modelId
stringlengths 5
122
| author
stringlengths 2
42
| last_modified
unknown | downloads
int64 0
738M
| likes
int64 0
11k
| library_name
stringclasses 245
values | tags
sequencelengths 1
4.05k
| pipeline_tag
stringclasses 48
values | createdAt
unknown | card
stringlengths 1
901k
|
---|---|---|---|---|---|---|---|---|---|
PrunaAI/bineric-NorskGPT-Llama-13B-v0.1-HQQ-2bit-smashed | PrunaAI | "2024-06-24T10:04:46Z" | 0 | 0 | transformers | [
"transformers",
"llama",
"text-generation",
"pruna-ai",
"conversational",
"base_model:bineric/NorskGPT-Llama-13B-v0.1",
"autotrain_compatible",
"endpoints_compatible",
"text-generation-inference",
"region:us"
] | text-generation | "2024-06-24T10:02:47Z" | ---
thumbnail: "https://assets-global.website-files.com/646b351987a8d8ce158d1940/64ec9e96b4334c0e1ac41504_Logo%20with%20white%20text.svg"
base_model: bineric/NorskGPT-Llama-13B-v0.1
metrics:
- memory_disk
- memory_inference
- inference_latency
- inference_throughput
- inference_CO2_emissions
- inference_energy_consumption
tags:
- pruna-ai
---
<!-- header start -->
<!-- 200823 -->
<div style="width: auto; margin-left: auto; margin-right: auto">
<a href="https://www.pruna.ai/" target="_blank" rel="noopener noreferrer">
<img src="https://i.imgur.com/eDAlcgk.png" alt="PrunaAI" style="width: 100%; min-width: 400px; display: block; margin: auto;">
</a>
</div>
<!-- header end -->
[](https://twitter.com/PrunaAI)
[](https://github.com/PrunaAI)
[](https://www.linkedin.com/company/93832878/admin/feed/posts/?feedType=following)
[](https://discord.gg/CP4VSgck)
# Simply make AI models cheaper, smaller, faster, and greener!
- Give a thumbs up if you like this model!
- Contact us and tell us which model to compress next [here](https://www.pruna.ai/contact).
- Request access to easily compress your *own* AI models [here](https://z0halsaff74.typeform.com/pruna-access?typeform-source=www.pruna.ai).
- Read the documentations to know more [here](https://pruna-ai-pruna.readthedocs-hosted.com/en/latest/)
- Join Pruna AI community on Discord [here](https://discord.gg/CP4VSgck) to share feedback/suggestions or get help.
## Results

**Frequently Asked Questions**
- ***How does the compression work?*** The model is compressed with hqq.
- ***How does the model quality change?*** The quality of the model output might vary compared to the base model.
- ***How is the model efficiency evaluated?*** These results were obtained on HARDWARE_NAME with configuration described in `model/smash_config.json` and are obtained after a hardware warmup. The smashed model is directly compared to the original base model. Efficiency results may vary in other settings (e.g. other hardware, image size, batch size, ...). We recommend to directly run them in the use-case conditions to know if the smashed model can benefit you.
- ***What is the model format?*** We use safetensors.
- ***What calibration data has been used?*** If needed by the compression method, we used WikiText as the calibration data.
- ***What is the naming convention for Pruna Huggingface models?*** We take the original model name and append "turbo", "tiny", or "green" if the smashed model has a measured inference speed, inference memory, or inference energy consumption which is less than 90% of the original base model.
- ***How to compress my own models?*** You can request premium access to more compression methods and tech support for your specific use-cases [here](https://z0halsaff74.typeform.com/pruna-access?typeform-source=www.pruna.ai).
- ***What are "first" metrics?*** Results mentioning "first" are obtained after the first run of the model. The first run might take more memory or be slower than the subsequent runs due cuda overheads.
- ***What are "Sync" and "Async" metrics?*** "Sync" metrics are obtained by syncing all GPU processes and stop measurement when all of them are executed. "Async" metrics are obtained without syncing all GPU processes and stop when the model output can be used by the CPU. We provide both metrics since both could be relevant depending on the use-case. We recommend to test the efficiency gains directly in your use-cases.
## Setup
You can run the smashed model with these steps:
0. Check requirements from the original repo bineric/NorskGPT-Llama-13B-v0.1 installed. In particular, check python, cuda, and transformers versions.
1. Make sure that you have installed quantization related packages.
```bash
pip install hqq
```
2. Load & run the model.
```python
from transformers import AutoModelForCausalLM, AutoTokenizer
from hqq.engine.hf import HQQModelForCausalLM
from hqq.models.hf.base import AutoHQQHFModel
try:
model = HQQModelForCausalLM.from_quantized("PrunaAI/bineric-NorskGPT-Llama-13B-v0.1-HQQ-2bit-smashed", device_map='auto')
except:
model = AutoHQQHFModel.from_quantized("PrunaAI/bineric-NorskGPT-Llama-13B-v0.1-HQQ-2bit-smashed")
tokenizer = AutoTokenizer.from_pretrained("bineric/NorskGPT-Llama-13B-v0.1")
input_ids = tokenizer("What is the color of prunes?,", return_tensors='pt').to(model.device)["input_ids"]
outputs = model.generate(input_ids, max_new_tokens=216)
tokenizer.decode(outputs[0])
```
## Configurations
The configuration info are in `smash_config.json`.
## Credits & License
The license of the smashed model follows the license of the original model. Please check the license of the original model bineric/NorskGPT-Llama-13B-v0.1 before using this model which provided the base model. The license of the `pruna-engine` is [here](https://pypi.org/project/pruna-engine/) on Pypi.
## Want to compress other models?
- Contact us and tell us which model to compress next [here](https://www.pruna.ai/contact).
- Request access to easily compress your own AI models [here](https://z0halsaff74.typeform.com/pruna-access?typeform-source=www.pruna.ai). |
PrunaAI/bineric-NorskGPT-Llama-13B-v0.1-HQQ-1bit-smashed | PrunaAI | "2024-06-24T10:04:11Z" | 0 | 0 | transformers | [
"transformers",
"llama",
"text-generation",
"pruna-ai",
"conversational",
"base_model:bineric/NorskGPT-Llama-13B-v0.1",
"autotrain_compatible",
"endpoints_compatible",
"text-generation-inference",
"region:us"
] | text-generation | "2024-06-24T10:02:50Z" | ---
thumbnail: "https://assets-global.website-files.com/646b351987a8d8ce158d1940/64ec9e96b4334c0e1ac41504_Logo%20with%20white%20text.svg"
base_model: bineric/NorskGPT-Llama-13B-v0.1
metrics:
- memory_disk
- memory_inference
- inference_latency
- inference_throughput
- inference_CO2_emissions
- inference_energy_consumption
tags:
- pruna-ai
---
<!-- header start -->
<!-- 200823 -->
<div style="width: auto; margin-left: auto; margin-right: auto">
<a href="https://www.pruna.ai/" target="_blank" rel="noopener noreferrer">
<img src="https://i.imgur.com/eDAlcgk.png" alt="PrunaAI" style="width: 100%; min-width: 400px; display: block; margin: auto;">
</a>
</div>
<!-- header end -->
[](https://twitter.com/PrunaAI)
[](https://github.com/PrunaAI)
[](https://www.linkedin.com/company/93832878/admin/feed/posts/?feedType=following)
[](https://discord.gg/CP4VSgck)
# Simply make AI models cheaper, smaller, faster, and greener!
- Give a thumbs up if you like this model!
- Contact us and tell us which model to compress next [here](https://www.pruna.ai/contact).
- Request access to easily compress your *own* AI models [here](https://z0halsaff74.typeform.com/pruna-access?typeform-source=www.pruna.ai).
- Read the documentations to know more [here](https://pruna-ai-pruna.readthedocs-hosted.com/en/latest/)
- Join Pruna AI community on Discord [here](https://discord.gg/CP4VSgck) to share feedback/suggestions or get help.
## Results

**Frequently Asked Questions**
- ***How does the compression work?*** The model is compressed with hqq.
- ***How does the model quality change?*** The quality of the model output might vary compared to the base model.
- ***How is the model efficiency evaluated?*** These results were obtained on HARDWARE_NAME with configuration described in `model/smash_config.json` and are obtained after a hardware warmup. The smashed model is directly compared to the original base model. Efficiency results may vary in other settings (e.g. other hardware, image size, batch size, ...). We recommend to directly run them in the use-case conditions to know if the smashed model can benefit you.
- ***What is the model format?*** We use safetensors.
- ***What calibration data has been used?*** If needed by the compression method, we used WikiText as the calibration data.
- ***What is the naming convention for Pruna Huggingface models?*** We take the original model name and append "turbo", "tiny", or "green" if the smashed model has a measured inference speed, inference memory, or inference energy consumption which is less than 90% of the original base model.
- ***How to compress my own models?*** You can request premium access to more compression methods and tech support for your specific use-cases [here](https://z0halsaff74.typeform.com/pruna-access?typeform-source=www.pruna.ai).
- ***What are "first" metrics?*** Results mentioning "first" are obtained after the first run of the model. The first run might take more memory or be slower than the subsequent runs due cuda overheads.
- ***What are "Sync" and "Async" metrics?*** "Sync" metrics are obtained by syncing all GPU processes and stop measurement when all of them are executed. "Async" metrics are obtained without syncing all GPU processes and stop when the model output can be used by the CPU. We provide both metrics since both could be relevant depending on the use-case. We recommend to test the efficiency gains directly in your use-cases.
## Setup
You can run the smashed model with these steps:
0. Check requirements from the original repo bineric/NorskGPT-Llama-13B-v0.1 installed. In particular, check python, cuda, and transformers versions.
1. Make sure that you have installed quantization related packages.
```bash
pip install hqq
```
2. Load & run the model.
```python
from transformers import AutoModelForCausalLM, AutoTokenizer
from hqq.engine.hf import HQQModelForCausalLM
from hqq.models.hf.base import AutoHQQHFModel
try:
model = HQQModelForCausalLM.from_quantized("PrunaAI/bineric-NorskGPT-Llama-13B-v0.1-HQQ-1bit-smashed", device_map='auto')
except:
model = AutoHQQHFModel.from_quantized("PrunaAI/bineric-NorskGPT-Llama-13B-v0.1-HQQ-1bit-smashed")
tokenizer = AutoTokenizer.from_pretrained("bineric/NorskGPT-Llama-13B-v0.1")
input_ids = tokenizer("What is the color of prunes?,", return_tensors='pt').to(model.device)["input_ids"]
outputs = model.generate(input_ids, max_new_tokens=216)
tokenizer.decode(outputs[0])
```
## Configurations
The configuration info are in `smash_config.json`.
## Credits & License
The license of the smashed model follows the license of the original model. Please check the license of the original model bineric/NorskGPT-Llama-13B-v0.1 before using this model which provided the base model. The license of the `pruna-engine` is [here](https://pypi.org/project/pruna-engine/) on Pypi.
## Want to compress other models?
- Contact us and tell us which model to compress next [here](https://www.pruna.ai/contact).
- Request access to easily compress your own AI models [here](https://z0halsaff74.typeform.com/pruna-access?typeform-source=www.pruna.ai). |
prabinpanta0/Fashion_MNIST | prabinpanta0 | "2024-06-24T10:24:36Z" | 0 | 1 | tensorflow | [
"tensorflow",
"keras",
"image-classification",
"Fasion_image-classification",
"neural-network",
"en",
"license:mit",
"region:us"
] | image-classification | "2024-06-24T10:03:04Z" | ---
license: mit
language: en
metrics: mean_squared_error
library_name: tensorflow
tags:
- image-classification
- Fasion_image-classification
- tensorflow
- neural-network
pipeline_tag: image-classification
---
This model was created as a practice exercise for the course "Intro to TensorFlow for Deep Learning" from Udacity, given by TensorFlow. It was trained on a dataset of TenserFlow Fashion MNIST. The model uses a small neural network built with TensorFlow.
## Model Input
**Dataset:** fashion_mnist tensorflow datasets


## Model Output




## License
This model is released under the MIT license. |
richardlastrucci/m2m100-tsn-eng | richardlastrucci | "2024-06-24T10:58:52Z" | 0 | 0 | transformers | [
"transformers",
"tensorboard",
"safetensors",
"m2m_100",
"text2text-generation",
"autotrain_compatible",
"endpoints_compatible",
"region:us"
] | text2text-generation | "2024-06-24T10:04:02Z" | Entry not found |
xxlrd/hansohee | xxlrd | "2024-06-25T03:05:59Z" | 0 | 0 | null | [
"region:us"
] | null | "2024-06-24T10:04:04Z" | https://civitai.com/models/144009/han-sohee |
mwz/llama-law-pk | mwz | "2024-06-24T10:04:05Z" | 0 | 0 | null | [
"region:us"
] | null | "2024-06-24T10:04:05Z" | Entry not found |
Reihaneh/wav2vec2_fy_nl_en_de_common_voice_45 | Reihaneh | "2024-06-24T10:04:59Z" | 0 | 0 | transformers | [
"transformers",
"arxiv:1910.09700",
"endpoints_compatible",
"region:us"
] | null | "2024-06-24T10:04:58Z" | ---
library_name: transformers
tags: []
---
# Model Card for Model ID
<!-- Provide a quick summary of what the model is/does. -->
## Model Details
### Model Description
<!-- Provide a longer summary of what this model is. -->
This is the model card of a 🤗 transformers model that has been pushed on the Hub. This model card has been automatically generated.
- **Developed by:** [More Information Needed]
- **Funded by [optional]:** [More Information Needed]
- **Shared by [optional]:** [More Information Needed]
- **Model type:** [More Information Needed]
- **Language(s) (NLP):** [More Information Needed]
- **License:** [More Information Needed]
- **Finetuned from model [optional]:** [More Information Needed]
### Model Sources [optional]
<!-- Provide the basic links for the model. -->
- **Repository:** [More Information Needed]
- **Paper [optional]:** [More Information Needed]
- **Demo [optional]:** [More Information Needed]
## Uses
<!-- Address questions around how the model is intended to be used, including the foreseeable users of the model and those affected by the model. -->
### Direct Use
<!-- This section is for the model use without fine-tuning or plugging into a larger ecosystem/app. -->
[More Information Needed]
### Downstream Use [optional]
<!-- This section is for the model use when fine-tuned for a task, or when plugged into a larger ecosystem/app -->
[More Information Needed]
### Out-of-Scope Use
<!-- This section addresses misuse, malicious use, and uses that the model will not work well for. -->
[More Information Needed]
## Bias, Risks, and Limitations
<!-- This section is meant to convey both technical and sociotechnical limitations. -->
[More Information Needed]
### Recommendations
<!-- This section is meant to convey recommendations with respect to the bias, risk, and technical limitations. -->
Users (both direct and downstream) should be made aware of the risks, biases and limitations of the model. More information needed for further recommendations.
## How to Get Started with the Model
Use the code below to get started with the model.
[More Information Needed]
## Training Details
### Training Data
<!-- This should link to a Dataset Card, perhaps with a short stub of information on what the training data is all about as well as documentation related to data pre-processing or additional filtering. -->
[More Information Needed]
### Training Procedure
<!-- This relates heavily to the Technical Specifications. Content here should link to that section when it is relevant to the training procedure. -->
#### Preprocessing [optional]
[More Information Needed]
#### Training Hyperparameters
- **Training regime:** [More Information Needed] <!--fp32, fp16 mixed precision, bf16 mixed precision, bf16 non-mixed precision, fp16 non-mixed precision, fp8 mixed precision -->
#### Speeds, Sizes, Times [optional]
<!-- This section provides information about throughput, start/end time, checkpoint size if relevant, etc. -->
[More Information Needed]
## Evaluation
<!-- This section describes the evaluation protocols and provides the results. -->
### Testing Data, Factors & Metrics
#### Testing Data
<!-- This should link to a Dataset Card if possible. -->
[More Information Needed]
#### Factors
<!-- These are the things the evaluation is disaggregating by, e.g., subpopulations or domains. -->
[More Information Needed]
#### Metrics
<!-- These are the evaluation metrics being used, ideally with a description of why. -->
[More Information Needed]
### Results
[More Information Needed]
#### Summary
## Model Examination [optional]
<!-- Relevant interpretability work for the model goes here -->
[More Information Needed]
## Environmental Impact
<!-- Total emissions (in grams of CO2eq) and additional considerations, such as electricity usage, go here. Edit the suggested text below accordingly -->
Carbon emissions can be estimated using the [Machine Learning Impact calculator](https://mlco2.github.io/impact#compute) presented in [Lacoste et al. (2019)](https://arxiv.org/abs/1910.09700).
- **Hardware Type:** [More Information Needed]
- **Hours used:** [More Information Needed]
- **Cloud Provider:** [More Information Needed]
- **Compute Region:** [More Information Needed]
- **Carbon Emitted:** [More Information Needed]
## Technical Specifications [optional]
### Model Architecture and Objective
[More Information Needed]
### Compute Infrastructure
[More Information Needed]
#### Hardware
[More Information Needed]
#### Software
[More Information Needed]
## Citation [optional]
<!-- If there is a paper or blog post introducing the model, the APA and Bibtex information for that should go in this section. -->
**BibTeX:**
[More Information Needed]
**APA:**
[More Information Needed]
## Glossary [optional]
<!-- If relevant, include terms and calculations in this section that can help readers understand the model or model card. -->
[More Information Needed]
## More Information [optional]
[More Information Needed]
## Model Card Authors [optional]
[More Information Needed]
## Model Card Contact
[More Information Needed] |
milenarmus/TTB_tallying_noisy_flipped_choice-model_noise0.0 | milenarmus | "2024-06-24T10:05:40Z" | 0 | 0 | null | [
"region:us"
] | null | "2024-06-24T10:05:40Z" | Entry not found |
PrunaAI/llmware-bling-phi-3-HQQ-1bit-smashed | PrunaAI | "2024-06-24T10:06:46Z" | 0 | 0 | transformers | [
"transformers",
"phi3",
"text-generation",
"pruna-ai",
"conversational",
"custom_code",
"base_model:llmware/bling-phi-3",
"autotrain_compatible",
"endpoints_compatible",
"region:us"
] | text-generation | "2024-06-24T10:06:15Z" | ---
thumbnail: "https://assets-global.website-files.com/646b351987a8d8ce158d1940/64ec9e96b4334c0e1ac41504_Logo%20with%20white%20text.svg"
base_model: llmware/bling-phi-3
metrics:
- memory_disk
- memory_inference
- inference_latency
- inference_throughput
- inference_CO2_emissions
- inference_energy_consumption
tags:
- pruna-ai
---
<!-- header start -->
<!-- 200823 -->
<div style="width: auto; margin-left: auto; margin-right: auto">
<a href="https://www.pruna.ai/" target="_blank" rel="noopener noreferrer">
<img src="https://i.imgur.com/eDAlcgk.png" alt="PrunaAI" style="width: 100%; min-width: 400px; display: block; margin: auto;">
</a>
</div>
<!-- header end -->
[](https://twitter.com/PrunaAI)
[](https://github.com/PrunaAI)
[](https://www.linkedin.com/company/93832878/admin/feed/posts/?feedType=following)
[](https://discord.gg/CP4VSgck)
# Simply make AI models cheaper, smaller, faster, and greener!
- Give a thumbs up if you like this model!
- Contact us and tell us which model to compress next [here](https://www.pruna.ai/contact).
- Request access to easily compress your *own* AI models [here](https://z0halsaff74.typeform.com/pruna-access?typeform-source=www.pruna.ai).
- Read the documentations to know more [here](https://pruna-ai-pruna.readthedocs-hosted.com/en/latest/)
- Join Pruna AI community on Discord [here](https://discord.gg/CP4VSgck) to share feedback/suggestions or get help.
## Results

**Frequently Asked Questions**
- ***How does the compression work?*** The model is compressed with hqq.
- ***How does the model quality change?*** The quality of the model output might vary compared to the base model.
- ***How is the model efficiency evaluated?*** These results were obtained on HARDWARE_NAME with configuration described in `model/smash_config.json` and are obtained after a hardware warmup. The smashed model is directly compared to the original base model. Efficiency results may vary in other settings (e.g. other hardware, image size, batch size, ...). We recommend to directly run them in the use-case conditions to know if the smashed model can benefit you.
- ***What is the model format?*** We use safetensors.
- ***What calibration data has been used?*** If needed by the compression method, we used WikiText as the calibration data.
- ***What is the naming convention for Pruna Huggingface models?*** We take the original model name and append "turbo", "tiny", or "green" if the smashed model has a measured inference speed, inference memory, or inference energy consumption which is less than 90% of the original base model.
- ***How to compress my own models?*** You can request premium access to more compression methods and tech support for your specific use-cases [here](https://z0halsaff74.typeform.com/pruna-access?typeform-source=www.pruna.ai).
- ***What are "first" metrics?*** Results mentioning "first" are obtained after the first run of the model. The first run might take more memory or be slower than the subsequent runs due cuda overheads.
- ***What are "Sync" and "Async" metrics?*** "Sync" metrics are obtained by syncing all GPU processes and stop measurement when all of them are executed. "Async" metrics are obtained without syncing all GPU processes and stop when the model output can be used by the CPU. We provide both metrics since both could be relevant depending on the use-case. We recommend to test the efficiency gains directly in your use-cases.
## Setup
You can run the smashed model with these steps:
0. Check requirements from the original repo llmware/bling-phi-3 installed. In particular, check python, cuda, and transformers versions.
1. Make sure that you have installed quantization related packages.
```bash
pip install hqq
```
2. Load & run the model.
```python
from transformers import AutoModelForCausalLM, AutoTokenizer
from hqq.engine.hf import HQQModelForCausalLM
from hqq.models.hf.base import AutoHQQHFModel
try:
model = HQQModelForCausalLM.from_quantized("PrunaAI/llmware-bling-phi-3-HQQ-1bit-smashed", device_map='auto')
except:
model = AutoHQQHFModel.from_quantized("PrunaAI/llmware-bling-phi-3-HQQ-1bit-smashed")
tokenizer = AutoTokenizer.from_pretrained("llmware/bling-phi-3")
input_ids = tokenizer("What is the color of prunes?,", return_tensors='pt').to(model.device)["input_ids"]
outputs = model.generate(input_ids, max_new_tokens=216)
tokenizer.decode(outputs[0])
```
## Configurations
The configuration info are in `smash_config.json`.
## Credits & License
The license of the smashed model follows the license of the original model. Please check the license of the original model llmware/bling-phi-3 before using this model which provided the base model. The license of the `pruna-engine` is [here](https://pypi.org/project/pruna-engine/) on Pypi.
## Want to compress other models?
- Contact us and tell us which model to compress next [here](https://www.pruna.ai/contact).
- Request access to easily compress your own AI models [here](https://z0halsaff74.typeform.com/pruna-access?typeform-source=www.pruna.ai). |
PrunaAI/hooking-dev-Monah-8b-Uncensored-HQQ-2bit-smashed | PrunaAI | "2024-06-24T10:08:17Z" | 0 | 0 | transformers | [
"transformers",
"llama",
"text-generation",
"pruna-ai",
"conversational",
"base_model:hooking-dev/Monah-8b-Uncensored",
"autotrain_compatible",
"endpoints_compatible",
"text-generation-inference",
"region:us"
] | text-generation | "2024-06-24T10:06:16Z" | ---
thumbnail: "https://assets-global.website-files.com/646b351987a8d8ce158d1940/64ec9e96b4334c0e1ac41504_Logo%20with%20white%20text.svg"
base_model: hooking-dev/Monah-8b-Uncensored
metrics:
- memory_disk
- memory_inference
- inference_latency
- inference_throughput
- inference_CO2_emissions
- inference_energy_consumption
tags:
- pruna-ai
---
<!-- header start -->
<!-- 200823 -->
<div style="width: auto; margin-left: auto; margin-right: auto">
<a href="https://www.pruna.ai/" target="_blank" rel="noopener noreferrer">
<img src="https://i.imgur.com/eDAlcgk.png" alt="PrunaAI" style="width: 100%; min-width: 400px; display: block; margin: auto;">
</a>
</div>
<!-- header end -->
[](https://twitter.com/PrunaAI)
[](https://github.com/PrunaAI)
[](https://www.linkedin.com/company/93832878/admin/feed/posts/?feedType=following)
[](https://discord.gg/CP4VSgck)
# Simply make AI models cheaper, smaller, faster, and greener!
- Give a thumbs up if you like this model!
- Contact us and tell us which model to compress next [here](https://www.pruna.ai/contact).
- Request access to easily compress your *own* AI models [here](https://z0halsaff74.typeform.com/pruna-access?typeform-source=www.pruna.ai).
- Read the documentations to know more [here](https://pruna-ai-pruna.readthedocs-hosted.com/en/latest/)
- Join Pruna AI community on Discord [here](https://discord.gg/CP4VSgck) to share feedback/suggestions or get help.
## Results

**Frequently Asked Questions**
- ***How does the compression work?*** The model is compressed with hqq.
- ***How does the model quality change?*** The quality of the model output might vary compared to the base model.
- ***How is the model efficiency evaluated?*** These results were obtained on HARDWARE_NAME with configuration described in `model/smash_config.json` and are obtained after a hardware warmup. The smashed model is directly compared to the original base model. Efficiency results may vary in other settings (e.g. other hardware, image size, batch size, ...). We recommend to directly run them in the use-case conditions to know if the smashed model can benefit you.
- ***What is the model format?*** We use safetensors.
- ***What calibration data has been used?*** If needed by the compression method, we used WikiText as the calibration data.
- ***What is the naming convention for Pruna Huggingface models?*** We take the original model name and append "turbo", "tiny", or "green" if the smashed model has a measured inference speed, inference memory, or inference energy consumption which is less than 90% of the original base model.
- ***How to compress my own models?*** You can request premium access to more compression methods and tech support for your specific use-cases [here](https://z0halsaff74.typeform.com/pruna-access?typeform-source=www.pruna.ai).
- ***What are "first" metrics?*** Results mentioning "first" are obtained after the first run of the model. The first run might take more memory or be slower than the subsequent runs due cuda overheads.
- ***What are "Sync" and "Async" metrics?*** "Sync" metrics are obtained by syncing all GPU processes and stop measurement when all of them are executed. "Async" metrics are obtained without syncing all GPU processes and stop when the model output can be used by the CPU. We provide both metrics since both could be relevant depending on the use-case. We recommend to test the efficiency gains directly in your use-cases.
## Setup
You can run the smashed model with these steps:
0. Check requirements from the original repo hooking-dev/Monah-8b-Uncensored installed. In particular, check python, cuda, and transformers versions.
1. Make sure that you have installed quantization related packages.
```bash
pip install hqq
```
2. Load & run the model.
```python
from transformers import AutoModelForCausalLM, AutoTokenizer
from hqq.engine.hf import HQQModelForCausalLM
from hqq.models.hf.base import AutoHQQHFModel
try:
model = HQQModelForCausalLM.from_quantized("PrunaAI/hooking-dev-Monah-8b-Uncensored-HQQ-2bit-smashed", device_map='auto')
except:
model = AutoHQQHFModel.from_quantized("PrunaAI/hooking-dev-Monah-8b-Uncensored-HQQ-2bit-smashed")
tokenizer = AutoTokenizer.from_pretrained("hooking-dev/Monah-8b-Uncensored")
input_ids = tokenizer("What is the color of prunes?,", return_tensors='pt').to(model.device)["input_ids"]
outputs = model.generate(input_ids, max_new_tokens=216)
tokenizer.decode(outputs[0])
```
## Configurations
The configuration info are in `smash_config.json`.
## Credits & License
The license of the smashed model follows the license of the original model. Please check the license of the original model hooking-dev/Monah-8b-Uncensored before using this model which provided the base model. The license of the `pruna-engine` is [here](https://pypi.org/project/pruna-engine/) on Pypi.
## Want to compress other models?
- Contact us and tell us which model to compress next [here](https://www.pruna.ai/contact).
- Request access to easily compress your own AI models [here](https://z0halsaff74.typeform.com/pruna-access?typeform-source=www.pruna.ai). |
PrunaAI/llmware-bling-phi-3-HQQ-2bit-smashed | PrunaAI | "2024-06-24T10:07:00Z" | 0 | 0 | transformers | [
"transformers",
"phi3",
"text-generation",
"pruna-ai",
"conversational",
"custom_code",
"base_model:llmware/bling-phi-3",
"autotrain_compatible",
"endpoints_compatible",
"region:us"
] | text-generation | "2024-06-24T10:06:17Z" | ---
thumbnail: "https://assets-global.website-files.com/646b351987a8d8ce158d1940/64ec9e96b4334c0e1ac41504_Logo%20with%20white%20text.svg"
base_model: llmware/bling-phi-3
metrics:
- memory_disk
- memory_inference
- inference_latency
- inference_throughput
- inference_CO2_emissions
- inference_energy_consumption
tags:
- pruna-ai
---
<!-- header start -->
<!-- 200823 -->
<div style="width: auto; margin-left: auto; margin-right: auto">
<a href="https://www.pruna.ai/" target="_blank" rel="noopener noreferrer">
<img src="https://i.imgur.com/eDAlcgk.png" alt="PrunaAI" style="width: 100%; min-width: 400px; display: block; margin: auto;">
</a>
</div>
<!-- header end -->
[](https://twitter.com/PrunaAI)
[](https://github.com/PrunaAI)
[](https://www.linkedin.com/company/93832878/admin/feed/posts/?feedType=following)
[](https://discord.gg/CP4VSgck)
# Simply make AI models cheaper, smaller, faster, and greener!
- Give a thumbs up if you like this model!
- Contact us and tell us which model to compress next [here](https://www.pruna.ai/contact).
- Request access to easily compress your *own* AI models [here](https://z0halsaff74.typeform.com/pruna-access?typeform-source=www.pruna.ai).
- Read the documentations to know more [here](https://pruna-ai-pruna.readthedocs-hosted.com/en/latest/)
- Join Pruna AI community on Discord [here](https://discord.gg/CP4VSgck) to share feedback/suggestions or get help.
## Results

**Frequently Asked Questions**
- ***How does the compression work?*** The model is compressed with hqq.
- ***How does the model quality change?*** The quality of the model output might vary compared to the base model.
- ***How is the model efficiency evaluated?*** These results were obtained on HARDWARE_NAME with configuration described in `model/smash_config.json` and are obtained after a hardware warmup. The smashed model is directly compared to the original base model. Efficiency results may vary in other settings (e.g. other hardware, image size, batch size, ...). We recommend to directly run them in the use-case conditions to know if the smashed model can benefit you.
- ***What is the model format?*** We use safetensors.
- ***What calibration data has been used?*** If needed by the compression method, we used WikiText as the calibration data.
- ***What is the naming convention for Pruna Huggingface models?*** We take the original model name and append "turbo", "tiny", or "green" if the smashed model has a measured inference speed, inference memory, or inference energy consumption which is less than 90% of the original base model.
- ***How to compress my own models?*** You can request premium access to more compression methods and tech support for your specific use-cases [here](https://z0halsaff74.typeform.com/pruna-access?typeform-source=www.pruna.ai).
- ***What are "first" metrics?*** Results mentioning "first" are obtained after the first run of the model. The first run might take more memory or be slower than the subsequent runs due cuda overheads.
- ***What are "Sync" and "Async" metrics?*** "Sync" metrics are obtained by syncing all GPU processes and stop measurement when all of them are executed. "Async" metrics are obtained without syncing all GPU processes and stop when the model output can be used by the CPU. We provide both metrics since both could be relevant depending on the use-case. We recommend to test the efficiency gains directly in your use-cases.
## Setup
You can run the smashed model with these steps:
0. Check requirements from the original repo llmware/bling-phi-3 installed. In particular, check python, cuda, and transformers versions.
1. Make sure that you have installed quantization related packages.
```bash
pip install hqq
```
2. Load & run the model.
```python
from transformers import AutoModelForCausalLM, AutoTokenizer
from hqq.engine.hf import HQQModelForCausalLM
from hqq.models.hf.base import AutoHQQHFModel
try:
model = HQQModelForCausalLM.from_quantized("PrunaAI/llmware-bling-phi-3-HQQ-2bit-smashed", device_map='auto')
except:
model = AutoHQQHFModel.from_quantized("PrunaAI/llmware-bling-phi-3-HQQ-2bit-smashed")
tokenizer = AutoTokenizer.from_pretrained("llmware/bling-phi-3")
input_ids = tokenizer("What is the color of prunes?,", return_tensors='pt').to(model.device)["input_ids"]
outputs = model.generate(input_ids, max_new_tokens=216)
tokenizer.decode(outputs[0])
```
## Configurations
The configuration info are in `smash_config.json`.
## Credits & License
The license of the smashed model follows the license of the original model. Please check the license of the original model llmware/bling-phi-3 before using this model which provided the base model. The license of the `pruna-engine` is [here](https://pypi.org/project/pruna-engine/) on Pypi.
## Want to compress other models?
- Contact us and tell us which model to compress next [here](https://www.pruna.ai/contact).
- Request access to easily compress your own AI models [here](https://z0halsaff74.typeform.com/pruna-access?typeform-source=www.pruna.ai). |
PrunaAI/llmware-bling-phi-3-HQQ-4bit-smashed | PrunaAI | "2024-06-24T10:07:29Z" | 0 | 0 | transformers | [
"transformers",
"phi3",
"text-generation",
"pruna-ai",
"conversational",
"custom_code",
"base_model:llmware/bling-phi-3",
"autotrain_compatible",
"endpoints_compatible",
"region:us"
] | text-generation | "2024-06-24T10:06:19Z" | ---
thumbnail: "https://assets-global.website-files.com/646b351987a8d8ce158d1940/64ec9e96b4334c0e1ac41504_Logo%20with%20white%20text.svg"
base_model: llmware/bling-phi-3
metrics:
- memory_disk
- memory_inference
- inference_latency
- inference_throughput
- inference_CO2_emissions
- inference_energy_consumption
tags:
- pruna-ai
---
<!-- header start -->
<!-- 200823 -->
<div style="width: auto; margin-left: auto; margin-right: auto">
<a href="https://www.pruna.ai/" target="_blank" rel="noopener noreferrer">
<img src="https://i.imgur.com/eDAlcgk.png" alt="PrunaAI" style="width: 100%; min-width: 400px; display: block; margin: auto;">
</a>
</div>
<!-- header end -->
[](https://twitter.com/PrunaAI)
[](https://github.com/PrunaAI)
[](https://www.linkedin.com/company/93832878/admin/feed/posts/?feedType=following)
[](https://discord.gg/CP4VSgck)
# Simply make AI models cheaper, smaller, faster, and greener!
- Give a thumbs up if you like this model!
- Contact us and tell us which model to compress next [here](https://www.pruna.ai/contact).
- Request access to easily compress your *own* AI models [here](https://z0halsaff74.typeform.com/pruna-access?typeform-source=www.pruna.ai).
- Read the documentations to know more [here](https://pruna-ai-pruna.readthedocs-hosted.com/en/latest/)
- Join Pruna AI community on Discord [here](https://discord.gg/CP4VSgck) to share feedback/suggestions or get help.
## Results

**Frequently Asked Questions**
- ***How does the compression work?*** The model is compressed with hqq.
- ***How does the model quality change?*** The quality of the model output might vary compared to the base model.
- ***How is the model efficiency evaluated?*** These results were obtained on HARDWARE_NAME with configuration described in `model/smash_config.json` and are obtained after a hardware warmup. The smashed model is directly compared to the original base model. Efficiency results may vary in other settings (e.g. other hardware, image size, batch size, ...). We recommend to directly run them in the use-case conditions to know if the smashed model can benefit you.
- ***What is the model format?*** We use safetensors.
- ***What calibration data has been used?*** If needed by the compression method, we used WikiText as the calibration data.
- ***What is the naming convention for Pruna Huggingface models?*** We take the original model name and append "turbo", "tiny", or "green" if the smashed model has a measured inference speed, inference memory, or inference energy consumption which is less than 90% of the original base model.
- ***How to compress my own models?*** You can request premium access to more compression methods and tech support for your specific use-cases [here](https://z0halsaff74.typeform.com/pruna-access?typeform-source=www.pruna.ai).
- ***What are "first" metrics?*** Results mentioning "first" are obtained after the first run of the model. The first run might take more memory or be slower than the subsequent runs due cuda overheads.
- ***What are "Sync" and "Async" metrics?*** "Sync" metrics are obtained by syncing all GPU processes and stop measurement when all of them are executed. "Async" metrics are obtained without syncing all GPU processes and stop when the model output can be used by the CPU. We provide both metrics since both could be relevant depending on the use-case. We recommend to test the efficiency gains directly in your use-cases.
## Setup
You can run the smashed model with these steps:
0. Check requirements from the original repo llmware/bling-phi-3 installed. In particular, check python, cuda, and transformers versions.
1. Make sure that you have installed quantization related packages.
```bash
pip install hqq
```
2. Load & run the model.
```python
from transformers import AutoModelForCausalLM, AutoTokenizer
from hqq.engine.hf import HQQModelForCausalLM
from hqq.models.hf.base import AutoHQQHFModel
try:
model = HQQModelForCausalLM.from_quantized("PrunaAI/llmware-bling-phi-3-HQQ-4bit-smashed", device_map='auto')
except:
model = AutoHQQHFModel.from_quantized("PrunaAI/llmware-bling-phi-3-HQQ-4bit-smashed")
tokenizer = AutoTokenizer.from_pretrained("llmware/bling-phi-3")
input_ids = tokenizer("What is the color of prunes?,", return_tensors='pt').to(model.device)["input_ids"]
outputs = model.generate(input_ids, max_new_tokens=216)
tokenizer.decode(outputs[0])
```
## Configurations
The configuration info are in `smash_config.json`.
## Credits & License
The license of the smashed model follows the license of the original model. Please check the license of the original model llmware/bling-phi-3 before using this model which provided the base model. The license of the `pruna-engine` is [here](https://pypi.org/project/pruna-engine/) on Pypi.
## Want to compress other models?
- Contact us and tell us which model to compress next [here](https://www.pruna.ai/contact).
- Request access to easily compress your own AI models [here](https://z0halsaff74.typeform.com/pruna-access?typeform-source=www.pruna.ai). |
PrunaAI/MysteriousAI-Mia-1B-HQQ-4bit-smashed | PrunaAI | "2024-06-24T10:07:12Z" | 0 | 0 | transformers | [
"transformers",
"llama",
"text-generation",
"pruna-ai",
"conversational",
"base_model:MysteriousAI/Mia-1B",
"autotrain_compatible",
"endpoints_compatible",
"text-generation-inference",
"region:us"
] | text-generation | "2024-06-24T10:06:42Z" | ---
thumbnail: "https://assets-global.website-files.com/646b351987a8d8ce158d1940/64ec9e96b4334c0e1ac41504_Logo%20with%20white%20text.svg"
base_model: MysteriousAI/Mia-1B
metrics:
- memory_disk
- memory_inference
- inference_latency
- inference_throughput
- inference_CO2_emissions
- inference_energy_consumption
tags:
- pruna-ai
---
<!-- header start -->
<!-- 200823 -->
<div style="width: auto; margin-left: auto; margin-right: auto">
<a href="https://www.pruna.ai/" target="_blank" rel="noopener noreferrer">
<img src="https://i.imgur.com/eDAlcgk.png" alt="PrunaAI" style="width: 100%; min-width: 400px; display: block; margin: auto;">
</a>
</div>
<!-- header end -->
[](https://twitter.com/PrunaAI)
[](https://github.com/PrunaAI)
[](https://www.linkedin.com/company/93832878/admin/feed/posts/?feedType=following)
[](https://discord.gg/CP4VSgck)
# Simply make AI models cheaper, smaller, faster, and greener!
- Give a thumbs up if you like this model!
- Contact us and tell us which model to compress next [here](https://www.pruna.ai/contact).
- Request access to easily compress your *own* AI models [here](https://z0halsaff74.typeform.com/pruna-access?typeform-source=www.pruna.ai).
- Read the documentations to know more [here](https://pruna-ai-pruna.readthedocs-hosted.com/en/latest/)
- Join Pruna AI community on Discord [here](https://discord.gg/CP4VSgck) to share feedback/suggestions or get help.
## Results

**Frequently Asked Questions**
- ***How does the compression work?*** The model is compressed with hqq.
- ***How does the model quality change?*** The quality of the model output might vary compared to the base model.
- ***How is the model efficiency evaluated?*** These results were obtained on HARDWARE_NAME with configuration described in `model/smash_config.json` and are obtained after a hardware warmup. The smashed model is directly compared to the original base model. Efficiency results may vary in other settings (e.g. other hardware, image size, batch size, ...). We recommend to directly run them in the use-case conditions to know if the smashed model can benefit you.
- ***What is the model format?*** We use safetensors.
- ***What calibration data has been used?*** If needed by the compression method, we used WikiText as the calibration data.
- ***What is the naming convention for Pruna Huggingface models?*** We take the original model name and append "turbo", "tiny", or "green" if the smashed model has a measured inference speed, inference memory, or inference energy consumption which is less than 90% of the original base model.
- ***How to compress my own models?*** You can request premium access to more compression methods and tech support for your specific use-cases [here](https://z0halsaff74.typeform.com/pruna-access?typeform-source=www.pruna.ai).
- ***What are "first" metrics?*** Results mentioning "first" are obtained after the first run of the model. The first run might take more memory or be slower than the subsequent runs due cuda overheads.
- ***What are "Sync" and "Async" metrics?*** "Sync" metrics are obtained by syncing all GPU processes and stop measurement when all of them are executed. "Async" metrics are obtained without syncing all GPU processes and stop when the model output can be used by the CPU. We provide both metrics since both could be relevant depending on the use-case. We recommend to test the efficiency gains directly in your use-cases.
## Setup
You can run the smashed model with these steps:
0. Check requirements from the original repo MysteriousAI/Mia-1B installed. In particular, check python, cuda, and transformers versions.
1. Make sure that you have installed quantization related packages.
```bash
pip install hqq
```
2. Load & run the model.
```python
from transformers import AutoModelForCausalLM, AutoTokenizer
from hqq.engine.hf import HQQModelForCausalLM
from hqq.models.hf.base import AutoHQQHFModel
try:
model = HQQModelForCausalLM.from_quantized("PrunaAI/MysteriousAI-Mia-1B-HQQ-4bit-smashed", device_map='auto')
except:
model = AutoHQQHFModel.from_quantized("PrunaAI/MysteriousAI-Mia-1B-HQQ-4bit-smashed")
tokenizer = AutoTokenizer.from_pretrained("MysteriousAI/Mia-1B")
input_ids = tokenizer("What is the color of prunes?,", return_tensors='pt').to(model.device)["input_ids"]
outputs = model.generate(input_ids, max_new_tokens=216)
tokenizer.decode(outputs[0])
```
## Configurations
The configuration info are in `smash_config.json`.
## Credits & License
The license of the smashed model follows the license of the original model. Please check the license of the original model MysteriousAI/Mia-1B before using this model which provided the base model. The license of the `pruna-engine` is [here](https://pypi.org/project/pruna-engine/) on Pypi.
## Want to compress other models?
- Contact us and tell us which model to compress next [here](https://www.pruna.ai/contact).
- Request access to easily compress your own AI models [here](https://z0halsaff74.typeform.com/pruna-access?typeform-source=www.pruna.ai). |
PrunaAI/MysteriousAI-Mia-1B-HQQ-2bit-smashed | PrunaAI | "2024-06-24T10:07:14Z" | 0 | 0 | transformers | [
"transformers",
"llama",
"text-generation",
"pruna-ai",
"conversational",
"base_model:MysteriousAI/Mia-1B",
"autotrain_compatible",
"endpoints_compatible",
"text-generation-inference",
"region:us"
] | text-generation | "2024-06-24T10:06:53Z" | ---
thumbnail: "https://assets-global.website-files.com/646b351987a8d8ce158d1940/64ec9e96b4334c0e1ac41504_Logo%20with%20white%20text.svg"
base_model: MysteriousAI/Mia-1B
metrics:
- memory_disk
- memory_inference
- inference_latency
- inference_throughput
- inference_CO2_emissions
- inference_energy_consumption
tags:
- pruna-ai
---
<!-- header start -->
<!-- 200823 -->
<div style="width: auto; margin-left: auto; margin-right: auto">
<a href="https://www.pruna.ai/" target="_blank" rel="noopener noreferrer">
<img src="https://i.imgur.com/eDAlcgk.png" alt="PrunaAI" style="width: 100%; min-width: 400px; display: block; margin: auto;">
</a>
</div>
<!-- header end -->
[](https://twitter.com/PrunaAI)
[](https://github.com/PrunaAI)
[](https://www.linkedin.com/company/93832878/admin/feed/posts/?feedType=following)
[](https://discord.gg/CP4VSgck)
# Simply make AI models cheaper, smaller, faster, and greener!
- Give a thumbs up if you like this model!
- Contact us and tell us which model to compress next [here](https://www.pruna.ai/contact).
- Request access to easily compress your *own* AI models [here](https://z0halsaff74.typeform.com/pruna-access?typeform-source=www.pruna.ai).
- Read the documentations to know more [here](https://pruna-ai-pruna.readthedocs-hosted.com/en/latest/)
- Join Pruna AI community on Discord [here](https://discord.gg/CP4VSgck) to share feedback/suggestions or get help.
## Results

