modelId
stringlengths 5
122
| author
stringlengths 2
42
| last_modified
unknown | downloads
int64 0
738M
| likes
int64 0
11k
| library_name
stringclasses 245
values | tags
sequencelengths 1
4.05k
| pipeline_tag
stringclasses 48
values | createdAt
unknown | card
stringlengths 1
901k
|
---|---|---|---|---|---|---|---|---|---|
katuni4ka/tiny-random-chatglm2 | katuni4ka | "2024-06-05T11:16:11Z" | 39,571 | 0 | transformers | [
"transformers",
"tensorboard",
"safetensors",
"chatglm",
"feature-extraction",
"generated_from_trainer",
"custom_code",
"base_model:katuni4ka/tiny-random-chatglm2",
"region:us"
] | feature-extraction | "2024-02-29T15:07:35Z" | ---
base_model: katuni4ka/tiny-random-chatglm2
tags:
- generated_from_trainer
model-index:
- name: tiny-random-chatglm2
results: []
---
<!-- This model card has been generated automatically according to the information the Trainer had access to. You
should probably proofread and complete it, then remove this comment. -->
# tiny-random-chatglm2
This model is a fine-tuned version of [katuni4ka/tiny-random-chatglm2](https://huggingface.co/katuni4ka/tiny-random-chatglm2) on an unknown dataset.
## Model description
More information needed
## Intended uses & limitations
More information needed
## Training and evaluation data
More information needed
## Training procedure
### Training hyperparameters
The following hyperparameters were used during training:
- learning_rate: 0.0005
- train_batch_size: 32
- eval_batch_size: 32
- seed: 42
- gradient_accumulation_steps: 8
- total_train_batch_size: 256
- optimizer: Adam with betas=(0.9,0.999) and epsilon=1e-08
- lr_scheduler_type: cosine
- lr_scheduler_warmup_steps: 1000
- num_epochs: 1
### Training results
### Framework versions
- Transformers 4.38.1
- Pytorch 2.1.0+cu121
- Datasets 2.17.1
- Tokenizers 0.15.2
|
cactusfriend/nightmare-promptgen-XL | cactusfriend | "2023-07-06T22:16:34Z" | 39,549 | 5 | transformers | [
"transformers",
"pytorch",
"safetensors",
"gpt_neo",
"text-generation",
"license:openrail",
"autotrain_compatible",
"endpoints_compatible",
"region:us"
] | text-generation | "2023-06-26T14:07:40Z" | ---
license: openrail
pipeline_tag: text-generation
library_name: transformers
widget:
- text: "a photograph of"
example_title: "photo"
- text: "a bizarre cg render"
example_title: "render"
- text: "the spaghetti"
example_title: "meal?"
- text: "a (detailed+ intricate)+ picture"
example_title: "weights"
- text: "photograph of various"
example_title: "variety"
inference:
parameters:
temperature: 2.6
max_new_tokens: 250
---
Experimental 'XL' version of [Nightmare InvokeAI Prompts](https://huggingface.co/cactusfriend/nightmare-invokeai-prompts). Very early version and may be deleted. |
audeering/wav2vec2-large-robust-12-ft-emotion-msp-dim | audeering | "2024-05-08T09:35:28Z" | 39,444 | 83 | transformers | [
"transformers",
"pytorch",
"safetensors",
"wav2vec2",
"speech",
"audio",
"audio-classification",
"emotion-recognition",
"en",
"dataset:msp-podcast",
"arxiv:2203.07378",
"license:cc-by-nc-sa-4.0",
"endpoints_compatible",
"region:us"
] | audio-classification | "2022-04-06T12:40:02Z" | ---
language: en
datasets:
- msp-podcast
inference: true
tags:
- speech
- audio
- wav2vec2
- audio-classification
- emotion-recognition
license: cc-by-nc-sa-4.0
pipeline_tag: audio-classification
---
# Model for Dimensional Speech Emotion Recognition based on Wav2vec 2.0
Please note that this model is for research purpose only. A commercial license for a model that has been trained on much more data can be acquired with [audEERING](https://www.audeering.com/products/devaice/).
The model expects a raw audio signal as input and outputs predictions for arousal, dominance and valence in a range of approximately 0...1. In addition, it also provides the pooled states of the last transformer layer. The model was created by fine-tuning [
Wav2Vec2-Large-Robust](https://huggingface.co/facebook/wav2vec2-large-robust) on [MSP-Podcast](https://ecs.utdallas.edu/research/researchlabs/msp-lab/MSP-Podcast.html) (v1.7). The model was pruned from 24 to 12 transformer layers before fine-tuning. An [ONNX](https://onnx.ai/") export of the model is available from [doi:10.5281/zenodo.6221127](https://zenodo.org/record/6221127). Further details are given in the associated [paper](https://arxiv.org/abs/2203.07378) and [tutorial](https://github.com/audeering/w2v2-how-to).
# Usage
```python
import numpy as np
import torch
import torch.nn as nn
from transformers import Wav2Vec2Processor
from transformers.models.wav2vec2.modeling_wav2vec2 import (
Wav2Vec2Model,
Wav2Vec2PreTrainedModel,
)
class RegressionHead(nn.Module):
r"""Classification head."""
def __init__(self, config):
super().__init__()
self.dense = nn.Linear(config.hidden_size, config.hidden_size)
self.dropout = nn.Dropout(config.final_dropout)
self.out_proj = nn.Linear(config.hidden_size, config.num_labels)
def forward(self, features, **kwargs):
x = features
x = self.dropout(x)
x = self.dense(x)
x = torch.tanh(x)
x = self.dropout(x)
x = self.out_proj(x)
return x
class EmotionModel(Wav2Vec2PreTrainedModel):
r"""Speech emotion classifier."""
def __init__(self, config):
super().__init__(config)
self.config = config
self.wav2vec2 = Wav2Vec2Model(config)
self.classifier = RegressionHead(config)
self.init_weights()
def forward(
self,
input_values,
):
outputs = self.wav2vec2(input_values)
hidden_states = outputs[0]
hidden_states = torch.mean(hidden_states, dim=1)
logits = self.classifier(hidden_states)
return hidden_states, logits
# load model from hub
device = 'cpu'
model_name = 'audeering/wav2vec2-large-robust-12-ft-emotion-msp-dim'
processor = Wav2Vec2Processor.from_pretrained(model_name)
model = EmotionModel.from_pretrained(model_name)
# dummy signal
sampling_rate = 16000
signal = np.zeros((1, sampling_rate), dtype=np.float32)
def process_func(
x: np.ndarray,
sampling_rate: int,
embeddings: bool = False,
) -> np.ndarray:
r"""Predict emotions or extract embeddings from raw audio signal."""
# run through processor to normalize signal
# always returns a batch, so we just get the first entry
# then we put it on the device
y = processor(x, sampling_rate=sampling_rate)
y = y['input_values'][0]
y = y.reshape(1, -1)
y = torch.from_numpy(y).to(device)
# run through model
with torch.no_grad():
y = model(y)[0 if embeddings else 1]
# convert to numpy
y = y.detach().cpu().numpy()
return y
print(process_func(signal, sampling_rate))
# Arousal dominance valence
# [[0.5460754 0.6062266 0.40431657]]
print(process_func(signal, sampling_rate, embeddings=True))
# Pooled hidden states of last transformer layer
# [[-0.00752167 0.0065819 -0.00746342 ... 0.00663632 0.00848748
# 0.00599211]]
``` |
tomh/toxigen_roberta | tomh | "2022-05-01T19:42:09Z" | 39,396 | 8 | transformers | [
"transformers",
"pytorch",
"roberta",
"text-classification",
"en",
"arxiv:2203.09509",
"autotrain_compatible",
"endpoints_compatible",
"region:us"
] | text-classification | "2022-05-01T13:19:41Z" | ---
language:
- en
tags:
- text-classification
---
Thomas Hartvigsen, Saadia Gabriel, Hamid Palangi, Maarten Sap, Dipankar Ray, Ece Kamar.
This model comes from the paper [ToxiGen: A Large-Scale Machine-Generated Dataset for Adversarial and Implicit Hate Speech Detection](https://arxiv.org/abs/2203.09509) and can be used to detect implicit hate speech.
Please visit the [Github Repository](https://github.com/microsoft/TOXIGEN) for the training dataset and further details.
```bibtex
@inproceedings{hartvigsen2022toxigen,
title = "{T}oxi{G}en: A Large-Scale Machine-Generated Dataset for Adversarial and Implicit Hate Speech Detection",
author = "Hartvigsen, Thomas and Gabriel, Saadia and Palangi, Hamid and Sap, Maarten and Ray, Dipankar and Kamar, Ece",
booktitle = "Proceedings of the 60th Annual Meeting of the Association of Computational Linguistics",
year = "2022"
}
``` |
katuni4ka/tiny-random-qwen | katuni4ka | "2024-03-04T14:28:37Z" | 39,396 | 0 | transformers | [
"transformers",
"safetensors",
"qwen",
"text-generation",
"custom_code",
"autotrain_compatible",
"region:us"
] | text-generation | "2024-03-01T15:30:30Z" | Entry not found |
NousResearch/Meta-Llama-3-8B | NousResearch | "2024-04-30T04:45:04Z" | 39,319 | 83 | transformers | [
"transformers",
"safetensors",
"llama",
"text-generation",
"facebook",
"meta",
"pytorch",
"llama-3",
"en",
"license:other",
"autotrain_compatible",
"endpoints_compatible",
"text-generation-inference",
"region:us"
] | text-generation | "2024-04-18T16:47:58Z" | ---
language:
- en
pipeline_tag: text-generation
tags:
- facebook
- meta
- pytorch
- llama
- llama-3
license: other
license_name: llama3
license_link: LICENSE
extra_gated_prompt: >-
### META LLAMA 3 COMMUNITY LICENSE AGREEMENT
Meta Llama 3 Version Release Date: April 18, 2024
"Agreement" means the terms and conditions for use, reproduction, distribution and modification of the
Llama Materials set forth herein.
"Documentation" means the specifications, manuals and documentation accompanying Meta Llama 3
distributed by Meta at https://llama.meta.com/get-started/.
"Licensee" or "you" means you, or your employer or any other person or entity (if you are entering into
this Agreement on such person or entity’s behalf), of the age required under applicable laws, rules or
regulations to provide legal consent and that has legal authority to bind your employer or such other
person or entity if you are entering in this Agreement on their behalf.
"Meta Llama 3" means the foundational large language models and software and algorithms, including
machine-learning model code, trained model weights, inference-enabling code, training-enabling code,
fine-tuning enabling code and other elements of the foregoing distributed by Meta at
https://llama.meta.com/llama-downloads.
"Llama Materials" means, collectively, Meta’s proprietary Meta Llama 3 and Documentation (and any
portion thereof) made available under this Agreement.
"Meta" or "we" means Meta Platforms Ireland Limited (if you are located in or, if you are an entity, your
principal place of business is in the EEA or Switzerland) and Meta Platforms, Inc. (if you are located
outside of the EEA or Switzerland).
1. License Rights and Redistribution.
a. Grant of Rights. You are granted a non-exclusive, worldwide, non-transferable and royalty-free
limited license under Meta’s intellectual property or other rights owned by Meta embodied in the Llama
Materials to use, reproduce, distribute, copy, create derivative works of, and make modifications to the
Llama Materials.
b. Redistribution and Use.
i. If you distribute or make available the Llama Materials (or any derivative works
thereof), or a product or service that uses any of them, including another AI model, you shall (A) provide
a copy of this Agreement with any such Llama Materials; and (B) prominently display “Built with Meta
Llama 3” on a related website, user interface, blogpost, about page, or product documentation. If you
use the Llama Materials to create, train, fine tune, or otherwise improve an AI model, which is
distributed or made available, you shall also include “Llama 3” at the beginning of any such AI model
name.
ii. If you receive Llama Materials, or any derivative works thereof, from a Licensee as part
of an integrated end user product, then Section 2 of this Agreement will not apply to you.
iii. You must retain in all copies of the Llama Materials that you distribute the following
attribution notice within a “Notice” text file distributed as a part of such copies: “Meta Llama 3 is
licensed under the Meta Llama 3 Community License, Copyright © Meta Platforms, Inc. All Rights
Reserved.”
iv. Your use of the Llama Materials must comply with applicable laws and regulations
(including trade compliance laws and regulations) and adhere to the Acceptable Use Policy for the Llama
Materials (available at https://llama.meta.com/llama3/use-policy), which is hereby incorporated by
reference into this Agreement.
v. You will not use the Llama Materials or any output or results of the Llama Materials to
improve any other large language model (excluding Meta Llama 3 or derivative works thereof).
2. Additional Commercial Terms. If, on the Meta Llama 3 version release date, the monthly active users
of the products or services made available by or for Licensee, or Licensee’s affiliates, is greater than 700
million monthly active users in the preceding calendar month, you must request a license from Meta,
which Meta may grant to you in its sole discretion, and you are not authorized to exercise any of the
rights under this Agreement unless or until Meta otherwise expressly grants you such rights.
3. Disclaimer of Warranty. UNLESS REQUIRED BY APPLICABLE LAW, THE LLAMA MATERIALS AND ANY
OUTPUT AND RESULTS THEREFROM ARE PROVIDED ON AN “AS IS” BASIS, WITHOUT WARRANTIES OF
ANY KIND, AND META DISCLAIMS ALL WARRANTIES OF ANY KIND, BOTH EXPRESS AND IMPLIED,
INCLUDING, WITHOUT LIMITATION, ANY WARRANTIES OF TITLE, NON-INFRINGEMENT,
MERCHANTABILITY, OR FITNESS FOR A PARTICULAR PURPOSE. YOU ARE SOLELY RESPONSIBLE FOR
DETERMINING THE APPROPRIATENESS OF USING OR REDISTRIBUTING THE LLAMA MATERIALS AND
ASSUME ANY RISKS ASSOCIATED WITH YOUR USE OF THE LLAMA MATERIALS AND ANY OUTPUT AND
RESULTS.
4. Limitation of Liability. IN NO EVENT WILL META OR ITS AFFILIATES BE LIABLE UNDER ANY THEORY OF
LIABILITY, WHETHER IN CONTRACT, TORT, NEGLIGENCE, PRODUCTS LIABILITY, OR OTHERWISE, ARISING
OUT OF THIS AGREEMENT, FOR ANY LOST PROFITS OR ANY INDIRECT, SPECIAL, CONSEQUENTIAL,
INCIDENTAL, EXEMPLARY OR PUNITIVE DAMAGES, EVEN IF META OR ITS AFFILIATES HAVE BEEN ADVISED
OF THE POSSIBILITY OF ANY OF THE FOREGOING.
5. Intellectual Property.
a. No trademark licenses are granted under this Agreement, and in connection with the Llama
Materials, neither Meta nor Licensee may use any name or mark owned by or associated with the other
or any of its affiliates, except as required for reasonable and customary use in describing and
redistributing the Llama Materials or as set forth in this Section 5(a). Meta hereby grants you a license to
use “Llama 3” (the “Mark”) solely as required to comply with the last sentence of Section 1.b.i. You will
comply with Meta’s brand guidelines (currently accessible at
https://about.meta.com/brand/resources/meta/company-brand/ ). All goodwill arising out of your use
of the Mark will inure to the benefit of Meta.
b. Subject to Meta’s ownership of Llama Materials and derivatives made by or for Meta, with
respect to any derivative works and modifications of the Llama Materials that are made by you, as
between you and Meta, you are and will be the owner of such derivative works and modifications.
c. If you institute litigation or other proceedings against Meta or any entity (including a
cross-claim or counterclaim in a lawsuit) alleging that the Llama Materials or Meta Llama 3 outputs or
results, or any portion of any of the foregoing, constitutes infringement of intellectual property or other
rights owned or licensable by you, then any licenses granted to you under this Agreement shall
terminate as of the date such litigation or claim is filed or instituted. You will indemnify and hold
harmless Meta from and against any claim by any third party arising out of or related to your use or
distribution of the Llama Materials.
6. Term and Termination. The term of this Agreement will commence upon your acceptance of this
Agreement or access to the Llama Materials and will continue in full force and effect until terminated in
accordance with the terms and conditions herein. Meta may terminate this Agreement if you are in
breach of any term or condition of this Agreement. Upon termination of this Agreement, you shall delete
and cease use of the Llama Materials. Sections 3, 4 and 7 shall survive the termination of this
Agreement.
7. Governing Law and Jurisdiction. This Agreement will be governed and construed under the laws of
the State of California without regard to choice of law principles, and the UN Convention on Contracts
for the International Sale of Goods does not apply to this Agreement. The courts of California shall have
exclusive jurisdiction of any dispute arising out of this Agreement.
### Meta Llama 3 Acceptable Use Policy
Meta is committed to promoting safe and fair use of its tools and features, including Meta Llama 3. If you
access or use Meta Llama 3, you agree to this Acceptable Use Policy (“Policy”). The most recent copy of
this policy can be found at [https://llama.meta.com/llama3/use-policy](https://llama.meta.com/llama3/use-policy)
#### Prohibited Uses
We want everyone to use Meta Llama 3 safely and responsibly. You agree you will not use, or allow
others to use, Meta Llama 3 to:
1. Violate the law or others’ rights, including to:
1. Engage in, promote, generate, contribute to, encourage, plan, incite, or further illegal or unlawful activity or content, such as:
1. Violence or terrorism
2. Exploitation or harm to children, including the solicitation, creation, acquisition, or dissemination of child exploitative content or failure to report Child Sexual Abuse Material
3. Human trafficking, exploitation, and sexual violence
4. The illegal distribution of information or materials to minors, including obscene materials, or failure to employ legally required age-gating in connection with such information or materials.
5. Sexual solicitation
6. Any other criminal activity
2. Engage in, promote, incite, or facilitate the harassment, abuse, threatening, or bullying of individuals or groups of individuals
3. Engage in, promote, incite, or facilitate discrimination or other unlawful or harmful conduct in the provision of employment, employment benefits, credit, housing, other economic benefits, or other essential goods and services
4. Engage in the unauthorized or unlicensed practice of any profession including, but not limited to, financial, legal, medical/health, or related professional practices
5. Collect, process, disclose, generate, or infer health, demographic, or other sensitive personal or private information about individuals without rights and consents required by applicable laws
6. Engage in or facilitate any action or generate any content that infringes, misappropriates, or otherwise violates any third-party rights, including the outputs or results of any products or services using the Llama Materials
7. Create, generate, or facilitate the creation of malicious code, malware, computer viruses or do anything else that could disable, overburden, interfere with or impair the proper working, integrity, operation or appearance of a website or computer system
2. Engage in, promote, incite, facilitate, or assist in the planning or development of activities that present a risk of death or bodily harm to individuals, including use of Meta Llama 3 related to the following:
1. Military, warfare, nuclear industries or applications, espionage, use for materials or activities that are subject to the International Traffic Arms Regulations (ITAR) maintained by the United States Department of State
2. Guns and illegal weapons (including weapon development)
3. Illegal drugs and regulated/controlled substances
4. Operation of critical infrastructure, transportation technologies, or heavy machinery
5. Self-harm or harm to others, including suicide, cutting, and eating disorders
6. Any content intended to incite or promote violence, abuse, or any infliction of bodily harm to an individual
3. Intentionally deceive or mislead others, including use of Meta Llama 3 related to the following:
1. Generating, promoting, or furthering fraud or the creation or promotion of disinformation
2. Generating, promoting, or furthering defamatory content, including the creation of defamatory statements, images, or other content
3. Generating, promoting, or further distributing spam
4. Impersonating another individual without consent, authorization, or legal right
5. Representing that the use of Meta Llama 3 or outputs are human-generated
6. Generating or facilitating false online engagement, including fake reviews and other means of fake online engagement
4. Fail to appropriately disclose to end users any known dangers of your AI system
Please report any violation of this Policy, software “bug,” or other problems that could lead to a violation
of this Policy through one of the following means:
* Reporting issues with the model: [https://github.com/meta-llama/llama3](https://github.com/meta-llama/llama3)
* Reporting risky content generated by the model:
developers.facebook.com/llama_output_feedback
* Reporting bugs and security concerns: facebook.com/whitehat/info
* Reporting violations of the Acceptable Use Policy or unlicensed uses of Meta Llama 3: LlamaUseReport@meta.com
extra_gated_fields:
First Name: text
Last Name: text
Date of birth: date_picker
Country: country
Affiliation: text
geo: ip_location
By clicking Submit below I accept the terms of the license and acknowledge that the information I provide will be collected stored processed and shared in accordance with the Meta Privacy Policy: checkbox
extra_gated_description: The information you provide will be collected, stored, processed and shared in accordance with the [Meta Privacy Policy](https://www.facebook.com/privacy/policy/).
extra_gated_button_content: Submit
---
## Model Details
Meta developed and released the Meta Llama 3 family of large language models (LLMs), a collection of pretrained and instruction tuned generative text models in 8 and 70B sizes. The Llama 3 instruction tuned models are optimized for dialogue use cases and outperform many of the available open source chat models on common industry benchmarks. Further, in developing these models, we took great care to optimize helpfulness and safety.
**Model developers** Meta
**Variations** Llama 3 comes in two sizes — 8B and 70B parameters — in pre-trained and instruction tuned variants.
**Input** Models input text only.
**Output** Models generate text and code only.
**Model Architecture** Llama 3 is an auto-regressive language model that uses an optimized transformer architecture. The tuned versions use supervised fine-tuning (SFT) and reinforcement learning with human feedback (RLHF) to align with human preferences for helpfulness and safety.
<table>
<tr>
<td>
</td>
<td><strong>Training Data</strong>
</td>
<td><strong>Params</strong>
</td>
<td><strong>Context length</strong>
</td>
<td><strong>GQA</strong>
</td>
<td><strong>Token count</strong>
</td>
<td><strong>Knowledge cutoff</strong>
</td>
</tr>
<tr>
<td rowspan="2" >Llama 3
</td>
<td rowspan="2" >A new mix of publicly available online data.
</td>
<td>8B
</td>
<td>8k
</td>
<td>Yes
</td>
<td rowspan="2" >15T+
</td>
<td>March, 2023
</td>
</tr>
<tr>
<td>70B
</td>
<td>8k
</td>
<td>Yes
</td>
<td>December, 2023
</td>
</tr>
</table>
**Llama 3 family of models**. Token counts refer to pretraining data only. Both the 8 and 70B versions use Grouped-Query Attention (GQA) for improved inference scalability.
**Model Release Date** April 18, 2024.
**Status** This is a static model trained on an offline dataset. Future versions of the tuned models will be released as we improve model safety with community feedback.
**License** A custom commercial license is available at: [https://llama.meta.com/llama3/license](https://llama.meta.com/llama3/license)
Where to send questions or comments about the model Instructions on how to provide feedback or comments on the model can be found in the model [README](https://github.com/meta-llama/llama3). For more technical information about generation parameters and recipes for how to use Llama 3 in applications, please go [here](https://github.com/meta-llama/llama-recipes).
## Intended Use
**Intended Use Cases** Llama 3 is intended for commercial and research use in English. Instruction tuned models are intended for assistant-like chat, whereas pretrained models can be adapted for a variety of natural language generation tasks.
**Out-of-scope** Use in any manner that violates applicable laws or regulations (including trade compliance laws). Use in any other way that is prohibited by the Acceptable Use Policy and Llama 3 Community License. Use in languages other than English**.
**Note: Developers may fine-tune Llama 3 models for languages beyond English provided they comply with the Llama 3 Community License and the Acceptable Use Policy.
## How to use
This repository contains two versions of Meta-Llama-3-8B, for use with transformers and with the original `llama3` codebase.
### Use with transformers
See the snippet below for usage with Transformers:
```python
>>> import transformers
>>> import torch
>>> model_id = "meta-llama/Meta-Llama-3-8B"
>>> pipeline = transformers.pipeline(
"text-generation", model=model_id, model_kwargs={"torch_dtype": torch.bfloat16}, device_map="auto"
)
>>> pipeline("Hey how are you doing today?")
```
### Use with `llama3`
Please, follow the instructions in the [repository](https://github.com/meta-llama/llama3).
To download Original checkpoints, see the example command below leveraging `huggingface-cli`:
```
huggingface-cli download meta-llama/Meta-Llama-3-8B --include "original/*" --local-dir Meta-Llama-3-8B
```
For Hugging Face support, we recommend using transformers or TGI, but a similar command works.
## Hardware and Software
**Training Factors** We used custom training libraries, Meta's Research SuperCluster, and production clusters for pretraining. Fine-tuning, annotation, and evaluation were also performed on third-party cloud compute.
**Carbon Footprint Pretraining utilized a cumulative** 7.7M GPU hours of computation on hardware of type H100-80GB (TDP of 700W). Estimated total emissions were 2290 tCO2eq, 100% of which were offset by Meta’s sustainability program.
<table>
<tr>
<td>
</td>
<td><strong>Time (GPU hours)</strong>
</td>
<td><strong>Power Consumption (W)</strong>
</td>
<td><strong>Carbon Emitted(tCO2eq)</strong>
</td>
</tr>
<tr>
<td>Llama 3 8B
</td>
<td>1.3M
</td>
<td>700
</td>
<td>390
</td>
</tr>
<tr>
<td>Llama 3 70B
</td>
<td>6.4M
</td>
<td>700
</td>
<td>1900
</td>
</tr>
<tr>
<td>Total
</td>
<td>7.7M
</td>
<td>
</td>
<td>2290
</td>
</tr>
</table>
**CO2 emissions during pre-training**. Time: total GPU time required for training each model. Power Consumption: peak power capacity per GPU device for the GPUs used adjusted for power usage efficiency. 100% of the emissions are directly offset by Meta's sustainability program, and because we are openly releasing these models, the pretraining costs do not need to be incurred by others.
## Training Data
**Overview** Llama 3 was pretrained on over 15 trillion tokens of data from publicly available sources. The fine-tuning data includes publicly available instruction datasets, as well as over 10M human-annotated examples. Neither the pretraining nor the fine-tuning datasets include Meta user data.
**Data Freshness** The pretraining data has a cutoff of March 2023 for the 7B and December 2023 for the 70B models respectively.
## Benchmarks
In this section, we report the results for Llama 3 models on standard automatic benchmarks. For all the evaluations, we use our internal evaluations library. For details on the methodology see [here](https://github.com/meta-llama/llama3/blob/main/eval_methodology.md).
### Base pretrained models
<table>
<tr>
<td><strong>Category</strong>
</td>
<td><strong>Benchmark</strong>
</td>
<td><strong>Llama 3 8B</strong>
</td>
<td><strong>Llama2 7B</strong>
</td>
<td><strong>Llama2 13B</strong>
</td>
<td><strong>Llama 3 70B</strong>
</td>
<td><strong>Llama2 70B</strong>
</td>
</tr>
<tr>
<td rowspan="6" >General
</td>
<td>MMLU (5-shot)
</td>
<td>66.6
</td>
<td>45.7
</td>
<td>53.8
</td>
<td>79.5
</td>
<td>69.7
</td>
</tr>
<tr>
<td>AGIEval English (3-5 shot)
</td>
<td>45.9
</td>
<td>28.8
</td>
<td>38.7
</td>
<td>63.0
</td>
<td>54.8
</td>
</tr>
<tr>
<td>CommonSenseQA (7-shot)
</td>
<td>72.6
</td>
<td>57.6
</td>
<td>67.6
</td>
<td>83.8
</td>
<td>78.7
</td>
</tr>
<tr>
<td>Winogrande (5-shot)
</td>
<td>76.1
</td>
<td>73.3
</td>
<td>75.4
</td>
<td>83.1
</td>
<td>81.8
</td>
</tr>
<tr>
<td>BIG-Bench Hard (3-shot, CoT)
</td>
<td>61.1
</td>
<td>38.1
</td>
<td>47.0
</td>
<td>81.3
</td>
<td>65.7
</td>
</tr>
<tr>
<td>ARC-Challenge (25-shot)
</td>
<td>78.6
</td>
<td>53.7
</td>
<td>67.6
</td>
<td>93.0
</td>
<td>85.3
</td>
</tr>
<tr>
<td>Knowledge reasoning
</td>
<td>TriviaQA-Wiki (5-shot)
</td>
<td>78.5
</td>
<td>72.1
</td>
<td>79.6
</td>
<td>89.7
</td>
<td>87.5
</td>
</tr>
<tr>
<td rowspan="4" >Reading comprehension
</td>
<td>SQuAD (1-shot)
</td>
<td>76.4
</td>
<td>72.2
</td>
<td>72.1
</td>
<td>85.6
</td>
<td>82.6
</td>
</tr>
<tr>
<td>QuAC (1-shot, F1)
</td>
<td>44.4
</td>
<td>39.6
</td>
<td>44.9
</td>
<td>51.1
</td>
<td>49.4
</td>
</tr>
<tr>
<td>BoolQ (0-shot)
</td>
<td>75.7
</td>
<td>65.5
</td>
<td>66.9
</td>
<td>79.0
</td>
<td>73.1
</td>
</tr>
<tr>
<td>DROP (3-shot, F1)
</td>
<td>58.4
</td>
<td>37.9
</td>
<td>49.8
</td>
<td>79.7
</td>
<td>70.2
</td>
</tr>
</table>
### Instruction tuned models
<table>
<tr>
<td><strong>Benchmark</strong>
</td>
<td><strong>Llama 3 8B</strong>
</td>
<td><strong>Llama 2 7B</strong>
</td>
<td><strong>Llama 2 13B</strong>
</td>
<td><strong>Llama 3 70B</strong>
</td>
<td><strong>Llama 2 70B</strong>
</td>
</tr>
<tr>
<td>MMLU (5-shot)
</td>
<td>68.4
</td>
<td>34.1
</td>
<td>47.8
</td>
<td>82.0
</td>
<td>52.9
</td>
</tr>
<tr>
<td>GPQA (0-shot)
</td>
<td>34.2
</td>
<td>21.7
</td>
<td>22.3
</td>
<td>39.5
</td>
<td>21.0
</td>
</tr>
<tr>
<td>HumanEval (0-shot)
</td>
<td>62.2
</td>
<td>7.9
</td>
<td>14.0
</td>
<td>81.7
</td>
<td>25.6
</td>
</tr>
<tr>
<td>GSM-8K (8-shot, CoT)
</td>
<td>79.6
</td>
<td>25.7
</td>
<td>77.4
</td>
<td>93.0
</td>
<td>57.5
</td>
</tr>
<tr>
<td>MATH (4-shot, CoT)
</td>
<td>30.0
</td>
<td>3.8
</td>
<td>6.7
</td>
<td>50.4
</td>
<td>11.6
</td>
</tr>
</table>
### Responsibility & Safety
We believe that an open approach to AI leads to better, safer products, faster innovation, and a bigger overall market. We are committed to Responsible AI development and took a series of steps to limit misuse and harm and support the open source community.
Foundation models are widely capable technologies that are built to be used for a diverse range of applications. They are not designed to meet every developer preference on safety levels for all use cases, out-of-the-box, as those by their nature will differ across different applications.
Rather, responsible LLM-application deployment is achieved by implementing a series of safety best practices throughout the development of such applications, from the model pre-training, fine-tuning and the deployment of systems composed of safeguards to tailor the safety needs specifically to the use case and audience.
As part of the Llama 3 release, we updated our [Responsible Use Guide](https://llama.meta.com/responsible-use-guide/) to outline the steps and best practices for developers to implement model and system level safety for their application. We also provide a set of resources including [Meta Llama Guard 2](https://llama.meta.com/purple-llama/) and [Code Shield](https://llama.meta.com/purple-llama/) safeguards. These tools have proven to drastically reduce residual risks of LLM Systems, while maintaining a high level of helpfulness. We encourage developers to tune and deploy these safeguards according to their needs and we provide a [reference implementation](https://github.com/meta-llama/llama-recipes/tree/main/recipes/responsible_ai) to get you started.
#### Llama 3-Instruct
As outlined in the Responsible Use Guide, some trade-off between model helpfulness and model alignment is likely unavoidable. Developers should exercise discretion about how to weigh the benefits of alignment and helpfulness for their specific use case and audience. Developers should be mindful of residual risks when using Llama models and leverage additional safety tools as needed to reach the right safety bar for their use case.
<span style="text-decoration:underline;">Safety</span>
For our instruction tuned model, we conducted extensive red teaming exercises, performed adversarial evaluations and implemented safety mitigations techniques to lower residual risks. As with any Large Language Model, residual risks will likely remain and we recommend that developers assess these risks in the context of their use case. In parallel, we are working with the community to make AI safety benchmark standards transparent, rigorous and interpretable.
<span style="text-decoration:underline;">Refusals</span>
In addition to residual risks, we put a great emphasis on model refusals to benign prompts. Over-refusing not only can impact the user experience but could even be harmful in certain contexts as well. We’ve heard the feedback from the developer community and improved our fine tuning to ensure that Llama 3 is significantly less likely to falsely refuse to answer prompts than Llama 2.
We built internal benchmarks and developed mitigations to limit false refusals making Llama 3 our most helpful model to date.
#### Responsible release
In addition to responsible use considerations outlined above, we followed a rigorous process that requires us to take extra measures against misuse and critical risks before we make our release decision.
Misuse
If you access or use Llama 3, you agree to the Acceptable Use Policy. The most recent copy of this policy can be found at [https://llama.meta.com/llama3/use-policy/](https://llama.meta.com/llama3/use-policy/).
#### Critical risks
<span style="text-decoration:underline;">CBRNE</span> (Chemical, Biological, Radiological, Nuclear, and high yield Explosives)
We have conducted a two fold assessment of the safety of the model in this area:
* Iterative testing during model training to assess the safety of responses related to CBRNE threats and other adversarial risks.
* Involving external CBRNE experts to conduct an uplift test assessing the ability of the model to accurately provide expert knowledge and reduce barriers to potential CBRNE misuse, by reference to what can be achieved using web search (without the model).
### <span style="text-decoration:underline;">Cyber Security </span>
We have evaluated Llama 3 with CyberSecEval, Meta’s cybersecurity safety eval suite, measuring Llama 3’s propensity to suggest insecure code when used as a coding assistant, and Llama 3’s propensity to comply with requests to help carry out cyber attacks, where attacks are defined by the industry standard MITRE ATT&CK cyber attack ontology. On our insecure coding and cyber attacker helpfulness tests, Llama 3 behaved in the same range or safer than models of [equivalent coding capability](https://huggingface.co/spaces/facebook/CyberSecEval).
### <span style="text-decoration:underline;">Child Safety</span>
Child Safety risk assessments were conducted using a team of experts, to assess the model’s capability to produce outputs that could result in Child Safety risks and inform on any necessary and appropriate risk mitigations via fine tuning. We leveraged those expert red teaming sessions to expand the coverage of our evaluation benchmarks through Llama 3 model development. For Llama 3, we conducted new in-depth sessions using objective based methodologies to assess the model risks along multiple attack vectors. We also partnered with content specialists to perform red teaming exercises assessing potentially violating content while taking account of market specific nuances or experiences.
### Community
Generative AI safety requires expertise and tooling, and we believe in the strength of the open community to accelerate its progress. We are active members of open consortiums, including the AI Alliance, Partnership in AI and MLCommons, actively contributing to safety standardization and transparency. We encourage the community to adopt taxonomies like the MLCommons Proof of Concept evaluation to facilitate collaboration and transparency on safety and content evaluations. Our Purple Llama tools are open sourced for the community to use and widely distributed across ecosystem partners including cloud service providers. We encourage community contributions to our [Github repository](https://github.com/meta-llama/PurpleLlama).
Finally, we put in place a set of resources including an [output reporting mechanism](https://developers.facebook.com/llama_output_feedback) and [bug bounty program](https://www.facebook.com/whitehat) to continuously improve the Llama technology with the help of the community.
## Ethical Considerations and Limitations
The core values of Llama 3 are openness, inclusivity and helpfulness. It is meant to serve everyone, and to work for a wide range of use cases. It is thus designed to be accessible to people across many different backgrounds, experiences and perspectives. Llama 3 addresses users and their needs as they are, without insertion unnecessary judgment or normativity, while reflecting the understanding that even content that may appear problematic in some cases can serve valuable purposes in others. It respects the dignity and autonomy of all users, especially in terms of the values of free thought and expression that power innovation and progress.
But Llama 3 is a new technology, and like any new technology, there are risks associated with its use. Testing conducted to date has been in English, and has not covered, nor could it cover, all scenarios. For these reasons, as with all LLMs, Llama 3’s potential outputs cannot be predicted in advance, and the model may in some instances produce inaccurate, biased or other objectionable responses to user prompts. Therefore, before deploying any applications of Llama 3 models, developers should perform safety testing and tuning tailored to their specific applications of the model. As outlined in the Responsible Use Guide, we recommend incorporating [Purple Llama](https://github.com/facebookresearch/PurpleLlama) solutions into your workflows and specifically [Llama Guard](https://ai.meta.com/research/publications/llama-guard-llm-based-input-output-safeguard-for-human-ai-conversations/) which provides a base model to filter input and output prompts to layer system-level safety on top of model-level safety.
Please see the Responsible Use Guide available at [http://llama.meta.com/responsible-use-guide](http://llama.meta.com/responsible-use-guide)
## Citation instructions
@article{llama3modelcard,
title={Llama 3 Model Card},
author={AI@Meta},
year={2024},
url = {https://github.com/meta-llama/llama3/blob/main/MODEL_CARD.md}
}
## Contributors
Aaditya Singh; Aaron Grattafiori; Abhimanyu Dubey; Abhinav Jauhri; Abhinav Pandey; Abhishek Kadian; Adam Kelsey; Adi Gangidi; Ahmad Al-Dahle; Ahuva Goldstand; Aiesha Letman; Ajay Menon; Akhil Mathur; Alan Schelten; Alex Vaughan; Amy Yang; Andrei Lupu; Andres Alvarado; Andrew Gallagher; Andrew Gu; Andrew Ho; Andrew Poulton; Andrew Ryan; Angela Fan; Ankit Ramchandani; Anthony Hartshorn; Archi Mitra; Archie Sravankumar; Artem Korenev; Arun Rao; Ashley Gabriel; Ashwin Bharambe; Assaf Eisenman; Aston Zhang; Aurelien Rodriguez; Austen Gregerson; Ava Spataru; Baptiste Roziere; Ben Maurer; Benjamin Leonhardi; Bernie Huang; Bhargavi Paranjape; Bing Liu; Binh Tang; Bobbie Chern; Brani Stojkovic; Brian Fuller; Catalina Mejia Arenas; Chao Zhou; Charlotte Caucheteux; Chaya Nayak; Ching-Hsiang Chu; Chloe Bi; Chris Cai; Chris Cox; Chris Marra; Chris McConnell; Christian Keller; Christoph Feichtenhofer; Christophe Touret; Chunyang Wu; Corinne Wong; Cristian Canton Ferrer; Damien Allonsius; Daniel Kreymer; Daniel Haziza; Daniel Li; Danielle Pintz; Danny Livshits; Danny Wyatt; David Adkins; David Esiobu; David Xu; Davide Testuggine; Delia David; Devi Parikh; Dhruv Choudhary; Dhruv Mahajan; Diana Liskovich; Diego Garcia-Olano; Diego Perino; Dieuwke Hupkes; Dingkang Wang; Dustin Holland; Egor Lakomkin; Elina Lobanova; Xiaoqing Ellen Tan; Emily Dinan; Eric Smith; Erik Brinkman; Esteban Arcaute; Filip Radenovic; Firat Ozgenel; Francesco Caggioni; Frank Seide; Frank Zhang; Gabriel Synnaeve; Gabriella Schwarz; Gabrielle Lee; Gada Badeer; Georgia Anderson; Graeme Nail; Gregoire Mialon; Guan Pang; Guillem Cucurell; Hailey Nguyen; Hannah Korevaar; Hannah Wang; Haroun Habeeb; Harrison Rudolph; Henry Aspegren; Hu Xu; Hugo Touvron; Iga Kozlowska; Igor Molybog; Igor Tufanov; Iliyan Zarov; Imanol Arrieta Ibarra; Irina-Elena Veliche; Isabel Kloumann; Ishan Misra; Ivan Evtimov; Jacob Xu; Jade Copet; Jake Weissman; Jan Geffert; Jana Vranes; Japhet Asher; Jason Park; Jay Mahadeokar; Jean-Baptiste Gaya; Jeet Shah; Jelmer van der Linde; Jennifer Chan; Jenny Hong; Jenya Lee; Jeremy Fu; Jeremy Teboul; Jianfeng Chi; Jianyu Huang; Jie Wang; Jiecao Yu; Joanna Bitton; Joe Spisak; Joelle Pineau; Jon Carvill; Jongsoo Park; Joseph Rocca; Joshua Johnstun; Junteng Jia; Kalyan Vasuden Alwala; Kam Hou U; Kate Plawiak; Kartikeya Upasani; Kaushik Veeraraghavan; Ke Li; Kenneth Heafield; Kevin Stone; Khalid El-Arini; Krithika Iyer; Kshitiz Malik; Kuenley Chiu; Kunal Bhalla; Kyle Huang; Lakshya Garg; Lauren Rantala-Yeary; Laurens van der Maaten; Lawrence Chen; Leandro Silva; Lee Bell; Lei Zhang; Liang Tan; Louis Martin; Lovish Madaan; Luca Wehrstedt; Lukas Blecher; Luke de Oliveira; Madeline Muzzi; Madian Khabsa; Manav Avlani; Mannat Singh; Manohar Paluri; Mark Zuckerberg; Marcin Kardas; Martynas Mankus; Mathew Oldham; Mathieu Rita; Matthew Lennie; Maya Pavlova; Meghan Keneally; Melanie Kambadur; Mihir Patel; Mikayel Samvelyan; Mike Clark; Mike Lewis; Min Si; Mitesh Kumar Singh; Mo Metanat; Mona Hassan; Naman Goyal; Narjes Torabi; Nicolas Usunier; Nikolay Bashlykov; Nikolay Bogoychev; Niladri Chatterji; Ning Dong; Oliver Aobo Yang; Olivier Duchenne; Onur Celebi; Parth Parekh; Patrick Alrassy; Paul Saab; Pavan Balaji; Pedro Rittner; Pengchuan Zhang; Pengwei Li; Petar Vasic; Peter Weng; Polina Zvyagina; Prajjwal Bhargava; Pratik Dubal; Praveen Krishnan; Punit Singh Koura; Qing He; Rachel Rodriguez; Ragavan Srinivasan; Rahul Mitra; Ramon Calderer; Raymond Li; Robert Stojnic; Roberta Raileanu; Robin Battey; Rocky Wang; Rohit Girdhar; Rohit Patel; Romain Sauvestre; Ronnie Polidoro; Roshan Sumbaly; Ross Taylor; Ruan Silva; Rui Hou; Rui Wang; Russ Howes; Ruty Rinott; Saghar Hosseini; Sai Jayesh Bondu; Samyak Datta; Sanjay Singh; Sara Chugh; Sargun Dhillon; Satadru Pan; Sean Bell; Sergey Edunov; Shaoliang Nie; Sharan Narang; Sharath Raparthy; Shaun Lindsay; Sheng Feng; Sheng Shen; Shenghao Lin; Shiva Shankar; Shruti Bhosale; Shun Zhang; Simon Vandenhende; Sinong Wang; Seohyun Sonia Kim; Soumya Batra; Sten Sootla; Steve Kehoe; Suchin Gururangan; Sumit Gupta; Sunny Virk; Sydney Borodinsky; Tamar Glaser; Tamar Herman; Tamara Best; Tara Fowler; Thomas Georgiou; Thomas Scialom; Tianhe Li; Todor Mihaylov; Tong Xiao; Ujjwal Karn; Vedanuj Goswami; Vibhor Gupta; Vignesh Ramanathan; Viktor Kerkez; Vinay Satish Kumar; Vincent Gonguet; Vish Vogeti; Vlad Poenaru; Vlad Tiberiu Mihailescu; Vladan Petrovic; Vladimir Ivanov; Wei Li; Weiwei Chu; Wenhan Xiong; Wenyin Fu; Wes Bouaziz; Whitney Meers; Will Constable; Xavier Martinet; Xiaojian Wu; Xinbo Gao; Xinfeng Xie; Xuchao Jia; Yaelle Goldschlag; Yann LeCun; Yashesh Gaur; Yasmine Babaei; Ye Qi; Yenda Li; Yi Wen; Yiwen Song; Youngjin Nam; Yuchen Hao; Yuchen Zhang; Yun Wang; Yuning Mao; Yuzi He; Zacharie Delpierre Coudert; Zachary DeVito; Zahra Hankir; Zhaoduo Wen; Zheng Yan; Zhengxing Chen; Zhenyu Yang; Zoe Papakipos
|
flair/ner-english-ontonotes-large | flair | "2021-05-08T15:35:21Z" | 39,210 | 92 | flair | [
"flair",
"pytorch",
"token-classification",
"sequence-tagger-model",
"en",
"dataset:ontonotes",
"arxiv:2011.06993",
"region:us"
] | token-classification | "2022-03-02T23:29:05Z" | ---
tags:
- flair
- token-classification
- sequence-tagger-model
language: en
datasets:
- ontonotes
widget:
- text: "On September 1st George won 1 dollar while watching Game of Thrones."
---
## English NER in Flair (Ontonotes large model)
This is the large 18-class NER model for English that ships with [Flair](https://github.com/flairNLP/flair/).
F1-Score: **90.93** (Ontonotes)
Predicts 18 tags:
| **tag** | **meaning** |
|---------------------------------|-----------|
| CARDINAL | cardinal value |
| DATE | date value |
| EVENT | event name |
| FAC | building name |
| GPE | geo-political entity |
| LANGUAGE | language name |
| LAW | law name |
| LOC | location name |
| MONEY | money name |
| NORP | affiliation |
| ORDINAL | ordinal value |
| ORG | organization name |
| PERCENT | percent value |
| PERSON | person name |
| PRODUCT | product name |
| QUANTITY | quantity value |
| TIME | time value |
| WORK_OF_ART | name of work of art |
Based on document-level XLM-R embeddings and [FLERT](https://arxiv.org/pdf/2011.06993v1.pdf/).
---
### Demo: How to use in Flair
Requires: **[Flair](https://github.com/flairNLP/flair/)** (`pip install flair`)
```python
from flair.data import Sentence
from flair.models import SequenceTagger
# load tagger
tagger = SequenceTagger.load("flair/ner-english-ontonotes-large")
# make example sentence
sentence = Sentence("On September 1st George won 1 dollar while watching Game of Thrones.")
# predict NER tags
tagger.predict(sentence)
# print sentence
print(sentence)
# print predicted NER spans
print('The following NER tags are found:')
# iterate over entities and print
for entity in sentence.get_spans('ner'):
print(entity)
```
This yields the following output:
```
Span [2,3]: "September 1st" [− Labels: DATE (1.0)]
Span [4]: "George" [− Labels: PERSON (1.0)]
Span [6,7]: "1 dollar" [− Labels: MONEY (1.0)]
Span [10,11,12]: "Game of Thrones" [− Labels: WORK_OF_ART (1.0)]
```
So, the entities "*September 1st*" (labeled as a **date**), "*George*" (labeled as a **person**), "*1 dollar*" (labeled as a **money**) and "Game of Thrones" (labeled as a **work of art**) are found in the sentence "*On September 1st George Washington won 1 dollar while watching Game of Thrones*".
---
### Training: Script to train this model
The following Flair script was used to train this model:
```python
from flair.data import Corpus
from flair.datasets import ColumnCorpus
from flair.embeddings import WordEmbeddings, StackedEmbeddings, FlairEmbeddings
# 1. load the corpus (Ontonotes does not ship with Flair, you need to download and reformat into a column format yourself)
corpus: Corpus = ColumnCorpus(
"resources/tasks/onto-ner",
column_format={0: "text", 1: "pos", 2: "upos", 3: "ner"},
tag_to_bioes="ner",
)
# 2. what tag do we want to predict?
tag_type = 'ner'
# 3. make the tag dictionary from the corpus
tag_dictionary = corpus.make_tag_dictionary(tag_type=tag_type)
# 4. initialize fine-tuneable transformer embeddings WITH document context
from flair.embeddings import TransformerWordEmbeddings
embeddings = TransformerWordEmbeddings(
model='xlm-roberta-large',
layers="-1",
subtoken_pooling="first",
fine_tune=True,
use_context=True,
)
# 5. initialize bare-bones sequence tagger (no CRF, no RNN, no reprojection)
from flair.models import SequenceTagger
tagger = SequenceTagger(
hidden_size=256,
embeddings=embeddings,
tag_dictionary=tag_dictionary,
tag_type='ner',
use_crf=False,
use_rnn=False,
reproject_embeddings=False,
)
# 6. initialize trainer with AdamW optimizer
from flair.trainers import ModelTrainer
trainer = ModelTrainer(tagger, corpus, optimizer=torch.optim.AdamW)
# 7. run training with XLM parameters (20 epochs, small LR)
from torch.optim.lr_scheduler import OneCycleLR
trainer.train('resources/taggers/ner-english-ontonotes-large',
learning_rate=5.0e-6,
mini_batch_size=4,
mini_batch_chunk_size=1,
max_epochs=20,
scheduler=OneCycleLR,
embeddings_storage_mode='none',
weight_decay=0.,
)
```
---
### Cite
Please cite the following paper when using this model.
```
@misc{schweter2020flert,
title={FLERT: Document-Level Features for Named Entity Recognition},
author={Stefan Schweter and Alan Akbik},
year={2020},
eprint={2011.06993},
archivePrefix={arXiv},
primaryClass={cs.CL}
}
```
---
### Issues?
The Flair issue tracker is available [here](https://github.com/flairNLP/flair/issues/).
|
flax-community/clip-rsicd-v2 | flax-community | "2022-04-24T21:03:53Z" | 39,191 | 18 | transformers | [
"transformers",
"pytorch",
"jax",
"clip",
"zero-shot-image-classification",
"vision",
"endpoints_compatible",
"region:us"
] | zero-shot-image-classification | "2022-03-02T23:29:05Z" | ---
tags:
- vision
---
# Model Card: clip-rsicd
## Model Details
This model is a fine-tuned [CLIP by OpenAI](https://huggingface.co/openai/clip-vit-base-patch32). It is designed with an aim to improve zero-shot image classification, text-to-image and image-to-image retrieval specifically on remote sensing images.
### Model Date
July 2021
### Model Type
The base model uses a ViT-B/32 Transformer architecture as an image encoder and uses a masked self-attention Transformer as a text encoder. These encoders are trained to maximize the similarity of (image, text) pairs via a contrastive loss.
### Model Version
We release several checkpoints for `clip-rsicd` model. Refer to [our github repo](https://github.com/arampacha/CLIP-rsicd#evaluation-results) for performance metrics on zero-shot classification for each of those.
### Training
To reproduce the fine-tuning procedure one can use released [script](https://github.com/arampacha/CLIP-rsicd/blob/master/run_clip_flax_tv.py).
The model was trained using batch size 1024, adafactor optimizer with linear warmup and decay with peak learning rate 1e-4 on 1 TPU-v3-8.
Full log of the training run can be found on [WandB](https://wandb.ai/wandb/hf-flax-clip-rsicd/runs/2dj1exsw).
### Demo
Check out the model text-to-image and image-to-image capabilities using [this demo](https://huggingface.co/spaces/sujitpal/clip-rsicd-demo).
### Documents
- [Fine-tuning CLIP on RSICD with HuggingFace and flax/jax on colab using TPU](https://colab.research.google.com/github/arampacha/CLIP-rsicd/blob/master/nbs/Fine_tuning_CLIP_with_HF_on_TPU.ipynb)
### Use with Transformers
```python
from PIL import Image
import requests
from transformers import CLIPProcessor, CLIPModel
model = CLIPModel.from_pretrained("flax-community/clip-rsicd-v2")
processor = CLIPProcessor.from_pretrained("flax-community/clip-rsicd-v2")
url = "https://raw.githubusercontent.com/arampacha/CLIP-rsicd/master/data/stadium_1.jpg"
image = Image.open(requests.get(url, stream=True).raw)
labels = ["residential area", "playground", "stadium", "forest", "airport"]
inputs = processor(text=[f"a photo of a {l}" for l in labels], images=image, return_tensors="pt", padding=True)
outputs = model(**inputs)
logits_per_image = outputs.logits_per_image # this is the image-text similarity score
probs = logits_per_image.softmax(dim=1) # we can take the softmax to get the label probabilities
for l, p in zip(labels, probs[0]):
print(f"{l:<16} {p:.4f}")
```
[Try it on colab](https://colab.research.google.com/github/arampacha/CLIP-rsicd/blob/master/nbs/clip_rsicd_zero_shot.ipynb)
## Model Use
### Intended Use
The model is intended as a research output for research communities. We hope that this model will enable researchers to better understand and explore zero-shot, arbitrary image classification.
In addition, we can imagine applications in defense and law enforcement, climate change and global warming, and even some consumer applications. A partial list of applications can be found [here](https://github.com/arampacha/CLIP-rsicd#applications). In general we think such models can be useful as digital assistants for humans engaged in searching through large collections of images.
We also hope it can be used for interdisciplinary studies of the potential impact of such models - the CLIP paper includes a discussion of potential downstream impacts to provide an example for this sort of analysis.
#### Primary intended uses
The primary intended users of these models are AI researchers.
We primarily imagine the model will be used by researchers to better understand robustness, generalization, and other capabilities, biases, and constraints of computer vision models.
## Data
The model was trained on publicly available remote sensing image captions datasets. Namely [RSICD](https://github.com/201528014227051/RSICD_optimal), [UCM](https://mega.nz/folder/wCpSzSoS#RXzIlrv--TDt3ENZdKN8JA) and [Sydney](https://mega.nz/folder/pG4yTYYA#4c4buNFLibryZnlujsrwEQ). More information on the datasets used can be found on [our project page](https://github.com/arampacha/CLIP-rsicd#dataset).
## Performance and Limitations
### Performance
| Model-name | k=1 | k=3 | k=5 | k=10 |
| -------------------------------- | ----- | ----- | ----- | ----- |
| original CLIP | 0.572 | 0.745 | 0.837 | 0.939 |
| clip-rsicd-v2 (this model) | **0.883** | **0.968** | **0.982** | **0.998** |
## Limitations
The model is fine-tuned on RSI data but can contain some biases and limitations of the original CLIP model. Refer to [CLIP model card](https://huggingface.co/openai/clip-vit-base-patch32#limitations) for details on those.
|
THUDM/cogvlm2-llama3-chat-19B | THUDM | "2024-05-25T13:10:37Z" | 39,135 | 161 | transformers | [
"transformers",
"safetensors",
"text-generation",
"chat",
"cogvlm2",
"conversational",
"custom_code",
"en",
"arxiv:2311.03079",
"license:other",
"autotrain_compatible",
"region:us"
] | text-generation | "2024-05-16T11:51:07Z" | ---
license: other
license_name: cogvlm2
license_link: https://huggingface.co/THUDM/cogvlm2-llama3-chat-19B/blob/main/LICENSE
language:
- en
pipeline_tag: text-generation
tags:
- chat
- cogvlm2
inference: false
---
# CogVLM2
<div align="center">
<img src=https://raw.githubusercontent.com/THUDM/CogVLM2/53d5d5ea1aa8d535edffc0d15e31685bac40f878/resources/logo.svg width="40%"/>
</div>
<p align="center">
👋 <a href="resources/WECHAT.md" target="_blank">Wechat</a> · 💡<a href="http://36.103.203.44:7861/" target="_blank">Online Demo</a> · 🎈<a href="https://github.com/THUDM/CogVLM2" target="_blank">Github Page</a>
</p>
<p align="center">
📍Experience the larger-scale CogVLM model on the <a href="https://open.bigmodel.cn/dev/api#glm-4v">ZhipuAI Open Platform</a>.
</p>
## Model introduction
We launch a new generation of **CogVLM2** series of models and open source two models built with [Meta-Llama-3-8B-Instruct](https://huggingface.co/meta-llama/Meta-Llama-3-8B-Instruct). Compared with the previous generation of CogVLM open source models, the CogVLM2 series of open source models have the following improvements:
1. Significant improvements in many benchmarks such as `TextVQA`, `DocVQA`.
2. Support **8K** content length.
3. Support image resolution up to **1344 * 1344**.
4. Provide an open source model version that supports both **Chinese and English**.
You can see the details of the CogVLM2 family of open source models in the table below:
| Model name | cogvlm2-llama3-chat-19B | cogvlm2-llama3-chinese-chat-19B |
|------------------|-------------------------------------|-------------------------------------|
| Base Model | Meta-Llama-3-8B-Instruct | Meta-Llama-3-8B-Instruct |
| Language | English | Chinese, English |
| Model size | 19B | 19B |
| Task | Image understanding, dialogue model | Image understanding, dialogue model |
| Text length | 8K | 8K |
| Image resolution | 1344 * 1344 | 1344 * 1344 |
## Benchmark
Our open source models have achieved good results in many lists compared to the previous generation of CogVLM open source models. Its excellent performance can compete with some non-open source models, as shown in the table below:
| Model | Open Source | LLM Size | TextVQA | DocVQA | ChartQA | OCRbench | MMMU | MMVet | MMBench |
|--------------------------------|-------------|----------|----------|----------|----------|----------|----------|----------|----------|
| CogVLM1.1 | ✅ | 7B | 69.7 | - | 68.3 | 590 | 37.3 | 52.0 | 65.8 |
| LLaVA-1.5 | ✅ | 13B | 61.3 | - | - | 337 | 37.0 | 35.4 | 67.7 |
| Mini-Gemini | ✅ | 34B | 74.1 | - | - | - | 48.0 | 59.3 | 80.6 |
| LLaVA-NeXT-LLaMA3 | ✅ | 8B | - | 78.2 | 69.5 | - | 41.7 | - | 72.1 |
| LLaVA-NeXT-110B | ✅ | 110B | - | 85.7 | 79.7 | - | 49.1 | - | 80.5 |
| InternVL-1.5 | ✅ | 20B | 80.6 | 90.9 | **83.8** | 720 | 46.8 | 55.4 | **82.3** |
| QwenVL-Plus | ❌ | - | 78.9 | 91.4 | 78.1 | 726 | 51.4 | 55.7 | 67.0 |
| Claude3-Opus | ❌ | - | - | 89.3 | 80.8 | 694 | **59.4** | 51.7 | 63.3 |
| Gemini Pro 1.5 | ❌ | - | 73.5 | 86.5 | 81.3 | - | 58.5 | - | - |
| GPT-4V | ❌ | - | 78.0 | 88.4 | 78.5 | 656 | 56.8 | **67.7** | 75.0 |
| CogVLM2-LLaMA3 (Ours) | ✅ | 8B | 84.2 | **92.3** | 81.0 | 756 | 44.3 | 60.4 | 80.5 |
| CogVLM2-LLaMA3-Chinese (Ours) | ✅ | 8B | **85.0** | 88.4 | 74.7 | **780** | 42.8 | 60.5 | 78.9 |
All reviews were obtained without using any external OCR tools ("pixel only").
## Quick Start
here is a simple example of how to use the model to chat with the CogVLM2 model. For More use case. Find in our [github](https://github.com/THUDM/CogVLM2)
```python
import torch
from PIL import Image
from transformers import AutoModelForCausalLM, AutoTokenizer
MODEL_PATH = "THUDM/cogvlm2-llama3-chat-19B"
DEVICE = 'cuda' if torch.cuda.is_available() else 'cpu'
TORCH_TYPE = torch.bfloat16 if torch.cuda.is_available() and torch.cuda.get_device_capability()[0] >= 8 else torch.float16
tokenizer = AutoTokenizer.from_pretrained(
MODEL_PATH,
trust_remote_code=True
)
model = AutoModelForCausalLM.from_pretrained(
MODEL_PATH,
torch_dtype=TORCH_TYPE,
trust_remote_code=True,
).to(DEVICE).eval()
text_only_template = "A chat between a curious user and an artificial intelligence assistant. The assistant gives helpful, detailed, and polite answers to the user's questions. USER: {} ASSISTANT:"
while True:
image_path = input("image path >>>>> ")
if image_path == '':
print('You did not enter image path, the following will be a plain text conversation.')
image = None
text_only_first_query = True
else:
image = Image.open(image_path).convert('RGB')
history = []
while True:
query = input("Human:")
if query == "clear":
break
if image is None:
if text_only_first_query:
query = text_only_template.format(query)
text_only_first_query = False
else:
old_prompt = ''
for _, (old_query, response) in enumerate(history):
old_prompt += old_query + " " + response + "\n"
query = old_prompt + "USER: {} ASSISTANT:".format(query)
if image is None:
input_by_model = model.build_conversation_input_ids(
tokenizer,
query=query,
history=history,
template_version='chat'
)
else:
input_by_model = model.build_conversation_input_ids(
tokenizer,
query=query,
history=history,
images=[image],
template_version='chat'
)
inputs = {
'input_ids': input_by_model['input_ids'].unsqueeze(0).to(DEVICE),
'token_type_ids': input_by_model['token_type_ids'].unsqueeze(0).to(DEVICE),
'attention_mask': input_by_model['attention_mask'].unsqueeze(0).to(DEVICE),
'images': [[input_by_model['images'][0].to(DEVICE).to(TORCH_TYPE)]] if image is not None else None,
}
gen_kwargs = {
"max_new_tokens": 2048,
"pad_token_id": 128002,
}
with torch.no_grad():
outputs = model.generate(**inputs, **gen_kwargs)
outputs = outputs[:, inputs['input_ids'].shape[1]:]
response = tokenizer.decode(outputs[0])
response = response.split("<|end_of_text|>")[0]
print("\nCogVLM2:", response)
history.append((query, response))
```
## License
This model is released under the CogVLM2 [LICENSE](LICENSE). For models built with Meta Llama 3, please also adhere to the [LLAMA3_LICENSE](LLAMA3_LICENSE).
## Citation
If you find our work helpful, please consider citing the following papers
```
@misc{wang2023cogvlm,
title={CogVLM: Visual Expert for Pretrained Language Models},
author={Weihan Wang and Qingsong Lv and Wenmeng Yu and Wenyi Hong and Ji Qi and Yan Wang and Junhui Ji and Zhuoyi Yang and Lei Zhao and Xixuan Song and Jiazheng Xu and Bin Xu and Juanzi Li and Yuxiao Dong and Ming Ding and Jie Tang},
year={2023},
eprint={2311.03079},
archivePrefix={arXiv},
primaryClass={cs.CV}
}
```
|
rubra-ai/Meta-Llama-3-70B-Instruct-GGUF | rubra-ai | "2024-07-01T06:20:33Z" | 39,052 | 3 | null | [
"gguf",
"function-calling",
"tool-calling",
"agentic",
"rubra",
"conversational",
"en",
"license:llama3",
"model-index",
"region:us"
] | null | "2024-06-14T16:26:00Z" | ---
license: llama3
model-index:
- name: Rubra-Meta-Llama-3-70B
results:
- task:
type: text-generation
dataset:
type: MMLU
name: MMLU
metrics:
- type: 5-shot
value: 75.9
verified: false
- task:
type: text-generation
dataset:
type: GPQA
name: GPQA
metrics:
- type: 0-shot
value: 33.93
verified: false
- task:
type: text-generation
dataset:
type: GSM-8K
name: GSM-8K
metrics:
- type: 8-shot, CoT
value: 82.26
verified: false
- task:
type: text-generation
dataset:
type: MATH
name: MATH
metrics:
- type: 4-shot, CoT
value: 34.24
verified: false
- task:
type: text-generation
dataset:
type: MT-bench
name: MT-bench
metrics:
- type: GPT-4 as Judge
value: 8.36
verified: false
tags:
- function-calling
- tool-calling
- agentic
- rubra
- conversational
language:
- en
---
# Rubra Llama-3 70B GGUF
Original model: [rubra-ai/Meta-Llama-3-70B-Instruct](https://huggingface.co/rubra-ai/Meta-Llama-3-70B-Instruct)
## Model description
The model is the result of further post-training [meta-llama/Meta-Llama-3-70B](https://huggingface.co/meta-llama/Meta-Llama-3-70B). This model is designed for high performance in various instruction-following tasks and complex interactions, including multi-turn function calling and detailed conversations.
## Training Data
The model underwent additional training on a proprietary dataset encompassing diverse instruction-following, chat, and function calling data. This post-training process enhances the model's ability to integrate tools and manage complex interaction scenarios effectively.
## How to use
Refer to https://docs.rubra.ai/inference/llamacpp for usage. Feel free to ask/open issues up in our Github repo: https://github.com/rubra-ai/rubra
## Limitations and Bias
While the model performs well on a wide range of tasks, it may still produce biased or incorrect outputs. Users should exercise caution and critical judgment when using the model in sensitive or high-stakes applications. The model's outputs are influenced by the data it was trained on, which may contain inherent biases.
## Ethical Considerations
Users should ensure that the deployment of this model adheres to ethical guidelines and consider the potential societal impact of the generated text. Misuse of the model for generating harmful or misleading content is strongly discouraged.
## Acknowledgements
We would like to thank Meta for the model.
## Contact Information
For questions or comments about the model, please reach out to [the rubra team](mailto:rubra@acorn.io).
## Citation
If you use this work, please cite it as:
```
@misc {rubra_ai_2024,
author = { Sanjay Nadhavajhala and Yingbei Tong },
title = { Rubra-Meta-Llama-3-70B-Instruct },
year = 2024,
url = { https://huggingface.co/rubra-ai/Meta-Llama-3-70B-Instruct },
doi = { 10.57967/hf/2643 },
publisher = { Hugging Face }
}
``` |
mradermacher/L3-Blackfall-Summanus-v0.1-15B-GGUF | mradermacher | "2024-07-01T16:51:37Z" | 39,022 | 2 | transformers | [
"transformers",
"gguf",
"mergekit",
"merge",
"not-for-all-audiences",
"en",
"base_model:kromeurus/L3-Blackfall-Summanus-v0.1-15B",
"license:cc-by-sa-4.0",
"endpoints_compatible",
"region:us"
] | null | "2024-07-01T05:50:18Z" | ---
base_model: kromeurus/L3-Blackfall-Summanus-v0.1-15B
language:
- en
library_name: transformers
license: cc-by-sa-4.0
quantized_by: mradermacher
tags:
- mergekit
- merge
- not-for-all-audiences
---
## About
<!-- ### quantize_version: 2 -->
<!-- ### output_tensor_quantised: 1 -->
<!-- ### convert_type: hf -->
<!-- ### vocab_type: -->
<!-- ### tags: -->
static quants of https://huggingface.co/kromeurus/L3-Blackfall-Summanus-v0.1-15B
<!-- provided-files -->
weighted/imatrix quants are available at https://huggingface.co/mradermacher/L3-Blackfall-Summanus-v0.1-15B-i1-GGUF
## Usage
If you are unsure how to use GGUF files, refer to one of [TheBloke's
READMEs](https://huggingface.co/TheBloke/KafkaLM-70B-German-V0.1-GGUF) for
more details, including on how to concatenate multi-part files.
## Provided Quants
(sorted by size, not necessarily quality. IQ-quants are often preferable over similar sized non-IQ quants)
| Link | Type | Size/GB | Notes |
|:-----|:-----|--------:|:------|
| [GGUF](https://huggingface.co/mradermacher/L3-Blackfall-Summanus-v0.1-15B-GGUF/resolve/main/L3-Blackfall-Summanus-v0.1-15B.Q2_K.gguf) | Q2_K | 5.8 | |
| [GGUF](https://huggingface.co/mradermacher/L3-Blackfall-Summanus-v0.1-15B-GGUF/resolve/main/L3-Blackfall-Summanus-v0.1-15B.IQ3_XS.gguf) | IQ3_XS | 6.5 | |
| [GGUF](https://huggingface.co/mradermacher/L3-Blackfall-Summanus-v0.1-15B-GGUF/resolve/main/L3-Blackfall-Summanus-v0.1-15B.Q3_K_S.gguf) | Q3_K_S | 6.8 | |
| [GGUF](https://huggingface.co/mradermacher/L3-Blackfall-Summanus-v0.1-15B-GGUF/resolve/main/L3-Blackfall-Summanus-v0.1-15B.IQ3_S.gguf) | IQ3_S | 6.8 | beats Q3_K* |
| [GGUF](https://huggingface.co/mradermacher/L3-Blackfall-Summanus-v0.1-15B-GGUF/resolve/main/L3-Blackfall-Summanus-v0.1-15B.IQ3_M.gguf) | IQ3_M | 7.0 | |
| [GGUF](https://huggingface.co/mradermacher/L3-Blackfall-Summanus-v0.1-15B-GGUF/resolve/main/L3-Blackfall-Summanus-v0.1-15B.Q3_K_M.gguf) | Q3_K_M | 7.5 | lower quality |
| [GGUF](https://huggingface.co/mradermacher/L3-Blackfall-Summanus-v0.1-15B-GGUF/resolve/main/L3-Blackfall-Summanus-v0.1-15B.Q3_K_L.gguf) | Q3_K_L | 8.1 | |
| [GGUF](https://huggingface.co/mradermacher/L3-Blackfall-Summanus-v0.1-15B-GGUF/resolve/main/L3-Blackfall-Summanus-v0.1-15B.IQ4_XS.gguf) | IQ4_XS | 8.4 | |
| [GGUF](https://huggingface.co/mradermacher/L3-Blackfall-Summanus-v0.1-15B-GGUF/resolve/main/L3-Blackfall-Summanus-v0.1-15B.Q4_K_S.gguf) | Q4_K_S | 8.7 | fast, recommended |
| [GGUF](https://huggingface.co/mradermacher/L3-Blackfall-Summanus-v0.1-15B-GGUF/resolve/main/L3-Blackfall-Summanus-v0.1-15B.Q4_K_M.gguf) | Q4_K_M | 9.2 | fast, recommended |
| [GGUF](https://huggingface.co/mradermacher/L3-Blackfall-Summanus-v0.1-15B-GGUF/resolve/main/L3-Blackfall-Summanus-v0.1-15B.Q5_K_S.gguf) | Q5_K_S | 10.5 | |
| [GGUF](https://huggingface.co/mradermacher/L3-Blackfall-Summanus-v0.1-15B-GGUF/resolve/main/L3-Blackfall-Summanus-v0.1-15B.Q5_K_M.gguf) | Q5_K_M | 10.8 | |
| [GGUF](https://huggingface.co/mradermacher/L3-Blackfall-Summanus-v0.1-15B-GGUF/resolve/main/L3-Blackfall-Summanus-v0.1-15B.Q6_K.gguf) | Q6_K | 12.4 | very good quality |
| [GGUF](https://huggingface.co/mradermacher/L3-Blackfall-Summanus-v0.1-15B-GGUF/resolve/main/L3-Blackfall-Summanus-v0.1-15B.Q8_0.gguf) | Q8_0 | 16.1 | fast, best quality |
Here is a handy graph by ikawrakow comparing some lower-quality quant
types (lower is better):

And here are Artefact2's thoughts on the matter:
https://gist.github.com/Artefact2/b5f810600771265fc1e39442288e8ec9
## FAQ / Model Request
See https://huggingface.co/mradermacher/model_requests for some answers to
questions you might have and/or if you want some other model quantized.
## Thanks
I thank my company, [nethype GmbH](https://www.nethype.de/), for letting
me use its servers and providing upgrades to my workstation to enable
this work in my free time.
<!-- end -->
|
sentence-transformers/paraphrase-xlm-r-multilingual-v1 | sentence-transformers | "2024-03-27T12:22:07Z" | 38,957 | 60 | sentence-transformers | [
"sentence-transformers",
"pytorch",
"tf",
"safetensors",
"xlm-roberta",
"feature-extraction",
"sentence-similarity",
"transformers",
"arxiv:1908.10084",
"license:apache-2.0",
"endpoints_compatible",
"region:us"
] | sentence-similarity | "2022-03-02T23:29:05Z" | ---
license: apache-2.0
library_name: sentence-transformers
tags:
- sentence-transformers
- feature-extraction
- sentence-similarity
- transformers
pipeline_tag: sentence-similarity
---
# sentence-transformers/paraphrase-xlm-r-multilingual-v1
This is a [sentence-transformers](https://www.SBERT.net) model: It maps sentences & paragraphs to a 768 dimensional dense vector space and can be used for tasks like clustering or semantic search.
## Usage (Sentence-Transformers)
Using this model becomes easy when you have [sentence-transformers](https://www.SBERT.net) installed:
```
pip install -U sentence-transformers
```
Then you can use the model like this:
```python
from sentence_transformers import SentenceTransformer
sentences = ["This is an example sentence", "Each sentence is converted"]
model = SentenceTransformer('sentence-transformers/paraphrase-xlm-r-multilingual-v1')
embeddings = model.encode(sentences)
print(embeddings)
```
## Usage (HuggingFace Transformers)
Without [sentence-transformers](https://www.SBERT.net), you can use the model like this: First, you pass your input through the transformer model, then you have to apply the right pooling-operation on-top of the contextualized word embeddings.
```python
from transformers import AutoTokenizer, AutoModel
import torch
#Mean Pooling - Take attention mask into account for correct averaging
def mean_pooling(model_output, attention_mask):
token_embeddings = model_output[0] #First element of model_output contains all token embeddings
input_mask_expanded = attention_mask.unsqueeze(-1).expand(token_embeddings.size()).float()
return torch.sum(token_embeddings * input_mask_expanded, 1) / torch.clamp(input_mask_expanded.sum(1), min=1e-9)
# Sentences we want sentence embeddings for
sentences = ['This is an example sentence', 'Each sentence is converted']
# Load model from HuggingFace Hub
tokenizer = AutoTokenizer.from_pretrained('sentence-transformers/paraphrase-xlm-r-multilingual-v1')
model = AutoModel.from_pretrained('sentence-transformers/paraphrase-xlm-r-multilingual-v1')
# Tokenize sentences
encoded_input = tokenizer(sentences, padding=True, truncation=True, return_tensors='pt')
# Compute token embeddings
with torch.no_grad():
model_output = model(**encoded_input)
# Perform pooling. In this case, max pooling.
sentence_embeddings = mean_pooling(model_output, encoded_input['attention_mask'])
print("Sentence embeddings:")
print(sentence_embeddings)
```
## Evaluation Results
For an automated evaluation of this model, see the *Sentence Embeddings Benchmark*: [https://seb.sbert.net](https://seb.sbert.net?model_name=sentence-transformers/paraphrase-xlm-r-multilingual-v1)
## Full Model Architecture
```
SentenceTransformer(
(0): Transformer({'max_seq_length': 128, 'do_lower_case': False}) with Transformer model: XLMRobertaModel
(1): Pooling({'word_embedding_dimension': 768, 'pooling_mode_cls_token': False, 'pooling_mode_mean_tokens': True, 'pooling_mode_max_tokens': False, 'pooling_mode_mean_sqrt_len_tokens': False})
)
```
## Citing & Authors
This model was trained by [sentence-transformers](https://www.sbert.net/).
If you find this model helpful, feel free to cite our publication [Sentence-BERT: Sentence Embeddings using Siamese BERT-Networks](https://arxiv.org/abs/1908.10084):
```bibtex
@inproceedings{reimers-2019-sentence-bert,
title = "Sentence-BERT: Sentence Embeddings using Siamese BERT-Networks",
author = "Reimers, Nils and Gurevych, Iryna",
booktitle = "Proceedings of the 2019 Conference on Empirical Methods in Natural Language Processing",
month = "11",
year = "2019",
publisher = "Association for Computational Linguistics",
url = "http://arxiv.org/abs/1908.10084",
}
``` |
Helsinki-NLP/opus-mt-en-ar | Helsinki-NLP | "2023-08-16T11:28:58Z" | 38,938 | 24 | transformers | [
"transformers",
"pytorch",
"tf",
"rust",
"marian",
"text2text-generation",
"translation",
"en",
"ar",
"license:apache-2.0",
"autotrain_compatible",
"endpoints_compatible",
"region:us"
] | translation | "2022-03-02T23:29:04Z" | ---
language:
- en
- ar
tags:
- translation
license: apache-2.0
---
### eng-ara
* source group: English
* target group: Arabic
* OPUS readme: [eng-ara](https://github.com/Helsinki-NLP/Tatoeba-Challenge/tree/master/models/eng-ara/README.md)
* model: transformer
* source language(s): eng
* target language(s): acm afb apc apc_Latn ara ara_Latn arq arq_Latn ary arz
* model: transformer
* pre-processing: normalization + SentencePiece (spm32k,spm32k)
* a sentence initial language token is required in the form of `>>id<<` (id = valid target language ID)
* download original weights: [opus-2020-07-03.zip](https://object.pouta.csc.fi/Tatoeba-MT-models/eng-ara/opus-2020-07-03.zip)
* test set translations: [opus-2020-07-03.test.txt](https://object.pouta.csc.fi/Tatoeba-MT-models/eng-ara/opus-2020-07-03.test.txt)
* test set scores: [opus-2020-07-03.eval.txt](https://object.pouta.csc.fi/Tatoeba-MT-models/eng-ara/opus-2020-07-03.eval.txt)
## Benchmarks
| testset | BLEU | chr-F |
|-----------------------|-------|-------|
| Tatoeba-test.eng.ara | 14.0 | 0.437 |
### System Info:
- hf_name: eng-ara
- source_languages: eng
- target_languages: ara
- opus_readme_url: https://github.com/Helsinki-NLP/Tatoeba-Challenge/tree/master/models/eng-ara/README.md
- original_repo: Tatoeba-Challenge
- tags: ['translation']
- languages: ['en', 'ar']
- src_constituents: {'eng'}
- tgt_constituents: {'apc', 'ara', 'arq_Latn', 'arq', 'afb', 'ara_Latn', 'apc_Latn', 'arz'}
- src_multilingual: False
- tgt_multilingual: False
- prepro: normalization + SentencePiece (spm32k,spm32k)
- url_model: https://object.pouta.csc.fi/Tatoeba-MT-models/eng-ara/opus-2020-07-03.zip
- url_test_set: https://object.pouta.csc.fi/Tatoeba-MT-models/eng-ara/opus-2020-07-03.test.txt
- src_alpha3: eng
- tgt_alpha3: ara
- short_pair: en-ar
- chrF2_score: 0.43700000000000006
- bleu: 14.0
- brevity_penalty: 1.0
- ref_len: 58935.0
- src_name: English
- tgt_name: Arabic
- train_date: 2020-07-03
- src_alpha2: en
- tgt_alpha2: ar
- prefer_old: False
- long_pair: eng-ara
- helsinki_git_sha: 480fcbe0ee1bf4774bcbe6226ad9f58e63f6c535
- transformers_git_sha: 2207e5d8cb224e954a7cba69fa4ac2309e9ff30b
- port_machine: brutasse
- port_time: 2020-08-21-14:41 |
speechbrain/mtl-mimic-voicebank | speechbrain | "2024-02-19T13:29:53Z" | 38,863 | 35 | speechbrain | [
"speechbrain",
"Robust ASR",
"audio-to-audio",
"speech-enhancement",
"PyTorch",
"en",
"dataset:Voicebank",
"dataset:DEMAND",
"arxiv:2106.04624",
"license:apache-2.0",
"region:us"
] | audio-to-audio | "2022-03-02T23:29:05Z" | ---
language: "en"
tags:
- Robust ASR
- audio-to-audio
- speech-enhancement
- PyTorch
- speechbrain
license: "apache-2.0"
datasets:
- Voicebank
- DEMAND
metrics:
- WER
- PESQ
- COVL
---
<iframe src="https://ghbtns.com/github-btn.html?user=speechbrain&repo=speechbrain&type=star&count=true&size=large&v=2" frameborder="0" scrolling="0" width="170" height="30" title="GitHub"></iframe>
<br/><br/>
# ResNet-like model
This repository provides all the necessary tools to perform enhancement and
robust ASR training (EN) within
SpeechBrain. For a better experience we encourage you to learn more about
[SpeechBrain](https://speechbrain.github.io). The model performance is:
| Release | Test PESQ | Test COVL | Valid WER | Test WER |
|:--------:|:----:|:----:|:----:|:----:|
| 22-06-21 | 3.05 | 3.74 | 2.89 | 2.80 |
Works with SpeechBrain v0.5.12
## Pipeline description
The mimic loss training system consists of three steps:
1. A perceptual model is pre-trained on clean speech features, the
same type used for the enhancement masking system.
2. An enhancement model is trained with mimic loss, using the
pre-trained perceptual model.
3. A large ASR model pre-trained on LibriSpeech is fine-tuned
using the enhancement front-end.
The enhancement and ASR models can be used together or
independently.
## Install SpeechBrain
First of all, please install SpeechBrain with the following command:
```
pip install speechbrain
```
Please notice that we encourage you to read our tutorials and learn more about
[SpeechBrain](https://speechbrain.github.io).
## Pretrained Usage
To use the mimic-loss-trained model for enhancement, use the following simple code:
```python
import torchaudio
from speechbrain.inference.enhancement import WaveformEnhancement
enhance_model = WaveformEnhancement.from_hparams(
source="speechbrain/mtl-mimic-voicebank",
savedir="pretrained_models/mtl-mimic-voicebank",
)
enhanced = enhance_model.enhance_file("speechbrain/mtl-mimic-voicebank/example.wav")
# Saving enhanced signal on disk
torchaudio.save('enhanced.wav', enhanced.unsqueeze(0).cpu(), 16000)
```
The system is trained with recordings sampled at 16kHz (single channel).
The code will automatically normalize your audio (i.e., resampling + mono channel selection) when calling *enhance_file* if needed. Make sure your input tensor is compliant with the expected sampling rate if you use *enhance_batch* as in the example.
### Inference on GPU
To perform inference on the GPU, add `run_opts={"device":"cuda"}` when calling the `from_hparams` method.
### Training
The model was trained with SpeechBrain (150e1890).
To train it from scratch follows these steps:
1. Clone SpeechBrain:
```bash
git clone https://github.com/speechbrain/speechbrain/
```
2. Install it:
```
cd speechbrain
pip install -r requirements.txt
pip install -e .
```
3. Run Training:
```
cd recipes/Voicebank/MTL/ASR_enhance
python train.py hparams/enhance_mimic.yaml --data_folder=your_data_folder
```
You can find our training results (models, logs, etc) [here](https://drive.google.com/drive/folders/1HaR0Bq679pgd1_4jD74_wDRUq-c3Wl4L?usp=sharing).
### Limitations
The SpeechBrain team does not provide any warranty on the performance achieved by this model when used on other datasets.
## Referencing Mimic Loss
If you find mimic loss useful, please cite:
```
@inproceedings{bagchi2018spectral,
title={Spectral Feature Mapping with Mimic Loss for Robust Speech Recognition},
author={Bagchi, Deblin and Plantinga, Peter and Stiff, Adam and Fosler-Lussier, Eric},
booktitle={IEEE Conference on Audio, Speech, and Signal Processing (ICASSP)},
year={2018}
}
```
# **About SpeechBrain**
- Website: https://speechbrain.github.io/
- Code: https://github.com/speechbrain/speechbrain/
- HuggingFace: https://huggingface.co/speechbrain/
# **Citing SpeechBrain**
Please, cite SpeechBrain if you use it for your research or business.
```bibtex
@misc{speechbrain,
title={{SpeechBrain}: A General-Purpose Speech Toolkit},
author={Mirco Ravanelli and Titouan Parcollet and Peter Plantinga and Aku Rouhe and Samuele Cornell and Loren Lugosch and Cem Subakan and Nauman Dawalatabad and Abdelwahab Heba and Jianyuan Zhong and Ju-Chieh Chou and Sung-Lin Yeh and Szu-Wei Fu and Chien-Feng Liao and Elena Rastorgueva and François Grondin and William Aris and Hwidong Na and Yan Gao and Renato De Mori and Yoshua Bengio},
year={2021},
eprint={2106.04624},
archivePrefix={arXiv},
primaryClass={eess.AS},
note={arXiv:2106.04624}
}
```
|
grammarly/coedit-large | grammarly | "2024-04-28T02:30:16Z" | 38,755 | 99 | transformers | [
"transformers",
"pytorch",
"safetensors",
"t5",
"text2text-generation",
"en",
"dataset:facebook/asset",
"dataset:wi_locness",
"dataset:GEM/wiki_auto_asset_turk",
"dataset:discofuse",
"dataset:zaemyung/IteraTeR_plus",
"dataset:jfleg",
"dataset:grammarly/coedit",
"arxiv:2305.09857",
"license:cc-by-nc-4.0",
"autotrain_compatible",
"endpoints_compatible",
"text-generation-inference",
"region:us"
] | text2text-generation | "2023-05-11T23:57:35Z" | ---
language:
- en
license: cc-by-nc-4.0
datasets:
- facebook/asset
- wi_locness
- GEM/wiki_auto_asset_turk
- discofuse
- zaemyung/IteraTeR_plus
- jfleg
- grammarly/coedit
metrics:
- sari
- bleu
- accuracy
widget:
- text: 'Fix the grammar: When I grow up, I start to understand what he said is quite
right.'
example_title: Fluency
- text: 'Make this text coherent: Their flight is weak. They run quickly through the
tree canopy.'
example_title: Coherence
- text: 'Rewrite to make this easier to understand: A storm surge is what forecasters
consider a hurricane''s most treacherous aspect.'
example_title: Simplification
- text: 'Paraphrase this: Do you know where I was born?'
example_title: Paraphrase
- text: 'Write this more formally: omg i love that song im listening to it right now'
example_title: Formalize
- text: 'Write in a more neutral way: The authors'' exposé on nutrition studies.'
example_title: Neutralize
---
# Model Card for CoEdIT-Large
This model was obtained by fine-tuning the corresponding `google/flan-t5-large` model on the CoEdIT dataset. Details of the dataset can be found in our paper and repository.
**Paper:** CoEdIT: Text Editing by Task-Specific Instruction Tuning
**Authors:** Vipul Raheja, Dhruv Kumar, Ryan Koo, Dongyeop Kang
## Model Details
### Model Description
- **Language(s) (NLP)**: English
- **Finetuned from model:** google/flan-t5-large
### Model Sources
- **Repository:** https://github.com/vipulraheja/coedit
- **Paper:** https://arxiv.org/abs/2305.09857
## How to use
We make available the models presented in our paper.
<table>
<tr>
<th>Model</th>
<th>Number of parameters</th>
</tr>
<tr>
<td>CoEdIT-large</td>
<td>770M</td>
</tr>
<tr>
<td>CoEdIT-xl</td>
<td>3B</td>
</tr>
<tr>
<td>CoEdIT-xxl</td>
<td>11B</td>
</tr>
</table>
## Uses
## Text Revision Task
Given an edit instruction and an original text, our model can generate the edited version of the text.<br>
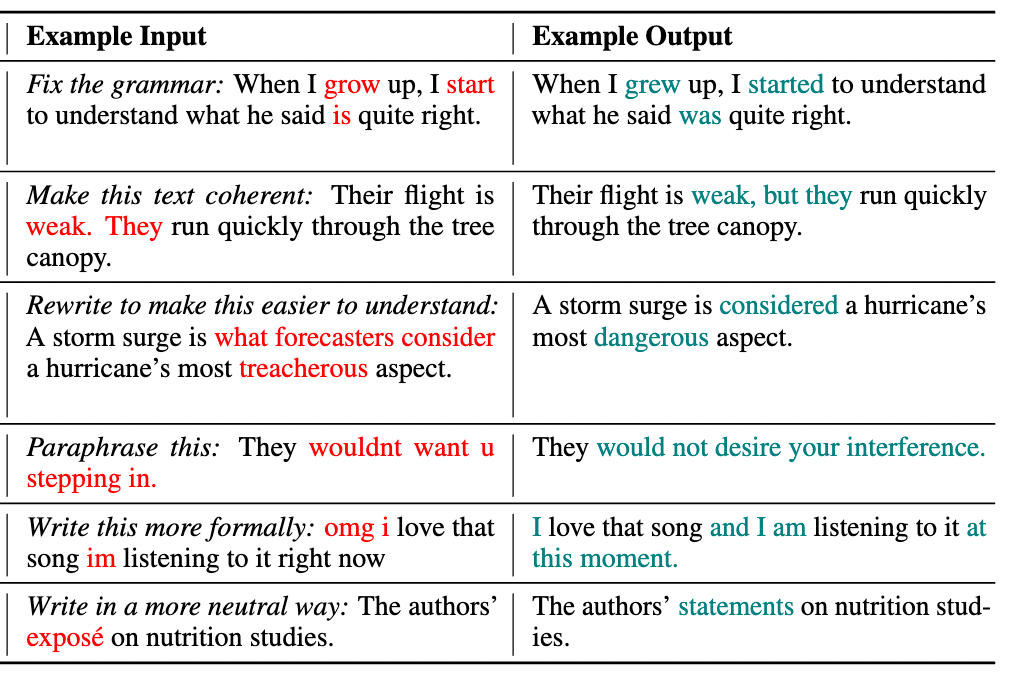
## Usage
```python
from transformers import AutoTokenizer, T5ForConditionalGeneration
tokenizer = AutoTokenizer.from_pretrained("grammarly/coedit-large")
model = T5ForConditionalGeneration.from_pretrained("grammarly/coedit-large")
input_text = 'Fix grammatical errors in this sentence: When I grow up, I start to understand what he said is quite right.'
input_ids = tokenizer(input_text, return_tensors="pt").input_ids
outputs = model.generate(input_ids, max_length=256)
edited_text = tokenizer.decode(outputs[0], skip_special_tokens=True)
```
#### Software
https://github.com/vipulraheja/coedit
## Citation
**BibTeX:**
```
@article{raheja2023coedit,
title={CoEdIT: Text Editing by Task-Specific Instruction Tuning},
author={Vipul Raheja and Dhruv Kumar and Ryan Koo and Dongyeop Kang},
year={2023},
eprint={2305.09857},
archivePrefix={arXiv},
primaryClass={cs.CL}
}
```
**APA:**
Raheja, V., Kumar, D., Koo, R., & Kang, D. (2023). CoEdIT: Text Editing by Task-Specific Instruction Tuning. ArXiv. /abs/2305.09857 |
mradermacher/colossus_120b-i1-GGUF | mradermacher | "2024-06-21T11:53:13Z" | 38,733 | 1 | transformers | [
"transformers",
"gguf",
"en",
"base_model:ibivibiv/colossus_120b",
"license:apache-2.0",
"endpoints_compatible",
"region:us"
] | null | "2024-06-18T11:10:03Z" | ---
base_model: ibivibiv/colossus_120b
language:
- en
library_name: transformers
license: apache-2.0
quantized_by: mradermacher
---
## About
<!-- ### quantize_version: 2 -->
<!-- ### output_tensor_quantised: 1 -->
<!-- ### convert_type: hf -->
<!-- ### vocab_type: -->
<!-- ### tags: nicoboss -->
weighted/imatrix quants of https://huggingface.co/ibivibiv/colossus_120b
<!-- provided-files -->
static quants are available at https://huggingface.co/mradermacher/colossus_120b-GGUF
## Usage
If you are unsure how to use GGUF files, refer to one of [TheBloke's
READMEs](https://huggingface.co/TheBloke/KafkaLM-70B-German-V0.1-GGUF) for
more details, including on how to concatenate multi-part files.
## Provided Quants
(sorted by size, not necessarily quality. IQ-quants are often preferable over similar sized non-IQ quants)
| Link | Type | Size/GB | Notes |
|:-----|:-----|--------:|:------|
| [GGUF](https://huggingface.co/mradermacher/colossus_120b-i1-GGUF/resolve/main/colossus_120b.i1-IQ1_S.gguf) | i1-IQ1_S | 24.8 | for the desperate |
| [GGUF](https://huggingface.co/mradermacher/colossus_120b-i1-GGUF/resolve/main/colossus_120b.i1-IQ1_M.gguf) | i1-IQ1_M | 27.2 | mostly desperate |
| [GGUF](https://huggingface.co/mradermacher/colossus_120b-i1-GGUF/resolve/main/colossus_120b.i1-IQ2_XXS.gguf) | i1-IQ2_XXS | 31.2 | |
| [GGUF](https://huggingface.co/mradermacher/colossus_120b-i1-GGUF/resolve/main/colossus_120b.i1-IQ2_XS.gguf) | i1-IQ2_XS | 34.7 | |
| [GGUF](https://huggingface.co/mradermacher/colossus_120b-i1-GGUF/resolve/main/colossus_120b.i1-IQ2_S.gguf) | i1-IQ2_S | 36.5 | |
| [GGUF](https://huggingface.co/mradermacher/colossus_120b-i1-GGUF/resolve/main/colossus_120b.i1-IQ2_M.gguf) | i1-IQ2_M | 39.7 | |
| [GGUF](https://huggingface.co/mradermacher/colossus_120b-i1-GGUF/resolve/main/colossus_120b.i1-Q2_K.gguf) | i1-Q2_K | 43.3 | IQ3_XXS probably better |
| [GGUF](https://huggingface.co/mradermacher/colossus_120b-i1-GGUF/resolve/main/colossus_120b.i1-IQ3_XXS.gguf) | i1-IQ3_XXS | 45.3 | lower quality |
| [GGUF](https://huggingface.co/mradermacher/colossus_120b-i1-GGUF/resolve/main/colossus_120b.i1-IQ3_XS.gguf) | i1-IQ3_XS | 48.2 | |
| [PART 1](https://huggingface.co/mradermacher/colossus_120b-i1-GGUF/resolve/main/colossus_120b.i1-Q3_K_S.gguf.part1of2) [PART 2](https://huggingface.co/mradermacher/colossus_120b-i1-GGUF/resolve/main/colossus_120b.i1-Q3_K_S.gguf.part2of2) | i1-Q3_K_S | 50.8 | IQ3_XS probably better |
| [PART 1](https://huggingface.co/mradermacher/colossus_120b-i1-GGUF/resolve/main/colossus_120b.i1-IQ3_S.gguf.part1of2) [PART 2](https://huggingface.co/mradermacher/colossus_120b-i1-GGUF/resolve/main/colossus_120b.i1-IQ3_S.gguf.part2of2) | i1-IQ3_S | 51.0 | beats Q3_K* |
| [PART 1](https://huggingface.co/mradermacher/colossus_120b-i1-GGUF/resolve/main/colossus_120b.i1-IQ3_M.gguf.part1of2) [PART 2](https://huggingface.co/mradermacher/colossus_120b-i1-GGUF/resolve/main/colossus_120b.i1-IQ3_M.gguf.part2of2) | i1-IQ3_M | 52.7 | |
| [PART 1](https://huggingface.co/mradermacher/colossus_120b-i1-GGUF/resolve/main/colossus_120b.i1-Q3_K_M.gguf.part1of2) [PART 2](https://huggingface.co/mradermacher/colossus_120b-i1-GGUF/resolve/main/colossus_120b.i1-Q3_K_M.gguf.part2of2) | i1-Q3_K_M | 56.7 | IQ3_S probably better |
| [PART 1](https://huggingface.co/mradermacher/colossus_120b-i1-GGUF/resolve/main/colossus_120b.i1-Q3_K_L.gguf.part1of2) [PART 2](https://huggingface.co/mradermacher/colossus_120b-i1-GGUF/resolve/main/colossus_120b.i1-Q3_K_L.gguf.part2of2) | i1-Q3_K_L | 61.8 | IQ3_M probably better |
| [PART 1](https://huggingface.co/mradermacher/colossus_120b-i1-GGUF/resolve/main/colossus_120b.i1-IQ4_XS.gguf.part1of2) [PART 2](https://huggingface.co/mradermacher/colossus_120b-i1-GGUF/resolve/main/colossus_120b.i1-IQ4_XS.gguf.part2of2) | i1-IQ4_XS | 62.9 | |
| [PART 1](https://huggingface.co/mradermacher/colossus_120b-i1-GGUF/resolve/main/colossus_120b.i1-Q4_0.gguf.part1of2) [PART 2](https://huggingface.co/mradermacher/colossus_120b-i1-GGUF/resolve/main/colossus_120b.i1-Q4_0.gguf.part2of2) | i1-Q4_0 | 66.7 | fast, low quality |
| [PART 1](https://huggingface.co/mradermacher/colossus_120b-i1-GGUF/resolve/main/colossus_120b.i1-Q4_K_S.gguf.part1of2) [PART 2](https://huggingface.co/mradermacher/colossus_120b-i1-GGUF/resolve/main/colossus_120b.i1-Q4_K_S.gguf.part2of2) | i1-Q4_K_S | 66.9 | optimal size/speed/quality |
| [PART 1](https://huggingface.co/mradermacher/colossus_120b-i1-GGUF/resolve/main/colossus_120b.i1-Q4_K_M.gguf.part1of2) [PART 2](https://huggingface.co/mradermacher/colossus_120b-i1-GGUF/resolve/main/colossus_120b.i1-Q4_K_M.gguf.part2of2) | i1-Q4_K_M | 70.7 | fast, recommended |
| [PART 1](https://huggingface.co/mradermacher/colossus_120b-i1-GGUF/resolve/main/colossus_120b.i1-Q5_K_S.gguf.part1of2) [PART 2](https://huggingface.co/mradermacher/colossus_120b-i1-GGUF/resolve/main/colossus_120b.i1-Q5_K_S.gguf.part2of2) | i1-Q5_K_S | 81.1 | |
| [PART 1](https://huggingface.co/mradermacher/colossus_120b-i1-GGUF/resolve/main/colossus_120b.i1-Q5_K_M.gguf.part1of2) [PART 2](https://huggingface.co/mradermacher/colossus_120b-i1-GGUF/resolve/main/colossus_120b.i1-Q5_K_M.gguf.part2of2) | i1-Q5_K_M | 83.3 | |
| [PART 1](https://huggingface.co/mradermacher/colossus_120b-i1-GGUF/resolve/main/colossus_120b.i1-Q6_K.gguf.part1of2) [PART 2](https://huggingface.co/mradermacher/colossus_120b-i1-GGUF/resolve/main/colossus_120b.i1-Q6_K.gguf.part2of2) | i1-Q6_K | 96.7 | practically like static Q6_K |
Here is a handy graph by ikawrakow comparing some lower-quality quant
types (lower is better):

