html_url
stringlengths 48
51
| title
stringlengths 5
280
| comments
stringlengths 63
51.8k
| body
stringlengths 0
36.2k
β | comment_length
int64 16
1.52k
| text
stringlengths 163
54.1k
|
---|---|---|---|---|---|
https://github.com/huggingface/datasets/issues/5874 | Using as_dataset on a "parquet" builder | Hi! You can refer to [this doc](https://huggingface.co/docs/datasets/filesystems#load-and-save-your-datasets-using-your-cloud-storage-filesystem) to see the intended usage (basically, it skips the Arrow -> Parquet conversion step in `ds = load_dataset(...); ds.to_parquet("path/to/parquet")`) and allows writing Parquet to remote storage unlike `to_parquet`).
> I guess I'd expect as_dataset to generate the dataset in arrow format if it has to, or to suggest an alternative way to load the dataset (I've also tried other methods with load_dataset to no avail, probably due to misunderstandings on my part).
`as_dataset` does not work with `file_format="parquet"` files as Parquet files cannot be memory-mapped, so I think we should just raise an error in that case.
| ### Describe the bug
I used a custom builder to ``download_and_prepare`` a dataset. The first (very minor) issue is that the doc seems to suggest ``download_and_prepare`` will return the dataset, while it does not ([builder.py](https://github.com/huggingface/datasets/blob/main/src/datasets/builder.py#L718-L738)).
```
>>> from datasets import load_dataset_builder
>>> builder = load_dataset_builder("rotten_tomatoes")
>>> ds = builder.download_and_prepare("./output_dir", file_format="parquet")
```
The main issue I am facing is loading the dataset from those parquet files. I used the `as_dataset` method suggested by the doc, however it returns:
`
FileNotFoundError: [Errno 2] Failed to open local file 'output_dir/__main__-train-00000-of-00245.arrow'. Detail:
[errno 2] No such file or directory.
`
### Steps to reproduce the bug
1. Create a custom builder of some sort: `builder = CustomBuilder()`.
2. Run `download_and_prepare` with the parquet format: `builder.download_and_prepare("./output_dir", file_format="parquet")`.
3. Run `dataset = builder.as_dataset()`.
### Expected behavior
I guess I'd expect `as_dataset` to generate the dataset in arrow format if it has to, or to suggest an alternative way to load the dataset (I've also tried other methods with `load_dataset` to no avail, probably due to misunderstandings on my part).
### Environment info
```
- `datasets` version: 2.12.0
- Platform: Linux-5.15.0-1027-gcp-x86_64-with-glibc2.31
- Python version: 3.10.0
- Huggingface_hub version: 0.14.1
- PyArrow version: 8.0.0
- Pandas version: 1.5.3
``` | 104 | Using as_dataset on a "parquet" builder
### Describe the bug
I used a custom builder to ``download_and_prepare`` a dataset. The first (very minor) issue is that the doc seems to suggest ``download_and_prepare`` will return the dataset, while it does not ([builder.py](https://github.com/huggingface/datasets/blob/main/src/datasets/builder.py#L718-L738)).
```
>>> from datasets import load_dataset_builder
>>> builder = load_dataset_builder("rotten_tomatoes")
>>> ds = builder.download_and_prepare("./output_dir", file_format="parquet")
```
The main issue I am facing is loading the dataset from those parquet files. I used the `as_dataset` method suggested by the doc, however it returns:
`
FileNotFoundError: [Errno 2] Failed to open local file 'output_dir/__main__-train-00000-of-00245.arrow'. Detail:
[errno 2] No such file or directory.
`
### Steps to reproduce the bug
1. Create a custom builder of some sort: `builder = CustomBuilder()`.
2. Run `download_and_prepare` with the parquet format: `builder.download_and_prepare("./output_dir", file_format="parquet")`.
3. Run `dataset = builder.as_dataset()`.
### Expected behavior
I guess I'd expect `as_dataset` to generate the dataset in arrow format if it has to, or to suggest an alternative way to load the dataset (I've also tried other methods with `load_dataset` to no avail, probably due to misunderstandings on my part).
### Environment info
```
- `datasets` version: 2.12.0
- Platform: Linux-5.15.0-1027-gcp-x86_64-with-glibc2.31
- Python version: 3.10.0
- Huggingface_hub version: 0.14.1
- PyArrow version: 8.0.0
- Pandas version: 1.5.3
```
Hi! You can refer to [this doc](https://huggingface.co/docs/datasets/filesystems#load-and-save-your-datasets-using-your-cloud-storage-filesystem) to see the intended usage (basically, it skips the Arrow -> Parquet conversion step in `ds = load_dataset(...); ds.to_parquet("path/to/parquet")`) and allows writing Parquet to remote storage unlike `to_parquet`).
> I guess I'd expect as_dataset to generate the dataset in arrow format if it has to, or to suggest an alternative way to load the dataset (I've also tried other methods with load_dataset to no avail, probably due to misunderstandings on my part).
`as_dataset` does not work with `file_format="parquet"` files as Parquet files cannot be memory-mapped, so I think we should just raise an error in that case.
|
https://github.com/huggingface/datasets/issues/5871 | data configuration hash suffix depends on uncanonicalized data_dir | Indeed, it makes sense to normalize `data_dir`. Feel free to submit a PR (this can be "fixed" [here](https://github.com/huggingface/datasets/blob/89f775226321ba94e5bf4670a323c0fb44f5f65c/src/datasets/builder.py#L173)) | ### Describe the bug
I am working with the `recipe_nlg` dataset, which requires manual download. Once it's downloaded, I've noticed that the hash in the custom data configuration is different if I add a trailing `/` to my `data_dir`. It took me a while to notice that the hashes were different, and to understand that that was the cause of my dataset being processed anew instead of the cached version being used.
### Steps to reproduce the bug
1. Follow the steps to manually download the `recipe_nlg` dataset to `/data/recipenlg`.
2. Load it using `load_dataset`, once without a trailing slash and once with one:
```python
>>> ds = load_dataset("recipe_nlg", data_dir="/data/recipenlg")
Using custom data configuration default-082278caeea85765
Downloading and preparing dataset recipe_nlg/default to /home/kyle/.cache/huggingface/datasets/recipe_nlg/default-082278caeea85765/1.0.0/aa4f120223637bedf7360cecb70a9bd108acfd64e38207ca90c9f385d21e5e74...
Dataset recipe_nlg downloaded and prepared to /home/kyle/.cache/huggingface/datasets/recipe_nlg/default-082278caeea85765/1.0.0/aa4f120223637bedf7360cecb70a9bd108acfd64e38207ca90c9f385d21e5e74. Subsequent calls will reuse this data.
100%|βββββββββββββββββββββββββββββββββββββββββββββββββββββββββββββββββββ| 1/1 [00:01<00:00, 1.10s/it]
DatasetDict({
train: Dataset({
features: ['id', 'title', 'ingredients', 'directions', 'link', 'source', 'ner'],
num_rows: 2231142
})
})
>>> ds = load_dataset("recipe_nlg", data_dir="/data/recipenlg/")
Using custom data configuration default-83e87680785d0493
Downloading and preparing dataset recipe_nlg/default to /home/user/.cache/huggingface/datasets/recipe_nlg/default-83e87680785d0493/1.0.0/aa4f120223637bedf7360cecb70a9bd108acfd64e38207ca90c9f385d21e5e74...
Generating train split: 1%| | 12701/2231142 [00:04<13:15, 2790.25 examples/s
^C
```
3. Observe that the hash suffix in the custom data configuration changes due to the altered string.
### Expected behavior
I think I would expect the hash to remain constant if it actually points to the same location on disk. I would expect the use of `os.path.normpath` to canonicalize the paths.
### Environment info
- `datasets` version: 2.8.0
- Platform: Linux-5.4.0-147-generic-x86_64-with-glibc2.31
- Python version: 3.10.8
- PyArrow version: 10.0.1
- Pandas version: 1.5.2 | 18 | data configuration hash suffix depends on uncanonicalized data_dir
### Describe the bug
I am working with the `recipe_nlg` dataset, which requires manual download. Once it's downloaded, I've noticed that the hash in the custom data configuration is different if I add a trailing `/` to my `data_dir`. It took me a while to notice that the hashes were different, and to understand that that was the cause of my dataset being processed anew instead of the cached version being used.
### Steps to reproduce the bug
1. Follow the steps to manually download the `recipe_nlg` dataset to `/data/recipenlg`.
2. Load it using `load_dataset`, once without a trailing slash and once with one:
```python
>>> ds = load_dataset("recipe_nlg", data_dir="/data/recipenlg")
Using custom data configuration default-082278caeea85765
Downloading and preparing dataset recipe_nlg/default to /home/kyle/.cache/huggingface/datasets/recipe_nlg/default-082278caeea85765/1.0.0/aa4f120223637bedf7360cecb70a9bd108acfd64e38207ca90c9f385d21e5e74...
Dataset recipe_nlg downloaded and prepared to /home/kyle/.cache/huggingface/datasets/recipe_nlg/default-082278caeea85765/1.0.0/aa4f120223637bedf7360cecb70a9bd108acfd64e38207ca90c9f385d21e5e74. Subsequent calls will reuse this data.
100%|βββββββββββββββββββββββββββββββββββββββββββββββββββββββββββββββββββ| 1/1 [00:01<00:00, 1.10s/it]
DatasetDict({
train: Dataset({
features: ['id', 'title', 'ingredients', 'directions', 'link', 'source', 'ner'],
num_rows: 2231142
})
})
>>> ds = load_dataset("recipe_nlg", data_dir="/data/recipenlg/")
Using custom data configuration default-83e87680785d0493
Downloading and preparing dataset recipe_nlg/default to /home/user/.cache/huggingface/datasets/recipe_nlg/default-83e87680785d0493/1.0.0/aa4f120223637bedf7360cecb70a9bd108acfd64e38207ca90c9f385d21e5e74...
Generating train split: 1%| | 12701/2231142 [00:04<13:15, 2790.25 examples/s
^C
```
3. Observe that the hash suffix in the custom data configuration changes due to the altered string.
### Expected behavior
I think I would expect the hash to remain constant if it actually points to the same location on disk. I would expect the use of `os.path.normpath` to canonicalize the paths.
### Environment info
- `datasets` version: 2.8.0
- Platform: Linux-5.4.0-147-generic-x86_64-with-glibc2.31
- Python version: 3.10.8
- PyArrow version: 10.0.1
- Pandas version: 1.5.2
Indeed, it makes sense to normalize `data_dir`. Feel free to submit a PR (this can be "fixed" [here](https://github.com/huggingface/datasets/blob/89f775226321ba94e5bf4670a323c0fb44f5f65c/src/datasets/builder.py#L173)) |
https://github.com/huggingface/datasets/issues/5870 | Behaviour difference between datasets.map and IterableDatasets.map | PS - some work is definitely needed for 'special cases' docs, not explanations, just usages of 'functions' under mixture of special cases, like a combination of custom databuilder + iterable dataset for large size + dynamic .map() application. | ### Describe the bug
All the examples in all the docs mentioned throughout huggingface datasets correspond to datasets object, and not IterableDatasets object. At one point of time, they might have been in sync, but the code for datasets version >=2.9.0 is very different as compared to the docs.
I basically need to .map() a transform on images in an iterable dataset, which was made using a custom databuilder config.
This works very good in map-styles datasets, but the .map() fails in IterableDatasets, show behvaiour as such:
"pixel_values" key not found, KeyError in examples object/dict passed into transform function for map, which works fine with map style, even as batch.
In iterable style, the object/dict passed into map() paramter callable function is completely different as what is mentioned in all examples.
Please look into this. Thank you
My databuilder class is inherited as such:
def _info(self):
print ("Config: ",self.config.__dict__.keys())
return datasets.DatasetInfo(
description=_DESCRIPTION,
features=datasets.Features(
{
"labels": datasets.Sequence(datasets.Value("uint16")),
# "labels_name": datasets.Value("string"),
# "pixel_values": datasets.Array3D(shape=(3, 1280, 960), dtype="float32"),
"pixel_values": datasets.Array3D(shape=(1280, 960, 3), dtype="uint8"),
"image_s3_path": datasets.Value("string"),
}
),
supervised_keys=None,
homepage="none",
citation="",
)
def _split_generators(self, dl_manager):
records_train = list(db.mini_set.find({'split':'train'},{'image_s3_path':1, 'ocwen_template_name':1}))[:10000]
records_val = list(db.mini_set.find({'split':'val'},{'image_s3_path':1, 'ocwen_template_name':1}))[:1000]
# print (len(records),self.config.num_shards)
# shard_size_train = len(records_train)//self.config.num_shards
# sharded_records_train = [records_train[i:i+shard_size_train] for i in range(0,len(records_train),shard_size_train)]
# shard_size_val = len(records_val)//self.config.num_shards
# sharded_records_val = [records_val[i:i+shard_size_val] for i in range(0,len(records_val),shard_size_val)]
return [
datasets.SplitGenerator(
name=datasets.Split.TRAIN, gen_kwargs={"records":records_train} # passing list of records, for sharding to take over
),
datasets.SplitGenerator(
name=datasets.Split.VALIDATION, gen_kwargs={"records":records_val} # passing list of records, for sharding to take over
),
]
def _generate_examples(self, records):
# print ("Generating examples for [{}] shards".format(len(shards)))
# initiate_db_connection()
# records = list(db.mini_set.find({'split':split},{'image_s3_path':1, 'ocwen_template_name':1}))[:10]
id_ = 0
# for records in shards:
for i,rec in enumerate(records):
img_local_path = fetch_file(rec['image_s3_path'],self.config.buffer_dir)
# t = self.config.processor(Image.open(img_local_path), random_padding=True, return_tensors="np").pixel_values.squeeze()
# print (t.shape, type(t),type(t[0][0][0]))
# sys.exit()
pvs = np.array(Image.open(img_local_path).resize((1280,960))) # image object is wxh, so resize as per that, numpy array of it is hxwxc, transposing to cxwxh
# pvs = self.config.processor(Image.open(img_local_path), random_padding=True, return_tensors="np").pixel_values.astype(np.float16).squeeze()
# print (type(pvs[0][0][0]))
lblids = self.config.processor.tokenizer('<s_class>'+rec['ocwen_template_name']+'</s_class>'+'</s>', add_special_tokens=False, padding=False, truncation=False, return_tensors="np")["input_ids"].squeeze(0) # take padding later, as per batch collating
# print (len(lblids),type(lblids[0]))
# print (type(pvs),pvs.shape,type(pvs[0][0][0]), type(lblids))
yield id_, {"labels":lblids,"pixel_values":pvs,"image_s3_path":rec['image_s3_path']}
id_+=1
os.remove(img_local_path)
and I load it inside my trainer script as such
`ds = load_dataset("/tmp/DonutDS/dataset/", split="train", streaming=True) # iterable dataset, where .map() falls`
or also as
`ds = load_from_disk('/tmp/DonutDS/dataset/') #map style dataset`
Thank you to the team for having such a great library, and for this bug fix in advance!
### Steps to reproduce the bug
Above config can allow one to reproduce the said bug
### Expected behavior
.map() should show some consistency b/w map-style and iterable-style datasets, or atleast the docs should address iterable-style datasets behaviour and examples. I honestly do not figure the use of such docs.
### Environment info
datasets==2.9.0
transformers==4.26.0 | 38 | Behaviour difference between datasets.map and IterableDatasets.map
### Describe the bug
All the examples in all the docs mentioned throughout huggingface datasets correspond to datasets object, and not IterableDatasets object. At one point of time, they might have been in sync, but the code for datasets version >=2.9.0 is very different as compared to the docs.
I basically need to .map() a transform on images in an iterable dataset, which was made using a custom databuilder config.
This works very good in map-styles datasets, but the .map() fails in IterableDatasets, show behvaiour as such:
"pixel_values" key not found, KeyError in examples object/dict passed into transform function for map, which works fine with map style, even as batch.
In iterable style, the object/dict passed into map() paramter callable function is completely different as what is mentioned in all examples.
Please look into this. Thank you
My databuilder class is inherited as such:
def _info(self):
print ("Config: ",self.config.__dict__.keys())
return datasets.DatasetInfo(
description=_DESCRIPTION,
features=datasets.Features(
{
"labels": datasets.Sequence(datasets.Value("uint16")),
# "labels_name": datasets.Value("string"),
# "pixel_values": datasets.Array3D(shape=(3, 1280, 960), dtype="float32"),
"pixel_values": datasets.Array3D(shape=(1280, 960, 3), dtype="uint8"),
"image_s3_path": datasets.Value("string"),
}
),
supervised_keys=None,
homepage="none",
citation="",
)
def _split_generators(self, dl_manager):
records_train = list(db.mini_set.find({'split':'train'},{'image_s3_path':1, 'ocwen_template_name':1}))[:10000]
records_val = list(db.mini_set.find({'split':'val'},{'image_s3_path':1, 'ocwen_template_name':1}))[:1000]
# print (len(records),self.config.num_shards)
# shard_size_train = len(records_train)//self.config.num_shards
# sharded_records_train = [records_train[i:i+shard_size_train] for i in range(0,len(records_train),shard_size_train)]
# shard_size_val = len(records_val)//self.config.num_shards
# sharded_records_val = [records_val[i:i+shard_size_val] for i in range(0,len(records_val),shard_size_val)]
return [
datasets.SplitGenerator(
name=datasets.Split.TRAIN, gen_kwargs={"records":records_train} # passing list of records, for sharding to take over
),
datasets.SplitGenerator(
name=datasets.Split.VALIDATION, gen_kwargs={"records":records_val} # passing list of records, for sharding to take over
),
]
def _generate_examples(self, records):
# print ("Generating examples for [{}] shards".format(len(shards)))
# initiate_db_connection()
# records = list(db.mini_set.find({'split':split},{'image_s3_path':1, 'ocwen_template_name':1}))[:10]
id_ = 0
# for records in shards:
for i,rec in enumerate(records):
img_local_path = fetch_file(rec['image_s3_path'],self.config.buffer_dir)
# t = self.config.processor(Image.open(img_local_path), random_padding=True, return_tensors="np").pixel_values.squeeze()
# print (t.shape, type(t),type(t[0][0][0]))
# sys.exit()
pvs = np.array(Image.open(img_local_path).resize((1280,960))) # image object is wxh, so resize as per that, numpy array of it is hxwxc, transposing to cxwxh
# pvs = self.config.processor(Image.open(img_local_path), random_padding=True, return_tensors="np").pixel_values.astype(np.float16).squeeze()
# print (type(pvs[0][0][0]))
lblids = self.config.processor.tokenizer('<s_class>'+rec['ocwen_template_name']+'</s_class>'+'</s>', add_special_tokens=False, padding=False, truncation=False, return_tensors="np")["input_ids"].squeeze(0) # take padding later, as per batch collating
# print (len(lblids),type(lblids[0]))
# print (type(pvs),pvs.shape,type(pvs[0][0][0]), type(lblids))
yield id_, {"labels":lblids,"pixel_values":pvs,"image_s3_path":rec['image_s3_path']}
id_+=1
os.remove(img_local_path)
and I load it inside my trainer script as such
`ds = load_dataset("/tmp/DonutDS/dataset/", split="train", streaming=True) # iterable dataset, where .map() falls`
or also as
`ds = load_from_disk('/tmp/DonutDS/dataset/') #map style dataset`
Thank you to the team for having such a great library, and for this bug fix in advance!
### Steps to reproduce the bug
Above config can allow one to reproduce the said bug
### Expected behavior
.map() should show some consistency b/w map-style and iterable-style datasets, or atleast the docs should address iterable-style datasets behaviour and examples. I honestly do not figure the use of such docs.
### Environment info
datasets==2.9.0
transformers==4.26.0
PS - some work is definitely needed for 'special cases' docs, not explanations, just usages of 'functions' under mixture of special cases, like a combination of custom databuilder + iterable dataset for large size + dynamic .map() application. |
https://github.com/huggingface/datasets/issues/5869 | Image Encoding Issue when submitting a Parquet Dataset | Hi @PhilippeMoussalli thanks for opening a detailed issue. It seems the issue is more related to the `datasets` library so I'll ping @lhoestq @mariosasko on this one :)
(edit: also can one of you move the issue to the datasets repo? Thanks in advance π) | ### Describe the bug
Hello,
I'd like to report an issue related to pushing a dataset represented as a Parquet file to a dataset repository using Dask. Here are the details:
We attempted to load an example dataset in Parquet format from the Hugging Face (HF) filesystem using Dask with the following code snippet:
```
import dask.dataframe as dd
df = dd.read_parquet("hf://datasets/lambdalabs/pokemon-blip-captions",index=False)
```
In this dataset, the "image" column is represented as a dictionary/struct with the format:
```
df = df.compute()
df["image"].iloc[0].keys()
-> dict_keys(['bytes', 'path'])
```
I think this is the format encoded by the [`Image`](https://huggingface.co/docs/datasets/v2.0.0/en/package_reference/main_classes#datasets.Image) feature extractor from datasets to format suitable for Arrow.
The next step was to push the dataset to a repository that I created:
```
dd.to_parquet(dask_df, path = "hf://datasets/philippemo/dummy_dataset/data")
```
However, after pushing the dataset using Dask, the "image" column is now represented as the encoded dictionary `(['bytes', 'path'])`, and the images are not properly visualized. You can find the dataset here: [Link to the problematic dataset](https://huggingface.co/datasets/philippemo/dummy_dataset).
It's worth noting that both the original dataset and the one submitted with Dask have the same schema with minor alterations related to metadata:
**[ Schema of original dummy example.](https://huggingface.co/datasets/lambdalabs/pokemon-blip-captions/blob/main/data/train-00000-of-00001-566cc9b19d7203f8.parquet)**
```
image: struct<bytes: binary, path: null>
child 0, bytes: binary
child 1, path: null
text: string
```
**[ Schema of pushed dataset with dask](https://huggingface.co/datasets/philippemo/dummy_dataset/blob/main/data/part.0.parquet)**
```
image: struct<bytes: binary, path: null>
child 0, bytes: binary
child 1, path: null
text: string
```
This issue seems to be related to an encoding type that occurs when pushing a model to the hub. Normally, models should be represented as an HF dataset before pushing, but we are working with an example where we need to push large datasets using Dask.
Could you please provide clarification on how to resolve this issue?
Thank you!
### Reproduction
To get the schema I downloaded the parquet files and used pyarrow.parquet to read the schema
```
import pyarrow.parquet
pyarrow.parquet.read_schema(<path_to_parquet>, memory_map=True)
```
### Logs
_No response_
### System info
```shell
- huggingface_hub version: 0.14.1
- Platform: Linux-5.19.0-41-generic-x86_64-with-glibc2.35
- Python version: 3.10.6
- Running in iPython ?: No
- Running in notebook ?: No
- Running in Google Colab ?: No
- Token path ?: /home/philippe/.cache/huggingface/token
- Has saved token ?: True
- Who am I ?: philippemo
- Configured git credential helpers: cache
- FastAI: N/A
- Tensorflow: N/A
- Torch: N/A
- Jinja2: 3.1.2
- Graphviz: N/A
- Pydot: N/A
- Pillow: 9.4.0
- hf_transfer: N/A
- gradio: N/A
- ENDPOINT: https://huggingface.co
- HUGGINGFACE_HUB_CACHE: /home/philippe/.cache/huggingface/hub
- HUGGINGFACE_ASSETS_CACHE: /home/philippe/.cache/huggingface/assets
- HF_TOKEN_PATH: /home/philippe/.cache/huggingface/token
- HF_HUB_OFFLINE: False
- HF_HUB_DISABLE_TELEMETRY: False
- HF_HUB_DISABLE_PROGRESS_BARS: None
- HF_HUB_DISABLE_SYMLINKS_WARNING: False
- HF_HUB_DISABLE_EXPERIMENTAL_WARNING: False
- HF_HUB_DISABLE_IMPLICIT_TOKEN: False
- HF_HUB_ENABLE_HF_TRANSFER: False
```
| 45 | Image Encoding Issue when submitting a Parquet Dataset
### Describe the bug
Hello,
I'd like to report an issue related to pushing a dataset represented as a Parquet file to a dataset repository using Dask. Here are the details:
We attempted to load an example dataset in Parquet format from the Hugging Face (HF) filesystem using Dask with the following code snippet:
```
import dask.dataframe as dd
df = dd.read_parquet("hf://datasets/lambdalabs/pokemon-blip-captions",index=False)
```
In this dataset, the "image" column is represented as a dictionary/struct with the format:
```
df = df.compute()
df["image"].iloc[0].keys()
-> dict_keys(['bytes', 'path'])
```
I think this is the format encoded by the [`Image`](https://huggingface.co/docs/datasets/v2.0.0/en/package_reference/main_classes#datasets.Image) feature extractor from datasets to format suitable for Arrow.
The next step was to push the dataset to a repository that I created:
```
dd.to_parquet(dask_df, path = "hf://datasets/philippemo/dummy_dataset/data")
```
However, after pushing the dataset using Dask, the "image" column is now represented as the encoded dictionary `(['bytes', 'path'])`, and the images are not properly visualized. You can find the dataset here: [Link to the problematic dataset](https://huggingface.co/datasets/philippemo/dummy_dataset).
It's worth noting that both the original dataset and the one submitted with Dask have the same schema with minor alterations related to metadata:
**[ Schema of original dummy example.](https://huggingface.co/datasets/lambdalabs/pokemon-blip-captions/blob/main/data/train-00000-of-00001-566cc9b19d7203f8.parquet)**
```
image: struct<bytes: binary, path: null>
child 0, bytes: binary
child 1, path: null
text: string
```
**[ Schema of pushed dataset with dask](https://huggingface.co/datasets/philippemo/dummy_dataset/blob/main/data/part.0.parquet)**
```
image: struct<bytes: binary, path: null>
child 0, bytes: binary
child 1, path: null
text: string
```
This issue seems to be related to an encoding type that occurs when pushing a model to the hub. Normally, models should be represented as an HF dataset before pushing, but we are working with an example where we need to push large datasets using Dask.
Could you please provide clarification on how to resolve this issue?
Thank you!
### Reproduction
To get the schema I downloaded the parquet files and used pyarrow.parquet to read the schema
```
import pyarrow.parquet
pyarrow.parquet.read_schema(<path_to_parquet>, memory_map=True)
```
### Logs
_No response_
### System info
```shell
- huggingface_hub version: 0.14.1
- Platform: Linux-5.19.0-41-generic-x86_64-with-glibc2.35
- Python version: 3.10.6
- Running in iPython ?: No
- Running in notebook ?: No
- Running in Google Colab ?: No
- Token path ?: /home/philippe/.cache/huggingface/token
- Has saved token ?: True
- Who am I ?: philippemo
- Configured git credential helpers: cache
- FastAI: N/A
- Tensorflow: N/A
- Torch: N/A
- Jinja2: 3.1.2
- Graphviz: N/A
- Pydot: N/A
- Pillow: 9.4.0
- hf_transfer: N/A
- gradio: N/A
- ENDPOINT: https://huggingface.co
- HUGGINGFACE_HUB_CACHE: /home/philippe/.cache/huggingface/hub
- HUGGINGFACE_ASSETS_CACHE: /home/philippe/.cache/huggingface/assets
- HF_TOKEN_PATH: /home/philippe/.cache/huggingface/token
- HF_HUB_OFFLINE: False
- HF_HUB_DISABLE_TELEMETRY: False
- HF_HUB_DISABLE_PROGRESS_BARS: None
- HF_HUB_DISABLE_SYMLINKS_WARNING: False
- HF_HUB_DISABLE_EXPERIMENTAL_WARNING: False
- HF_HUB_DISABLE_IMPLICIT_TOKEN: False
- HF_HUB_ENABLE_HF_TRANSFER: False
```
Hi @PhilippeMoussalli thanks for opening a detailed issue. It seems the issue is more related to the `datasets` library so I'll ping @lhoestq @mariosasko on this one :)
(edit: also can one of you move the issue to the datasets repo? Thanks in advance π) |
https://github.com/huggingface/datasets/issues/5869 | Image Encoding Issue when submitting a Parquet Dataset | Hi ! The `Image()` info is stored in the **schema metadata**. More precisely there should be a "huggingface" field in the schema metadata that contains the `datasets` feature type of each column.
To fix your issue, you can use the same schema as the original Parquet files to write the new ones. You can also get the schema with metadata from a `Features` object, e.g.
```python
from datasets import Features, Image, Value
features = Features({"image": Image(), "text": Value("string")})
schema = features.arrow_schema
print(schema.metadata)
# {b'huggingface': b'{"info": {"features": {"image": {"_type": "Image"}, "text": {"dtype": "string", "_type": "Value"}}}}'}
``` | ### Describe the bug
Hello,
I'd like to report an issue related to pushing a dataset represented as a Parquet file to a dataset repository using Dask. Here are the details:
We attempted to load an example dataset in Parquet format from the Hugging Face (HF) filesystem using Dask with the following code snippet:
```
import dask.dataframe as dd
df = dd.read_parquet("hf://datasets/lambdalabs/pokemon-blip-captions",index=False)
```
In this dataset, the "image" column is represented as a dictionary/struct with the format:
```
df = df.compute()
df["image"].iloc[0].keys()
-> dict_keys(['bytes', 'path'])
```
I think this is the format encoded by the [`Image`](https://huggingface.co/docs/datasets/v2.0.0/en/package_reference/main_classes#datasets.Image) feature extractor from datasets to format suitable for Arrow.
The next step was to push the dataset to a repository that I created:
```
dd.to_parquet(dask_df, path = "hf://datasets/philippemo/dummy_dataset/data")
```
However, after pushing the dataset using Dask, the "image" column is now represented as the encoded dictionary `(['bytes', 'path'])`, and the images are not properly visualized. You can find the dataset here: [Link to the problematic dataset](https://huggingface.co/datasets/philippemo/dummy_dataset).
It's worth noting that both the original dataset and the one submitted with Dask have the same schema with minor alterations related to metadata:
**[ Schema of original dummy example.](https://huggingface.co/datasets/lambdalabs/pokemon-blip-captions/blob/main/data/train-00000-of-00001-566cc9b19d7203f8.parquet)**
```
image: struct<bytes: binary, path: null>
child 0, bytes: binary
child 1, path: null
text: string
```
**[ Schema of pushed dataset with dask](https://huggingface.co/datasets/philippemo/dummy_dataset/blob/main/data/part.0.parquet)**
```
image: struct<bytes: binary, path: null>
child 0, bytes: binary
child 1, path: null
text: string
```
This issue seems to be related to an encoding type that occurs when pushing a model to the hub. Normally, models should be represented as an HF dataset before pushing, but we are working with an example where we need to push large datasets using Dask.
Could you please provide clarification on how to resolve this issue?
Thank you!
### Reproduction
To get the schema I downloaded the parquet files and used pyarrow.parquet to read the schema
```
import pyarrow.parquet
pyarrow.parquet.read_schema(<path_to_parquet>, memory_map=True)
```
### Logs
_No response_
### System info
```shell
- huggingface_hub version: 0.14.1
- Platform: Linux-5.19.0-41-generic-x86_64-with-glibc2.35
- Python version: 3.10.6
- Running in iPython ?: No
- Running in notebook ?: No
- Running in Google Colab ?: No
- Token path ?: /home/philippe/.cache/huggingface/token
- Has saved token ?: True
- Who am I ?: philippemo
- Configured git credential helpers: cache
- FastAI: N/A
- Tensorflow: N/A
- Torch: N/A
- Jinja2: 3.1.2
- Graphviz: N/A
- Pydot: N/A
- Pillow: 9.4.0
- hf_transfer: N/A
- gradio: N/A
- ENDPOINT: https://huggingface.co
- HUGGINGFACE_HUB_CACHE: /home/philippe/.cache/huggingface/hub
- HUGGINGFACE_ASSETS_CACHE: /home/philippe/.cache/huggingface/assets
- HF_TOKEN_PATH: /home/philippe/.cache/huggingface/token
- HF_HUB_OFFLINE: False
- HF_HUB_DISABLE_TELEMETRY: False
- HF_HUB_DISABLE_PROGRESS_BARS: None
- HF_HUB_DISABLE_SYMLINKS_WARNING: False
- HF_HUB_DISABLE_EXPERIMENTAL_WARNING: False
- HF_HUB_DISABLE_IMPLICIT_TOKEN: False
- HF_HUB_ENABLE_HF_TRANSFER: False
```
| 95 | Image Encoding Issue when submitting a Parquet Dataset
### Describe the bug
Hello,
I'd like to report an issue related to pushing a dataset represented as a Parquet file to a dataset repository using Dask. Here are the details:
We attempted to load an example dataset in Parquet format from the Hugging Face (HF) filesystem using Dask with the following code snippet:
```
import dask.dataframe as dd
df = dd.read_parquet("hf://datasets/lambdalabs/pokemon-blip-captions",index=False)
```
In this dataset, the "image" column is represented as a dictionary/struct with the format:
```
df = df.compute()
df["image"].iloc[0].keys()
-> dict_keys(['bytes', 'path'])
```
I think this is the format encoded by the [`Image`](https://huggingface.co/docs/datasets/v2.0.0/en/package_reference/main_classes#datasets.Image) feature extractor from datasets to format suitable for Arrow.
The next step was to push the dataset to a repository that I created:
```
dd.to_parquet(dask_df, path = "hf://datasets/philippemo/dummy_dataset/data")
```
However, after pushing the dataset using Dask, the "image" column is now represented as the encoded dictionary `(['bytes', 'path'])`, and the images are not properly visualized. You can find the dataset here: [Link to the problematic dataset](https://huggingface.co/datasets/philippemo/dummy_dataset).
It's worth noting that both the original dataset and the one submitted with Dask have the same schema with minor alterations related to metadata:
**[ Schema of original dummy example.](https://huggingface.co/datasets/lambdalabs/pokemon-blip-captions/blob/main/data/train-00000-of-00001-566cc9b19d7203f8.parquet)**
```
image: struct<bytes: binary, path: null>
child 0, bytes: binary
child 1, path: null
text: string
```
**[ Schema of pushed dataset with dask](https://huggingface.co/datasets/philippemo/dummy_dataset/blob/main/data/part.0.parquet)**
```
image: struct<bytes: binary, path: null>
child 0, bytes: binary
child 1, path: null
text: string
```
This issue seems to be related to an encoding type that occurs when pushing a model to the hub. Normally, models should be represented as an HF dataset before pushing, but we are working with an example where we need to push large datasets using Dask.
Could you please provide clarification on how to resolve this issue?
Thank you!
### Reproduction
To get the schema I downloaded the parquet files and used pyarrow.parquet to read the schema
```
import pyarrow.parquet
pyarrow.parquet.read_schema(<path_to_parquet>, memory_map=True)
```
### Logs
_No response_
### System info
```shell
- huggingface_hub version: 0.14.1
- Platform: Linux-5.19.0-41-generic-x86_64-with-glibc2.35
- Python version: 3.10.6
- Running in iPython ?: No
- Running in notebook ?: No
- Running in Google Colab ?: No
- Token path ?: /home/philippe/.cache/huggingface/token
- Has saved token ?: True
- Who am I ?: philippemo
- Configured git credential helpers: cache
- FastAI: N/A
- Tensorflow: N/A
- Torch: N/A
- Jinja2: 3.1.2
- Graphviz: N/A
- Pydot: N/A
- Pillow: 9.4.0
- hf_transfer: N/A
- gradio: N/A
- ENDPOINT: https://huggingface.co
- HUGGINGFACE_HUB_CACHE: /home/philippe/.cache/huggingface/hub
- HUGGINGFACE_ASSETS_CACHE: /home/philippe/.cache/huggingface/assets
- HF_TOKEN_PATH: /home/philippe/.cache/huggingface/token
- HF_HUB_OFFLINE: False
- HF_HUB_DISABLE_TELEMETRY: False
- HF_HUB_DISABLE_PROGRESS_BARS: None
- HF_HUB_DISABLE_SYMLINKS_WARNING: False
- HF_HUB_DISABLE_EXPERIMENTAL_WARNING: False
- HF_HUB_DISABLE_IMPLICIT_TOKEN: False
- HF_HUB_ENABLE_HF_TRANSFER: False
```
Hi ! The `Image()` info is stored in the **schema metadata**. More precisely there should be a "huggingface" field in the schema metadata that contains the `datasets` feature type of each column.
To fix your issue, you can use the same schema as the original Parquet files to write the new ones. You can also get the schema with metadata from a `Features` object, e.g.
```python
from datasets import Features, Image, Value
features = Features({"image": Image(), "text": Value("string")})
schema = features.arrow_schema
print(schema.metadata)
# {b'huggingface': b'{"info": {"features": {"image": {"_type": "Image"}, "text": {"dtype": "string", "_type": "Value"}}}}'}
``` |
https://github.com/huggingface/datasets/issues/5869 | Image Encoding Issue when submitting a Parquet Dataset | It appears that the parquet files at `hf://datasets/lambdalabs/pokemon-blip-captions` don't have this metadata, and it is defined in the dataset_infos.json instead (legacy).
You can get the right schema with the HF metadata this way:
```python
from datasets import load_dataset_builder
features = load_dataset_builder("lambdalabs/pokemon-blip-captions").info.features
schema = features.arrow_schema
``` | ### Describe the bug
Hello,
I'd like to report an issue related to pushing a dataset represented as a Parquet file to a dataset repository using Dask. Here are the details:
We attempted to load an example dataset in Parquet format from the Hugging Face (HF) filesystem using Dask with the following code snippet:
```
import dask.dataframe as dd
df = dd.read_parquet("hf://datasets/lambdalabs/pokemon-blip-captions",index=False)
```
In this dataset, the "image" column is represented as a dictionary/struct with the format:
```
df = df.compute()
df["image"].iloc[0].keys()
-> dict_keys(['bytes', 'path'])
```
I think this is the format encoded by the [`Image`](https://huggingface.co/docs/datasets/v2.0.0/en/package_reference/main_classes#datasets.Image) feature extractor from datasets to format suitable for Arrow.
The next step was to push the dataset to a repository that I created:
```
dd.to_parquet(dask_df, path = "hf://datasets/philippemo/dummy_dataset/data")
```
However, after pushing the dataset using Dask, the "image" column is now represented as the encoded dictionary `(['bytes', 'path'])`, and the images are not properly visualized. You can find the dataset here: [Link to the problematic dataset](https://huggingface.co/datasets/philippemo/dummy_dataset).
It's worth noting that both the original dataset and the one submitted with Dask have the same schema with minor alterations related to metadata:
**[ Schema of original dummy example.](https://huggingface.co/datasets/lambdalabs/pokemon-blip-captions/blob/main/data/train-00000-of-00001-566cc9b19d7203f8.parquet)**
```
image: struct<bytes: binary, path: null>
child 0, bytes: binary
child 1, path: null
text: string
```
**[ Schema of pushed dataset with dask](https://huggingface.co/datasets/philippemo/dummy_dataset/blob/main/data/part.0.parquet)**
```
image: struct<bytes: binary, path: null>
child 0, bytes: binary
child 1, path: null
text: string
```
This issue seems to be related to an encoding type that occurs when pushing a model to the hub. Normally, models should be represented as an HF dataset before pushing, but we are working with an example where we need to push large datasets using Dask.
Could you please provide clarification on how to resolve this issue?
Thank you!
### Reproduction
To get the schema I downloaded the parquet files and used pyarrow.parquet to read the schema
```
import pyarrow.parquet
pyarrow.parquet.read_schema(<path_to_parquet>, memory_map=True)
```
### Logs
_No response_
### System info
```shell
- huggingface_hub version: 0.14.1
- Platform: Linux-5.19.0-41-generic-x86_64-with-glibc2.35
- Python version: 3.10.6
- Running in iPython ?: No
- Running in notebook ?: No
- Running in Google Colab ?: No
- Token path ?: /home/philippe/.cache/huggingface/token
- Has saved token ?: True
- Who am I ?: philippemo
- Configured git credential helpers: cache
- FastAI: N/A
- Tensorflow: N/A
- Torch: N/A
- Jinja2: 3.1.2
- Graphviz: N/A
- Pydot: N/A
- Pillow: 9.4.0
- hf_transfer: N/A
- gradio: N/A
- ENDPOINT: https://huggingface.co
- HUGGINGFACE_HUB_CACHE: /home/philippe/.cache/huggingface/hub
- HUGGINGFACE_ASSETS_CACHE: /home/philippe/.cache/huggingface/assets
- HF_TOKEN_PATH: /home/philippe/.cache/huggingface/token
- HF_HUB_OFFLINE: False
- HF_HUB_DISABLE_TELEMETRY: False
- HF_HUB_DISABLE_PROGRESS_BARS: None
- HF_HUB_DISABLE_SYMLINKS_WARNING: False
- HF_HUB_DISABLE_EXPERIMENTAL_WARNING: False
- HF_HUB_DISABLE_IMPLICIT_TOKEN: False
- HF_HUB_ENABLE_HF_TRANSFER: False
```
| 45 | Image Encoding Issue when submitting a Parquet Dataset
### Describe the bug
Hello,
I'd like to report an issue related to pushing a dataset represented as a Parquet file to a dataset repository using Dask. Here are the details:
We attempted to load an example dataset in Parquet format from the Hugging Face (HF) filesystem using Dask with the following code snippet:
```
import dask.dataframe as dd
df = dd.read_parquet("hf://datasets/lambdalabs/pokemon-blip-captions",index=False)
```
In this dataset, the "image" column is represented as a dictionary/struct with the format:
```
df = df.compute()
df["image"].iloc[0].keys()
-> dict_keys(['bytes', 'path'])
```
I think this is the format encoded by the [`Image`](https://huggingface.co/docs/datasets/v2.0.0/en/package_reference/main_classes#datasets.Image) feature extractor from datasets to format suitable for Arrow.
The next step was to push the dataset to a repository that I created:
```
dd.to_parquet(dask_df, path = "hf://datasets/philippemo/dummy_dataset/data")
```
However, after pushing the dataset using Dask, the "image" column is now represented as the encoded dictionary `(['bytes', 'path'])`, and the images are not properly visualized. You can find the dataset here: [Link to the problematic dataset](https://huggingface.co/datasets/philippemo/dummy_dataset).
It's worth noting that both the original dataset and the one submitted with Dask have the same schema with minor alterations related to metadata:
**[ Schema of original dummy example.](https://huggingface.co/datasets/lambdalabs/pokemon-blip-captions/blob/main/data/train-00000-of-00001-566cc9b19d7203f8.parquet)**
```
image: struct<bytes: binary, path: null>
child 0, bytes: binary
child 1, path: null
text: string
```
**[ Schema of pushed dataset with dask](https://huggingface.co/datasets/philippemo/dummy_dataset/blob/main/data/part.0.parquet)**
```
image: struct<bytes: binary, path: null>
child 0, bytes: binary
child 1, path: null
text: string
```
This issue seems to be related to an encoding type that occurs when pushing a model to the hub. Normally, models should be represented as an HF dataset before pushing, but we are working with an example where we need to push large datasets using Dask.
Could you please provide clarification on how to resolve this issue?
Thank you!
### Reproduction
To get the schema I downloaded the parquet files and used pyarrow.parquet to read the schema
```
import pyarrow.parquet
pyarrow.parquet.read_schema(<path_to_parquet>, memory_map=True)
```
### Logs
_No response_
### System info
```shell
- huggingface_hub version: 0.14.1
- Platform: Linux-5.19.0-41-generic-x86_64-with-glibc2.35
- Python version: 3.10.6
- Running in iPython ?: No
- Running in notebook ?: No
- Running in Google Colab ?: No
- Token path ?: /home/philippe/.cache/huggingface/token
- Has saved token ?: True
- Who am I ?: philippemo
- Configured git credential helpers: cache
- FastAI: N/A
- Tensorflow: N/A
- Torch: N/A
- Jinja2: 3.1.2
- Graphviz: N/A
- Pydot: N/A
- Pillow: 9.4.0
- hf_transfer: N/A
- gradio: N/A
- ENDPOINT: https://huggingface.co
- HUGGINGFACE_HUB_CACHE: /home/philippe/.cache/huggingface/hub
- HUGGINGFACE_ASSETS_CACHE: /home/philippe/.cache/huggingface/assets
- HF_TOKEN_PATH: /home/philippe/.cache/huggingface/token
- HF_HUB_OFFLINE: False
- HF_HUB_DISABLE_TELEMETRY: False
- HF_HUB_DISABLE_PROGRESS_BARS: None
- HF_HUB_DISABLE_SYMLINKS_WARNING: False
- HF_HUB_DISABLE_EXPERIMENTAL_WARNING: False
- HF_HUB_DISABLE_IMPLICIT_TOKEN: False
- HF_HUB_ENABLE_HF_TRANSFER: False
```
It appears that the parquet files at `hf://datasets/lambdalabs/pokemon-blip-captions` don't have this metadata, and it is defined in the dataset_infos.json instead (legacy).
You can get the right schema with the HF metadata this way:
```python
from datasets import load_dataset_builder
features = load_dataset_builder("lambdalabs/pokemon-blip-captions").info.features
schema = features.arrow_schema
``` |
https://github.com/huggingface/datasets/issues/5869 | Image Encoding Issue when submitting a Parquet Dataset | Btw in the future we might add support for an dedicated Image extension type in Arrow so that you won't need to add the schema metadata anymore ;) | ### Describe the bug
Hello,
I'd like to report an issue related to pushing a dataset represented as a Parquet file to a dataset repository using Dask. Here are the details:
We attempted to load an example dataset in Parquet format from the Hugging Face (HF) filesystem using Dask with the following code snippet:
```
import dask.dataframe as dd
df = dd.read_parquet("hf://datasets/lambdalabs/pokemon-blip-captions",index=False)
```
In this dataset, the "image" column is represented as a dictionary/struct with the format:
```
df = df.compute()
df["image"].iloc[0].keys()
-> dict_keys(['bytes', 'path'])
```
I think this is the format encoded by the [`Image`](https://huggingface.co/docs/datasets/v2.0.0/en/package_reference/main_classes#datasets.Image) feature extractor from datasets to format suitable for Arrow.
The next step was to push the dataset to a repository that I created:
```
dd.to_parquet(dask_df, path = "hf://datasets/philippemo/dummy_dataset/data")
```
However, after pushing the dataset using Dask, the "image" column is now represented as the encoded dictionary `(['bytes', 'path'])`, and the images are not properly visualized. You can find the dataset here: [Link to the problematic dataset](https://huggingface.co/datasets/philippemo/dummy_dataset).
It's worth noting that both the original dataset and the one submitted with Dask have the same schema with minor alterations related to metadata:
**[ Schema of original dummy example.](https://huggingface.co/datasets/lambdalabs/pokemon-blip-captions/blob/main/data/train-00000-of-00001-566cc9b19d7203f8.parquet)**
```
image: struct<bytes: binary, path: null>
child 0, bytes: binary
child 1, path: null
text: string
```
**[ Schema of pushed dataset with dask](https://huggingface.co/datasets/philippemo/dummy_dataset/blob/main/data/part.0.parquet)**
```
image: struct<bytes: binary, path: null>
child 0, bytes: binary
child 1, path: null
text: string
```
This issue seems to be related to an encoding type that occurs when pushing a model to the hub. Normally, models should be represented as an HF dataset before pushing, but we are working with an example where we need to push large datasets using Dask.
Could you please provide clarification on how to resolve this issue?
Thank you!
### Reproduction
To get the schema I downloaded the parquet files and used pyarrow.parquet to read the schema
```
import pyarrow.parquet
pyarrow.parquet.read_schema(<path_to_parquet>, memory_map=True)
```
### Logs
_No response_
### System info
```shell
- huggingface_hub version: 0.14.1
- Platform: Linux-5.19.0-41-generic-x86_64-with-glibc2.35
- Python version: 3.10.6
- Running in iPython ?: No
- Running in notebook ?: No
- Running in Google Colab ?: No
- Token path ?: /home/philippe/.cache/huggingface/token
- Has saved token ?: True
- Who am I ?: philippemo
- Configured git credential helpers: cache
- FastAI: N/A
- Tensorflow: N/A
- Torch: N/A
- Jinja2: 3.1.2
- Graphviz: N/A
- Pydot: N/A
- Pillow: 9.4.0
- hf_transfer: N/A
- gradio: N/A
- ENDPOINT: https://huggingface.co
- HUGGINGFACE_HUB_CACHE: /home/philippe/.cache/huggingface/hub
- HUGGINGFACE_ASSETS_CACHE: /home/philippe/.cache/huggingface/assets
- HF_TOKEN_PATH: /home/philippe/.cache/huggingface/token
- HF_HUB_OFFLINE: False
- HF_HUB_DISABLE_TELEMETRY: False
- HF_HUB_DISABLE_PROGRESS_BARS: None
- HF_HUB_DISABLE_SYMLINKS_WARNING: False
- HF_HUB_DISABLE_EXPERIMENTAL_WARNING: False
- HF_HUB_DISABLE_IMPLICIT_TOKEN: False
- HF_HUB_ENABLE_HF_TRANSFER: False
```
| 28 | Image Encoding Issue when submitting a Parquet Dataset
### Describe the bug
Hello,
I'd like to report an issue related to pushing a dataset represented as a Parquet file to a dataset repository using Dask. Here are the details:
We attempted to load an example dataset in Parquet format from the Hugging Face (HF) filesystem using Dask with the following code snippet:
```
import dask.dataframe as dd
df = dd.read_parquet("hf://datasets/lambdalabs/pokemon-blip-captions",index=False)
```
In this dataset, the "image" column is represented as a dictionary/struct with the format:
```
df = df.compute()
df["image"].iloc[0].keys()
-> dict_keys(['bytes', 'path'])
```
I think this is the format encoded by the [`Image`](https://huggingface.co/docs/datasets/v2.0.0/en/package_reference/main_classes#datasets.Image) feature extractor from datasets to format suitable for Arrow.
The next step was to push the dataset to a repository that I created:
```
dd.to_parquet(dask_df, path = "hf://datasets/philippemo/dummy_dataset/data")
```
However, after pushing the dataset using Dask, the "image" column is now represented as the encoded dictionary `(['bytes', 'path'])`, and the images are not properly visualized. You can find the dataset here: [Link to the problematic dataset](https://huggingface.co/datasets/philippemo/dummy_dataset).
It's worth noting that both the original dataset and the one submitted with Dask have the same schema with minor alterations related to metadata:
**[ Schema of original dummy example.](https://huggingface.co/datasets/lambdalabs/pokemon-blip-captions/blob/main/data/train-00000-of-00001-566cc9b19d7203f8.parquet)**
```
image: struct<bytes: binary, path: null>
child 0, bytes: binary
child 1, path: null
text: string
```
**[ Schema of pushed dataset with dask](https://huggingface.co/datasets/philippemo/dummy_dataset/blob/main/data/part.0.parquet)**
```
image: struct<bytes: binary, path: null>
child 0, bytes: binary
child 1, path: null
text: string
```
This issue seems to be related to an encoding type that occurs when pushing a model to the hub. Normally, models should be represented as an HF dataset before pushing, but we are working with an example where we need to push large datasets using Dask.
Could you please provide clarification on how to resolve this issue?
Thank you!
### Reproduction
To get the schema I downloaded the parquet files and used pyarrow.parquet to read the schema
```
import pyarrow.parquet
pyarrow.parquet.read_schema(<path_to_parquet>, memory_map=True)
```
### Logs
_No response_
### System info
```shell
- huggingface_hub version: 0.14.1
- Platform: Linux-5.19.0-41-generic-x86_64-with-glibc2.35
- Python version: 3.10.6
- Running in iPython ?: No
- Running in notebook ?: No
- Running in Google Colab ?: No
- Token path ?: /home/philippe/.cache/huggingface/token
- Has saved token ?: True
- Who am I ?: philippemo
- Configured git credential helpers: cache
- FastAI: N/A
- Tensorflow: N/A
- Torch: N/A
- Jinja2: 3.1.2
- Graphviz: N/A
- Pydot: N/A
- Pillow: 9.4.0
- hf_transfer: N/A
- gradio: N/A
- ENDPOINT: https://huggingface.co
- HUGGINGFACE_HUB_CACHE: /home/philippe/.cache/huggingface/hub
- HUGGINGFACE_ASSETS_CACHE: /home/philippe/.cache/huggingface/assets
- HF_TOKEN_PATH: /home/philippe/.cache/huggingface/token
- HF_HUB_OFFLINE: False
- HF_HUB_DISABLE_TELEMETRY: False
- HF_HUB_DISABLE_PROGRESS_BARS: None
- HF_HUB_DISABLE_SYMLINKS_WARNING: False
- HF_HUB_DISABLE_EXPERIMENTAL_WARNING: False
- HF_HUB_DISABLE_IMPLICIT_TOKEN: False
- HF_HUB_ENABLE_HF_TRANSFER: False
```
Btw in the future we might add support for an dedicated Image extension type in Arrow so that you won't need to add the schema metadata anymore ;) |
https://github.com/huggingface/datasets/issues/5869 | Image Encoding Issue when submitting a Parquet Dataset | Thanks @Wauplin @lhoestq for the quick reply :)!
I tried your approach by passing the huggingface schema to the dask writer
```
from datasets import Features, Image, Value
df = dd.read_parquet(f"hf://datasets/lambdalabs/pokemon-blip-captions",index=False)
features = Features({"image": Image(), "text": Value("string")})
schema = features.arrow_schema
dd.to_parquet(df, path = "hf://datasets/philippemo/dummy_dataset/data", schema=schema)
```
At first it didn't work as I was not able to visualize the images, so then I manually added the `dataset_infos.json` from the example dataset and it worked :)
However, It's not very ideal since there are some metadata in that file that need to be computed in order to load the data properly such as `num_of_bytes` and `num_examples` which might be unknown in my use case.

Do you have any pointers there? you mentioned that `datasets_info.json` will be deprecated/legacy. Could you point me to some example image datasets on the hub that are stored as parquet and don't have the `datasets_info.json`?
| ### Describe the bug
Hello,
I'd like to report an issue related to pushing a dataset represented as a Parquet file to a dataset repository using Dask. Here are the details:
We attempted to load an example dataset in Parquet format from the Hugging Face (HF) filesystem using Dask with the following code snippet:
```
import dask.dataframe as dd
df = dd.read_parquet("hf://datasets/lambdalabs/pokemon-blip-captions",index=False)
```
In this dataset, the "image" column is represented as a dictionary/struct with the format:
```
df = df.compute()
df["image"].iloc[0].keys()
-> dict_keys(['bytes', 'path'])
```
I think this is the format encoded by the [`Image`](https://huggingface.co/docs/datasets/v2.0.0/en/package_reference/main_classes#datasets.Image) feature extractor from datasets to format suitable for Arrow.
The next step was to push the dataset to a repository that I created:
```
dd.to_parquet(dask_df, path = "hf://datasets/philippemo/dummy_dataset/data")
```
However, after pushing the dataset using Dask, the "image" column is now represented as the encoded dictionary `(['bytes', 'path'])`, and the images are not properly visualized. You can find the dataset here: [Link to the problematic dataset](https://huggingface.co/datasets/philippemo/dummy_dataset).
It's worth noting that both the original dataset and the one submitted with Dask have the same schema with minor alterations related to metadata:
**[ Schema of original dummy example.](https://huggingface.co/datasets/lambdalabs/pokemon-blip-captions/blob/main/data/train-00000-of-00001-566cc9b19d7203f8.parquet)**
```
image: struct<bytes: binary, path: null>
child 0, bytes: binary
child 1, path: null
text: string
```
**[ Schema of pushed dataset with dask](https://huggingface.co/datasets/philippemo/dummy_dataset/blob/main/data/part.0.parquet)**
```
image: struct<bytes: binary, path: null>
child 0, bytes: binary
child 1, path: null
text: string
```
This issue seems to be related to an encoding type that occurs when pushing a model to the hub. Normally, models should be represented as an HF dataset before pushing, but we are working with an example where we need to push large datasets using Dask.
Could you please provide clarification on how to resolve this issue?
Thank you!
### Reproduction
To get the schema I downloaded the parquet files and used pyarrow.parquet to read the schema
```
import pyarrow.parquet
pyarrow.parquet.read_schema(<path_to_parquet>, memory_map=True)
```
### Logs
_No response_
### System info
```shell
- huggingface_hub version: 0.14.1
- Platform: Linux-5.19.0-41-generic-x86_64-with-glibc2.35
- Python version: 3.10.6
- Running in iPython ?: No
- Running in notebook ?: No
- Running in Google Colab ?: No
- Token path ?: /home/philippe/.cache/huggingface/token
- Has saved token ?: True
- Who am I ?: philippemo
- Configured git credential helpers: cache
- FastAI: N/A
- Tensorflow: N/A
- Torch: N/A
- Jinja2: 3.1.2
- Graphviz: N/A
- Pydot: N/A
- Pillow: 9.4.0
- hf_transfer: N/A
- gradio: N/A
- ENDPOINT: https://huggingface.co
- HUGGINGFACE_HUB_CACHE: /home/philippe/.cache/huggingface/hub
- HUGGINGFACE_ASSETS_CACHE: /home/philippe/.cache/huggingface/assets
- HF_TOKEN_PATH: /home/philippe/.cache/huggingface/token
- HF_HUB_OFFLINE: False
- HF_HUB_DISABLE_TELEMETRY: False
- HF_HUB_DISABLE_PROGRESS_BARS: None
- HF_HUB_DISABLE_SYMLINKS_WARNING: False
- HF_HUB_DISABLE_EXPERIMENTAL_WARNING: False
- HF_HUB_DISABLE_IMPLICIT_TOKEN: False
- HF_HUB_ENABLE_HF_TRANSFER: False
```
| 152 | Image Encoding Issue when submitting a Parquet Dataset
### Describe the bug
Hello,
I'd like to report an issue related to pushing a dataset represented as a Parquet file to a dataset repository using Dask. Here are the details:
We attempted to load an example dataset in Parquet format from the Hugging Face (HF) filesystem using Dask with the following code snippet:
```
import dask.dataframe as dd
df = dd.read_parquet("hf://datasets/lambdalabs/pokemon-blip-captions",index=False)
```
In this dataset, the "image" column is represented as a dictionary/struct with the format:
```
df = df.compute()
df["image"].iloc[0].keys()
-> dict_keys(['bytes', 'path'])
```
I think this is the format encoded by the [`Image`](https://huggingface.co/docs/datasets/v2.0.0/en/package_reference/main_classes#datasets.Image) feature extractor from datasets to format suitable for Arrow.
The next step was to push the dataset to a repository that I created:
```
dd.to_parquet(dask_df, path = "hf://datasets/philippemo/dummy_dataset/data")
```
However, after pushing the dataset using Dask, the "image" column is now represented as the encoded dictionary `(['bytes', 'path'])`, and the images are not properly visualized. You can find the dataset here: [Link to the problematic dataset](https://huggingface.co/datasets/philippemo/dummy_dataset).
It's worth noting that both the original dataset and the one submitted with Dask have the same schema with minor alterations related to metadata:
**[ Schema of original dummy example.](https://huggingface.co/datasets/lambdalabs/pokemon-blip-captions/blob/main/data/train-00000-of-00001-566cc9b19d7203f8.parquet)**
```
image: struct<bytes: binary, path: null>
child 0, bytes: binary
child 1, path: null
text: string
```
**[ Schema of pushed dataset with dask](https://huggingface.co/datasets/philippemo/dummy_dataset/blob/main/data/part.0.parquet)**
```
image: struct<bytes: binary, path: null>
child 0, bytes: binary
child 1, path: null
text: string
```
This issue seems to be related to an encoding type that occurs when pushing a model to the hub. Normally, models should be represented as an HF dataset before pushing, but we are working with an example where we need to push large datasets using Dask.
Could you please provide clarification on how to resolve this issue?
Thank you!
### Reproduction
To get the schema I downloaded the parquet files and used pyarrow.parquet to read the schema
```
import pyarrow.parquet
pyarrow.parquet.read_schema(<path_to_parquet>, memory_map=True)
```
### Logs
_No response_
### System info
```shell
- huggingface_hub version: 0.14.1
- Platform: Linux-5.19.0-41-generic-x86_64-with-glibc2.35
- Python version: 3.10.6
- Running in iPython ?: No
- Running in notebook ?: No
- Running in Google Colab ?: No
- Token path ?: /home/philippe/.cache/huggingface/token
- Has saved token ?: True
- Who am I ?: philippemo
- Configured git credential helpers: cache
- FastAI: N/A
- Tensorflow: N/A
- Torch: N/A
- Jinja2: 3.1.2
- Graphviz: N/A
- Pydot: N/A
- Pillow: 9.4.0
- hf_transfer: N/A
- gradio: N/A
- ENDPOINT: https://huggingface.co
- HUGGINGFACE_HUB_CACHE: /home/philippe/.cache/huggingface/hub
- HUGGINGFACE_ASSETS_CACHE: /home/philippe/.cache/huggingface/assets
- HF_TOKEN_PATH: /home/philippe/.cache/huggingface/token
- HF_HUB_OFFLINE: False
- HF_HUB_DISABLE_TELEMETRY: False
- HF_HUB_DISABLE_PROGRESS_BARS: None
- HF_HUB_DISABLE_SYMLINKS_WARNING: False
- HF_HUB_DISABLE_EXPERIMENTAL_WARNING: False
- HF_HUB_DISABLE_IMPLICIT_TOKEN: False
- HF_HUB_ENABLE_HF_TRANSFER: False
```
Thanks @Wauplin @lhoestq for the quick reply :)!
I tried your approach by passing the huggingface schema to the dask writer
```
from datasets import Features, Image, Value
df = dd.read_parquet(f"hf://datasets/lambdalabs/pokemon-blip-captions",index=False)
features = Features({"image": Image(), "text": Value("string")})
schema = features.arrow_schema
dd.to_parquet(df, path = "hf://datasets/philippemo/dummy_dataset/data", schema=schema)
```
At first it didn't work as I was not able to visualize the images, so then I manually added the `dataset_infos.json` from the example dataset and it worked :)
However, It's not very ideal since there are some metadata in that file that need to be computed in order to load the data properly such as `num_of_bytes` and `num_examples` which might be unknown in my use case.

Do you have any pointers there? you mentioned that `datasets_info.json` will be deprecated/legacy. Could you point me to some example image datasets on the hub that are stored as parquet and don't have the `datasets_info.json`?
|
https://github.com/huggingface/datasets/issues/5869 | Image Encoding Issue when submitting a Parquet Dataset | You don't need the dataset_infos.json file as long as you have the schema with HF metadata ;)
I could also check that it works fine myself on the git revision without the dataset_infos.json file.
What made you think it didn't work ? | ### Describe the bug
Hello,
I'd like to report an issue related to pushing a dataset represented as a Parquet file to a dataset repository using Dask. Here are the details:
We attempted to load an example dataset in Parquet format from the Hugging Face (HF) filesystem using Dask with the following code snippet:
```
import dask.dataframe as dd
df = dd.read_parquet("hf://datasets/lambdalabs/pokemon-blip-captions",index=False)
```
In this dataset, the "image" column is represented as a dictionary/struct with the format:
```
df = df.compute()
df["image"].iloc[0].keys()
-> dict_keys(['bytes', 'path'])
```
I think this is the format encoded by the [`Image`](https://huggingface.co/docs/datasets/v2.0.0/en/package_reference/main_classes#datasets.Image) feature extractor from datasets to format suitable for Arrow.
The next step was to push the dataset to a repository that I created:
```
dd.to_parquet(dask_df, path = "hf://datasets/philippemo/dummy_dataset/data")
```
However, after pushing the dataset using Dask, the "image" column is now represented as the encoded dictionary `(['bytes', 'path'])`, and the images are not properly visualized. You can find the dataset here: [Link to the problematic dataset](https://huggingface.co/datasets/philippemo/dummy_dataset).
It's worth noting that both the original dataset and the one submitted with Dask have the same schema with minor alterations related to metadata:
**[ Schema of original dummy example.](https://huggingface.co/datasets/lambdalabs/pokemon-blip-captions/blob/main/data/train-00000-of-00001-566cc9b19d7203f8.parquet)**
```
image: struct<bytes: binary, path: null>
child 0, bytes: binary
child 1, path: null
text: string
```
**[ Schema of pushed dataset with dask](https://huggingface.co/datasets/philippemo/dummy_dataset/blob/main/data/part.0.parquet)**
```
image: struct<bytes: binary, path: null>
child 0, bytes: binary
child 1, path: null
text: string
```
This issue seems to be related to an encoding type that occurs when pushing a model to the hub. Normally, models should be represented as an HF dataset before pushing, but we are working with an example where we need to push large datasets using Dask.
Could you please provide clarification on how to resolve this issue?
Thank you!
### Reproduction
To get the schema I downloaded the parquet files and used pyarrow.parquet to read the schema
```
import pyarrow.parquet
pyarrow.parquet.read_schema(<path_to_parquet>, memory_map=True)
```
### Logs
_No response_
### System info
```shell
- huggingface_hub version: 0.14.1
- Platform: Linux-5.19.0-41-generic-x86_64-with-glibc2.35
- Python version: 3.10.6
- Running in iPython ?: No
- Running in notebook ?: No
- Running in Google Colab ?: No
- Token path ?: /home/philippe/.cache/huggingface/token
- Has saved token ?: True
- Who am I ?: philippemo
- Configured git credential helpers: cache
- FastAI: N/A
- Tensorflow: N/A
- Torch: N/A
- Jinja2: 3.1.2
- Graphviz: N/A
- Pydot: N/A
- Pillow: 9.4.0
- hf_transfer: N/A
- gradio: N/A
- ENDPOINT: https://huggingface.co
- HUGGINGFACE_HUB_CACHE: /home/philippe/.cache/huggingface/hub
- HUGGINGFACE_ASSETS_CACHE: /home/philippe/.cache/huggingface/assets
- HF_TOKEN_PATH: /home/philippe/.cache/huggingface/token
- HF_HUB_OFFLINE: False
- HF_HUB_DISABLE_TELEMETRY: False
- HF_HUB_DISABLE_PROGRESS_BARS: None
- HF_HUB_DISABLE_SYMLINKS_WARNING: False
- HF_HUB_DISABLE_EXPERIMENTAL_WARNING: False
- HF_HUB_DISABLE_IMPLICIT_TOKEN: False
- HF_HUB_ENABLE_HF_TRANSFER: False
```
| 42 | Image Encoding Issue when submitting a Parquet Dataset
### Describe the bug
Hello,
I'd like to report an issue related to pushing a dataset represented as a Parquet file to a dataset repository using Dask. Here are the details:
We attempted to load an example dataset in Parquet format from the Hugging Face (HF) filesystem using Dask with the following code snippet:
```
import dask.dataframe as dd
df = dd.read_parquet("hf://datasets/lambdalabs/pokemon-blip-captions",index=False)
```
In this dataset, the "image" column is represented as a dictionary/struct with the format:
```
df = df.compute()
df["image"].iloc[0].keys()
-> dict_keys(['bytes', 'path'])
```
I think this is the format encoded by the [`Image`](https://huggingface.co/docs/datasets/v2.0.0/en/package_reference/main_classes#datasets.Image) feature extractor from datasets to format suitable for Arrow.
The next step was to push the dataset to a repository that I created:
```
dd.to_parquet(dask_df, path = "hf://datasets/philippemo/dummy_dataset/data")
```
However, after pushing the dataset using Dask, the "image" column is now represented as the encoded dictionary `(['bytes', 'path'])`, and the images are not properly visualized. You can find the dataset here: [Link to the problematic dataset](https://huggingface.co/datasets/philippemo/dummy_dataset).
It's worth noting that both the original dataset and the one submitted with Dask have the same schema with minor alterations related to metadata:
**[ Schema of original dummy example.](https://huggingface.co/datasets/lambdalabs/pokemon-blip-captions/blob/main/data/train-00000-of-00001-566cc9b19d7203f8.parquet)**
```
image: struct<bytes: binary, path: null>
child 0, bytes: binary
child 1, path: null
text: string
```
**[ Schema of pushed dataset with dask](https://huggingface.co/datasets/philippemo/dummy_dataset/blob/main/data/part.0.parquet)**
```
image: struct<bytes: binary, path: null>
child 0, bytes: binary
child 1, path: null
text: string
```
This issue seems to be related to an encoding type that occurs when pushing a model to the hub. Normally, models should be represented as an HF dataset before pushing, but we are working with an example where we need to push large datasets using Dask.
Could you please provide clarification on how to resolve this issue?
Thank you!
### Reproduction
To get the schema I downloaded the parquet files and used pyarrow.parquet to read the schema
```
import pyarrow.parquet
pyarrow.parquet.read_schema(<path_to_parquet>, memory_map=True)
```
### Logs
_No response_
### System info
```shell
- huggingface_hub version: 0.14.1
- Platform: Linux-5.19.0-41-generic-x86_64-with-glibc2.35
- Python version: 3.10.6
- Running in iPython ?: No
- Running in notebook ?: No
- Running in Google Colab ?: No
- Token path ?: /home/philippe/.cache/huggingface/token
- Has saved token ?: True
- Who am I ?: philippemo
- Configured git credential helpers: cache
- FastAI: N/A
- Tensorflow: N/A
- Torch: N/A
- Jinja2: 3.1.2
- Graphviz: N/A
- Pydot: N/A
- Pillow: 9.4.0
- hf_transfer: N/A
- gradio: N/A
- ENDPOINT: https://huggingface.co
- HUGGINGFACE_HUB_CACHE: /home/philippe/.cache/huggingface/hub
- HUGGINGFACE_ASSETS_CACHE: /home/philippe/.cache/huggingface/assets
- HF_TOKEN_PATH: /home/philippe/.cache/huggingface/token
- HF_HUB_OFFLINE: False
- HF_HUB_DISABLE_TELEMETRY: False
- HF_HUB_DISABLE_PROGRESS_BARS: None
- HF_HUB_DISABLE_SYMLINKS_WARNING: False
- HF_HUB_DISABLE_EXPERIMENTAL_WARNING: False
- HF_HUB_DISABLE_IMPLICIT_TOKEN: False
- HF_HUB_ENABLE_HF_TRANSFER: False
```
You don't need the dataset_infos.json file as long as you have the schema with HF metadata ;)
I could also check that it works fine myself on the git revision without the dataset_infos.json file.
What made you think it didn't work ? |
https://github.com/huggingface/datasets/issues/5869 | Image Encoding Issue when submitting a Parquet Dataset | > You don't need the dataset_infos.json file as long as you have the schema with HF metadata ;) I could also check that it works fine myself on the git revision without the dataset_infos.json file.
>
> What made you think it didn't work ?
Those are two identical dataset repos where both were pushed with dask with the specified schema you mentioned above. I then uploaded the `dataset_infos.json` manually taken from the original example dataset into one of them.
* **With schema**: https://huggingface.co/datasets/philippemo/dummy_dataset_with_schema
* **Without schema**: https://huggingface.co/datasets/philippemo/dummy_dataset_without_schema
You can see that in the examples without schema the images fail to render properly. When loaded with `datasets` they return an dict and not a Pillow Image | ### Describe the bug
Hello,
I'd like to report an issue related to pushing a dataset represented as a Parquet file to a dataset repository using Dask. Here are the details:
We attempted to load an example dataset in Parquet format from the Hugging Face (HF) filesystem using Dask with the following code snippet:
```
import dask.dataframe as dd
df = dd.read_parquet("hf://datasets/lambdalabs/pokemon-blip-captions",index=False)
```
In this dataset, the "image" column is represented as a dictionary/struct with the format:
```
df = df.compute()
df["image"].iloc[0].keys()
-> dict_keys(['bytes', 'path'])
```
I think this is the format encoded by the [`Image`](https://huggingface.co/docs/datasets/v2.0.0/en/package_reference/main_classes#datasets.Image) feature extractor from datasets to format suitable for Arrow.
The next step was to push the dataset to a repository that I created:
```
dd.to_parquet(dask_df, path = "hf://datasets/philippemo/dummy_dataset/data")
```
However, after pushing the dataset using Dask, the "image" column is now represented as the encoded dictionary `(['bytes', 'path'])`, and the images are not properly visualized. You can find the dataset here: [Link to the problematic dataset](https://huggingface.co/datasets/philippemo/dummy_dataset).
It's worth noting that both the original dataset and the one submitted with Dask have the same schema with minor alterations related to metadata:
**[ Schema of original dummy example.](https://huggingface.co/datasets/lambdalabs/pokemon-blip-captions/blob/main/data/train-00000-of-00001-566cc9b19d7203f8.parquet)**
```
image: struct<bytes: binary, path: null>
child 0, bytes: binary
child 1, path: null
text: string
```
**[ Schema of pushed dataset with dask](https://huggingface.co/datasets/philippemo/dummy_dataset/blob/main/data/part.0.parquet)**
```
image: struct<bytes: binary, path: null>
child 0, bytes: binary
child 1, path: null
text: string
```
This issue seems to be related to an encoding type that occurs when pushing a model to the hub. Normally, models should be represented as an HF dataset before pushing, but we are working with an example where we need to push large datasets using Dask.
Could you please provide clarification on how to resolve this issue?
Thank you!
### Reproduction
To get the schema I downloaded the parquet files and used pyarrow.parquet to read the schema
```
import pyarrow.parquet
pyarrow.parquet.read_schema(<path_to_parquet>, memory_map=True)
```
### Logs
_No response_
### System info
```shell
- huggingface_hub version: 0.14.1
- Platform: Linux-5.19.0-41-generic-x86_64-with-glibc2.35
- Python version: 3.10.6
- Running in iPython ?: No
- Running in notebook ?: No
- Running in Google Colab ?: No
- Token path ?: /home/philippe/.cache/huggingface/token
- Has saved token ?: True
- Who am I ?: philippemo
- Configured git credential helpers: cache
- FastAI: N/A
- Tensorflow: N/A
- Torch: N/A
- Jinja2: 3.1.2
- Graphviz: N/A
- Pydot: N/A
- Pillow: 9.4.0
- hf_transfer: N/A
- gradio: N/A
- ENDPOINT: https://huggingface.co
- HUGGINGFACE_HUB_CACHE: /home/philippe/.cache/huggingface/hub
- HUGGINGFACE_ASSETS_CACHE: /home/philippe/.cache/huggingface/assets
- HF_TOKEN_PATH: /home/philippe/.cache/huggingface/token
- HF_HUB_OFFLINE: False
- HF_HUB_DISABLE_TELEMETRY: False
- HF_HUB_DISABLE_PROGRESS_BARS: None
- HF_HUB_DISABLE_SYMLINKS_WARNING: False
- HF_HUB_DISABLE_EXPERIMENTAL_WARNING: False
- HF_HUB_DISABLE_IMPLICIT_TOKEN: False
- HF_HUB_ENABLE_HF_TRANSFER: False
```
| 116 | Image Encoding Issue when submitting a Parquet Dataset
### Describe the bug
Hello,
I'd like to report an issue related to pushing a dataset represented as a Parquet file to a dataset repository using Dask. Here are the details:
We attempted to load an example dataset in Parquet format from the Hugging Face (HF) filesystem using Dask with the following code snippet:
```
import dask.dataframe as dd
df = dd.read_parquet("hf://datasets/lambdalabs/pokemon-blip-captions",index=False)
```
In this dataset, the "image" column is represented as a dictionary/struct with the format:
```
df = df.compute()
df["image"].iloc[0].keys()
-> dict_keys(['bytes', 'path'])
```
I think this is the format encoded by the [`Image`](https://huggingface.co/docs/datasets/v2.0.0/en/package_reference/main_classes#datasets.Image) feature extractor from datasets to format suitable for Arrow.
The next step was to push the dataset to a repository that I created:
```
dd.to_parquet(dask_df, path = "hf://datasets/philippemo/dummy_dataset/data")
```
However, after pushing the dataset using Dask, the "image" column is now represented as the encoded dictionary `(['bytes', 'path'])`, and the images are not properly visualized. You can find the dataset here: [Link to the problematic dataset](https://huggingface.co/datasets/philippemo/dummy_dataset).
It's worth noting that both the original dataset and the one submitted with Dask have the same schema with minor alterations related to metadata:
**[ Schema of original dummy example.](https://huggingface.co/datasets/lambdalabs/pokemon-blip-captions/blob/main/data/train-00000-of-00001-566cc9b19d7203f8.parquet)**
```
image: struct<bytes: binary, path: null>
child 0, bytes: binary
child 1, path: null
text: string
```
**[ Schema of pushed dataset with dask](https://huggingface.co/datasets/philippemo/dummy_dataset/blob/main/data/part.0.parquet)**
```
image: struct<bytes: binary, path: null>
child 0, bytes: binary
child 1, path: null
text: string
```
This issue seems to be related to an encoding type that occurs when pushing a model to the hub. Normally, models should be represented as an HF dataset before pushing, but we are working with an example where we need to push large datasets using Dask.
Could you please provide clarification on how to resolve this issue?
Thank you!
### Reproduction
To get the schema I downloaded the parquet files and used pyarrow.parquet to read the schema
```
import pyarrow.parquet
pyarrow.parquet.read_schema(<path_to_parquet>, memory_map=True)
```
### Logs
_No response_
### System info
```shell
- huggingface_hub version: 0.14.1
- Platform: Linux-5.19.0-41-generic-x86_64-with-glibc2.35
- Python version: 3.10.6
- Running in iPython ?: No
- Running in notebook ?: No
- Running in Google Colab ?: No
- Token path ?: /home/philippe/.cache/huggingface/token
- Has saved token ?: True
- Who am I ?: philippemo
- Configured git credential helpers: cache
- FastAI: N/A
- Tensorflow: N/A
- Torch: N/A
- Jinja2: 3.1.2
- Graphviz: N/A
- Pydot: N/A
- Pillow: 9.4.0
- hf_transfer: N/A
- gradio: N/A
- ENDPOINT: https://huggingface.co
- HUGGINGFACE_HUB_CACHE: /home/philippe/.cache/huggingface/hub
- HUGGINGFACE_ASSETS_CACHE: /home/philippe/.cache/huggingface/assets
- HF_TOKEN_PATH: /home/philippe/.cache/huggingface/token
- HF_HUB_OFFLINE: False
- HF_HUB_DISABLE_TELEMETRY: False
- HF_HUB_DISABLE_PROGRESS_BARS: None
- HF_HUB_DISABLE_SYMLINKS_WARNING: False
- HF_HUB_DISABLE_EXPERIMENTAL_WARNING: False
- HF_HUB_DISABLE_IMPLICIT_TOKEN: False
- HF_HUB_ENABLE_HF_TRANSFER: False
```
> You don't need the dataset_infos.json file as long as you have the schema with HF metadata ;) I could also check that it works fine myself on the git revision without the dataset_infos.json file.
>
> What made you think it didn't work ?
Those are two identical dataset repos where both were pushed with dask with the specified schema you mentioned above. I then uploaded the `dataset_infos.json` manually taken from the original example dataset into one of them.
* **With schema**: https://huggingface.co/datasets/philippemo/dummy_dataset_with_schema
* **Without schema**: https://huggingface.co/datasets/philippemo/dummy_dataset_without_schema
You can see that in the examples without schema the images fail to render properly. When loaded with `datasets` they return an dict and not a Pillow Image |
https://github.com/huggingface/datasets/issues/5869 | Image Encoding Issue when submitting a Parquet Dataset | I see ! I think it's a bug on our side - it should work without the metadata - let me investigate | ### Describe the bug
Hello,
I'd like to report an issue related to pushing a dataset represented as a Parquet file to a dataset repository using Dask. Here are the details:
We attempted to load an example dataset in Parquet format from the Hugging Face (HF) filesystem using Dask with the following code snippet:
```
import dask.dataframe as dd
df = dd.read_parquet("hf://datasets/lambdalabs/pokemon-blip-captions",index=False)
```
In this dataset, the "image" column is represented as a dictionary/struct with the format:
```
df = df.compute()
df["image"].iloc[0].keys()
-> dict_keys(['bytes', 'path'])
```
I think this is the format encoded by the [`Image`](https://huggingface.co/docs/datasets/v2.0.0/en/package_reference/main_classes#datasets.Image) feature extractor from datasets to format suitable for Arrow.
The next step was to push the dataset to a repository that I created:
```
dd.to_parquet(dask_df, path = "hf://datasets/philippemo/dummy_dataset/data")
```
However, after pushing the dataset using Dask, the "image" column is now represented as the encoded dictionary `(['bytes', 'path'])`, and the images are not properly visualized. You can find the dataset here: [Link to the problematic dataset](https://huggingface.co/datasets/philippemo/dummy_dataset).
It's worth noting that both the original dataset and the one submitted with Dask have the same schema with minor alterations related to metadata:
**[ Schema of original dummy example.](https://huggingface.co/datasets/lambdalabs/pokemon-blip-captions/blob/main/data/train-00000-of-00001-566cc9b19d7203f8.parquet)**
```
image: struct<bytes: binary, path: null>
child 0, bytes: binary
child 1, path: null
text: string
```
**[ Schema of pushed dataset with dask](https://huggingface.co/datasets/philippemo/dummy_dataset/blob/main/data/part.0.parquet)**
```
image: struct<bytes: binary, path: null>
child 0, bytes: binary
child 1, path: null
text: string
```
This issue seems to be related to an encoding type that occurs when pushing a model to the hub. Normally, models should be represented as an HF dataset before pushing, but we are working with an example where we need to push large datasets using Dask.
Could you please provide clarification on how to resolve this issue?
Thank you!
