
> from datasets import DownloadConfig, load_dataset
>
> load_dataset("<DATASET-NAME>", split="train", download_config=DownloadConfig(token="<TOKEN>"))
> ```
This did not work for me (there was some other error with the split being an unexpected size 0). Downgrading to 2.13 fixed it.... | ### Describe the bug
Since the release of `datasets` 2.14, private/gated datasets do not load when passing `token`: they raise `EmptyDatasetError`.
This is a non-planned backward incompatible breaking change.
Note that private datasets do load if instead `download_config` is passed:
```python
from datasets import DownloadConfig, load_dataset
ds = load_dataset("albertvillanova/tmp-private", split="train", download_config=DownloadConfig(token="<MY-TOKEN>"))
ds
```
gives
```
Dataset({
features: ['text'],
num_rows: 4
})
```
### Steps to reproduce the bug
```python
from datasets import load_dataset
ds = load_dataset("albertvillanova/tmp-private", split="train", token="<MY-TOKEN>")
```
gives
```
---------------------------------------------------------------------------
EmptyDatasetError Traceback (most recent call last)
[<ipython-input-2-25b48732107a>](https://localhost:8080/#) in <cell line: 3>()
1 from datasets import load_dataset
2
----> 3 ds = load_dataset("albertvillanova/tmp-private", split="train", token="<MY-TOKEN>")
5 frames
[/usr/local/lib/python3.10/dist-packages/datasets/load.py](https://localhost:8080/#) in load_dataset(path, name, data_dir, data_files, split, cache_dir, features, download_config, download_mode, verification_mode, ignore_verifications, keep_in_memory, save_infos, revision, token, use_auth_token, task, streaming, num_proc, storage_options, **config_kwargs)
2107
2108 # Create a dataset builder
-> 2109 builder_instance = load_dataset_builder(
2110 path=path,
2111 name=name,
[/usr/local/lib/python3.10/dist-packages/datasets/load.py](https://localhost:8080/#) in load_dataset_builder(path, name, data_dir, data_files, cache_dir, features, download_config, download_mode, revision, token, use_auth_token, storage_options, **config_kwargs)
1793 download_config = download_config.copy() if download_config else DownloadConfig()
1794 download_config.storage_options.update(storage_options)
-> 1795 dataset_module = dataset_module_factory(
1796 path,
1797 revision=revision,
[/usr/local/lib/python3.10/dist-packages/datasets/load.py](https://localhost:8080/#) in dataset_module_factory(path, revision, download_config, download_mode, dynamic_modules_path, data_dir, data_files, **download_kwargs)
1484 raise ConnectionError(f"Couldn't reach the Hugging Face Hub for dataset '{path}': {e1}") from None
1485 if isinstance(e1, EmptyDatasetError):
-> 1486 raise e1 from None
1487 if isinstance(e1, FileNotFoundError):
1488 raise FileNotFoundError(
[/usr/local/lib/python3.10/dist-packages/datasets/load.py](https://localhost:8080/#) in dataset_module_factory(path, revision, download_config, download_mode, dynamic_modules_path, data_dir, data_files, **download_kwargs)
1474 download_config=download_config,
1475 download_mode=download_mode,
-> 1476 ).get_module()
1477 except (
1478 Exception
[/usr/local/lib/python3.10/dist-packages/datasets/load.py](https://localhost:8080/#) in get_module(self)
1030 sanitize_patterns(self.data_files)
1031 if self.data_files is not None
-> 1032 else get_data_patterns(base_path, download_config=self.download_config)
1033 )
1034 data_files = DataFilesDict.from_patterns(
[/usr/local/lib/python3.10/dist-packages/datasets/data_files.py](https://localhost:8080/#) in get_data_patterns(base_path, download_config)
457 return _get_data_files_patterns(resolver)
458 except FileNotFoundError:
--> 459 raise EmptyDatasetError(f"The directory at {base_path} doesn't contain any data files") from None
460
461
EmptyDatasetError: The directory at hf://datasets/albertvillanova/tmp-private@79b9e4fe79670a9a050d6ebc385464891915a71d doesn't contain any data files
```
### Expected behavior
The dataset should load.
### Environment info
- `datasets` version: 2.14.3
- Platform: Linux-5.15.109+-x86_64-with-glibc2.35
- Python version: 3.10.12
- Huggingface_hub version: 0.16.4
- PyArrow version: 9.0.0
- Pandas version: 1.5.3 | 78 | Private datasets do not load when passing token
### Describe the bug
Since the release of `datasets` 2.14, private/gated datasets do not load when passing `token`: they raise `EmptyDatasetError`.
This is a non-planned backward incompatible breaking change.
Note that private datasets do load if instead `download_config` is passed:
```python
from datasets import DownloadConfig, load_dataset
ds = load_dataset("albertvillanova/tmp-private", split="train", download_config=DownloadConfig(token="<MY-TOKEN>"))
ds
```
gives
```
Dataset({
features: ['text'],
num_rows: 4
})
```
### Steps to reproduce the bug
```python
from datasets import load_dataset
ds = load_dataset("albertvillanova/tmp-private", split="train", token="<MY-TOKEN>")
```
gives
```
---------------------------------------------------------------------------
EmptyDatasetError Traceback (most recent call last)
[<ipython-input-2-25b48732107a>](https://localhost:8080/#) in <cell line: 3>()
1 from datasets import load_dataset
2
----> 3 ds = load_dataset("albertvillanova/tmp-private", split="train", token="<MY-TOKEN>")
5 frames
[/usr/local/lib/python3.10/dist-packages/datasets/load.py](https://localhost:8080/#) in load_dataset(path, name, data_dir, data_files, split, cache_dir, features, download_config, download_mode, verification_mode, ignore_verifications, keep_in_memory, save_infos, revision, token, use_auth_token, task, streaming, num_proc, storage_options, **config_kwargs)
2107
2108 # Create a dataset builder
-> 2109 builder_instance = load_dataset_builder(
2110 path=path,
2111 name=name,
[/usr/local/lib/python3.10/dist-packages/datasets/load.py](https://localhost:8080/#) in load_dataset_builder(path, name, data_dir, data_files, cache_dir, features, download_config, download_mode, revision, token, use_auth_token, storage_options, **config_kwargs)
1793 download_config = download_config.copy() if download_config else DownloadConfig()
1794 download_config.storage_options.update(storage_options)
-> 1795 dataset_module = dataset_module_factory(
1796 path,
1797 revision=revision,
[/usr/local/lib/python3.10/dist-packages/datasets/load.py](https://localhost:8080/#) in dataset_module_factory(path, revision, download_config, download_mode, dynamic_modules_path, data_dir, data_files, **download_kwargs)
1484 raise ConnectionError(f"Couldn't reach the Hugging Face Hub for dataset '{path}': {e1}") from None
1485 if isinstance(e1, EmptyDatasetError):
-> 1486 raise e1 from None
1487 if isinstance(e1, FileNotFoundError):
1488 raise FileNotFoundError(
[/usr/local/lib/python3.10/dist-packages/datasets/load.py](https://localhost:8080/#) in dataset_module_factory(path, revision, download_config, download_mode, dynamic_modules_path, data_dir, data_files, **download_kwargs)
1474 download_config=download_config,
1475 download_mode=download_mode,
-> 1476 ).get_module()
1477 except (
1478 Exception
[/usr/local/lib/python3.10/dist-packages/datasets/load.py](https://localhost:8080/#) in get_module(self)
1030 sanitize_patterns(self.data_files)
1031 if self.data_files is not None
-> 1032 else get_data_patterns(base_path, download_config=self.download_config)
1033 )
1034 data_files = DataFilesDict.from_patterns(
[/usr/local/lib/python3.10/dist-packages/datasets/data_files.py](https://localhost:8080/#) in get_data_patterns(base_path, download_config)
457 return _get_data_files_patterns(resolver)
458 except FileNotFoundError:
--> 459 raise EmptyDatasetError(f"The directory at {base_path} doesn't contain any data files") from None
460
461
EmptyDatasetError: The directory at hf://datasets/albertvillanova/tmp-private@79b9e4fe79670a9a050d6ebc385464891915a71d doesn't contain any data files
```
### Expected behavior
The dataset should load.
### Environment info
- `datasets` version: 2.14.3
- Platform: Linux-5.15.109+-x86_64-with-glibc2.35
- Python version: 3.10.12
- Huggingface_hub version: 0.16.4
- PyArrow version: 9.0.0
- Pandas version: 1.5.3
> We are planning to do a patch release today, after the merge of the fix:
>
> * [Fix authentication issues #6127](https://github.com/huggingface/datasets/pull/6127)
>
>
> In the meantime, the problem can be circumvented by passing `download_config` instead:
>
> ```python
> from datasets import DownloadConfig, load_dataset
>
> load_dataset("<DATASET-NAME>", split="train", download_config=DownloadConfig(token="<TOKEN>"))
> ```
This did not work for me (there was some other error with the split being an unexpected size 0). Downgrading to 2.13 fixed it.... |
https://github.com/huggingface/datasets/issues/6124 | Datasets crashing runs due to KeyError | i once had the same error and I could fix that by pushing a fake or a dummy commit on my hugging face dataset repo | ### Describe the bug
Hi all,
I have been running into a pretty persistent issue recently when trying to load datasets.
```python
train_dataset = load_dataset(
'llama-2-7b-tokenized',
split = 'train'
)
```
I receive a KeyError which crashes the runs.
```
Traceback (most recent call last):
main()
train_dataset = load_dataset(
^^^^^^^^^^^^^
builder_instance = load_dataset_builder(
^^^^^^^^^^^^^^^^^^^^^
dataset_module = dataset_module_factory(
^^^^^^^^^^^^^^^^^^^^^^^
raise e1 from None
).get_module()
^^^^^^^^^^^^
else get_data_patterns(base_path, download_config=self.download_config)
^^^^^^^^^^^^^^^^^^^^^^^^^^^^^^^^^^^^^^^^^^^^^^^^^^^^^^^^^^^^^^^^^^
return _get_data_files_patterns(resolver)
^^^^^^^^^^^^^^^^^^^^^^^^^^^^^^^^^^
data_files = pattern_resolver(pattern)
^^^^^^^^^^^^^^^^^^^^^^^^^
fs, _, _ = get_fs_token_paths(pattern, storage_options=storage_options)
^^^^^^^^^^^^^^^^^^^^^^^^^^^^^^^^^^^^^^^^^^^^^^^^^^^^^^^^^^^^
paths = [f for f in sorted(fs.glob(paths)) if not fs.isdir(f)]
^^^^^^^^^^^^^^
allpaths = self.find(root, maxdepth=depth, withdirs=True, detail=True, **kwargs)
^^^^^^^^^^^^^^^^^^^^^^^^^^^^^^^^^^^^^^^^^^^^^^^^^^^^^^^^^^^^^^^^^^^^^
for _, dirs, files in self.walk(path, maxdepth, detail=True, **kwargs):
listing = self.ls(path, detail=True, **kwargs)
^^^^^^^^^^^^^^^^^^^^^^^^^^^^^^^^^^^^
"last_modified": parse_datetime(tree_item["lastCommit"]["date"]),
~~~~~~~~~^^^^^^^^^^^^^^
KeyError: 'lastCommit'
```
Any help would be greatly appreciated.
Thank you,
Enrico
### Steps to reproduce the bug
Load the dataset from the Huggingface hub.
```python
train_dataset = load_dataset(
'llama-2-7b-tokenized',
split = 'train'
)
```
### Expected behavior
Loads the dataset.
### Environment info
datasets-2.14.3
CUDA 11.8
Python 3.11 | 25 | Datasets crashing runs due to KeyError
### Describe the bug
Hi all,
I have been running into a pretty persistent issue recently when trying to load datasets.
```python
train_dataset = load_dataset(
'llama-2-7b-tokenized',
split = 'train'
)
```
I receive a KeyError which crashes the runs.
```
Traceback (most recent call last):
main()
train_dataset = load_dataset(
^^^^^^^^^^^^^
builder_instance = load_dataset_builder(
^^^^^^^^^^^^^^^^^^^^^
dataset_module = dataset_module_factory(
^^^^^^^^^^^^^^^^^^^^^^^
raise e1 from None
).get_module()
^^^^^^^^^^^^
else get_data_patterns(base_path, download_config=self.download_config)
^^^^^^^^^^^^^^^^^^^^^^^^^^^^^^^^^^^^^^^^^^^^^^^^^^^^^^^^^^^^^^^^^^
return _get_data_files_patterns(resolver)
^^^^^^^^^^^^^^^^^^^^^^^^^^^^^^^^^^
data_files = pattern_resolver(pattern)
^^^^^^^^^^^^^^^^^^^^^^^^^
fs, _, _ = get_fs_token_paths(pattern, storage_options=storage_options)
^^^^^^^^^^^^^^^^^^^^^^^^^^^^^^^^^^^^^^^^^^^^^^^^^^^^^^^^^^^^
paths = [f for f in sorted(fs.glob(paths)) if not fs.isdir(f)]
^^^^^^^^^^^^^^
allpaths = self.find(root, maxdepth=depth, withdirs=True, detail=True, **kwargs)
^^^^^^^^^^^^^^^^^^^^^^^^^^^^^^^^^^^^^^^^^^^^^^^^^^^^^^^^^^^^^^^^^^^^^
for _, dirs, files in self.walk(path, maxdepth, detail=True, **kwargs):
listing = self.ls(path, detail=True, **kwargs)
^^^^^^^^^^^^^^^^^^^^^^^^^^^^^^^^^^^^
"last_modified": parse_datetime(tree_item["lastCommit"]["date"]),
~~~~~~~~~^^^^^^^^^^^^^^
KeyError: 'lastCommit'
```
Any help would be greatly appreciated.
Thank you,
Enrico
### Steps to reproduce the bug
Load the dataset from the Huggingface hub.
```python
train_dataset = load_dataset(
'llama-2-7b-tokenized',
split = 'train'
)
```
### Expected behavior
Loads the dataset.
### Environment info
datasets-2.14.3
CUDA 11.8
Python 3.11
i once had the same error and I could fix that by pushing a fake or a dummy commit on my hugging face dataset repo |
https://github.com/huggingface/datasets/issues/6124 | Datasets crashing runs due to KeyError | Hi! We need a reproducer to fix this. Can you provide a link to the dataset (if it's public)? | ### Describe the bug
Hi all,
I have been running into a pretty persistent issue recently when trying to load datasets.
```python
train_dataset = load_dataset(
'llama-2-7b-tokenized',
split = 'train'
)
```
I receive a KeyError which crashes the runs.
```
Traceback (most recent call last):
main()
train_dataset = load_dataset(
^^^^^^^^^^^^^
builder_instance = load_dataset_builder(
^^^^^^^^^^^^^^^^^^^^^
dataset_module = dataset_module_factory(
^^^^^^^^^^^^^^^^^^^^^^^
raise e1 from None
).get_module()
^^^^^^^^^^^^
else get_data_patterns(base_path, download_config=self.download_config)
^^^^^^^^^^^^^^^^^^^^^^^^^^^^^^^^^^^^^^^^^^^^^^^^^^^^^^^^^^^^^^^^^^
return _get_data_files_patterns(resolver)
^^^^^^^^^^^^^^^^^^^^^^^^^^^^^^^^^^
data_files = pattern_resolver(pattern)
^^^^^^^^^^^^^^^^^^^^^^^^^
fs, _, _ = get_fs_token_paths(pattern, storage_options=storage_options)
^^^^^^^^^^^^^^^^^^^^^^^^^^^^^^^^^^^^^^^^^^^^^^^^^^^^^^^^^^^^
paths = [f for f in sorted(fs.glob(paths)) if not fs.isdir(f)]
^^^^^^^^^^^^^^
allpaths = self.find(root, maxdepth=depth, withdirs=True, detail=True, **kwargs)
^^^^^^^^^^^^^^^^^^^^^^^^^^^^^^^^^^^^^^^^^^^^^^^^^^^^^^^^^^^^^^^^^^^^^
for _, dirs, files in self.walk(path, maxdepth, detail=True, **kwargs):
listing = self.ls(path, detail=True, **kwargs)
^^^^^^^^^^^^^^^^^^^^^^^^^^^^^^^^^^^^
"last_modified": parse_datetime(tree_item["lastCommit"]["date"]),
~~~~~~~~~^^^^^^^^^^^^^^
KeyError: 'lastCommit'
```
Any help would be greatly appreciated.
Thank you,
Enrico
### Steps to reproduce the bug
Load the dataset from the Huggingface hub.
```python
train_dataset = load_dataset(
'llama-2-7b-tokenized',
split = 'train'
)
```
### Expected behavior
Loads the dataset.
### Environment info
datasets-2.14.3
CUDA 11.8
Python 3.11 | 19 | Datasets crashing runs due to KeyError
### Describe the bug
Hi all,
I have been running into a pretty persistent issue recently when trying to load datasets.
```python
train_dataset = load_dataset(
'llama-2-7b-tokenized',
split = 'train'
)
```
I receive a KeyError which crashes the runs.
```
Traceback (most recent call last):
main()
train_dataset = load_dataset(
^^^^^^^^^^^^^
builder_instance = load_dataset_builder(
^^^^^^^^^^^^^^^^^^^^^
dataset_module = dataset_module_factory(
^^^^^^^^^^^^^^^^^^^^^^^
raise e1 from None
).get_module()
^^^^^^^^^^^^
else get_data_patterns(base_path, download_config=self.download_config)
^^^^^^^^^^^^^^^^^^^^^^^^^^^^^^^^^^^^^^^^^^^^^^^^^^^^^^^^^^^^^^^^^^
return _get_data_files_patterns(resolver)
^^^^^^^^^^^^^^^^^^^^^^^^^^^^^^^^^^
data_files = pattern_resolver(pattern)
^^^^^^^^^^^^^^^^^^^^^^^^^
fs, _, _ = get_fs_token_paths(pattern, storage_options=storage_options)
^^^^^^^^^^^^^^^^^^^^^^^^^^^^^^^^^^^^^^^^^^^^^^^^^^^^^^^^^^^^
paths = [f for f in sorted(fs.glob(paths)) if not fs.isdir(f)]
^^^^^^^^^^^^^^
allpaths = self.find(root, maxdepth=depth, withdirs=True, detail=True, **kwargs)
^^^^^^^^^^^^^^^^^^^^^^^^^^^^^^^^^^^^^^^^^^^^^^^^^^^^^^^^^^^^^^^^^^^^^
for _, dirs, files in self.walk(path, maxdepth, detail=True, **kwargs):
listing = self.ls(path, detail=True, **kwargs)
^^^^^^^^^^^^^^^^^^^^^^^^^^^^^^^^^^^^
"last_modified": parse_datetime(tree_item["lastCommit"]["date"]),
~~~~~~~~~^^^^^^^^^^^^^^
KeyError: 'lastCommit'
```
Any help would be greatly appreciated.
Thank you,
Enrico
### Steps to reproduce the bug
Load the dataset from the Huggingface hub.
```python
train_dataset = load_dataset(
'llama-2-7b-tokenized',
split = 'train'
)
```
### Expected behavior
Loads the dataset.
### Environment info
datasets-2.14.3
CUDA 11.8
Python 3.11
Hi! We need a reproducer to fix this. Can you provide a link to the dataset (if it's public)? |
https://github.com/huggingface/datasets/issues/6124 | Datasets crashing runs due to KeyError | > Hi! We need a reproducer to fix this. Can you provide a link to the dataset (if it's public)?
Hi Mario,
Unfortunately, the dataset in question is currently private until the model is trained and released.
This is not happening with one dataset but numerous hosted private datasets.
I am only loading the dataset and doing nothing else currently. It seems to happen completely sporadically.
Thank you,
Enrico | ### Describe the bug
Hi all,
I have been running into a pretty persistent issue recently when trying to load datasets.
```python
train_dataset = load_dataset(
'llama-2-7b-tokenized',
split = 'train'
)
```
I receive a KeyError which crashes the runs.
```
Traceback (most recent call last):
main()
train_dataset = load_dataset(
^^^^^^^^^^^^^
builder_instance = load_dataset_builder(
^^^^^^^^^^^^^^^^^^^^^
dataset_module = dataset_module_factory(
^^^^^^^^^^^^^^^^^^^^^^^
raise e1 from None
).get_module()
^^^^^^^^^^^^
else get_data_patterns(base_path, download_config=self.download_config)
^^^^^^^^^^^^^^^^^^^^^^^^^^^^^^^^^^^^^^^^^^^^^^^^^^^^^^^^^^^^^^^^^^
return _get_data_files_patterns(resolver)
^^^^^^^^^^^^^^^^^^^^^^^^^^^^^^^^^^
data_files = pattern_resolver(pattern)
^^^^^^^^^^^^^^^^^^^^^^^^^
fs, _, _ = get_fs_token_paths(pattern, storage_options=storage_options)
^^^^^^^^^^^^^^^^^^^^^^^^^^^^^^^^^^^^^^^^^^^^^^^^^^^^^^^^^^^^
paths = [f for f in sorted(fs.glob(paths)) if not fs.isdir(f)]
^^^^^^^^^^^^^^
allpaths = self.find(root, maxdepth=depth, withdirs=True, detail=True, **kwargs)
^^^^^^^^^^^^^^^^^^^^^^^^^^^^^^^^^^^^^^^^^^^^^^^^^^^^^^^^^^^^^^^^^^^^^
for _, dirs, files in self.walk(path, maxdepth, detail=True, **kwargs):
listing = self.ls(path, detail=True, **kwargs)
^^^^^^^^^^^^^^^^^^^^^^^^^^^^^^^^^^^^
"last_modified": parse_datetime(tree_item["lastCommit"]["date"]),
~~~~~~~~~^^^^^^^^^^^^^^
KeyError: 'lastCommit'
```
Any help would be greatly appreciated.
Thank you,
Enrico
### Steps to reproduce the bug
Load the dataset from the Huggingface hub.
```python
train_dataset = load_dataset(
'llama-2-7b-tokenized',
split = 'train'
)
```
### Expected behavior
Loads the dataset.
### Environment info
datasets-2.14.3
CUDA 11.8
Python 3.11 | 69 | Datasets crashing runs due to KeyError
### Describe the bug
Hi all,
I have been running into a pretty persistent issue recently when trying to load datasets.
```python
train_dataset = load_dataset(
'llama-2-7b-tokenized',
split = 'train'
)
```
I receive a KeyError which crashes the runs.
```
Traceback (most recent call last):
main()
train_dataset = load_dataset(
^^^^^^^^^^^^^
builder_instance = load_dataset_builder(
^^^^^^^^^^^^^^^^^^^^^
dataset_module = dataset_module_factory(
^^^^^^^^^^^^^^^^^^^^^^^
raise e1 from None
).get_module()
^^^^^^^^^^^^
else get_data_patterns(base_path, download_config=self.download_config)
^^^^^^^^^^^^^^^^^^^^^^^^^^^^^^^^^^^^^^^^^^^^^^^^^^^^^^^^^^^^^^^^^^
return _get_data_files_patterns(resolver)
^^^^^^^^^^^^^^^^^^^^^^^^^^^^^^^^^^
data_files = pattern_resolver(pattern)
^^^^^^^^^^^^^^^^^^^^^^^^^
fs, _, _ = get_fs_token_paths(pattern, storage_options=storage_options)
^^^^^^^^^^^^^^^^^^^^^^^^^^^^^^^^^^^^^^^^^^^^^^^^^^^^^^^^^^^^
paths = [f for f in sorted(fs.glob(paths)) if not fs.isdir(f)]
^^^^^^^^^^^^^^
allpaths = self.find(root, maxdepth=depth, withdirs=True, detail=True, **kwargs)
^^^^^^^^^^^^^^^^^^^^^^^^^^^^^^^^^^^^^^^^^^^^^^^^^^^^^^^^^^^^^^^^^^^^^
for _, dirs, files in self.walk(path, maxdepth, detail=True, **kwargs):
listing = self.ls(path, detail=True, **kwargs)
^^^^^^^^^^^^^^^^^^^^^^^^^^^^^^^^^^^^
"last_modified": parse_datetime(tree_item["lastCommit"]["date"]),
~~~~~~~~~^^^^^^^^^^^^^^
KeyError: 'lastCommit'
```
Any help would be greatly appreciated.
Thank you,
Enrico
### Steps to reproduce the bug
Load the dataset from the Huggingface hub.
```python
train_dataset = load_dataset(
'llama-2-7b-tokenized',
split = 'train'
)
```
### Expected behavior
Loads the dataset.
### Environment info
datasets-2.14.3
CUDA 11.8
Python 3.11
> Hi! We need a reproducer to fix this. Can you provide a link to the dataset (if it's public)?
Hi Mario,
Unfortunately, the dataset in question is currently private until the model is trained and released.
This is not happening with one dataset but numerous hosted private datasets.
I am only loading the dataset and doing nothing else currently. It seems to happen completely sporadically.
Thank you,
Enrico |
https://github.com/huggingface/datasets/issues/6123 | Inaccurate Bounding Boxes in "wildreceipt" Dataset | Hi! Thanks for the investigation, but we are not the authors of these datasets, so please report this on the Hub instead so that the actual authors can fix it. | ### Describe the bug
I would like to bring to your attention an issue related to the accuracy of bounding boxes within the "wildreceipt" dataset, which is made available through the Hugging Face API. Specifically, I have identified a discrepancy between the bounding boxes generated by the dataset loading commands, namely `load_dataset("Theivaprakasham/wildreceipt")` and `load_dataset("jinhybr/WildReceipt")`, and the actual labels and corresponding bounding boxes present in the dataset.
To illustrate this divergence, I've provided two examples in the form of screenshots. These screenshots highlight the contrasting outcomes between my personal implementation of the dataloader and the implementation offered by Hugging Face:
**Example 1:**



**Example 2:**
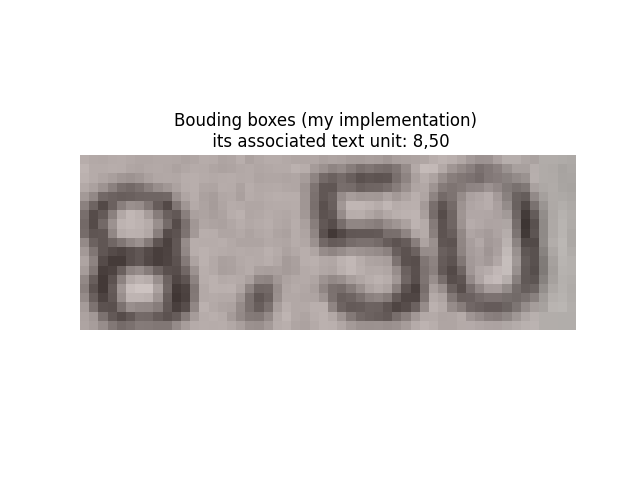


It's important to note that my dataloader implementation is based on the same dataset files as utilized in the Hugging Face implementation. For your reference, you can access the dataset files through this link: [wildreceipt dataset files](https://download.openmmlab.com/mmocr/data/wildreceipt.tar).
This inconsistency in bounding box accuracy warrants investigation and rectification for maintaining the integrity of the "wildreceipt" dataset. Your attention and assistance in addressing this matter would be greatly appreciated.
### Steps to reproduce the bug
```python
import matplotlib.pyplot as plt
from datasets import load_dataset
# Define functions to convert bounding box formats
def convert_format1(box):
x, y, w, h = box
x2, y2 = x + w, y + h
return [x, y, x2, y2]
def convert_format2(box):
x1, y1, x2, y2 = box
return [x1, y1, x2, y2]
def plot_cropped_image(image, box, title):
cropped_image = image.crop(box)
plt.imshow(cropped_image)
plt.title(title)
plt.axis('off')
plt.savefig(title+'.png')
plt.show()
doc_index = 1
word_index = 3
dataset = load_dataset("Theivaprakasham/wildreceipt")['train']
bbox_hugging_face = dataset[doc_index]['bboxes'][word_index]
text_unit_face = dataset[doc_index]['words'][word_index]
common_box_hugface_1 = convert_format1(bbox_hugging_face)
common_box_hugface_2 = convert_format2(bbox_hugging_face)
plot_cropped_image(image_hugging, common_box_hugface_1,
f'Hugging Face Bouding boxes (x,y,w,h format) \n its associated text unit: {text_unit_face}')
plot_cropped_image(image_hugging, common_box_hugface_2,
f'Hugging Face Bouding boxes (x1,y1,x2, y2 format) \n its associated text unit: {text_unit_face}')
```
### Expected behavior
The bounding boxes generated by the "wildreceipt" dataset in HuggingFace implementation loading commands should accurately match the actual labels and bounding boxes of the dataset.
### Environment info
- Python version: 3.8
- Hugging Face datasets version: 2.14.2
- Dataset file taken from this link: https://download.openmmlab.com/mmocr/data/wildreceipt.tar | 30 | Inaccurate Bounding Boxes in "wildreceipt" Dataset
### Describe the bug
I would like to bring to your attention an issue related to the accuracy of bounding boxes within the "wildreceipt" dataset, which is made available through the Hugging Face API. Specifically, I have identified a discrepancy between the bounding boxes generated by the dataset loading commands, namely `load_dataset("Theivaprakasham/wildreceipt")` and `load_dataset("jinhybr/WildReceipt")`, and the actual labels and corresponding bounding boxes present in the dataset.
To illustrate this divergence, I've provided two examples in the form of screenshots. These screenshots highlight the contrasting outcomes between my personal implementation of the dataloader and the implementation offered by Hugging Face:
**Example 1:**



**Example 2:**
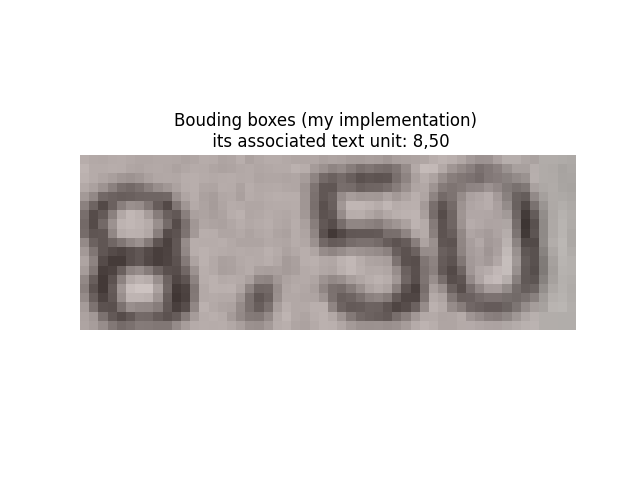


It's important to note that my dataloader implementation is based on the same dataset files as utilized in the Hugging Face implementation. For your reference, you can access the dataset files through this link: [wildreceipt dataset files](https://download.openmmlab.com/mmocr/data/wildreceipt.tar).
This inconsistency in bounding box accuracy warrants investigation and rectification for maintaining the integrity of the "wildreceipt" dataset. Your attention and assistance in addressing this matter would be greatly appreciated.
### Steps to reproduce the bug
```python
import matplotlib.pyplot as plt
from datasets import load_dataset
# Define functions to convert bounding box formats
def convert_format1(box):
x, y, w, h = box
x2, y2 = x + w, y + h
return [x, y, x2, y2]
def convert_format2(box):
x1, y1, x2, y2 = box
return [x1, y1, x2, y2]
def plot_cropped_image(image, box, title):
cropped_image = image.crop(box)
plt.imshow(cropped_image)
plt.title(title)
plt.axis('off')
plt.savefig(title+'.png')
plt.show()
doc_index = 1
word_index = 3
dataset = load_dataset("Theivaprakasham/wildreceipt")['train']
bbox_hugging_face = dataset[doc_index]['bboxes'][word_index]
text_unit_face = dataset[doc_index]['words'][word_index]
common_box_hugface_1 = convert_format1(bbox_hugging_face)
common_box_hugface_2 = convert_format2(bbox_hugging_face)
plot_cropped_image(image_hugging, common_box_hugface_1,
f'Hugging Face Bouding boxes (x,y,w,h format) \n its associated text unit: {text_unit_face}')
plot_cropped_image(image_hugging, common_box_hugface_2,
f'Hugging Face Bouding boxes (x1,y1,x2, y2 format) \n its associated text unit: {text_unit_face}')
```
### Expected behavior
The bounding boxes generated by the "wildreceipt" dataset in HuggingFace implementation loading commands should accurately match the actual labels and bounding boxes of the dataset.
### Environment info
- Python version: 3.8
- Hugging Face datasets version: 2.14.2
- Dataset file taken from this link: https://download.openmmlab.com/mmocr/data/wildreceipt.tar
Hi! Thanks for the investigation, but we are not the authors of these datasets, so please report this on the Hub instead so that the actual authors can fix it. |
https://github.com/huggingface/datasets/issues/6120 | Lookahead streaming support? | In which format is your dataset? We could expose the `pre_buffer` flag for Parquet to use PyArrow's background thread pool to speed up loading. | ### Feature request
From what I understand, streaming dataset currently pulls the data, and process the data as it is requested.
This can introduce significant latency delays when data is loaded into the training process, needing to wait for each segment.
While the delays might be dataset specific (or even mapping instruction/tokenizer specific)
Is it possible to introduce a `streaming_lookahead` parameter, which is used for predictable workloads (even shuffled dataset with fixed seed). As we can predict in advance what the next few datasamples will be. And fetch them while the current set is being trained.
With enough CPU & bandwidth to keep up with the training process, and a sufficiently large lookahead, this will reduce the various latency involved while waiting for the dataset to be ready between batches.
### Motivation
Faster streaming performance, while training over extra large TB sized datasets
### Your contribution
I currently use HF dataset, with pytorch lightning trainer for RWKV project, and would be able to help test this feature if supported. | 24 | Lookahead streaming support?
### Feature request
From what I understand, streaming dataset currently pulls the data, and process the data as it is requested.
This can introduce significant latency delays when data is loaded into the training process, needing to wait for each segment.
While the delays might be dataset specific (or even mapping instruction/tokenizer specific)
Is it possible to introduce a `streaming_lookahead` parameter, which is used for predictable workloads (even shuffled dataset with fixed seed). As we can predict in advance what the next few datasamples will be. And fetch them while the current set is being trained.
With enough CPU & bandwidth to keep up with the training process, and a sufficiently large lookahead, this will reduce the various latency involved while waiting for the dataset to be ready between batches.
### Motivation
Faster streaming performance, while training over extra large TB sized datasets
### Your contribution
I currently use HF dataset, with pytorch lightning trainer for RWKV project, and would be able to help test this feature if supported.
In which format is your dataset? We could expose the `pre_buffer` flag for Parquet to use PyArrow's background thread pool to speed up loading. |
https://github.com/huggingface/datasets/issues/6118 | IterableDataset.from_generator() fails with pickle error when provided a generator or iterator | Hi! `IterableDataset.from_generator` expects a generator function, not the object (to be consistent with `Dataset.from_generator`).
You can fix the above snippet as follows:
```python
train_dataset = IterableDataset.from_generator(line_generator, fn_kwargs={"files": model_training_files})
``` | ### Describe the bug
**Description**
Providing a generator in an instantiation of IterableDataset.from_generator() fails with `TypeError: cannot pickle 'generator' object` when the generator argument is supplied with a generator.
**Code example**
```
def line_generator(files: List[Path]):
if isinstance(files, str):
files = [Path(files)]
for file in files:
if isinstance(file, str):
file = Path(file)
yield from open(file,'r').readlines()
...
model_training_files = ['file1.txt', 'file2.txt', 'file3.txt']
train_dataset = IterableDataset.from_generator(generator=line_generator(model_training_files))
```
**Traceback**
Traceback (most recent call last):
File "/Library/Developer/CommandLineTools/Library/Frameworks/Python3.framework/Versions/3.9/lib/python3.9/contextlib.py", line 135, in __exit__
self.gen.throw(type, value, traceback)
File "/Users/d3p692/code/clem_bert/venv/lib/python3.9/site-packages/datasets/utils/py_utils.py", line 691, in _no_cache_fields
yield
File "/Users/d3p692/code/clem_bert/venv/lib/python3.9/site-packages/datasets/utils/py_utils.py", line 701, in dumps
dump(obj, file)
File "/Users/d3p692/code/clem_bert/venv/lib/python3.9/site-packages/datasets/utils/py_utils.py", line 676, in dump
Pickler(file, recurse=True).dump(obj)
File "/Users/d3p692/code/clem_bert/venv/lib/python3.9/site-packages/dill/_dill.py", line 394, in dump
StockPickler.dump(self, obj)
File "/Library/Developer/CommandLineTools/Library/Frameworks/Python3.framework/Versions/3.9/lib/python3.9/pickle.py", line 487, in dump
self.save(obj)
File "/Users/d3p692/code/clem_bert/venv/lib/python3.9/site-packages/datasets/utils/py_utils.py", line 666, in save
dill.Pickler.save(self, obj, save_persistent_id=save_persistent_id)
File "/Users/d3p692/code/clem_bert/venv/lib/python3.9/site-packages/dill/_dill.py", line 388, in save
StockPickler.save(self, obj, save_persistent_id)
File "/Library/Developer/CommandLineTools/Library/Frameworks/Python3.framework/Versions/3.9/lib/python3.9/pickle.py", line 560, in save
f(self, obj) # Call unbound method with explicit self
File "/Users/d3p692/code/clem_bert/venv/lib/python3.9/site-packages/dill/_dill.py", line 1186, in save_module_dict
StockPickler.save_dict(pickler, obj)
File "/Library/Developer/CommandLineTools/Library/Frameworks/Python3.framework/Versions/3.9/lib/python3.9/pickle.py", line 971, in save_dict
self._batch_setitems(obj.items())
File "/Library/Developer/CommandLineTools/Library/Frameworks/Python3.framework/Versions/3.9/lib/python3.9/pickle.py", line 997, in _batch_setitems
save(v)
File "/Users/d3p692/code/clem_bert/venv/lib/python3.9/site-packages/datasets/utils/py_utils.py", line 666, in save
dill.Pickler.save(self, obj, save_persistent_id=save_persistent_id)
File "/Users/d3p692/code/clem_bert/venv/lib/python3.9/site-packages/dill/_dill.py", line 388, in save
StockPickler.save(self, obj, save_persistent_id)
File "/Library/Developer/CommandLineTools/Library/Frameworks/Python3.framework/Versions/3.9/lib/python3.9/pickle.py", line 578, in save
rv = reduce(self.proto)
TypeError: cannot pickle 'generator' object
### Steps to reproduce the bug
1. Create a set of text files to iterate over.
2. Create a generator that returns the lines in each file until all files are exhausted.
3. Instantiate the dataset over the generator by instantiating an IterableDataset.from_generator().
4. Wait for the explosion.
### Expected behavior
I would expect that since the function claims to accept a generator that there would be no crash. Instead, I would expect the dataset to return all the lines in the files as queued up in the `line_generator()` function.
### Environment info
datasets.__version__ == '2.13.1'
Python 3.9.6
Platform: Darwin WE35261 22.5.0 Darwin Kernel Version 22.5.0: Thu Jun 8 22:22:22 PDT 2023; root:xnu-8796.121.3~7/RELEASE_X86_64 x86_64
| 29 | IterableDataset.from_generator() fails with pickle error when provided a generator or iterator
### Describe the bug
**Description**
Providing a generator in an instantiation of IterableDataset.from_generator() fails with `TypeError: cannot pickle 'generator' object` when the generator argument is supplied with a generator.
**Code example**
```
def line_generator(files: List[Path]):
if isinstance(files, str):
files = [Path(files)]
for file in files:
if isinstance(file, str):
file = Path(file)
yield from open(file,'r').readlines()
...
model_training_files = ['file1.txt', 'file2.txt', 'file3.txt']
train_dataset = IterableDataset.from_generator(generator=line_generator(model_training_files))
```
**Traceback**
Traceback (most recent call last):
File "/Library/Developer/CommandLineTools/Library/Frameworks/Python3.framework/Versions/3.9/lib/python3.9/contextlib.py", line 135, in __exit__
self.gen.throw(type, value, traceback)
File "/Users/d3p692/code/clem_bert/venv/lib/python3.9/site-packages/datasets/utils/py_utils.py", line 691, in _no_cache_fields
yield
File "/Users/d3p692/code/clem_bert/venv/lib/python3.9/site-packages/datasets/utils/py_utils.py", line 701, in dumps
dump(obj, file)
File "/Users/d3p692/code/clem_bert/venv/lib/python3.9/site-packages/datasets/utils/py_utils.py", line 676, in dump
Pickler(file, recurse=True).dump(obj)
File "/Users/d3p692/code/clem_bert/venv/lib/python3.9/site-packages/dill/_dill.py", line 394, in dump
StockPickler.dump(self, obj)
File "/Library/Developer/CommandLineTools/Library/Frameworks/Python3.framework/Versions/3.9/lib/python3.9/pickle.py", line 487, in dump
self.save(obj)
File "/Users/d3p692/code/clem_bert/venv/lib/python3.9/site-packages/datasets/utils/py_utils.py", line 666, in save
dill.Pickler.save(self, obj, save_persistent_id=save_persistent_id)
File "/Users/d3p692/code/clem_bert/venv/lib/python3.9/site-packages/dill/_dill.py", line 388, in save
StockPickler.save(self, obj, save_persistent_id)
File "/Library/Developer/CommandLineTools/Library/Frameworks/Python3.framework/Versions/3.9/lib/python3.9/pickle.py", line 560, in save
f(self, obj) # Call unbound method with explicit self
File "/Users/d3p692/code/clem_bert/venv/lib/python3.9/site-packages/dill/_dill.py", line 1186, in save_module_dict
StockPickler.save_dict(pickler, obj)
File "/Library/Developer/CommandLineTools/Library/Frameworks/Python3.framework/Versions/3.9/lib/python3.9/pickle.py", line 971, in save_dict
self._batch_setitems(obj.items())
File "/Library/Developer/CommandLineTools/Library/Frameworks/Python3.framework/Versions/3.9/lib/python3.9/pickle.py", line 997, in _batch_setitems
save(v)
File "/Users/d3p692/code/clem_bert/venv/lib/python3.9/site-packages/datasets/utils/py_utils.py", line 666, in save
dill.Pickler.save(self, obj, save_persistent_id=save_persistent_id)
File "/Users/d3p692/code/clem_bert/venv/lib/python3.9/site-packages/dill/_dill.py", line 388, in save
StockPickler.save(self, obj, save_persistent_id)
File "/Library/Developer/CommandLineTools/Library/Frameworks/Python3.framework/Versions/3.9/lib/python3.9/pickle.py", line 578, in save
rv = reduce(self.proto)
TypeError: cannot pickle 'generator' object
### Steps to reproduce the bug
1. Create a set of text files to iterate over.
2. Create a generator that returns the lines in each file until all files are exhausted.
3. Instantiate the dataset over the generator by instantiating an IterableDataset.from_generator().
4. Wait for the explosion.
### Expected behavior
I would expect that since the function claims to accept a generator that there would be no crash. Instead, I would expect the dataset to return all the lines in the files as queued up in the `line_generator()` function.
### Environment info
datasets.__version__ == '2.13.1'
Python 3.9.6
Platform: Darwin WE35261 22.5.0 Darwin Kernel Version 22.5.0: Thu Jun 8 22:22:22 PDT 2023; root:xnu-8796.121.3~7/RELEASE_X86_64 x86_64
Hi! `IterableDataset.from_generator` expects a generator function, not the object (to be consistent with `Dataset.from_generator`).
You can fix the above snippet as follows:
```python
train_dataset = IterableDataset.from_generator(line_generator, fn_kwargs={"files": model_training_files})
``` |
https://github.com/huggingface/datasets/issues/6114 | Cache not being used when loading commonvoice 8.0.0 | You can avoid this by using the `revision` parameter in `load_dataset` to always force downloading a specific commit (if not specified it defaults to HEAD, hence the redownload). | ### Describe the bug
I have commonvoice 8.0.0 downloaded in `~/.cache/huggingface/datasets/mozilla-foundation___common_voice_8_0/en/8.0.0/b2f8b72f8f30b2e98c41ccf855954d9e35a5fa498c43332df198534ff9797a4a`. The folder contains all the arrow files etc, and was used as the cached version last time I touched the ec2 instance I'm working on. Now, with the same command that downloaded it initially:
```
dataset = load_dataset("mozilla-foundation/common_voice_8_0", "en", use_auth_token="<mytoken>")
```
it tries to redownload the dataset to `~/.cache/huggingface/datasets/mozilla-foundation___common_voice_8_0/en/8.0.0/05bdc7940b0a336ceeaeef13470c89522c29a8e4494cbeece64fb472a87acb32`
### Steps to reproduce the bug
Steps to reproduce the behavior:
1. ```dataset = load_dataset("mozilla-foundation/common_voice_8_0", "en", use_auth_token="<mytoken>")```
2. dataset is updated by maintainers
3. ```dataset = load_dataset("mozilla-foundation/common_voice_8_0", "en", use_auth_token="<mytoken>")```
### Expected behavior
I expect that it uses the already downloaded data in `~/.cache/huggingface/datasets/mozilla-foundation___common_voice_8_0/en/8.0.0/b2f8b72f8f30b2e98c41ccf855954d9e35a5fa498c43332df198534ff9797a4a`.
