html_url
stringlengths 51
51
| title
stringlengths 6
280
| comments
stringlengths 67
24.7k
| body
stringlengths 51
36.2k
⌀ | comment_length
int64 16
1.45k
| text
stringlengths 159
38.3k
|
---|---|---|---|---|---|
https://github.com/huggingface/datasets/issues/3178 | "Property couldn't be hashed properly" even though fully picklable | Hi ! If your function is not picklable, then the fingerprint of the resulting dataset can't be computed. The fingerprint is a hash that is used by the cache to reload previously computed datasets: the dataset file is named `cache-<fingerprint>.arrow` in your dataset's cache directory.
As a workaround you can set the fingerprint that is going to be used by the cache:
```python
result = my_dataset.map(func, new_fingerprint=new_fingerprint)
```
Any future call to `map` with the same `new_fingerprint` will reload the result from the cache.
**Be careful using this though: if you change your `func`, be sure to change the `new_fingerprint` as well.** | ## Describe the bug
I am trying to tokenize a dataset with spaCy. I found that no matter what I do, the spaCy language object (`nlp`) prevents `datasets` from pickling correctly - or so the warning says - even though manually pickling is no issue. It should not be an issue either, since spaCy objects are picklable.
## Steps to reproduce the bug
Here is a [colab](https://colab.research.google.com/drive/1gt75LCBIzsmBMvvipEOvWulvyZseBiA7?usp=sharing) but for some reason I cannot reproduce it there. That may have to do with logging/tqdm on Colab, or with running things in notebooks. I tried below code on Windows and Ubuntu as a Python script and getting the same issue (warning below).
```python
import pickle
from datasets import load_dataset
import spacy
class Processor:
def __init__(self):
self.nlp = spacy.load("en_core_web_sm", disable=["tagger", "parser", "ner", "lemmatizer"])
@staticmethod
def collate(batch):
return [d["en"] for d in batch]
def parse(self, batch):
batch = batch["translation"]
return {"translation_tok": [{"en_tok": " ".join([t.text for t in doc])} for doc in self.nlp.pipe(self.collate(batch))]}
def process(self):
ds = load_dataset("wmt16", "de-en", split="train[:10%]")
ds = ds.map(self.parse, batched=True, num_proc=6)
if __name__ == '__main__':
pr = Processor()
# succeeds
with open("temp.pkl", "wb") as f:
pickle.dump(pr, f)
print("Successfully pickled!")
pr.process()
```
---
Here is a small change that includes `Hasher.hash` that shows that the hasher cannot seem to successfully pickle parts form the NLP object.
```python
from datasets.fingerprint import Hasher
import pickle
from datasets import load_dataset
import spacy
class Processor:
def __init__(self):
self.nlp = spacy.load("en_core_web_sm", disable=["tagger", "parser", "ner", "lemmatizer"])
@staticmethod
def collate(batch):
return [d["en"] for d in batch]
def parse(self, batch):
batch = batch["translation"]
return {"translation_tok": [{"en_tok": " ".join([t.text for t in doc])} for doc in self.nlp.pipe(self.collate(batch))]}
def process(self):
ds = load_dataset("wmt16", "de-en", split="train[:10]")
return ds.map(self.parse, batched=True)
if __name__ == '__main__':
pr = Processor()
# succeeds
with open("temp.pkl", "wb") as f:
pickle.dump(pr, f)
print("Successfully pickled class instance!")
# succeeds
with open("temp.pkl", "wb") as f:
pickle.dump(pr.nlp, f)
print("Successfully pickled nlp!")
# fails
print(Hasher.hash(pr.nlp))
pr.process()
```
## Expected results
This to be picklable, working (fingerprinted), and no warning.
## Actual results
In the first snippet, I get this warning
Parameter 'function'=<function Processor.parse at 0x7f44982247a0> of the transform datasets.arrow_dataset.Dataset._map_single couldn't be hashed properly, a random hash was used instead. Make sure your transforms and parameters are serializable with pickle or dill for the dataset fingerprinting and caching to work. If you reuse this transform, the caching mechanism will consider it to be different from the previous calls and recompute everything. This warning is only showed once. Subsequent hashing failures won't be showed.