### Reproduction
To get the schema I downloaded the parquet files and used pyarrow.parquet to read the schema
```
import pyarrow.parquet
pyarrow.parquet.read_schema(<path_to_parquet>, memory_map=True)
```
### Logs
_No response_
### System info
```shell
- huggingface_hub version: 0.14.1
- Platform: Linux-5.19.0-41-generic-x86_64-with-glibc2.35
- Python version: 3.10.6
- Running in iPython ?: No
- Running in notebook ?: No
- Running in Google Colab ?: No
- Token path ?: /home/philippe/.cache/huggingface/token
- Has saved token ?: True
- Who am I ?: philippemo
- Configured git credential helpers: cache
- FastAI: N/A
- Tensorflow: N/A
- Torch: N/A
- Jinja2: 3.1.2
- Graphviz: N/A
- Pydot: N/A
- Pillow: 9.4.0
- hf_transfer: N/A
- gradio: N/A
- ENDPOINT: https://huggingface.co
- HUGGINGFACE_HUB_CACHE: /home/philippe/.cache/huggingface/hub
- HUGGINGFACE_ASSETS_CACHE: /home/philippe/.cache/huggingface/assets
- HF_TOKEN_PATH: /home/philippe/.cache/huggingface/token
- HF_HUB_OFFLINE: False
- HF_HUB_DISABLE_TELEMETRY: False
- HF_HUB_DISABLE_PROGRESS_BARS: None
- HF_HUB_DISABLE_SYMLINKS_WARNING: False
- HF_HUB_DISABLE_EXPERIMENTAL_WARNING: False
- HF_HUB_DISABLE_IMPLICIT_TOKEN: False
- HF_HUB_ENABLE_HF_TRANSFER: False
```
| 22 | Image Encoding Issue when submitting a Parquet Dataset
### Describe the bug
Hello,
I'd like to report an issue related to pushing a dataset represented as a Parquet file to a dataset repository using Dask. Here are the details:
We attempted to load an example dataset in Parquet format from the Hugging Face (HF) filesystem using Dask with the following code snippet:
```
import dask.dataframe as dd
df = dd.read_parquet("hf://datasets/lambdalabs/pokemon-blip-captions",index=False)
```
In this dataset, the "image" column is represented as a dictionary/struct with the format:
```
df = df.compute()
df["image"].iloc[0].keys()
-> dict_keys(['bytes', 'path'])
```
I think this is the format encoded by the [`Image`](https://huggingface.co/docs/datasets/v2.0.0/en/package_reference/main_classes#datasets.Image) feature extractor from datasets to format suitable for Arrow.
The next step was to push the dataset to a repository that I created:
```
dd.to_parquet(dask_df, path = "hf://datasets/philippemo/dummy_dataset/data")
```
However, after pushing the dataset using Dask, the "image" column is now represented as the encoded dictionary `(['bytes', 'path'])`, and the images are not properly visualized. You can find the dataset here: [Link to the problematic dataset](https://huggingface.co/datasets/philippemo/dummy_dataset).
It's worth noting that both the original dataset and the one submitted with Dask have the same schema with minor alterations related to metadata:
**[ Schema of original dummy example.](https://huggingface.co/datasets/lambdalabs/pokemon-blip-captions/blob/main/data/train-00000-of-00001-566cc9b19d7203f8.parquet)**
```
image: struct<bytes: binary, path: null>
child 0, bytes: binary
child 1, path: null
text: string
```
**[ Schema of pushed dataset with dask](https://huggingface.co/datasets/philippemo/dummy_dataset/blob/main/data/part.0.parquet)**
```
image: struct<bytes: binary, path: null>
child 0, bytes: binary
child 1, path: null
text: string
```
This issue seems to be related to an encoding type that occurs when pushing a model to the hub. Normally, models should be represented as an HF dataset before pushing, but we are working with an example where we need to push large datasets using Dask.
Could you please provide clarification on how to resolve this issue?
Thank you!
### Reproduction
To get the schema I downloaded the parquet files and used pyarrow.parquet to read the schema
```
import pyarrow.parquet
pyarrow.parquet.read_schema(<path_to_parquet>, memory_map=True)
```
### Logs
_No response_
### System info
```shell
- huggingface_hub version: 0.14.1
- Platform: Linux-5.19.0-41-generic-x86_64-with-glibc2.35
- Python version: 3.10.6
- Running in iPython ?: No
- Running in notebook ?: No
- Running in Google Colab ?: No
- Token path ?: /home/philippe/.cache/huggingface/token
- Has saved token ?: True
- Who am I ?: philippemo
- Configured git credential helpers: cache
- FastAI: N/A
- Tensorflow: N/A
- Torch: N/A
- Jinja2: 3.1.2
- Graphviz: N/A
- Pydot: N/A
- Pillow: 9.4.0
- hf_transfer: N/A
- gradio: N/A
- ENDPOINT: https://huggingface.co
- HUGGINGFACE_HUB_CACHE: /home/philippe/.cache/huggingface/hub
- HUGGINGFACE_ASSETS_CACHE: /home/philippe/.cache/huggingface/assets
- HF_TOKEN_PATH: /home/philippe/.cache/huggingface/token
- HF_HUB_OFFLINE: False
- HF_HUB_DISABLE_TELEMETRY: False
- HF_HUB_DISABLE_PROGRESS_BARS: None
- HF_HUB_DISABLE_SYMLINKS_WARNING: False
- HF_HUB_DISABLE_EXPERIMENTAL_WARNING: False
- HF_HUB_DISABLE_IMPLICIT_TOKEN: False
- HF_HUB_ENABLE_HF_TRANSFER: False
```
I see ! I think it's a bug on our side - it should work without the metadata - let me investigate |
https://github.com/huggingface/datasets/issues/5869 | Image Encoding Issue when submitting a Parquet Dataset | Alright, it's fixed: https://huggingface.co/datasets/philippemo/dummy_dataset_without_schema
It shows the image correctly now - even without the extra metadata :) | ### Describe the bug
Hello,
I'd like to report an issue related to pushing a dataset represented as a Parquet file to a dataset repository using Dask. Here are the details:
We attempted to load an example dataset in Parquet format from the Hugging Face (HF) filesystem using Dask with the following code snippet:
```
import dask.dataframe as dd
df = dd.read_parquet("hf://datasets/lambdalabs/pokemon-blip-captions",index=False)
```
In this dataset, the "image" column is represented as a dictionary/struct with the format:
```
df = df.compute()
df["image"].iloc[0].keys()
-> dict_keys(['bytes', 'path'])
```
I think this is the format encoded by the [`Image`](https://huggingface.co/docs/datasets/v2.0.0/en/package_reference/main_classes#datasets.Image) feature extractor from datasets to format suitable for Arrow.
The next step was to push the dataset to a repository that I created:
```
dd.to_parquet(dask_df, path = "hf://datasets/philippemo/dummy_dataset/data")
```
However, after pushing the dataset using Dask, the "image" column is now represented as the encoded dictionary `(['bytes', 'path'])`, and the images are not properly visualized. You can find the dataset here: [Link to the problematic dataset](https://huggingface.co/datasets/philippemo/dummy_dataset).
It's worth noting that both the original dataset and the one submitted with Dask have the same schema with minor alterations related to metadata:
**[ Schema of original dummy example.](https://huggingface.co/datasets/lambdalabs/pokemon-blip-captions/blob/main/data/train-00000-of-00001-566cc9b19d7203f8.parquet)**
```
image: struct<bytes: binary, path: null>
child 0, bytes: binary
child 1, path: null
text: string
```
**[ Schema of pushed dataset with dask](https://huggingface.co/datasets/philippemo/dummy_dataset/blob/main/data/part.0.parquet)**
```
image: struct<bytes: binary, path: null>
child 0, bytes: binary
child 1, path: null
text: string
```
This issue seems to be related to an encoding type that occurs when pushing a model to the hub. Normally, models should be represented as an HF dataset before pushing, but we are working with an example where we need to push large datasets using Dask.
Could you please provide clarification on how to resolve this issue?
Thank you!
### Reproduction
To get the schema I downloaded the parquet files and used pyarrow.parquet to read the schema
```
import pyarrow.parquet
pyarrow.parquet.read_schema(<path_to_parquet>, memory_map=True)
```
### Logs
_No response_
### System info
```shell
- huggingface_hub version: 0.14.1
- Platform: Linux-5.19.0-41-generic-x86_64-with-glibc2.35
- Python version: 3.10.6
- Running in iPython ?: No
- Running in notebook ?: No
- Running in Google Colab ?: No
- Token path ?: /home/philippe/.cache/huggingface/token
- Has saved token ?: True
- Who am I ?: philippemo
- Configured git credential helpers: cache
- FastAI: N/A
- Tensorflow: N/A
- Torch: N/A
- Jinja2: 3.1.2
- Graphviz: N/A
- Pydot: N/A
- Pillow: 9.4.0
- hf_transfer: N/A
- gradio: N/A
- ENDPOINT: https://huggingface.co
- HUGGINGFACE_HUB_CACHE: /home/philippe/.cache/huggingface/hub
- HUGGINGFACE_ASSETS_CACHE: /home/philippe/.cache/huggingface/assets
- HF_TOKEN_PATH: /home/philippe/.cache/huggingface/token
- HF_HUB_OFFLINE: False
- HF_HUB_DISABLE_TELEMETRY: False
- HF_HUB_DISABLE_PROGRESS_BARS: None
- HF_HUB_DISABLE_SYMLINKS_WARNING: False
- HF_HUB_DISABLE_EXPERIMENTAL_WARNING: False
- HF_HUB_DISABLE_IMPLICIT_TOKEN: False
- HF_HUB_ENABLE_HF_TRANSFER: False
```
| 17 | Image Encoding Issue when submitting a Parquet Dataset
### Describe the bug
Hello,
I'd like to report an issue related to pushing a dataset represented as a Parquet file to a dataset repository using Dask. Here are the details:
We attempted to load an example dataset in Parquet format from the Hugging Face (HF) filesystem using Dask with the following code snippet:
```
import dask.dataframe as dd
df = dd.read_parquet("hf://datasets/lambdalabs/pokemon-blip-captions",index=False)
```
In this dataset, the "image" column is represented as a dictionary/struct with the format:
```
df = df.compute()
df["image"].iloc[0].keys()
-> dict_keys(['bytes', 'path'])
```
I think this is the format encoded by the [`Image`](https://huggingface.co/docs/datasets/v2.0.0/en/package_reference/main_classes#datasets.Image) feature extractor from datasets to format suitable for Arrow.
The next step was to push the dataset to a repository that I created:
```
dd.to_parquet(dask_df, path = "hf://datasets/philippemo/dummy_dataset/data")
```
However, after pushing the dataset using Dask, the "image" column is now represented as the encoded dictionary `(['bytes', 'path'])`, and the images are not properly visualized. You can find the dataset here: [Link to the problematic dataset](https://huggingface.co/datasets/philippemo/dummy_dataset).
It's worth noting that both the original dataset and the one submitted with Dask have the same schema with minor alterations related to metadata:
**[ Schema of original dummy example.](https://huggingface.co/datasets/lambdalabs/pokemon-blip-captions/blob/main/data/train-00000-of-00001-566cc9b19d7203f8.parquet)**
```
image: struct<bytes: binary, path: null>
child 0, bytes: binary
child 1, path: null
text: string
```
**[ Schema of pushed dataset with dask](https://huggingface.co/datasets/philippemo/dummy_dataset/blob/main/data/part.0.parquet)**
```
image: struct<bytes: binary, path: null>
child 0, bytes: binary
child 1, path: null
text: string
```
This issue seems to be related to an encoding type that occurs when pushing a model to the hub. Normally, models should be represented as an HF dataset before pushing, but we are working with an example where we need to push large datasets using Dask.
Could you please provide clarification on how to resolve this issue?
Thank you!
### Reproduction
To get the schema I downloaded the parquet files and used pyarrow.parquet to read the schema
```
import pyarrow.parquet
pyarrow.parquet.read_schema(<path_to_parquet>, memory_map=True)
```
### Logs
_No response_
### System info
```shell
- huggingface_hub version: 0.14.1
- Platform: Linux-5.19.0-41-generic-x86_64-with-glibc2.35
- Python version: 3.10.6
- Running in iPython ?: No
- Running in notebook ?: No
- Running in Google Colab ?: No
- Token path ?: /home/philippe/.cache/huggingface/token
- Has saved token ?: True
- Who am I ?: philippemo
- Configured git credential helpers: cache
- FastAI: N/A
- Tensorflow: N/A
- Torch: N/A
- Jinja2: 3.1.2
- Graphviz: N/A
- Pydot: N/A
- Pillow: 9.4.0
- hf_transfer: N/A
- gradio: N/A
- ENDPOINT: https://huggingface.co
- HUGGINGFACE_HUB_CACHE: /home/philippe/.cache/huggingface/hub
- HUGGINGFACE_ASSETS_CACHE: /home/philippe/.cache/huggingface/assets
- HF_TOKEN_PATH: /home/philippe/.cache/huggingface/token
- HF_HUB_OFFLINE: False
- HF_HUB_DISABLE_TELEMETRY: False
- HF_HUB_DISABLE_PROGRESS_BARS: None
- HF_HUB_DISABLE_SYMLINKS_WARNING: False
- HF_HUB_DISABLE_EXPERIMENTAL_WARNING: False
- HF_HUB_DISABLE_IMPLICIT_TOKEN: False
- HF_HUB_ENABLE_HF_TRANSFER: False
```
Alright, it's fixed: https://huggingface.co/datasets/philippemo/dummy_dataset_without_schema
It shows the image correctly now - even without the extra metadata :) |
https://github.com/huggingface/datasets/issues/5869 | Image Encoding Issue when submitting a Parquet Dataset | Thanks @lhoestq!
I tested pushing a dataset again without the metadata and it works perfectly!
I appreciate the help | ### Describe the bug
Hello,
I'd like to report an issue related to pushing a dataset represented as a Parquet file to a dataset repository using Dask. Here are the details:
We attempted to load an example dataset in Parquet format from the Hugging Face (HF) filesystem using Dask with the following code snippet:
```
import dask.dataframe as dd
df = dd.read_parquet("hf://datasets/lambdalabs/pokemon-blip-captions",index=False)
```
In this dataset, the "image" column is represented as a dictionary/struct with the format:
```
df = df.compute()
df["image"].iloc[0].keys()
-> dict_keys(['bytes', 'path'])
```
I think this is the format encoded by the [`Image`](https://huggingface.co/docs/datasets/v2.0.0/en/package_reference/main_classes#datasets.Image) feature extractor from datasets to format suitable for Arrow.
The next step was to push the dataset to a repository that I created:
```
dd.to_parquet(dask_df, path = "hf://datasets/philippemo/dummy_dataset/data")
```
However, after pushing the dataset using Dask, the "image" column is now represented as the encoded dictionary `(['bytes', 'path'])`, and the images are not properly visualized. You can find the dataset here: [Link to the problematic dataset](https://huggingface.co/datasets/philippemo/dummy_dataset).
It's worth noting that both the original dataset and the one submitted with Dask have the same schema with minor alterations related to metadata:
**[ Schema of original dummy example.](https://huggingface.co/datasets/lambdalabs/pokemon-blip-captions/blob/main/data/train-00000-of-00001-566cc9b19d7203f8.parquet)**
```
image: struct<bytes: binary, path: null>
child 0, bytes: binary
child 1, path: null
text: string
```
**[ Schema of pushed dataset with dask](https://huggingface.co/datasets/philippemo/dummy_dataset/blob/main/data/part.0.parquet)**
```
image: struct<bytes: binary, path: null>
child 0, bytes: binary
child 1, path: null
text: string
```
This issue seems to be related to an encoding type that occurs when pushing a model to the hub. Normally, models should be represented as an HF dataset before pushing, but we are working with an example where we need to push large datasets using Dask.
Could you please provide clarification on how to resolve this issue?
Thank you!
### Reproduction
To get the schema I downloaded the parquet files and used pyarrow.parquet to read the schema
```
import pyarrow.parquet
pyarrow.parquet.read_schema(<path_to_parquet>, memory_map=True)
```
### Logs
_No response_
### System info
```shell
- huggingface_hub version: 0.14.1
- Platform: Linux-5.19.0-41-generic-x86_64-with-glibc2.35
- Python version: 3.10.6
- Running in iPython ?: No
- Running in notebook ?: No
- Running in Google Colab ?: No
- Token path ?: /home/philippe/.cache/huggingface/token
- Has saved token ?: True
- Who am I ?: philippemo
- Configured git credential helpers: cache
- FastAI: N/A
- Tensorflow: N/A
- Torch: N/A
- Jinja2: 3.1.2
- Graphviz: N/A
- Pydot: N/A
- Pillow: 9.4.0
- hf_transfer: N/A
- gradio: N/A
- ENDPOINT: https://huggingface.co
- HUGGINGFACE_HUB_CACHE: /home/philippe/.cache/huggingface/hub
- HUGGINGFACE_ASSETS_CACHE: /home/philippe/.cache/huggingface/assets
- HF_TOKEN_PATH: /home/philippe/.cache/huggingface/token
- HF_HUB_OFFLINE: False
- HF_HUB_DISABLE_TELEMETRY: False
- HF_HUB_DISABLE_PROGRESS_BARS: None
- HF_HUB_DISABLE_SYMLINKS_WARNING: False
- HF_HUB_DISABLE_EXPERIMENTAL_WARNING: False
- HF_HUB_DISABLE_IMPLICIT_TOKEN: False
- HF_HUB_ENABLE_HF_TRANSFER: False
```
| 19 | Image Encoding Issue when submitting a Parquet Dataset
### Describe the bug
Hello,
I'd like to report an issue related to pushing a dataset represented as a Parquet file to a dataset repository using Dask. Here are the details:
We attempted to load an example dataset in Parquet format from the Hugging Face (HF) filesystem using Dask with the following code snippet:
```
import dask.dataframe as dd
df = dd.read_parquet("hf://datasets/lambdalabs/pokemon-blip-captions",index=False)
```
In this dataset, the "image" column is represented as a dictionary/struct with the format:
```
df = df.compute()
df["image"].iloc[0].keys()
-> dict_keys(['bytes', 'path'])
```
I think this is the format encoded by the [`Image`](https://huggingface.co/docs/datasets/v2.0.0/en/package_reference/main_classes#datasets.Image) feature extractor from datasets to format suitable for Arrow.
The next step was to push the dataset to a repository that I created:
```
dd.to_parquet(dask_df, path = "hf://datasets/philippemo/dummy_dataset/data")
```
However, after pushing the dataset using Dask, the "image" column is now represented as the encoded dictionary `(['bytes', 'path'])`, and the images are not properly visualized. You can find the dataset here: [Link to the problematic dataset](https://huggingface.co/datasets/philippemo/dummy_dataset).
It's worth noting that both the original dataset and the one submitted with Dask have the same schema with minor alterations related to metadata:
**[ Schema of original dummy example.](https://huggingface.co/datasets/lambdalabs/pokemon-blip-captions/blob/main/data/train-00000-of-00001-566cc9b19d7203f8.parquet)**
```
image: struct<bytes: binary, path: null>
child 0, bytes: binary
child 1, path: null
text: string
```
**[ Schema of pushed dataset with dask](https://huggingface.co/datasets/philippemo/dummy_dataset/blob/main/data/part.0.parquet)**
```
image: struct<bytes: binary, path: null>
child 0, bytes: binary
child 1, path: null
text: string
```
This issue seems to be related to an encoding type that occurs when pushing a model to the hub. Normally, models should be represented as an HF dataset before pushing, but we are working with an example where we need to push large datasets using Dask.
Could you please provide clarification on how to resolve this issue?
Thank you!
### Reproduction
To get the schema I downloaded the parquet files and used pyarrow.parquet to read the schema
```
import pyarrow.parquet
pyarrow.parquet.read_schema(<path_to_parquet>, memory_map=True)
```
### Logs
_No response_
### System info
```shell
- huggingface_hub version: 0.14.1
- Platform: Linux-5.19.0-41-generic-x86_64-with-glibc2.35
- Python version: 3.10.6
- Running in iPython ?: No
- Running in notebook ?: No
- Running in Google Colab ?: No
- Token path ?: /home/philippe/.cache/huggingface/token
- Has saved token ?: True
- Who am I ?: philippemo
- Configured git credential helpers: cache
- FastAI: N/A
- Tensorflow: N/A
- Torch: N/A
- Jinja2: 3.1.2
- Graphviz: N/A
- Pydot: N/A
- Pillow: 9.4.0
- hf_transfer: N/A
- gradio: N/A
- ENDPOINT: https://huggingface.co
- HUGGINGFACE_HUB_CACHE: /home/philippe/.cache/huggingface/hub
- HUGGINGFACE_ASSETS_CACHE: /home/philippe/.cache/huggingface/assets
- HF_TOKEN_PATH: /home/philippe/.cache/huggingface/token
- HF_HUB_OFFLINE: False
- HF_HUB_DISABLE_TELEMETRY: False
- HF_HUB_DISABLE_PROGRESS_BARS: None
- HF_HUB_DISABLE_SYMLINKS_WARNING: False
- HF_HUB_DISABLE_EXPERIMENTAL_WARNING: False
- HF_HUB_DISABLE_IMPLICIT_TOKEN: False
- HF_HUB_ENABLE_HF_TRANSFER: False
```
Thanks @lhoestq!
I tested pushing a dataset again without the metadata and it works perfectly!
I appreciate the help |
https://github.com/huggingface/datasets/issues/5869 | Image Encoding Issue when submitting a Parquet Dataset | Hi @lhoestq,
I'v tried pushing another dataset again and I think the issue reappeared again:
```
df = dd.read_parquet(f"hf://datasets/lambdalabs/pokemon-blip-captions")
features = datasets.Features({"image": datasets.Image(), "text": datasets.Value("string")})
schema = features.arrow_schema
dd.to_parquet(df, path = "hf://datasets/philippemo/dummy_dataset_without_schema_12_06/data", schema=schema)
```
Here is the dataset:
https://huggingface.co/datasets/philippemo/dummy_dataset_without_schema_12_06
The one that was working 2 weeks ago still seems to be intact though, it might be that It rendered properly when it was initially submitted and after this something was reverted from your side:
https://huggingface.co/datasets/philippemo/dummy_dataset_without_schema
It's weird because nothing really changed from the implementation, might be another issue in the hub backend. Do you have any pointers on how to resolve this? | ### Describe the bug
Hello,
I'd like to report an issue related to pushing a dataset represented as a Parquet file to a dataset repository using Dask. Here are the details:
We attempted to load an example dataset in Parquet format from the Hugging Face (HF) filesystem using Dask with the following code snippet:
```
import dask.dataframe as dd
df = dd.read_parquet("hf://datasets/lambdalabs/pokemon-blip-captions",index=False)
```
In this dataset, the "image" column is represented as a dictionary/struct with the format:
```
df = df.compute()
df["image"].iloc[0].keys()
-> dict_keys(['bytes', 'path'])
```
I think this is the format encoded by the [`Image`](https://huggingface.co/docs/datasets/v2.0.0/en/package_reference/main_classes#datasets.Image) feature extractor from datasets to format suitable for Arrow.
The next step was to push the dataset to a repository that I created:
```
dd.to_parquet(dask_df, path = "hf://datasets/philippemo/dummy_dataset/data")
```
However, after pushing the dataset using Dask, the "image" column is now represented as the encoded dictionary `(['bytes', 'path'])`, and the images are not properly visualized. You can find the dataset here: [Link to the problematic dataset](https://huggingface.co/datasets/philippemo/dummy_dataset).
It's worth noting that both the original dataset and the one submitted with Dask have the same schema with minor alterations related to metadata:
**[ Schema of original dummy example.](https://huggingface.co/datasets/lambdalabs/pokemon-blip-captions/blob/main/data/train-00000-of-00001-566cc9b19d7203f8.parquet)**
```
image: struct<bytes: binary, path: null>
child 0, bytes: binary
child 1, path: null
text: string
```
**[ Schema of pushed dataset with dask](https://huggingface.co/datasets/philippemo/dummy_dataset/blob/main/data/part.0.parquet)**
```
image: struct<bytes: binary, path: null>
child 0, bytes: binary
child 1, path: null
text: string
```
This issue seems to be related to an encoding type that occurs when pushing a model to the hub. Normally, models should be represented as an HF dataset before pushing, but we are working with an example where we need to push large datasets using Dask.
Could you please provide clarification on how to resolve this issue?
Thank you!
### Reproduction
To get the schema I downloaded the parquet files and used pyarrow.parquet to read the schema
```
import pyarrow.parquet
pyarrow.parquet.read_schema(<path_to_parquet>, memory_map=True)
```
### Logs
_No response_
### System info
```shell
- huggingface_hub version: 0.14.1
- Platform: Linux-5.19.0-41-generic-x86_64-with-glibc2.35
- Python version: 3.10.6
- Running in iPython ?: No
- Running in notebook ?: No
- Running in Google Colab ?: No
- Token path ?: /home/philippe/.cache/huggingface/token
- Has saved token ?: True
- Who am I ?: philippemo
- Configured git credential helpers: cache
- FastAI: N/A
- Tensorflow: N/A
- Torch: N/A
- Jinja2: 3.1.2
- Graphviz: N/A
- Pydot: N/A
- Pillow: 9.4.0
- hf_transfer: N/A
- gradio: N/A
- ENDPOINT: https://huggingface.co
- HUGGINGFACE_HUB_CACHE: /home/philippe/.cache/huggingface/hub
- HUGGINGFACE_ASSETS_CACHE: /home/philippe/.cache/huggingface/assets
- HF_TOKEN_PATH: /home/philippe/.cache/huggingface/token
- HF_HUB_OFFLINE: False
- HF_HUB_DISABLE_TELEMETRY: False
- HF_HUB_DISABLE_PROGRESS_BARS: None
- HF_HUB_DISABLE_SYMLINKS_WARNING: False
- HF_HUB_DISABLE_EXPERIMENTAL_WARNING: False
- HF_HUB_DISABLE_IMPLICIT_TOKEN: False
- HF_HUB_ENABLE_HF_TRANSFER: False
```
| 102 | Image Encoding Issue when submitting a Parquet Dataset
### Describe the bug
Hello,
I'd like to report an issue related to pushing a dataset represented as a Parquet file to a dataset repository using Dask. Here are the details:
We attempted to load an example dataset in Parquet format from the Hugging Face (HF) filesystem using Dask with the following code snippet:
```
import dask.dataframe as dd
df = dd.read_parquet("hf://datasets/lambdalabs/pokemon-blip-captions",index=False)
```
In this dataset, the "image" column is represented as a dictionary/struct with the format:
```
df = df.compute()
df["image"].iloc[0].keys()
-> dict_keys(['bytes', 'path'])
```
I think this is the format encoded by the [`Image`](https://huggingface.co/docs/datasets/v2.0.0/en/package_reference/main_classes#datasets.Image) feature extractor from datasets to format suitable for Arrow.
The next step was to push the dataset to a repository that I created:
```
dd.to_parquet(dask_df, path = "hf://datasets/philippemo/dummy_dataset/data")
```
However, after pushing the dataset using Dask, the "image" column is now represented as the encoded dictionary `(['bytes', 'path'])`, and the images are not properly visualized. You can find the dataset here: [Link to the problematic dataset](https://huggingface.co/datasets/philippemo/dummy_dataset).
It's worth noting that both the original dataset and the one submitted with Dask have the same schema with minor alterations related to metadata:
**[ Schema of original dummy example.](https://huggingface.co/datasets/lambdalabs/pokemon-blip-captions/blob/main/data/train-00000-of-00001-566cc9b19d7203f8.parquet)**
```
image: struct<bytes: binary, path: null>
child 0, bytes: binary
child 1, path: null
text: string
```
**[ Schema of pushed dataset with dask](https://huggingface.co/datasets/philippemo/dummy_dataset/blob/main/data/part.0.parquet)**
```
image: struct<bytes: binary, path: null>
child 0, bytes: binary
child 1, path: null
text: string
```
This issue seems to be related to an encoding type that occurs when pushing a model to the hub. Normally, models should be represented as an HF dataset before pushing, but we are working with an example where we need to push large datasets using Dask.
Could you please provide clarification on how to resolve this issue?
Thank you!
### Reproduction
To get the schema I downloaded the parquet files and used pyarrow.parquet to read the schema
```
import pyarrow.parquet
pyarrow.parquet.read_schema(<path_to_parquet>, memory_map=True)
```
### Logs
_No response_
### System info
```shell
- huggingface_hub version: 0.14.1
- Platform: Linux-5.19.0-41-generic-x86_64-with-glibc2.35
- Python version: 3.10.6
- Running in iPython ?: No
- Running in notebook ?: No
- Running in Google Colab ?: No
- Token path ?: /home/philippe/.cache/huggingface/token
- Has saved token ?: True
- Who am I ?: philippemo
- Configured git credential helpers: cache
- FastAI: N/A
- Tensorflow: N/A
- Torch: N/A
- Jinja2: 3.1.2
- Graphviz: N/A
- Pydot: N/A
- Pillow: 9.4.0
- hf_transfer: N/A
- gradio: N/A
- ENDPOINT: https://huggingface.co
- HUGGINGFACE_HUB_CACHE: /home/philippe/.cache/huggingface/hub
- HUGGINGFACE_ASSETS_CACHE: /home/philippe/.cache/huggingface/assets
- HF_TOKEN_PATH: /home/philippe/.cache/huggingface/token
- HF_HUB_OFFLINE: False
- HF_HUB_DISABLE_TELEMETRY: False
- HF_HUB_DISABLE_PROGRESS_BARS: None
- HF_HUB_DISABLE_SYMLINKS_WARNING: False
- HF_HUB_DISABLE_EXPERIMENTAL_WARNING: False
- HF_HUB_DISABLE_IMPLICIT_TOKEN: False
- HF_HUB_ENABLE_HF_TRANSFER: False
```
Hi @lhoestq,
I'v tried pushing another dataset again and I think the issue reappeared again:
```
df = dd.read_parquet(f"hf://datasets/lambdalabs/pokemon-blip-captions")
features = datasets.Features({"image": datasets.Image(), "text": datasets.Value("string")})
schema = features.arrow_schema
dd.to_parquet(df, path = "hf://datasets/philippemo/dummy_dataset_without_schema_12_06/data", schema=schema)
```
Here is the dataset:
https://huggingface.co/datasets/philippemo/dummy_dataset_without_schema_12_06
The one that was working 2 weeks ago still seems to be intact though, it might be that It rendered properly when it was initially submitted and after this something was reverted from your side:
https://huggingface.co/datasets/philippemo/dummy_dataset_without_schema
It's weird because nothing really changed from the implementation, might be another issue in the hub backend. Do you have any pointers on how to resolve this? |
https://github.com/huggingface/datasets/issues/5869 | Image Encoding Issue when submitting a Parquet Dataset | We're doing some changes in the way we're handling image parquet datasets right now. We'll include the fix from https://github.com/huggingface/datasets/pull/5921 in the new datasets-server version in the coming days | ### Describe the bug
Hello,
I'd like to report an issue related to pushing a dataset represented as a Parquet file to a dataset repository using Dask. Here are the details:
We attempted to load an example dataset in Parquet format from the Hugging Face (HF) filesystem using Dask with the following code snippet:
```
import dask.dataframe as dd
df = dd.read_parquet("hf://datasets/lambdalabs/pokemon-blip-captions",index=False)
```
In this dataset, the "image" column is represented as a dictionary/struct with the format:
```
df = df.compute()
df["image"].iloc[0].keys()
-> dict_keys(['bytes', 'path'])
```
I think this is the format encoded by the [`Image`](https://huggingface.co/docs/datasets/v2.0.0/en/package_reference/main_classes#datasets.Image) feature extractor from datasets to format suitable for Arrow.
The next step was to push the dataset to a repository that I created:
```
dd.to_parquet(dask_df, path = "hf://datasets/philippemo/dummy_dataset/data")
```
However, after pushing the dataset using Dask, the "image" column is now represented as the encoded dictionary `(['bytes', 'path'])`, and the images are not properly visualized. You can find the dataset here: [Link to the problematic dataset](https://huggingface.co/datasets/philippemo/dummy_dataset).
It's worth noting that both the original dataset and the one submitted with Dask have the same schema with minor alterations related to metadata:
**[ Schema of original dummy example.](https://huggingface.co/datasets/lambdalabs/pokemon-blip-captions/blob/main/data/train-00000-of-00001-566cc9b19d7203f8.parquet)**
```
image: struct<bytes: binary, path: null>
child 0, bytes: binary
child 1, path: null
text: string
```
**[ Schema of pushed dataset with dask](https://huggingface.co/datasets/philippemo/dummy_dataset/blob/main/data/part.0.parquet)**
```
image: struct<bytes: binary, path: null>
child 0, bytes: binary
child 1, path: null
text: string
```
This issue seems to be related to an encoding type that occurs when pushing a model to the hub. Normally, models should be represented as an HF dataset before pushing, but we are working with an example where we need to push large datasets using Dask.
Could you please provide clarification on how to resolve this issue?
Thank you!
### Reproduction
To get the schema I downloaded the parquet files and used pyarrow.parquet to read the schema
```
import pyarrow.parquet
pyarrow.parquet.read_schema(<path_to_parquet>, memory_map=True)
```
### Logs
_No response_
### System info
```shell
- huggingface_hub version: 0.14.1
- Platform: Linux-5.19.0-41-generic-x86_64-with-glibc2.35
- Python version: 3.10.6
- Running in iPython ?: No
- Running in notebook ?: No
- Running in Google Colab ?: No
- Token path ?: /home/philippe/.cache/huggingface/token
- Has saved token ?: True
- Who am I ?: philippemo
- Configured git credential helpers: cache
- FastAI: N/A
- Tensorflow: N/A
- Torch: N/A
- Jinja2: 3.1.2
- Graphviz: N/A
- Pydot: N/A
- Pillow: 9.4.0
- hf_transfer: N/A
- gradio: N/A
- ENDPOINT: https://huggingface.co
- HUGGINGFACE_HUB_CACHE: /home/philippe/.cache/huggingface/hub
- HUGGINGFACE_ASSETS_CACHE: /home/philippe/.cache/huggingface/assets
- HF_TOKEN_PATH: /home/philippe/.cache/huggingface/token
- HF_HUB_OFFLINE: False
- HF_HUB_DISABLE_TELEMETRY: False
- HF_HUB_DISABLE_PROGRESS_BARS: None
- HF_HUB_DISABLE_SYMLINKS_WARNING: False
- HF_HUB_DISABLE_EXPERIMENTAL_WARNING: False
- HF_HUB_DISABLE_IMPLICIT_TOKEN: False
- HF_HUB_ENABLE_HF_TRANSFER: False
```
| 29 | Image Encoding Issue when submitting a Parquet Dataset
### Describe the bug
Hello,
I'd like to report an issue related to pushing a dataset represented as a Parquet file to a dataset repository using Dask. Here are the details:
We attempted to load an example dataset in Parquet format from the Hugging Face (HF) filesystem using Dask with the following code snippet:
```
import dask.dataframe as dd
df = dd.read_parquet("hf://datasets/lambdalabs/pokemon-blip-captions",index=False)
```
In this dataset, the "image" column is represented as a dictionary/struct with the format:
```
df = df.compute()
df["image"].iloc[0].keys()
-> dict_keys(['bytes', 'path'])
```
I think this is the format encoded by the [`Image`](https://huggingface.co/docs/datasets/v2.0.0/en/package_reference/main_classes#datasets.Image) feature extractor from datasets to format suitable for Arrow.
The next step was to push the dataset to a repository that I created:
```
dd.to_parquet(dask_df, path = "hf://datasets/philippemo/dummy_dataset/data")
```
However, after pushing the dataset using Dask, the "image" column is now represented as the encoded dictionary `(['bytes', 'path'])`, and the images are not properly visualized. You can find the dataset here: [Link to the problematic dataset](https://huggingface.co/datasets/philippemo/dummy_dataset).
It's worth noting that both the original dataset and the one submitted with Dask have the same schema with minor alterations related to metadata:
**[ Schema of original dummy example.](https://huggingface.co/datasets/lambdalabs/pokemon-blip-captions/blob/main/data/train-00000-of-00001-566cc9b19d7203f8.parquet)**
```
image: struct<bytes: binary, path: null>
child 0, bytes: binary
child 1, path: null
text: string
```
**[ Schema of pushed dataset with dask](https://huggingface.co/datasets/philippemo/dummy_dataset/blob/main/data/part.0.parquet)**
```
image: struct<bytes: binary, path: null>
child 0, bytes: binary
child 1, path: null
text: string
```
This issue seems to be related to an encoding type that occurs when pushing a model to the hub. Normally, models should be represented as an HF dataset before pushing, but we are working with an example where we need to push large datasets using Dask.
Could you please provide clarification on how to resolve this issue?
Thank you!
### Reproduction
To get the schema I downloaded the parquet files and used pyarrow.parquet to read the schema
```
import pyarrow.parquet
pyarrow.parquet.read_schema(<path_to_parquet>, memory_map=True)
```
### Logs
_No response_
### System info
```shell
- huggingface_hub version: 0.14.1
- Platform: Linux-5.19.0-41-generic-x86_64-with-glibc2.35
- Python version: 3.10.6
- Running in iPython ?: No
- Running in notebook ?: No
- Running in Google Colab ?: No
- Token path ?: /home/philippe/.cache/huggingface/token
- Has saved token ?: True
- Who am I ?: philippemo
- Configured git credential helpers: cache
- FastAI: N/A
- Tensorflow: N/A
- Torch: N/A
- Jinja2: 3.1.2
- Graphviz: N/A
- Pydot: N/A
- Pillow: 9.4.0
- hf_transfer: N/A
- gradio: N/A
- ENDPOINT: https://huggingface.co
- HUGGINGFACE_HUB_CACHE: /home/philippe/.cache/huggingface/hub
- HUGGINGFACE_ASSETS_CACHE: /home/philippe/.cache/huggingface/assets
- HF_TOKEN_PATH: /home/philippe/.cache/huggingface/token
- HF_HUB_OFFLINE: False
- HF_HUB_DISABLE_TELEMETRY: False
- HF_HUB_DISABLE_PROGRESS_BARS: None
- HF_HUB_DISABLE_SYMLINKS_WARNING: False
- HF_HUB_DISABLE_EXPERIMENTAL_WARNING: False
- HF_HUB_DISABLE_IMPLICIT_TOKEN: False
- HF_HUB_ENABLE_HF_TRANSFER: False
```
We're doing some changes in the way we're handling image parquet datasets right now. We'll include the fix from https://github.com/huggingface/datasets/pull/5921 in the new datasets-server version in the coming days |
https://github.com/huggingface/datasets/issues/5869 | Image Encoding Issue when submitting a Parquet Dataset | alright thanks for the update :), would that be part of the new release of datasets or is it something separate? if so, where can I track it? | ### Describe the bug
Hello,
I'd like to report an issue related to pushing a dataset represented as a Parquet file to a dataset repository using Dask. Here are the details:
We attempted to load an example dataset in Parquet format from the Hugging Face (HF) filesystem using Dask with the following code snippet:
```
import dask.dataframe as dd
df = dd.read_parquet("hf://datasets/lambdalabs/pokemon-blip-captions",index=False)
```
In this dataset, the "image" column is represented as a dictionary/struct with the format:
```
df = df.compute()
df["image"].iloc[0].keys()
-> dict_keys(['bytes', 'path'])
```
I think this is the format encoded by the [`Image`](https://huggingface.co/docs/datasets/v2.0.0/en/package_reference/main_classes#datasets.Image) feature extractor from datasets to format suitable for Arrow.
The next step was to push the dataset to a repository that I created:
```
dd.to_parquet(dask_df, path = "hf://datasets/philippemo/dummy_dataset/data")
```
However, after pushing the dataset using Dask, the "image" column is now represented as the encoded dictionary `(['bytes', 'path'])`, and the images are not properly visualized. You can find the dataset here: [Link to the problematic dataset](https://huggingface.co/datasets/philippemo/dummy_dataset).
It's worth noting that both the original dataset and the one submitted with Dask have the same schema with minor alterations related to metadata:
**[ Schema of original dummy example.](https://huggingface.co/datasets/lambdalabs/pokemon-blip-captions/blob/main/data/train-00000-of-00001-566cc9b19d7203f8.parquet)**
```
image: struct<bytes: binary, path: null>
child 0, bytes: binary
child 1, path: null
text: string
```
**[ Schema of pushed dataset with dask](https://huggingface.co/datasets/philippemo/dummy_dataset/blob/main/data/part.0.parquet)**
```
image: struct<bytes: binary, path: null>
child 0, bytes: binary
child 1, path: null
text: string
```
This issue seems to be related to an encoding type that occurs when pushing a model to the hub. Normally, models should be represented as an HF dataset before pushing, but we are working with an example where we need to push large datasets using Dask.
Could you please provide clarification on how to resolve this issue?
Thank you!
### Reproduction
To get the schema I downloaded the parquet files and used pyarrow.parquet to read the schema
```
import pyarrow.parquet
pyarrow.parquet.read_schema(<path_to_parquet>, memory_map=True)
```
### Logs
_No response_
### System info
```shell
- huggingface_hub version: 0.14.1
- Platform: Linux-5.19.0-41-generic-x86_64-with-glibc2.35
- Python version: 3.10.6
- Running in iPython ?: No
- Running in notebook ?: No
- Running in Google Colab ?: No
- Token path ?: /home/philippe/.cache/huggingface/token
- Has saved token ?: True
- Who am I ?: philippemo
- Configured git credential helpers: cache
- FastAI: N/A
- Tensorflow: N/A
- Torch: N/A
- Jinja2: 3.1.2
- Graphviz: N/A
- Pydot: N/A
- Pillow: 9.4.0
- hf_transfer: N/A
- gradio: N/A
- ENDPOINT: https://huggingface.co
- HUGGINGFACE_HUB_CACHE: /home/philippe/.cache/huggingface/hub
- HUGGINGFACE_ASSETS_CACHE: /home/philippe/.cache/huggingface/assets
- HF_TOKEN_PATH: /home/philippe/.cache/huggingface/token
- HF_HUB_OFFLINE: False
- HF_HUB_DISABLE_TELEMETRY: False
- HF_HUB_DISABLE_PROGRESS_BARS: None
- HF_HUB_DISABLE_SYMLINKS_WARNING: False
- HF_HUB_DISABLE_EXPERIMENTAL_WARNING: False
- HF_HUB_DISABLE_IMPLICIT_TOKEN: False
- HF_HUB_ENABLE_HF_TRANSFER: False
```
| 28 | Image Encoding Issue when submitting a Parquet Dataset
### Describe the bug
Hello,
I'd like to report an issue related to pushing a dataset represented as a Parquet file to a dataset repository using Dask. Here are the details:
We attempted to load an example dataset in Parquet format from the Hugging Face (HF) filesystem using Dask with the following code snippet:
```
import dask.dataframe as dd
df = dd.read_parquet("hf://datasets/lambdalabs/pokemon-blip-captions",index=False)
```
In this dataset, the "image" column is represented as a dictionary/struct with the format:
```
df = df.compute()
df["image"].iloc[0].keys()
-> dict_keys(['bytes', 'path'])
```
I think this is the format encoded by the [`Image`](https://huggingface.co/docs/datasets/v2.0.0/en/package_reference/main_classes#datasets.Image) feature extractor from datasets to format suitable for Arrow.
The next step was to push the dataset to a repository that I created:
```
dd.to_parquet(dask_df, path = "hf://datasets/philippemo/dummy_dataset/data")
```
However, after pushing the dataset using Dask, the "image" column is now represented as the encoded dictionary `(['bytes', 'path'])`, and the images are not properly visualized. You can find the dataset here: [Link to the problematic dataset](https://huggingface.co/datasets/philippemo/dummy_dataset).
It's worth noting that both the original dataset and the one submitted with Dask have the same schema with minor alterations related to metadata:
**[ Schema of original dummy example.](https://huggingface.co/datasets/lambdalabs/pokemon-blip-captions/blob/main/data/train-00000-of-00001-566cc9b19d7203f8.parquet)**
```
image: struct<bytes: binary, path: null>
child 0, bytes: binary
child 1, path: null
text: string
```
**[ Schema of pushed dataset with dask](https://huggingface.co/datasets/philippemo/dummy_dataset/blob/main/data/part.0.parquet)**
```
image: struct<bytes: binary, path: null>
child 0, bytes: binary
child 1, path: null
text: string
```
This issue seems to be related to an encoding type that occurs when pushing a model to the hub. Normally, models should be represented as an HF dataset before pushing, but we are working with an example where we need to push large datasets using Dask.
Could you please provide clarification on how to resolve this issue?
Thank you!
### Reproduction
To get the schema I downloaded the parquet files and used pyarrow.parquet to read the schema
```
import pyarrow.parquet
pyarrow.parquet.read_schema(<path_to_parquet>, memory_map=True)
```
### Logs
_No response_
### System info
```shell
- huggingface_hub version: 0.14.1
- Platform: Linux-5.19.0-41-generic-x86_64-with-glibc2.35
- Python version: 3.10.6
- Running in iPython ?: No
- Running in notebook ?: No
- Running in Google Colab ?: No
- Token path ?: /home/philippe/.cache/huggingface/token
- Has saved token ?: True
- Who am I ?: philippemo
- Configured git credential helpers: cache
- FastAI: N/A
- Tensorflow: N/A
- Torch: N/A
- Jinja2: 3.1.2
- Graphviz: N/A
- Pydot: N/A
- Pillow: 9.4.0
- hf_transfer: N/A
- gradio: N/A
- ENDPOINT: https://huggingface.co
- HUGGINGFACE_HUB_CACHE: /home/philippe/.cache/huggingface/hub
- HUGGINGFACE_ASSETS_CACHE: /home/philippe/.cache/huggingface/assets
- HF_TOKEN_PATH: /home/philippe/.cache/huggingface/token
- HF_HUB_OFFLINE: False
- HF_HUB_DISABLE_TELEMETRY: False
- HF_HUB_DISABLE_PROGRESS_BARS: None
- HF_HUB_DISABLE_SYMLINKS_WARNING: False
- HF_HUB_DISABLE_EXPERIMENTAL_WARNING: False
- HF_HUB_DISABLE_IMPLICIT_TOKEN: False
- HF_HUB_ENABLE_HF_TRANSFER: False
```
alright thanks for the update :), would that be part of the new release of datasets or is it something separate? if so, where can I track it? |
https://github.com/huggingface/datasets/issues/5869 | Image Encoding Issue when submitting a Parquet Dataset | Once the new version of `datasets` is released (tomorrow probably) we'll open an issue on https://github.com/huggingface/datasets-server to update to this version :) | ### Describe the bug
Hello,
I'd like to report an issue related to pushing a dataset represented as a Parquet file to a dataset repository using Dask. Here are the details:
We attempted to load an example dataset in Parquet format from the Hugging Face (HF) filesystem using Dask with the following code snippet:
```
import dask.dataframe as dd
df = dd.read_parquet("hf://datasets/lambdalabs/pokemon-blip-captions",index=False)
```
In this dataset, the "image" column is represented as a dictionary/struct with the format:
```
df = df.compute()
df["image"].iloc[0].keys()
-> dict_keys(['bytes', 'path'])
```
I think this is the format encoded by the [`Image`](https://huggingface.co/docs/datasets/v2.0.0/en/package_reference/main_classes#datasets.Image) feature extractor from datasets to format suitable for Arrow.
The next step was to push the dataset to a repository that I created:
```
dd.to_parquet(dask_df, path = "hf://datasets/philippemo/dummy_dataset/data")
```
However, after pushing the dataset using Dask, the "image" column is now represented as the encoded dictionary `(['bytes', 'path'])`, and the images are not properly visualized. You can find the dataset here: [Link to the problematic dataset](https://huggingface.co/datasets/philippemo/dummy_dataset).
It's worth noting that both the original dataset and the one submitted with Dask have the same schema with minor alterations related to metadata:
**[ Schema of original dummy example.](https://huggingface.co/datasets/lambdalabs/pokemon-blip-captions/blob/main/data/train-00000-of-00001-566cc9b19d7203f8.parquet)**
```
image: struct<bytes: binary, path: null>
child 0, bytes: binary
child 1, path: null
text: string
```
**[ Schema of pushed dataset with dask](https://huggingface.co/datasets/philippemo/dummy_dataset/blob/main/data/part.0.parquet)**
```
image: struct<bytes: binary, path: null>
child 0, bytes: binary
child 1, path: null
text: string
```
This issue seems to be related to an encoding type that occurs when pushing a model to the hub. Normally, models should be represented as an HF dataset before pushing, but we are working with an example where we need to push large datasets using Dask.
Could you please provide clarification on how to resolve this issue?
Thank you!
### Reproduction
To get the schema I downloaded the parquet files and used pyarrow.parquet to read the schema
```
import pyarrow.parquet
pyarrow.parquet.read_schema(<path_to_parquet>, memory_map=True)
```
### Logs
_No response_
### System info
```shell
- huggingface_hub version: 0.14.1
- Platform: Linux-5.19.0-41-generic-x86_64-with-glibc2.35
- Python version: 3.10.6
- Running in iPython ?: No
- Running in notebook ?: No
- Running in Google Colab ?: No
- Token path ?: /home/philippe/.cache/huggingface/token
- Has saved token ?: True
- Who am I ?: philippemo
- Configured git credential helpers: cache
- FastAI: N/A
- Tensorflow: N/A
- Torch: N/A
- Jinja2: 3.1.2
- Graphviz: N/A
- Pydot: N/A
- Pillow: 9.4.0
- hf_transfer: N/A
- gradio: N/A
- ENDPOINT: https://huggingface.co
- HUGGINGFACE_HUB_CACHE: /home/philippe/.cache/huggingface/hub
- HUGGINGFACE_ASSETS_CACHE: /home/philippe/.cache/huggingface/assets
- HF_TOKEN_PATH: /home/philippe/.cache/huggingface/token
- HF_HUB_OFFLINE: False
- HF_HUB_DISABLE_TELEMETRY: False
- HF_HUB_DISABLE_PROGRESS_BARS: None
- HF_HUB_DISABLE_SYMLINKS_WARNING: False
- HF_HUB_DISABLE_EXPERIMENTAL_WARNING: False
- HF_HUB_DISABLE_IMPLICIT_TOKEN: False
- HF_HUB_ENABLE_HF_TRANSFER: False
```
| 22 | Image Encoding Issue when submitting a Parquet Dataset
### Describe the bug
Hello,
I'd like to report an issue related to pushing a dataset represented as a Parquet file to a dataset repository using Dask. Here are the details:
We attempted to load an example dataset in Parquet format from the Hugging Face (HF) filesystem using Dask with the following code snippet:
```
import dask.dataframe as dd
df = dd.read_parquet("hf://datasets/lambdalabs/pokemon-blip-captions",index=False)
```
In this dataset, the "image" column is represented as a dictionary/struct with the format:
```
df = df.compute()
df["image"].iloc[0].keys()
-> dict_keys(['bytes', 'path'])
```
I think this is the format encoded by the [`Image`](https://huggingface.co/docs/datasets/v2.0.0/en/package_reference/main_classes#datasets.Image) feature extractor from datasets to format suitable for Arrow.
The next step was to push the dataset to a repository that I created:
```
dd.to_parquet(dask_df, path = "hf://datasets/philippemo/dummy_dataset/data")
```
However, after pushing the dataset using Dask, the "image" column is now represented as the encoded dictionary `(['bytes', 'path'])`, and the images are not properly visualized. You can find the dataset here: [Link to the problematic dataset](https://huggingface.co/datasets/philippemo/dummy_dataset).
It's worth noting that both the original dataset and the one submitted with Dask have the same schema with minor alterations related to metadata:
**[ Schema of original dummy example.](https://huggingface.co/datasets/lambdalabs/pokemon-blip-captions/blob/main/data/train-00000-of-00001-566cc9b19d7203f8.parquet)**
```
image: struct<bytes: binary, path: null>
child 0, bytes: binary
child 1, path: null
text: string
```
**[ Schema of pushed dataset with dask](https://huggingface.co/datasets/philippemo/dummy_dataset/blob/main/data/part.0.parquet)**
```
image: struct<bytes: binary, path: null>
child 0, bytes: binary
child 1, path: null
text: string
```
This issue seems to be related to an encoding type that occurs when pushing a model to the hub. Normally, models should be represented as an HF dataset before pushing, but we are working with an example where we need to push large datasets using Dask.
Could you please provide clarification on how to resolve this issue?
Thank you!
### Reproduction
To get the schema I downloaded the parquet files and used pyarrow.parquet to read the schema
```
import pyarrow.parquet
pyarrow.parquet.read_schema(<path_to_parquet>, memory_map=True)
```
### Logs
_No response_
### System info
```shell
- huggingface_hub version: 0.14.1
- Platform: Linux-5.19.0-41-generic-x86_64-with-glibc2.35
- Python version: 3.10.6
- Running in iPython ?: No
- Running in notebook ?: No
- Running in Google Colab ?: No
- Token path ?: /home/philippe/.cache/huggingface/token
- Has saved token ?: True
- Who am I ?: philippemo
- Configured git credential helpers: cache
- FastAI: N/A
- Tensorflow: N/A
- Torch: N/A
- Jinja2: 3.1.2
- Graphviz: N/A
- Pydot: N/A
- Pillow: 9.4.0
- hf_transfer: N/A
- gradio: N/A
- ENDPOINT: https://huggingface.co
- HUGGINGFACE_HUB_CACHE: /home/philippe/.cache/huggingface/hub
- HUGGINGFACE_ASSETS_CACHE: /home/philippe/.cache/huggingface/assets
- HF_TOKEN_PATH: /home/philippe/.cache/huggingface/token
- HF_HUB_OFFLINE: False
- HF_HUB_DISABLE_TELEMETRY: False
- HF_HUB_DISABLE_PROGRESS_BARS: None
- HF_HUB_DISABLE_SYMLINKS_WARNING: False
- HF_HUB_DISABLE_EXPERIMENTAL_WARNING: False
- HF_HUB_DISABLE_IMPLICIT_TOKEN: False
- HF_HUB_ENABLE_HF_TRANSFER: False
```
Once the new version of `datasets` is released (tomorrow probably) we'll open an issue on https://github.com/huggingface/datasets-server to update to this version :) |
https://github.com/huggingface/datasets/issues/5868 | Is it possible to change a cached file and 're-cache' it instead of re-generating? | Arrow files/primitives (tables and arrays) are immutable, so re-generating them is the only option, I'm afraid. | ### Feature request
Hi,
I have a huge cached file using `map`(over 500GB), and I want to change an attribution of each element, is there possible to do it using some method instead of re-generating, because `map` takes over 24 hours
### Motivation
For large datasets, I think it is very important because we always face the problem which is changing something in the original cache without re-generating it.
### Your contribution
For now, I can't help, sorry. | 16 | Is it possible to change a cached file and 're-cache' it instead of re-generating?
### Feature request
Hi,
I have a huge cached file using `map`(over 500GB), and I want to change an attribution of each element, is there possible to do it using some method instead of re-generating, because `map` takes over 24 hours
### Motivation
For large datasets, I think it is very important because we always face the problem which is changing something in the original cache without re-generating it.
### Your contribution
For now, I can't help, sorry.
Arrow files/primitives (tables and arrays) are immutable, so re-generating them is the only option, I'm afraid. |
https://github.com/huggingface/datasets/issues/5864 | Slow iteration over Torch tensors | I am highly interested performance of dataset so I ran your example as a curious user.
```python
train_dataset.cast_column("x", Array3D(shape=img_shape, dtype="float32"))
```
have return values and "x" is a new column, it shoulde be
```python
ds=train_dataset.cast_column("img", Array3D(shape=(3,32,32), dtype="float32"))
```
I rewrite your example as
```python
train_dataset = load_dataset(
'cifar100',
split='train',
use_auth_token=True,
)
transform_func = torchvision.transforms.Compose([
ToTensor(),
Normalize(mean=[0.485, 0.456, 0.406], std= [0.229, 0.224, 0.225]),]
)
train_dataset = train_dataset.map(
desc=f"Preprocessing samples",
function=lambda x: {"img": transform_func(x["img"])},
)
ds=train_dataset.cast_column("img", Array3D(shape=(3,32,32), dtype="float32"))
for i in tqdm(ds):
pass
```
that require ~11s in my environment. While
```python
ds = load_dataset(
'cifar100',
split='train',
use_auth_token=True,
)
for i in tqdm(ds):
pass
```
only need ~6s. (So I guess it's still undesirable) | ### Describe the bug
I have a problem related to this [issue](https://github.com/huggingface/datasets/issues/5841): I get a way slower iteration when using a Torch dataloader if I use vanilla Numpy tensors or if I first apply a ToTensor transform to the input. In particular, it takes 5 seconds to iterate over the vanilla input and ~30s after the transformation.
### Steps to reproduce the bug
Here is the minimum code to reproduce the problem
```python
import numpy as np
from datasets import Dataset, DatasetDict, load_dataset, Array3D, Image, Features
from torch.utils.data import DataLoader
from tqdm import tqdm
import torchvision
from torchvision.transforms import ToTensor, Normalize
#################################
# Without transform
#################################
train_dataset = load_dataset(
'cifar100',
split='train',
use_auth_token=True,
)
train_dataset.set_format(type="numpy", columns=["img", "fine_label"])
train_loader= DataLoader(
train_dataset,
batch_size=100,
pin_memory=False,
shuffle=True,
num_workers=8,
)
for batch in tqdm(train_loader, desc="Loading data, no transform"):
pass
#################################
# With transform
#################################
transform_func = torchvision.transforms.Compose([
ToTensor(),
Normalize(mean=[0.485, 0.456, 0.406], std= [0.229, 0.224, 0.225]),]
)
train_dataset = train_dataset.map(
desc=f"Preprocessing samples",
function=lambda x: {"img": transform_func(x["img"])},
)
train_dataset.set_format(type="numpy", columns=["img", "fine_label"])
train_loader= DataLoader(
train_dataset,
batch_size=100,
pin_memory=False,
shuffle=True,
num_workers=8,
)
for batch in tqdm(train_loader, desc="Loading data after transform"):
pass
```
I have also tried converting the Image column to an Array3D
```python
img_shape = train_dataset[0]["img"].shape
features = train_dataset.features.copy()
features["x"] = Array3D(shape=img_shape, dtype="float32")
train_dataset = train_dataset.map(
desc=f"Preprocessing samples",
function=lambda x: {"x": np.array(x["img"], dtype=np.uint8)},
features=features,
)
train_dataset.cast_column("x", Array3D(shape=img_shape, dtype="float32"))
train_dataset.set_format(type="numpy", columns=["x", "fine_label"])
```
but to no avail. Any clue?
### Expected behavior
The iteration should take approximately the same time with or without the transformation, as it doesn't change the shape of the input. What may be the issue here?
### Environment info
```
- `datasets` version: 2.12.0
- Platform: Linux-5.4.0-137-generic-x86_64-with-glibc2.31
- Python version: 3.9.16
- Huggingface_hub version: 0.14.1
- PyArrow version: 12.0.0
- Pandas version: 2.0.1
``` | 112 | Slow iteration over Torch tensors
### Describe the bug
I have a problem related to this [issue](https://github.com/huggingface/datasets/issues/5841): I get a way slower iteration when using a Torch dataloader if I use vanilla Numpy tensors or if I first apply a ToTensor transform to the input. In particular, it takes 5 seconds to iterate over the vanilla input and ~30s after the transformation.
### Steps to reproduce the bug
Here is the minimum code to reproduce the problem
```python
import numpy as np
from datasets import Dataset, DatasetDict, load_dataset, Array3D, Image, Features
from torch.utils.data import DataLoader
from tqdm import tqdm
import torchvision
from torchvision.transforms import ToTensor, Normalize
#################################
# Without transform
#################################
train_dataset = load_dataset(
'cifar100',
split='train',
use_auth_token=True,
)
train_dataset.set_format(type="numpy", columns=["img", "fine_label"])
train_loader= DataLoader(
train_dataset,
batch_size=100,
pin_memory=False,
shuffle=True,
num_workers=8,
)
for batch in tqdm(train_loader, desc="Loading data, no transform"):
pass
#################################
# With transform
#################################
transform_func = torchvision.transforms.Compose([
ToTensor(),
Normalize(mean=[0.485, 0.456, 0.406], std= [0.229, 0.224, 0.225]),]
)
train_dataset = train_dataset.map(
desc=f"Preprocessing samples",
function=lambda x: {"img": transform_func(x["img"])},
)
train_dataset.set_format(type="numpy", columns=["img", "fine_label"])
train_loader= DataLoader(
train_dataset,
batch_size=100,
pin_memory=False,
shuffle=True,
num_workers=8,
)
for batch in tqdm(train_loader, desc="Loading data after transform"):
pass
```
I have also tried converting the Image column to an Array3D
```python
img_shape = train_dataset[0]["img"].shape
features = train_dataset.features.copy()
features["x"] = Array3D(shape=img_shape, dtype="float32")
train_dataset = train_dataset.map(
desc=f"Preprocessing samples",
function=lambda x: {"x": np.array(x["img"], dtype=np.uint8)},
features=features,
)
train_dataset.cast_column("x", Array3D(shape=img_shape, dtype="float32"))
train_dataset.set_format(type="numpy", columns=["x", "fine_label"])
```
but to no avail. Any clue?
### Expected behavior
The iteration should take approximately the same time with or without the transformation, as it doesn't change the shape of the input. What may be the issue here?
### Environment info
```
- `datasets` version: 2.12.0
- Platform: Linux-5.4.0-137-generic-x86_64-with-glibc2.31
- Python version: 3.9.16
- Huggingface_hub version: 0.14.1
- PyArrow version: 12.0.0
- Pandas version: 2.0.1
```
I am highly interested performance of dataset so I ran your example as a curious user.
```python
train_dataset.cast_column("x", Array3D(shape=img_shape, dtype="float32"))
```
have return values and "x" is a new column, it shoulde be
```python
ds=train_dataset.cast_column("img", Array3D(shape=(3,32,32), dtype="float32"))
```
I rewrite your example as
```python
train_dataset = load_dataset(
'cifar100',
split='train',
use_auth_token=True,
)
transform_func = torchvision.transforms.Compose([
ToTensor(),
Normalize(mean=[0.485, 0.456, 0.406], std= [0.229, 0.224, 0.225]),]
)
train_dataset = train_dataset.map(
desc=f"Preprocessing samples",
function=lambda x: {"img": transform_func(x["img"])},
)
ds=train_dataset.cast_column("img", Array3D(shape=(3,32,32), dtype="float32"))
for i in tqdm(ds):
pass
```
that require ~11s in my environment. While
```python
ds = load_dataset(
'cifar100',
split='train',
use_auth_token=True,
)
for i in tqdm(ds):
pass
```
only need ~6s. (So I guess it's still undesirable) |
https://github.com/huggingface/datasets/issues/5862 | IndexError: list index out of range with data hosted on Zenodo | This error is also raised when data is hosted on Google Drive:
- https://huggingface.co/datasets/docred/discussions/5
- https://huggingface.co/datasets/linnaeus/discussions/3
- https://huggingface.co/datasets/poleval2019_mt/discussions/3
- https://huggingface.co/datasets/reddit_tifu/discussions/2
- https://huggingface.co/datasets/species_800/discussions/3
- https://huggingface.co/datasets/wiki_lingua/discussions/1
- https://huggingface.co/datasets/yoruba_text_c3/discussions/1 | The dataset viewer sometimes raises an `IndexError`:
```
IndexError: list index out of range
```
See:
- huggingface/datasets-server#1151
- https://huggingface.co/datasets/reddit/discussions/5
- huggingface/datasets-server#1118
- https://huggingface.co/datasets/krr-oxford/OntoLAMA/discussions/1
- https://huggingface.co/datasets/hyperpartisan_news_detection/discussions/3
- https://huggingface.co/datasets/um005/discussions/2
- https://huggingface.co/datasets/tapaco/discussions/2
- https://huggingface.co/datasets/common_language/discussions/3
- https://huggingface.co/datasets/pass/discussions/1
After investigation:
- This happens with data files hosted on Zenodo
- Indeed, there is an underlying 429 HTTP error: Too Many Requests
Note that some time ago, it also happened with data files hosted on Google Drive. See:
- #4581
- #4580
The reason then was that there was a 403 HTTP error: Forbidden
| 26 | IndexError: list index out of range with data hosted on Zenodo
The dataset viewer sometimes raises an `IndexError`:
```
IndexError: list index out of range
```
See:
- huggingface/datasets-server#1151
- https://huggingface.co/datasets/reddit/discussions/5
- huggingface/datasets-server#1118
- https://huggingface.co/datasets/krr-oxford/OntoLAMA/discussions/1
- https://huggingface.co/datasets/hyperpartisan_news_detection/discussions/3
- https://huggingface.co/datasets/um005/discussions/2
- https://huggingface.co/datasets/tapaco/discussions/2
- https://huggingface.co/datasets/common_language/discussions/3
- https://huggingface.co/datasets/pass/discussions/1
After investigation:
- This happens with data files hosted on Zenodo
- Indeed, there is an underlying 429 HTTP error: Too Many Requests
Note that some time ago, it also happened with data files hosted on Google Drive. See:
- #4581
- #4580
The reason then was that there was a 403 HTTP error: Forbidden
This error is also raised when data is hosted on Google Drive:
- https://huggingface.co/datasets/docred/discussions/5
- https://huggingface.co/datasets/linnaeus/discussions/3
- https://huggingface.co/datasets/poleval2019_mt/discussions/3
- https://huggingface.co/datasets/reddit_tifu/discussions/2
- https://huggingface.co/datasets/species_800/discussions/3
- https://huggingface.co/datasets/wiki_lingua/discussions/1
- https://huggingface.co/datasets/yoruba_text_c3/discussions/1 |
https://github.com/huggingface/datasets/issues/5858 | Throw an error when dataset improperly indexed | Thanks for reporting, @sarahwie.
Please note that in `datasets` we do not have vectorized operation like `pandas`. Therefore, your equality comparisons above are `False`:
- For example: `squad['question']` returns a `list`, and this list is not equal to `"Who was the Norse leader?"`
The `False` value is equivalent to `0` when indexing a dataset, thus the reason why you get the first element (with index 0):
- For example: `squad[False]` is equivalent to `squad[0]`
Maybe we should an exception instead of assuming that `False` is equivalent to `0` (and `True` is equivalent to `1`) in the context of indexing. | ### Describe the bug
Pandas-style subset indexing on dataset does not throw an error, when maybe it should. Instead returns the first instance of the dataset regardless of index condition.
### Steps to reproduce the bug
Steps to reproduce the behavior:
1. `squad = datasets.load_dataset("squad_v2", split="validation")`
2. `item = squad[squad['question'] == "Who was the Norse leader?"]`
or `it = squad[squad['id'] == '56ddde6b9a695914005b962b']`
3. returns the first item in the dataset, which does not satisfy the above conditions:
`{'id': '56ddde6b9a695914005b9628', 'title': 'Normans', 'context': 'The Normans (Norman: Nourmands; French: Normands; Latin: Normanni) were the people who in the 10th and 11th centuries gave their name to Normandy, a region in France. They were descended from Norse ("Norman" comes from "Norseman") raiders and pirates from Denmark, Iceland and Norway who, under their leader Rollo, agreed to swear fealty to King Charles III of West Francia. Through generations of assimilation and mixing with the native Frankish and Roman-Gaulish populations, their descendants would gradually merge with the Carolingian-based cultures of West Francia. The distinct cultural and ethnic identity of the Normans emerged initially in the first half of the 10th century, and it continued to evolve over the succeeding centuries.', 'question': 'In what country is Normandy located?', 'answers': {'text': ['France', 'France', 'France', 'France'], 'answer_start': [159, 159, 159, 159]}}`
### Expected behavior
Should either throw an error message, or return the dataset item that satisfies the condition.
### Environment info
- `datasets` version: 2.9.0
- Platform: macOS-13.3.1-arm64-arm-64bit
- Python version: 3.10.8
- PyArrow version: 10.0.1
- Pandas version: 1.5.3 | 99 | Throw an error when dataset improperly indexed
### Describe the bug
Pandas-style subset indexing on dataset does not throw an error, when maybe it should. Instead returns the first instance of the dataset regardless of index condition.
### Steps to reproduce the bug
Steps to reproduce the behavior:
1. `squad = datasets.load_dataset("squad_v2", split="validation")`
2. `item = squad[squad['question'] == "Who was the Norse leader?"]`
or `it = squad[squad['id'] == '56ddde6b9a695914005b962b']`
3. returns the first item in the dataset, which does not satisfy the above conditions:
`{'id': '56ddde6b9a695914005b9628', 'title': 'Normans', 'context': 'The Normans (Norman: Nourmands; French: Normands; Latin: Normanni) were the people who in the 10th and 11th centuries gave their name to Normandy, a region in France. They were descended from Norse ("Norman" comes from "Norseman") raiders and pirates from Denmark, Iceland and Norway who, under their leader Rollo, agreed to swear fealty to King Charles III of West Francia. Through generations of assimilation and mixing with the native Frankish and Roman-Gaulish populations, their descendants would gradually merge with the Carolingian-based cultures of West Francia. The distinct cultural and ethnic identity of the Normans emerged initially in the first half of the 10th century, and it continued to evolve over the succeeding centuries.', 'question': 'In what country is Normandy located?', 'answers': {'text': ['France', 'France', 'France', 'France'], 'answer_start': [159, 159, 159, 159]}}`
### Expected behavior
Should either throw an error message, or return the dataset item that satisfies the condition.
### Environment info
- `datasets` version: 2.9.0
- Platform: macOS-13.3.1-arm64-arm-64bit
- Python version: 3.10.8
- PyArrow version: 10.0.1
- Pandas version: 1.5.3
Thanks for reporting, @sarahwie.
Please note that in `datasets` we do not have vectorized operation like `pandas`. Therefore, your equality comparisons above are `False`:
- For example: `squad['question']` returns a `list`, and this list is not equal to `"Who was the Norse leader?"`
The `False` value is equivalent to `0` when indexing a dataset, thus the reason why you get the first element (with index 0):
- For example: `squad[False]` is equivalent to `squad[0]`
Maybe we should an exception instead of assuming that `False` is equivalent to `0` (and `True` is equivalent to `1`) in the context of indexing. |
https://github.com/huggingface/datasets/issues/5857 | Adding chemistry dataset/models in huggingface | Hi!
This would be a nice addition to the Hub! You can find the existing chemistry datasets/models on the Hub (using the `chemistry` tag) [here](https://huggingface.co/search/full-text?q=chemistry&type=model&type=dataset).
Feel free to ping us here on the Hub if you need help adding the datasets.
| ### Feature request
Huggingface is really amazing platform for open science.
In addition to computer vision, video and NLP, would it be of interest to add chemistry/materials science dataset/models in Huggingface? Or, if its already done, can you provide some pointers.
We have been working on a comprehensive benchmark on this topic: [JARVIS-Leaderboard](https://pages.nist.gov/jarvis_leaderboard/) and I am wondering if we could contribute/integrate this project as a part of huggingface.
### Motivation
Similar to the main stream AI field, there is need of large scale benchmarks/models/infrastructure for chemistry/materials data.
### Your contribution
We can start adding datasets as our [benchmarks](https://github.com/usnistgov/jarvis_leaderboard/tree/main/jarvis_leaderboard/benchmarks) should be easily convertible to the dataset format. | 41 | Adding chemistry dataset/models in huggingface
### Feature request
Huggingface is really amazing platform for open science.
In addition to computer vision, video and NLP, would it be of interest to add chemistry/materials science dataset/models in Huggingface? Or, if its already done, can you provide some pointers.
We have been working on a comprehensive benchmark on this topic: [JARVIS-Leaderboard](https://pages.nist.gov/jarvis_leaderboard/) and I am wondering if we could contribute/integrate this project as a part of huggingface.
### Motivation
Similar to the main stream AI field, there is need of large scale benchmarks/models/infrastructure for chemistry/materials data.
### Your contribution
We can start adding datasets as our [benchmarks](https://github.com/usnistgov/jarvis_leaderboard/tree/main/jarvis_leaderboard/benchmarks) should be easily convertible to the dataset format.
Hi!
This would be a nice addition to the Hub! You can find the existing chemistry datasets/models on the Hub (using the `chemistry` tag) [here](https://huggingface.co/search/full-text?q=chemistry&type=model&type=dataset).
Feel free to ping us here on the Hub if you need help adding the datasets.
|
https://github.com/huggingface/datasets/issues/5856 | Error loading natural_questions | Hi! You can avoid this error by using the preprocessed version:
```python
import datasets
ds = datasets.load_dataset('natural_questions')
```
PS: Once we finish https://github.com/huggingface/datasets/pull/5364, this error will no longer be a problem. | ### Describe the bug
When try to load natural_questions through datasets == 2.12.0 with python == 3.8.9:
```python
import datasets
datasets.load_dataset('natural_questions',beam_runner='DirectRunner')
```
It failed with following info:
`pyarrow.lib.ArrowNotImplementedError: Nested data conversions not implemented for chunked array outputs`
### Steps to reproduce the bug
In python console:
```python
import datasets
datasets.load_dataset('natural_questions',beam_runner='DirectRunner')
```
Then the trace is:
```
Traceback (most recent call last):
File "<stdin>", line 1, in <module>
File "/home/nlp/.cache/pypoetry/virtualenvs/drg-W3LF4Ol9-py3.8/lib/python3.8/site-packages/datasets/load.py", line 1797, in load_dataset
builder_instance.download_and_prepare(
File "/home/nlp/.cache/pypoetry/virtualenvs/drg-W3LF4Ol9-py3.8/lib/python3.8/site-packages/datasets/builder.py", line 890, in download_and_prepare
self._download_and_prepare(
File "/home/nlp/.cache/pypoetry/virtualenvs/drg-W3LF4Ol9-py3.8/lib/python3.8/site-packages/datasets/builder.py", line 2019, in _download_and_prepare
num_examples, num_bytes = beam_writer.finalize(metrics.query(m_filter))
File "/home/nlp/.cache/pypoetry/virtualenvs/drg-W3LF4Ol9-py3.8/lib/python3.8/site-packages/datasets/arrow_writer.py", line 694, in finalize
shard_num_bytes, _ = parquet_to_arrow(source, destination)
File "/home/nlp/.cache/pypoetry/virtualenvs/drg-W3LF4Ol9-py3.8/lib/python3.8/site-packages/datasets/arrow_writer.py", line 737, in parquet_to_arrow
for record_batch in parquet_file.iter_batches():
File "pyarrow/_parquet.pyx", line 1323, in iter_batches
File "pyarrow/error.pxi", line 121, in pyarrow.lib.check_status
pyarrow.lib.ArrowNotImplementedError: Nested data conversions not implemented for chunked array outputs
```
### Expected behavior
load natural_question questions
### Environment info
```
- `datasets` version: 2.12.0
- Platform: Linux-3.10.0-1160.42.2.el7.x86_64-x86_64-with-glibc2.2.5
- Python version: 3.8.9
- Huggingface_hub version: 0.14.1
- PyArrow version: 11.0.0
- Pandas version: 2.0.1
``` | 31 | Error loading natural_questions
### Describe the bug
When try to load natural_questions through datasets == 2.12.0 with python == 3.8.9:
```python
import datasets
datasets.load_dataset('natural_questions',beam_runner='DirectRunner')
```
It failed with following info:
`pyarrow.lib.ArrowNotImplementedError: Nested data conversions not implemented for chunked array outputs`
### Steps to reproduce the bug
In python console:
```python
import datasets
datasets.load_dataset('natural_questions',beam_runner='DirectRunner')
```
Then the trace is:
```
Traceback (most recent call last):
File "<stdin>", line 1, in <module>
File "/home/nlp/.cache/pypoetry/virtualenvs/drg-W3LF4Ol9-py3.8/lib/python3.8/site-packages/datasets/load.py", line 1797, in load_dataset
builder_instance.download_and_prepare(
File "/home/nlp/.cache/pypoetry/virtualenvs/drg-W3LF4Ol9-py3.8/lib/python3.8/site-packages/datasets/builder.py", line 890, in download_and_prepare
self._download_and_prepare(
File "/home/nlp/.cache/pypoetry/virtualenvs/drg-W3LF4Ol9-py3.8/lib/python3.8/site-packages/datasets/builder.py", line 2019, in _download_and_prepare
num_examples, num_bytes = beam_writer.finalize(metrics.query(m_filter))
File "/home/nlp/.cache/pypoetry/virtualenvs/drg-W3LF4Ol9-py3.8/lib/python3.8/site-packages/datasets/arrow_writer.py", line 694, in finalize
shard_num_bytes, _ = parquet_to_arrow(source, destination)
File "/home/nlp/.cache/pypoetry/virtualenvs/drg-W3LF4Ol9-py3.8/lib/python3.8/site-packages/datasets/arrow_writer.py", line 737, in parquet_to_arrow
for record_batch in parquet_file.iter_batches():
File "pyarrow/_parquet.pyx", line 1323, in iter_batches
File "pyarrow/error.pxi", line 121, in pyarrow.lib.check_status
pyarrow.lib.ArrowNotImplementedError: Nested data conversions not implemented for chunked array outputs
```
### Expected behavior
load natural_question questions
### Environment info
```
- `datasets` version: 2.12.0
- Platform: Linux-3.10.0-1160.42.2.el7.x86_64-x86_64-with-glibc2.2.5
- Python version: 3.8.9
- Huggingface_hub version: 0.14.1
- PyArrow version: 11.0.0
- Pandas version: 2.0.1
```
Hi! You can avoid this error by using the preprocessed version:
```python
import datasets
ds = datasets.load_dataset('natural_questions')
```
PS: Once we finish https://github.com/huggingface/datasets/pull/5364, this error will no longer be a problem. |
https://github.com/huggingface/datasets/issues/5856 | Error loading natural_questions | > Hi! You can avoid this error by using the preprocessed version:
>
> ```python
> import datasets
> ds = datasets.load_dataset('natural_questions')
> ```
>
> PS: Once we finish #5364, this error will no longer be a problem.
Thanks, wish #5364 finish early | ### Describe the bug
When try to load natural_questions through datasets == 2.12.0 with python == 3.8.9:
```python
import datasets
datasets.load_dataset('natural_questions',beam_runner='DirectRunner')
```
It failed with following info:
`pyarrow.lib.ArrowNotImplementedError: Nested data conversions not implemented for chunked array outputs`
### Steps to reproduce the bug
In python console:
```python
import datasets
datasets.load_dataset('natural_questions',beam_runner='DirectRunner')
```
Then the trace is:
```
Traceback (most recent call last):
File "<stdin>", line 1, in <module>
File "/home/nlp/.cache/pypoetry/virtualenvs/drg-W3LF4Ol9-py3.8/lib/python3.8/site-packages/datasets/load.py", line 1797, in load_dataset
builder_instance.download_and_prepare(
File "/home/nlp/.cache/pypoetry/virtualenvs/drg-W3LF4Ol9-py3.8/lib/python3.8/site-packages/datasets/builder.py", line 890, in download_and_prepare
self._download_and_prepare(
File "/home/nlp/.cache/pypoetry/virtualenvs/drg-W3LF4Ol9-py3.8/lib/python3.8/site-packages/datasets/builder.py", line 2019, in _download_and_prepare
num_examples, num_bytes = beam_writer.finalize(metrics.query(m_filter))
File "/home/nlp/.cache/pypoetry/virtualenvs/drg-W3LF4Ol9-py3.8/lib/python3.8/site-packages/datasets/arrow_writer.py", line 694, in finalize
shard_num_bytes, _ = parquet_to_arrow(source, destination)
File "/home/nlp/.cache/pypoetry/virtualenvs/drg-W3LF4Ol9-py3.8/lib/python3.8/site-packages/datasets/arrow_writer.py", line 737, in parquet_to_arrow
for record_batch in parquet_file.iter_batches():
File "pyarrow/_parquet.pyx", line 1323, in iter_batches
File "pyarrow/error.pxi", line 121, in pyarrow.lib.check_status
pyarrow.lib.ArrowNotImplementedError: Nested data conversions not implemented for chunked array outputs
```
### Expected behavior
load natural_question questions
### Environment info
```
- `datasets` version: 2.12.0
- Platform: Linux-3.10.0-1160.42.2.el7.x86_64-x86_64-with-glibc2.2.5
- Python version: 3.8.9
- Huggingface_hub version: 0.14.1
- PyArrow version: 11.0.0
- Pandas version: 2.0.1
``` | 44 | Error loading natural_questions
### Describe the bug
When try to load natural_questions through datasets == 2.12.0 with python == 3.8.9:
```python
import datasets
datasets.load_dataset('natural_questions',beam_runner='DirectRunner')
```
It failed with following info:
`pyarrow.lib.ArrowNotImplementedError: Nested data conversions not implemented for chunked array outputs`
### Steps to reproduce the bug
In python console:
```python
import datasets
datasets.load_dataset('natural_questions',beam_runner='DirectRunner')
```
Then the trace is:
```
Traceback (most recent call last):
File "<stdin>", line 1, in <module>
File "/home/nlp/.cache/pypoetry/virtualenvs/drg-W3LF4Ol9-py3.8/lib/python3.8/site-packages/datasets/load.py", line 1797, in load_dataset
builder_instance.download_and_prepare(
File "/home/nlp/.cache/pypoetry/virtualenvs/drg-W3LF4Ol9-py3.8/lib/python3.8/site-packages/datasets/builder.py", line 890, in download_and_prepare
self._download_and_prepare(
File "/home/nlp/.cache/pypoetry/virtualenvs/drg-W3LF4Ol9-py3.8/lib/python3.8/site-packages/datasets/builder.py", line 2019, in _download_and_prepare
num_examples, num_bytes = beam_writer.finalize(metrics.query(m_filter))
File "/home/nlp/.cache/pypoetry/virtualenvs/drg-W3LF4Ol9-py3.8/lib/python3.8/site-packages/datasets/arrow_writer.py", line 694, in finalize
shard_num_bytes, _ = parquet_to_arrow(source, destination)
File "/home/nlp/.cache/pypoetry/virtualenvs/drg-W3LF4Ol9-py3.8/lib/python3.8/site-packages/datasets/arrow_writer.py", line 737, in parquet_to_arrow
for record_batch in parquet_file.iter_batches():
File "pyarrow/_parquet.pyx", line 1323, in iter_batches
File "pyarrow/error.pxi", line 121, in pyarrow.lib.check_status
pyarrow.lib.ArrowNotImplementedError: Nested data conversions not implemented for chunked array outputs
```
### Expected behavior
load natural_question questions
### Environment info
```
- `datasets` version: 2.12.0
- Platform: Linux-3.10.0-1160.42.2.el7.x86_64-x86_64-with-glibc2.2.5
- Python version: 3.8.9
- Huggingface_hub version: 0.14.1
- PyArrow version: 11.0.0
- Pandas version: 2.0.1
```
> Hi! You can avoid this error by using the preprocessed version:
>
> ```python
> import datasets
> ds = datasets.load_dataset('natural_questions')
> ```
>
> PS: Once we finish #5364, this error will no longer be a problem.