Not sure what's happening in 2. but if, say it's an issue with the dataset referenced by "mozilla-foundation/common_voice_8_0" being modified by the maintainers, how would I force datasets to point to the original version I downloaded?
EDIT: It was indeed that the maintainers had updated the dataset (v 8.0.0). However I still cant load the dataset from disk instead of redownloading, with for example:
```
load_dataset(".cache/huggingface/datasets/downloads/extracted/<hash>/cv-corpus-8.0-2022-01-19/en/", "en")
> ...
> File [~/miniconda3/envs/aa_torch2/lib/python3.10/site-packages/datasets/table.py:1938](.../ python3.10/site-packages/datasets/table.py:1938), in cast_array_to_feature(array, feature, allow_number_to_str)
1937 elif not isinstance(feature, (Sequence, dict, list, tuple)):
-> 1938 return array_cast(array, feature(), allow_number_to_str=allow_number_to_str)
...
1794 e = e.__context__
-> 1795 raise DatasetGenerationError("An error occurred while generating the dataset") from e
1797 yield job_id, True, (total_num_examples, total_num_bytes, writer._features, num_shards, shard_lengths)
DatasetGenerationError: An error occurred while generating the dataset
```
### Environment info
datasets==2.7.0
python==3.10.8
OS: AWS Linux | 28 | Cache not being used when loading commonvoice 8.0.0
### Describe the bug
I have commonvoice 8.0.0 downloaded in `~/.cache/huggingface/datasets/mozilla-foundation___common_voice_8_0/en/8.0.0/b2f8b72f8f30b2e98c41ccf855954d9e35a5fa498c43332df198534ff9797a4a`. The folder contains all the arrow files etc, and was used as the cached version last time I touched the ec2 instance I'm working on. Now, with the same command that downloaded it initially:
```
dataset = load_dataset("mozilla-foundation/common_voice_8_0", "en", use_auth_token="<mytoken>")
```
it tries to redownload the dataset to `~/.cache/huggingface/datasets/mozilla-foundation___common_voice_8_0/en/8.0.0/05bdc7940b0a336ceeaeef13470c89522c29a8e4494cbeece64fb472a87acb32`
### Steps to reproduce the bug
Steps to reproduce the behavior:
1. ```dataset = load_dataset("mozilla-foundation/common_voice_8_0", "en", use_auth_token="<mytoken>")```
2. dataset is updated by maintainers
3. ```dataset = load_dataset("mozilla-foundation/common_voice_8_0", "en", use_auth_token="<mytoken>")```
### Expected behavior
I expect that it uses the already downloaded data in `~/.cache/huggingface/datasets/mozilla-foundation___common_voice_8_0/en/8.0.0/b2f8b72f8f30b2e98c41ccf855954d9e35a5fa498c43332df198534ff9797a4a`.
Not sure what's happening in 2. but if, say it's an issue with the dataset referenced by "mozilla-foundation/common_voice_8_0" being modified by the maintainers, how would I force datasets to point to the original version I downloaded?
EDIT: It was indeed that the maintainers had updated the dataset (v 8.0.0). However I still cant load the dataset from disk instead of redownloading, with for example:
```
load_dataset(".cache/huggingface/datasets/downloads/extracted/<hash>/cv-corpus-8.0-2022-01-19/en/", "en")
> ...
> File [~/miniconda3/envs/aa_torch2/lib/python3.10/site-packages/datasets/table.py:1938](.../ python3.10/site-packages/datasets/table.py:1938), in cast_array_to_feature(array, feature, allow_number_to_str)
1937 elif not isinstance(feature, (Sequence, dict, list, tuple)):
-> 1938 return array_cast(array, feature(), allow_number_to_str=allow_number_to_str)
...
1794 e = e.__context__
-> 1795 raise DatasetGenerationError("An error occurred while generating the dataset") from e
1797 yield job_id, True, (total_num_examples, total_num_bytes, writer._features, num_shards, shard_lengths)
DatasetGenerationError: An error occurred while generating the dataset
```
### Environment info
datasets==2.7.0
python==3.10.8
OS: AWS Linux
You can avoid this by using the `revision` parameter in `load_dataset` to always force downloading a specific commit (if not specified it defaults to HEAD, hence the redownload). |
https://github.com/huggingface/datasets/issues/6114 | Cache not being used when loading commonvoice 8.0.0 | Thanks @mariosasko this works well, looks like I should have read the documentation a bit more carefully.
It is still a bit confusing which hash I should provide: passing `revision = c8fd66e85f086e3abb11eeee55b1737a3d1e8487` from https://huggingface.co/datasets/mozilla-foundation/common_voice_8_0/commits/main caused the cached version at `~/.cache/huggingface/datasets/mozilla-foundation___common_voice_8_0/en/8.0.0/b2f8b72f8f30b2e98c41ccf855954d9e35a5fa498c43332df198534ff9797a4a` to be loaded, so I had to know that it was the previous commit unless I've missed something else. | ### Describe the bug
I have commonvoice 8.0.0 downloaded in `~/.cache/huggingface/datasets/mozilla-foundation___common_voice_8_0/en/8.0.0/b2f8b72f8f30b2e98c41ccf855954d9e35a5fa498c43332df198534ff9797a4a`. The folder contains all the arrow files etc, and was used as the cached version last time I touched the ec2 instance I'm working on. Now, with the same command that downloaded it initially:
```
dataset = load_dataset("mozilla-foundation/common_voice_8_0", "en", use_auth_token="<mytoken>")
```
it tries to redownload the dataset to `~/.cache/huggingface/datasets/mozilla-foundation___common_voice_8_0/en/8.0.0/05bdc7940b0a336ceeaeef13470c89522c29a8e4494cbeece64fb472a87acb32`
### Steps to reproduce the bug
Steps to reproduce the behavior:
1. ```dataset = load_dataset("mozilla-foundation/common_voice_8_0", "en", use_auth_token="<mytoken>")```
2. dataset is updated by maintainers
3. ```dataset = load_dataset("mozilla-foundation/common_voice_8_0", "en", use_auth_token="<mytoken>")```
### Expected behavior
I expect that it uses the already downloaded data in `~/.cache/huggingface/datasets/mozilla-foundation___common_voice_8_0/en/8.0.0/b2f8b72f8f30b2e98c41ccf855954d9e35a5fa498c43332df198534ff9797a4a`.
Not sure what's happening in 2. but if, say it's an issue with the dataset referenced by "mozilla-foundation/common_voice_8_0" being modified by the maintainers, how would I force datasets to point to the original version I downloaded?
EDIT: It was indeed that the maintainers had updated the dataset (v 8.0.0). However I still cant load the dataset from disk instead of redownloading, with for example:
```
load_dataset(".cache/huggingface/datasets/downloads/extracted/<hash>/cv-corpus-8.0-2022-01-19/en/", "en")
> ...
> File [~/miniconda3/envs/aa_torch2/lib/python3.10/site-packages/datasets/table.py:1938](.../ python3.10/site-packages/datasets/table.py:1938), in cast_array_to_feature(array, feature, allow_number_to_str)
1937 elif not isinstance(feature, (Sequence, dict, list, tuple)):
-> 1938 return array_cast(array, feature(), allow_number_to_str=allow_number_to_str)
...
1794 e = e.__context__
-> 1795 raise DatasetGenerationError("An error occurred while generating the dataset") from e
1797 yield job_id, True, (total_num_examples, total_num_bytes, writer._features, num_shards, shard_lengths)
DatasetGenerationError: An error occurred while generating the dataset
```
### Environment info
datasets==2.7.0
python==3.10.8
OS: AWS Linux | 59 | Cache not being used when loading commonvoice 8.0.0
### Describe the bug
I have commonvoice 8.0.0 downloaded in `~/.cache/huggingface/datasets/mozilla-foundation___common_voice_8_0/en/8.0.0/b2f8b72f8f30b2e98c41ccf855954d9e35a5fa498c43332df198534ff9797a4a`. The folder contains all the arrow files etc, and was used as the cached version last time I touched the ec2 instance I'm working on. Now, with the same command that downloaded it initially:
```
dataset = load_dataset("mozilla-foundation/common_voice_8_0", "en", use_auth_token="<mytoken>")
```
it tries to redownload the dataset to `~/.cache/huggingface/datasets/mozilla-foundation___common_voice_8_0/en/8.0.0/05bdc7940b0a336ceeaeef13470c89522c29a8e4494cbeece64fb472a87acb32`
### Steps to reproduce the bug
Steps to reproduce the behavior:
1. ```dataset = load_dataset("mozilla-foundation/common_voice_8_0", "en", use_auth_token="<mytoken>")```
2. dataset is updated by maintainers
3. ```dataset = load_dataset("mozilla-foundation/common_voice_8_0", "en", use_auth_token="<mytoken>")```
### Expected behavior
I expect that it uses the already downloaded data in `~/.cache/huggingface/datasets/mozilla-foundation___common_voice_8_0/en/8.0.0/b2f8b72f8f30b2e98c41ccf855954d9e35a5fa498c43332df198534ff9797a4a`.
Not sure what's happening in 2. but if, say it's an issue with the dataset referenced by "mozilla-foundation/common_voice_8_0" being modified by the maintainers, how would I force datasets to point to the original version I downloaded?
EDIT: It was indeed that the maintainers had updated the dataset (v 8.0.0). However I still cant load the dataset from disk instead of redownloading, with for example:
```
load_dataset(".cache/huggingface/datasets/downloads/extracted/<hash>/cv-corpus-8.0-2022-01-19/en/", "en")
> ...
> File [~/miniconda3/envs/aa_torch2/lib/python3.10/site-packages/datasets/table.py:1938](.../ python3.10/site-packages/datasets/table.py:1938), in cast_array_to_feature(array, feature, allow_number_to_str)
1937 elif not isinstance(feature, (Sequence, dict, list, tuple)):
-> 1938 return array_cast(array, feature(), allow_number_to_str=allow_number_to_str)
...
1794 e = e.__context__
-> 1795 raise DatasetGenerationError("An error occurred while generating the dataset") from e
1797 yield job_id, True, (total_num_examples, total_num_bytes, writer._features, num_shards, shard_lengths)
DatasetGenerationError: An error occurred while generating the dataset
```
### Environment info
datasets==2.7.0
python==3.10.8
OS: AWS Linux
Thanks @mariosasko this works well, looks like I should have read the documentation a bit more carefully.
It is still a bit confusing which hash I should provide: passing `revision = c8fd66e85f086e3abb11eeee55b1737a3d1e8487` from https://huggingface.co/datasets/mozilla-foundation/common_voice_8_0/commits/main caused the cached version at `~/.cache/huggingface/datasets/mozilla-foundation___common_voice_8_0/en/8.0.0/b2f8b72f8f30b2e98c41ccf855954d9e35a5fa498c43332df198534ff9797a4a` to be loaded, so I had to know that it was the previous commit unless I've missed something else. |
https://github.com/huggingface/datasets/issues/6113 | load_dataset() fails with streamlit caching inside docker | Hi! This should be fixed in the latest (patch) release (run `pip install -U datasets` to install it). This behavior was due to a bug in our authentication logic. | ### Describe the bug
When calling `load_dataset` in a streamlit application running within a docker container, get a failure with the error message:
EmptyDatasetError: The directory at hf://datasets/fetch-rewards/inc-rings-2000@bea27cf60842b3641eae418f38864a2ec4cde684 doesn't contain any data files
Traceback:
File "/opt/conda/lib/python3.10/site-packages/streamlit/runtime/scriptrunner/script_runner.py", line 552, in _run_script
exec(code, module.__dict__)
File "/home/user/app/app.py", line 62, in <module>
dashboard()
File "/home/user/app/app.py", line 47, in dashboard
feat_dict, path_gml = load_data(hf_repo, model_gml_dict[selected_model], hf_token)
File "/opt/conda/lib/python3.10/site-packages/streamlit/runtime/caching/cache_utils.py", line 211, in wrapper
return cached_func(*args, **kwargs)
File "/opt/conda/lib/python3.10/site-packages/streamlit/runtime/caching/cache_utils.py", line 240, in __call__
return self._get_or_create_cached_value(args, kwargs)
File "/opt/conda/lib/python3.10/site-packages/streamlit/runtime/caching/cache_utils.py", line 266, in _get_or_create_cached_value
return self._handle_cache_miss(cache, value_key, func_args, func_kwargs)
File "/opt/conda/lib/python3.10/site-packages/streamlit/runtime/caching/cache_utils.py", line 320, in _handle_cache_miss
computed_value = self._info.func(*func_args, **func_kwargs)
File "/home/user/app/hf_interface.py", line 16, in load_data
hf_dataset = load_dataset(repo_id, use_auth_token=hf_token)
File "/opt/conda/lib/python3.10/site-packages/datasets/load.py", line 2109, in load_dataset
builder_instance = load_dataset_builder(
File "/opt/conda/lib/python3.10/site-packages/datasets/load.py", line 1795, in load_dataset_builder
dataset_module = dataset_module_factory(
File "/opt/conda/lib/python3.10/site-packages/datasets/load.py", line 1486, in dataset_module_factory
raise e1 from None
File "/opt/conda/lib/python3.10/site-packages/datasets/load.py", line 1476, in dataset_module_factory
).get_module()
File "/opt/conda/lib/python3.10/site-packages/datasets/load.py", line 1032, in get_module
else get_data_patterns(base_path, download_config=self.download_config)
File "/opt/conda/lib/python3.10/site-packages/datasets/data_files.py", line 458, in get_data_patterns
raise EmptyDatasetError(f"The directory at {base_path} doesn't contain any data files") from None
### Steps to reproduce the bug
```python
@st.cache_resource
def load_data(repo_id: str, hf_token=None):
"""Load data from HuggingFace Hub
"""
hf_dataset = load_dataset(repo_id, use_auth_token=hf_token)
hf_dataset = hf_dataset.map(lambda x: json.loads(x["ground_truth"]), remove_columns=["ground_truth"])
return hf_dataset
```
### Expected behavior
Expect to load.
Note: works fine with datasets==2.13.1
### Environment info
datasets==2.14.2,
Ubuntu bionic-based Docker container. | 29 | load_dataset() fails with streamlit caching inside docker
### Describe the bug
When calling `load_dataset` in a streamlit application running within a docker container, get a failure with the error message:
EmptyDatasetError: The directory at hf://datasets/fetch-rewards/inc-rings-2000@bea27cf60842b3641eae418f38864a2ec4cde684 doesn't contain any data files
Traceback:
File "/opt/conda/lib/python3.10/site-packages/streamlit/runtime/scriptrunner/script_runner.py", line 552, in _run_script
exec(code, module.__dict__)
File "/home/user/app/app.py", line 62, in <module>
dashboard()
File "/home/user/app/app.py", line 47, in dashboard
feat_dict, path_gml = load_data(hf_repo, model_gml_dict[selected_model], hf_token)
File "/opt/conda/lib/python3.10/site-packages/streamlit/runtime/caching/cache_utils.py", line 211, in wrapper
return cached_func(*args, **kwargs)
File "/opt/conda/lib/python3.10/site-packages/streamlit/runtime/caching/cache_utils.py", line 240, in __call__
return self._get_or_create_cached_value(args, kwargs)
File "/opt/conda/lib/python3.10/site-packages/streamlit/runtime/caching/cache_utils.py", line 266, in _get_or_create_cached_value
return self._handle_cache_miss(cache, value_key, func_args, func_kwargs)
File "/opt/conda/lib/python3.10/site-packages/streamlit/runtime/caching/cache_utils.py", line 320, in _handle_cache_miss
computed_value = self._info.func(*func_args, **func_kwargs)
File "/home/user/app/hf_interface.py", line 16, in load_data
hf_dataset = load_dataset(repo_id, use_auth_token=hf_token)
File "/opt/conda/lib/python3.10/site-packages/datasets/load.py", line 2109, in load_dataset
builder_instance = load_dataset_builder(
File "/opt/conda/lib/python3.10/site-packages/datasets/load.py", line 1795, in load_dataset_builder
dataset_module = dataset_module_factory(
File "/opt/conda/lib/python3.10/site-packages/datasets/load.py", line 1486, in dataset_module_factory
raise e1 from None
File "/opt/conda/lib/python3.10/site-packages/datasets/load.py", line 1476, in dataset_module_factory
).get_module()
File "/opt/conda/lib/python3.10/site-packages/datasets/load.py", line 1032, in get_module
else get_data_patterns(base_path, download_config=self.download_config)
File "/opt/conda/lib/python3.10/site-packages/datasets/data_files.py", line 458, in get_data_patterns
raise EmptyDatasetError(f"The directory at {base_path} doesn't contain any data files") from None
### Steps to reproduce the bug
```python
@st.cache_resource
def load_data(repo_id: str, hf_token=None):
"""Load data from HuggingFace Hub
"""
hf_dataset = load_dataset(repo_id, use_auth_token=hf_token)
hf_dataset = hf_dataset.map(lambda x: json.loads(x["ground_truth"]), remove_columns=["ground_truth"])
return hf_dataset
```
### Expected behavior
Expect to load.
Note: works fine with datasets==2.13.1
### Environment info
datasets==2.14.2,
Ubuntu bionic-based Docker container.
Hi! This should be fixed in the latest (patch) release (run `pip install -U datasets` to install it). This behavior was due to a bug in our authentication logic. |
https://github.com/huggingface/datasets/issues/6112 | yaml error using push_to_hub with generated README.md | Thanks for reporting! This is a bug in converting the `ArrayXD` types to YAML. It will be fixed soon. | ### Describe the bug
When I construct a dataset with the following features:
```
features = Features(
{
"pixel_values": Array3D(dtype="float64", shape=(3, 224, 224)),
"input_ids": Sequence(feature=Value(dtype="int64")),
"attention_mask": Sequence(Value(dtype="int64")),
"tokens": Sequence(Value(dtype="string")),
"bbox": Array2D(dtype="int64", shape=(512, 4)),
}
)
```
and run `push_to_hub`, the individual `*.parquet` files are pushed, but when trying to upload the auto-generated README, I run into the following error:
```
Traceback (most recent call last):
File "/Users/kevintee/.pyenv/versions/dev2/lib/python3.10/site-packages/huggingface_hub/utils/_errors.py", line 261, in hf_raise_for_status
response.raise_for_status()
File "/Users/kevintee/.pyenv/versions/dev2/lib/python3.10/site-packages/requests/models.py", line 1021, in raise_for_status
raise HTTPError(http_error_msg, response=self)
requests.exceptions.HTTPError: 400 Client Error: Bad Request for url: https://huggingface.co/api/datasets/looppayments/multitask_document_classification_dataset/commit/main
The above exception was the direct cause of the following exception:
Traceback (most recent call last):
File "/Users/kevintee/loop-payments/ml/src/ml/data_scripts/build_document_classification_training_data.py", line 297, in <module>
build_dataset()
File "/Users/kevintee/loop-payments/ml/src/ml/data_scripts/build_document_classification_training_data.py", line 290, in build_dataset
push_to_hub(dataset, "multitask_document_classification_dataset")
File "/Users/kevintee/loop-payments/ml/src/ml/data_scripts/build_document_classification_training_data.py", line 135, in push_to_hub
dataset.push_to_hub(f"looppayments/{dataset_name}", private=True)
File "/Users/kevintee/.pyenv/versions/dev2/lib/python3.10/site-packages/datasets/arrow_dataset.py", line 5577, in push_to_hub
HfApi(endpoint=config.HF_ENDPOINT).upload_file(
File "/Users/kevintee/.pyenv/versions/dev2/lib/python3.10/site-packages/huggingface_hub/utils/_validators.py", line 118, in _inner_fn
return fn(*args, **kwargs)
File "/Users/kevintee/.pyenv/versions/dev2/lib/python3.10/site-packages/huggingface_hub/hf_api.py", line 828, in _inner
return fn(self, *args, **kwargs)
File "/Users/kevintee/.pyenv/versions/dev2/lib/python3.10/site-packages/huggingface_hub/hf_api.py", line 3221, in upload_file
commit_info = self.create_commit(
File "/Users/kevintee/.pyenv/versions/dev2/lib/python3.10/site-packages/huggingface_hub/utils/_validators.py", line 118, in _inner_fn
return fn(*args, **kwargs)
File "/Users/kevintee/.pyenv/versions/dev2/lib/python3.10/site-packages/huggingface_hub/hf_api.py", line 828, in _inner
return fn(self, *args, **kwargs)
File "/Users/kevintee/.pyenv/versions/dev2/lib/python3.10/site-packages/huggingface_hub/hf_api.py", line 2728, in create_commit
hf_raise_for_status(commit_resp, endpoint_name="commit")
File "/Users/kevintee/.pyenv/versions/dev2/lib/python3.10/site-packages/huggingface_hub/utils/_errors.py", line 299, in hf_raise_for_status
raise BadRequestError(message, response=response) from e
huggingface_hub.utils._errors.BadRequestError: (Request ID: Root=1-64ca9c3d-2d2bbef354e102482a9a168e;bc00371c-8549-4859-9f41-43ff140ad36e)
Bad request for commit endpoint:
Invalid YAML in README.md: unknown tag !<tag:yaml.org,2002:python/tuple> (10:9)
7 | - 3
8 | - 224
9 | - 224
10 | dtype: float64
--------------^
11 | - name: input_ids
12 | sequence: int64
```
My guess is that the auto-generated yaml is unable to be parsed for some reason.
### Steps to reproduce the bug
The description contains most of what's needed to reproduce the issue, but I've added a shortened code snippet:
```
from datasets import Array2D, Array3D, ClassLabel, Dataset, Features, Sequence, Value
from PIL import Image
from transformers import AutoProcessor
features = Features(
{
"pixel_values": Array3D(dtype="float64", shape=(3, 224, 224)),
"input_ids": Sequence(feature=Value(dtype="int64")),
"attention_mask": Sequence(Value(dtype="int64")),
"tokens": Sequence(Value(dtype="string")),
"bbox": Array2D(dtype="int64", shape=(512, 4)),
}
)
processor = AutoProcessor.from_pretrained("microsoft/layoutlmv3-base", apply_ocr=False)
def preprocess_dataset(rows):
# Get images
images = [
Image.open(png_filename).convert("RGB") for png_filename in rows["png_filename"]
]
encoding = processor(
images,
rows["tokens"],
boxes=rows["bbox"],
truncation=True,
padding="max_length",
)
encoding["tokens"] = rows["tokens"]
return encoding
dataset = dataset.map(
preprocess_dataset,
batched=True,
batch_size=5,
features=features,
)
```
### Expected behavior
Using datasets==2.11.0, I'm able to succesfully push_to_hub, no issues, but with datasets==2.14.2, I run into the above error.
### Environment info
- `datasets` version: 2.14.2
- Platform: macOS-12.5-arm64-arm-64bit
- Python version: 3.10.12
- Huggingface_hub version: 0.16.4
- PyArrow version: 12.0.1
- Pandas version: 1.5.3 | 19 | yaml error using push_to_hub with generated README.md
### Describe the bug
When I construct a dataset with the following features:
```
features = Features(
{
"pixel_values": Array3D(dtype="float64", shape=(3, 224, 224)),
"input_ids": Sequence(feature=Value(dtype="int64")),
"attention_mask": Sequence(Value(dtype="int64")),
"tokens": Sequence(Value(dtype="string")),
"bbox": Array2D(dtype="int64", shape=(512, 4)),
}
)
```
and run `push_to_hub`, the individual `*.parquet` files are pushed, but when trying to upload the auto-generated README, I run into the following error:
```
Traceback (most recent call last):
File "/Users/kevintee/.pyenv/versions/dev2/lib/python3.10/site-packages/huggingface_hub/utils/_errors.py", line 261, in hf_raise_for_status
response.raise_for_status()
File "/Users/kevintee/.pyenv/versions/dev2/lib/python3.10/site-packages/requests/models.py", line 1021, in raise_for_status
raise HTTPError(http_error_msg, response=self)
requests.exceptions.HTTPError: 400 Client Error: Bad Request for url: https://huggingface.co/api/datasets/looppayments/multitask_document_classification_dataset/commit/main
The above exception was the direct cause of the following exception:
Traceback (most recent call last):
File "/Users/kevintee/loop-payments/ml/src/ml/data_scripts/build_document_classification_training_data.py", line 297, in <module>
build_dataset()
File "/Users/kevintee/loop-payments/ml/src/ml/data_scripts/build_document_classification_training_data.py", line 290, in build_dataset
push_to_hub(dataset, "multitask_document_classification_dataset")
File "/Users/kevintee/loop-payments/ml/src/ml/data_scripts/build_document_classification_training_data.py", line 135, in push_to_hub
dataset.push_to_hub(f"looppayments/{dataset_name}", private=True)
File "/Users/kevintee/.pyenv/versions/dev2/lib/python3.10/site-packages/datasets/arrow_dataset.py", line 5577, in push_to_hub
HfApi(endpoint=config.HF_ENDPOINT).upload_file(
File "/Users/kevintee/.pyenv/versions/dev2/lib/python3.10/site-packages/huggingface_hub/utils/_validators.py", line 118, in _inner_fn
return fn(*args, **kwargs)
File "/Users/kevintee/.pyenv/versions/dev2/lib/python3.10/site-packages/huggingface_hub/hf_api.py", line 828, in _inner
return fn(self, *args, **kwargs)
File "/Users/kevintee/.pyenv/versions/dev2/lib/python3.10/site-packages/huggingface_hub/hf_api.py", line 3221, in upload_file
commit_info = self.create_commit(
File "/Users/kevintee/.pyenv/versions/dev2/lib/python3.10/site-packages/huggingface_hub/utils/_validators.py", line 118, in _inner_fn
return fn(*args, **kwargs)
File "/Users/kevintee/.pyenv/versions/dev2/lib/python3.10/site-packages/huggingface_hub/hf_api.py", line 828, in _inner
return fn(self, *args, **kwargs)
File "/Users/kevintee/.pyenv/versions/dev2/lib/python3.10/site-packages/huggingface_hub/hf_api.py", line 2728, in create_commit
hf_raise_for_status(commit_resp, endpoint_name="commit")
File "/Users/kevintee/.pyenv/versions/dev2/lib/python3.10/site-packages/huggingface_hub/utils/_errors.py", line 299, in hf_raise_for_status
raise BadRequestError(message, response=response) from e
huggingface_hub.utils._errors.BadRequestError: (Request ID: Root=1-64ca9c3d-2d2bbef354e102482a9a168e;bc00371c-8549-4859-9f41-43ff140ad36e)
Bad request for commit endpoint:
Invalid YAML in README.md: unknown tag !<tag:yaml.org,2002:python/tuple> (10:9)
7 | - 3
8 | - 224
9 | - 224
10 | dtype: float64
--------------^
11 | - name: input_ids
12 | sequence: int64
```
My guess is that the auto-generated yaml is unable to be parsed for some reason.
### Steps to reproduce the bug
The description contains most of what's needed to reproduce the issue, but I've added a shortened code snippet:
```
from datasets import Array2D, Array3D, ClassLabel, Dataset, Features, Sequence, Value
from PIL import Image
from transformers import AutoProcessor
features = Features(
{
"pixel_values": Array3D(dtype="float64", shape=(3, 224, 224)),
"input_ids": Sequence(feature=Value(dtype="int64")),
"attention_mask": Sequence(Value(dtype="int64")),
"tokens": Sequence(Value(dtype="string")),
"bbox": Array2D(dtype="int64", shape=(512, 4)),
}
)
processor = AutoProcessor.from_pretrained("microsoft/layoutlmv3-base", apply_ocr=False)
def preprocess_dataset(rows):
# Get images
images = [
Image.open(png_filename).convert("RGB") for png_filename in rows["png_filename"]
]
encoding = processor(
images,
rows["tokens"],
boxes=rows["bbox"],
truncation=True,
padding="max_length",
)
encoding["tokens"] = rows["tokens"]
return encoding
dataset = dataset.map(
preprocess_dataset,
batched=True,
batch_size=5,
features=features,
)
```
### Expected behavior
Using datasets==2.11.0, I'm able to succesfully push_to_hub, no issues, but with datasets==2.14.2, I run into the above error.
### Environment info
- `datasets` version: 2.14.2
- Platform: macOS-12.5-arm64-arm-64bit
- Python version: 3.10.12
- Huggingface_hub version: 0.16.4
- PyArrow version: 12.0.1
- Pandas version: 1.5.3
Thanks for reporting! This is a bug in converting the `ArrayXD` types to YAML. It will be fixed soon. |
https://github.com/huggingface/datasets/issues/6111 | raise FileNotFoundError("Directory {dataset_path} is neither a `Dataset` directory nor a `DatasetDict` directory." ) | This should work: `load_dataset("path/to/downloaded_repo")`
`load_from_disk` is intended to be used on directories created with `Dataset.save_to_disk` or `DatasetDict.save_to_disk` | ### Describe the bug
For researchers in some countries or regions, it is usually the case that the download ability of `load_dataset` is disabled due to the complex network environment. People in these regions often prefer to use git clone or other programming tricks to manually download the files to the disk (for example, [How to elegantly download hf models, zhihu zhuanlan](https://zhuanlan.zhihu.com/p/475260268) proposed a crawlder based solution, and [Is there any mirror for hf_hub, zhihu answer](https://www.zhihu.com/question/371644077) provided some cloud based solutions, and [How to avoid pitfalls on Hugging face downloading, zhihu zhuanlan] gave some useful suggestions), and then use `load_from_disk` to get the dataset object.
However, when one finally has the local files on the disk, it is still buggy when trying to load the files into objects.
### Steps to reproduce the bug
Steps to reproduce the bug:
1. Found CIFAR dataset in hugging face: https://huggingface.co/datasets/cifar100/tree/main
2. Click ":" button to show "Clone repository" option, and then follow the prompts on the box:
```bash
cd my_directory_absolute
git lfs install
git clone https://huggingface.co/datasets/cifar100
ls my_directory_absolute/cifar100 # confirm that the directory exists and it is OK.
```
3. Write A python file to try to load the dataset
```python
from datasets import load_dataset, load_from_disk
dataset = load_from_disk("my_directory_absolute/cifar100")
```
Notice that according to issue #3700 , it is wrong to use load_dataset("my_directory_absolute/cifar100"), so we must use load_from_disk instead.
4. Then you will see the error reported:
```log
---------------------------------------------------------------------------
FileNotFoundError Traceback (most recent call last)
Cell In[5], line 9
1 from datasets import load_dataset, load_from_disk
----> 9 dataset = load_from_disk("my_directory_absolute/cifar100")
File [~/miniconda3/envs/ai/lib/python3.10/site-packages/datasets/load.py:2232), in load_from_disk(dataset_path, fs, keep_in_memory, storage_options)
2230 return DatasetDict.load_from_disk(dataset_path, keep_in_memory=keep_in_memory, storage_options=storage_options)
2231 else:
-> 2232 raise FileNotFoundError(
2233 f"Directory {dataset_path} is neither a `Dataset` directory nor a `DatasetDict` directory."
2234 )
FileNotFoundError: Directory my_directory_absolute/cifar100 is neither a `Dataset` directory nor a `DatasetDict` directory.
```
### Expected behavior
The dataset should be load successfully.
### Environment info
```bash
datasets-cli env
```
-> results:
```txt
Copy-and-paste the text below in your GitHub issue.
- `datasets` version: 2.14.2
- Platform: Linux-4.18.0-372.32.1.el8_6.x86_64-x86_64-with-glibc2.28
- Python version: 3.10.12
- Huggingface_hub version: 0.16.4
- PyArrow version: 12.0.1
- Pandas version: 2.0.3
``` | 17 | raise FileNotFoundError("Directory {dataset_path} is neither a `Dataset` directory nor a `DatasetDict` directory." )
### Describe the bug
For researchers in some countries or regions, it is usually the case that the download ability of `load_dataset` is disabled due to the complex network environment. People in these regions often prefer to use git clone or other programming tricks to manually download the files to the disk (for example, [How to elegantly download hf models, zhihu zhuanlan](https://zhuanlan.zhihu.com/p/475260268) proposed a crawlder based solution, and [Is there any mirror for hf_hub, zhihu answer](https://www.zhihu.com/question/371644077) provided some cloud based solutions, and [How to avoid pitfalls on Hugging face downloading, zhihu zhuanlan] gave some useful suggestions), and then use `load_from_disk` to get the dataset object.
However, when one finally has the local files on the disk, it is still buggy when trying to load the files into objects.
### Steps to reproduce the bug
Steps to reproduce the bug:
1. Found CIFAR dataset in hugging face: https://huggingface.co/datasets/cifar100/tree/main
2. Click ":" button to show "Clone repository" option, and then follow the prompts on the box:
```bash
cd my_directory_absolute
git lfs install
git clone https://huggingface.co/datasets/cifar100
ls my_directory_absolute/cifar100 # confirm that the directory exists and it is OK.
```
3. Write A python file to try to load the dataset
```python
from datasets import load_dataset, load_from_disk
dataset = load_from_disk("my_directory_absolute/cifar100")
```
Notice that according to issue #3700 , it is wrong to use load_dataset("my_directory_absolute/cifar100"), so we must use load_from_disk instead.
4. Then you will see the error reported:
```log
---------------------------------------------------------------------------
FileNotFoundError Traceback (most recent call last)
Cell In[5], line 9
1 from datasets import load_dataset, load_from_disk
----> 9 dataset = load_from_disk("my_directory_absolute/cifar100")
File [~/miniconda3/envs/ai/lib/python3.10/site-packages/datasets/load.py:2232), in load_from_disk(dataset_path, fs, keep_in_memory, storage_options)
2230 return DatasetDict.load_from_disk(dataset_path, keep_in_memory=keep_in_memory, storage_options=storage_options)
2231 else:
-> 2232 raise FileNotFoundError(
2233 f"Directory {dataset_path} is neither a `Dataset` directory nor a `DatasetDict` directory."
2234 )
FileNotFoundError: Directory my_directory_absolute/cifar100 is neither a `Dataset` directory nor a `DatasetDict` directory.
```
### Expected behavior
The dataset should be load successfully.
### Environment info
```bash
datasets-cli env
```
-> results:
```txt
Copy-and-paste the text below in your GitHub issue.
- `datasets` version: 2.14.2
- Platform: Linux-4.18.0-372.32.1.el8_6.x86_64-x86_64-with-glibc2.28
- Python version: 3.10.12
- Huggingface_hub version: 0.16.4
- PyArrow version: 12.0.1
- Pandas version: 2.0.3
```
This should work: `load_dataset("path/to/downloaded_repo")`
`load_from_disk` is intended to be used on directories created with `Dataset.save_to_disk` or `DatasetDict.save_to_disk` |
https://github.com/huggingface/datasets/issues/6110 | [BUG] Dataset initialized from in-memory data does not create cache. | This is expected behavior. You must provide `cache_file_name` when performing `.map` on an in-memory dataset for the result to be cached. | ### Describe the bug
`Dataset` initialized from in-memory data (dictionary in my case, haven't tested with other types) does not create cache when processed with the `map` method, unlike `Dataset` initialized by other methods such as `load_dataset`.
### Steps to reproduce the bug
```python
# below code was run the second time so the map function can be loaded from cache if exists
from datasets import load_dataset, Dataset
dataset = load_dataset("tatsu-lab/alpaca")['train']
dataset = dataset.map(lambda x: {'input': x['input'] + 'hi'}) # some random map
print(len(dataset.cache_files))
# 1
# copy the exact same data but initialize from a dictionary
memory_dataset = Dataset.from_dict({
'instruction': dataset['instruction'],
'input': dataset['input'],
'output': dataset['output'],
'text': dataset['text']})
memory_dataset = memory_dataset.map(lambda x: {'input': x['input'] + 'hi'}) # exact same map
print(len(memory_dataset.cache_files))
# Map: 100%|██████████| 52002[/52002]
# 0
```
### Expected behavior
The `map` function should create cache regardless of the method the `Dataset` was created.
### Environment info
- `datasets` version: 2.14.2
- Platform: Linux-5.15.0-41-generic-x86_64-with-glibc2.31
- Python version: 3.9.16
- Huggingface_hub version: 0.14.1
- PyArrow version: 11.0.0
- Pandas version: 1.5.3 | 21 | [BUG] Dataset initialized from in-memory data does not create cache.
### Describe the bug
`Dataset` initialized from in-memory data (dictionary in my case, haven't tested with other types) does not create cache when processed with the `map` method, unlike `Dataset` initialized by other methods such as `load_dataset`.
### Steps to reproduce the bug
```python
# below code was run the second time so the map function can be loaded from cache if exists
from datasets import load_dataset, Dataset
dataset = load_dataset("tatsu-lab/alpaca")['train']
dataset = dataset.map(lambda x: {'input': x['input'] + 'hi'}) # some random map
print(len(dataset.cache_files))
# 1
# copy the exact same data but initialize from a dictionary
memory_dataset = Dataset.from_dict({
'instruction': dataset['instruction'],
'input': dataset['input'],
'output': dataset['output'],
'text': dataset['text']})
memory_dataset = memory_dataset.map(lambda x: {'input': x['input'] + 'hi'}) # exact same map
print(len(memory_dataset.cache_files))
# Map: 100%|██████████| 52002[/52002]
# 0
```
### Expected behavior
The `map` function should create cache regardless of the method the `Dataset` was created.
### Environment info
- `datasets` version: 2.14.2
- Platform: Linux-5.15.0-41-generic-x86_64-with-glibc2.31
- Python version: 3.9.16
- Huggingface_hub version: 0.14.1
- PyArrow version: 11.0.0
- Pandas version: 1.5.3
This is expected behavior. You must provide `cache_file_name` when performing `.map` on an in-memory dataset for the result to be cached. |
https://github.com/huggingface/datasets/issues/6109 | Problems in downloading Amazon reviews from HF | Thanks for reporting, @610v4nn1.
Indeed, the source data files are no longer available. We have contacted the authors of the dataset and they report that Amazon has decided to stop distributing the multilingual reviews dataset.
We are adding a notification about this issue to the dataset card.
See: https://huggingface.co/datasets/amazon_reviews_multi/discussions/4#64c3898db63057f1fd3ce1a0 | ### Describe the bug
I have a script downloading `amazon_reviews_multi`.
When the download starts, I get
```
Downloading data files: 0%| | 0/1 [00:00<?, ?it/s]
Downloading data: 243B [00:00, 1.43MB/s]
Downloading data files: 100%|██████████| 1/1 [00:01<00:00, 1.54s/it]
Extracting data files: 100%|██████████| 1/1 [00:00<00:00, 842.40it/s]
Downloading data files: 0%| | 0/1 [00:00<?, ?it/s]
Downloading data: 243B [00:00, 928kB/s]
Downloading data files: 100%|██████████| 1/1 [00:01<00:00, 1.42s/it]
Extracting data files: 100%|██████████| 1/1 [00:00<00:00, 832.70it/s]
Downloading data files: 0%| | 0/1 [00:00<?, ?it/s]
Downloading data: 243B [00:00, 1.81MB/s]
Downloading data files: 100%|██████████| 1/1 [00:01<00:00, 1.40s/it]
Extracting data files: 100%|██████████| 1/1 [00:00<00:00, 1294.14it/s]
Generating train split: 0%| | 0/200000 [00:00<?, ? examples/s]
```
the file is clearly too small to contain the requested dataset, in fact it contains en error message:
```
<?xml version="1.0" encoding="UTF-8"?>
<Error><Code>AccessDenied</Code><Message>Access Denied</Message><RequestId>AGJWSY3ZADT2QVWE</RequestId><HostId>Gx1O2KXnxtQFqvzDLxyVSTq3+TTJuTnuVFnJL3SP89Yp8UzvYLPTVwd1PpniE4EvQzT3tCaqEJw=</HostId></Error>
```
obviously the script fails:
```
> raise DatasetGenerationError("An error occurred while generating the dataset") from e
E datasets.builder.DatasetGenerationError: An error occurred while generating the dataset
```
### Steps to reproduce the bug
1. load_dataset("amazon_reviews_multi", name="en", split="train", cache_dir="ADDYOURPATHHERE")
### Expected behavior
I would expect the dataset to be downloaded and processed
### Environment info
* The problem is present with both datasets 2.12.0 and 2.14.2
* python version 3.10.12 | 49 | Problems in downloading Amazon reviews from HF
### Describe the bug
I have a script downloading `amazon_reviews_multi`.
When the download starts, I get
```
Downloading data files: 0%| | 0/1 [00:00<?, ?it/s]
Downloading data: 243B [00:00, 1.43MB/s]
Downloading data files: 100%|██████████| 1/1 [00:01<00:00, 1.54s/it]
Extracting data files: 100%|██████████| 1/1 [00:00<00:00, 842.40it/s]
Downloading data files: 0%| | 0/1 [00:00<?, ?it/s]
Downloading data: 243B [00:00, 928kB/s]
Downloading data files: 100%|██████████| 1/1 [00:01<00:00, 1.42s/it]
Extracting data files: 100%|██████████| 1/1 [00:00<00:00, 832.70it/s]
Downloading data files: 0%| | 0/1 [00:00<?, ?it/s]
Downloading data: 243B [00:00, 1.81MB/s]
Downloading data files: 100%|██████████| 1/1 [00:01<00:00, 1.40s/it]
Extracting data files: 100%|██████████| 1/1 [00:00<00:00, 1294.14it/s]
Generating train split: 0%| | 0/200000 [00:00<?, ? examples/s]
```
the file is clearly too small to contain the requested dataset, in fact it contains en error message:
```
<?xml version="1.0" encoding="UTF-8"?>
<Error><Code>AccessDenied</Code><Message>Access Denied</Message><RequestId>AGJWSY3ZADT2QVWE</RequestId><HostId>Gx1O2KXnxtQFqvzDLxyVSTq3+TTJuTnuVFnJL3SP89Yp8UzvYLPTVwd1PpniE4EvQzT3tCaqEJw=</HostId></Error>
```
obviously the script fails:
```
> raise DatasetGenerationError("An error occurred while generating the dataset") from e
E datasets.builder.DatasetGenerationError: An error occurred while generating the dataset
```
### Steps to reproduce the bug
1. load_dataset("amazon_reviews_multi", name="en", split="train", cache_dir="ADDYOURPATHHERE")
### Expected behavior
I would expect the dataset to be downloaded and processed
### Environment info
* The problem is present with both datasets 2.12.0 and 2.14.2
* python version 3.10.12
Thanks for reporting, @610v4nn1.
Indeed, the source data files are no longer available. We have contacted the authors of the dataset and they report that Amazon has decided to stop distributing the multilingual reviews dataset.
We are adding a notification about this issue to the dataset card.