In the second, I get this traceback which directs to the `Hasher.hash` line.
```
Traceback (most recent call last):
File " \Python\Python36\lib\pickle.py", line 918, in save_global
obj2, parent = _getattribute(module, name)
File " \Python\Python36\lib\pickle.py", line 266, in _getattribute
.format(name, obj))
AttributeError: Can't get local attribute 'add_codes.<locals>.ErrorsWithCodes' on <function add_codes at 0x00000296FF606EA0>
During handling of the above exception, another exception occurred:
Traceback (most recent call last):
File " scratch_4.py", line 40, in <module>
print(Hasher.hash(pr.nlp))
File " \lib\site-packages\datasets\fingerprint.py", line 191, in hash
return cls.hash_default(value)
File " \lib\site-packages\datasets\fingerprint.py", line 184, in hash_default
return cls.hash_bytes(dumps(value))
File " \lib\site-packages\datasets\utils\py_utils.py", line 345, in dumps
dump(obj, file)
File " \lib\site-packages\datasets\utils\py_utils.py", line 320, in dump
Pickler(file, recurse=True).dump(obj)
File " \lib\site-packages\dill\_dill.py", line 498, in dump
StockPickler.dump(self, obj)
File " \Python\Python36\lib\pickle.py", line 409, in dump
self.save(obj)
File " \Python\Python36\lib\pickle.py", line 521, in save
self.save_reduce(obj=obj, *rv)
File " \Python\Python36\lib\pickle.py", line 634, in save_reduce
save(state)
File " \Python\Python36\lib\pickle.py", line 476, in save
f(self, obj) # Call unbound method with explicit self
File " \lib\site-packages\dill\_dill.py", line 990, in save_module_dict
StockPickler.save_dict(pickler, obj)
File " \Python\Python36\lib\pickle.py", line 821, in save_dict
self._batch_setitems(obj.items())
File " \Python\Python36\lib\pickle.py", line 847, in _batch_setitems
save(v)
File " \Python\Python36\lib\pickle.py", line 476, in save
f(self, obj) # Call unbound method with explicit self
File " \Python\Python36\lib\pickle.py", line 781, in save_list
self._batch_appends(obj)
File " \Python\Python36\lib\pickle.py", line 805, in _batch_appends
save(x)
File " \Python\Python36\lib\pickle.py", line 476, in save
f(self, obj) # Call unbound method with explicit self
File " \Python\Python36\lib\pickle.py", line 736, in save_tuple
save(element)
File " \Python\Python36\lib\pickle.py", line 521, in save
self.save_reduce(obj=obj, *rv)
File " \Python\Python36\lib\pickle.py", line 634, in save_reduce
save(state)
File " \Python\Python36\lib\pickle.py", line 476, in save
f(self, obj) # Call unbound method with explicit self
File " \Python\Python36\lib\pickle.py", line 736, in save_tuple
save(element)
File " \Python\Python36\lib\pickle.py", line 476, in save
f(self, obj) # Call unbound method with explicit self
File " \lib\site-packages\dill\_dill.py", line 990, in save_module_dict
StockPickler.save_dict(pickler, obj)
File " \Python\Python36\lib\pickle.py", line 821, in save_dict
self._batch_setitems(obj.items())
File " \Python\Python36\lib\pickle.py", line 847, in _batch_setitems
save(v)
File " \Python\Python36\lib\pickle.py", line 476, in save
f(self, obj) # Call unbound method with explicit self
File " \lib\site-packages\dill\_dill.py", line 1176, in save_instancemethod0
pickler.save_reduce(MethodType, (obj.__func__, obj.__self__), obj=obj)
File " \Python\Python36\lib\pickle.py", line 610, in save_reduce
save(args)
File " \Python\Python36\lib\pickle.py", line 476, in save
f(self, obj) # Call unbound method with explicit self