Thanks, wish #5364 finish early |
https://github.com/huggingface/datasets/issues/5855 | `to_tf_dataset` consumes too much memory | Cc @amyeroberts @Rocketknight1
Indded I think it's because it does something like this under the hood when there's no multiprocessing:
```python
tf_dataset = tf_dataset.shuffle(len(dataset))
```
PS: with multiprocessing it appears to be different:
```python
indices = np.arange(len(dataset))
if shuffle:
np.random.shuffle(indices)
``` | ### Describe the bug
Hi, I'm using `to_tf_dataset` to convert a _large_ dataset to `tf.data.Dataset`. I observed that the data loading *before* training took a lot of time and memory, even with `batch_size=1`.
After some digging, i believe the reason lies in the shuffle behavior. The [source code](https://github.com/huggingface/datasets/blob/main/src/datasets/utils/tf_utils.py#L185) uses `len(dataset)` as the `buffer_size`, which may load all the data into the memory, and the [tf.data doc](https://www.tensorflow.org/guide/data#randomly_shuffling_input_data) also states that "While large buffer_sizes shuffle more thoroughly, they can take a lot of memory, and significant time to fill".
### Steps to reproduce the bug
```python
from datasets import Dataset
def gen(): # some large data
for i in range(50000000):
yield {"data": i}
ds = Dataset.from_generator(gen, cache_dir="./huggingface")
tf_ds = ds.to_tf_dataset(
batch_size=64,
shuffle=False, # no shuffle
drop_remainder=False,
prefetch=True,
)
# fast and memory friendly π€
for batch in tf_ds:
...
tf_ds_shuffle = ds.to_tf_dataset(
batch_size=64,
shuffle=True,
drop_remainder=False,
prefetch=True,
)
# slow and memory hungry for simple iteration π±
for batch in tf_ds_shuffle:
...
```
### Expected behavior
Shuffling should not load all the data into the memory. Would adding a `buffer_size` parameter in the `to_tf_dataset` API alleviate the problem?
### Environment info
- `datasets` version: 2.11.0
- Platform: Linux-5.17.1-051701-generic-x86_64-with-glibc2.17
- Python version: 3.8.13
- Huggingface_hub version: 0.13.4
- PyArrow version: 11.0.0
- Pandas version: 1.4.3
| 41 | `to_tf_dataset` consumes too much memory
### Describe the bug
Hi, I'm using `to_tf_dataset` to convert a _large_ dataset to `tf.data.Dataset`. I observed that the data loading *before* training took a lot of time and memory, even with `batch_size=1`.
After some digging, i believe the reason lies in the shuffle behavior. The [source code](https://github.com/huggingface/datasets/blob/main/src/datasets/utils/tf_utils.py#L185) uses `len(dataset)` as the `buffer_size`, which may load all the data into the memory, and the [tf.data doc](https://www.tensorflow.org/guide/data#randomly_shuffling_input_data) also states that "While large buffer_sizes shuffle more thoroughly, they can take a lot of memory, and significant time to fill".
### Steps to reproduce the bug
```python
from datasets import Dataset
def gen(): # some large data
for i in range(50000000):
yield {"data": i}
ds = Dataset.from_generator(gen, cache_dir="./huggingface")
tf_ds = ds.to_tf_dataset(
batch_size=64,
shuffle=False, # no shuffle
drop_remainder=False,
prefetch=True,
)
# fast and memory friendly π€
for batch in tf_ds:
...
tf_ds_shuffle = ds.to_tf_dataset(
batch_size=64,
shuffle=True,
drop_remainder=False,
prefetch=True,
)
# slow and memory hungry for simple iteration π±
for batch in tf_ds_shuffle:
...
```
### Expected behavior
Shuffling should not load all the data into the memory. Would adding a `buffer_size` parameter in the `to_tf_dataset` API alleviate the problem?
### Environment info
- `datasets` version: 2.11.0
- Platform: Linux-5.17.1-051701-generic-x86_64-with-glibc2.17
- Python version: 3.8.13
- Huggingface_hub version: 0.13.4
- PyArrow version: 11.0.0
- Pandas version: 1.4.3
Cc @amyeroberts @Rocketknight1
Indded I think it's because it does something like this under the hood when there's no multiprocessing:
```python
tf_dataset = tf_dataset.shuffle(len(dataset))
```
PS: with multiprocessing it appears to be different:
```python
indices = np.arange(len(dataset))
if shuffle:
np.random.shuffle(indices)
``` |
https://github.com/huggingface/datasets/issues/5855 | `to_tf_dataset` consumes too much memory | Hi @massquantity, the dataset being shuffled there is not the full dataset. If you look at [the line above](https://github.com/huggingface/datasets/blob/main/src/datasets/utils/tf_utils.py#L182), the dataset is actually just a single indices array at that point, and that array is the only thing that gets fully loaded into memory and shuffled. We then load samples from the dataset by applying a transform function to the shuffled dataset, which fetches samples based on the indices it receives.
If your dataset is **really** gigantic, then this index tensor might be a memory issue, but since it's just an int64 tensor it will only use 1GB of memory per 125 million samples.
Still, if you're encountering memory issues, there might be another cause here - can you share some code to reproduce the error, or does it depend on some internal/proprietary dataset? | ### Describe the bug
Hi, I'm using `to_tf_dataset` to convert a _large_ dataset to `tf.data.Dataset`. I observed that the data loading *before* training took a lot of time and memory, even with `batch_size=1`.
After some digging, i believe the reason lies in the shuffle behavior. The [source code](https://github.com/huggingface/datasets/blob/main/src/datasets/utils/tf_utils.py#L185) uses `len(dataset)` as the `buffer_size`, which may load all the data into the memory, and the [tf.data doc](https://www.tensorflow.org/guide/data#randomly_shuffling_input_data) also states that "While large buffer_sizes shuffle more thoroughly, they can take a lot of memory, and significant time to fill".
### Steps to reproduce the bug
```python
from datasets import Dataset
def gen(): # some large data
for i in range(50000000):
yield {"data": i}
ds = Dataset.from_generator(gen, cache_dir="./huggingface")
tf_ds = ds.to_tf_dataset(
batch_size=64,
shuffle=False, # no shuffle
drop_remainder=False,
prefetch=True,
)
# fast and memory friendly π€
for batch in tf_ds:
...
tf_ds_shuffle = ds.to_tf_dataset(
batch_size=64,
shuffle=True,
drop_remainder=False,
prefetch=True,
)
# slow and memory hungry for simple iteration π±
for batch in tf_ds_shuffle:
...
```
### Expected behavior
Shuffling should not load all the data into the memory. Would adding a `buffer_size` parameter in the `to_tf_dataset` API alleviate the problem?
### Environment info
- `datasets` version: 2.11.0
- Platform: Linux-5.17.1-051701-generic-x86_64-with-glibc2.17
- Python version: 3.8.13
- Huggingface_hub version: 0.13.4
- PyArrow version: 11.0.0
- Pandas version: 1.4.3
| 134 | `to_tf_dataset` consumes too much memory
### Describe the bug
Hi, I'm using `to_tf_dataset` to convert a _large_ dataset to `tf.data.Dataset`. I observed that the data loading *before* training took a lot of time and memory, even with `batch_size=1`.
After some digging, i believe the reason lies in the shuffle behavior. The [source code](https://github.com/huggingface/datasets/blob/main/src/datasets/utils/tf_utils.py#L185) uses `len(dataset)` as the `buffer_size`, which may load all the data into the memory, and the [tf.data doc](https://www.tensorflow.org/guide/data#randomly_shuffling_input_data) also states that "While large buffer_sizes shuffle more thoroughly, they can take a lot of memory, and significant time to fill".
### Steps to reproduce the bug
```python
from datasets import Dataset
def gen(): # some large data
for i in range(50000000):
yield {"data": i}
ds = Dataset.from_generator(gen, cache_dir="./huggingface")
tf_ds = ds.to_tf_dataset(
batch_size=64,
shuffle=False, # no shuffle
drop_remainder=False,
prefetch=True,
)
# fast and memory friendly π€
for batch in tf_ds:
...
tf_ds_shuffle = ds.to_tf_dataset(
batch_size=64,
shuffle=True,
drop_remainder=False,
prefetch=True,
)
# slow and memory hungry for simple iteration π±
for batch in tf_ds_shuffle:
...
```
### Expected behavior
Shuffling should not load all the data into the memory. Would adding a `buffer_size` parameter in the `to_tf_dataset` API alleviate the problem?
### Environment info
- `datasets` version: 2.11.0
- Platform: Linux-5.17.1-051701-generic-x86_64-with-glibc2.17
- Python version: 3.8.13
- Huggingface_hub version: 0.13.4
- PyArrow version: 11.0.0
- Pandas version: 1.4.3
Hi @massquantity, the dataset being shuffled there is not the full dataset. If you look at [the line above](https://github.com/huggingface/datasets/blob/main/src/datasets/utils/tf_utils.py#L182), the dataset is actually just a single indices array at that point, and that array is the only thing that gets fully loaded into memory and shuffled. We then load samples from the dataset by applying a transform function to the shuffled dataset, which fetches samples based on the indices it receives.
If your dataset is **really** gigantic, then this index tensor might be a memory issue, but since it's just an int64 tensor it will only use 1GB of memory per 125 million samples.
Still, if you're encountering memory issues, there might be another cause here - can you share some code to reproduce the error, or does it depend on some internal/proprietary dataset? |
https://github.com/huggingface/datasets/issues/5855 | `to_tf_dataset` consumes too much memory | Hi @Rocketknight1, you're right and I also noticed that only indices are used in shuffling. My data has shape (50000000, 10), but really the problem doesn't relate to a specific dataset. Simply running the following code costs me 10GB of memory.
```python
from datasets import Dataset
def gen():
for i in range(50000000):
yield {"data": i}
ds = Dataset.from_generator(gen, cache_dir="./huggingface")
tf_ds = ds.to_tf_dataset(
batch_size=1,
shuffle=True,
drop_remainder=False,
prefetch=True,
)
tf_ds = iter(tf_ds)
next(tf_ds)
# {'data': <tf.Tensor: shape=(1,), dtype=int64, numpy=array([0])>}
```
I just realized maybe it was an issue from tensorflow (I'm using tf 2.12). So I tried the following code, and it used 10GB of memory too.
```python
import numpy as np
import tensorflow as tf
data_size = 50000000
tf_dataset = tf.data.Dataset.from_tensor_slices(np.arange(data_size))
tf_dataset = iter(tf_dataset.shuffle(data_size))
next(tf_dataset)
# <tf.Tensor: shape=(), dtype=int64, numpy=24774043>
```
By the way, as @lhoestq mentioned, multiprocessing uses numpy shuffling, and it uses less than 1 GB of memory:
```python
tf_ds_mp = ds.to_tf_dataset(
batch_size=1,
shuffle=True,
drop_remainder=False,
prefetch=True,
num_workers=2,
)
``` | ### Describe the bug
Hi, I'm using `to_tf_dataset` to convert a _large_ dataset to `tf.data.Dataset`. I observed that the data loading *before* training took a lot of time and memory, even with `batch_size=1`.
After some digging, i believe the reason lies in the shuffle behavior. The [source code](https://github.com/huggingface/datasets/blob/main/src/datasets/utils/tf_utils.py#L185) uses `len(dataset)` as the `buffer_size`, which may load all the data into the memory, and the [tf.data doc](https://www.tensorflow.org/guide/data#randomly_shuffling_input_data) also states that "While large buffer_sizes shuffle more thoroughly, they can take a lot of memory, and significant time to fill".
### Steps to reproduce the bug
```python
from datasets import Dataset
def gen(): # some large data
for i in range(50000000):
yield {"data": i}
ds = Dataset.from_generator(gen, cache_dir="./huggingface")
tf_ds = ds.to_tf_dataset(
batch_size=64,
shuffle=False, # no shuffle
drop_remainder=False,
prefetch=True,
)
# fast and memory friendly π€
for batch in tf_ds:
...
tf_ds_shuffle = ds.to_tf_dataset(
batch_size=64,
shuffle=True,
drop_remainder=False,
prefetch=True,
)
# slow and memory hungry for simple iteration π±
for batch in tf_ds_shuffle:
...
```
### Expected behavior
Shuffling should not load all the data into the memory. Would adding a `buffer_size` parameter in the `to_tf_dataset` API alleviate the problem?
### Environment info
- `datasets` version: 2.11.0
- Platform: Linux-5.17.1-051701-generic-x86_64-with-glibc2.17
- Python version: 3.8.13
- Huggingface_hub version: 0.13.4
- PyArrow version: 11.0.0
- Pandas version: 1.4.3
| 160 | `to_tf_dataset` consumes too much memory
### Describe the bug
Hi, I'm using `to_tf_dataset` to convert a _large_ dataset to `tf.data.Dataset`. I observed that the data loading *before* training took a lot of time and memory, even with `batch_size=1`.
After some digging, i believe the reason lies in the shuffle behavior. The [source code](https://github.com/huggingface/datasets/blob/main/src/datasets/utils/tf_utils.py#L185) uses `len(dataset)` as the `buffer_size`, which may load all the data into the memory, and the [tf.data doc](https://www.tensorflow.org/guide/data#randomly_shuffling_input_data) also states that "While large buffer_sizes shuffle more thoroughly, they can take a lot of memory, and significant time to fill".
### Steps to reproduce the bug
```python
from datasets import Dataset
def gen(): # some large data
for i in range(50000000):
yield {"data": i}
ds = Dataset.from_generator(gen, cache_dir="./huggingface")
tf_ds = ds.to_tf_dataset(
batch_size=64,
shuffle=False, # no shuffle
drop_remainder=False,
prefetch=True,
)
# fast and memory friendly π€
for batch in tf_ds:
...
tf_ds_shuffle = ds.to_tf_dataset(
batch_size=64,
shuffle=True,
drop_remainder=False,
prefetch=True,
)
# slow and memory hungry for simple iteration π±
for batch in tf_ds_shuffle:
...
```
### Expected behavior
Shuffling should not load all the data into the memory. Would adding a `buffer_size` parameter in the `to_tf_dataset` API alleviate the problem?
### Environment info
- `datasets` version: 2.11.0
- Platform: Linux-5.17.1-051701-generic-x86_64-with-glibc2.17
- Python version: 3.8.13
- Huggingface_hub version: 0.13.4
- PyArrow version: 11.0.0
- Pandas version: 1.4.3
Hi @Rocketknight1, you're right and I also noticed that only indices are used in shuffling. My data has shape (50000000, 10), but really the problem doesn't relate to a specific dataset. Simply running the following code costs me 10GB of memory.
```python
from datasets import Dataset
def gen():
for i in range(50000000):
yield {"data": i}
ds = Dataset.from_generator(gen, cache_dir="./huggingface")
tf_ds = ds.to_tf_dataset(
batch_size=1,
shuffle=True,
drop_remainder=False,
prefetch=True,
)
tf_ds = iter(tf_ds)
next(tf_ds)
# {'data': <tf.Tensor: shape=(1,), dtype=int64, numpy=array([0])>}
```
I just realized maybe it was an issue from tensorflow (I'm using tf 2.12). So I tried the following code, and it used 10GB of memory too.
```python
import numpy as np
import tensorflow as tf
data_size = 50000000
tf_dataset = tf.data.Dataset.from_tensor_slices(np.arange(data_size))
tf_dataset = iter(tf_dataset.shuffle(data_size))
next(tf_dataset)
# <tf.Tensor: shape=(), dtype=int64, numpy=24774043>
```
By the way, as @lhoestq mentioned, multiprocessing uses numpy shuffling, and it uses less than 1 GB of memory:
```python
tf_ds_mp = ds.to_tf_dataset(
batch_size=1,
shuffle=True,
drop_remainder=False,
prefetch=True,
num_workers=2,
)
``` |
https://github.com/huggingface/datasets/issues/5855 | `to_tf_dataset` consumes too much memory | Thanks for that reproduction script - I've confirmed the same issue is occurring for me. Investigating it now! | ### Describe the bug
Hi, I'm using `to_tf_dataset` to convert a _large_ dataset to `tf.data.Dataset`. I observed that the data loading *before* training took a lot of time and memory, even with `batch_size=1`.
After some digging, i believe the reason lies in the shuffle behavior. The [source code](https://github.com/huggingface/datasets/blob/main/src/datasets/utils/tf_utils.py#L185) uses `len(dataset)` as the `buffer_size`, which may load all the data into the memory, and the [tf.data doc](https://www.tensorflow.org/guide/data#randomly_shuffling_input_data) also states that "While large buffer_sizes shuffle more thoroughly, they can take a lot of memory, and significant time to fill".
### Steps to reproduce the bug
```python
from datasets import Dataset
def gen(): # some large data
for i in range(50000000):
yield {"data": i}
ds = Dataset.from_generator(gen, cache_dir="./huggingface")
tf_ds = ds.to_tf_dataset(
batch_size=64,
shuffle=False, # no shuffle
drop_remainder=False,
prefetch=True,
)
# fast and memory friendly π€
for batch in tf_ds:
...
tf_ds_shuffle = ds.to_tf_dataset(
batch_size=64,
shuffle=True,
drop_remainder=False,
prefetch=True,
)
# slow and memory hungry for simple iteration π±
for batch in tf_ds_shuffle:
...
```
### Expected behavior
Shuffling should not load all the data into the memory. Would adding a `buffer_size` parameter in the `to_tf_dataset` API alleviate the problem?
### Environment info
- `datasets` version: 2.11.0
- Platform: Linux-5.17.1-051701-generic-x86_64-with-glibc2.17
- Python version: 3.8.13
- Huggingface_hub version: 0.13.4
- PyArrow version: 11.0.0
- Pandas version: 1.4.3
| 18 | `to_tf_dataset` consumes too much memory
### Describe the bug
Hi, I'm using `to_tf_dataset` to convert a _large_ dataset to `tf.data.Dataset`. I observed that the data loading *before* training took a lot of time and memory, even with `batch_size=1`.
After some digging, i believe the reason lies in the shuffle behavior. The [source code](https://github.com/huggingface/datasets/blob/main/src/datasets/utils/tf_utils.py#L185) uses `len(dataset)` as the `buffer_size`, which may load all the data into the memory, and the [tf.data doc](https://www.tensorflow.org/guide/data#randomly_shuffling_input_data) also states that "While large buffer_sizes shuffle more thoroughly, they can take a lot of memory, and significant time to fill".
### Steps to reproduce the bug
```python
from datasets import Dataset
def gen(): # some large data
for i in range(50000000):
yield {"data": i}
ds = Dataset.from_generator(gen, cache_dir="./huggingface")
tf_ds = ds.to_tf_dataset(
batch_size=64,
shuffle=False, # no shuffle
drop_remainder=False,
prefetch=True,
)
# fast and memory friendly π€
for batch in tf_ds:
...
tf_ds_shuffle = ds.to_tf_dataset(
batch_size=64,
shuffle=True,
drop_remainder=False,
prefetch=True,
)
# slow and memory hungry for simple iteration π±
for batch in tf_ds_shuffle:
...
```
### Expected behavior
Shuffling should not load all the data into the memory. Would adding a `buffer_size` parameter in the `to_tf_dataset` API alleviate the problem?
### Environment info
- `datasets` version: 2.11.0
- Platform: Linux-5.17.1-051701-generic-x86_64-with-glibc2.17
- Python version: 3.8.13
- Huggingface_hub version: 0.13.4
- PyArrow version: 11.0.0
- Pandas version: 1.4.3
Thanks for that reproduction script - I've confirmed the same issue is occurring for me. Investigating it now! |
https://github.com/huggingface/datasets/issues/5855 | `to_tf_dataset` consumes too much memory | Update: The memory usage is occurring in creation of the index and shuffle buffer. You can reproduce it very simply with:
```python
import tensorflow as tf
indices = tf.range(50_000_000, dtype=tf.int64)
dataset = tf.data.Dataset.from_tensor_slices(indices)
dataset = dataset.shuffle(len(dataset))
print(next(iter(dataset))
```
When I wrote this code I thought `tf.data` had an optimization for shuffling an entire tensor that wouldn't create the entire shuffle buffer, but evidently it's just creating the enormous buffer in memory. I'll see if I can find a more efficient way to do this - we might end up moving everything to the `numpy` multiprocessing path to avoid it. | ### Describe the bug
Hi, I'm using `to_tf_dataset` to convert a _large_ dataset to `tf.data.Dataset`. I observed that the data loading *before* training took a lot of time and memory, even with `batch_size=1`.
After some digging, i believe the reason lies in the shuffle behavior. The [source code](https://github.com/huggingface/datasets/blob/main/src/datasets/utils/tf_utils.py#L185) uses `len(dataset)` as the `buffer_size`, which may load all the data into the memory, and the [tf.data doc](https://www.tensorflow.org/guide/data#randomly_shuffling_input_data) also states that "While large buffer_sizes shuffle more thoroughly, they can take a lot of memory, and significant time to fill".
### Steps to reproduce the bug
```python
from datasets import Dataset
def gen(): # some large data
for i in range(50000000):
yield {"data": i}
ds = Dataset.from_generator(gen, cache_dir="./huggingface")
tf_ds = ds.to_tf_dataset(
batch_size=64,
shuffle=False, # no shuffle
drop_remainder=False,
prefetch=True,
)
# fast and memory friendly π€
for batch in tf_ds:
...
tf_ds_shuffle = ds.to_tf_dataset(
batch_size=64,
shuffle=True,
drop_remainder=False,
prefetch=True,
)
# slow and memory hungry for simple iteration π±
for batch in tf_ds_shuffle:
...
```
### Expected behavior
Shuffling should not load all the data into the memory. Would adding a `buffer_size` parameter in the `to_tf_dataset` API alleviate the problem?
### Environment info
- `datasets` version: 2.11.0
- Platform: Linux-5.17.1-051701-generic-x86_64-with-glibc2.17
- Python version: 3.8.13
- Huggingface_hub version: 0.13.4
- PyArrow version: 11.0.0
- Pandas version: 1.4.3
| 99 | `to_tf_dataset` consumes too much memory
### Describe the bug
Hi, I'm using `to_tf_dataset` to convert a _large_ dataset to `tf.data.Dataset`. I observed that the data loading *before* training took a lot of time and memory, even with `batch_size=1`.
After some digging, i believe the reason lies in the shuffle behavior. The [source code](https://github.com/huggingface/datasets/blob/main/src/datasets/utils/tf_utils.py#L185) uses `len(dataset)` as the `buffer_size`, which may load all the data into the memory, and the [tf.data doc](https://www.tensorflow.org/guide/data#randomly_shuffling_input_data) also states that "While large buffer_sizes shuffle more thoroughly, they can take a lot of memory, and significant time to fill".
### Steps to reproduce the bug
```python
from datasets import Dataset
def gen(): # some large data
for i in range(50000000):
yield {"data": i}
ds = Dataset.from_generator(gen, cache_dir="./huggingface")
tf_ds = ds.to_tf_dataset(
batch_size=64,
shuffle=False, # no shuffle
drop_remainder=False,
prefetch=True,
)
# fast and memory friendly π€
for batch in tf_ds:
...
tf_ds_shuffle = ds.to_tf_dataset(
batch_size=64,
shuffle=True,
drop_remainder=False,
prefetch=True,
)
# slow and memory hungry for simple iteration π±
for batch in tf_ds_shuffle:
...
```
### Expected behavior
Shuffling should not load all the data into the memory. Would adding a `buffer_size` parameter in the `to_tf_dataset` API alleviate the problem?
### Environment info
- `datasets` version: 2.11.0
- Platform: Linux-5.17.1-051701-generic-x86_64-with-glibc2.17
- Python version: 3.8.13
- Huggingface_hub version: 0.13.4
- PyArrow version: 11.0.0
- Pandas version: 1.4.3
Update: The memory usage is occurring in creation of the index and shuffle buffer. You can reproduce it very simply with:
```python
import tensorflow as tf
indices = tf.range(50_000_000, dtype=tf.int64)
dataset = tf.data.Dataset.from_tensor_slices(indices)
dataset = dataset.shuffle(len(dataset))
print(next(iter(dataset))
```
When I wrote this code I thought `tf.data` had an optimization for shuffling an entire tensor that wouldn't create the entire shuffle buffer, but evidently it's just creating the enormous buffer in memory. I'll see if I can find a more efficient way to do this - we might end up moving everything to the `numpy` multiprocessing path to avoid it. |
https://github.com/huggingface/datasets/issues/5854 | Can not load audiofolder dataset on kaggle | Hi! `audiofolder` requires `datasets>=2.5.0`, so please update the `datasets`' installation (`pip install -U datasets`) in the environment (and restart the env for the update to take effect) to resolve the issue. | ### Describe the bug
It's crash log:
FileNotFoundError: Couldn't find a dataset script at /kaggle/working/audiofolder/audiofolder.py or any data file in the same directory. Couldn't find 'audiofolder' on the Hugging Face Hub either: FileNotFoundError: Couldn't find file at https://raw.githubusercontent.com/huggingface/datasets/master/datasets/audiofolder/audiofolder.py
### Steps to reproduce the bug

common_voice = load_dataset("audiofolder", data_dir="/kaggle/working/data")
### Expected behavior
load dataset without error. It works ok on colab, but on kaggle it happends.
### Environment info
- `datasets` version: 2.1.0
- Platform: Linux-5.15.109+-x86_64-with-glibc2.31
- Python version: 3.10.10
- PyArrow version: 9.0.0
- Pandas version: 1.5.3 | 31 | Can not load audiofolder dataset on kaggle
### Describe the bug
It's crash log:
FileNotFoundError: Couldn't find a dataset script at /kaggle/working/audiofolder/audiofolder.py or any data file in the same directory. Couldn't find 'audiofolder' on the Hugging Face Hub either: FileNotFoundError: Couldn't find file at https://raw.githubusercontent.com/huggingface/datasets/master/datasets/audiofolder/audiofolder.py
### Steps to reproduce the bug

common_voice = load_dataset("audiofolder", data_dir="/kaggle/working/data")
### Expected behavior
load dataset without error. It works ok on colab, but on kaggle it happends.
### Environment info
- `datasets` version: 2.1.0
- Platform: Linux-5.15.109+-x86_64-with-glibc2.31
- Python version: 3.10.10
- PyArrow version: 9.0.0
- Pandas version: 1.5.3
Hi! `audiofolder` requires `datasets>=2.5.0`, so please update the `datasets`' installation (`pip install -U datasets`) in the environment (and restart the env for the update to take effect) to resolve the issue. |
https://github.com/huggingface/datasets/issues/5854 | Can not load audiofolder dataset on kaggle | > Hi! `audiofolder` requires `datasets>=2.5.0`, so please update the `datasets`' installation (`pip install -U datasets`) in the environment to resolve the issue.
I don't think it is a problem of the version. It runs ok on colab or local machine. Only on kaggle will has this bug. | ### Describe the bug
It's crash log:
FileNotFoundError: Couldn't find a dataset script at /kaggle/working/audiofolder/audiofolder.py or any data file in the same directory. Couldn't find 'audiofolder' on the Hugging Face Hub either: FileNotFoundError: Couldn't find file at https://raw.githubusercontent.com/huggingface/datasets/master/datasets/audiofolder/audiofolder.py
### Steps to reproduce the bug

common_voice = load_dataset("audiofolder", data_dir="/kaggle/working/data")
### Expected behavior
load dataset without error. It works ok on colab, but on kaggle it happends.
### Environment info
- `datasets` version: 2.1.0
- Platform: Linux-5.15.109+-x86_64-with-glibc2.31
- Python version: 3.10.10
- PyArrow version: 9.0.0
- Pandas version: 1.5.3 | 47 | Can not load audiofolder dataset on kaggle
### Describe the bug
It's crash log:
FileNotFoundError: Couldn't find a dataset script at /kaggle/working/audiofolder/audiofolder.py or any data file in the same directory. Couldn't find 'audiofolder' on the Hugging Face Hub either: FileNotFoundError: Couldn't find file at https://raw.githubusercontent.com/huggingface/datasets/master/datasets/audiofolder/audiofolder.py
### Steps to reproduce the bug

common_voice = load_dataset("audiofolder", data_dir="/kaggle/working/data")
### Expected behavior
load dataset without error. It works ok on colab, but on kaggle it happends.
### Environment info
- `datasets` version: 2.1.0
- Platform: Linux-5.15.109+-x86_64-with-glibc2.31
- Python version: 3.10.10
- PyArrow version: 9.0.0
- Pandas version: 1.5.3
> Hi! `audiofolder` requires `datasets>=2.5.0`, so please update the `datasets`' installation (`pip install -U datasets`) in the environment to resolve the issue.
I don't think it is a problem of the version. It runs ok on colab or local machine. Only on kaggle will has this bug. |
https://github.com/huggingface/datasets/issues/5854 | Can not load audiofolder dataset on kaggle | Based on your dataset info, the installed version is `2.1.0`, which does not include `audiofolder`.
By default, Kaggle preinstalls `datasets` into a new env, but the version it installs is outdated and does not contain newer features such as `audiofolder` | ### Describe the bug
It's crash log:
FileNotFoundError: Couldn't find a dataset script at /kaggle/working/audiofolder/audiofolder.py or any data file in the same directory. Couldn't find 'audiofolder' on the Hugging Face Hub either: FileNotFoundError: Couldn't find file at https://raw.githubusercontent.com/huggingface/datasets/master/datasets/audiofolder/audiofolder.py
### Steps to reproduce the bug

common_voice = load_dataset("audiofolder", data_dir="/kaggle/working/data")
### Expected behavior
load dataset without error. It works ok on colab, but on kaggle it happends.
### Environment info
- `datasets` version: 2.1.0
- Platform: Linux-5.15.109+-x86_64-with-glibc2.31
- Python version: 3.10.10
- PyArrow version: 9.0.0
- Pandas version: 1.5.3 | 40 | Can not load audiofolder dataset on kaggle
### Describe the bug
It's crash log:
FileNotFoundError: Couldn't find a dataset script at /kaggle/working/audiofolder/audiofolder.py or any data file in the same directory. Couldn't find 'audiofolder' on the Hugging Face Hub either: FileNotFoundError: Couldn't find file at https://raw.githubusercontent.com/huggingface/datasets/master/datasets/audiofolder/audiofolder.py
### Steps to reproduce the bug

common_voice = load_dataset("audiofolder", data_dir="/kaggle/working/data")
### Expected behavior
load dataset without error. It works ok on colab, but on kaggle it happends.
### Environment info
- `datasets` version: 2.1.0
- Platform: Linux-5.15.109+-x86_64-with-glibc2.31
- Python version: 3.10.10
- PyArrow version: 9.0.0
- Pandas version: 1.5.3
Based on your dataset info, the installed version is `2.1.0`, which does not include `audiofolder`.
By default, Kaggle preinstalls `datasets` into a new env, but the version it installs is outdated and does not contain newer features such as `audiofolder` |
https://github.com/huggingface/datasets/issues/5847 | Streaming IterableDataset not working with translation pipeline | [`KeyDataset`](https://github.com/huggingface/transformers/blob/7f8b909189547944617741d8d3c6c84504701693/src/transformers/pipelines/pt_utils.py#L296) doesn't support iterable datasets, so you either need to implement a version that does (and also indexing nested (translation) fields):
```python
from torch.utils.data import Dataset, IterableDataset
def build_key_fetcher(key: str):
def _key_fetcher(item):
for sub_key in key.split("."):
item = item[sub_key]
return item
return _key_fetcher
class KeyDataset(Dataset):
def __new__(cls, dataset: Dataset, key: str):
cls = _KeyIterableDataset if isinstance(dataset, IterableDataset) else _KeyMapDataset
self = object.__new__(cls)
self.dataset = dataset
self.key = key
self._key_fetcher = build_key_fetcher(key)
return self
class _KeyMapDataset(KeyDataset):
def __getitem__(self, i):
return self._key_fetcher(self.dataset[i])
def __len__(self):
return len(self.dataset)
class _KeyIterableDataset(KeyDataset):
def __iter__(self):
for ex in self.dataset:
yield self._key_fetcher(ex)
ks = KeyDataset(ds, "translation.en")
```
or use `IterableDataset`'s `map`:
```python
def fetch_en_translation(ex):
return {"en": ex["translation"]["en"]}
ks = ds.map(fetch_en_translation, remove_columns=ds.column_names)
```
cc @sgugger: Perhaps the `KeyDataset` + PyTorch `IterableDataset` case should be supported by Transformers | ### Describe the bug
I'm trying to use a streaming dataset for translation inference to avoid downloading the training data.
I'm using a pipeline and a dataset, and following the guidance in the tutorial.
Instead I get an exception that IterableDataset has no len().
### Steps to reproduce the bug
CODE:
```
from transformers import pipeline
from transformers.pipelines.pt_utils import KeyDataset
from datasets import load_dataset
ds = load_dataset(path="wmt14", name="fr-en", split="test", streaming=True)
bs=1
mt = pipeline("translation_en_to_fr", model="t5-base", batch_size=bs)
#print(mt("hello")) THIS WORKS
ks = KeyDataset(ds, "translation")
print(f"{ks}")
xx= mt(ks)
for x in xx:
print(x)
```
RUN:
```
(watnlp) [jlquinn@bertdev01 hf]$ python ende.t5.pipe.py
2023-05-11 16:48:08.817572: I tensorflow/core/util/util.cc:169] oneDNN custom operations are on. You may see slightly different numerical results due to floating-point round-off errors from different computation orders. To turn them off, set the environment variable `TF_ENABLE_ONEDNN_OPTS=0`.
2023-05-11 16:48:08.821388: W tensorflow/stream_executor/platform/default/dso_loader.cc:64] Could not load dynamic library 'libcudart.so.11.0'; dlerror: libcudart.so.11.0: cannot open shared object file: No such file or directory
2023-05-11 16:48:08.821407: I tensorflow/stream_executor/cuda/cudart_stub.cc:29] Ignore above cudart dlerror if you do not have a GPU set up on your machine.
<transformers.pipelines.pt_utils.KeyDataset object at 0x7f61ed5da9d0>
Traceback (most recent call last):
File "/home/jlquinn/models/hf/ende.t5.pipe.py", line 11, in <module>
for x in xx:
File "/home/jlquinn/miniconda3/envs/watnlp/lib/python3.9/site-packages/transformers/pipelines/pt_utils.py", line 111, in __next__
item = next(self.iterator)
File "/home/jlquinn/miniconda3/envs/watnlp/lib/python3.9/site-packages/transformers/pipelines/pt_utils.py", line 111, in __next__
item = next(self.iterator)
File "/home/jlquinn/miniconda3/envs/watnlp/lib/python3.9/site-packages/torch/utils/data/dataloader.py", line 681, in __next__
data = self._next_data()
File "/home/jlquinn/miniconda3/envs/watnlp/lib/python3.9/site-packages/torch/utils/data/dataloader.py", line 720, in _next_data
index = self._next_index() # may raise StopIteration
File "/home/jlquinn/miniconda3/envs/watnlp/lib/python3.9/site-packages/torch/utils/data/dataloader.py", line 671, in _next_index
return next(self._sampler_iter) # may raise StopIteration
File "/home/jlquinn/miniconda3/envs/watnlp/lib/python3.9/site-packages/torch/utils/data/sampler.py", line 247, in __iter__
for idx in self.sampler:
File "/home/jlquinn/miniconda3/envs/watnlp/lib/python3.9/site-packages/torch/utils/data/sampler.py", line 76, in __iter__
return iter(range(len(self.data_source)))
File "/home/jlquinn/miniconda3/envs/watnlp/lib/python3.9/site-packages/transformers/pipelines/pt_utils.py", line 13, in __len__
return len(self.dataset)
File "/home/jlquinn/miniconda3/envs/watnlp/lib/python3.9/site-packages/transformers/pipelines/pt_utils.py", line 289, in __len__
return len(self.dataset)
TypeError: object of type 'IterableDataset' has no len()
```
### Expected behavior
I'm expecting french translations of the english test set to be printed.
### Environment info
Run on CPU with no GPU.
RHEL 8.7 x86_64
python 3.9.0
transformers 4.17.0
datasets 2.0.0
tokenizers 0.12.1
```
(watnlp) [jlquinn@bertdev01 hf]$ datasets-cli env
Copy-and-paste the text below in your GitHub issue.
- `datasets` version: 2.0.0
- Platform: Linux-4.18.0-372.19.1.el8_6.x86_64-x86_64-with-glibc2.28
- Python version: 3.9.0
- PyArrow version: 8.0.0
- Pandas version: 1.4.4
```
| 128 | Streaming IterableDataset not working with translation pipeline
### Describe the bug
I'm trying to use a streaming dataset for translation inference to avoid downloading the training data.
I'm using a pipeline and a dataset, and following the guidance in the tutorial.
Instead I get an exception that IterableDataset has no len().
### Steps to reproduce the bug
CODE:
```
from transformers import pipeline
from transformers.pipelines.pt_utils import KeyDataset
from datasets import load_dataset
ds = load_dataset(path="wmt14", name="fr-en", split="test", streaming=True)
bs=1
mt = pipeline("translation_en_to_fr", model="t5-base", batch_size=bs)
#print(mt("hello")) THIS WORKS
ks = KeyDataset(ds, "translation")
print(f"{ks}")
xx= mt(ks)
for x in xx:
print(x)
```
RUN:
```
(watnlp) [jlquinn@bertdev01 hf]$ python ende.t5.pipe.py
2023-05-11 16:48:08.817572: I tensorflow/core/util/util.cc:169] oneDNN custom operations are on. You may see slightly different numerical results due to floating-point round-off errors from different computation orders. To turn them off, set the environment variable `TF_ENABLE_ONEDNN_OPTS=0`.
2023-05-11 16:48:08.821388: W tensorflow/stream_executor/platform/default/dso_loader.cc:64] Could not load dynamic library 'libcudart.so.11.0'; dlerror: libcudart.so.11.0: cannot open shared object file: No such file or directory
2023-05-11 16:48:08.821407: I tensorflow/stream_executor/cuda/cudart_stub.cc:29] Ignore above cudart dlerror if you do not have a GPU set up on your machine.
<transformers.pipelines.pt_utils.KeyDataset object at 0x7f61ed5da9d0>
Traceback (most recent call last):
File "/home/jlquinn/models/hf/ende.t5.pipe.py", line 11, in <module>
for x in xx:
File "/home/jlquinn/miniconda3/envs/watnlp/lib/python3.9/site-packages/transformers/pipelines/pt_utils.py", line 111, in __next__
item = next(self.iterator)
File "/home/jlquinn/miniconda3/envs/watnlp/lib/python3.9/site-packages/transformers/pipelines/pt_utils.py", line 111, in __next__
item = next(self.iterator)
File "/home/jlquinn/miniconda3/envs/watnlp/lib/python3.9/site-packages/torch/utils/data/dataloader.py", line 681, in __next__
data = self._next_data()
File "/home/jlquinn/miniconda3/envs/watnlp/lib/python3.9/site-packages/torch/utils/data/dataloader.py", line 720, in _next_data
index = self._next_index() # may raise StopIteration
File "/home/jlquinn/miniconda3/envs/watnlp/lib/python3.9/site-packages/torch/utils/data/dataloader.py", line 671, in _next_index
return next(self._sampler_iter) # may raise StopIteration
File "/home/jlquinn/miniconda3/envs/watnlp/lib/python3.9/site-packages/torch/utils/data/sampler.py", line 247, in __iter__
for idx in self.sampler:
File "/home/jlquinn/miniconda3/envs/watnlp/lib/python3.9/site-packages/torch/utils/data/sampler.py", line 76, in __iter__
return iter(range(len(self.data_source)))
File "/home/jlquinn/miniconda3/envs/watnlp/lib/python3.9/site-packages/transformers/pipelines/pt_utils.py", line 13, in __len__
return len(self.dataset)
File "/home/jlquinn/miniconda3/envs/watnlp/lib/python3.9/site-packages/transformers/pipelines/pt_utils.py", line 289, in __len__
return len(self.dataset)
TypeError: object of type 'IterableDataset' has no len()
```
### Expected behavior
I'm expecting french translations of the english test set to be printed.
### Environment info
Run on CPU with no GPU.
RHEL 8.7 x86_64
python 3.9.0
transformers 4.17.0
datasets 2.0.0
tokenizers 0.12.1
```
(watnlp) [jlquinn@bertdev01 hf]$ datasets-cli env
Copy-and-paste the text below in your GitHub issue.
- `datasets` version: 2.0.0
- Platform: Linux-4.18.0-372.19.1.el8_6.x86_64-x86_64-with-glibc2.28
- Python version: 3.9.0
- PyArrow version: 8.0.0
- Pandas version: 1.4.4
```
[`KeyDataset`](https://github.com/huggingface/transformers/blob/7f8b909189547944617741d8d3c6c84504701693/src/transformers/pipelines/pt_utils.py#L296) doesn't support iterable datasets, so you either need to implement a version that does (and also indexing nested (translation) fields):
```python
from torch.utils.data import Dataset, IterableDataset
def build_key_fetcher(key: str):
def _key_fetcher(item):
for sub_key in key.split("."):
item = item[sub_key]
return item
return _key_fetcher
class KeyDataset(Dataset):
def __new__(cls, dataset: Dataset, key: str):
cls = _KeyIterableDataset if isinstance(dataset, IterableDataset) else _KeyMapDataset
self = object.__new__(cls)
self.dataset = dataset
self.key = key
self._key_fetcher = build_key_fetcher(key)
return self
class _KeyMapDataset(KeyDataset):
def __getitem__(self, i):
return self._key_fetcher(self.dataset[i])
def __len__(self):
return len(self.dataset)
class _KeyIterableDataset(KeyDataset):
def __iter__(self):
for ex in self.dataset:
yield self._key_fetcher(ex)
ks = KeyDataset(ds, "translation.en")
```
or use `IterableDataset`'s `map`:
```python
def fetch_en_translation(ex):
return {"en": ex["translation"]["en"]}
ks = ds.map(fetch_en_translation, remove_columns=ds.column_names)
```
cc @sgugger: Perhaps the `KeyDataset` + PyTorch `IterableDataset` case should be supported by Transformers |
https://github.com/huggingface/datasets/issues/5847 | Streaming IterableDataset not working with translation pipeline | @mariosasko The map snippet didn't quite work, but gave me enough of a clue to get it working. The following snippet does work:
```
def en_translation(x):
return {"en":x['translation']['en']}
ks = ds.map(en_translation, remove_columns=['translation'])
test=[]
for x in iter(ks):
test.append(x['en'])
xx= mt(test)
for x in xx:
print(x)
```
I tried just returning `x['translation']['en`]` in the helper function instead of the dict, but that didn't give me an iterator over strings that pipeline would work with either.
The snippet as is gives the following error:
```
Traceback (most recent call last):
File "/home/jlquinn/miniconda3/envs/watnlp/lib/python3.9/pdb.py", line 1704, in main
pdb._runscript(mainpyfile)
File "/home/jlquinn/miniconda3/envs/watnlp/lib/python3.9/pdb.py", line 1573, in _runscript
self.run(statement)
File "/home/jlquinn/miniconda3/envs/watnlp/lib/python3.9/bdb.py", line 580, in run
exec(cmd, globals, locals)
File "<string>", line 1, in <module>
File "/home/jlquinn/models/hf/ende.t5.pipe.py", line 1, in <module>
from transformers import pipeline
File "/home/jlquinn/miniconda3/envs/watnlp/lib/python3.9/site-packages/transformers/pipelines/text2text_generation.py", line 335, in __call__
return super().__call__(*args, **kwargs)
File "/home/jlquinn/miniconda3/envs/watnlp/lib/python3.9/site-packages/transformers/pipelines/text2text_generation.py", line 138, in __call__
result = super().__call__(*args, **kwargs)
File "/home/jlquinn/miniconda3/envs/watnlp/lib/python3.9/site-packages/transformers/pipelines/base.py", line 1027, in __call__
return self.run_single(inputs, preprocess_params, forward_params, postprocess_params)
File "/home/jlquinn/miniconda3/envs/watnlp/lib/python3.9/site-packages/transformers/pipelines/base.py", line 1033, in run_single
model_inputs = self.preprocess(inputs, **preprocess_params)
File "/home/jlquinn/miniconda3/envs/watnlp/lib/python3.9/site-packages/transformers/pipelines/text2text_generation.py", line 287, in preprocess
return super()._parse_and_tokenize(*args, truncation=truncation)
File "/home/jlquinn/miniconda3/envs/watnlp/lib/python3.9/site-packages/transformers/pipelines/text2text_generation.py", line 100, in _parse_and_tokenize
raise ValueError(
ValueError: `args[0]`: <datasets.iterable_dataset.IterableDataset object at 0x7f5fd38ef1c0> have the wrong format. The should be either of type `str` or type `list`
Uncaught exception. Entering post mortem debugging
Running 'cont' or 'step' will restart the program
```
| ### Describe the bug
I'm trying to use a streaming dataset for translation inference to avoid downloading the training data.
I'm using a pipeline and a dataset, and following the guidance in the tutorial.
Instead I get an exception that IterableDataset has no len().
### Steps to reproduce the bug
CODE:
```
from transformers import pipeline
from transformers.pipelines.pt_utils import KeyDataset
from datasets import load_dataset
ds = load_dataset(path="wmt14", name="fr-en", split="test", streaming=True)
bs=1
mt = pipeline("translation_en_to_fr", model="t5-base", batch_size=bs)
#print(mt("hello")) THIS WORKS
ks = KeyDataset(ds, "translation")
print(f"{ks}")
xx= mt(ks)
for x in xx:
print(x)
```
RUN:
```
(watnlp) [jlquinn@bertdev01 hf]$ python ende.t5.pipe.py
2023-05-11 16:48:08.817572: I tensorflow/core/util/util.cc:169] oneDNN custom operations are on. You may see slightly different numerical results due to floating-point round-off errors from different computation orders. To turn them off, set the environment variable `TF_ENABLE_ONEDNN_OPTS=0`.
2023-05-11 16:48:08.821388: W tensorflow/stream_executor/platform/default/dso_loader.cc:64] Could not load dynamic library 'libcudart.so.11.0'; dlerror: libcudart.so.11.0: cannot open shared object file: No such file or directory
2023-05-11 16:48:08.821407: I tensorflow/stream_executor/cuda/cudart_stub.cc:29] Ignore above cudart dlerror if you do not have a GPU set up on your machine.
<transformers.pipelines.pt_utils.KeyDataset object at 0x7f61ed5da9d0>
Traceback (most recent call last):
File "/home/jlquinn/models/hf/ende.t5.pipe.py", line 11, in <module>
for x in xx:
File "/home/jlquinn/miniconda3/envs/watnlp/lib/python3.9/site-packages/transformers/pipelines/pt_utils.py", line 111, in __next__
item = next(self.iterator)
File "/home/jlquinn/miniconda3/envs/watnlp/lib/python3.9/site-packages/transformers/pipelines/pt_utils.py", line 111, in __next__
item = next(self.iterator)
File "/home/jlquinn/miniconda3/envs/watnlp/lib/python3.9/site-packages/torch/utils/data/dataloader.py", line 681, in __next__
data = self._next_data()
File "/home/jlquinn/miniconda3/envs/watnlp/lib/python3.9/site-packages/torch/utils/data/dataloader.py", line 720, in _next_data
index = self._next_index() # may raise StopIteration
File "/home/jlquinn/miniconda3/envs/watnlp/lib/python3.9/site-packages/torch/utils/data/dataloader.py", line 671, in _next_index
return next(self._sampler_iter) # may raise StopIteration
File "/home/jlquinn/miniconda3/envs/watnlp/lib/python3.9/site-packages/torch/utils/data/sampler.py", line 247, in __iter__
for idx in self.sampler:
File "/home/jlquinn/miniconda3/envs/watnlp/lib/python3.9/site-packages/torch/utils/data/sampler.py", line 76, in __iter__
return iter(range(len(self.data_source)))
File "/home/jlquinn/miniconda3/envs/watnlp/lib/python3.9/site-packages/transformers/pipelines/pt_utils.py", line 13, in __len__
return len(self.dataset)
File "/home/jlquinn/miniconda3/envs/watnlp/lib/python3.9/site-packages/transformers/pipelines/pt_utils.py", line 289, in __len__
return len(self.dataset)
TypeError: object of type 'IterableDataset' has no len()
```
### Expected behavior
I'm expecting french translations of the english test set to be printed.
### Environment info
Run on CPU with no GPU.
RHEL 8.7 x86_64
python 3.9.0
transformers 4.17.0
datasets 2.0.0
tokenizers 0.12.1
```
(watnlp) [jlquinn@bertdev01 hf]$ datasets-cli env
Copy-and-paste the text below in your GitHub issue.
- `datasets` version: 2.0.0
- Platform: Linux-4.18.0-372.19.1.el8_6.x86_64-x86_64-with-glibc2.28
- Python version: 3.9.0
- PyArrow version: 8.0.0
- Pandas version: 1.4.4
```
| 219 | Streaming IterableDataset not working with translation pipeline
### Describe the bug
I'm trying to use a streaming dataset for translation inference to avoid downloading the training data.
I'm using a pipeline and a dataset, and following the guidance in the tutorial.
Instead I get an exception that IterableDataset has no len().
### Steps to reproduce the bug
CODE:
```
from transformers import pipeline
from transformers.pipelines.pt_utils import KeyDataset
from datasets import load_dataset
ds = load_dataset(path="wmt14", name="fr-en", split="test", streaming=True)
bs=1
mt = pipeline("translation_en_to_fr", model="t5-base", batch_size=bs)
#print(mt("hello")) THIS WORKS
ks = KeyDataset(ds, "translation")
print(f"{ks}")
xx= mt(ks)
for x in xx:
print(x)
```
RUN:
```
(watnlp) [jlquinn@bertdev01 hf]$ python ende.t5.pipe.py
2023-05-11 16:48:08.817572: I tensorflow/core/util/util.cc:169] oneDNN custom operations are on. You may see slightly different numerical results due to floating-point round-off errors from different computation orders. To turn them off, set the environment variable `TF_ENABLE_ONEDNN_OPTS=0`.
2023-05-11 16:48:08.821388: W tensorflow/stream_executor/platform/default/dso_loader.cc:64] Could not load dynamic library 'libcudart.so.11.0'; dlerror: libcudart.so.11.0: cannot open shared object file: No such file or directory
2023-05-11 16:48:08.821407: I tensorflow/stream_executor/cuda/cudart_stub.cc:29] Ignore above cudart dlerror if you do not have a GPU set up on your machine.
<transformers.pipelines.pt_utils.KeyDataset object at 0x7f61ed5da9d0>
Traceback (most recent call last):
File "/home/jlquinn/models/hf/ende.t5.pipe.py", line 11, in <module>
for x in xx:
File "/home/jlquinn/miniconda3/envs/watnlp/lib/python3.9/site-packages/transformers/pipelines/pt_utils.py", line 111, in __next__
item = next(self.iterator)
File "/home/jlquinn/miniconda3/envs/watnlp/lib/python3.9/site-packages/transformers/pipelines/pt_utils.py", line 111, in __next__
item = next(self.iterator)
File "/home/jlquinn/miniconda3/envs/watnlp/lib/python3.9/site-packages/torch/utils/data/dataloader.py", line 681, in __next__
data = self._next_data()
File "/home/jlquinn/miniconda3/envs/watnlp/lib/python3.9/site-packages/torch/utils/data/dataloader.py", line 720, in _next_data
index = self._next_index() # may raise StopIteration
File "/home/jlquinn/miniconda3/envs/watnlp/lib/python3.9/site-packages/torch/utils/data/dataloader.py", line 671, in _next_index
return next(self._sampler_iter) # may raise StopIteration
File "/home/jlquinn/miniconda3/envs/watnlp/lib/python3.9/site-packages/torch/utils/data/sampler.py", line 247, in __iter__
for idx in self.sampler:
File "/home/jlquinn/miniconda3/envs/watnlp/lib/python3.9/site-packages/torch/utils/data/sampler.py", line 76, in __iter__
return iter(range(len(self.data_source)))
File "/home/jlquinn/miniconda3/envs/watnlp/lib/python3.9/site-packages/transformers/pipelines/pt_utils.py", line 13, in __len__
return len(self.dataset)
File "/home/jlquinn/miniconda3/envs/watnlp/lib/python3.9/site-packages/transformers/pipelines/pt_utils.py", line 289, in __len__
return len(self.dataset)
TypeError: object of type 'IterableDataset' has no len()
```
### Expected behavior
I'm expecting french translations of the english test set to be printed.
### Environment info
Run on CPU with no GPU.
RHEL 8.7 x86_64
python 3.9.0
transformers 4.17.0
datasets 2.0.0
tokenizers 0.12.1
```
(watnlp) [jlquinn@bertdev01 hf]$ datasets-cli env
Copy-and-paste the text below in your GitHub issue.
- `datasets` version: 2.0.0
- Platform: Linux-4.18.0-372.19.1.el8_6.x86_64-x86_64-with-glibc2.28
- Python version: 3.9.0
- PyArrow version: 8.0.0
- Pandas version: 1.4.4
```
@mariosasko The map snippet didn't quite work, but gave me enough of a clue to get it working. The following snippet does work:
```
def en_translation(x):
return {"en":x['translation']['en']}
ks = ds.map(en_translation, remove_columns=['translation'])
test=[]
for x in iter(ks):
test.append(x['en'])
xx= mt(test)
for x in xx:
print(x)
```
I tried just returning `x['translation']['en`]` in the helper function instead of the dict, but that didn't give me an iterator over strings that pipeline would work with either.
The snippet as is gives the following error:
```
Traceback (most recent call last):
File "/home/jlquinn/miniconda3/envs/watnlp/lib/python3.9/pdb.py", line 1704, in main
pdb._runscript(mainpyfile)
File "/home/jlquinn/miniconda3/envs/watnlp/lib/python3.9/pdb.py", line 1573, in _runscript
self.run(statement)
File "/home/jlquinn/miniconda3/envs/watnlp/lib/python3.9/bdb.py", line 580, in run
exec(cmd, globals, locals)
File "<string>", line 1, in <module>
File "/home/jlquinn/models/hf/ende.t5.pipe.py", line 1, in <module>
from transformers import pipeline
File "/home/jlquinn/miniconda3/envs/watnlp/lib/python3.9/site-packages/transformers/pipelines/text2text_generation.py", line 335, in __call__
return super().__call__(*args, **kwargs)
File "/home/jlquinn/miniconda3/envs/watnlp/lib/python3.9/site-packages/transformers/pipelines/text2text_generation.py", line 138, in __call__
result = super().__call__(*args, **kwargs)
File "/home/jlquinn/miniconda3/envs/watnlp/lib/python3.9/site-packages/transformers/pipelines/base.py", line 1027, in __call__
return self.run_single(inputs, preprocess_params, forward_params, postprocess_params)
File "/home/jlquinn/miniconda3/envs/watnlp/lib/python3.9/site-packages/transformers/pipelines/base.py", line 1033, in run_single
model_inputs = self.preprocess(inputs, **preprocess_params)
File "/home/jlquinn/miniconda3/envs/watnlp/lib/python3.9/site-packages/transformers/pipelines/text2text_generation.py", line 287, in preprocess
return super()._parse_and_tokenize(*args, truncation=truncation)
File "/home/jlquinn/miniconda3/envs/watnlp/lib/python3.9/site-packages/transformers/pipelines/text2text_generation.py", line 100, in _parse_and_tokenize
raise ValueError(
ValueError: `args[0]`: <datasets.iterable_dataset.IterableDataset object at 0x7f5fd38ef1c0> have the wrong format. The should be either of type `str` or type `list`
Uncaught exception. Entering post mortem debugging
Running 'cont' or 'step' will restart the program
```
|
https://github.com/huggingface/datasets/issues/5847 | Streaming IterableDataset not working with translation pipeline | So perhaps there's no bug exactly, but I would love to see two things: 1) improve the documentation to better understand what's really getting returned. 2) update the example provided of using transformer pipeline with a dataset to include the oddball case that translation appears to be. | ### Describe the bug
I'm trying to use a streaming dataset for translation inference to avoid downloading the training data.
I'm using a pipeline and a dataset, and following the guidance in the tutorial.
Instead I get an exception that IterableDataset has no len().
### Steps to reproduce the bug
CODE:
```
from transformers import pipeline
from transformers.pipelines.pt_utils import KeyDataset
from datasets import load_dataset
ds = load_dataset(path="wmt14", name="fr-en", split="test", streaming=True)
bs=1
mt = pipeline("translation_en_to_fr", model="t5-base", batch_size=bs)
#print(mt("hello")) THIS WORKS
ks = KeyDataset(ds, "translation")
print(f"{ks}")
xx= mt(ks)
for x in xx:
print(x)
```
RUN:
```
(watnlp) [jlquinn@bertdev01 hf]$ python ende.t5.pipe.py
2023-05-11 16:48:08.817572: I tensorflow/core/util/util.cc:169] oneDNN custom operations are on. You may see slightly different numerical results due to floating-point round-off errors from different computation orders. To turn them off, set the environment variable `TF_ENABLE_ONEDNN_OPTS=0`.
2023-05-11 16:48:08.821388: W tensorflow/stream_executor/platform/default/dso_loader.cc:64] Could not load dynamic library 'libcudart.so.11.0'; dlerror: libcudart.so.11.0: cannot open shared object file: No such file or directory
2023-05-11 16:48:08.821407: I tensorflow/stream_executor/cuda/cudart_stub.cc:29] Ignore above cudart dlerror if you do not have a GPU set up on your machine.
<transformers.pipelines.pt_utils.KeyDataset object at 0x7f61ed5da9d0>
Traceback (most recent call last):
File "/home/jlquinn/models/hf/ende.t5.pipe.py", line 11, in <module>
for x in xx:
File "/home/jlquinn/miniconda3/envs/watnlp/lib/python3.9/site-packages/transformers/pipelines/pt_utils.py", line 111, in __next__
item = next(self.iterator)
File "/home/jlquinn/miniconda3/envs/watnlp/lib/python3.9/site-packages/transformers/pipelines/pt_utils.py", line 111, in __next__
item = next(self.iterator)
File "/home/jlquinn/miniconda3/envs/watnlp/lib/python3.9/site-packages/torch/utils/data/dataloader.py", line 681, in __next__
data = self._next_data()
File "/home/jlquinn/miniconda3/envs/watnlp/lib/python3.9/site-packages/torch/utils/data/dataloader.py", line 720, in _next_data
index = self._next_index() # may raise StopIteration
File "/home/jlquinn/miniconda3/envs/watnlp/lib/python3.9/site-packages/torch/utils/data/dataloader.py", line 671, in _next_index
return next(self._sampler_iter) # may raise StopIteration
File "/home/jlquinn/miniconda3/envs/watnlp/lib/python3.9/site-packages/torch/utils/data/sampler.py", line 247, in __iter__
for idx in self.sampler:
File "/home/jlquinn/miniconda3/envs/watnlp/lib/python3.9/site-packages/torch/utils/data/sampler.py", line 76, in __iter__
return iter(range(len(self.data_source)))
File "/home/jlquinn/miniconda3/envs/watnlp/lib/python3.9/site-packages/transformers/pipelines/pt_utils.py", line 13, in __len__
return len(self.dataset)
File "/home/jlquinn/miniconda3/envs/watnlp/lib/python3.9/site-packages/transformers/pipelines/pt_utils.py", line 289, in __len__
return len(self.dataset)
TypeError: object of type 'IterableDataset' has no len()
```
### Expected behavior
I'm expecting french translations of the english test set to be printed.
### Environment info
Run on CPU with no GPU.
RHEL 8.7 x86_64
python 3.9.0
transformers 4.17.0
datasets 2.0.0
tokenizers 0.12.1
```
(watnlp) [jlquinn@bertdev01 hf]$ datasets-cli env
Copy-and-paste the text below in your GitHub issue.
- `datasets` version: 2.0.0
- Platform: Linux-4.18.0-372.19.1.el8_6.x86_64-x86_64-with-glibc2.28
- Python version: 3.9.0
- PyArrow version: 8.0.0
- Pandas version: 1.4.4
```
| 47 | Streaming IterableDataset not working with translation pipeline
### Describe the bug
I'm trying to use a streaming dataset for translation inference to avoid downloading the training data.
I'm using a pipeline and a dataset, and following the guidance in the tutorial.
Instead I get an exception that IterableDataset has no len().
### Steps to reproduce the bug
CODE:
```
from transformers import pipeline
from transformers.pipelines.pt_utils import KeyDataset
from datasets import load_dataset
ds = load_dataset(path="wmt14", name="fr-en", split="test", streaming=True)
bs=1
mt = pipeline("translation_en_to_fr", model="t5-base", batch_size=bs)
#print(mt("hello")) THIS WORKS
ks = KeyDataset(ds, "translation")
print(f"{ks}")
xx= mt(ks)
for x in xx:
print(x)
```
RUN:
```
(watnlp) [jlquinn@bertdev01 hf]$ python ende.t5.pipe.py
2023-05-11 16:48:08.817572: I tensorflow/core/util/util.cc:169] oneDNN custom operations are on. You may see slightly different numerical results due to floating-point round-off errors from different computation orders. To turn them off, set the environment variable `TF_ENABLE_ONEDNN_OPTS=0`.
2023-05-11 16:48:08.821388: W tensorflow/stream_executor/platform/default/dso_loader.cc:64] Could not load dynamic library 'libcudart.so.11.0'; dlerror: libcudart.so.11.0: cannot open shared object file: No such file or directory
2023-05-11 16:48:08.821407: I tensorflow/stream_executor/cuda/cudart_stub.cc:29] Ignore above cudart dlerror if you do not have a GPU set up on your machine.
<transformers.pipelines.pt_utils.KeyDataset object at 0x7f61ed5da9d0>
Traceback (most recent call last):
File "/home/jlquinn/models/hf/ende.t5.pipe.py", line 11, in <module>
for x in xx:
File "/home/jlquinn/miniconda3/envs/watnlp/lib/python3.9/site-packages/transformers/pipelines/pt_utils.py", line 111, in __next__
item = next(self.iterator)
File "/home/jlquinn/miniconda3/envs/watnlp/lib/python3.9/site-packages/transformers/pipelines/pt_utils.py", line 111, in __next__
item = next(self.iterator)
File "/home/jlquinn/miniconda3/envs/watnlp/lib/python3.9/site-packages/torch/utils/data/dataloader.py", line 681, in __next__
data = self._next_data()
File "/home/jlquinn/miniconda3/envs/watnlp/lib/python3.9/site-packages/torch/utils/data/dataloader.py", line 720, in _next_data
index = self._next_index() # may raise StopIteration
File "/home/jlquinn/miniconda3/envs/watnlp/lib/python3.9/site-packages/torch/utils/data/dataloader.py", line 671, in _next_index
return next(self._sampler_iter) # may raise StopIteration
File "/home/jlquinn/miniconda3/envs/watnlp/lib/python3.9/site-packages/torch/utils/data/sampler.py", line 247, in __iter__
for idx in self.sampler:
File "/home/jlquinn/miniconda3/envs/watnlp/lib/python3.9/site-packages/torch/utils/data/sampler.py", line 76, in __iter__
return iter(range(len(self.data_source)))
File "/home/jlquinn/miniconda3/envs/watnlp/lib/python3.9/site-packages/transformers/pipelines/pt_utils.py", line 13, in __len__
return len(self.dataset)
File "/home/jlquinn/miniconda3/envs/watnlp/lib/python3.9/site-packages/transformers/pipelines/pt_utils.py", line 289, in __len__
return len(self.dataset)
TypeError: object of type 'IterableDataset' has no len()
```
### Expected behavior
I'm expecting french translations of the english test set to be printed.
### Environment info
Run on CPU with no GPU.
RHEL 8.7 x86_64
python 3.9.0
transformers 4.17.0
datasets 2.0.0
tokenizers 0.12.1
```
(watnlp) [jlquinn@bertdev01 hf]$ datasets-cli env
Copy-and-paste the text below in your GitHub issue.
- `datasets` version: 2.0.0
- Platform: Linux-4.18.0-372.19.1.el8_6.x86_64-x86_64-with-glibc2.28
- Python version: 3.9.0
- PyArrow version: 8.0.0
- Pandas version: 1.4.4
```
So perhaps there's no bug exactly, but I would love to see two things: 1) improve the documentation to better understand what's really getting returned. 2) update the example provided of using transformer pipeline with a dataset to include the oddball case that translation appears to be. |
https://github.com/huggingface/datasets/issues/5847 | Streaming IterableDataset not working with translation pipeline | Hi,
for the original snippet, the issue is that `streaming` datasets are not countable (they have no len) and therefore `KeyDataset` cannot work with them ( KeyDataset is a dataset and therefore requires a length).
I modified slightly the original snippet to make it work:
```python
from transformers import pipeline
from transformers.pipelines.pt_utils import KeyDataset
from datasets import load_dataset
ds = load_dataset(path="wmt14", name="fr-en", split="test", streaming=True)
bs = 1
mt = pipeline(
"translation_en_to_fr", model="hf-internal-testing/tiny-random-T5ForConditionalGeneration", batch_size=bs
)
def ks(ds):
for item in ds:
yield item["translation"]["en"]
# print(f"{ks}")
xx = mt(ks(ds))
for x in xx:
print(x)
```
This is what the first example in the docs suggests to use (as it's the most flexible): https://huggingface.co/docs/transformers/v4.29.1/en/pipeline_tutorial#using-pipelines-on-a-dataset
`KeyDataset` really exists only to get a `sized` dataset to work nicer with `tqdm` for instance.
@sgugger should we update the docs to remove `KeyDataset` entirely ? (We can add a note to pass manually the length of the data to tqdm so that the progress bar option can still be easy to use ?)
| ### Describe the bug
I'm trying to use a streaming dataset for translation inference to avoid downloading the training data.
I'm using a pipeline and a dataset, and following the guidance in the tutorial.
Instead I get an exception that IterableDataset has no len().
### Steps to reproduce the bug
CODE:
```
from transformers import pipeline
from transformers.pipelines.pt_utils import KeyDataset
from datasets import load_dataset
ds = load_dataset(path="wmt14", name="fr-en", split="test", streaming=True)
bs=1
mt = pipeline("translation_en_to_fr", model="t5-base", batch_size=bs)
#print(mt("hello")) THIS WORKS
ks = KeyDataset(ds, "translation")
print(f"{ks}")
xx= mt(ks)
for x in xx:
print(x)
```
RUN:
```
(watnlp) [jlquinn@bertdev01 hf]$ python ende.t5.pipe.py
2023-05-11 16:48:08.817572: I tensorflow/core/util/util.cc:169] oneDNN custom operations are on. You may see slightly different numerical results due to floating-point round-off errors from different computation orders. To turn them off, set the environment variable `TF_ENABLE_ONEDNN_OPTS=0`.
2023-05-11 16:48:08.821388: W tensorflow/stream_executor/platform/default/dso_loader.cc:64] Could not load dynamic library 'libcudart.so.11.0'; dlerror: libcudart.so.11.0: cannot open shared object file: No such file or directory
2023-05-11 16:48:08.821407: I tensorflow/stream_executor/cuda/cudart_stub.cc:29] Ignore above cudart dlerror if you do not have a GPU set up on your machine.
<transformers.pipelines.pt_utils.KeyDataset object at 0x7f61ed5da9d0>
Traceback (most recent call last):
File "/home/jlquinn/models/hf/ende.t5.pipe.py", line 11, in <module>
for x in xx:
File "/home/jlquinn/miniconda3/envs/watnlp/lib/python3.9/site-packages/transformers/pipelines/pt_utils.py", line 111, in __next__
item = next(self.iterator)
File "/home/jlquinn/miniconda3/envs/watnlp/lib/python3.9/site-packages/transformers/pipelines/pt_utils.py", line 111, in __next__
item = next(self.iterator)
File "/home/jlquinn/miniconda3/envs/watnlp/lib/python3.9/site-packages/torch/utils/data/dataloader.py", line 681, in __next__
data = self._next_data()
File "/home/jlquinn/miniconda3/envs/watnlp/lib/python3.9/site-packages/torch/utils/data/dataloader.py", line 720, in _next_data
index = self._next_index() # may raise StopIteration
File "/home/jlquinn/miniconda3/envs/watnlp/lib/python3.9/site-packages/torch/utils/data/dataloader.py", line 671, in _next_index
return next(self._sampler_iter) # may raise StopIteration
File "/home/jlquinn/miniconda3/envs/watnlp/lib/python3.9/site-packages/torch/utils/data/sampler.py", line 247, in __iter__
for idx in self.sampler:
File "/home/jlquinn/miniconda3/envs/watnlp/lib/python3.9/site-packages/torch/utils/data/sampler.py", line 76, in __iter__
return iter(range(len(self.data_source)))
File "/home/jlquinn/miniconda3/envs/watnlp/lib/python3.9/site-packages/transformers/pipelines/pt_utils.py", line 13, in __len__
return len(self.dataset)
File "/home/jlquinn/miniconda3/envs/watnlp/lib/python3.9/site-packages/transformers/pipelines/pt_utils.py", line 289, in __len__
return len(self.dataset)
TypeError: object of type 'IterableDataset' has no len()
```
### Expected behavior
I'm expecting french translations of the english test set to be printed.
### Environment info
Run on CPU with no GPU.
RHEL 8.7 x86_64
python 3.9.0
transformers 4.17.0
datasets 2.0.0
tokenizers 0.12.1
```
(watnlp) [jlquinn@bertdev01 hf]$ datasets-cli env
Copy-and-paste the text below in your GitHub issue.
- `datasets` version: 2.0.0
- Platform: Linux-4.18.0-372.19.1.el8_6.x86_64-x86_64-with-glibc2.28
- Python version: 3.9.0
- PyArrow version: 8.0.0
- Pandas version: 1.4.4
```
| 166 | Streaming IterableDataset not working with translation pipeline
### Describe the bug
I'm trying to use a streaming dataset for translation inference to avoid downloading the training data.
I'm using a pipeline and a dataset, and following the guidance in the tutorial.
Instead I get an exception that IterableDataset has no len().
### Steps to reproduce the bug
CODE:
```
from transformers import pipeline
from transformers.pipelines.pt_utils import KeyDataset
from datasets import load_dataset
ds = load_dataset(path="wmt14", name="fr-en", split="test", streaming=True)
bs=1
mt = pipeline("translation_en_to_fr", model="t5-base", batch_size=bs)
#print(mt("hello")) THIS WORKS
ks = KeyDataset(ds, "translation")
print(f"{ks}")
xx= mt(ks)
for x in xx:
print(x)
```
RUN:
```
(watnlp) [jlquinn@bertdev01 hf]$ python ende.t5.pipe.py
2023-05-11 16:48:08.817572: I tensorflow/core/util/util.cc:169] oneDNN custom operations are on. You may see slightly different numerical results due to floating-point round-off errors from different computation orders. To turn them off, set the environment variable `TF_ENABLE_ONEDNN_OPTS=0`.
2023-05-11 16:48:08.821388: W tensorflow/stream_executor/platform/default/dso_loader.cc:64] Could not load dynamic library 'libcudart.so.11.0'; dlerror: libcudart.so.11.0: cannot open shared object file: No such file or directory
2023-05-11 16:48:08.821407: I tensorflow/stream_executor/cuda/cudart_stub.cc:29] Ignore above cudart dlerror if you do not have a GPU set up on your machine.
<transformers.pipelines.pt_utils.KeyDataset object at 0x7f61ed5da9d0>
Traceback (most recent call last):
File "/home/jlquinn/models/hf/ende.t5.pipe.py", line 11, in <module>
for x in xx:
File "/home/jlquinn/miniconda3/envs/watnlp/lib/python3.9/site-packages/transformers/pipelines/pt_utils.py", line 111, in __next__
item = next(self.iterator)
File "/home/jlquinn/miniconda3/envs/watnlp/lib/python3.9/site-packages/transformers/pipelines/pt_utils.py", line 111, in __next__
item = next(self.iterator)
File "/home/jlquinn/miniconda3/envs/watnlp/lib/python3.9/site-packages/torch/utils/data/dataloader.py", line 681, in __next__
data = self._next_data()
File "/home/jlquinn/miniconda3/envs/watnlp/lib/python3.9/site-packages/torch/utils/data/dataloader.py", line 720, in _next_data
index = self._next_index() # may raise StopIteration
File "/home/jlquinn/miniconda3/envs/watnlp/lib/python3.9/site-packages/torch/utils/data/dataloader.py", line 671, in _next_index
return next(self._sampler_iter) # may raise StopIteration
File "/home/jlquinn/miniconda3/envs/watnlp/lib/python3.9/site-packages/torch/utils/data/sampler.py", line 247, in __iter__
for idx in self.sampler:
File "/home/jlquinn/miniconda3/envs/watnlp/lib/python3.9/site-packages/torch/utils/data/sampler.py", line 76, in __iter__
return iter(range(len(self.data_source)))
File "/home/jlquinn/miniconda3/envs/watnlp/lib/python3.9/site-packages/transformers/pipelines/pt_utils.py", line 13, in __len__
return len(self.dataset)
File "/home/jlquinn/miniconda3/envs/watnlp/lib/python3.9/site-packages/transformers/pipelines/pt_utils.py", line 289, in __len__
return len(self.dataset)
TypeError: object of type 'IterableDataset' has no len()
```
### Expected behavior
I'm expecting french translations of the english test set to be printed.
### Environment info
Run on CPU with no GPU.
RHEL 8.7 x86_64
python 3.9.0
transformers 4.17.0
datasets 2.0.0
tokenizers 0.12.1
```
(watnlp) [jlquinn@bertdev01 hf]$ datasets-cli env
Copy-and-paste the text below in your GitHub issue.
- `datasets` version: 2.0.0
- Platform: Linux-4.18.0-372.19.1.el8_6.x86_64-x86_64-with-glibc2.28
- Python version: 3.9.0
- PyArrow version: 8.0.0
- Pandas version: 1.4.4
```
Hi,
for the original snippet, the issue is that `streaming` datasets are not countable (they have no len) and therefore `KeyDataset` cannot work with them ( KeyDataset is a dataset and therefore requires a length).
I modified slightly the original snippet to make it work:
```python
from transformers import pipeline
from transformers.pipelines.pt_utils import KeyDataset
from datasets import load_dataset
ds = load_dataset(path="wmt14", name="fr-en", split="test", streaming=True)
bs = 1
mt = pipeline(
"translation_en_to_fr", model="hf-internal-testing/tiny-random-T5ForConditionalGeneration", batch_size=bs
)
def ks(ds):
for item in ds:
yield item["translation"]["en"]
# print(f"{ks}")
xx = mt(ks(ds))
for x in xx:
print(x)
```
This is what the first example in the docs suggests to use (as it's the most flexible): https://huggingface.co/docs/transformers/v4.29.1/en/pipeline_tutorial#using-pipelines-on-a-dataset
`KeyDataset` really exists only to get a `sized` dataset to work nicer with `tqdm` for instance.
@sgugger should we update the docs to remove `KeyDataset` entirely ? (We can add a note to pass manually the length of the data to tqdm so that the progress bar option can still be easy to use ?)
|
https://github.com/huggingface/datasets/issues/5847 | Streaming IterableDataset not working with translation pipeline | Maybe moving `KeyDataset` later on in the guide and specify it's mostly for streaming then? Or is it also necessary for batch_size>1 (which is what the current doc implies)? | ### Describe the bug
I'm trying to use a streaming dataset for translation inference to avoid downloading the training data.
I'm using a pipeline and a dataset, and following the guidance in the tutorial.
Instead I get an exception that IterableDataset has no len().
### Steps to reproduce the bug
CODE:
```
from transformers import pipeline
from transformers.pipelines.pt_utils import KeyDataset
from datasets import load_dataset
ds = load_dataset(path="wmt14", name="fr-en", split="test", streaming=True)
bs=1
mt = pipeline("translation_en_to_fr", model="t5-base", batch_size=bs)
#print(mt("hello")) THIS WORKS
ks = KeyDataset(ds, "translation")
print(f"{ks}")
xx= mt(ks)
for x in xx:
print(x)
```
RUN:
```
(watnlp) [jlquinn@bertdev01 hf]$ python ende.t5.pipe.py
2023-05-11 16:48:08.817572: I tensorflow/core/util/util.cc:169] oneDNN custom operations are on. You may see slightly different numerical results due to floating-point round-off errors from different computation orders. To turn them off, set the environment variable `TF_ENABLE_ONEDNN_OPTS=0`.
2023-05-11 16:48:08.821388: W tensorflow/stream_executor/platform/default/dso_loader.cc:64] Could not load dynamic library 'libcudart.so.11.0'; dlerror: libcudart.so.11.0: cannot open shared object file: No such file or directory
2023-05-11 16:48:08.821407: I tensorflow/stream_executor/cuda/cudart_stub.cc:29] Ignore above cudart dlerror if you do not have a GPU set up on your machine.
<transformers.pipelines.pt_utils.KeyDataset object at 0x7f61ed5da9d0>
Traceback (most recent call last):
File "/home/jlquinn/models/hf/ende.t5.pipe.py", line 11, in <module>
for x in xx:
File "/home/jlquinn/miniconda3/envs/watnlp/lib/python3.9/site-packages/transformers/pipelines/pt_utils.py", line 111, in __next__
item = next(self.iterator)
File "/home/jlquinn/miniconda3/envs/watnlp/lib/python3.9/site-packages/transformers/pipelines/pt_utils.py", line 111, in __next__
item = next(self.iterator)
File "/home/jlquinn/miniconda3/envs/watnlp/lib/python3.9/site-packages/torch/utils/data/dataloader.py", line 681, in __next__
data = self._next_data()
File "/home/jlquinn/miniconda3/envs/watnlp/lib/python3.9/site-packages/torch/utils/data/dataloader.py", line 720, in _next_data
index = self._next_index() # may raise StopIteration
File "/home/jlquinn/miniconda3/envs/watnlp/lib/python3.9/site-packages/torch/utils/data/dataloader.py", line 671, in _next_index
return next(self._sampler_iter) # may raise StopIteration
File "/home/jlquinn/miniconda3/envs/watnlp/lib/python3.9/site-packages/torch/utils/data/sampler.py", line 247, in __iter__
for idx in self.sampler:
File "/home/jlquinn/miniconda3/envs/watnlp/lib/python3.9/site-packages/torch/utils/data/sampler.py", line 76, in __iter__
return iter(range(len(self.data_source)))
File "/home/jlquinn/miniconda3/envs/watnlp/lib/python3.9/site-packages/transformers/pipelines/pt_utils.py", line 13, in __len__
return len(self.dataset)
File "/home/jlquinn/miniconda3/envs/watnlp/lib/python3.9/site-packages/transformers/pipelines/pt_utils.py", line 289, in __len__
return len(self.dataset)
TypeError: object of type 'IterableDataset' has no len()
```
### Expected behavior
I'm expecting french translations of the english test set to be printed.
### Environment info
Run on CPU with no GPU.
RHEL 8.7 x86_64
python 3.9.0
transformers 4.17.0
datasets 2.0.0
tokenizers 0.12.1
```
(watnlp) [jlquinn@bertdev01 hf]$ datasets-cli env
Copy-and-paste the text below in your GitHub issue.
- `datasets` version: 2.0.0
- Platform: Linux-4.18.0-372.19.1.el8_6.x86_64-x86_64-with-glibc2.28
- Python version: 3.9.0
- PyArrow version: 8.0.0
- Pandas version: 1.4.4
```
| 29 | Streaming IterableDataset not working with translation pipeline
### Describe the bug
I'm trying to use a streaming dataset for translation inference to avoid downloading the training data.
I'm using a pipeline and a dataset, and following the guidance in the tutorial.
Instead I get an exception that IterableDataset has no len().
### Steps to reproduce the bug
CODE:
```
from transformers import pipeline
from transformers.pipelines.pt_utils import KeyDataset
from datasets import load_dataset
ds = load_dataset(path="wmt14", name="fr-en", split="test", streaming=True)
bs=1
mt = pipeline("translation_en_to_fr", model="t5-base", batch_size=bs)
#print(mt("hello")) THIS WORKS
ks = KeyDataset(ds, "translation")
print(f"{ks}")
xx= mt(ks)
for x in xx:
print(x)
```
RUN:
```
(watnlp) [jlquinn@bertdev01 hf]$ python ende.t5.pipe.py
2023-05-11 16:48:08.817572: I tensorflow/core/util/util.cc:169] oneDNN custom operations are on. You may see slightly different numerical results due to floating-point round-off errors from different computation orders. To turn them off, set the environment variable `TF_ENABLE_ONEDNN_OPTS=0`.
2023-05-11 16:48:08.821388: W tensorflow/stream_executor/platform/default/dso_loader.cc:64] Could not load dynamic library 'libcudart.so.11.0'; dlerror: libcudart.so.11.0: cannot open shared object file: No such file or directory
2023-05-11 16:48:08.821407: I tensorflow/stream_executor/cuda/cudart_stub.cc:29] Ignore above cudart dlerror if you do not have a GPU set up on your machine.
<transformers.pipelines.pt_utils.KeyDataset object at 0x7f61ed5da9d0>
Traceback (most recent call last):
File "/home/jlquinn/models/hf/ende.t5.pipe.py", line 11, in <module>
for x in xx:
File "/home/jlquinn/miniconda3/envs/watnlp/lib/python3.9/site-packages/transformers/pipelines/pt_utils.py", line 111, in __next__
item = next(self.iterator)
File "/home/jlquinn/miniconda3/envs/watnlp/lib/python3.9/site-packages/transformers/pipelines/pt_utils.py", line 111, in __next__
item = next(self.iterator)
File "/home/jlquinn/miniconda3/envs/watnlp/lib/python3.9/site-packages/torch/utils/data/dataloader.py", line 681, in __next__
data = self._next_data()
File "/home/jlquinn/miniconda3/envs/watnlp/lib/python3.9/site-packages/torch/utils/data/dataloader.py", line 720, in _next_data
index = self._next_index() # may raise StopIteration
File "/home/jlquinn/miniconda3/envs/watnlp/lib/python3.9/site-packages/torch/utils/data/dataloader.py", line 671, in _next_index
return next(self._sampler_iter) # may raise StopIteration
File "/home/jlquinn/miniconda3/envs/watnlp/lib/python3.9/site-packages/torch/utils/data/sampler.py", line 247, in __iter__
for idx in self.sampler:
File "/home/jlquinn/miniconda3/envs/watnlp/lib/python3.9/site-packages/torch/utils/data/sampler.py", line 76, in __iter__
return iter(range(len(self.data_source)))
File "/home/jlquinn/miniconda3/envs/watnlp/lib/python3.9/site-packages/transformers/pipelines/pt_utils.py", line 13, in __len__
return len(self.dataset)
File "/home/jlquinn/miniconda3/envs/watnlp/lib/python3.9/site-packages/transformers/pipelines/pt_utils.py", line 289, in __len__
return len(self.dataset)
TypeError: object of type 'IterableDataset' has no len()
```
### Expected behavior
I'm expecting french translations of the english test set to be printed.