See: https://huggingface.co/datasets/amazon_reviews_multi/discussions/4#64c3898db63057f1fd3ce1a0 |
https://github.com/huggingface/datasets/issues/6108 | Loading local datasets got strangely stuck | Yesterday I waited for more than 12 hours to make sure it was really **stuck** instead of proceeding too slow. | ### Describe the bug
I try to use `load_dataset()` to load several local `.jsonl` files as a dataset. Every line of these files is a json structure only containing one key `text` (yeah it is a dataset for NLP model). The code snippet is as:
```python
ds = load_dataset("json", data_files=LIST_OF_FILE_PATHS, num_proc=16)['train']
```
However, I found that the loading process can get stuck -- the progress bar `Generating train split` no more proceed. When I was trying to find the cause and solution, I found a really strange behavior. If I load the dataset in this way:
```python
dlist = list()
for _ in LIST_OF_FILE_PATHS:
dlist.append(load_dataset("json", data_files=_)['train'])
ds = concatenate_datasets(dlist)
```
I can actually successfully load all the files despite its slow speed. But if I load them in batch like above, things go wrong. I did try to use Control-C to trace the stuck point but the program cannot be terminated in this way when `num_proc` is set to `None`. The only thing I can do is use Control-Z to hang it up then kill it. If I use more than 2 cpus, a Control-C would simply cause the following error:
```bash
^C
Process ForkPoolWorker-1:
Traceback (most recent call last):
File "/usr/local/lib/python3.10/dist-packages/multiprocess/process.py", line 314, in _bootstrap
self.run()
File "/usr/local/lib/python3.10/dist-packages/multiprocess/process.py", line 108, in run
self._target(*self._args, **self._kwargs)
File "/usr/local/lib/python3.10/dist-packages/multiprocess/pool.py", line 114, in worker
task = get()
File "/usr/local/lib/python3.10/dist-packages/multiprocess/queues.py", line 368, in get
res = self._reader.recv_bytes()
File "/usr/local/lib/python3.10/dist-packages/multiprocess/connection.py", line 224, in recv_bytes
buf = self._recv_bytes(maxlength)
File "/usr/local/lib/python3.10/dist-packages/multiprocess/connection.py", line 422, in _recv_bytes
buf = self._recv(4)
File "/usr/local/lib/python3.10/dist-packages/multiprocess/connection.py", line 387, in _recv
chunk = read(handle, remaining)
KeyboardInterrupt
Generating train split: 92431 examples [01:23, 1104.25 examples/s]
Traceback (most recent call last):
File "/usr/local/lib/python3.10/dist-packages/datasets/utils/py_utils.py", line 1373, in iflatmap_unordered
yield queue.get(timeout=0.05)
File "<string>", line 2, in get
File "/usr/local/lib/python3.10/dist-packages/multiprocess/managers.py", line 818, in _callmethod
kind, result = conn.recv()
File "/usr/local/lib/python3.10/dist-packages/multiprocess/connection.py", line 258, in recv
buf = self._recv_bytes()
File "/usr/local/lib/python3.10/dist-packages/multiprocess/connection.py", line 422, in _recv_bytes
buf = self._recv(4)
File "/usr/local/lib/python3.10/dist-packages/multiprocess/connection.py", line 387, in _recv
chunk = read(handle, remaining)
KeyboardInterrupt
During handling of the above exception, another exception occurred:
Traceback (most recent call last):
File "/mnt/data/liyongyuan/source/batch_load.py", line 11, in <module>
a = load_dataset(
File "/usr/local/lib/python3.10/dist-packages/datasets/load.py", line 2133, in load_dataset
builder_instance.download_and_prepare(
File "/usr/local/lib/python3.10/dist-packages/datasets/builder.py", line 954, in download_and_prepare
self._download_and_prepare(
File "/usr/local/lib/python3.10/dist-packages/datasets/builder.py", line 1049, in _download_and_prepare
self._prepare_split(split_generator, **prepare_split_kwargs)
File "/usr/local/lib/python3.10/dist-packages/datasets/builder.py", line 1842, in _prepare_split
for job_id, done, content in iflatmap_unordered(
File "/usr/local/lib/python3.10/dist-packages/datasets/utils/py_utils.py", line 1387, in iflatmap_unordered
[async_result.get(timeout=0.05) for async_result in async_results]
File "/usr/local/lib/python3.10/dist-packages/datasets/utils/py_utils.py", line 1387, in <listcomp>
[async_result.get(timeout=0.05) for async_result in async_results]
File "/usr/local/lib/python3.10/dist-packages/multiprocess/pool.py", line 770, in get
raise TimeoutError
multiprocess.context.TimeoutError
```
I have validated the basic correctness of these `.jsonl` files. They are correctly formatted (or they cannot be loaded singly by `load_dataset`) though some of the json may contain too long text (more than 1e7 characters). I do not know if this could be the problem. And there should not be any bottleneck in system's resource. The whole dataset is ~300GB, and I am using a cloud server with plenty of storage and 1TB ram.
Thanks for your efforts and patience! Any suggestion or help would be appreciated.
### Steps to reproduce the bug
1. use load_dataset() with `data_files = LIST_OF_FILES`
### Expected behavior
All the files should be smoothly loaded.
### Environment info
- Datasets: A private dataset. ~2500 `.jsonl` files. ~300GB in total. Each json structure only contains one key: `text`. Format checked.
- `datasets` version: 2.14.2
- Platform: Linux-4.19.91-014.kangaroo.alios7.x86_64-x86_64-with-glibc2.35
- Python version: 3.10.6
- Huggingface_hub version: 0.15.1
- PyArrow version: 10.0.1.dev0+ga6eabc2b.d20230609
- Pandas version: 1.5.2 | 20 | Loading local datasets got strangely stuck
### Describe the bug
I try to use `load_dataset()` to load several local `.jsonl` files as a dataset. Every line of these files is a json structure only containing one key `text` (yeah it is a dataset for NLP model). The code snippet is as:
```python
ds = load_dataset("json", data_files=LIST_OF_FILE_PATHS, num_proc=16)['train']
```
However, I found that the loading process can get stuck -- the progress bar `Generating train split` no more proceed. When I was trying to find the cause and solution, I found a really strange behavior. If I load the dataset in this way:
```python
dlist = list()
for _ in LIST_OF_FILE_PATHS:
dlist.append(load_dataset("json", data_files=_)['train'])
ds = concatenate_datasets(dlist)
```
I can actually successfully load all the files despite its slow speed. But if I load them in batch like above, things go wrong. I did try to use Control-C to trace the stuck point but the program cannot be terminated in this way when `num_proc` is set to `None`. The only thing I can do is use Control-Z to hang it up then kill it. If I use more than 2 cpus, a Control-C would simply cause the following error:
```bash
^C
Process ForkPoolWorker-1:
Traceback (most recent call last):
File "/usr/local/lib/python3.10/dist-packages/multiprocess/process.py", line 314, in _bootstrap
self.run()
File "/usr/local/lib/python3.10/dist-packages/multiprocess/process.py", line 108, in run
self._target(*self._args, **self._kwargs)
File "/usr/local/lib/python3.10/dist-packages/multiprocess/pool.py", line 114, in worker
task = get()
File "/usr/local/lib/python3.10/dist-packages/multiprocess/queues.py", line 368, in get
res = self._reader.recv_bytes()
File "/usr/local/lib/python3.10/dist-packages/multiprocess/connection.py", line 224, in recv_bytes
buf = self._recv_bytes(maxlength)
File "/usr/local/lib/python3.10/dist-packages/multiprocess/connection.py", line 422, in _recv_bytes
buf = self._recv(4)
File "/usr/local/lib/python3.10/dist-packages/multiprocess/connection.py", line 387, in _recv
chunk = read(handle, remaining)
KeyboardInterrupt
Generating train split: 92431 examples [01:23, 1104.25 examples/s]
Traceback (most recent call last):
File "/usr/local/lib/python3.10/dist-packages/datasets/utils/py_utils.py", line 1373, in iflatmap_unordered
yield queue.get(timeout=0.05)
File "<string>", line 2, in get
File "/usr/local/lib/python3.10/dist-packages/multiprocess/managers.py", line 818, in _callmethod
kind, result = conn.recv()
File "/usr/local/lib/python3.10/dist-packages/multiprocess/connection.py", line 258, in recv
buf = self._recv_bytes()
File "/usr/local/lib/python3.10/dist-packages/multiprocess/connection.py", line 422, in _recv_bytes
buf = self._recv(4)
File "/usr/local/lib/python3.10/dist-packages/multiprocess/connection.py", line 387, in _recv
chunk = read(handle, remaining)
KeyboardInterrupt
During handling of the above exception, another exception occurred:
Traceback (most recent call last):
File "/mnt/data/liyongyuan/source/batch_load.py", line 11, in <module>
a = load_dataset(
File "/usr/local/lib/python3.10/dist-packages/datasets/load.py", line 2133, in load_dataset
builder_instance.download_and_prepare(
File "/usr/local/lib/python3.10/dist-packages/datasets/builder.py", line 954, in download_and_prepare
self._download_and_prepare(
File "/usr/local/lib/python3.10/dist-packages/datasets/builder.py", line 1049, in _download_and_prepare
self._prepare_split(split_generator, **prepare_split_kwargs)
File "/usr/local/lib/python3.10/dist-packages/datasets/builder.py", line 1842, in _prepare_split
for job_id, done, content in iflatmap_unordered(
File "/usr/local/lib/python3.10/dist-packages/datasets/utils/py_utils.py", line 1387, in iflatmap_unordered
[async_result.get(timeout=0.05) for async_result in async_results]
File "/usr/local/lib/python3.10/dist-packages/datasets/utils/py_utils.py", line 1387, in <listcomp>
[async_result.get(timeout=0.05) for async_result in async_results]
File "/usr/local/lib/python3.10/dist-packages/multiprocess/pool.py", line 770, in get
raise TimeoutError
multiprocess.context.TimeoutError
```
I have validated the basic correctness of these `.jsonl` files. They are correctly formatted (or they cannot be loaded singly by `load_dataset`) though some of the json may contain too long text (more than 1e7 characters). I do not know if this could be the problem. And there should not be any bottleneck in system's resource. The whole dataset is ~300GB, and I am using a cloud server with plenty of storage and 1TB ram.
Thanks for your efforts and patience! Any suggestion or help would be appreciated.
### Steps to reproduce the bug
1. use load_dataset() with `data_files = LIST_OF_FILES`
### Expected behavior
All the files should be smoothly loaded.
### Environment info
- Datasets: A private dataset. ~2500 `.jsonl` files. ~300GB in total. Each json structure only contains one key: `text`. Format checked.
- `datasets` version: 2.14.2
- Platform: Linux-4.19.91-014.kangaroo.alios7.x86_64-x86_64-with-glibc2.35
- Python version: 3.10.6
- Huggingface_hub version: 0.15.1
- PyArrow version: 10.0.1.dev0+ga6eabc2b.d20230609
- Pandas version: 1.5.2
Yesterday I waited for more than 12 hours to make sure it was really **stuck** instead of proceeding too slow. |
https://github.com/huggingface/datasets/issues/6108 | Loading local datasets got strangely stuck | I've had similar weird issues with `load_dataset` as well. Not multiple files, but dataset is quite big, about 50G. | ### Describe the bug
I try to use `load_dataset()` to load several local `.jsonl` files as a dataset. Every line of these files is a json structure only containing one key `text` (yeah it is a dataset for NLP model). The code snippet is as:
```python
ds = load_dataset("json", data_files=LIST_OF_FILE_PATHS, num_proc=16)['train']
```
However, I found that the loading process can get stuck -- the progress bar `Generating train split` no more proceed. When I was trying to find the cause and solution, I found a really strange behavior. If I load the dataset in this way:
```python
dlist = list()
for _ in LIST_OF_FILE_PATHS:
dlist.append(load_dataset("json", data_files=_)['train'])
ds = concatenate_datasets(dlist)
```
I can actually successfully load all the files despite its slow speed. But if I load them in batch like above, things go wrong. I did try to use Control-C to trace the stuck point but the program cannot be terminated in this way when `num_proc` is set to `None`. The only thing I can do is use Control-Z to hang it up then kill it. If I use more than 2 cpus, a Control-C would simply cause the following error:
```bash
^C
Process ForkPoolWorker-1:
Traceback (most recent call last):
File "/usr/local/lib/python3.10/dist-packages/multiprocess/process.py", line 314, in _bootstrap
self.run()
File "/usr/local/lib/python3.10/dist-packages/multiprocess/process.py", line 108, in run
self._target(*self._args, **self._kwargs)
File "/usr/local/lib/python3.10/dist-packages/multiprocess/pool.py", line 114, in worker
task = get()
File "/usr/local/lib/python3.10/dist-packages/multiprocess/queues.py", line 368, in get
res = self._reader.recv_bytes()
File "/usr/local/lib/python3.10/dist-packages/multiprocess/connection.py", line 224, in recv_bytes
buf = self._recv_bytes(maxlength)
File "/usr/local/lib/python3.10/dist-packages/multiprocess/connection.py", line 422, in _recv_bytes
buf = self._recv(4)
File "/usr/local/lib/python3.10/dist-packages/multiprocess/connection.py", line 387, in _recv
chunk = read(handle, remaining)
KeyboardInterrupt
Generating train split: 92431 examples [01:23, 1104.25 examples/s]
Traceback (most recent call last):
File "/usr/local/lib/python3.10/dist-packages/datasets/utils/py_utils.py", line 1373, in iflatmap_unordered
yield queue.get(timeout=0.05)
File "<string>", line 2, in get
File "/usr/local/lib/python3.10/dist-packages/multiprocess/managers.py", line 818, in _callmethod
kind, result = conn.recv()
File "/usr/local/lib/python3.10/dist-packages/multiprocess/connection.py", line 258, in recv
buf = self._recv_bytes()
File "/usr/local/lib/python3.10/dist-packages/multiprocess/connection.py", line 422, in _recv_bytes
buf = self._recv(4)
File "/usr/local/lib/python3.10/dist-packages/multiprocess/connection.py", line 387, in _recv
chunk = read(handle, remaining)
KeyboardInterrupt
During handling of the above exception, another exception occurred:
Traceback (most recent call last):
File "/mnt/data/liyongyuan/source/batch_load.py", line 11, in <module>
a = load_dataset(
File "/usr/local/lib/python3.10/dist-packages/datasets/load.py", line 2133, in load_dataset
builder_instance.download_and_prepare(
File "/usr/local/lib/python3.10/dist-packages/datasets/builder.py", line 954, in download_and_prepare
self._download_and_prepare(
File "/usr/local/lib/python3.10/dist-packages/datasets/builder.py", line 1049, in _download_and_prepare
self._prepare_split(split_generator, **prepare_split_kwargs)
File "/usr/local/lib/python3.10/dist-packages/datasets/builder.py", line 1842, in _prepare_split
for job_id, done, content in iflatmap_unordered(
File "/usr/local/lib/python3.10/dist-packages/datasets/utils/py_utils.py", line 1387, in iflatmap_unordered
[async_result.get(timeout=0.05) for async_result in async_results]
File "/usr/local/lib/python3.10/dist-packages/datasets/utils/py_utils.py", line 1387, in <listcomp>
[async_result.get(timeout=0.05) for async_result in async_results]
File "/usr/local/lib/python3.10/dist-packages/multiprocess/pool.py", line 770, in get
raise TimeoutError
multiprocess.context.TimeoutError
```
I have validated the basic correctness of these `.jsonl` files. They are correctly formatted (or they cannot be loaded singly by `load_dataset`) though some of the json may contain too long text (more than 1e7 characters). I do not know if this could be the problem. And there should not be any bottleneck in system's resource. The whole dataset is ~300GB, and I am using a cloud server with plenty of storage and 1TB ram.
Thanks for your efforts and patience! Any suggestion or help would be appreciated.
### Steps to reproduce the bug
1. use load_dataset() with `data_files = LIST_OF_FILES`
### Expected behavior
All the files should be smoothly loaded.
### Environment info
- Datasets: A private dataset. ~2500 `.jsonl` files. ~300GB in total. Each json structure only contains one key: `text`. Format checked.
- `datasets` version: 2.14.2
- Platform: Linux-4.19.91-014.kangaroo.alios7.x86_64-x86_64-with-glibc2.35
- Python version: 3.10.6
- Huggingface_hub version: 0.15.1
- PyArrow version: 10.0.1.dev0+ga6eabc2b.d20230609
- Pandas version: 1.5.2 | 19 | Loading local datasets got strangely stuck
### Describe the bug
I try to use `load_dataset()` to load several local `.jsonl` files as a dataset. Every line of these files is a json structure only containing one key `text` (yeah it is a dataset for NLP model). The code snippet is as:
```python
ds = load_dataset("json", data_files=LIST_OF_FILE_PATHS, num_proc=16)['train']
```
However, I found that the loading process can get stuck -- the progress bar `Generating train split` no more proceed. When I was trying to find the cause and solution, I found a really strange behavior. If I load the dataset in this way:
```python
dlist = list()
for _ in LIST_OF_FILE_PATHS:
dlist.append(load_dataset("json", data_files=_)['train'])
ds = concatenate_datasets(dlist)
```
I can actually successfully load all the files despite its slow speed. But if I load them in batch like above, things go wrong. I did try to use Control-C to trace the stuck point but the program cannot be terminated in this way when `num_proc` is set to `None`. The only thing I can do is use Control-Z to hang it up then kill it. If I use more than 2 cpus, a Control-C would simply cause the following error:
```bash
^C
Process ForkPoolWorker-1:
Traceback (most recent call last):
File "/usr/local/lib/python3.10/dist-packages/multiprocess/process.py", line 314, in _bootstrap
self.run()
File "/usr/local/lib/python3.10/dist-packages/multiprocess/process.py", line 108, in run
self._target(*self._args, **self._kwargs)
File "/usr/local/lib/python3.10/dist-packages/multiprocess/pool.py", line 114, in worker
task = get()
File "/usr/local/lib/python3.10/dist-packages/multiprocess/queues.py", line 368, in get
res = self._reader.recv_bytes()
File "/usr/local/lib/python3.10/dist-packages/multiprocess/connection.py", line 224, in recv_bytes
buf = self._recv_bytes(maxlength)
File "/usr/local/lib/python3.10/dist-packages/multiprocess/connection.py", line 422, in _recv_bytes
buf = self._recv(4)
File "/usr/local/lib/python3.10/dist-packages/multiprocess/connection.py", line 387, in _recv
chunk = read(handle, remaining)
KeyboardInterrupt
Generating train split: 92431 examples [01:23, 1104.25 examples/s]
Traceback (most recent call last):
File "/usr/local/lib/python3.10/dist-packages/datasets/utils/py_utils.py", line 1373, in iflatmap_unordered
yield queue.get(timeout=0.05)
File "<string>", line 2, in get
File "/usr/local/lib/python3.10/dist-packages/multiprocess/managers.py", line 818, in _callmethod
kind, result = conn.recv()
File "/usr/local/lib/python3.10/dist-packages/multiprocess/connection.py", line 258, in recv
buf = self._recv_bytes()
File "/usr/local/lib/python3.10/dist-packages/multiprocess/connection.py", line 422, in _recv_bytes
buf = self._recv(4)
File "/usr/local/lib/python3.10/dist-packages/multiprocess/connection.py", line 387, in _recv
chunk = read(handle, remaining)
KeyboardInterrupt
During handling of the above exception, another exception occurred:
Traceback (most recent call last):
File "/mnt/data/liyongyuan/source/batch_load.py", line 11, in <module>
a = load_dataset(
File "/usr/local/lib/python3.10/dist-packages/datasets/load.py", line 2133, in load_dataset
builder_instance.download_and_prepare(
File "/usr/local/lib/python3.10/dist-packages/datasets/builder.py", line 954, in download_and_prepare
self._download_and_prepare(
File "/usr/local/lib/python3.10/dist-packages/datasets/builder.py", line 1049, in _download_and_prepare
self._prepare_split(split_generator, **prepare_split_kwargs)
File "/usr/local/lib/python3.10/dist-packages/datasets/builder.py", line 1842, in _prepare_split
for job_id, done, content in iflatmap_unordered(
File "/usr/local/lib/python3.10/dist-packages/datasets/utils/py_utils.py", line 1387, in iflatmap_unordered
[async_result.get(timeout=0.05) for async_result in async_results]
File "/usr/local/lib/python3.10/dist-packages/datasets/utils/py_utils.py", line 1387, in <listcomp>
[async_result.get(timeout=0.05) for async_result in async_results]
File "/usr/local/lib/python3.10/dist-packages/multiprocess/pool.py", line 770, in get
raise TimeoutError
multiprocess.context.TimeoutError
```
I have validated the basic correctness of these `.jsonl` files. They are correctly formatted (or they cannot be loaded singly by `load_dataset`) though some of the json may contain too long text (more than 1e7 characters). I do not know if this could be the problem. And there should not be any bottleneck in system's resource. The whole dataset is ~300GB, and I am using a cloud server with plenty of storage and 1TB ram.
Thanks for your efforts and patience! Any suggestion or help would be appreciated.
### Steps to reproduce the bug
1. use load_dataset() with `data_files = LIST_OF_FILES`
### Expected behavior
All the files should be smoothly loaded.
### Environment info
- Datasets: A private dataset. ~2500 `.jsonl` files. ~300GB in total. Each json structure only contains one key: `text`. Format checked.
- `datasets` version: 2.14.2
- Platform: Linux-4.19.91-014.kangaroo.alios7.x86_64-x86_64-with-glibc2.35
- Python version: 3.10.6
- Huggingface_hub version: 0.15.1
- PyArrow version: 10.0.1.dev0+ga6eabc2b.d20230609
- Pandas version: 1.5.2
I've had similar weird issues with `load_dataset` as well. Not multiple files, but dataset is quite big, about 50G. |
https://github.com/huggingface/datasets/issues/6108 | Loading local datasets got strangely stuck | We use a generic multiprocessing code, so there is little we can do about this - unfortunately, turning off multiprocessing seems to be the only solution. Multithreading would make our code easier to maintain and (most likely) avoid issues such as this one, but we cannot use it until the GIL is dropped (no-GIL Python should be released in 2024, so we can start exploring this then) | ### Describe the bug
I try to use `load_dataset()` to load several local `.jsonl` files as a dataset. Every line of these files is a json structure only containing one key `text` (yeah it is a dataset for NLP model). The code snippet is as:
```python
ds = load_dataset("json", data_files=LIST_OF_FILE_PATHS, num_proc=16)['train']
```
However, I found that the loading process can get stuck -- the progress bar `Generating train split` no more proceed. When I was trying to find the cause and solution, I found a really strange behavior. If I load the dataset in this way:
```python
dlist = list()
for _ in LIST_OF_FILE_PATHS:
dlist.append(load_dataset("json", data_files=_)['train'])
ds = concatenate_datasets(dlist)
```
I can actually successfully load all the files despite its slow speed. But if I load them in batch like above, things go wrong. I did try to use Control-C to trace the stuck point but the program cannot be terminated in this way when `num_proc` is set to `None`. The only thing I can do is use Control-Z to hang it up then kill it. If I use more than 2 cpus, a Control-C would simply cause the following error:
```bash
^C
Process ForkPoolWorker-1:
Traceback (most recent call last):
File "/usr/local/lib/python3.10/dist-packages/multiprocess/process.py", line 314, in _bootstrap
self.run()
File "/usr/local/lib/python3.10/dist-packages/multiprocess/process.py", line 108, in run
self._target(*self._args, **self._kwargs)
File "/usr/local/lib/python3.10/dist-packages/multiprocess/pool.py", line 114, in worker
task = get()
File "/usr/local/lib/python3.10/dist-packages/multiprocess/queues.py", line 368, in get
res = self._reader.recv_bytes()
File "/usr/local/lib/python3.10/dist-packages/multiprocess/connection.py", line 224, in recv_bytes
buf = self._recv_bytes(maxlength)
File "/usr/local/lib/python3.10/dist-packages/multiprocess/connection.py", line 422, in _recv_bytes
buf = self._recv(4)
File "/usr/local/lib/python3.10/dist-packages/multiprocess/connection.py", line 387, in _recv
chunk = read(handle, remaining)
KeyboardInterrupt
Generating train split: 92431 examples [01:23, 1104.25 examples/s]
Traceback (most recent call last):
File "/usr/local/lib/python3.10/dist-packages/datasets/utils/py_utils.py", line 1373, in iflatmap_unordered
yield queue.get(timeout=0.05)
File "<string>", line 2, in get
File "/usr/local/lib/python3.10/dist-packages/multiprocess/managers.py", line 818, in _callmethod
kind, result = conn.recv()
File "/usr/local/lib/python3.10/dist-packages/multiprocess/connection.py", line 258, in recv
buf = self._recv_bytes()
File "/usr/local/lib/python3.10/dist-packages/multiprocess/connection.py", line 422, in _recv_bytes
buf = self._recv(4)
File "/usr/local/lib/python3.10/dist-packages/multiprocess/connection.py", line 387, in _recv
chunk = read(handle, remaining)
KeyboardInterrupt
During handling of the above exception, another exception occurred:
Traceback (most recent call last):
File "/mnt/data/liyongyuan/source/batch_load.py", line 11, in <module>
a = load_dataset(
File "/usr/local/lib/python3.10/dist-packages/datasets/load.py", line 2133, in load_dataset
builder_instance.download_and_prepare(
File "/usr/local/lib/python3.10/dist-packages/datasets/builder.py", line 954, in download_and_prepare
self._download_and_prepare(
File "/usr/local/lib/python3.10/dist-packages/datasets/builder.py", line 1049, in _download_and_prepare
self._prepare_split(split_generator, **prepare_split_kwargs)
File "/usr/local/lib/python3.10/dist-packages/datasets/builder.py", line 1842, in _prepare_split
for job_id, done, content in iflatmap_unordered(
File "/usr/local/lib/python3.10/dist-packages/datasets/utils/py_utils.py", line 1387, in iflatmap_unordered
[async_result.get(timeout=0.05) for async_result in async_results]
File "/usr/local/lib/python3.10/dist-packages/datasets/utils/py_utils.py", line 1387, in <listcomp>
[async_result.get(timeout=0.05) for async_result in async_results]
File "/usr/local/lib/python3.10/dist-packages/multiprocess/pool.py", line 770, in get
raise TimeoutError
multiprocess.context.TimeoutError
```
I have validated the basic correctness of these `.jsonl` files. They are correctly formatted (or they cannot be loaded singly by `load_dataset`) though some of the json may contain too long text (more than 1e7 characters). I do not know if this could be the problem. And there should not be any bottleneck in system's resource. The whole dataset is ~300GB, and I am using a cloud server with plenty of storage and 1TB ram.
Thanks for your efforts and patience! Any suggestion or help would be appreciated.
### Steps to reproduce the bug
1. use load_dataset() with `data_files = LIST_OF_FILES`
### Expected behavior
All the files should be smoothly loaded.
### Environment info
- Datasets: A private dataset. ~2500 `.jsonl` files. ~300GB in total. Each json structure only contains one key: `text`. Format checked.
- `datasets` version: 2.14.2
- Platform: Linux-4.19.91-014.kangaroo.alios7.x86_64-x86_64-with-glibc2.35
- Python version: 3.10.6
- Huggingface_hub version: 0.15.1
- PyArrow version: 10.0.1.dev0+ga6eabc2b.d20230609
- Pandas version: 1.5.2 | 67 | Loading local datasets got strangely stuck
### Describe the bug
I try to use `load_dataset()` to load several local `.jsonl` files as a dataset. Every line of these files is a json structure only containing one key `text` (yeah it is a dataset for NLP model). The code snippet is as:
```python
ds = load_dataset("json", data_files=LIST_OF_FILE_PATHS, num_proc=16)['train']
```
However, I found that the loading process can get stuck -- the progress bar `Generating train split` no more proceed. When I was trying to find the cause and solution, I found a really strange behavior. If I load the dataset in this way:
```python
dlist = list()
for _ in LIST_OF_FILE_PATHS:
dlist.append(load_dataset("json", data_files=_)['train'])
ds = concatenate_datasets(dlist)
```
I can actually successfully load all the files despite its slow speed. But if I load them in batch like above, things go wrong. I did try to use Control-C to trace the stuck point but the program cannot be terminated in this way when `num_proc` is set to `None`. The only thing I can do is use Control-Z to hang it up then kill it. If I use more than 2 cpus, a Control-C would simply cause the following error:
```bash
^C
Process ForkPoolWorker-1:
Traceback (most recent call last):
File "/usr/local/lib/python3.10/dist-packages/multiprocess/process.py", line 314, in _bootstrap
self.run()
File "/usr/local/lib/python3.10/dist-packages/multiprocess/process.py", line 108, in run
self._target(*self._args, **self._kwargs)
File "/usr/local/lib/python3.10/dist-packages/multiprocess/pool.py", line 114, in worker
task = get()
File "/usr/local/lib/python3.10/dist-packages/multiprocess/queues.py", line 368, in get
res = self._reader.recv_bytes()
File "/usr/local/lib/python3.10/dist-packages/multiprocess/connection.py", line 224, in recv_bytes
buf = self._recv_bytes(maxlength)
File "/usr/local/lib/python3.10/dist-packages/multiprocess/connection.py", line 422, in _recv_bytes
buf = self._recv(4)
File "/usr/local/lib/python3.10/dist-packages/multiprocess/connection.py", line 387, in _recv
chunk = read(handle, remaining)
KeyboardInterrupt
Generating train split: 92431 examples [01:23, 1104.25 examples/s]
Traceback (most recent call last):
File "/usr/local/lib/python3.10/dist-packages/datasets/utils/py_utils.py", line 1373, in iflatmap_unordered
yield queue.get(timeout=0.05)
File "<string>", line 2, in get
File "/usr/local/lib/python3.10/dist-packages/multiprocess/managers.py", line 818, in _callmethod
kind, result = conn.recv()
File "/usr/local/lib/python3.10/dist-packages/multiprocess/connection.py", line 258, in recv
buf = self._recv_bytes()
File "/usr/local/lib/python3.10/dist-packages/multiprocess/connection.py", line 422, in _recv_bytes
buf = self._recv(4)
File "/usr/local/lib/python3.10/dist-packages/multiprocess/connection.py", line 387, in _recv
chunk = read(handle, remaining)
KeyboardInterrupt
During handling of the above exception, another exception occurred:
Traceback (most recent call last):
File "/mnt/data/liyongyuan/source/batch_load.py", line 11, in <module>
a = load_dataset(
File "/usr/local/lib/python3.10/dist-packages/datasets/load.py", line 2133, in load_dataset
builder_instance.download_and_prepare(
File "/usr/local/lib/python3.10/dist-packages/datasets/builder.py", line 954, in download_and_prepare
self._download_and_prepare(
File "/usr/local/lib/python3.10/dist-packages/datasets/builder.py", line 1049, in _download_and_prepare
self._prepare_split(split_generator, **prepare_split_kwargs)
File "/usr/local/lib/python3.10/dist-packages/datasets/builder.py", line 1842, in _prepare_split
for job_id, done, content in iflatmap_unordered(
File "/usr/local/lib/python3.10/dist-packages/datasets/utils/py_utils.py", line 1387, in iflatmap_unordered
[async_result.get(timeout=0.05) for async_result in async_results]
File "/usr/local/lib/python3.10/dist-packages/datasets/utils/py_utils.py", line 1387, in <listcomp>
[async_result.get(timeout=0.05) for async_result in async_results]
File "/usr/local/lib/python3.10/dist-packages/multiprocess/pool.py", line 770, in get
raise TimeoutError
multiprocess.context.TimeoutError
```
I have validated the basic correctness of these `.jsonl` files. They are correctly formatted (or they cannot be loaded singly by `load_dataset`) though some of the json may contain too long text (more than 1e7 characters). I do not know if this could be the problem. And there should not be any bottleneck in system's resource. The whole dataset is ~300GB, and I am using a cloud server with plenty of storage and 1TB ram.
Thanks for your efforts and patience! Any suggestion or help would be appreciated.
### Steps to reproduce the bug
1. use load_dataset() with `data_files = LIST_OF_FILES`
### Expected behavior
All the files should be smoothly loaded.
### Environment info
- Datasets: A private dataset. ~2500 `.jsonl` files. ~300GB in total. Each json structure only contains one key: `text`. Format checked.
- `datasets` version: 2.14.2
- Platform: Linux-4.19.91-014.kangaroo.alios7.x86_64-x86_64-with-glibc2.35
- Python version: 3.10.6
- Huggingface_hub version: 0.15.1
- PyArrow version: 10.0.1.dev0+ga6eabc2b.d20230609
- Pandas version: 1.5.2
We use a generic multiprocessing code, so there is little we can do about this - unfortunately, turning off multiprocessing seems to be the only solution. Multithreading would make our code easier to maintain and (most likely) avoid issues such as this one, but we cannot use it until the GIL is dropped (no-GIL Python should be released in 2024, so we can start exploring this then) |
https://github.com/huggingface/datasets/issues/6106 | load local json_file as dataset | Hi! We use PyArrow to read JSON files, and PyArrow doesn't allow different value types in the same column. #5776 should address this.
In the meantime, you can combine `Dataset.from_generator` with the above code to cast the values to the same type. | ### Describe the bug
I tried to load local json file as dataset but failed to parsing json file because some columns are 'float' type.
### Steps to reproduce the bug
1. load json file with certain columns are 'float' type. For example `data = load_data("json", data_files=JSON_PATH)`
2. Then, the error will be triggered like `ArrowInvalid: Could not convert '-0.2253' with type str: tried to convert to double
### Expected behavior
Should allow some columns are 'float' type, at least it should convert those columns to str type.
I tried to avoid the error by naively convert the float item to str:
```python
# if col type is not str, we need to convert it to str
mapping = {}
for col in keys:
if isinstance(dataset[0][col], str):
mapping[col] = [row.get(col) for row in dataset]
else:
mapping[col] = [str(row.get(col)) for row in dataset]
```
### Environment info
- `datasets` version: 2.14.2
- Platform: Linux-5.4.0-52-generic-x86_64-with-glibc2.31
- Python version: 3.9.16
- Huggingface_hub version: 0.16.4
- PyArrow version: 12.0.0
- Pandas version: 2.0.1 | 42 | load local json_file as dataset
### Describe the bug
I tried to load local json file as dataset but failed to parsing json file because some columns are 'float' type.
### Steps to reproduce the bug
1. load json file with certain columns are 'float' type. For example `data = load_data("json", data_files=JSON_PATH)`
2. Then, the error will be triggered like `ArrowInvalid: Could not convert '-0.2253' with type str: tried to convert to double
### Expected behavior
Should allow some columns are 'float' type, at least it should convert those columns to str type.
I tried to avoid the error by naively convert the float item to str:
```python
# if col type is not str, we need to convert it to str
mapping = {}
for col in keys:
if isinstance(dataset[0][col], str):
mapping[col] = [row.get(col) for row in dataset]
else:
mapping[col] = [str(row.get(col)) for row in dataset]
```
### Environment info
- `datasets` version: 2.14.2
- Platform: Linux-5.4.0-52-generic-x86_64-with-glibc2.31
- Python version: 3.9.16
- Huggingface_hub version: 0.16.4
- PyArrow version: 12.0.0
- Pandas version: 2.0.1
Hi! We use PyArrow to read JSON files, and PyArrow doesn't allow different value types in the same column. #5776 should address this.
In the meantime, you can combine `Dataset.from_generator` with the above code to cast the values to the same type. |
https://github.com/huggingface/datasets/issues/6099 | How do i get "amazon_us_reviews | Seems like the problem isn't with the library, but the dataset itself hosted on AWS S3.
Its [homepage](https://s3.amazonaws.com/amazon-reviews-pds/readme.html) returns an `AccessDenied` XML response, which is the same thing you get if you try to log the `record` that triggers the exception
```python
try:
example = self.info.features.encode_example(record) if self.info.features is not None else record
except Exception as e:
print(record)
```
⬇️
```
{'<?xml version="1.0" encoding="UTF-8"?>': '<Error><Code>AccessDenied</Code><Message>Access Denied</Message><RequestId>N2HFJ82ZV8SZW9BV</RequestId><HostId>Zw2DQ0V2GdRmvH5qWEpumK4uj5+W8YPcilQbN9fLBr3VqQOcKPHOhUZLG3LcM9X5fkOetxp48Os=</HostId></Error>'}
``` | ### Feature request
I have been trying to load 'amazon_us_dataset" but unable to do so.
`amazon_us_reviews = load_dataset('amazon_us_reviews')`
`print(amazon_us_reviews)`
> [ValueError: Config name is missing.
Please pick one among the available configs: ['Wireless_v1_00', 'Watches_v1_00', 'Video_Games_v1_00', 'Video_DVD_v1_00', 'Video_v1_00', 'Toys_v1_00', 'Tools_v1_00', 'Sports_v1_00', 'Software_v1_00', 'Shoes_v1_00', 'Pet_Products_v1_00', 'Personal_Care_Appliances_v1_00', 'PC_v1_00', 'Outdoors_v1_00', 'Office_Products_v1_00', 'Musical_Instruments_v1_00', 'Music_v1_00', 'Mobile_Electronics_v1_00', 'Mobile_Apps_v1_00', 'Major_Appliances_v1_00', 'Luggage_v1_00', 'Lawn_and_Garden_v1_00', 'Kitchen_v1_00', 'Jewelry_v1_00', 'Home_Improvement_v1_00', 'Home_Entertainment_v1_00', 'Home_v1_00', 'Health_Personal_Care_v1_00', 'Grocery_v1_00', 'Gift_Card_v1_00', 'Furniture_v1_00', 'Electronics_v1_00', 'Digital_Video_Games_v1_00', 'Digital_Video_Download_v1_00', 'Digital_Software_v1_00', 'Digital_Music_Purchase_v1_00', 'Digital_Ebook_Purchase_v1_00', 'Camera_v1_00', 'Books_v1_00', 'Beauty_v1_00', 'Baby_v1_00', 'Automotive_v1_00', 'Apparel_v1_00', 'Digital_Ebook_Purchase_v1_01', 'Books_v1_01', 'Books_v1_02']
Example of usage:
`load_dataset('amazon_us_reviews', 'Wireless_v1_00')`]
__________________________________________________________________________
`amazon_us_reviews = load_dataset('amazon_us_reviews', 'Watches_v1_00')
print(amazon_us_reviews)`
**ERROR**
`Generating` train split: 0%
0/960872 [00:00<?, ? examples/s]
---------------------------------------------------------------------------
KeyError Traceback (most recent call last)
/usr/local/lib/python3.10/dist-packages/datasets/builder.py in _prepare_split_single(self, gen_kwargs, fpath, file_format, max_shard_size, split_info, check_duplicate_keys, job_id)
1692 )
-> 1693 example = self.info.features.encode_example(record) if self.info.features is not None else record
1694 writer.write(example, key)
11 frames
KeyError: 'marketplace'
The above exception was the direct cause of the following exception:
DatasetGenerationError Traceback (most recent call last)
/usr/local/lib/python3.10/dist-packages/datasets/builder.py in _prepare_split_single(self, gen_kwargs, fpath, file_format, max_shard_size, split_info, check_duplicate_keys, job_id)
1710 if isinstance(e, SchemaInferenceError) and e.__context__ is not None:
1711 e = e.__context__
-> 1712 raise DatasetGenerationError("An error occurred while generating the dataset") from e
1713
1714 yield job_id, True, (total_num_examples, total_num_bytes, writer._features, num_shards, shard_lengths)
DatasetGenerationError: An error occurred while generating the dataset
### Motivation
The dataset I'm using
https://huggingface.co/datasets/amazon_us_reviews
### Your contribution
What is the best way to load this data | 67 | How do i get "amazon_us_reviews
### Feature request
I have been trying to load 'amazon_us_dataset" but unable to do so.
`amazon_us_reviews = load_dataset('amazon_us_reviews')`
`print(amazon_us_reviews)`
> [ValueError: Config name is missing.
Please pick one among the available configs: ['Wireless_v1_00', 'Watches_v1_00', 'Video_Games_v1_00', 'Video_DVD_v1_00', 'Video_v1_00', 'Toys_v1_00', 'Tools_v1_00', 'Sports_v1_00', 'Software_v1_00', 'Shoes_v1_00', 'Pet_Products_v1_00', 'Personal_Care_Appliances_v1_00', 'PC_v1_00', 'Outdoors_v1_00', 'Office_Products_v1_00', 'Musical_Instruments_v1_00', 'Music_v1_00', 'Mobile_Electronics_v1_00', 'Mobile_Apps_v1_00', 'Major_Appliances_v1_00', 'Luggage_v1_00', 'Lawn_and_Garden_v1_00', 'Kitchen_v1_00', 'Jewelry_v1_00', 'Home_Improvement_v1_00', 'Home_Entertainment_v1_00', 'Home_v1_00', 'Health_Personal_Care_v1_00', 'Grocery_v1_00', 'Gift_Card_v1_00', 'Furniture_v1_00', 'Electronics_v1_00', 'Digital_Video_Games_v1_00', 'Digital_Video_Download_v1_00', 'Digital_Software_v1_00', 'Digital_Music_Purchase_v1_00', 'Digital_Ebook_Purchase_v1_00', 'Camera_v1_00', 'Books_v1_00', 'Beauty_v1_00', 'Baby_v1_00', 'Automotive_v1_00', 'Apparel_v1_00', 'Digital_Ebook_Purchase_v1_01', 'Books_v1_01', 'Books_v1_02']
Example of usage:
`load_dataset('amazon_us_reviews', 'Wireless_v1_00')`]
__________________________________________________________________________
`amazon_us_reviews = load_dataset('amazon_us_reviews', 'Watches_v1_00')
print(amazon_us_reviews)`
**ERROR**
`Generating` train split: 0%
0/960872 [00:00<?, ? examples/s]
---------------------------------------------------------------------------
KeyError Traceback (most recent call last)
/usr/local/lib/python3.10/dist-packages/datasets/builder.py in _prepare_split_single(self, gen_kwargs, fpath, file_format, max_shard_size, split_info, check_duplicate_keys, job_id)
1692 )
-> 1693 example = self.info.features.encode_example(record) if self.info.features is not None else record
1694 writer.write(example, key)
11 frames
KeyError: 'marketplace'
The above exception was the direct cause of the following exception:
DatasetGenerationError Traceback (most recent call last)
/usr/local/lib/python3.10/dist-packages/datasets/builder.py in _prepare_split_single(self, gen_kwargs, fpath, file_format, max_shard_size, split_info, check_duplicate_keys, job_id)
1710 if isinstance(e, SchemaInferenceError) and e.__context__ is not None:
1711 e = e.__context__
-> 1712 raise DatasetGenerationError("An error occurred while generating the dataset") from e
1713
1714 yield job_id, True, (total_num_examples, total_num_bytes, writer._features, num_shards, shard_lengths)
DatasetGenerationError: An error occurred while generating the dataset
### Motivation
The dataset I'm using
https://huggingface.co/datasets/amazon_us_reviews
### Your contribution
What is the best way to load this data
Seems like the problem isn't with the library, but the dataset itself hosted on AWS S3.
Its [homepage](https://s3.amazonaws.com/amazon-reviews-pds/readme.html) returns an `AccessDenied` XML response, which is the same thing you get if you try to log the `record` that triggers the exception
```python
try:
example = self.info.features.encode_example(record) if self.info.features is not None else record
except Exception as e:
print(record)
```
⬇️
```
{'<?xml version="1.0" encoding="UTF-8"?>': '<Error><Code>AccessDenied</Code><Message>Access Denied</Message><RequestId>N2HFJ82ZV8SZW9BV</RequestId><HostId>Zw2DQ0V2GdRmvH5qWEpumK4uj5+W8YPcilQbN9fLBr3VqQOcKPHOhUZLG3LcM9X5fkOetxp48Os=</HostId></Error>'}
``` |
https://github.com/huggingface/datasets/issues/6099 | How do i get "amazon_us_reviews | I have figured it out. there was an option of **parquet formated files** i downloaded some from there. | ### Feature request
I have been trying to load 'amazon_us_dataset" but unable to do so.
`amazon_us_reviews = load_dataset('amazon_us_reviews')`
`print(amazon_us_reviews)`
> [ValueError: Config name is missing.