File " \Python\Python36\lib\pickle.py", line 736, in save_tuple
save(element)
File " \Python\Python36\lib\pickle.py", line 476, in save
f(self, obj) # Call unbound method with explicit self
File " \lib\site-packages\datasets\utils\py_utils.py", line 523, in save_function
obj=obj,
File " \Python\Python36\lib\pickle.py", line 610, in save_reduce
save(args)
File " \Python\Python36\lib\pickle.py", line 476, in save
f(self, obj) # Call unbound method with explicit self
File " \Python\Python36\lib\pickle.py", line 751, in save_tuple
save(element)
File " \Python\Python36\lib\pickle.py", line 476, in save
f(self, obj) # Call unbound method with explicit self
File " \lib\site-packages\dill\_dill.py", line 990, in save_module_dict
StockPickler.save_dict(pickler, obj)
File " \Python\Python36\lib\pickle.py", line 821, in save_dict
self._batch_setitems(obj.items())
File " \Python\Python36\lib\pickle.py", line 847, in _batch_setitems
save(v)
File " \Python\Python36\lib\pickle.py", line 521, in save
self.save_reduce(obj=obj, *rv)
File " \Python\Python36\lib\pickle.py", line 605, in save_reduce
save(cls)
File " \Python\Python36\lib\pickle.py", line 476, in save
f(self, obj) # Call unbound method with explicit self
File " \lib\site-packages\dill\_dill.py", line 1439, in save_type
StockPickler.save_global(pickler, obj, name=name)
File " \Python\Python36\lib\pickle.py", line 922, in save_global
(obj, module_name, name))
_pickle.PicklingError: Can't pickle <class 'spacy.errors.add_codes.<locals>.ErrorsWithCodes'>: it's not found as spacy.errors.add_codes.<locals>.ErrorsWithCodes
```
## Environment info
Tried on both Linux and Windows
- `datasets` version: 1.14.0
- Platform: Windows-10-10.0.19041-SP0 + Python 3.7.9; Linux-5.11.0-38-generic-x86_64-with-Ubuntu-20.04-focal + Python 3.7.12
- PyArrow version: 6.0.0
| 102 | "Property couldn't be hashed properly" even though fully picklable
## Describe the bug
I am trying to tokenize a dataset with spaCy. I found that no matter what I do, the spaCy language object (`nlp`) prevents `datasets` from pickling correctly - or so the warning says - even though manually pickling is no issue. It should not be an issue either, since spaCy objects are picklable.
## Steps to reproduce the bug
Here is a [colab](https://colab.research.google.com/drive/1gt75LCBIzsmBMvvipEOvWulvyZseBiA7?usp=sharing) but for some reason I cannot reproduce it there. That may have to do with logging/tqdm on Colab, or with running things in notebooks. I tried below code on Windows and Ubuntu as a Python script and getting the same issue (warning below).
```python
import pickle
from datasets import load_dataset
import spacy
class Processor:
def __init__(self):
self.nlp = spacy.load("en_core_web_sm", disable=["tagger", "parser", "ner", "lemmatizer"])
@staticmethod
def collate(batch):
return [d["en"] for d in batch]
def parse(self, batch):
batch = batch["translation"]
return {"translation_tok": [{"en_tok": " ".join([t.text for t in doc])} for doc in self.nlp.pipe(self.collate(batch))]}
def process(self):
ds = load_dataset("wmt16", "de-en", split="train[:10%]")
ds = ds.map(self.parse, batched=True, num_proc=6)
if __name__ == '__main__':
pr = Processor()
# succeeds
with open("temp.pkl", "wb") as f:
pickle.dump(pr, f)
print("Successfully pickled!")
pr.process()
```
---
Here is a small change that includes `Hasher.hash` that shows that the hasher cannot seem to successfully pickle parts form the NLP object.