### Environment info
Run on CPU with no GPU.
RHEL 8.7 x86_64
python 3.9.0
transformers 4.17.0
datasets 2.0.0
tokenizers 0.12.1
```
(watnlp) [jlquinn@bertdev01 hf]$ datasets-cli env
Copy-and-paste the text below in your GitHub issue.
- `datasets` version: 2.0.0
- Platform: Linux-4.18.0-372.19.1.el8_6.x86_64-x86_64-with-glibc2.28
- Python version: 3.9.0
- PyArrow version: 8.0.0
- Pandas version: 1.4.4
```
Maybe moving `KeyDataset` later on in the guide and specify it's mostly for streaming then? Or is it also necessary for batch_size>1 (which is what the current doc implies)? |
https://github.com/huggingface/datasets/issues/5847 | Streaming IterableDataset not working with translation pipeline | Hmm
Iterator (`yield`) :
- Not countable
- Super flexible
- Cannot use `num_workers>1` (threading requires indexing at the correct location, iterators require to iterate in order,so each thread would iterate over the full thing being genuinely a bad idea)
- Can batch
- tqdm doesn't show a nice progress bar (it has no total)
KeyDataset (Or any PyTorch like Dataset returning the correct object for the pipeline):
- Countable
- Less flexible (not applicable to datasets with streaming), can only work on single keys. But should be easy to read and write your own (like @mariosasko did)
- Works with `num_workers > 1` (Every worker can fetch exactly what's needed)
- Can batch
- tqdm shows a nice progress bar
In the docs, if we update all the examples to use iterators, and include an example with
```
for item in tqdm.tqdm(pipe(iterator(), total=len(dataset))))
```
We can save the biggest feature that doesn't work out of the box with iterators which is the tqdm progress bar.
`num_workers>1` we can mention it, but it tends to be an issues only on CPU intensive loads, like image (and maybe audio)
| ### Describe the bug
I'm trying to use a streaming dataset for translation inference to avoid downloading the training data.
I'm using a pipeline and a dataset, and following the guidance in the tutorial.
Instead I get an exception that IterableDataset has no len().
### Steps to reproduce the bug
CODE:
```
from transformers import pipeline
from transformers.pipelines.pt_utils import KeyDataset
from datasets import load_dataset
ds = load_dataset(path="wmt14", name="fr-en", split="test", streaming=True)
bs=1
mt = pipeline("translation_en_to_fr", model="t5-base", batch_size=bs)
#print(mt("hello")) THIS WORKS
ks = KeyDataset(ds, "translation")
print(f"{ks}")
xx= mt(ks)
for x in xx:
print(x)
```
RUN:
```
(watnlp) [jlquinn@bertdev01 hf]$ python ende.t5.pipe.py
2023-05-11 16:48:08.817572: I tensorflow/core/util/util.cc:169] oneDNN custom operations are on. You may see slightly different numerical results due to floating-point round-off errors from different computation orders. To turn them off, set the environment variable `TF_ENABLE_ONEDNN_OPTS=0`.
2023-05-11 16:48:08.821388: W tensorflow/stream_executor/platform/default/dso_loader.cc:64] Could not load dynamic library 'libcudart.so.11.0'; dlerror: libcudart.so.11.0: cannot open shared object file: No such file or directory
2023-05-11 16:48:08.821407: I tensorflow/stream_executor/cuda/cudart_stub.cc:29] Ignore above cudart dlerror if you do not have a GPU set up on your machine.
<transformers.pipelines.pt_utils.KeyDataset object at 0x7f61ed5da9d0>
Traceback (most recent call last):
File "/home/jlquinn/models/hf/ende.t5.pipe.py", line 11, in <module>
for x in xx:
File "/home/jlquinn/miniconda3/envs/watnlp/lib/python3.9/site-packages/transformers/pipelines/pt_utils.py", line 111, in __next__
item = next(self.iterator)
File "/home/jlquinn/miniconda3/envs/watnlp/lib/python3.9/site-packages/transformers/pipelines/pt_utils.py", line 111, in __next__
item = next(self.iterator)
File "/home/jlquinn/miniconda3/envs/watnlp/lib/python3.9/site-packages/torch/utils/data/dataloader.py", line 681, in __next__
data = self._next_data()
File "/home/jlquinn/miniconda3/envs/watnlp/lib/python3.9/site-packages/torch/utils/data/dataloader.py", line 720, in _next_data
index = self._next_index() # may raise StopIteration
File "/home/jlquinn/miniconda3/envs/watnlp/lib/python3.9/site-packages/torch/utils/data/dataloader.py", line 671, in _next_index
return next(self._sampler_iter) # may raise StopIteration
File "/home/jlquinn/miniconda3/envs/watnlp/lib/python3.9/site-packages/torch/utils/data/sampler.py", line 247, in __iter__
for idx in self.sampler:
File "/home/jlquinn/miniconda3/envs/watnlp/lib/python3.9/site-packages/torch/utils/data/sampler.py", line 76, in __iter__
return iter(range(len(self.data_source)))
File "/home/jlquinn/miniconda3/envs/watnlp/lib/python3.9/site-packages/transformers/pipelines/pt_utils.py", line 13, in __len__
return len(self.dataset)
File "/home/jlquinn/miniconda3/envs/watnlp/lib/python3.9/site-packages/transformers/pipelines/pt_utils.py", line 289, in __len__
return len(self.dataset)
TypeError: object of type 'IterableDataset' has no len()
```
### Expected behavior
I'm expecting french translations of the english test set to be printed.
### Environment info
Run on CPU with no GPU.
RHEL 8.7 x86_64
python 3.9.0
transformers 4.17.0
datasets 2.0.0
tokenizers 0.12.1
```
(watnlp) [jlquinn@bertdev01 hf]$ datasets-cli env
Copy-and-paste the text below in your GitHub issue.
- `datasets` version: 2.0.0
- Platform: Linux-4.18.0-372.19.1.el8_6.x86_64-x86_64-with-glibc2.28
- Python version: 3.9.0
- PyArrow version: 8.0.0
- Pandas version: 1.4.4
```
| 188 | Streaming IterableDataset not working with translation pipeline
### Describe the bug
I'm trying to use a streaming dataset for translation inference to avoid downloading the training data.
I'm using a pipeline and a dataset, and following the guidance in the tutorial.
Instead I get an exception that IterableDataset has no len().
### Steps to reproduce the bug
CODE:
```
from transformers import pipeline
from transformers.pipelines.pt_utils import KeyDataset
from datasets import load_dataset
ds = load_dataset(path="wmt14", name="fr-en", split="test", streaming=True)
bs=1
mt = pipeline("translation_en_to_fr", model="t5-base", batch_size=bs)
#print(mt("hello")) THIS WORKS
ks = KeyDataset(ds, "translation")
print(f"{ks}")
xx= mt(ks)
for x in xx:
print(x)
```
RUN:
```
(watnlp) [jlquinn@bertdev01 hf]$ python ende.t5.pipe.py
2023-05-11 16:48:08.817572: I tensorflow/core/util/util.cc:169] oneDNN custom operations are on. You may see slightly different numerical results due to floating-point round-off errors from different computation orders. To turn them off, set the environment variable `TF_ENABLE_ONEDNN_OPTS=0`.
2023-05-11 16:48:08.821388: W tensorflow/stream_executor/platform/default/dso_loader.cc:64] Could not load dynamic library 'libcudart.so.11.0'; dlerror: libcudart.so.11.0: cannot open shared object file: No such file or directory
2023-05-11 16:48:08.821407: I tensorflow/stream_executor/cuda/cudart_stub.cc:29] Ignore above cudart dlerror if you do not have a GPU set up on your machine.
<transformers.pipelines.pt_utils.KeyDataset object at 0x7f61ed5da9d0>
Traceback (most recent call last):
File "/home/jlquinn/models/hf/ende.t5.pipe.py", line 11, in <module>
for x in xx:
File "/home/jlquinn/miniconda3/envs/watnlp/lib/python3.9/site-packages/transformers/pipelines/pt_utils.py", line 111, in __next__
item = next(self.iterator)
File "/home/jlquinn/miniconda3/envs/watnlp/lib/python3.9/site-packages/transformers/pipelines/pt_utils.py", line 111, in __next__
item = next(self.iterator)
File "/home/jlquinn/miniconda3/envs/watnlp/lib/python3.9/site-packages/torch/utils/data/dataloader.py", line 681, in __next__
data = self._next_data()
File "/home/jlquinn/miniconda3/envs/watnlp/lib/python3.9/site-packages/torch/utils/data/dataloader.py", line 720, in _next_data
index = self._next_index() # may raise StopIteration
File "/home/jlquinn/miniconda3/envs/watnlp/lib/python3.9/site-packages/torch/utils/data/dataloader.py", line 671, in _next_index
return next(self._sampler_iter) # may raise StopIteration
File "/home/jlquinn/miniconda3/envs/watnlp/lib/python3.9/site-packages/torch/utils/data/sampler.py", line 247, in __iter__
for idx in self.sampler:
File "/home/jlquinn/miniconda3/envs/watnlp/lib/python3.9/site-packages/torch/utils/data/sampler.py", line 76, in __iter__
return iter(range(len(self.data_source)))
File "/home/jlquinn/miniconda3/envs/watnlp/lib/python3.9/site-packages/transformers/pipelines/pt_utils.py", line 13, in __len__
return len(self.dataset)
File "/home/jlquinn/miniconda3/envs/watnlp/lib/python3.9/site-packages/transformers/pipelines/pt_utils.py", line 289, in __len__
return len(self.dataset)
TypeError: object of type 'IterableDataset' has no len()
```
### Expected behavior
I'm expecting french translations of the english test set to be printed.
### Environment info
Run on CPU with no GPU.
RHEL 8.7 x86_64
python 3.9.0
transformers 4.17.0
datasets 2.0.0
tokenizers 0.12.1
```
(watnlp) [jlquinn@bertdev01 hf]$ datasets-cli env
Copy-and-paste the text below in your GitHub issue.
- `datasets` version: 2.0.0
- Platform: Linux-4.18.0-372.19.1.el8_6.x86_64-x86_64-with-glibc2.28
- Python version: 3.9.0
- PyArrow version: 8.0.0
- Pandas version: 1.4.4
```
Hmm
Iterator (`yield`) :
- Not countable
- Super flexible
- Cannot use `num_workers>1` (threading requires indexing at the correct location, iterators require to iterate in order,so each thread would iterate over the full thing being genuinely a bad idea)
- Can batch
- tqdm doesn't show a nice progress bar (it has no total)
KeyDataset (Or any PyTorch like Dataset returning the correct object for the pipeline):
- Countable
- Less flexible (not applicable to datasets with streaming), can only work on single keys. But should be easy to read and write your own (like @mariosasko did)
- Works with `num_workers > 1` (Every worker can fetch exactly what's needed)
- Can batch
- tqdm shows a nice progress bar
In the docs, if we update all the examples to use iterators, and include an example with
```
for item in tqdm.tqdm(pipe(iterator(), total=len(dataset))))
```
We can save the biggest feature that doesn't work out of the box with iterators which is the tqdm progress bar.
`num_workers>1` we can mention it, but it tends to be an issues only on CPU intensive loads, like image (and maybe audio)
|
https://github.com/huggingface/datasets/issues/5846 | load_dataset('bigcode/the-stack-dedup', streaming=True) very slow! | This is due to the slow resolution of the data files: https://github.com/huggingface/datasets/issues/5537.
We plan to switch to `huggingface_hub`'s `HfFileSystem` soon to make the resolution faster (will be up to 20x faster once we merge https://github.com/huggingface/huggingface_hub/pull/1443)
| ### Describe the bug
Running
```
import datasets
ds = datasets.load_dataset('bigcode/the-stack-dedup', streaming=True)
```
takes about 2.5 minutes!
I would expect this to be near instantaneous. With other datasets, the runtime is one or two seconds.
### Environment info
- `datasets` version: 2.11.0
- Platform: macOS-13.3.1-arm64-arm-64bit
- Python version: 3.10.10
- Huggingface_hub version: 0.13.4
- PyArrow version: 11.0.0
- Pandas version: 2.0.0 | 35 | load_dataset('bigcode/the-stack-dedup', streaming=True) very slow!
### Describe the bug
Running
```
import datasets
ds = datasets.load_dataset('bigcode/the-stack-dedup', streaming=True)
```
takes about 2.5 minutes!
I would expect this to be near instantaneous. With other datasets, the runtime is one or two seconds.
### Environment info
- `datasets` version: 2.11.0
- Platform: macOS-13.3.1-arm64-arm-64bit
- Python version: 3.10.10
- Huggingface_hub version: 0.13.4
- PyArrow version: 11.0.0
- Pandas version: 2.0.0
This is due to the slow resolution of the data files: https://github.com/huggingface/datasets/issues/5537.
We plan to switch to `huggingface_hub`'s `HfFileSystem` soon to make the resolution faster (will be up to 20x faster once we merge https://github.com/huggingface/huggingface_hub/pull/1443)
|
https://github.com/huggingface/datasets/issues/5846 | load_dataset('bigcode/the-stack-dedup', streaming=True) very slow! | You're right, when I try to parse more than 50GB of text data, I also get very slow, usually taking hours or even tens of hours. | ### Describe the bug
Running
```
import datasets
ds = datasets.load_dataset('bigcode/the-stack-dedup', streaming=True)
```
takes about 2.5 minutes!
I would expect this to be near instantaneous. With other datasets, the runtime is one or two seconds.
### Environment info
- `datasets` version: 2.11.0
- Platform: macOS-13.3.1-arm64-arm-64bit
- Python version: 3.10.10
- Huggingface_hub version: 0.13.4
- PyArrow version: 11.0.0
- Pandas version: 2.0.0 | 26 | load_dataset('bigcode/the-stack-dedup', streaming=True) very slow!
### Describe the bug
Running
```
import datasets
ds = datasets.load_dataset('bigcode/the-stack-dedup', streaming=True)
```
takes about 2.5 minutes!
I would expect this to be near instantaneous. With other datasets, the runtime is one or two seconds.
### Environment info
- `datasets` version: 2.11.0
- Platform: macOS-13.3.1-arm64-arm-64bit
- Python version: 3.10.10
- Huggingface_hub version: 0.13.4
- PyArrow version: 11.0.0
- Pandas version: 2.0.0
You're right, when I try to parse more than 50GB of text data, I also get very slow, usually taking hours or even tens of hours. |
https://github.com/huggingface/datasets/issues/5846 | load_dataset('bigcode/the-stack-dedup', streaming=True) very slow! | > You're right, when I try to parse more than 50GB of text data, I also get very slow, usually taking hours or even tens of hours.
That's unrelated to the problem discussed in this issue. | ### Describe the bug
Running
```
import datasets
ds = datasets.load_dataset('bigcode/the-stack-dedup', streaming=True)
```
takes about 2.5 minutes!
I would expect this to be near instantaneous. With other datasets, the runtime is one or two seconds.
### Environment info
- `datasets` version: 2.11.0
- Platform: macOS-13.3.1-arm64-arm-64bit
- Python version: 3.10.10
- Huggingface_hub version: 0.13.4
- PyArrow version: 11.0.0
- Pandas version: 2.0.0 | 36 | load_dataset('bigcode/the-stack-dedup', streaming=True) very slow!
### Describe the bug
Running
```
import datasets
ds = datasets.load_dataset('bigcode/the-stack-dedup', streaming=True)
```
takes about 2.5 minutes!
I would expect this to be near instantaneous. With other datasets, the runtime is one or two seconds.
### Environment info
- `datasets` version: 2.11.0
- Platform: macOS-13.3.1-arm64-arm-64bit
- Python version: 3.10.10
- Huggingface_hub version: 0.13.4
- PyArrow version: 11.0.0
- Pandas version: 2.0.0
> You're right, when I try to parse more than 50GB of text data, I also get very slow, usually taking hours or even tens of hours.
That's unrelated to the problem discussed in this issue. |
https://github.com/huggingface/datasets/issues/5846 | load_dataset('bigcode/the-stack-dedup', streaming=True) very slow! | > > You're right, when I try to parse more than 50GB of text data, I also get very slow, usually taking hours or even tens of hours.
>
> That's unrelated to the problem discussed in this issue.
Sorry, I misunderstood it. | ### Describe the bug
Running
```
import datasets
ds = datasets.load_dataset('bigcode/the-stack-dedup', streaming=True)
```
takes about 2.5 minutes!
I would expect this to be near instantaneous. With other datasets, the runtime is one or two seconds.
### Environment info
- `datasets` version: 2.11.0
- Platform: macOS-13.3.1-arm64-arm-64bit
- Python version: 3.10.10
- Huggingface_hub version: 0.13.4
- PyArrow version: 11.0.0
- Pandas version: 2.0.0 | 43 | load_dataset('bigcode/the-stack-dedup', streaming=True) very slow!
### Describe the bug
Running
```
import datasets
ds = datasets.load_dataset('bigcode/the-stack-dedup', streaming=True)
```
takes about 2.5 minutes!
I would expect this to be near instantaneous. With other datasets, the runtime is one or two seconds.
### Environment info
- `datasets` version: 2.11.0
- Platform: macOS-13.3.1-arm64-arm-64bit
- Python version: 3.10.10
- Huggingface_hub version: 0.13.4
- PyArrow version: 11.0.0
- Pandas version: 2.0.0
> > You're right, when I try to parse more than 50GB of text data, I also get very slow, usually taking hours or even tens of hours.
>
> That's unrelated to the problem discussed in this issue.
Sorry, I misunderstood it. |
https://github.com/huggingface/datasets/issues/5841 | Abusurdly slow on iteration | Hi ! You can try to use the [Image](https://huggingface.co/docs/datasets/v2.12.0/en/package_reference/main_classes#datasets.Image) type which [decodes images on-the-fly](https://huggingface.co/docs/datasets/v2.12.0/en/about_dataset_features#image-feature) into pytorch tensors :)
```python
ds = Dataset.from_dict({"tensor":a}).with_format("torch")
%time sum(1 for _ in ds)
# CPU times: user 5.04 s, sys: 96.5 ms, total: 5.14 s
# Wall time: 5.14 s
# 10000
```
```python
features = Features({"tensor": Image()})
ds = Dataset.from_dict({"tensor":a}, features=features).with_format("torch")
%time sum(1 for _ in ds)
# CPU times: user 1.86 s, sys: 49 ms, total: 1.91 s
# Wall time: 1.9 s
# 10000
```
-> Speed x2.7
And if you want to keep using arrays of integers, consider using the [Array2D](https://huggingface.co/docs/datasets/v2.12.0/en/package_reference/main_classes#datasets.Array2D) or [Array3D](https://huggingface.co/docs/datasets/v2.12.0/en/package_reference/main_classes#datasets.Array3D) types which are even faster (since it doesn't decode images):
```python
features = Features({"tensor": Array2D(shape=(100, 224), dtype="float32")})
ds = Dataset.from_dict({"tensor":a}, features=features).with_format("torch")
%time sum(1 for _ in ds)
# CPU times: user 828 ms, sys: 68.4 ms, total: 896 ms
# Wall time: 897 ms
# 10000
```
-> Speed x5.7
Batching also speeds up a lot
```python
from torch.utils.data import DataLoader
dl = DataLoader(ds, batch_size=100)
%time sum(1 for _ in dl)
# CPU times: user 564 ms, sys: 83.5 ms, total: 648 ms
# Wall time: 579 ms
# 100
```
-> Speed x8.9
```python
%time sum(1 for _ in ds.iter(batch_size=100))
# CPU times: user 119 ms, sys: 96.8 ms, total: 215 ms
# Wall time: 117 ms
# 100
```
-> Speed x46 | ### Describe the bug
I am attempting to iterate through an image dataset, but I am encountering a significant slowdown in the iteration speed. In order to investigate this issue, I conducted the following experiment:
```python
a=torch.randn(100,224)
a=torch.stack([a] * 10000)
a.shape
# %%
ds=Dataset.from_dict({"tensor":a})
for i in tqdm(ds.with_format("numpy")):
pass
for i in tqdm(ds.with_format("torch")):
pass
```
I noticed that the dataset in numpy format performs significantly faster than the one in torch format. My hypothesis is that the dataset undergoes a transformation process of torch->python->numpy(torch) in the background, which might be causing the slowdown. Is there any way to expedite the process by bypassing such transformations?
Furthermore, if I increase the size of a to an image shape, like:
```python
a=torch.randn(3,224,224)
```
the iteration speed becomes absurdly slow, around 100 iterations per second, whereas the speed with numpy format is approximately 250 iterations per second. This level of speed would be unacceptable for large image datasets, as it could take several hours just to iterate through a single epoch.
### Steps to reproduce the bug
```python
a=torch.randn(100,224)
a=torch.stack([a] * 10000)
a.shape
# %%
ds=Dataset.from_dict({"tensor":a})
for i in tqdm(ds.with_format("numpy")):
pass
for i in tqdm(ds.with_format("torch")):
pass
```
### Expected behavior
iteration faster
### Environment info
- `datasets` version: 2.11.0
- Platform: Linux-5.4.0-148-generic-x86_64-with-glibc2.10
- Python version: 3.8.16
- Huggingface_hub version: 0.13.4
- PyArrow version: 11.0.0
- Pandas version: 2.0.0 | 226 | Abusurdly slow on iteration
### Describe the bug
I am attempting to iterate through an image dataset, but I am encountering a significant slowdown in the iteration speed. In order to investigate this issue, I conducted the following experiment:
```python
a=torch.randn(100,224)
a=torch.stack([a] * 10000)
a.shape
# %%
ds=Dataset.from_dict({"tensor":a})
for i in tqdm(ds.with_format("numpy")):
pass
for i in tqdm(ds.with_format("torch")):
pass
```
I noticed that the dataset in numpy format performs significantly faster than the one in torch format. My hypothesis is that the dataset undergoes a transformation process of torch->python->numpy(torch) in the background, which might be causing the slowdown. Is there any way to expedite the process by bypassing such transformations?
Furthermore, if I increase the size of a to an image shape, like:
```python
a=torch.randn(3,224,224)
```
the iteration speed becomes absurdly slow, around 100 iterations per second, whereas the speed with numpy format is approximately 250 iterations per second. This level of speed would be unacceptable for large image datasets, as it could take several hours just to iterate through a single epoch.
### Steps to reproduce the bug
```python
a=torch.randn(100,224)
a=torch.stack([a] * 10000)
a.shape
# %%
ds=Dataset.from_dict({"tensor":a})
for i in tqdm(ds.with_format("numpy")):
pass
for i in tqdm(ds.with_format("torch")):
pass
```
### Expected behavior
iteration faster
### Environment info
- `datasets` version: 2.11.0
- Platform: Linux-5.4.0-148-generic-x86_64-with-glibc2.10
- Python version: 3.8.16
- Huggingface_hub version: 0.13.4
- PyArrow version: 11.0.0
- Pandas version: 2.0.0
Hi ! You can try to use the [Image](https://huggingface.co/docs/datasets/v2.12.0/en/package_reference/main_classes#datasets.Image) type which [decodes images on-the-fly](https://huggingface.co/docs/datasets/v2.12.0/en/about_dataset_features#image-feature) into pytorch tensors :)
```python
ds = Dataset.from_dict({"tensor":a}).with_format("torch")
%time sum(1 for _ in ds)
# CPU times: user 5.04 s, sys: 96.5 ms, total: 5.14 s
# Wall time: 5.14 s
# 10000
```
```python
features = Features({"tensor": Image()})
ds = Dataset.from_dict({"tensor":a}, features=features).with_format("torch")
%time sum(1 for _ in ds)
# CPU times: user 1.86 s, sys: 49 ms, total: 1.91 s
# Wall time: 1.9 s
# 10000
```
-> Speed x2.7
And if you want to keep using arrays of integers, consider using the [Array2D](https://huggingface.co/docs/datasets/v2.12.0/en/package_reference/main_classes#datasets.Array2D) or [Array3D](https://huggingface.co/docs/datasets/v2.12.0/en/package_reference/main_classes#datasets.Array3D) types which are even faster (since it doesn't decode images):
```python
features = Features({"tensor": Array2D(shape=(100, 224), dtype="float32")})
ds = Dataset.from_dict({"tensor":a}, features=features).with_format("torch")
%time sum(1 for _ in ds)
# CPU times: user 828 ms, sys: 68.4 ms, total: 896 ms
# Wall time: 897 ms
# 10000
```
-> Speed x5.7
Batching also speeds up a lot
```python
from torch.utils.data import DataLoader
dl = DataLoader(ds, batch_size=100)
%time sum(1 for _ in dl)
# CPU times: user 564 ms, sys: 83.5 ms, total: 648 ms
# Wall time: 579 ms
# 100
```
-> Speed x8.9
```python
%time sum(1 for _ in ds.iter(batch_size=100))
# CPU times: user 119 ms, sys: 96.8 ms, total: 215 ms
# Wall time: 117 ms
# 100
```
-> Speed x46 |
https://github.com/huggingface/datasets/issues/5841 | Abusurdly slow on iteration | Anyway, regarding the speed difference between numpy and pytorch, I think the issue is that we first convert numpy sub-arrays to pytorch and then consolidate into one tensor, while we should to the opposite. Indeed converting a numpy array to pytorch has a fix cost that seems to cause a slow down. The current pipeline is
```
arrow -> nested numpy arrays -> lists of torch tensors -> one torch tensor
```
and we should do
```
arrow -> nested numpy arrays -> one numpy array -> one torch tensor
``` | ### Describe the bug
I am attempting to iterate through an image dataset, but I am encountering a significant slowdown in the iteration speed. In order to investigate this issue, I conducted the following experiment:
```python
a=torch.randn(100,224)
a=torch.stack([a] * 10000)
a.shape
# %%
ds=Dataset.from_dict({"tensor":a})
for i in tqdm(ds.with_format("numpy")):
pass
for i in tqdm(ds.with_format("torch")):
pass
```
I noticed that the dataset in numpy format performs significantly faster than the one in torch format. My hypothesis is that the dataset undergoes a transformation process of torch->python->numpy(torch) in the background, which might be causing the slowdown. Is there any way to expedite the process by bypassing such transformations?
Furthermore, if I increase the size of a to an image shape, like:
```python
a=torch.randn(3,224,224)
```
the iteration speed becomes absurdly slow, around 100 iterations per second, whereas the speed with numpy format is approximately 250 iterations per second. This level of speed would be unacceptable for large image datasets, as it could take several hours just to iterate through a single epoch.
### Steps to reproduce the bug
```python
a=torch.randn(100,224)
a=torch.stack([a] * 10000)
a.shape
# %%
ds=Dataset.from_dict({"tensor":a})
for i in tqdm(ds.with_format("numpy")):
pass
for i in tqdm(ds.with_format("torch")):
pass
```
### Expected behavior
iteration faster
### Environment info
- `datasets` version: 2.11.0
- Platform: Linux-5.4.0-148-generic-x86_64-with-glibc2.10
- Python version: 3.8.16
- Huggingface_hub version: 0.13.4
- PyArrow version: 11.0.0
- Pandas version: 2.0.0 | 91 | Abusurdly slow on iteration
### Describe the bug
I am attempting to iterate through an image dataset, but I am encountering a significant slowdown in the iteration speed. In order to investigate this issue, I conducted the following experiment:
```python
a=torch.randn(100,224)
a=torch.stack([a] * 10000)
a.shape
# %%
ds=Dataset.from_dict({"tensor":a})
for i in tqdm(ds.with_format("numpy")):
pass
for i in tqdm(ds.with_format("torch")):
pass
```
I noticed that the dataset in numpy format performs significantly faster than the one in torch format. My hypothesis is that the dataset undergoes a transformation process of torch->python->numpy(torch) in the background, which might be causing the slowdown. Is there any way to expedite the process by bypassing such transformations?
Furthermore, if I increase the size of a to an image shape, like:
```python
a=torch.randn(3,224,224)
```
the iteration speed becomes absurdly slow, around 100 iterations per second, whereas the speed with numpy format is approximately 250 iterations per second. This level of speed would be unacceptable for large image datasets, as it could take several hours just to iterate through a single epoch.
### Steps to reproduce the bug
```python
a=torch.randn(100,224)
a=torch.stack([a] * 10000)
a.shape
# %%
ds=Dataset.from_dict({"tensor":a})
for i in tqdm(ds.with_format("numpy")):
pass
for i in tqdm(ds.with_format("torch")):
pass
```
### Expected behavior
iteration faster
### Environment info
- `datasets` version: 2.11.0
- Platform: Linux-5.4.0-148-generic-x86_64-with-glibc2.10
- Python version: 3.8.16
- Huggingface_hub version: 0.13.4
- PyArrow version: 11.0.0
- Pandas version: 2.0.0
Anyway, regarding the speed difference between numpy and pytorch, I think the issue is that we first convert numpy sub-arrays to pytorch and then consolidate into one tensor, while we should to the opposite. Indeed converting a numpy array to pytorch has a fix cost that seems to cause a slow down. The current pipeline is
```
arrow -> nested numpy arrays -> lists of torch tensors -> one torch tensor
```
and we should do
```
arrow -> nested numpy arrays -> one numpy array -> one torch tensor
``` |
https://github.com/huggingface/datasets/issues/5841 | Abusurdly slow on iteration | I have a similar issue: iterating over a dataset takes 5s without applying any transform, but takes ~30s after applying a transform.
Here is the minimum code to reproduce the problem
```python
import numpy as np
from datasets import Dataset, DatasetDict, load_dataset, Array3D, Image, Features
from torch.utils.data import DataLoader
from tqdm import tqdm
import torchvision
from torchvision.transforms import ToTensor, Normalize
#################################
# Without transform
#################################
train_dataset = load_dataset(
'cifar100',
split='train',
use_auth_token=True,
)
train_dataset.set_format(type="numpy", columns=["img", "fine_label"])
train_loader= DataLoader(
train_dataset,
batch_size=100,
pin_memory=False,
shuffle=True,
num_workers=8,
)
for batch in tqdm(train_loader, desc="Loading data, no transform"):
pass
#################################
# With transform
#################################
transform_func = torchvision.transforms.Compose([
ToTensor(),
Normalize(mean=[0.485, 0.456, 0.406], std= [0.229, 0.224, 0.225]),]
)
train_dataset = train_dataset.map(
desc=f"Preprocessing samples",
function=lambda x: {"img": transform_func(x["img"])},
)
train_dataset.set_format(type="numpy", columns=["img", "fine_label"])
train_loader= DataLoader(
train_dataset,
batch_size=100,
pin_memory=False,
shuffle=True,
num_workers=8,
)
for batch in tqdm(train_loader, desc="Loading data after transform"):
pass
```
I have also tried converting the Image column to an Array3D
```python
img_shape = train_dataset[0]["img"].shape
features = train_dataset.features.copy()
features["x"] = Array3D(shape=img_shape, dtype="float32")
train_dataset = train_dataset.map(
desc=f"Preprocessing samples",
function=lambda x: {"x": np.array(x["img"], dtype=np.uint8)},
features=features,
)
train_dataset.cast_column("x", Array3D(shape=img_shape, dtype="float32"))
train_dataset.set_format(type="numpy", columns=["x", "fine_label"])
```
but to no avail. Any clue? | ### Describe the bug
I am attempting to iterate through an image dataset, but I am encountering a significant slowdown in the iteration speed. In order to investigate this issue, I conducted the following experiment:
```python
a=torch.randn(100,224)
a=torch.stack([a] * 10000)
a.shape
# %%
ds=Dataset.from_dict({"tensor":a})
for i in tqdm(ds.with_format("numpy")):
pass
for i in tqdm(ds.with_format("torch")):
pass
```
I noticed that the dataset in numpy format performs significantly faster than the one in torch format. My hypothesis is that the dataset undergoes a transformation process of torch->python->numpy(torch) in the background, which might be causing the slowdown. Is there any way to expedite the process by bypassing such transformations?
Furthermore, if I increase the size of a to an image shape, like:
```python
a=torch.randn(3,224,224)
```
the iteration speed becomes absurdly slow, around 100 iterations per second, whereas the speed with numpy format is approximately 250 iterations per second. This level of speed would be unacceptable for large image datasets, as it could take several hours just to iterate through a single epoch.
### Steps to reproduce the bug
```python
a=torch.randn(100,224)
a=torch.stack([a] * 10000)
a.shape
# %%
ds=Dataset.from_dict({"tensor":a})
for i in tqdm(ds.with_format("numpy")):
pass
for i in tqdm(ds.with_format("torch")):
pass
```
### Expected behavior
iteration faster
### Environment info
- `datasets` version: 2.11.0
- Platform: Linux-5.4.0-148-generic-x86_64-with-glibc2.10
- Python version: 3.8.16
- Huggingface_hub version: 0.13.4
- PyArrow version: 11.0.0
- Pandas version: 2.0.0 | 187 | Abusurdly slow on iteration
### Describe the bug
I am attempting to iterate through an image dataset, but I am encountering a significant slowdown in the iteration speed. In order to investigate this issue, I conducted the following experiment:
```python
a=torch.randn(100,224)
a=torch.stack([a] * 10000)
a.shape
# %%
ds=Dataset.from_dict({"tensor":a})
for i in tqdm(ds.with_format("numpy")):
pass
for i in tqdm(ds.with_format("torch")):
pass
```
I noticed that the dataset in numpy format performs significantly faster than the one in torch format. My hypothesis is that the dataset undergoes a transformation process of torch->python->numpy(torch) in the background, which might be causing the slowdown. Is there any way to expedite the process by bypassing such transformations?
Furthermore, if I increase the size of a to an image shape, like:
```python
a=torch.randn(3,224,224)
```
the iteration speed becomes absurdly slow, around 100 iterations per second, whereas the speed with numpy format is approximately 250 iterations per second. This level of speed would be unacceptable for large image datasets, as it could take several hours just to iterate through a single epoch.
### Steps to reproduce the bug
```python
a=torch.randn(100,224)
a=torch.stack([a] * 10000)
a.shape
# %%
ds=Dataset.from_dict({"tensor":a})
for i in tqdm(ds.with_format("numpy")):
pass
for i in tqdm(ds.with_format("torch")):
pass
```
### Expected behavior
iteration faster
### Environment info
- `datasets` version: 2.11.0
- Platform: Linux-5.4.0-148-generic-x86_64-with-glibc2.10
- Python version: 3.8.16
- Huggingface_hub version: 0.13.4
- PyArrow version: 11.0.0
- Pandas version: 2.0.0
I have a similar issue: iterating over a dataset takes 5s without applying any transform, but takes ~30s after applying a transform.
Here is the minimum code to reproduce the problem
```python
import numpy as np
from datasets import Dataset, DatasetDict, load_dataset, Array3D, Image, Features
from torch.utils.data import DataLoader
from tqdm import tqdm
import torchvision
from torchvision.transforms import ToTensor, Normalize
#################################
# Without transform
#################################
train_dataset = load_dataset(
'cifar100',
split='train',
use_auth_token=True,
)
train_dataset.set_format(type="numpy", columns=["img", "fine_label"])
train_loader= DataLoader(
train_dataset,
batch_size=100,
pin_memory=False,
shuffle=True,
num_workers=8,
)
for batch in tqdm(train_loader, desc="Loading data, no transform"):
pass
#################################
# With transform
#################################
transform_func = torchvision.transforms.Compose([
ToTensor(),
Normalize(mean=[0.485, 0.456, 0.406], std= [0.229, 0.224, 0.225]),]
)
train_dataset = train_dataset.map(
desc=f"Preprocessing samples",
function=lambda x: {"img": transform_func(x["img"])},
)
train_dataset.set_format(type="numpy", columns=["img", "fine_label"])
train_loader= DataLoader(
train_dataset,
batch_size=100,
pin_memory=False,
shuffle=True,
num_workers=8,
)
for batch in tqdm(train_loader, desc="Loading data after transform"):
pass
```
I have also tried converting the Image column to an Array3D
```python
img_shape = train_dataset[0]["img"].shape
features = train_dataset.features.copy()
features["x"] = Array3D(shape=img_shape, dtype="float32")
train_dataset = train_dataset.map(
desc=f"Preprocessing samples",
function=lambda x: {"x": np.array(x["img"], dtype=np.uint8)},
features=features,
)
train_dataset.cast_column("x", Array3D(shape=img_shape, dtype="float32"))
train_dataset.set_format(type="numpy", columns=["x", "fine_label"])
```
but to no avail. Any clue? |
https://github.com/huggingface/datasets/issues/5842 | Remove columns in interable dataset | This method has been recently added to the `IterableDataset`, so you need to update the `datasets`' installation (`pip install -U datasets`) to use it. | ### Feature request
Right now, remove_columns() produces a NotImplementedError for iterable style datasets
### Motivation
It would be great to have the same functionality irrespective of whether one is using an iterable or a map-style dataset
### Your contribution
hope and courage. | 24 | Remove columns in interable dataset
### Feature request
Right now, remove_columns() produces a NotImplementedError for iterable style datasets
### Motivation
It would be great to have the same functionality irrespective of whether one is using an iterable or a map-style dataset
### Your contribution
hope and courage.
This method has been recently added to the `IterableDataset`, so you need to update the `datasets`' installation (`pip install -U datasets`) to use it. |
https://github.com/huggingface/datasets/issues/5838 | Streaming support for `load_from_disk` | As the name says, `load_from_disk` load the data from your disk. If the data is hosted on S3, it is first downloaded locally and then loaded from your disk.
There is a discussion on streaming data from S3 here though: #5281 | ### Feature request
Support for streaming datasets stored in object stores in `load_from_disk`.
### Motivation
The `load_from_disk` function supports fetching datasets stored in object stores such as `s3`. In many cases, the datasets that are stored in object stores are very large and being able to stream the data from the buckets becomes essential.
### Your contribution
I'd be happy to contribute this feature if I could get the guidance on how to do so. | 41 | Streaming support for `load_from_disk`
### Feature request
Support for streaming datasets stored in object stores in `load_from_disk`.
### Motivation
The `load_from_disk` function supports fetching datasets stored in object stores such as `s3`. In many cases, the datasets that are stored in object stores are very large and being able to stream the data from the buckets becomes essential.
### Your contribution
I'd be happy to contribute this feature if I could get the guidance on how to do so.
As the name says, `load_from_disk` load the data from your disk. If the data is hosted on S3, it is first downloaded locally and then loaded from your disk.
There is a discussion on streaming data from S3 here though: #5281 |
https://github.com/huggingface/datasets/issues/5838 | Streaming support for `load_from_disk` | @lhoestq
Thanks for your comment. I have checked out the discussion before and attempted at replicating the mentioned changes in the main branch (#5580). What I found was that if a dataset is saved using `save_to_disk`, it cannot be read by `load_dataset`. The error message asks me to to use `load_from_disk` instead. What would be the correct way of saving the data in this scenario? | ### Feature request
Support for streaming datasets stored in object stores in `load_from_disk`.
### Motivation
The `load_from_disk` function supports fetching datasets stored in object stores such as `s3`. In many cases, the datasets that are stored in object stores are very large and being able to stream the data from the buckets becomes essential.
### Your contribution
I'd be happy to contribute this feature if I could get the guidance on how to do so. | 65 | Streaming support for `load_from_disk`
### Feature request
Support for streaming datasets stored in object stores in `load_from_disk`.
### Motivation
The `load_from_disk` function supports fetching datasets stored in object stores such as `s3`. In many cases, the datasets that are stored in object stores are very large and being able to stream the data from the buckets becomes essential.
### Your contribution
I'd be happy to contribute this feature if I could get the guidance on how to do so.
@lhoestq
Thanks for your comment. I have checked out the discussion before and attempted at replicating the mentioned changes in the main branch (#5580). What I found was that if a dataset is saved using `save_to_disk`, it cannot be read by `load_dataset`. The error message asks me to to use `load_from_disk` instead. What would be the correct way of saving the data in this scenario? |
https://github.com/huggingface/datasets/issues/5838 | Streaming support for `load_from_disk` | Using `push_to_hub` you can save the dataset on the HF Hub as parquet files, and reload it / stream it using `load_dataset` :)
If you want to save your dataset somewhere else you can use `.to_parquet` to get a parquet file. If your dataset is big it's usually recommended to shard it into multi parquet files (around 1GB each). | ### Feature request
Support for streaming datasets stored in object stores in `load_from_disk`.
### Motivation
The `load_from_disk` function supports fetching datasets stored in object stores such as `s3`. In many cases, the datasets that are stored in object stores are very large and being able to stream the data from the buckets becomes essential.
### Your contribution
I'd be happy to contribute this feature if I could get the guidance on how to do so. | 59 | Streaming support for `load_from_disk`
### Feature request
Support for streaming datasets stored in object stores in `load_from_disk`.
### Motivation
The `load_from_disk` function supports fetching datasets stored in object stores such as `s3`. In many cases, the datasets that are stored in object stores are very large and being able to stream the data from the buckets becomes essential.
### Your contribution
I'd be happy to contribute this feature if I could get the guidance on how to do so.
Using `push_to_hub` you can save the dataset on the HF Hub as parquet files, and reload it / stream it using `load_dataset` :)
If you want to save your dataset somewhere else you can use `.to_parquet` to get a parquet file. If your dataset is big it's usually recommended to shard it into multi parquet files (around 1GB each). |
https://github.com/huggingface/datasets/issues/5838 | Streaming support for `load_from_disk` | @lhoestq
I tried the method you mentioned. This the current scenario I'm facing:
- The parquet file can be read from disk and streaming can be enabled.
- The parquet file can be read from `s3` (local MinIO).
- When `streaming=True` is enabled for `s3`, I get the error mentioned below:
```
File ~/.../lib/python3.8/site-packages/s3fs/core.py:502, in S3FileSystem.set_session(self, refresh, kwargs)
500 conf = AioConfig(**config_kwargs)
501 if self.session is None:
--> 502 self.session = aiobotocore.session.AioSession(**self.kwargs)
504 for parameters in (config_kwargs, self.kwargs, init_kwargs, client_kwargs):
505 for option in ("region_name", "endpoint_url"):
TypeError: __init__() got an unexpected keyword argument 'headers'
```
Does this mean there is a bug in the main branch? | ### Feature request
Support for streaming datasets stored in object stores in `load_from_disk`.
### Motivation
The `load_from_disk` function supports fetching datasets stored in object stores such as `s3`. In many cases, the datasets that are stored in object stores are very large and being able to stream the data from the buckets becomes essential.
### Your contribution
I'd be happy to contribute this feature if I could get the guidance on how to do so. | 106 | Streaming support for `load_from_disk`
### Feature request
Support for streaming datasets stored in object stores in `load_from_disk`.
### Motivation
The `load_from_disk` function supports fetching datasets stored in object stores such as `s3`. In many cases, the datasets that are stored in object stores are very large and being able to stream the data from the buckets becomes essential.
### Your contribution
I'd be happy to contribute this feature if I could get the guidance on how to do so.
@lhoestq
I tried the method you mentioned. This the current scenario I'm facing:
- The parquet file can be read from disk and streaming can be enabled.
- The parquet file can be read from `s3` (local MinIO).
- When `streaming=True` is enabled for `s3`, I get the error mentioned below:
```
File ~/.../lib/python3.8/site-packages/s3fs/core.py:502, in S3FileSystem.set_session(self, refresh, kwargs)
500 conf = AioConfig(**config_kwargs)
501 if self.session is None:
--> 502 self.session = aiobotocore.session.AioSession(**self.kwargs)
504 for parameters in (config_kwargs, self.kwargs, init_kwargs, client_kwargs):
505 for option in ("region_name", "endpoint_url"):
TypeError: __init__() got an unexpected keyword argument 'headers'
```
Does this mean there is a bug in the main branch? |
https://github.com/huggingface/datasets/issues/5838 | Streaming support for `load_from_disk` | Streaming from S3 is still experimental, there might be a few bugs unfortunately.
Can you share the full stack trace ? | ### Feature request
Support for streaming datasets stored in object stores in `load_from_disk`.
### Motivation
The `load_from_disk` function supports fetching datasets stored in object stores such as `s3`. In many cases, the datasets that are stored in object stores are very large and being able to stream the data from the buckets becomes essential.
### Your contribution
I'd be happy to contribute this feature if I could get the guidance on how to do so. | 21 | Streaming support for `load_from_disk`
### Feature request
Support for streaming datasets stored in object stores in `load_from_disk`.
### Motivation
The `load_from_disk` function supports fetching datasets stored in object stores such as `s3`. In many cases, the datasets that are stored in object stores are very large and being able to stream the data from the buckets becomes essential.
### Your contribution
I'd be happy to contribute this feature if I could get the guidance on how to do so.
Streaming from S3 is still experimental, there might be a few bugs unfortunately.
Can you share the full stack trace ? |
https://github.com/huggingface/datasets/issues/5838 | Streaming support for `load_from_disk` | @lhoestq
Sure, here you go:
```python
TypeError Traceback (most recent call last)
Cell In[8], line 1
----> 1 dataset = load_dataset("parquet", data_files=["s3://<bucket name>/<data folder>/data-parquet"], storage_options=fs.storage_options, streaming=True)
File ~/.../datasets/src/datasets/load.py:1790, in load_dataset(path, name, data_dir, data_files, split, cache_dir, features, download_config, download_mode, verification_mode, ignore_verifications, keep_in_memory, save_infos, revision, use_auth_token, task, streaming, num_proc, storage_options, **config_kwargs)
1788 # Return iterable dataset in case of streaming
1789 if streaming:
-> 1790 return builder_instance.as_streaming_dataset(split=split)
1792 # Some datasets are already processed on the HF google storage
1793 # Don't try downloading from Google storage for the packaged datasets as text, json, csv or pandas
1794 try_from_hf_gcs = path not in _PACKAGED_DATASETS_MODULES
File ~/.../datasets/src/datasets/builder.py:1264, in DatasetBuilder.as_streaming_dataset(self, split, base_path)
1257 dl_manager = StreamingDownloadManager(
1258 base_path=base_path or self.base_path,
1259 download_config=DownloadConfig(use_auth_token=self.use_auth_token, storage_options=self.storage_options),
1260 dataset_name=self.name,
1261 data_dir=self.config.data_dir,
1262 )
1263 self._check_manual_download(dl_manager)
-> 1264 splits_generators = {sg.name: sg for sg in self._split_generators(dl_manager)}
1265 # By default, return all splits
1266 if split is None:
File ~/.../datasets/src/datasets/packaged_modules/parquet/parquet.py:34, in Parquet._split_generators(self, dl_manager)
32 if not self.config.data_files:
33 raise ValueError(f"At least one data file must be specified, but got data_files={self.config.data_files}")
---> 34 data_files = dl_manager.download_and_extract(self.config.data_files)
35 if isinstance(data_files, (str, list, tuple)):
36 files = data_files
File ~/.../datasets/src/datasets/download/streaming_download_manager.py:1087, in StreamingDownloadManager.download_and_extract(self, url_or_urls)
1069 def download_and_extract(self, url_or_urls):
1070 """Prepare given `url_or_urls` for streaming (add extraction protocol).
1071
1072 This is the lazy version of `DownloadManager.download_and_extract` for streaming.
(...)
1085 url(s): (`str` or `list` or `dict`), URL(s) to stream data from matching the given input `url_or_urls`.
1086 """
-> 1087 return self.extract(self.download(url_or_urls))
File ~/.../datasets/src/datasets/download/streaming_download_manager.py:1039, in StreamingDownloadManager.extract(self, url_or_urls)
1020 def extract(self, url_or_urls):
1021 """Add extraction protocol for given url(s) for streaming.
1022
1023 This is the lazy version of `DownloadManager.extract` for streaming.
(...)
1037 ```
1038 """
-> 1039 urlpaths = map_nested(self._extract, url_or_urls, map_tuple=True)
1040 return urlpaths
File ~/.../datasets/src/datasets/utils/py_utils.py:443, in map_nested(function, data_struct, dict_only, map_list, map_tuple, map_numpy, num_proc, parallel_min_length, types, disable_tqdm, desc)
441 num_proc = 1
442 if num_proc <= 1 or len(iterable) < parallel_min_length:
--> 443 mapped = [
444 _single_map_nested((function, obj, types, None, True, None))
445 for obj in logging.tqdm(iterable, disable=disable_tqdm, desc=desc)
446 ]
447 else:
448 num_proc = num_proc if num_proc <= len(iterable) else len(iterable)
File ~/.../datasets/src/datasets/utils/py_utils.py:444, in <listcomp>(.0)
441 num_proc = 1
442 if num_proc <= 1 or len(iterable) < parallel_min_length:
443 mapped = [
--> 444 _single_map_nested((function, obj, types, None, True, None))
445 for obj in logging.tqdm(iterable, disable=disable_tqdm, desc=desc)
446 ]
447 else:
448 num_proc = num_proc if num_proc <= len(iterable) else len(iterable)
File ~/.../datasets/src/datasets/utils/py_utils.py:363, in _single_map_nested(args)
361 return {k: _single_map_nested((function, v, types, None, True, None)) for k, v in pbar}
362 else:
--> 363 mapped = [_single_map_nested((function, v, types, None, True, None)) for v in pbar]
364 if isinstance(data_struct, list):
365 return mapped
File ~/.../datasets/src/datasets/utils/py_utils.py:363, in <listcomp>(.0)
361 return {k: _single_map_nested((function, v, types, None, True, None)) for k, v in pbar}
362 else:
--> 363 mapped = [_single_map_nested((function, v, types, None, True, None)) for v in pbar]
364 if isinstance(data_struct, list):
365 return mapped
File ~/.../datasets/src/datasets/utils/py_utils.py:346, in _single_map_nested(args)
344 # Singleton first to spare some computation
345 if not isinstance(data_struct, dict) and not isinstance(data_struct, types):
--> 346 return function(data_struct)
348 # Reduce logging to keep things readable in multiprocessing with tqdm
349 if rank is not None and logging.get_verbosity() < logging.WARNING:
File ~/.../datasets/src/datasets/download/streaming_download_manager.py:1044, in StreamingDownloadManager._extract(self, urlpath)
1042 def _extract(self, urlpath: str) -> str:
1043 urlpath = str(urlpath)
-> 1044 protocol = _get_extraction_protocol(urlpath, use_auth_token=self.download_config.use_auth_token)
1045 # get inner file: zip://train-00000.json.gz::https://foo.bar/data.zip -> zip://train-00000.json.gz
1046 path = urlpath.split("::")[0]
File ~/.../datasets/src/datasets/download/streaming_download_manager.py:433, in _get_extraction_protocol(urlpath, use_auth_token)
431 else:
432 urlpath, kwargs = urlpath, {}
--> 433 with fsspec.open(urlpath, **kwargs) as f:
434 return _get_extraction_protocol_with_magic_number(f)
File ~/.../lib/python3.8/site-packages/fsspec/core.py:102, in OpenFile.__enter__(self)
99 def __enter__(self):
100 mode = self.mode.replace("t", "").replace("b", "") + "b"
--> 102 f = self.fs.open(self.path, mode=mode)
104 self.fobjects = [f]
106 if self.compression is not None:
File ~/.../lib/python3.8/site-packages/fsspec/spec.py:1199, in AbstractFileSystem.open(self, path, mode, block_size, cache_options, compression, **kwargs)
1197 else:
1198 ac = kwargs.pop("autocommit", not self._intrans)
-> 1199 f = self._open(
1200 path,
1201 mode=mode,
1202 block_size=block_size,
1203 autocommit=ac,
1204 cache_options=cache_options,
1205 **kwargs,
1206 )
1207 if compression is not None:
1208 from fsspec.compression import compr
File ~/.../lib/python3.8/site-packages/s3fs/core.py:659, in S3FileSystem._open(self, path, mode, block_size, acl, version_id, fill_cache, cache_type, autocommit, requester_pays, cache_options, **kwargs)
656 if cache_type is None:
657 cache_type = self.default_cache_type
--> 659 return S3File(
660 self,
661 path,
662 mode,
663 block_size=block_size,
664 acl=acl,
665 version_id=version_id,
666 fill_cache=fill_cache,
667 s3_additional_kwargs=kw,
668 cache_type=cache_type,
669 autocommit=autocommit,
670 requester_pays=requester_pays,
671 cache_options=cache_options,
672 )
File ~/.../lib/python3.8/site-packages/s3fs/core.py:2043, in S3File.__init__(self, s3, path, mode, block_size, acl, version_id, fill_cache, s3_additional_kwargs, autocommit, cache_type, requester_pays, cache_options)
2041 self.details = s3.info(path)
2042 self.version_id = self.details.get("VersionId")
-> 2043 super().__init__(
2044 s3,
2045 path,
2046 mode,
2047 block_size,
2048 autocommit=autocommit,
2049 cache_type=cache_type,
2050 cache_options=cache_options,
2051 )
2052 self.s3 = self.fs # compatibility
2054 # when not using autocommit we want to have transactional state to manage
File ~/.../lib/python3.8/site-packages/fsspec/spec.py:1555, in AbstractBufferedFile.__init__(self, fs, path, mode, block_size, autocommit, cache_type, cache_options, size, **kwargs)
1553 self.size = size
1554 else:
-> 1555 self.size = self.details["size"]
1556 self.cache = caches[cache_type](
1557 self.blocksize, self._fetch_range, self.size, **cache_options
1558 )
1559 else:
File ~/.../lib/python3.8/site-packages/fsspec/spec.py:1568, in AbstractBufferedFile.details(self)
1565 @property
1566 def details(self):
1567 if self._details is None:
-> 1568 self._details = self.fs.info(self.path)
1569 return self._details
File ~/.../lib/python3.8/site-packages/fsspec/asyn.py:115, in sync_wrapper.<locals>.wrapper(*args, **kwargs)
112 @functools.wraps(func)
113 def wrapper(*args, **kwargs):
114 self = obj or args[0]
--> 115 return sync(self.loop, func, *args, **kwargs)
File ~/.../lib/python3.8/site-packages/fsspec/asyn.py:100, in sync(loop, func, timeout, *args, **kwargs)
98 raise FSTimeoutError from return_result
99 elif isinstance(return_result, BaseException):
--> 100 raise return_result
101 else:
102 return return_result
File ~/.../lib/python3.8/site-packages/fsspec/asyn.py:55, in _runner(event, coro, result, timeout)
53 coro = asyncio.wait_for(coro, timeout=timeout)
54 try:
---> 55 result[0] = await coro
56 except Exception as ex:
57 result[0] = ex
File ~/.../lib/python3.8/site-packages/s3fs/core.py:1248, in S3FileSystem._info(self, path, bucket, key, refresh, version_id)
1246 if key:
1247 try:
-> 1248 out = await self._call_s3(
1249 "head_object",
1250 self.kwargs,
1251 Bucket=bucket,
1252 Key=key,
1253 **version_id_kw(version_id),
1254 **self.req_kw,
1255 )
1256 return {
1257 "ETag": out.get("ETag", ""),
1258 "LastModified": out["LastModified"],
(...)
1264 "ContentType": out.get("ContentType"),
1265 }
1266 except FileNotFoundError:
File ~/.../lib/python3.8/site-packages/s3fs/core.py:341, in S3FileSystem._call_s3(self, method, *akwarglist, **kwargs)
340 async def _call_s3(self, method, *akwarglist, **kwargs):
--> 341 await self.set_session()
342 s3 = await self.get_s3(kwargs.get("Bucket"))
343 method = getattr(s3, method)
File ~/.../lib/python3.8/site-packages/s3fs/core.py:502, in S3FileSystem.set_session(self, refresh, kwargs)
500 conf = AioConfig(**config_kwargs)
501 if self.session is None:
--> 502 self.session = aiobotocore.session.AioSession(**self.kwargs)
504 for parameters in (config_kwargs, self.kwargs, init_kwargs, client_kwargs):
505 for option in ("region_name", "endpoint_url"):
TypeError: __init__() got an unexpected keyword argument 'headers'
``` | ### Feature request
Support for streaming datasets stored in object stores in `load_from_disk`.
### Motivation
The `load_from_disk` function supports fetching datasets stored in object stores such as `s3`. In many cases, the datasets that are stored in object stores are very large and being able to stream the data from the buckets becomes essential.
### Your contribution
I'd be happy to contribute this feature if I could get the guidance on how to do so. | 1,037 | Streaming support for `load_from_disk`
### Feature request
Support for streaming datasets stored in object stores in `load_from_disk`.
### Motivation
The `load_from_disk` function supports fetching datasets stored in object stores such as `s3`. In many cases, the datasets that are stored in object stores are very large and being able to stream the data from the buckets becomes essential.
### Your contribution
I'd be happy to contribute this feature if I could get the guidance on how to do so.
@lhoestq
Sure, here you go:
```python
TypeError Traceback (most recent call last)
Cell In[8], line 1
----> 1 dataset = load_dataset("parquet", data_files=["s3://<bucket name>/<data folder>/data-parquet"], storage_options=fs.storage_options, streaming=True)
File ~/.../datasets/src/datasets/load.py:1790, in load_dataset(path, name, data_dir, data_files, split, cache_dir, features, download_config, download_mode, verification_mode, ignore_verifications, keep_in_memory, save_infos, revision, use_auth_token, task, streaming, num_proc, storage_options, **config_kwargs)
1788 # Return iterable dataset in case of streaming
1789 if streaming:
-> 1790 return builder_instance.as_streaming_dataset(split=split)
1792 # Some datasets are already processed on the HF google storage
1793 # Don't try downloading from Google storage for the packaged datasets as text, json, csv or pandas
1794 try_from_hf_gcs = path not in _PACKAGED_DATASETS_MODULES
File ~/.../datasets/src/datasets/builder.py:1264, in DatasetBuilder.as_streaming_dataset(self, split, base_path)
1257 dl_manager = StreamingDownloadManager(
1258 base_path=base_path or self.base_path,
1259 download_config=DownloadConfig(use_auth_token=self.use_auth_token, storage_options=self.storage_options),
1260 dataset_name=self.name,
1261 data_dir=self.config.data_dir,
1262 )
1263 self._check_manual_download(dl_manager)
-> 1264 splits_generators = {sg.name: sg for sg in self._split_generators(dl_manager)}
1265 # By default, return all splits
1266 if split is None:
File ~/.../datasets/src/datasets/packaged_modules/parquet/parquet.py:34, in Parquet._split_generators(self, dl_manager)
32 if not self.config.data_files:
33 raise ValueError(f"At least one data file must be specified, but got data_files={self.config.data_files}")
---> 34 data_files = dl_manager.download_and_extract(self.config.data_files)
35 if isinstance(data_files, (str, list, tuple)):
36 files = data_files
File ~/.../datasets/src/datasets/download/streaming_download_manager.py:1087, in StreamingDownloadManager.download_and_extract(self, url_or_urls)
1069 def download_and_extract(self, url_or_urls):
1070 """Prepare given `url_or_urls` for streaming (add extraction protocol).
1071
1072 This is the lazy version of `DownloadManager.download_and_extract` for streaming.
(...)
1085 url(s): (`str` or `list` or `dict`), URL(s) to stream data from matching the given input `url_or_urls`.
1086 """
-> 1087 return self.extract(self.download(url_or_urls))
File ~/.../datasets/src/datasets/download/streaming_download_manager.py:1039, in StreamingDownloadManager.extract(self, url_or_urls)
1020 def extract(self, url_or_urls):
1021 """Add extraction protocol for given url(s) for streaming.
1022
1023 This is the lazy version of `DownloadManager.extract` for streaming.
(...)
1037 ```
1038 """
-> 1039 urlpaths = map_nested(self._extract, url_or_urls, map_tuple=True)
1040 return urlpaths
File ~/.../datasets/src/datasets/utils/py_utils.py:443, in map_nested(function, data_struct, dict_only, map_list, map_tuple, map_numpy, num_proc, parallel_min_length, types, disable_tqdm, desc)
441 num_proc = 1
442 if num_proc <= 1 or len(iterable) < parallel_min_length:
--> 443 mapped = [
444 _single_map_nested((function, obj, types, None, True, None))
445 for obj in logging.tqdm(iterable, disable=disable_tqdm, desc=desc)
446 ]
447 else:
448 num_proc = num_proc if num_proc <= len(iterable) else len(iterable)
File ~/.../datasets/src/datasets/utils/py_utils.py:444, in <listcomp>(.0)
441 num_proc = 1
442 if num_proc <= 1 or len(iterable) < parallel_min_length:
443 mapped = [
--> 444 _single_map_nested((function, obj, types, None, True, None))
445 for obj in logging.tqdm(iterable, disable=disable_tqdm, desc=desc)
446 ]
447 else:
448 num_proc = num_proc if num_proc <= len(iterable) else len(iterable)
File ~/.../datasets/src/datasets/utils/py_utils.py:363, in _single_map_nested(args)
361 return {k: _single_map_nested((function, v, types, None, True, None)) for k, v in pbar}
362 else:
--> 363 mapped = [_single_map_nested((function, v, types, None, True, None)) for v in pbar]
364 if isinstance(data_struct, list):
365 return mapped
File ~/.../datasets/src/datasets/utils/py_utils.py:363, in <listcomp>(.0)
361 return {k: _single_map_nested((function, v, types, None, True, None)) for k, v in pbar}
362 else:
--> 363 mapped = [_single_map_nested((function, v, types, None, True, None)) for v in pbar]
364 if isinstance(data_struct, list):
365 return mapped
File ~/.../datasets/src/datasets/utils/py_utils.py:346, in _single_map_nested(args)
344 # Singleton first to spare some computation
345 if not isinstance(data_struct, dict) and not isinstance(data_struct, types):
--> 346 return function(data_struct)
348 # Reduce logging to keep things readable in multiprocessing with tqdm
349 if rank is not None and logging.get_verbosity() < logging.WARNING:
File ~/.../datasets/src/datasets/download/streaming_download_manager.py:1044, in StreamingDownloadManager._extract(self, urlpath)
1042 def _extract(self, urlpath: str) -> str:
1043 urlpath = str(urlpath)
-> 1044 protocol = _get_extraction_protocol(urlpath, use_auth_token=self.download_config.use_auth_token)
1045 # get inner file: zip://train-00000.json.gz::https://foo.bar/data.zip -> zip://train-00000.json.gz
1046 path = urlpath.split("::")[0]
File ~/.../datasets/src/datasets/download/streaming_download_manager.py:433, in _get_extraction_protocol(urlpath, use_auth_token)
431 else:
432 urlpath, kwargs = urlpath, {}
--> 433 with fsspec.open(urlpath, **kwargs) as f:
434 return _get_extraction_protocol_with_magic_number(f)
File ~/.../lib/python3.8/site-packages/fsspec/core.py:102, in OpenFile.__enter__(self)
99 def __enter__(self):
100 mode = self.mode.replace("t", "").replace("b", "") + "b"
--> 102 f = self.fs.open(self.path, mode=mode)
104 self.fobjects = [f]
106 if self.compression is not None:
File ~/.../lib/python3.8/site-packages/fsspec/spec.py:1199, in AbstractFileSystem.open(self, path, mode, block_size, cache_options, compression, **kwargs)
1197 else:
1198 ac = kwargs.pop("autocommit", not self._intrans)
-> 1199 f = self._open(
1200 path,
1201 mode=mode,
1202 block_size=block_size,
1203 autocommit=ac,
1204 cache_options=cache_options,
1205 **kwargs,
1206 )
1207 if compression is not None:
1208 from fsspec.compression import compr
File ~/.../lib/python3.8/site-packages/s3fs/core.py:659, in S3FileSystem._open(self, path, mode, block_size, acl, version_id, fill_cache, cache_type, autocommit, requester_pays, cache_options, **kwargs)
656 if cache_type is None:
657 cache_type = self.default_cache_type
--> 659 return S3File(
660 self,
661 path,
662 mode,
663 block_size=block_size,
664 acl=acl,
665 version_id=version_id,
666 fill_cache=fill_cache,
667 s3_additional_kwargs=kw,
668 cache_type=cache_type,
669 autocommit=autocommit,
670 requester_pays=requester_pays,
671 cache_options=cache_options,
672 )
File ~/.../lib/python3.8/site-packages/s3fs/core.py:2043, in S3File.__init__(self, s3, path, mode, block_size, acl, version_id, fill_cache, s3_additional_kwargs, autocommit, cache_type, requester_pays, cache_options)
2041 self.details = s3.info(path)
2042 self.version_id = self.details.get("VersionId")
-> 2043 super().__init__(
2044 s3,
2045 path,
2046 mode,
2047 block_size,
2048 autocommit=autocommit,
2049 cache_type=cache_type,
2050 cache_options=cache_options,
2051 )
2052 self.s3 = self.fs # compatibility
2054 # when not using autocommit we want to have transactional state to manage
File ~/.../lib/python3.8/site-packages/fsspec/spec.py:1555, in AbstractBufferedFile.__init__(self, fs, path, mode, block_size, autocommit, cache_type, cache_options, size, **kwargs)
1553 self.size = size
1554 else:
-> 1555 self.size = self.details["size"]
1556 self.cache = caches[cache_type](
1557 self.blocksize, self._fetch_range, self.size, **cache_options
1558 )
1559 else:
File ~/.../lib/python3.8/site-packages/fsspec/spec.py:1568, in AbstractBufferedFile.details(self)
1565 @property
1566 def details(self):
1567 if self._details is None:
-> 1568 self._details = self.fs.info(self.path)
1569 return self._details
File ~/.../lib/python3.8/site-packages/fsspec/asyn.py:115, in sync_wrapper.<locals>.wrapper(*args, **kwargs)
112 @functools.wraps(func)
113 def wrapper(*args, **kwargs):
114 self = obj or args[0]
--> 115 return sync(self.loop, func, *args, **kwargs)
File ~/.../lib/python3.8/site-packages/fsspec/asyn.py:100, in sync(loop, func, timeout, *args, **kwargs)
98 raise FSTimeoutError from return_result
99 elif isinstance(return_result, BaseException):
--> 100 raise return_result
101 else:
102 return return_result
File ~/.../lib/python3.8/site-packages/fsspec/asyn.py:55, in _runner(event, coro, result, timeout)
53 coro = asyncio.wait_for(coro, timeout=timeout)
54 try:
---> 55 result[0] = await coro
56 except Exception as ex:
57 result[0] = ex
File ~/.../lib/python3.8/site-packages/s3fs/core.py:1248, in S3FileSystem._info(self, path, bucket, key, refresh, version_id)
1246 if key:
1247 try:
-> 1248 out = await self._call_s3(
1249 "head_object",
1250 self.kwargs,
1251 Bucket=bucket,
1252 Key=key,
1253 **version_id_kw(version_id),
1254 **self.req_kw,
1255 )
1256 return {
1257 "ETag": out.get("ETag", ""),
1258 "LastModified": out["LastModified"],
(...)
1264 "ContentType": out.get("ContentType"),
1265 }
1266 except FileNotFoundError:
File ~/.../lib/python3.8/site-packages/s3fs/core.py:341, in S3FileSystem._call_s3(self, method, *akwarglist, **kwargs)
340 async def _call_s3(self, method, *akwarglist, **kwargs):
--> 341 await self.set_session()
342 s3 = await self.get_s3(kwargs.get("Bucket"))
343 method = getattr(s3, method)
File ~/.../lib/python3.8/site-packages/s3fs/core.py:502, in S3FileSystem.set_session(self, refresh, kwargs)
500 conf = AioConfig(**config_kwargs)
501 if self.session is None:
--> 502 self.session = aiobotocore.session.AioSession(**self.kwargs)
504 for parameters in (config_kwargs, self.kwargs, init_kwargs, client_kwargs):
505 for option in ("region_name", "endpoint_url"):
TypeError: __init__() got an unexpected keyword argument 'headers'
``` |
https://github.com/huggingface/datasets/issues/5838 | Streaming support for `load_from_disk` | Is `"data-parquet"` a file ? In `data_files` you should pass the paths to the parquet files (not to a directory). Glob patterns are not supported yet for S3 URLs.
The bug seems to happen because your provided data file has no extension. Because of that it tries to infer it from the file content, but fails because `_get_extraction_protocol` doesn't support S3 URLs yet.
| ### Feature request
Support for streaming datasets stored in object stores in `load_from_disk`.
### Motivation
The `load_from_disk` function supports fetching datasets stored in object stores such as `s3`. In many cases, the datasets that are stored in object stores are very large and being able to stream the data from the buckets becomes essential.
### Your contribution
I'd be happy to contribute this feature if I could get the guidance on how to do so. | 63 | Streaming support for `load_from_disk`
### Feature request
Support for streaming datasets stored in object stores in `load_from_disk`.
### Motivation
The `load_from_disk` function supports fetching datasets stored in object stores such as `s3`. In many cases, the datasets that are stored in object stores are very large and being able to stream the data from the buckets becomes essential.
### Your contribution
I'd be happy to contribute this feature if I could get the guidance on how to do so.
Is `"data-parquet"` a file ? In `data_files` you should pass the paths to the parquet files (not to a directory). Glob patterns are not supported yet for S3 URLs.
The bug seems to happen because your provided data file has no extension. Because of that it tries to infer it from the file content, but fails because `_get_extraction_protocol` doesn't support S3 URLs yet.
|
https://github.com/huggingface/datasets/issues/5838 | Streaming support for `load_from_disk` | @lhoestq
Thank you for your answer. Saving the file with `.parquet` extension solved the issue! This is really great! Really appreciate all the help!
Let me know if I should close the issue or feel free to close it if you want. | ### Feature request
Support for streaming datasets stored in object stores in `load_from_disk`.
### Motivation
The `load_from_disk` function supports fetching datasets stored in object stores such as `s3`. In many cases, the datasets that are stored in object stores are very large and being able to stream the data from the buckets becomes essential.
### Your contribution
I'd be happy to contribute this feature if I could get the guidance on how to do so. | 42 | Streaming support for `load_from_disk`
### Feature request
Support for streaming datasets stored in object stores in `load_from_disk`.
### Motivation
The `load_from_disk` function supports fetching datasets stored in object stores such as `s3`. In many cases, the datasets that are stored in object stores are very large and being able to stream the data from the buckets becomes essential.
### Your contribution
I'd be happy to contribute this feature if I could get the guidance on how to do so.
@lhoestq
Thank you for your answer. Saving the file with `.parquet` extension solved the issue! This is really great! Really appreciate all the help!
Let me know if I should close the issue or feel free to close it if you want. |
https://github.com/huggingface/datasets/issues/5838 | Streaming support for `load_from_disk` | Cool ! I'm glad it worked out :)
Sure feel free to close the issue, since the original question about streaming with load_from_disk has been answered anyway | ### Feature request
Support for streaming datasets stored in object stores in `load_from_disk`.
### Motivation
The `load_from_disk` function supports fetching datasets stored in object stores such as `s3`. In many cases, the datasets that are stored in object stores are very large and being able to stream the data from the buckets becomes essential.
### Your contribution
I'd be happy to contribute this feature if I could get the guidance on how to do so. | 27 | Streaming support for `load_from_disk`
### Feature request
Support for streaming datasets stored in object stores in `load_from_disk`.
### Motivation
The `load_from_disk` function supports fetching datasets stored in object stores such as `s3`. In many cases, the datasets that are stored in object stores are very large and being able to stream the data from the buckets becomes essential.
### Your contribution
I'd be happy to contribute this feature if I could get the guidance on how to do so.
Cool ! I'm glad it worked out :)
Sure feel free to close the issue, since the original question about streaming with load_from_disk has been answered anyway |
https://github.com/huggingface/datasets/issues/5837 | Use DeepSpeed load myself " .csv " dataset. | Hi ! Doing `load_dataset("path/to/data.csv")` is not supported yet, but you can do
```python
ds = load_dataset("csv", data_files=["path/to/data.csv"])
``` | ### Describe the bug
When I use DeepSpeed train a model with my own " XXX.csv" dataset I got the follow question:
Traceback (most recent call last):
File "<stdin>", line 1, in <module>
File "/home/fm001/.conda/envs/hzl/lib/python3.8/site-packages/datasets/load.py", line 1767, in load_dataset
builder_instance = load_dataset_builder(
File "/home/fm001/.conda/envs/hzl/lib/python3.8/site-packages/datasets/load.py", line 1498, in load_dataset_builder
dataset_module = dataset_module_factory(
File "/home/fm001/.conda/envs/hzl/lib/python3.8/site-packages/datasets/load.py", line 1217, in dataset_module_factory
raise FileNotFoundError(
FileNotFoundError: Couldn't find a dataset script at /home/fm001/hzl/Data/qa.csv/qa.csv.py or any data file in the same directory.
### Steps to reproduce the bug
my code is :
from datasets import load_dataset
mydata = load_dataset("/home/fm001/hzl/Data/qa.csv")
### Expected behavior
γγγ
### Environment info
γγγ | 18 | Use DeepSpeed load myself " .csv " dataset.
### Describe the bug
When I use DeepSpeed train a model with my own " XXX.csv" dataset I got the follow question:
Traceback (most recent call last):
File "<stdin>", line 1, in <module>
File "/home/fm001/.conda/envs/hzl/lib/python3.8/site-packages/datasets/load.py", line 1767, in load_dataset
builder_instance = load_dataset_builder(
File "/home/fm001/.conda/envs/hzl/lib/python3.8/site-packages/datasets/load.py", line 1498, in load_dataset_builder
dataset_module = dataset_module_factory(
File "/home/fm001/.conda/envs/hzl/lib/python3.8/site-packages/datasets/load.py", line 1217, in dataset_module_factory
raise FileNotFoundError(
FileNotFoundError: Couldn't find a dataset script at /home/fm001/hzl/Data/qa.csv/qa.csv.py or any data file in the same directory.
### Steps to reproduce the bug
my code is :
from datasets import load_dataset
mydata = load_dataset("/home/fm001/hzl/Data/qa.csv")
### Expected behavior
γγγ
### Environment info
γγγ
Hi ! Doing `load_dataset("path/to/data.csv")` is not supported yet, but you can do
```python
ds = load_dataset("csv", data_files=["path/to/data.csv"])
``` |
https://github.com/huggingface/datasets/issues/5837 | Use DeepSpeed load myself " .csv " dataset. | The other question:
Traceback (most recent call last):
File "<stdin>", line 1, in <module>
File "/home/fm001/.conda/envs/hzl/lib/python3.8/site-packages/datasets/load.py", line 1767, in load_dataset
builder_instance = load_dataset_builder(
File "/home/fm001/.conda/envs/hzl/lib/python3.8/site-packages/datasets/load.py", line 1498, in load_dataset_builder
dataset_module = dataset_module_factory(
File "/home/fm001/.conda/envs/hzl/lib/python3.8/site-packages/datasets/load.py", line 1127, in dataset_module_factory
return PackagedDatasetModuleFactory(
File "/home/fm001/.conda/envs/hzl/lib/python3.8/site-packages/datasets/load.py", line 708, in get_module
data_files = DataFilesDict.from_local_or_remote(
File "/home/fm001/.conda/envs/hzl/lib/python3.8/site-packages/datasets/data_files.py", line 796, in from_local_or_remote
DataFilesList.from_local_or_remote(
File "/home/fm001/.conda/envs/hzl/lib/python3.8/site-packages/datasets/data_files.py", line 764, in from_local_or_remote
data_files = resolve_patterns_locally_or_by_urls(base_path, patterns, allowed_extensions)
File "/home/fm001/.conda/envs/hzl/lib/python3.8/site-packages/datasets/data_files.py", line 362, in resolve_patterns_locally_or_by_urls
for path in _resolve_single_pattern_locally(base_path, pattern, allowed_extensions):
File "/home/fm001/.conda/envs/hzl/lib/python3.8/site-packages/datasets/data_files.py", line 306, in _resolve_single_pattern_locally
raise FileNotFoundError(error_msg)
FileNotFoundError: Unable to find '/home/fm001/hzl/Data/qa/' at /
>>> mydata = load_dataset("/home/fm001/hzl/Data/qa/")
Traceback (most recent call last):
File "<stdin>", line 1, in <module>
File "/home/fm001/.conda/envs/hzl/lib/python3.8/site-packages/datasets/load.py", line 1767, in load_dataset
builder_instance = load_dataset_builder(
File "/home/fm001/.conda/envs/hzl/lib/python3.8/site-packages/datasets/load.py", line 1508, in load_dataset_builder
builder_cls = import_main_class(dataset_module.module_path)
File "/home/fm001/.conda/envs/hzl/lib/python3.8/site-packages/datasets/load.py", line 115, in import_main_class
module = importlib.import_module(module_path)
File "/home/fm001/.conda/envs/hzl/lib/python3.8/importlib/__init__.py", line 127, in import_module
return _bootstrap._gcd_import(name[level:], package, level)
File "<frozen importlib._bootstrap>", line 1014, in _gcd_import
File "<frozen importlib._bootstrap>", line 991, in _find_and_load
File "<frozen importlib._bootstrap>", line 975, in _find_and_load_unlocked
File "<frozen importlib._bootstrap>", line 671, in _load_unlocked
File "<frozen importlib._bootstrap_external>", line 783, in exec_module
File "<frozen importlib._bootstrap>", line 219, in _call_with_frames_removed
File "/home/fm001/.cache/huggingface/modules/datasets_modules/datasets/qa/b8b9f481eff9d17b769b4b50f30a51da32b47c94d1af4d2bdffb9fc2c589513a/qa.py", line 2, in <module>
mydata = load_dataset("/home/fm001/hzl/Data/qa/")
File "/home/fm001/.conda/envs/hzl/lib/python3.8/site-packages/datasets/load.py", line 1767, in load_dataset
builder_instance = load_dataset_builder(
File "/home/fm001/.conda/envs/hzl/lib/python3.8/site-packages/datasets/load.py", line 1524, in load_dataset_builder
builder_instance: DatasetBuilder = builder_cls(
TypeError: 'NoneType' object is not callable
And I follow the setting with https://huggingface.co/docs/datasets/dataset_script | ### Describe the bug
When I use DeepSpeed train a model with my own " XXX.csv" dataset I got the follow question:
Traceback (most recent call last):
File "<stdin>", line 1, in <module>
File "/home/fm001/.conda/envs/hzl/lib/python3.8/site-packages/datasets/load.py", line 1767, in load_dataset
builder_instance = load_dataset_builder(
File "/home/fm001/.conda/envs/hzl/lib/python3.8/site-packages/datasets/load.py", line 1498, in load_dataset_builder
dataset_module = dataset_module_factory(
File "/home/fm001/.conda/envs/hzl/lib/python3.8/site-packages/datasets/load.py", line 1217, in dataset_module_factory
raise FileNotFoundError(
FileNotFoundError: Couldn't find a dataset script at /home/fm001/hzl/Data/qa.csv/qa.csv.py or any data file in the same directory.
### Steps to reproduce the bug
my code is :
from datasets import load_dataset
mydata = load_dataset("/home/fm001/hzl/Data/qa.csv")
### Expected behavior
γγγ
### Environment info
γγγ | 229 | Use DeepSpeed load myself " .csv " dataset.
### Describe the bug
When I use DeepSpeed train a model with my own " XXX.csv" dataset I got the follow question:
Traceback (most recent call last):
File "<stdin>", line 1, in <module>
File "/home/fm001/.conda/envs/hzl/lib/python3.8/site-packages/datasets/load.py", line 1767, in load_dataset
builder_instance = load_dataset_builder(
File "/home/fm001/.conda/envs/hzl/lib/python3.8/site-packages/datasets/load.py", line 1498, in load_dataset_builder
dataset_module = dataset_module_factory(
File "/home/fm001/.conda/envs/hzl/lib/python3.8/site-packages/datasets/load.py", line 1217, in dataset_module_factory
raise FileNotFoundError(
FileNotFoundError: Couldn't find a dataset script at /home/fm001/hzl/Data/qa.csv/qa.csv.py or any data file in the same directory.
### Steps to reproduce the bug
my code is :
from datasets import load_dataset
mydata = load_dataset("/home/fm001/hzl/Data/qa.csv")
### Expected behavior
γγγ
### Environment info
γγγ
The other question:
Traceback (most recent call last):
File "<stdin>", line 1, in <module>
File "/home/fm001/.conda/envs/hzl/lib/python3.8/site-packages/datasets/load.py", line 1767, in load_dataset
builder_instance = load_dataset_builder(
File "/home/fm001/.conda/envs/hzl/lib/python3.8/site-packages/datasets/load.py", line 1498, in load_dataset_builder
dataset_module = dataset_module_factory(
File "/home/fm001/.conda/envs/hzl/lib/python3.8/site-packages/datasets/load.py", line 1127, in dataset_module_factory
return PackagedDatasetModuleFactory(
File "/home/fm001/.conda/envs/hzl/lib/python3.8/site-packages/datasets/load.py", line 708, in get_module
data_files = DataFilesDict.from_local_or_remote(
File "/home/fm001/.conda/envs/hzl/lib/python3.8/site-packages/datasets/data_files.py", line 796, in from_local_or_remote
DataFilesList.from_local_or_remote(
File "/home/fm001/.conda/envs/hzl/lib/python3.8/site-packages/datasets/data_files.py", line 764, in from_local_or_remote
data_files = resolve_patterns_locally_or_by_urls(base_path, patterns, allowed_extensions)
File "/home/fm001/.conda/envs/hzl/lib/python3.8/site-packages/datasets/data_files.py", line 362, in resolve_patterns_locally_or_by_urls
for path in _resolve_single_pattern_locally(base_path, pattern, allowed_extensions):
File "/home/fm001/.conda/envs/hzl/lib/python3.8/site-packages/datasets/data_files.py", line 306, in _resolve_single_pattern_locally
raise FileNotFoundError(error_msg)
FileNotFoundError: Unable to find '/home/fm001/hzl/Data/qa/' at /
>>> mydata = load_dataset("/home/fm001/hzl/Data/qa/")
Traceback (most recent call last):
File "<stdin>", line 1, in <module>
File "/home/fm001/.conda/envs/hzl/lib/python3.8/site-packages/datasets/load.py", line 1767, in load_dataset
builder_instance = load_dataset_builder(
File "/home/fm001/.conda/envs/hzl/lib/python3.8/site-packages/datasets/load.py", line 1508, in load_dataset_builder
builder_cls = import_main_class(dataset_module.module_path)
File "/home/fm001/.conda/envs/hzl/lib/python3.8/site-packages/datasets/load.py", line 115, in import_main_class
module = importlib.import_module(module_path)
File "/home/fm001/.conda/envs/hzl/lib/python3.8/importlib/__init__.py", line 127, in import_module
return _bootstrap._gcd_import(name[level:], package, level)
File "<frozen importlib._bootstrap>", line 1014, in _gcd_import
File "<frozen importlib._bootstrap>", line 991, in _find_and_load
File "<frozen importlib._bootstrap>", line 975, in _find_and_load_unlocked
File "<frozen importlib._bootstrap>", line 671, in _load_unlocked
File "<frozen importlib._bootstrap_external>", line 783, in exec_module
File "<frozen importlib._bootstrap>", line 219, in _call_with_frames_removed
File "/home/fm001/.cache/huggingface/modules/datasets_modules/datasets/qa/b8b9f481eff9d17b769b4b50f30a51da32b47c94d1af4d2bdffb9fc2c589513a/qa.py", line 2, in <module>
mydata = load_dataset("/home/fm001/hzl/Data/qa/")
File "/home/fm001/.conda/envs/hzl/lib/python3.8/site-packages/datasets/load.py", line 1767, in load_dataset
builder_instance = load_dataset_builder(
File "/home/fm001/.conda/envs/hzl/lib/python3.8/site-packages/datasets/load.py", line 1524, in load_dataset_builder
builder_instance: DatasetBuilder = builder_cls(
TypeError: 'NoneType' object is not callable
And I follow the setting with https://huggingface.co/docs/datasets/dataset_script |
https://github.com/huggingface/datasets/issues/5834 | Is uint8 supported? | Hi ! The numpy formatting detaults to int64 and float32 - but you can use uint8 using
```python
ds = ds.with_format("numpy", dtype=np.uint8)
``` | ### Describe the bug
I expect the dataset to store the data in the `uint8` data type, but it's returning `int64` instead.