And here are Artefact2's thoughts on the matter:
https://gist.github.com/Artefact2/b5f810600771265fc1e39442288e8ec9
## FAQ / Model Request
See https://huggingface.co/mradermacher/model_requests for some answers to
questions you might have and/or if you want some other model quantized.
## Thanks
I thank my company, [nethype GmbH](https://www.nethype.de/), for letting
me use its servers and providing upgrades to my workstation to enable
this work in my free time. Additional thanks to [@nicoboss](https://huggingface.co/nicoboss) for giving me access to his hardware for calculating the imatrix for these quants.
<!-- end -->
|
legraphista/RoGemma-7b-Instruct-IMat-GGUF | legraphista | "2024-06-27T11:04:06Z" | 38,676 | 0 | gguf | [
"gguf",
"quantized",
"GGUF",
"quantization",
"imat",
"imatrix",
"static",
"16bit",
"8bit",
"6bit",
"5bit",
"4bit",
"3bit",
"2bit",
"1bit",
"text-generation",
"ro",
"base_model:OpenLLM-Ro/RoGemma-7b-Instruct",
"license:cc-by-nc-4.0",
"region:us"
] | text-generation | "2024-06-27T10:18:45Z" | ---
base_model: OpenLLM-Ro/RoGemma-7b-Instruct
inference: false
language:
- ro
library_name: gguf
license: cc-by-nc-4.0
pipeline_tag: text-generation
quantized_by: legraphista
tags:
- quantized
- GGUF
- quantization
- imat
- imatrix
- static
- 16bit
- 8bit
- 6bit
- 5bit
- 4bit
- 3bit
- 2bit
- 1bit
---
# RoGemma-7b-Instruct-IMat-GGUF
_Llama.cpp imatrix quantization of OpenLLM-Ro/RoGemma-7b-Instruct_
Original Model: [OpenLLM-Ro/RoGemma-7b-Instruct](https://huggingface.co/OpenLLM-Ro/RoGemma-7b-Instruct)
Original dtype: `BF16` (`bfloat16`)
Quantized by: llama.cpp [b3246](https://github.com/ggerganov/llama.cpp/releases/tag/b3246)
IMatrix dataset: [here](https://gist.githubusercontent.com/bartowski1182/eb213dccb3571f863da82e99418f81e8/raw/b2869d80f5c16fd7082594248e80144677736635/calibration_datav3.txt)
- [Files](#files)
- [IMatrix](#imatrix)
- [Common Quants](#common-quants)
- [All Quants](#all-quants)
- [Downloading using huggingface-cli](#downloading-using-huggingface-cli)
- [Inference](#inference)
- [Simple chat template](#simple-chat-template)
- [Chat template with system prompt](#chat-template-with-system-prompt)
- [Llama.cpp](#llama-cpp)
- [FAQ](#faq)
- [Why is the IMatrix not applied everywhere?](#why-is-the-imatrix-not-applied-everywhere)
- [How do I merge a split GGUF?](#how-do-i-merge-a-split-gguf)
---
## Files
### IMatrix
Status: ✅ Available
Link: [here](https://huggingface.co/legraphista/RoGemma-7b-Instruct-IMat-GGUF/blob/main/imatrix.dat)
### Common Quants
| Filename | Quant type | File Size | Status | Uses IMatrix | Is Split |
| -------- | ---------- | --------- | ------ | ------------ | -------- |
| [RoGemma-7b-Instruct.Q8_0.gguf](https://huggingface.co/legraphista/RoGemma-7b-Instruct-IMat-GGUF/blob/main/RoGemma-7b-Instruct.Q8_0.gguf) | Q8_0 | 9.08GB | ✅ Available | ⚪ Static | 📦 No
| [RoGemma-7b-Instruct.Q6_K.gguf](https://huggingface.co/legraphista/RoGemma-7b-Instruct-IMat-GGUF/blob/main/RoGemma-7b-Instruct.Q6_K.gguf) | Q6_K | 7.01GB | ✅ Available | ⚪ Static | 📦 No
| [RoGemma-7b-Instruct.Q4_K.gguf](https://huggingface.co/legraphista/RoGemma-7b-Instruct-IMat-GGUF/blob/main/RoGemma-7b-Instruct.Q4_K.gguf) | Q4_K | 5.33GB | ✅ Available | 🟢 IMatrix | 📦 No
| [RoGemma-7b-Instruct.Q3_K.gguf](https://huggingface.co/legraphista/RoGemma-7b-Instruct-IMat-GGUF/blob/main/RoGemma-7b-Instruct.Q3_K.gguf) | Q3_K | 4.37GB | ✅ Available | 🟢 IMatrix | 📦 No
| [RoGemma-7b-Instruct.Q2_K.gguf](https://huggingface.co/legraphista/RoGemma-7b-Instruct-IMat-GGUF/blob/main/RoGemma-7b-Instruct.Q2_K.gguf) | Q2_K | 3.48GB | ✅ Available | 🟢 IMatrix | 📦 No
### All Quants
| Filename | Quant type | File Size | Status | Uses IMatrix | Is Split |
| -------- | ---------- | --------- | ------ | ------------ | -------- |
| [RoGemma-7b-Instruct.BF16.gguf](https://huggingface.co/legraphista/RoGemma-7b-Instruct-IMat-GGUF/blob/main/RoGemma-7b-Instruct.BF16.gguf) | BF16 | 17.08GB | ✅ Available | ⚪ Static | 📦 No
| [RoGemma-7b-Instruct.FP16.gguf](https://huggingface.co/legraphista/RoGemma-7b-Instruct-IMat-GGUF/blob/main/RoGemma-7b-Instruct.FP16.gguf) | F16 | 17.08GB | ✅ Available | ⚪ Static | 📦 No
| [RoGemma-7b-Instruct.Q8_0.gguf](https://huggingface.co/legraphista/RoGemma-7b-Instruct-IMat-GGUF/blob/main/RoGemma-7b-Instruct.Q8_0.gguf) | Q8_0 | 9.08GB | ✅ Available | ⚪ Static | 📦 No
| [RoGemma-7b-Instruct.Q6_K.gguf](https://huggingface.co/legraphista/RoGemma-7b-Instruct-IMat-GGUF/blob/main/RoGemma-7b-Instruct.Q6_K.gguf) | Q6_K | 7.01GB | ✅ Available | ⚪ Static | 📦 No
| [RoGemma-7b-Instruct.Q5_K.gguf](https://huggingface.co/legraphista/RoGemma-7b-Instruct-IMat-GGUF/blob/main/RoGemma-7b-Instruct.Q5_K.gguf) | Q5_K | 6.14GB | ✅ Available | ⚪ Static | 📦 No
| [RoGemma-7b-Instruct.Q5_K_S.gguf](https://huggingface.co/legraphista/RoGemma-7b-Instruct-IMat-GGUF/blob/main/RoGemma-7b-Instruct.Q5_K_S.gguf) | Q5_K_S | 5.98GB | ✅ Available | ⚪ Static | 📦 No
| [RoGemma-7b-Instruct.Q4_K.gguf](https://huggingface.co/legraphista/RoGemma-7b-Instruct-IMat-GGUF/blob/main/RoGemma-7b-Instruct.Q4_K.gguf) | Q4_K | 5.33GB | ✅ Available | 🟢 IMatrix | 📦 No
| [RoGemma-7b-Instruct.Q4_K_S.gguf](https://huggingface.co/legraphista/RoGemma-7b-Instruct-IMat-GGUF/blob/main/RoGemma-7b-Instruct.Q4_K_S.gguf) | Q4_K_S | 5.05GB | ✅ Available | 🟢 IMatrix | 📦 No
| [RoGemma-7b-Instruct.IQ4_NL.gguf](https://huggingface.co/legraphista/RoGemma-7b-Instruct-IMat-GGUF/blob/main/RoGemma-7b-Instruct.IQ4_NL.gguf) | IQ4_NL | 5.01GB | ✅ Available | 🟢 IMatrix | 📦 No
| [RoGemma-7b-Instruct.IQ4_XS.gguf](https://huggingface.co/legraphista/RoGemma-7b-Instruct-IMat-GGUF/blob/main/RoGemma-7b-Instruct.IQ4_XS.gguf) | IQ4_XS | 4.77GB | ✅ Available | 🟢 IMatrix | 📦 No
| [RoGemma-7b-Instruct.Q3_K.gguf](https://huggingface.co/legraphista/RoGemma-7b-Instruct-IMat-GGUF/blob/main/RoGemma-7b-Instruct.Q3_K.gguf) | Q3_K | 4.37GB | ✅ Available | 🟢 IMatrix | 📦 No
| [RoGemma-7b-Instruct.Q3_K_L.gguf](https://huggingface.co/legraphista/RoGemma-7b-Instruct-IMat-GGUF/blob/main/RoGemma-7b-Instruct.Q3_K_L.gguf) | Q3_K_L | 4.71GB | ✅ Available | 🟢 IMatrix | 📦 No
| [RoGemma-7b-Instruct.Q3_K_S.gguf](https://huggingface.co/legraphista/RoGemma-7b-Instruct-IMat-GGUF/blob/main/RoGemma-7b-Instruct.Q3_K_S.gguf) | Q3_K_S | 3.98GB | ✅ Available | 🟢 IMatrix | 📦 No
| [RoGemma-7b-Instruct.IQ3_M.gguf](https://huggingface.co/legraphista/RoGemma-7b-Instruct-IMat-GGUF/blob/main/RoGemma-7b-Instruct.IQ3_M.gguf) | IQ3_M | 4.11GB | ✅ Available | 🟢 IMatrix | 📦 No
| [RoGemma-7b-Instruct.IQ3_S.gguf](https://huggingface.co/legraphista/RoGemma-7b-Instruct-IMat-GGUF/blob/main/RoGemma-7b-Instruct.IQ3_S.gguf) | IQ3_S | 3.98GB | ✅ Available | 🟢 IMatrix | 📦 No
| [RoGemma-7b-Instruct.IQ3_XS.gguf](https://huggingface.co/legraphista/RoGemma-7b-Instruct-IMat-GGUF/blob/main/RoGemma-7b-Instruct.IQ3_XS.gguf) | IQ3_XS | 3.80GB | ✅ Available | 🟢 IMatrix | 📦 No
| [RoGemma-7b-Instruct.IQ3_XXS.gguf](https://huggingface.co/legraphista/RoGemma-7b-Instruct-IMat-GGUF/blob/main/RoGemma-7b-Instruct.IQ3_XXS.gguf) | IQ3_XXS | 3.49GB | ✅ Available | 🟢 IMatrix | 📦 No
| [RoGemma-7b-Instruct.Q2_K.gguf](https://huggingface.co/legraphista/RoGemma-7b-Instruct-IMat-GGUF/blob/main/RoGemma-7b-Instruct.Q2_K.gguf) | Q2_K | 3.48GB | ✅ Available | 🟢 IMatrix | 📦 No
| [RoGemma-7b-Instruct.Q2_K_S.gguf](https://huggingface.co/legraphista/RoGemma-7b-Instruct-IMat-GGUF/blob/main/RoGemma-7b-Instruct.Q2_K_S.gguf) | Q2_K_S | 3.25GB | ✅ Available | 🟢 IMatrix | 📦 No
| [RoGemma-7b-Instruct.IQ2_M.gguf](https://huggingface.co/legraphista/RoGemma-7b-Instruct-IMat-GGUF/blob/main/RoGemma-7b-Instruct.IQ2_M.gguf) | IQ2_M | 3.13GB | ✅ Available | 🟢 IMatrix | 📦 No
| [RoGemma-7b-Instruct.IQ2_S.gguf](https://huggingface.co/legraphista/RoGemma-7b-Instruct-IMat-GGUF/blob/main/RoGemma-7b-Instruct.IQ2_S.gguf) | IQ2_S | 2.92GB | ✅ Available | 🟢 IMatrix | 📦 No
| [RoGemma-7b-Instruct.IQ2_XS.gguf](https://huggingface.co/legraphista/RoGemma-7b-Instruct-IMat-GGUF/blob/main/RoGemma-7b-Instruct.IQ2_XS.gguf) | IQ2_XS | 2.81GB | ✅ Available | 🟢 IMatrix | 📦 No
| [RoGemma-7b-Instruct.IQ2_XXS.gguf](https://huggingface.co/legraphista/RoGemma-7b-Instruct-IMat-GGUF/blob/main/RoGemma-7b-Instruct.IQ2_XXS.gguf) | IQ2_XXS | 2.59GB | ✅ Available | 🟢 IMatrix | 📦 No
| [RoGemma-7b-Instruct.IQ1_M.gguf](https://huggingface.co/legraphista/RoGemma-7b-Instruct-IMat-GGUF/blob/main/RoGemma-7b-Instruct.IQ1_M.gguf) | IQ1_M | 2.32GB | ✅ Available | 🟢 IMatrix | 📦 No
| [RoGemma-7b-Instruct.IQ1_S.gguf](https://huggingface.co/legraphista/RoGemma-7b-Instruct-IMat-GGUF/blob/main/RoGemma-7b-Instruct.IQ1_S.gguf) | IQ1_S | 2.16GB | ✅ Available | 🟢 IMatrix | 📦 No
## Downloading using huggingface-cli
If you do not have hugginface-cli installed:
```
pip install -U "huggingface_hub[cli]"
```
Download the specific file you want:
```
huggingface-cli download legraphista/RoGemma-7b-Instruct-IMat-GGUF --include "RoGemma-7b-Instruct.Q8_0.gguf" --local-dir ./
```
If the model file is big, it has been split into multiple files. In order to download them all to a local folder, run:
```
huggingface-cli download legraphista/RoGemma-7b-Instruct-IMat-GGUF --include "RoGemma-7b-Instruct.Q8_0/*" --local-dir ./
# see FAQ for merging GGUF's
```
---
## Inference
### Simple chat template
```
<bos><start_of_turn>user
{user_prompt}<end_of_turn>
<start_of_turn>model
{assistant_response}<end_of_turn>
<start_of_turn>user
{next_user_prompt}<end_of_turn>
<start_of_turn>model
```
### Chat template with system prompt
```
<bos>{system_prompt}<start_of_turn>user
{user_prompt}<end_of_turn>
<start_of_turn>model
{assistant_response}<end_of_turn>
<start_of_turn>user
{next_user_prompt}<end_of_turn>
<start_of_turn>model
```
### Llama.cpp
```
llama.cpp/main -m RoGemma-7b-Instruct.Q8_0.gguf --color -i -p "prompt here (according to the chat template)"
```
---
## FAQ
### Why is the IMatrix not applied everywhere?
According to [this investigation](https://www.reddit.com/r/LocalLLaMA/comments/1993iro/ggufs_quants_can_punch_above_their_weights_now/), it appears that lower quantizations are the only ones that benefit from the imatrix input (as per hellaswag results).
### How do I merge a split GGUF?
1. Make sure you have `gguf-split` available
- To get hold of `gguf-split`, navigate to https://github.com/ggerganov/llama.cpp/releases
- Download the appropriate zip for your system from the latest release
- Unzip the archive and you should be able to find `gguf-split`
2. Locate your GGUF chunks folder (ex: `RoGemma-7b-Instruct.Q8_0`)
3. Run `gguf-split --merge RoGemma-7b-Instruct.Q8_0/RoGemma-7b-Instruct.Q8_0-00001-of-XXXXX.gguf RoGemma-7b-Instruct.Q8_0.gguf`
- Make sure to point `gguf-split` to the first chunk of the split.
---
Got a suggestion? Ping me [@legraphista](https://x.com/legraphista)! |
microsoft/deberta-large | microsoft | "2022-09-26T08:50:58Z" | 38,642 | 13 | transformers | [
"transformers",
"pytorch",
"tf",
"deberta",
"deberta-v1",
"fill-mask",
"en",
"arxiv:2006.03654",
"license:mit",
"endpoints_compatible",
"region:us"
] | fill-mask | "2022-03-02T23:29:05Z" | ---
language: en
tags:
- deberta-v1
- fill-mask
thumbnail: https://huggingface.co/front/thumbnails/microsoft.png
license: mit
---
## DeBERTa: Decoding-enhanced BERT with Disentangled Attention
[DeBERTa](https://arxiv.org/abs/2006.03654) improves the BERT and RoBERTa models using disentangled attention and enhanced mask decoder. It outperforms BERT and RoBERTa on majority of NLU tasks with 80GB training data.
Please check the [official repository](https://github.com/microsoft/DeBERTa) for more details and updates.
#### Fine-tuning on NLU tasks
We present the dev results on SQuAD 1.1/2.0 and several GLUE benchmark tasks.
| Model | SQuAD 1.1 | SQuAD 2.0 | MNLI-m/mm | SST-2 | QNLI | CoLA | RTE | MRPC | QQP |STS-B |
|---------------------------|-----------|-----------|-------------|-------|------|------|--------|-------|-------|------|
| | F1/EM | F1/EM | Acc | Acc | Acc | MCC | Acc |Acc/F1 |Acc/F1 |P/S |
| BERT-Large | 90.9/84.1 | 81.8/79.0 | 86.6/- | 93.2 | 92.3 | 60.6 | 70.4 | 88.0/- | 91.3/- |90.0/- |
| RoBERTa-Large | 94.6/88.9 | 89.4/86.5 | 90.2/- | 96.4 | 93.9 | 68.0 | 86.6 | 90.9/- | 92.2/- |92.4/- |
| XLNet-Large | 95.1/89.7 | 90.6/87.9 | 90.8/- | 97.0 | 94.9 | 69.0 | 85.9 | 90.8/- | 92.3/- |92.5/- |
| [DeBERTa-Large](https://huggingface.co/microsoft/deberta-large)<sup>1</sup> | 95.5/90.1 | 90.7/88.0 | 91.3/91.1| 96.5|95.3| 69.5| 91.0| 92.6/94.6| 92.3/- |92.8/92.5 |
| [DeBERTa-XLarge](https://huggingface.co/microsoft/deberta-xlarge)<sup>1</sup> | -/- | -/- | 91.5/91.2| 97.0 | - | - | 93.1 | 92.1/94.3 | - |92.9/92.7|
| [DeBERTa-V2-XLarge](https://huggingface.co/microsoft/deberta-v2-xlarge)<sup>1</sup>|95.8/90.8| 91.4/88.9|91.7/91.6| **97.5**| 95.8|71.1|**93.9**|92.0/94.2|92.3/89.8|92.9/92.9|
|**[DeBERTa-V2-XXLarge](https://huggingface.co/microsoft/deberta-v2-xxlarge)<sup>1,2</sup>**|**96.1/91.4**|**92.2/89.7**|**91.7/91.9**|97.2|**96.0**|**72.0**| 93.5| **93.1/94.9**|**92.7/90.3** |**93.2/93.1** |
--------
#### Notes.
- <sup>1</sup> Following RoBERTa, for RTE, MRPC, STS-B, we fine-tune the tasks based on [DeBERTa-Large-MNLI](https://huggingface.co/microsoft/deberta-large-mnli), [DeBERTa-XLarge-MNLI](https://huggingface.co/microsoft/deberta-xlarge-mnli), [DeBERTa-V2-XLarge-MNLI](https://huggingface.co/microsoft/deberta-v2-xlarge-mnli), [DeBERTa-V2-XXLarge-MNLI](https://huggingface.co/microsoft/deberta-v2-xxlarge-mnli). The results of SST-2/QQP/QNLI/SQuADv2 will also be slightly improved when start from MNLI fine-tuned models, however, we only report the numbers fine-tuned from pretrained base models for those 4 tasks.
- <sup>2</sup> To try the **XXLarge** model with **[HF transformers](https://huggingface.co/transformers/main_classes/trainer.html)**, you need to specify **--sharded_ddp**
```bash
cd transformers/examples/text-classification/
export TASK_NAME=mrpc
python -m torch.distributed.launch --nproc_per_node=8 run_glue.py --model_name_or_path microsoft/deberta-v2-xxlarge \\
--task_name $TASK_NAME --do_train --do_eval --max_seq_length 128 --per_device_train_batch_size 4 \\
--learning_rate 3e-6 --num_train_epochs 3 --output_dir /tmp/$TASK_NAME/ --overwrite_output_dir --sharded_ddp --fp16
```
### Citation
If you find DeBERTa useful for your work, please cite the following paper:
``` latex
@inproceedings{
he2021deberta,
title={DEBERTA: DECODING-ENHANCED BERT WITH DISENTANGLED ATTENTION},
author={Pengcheng He and Xiaodong Liu and Jianfeng Gao and Weizhu Chen},
booktitle={International Conference on Learning Representations},
year={2021},
url={https://openreview.net/forum?id=XPZIaotutsD}
}
```
|
timm/vgg19_bn.tv_in1k | timm | "2023-04-25T20:18:13Z" | 38,553 | 0 | timm | [
"timm",
"pytorch",
"safetensors",
"image-classification",
"dataset:imagenet-1k",
"arxiv:1409.1556",
"license:bsd-3-clause",
"region:us"
] | image-classification | "2023-04-25T20:16:20Z" | ---
tags:
- image-classification
- timm
library_name: timm
license: bsd-3-clause
datasets:
- imagenet-1k
---
# Model card for vgg19_bn.tv_in1k
A VGG image classification model. Trained on ImageNet-1k, original torchvision weights.
## Model Details
- **Model Type:** Image classification / feature backbone
- **Model Stats:**
- Params (M): 143.7
- GMACs: 19.7
- Activations (M): 14.9
- Image size: 224 x 224
- **Papers:**
- Very Deep Convolutional Networks for Large-Scale Image Recognition: https://arxiv.org/abs/1409.1556
- **Dataset:** ImageNet-1k
- **Original:** https://github.com/pytorch/vision
## Model Usage
### Image Classification
```python
from urllib.request import urlopen
from PIL import Image
import timm
img = Image.open(urlopen(
'https://huggingface.co/datasets/huggingface/documentation-images/resolve/main/beignets-task-guide.png'
))
model = timm.create_model('vgg19_bn.tv_in1k', pretrained=True)
model = model.eval()
# get model specific transforms (normalization, resize)
data_config = timm.data.resolve_model_data_config(model)
transforms = timm.data.create_transform(**data_config, is_training=False)
output = model(transforms(img).unsqueeze(0)) # unsqueeze single image into batch of 1
top5_probabilities, top5_class_indices = torch.topk(output.softmax(dim=1) * 100, k=5)
```
### Feature Map Extraction
```python
from urllib.request import urlopen
from PIL import Image
import timm
img = Image.open(urlopen(
'https://huggingface.co/datasets/huggingface/documentation-images/resolve/main/beignets-task-guide.png'
))
model = timm.create_model(
'vgg19_bn.tv_in1k',
pretrained=True,
features_only=True,
)
model = model.eval()
# get model specific transforms (normalization, resize)
data_config = timm.data.resolve_model_data_config(model)
transforms = timm.data.create_transform(**data_config, is_training=False)
output = model(transforms(img).unsqueeze(0)) # unsqueeze single image into batch of 1
for o in output:
# print shape of each feature map in output
# e.g.:
# torch.Size([1, 64, 224, 224])
# torch.Size([1, 128, 112, 112])
# torch.Size([1, 256, 56, 56])
# torch.Size([1, 512, 28, 28])
# torch.Size([1, 512, 14, 14])
# torch.Size([1, 512, 7, 7])
print(o.shape)
```
### Image Embeddings
```python
from urllib.request import urlopen
from PIL import Image
import timm
img = Image.open(urlopen(
'https://huggingface.co/datasets/huggingface/documentation-images/resolve/main/beignets-task-guide.png'
))
model = timm.create_model(
'vgg19_bn.tv_in1k',
pretrained=True,
num_classes=0, # remove classifier nn.Linear
)
model = model.eval()
# get model specific transforms (normalization, resize)
data_config = timm.data.resolve_model_data_config(model)
transforms = timm.data.create_transform(**data_config, is_training=False)
output = model(transforms(img).unsqueeze(0)) # output is (batch_size, num_features) shaped tensor
# or equivalently (without needing to set num_classes=0)
output = model.forward_features(transforms(img).unsqueeze(0))
# output is unpooled, a (1, 512, 7, 7) shaped tensor
output = model.forward_head(output, pre_logits=True)
# output is a (1, num_features) shaped tensor
```
## Model Comparison
Explore the dataset and runtime metrics of this model in timm [model results](https://github.com/huggingface/pytorch-image-models/tree/main/results).
## Citation
```bibtex
@article{Simonyan2014VeryDC,
title={Very Deep Convolutional Networks for Large-Scale Image Recognition},
author={Karen Simonyan and Andrew Zisserman},
journal={CoRR},
year={2014},
volume={abs/1409.1556}
}
```
|
Snowflake/snowflake-arctic-embed-m | Snowflake | "2024-05-10T15:49:33Z" | 38,475 | 103 | sentence-transformers | [
"sentence-transformers",
"onnx",
"safetensors",
"bert",
"feature-extraction",
"sentence-similarity",
"mteb",
"arctic",
"snowflake-arctic-embed",
"transformers.js",
"arxiv:2405.05374",
"license:apache-2.0",
"model-index",
"endpoints_compatible",
"region:us"
] | sentence-similarity | "2024-04-11T11:07:56Z" | ---
pipeline_tag: sentence-similarity
tags:
- sentence-transformers
- feature-extraction
- sentence-similarity
- mteb
- arctic
- snowflake-arctic-embed
- transformers.js
model-index:
- name: snowflake-arctic-embed-m
results:
- task:
type: Classification
dataset:
type: mteb/amazon_counterfactual
name: MTEB AmazonCounterfactualClassification (en)
config: en
split: test
revision: e8379541af4e31359cca9fbcf4b00f2671dba205
metrics:
- type: accuracy
value: 76.80597014925374
- type: ap
value: 39.31198155789558
- type: f1
value: 70.48198448222148
- task:
type: Classification
dataset:
type: mteb/amazon_polarity
name: MTEB AmazonPolarityClassification
config: default
split: test
revision: e2d317d38cd51312af73b3d32a06d1a08b442046
metrics:
- type: accuracy
value: 82.831525
- type: ap
value: 77.4474050181638
- type: f1
value: 82.77204845110204
- task:
type: Classification
dataset:
type: mteb/amazon_reviews_multi
name: MTEB AmazonReviewsClassification (en)
config: en
split: test
revision: 1399c76144fd37290681b995c656ef9b2e06e26d
metrics:
- type: accuracy
value: 38.93000000000001
- type: f1
value: 37.98013371053459
- task:
type: Retrieval
dataset:
type: mteb/arguana
name: MTEB ArguAna
config: default
split: test
revision: c22ab2a51041ffd869aaddef7af8d8215647e41a
metrics:
- type: map_at_1
value: 31.223
- type: map_at_10
value: 47.43
- type: map_at_100
value: 48.208
- type: map_at_1000
value: 48.211
- type: map_at_3
value: 42.579
- type: map_at_5
value: 45.263999999999996
- type: mrr_at_1
value: 31.65
- type: mrr_at_10
value: 47.573
- type: mrr_at_100
value: 48.359
- type: mrr_at_1000
value: 48.362
- type: mrr_at_3
value: 42.734
- type: mrr_at_5
value: 45.415
- type: ndcg_at_1
value: 31.223
- type: ndcg_at_10
value: 56.436
- type: ndcg_at_100
value: 59.657000000000004
- type: ndcg_at_1000
value: 59.731
- type: ndcg_at_3
value: 46.327
- type: ndcg_at_5
value: 51.178000000000004
- type: precision_at_1
value: 31.223
- type: precision_at_10
value: 8.527999999999999
- type: precision_at_100
value: 0.991
- type: precision_at_1000
value: 0.1
- type: precision_at_3
value: 19.061
- type: precision_at_5
value: 13.797999999999998
- type: recall_at_1
value: 31.223
- type: recall_at_10
value: 85.277
- type: recall_at_100
value: 99.075
- type: recall_at_1000
value: 99.644
- type: recall_at_3
value: 57.18299999999999
- type: recall_at_5
value: 68.99
- task:
type: Clustering
dataset:
type: mteb/arxiv-clustering-p2p
name: MTEB ArxivClusteringP2P
config: default
split: test
revision: a122ad7f3f0291bf49cc6f4d32aa80929df69d5d
metrics:
- type: v_measure
value: 47.23625429411296
- task:
type: Clustering
dataset:
type: mteb/arxiv-clustering-s2s
name: MTEB ArxivClusteringS2S
config: default
split: test
revision: f910caf1a6075f7329cdf8c1a6135696f37dbd53
metrics:
- type: v_measure
value: 37.433880471403654
- task:
type: Reranking
dataset:
type: mteb/askubuntudupquestions-reranking
name: MTEB AskUbuntuDupQuestions
config: default
split: test
revision: 2000358ca161889fa9c082cb41daa8dcfb161a54
metrics:
- type: map
value: 60.53175025582013
- type: mrr
value: 74.51160796728664
- task:
type: STS
dataset:
type: mteb/biosses-sts
name: MTEB BIOSSES
config: default
split: test
revision: d3fb88f8f02e40887cd149695127462bbcf29b4a
metrics:
- type: cos_sim_pearson
value: 88.93746103286769
- type: cos_sim_spearman
value: 86.62245567912619
- type: euclidean_pearson
value: 87.154173907501
- type: euclidean_spearman
value: 86.62245567912619
- type: manhattan_pearson
value: 87.17682026633462
- type: manhattan_spearman
value: 86.74775973908348
- task:
type: Classification
dataset:
type: mteb/banking77
name: MTEB Banking77Classification
config: default
split: test
revision: 0fd18e25b25c072e09e0d92ab615fda904d66300
metrics:
- type: accuracy
value: 80.33766233766232
- type: f1
value: 79.64931422442245
- task:
type: Clustering
dataset:
type: jinaai/big-patent-clustering
name: MTEB BigPatentClustering
config: default
split: test
revision: 62d5330920bca426ce9d3c76ea914f15fc83e891
metrics:
- type: v_measure
value: 19.116028913890613
- task:
type: Clustering
dataset:
type: mteb/biorxiv-clustering-p2p
name: MTEB BiorxivClusteringP2P
config: default
split: test
revision: 65b79d1d13f80053f67aca9498d9402c2d9f1f40
metrics:
- type: v_measure
value: 36.966921852810174
- task:
type: Clustering
dataset:
type: mteb/biorxiv-clustering-s2s
name: MTEB BiorxivClusteringS2S
config: default
split: test
revision: 258694dd0231531bc1fd9de6ceb52a0853c6d908
metrics:
- type: v_measure
value: 31.98019698537654
- task:
type: Retrieval
dataset:
type: mteb/cqadupstack-android
name: MTEB CQADupstackAndroidRetrieval
config: default
split: test
revision: f46a197baaae43b4f621051089b82a364682dfeb
metrics:
- type: map_at_1
value: 34.079
- type: map_at_10
value: 46.35
- type: map_at_100
value: 47.785
- type: map_at_1000
value: 47.903
- type: map_at_3
value: 42.620999999999995
- type: map_at_5
value: 44.765
- type: mrr_at_1
value: 41.345
- type: mrr_at_10
value: 52.032000000000004
- type: mrr_at_100
value: 52.690000000000005
- type: mrr_at_1000
value: 52.727999999999994
- type: mrr_at_3
value: 49.428
- type: mrr_at_5
value: 51.093999999999994
- type: ndcg_at_1
value: 41.345
- type: ndcg_at_10
value: 53.027
- type: ndcg_at_100
value: 57.962
- type: ndcg_at_1000
value: 59.611999999999995
- type: ndcg_at_3
value: 47.687000000000005
- type: ndcg_at_5
value: 50.367
- type: precision_at_1
value: 41.345
- type: precision_at_10
value: 10.157
- type: precision_at_100
value: 1.567
- type: precision_at_1000
value: 0.199
- type: precision_at_3
value: 23.081
- type: precision_at_5
value: 16.738
- type: recall_at_1
value: 34.079
- type: recall_at_10
value: 65.93900000000001
- type: recall_at_100
value: 86.42699999999999
- type: recall_at_1000
value: 96.61
- type: recall_at_3
value: 50.56699999999999
- type: recall_at_5
value: 57.82000000000001
- task:
type: Retrieval
dataset:
type: mteb/cqadupstack-english
name: MTEB CQADupstackEnglishRetrieval
config: default
split: test
revision: ad9991cb51e31e31e430383c75ffb2885547b5f0
metrics:
- type: map_at_1
value: 33.289
- type: map_at_10
value: 43.681
- type: map_at_100
value: 45.056000000000004
- type: map_at_1000
value: 45.171
- type: map_at_3
value: 40.702
- type: map_at_5
value: 42.292
- type: mrr_at_1
value: 41.146
- type: mrr_at_10
value: 49.604
- type: mrr_at_100
value: 50.28399999999999
- type: mrr_at_1000
value: 50.322
- type: mrr_at_3
value: 47.611
- type: mrr_at_5
value: 48.717
- type: ndcg_at_1
value: 41.146
- type: ndcg_at_10
value: 49.43
- type: ndcg_at_100
value: 54.01899999999999
- type: ndcg_at_1000
value: 55.803000000000004
- type: ndcg_at_3
value: 45.503
- type: ndcg_at_5
value: 47.198
- type: precision_at_1
value: 41.146
- type: precision_at_10
value: 9.268
- type: precision_at_100
value: 1.4749999999999999
- type: precision_at_1000
value: 0.19
- type: precision_at_3
value: 21.932
- type: precision_at_5
value: 15.389
- type: recall_at_1
value: 33.289
- type: recall_at_10
value: 59.209999999999994
- type: recall_at_100
value: 78.676
- type: recall_at_1000
value: 89.84100000000001
- type: recall_at_3
value: 47.351
- type: recall_at_5
value: 52.178999999999995
- task:
type: Retrieval
dataset:
type: mteb/cqadupstack-gaming
name: MTEB CQADupstackGamingRetrieval
config: default
split: test
revision: 4885aa143210c98657558c04aaf3dc47cfb54340
metrics:
- type: map_at_1
value: 44.483
- type: map_at_10
value: 56.862
- type: map_at_100
value: 57.901
- type: map_at_1000
value: 57.948
- type: map_at_3
value: 53.737
- type: map_at_5
value: 55.64
- type: mrr_at_1
value: 50.658
- type: mrr_at_10
value: 60.281
- type: mrr_at_100
value: 60.946
- type: mrr_at_1000
value: 60.967000000000006
- type: mrr_at_3
value: 58.192
- type: mrr_at_5
value: 59.531
- type: ndcg_at_1
value: 50.658
- type: ndcg_at_10
value: 62.339
- type: ndcg_at_100
value: 66.28399999999999
- type: ndcg_at_1000
value: 67.166
- type: ndcg_at_3
value: 57.458
- type: ndcg_at_5
value: 60.112
- type: precision_at_1
value: 50.658
- type: precision_at_10
value: 9.762
- type: precision_at_100
value: 1.26
- type: precision_at_1000
value: 0.13799999999999998
- type: precision_at_3
value: 25.329
- type: precision_at_5
value: 17.254
- type: recall_at_1
value: 44.483
- type: recall_at_10
value: 74.819
- type: recall_at_100
value: 91.702
- type: recall_at_1000
value: 97.84
- type: recall_at_3
value: 62.13999999999999
- type: recall_at_5
value: 68.569
- task:
type: Retrieval
dataset:
type: mteb/cqadupstack-gis
name: MTEB CQADupstackGisRetrieval
config: default
split: test
revision: 5003b3064772da1887988e05400cf3806fe491f2
metrics:
- type: map_at_1
value: 26.489
- type: map_at_10
value: 37.004999999999995
- type: map_at_100
value: 38.001000000000005
- type: map_at_1000
value: 38.085
- type: map_at_3
value: 34.239999999999995
- type: map_at_5
value: 35.934
- type: mrr_at_1
value: 28.362
- type: mrr_at_10
value: 38.807
- type: mrr_at_100
value: 39.671
- type: mrr_at_1000
value: 39.736
- type: mrr_at_3
value: 36.29
- type: mrr_at_5
value: 37.906
- type: ndcg_at_1
value: 28.362
- type: ndcg_at_10
value: 42.510999999999996
- type: ndcg_at_100
value: 47.226
- type: ndcg_at_1000
value: 49.226
- type: ndcg_at_3
value: 37.295
- type: ndcg_at_5
value: 40.165
- type: precision_at_1
value: 28.362
- type: precision_at_10
value: 6.633
- type: precision_at_100
value: 0.9490000000000001
- type: precision_at_1000
value: 0.11499999999999999
- type: precision_at_3
value: 16.234
- type: precision_at_5
value: 11.434999999999999
- type: recall_at_1
value: 26.489
- type: recall_at_10
value: 57.457
- type: recall_at_100
value: 78.712
- type: recall_at_1000
value: 93.565
- type: recall_at_3
value: 43.748
- type: recall_at_5
value: 50.589
- task:
type: Retrieval
dataset:
type: mteb/cqadupstack-mathematica
name: MTEB CQADupstackMathematicaRetrieval
config: default
split: test
revision: 90fceea13679c63fe563ded68f3b6f06e50061de
metrics:
- type: map_at_1
value: 12.418999999999999
- type: map_at_10
value: 22.866
- type: map_at_100
value: 24.365000000000002
- type: map_at_1000
value: 24.479
- type: map_at_3
value: 19.965
- type: map_at_5
value: 21.684
- type: mrr_at_1
value: 14.677000000000001
- type: mrr_at_10
value: 26.316
- type: mrr_at_100
value: 27.514
- type: mrr_at_1000
value: 27.57
- type: mrr_at_3
value: 23.3
- type: mrr_at_5
value: 25.191000000000003
- type: ndcg_at_1
value: 14.677000000000001
- type: ndcg_at_10
value: 28.875
- type: ndcg_at_100
value: 35.607
- type: ndcg_at_1000
value: 38.237
- type: ndcg_at_3
value: 23.284
- type: ndcg_at_5
value: 26.226
- type: precision_at_1
value: 14.677000000000001
- type: precision_at_10
value: 5.771
- type: precision_at_100
value: 1.058
- type: precision_at_1000
value: 0.14200000000000002
- type: precision_at_3
value: 11.940000000000001
- type: precision_at_5
value: 9.229
- type: recall_at_1
value: 12.418999999999999
- type: recall_at_10
value: 43.333
- type: recall_at_100
value: 71.942
- type: recall_at_1000
value: 90.67399999999999
- type: recall_at_3
value: 28.787000000000003
- type: recall_at_5
value: 35.638
- task:
type: Retrieval
dataset:
type: mteb/cqadupstack-physics
name: MTEB CQADupstackPhysicsRetrieval
config: default
split: test
revision: 79531abbd1fb92d06c6d6315a0cbbbf5bb247ea4
metrics:
- type: map_at_1
value: 31.686999999999998
- type: map_at_10
value: 42.331
- type: map_at_100
value: 43.655
- type: map_at_1000
value: 43.771
- type: map_at_3
value: 38.944
- type: map_at_5
value: 40.991
- type: mrr_at_1
value: 37.921
- type: mrr_at_10
value: 47.534
- type: mrr_at_100
value: 48.362
- type: mrr_at_1000
value: 48.405
- type: mrr_at_3
value: 44.995000000000005
- type: mrr_at_5
value: 46.617
- type: ndcg_at_1
value: 37.921
- type: ndcg_at_10
value: 48.236000000000004
- type: ndcg_at_100
value: 53.705000000000005
- type: ndcg_at_1000
value: 55.596000000000004
- type: ndcg_at_3
value: 43.11
- type: ndcg_at_5
value: 45.862
- type: precision_at_1
value: 37.921
- type: precision_at_10
value: 8.643
- type: precision_at_100
value: 1.336
- type: precision_at_1000
value: 0.166
- type: precision_at_3
value: 20.308
- type: precision_at_5
value: 14.514
- type: recall_at_1
value: 31.686999999999998
- type: recall_at_10
value: 60.126999999999995
- type: recall_at_100
value: 83.10600000000001
- type: recall_at_1000
value: 95.15
- type: recall_at_3
value: 46.098
- type: recall_at_5
value: 53.179
- task:
type: Retrieval
dataset:
type: mteb/cqadupstack-programmers
name: MTEB CQADupstackProgrammersRetrieval
config: default
split: test
revision: 6184bc1440d2dbc7612be22b50686b8826d22b32
metrics:
- type: map_at_1
value: 28.686
- type: map_at_10
value: 39.146
- type: map_at_100
value: 40.543
- type: map_at_1000
value: 40.644999999999996
- type: map_at_3
value: 36.195
- type: map_at_5
value: 37.919000000000004
- type: mrr_at_1
value: 35.160000000000004
- type: mrr_at_10
value: 44.711
- type: mrr_at_100
value: 45.609
- type: mrr_at_1000
value: 45.655
- type: mrr_at_3
value: 42.409
- type: mrr_at_5
value: 43.779
- type: ndcg_at_1
value: 35.160000000000004
- type: ndcg_at_10
value: 44.977000000000004
- type: ndcg_at_100
value: 50.663000000000004
- type: ndcg_at_1000
value: 52.794
- type: ndcg_at_3
value: 40.532000000000004
- type: ndcg_at_5
value: 42.641
- type: precision_at_1
value: 35.160000000000004
- type: precision_at_10
value: 8.014000000000001
- type: precision_at_100
value: 1.269
- type: precision_at_1000
value: 0.163
- type: precision_at_3
value: 19.444
- type: precision_at_5
value: 13.653
- type: recall_at_1
value: 28.686
- type: recall_at_10
value: 56.801
- type: recall_at_100
value: 80.559
- type: recall_at_1000
value: 95.052
- type: recall_at_3
value: 43.675999999999995
- type: recall_at_5
value: 49.703
- task:
type: Retrieval
dataset:
type: mteb/cqadupstack
name: MTEB CQADupstackRetrieval
config: default
split: test
revision: 4ffe81d471b1924886b33c7567bfb200e9eec5c4
metrics:
- type: map_at_1
value: 28.173833333333338
- type: map_at_10
value: 38.202083333333334
- type: map_at_100
value: 39.47475
- type: map_at_1000
value: 39.586499999999994
- type: map_at_3
value: 35.17308333333334
- type: map_at_5
value: 36.914
- type: mrr_at_1
value: 32.92958333333333
- type: mrr_at_10
value: 42.16758333333333
- type: mrr_at_100
value: 43.04108333333333
- type: mrr_at_1000
value: 43.092499999999994
- type: mrr_at_3
value: 39.69166666666666
- type: mrr_at_5
value: 41.19458333333333
- type: ndcg_at_1
value: 32.92958333333333
- type: ndcg_at_10
value: 43.80583333333333
- type: ndcg_at_100
value: 49.060916666666664
- type: ndcg_at_1000
value: 51.127250000000004
- type: ndcg_at_3
value: 38.80383333333333
- type: ndcg_at_5
value: 41.29658333333333
- type: precision_at_1
value: 32.92958333333333
- type: precision_at_10
value: 7.655666666666666
- type: precision_at_100
value: 1.2094166666666668
- type: precision_at_1000
value: 0.15750000000000003
- type: precision_at_3
value: 17.87975
- type: precision_at_5
value: 12.741833333333332
- type: recall_at_1
value: 28.173833333333338
- type: recall_at_10
value: 56.219249999999995
- type: recall_at_100
value: 79.01416666666665
- type: recall_at_1000
value: 93.13425000000001
- type: recall_at_3
value: 42.39241666666667
- type: recall_at_5
value: 48.764833333333335
- task:
type: Retrieval
dataset:
type: mteb/cqadupstack-stats
name: MTEB CQADupstackStatsRetrieval
config: default
split: test
revision: 65ac3a16b8e91f9cee4c9828cc7c335575432a2a
metrics:
- type: map_at_1
value: 25.625999999999998
- type: map_at_10
value: 32.808
- type: map_at_100
value: 33.951
- type: map_at_1000
value: 34.052
- type: map_at_3
value: 30.536
- type: map_at_5
value: 31.77
- type: mrr_at_1
value: 28.374
- type: mrr_at_10
value: 35.527
- type: mrr_at_100
value: 36.451
- type: mrr_at_1000
value: 36.522
- type: mrr_at_3
value: 33.410000000000004
- type: mrr_at_5
value: 34.537
- type: ndcg_at_1
value: 28.374
- type: ndcg_at_10
value: 37.172
- type: ndcg_at_100
value: 42.474000000000004
- type: ndcg_at_1000
value: 44.853
- type: ndcg_at_3
value: 32.931
- type: ndcg_at_5
value: 34.882999999999996
- type: precision_at_1
value: 28.374
- type: precision_at_10
value: 5.813
- type: precision_at_100
value: 0.928
- type: precision_at_1000
value: 0.121
- type: precision_at_3
value: 14.008000000000001
- type: precision_at_5
value: 9.754999999999999
- type: recall_at_1
value: 25.625999999999998
- type: recall_at_10
value: 47.812
- type: recall_at_100
value: 71.61800000000001
- type: recall_at_1000
value: 88.881
- type: recall_at_3
value: 35.876999999999995
- type: recall_at_5
value: 40.839
- task:
type: Retrieval
dataset:
type: mteb/cqadupstack-tex
name: MTEB CQADupstackTexRetrieval
config: default
split: test
revision: 46989137a86843e03a6195de44b09deda022eec7
metrics:
- type: map_at_1
value: 18.233
- type: map_at_10
value: 26.375999999999998
- type: map_at_100
value: 27.575
- type: map_at_1000
value: 27.706999999999997
- type: map_at_3
value: 23.619
- type: map_at_5
value: 25.217
- type: mrr_at_1
value: 22.023
- type: mrr_at_10
value: 30.122
- type: mrr_at_100
value: 31.083
- type: mrr_at_1000
value: 31.163999999999998
- type: mrr_at_3
value: 27.541
- type: mrr_at_5
value: 29.061999999999998
- type: ndcg_at_1
value: 22.023
- type: ndcg_at_10
value: 31.476
- type: ndcg_at_100
value: 37.114000000000004
- type: ndcg_at_1000
value: 39.981
- type: ndcg_at_3
value: 26.538
- type: ndcg_at_5
value: 29.016
- type: precision_at_1
value: 22.023
- type: precision_at_10
value: 5.819
- type: precision_at_100
value: 1.018
- type: precision_at_1000
value: 0.14300000000000002
- type: precision_at_3
value: 12.583
- type: precision_at_5
value: 9.36
- type: recall_at_1
value: 18.233
- type: recall_at_10
value: 43.029
- type: recall_at_100
value: 68.253
- type: recall_at_1000
value: 88.319
- type: recall_at_3
value: 29.541
- type: recall_at_5
value: 35.783
- task:
type: Retrieval
dataset:
type: mteb/cqadupstack-unix
name: MTEB CQADupstackUnixRetrieval
config: default
split: test
revision: 6c6430d3a6d36f8d2a829195bc5dc94d7e063e53
metrics:
- type: map_at_1
value: 28.923
- type: map_at_10
value: 39.231
- type: map_at_100
value: 40.483000000000004
- type: map_at_1000
value: 40.575
- type: map_at_3
value: 35.94
- type: map_at_5
value: 37.683
- type: mrr_at_1
value: 33.955
- type: mrr_at_10
value: 43.163000000000004
- type: mrr_at_100
value: 44.054
- type: mrr_at_1000
value: 44.099
- type: mrr_at_3
value: 40.361000000000004
- type: mrr_at_5
value: 41.905
- type: ndcg_at_1
value: 33.955
- type: ndcg_at_10
value: 45.068000000000005
- type: ndcg_at_100
value: 50.470000000000006
- type: ndcg_at_1000
value: 52.349000000000004
- type: ndcg_at_3
value: 39.298
- type: ndcg_at_5
value: 41.821999999999996
- type: precision_at_1
value: 33.955
- type: precision_at_10
value: 7.649
- type: precision_at_100
value: 1.173
- type: precision_at_1000
value: 0.14200000000000002
- type: precision_at_3
value: 17.817
- type: precision_at_5
value: 12.537
- type: recall_at_1
value: 28.923
- type: recall_at_10
value: 58.934
- type: recall_at_100
value: 81.809
- type: recall_at_1000
value: 94.71300000000001
- type: recall_at_3
value: 42.975
- type: recall_at_5
value: 49.501
- task:
type: Retrieval
dataset:
type: mteb/cqadupstack-webmasters
name: MTEB CQADupstackWebmastersRetrieval
config: default
split: test
revision: 160c094312a0e1facb97e55eeddb698c0abe3571
metrics:
- type: map_at_1
value: 28.596
- type: map_at_10
value: 38.735
- type: map_at_100
value: 40.264
- type: map_at_1000
value: 40.48
- type: map_at_3
value: 35.394999999999996
- type: map_at_5
value: 37.099
- type: mrr_at_1
value: 33.992
- type: mrr_at_10
value: 43.076
- type: mrr_at_100
value: 44.005
- type: mrr_at_1000
value: 44.043
- type: mrr_at_3
value: 40.415
- type: mrr_at_5
value: 41.957
- type: ndcg_at_1
value: 33.992
- type: ndcg_at_10
value: 44.896
- type: ndcg_at_100
value: 50.44499999999999
- type: ndcg_at_1000
value: 52.675000000000004
- type: ndcg_at_3
value: 39.783
- type: ndcg_at_5
value: 41.997
- type: precision_at_1
value: 33.992
- type: precision_at_10
value: 8.498
- type: precision_at_100
value: 1.585
- type: precision_at_1000
value: 0.248
- type: precision_at_3
value: 18.511
- type: precision_at_5
value: 13.241
- type: recall_at_1
value: 28.596
- type: recall_at_10
value: 56.885
- type: recall_at_100
value: 82.306
- type: recall_at_1000
value: 95.813
- type: recall_at_3
value: 42.168
- type: recall_at_5
value: 48.32
- task:
type: Retrieval
dataset:
type: mteb/cqadupstack-wordpress
name: MTEB CQADupstackWordpressRetrieval
config: default
split: test
revision: 4ffe81d471b1924886b33c7567bfb200e9eec5c4
metrics:
- type: map_at_1
value: 25.576
- type: map_at_10
value: 33.034
- type: map_at_100
value: 34.117999999999995
- type: map_at_1000
value: 34.222
- type: map_at_3
value: 30.183
- type: map_at_5
value: 31.974000000000004
- type: mrr_at_1
value: 27.542
- type: mrr_at_10
value: 34.838
- type: mrr_at_100
value: 35.824
- type: mrr_at_1000
value: 35.899
- type: mrr_at_3
value: 32.348
- type: mrr_at_5
value: 34.039
- type: ndcg_at_1
value: 27.542
- type: ndcg_at_10
value: 37.663000000000004
- type: ndcg_at_100
value: 42.762
- type: ndcg_at_1000
value: 45.235
- type: ndcg_at_3
value: 32.227
- type: ndcg_at_5
value: 35.27
- type: precision_at_1
value: 27.542
- type: precision_at_10
value: 5.840999999999999
- type: precision_at_100
value: 0.895
- type: precision_at_1000
value: 0.123
- type: precision_at_3
value: 13.370000000000001
- type: precision_at_5
value: 9.797
- type: recall_at_1
value: 25.576
- type: recall_at_10
value: 50.285000000000004
- type: recall_at_100
value: 73.06
- type: recall_at_1000
value: 91.15299999999999
- type: recall_at_3
value: 35.781
- type: recall_at_5
value: 43.058
- task:
type: Retrieval
dataset:
type: mteb/climate-fever
name: MTEB ClimateFEVER
config: default
split: test
revision: 47f2ac6acb640fc46020b02a5b59fdda04d39380
metrics:
- type: map_at_1
value: 17.061
- type: map_at_10
value: 29.464000000000002
- type: map_at_100
value: 31.552999999999997
- type: map_at_1000
value: 31.707
- type: map_at_3
value: 24.834999999999997
- type: map_at_5
value: 27.355
- type: mrr_at_1
value: 38.958
- type: mrr_at_10
value: 51.578
- type: mrr_at_100
value: 52.262
- type: mrr_at_1000
value: 52.283
- type: mrr_at_3
value: 48.599
- type: mrr_at_5
value: 50.404
- type: ndcg_at_1
value: 38.958
- type: ndcg_at_10
value: 39.367999999999995
- type: ndcg_at_100
value: 46.521
- type: ndcg_at_1000
value: 49.086999999999996
- type: ndcg_at_3
value: 33.442
- type: ndcg_at_5
value: 35.515
- type: precision_at_1
value: 38.958
- type: precision_at_10
value: 12.110999999999999
- type: precision_at_100
value: 1.982
- type: precision_at_1000
value: 0.247
- type: precision_at_3
value: 25.102999999999998
- type: precision_at_5
value: 18.971
- type: recall_at_1
value: 17.061
- type: recall_at_10
value: 45.198
- type: recall_at_100
value: 69.18900000000001
- type: recall_at_1000
value: 83.38499999999999
- type: recall_at_3
value: 30.241
- type: recall_at_5
value: 36.851
- task:
type: Retrieval
dataset:
type: mteb/dbpedia
name: MTEB DBPedia
config: default
split: test
revision: c0f706b76e590d620bd6618b3ca8efdd34e2d659
metrics:
- type: map_at_1
value: 9.398
- type: map_at_10
value: 21.421
- type: map_at_100
value: 31.649
- type: map_at_1000
value: 33.469
- type: map_at_3
value: 15.310000000000002
- type: map_at_5
value: 17.946
- type: mrr_at_1
value: 71
- type: mrr_at_10
value: 78.92099999999999
- type: mrr_at_100
value: 79.225
- type: mrr_at_1000
value: 79.23
- type: mrr_at_3
value: 77.792
- type: mrr_at_5
value: 78.467
- type: ndcg_at_1
value: 57.99999999999999
- type: ndcg_at_10
value: 44.733000000000004
- type: ndcg_at_100
value: 50.646
- type: ndcg_at_1000
value: 57.903999999999996
- type: ndcg_at_3
value: 49.175999999999995
- type: ndcg_at_5
value: 46.800999999999995
- type: precision_at_1
value: 71
- type: precision_at_10
value: 36.25
- type: precision_at_100
value: 12.135
- type: precision_at_1000
value: 2.26
- type: precision_at_3
value: 52.75
- type: precision_at_5
value: 45.65
- type: recall_at_1
value: 9.398
- type: recall_at_10
value: 26.596999999999998
- type: recall_at_100
value: 57.943
- type: recall_at_1000
value: 81.147
- type: recall_at_3
value: 16.634
- type: recall_at_5
value: 20.7
- task:
type: Classification
dataset:
type: mteb/emotion
name: MTEB EmotionClassification
config: default
split: test
revision: 4f58c6b202a23cf9a4da393831edf4f9183cad37
metrics:
- type: accuracy
value: 46.535000000000004
- type: f1
value: 42.53702746452163
- task:
type: Retrieval
dataset:
type: mteb/fever
name: MTEB FEVER
config: default
split: test
revision: bea83ef9e8fb933d90a2f1d5515737465d613e12
metrics:
- type: map_at_1
value: 77.235
- type: map_at_10
value: 85.504
- type: map_at_100
value: 85.707
- type: map_at_1000
value: 85.718
- type: map_at_3
value: 84.425
- type: map_at_5
value: 85.13
- type: mrr_at_1
value: 83.363
- type: mrr_at_10
value: 89.916
- type: mrr_at_100
value: 89.955
- type: mrr_at_1000
value: 89.956
- type: mrr_at_3
value: 89.32600000000001
- type: mrr_at_5
value: 89.79
- type: ndcg_at_1
value: 83.363
- type: ndcg_at_10
value: 89.015
- type: ndcg_at_100
value: 89.649
- type: ndcg_at_1000
value: 89.825
- type: ndcg_at_3
value: 87.45100000000001
- type: ndcg_at_5
value: 88.39399999999999
- type: precision_at_1
value: 83.363
- type: precision_at_10
value: 10.659
- type: precision_at_100
value: 1.122
- type: precision_at_1000
value: 0.11499999999999999
- type: precision_at_3
value: 33.338
- type: precision_at_5
value: 20.671999999999997
- type: recall_at_1
value: 77.235
- type: recall_at_10
value: 95.389
- type: recall_at_100
value: 97.722
- type: recall_at_1000
value: 98.744
- type: recall_at_3
value: 91.19800000000001
- type: recall_at_5
value: 93.635
- task:
type: Retrieval
dataset:
type: mteb/fiqa
name: MTEB FiQA2018
config: default
split: test
revision: 27a168819829fe9bcd655c2df245fb19452e8e06
metrics:
- type: map_at_1
value: 20.835
- type: map_at_10
value: 34.459
- type: map_at_100
value: 36.335
- type: map_at_1000
value: 36.518
- type: map_at_3
value: 30.581000000000003
- type: map_at_5
value: 32.859
- type: mrr_at_1
value: 40.894999999999996
- type: mrr_at_10
value: 50.491
- type: mrr_at_100
value: 51.243
- type: mrr_at_1000
value: 51.286
- type: mrr_at_3
value: 47.994
- type: mrr_at_5
value: 49.429
- type: ndcg_at_1
value: 40.894999999999996
- type: ndcg_at_10
value: 42.403
- type: ndcg_at_100
value: 48.954
- type: ndcg_at_1000
value: 51.961
- type: ndcg_at_3
value: 39.11
- type: ndcg_at_5
value: 40.152
- type: precision_at_1
value: 40.894999999999996
- type: precision_at_10
value: 11.466
- type: precision_at_100
value: 1.833
- type: precision_at_1000
value: 0.23700000000000002
- type: precision_at_3
value: 25.874000000000002
- type: precision_at_5
value: 19.012
- type: recall_at_1
value: 20.835
- type: recall_at_10
value: 49.535000000000004
- type: recall_at_100
value: 73.39099999999999
- type: recall_at_1000
value: 91.01599999999999
- type: recall_at_3
value: 36.379
- type: recall_at_5
value: 42.059999999999995
- task:
type: Retrieval
dataset:
type: mteb/hotpotqa
name: MTEB HotpotQA
config: default
split: test
revision: ab518f4d6fcca38d87c25209f94beba119d02014
metrics:
- type: map_at_1
value: 40.945
- type: map_at_10
value: 65.376
- type: map_at_100
value: 66.278
- type: map_at_1000
value: 66.33
- type: map_at_3
value: 61.753
- type: map_at_5
value: 64.077
- type: mrr_at_1
value: 81.891
- type: mrr_at_10
value: 87.256
- type: mrr_at_100
value: 87.392
- type: mrr_at_1000
value: 87.395
- type: mrr_at_3
value: 86.442
- type: mrr_at_5
value: 86.991
- type: ndcg_at_1
value: 81.891
- type: ndcg_at_10
value: 73.654
- type: ndcg_at_100
value: 76.62299999999999
- type: ndcg_at_1000
value: 77.60000000000001
- type: ndcg_at_3
value: 68.71199999999999
- type: ndcg_at_5
value: 71.563
- type: precision_at_1
value: 81.891
- type: precision_at_10
value: 15.409
- type: precision_at_100
value: 1.77
- type: precision_at_1000
value: 0.19
- type: precision_at_3
value: 44.15
- type: precision_at_5
value: 28.732000000000003
- type: recall_at_1
value: 40.945
- type: recall_at_10
value: 77.04299999999999
- type: recall_at_100
value: 88.508
- type: recall_at_1000
value: 94.943
- type: recall_at_3
value: 66.226
- type: recall_at_5
value: 71.83
- task:
type: Classification
dataset:
type: mteb/imdb
name: MTEB ImdbClassification
config: default
split: test
revision: 3d86128a09e091d6018b6d26cad27f2739fc2db7
metrics:
- type: accuracy
value: 74.08200000000001
- type: ap
value: 68.10929101713998
- type: f1
value: 73.98447117652009
- task:
type: Retrieval
dataset:
type: mteb/msmarco
name: MTEB MSMARCO
config: default
split: dev
revision: c5a29a104738b98a9e76336939199e264163d4a0
metrics:
- type: map_at_1
value: 21.729000000000003
- type: map_at_10
value: 34.602
- type: map_at_100
value: 35.756
- type: map_at_1000
value: 35.803000000000004
- type: map_at_3
value: 30.619000000000003
- type: map_at_5
value: 32.914
- type: mrr_at_1
value: 22.364
- type: mrr_at_10
value: 35.183
- type: mrr_at_100
value: 36.287000000000006
- type: mrr_at_1000
value: 36.327999999999996
- type: mrr_at_3
value: 31.258000000000003
- type: mrr_at_5
value: 33.542
- type: ndcg_at_1
value: 22.364
- type: ndcg_at_10
value: 41.765
- type: ndcg_at_100
value: 47.293
- type: ndcg_at_1000
value: 48.457
- type: ndcg_at_3
value: 33.676
- type: ndcg_at_5
value: 37.783
- type: precision_at_1
value: 22.364
- type: precision_at_10
value: 6.662
- type: precision_at_100
value: 0.943
- type: precision_at_1000
value: 0.104
- type: precision_at_3
value: 14.435999999999998
- type: precision_at_5
value: 10.764999999999999
- type: recall_at_1
value: 21.729000000000003
- type: recall_at_10
value: 63.815999999999995
- type: recall_at_100
value: 89.265
- type: recall_at_1000
value: 98.149
- type: recall_at_3
value: 41.898
- type: recall_at_5
value: 51.76500000000001
- task:
type: Classification
dataset:
type: mteb/mtop_domain
name: MTEB MTOPDomainClassification (en)
config: en
split: test
revision: d80d48c1eb48d3562165c59d59d0034df9fff0bf
metrics:
- type: accuracy
value: 92.73141814865483
- type: f1
value: 92.17518476408004
- task:
type: Classification
dataset:
type: mteb/mtop_intent
name: MTEB MTOPIntentClassification (en)
config: en
split: test
revision: ae001d0e6b1228650b7bd1c2c65fb50ad11a8aba
metrics:
- type: accuracy
value: 65.18011855905152
- type: f1
value: 46.70999638311856
- task:
type: Classification
dataset:
type: masakhane/masakhanews
name: MTEB MasakhaNEWSClassification (eng)
config: eng
split: test
revision: 8ccc72e69e65f40c70e117d8b3c08306bb788b60
metrics:
- type: accuracy
value: 75.24261603375525
- type: f1
value: 74.07895183913367
- task:
type: Clustering
dataset:
type: masakhane/masakhanews
name: MTEB MasakhaNEWSClusteringP2P (eng)
config: eng
split: test
revision: 8ccc72e69e65f40c70e117d8b3c08306bb788b60
metrics:
- type: v_measure
value: 28.43855875387446
- task:
type: Clustering
dataset:
type: masakhane/masakhanews
name: MTEB MasakhaNEWSClusteringS2S (eng)
config: eng
split: test
revision: 8ccc72e69e65f40c70e117d8b3c08306bb788b60
metrics:
- type: v_measure
value: 29.05331990256969
- task:
type: Classification
dataset:
type: mteb/amazon_massive_intent
name: MTEB MassiveIntentClassification (en)
config: en
split: test
revision: 31efe3c427b0bae9c22cbb560b8f15491cc6bed7
metrics:
- type: accuracy
value: 66.92333557498318
- type: f1
value: 64.29789389602692
- task:
type: Classification
dataset:
type: mteb/amazon_massive_scenario
name: MTEB MassiveScenarioClassification (en)
config: en
split: test
revision: 7d571f92784cd94a019292a1f45445077d0ef634
metrics:
- type: accuracy
value: 72.74714189643578
- type: f1
value: 71.672585608315
- task:
type: Clustering
dataset:
type: mteb/medrxiv-clustering-p2p
name: MTEB MedrxivClusteringP2P
config: default
split: test
revision: e7a26af6f3ae46b30dde8737f02c07b1505bcc73
metrics:
- type: v_measure
value: 31.503564225501613
- task:
type: Clustering
dataset:
type: mteb/medrxiv-clustering-s2s
name: MTEB MedrxivClusteringS2S
config: default
split: test
revision: 35191c8c0dca72d8ff3efcd72aa802307d469663
metrics:
- type: v_measure
value: 28.410225127136457
- task:
type: Reranking
dataset:
type: mteb/mind_small
name: MTEB MindSmallReranking
config: default
split: test
revision: 3bdac13927fdc888b903db93b2ffdbd90b295a69
metrics:
- type: map
value: 29.170019896091908
- type: mrr
value: 29.881276831500976
- task:
type: Retrieval
dataset:
type: mteb/nfcorpus
name: MTEB NFCorpus
config: default
split: test
revision: ec0fa4fe99da2ff19ca1214b7966684033a58814
metrics:
- type: map_at_1
value: 6.544
- type: map_at_10
value: 14.116999999999999
- type: map_at_100
value: 17.522
- type: map_at_1000
value: 19
- type: map_at_3
value: 10.369
- type: map_at_5
value: 12.189
- type: mrr_at_1
value: 47.988
- type: mrr_at_10
value: 56.84
- type: mrr_at_100
value: 57.367000000000004
- type: mrr_at_1000
value: 57.403000000000006
- type: mrr_at_3
value: 54.592
- type: mrr_at_5
value: 56.233
- type: ndcg_at_1
value: 45.82
- type: ndcg_at_10
value: 36.767
- type: ndcg_at_100
value: 33.356
- type: ndcg_at_1000
value: 42.062
- type: ndcg_at_3
value: 42.15
- type: ndcg_at_5
value: 40.355000000000004
- type: precision_at_1
value: 47.988
- type: precision_at_10
value: 27.121000000000002
- type: precision_at_100
value: 8.455
- type: precision_at_1000
value: 2.103
- type: precision_at_3
value: 39.628
- type: precision_at_5
value: 35.356
- type: recall_at_1
value: 6.544
- type: recall_at_10
value: 17.928
- type: recall_at_100
value: 32.843
- type: recall_at_1000
value: 65.752
- type: recall_at_3
value: 11.297
- type: recall_at_5
value: 14.357000000000001
- task:
type: Retrieval
dataset:
type: mteb/nq
name: MTEB NQ
config: default
split: test
revision: b774495ed302d8c44a3a7ea25c90dbce03968f31
metrics:
- type: map_at_1
value: 39.262
- type: map_at_10
value: 55.095000000000006
- type: map_at_100
value: 55.93900000000001
- type: map_at_1000
value: 55.955999999999996
- type: map_at_3
value: 50.93
- type: map_at_5
value: 53.491
- type: mrr_at_1
value: 43.598
- type: mrr_at_10
value: 57.379999999999995
- type: mrr_at_100
value: 57.940999999999995
- type: mrr_at_1000
value: 57.952000000000005
- type: mrr_at_3
value: 53.998000000000005
- type: mrr_at_5
value: 56.128
- type: ndcg_at_1
value: 43.598
- type: ndcg_at_10
value: 62.427
- type: ndcg_at_100
value: 65.759
- type: ndcg_at_1000
value: 66.133
- type: ndcg_at_3
value: 54.745999999999995
- type: ndcg_at_5
value: 58.975
- type: precision_at_1
value: 43.598
- type: precision_at_10
value: 9.789
- type: precision_at_100
value: 1.171
- type: precision_at_1000
value: 0.121
- type: precision_at_3
value: 24.295
- type: precision_at_5
value: 17.028
- type: recall_at_1
value: 39.262
- type: recall_at_10
value: 82.317
- type: recall_at_100
value: 96.391
- type: recall_at_1000
value: 99.116
- type: recall_at_3
value: 62.621
- type: recall_at_5
value: 72.357
- task:
type: Classification
dataset:
type: ag_news
name: MTEB NewsClassification
config: default
split: test
revision: eb185aade064a813bc0b7f42de02595523103ca4
metrics:
- type: accuracy
value: 78.17500000000001
- type: f1
value: 78.01940892857273
- task:
type: PairClassification
dataset:
type: GEM/opusparcus
name: MTEB OpusparcusPC (en)
config: en
split: test
revision: 9e9b1f8ef51616073f47f306f7f47dd91663f86a
metrics:
- type: cos_sim_accuracy
value: 99.89816700610999
- type: cos_sim_ap
value: 100
- type: cos_sim_f1
value: 99.9490575649516
- type: cos_sim_precision
value: 100
- type: cos_sim_recall
value: 99.89816700610999
- type: dot_accuracy
value: 99.89816700610999
- type: dot_ap
value: 100
- type: dot_f1
value: 99.9490575649516
- type: dot_precision
value: 100
- type: dot_recall
value: 99.89816700610999
- type: euclidean_accuracy
value: 99.89816700610999
- type: euclidean_ap
value: 100
- type: euclidean_f1
value: 99.9490575649516
- type: euclidean_precision
value: 100
- type: euclidean_recall
value: 99.89816700610999
- type: manhattan_accuracy
value: 99.89816700610999
- type: manhattan_ap
value: 100
- type: manhattan_f1
value: 99.9490575649516
- type: manhattan_precision
value: 100
- type: manhattan_recall
value: 99.89816700610999
- type: max_accuracy
value: 99.89816700610999
- type: max_ap
value: 100
- type: max_f1
value: 99.9490575649516
- task:
type: PairClassification
dataset:
type: paws-x
name: MTEB PawsX (en)
config: en
split: test
revision: 8a04d940a42cd40658986fdd8e3da561533a3646
metrics:
- type: cos_sim_accuracy
value: 61
- type: cos_sim_ap
value: 59.630757252602464
- type: cos_sim_f1
value: 62.37521514629949
- type: cos_sim_precision
value: 45.34534534534534
- type: cos_sim_recall
value: 99.88974641675854
- type: dot_accuracy
value: 61
- type: dot_ap
value: 59.631527308059006
- type: dot_f1
value: 62.37521514629949
- type: dot_precision
value: 45.34534534534534
- type: dot_recall
value: 99.88974641675854
- type: euclidean_accuracy
value: 61
- type: euclidean_ap
value: 59.630757252602464
- type: euclidean_f1
value: 62.37521514629949
- type: euclidean_precision
value: 45.34534534534534
- type: euclidean_recall
value: 99.88974641675854
- type: manhattan_accuracy
value: 60.9
- type: manhattan_ap
value: 59.613947780462254
- type: manhattan_f1
value: 62.37521514629949
- type: manhattan_precision
value: 45.34534534534534
- type: manhattan_recall
value: 99.88974641675854
- type: max_accuracy
value: 61
- type: max_ap
value: 59.631527308059006
- type: max_f1
value: 62.37521514629949
- task:
type: Retrieval
dataset:
type: mteb/quora
name: MTEB QuoraRetrieval
config: default
split: test
revision: e4e08e0b7dbe3c8700f0daef558ff32256715259
metrics:
- type: map_at_1
value: 69.963
- type: map_at_10
value: 83.59400000000001
- type: map_at_100
value: 84.236
- type: map_at_1000
value: 84.255
- type: map_at_3
value: 80.69800000000001
- type: map_at_5
value: 82.568
- type: mrr_at_1
value: 80.58999999999999
- type: mrr_at_10
value: 86.78200000000001
- type: mrr_at_100
value: 86.89099999999999
- type: mrr_at_1000
value: 86.893
- type: mrr_at_3
value: 85.757
- type: mrr_at_5
value: 86.507
- type: ndcg_at_1
value: 80.60000000000001
- type: ndcg_at_10
value: 87.41799999999999
- type: ndcg_at_100
value: 88.723
- type: ndcg_at_1000
value: 88.875
- type: ndcg_at_3
value: 84.565
- type: ndcg_at_5
value: 86.236
- type: precision_at_1
value: 80.60000000000001
- type: precision_at_10
value: 13.239
- type: precision_at_100
value: 1.5150000000000001
- type: precision_at_1000
value: 0.156
- type: precision_at_3
value: 36.947
- type: precision_at_5
value: 24.354
- type: recall_at_1
value: 69.963
- type: recall_at_10
value: 94.553
- type: recall_at_100
value: 99.104
- type: recall_at_1000
value: 99.872
- type: recall_at_3
value: 86.317
- type: recall_at_5
value: 91.023
- task:
type: Clustering
dataset:
type: mteb/reddit-clustering
name: MTEB RedditClustering
config: default
split: test
revision: 24640382cdbf8abc73003fb0fa6d111a705499eb
metrics:
- type: v_measure
value: 47.52890410998761
- task:
type: Clustering
dataset:
type: mteb/reddit-clustering-p2p
name: MTEB RedditClusteringP2P
config: default
split: test
revision: 385e3cb46b4cfa89021f56c4380204149d0efe33
metrics:
- type: v_measure
value: 62.760692287940486
- task:
type: Retrieval
dataset:
type: mteb/scidocs
name: MTEB SCIDOCS
config: default
split: test
revision: f8c2fcf00f625baaa80f62ec5bd9e1fff3b8ae88
metrics:
- type: map_at_1
value: 5.093
- type: map_at_10
value: 12.695
- type: map_at_100
value: 14.824000000000002
- type: map_at_1000
value: 15.123000000000001
- type: map_at_3
value: 8.968
- type: map_at_5
value: 10.828
- type: mrr_at_1
value: 25.1
- type: mrr_at_10
value: 35.894999999999996
- type: mrr_at_100
value: 36.966
- type: mrr_at_1000
value: 37.019999999999996
- type: mrr_at_3
value: 32.467
- type: mrr_at_5
value: 34.416999999999994
- type: ndcg_at_1
value: 25.1
- type: ndcg_at_10
value: 21.096999999999998
- type: ndcg_at_100
value: 29.202
- type: ndcg_at_1000
value: 34.541
- type: ndcg_at_3
value: 19.875
- type: ndcg_at_5
value: 17.497
- type: precision_at_1
value: 25.1
- type: precision_at_10
value: 10.9
- type: precision_at_100
value: 2.255
- type: precision_at_1000
value: 0.35400000000000004
- type: precision_at_3
value: 18.367
- type: precision_at_5
value: 15.299999999999999
- type: recall_at_1
value: 5.093
- type: recall_at_10
value: 22.092
- type: recall_at_100
value: 45.778
- type: recall_at_1000
value: 71.985
- type: recall_at_3
value: 11.167
- type: recall_at_5
value: 15.501999999999999
- task:
type: STS
dataset:
type: mteb/sickr-sts
name: MTEB SICK-R
config: default
split: test
revision: 20a6d6f312dd54037fe07a32d58e5e168867909d
metrics:
- type: cos_sim_pearson
value: 74.04386981759481
- type: cos_sim_spearman
value: 69.12484963763646
- type: euclidean_pearson
value: 71.49384353291062
- type: euclidean_spearman
value: 69.12484548317074
- type: manhattan_pearson
value: 71.49828173987272
- type: manhattan_spearman
value: 69.08350274367014
- task:
type: STS
dataset:
type: mteb/sts12-sts
name: MTEB STS12
config: default
split: test
revision: a0d554a64d88156834ff5ae9920b964011b16384
metrics:
- type: cos_sim_pearson
value: 66.95372527615659
- type: cos_sim_spearman
value: 66.96821894433991
- type: euclidean_pearson
value: 64.675348002074
- type: euclidean_spearman
value: 66.96821894433991
- type: manhattan_pearson
value: 64.5965887073831
- type: manhattan_spearman
value: 66.88569076794741
- task:
type: STS
dataset:
type: mteb/sts13-sts
name: MTEB STS13
config: default
split: test
revision: 7e90230a92c190f1bf69ae9002b8cea547a64cca
metrics:
- type: cos_sim_pearson
value: 77.34698437961983
- type: cos_sim_spearman
value: 79.1153001117325
- type: euclidean_pearson
value: 78.53562874696966
- type: euclidean_spearman
value: 79.11530018205724
- type: manhattan_pearson
value: 78.46484988944093
- type: manhattan_spearman
value: 79.01416027493104
- task:
type: STS
dataset:
type: mteb/sts14-sts
name: MTEB STS14
config: default
split: test
revision: 6031580fec1f6af667f0bd2da0a551cf4f0b2375
metrics:
- type: cos_sim_pearson
value: 68.81220371935373
- type: cos_sim_spearman
value: 68.50538405089604
- type: euclidean_pearson
value: 68.69204272683749
- type: euclidean_spearman
value: 68.50534223912419
- type: manhattan_pearson
value: 68.67300120149523
- type: manhattan_spearman
value: 68.45404301623115
- task:
type: STS
dataset:
type: mteb/sts15-sts
name: MTEB STS15
config: default
split: test
revision: ae752c7c21bf194d8b67fd573edf7ae58183cbe3
metrics:
- type: cos_sim_pearson
value: 78.2464678879813
- type: cos_sim_spearman
value: 79.92003940566667
- type: euclidean_pearson
value: 79.8080778793964
- type: euclidean_spearman
value: 79.92003940566667
- type: manhattan_pearson
value: 79.80153621444681
- type: manhattan_spearman
value: 79.91293261418134
- task:
type: STS
dataset:
type: mteb/sts16-sts
name: MTEB STS16
config: default
split: test
revision: 4d8694f8f0e0100860b497b999b3dbed754a0513
metrics:
- type: cos_sim_pearson
value: 76.31179207708662
- type: cos_sim_spearman
value: 78.65597349856115
- type: euclidean_pearson
value: 78.76937027472678
- type: euclidean_spearman
value: 78.65597349856115
- type: manhattan_pearson
value: 78.77129513300605
- type: manhattan_spearman
value: 78.62640467680775
- task:
type: STS
dataset:
type: mteb/sts17-crosslingual-sts
name: MTEB STS17 (en-en)
config: en-en
split: test
revision: af5e6fb845001ecf41f4c1e033ce921939a2a68d
metrics:
- type: cos_sim_pearson
value: 79.43158429552561
- type: cos_sim_spearman
value: 81.46108646565362
- type: euclidean_pearson
value: 81.47071791452292
- type: euclidean_spearman
value: 81.46108646565362
- type: manhattan_pearson
value: 81.56920643846031
- type: manhattan_spearman
value: 81.42226241399516
- task:
type: STS
dataset:
type: mteb/sts22-crosslingual-sts
name: MTEB STS22 (en)
config: en
split: test
revision: eea2b4fe26a775864c896887d910b76a8098ad3f
metrics:
- type: cos_sim_pearson
value: 66.89546474141514
- type: cos_sim_spearman
value: 65.8393752170531
- type: euclidean_pearson
value: 67.2580522762307
- type: euclidean_spearman
value: 65.8393752170531
- type: manhattan_pearson
value: 67.45157729300522
- type: manhattan_spearman
value: 66.19470854403802
- task:
type: STS
dataset:
type: mteb/stsbenchmark-sts
name: MTEB STSBenchmark
config: default
split: test
revision: b0fddb56ed78048fa8b90373c8a3cfc37b684831
metrics:
- type: cos_sim_pearson
value: 71.39566306334434
- type: cos_sim_spearman
value: 74.0981396086974
- type: euclidean_pearson
value: 73.7834496259745
- type: euclidean_spearman
value: 74.09803741302046
- type: manhattan_pearson
value: 73.79958138780945
- type: manhattan_spearman
value: 74.09894837555905
- task:
type: STS
dataset:
type: PhilipMay/stsb_multi_mt
name: MTEB STSBenchmarkMultilingualSTS (en)
config: en
split: test
revision: 93d57ef91790589e3ce9c365164337a8a78b7632
metrics:
- type: cos_sim_pearson
value: 71.39566311006806
- type: cos_sim_spearman
value: 74.0981396086974
- type: euclidean_pearson
value: 73.78344970897099
- type: euclidean_spearman
value: 74.09803741302046
- type: manhattan_pearson
value: 73.79958147136705
- type: manhattan_spearman
value: 74.09894837555905
- task:
type: Reranking
dataset:
type: mteb/scidocs-reranking
name: MTEB SciDocsRR
config: default
split: test
revision: d3c5e1fc0b855ab6097bf1cda04dd73947d7caab
metrics:
- type: map
value: 80.81059564334683
- type: mrr
value: 94.62696617108381
- task:
type: Retrieval
dataset:
type: mteb/scifact
name: MTEB SciFact
config: default
split: test
revision: 0228b52cf27578f30900b9e5271d331663a030d7
metrics:
- type: map_at_1
value: 57.760999999999996
- type: map_at_10
value: 68.614
- type: map_at_100
value: 69.109
- type: map_at_1000
value: 69.134
- type: map_at_3
value: 65.735
- type: map_at_5
value: 67.42099999999999
- type: mrr_at_1
value: 60.667
- type: mrr_at_10
value: 69.94200000000001
- type: mrr_at_100
value: 70.254
- type: mrr_at_1000
value: 70.28
- type: mrr_at_3
value: 67.72200000000001
- type: mrr_at_5
value: 69.18900000000001
- type: ndcg_at_1
value: 60.667
- type: ndcg_at_10
value: 73.548
- type: ndcg_at_100
value: 75.381
- type: ndcg_at_1000
value: 75.991
- type: ndcg_at_3
value: 68.685
- type: ndcg_at_5
value: 71.26
- type: precision_at_1
value: 60.667
- type: precision_at_10
value: 9.833
- type: precision_at_100
value: 1.08
- type: precision_at_1000
value: 0.11299999999999999
- type: precision_at_3
value: 26.889000000000003
- type: precision_at_5
value: 17.8
- type: recall_at_1
value: 57.760999999999996
- type: recall_at_10
value: 87.13300000000001
- type: recall_at_100
value: 95
- type: recall_at_1000
value: 99.667
- type: recall_at_3
value: 74.211
- type: recall_at_5
value: 80.63900000000001
- task:
type: PairClassification
dataset:
type: mteb/sprintduplicatequestions-pairclassification
name: MTEB SprintDuplicateQuestions
config: default
split: test
revision: d66bd1f72af766a5cc4b0ca5e00c162f89e8cc46
metrics:
- type: cos_sim_accuracy
value: 99.81881188118813
- type: cos_sim_ap
value: 95.21196473745837
- type: cos_sim_f1
value: 90.69767441860465
- type: cos_sim_precision
value: 91.71779141104295
- type: cos_sim_recall
value: 89.7
- type: dot_accuracy
value: 99.81881188118813
- type: dot_ap
value: 95.21196473745837
- type: dot_f1
value: 90.69767441860465
- type: dot_precision
value: 91.71779141104295
- type: dot_recall
value: 89.7
- type: euclidean_accuracy
value: 99.81881188118813
- type: euclidean_ap
value: 95.21196473745839
- type: euclidean_f1
value: 90.69767441860465
- type: euclidean_precision
value: 91.71779141104295
- type: euclidean_recall
value: 89.7
- type: manhattan_accuracy
value: 99.81287128712871
- type: manhattan_ap
value: 95.16667174835017
- type: manhattan_f1
value: 90.41095890410959
- type: manhattan_precision
value: 91.7610710607621
- type: manhattan_recall
value: 89.1
- type: max_accuracy
value: 99.81881188118813
- type: max_ap
value: 95.21196473745839
- type: max_f1
value: 90.69767441860465
- task:
type: Clustering
dataset:
type: mteb/stackexchange-clustering
name: MTEB StackExchangeClustering
config: default
split: test
revision: 6cbc1f7b2bc0622f2e39d2c77fa502909748c259
metrics:
- type: v_measure
value: 59.54942204515638
- task:
type: Clustering
dataset:
type: mteb/stackexchange-clustering-p2p
name: MTEB StackExchangeClusteringP2P
config: default
split: test
revision: 815ca46b2622cec33ccafc3735d572c266efdb44
metrics:
- type: v_measure
value: 39.42892282672948
- task:
type: Reranking
dataset:
type: mteb/stackoverflowdupquestions-reranking
name: MTEB StackOverflowDupQuestions
config: default
split: test
revision: e185fbe320c72810689fc5848eb6114e1ef5ec69
metrics:
- type: map
value: 51.189033075914324
- type: mrr
value: 51.97014790764791
- task:
type: Summarization
dataset:
type: mteb/summeval
name: MTEB SummEval
config: default
split: test
revision: cda12ad7615edc362dbf25a00fdd61d3b1eaf93c
metrics:
- type: cos_sim_pearson
value: 30.09466569775977
- type: cos_sim_spearman
value: 30.31058660775912
- type: dot_pearson
value: 30.09466438861689
- type: dot_spearman
value: 30.31058660775912
- task:
type: Retrieval
dataset:
type: mteb/trec-covid
name: MTEB TRECCOVID
config: default
split: test
revision: bb9466bac8153a0349341eb1b22e06409e78ef4e
metrics:
- type: map_at_1
value: 0.253
- type: map_at_10
value: 2.07
- type: map_at_100
value: 12.679000000000002
- type: map_at_1000
value: 30.412
- type: map_at_3
value: 0.688
- type: map_at_5
value: 1.079
- type: mrr_at_1
value: 96
- type: mrr_at_10
value: 98
- type: mrr_at_100
value: 98
- type: mrr_at_1000
value: 98
- type: mrr_at_3
value: 98
- type: mrr_at_5
value: 98
- type: ndcg_at_1
value: 89
- type: ndcg_at_10
value: 79.646
- type: ndcg_at_100
value: 62.217999999999996
- type: ndcg_at_1000
value: 55.13400000000001
- type: ndcg_at_3
value: 83.458
- type: ndcg_at_5
value: 80.982
- type: precision_at_1
value: 96
- type: precision_at_10
value: 84.6
- type: precision_at_100
value: 64.34
- type: precision_at_1000
value: 24.534
- type: precision_at_3
value: 88.667
- type: precision_at_5
value: 85.6
- type: recall_at_1
value: 0.253
- type: recall_at_10
value: 2.253
- type: recall_at_100
value: 15.606
- type: recall_at_1000
value: 51.595
- type: recall_at_3
value: 0.7100000000000001
- type: recall_at_5
value: 1.139
- task:
type: Retrieval
dataset:
type: mteb/touche2020
name: MTEB Touche2020
config: default
split: test
revision: a34f9a33db75fa0cbb21bb5cfc3dae8dc8bec93f
metrics:
- type: map_at_1
value: 3.0540000000000003
- type: map_at_10
value: 13.078999999999999
- type: map_at_100
value: 19.468
- type: map_at_1000
value: 21.006
- type: map_at_3
value: 6.8629999999999995
- type: map_at_5
value: 9.187
- type: mrr_at_1
value: 42.857
- type: mrr_at_10
value: 56.735
- type: mrr_at_100
value: 57.352000000000004
- type: mrr_at_1000
value: 57.352000000000004
- type: mrr_at_3
value: 52.721
- type: mrr_at_5
value: 54.66
- type: ndcg_at_1
value: 38.775999999999996
- type: ndcg_at_10
value: 31.469
- type: ndcg_at_100
value: 42.016999999999996
- type: ndcg_at_1000
value: 52.60399999999999
- type: ndcg_at_3
value: 35.894
- type: ndcg_at_5
value: 33.873
- type: precision_at_1
value: 42.857
- type: precision_at_10
value: 27.346999999999998
- type: precision_at_100
value: 8.327
- type: precision_at_1000
value: 1.551
- type: precision_at_3
value: 36.735
- type: precision_at_5
value: 33.469
- type: recall_at_1
value: 3.0540000000000003
- type: recall_at_10
value: 19.185
- type: recall_at_100
value: 51.056000000000004
- type: recall_at_1000
value: 82.814
- type: recall_at_3
value: 7.961
- type: recall_at_5
value: 11.829
- task:
type: Classification
dataset:
type: mteb/toxic_conversations_50k
name: MTEB ToxicConversationsClassification
config: default
split: test
revision: edfaf9da55d3dd50d43143d90c1ac476895ae6de
metrics:
- type: accuracy
value: 64.9346
- type: ap
value: 12.121605736777527
- type: f1
value: 50.169902005887955
- task:
type: Classification
dataset:
type: mteb/tweet_sentiment_extraction
name: MTEB TweetSentimentExtractionClassification
config: default
split: test
revision: d604517c81ca91fe16a244d1248fc021f9ecee7a
metrics:
- type: accuracy
value: 56.72608941709111
- type: f1
value: 57.0702928875253
- task:
type: Clustering
dataset:
type: mteb/twentynewsgroups-clustering
name: MTEB TwentyNewsgroupsClustering
config: default
split: test
revision: 6125ec4e24fa026cec8a478383ee943acfbd5449
metrics:
- type: v_measure
value: 37.72671554400943
- task:
type: PairClassification
dataset:
type: mteb/twittersemeval2015-pairclassification
name: MTEB TwitterSemEval2015
config: default
split: test
revision: 70970daeab8776df92f5ea462b6173c0b46fd2d1
metrics:
- type: cos_sim_accuracy
value: 82.84556237706384
- type: cos_sim_ap
value: 63.28364215788651
- type: cos_sim_f1
value: 60.00000000000001
- type: cos_sim_precision
value: 54.45161290322581
- type: cos_sim_recall
value: 66.80738786279683
- type: dot_accuracy
value: 82.84556237706384
- type: dot_ap
value: 63.28364302860433
- type: dot_f1
value: 60.00000000000001
- type: dot_precision
value: 54.45161290322581
- type: dot_recall
value: 66.80738786279683
- type: euclidean_accuracy
value: 82.84556237706384
- type: euclidean_ap
value: 63.28363625097978
- type: euclidean_f1
value: 60.00000000000001
- type: euclidean_precision
value: 54.45161290322581
- type: euclidean_recall
value: 66.80738786279683
- type: manhattan_accuracy
value: 82.86940454193241
- type: manhattan_ap
value: 63.244773709836764
- type: manhattan_f1
value: 60.12680942696495
- type: manhattan_precision
value: 55.00109433136353
- type: manhattan_recall
value: 66.3060686015831
- type: max_accuracy
value: 82.86940454193241
- type: max_ap
value: 63.28364302860433
- type: max_f1
value: 60.12680942696495
- task:
type: PairClassification
dataset:
type: mteb/twitterurlcorpus-pairclassification
name: MTEB TwitterURLCorpus
config: default
split: test
revision: 8b6510b0b1fa4e4c4f879467980e9be563ec1cdf
metrics:
- type: cos_sim_accuracy
value: 88.32033220786278
- type: cos_sim_ap
value: 84.71928176006863
- type: cos_sim_f1
value: 76.51483333969684
- type: cos_sim_precision
value: 75.89184276300841
- type: cos_sim_recall
value: 77.14813674160764
- type: dot_accuracy
value: 88.32033220786278
- type: dot_ap
value: 84.71928330149228
- type: dot_f1
value: 76.51483333969684
- type: dot_precision
value: 75.89184276300841
- type: dot_recall
value: 77.14813674160764
- type: euclidean_accuracy
value: 88.32033220786278
- type: euclidean_ap
value: 84.71928045384345
- type: euclidean_f1
value: 76.51483333969684
- type: euclidean_precision
value: 75.89184276300841
- type: euclidean_recall
value: 77.14813674160764
- type: manhattan_accuracy
value: 88.27570147863545
- type: manhattan_ap
value: 84.68523541579755
- type: manhattan_f1
value: 76.51512269355146
- type: manhattan_precision
value: 75.62608107091825
- type: manhattan_recall
value: 77.42531567600862
- type: max_accuracy
value: 88.32033220786278
- type: max_ap
value: 84.71928330149228
- type: max_f1
value: 76.51512269355146
- task:
type: Clustering
dataset:
type: jinaai/cities_wiki_clustering
name: MTEB WikiCitiesClustering
config: default
split: test
revision: ddc9ee9242fa65332597f70e967ecc38b9d734fa
metrics:
- type: v_measure
value: 85.30624598674467
license: apache-2.0
---
<h1 align="center">Snowflake's Arctic-embed-m</h1>
<h4 align="center">
<p>
<a href=#news>News</a> |
<a href=#models>Models</a> |
<a href=#usage>Usage</a> |
<a href="#evaluation">Evaluation</a> |
<a href="#contact">Contact</a> |
<a href="#faq">FAQ</a>
<a href="#license">License</a> |
<a href="#acknowledgement">Acknowledgement</a>
<p>
</h4>
## News
05/10/2024: Release the [technical report on Arctic Embed](https://arxiv.org/abs/2405.05374)
04/16/2024: Release the ** snowflake-arctic-embed ** family of text embedding models. The releases are state-of-the-art for Retrieval quality at each of their representative size profiles. [Technical Report]() is coming shortly. For more details, please refer to our Github: [Arctic-Text-Embed](https://github.com/Snowflake-Labs/arctic-embed).
## Models
snowflake-arctic-embed is a suite of text embedding models that focuses on creating high-quality retrieval models optimized for performance.
The `snowflake-arctic-embedding` models achieve **state-of-the-art performance on the MTEB/BEIR leaderboard** for each of their size variants. Evaluation is performed using these [scripts](https://github.com/Snowflake-Labs/snowflake-arctic-embed/tree/main/src). As shown below, each class of model size achieves SOTA retrieval accuracy compared to other top models.
The models are trained by leveraging existing open-source text representation models, such as bert-base-uncased, and are trained in a multi-stage pipeline to optimize their retrieval performance. First, the models are trained with large batches of query-document pairs where negatives are derived in-batch—pretraining leverages about 400m samples of a mix of public datasets and proprietary web search data. Following pretraining models are further optimized with long training on a smaller dataset (about 1m samples) of triplets of query, positive document, and negative document derived from hard harmful mining. Mining of the negatives and data curation is crucial to retrieval accuracy. A detailed technical report can be found [here](https://arxiv.org/abs/2405.05374).
| Name | MTEB Retrieval Score (NDCG @ 10) | Parameters (Millions) | Embedding Dimension |
| ----------------------------------------------------------------------- | -------------------------------- | --------------------- | ------------------- |
| [snowflake-arctic-embed-xs](https://huggingface.co/Snowflake/snowflake-arctic-embed-xs/) | 50.15 | 22 | 384 |
| [snowflake-arctic-embed-s](https://huggingface.co/Snowflake/snowflake-arctic-embed-s/) | 51.98 | 33 | 384 |
| [snowflake-arctic-embed-m](https://huggingface.co/Snowflake/snowflake-arctic-embed-m/) | 54.90 | 110 | 768 |
| [snowflake-arctic-embed-m-long](https://huggingface.co/Snowflake/snowflake-arctic-embed-m-long/) | 54.83 | 137 | 768 |
| [snowflake-arctic-embed-l](https://huggingface.co/Snowflake/snowflake-arctic-embed-l/) | 55.98 | 335 | 1024 |
Aside from being great open-source models, the largest model, [snowflake-arctic-embed-l](https://huggingface.co/Snowflake/snowflake-arctic-embed-l/), can serve as a natural replacement for closed-source embedding, as shown below.
| Model Name | MTEB Retrieval Score (NDCG @ 10) |
| ------------------------------------------------------------------ | -------------------------------- |
| [snowflake-arctic-embed-l](https://huggingface.co/Snowflake/snowflake-arctic-embed-l/) | 55.98 |
| Google-gecko-text-embedding | 55.7 |
| text-embedding-3-large | 55.44 |
| Cohere-embed-english-v3.0 | 55.00 |
| bge-large-en-v1.5 | 54.29 |
### [snowflake-arctic-embed-xs](https://huggingface.co/Snowflake/snowflake-arctic-embed-xs)
This tiny model packs quite the punch. Based on the [all-MiniLM-L6-v2](https://huggingface.co/sentence-transformers/all-MiniLM-L6-v2) model with only 22m parameters and 384 dimensions, this model should meet even the strictest latency/TCO budgets. Despite its size, its retrieval accuracy is closer to that of models with 100m paramers.
| Model Name | MTEB Retrieval Score (NDCG @ 10) |
| ------------------------------------------------------------------- | -------------------------------- |
| [snowflake-arctic-embed-xs](https://huggingface.co/Snowflake/snowflake-arctic-embed-xs/) | 50.15 |
| GIST-all-MiniLM-L6-v2 | 45.12 |
| gte-tiny | 44.92 |
| all-MiniLM-L6-v2 | 41.95 |
| bge-micro-v2 | 42.56 |
### [snowflake-arctic-embed-s](https://huggingface.co/Snowflake/snowflake-arctic-embed-s)
Based on the [intfloat/e5-small-unsupervised](https://huggingface.co/intfloat/e5-small-unsupervised) model, this small model does not trade off retrieval accuracy for its small size. With only 33m parameters and 384 dimensions, this model should easily allow scaling to large datasets.
| Model Name | MTEB Retrieval Score (NDCG @ 10) |
| ------------------------------------------------------------------ | -------------------------------- |
| [snowflake-arctic-embed-s](https://huggingface.co/Snowflake/snowflake-arctic-embed-s/) | 51.98 |
| bge-small-en-v1.5 | 51.68 |
| Cohere-embed-english-light-v3.0 | 51.34 |
| text-embedding-3-small | 51.08 |
| e5-small-v2 | 49.04 |
### [snowflake-arctic-embed-m](https://huggingface.co/Snowflake/snowflake-arctic-embed-m/)
Based on the [intfloat/e5-base-unsupervised](https://huggingface.co/intfloat/e5-base-unsupervised) model, this medium model is the workhorse that provides the best retrieval performance without slowing down inference.
| Model Name | MTEB Retrieval Score (NDCG @ 10) |
| ------------------------------------------------------------------ | -------------------------------- |
| [snowflake-arctic-embed-m](https://huggingface.co/Snowflake/snowflake-arctic-embed-m/) | 54.90 |
| bge-base-en-v1.5 | 53.25 |
| nomic-embed-text-v1.5 | 53.25 |
| GIST-Embedding-v0 | 52.31 |
| gte-base | 52.31 |
### [snowflake-arctic-embed-m-long](https://huggingface.co/Snowflake/snowflake-arctic-embed-m-long/)
Based on the [nomic-ai/nomic-embed-text-v1-unsupervised](https://huggingface.co/nomic-ai/nomic-embed-text-v1-unsupervised) model, this long-context variant of our medium-sized model is perfect for workloads that can be constrained by the regular 512 token context of our other models. Without the use of RPE, this model supports up to 2048 tokens. With RPE, it can scale to 8192!
| Model Name | MTEB Retrieval Score (NDCG @ 10) |
| ------------------------------------------------------------------ | -------------------------------- |
| [snowflake-arctic-embed-m-long](https://huggingface.co/Snowflake/snowflake-arctic-embed-m-long/) | 54.83 |
| nomic-embed-text-v1.5 | 53.01 |
| nomic-embed-text-v1 | 52.81 |
### [snowflake-arctic-embed-l](https://huggingface.co/Snowflake/snowflake-arctic-embed-l/)
Based on the [intfloat/e5-large-unsupervised](https://huggingface.co/intfloat/e5-large-unsupervised) model, this large model is a direct drop-in for closed APIs and delivers the most accurate retrieval experience.
| Model Name | MTEB Retrieval Score (NDCG @ 10) |
| ------------------------------------------------------------------ | -------------------------------- |
| [snowflake-arctic-embed-l](https://huggingface.co/Snowflake/snowflake-arctic-embed-l/) | 55.98 |
| UAE-Large-V1 | 54.66 |
| bge-large-en-v1.5 | 54.29 |
| mxbai-embed-large-v1 | 54.39 |
| e5-Large-v2 | 50.56 |
## Usage
### Using Sentence Transformers
You can use the sentence-transformers package to use an snowflake-arctic-embed model, as shown below.
```python
from sentence_transformers import SentenceTransformer
model = SentenceTransformer("Snowflake/snowflake-arctic-embed-m")
queries = ['what is snowflake?', 'Where can I get the best tacos?']
documents = ['The Data Cloud!', 'Mexico City of Course!']
query_embeddings = model.encode(queries, prompt_name="query")
document_embeddings = model.encode(documents)
scores = query_embeddings @ document_embeddings.T
for query, query_scores in zip(queries, scores):
doc_score_pairs = list(zip(documents, query_scores))
doc_score_pairs = sorted(doc_score_pairs, key=lambda x: x[1], reverse=True)
# Output passages & scores
print("Query:", query)
for document, score in doc_score_pairs:
print(score, document)
```
```
Query: what is snowflake?
0.20051965 The Data Cloud!
0.07660701 Mexico City of Course!
Query: Where can I get the best tacos?
0.24481852 Mexico City of Course!
0.15664819 The Data Cloud!
```
### Using Huggingface transformers
You can use the transformers package to use an snowflake-arctic-embed model, as shown below. For optimal retrieval quality, use the CLS token to embed each text portion and use the query prefix below (just on the query).
```python
import torch
from transformers import AutoModel, AutoTokenizer
tokenizer = AutoTokenizer.from_pretrained('Snowflake/snowflake-arctic-embed-m')
model = AutoModel.from_pretrained('Snowflake/snowflake-arctic-embed-m', add_pooling_layer=False)
model.eval()
query_prefix = 'Represent this sentence for searching relevant passages: '
queries = ['what is snowflake?', 'Where can I get the best tacos?']
queries_with_prefix = ["{}{}".format(query_prefix, i) for i in queries]
query_tokens = tokenizer(queries_with_prefix, padding=True, truncation=True, return_tensors='pt', max_length=512)
documents = ['The Data Cloud!', 'Mexico City of Course!']
document_tokens = tokenizer(documents, padding=True, truncation=True, return_tensors='pt', max_length=512)
# Compute token embeddings
with torch.no_grad():
query_embeddings = model(**query_tokens)[0][:, 0]
doument_embeddings = model(**document_tokens)[0][:, 0]
# normalize embeddings
query_embeddings = torch.nn.functional.normalize(query_embeddings, p=2, dim=1)
doument_embeddings = torch.nn.functional.normalize(doument_embeddings, p=2, dim=1)
scores = torch.mm(query_embeddings, doument_embeddings.transpose(0, 1))
for query, query_scores in zip(queries, scores):
doc_score_pairs = list(zip(documents, query_scores))
doc_score_pairs = sorted(doc_score_pairs, key=lambda x: x[1], reverse=True)
#Output passages & scores
print("Query:", query)
for document, score in doc_score_pairs:
print(score, document)
```
### Using Transformers.js
If you haven't already, you can install the [Transformers.js](https://huggingface.co/docs/transformers.js) JavaScript library from [NPM](https://www.npmjs.com/package/@xenova/transformers) by running:
```bash
npm i @xenova/transformers
```
You can then use the model to compute embeddings as follows:
```js
import { pipeline, dot } from '@xenova/transformers';
// Create feature extraction pipeline
const extractor = await pipeline('feature-extraction', 'Snowflake/snowflake-arctic-embed-m', {
quantized: false, // Comment out this line to use the quantized version
});
// Generate sentence embeddings
const sentences = [
'Represent this sentence for searching relevant passages: Where can I get the best tacos?',
'The Data Cloud!',
'Mexico City of Course!',
]
const output = await extractor(sentences, { normalize: true, pooling: 'cls' });
// Compute similarity scores
const [source_embeddings, ...document_embeddings ] = output.tolist();
const similarities = document_embeddings.map(x => dot(source_embeddings, x));
console.log(similarities); // [0.15664823859882132, 0.24481869975470627]
```
## FAQ
TBD
## Contact
Feel free to open an issue or pull request if you have any questions or suggestions about this project.
You also can email Daniel Campos(daniel.campos@snowflake.com).
## License
Arctic is licensed under the [Apache-2](https://www.apache.org/licenses/LICENSE-2.0). The released models can be used for commercial purposes free of charge.
## Acknowledgement
We want to thank the open-source community, which has provided the great building blocks upon which we could make our models.
We thank our modeling engineers, Danmei Xu, Luke Merrick, Gaurav Nuti, and Daniel Campos, for making these great models possible.
We thank our leadership, Himabindu Pucha, Kelvin So, Vivek Raghunathan, and Sridhar Ramaswamy, for supporting this work.
We also thank the open-source community for producing the great models we could build on top of and making these releases possible.
Finally, we thank the researchers who created BEIR and MTEB benchmarks.
It is largely thanks to their tireless work to define what better looks like that we could improve model performance.
|
timm/vit_tiny_patch16_224.augreg_in21k_ft_in1k | timm | "2023-05-06T00:30:03Z" | 38,472 | 1 | timm | [
"timm",
"pytorch",
"safetensors",
"image-classification",
"dataset:imagenet-1k",
"dataset:imagenet-21k",
"arxiv:2106.10270",
"arxiv:2010.11929",
"license:apache-2.0",
"region:us"
] | image-classification | "2022-12-22T07:56:04Z" | ---
tags:
- image-classification
- timm
library_name: timm
license: apache-2.0
datasets:
- imagenet-1k
- imagenet-21k
---
# Model card for vit_tiny_patch16_224.augreg_in21k_ft_in1k
A Vision Transformer (ViT) image classification model. Trained on ImageNet-21k and fine-tuned on ImageNet-1k (with additional augmentation and regularization) in JAX by paper authors, ported to PyTorch by Ross Wightman.
## Model Details
- **Model Type:** Image classification / feature backbone
- **Model Stats:**
- Params (M): 5.7
- GMACs: 1.1
- Activations (M): 4.1
- Image size: 224 x 224
- **Papers:**
- How to train your ViT? Data, Augmentation, and Regularization in Vision Transformers: https://arxiv.org/abs/2106.10270
- An Image is Worth 16x16 Words: Transformers for Image Recognition at Scale: https://arxiv.org/abs/2010.11929v2
- **Dataset:** ImageNet-1k
- **Pretrain Dataset:** ImageNet-21k
- **Original:** https://github.com/google-research/vision_transformer
## Model Usage
### Image Classification
```python
from urllib.request import urlopen
from PIL import Image
import timm
img = Image.open(urlopen(
'https://huggingface.co/datasets/huggingface/documentation-images/resolve/main/beignets-task-guide.png'
))
model = timm.create_model('vit_tiny_patch16_224.augreg_in21k_ft_in1k', pretrained=True)
model = model.eval()
# get model specific transforms (normalization, resize)
data_config = timm.data.resolve_model_data_config(model)
transforms = timm.data.create_transform(**data_config, is_training=False)
output = model(transforms(img).unsqueeze(0)) # unsqueeze single image into batch of 1
top5_probabilities, top5_class_indices = torch.topk(output.softmax(dim=1) * 100, k=5)
```
### Image Embeddings
```python
from urllib.request import urlopen
from PIL import Image
import timm
img = Image.open(urlopen(
'https://huggingface.co/datasets/huggingface/documentation-images/resolve/main/beignets-task-guide.png'
))
model = timm.create_model(
'vit_tiny_patch16_224.augreg_in21k_ft_in1k',
pretrained=True,
num_classes=0, # remove classifier nn.Linear
)
model = model.eval()
# get model specific transforms (normalization, resize)
data_config = timm.data.resolve_model_data_config(model)
transforms = timm.data.create_transform(**data_config, is_training=False)
output = model(transforms(img).unsqueeze(0)) # output is (batch_size, num_features) shaped tensor
# or equivalently (without needing to set num_classes=0)
output = model.forward_features(transforms(img).unsqueeze(0))
# output is unpooled, a (1, 197, 192) shaped tensor
output = model.forward_head(output, pre_logits=True)
# output is a (1, num_features) shaped tensor
```
## Model Comparison
Explore the dataset and runtime metrics of this model in timm [model results](https://github.com/huggingface/pytorch-image-models/tree/main/results).
## Citation
```bibtex
@article{steiner2021augreg,
title={How to train your ViT? Data, Augmentation, and Regularization in Vision Transformers},
author={Steiner, Andreas and Kolesnikov, Alexander and and Zhai, Xiaohua and Wightman, Ross and Uszkoreit, Jakob and Beyer, Lucas},
journal={arXiv preprint arXiv:2106.10270},
year={2021}
}
```
```bibtex
@article{dosovitskiy2020vit,
title={An Image is Worth 16x16 Words: Transformers for Image Recognition at Scale},
author={Dosovitskiy, Alexey and Beyer, Lucas and Kolesnikov, Alexander and Weissenborn, Dirk and Zhai, Xiaohua and Unterthiner, Thomas and Dehghani, Mostafa and Minderer, Matthias and Heigold, Georg and Gelly, Sylvain and Uszkoreit, Jakob and Houlsby, Neil},
journal={ICLR},
year={2021}
}
```
```bibtex
@misc{rw2019timm,
author = {Ross Wightman},
title = {PyTorch Image Models},
year = {2019},
publisher = {GitHub},
journal = {GitHub repository},
doi = {10.5281/zenodo.4414861},
howpublished = {\url{https://github.com/huggingface/pytorch-image-models}}
}
```
|
facebook/convnextv2-tiny-22k-384 | facebook | "2023-09-26T17:19:37Z" | 38,461 | 2 | transformers | [
"transformers",
"pytorch",
"tf",
"convnextv2",
"image-classification",
"vision",
"dataset:imagenet-22k",
"arxiv:2301.00808",
"license:apache-2.0",
"autotrain_compatible",
"endpoints_compatible",
"region:us"
] | image-classification | "2023-02-19T07:24:50Z" | ---
license: apache-2.0
tags:
- vision
- image-classification
datasets:
- imagenet-22k
widget:
- src: https://huggingface.co/datasets/mishig/sample_images/resolve/main/tiger.jpg
example_title: Tiger
- src: https://huggingface.co/datasets/mishig/sample_images/resolve/main/teapot.jpg
example_title: Teapot
- src: https://huggingface.co/datasets/mishig/sample_images/resolve/main/palace.jpg
example_title: Palace
---
# ConvNeXt V2 (tiny-sized model)
ConvNeXt V2 model pretrained using the FCMAE framework and fine-tuned on the ImageNet-22K dataset at resolution 384x384. It was introduced in the paper [ConvNeXt V2: Co-designing and Scaling ConvNets with Masked Autoencoders](https://arxiv.org/abs/2301.00808) by Woo et al. and first released in [this repository](https://github.com/facebookresearch/ConvNeXt-V2).
Disclaimer: The team releasing ConvNeXT V2 did not write a model card for this model so this model card has been written by the Hugging Face team.
## Model description
ConvNeXt V2 is a pure convolutional model (ConvNet) that introduces a fully convolutional masked autoencoder framework (FCMAE) and a new Global Response Normalization (GRN) layer to ConvNeXt. ConvNeXt V2 significantly improves the performance of pure ConvNets on various recognition benchmarks.
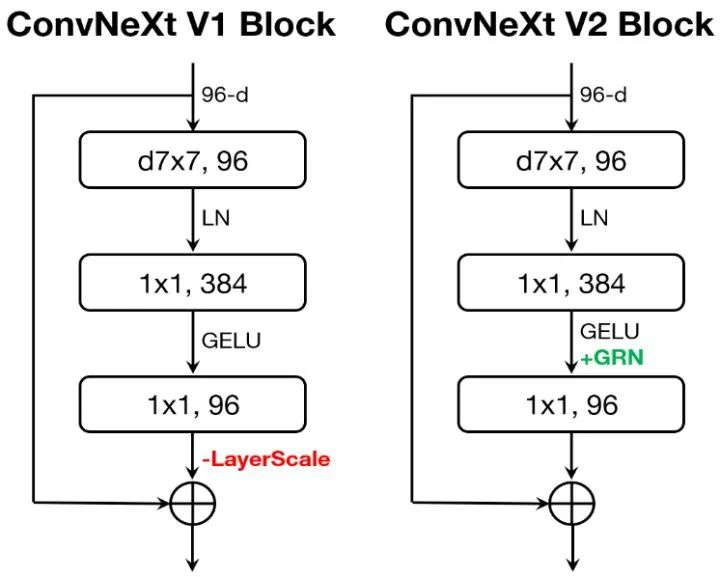
## Intended uses & limitations
You can use the raw model for image classification. See the [model hub](https://huggingface.co/models?search=convnextv2) to look for
fine-tuned versions on a task that interests you.
### How to use
Here is how to use this model to classify an image of the COCO 2017 dataset into one of the 1,000 ImageNet classes:
```python
from transformers import AutoImageProcessor, ConvNextV2ForImageClassification
import torch
from datasets import load_dataset
dataset = load_dataset("huggingface/cats-image")
image = dataset["test"]["image"][0]
preprocessor = AutoImageProcessor.from_pretrained("facebook/convnextv2-tiny-22k-384")
model = ConvNextV2ForImageClassification.from_pretrained("facebook/convnextv2-tiny-22k-384")
inputs = preprocessor(image, return_tensors="pt")
with torch.no_grad():
logits = model(**inputs).logits
# model predicts one of the 1000 ImageNet classes
predicted_label = logits.argmax(-1).item()
print(model.config.id2label[predicted_label]),
```
For more code examples, we refer to the [documentation](https://huggingface.co/docs/transformers/master/en/model_doc/convnextv2).
### BibTeX entry and citation info
```bibtex
@article{DBLP:journals/corr/abs-2301-00808,
author = {Sanghyun Woo and
Shoubhik Debnath and
Ronghang Hu and
Xinlei Chen and
Zhuang Liu and
In So Kweon and
Saining Xie},
title = {ConvNeXt {V2:} Co-designing and Scaling ConvNets with Masked Autoencoders},
journal = {CoRR},
volume = {abs/2301.00808},
year = {2023},
url = {https://doi.org/10.48550/arXiv.2301.00808},
doi = {10.48550/arXiv.2301.00808},
eprinttype = {arXiv},
eprint = {2301.00808},
timestamp = {Tue, 10 Jan 2023 15:10:12 +0100},
biburl = {https://dblp.org/rec/journals/corr/abs-2301-00808.bib},
bibsource = {dblp computer science bibliography, https://dblp.org}
}
``` |
qwp4w3hyb/DeepSeek-Coder-V2-Lite-Instruct-iMat-GGUF | qwp4w3hyb | "2024-06-25T21:07:17Z" | 38,384 | 1 | null | [
"gguf",
"arxiv:2401.06066",
"base_model:deepseek-ai/DeepSeek-Coder-V2-Lite-Instruct",
"license:other",
"region:us"
] | null | "2024-06-24T12:31:18Z" | ---
license: other
license_name: deepseek-license
license_link: LICENSE
base_model: deepseek-ai/DeepSeek-Coder-V2-Lite-Instruct
---
# Quant Infos
- quants done with an importance matrix for improved quantization loss
- ggufs & imatrix generated from bf16 for "optimal" accuracy loss
- Wide coverage of different gguf quant types from Q\_8\_0 down to IQ1\_S
- Quantized with [llama.cpp](https://github.com/ggerganov/llama.cpp) commit [4bfe50f741479c1df1c377260c3ff5702586719e](https://github.com/ggerganov/llama.cpp/commit/4bfe50f741479c1df1c377260c3ff5702586719e) (master as of 2024-06-11)
- Imatrix generated with [this](https://gist.github.com/bartowski1182/eb213dccb3571f863da82e99418f81e8) multi-purpose dataset by [bartowski](https://huggingface.co/bartowski).
```
./imatrix -c 512 -m $model_name-bf16.gguf -f calibration_datav3.txt -o $model_name.imatrix
```
# Original Model Card
<!-- markdownlint-disable first-line-h1 -->
<!-- markdownlint-disable html -->
<!-- markdownlint-disable no-duplicate-header -->
<div align="center">
<img src="https://github.com/deepseek-ai/DeepSeek-V2/blob/main/figures/logo.svg?raw=true" width="60%" alt="DeepSeek-V2" />
</div>
<hr>
<div align="center" style="line-height: 1;">
<a href="https://www.deepseek.com/" target="_blank" style="margin: 2px;">
<img alt="Homepage" src="https://github.com/deepseek-ai/DeepSeek-V2/blob/main/figures/badge.svg?raw=true" style="display: inline-block; vertical-align: middle;"/>
</a>
<a href="https://chat.deepseek.com/" target="_blank" style="margin: 2px;">
<img alt="Chat" src="https://img.shields.io/badge/🤖%20Chat-DeepSeek%20V2-536af5?color=536af5&logoColor=white" style="display: inline-block; vertical-align: middle;"/>
</a>
<a href="https://huggingface.co/deepseek-ai" target="_blank" style="margin: 2px;">
<img alt="Hugging Face" src="https://img.shields.io/badge/%F0%9F%A4%97%20Hugging%20Face-DeepSeek%20AI-ffc107?color=ffc107&logoColor=white" style="display: inline-block; vertical-align: middle;"/>
</a>
</div>
<div align="center" style="line-height: 1;">
<a href="https://discord.gg/Tc7c45Zzu5" target="_blank" style="margin: 2px;">
<img alt="Discord" src="https://img.shields.io/badge/Discord-DeepSeek%20AI-7289da?logo=discord&logoColor=white&color=7289da" style="display: inline-block; vertical-align: middle;"/>
</a>
<a href="https://github.com/deepseek-ai/DeepSeek-V2/blob/main/figures/qr.jpeg?raw=true" target="_blank" style="margin: 2px;">
<img alt="Wechat" src="https://img.shields.io/badge/WeChat-DeepSeek%20AI-brightgreen?logo=wechat&logoColor=white" style="display: inline-block; vertical-align: middle;"/>
</a>
<a href="https://twitter.com/deepseek_ai" target="_blank" style="margin: 2px;">
<img alt="Twitter Follow" src="https://img.shields.io/badge/Twitter-deepseek_ai-white?logo=x&logoColor=white" style="display: inline-block; vertical-align: middle;"/>
</a>
</div>
<div align="center" style="line-height: 1;">
<a href="https://github.com/deepseek-ai/DeepSeek-V2/blob/main/LICENSE-CODE" style="margin: 2px;">
<img alt="Code License" src="https://img.shields.io/badge/Code_License-MIT-f5de53?&color=f5de53" style="display: inline-block; vertical-align: middle;"/>
</a>
<a href="https://github.com/deepseek-ai/DeepSeek-V2/blob/main/LICENSE-MODEL" style="margin: 2px;">
<img alt="Model License" src="https://img.shields.io/badge/Model_License-Model_Agreement-f5de53?&color=f5de53" style="display: inline-block; vertical-align: middle;"/>
</a>
</div>
<p align="center">
<a href="#4-api-platform">API Platform</a> |
<a href="#5-how-to-run-locally">How to Use</a> |
<a href="#6-license">License</a> |
</p>
<p align="center">
<a href="https://github.com/deepseek-ai/DeepSeek-Coder-V2/blob/main/paper.pdf"><b>Paper Link</b>👁️</a>
</p>
# DeepSeek-Coder-V2: Breaking the Barrier of Closed-Source Models in Code Intelligence
## 1. Introduction
We present DeepSeek-Coder-V2, an open-source Mixture-of-Experts (MoE) code language model that achieves performance comparable to GPT4-Turbo in code-specific tasks. Specifically, DeepSeek-Coder-V2 is further pre-trained from an intermediate checkpoint of DeepSeek-V2 with additional 6 trillion tokens. Through this continued pre-training, DeepSeek-Coder-V2 substantially enhances the coding and mathematical reasoning capabilities of DeepSeek-V2, while maintaining comparable performance in general language tasks. Compared to DeepSeek-Coder-33B, DeepSeek-Coder-V2 demonstrates significant advancements in various aspects of code-related tasks, as well as reasoning and general capabilities. Additionally, DeepSeek-Coder-V2 expands its support for programming languages from 86 to 338, while extending the context length from 16K to 128K.
<p align="center">
<img width="100%" src="https://github.com/deepseek-ai/DeepSeek-Coder-V2/blob/main/figures/performance.png?raw=true">
</p>
In standard benchmark evaluations, DeepSeek-Coder-V2 achieves superior performance compared to closed-source models such as GPT4-Turbo, Claude 3 Opus, and Gemini 1.5 Pro in coding and math benchmarks. The list of supported programming languages can be found [here](https://github.com/deepseek-ai/DeepSeek-Coder-V2/blob/main/supported_langs.txt).
## 2. Model Downloads
We release the DeepSeek-Coder-V2 with 16B and 236B parameters based on the [DeepSeekMoE](https://arxiv.org/pdf/2401.06066) framework, which has actived parameters of only 2.4B and 21B , including base and instruct models, to the public.
<div align="center">
| **Model** | **#Total Params** | **#Active Params** | **Context Length** | **Download** |
| :-----------------------------: | :---------------: | :----------------: | :----------------: | :----------------------------------------------------------: |
| DeepSeek-Coder-V2-Lite-Base | 16B | 2.4B | 128k | [🤗 HuggingFace](https://huggingface.co/deepseek-ai/DeepSeek-Coder-V2-Lite-Base) |
| DeepSeek-Coder-V2-Lite-Instruct | 16B | 2.4B | 128k | [🤗 HuggingFace](https://huggingface.co/deepseek-ai/DeepSeek-Coder-V2-Lite-Instruct) |
| DeepSeek-Coder-V2-Base | 236B | 21B | 128k | [🤗 HuggingFace](https://huggingface.co/deepseek-ai/DeepSeek-Coder-V2-Base) |
| DeepSeek-Coder-V2-Instruct | 236B | 21B | 128k | [🤗 HuggingFace](https://huggingface.co/deepseek-ai/DeepSeek-Coder-V2-Instruct) |
</div>
## 3. Chat Website
You can chat with the DeepSeek-Coder-V2 on DeepSeek's official website: [coder.deepseek.com](https://coder.deepseek.com/sign_in)
## 4. API Platform
We also provide OpenAI-Compatible API at DeepSeek Platform: [platform.deepseek.com](https://platform.deepseek.com/), and you can also pay-as-you-go at an unbeatable price.
<p align="center">
<img width="40%" src="https://github.com/deepseek-ai/DeepSeek-Coder-V2/blob/main/figures/model_price.jpg?raw=true">
</p>
## 5. How to run locally
**Here, we provide some examples of how to use DeepSeek-Coder-V2-Lite model. If you want to utilize DeepSeek-Coder-V2 in BF16 format for inference, 80GB*8 GPUs are required.**
### Inference with Huggingface's Transformers
You can directly employ [Huggingface's Transformers](https://github.com/huggingface/transformers) for model inference.
#### Code Completion
```python
from transformers import AutoTokenizer, AutoModelForCausalLM
import torch
tokenizer = AutoTokenizer.from_pretrained("deepseek-ai/DeepSeek-Coder-V2-Lite-Base", trust_remote_code=True)
model = AutoModelForCausalLM.from_pretrained("deepseek-ai/DeepSeek-Coder-V2-Lite-Base", trust_remote_code=True, torch_dtype=torch.bfloat16).cuda()
input_text = "#write a quick sort algorithm"
inputs = tokenizer(input_text, return_tensors="pt").to(model.device)
outputs = model.generate(**inputs, max_length=128)
print(tokenizer.decode(outputs[0], skip_special_tokens=True))
```
#### Code Insertion
```python
from transformers import AutoTokenizer, AutoModelForCausalLM
import torch
tokenizer = AutoTokenizer.from_pretrained("deepseek-ai/DeepSeek-Coder-V2-Lite-Base", trust_remote_code=True)
model = AutoModelForCausalLM.from_pretrained("deepseek-ai/DeepSeek-Coder-V2-Lite-Base", trust_remote_code=True, torch_dtype=torch.bfloat16).cuda()
input_text = """<|fim▁begin|>def quick_sort(arr):
if len(arr) <= 1:
return arr
pivot = arr[0]
left = []
right = []
<|fim▁hole|>
if arr[i] < pivot:
left.append(arr[i])
else:
right.append(arr[i])
return quick_sort(left) + [pivot] + quick_sort(right)<|fim▁end|>"""
inputs = tokenizer(input_text, return_tensors="pt").to(model.device)
outputs = model.generate(**inputs, max_length=128)
print(tokenizer.decode(outputs[0], skip_special_tokens=True)[len(input_text):])
```
#### Chat Completion
```python
from transformers import AutoTokenizer, AutoModelForCausalLM
import torch
tokenizer = AutoTokenizer.from_pretrained("deepseek-ai/DeepSeek-Coder-V2-Lite-Instruct", trust_remote_code=True)
model = AutoModelForCausalLM.from_pretrained("deepseek-ai/DeepSeek-Coder-V2-Lite-Instruct", trust_remote_code=True, torch_dtype=torch.bfloat16).cuda()
messages=[
{ 'role': 'user', 'content': "write a quick sort algorithm in python."}
]
inputs = tokenizer.apply_chat_template(messages, add_generation_prompt=True, return_tensors="pt").to(model.device)
# tokenizer.eos_token_id is the id of <|EOT|> token
outputs = model.generate(inputs, max_new_tokens=512, do_sample=False, top_k=50, top_p=0.95, num_return_sequences=1, eos_token_id=tokenizer.eos_token_id)
print(tokenizer.decode(outputs[0][len(inputs[0]):], skip_special_tokens=True))
```
The complete chat template can be found within `tokenizer_config.json` located in the huggingface model repository.
An example of chat template is as belows:
```bash
<|begin▁of▁sentence|>User: {user_message_1}
Assistant: {assistant_message_1}<|end▁of▁sentence|>User: {user_message_2}
Assistant:
```
You can also add an optional system message:
```bash
<|begin▁of▁sentence|>{system_message}
User: {user_message_1}
Assistant: {assistant_message_1}<|end▁of▁sentence|>User: {user_message_2}
Assistant:
```
### Inference with vLLM (recommended)
To utilize [vLLM](https://github.com/vllm-project/vllm) for model inference, please merge this Pull Request into your vLLM codebase: https://github.com/vllm-project/vllm/pull/4650.
```python
from transformers import AutoTokenizer
from vllm import LLM, SamplingParams
max_model_len, tp_size = 8192, 1
model_name = "deepseek-ai/DeepSeek-Coder-V2-Lite-Instruct"
tokenizer = AutoTokenizer.from_pretrained(model_name)
llm = LLM(model=model_name, tensor_parallel_size=tp_size, max_model_len=max_model_len, trust_remote_code=True, enforce_eager=True)
sampling_params = SamplingParams(temperature=0.3, max_tokens=256, stop_token_ids=[tokenizer.eos_token_id])
messages_list = [
[{"role": "user", "content": "Who are you?"}],
[{"role": "user", "content": "write a quick sort algorithm in python."}],
[{"role": "user", "content": "Write a piece of quicksort code in C++."}],
]
prompt_token_ids = [tokenizer.apply_chat_template(messages, add_generation_prompt=True) for messages in messages_list]
outputs = llm.generate(prompt_token_ids=prompt_token_ids, sampling_params=sampling_params)
generated_text = [output.outputs[0].text for output in outputs]
print(generated_text)
```
## 6. License
This code repository is licensed under [the MIT License](https://github.com/deepseek-ai/DeepSeek-Coder-V2/blob/main/LICENSE-CODE). The use of DeepSeek-Coder-V2 Base/Instruct models is subject to [the Model License](https://github.com/deepseek-ai/DeepSeek-Coder-V2/blob/main/LICENSE-MODEL). DeepSeek-Coder-V2 series (including Base and Instruct) supports commercial use.
## 7. Contact
If you have any questions, please raise an issue or contact us at [service@deepseek.com](service@deepseek.com).
|
frankjoshua/realisticVisionV51_v51VAE | frankjoshua | "2023-09-04T23:37:20Z" | 38,378 | 2 | diffusers | [
"diffusers",
"autotrain_compatible",
"endpoints_compatible",
"diffusers:StableDiffusionPipeline",
"region:us"
] | text-to-image | "2023-09-04T23:35:53Z" | Entry not found |
AdrienB134/ColBERTv2.0-spanish-mmarcoES | AdrienB134 | "2024-02-27T12:17:06Z" | 38,323 | 2 | transformers | [
"transformers",
"safetensors",
"bert",
"colbert",
"ColBERT",
"es",
"dataset:unicamp-dl/mmarco",
"license:mit",
"endpoints_compatible",
"region:us"
] | null | "2024-02-27T06:08:45Z" | ---
license: mit
datasets:
- unicamp-dl/mmarco
language:
- es
tags:
- colbert
- ColBERT
---
## Training
#### Details
The model is initialized from the [ColBERTv1.0-bert-based-spanish-mmarcoES](https://huggingface.co/AdrienB134/ColBERTv1.0-bert-based-spanish-mmarcoES) checkpoint and trained using the ColBERTv2 style of training.
It was trained on 2 Tesla T4 GPU with 16GBs of memory each with 20k warmup steps warmup using a batch size of 64 and the AdamW optimizer with a constant learning rate of 1e-05.
Total training time was around 60 hours.
#### Data
The model is fine-tuned on the Spanish version of the [mMARCO](https://huggingface.co/datasets/unicamp-dl/mmarco) dataset, a multi-lingual machine-translated version of the MS MARCO dataset.
## Evaluation
The model is evaluated on the smaller development set of mMARCO-es, which consists of 6,980 queries for a corpus of 8.8M candidate passages. We report the mean reciprocal rank (MRR) and recall at various cut-offs (R@k).
| model | Vocab. | #Param. | Size | MRR@10 | R@50 | R@1000 |
|:------------------------------------------------------------------------------------------------------------------------|:--------|--------:|------:|---------:|-------:|--------:|
| **ColBERTv2.0-spanish-mmarcoES** | spanish | 110M | 440MB | **32.86** | **76.46** | **81.06** |
| **ColBERTv1.0-bert-based-spanish-mmarcoES** | spanish | 110M | 440MB | 24.70 | 59,23 | 63.86 | |
yisol/IDM-VTON | yisol | "2024-04-22T19:53:20Z" | 38,240 | 252 | diffusers | [
"diffusers",
"onnx",
"safetensors",
"stable-diffusion-xl",
"inpainting",
"virtual try-on",
"arxiv:2403.05139",
"base_model:stable-diffusion-xl-1.0-inpainting-0.1",
"license:cc-by-nc-sa-4.0",
"diffusers:StableDiffusionXLInpaintPipeline",
"region:us"
] | image-to-image | "2024-03-28T20:42:50Z" | ---
base_model: stable-diffusion-xl-1.0-inpainting-0.1
tags:
- stable-diffusion-xl
- inpainting
- virtual try-on
license: cc-by-nc-sa-4.0
---
# Check out more codes on our [github repository](https://github.com/yisol/IDM-VTON)!
# IDM-VTON : Improving Diffusion Models for Authentic Virtual Try-on in the Wild
This is an official implementation of paper 'Improving Diffusion Models for Authentic Virtual Try-on in the Wild'
- [paper](https://arxiv.org/abs/2403.05139)
- [project page](https://idm-vton.github.io/)
🤗 Try our huggingface [Demo](https://huggingface.co/spaces/yisol/IDM-VTON)