Please pick one among the available configs: ['Wireless_v1_00', 'Watches_v1_00', 'Video_Games_v1_00', 'Video_DVD_v1_00', 'Video_v1_00', 'Toys_v1_00', 'Tools_v1_00', 'Sports_v1_00', 'Software_v1_00', 'Shoes_v1_00', 'Pet_Products_v1_00', 'Personal_Care_Appliances_v1_00', 'PC_v1_00', 'Outdoors_v1_00', 'Office_Products_v1_00', 'Musical_Instruments_v1_00', 'Music_v1_00', 'Mobile_Electronics_v1_00', 'Mobile_Apps_v1_00', 'Major_Appliances_v1_00', 'Luggage_v1_00', 'Lawn_and_Garden_v1_00', 'Kitchen_v1_00', 'Jewelry_v1_00', 'Home_Improvement_v1_00', 'Home_Entertainment_v1_00', 'Home_v1_00', 'Health_Personal_Care_v1_00', 'Grocery_v1_00', 'Gift_Card_v1_00', 'Furniture_v1_00', 'Electronics_v1_00', 'Digital_Video_Games_v1_00', 'Digital_Video_Download_v1_00', 'Digital_Software_v1_00', 'Digital_Music_Purchase_v1_00', 'Digital_Ebook_Purchase_v1_00', 'Camera_v1_00', 'Books_v1_00', 'Beauty_v1_00', 'Baby_v1_00', 'Automotive_v1_00', 'Apparel_v1_00', 'Digital_Ebook_Purchase_v1_01', 'Books_v1_01', 'Books_v1_02']
Example of usage:
`load_dataset('amazon_us_reviews', 'Wireless_v1_00')`]
__________________________________________________________________________
`amazon_us_reviews = load_dataset('amazon_us_reviews', 'Watches_v1_00')
print(amazon_us_reviews)`
**ERROR**
`Generating` train split: 0%
0/960872 [00:00<?, ? examples/s]
---------------------------------------------------------------------------
KeyError Traceback (most recent call last)
/usr/local/lib/python3.10/dist-packages/datasets/builder.py in _prepare_split_single(self, gen_kwargs, fpath, file_format, max_shard_size, split_info, check_duplicate_keys, job_id)
1692 )
-> 1693 example = self.info.features.encode_example(record) if self.info.features is not None else record
1694 writer.write(example, key)
11 frames
KeyError: 'marketplace'
The above exception was the direct cause of the following exception:
DatasetGenerationError Traceback (most recent call last)
/usr/local/lib/python3.10/dist-packages/datasets/builder.py in _prepare_split_single(self, gen_kwargs, fpath, file_format, max_shard_size, split_info, check_duplicate_keys, job_id)
1710 if isinstance(e, SchemaInferenceError) and e.__context__ is not None:
1711 e = e.__context__
-> 1712 raise DatasetGenerationError("An error occurred while generating the dataset") from e
1713
1714 yield job_id, True, (total_num_examples, total_num_bytes, writer._features, num_shards, shard_lengths)
DatasetGenerationError: An error occurred while generating the dataset
### Motivation
The dataset I'm using
https://huggingface.co/datasets/amazon_us_reviews
### Your contribution
What is the best way to load this data | 18 | How do i get "amazon_us_reviews
### Feature request
I have been trying to load 'amazon_us_dataset" but unable to do so.
`amazon_us_reviews = load_dataset('amazon_us_reviews')`
`print(amazon_us_reviews)`
> [ValueError: Config name is missing.
Please pick one among the available configs: ['Wireless_v1_00', 'Watches_v1_00', 'Video_Games_v1_00', 'Video_DVD_v1_00', 'Video_v1_00', 'Toys_v1_00', 'Tools_v1_00', 'Sports_v1_00', 'Software_v1_00', 'Shoes_v1_00', 'Pet_Products_v1_00', 'Personal_Care_Appliances_v1_00', 'PC_v1_00', 'Outdoors_v1_00', 'Office_Products_v1_00', 'Musical_Instruments_v1_00', 'Music_v1_00', 'Mobile_Electronics_v1_00', 'Mobile_Apps_v1_00', 'Major_Appliances_v1_00', 'Luggage_v1_00', 'Lawn_and_Garden_v1_00', 'Kitchen_v1_00', 'Jewelry_v1_00', 'Home_Improvement_v1_00', 'Home_Entertainment_v1_00', 'Home_v1_00', 'Health_Personal_Care_v1_00', 'Grocery_v1_00', 'Gift_Card_v1_00', 'Furniture_v1_00', 'Electronics_v1_00', 'Digital_Video_Games_v1_00', 'Digital_Video_Download_v1_00', 'Digital_Software_v1_00', 'Digital_Music_Purchase_v1_00', 'Digital_Ebook_Purchase_v1_00', 'Camera_v1_00', 'Books_v1_00', 'Beauty_v1_00', 'Baby_v1_00', 'Automotive_v1_00', 'Apparel_v1_00', 'Digital_Ebook_Purchase_v1_01', 'Books_v1_01', 'Books_v1_02']
Example of usage:
`load_dataset('amazon_us_reviews', 'Wireless_v1_00')`]
__________________________________________________________________________
`amazon_us_reviews = load_dataset('amazon_us_reviews', 'Watches_v1_00')
print(amazon_us_reviews)`
**ERROR**
`Generating` train split: 0%
0/960872 [00:00<?, ? examples/s]
---------------------------------------------------------------------------
KeyError Traceback (most recent call last)
/usr/local/lib/python3.10/dist-packages/datasets/builder.py in _prepare_split_single(self, gen_kwargs, fpath, file_format, max_shard_size, split_info, check_duplicate_keys, job_id)
1692 )
-> 1693 example = self.info.features.encode_example(record) if self.info.features is not None else record
1694 writer.write(example, key)
11 frames
KeyError: 'marketplace'
The above exception was the direct cause of the following exception:
DatasetGenerationError Traceback (most recent call last)
/usr/local/lib/python3.10/dist-packages/datasets/builder.py in _prepare_split_single(self, gen_kwargs, fpath, file_format, max_shard_size, split_info, check_duplicate_keys, job_id)
1710 if isinstance(e, SchemaInferenceError) and e.__context__ is not None:
1711 e = e.__context__
-> 1712 raise DatasetGenerationError("An error occurred while generating the dataset") from e
1713
1714 yield job_id, True, (total_num_examples, total_num_bytes, writer._features, num_shards, shard_lengths)
DatasetGenerationError: An error occurred while generating the dataset
### Motivation
The dataset I'm using
https://huggingface.co/datasets/amazon_us_reviews
### Your contribution
What is the best way to load this data
I have figured it out. there was an option of **parquet formated files** i downloaded some from there. |
https://github.com/huggingface/datasets/issues/6099 | How do i get "amazon_us_reviews | Thanks for reporting, @IqraBaluch.
We contacted the authors and unfortunately they reported that Amazon has decided to stop distributing this dataset. | ### Feature request
I have been trying to load 'amazon_us_dataset" but unable to do so.
`amazon_us_reviews = load_dataset('amazon_us_reviews')`
`print(amazon_us_reviews)`
> [ValueError: Config name is missing.
Please pick one among the available configs: ['Wireless_v1_00', 'Watches_v1_00', 'Video_Games_v1_00', 'Video_DVD_v1_00', 'Video_v1_00', 'Toys_v1_00', 'Tools_v1_00', 'Sports_v1_00', 'Software_v1_00', 'Shoes_v1_00', 'Pet_Products_v1_00', 'Personal_Care_Appliances_v1_00', 'PC_v1_00', 'Outdoors_v1_00', 'Office_Products_v1_00', 'Musical_Instruments_v1_00', 'Music_v1_00', 'Mobile_Electronics_v1_00', 'Mobile_Apps_v1_00', 'Major_Appliances_v1_00', 'Luggage_v1_00', 'Lawn_and_Garden_v1_00', 'Kitchen_v1_00', 'Jewelry_v1_00', 'Home_Improvement_v1_00', 'Home_Entertainment_v1_00', 'Home_v1_00', 'Health_Personal_Care_v1_00', 'Grocery_v1_00', 'Gift_Card_v1_00', 'Furniture_v1_00', 'Electronics_v1_00', 'Digital_Video_Games_v1_00', 'Digital_Video_Download_v1_00', 'Digital_Software_v1_00', 'Digital_Music_Purchase_v1_00', 'Digital_Ebook_Purchase_v1_00', 'Camera_v1_00', 'Books_v1_00', 'Beauty_v1_00', 'Baby_v1_00', 'Automotive_v1_00', 'Apparel_v1_00', 'Digital_Ebook_Purchase_v1_01', 'Books_v1_01', 'Books_v1_02']
Example of usage:
`load_dataset('amazon_us_reviews', 'Wireless_v1_00')`]
__________________________________________________________________________
`amazon_us_reviews = load_dataset('amazon_us_reviews', 'Watches_v1_00')
print(amazon_us_reviews)`
**ERROR**
`Generating` train split: 0%
0/960872 [00:00<?, ? examples/s]
---------------------------------------------------------------------------
KeyError Traceback (most recent call last)
/usr/local/lib/python3.10/dist-packages/datasets/builder.py in _prepare_split_single(self, gen_kwargs, fpath, file_format, max_shard_size, split_info, check_duplicate_keys, job_id)
1692 )
-> 1693 example = self.info.features.encode_example(record) if self.info.features is not None else record
1694 writer.write(example, key)
11 frames
KeyError: 'marketplace'
The above exception was the direct cause of the following exception:
DatasetGenerationError Traceback (most recent call last)
/usr/local/lib/python3.10/dist-packages/datasets/builder.py in _prepare_split_single(self, gen_kwargs, fpath, file_format, max_shard_size, split_info, check_duplicate_keys, job_id)
1710 if isinstance(e, SchemaInferenceError) and e.__context__ is not None:
1711 e = e.__context__
-> 1712 raise DatasetGenerationError("An error occurred while generating the dataset") from e
1713
1714 yield job_id, True, (total_num_examples, total_num_bytes, writer._features, num_shards, shard_lengths)
DatasetGenerationError: An error occurred while generating the dataset
### Motivation
The dataset I'm using
https://huggingface.co/datasets/amazon_us_reviews
### Your contribution
What is the best way to load this data | 21 | How do i get "amazon_us_reviews
### Feature request
I have been trying to load 'amazon_us_dataset" but unable to do so.
`amazon_us_reviews = load_dataset('amazon_us_reviews')`
`print(amazon_us_reviews)`
> [ValueError: Config name is missing.
Please pick one among the available configs: ['Wireless_v1_00', 'Watches_v1_00', 'Video_Games_v1_00', 'Video_DVD_v1_00', 'Video_v1_00', 'Toys_v1_00', 'Tools_v1_00', 'Sports_v1_00', 'Software_v1_00', 'Shoes_v1_00', 'Pet_Products_v1_00', 'Personal_Care_Appliances_v1_00', 'PC_v1_00', 'Outdoors_v1_00', 'Office_Products_v1_00', 'Musical_Instruments_v1_00', 'Music_v1_00', 'Mobile_Electronics_v1_00', 'Mobile_Apps_v1_00', 'Major_Appliances_v1_00', 'Luggage_v1_00', 'Lawn_and_Garden_v1_00', 'Kitchen_v1_00', 'Jewelry_v1_00', 'Home_Improvement_v1_00', 'Home_Entertainment_v1_00', 'Home_v1_00', 'Health_Personal_Care_v1_00', 'Grocery_v1_00', 'Gift_Card_v1_00', 'Furniture_v1_00', 'Electronics_v1_00', 'Digital_Video_Games_v1_00', 'Digital_Video_Download_v1_00', 'Digital_Software_v1_00', 'Digital_Music_Purchase_v1_00', 'Digital_Ebook_Purchase_v1_00', 'Camera_v1_00', 'Books_v1_00', 'Beauty_v1_00', 'Baby_v1_00', 'Automotive_v1_00', 'Apparel_v1_00', 'Digital_Ebook_Purchase_v1_01', 'Books_v1_01', 'Books_v1_02']
Example of usage:
`load_dataset('amazon_us_reviews', 'Wireless_v1_00')`]
__________________________________________________________________________
`amazon_us_reviews = load_dataset('amazon_us_reviews', 'Watches_v1_00')
print(amazon_us_reviews)`
**ERROR**
`Generating` train split: 0%
0/960872 [00:00<?, ? examples/s]
---------------------------------------------------------------------------
KeyError Traceback (most recent call last)
/usr/local/lib/python3.10/dist-packages/datasets/builder.py in _prepare_split_single(self, gen_kwargs, fpath, file_format, max_shard_size, split_info, check_duplicate_keys, job_id)
1692 )
-> 1693 example = self.info.features.encode_example(record) if self.info.features is not None else record
1694 writer.write(example, key)
11 frames
KeyError: 'marketplace'
The above exception was the direct cause of the following exception:
DatasetGenerationError Traceback (most recent call last)
/usr/local/lib/python3.10/dist-packages/datasets/builder.py in _prepare_split_single(self, gen_kwargs, fpath, file_format, max_shard_size, split_info, check_duplicate_keys, job_id)
1710 if isinstance(e, SchemaInferenceError) and e.__context__ is not None:
1711 e = e.__context__
-> 1712 raise DatasetGenerationError("An error occurred while generating the dataset") from e
1713
1714 yield job_id, True, (total_num_examples, total_num_bytes, writer._features, num_shards, shard_lengths)
DatasetGenerationError: An error occurred while generating the dataset
### Motivation
The dataset I'm using
https://huggingface.co/datasets/amazon_us_reviews
### Your contribution
What is the best way to load this data
Thanks for reporting, @IqraBaluch.
We contacted the authors and unfortunately they reported that Amazon has decided to stop distributing this dataset. |
https://github.com/huggingface/datasets/issues/6099 | How do i get "amazon_us_reviews | I noticed that some book data is missing, we can only get Books_v1_02 data.
Is there any way we can get the Books_v1_00 and Books_v1_01?
Really appreciate !!! | ### Feature request
I have been trying to load 'amazon_us_dataset" but unable to do so.
`amazon_us_reviews = load_dataset('amazon_us_reviews')`
`print(amazon_us_reviews)`
> [ValueError: Config name is missing.
Please pick one among the available configs: ['Wireless_v1_00', 'Watches_v1_00', 'Video_Games_v1_00', 'Video_DVD_v1_00', 'Video_v1_00', 'Toys_v1_00', 'Tools_v1_00', 'Sports_v1_00', 'Software_v1_00', 'Shoes_v1_00', 'Pet_Products_v1_00', 'Personal_Care_Appliances_v1_00', 'PC_v1_00', 'Outdoors_v1_00', 'Office_Products_v1_00', 'Musical_Instruments_v1_00', 'Music_v1_00', 'Mobile_Electronics_v1_00', 'Mobile_Apps_v1_00', 'Major_Appliances_v1_00', 'Luggage_v1_00', 'Lawn_and_Garden_v1_00', 'Kitchen_v1_00', 'Jewelry_v1_00', 'Home_Improvement_v1_00', 'Home_Entertainment_v1_00', 'Home_v1_00', 'Health_Personal_Care_v1_00', 'Grocery_v1_00', 'Gift_Card_v1_00', 'Furniture_v1_00', 'Electronics_v1_00', 'Digital_Video_Games_v1_00', 'Digital_Video_Download_v1_00', 'Digital_Software_v1_00', 'Digital_Music_Purchase_v1_00', 'Digital_Ebook_Purchase_v1_00', 'Camera_v1_00', 'Books_v1_00', 'Beauty_v1_00', 'Baby_v1_00', 'Automotive_v1_00', 'Apparel_v1_00', 'Digital_Ebook_Purchase_v1_01', 'Books_v1_01', 'Books_v1_02']
Example of usage:
`load_dataset('amazon_us_reviews', 'Wireless_v1_00')`]
__________________________________________________________________________
`amazon_us_reviews = load_dataset('amazon_us_reviews', 'Watches_v1_00')
print(amazon_us_reviews)`
**ERROR**
`Generating` train split: 0%
0/960872 [00:00<?, ? examples/s]
---------------------------------------------------------------------------
KeyError Traceback (most recent call last)
/usr/local/lib/python3.10/dist-packages/datasets/builder.py in _prepare_split_single(self, gen_kwargs, fpath, file_format, max_shard_size, split_info, check_duplicate_keys, job_id)
1692 )
-> 1693 example = self.info.features.encode_example(record) if self.info.features is not None else record
1694 writer.write(example, key)
11 frames
KeyError: 'marketplace'
The above exception was the direct cause of the following exception:
DatasetGenerationError Traceback (most recent call last)
/usr/local/lib/python3.10/dist-packages/datasets/builder.py in _prepare_split_single(self, gen_kwargs, fpath, file_format, max_shard_size, split_info, check_duplicate_keys, job_id)
1710 if isinstance(e, SchemaInferenceError) and e.__context__ is not None:
1711 e = e.__context__
-> 1712 raise DatasetGenerationError("An error occurred while generating the dataset") from e
1713
1714 yield job_id, True, (total_num_examples, total_num_bytes, writer._features, num_shards, shard_lengths)
DatasetGenerationError: An error occurred while generating the dataset
### Motivation
The dataset I'm using
https://huggingface.co/datasets/amazon_us_reviews
### Your contribution
What is the best way to load this data | 28 | How do i get "amazon_us_reviews
### Feature request
I have been trying to load 'amazon_us_dataset" but unable to do so.
`amazon_us_reviews = load_dataset('amazon_us_reviews')`
`print(amazon_us_reviews)`
> [ValueError: Config name is missing.
Please pick one among the available configs: ['Wireless_v1_00', 'Watches_v1_00', 'Video_Games_v1_00', 'Video_DVD_v1_00', 'Video_v1_00', 'Toys_v1_00', 'Tools_v1_00', 'Sports_v1_00', 'Software_v1_00', 'Shoes_v1_00', 'Pet_Products_v1_00', 'Personal_Care_Appliances_v1_00', 'PC_v1_00', 'Outdoors_v1_00', 'Office_Products_v1_00', 'Musical_Instruments_v1_00', 'Music_v1_00', 'Mobile_Electronics_v1_00', 'Mobile_Apps_v1_00', 'Major_Appliances_v1_00', 'Luggage_v1_00', 'Lawn_and_Garden_v1_00', 'Kitchen_v1_00', 'Jewelry_v1_00', 'Home_Improvement_v1_00', 'Home_Entertainment_v1_00', 'Home_v1_00', 'Health_Personal_Care_v1_00', 'Grocery_v1_00', 'Gift_Card_v1_00', 'Furniture_v1_00', 'Electronics_v1_00', 'Digital_Video_Games_v1_00', 'Digital_Video_Download_v1_00', 'Digital_Software_v1_00', 'Digital_Music_Purchase_v1_00', 'Digital_Ebook_Purchase_v1_00', 'Camera_v1_00', 'Books_v1_00', 'Beauty_v1_00', 'Baby_v1_00', 'Automotive_v1_00', 'Apparel_v1_00', 'Digital_Ebook_Purchase_v1_01', 'Books_v1_01', 'Books_v1_02']
Example of usage:
`load_dataset('amazon_us_reviews', 'Wireless_v1_00')`]
__________________________________________________________________________
`amazon_us_reviews = load_dataset('amazon_us_reviews', 'Watches_v1_00')
print(amazon_us_reviews)`
**ERROR**
`Generating` train split: 0%
0/960872 [00:00<?, ? examples/s]
---------------------------------------------------------------------------
KeyError Traceback (most recent call last)
/usr/local/lib/python3.10/dist-packages/datasets/builder.py in _prepare_split_single(self, gen_kwargs, fpath, file_format, max_shard_size, split_info, check_duplicate_keys, job_id)
1692 )
-> 1693 example = self.info.features.encode_example(record) if self.info.features is not None else record
1694 writer.write(example, key)
11 frames
KeyError: 'marketplace'
The above exception was the direct cause of the following exception:
DatasetGenerationError Traceback (most recent call last)
/usr/local/lib/python3.10/dist-packages/datasets/builder.py in _prepare_split_single(self, gen_kwargs, fpath, file_format, max_shard_size, split_info, check_duplicate_keys, job_id)
1710 if isinstance(e, SchemaInferenceError) and e.__context__ is not None:
1711 e = e.__context__
-> 1712 raise DatasetGenerationError("An error occurred while generating the dataset") from e
1713
1714 yield job_id, True, (total_num_examples, total_num_bytes, writer._features, num_shards, shard_lengths)
DatasetGenerationError: An error occurred while generating the dataset
### Motivation
The dataset I'm using
https://huggingface.co/datasets/amazon_us_reviews
### Your contribution
What is the best way to load this data
I noticed that some book data is missing, we can only get Books_v1_02 data.
Is there any way we can get the Books_v1_00 and Books_v1_01?
Really appreciate !!! |
https://github.com/huggingface/datasets/issues/6099 | How do i get "amazon_us_reviews | @albertvillanova will this dataset be retired given the data are no longer hosted on S3? What is done in cases such as these? | ### Feature request
I have been trying to load 'amazon_us_dataset" but unable to do so.
`amazon_us_reviews = load_dataset('amazon_us_reviews')`
`print(amazon_us_reviews)`
> [ValueError: Config name is missing.
Please pick one among the available configs: ['Wireless_v1_00', 'Watches_v1_00', 'Video_Games_v1_00', 'Video_DVD_v1_00', 'Video_v1_00', 'Toys_v1_00', 'Tools_v1_00', 'Sports_v1_00', 'Software_v1_00', 'Shoes_v1_00', 'Pet_Products_v1_00', 'Personal_Care_Appliances_v1_00', 'PC_v1_00', 'Outdoors_v1_00', 'Office_Products_v1_00', 'Musical_Instruments_v1_00', 'Music_v1_00', 'Mobile_Electronics_v1_00', 'Mobile_Apps_v1_00', 'Major_Appliances_v1_00', 'Luggage_v1_00', 'Lawn_and_Garden_v1_00', 'Kitchen_v1_00', 'Jewelry_v1_00', 'Home_Improvement_v1_00', 'Home_Entertainment_v1_00', 'Home_v1_00', 'Health_Personal_Care_v1_00', 'Grocery_v1_00', 'Gift_Card_v1_00', 'Furniture_v1_00', 'Electronics_v1_00', 'Digital_Video_Games_v1_00', 'Digital_Video_Download_v1_00', 'Digital_Software_v1_00', 'Digital_Music_Purchase_v1_00', 'Digital_Ebook_Purchase_v1_00', 'Camera_v1_00', 'Books_v1_00', 'Beauty_v1_00', 'Baby_v1_00', 'Automotive_v1_00', 'Apparel_v1_00', 'Digital_Ebook_Purchase_v1_01', 'Books_v1_01', 'Books_v1_02']
Example of usage:
`load_dataset('amazon_us_reviews', 'Wireless_v1_00')`]
__________________________________________________________________________
`amazon_us_reviews = load_dataset('amazon_us_reviews', 'Watches_v1_00')
print(amazon_us_reviews)`
**ERROR**
`Generating` train split: 0%
0/960872 [00:00<?, ? examples/s]
---------------------------------------------------------------------------
KeyError Traceback (most recent call last)
/usr/local/lib/python3.10/dist-packages/datasets/builder.py in _prepare_split_single(self, gen_kwargs, fpath, file_format, max_shard_size, split_info, check_duplicate_keys, job_id)
1692 )
-> 1693 example = self.info.features.encode_example(record) if self.info.features is not None else record
1694 writer.write(example, key)
11 frames
KeyError: 'marketplace'
The above exception was the direct cause of the following exception:
DatasetGenerationError Traceback (most recent call last)
/usr/local/lib/python3.10/dist-packages/datasets/builder.py in _prepare_split_single(self, gen_kwargs, fpath, file_format, max_shard_size, split_info, check_duplicate_keys, job_id)
1710 if isinstance(e, SchemaInferenceError) and e.__context__ is not None:
1711 e = e.__context__
-> 1712 raise DatasetGenerationError("An error occurred while generating the dataset") from e
1713
1714 yield job_id, True, (total_num_examples, total_num_bytes, writer._features, num_shards, shard_lengths)
DatasetGenerationError: An error occurred while generating the dataset
### Motivation
The dataset I'm using
https://huggingface.co/datasets/amazon_us_reviews
### Your contribution
What is the best way to load this data | 23 | How do i get "amazon_us_reviews
### Feature request
I have been trying to load 'amazon_us_dataset" but unable to do so.
`amazon_us_reviews = load_dataset('amazon_us_reviews')`
`print(amazon_us_reviews)`
> [ValueError: Config name is missing.
Please pick one among the available configs: ['Wireless_v1_00', 'Watches_v1_00', 'Video_Games_v1_00', 'Video_DVD_v1_00', 'Video_v1_00', 'Toys_v1_00', 'Tools_v1_00', 'Sports_v1_00', 'Software_v1_00', 'Shoes_v1_00', 'Pet_Products_v1_00', 'Personal_Care_Appliances_v1_00', 'PC_v1_00', 'Outdoors_v1_00', 'Office_Products_v1_00', 'Musical_Instruments_v1_00', 'Music_v1_00', 'Mobile_Electronics_v1_00', 'Mobile_Apps_v1_00', 'Major_Appliances_v1_00', 'Luggage_v1_00', 'Lawn_and_Garden_v1_00', 'Kitchen_v1_00', 'Jewelry_v1_00', 'Home_Improvement_v1_00', 'Home_Entertainment_v1_00', 'Home_v1_00', 'Health_Personal_Care_v1_00', 'Grocery_v1_00', 'Gift_Card_v1_00', 'Furniture_v1_00', 'Electronics_v1_00', 'Digital_Video_Games_v1_00', 'Digital_Video_Download_v1_00', 'Digital_Software_v1_00', 'Digital_Music_Purchase_v1_00', 'Digital_Ebook_Purchase_v1_00', 'Camera_v1_00', 'Books_v1_00', 'Beauty_v1_00', 'Baby_v1_00', 'Automotive_v1_00', 'Apparel_v1_00', 'Digital_Ebook_Purchase_v1_01', 'Books_v1_01', 'Books_v1_02']
Example of usage:
`load_dataset('amazon_us_reviews', 'Wireless_v1_00')`]
__________________________________________________________________________
`amazon_us_reviews = load_dataset('amazon_us_reviews', 'Watches_v1_00')
print(amazon_us_reviews)`
**ERROR**
`Generating` train split: 0%
0/960872 [00:00<?, ? examples/s]
---------------------------------------------------------------------------
KeyError Traceback (most recent call last)
/usr/local/lib/python3.10/dist-packages/datasets/builder.py in _prepare_split_single(self, gen_kwargs, fpath, file_format, max_shard_size, split_info, check_duplicate_keys, job_id)
1692 )
-> 1693 example = self.info.features.encode_example(record) if self.info.features is not None else record
1694 writer.write(example, key)
11 frames
KeyError: 'marketplace'
The above exception was the direct cause of the following exception:
DatasetGenerationError Traceback (most recent call last)
/usr/local/lib/python3.10/dist-packages/datasets/builder.py in _prepare_split_single(self, gen_kwargs, fpath, file_format, max_shard_size, split_info, check_duplicate_keys, job_id)
1710 if isinstance(e, SchemaInferenceError) and e.__context__ is not None:
1711 e = e.__context__
-> 1712 raise DatasetGenerationError("An error occurred while generating the dataset") from e
1713
1714 yield job_id, True, (total_num_examples, total_num_bytes, writer._features, num_shards, shard_lengths)
DatasetGenerationError: An error occurred while generating the dataset
### Motivation
The dataset I'm using
https://huggingface.co/datasets/amazon_us_reviews
### Your contribution
What is the best way to load this data
@albertvillanova will this dataset be retired given the data are no longer hosted on S3? What is done in cases such as these? |
https://github.com/huggingface/datasets/issues/6089 | AssertionError: daemonic processes are not allowed to have children | We could add a "threads" parallel backend to `datasets.parallel.parallel_backend` to support downloading with threads but note that `download_and_extract` also decompresses archives, and this is a CPU-intensive task, which is not ideal for (Python) threads (good for IO-intensive tasks). | ### Describe the bug
When I load_dataset with num_proc > 0 in a deamon process, I got an error:
```python
File "/Users/codingl2k1/Work/datasets/src/datasets/download/download_manager.py", line 564, in download_and_extract
return self.extract(self.download(url_or_urls))
^^^^^^^^^^^^^^^^^
File "/Users/codingl2k1/Work/datasets/src/datasets/download/download_manager.py", line 427, in download
downloaded_path_or_paths = map_nested(
^^^^^^^^^^^^^^^^^
File "/Users/codingl2k1/Work/datasets/src/datasets/utils/py_utils.py", line 468, in map_nested
mapped = parallel_map(function, iterable, num_proc, types, disable_tqdm, desc, _single_map_nested)
^^^^^^^^^^^^^^^^^
File "/Users/codingl2k1/Work/datasets/src/datasets/utils/experimental.py", line 40, in _inner_fn
return fn(*args, **kwargs)
^^^^^^^^^^^^^^^^^
File "/Users/codingl2k1/Work/datasets/src/datasets/parallel/parallel.py", line 34, in parallel_map
return _map_with_multiprocessing_pool(
^^^^^^^^^^^^^^^^^
File "/Users/codingl2k1/Work/datasets/src/datasets/parallel/parallel.py", line 64, in _map_with_multiprocessing_pool
with Pool(num_proc, initargs=initargs, initializer=initializer) as pool:
^^^^^^^^^^^^^^^^^
File "/Users/codingl2k1/.pyenv/versions/3.11.4/lib/python3.11/multiprocessing/context.py", line 119, in Pool
return Pool(processes, initializer, initargs, maxtasksperchild,
^^^^^^^^^^^^^^^^^
File "/Users/codingl2k1/.pyenv/versions/3.11.4/lib/python3.11/multiprocessing/pool.py", line 215, in __init__
self._repopulate_pool()
^^^^^^^^^^^^^^^^^
File "/Users/codingl2k1/.pyenv/versions/3.11.4/lib/python3.11/multiprocessing/pool.py", line 306, in _repopulate_pool
return self._repopulate_pool_static(self._ctx, self.Process,
^^^^^^^^^^^^^^^^^
File "/Users/codingl2k1/.pyenv/versions/3.11.4/lib/python3.11/multiprocessing/pool.py", line 329, in _repopulate_pool_static
w.start()
File "/Users/codingl2k1/.pyenv/versions/3.11.4/lib/python3.11/multiprocessing/process.py", line 118, in start
assert not _current_process._config.get('daemon'), ^^^^^^^^^^^^^^^^^
AssertionError: daemonic processes are not allowed to have children
```
The download is io-intensive computing, may be datasets can replece the multi processing pool by a multi threading pool if in a deamon process.
### Steps to reproduce the bug
1. start a deamon process
2. run load_dataset with num_proc > 0
### Expected behavior
No error.
### Environment info
Python 3.11.4
datasets latest master | 38 | AssertionError: daemonic processes are not allowed to have children
### Describe the bug
When I load_dataset with num_proc > 0 in a deamon process, I got an error:
```python
File "/Users/codingl2k1/Work/datasets/src/datasets/download/download_manager.py", line 564, in download_and_extract
return self.extract(self.download(url_or_urls))
^^^^^^^^^^^^^^^^^
File "/Users/codingl2k1/Work/datasets/src/datasets/download/download_manager.py", line 427, in download
downloaded_path_or_paths = map_nested(
^^^^^^^^^^^^^^^^^
File "/Users/codingl2k1/Work/datasets/src/datasets/utils/py_utils.py", line 468, in map_nested
mapped = parallel_map(function, iterable, num_proc, types, disable_tqdm, desc, _single_map_nested)
^^^^^^^^^^^^^^^^^
File "/Users/codingl2k1/Work/datasets/src/datasets/utils/experimental.py", line 40, in _inner_fn
return fn(*args, **kwargs)
^^^^^^^^^^^^^^^^^
File "/Users/codingl2k1/Work/datasets/src/datasets/parallel/parallel.py", line 34, in parallel_map
return _map_with_multiprocessing_pool(
^^^^^^^^^^^^^^^^^
File "/Users/codingl2k1/Work/datasets/src/datasets/parallel/parallel.py", line 64, in _map_with_multiprocessing_pool
with Pool(num_proc, initargs=initargs, initializer=initializer) as pool:
^^^^^^^^^^^^^^^^^
File "/Users/codingl2k1/.pyenv/versions/3.11.4/lib/python3.11/multiprocessing/context.py", line 119, in Pool
return Pool(processes, initializer, initargs, maxtasksperchild,
^^^^^^^^^^^^^^^^^
File "/Users/codingl2k1/.pyenv/versions/3.11.4/lib/python3.11/multiprocessing/pool.py", line 215, in __init__
self._repopulate_pool()
^^^^^^^^^^^^^^^^^
File "/Users/codingl2k1/.pyenv/versions/3.11.4/lib/python3.11/multiprocessing/pool.py", line 306, in _repopulate_pool
return self._repopulate_pool_static(self._ctx, self.Process,
^^^^^^^^^^^^^^^^^
File "/Users/codingl2k1/.pyenv/versions/3.11.4/lib/python3.11/multiprocessing/pool.py", line 329, in _repopulate_pool_static
w.start()
File "/Users/codingl2k1/.pyenv/versions/3.11.4/lib/python3.11/multiprocessing/process.py", line 118, in start
assert not _current_process._config.get('daemon'), ^^^^^^^^^^^^^^^^^
AssertionError: daemonic processes are not allowed to have children
```
The download is io-intensive computing, may be datasets can replece the multi processing pool by a multi threading pool if in a deamon process.
### Steps to reproduce the bug
1. start a deamon process
2. run load_dataset with num_proc > 0
### Expected behavior
No error.
### Environment info
Python 3.11.4
datasets latest master
We could add a "threads" parallel backend to `datasets.parallel.parallel_backend` to support downloading with threads but note that `download_and_extract` also decompresses archives, and this is a CPU-intensive task, which is not ideal for (Python) threads (good for IO-intensive tasks). |
https://github.com/huggingface/datasets/issues/6089 | AssertionError: daemonic processes are not allowed to have children | > We could add a "threads" parallel backend to `datasets.parallel.parallel_backend` to support downloading with threads but note that `download_and_extract` also decompresses archives, and this is a CPU-intensive task, which is not ideal for (Python) threads (good for IO-intensive tasks).
Great! Download takes more time than extract, multiple threads can download in parallel, which can speed up a lot. | ### Describe the bug
When I load_dataset with num_proc > 0 in a deamon process, I got an error:
```python
File "/Users/codingl2k1/Work/datasets/src/datasets/download/download_manager.py", line 564, in download_and_extract
return self.extract(self.download(url_or_urls))
^^^^^^^^^^^^^^^^^
File "/Users/codingl2k1/Work/datasets/src/datasets/download/download_manager.py", line 427, in download
downloaded_path_or_paths = map_nested(
^^^^^^^^^^^^^^^^^
File "/Users/codingl2k1/Work/datasets/src/datasets/utils/py_utils.py", line 468, in map_nested
mapped = parallel_map(function, iterable, num_proc, types, disable_tqdm, desc, _single_map_nested)
^^^^^^^^^^^^^^^^^
File "/Users/codingl2k1/Work/datasets/src/datasets/utils/experimental.py", line 40, in _inner_fn
return fn(*args, **kwargs)
^^^^^^^^^^^^^^^^^
File "/Users/codingl2k1/Work/datasets/src/datasets/parallel/parallel.py", line 34, in parallel_map
return _map_with_multiprocessing_pool(
^^^^^^^^^^^^^^^^^
File "/Users/codingl2k1/Work/datasets/src/datasets/parallel/parallel.py", line 64, in _map_with_multiprocessing_pool
with Pool(num_proc, initargs=initargs, initializer=initializer) as pool:
^^^^^^^^^^^^^^^^^
File "/Users/codingl2k1/.pyenv/versions/3.11.4/lib/python3.11/multiprocessing/context.py", line 119, in Pool
return Pool(processes, initializer, initargs, maxtasksperchild,
^^^^^^^^^^^^^^^^^
File "/Users/codingl2k1/.pyenv/versions/3.11.4/lib/python3.11/multiprocessing/pool.py", line 215, in __init__
self._repopulate_pool()
^^^^^^^^^^^^^^^^^
File "/Users/codingl2k1/.pyenv/versions/3.11.4/lib/python3.11/multiprocessing/pool.py", line 306, in _repopulate_pool
return self._repopulate_pool_static(self._ctx, self.Process,
^^^^^^^^^^^^^^^^^
File "/Users/codingl2k1/.pyenv/versions/3.11.4/lib/python3.11/multiprocessing/pool.py", line 329, in _repopulate_pool_static
w.start()
File "/Users/codingl2k1/.pyenv/versions/3.11.4/lib/python3.11/multiprocessing/process.py", line 118, in start
assert not _current_process._config.get('daemon'), ^^^^^^^^^^^^^^^^^
AssertionError: daemonic processes are not allowed to have children
```
The download is io-intensive computing, may be datasets can replece the multi processing pool by a multi threading pool if in a deamon process.
### Steps to reproduce the bug
1. start a deamon process
2. run load_dataset with num_proc > 0
### Expected behavior
No error.
### Environment info
Python 3.11.4
datasets latest master | 58 | AssertionError: daemonic processes are not allowed to have children
### Describe the bug
When I load_dataset with num_proc > 0 in a deamon process, I got an error:
```python
File "/Users/codingl2k1/Work/datasets/src/datasets/download/download_manager.py", line 564, in download_and_extract
return self.extract(self.download(url_or_urls))
^^^^^^^^^^^^^^^^^
File "/Users/codingl2k1/Work/datasets/src/datasets/download/download_manager.py", line 427, in download
downloaded_path_or_paths = map_nested(
^^^^^^^^^^^^^^^^^
File "/Users/codingl2k1/Work/datasets/src/datasets/utils/py_utils.py", line 468, in map_nested
mapped = parallel_map(function, iterable, num_proc, types, disable_tqdm, desc, _single_map_nested)
^^^^^^^^^^^^^^^^^
File "/Users/codingl2k1/Work/datasets/src/datasets/utils/experimental.py", line 40, in _inner_fn
return fn(*args, **kwargs)
^^^^^^^^^^^^^^^^^
File "/Users/codingl2k1/Work/datasets/src/datasets/parallel/parallel.py", line 34, in parallel_map
return _map_with_multiprocessing_pool(
^^^^^^^^^^^^^^^^^
File "/Users/codingl2k1/Work/datasets/src/datasets/parallel/parallel.py", line 64, in _map_with_multiprocessing_pool
with Pool(num_proc, initargs=initargs, initializer=initializer) as pool:
^^^^^^^^^^^^^^^^^
File "/Users/codingl2k1/.pyenv/versions/3.11.4/lib/python3.11/multiprocessing/context.py", line 119, in Pool
return Pool(processes, initializer, initargs, maxtasksperchild,
^^^^^^^^^^^^^^^^^
File "/Users/codingl2k1/.pyenv/versions/3.11.4/lib/python3.11/multiprocessing/pool.py", line 215, in __init__
self._repopulate_pool()
^^^^^^^^^^^^^^^^^
File "/Users/codingl2k1/.pyenv/versions/3.11.4/lib/python3.11/multiprocessing/pool.py", line 306, in _repopulate_pool
return self._repopulate_pool_static(self._ctx, self.Process,
^^^^^^^^^^^^^^^^^
File "/Users/codingl2k1/.pyenv/versions/3.11.4/lib/python3.11/multiprocessing/pool.py", line 329, in _repopulate_pool_static
w.start()
File "/Users/codingl2k1/.pyenv/versions/3.11.4/lib/python3.11/multiprocessing/process.py", line 118, in start
assert not _current_process._config.get('daemon'), ^^^^^^^^^^^^^^^^^
AssertionError: daemonic processes are not allowed to have children
```
The download is io-intensive computing, may be datasets can replece the multi processing pool by a multi threading pool if in a deamon process.
### Steps to reproduce the bug
1. start a deamon process
2. run load_dataset with num_proc > 0
### Expected behavior
No error.
### Environment info
Python 3.11.4
datasets latest master
> We could add a "threads" parallel backend to `datasets.parallel.parallel_backend` to support downloading with threads but note that `download_and_extract` also decompresses archives, and this is a CPU-intensive task, which is not ideal for (Python) threads (good for IO-intensive tasks).
Great! Download takes more time than extract, multiple threads can download in parallel, which can speed up a lot. |
https://github.com/huggingface/datasets/issues/6086 | Support `fsspec` in `Dataset.to_<format>` methods | I'm assuming this should just cover `to_csv`, `to_parquet`, and `to_json`, right? As `to_list` and `to_dict` just return Python objects, `to_pandas` returns a `pandas.DataFrame` and `to_sql` just inserts into a SQL DB, is that right? | Supporting this should be fairly easy.
Requested on the forum [here](https://discuss.huggingface.co/t/how-can-i-convert-a-loaded-dataset-in-to-a-parquet-file-and-save-it-to-the-s3/48353). | 34 | Support `fsspec` in `Dataset.to_<format>` methods
Supporting this should be fairly easy.
Requested on the forum [here](https://discuss.huggingface.co/t/how-can-i-convert-a-loaded-dataset-in-to-a-parquet-file-and-save-it-to-the-s3/48353).
I'm assuming this should just cover `to_csv`, `to_parquet`, and `to_json`, right? As `to_list` and `to_dict` just return Python objects, `to_pandas` returns a `pandas.DataFrame` and `to_sql` just inserts into a SQL DB, is that right? |
https://github.com/huggingface/datasets/issues/6079 | Iterating over DataLoader based on HF datasets is stuck forever | When the process starts to hang, can you interrupt it with CTRL + C and paste the error stack trace here? | ### Describe the bug
I am using Amazon Sagemaker notebook (Amazon Linux 2) with python 3.10 based Conda environment.
I have a dataset in parquet format locally. When I try to iterate over it, the loader is stuck forever. Note that the same code is working for python 3.6 based conda environment seamlessly. What should be my next steps here?
### Steps to reproduce the bug
```
train_dataset = load_dataset(
"parquet", data_files = {'train': tr_data_path + '*.parquet'},
split = 'train',
collate_fn = streaming_data_collate_fn,
streaming = True
).with_format('torch')
train_dataloader = DataLoader(train_dataset, batch_size = 2, num_workers = 0)
t = time.time()
iter_ = 0
for batch in train_dataloader:
iter_ += 1
if iter_ == 1000:
break
print (time.time() - t)
```
### Expected behavior
The snippet should work normally and load the next batch of data.
### Environment info
datasets: '2.14.0'
pyarrow: '12.0.0'
torch: '2.0.0'
Python: 3.10.10 | packaged by conda-forge | (main, Mar 24 2023, 20:08:06) [GCC 11.3.0]
!uname -r
5.10.178-162.673.amzn2.x86_64 | 21 | Iterating over DataLoader based on HF datasets is stuck forever
### Describe the bug
I am using Amazon Sagemaker notebook (Amazon Linux 2) with python 3.10 based Conda environment.
I have a dataset in parquet format locally. When I try to iterate over it, the loader is stuck forever. Note that the same code is working for python 3.6 based conda environment seamlessly. What should be my next steps here?
### Steps to reproduce the bug
```
train_dataset = load_dataset(
"parquet", data_files = {'train': tr_data_path + '*.parquet'},
split = 'train',
collate_fn = streaming_data_collate_fn,
streaming = True
).with_format('torch')
train_dataloader = DataLoader(train_dataset, batch_size = 2, num_workers = 0)
t = time.time()
iter_ = 0
for batch in train_dataloader:
iter_ += 1
if iter_ == 1000:
break
print (time.time() - t)
```
### Expected behavior
The snippet should work normally and load the next batch of data.
### Environment info
datasets: '2.14.0'
pyarrow: '12.0.0'
torch: '2.0.0'
Python: 3.10.10 | packaged by conda-forge | (main, Mar 24 2023, 20:08:06) [GCC 11.3.0]
!uname -r
5.10.178-162.673.amzn2.x86_64
When the process starts to hang, can you interrupt it with CTRL + C and paste the error stack trace here? |
https://github.com/huggingface/datasets/issues/6079 | Iterating over DataLoader based on HF datasets is stuck forever | Thanks @mariosasko for your prompt response, here's the stack trace:
```
KeyboardInterrupt Traceback (most recent call last)
Cell In[12], line 4
2 t = time.time()
3 iter_ = 0
----> 4 for batch in train_dataloader:
5 #batch_proc = streaming_obj.collect_streaming_data_batch(batch)
6 iter_ += 1
8 if iter_ == 1:
File ~/anaconda3/envs/pytorch_p310/lib/python3.10/site-packages/torch/utils/data/dataloader.py:634, in _BaseDataLoaderIter.__next__(self)
631 if self._sampler_iter is None:
632 # TODO(https://github.com/pytorch/pytorch/issues/76750)
633 self._reset() # type: ignore[call-arg]
--> 634 data = self._next_data()
635 self._num_yielded += 1
636 if self._dataset_kind == _DatasetKind.Iterable and \
637 self._IterableDataset_len_called is not None and \
638 self._num_yielded > self._IterableDataset_len_called:
File ~/anaconda3/envs/pytorch_p310/lib/python3.10/site-packages/torch/utils/data/dataloader.py:678, in _SingleProcessDataLoaderIter._next_data(self)
676 def _next_data(self):
677 index = self._next_index() # may raise StopIteration
--> 678 data = self._dataset_fetcher.fetch(index) # may raise StopIteration
679 if self._pin_memory:
680 data = _utils.pin_memory.pin_memory(data, self._pin_memory_device)
File ~/anaconda3/envs/pytorch_p310/lib/python3.10/site-packages/torch/utils/data/_utils/fetch.py:32, in _IterableDatasetFetcher.fetch(self, possibly_batched_index)
30 for _ in possibly_batched_index:
31 try:
---> 32 data.append(next(self.dataset_iter))
33 except StopIteration:
34 self.ended = True
File ~/anaconda3/envs/pytorch_p310/lib/python3.10/site-packages/datasets/iterable_dataset.py:1353, in IterableDataset.__iter__(self)
1350 yield formatter.format_row(pa_table)
1351 return
-> 1353 for key, example in ex_iterable:
1354 if self.features:
1355 # `IterableDataset` automatically fills missing columns with None.