While I've found that `datasets` doesn't yet support float16 (https://github.com/huggingface/datasets/issues/4981), I'm wondering if this is the case for other data types as well.
Is there a way to store vector data as `uint8` and then upload it to the hub?
### Steps to reproduce the bug
```python
from datasets import Features, Dataset, Sequence, Value
import numpy as np
dataset = Dataset.from_dict(
{"vector": [np.array([0, 1, 2], dtype=np.uint8)]}, features=Features({"vector": Sequence(Value("uint8"))})
).with_format("numpy")
print(dataset[0]["vector"].dtype)
```
### Expected behavior
Expected: `uint8`
Actual: `int64`
### Environment info
- `datasets` version: 2.12.0
- Platform: macOS-12.1-x86_64-i386-64bit
- Python version: 3.8.12
- Huggingface_hub version: 0.12.1
- PyArrow version: 11.0.0
- Pandas version: 1.5.3 | 23 | Is uint8 supported?
### Describe the bug
I expect the dataset to store the data in the `uint8` data type, but it's returning `int64` instead.
While I've found that `datasets` doesn't yet support float16 (https://github.com/huggingface/datasets/issues/4981), I'm wondering if this is the case for other data types as well.
Is there a way to store vector data as `uint8` and then upload it to the hub?
### Steps to reproduce the bug
```python
from datasets import Features, Dataset, Sequence, Value
import numpy as np
dataset = Dataset.from_dict(
{"vector": [np.array([0, 1, 2], dtype=np.uint8)]}, features=Features({"vector": Sequence(Value("uint8"))})
).with_format("numpy")
print(dataset[0]["vector"].dtype)
```
### Expected behavior
Expected: `uint8`
Actual: `int64`
### Environment info
- `datasets` version: 2.12.0
- Platform: macOS-12.1-x86_64-i386-64bit
- Python version: 3.8.12
- Huggingface_hub version: 0.12.1
- PyArrow version: 11.0.0
- Pandas version: 1.5.3
Hi ! The numpy formatting detaults to int64 and float32 - but you can use uint8 using
```python
ds = ds.with_format("numpy", dtype=np.uint8)
``` |
https://github.com/huggingface/datasets/issues/5834 | Is uint8 supported? | Thank you!
By setting `ds.with_format("numpy", dtype=np.uint8)`, the dataset returns the data in `uint8`.
However, `with_format` and `set_format` seem to cast the data on-the-fly.
I want to reduce the dataset size by using `uint8` instead of `int64` and I observe no difference between using `int64` and `uint8` for the vector.
Is there any way to actually store the data in `uint8` and save the disk space and the downloading time when loaded from the hub?
| ### Describe the bug
I expect the dataset to store the data in the `uint8` data type, but it's returning `int64` instead.
While I've found that `datasets` doesn't yet support float16 (https://github.com/huggingface/datasets/issues/4981), I'm wondering if this is the case for other data types as well.
Is there a way to store vector data as `uint8` and then upload it to the hub?
### Steps to reproduce the bug
```python
from datasets import Features, Dataset, Sequence, Value
import numpy as np
dataset = Dataset.from_dict(
{"vector": [np.array([0, 1, 2], dtype=np.uint8)]}, features=Features({"vector": Sequence(Value("uint8"))})
).with_format("numpy")
print(dataset[0]["vector"].dtype)
```
### Expected behavior
Expected: `uint8`
Actual: `int64`
### Environment info
- `datasets` version: 2.12.0
- Platform: macOS-12.1-x86_64-i386-64bit
- Python version: 3.8.12
- Huggingface_hub version: 0.12.1
- PyArrow version: 11.0.0
- Pandas version: 1.5.3 | 74 | Is uint8 supported?
### Describe the bug
I expect the dataset to store the data in the `uint8` data type, but it's returning `int64` instead.
While I've found that `datasets` doesn't yet support float16 (https://github.com/huggingface/datasets/issues/4981), I'm wondering if this is the case for other data types as well.
Is there a way to store vector data as `uint8` and then upload it to the hub?
### Steps to reproduce the bug
```python
from datasets import Features, Dataset, Sequence, Value
import numpy as np
dataset = Dataset.from_dict(
{"vector": [np.array([0, 1, 2], dtype=np.uint8)]}, features=Features({"vector": Sequence(Value("uint8"))})
).with_format("numpy")
print(dataset[0]["vector"].dtype)
```
### Expected behavior
Expected: `uint8`
Actual: `int64`
### Environment info
- `datasets` version: 2.12.0
- Platform: macOS-12.1-x86_64-i386-64bit
- Python version: 3.8.12
- Huggingface_hub version: 0.12.1
- PyArrow version: 11.0.0
- Pandas version: 1.5.3
Thank you!
By setting `ds.with_format("numpy", dtype=np.uint8)`, the dataset returns the data in `uint8`.
However, `with_format` and `set_format` seem to cast the data on-the-fly.
I want to reduce the dataset size by using `uint8` instead of `int64` and I observe no difference between using `int64` and `uint8` for the vector.
Is there any way to actually store the data in `uint8` and save the disk space and the downloading time when loaded from the hub?
|
https://github.com/huggingface/datasets/issues/5834 | Is uint8 supported? | If the feature type is `Value("uint8")` then it's written an uint8 on disk using the uint8 Arrow dtype.
e.g.
```python
ds = Dataset.from_dict({"a": range(10)}, features=Features({"a": Value("uint8")}))
ds.data.nbytes
# 10
``` | ### Describe the bug
I expect the dataset to store the data in the `uint8` data type, but it's returning `int64` instead.
While I've found that `datasets` doesn't yet support float16 (https://github.com/huggingface/datasets/issues/4981), I'm wondering if this is the case for other data types as well.
Is there a way to store vector data as `uint8` and then upload it to the hub?
### Steps to reproduce the bug
```python
from datasets import Features, Dataset, Sequence, Value
import numpy as np
dataset = Dataset.from_dict(
{"vector": [np.array([0, 1, 2], dtype=np.uint8)]}, features=Features({"vector": Sequence(Value("uint8"))})
).with_format("numpy")
print(dataset[0]["vector"].dtype)
```
### Expected behavior
Expected: `uint8`
Actual: `int64`
### Environment info
- `datasets` version: 2.12.0
- Platform: macOS-12.1-x86_64-i386-64bit
- Python version: 3.8.12
- Huggingface_hub version: 0.12.1
- PyArrow version: 11.0.0
- Pandas version: 1.5.3 | 30 | Is uint8 supported?
### Describe the bug
I expect the dataset to store the data in the `uint8` data type, but it's returning `int64` instead.
While I've found that `datasets` doesn't yet support float16 (https://github.com/huggingface/datasets/issues/4981), I'm wondering if this is the case for other data types as well.
Is there a way to store vector data as `uint8` and then upload it to the hub?
### Steps to reproduce the bug
```python
from datasets import Features, Dataset, Sequence, Value
import numpy as np
dataset = Dataset.from_dict(
{"vector": [np.array([0, 1, 2], dtype=np.uint8)]}, features=Features({"vector": Sequence(Value("uint8"))})
).with_format("numpy")
print(dataset[0]["vector"].dtype)
```
### Expected behavior
Expected: `uint8`
Actual: `int64`
### Environment info
- `datasets` version: 2.12.0
- Platform: macOS-12.1-x86_64-i386-64bit
- Python version: 3.8.12
- Huggingface_hub version: 0.12.1
- PyArrow version: 11.0.0
- Pandas version: 1.5.3
If the feature type is `Value("uint8")` then it's written an uint8 on disk using the uint8 Arrow dtype.
e.g.
```python
ds = Dataset.from_dict({"a": range(10)}, features=Features({"a": Value("uint8")}))
ds.data.nbytes
# 10
``` |
https://github.com/huggingface/datasets/issues/5834 | Is uint8 supported? | Oh, I understand now.
The data was stored in `uint8` from the beginning (when the dataset returns `int64`).
Thank you for your time!
My question is fully resolved. | ### Describe the bug
I expect the dataset to store the data in the `uint8` data type, but it's returning `int64` instead.
While I've found that `datasets` doesn't yet support float16 (https://github.com/huggingface/datasets/issues/4981), I'm wondering if this is the case for other data types as well.
Is there a way to store vector data as `uint8` and then upload it to the hub?
### Steps to reproduce the bug
```python
from datasets import Features, Dataset, Sequence, Value
import numpy as np
dataset = Dataset.from_dict(
{"vector": [np.array([0, 1, 2], dtype=np.uint8)]}, features=Features({"vector": Sequence(Value("uint8"))})
).with_format("numpy")
print(dataset[0]["vector"].dtype)
```
### Expected behavior
Expected: `uint8`
Actual: `int64`
### Environment info
- `datasets` version: 2.12.0
- Platform: macOS-12.1-x86_64-i386-64bit
- Python version: 3.8.12
- Huggingface_hub version: 0.12.1
- PyArrow version: 11.0.0
- Pandas version: 1.5.3 | 28 | Is uint8 supported?
### Describe the bug
I expect the dataset to store the data in the `uint8` data type, but it's returning `int64` instead.
While I've found that `datasets` doesn't yet support float16 (https://github.com/huggingface/datasets/issues/4981), I'm wondering if this is the case for other data types as well.
Is there a way to store vector data as `uint8` and then upload it to the hub?
### Steps to reproduce the bug
```python
from datasets import Features, Dataset, Sequence, Value
import numpy as np
dataset = Dataset.from_dict(
{"vector": [np.array([0, 1, 2], dtype=np.uint8)]}, features=Features({"vector": Sequence(Value("uint8"))})
).with_format("numpy")
print(dataset[0]["vector"].dtype)
```
### Expected behavior
Expected: `uint8`
Actual: `int64`
### Environment info
- `datasets` version: 2.12.0
- Platform: macOS-12.1-x86_64-i386-64bit
- Python version: 3.8.12
- Huggingface_hub version: 0.12.1
- PyArrow version: 11.0.0
- Pandas version: 1.5.3
Oh, I understand now.
The data was stored in `uint8` from the beginning (when the dataset returns `int64`).
Thank you for your time!
My question is fully resolved. |
https://github.com/huggingface/datasets/issues/5833 | Unable to push dataset - `create_pr` problem | Thanks for reporting, @agombert.
In this case, I think the root issue is authentication: before pushing to Hub, you should authenticate. See our docs: https://huggingface.co/docs/datasets/upload_dataset#upload-with-python
> 2. To upload a dataset on the Hub in Python, you need to log in to your Hugging Face account:
```
huggingface-cli login
``` | ### Describe the bug
I can't upload to the hub the dataset I manually created locally (Image dataset). I have a problem when using the method `.push_to_hub` which asks for a `create_pr` attribute which is not compatible.
### Steps to reproduce the bug
here what I have:
```python
dataset.push_to_hub("agomberto/FrenchCensus-handwritten-texts")
```
Output:
```python
Pushing split train to the Hub.
Pushing dataset shards to the dataset hub: 0%| | 0/2 [00:00<?, ?it/s]
Creating parquet from Arrow format: 0%| | 0/3 [00:00<?, ?ba/s]
Creating parquet from Arrow format: 100%|βββββββββββββββββββββββββββββββββββββββββββββββββββββββββββββββββββββββββββββββββββββββββββββββββββββββββββββββββββββββββββββββββββββ| 3/3 [00:00<00:00, 12.70ba/s]
Pushing dataset shards to the dataset hub: 0%| | 0/2 [00:01<?, ?it/s]
---------------------------------------------------------------------------
HTTPError Traceback (most recent call last)
File ~/miniconda3/envs/hwocr/lib/python3.8/site-packages/huggingface_hub/utils/_errors.py:259, in hf_raise_for_status(response, endpoint_name)
258 try:
--> 259 response.raise_for_status()
260 except HTTPError as e:
File ~/miniconda3/envs/hwocr/lib/python3.8/site-packages/requests/models.py:1021, in Response.raise_for_status(self)
1020 if http_error_msg:
-> 1021 raise HTTPError(http_error_msg, response=self)
HTTPError: 403 Client Error: Forbidden for url: https://huggingface.co/api/datasets/agomberto/FrenchCensus-handwritten-texts/commit/main
The above exception was the direct cause of the following exception:
HfHubHTTPError Traceback (most recent call last)
Cell In[7], line 1
----> 1 dataset.push_to_hub("agomberto/FrenchCensus-handwritten-texts")
File ~/miniconda3/envs/hwocr/lib/python3.8/site-packages/datasets/dataset_dict.py:1583, in DatasetDict.push_to_hub(self, repo_id, private, token, branch, max_shard_size, num_shards, embed_external_files)
1581 logger.warning(f"Pushing split {split} to the Hub.")
1582 # The split=key needs to be removed before merging
-> 1583 repo_id, split, uploaded_size, dataset_nbytes, _, _ = self[split]._push_parquet_shards_to_hub(
1584 repo_id,
1585 split=split,
1586 private=private,
1587 token=token,
1588 branch=branch,
1589 max_shard_size=max_shard_size,
1590 num_shards=num_shards.get(split),
1591 embed_external_files=embed_external_files,
1592 )
1593 total_uploaded_size += uploaded_size
1594 total_dataset_nbytes += dataset_nbytes
File ~/miniconda3/envs/hwocr/lib/python3.8/site-packages/datasets/arrow_dataset.py:5275, in Dataset._push_parquet_shards_to_hub(self, repo_id, split, private, token, branch, max_shard_size, num_shards, embed_external_files)
5273 shard.to_parquet(buffer)
5274 uploaded_size += buffer.tell()
-> 5275 _retry(
5276 api.upload_file,
5277 func_kwargs={
5278 "path_or_fileobj": buffer.getvalue(),
5279 "path_in_repo": shard_path_in_repo,
5280 "repo_id": repo_id,
5281 "token": token,
5282 "repo_type": "dataset",
5283 "revision": branch,
5284 },
5285 exceptions=HTTPError,
5286 status_codes=[504],
5287 base_wait_time=2.0,
5288 max_retries=5,
5289 max_wait_time=20.0,
5290 )
5291 shards_path_in_repo.append(shard_path_in_repo)
5293 # Cleanup to remove unused files
File ~/miniconda3/envs/hwocr/lib/python3.8/site-packages/datasets/utils/file_utils.py:285, in _retry(func, func_args, func_kwargs, exceptions, status_codes, max_retries, base_wait_time, max_wait_time)
283 except exceptions as err:
284 if retry >= max_retries or (status_codes and err.response.status_code not in status_codes):
--> 285 raise err
286 else:
287 sleep_time = min(max_wait_time, base_wait_time * 2**retry) # Exponential backoff
File ~/miniconda3/envs/hwocr/lib/python3.8/site-packages/datasets/utils/file_utils.py:282, in _retry(func, func_args, func_kwargs, exceptions, status_codes, max_retries, base_wait_time, max_wait_time)
280 while True:
281 try:
--> 282 return func(*func_args, **func_kwargs)
283 except exceptions as err:
284 if retry >= max_retries or (status_codes and err.response.status_code not in status_codes):
File ~/miniconda3/envs/hwocr/lib/python3.8/site-packages/huggingface_hub/utils/_validators.py:120, in validate_hf_hub_args.<locals>._inner_fn(*args, **kwargs)
117 if check_use_auth_token:
118 kwargs = smoothly_deprecate_use_auth_token(fn_name=fn.__name__, has_token=has_token, kwargs=kwargs)
--> 120 return fn(*args, **kwargs)
File ~/miniconda3/envs/hwocr/lib/python3.8/site-packages/huggingface_hub/hf_api.py:2998, in HfApi.upload_file(self, path_or_fileobj, path_in_repo, repo_id, token, repo_type, revision, commit_message, commit_description, create_pr, parent_commit)
2990 commit_message = (
2991 commit_message if commit_message is not None else f"Upload {path_in_repo} with huggingface_hub"
2992 )
2993 operation = CommitOperationAdd(
2994 path_or_fileobj=path_or_fileobj,
2995 path_in_repo=path_in_repo,
2996 )
-> 2998 commit_info = self.create_commit(
2999 repo_id=repo_id,
3000 repo_type=repo_type,
3001 operations=[operation],
3002 commit_message=commit_message,
3003 commit_description=commit_description,
3004 token=token,
3005 revision=revision,
3006 create_pr=create_pr,
3007 parent_commit=parent_commit,
3008 )
3010 if commit_info.pr_url is not None:
3011 revision = quote(_parse_revision_from_pr_url(commit_info.pr_url), safe="")
File ~/miniconda3/envs/hwocr/lib/python3.8/site-packages/huggingface_hub/utils/_validators.py:120, in validate_hf_hub_args.<locals>._inner_fn(*args, **kwargs)
117 if check_use_auth_token:
118 kwargs = smoothly_deprecate_use_auth_token(fn_name=fn.__name__, has_token=has_token, kwargs=kwargs)
--> 120 return fn(*args, **kwargs)
File ~/miniconda3/envs/hwocr/lib/python3.8/site-packages/huggingface_hub/hf_api.py:2548, in HfApi.create_commit(self, repo_id, operations, commit_message, commit_description, token, repo_type, revision, create_pr, num_threads, parent_commit)
2546 try:
2547 commit_resp = get_session().post(url=commit_url, headers=headers, data=data, params=params)
-> 2548 hf_raise_for_status(commit_resp, endpoint_name="commit")
2549 except RepositoryNotFoundError as e:
2550 e.append_to_message(_CREATE_COMMIT_NO_REPO_ERROR_MESSAGE)
File ~/miniconda3/envs/hwocr/lib/python3.8/site-packages/huggingface_hub/utils/_errors.py:301, in hf_raise_for_status(response, endpoint_name)
297 raise BadRequestError(message, response=response) from e
299 # Convert `HTTPError` into a `HfHubHTTPError` to display request information
300 # as well (request id and/or server error message)
--> 301 raise HfHubHTTPError(str(e), response=response) from e
HfHubHTTPError: 403 Client Error: Forbidden for url: https://huggingface.co/api/datasets/agomberto/FrenchCensus-handwritten-texts/commit/main (Request ID: Root=1-645a66bf-255ad91602a6404e6cb70fba)
Forbidden: pass `create_pr=1` as a query parameter to create a Pull Request
```
And then when I do
```python
dataset.push_to_hub("agomberto/FrenchCensus-handwritten-texts", create_pr=1)
```
I get
```python
---------------------------------------------------------------------------
TypeError Traceback (most recent call last)
Cell In[8], line 1
----> 1 dataset.push_to_hub("agomberto/FrenchCensus-handwritten-texts", create_pr=1)
TypeError: push_to_hub() got an unexpected keyword argument 'create_pr'
```
### Expected behavior
I would like to have the dataset updloaded [here](https://huggingface.co/datasets/agomberto/FrenchCensus-handwritten-texts).
### Environment info
```bash
- `datasets` version: 2.12.0
- Platform: macOS-13.3.1-arm64-arm-64bit
- Python version: 3.8.16
- Huggingface_hub version: 0.14.1
- PyArrow version: 12.0.0
- Pandas version: 1.5.3
``` | 50 | Unable to push dataset - `create_pr` problem
### Describe the bug
I can't upload to the hub the dataset I manually created locally (Image dataset). I have a problem when using the method `.push_to_hub` which asks for a `create_pr` attribute which is not compatible.
### Steps to reproduce the bug
here what I have:
```python
dataset.push_to_hub("agomberto/FrenchCensus-handwritten-texts")
```
Output:
```python
Pushing split train to the Hub.
Pushing dataset shards to the dataset hub: 0%| | 0/2 [00:00<?, ?it/s]
Creating parquet from Arrow format: 0%| | 0/3 [00:00<?, ?ba/s]
Creating parquet from Arrow format: 100%|βββββββββββββββββββββββββββββββββββββββββββββββββββββββββββββββββββββββββββββββββββββββββββββββββββββββββββββββββββββββββββββββββββββ| 3/3 [00:00<00:00, 12.70ba/s]
Pushing dataset shards to the dataset hub: 0%| | 0/2 [00:01<?, ?it/s]
---------------------------------------------------------------------------
HTTPError Traceback (most recent call last)
File ~/miniconda3/envs/hwocr/lib/python3.8/site-packages/huggingface_hub/utils/_errors.py:259, in hf_raise_for_status(response, endpoint_name)
258 try:
--> 259 response.raise_for_status()
260 except HTTPError as e:
File ~/miniconda3/envs/hwocr/lib/python3.8/site-packages/requests/models.py:1021, in Response.raise_for_status(self)
1020 if http_error_msg:
-> 1021 raise HTTPError(http_error_msg, response=self)
HTTPError: 403 Client Error: Forbidden for url: https://huggingface.co/api/datasets/agomberto/FrenchCensus-handwritten-texts/commit/main
The above exception was the direct cause of the following exception:
HfHubHTTPError Traceback (most recent call last)
Cell In[7], line 1
----> 1 dataset.push_to_hub("agomberto/FrenchCensus-handwritten-texts")
File ~/miniconda3/envs/hwocr/lib/python3.8/site-packages/datasets/dataset_dict.py:1583, in DatasetDict.push_to_hub(self, repo_id, private, token, branch, max_shard_size, num_shards, embed_external_files)
1581 logger.warning(f"Pushing split {split} to the Hub.")
1582 # The split=key needs to be removed before merging
-> 1583 repo_id, split, uploaded_size, dataset_nbytes, _, _ = self[split]._push_parquet_shards_to_hub(
1584 repo_id,
1585 split=split,
1586 private=private,
1587 token=token,
1588 branch=branch,
1589 max_shard_size=max_shard_size,
1590 num_shards=num_shards.get(split),
1591 embed_external_files=embed_external_files,
1592 )
1593 total_uploaded_size += uploaded_size
1594 total_dataset_nbytes += dataset_nbytes
File ~/miniconda3/envs/hwocr/lib/python3.8/site-packages/datasets/arrow_dataset.py:5275, in Dataset._push_parquet_shards_to_hub(self, repo_id, split, private, token, branch, max_shard_size, num_shards, embed_external_files)
5273 shard.to_parquet(buffer)
5274 uploaded_size += buffer.tell()
-> 5275 _retry(
5276 api.upload_file,
5277 func_kwargs={
5278 "path_or_fileobj": buffer.getvalue(),
5279 "path_in_repo": shard_path_in_repo,
5280 "repo_id": repo_id,
5281 "token": token,
5282 "repo_type": "dataset",
5283 "revision": branch,
5284 },
5285 exceptions=HTTPError,
5286 status_codes=[504],
5287 base_wait_time=2.0,
5288 max_retries=5,
5289 max_wait_time=20.0,
5290 )
5291 shards_path_in_repo.append(shard_path_in_repo)
5293 # Cleanup to remove unused files
File ~/miniconda3/envs/hwocr/lib/python3.8/site-packages/datasets/utils/file_utils.py:285, in _retry(func, func_args, func_kwargs, exceptions, status_codes, max_retries, base_wait_time, max_wait_time)
283 except exceptions as err:
284 if retry >= max_retries or (status_codes and err.response.status_code not in status_codes):
--> 285 raise err
286 else:
287 sleep_time = min(max_wait_time, base_wait_time * 2**retry) # Exponential backoff
File ~/miniconda3/envs/hwocr/lib/python3.8/site-packages/datasets/utils/file_utils.py:282, in _retry(func, func_args, func_kwargs, exceptions, status_codes, max_retries, base_wait_time, max_wait_time)
280 while True:
281 try:
--> 282 return func(*func_args, **func_kwargs)
283 except exceptions as err:
284 if retry >= max_retries or (status_codes and err.response.status_code not in status_codes):
File ~/miniconda3/envs/hwocr/lib/python3.8/site-packages/huggingface_hub/utils/_validators.py:120, in validate_hf_hub_args.<locals>._inner_fn(*args, **kwargs)
117 if check_use_auth_token:
118 kwargs = smoothly_deprecate_use_auth_token(fn_name=fn.__name__, has_token=has_token, kwargs=kwargs)
--> 120 return fn(*args, **kwargs)
File ~/miniconda3/envs/hwocr/lib/python3.8/site-packages/huggingface_hub/hf_api.py:2998, in HfApi.upload_file(self, path_or_fileobj, path_in_repo, repo_id, token, repo_type, revision, commit_message, commit_description, create_pr, parent_commit)
2990 commit_message = (
2991 commit_message if commit_message is not None else f"Upload {path_in_repo} with huggingface_hub"
2992 )
2993 operation = CommitOperationAdd(
2994 path_or_fileobj=path_or_fileobj,
2995 path_in_repo=path_in_repo,
2996 )
-> 2998 commit_info = self.create_commit(
2999 repo_id=repo_id,
3000 repo_type=repo_type,
3001 operations=[operation],
3002 commit_message=commit_message,
3003 commit_description=commit_description,
3004 token=token,
3005 revision=revision,
3006 create_pr=create_pr,
3007 parent_commit=parent_commit,
3008 )
3010 if commit_info.pr_url is not None:
3011 revision = quote(_parse_revision_from_pr_url(commit_info.pr_url), safe="")
File ~/miniconda3/envs/hwocr/lib/python3.8/site-packages/huggingface_hub/utils/_validators.py:120, in validate_hf_hub_args.<locals>._inner_fn(*args, **kwargs)
117 if check_use_auth_token:
118 kwargs = smoothly_deprecate_use_auth_token(fn_name=fn.__name__, has_token=has_token, kwargs=kwargs)
--> 120 return fn(*args, **kwargs)
File ~/miniconda3/envs/hwocr/lib/python3.8/site-packages/huggingface_hub/hf_api.py:2548, in HfApi.create_commit(self, repo_id, operations, commit_message, commit_description, token, repo_type, revision, create_pr, num_threads, parent_commit)
2546 try:
2547 commit_resp = get_session().post(url=commit_url, headers=headers, data=data, params=params)
-> 2548 hf_raise_for_status(commit_resp, endpoint_name="commit")
2549 except RepositoryNotFoundError as e:
2550 e.append_to_message(_CREATE_COMMIT_NO_REPO_ERROR_MESSAGE)
File ~/miniconda3/envs/hwocr/lib/python3.8/site-packages/huggingface_hub/utils/_errors.py:301, in hf_raise_for_status(response, endpoint_name)
297 raise BadRequestError(message, response=response) from e
299 # Convert `HTTPError` into a `HfHubHTTPError` to display request information
300 # as well (request id and/or server error message)
--> 301 raise HfHubHTTPError(str(e), response=response) from e
HfHubHTTPError: 403 Client Error: Forbidden for url: https://huggingface.co/api/datasets/agomberto/FrenchCensus-handwritten-texts/commit/main (Request ID: Root=1-645a66bf-255ad91602a6404e6cb70fba)
Forbidden: pass `create_pr=1` as a query parameter to create a Pull Request
```
And then when I do
```python
dataset.push_to_hub("agomberto/FrenchCensus-handwritten-texts", create_pr=1)
```
I get
```python
---------------------------------------------------------------------------
TypeError Traceback (most recent call last)
Cell In[8], line 1
----> 1 dataset.push_to_hub("agomberto/FrenchCensus-handwritten-texts", create_pr=1)
TypeError: push_to_hub() got an unexpected keyword argument 'create_pr'
```
### Expected behavior
I would like to have the dataset updloaded [here](https://huggingface.co/datasets/agomberto/FrenchCensus-handwritten-texts).
### Environment info
```bash
- `datasets` version: 2.12.0
- Platform: macOS-13.3.1-arm64-arm-64bit
- Python version: 3.8.16
- Huggingface_hub version: 0.14.1
- PyArrow version: 12.0.0
- Pandas version: 1.5.3
```
Thanks for reporting, @agombert.
In this case, I think the root issue is authentication: before pushing to Hub, you should authenticate. See our docs: https://huggingface.co/docs/datasets/upload_dataset#upload-with-python
> 2. To upload a dataset on the Hub in Python, you need to log in to your Hugging Face account:
```
huggingface-cli login
``` |
https://github.com/huggingface/datasets/issues/5833 | Unable to push dataset - `create_pr` problem | Hey @albertvillanova well I actually did :D
<img width="1079" alt="Capture dβeΜcran 2023-05-09 aΜ 18 02 58" src="https://github.com/huggingface/datasets/assets/17645711/e091aa20-06b1-4dd3-bfdb-35e832c66f8d">
| ### Describe the bug
I can't upload to the hub the dataset I manually created locally (Image dataset). I have a problem when using the method `.push_to_hub` which asks for a `create_pr` attribute which is not compatible.
### Steps to reproduce the bug
here what I have:
```python
dataset.push_to_hub("agomberto/FrenchCensus-handwritten-texts")
```
Output:
```python
Pushing split train to the Hub.
Pushing dataset shards to the dataset hub: 0%| | 0/2 [00:00<?, ?it/s]
Creating parquet from Arrow format: 0%| | 0/3 [00:00<?, ?ba/s]
Creating parquet from Arrow format: 100%|βββββββββββββββββββββββββββββββββββββββββββββββββββββββββββββββββββββββββββββββββββββββββββββββββββββββββββββββββββββββββββββββββββββ| 3/3 [00:00<00:00, 12.70ba/s]
Pushing dataset shards to the dataset hub: 0%| | 0/2 [00:01<?, ?it/s]
---------------------------------------------------------------------------
HTTPError Traceback (most recent call last)
File ~/miniconda3/envs/hwocr/lib/python3.8/site-packages/huggingface_hub/utils/_errors.py:259, in hf_raise_for_status(response, endpoint_name)
258 try:
--> 259 response.raise_for_status()
260 except HTTPError as e:
File ~/miniconda3/envs/hwocr/lib/python3.8/site-packages/requests/models.py:1021, in Response.raise_for_status(self)
1020 if http_error_msg:
-> 1021 raise HTTPError(http_error_msg, response=self)
HTTPError: 403 Client Error: Forbidden for url: https://huggingface.co/api/datasets/agomberto/FrenchCensus-handwritten-texts/commit/main
The above exception was the direct cause of the following exception:
HfHubHTTPError Traceback (most recent call last)
Cell In[7], line 1
----> 1 dataset.push_to_hub("agomberto/FrenchCensus-handwritten-texts")
File ~/miniconda3/envs/hwocr/lib/python3.8/site-packages/datasets/dataset_dict.py:1583, in DatasetDict.push_to_hub(self, repo_id, private, token, branch, max_shard_size, num_shards, embed_external_files)
1581 logger.warning(f"Pushing split {split} to the Hub.")
1582 # The split=key needs to be removed before merging
-> 1583 repo_id, split, uploaded_size, dataset_nbytes, _, _ = self[split]._push_parquet_shards_to_hub(
1584 repo_id,
1585 split=split,
1586 private=private,
1587 token=token,
1588 branch=branch,
1589 max_shard_size=max_shard_size,
1590 num_shards=num_shards.get(split),
1591 embed_external_files=embed_external_files,
1592 )
1593 total_uploaded_size += uploaded_size
1594 total_dataset_nbytes += dataset_nbytes
File ~/miniconda3/envs/hwocr/lib/python3.8/site-packages/datasets/arrow_dataset.py:5275, in Dataset._push_parquet_shards_to_hub(self, repo_id, split, private, token, branch, max_shard_size, num_shards, embed_external_files)
5273 shard.to_parquet(buffer)
5274 uploaded_size += buffer.tell()
-> 5275 _retry(
5276 api.upload_file,
5277 func_kwargs={
5278 "path_or_fileobj": buffer.getvalue(),
5279 "path_in_repo": shard_path_in_repo,
5280 "repo_id": repo_id,
5281 "token": token,
5282 "repo_type": "dataset",
5283 "revision": branch,
5284 },
5285 exceptions=HTTPError,
5286 status_codes=[504],
5287 base_wait_time=2.0,
5288 max_retries=5,
5289 max_wait_time=20.0,
5290 )
5291 shards_path_in_repo.append(shard_path_in_repo)
5293 # Cleanup to remove unused files
File ~/miniconda3/envs/hwocr/lib/python3.8/site-packages/datasets/utils/file_utils.py:285, in _retry(func, func_args, func_kwargs, exceptions, status_codes, max_retries, base_wait_time, max_wait_time)
283 except exceptions as err:
284 if retry >= max_retries or (status_codes and err.response.status_code not in status_codes):
--> 285 raise err
286 else:
287 sleep_time = min(max_wait_time, base_wait_time * 2**retry) # Exponential backoff
File ~/miniconda3/envs/hwocr/lib/python3.8/site-packages/datasets/utils/file_utils.py:282, in _retry(func, func_args, func_kwargs, exceptions, status_codes, max_retries, base_wait_time, max_wait_time)
280 while True:
281 try:
--> 282 return func(*func_args, **func_kwargs)
283 except exceptions as err:
284 if retry >= max_retries or (status_codes and err.response.status_code not in status_codes):
File ~/miniconda3/envs/hwocr/lib/python3.8/site-packages/huggingface_hub/utils/_validators.py:120, in validate_hf_hub_args.<locals>._inner_fn(*args, **kwargs)
117 if check_use_auth_token:
118 kwargs = smoothly_deprecate_use_auth_token(fn_name=fn.__name__, has_token=has_token, kwargs=kwargs)
--> 120 return fn(*args, **kwargs)
File ~/miniconda3/envs/hwocr/lib/python3.8/site-packages/huggingface_hub/hf_api.py:2998, in HfApi.upload_file(self, path_or_fileobj, path_in_repo, repo_id, token, repo_type, revision, commit_message, commit_description, create_pr, parent_commit)
2990 commit_message = (
2991 commit_message if commit_message is not None else f"Upload {path_in_repo} with huggingface_hub"
2992 )
2993 operation = CommitOperationAdd(
2994 path_or_fileobj=path_or_fileobj,
2995 path_in_repo=path_in_repo,
2996 )
-> 2998 commit_info = self.create_commit(
2999 repo_id=repo_id,
3000 repo_type=repo_type,
3001 operations=[operation],
3002 commit_message=commit_message,
3003 commit_description=commit_description,
3004 token=token,
3005 revision=revision,
3006 create_pr=create_pr,
3007 parent_commit=parent_commit,
3008 )
3010 if commit_info.pr_url is not None:
3011 revision = quote(_parse_revision_from_pr_url(commit_info.pr_url), safe="")
File ~/miniconda3/envs/hwocr/lib/python3.8/site-packages/huggingface_hub/utils/_validators.py:120, in validate_hf_hub_args.<locals>._inner_fn(*args, **kwargs)
117 if check_use_auth_token:
118 kwargs = smoothly_deprecate_use_auth_token(fn_name=fn.__name__, has_token=has_token, kwargs=kwargs)
--> 120 return fn(*args, **kwargs)
File ~/miniconda3/envs/hwocr/lib/python3.8/site-packages/huggingface_hub/hf_api.py:2548, in HfApi.create_commit(self, repo_id, operations, commit_message, commit_description, token, repo_type, revision, create_pr, num_threads, parent_commit)
2546 try:
2547 commit_resp = get_session().post(url=commit_url, headers=headers, data=data, params=params)
-> 2548 hf_raise_for_status(commit_resp, endpoint_name="commit")
2549 except RepositoryNotFoundError as e:
2550 e.append_to_message(_CREATE_COMMIT_NO_REPO_ERROR_MESSAGE)
File ~/miniconda3/envs/hwocr/lib/python3.8/site-packages/huggingface_hub/utils/_errors.py:301, in hf_raise_for_status(response, endpoint_name)
297 raise BadRequestError(message, response=response) from e
299 # Convert `HTTPError` into a `HfHubHTTPError` to display request information
300 # as well (request id and/or server error message)
--> 301 raise HfHubHTTPError(str(e), response=response) from e
HfHubHTTPError: 403 Client Error: Forbidden for url: https://huggingface.co/api/datasets/agomberto/FrenchCensus-handwritten-texts/commit/main (Request ID: Root=1-645a66bf-255ad91602a6404e6cb70fba)
Forbidden: pass `create_pr=1` as a query parameter to create a Pull Request
```
And then when I do
```python
dataset.push_to_hub("agomberto/FrenchCensus-handwritten-texts", create_pr=1)
```
I get
```python
---------------------------------------------------------------------------
TypeError Traceback (most recent call last)
Cell In[8], line 1
----> 1 dataset.push_to_hub("agomberto/FrenchCensus-handwritten-texts", create_pr=1)
TypeError: push_to_hub() got an unexpected keyword argument 'create_pr'
```
### Expected behavior
I would like to have the dataset updloaded [here](https://huggingface.co/datasets/agomberto/FrenchCensus-handwritten-texts).
### Environment info
```bash
- `datasets` version: 2.12.0
- Platform: macOS-13.3.1-arm64-arm-64bit
- Python version: 3.8.16
- Huggingface_hub version: 0.14.1
- PyArrow version: 12.0.0
- Pandas version: 1.5.3
``` | 17 | Unable to push dataset - `create_pr` problem
### Describe the bug
I can't upload to the hub the dataset I manually created locally (Image dataset). I have a problem when using the method `.push_to_hub` which asks for a `create_pr` attribute which is not compatible.
### Steps to reproduce the bug
here what I have:
```python
dataset.push_to_hub("agomberto/FrenchCensus-handwritten-texts")
```
Output:
```python
Pushing split train to the Hub.
Pushing dataset shards to the dataset hub: 0%| | 0/2 [00:00<?, ?it/s]
Creating parquet from Arrow format: 0%| | 0/3 [00:00<?, ?ba/s]
Creating parquet from Arrow format: 100%|βββββββββββββββββββββββββββββββββββββββββββββββββββββββββββββββββββββββββββββββββββββββββββββββββββββββββββββββββββββββββββββββββββββ| 3/3 [00:00<00:00, 12.70ba/s]
Pushing dataset shards to the dataset hub: 0%| | 0/2 [00:01<?, ?it/s]
---------------------------------------------------------------------------
HTTPError Traceback (most recent call last)
File ~/miniconda3/envs/hwocr/lib/python3.8/site-packages/huggingface_hub/utils/_errors.py:259, in hf_raise_for_status(response, endpoint_name)
258 try:
--> 259 response.raise_for_status()
260 except HTTPError as e:
File ~/miniconda3/envs/hwocr/lib/python3.8/site-packages/requests/models.py:1021, in Response.raise_for_status(self)
1020 if http_error_msg:
-> 1021 raise HTTPError(http_error_msg, response=self)
HTTPError: 403 Client Error: Forbidden for url: https://huggingface.co/api/datasets/agomberto/FrenchCensus-handwritten-texts/commit/main
The above exception was the direct cause of the following exception:
HfHubHTTPError Traceback (most recent call last)
Cell In[7], line 1
----> 1 dataset.push_to_hub("agomberto/FrenchCensus-handwritten-texts")
File ~/miniconda3/envs/hwocr/lib/python3.8/site-packages/datasets/dataset_dict.py:1583, in DatasetDict.push_to_hub(self, repo_id, private, token, branch, max_shard_size, num_shards, embed_external_files)
1581 logger.warning(f"Pushing split {split} to the Hub.")
1582 # The split=key needs to be removed before merging
-> 1583 repo_id, split, uploaded_size, dataset_nbytes, _, _ = self[split]._push_parquet_shards_to_hub(
1584 repo_id,
1585 split=split,
1586 private=private,
1587 token=token,
1588 branch=branch,
1589 max_shard_size=max_shard_size,
1590 num_shards=num_shards.get(split),
1591 embed_external_files=embed_external_files,
1592 )
1593 total_uploaded_size += uploaded_size
1594 total_dataset_nbytes += dataset_nbytes
File ~/miniconda3/envs/hwocr/lib/python3.8/site-packages/datasets/arrow_dataset.py:5275, in Dataset._push_parquet_shards_to_hub(self, repo_id, split, private, token, branch, max_shard_size, num_shards, embed_external_files)
5273 shard.to_parquet(buffer)
5274 uploaded_size += buffer.tell()
-> 5275 _retry(
5276 api.upload_file,
5277 func_kwargs={
5278 "path_or_fileobj": buffer.getvalue(),
5279 "path_in_repo": shard_path_in_repo,
5280 "repo_id": repo_id,
5281 "token": token,
5282 "repo_type": "dataset",
5283 "revision": branch,
5284 },
5285 exceptions=HTTPError,
5286 status_codes=[504],
5287 base_wait_time=2.0,
5288 max_retries=5,
5289 max_wait_time=20.0,
5290 )
5291 shards_path_in_repo.append(shard_path_in_repo)
5293 # Cleanup to remove unused files
File ~/miniconda3/envs/hwocr/lib/python3.8/site-packages/datasets/utils/file_utils.py:285, in _retry(func, func_args, func_kwargs, exceptions, status_codes, max_retries, base_wait_time, max_wait_time)
283 except exceptions as err:
284 if retry >= max_retries or (status_codes and err.response.status_code not in status_codes):
--> 285 raise err
286 else:
287 sleep_time = min(max_wait_time, base_wait_time * 2**retry) # Exponential backoff
File ~/miniconda3/envs/hwocr/lib/python3.8/site-packages/datasets/utils/file_utils.py:282, in _retry(func, func_args, func_kwargs, exceptions, status_codes, max_retries, base_wait_time, max_wait_time)
280 while True:
281 try:
--> 282 return func(*func_args, **func_kwargs)
283 except exceptions as err:
284 if retry >= max_retries or (status_codes and err.response.status_code not in status_codes):
File ~/miniconda3/envs/hwocr/lib/python3.8/site-packages/huggingface_hub/utils/_validators.py:120, in validate_hf_hub_args.<locals>._inner_fn(*args, **kwargs)
117 if check_use_auth_token:
118 kwargs = smoothly_deprecate_use_auth_token(fn_name=fn.__name__, has_token=has_token, kwargs=kwargs)
--> 120 return fn(*args, **kwargs)
File ~/miniconda3/envs/hwocr/lib/python3.8/site-packages/huggingface_hub/hf_api.py:2998, in HfApi.upload_file(self, path_or_fileobj, path_in_repo, repo_id, token, repo_type, revision, commit_message, commit_description, create_pr, parent_commit)
2990 commit_message = (
2991 commit_message if commit_message is not None else f"Upload {path_in_repo} with huggingface_hub"
2992 )
2993 operation = CommitOperationAdd(
2994 path_or_fileobj=path_or_fileobj,
2995 path_in_repo=path_in_repo,
2996 )
-> 2998 commit_info = self.create_commit(
2999 repo_id=repo_id,
3000 repo_type=repo_type,
3001 operations=[operation],
3002 commit_message=commit_message,
3003 commit_description=commit_description,
3004 token=token,
3005 revision=revision,
3006 create_pr=create_pr,
3007 parent_commit=parent_commit,
3008 )
3010 if commit_info.pr_url is not None:
3011 revision = quote(_parse_revision_from_pr_url(commit_info.pr_url), safe="")
File ~/miniconda3/envs/hwocr/lib/python3.8/site-packages/huggingface_hub/utils/_validators.py:120, in validate_hf_hub_args.<locals>._inner_fn(*args, **kwargs)
117 if check_use_auth_token:
118 kwargs = smoothly_deprecate_use_auth_token(fn_name=fn.__name__, has_token=has_token, kwargs=kwargs)
--> 120 return fn(*args, **kwargs)
File ~/miniconda3/envs/hwocr/lib/python3.8/site-packages/huggingface_hub/hf_api.py:2548, in HfApi.create_commit(self, repo_id, operations, commit_message, commit_description, token, repo_type, revision, create_pr, num_threads, parent_commit)
2546 try:
2547 commit_resp = get_session().post(url=commit_url, headers=headers, data=data, params=params)
-> 2548 hf_raise_for_status(commit_resp, endpoint_name="commit")
2549 except RepositoryNotFoundError as e:
2550 e.append_to_message(_CREATE_COMMIT_NO_REPO_ERROR_MESSAGE)
File ~/miniconda3/envs/hwocr/lib/python3.8/site-packages/huggingface_hub/utils/_errors.py:301, in hf_raise_for_status(response, endpoint_name)
297 raise BadRequestError(message, response=response) from e
299 # Convert `HTTPError` into a `HfHubHTTPError` to display request information
300 # as well (request id and/or server error message)
--> 301 raise HfHubHTTPError(str(e), response=response) from e
HfHubHTTPError: 403 Client Error: Forbidden for url: https://huggingface.co/api/datasets/agomberto/FrenchCensus-handwritten-texts/commit/main (Request ID: Root=1-645a66bf-255ad91602a6404e6cb70fba)
Forbidden: pass `create_pr=1` as a query parameter to create a Pull Request
```
And then when I do
```python
dataset.push_to_hub("agomberto/FrenchCensus-handwritten-texts", create_pr=1)
```
I get
```python
---------------------------------------------------------------------------
TypeError Traceback (most recent call last)
Cell In[8], line 1
----> 1 dataset.push_to_hub("agomberto/FrenchCensus-handwritten-texts", create_pr=1)
TypeError: push_to_hub() got an unexpected keyword argument 'create_pr'
```
### Expected behavior
I would like to have the dataset updloaded [here](https://huggingface.co/datasets/agomberto/FrenchCensus-handwritten-texts).
### Environment info
```bash
- `datasets` version: 2.12.0
- Platform: macOS-13.3.1-arm64-arm-64bit
- Python version: 3.8.16
- Huggingface_hub version: 0.14.1
- PyArrow version: 12.0.0
- Pandas version: 1.5.3
```
Hey @albertvillanova well I actually did :D
<img width="1079" alt="Capture dβeΜcran 2023-05-09 aΜ 18 02 58" src="https://github.com/huggingface/datasets/assets/17645711/e091aa20-06b1-4dd3-bfdb-35e832c66f8d">
|
https://github.com/huggingface/datasets/issues/5833 | Unable to push dataset - `create_pr` problem | That is weird that you get a Forbidden error if you are properly authenticated...
Today we had a big outage issue affecting the Hugging Face Hub. Could you please retry to push_to_hub your dataset? Maybe that was the cause... | ### Describe the bug
I can't upload to the hub the dataset I manually created locally (Image dataset). I have a problem when using the method `.push_to_hub` which asks for a `create_pr` attribute which is not compatible.
### Steps to reproduce the bug
here what I have:
```python
dataset.push_to_hub("agomberto/FrenchCensus-handwritten-texts")
```
Output:
```python
Pushing split train to the Hub.
Pushing dataset shards to the dataset hub: 0%| | 0/2 [00:00<?, ?it/s]
Creating parquet from Arrow format: 0%| | 0/3 [00:00<?, ?ba/s]
Creating parquet from Arrow format: 100%|βββββββββββββββββββββββββββββββββββββββββββββββββββββββββββββββββββββββββββββββββββββββββββββββββββββββββββββββββββββββββββββββββββββ| 3/3 [00:00<00:00, 12.70ba/s]
Pushing dataset shards to the dataset hub: 0%| | 0/2 [00:01<?, ?it/s]
---------------------------------------------------------------------------
HTTPError Traceback (most recent call last)
File ~/miniconda3/envs/hwocr/lib/python3.8/site-packages/huggingface_hub/utils/_errors.py:259, in hf_raise_for_status(response, endpoint_name)
258 try:
--> 259 response.raise_for_status()
260 except HTTPError as e:
File ~/miniconda3/envs/hwocr/lib/python3.8/site-packages/requests/models.py:1021, in Response.raise_for_status(self)
1020 if http_error_msg:
-> 1021 raise HTTPError(http_error_msg, response=self)
HTTPError: 403 Client Error: Forbidden for url: https://huggingface.co/api/datasets/agomberto/FrenchCensus-handwritten-texts/commit/main
The above exception was the direct cause of the following exception:
HfHubHTTPError Traceback (most recent call last)
Cell In[7], line 1
----> 1 dataset.push_to_hub("agomberto/FrenchCensus-handwritten-texts")
File ~/miniconda3/envs/hwocr/lib/python3.8/site-packages/datasets/dataset_dict.py:1583, in DatasetDict.push_to_hub(self, repo_id, private, token, branch, max_shard_size, num_shards, embed_external_files)
1581 logger.warning(f"Pushing split {split} to the Hub.")
1582 # The split=key needs to be removed before merging
-> 1583 repo_id, split, uploaded_size, dataset_nbytes, _, _ = self[split]._push_parquet_shards_to_hub(
1584 repo_id,
1585 split=split,
1586 private=private,
1587 token=token,
1588 branch=branch,
1589 max_shard_size=max_shard_size,
1590 num_shards=num_shards.get(split),
1591 embed_external_files=embed_external_files,
1592 )
1593 total_uploaded_size += uploaded_size
1594 total_dataset_nbytes += dataset_nbytes
File ~/miniconda3/envs/hwocr/lib/python3.8/site-packages/datasets/arrow_dataset.py:5275, in Dataset._push_parquet_shards_to_hub(self, repo_id, split, private, token, branch, max_shard_size, num_shards, embed_external_files)
5273 shard.to_parquet(buffer)
5274 uploaded_size += buffer.tell()
-> 5275 _retry(
5276 api.upload_file,
5277 func_kwargs={
5278 "path_or_fileobj": buffer.getvalue(),
5279 "path_in_repo": shard_path_in_repo,
5280 "repo_id": repo_id,
5281 "token": token,
5282 "repo_type": "dataset",
5283 "revision": branch,
5284 },
5285 exceptions=HTTPError,
5286 status_codes=[504],
5287 base_wait_time=2.0,
5288 max_retries=5,
5289 max_wait_time=20.0,
5290 )
5291 shards_path_in_repo.append(shard_path_in_repo)
5293 # Cleanup to remove unused files
File ~/miniconda3/envs/hwocr/lib/python3.8/site-packages/datasets/utils/file_utils.py:285, in _retry(func, func_args, func_kwargs, exceptions, status_codes, max_retries, base_wait_time, max_wait_time)
283 except exceptions as err:
284 if retry >= max_retries or (status_codes and err.response.status_code not in status_codes):
--> 285 raise err
286 else:
287 sleep_time = min(max_wait_time, base_wait_time * 2**retry) # Exponential backoff
File ~/miniconda3/envs/hwocr/lib/python3.8/site-packages/datasets/utils/file_utils.py:282, in _retry(func, func_args, func_kwargs, exceptions, status_codes, max_retries, base_wait_time, max_wait_time)
280 while True:
281 try:
--> 282 return func(*func_args, **func_kwargs)
283 except exceptions as err:
284 if retry >= max_retries or (status_codes and err.response.status_code not in status_codes):
File ~/miniconda3/envs/hwocr/lib/python3.8/site-packages/huggingface_hub/utils/_validators.py:120, in validate_hf_hub_args.<locals>._inner_fn(*args, **kwargs)
117 if check_use_auth_token:
118 kwargs = smoothly_deprecate_use_auth_token(fn_name=fn.__name__, has_token=has_token, kwargs=kwargs)
--> 120 return fn(*args, **kwargs)
File ~/miniconda3/envs/hwocr/lib/python3.8/site-packages/huggingface_hub/hf_api.py:2998, in HfApi.upload_file(self, path_or_fileobj, path_in_repo, repo_id, token, repo_type, revision, commit_message, commit_description, create_pr, parent_commit)
2990 commit_message = (
2991 commit_message if commit_message is not None else f"Upload {path_in_repo} with huggingface_hub"
2992 )
2993 operation = CommitOperationAdd(
2994 path_or_fileobj=path_or_fileobj,
2995 path_in_repo=path_in_repo,
2996 )
-> 2998 commit_info = self.create_commit(
2999 repo_id=repo_id,
3000 repo_type=repo_type,
3001 operations=[operation],
3002 commit_message=commit_message,
3003 commit_description=commit_description,
3004 token=token,
3005 revision=revision,
3006 create_pr=create_pr,
3007 parent_commit=parent_commit,
3008 )
3010 if commit_info.pr_url is not None:
3011 revision = quote(_parse_revision_from_pr_url(commit_info.pr_url), safe="")
File ~/miniconda3/envs/hwocr/lib/python3.8/site-packages/huggingface_hub/utils/_validators.py:120, in validate_hf_hub_args.<locals>._inner_fn(*args, **kwargs)
117 if check_use_auth_token:
118 kwargs = smoothly_deprecate_use_auth_token(fn_name=fn.__name__, has_token=has_token, kwargs=kwargs)
--> 120 return fn(*args, **kwargs)
File ~/miniconda3/envs/hwocr/lib/python3.8/site-packages/huggingface_hub/hf_api.py:2548, in HfApi.create_commit(self, repo_id, operations, commit_message, commit_description, token, repo_type, revision, create_pr, num_threads, parent_commit)
2546 try:
2547 commit_resp = get_session().post(url=commit_url, headers=headers, data=data, params=params)
-> 2548 hf_raise_for_status(commit_resp, endpoint_name="commit")
2549 except RepositoryNotFoundError as e:
2550 e.append_to_message(_CREATE_COMMIT_NO_REPO_ERROR_MESSAGE)
File ~/miniconda3/envs/hwocr/lib/python3.8/site-packages/huggingface_hub/utils/_errors.py:301, in hf_raise_for_status(response, endpoint_name)
297 raise BadRequestError(message, response=response) from e
299 # Convert `HTTPError` into a `HfHubHTTPError` to display request information
300 # as well (request id and/or server error message)
--> 301 raise HfHubHTTPError(str(e), response=response) from e
HfHubHTTPError: 403 Client Error: Forbidden for url: https://huggingface.co/api/datasets/agomberto/FrenchCensus-handwritten-texts/commit/main (Request ID: Root=1-645a66bf-255ad91602a6404e6cb70fba)
Forbidden: pass `create_pr=1` as a query parameter to create a Pull Request
```
And then when I do
```python
dataset.push_to_hub("agomberto/FrenchCensus-handwritten-texts", create_pr=1)
```
I get
```python
---------------------------------------------------------------------------
TypeError Traceback (most recent call last)
Cell In[8], line 1
----> 1 dataset.push_to_hub("agomberto/FrenchCensus-handwritten-texts", create_pr=1)
TypeError: push_to_hub() got an unexpected keyword argument 'create_pr'
```
### Expected behavior
I would like to have the dataset updloaded [here](https://huggingface.co/datasets/agomberto/FrenchCensus-handwritten-texts).
### Environment info
```bash
- `datasets` version: 2.12.0
- Platform: macOS-13.3.1-arm64-arm-64bit
- Python version: 3.8.16
- Huggingface_hub version: 0.14.1
- PyArrow version: 12.0.0
- Pandas version: 1.5.3
``` | 39 | Unable to push dataset - `create_pr` problem
### Describe the bug
I can't upload to the hub the dataset I manually created locally (Image dataset). I have a problem when using the method `.push_to_hub` which asks for a `create_pr` attribute which is not compatible.
### Steps to reproduce the bug
here what I have:
```python
dataset.push_to_hub("agomberto/FrenchCensus-handwritten-texts")
```
Output:
```python
Pushing split train to the Hub.
Pushing dataset shards to the dataset hub: 0%| | 0/2 [00:00<?, ?it/s]
Creating parquet from Arrow format: 0%| | 0/3 [00:00<?, ?ba/s]
Creating parquet from Arrow format: 100%|βββββββββββββββββββββββββββββββββββββββββββββββββββββββββββββββββββββββββββββββββββββββββββββββββββββββββββββββββββββββββββββββββββββ| 3/3 [00:00<00:00, 12.70ba/s]
Pushing dataset shards to the dataset hub: 0%| | 0/2 [00:01<?, ?it/s]
---------------------------------------------------------------------------
HTTPError Traceback (most recent call last)
File ~/miniconda3/envs/hwocr/lib/python3.8/site-packages/huggingface_hub/utils/_errors.py:259, in hf_raise_for_status(response, endpoint_name)
258 try:
--> 259 response.raise_for_status()
260 except HTTPError as e:
File ~/miniconda3/envs/hwocr/lib/python3.8/site-packages/requests/models.py:1021, in Response.raise_for_status(self)
1020 if http_error_msg:
-> 1021 raise HTTPError(http_error_msg, response=self)
HTTPError: 403 Client Error: Forbidden for url: https://huggingface.co/api/datasets/agomberto/FrenchCensus-handwritten-texts/commit/main
The above exception was the direct cause of the following exception:
HfHubHTTPError Traceback (most recent call last)
Cell In[7], line 1
----> 1 dataset.push_to_hub("agomberto/FrenchCensus-handwritten-texts")
File ~/miniconda3/envs/hwocr/lib/python3.8/site-packages/datasets/dataset_dict.py:1583, in DatasetDict.push_to_hub(self, repo_id, private, token, branch, max_shard_size, num_shards, embed_external_files)
1581 logger.warning(f"Pushing split {split} to the Hub.")
1582 # The split=key needs to be removed before merging
-> 1583 repo_id, split, uploaded_size, dataset_nbytes, _, _ = self[split]._push_parquet_shards_to_hub(
1584 repo_id,
1585 split=split,
1586 private=private,
1587 token=token,
1588 branch=branch,
1589 max_shard_size=max_shard_size,
1590 num_shards=num_shards.get(split),
1591 embed_external_files=embed_external_files,
1592 )
1593 total_uploaded_size += uploaded_size
1594 total_dataset_nbytes += dataset_nbytes
File ~/miniconda3/envs/hwocr/lib/python3.8/site-packages/datasets/arrow_dataset.py:5275, in Dataset._push_parquet_shards_to_hub(self, repo_id, split, private, token, branch, max_shard_size, num_shards, embed_external_files)
5273 shard.to_parquet(buffer)
5274 uploaded_size += buffer.tell()
-> 5275 _retry(
5276 api.upload_file,
5277 func_kwargs={
5278 "path_or_fileobj": buffer.getvalue(),
5279 "path_in_repo": shard_path_in_repo,
5280 "repo_id": repo_id,
5281 "token": token,
5282 "repo_type": "dataset",
5283 "revision": branch,
5284 },
5285 exceptions=HTTPError,
5286 status_codes=[504],
5287 base_wait_time=2.0,
5288 max_retries=5,
5289 max_wait_time=20.0,
5290 )
5291 shards_path_in_repo.append(shard_path_in_repo)
5293 # Cleanup to remove unused files
File ~/miniconda3/envs/hwocr/lib/python3.8/site-packages/datasets/utils/file_utils.py:285, in _retry(func, func_args, func_kwargs, exceptions, status_codes, max_retries, base_wait_time, max_wait_time)
283 except exceptions as err:
284 if retry >= max_retries or (status_codes and err.response.status_code not in status_codes):
--> 285 raise err
286 else:
287 sleep_time = min(max_wait_time, base_wait_time * 2**retry) # Exponential backoff
File ~/miniconda3/envs/hwocr/lib/python3.8/site-packages/datasets/utils/file_utils.py:282, in _retry(func, func_args, func_kwargs, exceptions, status_codes, max_retries, base_wait_time, max_wait_time)
280 while True:
281 try:
--> 282 return func(*func_args, **func_kwargs)
283 except exceptions as err:
284 if retry >= max_retries or (status_codes and err.response.status_code not in status_codes):
File ~/miniconda3/envs/hwocr/lib/python3.8/site-packages/huggingface_hub/utils/_validators.py:120, in validate_hf_hub_args.<locals>._inner_fn(*args, **kwargs)
117 if check_use_auth_token:
118 kwargs = smoothly_deprecate_use_auth_token(fn_name=fn.__name__, has_token=has_token, kwargs=kwargs)
--> 120 return fn(*args, **kwargs)
File ~/miniconda3/envs/hwocr/lib/python3.8/site-packages/huggingface_hub/hf_api.py:2998, in HfApi.upload_file(self, path_or_fileobj, path_in_repo, repo_id, token, repo_type, revision, commit_message, commit_description, create_pr, parent_commit)
2990 commit_message = (
2991 commit_message if commit_message is not None else f"Upload {path_in_repo} with huggingface_hub"
2992 )
2993 operation = CommitOperationAdd(
2994 path_or_fileobj=path_or_fileobj,
2995 path_in_repo=path_in_repo,
2996 )
-> 2998 commit_info = self.create_commit(
2999 repo_id=repo_id,
3000 repo_type=repo_type,
3001 operations=[operation],
3002 commit_message=commit_message,
3003 commit_description=commit_description,
3004 token=token,
3005 revision=revision,
3006 create_pr=create_pr,
3007 parent_commit=parent_commit,
3008 )
3010 if commit_info.pr_url is not None:
3011 revision = quote(_parse_revision_from_pr_url(commit_info.pr_url), safe="")
File ~/miniconda3/envs/hwocr/lib/python3.8/site-packages/huggingface_hub/utils/_validators.py:120, in validate_hf_hub_args.<locals>._inner_fn(*args, **kwargs)
117 if check_use_auth_token:
118 kwargs = smoothly_deprecate_use_auth_token(fn_name=fn.__name__, has_token=has_token, kwargs=kwargs)
--> 120 return fn(*args, **kwargs)
File ~/miniconda3/envs/hwocr/lib/python3.8/site-packages/huggingface_hub/hf_api.py:2548, in HfApi.create_commit(self, repo_id, operations, commit_message, commit_description, token, repo_type, revision, create_pr, num_threads, parent_commit)
2546 try:
2547 commit_resp = get_session().post(url=commit_url, headers=headers, data=data, params=params)
-> 2548 hf_raise_for_status(commit_resp, endpoint_name="commit")
2549 except RepositoryNotFoundError as e:
2550 e.append_to_message(_CREATE_COMMIT_NO_REPO_ERROR_MESSAGE)
File ~/miniconda3/envs/hwocr/lib/python3.8/site-packages/huggingface_hub/utils/_errors.py:301, in hf_raise_for_status(response, endpoint_name)
297 raise BadRequestError(message, response=response) from e
299 # Convert `HTTPError` into a `HfHubHTTPError` to display request information
300 # as well (request id and/or server error message)
--> 301 raise HfHubHTTPError(str(e), response=response) from e
HfHubHTTPError: 403 Client Error: Forbidden for url: https://huggingface.co/api/datasets/agomberto/FrenchCensus-handwritten-texts/commit/main (Request ID: Root=1-645a66bf-255ad91602a6404e6cb70fba)
Forbidden: pass `create_pr=1` as a query parameter to create a Pull Request
```
And then when I do
```python
dataset.push_to_hub("agomberto/FrenchCensus-handwritten-texts", create_pr=1)
```
I get
```python
---------------------------------------------------------------------------
TypeError Traceback (most recent call last)
Cell In[8], line 1
----> 1 dataset.push_to_hub("agomberto/FrenchCensus-handwritten-texts", create_pr=1)
TypeError: push_to_hub() got an unexpected keyword argument 'create_pr'
```
### Expected behavior
I would like to have the dataset updloaded [here](https://huggingface.co/datasets/agomberto/FrenchCensus-handwritten-texts).
### Environment info
```bash
- `datasets` version: 2.12.0
- Platform: macOS-13.3.1-arm64-arm-64bit
- Python version: 3.8.16
- Huggingface_hub version: 0.14.1
- PyArrow version: 12.0.0
- Pandas version: 1.5.3
```
That is weird that you get a Forbidden error if you are properly authenticated...
Today we had a big outage issue affecting the Hugging Face Hub. Could you please retry to push_to_hub your dataset? Maybe that was the cause... |
https://github.com/huggingface/datasets/issues/5833 | Unable to push dataset - `create_pr` problem | Login successful but also got this error "Forbidden: pass `create_pr=1` as a query parameter to create a Pull Request" | ### Describe the bug
I can't upload to the hub the dataset I manually created locally (Image dataset). I have a problem when using the method `.push_to_hub` which asks for a `create_pr` attribute which is not compatible.
### Steps to reproduce the bug
here what I have:
```python
dataset.push_to_hub("agomberto/FrenchCensus-handwritten-texts")
```
Output:
```python
Pushing split train to the Hub.
Pushing dataset shards to the dataset hub: 0%| | 0/2 [00:00<?, ?it/s]
Creating parquet from Arrow format: 0%| | 0/3 [00:00<?, ?ba/s]
Creating parquet from Arrow format: 100%|βββββββββββββββββββββββββββββββββββββββββββββββββββββββββββββββββββββββββββββββββββββββββββββββββββββββββββββββββββββββββββββββββββββ| 3/3 [00:00<00:00, 12.70ba/s]
Pushing dataset shards to the dataset hub: 0%| | 0/2 [00:01<?, ?it/s]
---------------------------------------------------------------------------
HTTPError Traceback (most recent call last)
File ~/miniconda3/envs/hwocr/lib/python3.8/site-packages/huggingface_hub/utils/_errors.py:259, in hf_raise_for_status(response, endpoint_name)
258 try:
--> 259 response.raise_for_status()
260 except HTTPError as e:
File ~/miniconda3/envs/hwocr/lib/python3.8/site-packages/requests/models.py:1021, in Response.raise_for_status(self)
1020 if http_error_msg:
-> 1021 raise HTTPError(http_error_msg, response=self)
HTTPError: 403 Client Error: Forbidden for url: https://huggingface.co/api/datasets/agomberto/FrenchCensus-handwritten-texts/commit/main
The above exception was the direct cause of the following exception:
HfHubHTTPError Traceback (most recent call last)
Cell In[7], line 1
----> 1 dataset.push_to_hub("agomberto/FrenchCensus-handwritten-texts")
File ~/miniconda3/envs/hwocr/lib/python3.8/site-packages/datasets/dataset_dict.py:1583, in DatasetDict.push_to_hub(self, repo_id, private, token, branch, max_shard_size, num_shards, embed_external_files)
1581 logger.warning(f"Pushing split {split} to the Hub.")
1582 # The split=key needs to be removed before merging
-> 1583 repo_id, split, uploaded_size, dataset_nbytes, _, _ = self[split]._push_parquet_shards_to_hub(
1584 repo_id,
1585 split=split,
1586 private=private,
1587 token=token,
1588 branch=branch,
1589 max_shard_size=max_shard_size,
1590 num_shards=num_shards.get(split),
1591 embed_external_files=embed_external_files,
1592 )
1593 total_uploaded_size += uploaded_size
1594 total_dataset_nbytes += dataset_nbytes
File ~/miniconda3/envs/hwocr/lib/python3.8/site-packages/datasets/arrow_dataset.py:5275, in Dataset._push_parquet_shards_to_hub(self, repo_id, split, private, token, branch, max_shard_size, num_shards, embed_external_files)
5273 shard.to_parquet(buffer)
5274 uploaded_size += buffer.tell()
-> 5275 _retry(
5276 api.upload_file,
5277 func_kwargs={
5278 "path_or_fileobj": buffer.getvalue(),
5279 "path_in_repo": shard_path_in_repo,
5280 "repo_id": repo_id,
5281 "token": token,
5282 "repo_type": "dataset",
5283 "revision": branch,
5284 },
5285 exceptions=HTTPError,
5286 status_codes=[504],
5287 base_wait_time=2.0,
5288 max_retries=5,
5289 max_wait_time=20.0,
5290 )
5291 shards_path_in_repo.append(shard_path_in_repo)
5293 # Cleanup to remove unused files
File ~/miniconda3/envs/hwocr/lib/python3.8/site-packages/datasets/utils/file_utils.py:285, in _retry(func, func_args, func_kwargs, exceptions, status_codes, max_retries, base_wait_time, max_wait_time)
283 except exceptions as err:
284 if retry >= max_retries or (status_codes and err.response.status_code not in status_codes):
--> 285 raise err
286 else:
287 sleep_time = min(max_wait_time, base_wait_time * 2**retry) # Exponential backoff
File ~/miniconda3/envs/hwocr/lib/python3.8/site-packages/datasets/utils/file_utils.py:282, in _retry(func, func_args, func_kwargs, exceptions, status_codes, max_retries, base_wait_time, max_wait_time)
280 while True:
281 try:
--> 282 return func(*func_args, **func_kwargs)
283 except exceptions as err:
284 if retry >= max_retries or (status_codes and err.response.status_code not in status_codes):
File ~/miniconda3/envs/hwocr/lib/python3.8/site-packages/huggingface_hub/utils/_validators.py:120, in validate_hf_hub_args.<locals>._inner_fn(*args, **kwargs)
117 if check_use_auth_token:
118 kwargs = smoothly_deprecate_use_auth_token(fn_name=fn.__name__, has_token=has_token, kwargs=kwargs)
--> 120 return fn(*args, **kwargs)
File ~/miniconda3/envs/hwocr/lib/python3.8/site-packages/huggingface_hub/hf_api.py:2998, in HfApi.upload_file(self, path_or_fileobj, path_in_repo, repo_id, token, repo_type, revision, commit_message, commit_description, create_pr, parent_commit)
2990 commit_message = (
2991 commit_message if commit_message is not None else f"Upload {path_in_repo} with huggingface_hub"
2992 )
2993 operation = CommitOperationAdd(
2994 path_or_fileobj=path_or_fileobj,
2995 path_in_repo=path_in_repo,
2996 )
-> 2998 commit_info = self.create_commit(
2999 repo_id=repo_id,
3000 repo_type=repo_type,
3001 operations=[operation],
3002 commit_message=commit_message,
3003 commit_description=commit_description,
3004 token=token,
3005 revision=revision,
3006 create_pr=create_pr,
3007 parent_commit=parent_commit,
3008 )
3010 if commit_info.pr_url is not None:
3011 revision = quote(_parse_revision_from_pr_url(commit_info.pr_url), safe="")
File ~/miniconda3/envs/hwocr/lib/python3.8/site-packages/huggingface_hub/utils/_validators.py:120, in validate_hf_hub_args.<locals>._inner_fn(*args, **kwargs)
117 if check_use_auth_token:
118 kwargs = smoothly_deprecate_use_auth_token(fn_name=fn.__name__, has_token=has_token, kwargs=kwargs)
--> 120 return fn(*args, **kwargs)
File ~/miniconda3/envs/hwocr/lib/python3.8/site-packages/huggingface_hub/hf_api.py:2548, in HfApi.create_commit(self, repo_id, operations, commit_message, commit_description, token, repo_type, revision, create_pr, num_threads, parent_commit)
2546 try:
2547 commit_resp = get_session().post(url=commit_url, headers=headers, data=data, params=params)
-> 2548 hf_raise_for_status(commit_resp, endpoint_name="commit")
2549 except RepositoryNotFoundError as e:
2550 e.append_to_message(_CREATE_COMMIT_NO_REPO_ERROR_MESSAGE)
File ~/miniconda3/envs/hwocr/lib/python3.8/site-packages/huggingface_hub/utils/_errors.py:301, in hf_raise_for_status(response, endpoint_name)
297 raise BadRequestError(message, response=response) from e
299 # Convert `HTTPError` into a `HfHubHTTPError` to display request information
300 # as well (request id and/or server error message)
--> 301 raise HfHubHTTPError(str(e), response=response) from e
HfHubHTTPError: 403 Client Error: Forbidden for url: https://huggingface.co/api/datasets/agomberto/FrenchCensus-handwritten-texts/commit/main (Request ID: Root=1-645a66bf-255ad91602a6404e6cb70fba)
Forbidden: pass `create_pr=1` as a query parameter to create a Pull Request
```
And then when I do
```python
dataset.push_to_hub("agomberto/FrenchCensus-handwritten-texts", create_pr=1)
```
I get
```python
---------------------------------------------------------------------------
TypeError Traceback (most recent call last)
Cell In[8], line 1
----> 1 dataset.push_to_hub("agomberto/FrenchCensus-handwritten-texts", create_pr=1)
TypeError: push_to_hub() got an unexpected keyword argument 'create_pr'
```
### Expected behavior
I would like to have the dataset updloaded [here](https://huggingface.co/datasets/agomberto/FrenchCensus-handwritten-texts).
### Environment info
```bash
- `datasets` version: 2.12.0
- Platform: macOS-13.3.1-arm64-arm-64bit
- Python version: 3.8.16
- Huggingface_hub version: 0.14.1
- PyArrow version: 12.0.0
- Pandas version: 1.5.3
``` | 19 | Unable to push dataset - `create_pr` problem
### Describe the bug
I can't upload to the hub the dataset I manually created locally (Image dataset). I have a problem when using the method `.push_to_hub` which asks for a `create_pr` attribute which is not compatible.
### Steps to reproduce the bug
here what I have:
```python
dataset.push_to_hub("agomberto/FrenchCensus-handwritten-texts")
```
Output:
```python
Pushing split train to the Hub.
Pushing dataset shards to the dataset hub: 0%| | 0/2 [00:00<?, ?it/s]
Creating parquet from Arrow format: 0%| | 0/3 [00:00<?, ?ba/s]
Creating parquet from Arrow format: 100%|βββββββββββββββββββββββββββββββββββββββββββββββββββββββββββββββββββββββββββββββββββββββββββββββββββββββββββββββββββββββββββββββββββββ| 3/3 [00:00<00:00, 12.70ba/s]
Pushing dataset shards to the dataset hub: 0%| | 0/2 [00:01<?, ?it/s]
---------------------------------------------------------------------------
HTTPError Traceback (most recent call last)
File ~/miniconda3/envs/hwocr/lib/python3.8/site-packages/huggingface_hub/utils/_errors.py:259, in hf_raise_for_status(response, endpoint_name)
258 try:
--> 259 response.raise_for_status()
260 except HTTPError as e:
File ~/miniconda3/envs/hwocr/lib/python3.8/site-packages/requests/models.py:1021, in Response.raise_for_status(self)
1020 if http_error_msg:
-> 1021 raise HTTPError(http_error_msg, response=self)
HTTPError: 403 Client Error: Forbidden for url: https://huggingface.co/api/datasets/agomberto/FrenchCensus-handwritten-texts/commit/main
The above exception was the direct cause of the following exception:
HfHubHTTPError Traceback (most recent call last)
Cell In[7], line 1
----> 1 dataset.push_to_hub("agomberto/FrenchCensus-handwritten-texts")
File ~/miniconda3/envs/hwocr/lib/python3.8/site-packages/datasets/dataset_dict.py:1583, in DatasetDict.push_to_hub(self, repo_id, private, token, branch, max_shard_size, num_shards, embed_external_files)
1581 logger.warning(f"Pushing split {split} to the Hub.")
1582 # The split=key needs to be removed before merging
-> 1583 repo_id, split, uploaded_size, dataset_nbytes, _, _ = self[split]._push_parquet_shards_to_hub(
1584 repo_id,
1585 split=split,
1586 private=private,
1587 token=token,
1588 branch=branch,
1589 max_shard_size=max_shard_size,
1590 num_shards=num_shards.get(split),
1591 embed_external_files=embed_external_files,
1592 )
1593 total_uploaded_size += uploaded_size
1594 total_dataset_nbytes += dataset_nbytes
File ~/miniconda3/envs/hwocr/lib/python3.8/site-packages/datasets/arrow_dataset.py:5275, in Dataset._push_parquet_shards_to_hub(self, repo_id, split, private, token, branch, max_shard_size, num_shards, embed_external_files)
5273 shard.to_parquet(buffer)
5274 uploaded_size += buffer.tell()
-> 5275 _retry(
5276 api.upload_file,
5277 func_kwargs={
5278 "path_or_fileobj": buffer.getvalue(),
5279 "path_in_repo": shard_path_in_repo,
5280 "repo_id": repo_id,
5281 "token": token,
5282 "repo_type": "dataset",
5283 "revision": branch,
5284 },
5285 exceptions=HTTPError,
5286 status_codes=[504],
5287 base_wait_time=2.0,
5288 max_retries=5,
5289 max_wait_time=20.0,
5290 )
5291 shards_path_in_repo.append(shard_path_in_repo)
5293 # Cleanup to remove unused files
File ~/miniconda3/envs/hwocr/lib/python3.8/site-packages/datasets/utils/file_utils.py:285, in _retry(func, func_args, func_kwargs, exceptions, status_codes, max_retries, base_wait_time, max_wait_time)
283 except exceptions as err:
284 if retry >= max_retries or (status_codes and err.response.status_code not in status_codes):
--> 285 raise err
286 else:
287 sleep_time = min(max_wait_time, base_wait_time * 2**retry) # Exponential backoff
File ~/miniconda3/envs/hwocr/lib/python3.8/site-packages/datasets/utils/file_utils.py:282, in _retry(func, func_args, func_kwargs, exceptions, status_codes, max_retries, base_wait_time, max_wait_time)
280 while True:
281 try:
--> 282 return func(*func_args, **func_kwargs)
283 except exceptions as err:
284 if retry >= max_retries or (status_codes and err.response.status_code not in status_codes):
File ~/miniconda3/envs/hwocr/lib/python3.8/site-packages/huggingface_hub/utils/_validators.py:120, in validate_hf_hub_args.<locals>._inner_fn(*args, **kwargs)
117 if check_use_auth_token:
118 kwargs = smoothly_deprecate_use_auth_token(fn_name=fn.__name__, has_token=has_token, kwargs=kwargs)
--> 120 return fn(*args, **kwargs)
File ~/miniconda3/envs/hwocr/lib/python3.8/site-packages/huggingface_hub/hf_api.py:2998, in HfApi.upload_file(self, path_or_fileobj, path_in_repo, repo_id, token, repo_type, revision, commit_message, commit_description, create_pr, parent_commit)
2990 commit_message = (
2991 commit_message if commit_message is not None else f"Upload {path_in_repo} with huggingface_hub"
2992 )
2993 operation = CommitOperationAdd(
2994 path_or_fileobj=path_or_fileobj,
2995 path_in_repo=path_in_repo,
2996 )
-> 2998 commit_info = self.create_commit(
2999 repo_id=repo_id,
3000 repo_type=repo_type,
3001 operations=[operation],
3002 commit_message=commit_message,
3003 commit_description=commit_description,
3004 token=token,
3005 revision=revision,
3006 create_pr=create_pr,
3007 parent_commit=parent_commit,
3008 )
3010 if commit_info.pr_url is not None:
3011 revision = quote(_parse_revision_from_pr_url(commit_info.pr_url), safe="")
File ~/miniconda3/envs/hwocr/lib/python3.8/site-packages/huggingface_hub/utils/_validators.py:120, in validate_hf_hub_args.<locals>._inner_fn(*args, **kwargs)
117 if check_use_auth_token:
118 kwargs = smoothly_deprecate_use_auth_token(fn_name=fn.__name__, has_token=has_token, kwargs=kwargs)
--> 120 return fn(*args, **kwargs)
File ~/miniconda3/envs/hwocr/lib/python3.8/site-packages/huggingface_hub/hf_api.py:2548, in HfApi.create_commit(self, repo_id, operations, commit_message, commit_description, token, repo_type, revision, create_pr, num_threads, parent_commit)
2546 try:
2547 commit_resp = get_session().post(url=commit_url, headers=headers, data=data, params=params)
-> 2548 hf_raise_for_status(commit_resp, endpoint_name="commit")
2549 except RepositoryNotFoundError as e:
2550 e.append_to_message(_CREATE_COMMIT_NO_REPO_ERROR_MESSAGE)
File ~/miniconda3/envs/hwocr/lib/python3.8/site-packages/huggingface_hub/utils/_errors.py:301, in hf_raise_for_status(response, endpoint_name)
297 raise BadRequestError(message, response=response) from e
299 # Convert `HTTPError` into a `HfHubHTTPError` to display request information
300 # as well (request id and/or server error message)
--> 301 raise HfHubHTTPError(str(e), response=response) from e
HfHubHTTPError: 403 Client Error: Forbidden for url: https://huggingface.co/api/datasets/agomberto/FrenchCensus-handwritten-texts/commit/main (Request ID: Root=1-645a66bf-255ad91602a6404e6cb70fba)
Forbidden: pass `create_pr=1` as a query parameter to create a Pull Request
```
And then when I do
```python
dataset.push_to_hub("agomberto/FrenchCensus-handwritten-texts", create_pr=1)
```
I get
```python
---------------------------------------------------------------------------
TypeError Traceback (most recent call last)
Cell In[8], line 1
----> 1 dataset.push_to_hub("agomberto/FrenchCensus-handwritten-texts", create_pr=1)
TypeError: push_to_hub() got an unexpected keyword argument 'create_pr'
```
### Expected behavior
I would like to have the dataset updloaded [here](https://huggingface.co/datasets/agomberto/FrenchCensus-handwritten-texts).
### Environment info
```bash
- `datasets` version: 2.12.0
- Platform: macOS-13.3.1-arm64-arm-64bit
- Python version: 3.8.16
- Huggingface_hub version: 0.14.1
- PyArrow version: 12.0.0
- Pandas version: 1.5.3
```
Login successful but also got this error "Forbidden: pass `create_pr=1` as a query parameter to create a Pull Request" |
https://github.com/huggingface/datasets/issues/5833 | Unable to push dataset - `create_pr` problem | Make sure your API token has a `write` role. I had the same issue as you with the `read` token. Creating a `write` token and using that solved the issue. | ### Describe the bug
I can't upload to the hub the dataset I manually created locally (Image dataset). I have a problem when using the method `.push_to_hub` which asks for a `create_pr` attribute which is not compatible.