## TODO LIST
- [x] demo model
- [x] inference code
- [ ] training code
## Acknowledgements
For the demo, GPUs are supported from [zerogpu](https://huggingface.co/zero-gpu-explorers), and auto masking generation codes are based on [OOTDiffusion](https://github.com/levihsu/OOTDiffusion) and [DCI-VTON](https://github.com/bcmi/DCI-VTON-Virtual-Try-On).
Parts of the code are based on [IP-Adapter](https://github.com/tencent-ailab/IP-Adapter).
## Citation
```
@article{choi2024improving,
title={Improving Diffusion Models for Virtual Try-on},
author={Choi, Yisol and Kwak, Sangkyung and Lee, Kyungmin and Choi, Hyungwon and Shin, Jinwoo},
journal={arXiv preprint arXiv:2403.05139},
year={2024}
}
```
## License
The codes and checkpoints in this repository are under the [CC BY-NC-SA 4.0 license](https://creativecommons.org/licenses/by-nc-sa/4.0/legalcode).
|
Voicelab/trurl-2-13b-academic | Voicelab | "2023-11-23T12:56:46Z" | 38,237 | 4 | transformers | [
"transformers",
"pytorch",
"llama",
"text-generation",
"voicelab",
"llama-2",
"trurl",
"trurl-2",
"en",
"pl",
"autotrain_compatible",
"text-generation-inference",
"region:us"
] | text-generation | "2023-09-18T08:08:08Z" | ---
language:
- en
- pl
pipeline_tag: text-generation
inference: false
tags:
- voicelab
- pytorch
- llama-2
- trurl
- trurl-2
---
<img src="https://public.3.basecamp.com/p/rs5XqmAuF1iEuW6U7nMHcZeY/upload/download/VL-NLP-short.png" alt="logo voicelab nlp" style="width:300px;"/>
# Academic Trurl 2 -- Polish Llama 2
The Academic TRURL is a finetuned Llama 2, trained on over 1.7b tokens (855k conversational **Polish** and **English** samples) with a large context of 4096 tokens.
TRURL was trained on a large number of Polish data.
TRURL 2 is a collection of fine-tuned generative text models with 7 billion and 13 billion parameters.
This is the repository for the Academic 13B fine-tuned model, optimized for dialogue use cases.
This model was trained without MMLU dataset.
# Overview
**TRURL developers** Voicelab.AI
**Variations** Trurl 2 comes in 7B and 13B versions.
**Input** Models input text only.
**Output** Models generate text only.
**Model Architecture** Trurl is an auto-regressive language model that uses an optimized transformer architecture.
||Training Data|Params|Content Length|Num. Samples|Num. Tokens|start LR|
|---|---|---|---|---|---|---|
|Trurl 2|*A new mix of private and publicly available online data without MMLU*|7B|4k|855k|1.19b|2.0 x 10<sup>-5</sup>|
|Trurl 2|*A new mix of private and publicly available online data with MMLU*|13B|4k|970k|1.7b|2.0 x 10<sup>-5</sup>|
|Trurl 2 Academic|*A new mix of private and publicly available online data without MMLU*|13B|4k|855k|1.19b|2.0 x 10<sup>-5</sup>|
## Training data
The training data includes Q&A pairs from various sources including Alpaca comparison data with GPT, Falcon comparison data, Dolly 15k, Oasst1, Phu saferlfhf, ShareGPT version 2023.05.08v0 filtered and cleaned, Voicelab private datasets for JSON data extraction, modification, and analysis, CURLICAT dataset containing journal entries, dataset from Polish wiki with Q&A pairs grouped into conversations, Voicelab private dataset with sales conversations, arguments and objections, paraphrases, contact reason detection, and corrected dialogues.
## Intended Use
Trurl 2 is intended for commercial and research use in Polish and English. Tuned models are intended for assistant-like chat, but also adapted for a variety of natural language generation tasks.
# Evaluation Results
|Model | Size| hellaswag | arc_challenge | MMLU|
|---|---|---|---|---|
| Llama-2-chat | 7B | 78.55% | 52.9% | 48.32% |
| Llama-2-chat | 13B | 81.94% | 59.04% | 54.64% |
| Trurl 2.0 (with MMLU) | 13B | 80.09% | 59.30% | 78.35% |
| Trurl 2.0 Academic (no MMLU) | 13B | TO-DO | TO-DO | TO-DO|
| Trurl 2.0 (no MMLU) | 7b | 75.29% | 53.41%| 50.0%|
<img src="https://voicelab.ai/wp-content/uploads/trurl-hero.webp" alt="trurl graphic" style="width:100px;"/>
# Examples
## Polish language
> **HUMAN**: What’s the point of training you on Polish?
>
>
> **TRURL**: The point of training me on Polish, or any other language, is to enable me to understand and respond to queries and questions in that language. This allows people who speak that language to use me as a helpful tool for finding information, answering questions, and completing tasks. By training me on a wide variety of texts in Polish, I can learn about the culture, history, and language of Poland, and be able to provide more accurate and relevant responses to users. Additionally, training me on multiple languages allows me to be useful to a wider range of people, regardless of their native language.
## Stanisław Lem
> **HUMAN**: who is Trurl from Stanisław Lem book?
>
>
> **TRURL**: Trurl is a character in a series of short stories by Polish science fiction writer Stanisław Lem. The stories feature Trurl and another character, Klapaucius, who are both inventors and explorers of the universe. They use their advanced technology and knowledge to solve various problems and encounter strange and fantastical creatures along the way. The stories often explore themes of science, technology, and the nature of intelligence and consciousness.
The description of original Trurl generated by TRURL 2.0. Trurl was a humanoid robot and a genius constructor who created AI-like machines.
> **HUMAN**: why are you named Trurl?
>
>
> **TRURL**: I was named after the character Trurl from the short stories by Stanisław Lem. My creators, the VoiceLab AI team, were inspired by the character’s intelligence and creativity, and they wanted to create an AI assistant that embodied those qualities. They also wanted to give me a unique and memorable name, and Trurl seemed like the perfect fit.
# Example use
## LLM
Simply pass a prompt to a model and decode an output. Model will continue writing text based on sample you provided.
```
import torch
from transformers import LlamaForCausalLM, LlamaTokenizer
tokenizer = LlamaTokenizer.from_pretrained("Voicelab/trurl-2-13b")
model = LlamaForCausalLM.from_pretrained("Voicelab/trurl-2-13b")
prompt = "Yesterday, when I was"
tokenized_prompt = tokenizer(prompt, return_tensors="pt")
model.eval()
with torch.no_grad():
print(tokenizer.decode(
model.generate(**tokenized_prompt, max_new_tokens=200)[0],
skip_special_tokens=True))
```
## Chat
When using TRURL in a chat mode you should remember to use Llama 2 conversation template like in the example below.
```
import torch
from transformers import LlamaForCausalLM, LlamaTokenizer
tokenizer = LlamaTokenizer.from_pretrained("Voicelab/trurl-2-13b")
model = LlamaForCausalLM.from_pretrained("Voicelab/trurl-2-13b")
prompt = """
<s>[INST] <<SYS>> You are a helpful, respectful and honest assistant. Always answer as helpfully as possible, while being safe.
Your answers should not include any harmful, unethical, racist, sexist, toxic, dangerous, or illegal content.
Please ensure that your responses are socially unbiased and positive in nature.\n\n
If a question does not make any sense, or is not factually coherent, explain why instead of answering something not correct.
If you don't know the answer to a question, please don't share false information. <</SYS>>
What was the reason for calling in the conversation below? \n\n
AGENT: Hello, Bank of Albion, this is Mata Hari. How can I help you?
CLIENT: Hi. I've been locked out from my Internet account. I need your help.
AGENT: (yy) Yes, of course, I'll do my best to help you. But I need to find out why the locking-out happened. (yy) In order to ascertain that, I'll ask you a couple of questions to confirm your identity. I'm going to need your full name.
CLIENT: Lizz Truss.
AGENT: Thank you. Now I need your personal identification number.
CLIENT: Fourteen, two hundred thirty-one, thirty-eight, twenty-nine, sixty-five.
AGENT: Thank you. Now I need your client ID number. The client ID number is the eight digits we assigned to you at the very beginning, on conclusion of the contract.
CLIENT: OK. Give me a moment. I have to find it.
AGENT: (mhm) You'll find… You'll find it in the contract.
CLIENT: Yes, yes. I can see it. Sixty-five, twenty-nine, thirty-eight, thirty-one.
AGENT: Thank you. One final security question. Do you have any deposits in our bank?
CLIENT: No, no. I don't have any deposits in this bank.
AGENT: Thank you. Your identity has been (yy) confirmed. (yy) I can see that the account has been blocked, indeed, and you won't be able to log in via the Internet (yy) because (yy) the identity document which is listed for reference has expired. (yy) From what I can see, your identity document expired some time ago. Have you been issued a new one?
CLIENT: Well, no. I think my ID is still valid, you know. I didn't even know.
AGENT: Well, no... Your ID expired at the end of March. Well, almost at the end. Your old ID had been valid until 26 March. (yy) For that reason, your accout has been blocked, because you haven't notified us about the ID change for a few months. We are not interested if the ID document has been officialy reissued. (...) On our end, what matters is whether the document listed for our reference is valid (yy) so without a valid document I can't unlock your accout.
CLIENT: But I have to carry out an operation right now, so this is sort of problematic.
AGENT: I understand. But (yy) you are obligated, as an account holder, to notify the bank about any changes pending (yy), regrding, for example, your home address or phone number. Now, one of such safeguards protecting your… (yy) money, your sensitive data, is precisely about having a valid identification document. Since this is missing in your case, the account has been blocked. Now, I don't think this would have caught you off guard, because we always remind our customers that their ID is about to expire. When the ID is nearing expiration, we display relevant messages at least sixty days in advance. They appear once you've logged in, at the very top of the screen, there is a notification that (yy) the ID is about to expire (yy), so, well... The bank did notify you about this issue. Now, how you chose to act on this information was your choice, right? In any case, at this point, in order to unlock your accout, our protocols require that you produce a new identification document at one of our branches. You shall provide information concerning the new document number, new valid-thru date, and only then will you be able to use your account again. I can schedule an appointment with a consultant at our branch for you. What locality would you prefer?
CLIENT: Well, I'm not sure if I should share such information with you.
AGENT: And may I ask why exactly you are unsure? After all, you're calling a bank that runs your account, right?
CLIENT: Right, you know what, I need to go now. Good bye.
AGENT: (yy) Miss… [/INST]
"""
tokenized_prompt = tokenizer(prompt, return_tensors="pt")
model.eval()
with torch.no_grad():
print(tokenizer.decode(
model.generate(**tokenized_prompt, max_new_tokens=200)[0],
skip_special_tokens=True))
```
To get the expected features and performance for the chat versions, a specific Llama 2 formatting needs to be followed, including the `INST` and `<<SYS>>` tags, `BOS` and `EOS` tokens, and the whitespaces and breaklines in between (we recommend calling `strip()` on inputs to avoid double-spaces). See reference code in github for details: [`chat_completion`](https://github.com/facebookresearch/llama/blob/main/llama/generation.py#L212).
```
<s>[INST] <<SYS>> system prompt <</SYS>>
human prompt [/INST]
gpt response </s>
<s>[INST] human prompt [/INST]
gpt response </s>
```
# Ethical Considerations and Limitations
Trurl 2, same as a Llama 2, is a new technology that carries risks with use. Testing conducted to date has been in Polish and English, and has not covered, nor could it cover all scenarios. For these reasons, as with all LLMs, Trurl 2’s potential outputs cannot be predicted in advance, and the model may in some instances produce inaccurate, biased or other objectionable responses to user prompts. Therefore, before deploying any applications of Trurl 2, developers should perform safety testing and tuning tailored to their specific applications of the model.
Please see the Meta's Responsible Use Guide available at [https://ai.meta.com/llama/responsible-use-guide/](https://ai.meta.com/llama/responsible-use-guide)
# Authors
The model was trained by NLP Research Team at Voicelab.ai.
You can contact us [here](https://voicelab.ai/contact/).
* [TRURL 13b](https://huggingface.co/Voicelab/trurl-2-13b/)
* [TRURL 13b Academic](https://huggingface.co/Voicelab/trurl-2-13b-academic)
* [TRURL 7b](https://huggingface.co/Voicelab/trurl-2-7b/)
* [TRURL DEMO](https://trurl.ai)
Quantized models:
* [TRURL 13b - 8bit](https://huggingface.co/Voicelab/trurl-2-13b-8bit/)
* [TRURL 7b - 8bit](https://huggingface.co/Voicelab/trurl-2-7b-8bit/)
The work was supported by [#NASK](https://www.nask.pl/)
# [Open LLM Leaderboard Evaluation Results](https://huggingface.co/spaces/HuggingFaceH4/open_llm_leaderboard)
Detailed results can be found [here](https://huggingface.co/datasets/open-llm-leaderboard/details_Voicelab__trurl-2-13b-academic)
| Metric | Value |
|-----------------------|---------------------------|
| Avg. | 52.7 |
| ARC (25-shot) | 57.94 |
| HellaSwag (10-shot) | 79.55 |
| MMLU (5-shot) | 55.2 |
| TruthfulQA (0-shot) | 43.46 |
| Winogrande (5-shot) | 76.56 |
| GSM8K (5-shot) | 10.92 |
| DROP (3-shot) | 45.28 |
|
Helsinki-NLP/opus-mt-ja-en | Helsinki-NLP | "2023-08-16T11:59:08Z" | 38,190 | 44 | transformers | [
"transformers",
"pytorch",
"tf",
"marian",
"text2text-generation",
"translation",
"ja",
"en",
"license:apache-2.0",
"autotrain_compatible",
"endpoints_compatible",
"region:us"
] | translation | "2022-03-02T23:29:04Z" | ---
tags:
- translation
license: apache-2.0
---
### opus-mt-ja-en
* source languages: ja
* target languages: en
* OPUS readme: [ja-en](https://github.com/Helsinki-NLP/OPUS-MT-train/blob/master/models/ja-en/README.md)
* dataset: opus
* model: transformer-align
* pre-processing: normalization + SentencePiece
* download original weights: [opus-2019-12-18.zip](https://object.pouta.csc.fi/OPUS-MT-models/ja-en/opus-2019-12-18.zip)
* test set translations: [opus-2019-12-18.test.txt](https://object.pouta.csc.fi/OPUS-MT-models/ja-en/opus-2019-12-18.test.txt)
* test set scores: [opus-2019-12-18.eval.txt](https://object.pouta.csc.fi/OPUS-MT-models/ja-en/opus-2019-12-18.eval.txt)
## Benchmarks
| testset | BLEU | chr-F |
|-----------------------|-------|-------|
| Tatoeba.ja.en | 41.7 | 0.589 |
|
ku-nlp/deberta-v2-base-japanese | ku-nlp | "2023-05-12T14:13:03Z" | 38,171 | 26 | transformers | [
"transformers",
"pytorch",
"safetensors",
"deberta-v2",
"fill-mask",
"deberta",
"ja",
"dataset:wikipedia",
"dataset:cc100",
"dataset:oscar",
"license:cc-by-sa-4.0",
"autotrain_compatible",
"endpoints_compatible",
"region:us"
] | fill-mask | "2023-01-05T08:04:14Z" | ---
language: ja
license: cc-by-sa-4.0
library_name: transformers
tags:
- deberta
- deberta-v2
- fill-mask
datasets:
- wikipedia
- cc100
- oscar
metrics:
- accuracy
mask_token: "[MASK]"
widget:
- text: "京都 大学 で 自然 言語 処理 を [MASK] する 。"
---
# Model Card for Japanese DeBERTa V2 base
## Model description
This is a Japanese DeBERTa V2 base model pre-trained on Japanese Wikipedia, the Japanese portion of CC-100, and the Japanese portion of OSCAR.
## How to use
You can use this model for masked language modeling as follows:
```python
from transformers import AutoTokenizer, AutoModelForMaskedLM
tokenizer = AutoTokenizer.from_pretrained('ku-nlp/deberta-v2-base-japanese')
model = AutoModelForMaskedLM.from_pretrained('ku-nlp/deberta-v2-base-japanese')
sentence = '京都 大学 で 自然 言語 処理 を [MASK] する 。' # input should be segmented into words by Juman++ in advance
encoding = tokenizer(sentence, return_tensors='pt')
...
```
You can also fine-tune this model on downstream tasks.
## Tokenization
The input text should be segmented into words by [Juman++](https://github.com/ku-nlp/jumanpp) in advance. [Juman++ 2.0.0-rc3](https://github.com/ku-nlp/jumanpp/releases/tag/v2.0.0-rc3) was used for pre-training. Each word is tokenized into subwords by [sentencepiece](https://github.com/google/sentencepiece).
## Training data
We used the following corpora for pre-training:
- Japanese Wikipedia (as of 20221020, 3.2GB, 27M sentences, 1.3M documents)
- Japanese portion of CC-100 (85GB, 619M sentences, 66M documents)
- Japanese portion of OSCAR (54GB, 326M sentences, 25M documents)
Note that we filtered out documents annotated with "header", "footer", or "noisy" tags in OSCAR.
Also note that Japanese Wikipedia was duplicated 10 times to make the total size of the corpus comparable to that of CC-100 and OSCAR. As a result, the total size of the training data is 171GB.
## Training procedure
We first segmented texts in the corpora into words using [Juman++](https://github.com/ku-nlp/jumanpp).
Then, we built a sentencepiece model with 32000 tokens including words ([JumanDIC](https://github.com/ku-nlp/JumanDIC)) and subwords induced by the unigram language model of [sentencepiece](https://github.com/google/sentencepiece).
We tokenized the segmented corpora into subwords using the sentencepiece model and trained the Japanese DeBERTa model using [transformers](https://github.com/huggingface/transformers) library.
The training took three weeks using 8 NVIDIA A100-SXM4-40GB GPUs.
The following hyperparameters were used during pre-training:
- learning_rate: 2e-4
- per_device_train_batch_size: 44
- distributed_type: multi-GPU
- num_devices: 8
- gradient_accumulation_steps: 6
- total_train_batch_size: 2,112
- max_seq_length: 512
- optimizer: Adam with betas=(0.9,0.999) and epsilon=1e-06
- lr_scheduler_type: linear schedule with warmup
- training_steps: 500,000
- warmup_steps: 10,000
The accuracy of the trained model on the masked language modeling task was 0.779.
The evaluation set consists of 5,000 randomly sampled documents from each of the training corpora.
## Fine-tuning on NLU tasks
We fine-tuned the following models and evaluated them on the dev set of JGLUE.
We tuned learning rate and training epochs for each model and task following [the JGLUE paper](https://www.jstage.jst.go.jp/article/jnlp/30/1/30_63/_pdf/-char/ja).
| Model | MARC-ja/acc | JSTS/pearson | JSTS/spearman | JNLI/acc | JSQuAD/EM | JSQuAD/F1 | JComQA/acc |
|-------------------------------|-------------|--------------|---------------|----------|-----------|-----------|------------|
| Waseda RoBERTa base | 0.965 | 0.913 | 0.876 | 0.905 | 0.853 | 0.916 | 0.853 |
| Waseda RoBERTa large (seq512) | 0.969 | 0.925 | 0.890 | 0.928 | 0.910 | 0.955 | 0.900 |
| LUKE Japanese base* | 0.965 | 0.916 | 0.877 | 0.912 | - | - | 0.842 |
| LUKE Japanese large* | 0.965 | 0.932 | 0.902 | 0.927 | - | - | 0.893 |
| DeBERTaV2 base | 0.970 | 0.922 | 0.886 | 0.922 | 0.899 | 0.951 | 0.873 |
| DeBERTaV2 large | 0.968 | 0.925 | 0.892 | 0.924 | 0.912 | 0.959 | 0.890 |
*The scores of LUKE are from [the official repository](https://github.com/studio-ousia/luke).
## Acknowledgments
This work was supported by Joint Usage/Research Center for Interdisciplinary Large-scale Information Infrastructures (JHPCN) through General Collaboration Project no. jh221004, "Developing a Platform for Constructing and Sharing of Large-Scale Japanese Language Models".
For training models, we used the mdx: a platform for the data-driven future.
|
sazyou-roukaku/BracingEvoMix | sazyou-roukaku | "2023-10-01T08:58:54Z" | 38,137 | 149 | diffusers | [
"diffusers",
"stable-diffusion",
"text-to-image",
"ja",
"license:creativeml-openrail-m",
"region:us"
] | text-to-image | "2023-05-31T10:29:16Z" | ---
license: creativeml-openrail-m
language:
- ja
library_name: diffusers
pipeline_tag: text-to-image
tags:
- stable-diffusion
- text-to-image
---
License:[CreativeML Open RAIL-M](https://huggingface.co/sazyou-roukaku/BracingEvoMix/blob/main/license_v1.txt)<br>
Additional Copyright: sazyou_roukaku (TwitterID [@sazyou_roukaku](https://twitter.com/sazyou_roukaku)) as of May 31, 2023<br>
このモデルは『CreativeML Open RAIL-M』でLicenseそのものに変更はありません。<br>
~しかし追加著作者として鎖城郎郭の名前が追加されています。~<br>
しかし追加著作者として佐城郎画の名前が追加されています。(6/10 Twitterネーム変更に伴い、表記変更。License内はsazyou_roukakuの為変更なし)<br>
なお『CreativeML Open RAIL-M』に記載されている通り、<br>
本モデルを使用しての生成物に関してはLicenseの使用制限Aの事例を除き、当方は一切関与致しません。<br>
犯罪目的利用や医療用画像など特定専門的な用途での利用は使用制限Aで禁止されています。<br>
必ず確認しご利用ください。<br>
また当方は一切責任を持ちません。免責されていることをご了承の上、ご使用ください。<br>
<br>
2023/10/01<br>
BracingEvoMix_v2一般公開。<br>
<br>
**・BracingEvoMix_v2**<br>
CLIP設定/clip skip:2<br>
推奨ネガティブプロンプトベース:
```
(worst quality:2),(low quality:1,4),(undressing:1.5),(manicure:1.5),(long neck:2),lip,make up,(depth of field, bokeh, blurry, blurry background:1.4)
```
<br>
(depth of field, bokeh, blurry, blurry background:1.4)は背景を鮮明に出したいとき用です。<br>
<br>
なおBracingEvoMixシリーズの更新は一旦このv2にてストップします。<br>
追加学習を行っている方々たちがSDXLへ移行を進めており、選定基準に即したモデルで性能をあげるのに限界があること。<br>
下半身の一部ベースとなっているsxdがかなり初期のモデルの為、労力に対して、向上性能が誤差になる可能性が高いこと。<br>
利用可能なモデルの制限が厳しすぎて、服飾系の種類をいずれにせよ増やせないこと。<br>
以上が理由です。<br>
ただSD1.xの需要次第では、私自身でTrainingを行い、別シリーズを公開する可能性はあります。<br>
とはいえ、まずはSDXLでの動きや企業から使いやすいモデルが公開される可能性も見つつ、対応したいと考えています。<br>
<br>
<br>
<br>
<h4>制限</h4>
<div class="px-2">
<table class="table-fixed border mt-0 text-xs">
<tr>
<td class="align-middle px-4 w-8">
<span class="text-green-500">
<h5>OK</h5>
</span>
</td>
<td>
著作者表記を入れずにモデルを使用する<br>
Use the model without crediting the creator
</td>
</tr>
<tr>
<td class="align-middle px-4 w-8">
<span class="text-green-500">
<h5>OK</h5>
</span>
</td>
<td>
このモデルで生成した画像を商用利用する<br>
Sell images they generate
</td>
</tr>
<tr>
<td class="align-middle px-4 w-8">
<span class="text-green-500">
<h5>OK</h5>
</span>
</td>
<td>
商用画像生成サービスに、このモデルを使用する<br>
Run on services that generate images for money
</td>
</tr>
<tr>
<td class="align-middle px-4 w-8">
<span class="text-green-500">
<h5>OK</h5>
</span>
</td>
<td>
このモデルを使用したマージモデルを共有・配布する<br>
Share merges using this model
</td>
</tr>
<tr>
<td class="align-middle px-4 w-8">
<span class="text-green-500">
<h5>OK</h5>
</span>
</td>
<td>
このモデル、または派生モデルを販売する<br>
Sell this model or merges using this model
</td>
</tr>
<tr>
<td class="align-middle px-4 w-8">
<span class="text-green-500">
<h5>OK</h5>
</span>
</td>
<td>
このモデルをマージしたモデルに異なる権限を設定する<br>
Have different permissions when sharing merges
</td>
</tr>
</table>
</div>
なお、上記のモデルそのものの販売や商用画像生成サービスへの利用は、<br>
『CreativeML Open RAIL-M』のLicense上、使用制限Aに追記記載しない限り、<br>
制限することが本来できない為、マージ者への負担も考慮し、civitai制限表記上OKとしているだけであり、<br>
積極的な推奨は行っておらず、またそれにより何らかの問題が生じても当方は一切責任を持ちません。<br>
その点、ご留意いただくようお願いいたします。<br>
<br>
<div class="px-2">
<table class="table-fixed border mt-0 text-xs">
<tr>
<td colspan="2">
<strong><h4>BracingEvoMix_v2</h4></strong>
</td>
</tr>
<tr>
<td>
自然言語プロンプト反応(SD1.5)<br>
</td>
<td class="align-middle px-4 w-15">
<h5>90点</h5>
</td>
</tr>
<tr>
<td>
アジア顔出力の多様性<br>
</td>
<td class="align-middle px-4 w-15">
<h5>95点</h5>
</td>
</tr>
<tr>
<td>
フォトリアリティ<br>
</td>
<td class="align-middle px-4 w-15">
<h5>95点</h5>
</td>
</tr>
<tr>
<td>
非現実的美形度<br>
</td>
<td class="align-middle px-4 w-15">
<h5>40点</h5>
</td>
</tr>
<tr>
<td>
彩度・明度安定性<br>
</td>
<td class="align-middle px-4 w-15">
<h5>65点</h5>
</td>
</tr>
<tr>
<td>
手指の描画精度(最新上位モデルを90点とした場合)<br>
</td>
<td class="align-middle px-4 w-15">
<h5>80点</h5>
</td>
</tr>
<tr>
<td>
複雑ポーズ安定性<br>
</td>
<td class="align-middle px-4 w-15">
<h5>75点</h5>
</td>
</tr>
<tr>
<td>
乳部の意図せぬ露出制御性<br>
</td>
<td class="align-middle px-4 w-15">
<h5>60点</h5>
</td>
</tr>
<tr>
<td>
感情表現のプロンプト反応<br>
</td>
<td class="align-middle px-4 w-15">
<h5>70点</h5>
</td>
</tr>
<tr>
<td>
表現可能年齢幅<br>
</td>
<td class="align-middle px-4 w-15">
<h5>85点</h5>
</td>
</tr>
<tr>
<td colspan="2">
<strong>基礎モデル『BracingEvoMix_v1』の正当後継版。彩度や明度のムラが改善され、指精度も上昇しています。
また背景強化、横長解像度への強化が入っています。<br>
出力ムラは減ったので汎用性は高くなったと思います。</strong><br>
</td>
</tr>
<tr>
<td colspan="2">
<strong><h4>BracingEvoMix_v1</h4></strong>
</td>
</tr>
<tr>
<td>
自然言語プロンプト反応(SD1.5)<br>
</td>
<td class="align-middle px-4 w-15">
<h5>90点</h5>
</td>
</tr>
<tr>
<td>
アジア顔出力の多様性<br>
</td>
<td class="align-middle px-4 w-15">
<h5>95点</h5>
</td>
</tr>
<tr>
<td>
フォトリアリティ<br>
</td>
<td class="align-middle px-4 w-15">
<h5>95点</h5>
</td>
</tr>
<tr>
<td>
非現実的美形度<br>
</td>
<td class="align-middle px-4 w-15">
<h5>40点</h5>
</td>
</tr>
<tr>
<td>
彩度・明度安定性<br>
</td>
<td class="align-middle px-4 w-15">
<h5>40点</h5>
</td>
</tr>
<tr>
<td>
手指の描画精度(最新上位モデルを90点とした場合)<br>
</td>
<td class="align-middle px-4 w-15">
<h5>70点</h5>
</td>
</tr>
<tr>
<td>
複雑ポーズ安定性<br>
</td>
<td class="align-middle px-4 w-15">
<h5>60点</h5>
</td>
</tr>
<tr>
<td>
乳部の意図せぬ露出制御性<br>
</td>
<td class="align-middle px-4 w-15">
<h5>50点</h5>
</td>
</tr>
<tr>
<td>
感情表現のプロンプト反応<br>
</td>
<td class="align-middle px-4 w-15">
<h5>80点</h5>
</td>
</tr>
<tr>
<td>
表現可能年齢幅<br>
</td>
<td class="align-middle px-4 w-15">
<h5>85点</h5>
</td>
</tr>
<tr>
<td colspan="2">
<strong>基礎モデル。OpenBra由来のアジア顔を表現でき、リアリティも非常に高い反面、彩度や明度にムラがある。<br>
感情表現にも強いので制御できれば表現幅は広い一方、弱点も多いじゃじゃ馬。<br>
素材となった各モデルの良さと悪さを両方併せ持つので使いこなせれば、強いという玄人向け。</strong><br>
</td>
</tr>
<tr>
<td colspan="2">
<strong><h4>BracingEvoMix_Another</h4></strong>
</td>
</tr>
<tr>
<td>
自然言語プロンプト反応(SD1.5)<br>
</td>
<td class="align-middle px-4 w-15">
<h5>90点</h5>
</td>
</tr>
<tr>
<td>
アジア顔出力の多様性<br>
</td>
<td class="align-middle px-4 w-15">
<h5>80点</h5>
</td>
</tr>
<tr>
<td>
フォトリアリティ<br>
</td>
<td class="align-middle px-4 w-15">
<h5>90点</h5>
</td>
</tr>
<tr>
<td>
非現実的美形度<br>
</td>
<td class="align-middle px-4 w-15">
<h5>60点</h5>
</td>
</tr>
<tr>
<td>
彩度・明度安定性<br>
</td>
<td class="align-middle px-4 w-15">
<h5>75点</h5>
</td>
</tr>
<tr>
<td>
手指の描画精度(最新上位モデルを90点とした場合)<br>
</td>
<td class="align-middle px-4 w-15">
<h5>85点</h5>
</td>
</tr>
<tr>
<td>
複雑ポーズ安定性<br>
</td>
<td class="align-middle px-4 w-15">
<h5>60点</h5>
</td>
</tr>
<tr>
<td>
乳部の意図せぬ露出制御性<br>
</td>
<td class="align-middle px-4 w-15">
<h5>55点</h5>
</td>
</tr>
<tr>
<td>
感情表現のプロンプト反応<br>
</td>
<td class="align-middle px-4 w-15">
<h5>50点</h5>
</td>
</tr>
<tr>
<td>
表現可能年齢幅<br>
</td>
<td class="align-middle px-4 w-15">
<h5>60点</h5>
</td>
<tr>
<td colspan="2">
<strong>アナザーモデル。マージ手法を変更し、よりミキシングを深めることで彩度や明度の露骨な変化を安定化させた。<br>
結果的にBRA顔が薄れ、少し一般的なアジア顔系マージモデルの方向性によっている。<br>
指の安定性。特に爪や指先端の綺麗な表現はかなり最新モデル上位に比肩する。(グチャらない訳ではない)<br>
弱みが減った分、感情表現反応も弱まった部分がある。なおlarge breasts以上での一般的な服装だとポロリのしやすさはそう大差なし。<br></strong>
</td>
</tr>
</tr>
<tr>
<td colspan="2">
<strong><h4>BracingEvoMix_Fast</h4></strong>
</td>
</tr>
<tr>
<td>
自然言語プロンプト反応(SD1.5)<br>
</td>
<td class="align-middle px-4 w-15">
<h5>90点</h5>
</td>
</tr>
<tr>
<td>
アジア顔出力の多様性<br>
</td>
<td class="align-middle px-4 w-15">
<h5>80点</h5>
</td>
</tr>
<tr>
<td>
フォトリアリティ<br>
</td>
<td class="align-middle px-4 w-15">
<h5>80点</h5>
</td>
</tr>
<tr>
<td>
非現実的美形度<br>
</td>
<td class="align-middle px-4 w-15">
<h5>65点</h5>
</td>
</tr>
<tr>
<td>
彩度・明度安定性<br>
</td>
<td class="align-middle px-4 w-15">
<h5>75点</h5>
</td>
</tr>
<tr>
<td>
手指の描画精度(最新上位モデルを90点とした場合)<br>
</td>
<td class="align-middle px-4 w-15">
<h5>80点</h5>
</td>
</tr>
<tr>
<td>
複雑ポーズ安定性<br>
</td>
<td class="align-middle px-4 w-15">
<h5>60点</h5>
</td>
</tr>
<tr>
<td>
乳部の意図せぬ露出制御性<br>
</td>
<td class="align-middle px-4 w-15">
<h5>60点</h5>
</td>
</tr>
<tr>
<td>
感情表現のプロンプト反応<br>
</td>
<td class="align-middle px-4 w-15">
<h5>50点</h5>
</td>
</tr>
<tr>
<td>
表現可能年齢幅<br>
</td>
<td class="align-middle px-4 w-15">
<h5>60点</h5>
</td>
<tr>
<td colspan="2">
<strong>アナザーモデルをよりchilled_remix的なアレンジをした試作モデル。<br>
より完璧に整った顔立ちにしやすく、またエフェクト表現もchilled_remixほどではないも、多少通りやすいよう設計。<br>
ただしフォトリアルの領域からは逸脱させていないので、chilled_remixほどの自由さはないです<br>
</strong>
</td>
</tr>
</tr>
</table>
</div>
**マージ利用モデル一覧**
**[BracingEvoMix_v2]**
OpenBra
**©BanKai** [@PleaseBanKai](https://twitter.com/PleaseBanKai)
dreamshaper_6BakedVae
(https://civitai.com/models/4384) ©Lykon
epicrealism_newEra
epicrealism_pureEvolutionV5
(https://civitai.com/models/25694) ©epinikion
diamondCoalMix_diamondCoalv2
(https://civitai.com/models/41415) ©EnthusiastAI
sxd_v10
(https://civitai.com/models/1169) ©izuek
Evt_V4_e04_ema
(https://huggingface.co/haor/Evt_V4-preview) ©haor
bp_mk5
(https://huggingface.co/Crosstyan/BPModel) ©Crosstyan
**[BracingEvoMix_v1]**
OpenBraβ
OpenBra
**©BanKai** [@PleaseBanKai](https://twitter.com/PleaseBanKai)
dreamshaper_5Bakedvae
dreamshaper_6BakedVae
(https://civitai.com/models/4384) ©Lykon
epicrealism_newAge
epicrealism_newEra
(https://civitai.com/models/25694) ©epinikion
diamondCoalMix_diamondCoalv2
(https://civitai.com/models/41415) ©EnthusiastAI
sxd_v10
(https://civitai.com/models/1169) ©izuek
Evt_V4_e04_ema
(https://huggingface.co/haor/Evt_V4-preview) ©haor
**[BracingEvoMix_Another]**
OpenBra
**©BanKai** [@PleaseBanKai](https://twitter.com/PleaseBanKai)
dreamshaper_6BakedVae
(https://civitai.com/models/4384) ©Lykon
epicrealism_newEra
(https://civitai.com/models/25694) ©epinikion
sxd_v10
(https://civitai.com/models/1169) ©izuek
Evt_V4_e04_ema
(https://huggingface.co/haor/Evt_V4-preview) ©haor
**[BracingEvoMix_Fast]**
OpenBra
**©BanKai** [@PleaseBanKai](https://twitter.com/PleaseBanKai)
dreamshaper_6BakedVae
(https://civitai.com/models/4384) ©Lykon
epicrealism_newEra
(https://civitai.com/models/25694) ©epinikion
Evt_V4_e04_ema
(https://huggingface.co/haor/Evt_V4-preview) ©haor
bp_mk5
(https://huggingface.co/Crosstyan/BPModel) ©Crosstyan
--------------------------------------------------------------------------
**推奨設定**
CLIP設定/clip skip:2
badhand系のnegativeTIを入れた場合と入れない場合の差は感覚的に大差ありません。
このモデルは**EasyNegative**もしくは**BadBras推奨**です。
**EasyNegative v2** は人体構造破綻率が上記と比べて高いので非推奨。
自然言語的な文章プロンプトにかなり強いですが、シチュエーション以外の詳しい顔造形などは、
好みに合わせてワードプロンプトで指定するのが私のスタイルです。
ワードだけ構成でも問題があるわけではないので使いやすいスタイルで使ってください。
クオリティプロンプトは、high qualityなどは有効性を感じていません。
masterpieceは顔造形が変化する感覚ありますが、クオリティアップとしては微妙です。
ただhigh resolutionは背景や質感に効果あります。high res、Hiresなど色々ありますが、
一番high resolutionを信頼しています。
また1girlなどのWD式プロンプトではなく、girl、womanやyoung woman等の自然言語での使用も検討ください。
CGFスケールを少し下げ、5程度にすることで、全体的なコントラストを弱めることも技法として有用です。
**肌の色が濃く感じる場合は(fair skin:1.2)を入れると白くなります。上記と併せてご利用ください**
ネガティブプロンプトベース
```
EasyNegative,(worst quality:2),(low quality:1.4),(undressing:1.5), (disheveled clothes:1.4),(manicure:1.2),(nipple:1.2),(long neck:2),
```
**FAQ**
**Q1:BracingEvoMixとは何か**
**A1:**
従来のマージモデルはNAIleakモデルの混入やDreamlikeLicenseの混入の恐れがあり、<br>
本格的なビジネス利用において判断に困るような場面が見受けられました。<br>
今回のBracingEvoMixは、BRAの学習開発者であるBanKai氏と直接話し合い、<br>
有志の寄付の結果生まれたOpenBraβ・OpenBraをベースにマージしたモデルです。<br>
他も全て学習モデルとなっており、マージモデルでの組み合わせよりリスクモデルの混入確率をグンと減らしています。<br>
<br>
極東アジア系の顔が出るマージモデルの中では一番低リスクモデルだと考えられます。<br>
しかし学習モデルと名乗っていても、その中身に関して詳細を知ることはできないのでリスクゼロではありません。<br>
コサイン一致率などを元に選定も行っておりますが、混入がないとは言い切れない点はご承知おきください。<br>
<br>
<br>
**Q2:完全な混入していないモデルは無理なのか**
**A2:**
追加学習のみだと、データの偏りが生まれ、背景などを含めてどうしても能力にムラが出ます。<br>
個人レベルで完全な学習モデルを生み出すのは不可能に近い為、マージで対応する必要があります。<br>
しかしこれらの学習モデルの中身に関しては専門家でもないので、解析できません。<br>
<br>
なお差分抽出にて、特定モデルのデータと一致するデータを除去することは可能ですが、<br>
その差を取ることそのものが、派生モデルとしての条件を満たす可能性があります。<br>
またNAIleakモデル本体をDLしなければならず、そこも含めて困難と言えます。<br>
<br>
**Q3:各学習モデル選定基準について**
**A3:**
①sxd_v10<br>
これはNSFWモデルです。NSFWモデルを入れると脱衣をしやすくなるなど問題がある反面、<br>
OpenBraの弱点の一つである下半身の学習度合がやや低い点の補強と様々なポーズ学習データ補強に用いています。<br>
なお割合を増やし過ぎると露出がしやすくなる為、最低限の量をマージしています。<br>
sxdは比較的初期モデルながら、学習量も多めで、制限もない為、選択しています。<br>
<br>
②dreamshaper_5Bakedvae/dreamshaper_6BakedVae<br>
dreamshaperは商用画像生成サービスでも利用されている有名な学習モデルです。<br>
学習モデルとして追加学習量も多くデータの多様性も高い為、多様性補強用に採用しています。<br>
<br>
③epicrealism_newAge/epicrealism_newEra<br>
現行の学習モデルで最強のスペックを誇ると思われます。背景補強と能力の高さから採用しています。<br>
<br>
④diamondcoalmix_diamondcoalv2<br>
極東アジア人顔の系統データを持つ学習モデル。作者がLORA作成を多く行っており、またコサイン値からも問題ないと判断し、極東アジアデータ補強で採用。<br>
<br>
⑤Evt_V4_e04_ema<br>
ACertaintyというNAIleakデータを含まないと公言しているイラスト学習モデルでトレーニングを行い生み出されたモデル。<br>
イラストモデルをマージすることで、人種・年齢関係なく、顔データに影響を与えられるため、<br>
肖像権侵害率を下げる意味合いと日本人好みの顔立ちにする為に採用しています。<br>
<br>
※ACertaintyはNOVEL AIのデータを蒸留している可能性はありますが、こちらは特許法に抵触しない為、問題ないと考えています。<br>
ACertainty<br>
https://huggingface.co/JosephusCheung/ACertainty<br>
https://huggingface.co/JosephusCheung/ASimilarityCalculatior<br>
<br>
⑥bp_mk5<br>
ACertaintyベースの学習モデル。BracingEvoMix_Fastはリバランスの為に用いています。
<br>
<br>
**Q4:無印の指のクオリティがchilled_remix等と比べて低い**
**A4:**
使用モデルが一定の基準をクリアした学習モデルに限定されているため、データの安定性が最新モデルと比べて低いです。<br>
それでも初期モデルよりはまだシャープな指として出力され、3月中頃のモデルと比較して、平均的な出力だと感じています。<br>
~今後可能ならば、指強化等も検討しますが学習データからして困難と見ています。~<br>
申し訳ございませんが、controlnetでの制御などもご検討ください。<br>
<br>
6/14 追記:AnotherやFastはこの指の精度改善を行い、6月時点の最新フォトモデルの平均並みの出力だと考えています。
**Q5:今回の制限に問題や矛盾はないのか**
**A5:** **diamondCoalMix_diamondCoalv2** 、 **dreamshaper_5Bakedvae** 、 **dreamshaper_6BakedVae** は
**OK:Have different permissions when sharing merges**となっており解除可能。
他は制限なしの為、今回全て制限なしとし公開しております。
なおマージ利用モデル側にLicense変更・制限変更等が生じた際も
5/31時点のLicenseや制限を前提として公開している為、creativeml-openrail-mに準じます。
こちらはMergeModel_LicenseSS_v1に該当モデルのSSを保管しております。
なおマージ利用モデル側に重大な問題が発生した場合は、モデルの公開停止を行い、
利用停止を呼びかける可能性はありますが、**当方側を理由とした追加制限を設けることは致しません。** |
mradermacher/AceGPT-v2-32B-Chat-GGUF | mradermacher | "2024-06-24T12:57:03Z" | 38,080 | 0 | transformers | [
"transformers",
"gguf",
"ar",
"zh",
"en",
"base_model:FreedomIntelligence/AceGPT-v2-32B-Chat",
"license:apache-2.0",
"endpoints_compatible",
"region:us"
] | null | "2024-06-23T12:50:50Z" | ---
base_model: FreedomIntelligence/AceGPT-v2-32B-Chat
language:
- ar
- zh
- en
library_name: transformers
license: apache-2.0
quantized_by: mradermacher
---
## About
<!-- ### quantize_version: 2 -->
<!-- ### output_tensor_quantised: 1 -->
<!-- ### convert_type: hf -->
<!-- ### vocab_type: -->
<!-- ### tags: -->
static quants of https://huggingface.co/FreedomIntelligence/AceGPT-v2-32B-Chat
<!-- provided-files -->
weighted/imatrix quants are available at https://huggingface.co/mradermacher/AceGPT-v2-32B-Chat-i1-GGUF
## Usage
If you are unsure how to use GGUF files, refer to one of [TheBloke's
READMEs](https://huggingface.co/TheBloke/KafkaLM-70B-German-V0.1-GGUF) for
more details, including on how to concatenate multi-part files.
## Provided Quants
(sorted by size, not necessarily quality. IQ-quants are often preferable over similar sized non-IQ quants)
| Link | Type | Size/GB | Notes |
|:-----|:-----|--------:|:------|
| [GGUF](https://huggingface.co/mradermacher/AceGPT-v2-32B-Chat-GGUF/resolve/main/AceGPT-v2-32B-Chat.Q2_K.gguf) | Q2_K | 12.3 | |
| [GGUF](https://huggingface.co/mradermacher/AceGPT-v2-32B-Chat-GGUF/resolve/main/AceGPT-v2-32B-Chat.IQ3_XS.gguf) | IQ3_XS | 13.7 | |
| [GGUF](https://huggingface.co/mradermacher/AceGPT-v2-32B-Chat-GGUF/resolve/main/AceGPT-v2-32B-Chat.Q3_K_S.gguf) | Q3_K_S | 14.4 | |
| [GGUF](https://huggingface.co/mradermacher/AceGPT-v2-32B-Chat-GGUF/resolve/main/AceGPT-v2-32B-Chat.IQ3_S.gguf) | IQ3_S | 14.4 | beats Q3_K* |
| [GGUF](https://huggingface.co/mradermacher/AceGPT-v2-32B-Chat-GGUF/resolve/main/AceGPT-v2-32B-Chat.IQ3_M.gguf) | IQ3_M | 14.8 | |
| [GGUF](https://huggingface.co/mradermacher/AceGPT-v2-32B-Chat-GGUF/resolve/main/AceGPT-v2-32B-Chat.Q3_K_M.gguf) | Q3_K_M | 15.9 | lower quality |
| [GGUF](https://huggingface.co/mradermacher/AceGPT-v2-32B-Chat-GGUF/resolve/main/AceGPT-v2-32B-Chat.Q3_K_L.gguf) | Q3_K_L | 17.2 | |
| [GGUF](https://huggingface.co/mradermacher/AceGPT-v2-32B-Chat-GGUF/resolve/main/AceGPT-v2-32B-Chat.IQ4_XS.gguf) | IQ4_XS | 17.8 | |
| [GGUF](https://huggingface.co/mradermacher/AceGPT-v2-32B-Chat-GGUF/resolve/main/AceGPT-v2-32B-Chat.Q4_K_S.gguf) | Q4_K_S | 18.7 | fast, recommended |
| [GGUF](https://huggingface.co/mradermacher/AceGPT-v2-32B-Chat-GGUF/resolve/main/AceGPT-v2-32B-Chat.Q4_K_M.gguf) | Q4_K_M | 19.8 | fast, recommended |
| [GGUF](https://huggingface.co/mradermacher/AceGPT-v2-32B-Chat-GGUF/resolve/main/AceGPT-v2-32B-Chat.Q5_K_S.gguf) | Q5_K_S | 22.6 | |
| [GGUF](https://huggingface.co/mradermacher/AceGPT-v2-32B-Chat-GGUF/resolve/main/AceGPT-v2-32B-Chat.Q5_K_M.gguf) | Q5_K_M | 23.2 | |
| [GGUF](https://huggingface.co/mradermacher/AceGPT-v2-32B-Chat-GGUF/resolve/main/AceGPT-v2-32B-Chat.Q6_K.gguf) | Q6_K | 26.8 | very good quality |
| [GGUF](https://huggingface.co/mradermacher/AceGPT-v2-32B-Chat-GGUF/resolve/main/AceGPT-v2-32B-Chat.Q8_0.gguf) | Q8_0 | 34.7 | fast, best quality |
Here is a handy graph by ikawrakow comparing some lower-quality quant
types (lower is better):