1356 # This is done with `_apply_feature_types_on_example`.
1357 example = _apply_feature_types_on_example(
1358 example, self.features, token_per_repo_id=self._token_per_repo_id
1359 )
File ~/anaconda3/envs/pytorch_p310/lib/python3.10/site-packages/datasets/iterable_dataset.py:956, in BufferShuffledExamplesIterable.__iter__(self)
954 # this is the shuffle buffer that we keep in memory
955 mem_buffer = []
--> 956 for x in self.ex_iterable:
957 if len(mem_buffer) == buffer_size: # if the buffer is full, pick and example from it
958 i = next(indices_iterator)
File ~/anaconda3/envs/pytorch_p310/lib/python3.10/site-packages/datasets/iterable_dataset.py:296, in ShuffledDataSourcesArrowExamplesIterable.__iter__(self)
294 for key, pa_table in self.generate_tables_fn(**kwargs_with_shuffled_shards):
295 for pa_subtable in pa_table.to_reader(max_chunksize=config.ARROW_READER_BATCH_SIZE_IN_DATASET_ITER):
--> 296 formatted_batch = formatter.format_batch(pa_subtable)
297 for example in _batch_to_examples(formatted_batch):
298 yield key, example
File ~/anaconda3/envs/pytorch_p310/lib/python3.10/site-packages/datasets/formatting/formatting.py:448, in PythonFormatter.format_batch(self, pa_table)
446 if self.lazy:
447 return LazyBatch(pa_table, self)
--> 448 batch = self.python_arrow_extractor().extract_batch(pa_table)
449 batch = self.python_features_decoder.decode_batch(batch)
450 return batch
File ~/anaconda3/envs/pytorch_p310/lib/python3.10/site-packages/datasets/formatting/formatting.py:150, in PythonArrowExtractor.extract_batch(self, pa_table)
149 def extract_batch(self, pa_table: pa.Table) -> dict:
--> 150 return pa_table.to_pydict()
KeyboardInterrupt:
```
| ### Describe the bug
I am using Amazon Sagemaker notebook (Amazon Linux 2) with python 3.10 based Conda environment.
I have a dataset in parquet format locally. When I try to iterate over it, the loader is stuck forever. Note that the same code is working for python 3.6 based conda environment seamlessly. What should be my next steps here?
### Steps to reproduce the bug
```
train_dataset = load_dataset(
"parquet", data_files = {'train': tr_data_path + '*.parquet'},
split = 'train',
collate_fn = streaming_data_collate_fn,
streaming = True
).with_format('torch')
train_dataloader = DataLoader(train_dataset, batch_size = 2, num_workers = 0)
t = time.time()
iter_ = 0
for batch in train_dataloader:
iter_ += 1
if iter_ == 1000:
break
print (time.time() - t)
```
### Expected behavior
The snippet should work normally and load the next batch of data.
### Environment info
datasets: '2.14.0'
pyarrow: '12.0.0'
torch: '2.0.0'
Python: 3.10.10 | packaged by conda-forge | (main, Mar 24 2023, 20:08:06) [GCC 11.3.0]
!uname -r
5.10.178-162.673.amzn2.x86_64 | 308 | Iterating over DataLoader based on HF datasets is stuck forever
### Describe the bug
I am using Amazon Sagemaker notebook (Amazon Linux 2) with python 3.10 based Conda environment.
I have a dataset in parquet format locally. When I try to iterate over it, the loader is stuck forever. Note that the same code is working for python 3.6 based conda environment seamlessly. What should be my next steps here?
### Steps to reproduce the bug
```
train_dataset = load_dataset(
"parquet", data_files = {'train': tr_data_path + '*.parquet'},
split = 'train',
collate_fn = streaming_data_collate_fn,
streaming = True
).with_format('torch')
train_dataloader = DataLoader(train_dataset, batch_size = 2, num_workers = 0)
t = time.time()
iter_ = 0
for batch in train_dataloader:
iter_ += 1
if iter_ == 1000:
break
print (time.time() - t)
```
### Expected behavior
The snippet should work normally and load the next batch of data.
### Environment info
datasets: '2.14.0'
pyarrow: '12.0.0'
torch: '2.0.0'
Python: 3.10.10 | packaged by conda-forge | (main, Mar 24 2023, 20:08:06) [GCC 11.3.0]
!uname -r
5.10.178-162.673.amzn2.x86_64
Thanks @mariosasko for your prompt response, here's the stack trace:
```
KeyboardInterrupt Traceback (most recent call last)
Cell In[12], line 4
2 t = time.time()
3 iter_ = 0
----> 4 for batch in train_dataloader:
5 #batch_proc = streaming_obj.collect_streaming_data_batch(batch)
6 iter_ += 1
8 if iter_ == 1:
File ~/anaconda3/envs/pytorch_p310/lib/python3.10/site-packages/torch/utils/data/dataloader.py:634, in _BaseDataLoaderIter.__next__(self)
631 if self._sampler_iter is None:
632 # TODO(https://github.com/pytorch/pytorch/issues/76750)
633 self._reset() # type: ignore[call-arg]
--> 634 data = self._next_data()
635 self._num_yielded += 1
636 if self._dataset_kind == _DatasetKind.Iterable and \
637 self._IterableDataset_len_called is not None and \
638 self._num_yielded > self._IterableDataset_len_called:
File ~/anaconda3/envs/pytorch_p310/lib/python3.10/site-packages/torch/utils/data/dataloader.py:678, in _SingleProcessDataLoaderIter._next_data(self)
676 def _next_data(self):
677 index = self._next_index() # may raise StopIteration
--> 678 data = self._dataset_fetcher.fetch(index) # may raise StopIteration
679 if self._pin_memory:
680 data = _utils.pin_memory.pin_memory(data, self._pin_memory_device)
File ~/anaconda3/envs/pytorch_p310/lib/python3.10/site-packages/torch/utils/data/_utils/fetch.py:32, in _IterableDatasetFetcher.fetch(self, possibly_batched_index)
30 for _ in possibly_batched_index:
31 try:
---> 32 data.append(next(self.dataset_iter))
33 except StopIteration:
34 self.ended = True
File ~/anaconda3/envs/pytorch_p310/lib/python3.10/site-packages/datasets/iterable_dataset.py:1353, in IterableDataset.__iter__(self)
1350 yield formatter.format_row(pa_table)
1351 return
-> 1353 for key, example in ex_iterable:
1354 if self.features:
1355 # `IterableDataset` automatically fills missing columns with None.
1356 # This is done with `_apply_feature_types_on_example`.
1357 example = _apply_feature_types_on_example(
1358 example, self.features, token_per_repo_id=self._token_per_repo_id
1359 )
File ~/anaconda3/envs/pytorch_p310/lib/python3.10/site-packages/datasets/iterable_dataset.py:956, in BufferShuffledExamplesIterable.__iter__(self)
954 # this is the shuffle buffer that we keep in memory
955 mem_buffer = []
--> 956 for x in self.ex_iterable:
957 if len(mem_buffer) == buffer_size: # if the buffer is full, pick and example from it
958 i = next(indices_iterator)
File ~/anaconda3/envs/pytorch_p310/lib/python3.10/site-packages/datasets/iterable_dataset.py:296, in ShuffledDataSourcesArrowExamplesIterable.__iter__(self)
294 for key, pa_table in self.generate_tables_fn(**kwargs_with_shuffled_shards):
295 for pa_subtable in pa_table.to_reader(max_chunksize=config.ARROW_READER_BATCH_SIZE_IN_DATASET_ITER):
--> 296 formatted_batch = formatter.format_batch(pa_subtable)
297 for example in _batch_to_examples(formatted_batch):
298 yield key, example
File ~/anaconda3/envs/pytorch_p310/lib/python3.10/site-packages/datasets/formatting/formatting.py:448, in PythonFormatter.format_batch(self, pa_table)
446 if self.lazy:
447 return LazyBatch(pa_table, self)
--> 448 batch = self.python_arrow_extractor().extract_batch(pa_table)
449 batch = self.python_features_decoder.decode_batch(batch)
450 return batch
File ~/anaconda3/envs/pytorch_p310/lib/python3.10/site-packages/datasets/formatting/formatting.py:150, in PythonArrowExtractor.extract_batch(self, pa_table)
149 def extract_batch(self, pa_table: pa.Table) -> dict:
--> 150 return pa_table.to_pydict()
KeyboardInterrupt:
```
|
https://github.com/huggingface/datasets/issues/6079 | Iterating over DataLoader based on HF datasets is stuck forever | Update: If i let it run, it eventually fails with:
```
RuntimeError Traceback (most recent call last)
Cell In[16], line 4
2 t = time.time()
3 iter_ = 0
----> 4 for batch in train_dataloader:
5 #batch_proc = streaming_obj.collect_streaming_data_batch(batch)
6 iter_ += 1
8 if iter_ == 1:
File ~/anaconda3/envs/pytorch_p310/lib/python3.10/site-packages/torch/utils/data/dataloader.py:634, in _BaseDataLoaderIter.__next__(self)
631 if self._sampler_iter is None:
632 # TODO(https://github.com/pytorch/pytorch/issues/76750)
633 self._reset() # type: ignore[call-arg]
--> 634 data = self._next_data()
635 self._num_yielded += 1
636 if self._dataset_kind == _DatasetKind.Iterable and \
637 self._IterableDataset_len_called is not None and \
638 self._num_yielded > self._IterableDataset_len_called:
File ~/anaconda3/envs/pytorch_p310/lib/python3.10/site-packages/torch/utils/data/dataloader.py:678, in _SingleProcessDataLoaderIter._next_data(self)
676 def _next_data(self):
677 index = self._next_index() # may raise StopIteration
--> 678 data = self._dataset_fetcher.fetch(index) # may raise StopIteration
679 if self._pin_memory:
680 data = _utils.pin_memory.pin_memory(data, self._pin_memory_device)
File ~/anaconda3/envs/pytorch_p310/lib/python3.10/site-packages/torch/utils/data/_utils/fetch.py:32, in _IterableDatasetFetcher.fetch(self, possibly_batched_index)
30 for _ in possibly_batched_index:
31 try:
---> 32 data.append(next(self.dataset_iter))
33 except StopIteration:
34 self.ended = True
File ~/anaconda3/envs/pytorch_p310/lib/python3.10/site-packages/datasets/iterable_dataset.py:1360, in IterableDataset.__iter__(self)
1354 if self.features:
1355 # `IterableDataset` automatically fills missing columns with None.
1356 # This is done with `_apply_feature_types_on_example`.
1357 example = _apply_feature_types_on_example(
1358 example, self.features, token_per_repo_id=self._token_per_repo_id
1359 )
-> 1360 yield format_dict(example) if format_dict else example
File ~/anaconda3/envs/pytorch_p310/lib/python3.10/site-packages/datasets/formatting/torch_formatter.py:85, in TorchFormatter.recursive_tensorize(self, data_struct)
84 def recursive_tensorize(self, data_struct: dict):
---> 85 return map_nested(self._recursive_tensorize, data_struct, map_list=False)
File ~/anaconda3/envs/pytorch_p310/lib/python3.10/site-packages/datasets/utils/py_utils.py:463, in map_nested(function, data_struct, dict_only, map_list, map_tuple, map_numpy, num_proc, parallel_min_length, types, disable_tqdm, desc)
461 num_proc = 1
462 if num_proc != -1 and num_proc <= 1 or len(iterable) < parallel_min_length:
--> 463 mapped = [
464 _single_map_nested((function, obj, types, None, True, None))
465 for obj in logging.tqdm(iterable, disable=disable_tqdm, desc=desc)
466 ]
467 else:
468 mapped = parallel_map(function, iterable, num_proc, types, disable_tqdm, desc, _single_map_nested)
File ~/anaconda3/envs/pytorch_p310/lib/python3.10/site-packages/datasets/utils/py_utils.py:464, in <listcomp>(.0)
461 num_proc = 1
462 if num_proc != -1 and num_proc <= 1 or len(iterable) < parallel_min_length:
463 mapped = [
--> 464 _single_map_nested((function, obj, types, None, True, None))
465 for obj in logging.tqdm(iterable, disable=disable_tqdm, desc=desc)
466 ]
467 else:
468 mapped = parallel_map(function, iterable, num_proc, types, disable_tqdm, desc, _single_map_nested)
File ~/anaconda3/envs/pytorch_p310/lib/python3.10/site-packages/datasets/utils/py_utils.py:366, in _single_map_nested(args)
364 # Singleton first to spare some computation
365 if not isinstance(data_struct, dict) and not isinstance(data_struct, types):
--> 366 return function(data_struct)
368 # Reduce logging to keep things readable in multiprocessing with tqdm
369 if rank is not None and logging.get_verbosity() < logging.WARNING:
File ~/anaconda3/envs/pytorch_p310/lib/python3.10/site-packages/datasets/formatting/torch_formatter.py:82, in TorchFormatter._recursive_tensorize(self, data_struct)
80 elif isinstance(data_struct, (list, tuple)):
81 return self._consolidate([self.recursive_tensorize(substruct) for substruct in data_struct])
---> 82 return self._tensorize(data_struct)
File ~/anaconda3/envs/pytorch_p310/lib/python3.10/site-packages/datasets/formatting/torch_formatter.py:68, in TorchFormatter._tensorize(self, value)
66 if isinstance(value, PIL.Image.Image):
67 value = np.asarray(value)
---> 68 return torch.tensor(value, **{**default_dtype, **self.torch_tensor_kwargs})
RuntimeError: Could not infer dtype of decimal.Decimal
``` | ### Describe the bug
I am using Amazon Sagemaker notebook (Amazon Linux 2) with python 3.10 based Conda environment.
I have a dataset in parquet format locally. When I try to iterate over it, the loader is stuck forever. Note that the same code is working for python 3.6 based conda environment seamlessly. What should be my next steps here?
### Steps to reproduce the bug
```
train_dataset = load_dataset(
"parquet", data_files = {'train': tr_data_path + '*.parquet'},
split = 'train',
collate_fn = streaming_data_collate_fn,
streaming = True
).with_format('torch')
train_dataloader = DataLoader(train_dataset, batch_size = 2, num_workers = 0)
t = time.time()
iter_ = 0
for batch in train_dataloader:
iter_ += 1
if iter_ == 1000:
break
print (time.time() - t)
```
### Expected behavior
The snippet should work normally and load the next batch of data.
### Environment info
datasets: '2.14.0'
pyarrow: '12.0.0'
torch: '2.0.0'
Python: 3.10.10 | packaged by conda-forge | (main, Mar 24 2023, 20:08:06) [GCC 11.3.0]
!uname -r
5.10.178-162.673.amzn2.x86_64 | 416 | Iterating over DataLoader based on HF datasets is stuck forever
### Describe the bug
I am using Amazon Sagemaker notebook (Amazon Linux 2) with python 3.10 based Conda environment.
I have a dataset in parquet format locally. When I try to iterate over it, the loader is stuck forever. Note that the same code is working for python 3.6 based conda environment seamlessly. What should be my next steps here?
### Steps to reproduce the bug
```
train_dataset = load_dataset(
"parquet", data_files = {'train': tr_data_path + '*.parquet'},
split = 'train',
collate_fn = streaming_data_collate_fn,
streaming = True
).with_format('torch')
train_dataloader = DataLoader(train_dataset, batch_size = 2, num_workers = 0)
t = time.time()
iter_ = 0
for batch in train_dataloader:
iter_ += 1
if iter_ == 1000:
break
print (time.time() - t)
```
### Expected behavior
The snippet should work normally and load the next batch of data.
### Environment info
datasets: '2.14.0'
pyarrow: '12.0.0'
torch: '2.0.0'
Python: 3.10.10 | packaged by conda-forge | (main, Mar 24 2023, 20:08:06) [GCC 11.3.0]
!uname -r
5.10.178-162.673.amzn2.x86_64
Update: If i let it run, it eventually fails with:
```
RuntimeError Traceback (most recent call last)
Cell In[16], line 4
2 t = time.time()
3 iter_ = 0
----> 4 for batch in train_dataloader:
5 #batch_proc = streaming_obj.collect_streaming_data_batch(batch)
6 iter_ += 1
8 if iter_ == 1:
File ~/anaconda3/envs/pytorch_p310/lib/python3.10/site-packages/torch/utils/data/dataloader.py:634, in _BaseDataLoaderIter.__next__(self)
631 if self._sampler_iter is None:
632 # TODO(https://github.com/pytorch/pytorch/issues/76750)
633 self._reset() # type: ignore[call-arg]
--> 634 data = self._next_data()
635 self._num_yielded += 1
636 if self._dataset_kind == _DatasetKind.Iterable and \
637 self._IterableDataset_len_called is not None and \
638 self._num_yielded > self._IterableDataset_len_called:
File ~/anaconda3/envs/pytorch_p310/lib/python3.10/site-packages/torch/utils/data/dataloader.py:678, in _SingleProcessDataLoaderIter._next_data(self)
676 def _next_data(self):
677 index = self._next_index() # may raise StopIteration
--> 678 data = self._dataset_fetcher.fetch(index) # may raise StopIteration
679 if self._pin_memory:
680 data = _utils.pin_memory.pin_memory(data, self._pin_memory_device)
File ~/anaconda3/envs/pytorch_p310/lib/python3.10/site-packages/torch/utils/data/_utils/fetch.py:32, in _IterableDatasetFetcher.fetch(self, possibly_batched_index)
30 for _ in possibly_batched_index:
31 try:
---> 32 data.append(next(self.dataset_iter))
33 except StopIteration:
34 self.ended = True
File ~/anaconda3/envs/pytorch_p310/lib/python3.10/site-packages/datasets/iterable_dataset.py:1360, in IterableDataset.__iter__(self)
1354 if self.features:
1355 # `IterableDataset` automatically fills missing columns with None.
1356 # This is done with `_apply_feature_types_on_example`.
1357 example = _apply_feature_types_on_example(
1358 example, self.features, token_per_repo_id=self._token_per_repo_id
1359 )
-> 1360 yield format_dict(example) if format_dict else example
File ~/anaconda3/envs/pytorch_p310/lib/python3.10/site-packages/datasets/formatting/torch_formatter.py:85, in TorchFormatter.recursive_tensorize(self, data_struct)
84 def recursive_tensorize(self, data_struct: dict):
---> 85 return map_nested(self._recursive_tensorize, data_struct, map_list=False)
File ~/anaconda3/envs/pytorch_p310/lib/python3.10/site-packages/datasets/utils/py_utils.py:463, in map_nested(function, data_struct, dict_only, map_list, map_tuple, map_numpy, num_proc, parallel_min_length, types, disable_tqdm, desc)
461 num_proc = 1
462 if num_proc != -1 and num_proc <= 1 or len(iterable) < parallel_min_length:
--> 463 mapped = [
464 _single_map_nested((function, obj, types, None, True, None))
465 for obj in logging.tqdm(iterable, disable=disable_tqdm, desc=desc)
466 ]
467 else:
468 mapped = parallel_map(function, iterable, num_proc, types, disable_tqdm, desc, _single_map_nested)
File ~/anaconda3/envs/pytorch_p310/lib/python3.10/site-packages/datasets/utils/py_utils.py:464, in <listcomp>(.0)
461 num_proc = 1
462 if num_proc != -1 and num_proc <= 1 or len(iterable) < parallel_min_length:
463 mapped = [
--> 464 _single_map_nested((function, obj, types, None, True, None))
465 for obj in logging.tqdm(iterable, disable=disable_tqdm, desc=desc)
466 ]
467 else:
468 mapped = parallel_map(function, iterable, num_proc, types, disable_tqdm, desc, _single_map_nested)
File ~/anaconda3/envs/pytorch_p310/lib/python3.10/site-packages/datasets/utils/py_utils.py:366, in _single_map_nested(args)
364 # Singleton first to spare some computation
365 if not isinstance(data_struct, dict) and not isinstance(data_struct, types):
--> 366 return function(data_struct)
368 # Reduce logging to keep things readable in multiprocessing with tqdm
369 if rank is not None and logging.get_verbosity() < logging.WARNING:
File ~/anaconda3/envs/pytorch_p310/lib/python3.10/site-packages/datasets/formatting/torch_formatter.py:82, in TorchFormatter._recursive_tensorize(self, data_struct)
80 elif isinstance(data_struct, (list, tuple)):
81 return self._consolidate([self.recursive_tensorize(substruct) for substruct in data_struct])
---> 82 return self._tensorize(data_struct)
File ~/anaconda3/envs/pytorch_p310/lib/python3.10/site-packages/datasets/formatting/torch_formatter.py:68, in TorchFormatter._tensorize(self, value)
66 if isinstance(value, PIL.Image.Image):
67 value = np.asarray(value)
---> 68 return torch.tensor(value, **{**default_dtype, **self.torch_tensor_kwargs})
RuntimeError: Could not infer dtype of decimal.Decimal
``` |
https://github.com/huggingface/datasets/issues/6079 | Iterating over DataLoader based on HF datasets is stuck forever | PyTorch tensors cannot store `Decimal` objects. Casting the column with decimals to `float` should fix the issue. | ### Describe the bug
I am using Amazon Sagemaker notebook (Amazon Linux 2) with python 3.10 based Conda environment.
I have a dataset in parquet format locally. When I try to iterate over it, the loader is stuck forever. Note that the same code is working for python 3.6 based conda environment seamlessly. What should be my next steps here?
### Steps to reproduce the bug
```
train_dataset = load_dataset(
"parquet", data_files = {'train': tr_data_path + '*.parquet'},
split = 'train',
collate_fn = streaming_data_collate_fn,
streaming = True
).with_format('torch')
train_dataloader = DataLoader(train_dataset, batch_size = 2, num_workers = 0)
t = time.time()
iter_ = 0
for batch in train_dataloader:
iter_ += 1
if iter_ == 1000:
break
print (time.time() - t)
```
### Expected behavior
The snippet should work normally and load the next batch of data.
### Environment info
datasets: '2.14.0'
pyarrow: '12.0.0'
torch: '2.0.0'
Python: 3.10.10 | packaged by conda-forge | (main, Mar 24 2023, 20:08:06) [GCC 11.3.0]
!uname -r
5.10.178-162.673.amzn2.x86_64 | 17 | Iterating over DataLoader based on HF datasets is stuck forever
### Describe the bug
I am using Amazon Sagemaker notebook (Amazon Linux 2) with python 3.10 based Conda environment.
I have a dataset in parquet format locally. When I try to iterate over it, the loader is stuck forever. Note that the same code is working for python 3.6 based conda environment seamlessly. What should be my next steps here?
### Steps to reproduce the bug
```
train_dataset = load_dataset(
"parquet", data_files = {'train': tr_data_path + '*.parquet'},
split = 'train',
collate_fn = streaming_data_collate_fn,
streaming = True
).with_format('torch')
train_dataloader = DataLoader(train_dataset, batch_size = 2, num_workers = 0)
t = time.time()
iter_ = 0
for batch in train_dataloader:
iter_ += 1
if iter_ == 1000:
break
print (time.time() - t)
```
### Expected behavior
The snippet should work normally and load the next batch of data.
### Environment info
datasets: '2.14.0'
pyarrow: '12.0.0'
torch: '2.0.0'
Python: 3.10.10 | packaged by conda-forge | (main, Mar 24 2023, 20:08:06) [GCC 11.3.0]
!uname -r
5.10.178-162.673.amzn2.x86_64
PyTorch tensors cannot store `Decimal` objects. Casting the column with decimals to `float` should fix the issue. |
https://github.com/huggingface/datasets/issues/6079 | Iterating over DataLoader based on HF datasets is stuck forever | I already have cast in collate_fn, in which I perform .astype(float) for each numerical field.
On the same instance, I installed a conda env with python 3.6, and this works well.
Sample:
```
def streaming_data_collate_fn(batch):
df = pd.DataFrame.from_dict(batch)
feat_vals = torch.FloatTensor(np.nan_to_num(np.array(df[feats].astype(float))))
``` | ### Describe the bug
I am using Amazon Sagemaker notebook (Amazon Linux 2) with python 3.10 based Conda environment.
I have a dataset in parquet format locally. When I try to iterate over it, the loader is stuck forever. Note that the same code is working for python 3.6 based conda environment seamlessly. What should be my next steps here?
### Steps to reproduce the bug
```
train_dataset = load_dataset(
"parquet", data_files = {'train': tr_data_path + '*.parquet'},
split = 'train',
collate_fn = streaming_data_collate_fn,
streaming = True
).with_format('torch')
train_dataloader = DataLoader(train_dataset, batch_size = 2, num_workers = 0)
t = time.time()
iter_ = 0
for batch in train_dataloader:
iter_ += 1
if iter_ == 1000:
break
print (time.time() - t)
```
### Expected behavior
The snippet should work normally and load the next batch of data.
### Environment info
datasets: '2.14.0'
pyarrow: '12.0.0'
torch: '2.0.0'
Python: 3.10.10 | packaged by conda-forge | (main, Mar 24 2023, 20:08:06) [GCC 11.3.0]
!uname -r
5.10.178-162.673.amzn2.x86_64 | 42 | Iterating over DataLoader based on HF datasets is stuck forever
### Describe the bug
I am using Amazon Sagemaker notebook (Amazon Linux 2) with python 3.10 based Conda environment.
I have a dataset in parquet format locally. When I try to iterate over it, the loader is stuck forever. Note that the same code is working for python 3.6 based conda environment seamlessly. What should be my next steps here?
### Steps to reproduce the bug
```
train_dataset = load_dataset(
"parquet", data_files = {'train': tr_data_path + '*.parquet'},
split = 'train',
collate_fn = streaming_data_collate_fn,
streaming = True
).with_format('torch')
train_dataloader = DataLoader(train_dataset, batch_size = 2, num_workers = 0)
t = time.time()
iter_ = 0
for batch in train_dataloader:
iter_ += 1
if iter_ == 1000:
break
print (time.time() - t)
```
### Expected behavior
The snippet should work normally and load the next batch of data.
### Environment info
datasets: '2.14.0'
pyarrow: '12.0.0'
torch: '2.0.0'
Python: 3.10.10 | packaged by conda-forge | (main, Mar 24 2023, 20:08:06) [GCC 11.3.0]
!uname -r
5.10.178-162.673.amzn2.x86_64
I already have cast in collate_fn, in which I perform .astype(float) for each numerical field.
On the same instance, I installed a conda env with python 3.6, and this works well.
Sample:
```
def streaming_data_collate_fn(batch):
df = pd.DataFrame.from_dict(batch)
feat_vals = torch.FloatTensor(np.nan_to_num(np.array(df[feats].astype(float))))
``` |
https://github.com/huggingface/datasets/issues/6079 | Iterating over DataLoader based on HF datasets is stuck forever | `collate_fn` is applied after the `torch` formatting step, so I think the only option when working with an `IterableDataset` is to remove the `with_format` call and perform the conversion from Python values to PyTorch tensors in `collate_fn`. The standard `Dataset` supports `with_format("numpy")`, which should make this conversion faster. | ### Describe the bug
I am using Amazon Sagemaker notebook (Amazon Linux 2) with python 3.10 based Conda environment.
I have a dataset in parquet format locally. When I try to iterate over it, the loader is stuck forever. Note that the same code is working for python 3.6 based conda environment seamlessly. What should be my next steps here?
### Steps to reproduce the bug
```
train_dataset = load_dataset(
"parquet", data_files = {'train': tr_data_path + '*.parquet'},
split = 'train',
collate_fn = streaming_data_collate_fn,
streaming = True
).with_format('torch')
train_dataloader = DataLoader(train_dataset, batch_size = 2, num_workers = 0)
t = time.time()
iter_ = 0
for batch in train_dataloader:
iter_ += 1
if iter_ == 1000:
break
print (time.time() - t)
```
### Expected behavior
The snippet should work normally and load the next batch of data.
### Environment info
datasets: '2.14.0'
pyarrow: '12.0.0'
torch: '2.0.0'
Python: 3.10.10 | packaged by conda-forge | (main, Mar 24 2023, 20:08:06) [GCC 11.3.0]
!uname -r
5.10.178-162.673.amzn2.x86_64 | 48 | Iterating over DataLoader based on HF datasets is stuck forever
### Describe the bug
I am using Amazon Sagemaker notebook (Amazon Linux 2) with python 3.10 based Conda environment.
I have a dataset in parquet format locally. When I try to iterate over it, the loader is stuck forever. Note that the same code is working for python 3.6 based conda environment seamlessly. What should be my next steps here?
### Steps to reproduce the bug
```
train_dataset = load_dataset(
"parquet", data_files = {'train': tr_data_path + '*.parquet'},
split = 'train',
collate_fn = streaming_data_collate_fn,
streaming = True
).with_format('torch')
train_dataloader = DataLoader(train_dataset, batch_size = 2, num_workers = 0)
t = time.time()
iter_ = 0
for batch in train_dataloader:
iter_ += 1
if iter_ == 1000:
break
print (time.time() - t)
```
### Expected behavior
The snippet should work normally and load the next batch of data.
### Environment info
datasets: '2.14.0'
pyarrow: '12.0.0'
torch: '2.0.0'
Python: 3.10.10 | packaged by conda-forge | (main, Mar 24 2023, 20:08:06) [GCC 11.3.0]
!uname -r
5.10.178-162.673.amzn2.x86_64
`collate_fn` is applied after the `torch` formatting step, so I think the only option when working with an `IterableDataset` is to remove the `with_format` call and perform the conversion from Python values to PyTorch tensors in `collate_fn`. The standard `Dataset` supports `with_format("numpy")`, which should make this conversion faster. |
https://github.com/huggingface/datasets/issues/6079 | Iterating over DataLoader based on HF datasets is stuck forever | Thanks!
Python 3.10 conda-env: After replacing with_format("torch") with with_format("numpy"), the error went away. However, it was still taking over 2 minutes to load a very small batch of 64 samples with num_workers set to 32. Once I removed with_format call altogether, it is finishing in 11 seconds.
Python 3.6 based conda-env: When I switch the kernel , neither of the above work, and with_format("torch") is the only thing that works, and executes in 1.6 seconds.
I feel something else is also amiss here. | ### Describe the bug
I am using Amazon Sagemaker notebook (Amazon Linux 2) with python 3.10 based Conda environment.
I have a dataset in parquet format locally. When I try to iterate over it, the loader is stuck forever. Note that the same code is working for python 3.6 based conda environment seamlessly. What should be my next steps here?
### Steps to reproduce the bug
```
train_dataset = load_dataset(
"parquet", data_files = {'train': tr_data_path + '*.parquet'},
split = 'train',
collate_fn = streaming_data_collate_fn,
streaming = True
).with_format('torch')
train_dataloader = DataLoader(train_dataset, batch_size = 2, num_workers = 0)
t = time.time()
iter_ = 0
for batch in train_dataloader:
iter_ += 1
if iter_ == 1000:
break
print (time.time() - t)
```
### Expected behavior
The snippet should work normally and load the next batch of data.
### Environment info
datasets: '2.14.0'
pyarrow: '12.0.0'
torch: '2.0.0'
Python: 3.10.10 | packaged by conda-forge | (main, Mar 24 2023, 20:08:06) [GCC 11.3.0]
!uname -r
5.10.178-162.673.amzn2.x86_64 | 83 | Iterating over DataLoader based on HF datasets is stuck forever
### Describe the bug
I am using Amazon Sagemaker notebook (Amazon Linux 2) with python 3.10 based Conda environment.
I have a dataset in parquet format locally. When I try to iterate over it, the loader is stuck forever. Note that the same code is working for python 3.6 based conda environment seamlessly. What should be my next steps here?
### Steps to reproduce the bug
```
train_dataset = load_dataset(
"parquet", data_files = {'train': tr_data_path + '*.parquet'},
split = 'train',
collate_fn = streaming_data_collate_fn,
streaming = True
).with_format('torch')
train_dataloader = DataLoader(train_dataset, batch_size = 2, num_workers = 0)
t = time.time()
iter_ = 0
for batch in train_dataloader:
iter_ += 1
if iter_ == 1000:
break
print (time.time() - t)
```
### Expected behavior
The snippet should work normally and load the next batch of data.
### Environment info
datasets: '2.14.0'
pyarrow: '12.0.0'
torch: '2.0.0'
Python: 3.10.10 | packaged by conda-forge | (main, Mar 24 2023, 20:08:06) [GCC 11.3.0]
!uname -r
5.10.178-162.673.amzn2.x86_64
Thanks!
Python 3.10 conda-env: After replacing with_format("torch") with with_format("numpy"), the error went away. However, it was still taking over 2 minutes to load a very small batch of 64 samples with num_workers set to 32. Once I removed with_format call altogether, it is finishing in 11 seconds.
Python 3.6 based conda-env: When I switch the kernel , neither of the above work, and with_format("torch") is the only thing that works, and executes in 1.6 seconds.
I feel something else is also amiss here. |
https://github.com/huggingface/datasets/issues/6079 | Iterating over DataLoader based on HF datasets is stuck forever | Can you share the `datasets` and `torch` versions installed in these conda envs?
> Once I removed with_format call altogether, it is finishing in 11 seconds.
Hmm, that's surprising. What are your dataset's `.features`? | ### Describe the bug
I am using Amazon Sagemaker notebook (Amazon Linux 2) with python 3.10 based Conda environment.
I have a dataset in parquet format locally. When I try to iterate over it, the loader is stuck forever. Note that the same code is working for python 3.6 based conda environment seamlessly. What should be my next steps here?
### Steps to reproduce the bug
```
train_dataset = load_dataset(
"parquet", data_files = {'train': tr_data_path + '*.parquet'},
split = 'train',
collate_fn = streaming_data_collate_fn,
streaming = True
).with_format('torch')
train_dataloader = DataLoader(train_dataset, batch_size = 2, num_workers = 0)
t = time.time()
iter_ = 0
for batch in train_dataloader:
iter_ += 1
if iter_ == 1000:
break
print (time.time() - t)
```
### Expected behavior
The snippet should work normally and load the next batch of data.
### Environment info
datasets: '2.14.0'
pyarrow: '12.0.0'
torch: '2.0.0'
Python: 3.10.10 | packaged by conda-forge | (main, Mar 24 2023, 20:08:06) [GCC 11.3.0]
!uname -r
5.10.178-162.673.amzn2.x86_64 | 34 | Iterating over DataLoader based on HF datasets is stuck forever
### Describe the bug
I am using Amazon Sagemaker notebook (Amazon Linux 2) with python 3.10 based Conda environment.
I have a dataset in parquet format locally. When I try to iterate over it, the loader is stuck forever. Note that the same code is working for python 3.6 based conda environment seamlessly. What should be my next steps here?
### Steps to reproduce the bug
```
train_dataset = load_dataset(
"parquet", data_files = {'train': tr_data_path + '*.parquet'},
split = 'train',
collate_fn = streaming_data_collate_fn,
streaming = True
).with_format('torch')
train_dataloader = DataLoader(train_dataset, batch_size = 2, num_workers = 0)
t = time.time()
iter_ = 0
for batch in train_dataloader:
iter_ += 1
if iter_ == 1000:
break
print (time.time() - t)
```
### Expected behavior
The snippet should work normally and load the next batch of data.
### Environment info
datasets: '2.14.0'
pyarrow: '12.0.0'
torch: '2.0.0'
Python: 3.10.10 | packaged by conda-forge | (main, Mar 24 2023, 20:08:06) [GCC 11.3.0]
!uname -r
5.10.178-162.673.amzn2.x86_64
Can you share the `datasets` and `torch` versions installed in these conda envs?
> Once I removed with_format call altogether, it is finishing in 11 seconds.
Hmm, that's surprising. What are your dataset's `.features`? |
https://github.com/huggingface/datasets/issues/6079 | Iterating over DataLoader based on HF datasets is stuck forever | Python 3.6:
datasets.__version__ 2.4.0
torch.__version__ 1.10.1+cu102
Python 3.10:
datasets.__version__ 2.14.0
torch.__version__ 2.0.0
Anonymized features are of the form (subset shown here):
{
'string_feature_i': Value(dtype='string', id=None),
'numerical_feature_i': Value(dtype='decimal128(38, 0)', id=None),
'numerical_feature_series_i': Sequence(feature=Value(dtype='float64', id=None), length=-1, id=None),
}
There is no output from .features in python 3.6 kernel BTW. | ### Describe the bug
I am using Amazon Sagemaker notebook (Amazon Linux 2) with python 3.10 based Conda environment.
I have a dataset in parquet format locally. When I try to iterate over it, the loader is stuck forever. Note that the same code is working for python 3.6 based conda environment seamlessly. What should be my next steps here?
### Steps to reproduce the bug
```
train_dataset = load_dataset(
"parquet", data_files = {'train': tr_data_path + '*.parquet'},
split = 'train',
collate_fn = streaming_data_collate_fn,
streaming = True
).with_format('torch')
train_dataloader = DataLoader(train_dataset, batch_size = 2, num_workers = 0)
t = time.time()
iter_ = 0
for batch in train_dataloader:
iter_ += 1
if iter_ == 1000:
break
print (time.time() - t)
```
### Expected behavior
The snippet should work normally and load the next batch of data.
### Environment info
datasets: '2.14.0'
pyarrow: '12.0.0'
torch: '2.0.0'
Python: 3.10.10 | packaged by conda-forge | (main, Mar 24 2023, 20:08:06) [GCC 11.3.0]
!uname -r
5.10.178-162.673.amzn2.x86_64 | 46 | Iterating over DataLoader based on HF datasets is stuck forever
### Describe the bug
I am using Amazon Sagemaker notebook (Amazon Linux 2) with python 3.10 based Conda environment.
I have a dataset in parquet format locally. When I try to iterate over it, the loader is stuck forever. Note that the same code is working for python 3.6 based conda environment seamlessly. What should be my next steps here?
### Steps to reproduce the bug
```
train_dataset = load_dataset(
"parquet", data_files = {'train': tr_data_path + '*.parquet'},
split = 'train',
collate_fn = streaming_data_collate_fn,
streaming = True
).with_format('torch')
train_dataloader = DataLoader(train_dataset, batch_size = 2, num_workers = 0)
t = time.time()
iter_ = 0
for batch in train_dataloader:
iter_ += 1
if iter_ == 1000:
break
print (time.time() - t)
```
### Expected behavior
The snippet should work normally and load the next batch of data.
### Environment info
datasets: '2.14.0'
pyarrow: '12.0.0'
torch: '2.0.0'
Python: 3.10.10 | packaged by conda-forge | (main, Mar 24 2023, 20:08:06) [GCC 11.3.0]
!uname -r
5.10.178-162.673.amzn2.x86_64
Python 3.6:
datasets.__version__ 2.4.0
torch.__version__ 1.10.1+cu102
Python 3.10:
datasets.__version__ 2.14.0
torch.__version__ 2.0.0
Anonymized features are of the form (subset shown here):
{
'string_feature_i': Value(dtype='string', id=None),
'numerical_feature_i': Value(dtype='decimal128(38, 0)', id=None),
'numerical_feature_series_i': Sequence(feature=Value(dtype='float64', id=None), length=-1, id=None),
}
There is no output from .features in python 3.6 kernel BTW. |
https://github.com/huggingface/datasets/issues/6079 | Iterating over DataLoader based on HF datasets is stuck forever | One more thing, in python 3.10 based kernel, interestingly increasing num_workers seem to be increasing the runtime of iterating I was trying out. In python 3.10 kernel execution, I do not even see multiple CPU cores spiking unlike in 3.6.
512 batch size on 32 workers executes in 2.4 seconds on python 3.6 kernel, while it takes ~118 seconds on 3.10! | ### Describe the bug
I am using Amazon Sagemaker notebook (Amazon Linux 2) with python 3.10 based Conda environment.
I have a dataset in parquet format locally. When I try to iterate over it, the loader is stuck forever. Note that the same code is working for python 3.6 based conda environment seamlessly. What should be my next steps here?
### Steps to reproduce the bug
```
train_dataset = load_dataset(
"parquet", data_files = {'train': tr_data_path + '*.parquet'},
split = 'train',
collate_fn = streaming_data_collate_fn,
streaming = True
).with_format('torch')
train_dataloader = DataLoader(train_dataset, batch_size = 2, num_workers = 0)
t = time.time()
iter_ = 0
for batch in train_dataloader:
iter_ += 1
if iter_ == 1000:
break
print (time.time() - t)
```
### Expected behavior
The snippet should work normally and load the next batch of data.
### Environment info
datasets: '2.14.0'
pyarrow: '12.0.0'
torch: '2.0.0'
Python: 3.10.10 | packaged by conda-forge | (main, Mar 24 2023, 20:08:06) [GCC 11.3.0]
!uname -r
5.10.178-162.673.amzn2.x86_64 | 61 | Iterating over DataLoader based on HF datasets is stuck forever
### Describe the bug
I am using Amazon Sagemaker notebook (Amazon Linux 2) with python 3.10 based Conda environment.
I have a dataset in parquet format locally. When I try to iterate over it, the loader is stuck forever. Note that the same code is working for python 3.6 based conda environment seamlessly. What should be my next steps here?
### Steps to reproduce the bug
```
train_dataset = load_dataset(
"parquet", data_files = {'train': tr_data_path + '*.parquet'},
split = 'train',
collate_fn = streaming_data_collate_fn,
streaming = True
).with_format('torch')
train_dataloader = DataLoader(train_dataset, batch_size = 2, num_workers = 0)
t = time.time()
iter_ = 0
for batch in train_dataloader:
iter_ += 1
if iter_ == 1000:
break
print (time.time() - t)
```
### Expected behavior
The snippet should work normally and load the next batch of data.
### Environment info
datasets: '2.14.0'
pyarrow: '12.0.0'
torch: '2.0.0'
Python: 3.10.10 | packaged by conda-forge | (main, Mar 24 2023, 20:08:06) [GCC 11.3.0]
!uname -r
5.10.178-162.673.amzn2.x86_64
One more thing, in python 3.10 based kernel, interestingly increasing num_workers seem to be increasing the runtime of iterating I was trying out. In python 3.10 kernel execution, I do not even see multiple CPU cores spiking unlike in 3.6.
512 batch size on 32 workers executes in 2.4 seconds on python 3.6 kernel, while it takes ~118 seconds on 3.10! |
https://github.com/huggingface/datasets/issues/6079 | Iterating over DataLoader based on HF datasets is stuck forever | **Update**: It seems the latency part is more of a multiprocessing issue with torch and some host specific issue, and I had to scourge through relevant pytorch issues, when I stumbled across these threads:
1. https://github.com/pytorch/pytorch/issues/102494
2. https://github.com/pytorch/pytorch/issues/102269
3. https://github.com/pytorch/pytorch/issues/99625
Out of the suggested solutions, the one that worked in my case was:
```
os.environ['KMP_AFFINITY'] = "disabled"
```
It is working for now, though I have no clue why, just I hope it does not get stuck when I do actual model training, will update by tomorrow.
| ### Describe the bug
I am using Amazon Sagemaker notebook (Amazon Linux 2) with python 3.10 based Conda environment.
I have a dataset in parquet format locally. When I try to iterate over it, the loader is stuck forever. Note that the same code is working for python 3.6 based conda environment seamlessly. What should be my next steps here?
### Steps to reproduce the bug
```
train_dataset = load_dataset(
"parquet", data_files = {'train': tr_data_path + '*.parquet'},
split = 'train',
collate_fn = streaming_data_collate_fn,
streaming = True
).with_format('torch')
train_dataloader = DataLoader(train_dataset, batch_size = 2, num_workers = 0)
t = time.time()
iter_ = 0
for batch in train_dataloader:
iter_ += 1
if iter_ == 1000:
break
print (time.time() - t)
```
### Expected behavior
The snippet should work normally and load the next batch of data.
### Environment info
datasets: '2.14.0'
pyarrow: '12.0.0'
torch: '2.0.0'
Python: 3.10.10 | packaged by conda-forge | (main, Mar 24 2023, 20:08:06) [GCC 11.3.0]
!uname -r
5.10.178-162.673.amzn2.x86_64 | 87 | Iterating over DataLoader based on HF datasets is stuck forever
### Describe the bug
I am using Amazon Sagemaker notebook (Amazon Linux 2) with python 3.10 based Conda environment.