### Steps to reproduce the bug
here what I have:
```python
dataset.push_to_hub("agomberto/FrenchCensus-handwritten-texts")
```
Output:
```python
Pushing split train to the Hub.
Pushing dataset shards to the dataset hub: 0%| | 0/2 [00:00<?, ?it/s]
Creating parquet from Arrow format: 0%| | 0/3 [00:00<?, ?ba/s]
Creating parquet from Arrow format: 100%|βββββββββββββββββββββββββββββββββββββββββββββββββββββββββββββββββββββββββββββββββββββββββββββββββββββββββββββββββββββββββββββββββββββ| 3/3 [00:00<00:00, 12.70ba/s]
Pushing dataset shards to the dataset hub: 0%| | 0/2 [00:01<?, ?it/s]
---------------------------------------------------------------------------
HTTPError Traceback (most recent call last)
File ~/miniconda3/envs/hwocr/lib/python3.8/site-packages/huggingface_hub/utils/_errors.py:259, in hf_raise_for_status(response, endpoint_name)
258 try:
--> 259 response.raise_for_status()
260 except HTTPError as e:
File ~/miniconda3/envs/hwocr/lib/python3.8/site-packages/requests/models.py:1021, in Response.raise_for_status(self)
1020 if http_error_msg:
-> 1021 raise HTTPError(http_error_msg, response=self)
HTTPError: 403 Client Error: Forbidden for url: https://huggingface.co/api/datasets/agomberto/FrenchCensus-handwritten-texts/commit/main
The above exception was the direct cause of the following exception:
HfHubHTTPError Traceback (most recent call last)
Cell In[7], line 1
----> 1 dataset.push_to_hub("agomberto/FrenchCensus-handwritten-texts")
File ~/miniconda3/envs/hwocr/lib/python3.8/site-packages/datasets/dataset_dict.py:1583, in DatasetDict.push_to_hub(self, repo_id, private, token, branch, max_shard_size, num_shards, embed_external_files)
1581 logger.warning(f"Pushing split {split} to the Hub.")
1582 # The split=key needs to be removed before merging
-> 1583 repo_id, split, uploaded_size, dataset_nbytes, _, _ = self[split]._push_parquet_shards_to_hub(
1584 repo_id,
1585 split=split,
1586 private=private,
1587 token=token,
1588 branch=branch,
1589 max_shard_size=max_shard_size,
1590 num_shards=num_shards.get(split),
1591 embed_external_files=embed_external_files,
1592 )
1593 total_uploaded_size += uploaded_size
1594 total_dataset_nbytes += dataset_nbytes
File ~/miniconda3/envs/hwocr/lib/python3.8/site-packages/datasets/arrow_dataset.py:5275, in Dataset._push_parquet_shards_to_hub(self, repo_id, split, private, token, branch, max_shard_size, num_shards, embed_external_files)
5273 shard.to_parquet(buffer)
5274 uploaded_size += buffer.tell()
-> 5275 _retry(
5276 api.upload_file,
5277 func_kwargs={
5278 "path_or_fileobj": buffer.getvalue(),
5279 "path_in_repo": shard_path_in_repo,
5280 "repo_id": repo_id,
5281 "token": token,
5282 "repo_type": "dataset",
5283 "revision": branch,
5284 },
5285 exceptions=HTTPError,
5286 status_codes=[504],
5287 base_wait_time=2.0,
5288 max_retries=5,
5289 max_wait_time=20.0,
5290 )
5291 shards_path_in_repo.append(shard_path_in_repo)
5293 # Cleanup to remove unused files
File ~/miniconda3/envs/hwocr/lib/python3.8/site-packages/datasets/utils/file_utils.py:285, in _retry(func, func_args, func_kwargs, exceptions, status_codes, max_retries, base_wait_time, max_wait_time)
283 except exceptions as err:
284 if retry >= max_retries or (status_codes and err.response.status_code not in status_codes):
--> 285 raise err
286 else:
287 sleep_time = min(max_wait_time, base_wait_time * 2**retry) # Exponential backoff
File ~/miniconda3/envs/hwocr/lib/python3.8/site-packages/datasets/utils/file_utils.py:282, in _retry(func, func_args, func_kwargs, exceptions, status_codes, max_retries, base_wait_time, max_wait_time)
280 while True:
281 try:
--> 282 return func(*func_args, **func_kwargs)
283 except exceptions as err:
284 if retry >= max_retries or (status_codes and err.response.status_code not in status_codes):
File ~/miniconda3/envs/hwocr/lib/python3.8/site-packages/huggingface_hub/utils/_validators.py:120, in validate_hf_hub_args.<locals>._inner_fn(*args, **kwargs)
117 if check_use_auth_token:
118 kwargs = smoothly_deprecate_use_auth_token(fn_name=fn.__name__, has_token=has_token, kwargs=kwargs)
--> 120 return fn(*args, **kwargs)
File ~/miniconda3/envs/hwocr/lib/python3.8/site-packages/huggingface_hub/hf_api.py:2998, in HfApi.upload_file(self, path_or_fileobj, path_in_repo, repo_id, token, repo_type, revision, commit_message, commit_description, create_pr, parent_commit)
2990 commit_message = (
2991 commit_message if commit_message is not None else f"Upload {path_in_repo} with huggingface_hub"
2992 )
2993 operation = CommitOperationAdd(
2994 path_or_fileobj=path_or_fileobj,
2995 path_in_repo=path_in_repo,
2996 )
-> 2998 commit_info = self.create_commit(
2999 repo_id=repo_id,
3000 repo_type=repo_type,
3001 operations=[operation],
3002 commit_message=commit_message,
3003 commit_description=commit_description,
3004 token=token,
3005 revision=revision,
3006 create_pr=create_pr,
3007 parent_commit=parent_commit,
3008 )
3010 if commit_info.pr_url is not None:
3011 revision = quote(_parse_revision_from_pr_url(commit_info.pr_url), safe="")
File ~/miniconda3/envs/hwocr/lib/python3.8/site-packages/huggingface_hub/utils/_validators.py:120, in validate_hf_hub_args.<locals>._inner_fn(*args, **kwargs)
117 if check_use_auth_token:
118 kwargs = smoothly_deprecate_use_auth_token(fn_name=fn.__name__, has_token=has_token, kwargs=kwargs)
--> 120 return fn(*args, **kwargs)
File ~/miniconda3/envs/hwocr/lib/python3.8/site-packages/huggingface_hub/hf_api.py:2548, in HfApi.create_commit(self, repo_id, operations, commit_message, commit_description, token, repo_type, revision, create_pr, num_threads, parent_commit)
2546 try:
2547 commit_resp = get_session().post(url=commit_url, headers=headers, data=data, params=params)
-> 2548 hf_raise_for_status(commit_resp, endpoint_name="commit")
2549 except RepositoryNotFoundError as e:
2550 e.append_to_message(_CREATE_COMMIT_NO_REPO_ERROR_MESSAGE)
File ~/miniconda3/envs/hwocr/lib/python3.8/site-packages/huggingface_hub/utils/_errors.py:301, in hf_raise_for_status(response, endpoint_name)
297 raise BadRequestError(message, response=response) from e
299 # Convert `HTTPError` into a `HfHubHTTPError` to display request information
300 # as well (request id and/or server error message)
--> 301 raise HfHubHTTPError(str(e), response=response) from e
HfHubHTTPError: 403 Client Error: Forbidden for url: https://huggingface.co/api/datasets/agomberto/FrenchCensus-handwritten-texts/commit/main (Request ID: Root=1-645a66bf-255ad91602a6404e6cb70fba)
Forbidden: pass `create_pr=1` as a query parameter to create a Pull Request
```
And then when I do
```python
dataset.push_to_hub("agomberto/FrenchCensus-handwritten-texts", create_pr=1)
```
I get
```python
---------------------------------------------------------------------------
TypeError Traceback (most recent call last)
Cell In[8], line 1
----> 1 dataset.push_to_hub("agomberto/FrenchCensus-handwritten-texts", create_pr=1)
TypeError: push_to_hub() got an unexpected keyword argument 'create_pr'
```
### Expected behavior
I would like to have the dataset updloaded [here](https://huggingface.co/datasets/agomberto/FrenchCensus-handwritten-texts).
### Environment info
```bash
- `datasets` version: 2.12.0
- Platform: macOS-13.3.1-arm64-arm-64bit
- Python version: 3.8.16
- Huggingface_hub version: 0.14.1
- PyArrow version: 12.0.0
- Pandas version: 1.5.3
``` | 30 | Unable to push dataset - `create_pr` problem
### Describe the bug
I can't upload to the hub the dataset I manually created locally (Image dataset). I have a problem when using the method `.push_to_hub` which asks for a `create_pr` attribute which is not compatible.
### Steps to reproduce the bug
here what I have:
```python
dataset.push_to_hub("agomberto/FrenchCensus-handwritten-texts")
```
Output:
```python
Pushing split train to the Hub.
Pushing dataset shards to the dataset hub: 0%| | 0/2 [00:00<?, ?it/s]
Creating parquet from Arrow format: 0%| | 0/3 [00:00<?, ?ba/s]
Creating parquet from Arrow format: 100%|βββββββββββββββββββββββββββββββββββββββββββββββββββββββββββββββββββββββββββββββββββββββββββββββββββββββββββββββββββββββββββββββββββββ| 3/3 [00:00<00:00, 12.70ba/s]
Pushing dataset shards to the dataset hub: 0%| | 0/2 [00:01<?, ?it/s]
---------------------------------------------------------------------------
HTTPError Traceback (most recent call last)
File ~/miniconda3/envs/hwocr/lib/python3.8/site-packages/huggingface_hub/utils/_errors.py:259, in hf_raise_for_status(response, endpoint_name)
258 try:
--> 259 response.raise_for_status()
260 except HTTPError as e:
File ~/miniconda3/envs/hwocr/lib/python3.8/site-packages/requests/models.py:1021, in Response.raise_for_status(self)
1020 if http_error_msg:
-> 1021 raise HTTPError(http_error_msg, response=self)
HTTPError: 403 Client Error: Forbidden for url: https://huggingface.co/api/datasets/agomberto/FrenchCensus-handwritten-texts/commit/main
The above exception was the direct cause of the following exception:
HfHubHTTPError Traceback (most recent call last)
Cell In[7], line 1
----> 1 dataset.push_to_hub("agomberto/FrenchCensus-handwritten-texts")
File ~/miniconda3/envs/hwocr/lib/python3.8/site-packages/datasets/dataset_dict.py:1583, in DatasetDict.push_to_hub(self, repo_id, private, token, branch, max_shard_size, num_shards, embed_external_files)
1581 logger.warning(f"Pushing split {split} to the Hub.")
1582 # The split=key needs to be removed before merging
-> 1583 repo_id, split, uploaded_size, dataset_nbytes, _, _ = self[split]._push_parquet_shards_to_hub(
1584 repo_id,
1585 split=split,
1586 private=private,
1587 token=token,
1588 branch=branch,
1589 max_shard_size=max_shard_size,
1590 num_shards=num_shards.get(split),
1591 embed_external_files=embed_external_files,
1592 )
1593 total_uploaded_size += uploaded_size
1594 total_dataset_nbytes += dataset_nbytes
File ~/miniconda3/envs/hwocr/lib/python3.8/site-packages/datasets/arrow_dataset.py:5275, in Dataset._push_parquet_shards_to_hub(self, repo_id, split, private, token, branch, max_shard_size, num_shards, embed_external_files)
5273 shard.to_parquet(buffer)
5274 uploaded_size += buffer.tell()
-> 5275 _retry(
5276 api.upload_file,
5277 func_kwargs={
5278 "path_or_fileobj": buffer.getvalue(),
5279 "path_in_repo": shard_path_in_repo,
5280 "repo_id": repo_id,
5281 "token": token,
5282 "repo_type": "dataset",
5283 "revision": branch,
5284 },
5285 exceptions=HTTPError,
5286 status_codes=[504],
5287 base_wait_time=2.0,
5288 max_retries=5,
5289 max_wait_time=20.0,
5290 )
5291 shards_path_in_repo.append(shard_path_in_repo)
5293 # Cleanup to remove unused files
File ~/miniconda3/envs/hwocr/lib/python3.8/site-packages/datasets/utils/file_utils.py:285, in _retry(func, func_args, func_kwargs, exceptions, status_codes, max_retries, base_wait_time, max_wait_time)
283 except exceptions as err:
284 if retry >= max_retries or (status_codes and err.response.status_code not in status_codes):
--> 285 raise err
286 else:
287 sleep_time = min(max_wait_time, base_wait_time * 2**retry) # Exponential backoff
File ~/miniconda3/envs/hwocr/lib/python3.8/site-packages/datasets/utils/file_utils.py:282, in _retry(func, func_args, func_kwargs, exceptions, status_codes, max_retries, base_wait_time, max_wait_time)
280 while True:
281 try:
--> 282 return func(*func_args, **func_kwargs)
283 except exceptions as err:
284 if retry >= max_retries or (status_codes and err.response.status_code not in status_codes):
File ~/miniconda3/envs/hwocr/lib/python3.8/site-packages/huggingface_hub/utils/_validators.py:120, in validate_hf_hub_args.<locals>._inner_fn(*args, **kwargs)
117 if check_use_auth_token:
118 kwargs = smoothly_deprecate_use_auth_token(fn_name=fn.__name__, has_token=has_token, kwargs=kwargs)
--> 120 return fn(*args, **kwargs)
File ~/miniconda3/envs/hwocr/lib/python3.8/site-packages/huggingface_hub/hf_api.py:2998, in HfApi.upload_file(self, path_or_fileobj, path_in_repo, repo_id, token, repo_type, revision, commit_message, commit_description, create_pr, parent_commit)
2990 commit_message = (
2991 commit_message if commit_message is not None else f"Upload {path_in_repo} with huggingface_hub"
2992 )
2993 operation = CommitOperationAdd(
2994 path_or_fileobj=path_or_fileobj,
2995 path_in_repo=path_in_repo,
2996 )
-> 2998 commit_info = self.create_commit(
2999 repo_id=repo_id,
3000 repo_type=repo_type,
3001 operations=[operation],
3002 commit_message=commit_message,
3003 commit_description=commit_description,
3004 token=token,
3005 revision=revision,
3006 create_pr=create_pr,
3007 parent_commit=parent_commit,
3008 )
3010 if commit_info.pr_url is not None:
3011 revision = quote(_parse_revision_from_pr_url(commit_info.pr_url), safe="")
File ~/miniconda3/envs/hwocr/lib/python3.8/site-packages/huggingface_hub/utils/_validators.py:120, in validate_hf_hub_args.<locals>._inner_fn(*args, **kwargs)
117 if check_use_auth_token:
118 kwargs = smoothly_deprecate_use_auth_token(fn_name=fn.__name__, has_token=has_token, kwargs=kwargs)
--> 120 return fn(*args, **kwargs)
File ~/miniconda3/envs/hwocr/lib/python3.8/site-packages/huggingface_hub/hf_api.py:2548, in HfApi.create_commit(self, repo_id, operations, commit_message, commit_description, token, repo_type, revision, create_pr, num_threads, parent_commit)
2546 try:
2547 commit_resp = get_session().post(url=commit_url, headers=headers, data=data, params=params)
-> 2548 hf_raise_for_status(commit_resp, endpoint_name="commit")
2549 except RepositoryNotFoundError as e:
2550 e.append_to_message(_CREATE_COMMIT_NO_REPO_ERROR_MESSAGE)
File ~/miniconda3/envs/hwocr/lib/python3.8/site-packages/huggingface_hub/utils/_errors.py:301, in hf_raise_for_status(response, endpoint_name)
297 raise BadRequestError(message, response=response) from e
299 # Convert `HTTPError` into a `HfHubHTTPError` to display request information
300 # as well (request id and/or server error message)
--> 301 raise HfHubHTTPError(str(e), response=response) from e
HfHubHTTPError: 403 Client Error: Forbidden for url: https://huggingface.co/api/datasets/agomberto/FrenchCensus-handwritten-texts/commit/main (Request ID: Root=1-645a66bf-255ad91602a6404e6cb70fba)
Forbidden: pass `create_pr=1` as a query parameter to create a Pull Request
```
And then when I do
```python
dataset.push_to_hub("agomberto/FrenchCensus-handwritten-texts", create_pr=1)
```
I get
```python
---------------------------------------------------------------------------
TypeError Traceback (most recent call last)
Cell In[8], line 1
----> 1 dataset.push_to_hub("agomberto/FrenchCensus-handwritten-texts", create_pr=1)
TypeError: push_to_hub() got an unexpected keyword argument 'create_pr'
```
### Expected behavior
I would like to have the dataset updloaded [here](https://huggingface.co/datasets/agomberto/FrenchCensus-handwritten-texts).
### Environment info
```bash
- `datasets` version: 2.12.0
- Platform: macOS-13.3.1-arm64-arm-64bit
- Python version: 3.8.16
- Huggingface_hub version: 0.14.1
- PyArrow version: 12.0.0
- Pandas version: 1.5.3
```
Make sure your API token has a `write` role. I had the same issue as you with the `read` token. Creating a `write` token and using that solved the issue. |
https://github.com/huggingface/datasets/issues/5833 | Unable to push dataset - `create_pr` problem | > Make sure your API token has a `write` role. I had the same issue as you with the `read` token. Creating a `write` token and using that solved the issue.
I generate a token with write role. It works! thank you so much. | ### Describe the bug
I can't upload to the hub the dataset I manually created locally (Image dataset). I have a problem when using the method `.push_to_hub` which asks for a `create_pr` attribute which is not compatible.
### Steps to reproduce the bug
here what I have:
```python
dataset.push_to_hub("agomberto/FrenchCensus-handwritten-texts")
```
Output:
```python
Pushing split train to the Hub.
Pushing dataset shards to the dataset hub: 0%| | 0/2 [00:00<?, ?it/s]
Creating parquet from Arrow format: 0%| | 0/3 [00:00<?, ?ba/s]
Creating parquet from Arrow format: 100%|βββββββββββββββββββββββββββββββββββββββββββββββββββββββββββββββββββββββββββββββββββββββββββββββββββββββββββββββββββββββββββββββββββββ| 3/3 [00:00<00:00, 12.70ba/s]
Pushing dataset shards to the dataset hub: 0%| | 0/2 [00:01<?, ?it/s]
---------------------------------------------------------------------------
HTTPError Traceback (most recent call last)
File ~/miniconda3/envs/hwocr/lib/python3.8/site-packages/huggingface_hub/utils/_errors.py:259, in hf_raise_for_status(response, endpoint_name)
258 try:
--> 259 response.raise_for_status()
260 except HTTPError as e:
File ~/miniconda3/envs/hwocr/lib/python3.8/site-packages/requests/models.py:1021, in Response.raise_for_status(self)
1020 if http_error_msg:
-> 1021 raise HTTPError(http_error_msg, response=self)
HTTPError: 403 Client Error: Forbidden for url: https://huggingface.co/api/datasets/agomberto/FrenchCensus-handwritten-texts/commit/main
The above exception was the direct cause of the following exception:
HfHubHTTPError Traceback (most recent call last)
Cell In[7], line 1
----> 1 dataset.push_to_hub("agomberto/FrenchCensus-handwritten-texts")
File ~/miniconda3/envs/hwocr/lib/python3.8/site-packages/datasets/dataset_dict.py:1583, in DatasetDict.push_to_hub(self, repo_id, private, token, branch, max_shard_size, num_shards, embed_external_files)
1581 logger.warning(f"Pushing split {split} to the Hub.")
1582 # The split=key needs to be removed before merging
-> 1583 repo_id, split, uploaded_size, dataset_nbytes, _, _ = self[split]._push_parquet_shards_to_hub(
1584 repo_id,
1585 split=split,
1586 private=private,
1587 token=token,
1588 branch=branch,
1589 max_shard_size=max_shard_size,
1590 num_shards=num_shards.get(split),
1591 embed_external_files=embed_external_files,
1592 )
1593 total_uploaded_size += uploaded_size
1594 total_dataset_nbytes += dataset_nbytes
File ~/miniconda3/envs/hwocr/lib/python3.8/site-packages/datasets/arrow_dataset.py:5275, in Dataset._push_parquet_shards_to_hub(self, repo_id, split, private, token, branch, max_shard_size, num_shards, embed_external_files)
5273 shard.to_parquet(buffer)
5274 uploaded_size += buffer.tell()
-> 5275 _retry(
5276 api.upload_file,
5277 func_kwargs={
5278 "path_or_fileobj": buffer.getvalue(),
5279 "path_in_repo": shard_path_in_repo,
5280 "repo_id": repo_id,
5281 "token": token,
5282 "repo_type": "dataset",
5283 "revision": branch,
5284 },
5285 exceptions=HTTPError,
5286 status_codes=[504],
5287 base_wait_time=2.0,
5288 max_retries=5,
5289 max_wait_time=20.0,
5290 )
5291 shards_path_in_repo.append(shard_path_in_repo)
5293 # Cleanup to remove unused files
File ~/miniconda3/envs/hwocr/lib/python3.8/site-packages/datasets/utils/file_utils.py:285, in _retry(func, func_args, func_kwargs, exceptions, status_codes, max_retries, base_wait_time, max_wait_time)
283 except exceptions as err:
284 if retry >= max_retries or (status_codes and err.response.status_code not in status_codes):
--> 285 raise err
286 else:
287 sleep_time = min(max_wait_time, base_wait_time * 2**retry) # Exponential backoff
File ~/miniconda3/envs/hwocr/lib/python3.8/site-packages/datasets/utils/file_utils.py:282, in _retry(func, func_args, func_kwargs, exceptions, status_codes, max_retries, base_wait_time, max_wait_time)
280 while True:
281 try:
--> 282 return func(*func_args, **func_kwargs)
283 except exceptions as err:
284 if retry >= max_retries or (status_codes and err.response.status_code not in status_codes):
File ~/miniconda3/envs/hwocr/lib/python3.8/site-packages/huggingface_hub/utils/_validators.py:120, in validate_hf_hub_args.<locals>._inner_fn(*args, **kwargs)
117 if check_use_auth_token:
118 kwargs = smoothly_deprecate_use_auth_token(fn_name=fn.__name__, has_token=has_token, kwargs=kwargs)
--> 120 return fn(*args, **kwargs)
File ~/miniconda3/envs/hwocr/lib/python3.8/site-packages/huggingface_hub/hf_api.py:2998, in HfApi.upload_file(self, path_or_fileobj, path_in_repo, repo_id, token, repo_type, revision, commit_message, commit_description, create_pr, parent_commit)
2990 commit_message = (
2991 commit_message if commit_message is not None else f"Upload {path_in_repo} with huggingface_hub"
2992 )
2993 operation = CommitOperationAdd(
2994 path_or_fileobj=path_or_fileobj,
2995 path_in_repo=path_in_repo,
2996 )
-> 2998 commit_info = self.create_commit(
2999 repo_id=repo_id,
3000 repo_type=repo_type,
3001 operations=[operation],
3002 commit_message=commit_message,
3003 commit_description=commit_description,
3004 token=token,
3005 revision=revision,
3006 create_pr=create_pr,
3007 parent_commit=parent_commit,
3008 )
3010 if commit_info.pr_url is not None:
3011 revision = quote(_parse_revision_from_pr_url(commit_info.pr_url), safe="")
File ~/miniconda3/envs/hwocr/lib/python3.8/site-packages/huggingface_hub/utils/_validators.py:120, in validate_hf_hub_args.<locals>._inner_fn(*args, **kwargs)
117 if check_use_auth_token:
118 kwargs = smoothly_deprecate_use_auth_token(fn_name=fn.__name__, has_token=has_token, kwargs=kwargs)
--> 120 return fn(*args, **kwargs)
File ~/miniconda3/envs/hwocr/lib/python3.8/site-packages/huggingface_hub/hf_api.py:2548, in HfApi.create_commit(self, repo_id, operations, commit_message, commit_description, token, repo_type, revision, create_pr, num_threads, parent_commit)
2546 try:
2547 commit_resp = get_session().post(url=commit_url, headers=headers, data=data, params=params)
-> 2548 hf_raise_for_status(commit_resp, endpoint_name="commit")
2549 except RepositoryNotFoundError as e:
2550 e.append_to_message(_CREATE_COMMIT_NO_REPO_ERROR_MESSAGE)
File ~/miniconda3/envs/hwocr/lib/python3.8/site-packages/huggingface_hub/utils/_errors.py:301, in hf_raise_for_status(response, endpoint_name)
297 raise BadRequestError(message, response=response) from e
299 # Convert `HTTPError` into a `HfHubHTTPError` to display request information
300 # as well (request id and/or server error message)
--> 301 raise HfHubHTTPError(str(e), response=response) from e
HfHubHTTPError: 403 Client Error: Forbidden for url: https://huggingface.co/api/datasets/agomberto/FrenchCensus-handwritten-texts/commit/main (Request ID: Root=1-645a66bf-255ad91602a6404e6cb70fba)
Forbidden: pass `create_pr=1` as a query parameter to create a Pull Request
```
And then when I do
```python
dataset.push_to_hub("agomberto/FrenchCensus-handwritten-texts", create_pr=1)
```
I get
```python
---------------------------------------------------------------------------
TypeError Traceback (most recent call last)
Cell In[8], line 1
----> 1 dataset.push_to_hub("agomberto/FrenchCensus-handwritten-texts", create_pr=1)
TypeError: push_to_hub() got an unexpected keyword argument 'create_pr'
```
### Expected behavior
I would like to have the dataset updloaded [here](https://huggingface.co/datasets/agomberto/FrenchCensus-handwritten-texts).
### Environment info
```bash
- `datasets` version: 2.12.0
- Platform: macOS-13.3.1-arm64-arm-64bit
- Python version: 3.8.16
- Huggingface_hub version: 0.14.1
- PyArrow version: 12.0.0
- Pandas version: 1.5.3
``` | 44 | Unable to push dataset - `create_pr` problem
### Describe the bug
I can't upload to the hub the dataset I manually created locally (Image dataset). I have a problem when using the method `.push_to_hub` which asks for a `create_pr` attribute which is not compatible.
### Steps to reproduce the bug
here what I have:
```python
dataset.push_to_hub("agomberto/FrenchCensus-handwritten-texts")
```
Output:
```python
Pushing split train to the Hub.
Pushing dataset shards to the dataset hub: 0%| | 0/2 [00:00<?, ?it/s]
Creating parquet from Arrow format: 0%| | 0/3 [00:00<?, ?ba/s]
Creating parquet from Arrow format: 100%|βββββββββββββββββββββββββββββββββββββββββββββββββββββββββββββββββββββββββββββββββββββββββββββββββββββββββββββββββββββββββββββββββββββ| 3/3 [00:00<00:00, 12.70ba/s]
Pushing dataset shards to the dataset hub: 0%| | 0/2 [00:01<?, ?it/s]
---------------------------------------------------------------------------
HTTPError Traceback (most recent call last)
File ~/miniconda3/envs/hwocr/lib/python3.8/site-packages/huggingface_hub/utils/_errors.py:259, in hf_raise_for_status(response, endpoint_name)
258 try:
--> 259 response.raise_for_status()
260 except HTTPError as e:
File ~/miniconda3/envs/hwocr/lib/python3.8/site-packages/requests/models.py:1021, in Response.raise_for_status(self)
1020 if http_error_msg:
-> 1021 raise HTTPError(http_error_msg, response=self)
HTTPError: 403 Client Error: Forbidden for url: https://huggingface.co/api/datasets/agomberto/FrenchCensus-handwritten-texts/commit/main
The above exception was the direct cause of the following exception:
HfHubHTTPError Traceback (most recent call last)
Cell In[7], line 1
----> 1 dataset.push_to_hub("agomberto/FrenchCensus-handwritten-texts")
File ~/miniconda3/envs/hwocr/lib/python3.8/site-packages/datasets/dataset_dict.py:1583, in DatasetDict.push_to_hub(self, repo_id, private, token, branch, max_shard_size, num_shards, embed_external_files)
1581 logger.warning(f"Pushing split {split} to the Hub.")
1582 # The split=key needs to be removed before merging
-> 1583 repo_id, split, uploaded_size, dataset_nbytes, _, _ = self[split]._push_parquet_shards_to_hub(
1584 repo_id,
1585 split=split,
1586 private=private,
1587 token=token,
1588 branch=branch,
1589 max_shard_size=max_shard_size,
1590 num_shards=num_shards.get(split),
1591 embed_external_files=embed_external_files,
1592 )
1593 total_uploaded_size += uploaded_size
1594 total_dataset_nbytes += dataset_nbytes
File ~/miniconda3/envs/hwocr/lib/python3.8/site-packages/datasets/arrow_dataset.py:5275, in Dataset._push_parquet_shards_to_hub(self, repo_id, split, private, token, branch, max_shard_size, num_shards, embed_external_files)
5273 shard.to_parquet(buffer)
5274 uploaded_size += buffer.tell()
-> 5275 _retry(
5276 api.upload_file,
5277 func_kwargs={
5278 "path_or_fileobj": buffer.getvalue(),
5279 "path_in_repo": shard_path_in_repo,
5280 "repo_id": repo_id,
5281 "token": token,
5282 "repo_type": "dataset",
5283 "revision": branch,
5284 },
5285 exceptions=HTTPError,
5286 status_codes=[504],
5287 base_wait_time=2.0,
5288 max_retries=5,
5289 max_wait_time=20.0,
5290 )
5291 shards_path_in_repo.append(shard_path_in_repo)
5293 # Cleanup to remove unused files
File ~/miniconda3/envs/hwocr/lib/python3.8/site-packages/datasets/utils/file_utils.py:285, in _retry(func, func_args, func_kwargs, exceptions, status_codes, max_retries, base_wait_time, max_wait_time)
283 except exceptions as err:
284 if retry >= max_retries or (status_codes and err.response.status_code not in status_codes):
--> 285 raise err
286 else:
287 sleep_time = min(max_wait_time, base_wait_time * 2**retry) # Exponential backoff
File ~/miniconda3/envs/hwocr/lib/python3.8/site-packages/datasets/utils/file_utils.py:282, in _retry(func, func_args, func_kwargs, exceptions, status_codes, max_retries, base_wait_time, max_wait_time)
280 while True:
281 try:
--> 282 return func(*func_args, **func_kwargs)
283 except exceptions as err:
284 if retry >= max_retries or (status_codes and err.response.status_code not in status_codes):
File ~/miniconda3/envs/hwocr/lib/python3.8/site-packages/huggingface_hub/utils/_validators.py:120, in validate_hf_hub_args.<locals>._inner_fn(*args, **kwargs)
117 if check_use_auth_token:
118 kwargs = smoothly_deprecate_use_auth_token(fn_name=fn.__name__, has_token=has_token, kwargs=kwargs)
--> 120 return fn(*args, **kwargs)
File ~/miniconda3/envs/hwocr/lib/python3.8/site-packages/huggingface_hub/hf_api.py:2998, in HfApi.upload_file(self, path_or_fileobj, path_in_repo, repo_id, token, repo_type, revision, commit_message, commit_description, create_pr, parent_commit)
2990 commit_message = (
2991 commit_message if commit_message is not None else f"Upload {path_in_repo} with huggingface_hub"
2992 )
2993 operation = CommitOperationAdd(
2994 path_or_fileobj=path_or_fileobj,
2995 path_in_repo=path_in_repo,
2996 )
-> 2998 commit_info = self.create_commit(
2999 repo_id=repo_id,
3000 repo_type=repo_type,
3001 operations=[operation],
3002 commit_message=commit_message,
3003 commit_description=commit_description,
3004 token=token,
3005 revision=revision,
3006 create_pr=create_pr,
3007 parent_commit=parent_commit,
3008 )
3010 if commit_info.pr_url is not None:
3011 revision = quote(_parse_revision_from_pr_url(commit_info.pr_url), safe="")
File ~/miniconda3/envs/hwocr/lib/python3.8/site-packages/huggingface_hub/utils/_validators.py:120, in validate_hf_hub_args.<locals>._inner_fn(*args, **kwargs)
117 if check_use_auth_token:
118 kwargs = smoothly_deprecate_use_auth_token(fn_name=fn.__name__, has_token=has_token, kwargs=kwargs)
--> 120 return fn(*args, **kwargs)
File ~/miniconda3/envs/hwocr/lib/python3.8/site-packages/huggingface_hub/hf_api.py:2548, in HfApi.create_commit(self, repo_id, operations, commit_message, commit_description, token, repo_type, revision, create_pr, num_threads, parent_commit)
2546 try:
2547 commit_resp = get_session().post(url=commit_url, headers=headers, data=data, params=params)
-> 2548 hf_raise_for_status(commit_resp, endpoint_name="commit")
2549 except RepositoryNotFoundError as e:
2550 e.append_to_message(_CREATE_COMMIT_NO_REPO_ERROR_MESSAGE)
File ~/miniconda3/envs/hwocr/lib/python3.8/site-packages/huggingface_hub/utils/_errors.py:301, in hf_raise_for_status(response, endpoint_name)
297 raise BadRequestError(message, response=response) from e
299 # Convert `HTTPError` into a `HfHubHTTPError` to display request information
300 # as well (request id and/or server error message)
--> 301 raise HfHubHTTPError(str(e), response=response) from e
HfHubHTTPError: 403 Client Error: Forbidden for url: https://huggingface.co/api/datasets/agomberto/FrenchCensus-handwritten-texts/commit/main (Request ID: Root=1-645a66bf-255ad91602a6404e6cb70fba)
Forbidden: pass `create_pr=1` as a query parameter to create a Pull Request
```
And then when I do
```python
dataset.push_to_hub("agomberto/FrenchCensus-handwritten-texts", create_pr=1)
```
I get
```python
---------------------------------------------------------------------------
TypeError Traceback (most recent call last)
Cell In[8], line 1
----> 1 dataset.push_to_hub("agomberto/FrenchCensus-handwritten-texts", create_pr=1)
TypeError: push_to_hub() got an unexpected keyword argument 'create_pr'
```
### Expected behavior
I would like to have the dataset updloaded [here](https://huggingface.co/datasets/agomberto/FrenchCensus-handwritten-texts).
### Environment info
```bash
- `datasets` version: 2.12.0
- Platform: macOS-13.3.1-arm64-arm-64bit
- Python version: 3.8.16
- Huggingface_hub version: 0.14.1
- PyArrow version: 12.0.0
- Pandas version: 1.5.3
```
> Make sure your API token has a `write` role. I had the same issue as you with the `read` token. Creating a `write` token and using that solved the issue.
I generate a token with write role. It works! thank you so much. |
https://github.com/huggingface/datasets/issues/5833 | Unable to push dataset - `create_pr` problem | @dmitrijsk amazing thanks so much !
The error should be clearer when the token is read-only β I wasted a lot of time there.. | ### Describe the bug
I can't upload to the hub the dataset I manually created locally (Image dataset). I have a problem when using the method `.push_to_hub` which asks for a `create_pr` attribute which is not compatible.
### Steps to reproduce the bug
here what I have:
```python
dataset.push_to_hub("agomberto/FrenchCensus-handwritten-texts")
```
Output:
```python
Pushing split train to the Hub.
Pushing dataset shards to the dataset hub: 0%| | 0/2 [00:00<?, ?it/s]
Creating parquet from Arrow format: 0%| | 0/3 [00:00<?, ?ba/s]
Creating parquet from Arrow format: 100%|βββββββββββββββββββββββββββββββββββββββββββββββββββββββββββββββββββββββββββββββββββββββββββββββββββββββββββββββββββββββββββββββββββββ| 3/3 [00:00<00:00, 12.70ba/s]
Pushing dataset shards to the dataset hub: 0%| | 0/2 [00:01<?, ?it/s]
---------------------------------------------------------------------------
HTTPError Traceback (most recent call last)
File ~/miniconda3/envs/hwocr/lib/python3.8/site-packages/huggingface_hub/utils/_errors.py:259, in hf_raise_for_status(response, endpoint_name)
258 try:
--> 259 response.raise_for_status()
260 except HTTPError as e:
File ~/miniconda3/envs/hwocr/lib/python3.8/site-packages/requests/models.py:1021, in Response.raise_for_status(self)
1020 if http_error_msg:
-> 1021 raise HTTPError(http_error_msg, response=self)
HTTPError: 403 Client Error: Forbidden for url: https://huggingface.co/api/datasets/agomberto/FrenchCensus-handwritten-texts/commit/main
The above exception was the direct cause of the following exception:
HfHubHTTPError Traceback (most recent call last)
Cell In[7], line 1
----> 1 dataset.push_to_hub("agomberto/FrenchCensus-handwritten-texts")
File ~/miniconda3/envs/hwocr/lib/python3.8/site-packages/datasets/dataset_dict.py:1583, in DatasetDict.push_to_hub(self, repo_id, private, token, branch, max_shard_size, num_shards, embed_external_files)
1581 logger.warning(f"Pushing split {split} to the Hub.")
1582 # The split=key needs to be removed before merging
-> 1583 repo_id, split, uploaded_size, dataset_nbytes, _, _ = self[split]._push_parquet_shards_to_hub(
1584 repo_id,
1585 split=split,
1586 private=private,
1587 token=token,
1588 branch=branch,
1589 max_shard_size=max_shard_size,
1590 num_shards=num_shards.get(split),
1591 embed_external_files=embed_external_files,
1592 )
1593 total_uploaded_size += uploaded_size
1594 total_dataset_nbytes += dataset_nbytes
File ~/miniconda3/envs/hwocr/lib/python3.8/site-packages/datasets/arrow_dataset.py:5275, in Dataset._push_parquet_shards_to_hub(self, repo_id, split, private, token, branch, max_shard_size, num_shards, embed_external_files)
5273 shard.to_parquet(buffer)
5274 uploaded_size += buffer.tell()
-> 5275 _retry(
5276 api.upload_file,
5277 func_kwargs={
5278 "path_or_fileobj": buffer.getvalue(),
5279 "path_in_repo": shard_path_in_repo,
5280 "repo_id": repo_id,
5281 "token": token,
5282 "repo_type": "dataset",
5283 "revision": branch,
5284 },
5285 exceptions=HTTPError,
5286 status_codes=[504],
5287 base_wait_time=2.0,
5288 max_retries=5,
5289 max_wait_time=20.0,
5290 )
5291 shards_path_in_repo.append(shard_path_in_repo)
5293 # Cleanup to remove unused files
File ~/miniconda3/envs/hwocr/lib/python3.8/site-packages/datasets/utils/file_utils.py:285, in _retry(func, func_args, func_kwargs, exceptions, status_codes, max_retries, base_wait_time, max_wait_time)
283 except exceptions as err:
284 if retry >= max_retries or (status_codes and err.response.status_code not in status_codes):
--> 285 raise err
286 else:
287 sleep_time = min(max_wait_time, base_wait_time * 2**retry) # Exponential backoff
File ~/miniconda3/envs/hwocr/lib/python3.8/site-packages/datasets/utils/file_utils.py:282, in _retry(func, func_args, func_kwargs, exceptions, status_codes, max_retries, base_wait_time, max_wait_time)
280 while True:
281 try:
--> 282 return func(*func_args, **func_kwargs)
283 except exceptions as err:
284 if retry >= max_retries or (status_codes and err.response.status_code not in status_codes):
File ~/miniconda3/envs/hwocr/lib/python3.8/site-packages/huggingface_hub/utils/_validators.py:120, in validate_hf_hub_args.<locals>._inner_fn(*args, **kwargs)
117 if check_use_auth_token:
118 kwargs = smoothly_deprecate_use_auth_token(fn_name=fn.__name__, has_token=has_token, kwargs=kwargs)
--> 120 return fn(*args, **kwargs)
File ~/miniconda3/envs/hwocr/lib/python3.8/site-packages/huggingface_hub/hf_api.py:2998, in HfApi.upload_file(self, path_or_fileobj, path_in_repo, repo_id, token, repo_type, revision, commit_message, commit_description, create_pr, parent_commit)
2990 commit_message = (
2991 commit_message if commit_message is not None else f"Upload {path_in_repo} with huggingface_hub"
2992 )
2993 operation = CommitOperationAdd(
2994 path_or_fileobj=path_or_fileobj,
2995 path_in_repo=path_in_repo,
2996 )
-> 2998 commit_info = self.create_commit(
2999 repo_id=repo_id,
3000 repo_type=repo_type,
3001 operations=[operation],
3002 commit_message=commit_message,
3003 commit_description=commit_description,
3004 token=token,
3005 revision=revision,
3006 create_pr=create_pr,
3007 parent_commit=parent_commit,
3008 )
3010 if commit_info.pr_url is not None:
3011 revision = quote(_parse_revision_from_pr_url(commit_info.pr_url), safe="")
File ~/miniconda3/envs/hwocr/lib/python3.8/site-packages/huggingface_hub/utils/_validators.py:120, in validate_hf_hub_args.<locals>._inner_fn(*args, **kwargs)
117 if check_use_auth_token:
118 kwargs = smoothly_deprecate_use_auth_token(fn_name=fn.__name__, has_token=has_token, kwargs=kwargs)
--> 120 return fn(*args, **kwargs)
File ~/miniconda3/envs/hwocr/lib/python3.8/site-packages/huggingface_hub/hf_api.py:2548, in HfApi.create_commit(self, repo_id, operations, commit_message, commit_description, token, repo_type, revision, create_pr, num_threads, parent_commit)
2546 try:
2547 commit_resp = get_session().post(url=commit_url, headers=headers, data=data, params=params)
-> 2548 hf_raise_for_status(commit_resp, endpoint_name="commit")
2549 except RepositoryNotFoundError as e:
2550 e.append_to_message(_CREATE_COMMIT_NO_REPO_ERROR_MESSAGE)
File ~/miniconda3/envs/hwocr/lib/python3.8/site-packages/huggingface_hub/utils/_errors.py:301, in hf_raise_for_status(response, endpoint_name)
297 raise BadRequestError(message, response=response) from e
299 # Convert `HTTPError` into a `HfHubHTTPError` to display request information
300 # as well (request id and/or server error message)
--> 301 raise HfHubHTTPError(str(e), response=response) from e
HfHubHTTPError: 403 Client Error: Forbidden for url: https://huggingface.co/api/datasets/agomberto/FrenchCensus-handwritten-texts/commit/main (Request ID: Root=1-645a66bf-255ad91602a6404e6cb70fba)
Forbidden: pass `create_pr=1` as a query parameter to create a Pull Request
```
And then when I do
```python
dataset.push_to_hub("agomberto/FrenchCensus-handwritten-texts", create_pr=1)
```
I get
```python
---------------------------------------------------------------------------
TypeError Traceback (most recent call last)
Cell In[8], line 1
----> 1 dataset.push_to_hub("agomberto/FrenchCensus-handwritten-texts", create_pr=1)
TypeError: push_to_hub() got an unexpected keyword argument 'create_pr'
```
### Expected behavior
I would like to have the dataset updloaded [here](https://huggingface.co/datasets/agomberto/FrenchCensus-handwritten-texts).
### Environment info
```bash
- `datasets` version: 2.12.0
- Platform: macOS-13.3.1-arm64-arm-64bit
- Python version: 3.8.16
- Huggingface_hub version: 0.14.1
- PyArrow version: 12.0.0
- Pandas version: 1.5.3
``` | 24 | Unable to push dataset - `create_pr` problem
### Describe the bug
I can't upload to the hub the dataset I manually created locally (Image dataset). I have a problem when using the method `.push_to_hub` which asks for a `create_pr` attribute which is not compatible.
### Steps to reproduce the bug
here what I have:
```python
dataset.push_to_hub("agomberto/FrenchCensus-handwritten-texts")
```
Output:
```python
Pushing split train to the Hub.
Pushing dataset shards to the dataset hub: 0%| | 0/2 [00:00<?, ?it/s]
Creating parquet from Arrow format: 0%| | 0/3 [00:00<?, ?ba/s]
Creating parquet from Arrow format: 100%|βββββββββββββββββββββββββββββββββββββββββββββββββββββββββββββββββββββββββββββββββββββββββββββββββββββββββββββββββββββββββββββββββββββ| 3/3 [00:00<00:00, 12.70ba/s]
Pushing dataset shards to the dataset hub: 0%| | 0/2 [00:01<?, ?it/s]
---------------------------------------------------------------------------
HTTPError Traceback (most recent call last)
File ~/miniconda3/envs/hwocr/lib/python3.8/site-packages/huggingface_hub/utils/_errors.py:259, in hf_raise_for_status(response, endpoint_name)
258 try:
--> 259 response.raise_for_status()
260 except HTTPError as e:
File ~/miniconda3/envs/hwocr/lib/python3.8/site-packages/requests/models.py:1021, in Response.raise_for_status(self)
1020 if http_error_msg:
-> 1021 raise HTTPError(http_error_msg, response=self)
HTTPError: 403 Client Error: Forbidden for url: https://huggingface.co/api/datasets/agomberto/FrenchCensus-handwritten-texts/commit/main
The above exception was the direct cause of the following exception:
HfHubHTTPError Traceback (most recent call last)
Cell In[7], line 1
----> 1 dataset.push_to_hub("agomberto/FrenchCensus-handwritten-texts")
File ~/miniconda3/envs/hwocr/lib/python3.8/site-packages/datasets/dataset_dict.py:1583, in DatasetDict.push_to_hub(self, repo_id, private, token, branch, max_shard_size, num_shards, embed_external_files)
1581 logger.warning(f"Pushing split {split} to the Hub.")
1582 # The split=key needs to be removed before merging
-> 1583 repo_id, split, uploaded_size, dataset_nbytes, _, _ = self[split]._push_parquet_shards_to_hub(
1584 repo_id,
1585 split=split,
1586 private=private,
1587 token=token,
1588 branch=branch,
1589 max_shard_size=max_shard_size,
1590 num_shards=num_shards.get(split),
1591 embed_external_files=embed_external_files,
1592 )
1593 total_uploaded_size += uploaded_size
1594 total_dataset_nbytes += dataset_nbytes
File ~/miniconda3/envs/hwocr/lib/python3.8/site-packages/datasets/arrow_dataset.py:5275, in Dataset._push_parquet_shards_to_hub(self, repo_id, split, private, token, branch, max_shard_size, num_shards, embed_external_files)
5273 shard.to_parquet(buffer)
5274 uploaded_size += buffer.tell()
-> 5275 _retry(
5276 api.upload_file,
5277 func_kwargs={
5278 "path_or_fileobj": buffer.getvalue(),
5279 "path_in_repo": shard_path_in_repo,
5280 "repo_id": repo_id,
5281 "token": token,
5282 "repo_type": "dataset",
5283 "revision": branch,
5284 },
5285 exceptions=HTTPError,
5286 status_codes=[504],
5287 base_wait_time=2.0,
5288 max_retries=5,
5289 max_wait_time=20.0,
5290 )
5291 shards_path_in_repo.append(shard_path_in_repo)
5293 # Cleanup to remove unused files
File ~/miniconda3/envs/hwocr/lib/python3.8/site-packages/datasets/utils/file_utils.py:285, in _retry(func, func_args, func_kwargs, exceptions, status_codes, max_retries, base_wait_time, max_wait_time)
283 except exceptions as err:
284 if retry >= max_retries or (status_codes and err.response.status_code not in status_codes):
--> 285 raise err
286 else:
287 sleep_time = min(max_wait_time, base_wait_time * 2**retry) # Exponential backoff
File ~/miniconda3/envs/hwocr/lib/python3.8/site-packages/datasets/utils/file_utils.py:282, in _retry(func, func_args, func_kwargs, exceptions, status_codes, max_retries, base_wait_time, max_wait_time)
280 while True:
281 try:
--> 282 return func(*func_args, **func_kwargs)
283 except exceptions as err:
284 if retry >= max_retries or (status_codes and err.response.status_code not in status_codes):
File ~/miniconda3/envs/hwocr/lib/python3.8/site-packages/huggingface_hub/utils/_validators.py:120, in validate_hf_hub_args.<locals>._inner_fn(*args, **kwargs)
117 if check_use_auth_token:
118 kwargs = smoothly_deprecate_use_auth_token(fn_name=fn.__name__, has_token=has_token, kwargs=kwargs)
--> 120 return fn(*args, **kwargs)
File ~/miniconda3/envs/hwocr/lib/python3.8/site-packages/huggingface_hub/hf_api.py:2998, in HfApi.upload_file(self, path_or_fileobj, path_in_repo, repo_id, token, repo_type, revision, commit_message, commit_description, create_pr, parent_commit)
2990 commit_message = (
2991 commit_message if commit_message is not None else f"Upload {path_in_repo} with huggingface_hub"
2992 )
2993 operation = CommitOperationAdd(
2994 path_or_fileobj=path_or_fileobj,
2995 path_in_repo=path_in_repo,
2996 )
-> 2998 commit_info = self.create_commit(
2999 repo_id=repo_id,
3000 repo_type=repo_type,
3001 operations=[operation],
3002 commit_message=commit_message,
3003 commit_description=commit_description,
3004 token=token,
3005 revision=revision,
3006 create_pr=create_pr,
3007 parent_commit=parent_commit,
3008 )
3010 if commit_info.pr_url is not None:
3011 revision = quote(_parse_revision_from_pr_url(commit_info.pr_url), safe="")
File ~/miniconda3/envs/hwocr/lib/python3.8/site-packages/huggingface_hub/utils/_validators.py:120, in validate_hf_hub_args.<locals>._inner_fn(*args, **kwargs)
117 if check_use_auth_token:
118 kwargs = smoothly_deprecate_use_auth_token(fn_name=fn.__name__, has_token=has_token, kwargs=kwargs)
--> 120 return fn(*args, **kwargs)
File ~/miniconda3/envs/hwocr/lib/python3.8/site-packages/huggingface_hub/hf_api.py:2548, in HfApi.create_commit(self, repo_id, operations, commit_message, commit_description, token, repo_type, revision, create_pr, num_threads, parent_commit)
2546 try:
2547 commit_resp = get_session().post(url=commit_url, headers=headers, data=data, params=params)
-> 2548 hf_raise_for_status(commit_resp, endpoint_name="commit")
2549 except RepositoryNotFoundError as e:
2550 e.append_to_message(_CREATE_COMMIT_NO_REPO_ERROR_MESSAGE)
File ~/miniconda3/envs/hwocr/lib/python3.8/site-packages/huggingface_hub/utils/_errors.py:301, in hf_raise_for_status(response, endpoint_name)
297 raise BadRequestError(message, response=response) from e
299 # Convert `HTTPError` into a `HfHubHTTPError` to display request information
300 # as well (request id and/or server error message)
--> 301 raise HfHubHTTPError(str(e), response=response) from e
HfHubHTTPError: 403 Client Error: Forbidden for url: https://huggingface.co/api/datasets/agomberto/FrenchCensus-handwritten-texts/commit/main (Request ID: Root=1-645a66bf-255ad91602a6404e6cb70fba)
Forbidden: pass `create_pr=1` as a query parameter to create a Pull Request
```
And then when I do
```python
dataset.push_to_hub("agomberto/FrenchCensus-handwritten-texts", create_pr=1)
```
I get
```python
---------------------------------------------------------------------------
TypeError Traceback (most recent call last)
Cell In[8], line 1
----> 1 dataset.push_to_hub("agomberto/FrenchCensus-handwritten-texts", create_pr=1)
TypeError: push_to_hub() got an unexpected keyword argument 'create_pr'
```
### Expected behavior
I would like to have the dataset updloaded [here](https://huggingface.co/datasets/agomberto/FrenchCensus-handwritten-texts).
### Environment info
```bash
- `datasets` version: 2.12.0
- Platform: macOS-13.3.1-arm64-arm-64bit
- Python version: 3.8.16
- Huggingface_hub version: 0.14.1
- PyArrow version: 12.0.0
- Pandas version: 1.5.3
```
@dmitrijsk amazing thanks so much !
The error should be clearer when the token is read-only β I wasted a lot of time there.. |
https://github.com/huggingface/datasets/issues/5831 | [Bug]504 Server Error when loading dataset which was already cached | I am experiencing the same problem with the following environment:
* `datasets` version: 2.11.0
* Platform: `Linux 5.19.0-41-generic x86_64 GNU/Linux`
* Python version: `3.8.5`
* Huggingface_hub version: 0.13.3
* PyArrow version: `11.0.0`
* Pandas version: `1.5.3`
Trying to get some diagnostics, I got the following:
```python
>>> from huggingface_hub import scan_cache_dir
>>> sd = scan_cache_dir()
>>> sd
HFCacheInfo(size_on_disk=0, repos=frozenset(), warnings=[CorruptedCacheException('Repo path is not a directory: /home/myname/.cache/huggingface/hub/version_diffusers_cache.txt')])
```
However, that might also be because I had tried to manually specify the `cache_dir` and that resulted in trying to download the dataset again ... but into a folder one level higher up than it should have.
Note that my issue is with the `huggan/wikiart` dataset, so it is not a dataset-specific issue. | ### Describe the bug
I have already cached the dataset using:
```
dataset = load_dataset("databricks/databricks-dolly-15k",
cache_dir="/mnt/data/llm/datasets/databricks-dolly-15k")
```
After that, I tried to load it again using the same machine, I got this error:
```
Traceback (most recent call last):
File "/mnt/home/llm/pythia/train.py", line 16, in <module>
dataset = load_dataset("databricks/databricks-dolly-15k",
File "/mnt/data/conda/envs/pythia_ft/lib/python3.9/site-packages/datasets/load.py", line 1773, in load_dataset
builder_instance = load_dataset_builder(
File "/mnt/data/conda/envs/pythia_ft/lib/python3.9/site-packages/datasets/load.py", line 1502, in load_dataset_builder
dataset_module = dataset_module_factory(
File "/mnt/data/conda/envs/pythia_ft/lib/python3.9/site-packages/datasets/load.py", line 1219, in dataset_module_factory
raise e1 from None
File "/mnt/data/conda/envs/pythia_ft/lib/python3.9/site-packages/datasets/load.py", line 1186, in dataset_module_factory
raise e
File "/mnt/data/conda/envs/pythia_ft/lib/python3.9/site-packages/datasets/load.py", line 1160, in dataset_module_factory
dataset_info = hf_api.dataset_info(
File "/mnt/data/conda/envs/pythia_ft/lib/python3.9/site-packages/huggingface_hub/utils/_validators.py", line 120, in _inner_fn
return fn(*args, **kwargs)
File "/mnt/data/conda/envs/pythia_ft/lib/python3.9/site-packages/huggingface_hub/hf_api.py", line 1667, in dataset_info
hf_raise_for_status(r)
File "/mnt/data/conda/envs/pythia_ft/lib/python3.9/site-packages/huggingface_hub/utils/_errors.py", line 301, in hf_raise_for_status
raise HfHubHTTPError(str(e), response=response) from e
huggingface_hub.utils._errors.HfHubHTTPError: 504 Server Error: Gateway Time-out for url: https://huggingface.co/api/datasets/databricks/databricks-dolly-15k
```
### Steps to reproduce the bug
1. cache the databrick-dolly-15k dataset using load_dataset, setting a cache_dir
2. use load_dataset again, setting the same cache_dir
### Expected behavior
Dataset loaded succuessfully.
### Environment info
- `datasets` version: 2.12.0
- Platform: Linux-4.18.0-372.16.1.el8_6.x86_64-x86_64-with-glibc2.27
- Python version: 3.9.16
- Huggingface_hub version: 0.14.1
- PyArrow version: 11.0.0
- Pandas version: 1.5.3 | 120 | [Bug]504 Server Error when loading dataset which was already cached
### Describe the bug
I have already cached the dataset using:
```
dataset = load_dataset("databricks/databricks-dolly-15k",
cache_dir="/mnt/data/llm/datasets/databricks-dolly-15k")
```
After that, I tried to load it again using the same machine, I got this error:
```
Traceback (most recent call last):
File "/mnt/home/llm/pythia/train.py", line 16, in <module>
dataset = load_dataset("databricks/databricks-dolly-15k",
File "/mnt/data/conda/envs/pythia_ft/lib/python3.9/site-packages/datasets/load.py", line 1773, in load_dataset
builder_instance = load_dataset_builder(
File "/mnt/data/conda/envs/pythia_ft/lib/python3.9/site-packages/datasets/load.py", line 1502, in load_dataset_builder
dataset_module = dataset_module_factory(
File "/mnt/data/conda/envs/pythia_ft/lib/python3.9/site-packages/datasets/load.py", line 1219, in dataset_module_factory
raise e1 from None
File "/mnt/data/conda/envs/pythia_ft/lib/python3.9/site-packages/datasets/load.py", line 1186, in dataset_module_factory
raise e
File "/mnt/data/conda/envs/pythia_ft/lib/python3.9/site-packages/datasets/load.py", line 1160, in dataset_module_factory
dataset_info = hf_api.dataset_info(
File "/mnt/data/conda/envs/pythia_ft/lib/python3.9/site-packages/huggingface_hub/utils/_validators.py", line 120, in _inner_fn
return fn(*args, **kwargs)
File "/mnt/data/conda/envs/pythia_ft/lib/python3.9/site-packages/huggingface_hub/hf_api.py", line 1667, in dataset_info
hf_raise_for_status(r)
File "/mnt/data/conda/envs/pythia_ft/lib/python3.9/site-packages/huggingface_hub/utils/_errors.py", line 301, in hf_raise_for_status
raise HfHubHTTPError(str(e), response=response) from e
huggingface_hub.utils._errors.HfHubHTTPError: 504 Server Error: Gateway Time-out for url: https://huggingface.co/api/datasets/databricks/databricks-dolly-15k
```
### Steps to reproduce the bug
1. cache the databrick-dolly-15k dataset using load_dataset, setting a cache_dir
2. use load_dataset again, setting the same cache_dir
### Expected behavior
Dataset loaded succuessfully.
### Environment info
- `datasets` version: 2.12.0
- Platform: Linux-4.18.0-372.16.1.el8_6.x86_64-x86_64-with-glibc2.27
- Python version: 3.9.16
- Huggingface_hub version: 0.14.1
- PyArrow version: 11.0.0
- Pandas version: 1.5.3
I am experiencing the same problem with the following environment:
* `datasets` version: 2.11.0
* Platform: `Linux 5.19.0-41-generic x86_64 GNU/Linux`
* Python version: `3.8.5`
* Huggingface_hub version: 0.13.3
* PyArrow version: `11.0.0`
* Pandas version: `1.5.3`
Trying to get some diagnostics, I got the following:
```python
>>> from huggingface_hub import scan_cache_dir
>>> sd = scan_cache_dir()
>>> sd
HFCacheInfo(size_on_disk=0, repos=frozenset(), warnings=[CorruptedCacheException('Repo path is not a directory: /home/myname/.cache/huggingface/hub/version_diffusers_cache.txt')])
```
However, that might also be because I had tried to manually specify the `cache_dir` and that resulted in trying to download the dataset again ... but into a folder one level higher up than it should have.
Note that my issue is with the `huggan/wikiart` dataset, so it is not a dataset-specific issue. |
https://github.com/huggingface/datasets/issues/5831 | [Bug]504 Server Error when loading dataset which was already cached | same problem with a private dataset repo, seems the huggingface hub server got some connection problem? | ### Describe the bug
I have already cached the dataset using:
```
dataset = load_dataset("databricks/databricks-dolly-15k",
cache_dir="/mnt/data/llm/datasets/databricks-dolly-15k")
```
After that, I tried to load it again using the same machine, I got this error:
```
Traceback (most recent call last):
File "/mnt/home/llm/pythia/train.py", line 16, in <module>
dataset = load_dataset("databricks/databricks-dolly-15k",
File "/mnt/data/conda/envs/pythia_ft/lib/python3.9/site-packages/datasets/load.py", line 1773, in load_dataset
builder_instance = load_dataset_builder(
File "/mnt/data/conda/envs/pythia_ft/lib/python3.9/site-packages/datasets/load.py", line 1502, in load_dataset_builder
dataset_module = dataset_module_factory(
File "/mnt/data/conda/envs/pythia_ft/lib/python3.9/site-packages/datasets/load.py", line 1219, in dataset_module_factory
raise e1 from None
File "/mnt/data/conda/envs/pythia_ft/lib/python3.9/site-packages/datasets/load.py", line 1186, in dataset_module_factory
raise e
File "/mnt/data/conda/envs/pythia_ft/lib/python3.9/site-packages/datasets/load.py", line 1160, in dataset_module_factory
dataset_info = hf_api.dataset_info(
File "/mnt/data/conda/envs/pythia_ft/lib/python3.9/site-packages/huggingface_hub/utils/_validators.py", line 120, in _inner_fn
return fn(*args, **kwargs)
File "/mnt/data/conda/envs/pythia_ft/lib/python3.9/site-packages/huggingface_hub/hf_api.py", line 1667, in dataset_info
hf_raise_for_status(r)
File "/mnt/data/conda/envs/pythia_ft/lib/python3.9/site-packages/huggingface_hub/utils/_errors.py", line 301, in hf_raise_for_status
raise HfHubHTTPError(str(e), response=response) from e
huggingface_hub.utils._errors.HfHubHTTPError: 504 Server Error: Gateway Time-out for url: https://huggingface.co/api/datasets/databricks/databricks-dolly-15k
```
### Steps to reproduce the bug
1. cache the databrick-dolly-15k dataset using load_dataset, setting a cache_dir
2. use load_dataset again, setting the same cache_dir
### Expected behavior
Dataset loaded succuessfully.
### Environment info
- `datasets` version: 2.12.0
- Platform: Linux-4.18.0-372.16.1.el8_6.x86_64-x86_64-with-glibc2.27
- Python version: 3.9.16
- Huggingface_hub version: 0.14.1
- PyArrow version: 11.0.0
- Pandas version: 1.5.3 | 16 | [Bug]504 Server Error when loading dataset which was already cached
### Describe the bug
I have already cached the dataset using:
```
dataset = load_dataset("databricks/databricks-dolly-15k",
cache_dir="/mnt/data/llm/datasets/databricks-dolly-15k")
```
After that, I tried to load it again using the same machine, I got this error:
```
Traceback (most recent call last):
File "/mnt/home/llm/pythia/train.py", line 16, in <module>
dataset = load_dataset("databricks/databricks-dolly-15k",
File "/mnt/data/conda/envs/pythia_ft/lib/python3.9/site-packages/datasets/load.py", line 1773, in load_dataset
builder_instance = load_dataset_builder(
File "/mnt/data/conda/envs/pythia_ft/lib/python3.9/site-packages/datasets/load.py", line 1502, in load_dataset_builder
dataset_module = dataset_module_factory(
File "/mnt/data/conda/envs/pythia_ft/lib/python3.9/site-packages/datasets/load.py", line 1219, in dataset_module_factory
raise e1 from None
File "/mnt/data/conda/envs/pythia_ft/lib/python3.9/site-packages/datasets/load.py", line 1186, in dataset_module_factory
raise e
File "/mnt/data/conda/envs/pythia_ft/lib/python3.9/site-packages/datasets/load.py", line 1160, in dataset_module_factory
dataset_info = hf_api.dataset_info(
File "/mnt/data/conda/envs/pythia_ft/lib/python3.9/site-packages/huggingface_hub/utils/_validators.py", line 120, in _inner_fn
return fn(*args, **kwargs)
File "/mnt/data/conda/envs/pythia_ft/lib/python3.9/site-packages/huggingface_hub/hf_api.py", line 1667, in dataset_info
hf_raise_for_status(r)
File "/mnt/data/conda/envs/pythia_ft/lib/python3.9/site-packages/huggingface_hub/utils/_errors.py", line 301, in hf_raise_for_status
raise HfHubHTTPError(str(e), response=response) from e
huggingface_hub.utils._errors.HfHubHTTPError: 504 Server Error: Gateway Time-out for url: https://huggingface.co/api/datasets/databricks/databricks-dolly-15k
```
### Steps to reproduce the bug
1. cache the databrick-dolly-15k dataset using load_dataset, setting a cache_dir
2. use load_dataset again, setting the same cache_dir
### Expected behavior
Dataset loaded succuessfully.
### Environment info
- `datasets` version: 2.12.0
- Platform: Linux-4.18.0-372.16.1.el8_6.x86_64-x86_64-with-glibc2.27
- Python version: 3.9.16
- Huggingface_hub version: 0.14.1
- PyArrow version: 11.0.0
- Pandas version: 1.5.3
same problem with a private dataset repo, seems the huggingface hub server got some connection problem? |
https://github.com/huggingface/datasets/issues/5831 | [Bug]504 Server Error when loading dataset which was already cached | @SingL3 You can avoid this error by setting the [`HF_DATASETS_OFFLINE`](https://huggingface.co/docs/datasets/v2.12.0/en/loading#offline) env variable to 1. By default, if an internet connection is available, we check whether the cache of a cached dataset is up-to-date.
@lucidBrot `datasets`' cache is still not aligned with `huggigface_hub`'s. We plan to align it eventually. | ### Describe the bug
I have already cached the dataset using:
```
dataset = load_dataset("databricks/databricks-dolly-15k",
cache_dir="/mnt/data/llm/datasets/databricks-dolly-15k")
```
After that, I tried to load it again using the same machine, I got this error:
```
Traceback (most recent call last):
File "/mnt/home/llm/pythia/train.py", line 16, in <module>
dataset = load_dataset("databricks/databricks-dolly-15k",
File "/mnt/data/conda/envs/pythia_ft/lib/python3.9/site-packages/datasets/load.py", line 1773, in load_dataset
builder_instance = load_dataset_builder(
File "/mnt/data/conda/envs/pythia_ft/lib/python3.9/site-packages/datasets/load.py", line 1502, in load_dataset_builder
dataset_module = dataset_module_factory(
File "/mnt/data/conda/envs/pythia_ft/lib/python3.9/site-packages/datasets/load.py", line 1219, in dataset_module_factory
raise e1 from None
File "/mnt/data/conda/envs/pythia_ft/lib/python3.9/site-packages/datasets/load.py", line 1186, in dataset_module_factory
raise e
File "/mnt/data/conda/envs/pythia_ft/lib/python3.9/site-packages/datasets/load.py", line 1160, in dataset_module_factory
dataset_info = hf_api.dataset_info(
File "/mnt/data/conda/envs/pythia_ft/lib/python3.9/site-packages/huggingface_hub/utils/_validators.py", line 120, in _inner_fn
return fn(*args, **kwargs)
File "/mnt/data/conda/envs/pythia_ft/lib/python3.9/site-packages/huggingface_hub/hf_api.py", line 1667, in dataset_info
hf_raise_for_status(r)
File "/mnt/data/conda/envs/pythia_ft/lib/python3.9/site-packages/huggingface_hub/utils/_errors.py", line 301, in hf_raise_for_status
raise HfHubHTTPError(str(e), response=response) from e
huggingface_hub.utils._errors.HfHubHTTPError: 504 Server Error: Gateway Time-out for url: https://huggingface.co/api/datasets/databricks/databricks-dolly-15k
```
### Steps to reproduce the bug
1. cache the databrick-dolly-15k dataset using load_dataset, setting a cache_dir
2. use load_dataset again, setting the same cache_dir
### Expected behavior
Dataset loaded succuessfully.
### Environment info
- `datasets` version: 2.12.0
- Platform: Linux-4.18.0-372.16.1.el8_6.x86_64-x86_64-with-glibc2.27
- Python version: 3.9.16
- Huggingface_hub version: 0.14.1
- PyArrow version: 11.0.0
- Pandas version: 1.5.3 | 48 | [Bug]504 Server Error when loading dataset which was already cached
### Describe the bug
I have already cached the dataset using:
```
dataset = load_dataset("databricks/databricks-dolly-15k",
cache_dir="/mnt/data/llm/datasets/databricks-dolly-15k")
```
After that, I tried to load it again using the same machine, I got this error:
```
Traceback (most recent call last):
File "/mnt/home/llm/pythia/train.py", line 16, in <module>
dataset = load_dataset("databricks/databricks-dolly-15k",
File "/mnt/data/conda/envs/pythia_ft/lib/python3.9/site-packages/datasets/load.py", line 1773, in load_dataset
builder_instance = load_dataset_builder(
File "/mnt/data/conda/envs/pythia_ft/lib/python3.9/site-packages/datasets/load.py", line 1502, in load_dataset_builder
dataset_module = dataset_module_factory(
File "/mnt/data/conda/envs/pythia_ft/lib/python3.9/site-packages/datasets/load.py", line 1219, in dataset_module_factory
raise e1 from None
File "/mnt/data/conda/envs/pythia_ft/lib/python3.9/site-packages/datasets/load.py", line 1186, in dataset_module_factory
raise e
File "/mnt/data/conda/envs/pythia_ft/lib/python3.9/site-packages/datasets/load.py", line 1160, in dataset_module_factory
dataset_info = hf_api.dataset_info(
File "/mnt/data/conda/envs/pythia_ft/lib/python3.9/site-packages/huggingface_hub/utils/_validators.py", line 120, in _inner_fn
return fn(*args, **kwargs)
File "/mnt/data/conda/envs/pythia_ft/lib/python3.9/site-packages/huggingface_hub/hf_api.py", line 1667, in dataset_info
hf_raise_for_status(r)
File "/mnt/data/conda/envs/pythia_ft/lib/python3.9/site-packages/huggingface_hub/utils/_errors.py", line 301, in hf_raise_for_status
raise HfHubHTTPError(str(e), response=response) from e
huggingface_hub.utils._errors.HfHubHTTPError: 504 Server Error: Gateway Time-out for url: https://huggingface.co/api/datasets/databricks/databricks-dolly-15k
```
### Steps to reproduce the bug
1. cache the databrick-dolly-15k dataset using load_dataset, setting a cache_dir
2. use load_dataset again, setting the same cache_dir
### Expected behavior
Dataset loaded succuessfully.
### Environment info
- `datasets` version: 2.12.0
- Platform: Linux-4.18.0-372.16.1.el8_6.x86_64-x86_64-with-glibc2.27
- Python version: 3.9.16
- Huggingface_hub version: 0.14.1
- PyArrow version: 11.0.0
- Pandas version: 1.5.3
@SingL3 You can avoid this error by setting the [`HF_DATASETS_OFFLINE`](https://huggingface.co/docs/datasets/v2.12.0/en/loading#offline) env variable to 1. By default, if an internet connection is available, we check whether the cache of a cached dataset is up-to-date.
@lucidBrot `datasets`' cache is still not aligned with `huggigface_hub`'s. We plan to align it eventually. |
https://github.com/huggingface/datasets/issues/5831 | [Bug]504 Server Error when loading dataset which was already cached | Today we had a big issue affecting the Hugging Face Hub, thus all the `504 Server Error: Gateway Time-out` errors.
It is fixed now and loading your datasets should work as expected. | ### Describe the bug
I have already cached the dataset using:
```
dataset = load_dataset("databricks/databricks-dolly-15k",
cache_dir="/mnt/data/llm/datasets/databricks-dolly-15k")
```
After that, I tried to load it again using the same machine, I got this error:
```
Traceback (most recent call last):
File "/mnt/home/llm/pythia/train.py", line 16, in <module>
dataset = load_dataset("databricks/databricks-dolly-15k",
File "/mnt/data/conda/envs/pythia_ft/lib/python3.9/site-packages/datasets/load.py", line 1773, in load_dataset
builder_instance = load_dataset_builder(
File "/mnt/data/conda/envs/pythia_ft/lib/python3.9/site-packages/datasets/load.py", line 1502, in load_dataset_builder
dataset_module = dataset_module_factory(
File "/mnt/data/conda/envs/pythia_ft/lib/python3.9/site-packages/datasets/load.py", line 1219, in dataset_module_factory
raise e1 from None
File "/mnt/data/conda/envs/pythia_ft/lib/python3.9/site-packages/datasets/load.py", line 1186, in dataset_module_factory
raise e
File "/mnt/data/conda/envs/pythia_ft/lib/python3.9/site-packages/datasets/load.py", line 1160, in dataset_module_factory
dataset_info = hf_api.dataset_info(
File "/mnt/data/conda/envs/pythia_ft/lib/python3.9/site-packages/huggingface_hub/utils/_validators.py", line 120, in _inner_fn
return fn(*args, **kwargs)
File "/mnt/data/conda/envs/pythia_ft/lib/python3.9/site-packages/huggingface_hub/hf_api.py", line 1667, in dataset_info
hf_raise_for_status(r)
File "/mnt/data/conda/envs/pythia_ft/lib/python3.9/site-packages/huggingface_hub/utils/_errors.py", line 301, in hf_raise_for_status
raise HfHubHTTPError(str(e), response=response) from e
huggingface_hub.utils._errors.HfHubHTTPError: 504 Server Error: Gateway Time-out for url: https://huggingface.co/api/datasets/databricks/databricks-dolly-15k
```
### Steps to reproduce the bug
1. cache the databrick-dolly-15k dataset using load_dataset, setting a cache_dir
2. use load_dataset again, setting the same cache_dir
### Expected behavior
Dataset loaded succuessfully.
### Environment info
- `datasets` version: 2.12.0
- Platform: Linux-4.18.0-372.16.1.el8_6.x86_64-x86_64-with-glibc2.27
- Python version: 3.9.16
- Huggingface_hub version: 0.14.1
- PyArrow version: 11.0.0
- Pandas version: 1.5.3 | 32 | [Bug]504 Server Error when loading dataset which was already cached
### Describe the bug
I have already cached the dataset using:
```
dataset = load_dataset("databricks/databricks-dolly-15k",
cache_dir="/mnt/data/llm/datasets/databricks-dolly-15k")
```
After that, I tried to load it again using the same machine, I got this error:
```
Traceback (most recent call last):
File "/mnt/home/llm/pythia/train.py", line 16, in <module>
dataset = load_dataset("databricks/databricks-dolly-15k",
File "/mnt/data/conda/envs/pythia_ft/lib/python3.9/site-packages/datasets/load.py", line 1773, in load_dataset
builder_instance = load_dataset_builder(
File "/mnt/data/conda/envs/pythia_ft/lib/python3.9/site-packages/datasets/load.py", line 1502, in load_dataset_builder
dataset_module = dataset_module_factory(
File "/mnt/data/conda/envs/pythia_ft/lib/python3.9/site-packages/datasets/load.py", line 1219, in dataset_module_factory
raise e1 from None
File "/mnt/data/conda/envs/pythia_ft/lib/python3.9/site-packages/datasets/load.py", line 1186, in dataset_module_factory
raise e
File "/mnt/data/conda/envs/pythia_ft/lib/python3.9/site-packages/datasets/load.py", line 1160, in dataset_module_factory
dataset_info = hf_api.dataset_info(
File "/mnt/data/conda/envs/pythia_ft/lib/python3.9/site-packages/huggingface_hub/utils/_validators.py", line 120, in _inner_fn
return fn(*args, **kwargs)
File "/mnt/data/conda/envs/pythia_ft/lib/python3.9/site-packages/huggingface_hub/hf_api.py", line 1667, in dataset_info
hf_raise_for_status(r)
File "/mnt/data/conda/envs/pythia_ft/lib/python3.9/site-packages/huggingface_hub/utils/_errors.py", line 301, in hf_raise_for_status
raise HfHubHTTPError(str(e), response=response) from e
huggingface_hub.utils._errors.HfHubHTTPError: 504 Server Error: Gateway Time-out for url: https://huggingface.co/api/datasets/databricks/databricks-dolly-15k
```
### Steps to reproduce the bug
1. cache the databrick-dolly-15k dataset using load_dataset, setting a cache_dir
2. use load_dataset again, setting the same cache_dir
### Expected behavior
Dataset loaded succuessfully.
### Environment info
- `datasets` version: 2.12.0
- Platform: Linux-4.18.0-372.16.1.el8_6.x86_64-x86_64-with-glibc2.27
- Python version: 3.9.16
- Huggingface_hub version: 0.14.1
- PyArrow version: 11.0.0
- Pandas version: 1.5.3
Today we had a big issue affecting the Hugging Face Hub, thus all the `504 Server Error: Gateway Time-out` errors.
It is fixed now and loading your datasets should work as expected. |
https://github.com/huggingface/datasets/issues/5831 | [Bug]504 Server Error when loading dataset which was already cached | Hi, @albertvillanova.
If there is a locally cached version of datasets or something cache using huggingface_hub, when a network problem(either client or server) occurs, is it a better way to fallback to use the current cached version rather than raise a exception and exit? | ### Describe the bug
I have already cached the dataset using:
```
dataset = load_dataset("databricks/databricks-dolly-15k",
cache_dir="/mnt/data/llm/datasets/databricks-dolly-15k")
```
After that, I tried to load it again using the same machine, I got this error:
```
Traceback (most recent call last):
File "/mnt/home/llm/pythia/train.py", line 16, in <module>
dataset = load_dataset("databricks/databricks-dolly-15k",
File "/mnt/data/conda/envs/pythia_ft/lib/python3.9/site-packages/datasets/load.py", line 1773, in load_dataset
builder_instance = load_dataset_builder(
File "/mnt/data/conda/envs/pythia_ft/lib/python3.9/site-packages/datasets/load.py", line 1502, in load_dataset_builder
dataset_module = dataset_module_factory(
File "/mnt/data/conda/envs/pythia_ft/lib/python3.9/site-packages/datasets/load.py", line 1219, in dataset_module_factory
raise e1 from None
File "/mnt/data/conda/envs/pythia_ft/lib/python3.9/site-packages/datasets/load.py", line 1186, in dataset_module_factory
raise e
File "/mnt/data/conda/envs/pythia_ft/lib/python3.9/site-packages/datasets/load.py", line 1160, in dataset_module_factory
dataset_info = hf_api.dataset_info(
File "/mnt/data/conda/envs/pythia_ft/lib/python3.9/site-packages/huggingface_hub/utils/_validators.py", line 120, in _inner_fn
return fn(*args, **kwargs)
File "/mnt/data/conda/envs/pythia_ft/lib/python3.9/site-packages/huggingface_hub/hf_api.py", line 1667, in dataset_info
hf_raise_for_status(r)
File "/mnt/data/conda/envs/pythia_ft/lib/python3.9/site-packages/huggingface_hub/utils/_errors.py", line 301, in hf_raise_for_status
raise HfHubHTTPError(str(e), response=response) from e
huggingface_hub.utils._errors.HfHubHTTPError: 504 Server Error: Gateway Time-out for url: https://huggingface.co/api/datasets/databricks/databricks-dolly-15k
```
### Steps to reproduce the bug
1. cache the databrick-dolly-15k dataset using load_dataset, setting a cache_dir
2. use load_dataset again, setting the same cache_dir
### Expected behavior
Dataset loaded succuessfully.
### Environment info
- `datasets` version: 2.12.0
- Platform: Linux-4.18.0-372.16.1.el8_6.x86_64-x86_64-with-glibc2.27
- Python version: 3.9.16
- Huggingface_hub version: 0.14.1
- PyArrow version: 11.0.0
- Pandas version: 1.5.3 | 44 | [Bug]504 Server Error when loading dataset which was already cached
### Describe the bug
I have already cached the dataset using:
```
dataset = load_dataset("databricks/databricks-dolly-15k",
cache_dir="/mnt/data/llm/datasets/databricks-dolly-15k")
```
After that, I tried to load it again using the same machine, I got this error:
```
Traceback (most recent call last):
File "/mnt/home/llm/pythia/train.py", line 16, in <module>
dataset = load_dataset("databricks/databricks-dolly-15k",
File "/mnt/data/conda/envs/pythia_ft/lib/python3.9/site-packages/datasets/load.py", line 1773, in load_dataset
builder_instance = load_dataset_builder(
File "/mnt/data/conda/envs/pythia_ft/lib/python3.9/site-packages/datasets/load.py", line 1502, in load_dataset_builder
dataset_module = dataset_module_factory(
File "/mnt/data/conda/envs/pythia_ft/lib/python3.9/site-packages/datasets/load.py", line 1219, in dataset_module_factory
raise e1 from None
File "/mnt/data/conda/envs/pythia_ft/lib/python3.9/site-packages/datasets/load.py", line 1186, in dataset_module_factory
raise e
File "/mnt/data/conda/envs/pythia_ft/lib/python3.9/site-packages/datasets/load.py", line 1160, in dataset_module_factory
dataset_info = hf_api.dataset_info(
File "/mnt/data/conda/envs/pythia_ft/lib/python3.9/site-packages/huggingface_hub/utils/_validators.py", line 120, in _inner_fn
return fn(*args, **kwargs)
File "/mnt/data/conda/envs/pythia_ft/lib/python3.9/site-packages/huggingface_hub/hf_api.py", line 1667, in dataset_info
hf_raise_for_status(r)
File "/mnt/data/conda/envs/pythia_ft/lib/python3.9/site-packages/huggingface_hub/utils/_errors.py", line 301, in hf_raise_for_status
raise HfHubHTTPError(str(e), response=response) from e
huggingface_hub.utils._errors.HfHubHTTPError: 504 Server Error: Gateway Time-out for url: https://huggingface.co/api/datasets/databricks/databricks-dolly-15k
```
### Steps to reproduce the bug
1. cache the databrick-dolly-15k dataset using load_dataset, setting a cache_dir
2. use load_dataset again, setting the same cache_dir
### Expected behavior
Dataset loaded succuessfully.
### Environment info
- `datasets` version: 2.12.0
- Platform: Linux-4.18.0-372.16.1.el8_6.x86_64-x86_64-with-glibc2.27
- Python version: 3.9.16
- Huggingface_hub version: 0.14.1
- PyArrow version: 11.0.0
- Pandas version: 1.5.3
Hi, @albertvillanova.
If there is a locally cached version of datasets or something cache using huggingface_hub, when a network problem(either client or server) occurs, is it a better way to fallback to use the current cached version rather than raise a exception and exit? |
https://github.com/huggingface/datasets/issues/5829 | (mach-o file, but is an incompatible architecture (have 'arm64', need 'x86_64')) | That is weird. I can't reproduce it again after reboot.