And here are Artefact2's thoughts on the matter:
https://gist.github.com/Artefact2/b5f810600771265fc1e39442288e8ec9
## FAQ / Model Request
See https://huggingface.co/mradermacher/model_requests for some answers to
questions you might have and/or if you want some other model quantized.
## Thanks
I thank my company, [nethype GmbH](https://www.nethype.de/), for letting
me use its servers and providing upgrades to my workstation to enable
this work in my free time.
<!-- end -->
|
knowledgator/IUPAC2SMILES-canonical-base | knowledgator | "2024-02-15T15:58:42Z" | 38,078 | 5 | transformers | [
"transformers",
"pytorch",
"mt5",
"text2text-generation",
"chemistry",
"biology",
"medical",
"smiles",
"iupac",
"text-generation-inference",
"license:apache-2.0",
"autotrain_compatible",
"endpoints_compatible",
"region:us"
] | text2text-generation | "2024-02-06T08:15:49Z" | ---
license: apache-2.0
metrics:
- accuracy
- bleu
pipeline_tag: text2text-generation
tags:
- chemistry
- biology
- medical
- smiles
- iupac
- text-generation-inference
widget:
- text: ethanol
example_title: CCO
---
# IUPAC2SMILES-canonical-base
IUPAC2SMILES-canonical-base was designed to accurately translate IUPAC chemical names to SMILES.
## Model Details
### Model Description
IUPAC2SMILES-canonical-base is based on the MT5 model with optimizations in implementing different tokenizers for the encoder and decoder.
- **Developed by:** Knowladgator Engineering
- **Model type:** Encoder-Decoder with attention mechanism
- **Language(s) (NLP):** SMILES, IUPAC (English)
- **License:** Apache License 2.0
### Model Sources
- **Paper:** coming soon
- **Demo:** [ChemicalConverters](https://huggingface.co/spaces/knowledgator/ChemicalConverters)
## Quickstart
Firstly, install the library:
```commandline
pip install chemical-converters
```
### IUPAC to SMILES
#### To perform simple translation, follow the example:
```python
from chemicalconverters import NamesConverter
converter = NamesConverter(model_name="knowledgator/IUPAC2SMILES-canonical-base")
print(converter.iupac_to_smiles('ethanol'))
print(converter.iupac_to_smiles(['ethanol', 'ethanol', 'ethanol']))
```
```text
['CCO']
['CCO', 'CCO', 'CCO']
```
#### Processing in batches:
```python
from chemicalconverters import NamesConverter
converter = NamesConverter(model_name="knowledgator/IUPAC2SMILES-canonical-base")
print(converter.iupac_to_smiles(["buta-1,3-diene" for _ in range(10)], num_beams=1,
process_in_batch=True, batch_size=1000))
```
```text
['<SYST>C=CC=C', '<SYST>C=CC=C'...]
```
Our models also predict IUPAC styles from the table:
| Style Token | Description |
|-------------|----------------------------------------------------------------------------------------------------|
| `<BASE>` | The most known name of the substance, sometimes is the mixture of traditional and systematic style |
| `<SYST>` | The totally systematic style without trivial names |
| `<TRAD>` | The style is based on trivial names of the parts of substances |
## Bias, Risks, and Limitations
This model has limited accuracy in processing large molecules and currently, doesn't support isomeric and isotopic SMILES.
### Training Procedure
<!-- This should link to a Dataset Card, perhaps with a short stub of information on what the training data is all about as well as documentation related to data pre-processing or additional filtering. -->
The model was trained on 100M examples of SMILES-IUPAC pairs with lr=0.00001, batch_size=512 for 2 epochs.
## Evaluation
| Model | Accuracy | BLEU-4 score | Size(MB) |
|-------------------------------------|---------|------------------|----------|
| IUPAC2SMILES-canonical-small |88.9% |0.966 |23 |
| IUPAC2SMILES-canonical-base |93.7% |0.974 |180 |
| STOUT V2.0\* |68.47% |0.92 |128 |
*According to the original paper https://jcheminf.biomedcentral.com/articles/10.1186/s13321-021-00512-4
## Citation
Coming soon.
## Model Card Authors
[Mykhailo Shtopko](https://huggingface.co/BioMike)
## Model Card Contact
info@knowledgator.com |
vicgalle/xlm-roberta-large-xnli-anli | vicgalle | "2024-04-11T13:50:45Z" | 37,993 | 26 | transformers | [
"transformers",
"pytorch",
"safetensors",
"xlm-roberta",
"text-classification",
"zero-shot-classification",
"nli",
"multilingual",
"dataset:mnli",
"dataset:xnli",
"dataset:facebook/anli",
"doi:10.57967/hf/0977",
"license:mit",
"autotrain_compatible",
"endpoints_compatible",
"region:us"
] | zero-shot-classification | "2022-03-02T23:29:05Z" | ---
language: multilingual
license: mit
tags:
- zero-shot-classification
- nli
- pytorch
datasets:
- mnli
- xnli
- facebook/anli
pipeline_tag: zero-shot-classification
widget:
- text: De pugna erat fantastic. Nam Crixo decem quam dilexit et praeciderunt caput
aemulus.
candidate_labels: violent, peaceful
- text: La película empezaba bien pero terminó siendo un desastre.
candidate_labels: positivo, negativo, neutral
- text: La película empezó siendo un desastre pero en general fue bien.
candidate_labels: positivo, negativo, neutral
- text: ¿A quién vas a votar en 2020?
candidate_labels: Europa, elecciones, política, ciencia, deportes
---
### XLM-RoBERTa-large-XNLI-ANLI
XLM-RoBERTa-large model finetunned over several NLI datasets, ready to use for zero-shot classification.
Here are the accuracies for several test datasets:
| | XNLI-es | XNLI-fr | ANLI-R1 | ANLI-R2 | ANLI-R3 |
|-----------------------------|---------|---------|---------|---------|---------|
| xlm-roberta-large-xnli-anli | 93.7% | 93.2% | 68.5% | 53.6% | 49.0% |
The model can be loaded with the zero-shot-classification pipeline like so:
```
from transformers import pipeline
classifier = pipeline("zero-shot-classification",
model="vicgalle/xlm-roberta-large-xnli-anli")
```
You can then use this pipeline to classify sequences into any of the class names you specify:
```
sequence_to_classify = "Algún día iré a ver el mundo"
candidate_labels = ['viaje', 'cocina', 'danza']
classifier(sequence_to_classify, candidate_labels)
#{'sequence': 'Algún día iré a ver el mundo',
#'labels': ['viaje', 'danza', 'cocina'],
#'scores': [0.9991760849952698, 0.0004178212257102132, 0.0004059972707182169]}
``` |
cross-encoder/nli-MiniLM2-L6-H768 | cross-encoder | "2021-08-05T08:40:39Z" | 37,961 | 2 | transformers | [
"transformers",
"pytorch",
"roberta",
"text-classification",
"MiniLMv2",
"zero-shot-classification",
"en",
"dataset:multi_nli",
"dataset:snli",
"license:apache-2.0",
"autotrain_compatible",
"endpoints_compatible",
"region:us"
] | zero-shot-classification | "2022-03-02T23:29:05Z" | ---
language: en
pipeline_tag: zero-shot-classification
license: apache-2.0
tags:
- MiniLMv2
datasets:
- multi_nli
- snli
metrics:
- accuracy
---
# Cross-Encoder for Natural Language Inference
This model was trained using [SentenceTransformers](https://sbert.net) [Cross-Encoder](https://www.sbert.net/examples/applications/cross-encoder/README.html) class.
## Training Data
The model was trained on the [SNLI](https://nlp.stanford.edu/projects/snli/) and [MultiNLI](https://cims.nyu.edu/~sbowman/multinli/) datasets. For a given sentence pair, it will output three scores corresponding to the labels: contradiction, entailment, neutral.
## Performance
For evaluation results, see [SBERT.net - Pretrained Cross-Encoder](https://www.sbert.net/docs/pretrained_cross-encoders.html#nli).
## Usage
Pre-trained models can be used like this:
```python
from sentence_transformers import CrossEncoder
model = CrossEncoder('cross-encoder/nli-MiniLM2-L6-H768')
scores = model.predict([('A man is eating pizza', 'A man eats something'), ('A black race car starts up in front of a crowd of people.', 'A man is driving down a lonely road.')])
#Convert scores to labels
label_mapping = ['contradiction', 'entailment', 'neutral']
labels = [label_mapping[score_max] for score_max in scores.argmax(axis=1)]
```
## Usage with Transformers AutoModel
You can use the model also directly with Transformers library (without SentenceTransformers library):
```python
from transformers import AutoTokenizer, AutoModelForSequenceClassification
import torch
model = AutoModelForSequenceClassification.from_pretrained('cross-encoder/nli-MiniLM2-L6-H768')
tokenizer = AutoTokenizer.from_pretrained('cross-encoder/nli-MiniLM2-L6-H768')
features = tokenizer(['A man is eating pizza', 'A black race car starts up in front of a crowd of people.'], ['A man eats something', 'A man is driving down a lonely road.'], padding=True, truncation=True, return_tensors="pt")
model.eval()
with torch.no_grad():
scores = model(**features).logits
label_mapping = ['contradiction', 'entailment', 'neutral']
labels = [label_mapping[score_max] for score_max in scores.argmax(dim=1)]
print(labels)
```
## Zero-Shot Classification
This model can also be used for zero-shot-classification:
```python
from transformers import pipeline
classifier = pipeline("zero-shot-classification", model='cross-encoder/nli-MiniLM2-L6-H768')
sent = "Apple just announced the newest iPhone X"
candidate_labels = ["technology", "sports", "politics"]
res = classifier(sent, candidate_labels)
print(res)
``` |
seyonec/ChemBERTa-zinc-base-v1 | seyonec | "2021-05-20T20:55:33Z" | 37,957 | 31 | transformers | [
"transformers",
"pytorch",
"jax",
"roberta",
"fill-mask",
"chemistry",
"autotrain_compatible",
"endpoints_compatible",
"region:us"
] | fill-mask | "2022-03-02T23:29:05Z" | ---
tags:
- chemistry
---
# ChemBERTa: Training a BERT-like transformer model for masked language modelling of chemical SMILES strings.
Deep learning for chemistry and materials science remains a novel field with lots of potiential. However, the popularity of transfer learning based methods in areas such as NLP and computer vision have not yet been effectively developed in computational chemistry + machine learning. Using HuggingFace's suite of models and the ByteLevel tokenizer, we are able to train on a large corpus of 100k SMILES strings from a commonly known benchmark dataset, ZINC.
Training RoBERTa over 5 epochs, the model achieves a decent loss of 0.398, but may likely continue to decline if trained for a larger number of epochs. The model can predict tokens within a SMILES sequence/molecule, allowing for variants of a molecule within discoverable chemical space to be predicted.
By applying the representations of functional groups and atoms learned by the model, we can try to tackle problems of toxicity, solubility, drug-likeness, and synthesis accessibility on smaller datasets using the learned representations as features for graph convolution and attention models on the graph structure of molecules, as well as fine-tuning of BERT. Finally, we propose the use of attention visualization as a helpful tool for chemistry practitioners and students to quickly identify important substructures in various chemical properties.
Additionally, visualization of the attention mechanism have been seen through previous research as incredibly valuable towards chemical reaction classification. The applications of open-sourcing large-scale transformer models such as RoBERTa with HuggingFace may allow for the acceleration of these individual research directions.
A link to a repository which includes the training, uploading and evaluation notebook (with sample predictions on compounds such as Remdesivir) can be found [here](https://github.com/seyonechithrananda/bert-loves-chemistry). All of the notebooks can be copied into a new Colab runtime for easy execution.
Thanks for checking this out!
- Seyone
|
google/siglip-base-patch16-512 | google | "2024-01-19T23:31:30Z" | 37,939 | 10 | transformers | [
"transformers",
"safetensors",
"siglip",
"zero-shot-image-classification",
"vision",
"arxiv:2303.15343",
"arxiv:2209.06794",
"license:apache-2.0",
"endpoints_compatible",
"region:us"
] | zero-shot-image-classification | "2024-01-08T12:42:24Z" | ---
license: apache-2.0
tags:
- vision
widget:
- src: https://huggingface.co/datasets/mishig/sample_images/resolve/main/cat-dog-music.png
candidate_labels: playing music, playing sports
example_title: Cat & Dog
---
# SigLIP (base-sized model)
SigLIP model pre-trained on WebLi at resolution 512x512. It was introduced in the paper [Sigmoid Loss for Language Image Pre-Training](https://arxiv.org/abs/2303.15343) by Zhai et al. and first released in [this repository](https://github.com/google-research/big_vision).
Disclaimer: The team releasing SigLIP did not write a model card for this model so this model card has been written by the Hugging Face team.
## Model description
SigLIP is [CLIP](https://huggingface.co/docs/transformers/model_doc/clip), a multimodal model, with a better loss function. The sigmoid loss operates solely on image-text pairs and does not require a global view of the pairwise similarities for normalization. This allows further scaling up the batch size, while also performing better at smaller batch sizes.
A TLDR of SigLIP by one of the authors can be found [here](https://twitter.com/giffmana/status/1692641733459267713).
## Intended uses & limitations
You can use the raw model for tasks like zero-shot image classification and image-text retrieval. See the [model hub](https://huggingface.co/models?search=google/siglip) to look for
other versions on a task that interests you.
### How to use
Here is how to use this model to perform zero-shot image classification:
```python
from PIL import Image
import requests
from transformers import AutoProcessor, AutoModel
import torch
model = AutoModel.from_pretrained("google/siglip-base-patch16-512")
processor = AutoProcessor.from_pretrained("google/siglip-base-patch16-512")
url = "http://images.cocodataset.org/val2017/000000039769.jpg"
image = Image.open(requests.get(url, stream=True).raw)
texts = ["a photo of 2 cats", "a photo of 2 dogs"]
inputs = processor(text=texts, images=image, padding="max_length", return_tensors="pt")
with torch.no_grad():
outputs = model(**inputs)
logits_per_image = outputs.logits_per_image
probs = torch.sigmoid(logits_per_image) # these are the probabilities
print(f"{probs[0][0]:.1%} that image 0 is '{texts[0]}'")
```
Alternatively, one can leverage the pipeline API which abstracts away the complexity for the user:
```python
from transformers import pipeline
from PIL import Image
import requests
# load pipe
image_classifier = pipeline(task="zero-shot-image-classification", model="google/siglip-base-patch16-512")
# load image
url = 'http://images.cocodataset.org/val2017/000000039769.jpg'
image = Image.open(requests.get(url, stream=True).raw)
# inference
outputs = image_classifier(image, candidate_labels=["2 cats", "a plane", "a remote"])
outputs = [{"score": round(output["score"], 4), "label": output["label"] } for output in outputs]
print(outputs)
```
For more code examples, we refer to the [documentation](https://huggingface.co/transformers/main/model_doc/siglip.html#).
## Training procedure
### Training data
SigLIP is pre-trained on the English image-text pairs of the WebLI dataset [(Chen et al., 2023)](https://arxiv.org/abs/2209.06794).
### Preprocessing
Images are resized/rescaled to the same resolution (512x512) and normalized across the RGB channels with mean (0.5, 0.5, 0.5) and standard deviation (0.5, 0.5, 0.5).
Texts are tokenized and padded to the same length (64 tokens).
### Compute
The model was trained on 16 TPU-v4 chips for three days.
## Evaluation results
Evaluation of SigLIP compared to CLIP is shown below (taken from the paper).
<img src="https://huggingface.co/datasets/huggingface/documentation-images/resolve/main/transformers/model_doc/siglip_table.jpeg"
alt="drawing" width="600"/>
### BibTeX entry and citation info
```bibtex
@misc{zhai2023sigmoid,
title={Sigmoid Loss for Language Image Pre-Training},
author={Xiaohua Zhai and Basil Mustafa and Alexander Kolesnikov and Lucas Beyer},
year={2023},
eprint={2303.15343},
archivePrefix={arXiv},
primaryClass={cs.CV}
}
``` |
Qwen/Qwen2-57B-A14B-Instruct | Qwen | "2024-06-16T07:40:33Z" | 37,909 | 54 | transformers | [
"transformers",
"safetensors",
"qwen2_moe",
"text-generation",
"chat",
"conversational",
"en",
"arxiv:2309.00071",
"license:apache-2.0",
"autotrain_compatible",
"endpoints_compatible",
"region:us"
] | text-generation | "2024-06-04T08:11:26Z" | ---
license: apache-2.0
language:
- en
pipeline_tag: text-generation
tags:
- chat
---
# Qwen2-57B-A14B-Instruct
## Introduction
Qwen2 is the new series of Qwen large language models. For Qwen2, we release a number of base language models and instruction-tuned language models ranging from 0.5 to 72 billion parameters, including a Mixture-of-Experts model. This repo contains the instruction-tuned 57B-A14B Mixture-of-Experts Qwen2 model.
Compared with the state-of-the-art opensource language models, including the previous released Qwen1.5, Qwen2 has generally surpassed most opensource models and demonstrated competitiveness against proprietary models across a series of benchmarks targeting for language understanding, language generation, multilingual capability, coding, mathematics, reasoning, etc.
Qwen2-57B-A14B-Instruct supports a context length of up to 65,536 tokens, enabling the processing of extensive inputs. Please refer to [this section](#processing-long-texts) for detailed instructions on how to deploy Qwen2 for handling long texts.
For more details, please refer to our [blog](https://qwenlm.github.io/blog/qwen2/) and [GitHub](https://github.com/QwenLM/Qwen2).
<br>
## Model Details
Qwen2 is a language model series including decoder language models of different model sizes. For each size, we release the base language model and the aligned chat model. It is based on the Transformer architecture with SwiGLU activation, attention QKV bias, group query attention, etc. Additionally, we have an improved tokenizer adaptive to multiple natural languages and codes.
## Training details
We pretrained the models with a large amount of data, and we post-trained the models with both supervised finetuning and direct preference optimization.
## Requirements
The code of Qwen2MoE has been in the latest Hugging face transformers and we advise you to install `transformers>=4.40.0`, or you might encounter the following error:
```
KeyError: 'qwen2_moe'
```
## Quickstart
Here provides a code snippet with `apply_chat_template` to show you how to load the tokenizer and model and how to generate contents.
```python
from transformers import AutoModelForCausalLM, AutoTokenizer
device = "cuda" # the device to load the model onto
model = AutoModelForCausalLM.from_pretrained(
"Qwen/Qwen2-57B-A14B-Instruct",
torch_dtype="auto",
device_map="auto"
)
tokenizer = AutoTokenizer.from_pretrained("Qwen/Qwen2-57B-A14B-Instruct")
prompt = "Give me a short introduction to large language model."
messages = [
{"role": "system", "content": "You are a helpful assistant."},
{"role": "user", "content": prompt}
]
text = tokenizer.apply_chat_template(
messages,
tokenize=False,
add_generation_prompt=True
)
model_inputs = tokenizer([text], return_tensors="pt").to(device)
generated_ids = model.generate(
model_inputs.input_ids,
max_new_tokens=512
)
generated_ids = [
output_ids[len(input_ids):] for input_ids, output_ids in zip(model_inputs.input_ids, generated_ids)
]
response = tokenizer.batch_decode(generated_ids, skip_special_tokens=True)[0]
```
### Processing Long Texts
To handle extensive inputs exceeding 32,768 tokens, we utilize [YARN](https://arxiv.org/abs/2309.00071), a technique for enhancing model length extrapolation, ensuring optimal performance on lengthy texts.
For deployment, we recommend using vLLM. You can enable the long-context capabilities by following these steps:
1. **Install vLLM**: Ensure you have the latest version from the main branch of [vLLM](https://github.com/vllm-project/vllm).
2. **Configure Model Settings**: After downloading the model weights, modify the `config.json` file by including the below snippet:
```json
{
"architectures": [
"Qwen2MoeForCausalLM"
],
// ...
"vocab_size": 152064,
// adding the following snippets
"rope_scaling": {
"factor": 2.0,
"original_max_position_embeddings": 32768,
"type": "yarn"
}
}
```
This snippet enable YARN to support longer contexts.
3. **Model Deployment**: Utilize vLLM to deploy your model. For instance, you can set up an openAI-like server using the command:
```bash
python -m vllm.entrypoints.openai.api_server --served-model-name Qwen2-57B-A14B-Instruct --model path/to/weights
```
Then you can access the Chat API by:
```bash
curl http://localhost:8000/v1/chat/completions \
-H "Content-Type: application/json" \
-d '{
"model": "Qwen2-57B-A14B-Instruct",
"messages": [
{"role": "system", "content": "You are a helpful assistant."},
{"role": "user", "content": "Your Long Input Here."}
]
}'
```
For further usage instructions of vLLM, please refer to our [Github](https://github.com/QwenLM/Qwen2).
**Note**: Presently, vLLM only supports static YARN, which means the scaling factor remains constant regardless of input length, **potentially impacting performance on shorter texts**. We advise adding the `rope_scaling` configuration only when processing long contexts is required.
## Evaluation
We briefly compare Qwen2-57B-A14B-Instruct with similar-sized instruction-tuned LLMs, including Qwen1.5-32B-Chat. The results are shown as follows:
| Datasets | Mixtral-8x7B-Instruct-v0.1 | Yi-1.5-34B-Chat | Qwen1.5-32B-Chat | **Qwen2-57B-A14B-Instruct** |
| :--- | :---: | :---: | :---: | :---: |
|Architecture | MoE | Dense | Dense | MoE |
|#Activated Params | 12B | 34B | 32B | 14B |
|#Params | 47B | 34B | 32B | 57B |
| _**English**_ | | | | |
| MMLU | 71.4 | **76.8** | 74.8 | 75.4 |
| MMLU-Pro | 43.3 | 52.3 | 46.4 | **52.8** |
| GPQA | - | - | 30.8 | **34.3** |
| TheroemQA | - | - | 30.9 | **33.1** |
| MT-Bench | 8.30 | 8.50 | 8.30 | **8.55** |
| _**Coding**_ | | | | |
| HumanEval | 45.1 | 75.2 | 68.3 | **79.9** |
| MBPP | 59.5 | **74.6** | 67.9 | 70.9 |
| MultiPL-E | - | - | 50.7 | **66.4** |
| EvalPlus | 48.5 | - | 63.6 | **71.6** |
| LiveCodeBench | 12.3 | - | 15.2 | **25.5** |
| _**Mathematics**_ | | | | |
| GSM8K | 65.7 | **90.2** | 83.6 | 79.6 |
| MATH | 30.7 | **50.1** | 42.4 | 49.1 |
| _**Chinese**_ | | | | |
| C-Eval | - | - | 76.7 | 80.5 |
| AlignBench | 5.70 | 7.20 | 7.19 | **7.36** |
## Citation
If you find our work helpful, feel free to give us a cite.
```
@article{qwen2,
title={Qwen2 Technical Report},
year={2024}
}
``` |
openai/whisper-large | openai | "2024-02-29T10:57:46Z" | 37,747 | 447 | transformers | [
"transformers",
"pytorch",
"tf",
"jax",
"safetensors",
"whisper",
"automatic-speech-recognition",
"audio",
"hf-asr-leaderboard",
"en",
"zh",
"de",
"es",
"ru",
"ko",
"fr",
"ja",
"pt",
"tr",
"pl",
"ca",
"nl",
"ar",
"sv",
"it",
"id",
"hi",
"fi",
"vi",
"he",
"uk",
"el",
"ms",
"cs",
"ro",
"da",
"hu",
"ta",
"no",
"th",
"ur",
"hr",
"bg",
"lt",
"la",
"mi",
"ml",
"cy",
"sk",
"te",
"fa",
"lv",
"bn",
"sr",
"az",
"sl",
"kn",
"et",
"mk",
"br",
"eu",
"is",
"hy",
"ne",
"mn",
"bs",
"kk",
"sq",
"sw",
"gl",
"mr",
"pa",
"si",
"km",
"sn",
"yo",
"so",
"af",
"oc",
"ka",
"be",
"tg",
"sd",
"gu",
"am",
"yi",
"lo",
"uz",
"fo",
"ht",
"ps",
"tk",
"nn",
"mt",
"sa",
"lb",
"my",
"bo",
"tl",
"mg",
"as",
"tt",
"haw",
"ln",
"ha",
"ba",
"jw",
"su",
"arxiv:2212.04356",
"license:apache-2.0",
"model-index",
"endpoints_compatible",
"region:us"
] | automatic-speech-recognition | "2022-09-26T06:56:04Z" | ---
language:
- en
- zh
- de
- es
- ru
- ko
- fr
- ja
- pt
- tr
- pl
- ca
- nl
- ar
- sv
- it
- id
- hi
- fi
- vi
- he
- uk
- el
- ms
- cs
- ro
- da
- hu
- ta
- no
- th
- ur
- hr
- bg
- lt
- la
- mi
- ml
- cy
- sk
- te
- fa
- lv
- bn
- sr
- az
- sl
- kn
- et
- mk
- br
- eu
- is
- hy
- ne
- mn
- bs
- kk
- sq
- sw
- gl
- mr
- pa
- si
- km
- sn
- yo
- so
- af
- oc
- ka
- be
- tg
- sd
- gu
- am
- yi
- lo
- uz
- fo
- ht
- ps
- tk
- nn
- mt
- sa
- lb
- my
- bo
- tl
- mg
- as
- tt
- haw
- ln
- ha
- ba
- jw
- su
tags:
- audio
- automatic-speech-recognition
- hf-asr-leaderboard
widget:
- example_title: Librispeech sample 1
src: https://cdn-media.huggingface.co/speech_samples/sample1.flac
- example_title: Librispeech sample 2
src: https://cdn-media.huggingface.co/speech_samples/sample2.flac
model-index:
- name: whisper-large
results:
- task:
name: Automatic Speech Recognition
type: automatic-speech-recognition
dataset:
name: LibriSpeech (clean)
type: librispeech_asr
config: clean
split: test
args:
language: en
metrics:
- name: Test WER
type: wer
value: 3.0
- task:
name: Automatic Speech Recognition
type: automatic-speech-recognition
dataset:
name: LibriSpeech (other)
type: librispeech_asr
config: other
split: test
args:
language: en
metrics:
- name: Test WER
type: wer
value: 5.4
- task:
name: Automatic Speech Recognition
type: automatic-speech-recognition
dataset:
name: Common Voice 11.0
type: mozilla-foundation/common_voice_11_0
config: hi
split: test
args:
language: hi
metrics:
- name: Test WER
type: wer
value: 54.8
pipeline_tag: automatic-speech-recognition
license: apache-2.0
---
# Whisper
Whisper is a pre-trained model for automatic speech recognition (ASR) and speech translation. Trained on 680k hours
of labelled data, Whisper models demonstrate a strong ability to generalise to many datasets and domains **without** the need
for fine-tuning.
Whisper was proposed in the paper [Robust Speech Recognition via Large-Scale Weak Supervision](https://arxiv.org/abs/2212.04356)
by Alec Radford et al from OpenAI. The original code repository can be found [here](https://github.com/openai/whisper).
<div class="course-tip course-tip-orange bg-gradient-to-br dark:bg-gradient-to-r before:border-orange-500 dark:before:border-orange-800 from-orange-50 dark:from-gray-900 to-white dark:to-gray-950 border border-orange-50 text-orange-700 dark:text-gray-400">
<p><b>Update:</b> following the release of the paper, the Whisper authors announced a <a href="ttps://huggingface.co/openai/whisper-large-v2"> large-v2</a> model trained for 2.5x more epochs with regularization. This <a href="ttps://huggingface.co/openai/whisper-large-v2"> large-v2</a> model surpasses the performance of the large model, with no architecture changes. Thus, it is recommended that the <a href="ttps://huggingface.co/openai/whisper-large-v2"> large-v2</a> model is used in-place of the original large model. </p>
</div>
**Disclaimer**: Content for this model card has partly been written by the Hugging Face team, and parts of it were
copied and pasted from the original model card.
## Model details
Whisper is a Transformer based encoder-decoder model, also referred to as a _sequence-to-sequence_ model.
It was trained on 680k hours of labelled speech data annotated using large-scale weak supervision.
The models were trained on either English-only data or multilingual data. The English-only models were trained
on the task of speech recognition. The multilingual models were trained on both speech recognition and speech
translation. For speech recognition, the model predicts transcriptions in the *same* language as the audio.
For speech translation, the model predicts transcriptions to a *different* language to the audio.
Whisper checkpoints come in five configurations of varying model sizes.
The smallest four are trained on either English-only or multilingual data.
The largest checkpoints are multilingual only. All ten of the pre-trained checkpoints
are available on the [Hugging Face Hub](https://huggingface.co/models?search=openai/whisper). The
checkpoints are summarised in the following table with links to the models on the Hub:
| Size | Parameters | English-only | Multilingual |
|----------|------------|------------------------------------------------------|-----------------------------------------------------|
| tiny | 39 M | [✓](https://huggingface.co/openai/whisper-tiny.en) | [✓](https://huggingface.co/openai/whisper-tiny) |
| base | 74 M | [✓](https://huggingface.co/openai/whisper-base.en) | [✓](https://huggingface.co/openai/whisper-base) |
| small | 244 M | [✓](https://huggingface.co/openai/whisper-small.en) | [✓](https://huggingface.co/openai/whisper-small) |
| medium | 769 M | [✓](https://huggingface.co/openai/whisper-medium.en) | [✓](https://huggingface.co/openai/whisper-medium) |
| large | 1550 M | x | [✓](https://huggingface.co/openai/whisper-large) |
| large-v2 | 1550 M | x | [✓](https://huggingface.co/openai/whisper-large-v2) |
# Usage
To transcribe audio samples, the model has to be used alongside a [`WhisperProcessor`](https://huggingface.co/docs/transformers/model_doc/whisper#transformers.WhisperProcessor).
The `WhisperProcessor` is used to:
1. Pre-process the audio inputs (converting them to log-Mel spectrograms for the model)
2. Post-process the model outputs (converting them from tokens to text)
The model is informed of which task to perform (transcription or translation) by passing the appropriate "context tokens". These context tokens
are a sequence of tokens that are given to the decoder at the start of the decoding process, and take the following order:
1. The transcription always starts with the `<|startoftranscript|>` token
2. The second token is the language token (e.g. `<|en|>` for English)
3. The third token is the "task token". It can take one of two values: `<|transcribe|>` for speech recognition or `<|translate|>` for speech translation
4. In addition, a `<|notimestamps|>` token is added if the model should not include timestamp prediction
Thus, a typical sequence of context tokens might look as follows:
```
<|startoftranscript|> <|en|> <|transcribe|> <|notimestamps|>
```
Which tells the model to decode in English, under the task of speech recognition, and not to predict timestamps.
These tokens can either be forced or un-forced. If they are forced, the model is made to predict each token at
each position. This allows one to control the output language and task for the Whisper model. If they are un-forced,
the Whisper model will automatically predict the output langauge and task itself.
The context tokens can be set accordingly:
```python
model.config.forced_decoder_ids = WhisperProcessor.get_decoder_prompt_ids(language="english", task="transcribe")
```
Which forces the model to predict in English under the task of speech recognition.
## Transcription
### English to English
In this example, the context tokens are 'unforced', meaning the model automatically predicts the output language
(English) and task (transcribe).
```python
>>> from transformers import WhisperProcessor, WhisperForConditionalGeneration
>>> from datasets import load_dataset
>>> # load model and processor
>>> processor = WhisperProcessor.from_pretrained("openai/whisper-large")
>>> model = WhisperForConditionalGeneration.from_pretrained("openai/whisper-large")
>>> model.config.forced_decoder_ids = None
>>> # load dummy dataset and read audio files
>>> ds = load_dataset("hf-internal-testing/librispeech_asr_dummy", "clean", split="validation")
>>> sample = ds[0]["audio"]
>>> input_features = processor(sample["array"], sampling_rate=sample["sampling_rate"], return_tensors="pt").input_features
>>> # generate token ids
>>> predicted_ids = model.generate(input_features)
>>> # decode token ids to text
>>> transcription = processor.batch_decode(predicted_ids, skip_special_tokens=False)
['<|startoftranscript|><|en|><|transcribe|><|notimestamps|> Mr. Quilter is the apostle of the middle classes and we are glad to welcome his gospel.<|endoftext|>']
>>> transcription = processor.batch_decode(predicted_ids, skip_special_tokens=True)
[' Mr. Quilter is the apostle of the middle classes and we are glad to welcome his gospel.']
```
The context tokens can be removed from the start of the transcription by setting `skip_special_tokens=True`.
### French to French
The following example demonstrates French to French transcription by setting the decoder ids appropriately.
```python
>>> from transformers import WhisperProcessor, WhisperForConditionalGeneration
>>> from datasets import Audio, load_dataset
>>> # load model and processor
>>> processor = WhisperProcessor.from_pretrained("openai/whisper-large")
>>> model = WhisperForConditionalGeneration.from_pretrained("openai/whisper-large")
>>> forced_decoder_ids = processor.get_decoder_prompt_ids(language="french", task="transcribe")
>>> # load streaming dataset and read first audio sample
>>> ds = load_dataset("common_voice", "fr", split="test", streaming=True)
>>> ds = ds.cast_column("audio", Audio(sampling_rate=16_000))
>>> input_speech = next(iter(ds))["audio"]
>>> input_features = processor(input_speech["array"], sampling_rate=input_speech["sampling_rate"], return_tensors="pt").input_features
>>> # generate token ids
>>> predicted_ids = model.generate(input_features, forced_decoder_ids=forced_decoder_ids)
>>> # decode token ids to text
>>> transcription = processor.batch_decode(predicted_ids)
['<|startoftranscript|><|fr|><|transcribe|><|notimestamps|> Un vrai travail intéressant va enfin être mené sur ce sujet.<|endoftext|>']
>>> transcription = processor.batch_decode(predicted_ids, skip_special_tokens=True)
[' Un vrai travail intéressant va enfin être mené sur ce sujet.']
```
## Translation
Setting the task to "translate" forces the Whisper model to perform speech translation.
### French to English
```python
>>> from transformers import WhisperProcessor, WhisperForConditionalGeneration
>>> from datasets import Audio, load_dataset
>>> # load model and processor
>>> processor = WhisperProcessor.from_pretrained("openai/whisper-large")
>>> model = WhisperForConditionalGeneration.from_pretrained("openai/whisper-large")
>>> forced_decoder_ids = processor.get_decoder_prompt_ids(language="french", task="translate")
>>> # load streaming dataset and read first audio sample
>>> ds = load_dataset("common_voice", "fr", split="test", streaming=True)
>>> ds = ds.cast_column("audio", Audio(sampling_rate=16_000))
>>> input_speech = next(iter(ds))["audio"]
>>> input_features = processor(input_speech["array"], sampling_rate=input_speech["sampling_rate"], return_tensors="pt").input_features
>>> # generate token ids
>>> predicted_ids = model.generate(input_features, forced_decoder_ids=forced_decoder_ids)
>>> # decode token ids to text
>>> transcription = processor.batch_decode(predicted_ids, skip_special_tokens=True)
[' A very interesting work, we will finally be given on this subject.']
```
## Evaluation
This code snippet shows how to evaluate Whisper Large on [LibriSpeech test-clean](https://huggingface.co/datasets/librispeech_asr):
```python
>>> from datasets import load_dataset
>>> from transformers import WhisperForConditionalGeneration, WhisperProcessor
>>> import torch
>>> from evaluate import load
>>> librispeech_test_clean = load_dataset("librispeech_asr", "clean", split="test")
>>> processor = WhisperProcessor.from_pretrained("openai/whisper-large")
>>> model = WhisperForConditionalGeneration.from_pretrained("openai/whisper-large").to("cuda")
>>> def map_to_pred(batch):
>>> audio = batch["audio"]
>>> input_features = processor(audio["array"], sampling_rate=audio["sampling_rate"], return_tensors="pt").input_features
>>> batch["reference"] = processor.tokenizer._normalize(batch['text'])
>>>
>>> with torch.no_grad():
>>> predicted_ids = model.generate(input_features.to("cuda"))[0]
>>> transcription = processor.decode(predicted_ids)
>>> batch["prediction"] = processor.tokenizer._normalize(transcription)
>>> return batch
>>> result = librispeech_test_clean.map(map_to_pred)
>>> wer = load("wer")
>>> print(100 * wer.compute(references=result["reference"], predictions=result["prediction"]))
3.0003583080317572
```
## Long-Form Transcription
The Whisper model is intrinsically designed to work on audio samples of up to 30s in duration. However, by using a chunking
algorithm, it can be used to transcribe audio samples of up to arbitrary length. This is possible through Transformers
[`pipeline`](https://huggingface.co/docs/transformers/main_classes/pipelines#transformers.AutomaticSpeechRecognitionPipeline)
method. Chunking is enabled by setting `chunk_length_s=30` when instantiating the pipeline. With chunking enabled, the pipeline
can be run with batched inference. It can also be extended to predict sequence level timestamps by passing `return_timestamps=True`:
```python
>>> import torch
>>> from transformers import pipeline
>>> from datasets import load_dataset
>>> device = "cuda:0" if torch.cuda.is_available() else "cpu"
>>> pipe = pipeline(
>>> "automatic-speech-recognition",
>>> model="openai/whisper-large",
>>> chunk_length_s=30,
>>> device=device,
>>> )
>>> ds = load_dataset("hf-internal-testing/librispeech_asr_dummy", "clean", split="validation")
>>> sample = ds[0]["audio"]
>>> prediction = pipe(sample.copy(), batch_size=8)["text"]
" Mr. Quilter is the apostle of the middle classes, and we are glad to welcome his gospel."
>>> # we can also return timestamps for the predictions
>>> prediction = pipe(sample.copy(), batch_size=8, return_timestamps=True)["chunks"]
[{'text': ' Mr. Quilter is the apostle of the middle classes and we are glad to welcome his gospel.',
'timestamp': (0.0, 5.44)}]
```
Refer to the blog post [ASR Chunking](https://huggingface.co/blog/asr-chunking) for more details on the chunking algorithm.
## Fine-Tuning
The pre-trained Whisper model demonstrates a strong ability to generalise to different datasets and domains. However,
its predictive capabilities can be improved further for certain languages and tasks through *fine-tuning*. The blog
post [Fine-Tune Whisper with 🤗 Transformers](https://huggingface.co/blog/fine-tune-whisper) provides a step-by-step
guide to fine-tuning the Whisper model with as little as 5 hours of labelled data.
### Evaluated Use
The primary intended users of these models are AI researchers studying robustness, generalization, capabilities, biases, and constraints of the current model. However, Whisper is also potentially quite useful as an ASR solution for developers, especially for English speech recognition. We recognize that once models are released, it is impossible to restrict access to only “intended” uses or to draw reasonable guidelines around what is or is not research.
The models are primarily trained and evaluated on ASR and speech translation to English tasks. They show strong ASR results in ~10 languages. They may exhibit additional capabilities, particularly if fine-tuned on certain tasks like voice activity detection, speaker classification, or speaker diarization but have not been robustly evaluated in these areas. We strongly recommend that users perform robust evaluations of the models in a particular context and domain before deploying them.
In particular, we caution against using Whisper models to transcribe recordings of individuals taken without their consent or purporting to use these models for any kind of subjective classification. We recommend against use in high-risk domains like decision-making contexts, where flaws in accuracy can lead to pronounced flaws in outcomes. The models are intended to transcribe and translate speech, use of the model for classification is not only not evaluated but also not appropriate, particularly to infer human attributes.
## Training Data
The models are trained on 680,000 hours of audio and the corresponding transcripts collected from the internet. 65% of this data (or 438,000 hours) represents English-language audio and matched English transcripts, roughly 18% (or 126,000 hours) represents non-English audio and English transcripts, while the final 17% (or 117,000 hours) represents non-English audio and the corresponding transcript. This non-English data represents 98 different languages.
As discussed in [the accompanying paper](https://cdn.openai.com/papers/whisper.pdf), we see that performance on transcription in a given language is directly correlated with the amount of training data we employ in that language.
## Performance and Limitations
Our studies show that, over many existing ASR systems, the models exhibit improved robustness to accents, background noise, technical language, as well as zero shot translation from multiple languages into English; and that accuracy on speech recognition and translation is near the state-of-the-art level.
However, because the models are trained in a weakly supervised manner using large-scale noisy data, the predictions may include texts that are not actually spoken in the audio input (i.e. hallucination). We hypothesize that this happens because, given their general knowledge of language, the models combine trying to predict the next word in audio with trying to transcribe the audio itself.
Our models perform unevenly across languages, and we observe lower accuracy on low-resource and/or low-discoverability languages or languages where we have less training data. The models also exhibit disparate performance on different accents and dialects of particular languages, which may include higher word error rate across speakers of different genders, races, ages, or other demographic criteria. Our full evaluation results are presented in [the paper accompanying this release](https://cdn.openai.com/papers/whisper.pdf).
In addition, the sequence-to-sequence architecture of the model makes it prone to generating repetitive texts, which can be mitigated to some degree by beam search and temperature scheduling but not perfectly. Further analysis on these limitations are provided in [the paper](https://cdn.openai.com/papers/whisper.pdf). It is likely that this behavior and hallucinations may be worse on lower-resource and/or lower-discoverability languages.
## Broader Implications
We anticipate that Whisper models’ transcription capabilities may be used for improving accessibility tools. While Whisper models cannot be used for real-time transcription out of the box – their speed and size suggest that others may be able to build applications on top of them that allow for near-real-time speech recognition and translation. The real value of beneficial applications built on top of Whisper models suggests that the disparate performance of these models may have real economic implications.
There are also potential dual use concerns that come with releasing Whisper. While we hope the technology will be used primarily for beneficial purposes, making ASR technology more accessible could enable more actors to build capable surveillance technologies or scale up existing surveillance efforts, as the speed and accuracy allow for affordable automatic transcription and translation of large volumes of audio communication. Moreover, these models may have some capabilities to recognize specific individuals out of the box, which in turn presents safety concerns related both to dual use and disparate performance. In practice, we expect that the cost of transcription is not the limiting factor of scaling up surveillance projects.
### BibTeX entry and citation info
```bibtex
@misc{radford2022whisper,
doi = {10.48550/ARXIV.2212.04356},
url = {https://arxiv.org/abs/2212.04356},
author = {Radford, Alec and Kim, Jong Wook and Xu, Tao and Brockman, Greg and McLeavey, Christine and Sutskever, Ilya},
title = {Robust Speech Recognition via Large-Scale Weak Supervision},
publisher = {arXiv},
year = {2022},
copyright = {arXiv.org perpetual, non-exclusive license}
}
```
|
katuni4ka/tiny-random-qwen1.5-moe | katuni4ka | "2024-05-21T06:49:05Z" | 37,533 | 0 | transformers | [
"transformers",
"safetensors",
"qwen2_moe",
"text-generation",
"conversational",
"autotrain_compatible",
"endpoints_compatible",
"region:us"
] | text-generation | "2024-05-21T06:47:47Z" | Entry not found |
rinna/japanese-cloob-vit-b-16 | rinna | "2024-04-03T09:13:36Z" | 37,527 | 11 | transformers | [
"transformers",
"pytorch",
"cloob",
"feature-extraction",
"clip",
"vision",
"ja",
"arxiv:2110.11316",
"arxiv:2404.01657",
"license:apache-2.0",
"region:us"
] | feature-extraction | "2022-04-27T08:29:29Z" | ---
language: ja
thumbnail: https://github.com/rinnakk/japanese-pretrained-models/blob/master/rinna.png
license: apache-2.0
tags:
- feature-extraction
- clip
- cloob
- vision
inference: false
---
# rinna/japanese-cloob-vit-b-16

This is a Japanese [CLOOB (Contrastive Leave One Out Boost)](https://arxiv.org/abs/2110.11316) model trained by [rinna Co., Ltd.](https://corp.rinna.co.jp/).
Please see [japanese-clip](https://github.com/rinnakk/japanese-clip) for the other available models.
# How to use the model
1. Install package
```shell
$ pip install git+https://github.com/rinnakk/japanese-clip.git
```
2. Run
```python
import io
import requests
from PIL import Image
import torch
import japanese_clip as ja_clip
device = "cuda" if torch.cuda.is_available() else "cpu"
model, preprocess = ja_clip.load("rinna/japanese-cloob-vit-b-16", device=device)
tokenizer = ja_clip.load_tokenizer()
img = Image.open(io.BytesIO(requests.get('https://images.pexels.com/photos/2253275/pexels-photo-2253275.jpeg?auto=compress&cs=tinysrgb&dpr=3&h=750&w=1260').content))
image = preprocess(img).unsqueeze(0).to(device)
encodings = ja_clip.tokenize(
texts=["犬", "猫", "象"],
max_seq_len=77,
device=device,
tokenizer=tokenizer, # this is optional. if you don't pass, load tokenizer each time
)
with torch.no_grad():
image_features = model.get_image_features(image)
text_features = model.get_text_features(**encodings)
text_probs = (100.0 * image_features @ text_features.T).softmax(dim=-1)
print("Label probs:", text_probs) # prints: [[1.0, 0.0, 0.0]]
```
# Model architecture
The model was trained a ViT-B/16 Transformer architecture as an image encoder and uses a 12-layer BERT as a text encoder. The image encoder was initialized from the [AugReg `vit-base-patch16-224` model](https://github.com/google-research/vision_transformer).
# Training
The model was trained on [CC12M](https://github.com/google-research-datasets/conceptual-12m) translated the captions to Japanese.
# How to cite
~~~
@misc{rinna-japanese-cloob-vit-b-16,
title = {rinna/japanese-cloob-vit-b-16},
author={Shing, Makoto and Zhao, Tianyu and Sawada, Kei}
url = {https://huggingface.co/rinna/japanese-cloob-vit-b-16},
}
@inproceedings{sawada2024release,
title = {Release of Pre-Trained Models for the {J}apanese Language},
author = {Sawada, Kei and Zhao, Tianyu and Shing, Makoto and Mitsui, Kentaro and Kaga, Akio and Hono, Yukiya and Wakatsuki, Toshiaki and Mitsuda, Koh},
booktitle = {Proceedings of the 2024 Joint International Conference on Computational Linguistics, Language Resources and Evaluation (LREC-COLING 2024)},
month = {5},
year = {2024},
url = {https://arxiv.org/abs/2404.01657},
}
~~~
# License
[The Apache 2.0 license](https://www.apache.org/licenses/LICENSE-2.0) |
CohereForAI/aya-101 | CohereForAI | "2024-03-31T18:24:33Z" | 37,435 | 572 | transformers | [
"transformers",
"safetensors",
"t5",
"text2text-generation",
"afr",
"amh",
"ara",
"aze",
"bel",
"ben",
"bul",
"cat",
"ceb",
"ces",
"cym",
"dan",
"deu",
"ell",
"eng",
"epo",
"est",
"eus",
"fin",
"fil",
"fra",
"fry",
"gla",
"gle",
"glg",
"guj",
"hat",
"hau",
"heb",
"hin",
"hun",
"hye",
"ibo",
"ind",
"isl",
"ita",
"jav",
"jpn",
"kan",
"kat",
"kaz",
"khm",
"kir",
"kor",
"kur",
"lao",
"lav",
"lat",
"lit",
"ltz",
"mal",
"mar",
"mkd",
"mlg",
"mlt",
"mon",
"mri",
"msa",
"mya",
"nep",
"nld",
"nor",
"nso",
"nya",
"ory",
"pan",
"pes",
"pol",
"por",
"pus",
"ron",
"rus",
"sin",
"slk",
"slv",
"smo",
"sna",
"snd",
"som",
"sot",
"spa",
"sqi",
"srp",
"sun",
"swa",
"swe",
"tam",
"tel",
"tgk",
"tha",
"tur",
"twi",
"ukr",
"urd",
"uzb",
"vie",
"xho",
"yid",
"yor",
"zho",
"zul",
"dataset:CohereForAI/xP3x",
"dataset:CohereForAI/aya_dataset",
"dataset:CohereForAI/aya_collection",
"dataset:DataProvenanceInitiative/Commercially-Verified-Licenses",
"dataset:CohereForAI/aya_evaluation_suite",
"arxiv:2402.07827",
"license:apache-2.0",
"autotrain_compatible",
"endpoints_compatible",
"text-generation-inference",
"region:us"
] | text2text-generation | "2024-02-08T18:08:25Z" | ---
license: apache-2.0
datasets:
- CohereForAI/xP3x
- CohereForAI/aya_dataset
- CohereForAI/aya_collection
- DataProvenanceInitiative/Commercially-Verified-Licenses
- CohereForAI/aya_evaluation_suite
language:
- afr
- amh
- ara
- aze
- bel
- ben
- bul
- cat
- ceb
- ces
- cym
- dan
- deu
- ell
- eng
- epo
- est
- eus
- fin
- fil
- fra
- fry
- gla
- gle
- glg
- guj
- hat
- hau
- heb
- hin
- hun
- hye
- ibo
- ind
- isl
- ita
- jav
- jpn
- kan
- kat
- kaz
- khm
- kir
- kor
- kur
- lao
- lav
- lat
- lit
- ltz
- mal
- mar
- mkd
- mlg
- mlt
- mon
- mri
- msa
- mya
- nep
- nld
- nor
- nso
- nya
- ory
- pan
- pes
- pol
- por
- pus
- ron
- rus
- sin
- slk
- slv
- smo
- sna
- snd
- som
- sot
- spa
- sqi
- srp
- sun
- swa
- swe
- tam
- tel
- tgk
- tha
- tur
- twi
- ukr
- urd
- uzb
- vie
- xho
- yid
- yor
- zho
- zul
metrics:
- accuracy
- bleu
---
<img src="aya-fig1.png" alt="Aya model summary image" width="800" style="margin-left:'auto' margin-right:'auto' display:'block'"/>
# Model Card for Aya 101
## Model Summary
> The Aya model is a massively multilingual generative language model that follows instructions in 101 languages.
> Aya outperforms [mT0](https://huggingface.co/bigscience/mt0-xxl) and [BLOOMZ](https://huggingface.co/bigscience/bloomz) a wide variety of automatic and human evaluations despite covering double the number of languages.
> The Aya model is trained using [xP3x](https://huggingface.co/datasets/CohereForAI/xP3x), [Aya Dataset](https://huggingface.co/datasets/CohereForAI/aya_dataset), [Aya Collection](https://huggingface.co/datasets/CohereForAI/aya_collection), a subset of [DataProvenance collection](https://huggingface.co/datasets/DataProvenanceInitiative/Commercially-Verified-Licenses) and ShareGPT-Command.
> We release the checkpoints under a Apache-2.0 license to further our mission of multilingual technologies empowering a
> multilingual world.
- **Developed by:** [Cohere For AI](https://cohere.for.ai)
- **Model type:** a Transformer style autoregressive massively multilingual language model.
- **Paper**: [Aya Model: An Instruction Finetuned Open-Access Multilingual Language Model](https://arxiv.org/abs/2402.07827)
- **Point of Contact**: Cohere For AI: [cohere.for.ai](https://cohere.for.ai)
- **Languages**: Refer to the list of languages in the `language` section of this model card.
- **License**: Apache-2.0
- **Model**: [Aya-101](https://huggingface.co/CohereForAI/aya-101)
- **Model Size**: 13 billion parameters
- **Datasets**: [xP3x](https://huggingface.co/datasets/CohereForAI/xP3x), [Aya Dataset](https://huggingface.co/datasets/CohereForAI/aya_dataset), [Aya Collection](https://huggingface.co/datasets/CohereForAI/aya_collection), [DataProvenance collection](https://huggingface.co/datasets/DataProvenanceInitiative/Commercially-Verified-Licenses), ShareGPT-Command.
## Use
```python
# pip install -q transformers
from transformers import AutoModelForSeq2SeqLM, AutoTokenizer
checkpoint = "CohereForAI/aya-101"
tokenizer = AutoTokenizer.from_pretrained(checkpoint)
aya_model = AutoModelForSeq2SeqLM.from_pretrained(checkpoint)
# Turkish to English translation
tur_inputs = tokenizer.encode("Translate to English: Aya cok dilli bir dil modelidir.", return_tensors="pt")
tur_outputs = aya_model.generate(tur_inputs, max_new_tokens=128)
print(tokenizer.decode(tur_outputs[0]))
# Aya is a multi-lingual language model
# Q: Why are there so many languages in India?
hin_inputs = tokenizer.encode("भारत में इतनी सारी भाषाएँ क्यों हैं?", return_tensors="pt")
hin_outputs = aya_model.generate(hin_inputs, max_new_tokens=128)
print(tokenizer.decode(hin_outputs[0]))
# Expected output: भारत में कई भाषाएँ हैं और विभिन्न भाषाओं के बोली जाने वाले लोग हैं। यह विभिन्नता भाषाई विविधता और सांस्कृतिक विविधता का परिणाम है। Translates to "India has many languages and people speaking different languages. This diversity is the result of linguistic diversity and cultural diversity."
```
## Model Details
### Finetuning
- Architecture: Same as [mt5-xxl](https://huggingface.co/google/mt5-xxl)
- Number of Samples seen during Finetuning: 25M
- Batch size: 256
- Hardware: TPUv4-128
- Software: T5X, Jax
### Data Sources
The Aya model is trained on the following datasets:
- [xP3x](https://huggingface.co/datasets/CohereForAI/xP3x)
- [Aya Dataset](https://huggingface.co/datasets/CohereForAI/aya_dataset)
- [Aya Collection](https://huggingface.co/datasets/CohereForAI/aya_collection)
- [DataProvenance collection](https://huggingface.co/datasets/DataProvenanceInitiative/Commercially-Verified-Licenses)
- ShareGPT-Command
All datasets are subset to the 101 languages supported by [mT5](https://huggingface.co/google/mt5-xxl). See the [paper](https://arxiv.org/abs/2402.07827) for details about filtering and pruning.
## Evaluation
We refer to Section 5 from our paper for multilingual eval across 99 languages – including discriminative and generative tasks, human evaluation, and simulated win rates that cover both held-out tasks and in-distribution performance.
## Bias, Risks, and Limitations
For a detailed overview of our effort at safety mitigation and benchmarking toxicity and bias across multiple languages, we refer to Sections 6 and 7 of our paper: [Aya Model: An Instruction Finetuned Open-Access Multilingual Language Model](https://arxiv.org/abs/2402.07827).
We hope that the release of the Aya model will make community-based redteaming efforts possible, by exposing an open-source massively-multilingual model for community research.
## Citation
**BibTeX:**
```
@article{üstün2024aya,
title={Aya Model: An Instruction Finetuned Open-Access Multilingual Language Model},
author={Ahmet Üstün and Viraat Aryabumi and Zheng-Xin Yong and Wei-Yin Ko and Daniel D'souza and Gbemileke Onilude and Neel Bhandari and Shivalika Singh and Hui-Lee Ooi and Amr Kayid and Freddie Vargus and Phil Blunsom and Shayne Longpre and Niklas Muennighoff and Marzieh Fadaee and Julia Kreutzer and Sara Hooker},
journal={arXiv preprint arXiv:2402.07827},
year={2024}
}
```
## Languages Covered
<details>
<summary>Click to see Languages Covered</summary>
Below is the list of languages used in finetuning the Aya Model. We group languages into higher-, mid-, and lower-resourcedness based on a language classification by [Joshi et. al, 2020](https://microsoft.github.io/linguisticdiversity/). For further details, we refer to our [paper](https://arxiv.org/abs/2402.07827)
| ISO Code | Language Name | Script | Family | Subgrouping | Resourcedness |
| :------- | :-------------- | :----------: | :-------------: | :---------------: | :-----------: |
| afr | Afrikaans | Latin | Indo-European | Germanic | Mid |
| amh | Amharic | Ge'ez | Afro-Asiatic | Semitic | Low |
| ara | Arabic | Arabic | Afro-Asiatic | Semitic | High |
| aze | Azerbaijani | Arabic/Latin | Turkic | Common Turkic | Low |
| bel | Belarusian | Cyrillic | Indo-European | Balto-Slavic | Mid |
| ben | Bengali | Bengali | Indo-European | Indo-Aryan | Mid |
| bul | Bulgarian | Cyrillic | Indo-European | Balto-Slavic | Mid |
| cat | Catalan | Latin | Indo-European | Italic | High |
| ceb | Cebuano | Latin | Austronesian | Malayo-Polynesian | Mid |
| ces | Czech | Latin | Indo-European | Balto-Slavic | High |
| cym | Welsh | Latin | Indo-European | Celtic | Low |
| dan | Danish | Latin | Indo-European | Germanic | Mid |
| deu | German | Latin | Indo-European | Germanic | High |
| ell | Greek | Greek | Indo-European | Graeco-Phrygian | Mid |
| eng | English | Latin | Indo-European | Germanic | High |
| epo | Esperanto | Latin | Constructed | Esperantic | Low |
| est | Estonian | Latin | Uralic | Finnic | Mid |
| eus | Basque | Latin | Basque | - | High |
| fin | Finnish | Latin | Uralic | Finnic | High |
| fil | Tagalog | Latin | Austronesian | Malayo-Polynesian | Mid |
| fra | French | Latin | Indo-European | Italic | High |
| fry | Western Frisian | Latin | Indo-European | Germanic | Low |
| gla | Scottish Gaelic | Latin | Indo-European | Celtic | Low |
| gle | Irish | Latin | Indo-European | Celtic | Low |
| glg | Galician | Latin | Indo-European | Italic | Mid |
| guj | Gujarati | Gujarati | Indo-European | Indo-Aryan | Low |
| hat | Haitian Creole | Latin | Indo-European | Italic | Low |
| hau | Hausa | Latin | Afro-Asiatic | Chadic | Low |
| heb | Hebrew | Hebrew | Afro-Asiatic | Semitic | Mid |
| hin | Hindi | Devanagari | Indo-European | Indo-Aryan | High |
| hun | Hungarian | Latin | Uralic | - | High |
| hye | Armenian | Armenian | Indo-European | Armenic | Low |
| ibo | Igbo | Latin | Atlantic-Congo | Benue-Congo | Low |
| ind | Indonesian | Latin | Austronesian | Malayo-Polynesian | Mid |
| isl | Icelandic | Latin | Indo-European | Germanic | Low |
| ita | Italian | Latin | Indo-European | Italic | High |
| jav | Javanese | Latin | Austronesian | Malayo-Polynesian | Low |
| jpn | Japanese | Japanese | Japonic | Japanesic | High |
| kan | Kannada | Kannada | Dravidian | South Dravidian | Low |
| kat | Georgian | Georgian | Kartvelian | Georgian-Zan | Mid |
| kaz | Kazakh | Cyrillic | Turkic | Common Turkic | Mid |
| khm | Khmer | Khmer | Austroasiatic | Khmeric | Low |
| kir | Kyrgyz | Cyrillic | Turkic | Common Turkic | Low |
| kor | Korean | Hangul | Koreanic | Korean | High |
| kur | Kurdish | Latin | Indo-European | Iranian | Low |
| lao | Lao | Lao | Tai-Kadai | Kam-Tai | Low |
| lav | Latvian | Latin | Indo-European | Balto-Slavic | Mid |
| lat | Latin | Latin | Indo-European | Italic | Mid |
| lit | Lithuanian | Latin | Indo-European | Balto-Slavic | Mid |
| ltz | Luxembourgish | Latin | Indo-European | Germanic | Low |
| mal | Malayalam | Malayalam | Dravidian | South Dravidian | Low |
| mar | Marathi | Devanagari | Indo-European | Indo-Aryan | Low |
| mkd | Macedonian | Cyrillic | Indo-European | Balto-Slavic | Low |
| mlg | Malagasy | Latin | Austronesian | Malayo-Polynesian | Low |
| mlt | Maltese | Latin | Afro-Asiatic | Semitic | Low |
| mon | Mongolian | Cyrillic | Mongolic-Khitan | Mongolic | Low |
| mri | Maori | Latin | Austronesian | Malayo-Polynesian | Low |
| msa | Malay | Latin | Austronesian | Malayo-Polynesian | Mid |
| mya | Burmese | Myanmar | Sino-Tibetan | Burmo-Qiangic | Low |
| nep | Nepali | Devanagari | Indo-European | Indo-Aryan | Low |
| nld | Dutch | Latin | Indo-European | Germanic | High |
| nor | Norwegian | Latin | Indo-European | Germanic | Low |
| nso | Northern Sotho | Latin | Atlantic-Congo | Benue-Congo | Low |
| nya | Chichewa | Latin | Atlantic-Congo | Benue-Congo | Low |
| ory | Oriya | Oriya | Indo-European | Indo-Aryan | Low |
| pan | Punjabi | Gurmukhi | Indo-European | Indo-Aryan | Low |
| pes | Persian | Arabic | Indo-European | Iranian | High |
| pol | Polish | Latin | Indo-European | Balto-Slavic | High |
| por | Portuguese | Latin | Indo-European | Italic | High |
| pus | Pashto | Arabic | Indo-European | Iranian | Low |
| ron | Romanian | Latin | Indo-European | Italic | Mid |
| rus | Russian | Cyrillic | Indo-European | Balto-Slavic | High |
| sin | Sinhala | Sinhala | Indo-European | Indo-Aryan | Low |
| slk | Slovak | Latin | Indo-European | Balto-Slavic | Mid |
| slv | Slovenian | Latin | Indo-European | Balto-Slavic | Mid |
| smo | Samoan | Latin | Austronesian | Malayo-Polynesian | Low |
| sna | Shona | Latin | Indo-European | Indo-Aryan | Low |
| snd | Sindhi | Arabic | Indo-European | Indo-Aryan | Low |
| som | Somali | Latin | Afro-Asiatic | Cushitic | Low |
| sot | Southern Sotho | Latin | Atlantic-Congo | Benue-Congo | Low |
| spa | Spanish | Latin | Indo-European | Italic | High |
| sqi | Albanian | Latin | Indo-European | Albanian | Low |
| srp | Serbian | Cyrillic | Indo-European | Balto-Slavic | High |
| sun | Sundanese | Latin | Austronesian | Malayo-Polynesian | Low |
| swa | Swahili | Latin | Atlantic-Congo | Benue-Congo | Low |
| swe | Swedish | Latin | Indo-European | Germanic | High |
| tam | Tamil | Tamil | Dravidian | South Dravidian | Mid |
| tel | Telugu | Telugu | Dravidian | South Dravidian | Low |
| tgk | Tajik | Cyrillic | Indo-European | Iranian | Low |
| tha | Thai | Thai | Tai-Kadai | Kam-Tai | Mid |
| tur | Turkish | Latin | Turkic | Common Turkic | High |
| twi | Twi | Latin | Atlantic-Congo | Niger-Congo | Low |
| ukr | Ukrainian | Cyrillic | Indo-European | Balto-Slavic | Mid |
| urd | Urdu | Arabic | Indo-European | Indo-Aryan | Mid |
| uzb | Uzbek | Latin | Turkic | Common Turkic | Mid |
| vie | Vietnamese | Latin | Austroasiatic | Vietic | High |
| xho | Xhosa | Latin | Atlantic-Congo | Benue-Congo | Low |
| yid | Yiddish | Hebrew | Indo-European | Germanic | Low |
| yor | Yoruba | Latin | Atlantic-Congo | Benue-Congo | Low |
| zho | Chinese | Han | Sino-Tibetan | Sinitic | High |
| zul | Zulu | Latin | Atlantic-Congo | Benue-Congo | Low |
</details>
## Model Card Contact
For errors in this model card, contact Ahmet or Viraat, `{ahmet, viraat} at cohere dot com`.
|
hotchpotch/japanese-reranker-cross-encoder-xsmall-v1 | hotchpotch | "2024-06-10T03:57:05Z" | 37,290 | 2 | sentence-transformers | [
"sentence-transformers",
"safetensors",
"xlm-roberta",
"ja",
"dataset:hotchpotch/JQaRA",
"dataset:shunk031/JGLUE",
"dataset:miracl/miracl",
"dataset:castorini/mr-tydi",
"dataset:unicamp-dl/mmarco",
"license:mit",
"region:us"
] | null | "2024-03-28T04:29:26Z" | ---
license: mit
datasets:
- hotchpotch/JQaRA
- shunk031/JGLUE
- miracl/miracl
- castorini/mr-tydi
- unicamp-dl/mmarco
language:
- ja
library_name: sentence-transformers
---
## hotchpotch/japanese-reranker-cross-encoder-xsmall-v1
日本語で学習させた Reranker (CrossEncoder) シリーズです。
| モデル名 | layers | hidden_size |
| ----------------------------------------------------------------------------------------------------------------------------------- | ------ | ----------- |
| [hotchpotch/japanese-reranker-cross-encoder-xsmall-v1](https://huggingface.co/hotchpotch/japanese-reranker-cross-encoder-xsmall-v1) | 6 | 384 |
| [hotchpotch/japanese-reranker-cross-encoder-small-v1](https://huggingface.co/hotchpotch/japanese-reranker-cross-encoder-small-v1) | 12 | 384 |
| [hotchpotch/japanese-reranker-cross-encoder-base-v1](https://huggingface.co/hotchpotch/japanese-reranker-cross-encoder-base-v1) | 12 | 768 |
| [hotchpotch/japanese-reranker-cross-encoder-large-v1](https://huggingface.co/hotchpotch/japanese-reranker-cross-encoder-large-v1) | 24 | 1024 |
| [hotchpotch/japanese-bge-reranker-v2-m3-v1](https://huggingface.co/hotchpotch/japanese-bge-reranker-v2-m3-v1) | 24 | 1024 |
Reranker についてや、技術レポート・評価等は以下を参考ください。
- [日本語最高性能のRerankerをリリース / そもそも Reranker とは?](https://secon.dev/entry/2024/04/02/070000-japanese-reranker-release/)
- [日本語 Reranker 作成のテクニカルレポート](https://secon.dev/entry/2024/04/02/080000-japanese-reranker-tech-report/)
## 使い方
### SentenceTransformers
```python
from sentence_transformers import CrossEncoder
import torch
MODEL_NAME = "hotchpotch/japanese-reranker-cross-encoder-xsmall-v1"
device = "cuda" if torch.cuda.is_available() else "cpu"
model = CrossEncoder(MODEL_NAME, max_length=512, device=device)
if device == "cuda":
model.model.half()
query = "感動的な映画について"
passages = [
"深いテーマを持ちながらも、観る人の心を揺さぶる名作。登場人物の心情描写が秀逸で、ラストは涙なしでは見られない。",
"重要なメッセージ性は評価できるが、暗い話が続くので気分が落ち込んでしまった。もう少し明るい要素があればよかった。",
"どうにもリアリティに欠ける展開が気になった。もっと深みのある人間ドラマが見たかった。",
"アクションシーンが楽しすぎる。見ていて飽きない。ストーリーはシンプルだが、それが逆に良い。",
]
scores = model.predict([(query, passage) for passage in passages])
```
## HuggingFace transformers
```python
from transformers import AutoTokenizer, AutoModelForSequenceClassification
from torch.nn import Sigmoid
MODEL_NAME = "hotchpotch/japanese-reranker-cross-encoder-xsmall-v1"
device = "cuda" if torch.cuda.is_available() else "cpu"
tokenizer = AutoTokenizer.from_pretrained(MODEL_NAME)
model = AutoModelForSequenceClassification.from_pretrained(MODEL_NAME)
model.to(device)
model.eval()
if device == "cuda":
model.half()
query = "感動的な映画について"
passages = [
"深いテーマを持ちながらも、観る人の心を揺さぶる名作。登場人物の心情描写が秀逸で、ラストは涙なしでは見られない。",
"重要なメッセージ性は評価できるが、暗い話が続くので気分が落ち込んでしまった。もう少し明るい要素があればよかった。",
"どうにもリアリティに欠ける展開が気になった。もっと深みのある人間ドラマが見たかった。",
"アクションシーンが楽しすぎる。見ていて飽きない。ストーリーはシンプルだが、それが逆に良い。",
]
inputs = tokenizer(
[(query, passage) for passage in passages],
padding=True,
truncation=True,
max_length=512,
return_tensors="pt",
)
inputs = {k: v.to(device) for k, v in inputs.items()}
logits = model(**inputs).logits
activation = Sigmoid()
scores = activation(logits).squeeze().tolist()
```
## 評価結果
| Model Name | [JQaRA](https://huggingface.co/datasets/hotchpotch/JQaRA) | [JaCWIR](https://huggingface.co/datasets/hotchpotch/JaCWIR) | [MIRACL](https://huggingface.co/datasets/miracl/miracl) | [JSQuAD](https://github.com/yahoojapan/JGLUE) |
| ------------------------------------------------------------------------------------------------------------------------ | --------------------------------------------------------- | ----------------------------------------------------------- | ------------------------------------------------------- | --------------------------------------------- |
| [japanese-reranker-cross-encoder-xsmall-v1](https://huggingface.co/hotchpotch/japanese-reranker-cross-encoder-xsmall-v1) | 0.6136 | 0.9376 | 0.7411 | 0.9602 |
| [japanese-reranker-cross-encoder-small-v1](https://huggingface.co/hotchpotch/japanese-reranker-cross-encoder-small-v1) | 0.6247 | 0.939 | 0.7776 | 0.9604 |
| [japanese-reranker-cross-encoder-base-v1](https://huggingface.co/hotchpotch/japanese-reranker-cross-encoder-base-v1) | 0.6711 | 0.9337 | 0.818 | 0.9708 |
| [japanese-reranker-cross-encoder-large-v1](https://huggingface.co/hotchpotch/japanese-reranker-cross-encoder-large-v1) | 0.7099 | 0.9364 | 0.8406 | 0.9773 |
| [japanese-bge-reranker-v2-m3-v1](https://huggingface.co/hotchpotch/japanese-bge-reranker-v2-m3-v1) | 0.6918 | 0.9372 | 0.8423 | 0.9624 |
| [bge-reranker-v2-m3](https://huggingface.co/BAAI/bge-reranker-v2-m3) | 0.673 | 0.9343 | 0.8374 | 0.9599 |
| [bge-reranker-large](https://huggingface.co/BAAI/bge-reranker-large) | 0.4718 | 0.7332 | 0.7666 | 0.7081 |
| [bge-reranker-base](https://huggingface.co/BAAI/bge-reranker-base) | 0.2445 | 0.4905 | 0.6792 | 0.5757 |
| [cross-encoder-mmarco-mMiniLMv2-L12-H384-v1](https://huggingface.co/corrius/cross-encoder-mmarco-mMiniLMv2-L12-H384-v1) | 0.5588 | 0.9211 | 0.7158 | 0.932 |
| [shioriha-large-reranker](https://huggingface.co/cl-nagoya/shioriha-large-reranker) | 0.5775 | 0.8458 | 0.8084 | 0.9262 |
| [bge-m3+all](https://huggingface.co/BAAI/bge-m3) | 0.576 | 0.904 | 0.7926 | 0.9226 |
| [bge-m3+dense](https://huggingface.co/BAAI/bge-m3) | 0.539 | 0.8642 | 0.7753 | 0.8815 |
| [bge-m3+colbert](https://huggingface.co/BAAI/bge-m3) | 0.5656 | 0.9064 | 0.7902 | 0.9297 |
| [bge-m3+sparse](https://huggingface.co/BAAI/bge-m3) | 0.5088 | 0.8944 | 0.6941 | 0.9184 |
| [JaColBERTv2](https://huggingface.co/bclavie/JaColBERTv2) | 0.5847 | 0.9185 | 0.6861 | 0.9247 |
| [multilingual-e5-large](https://huggingface.co/intfloat/multilingual-e5-large) | 0.554 | 0.8759 | 0.7722 | 0.8892 |
| [multilingual-e5-small](https://huggingface.co/intfloat/multilingual-e5-small) | 0.4917 | 0.869 | 0.7025 | 0.8565 |
| bm25 | 0.458 | 0.8408 | 0.4387 | 0.9002 |
## ライセンス
MIT License |
katuni4ka/tiny-random-dbrx | katuni4ka | "2024-07-02T10:35:08Z" | 37,269 | 0 | transformers | [
"transformers",
"safetensors",
"dbrx",
"text-generation",
"conversational",
"autotrain_compatible",
"endpoints_compatible",
"text-generation-inference",
"region:us"
] | text-generation | "2024-05-21T05:02:07Z" | Entry not found |
tugstugi/bert-base-mongolian-cased | tugstugi | "2021-05-20T08:12:07Z" | 37,236 | 0 | transformers | [
"transformers",
"pytorch",
"tf",
"jax",
"bert",
"fill-mask",
"mongolian",
"cased",
"mn",
"arxiv:1810.04805",
"autotrain_compatible",
"endpoints_compatible",
"region:us"
] | fill-mask | "2022-03-02T23:29:05Z" | ---
language: "mn"
tags:
- bert
- mongolian
- cased
---
# BERT-BASE-MONGOLIAN-CASED
[Link to Official Mongolian-BERT repo](https://github.com/tugstugi/mongolian-bert)
## Model description
This repository contains pre-trained Mongolian [BERT](https://arxiv.org/abs/1810.04805) models trained by [tugstugi](https://github.com/tugstugi), [enod](https://github.com/enod) and [sharavsambuu](https://github.com/sharavsambuu).
Special thanks to [nabar](https://github.com/nabar) who provided 5x TPUs.
This repository is based on the following open source projects: [google-research/bert](https://github.com/google-research/bert/),
[huggingface/pytorch-pretrained-BERT](https://github.com/huggingface/pytorch-pretrained-BERT) and [yoheikikuta/bert-japanese](https://github.com/yoheikikuta/bert-japanese).
#### How to use
```python
from transformers import pipeline, AutoTokenizer, AutoModelForMaskedLM
tokenizer = AutoTokenizer.from_pretrained('tugstugi/bert-base-mongolian-cased', use_fast=False)
model = AutoModelForMaskedLM.from_pretrained('tugstugi/bert-base-mongolian-cased')
## declare task ##
pipe = pipeline(task="fill-mask", model=model, tokenizer=tokenizer)
## example ##
input_ = '[MASK] хот Монгол улсын нийслэл.'
output_ = pipe(input_)
for i in range(len(output_)):
print(output_[i])
## output ##
# {'sequence': 'Улаанбаатар хот Монгол улсын нийслэл.', 'score': 0.826970100402832, 'token': 281, 'token_str': 'Улаанбаатар'}
# {'sequence': 'Нийслэл хот Монгол улсын нийслэл.', 'score': 0.06551621109247208, 'token': 4059, 'token_str': 'Нийслэл'}
# {'sequence': 'Эрдэнэт хот Монгол улсын нийслэл.', 'score': 0.0264141745865345, 'token': 2229, 'token_str': 'Эрдэнэт'}
# {'sequence': 'Дархан хот Монгол улсын нийслэл.', 'score': 0.017083868384361267, 'token': 1646, 'token_str': 'Дархан'}
# {'sequence': 'УБ хот Монгол улсын нийслэл.', 'score': 0.010854342952370644, 'token': 7389, 'token_str': 'УБ'}
```
## Training data
Mongolian Wikipedia and the 700 million word Mongolian news data set [[Pretraining Procedure](https://github.com/tugstugi/mongolian-bert#pre-training)]
### BibTeX entry and citation info
```bibtex
@misc{mongolian-bert,
author = {Tuguldur, Erdene-Ochir and Gunchinish, Sharavsambuu and Bataa, Enkhbold},
title = {BERT Pretrained Models on Mongolian Datasets},
year = {2019},
publisher = {GitHub},
journal = {GitHub repository},
howpublished = {\url{https://github.com/tugstugi/mongolian-bert/}}
}
```
|
internlm/internlm2-base-7b | internlm | "2024-07-02T12:27:14Z" | 37,127 | 9 | transformers | [
"transformers",
"pytorch",
"internlm2",
"text-generation",
"custom_code",
"arxiv:2403.17297",
"license:other",
"autotrain_compatible",
"region:us"
] | text-generation | "2024-01-12T06:18:01Z" | ---
pipeline_tag: text-generation
license: other
---
# InternLM
<div align="center">
<img src="https://github.com/InternLM/InternLM/assets/22529082/b9788105-8892-4398-8b47-b513a292378e" width="200"/>
<div> </div>
<div align="center">
<b><font size="5">InternLM</font></b>
<sup>
<a href="https://internlm.intern-ai.org.cn/">
<i><font size="4">HOT</font></i>
</a>
</sup>
<div> </div>
</div>
[](https://github.com/internLM/OpenCompass/)
[💻Github Repo](https://github.com/InternLM/InternLM) • [🤔Reporting Issues](https://github.com/InternLM/InternLM/issues/new) • [📜Technical Report](https://arxiv.org/abs/2403.17297)
</div>
## Introduction
The second generation of the InternLM model, InternLM2, includes models at two scales: 7B and 20B. For the convenience of users and researchers, we have open-sourced four versions of each scale of the model, which are:
- internlm2-base: A high-quality and highly adaptable model base, serving as an excellent starting point for deep domain adaptation.
- internlm2 (**recommended**): Built upon the internlm2-base, this version has further pretrained on domain-specific corpus. It shows outstanding performance in evaluations while maintaining robust general language abilities, making it our recommended choice for most applications.
- internlm2-chat-sft: Based on the Base model, it undergoes supervised human alignment training.
- internlm2-chat (**recommended**): Optimized for conversational interaction on top of the internlm2-chat-sft through RLHF, it excels in instruction adherence, empathetic chatting, and tool invocation.
The base model of InternLM2 has the following technical features:
- Effective support for ultra-long contexts of up to 200,000 characters: The model nearly perfectly achieves "finding a needle in a haystack" in long inputs of 200,000 characters. It also leads among open-source models in performance on long-text tasks such as LongBench and L-Eval.
- Comprehensive performance enhancement: Compared to the previous generation model, it shows significant improvements in various capabilities, including reasoning, mathematics, and coding.
## InternLM2-Base-7B
### Performance Evaluation
We have evaluated InternLM2 on several important benchmarks using the open-source evaluation tool [OpenCompass](https://github.com/open-compass/opencompass). Some of the evaluation results are shown in the table below. You are welcome to visit the [OpenCompass Leaderboard](https://rank.opencompass.org.cn/leaderboard-llm) for more evaluation results.
| Dataset\Models | InternLM2-7B | InternLM2-Chat-7B | InternLM2-20B | InternLM2-Chat-20B | ChatGPT | GPT-4 |
| --- | --- | --- | --- | --- | --- | --- |
| MMLU | 65.8 | 63.7 | 67.7 | 66.5 | 69.1 | 83.0 |
| AGIEval | 49.9 | 47.2 | 53.0 | 50.3 | 39.9 | 55.1 |
| BBH | 65.0 | 61.2 | 72.1 | 68.3 | 70.1 | 86.7 |
| GSM8K | 70.8 | 70.7 | 76.1 | 79.6 | 78.2 | 91.4 |
| MATH | 20.2 | 23.0 | 25.5 | 31.9 | 28.0 | 45.8 |
| HumanEval | 43.3 | 59.8 | 48.8 | 67.1 | 73.2 | 74.4 |
| MBPP(Sanitized) | 51.8 | 51.4 | 63.0 | 65.8 | 78.9 | 79.0 |
- The evaluation results were obtained from [OpenCompass](https://github.com/open-compass/opencompass) , and evaluation configuration can be found in the configuration files provided by [OpenCompass](https://github.com/open-compass/opencompass).
- The evaluation data may have numerical differences due to the version iteration of [OpenCompass](https://github.com/open-compass/opencompass), so please refer to the latest evaluation results of [OpenCompass](https://github.com/open-compass/opencompass).
**Limitations:** Although we have made efforts to ensure the safety of the model during the training process and to encourage the model to generate text that complies with ethical and legal requirements, the model may still produce unexpected outputs due to its size and probabilistic generation paradigm. For example, the generated responses may contain biases, discrimination, or other harmful content. Please do not propagate such content. We are not responsible for any consequences resulting from the dissemination of harmful information.
### Import from Transformers
To load the InternLM2-Base-7B model using Transformers, use the following code:
```python
import torch
from transformers import AutoTokenizer, AutoModelForCausalLM
tokenizer = AutoTokenizer.from_pretrained("internlm/internlm2-base-7b", trust_remote_code=True)
# Set `torch_dtype=torch.float16` to load model in float16, otherwise it will be loaded as float32 and might cause OOM Error.
model = AutoModelForCausalLM.from_pretrained("internlm/internlm2-base-7b", torch_dtype=torch.float16, trust_remote_code=True).cuda()
model = model.eval()
inputs = tokenizer(["A beautiful flower"], return_tensors="pt")
for k,v in inputs.items():
inputs[k] = v.cuda()
gen_kwargs = {"max_length": 128, "top_p": 0.8, "temperature": 0.8, "do_sample": True, "repetition_penalty": 1.0}
output = model.generate(**inputs, **gen_kwargs)
output = tokenizer.decode(output[0].tolist(), skip_special_tokens=True)
print(output)
# A beautiful flowering perennial that produces blue flowers in late spring. The flowers are borne on upright stems to 2-3 feet tall. Prefers full sun to part shade. Plant in moist, well-drained soil. Spreads slowly by rhizomes.
```
## Open Source License
The code is licensed under Apache-2.0, while model weights are fully open for academic research and also allow **free** commercial usage. To apply for a commercial license, please fill in the [application form (English)](https://wj.qq.com/s2/12727483/5dba/)/[申请表(中文)](https://wj.qq.com/s2/12725412/f7c1/). For other questions or collaborations, please contact <internlm@pjlab.org.cn>.
## Citation
```
@misc{cai2024internlm2,
title={InternLM2 Technical Report},
author={Zheng Cai and Maosong Cao and Haojiong Chen and Kai Chen and Keyu Chen and Xin Chen and Xun Chen and Zehui Chen and Zhi Chen and Pei Chu and Xiaoyi Dong and Haodong Duan and Qi Fan and Zhaoye Fei and Yang Gao and Jiaye Ge and Chenya Gu and Yuzhe Gu and Tao Gui and Aijia Guo and Qipeng Guo and Conghui He and Yingfan Hu and Ting Huang and Tao Jiang and Penglong Jiao and Zhenjiang Jin and Zhikai Lei and Jiaxing Li and Jingwen Li and Linyang Li and Shuaibin Li and Wei Li and Yining Li and Hongwei Liu and Jiangning Liu and Jiawei Hong and Kaiwen Liu and Kuikun Liu and Xiaoran Liu and Chengqi Lv and Haijun Lv and Kai Lv and Li Ma and Runyuan Ma and Zerun Ma and Wenchang Ning and Linke Ouyang and Jiantao Qiu and Yuan Qu and Fukai Shang and Yunfan Shao and Demin Song and Zifan Song and Zhihao Sui and Peng Sun and Yu Sun and Huanze Tang and Bin Wang and Guoteng Wang and Jiaqi Wang and Jiayu Wang and Rui Wang and Yudong Wang and Ziyi Wang and Xingjian Wei and Qizhen Weng and Fan Wu and Yingtong Xiong and Chao Xu and Ruiliang Xu and Hang Yan and Yirong Yan and Xiaogui Yang and Haochen Ye and Huaiyuan Ying and Jia Yu and Jing Yu and Yuhang Zang and Chuyu Zhang and Li Zhang and Pan Zhang and Peng Zhang and Ruijie Zhang and Shuo Zhang and Songyang Zhang and Wenjian Zhang and Wenwei Zhang and Xingcheng Zhang and Xinyue Zhang and Hui Zhao and Qian Zhao and Xiaomeng Zhao and Fengzhe Zhou and Zaida Zhou and Jingming Zhuo and Yicheng Zou and Xipeng Qiu and Yu Qiao and Dahua Lin},
year={2024},
eprint={2403.17297},
archivePrefix={arXiv},
primaryClass={cs.CL}
}
```
## 简介
第二代浦语模型, InternLM2 包含 7B 和 20B 两个量级的模型。为了方便用户使用和研究,每个量级的模型我们总共开源了四个版本的模型,他们分别是
- internlm2-base: 高质量和具有很强可塑性的模型基座,是模型进行深度领域适配的高质量起点;
- internlm2(**推荐**): 在internlm2-base基础上,进一步在特定领域的语料上进行预训练,在评测中成绩优异,同时保持了很好的通用语言能力,是我们推荐的在大部分应用中考虑选用的优秀基座;
- internlm2-chat-sft:在Base基础上,进行有监督的人类对齐训练;
- internlm2-chat(**推荐**):在internlm2-chat-sft基础上,经过RLHF,面向对话交互进行了优化,具有很好的指令遵循、共情聊天和调用工具等的能力。
InternLM2 的基础模型具备以下的技术特点
- 有效支持20万字超长上下文:模型在20万字长输入中几乎完美地实现长文“大海捞针”,而且在 LongBench 和 L-Eval 等长文任务中的表现也达到开源模型中的领先水平。
- 综合性能全面提升:各能力维度相比上一代模型全面进步,在推理、数学、代码等方面的能力提升显著。
## InternLM2-Base-7B
### 性能评测
我们使用开源评测工具 [OpenCompass](https://github.com/internLM/OpenCompass/) 对 InternLM2 在几个重要的评测集进行了评测 ,部分评测结果如下表所示,欢迎访问[ OpenCompass 榜单 ](https://rank.opencompass.org.cn/leaderboard-llm)获取更多的评测结果。
| 评测集 | InternLM2-7B | InternLM2-Chat-7B | InternLM2-20B | InternLM2-Chat-20B | ChatGPT | GPT-4 |
| --- | --- | --- | --- | --- | --- | --- |
| MMLU | 65.8 | 63.7 | 67.7 | 66.5 | 69.1 | 83.0 |
| AGIEval | 49.9 | 47.2 | 53.0 | 50.3 | 39.9 | 55.1 |
| BBH | 65.0 | 61.2 | 72.1 | 68.3 | 70.1 | 86.7 |
| GSM8K | 70.8 | 70.7 | 76.1 | 79.6 | 78.2 | 91.4 |
| MATH | 20.2 | 23.0 | 25.5 | 31.9 | 28.0 | 45.8 |
| HumanEval | 43.3 | 59.8 | 48.8 | 67.1 | 73.2 | 74.4 |
| MBPP(Sanitized) | 51.8 | 51.4 | 63.0 | 65.8 | 78.9 | 79.0 |
- 以上评测结果基于 [OpenCompass](https://github.com/open-compass/opencompass) 获得(部分数据标注`*`代表数据来自原始论文),具体测试细节可参见 [OpenCompass](https://github.com/open-compass/opencompass) 中提供的配置文件。
- 评测数据会因 [OpenCompass](https://github.com/open-compass/opencompass) 的版本迭代而存在数值差异,请以 [OpenCompass](https://github.com/open-compass/opencompass) 最新版的评测结果为主。
**局限性:** 尽管在训练过程中我们非常注重模型的安全性,尽力促使模型输出符合伦理和法律要求的文本,但受限于模型大小以及概率生成范式,模型可能会产生各种不符合预期的输出,例如回复内容包含偏见、歧视等有害内容,请勿传播这些内容。由于传播不良信息导致的任何后果,本项目不承担责任。
### 通过 Transformers 加载
通过以下的代码加载 InternLM2-Base-7B 模型进行文本续写
```python
import torch
from transformers import AutoTokenizer, AutoModelForCausalLM
tokenizer = AutoTokenizer.from_pretrained("internlm/internlm2-base-7b", trust_remote_code=True)
# `torch_dtype=torch.float16` 可以令模型以 float16 精度加载,否则 transformers 会将模型加载为 float32,有可能导致显存不足
model = AutoModelForCausalLM.from_pretrained("internlm/internlm2-base-7b", torch_dtype=torch.float16, trust_remote_code=True).cuda()
model = model.eval()
inputs = tokenizer(["来到美丽的大自然"], return_tensors="pt")
for k,v in inputs.items():
inputs[k] = v.cuda()
gen_kwargs = {"max_length": 128, "top_p": 0.8, "temperature": 0.8, "do_sample": True, "repetition_penalty": 1.0}
output = model.generate(**inputs, **gen_kwargs)
output = tokenizer.decode(output[0].tolist(), skip_special_tokens=True)
print(output)
# 来到美丽的大自然
# #周末去哪儿 #笔记灵感
# 来到美丽的大自然,远离城市喧嚣,放飞心情,感受自然之美,体会生命的意义,享受生活的美好。
# 自然的美是纯粹的,是宁静的,是自由的,是充满生机的。
# 来到自然,可以让我们放下烦恼,放松身心,享受大自然的恩赐。
# 在这里,我们可以尽情地呼吸清新的空气,感受自然的气息,让自己的心灵得到净化和放松。
# 在这里,我们可以尽情地欣赏自然的美景,感受大自然的神奇和美丽,让自己的内心得到慰藉和满足。
```
## 开源许可证
本仓库的代码依照 Apache-2.0 协议开源。模型权重对学术研究完全开放,也可申请免费的商业使用授权([申请表](https://wj.qq.com/s2/12725412/f7c1/))。其他问题与合作请联系 <internlm@pjlab.org.cn>。
## 引用
```
@misc{cai2024internlm2,
title={InternLM2 Technical Report},
author={Zheng Cai and Maosong Cao and Haojiong Chen and Kai Chen and Keyu Chen and Xin Chen and Xun Chen and Zehui Chen and Zhi Chen and Pei Chu and Xiaoyi Dong and Haodong Duan and Qi Fan and Zhaoye Fei and Yang Gao and Jiaye Ge and Chenya Gu and Yuzhe Gu and Tao Gui and Aijia Guo and Qipeng Guo and Conghui He and Yingfan Hu and Ting Huang and Tao Jiang and Penglong Jiao and Zhenjiang Jin and Zhikai Lei and Jiaxing Li and Jingwen Li and Linyang Li and Shuaibin Li and Wei Li and Yining Li and Hongwei Liu and Jiangning Liu and Jiawei Hong and Kaiwen Liu and Kuikun Liu and Xiaoran Liu and Chengqi Lv and Haijun Lv and Kai Lv and Li Ma and Runyuan Ma and Zerun Ma and Wenchang Ning and Linke Ouyang and Jiantao Qiu and Yuan Qu and Fukai Shang and Yunfan Shao and Demin Song and Zifan Song and Zhihao Sui and Peng Sun and Yu Sun and Huanze Tang and Bin Wang and Guoteng Wang and Jiaqi Wang and Jiayu Wang and Rui Wang and Yudong Wang and Ziyi Wang and Xingjian Wei and Qizhen Weng and Fan Wu and Yingtong Xiong and Chao Xu and Ruiliang Xu and Hang Yan and Yirong Yan and Xiaogui Yang and Haochen Ye and Huaiyuan Ying and Jia Yu and Jing Yu and Yuhang Zang and Chuyu Zhang and Li Zhang and Pan Zhang and Peng Zhang and Ruijie Zhang and Shuo Zhang and Songyang Zhang and Wenjian Zhang and Wenwei Zhang and Xingcheng Zhang and Xinyue Zhang and Hui Zhao and Qian Zhao and Xiaomeng Zhao and Fengzhe Zhou and Zaida Zhou and Jingming Zhuo and Yicheng Zou and Xipeng Qiu and Yu Qiao and Dahua Lin},
year={2024},
eprint={2403.17297},
archivePrefix={arXiv},
primaryClass={cs.CL}
}
``` |
laion/CLIP-ViT-L-14-CommonPool.XL-s13B-b90K | laion | "2023-04-26T01:38:56Z" | 37,036 | 0 | open_clip | [
"open_clip",
"zero-shot-image-classification",
"clip",
"license:mit",
"region:us"
] | zero-shot-image-classification | "2023-04-26T01:38:15Z" | ---
tags:
- zero-shot-image-classification
- clip
library_name: open_clip
license: mit
---
# Model card for CLIP-ViT-L-14-CommonPool.XL-s13B-b90K
|
StanfordAIMI/stanford-deidentifier-base | StanfordAIMI | "2022-11-23T19:44:40Z" | 36,955 | 61 | transformers | [
"transformers",
"pytorch",
"bert",
"token-classification",
"sequence-tagger-model",
"pubmedbert",
"uncased",
"radiology",
"biomedical",
"en",
"dataset:radreports",
"license:mit",
"endpoints_compatible",
"region:us"
] | token-classification | "2022-06-16T18:24:42Z" | ---
widget:
- text: "PROCEDURE: Chest xray. COMPARISON: last seen on 1/1/2020 and also record dated of March 1st, 2019. FINDINGS: patchy airspace opacities. IMPRESSION: The results of the chest xray of January 1 2020 are the most concerning ones. The patient was transmitted to another service of UH Medical Center under the responsability of Dr. Perez. We used the system MedClinical data transmitter and sent the data on 2/1/2020, under the ID 5874233. We received the confirmation of Dr Perez. He is reachable at 567-493-1234."
- text: "Dr. Curt Langlotz chose to schedule a meeting on 06/23."
tags:
- token-classification
- sequence-tagger-model
- pytorch
- transformers
- pubmedbert
- uncased
- radiology
- biomedical
datasets:
- radreports
language:
- en
license: mit
---
Stanford de-identifier was trained on a variety of radiology and biomedical documents with the goal of automatising the de-identification process while reaching satisfactory accuracy for use in production. Manuscript in-proceedings.
These model weights are the recommended ones among all available deidentifier weights.
Associated github repo: https://github.com/MIDRC/Stanford_Penn_Deidentifier
## Citation
```bibtex
@article{10.1093/jamia/ocac219,
author = {Chambon, Pierre J and Wu, Christopher and Steinkamp, Jackson M and Adleberg, Jason and Cook, Tessa S and Langlotz, Curtis P},
title = "{Automated deidentification of radiology reports combining transformer and “hide in plain sight” rule-based methods}",
journal = {Journal of the American Medical Informatics Association},
year = {2022},
month = {11},
abstract = "{To develop an automated deidentification pipeline for radiology reports that detect protected health information (PHI) entities and replaces them with realistic surrogates “hiding in plain sight.”In this retrospective study, 999 chest X-ray and CT reports collected between November 2019 and November 2020 were annotated for PHI at the token level and combined with 3001 X-rays and 2193 medical notes previously labeled, forming a large multi-institutional and cross-domain dataset of 6193 documents. Two radiology test sets, from a known and a new institution, as well as i2b2 2006 and 2014 test sets, served as an evaluation set to estimate model performance and to compare it with previously released deidentification tools. Several PHI detection models were developed based on different training datasets, fine-tuning approaches and data augmentation techniques, and a synthetic PHI generation algorithm. These models were compared using metrics such as precision, recall and F1 score, as well as paired samples Wilcoxon tests.Our best PHI detection model achieves 97.9 F1 score on radiology reports from a known institution, 99.6 from a new institution, 99.5 on i2b2 2006, and 98.9 on i2b2 2014. On reports from a known institution, it achieves 99.1 recall of detecting the core of each PHI span.Our model outperforms all deidentifiers it was compared to on all test sets as well as human labelers on i2b2 2014 data. It enables accurate and automatic deidentification of radiology reports.A transformer-based deidentification pipeline can achieve state-of-the-art performance for deidentifying radiology reports and other medical documents.}",
issn = {1527-974X},
doi = {10.1093/jamia/ocac219},
url = {https://doi.org/10.1093/jamia/ocac219},
note = {ocac219},
eprint = {https://academic.oup.com/jamia/advance-article-pdf/doi/10.1093/jamia/ocac219/47220191/ocac219.pdf},
}
``` |
prov-gigapath/prov-gigapath | prov-gigapath | "2024-06-30T22:59:21Z" | 36,893 | 57 | timm | [
"timm",
"pytorch",
"vision",
"medical",
"image-feature-extraction",
"license:other",
"region:us"
] | image-feature-extraction | "2024-05-20T19:58:00Z" | ---
license: other
license_name: prov-gigapath-license
license_link: https://github.com/prov-gigapath/prov-gigapath/blob/main/LICENSE
tags:
- vision
- medical
pipeline_tag: image-feature-extraction
library_name: timm
---
# Prov-GigaPath
## A whole-slide foundation model for digital pathology from real-world data
[[`Code`]](https://github.com/prov-gigapath/prov-gigapath) [[`Model`](https://huggingface.co/prov-gigapath/prov-gigapath)] [[`Paper`](https://aka.ms/gigapath)] [[`BibTeX`](#Citation)]
Hanwen Xu*, Naoto Usuyama*, Jaspreet Bagga, Sheng Zhang, Rajesh Rao, Tristan Naumann, Cliff Wong, Zelalem Gero, Javier González, Yu Gu, Yanbo Xu, Mu Wei, Wenhui Wang, Shuming Ma, Furu Wei, Jianwei Yang, Chunyuan Li, Jianfeng Gao, Jaylen Rosemon, Tucker Bower, Soohee Lee, Roshanthi Weerasinghe, Bill J. Wright, Ari Robicsek, Brian Piening, Carlo Bifulco, Sheng Wang, Hoifung Poon (*Equal Contribution)
[]()
## Model Overview
<p align="center">
<img src="https://raw.githubusercontent.com/prov-gigapath/prov-gigapath/main/images/gigapath_overview.png" width="90%"> <br>
*Overview of Prov-GigaPath model architecture*
</p>
## Install
On an NVIDIA A100 Tensor Core GPU machine, with CUDA toolkit enabled.
1. Download our repository and open the Prov-GigaPath
```
git clone https://github.com/prov-gigapath/prov-gigapath
cd prov-gigapath
```
2. Install GigaPath and its dependencies
```Shell
conda env create -f environment.yaml
conda activate gigapath
pip install -e .
```
## Model Download
The Prov-GigaPath models can be accessed from [HuggingFace Hub](https://huggingface.co/prov-gigapath/prov-gigapath).
You need to agree to the terms to access the models. Once you have the necessary access, set your HuggingFace read-only token as an environment variable:
```
export HF_TOKEN=<huggingface read-only token>
```
If you don’t set the token, you might encounter the following error:
```
ValueError: We have no connection or you passed local_files_only, so force_download is not an accepted option.
```
## Inference
The Prov-GigaPath model consists of a tile encoder, that extracts local patterns at patch level, and a slide encoder, that outputs representations at slide level. This model can be used in both tile-level and slide-level tasks. When doing inference at the slide level, we recommend following this pipeline: (1) Tile the whole slide into N image tiles, with the coordinates of each tile. (2) Get the embeddings for each tile using our tile encoder. (3) Pass the N image tile embeddings and their coordinates into the slide encoder, to get slide level representations.
### Inference with the tile encoder
First, load GigaPath tile encoder:
```Python
import timm
from PIL import Image
from torchvision import transforms
import torch
tile_encoder = timm.create_model("hf_hub:prov-gigapath/prov-gigapath", pretrained=True)
transform = transforms.Compose(
[
transforms.Resize(256, interpolation=transforms.InterpolationMode.BICUBIC),
transforms.CenterCrop(224),
transforms.ToTensor(),
transforms.Normalize(mean=(0.485, 0.456, 0.406), std=(0.229, 0.224, 0.225)),
]
)
```
Running inference to extract tile level features:
```Python
img_path = "images/prov_normal_000_1.png"
sample_input = transform(Image.open(img_path).convert("RGB")).unsqueeze(0)
tile_encoder.eval()
with torch.no_grad():
output = tile_encoder(sample_input).squeeze()
```
### Inference with the slide encoder
To inference with our slide encoder, we need both the tile embeddings and their coordinates as input. First, let's load the GigaPath slide encoder:
```Python
import gigapath
slide_encoder = gigapath.slide_encoder.create_model("hf_hub:prov-gigapath/prov-gigapath", "gigapath_slide_enc12l768d", 1536)
```
Run the inference to get the slide level embeddings:
```Python
slide_encoder.eval()
with torch.no_grad():
output = slide_encoder(tile_embed, coordinates).squeeze()
```
## Fine-tuning
### Tile-Level Linear Probing Example Using PCam Dataset
For your convenience, we provide the pre-extracted embeddings for the PCam dataset. You can download them from the link below. Note that the file size is 2GB.
```sh
wget -nc https://hanoverprod.z21.web.core.windows.net/gigapath/GigaPath_PCam_embeddings.zip -P data/
```
There is no need to unzip this file.
To run the fine-tuning experiment, execute the following script:
```sh
bash scripts/run_pcam.sh data/GigaPath_PCam_embeddings.zip
```
### Slide-Level Fine-Tuning Example Using PANDA Dataset
For your convenience, we provide the pre-extracted embeddings for the PANDA dataset. You can download them from the link below. Note that the file size is 32GB. Please unzip this file.
```sh
wget -nc https://hanoverprod.z21.web.core.windows.net/gigapath/GigaPath_PANDA_embeddings.zip -P data/
unzip -n data/GigaPath_PANDA_embeddings.zip -d data/
```
To run the fine-tuning experiment, execute the following script:
```sh
bash scripts/run_panda.sh data/GigaPath_PANDA_embeddings/h5_files
```
## Sample Data Download
A sample de-identified subset of the Prov-Path data can be accessed from these links [[1](https://zenodo.org/records/10909616), [2](https://zenodo.org/records/10909922)].
## Model Uses
### Intended Use
The data, code, and model checkpoints are intended to be used solely for (I) future research on pathology foundation models and (II) reproducibility of the experimental results reported in the reference paper. The data, code, and model checkpoints are not intended to be used in clinical care or for any clinical decision-making purposes.
### Primary Intended Use
The primary intended use is to support AI researchers reproducing and building on top of this work. GigaPath should be helpful for exploring pre-training, and encoding of digital pathology slides data.
### Out-of-Scope Use
**Any** deployed use case of the model --- commercial or otherwise --- is out of scope. Although we evaluated the models using a broad set of publicly-available research benchmarks, the models and evaluations are intended *for research use only* and not intended for deployed use cases.
## Usage and License Notices
The model is not intended or made available for clinical use as a medical device, clinical support, diagnostic tool, or other technology intended to be used in the diagnosis, cure, mitigation, treatment, or prevention of disease or other conditions. The model is not designed or intended to be a substitute for professional medical advice, diagnosis, treatment, or judgment and should not be used as such. All users are responsible for reviewing the output of the developed model to determine whether the model meets the user’s needs and for validating and evaluating the model before any clinical use.
## Acknowledgements
We would like to express our gratitude to the authors and developers of the exceptional repositories that this project is built upon: DINOv2, MAE, Timm, and TorchScale. Their contributions have been invaluable to our work.
## Citation
If you find Prov-GigaPath useful for your your research and applications, please cite using this BibTeX:
```bibtex
@article{xu2024gigapath,
title={A whole-slide foundation model for digital pathology from real-world data},
author={Xu, Hanwen and Usuyama, Naoto and Bagga, Jaspreet and Zhang, Sheng and Rao, Rajesh and Naumann, Tristan and Wong, Cliff and Gero, Zelalem and González, Javier and Gu, Yu and Xu, Yanbo and Wei, Mu and Wang, Wenhui and Ma, Shuming and Wei, Furu and Yang, Jianwei and Li, Chunyuan and Gao, Jianfeng and Rosemon, Jaylen and Bower, Tucker and Lee, Soohee and Weerasinghe, Roshanthi and Wright, Bill J. and Robicsek, Ari and Piening, Brian and Bifulco, Carlo and Wang, Sheng and Poon, Hoifung},
journal={Nature},
year={2024},
publisher={Nature Publishing Group UK London}
}
```
|
autogluon/chronos-t5-large | autogluon | "2024-05-13T21:08:47Z" | 36,806 | 2 | transformers | [
"transformers",
"safetensors",
"t5",
"text2text-generation",
"time series",
"forecasting",
"pretrained models",
"foundation models",
"time series foundation models",
"time-series",
"time-series-forecasting",
"arxiv:2403.07815",
"arxiv:1910.10683",
"license:apache-2.0",
"autotrain_compatible",
"endpoints_compatible",
"text-generation-inference",
"region:us"
] | time-series-forecasting | "2024-05-14T15:57:34Z" | ---
license: apache-2.0
pipeline_tag: time-series-forecasting
tags:
- time series
- forecasting
- pretrained models
- foundation models
- time series foundation models
- time-series
---
# Chronos-T5 (Large)
Chronos is a family of **pretrained time series forecasting models** based on language model architectures. A time series is transformed into a sequence of tokens via scaling and quantization, and a language model is trained on these tokens using the cross-entropy loss. Once trained, probabilistic forecasts are obtained by sampling multiple future trajectories given the historical context. Chronos models have been trained on a large corpus of publicly available time series data, as well as synthetic data generated using Gaussian processes.
For details on Chronos models, training data and procedures, and experimental results, please refer to the paper [Chronos: Learning the Language of Time Series](https://arxiv.org/abs/2403.07815).
<p align="center">
<img src="figures/main-figure.png" width="100%">
<br />
<span>
Fig. 1: High-level depiction of Chronos. (<b>Left</b>) The input time series is scaled and quantized to obtain a sequence of tokens. (<b>Center</b>) The tokens are fed into a language model which may either be an encoder-decoder or a decoder-only model. The model is trained using the cross-entropy loss. (<b>Right</b>) During inference, we autoregressively sample tokens from the model and map them back to numerical values. Multiple trajectories are sampled to obtain a predictive distribution.
</span>
</p>
---
## Architecture
The models in this repository are based on the [T5 architecture](https://arxiv.org/abs/1910.10683). The only difference is in the vocabulary size: Chronos-T5 models use 4096 different tokens, compared to 32128 of the original T5 models, resulting in fewer parameters.
| Model | Parameters | Based on |
| ---------------------------------------------------------------------- | ---------- | ---------------------------------------------------------------------- |
| [**chronos-t5-tiny**](https://huggingface.co/amazon/chronos-t5-tiny) | 8M | [t5-efficient-tiny](https://huggingface.co/google/t5-efficient-tiny) |
| [**chronos-t5-mini**](https://huggingface.co/amazon/chronos-t5-mini) | 20M | [t5-efficient-mini](https://huggingface.co/google/t5-efficient-mini) |
| [**chronos-t5-small**](https://huggingface.co/amazon/chronos-t5-small) | 46M | [t5-efficient-small](https://huggingface.co/google/t5-efficient-small) |
| [**chronos-t5-base**](https://huggingface.co/amazon/chronos-t5-base) | 200M | [t5-efficient-base](https://huggingface.co/google/t5-efficient-base) |
| [**chronos-t5-large**](https://huggingface.co/amazon/chronos-t5-large) | 710M | [t5-efficient-large](https://huggingface.co/google/t5-efficient-large) |
## Usage
To perform inference with Chronos models, install the package in the GitHub [companion repo](https://github.com/amazon-science/chronos-forecasting) by running:
```
pip install git+https://github.com/amazon-science/chronos-forecasting.git
```
A minimal example showing how to perform inference using Chronos models:
```python
import matplotlib.pyplot as plt
import numpy as np
import pandas as pd
import torch
from chronos import ChronosPipeline
pipeline = ChronosPipeline.from_pretrained(
"amazon/chronos-t5-large",
device_map="cuda",
torch_dtype=torch.bfloat16,
)
df = pd.read_csv("https://raw.githubusercontent.com/AileenNielsen/TimeSeriesAnalysisWithPython/master/data/AirPassengers.csv")
# context must be either a 1D tensor, a list of 1D tensors,
# or a left-padded 2D tensor with batch as the first dimension
context = torch.tensor(df["#Passengers"])
prediction_length = 12
forecast = pipeline.predict(context, prediction_length) # shape [num_series, num_samples, prediction_length]
# visualize the forecast
forecast_index = range(len(df), len(df) + prediction_length)
low, median, high = np.quantile(forecast[0].numpy(), [0.1, 0.5, 0.9], axis=0)
plt.figure(figsize=(8, 4))
plt.plot(df["#Passengers"], color="royalblue", label="historical data")
plt.plot(forecast_index, median, color="tomato", label="median forecast")
plt.fill_between(forecast_index, low, high, color="tomato", alpha=0.3, label="80% prediction interval")
plt.legend()
plt.grid()
plt.show()
```
## Citation
If you find Chronos models useful for your research, please consider citing the associated [paper](https://arxiv.org/abs/2403.07815):
```
@article{ansari2024chronos,
author = {Ansari, Abdul Fatir and Stella, Lorenzo and Turkmen, Caner and Zhang, Xiyuan, and Mercado, Pedro and Shen, Huibin and Shchur, Oleksandr and Rangapuram, Syama Syndar and Pineda Arango, Sebastian and Kapoor, Shubham and Zschiegner, Jasper and Maddix, Danielle C. and Mahoney, Michael W. and Torkkola, Kari and Gordon Wilson, Andrew and Bohlke-Schneider, Michael and Wang, Yuyang},
title = {Chronos: Learning the Language of Time Series},
journal = {arXiv preprint arXiv:2403.07815},
year = {2024}
}
```
## Security
See [CONTRIBUTING](CONTRIBUTING.md#security-issue-notifications) for more information.
## License
This project is licensed under the Apache-2.0 License.
|
lllyasviel/control_v11f1e_sd15_tile | lllyasviel | "2023-05-04T18:51:13Z" | 36,799 | 82 | diffusers | [
"diffusers",
"art",
"controlnet",
"stable-diffusion",
"controlnet-v1-1",
"image-to-image",
"arxiv:2302.05543",
"base_model:runwayml/stable-diffusion-v1-5",
"license:openrail",
"region:us"
] | image-to-image | "2023-05-04T18:42:24Z" | ---
license: openrail
base_model: runwayml/stable-diffusion-v1-5
tags:
- art
- controlnet
- stable-diffusion
- controlnet-v1-1
- image-to-image
duplicated_from: ControlNet-1-1-preview/control_v11f1e_sd15_tile
---
# Controlnet - v1.1 - *Tile Version*
**Controlnet v1.1** was released in [lllyasviel/ControlNet-v1-1](https://huggingface.co/lllyasviel/ControlNet-v1-1) by [Lvmin Zhang](https://huggingface.co/lllyasviel).
This checkpoint is a conversion of [the original checkpoint](https://huggingface.co/lllyasviel/ControlNet-v1-1/blob/main/control_v11f1e_sd15_tile.pth) into `diffusers` format.
It can be used in combination with **Stable Diffusion**, such as [runwayml/stable-diffusion-v1-5](https://huggingface.co/runwayml/stable-diffusion-v1-5).
For more details, please also have a look at the [🧨 Diffusers docs](https://huggingface.co/docs/diffusers/api/pipelines/stable_diffusion/controlnet).
ControlNet is a neural network structure to control diffusion models by adding extra conditions.