**Frequently Asked Questions**
- ***How does the compression work?*** The model is compressed with hqq.
- ***How does the model quality change?*** The quality of the model output might vary compared to the base model.
- ***How is the model efficiency evaluated?*** These results were obtained on HARDWARE_NAME with configuration described in `model/smash_config.json` and are obtained after a hardware warmup. The smashed model is directly compared to the original base model. Efficiency results may vary in other settings (e.g. other hardware, image size, batch size, ...). We recommend to directly run them in the use-case conditions to know if the smashed model can benefit you.
- ***What is the model format?*** We use safetensors.
- ***What calibration data has been used?*** If needed by the compression method, we used WikiText as the calibration data.
- ***What is the naming convention for Pruna Huggingface models?*** We take the original model name and append "turbo", "tiny", or "green" if the smashed model has a measured inference speed, inference memory, or inference energy consumption which is less than 90% of the original base model.
- ***How to compress my own models?*** You can request premium access to more compression methods and tech support for your specific use-cases [here](https://z0halsaff74.typeform.com/pruna-access?typeform-source=www.pruna.ai).
- ***What are "first" metrics?*** Results mentioning "first" are obtained after the first run of the model. The first run might take more memory or be slower than the subsequent runs due cuda overheads.
- ***What are "Sync" and "Async" metrics?*** "Sync" metrics are obtained by syncing all GPU processes and stop measurement when all of them are executed. "Async" metrics are obtained without syncing all GPU processes and stop when the model output can be used by the CPU. We provide both metrics since both could be relevant depending on the use-case. We recommend to test the efficiency gains directly in your use-cases.
## Setup
You can run the smashed model with these steps:
0. Check requirements from the original repo MysteriousAI/Mia-1B installed. In particular, check python, cuda, and transformers versions.
1. Make sure that you have installed quantization related packages.
```bash
pip install hqq
```
2. Load & run the model.
```python
from transformers import AutoModelForCausalLM, AutoTokenizer
from hqq.engine.hf import HQQModelForCausalLM
from hqq.models.hf.base import AutoHQQHFModel
try:
model = HQQModelForCausalLM.from_quantized("PrunaAI/MysteriousAI-Mia-1B-HQQ-2bit-smashed", device_map='auto')
except:
model = AutoHQQHFModel.from_quantized("PrunaAI/MysteriousAI-Mia-1B-HQQ-2bit-smashed")
tokenizer = AutoTokenizer.from_pretrained("MysteriousAI/Mia-1B")
input_ids = tokenizer("What is the color of prunes?,", return_tensors='pt').to(model.device)["input_ids"]
outputs = model.generate(input_ids, max_new_tokens=216)
tokenizer.decode(outputs[0])
```
## Configurations
The configuration info are in `smash_config.json`.
## Credits & License
The license of the smashed model follows the license of the original model. Please check the license of the original model MysteriousAI/Mia-1B before using this model which provided the base model. The license of the `pruna-engine` is [here](https://pypi.org/project/pruna-engine/) on Pypi.
## Want to compress other models?
- Contact us and tell us which model to compress next [here](https://www.pruna.ai/contact).
- Request access to easily compress your own AI models [here](https://z0halsaff74.typeform.com/pruna-access?typeform-source=www.pruna.ai). |
Kalray/alexnet | Kalray | "2024-07-01T11:26:54Z" | 0 | 0 | null | [
"onnx",
"image-classification",
"dataset:ILSVRC/imagenet-1k",
"arxiv:1404.5997",
"license:mit",
"region:us"
] | image-classification | "2024-06-24T10:06:54Z" | ---
license: mit
datasets:
- ILSVRC/imagenet-1k
pipeline_tag: image-classification
---
# Introduction
This repository stores the model for Alexnet, compatible with Kalray's neural network API. </br>
Please see www.github.com/kalray/kann-models-zoo for details and proper usage. </br>
# Contents
- ONNX: alexnet-torch.onnx
# Lecture note reference
- ImageNet Classification with Deep Convolutional Neural Networks, https://papers.nips.cc/paper_files/paper/2012/file/c399862d3b9d6b76c8436e924a68c45b-Paper.pdf
- One weird trick for parallelizing convolutional neural networks, https://arxiv.org/pdf/1404.5997.pdf
# Repository or links references
- https://github.com/onnx/models/blob/main/vision/classification/alexnet/
- https://pytorch.org/vision/stable/models/generated/torchvision.models.alexnet.html#torchvision.models.alexnet
BibTeX entry and citation info
```
@article{ILSVRC15,
Author = {Olga Russakovsky and Jia Deng and Hao Su and Jonathan Krause and Sanjeev Satheesh and Sean Ma and Zhiheng Huang and Andrej Karpathy and Aditya Khosla and Michael Bernstein and Alexander C. Berg and Li Fei-Fei},
Title = {{ImageNet Large Scale Visual Recognition Challenge}},
Year = {2015},
journal = {International Journal of Computer Vision (IJCV)},
doi = {10.1007/s11263-015-0816-y},
volume={115},
number={3},
pages={211-252}
}
```
Author: nbouberbachene@kalrayinc.com |
nilcars/opencv_opencv_model | nilcars | "2024-06-24T10:53:40Z" | 0 | 0 | setfit | [
"setfit",
"safetensors",
"mpnet",
"sentence-transformers",
"text-classification",
"generated_from_setfit_trainer",
"arxiv:2209.11055",
"base_model:sentence-transformers/all-mpnet-base-v2",
"region:us"
] | text-classification | "2024-06-24T10:07:51Z" | ---
base_model: sentence-transformers/all-mpnet-base-v2
library_name: setfit
metrics:
- accuracy
pipeline_tag: text-classification
tags:
- setfit
- sentence-transformers
- text-classification
- generated_from_setfit_trainer
widget:
- text: "videowriter libraryLoad load opencv_videoio_ffmpeg460_64d.dll ### Descripe\
\ the feature and motivation\n\nCan I get opencv_videoio_ffmpeg460_64d.dll and\
\ opencv_videoio_ffmpeg460_64d.dll?(Shared libraries for debugging)Thank you!\n\
\n### Additional context\n\nI try to create video file by VideoWriter but failed,information\
\ like following:\r\nbackend_plugin.cpp (383) cv::impl::getPluginCandidates Found\
\ 3 plugin(s) for FFMPEG\r\nopencv-4.6.0\\\\modules\\\\core\\\\src\\\\utils\\\\\
plugin_loader.impl.hpp (67) cv::plugin::impl::DynamicLib::libraryLoad load D:\\\
\\develop\\\\opencv-4.6.0\\\\vs2017_x64\\\\bin\\\\Debug\\\\opencv_videoio_ffmpeg460_64d.dll\
\ => FAILED\r\nopencv-4.6.0\\\\modules\\\\core\\\\src\\\\utils\\\\plugin_loader.impl.hpp\
\ (67) cv::plugin::impl::DynamicLib::libraryLoad load opencv_videoio_ffmpeg460_64d.dll\
\ => FAILED\r\nopencv-4.6.0\\\\modules\\\\core\\\\src\\\\utils\\\\plugin_loader.impl.hpp\
\ (67) cv::plugin::impl::DynamicLib::libraryLoad load opencv_videoio_ffmpeg460_64.dll\
\ => FAILED\r\nopencv-4.6.0\\\\modules\\\\videoio\\\\src\\\\backend_plugin.cpp\
\ (383) cv::impl::getPluginCandidates Found 2 plugin(s) for INTEL_MFX\r\nopencv-4.6.0\\\
\\modules\\\\core\\\\src\\\\utils\\\\plugin_loader.impl.hpp (67) cv::plugin::impl::DynamicLib::libraryLoad\
\ load opencv-4.6.0\\\\vs2017_x64\\\\bin\\\\Debug\\\\opencv_videoio_intel_mfx460_64d.dll\
\ => FAILED\r\n[ INFO:0@51.519] \\\\opencv-4.6.0\\\\modules\\\\core\\\\src\\\\\
utils\\\\plugin_loader.impl.hpp (67) cv::plugin::impl::DynamicLib::libraryLoad\
\ load opencv_videoio_intel_mfx460_64d.dll => FAILED"
- text: "OpenCV solution for Visual Studio 2019 is not building opencv_core.lib when\
\ AVX2 is specified in (CPU_DISPATCH = SSE4_1;SSE4_2;AVX;FP16;AVX2) in x86 build\
\ ### System Information\n\nOpenCV solution for Visual Studio 2019 is not building\
\ opencv_core.lib when\r\nAVX2 is specified in (CPU_DISPATCH = SSE4_1;SSE4_2;AVX;FP16;AVX2)\
\ in x86 build\r\n\r\nOpenCV version: 4.8.0\r\nOperating System / Platform: Windows\
\ 10\r\nCompiler & compiler version: Visual Studio 2019\n\n### Detailed description\n\
\nBy default x64 CMake configuration for Visual Studio 2019 uses:\r\nCPU_BASELINE\
\ = SSE3;\r\nCPU_DISPATCH = SSE4_1;SSE4_2;AVX;FP16;AVX2\r\nand x86 configuration\
\ uses:\r\nCPU_BASELINE = SSE2;\r\nCPU_DISPATCH = SSE4_1;SSE4_2;AVX;FP16\r\n\r\
\nWhen I set the same flags in build for x86 as set in x64 build, building\r\n\
opencv_core module ends with failure:\r\n\r\nLogs from building opencv_core module:\r\
\nBuild started...\r\n1>------ Build started: Project: ZERO_CHECK, Configuration:\
\ Release Win32 ------\r\n1>Checking Build System\r\n2>------ Build started: Project:\
\ ippiw, Configuration: Release Win32 ------\r\n3>------ Build started: Project:\
\ ittnotify, Configuration: Release Win32 ------\r\n4>------ Build started: Project:\
\ opencv_core_SSE4_1, Configuration: Release Win32 ------\r\n5>------ Build started:\
\ Project: opencv_core_SSE4_2, Configuration: Release Win32 ------\r\n6>------\
\ Build started: Project: opencv_core_AVX, Configuration: Release Win32 ------\r\
\n7>------ Build started: Project: opencv_core_AVX2, Configuration: Release Win32\
\ ------\r\n8>------ Build started: Project: zlib, Configuration: Release Win32\
\ ------\r\n4>Building Custom Rule G:/opencv-4.8.0/modules/core/CMakeLists.txt\r\
\n2>Building Custom Rule G:/opencv-4.8.0/build_x86_AVX2/3rdparty/ippicv/ippicv_win/iw/CMakeLists.txt\r\
\n3>Building Custom Rule G:/opencv-4.8.0/3rdparty/ittnotify/CMakeLists.txt\r\n\
7>Building Custom Rule G:/opencv-4.8.0/modules/core/CMakeLists.txt\r\n6>Building\
\ Custom Rule G:/opencv-4.8.0/modules/core/CMakeLists.txt\r\n8>Building Custom\
\ Rule G:/opencv-4.8.0/3rdparty/zlib/CMakeLists.txt\r\n5>Building Custom Rule\
\ G:/opencv-4.8.0/modules/core/CMakeLists.txt\r\n2>iw_core.c\r\n6>mathfuncs_core.avx.cpp\r\
\n4>arithm.sse4_1.cpp\r\n2>iw_image.c\r\n2>iw_image_color_convert_all.c\r\n2>iw_image_color_convert_rgbs.c\r\
\n3>ittnotify_static.c\r\n2>iw_image_filter_bilateral.c\r\n2>iw_image_filter_box.c\r\
\n4>matmul.sse4_1.cpp\r\n3>jitprofiling.c\r\n2>iw_image_filter_canny.c\r\n2>iw_image_filter_gaussian.c\r\
\n5>stat.sse4_2.cpp\r\n7>mathfuncs_core.avx2.cpp\r\n7>stat.avx2.cpp\r\n8>adler32.c\r\
\n8>compress.c\r\n8>crc32.c\r\n8>deflate.c\r\n7>arithm.avx2.cpp\r\n7>convert.avx2.cpp\r\
\n8>gzclose.c\r\n8>gzlib.c\r\n8>gzread.c\r\n7>convert_scale.avx2.cpp\r\n7>count_non_zero.avx2.cpp\r\
\n7>has_non_zero.avx2.cpp\r\n7>matmul.avx2.cpp\r\n8>gzwrite.c\r\n2>iw_image_filter_general.c\r\
\n2>iw_image_filter_laplacian.c\r\n2>iw_image_filter_morphology.c\r\n8>inflate.c\r\
\n8>infback.c\r\n8>inftrees.c\r\n2>iw_image_filter_scharr.c\r\n2>iw_image_filter_sobel.c\r\
\n2>iw_image_op_copy.c\r\n2>iw_image_op_copy_channel.c\r\n2>iw_image_op_copy_make_border.c\r\
\n2>iw_image_op_copy_merge.c\r\n2>iw_image_op_copy_split.c\r\n2>iw_image_op_scale.c\r\
\n8>inffast.c\r\n8>trees.c\r\n8>uncompr.c\r\n8>zutil.c\r\n7>G:\\\\opencv-4.8.0\\\
\\modules\\\\core\\\\include\\\\opencv2/core/hal/intrin_avx.hpp(130,12): error\
\ C3861: '_mm256_extract_epi64': identifier not found (compiling source file G:\\\
\\opencv-4.8.0\\\\build_x86_AVX2\\\\modules\\\\core\\\\stat.avx2.cpp)\r\n7>G:\\\
\\opencv-4.8.0\\\\modules\\\\core\\\\include\\\\opencv2/core/hal/intrin_avx.hpp(2312):\
\ message : see reference to function template instantiation 'int64 cv::hal_AVX2::_v256_extract_epi64<3>(const\
\ __m256i &)' being compiled (compiling source file G:\\\\opencv-4.8.0\\\\build_x86_AVX2\\\
\\modules\\\\core\\\\stat.avx2.cpp)\r\n7>G:\\\\opencv-4.8.0\\\\modules\\\\core\\\
\\include\\\\opencv2/core/hal/intrin.hpp(1016): message : see reference to function\
\ template instantiation 'uint64 cv::hal_AVX2::v_extract_n<3>(cv::hal_AVX2::v_uint64x4)'\
\ being compiled (compiling source file G:\\\\opencv-4.8.0\\\\build_x86_AVX2\\\
\\modules\\\\core\\\\stat.avx2.cpp)\r\n7>mean.avx2.cpp\r\n2>iw_image_op_set.c\r\
\n2>iw_image_op_set_channel.c\r\n2>iw_image_op_swap_channels.c\r\n2>iw_image_transform_mirror.c\r\
\n2>iw_image_transform_resize.c\r\n2>iw_image_transform_rotate.c\r\n2>iw_image_transform_warpaffine.c\r\
\n2>iw_own.c\r\n7>G:\\\\opencv-4.8.0\\\\modules\\\\core\\\\include\\\\opencv2/core/hal/intrin_avx.hpp(130,12):\
\ error C3861: '_mm256_extract_epi64': identifier not found (compiling source\
\ file G:\\\\opencv-4.8.0\\\\build_x86_AVX2\\\\modules\\\\core\\\\arithm.avx2.cpp)\r\
\n7>G:\\\\opencv-4.8.0\\\\modules\\\\core\\\\include\\\\opencv2/core/hal/intrin_avx.hpp(2312):\
\ message : see reference to function template instantiation 'int64 cv::hal_AVX2::_v256_extract_epi64<3>(const\
\ __m256i &)' being compiled (compiling source file G:\\\\opencv-4.8.0\\\\build_x86_AVX2\\\
\\modules\\\\core\\\\arithm.avx2.cpp)\r\n7>G:\\\\opencv-4.8.0\\\\modules\\\\core\\\
\\include\\\\opencv2/core/hal/intrin.hpp(1016): message : see reference to function\
\ template instantiation 'uint64 cv::hal_AVX2::v_extract_n<3>(cv::hal_AVX2::v_uint64x4)'\
\ being compiled (compiling source file G:\\\\opencv-4.8.0\\\\build_x86_AVX2\\\
\\modules\\\\core\\\\arithm.avx2.cpp)\r\n7>merge.avx2.cpp\r\n7>G:\\\\opencv-4.8.0\\\
\\modules\\\\core\\\\include\\\\opencv2/core/hal/intrin_avx.hpp(130,12): error\
\ C3861: '_mm256_extract_epi64': identifier not found (compiling source file G:\\\
\\opencv-4.8.0\\\\build_x86_AVX2\\\\modules\\\\core\\\\mathfuncs_core.avx2.cpp)\r\
\n7>G:\\\\opencv-4.8.0\\\\modules\\\\core\\\\include\\\\opencv2/core/hal/intrin_avx.hpp(2312):\
\ message : see reference to function template instantiation 'int64 cv::hal_AVX2::_v256_extract_epi64<3>(const\
\ __m256i &)' being compiled (compiling source file G:\\\\opencv-4.8.0\\\\build_x86_AVX2\\\
\\modules\\\\core\\\\mathfuncs_core.avx2.cpp)\r\n7>G:\\\\opencv-4.8.0\\\\modules\\\
\\core\\\\include\\\\opencv2/core/hal/intrin.hpp(1016): message : see reference\
\ to function template instantiation 'uint64 cv::hal_AVX2::v_extract_n<3>(cv::hal_AVX2::v_uint64x4)'\
\ being compiled (compiling source file G:\\\\opencv-4.8.0\\\\build_x86_AVX2\\\
\\modules\\\\core\\\\mathfuncs_core.avx2.cpp)\r\n7>split.avx2.cpp\r\n7>G:\\\\\
opencv-4.8.0\\\\modules\\\\core\\\\include\\\\opencv2/core/hal/intrin_avx.hpp(130,12):\
\ error C3861: '_mm256_extract_epi64': identifier not found (compiling source\
\ file G:\\\\opencv-4.8.0\\\\build_x86_AVX2\\\\modules\\\\core\\\\count_non_zero.avx2.cpp)\r\
\n7>G:\\\\opencv-4.8.0\\\\modules\\\\core\\\\include\\\\opencv2/core/hal/intrin_avx.hpp(2312):\
\ message : see reference to function template instantiation 'int64 cv::hal_AVX2::_v256_extract_epi64<3>(const\
\ __m256i &)' being compiled (compiling source file G:\\\\opencv-4.8.0\\\\build_x86_AVX2\\\
\\modules\\\\core\\\\count_non_zero.avx2.cpp)\r\n7>G:\\\\opencv-4.8.0\\\\modules\\\
\\core\\\\include\\\\opencv2/core/hal/intrin.hpp(1016): message : see reference\
\ to function template instantiation 'uint64 cv::hal_AVX2::v_extract_n<3>(cv::hal_AVX2::v_uint64x4)'\
\ being compiled (compiling source file G:\\\\opencv-4.8.0\\\\build_x86_AVX2\\\
\\modules\\\\core\\\\count_non_zero.avx2.cpp)\r\n7>sum.avx2.cpp\r\n7>G:\\\\opencv-4.8.0\\\
\\modules\\\\core\\\\include\\\\opencv2/core/hal/intrin_avx.hpp(130,12): error\
\ C3861: '_mm256_extract_epi64': identifier not found (compiling source file G:\\\
\\opencv-4.8.0\\\\build_x86_AVX2\\\\modules\\\\core\\\\matmul.avx2.cpp)\r\n7>G:\\\
\\opencv-4.8.0\\\\modules\\\\core\\\\include\\\\opencv2/core/hal/intrin_avx.hpp(2312):\
\ message : see reference to function template instantiation 'int64 cv::hal_AVX2::_v256_extract_epi64<3>(const\
\ __m256i &)' being compiled (compiling source file G:\\\\opencv-4.8.0\\\\build_x86_AVX2\\\
\\modules\\\\core\\\\matmul.avx2.cpp)\r\n7>G:\\\\opencv-4.8.0\\\\modules\\\\core\\\
\\include\\\\opencv2/core/hal/intrin.hpp(1016): message : see reference to function\
\ template instantiation 'uint64 cv::hal_AVX2::v_extract_n<3>(cv::hal_AVX2::v_uint64x4)'\
\ being compiled (compiling source file G:\\\\opencv-4.8.0\\\\build_x86_AVX2\\\
\\modules\\\\core\\\\matmul.avx2.cpp)\r\n7>G:\\\\opencv-4.8.0\\\\modules\\\\core\\\
\\include\\\\opencv2/core/hal/intrin_avx.hpp(130,12): error C3861: '_mm256_extract_epi64':\
\ identifier not found (compiling source file G:\\\\opencv-4.8.0\\\\build_x86_AVX2\\\
\\modules\\\\core\\\\convert.avx2.cpp)\r\n7>G:\\\\opencv-4.8.0\\\\modules\\\\\
core\\\\include\\\\opencv2/core/hal/intrin_avx.hpp(2312): message : see reference\
\ to function template instantiation 'int64 cv::hal_AVX2::_v256_extract_epi64<3>(const\
\ __m256i &)' being compiled (compiling source file G:\\\\opencv-4.8.0\\\\build_x86_AVX2\\\
\\modules\\\\core\\\\convert.avx2.cpp)\r\n7>G:\\\\opencv-4.8.0\\\\modules\\\\\
core\\\\include\\\\opencv2/core/hal/intrin.hpp(1016): message : see reference\
\ to function template instantiation 'uint64 cv::hal_AVX2::v_extract_n<3>(cv::hal_AVX2::v_uint64x4)'\
\ being compiled (compiling source file G:\\\\opencv-4.8.0\\\\build_x86_AVX2\\\
\\modules\\\\core\\\\convert.avx2.cpp)\r\n7>G:\\\\opencv-4.8.0\\\\modules\\\\\
core\\\\include\\\\opencv2/core/hal/intrin_avx.hpp(130,12): error C3861: '_mm256_extract_epi64':\
\ identifier not found (compiling source file G:\\\\opencv-4.8.0\\\\build_x86_AVX2\\\
\\modules\\\\core\\\\convert_scale.avx2.cpp)\r\n7>G:\\\\opencv-4.8.0\\\\modules\\\
\\core\\\\include\\\\opencv2/core/hal/intrin_avx.hpp(2312): message : see reference\
\ to function template instantiation 'int64 cv::hal_AVX2::_v256_extract_epi64<3>(const\
\ __m256i &)' being compiled (compiling source file G:\\\\opencv-4.8.0\\\\build_x86_AVX2\\\
\\modules\\\\core\\\\convert_scale.avx2.cpp)\r\n7>G:\\\\opencv-4.8.0\\\\modules\\\
\\core\\\\include\\\\opencv2/core/hal/intrin.hpp(1016): message : see reference\
\ to function template instantiation 'uint64 cv::hal_AVX2::v_extract_n<3>(cv::hal_AVX2::v_uint64x4)'\
\ being compiled (compiling source file G:\\\\opencv-4.8.0\\\\build_x86_AVX2\\\
\\modules\\\\core\\\\convert_scale.avx2.cpp)\r\n7>G:\\\\opencv-4.8.0\\\\modules\\\
\\core\\\\include\\\\opencv2/core/hal/intrin_avx.hpp(130,12): error C3861: '_mm256_extract_epi64':\
\ identifier not found (compiling source file G:\\\\opencv-4.8.0\\\\build_x86_AVX2\\\
\\modules\\\\core\\\\has_non_zero.avx2.cpp)\r\n7>G:\\\\opencv-4.8.0\\\\modules\\\
\\core\\\\include\\\\opencv2/core/hal/intrin_avx.hpp(2312): message : see reference\
\ to function template instantiation 'int64 cv::hal_AVX2::_v256_extract_epi64<3>(const\
\ __m256i &)' being compiled (compiling source file G:\\\\opencv-4.8.0\\\\build_x86_AVX2\\\
\\modules\\\\core\\\\has_non_zero.avx2.cpp)\r\n7>G:\\\\opencv-4.8.0\\\\modules\\\
\\core\\\\include\\\\opencv2/core/hal/intrin.hpp(1016): message : see reference\
\ to function template instantiation 'uint64 cv::hal_AVX2::v_extract_n<3>(cv::hal_AVX2::v_uint64x4)'\
\ being compiled (compiling source file G:\\\\opencv-4.8.0\\\\build_x86_AVX2\\\
\\modules\\\\core\\\\has_non_zero.avx2.cpp)\r\n3>ittnotify.vcxproj -> G:\\\\opencv-4.8.0\\\
\\build_x86_AVX2\\\\3rdparty\\\\lib\\\\Release\\\\ittnotify.lib\r\n5>opencv_core_SSE4_2.vcxproj\
\ -> G:\\\\opencv-4.8.0\\\\build_x86_AVX2\\\\modules\\\\core\\\\opencv_core_SSE4_2.dir\\\
\\Release\\\\opencv_core_SSE4_2.lib\r\n2>ippiw.vcxproj -> G:\\\\opencv-4.8.0\\\
\\build_x86_AVX2\\\\3rdparty\\\\lib\\\\Release\\\\ippiw.lib\r\n8>zlib.vcxproj\
\ -> G:\\\\opencv-4.8.0\\\\build_x86_AVX2\\\\3rdparty\\\\lib\\\\Release\\\\zlib.lib\r\
\n6>opencv_core_AVX.vcxproj -> G:\\\\opencv-4.8.0\\\\build_x86_AVX2\\\\modules\\\
\\core\\\\opencv_core_AVX.dir\\\\Release\\\\opencv_core_AVX.lib\r\n4>opencv_core_SSE4_1.vcxproj\
\ -> G:\\\\opencv-4.8.0\\\\build_x86_AVX2\\\\modules\\\\core\\\\opencv_core_SSE4_1.dir\\\
\\Release\\\\opencv_core_SSE4_1.lib\r\n7>G:\\\\opencv-4.8.0\\\\modules\\\\core\\\
\\include\\\\opencv2/core/hal/intrin_avx.hpp(130,12): error C3861: '_mm256_extract_epi64':\
\ identifier not found (compiling source file G:\\\\opencv-4.8.0\\\\build_x86_AVX2\\\
\\modules\\\\core\\\\mean.avx2.cpp)\r\n7>G:\\\\opencv-4.8.0\\\\modules\\\\core\\\
\\include\\\\opencv2/core/hal/intrin_avx.hpp(2312): message : see reference to\
\ function template instantiation 'int64 cv::hal_AVX2::_v256_extract_epi64<3>(const\
\ __m256i &)' being compiled (compiling source file G:\\\\opencv-4.8.0\\\\build_x86_AVX2\\\
\\modules\\\\core\\\\mean.avx2.cpp)\r\n7>G:\\\\opencv-4.8.0\\\\modules\\\\core\\\
\\include\\\\opencv2/core/hal/intrin.hpp(1016): message : see reference to function\
\ template instantiation 'uint64 cv::hal_AVX2::v_extract_n<3>(cv::hal_AVX2::v_uint64x4)'\
\ being compiled (compiling source file G:\\\\opencv-4.8.0\\\\build_x86_AVX2\\\
\\modules\\\\core\\\\mean.avx2.cpp)\r\n7>G:\\\\opencv-4.8.0\\\\modules\\\\core\\\
\\include\\\\opencv2/core/hal/intrin_avx.hpp(130,12): error C3861: '_mm256_extract_epi64':\
\ identifier not found (compiling source file G:\\\\opencv-4.8.0\\\\build_x86_AVX2\\\
\\modules\\\\core\\\\merge.avx2.cpp)\r\n7>G:\\\\opencv-4.8.0\\\\modules\\\\core\\\
\\include\\\\opencv2/core/hal/intrin_avx.hpp(2312): message : see reference to\
\ function template instantiation 'int64 cv::hal_AVX2::_v256_extract_epi64<3>(const\
\ __m256i &)' being compiled (compiling source file G:\\\\opencv-4.8.0\\\\build_x86_AVX2\\\
\\modules\\\\core\\\\merge.avx2.cpp)\r\n7>G:\\\\opencv-4.8.0\\\\modules\\\\core\\\
\\include\\\\opencv2/core/hal/intrin.hpp(1016): message : see reference to function\
\ template instantiation 'uint64 cv::hal_AVX2::v_extract_n<3>(cv::hal_AVX2::v_uint64x4)'\
\ being compiled (compiling source file G:\\\\opencv-4.8.0\\\\build_x86_AVX2\\\
\\modules\\\\core\\\\merge.avx2.cpp)\r\n7>G:\\\\opencv-4.8.0\\\\modules\\\\core\\\
\\include\\\\opencv2/core/hal/intrin_avx.hpp(130,12): error C3861: '_mm256_extract_epi64':\
\ identifier not found (compiling source file G:\\\\opencv-4.8.0\\\\build_x86_AVX2\\\
\\modules\\\\core\\\\split.avx2.cpp)\r\n7>G:\\\\opencv-4.8.0\\\\modules\\\\core\\\
\\include\\\\opencv2/core/hal/intrin_avx.hpp(2312): message : see reference to\
\ function template instantiation 'int64 cv::hal_AVX2::_v256_extract_epi64<3>(const\
\ __m256i &)' being compiled (compiling source file G:\\\\opencv-4.8.0\\\\build_x86_AVX2\\\
\\modules\\\\core\\\\split.avx2.cpp)\r\n7>G:\\\\opencv-4.8.0\\\\modules\\\\core\\\
\\include\\\\opencv2/core/hal/intrin.hpp(1016): message : see reference to function\
\ template instantiation 'uint64 cv::hal_AVX2::v_extract_n<3>(cv::hal_AVX2::v_uint64x4)'\
\ being compiled (compiling source file G:\\\\opencv-4.8.0\\\\build_x86_AVX2\\\
\\modules\\\\core\\\\split.avx2.cpp)\r\n7>G:\\\\opencv-4.8.0\\\\modules\\\\core\\\
\\include\\\\opencv2/core/hal/intrin_avx.hpp(130,12): error C3861: '_mm256_extract_epi64':\
\ identifier not found (compiling source file G:\\\\opencv-4.8.0\\\\build_x86_AVX2\\\
\\modules\\\\core\\\\sum.avx2.cpp)\r\n7>G:\\\\opencv-4.8.0\\\\modules\\\\core\\\
\\include\\\\opencv2/core/hal/intrin_avx.hpp(2312): message : see reference to\
\ function template instantiation 'int64 cv::hal_AVX2::_v256_extract_epi64<3>(const\
\ __m256i &)' being compiled (compiling source file G:\\\\opencv-4.8.0\\\\build_x86_AVX2\\\
\\modules\\\\core\\\\sum.avx2.cpp)\r\n7>G:\\\\opencv-4.8.0\\\\modules\\\\core\\\
\\include\\\\opencv2/core/hal/intrin.hpp(1016): message : see reference to function\
\ template instantiation 'uint64 cv::hal_AVX2::v_extract_n<3>(cv::hal_AVX2::v_uint64x4)'\
\ being compiled (compiling source file G:\\\\opencv-4.8.0\\\\build_x86_AVX2\\\
\\modules\\\\core\\\\sum.avx2.cpp)\r\n7>Done building project \"opencv_core_AVX2.vcxproj\"\
\ -- FAILED.\r\n9>------ Build started: Project: opencv_core, Configuration: Release\
\ Win32 ------\r\n9>Processing OpenCL kernels (core)\r\n9>-- G:/opencv-4.8.0/build_x86_AVX2/modules/core/opencl_kernels_core.hpp\
\ contains the same content\r\n9>Building Custom Rule G:/opencv-4.8.0/modules/core/CMakeLists.txt\r\
\n9>cmake_pch.cxx\r\n9>algorithm.cpp\r\n9>arithm.cpp\r\n9>arithm.dispatch.cpp\r\
\n9>array.cpp\r\n9>async.cpp\r\n9>batch_distance.cpp\r\n9>bindings_utils.cpp\r\
\n9>buffer_area.cpp\r\n9>channels.cpp\r\n9>check.cpp\r\n9>command_line_parser.cpp\r\
\n9>conjugate_gradient.cpp\r\n9>convert.dispatch.cpp\r\n9>convert_c.cpp\r\n9>convert_scale.dispatch.cpp\r\
\n9>copy.cpp\r\n9>count_non_zero.dispatch.cpp\r\n9>cuda_gpu_mat.cpp\r\n9>cuda_gpu_mat_nd.cpp\r\
\n9>cuda_host_mem.cpp\r\n9>cuda_info.cpp\r\n9>cuda_stream.cpp\r\n9>datastructs.cpp\r\
\n9>directx.cpp\r\n9>downhill_simplex.cpp\r\n9>dxt.cpp\r\n9>gl_core_3_1.cpp\r\n\
9>glob.cpp\r\n9>hal_internal.cpp\r\n9>has_non_zero.dispatch.cpp\r\n9>kmeans.cpp\r\
\n9>lapack.cpp\r\n9>lda.cpp\r\n9>logger.cpp\r\n9>lpsolver.cpp\r\n9>lut.cpp\r\n\
9>mathfuncs.cpp\r\n9>mathfuncs_core.dispatch.cpp\r\n9>matmul.dispatch.cpp\r\n\
9>matrix.cpp\r\n9>matrix_c.cpp\r\n9>matrix_decomp.cpp\r\n9>matrix_expressions.cpp\r\
\n9>matrix_iterator.cpp\r\n9>matrix_operations.cpp\r\n9>matrix_sparse.cpp\r\n\
9>matrix_transform.cpp\r\n9>matrix_wrap.cpp\r\n9>mean.dispatch.cpp\r\n9>merge.dispatch.cpp\r\
\n9>minmax.cpp\r\n9>norm.cpp\r\n9>ocl.cpp\r\n9>opencl_clblas.cpp\r\n9>opencl_clfft.cpp\r\
\n9>opencl_core.cpp\r\n9>opengl.cpp\r\n9>out.cpp\r\n9>ovx.cpp\r\n9>parallel_openmp.cpp\r\
\n9>parallel_tbb.cpp\r\n9>parallel_impl.cpp\r\n9>pca.cpp\r\n9>persistence.cpp\r\
\n9>persistence_base64_encoding.cpp\r\n9>persistence_json.cpp\r\n9>persistence_types.cpp\r\
\n9>persistence_xml.cpp\r\n9>persistence_yml.cpp\r\n9>rand.cpp\r\n9>softfloat.cpp\r\
\n9>split.dispatch.cpp\r\n9>stat.dispatch.cpp\r\n9>stat_c.cpp\r\n9>stl.cpp\r\n\
9>sum.dispatch.cpp\r\n9>system.cpp\r\n9>tables.cpp\r\n9>trace.cpp\r\n9>types.cpp\r\
\n9>umatrix.cpp\r\n9>datafile.cpp\r\n9>filesystem.cpp\r\n9>logtagconfigparser.cpp\r\
\n9>logtagmanager.cpp\r\n9>samples.cpp\r\n9>va_intel.cpp\r\n9>opencl_kernels_core.cpp\r\
\n9>alloc.cpp\r\n9>parallel.cpp\r\n9>parallel.cpp\r\n9>LINK : fatal error LNK1181:\
\ cannot open input file 'G:\\\\opencv-4.8.0\\\\build_x86_AVX2\\\\modules\\\\\
core\\\\opencv_core_AVX2.dir\\\\Release\\\\mathfuncs_core.avx2.obj'\r\n9>Done\
\ building project \"opencv_core.vcxproj\" -- FAILED.\r\n========== Build: 7 succeeded,\
\ 2 failed, 0 up-to-date, 0 skipped ==========\r\n\n\n### Steps to reproduce\n\
\n1. Set Cmake configuration to Visual Studio 2019 x86\r\n2. Set this flags\r\n\
CPU_BASELINE = SSE3;\r\nCPU_DISPATCH = SSE4_1;SSE4_2;AVX;FP16;AVX2\r\nBUILD_SHARED_LIBS=OFF\r\
\n3. Build Visual Studio 2019 solution\r\n4. Open solution and build opencv_core\
\ module\r\n[CMakeCache.txt](https://github.com/opencv/opencv/files/11957266/CMakeCache.txt)\r\
\n\n\n### Issue submission checklist\n\n- [X] I report the issue, it's not a question\n\
- [X] I checked the problem with documentation, FAQ, open issues, forum.opencv.org,\
\ Stack Overflow, etc and have not found any solution\n- [X] I updated to the\
\ latest OpenCV version and the issue is still there\n- [X] There is reproducer\
\ code and related data files (videos, images, onnx, etc)"
- text: "dnn onnx: invalid shape dimension and wrong results in test case Test_ONNX_layers.OpenAI_CLIP_head\
\ ### System Information\n\nlatest opencv and lastest onnxruntime\n\n### Detailed\
\ description\n\nRelated PR: https://github.com/opencv/opencv/pull/23419 and https://github.com/opencv/opencv_extra/pull/1049.\r\
\n\r\nIn the above PR, several fixes are indtroduced for the clip head. Those\
\ fixes are fine, but the manually generated onnx model named \"clip-vit-base-head.onnx\"\
\ is invalid and **cannot be run by onnxruntime**:\r\n\r\n1. In the Expand operator,\
\ `shape` input should be of type `int64` instead of `int32`. Please use `onnx.checker.check_model(model)`\
\ (it checks operator definitions and so on) before saving a manually generated\
\ onnx model.\r\n2. Having that fixed, onnxruntime still complains invalid shape\
\ in the Expand operator. Changing the shape value from [1, 1, -1] to [1, 1, 1]\
\ fixes the problem. Please run the manually generated onnx model with onnxruntime\
\ to check whether it is all valid.\r\n\r\ncc @dkurt \n\n### Steps to reproduce\n\
\nInstall onnxruntime, then\r\n\r\n```\r\nimport numpy as np\r\nfrom onnxruntime\
\ import InferenceSession\r\n\r\n\r\nnet = InferenceSession(\"./clip-vit-base-head.onnx\"\
)\r\nout = net.run([], {\"input\": np.random.randn(1, 1, 3).astype(np.float32)})\r\
\nprint(out[0].shape)\r\n```\n\n### Issue submission checklist\n\n- [X] I report\
\ the issue, it's not a question\n- [X] I checked the problem with documentation,\
\ FAQ, open issues, forum.opencv.org, Stack Overflow, etc and have not found any\
\ solution\n- [X] I updated to the latest OpenCV version and the issue is still\
\ there\n- [X] There is reproducer code and related data files (videos, images,\
\ onnx, etc)"
- text: "OpenCV build - cmake don't recognize GTK2 or GTK3 ##### System information\
\ (version)\r\n\r\n- OpenCV => 4.7.0\r\n- Operating System / Platform => Ubuntu\
\ 20.04\r\n- Compiler => g++ (Ubuntu 9.4.0-1ubuntu1~20.04.1) 9.4.0\r\n- C++ Standard:\
\ 11\r\n\r\n##### Detailed description\r\n\r\nI am trying to create OpenCV with\
\ the GTK GUI. I have `GTK2` and `GTK3` installed on the system, but neither version\
\ is recognised by cmake. \r\n\r\n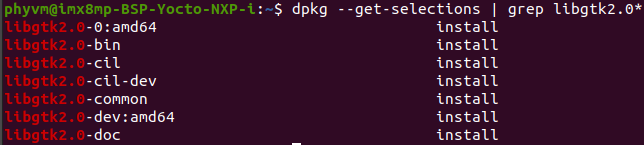\r\
\n\r\
\n\r\n\r\nThe output after executing cmake remains \r\n**GUI: NONE** \r\n**GTK+:NO**\r\
\n\r\nI execute the cmake command as follows:\r\n\r\n`cmake -DPYTHON_DEFAULT_EXECUTABLE=$(which\
\ python3) -DWITH_GTK=ON ../opencv`\r\n\r\nor\r\n\r\n`cmake -DPYTHON_DEFAULT_EXECUTABLE=$(which\
\ python3) -DWITH_GTK=ON -DWITH_GTK_2_X=ON ../opencv`\r\n\r\n##### Steps to reproduce\r\
\n\r\ngit clone https://github.com/opencv/opencv.git\r\nmkdir -p build && cd build\r\
\ncmake ../opencv (default, custom look above)\r\n\r\nThank you for your help\r\
\n"
- text: "How to confirm the version of the OpenCV 3rdParty libs <!--\r\nIf you have\
\ a question rather than reporting a bug please go to https://forum.opencv.org\
\ where you get much faster responses.\r\nIf you need further assistance please\
\ read [How To Contribute](https://github.com/opencv/opencv/wiki/How_to_contribute).\r\
\n\r\nThis is a template helping you to create an issue which can be processed\
\ as quickly as possible. This is the bug reporting section for the OpenCV library.\r\
\n-->\r\n\r\n##### System information (version)\r\n- OpenCV = 4.5.2\r\n- Operating\
\ System / Platform => Linux\r\n- Compiler => gcc version 4.8.5\r\n\r\n\r\n#####\
\ Detailed description\r\nI need to obtain each software version in the 3rdparty\
\ directory in the source code of OpenCV 4.5.2 to analyze whether OpenCV 4.5.2\
\ has dependency vulnerabilities.But the third-party software version is not found\
\ in the code repository or in the document.For example, the versions of open-source\
\ software such as carotene, cpufeatures, ippicv, and ittnotify cannot be confirmed.\r\
\n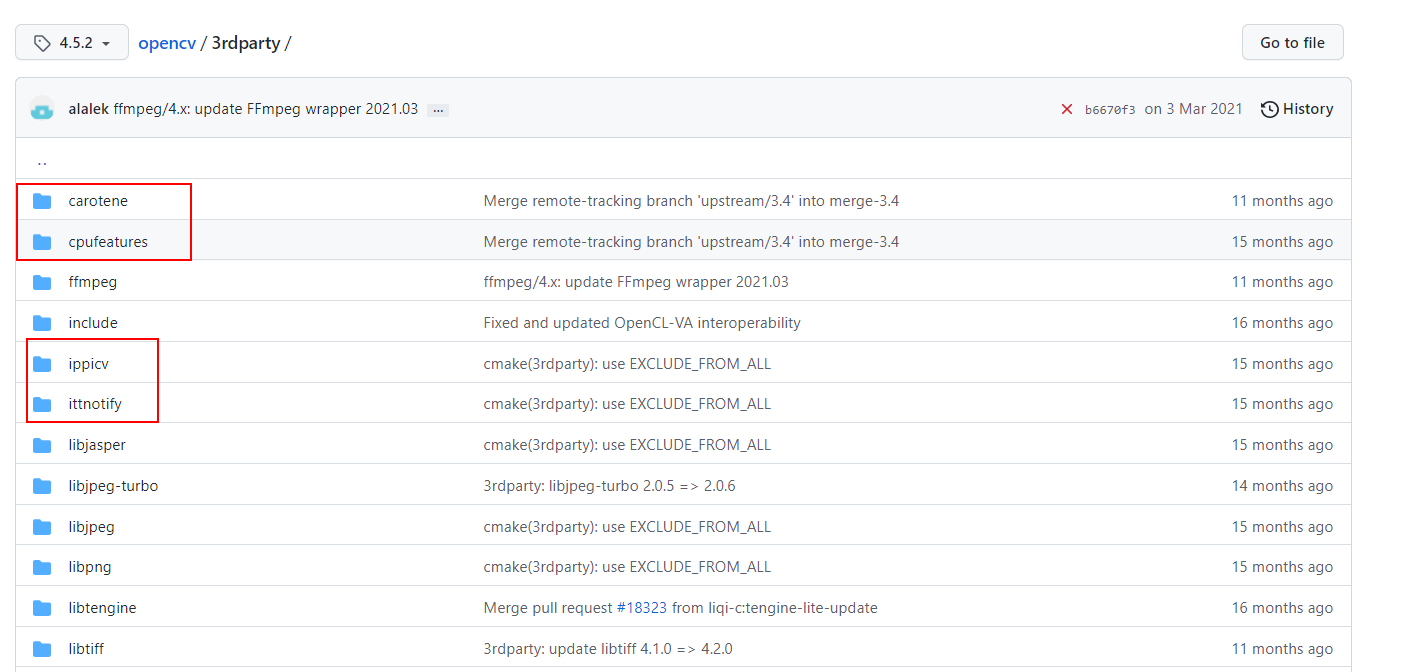\r\
\n\r\n\r\n\r\n##### Steps to reproduce\r\n\r\n\r\n##### Issue submission checklist\r\
\n\r\n - [x] I report the issue, it's not a question\r\n <!--\r\n OpenCV team\
\ works with forum.opencv.org, Stack Overflow and other communities\r\n to discuss\
\ problems. Tickets with questions without a real issue statement will be\r\n\
\ closed.\r\n -->\r\n - [x] I checked the problem with documentation, FAQ,\
\ open issues,\r\n forum.opencv.org, Stack Overflow, etc and have not found\
\ any solution\r\n <!--\r\n Places to check:\r\n * OpenCV documentation:\
\ https://docs.opencv.org\r\n * FAQ page: https://github.com/opencv/opencv/wiki/FAQ\r\
\n * OpenCV forum: https://forum.opencv.org\r\n * OpenCV issue tracker: https://github.com/opencv/opencv/issues?q=is%3Aissue\r\
\n * Stack Overflow branch: https://stackoverflow.com/questions/tagged/opencv\r\
\n -->\r\n - [x] I updated to the latest OpenCV version and the issue is still\
\ there\r\n <!--\r\n master branch for OpenCV 4.x and 3.4 branch for OpenCV\
\ 3.x releases.\r\n OpenCV team supports only the latest release for each branch.\r\
\n The ticket is closed if the problem is not reproduced with the modern version.\r\
\n -->\r\n - [ ] There is reproducer code and related data files: videos, images,\
\ onnx, etc\r\n <!--\r\n The best reproducer -- test case for OpenCV that\
\ we can add to the library.\r\n Recommendations for media files and binary\
\ files:\r\n * Try to reproduce the issue with images and videos in opencv_extra\
\ repository\r\n to reduce attachment size\r\n * Use PNG for images, if\
\ you report some CV related bug, but not image reader\r\n issue\r\n * Attach\
\ the image as an archive to the ticket, if you report some reader issue.\r\n\
\ Image hosting services compress images and it breaks the repro code.\r\n\
\ * Provide ONNX file for some public model or ONNX file with random weights,\r\
\n if you report ONNX parsing or handling issue. Architecture details diagram\r\
\n from netron tool can be very useful too. See https://lutzroeder.github.io/netron/\r\
\n -->\r\n"
inference: true
---
# SetFit with sentence-transformers/all-mpnet-base-v2
This is a [SetFit](https://github.com/huggingface/setfit) model that can be used for Text Classification. This SetFit model uses [sentence-transformers/all-mpnet-base-v2](https://huggingface.co/sentence-transformers/all-mpnet-base-v2) as the Sentence Transformer embedding model. A [LogisticRegression](https://scikit-learn.org/stable/modules/generated/sklearn.linear_model.LogisticRegression.html) instance is used for classification.
The model has been trained using an efficient few-shot learning technique that involves:
1. Fine-tuning a [Sentence Transformer](https://www.sbert.net) with contrastive learning.
2. Training a classification head with features from the fine-tuned Sentence Transformer.
## Model Details
### Model Description
- **Model Type:** SetFit
- **Sentence Transformer body:** [sentence-transformers/all-mpnet-base-v2](https://huggingface.co/sentence-transformers/all-mpnet-base-v2)
- **Classification head:** a [LogisticRegression](https://scikit-learn.org/stable/modules/generated/sklearn.linear_model.LogisticRegression.html) instance
- **Maximum Sequence Length:** 384 tokens
- **Number of Classes:** 3 classes
<!-- - **Training Dataset:** [Unknown](https://huggingface.co/datasets/unknown) -->
<!-- - **Language:** Unknown -->
<!-- - **License:** Unknown -->
### Model Sources
- **Repository:** [SetFit on GitHub](https://github.com/huggingface/setfit)
- **Paper:** [Efficient Few-Shot Learning Without Prompts](https://arxiv.org/abs/2209.11055)
- **Blogpost:** [SetFit: Efficient Few-Shot Learning Without Prompts](https://huggingface.co/blog/setfit)
### Model Labels
| Label | Examples |
|:---------|:-----------------------------------------------------------------------------------------------------------------------------------------------------------------------------------------------------------------------------------------------------------------------------------------------------------------------------------------------------------------------------------------------------------------------------------------------------------------------------------------------------------------------------------------------------------------------------------------------------------------------------------------------------------------------------------------------------------------------------------------------------------------------------------------------------------------------------------------------------------------------------------------------------------------------------------------------------------------------------------------------------------------------------------------------------------------------------------------------------------------------------------------------------------------------------------------------------------------------------------------------------------------------------------------------------------------------------------------------------------------------------------------------------------------------------------------------------------------------------------------------------------------------------------------------------------------------------------------------------------------------------------------------------------------------------------------------------------------------------------------------------------------------------------------------------------------------------------------------------------------------------------------------------------------------------------------------------------------------------------------------------------------------------------------------------------------------------------------------------------------------------------------------------------------------------------------------------------------------------------------------------------------------------------------------------------------------------------------------------------------------------------------------------------------------------------------------------------------------------------------------------------------------------------------------------------------------------------------------------------------------------------------------------------------------------------------------------------------------------------------------------------------------------------------------------------------------------------------------------------------------------------------------------------------------------------------------------------------------------------------------------------------------------------------------------------------------------------------------------------------------------------------------------------------------------------------------------------------------------------------------------------------------------------------------------------------------------------------------------------------------------------------------------------------------------------------------------------------------------------------------------------------------------------------------------------------------------------------------------------------------------------------------------------------------------------------------------------------------------------------------------------------------------------------------------------------------------------------------------------------------------------------------------------------------------------------------------------------------------------------------------------------------------------------------------------------------------------------------------------------------------------------------------------------------------------------------------------------------------------------------------------------------------------------------------------------------------------------------------------------------------------------------------------------------------------------------------------------------------------------------------------------------------------------------------------------------------------------------------------------------------------------------------------------------------------------------------------------------------------------------------------------------------------------------------------------------------------------------------------------------------------------------------------------------------------------------------------------------------------------------------------------------------------------------------------------------------------------------------------------------------------------------------------------------------------------------------------------------------------------------------------------------------------------------------------------------------------------------------------------------------------------------------------------------------------------------------------------------------------------------------------------------------------------------------------------------------------------------------------------------------------------------------------------------------------------------------------------------------------------------------------------------------------------------------------------------------------------------------------------------------------------------------------------------------------------------------------------------------------------------------------------------------------------------------------------------------------------------------------------------------------------------------------------------------------------------------------------------------------------------------------------------------------------------------------------------------------------------------------------------------------------------------------------------------------------------------------------------------------------------------------------------------------------------------------------------------------------------------------------------------------------------------------------------------------------------------------------------------------------------------------------------------------------------------------------------------------------------------------------------------------------------------------------------------------------------------------------------------------------------------------------------------------------------------------------------------------------------------------------------------------------------------------------------------------------------------------------------------------------------------------------------------------------------------------------------------------------------------------------------------------------------------------------------------------------------------------------------------------------------------------------------------------------------------------------------------------------------------------------------------------------------------------------------------------------------------------------------------------------------------------------------------------------------------------------------------------------------------------------------------------------------------------------------------------------------------------------------------------------------------------------------------------------------------------------------------------------------------------------------------------------------------------------------------------------------------------------------------------------------------------------------------------------------------------------------------------------------------------------------------------------|
| feature | <ul><li>'imshow速度太慢,如何能加快imshow ### Descripe the feature and motivation\n\nimshow速度太慢,如何能加快imshow\n\n### Additional context\n\n_No response_'</li><li>'Add H264 / H265 writter support for Android ### Pull Request Readiness Checklist\r\n\r\nSee details at https://github.com/opencv/opencv/wiki/How_to_contribute#making-a-good-pull-request\r\n\r\n- [x] I agree to contribute to the project under Apache 2 License.\r\n- [x] To the best of my knowledge, the proposed patch is not based on a code under GPL or another license that is incompatible with OpenCV\r\n- [x] The PR is proposed to the proper branch\r\n- [ ] There is a reference to the original bug report and related work\r\n- [ ] There is accuracy test, performance test and test data in opencv_extra repository, if applicable\r\n Patch to opencv_extra has the same branch name.\r\n- [ ] The feature is well documented and sample code can be built with the project CMake\r\n'</li><li>'4-bit_palette_color ### Pull Request Readiness Checklist\r\n\r\nSee details at https://github.com/opencv/opencv/wiki/How_to_contribute#making-a-good-pull-request\r\n\r\n- [x] I agree to contribute to the project under Apache 2 License.\r\n- [x] To the best of my knowledge, the proposed patch is not based on a code under GPL or another license that is incompatible with OpenCV\r\n- [x] The PR is proposed to the proper branch\r\n- [ ] There is a reference to the original bug report and related work\r\n- [x] There is accuracy test, performance test and test data in opencv_extra repository, if applicable\r\n Patch to opencv_extra has the same branch name.\r\n- [ ] The feature is well documented and sample code can be built with the project CMake\r\n'</li></ul> |
| bug | <ul><li>'DNN: support the split node of onnx opset >= 13 Merge with test case: https://github.com/opencv/opencv_extra/pull/1053.\r\n\r\nThe attribute of `split` in `Split layer` has been moved from `attribute` to `input`.\r\nRelated link: https://github.com/onnx/onnx/blob/main/docs/Operators.md#inputs-1---2-12\r\nThe purpose of this PR is to support the `split` with input type.\r\n\r\n### Pull Request Readiness Checklist\r\n\r\nSee details at https://github.com/opencv/opencv/wiki/How_to_contribute#making-a-good-pull-request\r\n\r\n- [x] I agree to contribute to the project under Apache 2 License.\r\n- [x] To the best of my knowledge, the proposed patch is not based on a code under GPL or another license that is incompatible with OpenCV\r\n- [x] The PR is proposed to the proper branch\r\n- [ ] There is a reference to the original bug report and related work\r\n- [ ] There is accuracy test, performance test and test data in opencv_extra repository, if applicable\r\n Patch to opencv_extra has the same branch name.\r\n- [ ] The feature is well documented and sample code can be built with the project CMake\r\n'</li><li>"Fixed bug when MSMF webcamera doesn't start when build with VIDEOIO_PLUGIN_ALL Fixed #23937 and #23056\r\n"</li><li>"Fixes pixel info color font for dark Qt themes For dark Qt themes, it is hard to read the pixel color information on the bottom left, like the coordinates or RGB values. This PR proposes a way on how the dynamically sets the font colors based on the system's theme.\r\nOriginal Example:\r\n\r\n\r\n\r\nWith patch:\r\n\r\n\r\n\r\n\r\nFor Windows, nothing is changed (tested on a windows 11 system), because the font color is #000000 when using the default Qt theme.\r\n\r\n### Pull Request Readiness Checklist\r\n\r\nSee details at https://github.com/opencv/opencv/wiki/How_to_contribute#making-a-good-pull-request\r\n\r\n- [x] I agree to contribute to the project under Apache 2 License.\r\n- [x] To the best of my knowledge, the proposed patch is not based on a code under GPL or another license that is incompatible with OpenCV\r\n- [x] The PR is proposed to the proper branch\r\n- [ ] There is a reference to the original bug report and related work\r\n- [ ] There is accuracy test, performance test and test data in opencv_extra repository, if applicable\r\n Patch to opencv_extra has the same branch name.\r\n- [ ] The feature is well documented and sample code can be built with the project CMake\r\n"</li></ul> |
| question | <ul><li>'In file included from /home/xxx/opencv/modules/python/src2/cv2.cpp:11:0: /home/xxx/opencv/build/modules/python_bindings_generator/pyopencv_generated_include.h:19:10: fatal error: opencv2/hdf/hdf5.hpp: no file or directory #include "opencv2/hdf/hdf5.hpp" ^~~~~~~~~~~~~~~~~~~~~~ I\'m building opencv-cuda,it happens at the end.'</li><li>"Opencv error with GPU backend <!--\r\nIf you have a question rather than reporting a bug please go to https://forum.opencv.org where you get much faster responses.\r\nIf you need further assistance please read [How To Contribute](https://github.com/opencv/opencv/wiki/How_to_contribute).\r\n\r\nThis is a template helping you to create an issue that can be processed as quickly as possible. This is the bug reporting section for the OpenCV library.\r\n-->\r\n\r\n##### System information (version)\r\n\r\n- OpenCV => 4.5.5-dev\r\n- Operating System / Platform => Ubuntu 20\r\n- Compiler => GCC\r\n\r\n##### Detailed description\r\n\r\nI cannot run anymore my cv2.dnn on GPU. \r\nI tried to run the code in CPU and its workings. I also tested with the older version of OpenCV and is working. I think the new commit merged in February changed the dnn output is an np.array[] and is not compatible with 4.5.5 release version. Could you please give an example of how to use the new output?\r\n\r\n##### Steps to reproduce\r\n ```.python\r\n //with CUDA enabled\r\n net = cv2.dnn.readNetFromONNX(model_yolo_v5))\r\n net.setInput(_image)\r\n results = net.forward()\r\n ```\r\n##### Issue submission checklist\r\n\r\n - [ ] I report the issue, it's not a question\r\n <!--\r\n OpenCV team works with forum.opencv.org, Stack Overflow and other communities\r\n to discuss problems. Tickets with questions without a real issue statement will be\r\n closed.\r\n -->\r\n - [x] I checked the problem with documentation, FAQ, open issues,\r\n forum.opencv.org, Stack Overflow, etc and have not found any solution\r\n <!--\r\n Places to check:\r\n * OpenCV documentation: https://docs.opencv.org\r\n * FAQ page: https://github.com/opencv/opencv/wiki/FAQ\r\n * OpenCV forum: https://forum.opencv.org\r\n * OpenCV issue tracker: https://github.com/opencv/opencv/issues?q=is%3Aissue\r\n * Stack Overflow branch: https://stackoverflow.com/questions/tagged/opencv\r\n -->\r\n - [x] I updated to the latest OpenCV version and the issue is still there\r\n <!--\r\n master branch for OpenCV 4.x and 3.4 branch for OpenCV 3.x releases.\r\n OpenCV team supports only the latest release for each branch.\r\n The ticket is closed if the problem is not reproduced with the modern version.\r\n -->\r\n - [ ] There is reproducer code and related data files: videos, images, onnx, etc\r\n <!--\r\n The best reproducer -- test case for OpenCV that we can add to the library.\r\n Recommendations for media files and binary files:\r\n * Try to reproduce the issue with images and videos in opencv_extra repository\r\n to reduce attachment size\r\n * Use PNG for images, if you report some CV related bug, but not image reader\r\n issue\r\n * Attach the image as an archive to the ticket, if you report some reader issue.\r\n Image hosting services compress images and it breaks the repro code.\r\n * Provide ONNX file for some public model or ONNX file with random weights,\r\n if you report ONNX parsing or handling issue. Architecture details diagram\r\n from netron tool can be very useful too. See https://lutzroeder.github.io/netron/\r\n -->\r\n"</li><li>'cv2.imread() fails when filenames have multiple dots (.) <!--\r\nIf you have a question rather than reporting a bug please go to https://forum.opencv.org where you get much faster responses.\r\nIf you need further assistance please read [How To Contribute](https://github.com/opencv/opencv/wiki/How_to_contribute).\r\n\r\nThis is a template helping you to create an issue which can be processed as quickly as possible. This is the bug reporting section for the OpenCV library.\r\n-->\r\n\r\n##### System information (version)\r\n<!-- Example\r\n- OpenCV => 4.2\r\n- Operating System / Platform => Windows 64 Bit\r\n- Compiler => Visual Studio 2017\r\n-->\r\n\r\n- OpenCV => 4.5.5.64\r\n- Operating System / Platform => Windows\r\n- Compiler => PyCharm Community Edition 2020.1.1\r\n\r\n##### Detailed description\r\n\r\nWhen the `cv2.imread()` method encounters a filename with multiple dots (.), a `NoneType` is returned. I think this might be because the file extension is assumed to be everything after the first dot, and not the last, but it could be something completely different.\r\n\r\nExample: \r\n\r\n##### Steps to reproduce\r\n\r\n<!-- to add code example fence it with triple backticks and optional file extension\r\n ```.cpp\r\n // C++ code example\r\n ```-->\r\nRunning the following code, with two images in the same directory,\r\n```.py\r\nimport cv2\r\n\r\nim = cv2.imread("C:/Users/.../Videos/Captures/test project – main.py 18_05_2022 15_44_50.png")\r\nim2 = cv2.imread("C:/Users/.../Videos/Captures/Screenshot 16_05_2022 23_40_07.png")\r\n\r\nprint(im)\r\nprint(im2)\r\n```\r\ngives the result:\r\n```.py\r\n[ WARN:0@0.221] global D:\\\\a\\\\opencv-python\\\\opencv-python\\\\opencv\\\\modules\\\\imgcodecs\\\\src\\\\loadsave.cpp (239) cv::findDecoder imread_(\'C:/Users/.../Videos/Captures/test project – main.py 18_05_2022 15_44_50.png\'): can\'t open/read file: check file path/integrity\r\nNone\r\n[[[255 255 255]\r\n [255 255 255]\r\n [255 255 255]\r\n ...\r\n [255 255 255]\r\n [255 255 255]\r\n [255 255 255]]\r\n\r\n [[255 255 255]\r\n [255 255 255]\r\n [255 255 255]\r\n ...\r\n [255 255 255]\r\n [255 255 255]\r\n [255 255 255]]\r\n\r\n [[153 139 140]\r\n [152 139 139]\r\n [151 139 138]\r\n ...\r\n [148 136 135]\r\n [150 138 138]\r\n [151 140 139]]]\r\n```\r\nwhich shows that the first image (one dot) loaded successfully, but the second one (with the multiple dots) failed.\r\n##### Issue submission checklist\r\n\r\n - [x] I report the issue, it\'s not a question\r\n <!--\r\n OpenCV team works with forum.opencv.org, Stack Overflow and other communities\r\n to discuss problems. Tickets with questions without a real issue statement will be\r\n closed.\r\n -->\r\n - [x] I checked the problem with documentation, FAQ, open issues,\r\n forum.opencv.org, Stack Overflow, etc and have not found any solution\r\n <!--\r\n Places to check:\r\n * OpenCV documentation: https://docs.opencv.org\r\n * FAQ page: https://github.com/opencv/opencv/wiki/FAQ\r\n * OpenCV forum: https://forum.opencv.org\r\n * OpenCV issue tracker: https://github.com/opencv/opencv/issues?q=is%3Aissue\r\n * Stack Overflow branch: https://stackoverflow.com/questions/tagged/opencv\r\n -->\r\n - [x] I updated to the latest OpenCV version and the issue is still there\r\n <!--\r\n master branch for OpenCV 4.x and 3.4 branch for OpenCV 3.x releases.\r\n OpenCV team supports only the latest release for each branch.\r\n The ticket is closed if the problem is not reproduced with the modern version.\r\n -->\r\n - [x] There is reproducer code and related data files: videos, images, onnx, etc\r\n <!--\r\n The best reproducer -- test case for OpenCV that we can add to the library.\r\n Recommendations for media files and binary files:\r\n * Try to reproduce the issue with images and videos in opencv_extra repository\r\n to reduce attachment size\r\n * Use PNG for images, if you report some CV related bug, but not image reader\r\n issue\r\n * Attach the image as an archive to the ticket, if you report some reader issue.\r\n Image hosting services compress images and it breaks the repro code.\r\n * Provide ONNX file for some public model or ONNX file with random weights,\r\n if you report ONNX parsing or handling issue. Architecture details diagram\r\n from netron tool can be very useful too. See https://lutzroeder.github.io/netron/\r\n -->\r\n'</li></ul> |
## Uses
### Direct Use for Inference
First install the SetFit library:
```bash
pip install setfit
```
Then you can load this model and run inference.
```python
from setfit import SetFitModel
# Download from the 🤗 Hub
model = SetFitModel.from_pretrained("setfit_model_id")
# Run inference
preds = model("OpenCV build - cmake don't recognize GTK2 or GTK3 ##### System information (version)
- OpenCV => 4.7.0
- Operating System / Platform => Ubuntu 20.04
- Compiler => g++ (Ubuntu 9.4.0-1ubuntu1~20.04.1) 9.4.0
- C++ Standard: 11
##### Detailed description
I am trying to create OpenCV with the GTK GUI. I have `GTK2` and `GTK3` installed on the system, but neither version is recognised by cmake.
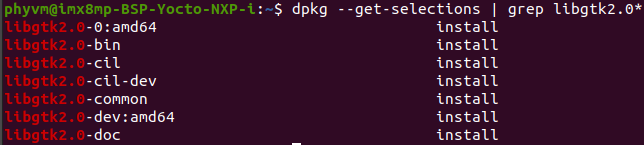

The output after executing cmake remains
**GUI: NONE**
**GTK+:NO**
I execute the cmake command as follows:
`cmake -DPYTHON_DEFAULT_EXECUTABLE=$(which python3) -DWITH_GTK=ON ../opencv`
or
`cmake -DPYTHON_DEFAULT_EXECUTABLE=$(which python3) -DWITH_GTK=ON -DWITH_GTK_2_X=ON ../opencv`
##### Steps to reproduce
git clone https://github.com/opencv/opencv.git
mkdir -p build && cd build
cmake ../opencv (default, custom look above)
Thank you for your help
")
```
<!--
### Downstream Use
*List how someone could finetune this model on their own dataset.*
-->
<!--
### Out-of-Scope Use
*List how the model may foreseeably be misused and address what users ought not to do with the model.*
-->
<!--
## Bias, Risks and Limitations
*What are the known or foreseeable issues stemming from this model? You could also flag here known failure cases or weaknesses of the model.*
-->
<!--
### Recommendations
*What are recommendations with respect to the foreseeable issues? For example, filtering explicit content.*
-->
## Training Details
### Training Set Metrics
| Training set | Min | Median | Max |
|:-------------|:----|:---------|:------|
| Word count | 2 | 393.0933 | 13648 |
| Label | Training Sample Count |
|:---------|:----------------------|
| bug | 200 |
| feature | 200 |
| question | 200 |
### Training Hyperparameters
- batch_size: (16, 2)
- num_epochs: (1, 1)
- max_steps: -1
- sampling_strategy: oversampling
- num_iterations: 20
- body_learning_rate: (2e-05, 1e-05)
- head_learning_rate: 0.01
- loss: CosineSimilarityLoss
- distance_metric: cosine_distance
- margin: 0.25
- end_to_end: False
- use_amp: False
- warmup_proportion: 0.1
- seed: 42
- eval_max_steps: -1
- load_best_model_at_end: False
### Training Results
| Epoch | Step | Training Loss | Validation Loss |
|:------:|:----:|:-------------:|:---------------:|
| 0.0007 | 1 | 0.2963 | - |
| 0.0067 | 10 | 0.2694 | - |
| 0.0133 | 20 | 0.2443 | - |
| 0.02 | 30 | 0.2872 | - |
| 0.0267 | 40 | 0.2828 | - |
| 0.0333 | 50 | 0.2279 | - |
| 0.04 | 60 | 0.1852 | - |
| 0.0467 | 70 | 0.1983 | - |
| 0.0533 | 80 | 0.224 | - |
| 0.06 | 90 | 0.2315 | - |
| 0.0667 | 100 | 0.2698 | - |
| 0.0733 | 110 | 0.1178 | - |
| 0.08 | 120 | 0.1216 | - |
| 0.0867 | 130 | 0.1065 | - |
| 0.0933 | 140 | 0.1519 | - |
| 0.1 | 150 | 0.073 | - |
| 0.1067 | 160 | 0.0858 | - |
| 0.1133 | 170 | 0.1697 | - |
| 0.12 | 180 | 0.1062 | - |
| 0.1267 | 190 | 0.0546 | - |
| 0.1333 | 200 | 0.139 | - |
| 0.14 | 210 | 0.0513 | - |
| 0.1467 | 220 | 0.1168 | - |
| 0.1533 | 230 | 0.0455 | - |
| 0.16 | 240 | 0.0521 | - |
| 0.1667 | 250 | 0.0307 | - |
| 0.1733 | 260 | 0.0618 | - |
| 0.18 | 270 | 0.0964 | - |
| 0.1867 | 280 | 0.0707 | - |
| 0.1933 | 290 | 0.0524 | - |
| 0.2 | 300 | 0.0358 | - |
| 0.2067 | 310 | 0.0238 | - |
| 0.2133 | 320 | 0.0759 | - |
| 0.22 | 330 | 0.0197 | - |
| 0.2267 | 340 | 0.0053 | - |
| 0.2333 | 350 | 0.0035 | - |
| 0.24 | 360 | 0.0036 | - |
| 0.2467 | 370 | 0.0079 | - |
| 0.2533 | 380 | 0.0033 | - |
| 0.26 | 390 | 0.0021 | - |
| 0.2667 | 400 | 0.0026 | - |
| 0.2733 | 410 | 0.0018 | - |
| 0.28 | 420 | 0.0014 | - |
| 0.2867 | 430 | 0.0019 | - |
| 0.2933 | 440 | 0.0027 | - |
| 0.3 | 450 | 0.0016 | - |
| 0.3067 | 460 | 0.0027 | - |
| 0.3133 | 470 | 0.0095 | - |
| 0.32 | 480 | 0.0005 | - |
| 0.3267 | 490 | 0.0006 | - |
| 0.3333 | 500 | 0.0006 | - |
| 0.34 | 510 | 0.0634 | - |
| 0.3467 | 520 | 0.0025 | - |
| 0.3533 | 530 | 0.0013 | - |
| 0.36 | 540 | 0.0007 | - |
| 0.3667 | 550 | 0.0007 | - |
| 0.3733 | 560 | 0.0003 | - |
| 0.38 | 570 | 0.0006 | - |
| 0.3867 | 580 | 0.0007 | - |
| 0.3933 | 590 | 0.0004 | - |
| 0.4 | 600 | 0.0006 | - |
| 0.4067 | 610 | 0.0007 | - |
| 0.4133 | 620 | 0.0005 | - |
| 0.42 | 630 | 0.0004 | - |
| 0.4267 | 640 | 0.0005 | - |
| 0.4333 | 650 | 0.0013 | - |
| 0.44 | 660 | 0.0005 | - |
| 0.4467 | 670 | 0.0007 | - |
| 0.4533 | 680 | 0.0008 | - |
| 0.46 | 690 | 0.0018 | - |
| 0.4667 | 700 | 0.0007 | - |
| 0.4733 | 710 | 0.0008 | - |
| 0.48 | 720 | 0.0007 | - |
| 0.4867 | 730 | 0.0007 | - |
| 0.4933 | 740 | 0.0002 | - |
| 0.5 | 750 | 0.0002 | - |
| 0.5067 | 760 | 0.0002 | - |
| 0.5133 | 770 | 0.0007 | - |
| 0.52 | 780 | 0.0004 | - |
| 0.5267 | 790 | 0.0003 | - |
| 0.5333 | 800 | 0.0007 | - |
| 0.54 | 810 | 0.0004 | - |
| 0.5467 | 820 | 0.0003 | - |
| 0.5533 | 830 | 0.0002 | - |
| 0.56 | 840 | 0.001 | - |
| 0.5667 | 850 | 0.008 | - |
| 0.5733 | 860 | 0.0003 | - |
| 0.58 | 870 | 0.0002 | - |
| 0.5867 | 880 | 0.0011 | - |
| 0.5933 | 890 | 0.0005 | - |
| 0.6 | 900 | 0.0004 | - |
| 0.6067 | 910 | 0.0003 | - |
| 0.6133 | 920 | 0.0002 | - |
| 0.62 | 930 | 0.0002 | - |
| 0.6267 | 940 | 0.0002 | - |
| 0.6333 | 950 | 0.0001 | - |
| 0.64 | 960 | 0.0002 | - |
| 0.6467 | 970 | 0.0003 | - |
| 0.6533 | 980 | 0.0002 | - |
| 0.66 | 990 | 0.0005 | - |
| 0.6667 | 1000 | 0.0003 | - |
| 0.6733 | 1010 | 0.0002 | - |
| 0.68 | 1020 | 0.0003 | - |
| 0.6867 | 1030 | 0.0008 | - |
| 0.6933 | 1040 | 0.0003 | - |
| 0.7 | 1050 | 0.0005 | - |
| 0.7067 | 1060 | 0.0012 | - |
| 0.7133 | 1070 | 0.0001 | - |
| 0.72 | 1080 | 0.0003 | - |
| 0.7267 | 1090 | 0.0002 | - |
| 0.7333 | 1100 | 0.0001 | - |
| 0.74 | 1110 | 0.0003 | - |
| 0.7467 | 1120 | 0.0002 | - |
| 0.7533 | 1130 | 0.0003 | - |
| 0.76 | 1140 | 0.0596 | - |
| 0.7667 | 1150 | 0.0012 | - |
| 0.7733 | 1160 | 0.0004 | - |
| 0.78 | 1170 | 0.0003 | - |
| 0.7867 | 1180 | 0.0003 | - |
| 0.7933 | 1190 | 0.0002 | - |
| 0.8 | 1200 | 0.0015 | - |
| 0.8067 | 1210 | 0.0002 | - |
| 0.8133 | 1220 | 0.0001 | - |
| 0.82 | 1230 | 0.0002 | - |
| 0.8267 | 1240 | 0.0002 | - |
| 0.8333 | 1250 | 0.0002 | - |
| 0.84 | 1260 | 0.0002 | - |
| 0.8467 | 1270 | 0.0003 | - |
| 0.8533 | 1280 | 0.0001 | - |
| 0.86 | 1290 | 0.0001 | - |
| 0.8667 | 1300 | 0.0002 | - |
| 0.8733 | 1310 | 0.0004 | - |
| 0.88 | 1320 | 0.0004 | - |
| 0.8867 | 1330 | 0.0004 | - |
| 0.8933 | 1340 | 0.0001 | - |
| 0.9 | 1350 | 0.0002 | - |
| 0.9067 | 1360 | 0.055 | - |
| 0.9133 | 1370 | 0.0002 | - |
| 0.92 | 1380 | 0.0004 | - |
| 0.9267 | 1390 | 0.0001 | - |
| 0.9333 | 1400 | 0.0002 | - |
| 0.94 | 1410 | 0.0002 | - |
| 0.9467 | 1420 | 0.0004 | - |
| 0.9533 | 1430 | 0.0009 | - |
| 0.96 | 1440 | 0.0003 | - |
| 0.9667 | 1450 | 0.0427 | - |
| 0.9733 | 1460 | 0.0004 | - |
| 0.98 | 1470 | 0.0001 | - |
| 0.9867 | 1480 | 0.0002 | - |
| 0.9933 | 1490 | 0.0002 | - |
| 1.0 | 1500 | 0.0002 | - |
### Framework Versions
- Python: 3.10.12
- SetFit: 1.0.3
- Sentence Transformers: 3.0.1
- Transformers: 4.39.0
- PyTorch: 2.3.0+cu121
- Datasets: 2.20.0
- Tokenizers: 0.15.2
## Citation
### BibTeX
```bibtex
@article{https://doi.org/10.48550/arxiv.2209.11055,
doi = {10.48550/ARXIV.2209.11055},
url = {https://arxiv.org/abs/2209.11055},
author = {Tunstall, Lewis and Reimers, Nils and Jo, Unso Eun Seo and Bates, Luke and Korat, Daniel and Wasserblat, Moshe and Pereg, Oren},
keywords = {Computation and Language (cs.CL), FOS: Computer and information sciences, FOS: Computer and information sciences},
title = {Efficient Few-Shot Learning Without Prompts},
publisher = {arXiv},
year = {2022},
copyright = {Creative Commons Attribution 4.0 International}
}
```
<!--
## Glossary
*Clearly define terms in order to be accessible across audiences.*
-->
<!--
## Model Card Authors
*Lists the people who create the model card, providing recognition and accountability for the detailed work that goes into its construction.*
-->
<!--
## Model Card Contact
*Provides a way for people who have updates to the Model Card, suggestions, or questions, to contact the Model Card authors.*
--> |
nilcars/bitcoin_bitcoin_model | nilcars | "2024-06-24T10:53:45Z" | 0 | 0 | setfit | [
"setfit",
"safetensors",
"mpnet",
"sentence-transformers",
"text-classification",
"generated_from_setfit_trainer",
"arxiv:2209.11055",
"base_model:sentence-transformers/all-mpnet-base-v2",
"region:us"
] | text-classification | "2024-06-24T10:08:13Z" | ---
base_model: sentence-transformers/all-mpnet-base-v2
library_name: setfit
metrics:
- accuracy
pipeline_tag: text-classification
tags:
- setfit
- sentence-transformers
- text-classification
- generated_from_setfit_trainer
widget:
- text: "[brainstorm] Improving `makeseeds.py` \r\nA. Filtering hosts with multiple\
\ ports can be removed IMO:\r\n\r\nhttps://github.com/bitcoin/bitcoin/blob/c44e734dca64a15fae92255a5d848c04adaad2fa/contrib/seeds/makeseeds.py#L215\r\
\n\r\n\r\nB. Tor v3 can also be included in the results.\r\n\r\nC. Recent observation\
\ which can be confirmed with:\r\n\r\n```\r\nwget https://gitlab.com/api/v4/projects/33695681/packages/generic/nrich/0.1.1/nrich_0.1.1_amd64.deb\r\
\nsudo dpkg -i nrich_0.1.1_amd64.deb\r\nhost -t a seed.bitcoin.sipa.be | sed -e\
\ 's/seed.bitcoin.sipa.be has address //g' | nrich -\r\n```\r\n\r\nPossible reasons\
\ for vulnerable machines used for bitcoin nodes:\r\n\r\n1. False positives\r\n\
2. Users not aware or don't care\r\n3. Attackers prefer using these for better\
\ results\r\n4. Honeypots\r\n5. Other reasons\r\n\r\nLeaving 1 which won't be\
\ true for all the results, filtering such nodes in `makeseeds.py` should make\
\ sense. Below is an example for one IP copied from [`suspicious_hosts.txt`](https://github.com/bitcoin/bitcoin/blob/master/contrib/seeds/suspicious_hosts.txt)\r\
\n\r\n\r\n```python\r\nip = '88.198.17.7'\r\n\r\nurl = 'https://internetdb.shodan.io/'\
\ + ip\r\nresponse = requests.get(url)\r\n\r\nif response.text.find('CVE') !=\
\ -1:\r\n print('vulnerable')\r\n```"
- text: Add "walletpassphrasechange" command in bitcoin-wallet.exe This is an underrated
useful tool. Dear devs, please add this "feature". Thanks.
- text: "qa: Intermittent failure in feature_segwit.py --descriptors https://api.cirrus-ci.com/v1/task/5763159330914304/logs/ci.log\r\
\n```\r\n5/238 - feature_segwit.py --descriptors failed, Duration: 11 s\r\n\r\n\
stdout:\r\n2022-03-16T18:38:33.242000Z TestFramework (INFO): Initializing test\
\ directory /tmp/cirrus-ci-build/ci/scratch/test_runner/test_runner_₿_\U0001F3C3\
_20220316_183814/feature_segwit_225\r\n2022-03-16T18:38:39.177000Z TestFramework\
\ (INFO): Verify sigops are counted in GBT with pre-BIP141 rules before the fork\r\
\n2022-03-16T18:38:43.168000Z TestFramework (INFO): Verify witness txs cannot\
\ be mined before the fork\r\n2022-03-16T18:38:43.200000Z TestFramework (INFO):\
\ Verify unsigned p2sh witness txs without a redeem script are invalid\r\n2022-03-16T18:38:43.630000Z\
\ TestFramework (ERROR): JSONRPC error\r\nTraceback (most recent call last):\r\
\n File \"/tmp/cirrus-ci-build/ci/scratch/build/bitcoin-i686-pc-linux-gnu/test/functional/test_framework/test_framework.py\"\
, line 132, in main\r\n self.run_test()\r\n File \"/tmp/cirrus-ci-build/ci/scratch/build/bitcoin-i686-pc-linux-gnu/test/functional/feature_segwit.py\"\
, line 210, in run_test\r\n self.generate(self.nodes[0], 4) # blocks 428-431\r\
\n File \"/tmp/cirrus-ci-build/ci/scratch/build/bitcoin-i686-pc-linux-gnu/test/functional/test_framework/test_framework.py\"\
, line 639, in generate\r\n blocks = generator.generate(*args, invalid_call=False,\
\ **kwargs)\r\n File \"/tmp/cirrus-ci-build/ci/scratch/build/bitcoin-i686-pc-linux-gnu/test/functional/test_framework/test_node.py\"\
, line 303, in generate\r\n return self.generatetoaddress(nblocks=nblocks,\
\ address=self.get_deterministic_priv_key().address, maxtries=maxtries, **kwargs)\r\
\n File \"/tmp/cirrus-ci-build/ci/scratch/build/bitcoin-i686-pc-linux-gnu/test/functional/test_framework/test_node.py\"\
, line 311, in generatetoaddress\r\n return self.__getattr__('generatetoaddress')(*args,\
\ **kwargs)\r\n File \"/tmp/cirrus-ci-build/ci/scratch/build/bitcoin-i686-pc-linux-gnu/test/functional/test_framework/coverage.py\"\
, line 49, in __call__\r\n return_val = self.auth_service_proxy_instance.__call__(*args,\
\ **kwargs)\r\n File \"/tmp/cirrus-ci-build/ci/scratch/build/bitcoin-i686-pc-linux-gnu/test/functional/test_framework/authproxy.py\"\
, line 144, in __call__\r\n raise JSONRPCException(response['error'], status)\r\
\ntest_framework.authproxy.JSONRPCException: CreateNewBlock: TestBlockValidity\
\ failed: unexpected-witness, ContextualCheckBlock : unexpected witness data found\
\ (-1)\r\n2022-03-16T18:38:43.682000Z TestFramework (INFO): Stopping nodes\r\n\
2022-03-16T18:38:43.856000Z TestFramework (WARNING): Not cleaning up dir /tmp/cirrus-ci-build/ci/scratch/test_runner/test_runner_₿_\U0001F3C3\
_20220316_183814/feature_segwit_225\r\n2022-03-16T18:38:43.856000Z TestFramework\
\ (ERROR): Test failed. Test logging available at /tmp/cirrus-ci-build/ci/scratch/test_runner/test_runner_₿_\U0001F3C3\
_20220316_183814/feature_segwit_225/test_framework.log\r\n2022-03-16T18:38:43.866000Z\
\ TestFramework (ERROR): \r\n2022-03-16T18:38:43.866000Z TestFramework (ERROR):\
\ Hint: Call /tmp/cirrus-ci-build/ci/scratch/build/bitcoin-i686-pc-linux-gnu/test/functional/combine_logs.py\
\ '/tmp/cirrus-ci-build/ci/scratch/test_runner/test_runner_₿_\U0001F3C3_20220316_183814/feature_segwit_225'\
\ to consolidate all logs\r\n2022-03-16T18:38:43.866000Z TestFramework (ERROR):\
\ \r\n2022-03-16T18:38:43.866000Z TestFramework (ERROR): If this failure happened\
\ unexpectedly or intermittently, please file a bug and provide a link or upload\
\ of the combined log.\r\n2022-03-16T18:38:43.867000Z TestFramework (ERROR): https://github.com/bitcoin/bitcoin/issues\r\
\n2022-03-16T18:38:43.867000Z TestFramework (ERROR): \r\n```"
- text: "Rpc not working on 0.17.1 ```\r\ncurl --user test:test --data-binary '{\"\
jsonrpc\": \"1.0\", \"id\":\"curltest\", \"method\": \"getbalance\", \"params\"\
: [\"*\", 1] }' -H 'content-type: text/plain;' http://127.0.0.1:8332/\r\ncurl:\
\ (7) Failed to connect to 127.0.0.1 port 8332: Connection refused\r\n\r\n```\r\
\nBut version 0.16 works "
- text: "guix: Prints \"g++: not found\" when building depends Steps to reproduce:\
\ Start a guix build on a system without gcc.\r\n\r\n\r\n```\r\n/bin/sh: 1: gcc:\
\ not found\r\n/bin/sh: 1: gcc: not found\r\n/bin/sh: 1: g++: not found\r\n/bin/sh:\
\ 1: g++: not found\r\nenv: '/home/micap/temp/scratch/guix/bitcoin/bitcoin/depends/x86_64-apple-darwin18/native/bin/clang':\
\ No such file or directory\r\nenv: '/home/micap/temp/scratch/guix/bitcoin/bitcoin/depends/x86_64-apple-darwin18/native/bin/clang':\
\ No such file or directory\r\nenv: '/home/micap/temp/scratch/guix/bitcoin/bitcoin/depends/x86_64-apple-darwin18/native/bin/clang++':\
\ No such file or directory\r\nenv: '/home/micap/temp/scratch/guix/bitcoin/bitcoin/depends/x86_64-apple-darwin18/native/bin/clang++':\
\ No such file or directory\r\nFound macOS SDK at '/home/micap/temp/scratch/guix/bitcoin/bitcoin/depends/SDKs/Xcode-11.3.1-11C505-extracted-SDK-with-libcxx-headers',\
\ using...\r\nmake: Entering directory '/home/micap/temp/scratch/guix/bitcoin/bitcoin/depends'\r\
\n/bin/sh: 1: gcc: not found\r\n/bin/sh: 1: gcc: not found\r\n/bin/sh: 1: g++:\
\ not found\r\n/bin/sh: 1: g++: not found\r\n/bin/sh: 1: gcc: not found\r\n/bin/sh:\
\ 1: gcc: not found\r\n/bin/sh: 1: g++: not found\r\n/bin/sh: 1: g++: not found\r\
\nmake[1]: Entering directory '/home/micap/temp/scratch/guix/bitcoin/bitcoin/depends'\r\
\n/bin/sh: 1: gcc: not found\r\n/bin/sh: 1: gcc: not found\r\n/bin/sh: 1: g++:\
\ not found\r\n/bin/sh: 1: g++: not found\r\nenv: '/home/micap/temp/scratch/guix/bitcoin/bitcoin/depends/x86_64-apple-darwin/native/bin/clang':\
\ No such file or directory\r\nenv: '/home/micap/temp/scratch/guix/bitcoin/bitcoin/depends/x86_64-apple-darwin/native/bin/clang':\
\ No such file or directory\r\nenv: '/home/micap/temp/scratch/guix/bitcoin/bitcoin/depends/x86_64-apple-darwin/native/bin/clang++':\
\ No such file or directory\r\nenv: '/home/micap/temp/scratch/guix/bitcoin/bitcoin/depends/x86_64-apple-darwin/native/bin/clang++':\
\ No such file or directory\r\nmake[1]: Leaving directory '/home/micap/temp/scratch/guix/bitcoin/bitcoin/depends'\r\
\nmake[1]: Entering directory '/home/micap/temp/scratch/guix/bitcoin/bitcoin/depends'\r\
\n/bin/sh: 1: gcc: not found\r\n/bin/sh: 1: gcc: not found\r\n/bin/sh: 1: g++:\
\ not found\r\n/bin/sh: 1: g++: not found\r\n/bin/sh: 1: gcc: not found\r\n/bin/sh:\
\ 1: gcc: not found\r\n/bin/sh: 1: g++: not found\r\n/bin/sh: 1: g++: not found\r\
\nmake[1]: Leaving directory '/home/micap/temp/scratch/guix/bitcoin/bitcoin/depends'\r\
\nmake[1]: Entering directory '/home/micap/temp/scratch/guix/bitcoin/bitcoin/depends'\r\
\n/bin/sh: 1: gcc: not found\r\n/bin/sh: 1: gcc: not found\r\n/bin/sh: 1: g++:\
\ not found\r\n/bin/sh: 1: g++: not found\r\n/bin/sh: 1: x86_64-w64-mingw32-gcc:\
\ not found\r\n/bin/sh: 1: x86_64-w64-mingw32-gcc: not found\r\n/bin/sh: 1: x86_64-w64-mingw32-g++:\
\ not found\r\n/bin/sh: 1: x86_64-w64-mingw32-g++: not found\r\nmake[1]: Leaving\
\ directory '/home/micap/temp/scratch/guix/bitcoin/bitcoin/depends'\r\nmake: Leaving\
\ directory '/home/micap/temp/scratch/guix/bitcoin/bitcoin/depends'\r\nINFO: Building\
\ commit a4903f747ccd for platform triple x86_64-linux-gnu:"
inference: true
---
# SetFit with sentence-transformers/all-mpnet-base-v2
This is a [SetFit](https://github.com/huggingface/setfit) model that can be used for Text Classification. This SetFit model uses [sentence-transformers/all-mpnet-base-v2](https://huggingface.co/sentence-transformers/all-mpnet-base-v2) as the Sentence Transformer embedding model. A [LogisticRegression](https://scikit-learn.org/stable/modules/generated/sklearn.linear_model.LogisticRegression.html) instance is used for classification.
The model has been trained using an efficient few-shot learning technique that involves:
1. Fine-tuning a [Sentence Transformer](https://www.sbert.net) with contrastive learning.
2. Training a classification head with features from the fine-tuned Sentence Transformer.
## Model Details
### Model Description
- **Model Type:** SetFit
- **Sentence Transformer body:** [sentence-transformers/all-mpnet-base-v2](https://huggingface.co/sentence-transformers/all-mpnet-base-v2)
- **Classification head:** a [LogisticRegression](https://scikit-learn.org/stable/modules/generated/sklearn.linear_model.LogisticRegression.html) instance
- **Maximum Sequence Length:** 384 tokens
- **Number of Classes:** 3 classes
<!-- - **Training Dataset:** [Unknown](https://huggingface.co/datasets/unknown) -->
<!-- - **Language:** Unknown -->
<!-- - **License:** Unknown -->
### Model Sources
- **Repository:** [SetFit on GitHub](https://github.com/huggingface/setfit)
- **Paper:** [Efficient Few-Shot Learning Without Prompts](https://arxiv.org/abs/2209.11055)
- **Blogpost:** [SetFit: Efficient Few-Shot Learning Without Prompts](https://huggingface.co/blog/setfit)
### Model Labels
| Label | Examples |
|:---------|:-------------------------------------------------------------------------------------------------------------------------------------------------------------------------------------------------------------------------------------------------------------------------------------------------------------------------------------------------------------------------------------------------------------------------------------------------------------------------------------------------------------------------------------------------------------------------------------------------------------------------------------------------------------------------------------------------------------------------------------------------------------------------------------------------------------------------------------------------------------------------------------------------------------------------------------------------------------------------------------------------------------------------------------------------------------------------------------------------------------------------------------------------------------------------------------------------------------------------------------------------------------------------------------------------------------------------------------------------------------------------------------------------------------------------------------------------------------------------------------------------------------------------------------------------------------------------------------------------------------------------------------------------------------------------------------------------------------------------------------------------------------------------------------------------------------------------------------------------------------------------------------------------------------------------------------------------------------------------------------------------------------------------------------------------------------------------------------------------------------------------------------------------------------------------------------------------------------------------------------------------------------------------------------------------------------------------------------------------------------------------------------------------------------------------------------------------------------------------------------------------------------------------------------------------------------------------------------------------------------------------------------------------------------------------------------------------------------------------------------------------------------------------------------------------------------------------------------------------------------------------------------------------------------------------------------------------------------------------------------------------------------------------------------------------------------------------------------------------------------------------------------------------------------------------------------------------------------------------------------------------------------------------------------------------------------------------------------------------------------------------------------------------------------------------------------------------------------------------------------------------------------------------------------------------------------------------------------------------------------------------------------------------------------------------------------------------------------------------------------------------------------------------------------------------------------------------------------------------------------------------------------------------------------------------------------------------------------------------------------------------------------------------------------------------------------------------------------------------------------------------------------------------------------------------------------------------------------------------------------------------------------------------------------------------------------------------------------------------------------------------------------------------------------------------------------------------------------------------------------------------------------------------------------------------------------------------------------------------------------------------------------------------------------------------------------------------------------------------------------------------------------------------------------------------------------------------------------------------------------------------------------------------------------------------------------------------------------------------------------------------------------------------------------------------------------------------------------------------------------------------------------------------------------------------------------------------------------------------------------------------------------------------------------------------------------------------------------------------------------------------------------------------------------------------------------------------------------------------------------------------------------------------------------------------------------------------------------------------------------------------------------------------------------------------------------------------------------------------------------------------------------------------------------------------------------------------------------------------------------------------------------------------------------------------------------------------------------------------------------------------------------------------------------------------------------------------------------------------------------------------------------------------------------------------------------------------------------------------------------------------------------------------------------------------------------------------------------------------------------------------------------------------------------------------------------------------------------------------------------------------------------------------------------------------------------------------------------------------------------------------------------------------------------------------------------------------------------------------------------------------------------------------------------------------------------------------------------------------------------------------------------------------------------------------------------------------------------------------------------------------------------------------------------------------------------------------------------------------------------------------------------------------------------------------------------------------------------------------------------------------------------------------------------------------------------------------------------------------------------------------------------------------------------------------------------------------------------------------------------------------------------------------------------------------------------------------------------------------------------------------------------------------------------------------------------------------------------------------------------------------------------------------------------------------------------------------------------------------------------------------------------------------------------------------------------------------------------------------------------------------------------------------------------------------------------------------------------------------------------------------------------------------------------------------------------------------------------------------------------------------------------------------------------------------------------------------------------------------------------------------------------------------------------------------------------------------------------------------------------------------------------------------------------------------------------------------------------------------------------------------------------------------------------------------------------------------------------------------------------------------------------------------------------------------------------------------------------------------------------------------------------------------------------------------------------------------------------------------------------------------------------------------------------------------------------------------------------------------------------------------------------------------------------------------------------------------------------------------------------------------------------------------------------------------------------------------------------------------------------------------------------------------------------------------------------------------------------------------------------------------------------------------------------------------------------------------------------------------------------------------------------------------------------------------------------------------------------------------------------------------------------------------------------------------------------------------------------------------------------------------------------------------------------------------------------------------------------------------------------------------------------------------------------------------------------------------------------------------------------------------------------------------------------------------------------------------------------------------------------------------------------------------------------------------------------------------------------------------------------------------------------------------------------------------------------------------------------------------------------------------------------------------------------------------------------------------------------------------------------------------------------------------------------------------------------------------------------------------------------------------------------------------------------------------------------------------------------------------------------------------------------------------------------------------------------------------------------------------------------------------------------------------------------------------------------------------------------------------------------------------------------------------------------------------------------------------------------------------------------------------------------------------------------------------------------------------------------------------------------------------------------------------------------------------------------------------------------------------------------------------------------------------------------------------------------------------------------------------------------------------------------------------------------------------------------------------------------------------------------------------------------------------------------------------------------------------------------------------------------------------------------------------------------------------------------------------------------------------------------------------------------------------------------------------------------------------------------------------------------------------------------------------------------------------------------------------------------------------------------------------------------------------------------------------------------------------------------------------------------------------------------------------------------------------------------------------------------------------------------------------------------------------------------------------------------------------------------------------------------------------------------------------------------------------------------------------------------------------------------------------------------------------------------------------------------------------------------------------------------------------------------------------------------------------------------------------------------------------------------------------------------------------------------------------------------------------------------------------------------------------------------------------------------------------------------------------------------------------------------------------------------------------------------------------------------------------------------------------------------------------------------------------------------------------------------------------------------------------------------------------------------------------------------------------------------------------------------------------------------------------------------------------------------------------------------------------------------------------------------------------------------------------------------------------------------------------------------------------------------------------------------------------------------------------------------------------------------------------------------------------------------------------------------------------------------------------------------------------------------------------------------------------------------------------------------------------------------------------------------------------------------------------------------------------------------------------------------------------------------------------------------------------------------------------------------------------------------------------------------------------------------------------------------------------------------------------------------------------------------------------------------------------------------------------------------------------------------------------------------------------------------------------------------------------------------------------------------------------------------------------------------------------------------------------------------------------------------------------------------------------------------------------------------------------------------------------------------------------------------------------------------------------------------------------------------------------------------------------------------------------------------------------------------------------------------------------------------------------------------------------------------------------------------------------------------------------------------------------------------------------------------------------------------------------------------------------------------------------------------------------------------------------------------------------------------------------------------------------------------------------------------------------------------------------------------------------------------------------------------------------------------------------------------------------------------------------------------------------------------------------------------------------------------------------------------------------------------------------------------------------------------------------------------------------------------------------------------------------------------------------------------------------------------------------------------------------------------------------------------------------------------------------------------------------------------------------------------------------------------------------------------------------------------------------------------------------------------------------------------------------------------------------------------------------------------------------------------------------------------------------------------------------------------------------------------------------------------------------------------------------------------------------------------------------------------------------------------------------------------------------------------------------------------------------------------------------------------------------------------------------------------------------------------------------------------------------------------------------------------------------------------------------------------------------------------------------------------------------------------------------------------------------------------------------------------------------------------------------------------------------------------------------------------------------------------------------------------------------------------------------------------------------------------------------------------------------------------------------------------------------------------------------------------------------------------------------------------------------------------------------------------------------------------------------------------------------------------------------------------------------------------------------------------------------------------------------------------------------------------------------------------------------------------------------------------------------------------------------------------------------------------------------------------------------------------------------------------------------------------------------------------------------------------------------------------------------------------------------------------------------------------------------------------------------------------------------------------------------------------------------------------------------------------------------------------------------------------------------------------------------------------------------------------------------------------------------------------------------------------------------------------------------------------------------------------------------------------------------------------------------------------------------------------------------------------------------------------------------------------------------------------------------------------------------------------------------------------------------------------------------------------------------------------------------------------------------------------------------------------------------------------------------------------------------------------------------------------------------------------------------------------------------------------------------------------------------------------------------------------------------------------------------------------------------------------------------------------------------------------------------------------------------------------------------------------------------------------------------------------------------------------------------------------------------------------------------------------------------------------------------------------------------------------------------------------------------------------------------------------------------------------------------------------------------------------------------------------------------------------------------------------------------------------------------------------------------------------------------------------------------------------------------------------------------------------------------------------------------------------------------------------------------------------------------------------------------------------------------------------------------------------------------------------------------------------------------------------------------------------------------------------------------------------------------------------------------------------------------------------------------------------------------------------------------------------------------------------------------------------------------------------------------------------------------------------------------------------------------------------------------------------------------------------------------------------------------------------------------------------------------------------------------------------------------------------------------------------------------------------------------------------------------------------------------------------------------------------------------------------------------------------------------------------------------------------------------------------------------------------------------------------------------------------------------------------------------------------------------------------------------------------------------------------------------------------------------------------------------------------------------------------------------------------------------------------------------------------------------------------------------------------------------------------------------------------------------------------------------------------------------------------------------------------------------------------------------------------------------------------------------------------------------------------------------------------------------------------------------------------------------------------------------------------------------------------------------------------------------------------------------------------------------------------------------------------------------------------------------------------------------------------------------------------------------------------------------------------------------------------------------------------------------------------------------------------------------------------------------------------------------------------------------------------------------------------------------------------------------------------------------------------------------------------------------------------------------------------------------------------------------------------------------------------------------------------------------------------------------------------------------------------------------------------------------------------------------------------------------------------------------------------------------------------------------------------------------------------------------------------------------------------------------------------------------------------------------------------------------------------------------------------------------------------------------------------------------------------------------------------------------------------------------------------------------------------------------------------------------------------------------------------------------------------------------------------------------------------------------------------------------------------------------------------------------------------------------------------------------------------------------------------------------------------------------------------------------------------------------------------------------------------------------------------------------------------------------------------------------------------------------------------------------------------------------------------------------------------------------------------------------------------------------------------------------------------------------------------------------------------------------------------------------------------------------------------------------------------------------------------------------------------------------------------------------------------------------------------------------------------------------------------------------------------------------------------------------------------------------------------------------------------------------------------------------------------------------------------------------------------------------------------------------------------------------------------------------------------------------------------------------------------------------------------------------------------------------------------------------------------------------------------------------------------------------------------------------------------------------------------------------------------------------------------------------------------------------------------------------------------------------------------------------------------------------------------------------------------------------------------------------------------------------------------------------------------------------------------------------------------------------------------------------------------------------------------------------------------------------------------------------------------------------------------------------------------------------------------------------------------------------------------------------------------------------------------------------------------------------------------------------------------------------------------------------------------------------------------------------------------------------------------------------------------------------------------------------------------------------------------------------------------------------------------------------------------------------------------------------------------------------------------------------------------------------------------------------------------------------------------------------------------------------------------------------------------|
| bug | <ul><li>"ThreadSanitizer: data race on vptr (ctor/dtor vs virtual call) in BaseIndex https://cirrus-ci.com/task/6564394053140480?logs=ci#L3875:\r\n```bash\r\nWARNING: ThreadSanitizer: data race on vptr (ctor/dtor vs virtual call) (pid=24158)\r\n Write of size 8 at 0x7ffe0efae9f8 by main thread:\r\n #0 BaseIndex::~BaseIndex() src/index/base.cpp:53:1 (test_bitcoin+0xcc6b69)\r\n #1 CoinStatsIndex::~CoinStatsIndex() src/./index/coinstatsindex.h:17:7 (test_bitcoin+0x3b9b21)\r\n #2 coinstatsindex_tests::coinstatsindex_initial_sync::test_method() src/test/coinstatsindex_tests.cpp:84:1 (test_bitcoin+0x3b9b21)\r\n #3 coinstatsindex_tests::coinstatsindex_initial_sync_invoker() src/test/coinstatsindex_tests.cpp:32:1 (test_bitcoin+0x3b814b)\r\n #4 boost::detail::function::void_function_invoker0<void (*)(), void>::invoke(boost::detail::function::function_buffer&) /tmp/cirrus-ci-build/depends/x86_64-pc-linux-gnu/include/boost/function/function_template.hpp:117:11 (test_bitcoin+0x2bbf1d)\r\n #5 boost::function0<void>::operator()() const /tmp/cirrus-ci-build/depends/x86_64-pc-linux-gnu/include/boost/function/function_template.hpp:763:14 (test_bitcoin+0x220877)\r\n #6 boost::detail::forward::operator()() /tmp/cirrus-ci-build/depends/x86_64-pc-linux-gnu/include/boost/test/impl/execution_monitor.ipp:1388:32 (test_bitcoin+0x220877)\r\n #7 boost::detail::function::function_obj_invoker0<boost::detail::forward, int>::invoke(boost::detail::function::function_buffer&) /tmp/cirrus-ci-build/depends/x86_64-pc-linux-gnu/include/boost/function/function_template.hpp:137:18 (test_bitcoin+0x220877)\r\n #8 boost::function0<int>::operator()() const /tmp/cirrus-ci-build/depends/x86_64-pc-linux-gnu/include/boost/function/function_template.hpp:763:14 (test_bitcoin+0x1ae59e)\r\n #9 int boost::detail::do_invoke<boost::shared_ptr<boost::detail::translator_holder_base>, boost::function<int ()> >(boost::shared_ptr<boost::detail::translator_holder_base> const&, boost::function<int ()> const&) /tmp/cirrus-ci-build/depends/x86_64-pc-linux-gnu/include/boost/test/impl/execution_monitor.ipp:301:30 (test_bitcoin+0x1ae59e)\r\n #10 boost::execution_monitor::catch_signals(boost::function<int ()> const&) /tmp/cirrus-ci-build/depends/x86_64-pc-linux-gnu/include/boost/test/impl/execution_monitor.ipp:903:16 (test_bitcoin+0x1ae59e)\r\n #11 boost::execution_monitor::execute(boost::function<int ()> const&) /tmp/cirrus-ci-build/depends/x86_64-pc-linux-gnu/include/boost/test/impl/execution_monitor.ipp:1301:16 (test_bitcoin+0x1ae8c0)\r\n #12 boost::execution_monitor::vexecute(boost::function<void ()> const&) /tmp/cirrus-ci-build/depends/x86_64-pc-linux-gnu/include/boost/test/impl/execution_monitor.ipp:1397:5 (test_bitcoin+0x1aa21b)\r\n #13 boost::unit_test::unit_test_monitor_t::execute_and_translate(boost::function<void ()> const&, unsigned long) /tmp/cirrus-ci-build/depends/x86_64-pc-linux-gnu/include/boost/test/impl/unit_test_monitor.ipp:49:9 (test_bitcoin+0x1aa21b)\r\n #14 boost::unit_test::framework::state::execute_test_tree(unsigned long, unsigned long, boost::unit_test::framework::state::random_generator_helper const*) /tmp/cirrus-ci-build/depends/x86_64-pc-linux-gnu/include/boost/test/impl/framework.ipp:815:44 (test_bitcoin+0x1ddb63)\r\n #15 boost::unit_test::framework::state::execute_test_tree(unsigned long, unsigned long, boost::unit_test::framework::state::random_generator_helper const*) /tmp/cirrus-ci-build/depends/x86_64-pc-linux-gnu/include/boost/test/impl/framework.ipp:784:58 (test_bitcoin+0x1de1d8)\r\n #16 boost::unit_test::framework::state::execute_test_tree(unsigned long, unsigned long, boost::unit_test::framework::state::random_generator_helper const*) /tmp/cirrus-ci-build/depends/x86_64-pc-linux-gnu/include/boost/test/impl/framework.ipp:784:58 (test_bitcoin+0x1de1d8)\r\n #17 boost::unit_test::framework::run(unsigned long, bool) /tmp/cirrus-ci-build/depends/x86_64-pc-linux-gnu/include/boost/test/impl/framework.ipp:1721:29 (test_bitcoin+0x1a8e66)\r\n #18 boost::unit_test::unit_test_main(boost::unit_test::test_suite* (*)(int, char**), int, char**) /tmp/cirrus-ci-build/depends/x86_64-pc-linux-gnu/include/boost/test/impl/unit_test_main.ipp:250:9 (test_bitcoin+0x1c19c6)\r\n #19 main /tmp/cirrus-ci-build/depends/x86_64-pc-linux-gnu/include/boost/test/impl/unit_test_main.ipp:306:12 (test_bitcoin+0x1c1ff6)\r\n Previous read of size 8 at 0x7ffe0efae9f8 by thread T1 (mutexes: write M603):\r\n #0 BaseIndex::SetBestBlockIndex(CBlockIndex const*)::$_1::operator()() const src/index/base.cpp:388:9 (test_bitcoin+0xcc74e6)\r\n #1 BaseIndex::SetBestBlockIndex(CBlockIndex const*) src/index/base.cpp:388:9 (test_bitcoin+0xcc74e6)\r\n #2 BaseIndex::BlockConnected(std::__1::shared_ptr<CBlock const> const&, CBlockIndex const*) src/index/base.cpp:273:9 (test_bitcoin+0xcc9759)\r\n #3 CMainSignals::BlockConnected(std::__1::shared_ptr<CBlock const> const&, CBlockIndex const*)::$_8::operator()() const::'lambda'(CValidationInterface&)::operator()(CValidationInterface&) const src/validationinterface.cpp:225:79 (test_bitcoin+0x10223a4)\r\n #4 void MainSignalsImpl::Iterate<CMainSignals::BlockConnected(std::__1::shared_ptr<CBlock const> const&, CBlockIndex const*)::$_8::operator()() const::'lambda'(CValidationInterface&)>(CMainSignals::BlockConnected(std::__1::shared_ptr<CBlock const> const&, CBlockIndex const*)::$_8::operator()() const::'lambda'(CValidationInterface&)&&) src/validationinterface.cpp:86:17 (test_bitcoin+0x10223a4)\r\n #5 CMainSignals::BlockConnected(std::__1::shared_ptr<CBlock const> const&, CBlockIndex const*)::$_8::operator()() const src/validationinterface.cpp:225:22 (test_bitcoin+0x10223a4)\r\n #6 CMainSignals::BlockConnected(std::__1::shared_ptr<CBlock const> const&, CBlockIndex const*)::$_9::operator()() const src/validationinterface.cpp:227:5 (test_bitcoin+0x10223a4)\r\n #7 decltype(static_cast<CMainSignals::BlockConnected(std::__1::shared_ptr<CBlock const> const&, CBlockIndex const*)::$_9&>(fp)()) std::__1::__invoke<CMainSignals::BlockConnected(std::__1::shared_ptr<CBlock const> const&, CBlockIndex const*)::$_9&>(CMainSignals::BlockConnected(std::__1::shared_ptr<CBlock const> const&, CBlockIndex const*)::$_9&) /usr/lib/llvm-13/bin/../include/c++/v1/type_traits:3918:1 (test_bitcoin+0x10223a4)\r\n #8 void std::__1::__invoke_void_return_wrapper<void, true>::__call<CMainSignals::BlockConnected(std::__1::shared_ptr<CBlock const> const&, CBlockIndex const*)::$_9&>(CMainSignals::BlockConnected(std::__1::shared_ptr<CBlock const> const&, CBlockIndex const*)::$_9&) /usr/lib/llvm-13/bin/../include/c++/v1/__functional/invoke.h:61:9 (test_bitcoin+0x10223a4)\r\n #9 std::__1::__function::__alloc_func<CMainSignals::BlockConnected(std::__1::shared_ptr<CBlock const> const&, CBlockIndex const*)::$_9, std::__1::allocator<CMainSignals::BlockConnected(std::__1::shared_ptr<CBlock const> const&, CBlockIndex const*)::$_9>, void ()>::operator()() /usr/lib/llvm-13/bin/../include/c++/v1/__functional/function.h:171:16 (test_bitcoin+0x10223a4)\r\n #10 std::__1::__function::__func<CMainSignals::BlockConnected(std::__1::shared_ptr<CBlock const> const&, CBlockIndex const*)::$_9, std::__1::allocator<CMainSignals::BlockConnected(std::__1::shared_ptr<CBlock const> const&, CBlockIndex const*)::$_9>, void ()>::operator()() /usr/lib/llvm-13/bin/../include/c++/v1/__functional/function.h:345:12 (test_bitcoin+0x10223a4)\r\n #11 std::__1::__function::__value_func<void ()>::operator()() const /usr/lib/llvm-13/bin/../include/c++/v1/__functional/function.h:498:16 (test_bitcoin+0x10b6b71)\r\n #12 std::__1::function<void ()>::operator()() const /usr/lib/llvm-13/bin/../include/c++/v1/__functional/function.h:1175:12 (test_bitcoin+0x10b6b71)\r\n #13 SingleThreadedSchedulerClient::ProcessQueue() src/scheduler.cpp:175:5 (test_bitcoin+0x10b6b71)\r\n #14 SingleThreadedSchedulerClient::MaybeScheduleProcessQueue()::$_1::operator()() const src/scheduler.cpp:144:41 (test_bitcoin+0x10b8875)\r\n #15 decltype(static_cast<SingleThreadedSchedulerClient::MaybeScheduleProcessQueue()::$_1&>(fp)()) std::__1::__invoke<SingleThreadedSchedulerClient::MaybeScheduleProcessQueue()::$_1&>(SingleThreadedSchedulerClient::MaybeScheduleProcessQueue()::$_1&) /usr/lib/llvm-13/bin/../include/c++/v1/type_traits:3918:1 (test_bitcoin+0x10b8875)\r\n #16 void std::__1::__invoke_void_return_wrapper<void, true>::__call<SingleThreadedSchedulerClient::MaybeScheduleProcessQueue()::$_1&>(SingleThreadedSchedulerClient::MaybeScheduleProcessQueue()::$_1&) /usr/lib/llvm-13/bin/../include/c++/v1/__functional/invoke.h:61:9 (test_bitcoin+0x10b8875)\r\n #17 std::__1::__function::__alloc_func<SingleThreadedSchedulerClient::MaybeScheduleProcessQueue()::$_1, std::__1::allocator<SingleThreadedSchedulerClient::MaybeScheduleProcessQueue()::$_1>, void ()>::operator()() /usr/lib/llvm-13/bin/../include/c++/v1/__functional/function.h:171:16 (test_bitcoin+0x10b8875)\r\n #18 std::__1::__function::__func<SingleThreadedSchedulerClient::MaybeScheduleProcessQueue()::$_1, std::__1::allocator<SingleThreadedSchedulerClient::MaybeScheduleProcessQueue()::$_1>, void ()>::operator()() /usr/lib/llvm-13/bin/../include/c++/v1/__functional/function.h:345:12 (test_bitcoin+0x10b8875)\r\n #19 std::__1::__function::__value_func<void ()>::operator()() const /usr/lib/llvm-13/bin/../include/c++/v1/__functional/function.h:498:16 (test_bitcoin+0x10b5b5c)\r\n #20 std::__1::function<void ()>::operator()() const /usr/lib/llvm-13/bin/../include/c++/v1/__functional/function.h:1175:12 (test_bitcoin+0x10b5b5c)\r\n #21 CScheduler::serviceQueue() src/scheduler.cpp:62:17 (test_bitcoin+0x10b5b5c)\r\n #22 ChainTestingSetup::ChainTestingSetup(std::__1::basic_string<char, std::__1::char_traits<char>, std::__1::allocator<char> > const&, std::__1::vector<char const*, std::__1::allocator<char const*> > const&)::$_0::operator()() const src/test/util/setup_common.cpp:160:110 (test_bitcoin+0xa4e7b8)\r\n #23 decltype(static_cast<ChainTestingSetup::ChainTestingSetup(std::__1::basic_string<char, std::__1::char_traits<char>, std::__1::allocator<char> > const&, std::__1::vector<char const*, std::__1::allocator<char const*> > const&)::$_0&>(fp)()) std::__1::__invoke<ChainTestingSetup::ChainTestingSetup(std::__1::basic_string<char, std::__1::char_traits<char>, std::__1::allocator<char> > const&, std::__1::vector<char const*, std::__1::allocator<char const*> > const&)::$_0&>(ChainTestingSetup::ChainTestingSetup(std::__1::basic_string<char, std::__1::char_traits<char>, std::__1::allocator<char> > const&, std::__1::vector<char const*, std::__1::allocator<char const*> > const&)::$_0&) /usr/lib/llvm-13/bin/../include/c++/v1/type_traits:3918:1 (test_bitcoin+0xa4e7b8)\r\n #24 void std::__1::__invoke_void_return_wrapper<void, true>::__call<ChainTestingSetup::ChainTestingSetup(std::__1::basic_string<char, std::__1::char_traits<char>, std::__1::allocator<char> > const&, std::__1::vector<char const*, std::__1::allocator<char const*> > const&)::$_0&>(ChainTestingSetup::ChainTestingSetup(std::__1::basic_string<char, std::__1::char_traits<char>, std::__1::allocator<char> > const&, std::__1::vector<char const*, std::__1::allocator<char const*> > const&)::$_0&) /usr/lib/llvm-13/bin/../include/c++/v1/__functional/invoke.h:61:9 (test_bitcoin+0xa4e7b8)\r\n #25 std::__1::__function::__alloc_func<ChainTestingSetup::ChainTestingSetup(std::__1::basic_string<char, std::__1::char_traits<char>, std::__1::allocator<char> > const&, std::__1::vector<char const*, std::__1::allocator<char const*> > const&)::$_0, std::__1::allocator<ChainTestingSetup::ChainTestingSetup(std::__1::basic_string<char, std::__1::char_traits<char>, std::__1::allocator<char> > const&, std::__1::vector<char const*, std::__1::allocator<char const*> > const&)::$_0>, void ()>::operator()() /usr/lib/llvm-13/bin/../include/c++/v1/__functional/function.h:171:16 (test_bitcoin+0xa4e7b8)\r\n #26 std::__1::__function::__func<ChainTestingSetup::ChainTestingSetup(std::__1::basic_string<char, std::__1::char_traits<char>, std::__1::allocator<char> > const&, std::__1::vector<char const*, std::__1::allocator<char const*> > const&)::$_0, std::__1::allocator<ChainTestingSetup::ChainTestingSetup(std::__1::basic_string<char, std::__1::char_traits<char>, std::__1::allocator<char> > const&, std::__1::vector<char const*, std::__1::allocator<char const*> > const&)::$_0>, void ()>::operator()() /usr/lib/llvm-13/bin/../include/c++/v1/__functional/function.h:345:12 (test_bitcoin+0xa4e7b8)\r\n #27 std::__1::__function::__value_func<void ()>::operator()() const /usr/lib/llvm-13/bin/../include/c++/v1/__functional/function.h:498:16 (test_bitcoin+0x115760f)\r\n #28 std::__1::function<void ()>::operator()() const /usr/lib/llvm-13/bin/../include/c++/v1/__functional/function.h:1175:12 (test_bitcoin+0x115760f)\r\n #29 util::TraceThread(char const*, std::__1::function<void ()>) src/util/thread.cpp:18:9 (test_bitcoin+0x115760f)\r\n #30 decltype(static_cast<void (*>(fp)(static_cast<char const*>(fp0), static_cast<ChainTestingSetup::ChainTestingSetup(std::__1::basic_string<char, std::__1::char_traits<char>, std::__1::allocator<char> > const&, std::__1::vector<char const*, std::__1::allocator<char const*> > const&)::$_0>(fp0))) std::__1::__invoke<void (*)(char const*, std::__1::function<void ()>), char const*, ChainTestingSetup::ChainTestingSetup(std::__1::basic_string<char, std::__1::char_traits<char>, std::__1::allocator<char> > const&, std::__1::vector<char const*, std::__1::allocator<char const*> > const&)::$_0>(void (*&&)(char const*, std::__1::function<void ()>), char const*&&, ChainTestingSetup::ChainTestingSetup(std::__1::basic_string<char, std::__1::char_traits<char>, std::__1::allocator<char> > const&, std::__1::vector<char const*, std::__1::allocator<char const*> > const&)::$_0&&) /usr/lib/llvm-13/bin/../include/c++/v1/type_traits:3918:1 (test_bitcoin+0xa4e3b1)\r\n #31 void std::__1::__thread_execute<std::__1::unique_ptr<std::__1::__thread_struct, std::__1::default_delete<std::__1::__thread_struct> >, void (*)(char const*, std::__1::function<void ()>), char const*, ChainTestingSetup::ChainTestingSetup(std::__1::basic_string<char, std::__1::char_traits<char>, std::__1::allocator<char> > const&, std::__1::vector<char const*, std::__1::allocator<char const*> > const&)::$_0, 2ul, 3ul>(std::__1::tuple<std::__1::unique_ptr<std::__1::__thread_struct, std::__1::default_delete<std::__1::__thread_struct> >, void (*)(char const*, std::__1::function<void ()>), char const*, ChainTestingSetup::ChainTestingSetup(std::__1::basic_string<char, std::__1::char_traits<char>, std::__1::allocator<char> > const&, std::__1::vector<char const*, std::__1::allocator<char const*> > const&)::$_0>&, std::__1::__tuple_indices<2ul, 3ul>) /usr/lib/llvm-13/bin/../include/c++/v1/thread:280:5 (test_bitcoin+0xa4e3b1)\r\n #32 void* std::__1::__thread_proxy<std::__1::tuple<std::__1::unique_ptr<std::__1::__thread_struct, std::__1::default_delete<std::__1::__thread_struct> >, void (*)(char const*, std::__1::function<void ()>), char const*, ChainTestingSetup::ChainTestingSetup(std::__1::basic_string<char, std::__1::char_traits<char>, std::__1::allocator<char> > const&, std::__1::vector<char const*, std::__1::allocator<char const*> > const&)::$_0> >(void*) /usr/lib/llvm-13/bin/../include/c++/v1/thread:291:5 (test_bitcoin+0xa4e3b1)\r\n Location is stack of main thread.\r\n Location is global '??' at 0x7ffe0ef91000 ([stack]+0x00000001d9f8)\r\n Mutex M603 (0x558df2c934a0) created at:\r\n #0 pthread_mutex_init <null> (test_bitcoin+0x11cf6f)\r\n #1 std::__1::recursive_mutex::recursive_mutex() <null> (libc++.so.1+0x49fb3)\r\n #2 __libc_start_main <null> (libc.so.6+0x29eba)\r\n Thread T1 'b-scheduler' (tid=24216, running) created by main thread at:\r\n #0 pthread_create <null> (test_bitcoin+0x11b7fd)\r\n #1 std::__1::__libcpp_thread_create(unsigned long*, void* (*)(void*), void*) /usr/lib/llvm-13/bin/../include/c++/v1/__threading_support:443:10 (test_bitcoin+0xa47a76)\r\n #2 std::__1::thread::thread<void (&)(char const*, std::__1::function<void ()>), char const (&) [10], ChainTestingSetup::ChainTestingSetup(std::__1::basic_string<char, std::__1::char_traits<char>, std::__1::allocator<char> > const&, std::__1::vector<char const*, std::__1::allocator<char const*> > const&)::$_0, void>(void (&)(char const*, std::__1::function<void ()>), char const (&) [10], ChainTestingSetup::ChainTestingSetup(std::__1::basic_string<char, std::__1::char_traits<char>, std::__1::allocator<char> > const&, std::__1::vector<char const*, std::__1::allocator<char const*> > const&)::$_0&&) /usr/lib/llvm-13/bin/../include/c++/v1/thread:307:16 (test_bitcoin+0xa47a76)\r\n #3 ChainTestingSetup::ChainTestingSetup(std::__1::basic_string<char, std::__1::char_traits<char>, std::__1::allocator<char> > const&, std::__1::vector<char const*, std::__1::allocator<char const*> > const&) src/test/util/setup_common.cpp:160:42 (test_bitcoin+0xa47a76)\r\n #4 TestingSetup::TestingSetup(std::__1::basic_string<char, std::__1::char_traits<char>, std::__1::allocator<char> > const&, std::__1::vector<char const*, std::__1::allocator<char const*> > const&) src/test/util/setup_common.cpp:198:7 (test_bitcoin+0xa47ed9)\r\n #5 TestChain100Setup::TestChain100Setup(std::__1::basic_string<char, std::__1::char_traits<char>, std::__1::allocator<char> > const&, std::__1::vector<char const*, std::__1::allocator<char const*> > const&) src/test/util/setup_common.cpp:246:7 (test_bitcoin+0xa48be3)\r\n #6 coinstatsindex_tests::coinstatsindex_initial_sync::coinstatsindex_initial_sync() src/test/coinstatsindex_tests.cpp:32:1 (test_bitcoin+0x3b7c8b)\r\n #7 coinstatsindex_tests::coinstatsindex_initial_sync_invoker() src/test/coinstatsindex_tests.cpp:32:1 (test_bitcoin+0x3b7c8b)\r\n #8 boost::detail::function::void_function_invoker0<void (*)(), void>::invoke(boost::detail::function::function_buffer&) /tmp/cirrus-ci-build/depends/x86_64-pc-linux-gnu/include/boost/function/function_template.hpp:117:11 (test_bitcoin+0x2bbf1d)\r\n #9 boost::function0<void>::operator()() const /tmp/cirrus-ci-build/depends/x86_64-pc-linux-gnu/include/boost/function/function_template.hpp:763:14 (test_bitcoin+0x220877)\r\n #10 boost::detail::forward::operator()() /tmp/cirrus-ci-build/depends/x86_64-pc-linux-gnu/include/boost/test/impl/execution_monitor.ipp:1388:32 (test_bitcoin+0x220877)\r\n #11 boost::detail::function::function_obj_invoker0<boost::detail::forward, int>::invoke(boost::detail::function::function_buffer&) /tmp/cirrus-ci-build/depends/x86_64-pc-linux-gnu/include/boost/function/function_template.hpp:137:18 (test_bitcoin+0x220877)\r\n #12 boost::function0<int>::operator()() const /tmp/cirrus-ci-build/depends/x86_64-pc-linux-gnu/include/boost/function/function_template.hpp:763:14 (test_bitcoin+0x1ae59e)\r\n #13 int boost::detail::do_invoke<boost::shared_ptr<boost::detail::translator_holder_base>, boost::function<int ()> >(boost::shared_ptr<boost::detail::translator_holder_base> const&, boost::function<int ()> const&) /tmp/cirrus-ci-build/depends/x86_64-pc-linux-gnu/include/boost/test/impl/execution_monitor.ipp:301:30 (test_bitcoin+0x1ae59e)\r\n #14 boost::execution_monitor::catch_signals(boost::function<int ()> const&) /tmp/cirrus-ci-build/depends/x86_64-pc-linux-gnu/include/boost/test/impl/execution_monitor.ipp:903:16 (test_bitcoin+0x1ae59e)\r\n #15 boost::execution_monitor::execute(boost::function<int ()> const&) /tmp/cirrus-ci-build/depends/x86_64-pc-linux-gnu/include/boost/test/impl/execution_monitor.ipp:1301:16 (test_bitcoin+0x1ae8c0)\r\n #16 boost::execution_monitor::vexecute(boost::function<void ()> const&) /tmp/cirrus-ci-build/depends/x86_64-pc-linux-gnu/include/boost/test/impl/execution_monitor.ipp:1397:5 (test_bitcoin+0x1aa21b)\r\n #17 boost::unit_test::unit_test_monitor_t::execute_and_translate(boost::function<void ()> const&, unsigned long) /tmp/cirrus-ci-build/depends/x86_64-pc-linux-gnu/include/boost/test/impl/unit_test_monitor.ipp:49:9 (test_bitcoin+0x1aa21b)\r\n #18 boost::unit_test::framework::state::execute_test_tree(unsigned long, unsigned long, boost::unit_test::framework::state::random_generator_helper const*) /tmp/cirrus-ci-build/depends/x86_64-pc-linux-gnu/include/boost/test/impl/framework.ipp:815:44 (test_bitcoin+0x1ddb63)\r\n #19 boost::unit_test::framework::state::execute_test_tree(unsigned long, unsigned long, boost::unit_test::framework::state::random_generator_helper const*) /tmp/cirrus-ci-build/depends/x86_64-pc-linux-gnu/include/boost/test/impl/framework.ipp:784:58 (test_bitcoin+0x1de1d8)\r\n #20 boost::unit_test::framework::state::execute_test_tree(unsigned long, unsigned long, boost::unit_test::framework::state::random_generator_helper const*) /tmp/cirrus-ci-build/depends/x86_64-pc-linux-gnu/include/boost/test/impl/framework.ipp:784:58 (test_bitcoin+0x1de1d8)\r\n #21 boost::unit_test::framework::run(unsigned long, bool) /tmp/cirrus-ci-build/depends/x86_64-pc-linux-gnu/include/boost/test/impl/framework.ipp:1721:29 (test_bitcoin+0x1a8e66)\r\n #22 boost::unit_test::unit_test_main(boost::unit_test::test_suite* (*)(int, char**), int, char**) /tmp/cirrus-ci-build/depends/x86_64-pc-linux-gnu/include/boost/test/impl/unit_test_main.ipp:250:9 (test_bitcoin+0x1c19c6)\r\n #23 main /tmp/cirrus-ci-build/depends/x86_64-pc-linux-gnu/include/boost/test/impl/unit_test_main.ipp:306:12 (test_bitcoin+0x1c1ff6)\r\nSUMMARY: ThreadSanitizer: data race on vptr (ctor/dtor vs virtual call) src/index/base.cpp:53:1 in BaseIndex::~BaseIndex()\r\n==================\r\nExit status: 2\r\n```"</li><li>'. .'</li><li>'Issue in `p2p_ibd_stalling.py` under Valgrind At 40c6c85c05812ee8bf824b639307b1ac17a001c4 with the native_valgrind job:\r\n```bash\r\n test 2023-03-05T21:26:43.074000Z TestFramework.node0 (DEBUG): Connecting to 127.0.0.1:12173 outbound-full-relay \r\n node0 2023-03-05T21:26:43.265731Z [msghand] [net_processing.cpp:5807] [SendMessages] [net] Requesting block 752405439cea869d584044084502582bc209e4ef97e4bf3b8c2ba3958acaf606 (21) peer=0 \r\n node0 2023-03-05T21:26:43.267295Z [msghand] [net.cpp:2816] [PushMessage] [net] sending getdata (37 bytes) peer=0 \r\n node0 2023-03-05T21:26:43.269862Z [http] [httpserver.cpp:239] [http_request_cb] [http] Received a POST request for / from 127.0.0.1:40916 \r\n node0 2023-03-05T21:26:43.271568Z [msghand] [net_processing.cpp:3169] [ProcessMessage] [net] received: headers (82947 bytes) peer=1 \r\n node0 2023-03-05T21:26:55.588032Z [httpworker.0] [rpc/request.cpp:179] [parse] [rpc] ThreadRPCServer method=addconnection user=__cookie__ \r\n node0 2023-03-05T21:26:55.709062Z [httpworker.0] [net.cpp:457] [ConnectNode] [net:debug] trying connection 127.0.0.1:12173 lastseen=0.0hrs \r\n node0 2023-03-05T21:26:55.731504Z [httpworker.0] [net.cpp:2803] [CNode] [net] Added connection peer=2 \r\n test 2023-03-05T21:27:43.097000Z TestFramework.utils (ERROR): wait_until() failed. Predicate: \'\'\'\' \r\n test_function = lambda: self.is_connected\r\n \'\'\'\r\n test 2023-03-05T21:27:43.097000Z TestFramework (ERROR): Assertion failed \r\n Traceback (most recent call last):\r\n File "/home/ubuntu/ci_scratch/ci/scratch/build/bitcoin-x86_64-pc-linux-gnu/test/functional/test_framework/test_framework.py", line 134, in main\r\n self.run_test()\r\n File "/home/ubuntu/ci_scratch/ci/scratch/build/bitcoin-x86_64-pc-linux-gnu/test/functional/p2p_ibd_stalling.py", line 77, in run_test\r\n peers.append(node.add_outbound_p2p_connection(P2PStaller(stall_block), p2p_idx=id, connection_type="outbound-full-relay"))\r\n File "/home/ubuntu/ci_scratch/ci/scratch/build/bitcoin-x86_64-pc-linux-gnu/test/functional/test_framework/test_node.py", line 663, in add_outbound_p2p_connection\r\n p2p_conn.wait_for_connect()\r\n File "/home/ubuntu/ci_scratch/ci/scratch/build/bitcoin-x86_64-pc-linux-gnu/test/functional/test_framework/p2p.py", line 467, in wait_for_connect\r\n wait_until_helper(test_function, timeout=timeout, lock=p2p_lock)\r\n File "/home/ubuntu/ci_scratch/ci/scratch/build/bitcoin-x86_64-pc-linux-gnu/test/functional/test_framework/util.py", line 281, in wait_until_helper\r\n raise AssertionError("Predicate {} not true after {} seconds".format(predicate_source, timeout))\r\n AssertionError: Predicate \'\'\'\'\r\n test_function = lambda: self.is_connected\r\n \'\'\' not true after 60.0 seconds\r\n test 2023-03-05T21:27:43.102000Z TestFramework (DEBUG): Closing down network thread \r\n test 2023-03-05T21:27:53.123000Z TestFramework.utils (ERROR): wait_until() failed. Predicate: \'\'\'\' \r\n wait_until_helper(lambda: not self.network_event_loop.is_running(), timeout=timeout)\r\n \'\'\'\r\n node0 2023-03-05T21:28:08.198208Z [msghand] [net_processing.cpp:2760] [UpdatePeerStateForReceivedHeaders] [net] Protecting outbound peer=1 from eviction \r\n node0 2023-03-05T21:28:08.201820Z [msghand] [net.cpp:2816] [PushMessage] [net] sending sendheaders (0 bytes) peer=1 \r\n```'</li></ul> |
| question | <ul><li>"bitcoin core's sync has stop for days! 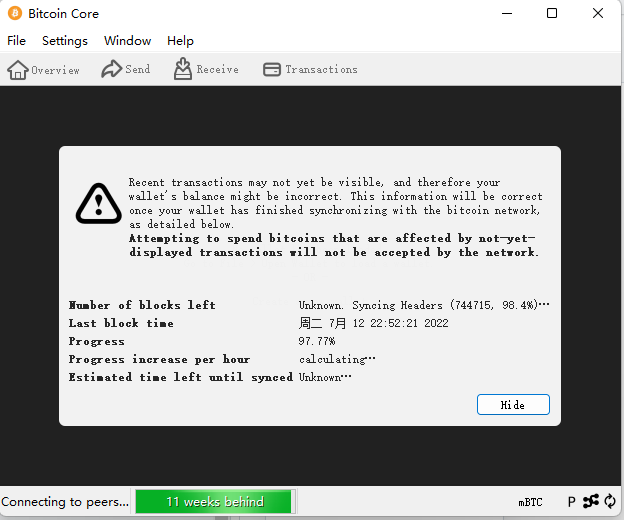\r\n\r\n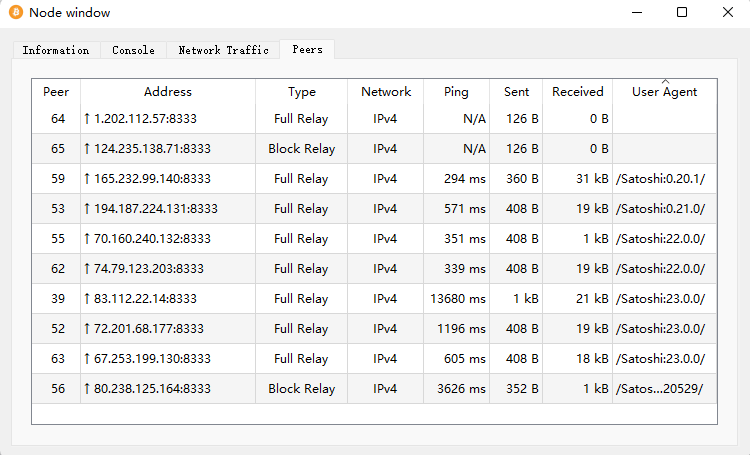\r\n\r\n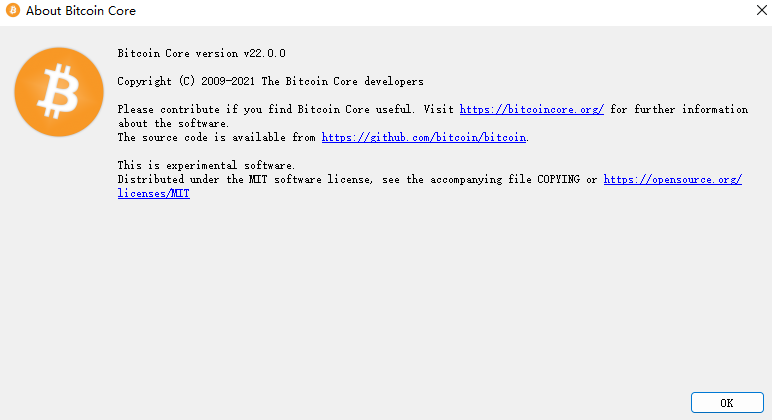\r\n\r\nOS info:\r\nwindows11 21H2\r\n\r\nwhat should i do?"</li><li>'Regtest mode loses unspents after day ### Is there an existing issue for this?\r\n\r\n- [X] I have searched the existing issues\r\n\r\n### Current behaviour\r\n\r\nTesting in regtest mode, I have noticed that after 1 day of send some funds from A wallet to B, the unspent inputs disappear from wallet B.\r\n\r\n**bitcoin.conf**\r\n\r\n```bash\r\n# Generated by https://jlopp.github.io/bitcoin-core-config-generator/\r\n\r\n# This config should be placed in following path:\r\n# ~/.bitcoin/bitcoin.conf\r\n\r\n# [chain]\r\n# Run this node on its own independent test network. Equivalent to -chain=regtest\r\nregtest=1\r\n\r\n# [core]\r\n# Specify a non-default location to store blockchain and other data.\r\ndatadir=/home/debian/.bitcoin\r\n# Reduce storage requirements by only storing most recent N MiB of block. This mode is incompatible with -txindex and -coinstatsindex. WARNING: Reverting this setting requires re-downloading th>\r\nprune=0\r\n\r\n# [wallet]\r\n# Bech32\r\naddresstype=bech32\r\n# Bech32\r\nchangetype=bech32\r\n# Specify wallet database path. Can be specified multiple times to load multiple wallets. Path is interpreted relative to <walletdir> if it is not absolute and will be created if it does not ex>\r\nwallet=default\r\n\r\n# [Sections]\r\n# Most options automatically apply to mainnet, testnet, and regtest networks.\r\n# If you want to confine an option to just one network, you should add it in the relevant section.\r\n# EXCEPTIONS: The options addnode, connect, port, bind, rpcport, rpcbind and wallet\r\n# only apply to mainnet unless they appear in the appropriate section below.\r\n\r\n# Options only for mainnet\r\n[main]\r\n\r\n# Options only for testnet\r\n[test]\r\n\r\n# Options only for regtest\r\n[regtest]\r\n# Accept command line and JSON-RPC commands.\r\nserver=1\r\n# Bind to given address to listen for JSON-RPC connections. This option is ignored unless -rpcallowip is also passed. Port is optional and overrides -rpcport. Use [host]:port notation for IPv6.>\r\nrpcbind=127.0.0.1\r\n# Listen for JSON-RPC connections on this port\r\nrpcport=10001\r\n# Allow JSON-RPC connections from specified source. Valid for <ip> are a single IP (e.g. 1.2.3.4), a network/netmask (e.g. 1.2.3.4/255.255.255.0) or a network/CIDR (e.g. 1.2.3.4/24). This optio>\r\nrpcallowip=127.0.0.1\r\n# Username and hashed password for JSON-RPC connections. The field <userpw> comes in the format: <USERNAME>:<SALT>$<HASH>. RPC clients connect using rpcuser=<USERNAME>/rpcpassword=<PASSWORD> ar>\r\nrpcauth=bitcoin:6e0efb08ebd20eff65959edc38d17bc4$9bc0e273f35e583d9b70071cfd71dc78034ff639d1900c780e3412d7011aab1f\r\n```\r\n\r\n### Expected behaviour\r\n\r\nObtain unspent inputs\r\n\r\n### Steps to reproduce\r\n\r\n```bash\r\n// Send unspent\r\nbitcoin-core.cli -named send outputs=\'{"bcrt1qugd904ne5z0ks45fgmdcne2qe37s2fv7jqra24": 0.034}\' fee_rate=25\r\n\r\n// Generate blocks\r\nbitcoin-core.cli generatetoaddress 6 bcrt1qpg03lyd93mfvz56p92rl6e0mxzasrfskccn77k\r\n\r\n// List unspent\r\nbitcoin-core.cli listunspent 0 9999999 [\\\\"bcrt1qugd904ne5z0ks45fgmdcne2qe37s2fv7jqra24\\\\"]\r\n```\r\n\r\nAfter day, trying again to list with `listunspent` and the result is empty.\r\n\r\n### Relevant log output\r\n\r\n_No response_\r\n\r\n### How did you obtain Bitcoin Core\r\n\r\nPackage manager\r\n\r\n### What version of Bitcoin Core are you using?\r\n\r\nv0.25\r\n\r\n### Operating system and version\r\n\r\nDebian 11\r\n\r\n### Machine specifications\r\n\r\n_No response_'</li><li>'bitcoin-cli does\'nt have "getnewaddress" method I have installed bitcoin core running on my local machine.\r\nI want to create a new address using bitcoin-cli, executing a command as follows then an error message was displayed.\r\nHow can I solve it?\r\n\r\n```\r\n$ bitcoin-cli getnewaddress\r\nerror code: -32601\r\nerror message:\r\nMethod not found\r\n```\r\n\r\nThe version of bitcoin-core is as follows.\r\n```\r\n$ bitcoin-cli -version\r\nBitcoin Core RPC client version v0.18.0.0-742f7dd97\r\n```\r\n\r\nThe machine running bitcoin core is virtual machine on VirtualBox on Windows7.\r\nThe guest OS is ubuntu server and version is as follows.\r\n```\r\n$ uname -a\r\nLinux ubuntu 4.15.0-45-generic #48-Ubuntu SMP Tue Jan 29 16:28:13 UTC 2019 x86_64 x86_64 x86_64 GNU/Linux\r\n```\r\n\r\n'</li></ul> |
| feature | <ul><li>"Validate user input and keep path in a separate argument for importwallet, createwallet and dumpwallet **Is your feature request related to a problem? Please describe.**\r\n\r\nWallets with weird names possible which can be exploited in vulnerable web applications that use Bitcoin Core and allow the users to create and import/export wallet\r\n\r\n`../testwallet` for Linux\r\n`..\\\\testwallet` for Windows\r\n\r\nTried on Bitcoin Core v 0.20.0\r\n\r\nhttps://github.com/bitcoin/bitcoin/pull/20080#issuecomment-706766527\r\n\r\n\r\n**Describe the solution you'd like**\r\n+ Keep two arguments: \r\n`wallet_name` and `wallet_path` for `createwallet` \r\n`filename` and `filepath` for `dumpwallet` `importwallet`\r\n\r\n+ Validate user input. Name should not contain special characters. Path should be optional and if not specified use a default value.\r\n\r\n**Describe alternatives you've considered**\r\nWeb developers should create secure web apps that use Bitcoin Core.\r\n\r\n**Additional context**\r\n\r\n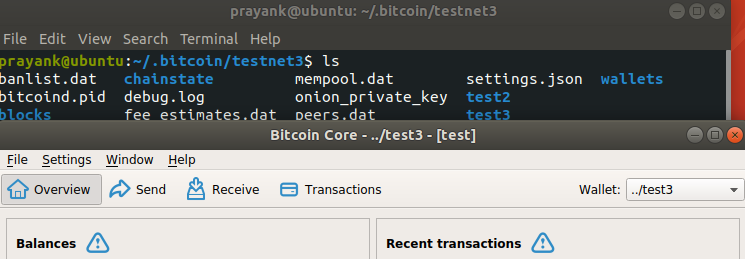\r\n\r\n\r\nI understand its not a vulnerability in Bitcoin Core and only affects vulnerable web apps that use Bitcoin Core. However we can at least consider it a bug that may affect something in future or other projects that use Bitcoin Core. Basic checks for user input can improve the security. In 2017 I had a website in which someone had reported a vulnerability that could be used to change price of flight tickets and book with almost zero bitcoin. It was an issue with the website and we had to fix it although nobody could exploit it because the third party APIs that we were using to book the fight tickets were validating all the things. So a ticket couldn't be processed after tampering and changing the price by attacker. Similarly, if any web developer makes a mistake and using Bitcoin Core for the web app would still be unaffected if Bitcoin Core itself doesn't allow such things for wallet names. \r\n\r\nThere have been lot of directory traversal related vulnerabilities, recently one was reported in Facebook android app:\r\n\r\nhttps://portswigger.net/daily-swig/vulnerability-in-facebook-android-app-nets-10k-bug-bounty\r\n\r\n"</li><li>'split policy/error consensus codes for CLEANSTACK, MINAMALIF Discussion here https://github.com/bitcoin/bitcoin/pull/20006#issuecomment-698487304\r\n\r\n'</li><li>'rpc: pipe support for submitblock If you use the `submitblock` RPC with a large enough block you\'ll run into "argument list too long". Using a pipe should fix this, at least on Linux / macOS.\r\n\r\nThe `submitblock` RPC can be useful to check validity of a stale block that never made it into the main chain (and that your node never fetched): call `invalidateblock` on its same-height-sibling and then feed it the raw block.\r\n\r\nEasiest workaround is to use a library that connects directly to `bitcoind` via RPC (be careful to avoid a line break character at the end). Using the GUI console fails with "Block decode failed" for me, but haven\'t tested that extensively. '</li></ul> |
## Uses
### Direct Use for Inference
First install the SetFit library:
```bash
pip install setfit
```
Then you can load this model and run inference.
```python
from setfit import SetFitModel
# Download from the 🤗 Hub
model = SetFitModel.from_pretrained("setfit_model_id")
# Run inference
preds = model("Add \"walletpassphrasechange\" command in bitcoin-wallet.exe This is an underrated useful tool. Dear devs, please add this \"feature\". Thanks.")
```
<!--
### Downstream Use
*List how someone could finetune this model on their own dataset.*
-->
<!--
### Out-of-Scope Use
*List how the model may foreseeably be misused and address what users ought not to do with the model.*
-->
<!--
## Bias, Risks and Limitations
*What are the known or foreseeable issues stemming from this model? You could also flag here known failure cases or weaknesses of the model.*
-->
<!--
### Recommendations
*What are recommendations with respect to the foreseeable issues? For example, filtering explicit content.*
-->
## Training Details
### Training Set Metrics
| Training set | Min | Median | Max |
|:-------------|:----|:---------|:------|
| Word count | 2 | 280.1983 | 19599 |
| Label | Training Sample Count |
|:---------|:----------------------|
| bug | 200 |
| feature | 200 |
| question | 200 |
### Training Hyperparameters
- batch_size: (16, 2)
- num_epochs: (1, 1)
- max_steps: -1
- sampling_strategy: oversampling
- num_iterations: 20
- body_learning_rate: (2e-05, 1e-05)
- head_learning_rate: 0.01
- loss: CosineSimilarityLoss
- distance_metric: cosine_distance
- margin: 0.25
- end_to_end: False
- use_amp: False
- warmup_proportion: 0.1
- seed: 42
- eval_max_steps: -1
- load_best_model_at_end: False
### Training Results
| Epoch | Step | Training Loss | Validation Loss |
|:------:|:----:|:-------------:|:---------------:|
| 0.0007 | 1 | 0.3844 | - |
| 0.0067 | 10 | 0.3318 | - |
| 0.0133 | 20 | 0.3378 | - |
| 0.02 | 30 | 0.2318 | - |
| 0.0267 | 40 | 0.2845 | - |
| 0.0333 | 50 | 0.2672 | - |
| 0.04 | 60 | 0.1494 | - |
| 0.0467 | 70 | 0.1949 | - |
| 0.0533 | 80 | 0.1737 | - |
| 0.06 | 90 | 0.1319 | - |
| 0.0667 | 100 | 0.1767 | - |
| 0.0733 | 110 | 0.1221 | - |
| 0.08 | 120 | 0.1747 | - |
| 0.0867 | 130 | 0.1264 | - |
| 0.0933 | 140 | 0.1445 | - |
| 0.1 | 150 | 0.2189 | - |
| 0.1067 | 160 | 0.0893 | - |
| 0.1133 | 170 | 0.1636 | - |
| 0.12 | 180 | 0.1663 | - |
| 0.1267 | 190 | 0.0792 | - |
| 0.1333 | 200 | 0.1131 | - |
| 0.14 | 210 | 0.1628 | - |
| 0.1467 | 220 | 0.1271 | - |
| 0.1533 | 230 | 0.175 | - |
| 0.16 | 240 | 0.1763 | - |
| 0.1667 | 250 | 0.1573 | - |
| 0.1733 | 260 | 0.0813 | - |
| 0.18 | 270 | 0.1035 | - |
| 0.1867 | 280 | 0.0076 | - |
| 0.1933 | 290 | 0.2546 | - |
| 0.2 | 300 | 0.0526 | - |
| 0.2067 | 310 | 0.014 | - |
| 0.2133 | 320 | 0.0211 | - |
| 0.22 | 330 | 0.0779 | - |
| 0.2267 | 340 | 0.0125 | - |
| 0.2333 | 350 | 0.0755 | - |
| 0.24 | 360 | 0.1108 | - |
| 0.2467 | 370 | 0.0351 | - |
| 0.2533 | 380 | 0.0096 | - |
| 0.26 | 390 | 0.0121 | - |
| 0.2667 | 400 | 0.0061 | - |
| 0.2733 | 410 | 0.0636 | - |
| 0.28 | 420 | 0.071 | - |
| 0.2867 | 430 | 0.0024 | - |
| 0.2933 | 440 | 0.0082 | - |
| 0.3 | 450 | 0.0084 | - |
| 0.3067 | 460 | 0.0427 | - |
| 0.3133 | 470 | 0.0027 | - |
| 0.32 | 480 | 0.0024 | - |
| 0.3267 | 490 | 0.001 | - |
| 0.3333 | 500 | 0.003 | - |
| 0.34 | 510 | 0.0019 | - |
| 0.3467 | 520 | 0.0077 | - |
| 0.3533 | 530 | 0.0014 | - |
| 0.36 | 540 | 0.0024 | - |
| 0.3667 | 550 | 0.0008 | - |
| 0.3733 | 560 | 0.0011 | - |
| 0.38 | 570 | 0.0037 | - |
| 0.3867 | 580 | 0.0117 | - |
| 0.3933 | 590 | 0.0004 | - |
| 0.4 | 600 | 0.0011 | - |
| 0.4067 | 610 | 0.0006 | - |
| 0.4133 | 620 | 0.0006 | - |
| 0.42 | 630 | 0.002 | - |
| 0.4267 | 640 | 0.0004 | - |
| 0.4333 | 650 | 0.0071 | - |
| 0.44 | 660 | 0.0002 | - |
| 0.4467 | 670 | 0.0006 | - |
| 0.4533 | 680 | 0.0003 | - |
| 0.46 | 690 | 0.0003 | - |
| 0.4667 | 700 | 0.0002 | - |
| 0.4733 | 710 | 0.0002 | - |
| 0.48 | 720 | 0.0002 | - |
| 0.4867 | 730 | 0.0003 | - |
| 0.4933 | 740 | 0.0046 | - |
| 0.5 | 750 | 0.0003 | - |
| 0.5067 | 760 | 0.0004 | - |
| 0.5133 | 770 | 0.0002 | - |
| 0.52 | 780 | 0.0003 | - |
| 0.5267 | 790 | 0.0001 | - |
| 0.5333 | 800 | 0.0002 | - |
| 0.54 | 810 | 0.0003 | - |
| 0.5467 | 820 | 0.0002 | - |
| 0.5533 | 830 | 0.0004 | - |
| 0.56 | 840 | 0.0004 | - |
| 0.5667 | 850 | 0.0006 | - |
| 0.5733 | 860 | 0.0001 | - |
| 0.58 | 870 | 0.0002 | - |
| 0.5867 | 880 | 0.0002 | - |
| 0.5933 | 890 | 0.0001 | - |
| 0.6 | 900 | 0.0002 | - |
| 0.6067 | 910 | 0.0001 | - |
| 0.6133 | 920 | 0.0001 | - |
| 0.62 | 930 | 0.0002 | - |
| 0.6267 | 940 | 0.0002 | - |
| 0.6333 | 950 | 0.0003 | - |
| 0.64 | 960 | 0.0498 | - |
| 0.6467 | 970 | 0.0002 | - |
| 0.6533 | 980 | 0.0001 | - |
| 0.66 | 990 | 0.0002 | - |
| 0.6667 | 1000 | 0.0002 | - |
| 0.6733 | 1010 | 0.0003 | - |
| 0.68 | 1020 | 0.0001 | - |
| 0.6867 | 1030 | 0.0001 | - |
| 0.6933 | 1040 | 0.0001 | - |
| 0.7 | 1050 | 0.0001 | - |
| 0.7067 | 1060 | 0.0001 | - |
| 0.7133 | 1070 | 0.0001 | - |
| 0.72 | 1080 | 0.0009 | - |
| 0.7267 | 1090 | 0.0001 | - |
| 0.7333 | 1100 | 0.044 | - |
| 0.74 | 1110 | 0.0001 | - |
| 0.7467 | 1120 | 0.0415 | - |
| 0.7533 | 1130 | 0.0003 | - |
| 0.76 | 1140 | 0.023 | - |
| 0.7667 | 1150 | 0.0002 | - |
| 0.7733 | 1160 | 0.0001 | - |
| 0.78 | 1170 | 0.0004 | - |
| 0.7867 | 1180 | 0.0001 | - |
| 0.7933 | 1190 | 0.0001 | - |
| 0.8 | 1200 | 0.0001 | - |
| 0.8067 | 1210 | 0.0001 | - |
| 0.8133 | 1220 | 0.0003 | - |
| 0.82 | 1230 | 0.0002 | - |
| 0.8267 | 1240 | 0.0003 | - |
| 0.8333 | 1250 | 0.0001 | - |
| 0.84 | 1260 | 0.0001 | - |
| 0.8467 | 1270 | 0.0001 | - |
| 0.8533 | 1280 | 0.0001 | - |
| 0.86 | 1290 | 0.0001 | - |
| 0.8667 | 1300 | 0.0001 | - |
| 0.8733 | 1310 | 0.0001 | - |
| 0.88 | 1320 | 0.0001 | - |
| 0.8867 | 1330 | 0.0001 | - |
| 0.8933 | 1340 | 0.0001 | - |
| 0.9 | 1350 | 0.0001 | - |
| 0.9067 | 1360 | 0.0001 | - |
| 0.9133 | 1370 | 0.0001 | - |
| 0.92 | 1380 | 0.0001 | - |
| 0.9267 | 1390 | 0.0001 | - |
| 0.9333 | 1400 | 0.0003 | - |
| 0.94 | 1410 | 0.0001 | - |
| 0.9467 | 1420 | 0.0001 | - |
| 0.9533 | 1430 | 0.0001 | - |
| 0.96 | 1440 | 0.0001 | - |
| 0.9667 | 1450 | 0.0002 | - |
| 0.9733 | 1460 | 0.0003 | - |
| 0.98 | 1470 | 0.0002 | - |
| 0.9867 | 1480 | 0.0002 | - |
| 0.9933 | 1490 | 0.0001 | - |
| 1.0 | 1500 | 0.0195 | - |
### Framework Versions
- Python: 3.10.12
- SetFit: 1.0.3
- Sentence Transformers: 3.0.1
- Transformers: 4.39.0
- PyTorch: 2.3.0+cu121
- Datasets: 2.20.0
- Tokenizers: 0.15.2
## Citation
### BibTeX
```bibtex
@article{https://doi.org/10.48550/arxiv.2209.11055,
doi = {10.48550/ARXIV.2209.11055},
url = {https://arxiv.org/abs/2209.11055},
author = {Tunstall, Lewis and Reimers, Nils and Jo, Unso Eun Seo and Bates, Luke and Korat, Daniel and Wasserblat, Moshe and Pereg, Oren},
keywords = {Computation and Language (cs.CL), FOS: Computer and information sciences, FOS: Computer and information sciences},
title = {Efficient Few-Shot Learning Without Prompts},
publisher = {arXiv},
year = {2022},
copyright = {Creative Commons Attribution 4.0 International}
}
```
<!--
## Glossary
*Clearly define terms in order to be accessible across audiences.*
-->
<!--
## Model Card Authors
*Lists the people who create the model card, providing recognition and accountability for the detailed work that goes into its construction.*
-->
<!--
## Model Card Contact
*Provides a way for people who have updates to the Model Card, suggestions, or questions, to contact the Model Card authors.*
--> |
PrunaAI/nickypro-tinyllama-15M-HQQ-2bit-smashed | PrunaAI | "2024-06-24T10:08:20Z" | 0 | 0 | transformers | [
"transformers",
"llama",
"text-generation",
"pruna-ai",
"base_model:nickypro/tinyllama-15M",
"autotrain_compatible",
"endpoints_compatible",
"text-generation-inference",
"region:us"
] | text-generation | "2024-06-24T10:08:14Z" | ---
thumbnail: "https://assets-global.website-files.com/646b351987a8d8ce158d1940/64ec9e96b4334c0e1ac41504_Logo%20with%20white%20text.svg"
base_model: nickypro/tinyllama-15M
metrics:
- memory_disk
- memory_inference
- inference_latency
- inference_throughput
- inference_CO2_emissions
- inference_energy_consumption
tags:
- pruna-ai
---
<!-- header start -->
<!-- 200823 -->
<div style="width: auto; margin-left: auto; margin-right: auto">
<a href="https://www.pruna.ai/" target="_blank" rel="noopener noreferrer">
<img src="https://i.imgur.com/eDAlcgk.png" alt="PrunaAI" style="width: 100%; min-width: 400px; display: block; margin: auto;">
</a>
</div>
<!-- header end -->
[](https://twitter.com/PrunaAI)
[](https://github.com/PrunaAI)
[](https://www.linkedin.com/company/93832878/admin/feed/posts/?feedType=following)
[](https://discord.gg/CP4VSgck)
# Simply make AI models cheaper, smaller, faster, and greener!
- Give a thumbs up if you like this model!
- Contact us and tell us which model to compress next [here](https://www.pruna.ai/contact).
- Request access to easily compress your *own* AI models [here](https://z0halsaff74.typeform.com/pruna-access?typeform-source=www.pruna.ai).
- Read the documentations to know more [here](https://pruna-ai-pruna.readthedocs-hosted.com/en/latest/)
- Join Pruna AI community on Discord [here](https://discord.gg/CP4VSgck) to share feedback/suggestions or get help.
## Results