I have a dataset in parquet format locally. When I try to iterate over it, the loader is stuck forever. Note that the same code is working for python 3.6 based conda environment seamlessly. What should be my next steps here?
### Steps to reproduce the bug
```
train_dataset = load_dataset(
"parquet", data_files = {'train': tr_data_path + '*.parquet'},
split = 'train',
collate_fn = streaming_data_collate_fn,
streaming = True
).with_format('torch')
train_dataloader = DataLoader(train_dataset, batch_size = 2, num_workers = 0)
t = time.time()
iter_ = 0
for batch in train_dataloader:
iter_ += 1
if iter_ == 1000:
break
print (time.time() - t)
```
### Expected behavior
The snippet should work normally and load the next batch of data.
### Environment info
datasets: '2.14.0'
pyarrow: '12.0.0'
torch: '2.0.0'
Python: 3.10.10 | packaged by conda-forge | (main, Mar 24 2023, 20:08:06) [GCC 11.3.0]
!uname -r
5.10.178-162.673.amzn2.x86_64
**Update**: It seems the latency part is more of a multiprocessing issue with torch and some host specific issue, and I had to scourge through relevant pytorch issues, when I stumbled across these threads:
1. https://github.com/pytorch/pytorch/issues/102494
2. https://github.com/pytorch/pytorch/issues/102269
3. https://github.com/pytorch/pytorch/issues/99625
Out of the suggested solutions, the one that worked in my case was:
```
os.environ['KMP_AFFINITY'] = "disabled"
```
It is working for now, though I have no clue why, just I hope it does not get stuck when I do actual model training, will update by tomorrow.
|
https://github.com/huggingface/datasets/issues/6078 | resume_download with streaming=True | Currently, it's not possible to efficiently resume streaming after an error. Eventually, we plan to support this for Parquet (see https://github.com/huggingface/datasets/issues/5380). | ### Describe the bug
I used:
```
dataset = load_dataset(
"oscar-corpus/OSCAR-2201",
token=True,
language="fr",
streaming=True,
split="train"
)
```
Unfortunately, the server had a problem during the training process. I saved the step my training stopped at.
But how can I resume download from step 1_000_´000 without re-streaming all the first 1 million docs of the dataset?
`download_config=DownloadConfig(resume_download=True)` seems to not work with streaming=True.
### Steps to reproduce the bug
```
from datasets import load_dataset, DownloadConfig
dataset = load_dataset(
"oscar-corpus/OSCAR-2201",
token=True,
language="fr",
streaming=True, # optional
split="train",
download_config=DownloadConfig(resume_download=True)
)
# interupt the run and try to relaunch it => this restart from scratch
```
### Expected behavior
I would expect a parameter to start streaming from a given index in the dataset.
### Environment info
- `datasets` version: 2.14.0
- Platform: Linux-5.19.0-45-generic-x86_64-with-glibc2.29
- Python version: 3.8.10
- Huggingface_hub version: 0.15.1
- PyArrow version: 12.0.1
- Pandas version: 2.0.0 | 21 | resume_download with streaming=True
### Describe the bug
I used:
```
dataset = load_dataset(
"oscar-corpus/OSCAR-2201",
token=True,
language="fr",
streaming=True,
split="train"
)
```
Unfortunately, the server had a problem during the training process. I saved the step my training stopped at.
But how can I resume download from step 1_000_´000 without re-streaming all the first 1 million docs of the dataset?
`download_config=DownloadConfig(resume_download=True)` seems to not work with streaming=True.
### Steps to reproduce the bug
```
from datasets import load_dataset, DownloadConfig
dataset = load_dataset(
"oscar-corpus/OSCAR-2201",
token=True,
language="fr",
streaming=True, # optional
split="train",
download_config=DownloadConfig(resume_download=True)
)
# interupt the run and try to relaunch it => this restart from scratch
```
### Expected behavior
I would expect a parameter to start streaming from a given index in the dataset.
### Environment info
- `datasets` version: 2.14.0
- Platform: Linux-5.19.0-45-generic-x86_64-with-glibc2.29
- Python version: 3.8.10
- Huggingface_hub version: 0.15.1
- PyArrow version: 12.0.1
- Pandas version: 2.0.0
Currently, it's not possible to efficiently resume streaming after an error. Eventually, we plan to support this for Parquet (see https://github.com/huggingface/datasets/issues/5380). |
https://github.com/huggingface/datasets/issues/6077 | Mapping gets stuck at 99% | The `MAX_MAP_BATCH_SIZE = 1_000_000_000` hack is bad as it loads the entire dataset into RAM when performing `.map`. Instead, it's best to use `.iter(batch_size)` to iterate over the data batches and compute `mean` for each column. (`stddev` can be computed in another pass).
Also, these arrays are big, so it makes sense to reduce `batch_size`/`writer_batch_size` to avoid RAM issues and slow IO. | ### Describe the bug
Hi !
I'm currently working with a large (~150GB) unnormalized dataset at work.
The dataset is available on a read-only filesystem internally, and I use a [loading script](https://huggingface.co/docs/datasets/dataset_script) to retreive it.
I want to normalize the features of the dataset, meaning I need to compute the mean and standard deviation metric for each feature of the entire dataset. I cannot load the entire dataset to RAM as it is too big, so following [this discussion on the huggingface discourse](https://discuss.huggingface.co/t/copy-columns-in-a-dataset-and-compute-statistics-for-a-column/22157) I am using a [map operation](https://huggingface.co/docs/datasets/v2.14.0/en/package_reference/main_classes#datasets.Dataset.map) to first compute the metrics and a second map operation to apply them on the dataset.
The problem lies in the second mapping, as it gets stuck at ~99%. By checking what the process does (using `htop` and `strace`) it seems to be doing a lot of I/O operations, and I'm not sure why.
Obviously, I could always normalize the dataset externally and then load it using a loading script. However, since the internal dataset is updated fairly frequently, using the library to perform normalization automatically would make it much easier for me.
### Steps to reproduce the bug
I'm able to reproduce the problem using the following scripts:
```python
# random_data.py
import datasets
import torch
_VERSION = "1.0.0"
class RandomDataset(datasets.GeneratorBasedBuilder):
def _info(self):
return datasets.DatasetInfo(
version=_VERSION,
supervised_keys=None,
features=datasets.Features(
{
"positions": datasets.Array2D(
shape=(30000, 3),
dtype="float32",
),
"normals": datasets.Array2D(
shape=(30000, 3),
dtype="float32",
),
"features": datasets.Array2D(
shape=(30000, 6),
dtype="float32",
),
"scalars": datasets.Sequence(
feature=datasets.Value("float32"),
length=20,
),
},
),
)
def _split_generators(self, dl_manager):
return [
datasets.SplitGenerator(
name=datasets.Split.TRAIN, # type: ignore
gen_kwargs={"nb_samples": 1000},
),
datasets.SplitGenerator(
name=datasets.Split.TEST, # type: ignore
gen_kwargs={"nb_samples": 100},
),
]
def _generate_examples(self, nb_samples: int):
for idx in range(nb_samples):
yield idx, {
"positions": torch.randn(30000, 3),
"normals": torch.randn(30000, 3),
"features": torch.randn(30000, 6),
"scalars": torch.randn(20),
}
```
```python
# main.py
import datasets
import torch
def apply_mean_std(
dataset: datasets.Dataset,
means: dict[str, torch.Tensor],
stds: dict[str, torch.Tensor],
) -> dict[str, torch.Tensor]:
"""Normalize the dataset using the mean and standard deviation of each feature.
Args:
dataset (`Dataset`): A huggingface dataset.
mean (`dict[str, Tensor]`): A dictionary containing the mean of each feature.
std (`dict[str, Tensor]`): A dictionary containing the standard deviation of each feature.
Returns:
dict: A dictionary containing the normalized dataset.
"""
result = {}
for key in means.keys():
# extract data from dataset
data: torch.Tensor = dataset[key] # type: ignore
# extract mean and std from dict
mean = means[key] # type: ignore
std = stds[key] # type: ignore
# normalize data
normalized_data = (data - mean) / std
result[key] = normalized_data
return result
# get dataset
ds = datasets.load_dataset(
path="random_data.py",
split="train",
).with_format("torch")
# compute mean (along last axis)
means = {key: torch.zeros(ds[key][0].shape[-1]) for key in ds.column_names}
means_sq = {key: torch.zeros(ds[key][0].shape[-1]) for key in ds.column_names}
for batch in ds.iter(batch_size=8):
for key in ds.column_names:
data = batch[key]
batch_size = data.shape[0]
data = data.reshape(-1, data.shape[-1])
means[key] += data.mean(dim=0) / len(ds) * batch_size
means_sq[key] += (data**2).mean(dim=0) / len(ds) * batch_size
# compute std (along last axis)
stds = {key: torch.sqrt(means_sq[key] - means[key] ** 2) for key in ds.column_names}
# normalize each feature of the dataset
ds_normalized = ds.map(
desc="Applying mean/std", # type: ignore
function=apply_mean_std,
batched=False,
fn_kwargs={
"means": means,
"stds": stds,
},
)
```
### Expected behavior
Using the previous scripts, the `ds_normalized` mapping completes in ~5 minutes, but any subsequent use of `ds_normalized` is really really slow, for example reapplying `apply_mean_std` to `ds_normalized` takes forever. This is very strange, I'm sure I must be missing something, but I would still expect this to be faster.
### Environment info
- `datasets` version: 2.13.1
- Platform: Linux-3.10.0-1160.66.1.el7.x86_64-x86_64-with-glibc2.17
- Python version: 3.10.12
- Huggingface_hub version: 0.15.1
- PyArrow version: 12.0.0
- Pandas version: 2.0.2 | 62 | Mapping gets stuck at 99%
### Describe the bug
Hi !
I'm currently working with a large (~150GB) unnormalized dataset at work.
The dataset is available on a read-only filesystem internally, and I use a [loading script](https://huggingface.co/docs/datasets/dataset_script) to retreive it.
I want to normalize the features of the dataset, meaning I need to compute the mean and standard deviation metric for each feature of the entire dataset. I cannot load the entire dataset to RAM as it is too big, so following [this discussion on the huggingface discourse](https://discuss.huggingface.co/t/copy-columns-in-a-dataset-and-compute-statistics-for-a-column/22157) I am using a [map operation](https://huggingface.co/docs/datasets/v2.14.0/en/package_reference/main_classes#datasets.Dataset.map) to first compute the metrics and a second map operation to apply them on the dataset.
The problem lies in the second mapping, as it gets stuck at ~99%. By checking what the process does (using `htop` and `strace`) it seems to be doing a lot of I/O operations, and I'm not sure why.
Obviously, I could always normalize the dataset externally and then load it using a loading script. However, since the internal dataset is updated fairly frequently, using the library to perform normalization automatically would make it much easier for me.
### Steps to reproduce the bug
I'm able to reproduce the problem using the following scripts:
```python
# random_data.py
import datasets
import torch
_VERSION = "1.0.0"
class RandomDataset(datasets.GeneratorBasedBuilder):
def _info(self):
return datasets.DatasetInfo(
version=_VERSION,
supervised_keys=None,
features=datasets.Features(
{
"positions": datasets.Array2D(
shape=(30000, 3),
dtype="float32",
),
"normals": datasets.Array2D(
shape=(30000, 3),
dtype="float32",
),
"features": datasets.Array2D(
shape=(30000, 6),
dtype="float32",
),
"scalars": datasets.Sequence(
feature=datasets.Value("float32"),
length=20,
),
},
),
)
def _split_generators(self, dl_manager):
return [
datasets.SplitGenerator(
name=datasets.Split.TRAIN, # type: ignore
gen_kwargs={"nb_samples": 1000},
),
datasets.SplitGenerator(
name=datasets.Split.TEST, # type: ignore
gen_kwargs={"nb_samples": 100},
),
]
def _generate_examples(self, nb_samples: int):
for idx in range(nb_samples):
yield idx, {
"positions": torch.randn(30000, 3),
"normals": torch.randn(30000, 3),
"features": torch.randn(30000, 6),
"scalars": torch.randn(20),
}
```
```python
# main.py
import datasets
import torch
def apply_mean_std(
dataset: datasets.Dataset,
means: dict[str, torch.Tensor],
stds: dict[str, torch.Tensor],
) -> dict[str, torch.Tensor]:
"""Normalize the dataset using the mean and standard deviation of each feature.
Args:
dataset (`Dataset`): A huggingface dataset.
mean (`dict[str, Tensor]`): A dictionary containing the mean of each feature.
std (`dict[str, Tensor]`): A dictionary containing the standard deviation of each feature.
Returns:
dict: A dictionary containing the normalized dataset.
"""
result = {}
for key in means.keys():
# extract data from dataset
data: torch.Tensor = dataset[key] # type: ignore
# extract mean and std from dict
mean = means[key] # type: ignore
std = stds[key] # type: ignore
# normalize data
normalized_data = (data - mean) / std
result[key] = normalized_data
return result
# get dataset
ds = datasets.load_dataset(
path="random_data.py",
split="train",
).with_format("torch")
# compute mean (along last axis)
means = {key: torch.zeros(ds[key][0].shape[-1]) for key in ds.column_names}
means_sq = {key: torch.zeros(ds[key][0].shape[-1]) for key in ds.column_names}
for batch in ds.iter(batch_size=8):
for key in ds.column_names:
data = batch[key]
batch_size = data.shape[0]
data = data.reshape(-1, data.shape[-1])
means[key] += data.mean(dim=0) / len(ds) * batch_size
means_sq[key] += (data**2).mean(dim=0) / len(ds) * batch_size
# compute std (along last axis)
stds = {key: torch.sqrt(means_sq[key] - means[key] ** 2) for key in ds.column_names}
# normalize each feature of the dataset
ds_normalized = ds.map(
desc="Applying mean/std", # type: ignore
function=apply_mean_std,
batched=False,
fn_kwargs={
"means": means,
"stds": stds,
},
)
```
### Expected behavior
Using the previous scripts, the `ds_normalized` mapping completes in ~5 minutes, but any subsequent use of `ds_normalized` is really really slow, for example reapplying `apply_mean_std` to `ds_normalized` takes forever. This is very strange, I'm sure I must be missing something, but I would still expect this to be faster.
### Environment info
- `datasets` version: 2.13.1
- Platform: Linux-3.10.0-1160.66.1.el7.x86_64-x86_64-with-glibc2.17
- Python version: 3.10.12
- Huggingface_hub version: 0.15.1
- PyArrow version: 12.0.0
- Pandas version: 2.0.2
The `MAX_MAP_BATCH_SIZE = 1_000_000_000` hack is bad as it loads the entire dataset into RAM when performing `.map`. Instead, it's best to use `.iter(batch_size)` to iterate over the data batches and compute `mean` for each column. (`stddev` can be computed in another pass).
Also, these arrays are big, so it makes sense to reduce `batch_size`/`writer_batch_size` to avoid RAM issues and slow IO. |
https://github.com/huggingface/datasets/issues/6077 | Mapping gets stuck at 99% | Hi @mariosasko !
I agree, it's an ugly hack, but it was convenient since the resulting `mean_std` could be cached by the library. For my large dataset (which doesn't fit in RAM), I'm actually using something similar to what you suggested. I got rid of the first mapping in the above scripts and replaced it with an iterator, but the issue with the second mapping still persists. | ### Describe the bug
Hi !
I'm currently working with a large (~150GB) unnormalized dataset at work.
The dataset is available on a read-only filesystem internally, and I use a [loading script](https://huggingface.co/docs/datasets/dataset_script) to retreive it.
I want to normalize the features of the dataset, meaning I need to compute the mean and standard deviation metric for each feature of the entire dataset. I cannot load the entire dataset to RAM as it is too big, so following [this discussion on the huggingface discourse](https://discuss.huggingface.co/t/copy-columns-in-a-dataset-and-compute-statistics-for-a-column/22157) I am using a [map operation](https://huggingface.co/docs/datasets/v2.14.0/en/package_reference/main_classes#datasets.Dataset.map) to first compute the metrics and a second map operation to apply them on the dataset.
The problem lies in the second mapping, as it gets stuck at ~99%. By checking what the process does (using `htop` and `strace`) it seems to be doing a lot of I/O operations, and I'm not sure why.
Obviously, I could always normalize the dataset externally and then load it using a loading script. However, since the internal dataset is updated fairly frequently, using the library to perform normalization automatically would make it much easier for me.
### Steps to reproduce the bug
I'm able to reproduce the problem using the following scripts:
```python
# random_data.py
import datasets
import torch
_VERSION = "1.0.0"
class RandomDataset(datasets.GeneratorBasedBuilder):
def _info(self):
return datasets.DatasetInfo(
version=_VERSION,
supervised_keys=None,
features=datasets.Features(
{
"positions": datasets.Array2D(
shape=(30000, 3),
dtype="float32",
),
"normals": datasets.Array2D(
shape=(30000, 3),
dtype="float32",
),
"features": datasets.Array2D(
shape=(30000, 6),
dtype="float32",
),
"scalars": datasets.Sequence(
feature=datasets.Value("float32"),
length=20,
),
},
),
)
def _split_generators(self, dl_manager):
return [
datasets.SplitGenerator(
name=datasets.Split.TRAIN, # type: ignore
gen_kwargs={"nb_samples": 1000},
),
datasets.SplitGenerator(
name=datasets.Split.TEST, # type: ignore
gen_kwargs={"nb_samples": 100},
),
]
def _generate_examples(self, nb_samples: int):
for idx in range(nb_samples):
yield idx, {
"positions": torch.randn(30000, 3),
"normals": torch.randn(30000, 3),
"features": torch.randn(30000, 6),
"scalars": torch.randn(20),
}
```
```python
# main.py
import datasets
import torch
def apply_mean_std(
dataset: datasets.Dataset,
means: dict[str, torch.Tensor],
stds: dict[str, torch.Tensor],
) -> dict[str, torch.Tensor]:
"""Normalize the dataset using the mean and standard deviation of each feature.
Args:
dataset (`Dataset`): A huggingface dataset.
mean (`dict[str, Tensor]`): A dictionary containing the mean of each feature.
std (`dict[str, Tensor]`): A dictionary containing the standard deviation of each feature.
Returns:
dict: A dictionary containing the normalized dataset.
"""
result = {}
for key in means.keys():
# extract data from dataset
data: torch.Tensor = dataset[key] # type: ignore
# extract mean and std from dict
mean = means[key] # type: ignore
std = stds[key] # type: ignore
# normalize data
normalized_data = (data - mean) / std
result[key] = normalized_data
return result
# get dataset
ds = datasets.load_dataset(
path="random_data.py",
split="train",
).with_format("torch")
# compute mean (along last axis)
means = {key: torch.zeros(ds[key][0].shape[-1]) for key in ds.column_names}
means_sq = {key: torch.zeros(ds[key][0].shape[-1]) for key in ds.column_names}
for batch in ds.iter(batch_size=8):
for key in ds.column_names:
data = batch[key]
batch_size = data.shape[0]
data = data.reshape(-1, data.shape[-1])
means[key] += data.mean(dim=0) / len(ds) * batch_size
means_sq[key] += (data**2).mean(dim=0) / len(ds) * batch_size
# compute std (along last axis)
stds = {key: torch.sqrt(means_sq[key] - means[key] ** 2) for key in ds.column_names}
# normalize each feature of the dataset
ds_normalized = ds.map(
desc="Applying mean/std", # type: ignore
function=apply_mean_std,
batched=False,
fn_kwargs={
"means": means,
"stds": stds,
},
)
```
### Expected behavior
Using the previous scripts, the `ds_normalized` mapping completes in ~5 minutes, but any subsequent use of `ds_normalized` is really really slow, for example reapplying `apply_mean_std` to `ds_normalized` takes forever. This is very strange, I'm sure I must be missing something, but I would still expect this to be faster.
### Environment info
- `datasets` version: 2.13.1
- Platform: Linux-3.10.0-1160.66.1.el7.x86_64-x86_64-with-glibc2.17
- Python version: 3.10.12
- Huggingface_hub version: 0.15.1
- PyArrow version: 12.0.0
- Pandas version: 2.0.2 | 67 | Mapping gets stuck at 99%
### Describe the bug
Hi !
I'm currently working with a large (~150GB) unnormalized dataset at work.
The dataset is available on a read-only filesystem internally, and I use a [loading script](https://huggingface.co/docs/datasets/dataset_script) to retreive it.
I want to normalize the features of the dataset, meaning I need to compute the mean and standard deviation metric for each feature of the entire dataset. I cannot load the entire dataset to RAM as it is too big, so following [this discussion on the huggingface discourse](https://discuss.huggingface.co/t/copy-columns-in-a-dataset-and-compute-statistics-for-a-column/22157) I am using a [map operation](https://huggingface.co/docs/datasets/v2.14.0/en/package_reference/main_classes#datasets.Dataset.map) to first compute the metrics and a second map operation to apply them on the dataset.
The problem lies in the second mapping, as it gets stuck at ~99%. By checking what the process does (using `htop` and `strace`) it seems to be doing a lot of I/O operations, and I'm not sure why.
Obviously, I could always normalize the dataset externally and then load it using a loading script. However, since the internal dataset is updated fairly frequently, using the library to perform normalization automatically would make it much easier for me.
### Steps to reproduce the bug
I'm able to reproduce the problem using the following scripts:
```python
# random_data.py
import datasets
import torch
_VERSION = "1.0.0"
class RandomDataset(datasets.GeneratorBasedBuilder):
def _info(self):
return datasets.DatasetInfo(
version=_VERSION,
supervised_keys=None,
features=datasets.Features(
{
"positions": datasets.Array2D(
shape=(30000, 3),
dtype="float32",
),
"normals": datasets.Array2D(
shape=(30000, 3),
dtype="float32",
),
"features": datasets.Array2D(
shape=(30000, 6),
dtype="float32",
),
"scalars": datasets.Sequence(
feature=datasets.Value("float32"),
length=20,
),
},
),
)
def _split_generators(self, dl_manager):
return [
datasets.SplitGenerator(
name=datasets.Split.TRAIN, # type: ignore
gen_kwargs={"nb_samples": 1000},
),
datasets.SplitGenerator(
name=datasets.Split.TEST, # type: ignore
gen_kwargs={"nb_samples": 100},
),
]
def _generate_examples(self, nb_samples: int):
for idx in range(nb_samples):
yield idx, {
"positions": torch.randn(30000, 3),
"normals": torch.randn(30000, 3),
"features": torch.randn(30000, 6),
"scalars": torch.randn(20),
}
```
```python
# main.py
import datasets
import torch
def apply_mean_std(
dataset: datasets.Dataset,
means: dict[str, torch.Tensor],
stds: dict[str, torch.Tensor],
) -> dict[str, torch.Tensor]:
"""Normalize the dataset using the mean and standard deviation of each feature.
Args:
dataset (`Dataset`): A huggingface dataset.
mean (`dict[str, Tensor]`): A dictionary containing the mean of each feature.
std (`dict[str, Tensor]`): A dictionary containing the standard deviation of each feature.
Returns:
dict: A dictionary containing the normalized dataset.
"""
result = {}
for key in means.keys():
# extract data from dataset
data: torch.Tensor = dataset[key] # type: ignore
# extract mean and std from dict
mean = means[key] # type: ignore
std = stds[key] # type: ignore
# normalize data
normalized_data = (data - mean) / std
result[key] = normalized_data
return result
# get dataset
ds = datasets.load_dataset(
path="random_data.py",
split="train",
).with_format("torch")
# compute mean (along last axis)
means = {key: torch.zeros(ds[key][0].shape[-1]) for key in ds.column_names}
means_sq = {key: torch.zeros(ds[key][0].shape[-1]) for key in ds.column_names}
for batch in ds.iter(batch_size=8):
for key in ds.column_names:
data = batch[key]
batch_size = data.shape[0]
data = data.reshape(-1, data.shape[-1])
means[key] += data.mean(dim=0) / len(ds) * batch_size
means_sq[key] += (data**2).mean(dim=0) / len(ds) * batch_size
# compute std (along last axis)
stds = {key: torch.sqrt(means_sq[key] - means[key] ** 2) for key in ds.column_names}
# normalize each feature of the dataset
ds_normalized = ds.map(
desc="Applying mean/std", # type: ignore
function=apply_mean_std,
batched=False,
fn_kwargs={
"means": means,
"stds": stds,
},
)
```
### Expected behavior
Using the previous scripts, the `ds_normalized` mapping completes in ~5 minutes, but any subsequent use of `ds_normalized` is really really slow, for example reapplying `apply_mean_std` to `ds_normalized` takes forever. This is very strange, I'm sure I must be missing something, but I would still expect this to be faster.
### Environment info
- `datasets` version: 2.13.1
- Platform: Linux-3.10.0-1160.66.1.el7.x86_64-x86_64-with-glibc2.17
- Python version: 3.10.12
- Huggingface_hub version: 0.15.1
- PyArrow version: 12.0.0
- Pandas version: 2.0.2
Hi @mariosasko !
I agree, it's an ugly hack, but it was convenient since the resulting `mean_std` could be cached by the library. For my large dataset (which doesn't fit in RAM), I'm actually using something similar to what you suggested. I got rid of the first mapping in the above scripts and replaced it with an iterator, but the issue with the second mapping still persists. |
https://github.com/huggingface/datasets/issues/6077 | Mapping gets stuck at 99% | Have you tried to reduce `batch_size`/`writer_batch_size` in the 2nd `.map`? Also, can you interrupt the process when it gets stuck and share the error stack trace? | ### Describe the bug
Hi !
I'm currently working with a large (~150GB) unnormalized dataset at work.
The dataset is available on a read-only filesystem internally, and I use a [loading script](https://huggingface.co/docs/datasets/dataset_script) to retreive it.
I want to normalize the features of the dataset, meaning I need to compute the mean and standard deviation metric for each feature of the entire dataset. I cannot load the entire dataset to RAM as it is too big, so following [this discussion on the huggingface discourse](https://discuss.huggingface.co/t/copy-columns-in-a-dataset-and-compute-statistics-for-a-column/22157) I am using a [map operation](https://huggingface.co/docs/datasets/v2.14.0/en/package_reference/main_classes#datasets.Dataset.map) to first compute the metrics and a second map operation to apply them on the dataset.
The problem lies in the second mapping, as it gets stuck at ~99%. By checking what the process does (using `htop` and `strace`) it seems to be doing a lot of I/O operations, and I'm not sure why.
Obviously, I could always normalize the dataset externally and then load it using a loading script. However, since the internal dataset is updated fairly frequently, using the library to perform normalization automatically would make it much easier for me.
### Steps to reproduce the bug
I'm able to reproduce the problem using the following scripts:
```python
# random_data.py
import datasets
import torch
_VERSION = "1.0.0"
class RandomDataset(datasets.GeneratorBasedBuilder):
def _info(self):
return datasets.DatasetInfo(
version=_VERSION,
supervised_keys=None,
features=datasets.Features(
{
"positions": datasets.Array2D(
shape=(30000, 3),
dtype="float32",
),
"normals": datasets.Array2D(
shape=(30000, 3),
dtype="float32",
),
"features": datasets.Array2D(
shape=(30000, 6),
dtype="float32",
),
"scalars": datasets.Sequence(
feature=datasets.Value("float32"),
length=20,
),
},
),
)
def _split_generators(self, dl_manager):
return [
datasets.SplitGenerator(
name=datasets.Split.TRAIN, # type: ignore
gen_kwargs={"nb_samples": 1000},
),
datasets.SplitGenerator(
name=datasets.Split.TEST, # type: ignore
gen_kwargs={"nb_samples": 100},
),
]
def _generate_examples(self, nb_samples: int):
for idx in range(nb_samples):
yield idx, {
"positions": torch.randn(30000, 3),
"normals": torch.randn(30000, 3),
"features": torch.randn(30000, 6),
"scalars": torch.randn(20),
}
```
```python
# main.py
import datasets
import torch
def apply_mean_std(
dataset: datasets.Dataset,
means: dict[str, torch.Tensor],
stds: dict[str, torch.Tensor],
) -> dict[str, torch.Tensor]:
"""Normalize the dataset using the mean and standard deviation of each feature.
Args:
dataset (`Dataset`): A huggingface dataset.
mean (`dict[str, Tensor]`): A dictionary containing the mean of each feature.
std (`dict[str, Tensor]`): A dictionary containing the standard deviation of each feature.
Returns:
dict: A dictionary containing the normalized dataset.
"""
result = {}
for key in means.keys():
# extract data from dataset
data: torch.Tensor = dataset[key] # type: ignore
# extract mean and std from dict
mean = means[key] # type: ignore
std = stds[key] # type: ignore
# normalize data
normalized_data = (data - mean) / std
result[key] = normalized_data
return result
# get dataset
ds = datasets.load_dataset(
path="random_data.py",
split="train",
).with_format("torch")
# compute mean (along last axis)
means = {key: torch.zeros(ds[key][0].shape[-1]) for key in ds.column_names}
means_sq = {key: torch.zeros(ds[key][0].shape[-1]) for key in ds.column_names}
for batch in ds.iter(batch_size=8):
for key in ds.column_names:
data = batch[key]
batch_size = data.shape[0]
data = data.reshape(-1, data.shape[-1])
means[key] += data.mean(dim=0) / len(ds) * batch_size
means_sq[key] += (data**2).mean(dim=0) / len(ds) * batch_size
# compute std (along last axis)
stds = {key: torch.sqrt(means_sq[key] - means[key] ** 2) for key in ds.column_names}
# normalize each feature of the dataset
ds_normalized = ds.map(
desc="Applying mean/std", # type: ignore
function=apply_mean_std,
batched=False,
fn_kwargs={
"means": means,
"stds": stds,
},
)
```
### Expected behavior
Using the previous scripts, the `ds_normalized` mapping completes in ~5 minutes, but any subsequent use of `ds_normalized` is really really slow, for example reapplying `apply_mean_std` to `ds_normalized` takes forever. This is very strange, I'm sure I must be missing something, but I would still expect this to be faster.
### Environment info
- `datasets` version: 2.13.1
- Platform: Linux-3.10.0-1160.66.1.el7.x86_64-x86_64-with-glibc2.17
- Python version: 3.10.12
- Huggingface_hub version: 0.15.1
- PyArrow version: 12.0.0
- Pandas version: 2.0.2 | 26 | Mapping gets stuck at 99%
### Describe the bug
Hi !
I'm currently working with a large (~150GB) unnormalized dataset at work.
The dataset is available on a read-only filesystem internally, and I use a [loading script](https://huggingface.co/docs/datasets/dataset_script) to retreive it.
I want to normalize the features of the dataset, meaning I need to compute the mean and standard deviation metric for each feature of the entire dataset. I cannot load the entire dataset to RAM as it is too big, so following [this discussion on the huggingface discourse](https://discuss.huggingface.co/t/copy-columns-in-a-dataset-and-compute-statistics-for-a-column/22157) I am using a [map operation](https://huggingface.co/docs/datasets/v2.14.0/en/package_reference/main_classes#datasets.Dataset.map) to first compute the metrics and a second map operation to apply them on the dataset.
The problem lies in the second mapping, as it gets stuck at ~99%. By checking what the process does (using `htop` and `strace`) it seems to be doing a lot of I/O operations, and I'm not sure why.
Obviously, I could always normalize the dataset externally and then load it using a loading script. However, since the internal dataset is updated fairly frequently, using the library to perform normalization automatically would make it much easier for me.
### Steps to reproduce the bug
I'm able to reproduce the problem using the following scripts:
```python
# random_data.py
import datasets
import torch
_VERSION = "1.0.0"
class RandomDataset(datasets.GeneratorBasedBuilder):
def _info(self):
return datasets.DatasetInfo(
version=_VERSION,
supervised_keys=None,
features=datasets.Features(
{
"positions": datasets.Array2D(
shape=(30000, 3),
dtype="float32",
),
"normals": datasets.Array2D(
shape=(30000, 3),
dtype="float32",
),
"features": datasets.Array2D(
shape=(30000, 6),
dtype="float32",
),
"scalars": datasets.Sequence(
feature=datasets.Value("float32"),
length=20,
),
},
),
)
def _split_generators(self, dl_manager):
return [
datasets.SplitGenerator(
name=datasets.Split.TRAIN, # type: ignore
gen_kwargs={"nb_samples": 1000},
),
datasets.SplitGenerator(
name=datasets.Split.TEST, # type: ignore
gen_kwargs={"nb_samples": 100},
),
]
def _generate_examples(self, nb_samples: int):
for idx in range(nb_samples):
yield idx, {
"positions": torch.randn(30000, 3),
"normals": torch.randn(30000, 3),
"features": torch.randn(30000, 6),
"scalars": torch.randn(20),
}
```
```python
# main.py
import datasets
import torch
def apply_mean_std(
dataset: datasets.Dataset,
means: dict[str, torch.Tensor],
stds: dict[str, torch.Tensor],
) -> dict[str, torch.Tensor]:
"""Normalize the dataset using the mean and standard deviation of each feature.
Args:
dataset (`Dataset`): A huggingface dataset.
mean (`dict[str, Tensor]`): A dictionary containing the mean of each feature.
std (`dict[str, Tensor]`): A dictionary containing the standard deviation of each feature.
Returns:
dict: A dictionary containing the normalized dataset.
"""
result = {}
for key in means.keys():
# extract data from dataset
data: torch.Tensor = dataset[key] # type: ignore
# extract mean and std from dict
mean = means[key] # type: ignore
std = stds[key] # type: ignore
# normalize data
normalized_data = (data - mean) / std
result[key] = normalized_data
return result
# get dataset
ds = datasets.load_dataset(
path="random_data.py",
split="train",
).with_format("torch")
# compute mean (along last axis)
means = {key: torch.zeros(ds[key][0].shape[-1]) for key in ds.column_names}
means_sq = {key: torch.zeros(ds[key][0].shape[-1]) for key in ds.column_names}
for batch in ds.iter(batch_size=8):
for key in ds.column_names:
data = batch[key]
batch_size = data.shape[0]
data = data.reshape(-1, data.shape[-1])
means[key] += data.mean(dim=0) / len(ds) * batch_size
means_sq[key] += (data**2).mean(dim=0) / len(ds) * batch_size
# compute std (along last axis)
stds = {key: torch.sqrt(means_sq[key] - means[key] ** 2) for key in ds.column_names}
# normalize each feature of the dataset
ds_normalized = ds.map(
desc="Applying mean/std", # type: ignore
function=apply_mean_std,
batched=False,
fn_kwargs={
"means": means,
"stds": stds,
},
)
```
### Expected behavior
Using the previous scripts, the `ds_normalized` mapping completes in ~5 minutes, but any subsequent use of `ds_normalized` is really really slow, for example reapplying `apply_mean_std` to `ds_normalized` takes forever. This is very strange, I'm sure I must be missing something, but I would still expect this to be faster.
### Environment info
- `datasets` version: 2.13.1
- Platform: Linux-3.10.0-1160.66.1.el7.x86_64-x86_64-with-glibc2.17
- Python version: 3.10.12
- Huggingface_hub version: 0.15.1
- PyArrow version: 12.0.0
- Pandas version: 2.0.2
Have you tried to reduce `batch_size`/`writer_batch_size` in the 2nd `.map`? Also, can you interrupt the process when it gets stuck and share the error stack trace? |
https://github.com/huggingface/datasets/issues/6077 | Mapping gets stuck at 99% | I think `batch_size/writer_batch_size` is already at its lowest in the 2nd `.map` since `batched=False` implies `batch_size=1` and `len(ds) = 1000 = writer_batch_size`.