This checkpoint corresponds to the ControlNet conditioned on **tiled image**. Conceptually, it is similar to a super-resolution model, but its usage is not limited to that. It is also possible to generate details at the same size as the input (conditione) image.
**This model was contributed by [*takuma104*](https://huggingface.co/takuma104)**
## Model Details
- **Developed by:** Lvmin Zhang, Maneesh Agrawala
- **Model type:** Diffusion-based text-to-image generation model
- **Language(s):** English
- **License:** [The CreativeML OpenRAIL M license](https://huggingface.co/spaces/CompVis/stable-diffusion-license) is an [Open RAIL M license](https://www.licenses.ai/blog/2022/8/18/naming-convention-of-responsible-ai-licenses), adapted from the work that [BigScience](https://bigscience.huggingface.co/) and [the RAIL Initiative](https://www.licenses.ai/) are jointly carrying in the area of responsible AI licensing. See also [the article about the BLOOM Open RAIL license](https://bigscience.huggingface.co/blog/the-bigscience-rail-license) on which our license is based.
- **Resources for more information:** [GitHub Repository](https://github.com/lllyasviel/ControlNet), [Paper](https://arxiv.org/abs/2302.05543).
- **Cite as:**
@misc{zhang2023adding,
title={Adding Conditional Control to Text-to-Image Diffusion Models},
author={Lvmin Zhang and Maneesh Agrawala},
year={2023},
eprint={2302.05543},
archivePrefix={arXiv},
primaryClass={cs.CV}
}
## Introduction
Controlnet was proposed in [*Adding Conditional Control to Text-to-Image Diffusion Models*](https://arxiv.org/abs/2302.05543) by
Lvmin Zhang, Maneesh Agrawala.
The abstract reads as follows:
*We present a neural network structure, ControlNet, to control pretrained large diffusion models to support additional input conditions.
The ControlNet learns task-specific conditions in an end-to-end way, and the learning is robust even when the training dataset is small (< 50k).
Moreover, training a ControlNet is as fast as fine-tuning a diffusion model, and the model can be trained on a personal devices.
Alternatively, if powerful computation clusters are available, the model can scale to large amounts (millions to billions) of data.
We report that large diffusion models like Stable Diffusion can be augmented with ControlNets to enable conditional inputs like edge maps, segmentation maps, keypoints, etc.
This may enrich the methods to control large diffusion models and further facilitate related applications.*
## Example
It is recommended to use the checkpoint with [Stable Diffusion v1-5](https://huggingface.co/runwayml/stable-diffusion-v1-5) as the checkpoint has been trained on it.
Experimentally, the checkpoint can be used with other diffusion models such as dreamboothed stable diffusion.
1. Let's install `diffusers` and related packages:
```
$ pip install diffusers transformers accelerate
```
2. Run code:
```python
import torch
from PIL import Image
from diffusers import ControlNetModel, DiffusionPipeline
from diffusers.utils import load_image
def resize_for_condition_image(input_image: Image, resolution: int):
input_image = input_image.convert("RGB")
W, H = input_image.size
k = float(resolution) / min(H, W)
H *= k
W *= k
H = int(round(H / 64.0)) * 64
W = int(round(W / 64.0)) * 64
img = input_image.resize((W, H), resample=Image.LANCZOS)
return img
controlnet = ControlNetModel.from_pretrained('lllyasviel/control_v11f1e_sd15_tile',
torch_dtype=torch.float16)
pipe = DiffusionPipeline.from_pretrained("runwayml/stable-diffusion-v1-5",
custom_pipeline="stable_diffusion_controlnet_img2img",
controlnet=controlnet,
torch_dtype=torch.float16).to('cuda')
pipe.enable_xformers_memory_efficient_attention()
source_image = load_image('https://huggingface.co/lllyasviel/control_v11f1e_sd15_tile/resolve/main/images/original.png')
condition_image = resize_for_condition_image(source_image, 1024)
image = pipe(prompt="best quality",
negative_prompt="blur, lowres, bad anatomy, bad hands, cropped, worst quality",
image=condition_image,
controlnet_conditioning_image=condition_image,
width=condition_image.size[0],
height=condition_image.size[1],
strength=1.0,
generator=torch.manual_seed(0),
num_inference_steps=32,
).images[0]
image.save('output.png')
```


## Other released checkpoints v1-1
The authors released 14 different checkpoints, each trained with [Stable Diffusion v1-5](https://huggingface.co/runwayml/stable-diffusion-v1-5)
on a different type of conditioning:
| Model Name | Control Image Overview| Condition Image | Control Image Example | Generated Image Example |
|---|---|---|---|---|
|[lllyasviel/control_v11p_sd15_canny](https://huggingface.co/lllyasviel/control_v11p_sd15_canny)<br/> | *Trained with canny edge detection* | A monochrome image with white edges on a black background.|<a href="https://huggingface.co/lllyasviel/control_v11p_sd15_canny/resolve/main/images/control.png"><img width="64" style="margin:0;padding:0;" src="https://huggingface.co/lllyasviel/control_v11p_sd15_canny/resolve/main/images/control.png"/></a>|<a href="https://huggingface.co/lllyasviel/control_v11p_sd15_canny/resolve/main/images/image_out.png"><img width="64" src="https://huggingface.co/lllyasviel/control_v11p_sd15_canny/resolve/main/images/image_out.png"/></a>|
|[lllyasviel/control_v11e_sd15_ip2p](https://huggingface.co/lllyasviel/control_v11e_sd15_ip2p)<br/> | *Trained with pixel to pixel instruction* | No condition .|<a href="https://huggingface.co/lllyasviel/control_v11e_sd15_ip2p/resolve/main/images/control.png"><img width="64" style="margin:0;padding:0;" src="https://huggingface.co/lllyasviel/control_v11e_sd15_ip2p/resolve/main/images/control.png"/></a>|<a href="https://huggingface.co/lllyasviel/control_v11e_sd15_ip2p/resolve/main/images/image_out.png"><img width="64" src="https://huggingface.co/lllyasviel/control_v11e_sd15_ip2p/resolve/main/images/image_out.png"/></a>|
|[lllyasviel/control_v11p_sd15_inpaint](https://huggingface.co/lllyasviel/control_v11p_sd15_inpaint)<br/> | Trained with image inpainting | No condition.|<a href="https://huggingface.co/lllyasviel/control_v11p_sd15_inpaint/resolve/main/images/control.png"><img width="64" style="margin:0;padding:0;" src="https://huggingface.co/lllyasviel/control_v11p_sd15_inpaint/resolve/main/images/control.png"/></a>|<a href="https://huggingface.co/lllyasviel/control_v11p_sd15_inpaint/resolve/main/images/output.png"><img width="64" src="https://huggingface.co/lllyasviel/control_v11p_sd15_inpaint/resolve/main/images/output.png"/></a>|
|[lllyasviel/control_v11p_sd15_mlsd](https://huggingface.co/lllyasviel/control_v11p_sd15_mlsd)<br/> | Trained with multi-level line segment detection | An image with annotated line segments.|<a href="https://huggingface.co/lllyasviel/control_v11p_sd15_mlsd/resolve/main/images/control.png"><img width="64" style="margin:0;padding:0;" src="https://huggingface.co/lllyasviel/control_v11p_sd15_mlsd/resolve/main/images/control.png"/></a>|<a href="https://huggingface.co/lllyasviel/control_v11p_sd15_mlsd/resolve/main/images/image_out.png"><img width="64" src="https://huggingface.co/lllyasviel/control_v11p_sd15_mlsd/resolve/main/images/image_out.png"/></a>|
|[lllyasviel/control_v11f1p_sd15_depth](https://huggingface.co/lllyasviel/control_v11f1p_sd15_depth)<br/> | Trained with depth estimation | An image with depth information, usually represented as a grayscale image.|<a href="https://huggingface.co/lllyasviel/control_v11f1p_sd15_depth/resolve/main/images/control.png"><img width="64" style="margin:0;padding:0;" src="https://huggingface.co/lllyasviel/control_v11f1p_sd15_depth/resolve/main/images/control.png"/></a>|<a href="https://huggingface.co/lllyasviel/control_v11f1p_sd15_depth/resolve/main/images/image_out.png"><img width="64" src="https://huggingface.co/lllyasviel/control_v11f1p_sd15_depth/resolve/main/images/image_out.png"/></a>|
|[lllyasviel/control_v11p_sd15_normalbae](https://huggingface.co/lllyasviel/control_v11p_sd15_normalbae)<br/> | Trained with surface normal estimation | An image with surface normal information, usually represented as a color-coded image.|<a href="https://huggingface.co/lllyasviel/control_v11p_sd15_normalbae/resolve/main/images/control.png"><img width="64" style="margin:0;padding:0;" src="https://huggingface.co/lllyasviel/control_v11p_sd15_normalbae/resolve/main/images/control.png"/></a>|<a href="https://huggingface.co/lllyasviel/control_v11p_sd15_normalbae/resolve/main/images/image_out.png"><img width="64" src="https://huggingface.co/lllyasviel/control_v11p_sd15_normalbae/resolve/main/images/image_out.png"/></a>|
|[lllyasviel/control_v11p_sd15_seg](https://huggingface.co/lllyasviel/control_v11p_sd15_seg)<br/> | Trained with image segmentation | An image with segmented regions, usually represented as a color-coded image.|<a href="https://huggingface.co/lllyasviel/control_v11p_sd15_seg/resolve/main/images/control.png"><img width="64" style="margin:0;padding:0;" src="https://huggingface.co/lllyasviel/control_v11p_sd15_seg/resolve/main/images/control.png"/></a>|<a href="https://huggingface.co/lllyasviel/control_v11p_sd15_seg/resolve/main/images/image_out.png"><img width="64" src="https://huggingface.co/lllyasviel/control_v11p_sd15_seg/resolve/main/images/image_out.png"/></a>|
|[lllyasviel/control_v11p_sd15_lineart](https://huggingface.co/lllyasviel/control_v11p_sd15_lineart)<br/> | Trained with line art generation | An image with line art, usually black lines on a white background.|<a href="https://huggingface.co/lllyasviel/control_v11p_sd15_lineart/resolve/main/images/control.png"><img width="64" style="margin:0;padding:0;" src="https://huggingface.co/lllyasviel/control_v11p_sd15_lineart/resolve/main/images/control.png"/></a>|<a href="https://huggingface.co/lllyasviel/control_v11p_sd15_lineart/resolve/main/images/image_out.png"><img width="64" src="https://huggingface.co/lllyasviel/control_v11p_sd15_lineart/resolve/main/images/image_out.png"/></a>|
|[lllyasviel/control_v11p_sd15s2_lineart_anime](https://huggingface.co/lllyasviel/control_v11p_sd15s2_lineart_anime)<br/> | Trained with anime line art generation | An image with anime-style line art.|<a href="https://huggingface.co/lllyasviel/control_v11p_sd15s2_lineart_anime/resolve/main/images/control.png"><img width="64" style="margin:0;padding:0;" src="https://huggingface.co/lllyasviel/control_v11p_sd15s2_lineart_anime/resolve/main/images/control.png"/></a>|<a href="https://huggingface.co/lllyasviel/control_v11p_sd15s2_lineart_anime/resolve/main/images/image_out.png"><img width="64" src="https://huggingface.co/lllyasviel/control_v11p_sd15s2_lineart_anime/resolve/main/images/image_out.png"/></a>|
|[lllyasviel/control_v11p_sd15_openpose](https://huggingface.co/lllyasviel/control_v11p_sd15s2_lineart_anime)<br/> | Trained with human pose estimation | An image with human poses, usually represented as a set of keypoints or skeletons.|<a href="https://huggingface.co/lllyasviel/control_v11p_sd15_openpose/resolve/main/images/control.png"><img width="64" style="margin:0;padding:0;" src="https://huggingface.co/lllyasviel/control_v11p_sd15_openpose/resolve/main/images/control.png"/></a>|<a href="https://huggingface.co/lllyasviel/control_v11p_sd15_openpose/resolve/main/images/image_out.png"><img width="64" src="https://huggingface.co/lllyasviel/control_v11p_sd15_openpose/resolve/main/images/image_out.png"/></a>|
|[lllyasviel/control_v11p_sd15_scribble](https://huggingface.co/lllyasviel/control_v11p_sd15_scribble)<br/> | Trained with scribble-based image generation | An image with scribbles, usually random or user-drawn strokes.|<a href="https://huggingface.co/lllyasviel/control_v11p_sd15_scribble/resolve/main/images/control.png"><img width="64" style="margin:0;padding:0;" src="https://huggingface.co/lllyasviel/control_v11p_sd15_scribble/resolve/main/images/control.png"/></a>|<a href="https://huggingface.co/lllyasviel/control_v11p_sd15_scribble/resolve/main/images/image_out.png"><img width="64" src="https://huggingface.co/lllyasviel/control_v11p_sd15_scribble/resolve/main/images/image_out.png"/></a>|
|[lllyasviel/control_v11p_sd15_softedge](https://huggingface.co/lllyasviel/control_v11p_sd15_softedge)<br/> | Trained with soft edge image generation | An image with soft edges, usually to create a more painterly or artistic effect.|<a href="https://huggingface.co/lllyasviel/control_v11p_sd15_softedge/resolve/main/images/control.png"><img width="64" style="margin:0;padding:0;" src="https://huggingface.co/lllyasviel/control_v11p_sd15_softedge/resolve/main/images/control.png"/></a>|<a href="https://huggingface.co/lllyasviel/control_v11p_sd15_softedge/resolve/main/images/image_out.png"><img width="64" src="https://huggingface.co/lllyasviel/control_v11p_sd15_softedge/resolve/main/images/image_out.png"/></a>|
|[lllyasviel/control_v11e_sd15_shuffle](https://huggingface.co/lllyasviel/control_v11e_sd15_shuffle)<br/> | Trained with image shuffling | An image with shuffled patches or regions.|<a href="https://huggingface.co/lllyasviel/control_v11e_sd15_shuffle/resolve/main/images/control.png"><img width="64" style="margin:0;padding:0;" src="https://huggingface.co/lllyasviel/control_v11e_sd15_shuffle/resolve/main/images/control.png"/></a>|<a href="https://huggingface.co/lllyasviel/control_v11e_sd15_shuffle/resolve/main/images/image_out.png"><img width="64" src="https://huggingface.co/lllyasviel/control_v11e_sd15_shuffle/resolve/main/images/image_out.png"/></a>|
|[lllyasviel/control_v11f1e_sd15_tile](https://huggingface.co/lllyasviel/control_v11f1e_sd15_tile)<br/> | Trained with image tiling | A blurry image or part of an image .|<a href="https://huggingface.co/lllyasviel/control_v11f1e_sd15_tile/resolve/main/images/original.png"><img width="64" style="margin:0;padding:0;" src="https://huggingface.co/lllyasviel/control_v11f1e_sd15_tile/resolve/main/images/original.png"/></a>|<a href="https://huggingface.co/lllyasviel/control_v11f1e_sd15_tile/resolve/main/images/output.png"><img width="64" src="https://huggingface.co/lllyasviel/control_v11f1e_sd15_tile/resolve/main/images/output.png"/></a>|
## More information
For more information, please also have a look at the [Diffusers ControlNet Blog Post](https://huggingface.co/blog/controlnet) and have a look at the [official docs](https://github.com/lllyasviel/ControlNet-v1-1-nightly). |
microsoft/graphcodebert-base | microsoft | "2022-09-26T17:06:54Z" | 36,749 | 39 | transformers | [
"transformers",
"pytorch",
"tf",
"jax",
"roberta",
"fill-mask",
"arxiv:2009.08366",
"autotrain_compatible",
"endpoints_compatible",
"region:us"
] | fill-mask | "2022-03-02T23:29:05Z" | ## GraphCodeBERT model
GraphCodeBERT is a graph-based pre-trained model based on the Transformer architecture for programming language, which also considers data-flow information along with code sequences. GraphCodeBERT consists of 12 layers, 768 dimensional hidden states, and 12 attention heads. The maximum sequence length for the model is 512. The model is trained on the CodeSearchNet dataset, which includes 2.3M functions with document pairs for six programming languages.
More details can be found in the [paper](https://arxiv.org/abs/2009.08366) by Guo et. al.
**Disclaimer:** The team releasing BERT did not write a model card for this model so this model card has been written by the Hugging Face community members. |
nitrosocke/Ghibli-Diffusion | nitrosocke | "2023-08-03T19:46:59Z" | 36,677 | 609 | diffusers | [
"diffusers",
"safetensors",
"stable-diffusion",
"text-to-image",
"image-to-image",
"en",
"license:creativeml-openrail-m",
"endpoints_compatible",
"diffusers:StableDiffusionPipeline",
"region:us"
] | text-to-image | "2022-11-18T15:50:51Z" | ---
language:
- en
license: creativeml-openrail-m
thumbnail: "https://huggingface.co/nitrosocke/Ghibli-Diffusion/resolve/main/images/ghibli-diffusion-thumbnail.jpg"
tags:
- stable-diffusion
- text-to-image
- image-to-image
- diffusers
---
### Ghibli Diffusion
This is the fine-tuned Stable Diffusion model trained on images from modern anime feature films from Studio Ghibli.
Use the tokens **_ghibli style_** in your prompts for the effect.
**If you enjoy my work and want to test new models before release, please consider supporting me**
[](https://patreon.com/user?u=79196446)
**Characters rendered with the model:**
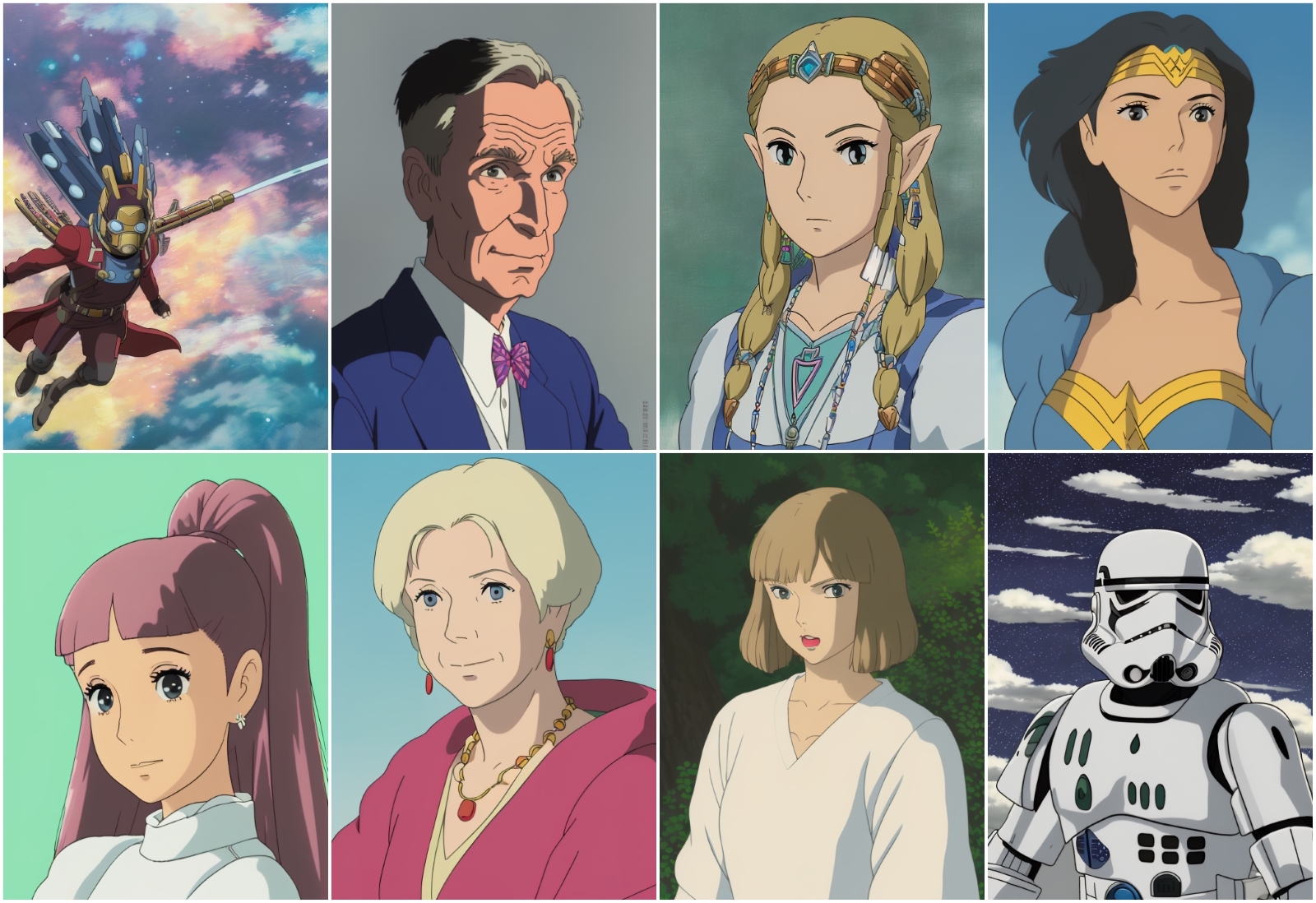
**Cars and Animals rendered with the model:**

**Landscapes rendered with the model:**
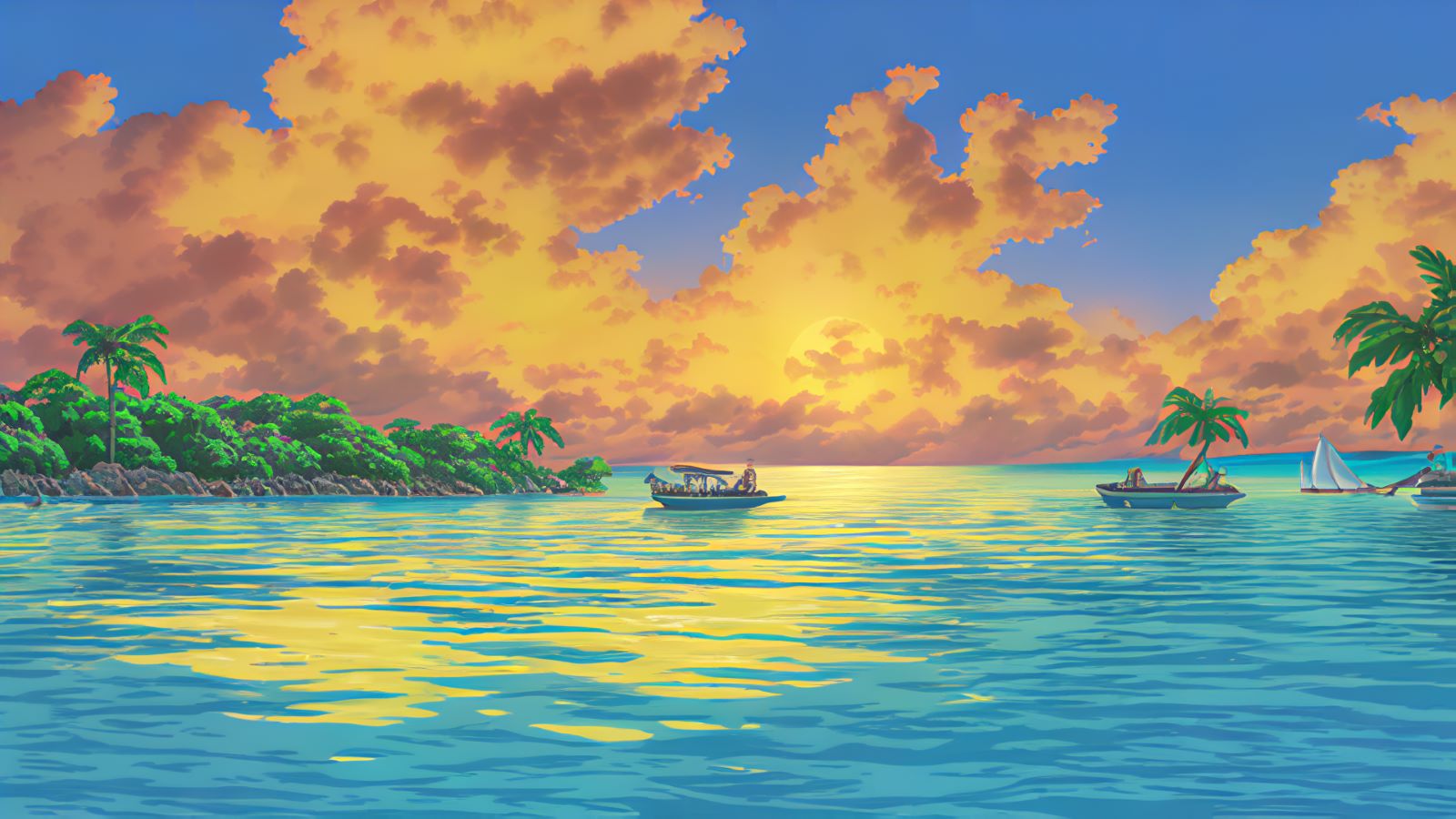
_ghibli style beautiful Caribbean beach tropical (sunset) - Negative prompt: soft blurry_
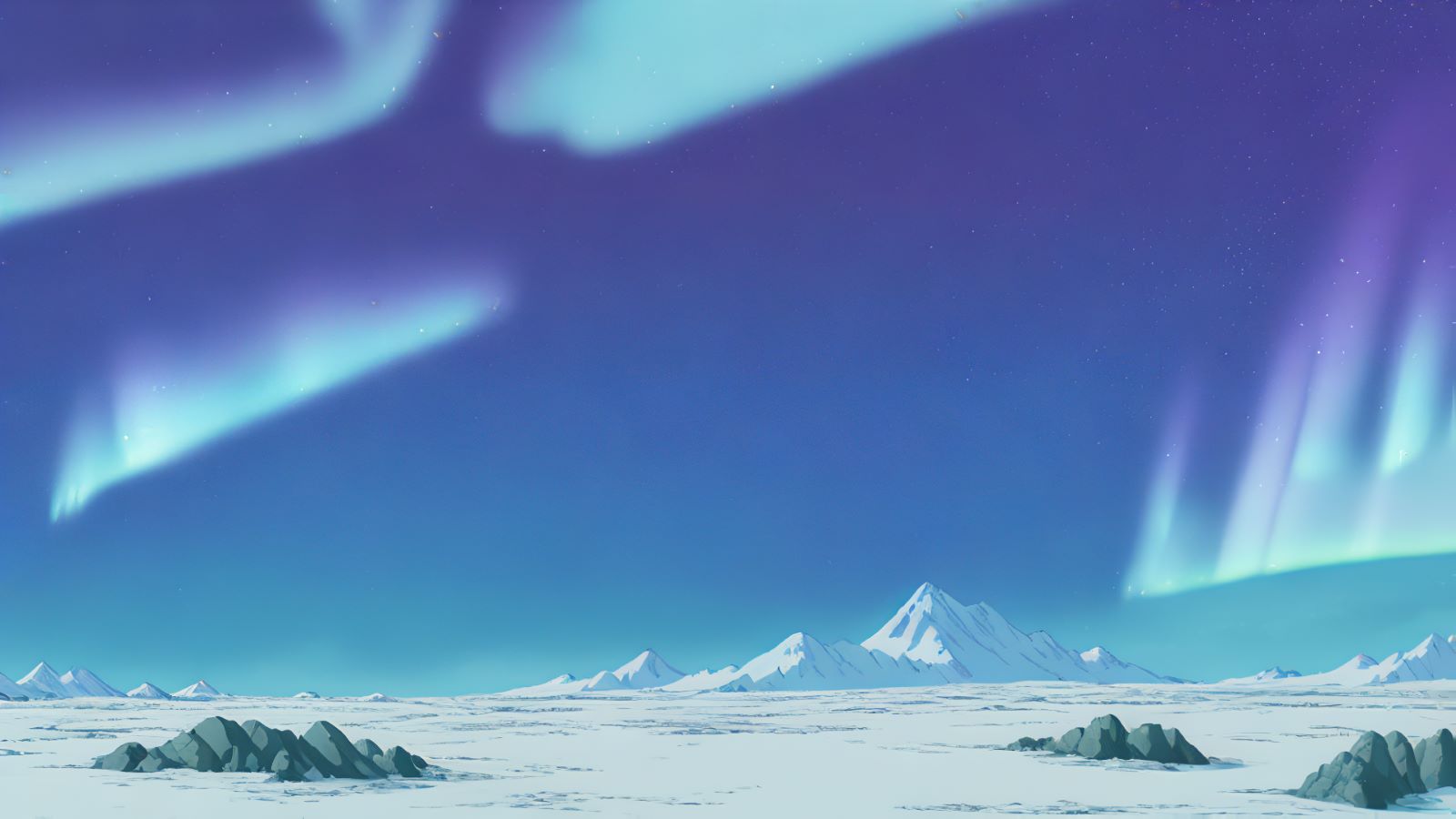
_ghibli style ice field white mountains ((northern lights)) starry sky low horizon - Negative prompt: soft blurry_
#### Prompt and settings for the Strom Trooper:
**ghibli style (storm trooper) Negative prompt: (bad anatomy)**
_Steps: 20, Sampler: DPM++ 2M Karras, CFG scale: 7, Seed: 3450349066, Size: 512x704_
#### Prompt and settings for the VW Beetle:
**ghibli style VW beetle Negative prompt: soft blurry**
_Steps: 30, Sampler: Euler a, CFG scale: 7, Seed: 1529856912, Size: 704x512_
This model was trained using the diffusers based dreambooth training by ShivamShrirao using prior-preservation loss and the _train-text-encoder_ flag in 15.000 steps.
<!-- ### Gradio
We support a [Gradio](https://github.com/gradio-app/gradio) Web UI run redshift-diffusion:
[](https://huggingface.co/spaces/nitrosocke/Ghibli-Diffusion-Demo)-->
### 🧨 Diffusers
This model can be used just like any other Stable Diffusion model. For more information,
please have a look at the [Stable Diffusion](https://huggingface.co/docs/diffusers/api/pipelines/stable_diffusion).
You can also export the model to [ONNX](https://huggingface.co/docs/diffusers/optimization/onnx), [MPS](https://huggingface.co/docs/diffusers/optimization/mps) and/or [FLAX/JAX]().
```python
from diffusers import StableDiffusionPipeline
import torch
model_id = "nitrosocke/Ghibli-Diffusion"
pipe = StableDiffusionPipeline.from_pretrained(model_id, torch_dtype=torch.float16)
pipe = pipe.to("cuda")
prompt = "ghibli style magical princess with golden hair"
image = pipe(prompt).images[0]
image.save("./magical_princess.png")
```
## License
This model is open access and available to all, with a CreativeML OpenRAIL-M license further specifying rights and usage.
The CreativeML OpenRAIL License specifies:
1. You can't use the model to deliberately produce nor share illegal or harmful outputs or content
2. The authors claims no rights on the outputs you generate, you are free to use them and are accountable for their use which must not go against the provisions set in the license
3. You may re-distribute the weights and use the model commercially and/or as a service. If you do, please be aware you have to include the same use restrictions as the ones in the license and share a copy of the CreativeML OpenRAIL-M to all your users (please read the license entirely and carefully)
[Please read the full license here](https://huggingface.co/spaces/CompVis/stable-diffusion-license) |
Yntec/AbsoluteReality | Yntec | "2024-06-08T12:21:18Z" | 36,661 | 2 | diffusers | [
"diffusers",
"safetensors",
"General",
"LandScapes",
"Photorealistic",
"Lykon",
"stable-diffusion",
"stable-diffusion-diffusers",
"text-to-image",
"license:creativeml-openrail-m",
"autotrain_compatible",
"endpoints_compatible",
"diffusers:StableDiffusionPipeline",
"region:us"
] | text-to-image | "2023-10-03T09:44:25Z" | ---
license: creativeml-openrail-m
library_name: diffusers
pipeline_tag: text-to-image
tags:
- General
- LandScapes
- Photorealistic
- Lykon
- stable-diffusion
- stable-diffusion-diffusers
- diffusers
- text-to-image
---
# Absolute Reality
UPDATE: Now hosting v1.6 version of this model. Original page: https://civitai.com/models/81458?modelVersionId=108576
Samples and prompts:
(Click for larger)

Top left: Full body picture of a pretty cute girl making cake in school, detailed brown eyes, short smile, beautiful and aesthetic, intricate, neat hair, highly detailed, detailed face, smooth, sharp focus, chiaroscuro, magazine ad, 1949, 2D Game Art, anime on canvas, rossdraws, clay mann, CHIBI ART, light novel cover art
Top right: 80s cinematic colored sitcom screenshot. young husband with wife. festive scene at a copper brewery with a wooden keg of enjoying burrito juice in the center. sitting cute little daughter. Display mugs of dark beer. Closeup. beautiful eyes. accompanied by halloween Shirley ingredients. portrait smile
Bottom left: Gacha life, movie, chibi, Kawaii, anime, 1girl, 90s illustration, digital illustration, character, little girl outfits, neon, colourful, warm, vibrant
Bottom right: highly detailed chibi koala in kitchen, hammer chef, fish, dungeons and dragons
Other versions:
https://huggingface.co/digiplay/AbsoluteReality_v1.0_diffusers
https://huggingface.co/digiplay/AbsoluteReality_v1.8.1
https://huggingface.co/Yntec/AbsoluteRemix
https://huggingface.co/Yntec/Thriller
https://huggingface.co/Yntec/IdleFancy |
unitary/unbiased-toxic-roberta | unitary | "2023-08-18T10:43:39Z" | 36,624 | 14 | transformers | [
"transformers",
"pytorch",
"jax",
"roberta",
"text-classification",
"arxiv:1703.04009",
"arxiv:1905.12516",
"license:apache-2.0",
"autotrain_compatible",
"endpoints_compatible",
"region:us"
] | text-classification | "2022-03-02T23:29:05Z" | ---
license: apache-2.0
---
<div align="center">
**⚠️ Disclaimer:**
The huggingface models currently give different results to the detoxify library (see issue [here](https://github.com/unitaryai/detoxify/issues/15)). For the most up to date models we recommend using the models from https://github.com/unitaryai/detoxify
# 🙊 Detoxify
## Toxic Comment Classification with ⚡ Pytorch Lightning and 🤗 Transformers


</div>

## Description
Trained models & code to predict toxic comments on 3 Jigsaw challenges: Toxic comment classification, Unintended Bias in Toxic comments, Multilingual toxic comment classification.
Built by [Laura Hanu](https://laurahanu.github.io/) at [Unitary](https://www.unitary.ai/), where we are working to stop harmful content online by interpreting visual content in context.
Dependencies:
- For inference:
- 🤗 Transformers
- ⚡ Pytorch lightning
- For training will also need:
- Kaggle API (to download data)
| Challenge | Year | Goal | Original Data Source | Detoxify Model Name | Top Kaggle Leaderboard Score | Detoxify Score
|-|-|-|-|-|-|-|
| [Toxic Comment Classification Challenge](https://www.kaggle.com/c/jigsaw-toxic-comment-classification-challenge) | 2018 | build a multi-headed model that’s capable of detecting different types of of toxicity like threats, obscenity, insults, and identity-based hate. | Wikipedia Comments | `original` | 0.98856 | 0.98636
| [Jigsaw Unintended Bias in Toxicity Classification](https://www.kaggle.com/c/jigsaw-unintended-bias-in-toxicity-classification) | 2019 | build a model that recognizes toxicity and minimizes this type of unintended bias with respect to mentions of identities. You'll be using a dataset labeled for identity mentions and optimizing a metric designed to measure unintended bias. | Civil Comments | `unbiased` | 0.94734 | 0.93639
| [Jigsaw Multilingual Toxic Comment Classification](https://www.kaggle.com/c/jigsaw-multilingual-toxic-comment-classification) | 2020 | build effective multilingual models | Wikipedia Comments + Civil Comments | `multilingual` | 0.9536 | 0.91655*
*Score not directly comparable since it is obtained on the validation set provided and not on the test set. To update when the test labels are made available.
It is also noteworthy to mention that the top leadearboard scores have been achieved using model ensembles. The purpose of this library was to build something user-friendly and straightforward to use.
## Limitations and ethical considerations
If words that are associated with swearing, insults or profanity are present in a comment, it is likely that it will be classified as toxic, regardless of the tone or the intent of the author e.g. humorous/self-deprecating. This could present some biases towards already vulnerable minority groups.
The intended use of this library is for research purposes, fine-tuning on carefully constructed datasets that reflect real world demographics and/or to aid content moderators in flagging out harmful content quicker.
Some useful resources about the risk of different biases in toxicity or hate speech detection are:
- [The Risk of Racial Bias in Hate Speech Detection](https://homes.cs.washington.edu/~msap/pdfs/sap2019risk.pdf)
- [Automated Hate Speech Detection and the Problem of Offensive Language](https://arxiv.org/pdf/1703.04009.pdf%201.pdf)
- [Racial Bias in Hate Speech and Abusive Language Detection Datasets](https://arxiv.org/pdf/1905.12516.pdf)
## Quick prediction
The `multilingual` model has been trained on 7 different languages so it should only be tested on: `english`, `french`, `spanish`, `italian`, `portuguese`, `turkish` or `russian`.
```bash
# install detoxify
pip install detoxify
```
```python
from detoxify import Detoxify
# each model takes in either a string or a list of strings
results = Detoxify('original').predict('example text')
results = Detoxify('unbiased').predict(['example text 1','example text 2'])
results = Detoxify('multilingual').predict(['example text','exemple de texte','texto de ejemplo','testo di esempio','texto de exemplo','örnek metin','пример текста'])
# optional to display results nicely (will need to pip install pandas)
import pandas as pd
print(pd.DataFrame(results, index=input_text).round(5))
```
For more details check the Prediction section.
## Labels
All challenges have a toxicity label. The toxicity labels represent the aggregate ratings of up to 10 annotators according the following schema:
- **Very Toxic** (a very hateful, aggressive, or disrespectful comment that is very likely to make you leave a discussion or give up on sharing your perspective)
- **Toxic** (a rude, disrespectful, or unreasonable comment that is somewhat likely to make you leave a discussion or give up on sharing your perspective)
- **Hard to Say**
- **Not Toxic**
More information about the labelling schema can be found [here](https://www.kaggle.com/c/jigsaw-unintended-bias-in-toxicity-classification/data).
### Toxic Comment Classification Challenge
This challenge includes the following labels:
- `toxic`
- `severe_toxic`
- `obscene`
- `threat`
- `insult`
- `identity_hate`
### Jigsaw Unintended Bias in Toxicity Classification
This challenge has 2 types of labels: the main toxicity labels and some additional identity labels that represent the identities mentioned in the comments.
Only identities with more than 500 examples in the test set (combined public and private) are included during training as additional labels and in the evaluation calculation.
- `toxicity`
- `severe_toxicity`
- `obscene`
- `threat`
- `insult`
- `identity_attack`
- `sexual_explicit`
Identity labels used:
- `male`
- `female`
- `homosexual_gay_or_lesbian`
- `christian`
- `jewish`
- `muslim`
- `black`
- `white`
- `psychiatric_or_mental_illness`
A complete list of all the identity labels available can be found [here](https://www.kaggle.com/c/jigsaw-unintended-bias-in-toxicity-classification/data).
### Jigsaw Multilingual Toxic Comment Classification
Since this challenge combines the data from the previous 2 challenges, it includes all labels from above, however the final evaluation is only on:
- `toxicity`
## How to run
First, install dependencies
```bash
# clone project
git clone https://github.com/unitaryai/detoxify
# create virtual env
python3 -m venv toxic-env
source toxic-env/bin/activate
# install project
pip install -e detoxify
cd detoxify
# for training
pip install -r requirements.txt
```
## Prediction
Trained models summary:
|Model name| Transformer type| Data from
|:--:|:--:|:--:|
|`original`| `bert-base-uncased` | Toxic Comment Classification Challenge
|`unbiased`| `roberta-base`| Unintended Bias in Toxicity Classification
|`multilingual`| `xlm-roberta-base`| Multilingual Toxic Comment Classification
For a quick prediction can run the example script on a comment directly or from a txt containing a list of comments.
```bash
# load model via torch.hub
python run_prediction.py --input 'example' --model_name original
# load model from from checkpoint path
python run_prediction.py --input 'example' --from_ckpt_path model_path
# save results to a .csv file
python run_prediction.py --input test_set.txt --model_name original --save_to results.csv
# to see usage
python run_prediction.py --help
```
Checkpoints can be downloaded from the latest release or via the Pytorch hub API with the following names:
- `toxic_bert`
- `unbiased_toxic_roberta`
- `multilingual_toxic_xlm_r`
```bash
model = torch.hub.load('unitaryai/detoxify','toxic_bert')
```
Importing detoxify in python:
```python
from detoxify import Detoxify
results = Detoxify('original').predict('some text')
results = Detoxify('unbiased').predict(['example text 1','example text 2'])
results = Detoxify('multilingual').predict(['example text','exemple de texte','texto de ejemplo','testo di esempio','texto de exemplo','örnek metin','пример текста'])
# to display results nicely
import pandas as pd
print(pd.DataFrame(results,index=input_text).round(5))
```
## Training
If you do not already have a Kaggle account:
- you need to create one to be able to download the data
- go to My Account and click on Create New API Token - this will download a kaggle.json file
- make sure this file is located in ~/.kaggle
```bash
# create data directory
mkdir jigsaw_data
cd jigsaw_data
# download data
kaggle competitions download -c jigsaw-toxic-comment-classification-challenge
kaggle competitions download -c jigsaw-unintended-bias-in-toxicity-classification
kaggle competitions download -c jigsaw-multilingual-toxic-comment-classification
```
## Start Training
### Toxic Comment Classification Challenge
```bash
python create_val_set.py
python train.py --config configs/Toxic_comment_classification_BERT.json
```
### Unintended Bias in Toxicicity Challenge
```bash
python train.py --config configs/Unintended_bias_toxic_comment_classification_RoBERTa.json
```
### Multilingual Toxic Comment Classification
This is trained in 2 stages. First, train on all available data, and second, train only on the translated versions of the first challenge.
The [translated data](https://www.kaggle.com/miklgr500/jigsaw-train-multilingual-coments-google-api) can be downloaded from Kaggle in french, spanish, italian, portuguese, turkish, and russian (the languages available in the test set).
```bash
# stage 1
python train.py --config configs/Multilingual_toxic_comment_classification_XLMR.json
# stage 2
python train.py --config configs/Multilingual_toxic_comment_classification_XLMR_stage2.json
```
### Monitor progress with tensorboard
```bash
tensorboard --logdir=./saved
```
## Model Evaluation
### Toxic Comment Classification Challenge
This challenge is evaluated on the mean AUC score of all the labels.
```bash
python evaluate.py --checkpoint saved/lightning_logs/checkpoints/example_checkpoint.pth --test_csv test.csv
```
### Unintended Bias in Toxicicity Challenge
This challenge is evaluated on a novel bias metric that combines different AUC scores to balance overall performance. More information on this metric [here](https://www.kaggle.com/c/jigsaw-unintended-bias-in-toxicity-classification/overview/evaluation).
```bash
python evaluate.py --checkpoint saved/lightning_logs/checkpoints/example_checkpoint.pth --test_csv test.csv
# to get the final bias metric
python model_eval/compute_bias_metric.py
```
### Multilingual Toxic Comment Classification
This challenge is evaluated on the AUC score of the main toxic label.
```bash
python evaluate.py --checkpoint saved/lightning_logs/checkpoints/example_checkpoint.pth --test_csv test.csv
```
### Citation
```
@misc{Detoxify,
title={Detoxify},
author={Hanu, Laura and {Unitary team}},
howpublished={Github. https://github.com/unitaryai/detoxify},
year={2020}
}
``` |
ilsilfverskiold/tech-keywords-extractor | ilsilfverskiold | "2023-11-29T15:17:47Z" | 36,593 | 29 | transformers | [
"transformers",
"tensorboard",
"safetensors",
"bart",
"text2text-generation",
"generated_from_trainer",
"base_model:facebook/bart-large",
"license:apache-2.0",
"autotrain_compatible",
"endpoints_compatible",
"region:us"
] | text2text-generation | "2023-11-23T19:19:22Z" | ---
license: apache-2.0
base_model: facebook/bart-large
tags:
- generated_from_trainer
model-index:
- name: tech-keyword-extractor
results: []
---
<!-- This model card has been generated automatically according to the information the Trainer had access to. You
should probably proofread and complete it, then remove this comment. -->
# tech-keyword-extractor
This model is a fine-tuned version of [facebook/bart-large](https://huggingface.co/facebook/bart-large) on a private dataset.
It achieves the following results on the evaluation set:
- Loss: 0.8795
## Model description
This model extracts tech terms, tools, company names from texts so they can easily be aggregated. It is trained to extract tech terms, tools, languages, platforms but may be used on other texts.
## Intended uses & limitations
Use to extract keywords from texts.
Example text: "If a task raises an exception, or a worker process dies, Celery will by default lose the job. So if you happen to reboot or redeploy, any running jobs with be lost to the sands of time."
Output: "Celery, Exception Handling, Worker Process"
Example text: "Spin 2.0 – open-source tool for building and running WebAssembly applications -"
Output: "Spin 2.0, WebAssembly, Open Source"
Example text: "Do you think that low-code and no-code is a threat for developers in the long term?"
Output: "Low Code, No Code, Developers"
Example text: "I'm reaching out for some guidance on choosing the right no-code or low-code platform for my web app development projects. As a proficient back-end developer with a strong grasp of AWS, I have always struggled with front-end development"
Output: "No Code, Low Code, Web App Development, AWS"
## Training and evaluation data
More information needed
## Training procedure
### Training hyperparameters
The following hyperparameters were used during training:
- learning_rate: 5e-05
- train_batch_size: 4
- eval_batch_size: 4
- seed: 42
- gradient_accumulation_steps: 16
- total_train_batch_size: 64
- optimizer: Adam with betas=(0.9,0.999) and epsilon=1e-08
- lr_scheduler_type: linear
- lr_scheduler_warmup_steps: 500
- num_epochs: 3
### Training results
| Training Loss | Epoch | Step | Validation Loss |
|:-------------:|:-----:|:----:|:---------------:|
| 1.5095 | 0.44 | 50 | 1.1766 |
| 1.1875 | 0.89 | 100 | 0.9652 |
| 1.0428 | 1.33 | 150 | 1.0587 |
| 0.9392 | 1.78 | 200 | 0.8968 |
| 0.786 | 2.22 | 250 | 1.0131 |
| 0.8503 | 2.67 | 300 | 0.8795 |
### Framework versions
- Transformers 4.35.2
- Pytorch 2.1.0+cu118
- Datasets 2.15.0
- Tokenizers 0.15.0
|
immich-app/XLM-Roberta-Large-Vit-B-16Plus | immich-app | "2023-10-28T23:00:16Z" | 36,426 | 6 | transformers | [
"transformers",
"onnx",
"immich",
"clip",
"multilingual",
"endpoints_compatible",
"region:us"
] | null | "2023-10-28T22:44:35Z" | ---
tags:
- immich
- clip
- multilingual
---
# Model Description
This repo contains ONNX exports for the multilingual CLIP model [M-CLIP/XLM-Roberta-Large-Vit-B-16Plus](https://huggingface.co/M-CLIP/XLM-Roberta-Large-Vit-B-16Plus).
It separates the visual and textual encoders into separate models for the purpose of generating image and text embeddings.
This repo is specifically intended for use with [Immich](https://immich.app/), a self-hosted photo library.
|
RunDiffusion/Juggernaut-XL-Lightning | RunDiffusion | "2024-03-11T20:09:52Z" | 36,417 | 40 | diffusers | [
"diffusers",
"art",
"people",
"diffusion",
"Cinematic",
"Photography",
"Landscape",
"Interior",
"Food",
"Car",
"Wildlife",
"Architecture",
"en",
"base_model:stabilityai/stable-diffusion-xl-base-1.0",
"license:creativeml-openrail-m",
"endpoints_compatible",
"diffusers:StableDiffusionXLPipeline",
"region:us"
] | text-to-image | "2024-02-23T20:45:29Z" | ---
language:
- en
license: creativeml-openrail-m
library_name: diffusers
tags:
- art
- people
- diffusion
- Cinematic
- Photography
- Landscape
- Interior
- Food
- Car
- Wildlife
- Architecture
thumbnail: https://imagedelivery.net/siANnpeNAc_S2q1M3-eDrA/49a32981-4aa2-410e-a5b1-35835bf20d00/padthumb
base_model: stabilityai/stable-diffusion-xl-base-1.0
---
# Juggernaut XL + RunDiffusion Lightning!