**Frequently Asked Questions**
- ***How does the compression work?*** The model is compressed with hqq.
- ***How does the model quality change?*** The quality of the model output might vary compared to the base model.
- ***How is the model efficiency evaluated?*** These results were obtained on HARDWARE_NAME with configuration described in `model/smash_config.json` and are obtained after a hardware warmup. The smashed model is directly compared to the original base model. Efficiency results may vary in other settings (e.g. other hardware, image size, batch size, ...). We recommend to directly run them in the use-case conditions to know if the smashed model can benefit you.
- ***What is the model format?*** We use safetensors.
- ***What calibration data has been used?*** If needed by the compression method, we used WikiText as the calibration data.
- ***What is the naming convention for Pruna Huggingface models?*** We take the original model name and append "turbo", "tiny", or "green" if the smashed model has a measured inference speed, inference memory, or inference energy consumption which is less than 90% of the original base model.
- ***How to compress my own models?*** You can request premium access to more compression methods and tech support for your specific use-cases [here](https://z0halsaff74.typeform.com/pruna-access?typeform-source=www.pruna.ai).
- ***What are "first" metrics?*** Results mentioning "first" are obtained after the first run of the model. The first run might take more memory or be slower than the subsequent runs due cuda overheads.
- ***What are "Sync" and "Async" metrics?*** "Sync" metrics are obtained by syncing all GPU processes and stop measurement when all of them are executed. "Async" metrics are obtained without syncing all GPU processes and stop when the model output can be used by the CPU. We provide both metrics since both could be relevant depending on the use-case. We recommend to test the efficiency gains directly in your use-cases.
## Setup
You can run the smashed model with these steps:
0. Check requirements from the original repo nickypro/tinyllama-15M installed. In particular, check python, cuda, and transformers versions.
1. Make sure that you have installed quantization related packages.
```bash
pip install hqq
```
2. Load & run the model.
```python
from transformers import AutoModelForCausalLM, AutoTokenizer
from hqq.engine.hf import HQQModelForCausalLM
from hqq.models.hf.base import AutoHQQHFModel
try:
model = HQQModelForCausalLM.from_quantized("PrunaAI/nickypro-tinyllama-15M-HQQ-2bit-smashed", device_map='auto')
except:
model = AutoHQQHFModel.from_quantized("PrunaAI/nickypro-tinyllama-15M-HQQ-2bit-smashed")
tokenizer = AutoTokenizer.from_pretrained("nickypro/tinyllama-15M")
input_ids = tokenizer("What is the color of prunes?,", return_tensors='pt').to(model.device)["input_ids"]
outputs = model.generate(input_ids, max_new_tokens=216)
tokenizer.decode(outputs[0])
```
## Configurations
The configuration info are in `smash_config.json`.
## Credits & License
The license of the smashed model follows the license of the original model. Please check the license of the original model nickypro/tinyllama-15M before using this model which provided the base model. The license of the `pruna-engine` is [here](https://pypi.org/project/pruna-engine/) on Pypi.
## Want to compress other models?
- Contact us and tell us which model to compress next [here](https://www.pruna.ai/contact).
- Request access to easily compress your own AI models [here](https://z0halsaff74.typeform.com/pruna-access?typeform-source=www.pruna.ai). |
PrunaAI/Desm0nt-Phi-3-HornyVision-128k-instruct-HQQ-1bit-smashed | PrunaAI | "2024-06-24T10:11:25Z" | 0 | 0 | transformers | [
"transformers",
"phi3_v",
"text-generation",
"pruna-ai",
"conversational",
"custom_code",
"base_model:Desm0nt/Phi-3-HornyVision-128k-instruct",
"autotrain_compatible",
"region:us"
] | text-generation | "2024-06-24T10:10:48Z" | ---
thumbnail: "https://assets-global.website-files.com/646b351987a8d8ce158d1940/64ec9e96b4334c0e1ac41504_Logo%20with%20white%20text.svg"
base_model: Desm0nt/Phi-3-HornyVision-128k-instruct
metrics:
- memory_disk
- memory_inference
- inference_latency
- inference_throughput
- inference_CO2_emissions
- inference_energy_consumption
tags:
- pruna-ai
---
<!-- header start -->
<!-- 200823 -->
<div style="width: auto; margin-left: auto; margin-right: auto">
<a href="https://www.pruna.ai/" target="_blank" rel="noopener noreferrer">
<img src="https://i.imgur.com/eDAlcgk.png" alt="PrunaAI" style="width: 100%; min-width: 400px; display: block; margin: auto;">
</a>
</div>
<!-- header end -->
[](https://twitter.com/PrunaAI)
[](https://github.com/PrunaAI)
[](https://www.linkedin.com/company/93832878/admin/feed/posts/?feedType=following)
[](https://discord.gg/CP4VSgck)
# Simply make AI models cheaper, smaller, faster, and greener!
- Give a thumbs up if you like this model!
- Contact us and tell us which model to compress next [here](https://www.pruna.ai/contact).
- Request access to easily compress your *own* AI models [here](https://z0halsaff74.typeform.com/pruna-access?typeform-source=www.pruna.ai).
- Read the documentations to know more [here](https://pruna-ai-pruna.readthedocs-hosted.com/en/latest/)
- Join Pruna AI community on Discord [here](https://discord.gg/CP4VSgck) to share feedback/suggestions or get help.
## Results