```python
from datasets.fingerprint import Hasher
import pickle
from datasets import load_dataset
import spacy
class Processor:
def __init__(self):
self.nlp = spacy.load("en_core_web_sm", disable=["tagger", "parser", "ner", "lemmatizer"])
@staticmethod
def collate(batch):
return [d["en"] for d in batch]
def parse(self, batch):
batch = batch["translation"]
return {"translation_tok": [{"en_tok": " ".join([t.text for t in doc])} for doc in self.nlp.pipe(self.collate(batch))]}
def process(self):
ds = load_dataset("wmt16", "de-en", split="train[:10]")
return ds.map(self.parse, batched=True)
if __name__ == '__main__':
pr = Processor()
# succeeds
with open("temp.pkl", "wb") as f:
pickle.dump(pr, f)
print("Successfully pickled class instance!")
# succeeds
with open("temp.pkl", "wb") as f:
pickle.dump(pr.nlp, f)
print("Successfully pickled nlp!")
# fails
print(Hasher.hash(pr.nlp))
pr.process()
```
## Expected results
This to be picklable, working (fingerprinted), and no warning.
## Actual results
In the first snippet, I get this warning
Parameter 'function'=<function Processor.parse at 0x7f44982247a0> of the transform datasets.arrow_dataset.Dataset._map_single couldn't be hashed properly, a random hash was used instead. Make sure your transforms and parameters are serializable with pickle or dill for the dataset fingerprinting and caching to work. If you reuse this transform, the caching mechanism will consider it to be different from the previous calls and recompute everything. This warning is only showed once. Subsequent hashing failures won't be showed.
In the second, I get this traceback which directs to the `Hasher.hash` line.
```
Traceback (most recent call last):
File " \Python\Python36\lib\pickle.py", line 918, in save_global
obj2, parent = _getattribute(module, name)
File " \Python\Python36\lib\pickle.py", line 266, in _getattribute
.format(name, obj))
AttributeError: Can't get local attribute 'add_codes.<locals>.ErrorsWithCodes' on <function add_codes at 0x00000296FF606EA0>
During handling of the above exception, another exception occurred:
Traceback (most recent call last):
File " scratch_4.py", line 40, in <module>
print(Hasher.hash(pr.nlp))
File " \lib\site-packages\datasets\fingerprint.py", line 191, in hash
return cls.hash_default(value)
File " \lib\site-packages\datasets\fingerprint.py", line 184, in hash_default
return cls.hash_bytes(dumps(value))
File " \lib\site-packages\datasets\utils\py_utils.py", line 345, in dumps
dump(obj, file)
File " \lib\site-packages\datasets\utils\py_utils.py", line 320, in dump
Pickler(file, recurse=True).dump(obj)
File " \lib\site-packages\dill\_dill.py", line 498, in dump
StockPickler.dump(self, obj)
File " \Python\Python36\lib\pickle.py", line 409, in dump
self.save(obj)
File " \Python\Python36\lib\pickle.py", line 521, in save
self.save_reduce(obj=obj, *rv)
File " \Python\Python36\lib\pickle.py", line 634, in save_reduce
save(state)
File " \Python\Python36\lib\pickle.py", line 476, in save
f(self, obj) # Call unbound method with explicit self
File " \lib\site-packages\dill\_dill.py", line 990, in save_module_dict
StockPickler.save_dict(pickler, obj)
File " \Python\Python36\lib\pickle.py", line 821, in save_dict
self._batch_setitems(obj.items())
File " \Python\Python36\lib\pickle.py", line 847, in _batch_setitems
save(v)
File " \Python\Python36\lib\pickle.py", line 476, in save
f(self, obj) # Call unbound method with explicit self
File " \Python\Python36\lib\pickle.py", line 781, in save_list
self._batch_appends(obj)
File " \Python\Python36\lib\pickle.py", line 805, in _batch_appends
save(x)
File " \Python\Python36\lib\pickle.py", line 476, in save
f(self, obj) # Call unbound method with explicit self
File " \Python\Python36\lib\pickle.py", line 736, in save_tuple
save(element)
File " \Python\Python36\lib\pickle.py", line 521, in save