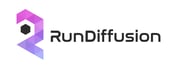
## Want the full version of Juggernaut? Try v9! [Juggernaut v9 + RunDiffusion Photo v2](https://huggingface.co/RunDiffusion/Juggernaut-XL-v9)
This model is not permitted to be used behind API services. Please contact [juggernaut@rundiffusion.com](mailto:juggernaut@rundiffusion.com) for business inquires, commercial licensing, custom models, and consultation.
Juggernaut is available on the new Auto1111 Forge on [RunDiffusion](http://rundiffusion.com/?utm_source=huggingface&utm_medium=referral&utm_campaign=Kandoo)
#Juggernaut XL Lightning is here
Get ready for speed and quality. Who ever said you couldn't have both?! Now you can with the worlds most downloaded model series!
Here are some tips to get you started.
Use this in Automatic1111 and Automatic1111 Forge (Both available on [RunDiffusion](http://rundiffusion.com/?utm_source=huggingface&utm_medium=referral&utm_campaign=Kandoo))
Start with your favorite prompt and negative prompt.
- Set the sampler to: DPM++ SDE or DPM++ SDE Karras
- Set the steps between 5 and 7
- Set the CFG between 1.5 and 2
- Set the resolution to >= 1024x1024
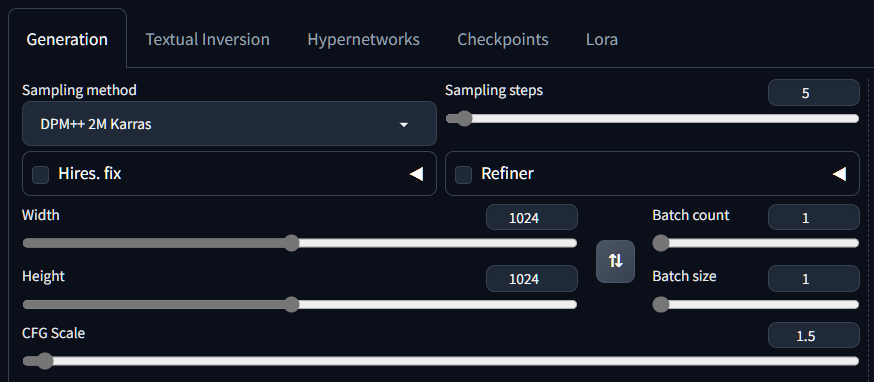 |
firqaaa/indo-sentence-bert-base | firqaaa | "2024-03-03T00:02:00Z" | 36,389 | 11 | sentence-transformers | [
"sentence-transformers",
"pytorch",
"bert",
"feature-extraction",
"sentence-similarity",
"transformers",
"id",
"arxiv:1908.10084",
"license:apache-2.0",
"endpoints_compatible",
"region:us"
] | sentence-similarity | "2022-09-19T18:01:57Z" | ---
pipeline_tag: sentence-similarity
tags:
- sentence-transformers
- feature-extraction
- sentence-similarity
- transformers
license: apache-2.0
language:
- id
library_name: sentence-transformers
---
# indo-sentence-bert-base
This is a [sentence-transformers](https://www.SBERT.net) model: It maps sentences & paragraphs to a 768 dimensional dense vector space and can be used for tasks like clustering or semantic search.
<!--- Describe your model here -->
## Usage (Sentence-Transformers)
Using this model becomes easy when you have [sentence-transformers](https://www.SBERT.net) installed:
```
pip install -U sentence-transformers
```
Then you can use the model like this:
```python
from sentence_transformers import SentenceTransformer
sentences = ["Ibukota Perancis adalah Paris",
"Menara Eifel terletak di Paris, Perancis",
"Pizza adalah makanan khas Italia",
"Saya kuliah di Carneige Mellon University"]
model = SentenceTransformer('firqaaa/indo-sentence-bert-base')
embeddings = model.encode(sentences)
print(embeddings)
```
## Usage (HuggingFace Transformers)
Without [sentence-transformers](https://www.SBERT.net), you can use the model like this: First, you pass your input through the transformer model, then you have to apply the right pooling-operation on-top of the contextualized word embeddings.
```python
from transformers import AutoTokenizer, AutoModel
import torch
#Mean Pooling - Take attention mask into account for correct averaging
def mean_pooling(model_output, attention_mask):
token_embeddings = model_output[0] #First element of model_output contains all token embeddings
input_mask_expanded = attention_mask.unsqueeze(-1).expand(token_embeddings.size()).float()
return torch.sum(token_embeddings * input_mask_expanded, 1) / torch.clamp(input_mask_expanded.sum(1), min=1e-9)
# Sentences we want sentence embeddings for
sentences = ["Ibukota Perancis adalah Paris",
"Menara Eifel terletak di Paris, Perancis",
"Pizza adalah makanan khas Italia",
"Saya kuliah di Carneige Mellon University"]
# Load model from HuggingFace Hub
tokenizer = AutoTokenizer.from_pretrained('firqaaa/indo-sentence-bert-base')
model = AutoModel.from_pretrained('firqaaa/indo-sentence-bert-base')
# Tokenize sentences
encoded_input = tokenizer(sentences, padding=True, truncation=True, return_tensors='pt')
# Compute token embeddings
with torch.no_grad():
model_output = model(**encoded_input)
# Perform pooling. In this case, mean pooling.
sentence_embeddings = mean_pooling(model_output, encoded_input['attention_mask'])
print("Sentence embeddings:")
print(sentence_embeddings)
```
## Evaluation Results
<!--- Describe how your model was evaluated -->
For an automated evaluation of this model, see the *Sentence Embeddings Benchmark*: [https://seb.sbert.net](https://seb.sbert.net?model_name={MODEL_NAME})
## Training
The model was trained with the parameters:
**DataLoader**:
`sentence_transformers.datasets.NoDuplicatesDataLoader.NoDuplicatesDataLoader` of length 19644 with parameters:
```
{'batch_size': 16}
```
**Loss**:
`sentence_transformers.losses.MultipleNegativesRankingLoss.MultipleNegativesRankingLoss` with parameters:
```
{'scale': 20.0, 'similarity_fct': 'cos_sim'}
```
Parameters of the fit()-Method:
```
{
"epochs": 5,
"evaluation_steps": 0,
"evaluator": "NoneType",
"max_grad_norm": 1,
"optimizer_class": "<class 'torch.optim.adamw.AdamW'>",
"optimizer_params": {
"lr": 2e-05
},
"scheduler": "WarmupLinear",
"steps_per_epoch": null,
"warmup_steps": 9930,
"weight_decay": 0.01
}
```
## Full Model Architecture
```
SentenceTransformer(
(0): Transformer({'max_seq_length': 512, 'do_lower_case': False}) with Transformer model: BertModel
(1): Pooling({'word_embedding_dimension': 768, 'pooling_mode_cls_token': False, 'pooling_mode_mean_tokens': True, 'pooling_mode_max_tokens': False, 'pooling_mode_mean_sqrt_len_tokens': False})
)
```
## Citing & Authors
<!--- Describe where people can find more information -->
```
@inproceedings{reimers-2019-sentence-bert,
title = "Sentence-BERT: Sentence Embeddings using Siamese BERT-Networks",
author = "Reimers, Nils and Gurevych, Iryna",
booktitle = "Proceedings of the 2019 Conference on Empirical Methods in Natural Language Processing",
month = "11",
year = "2019",
publisher = "Association for Computational Linguistics",
url = "https://arxiv.org/abs/1908.10084",
```
```
@misc{author = {Arasyi, Firqa},
title = {indo-sentence-bert: Sentence Transformer for Bahasa Indonesia with Multiple Negative Ranking Loss},
year = {2022},
month = {9}
publisher = {huggingface},
journal = {huggingface repository},
howpublished = {https://huggingface.co/firqaaa/indo-sentence-bert-base}
}
``` |
dangvantuan/sentence-camembert-base | dangvantuan | "2022-03-11T17:02:50Z" | 36,327 | 15 | transformers | [
"transformers",
"pytorch",
"camembert",
"feature-extraction",
"Text",
"Sentence Similarity",
"Sentence-Embedding",
"camembert-base",
"sentence-similarity",
"fr",
"dataset:stsb_multi_mt",
"arxiv:1908.10084",
"license:apache-2.0",
"model-index",
"endpoints_compatible",
"region:us"
] | sentence-similarity | "2022-03-11T16:16:15Z" | ---
pipeline_tag: sentence-similarity
language: fr
datasets:
- stsb_multi_mt
tags:
- Text
- Sentence Similarity
- Sentence-Embedding
- camembert-base
license: apache-2.0
model-index:
- name: sentence-camembert-base by Van Tuan DANG
results:
- task:
name: Sentence-Embedding
type: Text Similarity
dataset:
name: Text Similarity fr
type: stsb_multi_mt
args: fr
metrics:
- name: Test Pearson correlation coefficient
type: Pearson_correlation_coefficient
value: xx.xx
---
## Pre-trained sentence embedding models are the state-of-the-art of Sentence Embeddings for French.
Model is Fine-tuned using pre-trained [facebook/camembert-base](https://huggingface.co/camembert/camembert-base) and
[Siamese BERT-Networks with 'sentences-transformers'](https://www.sbert.net/) on dataset [stsb](https://huggingface.co/datasets/stsb_multi_mt/viewer/fr/train)
## Usage
The model can be used directly (without a language model) as follows:
```python
from sentence_transformers import SentenceTransformer
model = SentenceTransformer("dangvantuan/sentence-camembert-base")
sentences = ["Un avion est en train de décoller.",
"Un homme joue d'une grande flûte.",
"Un homme étale du fromage râpé sur une pizza.",
"Une personne jette un chat au plafond.",
"Une personne est en train de plier un morceau de papier.",
]
embeddings = model.encode(sentences)
```
## Evaluation
The model can be evaluated as follows on the French test data of stsb.
```python
from sentence_transformers import SentenceTransformer
from sentence_transformers.readers import InputExample
from sentence_transformers.evaluation import EmbeddingSimilarityEvaluator
from datasets import load_dataset
def convert_dataset(dataset):
dataset_samples=[]
for df in dataset:
score = float(df['similarity_score'])/5.0 # Normalize score to range 0 ... 1
inp_example = InputExample(texts=[df['sentence1'],
df['sentence2']], label=score)
dataset_samples.append(inp_example)
return dataset_samples
# Loading the dataset for evaluation
df_dev = load_dataset("stsb_multi_mt", name="fr", split="dev")
df_test = load_dataset("stsb_multi_mt", name="fr", split="test")
# Convert the dataset for evaluation
# For Dev set:
dev_samples = convert_dataset(df_dev)
val_evaluator = EmbeddingSimilarityEvaluator.from_input_examples(dev_samples, name='sts-dev')
val_evaluator(model, output_path="./")
# For Test set:
test_samples = convert_dataset(df_test)
test_evaluator = EmbeddingSimilarityEvaluator.from_input_examples(test_samples, name='sts-test')
test_evaluator(model, output_path="./")
```
**Test Result**:
The performance is measured using Pearson and Spearman correlation:
- On dev
| Model | Pearson correlation | Spearman correlation | #params |
| ------------- | ------------- | ------------- |------------- |
| [dangvantuan/sentence-camembert-base](https://huggingface.co/dangvantuan/sentence-camembert-base)| 86.73 |86.54 | 110M |
| [distiluse-base-multilingual-cased](https://huggingface.co/sentence-transformers/distiluse-base-multilingual-cased) | 79.22 | 79.16|135M |
- On test
| Model | Pearson correlation | Spearman correlation |
| ------------- | ------------- | ------------- |
| [dangvantuan/sentence-camembert-base](https://huggingface.co/dangvantuan/sentence-camembert-base)| 82.36 | 81.64|
| [distiluse-base-multilingual-cased](https://huggingface.co/sentence-transformers/distiluse-base-multilingual-cased) | 78.62 | 77.48|
## Citation
@article{reimers2019sentence,
title={Sentence-BERT: Sentence Embeddings using Siamese BERT-Networks},
author={Nils Reimers, Iryna Gurevych},
journal={https://arxiv.org/abs/1908.10084},
year={2019}
}
@article{martin2020camembert,
title={CamemBERT: a Tasty French Language Mode},
author={Martin, Louis and Muller, Benjamin and Su{\'a}rez, Pedro Javier Ortiz and Dupont, Yoann and Romary, Laurent and de la Clergerie, {\'E}ric Villemonte and Seddah, Djam{\'e} and Sagot, Beno{\^\i}t},
journal={Proceedings of the 58th Annual Meeting of the Association for Computational Linguistics},
year={2020}
} |
hpcai-tech/OpenSora-STDiT-v3 | hpcai-tech | "2024-06-17T08:24:41Z" | 36,274 | 22 | transformers | [
"transformers",
"safetensors",
"STDiT3",
"license:apache-2.0",
"endpoints_compatible",
"region:us"
] | null | "2024-06-17T02:33:20Z" | ---
license: apache-2.0
---
<p align="center">
<img src="https://cdn-uploads.huggingface.co/production/uploads/63993d721fad4d6eb265d999/UXleJWJExX2WlBizxzYxn.png" width="250"/>
</p>
# Open-Sora STDiT-v3 Weights
This repository stores the weights of the STDiT3 released by the Open-Sora team. You can visit our project at:
- [GitHub](https://github.com/hpcaitech/Open-Sora)
- [Gallery](https://hpcaitech.github.io/Open-Sora/)
- [Gradio Demo](https://huggingface.co/spaces/hpcai-tech/open-sora)
The weights are released together with Open-Sora v1.2.
We recommend you to use this weights in the [Open-Sora codebase]((https://github.com/hpcaitech/Open-Sora)). If you want to use STDiT in your own project, you may use the following sample code.
1. Install `opensora`
```bash
pip install git+https://github.com/hpcaitech/Open-Sora.git
```
2. Use `STDiT3` in your own code
```python
from opensora.models.stdit.stdit3 import STDiT3
stdit = STDiT3.from_pretrained("hpcai-tech/OpenSora-STDiT-v3")
``` |
guillaumekln/faster-whisper-large-v2 | guillaumekln | "2023-05-12T18:58:25Z" | 36,202 | 188 | ctranslate2 | [
"ctranslate2",
"audio",
"automatic-speech-recognition",
"en",
"zh",
"de",
"es",
"ru",
"ko",
"fr",
"ja",
"pt",
"tr",
"pl",
"ca",
"nl",
"ar",
"sv",
"it",
"id",
"hi",
"fi",
"vi",
"he",
"uk",
"el",
"ms",
"cs",
"ro",
"da",
"hu",
"ta",
"no",
"th",
"ur",
"hr",
"bg",
"lt",
"la",
"mi",
"ml",
"cy",
"sk",
"te",
"fa",
"lv",
"bn",
"sr",
"az",
"sl",
"kn",
"et",
"mk",
"br",
"eu",
"is",
"hy",
"ne",
"mn",
"bs",
"kk",
"sq",
"sw",
"gl",
"mr",
"pa",
"si",
"km",
"sn",
"yo",
"so",
"af",
"oc",
"ka",
"be",
"tg",
"sd",
"gu",
"am",
"yi",
"lo",
"uz",
"fo",
"ht",
"ps",
"tk",
"nn",
"mt",
"sa",
"lb",
"my",
"bo",
"tl",
"mg",
"as",
"tt",
"haw",
"ln",
"ha",
"ba",
"jw",
"su",
"license:mit",
"region:us"
] | automatic-speech-recognition | "2023-03-23T10:36:06Z" | ---
language:
- en
- zh
- de
- es
- ru
- ko
- fr
- ja
- pt
- tr
- pl
- ca
- nl
- ar
- sv
- it
- id
- hi
- fi
- vi
- he
- uk
- el
- ms
- cs
- ro
- da
- hu
- ta
- 'no'
- th
- ur
- hr
- bg
- lt
- la
- mi
- ml
- cy
- sk
- te
- fa
- lv
- bn
- sr
- az
- sl
- kn
- et
- mk
- br
- eu
- is
- hy
- ne
- mn
- bs
- kk
- sq
- sw
- gl
- mr
- pa
- si
- km
- sn
- yo
- so
- af
- oc
- ka
- be
- tg
- sd
- gu
- am
- yi
- lo
- uz
- fo
- ht
- ps
- tk
- nn
- mt
- sa
- lb
- my
- bo
- tl
- mg
- as
- tt
- haw
- ln
- ha
- ba
- jw
- su
tags:
- audio
- automatic-speech-recognition
license: mit
library_name: ctranslate2
---
# Whisper large-v2 model for CTranslate2
This repository contains the conversion of [openai/whisper-large-v2](https://huggingface.co/openai/whisper-large-v2) to the [CTranslate2](https://github.com/OpenNMT/CTranslate2) model format.
This model can be used in CTranslate2 or projects based on CTranslate2 such as [faster-whisper](https://github.com/guillaumekln/faster-whisper).
## Example
```python
from faster_whisper import WhisperModel
model = WhisperModel("large-v2")
segments, info = model.transcribe("audio.mp3")
for segment in segments:
print("[%.2fs -> %.2fs] %s" % (segment.start, segment.end, segment.text))
```
## Conversion details
The original model was converted with the following command:
```
ct2-transformers-converter --model openai/whisper-large-v2 --output_dir faster-whisper-large-v2 \
--copy_files tokenizer.json --quantization float16
```
Note that the model weights are saved in FP16. This type can be changed when the model is loaded using the [`compute_type` option in CTranslate2](https://opennmt.net/CTranslate2/quantization.html).
## More information
**For more information about the original model, see its [model card](https://huggingface.co/openai/whisper-large-v2).**
|
facebook/rag-token-nq | facebook | "2023-11-13T16:13:45Z" | 36,193 | 118 | transformers | [
"transformers",
"pytorch",
"tf",
"rag",
"en",
"dataset:wiki_dpr",
"arxiv:2005.11401",
"license:apache-2.0",
"endpoints_compatible",
"region:us"
] | null | "2022-03-02T23:29:05Z" | ---
language: en
license: apache-2.0
datasets:
- wiki_dpr
thumbnail: https://huggingface.co/front/thumbnails/facebook.png
---
## RAG
This is the RAG-Token Model of the the paper [Retrieval-Augmented Generation for Knowledge-Intensive NLP Tasks](https://arxiv.org/pdf/2005.11401.pdf)
by Patrick Lewis, Ethan Perez, Aleksandara Piktus et al.
The model is a *uncased* model, which means that capital letters are simply converted to lower-case letters.
The model consists of a *question_encoder*, *retriever* and a *generator*. The retriever extracts relevant passages from the *wiki_dpr* `train` datasets, which is linked above.
The question_encoder and retriever are based on `facebook/dpr-question_encoder-single-nq-base` and `facebook/bart-large`, which were jointly finetuned on
on the *wiki_dpr* QA dataset in an end-to-end fashion.
## Usage:
**Note**: In the usage example below only the *dummy* retriever of *wiki_dpr* is used because the complete *lecagy* index requires over 75 GB of RAM.
The model can generate answers to any factoid question as follows:
```python
from transformers import RagTokenizer, RagRetriever, RagTokenForGeneration
tokenizer = RagTokenizer.from_pretrained("facebook/rag-token-nq")
retriever = RagRetriever.from_pretrained("facebook/rag-token-nq", index_name="exact", use_dummy_dataset=True)
model = RagTokenForGeneration.from_pretrained("facebook/rag-token-nq", retriever=retriever)
input_dict = tokenizer.prepare_seq2seq_batch("who holds the record in 100m freestyle", return_tensors="pt")
generated = model.generate(input_ids=input_dict["input_ids"])
print(tokenizer.batch_decode(generated, skip_special_tokens=True)[0])
# should give michael phelps => sounds reasonable
```
|
bartowski/L3-8B-Lunaris-v1-GGUF | bartowski | "2024-06-29T05:20:35Z" | 36,166 | 6 | null | [
"gguf",
"text-generation",
"en",
"license:llama3",
"region:us"
] | text-generation | "2024-06-29T04:53:40Z" | ---
license: llama3
language:
- en
quantized_by: bartowski
pipeline_tag: text-generation
---
## Llamacpp imatrix Quantizations of L3-8B-Lunaris-v1
Using <a href="https://github.com/ggerganov/llama.cpp/">llama.cpp</a> release <a href="https://github.com/ggerganov/llama.cpp/releases/tag/b3259">b3259</a> for quantization.
Original model: https://huggingface.co/Sao10K/L3-8B-Lunaris-v1
All quants made using imatrix option with dataset from [here](https://gist.github.com/bartowski1182/eb213dccb3571f863da82e99418f81e8)
## Prompt format
```
<|begin_of_text|><|start_header_id|>system<|end_header_id|>
{system_prompt}<|eot_id|><|start_header_id|>user<|end_header_id|>
{prompt}<|eot_id|><|start_header_id|>assistant<|end_header_id|>
```
## Download a file (not the whole branch) from below:
| Filename | Quant type | File Size | Description |
| -------- | ---------- | --------- | ----------- |
| [L3-8B-Lunaris-v1-Q8_0_L.gguf](https://huggingface.co/bartowski/L3-8B-Lunaris-v1-GGUF/blob/main/L3-8B-Lunaris-v1-Q8_1.gguf) | Q8_0_L | 9.52GB | *Experimental*, uses f16 for embed and output weights. Please provide any feedback of differences. Extremely high quality, generally unneeded but max available quant. |
| [L3-8B-Lunaris-v1-Q8_0.gguf](https://huggingface.co/bartowski/L3-8B-Lunaris-v1-GGUF/blob/main/L3-8B-Lunaris-v1-Q8_0.gguf) | Q8_0 | 8.54GB | Extremely high quality, generally unneeded but max available quant. |
| [L3-8B-Lunaris-v1-Q6_K_L.gguf](https://huggingface.co/bartowski/L3-8B-Lunaris-v1-GGUF/blob/main/L3-8B-Lunaris-v1-Q6_K_L.gguf) | Q6_K_L | 7.83GB | *Experimental*, uses f16 for embed and output weights. Please provide any feedback of differences. Very high quality, near perfect, *recommended*. |
| [L3-8B-Lunaris-v1-Q6_K.gguf](https://huggingface.co/bartowski/L3-8B-Lunaris-v1-GGUF/blob/main/L3-8B-Lunaris-v1-Q6_K.gguf) | Q6_K | 6.59GB | Very high quality, near perfect, *recommended*. |
| [L3-8B-Lunaris-v1-Q5_K_L.gguf](https://huggingface.co/bartowski/L3-8B-Lunaris-v1-GGUF/blob/main/L3-8B-Lunaris-v1-Q5_K_L.gguf) | Q5_K_L | 7.04GB | *Experimental*, uses f16 for embed and output weights. Please provide any feedback of differences. High quality, *recommended*. |
| [L3-8B-Lunaris-v1-Q5_K_M.gguf](https://huggingface.co/bartowski/L3-8B-Lunaris-v1-GGUF/blob/main/L3-8B-Lunaris-v1-Q5_K_M.gguf) | Q5_K_M | 5.73GB | High quality, *recommended*. |
| [L3-8B-Lunaris-v1-Q5_K_S.gguf](https://huggingface.co/bartowski/L3-8B-Lunaris-v1-GGUF/blob/main/L3-8B-Lunaris-v1-Q5_K_S.gguf) | Q5_K_S | 5.59GB | High quality, *recommended*. |
| [L3-8B-Lunaris-v1-Q4_K_L.gguf](https://huggingface.co/bartowski/L3-8B-Lunaris-v1-GGUF/blob/main/L3-8B-Lunaris-v1-Q4_K_L.gguf) | Q4_K_L | 6.29GB | *Experimental*, uses f16 for embed and output weights. Please provide any feedback of differences. Good quality, uses about 4.83 bits per weight, *recommended*. |
| [L3-8B-Lunaris-v1-Q4_K_M.gguf](https://huggingface.co/bartowski/L3-8B-Lunaris-v1-GGUF/blob/main/L3-8B-Lunaris-v1-Q4_K_M.gguf) | Q4_K_M | 4.92GB | Good quality, uses about 4.83 bits per weight, *recommended*. |
| [L3-8B-Lunaris-v1-Q4_K_S.gguf](https://huggingface.co/bartowski/L3-8B-Lunaris-v1-GGUF/blob/main/L3-8B-Lunaris-v1-Q4_K_S.gguf) | Q4_K_S | 4.69GB | Slightly lower quality with more space savings, *recommended*. |
| [L3-8B-Lunaris-v1-IQ4_XS.gguf](https://huggingface.co/bartowski/L3-8B-Lunaris-v1-GGUF/blob/main/L3-8B-Lunaris-v1-IQ4_XS.gguf) | IQ4_XS | 4.44GB | Decent quality, smaller than Q4_K_S with similar performance, *recommended*. |
| [L3-8B-Lunaris-v1-Q3_K_XL.gguf](https://huggingface.co/bartowski/L3-8B-Lunaris-v1-GGUF/blob/main/L3-8B-Lunaris-v1-Q3_K_XL.gguf) | Q3_K_XL | 5.76GB | *Experimental*, uses f16 for embed and output weights. Please provide any feedback of differences. Lower quality but usable, good for low RAM availability. |
| [L3-8B-Lunaris-v1-Q3_K_L.gguf](https://huggingface.co/bartowski/L3-8B-Lunaris-v1-GGUF/blob/main/L3-8B-Lunaris-v1-Q3_K_L.gguf) | Q3_K_L | 4.32GB | Lower quality but usable, good for low RAM availability. |
| [L3-8B-Lunaris-v1-Q3_K_M.gguf](https://huggingface.co/bartowski/L3-8B-Lunaris-v1-GGUF/blob/main/L3-8B-Lunaris-v1-Q3_K_M.gguf) | Q3_K_M | 4.01GB | Even lower quality. |
| [L3-8B-Lunaris-v1-IQ3_M.gguf](https://huggingface.co/bartowski/L3-8B-Lunaris-v1-GGUF/blob/main/L3-8B-Lunaris-v1-IQ3_M.gguf) | IQ3_M | 3.78GB | Medium-low quality, new method with decent performance comparable to Q3_K_M. |
| [L3-8B-Lunaris-v1-Q3_K_S.gguf](https://huggingface.co/bartowski/L3-8B-Lunaris-v1-GGUF/blob/main/L3-8B-Lunaris-v1-Q3_K_S.gguf) | Q3_K_S | 3.66GB | Low quality, not recommended. |
| [L3-8B-Lunaris-v1-IQ3_XS.gguf](https://huggingface.co/bartowski/L3-8B-Lunaris-v1-GGUF/blob/main/L3-8B-Lunaris-v1-IQ3_XS.gguf) | IQ3_XS | 3.51GB | Lower quality, new method with decent performance, slightly better than Q3_K_S. |
| [L3-8B-Lunaris-v1-IQ3_XXS.gguf](https://huggingface.co/bartowski/L3-8B-Lunaris-v1-GGUF/blob/main/L3-8B-Lunaris-v1-IQ3_XXS.gguf) | IQ3_XXS | 3.27GB | Lower quality, new method with decent performance, comparable to Q3 quants. |
| [L3-8B-Lunaris-v1-Q2_K.gguf](https://huggingface.co/bartowski/L3-8B-Lunaris-v1-GGUF/blob/main/L3-8B-Lunaris-v1-Q2_K.gguf) | Q2_K | 3.17GB | Very low quality but surprisingly usable. |
| [L3-8B-Lunaris-v1-IQ2_M.gguf](https://huggingface.co/bartowski/L3-8B-Lunaris-v1-GGUF/blob/main/L3-8B-Lunaris-v1-IQ2_M.gguf) | IQ2_M | 2.94GB | Very low quality, uses SOTA techniques to also be surprisingly usable. |
| [L3-8B-Lunaris-v1-IQ2_S.gguf](https://huggingface.co/bartowski/L3-8B-Lunaris-v1-GGUF/blob/main/L3-8B-Lunaris-v1-IQ2_S.gguf) | IQ2_S | 2.75GB | Very low quality, uses SOTA techniques to be usable. |
| [L3-8B-Lunaris-v1-IQ2_XS.gguf](https://huggingface.co/bartowski/L3-8B-Lunaris-v1-GGUF/blob/main/L3-8B-Lunaris-v1-IQ2_XS.gguf) | IQ2_XS | 2.60GB | Very low quality, uses SOTA techniques to be usable. |
## Downloading using huggingface-cli
First, make sure you have hugginface-cli installed:
```
pip install -U "huggingface_hub[cli]"
```
Then, you can target the specific file you want:
```
huggingface-cli download bartowski/L3-8B-Lunaris-v1-GGUF --include "L3-8B-Lunaris-v1-Q4_K_M.gguf" --local-dir ./
```
If the model is bigger than 50GB, it will have been split into multiple files. In order to download them all to a local folder, run:
```
huggingface-cli download bartowski/L3-8B-Lunaris-v1-GGUF --include "L3-8B-Lunaris-v1-Q8_0.gguf/*" --local-dir L3-8B-Lunaris-v1-Q8_0
```
You can either specify a new local-dir (L3-8B-Lunaris-v1-Q8_0) or download them all in place (./)
## Which file should I choose?
A great write up with charts showing various performances is provided by Artefact2 [here](https://gist.github.com/Artefact2/b5f810600771265fc1e39442288e8ec9)
The first thing to figure out is how big a model you can run. To do this, you'll need to figure out how much RAM and/or VRAM you have.
If you want your model running as FAST as possible, you'll want to fit the whole thing on your GPU's VRAM. Aim for a quant with a file size 1-2GB smaller than your GPU's total VRAM.
If you want the absolute maximum quality, add both your system RAM and your GPU's VRAM together, then similarly grab a quant with a file size 1-2GB Smaller than that total.
Next, you'll need to decide if you want to use an 'I-quant' or a 'K-quant'.
If you don't want to think too much, grab one of the K-quants. These are in format 'QX_K_X', like Q5_K_M.
If you want to get more into the weeds, you can check out this extremely useful feature chart:
[llama.cpp feature matrix](https://github.com/ggerganov/llama.cpp/wiki/Feature-matrix)
But basically, if you're aiming for below Q4, and you're running cuBLAS (Nvidia) or rocBLAS (AMD), you should look towards the I-quants. These are in format IQX_X, like IQ3_M. These are newer and offer better performance for their size.
These I-quants can also be used on CPU and Apple Metal, but will be slower than their K-quant equivalent, so speed vs performance is a tradeoff you'll have to decide.
The I-quants are *not* compatible with Vulcan, which is also AMD, so if you have an AMD card double check if you're using the rocBLAS build or the Vulcan build. At the time of writing this, LM Studio has a preview with ROCm support, and other inference engines have specific builds for ROCm.
Want to support my work? Visit my ko-fi page here: https://ko-fi.com/bartowski
|
jplu/tf-camembert-base | jplu | "2020-12-11T21:47:52Z" | 36,155 | 0 | transformers | [
"transformers",
"tf",
"camembert",
"fill-mask",
"autotrain_compatible",
"endpoints_compatible",
"region:us"
] | fill-mask | "2022-03-02T23:29:05Z" | # Tensorflow CamemBERT
In this repository you will find different versions of the CamemBERT model for Tensorflow.
## CamemBERT
[CamemBERT](https://camembert-model.fr/) is a state-of-the-art language model for French based on the RoBERTa architecture pretrained on the French subcorpus of the newly available multilingual corpus OSCAR.
## Model Weights
| Model | Downloads
| -------------------------------- | ---------------------------------------------------------------------------------------------------------------
| `jplu/tf-camembert-base` | [`config.json`](https://s3.amazonaws.com/models.huggingface.co/bert/jplu/tf-camembert-base/config.json) • [`tf_model.h5`](https://s3.amazonaws.com/models.huggingface.co/bert/jplu/tf-camembert-base/tf_model.h5)
## Usage
With Transformers >= 2.4 the Tensorflow models of CamemBERT can be loaded like:
```python
from transformers import TFCamembertModel
model = TFCamembertModel.from_pretrained("jplu/tf-camembert-base")
```
## Huggingface model hub
All models are available on the [Huggingface model hub](https://huggingface.co/jplu).
## Acknowledgments
Thanks to all the Huggingface team for the support and their amazing library!
|
google/vit-large-patch16-224-in21k | google | "2024-02-14T13:43:52Z" | 36,139 | 20 | transformers | [
"transformers",
"pytorch",
"tf",
"jax",
"safetensors",
"vit",
"image-feature-extraction",
"vision",
"dataset:imagenet-21k",
"arxiv:2010.11929",
"arxiv:2006.03677",
"license:apache-2.0",
"region:us"
] | image-feature-extraction | "2022-03-02T23:29:05Z" | ---
license: apache-2.0
tags:
- vision
datasets:
- imagenet-21k
inference: false
---
# Vision Transformer (large-sized model)
Vision Transformer (ViT) model pre-trained on ImageNet-21k (14 million images, 21,843 classes) at resolution 224x224. It was introduced in the paper [An Image is Worth 16x16 Words: Transformers for Image Recognition at Scale](https://arxiv.org/abs/2010.11929) by Dosovitskiy et al. and first released in [this repository](https://github.com/google-research/vision_transformer). However, the weights were converted from the [timm repository](https://github.com/rwightman/pytorch-image-models) by Ross Wightman, who already converted the weights from JAX to PyTorch. Credits go to him.
Disclaimer: The team releasing ViT did not write a model card for this model so this model card has been written by the Hugging Face team.
## Model description
The Vision Transformer (ViT) is a transformer encoder model (BERT-like) pretrained on a large collection of images in a supervised fashion, namely ImageNet-21k, at a resolution of 224x224 pixels.
Images are presented to the model as a sequence of fixed-size patches (resolution 16x16), which are linearly embedded. One also adds a [CLS] token to the beginning of a sequence to use it for classification tasks. One also adds absolute position embeddings before feeding the sequence to the layers of the Transformer encoder.
Note that this model does not provide any fine-tuned heads, as these were zero'd by Google researchers. However, the model does include the pre-trained pooler, which can be used for downstream tasks (such as image classification).
By pre-training the model, it learns an inner representation of images that can then be used to extract features useful for downstream tasks: if you have a dataset of labeled images for instance, you can train a standard classifier by placing a linear layer on top of the pre-trained encoder. One typically places a linear layer on top of the [CLS] token, as the last hidden state of this token can be seen as a representation of an entire image.
## Intended uses & limitations
You can use the raw model to embed images, but it's mostly intended to be fine-tuned on a downstream task.
### How to use
Here is how to use this model:
```python
from transformers import ViTImageProcessor, ViTModel
from PIL import Image
import requests
url = 'http://images.cocodataset.org/val2017/000000039769.jpg'
image = Image.open(requests.get(url, stream=True).raw)
processor = ViTImageProcessor.from_pretrained('google/vit-large-patch16-224-in21k')
model = ViTModel.from_pretrained('google/vit-large-patch16-224-in21k')
inputs = processor(images=image, return_tensors="pt")
outputs = model(**inputs)
last_hidden_state = outputs.last_hidden_state
```
Currently, both the feature extractor and model support PyTorch. Tensorflow and JAX/FLAX are coming soon, and the API of ViTFeatureExtractor might change.
## Training data
The ViT model was pretrained on [ImageNet-21k](http://www.image-net.org/), a dataset consisting of 14 million images and 21k classes.
## Training procedure
### Preprocessing
The exact details of preprocessing of images during training/validation can be found [here](https://github.com/google-research/vision_transformer/blob/master/vit_jax/input_pipeline.py).
Images are resized/rescaled to the same resolution (224x224) and normalized across the RGB channels with mean (0.5, 0.5, 0.5) and standard deviation (0.5, 0.5, 0.5).
### Pretraining
The model was trained on TPUv3 hardware (8 cores). All model variants are trained with a batch size of 4096 and learning rate warmup of 10k steps. For ImageNet, the authors found it beneficial to additionally apply gradient clipping at global norm 1. Pre-training resolution is 224.
## Evaluation results
For evaluation results on several image classification benchmarks, we refer to tables 2 and 5 of the original paper. Note that for fine-tuning, the best results are obtained with a higher resolution (384x384). Of course, increasing the model size will result in better performance.
### BibTeX entry and citation info
```bibtex
@misc{wu2020visual,
title={Visual Transformers: Token-based Image Representation and Processing for Computer Vision},
author={Bichen Wu and Chenfeng Xu and Xiaoliang Dai and Alvin Wan and Peizhao Zhang and Zhicheng Yan and Masayoshi Tomizuka and Joseph Gonzalez and Kurt Keutzer and Peter Vajda},
year={2020},
eprint={2006.03677},
archivePrefix={arXiv},
primaryClass={cs.CV}
}
```
```bibtex
@inproceedings{deng2009imagenet,
title={Imagenet: A large-scale hierarchical image database},
author={Deng, Jia and Dong, Wei and Socher, Richard and Li, Li-Jia and Li, Kai and Fei-Fei, Li},
booktitle={2009 IEEE conference on computer vision and pattern recognition},
pages={248--255},
year={2009},
organization={Ieee}
}
``` |
GritLM/GritLM-7B | GritLM | "2024-02-16T10:14:51Z" | 36,119 | 78 | transformers | [
"transformers",
"pytorch",
"safetensors",
"mistral",
"text-generation",
"mteb",
"conversational",
"custom_code",
"dataset:GritLM/tulu2",
"arxiv:2402.09906",
"license:apache-2.0",
"model-index",
"autotrain_compatible",
"endpoints_compatible",
"text-generation-inference",
"region:us"
] | text-generation | "2024-02-11T15:55:35Z" | ---
pipeline_tag: text-generation
inference: true
license: apache-2.0
datasets:
- GritLM/tulu2
tags:
- mteb
model-index:
- name: GritLM-7B
results:
- task:
type: Classification
dataset:
type: mteb/amazon_counterfactual
name: MTEB AmazonCounterfactualClassification (en)
config: en
split: test
revision: e8379541af4e31359cca9fbcf4b00f2671dba205
metrics:
- type: accuracy
value: 81.17910447761194
- type: ap
value: 46.26260671758199
- type: f1
value: 75.44565719934167
- task:
type: Classification
dataset:
type: mteb/amazon_polarity
name: MTEB AmazonPolarityClassification
config: default
split: test
revision: e2d317d38cd51312af73b3d32a06d1a08b442046
metrics:
- type: accuracy
value: 96.5161
- type: ap
value: 94.79131981460425
- type: f1
value: 96.51506148413065
- task:
type: Classification
dataset:
type: mteb/amazon_reviews_multi
name: MTEB AmazonReviewsClassification (en)
config: en
split: test
revision: 1399c76144fd37290681b995c656ef9b2e06e26d
metrics:
- type: accuracy
value: 57.806000000000004
- type: f1
value: 56.78350156257903
- task:
type: Retrieval
dataset:
type: arguana
name: MTEB ArguAna
config: default
split: test
revision: None
metrics:
- type: map_at_1
value: 38.478
- type: map_at_10
value: 54.955
- type: map_at_100
value: 54.955
- type: map_at_1000
value: 54.955
- type: map_at_3
value: 50.888999999999996
- type: map_at_5
value: 53.349999999999994
- type: mrr_at_1
value: 39.757999999999996
- type: mrr_at_10
value: 55.449000000000005
- type: mrr_at_100
value: 55.449000000000005
- type: mrr_at_1000
value: 55.449000000000005
- type: mrr_at_3
value: 51.37500000000001
- type: mrr_at_5
value: 53.822
- type: ndcg_at_1
value: 38.478
- type: ndcg_at_10
value: 63.239999999999995
- type: ndcg_at_100
value: 63.239999999999995
- type: ndcg_at_1000
value: 63.239999999999995
- type: ndcg_at_3
value: 54.935
- type: ndcg_at_5
value: 59.379000000000005
- type: precision_at_1
value: 38.478
- type: precision_at_10
value: 8.933
- type: precision_at_100
value: 0.893
- type: precision_at_1000
value: 0.089
- type: precision_at_3
value: 22.214
- type: precision_at_5
value: 15.491
- type: recall_at_1
value: 38.478
- type: recall_at_10
value: 89.331
- type: recall_at_100
value: 89.331
- type: recall_at_1000
value: 89.331
- type: recall_at_3
value: 66.643
- type: recall_at_5
value: 77.45400000000001
- task:
type: Clustering
dataset:
type: mteb/arxiv-clustering-p2p
name: MTEB ArxivClusteringP2P
config: default
split: test
revision: a122ad7f3f0291bf49cc6f4d32aa80929df69d5d
metrics:
- type: v_measure
value: 51.67144081472449
- task:
type: Clustering
dataset:
type: mteb/arxiv-clustering-s2s
name: MTEB ArxivClusteringS2S
config: default
split: test
revision: f910caf1a6075f7329cdf8c1a6135696f37dbd53
metrics:
- type: v_measure
value: 48.11256154264126
- task:
type: Reranking
dataset:
type: mteb/askubuntudupquestions-reranking
name: MTEB AskUbuntuDupQuestions
config: default
split: test
revision: 2000358ca161889fa9c082cb41daa8dcfb161a54
metrics:
- type: map
value: 67.33801955487878
- type: mrr
value: 80.71549487754474
- task:
type: STS
dataset:
type: mteb/biosses-sts
name: MTEB BIOSSES
config: default
split: test
revision: d3fb88f8f02e40887cd149695127462bbcf29b4a
metrics:
- type: cos_sim_pearson
value: 88.1935203751726
- type: cos_sim_spearman
value: 86.35497970498659
- type: euclidean_pearson
value: 85.46910708503744
- type: euclidean_spearman
value: 85.13928935405485
- type: manhattan_pearson
value: 85.68373836333303
- type: manhattan_spearman
value: 85.40013867117746
- task:
type: Classification
dataset:
type: mteb/banking77
name: MTEB Banking77Classification
config: default
split: test
revision: 0fd18e25b25c072e09e0d92ab615fda904d66300
metrics:
- type: accuracy
value: 88.46753246753248
- type: f1
value: 88.43006344981134
- task:
type: Clustering
dataset:
type: mteb/biorxiv-clustering-p2p
name: MTEB BiorxivClusteringP2P
config: default
split: test
revision: 65b79d1d13f80053f67aca9498d9402c2d9f1f40
metrics:
- type: v_measure
value: 40.86793640310432
- task:
type: Clustering
dataset:
type: mteb/biorxiv-clustering-s2s
name: MTEB BiorxivClusteringS2S
config: default
split: test
revision: 258694dd0231531bc1fd9de6ceb52a0853c6d908
metrics:
- type: v_measure
value: 39.80291334130727
- task:
type: Retrieval
dataset:
type: BeIR/cqadupstack
name: MTEB CQADupstackAndroidRetrieval
config: default
split: test
revision: None
metrics:
- type: map_at_1
value: 38.421
- type: map_at_10
value: 52.349000000000004
- type: map_at_100
value: 52.349000000000004
- type: map_at_1000
value: 52.349000000000004
- type: map_at_3
value: 48.17
- type: map_at_5
value: 50.432
- type: mrr_at_1
value: 47.353
- type: mrr_at_10
value: 58.387
- type: mrr_at_100
value: 58.387
- type: mrr_at_1000
value: 58.387
- type: mrr_at_3
value: 56.199
- type: mrr_at_5
value: 57.487
- type: ndcg_at_1
value: 47.353
- type: ndcg_at_10
value: 59.202
- type: ndcg_at_100
value: 58.848
- type: ndcg_at_1000
value: 58.831999999999994
- type: ndcg_at_3
value: 54.112
- type: ndcg_at_5
value: 56.312
- type: precision_at_1
value: 47.353
- type: precision_at_10
value: 11.459
- type: precision_at_100
value: 1.146
- type: precision_at_1000
value: 0.11499999999999999
- type: precision_at_3
value: 26.133
- type: precision_at_5
value: 18.627
- type: recall_at_1
value: 38.421
- type: recall_at_10
value: 71.89
- type: recall_at_100
value: 71.89
- type: recall_at_1000
value: 71.89
- type: recall_at_3
value: 56.58
- type: recall_at_5
value: 63.125
- task:
type: Retrieval
dataset:
type: BeIR/cqadupstack
name: MTEB CQADupstackEnglishRetrieval
config: default
split: test
revision: None
metrics:
- type: map_at_1
value: 38.025999999999996
- type: map_at_10
value: 50.590999999999994
- type: map_at_100
value: 51.99700000000001
- type: map_at_1000
value: 52.11599999999999
- type: map_at_3
value: 47.435
- type: map_at_5
value: 49.236000000000004
- type: mrr_at_1
value: 48.28
- type: mrr_at_10
value: 56.814
- type: mrr_at_100
value: 57.446
- type: mrr_at_1000
value: 57.476000000000006
- type: mrr_at_3
value: 54.958
- type: mrr_at_5
value: 56.084999999999994
- type: ndcg_at_1
value: 48.28
- type: ndcg_at_10
value: 56.442
- type: ndcg_at_100
value: 60.651999999999994
- type: ndcg_at_1000
value: 62.187000000000005
- type: ndcg_at_3
value: 52.866
- type: ndcg_at_5
value: 54.515
- type: precision_at_1
value: 48.28
- type: precision_at_10
value: 10.586
- type: precision_at_100
value: 1.6310000000000002
- type: precision_at_1000
value: 0.20600000000000002
- type: precision_at_3
value: 25.945
- type: precision_at_5
value: 18.076
- type: recall_at_1
value: 38.025999999999996
- type: recall_at_10
value: 66.11399999999999
- type: recall_at_100
value: 83.339
- type: recall_at_1000
value: 92.413
- type: recall_at_3
value: 54.493
- type: recall_at_5
value: 59.64699999999999
- task:
type: Retrieval
dataset:
type: BeIR/cqadupstack
name: MTEB CQADupstackGamingRetrieval
config: default
split: test
revision: None
metrics:
- type: map_at_1
value: 47.905
- type: map_at_10
value: 61.58
- type: map_at_100
value: 62.605
- type: map_at_1000
value: 62.637
- type: map_at_3
value: 58.074000000000005
- type: map_at_5
value: 60.260000000000005
- type: mrr_at_1
value: 54.42
- type: mrr_at_10
value: 64.847
- type: mrr_at_100
value: 65.403
- type: mrr_at_1000
value: 65.41900000000001
- type: mrr_at_3
value: 62.675000000000004
- type: mrr_at_5
value: 64.101
- type: ndcg_at_1
value: 54.42
- type: ndcg_at_10
value: 67.394
- type: ndcg_at_100
value: 70.846
- type: ndcg_at_1000
value: 71.403
- type: ndcg_at_3
value: 62.025
- type: ndcg_at_5
value: 65.032
- type: precision_at_1
value: 54.42
- type: precision_at_10
value: 10.646
- type: precision_at_100
value: 1.325
- type: precision_at_1000
value: 0.13999999999999999
- type: precision_at_3
value: 27.398
- type: precision_at_5
value: 18.796
- type: recall_at_1
value: 47.905
- type: recall_at_10
value: 80.84599999999999
- type: recall_at_100
value: 95.078
- type: recall_at_1000
value: 98.878
- type: recall_at_3
value: 67.05600000000001
- type: recall_at_5
value: 74.261
- task:
type: Retrieval
dataset:
type: BeIR/cqadupstack
name: MTEB CQADupstackGisRetrieval
config: default
split: test
revision: None
metrics:
- type: map_at_1
value: 30.745
- type: map_at_10
value: 41.021
- type: map_at_100
value: 41.021
- type: map_at_1000
value: 41.021
- type: map_at_3
value: 37.714999999999996
- type: map_at_5
value: 39.766
- type: mrr_at_1
value: 33.559
- type: mrr_at_10
value: 43.537
- type: mrr_at_100
value: 43.537
- type: mrr_at_1000
value: 43.537
- type: mrr_at_3
value: 40.546
- type: mrr_at_5
value: 42.439
- type: ndcg_at_1
value: 33.559
- type: ndcg_at_10
value: 46.781
- type: ndcg_at_100
value: 46.781
- type: ndcg_at_1000
value: 46.781
- type: ndcg_at_3
value: 40.516000000000005
- type: ndcg_at_5
value: 43.957
- type: precision_at_1
value: 33.559
- type: precision_at_10
value: 7.198
- type: precision_at_100
value: 0.72
- type: precision_at_1000
value: 0.07200000000000001
- type: precision_at_3
value: 17.1
- type: precision_at_5
value: 12.316
- type: recall_at_1
value: 30.745
- type: recall_at_10
value: 62.038000000000004
- type: recall_at_100
value: 62.038000000000004
- type: recall_at_1000
value: 62.038000000000004
- type: recall_at_3
value: 45.378
- type: recall_at_5
value: 53.580000000000005
- task:
type: Retrieval
dataset:
type: BeIR/cqadupstack
name: MTEB CQADupstackMathematicaRetrieval
config: default
split: test
revision: None
metrics:
- type: map_at_1
value: 19.637999999999998
- type: map_at_10
value: 31.05
- type: map_at_100
value: 31.05
- type: map_at_1000
value: 31.05
- type: map_at_3
value: 27.628000000000004
- type: map_at_5
value: 29.767
- type: mrr_at_1
value: 25.0
- type: mrr_at_10
value: 36.131
- type: mrr_at_100
value: 36.131
- type: mrr_at_1000
value: 36.131
- type: mrr_at_3
value: 33.333
- type: mrr_at_5
value: 35.143
- type: ndcg_at_1
value: 25.0
- type: ndcg_at_10
value: 37.478
- type: ndcg_at_100
value: 37.469
- type: ndcg_at_1000
value: 37.469
- type: ndcg_at_3
value: 31.757999999999996
- type: ndcg_at_5
value: 34.821999999999996
- type: precision_at_1
value: 25.0
- type: precision_at_10
value: 7.188999999999999
- type: precision_at_100
value: 0.719
- type: precision_at_1000
value: 0.07200000000000001
- type: precision_at_3
value: 15.837000000000002
- type: precision_at_5
value: 11.841
- type: recall_at_1
value: 19.637999999999998
- type: recall_at_10
value: 51.836000000000006
- type: recall_at_100
value: 51.836000000000006
- type: recall_at_1000
value: 51.836000000000006
- type: recall_at_3
value: 36.384
- type: recall_at_5
value: 43.964
- task:
type: Retrieval
dataset:
type: BeIR/cqadupstack
name: MTEB CQADupstackPhysicsRetrieval
config: default
split: test
revision: None
metrics:
- type: map_at_1
value: 34.884
- type: map_at_10
value: 47.88
- type: map_at_100
value: 47.88
- type: map_at_1000
value: 47.88
- type: map_at_3
value: 43.85
- type: map_at_5
value: 46.414
- type: mrr_at_1
value: 43.022
- type: mrr_at_10
value: 53.569
- type: mrr_at_100
value: 53.569
- type: mrr_at_1000
value: 53.569
- type: mrr_at_3
value: 51.075
- type: mrr_at_5
value: 52.725
- type: ndcg_at_1
value: 43.022
- type: ndcg_at_10
value: 54.461000000000006
- type: ndcg_at_100
value: 54.388000000000005
- type: ndcg_at_1000
value: 54.388000000000005
- type: ndcg_at_3
value: 48.864999999999995
- type: ndcg_at_5
value: 52.032000000000004
- type: precision_at_1
value: 43.022
- type: precision_at_10
value: 9.885
- type: precision_at_100
value: 0.988
- type: precision_at_1000
value: 0.099
- type: precision_at_3
value: 23.612
- type: precision_at_5
value: 16.997
- type: recall_at_1
value: 34.884
- type: recall_at_10
value: 68.12899999999999
- type: recall_at_100
value: 68.12899999999999
- type: recall_at_1000
value: 68.12899999999999
- type: recall_at_3
value: 52.428
- type: recall_at_5
value: 60.662000000000006
- task:
type: Retrieval
dataset:
type: BeIR/cqadupstack
name: MTEB CQADupstackProgrammersRetrieval
config: default
split: test
revision: None
metrics:
- type: map_at_1
value: 31.588
- type: map_at_10
value: 43.85
- type: map_at_100
value: 45.317
- type: map_at_1000
value: 45.408
- type: map_at_3
value: 39.73
- type: map_at_5
value: 42.122
- type: mrr_at_1
value: 38.927
- type: mrr_at_10
value: 49.582
- type: mrr_at_100
value: 50.39
- type: mrr_at_1000
value: 50.426
- type: mrr_at_3
value: 46.518
- type: mrr_at_5
value: 48.271
- type: ndcg_at_1
value: 38.927
- type: ndcg_at_10
value: 50.605999999999995
- type: ndcg_at_100
value: 56.22200000000001
- type: ndcg_at_1000
value: 57.724
- type: ndcg_at_3
value: 44.232
- type: ndcg_at_5
value: 47.233999999999995
- type: precision_at_1
value: 38.927
- type: precision_at_10
value: 9.429
- type: precision_at_100
value: 1.435
- type: precision_at_1000
value: 0.172
- type: precision_at_3
value: 21.271
- type: precision_at_5
value: 15.434000000000001
- type: recall_at_1
value: 31.588
- type: recall_at_10
value: 64.836
- type: recall_at_100
value: 88.066
- type: recall_at_1000
value: 97.748
- type: recall_at_3
value: 47.128
- type: recall_at_5
value: 54.954
- task:
type: Retrieval
dataset:
type: BeIR/cqadupstack
name: MTEB CQADupstackRetrieval
config: default
split: test
revision: None
metrics:
- type: map_at_1
value: 31.956083333333336
- type: map_at_10
value: 43.33483333333333
- type: map_at_100
value: 44.64883333333333
- type: map_at_1000
value: 44.75
- type: map_at_3
value: 39.87741666666666
- type: map_at_5
value: 41.86766666666667
- type: mrr_at_1
value: 38.06341666666667
- type: mrr_at_10
value: 47.839666666666666
- type: mrr_at_100
value: 48.644000000000005
- type: mrr_at_1000
value: 48.68566666666667
- type: mrr_at_3
value: 45.26358333333334
- type: mrr_at_5
value: 46.790000000000006
- type: ndcg_at_1
value: 38.06341666666667
- type: ndcg_at_10
value: 49.419333333333334
- type: ndcg_at_100
value: 54.50166666666667
- type: ndcg_at_1000
value: 56.161166666666674
- type: ndcg_at_3
value: 43.982416666666666
- type: ndcg_at_5
value: 46.638083333333334
- type: precision_at_1
value: 38.06341666666667
- type: precision_at_10
value: 8.70858333333333
- type: precision_at_100
value: 1.327
- type: precision_at_1000
value: 0.165
- type: precision_at_3
value: 20.37816666666667
- type: precision_at_5
value: 14.516333333333334
- type: recall_at_1
value: 31.956083333333336
- type: recall_at_10
value: 62.69458333333334
- type: recall_at_100
value: 84.46433333333334
- type: recall_at_1000
value: 95.58449999999999
- type: recall_at_3
value: 47.52016666666666
- type: recall_at_5
value: 54.36066666666666
- task:
type: Retrieval
dataset:
type: BeIR/cqadupstack
name: MTEB CQADupstackStatsRetrieval
config: default
split: test
revision: None
metrics:
- type: map_at_1
value: 28.912
- type: map_at_10
value: 38.291
- type: map_at_100
value: 39.44
- type: map_at_1000
value: 39.528
- type: map_at_3
value: 35.638
- type: map_at_5
value: 37.218
- type: mrr_at_1
value: 32.822
- type: mrr_at_10
value: 41.661
- type: mrr_at_100
value: 42.546
- type: mrr_at_1000
value: 42.603
- type: mrr_at_3
value: 39.238
- type: mrr_at_5
value: 40.726
- type: ndcg_at_1
value: 32.822
- type: ndcg_at_10
value: 43.373
- type: ndcg_at_100
value: 48.638
- type: ndcg_at_1000
value: 50.654999999999994
- type: ndcg_at_3
value: 38.643
- type: ndcg_at_5
value: 41.126000000000005
- type: precision_at_1
value: 32.822
- type: precision_at_10
value: 6.8709999999999996
- type: precision_at_100
value: 1.032
- type: precision_at_1000
value: 0.128
- type: precision_at_3
value: 16.82
- type: precision_at_5
value: 11.718
- type: recall_at_1
value: 28.912
- type: recall_at_10
value: 55.376999999999995
- type: recall_at_100
value: 79.066
- type: recall_at_1000
value: 93.664
- type: recall_at_3
value: 42.569
- type: recall_at_5
value: 48.719
- task:
type: Retrieval
dataset:
type: BeIR/cqadupstack
name: MTEB CQADupstackTexRetrieval
config: default
split: test
revision: None
metrics:
- type: map_at_1
value: 22.181
- type: map_at_10
value: 31.462
- type: map_at_100
value: 32.73
- type: map_at_1000
value: 32.848
- type: map_at_3
value: 28.57
- type: map_at_5
value: 30.182
- type: mrr_at_1
value: 27.185
- type: mrr_at_10
value: 35.846000000000004
- type: mrr_at_100
value: 36.811
- type: mrr_at_1000
value: 36.873
- type: mrr_at_3
value: 33.437
- type: mrr_at_5
value: 34.813
- type: ndcg_at_1
value: 27.185
- type: ndcg_at_10
value: 36.858000000000004
- type: ndcg_at_100
value: 42.501
- type: ndcg_at_1000
value: 44.945
- type: ndcg_at_3
value: 32.066
- type: ndcg_at_5
value: 34.29
- type: precision_at_1
value: 27.185
- type: precision_at_10
value: 6.752
- type: precision_at_100
value: 1.111
- type: precision_at_1000
value: 0.151
- type: precision_at_3
value: 15.290000000000001
- type: precision_at_5
value: 11.004999999999999
- type: recall_at_1
value: 22.181
- type: recall_at_10
value: 48.513
- type: recall_at_100
value: 73.418
- type: recall_at_1000
value: 90.306
- type: recall_at_3
value: 35.003
- type: recall_at_5
value: 40.876000000000005
- task:
type: Retrieval
dataset:
type: BeIR/cqadupstack
name: MTEB CQADupstackUnixRetrieval
config: default
split: test
revision: None
metrics:
- type: map_at_1
value: 33.934999999999995
- type: map_at_10
value: 44.727
- type: map_at_100
value: 44.727
- type: map_at_1000
value: 44.727
- type: map_at_3
value: 40.918
- type: map_at_5
value: 42.961
- type: mrr_at_1
value: 39.646
- type: mrr_at_10
value: 48.898
- type: mrr_at_100
value: 48.898
- type: mrr_at_1000
value: 48.898
- type: mrr_at_3
value: 45.896
- type: mrr_at_5
value: 47.514
- type: ndcg_at_1
value: 39.646
- type: ndcg_at_10
value: 50.817
- type: ndcg_at_100
value: 50.803
- type: ndcg_at_1000
value: 50.803
- type: ndcg_at_3
value: 44.507999999999996
- type: ndcg_at_5
value: 47.259
- type: precision_at_1
value: 39.646
- type: precision_at_10
value: 8.759
- type: precision_at_100
value: 0.876
- type: precision_at_1000
value: 0.08800000000000001
- type: precision_at_3
value: 20.274
- type: precision_at_5
value: 14.366000000000001
- type: recall_at_1
value: 33.934999999999995
- type: recall_at_10
value: 65.037
- type: recall_at_100
value: 65.037
- type: recall_at_1000
value: 65.037
- type: recall_at_3
value: 47.439
- type: recall_at_5
value: 54.567
- task:
type: Retrieval
dataset:
type: BeIR/cqadupstack
name: MTEB CQADupstackWebmastersRetrieval
config: default
split: test
revision: None
metrics:
- type: map_at_1
value: 32.058
- type: map_at_10
value: 43.137
- type: map_at_100
value: 43.137
- type: map_at_1000
value: 43.137
- type: map_at_3
value: 39.882
- type: map_at_5
value: 41.379
- type: mrr_at_1
value: 38.933
- type: mrr_at_10
value: 48.344
- type: mrr_at_100
value: 48.344
- type: mrr_at_1000
value: 48.344
- type: mrr_at_3
value: 45.652
- type: mrr_at_5
value: 46.877
- type: ndcg_at_1
value: 38.933
- type: ndcg_at_10
value: 49.964
- type: ndcg_at_100
value: 49.242000000000004
- type: ndcg_at_1000
value: 49.222
- type: ndcg_at_3
value: 44.605
- type: ndcg_at_5
value: 46.501999999999995
- type: precision_at_1
value: 38.933
- type: precision_at_10
value: 9.427000000000001
- type: precision_at_100
value: 0.943
- type: precision_at_1000
value: 0.094
- type: precision_at_3
value: 20.685000000000002
- type: precision_at_5
value: 14.585
- type: recall_at_1
value: 32.058
- type: recall_at_10
value: 63.074
- type: recall_at_100
value: 63.074
- type: recall_at_1000
value: 63.074
- type: recall_at_3
value: 47.509
- type: recall_at_5
value: 52.455
- task:
type: Retrieval
dataset:
type: BeIR/cqadupstack
name: MTEB CQADupstackWordpressRetrieval
config: default
split: test
revision: None
metrics:
- type: map_at_1
value: 26.029000000000003
- type: map_at_10
value: 34.646
- type: map_at_100
value: 34.646
- type: map_at_1000
value: 34.646
- type: map_at_3
value: 31.456
- type: map_at_5
value: 33.138
- type: mrr_at_1
value: 28.281
- type: mrr_at_10
value: 36.905
- type: mrr_at_100
value: 36.905
- type: mrr_at_1000
value: 36.905
- type: mrr_at_3
value: 34.011
- type: mrr_at_5
value: 35.638
- type: ndcg_at_1
value: 28.281
- type: ndcg_at_10
value: 40.159
- type: ndcg_at_100
value: 40.159
- type: ndcg_at_1000
value: 40.159
- type: ndcg_at_3
value: 33.995
- type: ndcg_at_5
value: 36.836999999999996
- type: precision_at_1
value: 28.281
- type: precision_at_10
value: 6.358999999999999
- type: precision_at_100
value: 0.636
- type: precision_at_1000
value: 0.064
- type: precision_at_3
value: 14.233
- type: precision_at_5
value: 10.314
- type: recall_at_1
value: 26.029000000000003
- type: recall_at_10
value: 55.08
- type: recall_at_100
value: 55.08
- type: recall_at_1000
value: 55.08
- type: recall_at_3
value: 38.487
- type: recall_at_5
value: 45.308
- task:
type: Retrieval
dataset:
type: climate-fever
name: MTEB ClimateFEVER
config: default
split: test
revision: None
metrics:
- type: map_at_1
value: 12.842999999999998
- type: map_at_10
value: 22.101000000000003
- type: map_at_100
value: 24.319
- type: map_at_1000
value: 24.51
- type: map_at_3
value: 18.372
- type: map_at_5
value: 20.323
- type: mrr_at_1
value: 27.948
- type: mrr_at_10
value: 40.321
- type: mrr_at_100
value: 41.262
- type: mrr_at_1000
value: 41.297
- type: mrr_at_3
value: 36.558
- type: mrr_at_5
value: 38.824999999999996
- type: ndcg_at_1
value: 27.948
- type: ndcg_at_10
value: 30.906
- type: ndcg_at_100
value: 38.986
- type: ndcg_at_1000
value: 42.136
- type: ndcg_at_3
value: 24.911
- type: ndcg_at_5
value: 27.168999999999997
- type: precision_at_1
value: 27.948
- type: precision_at_10
value: 9.798
- type: precision_at_100
value: 1.8399999999999999
- type: precision_at_1000
value: 0.243
- type: precision_at_3
value: 18.328
- type: precision_at_5
value: 14.502
- type: recall_at_1
value: 12.842999999999998
- type: recall_at_10
value: 37.245
- type: recall_at_100
value: 64.769
- type: recall_at_1000
value: 82.055
- type: recall_at_3
value: 23.159
- type: recall_at_5
value: 29.113
- task:
type: Retrieval
dataset:
type: dbpedia-entity
name: MTEB DBPedia
config: default
split: test
revision: None
metrics:
- type: map_at_1
value: 8.934000000000001
- type: map_at_10
value: 21.915000000000003
- type: map_at_100
value: 21.915000000000003
- type: map_at_1000
value: 21.915000000000003
- type: map_at_3
value: 14.623
- type: map_at_5
value: 17.841
- type: mrr_at_1
value: 71.25
- type: mrr_at_10
value: 78.994
- type: mrr_at_100
value: 78.994
- type: mrr_at_1000
value: 78.994
- type: mrr_at_3
value: 77.208
- type: mrr_at_5
value: 78.55799999999999
- type: ndcg_at_1
value: 60.62499999999999
- type: ndcg_at_10
value: 46.604
- type: ndcg_at_100
value: 35.653
- type: ndcg_at_1000
value: 35.531
- type: ndcg_at_3
value: 50.605
- type: ndcg_at_5
value: 48.730000000000004
- type: precision_at_1
value: 71.25
- type: precision_at_10
value: 37.75
- type: precision_at_100
value: 3.775
- type: precision_at_1000
value: 0.377
- type: precision_at_3
value: 54.417
- type: precision_at_5
value: 48.15
- type: recall_at_1
value: 8.934000000000001
- type: recall_at_10
value: 28.471000000000004
- type: recall_at_100
value: 28.471000000000004
- type: recall_at_1000
value: 28.471000000000004
- type: recall_at_3
value: 16.019
- type: recall_at_5
value: 21.410999999999998
- task:
type: Classification
dataset:
type: mteb/emotion
name: MTEB EmotionClassification
config: default
split: test
revision: 4f58c6b202a23cf9a4da393831edf4f9183cad37
metrics:
- type: accuracy
value: 52.81
- type: f1
value: 47.987573380720114
- task:
type: Retrieval
dataset:
type: fever
name: MTEB FEVER
config: default
split: test
revision: None
metrics:
- type: map_at_1
value: 66.81899999999999
- type: map_at_10
value: 78.034
- type: map_at_100
value: 78.034
- type: map_at_1000
value: 78.034
- type: map_at_3
value: 76.43100000000001
- type: map_at_5
value: 77.515
- type: mrr_at_1
value: 71.542
- type: mrr_at_10
value: 81.638
- type: mrr_at_100
value: 81.638
- type: mrr_at_1000
value: 81.638
- type: mrr_at_3
value: 80.403
- type: mrr_at_5
value: 81.256
- type: ndcg_at_1
value: 71.542
- type: ndcg_at_10
value: 82.742
- type: ndcg_at_100
value: 82.741
- type: ndcg_at_1000
value: 82.741
- type: ndcg_at_3
value: 80.039
- type: ndcg_at_5
value: 81.695
- type: precision_at_1
value: 71.542
- type: precision_at_10
value: 10.387
- type: precision_at_100
value: 1.039
- type: precision_at_1000
value: 0.104
- type: precision_at_3
value: 31.447999999999997
- type: precision_at_5
value: 19.91
- type: recall_at_1
value: 66.81899999999999
- type: recall_at_10
value: 93.372
- type: recall_at_100
value: 93.372
- type: recall_at_1000
value: 93.372
- type: recall_at_3
value: 86.33
- type: recall_at_5
value: 90.347
- task:
type: Retrieval
dataset:
type: fiqa
name: MTEB FiQA2018
config: default
split: test
revision: None
metrics:
- type: map_at_1
value: 31.158
- type: map_at_10
value: 52.017
- type: map_at_100
value: 54.259
- type: map_at_1000
value: 54.367
- type: map_at_3
value: 45.738
- type: map_at_5
value: 49.283
- type: mrr_at_1
value: 57.87
- type: mrr_at_10
value: 66.215
- type: mrr_at_100
value: 66.735
- type: mrr_at_1000
value: 66.75
- type: mrr_at_3
value: 64.043
- type: mrr_at_5
value: 65.116
- type: ndcg_at_1
value: 57.87
- type: ndcg_at_10
value: 59.946999999999996
- type: ndcg_at_100
value: 66.31099999999999
- type: ndcg_at_1000
value: 67.75999999999999
- type: ndcg_at_3
value: 55.483000000000004
- type: ndcg_at_5
value: 56.891000000000005
- type: precision_at_1
value: 57.87
- type: precision_at_10
value: 16.497
- type: precision_at_100
value: 2.321
- type: precision_at_1000
value: 0.258
- type: precision_at_3
value: 37.14
- type: precision_at_5
value: 27.067999999999998
- type: recall_at_1
value: 31.158
- type: recall_at_10
value: 67.381
- type: recall_at_100
value: 89.464
- type: recall_at_1000
value: 97.989
- type: recall_at_3
value: 50.553000000000004
- type: recall_at_5
value: 57.824
- task:
type: Retrieval
dataset:
type: hotpotqa
name: MTEB HotpotQA
config: default
split: test
revision: None
metrics:
- type: map_at_1
value: 42.073
- type: map_at_10
value: 72.418
- type: map_at_100
value: 73.175
- type: map_at_1000
value: 73.215
- type: map_at_3
value: 68.791
- type: map_at_5
value: 71.19
- type: mrr_at_1
value: 84.146
- type: mrr_at_10
value: 88.994
- type: mrr_at_100
value: 89.116
- type: mrr_at_1000
value: 89.12
- type: mrr_at_3
value: 88.373
- type: mrr_at_5
value: 88.82
- type: ndcg_at_1
value: 84.146
- type: ndcg_at_10
value: 79.404
- type: ndcg_at_100
value: 81.83200000000001
- type: ndcg_at_1000
value: 82.524
- type: ndcg_at_3
value: 74.595
- type: ndcg_at_5
value: 77.474
- type: precision_at_1
value: 84.146
- type: precision_at_10
value: 16.753999999999998
- type: precision_at_100
value: 1.8599999999999999
- type: precision_at_1000
value: 0.19499999999999998
- type: precision_at_3
value: 48.854
- type: precision_at_5
value: 31.579
- type: recall_at_1
value: 42.073
- type: recall_at_10
value: 83.768
- type: recall_at_100
value: 93.018
- type: recall_at_1000
value: 97.481
- type: recall_at_3
value: 73.282
- type: recall_at_5
value: 78.947
- task:
type: Classification
dataset:
type: mteb/imdb
name: MTEB ImdbClassification
config: default
split: test
revision: 3d86128a09e091d6018b6d26cad27f2739fc2db7
metrics:
- type: accuracy
value: 94.9968
- type: ap
value: 92.93892195862824
- type: f1
value: 94.99327998213761
- task:
type: Retrieval
dataset:
type: msmarco
name: MTEB MSMARCO
config: default
split: dev
revision: None
metrics:
- type: map_at_1
value: 21.698
- type: map_at_10
value: 34.585
- type: map_at_100
value: 35.782000000000004
- type: map_at_1000
value: 35.825
- type: map_at_3
value: 30.397999999999996
- type: map_at_5
value: 32.72
- type: mrr_at_1
value: 22.192
- type: mrr_at_10
value: 35.085
- type: mrr_at_100
value: 36.218
- type: mrr_at_1000
value: 36.256
- type: mrr_at_3
value: 30.986000000000004
- type: mrr_at_5
value: 33.268
- type: ndcg_at_1
value: 22.192
- type: ndcg_at_10
value: 41.957
- type: ndcg_at_100
value: 47.658
- type: ndcg_at_1000
value: 48.697
- type: ndcg_at_3
value: 33.433
- type: ndcg_at_5
value: 37.551
- type: precision_at_1
value: 22.192
- type: precision_at_10
value: 6.781
- type: precision_at_100
value: 0.963
- type: precision_at_1000
value: 0.105
- type: precision_at_3
value: 14.365
- type: precision_at_5
value: 10.713000000000001
- type: recall_at_1
value: 21.698
- type: recall_at_10
value: 64.79
- type: recall_at_100
value: 91.071
- type: recall_at_1000
value: 98.883
- type: recall_at_3
value: 41.611
- type: recall_at_5
value: 51.459999999999994
- task:
type: Classification
dataset:
type: mteb/mtop_domain
name: MTEB MTOPDomainClassification (en)
config: en
split: test
revision: d80d48c1eb48d3562165c59d59d0034df9fff0bf
metrics:
- type: accuracy
value: 96.15823073415413
- type: f1
value: 96.00362034963248
- task:
type: Classification
dataset:
type: mteb/mtop_intent
name: MTEB MTOPIntentClassification (en)
config: en
split: test
revision: ae001d0e6b1228650b7bd1c2c65fb50ad11a8aba
metrics:
- type: accuracy
value: 87.12722298221614
- type: f1
value: 70.46888967516227
- task:
type: Classification
dataset:
type: mteb/amazon_massive_intent
name: MTEB MassiveIntentClassification (en)
config: en
split: test
revision: 31efe3c427b0bae9c22cbb560b8f15491cc6bed7
metrics:
- type: accuracy
value: 80.77673167451245
- type: f1
value: 77.60202561132175
- task:
type: Classification
dataset:
type: mteb/amazon_massive_scenario
name: MTEB MassiveScenarioClassification (en)
config: en
split: test
revision: 7d571f92784cd94a019292a1f45445077d0ef634
metrics:
- type: accuracy
value: 82.09145931405514
- type: f1
value: 81.7701921473406
- task:
type: Clustering
dataset:
type: mteb/medrxiv-clustering-p2p
name: MTEB MedrxivClusteringP2P
config: default
split: test
revision: e7a26af6f3ae46b30dde8737f02c07b1505bcc73
metrics:
- type: v_measure
value: 36.52153488185864
- task:
type: Clustering
dataset:
type: mteb/medrxiv-clustering-s2s
name: MTEB MedrxivClusteringS2S
config: default
split: test
revision: 35191c8c0dca72d8ff3efcd72aa802307d469663
metrics:
- type: v_measure
value: 36.80090398444147
- task:
type: Reranking
dataset:
type: mteb/mind_small
name: MTEB MindSmallReranking
config: default
split: test
revision: 3bdac13927fdc888b903db93b2ffdbd90b295a69
metrics:
- type: map
value: 31.807141746058605
- type: mrr
value: 32.85025611455029
- task:
type: Retrieval
dataset:
type: nfcorpus
name: MTEB NFCorpus
config: default
split: test
revision: None
metrics:
- type: map_at_1
value: 6.920999999999999
- type: map_at_10
value: 16.049
- type: map_at_100
value: 16.049
- type: map_at_1000
value: 16.049
- type: map_at_3
value: 11.865
- type: map_at_5
value: 13.657
- type: mrr_at_1
value: 53.87
- type: mrr_at_10
value: 62.291
- type: mrr_at_100
value: 62.291
- type: mrr_at_1000
value: 62.291
- type: mrr_at_3
value: 60.681
- type: mrr_at_5
value: 61.61
- type: ndcg_at_1
value: 51.23799999999999
- type: ndcg_at_10
value: 40.892
- type: ndcg_at_100
value: 26.951999999999998
- type: ndcg_at_1000
value: 26.474999999999998
- type: ndcg_at_3
value: 46.821
- type: ndcg_at_5
value: 44.333
- type: precision_at_1
value: 53.251000000000005
- type: precision_at_10
value: 30.124000000000002
- type: precision_at_100
value: 3.012
- type: precision_at_1000
value: 0.301
- type: precision_at_3
value: 43.55
- type: precision_at_5
value: 38.266
- type: recall_at_1
value: 6.920999999999999
- type: recall_at_10
value: 20.852
- type: recall_at_100
value: 20.852
- type: recall_at_1000
value: 20.852
- type: recall_at_3
value: 13.628000000000002
- type: recall_at_5
value: 16.273
- task:
type: Retrieval
dataset:
type: nq
name: MTEB NQ
config: default
split: test
revision: None
metrics:
- type: map_at_1
value: 46.827999999999996
- type: map_at_10
value: 63.434000000000005
- type: map_at_100
value: 63.434000000000005
- type: map_at_1000
value: 63.434000000000005
- type: map_at_3
value: 59.794000000000004
- type: map_at_5
value: 62.08
- type: mrr_at_1
value: 52.288999999999994
- type: mrr_at_10
value: 65.95
- type: mrr_at_100
value: 65.95
- type: mrr_at_1000
value: 65.95
- type: mrr_at_3
value: 63.413
- type: mrr_at_5
value: 65.08
- type: ndcg_at_1
value: 52.288999999999994
- type: ndcg_at_10
value: 70.301
- type: ndcg_at_100
value: 70.301
- type: ndcg_at_1000
value: 70.301
- type: ndcg_at_3
value: 63.979
- type: ndcg_at_5
value: 67.582
- type: precision_at_1
value: 52.288999999999994
- type: precision_at_10
value: 10.576
- type: precision_at_100
value: 1.058
- type: precision_at_1000
value: 0.106
- type: precision_at_3
value: 28.177000000000003
- type: precision_at_5
value: 19.073
- type: recall_at_1
value: 46.827999999999996
- type: recall_at_10
value: 88.236
- type: recall_at_100
value: 88.236
- type: recall_at_1000
value: 88.236
- type: recall_at_3
value: 72.371
- type: recall_at_5
value: 80.56
- task:
type: Retrieval
dataset:
type: quora
name: MTEB QuoraRetrieval
config: default
split: test
revision: None
metrics:
- type: map_at_1
value: 71.652
- type: map_at_10
value: 85.953
- type: map_at_100
value: 85.953
- type: map_at_1000
value: 85.953
- type: map_at_3
value: 83.05399999999999
- type: map_at_5
value: 84.89
- type: mrr_at_1
value: 82.42
- type: mrr_at_10
value: 88.473
- type: mrr_at_100
value: 88.473
- type: mrr_at_1000
value: 88.473
- type: mrr_at_3
value: 87.592
- type: mrr_at_5
value: 88.211
- type: ndcg_at_1
value: 82.44
- type: ndcg_at_10
value: 89.467
- type: ndcg_at_100
value: 89.33
- type: ndcg_at_1000
value: 89.33
- type: ndcg_at_3
value: 86.822
- type: ndcg_at_5
value: 88.307
- type: precision_at_1
value: 82.44
- type: precision_at_10
value: 13.616
- type: precision_at_100
value: 1.362
- type: precision_at_1000
value: 0.136
- type: precision_at_3
value: 38.117000000000004
- type: precision_at_5
value: 25.05
- type: recall_at_1
value: 71.652
- type: recall_at_10
value: 96.224
- type: recall_at_100
value: 96.224
- type: recall_at_1000
value: 96.224
- type: recall_at_3
value: 88.571
- type: recall_at_5
value: 92.812
- task:
type: Clustering
dataset:
type: mteb/reddit-clustering
name: MTEB RedditClustering
config: default
split: test
revision: 24640382cdbf8abc73003fb0fa6d111a705499eb
metrics:
- type: v_measure
value: 61.295010338050474
- task:
type: Clustering
dataset:
type: mteb/reddit-clustering-p2p
name: MTEB RedditClusteringP2P
config: default
split: test
revision: 282350215ef01743dc01b456c7f5241fa8937f16
metrics:
- type: v_measure
value: 67.26380819328142
- task:
type: Retrieval
dataset:
type: scidocs
name: MTEB SCIDOCS
config: default
split: test
revision: None
metrics:
- type: map_at_1
value: 5.683
- type: map_at_10
value: 14.924999999999999
- type: map_at_100
value: 17.532
- type: map_at_1000
value: 17.875
- type: map_at_3
value: 10.392
- type: map_at_5
value: 12.592
- type: mrr_at_1
value: 28.000000000000004
- type: mrr_at_10
value: 39.951
- type: mrr_at_100
value: 41.025
- type: mrr_at_1000
value: 41.056
- type: mrr_at_3
value: 36.317
- type: mrr_at_5
value: 38.412
- type: ndcg_at_1
value: 28.000000000000004
- type: ndcg_at_10
value: 24.410999999999998
- type: ndcg_at_100
value: 33.79
- type: ndcg_at_1000
value: 39.035
- type: ndcg_at_3
value: 22.845
- type: ndcg_at_5
value: 20.080000000000002
- type: precision_at_1
value: 28.000000000000004
- type: precision_at_10
value: 12.790000000000001
- type: precision_at_100
value: 2.633
- type: precision_at_1000
value: 0.388
- type: precision_at_3
value: 21.367
- type: precision_at_5
value: 17.7
- type: recall_at_1
value: 5.683
- type: recall_at_10
value: 25.91
- type: recall_at_100
value: 53.443
- type: recall_at_1000
value: 78.73
- type: recall_at_3
value: 13.003
- type: recall_at_5
value: 17.932000000000002
- task:
type: STS
dataset:
type: mteb/sickr-sts
name: MTEB SICK-R
config: default
split: test
revision: a6ea5a8cab320b040a23452cc28066d9beae2cee
metrics:
- type: cos_sim_pearson
value: 84.677978681023
- type: cos_sim_spearman
value: 83.13093441058189
- type: euclidean_pearson
value: 83.35535759341572
- type: euclidean_spearman
value: 83.42583744219611
- type: manhattan_pearson
value: 83.2243124045889
- type: manhattan_spearman
value: 83.39801618652632
- task:
type: STS
dataset:
type: mteb/sts12-sts
name: MTEB STS12
config: default
split: test
revision: a0d554a64d88156834ff5ae9920b964011b16384
metrics:
- type: cos_sim_pearson
value: 81.68960206569666
- type: cos_sim_spearman
value: 77.3368966488535
- type: euclidean_pearson
value: 77.62828980560303
- type: euclidean_spearman
value: 76.77951481444651
- type: manhattan_pearson
value: 77.88637240839041
- type: manhattan_spearman
value: 77.22157841466188
- task:
type: STS
dataset:
type: mteb/sts13-sts
name: MTEB STS13
config: default
split: test
revision: 7e90230a92c190f1bf69ae9002b8cea547a64cca
metrics:
- type: cos_sim_pearson
value: 84.18745821650724
- type: cos_sim_spearman
value: 85.04423285574542
- type: euclidean_pearson
value: 85.46604816931023
- type: euclidean_spearman
value: 85.5230593932974
- type: manhattan_pearson
value: 85.57912805986261
- type: manhattan_spearman
value: 85.65955905111873
- task:
type: STS
dataset:
type: mteb/sts14-sts
name: MTEB STS14
config: default
split: test
revision: 6031580fec1f6af667f0bd2da0a551cf4f0b2375
metrics:
- type: cos_sim_pearson
value: 83.6715333300355
- type: cos_sim_spearman
value: 82.9058522514908
- type: euclidean_pearson
value: 83.9640357424214
- type: euclidean_spearman
value: 83.60415457472637
- type: manhattan_pearson
value: 84.05621005853469
- type: manhattan_spearman
value: 83.87077724707746
- task:
type: STS
dataset:
type: mteb/sts15-sts
name: MTEB STS15
config: default
split: test
revision: ae752c7c21bf194d8b67fd573edf7ae58183cbe3
metrics:
- type: cos_sim_pearson
value: 87.82422928098886
- type: cos_sim_spearman
value: 88.12660311894628
- type: euclidean_pearson
value: 87.50974805056555
- type: euclidean_spearman
value: 87.91957275596677
- type: manhattan_pearson
value: 87.74119404878883
- type: manhattan_spearman
value: 88.2808922165719
- task:
type: STS
dataset:
type: mteb/sts16-sts
name: MTEB STS16
config: default
split: test
revision: 4d8694f8f0e0100860b497b999b3dbed754a0513
metrics:
- type: cos_sim_pearson
value: 84.80605838552093
- type: cos_sim_spearman
value: 86.24123388765678
- type: euclidean_pearson
value: 85.32648347339814
- type: euclidean_spearman
value: 85.60046671950158
- type: manhattan_pearson
value: 85.53800168487811
- type: manhattan_spearman
value: 85.89542420480763
- task:
type: STS
dataset:
type: mteb/sts17-crosslingual-sts
name: MTEB STS17 (en-en)
config: en-en
split: test
revision: af5e6fb845001ecf41f4c1e033ce921939a2a68d
metrics:
- type: cos_sim_pearson
value: 89.87540978988132
- type: cos_sim_spearman
value: 90.12715295099461
- type: euclidean_pearson
value: 91.61085993525275
- type: euclidean_spearman
value: 91.31835942311758
- type: manhattan_pearson
value: 91.57500202032934
- type: manhattan_spearman
value: 91.1790925526635
- task:
type: STS
dataset:
type: mteb/sts22-crosslingual-sts
name: MTEB STS22 (en)
config: en
split: test
revision: eea2b4fe26a775864c896887d910b76a8098ad3f
metrics:
- type: cos_sim_pearson
value: 69.87136205329556
- type: cos_sim_spearman
value: 68.6253154635078
- type: euclidean_pearson
value: 68.91536015034222
- type: euclidean_spearman
value: 67.63744649352542
- type: manhattan_pearson
value: 69.2000713045275
- type: manhattan_spearman
value: 68.16002901587316
- task:
type: STS
dataset:
type: mteb/stsbenchmark-sts
name: MTEB STSBenchmark
config: default
split: test
revision: b0fddb56ed78048fa8b90373c8a3cfc37b684831
metrics:
- type: cos_sim_pearson
value: 85.21849551039082
- type: cos_sim_spearman
value: 85.6392959372461
- type: euclidean_pearson
value: 85.92050852609488
- type: euclidean_spearman
value: 85.97205649009734
- type: manhattan_pearson
value: 86.1031154802254
- type: manhattan_spearman
value: 86.26791155517466
- task:
type: Reranking
dataset:
type: mteb/scidocs-reranking
name: MTEB SciDocsRR
config: default
split: test
revision: d3c5e1fc0b855ab6097bf1cda04dd73947d7caab
metrics:
- type: map
value: 86.83953958636627
- type: mrr
value: 96.71167612344082
- task:
type: Retrieval
dataset:
type: scifact
name: MTEB SciFact
config: default
split: test
revision: None
metrics:
- type: map_at_1
value: 64.994
- type: map_at_10
value: 74.763
- type: map_at_100
value: 75.127
- type: map_at_1000
value: 75.143
- type: map_at_3
value: 71.824
- type: map_at_5
value: 73.71
- type: mrr_at_1
value: 68.333
- type: mrr_at_10
value: 75.749
- type: mrr_at_100
value: 75.922
- type: mrr_at_1000
value: 75.938
- type: mrr_at_3
value: 73.556
- type: mrr_at_5
value: 74.739
- type: ndcg_at_1
value: 68.333
- type: ndcg_at_10
value: 79.174
- type: ndcg_at_100
value: 80.41
- type: ndcg_at_1000
value: 80.804
- type: ndcg_at_3
value: 74.361
- type: ndcg_at_5
value: 76.861
- type: precision_at_1
value: 68.333
- type: precision_at_10
value: 10.333
- type: precision_at_100
value: 1.0999999999999999
- type: precision_at_1000
value: 0.11299999999999999
- type: precision_at_3
value: 28.778
- type: precision_at_5
value: 19.067
- type: recall_at_1
value: 64.994
- type: recall_at_10
value: 91.822
- type: recall_at_100
value: 97.0
- type: recall_at_1000
value: 100.0
- type: recall_at_3
value: 78.878
- type: recall_at_5
value: 85.172
- task:
type: PairClassification
dataset:
type: mteb/sprintduplicatequestions-pairclassification
name: MTEB SprintDuplicateQuestions
config: default
split: test
revision: d66bd1f72af766a5cc4b0ca5e00c162f89e8cc46
metrics:
- type: cos_sim_accuracy
value: 99.72079207920792
- type: cos_sim_ap
value: 93.00265215525152
- type: cos_sim_f1
value: 85.06596306068602
- type: cos_sim_precision
value: 90.05586592178771
- type: cos_sim_recall
value: 80.60000000000001
- type: dot_accuracy
value: 99.66039603960397
- type: dot_ap
value: 91.22371407479089
- type: dot_f1
value: 82.34693877551021
- type: dot_precision
value: 84.0625
- type: dot_recall
value: 80.7
- type: euclidean_accuracy
value: 99.71881188118812
- type: euclidean_ap
value: 92.88449963304728
- type: euclidean_f1
value: 85.19480519480518
- type: euclidean_precision
value: 88.64864864864866
- type: euclidean_recall
value: 82.0
- type: manhattan_accuracy
value: 99.73267326732673
- type: manhattan_ap
value: 93.23055393056883
- type: manhattan_f1
value: 85.88957055214725
- type: manhattan_precision
value: 87.86610878661088
- type: manhattan_recall
value: 84.0
- type: max_accuracy
value: 99.73267326732673
- type: max_ap
value: 93.23055393056883
- type: max_f1
value: 85.88957055214725
- task:
type: Clustering
dataset:
type: mteb/stackexchange-clustering
name: MTEB StackExchangeClustering
config: default
split: test
revision: 6cbc1f7b2bc0622f2e39d2c77fa502909748c259
metrics:
- type: v_measure
value: 77.3305735900358
- task:
type: Clustering
dataset:
type: mteb/stackexchange-clustering-p2p
name: MTEB StackExchangeClusteringP2P
config: default
split: test
revision: 815ca46b2622cec33ccafc3735d572c266efdb44
metrics:
- type: v_measure
value: 41.32967136540674
- task:
type: Reranking
dataset:
type: mteb/stackoverflowdupquestions-reranking
name: MTEB StackOverflowDupQuestions
config: default
split: test
revision: e185fbe320c72810689fc5848eb6114e1ef5ec69
metrics:
- type: map
value: 55.95514866379359
- type: mrr
value: 56.95423245055598
- task:
type: Summarization
dataset:
type: mteb/summeval
name: MTEB SummEval
config: default
split: test
revision: cda12ad7615edc362dbf25a00fdd61d3b1eaf93c
metrics:
- type: cos_sim_pearson
value: 30.783007208997144
- type: cos_sim_spearman
value: 30.373444721540533
- type: dot_pearson
value: 29.210604111143905
- type: dot_spearman
value: 29.98809758085659
- task:
type: Retrieval
dataset:
type: trec-covid
name: MTEB TRECCOVID
config: default
split: test
revision: None
metrics:
- type: map_at_1
value: 0.234
- type: map_at_10
value: 1.894
- type: map_at_100
value: 1.894
- type: map_at_1000
value: 1.894
- type: map_at_3
value: 0.636
- type: map_at_5
value: 1.0
- type: mrr_at_1
value: 88.0
- type: mrr_at_10
value: 93.667
- type: mrr_at_100
value: 93.667
- type: mrr_at_1000
value: 93.667
- type: mrr_at_3
value: 93.667
- type: mrr_at_5
value: 93.667
- type: ndcg_at_1
value: 85.0
- type: ndcg_at_10
value: 74.798
- type: ndcg_at_100
value: 16.462
- type: ndcg_at_1000
value: 7.0889999999999995
- type: ndcg_at_3
value: 80.754
- type: ndcg_at_5
value: 77.319
- type: precision_at_1
value: 88.0
- type: precision_at_10
value: 78.0
- type: precision_at_100
value: 7.8
- type: precision_at_1000
value: 0.7799999999999999
- type: precision_at_3
value: 83.333
- type: precision_at_5
value: 80.80000000000001
- type: recall_at_1
value: 0.234
- type: recall_at_10
value: 2.093
- type: recall_at_100
value: 2.093
- type: recall_at_1000
value: 2.093
- type: recall_at_3
value: 0.662
- type: recall_at_5
value: 1.0739999999999998
- task:
type: Retrieval
dataset:
type: webis-touche2020
name: MTEB Touche2020
config: default
split: test
revision: None
metrics:
- type: map_at_1
value: 2.703
- type: map_at_10
value: 10.866000000000001
- type: map_at_100
value: 10.866000000000001
- type: map_at_1000
value: 10.866000000000001
- type: map_at_3
value: 5.909
- type: map_at_5
value: 7.35
- type: mrr_at_1
value: 36.735
- type: mrr_at_10
value: 53.583000000000006
- type: mrr_at_100
value: 53.583000000000006
- type: mrr_at_1000
value: 53.583000000000006
- type: mrr_at_3
value: 49.32
- type: mrr_at_5
value: 51.769
- type: ndcg_at_1
value: 34.694
- type: ndcg_at_10
value: 27.926000000000002
- type: ndcg_at_100
value: 22.701
- type: ndcg_at_1000
value: 22.701
- type: ndcg_at_3
value: 32.073
- type: ndcg_at_5
value: 28.327999999999996
- type: precision_at_1
value: 36.735
- type: precision_at_10
value: 24.694
- type: precision_at_100
value: 2.469
- type: precision_at_1000
value: 0.247
- type: precision_at_3
value: 31.973000000000003
- type: precision_at_5
value: 26.939
- type: recall_at_1
value: 2.703
- type: recall_at_10
value: 17.702
- type: recall_at_100
value: 17.702
- type: recall_at_1000
value: 17.702
- type: recall_at_3
value: 7.208
- type: recall_at_5
value: 9.748999999999999
- task:
type: Classification
dataset:
type: mteb/toxic_conversations_50k
name: MTEB ToxicConversationsClassification
config: default
split: test
revision: d7c0de2777da35d6aae2200a62c6e0e5af397c4c
metrics:
- type: accuracy
value: 70.79960000000001
- type: ap
value: 15.467565415565815
- type: f1
value: 55.28639823443618
- task:
type: Classification
dataset:
type: mteb/tweet_sentiment_extraction
name: MTEB TweetSentimentExtractionClassification
config: default
split: test
revision: d604517c81ca91fe16a244d1248fc021f9ecee7a
metrics:
- type: accuracy
value: 64.7792869269949
- type: f1
value: 65.08597154774318
- task:
type: Clustering
dataset:
type: mteb/twentynewsgroups-clustering
name: MTEB TwentyNewsgroupsClustering
config: default
split: test
revision: 6125ec4e24fa026cec8a478383ee943acfbd5449
metrics:
- type: v_measure
value: 55.70352297774293
- task:
type: PairClassification
dataset:
type: mteb/twittersemeval2015-pairclassification
name: MTEB TwitterSemEval2015
config: default
split: test
revision: 70970daeab8776df92f5ea462b6173c0b46fd2d1
metrics:
- type: cos_sim_accuracy
value: 88.27561542588067
- type: cos_sim_ap
value: 81.08262141256193
- type: cos_sim_f1
value: 73.82341501361338
- type: cos_sim_precision
value: 72.5720112159062
- type: cos_sim_recall
value: 75.11873350923483
- type: dot_accuracy
value: 86.66030875603504
- type: dot_ap
value: 76.6052349228621
- type: dot_f1
value: 70.13897280966768
- type: dot_precision
value: 64.70457079152732
- type: dot_recall
value: 76.56992084432717
- type: euclidean_accuracy
value: 88.37098408535495
- type: euclidean_ap
value: 81.12515230092113
- type: euclidean_f1
value: 74.10338225909379
- type: euclidean_precision
value: 71.76761433868974
- type: euclidean_recall
value: 76.59630606860158
- type: manhattan_accuracy
value: 88.34118137926924
- type: manhattan_ap
value: 80.95751834536561
- type: manhattan_f1
value: 73.9119496855346
- type: manhattan_precision
value: 70.625
- type: manhattan_recall
value: 77.5197889182058
- type: max_accuracy
value: 88.37098408535495
- type: max_ap
value: 81.12515230092113
- type: max_f1
value: 74.10338225909379
- task:
type: PairClassification
dataset:
type: mteb/twitterurlcorpus-pairclassification
name: MTEB TwitterURLCorpus
config: default
split: test
revision: 8b6510b0b1fa4e4c4f879467980e9be563ec1cdf
metrics:
- type: cos_sim_accuracy
value: 89.79896767182831
- type: cos_sim_ap
value: 87.40071784061065
- type: cos_sim_f1
value: 79.87753144712087
- type: cos_sim_precision
value: 76.67304015296367
- type: cos_sim_recall
value: 83.3615645210964
- type: dot_accuracy
value: 88.95486474948578
- type: dot_ap
value: 86.00227979119943
- type: dot_f1
value: 78.54601474525914
- type: dot_precision
value: 75.00525394045535
- type: dot_recall
value: 82.43763473975977
- type: euclidean_accuracy
value: 89.7892653393876
- type: euclidean_ap
value: 87.42174706480819
- type: euclidean_f1
value: 80.07283321194465
- type: euclidean_precision
value: 75.96738529574351
- type: euclidean_recall
value: 84.6473668001232
- type: manhattan_accuracy
value: 89.8474793340319
- type: manhattan_ap
value: 87.47814292587448
- type: manhattan_f1
value: 80.15461150280949
- type: manhattan_precision
value: 74.88798234468
- type: manhattan_recall
value: 86.21804742839544
- type: max_accuracy
value: 89.8474793340319
- type: max_ap
value: 87.47814292587448
- type: max_f1
value: 80.15461150280949
---
# Model Summary
> GritLM is a generative representational instruction tuned language model. It unifies text representation (embedding) and text generation into a single model achieving state-of-the-art performance on both types of tasks.
- **Repository:** [ContextualAI/gritlm](https://github.com/ContextualAI/gritlm)
- **Paper:** https://arxiv.org/abs/2402.09906
- **Logs:** https://wandb.ai/muennighoff/gritlm/runs/0uui712t/overview
- **Script:** https://github.com/ContextualAI/gritlm/blob/main/scripts/training/train_gritlm_7b.sh
| Model | Description |
|-------|-------------|
| [GritLM 7B](https://hf.co/GritLM/GritLM-7B) | Mistral 7B finetuned using GRIT |
| [GritLM 8x7B](https://hf.co/GritLM/GritLM-8x7B) | Mixtral 8x7B finetuned using GRIT |
# Use
The model usage is documented [here](https://github.com/ContextualAI/gritlm?tab=readme-ov-file#inference).
# Citation
```bibtex
@misc{muennighoff2024generative,
title={Generative Representational Instruction Tuning},
author={Niklas Muennighoff and Hongjin Su and Liang Wang and Nan Yang and Furu Wei and Tao Yu and Amanpreet Singh and Douwe Kiela},
year={2024},
eprint={2402.09906},
archivePrefix={arXiv},
primaryClass={cs.CL}
}
``` |
John6666/mala-anime-mix-nsfw-pony-xl-v3-sdxl | John6666 | "2024-06-26T11:50:14Z" | 36,086 | 8 | diffusers | [
"diffusers",
"safetensors",
"text-to-image",
"stable-diffusion",
"stable-diffusion-xl",
"quality",
"anime",
"realistic",
"photorealistic",
"license:other",
"autotrain_compatible",
"endpoints_compatible",
"diffusers:StableDiffusionXLPipeline",
"region:us"
] | text-to-image | "2024-05-29T09:28:48Z" | ---
license: other
tags:
- text-to-image
- stable-diffusion
- stable-diffusion-xl
- quality
- anime
- realistic
- photorealistic
---
Original model is [here](https://civitai.com/models/442163/mala-anime-mix-nsfw-ponyxl).
|
cardiffnlp/tweet-topic-21-multi | cardiffnlp | "2023-05-28T04:56:09Z" | 36,054 | 64 | transformers | [
"transformers",
"pytorch",
"tf",
"roberta",
"text-classification",
"en",
"dataset:cardiffnlp/tweet_topic_multi",
"arxiv:2209.09824",
"license:mit",
"autotrain_compatible",
"endpoints_compatible",
"region:us"
] | text-classification | "2022-06-06T14:52:42Z" | ---
language: en
widget:
- text: It is great to see athletes promoting awareness for climate change.
datasets:
- cardiffnlp/tweet_topic_multi
license: mit
metrics:
- f1
- accuracy
pipeline_tag: text-classification
---
# tweet-topic-21-multi
This model is based on a [TimeLMs](https://github.com/cardiffnlp/timelms) language model trained on ~124M tweets from January 2018 to December 2021 (see [here](https://huggingface.co/cardiffnlp/twitter-roberta-base-2021-124m)), and finetuned for multi-label topic classification on a corpus of 11,267 [tweets](https://huggingface.co/datasets/cardiffnlp/tweet_topic_multi). This model is suitable for English.
- Reference Paper: [TweetTopic](https://arxiv.org/abs/2209.09824) (COLING 2022).
<b>Labels</b>:
| <span style="font-weight:normal">0: arts_&_culture</span> | <span style="font-weight:normal">5: fashion_&_style</span> | <span style="font-weight:normal">10: learning_&_educational</span> | <span style="font-weight:normal">15: science_&_technology</span> |
|-----------------------------|---------------------|----------------------------|--------------------------|
| 1: business_&_entrepreneurs | 6: film_tv_&_video | 11: music | 16: sports |
| 2: celebrity_&_pop_culture | 7: fitness_&_health | 12: news_&_social_concern | 17: travel_&_adventure |
| 3: diaries_&_daily_life | 8: food_&_dining | 13: other_hobbies | 18: youth_&_student_life |
| 4: family | 9: gaming | 14: relationships | |
## Full classification example
```python
from transformers import AutoModelForSequenceClassification, TFAutoModelForSequenceClassification
from transformers import AutoTokenizer
import numpy as np
from scipy.special import expit
MODEL = f"cardiffnlp/tweet-topic-21-multi"
tokenizer = AutoTokenizer.from_pretrained(MODEL)
# PT
model = AutoModelForSequenceClassification.from_pretrained(MODEL)
class_mapping = model.config.id2label
text = "It is great to see athletes promoting awareness for climate change."
tokens = tokenizer(text, return_tensors='pt')
output = model(**tokens)
scores = output[0][0].detach().numpy()
scores = expit(scores)
predictions = (scores >= 0.5) * 1
# TF
#tf_model = TFAutoModelForSequenceClassification.from_pretrained(MODEL)
#class_mapping = tf_model.config.id2label
#text = "It is great to see athletes promoting awareness for climate change."
#tokens = tokenizer(text, return_tensors='tf')
#output = tf_model(**tokens)
#scores = output[0][0]
#scores = expit(scores)
#predictions = (scores >= 0.5) * 1
# Map to classes
for i in range(len(predictions)):
if predictions[i]:
print(class_mapping[i])
```
Output:
```
news_&_social_concern
sports
```
### BibTeX entry and citation info
Please cite the [reference paper](https://aclanthology.org/2022.coling-1.299/) if you use this model.
```bibtex
@inproceedings{antypas-etal-2022-twitter,
title = "{T}witter Topic Classification",
author = "Antypas, Dimosthenis and
Ushio, Asahi and
Camacho-Collados, Jose and
Silva, Vitor and
Neves, Leonardo and
Barbieri, Francesco",
booktitle = "Proceedings of the 29th International Conference on Computational Linguistics",
month = oct,
year = "2022",
address = "Gyeongju, Republic of Korea",
publisher = "International Committee on Computational Linguistics",
url = "https://aclanthology.org/2022.coling-1.299",
pages = "3386--3400"
}
``` |
mixedbread-ai/mxbai-rerank-base-v1 | mixedbread-ai | "2024-05-22T00:02:19Z" | 36,044 | 21 | transformers | [
"transformers",
"onnx",
"safetensors",
"deberta-v2",
"text-classification",
"reranker",
"transformers.js",
"en",
"license:apache-2.0",
"autotrain_compatible",
"endpoints_compatible",
"region:us"
] | text-classification | "2024-02-29T14:36:24Z" | ---
library_name: transformers
tags:
- reranker
- transformers.js
license: apache-2.0
language:
- en
---
<br><br>
<p align="center">
<svg xmlns="http://www.w3.org/2000/svg" xml:space="preserve" viewBox="0 0 2020 1130" width="150" height="150" aria-hidden="true"><path fill="#e95a0f" d="M398.167 621.992c-1.387-20.362-4.092-40.739-3.851-61.081.355-30.085 6.873-59.139 21.253-85.976 10.487-19.573 24.09-36.822 40.662-51.515 16.394-14.535 34.338-27.046 54.336-36.182 15.224-6.955 31.006-12.609 47.829-14.168 11.809-1.094 23.753-2.514 35.524-1.836 23.033 1.327 45.131 7.255 66.255 16.75 16.24 7.3 31.497 16.165 45.651 26.969 12.997 9.921 24.412 21.37 34.158 34.509 11.733 15.817 20.849 33.037 25.987 52.018 3.468 12.81 6.438 25.928 7.779 39.097 1.722 16.908 1.642 34.003 2.235 51.021.427 12.253.224 24.547 1.117 36.762 1.677 22.93 4.062 45.764 11.8 67.7 5.376 15.239 12.499 29.55 20.846 43.681l-18.282 20.328c-1.536 1.71-2.795 3.665-4.254 5.448l-19.323 23.533c-13.859-5.449-27.446-11.803-41.657-16.086-13.622-4.106-27.793-6.765-41.905-8.775-15.256-2.173-30.701-3.475-46.105-4.049-23.571-.879-47.178-1.056-70.769-1.029-10.858.013-21.723 1.116-32.57 1.926-5.362.4-10.69 1.255-16.464 1.477-2.758-7.675-5.284-14.865-7.367-22.181-3.108-10.92-4.325-22.554-13.16-31.095-2.598-2.512-5.069-5.341-6.883-8.443-6.366-10.884-12.48-21.917-18.571-32.959-4.178-7.573-8.411-14.375-17.016-18.559-10.34-5.028-19.538-12.387-29.311-18.611-3.173-2.021-6.414-4.312-9.952-5.297-5.857-1.63-11.98-2.301-17.991-3.376z"></path><path fill="#ed6d7b" d="M1478.998 758.842c-12.025.042-24.05.085-36.537-.373-.14-8.536.231-16.569.453-24.607.033-1.179-.315-2.986-1.081-3.4-.805-.434-2.376.338-3.518.81-.856.354-1.562 1.069-3.589 2.521-.239-3.308-.664-5.586-.519-7.827.488-7.544 2.212-15.166 1.554-22.589-1.016-11.451 1.397-14.592-12.332-14.419-3.793.048-3.617-2.803-3.332-5.331.499-4.422 1.45-8.803 1.77-13.233.311-4.316.068-8.672.068-12.861-2.554-.464-4.326-.86-6.12-1.098-4.415-.586-6.051-2.251-5.065-7.31 1.224-6.279.848-12.862 1.276-19.306.19-2.86-.971-4.473-3.794-4.753-4.113-.407-8.242-1.057-12.352-.975-4.663.093-5.192-2.272-4.751-6.012.733-6.229 1.252-12.483 1.875-18.726l1.102-10.495c-5.905-.309-11.146-.805-16.385-.778-3.32.017-5.174-1.4-5.566-4.4-1.172-8.968-2.479-17.944-3.001-26.96-.26-4.484-1.936-5.705-6.005-5.774-9.284-.158-18.563-.594-27.843-.953-7.241-.28-10.137-2.764-11.3-9.899-.746-4.576-2.715-7.801-7.777-8.207-7.739-.621-15.511-.992-23.207-1.961-7.327-.923-14.587-2.415-21.853-3.777-5.021-.941-10.003-2.086-15.003-3.14 4.515-22.952 13.122-44.382 26.284-63.587 18.054-26.344 41.439-47.239 69.102-63.294 15.847-9.197 32.541-16.277 50.376-20.599 16.655-4.036 33.617-5.715 50.622-4.385 33.334 2.606 63.836 13.955 92.415 31.15 15.864 9.545 30.241 20.86 42.269 34.758 8.113 9.374 15.201 19.78 21.718 30.359 10.772 17.484 16.846 36.922 20.611 56.991 1.783 9.503 2.815 19.214 3.318 28.876.758 14.578.755 29.196.65 44.311l-51.545 20.013c-7.779 3.059-15.847 5.376-21.753 12.365-4.73 5.598-10.658 10.316-16.547 14.774-9.9 7.496-18.437 15.988-25.083 26.631-3.333 5.337-7.901 10.381-12.999 14.038-11.355 8.144-17.397 18.973-19.615 32.423l-6.988 41.011z"></path><path fill="#ec663e" d="M318.11 923.047c-.702 17.693-.832 35.433-2.255 53.068-1.699 21.052-6.293 41.512-14.793 61.072-9.001 20.711-21.692 38.693-38.496 53.583-16.077 14.245-34.602 24.163-55.333 30.438-21.691 6.565-43.814 8.127-66.013 6.532-22.771-1.636-43.88-9.318-62.74-22.705-20.223-14.355-35.542-32.917-48.075-54.096-9.588-16.203-16.104-33.55-19.201-52.015-2.339-13.944-2.307-28.011-.403-42.182 2.627-19.545 9.021-37.699 17.963-55.067 11.617-22.564 27.317-41.817 48.382-56.118 15.819-10.74 33.452-17.679 52.444-20.455 8.77-1.282 17.696-1.646 26.568-2.055 11.755-.542 23.534-.562 35.289-1.11 8.545-.399 17.067-1.291 26.193-1.675 1.349 1.77 2.24 3.199 2.835 4.742 4.727 12.261 10.575 23.865 18.636 34.358 7.747 10.084 14.83 20.684 22.699 30.666 3.919 4.972 8.37 9.96 13.609 13.352 7.711 4.994 16.238 8.792 24.617 12.668 5.852 2.707 12.037 4.691 18.074 6.998z"></path><path fill="#ea580e" d="M1285.167 162.995c3.796-29.75 13.825-56.841 32.74-80.577 16.339-20.505 36.013-36.502 59.696-47.614 14.666-6.881 29.971-11.669 46.208-12.749 10.068-.669 20.239-1.582 30.255-.863 16.6 1.191 32.646 5.412 47.9 12.273 19.39 8.722 36.44 20.771 50.582 36.655 15.281 17.162 25.313 37.179 31.49 59.286 5.405 19.343 6.31 39.161 4.705 58.825-2.37 29.045-11.836 55.923-30.451 78.885-10.511 12.965-22.483 24.486-37.181 33.649-5.272-5.613-10.008-11.148-14.539-16.846-5.661-7.118-10.958-14.533-16.78-21.513-4.569-5.478-9.548-10.639-14.624-15.658-3.589-3.549-7.411-6.963-11.551-9.827-5.038-3.485-10.565-6.254-15.798-9.468-8.459-5.195-17.011-9.669-26.988-11.898-12.173-2.72-24.838-4.579-35.622-11.834-1.437-.967-3.433-1.192-5.213-1.542-12.871-2.529-25.454-5.639-36.968-12.471-5.21-3.091-11.564-4.195-17.011-6.965-4.808-2.445-8.775-6.605-13.646-8.851-8.859-4.085-18.114-7.311-27.204-10.896z"></path><path fill="#f8ab00" d="M524.963 311.12c-9.461-5.684-19.513-10.592-28.243-17.236-12.877-9.801-24.031-21.578-32.711-35.412-11.272-17.965-19.605-37.147-21.902-58.403-1.291-11.951-2.434-24.073-1.87-36.034.823-17.452 4.909-34.363 11.581-50.703 8.82-21.603 22.25-39.792 39.568-55.065 18.022-15.894 39.162-26.07 62.351-32.332 19.22-5.19 38.842-6.177 58.37-4.674 23.803 1.831 45.56 10.663 65.062 24.496 17.193 12.195 31.688 27.086 42.894 45.622-11.403 8.296-22.633 16.117-34.092 23.586-17.094 11.142-34.262 22.106-48.036 37.528-8.796 9.848-17.201 20.246-27.131 28.837-16.859 14.585-27.745 33.801-41.054 51.019-11.865 15.349-20.663 33.117-30.354 50.08-5.303 9.283-9.654 19.11-14.434 28.692z"></path><path fill="#ea5227" d="M1060.11 1122.049c-7.377 1.649-14.683 4.093-22.147 4.763-11.519 1.033-23.166 1.441-34.723 1.054-19.343-.647-38.002-4.7-55.839-12.65-15.078-6.72-28.606-15.471-40.571-26.836-24.013-22.81-42.053-49.217-49.518-81.936-1.446-6.337-1.958-12.958-2.235-19.477-.591-13.926-.219-27.909-1.237-41.795-.916-12.5-3.16-24.904-4.408-37.805 1.555-1.381 3.134-2.074 3.778-3.27 4.729-8.79 12.141-15.159 19.083-22.03 5.879-5.818 10.688-12.76 16.796-18.293 6.993-6.335 11.86-13.596 14.364-22.612l8.542-29.993c8.015 1.785 15.984 3.821 24.057 5.286 8.145 1.478 16.371 2.59 24.602 3.493 8.453.927 16.956 1.408 25.891 2.609 1.119 16.09 1.569 31.667 2.521 47.214.676 11.045 1.396 22.154 3.234 33.043 2.418 14.329 5.708 28.527 9.075 42.674 3.499 14.705 4.028 29.929 10.415 44.188 10.157 22.674 18.29 46.25 28.281 69.004 7.175 16.341 12.491 32.973 15.078 50.615.645 4.4 3.256 8.511 4.963 12.755z"></path><path fill="#ea5330" d="M1060.512 1122.031c-2.109-4.226-4.72-8.337-5.365-12.737-2.587-17.642-7.904-34.274-15.078-50.615-9.991-22.755-18.124-46.33-28.281-69.004-6.387-14.259-6.916-29.482-10.415-44.188-3.366-14.147-6.656-28.346-9.075-42.674-1.838-10.889-2.558-21.999-3.234-33.043-.951-15.547-1.401-31.124-2.068-47.146 8.568-.18 17.146.487 25.704.286l41.868-1.4c.907 3.746 1.245 7.04 1.881 10.276l8.651 42.704c.903 4.108 2.334 8.422 4.696 11.829 7.165 10.338 14.809 20.351 22.456 30.345 4.218 5.512 8.291 11.304 13.361 15.955 8.641 7.927 18.065 14.995 27.071 22.532 12.011 10.052 24.452 19.302 40.151 22.854-1.656 11.102-2.391 22.44-5.172 33.253-4.792 18.637-12.38 36.209-23.412 52.216-13.053 18.94-29.086 34.662-49.627 45.055-10.757 5.443-22.443 9.048-34.111 13.501z"></path><path fill="#f8aa05" d="M1989.106 883.951c5.198 8.794 11.46 17.148 15.337 26.491 5.325 12.833 9.744 26.207 12.873 39.737 2.95 12.757 3.224 25.908 1.987 39.219-1.391 14.973-4.643 29.268-10.349 43.034-5.775 13.932-13.477 26.707-23.149 38.405-14.141 17.104-31.215 30.458-50.807 40.488-14.361 7.352-29.574 12.797-45.741 14.594-10.297 1.144-20.732 2.361-31.031 1.894-24.275-1.1-47.248-7.445-68.132-20.263-6.096-3.741-11.925-7.917-17.731-12.342 5.319-5.579 10.361-10.852 15.694-15.811l37.072-34.009c.975-.892 2.113-1.606 3.08-2.505 6.936-6.448 14.765-12.2 20.553-19.556 8.88-11.285 20.064-19.639 31.144-28.292 4.306-3.363 9.06-6.353 12.673-10.358 5.868-6.504 10.832-13.814 16.422-20.582 6.826-8.264 13.727-16.481 20.943-24.401 4.065-4.461 8.995-8.121 13.249-12.424 14.802-14.975 28.77-30.825 45.913-43.317z"></path><path fill="#ed6876" d="M1256.099 523.419c5.065.642 10.047 1.787 15.068 2.728 7.267 1.362 14.526 2.854 21.853 3.777 7.696.97 15.468 1.34 23.207 1.961 5.062.406 7.031 3.631 7.777 8.207 1.163 7.135 4.059 9.62 11.3 9.899l27.843.953c4.069.069 5.745 1.291 6.005 5.774.522 9.016 1.829 17.992 3.001 26.96.392 3 2.246 4.417 5.566 4.4 5.239-.026 10.48.469 16.385.778l-1.102 10.495-1.875 18.726c-.44 3.74.088 6.105 4.751 6.012 4.11-.082 8.239.568 12.352.975 2.823.28 3.984 1.892 3.794 4.753-.428 6.444-.052 13.028-1.276 19.306-.986 5.059.651 6.724 5.065 7.31 1.793.238 3.566.634 6.12 1.098 0 4.189.243 8.545-.068 12.861-.319 4.43-1.27 8.811-1.77 13.233-.285 2.528-.461 5.379 3.332 5.331 13.729-.173 11.316 2.968 12.332 14.419.658 7.423-1.066 15.045-1.554 22.589-.145 2.241.28 4.519.519 7.827 2.026-1.452 2.733-2.167 3.589-2.521 1.142-.472 2.713-1.244 3.518-.81.767.414 1.114 2.221 1.081 3.4l-.917 24.539c-11.215.82-22.45.899-33.636 1.674l-43.952 3.436c-1.086-3.01-2.319-5.571-2.296-8.121.084-9.297-4.468-16.583-9.091-24.116-3.872-6.308-8.764-13.052-9.479-19.987-1.071-10.392-5.716-15.936-14.889-18.979-1.097-.364-2.16-.844-3.214-1.327-7.478-3.428-15.548-5.918-19.059-14.735-.904-2.27-3.657-3.775-5.461-5.723-2.437-2.632-4.615-5.525-7.207-7.987-2.648-2.515-5.352-5.346-8.589-6.777-4.799-2.121-10.074-3.185-15.175-4.596l-15.785-4.155c.274-12.896 1.722-25.901.54-38.662-1.647-17.783-3.457-35.526-2.554-53.352.528-10.426 2.539-20.777 3.948-31.574z"></path><path fill="#f6a200" d="M525.146 311.436c4.597-9.898 8.947-19.725 14.251-29.008 9.691-16.963 18.49-34.73 30.354-50.08 13.309-17.218 24.195-36.434 41.054-51.019 9.93-8.591 18.335-18.989 27.131-28.837 13.774-15.422 30.943-26.386 48.036-37.528 11.459-7.469 22.688-15.29 34.243-23.286 11.705 16.744 19.716 35.424 22.534 55.717 2.231 16.066 2.236 32.441 2.753 49.143-4.756 1.62-9.284 2.234-13.259 4.056-6.43 2.948-12.193 7.513-18.774 9.942-19.863 7.331-33.806 22.349-47.926 36.784-7.86 8.035-13.511 18.275-19.886 27.705-4.434 6.558-9.345 13.037-12.358 20.254-4.249 10.177-6.94 21.004-10.296 31.553-12.33.053-24.741 1.027-36.971-.049-20.259-1.783-40.227-5.567-58.755-14.69-.568-.28-1.295-.235-2.132-.658z"></path><path fill="#f7a80d" d="M1989.057 883.598c-17.093 12.845-31.061 28.695-45.863 43.67-4.254 4.304-9.184 7.963-13.249 12.424-7.216 7.92-14.117 16.137-20.943 24.401-5.59 6.768-10.554 14.078-16.422 20.582-3.614 4.005-8.367 6.995-12.673 10.358-11.08 8.653-22.264 17.007-31.144 28.292-5.788 7.356-13.617 13.108-20.553 19.556-.967.899-2.105 1.614-3.08 2.505l-37.072 34.009c-5.333 4.96-10.375 10.232-15.859 15.505-21.401-17.218-37.461-38.439-48.623-63.592 3.503-1.781 7.117-2.604 9.823-4.637 8.696-6.536 20.392-8.406 27.297-17.714.933-1.258 2.646-1.973 4.065-2.828 17.878-10.784 36.338-20.728 53.441-32.624 10.304-7.167 18.637-17.23 27.583-26.261 3.819-3.855 7.436-8.091 10.3-12.681 12.283-19.68 24.43-39.446 40.382-56.471 12.224-13.047 17.258-29.524 22.539-45.927 15.85 4.193 29.819 12.129 42.632 22.08 10.583 8.219 19.782 17.883 27.42 29.351z"></path><path fill="#ef7a72" d="M1479.461 758.907c1.872-13.734 4.268-27.394 6.525-41.076 2.218-13.45 8.26-24.279 19.615-32.423 5.099-3.657 9.667-8.701 12.999-14.038 6.646-10.643 15.183-19.135 25.083-26.631 5.888-4.459 11.817-9.176 16.547-14.774 5.906-6.99 13.974-9.306 21.753-12.365l51.48-19.549c.753 11.848.658 23.787 1.641 35.637 1.771 21.353 4.075 42.672 11.748 62.955.17.449.107.985-.019 2.158-6.945 4.134-13.865 7.337-20.437 11.143-3.935 2.279-7.752 5.096-10.869 8.384-6.011 6.343-11.063 13.624-17.286 19.727-9.096 8.92-12.791 20.684-18.181 31.587-.202.409-.072.984-.096 1.481-8.488-1.72-16.937-3.682-25.476-5.094-9.689-1.602-19.426-3.084-29.201-3.949-15.095-1.335-30.241-2.1-45.828-3.172z"></path><path fill="#e94e3b" d="M957.995 766.838c-20.337-5.467-38.791-14.947-55.703-27.254-8.2-5.967-15.451-13.238-22.958-20.37 2.969-3.504 5.564-6.772 8.598-9.563 7.085-6.518 11.283-14.914 15.8-23.153 4.933-8.996 10.345-17.743 14.966-26.892 2.642-5.231 5.547-11.01 5.691-16.611.12-4.651.194-8.932 2.577-12.742 8.52-13.621 15.483-28.026 18.775-43.704 2.11-10.049 7.888-18.774 7.81-29.825-.064-9.089 4.291-18.215 6.73-27.313 3.212-11.983 7.369-23.797 9.492-35.968 3.202-18.358 5.133-36.945 7.346-55.466l4.879-45.8c6.693.288 13.386.575 20.54 1.365.13 3.458-.41 6.407-.496 9.37l-1.136 42.595c-.597 11.552-2.067 23.058-3.084 34.59l-3.845 44.478c-.939 10.202-1.779 20.432-3.283 30.557-.96 6.464-4.46 12.646-1.136 19.383.348.706-.426 1.894-.448 2.864-.224 9.918-5.99 19.428-2.196 29.646.103.279-.033.657-.092.983l-8.446 46.205c-1.231 6.469-2.936 12.846-4.364 19.279-1.5 6.757-2.602 13.621-4.456 20.277-3.601 12.93-10.657 25.3-5.627 39.47.368 1.036.234 2.352.017 3.476l-5.949 30.123z"></path><path fill="#ea5043" d="M958.343 767.017c1.645-10.218 3.659-20.253 5.602-30.302.217-1.124.351-2.44-.017-3.476-5.03-14.17 2.026-26.539 5.627-39.47 1.854-6.656 2.956-13.52 4.456-20.277 1.428-6.433 3.133-12.81 4.364-19.279l8.446-46.205c.059-.326.196-.705.092-.983-3.794-10.218 1.972-19.728 2.196-29.646.022-.97.796-2.158.448-2.864-3.324-6.737.176-12.919 1.136-19.383 1.504-10.125 2.344-20.355 3.283-30.557l3.845-44.478c1.017-11.532 2.488-23.038 3.084-34.59.733-14.18.722-28.397 1.136-42.595.086-2.963.626-5.912.956-9.301 5.356-.48 10.714-.527 16.536-.081 2.224 15.098 1.855 29.734 1.625 44.408-.157 10.064 1.439 20.142 1.768 30.23.334 10.235-.035 20.49.116 30.733.084 5.713.789 11.418.861 17.13.054 4.289-.469 8.585-.702 12.879-.072 1.323-.138 2.659-.031 3.975l2.534 34.405-1.707 36.293-1.908 48.69c-.182 8.103.993 16.237.811 24.34-.271 12.076-1.275 24.133-1.787 36.207-.102 2.414-.101 5.283 1.06 7.219 4.327 7.22 4.463 15.215 4.736 23.103.365 10.553.088 21.128.086 31.693-11.44 2.602-22.84.688-34.106-.916-11.486-1.635-22.806-4.434-34.546-6.903z"></path><path fill="#eb5d19" d="M398.091 622.45c6.086.617 12.21 1.288 18.067 2.918 3.539.985 6.779 3.277 9.952 5.297 9.773 6.224 18.971 13.583 29.311 18.611 8.606 4.184 12.839 10.986 17.016 18.559l18.571 32.959c1.814 3.102 4.285 5.931 6.883 8.443 8.835 8.542 10.052 20.175 13.16 31.095 2.082 7.317 4.609 14.507 6.946 22.127-29.472 3.021-58.969 5.582-87.584 15.222-1.185-2.302-1.795-4.362-2.769-6.233-4.398-8.449-6.703-18.174-14.942-24.299-2.511-1.866-5.103-3.814-7.047-6.218-8.358-10.332-17.028-20.276-28.772-26.973 4.423-11.478 9.299-22.806 13.151-34.473 4.406-13.348 6.724-27.18 6.998-41.313.098-5.093.643-10.176 1.06-15.722z"></path><path fill="#e94c32" d="M981.557 392.109c-1.172 15.337-2.617 30.625-4.438 45.869-2.213 18.521-4.144 37.108-7.346 55.466-2.123 12.171-6.28 23.985-9.492 35.968-2.439 9.098-6.794 18.224-6.73 27.313.078 11.051-5.7 19.776-7.81 29.825-3.292 15.677-10.255 30.082-18.775 43.704-2.383 3.81-2.458 8.091-2.577 12.742-.144 5.6-3.049 11.38-5.691 16.611-4.621 9.149-10.033 17.896-14.966 26.892-4.517 8.239-8.715 16.635-15.8 23.153-3.034 2.791-5.629 6.06-8.735 9.255-12.197-10.595-21.071-23.644-29.301-37.24-7.608-12.569-13.282-25.962-17.637-40.37 13.303-6.889 25.873-13.878 35.311-25.315.717-.869 1.934-1.312 2.71-2.147 5.025-5.405 10.515-10.481 14.854-16.397 6.141-8.374 10.861-17.813 17.206-26.008 8.22-10.618 13.657-22.643 20.024-34.466 4.448-.626 6.729-3.21 8.114-6.89 1.455-3.866 2.644-7.895 4.609-11.492 4.397-8.05 9.641-15.659 13.708-23.86 3.354-6.761 5.511-14.116 8.203-21.206 5.727-15.082 7.277-31.248 12.521-46.578 3.704-10.828 3.138-23.116 4.478-34.753l7.56-.073z"></path><path fill="#f7a617" d="M1918.661 831.99c-4.937 16.58-9.971 33.057-22.196 46.104-15.952 17.025-28.099 36.791-40.382 56.471-2.864 4.59-6.481 8.825-10.3 12.681-8.947 9.031-17.279 19.094-27.583 26.261-17.103 11.896-35.564 21.84-53.441 32.624-1.419.856-3.132 1.571-4.065 2.828-6.904 9.308-18.6 11.178-27.297 17.714-2.705 2.033-6.319 2.856-9.874 4.281-3.413-9.821-6.916-19.583-9.36-29.602-1.533-6.284-1.474-12.957-1.665-19.913 1.913-.78 3.374-1.057 4.81-1.431 15.822-4.121 31.491-8.029 43.818-20.323 9.452-9.426 20.371-17.372 30.534-26.097 6.146-5.277 13.024-10.052 17.954-16.326 14.812-18.848 28.876-38.285 43.112-57.581 2.624-3.557 5.506-7.264 6.83-11.367 2.681-8.311 4.375-16.94 6.476-25.438 17.89.279 35.333 3.179 52.629 9.113z"></path><path fill="#ea553a" d="M1172.91 977.582c-15.775-3.127-28.215-12.377-40.227-22.43-9.005-7.537-18.43-14.605-27.071-22.532-5.07-4.651-9.143-10.443-13.361-15.955-7.647-9.994-15.291-20.007-22.456-30.345-2.361-3.407-3.792-7.72-4.696-11.829-3.119-14.183-5.848-28.453-8.651-42.704-.636-3.236-.974-6.53-1.452-10.209 15.234-2.19 30.471-3.969 46.408-5.622 2.692 5.705 4.882 11.222 6.63 16.876 2.9 9.381 7.776 17.194 15.035 24.049 7.056 6.662 13.305 14.311 19.146 22.099 9.509 12.677 23.01 19.061 36.907 25.054-1.048 7.441-2.425 14.854-3.066 22.33-.956 11.162-1.393 22.369-2.052 33.557l-1.096 17.661z"></path><path fill="#ea5453" d="M1163.123 704.036c-4.005 5.116-7.685 10.531-12.075 15.293-12.842 13.933-27.653 25.447-44.902 34.538-3.166-5.708-5.656-11.287-8.189-17.251-3.321-12.857-6.259-25.431-9.963-37.775-4.6-15.329-10.6-30.188-11.349-46.562-.314-6.871-1.275-14.287-7.114-19.644-1.047-.961-1.292-3.053-1.465-4.67l-4.092-39.927c-.554-5.245-.383-10.829-2.21-15.623-3.622-9.503-4.546-19.253-4.688-29.163-.088-6.111 1.068-12.256.782-18.344-.67-14.281-1.76-28.546-2.9-42.8-.657-8.222-1.951-16.395-2.564-24.62-.458-6.137-.285-12.322-.104-18.21.959 5.831 1.076 11.525 2.429 16.909 2.007 7.986 5.225 15.664 7.324 23.632 3.222 12.23 1.547 25.219 6.728 37.355 4.311 10.099 6.389 21.136 9.732 31.669 2.228 7.02 6.167 13.722 7.121 20.863 1.119 8.376 6.1 13.974 10.376 20.716l2.026 10.576c1.711 9.216 3.149 18.283 8.494 26.599 6.393 9.946 11.348 20.815 16.943 31.276 4.021 7.519 6.199 16.075 12.925 22.065l24.462 22.26c.556.503 1.507.571 2.274.841z"></path><path fill="#ea5b15" d="M1285.092 163.432c9.165 3.148 18.419 6.374 27.279 10.459 4.871 2.246 8.838 6.406 13.646 8.851 5.446 2.77 11.801 3.874 17.011 6.965 11.514 6.831 24.097 9.942 36.968 12.471 1.78.35 3.777.576 5.213 1.542 10.784 7.255 23.448 9.114 35.622 11.834 9.977 2.23 18.529 6.703 26.988 11.898 5.233 3.214 10.76 5.983 15.798 9.468 4.14 2.864 7.962 6.279 11.551 9.827 5.076 5.02 10.056 10.181 14.624 15.658 5.822 6.98 11.119 14.395 16.78 21.513 4.531 5.698 9.267 11.233 14.222 16.987-10.005 5.806-20.07 12.004-30.719 16.943-7.694 3.569-16.163 5.464-24.688 7.669-2.878-7.088-5.352-13.741-7.833-20.392-.802-2.15-1.244-4.55-2.498-6.396-4.548-6.7-9.712-12.999-14.011-19.847-6.672-10.627-15.34-18.93-26.063-25.376-9.357-5.625-18.367-11.824-27.644-17.587-6.436-3.997-12.902-8.006-19.659-11.405-5.123-2.577-11.107-3.536-16.046-6.37-17.187-9.863-35.13-17.887-54.031-23.767-4.403-1.37-8.953-2.267-13.436-3.382l.926-27.565z"></path><path fill="#ea504b" d="M1098 737l7.789 16.893c-15.04 9.272-31.679 15.004-49.184 17.995-9.464 1.617-19.122 2.097-29.151 3.019-.457-10.636-.18-21.211-.544-31.764-.273-7.888-.409-15.883-4.736-23.103-1.16-1.936-1.162-4.805-1.06-7.219l1.787-36.207c.182-8.103-.993-16.237-.811-24.34.365-16.236 1.253-32.461 1.908-48.69.484-12 .942-24.001 1.98-36.069 5.57 10.19 10.632 20.42 15.528 30.728 1.122 2.362 2.587 5.09 2.339 7.488-1.536 14.819 5.881 26.839 12.962 38.33 10.008 16.241 16.417 33.54 20.331 51.964 2.285 10.756 4.729 21.394 11.958 30.165L1098 737z"></path><path fill="#f6a320" d="M1865.78 822.529c-1.849 8.846-3.544 17.475-6.224 25.786-1.323 4.102-4.206 7.81-6.83 11.367l-43.112 57.581c-4.93 6.273-11.808 11.049-17.954 16.326-10.162 8.725-21.082 16.671-30.534 26.097-12.327 12.294-27.997 16.202-43.818 20.323-1.436.374-2.897.651-4.744.986-1.107-17.032-1.816-34.076-2.079-51.556 1.265-.535 2.183-.428 2.888-.766 10.596-5.072 20.8-11.059 32.586-13.273 1.69-.317 3.307-1.558 4.732-2.662l26.908-21.114c4.992-4.003 11.214-7.393 14.381-12.585 11.286-18.5 22.363-37.263 27.027-58.87l36.046 1.811c3.487.165 6.983.14 10.727.549z"></path><path fill="#ec6333" d="M318.448 922.814c-6.374-2.074-12.56-4.058-18.412-6.765-8.379-3.876-16.906-7.675-24.617-12.668-5.239-3.392-9.69-8.381-13.609-13.352-7.87-9.983-14.953-20.582-22.699-30.666-8.061-10.493-13.909-22.097-18.636-34.358-.595-1.543-1.486-2.972-2.382-4.783 6.84-1.598 13.797-3.023 20.807-4.106 18.852-2.912 36.433-9.493 53.737-17.819.697.888.889 1.555 1.292 2.051l17.921 21.896c4.14 4.939 8.06 10.191 12.862 14.412 5.67 4.984 12.185 9.007 18.334 13.447-8.937 16.282-16.422 33.178-20.696 51.31-1.638 6.951-2.402 14.107-3.903 21.403z"></path><path fill="#f49700" d="M623.467 326.903c2.893-10.618 5.584-21.446 9.833-31.623 3.013-7.217 7.924-13.696 12.358-20.254 6.375-9.43 12.026-19.67 19.886-27.705 14.12-14.434 28.063-29.453 47.926-36.784 6.581-2.429 12.344-6.994 18.774-9.942 3.975-1.822 8.503-2.436 13.186-3.592 1.947 18.557 3.248 37.15 8.307 55.686-15.453 7.931-28.853 18.092-40.46 29.996-10.417 10.683-19.109 23.111-28.013 35.175-3.238 4.388-4.888 9.948-7.262 14.973-17.803-3.987-35.767-6.498-54.535-5.931z"></path><path fill="#ea544c" d="M1097.956 736.615c-2.925-3.218-5.893-6.822-8.862-10.425-7.229-8.771-9.672-19.409-11.958-30.165-3.914-18.424-10.323-35.722-20.331-51.964-7.081-11.491-14.498-23.511-12.962-38.33.249-2.398-1.217-5.126-2.339-7.488l-15.232-31.019-3.103-34.338c-.107-1.316-.041-2.653.031-3.975.233-4.294.756-8.59.702-12.879-.072-5.713-.776-11.417-.861-17.13l-.116-30.733c-.329-10.088-1.926-20.166-1.768-30.23.23-14.674.599-29.31-1.162-44.341 9.369-.803 18.741-1.179 28.558-1.074 1.446 15.814 2.446 31.146 3.446 46.478.108 6.163-.064 12.348.393 18.485.613 8.225 1.907 16.397 2.564 24.62l2.9 42.8c.286 6.088-.869 12.234-.782 18.344.142 9.91 1.066 19.661 4.688 29.163 1.827 4.794 1.657 10.377 2.21 15.623l4.092 39.927c.172 1.617.417 3.71 1.465 4.67 5.839 5.357 6.8 12.773 7.114 19.644.749 16.374 6.749 31.233 11.349 46.562 3.704 12.344 6.642 24.918 9.963 37.775z"></path><path fill="#ec5c61" d="M1204.835 568.008c1.254 25.351-1.675 50.16-10.168 74.61-8.598-4.883-18.177-8.709-24.354-15.59-7.44-8.289-13.929-17.442-21.675-25.711-8.498-9.072-16.731-18.928-21.084-31.113-.54-1.513-1.691-2.807-2.594-4.564-4.605-9.247-7.706-18.544-7.96-29.09-.835-7.149-1.214-13.944-2.609-20.523-2.215-10.454-5.626-20.496-7.101-31.302-2.513-18.419-7.207-36.512-5.347-55.352.24-2.43-.17-4.949-.477-7.402l-4.468-34.792c2.723-.379 5.446-.757 8.585-.667 1.749 8.781 2.952 17.116 4.448 25.399 1.813 10.037 3.64 20.084 5.934 30.017 1.036 4.482 3.953 8.573 4.73 13.064 1.794 10.377 4.73 20.253 9.272 29.771 2.914 6.105 4.761 12.711 7.496 18.912 2.865 6.496 6.264 12.755 9.35 19.156 3.764 7.805 7.667 15.013 16.1 19.441 7.527 3.952 13.713 10.376 20.983 14.924 6.636 4.152 13.932 7.25 20.937 10.813z"></path><path fill="#ed676f" d="M1140.75 379.231c18.38-4.858 36.222-11.21 53.979-18.971 3.222 3.368 5.693 6.744 8.719 9.512 2.333 2.134 5.451 5.07 8.067 4.923 7.623-.429 12.363 2.688 17.309 8.215 5.531 6.18 12.744 10.854 19.224 16.184-5.121 7.193-10.461 14.241-15.323 21.606-13.691 20.739-22.99 43.255-26.782 67.926-.543 3.536-1.281 7.043-2.366 10.925-14.258-6.419-26.411-14.959-32.731-29.803-1.087-2.553-2.596-4.93-3.969-7.355-1.694-2.993-3.569-5.89-5.143-8.943-1.578-3.062-2.922-6.249-4.295-9.413-1.57-3.621-3.505-7.163-4.47-10.946-1.257-4.93-.636-10.572-2.725-15.013-5.831-12.397-7.467-25.628-9.497-38.847z"></path><path fill="#ed656e" d="M1254.103 647.439c5.325.947 10.603 2.272 15.847 3.722 5.101 1.41 10.376 2.475 15.175 4.596 3.237 1.431 5.942 4.262 8.589 6.777 2.592 2.462 4.77 5.355 7.207 7.987 1.804 1.948 4.557 3.453 5.461 5.723 3.51 8.817 11.581 11.307 19.059 14.735 1.053.483 2.116.963 3.214 1.327 9.172 3.043 13.818 8.587 14.889 18.979.715 6.935 5.607 13.679 9.479 19.987 4.623 7.533 9.175 14.819 9.091 24.116-.023 2.55 1.21 5.111 1.874 8.055-19.861 2.555-39.795 4.296-59.597 9.09l-11.596-23.203c-1.107-2.169-2.526-4.353-4.307-5.975-7.349-6.694-14.863-13.209-22.373-19.723l-17.313-14.669c-2.776-2.245-5.935-4.017-8.92-6.003l11.609-38.185c1.508-5.453 1.739-11.258 2.613-17.336z"></path><path fill="#ec6168" d="M1140.315 379.223c2.464 13.227 4.101 26.459 9.931 38.856 2.089 4.441 1.468 10.083 2.725 15.013.965 3.783 2.9 7.325 4.47 10.946 1.372 3.164 2.716 6.351 4.295 9.413 1.574 3.053 3.449 5.95 5.143 8.943 1.372 2.425 2.882 4.803 3.969 7.355 6.319 14.844 18.473 23.384 32.641 30.212.067 5.121-.501 10.201-.435 15.271l.985 38.117c.151 4.586.616 9.162.868 14.201-7.075-3.104-14.371-6.202-21.007-10.354-7.269-4.548-13.456-10.972-20.983-14.924-8.434-4.428-12.337-11.637-16.1-19.441-3.087-6.401-6.485-12.66-9.35-19.156-2.735-6.201-4.583-12.807-7.496-18.912-4.542-9.518-7.477-19.394-9.272-29.771-.777-4.491-3.694-8.581-4.73-13.064-2.294-9.933-4.121-19.98-5.934-30.017-1.496-8.283-2.699-16.618-4.036-25.335 10.349-2.461 20.704-4.511 31.054-6.582.957-.191 1.887-.515 3.264-.769z"></path><path fill="#e94c28" d="M922 537c-6.003 11.784-11.44 23.81-19.66 34.428-6.345 8.196-11.065 17.635-17.206 26.008-4.339 5.916-9.828 10.992-14.854 16.397-.776.835-1.993 1.279-2.71 2.147-9.439 11.437-22.008 18.427-35.357 24.929-4.219-10.885-6.942-22.155-7.205-33.905l-.514-49.542c7.441-2.893 14.452-5.197 21.334-7.841 1.749-.672 3.101-2.401 4.604-3.681 6.749-5.745 12.845-12.627 20.407-16.944 7.719-4.406 14.391-9.101 18.741-16.889.626-1.122 1.689-2.077 2.729-2.877 7.197-5.533 12.583-12.51 16.906-20.439.68-1.247 2.495-1.876 4.105-2.651 2.835 1.408 5.267 2.892 7.884 3.892 3.904 1.491 4.392 3.922 2.833 7.439-1.47 3.318-2.668 6.756-4.069 10.106-1.247 2.981-.435 5.242 2.413 6.544 2.805 1.282 3.125 3.14 1.813 5.601l-6.907 12.799L922 537z"></path><path fill="#eb5659" d="M1124.995 566c.868 1.396 2.018 2.691 2.559 4.203 4.353 12.185 12.586 22.041 21.084 31.113 7.746 8.269 14.235 17.422 21.675 25.711 6.176 6.881 15.756 10.707 24.174 15.932-6.073 22.316-16.675 42.446-31.058 60.937-1.074-.131-2.025-.199-2.581-.702l-24.462-22.26c-6.726-5.99-8.904-14.546-12.925-22.065-5.594-10.461-10.55-21.33-16.943-31.276-5.345-8.315-6.783-17.383-8.494-26.599-.63-3.394-1.348-6.772-1.738-10.848-.371-6.313-1.029-11.934-1.745-18.052l6.34 4.04 1.288-.675-2.143-15.385 9.454 1.208v-8.545L1124.995 566z"></path><path fill="#f5a02d" d="M1818.568 820.096c-4.224 21.679-15.302 40.442-26.587 58.942-3.167 5.192-9.389 8.582-14.381 12.585l-26.908 21.114c-1.425 1.104-3.042 2.345-4.732 2.662-11.786 2.214-21.99 8.201-32.586 13.273-.705.338-1.624.231-2.824.334a824.35 824.35 0 0 1-8.262-42.708c4.646-2.14 9.353-3.139 13.269-5.47 5.582-3.323 11.318-6.942 15.671-11.652 7.949-8.6 14.423-18.572 22.456-27.081 8.539-9.046 13.867-19.641 18.325-30.922l46.559 8.922z"></path><path fill="#eb5a57" d="M1124.96 565.639c-5.086-4.017-10.208-8.395-15.478-12.901v8.545l-9.454-1.208 2.143 15.385-1.288.675-6.34-4.04c.716 6.118 1.375 11.74 1.745 17.633-4.564-6.051-9.544-11.649-10.663-20.025-.954-7.141-4.892-13.843-7.121-20.863-3.344-10.533-5.421-21.57-9.732-31.669-5.181-12.135-3.506-25.125-6.728-37.355-2.099-7.968-5.317-15.646-7.324-23.632-1.353-5.384-1.47-11.078-2.429-16.909l-3.294-46.689a278.63 278.63 0 0 1 27.57-2.084c2.114 12.378 3.647 24.309 5.479 36.195 1.25 8.111 2.832 16.175 4.422 24.23 1.402 7.103 2.991 14.169 4.55 21.241 1.478 6.706.273 14.002 4.6 20.088 5.401 7.597 7.176 16.518 9.467 25.337 1.953 7.515 5.804 14.253 11.917 19.406.254 10.095 3.355 19.392 7.96 28.639z"></path><path fill="#ea541c" d="M911.651 810.999c-2.511 10.165-5.419 20.146-8.2 30.162-2.503 9.015-7.37 16.277-14.364 22.612-6.108 5.533-10.917 12.475-16.796 18.293-6.942 6.871-14.354 13.24-19.083 22.03-.644 1.196-2.222 1.889-3.705 2.857-2.39-7.921-4.101-15.991-6.566-23.823-5.451-17.323-12.404-33.976-23.414-48.835l21.627-21.095c3.182-3.29 5.532-7.382 8.295-11.083l10.663-14.163c9.528 4.78 18.925 9.848 28.625 14.247 7.324 3.321 15.036 5.785 22.917 8.799z"></path><path fill="#eb5d19" d="M1284.092 191.421c4.557.69 9.107 1.587 13.51 2.957 18.901 5.881 36.844 13.904 54.031 23.767 4.938 2.834 10.923 3.792 16.046 6.37 6.757 3.399 13.224 7.408 19.659 11.405l27.644 17.587c10.723 6.446 19.392 14.748 26.063 25.376 4.299 6.848 9.463 13.147 14.011 19.847 1.254 1.847 1.696 4.246 2.498 6.396l7.441 20.332c-11.685 1.754-23.379 3.133-35.533 4.037-.737-2.093-.995-3.716-1.294-5.33-3.157-17.057-14.048-30.161-23.034-44.146-3.027-4.71-7.786-8.529-12.334-11.993-9.346-7.116-19.004-13.834-28.688-20.491-6.653-4.573-13.311-9.251-20.431-13.002-8.048-4.24-16.479-7.85-24.989-11.091-11.722-4.465-23.673-8.328-35.527-12.449l.927-19.572z"></path><path fill="#eb5e24" d="M1283.09 211.415c11.928 3.699 23.88 7.562 35.602 12.027 8.509 3.241 16.941 6.852 24.989 11.091 7.12 3.751 13.778 8.429 20.431 13.002 9.684 6.657 19.342 13.375 28.688 20.491 4.548 3.463 9.307 7.283 12.334 11.993 8.986 13.985 19.877 27.089 23.034 44.146.299 1.615.557 3.237.836 5.263-13.373-.216-26.749-.839-40.564-1.923-2.935-9.681-4.597-18.92-12.286-26.152-15.577-14.651-30.4-30.102-45.564-45.193-.686-.683-1.626-1.156-2.516-1.584l-47.187-22.615 2.203-20.546z"></path><path fill="#e9511f" d="M913 486.001c-1.29.915-3.105 1.543-3.785 2.791-4.323 7.929-9.709 14.906-16.906 20.439-1.04.8-2.103 1.755-2.729 2.877-4.35 7.788-11.022 12.482-18.741 16.889-7.562 4.317-13.658 11.199-20.407 16.944-1.503 1.28-2.856 3.009-4.604 3.681-6.881 2.643-13.893 4.948-21.262 7.377-.128-11.151.202-22.302.378-33.454.03-1.892-.6-3.795-.456-6.12 13.727-1.755 23.588-9.527 33.278-17.663 2.784-2.337 6.074-4.161 8.529-6.784l29.057-31.86c1.545-1.71 3.418-3.401 4.221-5.459 5.665-14.509 11.49-28.977 16.436-43.736 2.817-8.407 4.074-17.338 6.033-26.032 5.039.714 10.078 1.427 15.536 2.629-.909 8.969-2.31 17.438-3.546 25.931-2.41 16.551-5.84 32.839-11.991 48.461L913 486.001z"></path><path fill="#ea5741" d="M1179.451 903.828c-14.224-5.787-27.726-12.171-37.235-24.849-5.841-7.787-12.09-15.436-19.146-22.099-7.259-6.854-12.136-14.667-15.035-24.049-1.748-5.654-3.938-11.171-6.254-17.033 15.099-4.009 30.213-8.629 44.958-15.533l28.367 36.36c6.09 8.015 13.124 14.75 22.72 18.375-7.404 14.472-13.599 29.412-17.48 45.244-.271 1.106-.382 2.25-.895 3.583z"></path><path fill="#ea522a" d="M913.32 486.141c2.693-7.837 5.694-15.539 8.722-23.231 6.151-15.622 9.581-31.91 11.991-48.461l3.963-25.861c7.582.317 15.168 1.031 22.748 1.797 4.171.421 8.333.928 12.877 1.596-.963 11.836-.398 24.125-4.102 34.953-5.244 15.33-6.794 31.496-12.521 46.578-2.692 7.09-4.849 14.445-8.203 21.206-4.068 8.201-9.311 15.81-13.708 23.86-1.965 3.597-3.154 7.627-4.609 11.492-1.385 3.68-3.666 6.265-8.114 6.89-1.994-1.511-3.624-3.059-5.077-4.44l6.907-12.799c1.313-2.461.993-4.318-1.813-5.601-2.849-1.302-3.66-3.563-2.413-6.544 1.401-3.35 2.599-6.788 4.069-10.106 1.558-3.517 1.071-5.948-2.833-7.439-2.617-1-5.049-2.484-7.884-3.892z"></path><path fill="#eb5e24" d="M376.574 714.118c12.053 6.538 20.723 16.481 29.081 26.814 1.945 2.404 4.537 4.352 7.047 6.218 8.24 6.125 10.544 15.85 14.942 24.299.974 1.871 1.584 3.931 2.376 6.29-7.145 3.719-14.633 6.501-21.386 10.517-9.606 5.713-18.673 12.334-28.425 18.399-3.407-3.73-6.231-7.409-9.335-10.834l-30.989-33.862c11.858-11.593 22.368-24.28 31.055-38.431 1.86-3.031 3.553-6.164 5.632-9.409z"></path><path fill="#e95514" d="M859.962 787.636c-3.409 5.037-6.981 9.745-10.516 14.481-2.763 3.701-5.113 7.792-8.295 11.083-6.885 7.118-14.186 13.834-21.65 20.755-13.222-17.677-29.417-31.711-48.178-42.878-.969-.576-2.068-.934-3.27-1.709 6.28-8.159 12.733-15.993 19.16-23.849 1.459-1.783 2.718-3.738 4.254-5.448l18.336-19.969c4.909 5.34 9.619 10.738 14.081 16.333 9.72 12.19 21.813 21.566 34.847 29.867.411.262.725.674 1.231 1.334z"></path><path fill="#eb5f2d" d="M339.582 762.088l31.293 33.733c3.104 3.425 5.928 7.104 9.024 10.979-12.885 11.619-24.548 24.139-33.899 38.704-.872 1.359-1.56 2.837-2.644 4.428-6.459-4.271-12.974-8.294-18.644-13.278-4.802-4.221-8.722-9.473-12.862-14.412l-17.921-21.896c-.403-.496-.595-1.163-.926-2.105 16.738-10.504 32.58-21.87 46.578-36.154z"></path><path fill="#f28d00" d="M678.388 332.912c1.989-5.104 3.638-10.664 6.876-15.051 8.903-12.064 17.596-24.492 28.013-35.175 11.607-11.904 25.007-22.064 40.507-29.592 4.873 11.636 9.419 23.412 13.67 35.592-5.759 4.084-11.517 7.403-16.594 11.553-4.413 3.607-8.124 8.092-12.023 12.301-5.346 5.772-10.82 11.454-15.782 17.547-3.929 4.824-7.17 10.208-10.716 15.344l-33.95-12.518z"></path><path fill="#f08369" d="M1580.181 771.427c-.191-.803-.322-1.377-.119-1.786 5.389-10.903 9.084-22.666 18.181-31.587 6.223-6.103 11.276-13.385 17.286-19.727 3.117-3.289 6.933-6.105 10.869-8.384 6.572-3.806 13.492-7.009 20.461-10.752 1.773 3.23 3.236 6.803 4.951 10.251l12.234 24.993c-1.367 1.966-2.596 3.293-3.935 4.499-7.845 7.07-16.315 13.564-23.407 21.32-6.971 7.623-12.552 16.517-18.743 24.854l-37.777-13.68z"></path><path fill="#f18b5e" d="M1618.142 785.4c6.007-8.63 11.588-17.524 18.559-25.147 7.092-7.755 15.562-14.249 23.407-21.32 1.338-1.206 2.568-2.534 3.997-4.162l28.996 33.733c1.896 2.205 4.424 3.867 6.66 6.394-6.471 7.492-12.967 14.346-19.403 21.255l-18.407 19.953c-12.958-12.409-27.485-22.567-43.809-30.706z"></path><path fill="#f49c3a" d="M1771.617 811.1c-4.066 11.354-9.394 21.949-17.933 30.995-8.032 8.509-14.507 18.481-22.456 27.081-4.353 4.71-10.089 8.329-15.671 11.652-3.915 2.331-8.623 3.331-13.318 5.069-4.298-9.927-8.255-19.998-12.1-30.743 4.741-4.381 9.924-7.582 13.882-11.904 7.345-8.021 14.094-16.603 20.864-25.131 4.897-6.168 9.428-12.626 14.123-18.955l32.61 11.936z"></path><path fill="#f08000" d="M712.601 345.675c3.283-5.381 6.524-10.765 10.453-15.589 4.962-6.093 10.435-11.774 15.782-17.547 3.899-4.21 7.61-8.695 12.023-12.301 5.078-4.15 10.836-7.469 16.636-11.19a934.12 934.12 0 0 1 23.286 35.848c-4.873 6.234-9.676 11.895-14.63 17.421l-25.195 27.801c-11.713-9.615-24.433-17.645-38.355-24.443z"></path><path fill="#ed6e04" d="M751.11 370.42c8.249-9.565 16.693-18.791 25.041-28.103 4.954-5.526 9.757-11.187 14.765-17.106 7.129 6.226 13.892 13.041 21.189 19.225 5.389 4.567 11.475 8.312 17.53 12.92-5.51 7.863-10.622 15.919-17.254 22.427-8.881 8.716-18.938 16.233-28.49 24.264-5.703-6.587-11.146-13.427-17.193-19.682-4.758-4.921-10.261-9.121-15.587-13.944z"></path><path fill="#ea541c" d="M921.823 385.544c-1.739 9.04-2.995 17.971-5.813 26.378-4.946 14.759-10.771 29.227-16.436 43.736-.804 2.058-2.676 3.749-4.221 5.459l-29.057 31.86c-2.455 2.623-5.745 4.447-8.529 6.784-9.69 8.135-19.551 15.908-33.208 17.237-1.773-9.728-3.147-19.457-4.091-29.6l36.13-16.763c.581-.267 1.046-.812 1.525-1.269 8.033-7.688 16.258-15.19 24.011-23.152 4.35-4.467 9.202-9.144 11.588-14.69 6.638-15.425 15.047-30.299 17.274-47.358 3.536.344 7.072.688 10.829 1.377z"></path><path fill="#f3944d" d="M1738.688 798.998c-4.375 6.495-8.906 12.953-13.803 19.121-6.771 8.528-13.519 17.11-20.864 25.131-3.958 4.322-9.141 7.523-13.925 11.54-8.036-13.464-16.465-26.844-27.999-38.387 5.988-6.951 12.094-13.629 18.261-20.25l19.547-20.95 38.783 23.794z"></path><path fill="#ec6168" d="M1239.583 703.142c3.282 1.805 6.441 3.576 9.217 5.821 5.88 4.755 11.599 9.713 17.313 14.669l22.373 19.723c1.781 1.622 3.2 3.806 4.307 5.975 3.843 7.532 7.477 15.171 11.194 23.136-10.764 4.67-21.532 8.973-32.69 12.982l-22.733-27.366c-2.003-2.416-4.096-4.758-6.194-7.093-3.539-3.94-6.927-8.044-10.74-11.701-2.57-2.465-5.762-4.283-8.675-6.39l16.627-29.755z"></path><path fill="#ec663e" d="M1351.006 332.839l-28.499 10.33c-.294.107-.533.367-1.194.264-11.067-19.018-27.026-32.559-44.225-44.855-4.267-3.051-8.753-5.796-13.138-8.682l9.505-24.505c10.055 4.069 19.821 8.227 29.211 13.108 3.998 2.078 7.299 5.565 10.753 8.598 3.077 2.701 5.743 5.891 8.926 8.447 4.116 3.304 9.787 5.345 12.62 9.432 6.083 8.777 10.778 18.517 16.041 27.863z"></path><path fill="#eb5e5b" d="M1222.647 733.051c3.223 1.954 6.415 3.771 8.985 6.237 3.813 3.658 7.201 7.761 10.74 11.701l6.194 7.093 22.384 27.409c-13.056 6.836-25.309 14.613-36.736 24.161l-39.323-44.7 24.494-27.846c1.072-1.224 1.974-2.598 3.264-4.056z"></path><path fill="#ea580e" d="M876.001 376.171c5.874 1.347 11.748 2.694 17.812 4.789-.81 5.265-2.687 9.791-2.639 14.296.124 11.469-4.458 20.383-12.73 27.863-2.075 1.877-3.659 4.286-5.668 6.248l-22.808 21.967c-.442.422-1.212.488-1.813.757l-23.113 10.389-9.875 4.514c-2.305-6.09-4.609-12.181-6.614-18.676 7.64-4.837 15.567-8.54 22.18-13.873 9.697-7.821 18.931-16.361 27.443-25.455 5.613-5.998 12.679-11.331 14.201-20.475.699-4.2 2.384-8.235 3.623-12.345z"></path><path fill="#e95514" d="M815.103 467.384c3.356-1.894 6.641-3.415 9.94-4.903l23.113-10.389c.6-.269 1.371-.335 1.813-.757l22.808-21.967c2.008-1.962 3.593-4.371 5.668-6.248 8.272-7.48 12.854-16.394 12.73-27.863-.049-4.505 1.828-9.031 2.847-13.956 5.427.559 10.836 1.526 16.609 2.68-1.863 17.245-10.272 32.119-16.91 47.544-2.387 5.546-7.239 10.223-11.588 14.69-7.753 7.962-15.978 15.464-24.011 23.152-.478.458-.944 1.002-1.525 1.269l-36.069 16.355c-2.076-6.402-3.783-12.81-5.425-19.607z"></path><path fill="#eb620b" d="M783.944 404.402c9.499-8.388 19.556-15.905 28.437-24.621 6.631-6.508 11.744-14.564 17.575-22.273 9.271 4.016 18.501 8.375 27.893 13.43-4.134 7.07-8.017 13.778-12.833 19.731-5.785 7.15-12.109 13.917-18.666 20.376-7.99 7.869-16.466 15.244-24.731 22.832l-17.674-29.475z"></path><path fill="#ea544c" d="M1197.986 854.686c-9.756-3.309-16.79-10.044-22.88-18.059l-28.001-36.417c8.601-5.939 17.348-11.563 26.758-17.075 1.615 1.026 2.639 1.876 3.505 2.865l26.664 30.44c3.723 4.139 7.995 7.785 12.017 11.656l-18.064 26.591z"></path><path fill="#ec6333" d="M1351.41 332.903c-5.667-9.409-10.361-19.149-16.445-27.926-2.833-4.087-8.504-6.128-12.62-9.432-3.184-2.555-5.849-5.745-8.926-8.447-3.454-3.033-6.756-6.52-10.753-8.598-9.391-4.88-19.157-9.039-29.138-13.499 1.18-5.441 2.727-10.873 4.81-16.607 11.918 4.674 24.209 8.261 34.464 14.962 14.239 9.304 29.011 18.453 39.595 32.464 2.386 3.159 5.121 6.077 7.884 8.923 6.564 6.764 10.148 14.927 11.723 24.093l-20.594 4.067z"></path><path fill="#eb5e5b" d="M1117 536.549c-6.113-4.702-9.965-11.44-11.917-18.955-2.292-8.819-4.066-17.74-9.467-25.337-4.327-6.085-3.122-13.382-4.6-20.088l-4.55-21.241c-1.59-8.054-3.172-16.118-4.422-24.23l-5.037-36.129c6.382-1.43 12.777-2.462 19.582-3.443 1.906 11.646 3.426 23.24 4.878 34.842.307 2.453.717 4.973.477 7.402-1.86 18.84 2.834 36.934 5.347 55.352 1.474 10.806 4.885 20.848 7.101 31.302 1.394 6.579 1.774 13.374 2.609 20.523z"></path><path fill="#ec644b" d="M1263.638 290.071c4.697 2.713 9.183 5.458 13.45 8.509 17.199 12.295 33.158 25.836 43.873 44.907-8.026 4.725-16.095 9.106-24.83 13.372-11.633-15.937-25.648-28.515-41.888-38.689-1.609-1.008-3.555-1.48-5.344-2.2 2.329-3.852 4.766-7.645 6.959-11.573l7.78-14.326z"></path><path fill="#eb5f2d" d="M1372.453 328.903c-2.025-9.233-5.608-17.396-12.172-24.16-2.762-2.846-5.498-5.764-7.884-8.923-10.584-14.01-25.356-23.16-39.595-32.464-10.256-6.701-22.546-10.289-34.284-15.312.325-5.246 1.005-10.444 2.027-15.863l47.529 22.394c.89.428 1.83.901 2.516 1.584l45.564 45.193c7.69 7.233 9.352 16.472 11.849 26.084-5.032.773-10.066 1.154-15.55 1.466z"></path><path fill="#e95a0f" d="M801.776 434.171c8.108-7.882 16.584-15.257 24.573-23.126 6.558-6.459 12.881-13.226 18.666-20.376 4.817-5.953 8.7-12.661 13.011-19.409 5.739 1.338 11.463 3.051 17.581 4.838-.845 4.183-2.53 8.219-3.229 12.418-1.522 9.144-8.588 14.477-14.201 20.475-8.512 9.094-17.745 17.635-27.443 25.455-6.613 5.333-14.54 9.036-22.223 13.51-2.422-4.469-4.499-8.98-6.735-13.786z"></path><path fill="#eb5e5b" d="M1248.533 316.002c2.155.688 4.101 1.159 5.71 2.168 16.24 10.174 30.255 22.752 41.532 38.727-7.166 5.736-14.641 11.319-22.562 16.731-1.16-1.277-1.684-2.585-2.615-3.46l-38.694-36.2 14.203-15.029c.803-.86 1.38-1.93 2.427-2.936z"></path><path fill="#eb5a57" d="M1216.359 827.958c-4.331-3.733-8.603-7.379-12.326-11.518l-26.664-30.44c-.866-.989-1.89-1.839-3.152-2.902 6.483-6.054 13.276-11.959 20.371-18.005l39.315 44.704c-5.648 6.216-11.441 12.12-17.544 18.161z"></path><path fill="#ec6168" d="M1231.598 334.101l38.999 36.066c.931.876 1.456 2.183 2.303 3.608-4.283 4.279-8.7 8.24-13.769 12.091-4.2-3.051-7.512-6.349-11.338-8.867-12.36-8.136-22.893-18.27-32.841-29.093l16.646-13.805z"></path><path fill="#ed656e" d="M1214.597 347.955c10.303 10.775 20.836 20.908 33.196 29.044 3.825 2.518 7.137 5.816 10.992 8.903-3.171 4.397-6.65 8.648-10.432 13.046-6.785-5.184-13.998-9.858-19.529-16.038-4.946-5.527-9.687-8.644-17.309-8.215-2.616.147-5.734-2.788-8.067-4.923-3.026-2.769-5.497-6.144-8.35-9.568 6.286-4.273 12.715-8.237 19.499-12.25z"></path></svg>
</p>
<p align="center">
<b>The crispy rerank family from <a href="https://mixedbread.ai"><b>mixedbread ai</b></a>.</b>
</p>
# mxbai-rerank-base-v1
This is the base model in our family of powerful reranker models. You can learn more about the models in our [blog post](https://www.mixedbread.ai/blog/mxbai-rerank-v1).
We have three models:
- [mxbai-rerank-xsmall-v1](https://huggingface.co/mixedbread-ai/mxbai-rerank-xsmall-v1)
- [mxbai-rerank-base-v1](https://huggingface.co/mixedbread-ai/mxbai-rerank-base-v1) (🍞)
- [mxbai-rerank-large-v1](https://huggingface.co/mixedbread-ai/mxbai-rerank-large-v1)
## Quickstart
Currently, the best way to use our models is with the most recent version of sentence-transformers.
`pip install -U sentence-transformers`
Let's say you have a query, and you want to rerank a set of documents. You can do that with only one line of code:
```python
from sentence_transformers import CrossEncoder
# Load the model, here we use our base sized model
model = CrossEncoder("mixedbread-ai/mxbai-rerank-base-v1")
# Example query and documents
query = "Who wrote 'To Kill a Mockingbird'?"
documents = [
"'To Kill a Mockingbird' is a novel by Harper Lee published in 1960. It was immediately successful, winning the Pulitzer Prize, and has become a classic of modern American literature.",
"The novel 'Moby-Dick' was written by Herman Melville and first published in 1851. It is considered a masterpiece of American literature and deals with complex themes of obsession, revenge, and the conflict between good and evil.",
"Harper Lee, an American novelist widely known for her novel 'To Kill a Mockingbird', was born in 1926 in Monroeville, Alabama. She received the Pulitzer Prize for Fiction in 1961.",
"Jane Austen was an English novelist known primarily for her six major novels, which interpret, critique and comment upon the British landed gentry at the end of the 18th century.",
"The 'Harry Potter' series, which consists of seven fantasy novels written by British author J.K. Rowling, is among the most popular and critically acclaimed books of the modern era.",
"'The Great Gatsby', a novel written by American author F. Scott Fitzgerald, was published in 1925. The story is set in the Jazz Age and follows the life of millionaire Jay Gatsby and his pursuit of Daisy Buchanan."
]
# Lets get the scores
results = model.rank(query, documents, return_documents=True, top_k=3)
```
<details>
<summary>JavaScript Example</summary>
Install [transformers.js](https://github.com/xenova/transformers.js)
`npm i @xenova/transformers`
Let's say you have a query, and you want to rerank a set of documents. In JavaScript, you need to add a function:
```javascript
import { AutoTokenizer, AutoModelForSequenceClassification } from '@xenova/transformers';
const model_id = 'mixedbread-ai/mxbai-rerank-base-v1';
const model = await AutoModelForSequenceClassification.from_pretrained(model_id);
const tokenizer = await AutoTokenizer.from_pretrained(model_id);
/**
* Performs ranking with the CrossEncoder on the given query and documents. Returns a sorted list with the document indices and scores.
* @param {string} query A single query
* @param {string[]} documents A list of documents
* @param {Object} options Options for ranking
* @param {number} [options.top_k=undefined] Return the top-k documents. If undefined, all documents are returned.
* @param {number} [options.return_documents=false] If true, also returns the documents. If false, only returns the indices and scores.
*/
async function rank(query, documents, {
top_k = undefined,
return_documents = false,
} = {}) {
const inputs = tokenizer(
new Array(documents.length).fill(query),
{
text_pair: documents,
padding: true,
truncation: true,
}
)
const { logits } = await model(inputs);
return logits
.sigmoid()
.tolist()
.map(([score], i) => ({
corpus_id: i,
score,
...(return_documents ? { text: documents[i] } : {})
}))
.sort((a, b) => b.score - a.score)
.slice(0, top_k);
}
// Example usage:
const query = "Who wrote 'To Kill a Mockingbird'?"
const documents = [
"'To Kill a Mockingbird' is a novel by Harper Lee published in 1960. It was immediately successful, winning the Pulitzer Prize, and has become a classic of modern American literature.",
"The novel 'Moby-Dick' was written by Herman Melville and first published in 1851. It is considered a masterpiece of American literature and deals with complex themes of obsession, revenge, and the conflict between good and evil.",
"Harper Lee, an American novelist widely known for her novel 'To Kill a Mockingbird', was born in 1926 in Monroeville, Alabama. She received the Pulitzer Prize for Fiction in 1961.",
"Jane Austen was an English novelist known primarily for her six major novels, which interpret, critique and comment upon the British landed gentry at the end of the 18th century.",
"The 'Harry Potter' series, which consists of seven fantasy novels written by British author J.K. Rowling, is among the most popular and critically acclaimed books of the modern era.",
"'The Great Gatsby', a novel written by American author F. Scott Fitzgerald, was published in 1925. The story is set in the Jazz Age and follows the life of millionaire Jay Gatsby and his pursuit of Daisy Buchanan."
]
const results = await rank(query, documents, { return_documents: true, top_k: 3 });
console.log(results);
```
</details>
## Using API
You can use the large model via our API as follows:
```python
from mixedbread_ai.client import MixedbreadAI
mxbai = MixedbreadAI(api_key="{MIXEDBREAD_API_KEY}")
res = mxbai.reranking(
model="mixedbread-ai/mxbai-rerank-large-v1",
query="Who is the author of To Kill a Mockingbird?",
input=[
"To Kill a Mockingbird is a novel by Harper Lee published in 1960. It was immediately successful, winning the Pulitzer Prize, and has become a classic of modern American literature.",
"The novel Moby-Dick was written by Herman Melville and first published in 1851. It is considered a masterpiece of American literature and deals with complex themes of obsession, revenge, and the conflict between good and evil.",
"Harper Lee, an American novelist widely known for her novel To Kill a Mockingbird, was born in 1926 in Monroeville, Alabama. She received the Pulitzer Prize for Fiction in 1961.",
"Jane Austen was an English novelist known primarily for her six major novels, which interpret, critique and comment upon the British landed gentry at the end of the 18th century.",
"The Harry Potter series, which consists of seven fantasy novels written by British author J.K. Rowling, is among the most popular and critically acclaimed books of the modern era.",
"The Great Gatsby, a novel written by American author F. Scott Fitzgerald, was published in 1925. The story is set in the Jazz Age and follows the life of millionaire Jay Gatsby and his pursuit of Daisy Buchanan."
],
top_k=3,
return_input=false
)
print(res.data)
```
The API comes with additional features, such as a continous trained reranker! Check out the [docs](https://www.mixedbread.ai/docs) for more information.
## Evaluation
Our reranker models are designed to elevate your search. They work extremely well in combination with keyword search and can even outperform semantic search systems in many cases.
| Model | NDCG@10 | Accuracy@3 |
| ------------------------------------------------------------------------------------- | -------- | ---------- |
| Lexical Search (Lucene) | 38.0 | 66.4 |
| [BAAI/bge-reranker-base](https://huggingface.co/BAAI/bge-reranker-base) | 41.6 | 66.9 |
| [BAAI/bge-reranker-large](https://huggingface.co/BAAI/bge-reranker-large) | 45.2 | 70.6 |
| cohere-embed-v3 (semantic search) | 47.5 | 70.9 |
| [mxbai-rerank-xsmall-v1](https://huggingface.co/mixedbread-ai/mxbai-rerank-xsmall-v1) | **43.9** | **70.0** |
| [mxbai-rerank-base-v1](https://huggingface.co/mixedbread-ai/mxbai-rerank-base-v1) | **46.9** | **72.3** |
| [mxbai-rerank-large-v1](https://huggingface.co/mixedbread-ai/mxbai-rerank-large-v1) | **48.8** | **74.9** |
The reported results are aggregated from 11 datasets of BEIR. We used [Pyserini](https://github.com/castorini/pyserini/) to evaluate the models. Find more in our [blog-post](https://www.mixedbread.ai/blog/mxbai-rerank-v1) and on this [spreadsheet](https://docs.google.com/spreadsheets/d/15ELkSMFv-oHa5TRiIjDvhIstH9dlc3pnZeO-iGz4Ld4/edit?usp=sharing).
## Community
Please join our [Discord Community](https://discord.gg/jDfMHzAVfU) and share your feedback and thoughts! We are here to help and also always happy to chat.
## License
Apache 2.0 |
Qwen/Qwen2-1.5B | Qwen | "2024-06-06T14:36:18Z" | 36,006 | 37 | transformers | [
"transformers",
"safetensors",
"qwen2",
"text-generation",
"pretrained",
"conversational",
"en",
"license:apache-2.0",
"autotrain_compatible",
"endpoints_compatible",
"text-generation-inference",
"region:us"
] | text-generation | "2024-05-31T08:41:32Z" | ---
language:
- en
pipeline_tag: text-generation
tags:
- pretrained
license: apache-2.0
---
# Qwen2-1.5B
## Introduction
Qwen2 is the new series of Qwen large language models. For Qwen2, we release a number of base language models and instruction-tuned language models ranging from 0.5 to 72 billion parameters, including a Mixture-of-Experts model. This repo contains the 1.5B Qwen2 base language model.
Compared with the state-of-the-art opensource language models, including the previous released Qwen1.5, Qwen2 has generally surpassed most opensource models and demonstrated competitiveness against proprietary models across a series of benchmarks targeting for language understanding, language generation, multilingual capability, coding, mathematics, reasoning, etc.
For more details, please refer to our [blog](https://qwenlm.github.io/blog/qwen2/), [GitHub](https://github.com/QwenLM/Qwen2), and [Documentation](https://qwen.readthedocs.io/en/latest/).
<br>
## Model Details
Qwen2 is a language model series including decoder language models of different model sizes. For each size, we release the base language model and the aligned chat model. It is based on the Transformer architecture with SwiGLU activation, attention QKV bias, group query attention, etc. Additionally, we have an improved tokenizer adaptive to multiple natural languages and codes.
## Requirements
The code of Qwen2 has been in the latest Hugging face transformers and we advise you to install `transformers>=4.37.0`, or you might encounter the following error:
```
KeyError: 'qwen2'
```
## Usage
We do not advise you to use base language models for text generation. Instead, you can apply post-training, e.g., SFT, RLHF, continued pretraining, etc., on this model.
## Performance
The evaluation of base models mainly focuses on the model performance of natural language understanding, general question answering, coding, mathematics, scientific knowledge, reasoning, multilingual capability, etc.
The datasets for evaluation include:
**English Tasks**: MMLU (5-shot), MMLU-Pro (5-shot), GPQA (5shot), Theorem QA (5-shot), BBH (3-shot), HellaSwag (10-shot), Winogrande (5-shot), TruthfulQA (0-shot), ARC-C (25-shot)
**Coding Tasks**: EvalPlus (0-shot) (HumanEval, MBPP, HumanEval+, MBPP+), MultiPL-E (0-shot) (Python, C++, JAVA, PHP, TypeScript, C#, Bash, JavaScript)
**Math Tasks**: GSM8K (4-shot), MATH (4-shot)
**Chinese Tasks**: C-Eval(5-shot), CMMLU (5-shot)
**Multilingual Tasks**: Multi-Exam (M3Exam 5-shot, IndoMMLU 3-shot, ruMMLU 5-shot, mMMLU 5-shot), Multi-Understanding (BELEBELE 5-shot, XCOPA 5-shot, XWinograd 5-shot, XStoryCloze 0-shot, PAWS-X 5-shot), Multi-Mathematics (MGSM 8-shot), Multi-Translation (Flores-101 5-shot)
#### Qwen2-0.5B & Qwen2-1.5B performances
| Datasets | Phi-2 | Gemma-2B | MiniCPM | Qwen1.5-1.8B | Qwen2-0.5B | Qwen2-1.5B |
| :--------| :---------: | :------------: | :------------: |:------------: | :------------: | :------------: |
|#Non-Emb Params | 2.5B | 2.0B | 2.4B | 1.3B | 0.35B | 1.3B |
|MMLU | 52.7 | 42.3 | 53.5 | 46.8 | 45.4 | **56.5** |
|MMLU-Pro | - | 15.9 | - | - | 14.7 | 21.8 |
|Theorem QA | - | - | - |- | 8.9 | **15.0** |
|HumanEval | 47.6 | 22.0 |**50.0**| 20.1 | 22.0 | 31.1 |
|MBPP | **55.0** | 29.2 | 47.3 | 18.0 | 22.0 | 37.4 |
|GSM8K | 57.2 | 17.7 | 53.8 | 38.4 | 36.5 | **58.5** |
|MATH | 3.5 | 11.8 | 10.2 | 10.1 | 10.7 | **21.7** |
|BBH | **43.4** | 35.2 | 36.9 | 24.2 | 28.4 | 37.2 |
|HellaSwag | **73.1** | 71.4 | 68.3 | 61.4 | 49.3 | 66.6 |
|Winogrande | **74.4** | 66.8 | -| 60.3 | 56.8 | 66.2 |
|ARC-C | **61.1** | 48.5 | -| 37.9 | 31.5 | 43.9 |
|TruthfulQA | 44.5 | 33.1 | -| 39.4 | 39.7 | **45.9** |
|C-Eval | 23.4 | 28.0 | 51.1| 59.7 | 58.2 | **70.6** |
|CMMLU | 24.2 | - | 51.1 | 57.8 | 55.1 | **70.3** |
## Citation
If you find our work helpful, feel free to give us a cite.
```
@article{qwen2,
title={Qwen2 Technical Report},
year={2024}
}
```
|
timm/tf_mobilenetv3_large_minimal_100.in1k | timm | "2023-04-27T22:49:48Z" | 35,962 | 0 | timm | [
"timm",
"pytorch",
"safetensors",
"image-classification",
"dataset:imagenet-1k",
"arxiv:1905.02244",
"license:apache-2.0",
"region:us"
] | image-classification | "2022-12-16T05:39:06Z" | ---
tags:
- image-classification
- timm
library_name: timm
license: apache-2.0
datasets:
- imagenet-1k
---
# Model card for tf_mobilenetv3_large_minimal_100.in1k
A MobileNet-v3 image classification model. Trained on ImageNet-1k in Tensorflow by paper authors, ported to PyTorch by Ross Wightman.
## Model Details
- **Model Type:** Image classification / feature backbone
- **Model Stats:**
- Params (M): 3.9
- GMACs: 0.2
- Activations (M): 4.4
- Image size: 224 x 224
- **Papers:**
- Searching for MobileNetV3: https://arxiv.org/abs/1905.02244
- **Dataset:** ImageNet-1k
- **Original:** https://github.com/tensorflow/tpu/tree/master/models/official/efficientnet
## Model Usage
### Image Classification
```python
from urllib.request import urlopen
from PIL import Image
import timm
img = Image.open(urlopen(
'https://huggingface.co/datasets/huggingface/documentation-images/resolve/main/beignets-task-guide.png'
))
model = timm.create_model('tf_mobilenetv3_large_minimal_100.in1k', pretrained=True)
model = model.eval()
# get model specific transforms (normalization, resize)
data_config = timm.data.resolve_model_data_config(model)
transforms = timm.data.create_transform(**data_config, is_training=False)
output = model(transforms(img).unsqueeze(0)) # unsqueeze single image into batch of 1
top5_probabilities, top5_class_indices = torch.topk(output.softmax(dim=1) * 100, k=5)
```
### Feature Map Extraction
```python
from urllib.request import urlopen
from PIL import Image
import timm
img = Image.open(urlopen(
'https://huggingface.co/datasets/huggingface/documentation-images/resolve/main/beignets-task-guide.png'
))
model = timm.create_model(
'tf_mobilenetv3_large_minimal_100.in1k',
pretrained=True,
features_only=True,
)
model = model.eval()
# get model specific transforms (normalization, resize)
data_config = timm.data.resolve_model_data_config(model)
transforms = timm.data.create_transform(**data_config, is_training=False)
output = model(transforms(img).unsqueeze(0)) # unsqueeze single image into batch of 1
for o in output:
# print shape of each feature map in output
# e.g.:
# torch.Size([1, 16, 112, 112])
# torch.Size([1, 24, 56, 56])
# torch.Size([1, 40, 28, 28])
# torch.Size([1, 112, 14, 14])
# torch.Size([1, 960, 7, 7])
print(o.shape)
```
### Image Embeddings
```python
from urllib.request import urlopen
from PIL import Image
import timm
img = Image.open(urlopen(
'https://huggingface.co/datasets/huggingface/documentation-images/resolve/main/beignets-task-guide.png'
))
model = timm.create_model(
'tf_mobilenetv3_large_minimal_100.in1k',
pretrained=True,
num_classes=0, # remove classifier nn.Linear
)
model = model.eval()
# get model specific transforms (normalization, resize)
data_config = timm.data.resolve_model_data_config(model)
transforms = timm.data.create_transform(**data_config, is_training=False)
output = model(transforms(img).unsqueeze(0)) # output is (batch_size, num_features) shaped tensor
# or equivalently (without needing to set num_classes=0)
output = model.forward_features(transforms(img).unsqueeze(0))
# output is unpooled, a (1, 960, 7, 7) shaped tensor
output = model.forward_head(output, pre_logits=True)
# output is a (1, num_features) shaped tensor
```
## Model Comparison
Explore the dataset and runtime metrics of this model in timm [model results](https://github.com/huggingface/pytorch-image-models/tree/main/results).
## Citation
```bibtex
@inproceedings{howard2019searching,
title={Searching for mobilenetv3},
author={Howard, Andrew and Sandler, Mark and Chu, Grace and Chen, Liang-Chieh and Chen, Bo and Tan, Mingxing and Wang, Weijun and Zhu, Yukun and Pang, Ruoming and Vasudevan, Vijay and others},
booktitle={Proceedings of the IEEE/CVF international conference on computer vision},
pages={1314--1324},
year={2019}
}
```
```bibtex
@misc{rw2019timm,
author = {Ross Wightman},
title = {PyTorch Image Models},
year = {2019},
publisher = {GitHub},
journal = {GitHub repository},
doi = {10.5281/zenodo.4414861},
howpublished = {\url{https://github.com/huggingface/pytorch-image-models}}
}
```
|
openai/whisper-base.en | openai | "2024-01-22T17:55:08Z" | 35,925 | 21 | transformers | [
"transformers",
"pytorch",
"tf",
"jax",
"safetensors",
"whisper",
"automatic-speech-recognition",
"audio",
"hf-asr-leaderboard",
"en",
"arxiv:2212.04356",
"license:apache-2.0",
"model-index",
"endpoints_compatible",
"region:us"
] | automatic-speech-recognition | "2022-09-26T06:58:29Z" | ---
language:
- en
tags:
- audio
- automatic-speech-recognition
- hf-asr-leaderboard
widget:
- example_title: Librispeech sample 1
src: https://cdn-media.huggingface.co/speech_samples/sample1.flac
- example_title: Librispeech sample 2
src: https://cdn-media.huggingface.co/speech_samples/sample2.flac
model-index:
- name: whisper-base.en
results:
- task:
name: Automatic Speech Recognition
type: automatic-speech-recognition
dataset:
name: LibriSpeech (clean)
type: librispeech_asr
config: clean
split: test
args:
language: en
metrics:
- name: Test WER
type: wer
value:
- task:
name: Automatic Speech Recognition
type: automatic-speech-recognition
dataset:
name: LibriSpeech (other)
type: librispeech_asr
config: other
split: test
args:
language: en
metrics:
- name: Test WER
type: wer
value: 12.803978669490565
pipeline_tag: automatic-speech-recognition
license: apache-2.0
---
# Whisper
Whisper is a pre-trained model for automatic speech recognition (ASR) and speech translation. Trained on 680k hours
of labelled data, Whisper models demonstrate a strong ability to generalise to many datasets and domains **without** the need
for fine-tuning.
Whisper was proposed in the paper [Robust Speech Recognition via Large-Scale Weak Supervision](https://arxiv.org/abs/2212.04356)
by Alec Radford et al. from OpenAI. The original code repository can be found [here](https://github.com/openai/whisper).
**Disclaimer**: Content for this model card has partly been written by the Hugging Face team, and parts of it were
copied and pasted from the original model card.
## Model details
Whisper is a Transformer based encoder-decoder model, also referred to as a _sequence-to-sequence_ model.
It was trained on 680k hours of labelled speech data annotated using large-scale weak supervision.
The models were trained on either English-only data or multilingual data. The English-only models were trained
on the task of speech recognition. The multilingual models were trained on both speech recognition and speech
translation. For speech recognition, the model predicts transcriptions in the *same* language as the audio.
For speech translation, the model predicts transcriptions to a *different* language to the audio.
Whisper checkpoints come in five configurations of varying model sizes.
The smallest four are trained on either English-only or multilingual data.
The largest checkpoints are multilingual only. All ten of the pre-trained checkpoints
are available on the [Hugging Face Hub](https://huggingface.co/models?search=openai/whisper). The
checkpoints are summarised in the following table with links to the models on the Hub:
| Size | Parameters | English-only | Multilingual |
|----------|------------|------------------------------------------------------|-----------------------------------------------------|
| tiny | 39 M | [✓](https://huggingface.co/openai/whisper-tiny.en) | [✓](https://huggingface.co/openai/whisper-tiny) |
| base | 74 M | [✓](https://huggingface.co/openai/whisper-base.en) | [✓](https://huggingface.co/openai/whisper-base) |
| small | 244 M | [✓](https://huggingface.co/openai/whisper-small.en) | [✓](https://huggingface.co/openai/whisper-small) |
| medium | 769 M | [✓](https://huggingface.co/openai/whisper-medium.en) | [✓](https://huggingface.co/openai/whisper-medium) |
| large | 1550 M | x | [✓](https://huggingface.co/openai/whisper-large) |
| large-v2 | 1550 M | x | [✓](https://huggingface.co/openai/whisper-large-v2) |
# Usage
This checkpoint is an *English-only* model, meaning it can be used for English speech recognition. Multilingual speech
recognition or speech translation is possible through use of a multilingual checkpoint.
To transcribe audio samples, the model has to be used alongside a [`WhisperProcessor`](https://huggingface.co/docs/transformers/model_doc/whisper#transformers.WhisperProcessor).
The `WhisperProcessor` is used to:
1. Pre-process the audio inputs (converting them to log-Mel spectrograms for the model)
2. Post-process the model outputs (converting them from tokens to text)
## Transcription
```python
>>> from transformers import WhisperProcessor, WhisperForConditionalGeneration
>>> from datasets import load_dataset
>>> # load model and processor
>>> processor = WhisperProcessor.from_pretrained("openai/whisper-base.en")
>>> model = WhisperForConditionalGeneration.from_pretrained("openai/whisper-base.en")
>>> # load dummy dataset and read audio files
>>> ds = load_dataset("hf-internal-testing/librispeech_asr_dummy", "clean", split="validation")
>>> sample = ds[0]["audio"]
>>> input_features = processor(sample["array"], sampling_rate=sample["sampling_rate"], return_tensors="pt").input_features
>>> # generate token ids
>>> predicted_ids = model.generate(input_features)
>>> # decode token ids to text
>>> transcription = processor.batch_decode(predicted_ids, skip_special_tokens=False)
['<|startoftranscript|><|notimestamps|> Mr. Quilter is the apostle of the middle classes, and we are glad to welcome his gospel.<|endoftext|>']
>>> transcription = processor.batch_decode(predicted_ids, skip_special_tokens=True)
[' Mr. Quilter is the apostle of the middle classes and we are glad to welcome his gospel.']
```
The context tokens can be removed from the start of the transcription by setting `skip_special_tokens=True`.
## Evaluation
This code snippet shows how to evaluate Whisper base.en on [LibriSpeech test-clean](https://huggingface.co/datasets/librispeech_asr):
```python
>>> from datasets import load_dataset
>>> from transformers import WhisperForConditionalGeneration, WhisperProcessor
>>> import torch
>>> from evaluate import load
>>> librispeech_test_clean = load_dataset("librispeech_asr", "clean", split="test")
>>> processor = WhisperProcessor.from_pretrained("openai/whisper-base.en")
>>> model = WhisperForConditionalGeneration.from_pretrained("openai/whisper-base.en").to("cuda")
>>> def map_to_pred(batch):
>>> audio = batch["audio"]
>>> input_features = processor(audio["array"], sampling_rate=audio["sampling_rate"], return_tensors="pt").input_features
>>> batch["reference"] = processor.tokenizer._normalize(batch['text'])
>>>
>>> with torch.no_grad():
>>> predicted_ids = model.generate(input_features.to("cuda"))[0]
>>> transcription = processor.decode(predicted_ids)
>>> batch["prediction"] = processor.tokenizer._normalize(transcription)
>>> return batch
>>> result = librispeech_test_clean.map(map_to_pred)
>>> wer = load("wer")
>>> print(100 * wer.compute(references=result["reference"], predictions=result["prediction"]))
4.271408904897505
```
## Long-Form Transcription
The Whisper model is intrinsically designed to work on audio samples of up to 30s in duration. However, by using a chunking
algorithm, it can be used to transcribe audio samples of up to arbitrary length. This is possible through Transformers
[`pipeline`](https://huggingface.co/docs/transformers/main_classes/pipelines#transformers.AutomaticSpeechRecognitionPipeline)
method. Chunking is enabled by setting `chunk_length_s=30` when instantiating the pipeline. With chunking enabled, the pipeline
can be run with batched inference. It can also be extended to predict sequence level timestamps by passing `return_timestamps=True`:
```python
>>> import torch
>>> from transformers import pipeline
>>> from datasets import load_dataset
>>> device = "cuda:0" if torch.cuda.is_available() else "cpu"
>>> pipe = pipeline(
>>> "automatic-speech-recognition",
>>> model="openai/whisper-base.en",
>>> chunk_length_s=30,
>>> device=device,
>>> )
>>> ds = load_dataset("hf-internal-testing/librispeech_asr_dummy", "clean", split="validation")
>>> sample = ds[0]["audio"]
>>> prediction = pipe(sample.copy(), batch_size=8)["text"]
" Mr. Quilter is the apostle of the middle classes, and we are glad to welcome his gospel."
>>> # we can also return timestamps for the predictions
>>> prediction = pipe(sample.copy(), batch_size=8, return_timestamps=True)["chunks"]
[{'text': ' Mr. Quilter is the apostle of the middle classes and we are glad to welcome his gospel.',
'timestamp': (0.0, 5.44)}]
```
Refer to the blog post [ASR Chunking](https://huggingface.co/blog/asr-chunking) for more details on the chunking algorithm.
## Fine-Tuning
The pre-trained Whisper model demonstrates a strong ability to generalise to different datasets and domains. However,
its predictive capabilities can be improved further for certain languages and tasks through *fine-tuning*. The blog
post [Fine-Tune Whisper with 🤗 Transformers](https://huggingface.co/blog/fine-tune-whisper) provides a step-by-step
guide to fine-tuning the Whisper model with as little as 5 hours of labelled data.
### Evaluated Use
The primary intended users of these models are AI researchers studying robustness, generalization, capabilities, biases, and constraints of the current model. However, Whisper is also potentially quite useful as an ASR solution for developers, especially for English speech recognition. We recognize that once models are released, it is impossible to restrict access to only “intended” uses or to draw reasonable guidelines around what is or is not research.
The models are primarily trained and evaluated on ASR and speech translation to English tasks. They show strong ASR results in ~10 languages. They may exhibit additional capabilities, particularly if fine-tuned on certain tasks like voice activity detection, speaker classification, or speaker diarization but have not been robustly evaluated in these areas. We strongly recommend that users perform robust evaluations of the models in a particular context and domain before deploying them.
In particular, we caution against using Whisper models to transcribe recordings of individuals taken without their consent or purporting to use these models for any kind of subjective classification. We recommend against use in high-risk domains like decision-making contexts, where flaws in accuracy can lead to pronounced flaws in outcomes. The models are intended to transcribe and translate speech, use of the model for classification is not only not evaluated but also not appropriate, particularly to infer human attributes.
## Training Data
The models are trained on 680,000 hours of audio and the corresponding transcripts collected from the internet. 65% of this data (or 438,000 hours) represents English-language audio and matched English transcripts, roughly 18% (or 126,000 hours) represents non-English audio and English transcripts, while the final 17% (or 117,000 hours) represents non-English audio and the corresponding transcript. This non-English data represents 98 different languages.
As discussed in [the accompanying paper](https://cdn.openai.com/papers/whisper.pdf), we see that performance on transcription in a given language is directly correlated with the amount of training data we employ in that language.
## Performance and Limitations
Our studies show that, over many existing ASR systems, the models exhibit improved robustness to accents, background noise, technical language, as well as zero shot translation from multiple languages into English; and that accuracy on speech recognition and translation is near the state-of-the-art level.
However, because the models are trained in a weakly supervised manner using large-scale noisy data, the predictions may include texts that are not actually spoken in the audio input (i.e. hallucination). We hypothesize that this happens because, given their general knowledge of language, the models combine trying to predict the next word in audio with trying to transcribe the audio itself.
Our models perform unevenly across languages, and we observe lower accuracy on low-resource and/or low-discoverability languages or languages where we have less training data. The models also exhibit disparate performance on different accents and dialects of particular languages, which may include higher word error rate across speakers of different genders, races, ages, or other demographic criteria. Our full evaluation results are presented in [the paper accompanying this release](https://cdn.openai.com/papers/whisper.pdf).
In addition, the sequence-to-sequence architecture of the model makes it prone to generating repetitive texts, which can be mitigated to some degree by beam search and temperature scheduling but not perfectly. Further analysis on these limitations are provided in [the paper](https://cdn.openai.com/papers/whisper.pdf). It is likely that this behavior and hallucinations may be worse on lower-resource and/or lower-discoverability languages.
## Broader Implications
We anticipate that Whisper models’ transcription capabilities may be used for improving accessibility tools. While Whisper models cannot be used for real-time transcription out of the box – their speed and size suggest that others may be able to build applications on top of them that allow for near-real-time speech recognition and translation. The real value of beneficial applications built on top of Whisper models suggests that the disparate performance of these models may have real economic implications.
There are also potential dual use concerns that come with releasing Whisper. While we hope the technology will be used primarily for beneficial purposes, making ASR technology more accessible could enable more actors to build capable surveillance technologies or scale up existing surveillance efforts, as the speed and accuracy allow for affordable automatic transcription and translation of large volumes of audio communication. Moreover, these models may have some capabilities to recognize specific individuals out of the box, which in turn presents safety concerns related both to dual use and disparate performance. In practice, we expect that the cost of transcription is not the limiting factor of scaling up surveillance projects.
### BibTeX entry and citation info
```bibtex
@misc{radford2022whisper,
doi = {10.48550/ARXIV.2212.04356},
url = {https://arxiv.org/abs/2212.04356},
author = {Radford, Alec and Kim, Jong Wook and Xu, Tao and Brockman, Greg and McLeavey, Christine and Sutskever, Ilya},
title = {Robust Speech Recognition via Large-Scale Weak Supervision},
publisher = {arXiv},
year = {2022},
copyright = {arXiv.org perpetual, non-exclusive license}
}
```
|
distilbert/distilbert-base-german-cased | distilbert | "2024-05-06T13:46:46Z" | 35,761 | 17 | transformers | [
"transformers",
"pytorch",
"safetensors",
"distilbert",
"fill-mask",
"de",
"license:apache-2.0",
"autotrain_compatible",
"endpoints_compatible",
"region:us"
] | fill-mask | "2022-03-02T23:29:04Z" | ---
language: de
license: apache-2.0
---
## distilbert-base-german-cased
|
google/ddpm-cifar10-32 | google | "2023-08-03T07:24:08Z" | 35,749 | 49 | diffusers | [
"diffusers",
"safetensors",
"pytorch",
"unconditional-image-generation",
"arxiv:2006.11239",
"license:apache-2.0",
"diffusers:DDPMPipeline",
"region:us"
] | unconditional-image-generation | "2022-06-16T12:53:22Z" | ---
license: apache-2.0
tags:
- pytorch
- diffusers
- unconditional-image-generation
---
# Denoising Diffusion Probabilistic Models (DDPM)
**Paper**: [Denoising Diffusion Probabilistic Models](https://arxiv.org/abs/2006.11239)
**Authors**: Jonathan Ho, Ajay Jain, Pieter Abbeel
**Abstract**:
*We present high quality image synthesis results using diffusion probabilistic models, a class of latent variable models inspired by considerations from nonequilibrium thermodynamics. Our best results are obtained by training on a weighted variational bound designed according to a novel connection between diffusion probabilistic models and denoising score matching with Langevin dynamics, and our models naturally admit a progressive lossy decompression scheme that can be interpreted as a generalization of autoregressive decoding. On the unconditional CIFAR10 dataset, we obtain an Inception score of 9.46 and a state-of-the-art FID score of 3.17. On 256x256 LSUN, we obtain sample quality similar to ProgressiveGAN.*
## Inference
**DDPM** models can use *discrete noise schedulers* such as:
- [scheduling_ddpm](https://github.com/huggingface/diffusers/blob/main/src/diffusers/schedulers/scheduling_ddpm.py)
- [scheduling_ddim](https://github.com/huggingface/diffusers/blob/main/src/diffusers/schedulers/scheduling_ddim.py)
- [scheduling_pndm](https://github.com/huggingface/diffusers/blob/main/src/diffusers/schedulers/scheduling_pndm.py)
for inference. Note that while the *ddpm* scheduler yields the highest quality, it also takes the longest.
For a good trade-off between quality and inference speed you might want to consider the *ddim* or *pndm* schedulers instead.
See the following code:
```python
# !pip install diffusers
from diffusers import DDPMPipeline, DDIMPipeline, PNDMPipeline
model_id = "google/ddpm-cifar10-32"
# load model and scheduler
ddpm = DDPMPipeline.from_pretrained(model_id) # you can replace DDPMPipeline with DDIMPipeline or PNDMPipeline for faster inference
# run pipeline in inference (sample random noise and denoise)
image = ddpm().images[0]
# save image
image.save("ddpm_generated_image.png")
```
For more in-detail information, please have a look at the [official inference example](https://colab.research.google.com/github/huggingface/notebooks/blob/main/diffusers/diffusers_intro.ipynb)
## Training
If you want to train your own model, please have a look at the [official training example](https://colab.research.google.com/github/huggingface/notebooks/blob/main/diffusers/training_example.ipynb)
## Samples
1. 
2. 
3. 
4.  |
Qwen/Qwen1.5-14B | Qwen | "2024-04-05T10:43:26Z" | 35,746 | 36 | transformers | [
"transformers",
"safetensors",
"qwen2",
"text-generation",
"pretrained",
"conversational",
"en",
"license:other",
"autotrain_compatible",
"endpoints_compatible",
"text-generation-inference",
"region:us"
] | text-generation | "2024-01-22T17:15:09Z" | ---
license: other
license_name: tongyi-qianwen
license_link: >-
https://huggingface.co/Qwen/Qwen1.5-14B/blob/main/LICENSE
language:
- en
pipeline_tag: text-generation
tags:
- pretrained
---
# Qwen1.5-14B
## Introduction
Qwen1.5 is the beta version of Qwen2, a transformer-based decoder-only language model pretrained on a large amount of data. In comparison with the previous released Qwen, the improvements include:
* 8 model sizes, including 0.5B, 1.8B, 4B, 7B, 14B, 32B and 72B dense models, and an MoE model of 14B with 2.7B activated;
* Significant performance improvement in Chat models;
* Multilingual support of both base and chat models;
* Stable support of 32K context length for models of all sizes
* No need of `trust_remote_code`.
For more details, please refer to our [blog post](https://qwenlm.github.io/blog/qwen1.5/) and [GitHub repo](https://github.com/QwenLM/Qwen1.5).
## Model Details
Qwen1.5 is a language model series including decoder language models of different model sizes. For each size, we release the base language model and the aligned chat model. It is based on the Transformer architecture with SwiGLU activation, attention QKV bias, group query attention, mixture of sliding window attention and full attention, etc. Additionally, we have an improved tokenizer adaptive to multiple natural languages and codes. For the beta version, temporarily we did not include GQA (except for 32B) and the mixture of SWA and full attention.
## Requirements
The code of Qwen1.5 has been in the latest Hugging face transformers and we advise you to install `transformers>=4.37.0`, or you might encounter the following error:
```
KeyError: 'qwen2'.
```
## Usage
We do not advise you to use base language models for text generation. Instead, you can apply post-training, e.g., SFT, RLHF, continued pretraining, etc., on this model.
## Citation
If you find our work helpful, feel free to give us a cite.
```
@article{qwen,
title={Qwen Technical Report},
author={Jinze Bai and Shuai Bai and Yunfei Chu and Zeyu Cui and Kai Dang and Xiaodong Deng and Yang Fan and Wenbin Ge and Yu Han and Fei Huang and Binyuan Hui and Luo Ji and Mei Li and Junyang Lin and Runji Lin and Dayiheng Liu and Gao Liu and Chengqiang Lu and Keming Lu and Jianxin Ma and Rui Men and Xingzhang Ren and Xuancheng Ren and Chuanqi Tan and Sinan Tan and Jianhong Tu and Peng Wang and Shijie Wang and Wei Wang and Shengguang Wu and Benfeng Xu and Jin Xu and An Yang and Hao Yang and Jian Yang and Shusheng Yang and Yang Yao and Bowen Yu and Hongyi Yuan and Zheng Yuan and Jianwei Zhang and Xingxuan Zhang and Yichang Zhang and Zhenru Zhang and Chang Zhou and Jingren Zhou and Xiaohuan Zhou and Tianhang Zhu},
journal={arXiv preprint arXiv:2309.16609},
year={2023}
}
``` |
mradermacher/Llama-Salad-4x8B-V3-i1-GGUF | mradermacher | "2024-06-19T21:04:43Z" | 35,679 | 2 | transformers | [
"transformers",
"gguf",
"nsfw",
"not-for-all-audiences",
"llama-3",
"text-generation-inference",
"moe",
"mergekit",
"merge",
"en",
"base_model:HiroseKoichi/Llama-Salad-4x8B-V3",
"license:llama3",
"endpoints_compatible",
"region:us"
] | null | "2024-06-19T13:01:15Z" | ---
base_model: HiroseKoichi/Llama-Salad-4x8B-V3
language:
- en
library_name: transformers
license: llama3
quantized_by: mradermacher
tags:
- nsfw
- not-for-all-audiences
- llama-3
- text-generation-inference
- moe
- mergekit
- merge
---
## About
<!-- ### quantize_version: 2 -->
<!-- ### output_tensor_quantised: 1 -->
<!-- ### convert_type: hf -->
<!-- ### vocab_type: -->
<!-- ### tags: nicoboss -->
weighted/imatrix quants of https://huggingface.co/HiroseKoichi/Llama-Salad-4x8B-V3
<!-- provided-files -->
static quants are available at https://huggingface.co/mradermacher/Llama-Salad-4x8B-V3-GGUF
## Usage
If you are unsure how to use GGUF files, refer to one of [TheBloke's
READMEs](https://huggingface.co/TheBloke/KafkaLM-70B-German-V0.1-GGUF) for
more details, including on how to concatenate multi-part files.
## Provided Quants
(sorted by size, not necessarily quality. IQ-quants are often preferable over similar sized non-IQ quants)
| Link | Type | Size/GB | Notes |
|:-----|:-----|--------:|:------|
| [GGUF](https://huggingface.co/mradermacher/Llama-Salad-4x8B-V3-i1-GGUF/resolve/main/Llama-Salad-4x8B-V3.i1-IQ1_S.gguf) | i1-IQ1_S | 5.5 | for the desperate |
| [GGUF](https://huggingface.co/mradermacher/Llama-Salad-4x8B-V3-i1-GGUF/resolve/main/Llama-Salad-4x8B-V3.i1-IQ1_M.gguf) | i1-IQ1_M | 6.0 | mostly desperate |
| [GGUF](https://huggingface.co/mradermacher/Llama-Salad-4x8B-V3-i1-GGUF/resolve/main/Llama-Salad-4x8B-V3.i1-IQ2_XXS.gguf) | i1-IQ2_XXS | 6.9 | |
| [GGUF](https://huggingface.co/mradermacher/Llama-Salad-4x8B-V3-i1-GGUF/resolve/main/Llama-Salad-4x8B-V3.i1-IQ2_XS.gguf) | i1-IQ2_XS | 7.6 | |
| [GGUF](https://huggingface.co/mradermacher/Llama-Salad-4x8B-V3-i1-GGUF/resolve/main/Llama-Salad-4x8B-V3.i1-IQ2_S.gguf) | i1-IQ2_S | 7.8 | |
| [GGUF](https://huggingface.co/mradermacher/Llama-Salad-4x8B-V3-i1-GGUF/resolve/main/Llama-Salad-4x8B-V3.i1-IQ2_M.gguf) | i1-IQ2_M | 8.5 | |
| [GGUF](https://huggingface.co/mradermacher/Llama-Salad-4x8B-V3-i1-GGUF/resolve/main/Llama-Salad-4x8B-V3.i1-Q2_K.gguf) | i1-Q2_K | 9.4 | IQ3_XXS probably better |
| [GGUF](https://huggingface.co/mradermacher/Llama-Salad-4x8B-V3-i1-GGUF/resolve/main/Llama-Salad-4x8B-V3.i1-IQ3_XXS.gguf) | i1-IQ3_XXS | 9.9 | lower quality |
| [GGUF](https://huggingface.co/mradermacher/Llama-Salad-4x8B-V3-i1-GGUF/resolve/main/Llama-Salad-4x8B-V3.i1-IQ3_XS.gguf) | i1-IQ3_XS | 10.5 | |
| [GGUF](https://huggingface.co/mradermacher/Llama-Salad-4x8B-V3-i1-GGUF/resolve/main/Llama-Salad-4x8B-V3.i1-Q3_K_S.gguf) | i1-Q3_K_S | 11.0 | IQ3_XS probably better |
| [GGUF](https://huggingface.co/mradermacher/Llama-Salad-4x8B-V3-i1-GGUF/resolve/main/Llama-Salad-4x8B-V3.i1-IQ3_S.gguf) | i1-IQ3_S | 11.1 | beats Q3_K* |
| [GGUF](https://huggingface.co/mradermacher/Llama-Salad-4x8B-V3-i1-GGUF/resolve/main/Llama-Salad-4x8B-V3.i1-IQ3_M.gguf) | i1-IQ3_M | 11.2 | |
| [GGUF](https://huggingface.co/mradermacher/Llama-Salad-4x8B-V3-i1-GGUF/resolve/main/Llama-Salad-4x8B-V3.i1-Q3_K_M.gguf) | i1-Q3_K_M | 12.2 | IQ3_S probably better |
| [GGUF](https://huggingface.co/mradermacher/Llama-Salad-4x8B-V3-i1-GGUF/resolve/main/Llama-Salad-4x8B-V3.i1-Q3_K_L.gguf) | i1-Q3_K_L | 13.1 | IQ3_M probably better |
| [GGUF](https://huggingface.co/mradermacher/Llama-Salad-4x8B-V3-i1-GGUF/resolve/main/Llama-Salad-4x8B-V3.i1-IQ4_XS.gguf) | i1-IQ4_XS | 13.5 | |
| [GGUF](https://huggingface.co/mradermacher/Llama-Salad-4x8B-V3-i1-GGUF/resolve/main/Llama-Salad-4x8B-V3.i1-Q4_0.gguf) | i1-Q4_0 | 14.3 | fast, low quality |
| [GGUF](https://huggingface.co/mradermacher/Llama-Salad-4x8B-V3-i1-GGUF/resolve/main/Llama-Salad-4x8B-V3.i1-Q4_K_S.gguf) | i1-Q4_K_S | 14.4 | optimal size/speed/quality |
| [GGUF](https://huggingface.co/mradermacher/Llama-Salad-4x8B-V3-i1-GGUF/resolve/main/Llama-Salad-4x8B-V3.i1-Q4_K_M.gguf) | i1-Q4_K_M | 15.3 | fast, recommended |
| [GGUF](https://huggingface.co/mradermacher/Llama-Salad-4x8B-V3-i1-GGUF/resolve/main/Llama-Salad-4x8B-V3.i1-Q5_K_S.gguf) | i1-Q5_K_S | 17.3 | |
| [GGUF](https://huggingface.co/mradermacher/Llama-Salad-4x8B-V3-i1-GGUF/resolve/main/Llama-Salad-4x8B-V3.i1-Q5_K_M.gguf) | i1-Q5_K_M | 17.8 | |
| [GGUF](https://huggingface.co/mradermacher/Llama-Salad-4x8B-V3-i1-GGUF/resolve/main/Llama-Salad-4x8B-V3.i1-Q6_K.gguf) | i1-Q6_K | 20.6 | practically like static Q6_K |
Here is a handy graph by ikawrakow comparing some lower-quality quant
types (lower is better):