**Frequently Asked Questions**
- ***How does the compression work?*** The model is compressed with hqq.
- ***How does the model quality change?*** The quality of the model output might vary compared to the base model.
- ***How is the model efficiency evaluated?*** These results were obtained on HARDWARE_NAME with configuration described in `model/smash_config.json` and are obtained after a hardware warmup. The smashed model is directly compared to the original base model. Efficiency results may vary in other settings (e.g. other hardware, image size, batch size, ...). We recommend to directly run them in the use-case conditions to know if the smashed model can benefit you.
- ***What is the model format?*** We use safetensors.
- ***What calibration data has been used?*** If needed by the compression method, we used WikiText as the calibration data.
- ***What is the naming convention for Pruna Huggingface models?*** We take the original model name and append "turbo", "tiny", or "green" if the smashed model has a measured inference speed, inference memory, or inference energy consumption which is less than 90% of the original base model.
- ***How to compress my own models?*** You can request premium access to more compression methods and tech support for your specific use-cases [here](https://z0halsaff74.typeform.com/pruna-access?typeform-source=www.pruna.ai).
- ***What are "first" metrics?*** Results mentioning "first" are obtained after the first run of the model. The first run might take more memory or be slower than the subsequent runs due cuda overheads.
- ***What are "Sync" and "Async" metrics?*** "Sync" metrics are obtained by syncing all GPU processes and stop measurement when all of them are executed. "Async" metrics are obtained without syncing all GPU processes and stop when the model output can be used by the CPU. We provide both metrics since both could be relevant depending on the use-case. We recommend to test the efficiency gains directly in your use-cases.
## Setup
You can run the smashed model with these steps:
0. Check requirements from the original repo Desm0nt/Phi-3-HornyVision-128k-instruct installed. In particular, check python, cuda, and transformers versions.
1. Make sure that you have installed quantization related packages.
```bash
pip install hqq
```
2. Load & run the model.
```python
from transformers import AutoModelForCausalLM, AutoTokenizer
from hqq.engine.hf import HQQModelForCausalLM
from hqq.models.hf.base import AutoHQQHFModel
try:
model = HQQModelForCausalLM.from_quantized("PrunaAI/Desm0nt-Phi-3-HornyVision-128k-instruct-HQQ-1bit-smashed", device_map='auto')
except:
model = AutoHQQHFModel.from_quantized("PrunaAI/Desm0nt-Phi-3-HornyVision-128k-instruct-HQQ-1bit-smashed")
tokenizer = AutoTokenizer.from_pretrained("Desm0nt/Phi-3-HornyVision-128k-instruct")
input_ids = tokenizer("What is the color of prunes?,", return_tensors='pt').to(model.device)["input_ids"]
outputs = model.generate(input_ids, max_new_tokens=216)
tokenizer.decode(outputs[0])
```
## Configurations
The configuration info are in `smash_config.json`.
## Credits & License
The license of the smashed model follows the license of the original model. Please check the license of the original model Desm0nt/Phi-3-HornyVision-128k-instruct before using this model which provided the base model. The license of the `pruna-engine` is [here](https://pypi.org/project/pruna-engine/) on Pypi.
## Want to compress other models?
- Contact us and tell us which model to compress next [here](https://www.pruna.ai/contact).
- Request access to easily compress your own AI models [here](https://z0halsaff74.typeform.com/pruna-access?typeform-source=www.pruna.ai). |
PrunaAI/Desm0nt-Phi-3-HornyVision-128k-instruct-HQQ-2bit-smashed | PrunaAI | "2024-06-24T10:11:42Z" | 0 | 0 | transformers | [
"transformers",
"phi3_v",
"text-generation",
"pruna-ai",
"conversational",
"custom_code",
"base_model:Desm0nt/Phi-3-HornyVision-128k-instruct",
"autotrain_compatible",
"region:us"
] | text-generation | "2024-06-24T10:10:55Z" | ---
thumbnail: "https://assets-global.website-files.com/646b351987a8d8ce158d1940/64ec9e96b4334c0e1ac41504_Logo%20with%20white%20text.svg"
base_model: Desm0nt/Phi-3-HornyVision-128k-instruct
metrics:
- memory_disk
- memory_inference
- inference_latency
- inference_throughput
- inference_CO2_emissions
- inference_energy_consumption
tags:
- pruna-ai
---
<!-- header start -->
<!-- 200823 -->
<div style="width: auto; margin-left: auto; margin-right: auto">
<a href="https://www.pruna.ai/" target="_blank" rel="noopener noreferrer">
<img src="https://i.imgur.com/eDAlcgk.png" alt="PrunaAI" style="width: 100%; min-width: 400px; display: block; margin: auto;">
</a>
</div>
<!-- header end -->
[](https://twitter.com/PrunaAI)
[](https://github.com/PrunaAI)
[](https://www.linkedin.com/company/93832878/admin/feed/posts/?feedType=following)
[](https://discord.gg/CP4VSgck)
# Simply make AI models cheaper, smaller, faster, and greener!
- Give a thumbs up if you like this model!
- Contact us and tell us which model to compress next [here](https://www.pruna.ai/contact).
- Request access to easily compress your *own* AI models [here](https://z0halsaff74.typeform.com/pruna-access?typeform-source=www.pruna.ai).
- Read the documentations to know more [here](https://pruna-ai-pruna.readthedocs-hosted.com/en/latest/)
- Join Pruna AI community on Discord [here](https://discord.gg/CP4VSgck) to share feedback/suggestions or get help.
## Results

**Frequently Asked Questions**
- ***How does the compression work?*** The model is compressed with hqq.
- ***How does the model quality change?*** The quality of the model output might vary compared to the base model.
- ***How is the model efficiency evaluated?*** These results were obtained on HARDWARE_NAME with configuration described in `model/smash_config.json` and are obtained after a hardware warmup. The smashed model is directly compared to the original base model. Efficiency results may vary in other settings (e.g. other hardware, image size, batch size, ...). We recommend to directly run them in the use-case conditions to know if the smashed model can benefit you.
- ***What is the model format?*** We use safetensors.
- ***What calibration data has been used?*** If needed by the compression method, we used WikiText as the calibration data.
- ***What is the naming convention for Pruna Huggingface models?*** We take the original model name and append "turbo", "tiny", or "green" if the smashed model has a measured inference speed, inference memory, or inference energy consumption which is less than 90% of the original base model.
- ***How to compress my own models?*** You can request premium access to more compression methods and tech support for your specific use-cases [here](https://z0halsaff74.typeform.com/pruna-access?typeform-source=www.pruna.ai).
- ***What are "first" metrics?*** Results mentioning "first" are obtained after the first run of the model. The first run might take more memory or be slower than the subsequent runs due cuda overheads.
- ***What are "Sync" and "Async" metrics?*** "Sync" metrics are obtained by syncing all GPU processes and stop measurement when all of them are executed. "Async" metrics are obtained without syncing all GPU processes and stop when the model output can be used by the CPU. We provide both metrics since both could be relevant depending on the use-case. We recommend to test the efficiency gains directly in your use-cases.
## Setup
You can run the smashed model with these steps:
0. Check requirements from the original repo Desm0nt/Phi-3-HornyVision-128k-instruct installed. In particular, check python, cuda, and transformers versions.
1. Make sure that you have installed quantization related packages.
```bash
pip install hqq
```
2. Load & run the model.
```python
from transformers import AutoModelForCausalLM, AutoTokenizer
from hqq.engine.hf import HQQModelForCausalLM
from hqq.models.hf.base import AutoHQQHFModel
try:
model = HQQModelForCausalLM.from_quantized("PrunaAI/Desm0nt-Phi-3-HornyVision-128k-instruct-HQQ-2bit-smashed", device_map='auto')
except:
model = AutoHQQHFModel.from_quantized("PrunaAI/Desm0nt-Phi-3-HornyVision-128k-instruct-HQQ-2bit-smashed")
tokenizer = AutoTokenizer.from_pretrained("Desm0nt/Phi-3-HornyVision-128k-instruct")
input_ids = tokenizer("What is the color of prunes?,", return_tensors='pt').to(model.device)["input_ids"]
outputs = model.generate(input_ids, max_new_tokens=216)
tokenizer.decode(outputs[0])
```
## Configurations
The configuration info are in `smash_config.json`.
## Credits & License
The license of the smashed model follows the license of the original model. Please check the license of the original model Desm0nt/Phi-3-HornyVision-128k-instruct before using this model which provided the base model. The license of the `pruna-engine` is [here](https://pypi.org/project/pruna-engine/) on Pypi.
## Want to compress other models?
- Contact us and tell us which model to compress next [here](https://www.pruna.ai/contact).
- Request access to easily compress your own AI models [here](https://z0halsaff74.typeform.com/pruna-access?typeform-source=www.pruna.ai). |
tchen175/Mistral-7B-dolly-dataset-0001 | tchen175 | "2024-06-24T10:11:26Z" | 0 | 0 | null | [
"region:us"
] | null | "2024-06-24T10:11:26Z" | Entry not found |
FancyCat260/Minecraft | FancyCat260 | "2024-06-24T10:13:15Z" | 0 | 0 | null | [
"region:us"
] | null | "2024-06-24T10:11:48Z" | Entry not found |
limaatulya/my_awesome_billsum_model_90 | limaatulya | "2024-06-24T10:13:24Z" | 0 | 0 | transformers | [
"transformers",
"tensorboard",
"safetensors",
"t5",
"text2text-generation",
"generated_from_trainer",
"base_model:google-t5/t5-small",
"license:apache-2.0",
"autotrain_compatible",
"endpoints_compatible",
"text-generation-inference",
"region:us"
] | text2text-generation | "2024-06-24T10:13:14Z" | ---
license: apache-2.0
base_model: google-t5/t5-small
tags:
- generated_from_trainer
model-index:
- name: my_awesome_billsum_model_90
results: []
---
<!-- This model card has been generated automatically according to the information the Trainer had access to. You
should probably proofread and complete it, then remove this comment. -->
# my_awesome_billsum_model_90
This model is a fine-tuned version of [google-t5/t5-small](https://huggingface.co/google-t5/t5-small) on the None dataset.
## Model description
More information needed
## Intended uses & limitations
More information needed
## Training and evaluation data
More information needed
## Training procedure
### Training hyperparameters
The following hyperparameters were used during training:
- learning_rate: 2e-05
- train_batch_size: 8
- eval_batch_size: 8
- seed: 42
- optimizer: Adam with betas=(0.9,0.999) and epsilon=1e-08
- lr_scheduler_type: linear
- num_epochs: 3.0
### Training results
### Framework versions
- Transformers 4.41.2
- Pytorch 2.3.0+cu121
- Datasets 2.20.0
- Tokenizers 0.19.1
|
cahya/TestLM | cahya | "2024-06-24T10:17:20Z" | 0 | 0 | transformers | [
"transformers",
"safetensors",
"rwkv5",
"text-generation",
"custom_code",
"en",
"dataset:teknium/OpenHermes-2.5",
"license:apache-2.0",
"autotrain_compatible",
"region:us"
] | text-generation | "2024-06-24T10:13:51Z" | ---
license: apache-2.0
datasets:
- teknium/OpenHermes-2.5
language:
- en
---
This is just a place holder to test the tokenizer.
The chat format is identical to the default HuggingFace format, **EXCEPT** that we replace the `<|im_start|>` and `<|im_end|>` special tokens with the unused ASCII codes `"\x16"` and `"\x17"` respectively. This was done to ensure complete compatibility withe standard RWKV World tokenizer.
An example interaction:
```
\x16user
Write a poem about the beauty of mathematics in the style of Emily Dickinson.\x17
\x16assistant
In the heart of Numbers, so precise,
A Beauty dwells - both cool and nice.
An elegance that sings in sums,
And dances to algebraic drums.
Addition's grace, subtraction's might,
Multiplication's sheer delight,
Division's firm yet gentle hand,
All part of this enchanted land.
Infinity, a lover bold,
To whom the stars their secrets told,
While Zero, cloaked in mystery,
Unlocks each mathematical decree.
Fractals bloom like flowers wild,
Geometry, Nature's favored child,
Calculus, with steady gaze,
Maps out life's intricate maze.
Pi, an endless serenade,
In circles' arms forever laid,
And prime numbers,
standing lone,
Make the universe their own.
Each equation, theorem scribed,
In
parchment old or computer imbibed,
Is but a whisper from the skies,
A cosmic truth in disguise.\x17
```
In the above, newlines are printed without escaping, while ASCII codes are escaped for readability.
|
SDFT1122/trained-sd3-lora | SDFT1122 | "2024-06-24T10:13:53Z" | 0 | 0 | null | [
"region:us"
] | null | "2024-06-24T10:13:53Z" | Entry not found |
PrunaAI/simecek-cswikimistral_0.1-HQQ-1bit-smashed | PrunaAI | "2024-06-24T10:14:46Z" | 0 | 0 | transformers | [
"transformers",
"mistral",
"text-generation",
"pruna-ai",
"base_model:simecek/cswikimistral_0.1",
"autotrain_compatible",
"endpoints_compatible",
"text-generation-inference",
"region:us"
] | text-generation | "2024-06-24T10:13:55Z" | ---
thumbnail: "https://assets-global.website-files.com/646b351987a8d8ce158d1940/64ec9e96b4334c0e1ac41504_Logo%20with%20white%20text.svg"
base_model: simecek/cswikimistral_0.1
metrics:
- memory_disk
- memory_inference
- inference_latency
- inference_throughput
- inference_CO2_emissions
- inference_energy_consumption
tags:
- pruna-ai
---
<!-- header start -->
<!-- 200823 -->
<div style="width: auto; margin-left: auto; margin-right: auto">
<a href="https://www.pruna.ai/" target="_blank" rel="noopener noreferrer">
<img src="https://i.imgur.com/eDAlcgk.png" alt="PrunaAI" style="width: 100%; min-width: 400px; display: block; margin: auto;">
</a>
</div>
<!-- header end -->
[](https://twitter.com/PrunaAI)
[](https://github.com/PrunaAI)
[](https://www.linkedin.com/company/93832878/admin/feed/posts/?feedType=following)
[](https://discord.gg/CP4VSgck)
# Simply make AI models cheaper, smaller, faster, and greener!
- Give a thumbs up if you like this model!
- Contact us and tell us which model to compress next [here](https://www.pruna.ai/contact).
- Request access to easily compress your *own* AI models [here](https://z0halsaff74.typeform.com/pruna-access?typeform-source=www.pruna.ai).
- Read the documentations to know more [here](https://pruna-ai-pruna.readthedocs-hosted.com/en/latest/)
- Join Pruna AI community on Discord [here](https://discord.gg/CP4VSgck) to share feedback/suggestions or get help.
## Results

**Frequently Asked Questions**
- ***How does the compression work?*** The model is compressed with hqq.
- ***How does the model quality change?*** The quality of the model output might vary compared to the base model.
- ***How is the model efficiency evaluated?*** These results were obtained on HARDWARE_NAME with configuration described in `model/smash_config.json` and are obtained after a hardware warmup. The smashed model is directly compared to the original base model. Efficiency results may vary in other settings (e.g. other hardware, image size, batch size, ...). We recommend to directly run them in the use-case conditions to know if the smashed model can benefit you.
- ***What is the model format?*** We use safetensors.
- ***What calibration data has been used?*** If needed by the compression method, we used WikiText as the calibration data.
- ***What is the naming convention for Pruna Huggingface models?*** We take the original model name and append "turbo", "tiny", or "green" if the smashed model has a measured inference speed, inference memory, or inference energy consumption which is less than 90% of the original base model.
- ***How to compress my own models?*** You can request premium access to more compression methods and tech support for your specific use-cases [here](https://z0halsaff74.typeform.com/pruna-access?typeform-source=www.pruna.ai).
- ***What are "first" metrics?*** Results mentioning "first" are obtained after the first run of the model. The first run might take more memory or be slower than the subsequent runs due cuda overheads.
- ***What are "Sync" and "Async" metrics?*** "Sync" metrics are obtained by syncing all GPU processes and stop measurement when all of them are executed. "Async" metrics are obtained without syncing all GPU processes and stop when the model output can be used by the CPU. We provide both metrics since both could be relevant depending on the use-case. We recommend to test the efficiency gains directly in your use-cases.
## Setup
You can run the smashed model with these steps:
0. Check requirements from the original repo simecek/cswikimistral_0.1 installed. In particular, check python, cuda, and transformers versions.
1. Make sure that you have installed quantization related packages.
```bash
pip install hqq
```
2. Load & run the model.
```python
from transformers import AutoModelForCausalLM, AutoTokenizer
from hqq.engine.hf import HQQModelForCausalLM
from hqq.models.hf.base import AutoHQQHFModel
try:
model = HQQModelForCausalLM.from_quantized("PrunaAI/simecek-cswikimistral_0.1-HQQ-1bit-smashed", device_map='auto')
except:
model = AutoHQQHFModel.from_quantized("PrunaAI/simecek-cswikimistral_0.1-HQQ-1bit-smashed")
tokenizer = AutoTokenizer.from_pretrained("simecek/cswikimistral_0.1")
input_ids = tokenizer("What is the color of prunes?,", return_tensors='pt').to(model.device)["input_ids"]
outputs = model.generate(input_ids, max_new_tokens=216)
tokenizer.decode(outputs[0])
```
## Configurations
The configuration info are in `smash_config.json`.
## Credits & License
The license of the smashed model follows the license of the original model. Please check the license of the original model simecek/cswikimistral_0.1 before using this model which provided the base model. The license of the `pruna-engine` is [here](https://pypi.org/project/pruna-engine/) on Pypi.
## Want to compress other models?
- Contact us and tell us which model to compress next [here](https://www.pruna.ai/contact).
- Request access to easily compress your own AI models [here](https://z0halsaff74.typeform.com/pruna-access?typeform-source=www.pruna.ai). |
xxlrd/iu | xxlrd | "2024-06-25T03:06:26Z" | 0 | 0 | null | [
"region:us"
] | null | "2024-06-24T10:14:15Z" | https://civitai.com/models/11722/iu |
PrunaAI/simecek-cswikimistral_0.1-HQQ-2bit-smashed | PrunaAI | "2024-06-24T10:15:46Z" | 0 | 0 | transformers | [
"transformers",
"mistral",
"text-generation",
"pruna-ai",
"base_model:simecek/cswikimistral_0.1",
"autotrain_compatible",
"endpoints_compatible",
"text-generation-inference",
"region:us"
] | text-generation | "2024-06-24T10:14:36Z" | ---
thumbnail: "https://assets-global.website-files.com/646b351987a8d8ce158d1940/64ec9e96b4334c0e1ac41504_Logo%20with%20white%20text.svg"
base_model: simecek/cswikimistral_0.1
metrics:
- memory_disk
- memory_inference
- inference_latency
- inference_throughput
- inference_CO2_emissions
- inference_energy_consumption
tags:
- pruna-ai
---
<!-- header start -->
<!-- 200823 -->
<div style="width: auto; margin-left: auto; margin-right: auto">
<a href="https://www.pruna.ai/" target="_blank" rel="noopener noreferrer">
<img src="https://i.imgur.com/eDAlcgk.png" alt="PrunaAI" style="width: 100%; min-width: 400px; display: block; margin: auto;">
</a>
</div>
<!-- header end -->
[](https://twitter.com/PrunaAI)
[](https://github.com/PrunaAI)
[](https://www.linkedin.com/company/93832878/admin/feed/posts/?feedType=following)
[](https://discord.gg/CP4VSgck)
# Simply make AI models cheaper, smaller, faster, and greener!
- Give a thumbs up if you like this model!
- Contact us and tell us which model to compress next [here](https://www.pruna.ai/contact).
- Request access to easily compress your *own* AI models [here](https://z0halsaff74.typeform.com/pruna-access?typeform-source=www.pruna.ai).
- Read the documentations to know more [here](https://pruna-ai-pruna.readthedocs-hosted.com/en/latest/)
- Join Pruna AI community on Discord [here](https://discord.gg/CP4VSgck) to share feedback/suggestions or get help.
## Results

**Frequently Asked Questions**
- ***How does the compression work?*** The model is compressed with hqq.
- ***How does the model quality change?*** The quality of the model output might vary compared to the base model.
- ***How is the model efficiency evaluated?*** These results were obtained on HARDWARE_NAME with configuration described in `model/smash_config.json` and are obtained after a hardware warmup. The smashed model is directly compared to the original base model. Efficiency results may vary in other settings (e.g. other hardware, image size, batch size, ...). We recommend to directly run them in the use-case conditions to know if the smashed model can benefit you.
- ***What is the model format?*** We use safetensors.
- ***What calibration data has been used?*** If needed by the compression method, we used WikiText as the calibration data.
- ***What is the naming convention for Pruna Huggingface models?*** We take the original model name and append "turbo", "tiny", or "green" if the smashed model has a measured inference speed, inference memory, or inference energy consumption which is less than 90% of the original base model.
- ***How to compress my own models?*** You can request premium access to more compression methods and tech support for your specific use-cases [here](https://z0halsaff74.typeform.com/pruna-access?typeform-source=www.pruna.ai).
- ***What are "first" metrics?*** Results mentioning "first" are obtained after the first run of the model. The first run might take more memory or be slower than the subsequent runs due cuda overheads.
- ***What are "Sync" and "Async" metrics?*** "Sync" metrics are obtained by syncing all GPU processes and stop measurement when all of them are executed. "Async" metrics are obtained without syncing all GPU processes and stop when the model output can be used by the CPU. We provide both metrics since both could be relevant depending on the use-case. We recommend to test the efficiency gains directly in your use-cases.
## Setup
You can run the smashed model with these steps:
0. Check requirements from the original repo simecek/cswikimistral_0.1 installed. In particular, check python, cuda, and transformers versions.
1. Make sure that you have installed quantization related packages.
```bash
pip install hqq
```
2. Load & run the model.
```python
from transformers import AutoModelForCausalLM, AutoTokenizer
from hqq.engine.hf import HQQModelForCausalLM
from hqq.models.hf.base import AutoHQQHFModel
try:
model = HQQModelForCausalLM.from_quantized("PrunaAI/simecek-cswikimistral_0.1-HQQ-2bit-smashed", device_map='auto')
except:
model = AutoHQQHFModel.from_quantized("PrunaAI/simecek-cswikimistral_0.1-HQQ-2bit-smashed")
tokenizer = AutoTokenizer.from_pretrained("simecek/cswikimistral_0.1")
input_ids = tokenizer("What is the color of prunes?,", return_tensors='pt').to(model.device)["input_ids"]
outputs = model.generate(input_ids, max_new_tokens=216)
tokenizer.decode(outputs[0])
```
## Configurations
The configuration info are in `smash_config.json`.
## Credits & License
The license of the smashed model follows the license of the original model. Please check the license of the original model simecek/cswikimistral_0.1 before using this model which provided the base model. The license of the `pruna-engine` is [here](https://pypi.org/project/pruna-engine/) on Pypi.
## Want to compress other models?
- Contact us and tell us which model to compress next [here](https://www.pruna.ai/contact).
- Request access to easily compress your own AI models [here](https://z0halsaff74.typeform.com/pruna-access?typeform-source=www.pruna.ai). |
PrunaAI/THU-KEG-ADELIE-SFT-HQQ-2bit-smashed | PrunaAI | "2024-06-24T10:16:57Z" | 0 | 0 | transformers | [
"transformers",
"llama",
"text-generation",
"pruna-ai",
"base_model:THU-KEG/ADELIE-SFT",
"autotrain_compatible",
"endpoints_compatible",
"text-generation-inference",
"region:us"
] | text-generation | "2024-06-24T10:15:49Z" | ---
thumbnail: "https://assets-global.website-files.com/646b351987a8d8ce158d1940/64ec9e96b4334c0e1ac41504_Logo%20with%20white%20text.svg"
base_model: THU-KEG/ADELIE-SFT
metrics:
- memory_disk
- memory_inference
- inference_latency
- inference_throughput
- inference_CO2_emissions
- inference_energy_consumption
tags:
- pruna-ai
---
<!-- header start -->
<!-- 200823 -->
<div style="width: auto; margin-left: auto; margin-right: auto">
<a href="https://www.pruna.ai/" target="_blank" rel="noopener noreferrer">
<img src="https://i.imgur.com/eDAlcgk.png" alt="PrunaAI" style="width: 100%; min-width: 400px; display: block; margin: auto;">
</a>
</div>
<!-- header end -->
[](https://twitter.com/PrunaAI)
[](https://github.com/PrunaAI)
[](https://www.linkedin.com/company/93832878/admin/feed/posts/?feedType=following)
[](https://discord.gg/CP4VSgck)
# Simply make AI models cheaper, smaller, faster, and greener!
- Give a thumbs up if you like this model!
- Contact us and tell us which model to compress next [here](https://www.pruna.ai/contact).
- Request access to easily compress your *own* AI models [here](https://z0halsaff74.typeform.com/pruna-access?typeform-source=www.pruna.ai).
- Read the documentations to know more [here](https://pruna-ai-pruna.readthedocs-hosted.com/en/latest/)
- Join Pruna AI community on Discord [here](https://discord.gg/CP4VSgck) to share feedback/suggestions or get help.
## Results

**Frequently Asked Questions**
- ***How does the compression work?*** The model is compressed with hqq.
- ***How does the model quality change?*** The quality of the model output might vary compared to the base model.
- ***How is the model efficiency evaluated?*** These results were obtained on HARDWARE_NAME with configuration described in `model/smash_config.json` and are obtained after a hardware warmup. The smashed model is directly compared to the original base model. Efficiency results may vary in other settings (e.g. other hardware, image size, batch size, ...). We recommend to directly run them in the use-case conditions to know if the smashed model can benefit you.
- ***What is the model format?*** We use safetensors.
- ***What calibration data has been used?*** If needed by the compression method, we used WikiText as the calibration data.
- ***What is the naming convention for Pruna Huggingface models?*** We take the original model name and append "turbo", "tiny", or "green" if the smashed model has a measured inference speed, inference memory, or inference energy consumption which is less than 90% of the original base model.
- ***How to compress my own models?*** You can request premium access to more compression methods and tech support for your specific use-cases [here](https://z0halsaff74.typeform.com/pruna-access?typeform-source=www.pruna.ai).
- ***What are "first" metrics?*** Results mentioning "first" are obtained after the first run of the model. The first run might take more memory or be slower than the subsequent runs due cuda overheads.
- ***What are "Sync" and "Async" metrics?*** "Sync" metrics are obtained by syncing all GPU processes and stop measurement when all of them are executed. "Async" metrics are obtained without syncing all GPU processes and stop when the model output can be used by the CPU. We provide both metrics since both could be relevant depending on the use-case. We recommend to test the efficiency gains directly in your use-cases.
## Setup
You can run the smashed model with these steps:
0. Check requirements from the original repo THU-KEG/ADELIE-SFT installed. In particular, check python, cuda, and transformers versions.
1. Make sure that you have installed quantization related packages.
```bash
pip install hqq
```
2. Load & run the model.
```python
from transformers import AutoModelForCausalLM, AutoTokenizer
from hqq.engine.hf import HQQModelForCausalLM
from hqq.models.hf.base import AutoHQQHFModel
try:
model = HQQModelForCausalLM.from_quantized("PrunaAI/THU-KEG-ADELIE-SFT-HQQ-2bit-smashed", device_map='auto')
except:
model = AutoHQQHFModel.from_quantized("PrunaAI/THU-KEG-ADELIE-SFT-HQQ-2bit-smashed")
tokenizer = AutoTokenizer.from_pretrained("THU-KEG/ADELIE-SFT")
input_ids = tokenizer("What is the color of prunes?,", return_tensors='pt').to(model.device)["input_ids"]
outputs = model.generate(input_ids, max_new_tokens=216)
tokenizer.decode(outputs[0])
```
## Configurations
The configuration info are in `smash_config.json`.
## Credits & License
The license of the smashed model follows the license of the original model. Please check the license of the original model THU-KEG/ADELIE-SFT before using this model which provided the base model. The license of the `pruna-engine` is [here](https://pypi.org/project/pruna-engine/) on Pypi.
## Want to compress other models?
- Contact us and tell us which model to compress next [here](https://www.pruna.ai/contact).
- Request access to easily compress your own AI models [here](https://z0halsaff74.typeform.com/pruna-access?typeform-source=www.pruna.ai). |
PrunaAI/THU-KEG-ADELIE-SFT-HQQ-1bit-smashed | PrunaAI | "2024-06-24T10:16:58Z" | 0 | 0 | transformers | [
"transformers",
"llama",
"text-generation",
"pruna-ai",
"base_model:THU-KEG/ADELIE-SFT",
"autotrain_compatible",
"endpoints_compatible",
"text-generation-inference",
"region:us"
] | text-generation | "2024-06-24T10:16:14Z" | ---
thumbnail: "https://assets-global.website-files.com/646b351987a8d8ce158d1940/64ec9e96b4334c0e1ac41504_Logo%20with%20white%20text.svg"
base_model: THU-KEG/ADELIE-SFT
metrics:
- memory_disk
- memory_inference
- inference_latency
- inference_throughput
- inference_CO2_emissions
- inference_energy_consumption
tags:
- pruna-ai
---
<!-- header start -->
<!-- 200823 -->
<div style="width: auto; margin-left: auto; margin-right: auto">
<a href="https://www.pruna.ai/" target="_blank" rel="noopener noreferrer">
<img src="https://i.imgur.com/eDAlcgk.png" alt="PrunaAI" style="width: 100%; min-width: 400px; display: block; margin: auto;">
</a>
</div>
<!-- header end -->
[](https://twitter.com/PrunaAI)
[](https://github.com/PrunaAI)
[](https://www.linkedin.com/company/93832878/admin/feed/posts/?feedType=following)
[](https://discord.gg/CP4VSgck)
# Simply make AI models cheaper, smaller, faster, and greener!
- Give a thumbs up if you like this model!
- Contact us and tell us which model to compress next [here](https://www.pruna.ai/contact).
- Request access to easily compress your *own* AI models [here](https://z0halsaff74.typeform.com/pruna-access?typeform-source=www.pruna.ai).
- Read the documentations to know more [here](https://pruna-ai-pruna.readthedocs-hosted.com/en/latest/)
- Join Pruna AI community on Discord [here](https://discord.gg/CP4VSgck) to share feedback/suggestions or get help.
## Results

**Frequently Asked Questions**
- ***How does the compression work?*** The model is compressed with hqq.
- ***How does the model quality change?*** The quality of the model output might vary compared to the base model.
- ***How is the model efficiency evaluated?*** These results were obtained on HARDWARE_NAME with configuration described in `model/smash_config.json` and are obtained after a hardware warmup. The smashed model is directly compared to the original base model. Efficiency results may vary in other settings (e.g. other hardware, image size, batch size, ...). We recommend to directly run them in the use-case conditions to know if the smashed model can benefit you.
- ***What is the model format?*** We use safetensors.
- ***What calibration data has been used?*** If needed by the compression method, we used WikiText as the calibration data.
- ***What is the naming convention for Pruna Huggingface models?*** We take the original model name and append "turbo", "tiny", or "green" if the smashed model has a measured inference speed, inference memory, or inference energy consumption which is less than 90% of the original base model.
- ***How to compress my own models?*** You can request premium access to more compression methods and tech support for your specific use-cases [here](https://z0halsaff74.typeform.com/pruna-access?typeform-source=www.pruna.ai).
- ***What are "first" metrics?*** Results mentioning "first" are obtained after the first run of the model. The first run might take more memory or be slower than the subsequent runs due cuda overheads.
- ***What are "Sync" and "Async" metrics?*** "Sync" metrics are obtained by syncing all GPU processes and stop measurement when all of them are executed. "Async" metrics are obtained without syncing all GPU processes and stop when the model output can be used by the CPU. We provide both metrics since both could be relevant depending on the use-case. We recommend to test the efficiency gains directly in your use-cases.
## Setup
You can run the smashed model with these steps:
0. Check requirements from the original repo THU-KEG/ADELIE-SFT installed. In particular, check python, cuda, and transformers versions.
1. Make sure that you have installed quantization related packages.
```bash
pip install hqq
```
2. Load & run the model.
```python
from transformers import AutoModelForCausalLM, AutoTokenizer
from hqq.engine.hf import HQQModelForCausalLM
from hqq.models.hf.base import AutoHQQHFModel
try:
model = HQQModelForCausalLM.from_quantized("PrunaAI/THU-KEG-ADELIE-SFT-HQQ-1bit-smashed", device_map='auto')
except:
model = AutoHQQHFModel.from_quantized("PrunaAI/THU-KEG-ADELIE-SFT-HQQ-1bit-smashed")
tokenizer = AutoTokenizer.from_pretrained("THU-KEG/ADELIE-SFT")
input_ids = tokenizer("What is the color of prunes?,", return_tensors='pt').to(model.device)["input_ids"]
outputs = model.generate(input_ids, max_new_tokens=216)
tokenizer.decode(outputs[0])
```
## Configurations
The configuration info are in `smash_config.json`.
## Credits & License
The license of the smashed model follows the license of the original model. Please check the license of the original model THU-KEG/ADELIE-SFT before using this model which provided the base model. The license of the `pruna-engine` is [here](https://pypi.org/project/pruna-engine/) on Pypi.
## Want to compress other models?
- Contact us and tell us which model to compress next [here](https://www.pruna.ai/contact).
- Request access to easily compress your own AI models [here](https://z0halsaff74.typeform.com/pruna-access?typeform-source=www.pruna.ai). |
PrunaAI/THU-KEG-ADELIE-SFT-HQQ-4bit-smashed | PrunaAI | "2024-06-24T10:18:18Z" | 0 | 0 | transformers | [
"transformers",
"llama",
"text-generation",
"pruna-ai",
"base_model:THU-KEG/ADELIE-SFT",
"autotrain_compatible",
"endpoints_compatible",
"text-generation-inference",
"region:us"
] | text-generation | "2024-06-24T10:16:28Z" | ---
thumbnail: "https://assets-global.website-files.com/646b351987a8d8ce158d1940/64ec9e96b4334c0e1ac41504_Logo%20with%20white%20text.svg"
base_model: THU-KEG/ADELIE-SFT
metrics:
- memory_disk
- memory_inference
- inference_latency
- inference_throughput
- inference_CO2_emissions
- inference_energy_consumption
tags:
- pruna-ai
---
<!-- header start -->
<!-- 200823 -->
<div style="width: auto; margin-left: auto; margin-right: auto">
<a href="https://www.pruna.ai/" target="_blank" rel="noopener noreferrer">
<img src="https://i.imgur.com/eDAlcgk.png" alt="PrunaAI" style="width: 100%; min-width: 400px; display: block; margin: auto;">
</a>
</div>
<!-- header end -->
[](https://twitter.com/PrunaAI)
[](https://github.com/PrunaAI)
[](https://www.linkedin.com/company/93832878/admin/feed/posts/?feedType=following)
[](https://discord.gg/CP4VSgck)
# Simply make AI models cheaper, smaller, faster, and greener!
- Give a thumbs up if you like this model!
- Contact us and tell us which model to compress next [here](https://www.pruna.ai/contact).
- Request access to easily compress your *own* AI models [here](https://z0halsaff74.typeform.com/pruna-access?typeform-source=www.pruna.ai).
- Read the documentations to know more [here](https://pruna-ai-pruna.readthedocs-hosted.com/en/latest/)
- Join Pruna AI community on Discord [here](https://discord.gg/CP4VSgck) to share feedback/suggestions or get help.
## Results

**Frequently Asked Questions**
- ***How does the compression work?*** The model is compressed with hqq.
- ***How does the model quality change?*** The quality of the model output might vary compared to the base model.
- ***How is the model efficiency evaluated?*** These results were obtained on HARDWARE_NAME with configuration described in `model/smash_config.json` and are obtained after a hardware warmup. The smashed model is directly compared to the original base model. Efficiency results may vary in other settings (e.g. other hardware, image size, batch size, ...). We recommend to directly run them in the use-case conditions to know if the smashed model can benefit you.
- ***What is the model format?*** We use safetensors.
- ***What calibration data has been used?*** If needed by the compression method, we used WikiText as the calibration data.
- ***What is the naming convention for Pruna Huggingface models?*** We take the original model name and append "turbo", "tiny", or "green" if the smashed model has a measured inference speed, inference memory, or inference energy consumption which is less than 90% of the original base model.
- ***How to compress my own models?*** You can request premium access to more compression methods and tech support for your specific use-cases [here](https://z0halsaff74.typeform.com/pruna-access?typeform-source=www.pruna.ai).
- ***What are "first" metrics?*** Results mentioning "first" are obtained after the first run of the model. The first run might take more memory or be slower than the subsequent runs due cuda overheads.
- ***What are "Sync" and "Async" metrics?*** "Sync" metrics are obtained by syncing all GPU processes and stop measurement when all of them are executed. "Async" metrics are obtained without syncing all GPU processes and stop when the model output can be used by the CPU. We provide both metrics since both could be relevant depending on the use-case. We recommend to test the efficiency gains directly in your use-cases.
## Setup
You can run the smashed model with these steps:
0. Check requirements from the original repo THU-KEG/ADELIE-SFT installed. In particular, check python, cuda, and transformers versions.
1. Make sure that you have installed quantization related packages.
```bash
pip install hqq
```
2. Load & run the model.
```python
from transformers import AutoModelForCausalLM, AutoTokenizer
from hqq.engine.hf import HQQModelForCausalLM
from hqq.models.hf.base import AutoHQQHFModel
try:
model = HQQModelForCausalLM.from_quantized("PrunaAI/THU-KEG-ADELIE-SFT-HQQ-4bit-smashed", device_map='auto')
except:
model = AutoHQQHFModel.from_quantized("PrunaAI/THU-KEG-ADELIE-SFT-HQQ-4bit-smashed")
tokenizer = AutoTokenizer.from_pretrained("THU-KEG/ADELIE-SFT")
input_ids = tokenizer("What is the color of prunes?,", return_tensors='pt').to(model.device)["input_ids"]
outputs = model.generate(input_ids, max_new_tokens=216)
tokenizer.decode(outputs[0])
```
## Configurations
The configuration info are in `smash_config.json`.
## Credits & License
The license of the smashed model follows the license of the original model. Please check the license of the original model THU-KEG/ADELIE-SFT before using this model which provided the base model. The license of the `pruna-engine` is [here](https://pypi.org/project/pruna-engine/) on Pypi.
## Want to compress other models?
- Contact us and tell us which model to compress next [here](https://www.pruna.ai/contact).
- Request access to easily compress your own AI models [here](https://z0halsaff74.typeform.com/pruna-access?typeform-source=www.pruna.ai). |
akemimedrano100/asriel | akemimedrano100 | "2024-06-24T10:17:44Z" | 0 | 0 | null | [
"region:us"
] | null | "2024-06-24T10:16:39Z" | Entry not found |
PrunaAI/RESMPDEV-Gemma-Wukong-2b-HQQ-1bit-smashed | PrunaAI | "2024-06-24T10:18:37Z" | 0 | 0 | transformers | [
"transformers",
"gemma",
"text-generation",
"pruna-ai",
"conversational",
"base_model:RESMPDEV/Gemma-Wukong-2b",
"autotrain_compatible",
"endpoints_compatible",
"text-generation-inference",
"region:us"
] | text-generation | "2024-06-24T10:17:53Z" | ---
thumbnail: "https://assets-global.website-files.com/646b351987a8d8ce158d1940/64ec9e96b4334c0e1ac41504_Logo%20with%20white%20text.svg"
base_model: RESMPDEV/Gemma-Wukong-2b
metrics:
- memory_disk
- memory_inference
- inference_latency
- inference_throughput
- inference_CO2_emissions
- inference_energy_consumption
tags:
- pruna-ai
---
<!-- header start -->
<!-- 200823 -->
<div style="width: auto; margin-left: auto; margin-right: auto">
<a href="https://www.pruna.ai/" target="_blank" rel="noopener noreferrer">
<img src="https://i.imgur.com/eDAlcgk.png" alt="PrunaAI" style="width: 100%; min-width: 400px; display: block; margin: auto;">
</a>
</div>
<!-- header end -->
[](https://twitter.com/PrunaAI)
[](https://github.com/PrunaAI)
[](https://www.linkedin.com/company/93832878/admin/feed/posts/?feedType=following)
[](https://discord.gg/CP4VSgck)
# Simply make AI models cheaper, smaller, faster, and greener!
- Give a thumbs up if you like this model!
- Contact us and tell us which model to compress next [here](https://www.pruna.ai/contact).
- Request access to easily compress your *own* AI models [here](https://z0halsaff74.typeform.com/pruna-access?typeform-source=www.pruna.ai).
- Read the documentations to know more [here](https://pruna-ai-pruna.readthedocs-hosted.com/en/latest/)
- Join Pruna AI community on Discord [here](https://discord.gg/CP4VSgck) to share feedback/suggestions or get help.
## Results

**Frequently Asked Questions**
- ***How does the compression work?*** The model is compressed with hqq.
- ***How does the model quality change?*** The quality of the model output might vary compared to the base model.
- ***How is the model efficiency evaluated?*** These results were obtained on HARDWARE_NAME with configuration described in `model/smash_config.json` and are obtained after a hardware warmup. The smashed model is directly compared to the original base model. Efficiency results may vary in other settings (e.g. other hardware, image size, batch size, ...). We recommend to directly run them in the use-case conditions to know if the smashed model can benefit you.
- ***What is the model format?*** We use safetensors.
- ***What calibration data has been used?*** If needed by the compression method, we used WikiText as the calibration data.
- ***What is the naming convention for Pruna Huggingface models?*** We take the original model name and append "turbo", "tiny", or "green" if the smashed model has a measured inference speed, inference memory, or inference energy consumption which is less than 90% of the original base model.
- ***How to compress my own models?*** You can request premium access to more compression methods and tech support for your specific use-cases [here](https://z0halsaff74.typeform.com/pruna-access?typeform-source=www.pruna.ai).
- ***What are "first" metrics?*** Results mentioning "first" are obtained after the first run of the model. The first run might take more memory or be slower than the subsequent runs due cuda overheads.
- ***What are "Sync" and "Async" metrics?*** "Sync" metrics are obtained by syncing all GPU processes and stop measurement when all of them are executed. "Async" metrics are obtained without syncing all GPU processes and stop when the model output can be used by the CPU. We provide both metrics since both could be relevant depending on the use-case. We recommend to test the efficiency gains directly in your use-cases.
## Setup
You can run the smashed model with these steps:
0. Check requirements from the original repo RESMPDEV/Gemma-Wukong-2b installed. In particular, check python, cuda, and transformers versions.
1. Make sure that you have installed quantization related packages.
```bash
pip install hqq
```
2. Load & run the model.
```python
from transformers import AutoModelForCausalLM, AutoTokenizer
from hqq.engine.hf import HQQModelForCausalLM
from hqq.models.hf.base import AutoHQQHFModel
try:
model = HQQModelForCausalLM.from_quantized("PrunaAI/RESMPDEV-Gemma-Wukong-2b-HQQ-1bit-smashed", device_map='auto')
except:
model = AutoHQQHFModel.from_quantized("PrunaAI/RESMPDEV-Gemma-Wukong-2b-HQQ-1bit-smashed")
tokenizer = AutoTokenizer.from_pretrained("RESMPDEV/Gemma-Wukong-2b")
input_ids = tokenizer("What is the color of prunes?,", return_tensors='pt').to(model.device)["input_ids"]
outputs = model.generate(input_ids, max_new_tokens=216)
tokenizer.decode(outputs[0])
```
## Configurations
The configuration info are in `smash_config.json`.
## Credits & License
The license of the smashed model follows the license of the original model. Please check the license of the original model RESMPDEV/Gemma-Wukong-2b before using this model which provided the base model. The license of the `pruna-engine` is [here](https://pypi.org/project/pruna-engine/) on Pypi.
## Want to compress other models?
- Contact us and tell us which model to compress next [here](https://www.pruna.ai/contact).
- Request access to easily compress your own AI models [here](https://z0halsaff74.typeform.com/pruna-access?typeform-source=www.pruna.ai). |
PrunaAI/RESMPDEV-Wukong-0.1-Mistral-7B-v0.2-HQQ-1bit-smashed | PrunaAI | "2024-06-24T10:18:46Z" | 0 | 0 | transformers | [
"transformers",
"mistral",
"text-generation",
"pruna-ai",
"base_model:RESMPDEV/Wukong-0.1-Mistral-7B-v0.2",
"autotrain_compatible",
"endpoints_compatible",
"text-generation-inference",
"region:us"
] | text-generation | "2024-06-24T10:17:56Z" | ---
thumbnail: "https://assets-global.website-files.com/646b351987a8d8ce158d1940/64ec9e96b4334c0e1ac41504_Logo%20with%20white%20text.svg"
base_model: RESMPDEV/Wukong-0.1-Mistral-7B-v0.2
metrics:
- memory_disk
- memory_inference
- inference_latency
- inference_throughput
- inference_CO2_emissions
- inference_energy_consumption
tags:
- pruna-ai
---
<!-- header start -->
<!-- 200823 -->
<div style="width: auto; margin-left: auto; margin-right: auto">
<a href="https://www.pruna.ai/" target="_blank" rel="noopener noreferrer">
<img src="https://i.imgur.com/eDAlcgk.png" alt="PrunaAI" style="width: 100%; min-width: 400px; display: block; margin: auto;">
</a>
</div>
<!-- header end -->
[](https://twitter.com/PrunaAI)
[](https://github.com/PrunaAI)
[](https://www.linkedin.com/company/93832878/admin/feed/posts/?feedType=following)
[](https://discord.gg/CP4VSgck)
# Simply make AI models cheaper, smaller, faster, and greener!
- Give a thumbs up if you like this model!
- Contact us and tell us which model to compress next [here](https://www.pruna.ai/contact).
- Request access to easily compress your *own* AI models [here](https://z0halsaff74.typeform.com/pruna-access?typeform-source=www.pruna.ai).
- Read the documentations to know more [here](https://pruna-ai-pruna.readthedocs-hosted.com/en/latest/)
- Join Pruna AI community on Discord [here](https://discord.gg/CP4VSgck) to share feedback/suggestions or get help.
## Results

**Frequently Asked Questions**
- ***How does the compression work?*** The model is compressed with hqq.
- ***How does the model quality change?*** The quality of the model output might vary compared to the base model.
- ***How is the model efficiency evaluated?*** These results were obtained on HARDWARE_NAME with configuration described in `model/smash_config.json` and are obtained after a hardware warmup. The smashed model is directly compared to the original base model. Efficiency results may vary in other settings (e.g. other hardware, image size, batch size, ...). We recommend to directly run them in the use-case conditions to know if the smashed model can benefit you.
- ***What is the model format?*** We use safetensors.
- ***What calibration data has been used?*** If needed by the compression method, we used WikiText as the calibration data.
- ***What is the naming convention for Pruna Huggingface models?*** We take the original model name and append "turbo", "tiny", or "green" if the smashed model has a measured inference speed, inference memory, or inference energy consumption which is less than 90% of the original base model.
- ***How to compress my own models?*** You can request premium access to more compression methods and tech support for your specific use-cases [here](https://z0halsaff74.typeform.com/pruna-access?typeform-source=www.pruna.ai).
- ***What are "first" metrics?*** Results mentioning "first" are obtained after the first run of the model. The first run might take more memory or be slower than the subsequent runs due cuda overheads.
- ***What are "Sync" and "Async" metrics?*** "Sync" metrics are obtained by syncing all GPU processes and stop measurement when all of them are executed. "Async" metrics are obtained without syncing all GPU processes and stop when the model output can be used by the CPU. We provide both metrics since both could be relevant depending on the use-case. We recommend to test the efficiency gains directly in your use-cases.
## Setup
You can run the smashed model with these steps:
0. Check requirements from the original repo RESMPDEV/Wukong-0.1-Mistral-7B-v0.2 installed. In particular, check python, cuda, and transformers versions.
1. Make sure that you have installed quantization related packages.
```bash
pip install hqq
```
2. Load & run the model.
```python
from transformers import AutoModelForCausalLM, AutoTokenizer
from hqq.engine.hf import HQQModelForCausalLM
from hqq.models.hf.base import AutoHQQHFModel
try:
model = HQQModelForCausalLM.from_quantized("PrunaAI/RESMPDEV-Wukong-0.1-Mistral-7B-v0.2-HQQ-1bit-smashed", device_map='auto')
except:
model = AutoHQQHFModel.from_quantized("PrunaAI/RESMPDEV-Wukong-0.1-Mistral-7B-v0.2-HQQ-1bit-smashed")
tokenizer = AutoTokenizer.from_pretrained("RESMPDEV/Wukong-0.1-Mistral-7B-v0.2")
input_ids = tokenizer("What is the color of prunes?,", return_tensors='pt').to(model.device)["input_ids"]
outputs = model.generate(input_ids, max_new_tokens=216)
tokenizer.decode(outputs[0])
```
## Configurations
The configuration info are in `smash_config.json`.
## Credits & License
The license of the smashed model follows the license of the original model. Please check the license of the original model RESMPDEV/Wukong-0.1-Mistral-7B-v0.2 before using this model which provided the base model. The license of the `pruna-engine` is [here](https://pypi.org/project/pruna-engine/) on Pypi.
## Want to compress other models?
- Contact us and tell us which model to compress next [here](https://www.pruna.ai/contact).
- Request access to easily compress your own AI models [here](https://z0halsaff74.typeform.com/pruna-access?typeform-source=www.pruna.ai). |
PrunaAI/RESMPDEV-Gemma-Wukong-2b-HQQ-4bit-smashed | PrunaAI | "2024-06-24T10:19:05Z" | 0 | 0 | transformers | [
"transformers",
"gemma",
"text-generation",
"pruna-ai",
"conversational",
"base_model:RESMPDEV/Gemma-Wukong-2b",
"autotrain_compatible",
"endpoints_compatible",
"text-generation-inference",
"region:us"
] | text-generation | "2024-06-24T10:18:02Z" | ---
thumbnail: "https://assets-global.website-files.com/646b351987a8d8ce158d1940/64ec9e96b4334c0e1ac41504_Logo%20with%20white%20text.svg"
base_model: RESMPDEV/Gemma-Wukong-2b
metrics:
- memory_disk
- memory_inference
- inference_latency
- inference_throughput
- inference_CO2_emissions
- inference_energy_consumption
tags:
- pruna-ai
---
<!-- header start -->
<!-- 200823 -->
<div style="width: auto; margin-left: auto; margin-right: auto">
<a href="https://www.pruna.ai/" target="_blank" rel="noopener noreferrer">
<img src="https://i.imgur.com/eDAlcgk.png" alt="PrunaAI" style="width: 100%; min-width: 400px; display: block; margin: auto;">
</a>
</div>
<!-- header end -->
[](https://twitter.com/PrunaAI)
[](https://github.com/PrunaAI)
[](https://www.linkedin.com/company/93832878/admin/feed/posts/?feedType=following)
[](https://discord.gg/CP4VSgck)
# Simply make AI models cheaper, smaller, faster, and greener!
- Give a thumbs up if you like this model!
- Contact us and tell us which model to compress next [here](https://www.pruna.ai/contact).
- Request access to easily compress your *own* AI models [here](https://z0halsaff74.typeform.com/pruna-access?typeform-source=www.pruna.ai).
- Read the documentations to know more [here](https://pruna-ai-pruna.readthedocs-hosted.com/en/latest/)
- Join Pruna AI community on Discord [here](https://discord.gg/CP4VSgck) to share feedback/suggestions or get help.
## Results

**Frequently Asked Questions**
- ***How does the compression work?*** The model is compressed with hqq.
- ***How does the model quality change?*** The quality of the model output might vary compared to the base model.
- ***How is the model efficiency evaluated?*** These results were obtained on HARDWARE_NAME with configuration described in `model/smash_config.json` and are obtained after a hardware warmup. The smashed model is directly compared to the original base model. Efficiency results may vary in other settings (e.g. other hardware, image size, batch size, ...). We recommend to directly run them in the use-case conditions to know if the smashed model can benefit you.
- ***What is the model format?*** We use safetensors.
- ***What calibration data has been used?*** If needed by the compression method, we used WikiText as the calibration data.
- ***What is the naming convention for Pruna Huggingface models?*** We take the original model name and append "turbo", "tiny", or "green" if the smashed model has a measured inference speed, inference memory, or inference energy consumption which is less than 90% of the original base model.
- ***How to compress my own models?*** You can request premium access to more compression methods and tech support for your specific use-cases [here](https://z0halsaff74.typeform.com/pruna-access?typeform-source=www.pruna.ai).
- ***What are "first" metrics?*** Results mentioning "first" are obtained after the first run of the model. The first run might take more memory or be slower than the subsequent runs due cuda overheads.
- ***What are "Sync" and "Async" metrics?*** "Sync" metrics are obtained by syncing all GPU processes and stop measurement when all of them are executed. "Async" metrics are obtained without syncing all GPU processes and stop when the model output can be used by the CPU. We provide both metrics since both could be relevant depending on the use-case. We recommend to test the efficiency gains directly in your use-cases.
## Setup
You can run the smashed model with these steps:
0. Check requirements from the original repo RESMPDEV/Gemma-Wukong-2b installed. In particular, check python, cuda, and transformers versions.
1. Make sure that you have installed quantization related packages.
```bash
pip install hqq
```
2. Load & run the model.
```python
from transformers import AutoModelForCausalLM, AutoTokenizer
from hqq.engine.hf import HQQModelForCausalLM
from hqq.models.hf.base import AutoHQQHFModel
try:
model = HQQModelForCausalLM.from_quantized("PrunaAI/RESMPDEV-Gemma-Wukong-2b-HQQ-4bit-smashed", device_map='auto')
except:
model = AutoHQQHFModel.from_quantized("PrunaAI/RESMPDEV-Gemma-Wukong-2b-HQQ-4bit-smashed")
tokenizer = AutoTokenizer.from_pretrained("RESMPDEV/Gemma-Wukong-2b")
input_ids = tokenizer("What is the color of prunes?,", return_tensors='pt').to(model.device)["input_ids"]
outputs = model.generate(input_ids, max_new_tokens=216)
tokenizer.decode(outputs[0])
```
## Configurations
The configuration info are in `smash_config.json`.
## Credits & License
The license of the smashed model follows the license of the original model. Please check the license of the original model RESMPDEV/Gemma-Wukong-2b before using this model which provided the base model. The license of the `pruna-engine` is [here](https://pypi.org/project/pruna-engine/) on Pypi.
## Want to compress other models?
- Contact us and tell us which model to compress next [here](https://www.pruna.ai/contact).
- Request access to easily compress your own AI models [here](https://z0halsaff74.typeform.com/pruna-access?typeform-source=www.pruna.ai). |
PrunaAI/RESMPDEV-Wukong-0.1-Mistral-7B-v0.2-HQQ-2bit-smashed | PrunaAI | "2024-06-24T10:19:22Z" | 0 | 0 | transformers | [
"transformers",
"mistral",
"text-generation",
"pruna-ai",
"base_model:RESMPDEV/Wukong-0.1-Mistral-7B-v0.2",
"autotrain_compatible",
"endpoints_compatible",
"text-generation-inference",
"region:us"
] | text-generation | "2024-06-24T10:18:09Z" | ---
thumbnail: "https://assets-global.website-files.com/646b351987a8d8ce158d1940/64ec9e96b4334c0e1ac41504_Logo%20with%20white%20text.svg"
base_model: RESMPDEV/Wukong-0.1-Mistral-7B-v0.2
metrics:
- memory_disk
- memory_inference
- inference_latency
- inference_throughput
- inference_CO2_emissions
- inference_energy_consumption
tags:
- pruna-ai
---
<!-- header start -->
<!-- 200823 -->
<div style="width: auto; margin-left: auto; margin-right: auto">
<a href="https://www.pruna.ai/" target="_blank" rel="noopener noreferrer">
<img src="https://i.imgur.com/eDAlcgk.png" alt="PrunaAI" style="width: 100%; min-width: 400px; display: block; margin: auto;">
</a>
</div>
<!-- header end -->
[](https://twitter.com/PrunaAI)
[](https://github.com/PrunaAI)
[](https://www.linkedin.com/company/93832878/admin/feed/posts/?feedType=following)
[](https://discord.gg/CP4VSgck)
# Simply make AI models cheaper, smaller, faster, and greener!
- Give a thumbs up if you like this model!
- Contact us and tell us which model to compress next [here](https://www.pruna.ai/contact).
- Request access to easily compress your *own* AI models [here](https://z0halsaff74.typeform.com/pruna-access?typeform-source=www.pruna.ai).
- Read the documentations to know more [here](https://pruna-ai-pruna.readthedocs-hosted.com/en/latest/)
- Join Pruna AI community on Discord [here](https://discord.gg/CP4VSgck) to share feedback/suggestions or get help.
## Results