Here is also a bunch of stack traces when I interrupted the process:
<details>
<summary>stack trace 1</summary>
```python
(pyg)[d623204@rosetta-bigviz01 stage-laurent-f]$ python src/random_scripts/uses_random_data.py
Found cached dataset random_data (/local_scratch/lfainsin/.cache/huggingface/datasets/random_data/default/0.0.0/444e214e1d0e6298cfd3f2368323ec37073dc1439f618e19395b1f421c69b066)
Applying mean/std: 97%|████████████████████████████████████████████████████████████████████████████████████████████████████████████████████████████████████████████████ | 967/1000 [00:01<00:00, 534.87 examples/s]Traceback (most recent call last):
File "/local_scratch/lfainsin/.conda/envs/pyg/lib/python3.10/site-packages/datasets/arrow_writer.py", line 179, in __arrow_array__
storage = to_pyarrow_listarray(data, pa_type)
File "/local_scratch/lfainsin/.conda/envs/pyg/lib/python3.10/site-packages/datasets/features/features.py", line 1466, in to_pyarrow_listarray
return pa.array(data, pa_type.storage_dtype)
File "pyarrow/array.pxi", line 320, in pyarrow.lib.array
File "pyarrow/array.pxi", line 39, in pyarrow.lib._sequence_to_array
File "pyarrow/error.pxi", line 144, in pyarrow.lib.pyarrow_internal_check_status
File "pyarrow/error.pxi", line 123, in pyarrow.lib.check_status
pyarrow.lib.ArrowTypeError: Could not convert tensor([[-1.0273, -0.8037, -0.6860],
[-0.5034, -1.2685, -0.0558],
[-1.0908, -1.1820, -0.3178],
...,
[-0.8171, 0.1781, -0.5903],
[ 0.4370, 1.9305, 0.5899],
[-0.1426, 0.9053, -1.7559]]) with type Tensor: was not a sequence or recognized null for conversion to list type
During handling of the above exception, another exception occurred:
Traceback (most recent call last):
File "/local_scratch/lfainsin/.conda/envs/pyg/lib/python3.10/site-packages/datasets/arrow_dataset.py", line 3449, in _map_single
writer.write(example)
File "/local_scratch/lfainsin/.conda/envs/pyg/lib/python3.10/site-packages/datasets/arrow_writer.py", line 490, in write
self.write_examples_on_file()
File "/local_scratch/lfainsin/.conda/envs/pyg/lib/python3.10/site-packages/datasets/arrow_writer.py", line 448, in write_examples_on_file
self.write_batch(batch_examples=batch_examples)
File "/local_scratch/lfainsin/.conda/envs/pyg/lib/python3.10/site-packages/datasets/arrow_writer.py", line 553, in write_batch
arrays.append(pa.array(typed_sequence))
File "pyarrow/array.pxi", line 236, in pyarrow.lib.array
File "pyarrow/array.pxi", line 110, in pyarrow.lib._handle_arrow_array_protocol
File "/local_scratch/lfainsin/.conda/envs/pyg/lib/python3.10/site-packages/datasets/arrow_writer.py", line 223, in __arrow_array__
return pa.array(cast_to_python_objects(data, only_1d_for_numpy=True))
File "/local_scratch/lfainsin/.conda/envs/pyg/lib/python3.10/site-packages/datasets/features/features.py", line 446, in cast_to_python_objects
return _cast_to_python_objects(
File "/local_scratch/lfainsin/.conda/envs/pyg/lib/python3.10/site-packages/datasets/features/features.py", line 407, in _cast_to_python_objects
[
File "/local_scratch/lfainsin/.conda/envs/pyg/lib/python3.10/site-packages/datasets/features/features.py", line 408, in <listcomp>
_cast_to_python_objects(
File "/local_scratch/lfainsin/.conda/envs/pyg/lib/python3.10/site-packages/datasets/features/features.py", line 319, in _cast_to_python_objects
[
File "/local_scratch/lfainsin/.conda/envs/pyg/lib/python3.10/site-packages/datasets/features/features.py", line 320, in <listcomp>
_cast_to_python_objects(
File "/local_scratch/lfainsin/.conda/envs/pyg/lib/python3.10/site-packages/datasets/features/features.py", line 263, in _cast_to_python_objects
def _cast_to_python_objects(obj: Any, only_1d_for_numpy: bool, optimize_list_casting: bool) -> Tuple[Any, bool]:
KeyboardInterrupt
During handling of the above exception, another exception occurred:
Traceback (most recent call last):
File "/local_scratch/lfainsin/.conda/envs/pyg/lib/python3.10/site-packages/datasets/arrow_writer.py", line 179, in __arrow_array__
storage = to_pyarrow_listarray(data, pa_type)
File "/local_scratch/lfainsin/.conda/envs/pyg/lib/python3.10/site-packages/datasets/features/features.py", line 1466, in to_pyarrow_listarray
return pa.array(data, pa_type.storage_dtype)
File "pyarrow/array.pxi", line 320, in pyarrow.lib.array
File "pyarrow/array.pxi", line 39, in pyarrow.lib._sequence_to_array
File "pyarrow/error.pxi", line 144, in pyarrow.lib.pyarrow_internal_check_status
File "pyarrow/error.pxi", line 123, in pyarrow.lib.check_status
pyarrow.lib.ArrowTypeError: Could not convert tensor([[-1.0273, -0.8037, -0.6860],
[-0.5034, -1.2685, -0.0558],
[-1.0908, -1.1820, -0.3178],
...,
[-0.8171, 0.1781, -0.5903],
[ 0.4370, 1.9305, 0.5899],
[-0.1426, 0.9053, -1.7559]]) with type Tensor: was not a sequence or recognized null for conversion to list type
During handling of the above exception, another exception occurred:
Traceback (most recent call last):
File "/gpfs_new/data/users/lfainsin/stage-laurent-f/src/random_scripts/uses_random_data.py", line 62, in <module>
ds_normalized = ds.map(
File "/local_scratch/lfainsin/.conda/envs/pyg/lib/python3.10/site-packages/datasets/arrow_dataset.py", line 580, in wrapper
out: Union["Dataset", "DatasetDict"] = func(self, *args, **kwargs)
File "/local_scratch/lfainsin/.conda/envs/pyg/lib/python3.10/site-packages/datasets/arrow_dataset.py", line 545, in wrapper
out: Union["Dataset", "DatasetDict"] = func(self, *args, **kwargs)
File "/local_scratch/lfainsin/.conda/envs/pyg/lib/python3.10/site-packages/datasets/arrow_dataset.py", line 3087, in map
for rank, done, content in Dataset._map_single(**dataset_kwargs):
File "/local_scratch/lfainsin/.conda/envs/pyg/lib/python3.10/site-packages/datasets/arrow_dataset.py", line 3492, in _map_single
writer.finalize()
File "/local_scratch/lfainsin/.conda/envs/pyg/lib/python3.10/site-packages/datasets/arrow_writer.py", line 584, in finalize
self.write_examples_on_file()
File "/local_scratch/lfainsin/.conda/envs/pyg/lib/python3.10/site-packages/datasets/arrow_writer.py", line 448, in write_examples_on_file
self.write_batch(batch_examples=batch_examples)
File "/local_scratch/lfainsin/.conda/envs/pyg/lib/python3.10/site-packages/datasets/arrow_writer.py", line 553, in write_batch
arrays.append(pa.array(typed_sequence))
File "pyarrow/array.pxi", line 236, in pyarrow.lib.array
File "pyarrow/array.pxi", line 110, in pyarrow.lib._handle_arrow_array_protocol
File "/local_scratch/lfainsin/.conda/envs/pyg/lib/python3.10/site-packages/datasets/arrow_writer.py", line 223, in __arrow_array__
return pa.array(cast_to_python_objects(data, only_1d_for_numpy=True))
File "/local_scratch/lfainsin/.conda/envs/pyg/lib/python3.10/site-packages/datasets/features/features.py", line 446, in cast_to_python_objects
return _cast_to_python_objects(
File "/local_scratch/lfainsin/.conda/envs/pyg/lib/python3.10/site-packages/datasets/features/features.py", line 407, in _cast_to_python_objects
[
File "/local_scratch/lfainsin/.conda/envs/pyg/lib/python3.10/site-packages/datasets/features/features.py", line 408, in <listcomp>
_cast_to_python_objects(
File "/local_scratch/lfainsin/.conda/envs/pyg/lib/python3.10/site-packages/datasets/features/features.py", line 319, in _cast_to_python_objects
[
File "/local_scratch/lfainsin/.conda/envs/pyg/lib/python3.10/site-packages/datasets/features/features.py", line 319, in <listcomp>
[
KeyboardInterrupt
```
</details>
<details>
<summary>stack trace 2</summary>
```python
(pyg)[d623204@rosetta-bigviz01 stage-laurent-f]$ python src/random_scripts/uses_random_data.py
Found cached dataset random_data (/local_scratch/lfainsin/.cache/huggingface/datasets/random_data/default/0.0.0/444e214e1d0e6298cfd3f2368323ec37073dc1439f618e19395b1f421c69b066)
Applying mean/std: 99%|███████████████████████████████████████████████████████████████████████████████████████████████████████████████████████████████████████████████████▏ | 988/1000 [00:20<00:00, 526.19 examples/s]Applying mean/std: 100%|█████████████████████████████████████████████████████████████████████████████████████████████████████████████████████████████████████████████████████▊| 999/1000 [00:21<00:00, 9.66 examples/s]Traceback (most recent call last):
File "/local_scratch/lfainsin/.conda/envs/pyg/lib/python3.10/site-packages/datasets/arrow_writer.py", line 179, in __arrow_array__
storage = to_pyarrow_listarray(data, pa_type)
File "/local_scratch/lfainsin/.conda/envs/pyg/lib/python3.10/site-packages/datasets/features/features.py", line 1466, in to_pyarrow_listarray
return pa.array(data, pa_type.storage_dtype)
File "pyarrow/array.pxi", line 320, in pyarrow.lib.array
File "pyarrow/array.pxi", line 39, in pyarrow.lib._sequence_to_array
File "pyarrow/error.pxi", line 144, in pyarrow.lib.pyarrow_internal_check_status
File "pyarrow/error.pxi", line 123, in pyarrow.lib.check_status
pyarrow.lib.ArrowTypeError: Could not convert tensor([[-1.0273, -0.8037, -0.6860],
[-0.5034, -1.2685, -0.0558],
[-1.0908, -1.1820, -0.3178],
...,
[-0.8171, 0.1781, -0.5903],
[ 0.4370, 1.9305, 0.5899],
[-0.1426, 0.9053, -1.7559]]) with type Tensor: was not a sequence or recognized null for conversion to list type
During handling of the above exception, another exception occurred:
Traceback (most recent call last):
File "/local_scratch/lfainsin/.conda/envs/pyg/lib/python3.10/site-packages/datasets/arrow_dataset.py", line 3449, in _map_single
writer.write(example)
File "/local_scratch/lfainsin/.conda/envs/pyg/lib/python3.10/site-packages/datasets/arrow_writer.py", line 490, in write
self.write_examples_on_file()
File "/local_scratch/lfainsin/.conda/envs/pyg/lib/python3.10/site-packages/datasets/arrow_writer.py", line 448, in write_examples_on_file
self.write_batch(batch_examples=batch_examples)
File "/local_scratch/lfainsin/.conda/envs/pyg/lib/python3.10/site-packages/datasets/arrow_writer.py", line 553, in write_batch
arrays.append(pa.array(typed_sequence))
File "pyarrow/array.pxi", line 236, in pyarrow.lib.array
File "pyarrow/array.pxi", line 110, in pyarrow.lib._handle_arrow_array_protocol
File "/local_scratch/lfainsin/.conda/envs/pyg/lib/python3.10/site-packages/datasets/arrow_writer.py", line 223, in __arrow_array__
return pa.array(cast_to_python_objects(data, only_1d_for_numpy=True))
File "/local_scratch/lfainsin/.conda/envs/pyg/lib/python3.10/site-packages/datasets/features/features.py", line 446, in cast_to_python_objects
return _cast_to_python_objects(
File "/local_scratch/lfainsin/.conda/envs/pyg/lib/python3.10/site-packages/datasets/features/features.py", line 407, in _cast_to_python_objects
[
File "/local_scratch/lfainsin/.conda/envs/pyg/lib/python3.10/site-packages/datasets/features/features.py", line 408, in <listcomp>
_cast_to_python_objects(
File "/local_scratch/lfainsin/.conda/envs/pyg/lib/python3.10/site-packages/datasets/features/features.py", line 319, in _cast_to_python_objects
[
File "/local_scratch/lfainsin/.conda/envs/pyg/lib/python3.10/site-packages/datasets/features/features.py", line 320, in <listcomp>
_cast_to_python_objects(
File "/local_scratch/lfainsin/.conda/envs/pyg/lib/python3.10/site-packages/datasets/features/features.py", line 263, in _cast_to_python_objects
def _cast_to_python_objects(obj: Any, only_1d_for_numpy: bool, optimize_list_casting: bool) -> Tuple[Any, bool]:
KeyboardInterrupt
During handling of the above exception, another exception occurred:
Traceback (most recent call last):
File "/local_scratch/lfainsin/.conda/envs/pyg/lib/python3.10/site-packages/datasets/arrow_writer.py", line 179, in __arrow_array__
storage = to_pyarrow_listarray(data, pa_type)
File "/local_scratch/lfainsin/.conda/envs/pyg/lib/python3.10/site-packages/datasets/features/features.py", line 1466, in to_pyarrow_listarray
return pa.array(data, pa_type.storage_dtype)
File "pyarrow/array.pxi", line 320, in pyarrow.lib.array
File "pyarrow/array.pxi", line 39, in pyarrow.lib._sequence_to_array
File "pyarrow/error.pxi", line 144, in pyarrow.lib.pyarrow_internal_check_status
File "pyarrow/error.pxi", line 123, in pyarrow.lib.check_status
pyarrow.lib.ArrowTypeError: Could not convert tensor([[-1.0273, -0.8037, -0.6860],
[-0.5034, -1.2685, -0.0558],
[-1.0908, -1.1820, -0.3178],
...,
[-0.8171, 0.1781, -0.5903],
[ 0.4370, 1.9305, 0.5899],
[-0.1426, 0.9053, -1.7559]]) with type Tensor: was not a sequence or recognized null for conversion to list type
During handling of the above exception, another exception occurred:
Traceback (most recent call last):
File "/gpfs_new/data/users/lfainsin/stage-laurent-f/src/random_scripts/uses_random_data.py", line 62, in <module>
ds_normalized = ds.map(
File "/local_scratch/lfainsin/.conda/envs/pyg/lib/python3.10/site-packages/datasets/arrow_dataset.py", line 580, in wrapper
out: Union["Dataset", "DatasetDict"] = func(self, *args, **kwargs)
File "/local_scratch/lfainsin/.conda/envs/pyg/lib/python3.10/site-packages/datasets/arrow_dataset.py", line 545, in wrapper
out: Union["Dataset", "DatasetDict"] = func(self, *args, **kwargs)
File "/local_scratch/lfainsin/.conda/envs/pyg/lib/python3.10/site-packages/datasets/arrow_dataset.py", line 3087, in map
for rank, done, content in Dataset._map_single(**dataset_kwargs):
File "/local_scratch/lfainsin/.conda/envs/pyg/lib/python3.10/site-packages/datasets/arrow_dataset.py", line 3492, in _map_single
writer.finalize()
File "/local_scratch/lfainsin/.conda/envs/pyg/lib/python3.10/site-packages/datasets/arrow_writer.py", line 584, in finalize
self.write_examples_on_file()
File "/local_scratch/lfainsin/.conda/envs/pyg/lib/python3.10/site-packages/datasets/arrow_writer.py", line 448, in write_examples_on_file
self.write_batch(batch_examples=batch_examples)
File "/local_scratch/lfainsin/.conda/envs/pyg/lib/python3.10/site-packages/datasets/arrow_writer.py", line 553, in write_batch
arrays.append(pa.array(typed_sequence))
File "pyarrow/array.pxi", line 236, in pyarrow.lib.array
File "pyarrow/array.pxi", line 110, in pyarrow.lib._handle_arrow_array_protocol
File "/local_scratch/lfainsin/.conda/envs/pyg/lib/python3.10/site-packages/datasets/arrow_writer.py", line 223, in __arrow_array__
return pa.array(cast_to_python_objects(data, only_1d_for_numpy=True))
File "/local_scratch/lfainsin/.conda/envs/pyg/lib/python3.10/site-packages/datasets/features/features.py", line 446, in cast_to_python_objects
return _cast_to_python_objects(
File "/local_scratch/lfainsin/.conda/envs/pyg/lib/python3.10/site-packages/datasets/features/features.py", line 407, in _cast_to_python_objects
[
File "/local_scratch/lfainsin/.conda/envs/pyg/lib/python3.10/site-packages/datasets/features/features.py", line 408, in <listcomp>
_cast_to_python_objects(
File "/local_scratch/lfainsin/.conda/envs/pyg/lib/python3.10/site-packages/datasets/features/features.py", line 319, in _cast_to_python_objects
[
File "/local_scratch/lfainsin/.conda/envs/pyg/lib/python3.10/site-packages/datasets/features/features.py", line 320, in <listcomp>
_cast_to_python_objects(
File "/local_scratch/lfainsin/.conda/envs/pyg/lib/python3.10/site-packages/datasets/features/features.py", line 291, in _cast_to_python_objects
if config.JAX_AVAILABLE and "jax" in sys.modules:
KeyboardInterrupt
```
</details>
<details>
<summary>stack trace 3</summary>
```python
(pyg)[d623204@rosetta-bigviz01 stage-laurent-f]$ python src/random_scripts/uses_random_data.py
Found cached dataset random_data (/local_scratch/lfainsin/.cache/huggingface/datasets/random_data/default/0.0.0/444e214e1d0e6298cfd3f2368323ec37073dc1439f618e19395b1f421c69b066)
Applying mean/std: 99%|███████████████████████████████████████████████████████████████████████████████████████████████████████████████████████████████████████████████████▎ | 989/1000 [00:01<00:00, 504.80 examples/s]Traceback (most recent call last):
File "/local_scratch/lfainsin/.conda/envs/pyg/lib/python3.10/site-packages/datasets/arrow_writer.py", line 179, in __arrow_array__
storage = to_pyarrow_listarray(data, pa_type)
File "/local_scratch/lfainsin/.conda/envs/pyg/lib/python3.10/site-packages/datasets/features/features.py", line 1466, in to_pyarrow_listarray
return pa.array(data, pa_type.storage_dtype)
File "pyarrow/array.pxi", line 320, in pyarrow.lib.array
File "pyarrow/array.pxi", line 39, in pyarrow.lib._sequence_to_array
File "pyarrow/error.pxi", line 144, in pyarrow.lib.pyarrow_internal_check_status
File "pyarrow/error.pxi", line 123, in pyarrow.lib.check_status
pyarrow.lib.ArrowTypeError: Could not convert tensor([[-1.0273, -0.8037, -0.6860],
[-0.5034, -1.2685, -0.0558],
[-1.0908, -1.1820, -0.3178],
...,
[-0.8171, 0.1781, -0.5903],
[ 0.4370, 1.9305, 0.5899],
[-0.1426, 0.9053, -1.7559]]) with type Tensor: was not a sequence or recognized null for conversion to list type
During handling of the above exception, another exception occurred:
Traceback (most recent call last):
File "/local_scratch/lfainsin/.conda/envs/pyg/lib/python3.10/site-packages/datasets/arrow_dataset.py", line 3449, in _map_single
writer.write(example)
File "/local_scratch/lfainsin/.conda/envs/pyg/lib/python3.10/site-packages/datasets/arrow_writer.py", line 490, in write
self.write_examples_on_file()
File "/local_scratch/lfainsin/.conda/envs/pyg/lib/python3.10/site-packages/datasets/arrow_writer.py", line 448, in write_examples_on_file
self.write_batch(batch_examples=batch_examples)
File "/local_scratch/lfainsin/.conda/envs/pyg/lib/python3.10/site-packages/datasets/arrow_writer.py", line 553, in write_batch
arrays.append(pa.array(typed_sequence))
File "pyarrow/array.pxi", line 236, in pyarrow.lib.array
File "pyarrow/array.pxi", line 110, in pyarrow.lib._handle_arrow_array_protocol
File "/local_scratch/lfainsin/.conda/envs/pyg/lib/python3.10/site-packages/datasets/arrow_writer.py", line 223, in __arrow_array__
return pa.array(cast_to_python_objects(data, only_1d_for_numpy=True))
File "/local_scratch/lfainsin/.conda/envs/pyg/lib/python3.10/site-packages/datasets/features/features.py", line 446, in cast_to_python_objects
return _cast_to_python_objects(
File "/local_scratch/lfainsin/.conda/envs/pyg/lib/python3.10/site-packages/datasets/features/features.py", line 407, in _cast_to_python_objects
[
File "/local_scratch/lfainsin/.conda/envs/pyg/lib/python3.10/site-packages/datasets/features/features.py", line 408, in <listcomp>
_cast_to_python_objects(
File "/local_scratch/lfainsin/.conda/envs/pyg/lib/python3.10/site-packages/datasets/features/features.py", line 319, in _cast_to_python_objects
[
File "/local_scratch/lfainsin/.conda/envs/pyg/lib/python3.10/site-packages/datasets/features/features.py", line 320, in <listcomp>
_cast_to_python_objects(
KeyboardInterrupt
During handling of the above exception, another exception occurred:
Traceback (most recent call last):
File "/local_scratch/lfainsin/.conda/envs/pyg/lib/python3.10/site-packages/datasets/arrow_writer.py", line 179, in __arrow_array__
storage = to_pyarrow_listarray(data, pa_type)
File "/local_scratch/lfainsin/.conda/envs/pyg/lib/python3.10/site-packages/datasets/features/features.py", line 1466, in to_pyarrow_listarray
return pa.array(data, pa_type.storage_dtype)
File "pyarrow/array.pxi", line 320, in pyarrow.lib.array
File "pyarrow/array.pxi", line 39, in pyarrow.lib._sequence_to_array
File "pyarrow/error.pxi", line 144, in pyarrow.lib.pyarrow_internal_check_status
File "pyarrow/error.pxi", line 123, in pyarrow.lib.check_status
pyarrow.lib.ArrowTypeError: Could not convert tensor([[-1.0273, -0.8037, -0.6860],
[-0.5034, -1.2685, -0.0558],
[-1.0908, -1.1820, -0.3178],
...,
[-0.8171, 0.1781, -0.5903],
[ 0.4370, 1.9305, 0.5899],
[-0.1426, 0.9053, -1.7559]]) with type Tensor: was not a sequence or recognized null for conversion to list type
During handling of the above exception, another exception occurred:
Traceback (most recent call last):
File "/gpfs_new/data/users/lfainsin/stage-laurent-f/src/random_scripts/uses_random_data.py", line 62, in <module>
ds_normalized = ds.map(
File "/local_scratch/lfainsin/.conda/envs/pyg/lib/python3.10/site-packages/datasets/arrow_dataset.py", line 580, in wrapper
out: Union["Dataset", "DatasetDict"] = func(self, *args, **kwargs)
File "/local_scratch/lfainsin/.conda/envs/pyg/lib/python3.10/site-packages/datasets/arrow_dataset.py", line 545, in wrapper
out: Union["Dataset", "DatasetDict"] = func(self, *args, **kwargs)
File "/local_scratch/lfainsin/.conda/envs/pyg/lib/python3.10/site-packages/datasets/arrow_dataset.py", line 3087, in map
for rank, done, content in Dataset._map_single(**dataset_kwargs):
File "/local_scratch/lfainsin/.conda/envs/pyg/lib/python3.10/site-packages/datasets/arrow_dataset.py", line 3492, in _map_single
writer.finalize()
File "/local_scratch/lfainsin/.conda/envs/pyg/lib/python3.10/site-packages/datasets/arrow_writer.py", line 584, in finalize
self.write_examples_on_file()
File "/local_scratch/lfainsin/.conda/envs/pyg/lib/python3.10/site-packages/datasets/arrow_writer.py", line 448, in write_examples_on_file
self.write_batch(batch_examples=batch_examples)
File "/local_scratch/lfainsin/.conda/envs/pyg/lib/python3.10/site-packages/datasets/arrow_writer.py", line 553, in write_batch
arrays.append(pa.array(typed_sequence))
File "pyarrow/array.pxi", line 236, in pyarrow.lib.array
File "pyarrow/array.pxi", line 110, in pyarrow.lib._handle_arrow_array_protocol
File "/local_scratch/lfainsin/.conda/envs/pyg/lib/python3.10/site-packages/datasets/arrow_writer.py", line 223, in __arrow_array__
return pa.array(cast_to_python_objects(data, only_1d_for_numpy=True))
File "/local_scratch/lfainsin/.conda/envs/pyg/lib/python3.10/site-packages/datasets/features/features.py", line 446, in cast_to_python_objects
return _cast_to_python_objects(
File "/local_scratch/lfainsin/.conda/envs/pyg/lib/python3.10/site-packages/datasets/features/features.py", line 407, in _cast_to_python_objects
[
File "/local_scratch/lfainsin/.conda/envs/pyg/lib/python3.10/site-packages/datasets/features/features.py", line 408, in <listcomp>
_cast_to_python_objects(
File "/local_scratch/lfainsin/.conda/envs/pyg/lib/python3.10/site-packages/datasets/features/features.py", line 319, in _cast_to_python_objects
[
File "/local_scratch/lfainsin/.conda/envs/pyg/lib/python3.10/site-packages/datasets/features/features.py", line 320, in <listcomp>
_cast_to_python_objects(
File "/local_scratch/lfainsin/.conda/envs/pyg/lib/python3.10/site-packages/datasets/features/features.py", line 298, in _cast_to_python_objects
if obj.ndim == 0:
KeyboardInterrupt
```
</details>
| ### Describe the bug
Hi !
I'm currently working with a large (~150GB) unnormalized dataset at work.
The dataset is available on a read-only filesystem internally, and I use a [loading script](https://huggingface.co/docs/datasets/dataset_script) to retreive it.
I want to normalize the features of the dataset, meaning I need to compute the mean and standard deviation metric for each feature of the entire dataset. I cannot load the entire dataset to RAM as it is too big, so following [this discussion on the huggingface discourse](https://discuss.huggingface.co/t/copy-columns-in-a-dataset-and-compute-statistics-for-a-column/22157) I am using a [map operation](https://huggingface.co/docs/datasets/v2.14.0/en/package_reference/main_classes#datasets.Dataset.map) to first compute the metrics and a second map operation to apply them on the dataset.
The problem lies in the second mapping, as it gets stuck at ~99%. By checking what the process does (using `htop` and `strace`) it seems to be doing a lot of I/O operations, and I'm not sure why.
Obviously, I could always normalize the dataset externally and then load it using a loading script. However, since the internal dataset is updated fairly frequently, using the library to perform normalization automatically would make it much easier for me.
### Steps to reproduce the bug
I'm able to reproduce the problem using the following scripts:
```python
# random_data.py
import datasets
import torch
_VERSION = "1.0.0"
class RandomDataset(datasets.GeneratorBasedBuilder):
def _info(self):
return datasets.DatasetInfo(
version=_VERSION,
supervised_keys=None,
features=datasets.Features(
{
"positions": datasets.Array2D(
shape=(30000, 3),
dtype="float32",
),
"normals": datasets.Array2D(
shape=(30000, 3),
dtype="float32",
),
"features": datasets.Array2D(
shape=(30000, 6),
dtype="float32",
),
"scalars": datasets.Sequence(
feature=datasets.Value("float32"),
length=20,
),
},
),
)
def _split_generators(self, dl_manager):
return [
datasets.SplitGenerator(
name=datasets.Split.TRAIN, # type: ignore
gen_kwargs={"nb_samples": 1000},
),
datasets.SplitGenerator(
name=datasets.Split.TEST, # type: ignore
gen_kwargs={"nb_samples": 100},
),
]
def _generate_examples(self, nb_samples: int):
for idx in range(nb_samples):
yield idx, {
"positions": torch.randn(30000, 3),
"normals": torch.randn(30000, 3),
"features": torch.randn(30000, 6),
"scalars": torch.randn(20),
}
```
```python
# main.py
import datasets
import torch
def apply_mean_std(
dataset: datasets.Dataset,
means: dict[str, torch.Tensor],
stds: dict[str, torch.Tensor],
) -> dict[str, torch.Tensor]:
"""Normalize the dataset using the mean and standard deviation of each feature.
Args:
dataset (`Dataset`): A huggingface dataset.
mean (`dict[str, Tensor]`): A dictionary containing the mean of each feature.
std (`dict[str, Tensor]`): A dictionary containing the standard deviation of each feature.
Returns:
dict: A dictionary containing the normalized dataset.
"""
result = {}
for key in means.keys():
# extract data from dataset
data: torch.Tensor = dataset[key] # type: ignore
# extract mean and std from dict
mean = means[key] # type: ignore
std = stds[key] # type: ignore
# normalize data
normalized_data = (data - mean) / std
result[key] = normalized_data
return result
# get dataset
ds = datasets.load_dataset(
path="random_data.py",
split="train",
).with_format("torch")
# compute mean (along last axis)
means = {key: torch.zeros(ds[key][0].shape[-1]) for key in ds.column_names}
means_sq = {key: torch.zeros(ds[key][0].shape[-1]) for key in ds.column_names}
for batch in ds.iter(batch_size=8):
for key in ds.column_names:
data = batch[key]
batch_size = data.shape[0]
data = data.reshape(-1, data.shape[-1])
means[key] += data.mean(dim=0) / len(ds) * batch_size
means_sq[key] += (data**2).mean(dim=0) / len(ds) * batch_size
# compute std (along last axis)
stds = {key: torch.sqrt(means_sq[key] - means[key] ** 2) for key in ds.column_names}
# normalize each feature of the dataset
ds_normalized = ds.map(
desc="Applying mean/std", # type: ignore
function=apply_mean_std,
batched=False,
fn_kwargs={
"means": means,
"stds": stds,
},
)
```
### Expected behavior
Using the previous scripts, the `ds_normalized` mapping completes in ~5 minutes, but any subsequent use of `ds_normalized` is really really slow, for example reapplying `apply_mean_std` to `ds_normalized` takes forever. This is very strange, I'm sure I must be missing something, but I would still expect this to be faster.
### Environment info
- `datasets` version: 2.13.1
- Platform: Linux-3.10.0-1160.66.1.el7.x86_64-x86_64-with-glibc2.17
- Python version: 3.10.12
- Huggingface_hub version: 0.15.1
- PyArrow version: 12.0.0
- Pandas version: 2.0.2 | 1,454 | Mapping gets stuck at 99%
### Describe the bug
Hi !
I'm currently working with a large (~150GB) unnormalized dataset at work.
The dataset is available on a read-only filesystem internally, and I use a [loading script](https://huggingface.co/docs/datasets/dataset_script) to retreive it.
I want to normalize the features of the dataset, meaning I need to compute the mean and standard deviation metric for each feature of the entire dataset. I cannot load the entire dataset to RAM as it is too big, so following [this discussion on the huggingface discourse](https://discuss.huggingface.co/t/copy-columns-in-a-dataset-and-compute-statistics-for-a-column/22157) I am using a [map operation](https://huggingface.co/docs/datasets/v2.14.0/en/package_reference/main_classes#datasets.Dataset.map) to first compute the metrics and a second map operation to apply them on the dataset.
The problem lies in the second mapping, as it gets stuck at ~99%. By checking what the process does (using `htop` and `strace`) it seems to be doing a lot of I/O operations, and I'm not sure why.
Obviously, I could always normalize the dataset externally and then load it using a loading script. However, since the internal dataset is updated fairly frequently, using the library to perform normalization automatically would make it much easier for me.
### Steps to reproduce the bug
I'm able to reproduce the problem using the following scripts:
```python
# random_data.py
import datasets
import torch
_VERSION = "1.0.0"
class RandomDataset(datasets.GeneratorBasedBuilder):
def _info(self):
return datasets.DatasetInfo(
version=_VERSION,
supervised_keys=None,
features=datasets.Features(
{
"positions": datasets.Array2D(
shape=(30000, 3),
dtype="float32",
),
"normals": datasets.Array2D(
shape=(30000, 3),
dtype="float32",
),
"features": datasets.Array2D(
shape=(30000, 6),
dtype="float32",
),
"scalars": datasets.Sequence(
feature=datasets.Value("float32"),
length=20,
),
},
),
)
def _split_generators(self, dl_manager):
return [
datasets.SplitGenerator(
name=datasets.Split.TRAIN, # type: ignore
gen_kwargs={"nb_samples": 1000},
),
datasets.SplitGenerator(
name=datasets.Split.TEST, # type: ignore
gen_kwargs={"nb_samples": 100},
),
]
def _generate_examples(self, nb_samples: int):
for idx in range(nb_samples):
yield idx, {
"positions": torch.randn(30000, 3),
"normals": torch.randn(30000, 3),
"features": torch.randn(30000, 6),
"scalars": torch.randn(20),
}
```
```python
# main.py
import datasets
import torch
def apply_mean_std(
dataset: datasets.Dataset,
means: dict[str, torch.Tensor],
stds: dict[str, torch.Tensor],
) -> dict[str, torch.Tensor]:
"""Normalize the dataset using the mean and standard deviation of each feature.
Args:
dataset (`Dataset`): A huggingface dataset.
mean (`dict[str, Tensor]`): A dictionary containing the mean of each feature.
std (`dict[str, Tensor]`): A dictionary containing the standard deviation of each feature.
Returns:
dict: A dictionary containing the normalized dataset.
"""
result = {}
for key in means.keys():
# extract data from dataset
data: torch.Tensor = dataset[key] # type: ignore
# extract mean and std from dict
mean = means[key] # type: ignore
std = stds[key] # type: ignore
# normalize data
normalized_data = (data - mean) / std
result[key] = normalized_data
return result
# get dataset
ds = datasets.load_dataset(
path="random_data.py",
split="train",
).with_format("torch")
# compute mean (along last axis)
means = {key: torch.zeros(ds[key][0].shape[-1]) for key in ds.column_names}
means_sq = {key: torch.zeros(ds[key][0].shape[-1]) for key in ds.column_names}
for batch in ds.iter(batch_size=8):
for key in ds.column_names:
data = batch[key]
batch_size = data.shape[0]
data = data.reshape(-1, data.shape[-1])
means[key] += data.mean(dim=0) / len(ds) * batch_size
means_sq[key] += (data**2).mean(dim=0) / len(ds) * batch_size
# compute std (along last axis)
stds = {key: torch.sqrt(means_sq[key] - means[key] ** 2) for key in ds.column_names}
# normalize each feature of the dataset
ds_normalized = ds.map(
desc="Applying mean/std", # type: ignore
function=apply_mean_std,
batched=False,
fn_kwargs={
"means": means,
"stds": stds,
},
)
```
### Expected behavior
Using the previous scripts, the `ds_normalized` mapping completes in ~5 minutes, but any subsequent use of `ds_normalized` is really really slow, for example reapplying `apply_mean_std` to `ds_normalized` takes forever. This is very strange, I'm sure I must be missing something, but I would still expect this to be faster.
### Environment info
- `datasets` version: 2.13.1
- Platform: Linux-3.10.0-1160.66.1.el7.x86_64-x86_64-with-glibc2.17
- Python version: 3.10.12
- Huggingface_hub version: 0.15.1
- PyArrow version: 12.0.0
- Pandas version: 2.0.2
I think `batch_size/writer_batch_size` is already at its lowest in the 2nd `.map` since `batched=False` implies `batch_size=1` and `len(ds) = 1000 = writer_batch_size`.
Here is also a bunch of stack traces when I interrupted the process:
<details>
<summary>stack trace 1</summary>
```python
(pyg)[d623204@rosetta-bigviz01 stage-laurent-f]$ python src/random_scripts/uses_random_data.py
Found cached dataset random_data (/local_scratch/lfainsin/.cache/huggingface/datasets/random_data/default/0.0.0/444e214e1d0e6298cfd3f2368323ec37073dc1439f618e19395b1f421c69b066)
Applying mean/std: 97%|████████████████████████████████████████████████████████████████████████████████████████████████████████████████████████████████████████████████ | 967/1000 [00:01<00:00, 534.87 examples/s]Traceback (most recent call last):
File "/local_scratch/lfainsin/.conda/envs/pyg/lib/python3.10/site-packages/datasets/arrow_writer.py", line 179, in __arrow_array__
storage = to_pyarrow_listarray(data, pa_type)
File "/local_scratch/lfainsin/.conda/envs/pyg/lib/python3.10/site-packages/datasets/features/features.py", line 1466, in to_pyarrow_listarray
return pa.array(data, pa_type.storage_dtype)
File "pyarrow/array.pxi", line 320, in pyarrow.lib.array
File "pyarrow/array.pxi", line 39, in pyarrow.lib._sequence_to_array
File "pyarrow/error.pxi", line 144, in pyarrow.lib.pyarrow_internal_check_status
File "pyarrow/error.pxi", line 123, in pyarrow.lib.check_status
pyarrow.lib.ArrowTypeError: Could not convert tensor([[-1.0273, -0.8037, -0.6860],
[-0.5034, -1.2685, -0.0558],
[-1.0908, -1.1820, -0.3178],
...,
[-0.8171, 0.1781, -0.5903],
[ 0.4370, 1.9305, 0.5899],
[-0.1426, 0.9053, -1.7559]]) with type Tensor: was not a sequence or recognized null for conversion to list type
During handling of the above exception, another exception occurred:
Traceback (most recent call last):
File "/local_scratch/lfainsin/.conda/envs/pyg/lib/python3.10/site-packages/datasets/arrow_dataset.py", line 3449, in _map_single
writer.write(example)
File "/local_scratch/lfainsin/.conda/envs/pyg/lib/python3.10/site-packages/datasets/arrow_writer.py", line 490, in write
self.write_examples_on_file()
File "/local_scratch/lfainsin/.conda/envs/pyg/lib/python3.10/site-packages/datasets/arrow_writer.py", line 448, in write_examples_on_file
self.write_batch(batch_examples=batch_examples)
File "/local_scratch/lfainsin/.conda/envs/pyg/lib/python3.10/site-packages/datasets/arrow_writer.py", line 553, in write_batch
arrays.append(pa.array(typed_sequence))
File "pyarrow/array.pxi", line 236, in pyarrow.lib.array
File "pyarrow/array.pxi", line 110, in pyarrow.lib._handle_arrow_array_protocol
File "/local_scratch/lfainsin/.conda/envs/pyg/lib/python3.10/site-packages/datasets/arrow_writer.py", line 223, in __arrow_array__
return pa.array(cast_to_python_objects(data, only_1d_for_numpy=True))
File "/local_scratch/lfainsin/.conda/envs/pyg/lib/python3.10/site-packages/datasets/features/features.py", line 446, in cast_to_python_objects
return _cast_to_python_objects(
File "/local_scratch/lfainsin/.conda/envs/pyg/lib/python3.10/site-packages/datasets/features/features.py", line 407, in _cast_to_python_objects
[
File "/local_scratch/lfainsin/.conda/envs/pyg/lib/python3.10/site-packages/datasets/features/features.py", line 408, in <listcomp>
_cast_to_python_objects(
File "/local_scratch/lfainsin/.conda/envs/pyg/lib/python3.10/site-packages/datasets/features/features.py", line 319, in _cast_to_python_objects
[
File "/local_scratch/lfainsin/.conda/envs/pyg/lib/python3.10/site-packages/datasets/features/features.py", line 320, in <listcomp>
_cast_to_python_objects(
File "/local_scratch/lfainsin/.conda/envs/pyg/lib/python3.10/site-packages/datasets/features/features.py", line 263, in _cast_to_python_objects
def _cast_to_python_objects(obj: Any, only_1d_for_numpy: bool, optimize_list_casting: bool) -> Tuple[Any, bool]:
KeyboardInterrupt
During handling of the above exception, another exception occurred:
Traceback (most recent call last):
File "/local_scratch/lfainsin/.conda/envs/pyg/lib/python3.10/site-packages/datasets/arrow_writer.py", line 179, in __arrow_array__
storage = to_pyarrow_listarray(data, pa_type)
File "/local_scratch/lfainsin/.conda/envs/pyg/lib/python3.10/site-packages/datasets/features/features.py", line 1466, in to_pyarrow_listarray
return pa.array(data, pa_type.storage_dtype)
File "pyarrow/array.pxi", line 320, in pyarrow.lib.array
File "pyarrow/array.pxi", line 39, in pyarrow.lib._sequence_to_array
File "pyarrow/error.pxi", line 144, in pyarrow.lib.pyarrow_internal_check_status
File "pyarrow/error.pxi", line 123, in pyarrow.lib.check_status
pyarrow.lib.ArrowTypeError: Could not convert tensor([[-1.0273, -0.8037, -0.6860],
[-0.5034, -1.2685, -0.0558],
[-1.0908, -1.1820, -0.3178],
...,
[-0.8171, 0.1781, -0.5903],
[ 0.4370, 1.9305, 0.5899],
[-0.1426, 0.9053, -1.7559]]) with type Tensor: was not a sequence or recognized null for conversion to list type
During handling of the above exception, another exception occurred:
Traceback (most recent call last):
File "/gpfs_new/data/users/lfainsin/stage-laurent-f/src/random_scripts/uses_random_data.py", line 62, in <module>
ds_normalized = ds.map(
File "/local_scratch/lfainsin/.conda/envs/pyg/lib/python3.10/site-packages/datasets/arrow_dataset.py", line 580, in wrapper
out: Union["Dataset", "DatasetDict"] = func(self, *args, **kwargs)
File "/local_scratch/lfainsin/.conda/envs/pyg/lib/python3.10/site-packages/datasets/arrow_dataset.py", line 545, in wrapper
out: Union["Dataset", "DatasetDict"] = func(self, *args, **kwargs)
File "/local_scratch/lfainsin/.conda/envs/pyg/lib/python3.10/site-packages/datasets/arrow_dataset.py", line 3087, in map
for rank, done, content in Dataset._map_single(**dataset_kwargs):
File "/local_scratch/lfainsin/.conda/envs/pyg/lib/python3.10/site-packages/datasets/arrow_dataset.py", line 3492, in _map_single
writer.finalize()
File "/local_scratch/lfainsin/.conda/envs/pyg/lib/python3.10/site-packages/datasets/arrow_writer.py", line 584, in finalize
self.write_examples_on_file()
File "/local_scratch/lfainsin/.conda/envs/pyg/lib/python3.10/site-packages/datasets/arrow_writer.py", line 448, in write_examples_on_file
self.write_batch(batch_examples=batch_examples)
File "/local_scratch/lfainsin/.conda/envs/pyg/lib/python3.10/site-packages/datasets/arrow_writer.py", line 553, in write_batch
arrays.append(pa.array(typed_sequence))
File "pyarrow/array.pxi", line 236, in pyarrow.lib.array
File "pyarrow/array.pxi", line 110, in pyarrow.lib._handle_arrow_array_protocol
File "/local_scratch/lfainsin/.conda/envs/pyg/lib/python3.10/site-packages/datasets/arrow_writer.py", line 223, in __arrow_array__
return pa.array(cast_to_python_objects(data, only_1d_for_numpy=True))
File "/local_scratch/lfainsin/.conda/envs/pyg/lib/python3.10/site-packages/datasets/features/features.py", line 446, in cast_to_python_objects
return _cast_to_python_objects(
File "/local_scratch/lfainsin/.conda/envs/pyg/lib/python3.10/site-packages/datasets/features/features.py", line 407, in _cast_to_python_objects
[
File "/local_scratch/lfainsin/.conda/envs/pyg/lib/python3.10/site-packages/datasets/features/features.py", line 408, in <listcomp>
_cast_to_python_objects(
File "/local_scratch/lfainsin/.conda/envs/pyg/lib/python3.10/site-packages/datasets/features/features.py", line 319, in _cast_to_python_objects
[
File "/local_scratch/lfainsin/.conda/envs/pyg/lib/python3.10/site-packages/datasets/features/features.py", line 319, in <listcomp>
[
KeyboardInterrupt
```
</details>
<details>
<summary>stack trace 2</summary>
```python
(pyg)[d623204@rosetta-bigviz01 stage-laurent-f]$ python src/random_scripts/uses_random_data.py
Found cached dataset random_data (/local_scratch/lfainsin/.cache/huggingface/datasets/random_data/default/0.0.0/444e214e1d0e6298cfd3f2368323ec37073dc1439f618e19395b1f421c69b066)
Applying mean/std: 99%|███████████████████████████████████████████████████████████████████████████████████████████████████████████████████████████████████████████████████▏ | 988/1000 [00:20<00:00, 526.19 examples/s]Applying mean/std: 100%|█████████████████████████████████████████████████████████████████████████████████████████████████████████████████████████████████████████████████████▊| 999/1000 [00:21<00:00, 9.66 examples/s]Traceback (most recent call last):
File "/local_scratch/lfainsin/.conda/envs/pyg/lib/python3.10/site-packages/datasets/arrow_writer.py", line 179, in __arrow_array__
storage = to_pyarrow_listarray(data, pa_type)
File "/local_scratch/lfainsin/.conda/envs/pyg/lib/python3.10/site-packages/datasets/features/features.py", line 1466, in to_pyarrow_listarray
return pa.array(data, pa_type.storage_dtype)
File "pyarrow/array.pxi", line 320, in pyarrow.lib.array
File "pyarrow/array.pxi", line 39, in pyarrow.lib._sequence_to_array
File "pyarrow/error.pxi", line 144, in pyarrow.lib.pyarrow_internal_check_status
File "pyarrow/error.pxi", line 123, in pyarrow.lib.check_status
pyarrow.lib.ArrowTypeError: Could not convert tensor([[-1.0273, -0.8037, -0.6860],
[-0.5034, -1.2685, -0.0558],
[-1.0908, -1.1820, -0.3178],
...,
[-0.8171, 0.1781, -0.5903],
[ 0.4370, 1.9305, 0.5899],
[-0.1426, 0.9053, -1.7559]]) with type Tensor: was not a sequence or recognized null for conversion to list type
During handling of the above exception, another exception occurred:
Traceback (most recent call last):
File "/local_scratch/lfainsin/.conda/envs/pyg/lib/python3.10/site-packages/datasets/arrow_dataset.py", line 3449, in _map_single
writer.write(example)
File "/local_scratch/lfainsin/.conda/envs/pyg/lib/python3.10/site-packages/datasets/arrow_writer.py", line 490, in write
self.write_examples_on_file()
File "/local_scratch/lfainsin/.conda/envs/pyg/lib/python3.10/site-packages/datasets/arrow_writer.py", line 448, in write_examples_on_file
self.write_batch(batch_examples=batch_examples)
File "/local_scratch/lfainsin/.conda/envs/pyg/lib/python3.10/site-packages/datasets/arrow_writer.py", line 553, in write_batch
arrays.append(pa.array(typed_sequence))
File "pyarrow/array.pxi", line 236, in pyarrow.lib.array
File "pyarrow/array.pxi", line 110, in pyarrow.lib._handle_arrow_array_protocol
File "/local_scratch/lfainsin/.conda/envs/pyg/lib/python3.10/site-packages/datasets/arrow_writer.py", line 223, in __arrow_array__
return pa.array(cast_to_python_objects(data, only_1d_for_numpy=True))
File "/local_scratch/lfainsin/.conda/envs/pyg/lib/python3.10/site-packages/datasets/features/features.py", line 446, in cast_to_python_objects
return _cast_to_python_objects(
File "/local_scratch/lfainsin/.conda/envs/pyg/lib/python3.10/site-packages/datasets/features/features.py", line 407, in _cast_to_python_objects
[
File "/local_scratch/lfainsin/.conda/envs/pyg/lib/python3.10/site-packages/datasets/features/features.py", line 408, in <listcomp>
_cast_to_python_objects(
File "/local_scratch/lfainsin/.conda/envs/pyg/lib/python3.10/site-packages/datasets/features/features.py", line 319, in _cast_to_python_objects
[
File "/local_scratch/lfainsin/.conda/envs/pyg/lib/python3.10/site-packages/datasets/features/features.py", line 320, in <listcomp>
_cast_to_python_objects(
File "/local_scratch/lfainsin/.conda/envs/pyg/lib/python3.10/site-packages/datasets/features/features.py", line 263, in _cast_to_python_objects
def _cast_to_python_objects(obj: Any, only_1d_for_numpy: bool, optimize_list_casting: bool) -> Tuple[Any, bool]:
KeyboardInterrupt
During handling of the above exception, another exception occurred:
Traceback (most recent call last):
File "/local_scratch/lfainsin/.conda/envs/pyg/lib/python3.10/site-packages/datasets/arrow_writer.py", line 179, in __arrow_array__
storage = to_pyarrow_listarray(data, pa_type)
File "/local_scratch/lfainsin/.conda/envs/pyg/lib/python3.10/site-packages/datasets/features/features.py", line 1466, in to_pyarrow_listarray
return pa.array(data, pa_type.storage_dtype)
File "pyarrow/array.pxi", line 320, in pyarrow.lib.array
File "pyarrow/array.pxi", line 39, in pyarrow.lib._sequence_to_array
File "pyarrow/error.pxi", line 144, in pyarrow.lib.pyarrow_internal_check_status
File "pyarrow/error.pxi", line 123, in pyarrow.lib.check_status
pyarrow.lib.ArrowTypeError: Could not convert tensor([[-1.0273, -0.8037, -0.6860],
[-0.5034, -1.2685, -0.0558],
[-1.0908, -1.1820, -0.3178],
...,
[-0.8171, 0.1781, -0.5903],
[ 0.4370, 1.9305, 0.5899],
[-0.1426, 0.9053, -1.7559]]) with type Tensor: was not a sequence or recognized null for conversion to list type
During handling of the above exception, another exception occurred:
Traceback (most recent call last):
File "/gpfs_new/data/users/lfainsin/stage-laurent-f/src/random_scripts/uses_random_data.py", line 62, in <module>
ds_normalized = ds.map(
File "/local_scratch/lfainsin/.conda/envs/pyg/lib/python3.10/site-packages/datasets/arrow_dataset.py", line 580, in wrapper
out: Union["Dataset", "DatasetDict"] = func(self, *args, **kwargs)
File "/local_scratch/lfainsin/.conda/envs/pyg/lib/python3.10/site-packages/datasets/arrow_dataset.py", line 545, in wrapper
out: Union["Dataset", "DatasetDict"] = func(self, *args, **kwargs)
File "/local_scratch/lfainsin/.conda/envs/pyg/lib/python3.10/site-packages/datasets/arrow_dataset.py", line 3087, in map
for rank, done, content in Dataset._map_single(**dataset_kwargs):
File "/local_scratch/lfainsin/.conda/envs/pyg/lib/python3.10/site-packages/datasets/arrow_dataset.py", line 3492, in _map_single
writer.finalize()
File "/local_scratch/lfainsin/.conda/envs/pyg/lib/python3.10/site-packages/datasets/arrow_writer.py", line 584, in finalize
self.write_examples_on_file()
File "/local_scratch/lfainsin/.conda/envs/pyg/lib/python3.10/site-packages/datasets/arrow_writer.py", line 448, in write_examples_on_file
self.write_batch(batch_examples=batch_examples)
File "/local_scratch/lfainsin/.conda/envs/pyg/lib/python3.10/site-packages/datasets/arrow_writer.py", line 553, in write_batch
arrays.append(pa.array(typed_sequence))
File "pyarrow/array.pxi", line 236, in pyarrow.lib.array
File "pyarrow/array.pxi", line 110, in pyarrow.lib._handle_arrow_array_protocol
File "/local_scratch/lfainsin/.conda/envs/pyg/lib/python3.10/site-packages/datasets/arrow_writer.py", line 223, in __arrow_array__
return pa.array(cast_to_python_objects(data, only_1d_for_numpy=True))
File "/local_scratch/lfainsin/.conda/envs/pyg/lib/python3.10/site-packages/datasets/features/features.py", line 446, in cast_to_python_objects
return _cast_to_python_objects(
File "/local_scratch/lfainsin/.conda/envs/pyg/lib/python3.10/site-packages/datasets/features/features.py", line 407, in _cast_to_python_objects
[
File "/local_scratch/lfainsin/.conda/envs/pyg/lib/python3.10/site-packages/datasets/features/features.py", line 408, in <listcomp>
_cast_to_python_objects(
File "/local_scratch/lfainsin/.conda/envs/pyg/lib/python3.10/site-packages/datasets/features/features.py", line 319, in _cast_to_python_objects
[
File "/local_scratch/lfainsin/.conda/envs/pyg/lib/python3.10/site-packages/datasets/features/features.py", line 320, in <listcomp>
_cast_to_python_objects(
File "/local_scratch/lfainsin/.conda/envs/pyg/lib/python3.10/site-packages/datasets/features/features.py", line 291, in _cast_to_python_objects
if config.JAX_AVAILABLE and "jax" in sys.modules:
KeyboardInterrupt
```
</details>
<details>
<summary>stack trace 3</summary>
```python
(pyg)[d623204@rosetta-bigviz01 stage-laurent-f]$ python src/random_scripts/uses_random_data.py
Found cached dataset random_data (/local_scratch/lfainsin/.cache/huggingface/datasets/random_data/default/0.0.0/444e214e1d0e6298cfd3f2368323ec37073dc1439f618e19395b1f421c69b066)
Applying mean/std: 99%|███████████████████████████████████████████████████████████████████████████████████████████████████████████████████████████████████████████████████▎ | 989/1000 [00:01<00:00, 504.80 examples/s]Traceback (most recent call last):
File "/local_scratch/lfainsin/.conda/envs/pyg/lib/python3.10/site-packages/datasets/arrow_writer.py", line 179, in __arrow_array__
storage = to_pyarrow_listarray(data, pa_type)
File "/local_scratch/lfainsin/.conda/envs/pyg/lib/python3.10/site-packages/datasets/features/features.py", line 1466, in to_pyarrow_listarray
return pa.array(data, pa_type.storage_dtype)
File "pyarrow/array.pxi", line 320, in pyarrow.lib.array
File "pyarrow/array.pxi", line 39, in pyarrow.lib._sequence_to_array
File "pyarrow/error.pxi", line 144, in pyarrow.lib.pyarrow_internal_check_status
File "pyarrow/error.pxi", line 123, in pyarrow.lib.check_status
pyarrow.lib.ArrowTypeError: Could not convert tensor([[-1.0273, -0.8037, -0.6860],
[-0.5034, -1.2685, -0.0558],
[-1.0908, -1.1820, -0.3178],
...,
[-0.8171, 0.1781, -0.5903],
[ 0.4370, 1.9305, 0.5899],
[-0.1426, 0.9053, -1.7559]]) with type Tensor: was not a sequence or recognized null for conversion to list type
During handling of the above exception, another exception occurred:
Traceback (most recent call last):
File "/local_scratch/lfainsin/.conda/envs/pyg/lib/python3.10/site-packages/datasets/arrow_dataset.py", line 3449, in _map_single
writer.write(example)
File "/local_scratch/lfainsin/.conda/envs/pyg/lib/python3.10/site-packages/datasets/arrow_writer.py", line 490, in write
self.write_examples_on_file()
File "/local_scratch/lfainsin/.conda/envs/pyg/lib/python3.10/site-packages/datasets/arrow_writer.py", line 448, in write_examples_on_file
self.write_batch(batch_examples=batch_examples)
File "/local_scratch/lfainsin/.conda/envs/pyg/lib/python3.10/site-packages/datasets/arrow_writer.py", line 553, in write_batch
arrays.append(pa.array(typed_sequence))
File "pyarrow/array.pxi", line 236, in pyarrow.lib.array
File "pyarrow/array.pxi", line 110, in pyarrow.lib._handle_arrow_array_protocol
File "/local_scratch/lfainsin/.conda/envs/pyg/lib/python3.10/site-packages/datasets/arrow_writer.py", line 223, in __arrow_array__
return pa.array(cast_to_python_objects(data, only_1d_for_numpy=True))
File "/local_scratch/lfainsin/.conda/envs/pyg/lib/python3.10/site-packages/datasets/features/features.py", line 446, in cast_to_python_objects
return _cast_to_python_objects(
File "/local_scratch/lfainsin/.conda/envs/pyg/lib/python3.10/site-packages/datasets/features/features.py", line 407, in _cast_to_python_objects
[
File "/local_scratch/lfainsin/.conda/envs/pyg/lib/python3.10/site-packages/datasets/features/features.py", line 408, in <listcomp>
_cast_to_python_objects(
File "/local_scratch/lfainsin/.conda/envs/pyg/lib/python3.10/site-packages/datasets/features/features.py", line 319, in _cast_to_python_objects
[
File "/local_scratch/lfainsin/.conda/envs/pyg/lib/python3.10/site-packages/datasets/features/features.py", line 320, in <listcomp>
_cast_to_python_objects(
KeyboardInterrupt
During handling of the above exception, another exception occurred:
Traceback (most recent call last):
File "/local_scratch/lfainsin/.conda/envs/pyg/lib/python3.10/site-packages/datasets/arrow_writer.py", line 179, in __arrow_array__
storage = to_pyarrow_listarray(data, pa_type)
File "/local_scratch/lfainsin/.conda/envs/pyg/lib/python3.10/site-packages/datasets/features/features.py", line 1466, in to_pyarrow_listarray
return pa.array(data, pa_type.storage_dtype)
File "pyarrow/array.pxi", line 320, in pyarrow.lib.array
File "pyarrow/array.pxi", line 39, in pyarrow.lib._sequence_to_array
File "pyarrow/error.pxi", line 144, in pyarrow.lib.pyarrow_internal_check_status
File "pyarrow/error.pxi", line 123, in pyarrow.lib.check_status
pyarrow.lib.ArrowTypeError: Could not convert tensor([[-1.0273, -0.8037, -0.6860],
[-0.5034, -1.2685, -0.0558],
[-1.0908, -1.1820, -0.3178],
...,
[-0.8171, 0.1781, -0.5903],
[ 0.4370, 1.9305, 0.5899],
[-0.1426, 0.9053, -1.7559]]) with type Tensor: was not a sequence or recognized null for conversion to list type
During handling of the above exception, another exception occurred:
Traceback (most recent call last):
File "/gpfs_new/data/users/lfainsin/stage-laurent-f/src/random_scripts/uses_random_data.py", line 62, in <module>
ds_normalized = ds.map(
File "/local_scratch/lfainsin/.conda/envs/pyg/lib/python3.10/site-packages/datasets/arrow_dataset.py", line 580, in wrapper
out: Union["Dataset", "DatasetDict"] = func(self, *args, **kwargs)
File "/local_scratch/lfainsin/.conda/envs/pyg/lib/python3.10/site-packages/datasets/arrow_dataset.py", line 545, in wrapper
out: Union["Dataset", "DatasetDict"] = func(self, *args, **kwargs)
File "/local_scratch/lfainsin/.conda/envs/pyg/lib/python3.10/site-packages/datasets/arrow_dataset.py", line 3087, in map
for rank, done, content in Dataset._map_single(**dataset_kwargs):
File "/local_scratch/lfainsin/.conda/envs/pyg/lib/python3.10/site-packages/datasets/arrow_dataset.py", line 3492, in _map_single
writer.finalize()
File "/local_scratch/lfainsin/.conda/envs/pyg/lib/python3.10/site-packages/datasets/arrow_writer.py", line 584, in finalize
self.write_examples_on_file()
File "/local_scratch/lfainsin/.conda/envs/pyg/lib/python3.10/site-packages/datasets/arrow_writer.py", line 448, in write_examples_on_file
self.write_batch(batch_examples=batch_examples)
File "/local_scratch/lfainsin/.conda/envs/pyg/lib/python3.10/site-packages/datasets/arrow_writer.py", line 553, in write_batch
arrays.append(pa.array(typed_sequence))
File "pyarrow/array.pxi", line 236, in pyarrow.lib.array
File "pyarrow/array.pxi", line 110, in pyarrow.lib._handle_arrow_array_protocol
File "/local_scratch/lfainsin/.conda/envs/pyg/lib/python3.10/site-packages/datasets/arrow_writer.py", line 223, in __arrow_array__
return pa.array(cast_to_python_objects(data, only_1d_for_numpy=True))
File "/local_scratch/lfainsin/.conda/envs/pyg/lib/python3.10/site-packages/datasets/features/features.py", line 446, in cast_to_python_objects
return _cast_to_python_objects(
File "/local_scratch/lfainsin/.conda/envs/pyg/lib/python3.10/site-packages/datasets/features/features.py", line 407, in _cast_to_python_objects
[
File "/local_scratch/lfainsin/.conda/envs/pyg/lib/python3.10/site-packages/datasets/features/features.py", line 408, in <listcomp>
_cast_to_python_objects(
File "/local_scratch/lfainsin/.conda/envs/pyg/lib/python3.10/site-packages/datasets/features/features.py", line 319, in _cast_to_python_objects
[
File "/local_scratch/lfainsin/.conda/envs/pyg/lib/python3.10/site-packages/datasets/features/features.py", line 320, in <listcomp>
_cast_to_python_objects(
File "/local_scratch/lfainsin/.conda/envs/pyg/lib/python3.10/site-packages/datasets/features/features.py", line 298, in _cast_to_python_objects
if obj.ndim == 0:
KeyboardInterrupt
```
</details>
|
https://github.com/huggingface/datasets/issues/6075 | Error loading music files using `load_dataset` | This code behaves as expected on my local machine or in Colab. Which version of `soundfile` do you have installed? MP3 requires `soundfile>=0.12.1`. | ### Describe the bug
I tried to load a music file using `datasets.load_dataset()` from the repository - https://huggingface.co/datasets/susnato/pop2piano_real_music_test
I got the following error -
```
Traceback (most recent call last):
File "<stdin>", line 1, in <module>
File "/home/susnato/anaconda3/envs/p2p/lib/python3.9/site-packages/datasets/arrow_dataset.py", line 2803, in __getitem__
return self._getitem(key)
File "/home/susnato/anaconda3/envs/p2p/lib/python3.9/site-packages/datasets/arrow_dataset.py", line 2788, in _getitem
formatted_output = format_table(
File "/home/susnato/anaconda3/envs/p2p/lib/python3.9/site-packages/datasets/formatting/formatting.py", line 629, in format_table
return formatter(pa_table, query_type=query_type)
File "/home/susnato/anaconda3/envs/p2p/lib/python3.9/site-packages/datasets/formatting/formatting.py", line 398, in __call__
return self.format_column(pa_table)
File "/home/susnato/anaconda3/envs/p2p/lib/python3.9/site-packages/datasets/formatting/formatting.py", line 442, in format_column
column = self.python_features_decoder.decode_column(column, pa_table.column_names[0])
File "/home/susnato/anaconda3/envs/p2p/lib/python3.9/site-packages/datasets/formatting/formatting.py", line 218, in decode_column
return self.features.decode_column(column, column_name) if self.features else column
File "/home/susnato/anaconda3/envs/p2p/lib/python3.9/site-packages/datasets/features/features.py", line 1924, in decode_column
[decode_nested_example(self[column_name], value) if value is not None else None for value in column]
File "/home/susnato/anaconda3/envs/p2p/lib/python3.9/site-packages/datasets/features/features.py", line 1924, in <listcomp>
[decode_nested_example(self[column_name], value) if value is not None else None for value in column]
File "/home/susnato/anaconda3/envs/p2p/lib/python3.9/site-packages/datasets/features/features.py", line 1325, in decode_nested_example
return schema.decode_example(obj, token_per_repo_id=token_per_repo_id)
File "/home/susnato/anaconda3/envs/p2p/lib/python3.9/site-packages/datasets/features/audio.py", line 184, in decode_example
array, sampling_rate = sf.read(f)
File "/home/susnato/anaconda3/envs/p2p/lib/python3.9/site-packages/soundfile.py", line 372, in read
with SoundFile(file, 'r', samplerate, channels,
File "/home/susnato/anaconda3/envs/p2p/lib/python3.9/site-packages/soundfile.py", line 740, in __init__
self._file = self._open(file, mode_int, closefd)
File "/home/susnato/anaconda3/envs/p2p/lib/python3.9/site-packages/soundfile.py", line 1264, in _open
_error_check(_snd.sf_error(file_ptr),
File "/home/susnato/anaconda3/envs/p2p/lib/python3.9/site-packages/soundfile.py", line 1455, in _error_check
raise RuntimeError(prefix + _ffi.string(err_str).decode('utf-8', 'replace'))
RuntimeError: Error opening <_io.BufferedReader name='/home/susnato/.cache/huggingface/datasets/downloads/d2b09cb974b967b13f91553297c40c0f02f3c0d4c8356350743598ff48d6f29e'>: Format not recognised.