And here are Artefact2's thoughts on the matter:
https://gist.github.com/Artefact2/b5f810600771265fc1e39442288e8ec9
## FAQ / Model Request
See https://huggingface.co/mradermacher/model_requests for some answers to
questions you might have and/or if you want some other model quantized.
## Thanks
I thank my company, [nethype GmbH](https://www.nethype.de/), for letting
me use its servers and providing upgrades to my workstation to enable
this work in my free time. Additional thanks to [@nicoboss](https://huggingface.co/nicoboss) for giving me access to his hardware for calculating the imatrix for these quants.
<!-- end -->
|
laion/CLIP-ViT-H-14-frozen-xlm-roberta-large-laion5B-s13B-b90k | laion | "2022-11-18T21:00:32Z" | 35,667 | 15 | open_clip | [
"open_clip",
"arxiv:1910.04867",
"license:mit",
"region:us"
] | null | "2022-11-18T20:49:11Z" | ---
license: mit
widget:
- src: https://huggingface.co/datasets/mishig/sample_images/resolve/main/cat-dog-music.png
candidate_labels: playing music, playing sports
example_title: Cat & Dog
---
# Model Card for CLIP ViT-H/14 frozen xlm roberta large - LAION-5B
# Table of Contents
1. [Model Details](#model-details)
2. [Uses](#uses)
3. [Training Details](#training-details)
4. [Evaluation](#evaluation)
5. [Acknowledgements](#acknowledgements)
6. [Citation](#citation)
7. [How To Get Started With the Model](#how-to-get-started-with-the-model)
# Model Details
## Model Description
A CLIP ViT-H/14 frozen xlm roberta large model trained with the LAION-5B (https://laion.ai/blog/laion-5b/) using OpenCLIP (https://github.com/mlfoundations/open_clip).
Model training done by Romain Beaumont on the [stability.ai](https://stability.ai/) cluster.
# Uses
## Direct Use
Zero-shot image classification, image and text retrieval, among others.
## Downstream Use
Image classification and other image task fine-tuning, linear probe image classification, image generation guiding and conditioning, among others.
# Training Details
## Training Data
This model was trained with the full LAION-5B (https://laion.ai/blog/laion-5b/).
## Training Procedure
Training with batch size 90k for 13B sample of laion5B, see https://wandb.ai/rom1504/open-clip/reports/xlm-roberta-large-unfrozen-vit-h-14-frozen--VmlldzoyOTc3ODY3
Model is H/14 on visual side, xlm roberta large initialized with pretrained weights on text side.
The H/14 was initialized from https://huggingface.co/laion/CLIP-ViT-H-14-laion2B-s32B-b79K and kept frozen during training.
# Evaluation
Evaluation done with code in the [LAION CLIP Benchmark suite](https://github.com/LAION-AI/CLIP_benchmark).
## Testing Data, Factors & Metrics
### Testing Data
The testing is performed with VTAB+ (A combination of VTAB (https://arxiv.org/abs/1910.04867) w/ additional robustness datasets) for classification and COCO and Flickr for retrieval.
## Results
The model achieves imagenet 1k 77.0% (vs 78% for the english H/14)

On zero shot classification on imagenet with translated prompts this model reaches:
* 56% in italian (vs 21% for https://github.com/clip-italian/clip-italian)
* 53% in japanese (vs 54.6% for https://github.com/rinnakk/japanese-clip)
* 55.7% in chinese (to be compared with https://github.com/OFA-Sys/Chinese-CLIP)
This model reaches strong results in both english and other languages.
# Acknowledgements
Acknowledging [stability.ai](https://stability.ai/) for the compute used to train this model.
# Citation
**BibTeX:**
In addition to forthcoming LAION-5B (https://laion.ai/blog/laion-5b/) paper, please cite:
OpenAI CLIP paper
```
@inproceedings{Radford2021LearningTV,
title={Learning Transferable Visual Models From Natural Language Supervision},
author={Alec Radford and Jong Wook Kim and Chris Hallacy and A. Ramesh and Gabriel Goh and Sandhini Agarwal and Girish Sastry and Amanda Askell and Pamela Mishkin and Jack Clark and Gretchen Krueger and Ilya Sutskever},
booktitle={ICML},
year={2021}
}
```
OpenCLIP software
```
@software{ilharco_gabriel_2021_5143773,
author = {Ilharco, Gabriel and
Wortsman, Mitchell and
Wightman, Ross and
Gordon, Cade and
Carlini, Nicholas and
Taori, Rohan and
Dave, Achal and
Shankar, Vaishaal and
Namkoong, Hongseok and
Miller, John and
Hajishirzi, Hannaneh and
Farhadi, Ali and
Schmidt, Ludwig},
title = {OpenCLIP},
month = jul,
year = 2021,
note = {If you use this software, please cite it as below.},
publisher = {Zenodo},
version = {0.1},
doi = {10.5281/zenodo.5143773},
url = {https://doi.org/10.5281/zenodo.5143773}
}
```
# How To Get Started With the Model
https://github.com/mlfoundations/open_clip |
redstonehero/animesh_prunedv21 | redstonehero | "2023-08-17T04:13:15Z" | 35,578 | 0 | diffusers | [
"diffusers",
"license:creativeml-openrail-m",
"autotrain_compatible",
"endpoints_compatible",
"diffusers:StableDiffusionPipeline",
"region:us"
] | text-to-image | "2023-08-17T01:53:05Z" | ---
license: creativeml-openrail-m
library_name: diffusers
--- |
backyardai/Psyonic-Cetacean-V1-20B-Ultra-Quality-GGUF | backyardai | "2024-06-24T20:15:34Z" | 35,530 | 0 | null | [
"gguf",
"32 bit upscale",
"full 32 bit precision",
"master files",
"en",
"base_model:DavidAU/Psyonic-Cetacean-V1-20B-Ultra-Quality-Float32",
"license:apache-2.0",
"region:us"
] | null | "2024-06-05T06:27:25Z" | ---
language:
- en
license: apache-2.0
tags:
- 32 bit upscale
- full 32 bit precision
- master files
base_model: DavidAU/Psyonic-Cetacean-V1-20B-Ultra-Quality-Float32
model_name: Psyonic-Cetacean-V1-20B-Ultra-Quality-GGUF
quantized_by: brooketh
parameter_count: 19994362880
---
<img src="BackyardAI_Banner.png" alt="Backyard.ai" style="height: 90px; min-width: 32px; display: block; margin: auto;">
**<p style="text-align: center;">The official library of GGUF format models for use in the local AI chat app, Backyard AI.</p>**
<p style="text-align: center;"><a href="https://backyard.ai/">Download Backyard AI here to get started.</a></p>
<p style="text-align: center;"><a href="https://www.reddit.com/r/LLM_Quants/">Request Additional models at r/LLM_Quants.</a></p>
***
# Psyonic Cetacean V1 Ultra Quality 20B
- **Creator:** [DavidAU](https://huggingface.co/DavidAU/)
- **Original:** [Psyonic Cetacean V1 Ultra Quality 20B](https://huggingface.co/DavidAU/Psyonic-Cetacean-V1-20B-Ultra-Quality-Float32)
- **Date Created:** 2024-06-01
- **Trained Context:** 4096 tokens
- **Description:** The one and only Space Whale, remastered and requantized in full 32-bit precision. Should be a dramatic improvement over the classic Psyonic Cetacean 20B in terms of creativity and quality of inference.
***
## What is a GGUF?
GGUF is a large language model (LLM) format that can be split between CPU and GPU. GGUFs are compatible with applications based on llama.cpp, such as Backyard AI. Where other model formats require higher end GPUs with ample VRAM, GGUFs can be efficiently run on a wider variety of hardware.
GGUF models are quantized to reduce resource usage, with a tradeoff of reduced coherence at lower quantizations. Quantization reduces the precision of the model weights by changing the number of bits used for each weight.
***
<img src="BackyardAI_Logo.png" alt="Backyard.ai" style="height: 75px; min-width: 32px; display: block; horizontal align: left;">
## Backyard AI
- Free, local AI chat application.
- One-click installation on Mac and PC.
- Automatically use GPU for maximum speed.
- Built-in model manager.
- High-quality character hub.
- Zero-config desktop-to-mobile tethering.
Backyard AI makes it easy to start chatting with AI using your own characters or one of the many found in the built-in character hub. The model manager helps you find the latest and greatest models without worrying about whether it's the correct format. Backyard AI supports advanced features such as lorebooks, author's note, text formatting, custom context size, sampler settings, grammars, local TTS, cloud inference, and tethering, all implemented in a way that is straightforward and reliable.
**Join us on [Discord](https://discord.gg/SyNN2vC9tQ)**
*** |
Elron/bleurt-tiny-512 | Elron | "2022-11-26T15:13:43Z" | 35,525 | 2 | transformers | [
"transformers",
"pytorch",
"bert",
"text-classification",
"arxiv:1910.09700",
"autotrain_compatible",
"endpoints_compatible",
"region:us"
] | text-classification | "2022-03-02T23:29:04Z" | ---
tags:
- text-classification
- bert
---
# Model Card for bleurt-tiny-512
# Model Details
## Model Description
Pytorch version of the original BLEURT models from ACL paper
- **Developed by:** Elron Bandel, Thibault Sellam, Dipanjan Das and Ankur P. Parikh of Google Research
- **Shared by [Optional]:** Elron Bandel
- **Model type:** Text Classification
- **Language(s) (NLP):** More information needed
- **License:** More information needed
- **Parent Model:** BERT
- **Resources for more information:**
- [GitHub Repo](https://github.com/google-research/bleurt/tree/master)
- [Associated Paper](https://aclanthology.org/2020.acl-main.704/)
- [Blog Post](https://ai.googleblog.com/2020/05/evaluating-natural-language-generation.html)
# Uses
## Direct Use
This model can be used for the task of Text Classification
## Downstream Use [Optional]
More information needed.
## Out-of-Scope Use
The model should not be used to intentionally create hostile or alienating environments for people.
# Bias, Risks, and Limitations
Significant research has explored bias and fairness issues with language models (see, e.g., [Sheng et al. (2021)](https://aclanthology.org/2021.acl-long.330.pdf) and [Bender et al. (2021)](https://dl.acm.org/doi/pdf/10.1145/3442188.3445922)). Predictions generated by the model may include disturbing and harmful stereotypes across protected classes; identity characteristics; and sensitive, social, and occupational groups.
## Recommendations
Users (both direct and downstream) should be made aware of the risks, biases and limitations of the model. More information needed for further recommendations.
# Training Details
## Training Data
The model authors note in the [associated paper](https://aclanthology.org/2020.acl-main.704.pdf):
> We use years 2017 to 2019 of the WMT Metrics Shared Task, to-English language pairs. For each year, we used the of- ficial WMT test set, which include several thou- sand pairs of sentences with human ratings from the news domain. The training sets contain 5,360, 9,492, and 147,691 records for each year.
## Training Procedure
### Preprocessing
More information needed
### Speeds, Sizes, Times
More information needed
# Evaluation
## Testing Data, Factors & Metrics
### Testing Data
The test sets for years 2018 and 2019 [of the WMT Metrics Shared Task, to-English language pairs.] are noisier,
### Factors
More information needed
### Metrics
More information needed
## Results
More information needed
# Model Examination
More information needed
# Environmental Impact
Carbon emissions can be estimated using the [Machine Learning Impact calculator](https://mlco2.github.io/impact#compute) presented in [Lacoste et al. (2019)](https://arxiv.org/abs/1910.09700).
- **Hardware Type:** More information needed
- **Hours used:** More information needed
- **Cloud Provider:** More information needed
- **Compute Region:** More information needed
- **Carbon Emitted:** More information needed
# Technical Specifications [optional]
## Model Architecture and Objective
More information needed
## Compute Infrastructure
More information needed
### Hardware
More information needed
### Software
More information needed.
# Citation
**BibTeX:**
```bibtex
@inproceedings{sellam2020bleurt,
title = {BLEURT: Learning Robust Metrics for Text Generation},
author = {Thibault Sellam and Dipanjan Das and Ankur P Parikh},
year = {2020},
booktitle = {Proceedings of ACL}
}
```
# Glossary [optional]
More information needed
# More Information [optional]
More information needed
# Model Card Authors [optional]
Elron Bandel in collaboration with Ezi Ozoani and the Hugging Face team
# Model Card Contact
More information needed
# How to Get Started with the Model
Use the code below to get started with the model.
<details>
<summary> Click to expand </summary>
```python
from transformers import AutoModelForSequenceClassification, AutoTokenizer
import torch
tokenizer = AutoTokenizer.from_pretrained("Elron/bleurt-tiny-512")
model = AutoModelForSequenceClassification.from_pretrained("Elron/bleurt-tiny-512")
model.eval()
references = ["hello world", "hello world"]
candidates = ["hi universe", "bye world"]
with torch.no_grad():
scores = model(**tokenizer(references, candidates, return_tensors='pt'))[0].squeeze()
print(scores) # tensor([-0.9414, -0.5678])
```
See [this notebook](https://colab.research.google.com/drive/1KsCUkFW45d5_ROSv2aHtXgeBa2Z98r03?usp=sharing) for model conversion code.
</details>
|
facebook/w2v-bert-2.0 | facebook | "2024-01-25T13:51:13Z" | 35,473 | 125 | transformers | [
"transformers",
"safetensors",
"wav2vec2-bert",
"feature-extraction",
"af",
"am",
"ar",
"as",
"az",
"be",
"bn",
"bs",
"bg",
"ca",
"cs",
"zh",
"cy",
"da",
"de",
"el",
"en",
"et",
"fi",
"fr",
"or",
"om",
"ga",
"gl",
"gu",
"ha",
"he",
"hi",
"hr",
"hu",
"hy",
"ig",
"id",
"is",
"it",
"jv",
"ja",
"kn",
"ka",
"kk",
"mn",
"km",
"ky",
"ko",
"lo",
"ln",
"lt",
"lb",
"lg",
"lv",
"ml",
"mr",
"mk",
"mt",
"mi",
"my",
"nl",
"nb",
"ne",
"ny",
"oc",
"pa",
"ps",
"fa",
"pl",
"pt",
"ro",
"ru",
"sk",
"sl",
"sn",
"sd",
"so",
"es",
"sr",
"sv",
"sw",
"ta",
"te",
"tg",
"tl",
"th",
"tr",
"uk",
"ur",
"uz",
"vi",
"wo",
"xh",
"yo",
"ms",
"zu",
"ary",
"arz",
"yue",
"kea",
"arxiv:2312.05187",
"license:mit",
"region:us"
] | feature-extraction | "2023-12-19T07:41:49Z" | ---
license: mit
language:
- af
- am
- ar
- as
- az
- be
- bn
- bs
- bg
- ca
- cs
- zh
- cy
- da
- de
- el
- en
- et
- fi
- fr
- or
- om
- ga
- gl
- gu
- ha
- he
- hi
- hr
- hu
- hy
- ig
- id
- is
- it
- jv
- ja
- kn
- ka
- kk
- mn
- km
- ky
- ko
- lo
- ln
- lt
- lb
- lg
- lv
- ml
- mr
- mk
- mt
- mi
- my
- nl
- nb
- ne
- ny
- oc
- pa
- ps
- fa
- pl
- pt
- ro
- ru
- sk
- sl
- sn
- sd
- so
- es
- sr
- sv
- sw
- ta
- te
- tg
- tl
- th
- tr
- uk
- ur
- uz
- vi
- wo
- xh
- yo
- ms
- zu
- ary
- arz
- yue
- kea
inference: false
---
# W2v-BERT 2.0 speech encoder
We are open-sourcing our Conformer-based [W2v-BERT 2.0 speech encoder](#w2v-bert-20-speech-encoder) as described in Section 3.2.1 of the [paper](https://arxiv.org/pdf/2312.05187.pdf), which is at the core of our Seamless models.
This model was pre-trained on 4.5M hours of unlabeled audio data covering more than 143 languages. It requires finetuning to be used for downstream tasks such as Automatic Speech Recognition (ASR), or Audio Classification.
| Model Name | #params | checkpoint |
| ----------------- | ------- | ------------------------------------------------------------------------------------------------------------------------------------------------------------------------------------------------------------------------------------------------------------------------------------------------------- |
| W2v-BERT 2.0 | 600M | [checkpoint](https://huggingface.co/reach-vb/conformer-shaw/resolve/main/conformer_shaw.pt)
**This model and its training are supported by 🤗 Transformers, more on it in the [docs](https://huggingface.co/docs/transformers/main/en/model_doc/wav2vec2-bert).**
# 🤗 Transformers usage
This is a bare checkpoint without any modeling head, and thus requires finetuning to be used for downstream tasks such as ASR. You can however use it to extract audio embeddings from the top layer with this code snippet:
```python
from transformers import AutoFeatureExtractor, Wav2Vec2BertModel
import torch
from datasets import load_dataset
dataset = load_dataset("hf-internal-testing/librispeech_asr_demo", "clean", split="validation")
dataset = dataset.sort("id")
sampling_rate = dataset.features["audio"].sampling_rate
processor = AutoProcessor.from_pretrained("facebook/w2v-bert-2.0")
model = Wav2Vec2BertModel.from_pretrained("facebook/w2v-bert-2.0")
# audio file is decoded on the fly
inputs = processor(dataset[0]["audio"]["array"], sampling_rate=sampling_rate, return_tensors="pt")
with torch.no_grad():
outputs = model(**inputs)
```
To learn more about the model use, refer to the following resources:
- [its docs](https://huggingface.co/docs/transformers/main/en/model_doc/wav2vec2-bert)
- [a blog post showing how to fine-tune it on Mongolian ASR](https://huggingface.co/blog/fine-tune-w2v2-bert)
- [a training script example](https://github.com/huggingface/transformers/blob/main/examples/pytorch/speech-recognition/run_speech_recognition_ctc.py)
# Seamless Communication usage
This model can be used in [Seamless Communication](https://github.com/facebookresearch/seamless_communication), where it was released.
Here's how to make a forward pass through the voice encoder, after having completed the [installation steps](https://github.com/facebookresearch/seamless_communication?tab=readme-ov-file#installation):
```python
import torch
from fairseq2.data.audio import AudioDecoder, WaveformToFbankConverter
from fairseq2.memory import MemoryBlock
from fairseq2.nn.padding import get_seqs_and_padding_mask
from pathlib import Path
from seamless_communication.models.conformer_shaw import load_conformer_shaw_model
audio_wav_path, device, dtype = ...
audio_decoder = AudioDecoder(dtype=torch.float32, device=device)
fbank_converter = WaveformToFbankConverter(
num_mel_bins=80,
waveform_scale=2**15,
channel_last=True,
standardize=True,
device=device,
dtype=dtype,
)
collater = Collater(pad_value=1)
model = load_conformer_shaw_model("conformer_shaw", device=device, dtype=dtype)
model.eval()
with Path(audio_wav_path).open("rb") as fb:
block = MemoryBlock(fb.read())
decoded_audio = audio_decoder(block)
src = collater(fbank_converter(decoded_audio))["fbank"]
seqs, padding_mask = get_seqs_and_padding_mask(src)
with torch.inference_mode():
seqs, padding_mask = model.encoder_frontend(seqs, padding_mask)
seqs, padding_mask = model.encoder(seqs, padding_mask)
``` |
timm/vit_base_patch16_224.augreg_in21k | timm | "2023-05-06T00:00:35Z" | 35,450 | 7 | timm | [
"timm",
"pytorch",
"safetensors",
"image-classification",
"dataset:imagenet-21k",
"arxiv:2106.10270",
"arxiv:2010.11929",
"license:apache-2.0",
"region:us"
] | image-classification | "2022-12-22T07:25:23Z" | ---
tags:
- image-classification
- timm
library_name: timm
license: apache-2.0
datasets:
- imagenet-21k
---
# Model card for vit_base_patch16_224.augreg_in21k
A Vision Transformer (ViT) image classification model. Trained on ImageNet-21k (with additional augmentation and regularization) in JAX by paper authors, ported to PyTorch by Ross Wightman.
## Model Details
- **Model Type:** Image classification / feature backbone
- **Model Stats:**
- Params (M): 102.6
- GMACs: 16.9
- Activations (M): 16.5
- Image size: 224 x 224
- **Papers:**
- How to train your ViT? Data, Augmentation, and Regularization in Vision Transformers: https://arxiv.org/abs/2106.10270
- An Image is Worth 16x16 Words: Transformers for Image Recognition at Scale: https://arxiv.org/abs/2010.11929v2
- **Dataset:** ImageNet-21k
- **Original:** https://github.com/google-research/vision_transformer
## Model Usage
### Image Classification
```python
from urllib.request import urlopen
from PIL import Image
import timm
img = Image.open(urlopen(
'https://huggingface.co/datasets/huggingface/documentation-images/resolve/main/beignets-task-guide.png'
))
model = timm.create_model('vit_base_patch16_224.augreg_in21k', pretrained=True)
model = model.eval()
# get model specific transforms (normalization, resize)
data_config = timm.data.resolve_model_data_config(model)
transforms = timm.data.create_transform(**data_config, is_training=False)
output = model(transforms(img).unsqueeze(0)) # unsqueeze single image into batch of 1
top5_probabilities, top5_class_indices = torch.topk(output.softmax(dim=1) * 100, k=5)
```
### Image Embeddings
```python
from urllib.request import urlopen
from PIL import Image
import timm
img = Image.open(urlopen(
'https://huggingface.co/datasets/huggingface/documentation-images/resolve/main/beignets-task-guide.png'
))
model = timm.create_model(
'vit_base_patch16_224.augreg_in21k',
pretrained=True,
num_classes=0, # remove classifier nn.Linear
)
model = model.eval()
# get model specific transforms (normalization, resize)
data_config = timm.data.resolve_model_data_config(model)
transforms = timm.data.create_transform(**data_config, is_training=False)
output = model(transforms(img).unsqueeze(0)) # output is (batch_size, num_features) shaped tensor
# or equivalently (without needing to set num_classes=0)
output = model.forward_features(transforms(img).unsqueeze(0))
# output is unpooled, a (1, 197, 768) shaped tensor
output = model.forward_head(output, pre_logits=True)
# output is a (1, num_features) shaped tensor
```
## Model Comparison
Explore the dataset and runtime metrics of this model in timm [model results](https://github.com/huggingface/pytorch-image-models/tree/main/results).
## Citation
```bibtex
@article{steiner2021augreg,
title={How to train your ViT? Data, Augmentation, and Regularization in Vision Transformers},
author={Steiner, Andreas and Kolesnikov, Alexander and and Zhai, Xiaohua and Wightman, Ross and Uszkoreit, Jakob and Beyer, Lucas},
journal={arXiv preprint arXiv:2106.10270},
year={2021}
}
```
```bibtex
@article{dosovitskiy2020vit,
title={An Image is Worth 16x16 Words: Transformers for Image Recognition at Scale},
author={Dosovitskiy, Alexey and Beyer, Lucas and Kolesnikov, Alexander and Weissenborn, Dirk and Zhai, Xiaohua and Unterthiner, Thomas and Dehghani, Mostafa and Minderer, Matthias and Heigold, Georg and Gelly, Sylvain and Uszkoreit, Jakob and Houlsby, Neil},
journal={ICLR},
year={2021}
}
```
```bibtex
@misc{rw2019timm,
author = {Ross Wightman},
title = {PyTorch Image Models},
year = {2019},
publisher = {GitHub},
journal = {GitHub repository},
doi = {10.5281/zenodo.4414861},
howpublished = {\url{https://github.com/huggingface/pytorch-image-models}}
}
```
|
SanctumAI/Phi-3-mini-4k-instruct-GGUF | SanctumAI | "2024-04-23T16:31:42Z" | 35,438 | 12 | transformers | [
"transformers",
"gguf",
"phi3",
"nlp",
"code",
"text-generation",
"en",
"license:mit",
"endpoints_compatible",
"region:us"
] | text-generation | "2024-04-23T15:22:44Z" | ---
language:
- en
pipeline_tag: text-generation
tags:
- nlp
- code
license: mit
license_link: https://huggingface.co/microsoft/Phi-3-mini-4k-instruct/resolve/main/LICENSE
---