**Frequently Asked Questions**
- ***How does the compression work?*** The model is compressed with hqq.
- ***How does the model quality change?*** The quality of the model output might vary compared to the base model.
- ***How is the model efficiency evaluated?*** These results were obtained on HARDWARE_NAME with configuration described in `model/smash_config.json` and are obtained after a hardware warmup. The smashed model is directly compared to the original base model. Efficiency results may vary in other settings (e.g. other hardware, image size, batch size, ...). We recommend to directly run them in the use-case conditions to know if the smashed model can benefit you.
- ***What is the model format?*** We use safetensors.
- ***What calibration data has been used?*** If needed by the compression method, we used WikiText as the calibration data.
- ***What is the naming convention for Pruna Huggingface models?*** We take the original model name and append "turbo", "tiny", or "green" if the smashed model has a measured inference speed, inference memory, or inference energy consumption which is less than 90% of the original base model.
- ***How to compress my own models?*** You can request premium access to more compression methods and tech support for your specific use-cases [here](https://z0halsaff74.typeform.com/pruna-access?typeform-source=www.pruna.ai).
- ***What are "first" metrics?*** Results mentioning "first" are obtained after the first run of the model. The first run might take more memory or be slower than the subsequent runs due cuda overheads.
- ***What are "Sync" and "Async" metrics?*** "Sync" metrics are obtained by syncing all GPU processes and stop measurement when all of them are executed. "Async" metrics are obtained without syncing all GPU processes and stop when the model output can be used by the CPU. We provide both metrics since both could be relevant depending on the use-case. We recommend to test the efficiency gains directly in your use-cases.
## Setup
You can run the smashed model with these steps:
0. Check requirements from the original repo RESMPDEV/Wukong-0.1-Mistral-7B-v0.2 installed. In particular, check python, cuda, and transformers versions.
1. Make sure that you have installed quantization related packages.
```bash
pip install hqq
```
2. Load & run the model.
```python
from transformers import AutoModelForCausalLM, AutoTokenizer
from hqq.engine.hf import HQQModelForCausalLM
from hqq.models.hf.base import AutoHQQHFModel
try:
model = HQQModelForCausalLM.from_quantized("PrunaAI/RESMPDEV-Wukong-0.1-Mistral-7B-v0.2-HQQ-2bit-smashed", device_map='auto')
except:
model = AutoHQQHFModel.from_quantized("PrunaAI/RESMPDEV-Wukong-0.1-Mistral-7B-v0.2-HQQ-2bit-smashed")
tokenizer = AutoTokenizer.from_pretrained("RESMPDEV/Wukong-0.1-Mistral-7B-v0.2")
input_ids = tokenizer("What is the color of prunes?,", return_tensors='pt').to(model.device)["input_ids"]
outputs = model.generate(input_ids, max_new_tokens=216)
tokenizer.decode(outputs[0])
```
## Configurations
The configuration info are in `smash_config.json`.
## Credits & License
The license of the smashed model follows the license of the original model. Please check the license of the original model RESMPDEV/Wukong-0.1-Mistral-7B-v0.2 before using this model which provided the base model. The license of the `pruna-engine` is [here](https://pypi.org/project/pruna-engine/) on Pypi.
## Want to compress other models?
- Contact us and tell us which model to compress next [here](https://www.pruna.ai/contact).
- Request access to easily compress your own AI models [here](https://z0halsaff74.typeform.com/pruna-access?typeform-source=www.pruna.ai). |
PrunaAI/RESMPDEV-Gemma-Wukong-2b-HQQ-2bit-smashed | PrunaAI | "2024-06-24T10:19:06Z" | 0 | 0 | transformers | [
"transformers",
"gemma",
"text-generation",
"pruna-ai",
"conversational",
"base_model:RESMPDEV/Gemma-Wukong-2b",
"autotrain_compatible",
"endpoints_compatible",
"text-generation-inference",
"region:us"
] | text-generation | "2024-06-24T10:18:20Z" | ---
thumbnail: "https://assets-global.website-files.com/646b351987a8d8ce158d1940/64ec9e96b4334c0e1ac41504_Logo%20with%20white%20text.svg"
base_model: RESMPDEV/Gemma-Wukong-2b
metrics:
- memory_disk
- memory_inference
- inference_latency
- inference_throughput
- inference_CO2_emissions
- inference_energy_consumption
tags:
- pruna-ai
---
<!-- header start -->
<!-- 200823 -->
<div style="width: auto; margin-left: auto; margin-right: auto">
<a href="https://www.pruna.ai/" target="_blank" rel="noopener noreferrer">
<img src="https://i.imgur.com/eDAlcgk.png" alt="PrunaAI" style="width: 100%; min-width: 400px; display: block; margin: auto;">
</a>
</div>
<!-- header end -->
[](https://twitter.com/PrunaAI)
[](https://github.com/PrunaAI)
[](https://www.linkedin.com/company/93832878/admin/feed/posts/?feedType=following)
[](https://discord.gg/CP4VSgck)
# Simply make AI models cheaper, smaller, faster, and greener!
- Give a thumbs up if you like this model!
- Contact us and tell us which model to compress next [here](https://www.pruna.ai/contact).
- Request access to easily compress your *own* AI models [here](https://z0halsaff74.typeform.com/pruna-access?typeform-source=www.pruna.ai).
- Read the documentations to know more [here](https://pruna-ai-pruna.readthedocs-hosted.com/en/latest/)
- Join Pruna AI community on Discord [here](https://discord.gg/CP4VSgck) to share feedback/suggestions or get help.
## Results

**Frequently Asked Questions**
- ***How does the compression work?*** The model is compressed with hqq.
- ***How does the model quality change?*** The quality of the model output might vary compared to the base model.
- ***How is the model efficiency evaluated?*** These results were obtained on HARDWARE_NAME with configuration described in `model/smash_config.json` and are obtained after a hardware warmup. The smashed model is directly compared to the original base model. Efficiency results may vary in other settings (e.g. other hardware, image size, batch size, ...). We recommend to directly run them in the use-case conditions to know if the smashed model can benefit you.
- ***What is the model format?*** We use safetensors.
- ***What calibration data has been used?*** If needed by the compression method, we used WikiText as the calibration data.
- ***What is the naming convention for Pruna Huggingface models?*** We take the original model name and append "turbo", "tiny", or "green" if the smashed model has a measured inference speed, inference memory, or inference energy consumption which is less than 90% of the original base model.
- ***How to compress my own models?*** You can request premium access to more compression methods and tech support for your specific use-cases [here](https://z0halsaff74.typeform.com/pruna-access?typeform-source=www.pruna.ai).
- ***What are "first" metrics?*** Results mentioning "first" are obtained after the first run of the model. The first run might take more memory or be slower than the subsequent runs due cuda overheads.
- ***What are "Sync" and "Async" metrics?*** "Sync" metrics are obtained by syncing all GPU processes and stop measurement when all of them are executed. "Async" metrics are obtained without syncing all GPU processes and stop when the model output can be used by the CPU. We provide both metrics since both could be relevant depending on the use-case. We recommend to test the efficiency gains directly in your use-cases.
## Setup
You can run the smashed model with these steps:
0. Check requirements from the original repo RESMPDEV/Gemma-Wukong-2b installed. In particular, check python, cuda, and transformers versions.
1. Make sure that you have installed quantization related packages.
```bash
pip install hqq
```
2. Load & run the model.
```python
from transformers import AutoModelForCausalLM, AutoTokenizer
from hqq.engine.hf import HQQModelForCausalLM
from hqq.models.hf.base import AutoHQQHFModel
try:
model = HQQModelForCausalLM.from_quantized("PrunaAI/RESMPDEV-Gemma-Wukong-2b-HQQ-2bit-smashed", device_map='auto')
except:
model = AutoHQQHFModel.from_quantized("PrunaAI/RESMPDEV-Gemma-Wukong-2b-HQQ-2bit-smashed")
tokenizer = AutoTokenizer.from_pretrained("RESMPDEV/Gemma-Wukong-2b")
input_ids = tokenizer("What is the color of prunes?,", return_tensors='pt').to(model.device)["input_ids"]
outputs = model.generate(input_ids, max_new_tokens=216)
tokenizer.decode(outputs[0])
```
## Configurations
The configuration info are in `smash_config.json`.
## Credits & License
The license of the smashed model follows the license of the original model. Please check the license of the original model RESMPDEV/Gemma-Wukong-2b before using this model which provided the base model. The license of the `pruna-engine` is [here](https://pypi.org/project/pruna-engine/) on Pypi.
## Want to compress other models?
- Contact us and tell us which model to compress next [here](https://www.pruna.ai/contact).
- Request access to easily compress your own AI models [here](https://z0halsaff74.typeform.com/pruna-access?typeform-source=www.pruna.ai). |
KasuleTrevor/wav2vec2-large-xls-r-300m-lg-10hr-v2 | KasuleTrevor | "2024-06-24T14:49:14Z" | 0 | 0 | transformers | [
"transformers",
"safetensors",
"wav2vec2",
"automatic-speech-recognition",
"arxiv:1910.09700",
"endpoints_compatible",
"region:us"
] | automatic-speech-recognition | "2024-06-24T10:18:32Z" | ---
library_name: transformers
tags: []
---
# Model Card for Model ID
<!-- Provide a quick summary of what the model is/does. -->
## Model Details
### Model Description
<!-- Provide a longer summary of what this model is. -->
This is the model card of a 🤗 transformers model that has been pushed on the Hub. This model card has been automatically generated.
- **Developed by:** [More Information Needed]
- **Funded by [optional]:** [More Information Needed]
- **Shared by [optional]:** [More Information Needed]
- **Model type:** [More Information Needed]
- **Language(s) (NLP):** [More Information Needed]
- **License:** [More Information Needed]
- **Finetuned from model [optional]:** [More Information Needed]
### Model Sources [optional]
<!-- Provide the basic links for the model. -->
- **Repository:** [More Information Needed]
- **Paper [optional]:** [More Information Needed]
- **Demo [optional]:** [More Information Needed]
## Uses
<!-- Address questions around how the model is intended to be used, including the foreseeable users of the model and those affected by the model. -->
### Direct Use
<!-- This section is for the model use without fine-tuning or plugging into a larger ecosystem/app. -->
[More Information Needed]
### Downstream Use [optional]
<!-- This section is for the model use when fine-tuned for a task, or when plugged into a larger ecosystem/app -->
[More Information Needed]
### Out-of-Scope Use
<!-- This section addresses misuse, malicious use, and uses that the model will not work well for. -->
[More Information Needed]
## Bias, Risks, and Limitations
<!-- This section is meant to convey both technical and sociotechnical limitations. -->
[More Information Needed]
### Recommendations
<!-- This section is meant to convey recommendations with respect to the bias, risk, and technical limitations. -->
Users (both direct and downstream) should be made aware of the risks, biases and limitations of the model. More information needed for further recommendations.
## How to Get Started with the Model
Use the code below to get started with the model.
[More Information Needed]
## Training Details
### Training Data
<!-- This should link to a Dataset Card, perhaps with a short stub of information on what the training data is all about as well as documentation related to data pre-processing or additional filtering. -->
[More Information Needed]
### Training Procedure
<!-- This relates heavily to the Technical Specifications. Content here should link to that section when it is relevant to the training procedure. -->
#### Preprocessing [optional]
[More Information Needed]
#### Training Hyperparameters
- **Training regime:** [More Information Needed] <!--fp32, fp16 mixed precision, bf16 mixed precision, bf16 non-mixed precision, fp16 non-mixed precision, fp8 mixed precision -->
#### Speeds, Sizes, Times [optional]
<!-- This section provides information about throughput, start/end time, checkpoint size if relevant, etc. -->
[More Information Needed]
## Evaluation
<!-- This section describes the evaluation protocols and provides the results. -->
### Testing Data, Factors & Metrics
#### Testing Data
<!-- This should link to a Dataset Card if possible. -->
[More Information Needed]
#### Factors
<!-- These are the things the evaluation is disaggregating by, e.g., subpopulations or domains. -->
[More Information Needed]
#### Metrics
<!-- These are the evaluation metrics being used, ideally with a description of why. -->
[More Information Needed]
### Results
[More Information Needed]
#### Summary
## Model Examination [optional]
<!-- Relevant interpretability work for the model goes here -->
[More Information Needed]
## Environmental Impact
<!-- Total emissions (in grams of CO2eq) and additional considerations, such as electricity usage, go here. Edit the suggested text below accordingly -->
Carbon emissions can be estimated using the [Machine Learning Impact calculator](https://mlco2.github.io/impact#compute) presented in [Lacoste et al. (2019)](https://arxiv.org/abs/1910.09700).
- **Hardware Type:** [More Information Needed]
- **Hours used:** [More Information Needed]
- **Cloud Provider:** [More Information Needed]
- **Compute Region:** [More Information Needed]
- **Carbon Emitted:** [More Information Needed]
## Technical Specifications [optional]
### Model Architecture and Objective
[More Information Needed]
### Compute Infrastructure
[More Information Needed]
#### Hardware
[More Information Needed]
#### Software
[More Information Needed]
## Citation [optional]
<!-- If there is a paper or blog post introducing the model, the APA and Bibtex information for that should go in this section. -->
**BibTeX:**
[More Information Needed]
**APA:**
[More Information Needed]
## Glossary [optional]
<!-- If relevant, include terms and calculations in this section that can help readers understand the model or model card. -->
[More Information Needed]
## More Information [optional]
[More Information Needed]
## Model Card Authors [optional]
[More Information Needed]
## Model Card Contact
[More Information Needed] |
yemen2016/memo3_NC_2 | yemen2016 | "2024-06-24T10:33:02Z" | 0 | 0 | transformers | [
"transformers",
"tensorboard",
"safetensors",
"xlm-roberta",
"text-classification",
"autotrain_compatible",
"endpoints_compatible",
"region:us"
] | text-classification | "2024-06-24T10:18:35Z" | Entry not found |
PrunaAI/RESMPDEV-Wukong-0.1-Mistral-7B-v0.2-HQQ-4bit-smashed | PrunaAI | "2024-06-24T10:20:45Z" | 0 | 0 | transformers | [
"transformers",
"mistral",
"text-generation",
"pruna-ai",
"base_model:RESMPDEV/Wukong-0.1-Mistral-7B-v0.2",
"autotrain_compatible",
"endpoints_compatible",
"text-generation-inference",
"region:us"
] | text-generation | "2024-06-24T10:18:48Z" | ---
thumbnail: "https://assets-global.website-files.com/646b351987a8d8ce158d1940/64ec9e96b4334c0e1ac41504_Logo%20with%20white%20text.svg"
base_model: RESMPDEV/Wukong-0.1-Mistral-7B-v0.2
metrics:
- memory_disk
- memory_inference
- inference_latency
- inference_throughput
- inference_CO2_emissions
- inference_energy_consumption
tags:
- pruna-ai
---
<!-- header start -->
<!-- 200823 -->
<div style="width: auto; margin-left: auto; margin-right: auto">
<a href="https://www.pruna.ai/" target="_blank" rel="noopener noreferrer">
<img src="https://i.imgur.com/eDAlcgk.png" alt="PrunaAI" style="width: 100%; min-width: 400px; display: block; margin: auto;">
</a>
</div>
<!-- header end -->
[](https://twitter.com/PrunaAI)
[](https://github.com/PrunaAI)
[](https://www.linkedin.com/company/93832878/admin/feed/posts/?feedType=following)
[](https://discord.gg/CP4VSgck)
# Simply make AI models cheaper, smaller, faster, and greener!
- Give a thumbs up if you like this model!
- Contact us and tell us which model to compress next [here](https://www.pruna.ai/contact).
- Request access to easily compress your *own* AI models [here](https://z0halsaff74.typeform.com/pruna-access?typeform-source=www.pruna.ai).
- Read the documentations to know more [here](https://pruna-ai-pruna.readthedocs-hosted.com/en/latest/)
- Join Pruna AI community on Discord [here](https://discord.gg/CP4VSgck) to share feedback/suggestions or get help.
## Results

**Frequently Asked Questions**
- ***How does the compression work?*** The model is compressed with hqq.
- ***How does the model quality change?*** The quality of the model output might vary compared to the base model.
- ***How is the model efficiency evaluated?*** These results were obtained on HARDWARE_NAME with configuration described in `model/smash_config.json` and are obtained after a hardware warmup. The smashed model is directly compared to the original base model. Efficiency results may vary in other settings (e.g. other hardware, image size, batch size, ...). We recommend to directly run them in the use-case conditions to know if the smashed model can benefit you.
- ***What is the model format?*** We use safetensors.
- ***What calibration data has been used?*** If needed by the compression method, we used WikiText as the calibration data.
- ***What is the naming convention for Pruna Huggingface models?*** We take the original model name and append "turbo", "tiny", or "green" if the smashed model has a measured inference speed, inference memory, or inference energy consumption which is less than 90% of the original base model.
- ***How to compress my own models?*** You can request premium access to more compression methods and tech support for your specific use-cases [here](https://z0halsaff74.typeform.com/pruna-access?typeform-source=www.pruna.ai).
- ***What are "first" metrics?*** Results mentioning "first" are obtained after the first run of the model. The first run might take more memory or be slower than the subsequent runs due cuda overheads.
- ***What are "Sync" and "Async" metrics?*** "Sync" metrics are obtained by syncing all GPU processes and stop measurement when all of them are executed. "Async" metrics are obtained without syncing all GPU processes and stop when the model output can be used by the CPU. We provide both metrics since both could be relevant depending on the use-case. We recommend to test the efficiency gains directly in your use-cases.
## Setup
You can run the smashed model with these steps:
0. Check requirements from the original repo RESMPDEV/Wukong-0.1-Mistral-7B-v0.2 installed. In particular, check python, cuda, and transformers versions.
1. Make sure that you have installed quantization related packages.
```bash
pip install hqq
```
2. Load & run the model.
```python
from transformers import AutoModelForCausalLM, AutoTokenizer
from hqq.engine.hf import HQQModelForCausalLM
from hqq.models.hf.base import AutoHQQHFModel
try:
model = HQQModelForCausalLM.from_quantized("PrunaAI/RESMPDEV-Wukong-0.1-Mistral-7B-v0.2-HQQ-4bit-smashed", device_map='auto')
except:
model = AutoHQQHFModel.from_quantized("PrunaAI/RESMPDEV-Wukong-0.1-Mistral-7B-v0.2-HQQ-4bit-smashed")
tokenizer = AutoTokenizer.from_pretrained("RESMPDEV/Wukong-0.1-Mistral-7B-v0.2")
input_ids = tokenizer("What is the color of prunes?,", return_tensors='pt').to(model.device)["input_ids"]
outputs = model.generate(input_ids, max_new_tokens=216)
tokenizer.decode(outputs[0])
```
## Configurations
The configuration info are in `smash_config.json`.
## Credits & License
The license of the smashed model follows the license of the original model. Please check the license of the original model RESMPDEV/Wukong-0.1-Mistral-7B-v0.2 before using this model which provided the base model. The license of the `pruna-engine` is [here](https://pypi.org/project/pruna-engine/) on Pypi.
## Want to compress other models?
- Contact us and tell us which model to compress next [here](https://www.pruna.ai/contact).
- Request access to easily compress your own AI models [here](https://z0halsaff74.typeform.com/pruna-access?typeform-source=www.pruna.ai). |
rammafitra/finetuning-sentiment-model-3000-samples | rammafitra | "2024-06-24T10:19:18Z" | 0 | 0 | null | [
"region:us"
] | null | "2024-06-24T10:19:18Z" | Entry not found |
PrunaAI/ajibawa-2023-Scarlett-Llama-3-8B-HQQ-1bit-smashed | PrunaAI | "2024-06-24T10:21:56Z" | 0 | 0 | transformers | [
"transformers",
"llama",
"text-generation",
"pruna-ai",
"conversational",
"base_model:ajibawa-2023/Scarlett-Llama-3-8B",
"autotrain_compatible",
"endpoints_compatible",
"text-generation-inference",
"region:us"
] | text-generation | "2024-06-24T10:20:26Z" | ---
thumbnail: "https://assets-global.website-files.com/646b351987a8d8ce158d1940/64ec9e96b4334c0e1ac41504_Logo%20with%20white%20text.svg"
base_model: ajibawa-2023/Scarlett-Llama-3-8B
metrics:
- memory_disk
- memory_inference
- inference_latency
- inference_throughput
- inference_CO2_emissions
- inference_energy_consumption
tags:
- pruna-ai
---
<!-- header start -->
<!-- 200823 -->
<div style="width: auto; margin-left: auto; margin-right: auto">
<a href="https://www.pruna.ai/" target="_blank" rel="noopener noreferrer">
<img src="https://i.imgur.com/eDAlcgk.png" alt="PrunaAI" style="width: 100%; min-width: 400px; display: block; margin: auto;">
</a>
</div>
<!-- header end -->
[](https://twitter.com/PrunaAI)
[](https://github.com/PrunaAI)
[](https://www.linkedin.com/company/93832878/admin/feed/posts/?feedType=following)
[](https://discord.gg/CP4VSgck)
# Simply make AI models cheaper, smaller, faster, and greener!
- Give a thumbs up if you like this model!
- Contact us and tell us which model to compress next [here](https://www.pruna.ai/contact).
- Request access to easily compress your *own* AI models [here](https://z0halsaff74.typeform.com/pruna-access?typeform-source=www.pruna.ai).
- Read the documentations to know more [here](https://pruna-ai-pruna.readthedocs-hosted.com/en/latest/)
- Join Pruna AI community on Discord [here](https://discord.gg/CP4VSgck) to share feedback/suggestions or get help.
## Results

**Frequently Asked Questions**
- ***How does the compression work?*** The model is compressed with hqq.
- ***How does the model quality change?*** The quality of the model output might vary compared to the base model.
- ***How is the model efficiency evaluated?*** These results were obtained on HARDWARE_NAME with configuration described in `model/smash_config.json` and are obtained after a hardware warmup. The smashed model is directly compared to the original base model. Efficiency results may vary in other settings (e.g. other hardware, image size, batch size, ...). We recommend to directly run them in the use-case conditions to know if the smashed model can benefit you.
- ***What is the model format?*** We use safetensors.
- ***What calibration data has been used?*** If needed by the compression method, we used WikiText as the calibration data.
- ***What is the naming convention for Pruna Huggingface models?*** We take the original model name and append "turbo", "tiny", or "green" if the smashed model has a measured inference speed, inference memory, or inference energy consumption which is less than 90% of the original base model.
- ***How to compress my own models?*** You can request premium access to more compression methods and tech support for your specific use-cases [here](https://z0halsaff74.typeform.com/pruna-access?typeform-source=www.pruna.ai).
- ***What are "first" metrics?*** Results mentioning "first" are obtained after the first run of the model. The first run might take more memory or be slower than the subsequent runs due cuda overheads.
- ***What are "Sync" and "Async" metrics?*** "Sync" metrics are obtained by syncing all GPU processes and stop measurement when all of them are executed. "Async" metrics are obtained without syncing all GPU processes and stop when the model output can be used by the CPU. We provide both metrics since both could be relevant depending on the use-case. We recommend to test the efficiency gains directly in your use-cases.
## Setup
You can run the smashed model with these steps:
0. Check requirements from the original repo ajibawa-2023/Scarlett-Llama-3-8B installed. In particular, check python, cuda, and transformers versions.
1. Make sure that you have installed quantization related packages.
```bash
pip install hqq
```
2. Load & run the model.
```python
from transformers import AutoModelForCausalLM, AutoTokenizer
from hqq.engine.hf import HQQModelForCausalLM
from hqq.models.hf.base import AutoHQQHFModel
try:
model = HQQModelForCausalLM.from_quantized("PrunaAI/ajibawa-2023-Scarlett-Llama-3-8B-HQQ-1bit-smashed", device_map='auto')
except:
model = AutoHQQHFModel.from_quantized("PrunaAI/ajibawa-2023-Scarlett-Llama-3-8B-HQQ-1bit-smashed")
tokenizer = AutoTokenizer.from_pretrained("ajibawa-2023/Scarlett-Llama-3-8B")
input_ids = tokenizer("What is the color of prunes?,", return_tensors='pt').to(model.device)["input_ids"]
outputs = model.generate(input_ids, max_new_tokens=216)
tokenizer.decode(outputs[0])
```
## Configurations
The configuration info are in `smash_config.json`.
## Credits & License
The license of the smashed model follows the license of the original model. Please check the license of the original model ajibawa-2023/Scarlett-Llama-3-8B before using this model which provided the base model. The license of the `pruna-engine` is [here](https://pypi.org/project/pruna-engine/) on Pypi.
## Want to compress other models?
- Contact us and tell us which model to compress next [here](https://www.pruna.ai/contact).
- Request access to easily compress your own AI models [here](https://z0halsaff74.typeform.com/pruna-access?typeform-source=www.pruna.ai). |
PrunaAI/ajibawa-2023-Scarlett-Llama-3-8B-HQQ-2bit-smashed | PrunaAI | "2024-06-24T10:22:39Z" | 0 | 0 | transformers | [
"transformers",
"llama",
"text-generation",
"pruna-ai",
"conversational",
"base_model:ajibawa-2023/Scarlett-Llama-3-8B",
"autotrain_compatible",
"endpoints_compatible",
"text-generation-inference",
"region:us"
] | text-generation | "2024-06-24T10:20:39Z" | ---
thumbnail: "https://assets-global.website-files.com/646b351987a8d8ce158d1940/64ec9e96b4334c0e1ac41504_Logo%20with%20white%20text.svg"
base_model: ajibawa-2023/Scarlett-Llama-3-8B
metrics:
- memory_disk
- memory_inference
- inference_latency
- inference_throughput
- inference_CO2_emissions
- inference_energy_consumption
tags:
- pruna-ai
---
<!-- header start -->
<!-- 200823 -->
<div style="width: auto; margin-left: auto; margin-right: auto">
<a href="https://www.pruna.ai/" target="_blank" rel="noopener noreferrer">
<img src="https://i.imgur.com/eDAlcgk.png" alt="PrunaAI" style="width: 100%; min-width: 400px; display: block; margin: auto;">
</a>
</div>
<!-- header end -->
[](https://twitter.com/PrunaAI)
[](https://github.com/PrunaAI)
[](https://www.linkedin.com/company/93832878/admin/feed/posts/?feedType=following)
[](https://discord.gg/CP4VSgck)
# Simply make AI models cheaper, smaller, faster, and greener!
- Give a thumbs up if you like this model!
- Contact us and tell us which model to compress next [here](https://www.pruna.ai/contact).
- Request access to easily compress your *own* AI models [here](https://z0halsaff74.typeform.com/pruna-access?typeform-source=www.pruna.ai).
- Read the documentations to know more [here](https://pruna-ai-pruna.readthedocs-hosted.com/en/latest/)
- Join Pruna AI community on Discord [here](https://discord.gg/CP4VSgck) to share feedback/suggestions or get help.
## Results

**Frequently Asked Questions**
- ***How does the compression work?*** The model is compressed with hqq.
- ***How does the model quality change?*** The quality of the model output might vary compared to the base model.
- ***How is the model efficiency evaluated?*** These results were obtained on HARDWARE_NAME with configuration described in `model/smash_config.json` and are obtained after a hardware warmup. The smashed model is directly compared to the original base model. Efficiency results may vary in other settings (e.g. other hardware, image size, batch size, ...). We recommend to directly run them in the use-case conditions to know if the smashed model can benefit you.
- ***What is the model format?*** We use safetensors.
- ***What calibration data has been used?*** If needed by the compression method, we used WikiText as the calibration data.
- ***What is the naming convention for Pruna Huggingface models?*** We take the original model name and append "turbo", "tiny", or "green" if the smashed model has a measured inference speed, inference memory, or inference energy consumption which is less than 90% of the original base model.
- ***How to compress my own models?*** You can request premium access to more compression methods and tech support for your specific use-cases [here](https://z0halsaff74.typeform.com/pruna-access?typeform-source=www.pruna.ai).
- ***What are "first" metrics?*** Results mentioning "first" are obtained after the first run of the model. The first run might take more memory or be slower than the subsequent runs due cuda overheads.
- ***What are "Sync" and "Async" metrics?*** "Sync" metrics are obtained by syncing all GPU processes and stop measurement when all of them are executed. "Async" metrics are obtained without syncing all GPU processes and stop when the model output can be used by the CPU. We provide both metrics since both could be relevant depending on the use-case. We recommend to test the efficiency gains directly in your use-cases.
## Setup
You can run the smashed model with these steps:
0. Check requirements from the original repo ajibawa-2023/Scarlett-Llama-3-8B installed. In particular, check python, cuda, and transformers versions.
1. Make sure that you have installed quantization related packages.
```bash
pip install hqq
```
2. Load & run the model.
```python
from transformers import AutoModelForCausalLM, AutoTokenizer
from hqq.engine.hf import HQQModelForCausalLM
from hqq.models.hf.base import AutoHQQHFModel
try:
model = HQQModelForCausalLM.from_quantized("PrunaAI/ajibawa-2023-Scarlett-Llama-3-8B-HQQ-2bit-smashed", device_map='auto')
except:
model = AutoHQQHFModel.from_quantized("PrunaAI/ajibawa-2023-Scarlett-Llama-3-8B-HQQ-2bit-smashed")
tokenizer = AutoTokenizer.from_pretrained("ajibawa-2023/Scarlett-Llama-3-8B")
input_ids = tokenizer("What is the color of prunes?,", return_tensors='pt').to(model.device)["input_ids"]
outputs = model.generate(input_ids, max_new_tokens=216)
tokenizer.decode(outputs[0])
```
## Configurations
The configuration info are in `smash_config.json`.
## Credits & License
The license of the smashed model follows the license of the original model. Please check the license of the original model ajibawa-2023/Scarlett-Llama-3-8B before using this model which provided the base model. The license of the `pruna-engine` is [here](https://pypi.org/project/pruna-engine/) on Pypi.
## Want to compress other models?
- Contact us and tell us which model to compress next [here](https://www.pruna.ai/contact).
- Request access to easily compress your own AI models [here](https://z0halsaff74.typeform.com/pruna-access?typeform-source=www.pruna.ai). |
PrunaAI/ajibawa-2023-Scarlett-Llama-3-8B-HQQ-4bit-smashed | PrunaAI | "2024-06-24T10:23:28Z" | 0 | 0 | transformers | [
"transformers",
"llama",
"text-generation",
"pruna-ai",
"conversational",
"base_model:ajibawa-2023/Scarlett-Llama-3-8B",
"autotrain_compatible",
"endpoints_compatible",
"text-generation-inference",
"region:us"
] | text-generation | "2024-06-24T10:20:43Z" | ---
thumbnail: "https://assets-global.website-files.com/646b351987a8d8ce158d1940/64ec9e96b4334c0e1ac41504_Logo%20with%20white%20text.svg"
base_model: ajibawa-2023/Scarlett-Llama-3-8B
metrics:
- memory_disk
- memory_inference
- inference_latency
- inference_throughput
- inference_CO2_emissions
- inference_energy_consumption
tags:
- pruna-ai
---
<!-- header start -->
<!-- 200823 -->
<div style="width: auto; margin-left: auto; margin-right: auto">
<a href="https://www.pruna.ai/" target="_blank" rel="noopener noreferrer">
<img src="https://i.imgur.com/eDAlcgk.png" alt="PrunaAI" style="width: 100%; min-width: 400px; display: block; margin: auto;">
</a>
</div>
<!-- header end -->
[](https://twitter.com/PrunaAI)
[](https://github.com/PrunaAI)
[](https://www.linkedin.com/company/93832878/admin/feed/posts/?feedType=following)
[](https://discord.gg/CP4VSgck)
# Simply make AI models cheaper, smaller, faster, and greener!
- Give a thumbs up if you like this model!
- Contact us and tell us which model to compress next [here](https://www.pruna.ai/contact).
- Request access to easily compress your *own* AI models [here](https://z0halsaff74.typeform.com/pruna-access?typeform-source=www.pruna.ai).
- Read the documentations to know more [here](https://pruna-ai-pruna.readthedocs-hosted.com/en/latest/)
- Join Pruna AI community on Discord [here](https://discord.gg/CP4VSgck) to share feedback/suggestions or get help.
## Results

**Frequently Asked Questions**
- ***How does the compression work?*** The model is compressed with hqq.
- ***How does the model quality change?*** The quality of the model output might vary compared to the base model.
- ***How is the model efficiency evaluated?*** These results were obtained on HARDWARE_NAME with configuration described in `model/smash_config.json` and are obtained after a hardware warmup. The smashed model is directly compared to the original base model. Efficiency results may vary in other settings (e.g. other hardware, image size, batch size, ...). We recommend to directly run them in the use-case conditions to know if the smashed model can benefit you.
- ***What is the model format?*** We use safetensors.
- ***What calibration data has been used?*** If needed by the compression method, we used WikiText as the calibration data.
- ***What is the naming convention for Pruna Huggingface models?*** We take the original model name and append "turbo", "tiny", or "green" if the smashed model has a measured inference speed, inference memory, or inference energy consumption which is less than 90% of the original base model.
- ***How to compress my own models?*** You can request premium access to more compression methods and tech support for your specific use-cases [here](https://z0halsaff74.typeform.com/pruna-access?typeform-source=www.pruna.ai).
- ***What are "first" metrics?*** Results mentioning "first" are obtained after the first run of the model. The first run might take more memory or be slower than the subsequent runs due cuda overheads.
- ***What are "Sync" and "Async" metrics?*** "Sync" metrics are obtained by syncing all GPU processes and stop measurement when all of them are executed. "Async" metrics are obtained without syncing all GPU processes and stop when the model output can be used by the CPU. We provide both metrics since both could be relevant depending on the use-case. We recommend to test the efficiency gains directly in your use-cases.
## Setup
You can run the smashed model with these steps:
0. Check requirements from the original repo ajibawa-2023/Scarlett-Llama-3-8B installed. In particular, check python, cuda, and transformers versions.
1. Make sure that you have installed quantization related packages.
```bash
pip install hqq
```
2. Load & run the model.
```python
from transformers import AutoModelForCausalLM, AutoTokenizer
from hqq.engine.hf import HQQModelForCausalLM
from hqq.models.hf.base import AutoHQQHFModel
try:
model = HQQModelForCausalLM.from_quantized("PrunaAI/ajibawa-2023-Scarlett-Llama-3-8B-HQQ-4bit-smashed", device_map='auto')
except:
model = AutoHQQHFModel.from_quantized("PrunaAI/ajibawa-2023-Scarlett-Llama-3-8B-HQQ-4bit-smashed")
tokenizer = AutoTokenizer.from_pretrained("ajibawa-2023/Scarlett-Llama-3-8B")
input_ids = tokenizer("What is the color of prunes?,", return_tensors='pt').to(model.device)["input_ids"]
outputs = model.generate(input_ids, max_new_tokens=216)
tokenizer.decode(outputs[0])
```
## Configurations
The configuration info are in `smash_config.json`.
## Credits & License
The license of the smashed model follows the license of the original model. Please check the license of the original model ajibawa-2023/Scarlett-Llama-3-8B before using this model which provided the base model. The license of the `pruna-engine` is [here](https://pypi.org/project/pruna-engine/) on Pypi.
## Want to compress other models?
- Contact us and tell us which model to compress next [here](https://www.pruna.ai/contact).
- Request access to easily compress your own AI models [here](https://z0halsaff74.typeform.com/pruna-access?typeform-source=www.pruna.ai). |
PrunaAI/openbmb-MiniCPM-2B-128k-HQQ-1bit-smashed | PrunaAI | "2024-06-24T10:21:35Z" | 0 | 0 | transformers | [
"transformers",
"minicpm",
"text-generation",
"pruna-ai",
"conversational",
"custom_code",
"base_model:openbmb/MiniCPM-2B-128k",
"autotrain_compatible",
"region:us"
] | text-generation | "2024-06-24T10:20:45Z" | ---
thumbnail: "https://assets-global.website-files.com/646b351987a8d8ce158d1940/64ec9e96b4334c0e1ac41504_Logo%20with%20white%20text.svg"
base_model: openbmb/MiniCPM-2B-128k
metrics:
- memory_disk
- memory_inference
- inference_latency
- inference_throughput
- inference_CO2_emissions
- inference_energy_consumption
tags:
- pruna-ai
---
<!-- header start -->
<!-- 200823 -->
<div style="width: auto; margin-left: auto; margin-right: auto">
<a href="https://www.pruna.ai/" target="_blank" rel="noopener noreferrer">
<img src="https://i.imgur.com/eDAlcgk.png" alt="PrunaAI" style="width: 100%; min-width: 400px; display: block; margin: auto;">
</a>
</div>
<!-- header end -->
[](https://twitter.com/PrunaAI)
[](https://github.com/PrunaAI)
[](https://www.linkedin.com/company/93832878/admin/feed/posts/?feedType=following)
[](https://discord.gg/CP4VSgck)
# Simply make AI models cheaper, smaller, faster, and greener!
- Give a thumbs up if you like this model!
- Contact us and tell us which model to compress next [here](https://www.pruna.ai/contact).
- Request access to easily compress your *own* AI models [here](https://z0halsaff74.typeform.com/pruna-access?typeform-source=www.pruna.ai).
- Read the documentations to know more [here](https://pruna-ai-pruna.readthedocs-hosted.com/en/latest/)
- Join Pruna AI community on Discord [here](https://discord.gg/CP4VSgck) to share feedback/suggestions or get help.
## Results

**Frequently Asked Questions**
- ***How does the compression work?*** The model is compressed with hqq.
- ***How does the model quality change?*** The quality of the model output might vary compared to the base model.
- ***How is the model efficiency evaluated?*** These results were obtained on HARDWARE_NAME with configuration described in `model/smash_config.json` and are obtained after a hardware warmup. The smashed model is directly compared to the original base model. Efficiency results may vary in other settings (e.g. other hardware, image size, batch size, ...). We recommend to directly run them in the use-case conditions to know if the smashed model can benefit you.
- ***What is the model format?*** We use safetensors.
- ***What calibration data has been used?*** If needed by the compression method, we used WikiText as the calibration data.
- ***What is the naming convention for Pruna Huggingface models?*** We take the original model name and append "turbo", "tiny", or "green" if the smashed model has a measured inference speed, inference memory, or inference energy consumption which is less than 90% of the original base model.
- ***How to compress my own models?*** You can request premium access to more compression methods and tech support for your specific use-cases [here](https://z0halsaff74.typeform.com/pruna-access?typeform-source=www.pruna.ai).
- ***What are "first" metrics?*** Results mentioning "first" are obtained after the first run of the model. The first run might take more memory or be slower than the subsequent runs due cuda overheads.
- ***What are "Sync" and "Async" metrics?*** "Sync" metrics are obtained by syncing all GPU processes and stop measurement when all of them are executed. "Async" metrics are obtained without syncing all GPU processes and stop when the model output can be used by the CPU. We provide both metrics since both could be relevant depending on the use-case. We recommend to test the efficiency gains directly in your use-cases.
## Setup
You can run the smashed model with these steps:
0. Check requirements from the original repo openbmb/MiniCPM-2B-128k installed. In particular, check python, cuda, and transformers versions.
1. Make sure that you have installed quantization related packages.
```bash
pip install hqq
```
2. Load & run the model.
```python
from transformers import AutoModelForCausalLM, AutoTokenizer
from hqq.engine.hf import HQQModelForCausalLM
from hqq.models.hf.base import AutoHQQHFModel
try:
model = HQQModelForCausalLM.from_quantized("PrunaAI/openbmb-MiniCPM-2B-128k-HQQ-1bit-smashed", device_map='auto')
except:
model = AutoHQQHFModel.from_quantized("PrunaAI/openbmb-MiniCPM-2B-128k-HQQ-1bit-smashed")
tokenizer = AutoTokenizer.from_pretrained("openbmb/MiniCPM-2B-128k")
input_ids = tokenizer("What is the color of prunes?,", return_tensors='pt').to(model.device)["input_ids"]
outputs = model.generate(input_ids, max_new_tokens=216)
tokenizer.decode(outputs[0])
```
## Configurations
The configuration info are in `smash_config.json`.
## Credits & License
The license of the smashed model follows the license of the original model. Please check the license of the original model openbmb/MiniCPM-2B-128k before using this model which provided the base model. The license of the `pruna-engine` is [here](https://pypi.org/project/pruna-engine/) on Pypi.
## Want to compress other models?
- Contact us and tell us which model to compress next [here](https://www.pruna.ai/contact).
- Request access to easily compress your own AI models [here](https://z0halsaff74.typeform.com/pruna-access?typeform-source=www.pruna.ai). |
PrunaAI/openbmb-MiniCPM-2B-128k-HQQ-2bit-smashed | PrunaAI | "2024-06-24T10:22:15Z" | 0 | 0 | transformers | [
"transformers",
"minicpm",
"text-generation",
"pruna-ai",
"conversational",
"custom_code",
"base_model:openbmb/MiniCPM-2B-128k",
"autotrain_compatible",
"region:us"
] | text-generation | "2024-06-24T10:21:17Z" | ---
thumbnail: "https://assets-global.website-files.com/646b351987a8d8ce158d1940/64ec9e96b4334c0e1ac41504_Logo%20with%20white%20text.svg"
base_model: openbmb/MiniCPM-2B-128k
metrics:
- memory_disk
- memory_inference
- inference_latency
- inference_throughput
- inference_CO2_emissions
- inference_energy_consumption
tags:
- pruna-ai
---
<!-- header start -->
<!-- 200823 -->
<div style="width: auto; margin-left: auto; margin-right: auto">
<a href="https://www.pruna.ai/" target="_blank" rel="noopener noreferrer">
<img src="https://i.imgur.com/eDAlcgk.png" alt="PrunaAI" style="width: 100%; min-width: 400px; display: block; margin: auto;">
</a>
</div>
<!-- header end -->
[](https://twitter.com/PrunaAI)
[](https://github.com/PrunaAI)
[](https://www.linkedin.com/company/93832878/admin/feed/posts/?feedType=following)
[](https://discord.gg/CP4VSgck)
# Simply make AI models cheaper, smaller, faster, and greener!
- Give a thumbs up if you like this model!
- Contact us and tell us which model to compress next [here](https://www.pruna.ai/contact).
- Request access to easily compress your *own* AI models [here](https://z0halsaff74.typeform.com/pruna-access?typeform-source=www.pruna.ai).
- Read the documentations to know more [here](https://pruna-ai-pruna.readthedocs-hosted.com/en/latest/)
- Join Pruna AI community on Discord [here](https://discord.gg/CP4VSgck) to share feedback/suggestions or get help.
## Results

**Frequently Asked Questions**
- ***How does the compression work?*** The model is compressed with hqq.
- ***How does the model quality change?*** The quality of the model output might vary compared to the base model.
- ***How is the model efficiency evaluated?*** These results were obtained on HARDWARE_NAME with configuration described in `model/smash_config.json` and are obtained after a hardware warmup. The smashed model is directly compared to the original base model. Efficiency results may vary in other settings (e.g. other hardware, image size, batch size, ...). We recommend to directly run them in the use-case conditions to know if the smashed model can benefit you.
- ***What is the model format?*** We use safetensors.
- ***What calibration data has been used?*** If needed by the compression method, we used WikiText as the calibration data.
- ***What is the naming convention for Pruna Huggingface models?*** We take the original model name and append "turbo", "tiny", or "green" if the smashed model has a measured inference speed, inference memory, or inference energy consumption which is less than 90% of the original base model.
- ***How to compress my own models?*** You can request premium access to more compression methods and tech support for your specific use-cases [here](https://z0halsaff74.typeform.com/pruna-access?typeform-source=www.pruna.ai).
- ***What are "first" metrics?*** Results mentioning "first" are obtained after the first run of the model. The first run might take more memory or be slower than the subsequent runs due cuda overheads.
- ***What are "Sync" and "Async" metrics?*** "Sync" metrics are obtained by syncing all GPU processes and stop measurement when all of them are executed. "Async" metrics are obtained without syncing all GPU processes and stop when the model output can be used by the CPU. We provide both metrics since both could be relevant depending on the use-case. We recommend to test the efficiency gains directly in your use-cases.
## Setup
You can run the smashed model with these steps:
0. Check requirements from the original repo openbmb/MiniCPM-2B-128k installed. In particular, check python, cuda, and transformers versions.
1. Make sure that you have installed quantization related packages.
```bash
pip install hqq
```
2. Load & run the model.
```python
from transformers import AutoModelForCausalLM, AutoTokenizer
from hqq.engine.hf import HQQModelForCausalLM
from hqq.models.hf.base import AutoHQQHFModel
try:
model = HQQModelForCausalLM.from_quantized("PrunaAI/openbmb-MiniCPM-2B-128k-HQQ-2bit-smashed", device_map='auto')
except:
model = AutoHQQHFModel.from_quantized("PrunaAI/openbmb-MiniCPM-2B-128k-HQQ-2bit-smashed")
tokenizer = AutoTokenizer.from_pretrained("openbmb/MiniCPM-2B-128k")
input_ids = tokenizer("What is the color of prunes?,", return_tensors='pt').to(model.device)["input_ids"]
outputs = model.generate(input_ids, max_new_tokens=216)
tokenizer.decode(outputs[0])
```
## Configurations
The configuration info are in `smash_config.json`.
## Credits & License
The license of the smashed model follows the license of the original model. Please check the license of the original model openbmb/MiniCPM-2B-128k before using this model which provided the base model. The license of the `pruna-engine` is [here](https://pypi.org/project/pruna-engine/) on Pypi.
## Want to compress other models?
- Contact us and tell us which model to compress next [here](https://www.pruna.ai/contact).
- Request access to easily compress your own AI models [here](https://z0halsaff74.typeform.com/pruna-access?typeform-source=www.pruna.ai). |
PrunaAI/openbmb-MiniCPM-2B-128k-HQQ-4bit-smashed | PrunaAI | "2024-06-24T10:22:39Z" | 0 | 0 | transformers | [
"transformers",
"minicpm",
"text-generation",
"pruna-ai",
"conversational",
"custom_code",
"base_model:openbmb/MiniCPM-2B-128k",
"autotrain_compatible",
"region:us"
] | text-generation | "2024-06-24T10:21:22Z" | ---
thumbnail: "https://assets-global.website-files.com/646b351987a8d8ce158d1940/64ec9e96b4334c0e1ac41504_Logo%20with%20white%20text.svg"
base_model: openbmb/MiniCPM-2B-128k
metrics:
- memory_disk
- memory_inference
- inference_latency
- inference_throughput
- inference_CO2_emissions
- inference_energy_consumption
tags:
- pruna-ai
---
<!-- header start -->
<!-- 200823 -->
<div style="width: auto; margin-left: auto; margin-right: auto">
<a href="https://www.pruna.ai/" target="_blank" rel="noopener noreferrer">
<img src="https://i.imgur.com/eDAlcgk.png" alt="PrunaAI" style="width: 100%; min-width: 400px; display: block; margin: auto;">
</a>
</div>
<!-- header end -->
[](https://twitter.com/PrunaAI)
[](https://github.com/PrunaAI)
[](https://www.linkedin.com/company/93832878/admin/feed/posts/?feedType=following)
[](https://discord.gg/CP4VSgck)
# Simply make AI models cheaper, smaller, faster, and greener!
- Give a thumbs up if you like this model!
- Contact us and tell us which model to compress next [here](https://www.pruna.ai/contact).
- Request access to easily compress your *own* AI models [here](https://z0halsaff74.typeform.com/pruna-access?typeform-source=www.pruna.ai).
- Read the documentations to know more [here](https://pruna-ai-pruna.readthedocs-hosted.com/en/latest/)
- Join Pruna AI community on Discord [here](https://discord.gg/CP4VSgck) to share feedback/suggestions or get help.
## Results

**Frequently Asked Questions**
- ***How does the compression work?*** The model is compressed with hqq.
- ***How does the model quality change?*** The quality of the model output might vary compared to the base model.
- ***How is the model efficiency evaluated?*** These results were obtained on HARDWARE_NAME with configuration described in `model/smash_config.json` and are obtained after a hardware warmup. The smashed model is directly compared to the original base model. Efficiency results may vary in other settings (e.g. other hardware, image size, batch size, ...). We recommend to directly run them in the use-case conditions to know if the smashed model can benefit you.
- ***What is the model format?*** We use safetensors.
- ***What calibration data has been used?*** If needed by the compression method, we used WikiText as the calibration data.
- ***What is the naming convention for Pruna Huggingface models?*** We take the original model name and append "turbo", "tiny", or "green" if the smashed model has a measured inference speed, inference memory, or inference energy consumption which is less than 90% of the original base model.
- ***How to compress my own models?*** You can request premium access to more compression methods and tech support for your specific use-cases [here](https://z0halsaff74.typeform.com/pruna-access?typeform-source=www.pruna.ai).
- ***What are "first" metrics?*** Results mentioning "first" are obtained after the first run of the model. The first run might take more memory or be slower than the subsequent runs due cuda overheads.
- ***What are "Sync" and "Async" metrics?*** "Sync" metrics are obtained by syncing all GPU processes and stop measurement when all of them are executed. "Async" metrics are obtained without syncing all GPU processes and stop when the model output can be used by the CPU. We provide both metrics since both could be relevant depending on the use-case. We recommend to test the efficiency gains directly in your use-cases.
## Setup
You can run the smashed model with these steps:
0. Check requirements from the original repo openbmb/MiniCPM-2B-128k installed. In particular, check python, cuda, and transformers versions.
1. Make sure that you have installed quantization related packages.
```bash
pip install hqq
```
2. Load & run the model.
```python
from transformers import AutoModelForCausalLM, AutoTokenizer
from hqq.engine.hf import HQQModelForCausalLM
from hqq.models.hf.base import AutoHQQHFModel
try:
model = HQQModelForCausalLM.from_quantized("PrunaAI/openbmb-MiniCPM-2B-128k-HQQ-4bit-smashed", device_map='auto')
except:
model = AutoHQQHFModel.from_quantized("PrunaAI/openbmb-MiniCPM-2B-128k-HQQ-4bit-smashed")
tokenizer = AutoTokenizer.from_pretrained("openbmb/MiniCPM-2B-128k")
input_ids = tokenizer("What is the color of prunes?,", return_tensors='pt').to(model.device)["input_ids"]
outputs = model.generate(input_ids, max_new_tokens=216)
tokenizer.decode(outputs[0])
```
## Configurations
The configuration info are in `smash_config.json`.
## Credits & License
The license of the smashed model follows the license of the original model. Please check the license of the original model openbmb/MiniCPM-2B-128k before using this model which provided the base model. The license of the `pruna-engine` is [here](https://pypi.org/project/pruna-engine/) on Pypi.
## Want to compress other models?
- Contact us and tell us which model to compress next [here](https://www.pruna.ai/contact).
- Request access to easily compress your own AI models [here](https://z0halsaff74.typeform.com/pruna-access?typeform-source=www.pruna.ai). |
afila/mistrella | afila | "2024-06-24T10:22:34Z" | 0 | 0 | null | [
"region:us"
] | null | "2024-06-24T10:22:34Z" | Entry not found |
PrunaAI/PipableAI-pip-library-etl-1.3b-HQQ-1bit-smashed | PrunaAI | "2024-06-24T10:23:21Z" | 0 | 0 | transformers | [
"transformers",
"llama",
"text-generation",
"pruna-ai",
"conversational",
"base_model:PipableAI/pip-library-etl-1.3b",
"autotrain_compatible",
"endpoints_compatible",
"text-generation-inference",
"region:us"
] | text-generation | "2024-06-24T10:23:03Z" | ---
thumbnail: "https://assets-global.website-files.com/646b351987a8d8ce158d1940/64ec9e96b4334c0e1ac41504_Logo%20with%20white%20text.svg"
base_model: PipableAI/pip-library-etl-1.3b
metrics:
- memory_disk
- memory_inference
- inference_latency
- inference_throughput
- inference_CO2_emissions
- inference_energy_consumption
tags:
- pruna-ai
---
<!-- header start -->
<!-- 200823 -->
<div style="width: auto; margin-left: auto; margin-right: auto">
<a href="https://www.pruna.ai/" target="_blank" rel="noopener noreferrer">
<img src="https://i.imgur.com/eDAlcgk.png" alt="PrunaAI" style="width: 100%; min-width: 400px; display: block; margin: auto;">
</a>
</div>
<!-- header end -->
[](https://twitter.com/PrunaAI)
[](https://github.com/PrunaAI)
[](https://www.linkedin.com/company/93832878/admin/feed/posts/?feedType=following)
[](https://discord.gg/CP4VSgck)
# Simply make AI models cheaper, smaller, faster, and greener!
- Give a thumbs up if you like this model!
- Contact us and tell us which model to compress next [here](https://www.pruna.ai/contact).
- Request access to easily compress your *own* AI models [here](https://z0halsaff74.typeform.com/pruna-access?typeform-source=www.pruna.ai).
- Read the documentations to know more [here](https://pruna-ai-pruna.readthedocs-hosted.com/en/latest/)
- Join Pruna AI community on Discord [here](https://discord.gg/CP4VSgck) to share feedback/suggestions or get help.
## Results

**Frequently Asked Questions**
- ***How does the compression work?*** The model is compressed with hqq.
- ***How does the model quality change?*** The quality of the model output might vary compared to the base model.
- ***How is the model efficiency evaluated?*** These results were obtained on HARDWARE_NAME with configuration described in `model/smash_config.json` and are obtained after a hardware warmup. The smashed model is directly compared to the original base model. Efficiency results may vary in other settings (e.g. other hardware, image size, batch size, ...). We recommend to directly run them in the use-case conditions to know if the smashed model can benefit you.
- ***What is the model format?*** We use safetensors.
- ***What calibration data has been used?*** If needed by the compression method, we used WikiText as the calibration data.
- ***What is the naming convention for Pruna Huggingface models?*** We take the original model name and append "turbo", "tiny", or "green" if the smashed model has a measured inference speed, inference memory, or inference energy consumption which is less than 90% of the original base model.
- ***How to compress my own models?*** You can request premium access to more compression methods and tech support for your specific use-cases [here](https://z0halsaff74.typeform.com/pruna-access?typeform-source=www.pruna.ai).
- ***What are "first" metrics?*** Results mentioning "first" are obtained after the first run of the model. The first run might take more memory or be slower than the subsequent runs due cuda overheads.
- ***What are "Sync" and "Async" metrics?*** "Sync" metrics are obtained by syncing all GPU processes and stop measurement when all of them are executed. "Async" metrics are obtained without syncing all GPU processes and stop when the model output can be used by the CPU. We provide both metrics since both could be relevant depending on the use-case. We recommend to test the efficiency gains directly in your use-cases.
## Setup
You can run the smashed model with these steps:
0. Check requirements from the original repo PipableAI/pip-library-etl-1.3b installed. In particular, check python, cuda, and transformers versions.
1. Make sure that you have installed quantization related packages.
```bash
pip install hqq
```
2. Load & run the model.
```python
from transformers import AutoModelForCausalLM, AutoTokenizer
from hqq.engine.hf import HQQModelForCausalLM
from hqq.models.hf.base import AutoHQQHFModel
try:
model = HQQModelForCausalLM.from_quantized("PrunaAI/PipableAI-pip-library-etl-1.3b-HQQ-1bit-smashed", device_map='auto')
except:
model = AutoHQQHFModel.from_quantized("PrunaAI/PipableAI-pip-library-etl-1.3b-HQQ-1bit-smashed")
tokenizer = AutoTokenizer.from_pretrained("PipableAI/pip-library-etl-1.3b")
input_ids = tokenizer("What is the color of prunes?,", return_tensors='pt').to(model.device)["input_ids"]
outputs = model.generate(input_ids, max_new_tokens=216)
tokenizer.decode(outputs[0])
```
## Configurations
The configuration info are in `smash_config.json`.
## Credits & License
The license of the smashed model follows the license of the original model. Please check the license of the original model PipableAI/pip-library-etl-1.3b before using this model which provided the base model. The license of the `pruna-engine` is [here](https://pypi.org/project/pruna-engine/) on Pypi.
## Want to compress other models?
- Contact us and tell us which model to compress next [here](https://www.pruna.ai/contact).
- Request access to easily compress your own AI models [here](https://z0halsaff74.typeform.com/pruna-access?typeform-source=www.pruna.ai). |
afila/mistral | afila | "2024-06-24T10:23:03Z" | 0 | 0 | null | [
"region:us"
] | null | "2024-06-24T10:23:03Z" | Entry not found |
PrunaAI/PipableAI-pip-library-etl-1.3b-HQQ-2bit-smashed | PrunaAI | "2024-06-24T10:23:36Z" | 0 | 0 | transformers | [
"transformers",
"llama",
"text-generation",
"pruna-ai",
"conversational",
"base_model:PipableAI/pip-library-etl-1.3b",
"autotrain_compatible",
"endpoints_compatible",
"text-generation-inference",
"region:us"
] | text-generation | "2024-06-24T10:23:13Z" | ---
thumbnail: "https://assets-global.website-files.com/646b351987a8d8ce158d1940/64ec9e96b4334c0e1ac41504_Logo%20with%20white%20text.svg"
base_model: PipableAI/pip-library-etl-1.3b
metrics:
- memory_disk
- memory_inference
- inference_latency
- inference_throughput
- inference_CO2_emissions
- inference_energy_consumption
tags:
- pruna-ai
---
<!-- header start -->
<!-- 200823 -->
<div style="width: auto; margin-left: auto; margin-right: auto">
<a href="https://www.pruna.ai/" target="_blank" rel="noopener noreferrer">
<img src="https://i.imgur.com/eDAlcgk.png" alt="PrunaAI" style="width: 100%; min-width: 400px; display: block; margin: auto;">
</a>
</div>
<!-- header end -->
[](https://twitter.com/PrunaAI)
[](https://github.com/PrunaAI)
[](https://www.linkedin.com/company/93832878/admin/feed/posts/?feedType=following)
[](https://discord.gg/CP4VSgck)
# Simply make AI models cheaper, smaller, faster, and greener!
- Give a thumbs up if you like this model!
- Contact us and tell us which model to compress next [here](https://www.pruna.ai/contact).
- Request access to easily compress your *own* AI models [here](https://z0halsaff74.typeform.com/pruna-access?typeform-source=www.pruna.ai).
- Read the documentations to know more [here](https://pruna-ai-pruna.readthedocs-hosted.com/en/latest/)
- Join Pruna AI community on Discord [here](https://discord.gg/CP4VSgck) to share feedback/suggestions or get help.
## Results

**Frequently Asked Questions**
- ***How does the compression work?*** The model is compressed with hqq.
- ***How does the model quality change?*** The quality of the model output might vary compared to the base model.
- ***How is the model efficiency evaluated?*** These results were obtained on HARDWARE_NAME with configuration described in `model/smash_config.json` and are obtained after a hardware warmup. The smashed model is directly compared to the original base model. Efficiency results may vary in other settings (e.g. other hardware, image size, batch size, ...). We recommend to directly run them in the use-case conditions to know if the smashed model can benefit you.
- ***What is the model format?*** We use safetensors.
- ***What calibration data has been used?*** If needed by the compression method, we used WikiText as the calibration data.
- ***What is the naming convention for Pruna Huggingface models?*** We take the original model name and append "turbo", "tiny", or "green" if the smashed model has a measured inference speed, inference memory, or inference energy consumption which is less than 90% of the original base model.
- ***How to compress my own models?*** You can request premium access to more compression methods and tech support for your specific use-cases [here](https://z0halsaff74.typeform.com/pruna-access?typeform-source=www.pruna.ai).
- ***What are "first" metrics?*** Results mentioning "first" are obtained after the first run of the model. The first run might take more memory or be slower than the subsequent runs due cuda overheads.
- ***What are "Sync" and "Async" metrics?*** "Sync" metrics are obtained by syncing all GPU processes and stop measurement when all of them are executed. "Async" metrics are obtained without syncing all GPU processes and stop when the model output can be used by the CPU. We provide both metrics since both could be relevant depending on the use-case. We recommend to test the efficiency gains directly in your use-cases.
## Setup
You can run the smashed model with these steps:
0. Check requirements from the original repo PipableAI/pip-library-etl-1.3b installed. In particular, check python, cuda, and transformers versions.
1. Make sure that you have installed quantization related packages.
```bash
pip install hqq
```
2. Load & run the model.
```python
from transformers import AutoModelForCausalLM, AutoTokenizer
from hqq.engine.hf import HQQModelForCausalLM
from hqq.models.hf.base import AutoHQQHFModel
try:
model = HQQModelForCausalLM.from_quantized("PrunaAI/PipableAI-pip-library-etl-1.3b-HQQ-2bit-smashed", device_map='auto')
except:
model = AutoHQQHFModel.from_quantized("PrunaAI/PipableAI-pip-library-etl-1.3b-HQQ-2bit-smashed")
tokenizer = AutoTokenizer.from_pretrained("PipableAI/pip-library-etl-1.3b")
input_ids = tokenizer("What is the color of prunes?,", return_tensors='pt').to(model.device)["input_ids"]
outputs = model.generate(input_ids, max_new_tokens=216)
tokenizer.decode(outputs[0])
```
## Configurations
The configuration info are in `smash_config.json`.
## Credits & License
The license of the smashed model follows the license of the original model. Please check the license of the original model PipableAI/pip-library-etl-1.3b before using this model which provided the base model. The license of the `pruna-engine` is [here](https://pypi.org/project/pruna-engine/) on Pypi.
## Want to compress other models?
- Contact us and tell us which model to compress next [here](https://www.pruna.ai/contact).
- Request access to easily compress your own AI models [here](https://z0halsaff74.typeform.com/pruna-access?typeform-source=www.pruna.ai). |
PrunaAI/PipableAI-pip-library-etl-1.3b-HQQ-4bit-smashed | PrunaAI | "2024-06-24T10:23:54Z" | 0 | 0 | transformers | [
"transformers",
"llama",
"text-generation",
"pruna-ai",
"conversational",
"base_model:PipableAI/pip-library-etl-1.3b",
"autotrain_compatible",
"endpoints_compatible",
"text-generation-inference",
"region:us"
] | text-generation | "2024-06-24T10:23:24Z" | ---
thumbnail: "https://assets-global.website-files.com/646b351987a8d8ce158d1940/64ec9e96b4334c0e1ac41504_Logo%20with%20white%20text.svg"
base_model: PipableAI/pip-library-etl-1.3b
metrics:
- memory_disk
- memory_inference
- inference_latency
- inference_throughput
- inference_CO2_emissions
- inference_energy_consumption
tags:
- pruna-ai
---
<!-- header start -->
<!-- 200823 -->
<div style="width: auto; margin-left: auto; margin-right: auto">
<a href="https://www.pruna.ai/" target="_blank" rel="noopener noreferrer">
<img src="https://i.imgur.com/eDAlcgk.png" alt="PrunaAI" style="width: 100%; min-width: 400px; display: block; margin: auto;">
</a>
</div>
<!-- header end -->
[](https://twitter.com/PrunaAI)
[](https://github.com/PrunaAI)
[](https://www.linkedin.com/company/93832878/admin/feed/posts/?feedType=following)
[](https://discord.gg/CP4VSgck)
# Simply make AI models cheaper, smaller, faster, and greener!
- Give a thumbs up if you like this model!
- Contact us and tell us which model to compress next [here](https://www.pruna.ai/contact).
- Request access to easily compress your *own* AI models [here](https://z0halsaff74.typeform.com/pruna-access?typeform-source=www.pruna.ai).
- Read the documentations to know more [here](https://pruna-ai-pruna.readthedocs-hosted.com/en/latest/)
- Join Pruna AI community on Discord [here](https://discord.gg/CP4VSgck) to share feedback/suggestions or get help.
## Results