```python
In [2]: import platform
In [3]: platform.platform()
Out[3]: 'macOS-13.2-arm64-arm-64bit'
In [4]: from datasets import load_dataset
...:
...: jazzy = load_dataset("nomic-ai/gpt4all-j-prompt-generations", revision='v1.2-jazzy')
Found cached dataset parquet (/Users/sarit/.cache/huggingface/datasets/nomic-ai___parquet/nomic-ai--gpt4all-j-prompt-generations-a3b62015e2e52043/0.0.0/2a3b91fbd88a2c90d1dbbb32b460cf621d31bd5b05b934492fdef7d8d6f236ec)
100%|βββββββββββββββββββββββββββββββββββββββββββββββββββββββββββββββββββββββββββββββββββββββββββββββββββββββββββββββββββββββββββββββββββββββ| 1/1 [00:00<00:00, 63.25it/s]
``` | ### Describe the bug
M2 MBP can't run
```python
from datasets import load_dataset
jazzy = load_dataset("nomic-ai/gpt4all-j-prompt-generations", revision='v1.2-jazzy')
```
### Steps to reproduce the bug
1. Use M2 MBP
2. Python 3.10.10 from pyenv
3. Run
```
from datasets import load_dataset
jazzy = load_dataset("nomic-ai/gpt4all-j-prompt-generations", revision='v1.2-jazzy')
```
### Expected behavior
Be able to run normally
### Environment info
```
from datasets import load_dataset
jazzy = load_dataset("nomic-ai/gpt4all-j-prompt-generations", revision='v1.2-jazzy')
```
OSX: 13.2
CPU: M2
| 42 | (mach-o file, but is an incompatible architecture (have 'arm64', need 'x86_64'))
### Describe the bug
M2 MBP can't run
```python
from datasets import load_dataset
jazzy = load_dataset("nomic-ai/gpt4all-j-prompt-generations", revision='v1.2-jazzy')
```
### Steps to reproduce the bug
1. Use M2 MBP
2. Python 3.10.10 from pyenv
3. Run
```
from datasets import load_dataset
jazzy = load_dataset("nomic-ai/gpt4all-j-prompt-generations", revision='v1.2-jazzy')
```
### Expected behavior
Be able to run normally
### Environment info
```
from datasets import load_dataset
jazzy = load_dataset("nomic-ai/gpt4all-j-prompt-generations", revision='v1.2-jazzy')
```
OSX: 13.2
CPU: M2
That is weird. I can't reproduce it again after reboot.
```python
In [2]: import platform
In [3]: platform.platform()
Out[3]: 'macOS-13.2-arm64-arm-64bit'
In [4]: from datasets import load_dataset
...:
...: jazzy = load_dataset("nomic-ai/gpt4all-j-prompt-generations", revision='v1.2-jazzy')
Found cached dataset parquet (/Users/sarit/.cache/huggingface/datasets/nomic-ai___parquet/nomic-ai--gpt4all-j-prompt-generations-a3b62015e2e52043/0.0.0/2a3b91fbd88a2c90d1dbbb32b460cf621d31bd5b05b934492fdef7d8d6f236ec)
100%|βββββββββββββββββββββββββββββββββββββββββββββββββββββββββββββββββββββββββββββββββββββββββββββββββββββββββββββββββββββββββββββββββββββββ| 1/1 [00:00<00:00, 63.25it/s]
``` |
https://github.com/huggingface/datasets/issues/5828 | Stream data concatenation issue | Hi!
You can call `map` as follows to avoid the error:
```python
augmented_dataset_cln = dataset_cln['train'].map(augment_dataset, features=dataset_cln['train'].features)
``` | ### Describe the bug
I am not able to concatenate the augmentation of the stream data. I am using the latest version of dataset.
ValueError: The features can't be aligned because the key audio of features {'audio_id': Value(dtype='string',
id=None), 'audio': {'array': Sequence(feature=Value(dtype='float32', id=None), length=-1, id=None), 'path':
Value(dtype='null', id=None), 'sampling_rate': Value(dtype='int64', id=None)}, 'transcript': Value(dtype='string',
id=None)} has unexpected type - {'array': Sequence(feature=Value(dtype='float32', id=None), length=-1, id=None),
'path': Value(dtype='null', id=None), 'sampling_rate': Value(dtype='int64', id=None)} (expected either
Audio(sampling_rate=16000, mono=True, decode=True, id=None) or Value("null").
### Steps to reproduce the bug
dataset = load_dataset("tobiolatunji/afrispeech-200", "all", streaming=True).shuffle(seed=42)
dataset_cln = dataset.remove_columns(['speaker_id', 'path', 'age_group', 'gender', 'accent', 'domain', 'country', 'duration'])
dataset_cln = dataset_cln.cast_column("audio", Audio(sampling_rate=16000))
from audiomentations import AddGaussianNoise,Compose,Gain,OneOf,PitchShift,PolarityInversion,TimeStretch
augmentation = Compose([
AddGaussianNoise(min_amplitude=0.005, max_amplitude=0.015, p=0.2)
])
def augment_dataset(batch):
audio = batch["audio"]
audio["array"] = augmentation(audio["array"], sample_rate=audio["sampling_rate"])
return batch
augmented_dataset_cln = dataset_cln['train'].map(augment_dataset)
dataset_cln['train'] = interleave_datasets([dataset_cln['train'], augmented_dataset_cln])
dataset_cln['train'] = dataset_cln['train'].shuffle(seed=42)
### Expected behavior
I should be able to merge as sampling rate is same.
### Environment info
import datasets
import transformers
import accelerate
print(datasets.__version__)
print(transformers.__version__)
print(torch.__version__)
print(evaluate.__version__)
print(accelerate.__version__)
2.12.0
4.28.1
2.0.0
0.4.0
0.18.0 | 17 | Stream data concatenation issue
### Describe the bug
I am not able to concatenate the augmentation of the stream data. I am using the latest version of dataset.
ValueError: The features can't be aligned because the key audio of features {'audio_id': Value(dtype='string',
id=None), 'audio': {'array': Sequence(feature=Value(dtype='float32', id=None), length=-1, id=None), 'path':
Value(dtype='null', id=None), 'sampling_rate': Value(dtype='int64', id=None)}, 'transcript': Value(dtype='string',
id=None)} has unexpected type - {'array': Sequence(feature=Value(dtype='float32', id=None), length=-1, id=None),
'path': Value(dtype='null', id=None), 'sampling_rate': Value(dtype='int64', id=None)} (expected either
Audio(sampling_rate=16000, mono=True, decode=True, id=None) or Value("null").
### Steps to reproduce the bug
dataset = load_dataset("tobiolatunji/afrispeech-200", "all", streaming=True).shuffle(seed=42)
dataset_cln = dataset.remove_columns(['speaker_id', 'path', 'age_group', 'gender', 'accent', 'domain', 'country', 'duration'])
dataset_cln = dataset_cln.cast_column("audio", Audio(sampling_rate=16000))
from audiomentations import AddGaussianNoise,Compose,Gain,OneOf,PitchShift,PolarityInversion,TimeStretch
augmentation = Compose([
AddGaussianNoise(min_amplitude=0.005, max_amplitude=0.015, p=0.2)
])
def augment_dataset(batch):
audio = batch["audio"]
audio["array"] = augmentation(audio["array"], sample_rate=audio["sampling_rate"])
return batch
augmented_dataset_cln = dataset_cln['train'].map(augment_dataset)
dataset_cln['train'] = interleave_datasets([dataset_cln['train'], augmented_dataset_cln])
dataset_cln['train'] = dataset_cln['train'].shuffle(seed=42)
### Expected behavior
I should be able to merge as sampling rate is same.
### Environment info
import datasets
import transformers
import accelerate
print(datasets.__version__)
print(transformers.__version__)
print(torch.__version__)
print(evaluate.__version__)
print(accelerate.__version__)
2.12.0
4.28.1
2.0.0
0.4.0
0.18.0
Hi!
You can call `map` as follows to avoid the error:
```python
augmented_dataset_cln = dataset_cln['train'].map(augment_dataset, features=dataset_cln['train'].features)
``` |
https://github.com/huggingface/datasets/issues/5828 | Stream data concatenation issue | Hi!
I have run into the same problem with you. Could you please let me know how you solve it? Thanks! | ### Describe the bug
I am not able to concatenate the augmentation of the stream data. I am using the latest version of dataset.
ValueError: The features can't be aligned because the key audio of features {'audio_id': Value(dtype='string',
id=None), 'audio': {'array': Sequence(feature=Value(dtype='float32', id=None), length=-1, id=None), 'path':
Value(dtype='null', id=None), 'sampling_rate': Value(dtype='int64', id=None)}, 'transcript': Value(dtype='string',
id=None)} has unexpected type - {'array': Sequence(feature=Value(dtype='float32', id=None), length=-1, id=None),
'path': Value(dtype='null', id=None), 'sampling_rate': Value(dtype='int64', id=None)} (expected either
Audio(sampling_rate=16000, mono=True, decode=True, id=None) or Value("null").
### Steps to reproduce the bug
dataset = load_dataset("tobiolatunji/afrispeech-200", "all", streaming=True).shuffle(seed=42)
dataset_cln = dataset.remove_columns(['speaker_id', 'path', 'age_group', 'gender', 'accent', 'domain', 'country', 'duration'])
dataset_cln = dataset_cln.cast_column("audio", Audio(sampling_rate=16000))
from audiomentations import AddGaussianNoise,Compose,Gain,OneOf,PitchShift,PolarityInversion,TimeStretch
augmentation = Compose([
AddGaussianNoise(min_amplitude=0.005, max_amplitude=0.015, p=0.2)
])
def augment_dataset(batch):
audio = batch["audio"]
audio["array"] = augmentation(audio["array"], sample_rate=audio["sampling_rate"])
return batch
augmented_dataset_cln = dataset_cln['train'].map(augment_dataset)
dataset_cln['train'] = interleave_datasets([dataset_cln['train'], augmented_dataset_cln])
dataset_cln['train'] = dataset_cln['train'].shuffle(seed=42)
### Expected behavior
I should be able to merge as sampling rate is same.
### Environment info
import datasets
import transformers
import accelerate
print(datasets.__version__)
print(transformers.__version__)
print(torch.__version__)
print(evaluate.__version__)
print(accelerate.__version__)
2.12.0
4.28.1
2.0.0
0.4.0
0.18.0 | 21 | Stream data concatenation issue
### Describe the bug
I am not able to concatenate the augmentation of the stream data. I am using the latest version of dataset.
ValueError: The features can't be aligned because the key audio of features {'audio_id': Value(dtype='string',
id=None), 'audio': {'array': Sequence(feature=Value(dtype='float32', id=None), length=-1, id=None), 'path':
Value(dtype='null', id=None), 'sampling_rate': Value(dtype='int64', id=None)}, 'transcript': Value(dtype='string',
id=None)} has unexpected type - {'array': Sequence(feature=Value(dtype='float32', id=None), length=-1, id=None),
'path': Value(dtype='null', id=None), 'sampling_rate': Value(dtype='int64', id=None)} (expected either
Audio(sampling_rate=16000, mono=True, decode=True, id=None) or Value("null").
### Steps to reproduce the bug
dataset = load_dataset("tobiolatunji/afrispeech-200", "all", streaming=True).shuffle(seed=42)
dataset_cln = dataset.remove_columns(['speaker_id', 'path', 'age_group', 'gender', 'accent', 'domain', 'country', 'duration'])
dataset_cln = dataset_cln.cast_column("audio", Audio(sampling_rate=16000))
from audiomentations import AddGaussianNoise,Compose,Gain,OneOf,PitchShift,PolarityInversion,TimeStretch
augmentation = Compose([
AddGaussianNoise(min_amplitude=0.005, max_amplitude=0.015, p=0.2)
])
def augment_dataset(batch):
audio = batch["audio"]
audio["array"] = augmentation(audio["array"], sample_rate=audio["sampling_rate"])
return batch
augmented_dataset_cln = dataset_cln['train'].map(augment_dataset)
dataset_cln['train'] = interleave_datasets([dataset_cln['train'], augmented_dataset_cln])
dataset_cln['train'] = dataset_cln['train'].shuffle(seed=42)
### Expected behavior
I should be able to merge as sampling rate is same.
### Environment info
import datasets
import transformers
import accelerate
print(datasets.__version__)
print(transformers.__version__)
print(torch.__version__)
print(evaluate.__version__)
print(accelerate.__version__)
2.12.0
4.28.1
2.0.0
0.4.0
0.18.0
Hi!
I have run into the same problem with you. Could you please let me know how you solve it? Thanks! |
https://github.com/huggingface/datasets/issues/5827 | load json dataset interrupt when dtype cast problem occured | Indeed the JSON dataset builder raises an error when it encounters an unexpected type.
There's an old PR open to add away to ignore such elements though, if it can help: https://github.com/huggingface/datasets/pull/2838 | ### Describe the bug
i have a json like this:
[
{"id": 1, "name": 1},
{"id": 2, "name": "Nan"},
{"id": 3, "name": 3},
....
]
οΌwhich have several problematic rows data like row 2, then i load it with datasets.load_dataset('json', data_files=['xx.json'], split='train'), it will report like this:
Generating train split: 0 examples [00:00, ? examples/s]Failed to read file 'C:\Users\gawinjunwu\Downloads\test\data\a.json' with error <class 'pyarrow.lib.ArrowInvalid'>: Could not convert '2' with type str: tried to convert to int64
Traceback (most recent call last):
File "D:\Python3.9\lib\site-packages\datasets\builder.py", line 1858, in _prepare_split_single
for _, table in generator:
File "D:\Python3.9\lib\site-packages\datasets\packaged_modules\json\json.py", line 146, in _generate_tables
raise ValueError(f"Not able to read records in the JSON file at {file}.") from None
ValueError: Not able to read records in the JSON file at C:\Users\gawinjunwu\Downloads\test\data\a.json.
The above exception was the direct cause of the following exception:
Traceback (most recent call last):
File "c:\Users\gawinjunwu\Downloads\test\scripts\a.py", line 4, in <module>
ds = load_dataset('json', data_dir='data', split='train')
File "D:\Python3.9\lib\site-packages\datasets\load.py", line 1797, in load_dataset
builder_instance.download_and_prepare(
File "D:\Python3.9\lib\site-packages\datasets\builder.py", line 890, in download_and_prepare
self._download_and_prepare(
File "D:\Python3.9\lib\site-packages\datasets\builder.py", line 985, in _download_and_prepare
self._prepare_split(split_generator, **prepare_split_kwargs)
File "D:\Python3.9\lib\site-packages\datasets\builder.py", line 1746, in _prepare_split
for job_id, done, content in self._prepare_split_single(
File "D:\Python3.9\lib\site-packages\datasets\builder.py", line 1891, in _prepare_split_single
raise DatasetGenerationError("An error occurred while generating the dataset") from e
datasets.builder.DatasetGenerationError: An error occurred while generating the dataset.
Could datasets skip those problematic data row?
### Steps to reproduce the bug
prepare a json file like this:
[
{"id": 1, "name": 1},
{"id": 2, "name": "Nan"},
{"id": 3, "name": 3}
]
then use datasets.load_dataset('json', dir_files=['xxx.json']) to load the json file
### Expected behavior
skip the problematic data row and load row1 and row3
### Environment info
python3.9 | 32 | load json dataset interrupt when dtype cast problem occured
### Describe the bug
i have a json like this:
[
{"id": 1, "name": 1},
{"id": 2, "name": "Nan"},
{"id": 3, "name": 3},
....
]
οΌwhich have several problematic rows data like row 2, then i load it with datasets.load_dataset('json', data_files=['xx.json'], split='train'), it will report like this:
Generating train split: 0 examples [00:00, ? examples/s]Failed to read file 'C:\Users\gawinjunwu\Downloads\test\data\a.json' with error <class 'pyarrow.lib.ArrowInvalid'>: Could not convert '2' with type str: tried to convert to int64
Traceback (most recent call last):
File "D:\Python3.9\lib\site-packages\datasets\builder.py", line 1858, in _prepare_split_single
for _, table in generator:
File "D:\Python3.9\lib\site-packages\datasets\packaged_modules\json\json.py", line 146, in _generate_tables
raise ValueError(f"Not able to read records in the JSON file at {file}.") from None
ValueError: Not able to read records in the JSON file at C:\Users\gawinjunwu\Downloads\test\data\a.json.
The above exception was the direct cause of the following exception:
Traceback (most recent call last):
File "c:\Users\gawinjunwu\Downloads\test\scripts\a.py", line 4, in <module>
ds = load_dataset('json', data_dir='data', split='train')
File "D:\Python3.9\lib\site-packages\datasets\load.py", line 1797, in load_dataset
builder_instance.download_and_prepare(
File "D:\Python3.9\lib\site-packages\datasets\builder.py", line 890, in download_and_prepare
self._download_and_prepare(
File "D:\Python3.9\lib\site-packages\datasets\builder.py", line 985, in _download_and_prepare
self._prepare_split(split_generator, **prepare_split_kwargs)
File "D:\Python3.9\lib\site-packages\datasets\builder.py", line 1746, in _prepare_split
for job_id, done, content in self._prepare_split_single(
File "D:\Python3.9\lib\site-packages\datasets\builder.py", line 1891, in _prepare_split_single
raise DatasetGenerationError("An error occurred while generating the dataset") from e
datasets.builder.DatasetGenerationError: An error occurred while generating the dataset.
Could datasets skip those problematic data row?
### Steps to reproduce the bug
prepare a json file like this:
[
{"id": 1, "name": 1},
{"id": 2, "name": "Nan"},
{"id": 3, "name": 3}
]
then use datasets.load_dataset('json', dir_files=['xxx.json']) to load the json file
### Expected behavior
skip the problematic data row and load row1 and row3
### Environment info
python3.9
Indeed the JSON dataset builder raises an error when it encounters an unexpected type.
There's an old PR open to add away to ignore such elements though, if it can help: https://github.com/huggingface/datasets/pull/2838 |
https://github.com/huggingface/datasets/issues/5825 | FileNotFound even though exists | Hi!
This would only work if `bigscience/xP3` was a no-code dataset, but it isn't (it has a Python builder script).
But this should work:
```python
load_dataset("json", data_files="https://huggingface.co/datasets/bigscience/xP3/resolve/main/ur/xp3_facebook_flores_spa_Latn-urd_Arab_devtest_ab-spa_Latn-urd_Arab.jsonl")
```
| ### Describe the bug
I'm trying to download https://huggingface.co/datasets/bigscience/xP3/resolve/main/ur/xp3_facebook_flores_spa_Latn-urd_Arab_devtest_ab-spa_Latn-urd_Arab.jsonl which works fine in my webbrowser, but somehow not with datasets. Am I doing sth wrong?
```
Downloading builder script: 100%
2.82k/2.82k [00:00<00:00, 64.2kB/s]
Downloading readme: 100%
12.6k/12.6k [00:00<00:00, 585kB/s]
---------------------------------------------------------------------------
FileNotFoundError Traceback (most recent call last)
[<ipython-input-2-4b45446a91d5>](https://localhost:8080/#) in <cell line: 4>()
2 lang = "ur"
3 fname = "xp3_facebook_flores_spa_Latn-urd_Arab_devtest_ab-spa_Latn-urd_Arab.jsonl"
----> 4 dataset = load_dataset("bigscience/xP3", data_files=f"{lang}/{fname}")
6 frames
[/usr/local/lib/python3.10/dist-packages/datasets/data_files.py](https://localhost:8080/#) in _resolve_single_pattern_locally(base_path, pattern, allowed_extensions)
291 if allowed_extensions is not None:
292 error_msg += f" with any supported extension {list(allowed_extensions)}"
--> 293 raise FileNotFoundError(error_msg)
294 return sorted(out)
295
FileNotFoundError: Unable to find 'https://huggingface.co/datasets/bigscience/xP3/resolve/main/ur/xp3_facebook_flores_spa_Latn-urd_Arab_devtest_ab-spa_Latn-urd_Arab.jsonl' at /content/https:/huggingface.co/datasets/bigscience/xP3/resolve/main
```
### Steps to reproduce the bug
```
!pip install -q datasets
from datasets import load_dataset
lang = "ur"
fname = "xp3_facebook_flores_spa_Latn-urd_Arab_devtest_ab-spa_Latn-urd_Arab.jsonl"
dataset = load_dataset("bigscience/xP3", data_files=f"{lang}/{fname}")
```
### Expected behavior
Correctly downloads
### Environment info
latest versions | 28 | FileNotFound even though exists
### Describe the bug
I'm trying to download https://huggingface.co/datasets/bigscience/xP3/resolve/main/ur/xp3_facebook_flores_spa_Latn-urd_Arab_devtest_ab-spa_Latn-urd_Arab.jsonl which works fine in my webbrowser, but somehow not with datasets. Am I doing sth wrong?
```
Downloading builder script: 100%
2.82k/2.82k [00:00<00:00, 64.2kB/s]
Downloading readme: 100%
12.6k/12.6k [00:00<00:00, 585kB/s]
---------------------------------------------------------------------------
FileNotFoundError Traceback (most recent call last)
[<ipython-input-2-4b45446a91d5>](https://localhost:8080/#) in <cell line: 4>()
2 lang = "ur"
3 fname = "xp3_facebook_flores_spa_Latn-urd_Arab_devtest_ab-spa_Latn-urd_Arab.jsonl"
----> 4 dataset = load_dataset("bigscience/xP3", data_files=f"{lang}/{fname}")
6 frames
[/usr/local/lib/python3.10/dist-packages/datasets/data_files.py](https://localhost:8080/#) in _resolve_single_pattern_locally(base_path, pattern, allowed_extensions)
291 if allowed_extensions is not None:
292 error_msg += f" with any supported extension {list(allowed_extensions)}"
--> 293 raise FileNotFoundError(error_msg)
294 return sorted(out)
295
FileNotFoundError: Unable to find 'https://huggingface.co/datasets/bigscience/xP3/resolve/main/ur/xp3_facebook_flores_spa_Latn-urd_Arab_devtest_ab-spa_Latn-urd_Arab.jsonl' at /content/https:/huggingface.co/datasets/bigscience/xP3/resolve/main
```
### Steps to reproduce the bug
```
!pip install -q datasets
from datasets import load_dataset
lang = "ur"
fname = "xp3_facebook_flores_spa_Latn-urd_Arab_devtest_ab-spa_Latn-urd_Arab.jsonl"
dataset = load_dataset("bigscience/xP3", data_files=f"{lang}/{fname}")
```
### Expected behavior
Correctly downloads
### Environment info
latest versions
Hi!
This would only work if `bigscience/xP3` was a no-code dataset, but it isn't (it has a Python builder script).
But this should work:
```python
load_dataset("json", data_files="https://huggingface.co/datasets/bigscience/xP3/resolve/main/ur/xp3_facebook_flores_spa_Latn-urd_Arab_devtest_ab-spa_Latn-urd_Arab.jsonl")
```
|
https://github.com/huggingface/datasets/issues/5825 | FileNotFound even though exists | > I see, it's not compatible w/ regex right? e.g. `load_dataset("json", data_files="https://huggingface.co/datasets/bigscience/xP3/resolve/main/ur/*")`
It should work for patterns that "reference" the local filesystem, but to make this work with the Hub, we must implement https://github.com/huggingface/datasets/issues/5281 first.
In the meantime, you can fetch these glob files with `HfFileSystem` and pass them as a list to `load_dataset`:
```python
from datasets import load_dataset
from huggingface_hub import HfFileSystem, hf_hub_url # `HfFileSystem` requires the latest version of `huggingface_hub`
fs = HfFileSystem()
glob_files = fs.glob("datasets/bigscience/xP3/ur/*")
# convert fsspec URLs to HTTP URLs
resolved_paths = [fs.resolve_path(file) for file in glob_files]
data_files = [hf_hub_url(resolved_path.repo_id, resolved_path.path_in_repo, repo_type=resolved_path.repo_type) for resolved_path in resolved_paths]
ds = load_dataset("json", data_files=data_files)
``` | ### Describe the bug
I'm trying to download https://huggingface.co/datasets/bigscience/xP3/resolve/main/ur/xp3_facebook_flores_spa_Latn-urd_Arab_devtest_ab-spa_Latn-urd_Arab.jsonl which works fine in my webbrowser, but somehow not with datasets. Am I doing sth wrong?
```
Downloading builder script: 100%
2.82k/2.82k [00:00<00:00, 64.2kB/s]
Downloading readme: 100%
12.6k/12.6k [00:00<00:00, 585kB/s]
---------------------------------------------------------------------------
FileNotFoundError Traceback (most recent call last)
[<ipython-input-2-4b45446a91d5>](https://localhost:8080/#) in <cell line: 4>()
2 lang = "ur"
3 fname = "xp3_facebook_flores_spa_Latn-urd_Arab_devtest_ab-spa_Latn-urd_Arab.jsonl"
----> 4 dataset = load_dataset("bigscience/xP3", data_files=f"{lang}/{fname}")
6 frames
[/usr/local/lib/python3.10/dist-packages/datasets/data_files.py](https://localhost:8080/#) in _resolve_single_pattern_locally(base_path, pattern, allowed_extensions)
291 if allowed_extensions is not None:
292 error_msg += f" with any supported extension {list(allowed_extensions)}"
--> 293 raise FileNotFoundError(error_msg)
294 return sorted(out)
295
FileNotFoundError: Unable to find 'https://huggingface.co/datasets/bigscience/xP3/resolve/main/ur/xp3_facebook_flores_spa_Latn-urd_Arab_devtest_ab-spa_Latn-urd_Arab.jsonl' at /content/https:/huggingface.co/datasets/bigscience/xP3/resolve/main
```
### Steps to reproduce the bug
```
!pip install -q datasets
from datasets import load_dataset
lang = "ur"
fname = "xp3_facebook_flores_spa_Latn-urd_Arab_devtest_ab-spa_Latn-urd_Arab.jsonl"
dataset = load_dataset("bigscience/xP3", data_files=f"{lang}/{fname}")
```
### Expected behavior
Correctly downloads
### Environment info
latest versions | 106 | FileNotFound even though exists
### Describe the bug
I'm trying to download https://huggingface.co/datasets/bigscience/xP3/resolve/main/ur/xp3_facebook_flores_spa_Latn-urd_Arab_devtest_ab-spa_Latn-urd_Arab.jsonl which works fine in my webbrowser, but somehow not with datasets. Am I doing sth wrong?
```
Downloading builder script: 100%
2.82k/2.82k [00:00<00:00, 64.2kB/s]
Downloading readme: 100%
12.6k/12.6k [00:00<00:00, 585kB/s]
---------------------------------------------------------------------------
FileNotFoundError Traceback (most recent call last)
[<ipython-input-2-4b45446a91d5>](https://localhost:8080/#) in <cell line: 4>()
2 lang = "ur"
3 fname = "xp3_facebook_flores_spa_Latn-urd_Arab_devtest_ab-spa_Latn-urd_Arab.jsonl"
----> 4 dataset = load_dataset("bigscience/xP3", data_files=f"{lang}/{fname}")
6 frames
[/usr/local/lib/python3.10/dist-packages/datasets/data_files.py](https://localhost:8080/#) in _resolve_single_pattern_locally(base_path, pattern, allowed_extensions)
291 if allowed_extensions is not None:
292 error_msg += f" with any supported extension {list(allowed_extensions)}"
--> 293 raise FileNotFoundError(error_msg)
294 return sorted(out)
295
FileNotFoundError: Unable to find 'https://huggingface.co/datasets/bigscience/xP3/resolve/main/ur/xp3_facebook_flores_spa_Latn-urd_Arab_devtest_ab-spa_Latn-urd_Arab.jsonl' at /content/https:/huggingface.co/datasets/bigscience/xP3/resolve/main
```
### Steps to reproduce the bug
```
!pip install -q datasets
from datasets import load_dataset
lang = "ur"
fname = "xp3_facebook_flores_spa_Latn-urd_Arab_devtest_ab-spa_Latn-urd_Arab.jsonl"
dataset = load_dataset("bigscience/xP3", data_files=f"{lang}/{fname}")
```
### Expected behavior
Correctly downloads
### Environment info
latest versions
> I see, it's not compatible w/ regex right? e.g. `load_dataset("json", data_files="https://huggingface.co/datasets/bigscience/xP3/resolve/main/ur/*")`
It should work for patterns that "reference" the local filesystem, but to make this work with the Hub, we must implement https://github.com/huggingface/datasets/issues/5281 first.
In the meantime, you can fetch these glob files with `HfFileSystem` and pass them as a list to `load_dataset`:
```python
from datasets import load_dataset
from huggingface_hub import HfFileSystem, hf_hub_url # `HfFileSystem` requires the latest version of `huggingface_hub`
fs = HfFileSystem()
glob_files = fs.glob("datasets/bigscience/xP3/ur/*")
# convert fsspec URLs to HTTP URLs
resolved_paths = [fs.resolve_path(file) for file in glob_files]
data_files = [hf_hub_url(resolved_path.repo_id, resolved_path.path_in_repo, repo_type=resolved_path.repo_type) for resolved_path in resolved_paths]
ds = load_dataset("json", data_files=data_files)
``` |
https://github.com/huggingface/datasets/issues/5820 | Incomplete docstring for `BuilderConfig` | Thanks for reporting! You are more than welcome to improve `BuilderConfig`'s docstring.
This class serves an identical purpose as `tensorflow_datasets`'s `BuilderConfig`, and its docstring is [here](https://github.com/tensorflow/datasets/blob/a95e38b5bb018312c3d3720619c2a8ef83ebf57f/tensorflow_datasets/core/dataset_builder.py#L81), so feel free to re-use parts of it. | Hi guys !
I stumbled upon this docstring while working on a project.
Some of the attributes have missing descriptions.
https://github.com/huggingface/datasets/blob/bc5fef5b6d91f009e4101684adcb374df2c170f6/src/datasets/builder.py#L104-L117 | 34 | Incomplete docstring for `BuilderConfig`
Hi guys !
I stumbled upon this docstring while working on a project.
Some of the attributes have missing descriptions.
https://github.com/huggingface/datasets/blob/bc5fef5b6d91f009e4101684adcb374df2c170f6/src/datasets/builder.py#L104-L117
Thanks for reporting! You are more than welcome to improve `BuilderConfig`'s docstring.
This class serves an identical purpose as `tensorflow_datasets`'s `BuilderConfig`, and its docstring is [here](https://github.com/tensorflow/datasets/blob/a95e38b5bb018312c3d3720619c2a8ef83ebf57f/tensorflow_datasets/core/dataset_builder.py#L81), so feel free to re-use parts of it. |
https://github.com/huggingface/datasets/issues/5819 | Cannot pickle error in Dataset.from_generator() | Hi! It should work if you put `model = torch.compile(model)` inside the `generate_data` function. If a referenced object is outside, it needs to be pickable, and that's not the case for the compiled models (or functions). | ### Describe the bug
I'm trying to use Dataset.from_generator() to generate a large dataset.
### Steps to reproduce the bug
Code to reproduce:
```
from transformers import T5Tokenizer, T5ForConditionalGeneration, GenerationConfig
import torch
from tqdm import tqdm
from datasets import load_dataset
tokenizer = T5Tokenizer.from_pretrained("google/flan-t5-small")
model = T5ForConditionalGeneration.from_pretrained("google/flan-t5-small", device_map="auto")
model = torch.compile(model)
def generate_data(data_loader):
model.eval()
for batch in tqdm(data_loader):
input_ids = tokenizer(batch['instruction'], return_tensors='pt', padding=True, truncation=True).input_ids.to("cuda:0")
with torch.no_grad():
outputs = model.generate(input_ids, generation_config=generation_config)
decoder_hidden_states = outputs.decoder_hidden_states
for i, h in zip(batch['instruction'], decoder_hidden_states):
yield {"instruction": i, "decoder_hidden_states": h}
generation_config = GenerationConfig(
temperature=1,
max_new_tokens=1024,
do_sample=False,
num_return_sequences=1,
return_dict_in_generate=True,
output_scores=True,
output_hidden_states=True,
)
from datasets import Dataset, load_dataset
from torch.utils.data import DataLoader
dataset = load_dataset("HuggingFaceH4/databricks_dolly_15k")
train_loader = DataLoader(dataset['train'], batch_size=2, shuffle=True)
dataset = Dataset.from_generator(generator=generate_data, gen_kwargs={"data_loader": train_loader})
dataset.save_to_disk("data/flant5_small_generation")
```
### Expected behavior
The dataset should be generated and saved.
But the following error occurred:
```
Traceback (most recent call last):
File "/remote-home/xhwang/alpaca-lora/data_collection_t5.py", line 46, in <module>
dataset = Dataset.from_generator(generator=generate_data, gen_kwargs={"data_loader": train_loader})
File "/remote-home/xhwang/anaconda3/envs/alpaca-lora/lib/python3.10/site-packages/datasets/arrow_dataset.py", line 1035, in from_generator
return GeneratorDatasetInputStream(
File "/remote-home/xhwang/anaconda3/envs/alpaca-lora/lib/python3.10/site-packages/datasets/io/generator.py", line 28, in __init__
self.builder = Generator(
File "/remote-home/xhwang/anaconda3/envs/alpaca-lora/lib/python3.10/site-packages/datasets/builder.py", line 336, in __init__
self.config, self.config_id = self._create_builder_config(
File "/remote-home/xhwang/anaconda3/envs/alpaca-lora/lib/python3.10/site-packages/datasets/builder.py", line 505, in _create_builder_config
config_id = builder_config.create_config_id(
File "/remote-home/xhwang/anaconda3/envs/alpaca-lora/lib/python3.10/site-packages/datasets/builder.py", line 179, in create_config_id
suffix = Hasher.hash(config_kwargs_to_add_to_suffix)
File "/remote-home/xhwang/anaconda3/envs/alpaca-lora/lib/python3.10/site-packages/datasets/fingerprint.py", line 236, in hash
return cls.hash_default(value)
File "/remote-home/xhwang/anaconda3/envs/alpaca-lora/lib/python3.10/site-packages/datasets/fingerprint.py", line 229, in hash_default
return cls.hash_bytes(dumps(value))
File "/remote-home/xhwang/anaconda3/envs/alpaca-lora/lib/python3.10/site-packages/datasets/utils/py_utils.py", line 726, in dumps
dump(obj, file)
File "/remote-home/xhwang/anaconda3/envs/alpaca-lora/lib/python3.10/site-packages/datasets/utils/py_utils.py", line 701, in dump
Pickler(file, recurse=True).dump(obj)
File "/remote-home/xhwang/anaconda3/envs/alpaca-lora/lib/python3.10/site-packages/dill/_dill.py", line 394, in dump
StockPickler.dump(self, obj)
File "/remote-home/xhwang/anaconda3/envs/alpaca-lora/lib/python3.10/pickle.py", line 487, in dump
self.save(obj)
File "/remote-home/xhwang/anaconda3/envs/alpaca-lora/lib/python3.10/site-packages/datasets/utils/py_utils.py", line 691, in save
dill.Pickler.save(self, obj, save_persistent_id=save_persistent_id)
File "/remote-home/xhwang/anaconda3/envs/alpaca-lora/lib/python3.10/site-packages/dill/_dill.py", line 388, in save
StockPickler.save(self, obj, save_persistent_id)
File "/remote-home/xhwang/anaconda3/envs/alpaca-lora/lib/python3.10/pickle.py", line 560, in save
f(self, obj) # Call unbound method with explicit self
File "/remote-home/xhwang/anaconda3/envs/alpaca-lora/lib/python3.10/site-packages/dill/_dill.py", line 1186, in save_module_dict
StockPickler.save_dict(pickler, obj)
File "/remote-home/xhwang/anaconda3/envs/alpaca-lora/lib/python3.10/pickle.py", line 972, in save_dict
self._batch_setitems(obj.items())
File "/remote-home/xhwang/anaconda3/envs/alpaca-lora/lib/python3.10/pickle.py", line 998, in _batch_setitems
save(v)
File "/remote-home/xhwang/anaconda3/envs/alpaca-lora/lib/python3.10/site-packages/datasets/utils/py_utils.py", line 691, in save
dill.Pickler.save(self, obj, save_persistent_id=save_persistent_id)
File "/remote-home/xhwang/anaconda3/envs/alpaca-lora/lib/python3.10/site-packages/dill/_dill.py", line 388, in save
StockPickler.save(self, obj, save_persistent_id)
File "/remote-home/xhwang/anaconda3/envs/alpaca-lora/lib/python3.10/pickle.py", line 560, in save
f(self, obj) # Call unbound method with explicit self
File "/remote-home/xhwang/anaconda3/envs/alpaca-lora/lib/python3.10/site-packages/datasets/utils/py_utils.py", line 1311, in save_function
dill._dill._save_with_postproc(
File "/remote-home/xhwang/anaconda3/envs/alpaca-lora/lib/python3.10/site-packages/dill/_dill.py", line 1084, in _save_with_postproc
pickler._batch_setitems(iter(source.items()))
File "/remote-home/xhwang/anaconda3/envs/alpaca-lora/lib/python3.10/pickle.py", line 998, in _batch_setitems
save(v)
File "/remote-home/xhwang/anaconda3/envs/alpaca-lora/lib/python3.10/site-packages/datasets/utils/py_utils.py", line 691, in save
dill.Pickler.save(self, obj, save_persistent_id=save_persistent_id)
File "/remote-home/xhwang/anaconda3/envs/alpaca-lora/lib/python3.10/site-packages/dill/_dill.py", line 388, in save
StockPickler.save(self, obj, save_persistent_id)
File "/remote-home/xhwang/anaconda3/envs/alpaca-lora/lib/python3.10/pickle.py", line 603, in save
self.save_reduce(obj=obj, *rv)
File "/remote-home/xhwang/anaconda3/envs/alpaca-lora/lib/python3.10/pickle.py", line 717, in save_reduce
save(state)
File "/remote-home/xhwang/anaconda3/envs/alpaca-lora/lib/python3.10/site-packages/datasets/utils/py_utils.py", line 691, in save
dill.Pickler.save(self, obj, save_persistent_id=save_persistent_id)
File "/remote-home/xhwang/anaconda3/envs/alpaca-lora/lib/python3.10/site-packages/dill/_dill.py", line 388, in save
StockPickler.save(self, obj, save_persistent_id)
File "/remote-home/xhwang/anaconda3/envs/alpaca-lora/lib/python3.10/pickle.py", line 560, in save
f(self, obj) # Call unbound method with explicit self
File "/remote-home/xhwang/anaconda3/envs/alpaca-lora/lib/python3.10/site-packages/dill/_dill.py", line 1186, in save_module_dict
StockPickler.save_dict(pickler, obj)
File "/remote-home/xhwang/anaconda3/envs/alpaca-lora/lib/python3.10/pickle.py", line 972, in save_dict
self._batch_setitems(obj.items())
File "/remote-home/xhwang/anaconda3/envs/alpaca-lora/lib/python3.10/pickle.py", line 998, in _batch_setitems
save(v)
File "/remote-home/xhwang/anaconda3/envs/alpaca-lora/lib/python3.10/site-packages/datasets/utils/py_utils.py", line 691, in save
dill.Pickler.save(self, obj, save_persistent_id=save_persistent_id)
File "/remote-home/xhwang/anaconda3/envs/alpaca-lora/lib/python3.10/site-packages/dill/_dill.py", line 388, in save
StockPickler.save(self, obj, save_persistent_id)
File "/remote-home/xhwang/anaconda3/envs/alpaca-lora/lib/python3.10/pickle.py", line 603, in save
self.save_reduce(obj=obj, *rv)
File "/remote-home/xhwang/anaconda3/envs/alpaca-lora/lib/python3.10/pickle.py", line 717, in save_reduce
save(state)
File "/remote-home/xhwang/anaconda3/envs/alpaca-lora/lib/python3.10/site-packages/datasets/utils/py_utils.py", line 691, in save
dill.Pickler.save(self, obj, save_persistent_id=save_persistent_id)
File "/remote-home/xhwang/anaconda3/envs/alpaca-lora/lib/python3.10/site-packages/dill/_dill.py", line 388, in save
StockPickler.save(self, obj, save_persistent_id)
File "/remote-home/xhwang/anaconda3/envs/alpaca-lora/lib/python3.10/pickle.py", line 560, in save
f(self, obj) # Call unbound method with explicit self
File "/remote-home/xhwang/anaconda3/envs/alpaca-lora/lib/python3.10/site-packages/dill/_dill.py", line 1186, in save_module_dict
StockPickler.save_dict(pickler, obj)
File "/remote-home/xhwang/anaconda3/envs/alpaca-lora/lib/python3.10/pickle.py", line 972, in save_dict
self._batch_setitems(obj.items())
File "/remote-home/xhwang/anaconda3/envs/alpaca-lora/lib/python3.10/pickle.py", line 998, in _batch_setitems
save(v)
File "/remote-home/xhwang/anaconda3/envs/alpaca-lora/lib/python3.10/site-packages/datasets/utils/py_utils.py", line 691, in save
dill.Pickler.save(self, obj, save_persistent_id=save_persistent_id)
File "/remote-home/xhwang/anaconda3/envs/alpaca-lora/lib/python3.10/site-packages/dill/_dill.py", line 388, in save
StockPickler.save(self, obj, save_persistent_id)
File "/remote-home/xhwang/anaconda3/envs/alpaca-lora/lib/python3.10/pickle.py", line 560, in save
f(self, obj) # Call unbound method with explicit self
File "/remote-home/xhwang/anaconda3/envs/alpaca-lora/lib/python3.10/site-packages/datasets/utils/py_utils.py", line 1311, in save_function
dill._dill._save_with_postproc(
File "/remote-home/xhwang/anaconda3/envs/alpaca-lora/lib/python3.10/site-packages/dill/_dill.py", line 1070, in _save_with_postproc
pickler.save_reduce(*reduction, obj=obj)
File "/remote-home/xhwang/anaconda3/envs/alpaca-lora/lib/python3.10/pickle.py", line 717, in save_reduce
save(state)
File "/remote-home/xhwang/anaconda3/envs/alpaca-lora/lib/python3.10/site-packages/datasets/utils/py_utils.py", line 691, in save
dill.Pickler.save(self, obj, save_persistent_id=save_persistent_id)
File "/remote-home/xhwang/anaconda3/envs/alpaca-lora/lib/python3.10/site-packages/dill/_dill.py", line 388, in save
StockPickler.save(self, obj, save_persistent_id)
File "/remote-home/xhwang/anaconda3/envs/alpaca-lora/lib/python3.10/pickle.py", line 560, in save
f(self, obj) # Call unbound method with explicit self
File "/remote-home/xhwang/anaconda3/envs/alpaca-lora/lib/python3.10/pickle.py", line 887, in save_tuple
save(element)
File "/remote-home/xhwang/anaconda3/envs/alpaca-lora/lib/python3.10/site-packages/datasets/utils/py_utils.py", line 691, in save
dill.Pickler.save(self, obj, save_persistent_id=save_persistent_id)
File "/remote-home/xhwang/anaconda3/envs/alpaca-lora/lib/python3.10/site-packages/dill/_dill.py", line 388, in save
StockPickler.save(self, obj, save_persistent_id)
File "/remote-home/xhwang/anaconda3/envs/alpaca-lora/lib/python3.10/pickle.py", line 560, in save
f(self, obj) # Call unbound method with explicit self
File "/remote-home/xhwang/anaconda3/envs/alpaca-lora/lib/python3.10/site-packages/dill/_dill.py", line 1186, in save_module_dict
StockPickler.save_dict(pickler, obj)
File "/remote-home/xhwang/anaconda3/envs/alpaca-lora/lib/python3.10/pickle.py", line 972, in save_dict
self._batch_setitems(obj.items())
File "/remote-home/xhwang/anaconda3/envs/alpaca-lora/lib/python3.10/pickle.py", line 998, in _batch_setitems
save(v)
File "/remote-home/xhwang/anaconda3/envs/alpaca-lora/lib/python3.10/site-packages/datasets/utils/py_utils.py", line 691, in save
dill.Pickler.save(self, obj, save_persistent_id=save_persistent_id)
File "/remote-home/xhwang/anaconda3/envs/alpaca-lora/lib/python3.10/site-packages/dill/_dill.py", line 388, in save
StockPickler.save(self, obj, save_persistent_id)
File "/remote-home/xhwang/anaconda3/envs/alpaca-lora/lib/python3.10/pickle.py", line 560, in save
f(self, obj) # Call unbound method with explicit self
File "/remote-home/xhwang/anaconda3/envs/alpaca-lora/lib/python3.10/site-packages/datasets/utils/py_utils.py", line 1311, in save_function
dill._dill._save_with_postproc(
File "/remote-home/xhwang/anaconda3/envs/alpaca-lora/lib/python3.10/site-packages/dill/_dill.py", line 1070, in _save_with_postproc
pickler.save_reduce(*reduction, obj=obj)
File "/remote-home/xhwang/anaconda3/envs/alpaca-lora/lib/python3.10/pickle.py", line 717, in save_reduce
save(state)
File "/remote-home/xhwang/anaconda3/envs/alpaca-lora/lib/python3.10/site-packages/datasets/utils/py_utils.py", line 691, in save
dill.Pickler.save(self, obj, save_persistent_id=save_persistent_id)
File "/remote-home/xhwang/anaconda3/envs/alpaca-lora/lib/python3.10/site-packages/dill/_dill.py", line 388, in save
StockPickler.save(self, obj, save_persistent_id)
File "/remote-home/xhwang/anaconda3/envs/alpaca-lora/lib/python3.10/pickle.py", line 560, in save
f(self, obj) # Call unbound method with explicit self
File "/remote-home/xhwang/anaconda3/envs/alpaca-lora/lib/python3.10/pickle.py", line 887, in save_tuple
save(element)
File "/remote-home/xhwang/anaconda3/envs/alpaca-lora/lib/python3.10/site-packages/datasets/utils/py_utils.py", line 691, in save
dill.Pickler.save(self, obj, save_persistent_id=save_persistent_id)
File "/remote-home/xhwang/anaconda3/envs/alpaca-lora/lib/python3.10/site-packages/dill/_dill.py", line 388, in save
StockPickler.save(self, obj, save_persistent_id)
File "/remote-home/xhwang/anaconda3/envs/alpaca-lora/lib/python3.10/pickle.py", line 560, in save
f(self, obj) # Call unbound method with explicit self
File "/remote-home/xhwang/anaconda3/envs/alpaca-lora/lib/python3.10/site-packages/dill/_dill.py", line 1186, in save_module_dict
StockPickler.save_dict(pickler, obj)
File "/remote-home/xhwang/anaconda3/envs/alpaca-lora/lib/python3.10/pickle.py", line 972, in save_dict
self._batch_setitems(obj.items())
File "/remote-home/xhwang/anaconda3/envs/alpaca-lora/lib/python3.10/pickle.py", line 1003, in _batch_setitems
save(v)
File "/remote-home/xhwang/anaconda3/envs/alpaca-lora/lib/python3.10/site-packages/datasets/utils/py_utils.py", line 691, in save
dill.Pickler.save(self, obj, save_persistent_id=save_persistent_id)
File "/remote-home/xhwang/anaconda3/envs/alpaca-lora/lib/python3.10/site-packages/dill/_dill.py", line 388, in save
StockPickler.save(self, obj, save_persistent_id)
File "/remote-home/xhwang/anaconda3/envs/alpaca-lora/lib/python3.10/pickle.py", line 560, in save
f(self, obj) # Call unbound method with explicit self
File "/remote-home/xhwang/anaconda3/envs/alpaca-lora/lib/python3.10/site-packages/datasets/utils/py_utils.py", line 1311, in save_function
dill._dill._save_with_postproc(
File "/remote-home/xhwang/anaconda3/envs/alpaca-lora/lib/python3.10/site-packages/dill/_dill.py", line 1084, in _save_with_postproc
pickler._batch_setitems(iter(source.items()))
File "/remote-home/xhwang/anaconda3/envs/alpaca-lora/lib/python3.10/pickle.py", line 998, in _batch_setitems
save(v)
File "/remote-home/xhwang/anaconda3/envs/alpaca-lora/lib/python3.10/site-packages/datasets/utils/py_utils.py", line 691, in save
dill.Pickler.save(self, obj, save_persistent_id=save_persistent_id)
File "/remote-home/xhwang/anaconda3/envs/alpaca-lora/lib/python3.10/site-packages/dill/_dill.py", line 388, in save
StockPickler.save(self, obj, save_persistent_id)
File "/remote-home/xhwang/anaconda3/envs/alpaca-lora/lib/python3.10/pickle.py", line 578, in save
rv = reduce(self.proto)
TypeError: cannot pickle 'ConfigModuleInstance' object
```
### Environment info
- `datasets` version: 2.11.0
- Platform: Linux-4.15.0-156-generic-x86_64-with-glibc2.31
- Python version: 3.10.10
- Huggingface_hub version: 0.13.2
- PyArrow version: 11.0.0
- Pandas version: 1.5.3 | 36 | Cannot pickle error in Dataset.from_generator()
### Describe the bug
I'm trying to use Dataset.from_generator() to generate a large dataset.
### Steps to reproduce the bug
Code to reproduce:
```
from transformers import T5Tokenizer, T5ForConditionalGeneration, GenerationConfig
import torch
from tqdm import tqdm
from datasets import load_dataset
tokenizer = T5Tokenizer.from_pretrained("google/flan-t5-small")
model = T5ForConditionalGeneration.from_pretrained("google/flan-t5-small", device_map="auto")
model = torch.compile(model)
def generate_data(data_loader):
model.eval()
for batch in tqdm(data_loader):
input_ids = tokenizer(batch['instruction'], return_tensors='pt', padding=True, truncation=True).input_ids.to("cuda:0")
with torch.no_grad():
outputs = model.generate(input_ids, generation_config=generation_config)
decoder_hidden_states = outputs.decoder_hidden_states
for i, h in zip(batch['instruction'], decoder_hidden_states):
yield {"instruction": i, "decoder_hidden_states": h}
generation_config = GenerationConfig(
temperature=1,
max_new_tokens=1024,
do_sample=False,
num_return_sequences=1,
return_dict_in_generate=True,
output_scores=True,
output_hidden_states=True,
)
from datasets import Dataset, load_dataset
from torch.utils.data import DataLoader
dataset = load_dataset("HuggingFaceH4/databricks_dolly_15k")
train_loader = DataLoader(dataset['train'], batch_size=2, shuffle=True)
dataset = Dataset.from_generator(generator=generate_data, gen_kwargs={"data_loader": train_loader})
dataset.save_to_disk("data/flant5_small_generation")
```
### Expected behavior
The dataset should be generated and saved.
But the following error occurred:
```
Traceback (most recent call last):
File "/remote-home/xhwang/alpaca-lora/data_collection_t5.py", line 46, in <module>
dataset = Dataset.from_generator(generator=generate_data, gen_kwargs={"data_loader": train_loader})
File "/remote-home/xhwang/anaconda3/envs/alpaca-lora/lib/python3.10/site-packages/datasets/arrow_dataset.py", line 1035, in from_generator
return GeneratorDatasetInputStream(
File "/remote-home/xhwang/anaconda3/envs/alpaca-lora/lib/python3.10/site-packages/datasets/io/generator.py", line 28, in __init__
self.builder = Generator(
File "/remote-home/xhwang/anaconda3/envs/alpaca-lora/lib/python3.10/site-packages/datasets/builder.py", line 336, in __init__
self.config, self.config_id = self._create_builder_config(
File "/remote-home/xhwang/anaconda3/envs/alpaca-lora/lib/python3.10/site-packages/datasets/builder.py", line 505, in _create_builder_config
config_id = builder_config.create_config_id(
File "/remote-home/xhwang/anaconda3/envs/alpaca-lora/lib/python3.10/site-packages/datasets/builder.py", line 179, in create_config_id
suffix = Hasher.hash(config_kwargs_to_add_to_suffix)
File "/remote-home/xhwang/anaconda3/envs/alpaca-lora/lib/python3.10/site-packages/datasets/fingerprint.py", line 236, in hash
return cls.hash_default(value)
File "/remote-home/xhwang/anaconda3/envs/alpaca-lora/lib/python3.10/site-packages/datasets/fingerprint.py", line 229, in hash_default
return cls.hash_bytes(dumps(value))
File "/remote-home/xhwang/anaconda3/envs/alpaca-lora/lib/python3.10/site-packages/datasets/utils/py_utils.py", line 726, in dumps
dump(obj, file)
File "/remote-home/xhwang/anaconda3/envs/alpaca-lora/lib/python3.10/site-packages/datasets/utils/py_utils.py", line 701, in dump
Pickler(file, recurse=True).dump(obj)
File "/remote-home/xhwang/anaconda3/envs/alpaca-lora/lib/python3.10/site-packages/dill/_dill.py", line 394, in dump
StockPickler.dump(self, obj)
File "/remote-home/xhwang/anaconda3/envs/alpaca-lora/lib/python3.10/pickle.py", line 487, in dump
self.save(obj)
File "/remote-home/xhwang/anaconda3/envs/alpaca-lora/lib/python3.10/site-packages/datasets/utils/py_utils.py", line 691, in save
dill.Pickler.save(self, obj, save_persistent_id=save_persistent_id)
File "/remote-home/xhwang/anaconda3/envs/alpaca-lora/lib/python3.10/site-packages/dill/_dill.py", line 388, in save
StockPickler.save(self, obj, save_persistent_id)
File "/remote-home/xhwang/anaconda3/envs/alpaca-lora/lib/python3.10/pickle.py", line 560, in save
f(self, obj) # Call unbound method with explicit self
File "/remote-home/xhwang/anaconda3/envs/alpaca-lora/lib/python3.10/site-packages/dill/_dill.py", line 1186, in save_module_dict
StockPickler.save_dict(pickler, obj)
File "/remote-home/xhwang/anaconda3/envs/alpaca-lora/lib/python3.10/pickle.py", line 972, in save_dict
self._batch_setitems(obj.items())
File "/remote-home/xhwang/anaconda3/envs/alpaca-lora/lib/python3.10/pickle.py", line 998, in _batch_setitems
save(v)
File "/remote-home/xhwang/anaconda3/envs/alpaca-lora/lib/python3.10/site-packages/datasets/utils/py_utils.py", line 691, in save
dill.Pickler.save(self, obj, save_persistent_id=save_persistent_id)
File "/remote-home/xhwang/anaconda3/envs/alpaca-lora/lib/python3.10/site-packages/dill/_dill.py", line 388, in save
StockPickler.save(self, obj, save_persistent_id)
File "/remote-home/xhwang/anaconda3/envs/alpaca-lora/lib/python3.10/pickle.py", line 560, in save
f(self, obj) # Call unbound method with explicit self
File "/remote-home/xhwang/anaconda3/envs/alpaca-lora/lib/python3.10/site-packages/datasets/utils/py_utils.py", line 1311, in save_function
dill._dill._save_with_postproc(
File "/remote-home/xhwang/anaconda3/envs/alpaca-lora/lib/python3.10/site-packages/dill/_dill.py", line 1084, in _save_with_postproc
pickler._batch_setitems(iter(source.items()))
File "/remote-home/xhwang/anaconda3/envs/alpaca-lora/lib/python3.10/pickle.py", line 998, in _batch_setitems
save(v)
File "/remote-home/xhwang/anaconda3/envs/alpaca-lora/lib/python3.10/site-packages/datasets/utils/py_utils.py", line 691, in save
dill.Pickler.save(self, obj, save_persistent_id=save_persistent_id)
File "/remote-home/xhwang/anaconda3/envs/alpaca-lora/lib/python3.10/site-packages/dill/_dill.py", line 388, in save
StockPickler.save(self, obj, save_persistent_id)
File "/remote-home/xhwang/anaconda3/envs/alpaca-lora/lib/python3.10/pickle.py", line 603, in save
self.save_reduce(obj=obj, *rv)
File "/remote-home/xhwang/anaconda3/envs/alpaca-lora/lib/python3.10/pickle.py", line 717, in save_reduce
save(state)
File "/remote-home/xhwang/anaconda3/envs/alpaca-lora/lib/python3.10/site-packages/datasets/utils/py_utils.py", line 691, in save
dill.Pickler.save(self, obj, save_persistent_id=save_persistent_id)
File "/remote-home/xhwang/anaconda3/envs/alpaca-lora/lib/python3.10/site-packages/dill/_dill.py", line 388, in save
StockPickler.save(self, obj, save_persistent_id)
File "/remote-home/xhwang/anaconda3/envs/alpaca-lora/lib/python3.10/pickle.py", line 560, in save
f(self, obj) # Call unbound method with explicit self
File "/remote-home/xhwang/anaconda3/envs/alpaca-lora/lib/python3.10/site-packages/dill/_dill.py", line 1186, in save_module_dict
StockPickler.save_dict(pickler, obj)
File "/remote-home/xhwang/anaconda3/envs/alpaca-lora/lib/python3.10/pickle.py", line 972, in save_dict
self._batch_setitems(obj.items())
File "/remote-home/xhwang/anaconda3/envs/alpaca-lora/lib/python3.10/pickle.py", line 998, in _batch_setitems
save(v)
File "/remote-home/xhwang/anaconda3/envs/alpaca-lora/lib/python3.10/site-packages/datasets/utils/py_utils.py", line 691, in save
dill.Pickler.save(self, obj, save_persistent_id=save_persistent_id)
File "/remote-home/xhwang/anaconda3/envs/alpaca-lora/lib/python3.10/site-packages/dill/_dill.py", line 388, in save
StockPickler.save(self, obj, save_persistent_id)
File "/remote-home/xhwang/anaconda3/envs/alpaca-lora/lib/python3.10/pickle.py", line 603, in save
self.save_reduce(obj=obj, *rv)
File "/remote-home/xhwang/anaconda3/envs/alpaca-lora/lib/python3.10/pickle.py", line 717, in save_reduce
save(state)
File "/remote-home/xhwang/anaconda3/envs/alpaca-lora/lib/python3.10/site-packages/datasets/utils/py_utils.py", line 691, in save
dill.Pickler.save(self, obj, save_persistent_id=save_persistent_id)
File "/remote-home/xhwang/anaconda3/envs/alpaca-lora/lib/python3.10/site-packages/dill/_dill.py", line 388, in save
StockPickler.save(self, obj, save_persistent_id)
File "/remote-home/xhwang/anaconda3/envs/alpaca-lora/lib/python3.10/pickle.py", line 560, in save
f(self, obj) # Call unbound method with explicit self
File "/remote-home/xhwang/anaconda3/envs/alpaca-lora/lib/python3.10/site-packages/dill/_dill.py", line 1186, in save_module_dict
StockPickler.save_dict(pickler, obj)
File "/remote-home/xhwang/anaconda3/envs/alpaca-lora/lib/python3.10/pickle.py", line 972, in save_dict
self._batch_setitems(obj.items())
File "/remote-home/xhwang/anaconda3/envs/alpaca-lora/lib/python3.10/pickle.py", line 998, in _batch_setitems
save(v)
File "/remote-home/xhwang/anaconda3/envs/alpaca-lora/lib/python3.10/site-packages/datasets/utils/py_utils.py", line 691, in save
dill.Pickler.save(self, obj, save_persistent_id=save_persistent_id)
File "/remote-home/xhwang/anaconda3/envs/alpaca-lora/lib/python3.10/site-packages/dill/_dill.py", line 388, in save
StockPickler.save(self, obj, save_persistent_id)
File "/remote-home/xhwang/anaconda3/envs/alpaca-lora/lib/python3.10/pickle.py", line 560, in save
f(self, obj) # Call unbound method with explicit self
File "/remote-home/xhwang/anaconda3/envs/alpaca-lora/lib/python3.10/site-packages/datasets/utils/py_utils.py", line 1311, in save_function
dill._dill._save_with_postproc(
File "/remote-home/xhwang/anaconda3/envs/alpaca-lora/lib/python3.10/site-packages/dill/_dill.py", line 1070, in _save_with_postproc
pickler.save_reduce(*reduction, obj=obj)
File "/remote-home/xhwang/anaconda3/envs/alpaca-lora/lib/python3.10/pickle.py", line 717, in save_reduce
save(state)
File "/remote-home/xhwang/anaconda3/envs/alpaca-lora/lib/python3.10/site-packages/datasets/utils/py_utils.py", line 691, in save
dill.Pickler.save(self, obj, save_persistent_id=save_persistent_id)
File "/remote-home/xhwang/anaconda3/envs/alpaca-lora/lib/python3.10/site-packages/dill/_dill.py", line 388, in save
StockPickler.save(self, obj, save_persistent_id)
File "/remote-home/xhwang/anaconda3/envs/alpaca-lora/lib/python3.10/pickle.py", line 560, in save
f(self, obj) # Call unbound method with explicit self
File "/remote-home/xhwang/anaconda3/envs/alpaca-lora/lib/python3.10/pickle.py", line 887, in save_tuple
save(element)
File "/remote-home/xhwang/anaconda3/envs/alpaca-lora/lib/python3.10/site-packages/datasets/utils/py_utils.py", line 691, in save
dill.Pickler.save(self, obj, save_persistent_id=save_persistent_id)
File "/remote-home/xhwang/anaconda3/envs/alpaca-lora/lib/python3.10/site-packages/dill/_dill.py", line 388, in save
StockPickler.save(self, obj, save_persistent_id)
File "/remote-home/xhwang/anaconda3/envs/alpaca-lora/lib/python3.10/pickle.py", line 560, in save
f(self, obj) # Call unbound method with explicit self
File "/remote-home/xhwang/anaconda3/envs/alpaca-lora/lib/python3.10/site-packages/dill/_dill.py", line 1186, in save_module_dict
StockPickler.save_dict(pickler, obj)
File "/remote-home/xhwang/anaconda3/envs/alpaca-lora/lib/python3.10/pickle.py", line 972, in save_dict
self._batch_setitems(obj.items())
File "/remote-home/xhwang/anaconda3/envs/alpaca-lora/lib/python3.10/pickle.py", line 998, in _batch_setitems
save(v)
File "/remote-home/xhwang/anaconda3/envs/alpaca-lora/lib/python3.10/site-packages/datasets/utils/py_utils.py", line 691, in save
dill.Pickler.save(self, obj, save_persistent_id=save_persistent_id)
File "/remote-home/xhwang/anaconda3/envs/alpaca-lora/lib/python3.10/site-packages/dill/_dill.py", line 388, in save
StockPickler.save(self, obj, save_persistent_id)
File "/remote-home/xhwang/anaconda3/envs/alpaca-lora/lib/python3.10/pickle.py", line 560, in save
f(self, obj) # Call unbound method with explicit self
File "/remote-home/xhwang/anaconda3/envs/alpaca-lora/lib/python3.10/site-packages/datasets/utils/py_utils.py", line 1311, in save_function
dill._dill._save_with_postproc(
File "/remote-home/xhwang/anaconda3/envs/alpaca-lora/lib/python3.10/site-packages/dill/_dill.py", line 1070, in _save_with_postproc
pickler.save_reduce(*reduction, obj=obj)
File "/remote-home/xhwang/anaconda3/envs/alpaca-lora/lib/python3.10/pickle.py", line 717, in save_reduce
save(state)
File "/remote-home/xhwang/anaconda3/envs/alpaca-lora/lib/python3.10/site-packages/datasets/utils/py_utils.py", line 691, in save
dill.Pickler.save(self, obj, save_persistent_id=save_persistent_id)
File "/remote-home/xhwang/anaconda3/envs/alpaca-lora/lib/python3.10/site-packages/dill/_dill.py", line 388, in save
StockPickler.save(self, obj, save_persistent_id)
File "/remote-home/xhwang/anaconda3/envs/alpaca-lora/lib/python3.10/pickle.py", line 560, in save
f(self, obj) # Call unbound method with explicit self
File "/remote-home/xhwang/anaconda3/envs/alpaca-lora/lib/python3.10/pickle.py", line 887, in save_tuple
save(element)
File "/remote-home/xhwang/anaconda3/envs/alpaca-lora/lib/python3.10/site-packages/datasets/utils/py_utils.py", line 691, in save
dill.Pickler.save(self, obj, save_persistent_id=save_persistent_id)
File "/remote-home/xhwang/anaconda3/envs/alpaca-lora/lib/python3.10/site-packages/dill/_dill.py", line 388, in save
StockPickler.save(self, obj, save_persistent_id)
File "/remote-home/xhwang/anaconda3/envs/alpaca-lora/lib/python3.10/pickle.py", line 560, in save
f(self, obj) # Call unbound method with explicit self
File "/remote-home/xhwang/anaconda3/envs/alpaca-lora/lib/python3.10/site-packages/dill/_dill.py", line 1186, in save_module_dict
StockPickler.save_dict(pickler, obj)
File "/remote-home/xhwang/anaconda3/envs/alpaca-lora/lib/python3.10/pickle.py", line 972, in save_dict
self._batch_setitems(obj.items())
File "/remote-home/xhwang/anaconda3/envs/alpaca-lora/lib/python3.10/pickle.py", line 1003, in _batch_setitems
save(v)
File "/remote-home/xhwang/anaconda3/envs/alpaca-lora/lib/python3.10/site-packages/datasets/utils/py_utils.py", line 691, in save
dill.Pickler.save(self, obj, save_persistent_id=save_persistent_id)
File "/remote-home/xhwang/anaconda3/envs/alpaca-lora/lib/python3.10/site-packages/dill/_dill.py", line 388, in save
StockPickler.save(self, obj, save_persistent_id)
File "/remote-home/xhwang/anaconda3/envs/alpaca-lora/lib/python3.10/pickle.py", line 560, in save
f(self, obj) # Call unbound method with explicit self
File "/remote-home/xhwang/anaconda3/envs/alpaca-lora/lib/python3.10/site-packages/datasets/utils/py_utils.py", line 1311, in save_function
dill._dill._save_with_postproc(
File "/remote-home/xhwang/anaconda3/envs/alpaca-lora/lib/python3.10/site-packages/dill/_dill.py", line 1084, in _save_with_postproc
pickler._batch_setitems(iter(source.items()))
File "/remote-home/xhwang/anaconda3/envs/alpaca-lora/lib/python3.10/pickle.py", line 998, in _batch_setitems
save(v)
File "/remote-home/xhwang/anaconda3/envs/alpaca-lora/lib/python3.10/site-packages/datasets/utils/py_utils.py", line 691, in save
dill.Pickler.save(self, obj, save_persistent_id=save_persistent_id)
File "/remote-home/xhwang/anaconda3/envs/alpaca-lora/lib/python3.10/site-packages/dill/_dill.py", line 388, in save
StockPickler.save(self, obj, save_persistent_id)
File "/remote-home/xhwang/anaconda3/envs/alpaca-lora/lib/python3.10/pickle.py", line 578, in save
rv = reduce(self.proto)
TypeError: cannot pickle 'ConfigModuleInstance' object
```
### Environment info
- `datasets` version: 2.11.0
- Platform: Linux-4.15.0-156-generic-x86_64-with-glibc2.31
- Python version: 3.10.10
- Huggingface_hub version: 0.13.2
- PyArrow version: 11.0.0
- Pandas version: 1.5.3
Hi! It should work if you put `model = torch.compile(model)` inside the `generate_data` function. If a referenced object is outside, it needs to be pickable, and that's not the case for the compiled models (or functions). |
https://github.com/huggingface/datasets/issues/5819 | Cannot pickle error in Dataset.from_generator() | > Hi! It should work if you put `model = torch.compile(model)` inside the `generate_data` function. If a referenced object is outside, it needs to be pickable, and that's not the case for the compiled models (or functions).
Hi! Thank you for your reply! Everything works perfectly with your suggestion!
Closing the issue.
| ### Describe the bug
I'm trying to use Dataset.from_generator() to generate a large dataset.
### Steps to reproduce the bug
Code to reproduce:
```
from transformers import T5Tokenizer, T5ForConditionalGeneration, GenerationConfig
import torch
from tqdm import tqdm
from datasets import load_dataset
tokenizer = T5Tokenizer.from_pretrained("google/flan-t5-small")
model = T5ForConditionalGeneration.from_pretrained("google/flan-t5-small", device_map="auto")
model = torch.compile(model)
def generate_data(data_loader):
model.eval()
for batch in tqdm(data_loader):
input_ids = tokenizer(batch['instruction'], return_tensors='pt', padding=True, truncation=True).input_ids.to("cuda:0")
with torch.no_grad():
outputs = model.generate(input_ids, generation_config=generation_config)
decoder_hidden_states = outputs.decoder_hidden_states
for i, h in zip(batch['instruction'], decoder_hidden_states):
yield {"instruction": i, "decoder_hidden_states": h}
generation_config = GenerationConfig(
temperature=1,
max_new_tokens=1024,
do_sample=False,
num_return_sequences=1,
return_dict_in_generate=True,
output_scores=True,
output_hidden_states=True,
)
from datasets import Dataset, load_dataset
from torch.utils.data import DataLoader
dataset = load_dataset("HuggingFaceH4/databricks_dolly_15k")
train_loader = DataLoader(dataset['train'], batch_size=2, shuffle=True)
dataset = Dataset.from_generator(generator=generate_data, gen_kwargs={"data_loader": train_loader})
dataset.save_to_disk("data/flant5_small_generation")
```
### Expected behavior
The dataset should be generated and saved.
But the following error occurred:
```
Traceback (most recent call last):
File "/remote-home/xhwang/alpaca-lora/data_collection_t5.py", line 46, in <module>
dataset = Dataset.from_generator(generator=generate_data, gen_kwargs={"data_loader": train_loader})
File "/remote-home/xhwang/anaconda3/envs/alpaca-lora/lib/python3.10/site-packages/datasets/arrow_dataset.py", line 1035, in from_generator
return GeneratorDatasetInputStream(
File "/remote-home/xhwang/anaconda3/envs/alpaca-lora/lib/python3.10/site-packages/datasets/io/generator.py", line 28, in __init__
self.builder = Generator(
File "/remote-home/xhwang/anaconda3/envs/alpaca-lora/lib/python3.10/site-packages/datasets/builder.py", line 336, in __init__
self.config, self.config_id = self._create_builder_config(
File "/remote-home/xhwang/anaconda3/envs/alpaca-lora/lib/python3.10/site-packages/datasets/builder.py", line 505, in _create_builder_config
config_id = builder_config.create_config_id(
File "/remote-home/xhwang/anaconda3/envs/alpaca-lora/lib/python3.10/site-packages/datasets/builder.py", line 179, in create_config_id
suffix = Hasher.hash(config_kwargs_to_add_to_suffix)
File "/remote-home/xhwang/anaconda3/envs/alpaca-lora/lib/python3.10/site-packages/datasets/fingerprint.py", line 236, in hash
return cls.hash_default(value)
File "/remote-home/xhwang/anaconda3/envs/alpaca-lora/lib/python3.10/site-packages/datasets/fingerprint.py", line 229, in hash_default
return cls.hash_bytes(dumps(value))
File "/remote-home/xhwang/anaconda3/envs/alpaca-lora/lib/python3.10/site-packages/datasets/utils/py_utils.py", line 726, in dumps
dump(obj, file)
File "/remote-home/xhwang/anaconda3/envs/alpaca-lora/lib/python3.10/site-packages/datasets/utils/py_utils.py", line 701, in dump
Pickler(file, recurse=True).dump(obj)
File "/remote-home/xhwang/anaconda3/envs/alpaca-lora/lib/python3.10/site-packages/dill/_dill.py", line 394, in dump
StockPickler.dump(self, obj)
File "/remote-home/xhwang/anaconda3/envs/alpaca-lora/lib/python3.10/pickle.py", line 487, in dump
self.save(obj)
File "/remote-home/xhwang/anaconda3/envs/alpaca-lora/lib/python3.10/site-packages/datasets/utils/py_utils.py", line 691, in save
dill.Pickler.save(self, obj, save_persistent_id=save_persistent_id)
File "/remote-home/xhwang/anaconda3/envs/alpaca-lora/lib/python3.10/site-packages/dill/_dill.py", line 388, in save
StockPickler.save(self, obj, save_persistent_id)
File "/remote-home/xhwang/anaconda3/envs/alpaca-lora/lib/python3.10/pickle.py", line 560, in save
f(self, obj) # Call unbound method with explicit self
File "/remote-home/xhwang/anaconda3/envs/alpaca-lora/lib/python3.10/site-packages/dill/_dill.py", line 1186, in save_module_dict
StockPickler.save_dict(pickler, obj)
File "/remote-home/xhwang/anaconda3/envs/alpaca-lora/lib/python3.10/pickle.py", line 972, in save_dict
self._batch_setitems(obj.items())
File "/remote-home/xhwang/anaconda3/envs/alpaca-lora/lib/python3.10/pickle.py", line 998, in _batch_setitems
save(v)
File "/remote-home/xhwang/anaconda3/envs/alpaca-lora/lib/python3.10/site-packages/datasets/utils/py_utils.py", line 691, in save
dill.Pickler.save(self, obj, save_persistent_id=save_persistent_id)
File "/remote-home/xhwang/anaconda3/envs/alpaca-lora/lib/python3.10/site-packages/dill/_dill.py", line 388, in save
StockPickler.save(self, obj, save_persistent_id)
File "/remote-home/xhwang/anaconda3/envs/alpaca-lora/lib/python3.10/pickle.py", line 560, in save
f(self, obj) # Call unbound method with explicit self
File "/remote-home/xhwang/anaconda3/envs/alpaca-lora/lib/python3.10/site-packages/datasets/utils/py_utils.py", line 1311, in save_function
dill._dill._save_with_postproc(
File "/remote-home/xhwang/anaconda3/envs/alpaca-lora/lib/python3.10/site-packages/dill/_dill.py", line 1084, in _save_with_postproc
pickler._batch_setitems(iter(source.items()))
File "/remote-home/xhwang/anaconda3/envs/alpaca-lora/lib/python3.10/pickle.py", line 998, in _batch_setitems
save(v)
File "/remote-home/xhwang/anaconda3/envs/alpaca-lora/lib/python3.10/site-packages/datasets/utils/py_utils.py", line 691, in save
dill.Pickler.save(self, obj, save_persistent_id=save_persistent_id)
File "/remote-home/xhwang/anaconda3/envs/alpaca-lora/lib/python3.10/site-packages/dill/_dill.py", line 388, in save
StockPickler.save(self, obj, save_persistent_id)
File "/remote-home/xhwang/anaconda3/envs/alpaca-lora/lib/python3.10/pickle.py", line 603, in save
self.save_reduce(obj=obj, *rv)
File "/remote-home/xhwang/anaconda3/envs/alpaca-lora/lib/python3.10/pickle.py", line 717, in save_reduce
save(state)
File "/remote-home/xhwang/anaconda3/envs/alpaca-lora/lib/python3.10/site-packages/datasets/utils/py_utils.py", line 691, in save
dill.Pickler.save(self, obj, save_persistent_id=save_persistent_id)
File "/remote-home/xhwang/anaconda3/envs/alpaca-lora/lib/python3.10/site-packages/dill/_dill.py", line 388, in save
StockPickler.save(self, obj, save_persistent_id)
File "/remote-home/xhwang/anaconda3/envs/alpaca-lora/lib/python3.10/pickle.py", line 560, in save
f(self, obj) # Call unbound method with explicit self
File "/remote-home/xhwang/anaconda3/envs/alpaca-lora/lib/python3.10/site-packages/dill/_dill.py", line 1186, in save_module_dict
StockPickler.save_dict(pickler, obj)
File "/remote-home/xhwang/anaconda3/envs/alpaca-lora/lib/python3.10/pickle.py", line 972, in save_dict
self._batch_setitems(obj.items())
File "/remote-home/xhwang/anaconda3/envs/alpaca-lora/lib/python3.10/pickle.py", line 998, in _batch_setitems
save(v)
File "/remote-home/xhwang/anaconda3/envs/alpaca-lora/lib/python3.10/site-packages/datasets/utils/py_utils.py", line 691, in save
dill.Pickler.save(self, obj, save_persistent_id=save_persistent_id)
File "/remote-home/xhwang/anaconda3/envs/alpaca-lora/lib/python3.10/site-packages/dill/_dill.py", line 388, in save
StockPickler.save(self, obj, save_persistent_id)
File "/remote-home/xhwang/anaconda3/envs/alpaca-lora/lib/python3.10/pickle.py", line 603, in save
self.save_reduce(obj=obj, *rv)
File "/remote-home/xhwang/anaconda3/envs/alpaca-lora/lib/python3.10/pickle.py", line 717, in save_reduce
save(state)
File "/remote-home/xhwang/anaconda3/envs/alpaca-lora/lib/python3.10/site-packages/datasets/utils/py_utils.py", line 691, in save
dill.Pickler.save(self, obj, save_persistent_id=save_persistent_id)
File "/remote-home/xhwang/anaconda3/envs/alpaca-lora/lib/python3.10/site-packages/dill/_dill.py", line 388, in save
StockPickler.save(self, obj, save_persistent_id)
File "/remote-home/xhwang/anaconda3/envs/alpaca-lora/lib/python3.10/pickle.py", line 560, in save
f(self, obj) # Call unbound method with explicit self
File "/remote-home/xhwang/anaconda3/envs/alpaca-lora/lib/python3.10/site-packages/dill/_dill.py", line 1186, in save_module_dict
StockPickler.save_dict(pickler, obj)
File "/remote-home/xhwang/anaconda3/envs/alpaca-lora/lib/python3.10/pickle.py", line 972, in save_dict
self._batch_setitems(obj.items())
File "/remote-home/xhwang/anaconda3/envs/alpaca-lora/lib/python3.10/pickle.py", line 998, in _batch_setitems
save(v)
File "/remote-home/xhwang/anaconda3/envs/alpaca-lora/lib/python3.10/site-packages/datasets/utils/py_utils.py", line 691, in save
dill.Pickler.save(self, obj, save_persistent_id=save_persistent_id)
File "/remote-home/xhwang/anaconda3/envs/alpaca-lora/lib/python3.10/site-packages/dill/_dill.py", line 388, in save
StockPickler.save(self, obj, save_persistent_id)
File "/remote-home/xhwang/anaconda3/envs/alpaca-lora/lib/python3.10/pickle.py", line 560, in save
f(self, obj) # Call unbound method with explicit self
File "/remote-home/xhwang/anaconda3/envs/alpaca-lora/lib/python3.10/site-packages/datasets/utils/py_utils.py", line 1311, in save_function
dill._dill._save_with_postproc(
File "/remote-home/xhwang/anaconda3/envs/alpaca-lora/lib/python3.10/site-packages/dill/_dill.py", line 1070, in _save_with_postproc
pickler.save_reduce(*reduction, obj=obj)
File "/remote-home/xhwang/anaconda3/envs/alpaca-lora/lib/python3.10/pickle.py", line 717, in save_reduce
save(state)
File "/remote-home/xhwang/anaconda3/envs/alpaca-lora/lib/python3.10/site-packages/datasets/utils/py_utils.py", line 691, in save
dill.Pickler.save(self, obj, save_persistent_id=save_persistent_id)
File "/remote-home/xhwang/anaconda3/envs/alpaca-lora/lib/python3.10/site-packages/dill/_dill.py", line 388, in save
StockPickler.save(self, obj, save_persistent_id)
File "/remote-home/xhwang/anaconda3/envs/alpaca-lora/lib/python3.10/pickle.py", line 560, in save
f(self, obj) # Call unbound method with explicit self
File "/remote-home/xhwang/anaconda3/envs/alpaca-lora/lib/python3.10/pickle.py", line 887, in save_tuple
save(element)
File "/remote-home/xhwang/anaconda3/envs/alpaca-lora/lib/python3.10/site-packages/datasets/utils/py_utils.py", line 691, in save
dill.Pickler.save(self, obj, save_persistent_id=save_persistent_id)
File "/remote-home/xhwang/anaconda3/envs/alpaca-lora/lib/python3.10/site-packages/dill/_dill.py", line 388, in save
StockPickler.save(self, obj, save_persistent_id)
File "/remote-home/xhwang/anaconda3/envs/alpaca-lora/lib/python3.10/pickle.py", line 560, in save
f(self, obj) # Call unbound method with explicit self
File "/remote-home/xhwang/anaconda3/envs/alpaca-lora/lib/python3.10/site-packages/dill/_dill.py", line 1186, in save_module_dict
StockPickler.save_dict(pickler, obj)
File "/remote-home/xhwang/anaconda3/envs/alpaca-lora/lib/python3.10/pickle.py", line 972, in save_dict
self._batch_setitems(obj.items())
File "/remote-home/xhwang/anaconda3/envs/alpaca-lora/lib/python3.10/pickle.py", line 998, in _batch_setitems
save(v)
File "/remote-home/xhwang/anaconda3/envs/alpaca-lora/lib/python3.10/site-packages/datasets/utils/py_utils.py", line 691, in save
dill.Pickler.save(self, obj, save_persistent_id=save_persistent_id)
File "/remote-home/xhwang/anaconda3/envs/alpaca-lora/lib/python3.10/site-packages/dill/_dill.py", line 388, in save
StockPickler.save(self, obj, save_persistent_id)
File "/remote-home/xhwang/anaconda3/envs/alpaca-lora/lib/python3.10/pickle.py", line 560, in save
f(self, obj) # Call unbound method with explicit self
File "/remote-home/xhwang/anaconda3/envs/alpaca-lora/lib/python3.10/site-packages/datasets/utils/py_utils.py", line 1311, in save_function
dill._dill._save_with_postproc(
File "/remote-home/xhwang/anaconda3/envs/alpaca-lora/lib/python3.10/site-packages/dill/_dill.py", line 1070, in _save_with_postproc
pickler.save_reduce(*reduction, obj=obj)
File "/remote-home/xhwang/anaconda3/envs/alpaca-lora/lib/python3.10/pickle.py", line 717, in save_reduce
save(state)
File "/remote-home/xhwang/anaconda3/envs/alpaca-lora/lib/python3.10/site-packages/datasets/utils/py_utils.py", line 691, in save
dill.Pickler.save(self, obj, save_persistent_id=save_persistent_id)
File "/remote-home/xhwang/anaconda3/envs/alpaca-lora/lib/python3.10/site-packages/dill/_dill.py", line 388, in save
StockPickler.save(self, obj, save_persistent_id)
File "/remote-home/xhwang/anaconda3/envs/alpaca-lora/lib/python3.10/pickle.py", line 560, in save
f(self, obj) # Call unbound method with explicit self
File "/remote-home/xhwang/anaconda3/envs/alpaca-lora/lib/python3.10/pickle.py", line 887, in save_tuple
save(element)
File "/remote-home/xhwang/anaconda3/envs/alpaca-lora/lib/python3.10/site-packages/datasets/utils/py_utils.py", line 691, in save
dill.Pickler.save(self, obj, save_persistent_id=save_persistent_id)
File "/remote-home/xhwang/anaconda3/envs/alpaca-lora/lib/python3.10/site-packages/dill/_dill.py", line 388, in save
StockPickler.save(self, obj, save_persistent_id)
File "/remote-home/xhwang/anaconda3/envs/alpaca-lora/lib/python3.10/pickle.py", line 560, in save
f(self, obj) # Call unbound method with explicit self
File "/remote-home/xhwang/anaconda3/envs/alpaca-lora/lib/python3.10/site-packages/dill/_dill.py", line 1186, in save_module_dict
StockPickler.save_dict(pickler, obj)
File "/remote-home/xhwang/anaconda3/envs/alpaca-lora/lib/python3.10/pickle.py", line 972, in save_dict
self._batch_setitems(obj.items())
File "/remote-home/xhwang/anaconda3/envs/alpaca-lora/lib/python3.10/pickle.py", line 1003, in _batch_setitems
save(v)
File "/remote-home/xhwang/anaconda3/envs/alpaca-lora/lib/python3.10/site-packages/datasets/utils/py_utils.py", line 691, in save
dill.Pickler.save(self, obj, save_persistent_id=save_persistent_id)
File "/remote-home/xhwang/anaconda3/envs/alpaca-lora/lib/python3.10/site-packages/dill/_dill.py", line 388, in save
StockPickler.save(self, obj, save_persistent_id)
File "/remote-home/xhwang/anaconda3/envs/alpaca-lora/lib/python3.10/pickle.py", line 560, in save
f(self, obj) # Call unbound method with explicit self
File "/remote-home/xhwang/anaconda3/envs/alpaca-lora/lib/python3.10/site-packages/datasets/utils/py_utils.py", line 1311, in save_function
dill._dill._save_with_postproc(
File "/remote-home/xhwang/anaconda3/envs/alpaca-lora/lib/python3.10/site-packages/dill/_dill.py", line 1084, in _save_with_postproc
pickler._batch_setitems(iter(source.items()))
File "/remote-home/xhwang/anaconda3/envs/alpaca-lora/lib/python3.10/pickle.py", line 998, in _batch_setitems
save(v)
File "/remote-home/xhwang/anaconda3/envs/alpaca-lora/lib/python3.10/site-packages/datasets/utils/py_utils.py", line 691, in save
dill.Pickler.save(self, obj, save_persistent_id=save_persistent_id)
File "/remote-home/xhwang/anaconda3/envs/alpaca-lora/lib/python3.10/site-packages/dill/_dill.py", line 388, in save
StockPickler.save(self, obj, save_persistent_id)
File "/remote-home/xhwang/anaconda3/envs/alpaca-lora/lib/python3.10/pickle.py", line 578, in save
rv = reduce(self.proto)
TypeError: cannot pickle 'ConfigModuleInstance' object
```
### Environment info
- `datasets` version: 2.11.0
- Platform: Linux-4.15.0-156-generic-x86_64-with-glibc2.31
- Python version: 3.10.10
- Huggingface_hub version: 0.13.2
- PyArrow version: 11.0.0
- Pandas version: 1.5.3 | 52 | Cannot pickle error in Dataset.from_generator()
### Describe the bug
I'm trying to use Dataset.from_generator() to generate a large dataset.