```
### Steps to reproduce the bug
Code to reproduce the error -
```python
from datasets import load_dataset
ds = load_dataset("susnato/pop2piano_real_music_test", split="test")
print(ds[0])
```
### Expected behavior
I should be able to read the music file without any error.
### Environment info
- `datasets` version: 2.14.0
- Platform: Linux-5.19.0-50-generic-x86_64-with-glibc2.35
- Python version: 3.9.16
- Huggingface_hub version: 0.15.1
- PyArrow version: 11.0.0
- Pandas version: 1.5.3
| 23 | Error loading music files using `load_dataset`
### Describe the bug
I tried to load a music file using `datasets.load_dataset()` from the repository - https://huggingface.co/datasets/susnato/pop2piano_real_music_test
I got the following error -
```
Traceback (most recent call last):
File "<stdin>", line 1, in <module>
File "/home/susnato/anaconda3/envs/p2p/lib/python3.9/site-packages/datasets/arrow_dataset.py", line 2803, in __getitem__
return self._getitem(key)
File "/home/susnato/anaconda3/envs/p2p/lib/python3.9/site-packages/datasets/arrow_dataset.py", line 2788, in _getitem
formatted_output = format_table(
File "/home/susnato/anaconda3/envs/p2p/lib/python3.9/site-packages/datasets/formatting/formatting.py", line 629, in format_table
return formatter(pa_table, query_type=query_type)
File "/home/susnato/anaconda3/envs/p2p/lib/python3.9/site-packages/datasets/formatting/formatting.py", line 398, in __call__
return self.format_column(pa_table)
File "/home/susnato/anaconda3/envs/p2p/lib/python3.9/site-packages/datasets/formatting/formatting.py", line 442, in format_column
column = self.python_features_decoder.decode_column(column, pa_table.column_names[0])
File "/home/susnato/anaconda3/envs/p2p/lib/python3.9/site-packages/datasets/formatting/formatting.py", line 218, in decode_column
return self.features.decode_column(column, column_name) if self.features else column
File "/home/susnato/anaconda3/envs/p2p/lib/python3.9/site-packages/datasets/features/features.py", line 1924, in decode_column
[decode_nested_example(self[column_name], value) if value is not None else None for value in column]
File "/home/susnato/anaconda3/envs/p2p/lib/python3.9/site-packages/datasets/features/features.py", line 1924, in <listcomp>
[decode_nested_example(self[column_name], value) if value is not None else None for value in column]
File "/home/susnato/anaconda3/envs/p2p/lib/python3.9/site-packages/datasets/features/features.py", line 1325, in decode_nested_example
return schema.decode_example(obj, token_per_repo_id=token_per_repo_id)
File "/home/susnato/anaconda3/envs/p2p/lib/python3.9/site-packages/datasets/features/audio.py", line 184, in decode_example
array, sampling_rate = sf.read(f)
File "/home/susnato/anaconda3/envs/p2p/lib/python3.9/site-packages/soundfile.py", line 372, in read
with SoundFile(file, 'r', samplerate, channels,
File "/home/susnato/anaconda3/envs/p2p/lib/python3.9/site-packages/soundfile.py", line 740, in __init__
self._file = self._open(file, mode_int, closefd)
File "/home/susnato/anaconda3/envs/p2p/lib/python3.9/site-packages/soundfile.py", line 1264, in _open
_error_check(_snd.sf_error(file_ptr),
File "/home/susnato/anaconda3/envs/p2p/lib/python3.9/site-packages/soundfile.py", line 1455, in _error_check
raise RuntimeError(prefix + _ffi.string(err_str).decode('utf-8', 'replace'))
RuntimeError: Error opening <_io.BufferedReader name='/home/susnato/.cache/huggingface/datasets/downloads/d2b09cb974b967b13f91553297c40c0f02f3c0d4c8356350743598ff48d6f29e'>: Format not recognised.
```
### Steps to reproduce the bug
Code to reproduce the error -
```python
from datasets import load_dataset
ds = load_dataset("susnato/pop2piano_real_music_test", split="test")
print(ds[0])
```
### Expected behavior
I should be able to read the music file without any error.
### Environment info
- `datasets` version: 2.14.0
- Platform: Linux-5.19.0-50-generic-x86_64-with-glibc2.35
- Python version: 3.9.16
- Huggingface_hub version: 0.15.1
- PyArrow version: 11.0.0
- Pandas version: 1.5.3
This code behaves as expected on my local machine or in Colab. Which version of `soundfile` do you have installed? MP3 requires `soundfile>=0.12.1`. |
https://github.com/huggingface/datasets/issues/6176 | how to limit the size of memory mapped file? | Hi! Can you share the error this reproducer throws in your environment? `streaming=True` streams the dataset as it's iterated over without creating a memory-map file. | ### Describe the bug
Huggingface datasets use memory-mapped file to map large datasets in memory for fast access.
However, it seems like huggingface will occupy all the memory for memory-mapped files, which makes a troublesome situation since we cluster will distribute a small portion of memory to me (once it's over the limit, memory cannot be allocated), however, when the dataset checks the total memory, all of the memory will be taken into account which makes huggingface dataset try to allocate more memory than allowed.
So is there a way to explicitly limit the size of memory mapped file?
### Steps to reproduce the bug
python
>>> from datasets import load_dataset
>>> dataset = load_dataset("c4", "en", streaming=True)
### Expected behavior
In a normal environment, this will not have any problem.
However, when the system allocates a portion of the memory to the program and when the dataset checks the total memory, all of the memory will be taken into account which makes huggingface dataset try to allocate more memory than allowed.
### Environment info
linux cluster with SGE(Sun Grid Engine) | 25 | how to limit the size of memory mapped file?
### Describe the bug
Huggingface datasets use memory-mapped file to map large datasets in memory for fast access.
However, it seems like huggingface will occupy all the memory for memory-mapped files, which makes a troublesome situation since we cluster will distribute a small portion of memory to me (once it's over the limit, memory cannot be allocated), however, when the dataset checks the total memory, all of the memory will be taken into account which makes huggingface dataset try to allocate more memory than allowed.
So is there a way to explicitly limit the size of memory mapped file?
### Steps to reproduce the bug
python
>>> from datasets import load_dataset
>>> dataset = load_dataset("c4", "en", streaming=True)
### Expected behavior
In a normal environment, this will not have any problem.
However, when the system allocates a portion of the memory to the program and when the dataset checks the total memory, all of the memory will be taken into account which makes huggingface dataset try to allocate more memory than allowed.
### Environment info
linux cluster with SGE(Sun Grid Engine)
Hi! Can you share the error this reproducer throws in your environment? `streaming=True` streams the dataset as it's iterated over without creating a memory-map file. |
https://github.com/huggingface/datasets/issues/6176 | how to limit the size of memory mapped file? | The trace of the error. Streaming works but is slower.
```
Root Cause (first observed failure):
[0]:
time : 2023-08-24_06:06:01
host : compute-126.cm.cluster
rank : 0 (local_rank: 0)
exitcode : 1 (pid: 48442)
error_file: /tmp/torchelastic_4fqzcuuz/none_rx2470jl/attempt_0/0/error.json
traceback : Traceback (most recent call last):
File "/users/yli7/.conda/envs/pytorch2.0/lib/python3.8/site-packages/torch/distributed/elastic/multiprocessing/errors/__init__.py", line 346, in wrapper
return f(*args, **kwargs)
File "Pretrain.py", line 214, in main
pair_dataset, c4_dataset = create_dataset('pretrain', config)
File "/dcs05/qiao/data/william/project/DaVinci/dataset/__init__.py", line 109, in create_dataset
c4_dataset = load_dataset("c4", "en", split="train").to_iterable_dataset(num_shards=1024).map(pre_caption_huggingface)
File "/users/yli7/.local/lib/python3.8/site-packages/datasets/load.py", line 1810, in load_dataset
ds = builder_instance.as_dataset(split=split, verification_mode=verification_mode, in_memory=keep_in_memory)
File "/users/yli7/.local/lib/python3.8/site-packages/datasets/builder.py", line 1145, in as_dataset
datasets = map_nested(
File "/users/yli7/.local/lib/python3.8/site-packages/datasets/utils/py_utils.py", line 436, in map_nested
return function(data_struct)
File "/users/yli7/.local/lib/python3.8/site-packages/datasets/builder.py", line 1175, in _build_single_dataset
ds = self._as_dataset(
File "/users/yli7/.local/lib/python3.8/site-packages/datasets/builder.py", line 1246, in _as_dataset
dataset_kwargs = ArrowReader(cache_dir, self.info).read(
File "/users/yli7/.local/lib/python3.8/site-packages/datasets/arrow_reader.py", line 244, in read
return self.read_files(files=files, original_instructions=instructions, in_memory=in_memory)
File "/users/yli7/.local/lib/python3.8/site-packages/datasets/arrow_reader.py", line 265, in read_files
pa_table = self._read_files(files, in_memory=in_memory)
File "/users/yli7/.local/lib/python3.8/site-packages/datasets/arrow_reader.py", line 200, in _read_files
pa_table: Table = self._get_table_from_filename(f_dict, in_memory=in_memory)
File "/users/yli7/.local/lib/python3.8/site-packages/datasets/arrow_reader.py", line 336, in _get_table_from_filename
table = ArrowReader.read_table(filename, in_memory=in_memory)
File "/users/yli7/.local/lib/python3.8/site-packages/datasets/arrow_reader.py", line 357, in read_table
return table_cls.from_file(filename)
File "/users/yli7/.local/lib/python3.8/site-packages/datasets/table.py", line 1059, in from_file
table = _memory_mapped_arrow_table_from_file(filename)
File "/users/yli7/.local/lib/python3.8/site-packages/datasets/table.py", line 65, in _memory_mapped_arrow_table_from_file
opened_stream = _memory_mapped_record_batch_reader_from_file(filename)
File "/users/yli7/.local/lib/python3.8/site-packages/datasets/table.py", line 50, in _memory_mapped_record_batch_reader_from_file
memory_mapped_stream = pa.memory_map(filename)
File "pyarrow/io.pxi", line 1009, in pyarrow.lib.memory_map
File "pyarrow/io.pxi", line 956, in pyarrow.lib.MemoryMappedFile._open
File "pyarrow/error.pxi", line 144, in pyarrow.lib.pyarrow_internal_check_status
File "pyarrow/error.pxi", line 115, in pyarrow.lib.check_status
OSError: Memory mapping file failed: Cannot allocate memory
``` | ### Describe the bug
Huggingface datasets use memory-mapped file to map large datasets in memory for fast access.
However, it seems like huggingface will occupy all the memory for memory-mapped files, which makes a troublesome situation since we cluster will distribute a small portion of memory to me (once it's over the limit, memory cannot be allocated), however, when the dataset checks the total memory, all of the memory will be taken into account which makes huggingface dataset try to allocate more memory than allowed.
So is there a way to explicitly limit the size of memory mapped file?
### Steps to reproduce the bug
python
>>> from datasets import load_dataset
>>> dataset = load_dataset("c4", "en", streaming=True)
### Expected behavior
In a normal environment, this will not have any problem.
However, when the system allocates a portion of the memory to the program and when the dataset checks the total memory, all of the memory will be taken into account which makes huggingface dataset try to allocate more memory than allowed.
### Environment info
linux cluster with SGE(Sun Grid Engine) | 229 | how to limit the size of memory mapped file?
### Describe the bug
Huggingface datasets use memory-mapped file to map large datasets in memory for fast access.
However, it seems like huggingface will occupy all the memory for memory-mapped files, which makes a troublesome situation since we cluster will distribute a small portion of memory to me (once it's over the limit, memory cannot be allocated), however, when the dataset checks the total memory, all of the memory will be taken into account which makes huggingface dataset try to allocate more memory than allowed.
So is there a way to explicitly limit the size of memory mapped file?
### Steps to reproduce the bug
python
>>> from datasets import load_dataset
>>> dataset = load_dataset("c4", "en", streaming=True)
### Expected behavior
In a normal environment, this will not have any problem.
However, when the system allocates a portion of the memory to the program and when the dataset checks the total memory, all of the memory will be taken into account which makes huggingface dataset try to allocate more memory than allowed.
### Environment info
linux cluster with SGE(Sun Grid Engine)
The trace of the error. Streaming works but is slower.
```
Root Cause (first observed failure):
[0]:
time : 2023-08-24_06:06:01
host : compute-126.cm.cluster
rank : 0 (local_rank: 0)
exitcode : 1 (pid: 48442)
error_file: /tmp/torchelastic_4fqzcuuz/none_rx2470jl/attempt_0/0/error.json
traceback : Traceback (most recent call last):
File "/users/yli7/.conda/envs/pytorch2.0/lib/python3.8/site-packages/torch/distributed/elastic/multiprocessing/errors/__init__.py", line 346, in wrapper
return f(*args, **kwargs)
File "Pretrain.py", line 214, in main
pair_dataset, c4_dataset = create_dataset('pretrain', config)
File "/dcs05/qiao/data/william/project/DaVinci/dataset/__init__.py", line 109, in create_dataset
c4_dataset = load_dataset("c4", "en", split="train").to_iterable_dataset(num_shards=1024).map(pre_caption_huggingface)
File "/users/yli7/.local/lib/python3.8/site-packages/datasets/load.py", line 1810, in load_dataset
ds = builder_instance.as_dataset(split=split, verification_mode=verification_mode, in_memory=keep_in_memory)
File "/users/yli7/.local/lib/python3.8/site-packages/datasets/builder.py", line 1145, in as_dataset
datasets = map_nested(
File "/users/yli7/.local/lib/python3.8/site-packages/datasets/utils/py_utils.py", line 436, in map_nested
return function(data_struct)
File "/users/yli7/.local/lib/python3.8/site-packages/datasets/builder.py", line 1175, in _build_single_dataset
ds = self._as_dataset(
File "/users/yli7/.local/lib/python3.8/site-packages/datasets/builder.py", line 1246, in _as_dataset
dataset_kwargs = ArrowReader(cache_dir, self.info).read(
File "/users/yli7/.local/lib/python3.8/site-packages/datasets/arrow_reader.py", line 244, in read
return self.read_files(files=files, original_instructions=instructions, in_memory=in_memory)
File "/users/yli7/.local/lib/python3.8/site-packages/datasets/arrow_reader.py", line 265, in read_files
pa_table = self._read_files(files, in_memory=in_memory)
File "/users/yli7/.local/lib/python3.8/site-packages/datasets/arrow_reader.py", line 200, in _read_files
pa_table: Table = self._get_table_from_filename(f_dict, in_memory=in_memory)
File "/users/yli7/.local/lib/python3.8/site-packages/datasets/arrow_reader.py", line 336, in _get_table_from_filename
table = ArrowReader.read_table(filename, in_memory=in_memory)
File "/users/yli7/.local/lib/python3.8/site-packages/datasets/arrow_reader.py", line 357, in read_table
return table_cls.from_file(filename)
File "/users/yli7/.local/lib/python3.8/site-packages/datasets/table.py", line 1059, in from_file
table = _memory_mapped_arrow_table_from_file(filename)
File "/users/yli7/.local/lib/python3.8/site-packages/datasets/table.py", line 65, in _memory_mapped_arrow_table_from_file
opened_stream = _memory_mapped_record_batch_reader_from_file(filename)
File "/users/yli7/.local/lib/python3.8/site-packages/datasets/table.py", line 50, in _memory_mapped_record_batch_reader_from_file
memory_mapped_stream = pa.memory_map(filename)
File "pyarrow/io.pxi", line 1009, in pyarrow.lib.memory_map
File "pyarrow/io.pxi", line 956, in pyarrow.lib.MemoryMappedFile._open
File "pyarrow/error.pxi", line 144, in pyarrow.lib.pyarrow_internal_check_status
File "pyarrow/error.pxi", line 115, in pyarrow.lib.check_status
OSError: Memory mapping file failed: Cannot allocate memory
``` |
https://github.com/huggingface/datasets/issues/6176 | how to limit the size of memory mapped file? | This issue has previously been reported here: https://github.com/huggingface/datasets/issues/5710. Reporting it in the Arrow repo makes more sense as they have control over memory mapping.
PS: this is the API to reduce the size of the generated Arrow file:
```python
from datasets import load_dataset
builder = load_dataset_builder("c4", "en")
builder.download_and_prepare(max_shard_size="5GB")
dataset = builder.as_dataset()
```
If this resolves the issue, we can consider exposing `max_shard_size` in `load_dataset`. | ### Describe the bug
Huggingface datasets use memory-mapped file to map large datasets in memory for fast access.
However, it seems like huggingface will occupy all the memory for memory-mapped files, which makes a troublesome situation since we cluster will distribute a small portion of memory to me (once it's over the limit, memory cannot be allocated), however, when the dataset checks the total memory, all of the memory will be taken into account which makes huggingface dataset try to allocate more memory than allowed.
So is there a way to explicitly limit the size of memory mapped file?
### Steps to reproduce the bug
python
>>> from datasets import load_dataset
>>> dataset = load_dataset("c4", "en", streaming=True)
### Expected behavior
In a normal environment, this will not have any problem.
However, when the system allocates a portion of the memory to the program and when the dataset checks the total memory, all of the memory will be taken into account which makes huggingface dataset try to allocate more memory than allowed.
### Environment info
linux cluster with SGE(Sun Grid Engine) | 64 | how to limit the size of memory mapped file?
### Describe the bug
Huggingface datasets use memory-mapped file to map large datasets in memory for fast access.
However, it seems like huggingface will occupy all the memory for memory-mapped files, which makes a troublesome situation since we cluster will distribute a small portion of memory to me (once it's over the limit, memory cannot be allocated), however, when the dataset checks the total memory, all of the memory will be taken into account which makes huggingface dataset try to allocate more memory than allowed.
So is there a way to explicitly limit the size of memory mapped file?
### Steps to reproduce the bug
python
>>> from datasets import load_dataset
>>> dataset = load_dataset("c4", "en", streaming=True)
### Expected behavior
In a normal environment, this will not have any problem.
However, when the system allocates a portion of the memory to the program and when the dataset checks the total memory, all of the memory will be taken into account which makes huggingface dataset try to allocate more memory than allowed.
### Environment info
linux cluster with SGE(Sun Grid Engine)
This issue has previously been reported here: https://github.com/huggingface/datasets/issues/5710. Reporting it in the Arrow repo makes more sense as they have control over memory mapping.
PS: this is the API to reduce the size of the generated Arrow file:
```python
from datasets import load_dataset
builder = load_dataset_builder("c4", "en")
builder.download_and_prepare(max_shard_size="5GB")
dataset = builder.as_dataset()
```
If this resolves the issue, we can consider exposing `max_shard_size` in `load_dataset`. |
https://github.com/huggingface/datasets/issues/6172 | Make Dataset streaming queries retryable | Hi! The streaming mode also retries requests - `datasets.config.STREAMING_READ_MAX_RETRIES` (20 sec by default) controls the number of retries and `datasets.config.STREAMING_READ_RETRY_INTERVAL` (5 sec) the sleep time between retries.
> At step 1800 I got a 504 HTTP status code error from Huggingface hub for my pytorch dataloader
A minor Hub outage that we experienced yesterday could be the cause. | ### Feature request
Streaming datasets, as intended, do not load the entire dataset in memory or disk. However, while querying the next data chunk from the remote, sometimes it is possible that the service is down or there might be other issues that may cause the query to fail. In such a scenario, it would be nice to make these queries retryable (perhaps with a backoff strategy).
### Motivation
I was working on a model and the model checkpoints after every 1000 steps. At step 1800 I got a 504 HTTP status code error from Huggingface hub for my pytorch `dataloader`. Given the size of my model and data, it took around 2 hours to reach 1800 steps and now it will take about an hour to recover the lost 800. It would be better to get a retryable querying strategy.
### Your contribution
It would be better if someone having experience in this area takes this up as this would require some testing. | 58 | Make Dataset streaming queries retryable
### Feature request
Streaming datasets, as intended, do not load the entire dataset in memory or disk. However, while querying the next data chunk from the remote, sometimes it is possible that the service is down or there might be other issues that may cause the query to fail. In such a scenario, it would be nice to make these queries retryable (perhaps with a backoff strategy).
### Motivation
I was working on a model and the model checkpoints after every 1000 steps. At step 1800 I got a 504 HTTP status code error from Huggingface hub for my pytorch `dataloader`. Given the size of my model and data, it took around 2 hours to reach 1800 steps and now it will take about an hour to recover the lost 800. It would be better to get a retryable querying strategy.
### Your contribution
It would be better if someone having experience in this area takes this up as this would require some testing.
Hi! The streaming mode also retries requests - `datasets.config.STREAMING_READ_MAX_RETRIES` (20 sec by default) controls the number of retries and `datasets.config.STREAMING_READ_RETRY_INTERVAL` (5 sec) the sleep time between retries.
> At step 1800 I got a 504 HTTP status code error from Huggingface hub for my pytorch dataloader
A minor Hub outage that we experienced yesterday could be the cause. |
https://github.com/huggingface/datasets/issues/6169 | Configurations in yaml not working | Unfortunately, I cannot reproduce this behavior on my machine or Colab - the reproducer returns `['main_data', 'additional_data']` as expected. | ### Dataset configurations cannot be created in YAML/README
Hello! I'm trying to follow the docs here in order to create structure in my dataset as added from here (#5331): https://github.com/huggingface/datasets/blob/8b8e6ee067eb74e7965ca2a6768f15f9398cb7c8/docs/source/repository_structure.mdx#L110-L118
I have the exact example in my config file for [my data repo](https://huggingface.co/datasets/tsor13/test):
```
configs:
- config_name: main_data
data_files: "main_data.csv"
- config_name: additional_data
data_files: "additional_data.csv"
```
Yet, I'm unable to load different configurations:
```
from datasets import get_dataset_config_names
get_dataset_config_names('tsor13/test', use_auth_token=True)
```
returns a single split, `['tsor13--test']`
Does anyone have any insights?
@polinaeterna thank you for adding this feature, it is super useful. Do you happen to have any ideas?
### Steps to reproduce the bug
from datasets import get_dataset_config_names
get_dataset_config_names('tsor13/test')
### Expected behavior
I would expect there to be two splits, `main_data` and `additional_data`. However, only `['tsor13--test']` test is returned.
### Environment info
- `datasets` version: 2.14.4
- Platform: macOS-13.4-arm64-arm-64bit
- Python version: 3.11.4
- Huggingface_hub version: 0.16.4
- PyArrow version: 12.0.1
- Pandas version: 1.5.1 | 19 | Configurations in yaml not working
### Dataset configurations cannot be created in YAML/README
Hello! I'm trying to follow the docs here in order to create structure in my dataset as added from here (#5331): https://github.com/huggingface/datasets/blob/8b8e6ee067eb74e7965ca2a6768f15f9398cb7c8/docs/source/repository_structure.mdx#L110-L118
I have the exact example in my config file for [my data repo](https://huggingface.co/datasets/tsor13/test):
```
configs:
- config_name: main_data
data_files: "main_data.csv"
- config_name: additional_data
data_files: "additional_data.csv"
```
Yet, I'm unable to load different configurations:
```
from datasets import get_dataset_config_names
get_dataset_config_names('tsor13/test', use_auth_token=True)
```
returns a single split, `['tsor13--test']`
Does anyone have any insights?
@polinaeterna thank you for adding this feature, it is super useful. Do you happen to have any ideas?
### Steps to reproduce the bug
from datasets import get_dataset_config_names
get_dataset_config_names('tsor13/test')
### Expected behavior
I would expect there to be two splits, `main_data` and `additional_data`. However, only `['tsor13--test']` test is returned.
### Environment info
- `datasets` version: 2.14.4
- Platform: macOS-13.4-arm64-arm-64bit
- Python version: 3.11.4
- Huggingface_hub version: 0.16.4
- PyArrow version: 12.0.1
- Pandas version: 1.5.1
Unfortunately, I cannot reproduce this behavior on my machine or Colab - the reproducer returns `['main_data', 'additional_data']` as expected. |
https://github.com/huggingface/datasets/issues/6169 | Configurations in yaml not working | Thank you for looking into this, Mario. Is this on [my repository](https://huggingface.co/datasets/tsor13/test), or on another one that you have reproduced? Would you mind pointing me to it if so? | ### Dataset configurations cannot be created in YAML/README
Hello! I'm trying to follow the docs here in order to create structure in my dataset as added from here (#5331): https://github.com/huggingface/datasets/blob/8b8e6ee067eb74e7965ca2a6768f15f9398cb7c8/docs/source/repository_structure.mdx#L110-L118
I have the exact example in my config file for [my data repo](https://huggingface.co/datasets/tsor13/test):
```
configs:
- config_name: main_data
data_files: "main_data.csv"
- config_name: additional_data
data_files: "additional_data.csv"
```
Yet, I'm unable to load different configurations:
```
from datasets import get_dataset_config_names
get_dataset_config_names('tsor13/test', use_auth_token=True)
```
returns a single split, `['tsor13--test']`
Does anyone have any insights?
@polinaeterna thank you for adding this feature, it is super useful. Do you happen to have any ideas?
### Steps to reproduce the bug
from datasets import get_dataset_config_names
get_dataset_config_names('tsor13/test')
### Expected behavior
I would expect there to be two splits, `main_data` and `additional_data`. However, only `['tsor13--test']` test is returned.
### Environment info
- `datasets` version: 2.14.4
- Platform: macOS-13.4-arm64-arm-64bit
- Python version: 3.11.4
- Huggingface_hub version: 0.16.4
- PyArrow version: 12.0.1
- Pandas version: 1.5.1 | 29 | Configurations in yaml not working
### Dataset configurations cannot be created in YAML/README
Hello! I'm trying to follow the docs here in order to create structure in my dataset as added from here (#5331): https://github.com/huggingface/datasets/blob/8b8e6ee067eb74e7965ca2a6768f15f9398cb7c8/docs/source/repository_structure.mdx#L110-L118
I have the exact example in my config file for [my data repo](https://huggingface.co/datasets/tsor13/test):
```
configs:
- config_name: main_data
data_files: "main_data.csv"
- config_name: additional_data
data_files: "additional_data.csv"
```
Yet, I'm unable to load different configurations:
```
from datasets import get_dataset_config_names
get_dataset_config_names('tsor13/test', use_auth_token=True)
```
returns a single split, `['tsor13--test']`
Does anyone have any insights?
@polinaeterna thank you for adding this feature, it is super useful. Do you happen to have any ideas?
### Steps to reproduce the bug
from datasets import get_dataset_config_names
get_dataset_config_names('tsor13/test')
### Expected behavior
I would expect there to be two splits, `main_data` and `additional_data`. However, only `['tsor13--test']` test is returned.
### Environment info
- `datasets` version: 2.14.4
- Platform: macOS-13.4-arm64-arm-64bit
- Python version: 3.11.4
- Huggingface_hub version: 0.16.4
- PyArrow version: 12.0.1
- Pandas version: 1.5.1
Thank you for looking into this, Mario. Is this on [my repository](https://huggingface.co/datasets/tsor13/test), or on another one that you have reproduced? Would you mind pointing me to it if so? |
https://github.com/huggingface/datasets/issues/6169 | Configurations in yaml not working | Whoa, in colab I received the correct behavior using my dataset. It must have something to do with my local copy of `datasets` (which again just failed).
I've tried uninstalling/reinstnalling to no avail | ### Dataset configurations cannot be created in YAML/README
Hello! I'm trying to follow the docs here in order to create structure in my dataset as added from here (#5331): https://github.com/huggingface/datasets/blob/8b8e6ee067eb74e7965ca2a6768f15f9398cb7c8/docs/source/repository_structure.mdx#L110-L118
I have the exact example in my config file for [my data repo](https://huggingface.co/datasets/tsor13/test):
```
configs:
- config_name: main_data
data_files: "main_data.csv"
- config_name: additional_data
data_files: "additional_data.csv"
```
Yet, I'm unable to load different configurations:
```
from datasets import get_dataset_config_names
get_dataset_config_names('tsor13/test', use_auth_token=True)
```
returns a single split, `['tsor13--test']`
Does anyone have any insights?
@polinaeterna thank you for adding this feature, it is super useful. Do you happen to have any ideas?
### Steps to reproduce the bug
from datasets import get_dataset_config_names
get_dataset_config_names('tsor13/test')
### Expected behavior
I would expect there to be two splits, `main_data` and `additional_data`. However, only `['tsor13--test']` test is returned.
### Environment info
- `datasets` version: 2.14.4
- Platform: macOS-13.4-arm64-arm-64bit
- Python version: 3.11.4
- Huggingface_hub version: 0.16.4
- PyArrow version: 12.0.1
- Pandas version: 1.5.1 | 33 | Configurations in yaml not working
### Dataset configurations cannot be created in YAML/README
Hello! I'm trying to follow the docs here in order to create structure in my dataset as added from here (#5331): https://github.com/huggingface/datasets/blob/8b8e6ee067eb74e7965ca2a6768f15f9398cb7c8/docs/source/repository_structure.mdx#L110-L118
I have the exact example in my config file for [my data repo](https://huggingface.co/datasets/tsor13/test):
```
configs:
- config_name: main_data
data_files: "main_data.csv"
- config_name: additional_data
data_files: "additional_data.csv"
```
Yet, I'm unable to load different configurations:
```
from datasets import get_dataset_config_names
get_dataset_config_names('tsor13/test', use_auth_token=True)
```
returns a single split, `['tsor13--test']`
Does anyone have any insights?
@polinaeterna thank you for adding this feature, it is super useful. Do you happen to have any ideas?
### Steps to reproduce the bug
from datasets import get_dataset_config_names
get_dataset_config_names('tsor13/test')
### Expected behavior
I would expect there to be two splits, `main_data` and `additional_data`. However, only `['tsor13--test']` test is returned.
### Environment info
- `datasets` version: 2.14.4
- Platform: macOS-13.4-arm64-arm-64bit
- Python version: 3.11.4
- Huggingface_hub version: 0.16.4
- PyArrow version: 12.0.1
- Pandas version: 1.5.1
Whoa, in colab I received the correct behavior using my dataset. It must have something to do with my local copy of `datasets` (which again just failed).
I've tried uninstalling/reinstnalling to no avail |
https://github.com/huggingface/datasets/issues/6169 | Configurations in yaml not working | hi @tsor13 , I haven't been able to reproduce your issue on `tsor13/test` dataset locally either. reinstalling doesn't help? | ### Dataset configurations cannot be created in YAML/README
Hello! I'm trying to follow the docs here in order to create structure in my dataset as added from here (#5331): https://github.com/huggingface/datasets/blob/8b8e6ee067eb74e7965ca2a6768f15f9398cb7c8/docs/source/repository_structure.mdx#L110-L118
I have the exact example in my config file for [my data repo](https://huggingface.co/datasets/tsor13/test):
```
configs:
- config_name: main_data
data_files: "main_data.csv"
- config_name: additional_data
data_files: "additional_data.csv"
```
Yet, I'm unable to load different configurations:
```
from datasets import get_dataset_config_names
get_dataset_config_names('tsor13/test', use_auth_token=True)
```
returns a single split, `['tsor13--test']`
Does anyone have any insights?
@polinaeterna thank you for adding this feature, it is super useful. Do you happen to have any ideas?
### Steps to reproduce the bug
from datasets import get_dataset_config_names
get_dataset_config_names('tsor13/test')
### Expected behavior
I would expect there to be two splits, `main_data` and `additional_data`. However, only `['tsor13--test']` test is returned.
### Environment info
- `datasets` version: 2.14.4
- Platform: macOS-13.4-arm64-arm-64bit
- Python version: 3.11.4
- Huggingface_hub version: 0.16.4
- PyArrow version: 12.0.1
- Pandas version: 1.5.1 | 19 | Configurations in yaml not working
### Dataset configurations cannot be created in YAML/README
Hello! I'm trying to follow the docs here in order to create structure in my dataset as added from here (#5331): https://github.com/huggingface/datasets/blob/8b8e6ee067eb74e7965ca2a6768f15f9398cb7c8/docs/source/repository_structure.mdx#L110-L118
I have the exact example in my config file for [my data repo](https://huggingface.co/datasets/tsor13/test):
```
configs:
- config_name: main_data
data_files: "main_data.csv"
- config_name: additional_data
data_files: "additional_data.csv"
```
Yet, I'm unable to load different configurations:
```
from datasets import get_dataset_config_names
get_dataset_config_names('tsor13/test', use_auth_token=True)
```
returns a single split, `['tsor13--test']`
Does anyone have any insights?
@polinaeterna thank you for adding this feature, it is super useful. Do you happen to have any ideas?
### Steps to reproduce the bug
from datasets import get_dataset_config_names
get_dataset_config_names('tsor13/test')
### Expected behavior
I would expect there to be two splits, `main_data` and `additional_data`. However, only `['tsor13--test']` test is returned.
### Environment info
- `datasets` version: 2.14.4
- Platform: macOS-13.4-arm64-arm-64bit
- Python version: 3.11.4
- Huggingface_hub version: 0.16.4
- PyArrow version: 12.0.1
- Pandas version: 1.5.1
hi @tsor13 , I haven't been able to reproduce your issue on `tsor13/test` dataset locally either. reinstalling doesn't help? |