**Frequently Asked Questions**
- ***How does the compression work?*** The model is compressed with hqq.
- ***How does the model quality change?*** The quality of the model output might vary compared to the base model.
- ***How is the model efficiency evaluated?*** These results were obtained on HARDWARE_NAME with configuration described in `model/smash_config.json` and are obtained after a hardware warmup. The smashed model is directly compared to the original base model. Efficiency results may vary in other settings (e.g. other hardware, image size, batch size, ...). We recommend to directly run them in the use-case conditions to know if the smashed model can benefit you.
- ***What is the model format?*** We use safetensors.
- ***What calibration data has been used?*** If needed by the compression method, we used WikiText as the calibration data.
- ***What is the naming convention for Pruna Huggingface models?*** We take the original model name and append "turbo", "tiny", or "green" if the smashed model has a measured inference speed, inference memory, or inference energy consumption which is less than 90% of the original base model.
- ***How to compress my own models?*** You can request premium access to more compression methods and tech support for your specific use-cases [here](https://z0halsaff74.typeform.com/pruna-access?typeform-source=www.pruna.ai).
- ***What are "first" metrics?*** Results mentioning "first" are obtained after the first run of the model. The first run might take more memory or be slower than the subsequent runs due cuda overheads.
- ***What are "Sync" and "Async" metrics?*** "Sync" metrics are obtained by syncing all GPU processes and stop measurement when all of them are executed. "Async" metrics are obtained without syncing all GPU processes and stop when the model output can be used by the CPU. We provide both metrics since both could be relevant depending on the use-case. We recommend to test the efficiency gains directly in your use-cases.
## Setup
You can run the smashed model with these steps:
0. Check requirements from the original repo PipableAI/pip-library-etl-1.3b installed. In particular, check python, cuda, and transformers versions.
1. Make sure that you have installed quantization related packages.
```bash
pip install hqq
```
2. Load & run the model.
```python
from transformers import AutoModelForCausalLM, AutoTokenizer
from hqq.engine.hf import HQQModelForCausalLM
from hqq.models.hf.base import AutoHQQHFModel
try:
model = HQQModelForCausalLM.from_quantized("PrunaAI/PipableAI-pip-library-etl-1.3b-HQQ-4bit-smashed", device_map='auto')
except:
model = AutoHQQHFModel.from_quantized("PrunaAI/PipableAI-pip-library-etl-1.3b-HQQ-4bit-smashed")
tokenizer = AutoTokenizer.from_pretrained("PipableAI/pip-library-etl-1.3b")
input_ids = tokenizer("What is the color of prunes?,", return_tensors='pt').to(model.device)["input_ids"]
outputs = model.generate(input_ids, max_new_tokens=216)
tokenizer.decode(outputs[0])
```
## Configurations
The configuration info are in `smash_config.json`.
## Credits & License
The license of the smashed model follows the license of the original model. Please check the license of the original model PipableAI/pip-library-etl-1.3b before using this model which provided the base model. The license of the `pruna-engine` is [here](https://pypi.org/project/pruna-engine/) on Pypi.
## Want to compress other models?
- Contact us and tell us which model to compress next [here](https://www.pruna.ai/contact).
- Request access to easily compress your own AI models [here](https://z0halsaff74.typeform.com/pruna-access?typeform-source=www.pruna.ai). |
PrunaAI/aisquared-dlite-v2-774m-HQQ-1bit-smashed | PrunaAI | "2024-06-24T10:24:25Z" | 0 | 0 | transformers | [
"transformers",
"gpt2",
"text-generation",
"pruna-ai",
"base_model:aisquared/dlite-v2-774m",
"autotrain_compatible",
"endpoints_compatible",
"text-generation-inference",
"region:us"
] | text-generation | "2024-06-24T10:23:36Z" | ---
thumbnail: "https://assets-global.website-files.com/646b351987a8d8ce158d1940/64ec9e96b4334c0e1ac41504_Logo%20with%20white%20text.svg"
base_model: aisquared/dlite-v2-774m
metrics:
- memory_disk
- memory_inference
- inference_latency
- inference_throughput
- inference_CO2_emissions
- inference_energy_consumption
tags:
- pruna-ai
---
<!-- header start -->
<!-- 200823 -->
<div style="width: auto; margin-left: auto; margin-right: auto">
<a href="https://www.pruna.ai/" target="_blank" rel="noopener noreferrer">
<img src="https://i.imgur.com/eDAlcgk.png" alt="PrunaAI" style="width: 100%; min-width: 400px; display: block; margin: auto;">
</a>
</div>
<!-- header end -->
[](https://twitter.com/PrunaAI)
[](https://github.com/PrunaAI)
[](https://www.linkedin.com/company/93832878/admin/feed/posts/?feedType=following)
[](https://discord.gg/CP4VSgck)
# Simply make AI models cheaper, smaller, faster, and greener!
- Give a thumbs up if you like this model!
- Contact us and tell us which model to compress next [here](https://www.pruna.ai/contact).
- Request access to easily compress your *own* AI models [here](https://z0halsaff74.typeform.com/pruna-access?typeform-source=www.pruna.ai).
- Read the documentations to know more [here](https://pruna-ai-pruna.readthedocs-hosted.com/en/latest/)
- Join Pruna AI community on Discord [here](https://discord.gg/CP4VSgck) to share feedback/suggestions or get help.
## Results

**Frequently Asked Questions**
- ***How does the compression work?*** The model is compressed with hqq.
- ***How does the model quality change?*** The quality of the model output might vary compared to the base model.
- ***How is the model efficiency evaluated?*** These results were obtained on HARDWARE_NAME with configuration described in `model/smash_config.json` and are obtained after a hardware warmup. The smashed model is directly compared to the original base model. Efficiency results may vary in other settings (e.g. other hardware, image size, batch size, ...). We recommend to directly run them in the use-case conditions to know if the smashed model can benefit you.
- ***What is the model format?*** We use safetensors.
- ***What calibration data has been used?*** If needed by the compression method, we used WikiText as the calibration data.
- ***What is the naming convention for Pruna Huggingface models?*** We take the original model name and append "turbo", "tiny", or "green" if the smashed model has a measured inference speed, inference memory, or inference energy consumption which is less than 90% of the original base model.
- ***How to compress my own models?*** You can request premium access to more compression methods and tech support for your specific use-cases [here](https://z0halsaff74.typeform.com/pruna-access?typeform-source=www.pruna.ai).
- ***What are "first" metrics?*** Results mentioning "first" are obtained after the first run of the model. The first run might take more memory or be slower than the subsequent runs due cuda overheads.
- ***What are "Sync" and "Async" metrics?*** "Sync" metrics are obtained by syncing all GPU processes and stop measurement when all of them are executed. "Async" metrics are obtained without syncing all GPU processes and stop when the model output can be used by the CPU. We provide both metrics since both could be relevant depending on the use-case. We recommend to test the efficiency gains directly in your use-cases.
## Setup
You can run the smashed model with these steps:
0. Check requirements from the original repo aisquared/dlite-v2-774m installed. In particular, check python, cuda, and transformers versions.
1. Make sure that you have installed quantization related packages.
```bash
pip install hqq
```
2. Load & run the model.
```python
from transformers import AutoModelForCausalLM, AutoTokenizer
from hqq.engine.hf import HQQModelForCausalLM
from hqq.models.hf.base import AutoHQQHFModel
try:
model = HQQModelForCausalLM.from_quantized("PrunaAI/aisquared-dlite-v2-774m-HQQ-1bit-smashed", device_map='auto')
except:
model = AutoHQQHFModel.from_quantized("PrunaAI/aisquared-dlite-v2-774m-HQQ-1bit-smashed")
tokenizer = AutoTokenizer.from_pretrained("aisquared/dlite-v2-774m")
input_ids = tokenizer("What is the color of prunes?,", return_tensors='pt').to(model.device)["input_ids"]
outputs = model.generate(input_ids, max_new_tokens=216)
tokenizer.decode(outputs[0])
```
## Configurations
The configuration info are in `smash_config.json`.
## Credits & License
The license of the smashed model follows the license of the original model. Please check the license of the original model aisquared/dlite-v2-774m before using this model which provided the base model. The license of the `pruna-engine` is [here](https://pypi.org/project/pruna-engine/) on Pypi.
## Want to compress other models?
- Contact us and tell us which model to compress next [here](https://www.pruna.ai/contact).
- Request access to easily compress your own AI models [here](https://z0halsaff74.typeform.com/pruna-access?typeform-source=www.pruna.ai). |
PrunaAI/aisquared-dlite-v2-774m-HQQ-2bit-smashed | PrunaAI | "2024-06-24T10:24:37Z" | 0 | 0 | transformers | [
"transformers",
"gpt2",
"text-generation",
"pruna-ai",
"base_model:aisquared/dlite-v2-774m",
"autotrain_compatible",
"endpoints_compatible",
"text-generation-inference",
"region:us"
] | text-generation | "2024-06-24T10:23:50Z" | ---
thumbnail: "https://assets-global.website-files.com/646b351987a8d8ce158d1940/64ec9e96b4334c0e1ac41504_Logo%20with%20white%20text.svg"
base_model: aisquared/dlite-v2-774m
metrics:
- memory_disk
- memory_inference
- inference_latency
- inference_throughput
- inference_CO2_emissions
- inference_energy_consumption
tags:
- pruna-ai
---
<!-- header start -->
<!-- 200823 -->
<div style="width: auto; margin-left: auto; margin-right: auto">
<a href="https://www.pruna.ai/" target="_blank" rel="noopener noreferrer">
<img src="https://i.imgur.com/eDAlcgk.png" alt="PrunaAI" style="width: 100%; min-width: 400px; display: block; margin: auto;">
</a>
</div>
<!-- header end -->
[](https://twitter.com/PrunaAI)
[](https://github.com/PrunaAI)
[](https://www.linkedin.com/company/93832878/admin/feed/posts/?feedType=following)
[](https://discord.gg/CP4VSgck)
# Simply make AI models cheaper, smaller, faster, and greener!
- Give a thumbs up if you like this model!
- Contact us and tell us which model to compress next [here](https://www.pruna.ai/contact).
- Request access to easily compress your *own* AI models [here](https://z0halsaff74.typeform.com/pruna-access?typeform-source=www.pruna.ai).
- Read the documentations to know more [here](https://pruna-ai-pruna.readthedocs-hosted.com/en/latest/)
- Join Pruna AI community on Discord [here](https://discord.gg/CP4VSgck) to share feedback/suggestions or get help.
## Results

**Frequently Asked Questions**
- ***How does the compression work?*** The model is compressed with hqq.
- ***How does the model quality change?*** The quality of the model output might vary compared to the base model.
- ***How is the model efficiency evaluated?*** These results were obtained on HARDWARE_NAME with configuration described in `model/smash_config.json` and are obtained after a hardware warmup. The smashed model is directly compared to the original base model. Efficiency results may vary in other settings (e.g. other hardware, image size, batch size, ...). We recommend to directly run them in the use-case conditions to know if the smashed model can benefit you.
- ***What is the model format?*** We use safetensors.
- ***What calibration data has been used?*** If needed by the compression method, we used WikiText as the calibration data.
- ***What is the naming convention for Pruna Huggingface models?*** We take the original model name and append "turbo", "tiny", or "green" if the smashed model has a measured inference speed, inference memory, or inference energy consumption which is less than 90% of the original base model.
- ***How to compress my own models?*** You can request premium access to more compression methods and tech support for your specific use-cases [here](https://z0halsaff74.typeform.com/pruna-access?typeform-source=www.pruna.ai).
- ***What are "first" metrics?*** Results mentioning "first" are obtained after the first run of the model. The first run might take more memory or be slower than the subsequent runs due cuda overheads.
- ***What are "Sync" and "Async" metrics?*** "Sync" metrics are obtained by syncing all GPU processes and stop measurement when all of them are executed. "Async" metrics are obtained without syncing all GPU processes and stop when the model output can be used by the CPU. We provide both metrics since both could be relevant depending on the use-case. We recommend to test the efficiency gains directly in your use-cases.
## Setup
You can run the smashed model with these steps:
0. Check requirements from the original repo aisquared/dlite-v2-774m installed. In particular, check python, cuda, and transformers versions.
1. Make sure that you have installed quantization related packages.
```bash
pip install hqq
```
2. Load & run the model.
```python
from transformers import AutoModelForCausalLM, AutoTokenizer
from hqq.engine.hf import HQQModelForCausalLM
from hqq.models.hf.base import AutoHQQHFModel
try:
model = HQQModelForCausalLM.from_quantized("PrunaAI/aisquared-dlite-v2-774m-HQQ-2bit-smashed", device_map='auto')
except:
model = AutoHQQHFModel.from_quantized("PrunaAI/aisquared-dlite-v2-774m-HQQ-2bit-smashed")
tokenizer = AutoTokenizer.from_pretrained("aisquared/dlite-v2-774m")
input_ids = tokenizer("What is the color of prunes?,", return_tensors='pt').to(model.device)["input_ids"]
outputs = model.generate(input_ids, max_new_tokens=216)
tokenizer.decode(outputs[0])
```
## Configurations
The configuration info are in `smash_config.json`.
## Credits & License
The license of the smashed model follows the license of the original model. Please check the license of the original model aisquared/dlite-v2-774m before using this model which provided the base model. The license of the `pruna-engine` is [here](https://pypi.org/project/pruna-engine/) on Pypi.
## Want to compress other models?
- Contact us and tell us which model to compress next [here](https://www.pruna.ai/contact).
- Request access to easily compress your own AI models [here](https://z0halsaff74.typeform.com/pruna-access?typeform-source=www.pruna.ai). |
9rofe/Wernicke-AI2 | 9rofe | "2024-06-24T10:23:58Z" | 0 | 0 | transformers | [
"transformers",
"safetensors",
"arxiv:1910.09700",
"endpoints_compatible",
"region:us"
] | null | "2024-06-24T10:23:55Z" | ---
library_name: transformers
tags: []
---
# Model Card for Model ID
<!-- Provide a quick summary of what the model is/does. -->
## Model Details
### Model Description
<!-- Provide a longer summary of what this model is. -->
This is the model card of a 🤗 transformers model that has been pushed on the Hub. This model card has been automatically generated.
- **Developed by:** [More Information Needed]
- **Funded by [optional]:** [More Information Needed]
- **Shared by [optional]:** [More Information Needed]
- **Model type:** [More Information Needed]
- **Language(s) (NLP):** [More Information Needed]
- **License:** [More Information Needed]
- **Finetuned from model [optional]:** [More Information Needed]
### Model Sources [optional]
<!-- Provide the basic links for the model. -->
- **Repository:** [More Information Needed]
- **Paper [optional]:** [More Information Needed]
- **Demo [optional]:** [More Information Needed]
## Uses
<!-- Address questions around how the model is intended to be used, including the foreseeable users of the model and those affected by the model. -->
### Direct Use
<!-- This section is for the model use without fine-tuning or plugging into a larger ecosystem/app. -->
[More Information Needed]
### Downstream Use [optional]
<!-- This section is for the model use when fine-tuned for a task, or when plugged into a larger ecosystem/app -->
[More Information Needed]
### Out-of-Scope Use
<!-- This section addresses misuse, malicious use, and uses that the model will not work well for. -->
[More Information Needed]
## Bias, Risks, and Limitations
<!-- This section is meant to convey both technical and sociotechnical limitations. -->
[More Information Needed]
### Recommendations
<!-- This section is meant to convey recommendations with respect to the bias, risk, and technical limitations. -->
Users (both direct and downstream) should be made aware of the risks, biases and limitations of the model. More information needed for further recommendations.
## How to Get Started with the Model
Use the code below to get started with the model.
[More Information Needed]
## Training Details
### Training Data
<!-- This should link to a Dataset Card, perhaps with a short stub of information on what the training data is all about as well as documentation related to data pre-processing or additional filtering. -->
[More Information Needed]
### Training Procedure
<!-- This relates heavily to the Technical Specifications. Content here should link to that section when it is relevant to the training procedure. -->
#### Preprocessing [optional]
[More Information Needed]
#### Training Hyperparameters
- **Training regime:** [More Information Needed] <!--fp32, fp16 mixed precision, bf16 mixed precision, bf16 non-mixed precision, fp16 non-mixed precision, fp8 mixed precision -->
#### Speeds, Sizes, Times [optional]
<!-- This section provides information about throughput, start/end time, checkpoint size if relevant, etc. -->
[More Information Needed]
## Evaluation
<!-- This section describes the evaluation protocols and provides the results. -->
### Testing Data, Factors & Metrics
#### Testing Data
<!-- This should link to a Dataset Card if possible. -->
[More Information Needed]
#### Factors
<!-- These are the things the evaluation is disaggregating by, e.g., subpopulations or domains. -->
[More Information Needed]
#### Metrics
<!-- These are the evaluation metrics being used, ideally with a description of why. -->
[More Information Needed]
### Results
[More Information Needed]
#### Summary
## Model Examination [optional]
<!-- Relevant interpretability work for the model goes here -->
[More Information Needed]
## Environmental Impact
<!-- Total emissions (in grams of CO2eq) and additional considerations, such as electricity usage, go here. Edit the suggested text below accordingly -->
Carbon emissions can be estimated using the [Machine Learning Impact calculator](https://mlco2.github.io/impact#compute) presented in [Lacoste et al. (2019)](https://arxiv.org/abs/1910.09700).
- **Hardware Type:** [More Information Needed]
- **Hours used:** [More Information Needed]
- **Cloud Provider:** [More Information Needed]
- **Compute Region:** [More Information Needed]
- **Carbon Emitted:** [More Information Needed]
## Technical Specifications [optional]
### Model Architecture and Objective
[More Information Needed]
### Compute Infrastructure
[More Information Needed]
#### Hardware
[More Information Needed]
#### Software
[More Information Needed]
## Citation [optional]
<!-- If there is a paper or blog post introducing the model, the APA and Bibtex information for that should go in this section. -->
**BibTeX:**
[More Information Needed]
**APA:**
[More Information Needed]
## Glossary [optional]
<!-- If relevant, include terms and calculations in this section that can help readers understand the model or model card. -->
[More Information Needed]
## More Information [optional]
[More Information Needed]
## Model Card Authors [optional]
[More Information Needed]
## Model Card Contact
[More Information Needed] |
PrunaAI/aisquared-dlite-v2-774m-HQQ-4bit-smashed | PrunaAI | "2024-06-24T10:24:48Z" | 0 | 0 | transformers | [
"transformers",
"gpt2",
"text-generation",
"pruna-ai",
"base_model:aisquared/dlite-v2-774m",
"autotrain_compatible",
"endpoints_compatible",
"text-generation-inference",
"region:us"
] | text-generation | "2024-06-24T10:23:59Z" | ---
thumbnail: "https://assets-global.website-files.com/646b351987a8d8ce158d1940/64ec9e96b4334c0e1ac41504_Logo%20with%20white%20text.svg"
base_model: aisquared/dlite-v2-774m
metrics:
- memory_disk
- memory_inference
- inference_latency
- inference_throughput
- inference_CO2_emissions
- inference_energy_consumption
tags:
- pruna-ai
---
<!-- header start -->
<!-- 200823 -->
<div style="width: auto; margin-left: auto; margin-right: auto">
<a href="https://www.pruna.ai/" target="_blank" rel="noopener noreferrer">
<img src="https://i.imgur.com/eDAlcgk.png" alt="PrunaAI" style="width: 100%; min-width: 400px; display: block; margin: auto;">
</a>
</div>
<!-- header end -->
[](https://twitter.com/PrunaAI)
[](https://github.com/PrunaAI)
[](https://www.linkedin.com/company/93832878/admin/feed/posts/?feedType=following)
[](https://discord.gg/CP4VSgck)
# Simply make AI models cheaper, smaller, faster, and greener!
- Give a thumbs up if you like this model!
- Contact us and tell us which model to compress next [here](https://www.pruna.ai/contact).
- Request access to easily compress your *own* AI models [here](https://z0halsaff74.typeform.com/pruna-access?typeform-source=www.pruna.ai).
- Read the documentations to know more [here](https://pruna-ai-pruna.readthedocs-hosted.com/en/latest/)
- Join Pruna AI community on Discord [here](https://discord.gg/CP4VSgck) to share feedback/suggestions or get help.
## Results

**Frequently Asked Questions**
- ***How does the compression work?*** The model is compressed with hqq.
- ***How does the model quality change?*** The quality of the model output might vary compared to the base model.
- ***How is the model efficiency evaluated?*** These results were obtained on HARDWARE_NAME with configuration described in `model/smash_config.json` and are obtained after a hardware warmup. The smashed model is directly compared to the original base model. Efficiency results may vary in other settings (e.g. other hardware, image size, batch size, ...). We recommend to directly run them in the use-case conditions to know if the smashed model can benefit you.
- ***What is the model format?*** We use safetensors.
- ***What calibration data has been used?*** If needed by the compression method, we used WikiText as the calibration data.
- ***What is the naming convention for Pruna Huggingface models?*** We take the original model name and append "turbo", "tiny", or "green" if the smashed model has a measured inference speed, inference memory, or inference energy consumption which is less than 90% of the original base model.
- ***How to compress my own models?*** You can request premium access to more compression methods and tech support for your specific use-cases [here](https://z0halsaff74.typeform.com/pruna-access?typeform-source=www.pruna.ai).
- ***What are "first" metrics?*** Results mentioning "first" are obtained after the first run of the model. The first run might take more memory or be slower than the subsequent runs due cuda overheads.
- ***What are "Sync" and "Async" metrics?*** "Sync" metrics are obtained by syncing all GPU processes and stop measurement when all of them are executed. "Async" metrics are obtained without syncing all GPU processes and stop when the model output can be used by the CPU. We provide both metrics since both could be relevant depending on the use-case. We recommend to test the efficiency gains directly in your use-cases.
## Setup
You can run the smashed model with these steps:
0. Check requirements from the original repo aisquared/dlite-v2-774m installed. In particular, check python, cuda, and transformers versions.
1. Make sure that you have installed quantization related packages.
```bash
pip install hqq
```
2. Load & run the model.
```python
from transformers import AutoModelForCausalLM, AutoTokenizer
from hqq.engine.hf import HQQModelForCausalLM
from hqq.models.hf.base import AutoHQQHFModel
try:
model = HQQModelForCausalLM.from_quantized("PrunaAI/aisquared-dlite-v2-774m-HQQ-4bit-smashed", device_map='auto')
except:
model = AutoHQQHFModel.from_quantized("PrunaAI/aisquared-dlite-v2-774m-HQQ-4bit-smashed")
tokenizer = AutoTokenizer.from_pretrained("aisquared/dlite-v2-774m")
input_ids = tokenizer("What is the color of prunes?,", return_tensors='pt').to(model.device)["input_ids"]
outputs = model.generate(input_ids, max_new_tokens=216)
tokenizer.decode(outputs[0])
```
## Configurations
The configuration info are in `smash_config.json`.
## Credits & License
The license of the smashed model follows the license of the original model. Please check the license of the original model aisquared/dlite-v2-774m before using this model which provided the base model. The license of the `pruna-engine` is [here](https://pypi.org/project/pruna-engine/) on Pypi.
## Want to compress other models?
- Contact us and tell us which model to compress next [here](https://www.pruna.ai/contact).
- Request access to easily compress your own AI models [here](https://z0halsaff74.typeform.com/pruna-access?typeform-source=www.pruna.ai). |
dmm771/code-llama-7b-text-to-sql | dmm771 | "2024-06-24T10:24:17Z" | 0 | 0 | null | [
"region:us"
] | null | "2024-06-24T10:24:17Z" | Entry not found |
PrunaAI/Xwin-LM-Xwin-Math-7B-V1.1-HQQ-1bit-smashed | PrunaAI | "2024-06-24T10:27:03Z" | 0 | 0 | transformers | [
"transformers",
"llama",
"text-generation",
"pruna-ai",
"base_model:Xwin-LM/Xwin-Math-7B-V1.1",
"autotrain_compatible",
"endpoints_compatible",
"text-generation-inference",
"region:us"
] | text-generation | "2024-06-24T10:26:19Z" | ---
thumbnail: "https://assets-global.website-files.com/646b351987a8d8ce158d1940/64ec9e96b4334c0e1ac41504_Logo%20with%20white%20text.svg"
base_model: Xwin-LM/Xwin-Math-7B-V1.1
metrics:
- memory_disk
- memory_inference
- inference_latency
- inference_throughput
- inference_CO2_emissions
- inference_energy_consumption
tags:
- pruna-ai
---
<!-- header start -->
<!-- 200823 -->
<div style="width: auto; margin-left: auto; margin-right: auto">
<a href="https://www.pruna.ai/" target="_blank" rel="noopener noreferrer">
<img src="https://i.imgur.com/eDAlcgk.png" alt="PrunaAI" style="width: 100%; min-width: 400px; display: block; margin: auto;">
</a>
</div>
<!-- header end -->
[](https://twitter.com/PrunaAI)
[](https://github.com/PrunaAI)
[](https://www.linkedin.com/company/93832878/admin/feed/posts/?feedType=following)
[](https://discord.gg/CP4VSgck)
# Simply make AI models cheaper, smaller, faster, and greener!
- Give a thumbs up if you like this model!
- Contact us and tell us which model to compress next [here](https://www.pruna.ai/contact).
- Request access to easily compress your *own* AI models [here](https://z0halsaff74.typeform.com/pruna-access?typeform-source=www.pruna.ai).
- Read the documentations to know more [here](https://pruna-ai-pruna.readthedocs-hosted.com/en/latest/)
- Join Pruna AI community on Discord [here](https://discord.gg/CP4VSgck) to share feedback/suggestions or get help.
## Results

**Frequently Asked Questions**
- ***How does the compression work?*** The model is compressed with hqq.
- ***How does the model quality change?*** The quality of the model output might vary compared to the base model.
- ***How is the model efficiency evaluated?*** These results were obtained on HARDWARE_NAME with configuration described in `model/smash_config.json` and are obtained after a hardware warmup. The smashed model is directly compared to the original base model. Efficiency results may vary in other settings (e.g. other hardware, image size, batch size, ...). We recommend to directly run them in the use-case conditions to know if the smashed model can benefit you.
- ***What is the model format?*** We use safetensors.
- ***What calibration data has been used?*** If needed by the compression method, we used WikiText as the calibration data.
- ***What is the naming convention for Pruna Huggingface models?*** We take the original model name and append "turbo", "tiny", or "green" if the smashed model has a measured inference speed, inference memory, or inference energy consumption which is less than 90% of the original base model.
- ***How to compress my own models?*** You can request premium access to more compression methods and tech support for your specific use-cases [here](https://z0halsaff74.typeform.com/pruna-access?typeform-source=www.pruna.ai).
- ***What are "first" metrics?*** Results mentioning "first" are obtained after the first run of the model. The first run might take more memory or be slower than the subsequent runs due cuda overheads.
- ***What are "Sync" and "Async" metrics?*** "Sync" metrics are obtained by syncing all GPU processes and stop measurement when all of them are executed. "Async" metrics are obtained without syncing all GPU processes and stop when the model output can be used by the CPU. We provide both metrics since both could be relevant depending on the use-case. We recommend to test the efficiency gains directly in your use-cases.
## Setup
You can run the smashed model with these steps:
0. Check requirements from the original repo Xwin-LM/Xwin-Math-7B-V1.1 installed. In particular, check python, cuda, and transformers versions.
1. Make sure that you have installed quantization related packages.
```bash
pip install hqq
```
2. Load & run the model.
```python
from transformers import AutoModelForCausalLM, AutoTokenizer
from hqq.engine.hf import HQQModelForCausalLM
from hqq.models.hf.base import AutoHQQHFModel
try:
model = HQQModelForCausalLM.from_quantized("PrunaAI/Xwin-LM-Xwin-Math-7B-V1.1-HQQ-1bit-smashed", device_map='auto')
except:
model = AutoHQQHFModel.from_quantized("PrunaAI/Xwin-LM-Xwin-Math-7B-V1.1-HQQ-1bit-smashed")
tokenizer = AutoTokenizer.from_pretrained("Xwin-LM/Xwin-Math-7B-V1.1")
input_ids = tokenizer("What is the color of prunes?,", return_tensors='pt').to(model.device)["input_ids"]
outputs = model.generate(input_ids, max_new_tokens=216)
tokenizer.decode(outputs[0])
```
## Configurations
The configuration info are in `smash_config.json`.
## Credits & License
The license of the smashed model follows the license of the original model. Please check the license of the original model Xwin-LM/Xwin-Math-7B-V1.1 before using this model which provided the base model. The license of the `pruna-engine` is [here](https://pypi.org/project/pruna-engine/) on Pypi.
## Want to compress other models?
- Contact us and tell us which model to compress next [here](https://www.pruna.ai/contact).
- Request access to easily compress your own AI models [here](https://z0halsaff74.typeform.com/pruna-access?typeform-source=www.pruna.ai). |
PrunaAI/Xwin-LM-Xwin-Math-7B-V1.1-HQQ-2bit-smashed | PrunaAI | "2024-06-24T10:27:56Z" | 0 | 0 | transformers | [
"transformers",
"llama",
"text-generation",
"pruna-ai",
"base_model:Xwin-LM/Xwin-Math-7B-V1.1",
"autotrain_compatible",
"endpoints_compatible",
"text-generation-inference",
"region:us"
] | text-generation | "2024-06-24T10:26:32Z" | ---
thumbnail: "https://assets-global.website-files.com/646b351987a8d8ce158d1940/64ec9e96b4334c0e1ac41504_Logo%20with%20white%20text.svg"
base_model: Xwin-LM/Xwin-Math-7B-V1.1
metrics:
- memory_disk
- memory_inference
- inference_latency
- inference_throughput
- inference_CO2_emissions
- inference_energy_consumption
tags:
- pruna-ai
---
<!-- header start -->
<!-- 200823 -->
<div style="width: auto; margin-left: auto; margin-right: auto">
<a href="https://www.pruna.ai/" target="_blank" rel="noopener noreferrer">
<img src="https://i.imgur.com/eDAlcgk.png" alt="PrunaAI" style="width: 100%; min-width: 400px; display: block; margin: auto;">
</a>
</div>
<!-- header end -->
[](https://twitter.com/PrunaAI)
[](https://github.com/PrunaAI)
[](https://www.linkedin.com/company/93832878/admin/feed/posts/?feedType=following)
[](https://discord.gg/CP4VSgck)
# Simply make AI models cheaper, smaller, faster, and greener!
- Give a thumbs up if you like this model!
- Contact us and tell us which model to compress next [here](https://www.pruna.ai/contact).
- Request access to easily compress your *own* AI models [here](https://z0halsaff74.typeform.com/pruna-access?typeform-source=www.pruna.ai).
- Read the documentations to know more [here](https://pruna-ai-pruna.readthedocs-hosted.com/en/latest/)
- Join Pruna AI community on Discord [here](https://discord.gg/CP4VSgck) to share feedback/suggestions or get help.
## Results

**Frequently Asked Questions**
- ***How does the compression work?*** The model is compressed with hqq.
- ***How does the model quality change?*** The quality of the model output might vary compared to the base model.
- ***How is the model efficiency evaluated?*** These results were obtained on HARDWARE_NAME with configuration described in `model/smash_config.json` and are obtained after a hardware warmup. The smashed model is directly compared to the original base model. Efficiency results may vary in other settings (e.g. other hardware, image size, batch size, ...). We recommend to directly run them in the use-case conditions to know if the smashed model can benefit you.
- ***What is the model format?*** We use safetensors.
- ***What calibration data has been used?*** If needed by the compression method, we used WikiText as the calibration data.
- ***What is the naming convention for Pruna Huggingface models?*** We take the original model name and append "turbo", "tiny", or "green" if the smashed model has a measured inference speed, inference memory, or inference energy consumption which is less than 90% of the original base model.
- ***How to compress my own models?*** You can request premium access to more compression methods and tech support for your specific use-cases [here](https://z0halsaff74.typeform.com/pruna-access?typeform-source=www.pruna.ai).
- ***What are "first" metrics?*** Results mentioning "first" are obtained after the first run of the model. The first run might take more memory or be slower than the subsequent runs due cuda overheads.
- ***What are "Sync" and "Async" metrics?*** "Sync" metrics are obtained by syncing all GPU processes and stop measurement when all of them are executed. "Async" metrics are obtained without syncing all GPU processes and stop when the model output can be used by the CPU. We provide both metrics since both could be relevant depending on the use-case. We recommend to test the efficiency gains directly in your use-cases.
## Setup
You can run the smashed model with these steps:
0. Check requirements from the original repo Xwin-LM/Xwin-Math-7B-V1.1 installed. In particular, check python, cuda, and transformers versions.
1. Make sure that you have installed quantization related packages.
```bash
pip install hqq
```
2. Load & run the model.
```python
from transformers import AutoModelForCausalLM, AutoTokenizer
from hqq.engine.hf import HQQModelForCausalLM
from hqq.models.hf.base import AutoHQQHFModel
try:
model = HQQModelForCausalLM.from_quantized("PrunaAI/Xwin-LM-Xwin-Math-7B-V1.1-HQQ-2bit-smashed", device_map='auto')
except:
model = AutoHQQHFModel.from_quantized("PrunaAI/Xwin-LM-Xwin-Math-7B-V1.1-HQQ-2bit-smashed")
tokenizer = AutoTokenizer.from_pretrained("Xwin-LM/Xwin-Math-7B-V1.1")
input_ids = tokenizer("What is the color of prunes?,", return_tensors='pt').to(model.device)["input_ids"]
outputs = model.generate(input_ids, max_new_tokens=216)
tokenizer.decode(outputs[0])
```
## Configurations
The configuration info are in `smash_config.json`.
## Credits & License
The license of the smashed model follows the license of the original model. Please check the license of the original model Xwin-LM/Xwin-Math-7B-V1.1 before using this model which provided the base model. The license of the `pruna-engine` is [here](https://pypi.org/project/pruna-engine/) on Pypi.
## Want to compress other models?
- Contact us and tell us which model to compress next [here](https://www.pruna.ai/contact).
- Request access to easily compress your own AI models [here](https://z0halsaff74.typeform.com/pruna-access?typeform-source=www.pruna.ai). |
PrunaAI/Xwin-LM-Xwin-Math-7B-V1.1-HQQ-4bit-smashed | PrunaAI | "2024-06-24T10:29:00Z" | 0 | 0 | transformers | [
"transformers",
"llama",
"text-generation",
"pruna-ai",
"base_model:Xwin-LM/Xwin-Math-7B-V1.1",
"autotrain_compatible",
"endpoints_compatible",
"text-generation-inference",
"region:us"
] | text-generation | "2024-06-24T10:27:10Z" | ---
thumbnail: "https://assets-global.website-files.com/646b351987a8d8ce158d1940/64ec9e96b4334c0e1ac41504_Logo%20with%20white%20text.svg"
base_model: Xwin-LM/Xwin-Math-7B-V1.1
metrics:
- memory_disk
- memory_inference
- inference_latency
- inference_throughput
- inference_CO2_emissions
- inference_energy_consumption
tags:
- pruna-ai
---
<!-- header start -->
<!-- 200823 -->
<div style="width: auto; margin-left: auto; margin-right: auto">
<a href="https://www.pruna.ai/" target="_blank" rel="noopener noreferrer">
<img src="https://i.imgur.com/eDAlcgk.png" alt="PrunaAI" style="width: 100%; min-width: 400px; display: block; margin: auto;">
</a>
</div>
<!-- header end -->
[](https://twitter.com/PrunaAI)
[](https://github.com/PrunaAI)
[](https://www.linkedin.com/company/93832878/admin/feed/posts/?feedType=following)
[](https://discord.gg/CP4VSgck)
# Simply make AI models cheaper, smaller, faster, and greener!
- Give a thumbs up if you like this model!
- Contact us and tell us which model to compress next [here](https://www.pruna.ai/contact).
- Request access to easily compress your *own* AI models [here](https://z0halsaff74.typeform.com/pruna-access?typeform-source=www.pruna.ai).
- Read the documentations to know more [here](https://pruna-ai-pruna.readthedocs-hosted.com/en/latest/)
- Join Pruna AI community on Discord [here](https://discord.gg/CP4VSgck) to share feedback/suggestions or get help.
## Results

**Frequently Asked Questions**
- ***How does the compression work?*** The model is compressed with hqq.
- ***How does the model quality change?*** The quality of the model output might vary compared to the base model.
- ***How is the model efficiency evaluated?*** These results were obtained on HARDWARE_NAME with configuration described in `model/smash_config.json` and are obtained after a hardware warmup. The smashed model is directly compared to the original base model. Efficiency results may vary in other settings (e.g. other hardware, image size, batch size, ...). We recommend to directly run them in the use-case conditions to know if the smashed model can benefit you.
- ***What is the model format?*** We use safetensors.
- ***What calibration data has been used?*** If needed by the compression method, we used WikiText as the calibration data.
- ***What is the naming convention for Pruna Huggingface models?*** We take the original model name and append "turbo", "tiny", or "green" if the smashed model has a measured inference speed, inference memory, or inference energy consumption which is less than 90% of the original base model.
- ***How to compress my own models?*** You can request premium access to more compression methods and tech support for your specific use-cases [here](https://z0halsaff74.typeform.com/pruna-access?typeform-source=www.pruna.ai).
- ***What are "first" metrics?*** Results mentioning "first" are obtained after the first run of the model. The first run might take more memory or be slower than the subsequent runs due cuda overheads.
- ***What are "Sync" and "Async" metrics?*** "Sync" metrics are obtained by syncing all GPU processes and stop measurement when all of them are executed. "Async" metrics are obtained without syncing all GPU processes and stop when the model output can be used by the CPU. We provide both metrics since both could be relevant depending on the use-case. We recommend to test the efficiency gains directly in your use-cases.
## Setup
You can run the smashed model with these steps:
0. Check requirements from the original repo Xwin-LM/Xwin-Math-7B-V1.1 installed. In particular, check python, cuda, and transformers versions.
1. Make sure that you have installed quantization related packages.
```bash
pip install hqq
```
2. Load & run the model.
```python
from transformers import AutoModelForCausalLM, AutoTokenizer
from hqq.engine.hf import HQQModelForCausalLM
from hqq.models.hf.base import AutoHQQHFModel
try:
model = HQQModelForCausalLM.from_quantized("PrunaAI/Xwin-LM-Xwin-Math-7B-V1.1-HQQ-4bit-smashed", device_map='auto')
except:
model = AutoHQQHFModel.from_quantized("PrunaAI/Xwin-LM-Xwin-Math-7B-V1.1-HQQ-4bit-smashed")
tokenizer = AutoTokenizer.from_pretrained("Xwin-LM/Xwin-Math-7B-V1.1")
input_ids = tokenizer("What is the color of prunes?,", return_tensors='pt').to(model.device)["input_ids"]
outputs = model.generate(input_ids, max_new_tokens=216)
tokenizer.decode(outputs[0])
```
## Configurations
The configuration info are in `smash_config.json`.
## Credits & License
The license of the smashed model follows the license of the original model. Please check the license of the original model Xwin-LM/Xwin-Math-7B-V1.1 before using this model which provided the base model. The license of the `pruna-engine` is [here](https://pypi.org/project/pruna-engine/) on Pypi.
## Want to compress other models?
- Contact us and tell us which model to compress next [here](https://www.pruna.ai/contact).
- Request access to easily compress your own AI models [here](https://z0halsaff74.typeform.com/pruna-access?typeform-source=www.pruna.ai). |
mogmyij/Llama2-7b-BoolQ-layers-20-31 | mogmyij | "2024-06-24T10:28:01Z" | 0 | 0 | peft | [
"peft",
"safetensors",
"trl",
"sft",
"generated_from_trainer",
"base_model:meta-llama/Llama-2-7b-hf",
"license:llama2",
"region:us"
] | null | "2024-06-24T10:27:56Z" | ---
license: llama2
library_name: peft
tags:
- trl
- sft
- generated_from_trainer
base_model: meta-llama/Llama-2-7b-hf
model-index:
- name: Llama2-7b-BoolQ-layers-20-31
results: []
---
<!-- This model card has been generated automatically according to the information the Trainer had access to. You
should probably proofread and complete it, then remove this comment. -->
# Llama2-7b-BoolQ-layers-20-31
This model is a fine-tuned version of [meta-llama/Llama-2-7b-hf](https://huggingface.co/meta-llama/Llama-2-7b-hf) on an unknown dataset.
It achieves the following results on the evaluation set:
- Loss: 0.5920
## Model description
More information needed
## Intended uses & limitations
More information needed
## Training and evaluation data
More information needed
## Training procedure
### Training hyperparameters
The following hyperparameters were used during training:
- learning_rate: 0.0001
- train_batch_size: 2
- eval_batch_size: 8
- seed: 42
- gradient_accumulation_steps: 4
- total_train_batch_size: 8
- optimizer: Adam with betas=(0.9,0.999) and epsilon=1e-08
- lr_scheduler_type: cosine
- lr_scheduler_warmup_ratio: 0.05
- num_epochs: 3
- mixed_precision_training: Native AMP
### Training results
| Training Loss | Epoch | Step | Validation Loss |
|:-------------:|:------:|:----:|:---------------:|
| 0.443 | 0.9996 | 1178 | 0.5994 |
| 0.1443 | 2.0 | 2357 | 0.5665 |
| 0.6133 | 2.9987 | 3534 | 0.5920 |
### Framework versions
- PEFT 0.11.1
- Transformers 4.41.1
- Pytorch 2.3.0
- Datasets 2.19.1
- Tokenizers 0.19.1 |
PrunaAI/snorkelai-Snorkel-Mistral-PairRM-DPO-HQQ-4bit-smashed | PrunaAI | "2024-06-24T10:30:13Z" | 0 | 0 | transformers | [
"transformers",
"mistral",
"text-generation",
"pruna-ai",
"conversational",
"base_model:snorkelai/Snorkel-Mistral-PairRM-DPO",
"autotrain_compatible",
"endpoints_compatible",
"text-generation-inference",
"region:us"
] | text-generation | "2024-06-24T10:28:00Z" | ---
thumbnail: "https://assets-global.website-files.com/646b351987a8d8ce158d1940/64ec9e96b4334c0e1ac41504_Logo%20with%20white%20text.svg"
base_model: snorkelai/Snorkel-Mistral-PairRM-DPO
metrics:
- memory_disk
- memory_inference
- inference_latency
- inference_throughput
- inference_CO2_emissions
- inference_energy_consumption
tags:
- pruna-ai
---
<!-- header start -->
<!-- 200823 -->
<div style="width: auto; margin-left: auto; margin-right: auto">
<a href="https://www.pruna.ai/" target="_blank" rel="noopener noreferrer">
<img src="https://i.imgur.com/eDAlcgk.png" alt="PrunaAI" style="width: 100%; min-width: 400px; display: block; margin: auto;">
</a>
</div>
<!-- header end -->
[](https://twitter.com/PrunaAI)
[](https://github.com/PrunaAI)
[](https://www.linkedin.com/company/93832878/admin/feed/posts/?feedType=following)
[](https://discord.gg/CP4VSgck)
# Simply make AI models cheaper, smaller, faster, and greener!
- Give a thumbs up if you like this model!
- Contact us and tell us which model to compress next [here](https://www.pruna.ai/contact).
- Request access to easily compress your *own* AI models [here](https://z0halsaff74.typeform.com/pruna-access?typeform-source=www.pruna.ai).
- Read the documentations to know more [here](https://pruna-ai-pruna.readthedocs-hosted.com/en/latest/)
- Join Pruna AI community on Discord [here](https://discord.gg/CP4VSgck) to share feedback/suggestions or get help.
## Results

**Frequently Asked Questions**
- ***How does the compression work?*** The model is compressed with hqq.
- ***How does the model quality change?*** The quality of the model output might vary compared to the base model.
- ***How is the model efficiency evaluated?*** These results were obtained on HARDWARE_NAME with configuration described in `model/smash_config.json` and are obtained after a hardware warmup. The smashed model is directly compared to the original base model. Efficiency results may vary in other settings (e.g. other hardware, image size, batch size, ...). We recommend to directly run them in the use-case conditions to know if the smashed model can benefit you.
- ***What is the model format?*** We use safetensors.
- ***What calibration data has been used?*** If needed by the compression method, we used WikiText as the calibration data.
- ***What is the naming convention for Pruna Huggingface models?*** We take the original model name and append "turbo", "tiny", or "green" if the smashed model has a measured inference speed, inference memory, or inference energy consumption which is less than 90% of the original base model.
- ***How to compress my own models?*** You can request premium access to more compression methods and tech support for your specific use-cases [here](https://z0halsaff74.typeform.com/pruna-access?typeform-source=www.pruna.ai).
- ***What are "first" metrics?*** Results mentioning "first" are obtained after the first run of the model. The first run might take more memory or be slower than the subsequent runs due cuda overheads.
- ***What are "Sync" and "Async" metrics?*** "Sync" metrics are obtained by syncing all GPU processes and stop measurement when all of them are executed. "Async" metrics are obtained without syncing all GPU processes and stop when the model output can be used by the CPU. We provide both metrics since both could be relevant depending on the use-case. We recommend to test the efficiency gains directly in your use-cases.
## Setup
You can run the smashed model with these steps:
0. Check requirements from the original repo snorkelai/Snorkel-Mistral-PairRM-DPO installed. In particular, check python, cuda, and transformers versions.
1. Make sure that you have installed quantization related packages.
```bash
pip install hqq
```
2. Load & run the model.
```python
from transformers import AutoModelForCausalLM, AutoTokenizer
from hqq.engine.hf import HQQModelForCausalLM
from hqq.models.hf.base import AutoHQQHFModel
try:
model = HQQModelForCausalLM.from_quantized("PrunaAI/snorkelai-Snorkel-Mistral-PairRM-DPO-HQQ-4bit-smashed", device_map='auto')
except:
model = AutoHQQHFModel.from_quantized("PrunaAI/snorkelai-Snorkel-Mistral-PairRM-DPO-HQQ-4bit-smashed")
tokenizer = AutoTokenizer.from_pretrained("snorkelai/Snorkel-Mistral-PairRM-DPO")
input_ids = tokenizer("What is the color of prunes?,", return_tensors='pt').to(model.device)["input_ids"]
outputs = model.generate(input_ids, max_new_tokens=216)
tokenizer.decode(outputs[0])
```
## Configurations
The configuration info are in `smash_config.json`.
## Credits & License
The license of the smashed model follows the license of the original model. Please check the license of the original model snorkelai/Snorkel-Mistral-PairRM-DPO before using this model which provided the base model. The license of the `pruna-engine` is [here](https://pypi.org/project/pruna-engine/) on Pypi.
## Want to compress other models?
- Contact us and tell us which model to compress next [here](https://www.pruna.ai/contact).
- Request access to easily compress your own AI models [here](https://z0halsaff74.typeform.com/pruna-access?typeform-source=www.pruna.ai). |
PrunaAI/snorkelai-Snorkel-Mistral-PairRM-DPO-HQQ-1bit-smashed | PrunaAI | "2024-06-24T10:29:00Z" | 0 | 0 | transformers | [
"transformers",
"mistral",
"text-generation",
"pruna-ai",
"conversational",
"base_model:snorkelai/Snorkel-Mistral-PairRM-DPO",
"autotrain_compatible",
"endpoints_compatible",
"text-generation-inference",
"region:us"
] | text-generation | "2024-06-24T10:28:12Z" | ---
thumbnail: "https://assets-global.website-files.com/646b351987a8d8ce158d1940/64ec9e96b4334c0e1ac41504_Logo%20with%20white%20text.svg"
base_model: snorkelai/Snorkel-Mistral-PairRM-DPO
metrics:
- memory_disk
- memory_inference
- inference_latency
- inference_throughput
- inference_CO2_emissions
- inference_energy_consumption
tags:
- pruna-ai
---
<!-- header start -->
<!-- 200823 -->
<div style="width: auto; margin-left: auto; margin-right: auto">
<a href="https://www.pruna.ai/" target="_blank" rel="noopener noreferrer">
<img src="https://i.imgur.com/eDAlcgk.png" alt="PrunaAI" style="width: 100%; min-width: 400px; display: block; margin: auto;">
</a>
</div>
<!-- header end -->
[](https://twitter.com/PrunaAI)
[](https://github.com/PrunaAI)
[](https://www.linkedin.com/company/93832878/admin/feed/posts/?feedType=following)
[](https://discord.gg/CP4VSgck)
# Simply make AI models cheaper, smaller, faster, and greener!
- Give a thumbs up if you like this model!
- Contact us and tell us which model to compress next [here](https://www.pruna.ai/contact).
- Request access to easily compress your *own* AI models [here](https://z0halsaff74.typeform.com/pruna-access?typeform-source=www.pruna.ai).
- Read the documentations to know more [here](https://pruna-ai-pruna.readthedocs-hosted.com/en/latest/)
- Join Pruna AI community on Discord [here](https://discord.gg/CP4VSgck) to share feedback/suggestions or get help.
## Results

**Frequently Asked Questions**
- ***How does the compression work?*** The model is compressed with hqq.
- ***How does the model quality change?*** The quality of the model output might vary compared to the base model.
- ***How is the model efficiency evaluated?*** These results were obtained on HARDWARE_NAME with configuration described in `model/smash_config.json` and are obtained after a hardware warmup. The smashed model is directly compared to the original base model. Efficiency results may vary in other settings (e.g. other hardware, image size, batch size, ...). We recommend to directly run them in the use-case conditions to know if the smashed model can benefit you.
- ***What is the model format?*** We use safetensors.
- ***What calibration data has been used?*** If needed by the compression method, we used WikiText as the calibration data.
- ***What is the naming convention for Pruna Huggingface models?*** We take the original model name and append "turbo", "tiny", or "green" if the smashed model has a measured inference speed, inference memory, or inference energy consumption which is less than 90% of the original base model.
- ***How to compress my own models?*** You can request premium access to more compression methods and tech support for your specific use-cases [here](https://z0halsaff74.typeform.com/pruna-access?typeform-source=www.pruna.ai).
- ***What are "first" metrics?*** Results mentioning "first" are obtained after the first run of the model. The first run might take more memory or be slower than the subsequent runs due cuda overheads.
- ***What are "Sync" and "Async" metrics?*** "Sync" metrics are obtained by syncing all GPU processes and stop measurement when all of them are executed. "Async" metrics are obtained without syncing all GPU processes and stop when the model output can be used by the CPU. We provide both metrics since both could be relevant depending on the use-case. We recommend to test the efficiency gains directly in your use-cases.
## Setup
You can run the smashed model with these steps:
0. Check requirements from the original repo snorkelai/Snorkel-Mistral-PairRM-DPO installed. In particular, check python, cuda, and transformers versions.
1. Make sure that you have installed quantization related packages.
```bash
pip install hqq
```
2. Load & run the model.
```python
from transformers import AutoModelForCausalLM, AutoTokenizer
from hqq.engine.hf import HQQModelForCausalLM
from hqq.models.hf.base import AutoHQQHFModel
try:
model = HQQModelForCausalLM.from_quantized("PrunaAI/snorkelai-Snorkel-Mistral-PairRM-DPO-HQQ-1bit-smashed", device_map='auto')
except:
model = AutoHQQHFModel.from_quantized("PrunaAI/snorkelai-Snorkel-Mistral-PairRM-DPO-HQQ-1bit-smashed")
tokenizer = AutoTokenizer.from_pretrained("snorkelai/Snorkel-Mistral-PairRM-DPO")
input_ids = tokenizer("What is the color of prunes?,", return_tensors='pt').to(model.device)["input_ids"]
outputs = model.generate(input_ids, max_new_tokens=216)
tokenizer.decode(outputs[0])
```
## Configurations
The configuration info are in `smash_config.json`.
## Credits & License
The license of the smashed model follows the license of the original model. Please check the license of the original model snorkelai/Snorkel-Mistral-PairRM-DPO before using this model which provided the base model. The license of the `pruna-engine` is [here](https://pypi.org/project/pruna-engine/) on Pypi.
## Want to compress other models?
- Contact us and tell us which model to compress next [here](https://www.pruna.ai/contact).
- Request access to easily compress your own AI models [here](https://z0halsaff74.typeform.com/pruna-access?typeform-source=www.pruna.ai). |
PrunaAI/snorkelai-Snorkel-Mistral-PairRM-DPO-HQQ-2bit-smashed | PrunaAI | "2024-06-24T10:29:43Z" | 0 | 0 | transformers | [
"transformers",
"mistral",
"text-generation",
"pruna-ai",
"conversational",
"base_model:snorkelai/Snorkel-Mistral-PairRM-DPO",
"autotrain_compatible",
"endpoints_compatible",
"text-generation-inference",
"region:us"
] | text-generation | "2024-06-24T10:28:33Z" | ---
thumbnail: "https://assets-global.website-files.com/646b351987a8d8ce158d1940/64ec9e96b4334c0e1ac41504_Logo%20with%20white%20text.svg"
base_model: snorkelai/Snorkel-Mistral-PairRM-DPO
metrics:
- memory_disk
- memory_inference
- inference_latency
- inference_throughput
- inference_CO2_emissions
- inference_energy_consumption
tags:
- pruna-ai
---
<!-- header start -->
<!-- 200823 -->
<div style="width: auto; margin-left: auto; margin-right: auto">
<a href="https://www.pruna.ai/" target="_blank" rel="noopener noreferrer">
<img src="https://i.imgur.com/eDAlcgk.png" alt="PrunaAI" style="width: 100%; min-width: 400px; display: block; margin: auto;">
</a>
</div>
<!-- header end -->
[](https://twitter.com/PrunaAI)
[](https://github.com/PrunaAI)
[](https://www.linkedin.com/company/93832878/admin/feed/posts/?feedType=following)
[](https://discord.gg/CP4VSgck)
# Simply make AI models cheaper, smaller, faster, and greener!
- Give a thumbs up if you like this model!
- Contact us and tell us which model to compress next [here](https://www.pruna.ai/contact).
- Request access to easily compress your *own* AI models [here](https://z0halsaff74.typeform.com/pruna-access?typeform-source=www.pruna.ai).
- Read the documentations to know more [here](https://pruna-ai-pruna.readthedocs-hosted.com/en/latest/)
- Join Pruna AI community on Discord [here](https://discord.gg/CP4VSgck) to share feedback/suggestions or get help.
## Results

**Frequently Asked Questions**
- ***How does the compression work?*** The model is compressed with hqq.
- ***How does the model quality change?*** The quality of the model output might vary compared to the base model.
- ***How is the model efficiency evaluated?*** These results were obtained on HARDWARE_NAME with configuration described in `model/smash_config.json` and are obtained after a hardware warmup. The smashed model is directly compared to the original base model. Efficiency results may vary in other settings (e.g. other hardware, image size, batch size, ...). We recommend to directly run them in the use-case conditions to know if the smashed model can benefit you.
- ***What is the model format?*** We use safetensors.
- ***What calibration data has been used?*** If needed by the compression method, we used WikiText as the calibration data.
- ***What is the naming convention for Pruna Huggingface models?*** We take the original model name and append "turbo", "tiny", or "green" if the smashed model has a measured inference speed, inference memory, or inference energy consumption which is less than 90% of the original base model.
- ***How to compress my own models?*** You can request premium access to more compression methods and tech support for your specific use-cases [here](https://z0halsaff74.typeform.com/pruna-access?typeform-source=www.pruna.ai).
- ***What are "first" metrics?*** Results mentioning "first" are obtained after the first run of the model. The first run might take more memory or be slower than the subsequent runs due cuda overheads.
- ***What are "Sync" and "Async" metrics?*** "Sync" metrics are obtained by syncing all GPU processes and stop measurement when all of them are executed. "Async" metrics are obtained without syncing all GPU processes and stop when the model output can be used by the CPU. We provide both metrics since both could be relevant depending on the use-case. We recommend to test the efficiency gains directly in your use-cases.
## Setup
You can run the smashed model with these steps:
0. Check requirements from the original repo snorkelai/Snorkel-Mistral-PairRM-DPO installed. In particular, check python, cuda, and transformers versions.
1. Make sure that you have installed quantization related packages.
```bash
pip install hqq
```
2. Load & run the model.
```python
from transformers import AutoModelForCausalLM, AutoTokenizer
from hqq.engine.hf import HQQModelForCausalLM
from hqq.models.hf.base import AutoHQQHFModel
try:
model = HQQModelForCausalLM.from_quantized("PrunaAI/snorkelai-Snorkel-Mistral-PairRM-DPO-HQQ-2bit-smashed", device_map='auto')
except:
model = AutoHQQHFModel.from_quantized("PrunaAI/snorkelai-Snorkel-Mistral-PairRM-DPO-HQQ-2bit-smashed")
tokenizer = AutoTokenizer.from_pretrained("snorkelai/Snorkel-Mistral-PairRM-DPO")
input_ids = tokenizer("What is the color of prunes?,", return_tensors='pt').to(model.device)["input_ids"]
outputs = model.generate(input_ids, max_new_tokens=216)
tokenizer.decode(outputs[0])
```
## Configurations
The configuration info are in `smash_config.json`.
## Credits & License
The license of the smashed model follows the license of the original model. Please check the license of the original model snorkelai/Snorkel-Mistral-PairRM-DPO before using this model which provided the base model. The license of the `pruna-engine` is [here](https://pypi.org/project/pruna-engine/) on Pypi.
## Want to compress other models?
- Contact us and tell us which model to compress next [here](https://www.pruna.ai/contact).
- Request access to easily compress your own AI models [here](https://z0halsaff74.typeform.com/pruna-access?typeform-source=www.pruna.ai). |
Mikeshu/__ | Mikeshu | "2024-06-24T10:30:39Z" | 0 | 0 | diffusers | [
"diffusers",
"not-for-all-audiences",
"ar",
"dataset:HuggingFaceFW/fineweb-edu",
"region:us"
] | null | "2024-06-24T10:29:50Z" | ---
datasets:
- HuggingFaceFW/fineweb-edu
language:
- ar
library_name: diffusers
tags:
- not-for-all-audiences
--- |
PrunaAI/beomi-Llama-3-KoEn-8B-HQQ-4bit-smashed | PrunaAI | "2024-06-24T10:35:18Z" | 0 | 0 | transformers | [
"transformers",
"llama",
"text-generation",
"pruna-ai",
"conversational",
"base_model:beomi/Llama-3-KoEn-8B",
"autotrain_compatible",
"endpoints_compatible",
"text-generation-inference",
"region:us"
] | text-generation | "2024-06-24T10:32:31Z" | ---
thumbnail: "https://assets-global.website-files.com/646b351987a8d8ce158d1940/64ec9e96b4334c0e1ac41504_Logo%20with%20white%20text.svg"
base_model: beomi/Llama-3-KoEn-8B
metrics:
- memory_disk
- memory_inference
- inference_latency
- inference_throughput
- inference_CO2_emissions
- inference_energy_consumption
tags:
- pruna-ai
---
<!-- header start -->
<!-- 200823 -->
<div style="width: auto; margin-left: auto; margin-right: auto">
<a href="https://www.pruna.ai/" target="_blank" rel="noopener noreferrer">
<img src="https://i.imgur.com/eDAlcgk.png" alt="PrunaAI" style="width: 100%; min-width: 400px; display: block; margin: auto;">
</a>
</div>
<!-- header end -->
[](https://twitter.com/PrunaAI)
[](https://github.com/PrunaAI)
[](https://www.linkedin.com/company/93832878/admin/feed/posts/?feedType=following)
[](https://discord.gg/CP4VSgck)
# Simply make AI models cheaper, smaller, faster, and greener!
- Give a thumbs up if you like this model!
- Contact us and tell us which model to compress next [here](https://www.pruna.ai/contact).
- Request access to easily compress your *own* AI models [here](https://z0halsaff74.typeform.com/pruna-access?typeform-source=www.pruna.ai).
- Read the documentations to know more [here](https://pruna-ai-pruna.readthedocs-hosted.com/en/latest/)
- Join Pruna AI community on Discord [here](https://discord.gg/CP4VSgck) to share feedback/suggestions or get help.
## Results

**Frequently Asked Questions**
- ***How does the compression work?*** The model is compressed with hqq.
- ***How does the model quality change?*** The quality of the model output might vary compared to the base model.
- ***How is the model efficiency evaluated?*** These results were obtained on HARDWARE_NAME with configuration described in `model/smash_config.json` and are obtained after a hardware warmup. The smashed model is directly compared to the original base model. Efficiency results may vary in other settings (e.g. other hardware, image size, batch size, ...). We recommend to directly run them in the use-case conditions to know if the smashed model can benefit you.
- ***What is the model format?*** We use safetensors.
- ***What calibration data has been used?*** If needed by the compression method, we used WikiText as the calibration data.
- ***What is the naming convention for Pruna Huggingface models?*** We take the original model name and append "turbo", "tiny", or "green" if the smashed model has a measured inference speed, inference memory, or inference energy consumption which is less than 90% of the original base model.
- ***How to compress my own models?*** You can request premium access to more compression methods and tech support for your specific use-cases [here](https://z0halsaff74.typeform.com/pruna-access?typeform-source=www.pruna.ai).
- ***What are "first" metrics?*** Results mentioning "first" are obtained after the first run of the model. The first run might take more memory or be slower than the subsequent runs due cuda overheads.
- ***What are "Sync" and "Async" metrics?*** "Sync" metrics are obtained by syncing all GPU processes and stop measurement when all of them are executed. "Async" metrics are obtained without syncing all GPU processes and stop when the model output can be used by the CPU. We provide both metrics since both could be relevant depending on the use-case. We recommend to test the efficiency gains directly in your use-cases.
## Setup
You can run the smashed model with these steps:
0. Check requirements from the original repo beomi/Llama-3-KoEn-8B installed. In particular, check python, cuda, and transformers versions.
1. Make sure that you have installed quantization related packages.
```bash
pip install hqq
```
2. Load & run the model.
```python
from transformers import AutoModelForCausalLM, AutoTokenizer
from hqq.engine.hf import HQQModelForCausalLM
from hqq.models.hf.base import AutoHQQHFModel
try:
model = HQQModelForCausalLM.from_quantized("PrunaAI/beomi-Llama-3-KoEn-8B-HQQ-4bit-smashed", device_map='auto')
except:
model = AutoHQQHFModel.from_quantized("PrunaAI/beomi-Llama-3-KoEn-8B-HQQ-4bit-smashed")
tokenizer = AutoTokenizer.from_pretrained("beomi/Llama-3-KoEn-8B")
input_ids = tokenizer("What is the color of prunes?,", return_tensors='pt').to(model.device)["input_ids"]
outputs = model.generate(input_ids, max_new_tokens=216)
tokenizer.decode(outputs[0])
```
## Configurations
The configuration info are in `smash_config.json`.
## Credits & License
The license of the smashed model follows the license of the original model. Please check the license of the original model beomi/Llama-3-KoEn-8B before using this model which provided the base model. The license of the `pruna-engine` is [here](https://pypi.org/project/pruna-engine/) on Pypi.
## Want to compress other models?
- Contact us and tell us which model to compress next [here](https://www.pruna.ai/contact).
- Request access to easily compress your own AI models [here](https://z0halsaff74.typeform.com/pruna-access?typeform-source=www.pruna.ai). |
PrunaAI/beomi-Llama-3-KoEn-8B-HQQ-1bit-smashed | PrunaAI | "2024-06-24T10:34:25Z" | 0 | 0 | transformers | [
"transformers",
"llama",
"text-generation",
"pruna-ai",
"conversational",
"base_model:beomi/Llama-3-KoEn-8B",
"autotrain_compatible",
"endpoints_compatible",
"text-generation-inference",
"region:us"
] | text-generation | "2024-06-24T10:32:47Z" | ---
thumbnail: "https://assets-global.website-files.com/646b351987a8d8ce158d1940/64ec9e96b4334c0e1ac41504_Logo%20with%20white%20text.svg"
base_model: beomi/Llama-3-KoEn-8B
metrics:
- memory_disk
- memory_inference
- inference_latency
- inference_throughput
- inference_CO2_emissions
- inference_energy_consumption
tags:
- pruna-ai
---
<!-- header start -->
<!-- 200823 -->
<div style="width: auto; margin-left: auto; margin-right: auto">
<a href="https://www.pruna.ai/" target="_blank" rel="noopener noreferrer">
<img src="https://i.imgur.com/eDAlcgk.png" alt="PrunaAI" style="width: 100%; min-width: 400px; display: block; margin: auto;">
</a>
</div>
<!-- header end -->
[](https://twitter.com/PrunaAI)
[](https://github.com/PrunaAI)
[](https://www.linkedin.com/company/93832878/admin/feed/posts/?feedType=following)
[](https://discord.gg/CP4VSgck)
# Simply make AI models cheaper, smaller, faster, and greener!
- Give a thumbs up if you like this model!
- Contact us and tell us which model to compress next [here](https://www.pruna.ai/contact).
- Request access to easily compress your *own* AI models [here](https://z0halsaff74.typeform.com/pruna-access?typeform-source=www.pruna.ai).
- Read the documentations to know more [here](https://pruna-ai-pruna.readthedocs-hosted.com/en/latest/)
- Join Pruna AI community on Discord [here](https://discord.gg/CP4VSgck) to share feedback/suggestions or get help.
## Results