### Steps to reproduce the bug
Code to reproduce:
```
from transformers import T5Tokenizer, T5ForConditionalGeneration, GenerationConfig
import torch
from tqdm import tqdm
from datasets import load_dataset
tokenizer = T5Tokenizer.from_pretrained("google/flan-t5-small")
model = T5ForConditionalGeneration.from_pretrained("google/flan-t5-small", device_map="auto")
model = torch.compile(model)
def generate_data(data_loader):
model.eval()
for batch in tqdm(data_loader):
input_ids = tokenizer(batch['instruction'], return_tensors='pt', padding=True, truncation=True).input_ids.to("cuda:0")
with torch.no_grad():
outputs = model.generate(input_ids, generation_config=generation_config)
decoder_hidden_states = outputs.decoder_hidden_states
for i, h in zip(batch['instruction'], decoder_hidden_states):
yield {"instruction": i, "decoder_hidden_states": h}
generation_config = GenerationConfig(
temperature=1,
max_new_tokens=1024,
do_sample=False,
num_return_sequences=1,
return_dict_in_generate=True,
output_scores=True,
output_hidden_states=True,
)
from datasets import Dataset, load_dataset
from torch.utils.data import DataLoader
dataset = load_dataset("HuggingFaceH4/databricks_dolly_15k")
train_loader = DataLoader(dataset['train'], batch_size=2, shuffle=True)
dataset = Dataset.from_generator(generator=generate_data, gen_kwargs={"data_loader": train_loader})
dataset.save_to_disk("data/flant5_small_generation")
```
### Expected behavior
The dataset should be generated and saved.
But the following error occurred:
```
Traceback (most recent call last):
File "/remote-home/xhwang/alpaca-lora/data_collection_t5.py", line 46, in <module>
dataset = Dataset.from_generator(generator=generate_data, gen_kwargs={"data_loader": train_loader})
File "/remote-home/xhwang/anaconda3/envs/alpaca-lora/lib/python3.10/site-packages/datasets/arrow_dataset.py", line 1035, in from_generator
return GeneratorDatasetInputStream(
File "/remote-home/xhwang/anaconda3/envs/alpaca-lora/lib/python3.10/site-packages/datasets/io/generator.py", line 28, in __init__
self.builder = Generator(
File "/remote-home/xhwang/anaconda3/envs/alpaca-lora/lib/python3.10/site-packages/datasets/builder.py", line 336, in __init__
self.config, self.config_id = self._create_builder_config(
File "/remote-home/xhwang/anaconda3/envs/alpaca-lora/lib/python3.10/site-packages/datasets/builder.py", line 505, in _create_builder_config
config_id = builder_config.create_config_id(
File "/remote-home/xhwang/anaconda3/envs/alpaca-lora/lib/python3.10/site-packages/datasets/builder.py", line 179, in create_config_id
suffix = Hasher.hash(config_kwargs_to_add_to_suffix)
File "/remote-home/xhwang/anaconda3/envs/alpaca-lora/lib/python3.10/site-packages/datasets/fingerprint.py", line 236, in hash
return cls.hash_default(value)
File "/remote-home/xhwang/anaconda3/envs/alpaca-lora/lib/python3.10/site-packages/datasets/fingerprint.py", line 229, in hash_default
return cls.hash_bytes(dumps(value))
File "/remote-home/xhwang/anaconda3/envs/alpaca-lora/lib/python3.10/site-packages/datasets/utils/py_utils.py", line 726, in dumps
dump(obj, file)
File "/remote-home/xhwang/anaconda3/envs/alpaca-lora/lib/python3.10/site-packages/datasets/utils/py_utils.py", line 701, in dump
Pickler(file, recurse=True).dump(obj)
File "/remote-home/xhwang/anaconda3/envs/alpaca-lora/lib/python3.10/site-packages/dill/_dill.py", line 394, in dump
StockPickler.dump(self, obj)
File "/remote-home/xhwang/anaconda3/envs/alpaca-lora/lib/python3.10/pickle.py", line 487, in dump
self.save(obj)
File "/remote-home/xhwang/anaconda3/envs/alpaca-lora/lib/python3.10/site-packages/datasets/utils/py_utils.py", line 691, in save
dill.Pickler.save(self, obj, save_persistent_id=save_persistent_id)
File "/remote-home/xhwang/anaconda3/envs/alpaca-lora/lib/python3.10/site-packages/dill/_dill.py", line 388, in save
StockPickler.save(self, obj, save_persistent_id)
File "/remote-home/xhwang/anaconda3/envs/alpaca-lora/lib/python3.10/pickle.py", line 560, in save
f(self, obj) # Call unbound method with explicit self
File "/remote-home/xhwang/anaconda3/envs/alpaca-lora/lib/python3.10/site-packages/dill/_dill.py", line 1186, in save_module_dict
StockPickler.save_dict(pickler, obj)
File "/remote-home/xhwang/anaconda3/envs/alpaca-lora/lib/python3.10/pickle.py", line 972, in save_dict
self._batch_setitems(obj.items())
File "/remote-home/xhwang/anaconda3/envs/alpaca-lora/lib/python3.10/pickle.py", line 998, in _batch_setitems
save(v)
File "/remote-home/xhwang/anaconda3/envs/alpaca-lora/lib/python3.10/site-packages/datasets/utils/py_utils.py", line 691, in save
dill.Pickler.save(self, obj, save_persistent_id=save_persistent_id)
File "/remote-home/xhwang/anaconda3/envs/alpaca-lora/lib/python3.10/site-packages/dill/_dill.py", line 388, in save
StockPickler.save(self, obj, save_persistent_id)
File "/remote-home/xhwang/anaconda3/envs/alpaca-lora/lib/python3.10/pickle.py", line 560, in save
f(self, obj) # Call unbound method with explicit self
File "/remote-home/xhwang/anaconda3/envs/alpaca-lora/lib/python3.10/site-packages/datasets/utils/py_utils.py", line 1311, in save_function
dill._dill._save_with_postproc(
File "/remote-home/xhwang/anaconda3/envs/alpaca-lora/lib/python3.10/site-packages/dill/_dill.py", line 1084, in _save_with_postproc
pickler._batch_setitems(iter(source.items()))
File "/remote-home/xhwang/anaconda3/envs/alpaca-lora/lib/python3.10/pickle.py", line 998, in _batch_setitems
save(v)
File "/remote-home/xhwang/anaconda3/envs/alpaca-lora/lib/python3.10/site-packages/datasets/utils/py_utils.py", line 691, in save
dill.Pickler.save(self, obj, save_persistent_id=save_persistent_id)
File "/remote-home/xhwang/anaconda3/envs/alpaca-lora/lib/python3.10/site-packages/dill/_dill.py", line 388, in save
StockPickler.save(self, obj, save_persistent_id)
File "/remote-home/xhwang/anaconda3/envs/alpaca-lora/lib/python3.10/pickle.py", line 603, in save
self.save_reduce(obj=obj, *rv)
File "/remote-home/xhwang/anaconda3/envs/alpaca-lora/lib/python3.10/pickle.py", line 717, in save_reduce
save(state)
File "/remote-home/xhwang/anaconda3/envs/alpaca-lora/lib/python3.10/site-packages/datasets/utils/py_utils.py", line 691, in save
dill.Pickler.save(self, obj, save_persistent_id=save_persistent_id)
File "/remote-home/xhwang/anaconda3/envs/alpaca-lora/lib/python3.10/site-packages/dill/_dill.py", line 388, in save
StockPickler.save(self, obj, save_persistent_id)
File "/remote-home/xhwang/anaconda3/envs/alpaca-lora/lib/python3.10/pickle.py", line 560, in save
f(self, obj) # Call unbound method with explicit self
File "/remote-home/xhwang/anaconda3/envs/alpaca-lora/lib/python3.10/site-packages/dill/_dill.py", line 1186, in save_module_dict
StockPickler.save_dict(pickler, obj)
File "/remote-home/xhwang/anaconda3/envs/alpaca-lora/lib/python3.10/pickle.py", line 972, in save_dict
self._batch_setitems(obj.items())
File "/remote-home/xhwang/anaconda3/envs/alpaca-lora/lib/python3.10/pickle.py", line 998, in _batch_setitems
save(v)
File "/remote-home/xhwang/anaconda3/envs/alpaca-lora/lib/python3.10/site-packages/datasets/utils/py_utils.py", line 691, in save
dill.Pickler.save(self, obj, save_persistent_id=save_persistent_id)
File "/remote-home/xhwang/anaconda3/envs/alpaca-lora/lib/python3.10/site-packages/dill/_dill.py", line 388, in save
StockPickler.save(self, obj, save_persistent_id)
File "/remote-home/xhwang/anaconda3/envs/alpaca-lora/lib/python3.10/pickle.py", line 603, in save
self.save_reduce(obj=obj, *rv)
File "/remote-home/xhwang/anaconda3/envs/alpaca-lora/lib/python3.10/pickle.py", line 717, in save_reduce
save(state)
File "/remote-home/xhwang/anaconda3/envs/alpaca-lora/lib/python3.10/site-packages/datasets/utils/py_utils.py", line 691, in save
dill.Pickler.save(self, obj, save_persistent_id=save_persistent_id)
File "/remote-home/xhwang/anaconda3/envs/alpaca-lora/lib/python3.10/site-packages/dill/_dill.py", line 388, in save
StockPickler.save(self, obj, save_persistent_id)
File "/remote-home/xhwang/anaconda3/envs/alpaca-lora/lib/python3.10/pickle.py", line 560, in save
f(self, obj) # Call unbound method with explicit self
File "/remote-home/xhwang/anaconda3/envs/alpaca-lora/lib/python3.10/site-packages/dill/_dill.py", line 1186, in save_module_dict
StockPickler.save_dict(pickler, obj)
File "/remote-home/xhwang/anaconda3/envs/alpaca-lora/lib/python3.10/pickle.py", line 972, in save_dict
self._batch_setitems(obj.items())
File "/remote-home/xhwang/anaconda3/envs/alpaca-lora/lib/python3.10/pickle.py", line 998, in _batch_setitems
save(v)
File "/remote-home/xhwang/anaconda3/envs/alpaca-lora/lib/python3.10/site-packages/datasets/utils/py_utils.py", line 691, in save
dill.Pickler.save(self, obj, save_persistent_id=save_persistent_id)
File "/remote-home/xhwang/anaconda3/envs/alpaca-lora/lib/python3.10/site-packages/dill/_dill.py", line 388, in save
StockPickler.save(self, obj, save_persistent_id)
File "/remote-home/xhwang/anaconda3/envs/alpaca-lora/lib/python3.10/pickle.py", line 560, in save
f(self, obj) # Call unbound method with explicit self
File "/remote-home/xhwang/anaconda3/envs/alpaca-lora/lib/python3.10/site-packages/datasets/utils/py_utils.py", line 1311, in save_function
dill._dill._save_with_postproc(
File "/remote-home/xhwang/anaconda3/envs/alpaca-lora/lib/python3.10/site-packages/dill/_dill.py", line 1070, in _save_with_postproc
pickler.save_reduce(*reduction, obj=obj)
File "/remote-home/xhwang/anaconda3/envs/alpaca-lora/lib/python3.10/pickle.py", line 717, in save_reduce
save(state)
File "/remote-home/xhwang/anaconda3/envs/alpaca-lora/lib/python3.10/site-packages/datasets/utils/py_utils.py", line 691, in save
dill.Pickler.save(self, obj, save_persistent_id=save_persistent_id)
File "/remote-home/xhwang/anaconda3/envs/alpaca-lora/lib/python3.10/site-packages/dill/_dill.py", line 388, in save
StockPickler.save(self, obj, save_persistent_id)
File "/remote-home/xhwang/anaconda3/envs/alpaca-lora/lib/python3.10/pickle.py", line 560, in save
f(self, obj) # Call unbound method with explicit self
File "/remote-home/xhwang/anaconda3/envs/alpaca-lora/lib/python3.10/pickle.py", line 887, in save_tuple
save(element)
File "/remote-home/xhwang/anaconda3/envs/alpaca-lora/lib/python3.10/site-packages/datasets/utils/py_utils.py", line 691, in save
dill.Pickler.save(self, obj, save_persistent_id=save_persistent_id)
File "/remote-home/xhwang/anaconda3/envs/alpaca-lora/lib/python3.10/site-packages/dill/_dill.py", line 388, in save
StockPickler.save(self, obj, save_persistent_id)
File "/remote-home/xhwang/anaconda3/envs/alpaca-lora/lib/python3.10/pickle.py", line 560, in save
f(self, obj) # Call unbound method with explicit self
File "/remote-home/xhwang/anaconda3/envs/alpaca-lora/lib/python3.10/site-packages/dill/_dill.py", line 1186, in save_module_dict
StockPickler.save_dict(pickler, obj)
File "/remote-home/xhwang/anaconda3/envs/alpaca-lora/lib/python3.10/pickle.py", line 972, in save_dict
self._batch_setitems(obj.items())
File "/remote-home/xhwang/anaconda3/envs/alpaca-lora/lib/python3.10/pickle.py", line 998, in _batch_setitems
save(v)
File "/remote-home/xhwang/anaconda3/envs/alpaca-lora/lib/python3.10/site-packages/datasets/utils/py_utils.py", line 691, in save
dill.Pickler.save(self, obj, save_persistent_id=save_persistent_id)
File "/remote-home/xhwang/anaconda3/envs/alpaca-lora/lib/python3.10/site-packages/dill/_dill.py", line 388, in save
StockPickler.save(self, obj, save_persistent_id)
File "/remote-home/xhwang/anaconda3/envs/alpaca-lora/lib/python3.10/pickle.py", line 560, in save
f(self, obj) # Call unbound method with explicit self
File "/remote-home/xhwang/anaconda3/envs/alpaca-lora/lib/python3.10/site-packages/datasets/utils/py_utils.py", line 1311, in save_function
dill._dill._save_with_postproc(
File "/remote-home/xhwang/anaconda3/envs/alpaca-lora/lib/python3.10/site-packages/dill/_dill.py", line 1070, in _save_with_postproc
pickler.save_reduce(*reduction, obj=obj)
File "/remote-home/xhwang/anaconda3/envs/alpaca-lora/lib/python3.10/pickle.py", line 717, in save_reduce
save(state)
File "/remote-home/xhwang/anaconda3/envs/alpaca-lora/lib/python3.10/site-packages/datasets/utils/py_utils.py", line 691, in save
dill.Pickler.save(self, obj, save_persistent_id=save_persistent_id)
File "/remote-home/xhwang/anaconda3/envs/alpaca-lora/lib/python3.10/site-packages/dill/_dill.py", line 388, in save
StockPickler.save(self, obj, save_persistent_id)
File "/remote-home/xhwang/anaconda3/envs/alpaca-lora/lib/python3.10/pickle.py", line 560, in save
f(self, obj) # Call unbound method with explicit self
File "/remote-home/xhwang/anaconda3/envs/alpaca-lora/lib/python3.10/pickle.py", line 887, in save_tuple
save(element)
File "/remote-home/xhwang/anaconda3/envs/alpaca-lora/lib/python3.10/site-packages/datasets/utils/py_utils.py", line 691, in save
dill.Pickler.save(self, obj, save_persistent_id=save_persistent_id)
File "/remote-home/xhwang/anaconda3/envs/alpaca-lora/lib/python3.10/site-packages/dill/_dill.py", line 388, in save
StockPickler.save(self, obj, save_persistent_id)
File "/remote-home/xhwang/anaconda3/envs/alpaca-lora/lib/python3.10/pickle.py", line 560, in save
f(self, obj) # Call unbound method with explicit self
File "/remote-home/xhwang/anaconda3/envs/alpaca-lora/lib/python3.10/site-packages/dill/_dill.py", line 1186, in save_module_dict
StockPickler.save_dict(pickler, obj)
File "/remote-home/xhwang/anaconda3/envs/alpaca-lora/lib/python3.10/pickle.py", line 972, in save_dict
self._batch_setitems(obj.items())
File "/remote-home/xhwang/anaconda3/envs/alpaca-lora/lib/python3.10/pickle.py", line 1003, in _batch_setitems
save(v)
File "/remote-home/xhwang/anaconda3/envs/alpaca-lora/lib/python3.10/site-packages/datasets/utils/py_utils.py", line 691, in save
dill.Pickler.save(self, obj, save_persistent_id=save_persistent_id)
File "/remote-home/xhwang/anaconda3/envs/alpaca-lora/lib/python3.10/site-packages/dill/_dill.py", line 388, in save
StockPickler.save(self, obj, save_persistent_id)
File "/remote-home/xhwang/anaconda3/envs/alpaca-lora/lib/python3.10/pickle.py", line 560, in save
f(self, obj) # Call unbound method with explicit self
File "/remote-home/xhwang/anaconda3/envs/alpaca-lora/lib/python3.10/site-packages/datasets/utils/py_utils.py", line 1311, in save_function
dill._dill._save_with_postproc(
File "/remote-home/xhwang/anaconda3/envs/alpaca-lora/lib/python3.10/site-packages/dill/_dill.py", line 1084, in _save_with_postproc
pickler._batch_setitems(iter(source.items()))
File "/remote-home/xhwang/anaconda3/envs/alpaca-lora/lib/python3.10/pickle.py", line 998, in _batch_setitems
save(v)
File "/remote-home/xhwang/anaconda3/envs/alpaca-lora/lib/python3.10/site-packages/datasets/utils/py_utils.py", line 691, in save
dill.Pickler.save(self, obj, save_persistent_id=save_persistent_id)
File "/remote-home/xhwang/anaconda3/envs/alpaca-lora/lib/python3.10/site-packages/dill/_dill.py", line 388, in save
StockPickler.save(self, obj, save_persistent_id)
File "/remote-home/xhwang/anaconda3/envs/alpaca-lora/lib/python3.10/pickle.py", line 578, in save
rv = reduce(self.proto)
TypeError: cannot pickle 'ConfigModuleInstance' object
```
### Environment info
- `datasets` version: 2.11.0
- Platform: Linux-4.15.0-156-generic-x86_64-with-glibc2.31
- Python version: 3.10.10
- Huggingface_hub version: 0.13.2
- PyArrow version: 11.0.0
- Pandas version: 1.5.3
> Hi! It should work if you put `model = torch.compile(model)` inside the `generate_data` function. If a referenced object is outside, it needs to be pickable, and that's not the case for the compiled models (or functions).
Hi! Thank you for your reply! Everything works perfectly with your suggestion!
Closing the issue.
|
https://github.com/huggingface/datasets/issues/5818 | Ability to update a dataset | In this case, I think we can avoid the `PermissionError` by unpacking the underlying `ConcatenationTable` and saving only the newly added data blocks (in new files). | ### Feature request
The ability to load a dataset, add or change something, and save it back to disk.
Maybe it's possible, but I can't work out how to do it, e.g. this fails:
```py
import datasets
dataset = datasets.load_from_disk("data/test1")
dataset = dataset.add_item({"text": "A new item"})
dataset.save_to_disk("data/test1")
```
With the error:
```
PermissionError: Tried to overwrite /mnt/c/Users/david/py/learning/mini_projects/data_sorting_and_filtering/data/test1 but a dataset can't overwrite itself.
```
### Motivation
My use case is that I want to process a dataset in a particular way but it doesn't fit in memory if I do it in one go. So I want to perform a loop and at each step in the loop, process one shard and append it to an ever-growing dataset. The code in the loop will load a dataset, add some rows, then save it again.
Maybe I'm just thinking about things incorrectly and there's a better approach. FWIW I can't use `dataset.map()` to do the task because that doesn't work with `num_proc` when adding rows, so is confined to a single process which is too slow.
The only other way I can think of is to create a new file each time, but surely that's not how people do this sort of thing.
### Your contribution
na | 26 | Ability to update a dataset
### Feature request
The ability to load a dataset, add or change something, and save it back to disk.
Maybe it's possible, but I can't work out how to do it, e.g. this fails:
```py
import datasets
dataset = datasets.load_from_disk("data/test1")
dataset = dataset.add_item({"text": "A new item"})
dataset.save_to_disk("data/test1")
```
With the error:
```
PermissionError: Tried to overwrite /mnt/c/Users/david/py/learning/mini_projects/data_sorting_and_filtering/data/test1 but a dataset can't overwrite itself.
```
### Motivation
My use case is that I want to process a dataset in a particular way but it doesn't fit in memory if I do it in one go. So I want to perform a loop and at each step in the loop, process one shard and append it to an ever-growing dataset. The code in the loop will load a dataset, add some rows, then save it again.
Maybe I'm just thinking about things incorrectly and there's a better approach. FWIW I can't use `dataset.map()` to do the task because that doesn't work with `num_proc` when adding rows, so is confined to a single process which is too slow.
The only other way I can think of is to create a new file each time, but surely that's not how people do this sort of thing.
### Your contribution
na
In this case, I think we can avoid the `PermissionError` by unpacking the underlying `ConcatenationTable` and saving only the newly added data blocks (in new files). |
https://github.com/huggingface/datasets/issues/5818 | Ability to update a dataset | Thanks @stevhliu and @mariosasko , so saving to individual files then loading them later, concatenating again and saving again is the recommended way. Good to know.
Question that I hope doesn't sound rude: is this sort of thing (processing a dataset that doesn't fit in memory) outside of `datasets`'s core area of focus? Are there other tools you would recommend to do this sort of thing that play nice with `datasets`? Or is it just that I've found myself in a niche situation that hasn't specifically been catered for? | ### Feature request
The ability to load a dataset, add or change something, and save it back to disk.
Maybe it's possible, but I can't work out how to do it, e.g. this fails:
```py
import datasets
dataset = datasets.load_from_disk("data/test1")
dataset = dataset.add_item({"text": "A new item"})
dataset.save_to_disk("data/test1")
```
With the error:
```
PermissionError: Tried to overwrite /mnt/c/Users/david/py/learning/mini_projects/data_sorting_and_filtering/data/test1 but a dataset can't overwrite itself.
```
### Motivation
My use case is that I want to process a dataset in a particular way but it doesn't fit in memory if I do it in one go. So I want to perform a loop and at each step in the loop, process one shard and append it to an ever-growing dataset. The code in the loop will load a dataset, add some rows, then save it again.
Maybe I'm just thinking about things incorrectly and there's a better approach. FWIW I can't use `dataset.map()` to do the task because that doesn't work with `num_proc` when adding rows, so is confined to a single process which is too slow.
The only other way I can think of is to create a new file each time, but surely that's not how people do this sort of thing.
### Your contribution
na | 89 | Ability to update a dataset
### Feature request
The ability to load a dataset, add or change something, and save it back to disk.
Maybe it's possible, but I can't work out how to do it, e.g. this fails:
```py
import datasets
dataset = datasets.load_from_disk("data/test1")
dataset = dataset.add_item({"text": "A new item"})
dataset.save_to_disk("data/test1")
```
With the error:
```
PermissionError: Tried to overwrite /mnt/c/Users/david/py/learning/mini_projects/data_sorting_and_filtering/data/test1 but a dataset can't overwrite itself.
```
### Motivation
My use case is that I want to process a dataset in a particular way but it doesn't fit in memory if I do it in one go. So I want to perform a loop and at each step in the loop, process one shard and append it to an ever-growing dataset. The code in the loop will load a dataset, add some rows, then save it again.
Maybe I'm just thinking about things incorrectly and there's a better approach. FWIW I can't use `dataset.map()` to do the task because that doesn't work with `num_proc` when adding rows, so is confined to a single process which is too slow.
The only other way I can think of is to create a new file each time, but surely that's not how people do this sort of thing.
### Your contribution
na
Thanks @stevhliu and @mariosasko , so saving to individual files then loading them later, concatenating again and saving again is the recommended way. Good to know.
Question that I hope doesn't sound rude: is this sort of thing (processing a dataset that doesn't fit in memory) outside of `datasets`'s core area of focus? Are there other tools you would recommend to do this sort of thing that play nice with `datasets`? Or is it just that I've found myself in a niche situation that hasn't specifically been catered for? |
https://github.com/huggingface/datasets/issues/5817 | Setting `num_proc` errors when `.map` returns additional items. | Hi ! Unfortunately I couldn't reproduce on my side locally and with datasets 2.11 and python 3.10.11 on colab.
What version of `multiprocess` are you using ? | ### Describe the bug
I'm using a map function that returns more rows than are passed in.
If I try to use `num_proc` I get:
```
File "/home/davidg/.virtualenvs/learning/lib/python3.10/site-packages/datasets/arrow_dataset.py", line 563, in wrapper
out: Union["Dataset", "DatasetDict"] = func(self, *args, **kwargs)
File "/home/davidg/.virtualenvs/learning/lib/python3.10/site-packages/datasets/arrow_dataset.py", line 528, in wrapper
out: Union["Dataset", "DatasetDict"] = func(self, *args, **kwargs)
File "/home/davidg/.virtualenvs/learning/lib/python3.10/site-packages/datasets/arrow_dataset.py", line 3097, in map
for rank, done, content in iflatmap_unordered(
File "/home/davidg/.virtualenvs/learning/lib/python3.10/site-packages/datasets/utils/py_utils.py", line 1372, in iflatmap_unordered
yield queue.get(timeout=0.05)
File "<string>", line 2, in get
File "/home/davidg/.virtualenvs/learning/lib/python3.10/site-packages/multiprocess/managers.py", line 818, in _callmethod
kind, result = conn.recv()
File "/home/davidg/.virtualenvs/learning/lib/python3.10/site-packages/multiprocess/connection.py", line 258, in recv
buf = self._recv_bytes()
File "/home/davidg/.virtualenvs/learning/lib/python3.10/site-packages/multiprocess/connection.py", line 422, in _recv_bytes
buf = self._recv(4)
File "/home/davidg/.virtualenvs/learning/lib/python3.10/site-packages/multiprocess/connection.py", line 391, in _recv
raise EOFError
EOFError
```
### Steps to reproduce the bug
This is copied from the [Datasets docs](https://huggingface.co/docs/datasets/v2.12.0/en/process#batch-processing), with `num_proc` added, and will error.
```py
import datasets
dataset = ... # any old dataset
def chunk_examples(examples):
chunks = []
for sentence in examples["text"]:
chunks += [sentence[i : i + 50] for i in range(0, len(sentence), 50)]
return {"chunks": chunks}
chunked_dataset = dataset.map(
chunk_examples,
batched=True,
remove_columns=dataset.column_names,
num_proc=2, # Remove and it works
)
```
### Expected behavior
Should work fine. On a related note, multi-processing also fails if there is a Meta class anywhere in scope (and there are plenty in the standard library). This is the fault of `dill` and is a long standing issue.
Have you considered using Loky for multiprocessing? I've found that the built-in `datasets` multi-processing breaks more than it works so have written my own function using `loky`, for reference:
```py
import datasets
import loky
def fast_loop(dataset: datasets.Dataset, func, num_proc=None):
if num_proc is None:
import os
num_proc = len(os.sched_getaffinity(0))
shards = [
dataset.shard(num_shards=num_proc, index=i, contiguous=True)
for i in range(num_proc)
]
executor = loky.get_reusable_executor(max_workers=num_proc)
results = executor.map(func, shards)
return datasets.combine.concatenate_datasets(list(results))
```
### Environment info
- `datasets` version: 2.11.0
- Platform: Linux-5.15.90.1-microsoft-standard-WSL2-x86_64-with-glibc2.31
- Python version: 3.10.8
- Huggingface_hub version: 0.12.1
- PyArrow version: 11.0.0
- Pandas version: 2.0.1 | 27 | Setting `num_proc` errors when `.map` returns additional items.
### Describe the bug
I'm using a map function that returns more rows than are passed in.
If I try to use `num_proc` I get:
```
File "/home/davidg/.virtualenvs/learning/lib/python3.10/site-packages/datasets/arrow_dataset.py", line 563, in wrapper
out: Union["Dataset", "DatasetDict"] = func(self, *args, **kwargs)
File "/home/davidg/.virtualenvs/learning/lib/python3.10/site-packages/datasets/arrow_dataset.py", line 528, in wrapper
out: Union["Dataset", "DatasetDict"] = func(self, *args, **kwargs)
File "/home/davidg/.virtualenvs/learning/lib/python3.10/site-packages/datasets/arrow_dataset.py", line 3097, in map
for rank, done, content in iflatmap_unordered(
File "/home/davidg/.virtualenvs/learning/lib/python3.10/site-packages/datasets/utils/py_utils.py", line 1372, in iflatmap_unordered
yield queue.get(timeout=0.05)
File "<string>", line 2, in get
File "/home/davidg/.virtualenvs/learning/lib/python3.10/site-packages/multiprocess/managers.py", line 818, in _callmethod
kind, result = conn.recv()
File "/home/davidg/.virtualenvs/learning/lib/python3.10/site-packages/multiprocess/connection.py", line 258, in recv
buf = self._recv_bytes()
File "/home/davidg/.virtualenvs/learning/lib/python3.10/site-packages/multiprocess/connection.py", line 422, in _recv_bytes
buf = self._recv(4)
File "/home/davidg/.virtualenvs/learning/lib/python3.10/site-packages/multiprocess/connection.py", line 391, in _recv
raise EOFError
EOFError
```
### Steps to reproduce the bug
This is copied from the [Datasets docs](https://huggingface.co/docs/datasets/v2.12.0/en/process#batch-processing), with `num_proc` added, and will error.
```py
import datasets
dataset = ... # any old dataset
def chunk_examples(examples):
chunks = []
for sentence in examples["text"]:
chunks += [sentence[i : i + 50] for i in range(0, len(sentence), 50)]
return {"chunks": chunks}
chunked_dataset = dataset.map(
chunk_examples,
batched=True,
remove_columns=dataset.column_names,
num_proc=2, # Remove and it works
)
```
### Expected behavior
Should work fine. On a related note, multi-processing also fails if there is a Meta class anywhere in scope (and there are plenty in the standard library). This is the fault of `dill` and is a long standing issue.
Have you considered using Loky for multiprocessing? I've found that the built-in `datasets` multi-processing breaks more than it works so have written my own function using `loky`, for reference:
```py
import datasets
import loky
def fast_loop(dataset: datasets.Dataset, func, num_proc=None):
if num_proc is None:
import os
num_proc = len(os.sched_getaffinity(0))
shards = [
dataset.shard(num_shards=num_proc, index=i, contiguous=True)
for i in range(num_proc)
]
executor = loky.get_reusable_executor(max_workers=num_proc)
results = executor.map(func, shards)
return datasets.combine.concatenate_datasets(list(results))
```
### Environment info
- `datasets` version: 2.11.0
- Platform: Linux-5.15.90.1-microsoft-standard-WSL2-x86_64-with-glibc2.31
- Python version: 3.10.8
- Huggingface_hub version: 0.12.1
- PyArrow version: 11.0.0
- Pandas version: 2.0.1
Hi ! Unfortunately I couldn't reproduce on my side locally and with datasets 2.11 and python 3.10.11 on colab.
What version of `multiprocess` are you using ? |
https://github.com/huggingface/datasets/issues/5817 | Setting `num_proc` errors when `.map` returns additional items. | I've got `multiprocess` version `0.70.14`.
I've done some more testing and the error only occurs in PyCharm's Python Console. It seems to be [this PyCharm bug](https://youtrack.jetbrains.com/issue/PY-51922/Multiprocessing-bug.-Can-only-run-in-debugger.), I'll close this. | ### Describe the bug
I'm using a map function that returns more rows than are passed in.
If I try to use `num_proc` I get:
```
File "/home/davidg/.virtualenvs/learning/lib/python3.10/site-packages/datasets/arrow_dataset.py", line 563, in wrapper
out: Union["Dataset", "DatasetDict"] = func(self, *args, **kwargs)
File "/home/davidg/.virtualenvs/learning/lib/python3.10/site-packages/datasets/arrow_dataset.py", line 528, in wrapper
out: Union["Dataset", "DatasetDict"] = func(self, *args, **kwargs)
File "/home/davidg/.virtualenvs/learning/lib/python3.10/site-packages/datasets/arrow_dataset.py", line 3097, in map
for rank, done, content in iflatmap_unordered(
File "/home/davidg/.virtualenvs/learning/lib/python3.10/site-packages/datasets/utils/py_utils.py", line 1372, in iflatmap_unordered
yield queue.get(timeout=0.05)
File "<string>", line 2, in get
File "/home/davidg/.virtualenvs/learning/lib/python3.10/site-packages/multiprocess/managers.py", line 818, in _callmethod
kind, result = conn.recv()
File "/home/davidg/.virtualenvs/learning/lib/python3.10/site-packages/multiprocess/connection.py", line 258, in recv
buf = self._recv_bytes()
File "/home/davidg/.virtualenvs/learning/lib/python3.10/site-packages/multiprocess/connection.py", line 422, in _recv_bytes
buf = self._recv(4)
File "/home/davidg/.virtualenvs/learning/lib/python3.10/site-packages/multiprocess/connection.py", line 391, in _recv
raise EOFError
EOFError
```
### Steps to reproduce the bug
This is copied from the [Datasets docs](https://huggingface.co/docs/datasets/v2.12.0/en/process#batch-processing), with `num_proc` added, and will error.
```py
import datasets
dataset = ... # any old dataset
def chunk_examples(examples):
chunks = []
for sentence in examples["text"]:
chunks += [sentence[i : i + 50] for i in range(0, len(sentence), 50)]
return {"chunks": chunks}
chunked_dataset = dataset.map(
chunk_examples,
batched=True,
remove_columns=dataset.column_names,
num_proc=2, # Remove and it works
)
```
### Expected behavior
Should work fine. On a related note, multi-processing also fails if there is a Meta class anywhere in scope (and there are plenty in the standard library). This is the fault of `dill` and is a long standing issue.
Have you considered using Loky for multiprocessing? I've found that the built-in `datasets` multi-processing breaks more than it works so have written my own function using `loky`, for reference:
```py
import datasets
import loky
def fast_loop(dataset: datasets.Dataset, func, num_proc=None):
if num_proc is None:
import os
num_proc = len(os.sched_getaffinity(0))
shards = [
dataset.shard(num_shards=num_proc, index=i, contiguous=True)
for i in range(num_proc)
]
executor = loky.get_reusable_executor(max_workers=num_proc)
results = executor.map(func, shards)
return datasets.combine.concatenate_datasets(list(results))
```
### Environment info
- `datasets` version: 2.11.0
- Platform: Linux-5.15.90.1-microsoft-standard-WSL2-x86_64-with-glibc2.31
- Python version: 3.10.8
- Huggingface_hub version: 0.12.1
- PyArrow version: 11.0.0
- Pandas version: 2.0.1 | 29 | Setting `num_proc` errors when `.map` returns additional items.
### Describe the bug
I'm using a map function that returns more rows than are passed in.
If I try to use `num_proc` I get:
```
File "/home/davidg/.virtualenvs/learning/lib/python3.10/site-packages/datasets/arrow_dataset.py", line 563, in wrapper
out: Union["Dataset", "DatasetDict"] = func(self, *args, **kwargs)
File "/home/davidg/.virtualenvs/learning/lib/python3.10/site-packages/datasets/arrow_dataset.py", line 528, in wrapper
out: Union["Dataset", "DatasetDict"] = func(self, *args, **kwargs)
File "/home/davidg/.virtualenvs/learning/lib/python3.10/site-packages/datasets/arrow_dataset.py", line 3097, in map
for rank, done, content in iflatmap_unordered(
File "/home/davidg/.virtualenvs/learning/lib/python3.10/site-packages/datasets/utils/py_utils.py", line 1372, in iflatmap_unordered
yield queue.get(timeout=0.05)
File "<string>", line 2, in get
File "/home/davidg/.virtualenvs/learning/lib/python3.10/site-packages/multiprocess/managers.py", line 818, in _callmethod
kind, result = conn.recv()
File "/home/davidg/.virtualenvs/learning/lib/python3.10/site-packages/multiprocess/connection.py", line 258, in recv
buf = self._recv_bytes()
File "/home/davidg/.virtualenvs/learning/lib/python3.10/site-packages/multiprocess/connection.py", line 422, in _recv_bytes
buf = self._recv(4)
File "/home/davidg/.virtualenvs/learning/lib/python3.10/site-packages/multiprocess/connection.py", line 391, in _recv
raise EOFError
EOFError
```
### Steps to reproduce the bug
This is copied from the [Datasets docs](https://huggingface.co/docs/datasets/v2.12.0/en/process#batch-processing), with `num_proc` added, and will error.
```py
import datasets
dataset = ... # any old dataset
def chunk_examples(examples):
chunks = []
for sentence in examples["text"]:
chunks += [sentence[i : i + 50] for i in range(0, len(sentence), 50)]
return {"chunks": chunks}
chunked_dataset = dataset.map(
chunk_examples,
batched=True,
remove_columns=dataset.column_names,
num_proc=2, # Remove and it works
)
```
### Expected behavior
Should work fine. On a related note, multi-processing also fails if there is a Meta class anywhere in scope (and there are plenty in the standard library). This is the fault of `dill` and is a long standing issue.
Have you considered using Loky for multiprocessing? I've found that the built-in `datasets` multi-processing breaks more than it works so have written my own function using `loky`, for reference:
```py
import datasets
import loky
def fast_loop(dataset: datasets.Dataset, func, num_proc=None):
if num_proc is None:
import os
num_proc = len(os.sched_getaffinity(0))
shards = [
dataset.shard(num_shards=num_proc, index=i, contiguous=True)
for i in range(num_proc)
]
executor = loky.get_reusable_executor(max_workers=num_proc)
results = executor.map(func, shards)
return datasets.combine.concatenate_datasets(list(results))
```
### Environment info
- `datasets` version: 2.11.0
- Platform: Linux-5.15.90.1-microsoft-standard-WSL2-x86_64-with-glibc2.31
- Python version: 3.10.8
- Huggingface_hub version: 0.12.1
- PyArrow version: 11.0.0
- Pandas version: 2.0.1
I've got `multiprocess` version `0.70.14`.
I've done some more testing and the error only occurs in PyCharm's Python Console. It seems to be [this PyCharm bug](https://youtrack.jetbrains.com/issue/PY-51922/Multiprocessing-bug.-Can-only-run-in-debugger.), I'll close this. |
https://github.com/huggingface/datasets/issues/5817 | Setting `num_proc` errors when `.map` returns additional items. | For other users facing this, my workaround is to conditionally set `num_proc` so I can work interactively in the PyCharm Python Console while developing, then when I'm ready to run on the whole dataset, run it as a script and use multiprocessing.
```py
mapped_ds = ds.map(
my_map_function,
batched=True,
remove_columns=ds.column_names,
num_proc=1 if "PYCHARM_HOSTED" in os.environ else 8,
)
``` | ### Describe the bug
I'm using a map function that returns more rows than are passed in.
If I try to use `num_proc` I get:
```
File "/home/davidg/.virtualenvs/learning/lib/python3.10/site-packages/datasets/arrow_dataset.py", line 563, in wrapper
out: Union["Dataset", "DatasetDict"] = func(self, *args, **kwargs)
File "/home/davidg/.virtualenvs/learning/lib/python3.10/site-packages/datasets/arrow_dataset.py", line 528, in wrapper
out: Union["Dataset", "DatasetDict"] = func(self, *args, **kwargs)
File "/home/davidg/.virtualenvs/learning/lib/python3.10/site-packages/datasets/arrow_dataset.py", line 3097, in map
for rank, done, content in iflatmap_unordered(
File "/home/davidg/.virtualenvs/learning/lib/python3.10/site-packages/datasets/utils/py_utils.py", line 1372, in iflatmap_unordered
yield queue.get(timeout=0.05)
File "<string>", line 2, in get
File "/home/davidg/.virtualenvs/learning/lib/python3.10/site-packages/multiprocess/managers.py", line 818, in _callmethod
kind, result = conn.recv()
File "/home/davidg/.virtualenvs/learning/lib/python3.10/site-packages/multiprocess/connection.py", line 258, in recv
buf = self._recv_bytes()
File "/home/davidg/.virtualenvs/learning/lib/python3.10/site-packages/multiprocess/connection.py", line 422, in _recv_bytes
buf = self._recv(4)
File "/home/davidg/.virtualenvs/learning/lib/python3.10/site-packages/multiprocess/connection.py", line 391, in _recv
raise EOFError
EOFError
```
### Steps to reproduce the bug
This is copied from the [Datasets docs](https://huggingface.co/docs/datasets/v2.12.0/en/process#batch-processing), with `num_proc` added, and will error.
```py
import datasets
dataset = ... # any old dataset
def chunk_examples(examples):
chunks = []
for sentence in examples["text"]:
chunks += [sentence[i : i + 50] for i in range(0, len(sentence), 50)]
return {"chunks": chunks}
chunked_dataset = dataset.map(
chunk_examples,
batched=True,
remove_columns=dataset.column_names,
num_proc=2, # Remove and it works
)
```
### Expected behavior
Should work fine. On a related note, multi-processing also fails if there is a Meta class anywhere in scope (and there are plenty in the standard library). This is the fault of `dill` and is a long standing issue.
Have you considered using Loky for multiprocessing? I've found that the built-in `datasets` multi-processing breaks more than it works so have written my own function using `loky`, for reference:
```py
import datasets
import loky
def fast_loop(dataset: datasets.Dataset, func, num_proc=None):
if num_proc is None:
import os
num_proc = len(os.sched_getaffinity(0))
shards = [
dataset.shard(num_shards=num_proc, index=i, contiguous=True)
for i in range(num_proc)
]
executor = loky.get_reusable_executor(max_workers=num_proc)
results = executor.map(func, shards)
return datasets.combine.concatenate_datasets(list(results))
```
### Environment info
- `datasets` version: 2.11.0
- Platform: Linux-5.15.90.1-microsoft-standard-WSL2-x86_64-with-glibc2.31
- Python version: 3.10.8
- Huggingface_hub version: 0.12.1
- PyArrow version: 11.0.0
- Pandas version: 2.0.1 | 58 | Setting `num_proc` errors when `.map` returns additional items.
### Describe the bug
I'm using a map function that returns more rows than are passed in.
If I try to use `num_proc` I get:
```
File "/home/davidg/.virtualenvs/learning/lib/python3.10/site-packages/datasets/arrow_dataset.py", line 563, in wrapper
out: Union["Dataset", "DatasetDict"] = func(self, *args, **kwargs)
File "/home/davidg/.virtualenvs/learning/lib/python3.10/site-packages/datasets/arrow_dataset.py", line 528, in wrapper
out: Union["Dataset", "DatasetDict"] = func(self, *args, **kwargs)
File "/home/davidg/.virtualenvs/learning/lib/python3.10/site-packages/datasets/arrow_dataset.py", line 3097, in map
for rank, done, content in iflatmap_unordered(
File "/home/davidg/.virtualenvs/learning/lib/python3.10/site-packages/datasets/utils/py_utils.py", line 1372, in iflatmap_unordered
yield queue.get(timeout=0.05)
File "<string>", line 2, in get
File "/home/davidg/.virtualenvs/learning/lib/python3.10/site-packages/multiprocess/managers.py", line 818, in _callmethod
kind, result = conn.recv()
File "/home/davidg/.virtualenvs/learning/lib/python3.10/site-packages/multiprocess/connection.py", line 258, in recv
buf = self._recv_bytes()
File "/home/davidg/.virtualenvs/learning/lib/python3.10/site-packages/multiprocess/connection.py", line 422, in _recv_bytes
buf = self._recv(4)
File "/home/davidg/.virtualenvs/learning/lib/python3.10/site-packages/multiprocess/connection.py", line 391, in _recv
raise EOFError
EOFError
```
### Steps to reproduce the bug
This is copied from the [Datasets docs](https://huggingface.co/docs/datasets/v2.12.0/en/process#batch-processing), with `num_proc` added, and will error.
```py
import datasets
dataset = ... # any old dataset
def chunk_examples(examples):
chunks = []
for sentence in examples["text"]:
chunks += [sentence[i : i + 50] for i in range(0, len(sentence), 50)]
return {"chunks": chunks}
chunked_dataset = dataset.map(
chunk_examples,
batched=True,
remove_columns=dataset.column_names,
num_proc=2, # Remove and it works
)
```
### Expected behavior
Should work fine. On a related note, multi-processing also fails if there is a Meta class anywhere in scope (and there are plenty in the standard library). This is the fault of `dill` and is a long standing issue.
Have you considered using Loky for multiprocessing? I've found that the built-in `datasets` multi-processing breaks more than it works so have written my own function using `loky`, for reference:
```py
import datasets
import loky
def fast_loop(dataset: datasets.Dataset, func, num_proc=None):
if num_proc is None:
import os
num_proc = len(os.sched_getaffinity(0))
shards = [
dataset.shard(num_shards=num_proc, index=i, contiguous=True)
for i in range(num_proc)
]
executor = loky.get_reusable_executor(max_workers=num_proc)
results = executor.map(func, shards)
return datasets.combine.concatenate_datasets(list(results))
```
### Environment info
- `datasets` version: 2.11.0
- Platform: Linux-5.15.90.1-microsoft-standard-WSL2-x86_64-with-glibc2.31
- Python version: 3.10.8
- Huggingface_hub version: 0.12.1
- PyArrow version: 11.0.0
- Pandas version: 2.0.1
For other users facing this, my workaround is to conditionally set `num_proc` so I can work interactively in the PyCharm Python Console while developing, then when I'm ready to run on the whole dataset, run it as a script and use multiprocessing.
```py
mapped_ds = ds.map(
my_map_function,
batched=True,
remove_columns=ds.column_names,
num_proc=1 if "PYCHARM_HOSTED" in os.environ else 8,
)
``` |
https://github.com/huggingface/datasets/issues/5815 | Easy way to create a Kaggle dataset from a Huggingface dataset? | Hi @hrbigelow , I'm no expert for such a question so I'll ping @lhoestq from the `datasets` library (also this issue could be moved there if someone with permission can do it :) ) | I'm not sure whether this is more appropriately addressed with HuggingFace or Kaggle. I would like to somehow directly create a Kaggle dataset from a HuggingFace Dataset.
While Kaggle does provide the option to create a dataset from a URI, that URI must point to a single file. For example:
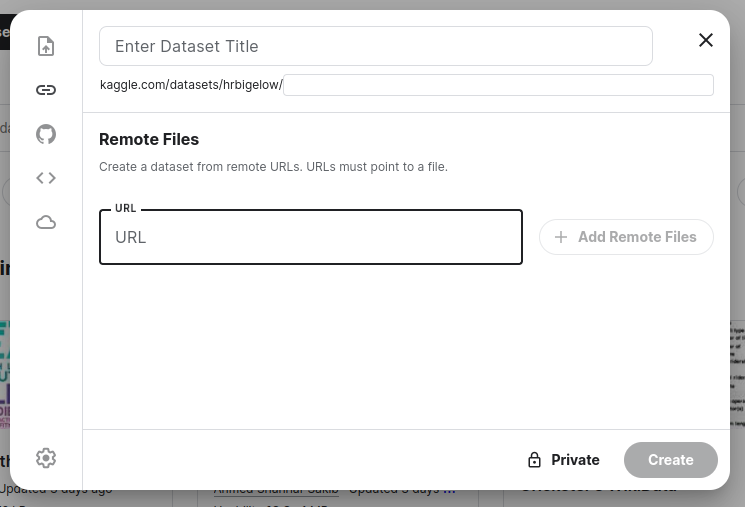
Is there some mechanism from huggingface to represent a dataset (such as that from `load_dataset('wmt14', 'de-en', split='train')` as a single file? Or, some other way to get that into a Kaggle dataset so that I can use the huggingface `datasets` module to process and consume it inside of a Kaggle notebook?
Thanks in advance!
| 34 | Easy way to create a Kaggle dataset from a Huggingface dataset?
I'm not sure whether this is more appropriately addressed with HuggingFace or Kaggle. I would like to somehow directly create a Kaggle dataset from a HuggingFace Dataset.
While Kaggle does provide the option to create a dataset from a URI, that URI must point to a single file. For example:
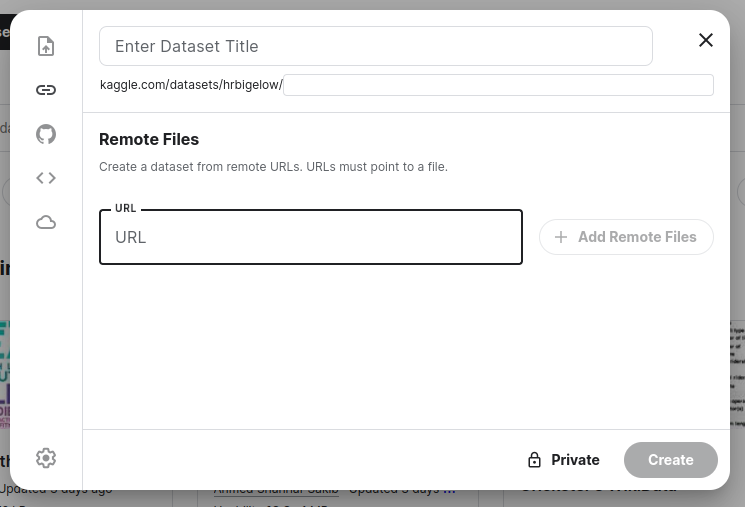
Is there some mechanism from huggingface to represent a dataset (such as that from `load_dataset('wmt14', 'de-en', split='train')` as a single file? Or, some other way to get that into a Kaggle dataset so that I can use the huggingface `datasets` module to process and consume it inside of a Kaggle notebook?
Thanks in advance!
Hi @hrbigelow , I'm no expert for such a question so I'll ping @lhoestq from the `datasets` library (also this issue could be moved there if someone with permission can do it :) ) |
https://github.com/huggingface/datasets/issues/5815 | Easy way to create a Kaggle dataset from a Huggingface dataset? | Hi ! Many datasets are made of several files, and how they are parsed often requires a python script. Because of that, datasets like wmt14 are not available as a single file on HF. Though you can create this file using `datasets`:
```python
from datasets import load_dataset
ds = load_dataset("wmt14", "de-en", split="train")
ds.to_json("wmt14-train.json")
# OR to parquet, which is compressed:
# ds.to_parquet("wmt14-train.parquet")
```
We are also working on providing parquet exports for all datasets, but wmt14 is not supported yet (we're rolling it out for datasets <1GB first). They're usually available in the `refs/convert/parquet` branch (empty for wmt14):
<img width="267" alt="image" src="https://user-images.githubusercontent.com/42851186/235878909-7339f5a4-be19-4ada-85d8-8a50d23acf35.png">
| I'm not sure whether this is more appropriately addressed with HuggingFace or Kaggle. I would like to somehow directly create a Kaggle dataset from a HuggingFace Dataset.
While Kaggle does provide the option to create a dataset from a URI, that URI must point to a single file. For example:
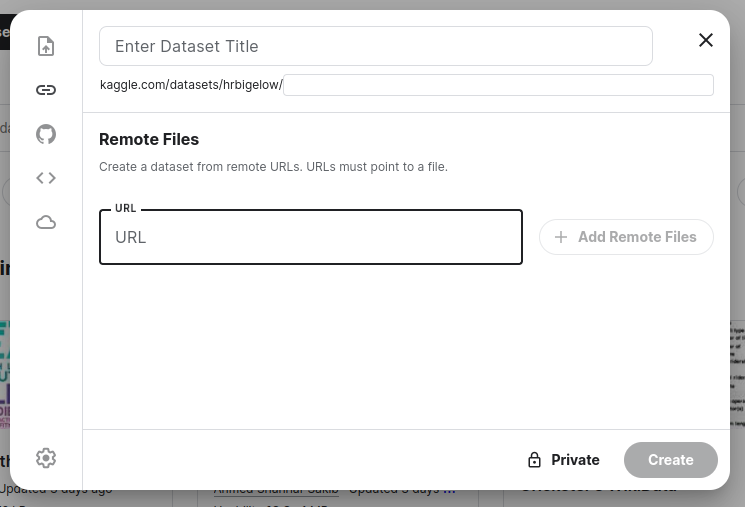
Is there some mechanism from huggingface to represent a dataset (such as that from `load_dataset('wmt14', 'de-en', split='train')` as a single file? Or, some other way to get that into a Kaggle dataset so that I can use the huggingface `datasets` module to process and consume it inside of a Kaggle notebook?
Thanks in advance!
| 102 | Easy way to create a Kaggle dataset from a Huggingface dataset?
I'm not sure whether this is more appropriately addressed with HuggingFace or Kaggle. I would like to somehow directly create a Kaggle dataset from a HuggingFace Dataset.
While Kaggle does provide the option to create a dataset from a URI, that URI must point to a single file. For example:
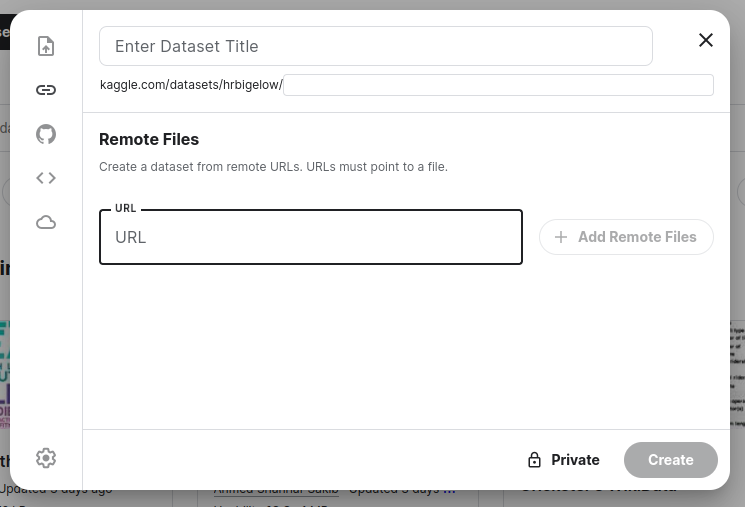
Is there some mechanism from huggingface to represent a dataset (such as that from `load_dataset('wmt14', 'de-en', split='train')` as a single file? Or, some other way to get that into a Kaggle dataset so that I can use the huggingface `datasets` module to process and consume it inside of a Kaggle notebook?
Thanks in advance!
Hi ! Many datasets are made of several files, and how they are parsed often requires a python script. Because of that, datasets like wmt14 are not available as a single file on HF. Though you can create this file using `datasets`:
```python
from datasets import load_dataset
ds = load_dataset("wmt14", "de-en", split="train")
ds.to_json("wmt14-train.json")
# OR to parquet, which is compressed:
# ds.to_parquet("wmt14-train.parquet")
```
We are also working on providing parquet exports for all datasets, but wmt14 is not supported yet (we're rolling it out for datasets <1GB first). They're usually available in the `refs/convert/parquet` branch (empty for wmt14):
<img width="267" alt="image" src="https://user-images.githubusercontent.com/42851186/235878909-7339f5a4-be19-4ada-85d8-8a50d23acf35.png">
|
https://github.com/huggingface/datasets/issues/5815 | Easy way to create a Kaggle dataset from a Huggingface dataset? | I've requested support for creating a Kaggle dataset from an imported HF dataset repo on their "forum" here: https://www.kaggle.com/discussions/product-feedback/427142 (upvotes appreciated π) | I'm not sure whether this is more appropriately addressed with HuggingFace or Kaggle. I would like to somehow directly create a Kaggle dataset from a HuggingFace Dataset.
While Kaggle does provide the option to create a dataset from a URI, that URI must point to a single file. For example:
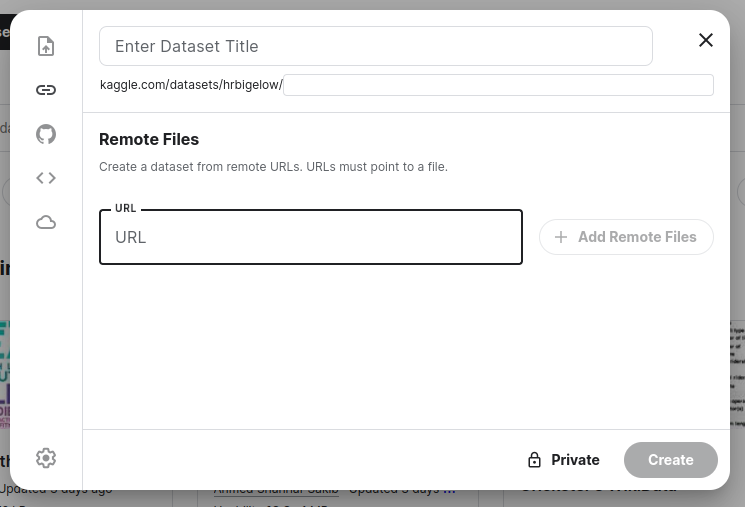
Is there some mechanism from huggingface to represent a dataset (such as that from `load_dataset('wmt14', 'de-en', split='train')` as a single file? Or, some other way to get that into a Kaggle dataset so that I can use the huggingface `datasets` module to process and consume it inside of a Kaggle notebook?
Thanks in advance!
| 22 | Easy way to create a Kaggle dataset from a Huggingface dataset?
I'm not sure whether this is more appropriately addressed with HuggingFace or Kaggle. I would like to somehow directly create a Kaggle dataset from a HuggingFace Dataset.
While Kaggle does provide the option to create a dataset from a URI, that URI must point to a single file. For example:
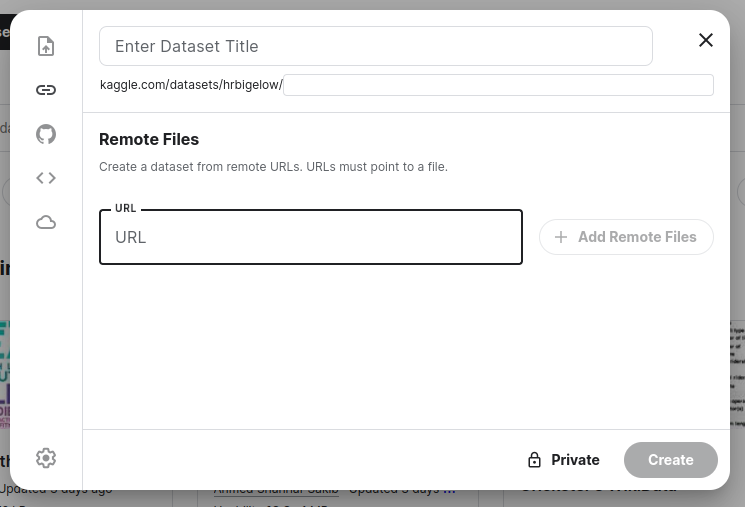
Is there some mechanism from huggingface to represent a dataset (such as that from `load_dataset('wmt14', 'de-en', split='train')` as a single file? Or, some other way to get that into a Kaggle dataset so that I can use the huggingface `datasets` module to process and consume it inside of a Kaggle notebook?
Thanks in advance!
I've requested support for creating a Kaggle dataset from an imported HF dataset repo on their "forum" here: https://www.kaggle.com/discussions/product-feedback/427142 (upvotes appreciated π) |
https://github.com/huggingface/datasets/issues/5811 | load_dataset: TypeError: 'NoneType' object is not callable, on local dataset filename changes | This error means a `DatasetBuilder` subclass that generates the dataset could not be found inside the script, so make sure `dushowxa-characters/dushowxa-characters.py `is a valid dataset script (assuming `path_or_dataset` is `dushowxa-characters`)
Also, we should improve the error to make it more obvious what the problem is. | ### Describe the bug
I've adapted Databrick's [train_dolly.py](/databrickslabs/dolly/blob/master/train_dolly.py) to train using a local dataset, which has been working. Upon changing the filenames of the `.json` & `.py` files in my local dataset directory, `dataset = load_dataset(path_or_dataset)["train"]` throws the error:
```python
2023-04-30 09:10:52 INFO [training.trainer] Loading dataset from dushowxa-characters
Traceback (most recent call last):
File "/data/dushowxa-dolly/train_dushowxa.py", line 26, in <module>
load_training_dataset()
File "/data/dushowxa-dolly/training/trainer.py", line 89, in load_training_dataset
dataset = load_dataset(path_or_dataset)["train"]
File "/data/dushowxa-dolly/.venv/lib/python3.10/site-packages/datasets/load.py", line 1773, in load_dataset
builder_instance = load_dataset_builder(
File "/data/dushowxa-dolly/.venv/lib/python3.10/site-packages/datasets/load.py", line 1528, in load_dataset_builder
builder_instance: DatasetBuilder = builder_cls(
TypeError: 'NoneType' object is not callable
```
The local dataset filenames were of the form `dushowxa-characters/expanse-dushowxa-characters.json` and are now of the form `dushowxa-characters/dushowxa-characters.json` (the word `expanse-` was removed from the filenames). Is this perhaps a dataset caching issue?
I have attempted to manually clear caches, but to no effect:
```sh
rm -rfv ~/.cache/huggingface/datasets/*
rm -rfv ~/.cache/huggingface/modules/*
```
### Steps to reproduce the bug
Run `python3 train_dushowxa.py` (adapted from Databrick's [train_dolly.py](/databrickslabs/dolly/blob/master/train_dolly.py)).
### Expected behavior
Training succeeds as before local dataset filenames were changed.
### Environment info
Ubuntu 22.04, Python 3.10.6, venv
```python
accelerate>=0.16.0,<1
click>=8.0.4,<9
datasets>=2.10.0,<3
deepspeed>=0.9.0,<1
transformers[torch]>=4.28.1,<5
langchain>=0.0.139
``` | 45 | load_dataset: TypeError: 'NoneType' object is not callable, on local dataset filename changes
### Describe the bug
I've adapted Databrick's [train_dolly.py](/databrickslabs/dolly/blob/master/train_dolly.py) to train using a local dataset, which has been working. Upon changing the filenames of the `.json` & `.py` files in my local dataset directory, `dataset = load_dataset(path_or_dataset)["train"]` throws the error:
```python
2023-04-30 09:10:52 INFO [training.trainer] Loading dataset from dushowxa-characters
Traceback (most recent call last):
File "/data/dushowxa-dolly/train_dushowxa.py", line 26, in <module>
load_training_dataset()
File "/data/dushowxa-dolly/training/trainer.py", line 89, in load_training_dataset
dataset = load_dataset(path_or_dataset)["train"]
File "/data/dushowxa-dolly/.venv/lib/python3.10/site-packages/datasets/load.py", line 1773, in load_dataset
builder_instance = load_dataset_builder(
File "/data/dushowxa-dolly/.venv/lib/python3.10/site-packages/datasets/load.py", line 1528, in load_dataset_builder
builder_instance: DatasetBuilder = builder_cls(
TypeError: 'NoneType' object is not callable
```
The local dataset filenames were of the form `dushowxa-characters/expanse-dushowxa-characters.json` and are now of the form `dushowxa-characters/dushowxa-characters.json` (the word `expanse-` was removed from the filenames). Is this perhaps a dataset caching issue?
I have attempted to manually clear caches, but to no effect:
```sh
rm -rfv ~/.cache/huggingface/datasets/*
rm -rfv ~/.cache/huggingface/modules/*
```
### Steps to reproduce the bug
Run `python3 train_dushowxa.py` (adapted from Databrick's [train_dolly.py](/databrickslabs/dolly/blob/master/train_dolly.py)).
### Expected behavior
Training succeeds as before local dataset filenames were changed.
### Environment info
Ubuntu 22.04, Python 3.10.6, venv
```python
accelerate>=0.16.0,<1
click>=8.0.4,<9
datasets>=2.10.0,<3
deepspeed>=0.9.0,<1
transformers[torch]>=4.28.1,<5
langchain>=0.0.139
```
This error means a `DatasetBuilder` subclass that generates the dataset could not be found inside the script, so make sure `dushowxa-characters/dushowxa-characters.py `is a valid dataset script (assuming `path_or_dataset` is `dushowxa-characters`)
Also, we should improve the error to make it more obvious what the problem is. |
https://github.com/huggingface/datasets/issues/5809 | wiki_dpr details for Open Domain Question Answering tasks | Hi ! I don't remember exactly how it was done, but maybe you have to embed `f"{title}<sep>{text}"` ?
Using a HF tokenizer it corresponds to doing
```python
tokenized = tokenizer(titles, texts)
``` | Hey guys!
Thanks for creating the wiki_dpr dataset!
I am currently trying to combine wiki_dpr and my own datasets. but I don't know how to make the embedding value the same way as wiki_dpr.
As an experiment, I embeds the text of id="7" of wiki_dpr, but this result was very different from wiki_dpr. | 32 | wiki_dpr details for Open Domain Question Answering tasks
Hey guys!
Thanks for creating the wiki_dpr dataset!
I am currently trying to combine wiki_dpr and my own datasets. but I don't know how to make the embedding value the same way as wiki_dpr.
As an experiment, I embeds the text of id="7" of wiki_dpr, but this result was very different from wiki_dpr.
Hi ! I don't remember exactly how it was done, but maybe you have to embed `f"{title}<sep>{text}"` ?
Using a HF tokenizer it corresponds to doing
```python
tokenized = tokenizer(titles, texts)
``` |
https://github.com/huggingface/datasets/issues/5806 | Return the name of the currently loaded file in the load_dataset function. | Implementing this makes sense (e.g., `tensorflow_datasets`' imagefolder returns image filenames). Also, in Datasets 3.0, we plan only to store the bytes of an image/audio, not its path, so this feature would be useful when the path info is still needed. | ### Feature request
Add an optional parameter return_file_name in the load_dataset function. When it is set to True, the function will include the name of the file corresponding to the current line as a feature in the returned output.
### Motivation
When training large language models, machine problems may interrupt the training process. In such cases, it is common to load a previously saved checkpoint to resume training. I would like to be able to obtain the names of the previously trained data shards, so that I can skip these parts of the data during continued training to avoid overfitting and redundant training time.
### Your contribution
I currently use a dataset in jsonl format, so I am primarily interested in the json format. I suggest adding the file name to the returned table here https://github.com/huggingface/datasets/blob/main/src/datasets/packaged_modules/json/json.py#L92. | 40 | Return the name of the currently loaded file in the load_dataset function.
### Feature request
Add an optional parameter return_file_name in the load_dataset function. When it is set to True, the function will include the name of the file corresponding to the current line as a feature in the returned output.
### Motivation
When training large language models, machine problems may interrupt the training process. In such cases, it is common to load a previously saved checkpoint to resume training. I would like to be able to obtain the names of the previously trained data shards, so that I can skip these parts of the data during continued training to avoid overfitting and redundant training time.
### Your contribution
I currently use a dataset in jsonl format, so I am primarily interested in the json format. I suggest adding the file name to the returned table here https://github.com/huggingface/datasets/blob/main/src/datasets/packaged_modules/json/json.py#L92.
Implementing this makes sense (e.g., `tensorflow_datasets`' imagefolder returns image filenames). Also, in Datasets 3.0, we plan only to store the bytes of an image/audio, not its path, so this feature would be useful when the path info is still needed. |
https://github.com/huggingface/datasets/issues/5806 | Return the name of the currently loaded file in the load_dataset function. | Hey @mariosasko, Can I work on this issue, this one seems interesting to implement. I have contributed to jupyterlab recently, and would love to contribute here as well. | ### Feature request
Add an optional parameter return_file_name in the load_dataset function. When it is set to True, the function will include the name of the file corresponding to the current line as a feature in the returned output.
### Motivation
When training large language models, machine problems may interrupt the training process. In such cases, it is common to load a previously saved checkpoint to resume training. I would like to be able to obtain the names of the previously trained data shards, so that I can skip these parts of the data during continued training to avoid overfitting and redundant training time.
### Your contribution
I currently use a dataset in jsonl format, so I am primarily interested in the json format. I suggest adding the file name to the returned table here https://github.com/huggingface/datasets/blob/main/src/datasets/packaged_modules/json/json.py#L92. | 28 | Return the name of the currently loaded file in the load_dataset function.
### Feature request
Add an optional parameter return_file_name in the load_dataset function. When it is set to True, the function will include the name of the file corresponding to the current line as a feature in the returned output.
### Motivation
When training large language models, machine problems may interrupt the training process. In such cases, it is common to load a previously saved checkpoint to resume training. I would like to be able to obtain the names of the previously trained data shards, so that I can skip these parts of the data during continued training to avoid overfitting and redundant training time.
### Your contribution
I currently use a dataset in jsonl format, so I am primarily interested in the json format. I suggest adding the file name to the returned table here https://github.com/huggingface/datasets/blob/main/src/datasets/packaged_modules/json/json.py#L92.
Hey @mariosasko, Can I work on this issue, this one seems interesting to implement. I have contributed to jupyterlab recently, and would love to contribute here as well. |
https://github.com/huggingface/datasets/issues/5806 | Return the name of the currently loaded file in the load_dataset function. | @tsabbir96 if you are planning to start working on this, you can take on this issue by writing a comment with only the keyword: #self-assign | ### Feature request
Add an optional parameter return_file_name in the load_dataset function. When it is set to True, the function will include the name of the file corresponding to the current line as a feature in the returned output.
### Motivation
When training large language models, machine problems may interrupt the training process. In such cases, it is common to load a previously saved checkpoint to resume training. I would like to be able to obtain the names of the previously trained data shards, so that I can skip these parts of the data during continued training to avoid overfitting and redundant training time.
### Your contribution
I currently use a dataset in jsonl format, so I am primarily interested in the json format. I suggest adding the file name to the returned table here https://github.com/huggingface/datasets/blob/main/src/datasets/packaged_modules/json/json.py#L92. | 25 | Return the name of the currently loaded file in the load_dataset function.
### Feature request
Add an optional parameter return_file_name in the load_dataset function. When it is set to True, the function will include the name of the file corresponding to the current line as a feature in the returned output.
### Motivation
When training large language models, machine problems may interrupt the training process. In such cases, it is common to load a previously saved checkpoint to resume training. I would like to be able to obtain the names of the previously trained data shards, so that I can skip these parts of the data during continued training to avoid overfitting and redundant training time.
### Your contribution
I currently use a dataset in jsonl format, so I am primarily interested in the json format. I suggest adding the file name to the returned table here https://github.com/huggingface/datasets/blob/main/src/datasets/packaged_modules/json/json.py#L92.
@tsabbir96 if you are planning to start working on this, you can take on this issue by writing a comment with only the keyword: #self-assign |
https://github.com/huggingface/datasets/issues/5806 | Return the name of the currently loaded file in the load_dataset function. | @albertvillanova thank you for letting me contribute here.
@albertvillanova @mariosasko As I am totally new to this repo, could you tell me something more about this issue or perhaps give me some idea on how I can proceed with it? Thanks! | ### Feature request
Add an optional parameter return_file_name in the load_dataset function. When it is set to True, the function will include the name of the file corresponding to the current line as a feature in the returned output.
### Motivation
When training large language models, machine problems may interrupt the training process. In such cases, it is common to load a previously saved checkpoint to resume training. I would like to be able to obtain the names of the previously trained data shards, so that I can skip these parts of the data during continued training to avoid overfitting and redundant training time.
### Your contribution
I currently use a dataset in jsonl format, so I am primarily interested in the json format. I suggest adding the file name to the returned table here https://github.com/huggingface/datasets/blob/main/src/datasets/packaged_modules/json/json.py#L92. | 41 | Return the name of the currently loaded file in the load_dataset function.
### Feature request
Add an optional parameter return_file_name in the load_dataset function. When it is set to True, the function will include the name of the file corresponding to the current line as a feature in the returned output.
### Motivation
When training large language models, machine problems may interrupt the training process. In such cases, it is common to load a previously saved checkpoint to resume training. I would like to be able to obtain the names of the previously trained data shards, so that I can skip these parts of the data during continued training to avoid overfitting and redundant training time.
### Your contribution
I currently use a dataset in jsonl format, so I am primarily interested in the json format. I suggest adding the file name to the returned table here https://github.com/huggingface/datasets/blob/main/src/datasets/packaged_modules/json/json.py#L92.
@albertvillanova thank you for letting me contribute here.
@albertvillanova @mariosasko As I am totally new to this repo, could you tell me something more about this issue or perhaps give me some idea on how I can proceed with it? Thanks! |
https://github.com/huggingface/datasets/issues/5806 | Return the name of the currently loaded file in the load_dataset function. | Hello there, is this issue resolved? @tsabbir96 are you still working on it? Otherwise I would love to give it a try | ### Feature request
Add an optional parameter return_file_name in the load_dataset function. When it is set to True, the function will include the name of the file corresponding to the current line as a feature in the returned output.
### Motivation
When training large language models, machine problems may interrupt the training process. In such cases, it is common to load a previously saved checkpoint to resume training. I would like to be able to obtain the names of the previously trained data shards, so that I can skip these parts of the data during continued training to avoid overfitting and redundant training time.
### Your contribution
I currently use a dataset in jsonl format, so I am primarily interested in the json format. I suggest adding the file name to the returned table here https://github.com/huggingface/datasets/blob/main/src/datasets/packaged_modules/json/json.py#L92. | 22 | Return the name of the currently loaded file in the load_dataset function.
### Feature request
Add an optional parameter return_file_name in the load_dataset function. When it is set to True, the function will include the name of the file corresponding to the current line as a feature in the returned output.
### Motivation
When training large language models, machine problems may interrupt the training process. In such cases, it is common to load a previously saved checkpoint to resume training. I would like to be able to obtain the names of the previously trained data shards, so that I can skip these parts of the data during continued training to avoid overfitting and redundant training time.
### Your contribution
I currently use a dataset in jsonl format, so I am primarily interested in the json format. I suggest adding the file name to the returned table here https://github.com/huggingface/datasets/blob/main/src/datasets/packaged_modules/json/json.py#L92.
Hello there, is this issue resolved? @tsabbir96 are you still working on it? Otherwise I would love to give it a try |
https://github.com/huggingface/datasets/issues/5806 | Return the name of the currently loaded file in the load_dataset function. | Hey @mariosasko, I've taken the time to take a look at how we load the datasets usually. My main question now is about the final solution.
So the idea is that whenever we load the datasets we also add a new column in the _generate_tables() method from the builders called filename (or file_name) that should be related files contained in each split, right?
Do you have any suggestions of where I could add that? | ### Feature request
Add an optional parameter return_file_name in the load_dataset function. When it is set to True, the function will include the name of the file corresponding to the current line as a feature in the returned output.
### Motivation
When training large language models, machine problems may interrupt the training process. In such cases, it is common to load a previously saved checkpoint to resume training. I would like to be able to obtain the names of the previously trained data shards, so that I can skip these parts of the data during continued training to avoid overfitting and redundant training time.
### Your contribution
I currently use a dataset in jsonl format, so I am primarily interested in the json format. I suggest adding the file name to the returned table here https://github.com/huggingface/datasets/blob/main/src/datasets/packaged_modules/json/json.py#L92. | 74 | Return the name of the currently loaded file in the load_dataset function.
### Feature request
Add an optional parameter return_file_name in the load_dataset function. When it is set to True, the function will include the name of the file corresponding to the current line as a feature in the returned output.
### Motivation
When training large language models, machine problems may interrupt the training process. In such cases, it is common to load a previously saved checkpoint to resume training. I would like to be able to obtain the names of the previously trained data shards, so that I can skip these parts of the data during continued training to avoid overfitting and redundant training time.
### Your contribution
I currently use a dataset in jsonl format, so I am primarily interested in the json format. I suggest adding the file name to the returned table here https://github.com/huggingface/datasets/blob/main/src/datasets/packaged_modules/json/json.py#L92.
Hey @mariosasko, I've taken the time to take a look at how we load the datasets usually. My main question now is about the final solution.
So the idea is that whenever we load the datasets we also add a new column in the _generate_tables() method from the builders called filename (or file_name) that should be related files contained in each split, right?
Do you have any suggestions of where I could add that? |
https://github.com/huggingface/datasets/issues/5805 | Improve `Create a dataset` tutorial | I can work on this. The link to the tutorial seems to be broken though @polinaeterna. | Our [tutorial on how to create a dataset](https://huggingface.co/docs/datasets/create_dataset) is a bit misleading.
1. In **Folder-based builders** section it says that we have two folder-based builders as standard builders, but we also have similar builders (that can be created from directory with data of required format) for `csv`, `json/jsonl`, `parquet` and `txt` files. We have info about these loaders in separate [guide for loading](https://huggingface.co/docs/datasets/loading#local-and-remote-files) but it's worth briefly mentioning them in the beginning tutorial because they are more common and for consistency. Would be helpful to add the link to the full guide.
2. **From local files** section lists methods for creating a dataset from in-memory data which are also described in [loading guide](https://huggingface.co/docs/datasets/loading#inmemory-data).
Maybe we should actually rethink and restructure this tutorial somehow. | 16 | Improve `Create a dataset` tutorial
Our [tutorial on how to create a dataset](https://huggingface.co/docs/datasets/create_dataset) is a bit misleading.
1. In **Folder-based builders** section it says that we have two folder-based builders as standard builders, but we also have similar builders (that can be created from directory with data of required format) for `csv`, `json/jsonl`, `parquet` and `txt` files. We have info about these loaders in separate [guide for loading](https://huggingface.co/docs/datasets/loading#local-and-remote-files) but it's worth briefly mentioning them in the beginning tutorial because they are more common and for consistency. Would be helpful to add the link to the full guide.
2. **From local files** section lists methods for creating a dataset from in-memory data which are also described in [loading guide](https://huggingface.co/docs/datasets/loading#inmemory-data).
Maybe we should actually rethink and restructure this tutorial somehow.
I can work on this. The link to the tutorial seems to be broken though @polinaeterna. |
https://github.com/huggingface/datasets/issues/5805 | Improve `Create a dataset` tutorial | @isunitha98selvan would be great, thank you! which link are you talking about? I think it should work: https://huggingface.co/docs/datasets/create_dataset | Our [tutorial on how to create a dataset](https://huggingface.co/docs/datasets/create_dataset) is a bit misleading.
1. In **Folder-based builders** section it says that we have two folder-based builders as standard builders, but we also have similar builders (that can be created from directory with data of required format) for `csv`, `json/jsonl`, `parquet` and `txt` files. We have info about these loaders in separate [guide for loading](https://huggingface.co/docs/datasets/loading#local-and-remote-files) but it's worth briefly mentioning them in the beginning tutorial because they are more common and for consistency. Would be helpful to add the link to the full guide.
2. **From local files** section lists methods for creating a dataset from in-memory data which are also described in [loading guide](https://huggingface.co/docs/datasets/loading#inmemory-data).
Maybe we should actually rethink and restructure this tutorial somehow. | 18 | Improve `Create a dataset` tutorial
Our [tutorial on how to create a dataset](https://huggingface.co/docs/datasets/create_dataset) is a bit misleading.
1. In **Folder-based builders** section it says that we have two folder-based builders as standard builders, but we also have similar builders (that can be created from directory with data of required format) for `csv`, `json/jsonl`, `parquet` and `txt` files. We have info about these loaders in separate [guide for loading](https://huggingface.co/docs/datasets/loading#local-and-remote-files) but it's worth briefly mentioning them in the beginning tutorial because they are more common and for consistency. Would be helpful to add the link to the full guide.
2. **From local files** section lists methods for creating a dataset from in-memory data which are also described in [loading guide](https://huggingface.co/docs/datasets/loading#inmemory-data).
Maybe we should actually rethink and restructure this tutorial somehow.
@isunitha98selvan would be great, thank you! which link are you talking about? I think it should work: https://huggingface.co/docs/datasets/create_dataset |