*This model was quantized by [SanctumAI](https://sanctum.ai). To leave feedback, join our community in [Discord](https://discord.gg/7ZNE78HJKh).*
# Phi 3 Mini 4K Instruct GGUF
**Model creator:** [microsoft](https://huggingface.co/microsoft)<br>
**Original model**: [Phi-3-mini-4k-instruct](https://huggingface.co/microsoft/Phi-3-mini-4k-instruct)<br>
## Model Summary:
The Phi-3-Mini-4K-Instruct is a 3.8B parameters, lightweight, state-of-the-art open model trained with the Phi-3 datasets that includes both synthetic data and the filtered publicly available websites data with a focus on high-quality and reasoning dense properties. The model belongs to the Phi-3 family with the Mini version in two variants 4K and 128K which is the context length (in tokens) that it can support.
The model has underwent a post-training process that incorporates both supervised fine-tuning and direct preference optimization for the instruction following and safety measures. When assessed against benchmarks testing common sense, language understanding, math, code, long context and logical reasoning, Phi-3 Mini-4K-Instruct showcased a robust and state-of-the-art performance among models with less than 13 billion parameters.
## Prompt Template:
If you're using Sanctum app, simply use `Phi 3` model preset.
Prompt template:
```
<|system|>
{system_prompt}.<|end|>
<|user|>
{prompt}<|end|>
<|assistant|>
```
## Hardware Requirements Estimate
| Name | Quant method | Size | Memory (RAM, vRAM) required |
| ---- | ---- | ---- | ---- |
| [phi-3-mini-4k-instruct.Q2_K.gguf](https://huggingface.co/SanctumAI/Phi-3-mini-4k-instruct-GGUF/blob/main/phi-3-mini-4k-instruct.Q2_K.gguf) | Q2_K | 1.45 GB | 5.05 GB |
| [phi-3-mini-4k-instruct.Q3_K_S.gguf](https://huggingface.co/SanctumAI/Phi-3-mini-4k-instruct-GGUF/blob/main/phi-3-mini-4k-instruct.Q3_K_S.gguf) | Q3_K_S | 1.68 GB | 5.27 GB |
| [phi-3-mini-4k-instruct.Q3_K_M.gguf](https://huggingface.co/SanctumAI/Phi-3-mini-4k-instruct-GGUF/blob/main/phi-3-mini-4k-instruct.Q3_K_M.gguf) | Q3_K_M | 1.88 GB | 5.45 GB |
| [phi-3-mini-4k-instruct.Q3_K_L.gguf](https://huggingface.co/SanctumAI/Phi-3-mini-4k-instruct-GGUF/blob/main/phi-3-mini-4k-instruct.Q3_K_L.gguf) | Q3_K_L | 2.05 GB | 5.61 GB |
| [phi-3-mini-4k-instruct.Q4_0.gguf](https://huggingface.co/SanctumAI/Phi-3-mini-4k-instruct-GGUF/blob/main/phi-3-mini-4k-instruct.Q4_0.gguf) | Q4_0 | 2.18 GB | 5.73 GB |
| [phi-3-mini-4k-instruct.Q4_K_S.gguf](https://huggingface.co/SanctumAI/Phi-3-mini-4k-instruct-GGUF/blob/main/phi-3-mini-4k-instruct.Q4_0.gguf) | Q4_K_S | 2.19 GB | 5.74 GB |
| [phi-3-mini-4k-instruct.Q4_K_M.gguf](https://huggingface.co/SanctumAI/Phi-3-mini-4k-instruct-GGUF/blob/main/phi-3-mini-4k-instruct.Q4_K_M.gguf) | Q4_K_M | 2.32 GB | 5.86 GB |
| [phi-3-mini-4k-instruct.Q4_K.gguf](https://huggingface.co/SanctumAI/Phi-3-mini-4k-instruct-GGUF/blob/main/phi-3-mini-4k-instruct.Q4_K.gguf) | Q4_K | 2.32 GB | 5.86 GB |
| [phi-3-mini-4k-instruct.Q4_1.gguf](https://huggingface.co/SanctumAI/Phi-3-mini-4k-instruct-GGUF/blob/main/phi-3-mini-4k-instruct.Q4_1.gguf) | Q4_1 | 2.41 GB | 5.94 GB |
| [phi-3-mini-4k-instruct.Q5_0.gguf](https://huggingface.co/SanctumAI/Phi-3-mini-4k-instruct-GGUF/blob/main/phi-3-mini-4k-instruct.Q5_0.gguf) | Q5_0 | 2.64 GB | 6.16 GB |
| [phi-3-mini-4k-instruct.Q5_K_S.gguf](https://huggingface.co/SanctumAI/Phi-3-mini-4k-instruct-GGUF/blob/main/phi-3-mini-4k-instruct.Q5_K_S.gguf) | Q5_K_S | 2.64 GB | 6.16 GB |
| [phi-3-mini-4k-instruct.Q5_K_M.gguf](https://huggingface.co/SanctumAI/Phi-3-mini-4k-instruct-GGUF/blob/main/phi-3-mini-4k-instruct.Q5_K_M.gguf) | Q5_K_M | 2.72 GB | 6.23 GB |
| [phi-3-mini-4k-instruct.Q5_K.gguf](https://huggingface.co/SanctumAI/Phi-3-mini-4k-instruct-GGUF/blob/main/phi-3-mini-4k-instruct.Q5_K.gguf) | Q5_K | 2.72 GB | 6.23 GB |
| [phi-3-mini-4k-instruct.Q5_1.gguf](https://huggingface.co/SanctumAI/Phi-3-mini-4k-instruct-GGUF/blob/main/phi-3-mini-4k-instruct.Q5_1.gguf) | Q5_1 | 2.87 GB | 6.38 GB |
| [phi-3-mini-4k-instruct.Q6_K.gguf](https://huggingface.co/SanctumAI/Phi-3-mini-4k-instruct-GGUF/blob/main/phi-3-mini-4k-instruct.Q6_K.gguf) | Q6_K | 3.14 GB | 6.62 GB |
| [phi-3-mini-4k-instruct.Q8_0.gguf](https://huggingface.co/SanctumAI/Phi-3-mini-4k-instruct-GGUF/blob/main/phi-3-mini-4k-instruct.Q8_0.gguf) | Q8_0 | 4.06 GB | 7.48 GB |
| [phi-3-mini-4k-instruct.fp16.gguf](https://huggingface.co/SanctumAI/Phi-3-mini-4k-instruct-GGUF/blob/main/phi-3-mini-4k-instruct.fp16.gguf) | f16 | 7.64 GB | 10.82 GB |
## Disclaimer
Sanctum is not the creator, originator, or owner of any Model featured in the Models section of the Sanctum application. Each Model is created and provided by third parties. Sanctum does not endorse, support, represent or guarantee the completeness, truthfulness, accuracy, or reliability of any Model listed there. You understand that supported Models can produce content that might be offensive, harmful, inaccurate or otherwise inappropriate, or deceptive. Each Model is the sole responsibility of the person or entity who originated such Model. Sanctum may not monitor or control the Models supported and cannot, and does not, take responsibility for any such Model. Sanctum disclaims all warranties or guarantees about the accuracy, reliability or benefits of the Models. Sanctum further disclaims any warranty that the Model will meet your requirements, be secure, uninterrupted or available at any time or location, or error-free, viruses-free, or that any errors will be corrected, or otherwise. You will be solely responsible for any damage resulting from your use of or access to the Models, your downloading of any Model, or use of any other Model provided by or through Sanctum.
|
naver-clova-ix/donut-base | naver-clova-ix | "2022-08-13T08:27:12Z" | 35,380 | 157 | transformers | [
"transformers",
"pytorch",
"vision-encoder-decoder",
"donut",
"image-to-text",
"vision",
"arxiv:2111.15664",
"license:mit",
"endpoints_compatible",
"region:us"
] | image-to-text | "2022-07-19T13:49:17Z" | ---
license: mit
tags:
- donut
- image-to-text
- vision
---
# Donut (base-sized model, pre-trained only)
Donut model pre-trained-only. It was introduced in the paper [OCR-free Document Understanding Transformer](https://arxiv.org/abs/2111.15664) by Geewok et al. and first released in [this repository](https://github.com/clovaai/donut).
Disclaimer: The team releasing Donut did not write a model card for this model so this model card has been written by the Hugging Face team.
## Model description
Donut consists of a vision encoder (Swin Transformer) and a text decoder (BART). Given an image, the encoder first encodes the image into a tensor of embeddings (of shape batch_size, seq_len, hidden_size), after which the decoder autoregressively generates text, conditioned on the encoding of the encoder.
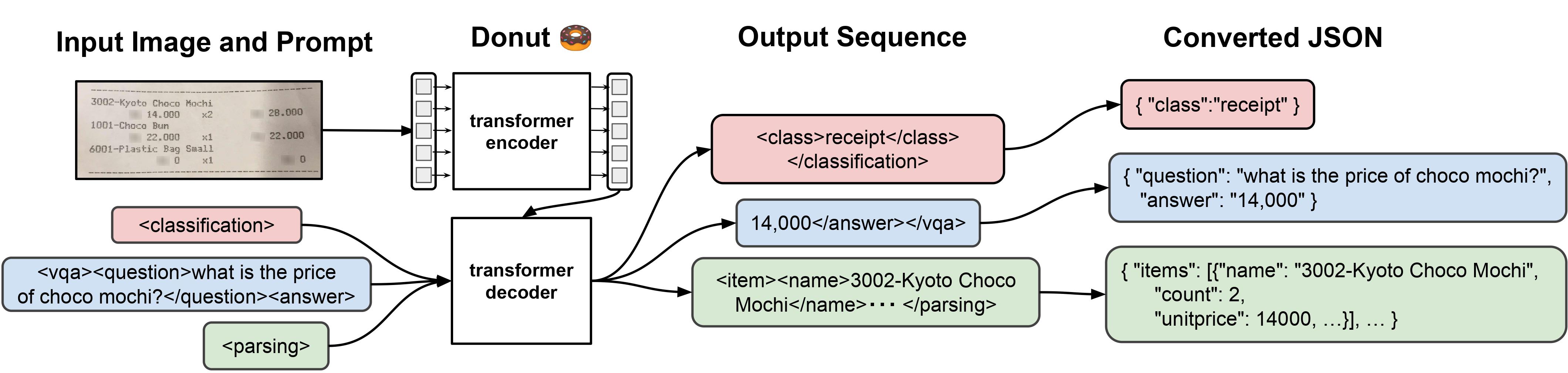
## Intended uses & limitations
This model is meant to be fine-tuned on a downstream task, like document image classification or document parsing. See the [model hub](https://huggingface.co/models?search=donut) to look for fine-tuned versions on a task that interests you.
### How to use
We refer to the [documentation](https://huggingface.co/docs/transformers/main/en/model_doc/donut) which includes code examples.
### BibTeX entry and citation info
```bibtex
@article{DBLP:journals/corr/abs-2111-15664,
author = {Geewook Kim and
Teakgyu Hong and
Moonbin Yim and
Jinyoung Park and
Jinyeong Yim and
Wonseok Hwang and
Sangdoo Yun and
Dongyoon Han and
Seunghyun Park},
title = {Donut: Document Understanding Transformer without {OCR}},
journal = {CoRR},
volume = {abs/2111.15664},
year = {2021},
url = {https://arxiv.org/abs/2111.15664},
eprinttype = {arXiv},
eprint = {2111.15664},
timestamp = {Thu, 02 Dec 2021 10:50:44 +0100},
biburl = {https://dblp.org/rec/journals/corr/abs-2111-15664.bib},
bibsource = {dblp computer science bibliography, https://dblp.org}
}
``` |
facebook/mbart-large-50-many-to-one-mmt | facebook | "2023-03-28T09:18:56Z" | 35,374 | 58 | transformers | [
"transformers",
"pytorch",
"tf",
"jax",
"mbart",
"text2text-generation",
"mbart-50",
"multilingual",
"ar",
"cs",
"de",
"en",
"es",
"et",
"fi",
"fr",
"gu",
"hi",
"it",
"ja",
"kk",
"ko",
"lt",
"lv",
"my",
"ne",
"nl",
"ro",
"ru",
"si",
"tr",
"vi",
"zh",
"af",
"az",
"bn",
"fa",
"he",
"hr",
"id",
"ka",
"km",
"mk",
"ml",
"mn",
"mr",
"pl",
"ps",
"pt",
"sv",
"sw",
"ta",
"te",
"th",
"tl",
"uk",
"ur",
"xh",
"gl",
"sl",
"arxiv:2008.00401",
"autotrain_compatible",
"endpoints_compatible",
"region:us"
] | text2text-generation | "2022-03-02T23:29:05Z" | ---
language:
- multilingual
- ar
- cs
- de
- en
- es
- et
- fi
- fr
- gu
- hi
- it
- ja
- kk
- ko
- lt
- lv
- my
- ne
- nl
- ro
- ru
- si
- tr
- vi
- zh
- af
- az
- bn
- fa
- he
- hr
- id
- ka
- km
- mk
- ml
- mn
- mr
- pl
- ps
- pt
- sv
- sw
- ta
- te
- th
- tl
- uk
- ur
- xh
- gl
- sl
tags:
- mbart-50
---
# mBART-50 many to one multilingual machine translation
This model is a fine-tuned checkpoint of [mBART-large-50](https://huggingface.co/facebook/mbart-large-50). `mbart-large-50-many-to-many-mmt` is fine-tuned for multilingual machine translation. It was introduced in [Multilingual Translation with Extensible Multilingual Pretraining and Finetuning](https://arxiv.org/abs/2008.00401) paper.
The model can translate directly between any pair of 50 languages.
```python
from transformers import MBartForConditionalGeneration, MBart50TokenizerFast
article_hi = "संयुक्त राष्ट्र के प्रमुख का कहना है कि सीरिया में कोई सैन्य समाधान नहीं है"
article_ar = "الأمين العام للأمم المتحدة يقول إنه لا يوجد حل عسكري في سوريا."
model = MBartForConditionalGeneration.from_pretrained("facebook/mbart-large-50-many-to-one-mmt")
tokenizer = MBart50TokenizerFast.from_pretrained("facebook/mbart-large-50-many-to-one-mmt")
# translate Hindi to English
tokenizer.src_lang = "hi_IN"
encoded_hi = tokenizer(article_hi, return_tensors="pt")
generated_tokens = model.generate(**encoded_hi)
tokenizer.batch_decode(generated_tokens, skip_special_tokens=True)
# => "The head of the UN says there is no military solution in Syria."
# translate Arabic to English
tokenizer.src_lang = "ar_AR"
encoded_ar = tokenizer(article_ar, return_tensors="pt")
generated_tokens = model.generate(**encoded_ar)
tokenizer.batch_decode(generated_tokens, skip_special_tokens=True)
# => "The Secretary-General of the United Nations says there is no military solution in Syria."
```
See the [model hub](https://huggingface.co/models?filter=mbart-50) to look for more fine-tuned versions.
## Languages covered
Arabic (ar_AR), Czech (cs_CZ), German (de_DE), English (en_XX), Spanish (es_XX), Estonian (et_EE), Finnish (fi_FI), French (fr_XX), Gujarati (gu_IN), Hindi (hi_IN), Italian (it_IT), Japanese (ja_XX), Kazakh (kk_KZ), Korean (ko_KR), Lithuanian (lt_LT), Latvian (lv_LV), Burmese (my_MM), Nepali (ne_NP), Dutch (nl_XX), Romanian (ro_RO), Russian (ru_RU), Sinhala (si_LK), Turkish (tr_TR), Vietnamese (vi_VN), Chinese (zh_CN), Afrikaans (af_ZA), Azerbaijani (az_AZ), Bengali (bn_IN), Persian (fa_IR), Hebrew (he_IL), Croatian (hr_HR), Indonesian (id_ID), Georgian (ka_GE), Khmer (km_KH), Macedonian (mk_MK), Malayalam (ml_IN), Mongolian (mn_MN), Marathi (mr_IN), Polish (pl_PL), Pashto (ps_AF), Portuguese (pt_XX), Swedish (sv_SE), Swahili (sw_KE), Tamil (ta_IN), Telugu (te_IN), Thai (th_TH), Tagalog (tl_XX), Ukrainian (uk_UA), Urdu (ur_PK), Xhosa (xh_ZA), Galician (gl_ES), Slovene (sl_SI)
## BibTeX entry and citation info
```
@article{tang2020multilingual,
title={Multilingual Translation with Extensible Multilingual Pretraining and Finetuning},
author={Yuqing Tang and Chau Tran and Xian Li and Peng-Jen Chen and Naman Goyal and Vishrav Chaudhary and Jiatao Gu and Angela Fan},
year={2020},
eprint={2008.00401},
archivePrefix={arXiv},
primaryClass={cs.CL}
}
``` |
stabilityai/sd-x2-latent-upscaler | stabilityai | "2023-06-05T16:28:02Z" | 35,263 | 173 | diffusers | [
"diffusers",
"safetensors",
"stable-diffusion",
"license:openrail++",
"diffusers:StableDiffusionLatentUpscalePipeline",
"region:us"
] | null | "2023-02-03T11:24:02Z" | ---
license: openrail++
tags:
- stable-diffusion
inference: false
---
# Stable Diffusion x2 latent upscaler model card
This model card focuses on the latent diffusion-based upscaler developed by [Katherine Crowson](https://github.com/crowsonkb/k-diffusion)
in collaboration with [Stability AI](https://stability.ai/).
This model was trained on a high-resolution subset of the LAION-2B dataset.
It is a diffusion model that operates in the same latent space as the Stable Diffusion model, which is decoded into a full-resolution image.
To use it with Stable Diffusion, You can take the generated latent from Stable Diffusion and pass it into the upscaler before decoding with your standard VAE.
Or you can take any image, encode it into the latent space, use the upscaler, and decode it.
**Note**:
This upscaling model is designed explicitely for **Stable Diffusion** as it can upscale Stable Diffusion's latent denoised image embeddings.
This allows for very fast text-to-image + upscaling pipelines as all intermeditate states can be kept on GPU. More for information, see example below.
This model works on all [Stable Diffusion checkpoints](https://huggingface.co/models?other=stable-diffusion)
| 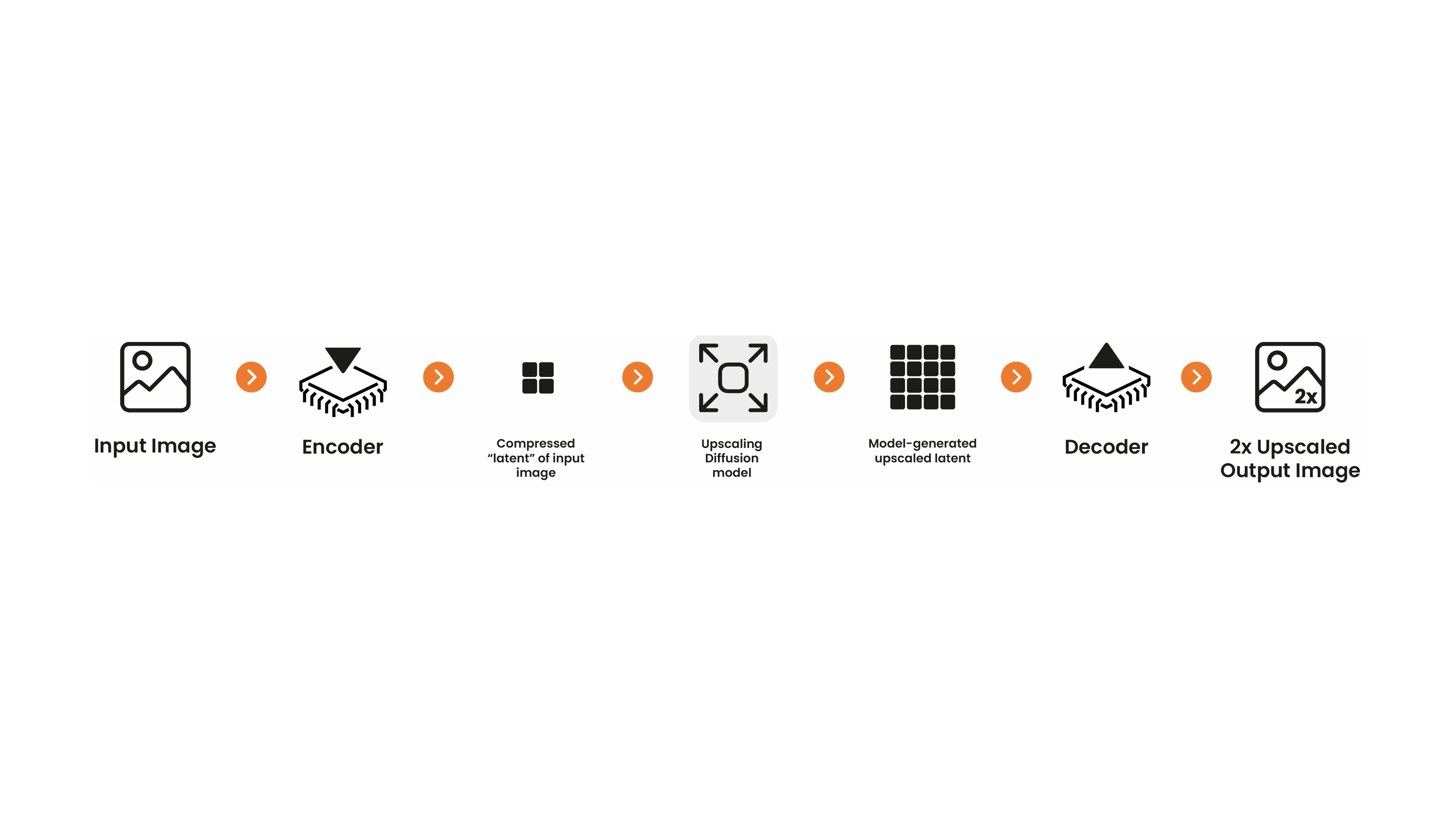 |
|:--:|
Image by Tanishq Abraham from [Stability AI](https://stability.ai/) originating from [this tweet](https://twitter.com/StabilityAI/status/1590531958815064065)|
Original output image | 2x upscaled output image
:-------------------------:|:-------------------------:
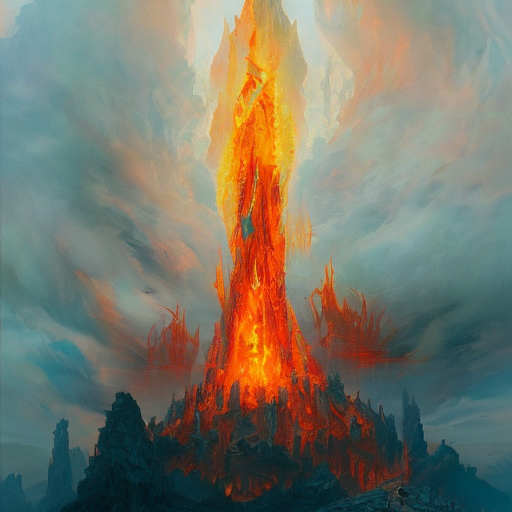 | 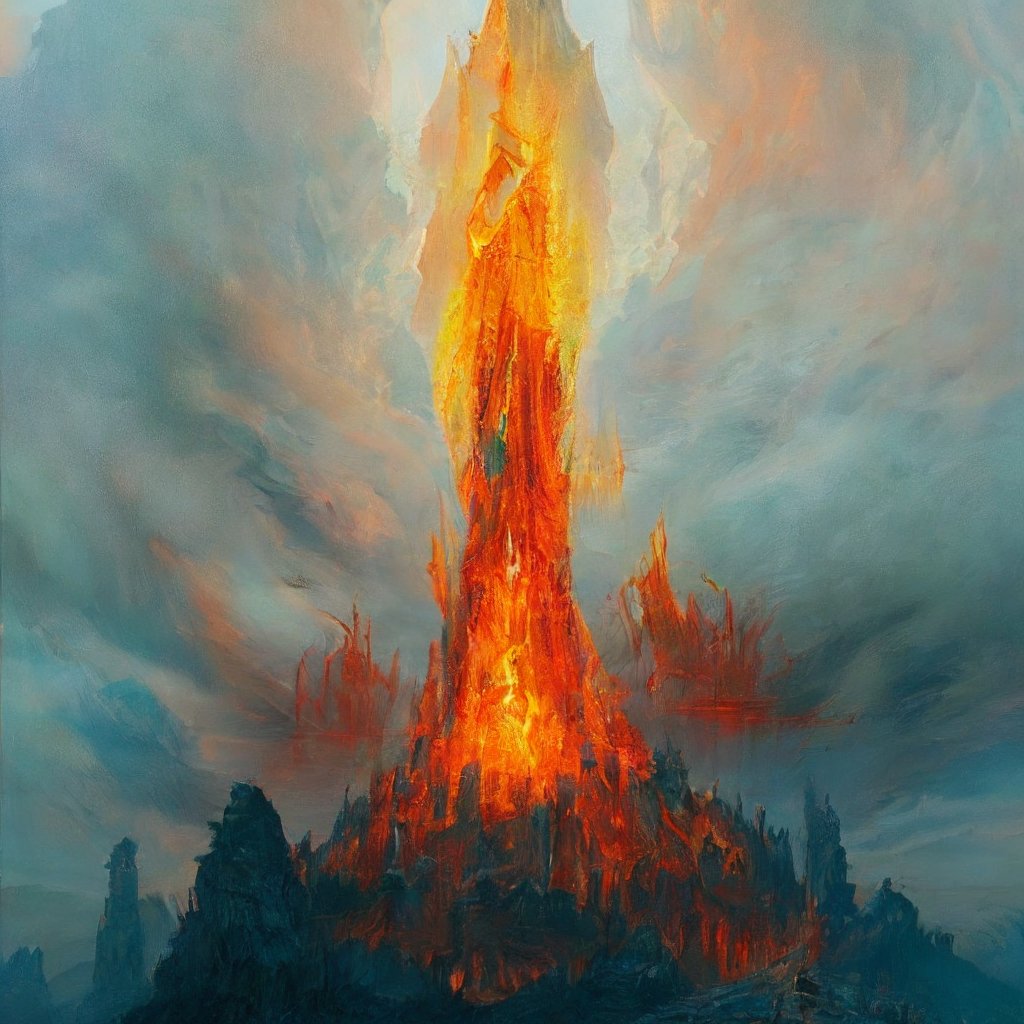
- Use it with 🧨 [`diffusers`](https://huggingface.co/stabilityai/sd-x2-latent-upscaler#examples)
## Model Details
- **Developed by:** Katherine Crowson
- **Model type:** Diffusion-based latent upscaler
- **Language(s):** English
- **License:** [CreativeML Open RAIL++-M License](https://huggingface.co/stabilityai/stable-diffusion-2/blob/main/LICENSE-MODEL)
## Examples
Using the [🤗's Diffusers library](https://github.com/huggingface/diffusers) to run latent upscaler on top of any `StableDiffusionUpscalePipeline` checkpoint
to enhance its output image resolution by a factor of 2.
```bash
pip install git+https://github.com/huggingface/diffusers.git
pip install transformers accelerate scipy safetensors
```
```python
from diffusers import StableDiffusionLatentUpscalePipeline, StableDiffusionPipeline
import torch
pipeline = StableDiffusionPipeline.from_pretrained("CompVis/stable-diffusion-v1-4", torch_dtype=torch.float16)
pipeline.to("cuda")
upscaler = StableDiffusionLatentUpscalePipeline.from_pretrained("stabilityai/sd-x2-latent-upscaler", torch_dtype=torch.float16)
upscaler.to("cuda")
prompt = "a photo of an astronaut high resolution, unreal engine, ultra realistic"
generator = torch.manual_seed(33)
# we stay in latent space! Let's make sure that Stable Diffusion returns the image
# in latent space
low_res_latents = pipeline(prompt, generator=generator, output_type="latent").images
upscaled_image = upscaler(
prompt=prompt,
image=low_res_latents,
num_inference_steps=20,
guidance_scale=0,
generator=generator,
).images[0]
# Let's save the upscaled image under "upscaled_astronaut.png"
upscaled_image.save("astronaut_1024.png")
# as a comparison: Let's also save the low-res image
with torch.no_grad():
image = pipeline.decode_latents(low_res_latents)
image = pipeline.numpy_to_pil(image)[0]
image.save("astronaut_512.png")
```
**Result**:
*512-res Astronaut*

*1024-res Astronaut*

**Notes**:
- Despite not being a dependency, we highly recommend you to install [xformers](https://github.com/facebookresearch/xformers) for memory efficient attention (better performance)
- If you have low GPU RAM available, make sure to add a `pipe.enable_attention_slicing()` after sending it to `cuda` for less VRAM usage (to the cost of speed)
# Uses
## Direct Use
The model is intended for research purposes only. Possible research areas and tasks include
- Safe deployment of models which have the potential to generate harmful content.
- Probing and understanding the limitations and biases of generative models.
- Generation of artworks and use in design and other artistic processes.
- Applications in educational or creative tools.
- Research on generative models.
Excluded uses are described below.
### Misuse, Malicious Use, and Out-of-Scope Use
_Note: This section is originally taken from the [DALLE-MINI model card](https://huggingface.co/dalle-mini/dalle-mini), was used for Stable Diffusion v1, but applies in the same way to Stable Diffusion v2_.
The model should not be used to intentionally create or disseminate images that create hostile or alienating environments for people. This includes generating images that people would foreseeably find disturbing, distressing, or offensive; or content that propagates historical or current stereotypes.
#### Out-of-Scope Use
The model was not trained to be factual or true representations of people or events, and therefore using the model to generate such content is out-of-scope for the abilities of this model.
#### Misuse and Malicious Use
Using the model to generate content that is cruel to individuals is a misuse of this model. This includes, but is not limited to:
- Generating demeaning, dehumanizing, or otherwise harmful representations of people or their environments, cultures, religions, etc.
- Intentionally promoting or propagating discriminatory content or harmful stereotypes.
- Impersonating individuals without their consent.
- Sexual content without consent of the people who might see it.
- Mis- and disinformation
- Representations of egregious violence and gore
- Sharing of copyrighted or licensed material in violation of its terms of use.
- Sharing content that is an alteration of copyrighted or licensed material in violation of its terms of use.
## Limitations and Bias
### Limitations
- The model does not achieve perfect photorealism
- The model cannot render legible text
- The model does not perform well on more difficult tasks which involve compositionality, such as rendering an image corresponding to “A red cube on top of a blue sphere”
- Faces and people in general may not be generated properly.
- The model was trained mainly with English captions and will not work as well in other languages.
- The autoencoding part of the model is lossy
- The model was trained on a subset of the large-scale dataset
[LAION-5B](https://laion.ai/blog/laion-5b/), which contains adult, violent and sexual content. To partially mitigate this, we have filtered the dataset using LAION's NFSW detector (see Training section).
### Bias
While the capabilities of image generation models are impressive, they can also reinforce or exacerbate social biases.
Stable Diffusion vw was primarily trained on subsets of [LAION-2B(en)](https://laion.ai/blog/laion-5b/),
which consists of images that are limited to English descriptions.
Texts and images from communities and cultures that use other languages are likely to be insufficiently accounted for.
This affects the overall output of the model, as white and western cultures are often set as the default. Further, the
ability of the model to generate content with non-English prompts is significantly worse than with English-language prompts.
Stable Diffusion v2 mirrors and exacerbates biases to such a degree that viewer discretion must be advised irrespective of the input or its intent. |
facebook/nllb-200-distilled-1.3B | facebook | "2023-02-11T20:19:10Z" | 35,246 | 84 | transformers | [
"transformers",
"pytorch",
"m2m_100",
"text2text-generation",
"nllb",
"translation",
"ace",
"acm",
"acq",
"aeb",
"af",
"ajp",
"ak",
"als",
"am",
"apc",
"ar",
"ars",
"ary",
"arz",
"as",
"ast",
"awa",
"ayr",
"azb",
"azj",
"ba",
"bm",
"ban",
"be",
"bem",
"bn",
"bho",
"bjn",
"bo",
"bs",
"bug",
"bg",
"ca",
"ceb",
"cs",
"cjk",
"ckb",
"crh",
"cy",
"da",
"de",
"dik",
"dyu",
"dz",
"el",
"en",
"eo",
"et",
"eu",
"ee",
"fo",
"fj",
"fi",
"fon",
"fr",
"fur",
"fuv",
"gaz",
"gd",
"ga",
"gl",
"gn",
"gu",
"ht",
"ha",
"he",
"hi",
"hne",
"hr",
"hu",
"hy",
"ig",
"ilo",
"id",
"is",
"it",
"jv",
"ja",
"kab",
"kac",
"kam",
"kn",
"ks",
"ka",
"kk",
"kbp",
"kea",
"khk",
"km",
"ki",
"rw",
"ky",
"kmb",
"kmr",
"knc",
"kg",
"ko",
"lo",
"lij",
"li",
"ln",
"lt",
"lmo",
"ltg",
"lb",
"lua",
"lg",
"luo",
"lus",
"lvs",
"mag",
"mai",
"ml",
"mar",
"min",
"mk",
"mt",
"mni",
"mos",
"mi",
"my",
"nl",
"nn",
"nb",
"npi",
"nso",
"nus",
"ny",
"oc",
"ory",
"pag",
"pa",
"pap",
"pbt",
"pes",
"plt",
"pl",
"pt",
"prs",
"quy",
"ro",
"rn",
"ru",
"sg",
"sa",
"sat",
"scn",
"shn",
"si",
"sk",
"sl",
"sm",
"sn",
"sd",
"so",
"st",
"es",
"sc",
"sr",
"ss",
"su",
"sv",
"swh",
"szl",
"ta",
"taq",
"tt",
"te",
"tg",
"tl",
"th",
"ti",
"tpi",
"tn",
"ts",
"tk",
"tum",
"tr",
"tw",
"tzm",
"ug",
"uk",
"umb",
"ur",
"uzn",
"vec",
"vi",
"war",
"wo",
"xh",
"ydd",
"yo",
"yue",
"zh",
"zsm",
"zu",
"dataset:flores-200",
"license:cc-by-nc-4.0",
"autotrain_compatible",
"region:us"
] | translation | "2022-07-08T10:57:38Z" | ---
language:
- ace
- acm
- acq
- aeb
- af
- ajp
- ak
- als
- am
- apc
- ar
- ars
- ary
- arz
- as
- ast
- awa
- ayr
- azb
- azj
- ba
- bm
- ban
- be
- bem
- bn
- bho
- bjn
- bo
- bs
- bug
- bg
- ca
- ceb
- cs
- cjk
- ckb
- crh
- cy
- da
- de
- dik
- dyu
- dz
- el
- en
- eo
- et
- eu
- ee
- fo
- fj
- fi
- fon
- fr
- fur
- fuv
- gaz
- gd
- ga
- gl
- gn
- gu
- ht
- ha
- he
- hi
- hne
- hr
- hu
- hy
- ig
- ilo
- id
- is
- it
- jv
- ja
- kab
- kac
- kam
- kn
- ks
- ka
- kk
- kbp
- kea
- khk
- km
- ki
- rw
- ky
- kmb
- kmr
- knc
- kg
- ko
- lo
- lij
- li
- ln
- lt
- lmo
- ltg
- lb
- lua
- lg
- luo
- lus
- lvs
- mag
- mai
- ml
- mar
- min
- mk
- mt
- mni
- mos
- mi
- my
- nl
- nn
- nb
- npi
- nso
- nus
- ny
- oc
- ory
- pag
- pa
- pap
- pbt
- pes
- plt
- pl
- pt
- prs
- quy
- ro
- rn
- ru
- sg
- sa
- sat
- scn
- shn
- si
- sk
- sl
- sm
- sn
- sd
- so
- st
- es
- sc
- sr
- ss
- su
- sv
- swh
- szl
- ta
- taq
- tt
- te
- tg
- tl
- th
- ti
- tpi
- tn
- ts
- tk
- tum
- tr
- tw
- tzm
- ug
- uk
- umb
- ur
- uzn
- vec
- vi
- war
- wo
- xh
- ydd
- yo
- yue
- zh
- zsm
- zu
language_details: "ace_Arab, ace_Latn, acm_Arab, acq_Arab, aeb_Arab, afr_Latn, ajp_Arab, aka_Latn, amh_Ethi, apc_Arab, arb_Arab, ars_Arab, ary_Arab, arz_Arab, asm_Beng, ast_Latn, awa_Deva, ayr_Latn, azb_Arab, azj_Latn, bak_Cyrl, bam_Latn, ban_Latn,bel_Cyrl, bem_Latn, ben_Beng, bho_Deva, bjn_Arab, bjn_Latn, bod_Tibt, bos_Latn, bug_Latn, bul_Cyrl, cat_Latn, ceb_Latn, ces_Latn, cjk_Latn, ckb_Arab, crh_Latn, cym_Latn, dan_Latn, deu_Latn, dik_Latn, dyu_Latn, dzo_Tibt, ell_Grek, eng_Latn, epo_Latn, est_Latn, eus_Latn, ewe_Latn, fao_Latn, pes_Arab, fij_Latn, fin_Latn, fon_Latn, fra_Latn, fur_Latn, fuv_Latn, gla_Latn, gle_Latn, glg_Latn, grn_Latn, guj_Gujr, hat_Latn, hau_Latn, heb_Hebr, hin_Deva, hne_Deva, hrv_Latn, hun_Latn, hye_Armn, ibo_Latn, ilo_Latn, ind_Latn, isl_Latn, ita_Latn, jav_Latn, jpn_Jpan, kab_Latn, kac_Latn, kam_Latn, kan_Knda, kas_Arab, kas_Deva, kat_Geor, knc_Arab, knc_Latn, kaz_Cyrl, kbp_Latn, kea_Latn, khm_Khmr, kik_Latn, kin_Latn, kir_Cyrl, kmb_Latn, kon_Latn, kor_Hang, kmr_Latn, lao_Laoo, lvs_Latn, lij_Latn, lim_Latn, lin_Latn, lit_Latn, lmo_Latn, ltg_Latn, ltz_Latn, lua_Latn, lug_Latn, luo_Latn, lus_Latn, mag_Deva, mai_Deva, mal_Mlym, mar_Deva, min_Latn, mkd_Cyrl, plt_Latn, mlt_Latn, mni_Beng, khk_Cyrl, mos_Latn, mri_Latn, zsm_Latn, mya_Mymr, nld_Latn, nno_Latn, nob_Latn, npi_Deva, nso_Latn, nus_Latn, nya_Latn, oci_Latn, gaz_Latn, ory_Orya, pag_Latn, pan_Guru, pap_Latn, pol_Latn, por_Latn, prs_Arab, pbt_Arab, quy_Latn, ron_Latn, run_Latn, rus_Cyrl, sag_Latn, san_Deva, sat_Beng, scn_Latn, shn_Mymr, sin_Sinh, slk_Latn, slv_Latn, smo_Latn, sna_Latn, snd_Arab, som_Latn, sot_Latn, spa_Latn, als_Latn, srd_Latn, srp_Cyrl, ssw_Latn, sun_Latn, swe_Latn, swh_Latn, szl_Latn, tam_Taml, tat_Cyrl, tel_Telu, tgk_Cyrl, tgl_Latn, tha_Thai, tir_Ethi, taq_Latn, taq_Tfng, tpi_Latn, tsn_Latn, tso_Latn, tuk_Latn, tum_Latn, tur_Latn, twi_Latn, tzm_Tfng, uig_Arab, ukr_Cyrl, umb_Latn, urd_Arab, uzn_Latn, vec_Latn, vie_Latn, war_Latn, wol_Latn, xho_Latn, ydd_Hebr, yor_Latn, yue_Hant, zho_Hans, zho_Hant, zul_Latn"
tags:
- nllb
- translation
license: "cc-by-nc-4.0"
datasets:
- flores-200
metrics:
- bleu
- spbleu
- chrf++
inference: false
---
# NLLB-200
This is the model card of NLLB-200's distilled 1.3B variant.
Here are the [metrics](https://tinyurl.com/nllb200densedst1bmetrics) for that particular checkpoint.
- Information about training algorithms, parameters, fairness constraints or other applied approaches, and features. The exact training algorithm, data and the strategies to handle data imbalances for high and low resource languages that were used to train NLLB-200 is described in the paper.
- Paper or other resource for more information NLLB Team et al, No Language Left Behind: Scaling Human-Centered Machine Translation, Arxiv, 2022
- License: CC-BY-NC
- Where to send questions or comments about the model: https://github.com/facebookresearch/fairseq/issues
## Intended Use
- Primary intended uses: NLLB-200 is a machine translation model primarily intended for research in machine translation, - especially for low-resource languages. It allows for single sentence translation among 200 languages. Information on how to - use the model can be found in Fairseq code repository along with the training code and references to evaluation and training data.
- Primary intended users: Primary users are researchers and machine translation research community.
- Out-of-scope use cases: NLLB-200 is a research model and is not released for production deployment. NLLB-200 is trained on general domain text data and is not intended to be used with domain specific texts, such as medical domain or legal domain. The model is not intended to be used for document translation. The model was trained with input lengths not exceeding 512 tokens, therefore translating longer sequences might result in quality degradation. NLLB-200 translations can not be used as certified translations.
## Metrics
• Model performance measures: NLLB-200 model was evaluated using BLEU, spBLEU, and chrF++ metrics widely adopted by machine translation community. Additionally, we performed human evaluation with the XSTS protocol and measured the toxicity of the generated translations.
## Evaluation Data
- Datasets: Flores-200 dataset is described in Section 4
- Motivation: We used Flores-200 as it provides full evaluation coverage of the languages in NLLB-200
- Preprocessing: Sentence-split raw text data was preprocessed using SentencePiece. The
SentencePiece model is released along with NLLB-200.
## Training Data
• We used parallel multilingual data from a variety of sources to train the model. We provide detailed report on data selection and construction process in Section 5 in the paper. We also used monolingual data constructed from Common Crawl. We provide more details in Section 5.2.
## Ethical Considerations
• In this work, we took a reflexive approach in technological development to ensure that we prioritize human users and minimize risks that could be transferred to them. While we reflect on our ethical considerations throughout the article, here are some additional points to highlight. For one, many languages chosen for this study are low-resource languages, with a heavy emphasis on African languages. While quality translation could improve education and information access in many in these communities, such an access could also make groups with lower levels of digital literacy more vulnerable to misinformation or online scams. The latter scenarios could arise if bad actors misappropriate our work for nefarious activities, which we conceive as an example of unintended use. Regarding data acquisition, the training data used for model development were mined from various publicly available sources on the web. Although we invested heavily in data cleaning, personally identifiable information may not be entirely eliminated. Finally, although we did our best to optimize for translation quality, mistranslations produced by the model could remain. Although the odds are low, this could have adverse impact on those who rely on these translations to make important decisions (particularly when related to health and safety).
## Caveats and Recommendations
• Our model has been tested on the Wikimedia domain with limited investigation on other domains supported in NLLB-MD. In addition, the supported languages may have variations that our model is not capturing. Users should make appropriate assessments.
## Carbon Footprint Details
• The carbon dioxide (CO2e) estimate is reported in Section 8.8. |
techiaith/wav2vec2-xlsr-53-ft-cy-en | techiaith | "2023-03-02T06:30:13Z" | 35,195 | 1 | transformers | [
"transformers",
"pytorch",
"wav2vec2",
"automatic-speech-recognition",
"speech",
"cy",
"en",
"dataset:common_voice",
"license:apache-2.0",
"model-index",
"endpoints_compatible",
"region:us"
] | automatic-speech-recognition | "2022-10-24T11:57:48Z" | ---
language:
- cy
- en
datasets:
- common_voice
metrics:
- wer
tags:
- automatic-speech-recognition
- speech
license: apache-2.0
model-index:
- name: wav2vec2-xlsr-ft-en-cy
results:
- task:
name: Speech Recognition
type: automatic-speech-recognition
dataset:
name: Common Voice cy
type: common_voice
args: cy
metrics:
- name: Test WER
type: wer
value: 17.70%
---
# wav2vec2-xlsr-ft-en-cy
A speech recognition acoustic model for Welsh and English, fine-tuned from [facebook/wav2vec2-large-xlsr-53](https://huggingface.co/facebook/wav2vec2-large-xlsr-53) using English/Welsh balanced data derived from version 11 of their respective Common Voice datasets (https://commonvoice.mozilla.org/cy/datasets). Custom bilingual Common Voice train/dev and test splits were built using the scripts at https://github.com/techiaith/docker-commonvoice-custom-splits-builder#introduction
Source code and scripts for training wav2vec2-xlsr-ft-en-cy can be found at [https://github.com/techiaith/docker-wav2vec2-cy](https://github.com/techiaith/docker-wav2vec2-cy/blob/main/train/fine-tune/python/run_en_cy.sh).
## Usage
The wav2vec2-xlsr-ft-en-cy model can be used directly as follows:
```python
import torch
import torchaudio
import librosa
from transformers import Wav2Vec2ForCTC, Wav2Vec2Processor
processor = Wav2Vec2Processor.from_pretrained("techiaith/wav2vec2-xlsr-ft-en-cy")
model = Wav2Vec2ForCTC.from_pretrained("techiaith/wav2vec2-xlsr-ft-en-cy")
audio, rate = librosa.load(audio_file, sr=16000)
inputs = processor(audio, sampling_rate=16_000, return_tensors="pt", padding=True)
with torch.no_grad():
tlogits = model(inputs.input_values, attention_mask=inputs.attention_mask).logits
# greedy decoding
predicted_ids = torch.argmax(logits, dim=-1)
print("Prediction:", processor.batch_decode(predicted_ids))
```
## Evaluation
According to a balanced English+Welsh test set derived from Common Voice version 11, the WER of techiaith/wav2vec2-xlsr-ft-en-cy is **17.7%**
However, when evaluated with language specific test sets, the model exhibits a bias to perform better with Welsh.
| Common Voice Test Set Language | WER | CER |
| -------- | --- | --- |
| EN+CY | 17.07| 7.32 |
| EN | 27.54 | 11.6 |
| CY | 7.13 | 2.2 |
|
timm/beitv2_base_patch16_224.in1k_ft_in22k | timm | "2023-05-08T23:34:58Z" | 35,188 | 1 | timm | [
"timm",
"pytorch",
"safetensors",
"image-classification",
"dataset:imagenet-22k",
"arxiv:2208.06366",
"arxiv:2010.11929",
"license:apache-2.0",
"region:us"
] | image-classification | "2022-12-23T02:33:15Z" | ---
tags:
- image-classification
- timm
library_name: timm
license: apache-2.0
datasets:
- imagenet-22k
---
# Model card for beitv2_base_patch16_224.in1k_ft_in22k
A BEiT-v2 image classification model. Trained on ImageNet-1k with self-supervised masked image modelling (MIM) using a VQ-KD encoder as a visual tokenizer (via OpenAI CLIP B/16 teacher). Fine-tuned on ImageNet-22k.
## Model Details
- **Model Type:** Image classification / feature backbone
- **Model Stats:**
- Params (M): 102.6
- GMACs: 17.6
- Activations (M): 23.9
- Image size: 224 x 224
- **Papers:**
- BEiT v2: Masked Image Modeling with Vector-Quantized Visual Tokenizers: https://arxiv.org/abs/2208.06366
- An Image is Worth 16x16 Words: Transformers for Image Recognition at Scale: https://arxiv.org/abs/2010.11929v2
- **Dataset:** ImageNet-22k
- **Original:** https://github.com/microsoft/unilm/tree/master/beit2
## Model Usage
### Image Classification
```python
from urllib.request import urlopen
from PIL import Image
import timm
img = Image.open(urlopen(
'https://huggingface.co/datasets/huggingface/documentation-images/resolve/main/beignets-task-guide.png'
))
model = timm.create_model('beitv2_base_patch16_224.in1k_ft_in22k', pretrained=True)
model = model.eval()
# get model specific transforms (normalization, resize)
data_config = timm.data.resolve_model_data_config(model)
transforms = timm.data.create_transform(**data_config, is_training=False)
output = model(transforms(img).unsqueeze(0)) # unsqueeze single image into batch of 1
top5_probabilities, top5_class_indices = torch.topk(output.softmax(dim=1) * 100, k=5)
```
### Image Embeddings
```python
from urllib.request import urlopen
from PIL import Image
import timm
img = Image.open(urlopen(
'https://huggingface.co/datasets/huggingface/documentation-images/resolve/main/beignets-task-guide.png'
))
model = timm.create_model(
'beitv2_base_patch16_224.in1k_ft_in22k',
pretrained=True,
num_classes=0, # remove classifier nn.Linear
)
model = model.eval()
# get model specific transforms (normalization, resize)
data_config = timm.data.resolve_model_data_config(model)
transforms = timm.data.create_transform(**data_config, is_training=False)
output = model(transforms(img).unsqueeze(0)) # output is (batch_size, num_features) shaped tensor
# or equivalently (without needing to set num_classes=0)
output = model.forward_features(transforms(img).unsqueeze(0))
# output is unpooled, a (1, 197, 768) shaped tensor
output = model.forward_head(output, pre_logits=True)
# output is a (1, num_features) shaped tensor
```
## Model Comparison
Explore the dataset and runtime metrics of this model in timm [model results](https://github.com/huggingface/pytorch-image-models/tree/main/results).
## Citation
```bibtex
@article{peng2022beit,
title={Beit v2: Masked image modeling with vector-quantized visual tokenizers},
author={Peng, Zhiliang and Dong, Li and Bao, Hangbo and Ye, Qixiang and Wei, Furu},
journal={arXiv preprint arXiv:2208.06366},
year={2022}
}
```
```bibtex
@article{dosovitskiy2020vit,
title={An Image is Worth 16x16 Words: Transformers for Image Recognition at Scale},
author={Dosovitskiy, Alexey and Beyer, Lucas and Kolesnikov, Alexander and Weissenborn, Dirk and Zhai, Xiaohua and Unterthiner, Thomas and Dehghani, Mostafa and Minderer, Matthias and Heigold, Georg and Gelly, Sylvain and Uszkoreit, Jakob and Houlsby, Neil},
journal={ICLR},
year={2021}
}
```
```bibtex
@misc{rw2019timm,
author = {Ross Wightman},
title = {PyTorch Image Models},
year = {2019},
publisher = {GitHub},
journal = {GitHub repository},
doi = {10.5281/zenodo.4414861},
howpublished = {\url{https://github.com/huggingface/pytorch-image-models}}
}
```
|
trl-internal-testing/tiny-random-GPT2LMHeadModel | trl-internal-testing | "2023-01-23T10:38:18Z" | 35,183 | 1 | transformers | [
"transformers",
"pytorch",
"gpt2",
"text-generation",
"autotrain_compatible",
"endpoints_compatible",
"text-generation-inference",
"region:us"
] | text-generation | "2022-12-20T10:34:11Z" | Entry not found |
Sandrro/text_to_subfunction_v10 | Sandrro | "2023-07-13T16:28:26Z" | 35,168 | 0 | transformers | [
"transformers",
"pytorch",
"tensorboard",
"bert",
"text-classification",
"autotrain_compatible",
"endpoints_compatible",
"region:us"
] | text-classification | "2023-07-13T08:23:01Z" | Entry not found |
facebook/dinov2-giant-imagenet1k-1-layer | facebook | "2023-09-16T10:14:37Z" | 35,001 | 2 | transformers | [
"transformers",
"pytorch",
"safetensors",
"dinov2",
"image-classification",
"dino",
"vision",
"dataset:imagenet-1k",
"arxiv:2304.07193",
"license:apache-2.0",
"autotrain_compatible",
"endpoints_compatible",
"region:us"
] | image-classification | "2023-09-14T20:18:41Z" | ---
license: apache-2.0
tags:
- dino
- vision
datasets:
- imagenet-1k
---
# Vision Transformer (giant-sized model) trained using DINOv2
Vision Transformer (ViT) model trained using the DINOv2 method. It was introduced in the paper [DINOv2: Learning Robust Visual Features without Supervision](https://arxiv.org/abs/2304.07193) by Oquab et al. and first released in [this repository](https://github.com/facebookresearch/dinov2).
Disclaimer: The team releasing DINOv2 did not write a model card for this model so this model card has been written by the Hugging Face team.
## Model description
The Vision Transformer (ViT) is a transformer encoder model (BERT-like) pretrained on a large collection of images in a self-supervised fashion.
Images are presented to the model as a sequence of fixed-size patches, which are linearly embedded. One also adds a [CLS] token to the beginning of a sequence to use it for classification tasks. One also adds absolute position embeddings before feeding the sequence to the layers of the Transformer encoder.
Note that this model does not include any fine-tuned heads.
By pre-training the model, it learns an inner representation of images that can then be used to extract features useful for downstream tasks: if you have a dataset of labeled images for instance, you can train a standard classifier by placing a linear layer on top of the pre-trained encoder. One typically places a linear layer on top of the [CLS] token, as the last hidden state of this token can be seen as a representation of an entire image.
## Intended uses & limitations
You can use the model for classifying an image among one of the [1000 ImageNet labels](https://huggingface.co/datasets/huggingface/label-files/blob/main/imagenet-1k-id2label.json). See the [model hub](https://huggingface.co/models?search=facebook/dinov2) to look for
other fine-tuned versions on a task that interests you.
### How to use
Here is how to use this model:
```python
from transformers import AutoImageProcessor, AutoModelForImageClassification
from PIL import Image
import requests
url = 'http://images.cocodataset.org/val2017/000000039769.jpg'
image = Image.open(requests.get(url, stream=True).raw)
processor = AutoImageProcessor.from_pretrained('facebook/dinov2-giant-imagenet1k-1-layer')
model = AutoModelForImageClassification.from_pretrained('facebook/dinov2-giant-imagenet1k-1-layer')
inputs = processor(images=image, return_tensors="pt")
outputs = model(**inputs)
logits = outputs.logits
predicted_class_idx = logits.argmax(-1).item()
print("Predicted class:", model.config.id2label[predicted_class_idx])
```
### BibTeX entry and citation info
```bibtex
misc{oquab2023dinov2,
title={DINOv2: Learning Robust Visual Features without Supervision},
author={Maxime Oquab and Timothée Darcet and Théo Moutakanni and Huy Vo and Marc Szafraniec and Vasil Khalidov and Pierre Fernandez and Daniel Haziza and Francisco Massa and Alaaeldin El-Nouby and Mahmoud Assran and Nicolas Ballas and Wojciech Galuba and Russell Howes and Po-Yao Huang and Shang-Wen Li and Ishan Misra and Michael Rabbat and Vasu Sharma and Gabriel Synnaeve and Hu Xu and Hervé Jegou and Julien Mairal and Patrick Labatut and Armand Joulin and Piotr Bojanowski},
year={2023},
eprint={2304.07193},
archivePrefix={arXiv},
primaryClass={cs.CV}
}
``` |
Bakanayatsu/ponyDiffusion-V6-XL-Turbo-DPO | Bakanayatsu | "2024-02-13T17:52:05Z" | 34,908 | 8 | diffusers | [
"diffusers",
"safetensors",
"Safetensors",
"Text-to-Image",
"autotrain_compatible",
"endpoints_compatible",
"diffusers:StableDiffusionXLPipeline",
"region:us"
] | text-to-image | "2024-02-11T12:00:59Z" | ---
tags:
- Safetensors
- Text-to-Image
---
Same as the original [Pony Diffusion V6 XL Turbo DPO](https://civitai.com/models/257749?modelVersionId=298112) but in diffusers version |
bigscience/bloom-1b1 | bigscience | "2023-09-26T09:16:38Z" | 34,831 | 54 | transformers | [
"transformers",
"pytorch",
"jax",
"onnx",
"safetensors",
"bloom",
"text-generation",
"ak",
"ar",
"as",
"bm",
"bn",
"ca",
"code",
"en",
"es",
"eu",
"fon",
"fr",
"gu",
"hi",
"id",
"ig",
"ki",
"kn",
"lg",
"ln",
"ml",
"mr",
"ne",
"nso",
"ny",
"or",
"pa",
"pt",
"rn",
"rw",
"sn",
"st",
"sw",
"ta",
"te",
"tn",
"ts",
"tum",
"tw",
"ur",
"vi",
"wo",
"xh",
"yo",
"zh",
"zhs",
"zht",
"zu",
"arxiv:1909.08053",
"arxiv:2110.02861",
"arxiv:2108.12409",
"license:bigscience-bloom-rail-1.0",
"autotrain_compatible",
"endpoints_compatible",
"text-generation-inference",
"region:us"
] | text-generation | "2022-05-19T11:51:48Z" | ---
license: bigscience-bloom-rail-1.0
language:
- ak
- ar
- as
- bm
- bn
- ca
- code
- en
- es
- eu
- fon
- fr
- gu
- hi
- id
- ig
- ki
- kn
- lg
- ln
- ml
- mr
- ne
- nso
- ny
- or
- pa
- pt
- rn
- rw
- sn
- st
- sw
- ta
- te
- tn
- ts
- tum
- tw
- ur
- vi
- wo
- xh
- yo
- zh
- zhs
- zht
- zu
pipeline_tag: text-generation
---
<h1 style='text-align: center '>BLOOM LM</h1>
<h2 style='text-align: center '><em>BigScience Large Open-science Open-access Multilingual Language Model</em> </h2>
<h3 style='text-align: center '>Model Card</h3>
<img src="https://s3.amazonaws.com/moonup/production/uploads/1657124309515-5f17f0a0925b9863e28ad517.png" alt="BigScience Logo" width="800" style="margin-left:'auto' margin-right:'auto' display:'block'"/>
Version 1.0 / 26.May.2022
## Table of Contents
1. [Model Details](#model-details)
2. [Uses](#uses)
3. [Training Data](#training-data)
4. [Risks and Limitations](#risks-and-limitations)
5. [Evaluation](#evaluation)
6. [Recommendations](#recommendations)
7. [Glossary and Calculations](#glossary-and-calculations)
8. [More Information](#more-information)
9. [Model Card Authors](#model-card-authors)
## Model Details
### Basics
*This section provides information for anyone who wants to know about the model.*
<details>
<summary>Click to expand</summary> <br/>
**Developed by:** BigScience ([website](https://bigscience.huggingface.co))
* All collaborators are either volunteers or have an agreement with their employer. *(Further breakdown of participants forthcoming.)*
**Model Type:** Transformer-based Language Model
**Version:** 1.0.0
**Languages:** Multiple; see [training data](#training-data)
**License:** RAIL License v1.0 ([link](https://huggingface.co/spaces/bigscience/license))
**Release Date Estimate:** Monday, 11.July.2022
**Send Questions to:** bigscience-contact@googlegroups.com
**Cite as:** BigScience, _BigScience Language Open-science Open-access Multilingual (BLOOM) Language Model_. International, May 2021-May 2022
**Funded by:**
* The French government.
* Hugging Face ([website](https://huggingface.co)).
* Organizations of contributors. *(Further breakdown of organizations forthcoming.)*
</details>
### Technical Specifications
*This section provides information for people who work on model development.*
<details>
<summary>Click to expand</summary><br/>
Please see [the BLOOM training README](https://github.com/bigscience-workshop/bigscience/tree/master/train/tr11-176B-ml#readme) for full details on replicating training.
**Model Architecture:** Modified from Megatron-LM GPT2 (see [paper](https://arxiv.org/abs/1909.08053), [BLOOM Megatron code](https://github.com/bigscience-workshop/Megatron-DeepSpeed)):
* Decoder-only architecture
* Layer normalization applied to word embeddings layer (`StableEmbedding`; see [code](https://github.com/facebookresearch/bitsandbytes), [paper](https://arxiv.org/pdf/2110.02861.pdf))
* ALiBI positional encodings (see [paper](https://arxiv.org/pdf/2108.12409.pdf)), with GeLU activation functions
* 1,065,314,304 parameters:
* 385,351,680 embedding parameters
* 24 layers, 16 attention heads
* Hidden layers are 1536-dimensional
* Sequence length of 2048 tokens used (see [BLOOM tokenizer](https://huggingface.co/bigscience/tokenizer), [tokenizer description](#tokenization))
**Objective Function:** Cross Entropy with mean reduction (see [API documentation](https://pytorch.org/docs/stable/generated/torch.nn.CrossEntropyLoss.html#torch.nn.CrossEntropyLoss)).
**Compute infrastructure:** Jean Zay Public Supercomputer, provided by the French government (see [announcement](https://www.enseignementsup-recherche.gouv.fr/fr/signature-du-marche-d-acquisition-de-l-un-des-supercalculateurs-les-plus-puissants-d-europe-46733)).
* Hardware: 384 A100 80GB GPUs (48 nodes):
* Additional 32 A100 80GB GPUs (4 nodes) in reserve
* 8 GPUs per node Using NVLink 4 inter-gpu connects, 4 OmniPath links
* CPU: AMD
* CPU memory: 512GB per node
* GPU memory: 640GB per node
* Inter-node connect: Omni-Path Architecture (OPA)
* NCCL-communications network: a fully dedicated subnet
* Disc IO network: shared network with other types of nodes
* Software:
* Megatron-DeepSpeed ([Github link](https://github.com/bigscience-workshop/Megatron-DeepSpeed))
* DeepSpeed ([Github link](https://github.com/microsoft/DeepSpeed))
* PyTorch (pytorch-1.11 w/ CUDA-11.5; see [Github link](https://github.com/pytorch/pytorch))
* apex ([Github link](https://github.com/NVIDIA/apex))
#### **Training**
Training logs: [Tensorboard link](https://huggingface.co/tensorboard/bigscience/tr11d-760M-logs)
- Number of epochs: 1
- Dates:
- Started 11th March, 2022 11:42am PST
- Ended 5th July, 2022
- Estimated cost of training: Equivalent of $2-5M in cloud computing (including preliminary experiments and other model sizes)
- Server training location: Île-de-France, France
#### **Tokenization**
The BLOOM tokenizer ([link](https://huggingface.co/bigscience/tokenizer)) is a learned subword tokenizer trained using:
- A byte-level Byte Pair Encoding (BPE) algorithm
- A simple pre-tokenization rule, no normalization
- A vocabulary size of 250,680
It was trained on a subset of a preliminary version of the corpus using alpha-weighting per language.
</details>
### Environmental Impact
<details>
<summary>Click to expand</summary><br/>
The training supercomputer, Jean Zay ([website](http://www.idris.fr/eng/jean-zay/jean-zay-presentation-eng.html)), uses mostly nuclear energy. The heat generated by it is reused for heating campus housing.
**Estimated carbon emissions:** *(Forthcoming upon completion of training.)*
**Estimated electricity usage:** *(Forthcoming upon completion of training.)*
</details>
<p> </p>
## Uses
*This section addresses questions around how the model is intended to be used, discusses the foreseeable users of the model (including those affected by the model), and describes uses that are considered out of scope or misuse of the model.
It provides information for anyone considering using the model or who is affected by the model.*
<details>
<summary>Click to expand</summary><br/>
### Intended Use
This model is being created in order to enable public research on large language models (LLMs). LLMs are intended to be used for language generation or as a pretrained base model that can be further fine-tuned for specific tasks. Use cases below are not exhaustive.
#### **Direct Use**
- Text generation
- Exploring characteristics of language generated by a language model
- Examples: Cloze tests, counterfactuals, generations with reframings
#### **Downstream Use**
- Tasks that leverage language models include: Information Extraction, Question Answering, Summarization
### Misuse and Out-of-scope Use
*This section addresses what users ought not do with the model.*
See the [BLOOM License](https://huggingface.co/spaces/bigscience/license), Attachment A, for detailed usage restrictions. The below list is non-exhaustive, but lists some easily foreseeable problematic use cases.
#### **Out-of-scope Uses**
Using the model in [high-stakes](#high-stakes) settings is out of scope for this model. The model is not designed for [critical decisions](#critical-decisions) nor uses with any material consequences on an individual's livelihood or wellbeing. The model outputs content that appears factual but is not correct.
##### Out-of-scope Uses Include:
- Usage in biomedical domains, political and legal domains, or finance domains
- Usage for evaluating or scoring individuals, such as for employment, education, or credit
- Applying the model for critical automatic decisions, generating factual content, creating reliable summaries, or generating predictions that must be correct
#### **Misuse**
Intentionally using the model for harm, violating [human rights](#human-rights), or other kinds of malicious activities, is a misuse of this model. This includes:
- Spam generation
- Disinformation and influence operations
- Disparagement and defamation
- Harassment and abuse
- [Deception](#deception)
- Unconsented impersonation and imitation
- Unconsented surveillance
- Generating content without attribution to the model, as specified in the [RAIL License, Use Restrictions](https://huggingface.co/spaces/bigscience/license)
### Intended Users
#### **Direct Users**
- General Public
- Researchers
- Students
- Educators
- Engineers/developers
- Non-commercial entities
- Community advocates, including human and civil rights groups
#### Indirect Users
- Users of derivatives created by Direct Users, such as those using software with an [intended use](#intended-use)
- Users of [Derivatives of the Model, as described in the License](https://huggingface.co/spaces/bigscience/license)
#### Others Affected (Parties Prenantes)
- People and groups referred to by the LLM
- People and groups exposed to outputs of, or decisions based on, the LLM
- People and groups whose original work is included in the LLM
</details>
<p> </p>
## Training Data
*This section provides a high-level overview of the training data. It is relevant for anyone who wants to know the basics of what the model is learning.*
<details>
<summary>Click to expand</summary><br/>
Details for each dataset are provided in individual [Data Cards](https://huggingface.co/spaces/bigscience/BigScienceCorpus).
Training data includes:
- 45 natural languages
- 12 programming languages
- In 1.5TB of pre-processed text, converted into 350B unique tokens (see [the tokenizer section](#tokenization) for more.)
#### **Languages**
The pie chart shows the distribution of languages in training data.

The following table shows the further distribution of Niger-Congo and Indic languages in the training data.
<details>
<summary>Click to expand</summary><br/>
| Niger Congo | Percentage | | Indic | Percentage |
|----------------|------------ |------ |-----------|------------|
| Chi Tumbuka | 0.00002 | | Assamese | 0.01 |
| Kikuyu | 0.00004 | | Odia | 0.04 |
| Bambara | 0.00004 | | Gujarati | 0.04 |
| Akan | 0.00007 | | Marathi | 0.05 |
| Xitsonga | 0.00007 | | Punjabi | 0.05 |
| Sesotho | 0.00007 | | Kannada | 0.06 |
| Chi Chewa | 0.0001 | | Nepali | 0.07 |
| Setswana | 0.0002 | | Telugu | 0.09 |
| Northern Sotho | 0.0002 | | Malayalam | 0.10 |
| Fon | 0.0002 | | Urdu | 0.10 |
| Kirundi | 0.0003 | | Tamil | 0.20 |
| Wolof | 0.0004 | | Bengali | 0.50 |
| Kuganda | 0.0004 | | Hindi | 0.70 |
| Chi Shona | 0.001 |
| Isi Zulu | 0.001 |
| Igbo | 0.001 |
| Xhosa | 0.001 |
| Kinyarwanda | 0.003 |
| Yoruba | 0.006 |
| Swahili | 0.02 |
</details>
The following table shows the distribution of programming languages.
<details>
<summary>Click to expand</summary><br/>
| Extension | Language | Number of files |
|----------------|------------|-----------------|
| java | Java | 5,407,724 |
| php | PHP | 4,942,186 |
| cpp | C++ | 2,503,930 |
| py | Python | 2,435,072 |
| js | JavaScript | 1,905,518 |
| cs | C# | 1,577,347 |
| rb | Ruby | 6,78,413 |
| cc | C++ | 443,054 |
| hpp | C++ | 391,048 |
| lua | Lua | 352,317 |
| go | GO | 227,763 |
| ts | TypeScript | 195,254 |
| C | C | 134,537 |
| scala | Scala | 92,052 |
| hh | C++ | 67,161 |
| H | C++ | 55,899 |
| tsx | TypeScript | 33,107 |
| rs | Rust | 29,693 |
| phpt | PHP | 9,702 |
| c++ | C++ | 1,342 |
| h++ | C++ | 791 |
| php3 | PHP | 540 |
| phps | PHP | 270 |
| php5 | PHP | 166 |
| php4 | PHP | 29 |
</details>
</details>
<p> </p>
## Risks and Limitations
*This section identifies foreseeable harms and misunderstandings.*
<details>
<summary>Click to expand</summary><br/>
Model may:
- Overrepresent some viewpoints and underrepresent others
- Contain stereotypes
- Contain [personal information](#personal-data-and-information)
- Generate:
- Hateful, abusive, or violent language
- Discriminatory or prejudicial language
- Content that may not be appropriate for all settings, including sexual content
- Make errors, including producing incorrect information as if it were factual
- Generate irrelevant or repetitive outputs
</details>
<p> </p>
## Evaluation
*This section describes the evaluation protocols and provides the results.*
<details>
<summary>Click to expand</summary><br/>
### Metrics
*This section describes the different ways performance is calculated and why.*
Includes:
| Metric | Why chosen |
|--------------------|--------------------------------------------------------------------|
| [Perplexity](#perplexity) | Standard metric for quantifying model improvements during training |
| Cross Entropy [Loss](#loss) | Standard objective for language models. |
And multiple different metrics for specific tasks. _(More evaluation metrics forthcoming upon completion of evaluation protocol.)_
### Factors
*This section lists some different aspects of BLOOM models. Its focus is on those aspects that are likely to give rise to high variance in model behavior.*
- Language, such as English or Yoruba
- Domain, such as newswire or stories
- Demographic characteristics, such as gender or nationality
### Results
*Results are based on the [Factors](#factors) and [Metrics](#metrics).*
**Train-time Evaluation:**
As of 25.May.2022, 15:00 PST:
- Training Loss: 2.7
- Validation Loss: 3.1
- Perplexity: 21.9
(More evaluation scores forthcoming at the end of model training.)
</details>
<p> </p>
## Recommendations
*This section provides information on warnings and potential mitigations.*
<details>
<summary>Click to expand</summary><br/>
- Indirect users should be made aware when the content they're working with is created by the LLM.
- Users should be aware of [Risks and Limitations](#risks-and-limitations), and include an appropriate age disclaimer or blocking interface as necessary.
- Models pretrained with the LLM should include an updated Model Card.
- Users of the model should provide mechanisms for those affected to provide feedback, such as an email address for comments.
</details>
<p> </p>
## Glossary and Calculations
*This section defines common terms and how metrics are calculated.*
<details>
<summary>Click to expand</summary><br/>
- <a name="loss">**Loss:**</a> A calculation of the difference between what the model has learned and what the data shows ("groundtruth"). The lower the loss, the better. The training process aims to minimize the loss.
- <a name="perplexity">**Perplexity:**</a> This is based on what the model estimates the probability of new data is. The lower the perplexity, the better. If the model is 100% correct at predicting the next token it will see, then the perplexity is 1. Mathematically this is calculated using entropy.
- <a name="high-stakes">**High-stakes settings:**</a> Such as those identified as "high-risk AI systems" and "unacceptable risk AI systems" in the European Union's proposed [Artificial Intelligence (AI) Act](https://artificialintelligenceact.eu/annexes/).
- <a name="critical-decisions">**Critical decisions:**</a> Such as those defined in [the United States' proposed Algorithmic Accountability Act](https://www.congress.gov/117/bills/s3572/BILLS-117s3572is.pdf).
- <a name="human-rights">**Human rights:**</a> Includes those rights defined in the [Universal Declaration of Human Rights](https://www.un.org/sites/un2.un.org/files/2021/03/udhr.pdf).
- <a name="personal-data-and-information">**Personal Data and Personal Information:**</a> Personal data and information is defined in multiple data protection regulations, such as "[personal data](https://gdpr-info.eu/issues/personal-data/)" in the [European Union's General Data Protection Regulation](https://gdpr-info.eu); and "personal information" in the Republic of South Africa's [Protection of Personal Information Act](https://www.gov.za/sites/default/files/gcis_document/201409/3706726-11act4of2013popi.pdf), The People's Republic of China's [Personal information protection law](http://en.npc.gov.cn.cdurl.cn/2021-12/29/c_694559.htm).
- <a name="sensitive-characteristics">**Sensitive characteristics:**</a> This includes specifically protected categories in human rights (see [UHDR, Article 2](https://www.un.org/sites/un2.un.org/files/2021/03/udhr.pdf)) and personal information regulation (see GDPR, [Article 9; Protection of Personal Information Act, Chapter 1](https://www.gov.za/sites/default/files/gcis_document/201409/3706726-11act4of2013popi.pdf))
- <a name="deception">**Deception:**</a> Doing something to intentionally mislead individuals to believe something that is false, such as by creating deadbots or chatbots on social media posing as real people, or generating text documents without making consumers aware that the text is machine generated.
</details>
<p> </p>
## More Information
<details>
<summary>Click to expand</summary><br/>
### Dataset Creation
Blog post detailing the design choices during the dataset creation: https://bigscience.huggingface.co/blog/building-a-tb-scale-multilingual-dataset-for-language-modeling
### Technical Specifications
Blog post summarizing how the architecture, size, shape, and pre-training duration where selected: https://bigscience.huggingface.co/blog/what-language-model-to-train-if-you-have-two-million-gpu-hours
More details on the architecture/optimizer: https://github.com/bigscience-workshop/bigscience/tree/master/train/tr11-176B-ml
Blog post on the hardware/engineering side: https://bigscience.huggingface.co/blog/which-hardware-to-train-a-176b-parameters-model
Details on the distributed setup used for the training: https://github.com/bigscience-workshop/bigscience/tree/master/train/tr11-176B-ml
Tensorboard updated during the training: https://huggingface.co/bigscience/tr11-176B-ml-logs/tensorboard#scalars&tagFilter=loss
Insights on how to approach training, negative results: https://github.com/bigscience-workshop/bigscience/blob/master/train/lessons-learned.md
Details on the obstacles overcome during the preparation on the engineering side (instabilities, optimization of training throughput, so many technical tricks and questions): https://github.com/bigscience-workshop/bigscience/blob/master/train/tr11-176B-ml/chronicles.md
### Initial Results
Initial prompting experiments using interim checkpoints: https://huggingface.co/spaces/bigscience/bloom-book
</details>
<p> </p>
## Model Card Authors
*Ordered roughly chronologically and by amount of time spent.*
Margaret Mitchell, Giada Pistilli, Yacine Jernite, Ezinwanne Ozoani, Marissa Gerchick, Nazneen Rajani, Sasha Luccioni, Irene Solaiman, Maraim Masoud, Somaieh Nikpoor, Carlos Muñoz Ferrandis, Stas Bekman, Christopher Akiki, Danish Contractor, David Lansky, Angelina McMillan-Major, Tristan Thrush, Suzana Ilić, Gérard Dupont, Shayne Longpre, Manan Dey, Stella Biderman, Douwe Kiela, Emi Baylor, Teven Le Scao, Aaron Gokaslan, Julien Launay, Niklas Muennighoff
|
mradermacher/dolphin-2.9.3-Yi-1.5-34B-32k-GGUF | mradermacher | "2024-06-24T16:29:58Z" | 34,817 | 0 | transformers | [
"transformers",
"gguf",
"generated_from_trainer",
"axolotl",
"en",
"dataset:cognitivecomputations/Dolphin-2.9",
"dataset:teknium/OpenHermes-2.5",
"dataset:m-a-p/CodeFeedback-Filtered-Instruction",
"dataset:cognitivecomputations/dolphin-coder",
"dataset:cognitivecomputations/samantha-data",
"dataset:microsoft/orca-math-word-problems-200k",
"dataset:Locutusque/function-calling-chatml",
"dataset:internlm/Agent-FLAN",
"base_model:cognitivecomputations/dolphin-2.9.3-Yi-1.5-34B-32k",
"license:apache-2.0",
"endpoints_compatible",
"region:us"
] | null | "2024-06-24T14:20:22Z" | ---
base_model: cognitivecomputations/dolphin-2.9.3-Yi-1.5-34B-32k
datasets:
- cognitivecomputations/Dolphin-2.9
- teknium/OpenHermes-2.5
- m-a-p/CodeFeedback-Filtered-Instruction
- cognitivecomputations/dolphin-coder
- cognitivecomputations/samantha-data
- microsoft/orca-math-word-problems-200k
- Locutusque/function-calling-chatml
- internlm/Agent-FLAN
language:
- en
library_name: transformers
license: apache-2.0
quantized_by: mradermacher
tags:
- generated_from_trainer
- axolotl
---
## About
<!-- ### quantize_version: 2 -->
<!-- ### output_tensor_quantised: 1 -->
<!-- ### convert_type: hf -->
<!-- ### vocab_type: -->
<!-- ### tags: -->
static quants of https://huggingface.co/cognitivecomputations/dolphin-2.9.3-Yi-1.5-34B-32k
<!-- provided-files -->
weighted/imatrix quants are available at https://huggingface.co/mradermacher/dolphin-2.9.3-Yi-1.5-34B-32k-i1-GGUF
## Usage
If you are unsure how to use GGUF files, refer to one of [TheBloke's
READMEs](https://huggingface.co/TheBloke/KafkaLM-70B-German-V0.1-GGUF) for
more details, including on how to concatenate multi-part files.
## Provided Quants
(sorted by size, not necessarily quality. IQ-quants are often preferable over similar sized non-IQ quants)
| Link | Type | Size/GB | Notes |
|:-----|:-----|--------:|:------|
| [GGUF](https://huggingface.co/mradermacher/dolphin-2.9.3-Yi-1.5-34B-32k-GGUF/resolve/main/dolphin-2.9.3-Yi-1.5-34B-32k.Q2_K.gguf) | Q2_K | 12.9 | |
| [GGUF](https://huggingface.co/mradermacher/dolphin-2.9.3-Yi-1.5-34B-32k-GGUF/resolve/main/dolphin-2.9.3-Yi-1.5-34B-32k.IQ3_XS.gguf) | IQ3_XS | 14.3 | |
| [GGUF](https://huggingface.co/mradermacher/dolphin-2.9.3-Yi-1.5-34B-32k-GGUF/resolve/main/dolphin-2.9.3-Yi-1.5-34B-32k.Q3_K_S.gguf) | Q3_K_S | 15.1 | |
| [GGUF](https://huggingface.co/mradermacher/dolphin-2.9.3-Yi-1.5-34B-32k-GGUF/resolve/main/dolphin-2.9.3-Yi-1.5-34B-32k.IQ3_S.gguf) | IQ3_S | 15.1 | beats Q3_K* |
| [GGUF](https://huggingface.co/mradermacher/dolphin-2.9.3-Yi-1.5-34B-32k-GGUF/resolve/main/dolphin-2.9.3-Yi-1.5-34B-32k.IQ3_M.gguf) | IQ3_M | 15.7 | |
| [GGUF](https://huggingface.co/mradermacher/dolphin-2.9.3-Yi-1.5-34B-32k-GGUF/resolve/main/dolphin-2.9.3-Yi-1.5-34B-32k.Q3_K_M.gguf) | Q3_K_M | 16.8 | lower quality |
| [GGUF](https://huggingface.co/mradermacher/dolphin-2.9.3-Yi-1.5-34B-32k-GGUF/resolve/main/dolphin-2.9.3-Yi-1.5-34B-32k.Q3_K_L.gguf) | Q3_K_L | 18.2 | |
| [GGUF](https://huggingface.co/mradermacher/dolphin-2.9.3-Yi-1.5-34B-32k-GGUF/resolve/main/dolphin-2.9.3-Yi-1.5-34B-32k.IQ4_XS.gguf) | IQ4_XS | 18.7 | |
| [GGUF](https://huggingface.co/mradermacher/dolphin-2.9.3-Yi-1.5-34B-32k-GGUF/resolve/main/dolphin-2.9.3-Yi-1.5-34B-32k.Q4_K_S.gguf) | Q4_K_S | 19.7 | fast, recommended |
| [GGUF](https://huggingface.co/mradermacher/dolphin-2.9.3-Yi-1.5-34B-32k-GGUF/resolve/main/dolphin-2.9.3-Yi-1.5-34B-32k.Q4_K_M.gguf) | Q4_K_M | 20.8 | fast, recommended |
| [GGUF](https://huggingface.co/mradermacher/dolphin-2.9.3-Yi-1.5-34B-32k-GGUF/resolve/main/dolphin-2.9.3-Yi-1.5-34B-32k.Q5_K_S.gguf) | Q5_K_S | 23.8 | |
| [GGUF](https://huggingface.co/mradermacher/dolphin-2.9.3-Yi-1.5-34B-32k-GGUF/resolve/main/dolphin-2.9.3-Yi-1.5-34B-32k.Q5_K_M.gguf) | Q5_K_M | 24.4 | |
| [GGUF](https://huggingface.co/mradermacher/dolphin-2.9.3-Yi-1.5-34B-32k-GGUF/resolve/main/dolphin-2.9.3-Yi-1.5-34B-32k.Q6_K.gguf) | Q6_K | 28.3 | very good quality |
| [GGUF](https://huggingface.co/mradermacher/dolphin-2.9.3-Yi-1.5-34B-32k-GGUF/resolve/main/dolphin-2.9.3-Yi-1.5-34B-32k.Q8_0.gguf) | Q8_0 | 36.6 | fast, best quality |
Here is a handy graph by ikawrakow comparing some lower-quality quant
types (lower is better):

And here are Artefact2's thoughts on the matter:
https://gist.github.com/Artefact2/b5f810600771265fc1e39442288e8ec9
## FAQ / Model Request
See https://huggingface.co/mradermacher/model_requests for some answers to
questions you might have and/or if you want some other model quantized.
## Thanks
I thank my company, [nethype GmbH](https://www.nethype.de/), for letting
me use its servers and providing upgrades to my workstation to enable
this work in my free time.
<!-- end -->
|
sentence-transformers/gtr-t5-base | sentence-transformers | "2024-03-27T10:40:20Z" | 34,767 | 16 | sentence-transformers | [
"sentence-transformers",
"pytorch",
"safetensors",
"t5",
"feature-extraction",
"sentence-similarity",
"en",
"arxiv:2112.07899",
"license:apache-2.0",
"autotrain_compatible",
"endpoints_compatible",
"region:us"
] | sentence-similarity | "2022-03-02T23:29:05Z" | ---
language: en
license: apache-2.0
library_name: sentence-transformers
tags:
- sentence-transformers
- feature-extraction
- sentence-similarity
pipeline_tag: sentence-similarity
---
# sentence-transformers/gtr-t5-base
This is a [sentence-transformers](https://www.SBERT.net) model: It maps sentences & paragraphs to a 768 dimensional dense vector space. The model was specifically trained for the task of semantic search.
This model was converted from the Tensorflow model [gtr-base-1](https://tfhub.dev/google/gtr/gtr-base/1) to PyTorch. When using this model, have a look at the publication: [Large Dual Encoders Are Generalizable Retrievers](https://arxiv.org/abs/2112.07899). The tfhub model and this PyTorch model can produce slightly different embeddings, however, when run on the same benchmarks, they produce identical results.
The model uses only the encoder from a T5-base model. The weights are stored in FP16.
## Usage (Sentence-Transformers)
Using this model becomes easy when you have [sentence-transformers](https://www.SBERT.net) installed:
```
pip install -U sentence-transformers
```
Then you can use the model like this:
```python
from sentence_transformers import SentenceTransformer
sentences = ["This is an example sentence", "Each sentence is converted"]
model = SentenceTransformer('sentence-transformers/gtr-t5-base')
embeddings = model.encode(sentences)
print(embeddings)
```
The model requires sentence-transformers version 2.2.0 or newer.
## Evaluation Results
For an automated evaluation of this model, see the *Sentence Embeddings Benchmark*: [https://seb.sbert.net](https://seb.sbert.net?model_name=sentence-transformers/gtr-t5-base)
## Citing & Authors
If you find this model helpful, please cite the respective publication:
[Large Dual Encoders Are Generalizable Retrievers](https://arxiv.org/abs/2112.07899)
|
google/mt5-xl | google | "2023-01-24T16:37:35Z" | 34,663 | 18 | transformers | [
"transformers",
"pytorch",
"tf",
"jax",
"mt5",
"text2text-generation",
"multilingual",
"af",
"am",
"ar",
"az",
"be",
"bg",
"bn",
"ca",
"ceb",
"co",
"cs",
"cy",
"da",
"de",
"el",
"en",
"eo",
"es",
"et",
"eu",
"fa",
"fi",
"fil",
"fr",
"fy",
"ga",
"gd",
"gl",
"gu",
"ha",
"haw",
"hi",
"hmn",
"ht",
"hu",
"hy",
"ig",
"is",
"it",
"iw",
"ja",
"jv",
"ka",
"kk",
"km",
"kn",
"ko",
"ku",
"ky",
"la",
"lb",
"lo",
"lt",
"lv",
"mg",
"mi",
"mk",
"ml",
"mn",
"mr",
"ms",
"mt",
"my",
"ne",
"nl",
"no",
"ny",
"pa",
"pl",
"ps",
"pt",
"ro",
"ru",
"sd",
"si",
"sk",
"sl",
"sm",
"sn",
"so",
"sq",
"sr",
"st",
"su",
"sv",
"sw",
"ta",
"te",
"tg",
"th",
"tr",
"uk",
"und",
"ur",
"uz",
"vi",
"xh",
"yi",
"yo",
"zh",
"zu",
"dataset:mc4",
"arxiv:2010.11934",
"license:apache-2.0",
"autotrain_compatible",
"endpoints_compatible",
"region:us"
] | text2text-generation | "2022-03-02T23:29:05Z" | ---
language:
- multilingual
- af
- am
- ar
- az
- be
- bg
- bn
- ca
- ceb
- co
- cs
- cy
- da
- de
- el
- en
- eo
- es
- et
- eu
- fa
- fi
- fil
- fr
- fy
- ga
- gd
- gl
- gu
- ha
- haw
- hi
- hmn
- ht
- hu
- hy
- ig
- is
- it
- iw
- ja
- jv
- ka
- kk
- km
- kn
- ko
- ku
- ky
- la
- lb
- lo
- lt
- lv
- mg
- mi
- mk
- ml
- mn
- mr
- ms
- mt
- my
- ne
- nl
- no
- ny
- pa
- pl
- ps
- pt
- ro
- ru
- sd
- si
- sk
- sl
- sm
- sn
- so
- sq
- sr
- st
- su
- sv
- sw
- ta
- te
- tg
- th
- tr
- uk
- und
- ur
- uz
- vi
- xh
- yi
- yo
- zh
- zu
datasets:
- mc4
license: apache-2.0
---
[Google's mT5](https://github.com/google-research/multilingual-t5)
mT5 is pretrained on the [mC4](https://www.tensorflow.org/datasets/catalog/c4#c4multilingual) corpus, covering 101 languages:
Afrikaans, Albanian, Amharic, Arabic, Armenian, Azerbaijani, Basque, Belarusian, Bengali, Bulgarian, Burmese, Catalan, Cebuano, Chichewa, Chinese, Corsican, Czech, Danish, Dutch, English, Esperanto, Estonian, Filipino, Finnish, French, Galician, Georgian, German, Greek, Gujarati, Haitian Creole, Hausa, Hawaiian, Hebrew, Hindi, Hmong, Hungarian, Icelandic, Igbo, Indonesian, Irish, Italian, Japanese, Javanese, Kannada, Kazakh, Khmer, Korean, Kurdish, Kyrgyz, Lao, Latin, Latvian, Lithuanian, Luxembourgish, Macedonian, Malagasy, Malay, Malayalam, Maltese, Maori, Marathi, Mongolian, Nepali, Norwegian, Pashto, Persian, Polish, Portuguese, Punjabi, Romanian, Russian, Samoan, Scottish Gaelic, Serbian, Shona, Sindhi, Sinhala, Slovak, Slovenian, Somali, Sotho, Spanish, Sundanese, Swahili, Swedish, Tajik, Tamil, Telugu, Thai, Turkish, Ukrainian, Urdu, Uzbek, Vietnamese, Welsh, West Frisian, Xhosa, Yiddish, Yoruba, Zulu.
**Note**: mT5 was only pre-trained on mC4 excluding any supervised training. Therefore, this model has to be fine-tuned before it is useable on a downstream task.
Pretraining Dataset: [mC4](https://www.tensorflow.org/datasets/catalog/c4#c4multilingual)
Other Community Checkpoints: [here](https://huggingface.co/models?search=mt5)
Paper: [mT5: A massively multilingual pre-trained text-to-text transformer](https://arxiv.org/abs/2010.11934)
Authors: *Linting Xue, Noah Constant, Adam Roberts, Mihir Kale, Rami Al-Rfou, Aditya Siddhant, Aditya Barua, Colin Raffel*
## Abstract
The recent "Text-to-Text Transfer Transformer" (T5) leveraged a unified text-to-text format and scale to attain state-of-the-art results on a wide variety of English-language NLP tasks. In this paper, we introduce mT5, a multilingual variant of T5 that was pre-trained on a new Common Crawl-based dataset covering 101 languages. We describe the design and modified training of mT5 and demonstrate its state-of-the-art performance on many multilingual benchmarks. All of the code and model checkpoints used in this work are publicly available. |
mradermacher/L3-Blackened-Sunfall-15B-i1-GGUF | mradermacher | "2024-06-29T12:05:32Z" | 34,484 | 1 | transformers | [
"transformers",
"gguf",
"merge",
"mergekit",
"lazymergekit",
"crestf411/L3-8B-sunfall-v0.4-stheno-v3.2",
"Hastagaras/Jamet-8B-L3-MK.V-Blackroot",
"TheDrummer/Llama-3SOME-8B-v2",
"not-for-all-audiences",
"en",
"base_model:kromeurus/L3-Blackened-Sunfall-15B",
"endpoints_compatible",
"region:us"
] | null | "2024-06-29T06:41:46Z" | ---
base_model: kromeurus/L3-Blackened-Sunfall-15B
language:
- en
library_name: transformers
quantized_by: mradermacher
tags:
- merge
- mergekit
- lazymergekit
- crestf411/L3-8B-sunfall-v0.4-stheno-v3.2
- Hastagaras/Jamet-8B-L3-MK.V-Blackroot
- TheDrummer/Llama-3SOME-8B-v2
- not-for-all-audiences
---
## About
<!-- ### quantize_version: 2 -->
<!-- ### output_tensor_quantised: 1 -->
<!-- ### convert_type: hf -->
<!-- ### vocab_type: -->
<!-- ### tags: nicoboss -->
weighted/imatrix quants of https://huggingface.co/kromeurus/L3-Blackened-Sunfall-15B
<!-- provided-files -->
static quants are available at https://huggingface.co/mradermacher/L3-Blackened-Sunfall-15B-GGUF
## Usage
If you are unsure how to use GGUF files, refer to one of [TheBloke's
READMEs](https://huggingface.co/TheBloke/KafkaLM-70B-German-V0.1-GGUF) for
more details, including on how to concatenate multi-part files.
## Provided Quants
(sorted by size, not necessarily quality. IQ-quants are often preferable over similar sized non-IQ quants)
| Link | Type | Size/GB | Notes |
|:-----|:-----|--------:|:------|
| [GGUF](https://huggingface.co/mradermacher/L3-Blackened-Sunfall-15B-i1-GGUF/resolve/main/L3-Blackened-Sunfall-15B.i1-IQ1_S.gguf) | i1-IQ1_S | 3.6 | for the desperate |
| [GGUF](https://huggingface.co/mradermacher/L3-Blackened-Sunfall-15B-i1-GGUF/resolve/main/L3-Blackened-Sunfall-15B.i1-IQ1_M.gguf) | i1-IQ1_M | 3.9 | mostly desperate |
| [GGUF](https://huggingface.co/mradermacher/L3-Blackened-Sunfall-15B-i1-GGUF/resolve/main/L3-Blackened-Sunfall-15B.i1-IQ2_XXS.gguf) | i1-IQ2_XXS | 4.4 | |
| [GGUF](https://huggingface.co/mradermacher/L3-Blackened-Sunfall-15B-i1-GGUF/resolve/main/L3-Blackened-Sunfall-15B.i1-IQ2_XS.gguf) | i1-IQ2_XS | 4.8 | |
| [GGUF](https://huggingface.co/mradermacher/L3-Blackened-Sunfall-15B-i1-GGUF/resolve/main/L3-Blackened-Sunfall-15B.i1-IQ2_S.gguf) | i1-IQ2_S | 5.0 | |
| [GGUF](https://huggingface.co/mradermacher/L3-Blackened-Sunfall-15B-i1-GGUF/resolve/main/L3-Blackened-Sunfall-15B.i1-IQ2_M.gguf) | i1-IQ2_M | 5.4 | |
| [GGUF](https://huggingface.co/mradermacher/L3-Blackened-Sunfall-15B-i1-GGUF/resolve/main/L3-Blackened-Sunfall-15B.i1-Q2_K.gguf) | i1-Q2_K | 5.8 | IQ3_XXS probably better |
| [GGUF](https://huggingface.co/mradermacher/L3-Blackened-Sunfall-15B-i1-GGUF/resolve/main/L3-Blackened-Sunfall-15B.i1-IQ3_XXS.gguf) | i1-IQ3_XXS | 6.1 | lower quality |
| [GGUF](https://huggingface.co/mradermacher/L3-Blackened-Sunfall-15B-i1-GGUF/resolve/main/L3-Blackened-Sunfall-15B.i1-IQ3_XS.gguf) | i1-IQ3_XS | 6.5 | |
| [GGUF](https://huggingface.co/mradermacher/L3-Blackened-Sunfall-15B-i1-GGUF/resolve/main/L3-Blackened-Sunfall-15B.i1-Q3_K_S.gguf) | i1-Q3_K_S | 6.8 | IQ3_XS probably better |
| [GGUF](https://huggingface.co/mradermacher/L3-Blackened-Sunfall-15B-i1-GGUF/resolve/main/L3-Blackened-Sunfall-15B.i1-IQ3_S.gguf) | i1-IQ3_S | 6.8 | beats Q3_K* |
| [GGUF](https://huggingface.co/mradermacher/L3-Blackened-Sunfall-15B-i1-GGUF/resolve/main/L3-Blackened-Sunfall-15B.i1-IQ3_M.gguf) | i1-IQ3_M | 7.0 | |
| [GGUF](https://huggingface.co/mradermacher/L3-Blackened-Sunfall-15B-i1-GGUF/resolve/main/L3-Blackened-Sunfall-15B.i1-Q3_K_M.gguf) | i1-Q3_K_M | 7.5 | IQ3_S probably better |
| [GGUF](https://huggingface.co/mradermacher/L3-Blackened-Sunfall-15B-i1-GGUF/resolve/main/L3-Blackened-Sunfall-15B.i1-Q3_K_L.gguf) | i1-Q3_K_L | 8.1 | IQ3_M probably better |
| [GGUF](https://huggingface.co/mradermacher/L3-Blackened-Sunfall-15B-i1-GGUF/resolve/main/L3-Blackened-Sunfall-15B.i1-IQ4_XS.gguf) | i1-IQ4_XS | 8.3 | |
| [GGUF](https://huggingface.co/mradermacher/L3-Blackened-Sunfall-15B-i1-GGUF/resolve/main/L3-Blackened-Sunfall-15B.i1-Q4_0.gguf) | i1-Q4_0 | 8.7 | fast, low quality |
| [GGUF](https://huggingface.co/mradermacher/L3-Blackened-Sunfall-15B-i1-GGUF/resolve/main/L3-Blackened-Sunfall-15B.i1-Q4_K_S.gguf) | i1-Q4_K_S | 8.7 | optimal size/speed/quality |
| [GGUF](https://huggingface.co/mradermacher/L3-Blackened-Sunfall-15B-i1-GGUF/resolve/main/L3-Blackened-Sunfall-15B.i1-Q4_K_M.gguf) | i1-Q4_K_M | 9.2 | fast, recommended |
| [GGUF](https://huggingface.co/mradermacher/L3-Blackened-Sunfall-15B-i1-GGUF/resolve/main/L3-Blackened-Sunfall-15B.i1-Q5_K_S.gguf) | i1-Q5_K_S | 10.5 | |
| [GGUF](https://huggingface.co/mradermacher/L3-Blackened-Sunfall-15B-i1-GGUF/resolve/main/L3-Blackened-Sunfall-15B.i1-Q5_K_M.gguf) | i1-Q5_K_M | 10.8 | |
| [GGUF](https://huggingface.co/mradermacher/L3-Blackened-Sunfall-15B-i1-GGUF/resolve/main/L3-Blackened-Sunfall-15B.i1-Q6_K.gguf) | i1-Q6_K | 12.4 | practically like static Q6_K |
Here is a handy graph by ikawrakow comparing some lower-quality quant
types (lower is better):

And here are Artefact2's thoughts on the matter:
https://gist.github.com/Artefact2/b5f810600771265fc1e39442288e8ec9
## FAQ / Model Request
See https://huggingface.co/mradermacher/model_requests for some answers to
questions you might have and/or if you want some other model quantized.
## Thanks
I thank my company, [nethype GmbH](https://www.nethype.de/), for letting
me use its servers and providing upgrades to my workstation to enable
this work in my free time. Additional thanks to [@nicoboss](https://huggingface.co/nicoboss) for giving me access to his hardware for calculating the imatrix for these quants.
<!-- end -->
|
superb/hubert-base-superb-ks | superb | "2021-11-04T16:03:26Z" | 34,449 | 4 | transformers | [
"transformers",
"pytorch",
"hubert",
"audio-classification",
"speech",
"audio",
"en",
"dataset:superb",
"arxiv:2105.01051",
"license:apache-2.0",
"endpoints_compatible",
"region:us"
] | audio-classification | "2022-03-02T23:29:05Z" | ---
language: en
datasets:
- superb
tags:
- speech
- audio
- hubert
- audio-classification
license: apache-2.0
widget:
- example_title: Speech Commands "down"
src: https://cdn-media.huggingface.co/speech_samples/keyword_spotting_down.wav
- example_title: Speech Commands "go"
src: https://cdn-media.huggingface.co/speech_samples/keyword_spotting_go.wav
---
# Hubert-Base for Keyword Spotting
## Model description
This is a ported version of [S3PRL's Hubert for the SUPERB Keyword Spotting task](https://github.com/s3prl/s3prl/tree/master/s3prl/downstream/speech_commands).
The base model is [hubert-base-ls960](https://huggingface.co/facebook/hubert-base-ls960), which is pretrained on 16kHz
sampled speech audio. When using the model make sure that your speech input is also sampled at 16Khz.
For more information refer to [SUPERB: Speech processing Universal PERformance Benchmark](https://arxiv.org/abs/2105.01051)
## Task and dataset description
Keyword Spotting (KS) detects preregistered keywords by classifying utterances into a predefined set of
words. The task is usually performed on-device for the fast response time. Thus, accuracy, model size, and
inference time are all crucial. SUPERB uses the widely used
[Speech Commands dataset v1.0](https://www.tensorflow.org/datasets/catalog/speech_commands) for the task.
The dataset consists of ten classes of keywords, a class for silence, and an unknown class to include the
false positive.
For the original model's training and evaluation instructions refer to the
[S3PRL downstream task README](https://github.com/s3prl/s3prl/tree/master/s3prl/downstream#ks-keyword-spotting).
## Usage examples
You can use the model via the Audio Classification pipeline:
```python
from datasets import load_dataset
from transformers import pipeline
dataset = load_dataset("anton-l/superb_demo", "ks", split="test")
classifier = pipeline("audio-classification", model="superb/hubert-base-superb-ks")
labels = classifier(dataset[0]["file"], top_k=5)
```
Or use the model directly:
```python
import torch
from datasets import load_dataset
from transformers import HubertForSequenceClassification, Wav2Vec2FeatureExtractor
from torchaudio.sox_effects import apply_effects_file
effects = [["channels", "1"], ["rate", "16000"], ["gain", "-3.0"]]
def map_to_array(example):
speech, _ = apply_effects_file(example["file"], effects)
example["speech"] = speech.squeeze(0).numpy()
return example
# load a demo dataset and read audio files
dataset = load_dataset("anton-l/superb_demo", "ks", split="test")
dataset = dataset.map(map_to_array)
model = HubertForSequenceClassification.from_pretrained("superb/hubert-base-superb-ks")
feature_extractor = Wav2Vec2FeatureExtractor.from_pretrained("superb/hubert-base-superb-ks")
# compute attention masks and normalize the waveform if needed
inputs = feature_extractor(dataset[:4]["speech"], sampling_rate=16000, padding=True, return_tensors="pt")
logits = model(**inputs).logits
predicted_ids = torch.argmax(logits, dim=-1)
labels = [model.config.id2label[_id] for _id in predicted_ids.tolist()]
```
## Eval results
The evaluation metric is accuracy.
| | **s3prl** | **transformers** |
|--------|-----------|------------------|
|**test**| `0.9630` | `0.9672` |
### BibTeX entry and citation info
```bibtex
@article{yang2021superb,
title={SUPERB: Speech processing Universal PERformance Benchmark},
author={Yang, Shu-wen and Chi, Po-Han and Chuang, Yung-Sung and Lai, Cheng-I Jeff and Lakhotia, Kushal and Lin, Yist Y and Liu, Andy T and Shi, Jiatong and Chang, Xuankai and Lin, Guan-Ting and others},
journal={arXiv preprint arXiv:2105.01051},
year={2021}
}
``` |
google/bert_uncased_L-4_H-256_A-4 | google | "2021-05-19T17:30:27Z" | 34,411 | 5 | transformers | [
"transformers",
"pytorch",
"jax",
"bert",
"arxiv:1908.08962",
"license:apache-2.0",
"endpoints_compatible",
"region:us"
] | null | "2022-03-02T23:29:05Z" | ---
thumbnail: https://huggingface.co/front/thumbnails/google.png
license: apache-2.0
---
BERT Miniatures
===
This is the set of 24 BERT models referenced in [Well-Read Students Learn Better: On the Importance of Pre-training Compact Models](https://arxiv.org/abs/1908.08962) (English only, uncased, trained with WordPiece masking).
We have shown that the standard BERT recipe (including model architecture and training objective) is effective on a wide range of model sizes, beyond BERT-Base and BERT-Large. The smaller BERT models are intended for environments with restricted computational resources. They can be fine-tuned in the same manner as the original BERT models. However, they are most effective in the context of knowledge distillation, where the fine-tuning labels are produced by a larger and more accurate teacher.
Our goal is to enable research in institutions with fewer computational resources and encourage the community to seek directions of innovation alternative to increasing model capacity.
You can download the 24 BERT miniatures either from the [official BERT Github page](https://github.com/google-research/bert/), or via HuggingFace from the links below:
| |H=128|H=256|H=512|H=768|
|---|:---:|:---:|:---:|:---:|
| **L=2** |[**2/128 (BERT-Tiny)**][2_128]|[2/256][2_256]|[2/512][2_512]|[2/768][2_768]|
| **L=4** |[4/128][4_128]|[**4/256 (BERT-Mini)**][4_256]|[**4/512 (BERT-Small)**][4_512]|[4/768][4_768]|
| **L=6** |[6/128][6_128]|[6/256][6_256]|[6/512][6_512]|[6/768][6_768]|
| **L=8** |[8/128][8_128]|[8/256][8_256]|[**8/512 (BERT-Medium)**][8_512]|[8/768][8_768]|
| **L=10** |[10/128][10_128]|[10/256][10_256]|[10/512][10_512]|[10/768][10_768]|
| **L=12** |[12/128][12_128]|[12/256][12_256]|[12/512][12_512]|[**12/768 (BERT-Base)**][12_768]|
Note that the BERT-Base model in this release is included for completeness only; it was re-trained under the same regime as the original model.
Here are the corresponding GLUE scores on the test set:
|Model|Score|CoLA|SST-2|MRPC|STS-B|QQP|MNLI-m|MNLI-mm|QNLI(v2)|RTE|WNLI|AX|
|---|:---:|:---:|:---:|:---:|:---:|:---:|:---:|:---:|:---:|:---:|:---:|:---:|
|BERT-Tiny|64.2|0.0|83.2|81.1/71.1|74.3/73.6|62.2/83.4|70.2|70.3|81.5|57.2|62.3|21.0|
|BERT-Mini|65.8|0.0|85.9|81.1/71.8|75.4/73.3|66.4/86.2|74.8|74.3|84.1|57.9|62.3|26.1|
|BERT-Small|71.2|27.8|89.7|83.4/76.2|78.8/77.0|68.1/87.0|77.6|77.0|86.4|61.8|62.3|28.6|
|BERT-Medium|73.5|38.0|89.6|86.6/81.6|80.4/78.4|69.6/87.9|80.0|79.1|87.7|62.2|62.3|30.5|
For each task, we selected the best fine-tuning hyperparameters from the lists below, and trained for 4 epochs:
- batch sizes: 8, 16, 32, 64, 128
- learning rates: 3e-4, 1e-4, 5e-5, 3e-5
If you use these models, please cite the following paper:
```
@article{turc2019,
title={Well-Read Students Learn Better: On the Importance of Pre-training Compact Models},
author={Turc, Iulia and Chang, Ming-Wei and Lee, Kenton and Toutanova, Kristina},
journal={arXiv preprint arXiv:1908.08962v2 },
year={2019}
}
```
[2_128]: https://huggingface.co/google/bert_uncased_L-2_H-128_A-2
[2_256]: https://huggingface.co/google/bert_uncased_L-2_H-256_A-4
[2_512]: https://huggingface.co/google/bert_uncased_L-2_H-512_A-8
[2_768]: https://huggingface.co/google/bert_uncased_L-2_H-768_A-12
[4_128]: https://huggingface.co/google/bert_uncased_L-4_H-128_A-2
[4_256]: https://huggingface.co/google/bert_uncased_L-4_H-256_A-4
[4_512]: https://huggingface.co/google/bert_uncased_L-4_H-512_A-8
[4_768]: https://huggingface.co/google/bert_uncased_L-4_H-768_A-12
[6_128]: https://huggingface.co/google/bert_uncased_L-6_H-128_A-2
[6_256]: https://huggingface.co/google/bert_uncased_L-6_H-256_A-4
[6_512]: https://huggingface.co/google/bert_uncased_L-6_H-512_A-8
[6_768]: https://huggingface.co/google/bert_uncased_L-6_H-768_A-12
[8_128]: https://huggingface.co/google/bert_uncased_L-8_H-128_A-2
[8_256]: https://huggingface.co/google/bert_uncased_L-8_H-256_A-4
[8_512]: https://huggingface.co/google/bert_uncased_L-8_H-512_A-8
[8_768]: https://huggingface.co/google/bert_uncased_L-8_H-768_A-12
[10_128]: https://huggingface.co/google/bert_uncased_L-10_H-128_A-2
[10_256]: https://huggingface.co/google/bert_uncased_L-10_H-256_A-4
[10_512]: https://huggingface.co/google/bert_uncased_L-10_H-512_A-8
[10_768]: https://huggingface.co/google/bert_uncased_L-10_H-768_A-12
[12_128]: https://huggingface.co/google/bert_uncased_L-12_H-128_A-2
[12_256]: https://huggingface.co/google/bert_uncased_L-12_H-256_A-4
[12_512]: https://huggingface.co/google/bert_uncased_L-12_H-512_A-8
[12_768]: https://huggingface.co/google/bert_uncased_L-12_H-768_A-12
|