**Frequently Asked Questions**
- ***How does the compression work?*** The model is compressed with hqq.
- ***How does the model quality change?*** The quality of the model output might vary compared to the base model.
- ***How is the model efficiency evaluated?*** These results were obtained on HARDWARE_NAME with configuration described in `model/smash_config.json` and are obtained after a hardware warmup. The smashed model is directly compared to the original base model. Efficiency results may vary in other settings (e.g. other hardware, image size, batch size, ...). We recommend to directly run them in the use-case conditions to know if the smashed model can benefit you.
- ***What is the model format?*** We use safetensors.
- ***What calibration data has been used?*** If needed by the compression method, we used WikiText as the calibration data.
- ***What is the naming convention for Pruna Huggingface models?*** We take the original model name and append "turbo", "tiny", or "green" if the smashed model has a measured inference speed, inference memory, or inference energy consumption which is less than 90% of the original base model.
- ***How to compress my own models?*** You can request premium access to more compression methods and tech support for your specific use-cases [here](https://z0halsaff74.typeform.com/pruna-access?typeform-source=www.pruna.ai).
- ***What are "first" metrics?*** Results mentioning "first" are obtained after the first run of the model. The first run might take more memory or be slower than the subsequent runs due cuda overheads.
- ***What are "Sync" and "Async" metrics?*** "Sync" metrics are obtained by syncing all GPU processes and stop measurement when all of them are executed. "Async" metrics are obtained without syncing all GPU processes and stop when the model output can be used by the CPU. We provide both metrics since both could be relevant depending on the use-case. We recommend to test the efficiency gains directly in your use-cases.
## Setup
You can run the smashed model with these steps:
0. Check requirements from the original repo beomi/Llama-3-KoEn-8B installed. In particular, check python, cuda, and transformers versions.
1. Make sure that you have installed quantization related packages.
```bash
pip install hqq
```
2. Load & run the model.
```python
from transformers import AutoModelForCausalLM, AutoTokenizer
from hqq.engine.hf import HQQModelForCausalLM
from hqq.models.hf.base import AutoHQQHFModel
try:
model = HQQModelForCausalLM.from_quantized("PrunaAI/beomi-Llama-3-KoEn-8B-HQQ-1bit-smashed", device_map='auto')
except:
model = AutoHQQHFModel.from_quantized("PrunaAI/beomi-Llama-3-KoEn-8B-HQQ-1bit-smashed")
tokenizer = AutoTokenizer.from_pretrained("beomi/Llama-3-KoEn-8B")
input_ids = tokenizer("What is the color of prunes?,", return_tensors='pt').to(model.device)["input_ids"]
outputs = model.generate(input_ids, max_new_tokens=216)
tokenizer.decode(outputs[0])
```
## Configurations
The configuration info are in `smash_config.json`.
## Credits & License
The license of the smashed model follows the license of the original model. Please check the license of the original model beomi/Llama-3-KoEn-8B before using this model which provided the base model. The license of the `pruna-engine` is [here](https://pypi.org/project/pruna-engine/) on Pypi.
## Want to compress other models?
- Contact us and tell us which model to compress next [here](https://www.pruna.ai/contact).
- Request access to easily compress your own AI models [here](https://z0halsaff74.typeform.com/pruna-access?typeform-source=www.pruna.ai). |
PrunaAI/beomi-Llama-3-KoEn-8B-HQQ-2bit-smashed | PrunaAI | "2024-06-24T10:35:03Z" | 0 | 0 | transformers | [
"transformers",
"llama",
"text-generation",
"pruna-ai",
"conversational",
"base_model:beomi/Llama-3-KoEn-8B",
"autotrain_compatible",
"endpoints_compatible",
"text-generation-inference",
"region:us"
] | text-generation | "2024-06-24T10:32:51Z" | ---
thumbnail: "https://assets-global.website-files.com/646b351987a8d8ce158d1940/64ec9e96b4334c0e1ac41504_Logo%20with%20white%20text.svg"
base_model: beomi/Llama-3-KoEn-8B
metrics:
- memory_disk
- memory_inference
- inference_latency
- inference_throughput
- inference_CO2_emissions
- inference_energy_consumption
tags:
- pruna-ai
---
<!-- header start -->
<!-- 200823 -->
<div style="width: auto; margin-left: auto; margin-right: auto">
<a href="https://www.pruna.ai/" target="_blank" rel="noopener noreferrer">
<img src="https://i.imgur.com/eDAlcgk.png" alt="PrunaAI" style="width: 100%; min-width: 400px; display: block; margin: auto;">
</a>
</div>
<!-- header end -->
[](https://twitter.com/PrunaAI)
[](https://github.com/PrunaAI)
[](https://www.linkedin.com/company/93832878/admin/feed/posts/?feedType=following)
[](https://discord.gg/CP4VSgck)
# Simply make AI models cheaper, smaller, faster, and greener!
- Give a thumbs up if you like this model!
- Contact us and tell us which model to compress next [here](https://www.pruna.ai/contact).
- Request access to easily compress your *own* AI models [here](https://z0halsaff74.typeform.com/pruna-access?typeform-source=www.pruna.ai).
- Read the documentations to know more [here](https://pruna-ai-pruna.readthedocs-hosted.com/en/latest/)
- Join Pruna AI community on Discord [here](https://discord.gg/CP4VSgck) to share feedback/suggestions or get help.
## Results

**Frequently Asked Questions**
- ***How does the compression work?*** The model is compressed with hqq.
- ***How does the model quality change?*** The quality of the model output might vary compared to the base model.
- ***How is the model efficiency evaluated?*** These results were obtained on HARDWARE_NAME with configuration described in `model/smash_config.json` and are obtained after a hardware warmup. The smashed model is directly compared to the original base model. Efficiency results may vary in other settings (e.g. other hardware, image size, batch size, ...). We recommend to directly run them in the use-case conditions to know if the smashed model can benefit you.
- ***What is the model format?*** We use safetensors.
- ***What calibration data has been used?*** If needed by the compression method, we used WikiText as the calibration data.
- ***What is the naming convention for Pruna Huggingface models?*** We take the original model name and append "turbo", "tiny", or "green" if the smashed model has a measured inference speed, inference memory, or inference energy consumption which is less than 90% of the original base model.
- ***How to compress my own models?*** You can request premium access to more compression methods and tech support for your specific use-cases [here](https://z0halsaff74.typeform.com/pruna-access?typeform-source=www.pruna.ai).
- ***What are "first" metrics?*** Results mentioning "first" are obtained after the first run of the model. The first run might take more memory or be slower than the subsequent runs due cuda overheads.
- ***What are "Sync" and "Async" metrics?*** "Sync" metrics are obtained by syncing all GPU processes and stop measurement when all of them are executed. "Async" metrics are obtained without syncing all GPU processes and stop when the model output can be used by the CPU. We provide both metrics since both could be relevant depending on the use-case. We recommend to test the efficiency gains directly in your use-cases.
## Setup
You can run the smashed model with these steps:
0. Check requirements from the original repo beomi/Llama-3-KoEn-8B installed. In particular, check python, cuda, and transformers versions.
1. Make sure that you have installed quantization related packages.
```bash
pip install hqq
```
2. Load & run the model.
```python
from transformers import AutoModelForCausalLM, AutoTokenizer
from hqq.engine.hf import HQQModelForCausalLM
from hqq.models.hf.base import AutoHQQHFModel
try:
model = HQQModelForCausalLM.from_quantized("PrunaAI/beomi-Llama-3-KoEn-8B-HQQ-2bit-smashed", device_map='auto')
except:
model = AutoHQQHFModel.from_quantized("PrunaAI/beomi-Llama-3-KoEn-8B-HQQ-2bit-smashed")
tokenizer = AutoTokenizer.from_pretrained("beomi/Llama-3-KoEn-8B")
input_ids = tokenizer("What is the color of prunes?,", return_tensors='pt').to(model.device)["input_ids"]
outputs = model.generate(input_ids, max_new_tokens=216)
tokenizer.decode(outputs[0])
```
## Configurations
The configuration info are in `smash_config.json`.
## Credits & License
The license of the smashed model follows the license of the original model. Please check the license of the original model beomi/Llama-3-KoEn-8B before using this model which provided the base model. The license of the `pruna-engine` is [here](https://pypi.org/project/pruna-engine/) on Pypi.
## Want to compress other models?
- Contact us and tell us which model to compress next [here](https://www.pruna.ai/contact).
- Request access to easily compress your own AI models [here](https://z0halsaff74.typeform.com/pruna-access?typeform-source=www.pruna.ai). |
PrunaAI/openchat-openchat_3.5-HQQ-1bit-smashed | PrunaAI | "2024-06-24T10:34:11Z" | 0 | 0 | transformers | [
"transformers",
"mistral",
"text-generation",
"pruna-ai",
"conversational",
"base_model:openchat/openchat_3.5",
"autotrain_compatible",
"endpoints_compatible",
"text-generation-inference",
"region:us"
] | text-generation | "2024-06-24T10:33:18Z" | ---
thumbnail: "https://assets-global.website-files.com/646b351987a8d8ce158d1940/64ec9e96b4334c0e1ac41504_Logo%20with%20white%20text.svg"
base_model: openchat/openchat_3.5
metrics:
- memory_disk
- memory_inference
- inference_latency
- inference_throughput
- inference_CO2_emissions
- inference_energy_consumption
tags:
- pruna-ai
---
<!-- header start -->
<!-- 200823 -->
<div style="width: auto; margin-left: auto; margin-right: auto">
<a href="https://www.pruna.ai/" target="_blank" rel="noopener noreferrer">
<img src="https://i.imgur.com/eDAlcgk.png" alt="PrunaAI" style="width: 100%; min-width: 400px; display: block; margin: auto;">
</a>
</div>
<!-- header end -->
[](https://twitter.com/PrunaAI)
[](https://github.com/PrunaAI)
[](https://www.linkedin.com/company/93832878/admin/feed/posts/?feedType=following)
[](https://discord.gg/CP4VSgck)
# Simply make AI models cheaper, smaller, faster, and greener!
- Give a thumbs up if you like this model!
- Contact us and tell us which model to compress next [here](https://www.pruna.ai/contact).
- Request access to easily compress your *own* AI models [here](https://z0halsaff74.typeform.com/pruna-access?typeform-source=www.pruna.ai).
- Read the documentations to know more [here](https://pruna-ai-pruna.readthedocs-hosted.com/en/latest/)
- Join Pruna AI community on Discord [here](https://discord.gg/CP4VSgck) to share feedback/suggestions or get help.
## Results

**Frequently Asked Questions**
- ***How does the compression work?*** The model is compressed with hqq.
- ***How does the model quality change?*** The quality of the model output might vary compared to the base model.
- ***How is the model efficiency evaluated?*** These results were obtained on HARDWARE_NAME with configuration described in `model/smash_config.json` and are obtained after a hardware warmup. The smashed model is directly compared to the original base model. Efficiency results may vary in other settings (e.g. other hardware, image size, batch size, ...). We recommend to directly run them in the use-case conditions to know if the smashed model can benefit you.
- ***What is the model format?*** We use safetensors.
- ***What calibration data has been used?*** If needed by the compression method, we used WikiText as the calibration data.
- ***What is the naming convention for Pruna Huggingface models?*** We take the original model name and append "turbo", "tiny", or "green" if the smashed model has a measured inference speed, inference memory, or inference energy consumption which is less than 90% of the original base model.
- ***How to compress my own models?*** You can request premium access to more compression methods and tech support for your specific use-cases [here](https://z0halsaff74.typeform.com/pruna-access?typeform-source=www.pruna.ai).
- ***What are "first" metrics?*** Results mentioning "first" are obtained after the first run of the model. The first run might take more memory or be slower than the subsequent runs due cuda overheads.
- ***What are "Sync" and "Async" metrics?*** "Sync" metrics are obtained by syncing all GPU processes and stop measurement when all of them are executed. "Async" metrics are obtained without syncing all GPU processes and stop when the model output can be used by the CPU. We provide both metrics since both could be relevant depending on the use-case. We recommend to test the efficiency gains directly in your use-cases.
## Setup
You can run the smashed model with these steps:
0. Check requirements from the original repo openchat/openchat_3.5 installed. In particular, check python, cuda, and transformers versions.
1. Make sure that you have installed quantization related packages.
```bash
pip install hqq
```
2. Load & run the model.
```python
from transformers import AutoModelForCausalLM, AutoTokenizer
from hqq.engine.hf import HQQModelForCausalLM
from hqq.models.hf.base import AutoHQQHFModel
try:
model = HQQModelForCausalLM.from_quantized("PrunaAI/openchat-openchat_3.5-HQQ-1bit-smashed", device_map='auto')
except:
model = AutoHQQHFModel.from_quantized("PrunaAI/openchat-openchat_3.5-HQQ-1bit-smashed")
tokenizer = AutoTokenizer.from_pretrained("openchat/openchat_3.5")
input_ids = tokenizer("What is the color of prunes?,", return_tensors='pt').to(model.device)["input_ids"]
outputs = model.generate(input_ids, max_new_tokens=216)
tokenizer.decode(outputs[0])
```
## Configurations
The configuration info are in `smash_config.json`.
## Credits & License
The license of the smashed model follows the license of the original model. Please check the license of the original model openchat/openchat_3.5 before using this model which provided the base model. The license of the `pruna-engine` is [here](https://pypi.org/project/pruna-engine/) on Pypi.
## Want to compress other models?
- Contact us and tell us which model to compress next [here](https://www.pruna.ai/contact).
- Request access to easily compress your own AI models [here](https://z0halsaff74.typeform.com/pruna-access?typeform-source=www.pruna.ai). |
gaurav1033/mistral_7b_guanaco | gaurav1033 | "2024-06-24T10:33:38Z" | 0 | 0 | transformers | [
"transformers",
"safetensors",
"arxiv:1910.09700",
"endpoints_compatible",
"region:us"
] | null | "2024-06-24T10:33:30Z" | ---
library_name: transformers
tags: []
---
# Model Card for Model ID
<!-- Provide a quick summary of what the model is/does. -->
## Model Details
### Model Description
<!-- Provide a longer summary of what this model is. -->
This is the model card of a 🤗 transformers model that has been pushed on the Hub. This model card has been automatically generated.
- **Developed by:** [More Information Needed]
- **Funded by [optional]:** [More Information Needed]
- **Shared by [optional]:** [More Information Needed]
- **Model type:** [More Information Needed]
- **Language(s) (NLP):** [More Information Needed]
- **License:** [More Information Needed]
- **Finetuned from model [optional]:** [More Information Needed]
### Model Sources [optional]
<!-- Provide the basic links for the model. -->
- **Repository:** [More Information Needed]
- **Paper [optional]:** [More Information Needed]
- **Demo [optional]:** [More Information Needed]
## Uses
<!-- Address questions around how the model is intended to be used, including the foreseeable users of the model and those affected by the model. -->
### Direct Use
<!-- This section is for the model use without fine-tuning or plugging into a larger ecosystem/app. -->
[More Information Needed]
### Downstream Use [optional]
<!-- This section is for the model use when fine-tuned for a task, or when plugged into a larger ecosystem/app -->
[More Information Needed]
### Out-of-Scope Use
<!-- This section addresses misuse, malicious use, and uses that the model will not work well for. -->
[More Information Needed]
## Bias, Risks, and Limitations
<!-- This section is meant to convey both technical and sociotechnical limitations. -->
[More Information Needed]
### Recommendations
<!-- This section is meant to convey recommendations with respect to the bias, risk, and technical limitations. -->
Users (both direct and downstream) should be made aware of the risks, biases and limitations of the model. More information needed for further recommendations.
## How to Get Started with the Model
Use the code below to get started with the model.
[More Information Needed]
## Training Details
### Training Data
<!-- This should link to a Dataset Card, perhaps with a short stub of information on what the training data is all about as well as documentation related to data pre-processing or additional filtering. -->
[More Information Needed]
### Training Procedure
<!-- This relates heavily to the Technical Specifications. Content here should link to that section when it is relevant to the training procedure. -->
#### Preprocessing [optional]
[More Information Needed]
#### Training Hyperparameters
- **Training regime:** [More Information Needed] <!--fp32, fp16 mixed precision, bf16 mixed precision, bf16 non-mixed precision, fp16 non-mixed precision, fp8 mixed precision -->
#### Speeds, Sizes, Times [optional]
<!-- This section provides information about throughput, start/end time, checkpoint size if relevant, etc. -->
[More Information Needed]
## Evaluation
<!-- This section describes the evaluation protocols and provides the results. -->
### Testing Data, Factors & Metrics
#### Testing Data
<!-- This should link to a Dataset Card if possible. -->
[More Information Needed]
#### Factors
<!-- These are the things the evaluation is disaggregating by, e.g., subpopulations or domains. -->
[More Information Needed]
#### Metrics
<!-- These are the evaluation metrics being used, ideally with a description of why. -->
[More Information Needed]
### Results
[More Information Needed]
#### Summary
## Model Examination [optional]
<!-- Relevant interpretability work for the model goes here -->
[More Information Needed]
## Environmental Impact
<!-- Total emissions (in grams of CO2eq) and additional considerations, such as electricity usage, go here. Edit the suggested text below accordingly -->
Carbon emissions can be estimated using the [Machine Learning Impact calculator](https://mlco2.github.io/impact#compute) presented in [Lacoste et al. (2019)](https://arxiv.org/abs/1910.09700).
- **Hardware Type:** [More Information Needed]
- **Hours used:** [More Information Needed]
- **Cloud Provider:** [More Information Needed]
- **Compute Region:** [More Information Needed]
- **Carbon Emitted:** [More Information Needed]
## Technical Specifications [optional]
### Model Architecture and Objective
[More Information Needed]
### Compute Infrastructure
[More Information Needed]
#### Hardware
[More Information Needed]
#### Software
[More Information Needed]
## Citation [optional]
<!-- If there is a paper or blog post introducing the model, the APA and Bibtex information for that should go in this section. -->
**BibTeX:**
[More Information Needed]
**APA:**
[More Information Needed]
## Glossary [optional]
<!-- If relevant, include terms and calculations in this section that can help readers understand the model or model card. -->
[More Information Needed]
## More Information [optional]
[More Information Needed]
## Model Card Authors [optional]
[More Information Needed]
## Model Card Contact
[More Information Needed] |
Pandita-IA/Reinforce-Pixelcopter-PLE-v0 | Pandita-IA | "2024-06-24T10:33:54Z" | 0 | 0 | null | [
"Pixelcopter-PLE-v0",
"reinforce",
"reinforcement-learning",
"custom-implementation",
"deep-rl-class",
"model-index",
"region:us"
] | reinforcement-learning | "2024-06-24T10:33:30Z" | ---
tags:
- Pixelcopter-PLE-v0
- reinforce
- reinforcement-learning
- custom-implementation
- deep-rl-class
model-index:
- name: Reinforce-Pixelcopter-PLE-v0
results:
- task:
type: reinforcement-learning
name: reinforcement-learning
dataset:
name: Pixelcopter-PLE-v0
type: Pixelcopter-PLE-v0
metrics:
- type: mean_reward
value: 33.70 +/- 25.85
name: mean_reward
verified: false
---
# **Reinforce** Agent playing **Pixelcopter-PLE-v0**
This is a trained model of a **Reinforce** agent playing **Pixelcopter-PLE-v0** .
To learn to use this model and train yours check Unit 4 of the Deep Reinforcement Learning Course: https://huggingface.co/deep-rl-course/unit4/introduction
|
PrunaAI/openchat-openchat_3.5-HQQ-2bit-smashed | PrunaAI | "2024-06-24T10:35:07Z" | 0 | 0 | transformers | [
"transformers",
"mistral",
"text-generation",
"pruna-ai",
"conversational",
"base_model:openchat/openchat_3.5",
"autotrain_compatible",
"endpoints_compatible",
"text-generation-inference",
"region:us"
] | text-generation | "2024-06-24T10:33:47Z" | ---
thumbnail: "https://assets-global.website-files.com/646b351987a8d8ce158d1940/64ec9e96b4334c0e1ac41504_Logo%20with%20white%20text.svg"
base_model: openchat/openchat_3.5
metrics:
- memory_disk
- memory_inference
- inference_latency
- inference_throughput
- inference_CO2_emissions
- inference_energy_consumption
tags:
- pruna-ai
---
<!-- header start -->
<!-- 200823 -->
<div style="width: auto; margin-left: auto; margin-right: auto">
<a href="https://www.pruna.ai/" target="_blank" rel="noopener noreferrer">
<img src="https://i.imgur.com/eDAlcgk.png" alt="PrunaAI" style="width: 100%; min-width: 400px; display: block; margin: auto;">
</a>
</div>
<!-- header end -->
[](https://twitter.com/PrunaAI)
[](https://github.com/PrunaAI)
[](https://www.linkedin.com/company/93832878/admin/feed/posts/?feedType=following)
[](https://discord.gg/CP4VSgck)
# Simply make AI models cheaper, smaller, faster, and greener!
- Give a thumbs up if you like this model!
- Contact us and tell us which model to compress next [here](https://www.pruna.ai/contact).
- Request access to easily compress your *own* AI models [here](https://z0halsaff74.typeform.com/pruna-access?typeform-source=www.pruna.ai).
- Read the documentations to know more [here](https://pruna-ai-pruna.readthedocs-hosted.com/en/latest/)
- Join Pruna AI community on Discord [here](https://discord.gg/CP4VSgck) to share feedback/suggestions or get help.
## Results

**Frequently Asked Questions**
- ***How does the compression work?*** The model is compressed with hqq.
- ***How does the model quality change?*** The quality of the model output might vary compared to the base model.
- ***How is the model efficiency evaluated?*** These results were obtained on HARDWARE_NAME with configuration described in `model/smash_config.json` and are obtained after a hardware warmup. The smashed model is directly compared to the original base model. Efficiency results may vary in other settings (e.g. other hardware, image size, batch size, ...). We recommend to directly run them in the use-case conditions to know if the smashed model can benefit you.
- ***What is the model format?*** We use safetensors.
- ***What calibration data has been used?*** If needed by the compression method, we used WikiText as the calibration data.
- ***What is the naming convention for Pruna Huggingface models?*** We take the original model name and append "turbo", "tiny", or "green" if the smashed model has a measured inference speed, inference memory, or inference energy consumption which is less than 90% of the original base model.
- ***How to compress my own models?*** You can request premium access to more compression methods and tech support for your specific use-cases [here](https://z0halsaff74.typeform.com/pruna-access?typeform-source=www.pruna.ai).
- ***What are "first" metrics?*** Results mentioning "first" are obtained after the first run of the model. The first run might take more memory or be slower than the subsequent runs due cuda overheads.
- ***What are "Sync" and "Async" metrics?*** "Sync" metrics are obtained by syncing all GPU processes and stop measurement when all of them are executed. "Async" metrics are obtained without syncing all GPU processes and stop when the model output can be used by the CPU. We provide both metrics since both could be relevant depending on the use-case. We recommend to test the efficiency gains directly in your use-cases.
## Setup
You can run the smashed model with these steps:
0. Check requirements from the original repo openchat/openchat_3.5 installed. In particular, check python, cuda, and transformers versions.
1. Make sure that you have installed quantization related packages.
```bash
pip install hqq
```
2. Load & run the model.
```python
from transformers import AutoModelForCausalLM, AutoTokenizer
from hqq.engine.hf import HQQModelForCausalLM
from hqq.models.hf.base import AutoHQQHFModel
try:
model = HQQModelForCausalLM.from_quantized("PrunaAI/openchat-openchat_3.5-HQQ-2bit-smashed", device_map='auto')
except:
model = AutoHQQHFModel.from_quantized("PrunaAI/openchat-openchat_3.5-HQQ-2bit-smashed")
tokenizer = AutoTokenizer.from_pretrained("openchat/openchat_3.5")
input_ids = tokenizer("What is the color of prunes?,", return_tensors='pt').to(model.device)["input_ids"]
outputs = model.generate(input_ids, max_new_tokens=216)
tokenizer.decode(outputs[0])
```
## Configurations
The configuration info are in `smash_config.json`.
## Credits & License
The license of the smashed model follows the license of the original model. Please check the license of the original model openchat/openchat_3.5 before using this model which provided the base model. The license of the `pruna-engine` is [here](https://pypi.org/project/pruna-engine/) on Pypi.
## Want to compress other models?
- Contact us and tell us which model to compress next [here](https://www.pruna.ai/contact).
- Request access to easily compress your own AI models [here](https://z0halsaff74.typeform.com/pruna-access?typeform-source=www.pruna.ai). |
Qamatrix/Instanbul | Qamatrix | "2024-06-24T10:33:55Z" | 0 | 0 | null | [
"region:us"
] | null | "2024-06-24T10:33:55Z" | Entry not found |
Mikeshu/___ | Mikeshu | "2024-06-24T10:33:59Z" | 0 | 0 | null | [
"region:us"
] | null | "2024-06-24T10:33:59Z" | Entry not found |
PrunaAI/allenai-tulu-2-dpo-13b-HQQ-2bit-smashed | PrunaAI | "2024-06-24T10:37:15Z" | 0 | 0 | transformers | [
"transformers",
"llama",
"text-generation",
"pruna-ai",
"conversational",
"base_model:allenai/tulu-2-dpo-13b",
"autotrain_compatible",
"endpoints_compatible",
"text-generation-inference",
"region:us"
] | text-generation | "2024-06-24T10:35:16Z" | ---
thumbnail: "https://assets-global.website-files.com/646b351987a8d8ce158d1940/64ec9e96b4334c0e1ac41504_Logo%20with%20white%20text.svg"
base_model: allenai/tulu-2-dpo-13b
metrics:
- memory_disk
- memory_inference
- inference_latency
- inference_throughput
- inference_CO2_emissions
- inference_energy_consumption
tags:
- pruna-ai
---
<!-- header start -->
<!-- 200823 -->
<div style="width: auto; margin-left: auto; margin-right: auto">
<a href="https://www.pruna.ai/" target="_blank" rel="noopener noreferrer">
<img src="https://i.imgur.com/eDAlcgk.png" alt="PrunaAI" style="width: 100%; min-width: 400px; display: block; margin: auto;">
</a>
</div>
<!-- header end -->
[](https://twitter.com/PrunaAI)
[](https://github.com/PrunaAI)
[](https://www.linkedin.com/company/93832878/admin/feed/posts/?feedType=following)
[](https://discord.gg/CP4VSgck)
# Simply make AI models cheaper, smaller, faster, and greener!
- Give a thumbs up if you like this model!
- Contact us and tell us which model to compress next [here](https://www.pruna.ai/contact).
- Request access to easily compress your *own* AI models [here](https://z0halsaff74.typeform.com/pruna-access?typeform-source=www.pruna.ai).
- Read the documentations to know more [here](https://pruna-ai-pruna.readthedocs-hosted.com/en/latest/)
- Join Pruna AI community on Discord [here](https://discord.gg/CP4VSgck) to share feedback/suggestions or get help.
## Results

**Frequently Asked Questions**
- ***How does the compression work?*** The model is compressed with hqq.
- ***How does the model quality change?*** The quality of the model output might vary compared to the base model.
- ***How is the model efficiency evaluated?*** These results were obtained on HARDWARE_NAME with configuration described in `model/smash_config.json` and are obtained after a hardware warmup. The smashed model is directly compared to the original base model. Efficiency results may vary in other settings (e.g. other hardware, image size, batch size, ...). We recommend to directly run them in the use-case conditions to know if the smashed model can benefit you.
- ***What is the model format?*** We use safetensors.
- ***What calibration data has been used?*** If needed by the compression method, we used WikiText as the calibration data.
- ***What is the naming convention for Pruna Huggingface models?*** We take the original model name and append "turbo", "tiny", or "green" if the smashed model has a measured inference speed, inference memory, or inference energy consumption which is less than 90% of the original base model.
- ***How to compress my own models?*** You can request premium access to more compression methods and tech support for your specific use-cases [here](https://z0halsaff74.typeform.com/pruna-access?typeform-source=www.pruna.ai).
- ***What are "first" metrics?*** Results mentioning "first" are obtained after the first run of the model. The first run might take more memory or be slower than the subsequent runs due cuda overheads.
- ***What are "Sync" and "Async" metrics?*** "Sync" metrics are obtained by syncing all GPU processes and stop measurement when all of them are executed. "Async" metrics are obtained without syncing all GPU processes and stop when the model output can be used by the CPU. We provide both metrics since both could be relevant depending on the use-case. We recommend to test the efficiency gains directly in your use-cases.
## Setup
You can run the smashed model with these steps:
0. Check requirements from the original repo allenai/tulu-2-dpo-13b installed. In particular, check python, cuda, and transformers versions.
1. Make sure that you have installed quantization related packages.
```bash
pip install hqq
```
2. Load & run the model.
```python
from transformers import AutoModelForCausalLM, AutoTokenizer
from hqq.engine.hf import HQQModelForCausalLM
from hqq.models.hf.base import AutoHQQHFModel
try:
model = HQQModelForCausalLM.from_quantized("PrunaAI/allenai-tulu-2-dpo-13b-HQQ-2bit-smashed", device_map='auto')
except:
model = AutoHQQHFModel.from_quantized("PrunaAI/allenai-tulu-2-dpo-13b-HQQ-2bit-smashed")
tokenizer = AutoTokenizer.from_pretrained("allenai/tulu-2-dpo-13b")
input_ids = tokenizer("What is the color of prunes?,", return_tensors='pt').to(model.device)["input_ids"]
outputs = model.generate(input_ids, max_new_tokens=216)
tokenizer.decode(outputs[0])
```
## Configurations
The configuration info are in `smash_config.json`.
## Credits & License
The license of the smashed model follows the license of the original model. Please check the license of the original model allenai/tulu-2-dpo-13b before using this model which provided the base model. The license of the `pruna-engine` is [here](https://pypi.org/project/pruna-engine/) on Pypi.
## Want to compress other models?
- Contact us and tell us which model to compress next [here](https://www.pruna.ai/contact).
- Request access to easily compress your own AI models [here](https://z0halsaff74.typeform.com/pruna-access?typeform-source=www.pruna.ai). |
itay-nakash/model_6e99ce7442_sweep_skilled-flower-912 | itay-nakash | "2024-06-24T10:36:14Z" | 0 | 0 | null | [
"region:us"
] | null | "2024-06-24T10:36:14Z" | Entry not found |
PrunaAI/allenai-tulu-2-dpo-13b-HQQ-4bit-smashed | PrunaAI | "2024-06-24T10:39:45Z" | 0 | 0 | transformers | [
"transformers",
"llama",
"text-generation",
"pruna-ai",
"conversational",
"base_model:allenai/tulu-2-dpo-13b",
"autotrain_compatible",
"endpoints_compatible",
"text-generation-inference",
"region:us"
] | text-generation | "2024-06-24T10:36:15Z" | ---
thumbnail: "https://assets-global.website-files.com/646b351987a8d8ce158d1940/64ec9e96b4334c0e1ac41504_Logo%20with%20white%20text.svg"
base_model: allenai/tulu-2-dpo-13b
metrics:
- memory_disk
- memory_inference
- inference_latency
- inference_throughput
- inference_CO2_emissions
- inference_energy_consumption
tags:
- pruna-ai
---
<!-- header start -->
<!-- 200823 -->
<div style="width: auto; margin-left: auto; margin-right: auto">
<a href="https://www.pruna.ai/" target="_blank" rel="noopener noreferrer">
<img src="https://i.imgur.com/eDAlcgk.png" alt="PrunaAI" style="width: 100%; min-width: 400px; display: block; margin: auto;">
</a>
</div>
<!-- header end -->
[](https://twitter.com/PrunaAI)
[](https://github.com/PrunaAI)
[](https://www.linkedin.com/company/93832878/admin/feed/posts/?feedType=following)
[](https://discord.gg/CP4VSgck)
# Simply make AI models cheaper, smaller, faster, and greener!
- Give a thumbs up if you like this model!
- Contact us and tell us which model to compress next [here](https://www.pruna.ai/contact).
- Request access to easily compress your *own* AI models [here](https://z0halsaff74.typeform.com/pruna-access?typeform-source=www.pruna.ai).
- Read the documentations to know more [here](https://pruna-ai-pruna.readthedocs-hosted.com/en/latest/)
- Join Pruna AI community on Discord [here](https://discord.gg/CP4VSgck) to share feedback/suggestions or get help.
## Results

**Frequently Asked Questions**
- ***How does the compression work?*** The model is compressed with hqq.
- ***How does the model quality change?*** The quality of the model output might vary compared to the base model.
- ***How is the model efficiency evaluated?*** These results were obtained on HARDWARE_NAME with configuration described in `model/smash_config.json` and are obtained after a hardware warmup. The smashed model is directly compared to the original base model. Efficiency results may vary in other settings (e.g. other hardware, image size, batch size, ...). We recommend to directly run them in the use-case conditions to know if the smashed model can benefit you.
- ***What is the model format?*** We use safetensors.
- ***What calibration data has been used?*** If needed by the compression method, we used WikiText as the calibration data.
- ***What is the naming convention for Pruna Huggingface models?*** We take the original model name and append "turbo", "tiny", or "green" if the smashed model has a measured inference speed, inference memory, or inference energy consumption which is less than 90% of the original base model.
- ***How to compress my own models?*** You can request premium access to more compression methods and tech support for your specific use-cases [here](https://z0halsaff74.typeform.com/pruna-access?typeform-source=www.pruna.ai).
- ***What are "first" metrics?*** Results mentioning "first" are obtained after the first run of the model. The first run might take more memory or be slower than the subsequent runs due cuda overheads.
- ***What are "Sync" and "Async" metrics?*** "Sync" metrics are obtained by syncing all GPU processes and stop measurement when all of them are executed. "Async" metrics are obtained without syncing all GPU processes and stop when the model output can be used by the CPU. We provide both metrics since both could be relevant depending on the use-case. We recommend to test the efficiency gains directly in your use-cases.
## Setup
You can run the smashed model with these steps:
0. Check requirements from the original repo allenai/tulu-2-dpo-13b installed. In particular, check python, cuda, and transformers versions.
1. Make sure that you have installed quantization related packages.
```bash
pip install hqq
```
2. Load & run the model.
```python
from transformers import AutoModelForCausalLM, AutoTokenizer
from hqq.engine.hf import HQQModelForCausalLM
from hqq.models.hf.base import AutoHQQHFModel
try:
model = HQQModelForCausalLM.from_quantized("PrunaAI/allenai-tulu-2-dpo-13b-HQQ-4bit-smashed", device_map='auto')
except:
model = AutoHQQHFModel.from_quantized("PrunaAI/allenai-tulu-2-dpo-13b-HQQ-4bit-smashed")
tokenizer = AutoTokenizer.from_pretrained("allenai/tulu-2-dpo-13b")
input_ids = tokenizer("What is the color of prunes?,", return_tensors='pt').to(model.device)["input_ids"]
outputs = model.generate(input_ids, max_new_tokens=216)
tokenizer.decode(outputs[0])
```
## Configurations
The configuration info are in `smash_config.json`.
## Credits & License
The license of the smashed model follows the license of the original model. Please check the license of the original model allenai/tulu-2-dpo-13b before using this model which provided the base model. The license of the `pruna-engine` is [here](https://pypi.org/project/pruna-engine/) on Pypi.
## Want to compress other models?
- Contact us and tell us which model to compress next [here](https://www.pruna.ai/contact).
- Request access to easily compress your own AI models [here](https://z0halsaff74.typeform.com/pruna-access?typeform-source=www.pruna.ai). |
PrunaAI/rinna-bilingual-gpt-neox-4b-HQQ-2bit-smashed | PrunaAI | "2024-06-24T10:37:02Z" | 0 | 0 | transformers | [
"transformers",
"gpt_neox",
"text-generation",
"pruna-ai",
"base_model:rinna/bilingual-gpt-neox-4b",
"autotrain_compatible",
"endpoints_compatible",
"text-generation-inference",
"region:us"
] | text-generation | "2024-06-24T10:36:21Z" | ---
thumbnail: "https://assets-global.website-files.com/646b351987a8d8ce158d1940/64ec9e96b4334c0e1ac41504_Logo%20with%20white%20text.svg"
base_model: rinna/bilingual-gpt-neox-4b
metrics:
- memory_disk
- memory_inference
- inference_latency
- inference_throughput
- inference_CO2_emissions
- inference_energy_consumption
tags:
- pruna-ai
---
<!-- header start -->
<!-- 200823 -->
<div style="width: auto; margin-left: auto; margin-right: auto">
<a href="https://www.pruna.ai/" target="_blank" rel="noopener noreferrer">
<img src="https://i.imgur.com/eDAlcgk.png" alt="PrunaAI" style="width: 100%; min-width: 400px; display: block; margin: auto;">
</a>
</div>
<!-- header end -->
[](https://twitter.com/PrunaAI)
[](https://github.com/PrunaAI)
[](https://www.linkedin.com/company/93832878/admin/feed/posts/?feedType=following)
[](https://discord.gg/CP4VSgck)
# Simply make AI models cheaper, smaller, faster, and greener!
- Give a thumbs up if you like this model!
- Contact us and tell us which model to compress next [here](https://www.pruna.ai/contact).
- Request access to easily compress your *own* AI models [here](https://z0halsaff74.typeform.com/pruna-access?typeform-source=www.pruna.ai).
- Read the documentations to know more [here](https://pruna-ai-pruna.readthedocs-hosted.com/en/latest/)
- Join Pruna AI community on Discord [here](https://discord.gg/CP4VSgck) to share feedback/suggestions or get help.
## Results

**Frequently Asked Questions**
- ***How does the compression work?*** The model is compressed with hqq.
- ***How does the model quality change?*** The quality of the model output might vary compared to the base model.
- ***How is the model efficiency evaluated?*** These results were obtained on HARDWARE_NAME with configuration described in `model/smash_config.json` and are obtained after a hardware warmup. The smashed model is directly compared to the original base model. Efficiency results may vary in other settings (e.g. other hardware, image size, batch size, ...). We recommend to directly run them in the use-case conditions to know if the smashed model can benefit you.
- ***What is the model format?*** We use safetensors.
- ***What calibration data has been used?*** If needed by the compression method, we used WikiText as the calibration data.
- ***What is the naming convention for Pruna Huggingface models?*** We take the original model name and append "turbo", "tiny", or "green" if the smashed model has a measured inference speed, inference memory, or inference energy consumption which is less than 90% of the original base model.
- ***How to compress my own models?*** You can request premium access to more compression methods and tech support for your specific use-cases [here](https://z0halsaff74.typeform.com/pruna-access?typeform-source=www.pruna.ai).
- ***What are "first" metrics?*** Results mentioning "first" are obtained after the first run of the model. The first run might take more memory or be slower than the subsequent runs due cuda overheads.
- ***What are "Sync" and "Async" metrics?*** "Sync" metrics are obtained by syncing all GPU processes and stop measurement when all of them are executed. "Async" metrics are obtained without syncing all GPU processes and stop when the model output can be used by the CPU. We provide both metrics since both could be relevant depending on the use-case. We recommend to test the efficiency gains directly in your use-cases.
## Setup
You can run the smashed model with these steps:
0. Check requirements from the original repo rinna/bilingual-gpt-neox-4b installed. In particular, check python, cuda, and transformers versions.
1. Make sure that you have installed quantization related packages.
```bash
pip install hqq
```
2. Load & run the model.
```python
from transformers import AutoModelForCausalLM, AutoTokenizer
from hqq.engine.hf import HQQModelForCausalLM
from hqq.models.hf.base import AutoHQQHFModel
try:
model = HQQModelForCausalLM.from_quantized("PrunaAI/rinna-bilingual-gpt-neox-4b-HQQ-2bit-smashed", device_map='auto')
except:
model = AutoHQQHFModel.from_quantized("PrunaAI/rinna-bilingual-gpt-neox-4b-HQQ-2bit-smashed")
tokenizer = AutoTokenizer.from_pretrained("rinna/bilingual-gpt-neox-4b")
input_ids = tokenizer("What is the color of prunes?,", return_tensors='pt').to(model.device)["input_ids"]
outputs = model.generate(input_ids, max_new_tokens=216)
tokenizer.decode(outputs[0])
```
## Configurations
The configuration info are in `smash_config.json`.
## Credits & License
The license of the smashed model follows the license of the original model. Please check the license of the original model rinna/bilingual-gpt-neox-4b before using this model which provided the base model. The license of the `pruna-engine` is [here](https://pypi.org/project/pruna-engine/) on Pypi.
## Want to compress other models?
- Contact us and tell us which model to compress next [here](https://www.pruna.ai/contact).
- Request access to easily compress your own AI models [here](https://z0halsaff74.typeform.com/pruna-access?typeform-source=www.pruna.ai). |
PrunaAI/rinna-bilingual-gpt-neox-4b-HQQ-4bit-smashed | PrunaAI | "2024-06-24T10:37:32Z" | 0 | 0 | transformers | [
"transformers",
"gpt_neox",
"text-generation",
"pruna-ai",
"base_model:rinna/bilingual-gpt-neox-4b",
"autotrain_compatible",
"endpoints_compatible",
"text-generation-inference",
"region:us"
] | text-generation | "2024-06-24T10:36:23Z" | ---
thumbnail: "https://assets-global.website-files.com/646b351987a8d8ce158d1940/64ec9e96b4334c0e1ac41504_Logo%20with%20white%20text.svg"
base_model: rinna/bilingual-gpt-neox-4b
metrics:
- memory_disk
- memory_inference
- inference_latency
- inference_throughput
- inference_CO2_emissions
- inference_energy_consumption
tags:
- pruna-ai
---
<!-- header start -->
<!-- 200823 -->
<div style="width: auto; margin-left: auto; margin-right: auto">
<a href="https://www.pruna.ai/" target="_blank" rel="noopener noreferrer">
<img src="https://i.imgur.com/eDAlcgk.png" alt="PrunaAI" style="width: 100%; min-width: 400px; display: block; margin: auto;">
</a>
</div>
<!-- header end -->
[](https://twitter.com/PrunaAI)
[](https://github.com/PrunaAI)
[](https://www.linkedin.com/company/93832878/admin/feed/posts/?feedType=following)
[](https://discord.gg/CP4VSgck)
# Simply make AI models cheaper, smaller, faster, and greener!
- Give a thumbs up if you like this model!
- Contact us and tell us which model to compress next [here](https://www.pruna.ai/contact).
- Request access to easily compress your *own* AI models [here](https://z0halsaff74.typeform.com/pruna-access?typeform-source=www.pruna.ai).
- Read the documentations to know more [here](https://pruna-ai-pruna.readthedocs-hosted.com/en/latest/)
- Join Pruna AI community on Discord [here](https://discord.gg/CP4VSgck) to share feedback/suggestions or get help.
## Results

**Frequently Asked Questions**
- ***How does the compression work?*** The model is compressed with hqq.
- ***How does the model quality change?*** The quality of the model output might vary compared to the base model.
- ***How is the model efficiency evaluated?*** These results were obtained on HARDWARE_NAME with configuration described in `model/smash_config.json` and are obtained after a hardware warmup. The smashed model is directly compared to the original base model. Efficiency results may vary in other settings (e.g. other hardware, image size, batch size, ...). We recommend to directly run them in the use-case conditions to know if the smashed model can benefit you.
- ***What is the model format?*** We use safetensors.
- ***What calibration data has been used?*** If needed by the compression method, we used WikiText as the calibration data.
- ***What is the naming convention for Pruna Huggingface models?*** We take the original model name and append "turbo", "tiny", or "green" if the smashed model has a measured inference speed, inference memory, or inference energy consumption which is less than 90% of the original base model.
- ***How to compress my own models?*** You can request premium access to more compression methods and tech support for your specific use-cases [here](https://z0halsaff74.typeform.com/pruna-access?typeform-source=www.pruna.ai).
- ***What are "first" metrics?*** Results mentioning "first" are obtained after the first run of the model. The first run might take more memory or be slower than the subsequent runs due cuda overheads.
- ***What are "Sync" and "Async" metrics?*** "Sync" metrics are obtained by syncing all GPU processes and stop measurement when all of them are executed. "Async" metrics are obtained without syncing all GPU processes and stop when the model output can be used by the CPU. We provide both metrics since both could be relevant depending on the use-case. We recommend to test the efficiency gains directly in your use-cases.
## Setup
You can run the smashed model with these steps:
0. Check requirements from the original repo rinna/bilingual-gpt-neox-4b installed. In particular, check python, cuda, and transformers versions.
1. Make sure that you have installed quantization related packages.
```bash
pip install hqq
```
2. Load & run the model.
```python
from transformers import AutoModelForCausalLM, AutoTokenizer
from hqq.engine.hf import HQQModelForCausalLM
from hqq.models.hf.base import AutoHQQHFModel
try:
model = HQQModelForCausalLM.from_quantized("PrunaAI/rinna-bilingual-gpt-neox-4b-HQQ-4bit-smashed", device_map='auto')
except:
model = AutoHQQHFModel.from_quantized("PrunaAI/rinna-bilingual-gpt-neox-4b-HQQ-4bit-smashed")
tokenizer = AutoTokenizer.from_pretrained("rinna/bilingual-gpt-neox-4b")
input_ids = tokenizer("What is the color of prunes?,", return_tensors='pt').to(model.device)["input_ids"]
outputs = model.generate(input_ids, max_new_tokens=216)
tokenizer.decode(outputs[0])
```
## Configurations
The configuration info are in `smash_config.json`.
## Credits & License
The license of the smashed model follows the license of the original model. Please check the license of the original model rinna/bilingual-gpt-neox-4b before using this model which provided the base model. The license of the `pruna-engine` is [here](https://pypi.org/project/pruna-engine/) on Pypi.
## Want to compress other models?
- Contact us and tell us which model to compress next [here](https://www.pruna.ai/contact).
- Request access to easily compress your own AI models [here](https://z0halsaff74.typeform.com/pruna-access?typeform-source=www.pruna.ai). |
PrunaAI/rinna-bilingual-gpt-neox-4b-HQQ-1bit-smashed | PrunaAI | "2024-06-24T10:37:02Z" | 0 | 0 | transformers | [
"transformers",
"gpt_neox",
"text-generation",
"pruna-ai",
"base_model:rinna/bilingual-gpt-neox-4b",
"autotrain_compatible",
"endpoints_compatible",
"text-generation-inference",
"region:us"
] | text-generation | "2024-06-24T10:36:29Z" | ---
thumbnail: "https://assets-global.website-files.com/646b351987a8d8ce158d1940/64ec9e96b4334c0e1ac41504_Logo%20with%20white%20text.svg"
base_model: rinna/bilingual-gpt-neox-4b
metrics:
- memory_disk
- memory_inference
- inference_latency
- inference_throughput
- inference_CO2_emissions
- inference_energy_consumption
tags:
- pruna-ai
---
<!-- header start -->
<!-- 200823 -->
<div style="width: auto; margin-left: auto; margin-right: auto">
<a href="https://www.pruna.ai/" target="_blank" rel="noopener noreferrer">
<img src="https://i.imgur.com/eDAlcgk.png" alt="PrunaAI" style="width: 100%; min-width: 400px; display: block; margin: auto;">
</a>
</div>
<!-- header end -->
[](https://twitter.com/PrunaAI)
[](https://github.com/PrunaAI)
[](https://www.linkedin.com/company/93832878/admin/feed/posts/?feedType=following)
[](https://discord.gg/CP4VSgck)
# Simply make AI models cheaper, smaller, faster, and greener!
- Give a thumbs up if you like this model!
- Contact us and tell us which model to compress next [here](https://www.pruna.ai/contact).
- Request access to easily compress your *own* AI models [here](https://z0halsaff74.typeform.com/pruna-access?typeform-source=www.pruna.ai).
- Read the documentations to know more [here](https://pruna-ai-pruna.readthedocs-hosted.com/en/latest/)
- Join Pruna AI community on Discord [here](https://discord.gg/CP4VSgck) to share feedback/suggestions or get help.
## Results

**Frequently Asked Questions**
- ***How does the compression work?*** The model is compressed with hqq.
- ***How does the model quality change?*** The quality of the model output might vary compared to the base model.
- ***How is the model efficiency evaluated?*** These results were obtained on HARDWARE_NAME with configuration described in `model/smash_config.json` and are obtained after a hardware warmup. The smashed model is directly compared to the original base model. Efficiency results may vary in other settings (e.g. other hardware, image size, batch size, ...). We recommend to directly run them in the use-case conditions to know if the smashed model can benefit you.
- ***What is the model format?*** We use safetensors.
- ***What calibration data has been used?*** If needed by the compression method, we used WikiText as the calibration data.
- ***What is the naming convention for Pruna Huggingface models?*** We take the original model name and append "turbo", "tiny", or "green" if the smashed model has a measured inference speed, inference memory, or inference energy consumption which is less than 90% of the original base model.
- ***How to compress my own models?*** You can request premium access to more compression methods and tech support for your specific use-cases [here](https://z0halsaff74.typeform.com/pruna-access?typeform-source=www.pruna.ai).
- ***What are "first" metrics?*** Results mentioning "first" are obtained after the first run of the model. The first run might take more memory or be slower than the subsequent runs due cuda overheads.
- ***What are "Sync" and "Async" metrics?*** "Sync" metrics are obtained by syncing all GPU processes and stop measurement when all of them are executed. "Async" metrics are obtained without syncing all GPU processes and stop when the model output can be used by the CPU. We provide both metrics since both could be relevant depending on the use-case. We recommend to test the efficiency gains directly in your use-cases.
## Setup
You can run the smashed model with these steps:
0. Check requirements from the original repo rinna/bilingual-gpt-neox-4b installed. In particular, check python, cuda, and transformers versions.
1. Make sure that you have installed quantization related packages.
```bash
pip install hqq
```
2. Load & run the model.
```python
from transformers import AutoModelForCausalLM, AutoTokenizer
from hqq.engine.hf import HQQModelForCausalLM
from hqq.models.hf.base import AutoHQQHFModel
try:
model = HQQModelForCausalLM.from_quantized("PrunaAI/rinna-bilingual-gpt-neox-4b-HQQ-1bit-smashed", device_map='auto')
except:
model = AutoHQQHFModel.from_quantized("PrunaAI/rinna-bilingual-gpt-neox-4b-HQQ-1bit-smashed")
tokenizer = AutoTokenizer.from_pretrained("rinna/bilingual-gpt-neox-4b")
input_ids = tokenizer("What is the color of prunes?,", return_tensors='pt').to(model.device)["input_ids"]
outputs = model.generate(input_ids, max_new_tokens=216)
tokenizer.decode(outputs[0])
```
## Configurations
The configuration info are in `smash_config.json`.
## Credits & License
The license of the smashed model follows the license of the original model. Please check the license of the original model rinna/bilingual-gpt-neox-4b before using this model which provided the base model. The license of the `pruna-engine` is [here](https://pypi.org/project/pruna-engine/) on Pypi.
## Want to compress other models?
- Contact us and tell us which model to compress next [here](https://www.pruna.ai/contact).
- Request access to easily compress your own AI models [here](https://z0halsaff74.typeform.com/pruna-access?typeform-source=www.pruna.ai). |
SeyiClover/whisper-small-ko | SeyiClover | "2024-07-02T12:07:14Z" | 0 | 0 | transformers | [
"transformers",
"tensorboard",
"safetensors",
"whisper",
"automatic-speech-recognition",
"endpoints_compatible",
"region:us"
] | automatic-speech-recognition | "2024-06-24T10:36:31Z" | Entry not found |
PrunaAI/allenai-tulu-2-dpo-13b-HQQ-1bit-smashed | PrunaAI | "2024-06-24T10:37:48Z" | 0 | 0 | transformers | [
"transformers",
"llama",
"text-generation",
"pruna-ai",
"conversational",
"base_model:allenai/tulu-2-dpo-13b",
"autotrain_compatible",
"endpoints_compatible",
"text-generation-inference",
"region:us"
] | text-generation | "2024-06-24T10:36:32Z" | ---
thumbnail: "https://assets-global.website-files.com/646b351987a8d8ce158d1940/64ec9e96b4334c0e1ac41504_Logo%20with%20white%20text.svg"
base_model: allenai/tulu-2-dpo-13b
metrics:
- memory_disk
- memory_inference
- inference_latency
- inference_throughput
- inference_CO2_emissions
- inference_energy_consumption
tags:
- pruna-ai
---
<!-- header start -->
<!-- 200823 -->
<div style="width: auto; margin-left: auto; margin-right: auto">
<a href="https://www.pruna.ai/" target="_blank" rel="noopener noreferrer">
<img src="https://i.imgur.com/eDAlcgk.png" alt="PrunaAI" style="width: 100%; min-width: 400px; display: block; margin: auto;">
</a>
</div>
<!-- header end -->
[](https://twitter.com/PrunaAI)
[](https://github.com/PrunaAI)
[](https://www.linkedin.com/company/93832878/admin/feed/posts/?feedType=following)
[](https://discord.gg/CP4VSgck)
# Simply make AI models cheaper, smaller, faster, and greener!
- Give a thumbs up if you like this model!
- Contact us and tell us which model to compress next [here](https://www.pruna.ai/contact).
- Request access to easily compress your *own* AI models [here](https://z0halsaff74.typeform.com/pruna-access?typeform-source=www.pruna.ai).
- Read the documentations to know more [here](https://pruna-ai-pruna.readthedocs-hosted.com/en/latest/)
- Join Pruna AI community on Discord [here](https://discord.gg/CP4VSgck) to share feedback/suggestions or get help.
## Results

**Frequently Asked Questions**
- ***How does the compression work?*** The model is compressed with hqq.
- ***How does the model quality change?*** The quality of the model output might vary compared to the base model.
- ***How is the model efficiency evaluated?*** These results were obtained on HARDWARE_NAME with configuration described in `model/smash_config.json` and are obtained after a hardware warmup. The smashed model is directly compared to the original base model. Efficiency results may vary in other settings (e.g. other hardware, image size, batch size, ...). We recommend to directly run them in the use-case conditions to know if the smashed model can benefit you.
- ***What is the model format?*** We use safetensors.
- ***What calibration data has been used?*** If needed by the compression method, we used WikiText as the calibration data.
- ***What is the naming convention for Pruna Huggingface models?*** We take the original model name and append "turbo", "tiny", or "green" if the smashed model has a measured inference speed, inference memory, or inference energy consumption which is less than 90% of the original base model.
- ***How to compress my own models?*** You can request premium access to more compression methods and tech support for your specific use-cases [here](https://z0halsaff74.typeform.com/pruna-access?typeform-source=www.pruna.ai).
- ***What are "first" metrics?*** Results mentioning "first" are obtained after the first run of the model. The first run might take more memory or be slower than the subsequent runs due cuda overheads.
- ***What are "Sync" and "Async" metrics?*** "Sync" metrics are obtained by syncing all GPU processes and stop measurement when all of them are executed. "Async" metrics are obtained without syncing all GPU processes and stop when the model output can be used by the CPU. We provide both metrics since both could be relevant depending on the use-case. We recommend to test the efficiency gains directly in your use-cases.
## Setup
You can run the smashed model with these steps:
0. Check requirements from the original repo allenai/tulu-2-dpo-13b installed. In particular, check python, cuda, and transformers versions.
1. Make sure that you have installed quantization related packages.
```bash
pip install hqq
```
2. Load & run the model.
```python
from transformers import AutoModelForCausalLM, AutoTokenizer
from hqq.engine.hf import HQQModelForCausalLM
from hqq.models.hf.base import AutoHQQHFModel
try:
model = HQQModelForCausalLM.from_quantized("PrunaAI/allenai-tulu-2-dpo-13b-HQQ-1bit-smashed", device_map='auto')
except:
model = AutoHQQHFModel.from_quantized("PrunaAI/allenai-tulu-2-dpo-13b-HQQ-1bit-smashed")
tokenizer = AutoTokenizer.from_pretrained("allenai/tulu-2-dpo-13b")
input_ids = tokenizer("What is the color of prunes?,", return_tensors='pt').to(model.device)["input_ids"]
outputs = model.generate(input_ids, max_new_tokens=216)
tokenizer.decode(outputs[0])
```
## Configurations
The configuration info are in `smash_config.json`.
## Credits & License
The license of the smashed model follows the license of the original model. Please check the license of the original model allenai/tulu-2-dpo-13b before using this model which provided the base model. The license of the `pruna-engine` is [here](https://pypi.org/project/pruna-engine/) on Pypi.
## Want to compress other models?
- Contact us and tell us which model to compress next [here](https://www.pruna.ai/contact).
- Request access to easily compress your own AI models [here](https://z0halsaff74.typeform.com/pruna-access?typeform-source=www.pruna.ai). |
mmtg/whisper-base-fine | mmtg | "2024-06-24T11:34:30Z" | 0 | 0 | transformers | [
"transformers",
"tensorboard",
"safetensors",
"whisper",
"automatic-speech-recognition",
"endpoints_compatible",
"region:us"
] | automatic-speech-recognition | "2024-06-24T10:37:46Z" | Entry not found |
PrunaAI/h2oai-h2ogpt-gm-oasst1-en-2048-falcon-7b-v3-HQQ-1bit-smashed | PrunaAI | "2024-06-24T10:44:51Z" | 0 | 0 | transformers | [
"transformers",
"RefinedWebModel",
"text-generation",
"pruna-ai",
"custom_code",
"base_model:h2oai/h2ogpt-gm-oasst1-en-2048-falcon-7b-v3",
"autotrain_compatible",
"text-generation-inference",
"region:us"
] | text-generation | "2024-06-24T10:38:28Z" | ---
thumbnail: "https://assets-global.website-files.com/646b351987a8d8ce158d1940/64ec9e96b4334c0e1ac41504_Logo%20with%20white%20text.svg"
base_model: h2oai/h2ogpt-gm-oasst1-en-2048-falcon-7b-v3
metrics:
- memory_disk
- memory_inference
- inference_latency
- inference_throughput
- inference_CO2_emissions
- inference_energy_consumption
tags:
- pruna-ai
---
<!-- header start -->
<!-- 200823 -->
<div style="width: auto; margin-left: auto; margin-right: auto">
<a href="https://www.pruna.ai/" target="_blank" rel="noopener noreferrer">
<img src="https://i.imgur.com/eDAlcgk.png" alt="PrunaAI" style="width: 100%; min-width: 400px; display: block; margin: auto;">
</a>
</div>
<!-- header end -->
[](https://twitter.com/PrunaAI)
[](https://github.com/PrunaAI)
[](https://www.linkedin.com/company/93832878/admin/feed/posts/?feedType=following)
[](https://discord.gg/CP4VSgck)
# Simply make AI models cheaper, smaller, faster, and greener!
- Give a thumbs up if you like this model!
- Contact us and tell us which model to compress next [here](https://www.pruna.ai/contact).
- Request access to easily compress your *own* AI models [here](https://z0halsaff74.typeform.com/pruna-access?typeform-source=www.pruna.ai).
- Read the documentations to know more [here](https://pruna-ai-pruna.readthedocs-hosted.com/en/latest/)
- Join Pruna AI community on Discord [here](https://discord.gg/CP4VSgck) to share feedback/suggestions or get help.
## Results

**Frequently Asked Questions**
- ***How does the compression work?*** The model is compressed with hqq.
- ***How does the model quality change?*** The quality of the model output might vary compared to the base model.
- ***How is the model efficiency evaluated?*** These results were obtained on HARDWARE_NAME with configuration described in `model/smash_config.json` and are obtained after a hardware warmup. The smashed model is directly compared to the original base model. Efficiency results may vary in other settings (e.g. other hardware, image size, batch size, ...). We recommend to directly run them in the use-case conditions to know if the smashed model can benefit you.
- ***What is the model format?*** We use safetensors.
- ***What calibration data has been used?*** If needed by the compression method, we used WikiText as the calibration data.
- ***What is the naming convention for Pruna Huggingface models?*** We take the original model name and append "turbo", "tiny", or "green" if the smashed model has a measured inference speed, inference memory, or inference energy consumption which is less than 90% of the original base model.
- ***How to compress my own models?*** You can request premium access to more compression methods and tech support for your specific use-cases [here](https://z0halsaff74.typeform.com/pruna-access?typeform-source=www.pruna.ai).
- ***What are "first" metrics?*** Results mentioning "first" are obtained after the first run of the model. The first run might take more memory or be slower than the subsequent runs due cuda overheads.
- ***What are "Sync" and "Async" metrics?*** "Sync" metrics are obtained by syncing all GPU processes and stop measurement when all of them are executed. "Async" metrics are obtained without syncing all GPU processes and stop when the model output can be used by the CPU. We provide both metrics since both could be relevant depending on the use-case. We recommend to test the efficiency gains directly in your use-cases.
## Setup
You can run the smashed model with these steps:
0. Check requirements from the original repo h2oai/h2ogpt-gm-oasst1-en-2048-falcon-7b-v3 installed. In particular, check python, cuda, and transformers versions.
1. Make sure that you have installed quantization related packages.
```bash
pip install hqq
```
2. Load & run the model.
```python
from transformers import AutoModelForCausalLM, AutoTokenizer
from hqq.engine.hf import HQQModelForCausalLM
from hqq.models.hf.base import AutoHQQHFModel
try:
model = HQQModelForCausalLM.from_quantized("PrunaAI/h2oai-h2ogpt-gm-oasst1-en-2048-falcon-7b-v3-HQQ-1bit-smashed", device_map='auto')
except:
model = AutoHQQHFModel.from_quantized("PrunaAI/h2oai-h2ogpt-gm-oasst1-en-2048-falcon-7b-v3-HQQ-1bit-smashed")
tokenizer = AutoTokenizer.from_pretrained("h2oai/h2ogpt-gm-oasst1-en-2048-falcon-7b-v3")
input_ids = tokenizer("What is the color of prunes?,", return_tensors='pt').to(model.device)["input_ids"]
outputs = model.generate(input_ids, max_new_tokens=216)
tokenizer.decode(outputs[0])
```
## Configurations
The configuration info are in `smash_config.json`.
## Credits & License
The license of the smashed model follows the license of the original model. Please check the license of the original model h2oai/h2ogpt-gm-oasst1-en-2048-falcon-7b-v3 before using this model which provided the base model. The license of the `pruna-engine` is [here](https://pypi.org/project/pruna-engine/) on Pypi.
## Want to compress other models?
- Contact us and tell us which model to compress next [here](https://www.pruna.ai/contact).
- Request access to easily compress your own AI models [here](https://z0halsaff74.typeform.com/pruna-access?typeform-source=www.pruna.ai). |
PrunaAI/ytu-ce-cosmos-turkish-gpt2-large-HQQ-2bit-smashed | PrunaAI | "2024-06-24T10:40:04Z" | 0 | 0 | transformers | [
"transformers",
"gpt2",
"text-generation",
"pruna-ai",
"base_model:ytu-ce-cosmos/turkish-gpt2-large",
"autotrain_compatible",
"endpoints_compatible",
"text-generation-inference",
"region:us"
] | text-generation | "2024-06-24T10:39:15Z" | ---
thumbnail: "https://assets-global.website-files.com/646b351987a8d8ce158d1940/64ec9e96b4334c0e1ac41504_Logo%20with%20white%20text.svg"
base_model: ytu-ce-cosmos/turkish-gpt2-large
metrics:
- memory_disk
- memory_inference
- inference_latency
- inference_throughput
- inference_CO2_emissions
- inference_energy_consumption
tags:
- pruna-ai
---
<!-- header start -->
<!-- 200823 -->
<div style="width: auto; margin-left: auto; margin-right: auto">
<a href="https://www.pruna.ai/" target="_blank" rel="noopener noreferrer">
<img src="https://i.imgur.com/eDAlcgk.png" alt="PrunaAI" style="width: 100%; min-width: 400px; display: block; margin: auto;">
</a>
</div>
<!-- header end -->
[](https://twitter.com/PrunaAI)
[](https://github.com/PrunaAI)
[](https://www.linkedin.com/company/93832878/admin/feed/posts/?feedType=following)
[](https://discord.gg/CP4VSgck)
# Simply make AI models cheaper, smaller, faster, and greener!
- Give a thumbs up if you like this model!
- Contact us and tell us which model to compress next [here](https://www.pruna.ai/contact).
- Request access to easily compress your *own* AI models [here](https://z0halsaff74.typeform.com/pruna-access?typeform-source=www.pruna.ai).
- Read the documentations to know more [here](https://pruna-ai-pruna.readthedocs-hosted.com/en/latest/)
- Join Pruna AI community on Discord [here](https://discord.gg/CP4VSgck) to share feedback/suggestions or get help.
## Results

**Frequently Asked Questions**
- ***How does the compression work?*** The model is compressed with hqq.
- ***How does the model quality change?*** The quality of the model output might vary compared to the base model.
- ***How is the model efficiency evaluated?*** These results were obtained on HARDWARE_NAME with configuration described in `model/smash_config.json` and are obtained after a hardware warmup. The smashed model is directly compared to the original base model. Efficiency results may vary in other settings (e.g. other hardware, image size, batch size, ...). We recommend to directly run them in the use-case conditions to know if the smashed model can benefit you.
- ***What is the model format?*** We use safetensors.
- ***What calibration data has been used?*** If needed by the compression method, we used WikiText as the calibration data.
- ***What is the naming convention for Pruna Huggingface models?*** We take the original model name and append "turbo", "tiny", or "green" if the smashed model has a measured inference speed, inference memory, or inference energy consumption which is less than 90% of the original base model.
- ***How to compress my own models?*** You can request premium access to more compression methods and tech support for your specific use-cases [here](https://z0halsaff74.typeform.com/pruna-access?typeform-source=www.pruna.ai).
- ***What are "first" metrics?*** Results mentioning "first" are obtained after the first run of the model. The first run might take more memory or be slower than the subsequent runs due cuda overheads.
- ***What are "Sync" and "Async" metrics?*** "Sync" metrics are obtained by syncing all GPU processes and stop measurement when all of them are executed. "Async" metrics are obtained without syncing all GPU processes and stop when the model output can be used by the CPU. We provide both metrics since both could be relevant depending on the use-case. We recommend to test the efficiency gains directly in your use-cases.
## Setup
You can run the smashed model with these steps:
0. Check requirements from the original repo ytu-ce-cosmos/turkish-gpt2-large installed. In particular, check python, cuda, and transformers versions.
1. Make sure that you have installed quantization related packages.
```bash
pip install hqq
```
2. Load & run the model.
```python
from transformers import AutoModelForCausalLM, AutoTokenizer
from hqq.engine.hf import HQQModelForCausalLM
from hqq.models.hf.base import AutoHQQHFModel
try:
model = HQQModelForCausalLM.from_quantized("PrunaAI/ytu-ce-cosmos-turkish-gpt2-large-HQQ-2bit-smashed", device_map='auto')
except:
model = AutoHQQHFModel.from_quantized("PrunaAI/ytu-ce-cosmos-turkish-gpt2-large-HQQ-2bit-smashed")
tokenizer = AutoTokenizer.from_pretrained("ytu-ce-cosmos/turkish-gpt2-large")
input_ids = tokenizer("What is the color of prunes?,", return_tensors='pt').to(model.device)["input_ids"]
outputs = model.generate(input_ids, max_new_tokens=216)
tokenizer.decode(outputs[0])
```
## Configurations
The configuration info are in `smash_config.json`.
## Credits & License
The license of the smashed model follows the license of the original model. Please check the license of the original model ytu-ce-cosmos/turkish-gpt2-large before using this model which provided the base model. The license of the `pruna-engine` is [here](https://pypi.org/project/pruna-engine/) on Pypi.
## Want to compress other models?
- Contact us and tell us which model to compress next [here](https://www.pruna.ai/contact).
- Request access to easily compress your own AI models [here](https://z0halsaff74.typeform.com/pruna-access?typeform-source=www.pruna.ai). |
PrunaAI/h2oai-h2ogpt-gm-oasst1-en-2048-falcon-7b-v3-HQQ-4bit-smashed | PrunaAI | "2024-06-24T10:46:33Z" | 0 | 0 | transformers | [
"transformers",
"RefinedWebModel",
"text-generation",
"pruna-ai",
"custom_code",
"base_model:h2oai/h2ogpt-gm-oasst1-en-2048-falcon-7b-v3",
"autotrain_compatible",
"text-generation-inference",
"region:us"
] | text-generation | "2024-06-24T10:39:32Z" | ---
thumbnail: "https://assets-global.website-files.com/646b351987a8d8ce158d1940/64ec9e96b4334c0e1ac41504_Logo%20with%20white%20text.svg"
base_model: h2oai/h2ogpt-gm-oasst1-en-2048-falcon-7b-v3
metrics:
- memory_disk
- memory_inference
- inference_latency
- inference_throughput
- inference_CO2_emissions
- inference_energy_consumption
tags:
- pruna-ai
---
<!-- header start -->
<!-- 200823 -->
<div style="width: auto; margin-left: auto; margin-right: auto">
<a href="https://www.pruna.ai/" target="_blank" rel="noopener noreferrer">
<img src="https://i.imgur.com/eDAlcgk.png" alt="PrunaAI" style="width: 100%; min-width: 400px; display: block; margin: auto;">
</a>
</div>
<!-- header end -->
[](https://twitter.com/PrunaAI)
[](https://github.com/PrunaAI)
[](https://www.linkedin.com/company/93832878/admin/feed/posts/?feedType=following)
[](https://discord.gg/CP4VSgck)
# Simply make AI models cheaper, smaller, faster, and greener!
- Give a thumbs up if you like this model!
- Contact us and tell us which model to compress next [here](https://www.pruna.ai/contact).
- Request access to easily compress your *own* AI models [here](https://z0halsaff74.typeform.com/pruna-access?typeform-source=www.pruna.ai).
- Read the documentations to know more [here](https://pruna-ai-pruna.readthedocs-hosted.com/en/latest/)
- Join Pruna AI community on Discord [here](https://discord.gg/CP4VSgck) to share feedback/suggestions or get help.
## Results

**Frequently Asked Questions**
- ***How does the compression work?*** The model is compressed with hqq.
- ***How does the model quality change?*** The quality of the model output might vary compared to the base model.
- ***How is the model efficiency evaluated?*** These results were obtained on HARDWARE_NAME with configuration described in `model/smash_config.json` and are obtained after a hardware warmup. The smashed model is directly compared to the original base model. Efficiency results may vary in other settings (e.g. other hardware, image size, batch size, ...). We recommend to directly run them in the use-case conditions to know if the smashed model can benefit you.
- ***What is the model format?*** We use safetensors.
- ***What calibration data has been used?*** If needed by the compression method, we used WikiText as the calibration data.
- ***What is the naming convention for Pruna Huggingface models?*** We take the original model name and append "turbo", "tiny", or "green" if the smashed model has a measured inference speed, inference memory, or inference energy consumption which is less than 90% of the original base model.
- ***How to compress my own models?*** You can request premium access to more compression methods and tech support for your specific use-cases [here](https://z0halsaff74.typeform.com/pruna-access?typeform-source=www.pruna.ai).
- ***What are "first" metrics?*** Results mentioning "first" are obtained after the first run of the model. The first run might take more memory or be slower than the subsequent runs due cuda overheads.
- ***What are "Sync" and "Async" metrics?*** "Sync" metrics are obtained by syncing all GPU processes and stop measurement when all of them are executed. "Async" metrics are obtained without syncing all GPU processes and stop when the model output can be used by the CPU. We provide both metrics since both could be relevant depending on the use-case. We recommend to test the efficiency gains directly in your use-cases.
## Setup
You can run the smashed model with these steps:
0. Check requirements from the original repo h2oai/h2ogpt-gm-oasst1-en-2048-falcon-7b-v3 installed. In particular, check python, cuda, and transformers versions.
1. Make sure that you have installed quantization related packages.
```bash
pip install hqq
```
2. Load & run the model.
```python
from transformers import AutoModelForCausalLM, AutoTokenizer
from hqq.engine.hf import HQQModelForCausalLM
from hqq.models.hf.base import AutoHQQHFModel
try:
model = HQQModelForCausalLM.from_quantized("PrunaAI/h2oai-h2ogpt-gm-oasst1-en-2048-falcon-7b-v3-HQQ-4bit-smashed", device_map='auto')
except:
model = AutoHQQHFModel.from_quantized("PrunaAI/h2oai-h2ogpt-gm-oasst1-en-2048-falcon-7b-v3-HQQ-4bit-smashed")
tokenizer = AutoTokenizer.from_pretrained("h2oai/h2ogpt-gm-oasst1-en-2048-falcon-7b-v3")
input_ids = tokenizer("What is the color of prunes?,", return_tensors='pt').to(model.device)["input_ids"]
outputs = model.generate(input_ids, max_new_tokens=216)
tokenizer.decode(outputs[0])
```
## Configurations
The configuration info are in `smash_config.json`.
## Credits & License
The license of the smashed model follows the license of the original model. Please check the license of the original model h2oai/h2ogpt-gm-oasst1-en-2048-falcon-7b-v3 before using this model which provided the base model. The license of the `pruna-engine` is [here](https://pypi.org/project/pruna-engine/) on Pypi.
## Want to compress other models?
- Contact us and tell us which model to compress next [here](https://www.pruna.ai/contact).
- Request access to easily compress your own AI models [here](https://z0halsaff74.typeform.com/pruna-access?typeform-source=www.pruna.ai). |
PrunaAI/h2oai-h2ogpt-gm-oasst1-en-2048-falcon-7b-v3-HQQ-2bit-smashed | PrunaAI | "2024-06-24T10:46:28Z" | 0 | 0 | transformers | [
"transformers",
"RefinedWebModel",
"text-generation",
"pruna-ai",
"custom_code",
"base_model:h2oai/h2ogpt-gm-oasst1-en-2048-falcon-7b-v3",
"autotrain_compatible",
"text-generation-inference",
"region:us"
] | text-generation | "2024-06-24T10:39:32Z" | ---
thumbnail: "https://assets-global.website-files.com/646b351987a8d8ce158d1940/64ec9e96b4334c0e1ac41504_Logo%20with%20white%20text.svg"
base_model: h2oai/h2ogpt-gm-oasst1-en-2048-falcon-7b-v3
metrics:
- memory_disk
- memory_inference
- inference_latency
- inference_throughput
- inference_CO2_emissions
- inference_energy_consumption
tags:
- pruna-ai
---
<!-- header start -->
<!-- 200823 -->
<div style="width: auto; margin-left: auto; margin-right: auto">
<a href="https://www.pruna.ai/" target="_blank" rel="noopener noreferrer">
<img src="https://i.imgur.com/eDAlcgk.png" alt="PrunaAI" style="width: 100%; min-width: 400px; display: block; margin: auto;">
</a>
</div>
<!-- header end -->
[](https://twitter.com/PrunaAI)
[](https://github.com/PrunaAI)
[](https://www.linkedin.com/company/93832878/admin/feed/posts/?feedType=following)
[](https://discord.gg/CP4VSgck)
# Simply make AI models cheaper, smaller, faster, and greener!
- Give a thumbs up if you like this model!
- Contact us and tell us which model to compress next [here](https://www.pruna.ai/contact).
- Request access to easily compress your *own* AI models [here](https://z0halsaff74.typeform.com/pruna-access?typeform-source=www.pruna.ai).
- Read the documentations to know more [here](https://pruna-ai-pruna.readthedocs-hosted.com/en/latest/)
- Join Pruna AI community on Discord [here](https://discord.gg/CP4VSgck) to share feedback/suggestions or get help.
## Results

**Frequently Asked Questions**
- ***How does the compression work?*** The model is compressed with hqq.
- ***How does the model quality change?*** The quality of the model output might vary compared to the base model.
- ***How is the model efficiency evaluated?*** These results were obtained on HARDWARE_NAME with configuration described in `model/smash_config.json` and are obtained after a hardware warmup. The smashed model is directly compared to the original base model. Efficiency results may vary in other settings (e.g. other hardware, image size, batch size, ...). We recommend to directly run them in the use-case conditions to know if the smashed model can benefit you.
- ***What is the model format?*** We use safetensors.
- ***What calibration data has been used?*** If needed by the compression method, we used WikiText as the calibration data.
- ***What is the naming convention for Pruna Huggingface models?*** We take the original model name and append "turbo", "tiny", or "green" if the smashed model has a measured inference speed, inference memory, or inference energy consumption which is less than 90% of the original base model.
- ***How to compress my own models?*** You can request premium access to more compression methods and tech support for your specific use-cases [here](https://z0halsaff74.typeform.com/pruna-access?typeform-source=www.pruna.ai).
- ***What are "first" metrics?*** Results mentioning "first" are obtained after the first run of the model. The first run might take more memory or be slower than the subsequent runs due cuda overheads.
- ***What are "Sync" and "Async" metrics?*** "Sync" metrics are obtained by syncing all GPU processes and stop measurement when all of them are executed. "Async" metrics are obtained without syncing all GPU processes and stop when the model output can be used by the CPU. We provide both metrics since both could be relevant depending on the use-case. We recommend to test the efficiency gains directly in your use-cases.
## Setup
You can run the smashed model with these steps:
0. Check requirements from the original repo h2oai/h2ogpt-gm-oasst1-en-2048-falcon-7b-v3 installed. In particular, check python, cuda, and transformers versions.
1. Make sure that you have installed quantization related packages.
```bash
pip install hqq
```
2. Load & run the model.
```python
from transformers import AutoModelForCausalLM, AutoTokenizer
from hqq.engine.hf import HQQModelForCausalLM
from hqq.models.hf.base import AutoHQQHFModel
try:
model = HQQModelForCausalLM.from_quantized("PrunaAI/h2oai-h2ogpt-gm-oasst1-en-2048-falcon-7b-v3-HQQ-2bit-smashed", device_map='auto')
except:
model = AutoHQQHFModel.from_quantized("PrunaAI/h2oai-h2ogpt-gm-oasst1-en-2048-falcon-7b-v3-HQQ-2bit-smashed")
tokenizer = AutoTokenizer.from_pretrained("h2oai/h2ogpt-gm-oasst1-en-2048-falcon-7b-v3")
input_ids = tokenizer("What is the color of prunes?,", return_tensors='pt').to(model.device)["input_ids"]
outputs = model.generate(input_ids, max_new_tokens=216)
tokenizer.decode(outputs[0])
```
## Configurations
The configuration info are in `smash_config.json`.
## Credits & License
The license of the smashed model follows the license of the original model. Please check the license of the original model h2oai/h2ogpt-gm-oasst1-en-2048-falcon-7b-v3 before using this model which provided the base model. The license of the `pruna-engine` is [here](https://pypi.org/project/pruna-engine/) on Pypi.
## Want to compress other models?
- Contact us and tell us which model to compress next [here](https://www.pruna.ai/contact).
- Request access to easily compress your own AI models [here](https://z0halsaff74.typeform.com/pruna-access?typeform-source=www.pruna.ai). |
PrunaAI/M4-ai-tau-0.5B-HQQ-1bit-smashed | PrunaAI | "2024-06-24T10:39:54Z" | 0 | 0 | transformers | [
"transformers",
"qwen2",
"text-generation",
"pruna-ai",
"conversational",
"base_model:M4-ai/tau-0.5B",
"autotrain_compatible",
"endpoints_compatible",
"text-generation-inference",
"region:us"
] | text-generation | "2024-06-24T10:39:36Z" | ---
thumbnail: "https://assets-global.website-files.com/646b351987a8d8ce158d1940/64ec9e96b4334c0e1ac41504_Logo%20with%20white%20text.svg"
base_model: M4-ai/tau-0.5B
metrics:
- memory_disk
- memory_inference
- inference_latency
- inference_throughput
- inference_CO2_emissions
- inference_energy_consumption
tags:
- pruna-ai
---
<!-- header start -->
<!-- 200823 -->
<div style="width: auto; margin-left: auto; margin-right: auto">
<a href="https://www.pruna.ai/" target="_blank" rel="noopener noreferrer">
<img src="https://i.imgur.com/eDAlcgk.png" alt="PrunaAI" style="width: 100%; min-width: 400px; display: block; margin: auto;">
</a>
</div>
<!-- header end -->
[](https://twitter.com/PrunaAI)
[](https://github.com/PrunaAI)
[](https://www.linkedin.com/company/93832878/admin/feed/posts/?feedType=following)
[](https://discord.gg/CP4VSgck)
# Simply make AI models cheaper, smaller, faster, and greener!
- Give a thumbs up if you like this model!
- Contact us and tell us which model to compress next [here](https://www.pruna.ai/contact).
- Request access to easily compress your *own* AI models [here](https://z0halsaff74.typeform.com/pruna-access?typeform-source=www.pruna.ai).
- Read the documentations to know more [here](https://pruna-ai-pruna.readthedocs-hosted.com/en/latest/)
- Join Pruna AI community on Discord [here](https://discord.gg/CP4VSgck) to share feedback/suggestions or get help.
## Results

**Frequently Asked Questions**
- ***How does the compression work?*** The model is compressed with hqq.
- ***How does the model quality change?*** The quality of the model output might vary compared to the base model.
- ***How is the model efficiency evaluated?*** These results were obtained on HARDWARE_NAME with configuration described in `model/smash_config.json` and are obtained after a hardware warmup. The smashed model is directly compared to the original base model. Efficiency results may vary in other settings (e.g. other hardware, image size, batch size, ...). We recommend to directly run them in the use-case conditions to know if the smashed model can benefit you.
- ***What is the model format?*** We use safetensors.
- ***What calibration data has been used?*** If needed by the compression method, we used WikiText as the calibration data.
- ***What is the naming convention for Pruna Huggingface models?*** We take the original model name and append "turbo", "tiny", or "green" if the smashed model has a measured inference speed, inference memory, or inference energy consumption which is less than 90% of the original base model.
- ***How to compress my own models?*** You can request premium access to more compression methods and tech support for your specific use-cases [here](https://z0halsaff74.typeform.com/pruna-access?typeform-source=www.pruna.ai).
- ***What are "first" metrics?*** Results mentioning "first" are obtained after the first run of the model. The first run might take more memory or be slower than the subsequent runs due cuda overheads.
- ***What are "Sync" and "Async" metrics?*** "Sync" metrics are obtained by syncing all GPU processes and stop measurement when all of them are executed. "Async" metrics are obtained without syncing all GPU processes and stop when the model output can be used by the CPU. We provide both metrics since both could be relevant depending on the use-case. We recommend to test the efficiency gains directly in your use-cases.
## Setup
You can run the smashed model with these steps:
0. Check requirements from the original repo M4-ai/tau-0.5B installed. In particular, check python, cuda, and transformers versions.
1. Make sure that you have installed quantization related packages.
```bash
pip install hqq
```
2. Load & run the model.
```python
from transformers import AutoModelForCausalLM, AutoTokenizer
from hqq.engine.hf import HQQModelForCausalLM
from hqq.models.hf.base import AutoHQQHFModel
try:
model = HQQModelForCausalLM.from_quantized("PrunaAI/M4-ai-tau-0.5B-HQQ-1bit-smashed", device_map='auto')
except:
model = AutoHQQHFModel.from_quantized("PrunaAI/M4-ai-tau-0.5B-HQQ-1bit-smashed")
tokenizer = AutoTokenizer.from_pretrained("M4-ai/tau-0.5B")
input_ids = tokenizer("What is the color of prunes?,", return_tensors='pt').to(model.device)["input_ids"]
outputs = model.generate(input_ids, max_new_tokens=216)
tokenizer.decode(outputs[0])
```
## Configurations
The configuration info are in `smash_config.json`.
## Credits & License
The license of the smashed model follows the license of the original model. Please check the license of the original model M4-ai/tau-0.5B before using this model which provided the base model. The license of the `pruna-engine` is [here](https://pypi.org/project/pruna-engine/) on Pypi.
## Want to compress other models?
- Contact us and tell us which model to compress next [here](https://www.pruna.ai/contact).
- Request access to easily compress your own AI models [here](https://z0halsaff74.typeform.com/pruna-access?typeform-source=www.pruna.ai). |
PrunaAI/M4-ai-tau-0.5B-HQQ-2bit-smashed | PrunaAI | "2024-06-24T10:39:58Z" | 0 | 0 | transformers | [
"transformers",
"qwen2",
"text-generation",
"pruna-ai",
"conversational",
"base_model:M4-ai/tau-0.5B",
"autotrain_compatible",
"endpoints_compatible",
"text-generation-inference",
"region:us"
] | text-generation | "2024-06-24T10:39:38Z" | ---
thumbnail: "https://assets-global.website-files.com/646b351987a8d8ce158d1940/64ec9e96b4334c0e1ac41504_Logo%20with%20white%20text.svg"
base_model: M4-ai/tau-0.5B
metrics:
- memory_disk
- memory_inference
- inference_latency
- inference_throughput
- inference_CO2_emissions
- inference_energy_consumption
tags:
- pruna-ai
---
<!-- header start -->
<!-- 200823 -->
<div style="width: auto; margin-left: auto; margin-right: auto">
<a href="https://www.pruna.ai/" target="_blank" rel="noopener noreferrer">
<img src="https://i.imgur.com/eDAlcgk.png" alt="PrunaAI" style="width: 100%; min-width: 400px; display: block; margin: auto;">
</a>
</div>
<!-- header end -->
[](https://twitter.com/PrunaAI)
[](https://github.com/PrunaAI)
[](https://www.linkedin.com/company/93832878/admin/feed/posts/?feedType=following)
[](https://discord.gg/CP4VSgck)
# Simply make AI models cheaper, smaller, faster, and greener!
- Give a thumbs up if you like this model!
- Contact us and tell us which model to compress next [here](https://www.pruna.ai/contact).
- Request access to easily compress your *own* AI models [here](https://z0halsaff74.typeform.com/pruna-access?typeform-source=www.pruna.ai).
- Read the documentations to know more [here](https://pruna-ai-pruna.readthedocs-hosted.com/en/latest/)
- Join Pruna AI community on Discord [here](https://discord.gg/CP4VSgck) to share feedback/suggestions or get help.
## Results

**Frequently Asked Questions**
- ***How does the compression work?*** The model is compressed with hqq.
- ***How does the model quality change?*** The quality of the model output might vary compared to the base model.
- ***How is the model efficiency evaluated?*** These results were obtained on HARDWARE_NAME with configuration described in `model/smash_config.json` and are obtained after a hardware warmup. The smashed model is directly compared to the original base model. Efficiency results may vary in other settings (e.g. other hardware, image size, batch size, ...). We recommend to directly run them in the use-case conditions to know if the smashed model can benefit you.
- ***What is the model format?*** We use safetensors.
- ***What calibration data has been used?*** If needed by the compression method, we used WikiText as the calibration data.
- ***What is the naming convention for Pruna Huggingface models?*** We take the original model name and append "turbo", "tiny", or "green" if the smashed model has a measured inference speed, inference memory, or inference energy consumption which is less than 90% of the original base model.
- ***How to compress my own models?*** You can request premium access to more compression methods and tech support for your specific use-cases [here](https://z0halsaff74.typeform.com/pruna-access?typeform-source=www.pruna.ai).
- ***What are "first" metrics?*** Results mentioning "first" are obtained after the first run of the model. The first run might take more memory or be slower than the subsequent runs due cuda overheads.
- ***What are "Sync" and "Async" metrics?*** "Sync" metrics are obtained by syncing all GPU processes and stop measurement when all of them are executed. "Async" metrics are obtained without syncing all GPU processes and stop when the model output can be used by the CPU. We provide both metrics since both could be relevant depending on the use-case. We recommend to test the efficiency gains directly in your use-cases.
## Setup
You can run the smashed model with these steps:
0. Check requirements from the original repo M4-ai/tau-0.5B installed. In particular, check python, cuda, and transformers versions.
1. Make sure that you have installed quantization related packages.
```bash
pip install hqq
```
2. Load & run the model.
```python
from transformers import AutoModelForCausalLM, AutoTokenizer
from hqq.engine.hf import HQQModelForCausalLM
from hqq.models.hf.base import AutoHQQHFModel
try:
model = HQQModelForCausalLM.from_quantized("PrunaAI/M4-ai-tau-0.5B-HQQ-2bit-smashed", device_map='auto')
except:
model = AutoHQQHFModel.from_quantized("PrunaAI/M4-ai-tau-0.5B-HQQ-2bit-smashed")
tokenizer = AutoTokenizer.from_pretrained("M4-ai/tau-0.5B")
input_ids = tokenizer("What is the color of prunes?,", return_tensors='pt').to(model.device)["input_ids"]
outputs = model.generate(input_ids, max_new_tokens=216)
tokenizer.decode(outputs[0])
```
## Configurations
The configuration info are in `smash_config.json`.
## Credits & License
The license of the smashed model follows the license of the original model. Please check the license of the original model M4-ai/tau-0.5B before using this model which provided the base model. The license of the `pruna-engine` is [here](https://pypi.org/project/pruna-engine/) on Pypi.
## Want to compress other models?
- Contact us and tell us which model to compress next [here](https://www.pruna.ai/contact).
- Request access to easily compress your own AI models [here](https://z0halsaff74.typeform.com/pruna-access?typeform-source=www.pruna.ai). |
PrunaAI/M4-ai-tau-0.5B-HQQ-4bit-smashed | PrunaAI | "2024-06-24T10:40:15Z" | 0 | 0 | transformers | [
"transformers",
"qwen2",
"text-generation",
"pruna-ai",
"conversational",
"base_model:M4-ai/tau-0.5B",
"autotrain_compatible",
"endpoints_compatible",
"text-generation-inference",
"region:us"
] | text-generation | "2024-06-24T10:39:53Z" | ---
thumbnail: "https://assets-global.website-files.com/646b351987a8d8ce158d1940/64ec9e96b4334c0e1ac41504_Logo%20with%20white%20text.svg"
base_model: M4-ai/tau-0.5B
metrics:
- memory_disk
- memory_inference
- inference_latency
- inference_throughput
- inference_CO2_emissions
- inference_energy_consumption
tags:
- pruna-ai
---
<!-- header start -->
<!-- 200823 -->
<div style="width: auto; margin-left: auto; margin-right: auto">
<a href="https://www.pruna.ai/" target="_blank" rel="noopener noreferrer">
<img src="https://i.imgur.com/eDAlcgk.png" alt="PrunaAI" style="width: 100%; min-width: 400px; display: block; margin: auto;">
</a>
</div>
<!-- header end -->
[](https://twitter.com/PrunaAI)
[](https://github.com/PrunaAI)
[](https://www.linkedin.com/company/93832878/admin/feed/posts/?feedType=following)
[](https://discord.gg/CP4VSgck)
# Simply make AI models cheaper, smaller, faster, and greener!
- Give a thumbs up if you like this model!
- Contact us and tell us which model to compress next [here](https://www.pruna.ai/contact).
- Request access to easily compress your *own* AI models [here](https://z0halsaff74.typeform.com/pruna-access?typeform-source=www.pruna.ai).
- Read the documentations to know more [here](https://pruna-ai-pruna.readthedocs-hosted.com/en/latest/)
- Join Pruna AI community on Discord [here](https://discord.gg/CP4VSgck) to share feedback/suggestions or get help.
## Results

**Frequently Asked Questions**
- ***How does the compression work?*** The model is compressed with hqq.
- ***How does the model quality change?*** The quality of the model output might vary compared to the base model.
- ***How is the model efficiency evaluated?*** These results were obtained on HARDWARE_NAME with configuration described in `model/smash_config.json` and are obtained after a hardware warmup. The smashed model is directly compared to the original base model. Efficiency results may vary in other settings (e.g. other hardware, image size, batch size, ...). We recommend to directly run them in the use-case conditions to know if the smashed model can benefit you.
- ***What is the model format?*** We use safetensors.
- ***What calibration data has been used?*** If needed by the compression method, we used WikiText as the calibration data.
- ***What is the naming convention for Pruna Huggingface models?*** We take the original model name and append "turbo", "tiny", or "green" if the smashed model has a measured inference speed, inference memory, or inference energy consumption which is less than 90% of the original base model.
- ***How to compress my own models?*** You can request premium access to more compression methods and tech support for your specific use-cases [here](https://z0halsaff74.typeform.com/pruna-access?typeform-source=www.pruna.ai).
- ***What are "first" metrics?*** Results mentioning "first" are obtained after the first run of the model. The first run might take more memory or be slower than the subsequent runs due cuda overheads.
- ***What are "Sync" and "Async" metrics?*** "Sync" metrics are obtained by syncing all GPU processes and stop measurement when all of them are executed. "Async" metrics are obtained without syncing all GPU processes and stop when the model output can be used by the CPU. We provide both metrics since both could be relevant depending on the use-case. We recommend to test the efficiency gains directly in your use-cases.
## Setup
You can run the smashed model with these steps:
0. Check requirements from the original repo M4-ai/tau-0.5B installed. In particular, check python, cuda, and transformers versions.
1. Make sure that you have installed quantization related packages.
```bash
pip install hqq
```
2. Load & run the model.
```python
from transformers import AutoModelForCausalLM, AutoTokenizer
from hqq.engine.hf import HQQModelForCausalLM
from hqq.models.hf.base import AutoHQQHFModel
try:
model = HQQModelForCausalLM.from_quantized("PrunaAI/M4-ai-tau-0.5B-HQQ-4bit-smashed", device_map='auto')
except:
model = AutoHQQHFModel.from_quantized("PrunaAI/M4-ai-tau-0.5B-HQQ-4bit-smashed")
tokenizer = AutoTokenizer.from_pretrained("M4-ai/tau-0.5B")
input_ids = tokenizer("What is the color of prunes?,", return_tensors='pt').to(model.device)["input_ids"]
outputs = model.generate(input_ids, max_new_tokens=216)
tokenizer.decode(outputs[0])
```
## Configurations
The configuration info are in `smash_config.json`.
## Credits & License
The license of the smashed model follows the license of the original model. Please check the license of the original model M4-ai/tau-0.5B before using this model which provided the base model. The license of the `pruna-engine` is [here](https://pypi.org/project/pruna-engine/) on Pypi.
## Want to compress other models?
- Contact us and tell us which model to compress next [here](https://www.pruna.ai/contact).
- Request access to easily compress your own AI models [here](https://z0halsaff74.typeform.com/pruna-access?typeform-source=www.pruna.ai). |
PrunaAI/ytu-ce-cosmos-turkish-gpt2-large-HQQ-4bit-smashed | PrunaAI | "2024-06-24T10:40:45Z" | 0 | 0 | transformers | [
"transformers",
"gpt2",
"text-generation",
"pruna-ai",
"base_model:ytu-ce-cosmos/turkish-gpt2-large",
"autotrain_compatible",
"endpoints_compatible",
"text-generation-inference",
"region:us"
] | text-generation | "2024-06-24T10:39:54Z" | ---
thumbnail: "https://assets-global.website-files.com/646b351987a8d8ce158d1940/64ec9e96b4334c0e1ac41504_Logo%20with%20white%20text.svg"
base_model: ytu-ce-cosmos/turkish-gpt2-large
metrics:
- memory_disk
- memory_inference
- inference_latency
- inference_throughput
- inference_CO2_emissions
- inference_energy_consumption
tags:
- pruna-ai
---
<!-- header start -->
<!-- 200823 -->
<div style="width: auto; margin-left: auto; margin-right: auto">
<a href="https://www.pruna.ai/" target="_blank" rel="noopener noreferrer">
<img src="https://i.imgur.com/eDAlcgk.png" alt="PrunaAI" style="width: 100%; min-width: 400px; display: block; margin: auto;">
</a>
</div>
<!-- header end -->
[](https://twitter.com/PrunaAI)
[](https://github.com/PrunaAI)
[](https://www.linkedin.com/company/93832878/admin/feed/posts/?feedType=following)
[](https://discord.gg/CP4VSgck)
# Simply make AI models cheaper, smaller, faster, and greener!
- Give a thumbs up if you like this model!
- Contact us and tell us which model to compress next [here](https://www.pruna.ai/contact).
- Request access to easily compress your *own* AI models [here](https://z0halsaff74.typeform.com/pruna-access?typeform-source=www.pruna.ai).
- Read the documentations to know more [here](https://pruna-ai-pruna.readthedocs-hosted.com/en/latest/)
- Join Pruna AI community on Discord [here](https://discord.gg/CP4VSgck) to share feedback/suggestions or get help.
## Results

**Frequently Asked Questions**
- ***How does the compression work?*** The model is compressed with hqq.
- ***How does the model quality change?*** The quality of the model output might vary compared to the base model.
- ***How is the model efficiency evaluated?*** These results were obtained on HARDWARE_NAME with configuration described in `model/smash_config.json` and are obtained after a hardware warmup. The smashed model is directly compared to the original base model. Efficiency results may vary in other settings (e.g. other hardware, image size, batch size, ...). We recommend to directly run them in the use-case conditions to know if the smashed model can benefit you.
- ***What is the model format?*** We use safetensors.
- ***What calibration data has been used?*** If needed by the compression method, we used WikiText as the calibration data.
- ***What is the naming convention for Pruna Huggingface models?*** We take the original model name and append "turbo", "tiny", or "green" if the smashed model has a measured inference speed, inference memory, or inference energy consumption which is less than 90% of the original base model.
- ***How to compress my own models?*** You can request premium access to more compression methods and tech support for your specific use-cases [here](https://z0halsaff74.typeform.com/pruna-access?typeform-source=www.pruna.ai).
- ***What are "first" metrics?*** Results mentioning "first" are obtained after the first run of the model. The first run might take more memory or be slower than the subsequent runs due cuda overheads.
- ***What are "Sync" and "Async" metrics?*** "Sync" metrics are obtained by syncing all GPU processes and stop measurement when all of them are executed. "Async" metrics are obtained without syncing all GPU processes and stop when the model output can be used by the CPU. We provide both metrics since both could be relevant depending on the use-case. We recommend to test the efficiency gains directly in your use-cases.
## Setup
You can run the smashed model with these steps:
0. Check requirements from the original repo ytu-ce-cosmos/turkish-gpt2-large installed. In particular, check python, cuda, and transformers versions.
1. Make sure that you have installed quantization related packages.
```bash
pip install hqq
```
2. Load & run the model.
```python
from transformers import AutoModelForCausalLM, AutoTokenizer
from hqq.engine.hf import HQQModelForCausalLM
from hqq.models.hf.base import AutoHQQHFModel
try:
model = HQQModelForCausalLM.from_quantized("PrunaAI/ytu-ce-cosmos-turkish-gpt2-large-HQQ-4bit-smashed", device_map='auto')
except:
model = AutoHQQHFModel.from_quantized("PrunaAI/ytu-ce-cosmos-turkish-gpt2-large-HQQ-4bit-smashed")
tokenizer = AutoTokenizer.from_pretrained("ytu-ce-cosmos/turkish-gpt2-large")
input_ids = tokenizer("What is the color of prunes?,", return_tensors='pt').to(model.device)["input_ids"]
outputs = model.generate(input_ids, max_new_tokens=216)
tokenizer.decode(outputs[0])
```
## Configurations
The configuration info are in `smash_config.json`.
## Credits & License
The license of the smashed model follows the license of the original model. Please check the license of the original model ytu-ce-cosmos/turkish-gpt2-large before using this model which provided the base model. The license of the `pruna-engine` is [here](https://pypi.org/project/pruna-engine/) on Pypi.
## Want to compress other models?
- Contact us and tell us which model to compress next [here](https://www.pruna.ai/contact).
- Request access to easily compress your own AI models [here](https://z0halsaff74.typeform.com/pruna-access?typeform-source=www.pruna.ai). |
PrunaAI/ytu-ce-cosmos-turkish-gpt2-large-HQQ-1bit-smashed | PrunaAI | "2024-06-24T10:41:05Z" | 0 | 0 | transformers | [
"transformers",
"gpt2",
"text-generation",
"pruna-ai",
"base_model:ytu-ce-cosmos/turkish-gpt2-large",
"autotrain_compatible",
"endpoints_compatible",
"text-generation-inference",
"region:us"
] | text-generation | "2024-06-24T10:40:15Z" | ---
thumbnail: "https://assets-global.website-files.com/646b351987a8d8ce158d1940/64ec9e96b4334c0e1ac41504_Logo%20with%20white%20text.svg"
base_model: ytu-ce-cosmos/turkish-gpt2-large
metrics:
- memory_disk
- memory_inference
- inference_latency
- inference_throughput
- inference_CO2_emissions
- inference_energy_consumption
tags:
- pruna-ai
---
<!-- header start -->
<!-- 200823 -->
<div style="width: auto; margin-left: auto; margin-right: auto">
<a href="https://www.pruna.ai/" target="_blank" rel="noopener noreferrer">
<img src="https://i.imgur.com/eDAlcgk.png" alt="PrunaAI" style="width: 100%; min-width: 400px; display: block; margin: auto;">
</a>
</div>
<!-- header end -->
[](https://twitter.com/PrunaAI)
[](https://github.com/PrunaAI)
[](https://www.linkedin.com/company/93832878/admin/feed/posts/?feedType=following)
[](https://discord.gg/CP4VSgck)
# Simply make AI models cheaper, smaller, faster, and greener!
- Give a thumbs up if you like this model!
- Contact us and tell us which model to compress next [here](https://www.pruna.ai/contact).
- Request access to easily compress your *own* AI models [here](https://z0halsaff74.typeform.com/pruna-access?typeform-source=www.pruna.ai).
- Read the documentations to know more [here](https://pruna-ai-pruna.readthedocs-hosted.com/en/latest/)
- Join Pruna AI community on Discord [here](https://discord.gg/CP4VSgck) to share feedback/suggestions or get help.
## Results

**Frequently Asked Questions**
- ***How does the compression work?*** The model is compressed with hqq.
- ***How does the model quality change?*** The quality of the model output might vary compared to the base model.
- ***How is the model efficiency evaluated?*** These results were obtained on HARDWARE_NAME with configuration described in `model/smash_config.json` and are obtained after a hardware warmup. The smashed model is directly compared to the original base model. Efficiency results may vary in other settings (e.g. other hardware, image size, batch size, ...). We recommend to directly run them in the use-case conditions to know if the smashed model can benefit you.
- ***What is the model format?*** We use safetensors.
- ***What calibration data has been used?*** If needed by the compression method, we used WikiText as the calibration data.
- ***What is the naming convention for Pruna Huggingface models?*** We take the original model name and append "turbo", "tiny", or "green" if the smashed model has a measured inference speed, inference memory, or inference energy consumption which is less than 90% of the original base model.
- ***How to compress my own models?*** You can request premium access to more compression methods and tech support for your specific use-cases [here](https://z0halsaff74.typeform.com/pruna-access?typeform-source=www.pruna.ai).
- ***What are "first" metrics?*** Results mentioning "first" are obtained after the first run of the model. The first run might take more memory or be slower than the subsequent runs due cuda overheads.
- ***What are "Sync" and "Async" metrics?*** "Sync" metrics are obtained by syncing all GPU processes and stop measurement when all of them are executed. "Async" metrics are obtained without syncing all GPU processes and stop when the model output can be used by the CPU. We provide both metrics since both could be relevant depending on the use-case. We recommend to test the efficiency gains directly in your use-cases.
## Setup
You can run the smashed model with these steps:
0. Check requirements from the original repo ytu-ce-cosmos/turkish-gpt2-large installed. In particular, check python, cuda, and transformers versions.
1. Make sure that you have installed quantization related packages.
```bash
pip install hqq
```
2. Load & run the model.
```python
from transformers import AutoModelForCausalLM, AutoTokenizer
from hqq.engine.hf import HQQModelForCausalLM
from hqq.models.hf.base import AutoHQQHFModel
try:
model = HQQModelForCausalLM.from_quantized("PrunaAI/ytu-ce-cosmos-turkish-gpt2-large-HQQ-1bit-smashed", device_map='auto')
except:
model = AutoHQQHFModel.from_quantized("PrunaAI/ytu-ce-cosmos-turkish-gpt2-large-HQQ-1bit-smashed")
tokenizer = AutoTokenizer.from_pretrained("ytu-ce-cosmos/turkish-gpt2-large")
input_ids = tokenizer("What is the color of prunes?,", return_tensors='pt').to(model.device)["input_ids"]
outputs = model.generate(input_ids, max_new_tokens=216)
tokenizer.decode(outputs[0])
```
## Configurations
The configuration info are in `smash_config.json`.
## Credits & License
The license of the smashed model follows the license of the original model. Please check the license of the original model ytu-ce-cosmos/turkish-gpt2-large before using this model which provided the base model. The license of the `pruna-engine` is [here](https://pypi.org/project/pruna-engine/) on Pypi.
## Want to compress other models?
- Contact us and tell us which model to compress next [here](https://www.pruna.ai/contact).
- Request access to easily compress your own AI models [here](https://z0halsaff74.typeform.com/pruna-access?typeform-source=www.pruna.ai). |
zaidulhassan/myai2 | zaidulhassan | "2024-06-24T10:41:09Z" | 0 | 0 | null | [
"license:mit",
"region:us"
] | null | "2024-06-24T10:41:09Z" | ---
license: mit
---
|
HyperdustProtocol/HyperAuto-cog-llama2-7b-3686 | HyperdustProtocol | "2024-06-24T10:41:32Z" | 0 | 0 | transformers | [
"transformers",
"safetensors",
"text-generation-inference",
"unsloth",
"llama",
"trl",
"en",
"base_model:unsloth/llama-2-7b-bnb-4bit",
"license:apache-2.0",
"endpoints_compatible",
"region:us"
] | null | "2024-06-24T10:41:18Z" | ---
base_model: unsloth/llama-2-7b-bnb-4bit
language:
- en
license: apache-2.0
tags:
- text-generation-inference
- transformers
- unsloth
- llama
- trl
---
# Uploaded model
- **Developed by:** HyperdustProtocol
- **License:** apache-2.0
- **Finetuned from model :** unsloth/llama-2-7b-bnb-4bit
This llama model was trained 2x faster with [Unsloth](https://github.com/unslothai/unsloth) and Huggingface's TRL library.
[<img src="https://raw.githubusercontent.com/unslothai/unsloth/main/images/unsloth%20made%20with%20love.png" width="200"/>](https://github.com/unslothai/unsloth)
|
itay-nakash/model_6e99ce7442_sweep_polar-pyramid-913 | itay-nakash | "2024-06-24T10:44:27Z" | 0 | 0 | null | [
"region:us"
] | null | "2024-06-24T10:44:27Z" | Entry not found |
PrunaAI/alfredplpl-Llama-3-8B-Instruct-Ja-HQQ-1bit-smashed | PrunaAI | "2024-06-24T10:46:32Z" | 0 | 0 | transformers | [
"transformers",
"llama",
"text-generation",
"pruna-ai",
"conversational",
"base_model:alfredplpl/Llama-3-8B-Instruct-Ja",
"autotrain_compatible",
"endpoints_compatible",
"text-generation-inference",
"region:us"
] | text-generation | "2024-06-24T10:45:03Z" | ---
thumbnail: "https://assets-global.website-files.com/646b351987a8d8ce158d1940/64ec9e96b4334c0e1ac41504_Logo%20with%20white%20text.svg"
base_model: alfredplpl/Llama-3-8B-Instruct-Ja
metrics:
- memory_disk
- memory_inference
- inference_latency
- inference_throughput
- inference_CO2_emissions
- inference_energy_consumption
tags:
- pruna-ai
---
<!-- header start -->
<!-- 200823 -->
<div style="width: auto; margin-left: auto; margin-right: auto">
<a href="https://www.pruna.ai/" target="_blank" rel="noopener noreferrer">
<img src="https://i.imgur.com/eDAlcgk.png" alt="PrunaAI" style="width: 100%; min-width: 400px; display: block; margin: auto;">
</a>
</div>
<!-- header end -->
[](https://twitter.com/PrunaAI)
[](https://github.com/PrunaAI)
[](https://www.linkedin.com/company/93832878/admin/feed/posts/?feedType=following)
[](https://discord.gg/CP4VSgck)
# Simply make AI models cheaper, smaller, faster, and greener!
- Give a thumbs up if you like this model!
- Contact us and tell us which model to compress next [here](https://www.pruna.ai/contact).
- Request access to easily compress your *own* AI models [here](https://z0halsaff74.typeform.com/pruna-access?typeform-source=www.pruna.ai).
- Read the documentations to know more [here](https://pruna-ai-pruna.readthedocs-hosted.com/en/latest/)
- Join Pruna AI community on Discord [here](https://discord.gg/CP4VSgck) to share feedback/suggestions or get help.
## Results

**Frequently Asked Questions**
- ***How does the compression work?*** The model is compressed with hqq.
- ***How does the model quality change?*** The quality of the model output might vary compared to the base model.
- ***How is the model efficiency evaluated?*** These results were obtained on HARDWARE_NAME with configuration described in `model/smash_config.json` and are obtained after a hardware warmup. The smashed model is directly compared to the original base model. Efficiency results may vary in other settings (e.g. other hardware, image size, batch size, ...). We recommend to directly run them in the use-case conditions to know if the smashed model can benefit you.
- ***What is the model format?*** We use safetensors.
- ***What calibration data has been used?*** If needed by the compression method, we used WikiText as the calibration data.
- ***What is the naming convention for Pruna Huggingface models?*** We take the original model name and append "turbo", "tiny", or "green" if the smashed model has a measured inference speed, inference memory, or inference energy consumption which is less than 90% of the original base model.
- ***How to compress my own models?*** You can request premium access to more compression methods and tech support for your specific use-cases [here](https://z0halsaff74.typeform.com/pruna-access?typeform-source=www.pruna.ai).
- ***What are "first" metrics?*** Results mentioning "first" are obtained after the first run of the model. The first run might take more memory or be slower than the subsequent runs due cuda overheads.
- ***What are "Sync" and "Async" metrics?*** "Sync" metrics are obtained by syncing all GPU processes and stop measurement when all of them are executed. "Async" metrics are obtained without syncing all GPU processes and stop when the model output can be used by the CPU. We provide both metrics since both could be relevant depending on the use-case. We recommend to test the efficiency gains directly in your use-cases.
## Setup
You can run the smashed model with these steps:
0. Check requirements from the original repo alfredplpl/Llama-3-8B-Instruct-Ja installed. In particular, check python, cuda, and transformers versions.
1. Make sure that you have installed quantization related packages.
```bash
pip install hqq
```
2. Load & run the model.
```python
from transformers import AutoModelForCausalLM, AutoTokenizer
from hqq.engine.hf import HQQModelForCausalLM
from hqq.models.hf.base import AutoHQQHFModel
try:
model = HQQModelForCausalLM.from_quantized("PrunaAI/alfredplpl-Llama-3-8B-Instruct-Ja-HQQ-1bit-smashed", device_map='auto')
except:
model = AutoHQQHFModel.from_quantized("PrunaAI/alfredplpl-Llama-3-8B-Instruct-Ja-HQQ-1bit-smashed")
tokenizer = AutoTokenizer.from_pretrained("alfredplpl/Llama-3-8B-Instruct-Ja")
input_ids = tokenizer("What is the color of prunes?,", return_tensors='pt').to(model.device)["input_ids"]
outputs = model.generate(input_ids, max_new_tokens=216)
tokenizer.decode(outputs[0])
```
## Configurations
The configuration info are in `smash_config.json`.
## Credits & License
The license of the smashed model follows the license of the original model. Please check the license of the original model alfredplpl/Llama-3-8B-Instruct-Ja before using this model which provided the base model. The license of the `pruna-engine` is [here](https://pypi.org/project/pruna-engine/) on Pypi.
## Want to compress other models?
- Contact us and tell us which model to compress next [here](https://www.pruna.ai/contact).
- Request access to easily compress your own AI models [here](https://z0halsaff74.typeform.com/pruna-access?typeform-source=www.pruna.ai). |
PrunaAI/alfredplpl-Llama-3-8B-Instruct-Ja-HQQ-4bit-smashed | PrunaAI | "2024-06-24T10:47:36Z" | 0 | 0 | transformers | [
"transformers",
"llama",
"text-generation",
"pruna-ai",
"conversational",
"base_model:alfredplpl/Llama-3-8B-Instruct-Ja",
"autotrain_compatible",
"endpoints_compatible",
"text-generation-inference",
"region:us"
] | text-generation | "2024-06-24T10:45:05Z" | ---
thumbnail: "https://assets-global.website-files.com/646b351987a8d8ce158d1940/64ec9e96b4334c0e1ac41504_Logo%20with%20white%20text.svg"
base_model: alfredplpl/Llama-3-8B-Instruct-Ja
metrics:
- memory_disk
- memory_inference
- inference_latency
- inference_throughput
- inference_CO2_emissions
- inference_energy_consumption
tags:
- pruna-ai
---
<!-- header start -->
<!-- 200823 -->
<div style="width: auto; margin-left: auto; margin-right: auto">
<a href="https://www.pruna.ai/" target="_blank" rel="noopener noreferrer">
<img src="https://i.imgur.com/eDAlcgk.png" alt="PrunaAI" style="width: 100%; min-width: 400px; display: block; margin: auto;">
</a>
</div>
<!-- header end -->
[](https://twitter.com/PrunaAI)
[](https://github.com/PrunaAI)
[](https://www.linkedin.com/company/93832878/admin/feed/posts/?feedType=following)
[](https://discord.gg/CP4VSgck)
# Simply make AI models cheaper, smaller, faster, and greener!
- Give a thumbs up if you like this model!
- Contact us and tell us which model to compress next [here](https://www.pruna.ai/contact).
- Request access to easily compress your *own* AI models [here](https://z0halsaff74.typeform.com/pruna-access?typeform-source=www.pruna.ai).
- Read the documentations to know more [here](https://pruna-ai-pruna.readthedocs-hosted.com/en/latest/)
- Join Pruna AI community on Discord [here](https://discord.gg/CP4VSgck) to share feedback/suggestions or get help.
## Results

**Frequently Asked Questions**
- ***How does the compression work?*** The model is compressed with hqq.
- ***How does the model quality change?*** The quality of the model output might vary compared to the base model.
- ***How is the model efficiency evaluated?*** These results were obtained on HARDWARE_NAME with configuration described in `model/smash_config.json` and are obtained after a hardware warmup. The smashed model is directly compared to the original base model. Efficiency results may vary in other settings (e.g. other hardware, image size, batch size, ...). We recommend to directly run them in the use-case conditions to know if the smashed model can benefit you.
- ***What is the model format?*** We use safetensors.
- ***What calibration data has been used?*** If needed by the compression method, we used WikiText as the calibration data.
- ***What is the naming convention for Pruna Huggingface models?*** We take the original model name and append "turbo", "tiny", or "green" if the smashed model has a measured inference speed, inference memory, or inference energy consumption which is less than 90% of the original base model.
- ***How to compress my own models?*** You can request premium access to more compression methods and tech support for your specific use-cases [here](https://z0halsaff74.typeform.com/pruna-access?typeform-source=www.pruna.ai).
- ***What are "first" metrics?*** Results mentioning "first" are obtained after the first run of the model. The first run might take more memory or be slower than the subsequent runs due cuda overheads.
- ***What are "Sync" and "Async" metrics?*** "Sync" metrics are obtained by syncing all GPU processes and stop measurement when all of them are executed. "Async" metrics are obtained without syncing all GPU processes and stop when the model output can be used by the CPU. We provide both metrics since both could be relevant depending on the use-case. We recommend to test the efficiency gains directly in your use-cases.
## Setup
You can run the smashed model with these steps:
0. Check requirements from the original repo alfredplpl/Llama-3-8B-Instruct-Ja installed. In particular, check python, cuda, and transformers versions.
1. Make sure that you have installed quantization related packages.
```bash
pip install hqq
```
2. Load & run the model.
```python
from transformers import AutoModelForCausalLM, AutoTokenizer
from hqq.engine.hf import HQQModelForCausalLM
from hqq.models.hf.base import AutoHQQHFModel
try:
model = HQQModelForCausalLM.from_quantized("PrunaAI/alfredplpl-Llama-3-8B-Instruct-Ja-HQQ-4bit-smashed", device_map='auto')
except:
model = AutoHQQHFModel.from_quantized("PrunaAI/alfredplpl-Llama-3-8B-Instruct-Ja-HQQ-4bit-smashed")
tokenizer = AutoTokenizer.from_pretrained("alfredplpl/Llama-3-8B-Instruct-Ja")
input_ids = tokenizer("What is the color of prunes?,", return_tensors='pt').to(model.device)["input_ids"]
outputs = model.generate(input_ids, max_new_tokens=216)
tokenizer.decode(outputs[0])
```
## Configurations
The configuration info are in `smash_config.json`.
## Credits & License
The license of the smashed model follows the license of the original model. Please check the license of the original model alfredplpl/Llama-3-8B-Instruct-Ja before using this model which provided the base model. The license of the `pruna-engine` is [here](https://pypi.org/project/pruna-engine/) on Pypi.
## Want to compress other models?
- Contact us and tell us which model to compress next [here](https://www.pruna.ai/contact).
- Request access to easily compress your own AI models [here](https://z0halsaff74.typeform.com/pruna-access?typeform-source=www.pruna.ai). |
marsggbo/t2-small-token-pattern-predictor-switch128-xsum | marsggbo | "2024-06-24T10:47:26Z" | 0 | 0 | transformers | [
"transformers",
"safetensors",
"t5",
"text2text-generation",
"arxiv:1910.09700",
"autotrain_compatible",
"endpoints_compatible",
"text-generation-inference",
"region:us"
] | text2text-generation | "2024-06-24T10:46:53Z" | ---
library_name: transformers
tags: []
---
# Model Card for Model ID
<!-- Provide a quick summary of what the model is/does. -->
## Model Details
### Model Description
<!-- Provide a longer summary of what this model is. -->
This is the model card of a 🤗 transformers model that has been pushed on the Hub. This model card has been automatically generated.
- **Developed by:** [More Information Needed]
- **Funded by [optional]:** [More Information Needed]
- **Shared by [optional]:** [More Information Needed]
- **Model type:** [More Information Needed]
- **Language(s) (NLP):** [More Information Needed]
- **License:** [More Information Needed]
- **Finetuned from model [optional]:** [More Information Needed]
### Model Sources [optional]
<!-- Provide the basic links for the model. -->
- **Repository:** [More Information Needed]
- **Paper [optional]:** [More Information Needed]
- **Demo [optional]:** [More Information Needed]
## Uses
<!-- Address questions around how the model is intended to be used, including the foreseeable users of the model and those affected by the model. -->
### Direct Use
<!-- This section is for the model use without fine-tuning or plugging into a larger ecosystem/app. -->
[More Information Needed]
### Downstream Use [optional]
<!-- This section is for the model use when fine-tuned for a task, or when plugged into a larger ecosystem/app -->
[More Information Needed]
### Out-of-Scope Use
<!-- This section addresses misuse, malicious use, and uses that the model will not work well for. -->
[More Information Needed]
## Bias, Risks, and Limitations
<!-- This section is meant to convey both technical and sociotechnical limitations. -->
[More Information Needed]
### Recommendations
<!-- This section is meant to convey recommendations with respect to the bias, risk, and technical limitations. -->
Users (both direct and downstream) should be made aware of the risks, biases and limitations of the model. More information needed for further recommendations.
## How to Get Started with the Model
Use the code below to get started with the model.
[More Information Needed]
## Training Details
### Training Data
<!-- This should link to a Dataset Card, perhaps with a short stub of information on what the training data is all about as well as documentation related to data pre-processing or additional filtering. -->
[More Information Needed]
### Training Procedure
<!-- This relates heavily to the Technical Specifications. Content here should link to that section when it is relevant to the training procedure. -->
#### Preprocessing [optional]
[More Information Needed]
#### Training Hyperparameters
- **Training regime:** [More Information Needed] <!--fp32, fp16 mixed precision, bf16 mixed precision, bf16 non-mixed precision, fp16 non-mixed precision, fp8 mixed precision -->
#### Speeds, Sizes, Times [optional]
<!-- This section provides information about throughput, start/end time, checkpoint size if relevant, etc. -->
[More Information Needed]
## Evaluation
<!-- This section describes the evaluation protocols and provides the results. -->
### Testing Data, Factors & Metrics
#### Testing Data
<!-- This should link to a Dataset Card if possible. -->
[More Information Needed]
#### Factors
<!-- These are the things the evaluation is disaggregating by, e.g., subpopulations or domains. -->
[More Information Needed]
#### Metrics
<!-- These are the evaluation metrics being used, ideally with a description of why. -->
[More Information Needed]
### Results
[More Information Needed]
#### Summary
## Model Examination [optional]
<!-- Relevant interpretability work for the model goes here -->
[More Information Needed]
## Environmental Impact
<!-- Total emissions (in grams of CO2eq) and additional considerations, such as electricity usage, go here. Edit the suggested text below accordingly -->
Carbon emissions can be estimated using the [Machine Learning Impact calculator](https://mlco2.github.io/impact#compute) presented in [Lacoste et al. (2019)](https://arxiv.org/abs/1910.09700).
- **Hardware Type:** [More Information Needed]
- **Hours used:** [More Information Needed]
- **Cloud Provider:** [More Information Needed]
- **Compute Region:** [More Information Needed]
- **Carbon Emitted:** [More Information Needed]
## Technical Specifications [optional]
### Model Architecture and Objective
[More Information Needed]
### Compute Infrastructure
[More Information Needed]
#### Hardware
[More Information Needed]
#### Software
[More Information Needed]
## Citation [optional]
<!-- If there is a paper or blog post introducing the model, the APA and Bibtex information for that should go in this section. -->
**BibTeX:**
[More Information Needed]
**APA:**
[More Information Needed]
## Glossary [optional]
<!-- If relevant, include terms and calculations in this section that can help readers understand the model or model card. -->
[More Information Needed]
## More Information [optional]
[More Information Needed]
## Model Card Authors [optional]
[More Information Needed]
## Model Card Contact
[More Information Needed] |
PrunaAI/NousResearch-Llama-2-13b-hf-HQQ-1bit-smashed | PrunaAI | "2024-06-24T10:50:06Z" | 0 | 0 | transformers | [
"transformers",
"llama",
"text-generation",
"pruna-ai",
"base_model:NousResearch/Llama-2-13b-hf",
"autotrain_compatible",
"endpoints_compatible",
"text-generation-inference",
"region:us"
] | text-generation | "2024-06-24T10:48:45Z" | ---
thumbnail: "https://assets-global.website-files.com/646b351987a8d8ce158d1940/64ec9e96b4334c0e1ac41504_Logo%20with%20white%20text.svg"
base_model: NousResearch/Llama-2-13b-hf
metrics:
- memory_disk
- memory_inference
- inference_latency
- inference_throughput
- inference_CO2_emissions
- inference_energy_consumption
tags:
- pruna-ai
---
<!-- header start -->
<!-- 200823 -->
<div style="width: auto; margin-left: auto; margin-right: auto">
<a href="https://www.pruna.ai/" target="_blank" rel="noopener noreferrer">
<img src="https://i.imgur.com/eDAlcgk.png" alt="PrunaAI" style="width: 100%; min-width: 400px; display: block; margin: auto;">
</a>
</div>
<!-- header end -->
[](https://twitter.com/PrunaAI)
[](https://github.com/PrunaAI)
[](https://www.linkedin.com/company/93832878/admin/feed/posts/?feedType=following)
[](https://discord.gg/CP4VSgck)
# Simply make AI models cheaper, smaller, faster, and greener!
- Give a thumbs up if you like this model!
- Contact us and tell us which model to compress next [here](https://www.pruna.ai/contact).
- Request access to easily compress your *own* AI models [here](https://z0halsaff74.typeform.com/pruna-access?typeform-source=www.pruna.ai).
- Read the documentations to know more [here](https://pruna-ai-pruna.readthedocs-hosted.com/en/latest/)
- Join Pruna AI community on Discord [here](https://discord.gg/CP4VSgck) to share feedback/suggestions or get help.
## Results

**Frequently Asked Questions**
- ***How does the compression work?*** The model is compressed with hqq.
- ***How does the model quality change?*** The quality of the model output might vary compared to the base model.
- ***How is the model efficiency evaluated?*** These results were obtained on HARDWARE_NAME with configuration described in `model/smash_config.json` and are obtained after a hardware warmup. The smashed model is directly compared to the original base model. Efficiency results may vary in other settings (e.g. other hardware, image size, batch size, ...). We recommend to directly run them in the use-case conditions to know if the smashed model can benefit you.
- ***What is the model format?*** We use safetensors.
- ***What calibration data has been used?*** If needed by the compression method, we used WikiText as the calibration data.
- ***What is the naming convention for Pruna Huggingface models?*** We take the original model name and append "turbo", "tiny", or "green" if the smashed model has a measured inference speed, inference memory, or inference energy consumption which is less than 90% of the original base model.
- ***How to compress my own models?*** You can request premium access to more compression methods and tech support for your specific use-cases [here](https://z0halsaff74.typeform.com/pruna-access?typeform-source=www.pruna.ai).
- ***What are "first" metrics?*** Results mentioning "first" are obtained after the first run of the model. The first run might take more memory or be slower than the subsequent runs due cuda overheads.
- ***What are "Sync" and "Async" metrics?*** "Sync" metrics are obtained by syncing all GPU processes and stop measurement when all of them are executed. "Async" metrics are obtained without syncing all GPU processes and stop when the model output can be used by the CPU. We provide both metrics since both could be relevant depending on the use-case. We recommend to test the efficiency gains directly in your use-cases.
## Setup
You can run the smashed model with these steps:
0. Check requirements from the original repo NousResearch/Llama-2-13b-hf installed. In particular, check python, cuda, and transformers versions.
1. Make sure that you have installed quantization related packages.
```bash
pip install hqq
```
2. Load & run the model.
```python
from transformers import AutoModelForCausalLM, AutoTokenizer
from hqq.engine.hf import HQQModelForCausalLM
from hqq.models.hf.base import AutoHQQHFModel
try:
model = HQQModelForCausalLM.from_quantized("PrunaAI/NousResearch-Llama-2-13b-hf-HQQ-1bit-smashed", device_map='auto')
except:
model = AutoHQQHFModel.from_quantized("PrunaAI/NousResearch-Llama-2-13b-hf-HQQ-1bit-smashed")
tokenizer = AutoTokenizer.from_pretrained("NousResearch/Llama-2-13b-hf")
input_ids = tokenizer("What is the color of prunes?,", return_tensors='pt').to(model.device)["input_ids"]
outputs = model.generate(input_ids, max_new_tokens=216)
tokenizer.decode(outputs[0])
```
## Configurations
The configuration info are in `smash_config.json`.
## Credits & License
The license of the smashed model follows the license of the original model. Please check the license of the original model NousResearch/Llama-2-13b-hf before using this model which provided the base model. The license of the `pruna-engine` is [here](https://pypi.org/project/pruna-engine/) on Pypi.
## Want to compress other models?
- Contact us and tell us which model to compress next [here](https://www.pruna.ai/contact).
- Request access to easily compress your own AI models [here](https://z0halsaff74.typeform.com/pruna-access?typeform-source=www.pruna.ai). |
achamajames/openai-whisper-large-v2__colab1 | achamajames | "2024-06-24T10:50:12Z" | 0 | 0 | transformers | [
"transformers",
"safetensors",
"arxiv:1910.09700",
"endpoints_compatible",
"region:us"
] | null | "2024-06-24T10:50:07Z" | ---
library_name: transformers
tags: []
---
# Model Card for Model ID
<!-- Provide a quick summary of what the model is/does. -->
## Model Details
### Model Description
<!-- Provide a longer summary of what this model is. -->
This is the model card of a 🤗 transformers model that has been pushed on the Hub. This model card has been automatically generated.
- **Developed by:** [More Information Needed]
- **Funded by [optional]:** [More Information Needed]
- **Shared by [optional]:** [More Information Needed]
- **Model type:** [More Information Needed]
- **Language(s) (NLP):** [More Information Needed]
- **License:** [More Information Needed]
- **Finetuned from model [optional]:** [More Information Needed]
### Model Sources [optional]
<!-- Provide the basic links for the model. -->
- **Repository:** [More Information Needed]
- **Paper [optional]:** [More Information Needed]
- **Demo [optional]:** [More Information Needed]
## Uses
<!-- Address questions around how the model is intended to be used, including the foreseeable users of the model and those affected by the model. -->
### Direct Use
<!-- This section is for the model use without fine-tuning or plugging into a larger ecosystem/app. -->
[More Information Needed]
### Downstream Use [optional]
<!-- This section is for the model use when fine-tuned for a task, or when plugged into a larger ecosystem/app -->
[More Information Needed]
### Out-of-Scope Use
<!-- This section addresses misuse, malicious use, and uses that the model will not work well for. -->
[More Information Needed]
## Bias, Risks, and Limitations
<!-- This section is meant to convey both technical and sociotechnical limitations. -->
[More Information Needed]
### Recommendations
<!-- This section is meant to convey recommendations with respect to the bias, risk, and technical limitations. -->
Users (both direct and downstream) should be made aware of the risks, biases and limitations of the model. More information needed for further recommendations.
## How to Get Started with the Model
Use the code below to get started with the model.
[More Information Needed]
## Training Details
### Training Data
<!-- This should link to a Dataset Card, perhaps with a short stub of information on what the training data is all about as well as documentation related to data pre-processing or additional filtering. -->
[More Information Needed]
### Training Procedure
<!-- This relates heavily to the Technical Specifications. Content here should link to that section when it is relevant to the training procedure. -->
#### Preprocessing [optional]
[More Information Needed]
#### Training Hyperparameters
- **Training regime:** [More Information Needed] <!--fp32, fp16 mixed precision, bf16 mixed precision, bf16 non-mixed precision, fp16 non-mixed precision, fp8 mixed precision -->
#### Speeds, Sizes, Times [optional]
<!-- This section provides information about throughput, start/end time, checkpoint size if relevant, etc. -->
[More Information Needed]
## Evaluation
<!-- This section describes the evaluation protocols and provides the results. -->
### Testing Data, Factors & Metrics
#### Testing Data
<!-- This should link to a Dataset Card if possible. -->
[More Information Needed]
#### Factors
<!-- These are the things the evaluation is disaggregating by, e.g., subpopulations or domains. -->
[More Information Needed]
#### Metrics
<!-- These are the evaluation metrics being used, ideally with a description of why. -->
[More Information Needed]
### Results
[More Information Needed]
#### Summary
## Model Examination [optional]
<!-- Relevant interpretability work for the model goes here -->
[More Information Needed]
## Environmental Impact
<!-- Total emissions (in grams of CO2eq) and additional considerations, such as electricity usage, go here. Edit the suggested text below accordingly -->
Carbon emissions can be estimated using the [Machine Learning Impact calculator](https://mlco2.github.io/impact#compute) presented in [Lacoste et al. (2019)](https://arxiv.org/abs/1910.09700).
- **Hardware Type:** [More Information Needed]
- **Hours used:** [More Information Needed]
- **Cloud Provider:** [More Information Needed]
- **Compute Region:** [More Information Needed]
- **Carbon Emitted:** [More Information Needed]
## Technical Specifications [optional]
### Model Architecture and Objective
[More Information Needed]
### Compute Infrastructure
[More Information Needed]
#### Hardware
[More Information Needed]
#### Software
[More Information Needed]
## Citation [optional]
<!-- If there is a paper or blog post introducing the model, the APA and Bibtex information for that should go in this section. -->
**BibTeX:**
[More Information Needed]
**APA:**
[More Information Needed]
## Glossary [optional]
<!-- If relevant, include terms and calculations in this section that can help readers understand the model or model card. -->
[More Information Needed]
## More Information [optional]
[More Information Needed]
## Model Card Authors [optional]
[More Information Needed]
## Model Card Contact
[More Information Needed] |