self.save_reduce(obj=obj, *rv)
File " \Python\Python36\lib\pickle.py", line 634, in save_reduce
save(state)
File " \Python\Python36\lib\pickle.py", line 476, in save
f(self, obj) # Call unbound method with explicit self
File " \Python\Python36\lib\pickle.py", line 736, in save_tuple
save(element)
File " \Python\Python36\lib\pickle.py", line 476, in save
f(self, obj) # Call unbound method with explicit self
File " \lib\site-packages\dill\_dill.py", line 990, in save_module_dict
StockPickler.save_dict(pickler, obj)
File " \Python\Python36\lib\pickle.py", line 821, in save_dict
self._batch_setitems(obj.items())
File " \Python\Python36\lib\pickle.py", line 847, in _batch_setitems
save(v)
File " \Python\Python36\lib\pickle.py", line 476, in save
f(self, obj) # Call unbound method with explicit self
File " \lib\site-packages\dill\_dill.py", line 1176, in save_instancemethod0
pickler.save_reduce(MethodType, (obj.__func__, obj.__self__), obj=obj)
File " \Python\Python36\lib\pickle.py", line 610, in save_reduce
save(args)
File " \Python\Python36\lib\pickle.py", line 476, in save
f(self, obj) # Call unbound method with explicit self
File " \Python\Python36\lib\pickle.py", line 736, in save_tuple
save(element)
File " \Python\Python36\lib\pickle.py", line 476, in save
f(self, obj) # Call unbound method with explicit self
File " \lib\site-packages\datasets\utils\py_utils.py", line 523, in save_function
obj=obj,
File " \Python\Python36\lib\pickle.py", line 610, in save_reduce
save(args)
File " \Python\Python36\lib\pickle.py", line 476, in save
f(self, obj) # Call unbound method with explicit self
File " \Python\Python36\lib\pickle.py", line 751, in save_tuple
save(element)
File " \Python\Python36\lib\pickle.py", line 476, in save
f(self, obj) # Call unbound method with explicit self
File " \lib\site-packages\dill\_dill.py", line 990, in save_module_dict
StockPickler.save_dict(pickler, obj)
File " \Python\Python36\lib\pickle.py", line 821, in save_dict
self._batch_setitems(obj.items())
File " \Python\Python36\lib\pickle.py", line 847, in _batch_setitems
save(v)
File " \Python\Python36\lib\pickle.py", line 521, in save
self.save_reduce(obj=obj, *rv)
File " \Python\Python36\lib\pickle.py", line 605, in save_reduce
save(cls)
File " \Python\Python36\lib\pickle.py", line 476, in save
f(self, obj) # Call unbound method with explicit self
File " \lib\site-packages\dill\_dill.py", line 1439, in save_type
StockPickler.save_global(pickler, obj, name=name)
File " \Python\Python36\lib\pickle.py", line 922, in save_global
(obj, module_name, name))
_pickle.PicklingError: Can't pickle <class 'spacy.errors.add_codes.<locals>.ErrorsWithCodes'>: it's not found as spacy.errors.add_codes.<locals>.ErrorsWithCodes
```
## Environment info
Tried on both Linux and Windows
- `datasets` version: 1.14.0
- Platform: Windows-10-10.0.19041-SP0 + Python 3.7.9; Linux-5.11.0-38-generic-x86_64-with-Ubuntu-20.04-focal + Python 3.7.12
- PyArrow version: 6.0.0
Hi ! If your function is not picklable, then the fingerprint of the resulting dataset can't be computed. The fingerprint is a hash that is used by the cache to reload previously computed datasets: the dataset file is named `cache-<fingerprint>.arrow` in your dataset's cache directory.
As a workaround you can set the fingerprint that is going to be used by the cache:
```python
result = my_dataset.map(func, new_fingerprint=new_fingerprint)
```
Any future call to `map` with the same `new_fingerprint` will reload the result from the cache.
**Be careful using this though: if you change your `func`, be sure to change the `new_fingerprint` as well.** |
https://github.com/huggingface/datasets/issues/3178 | "Property couldn't be hashed properly" even though fully picklable | I've been having an issue that might be related to this when trying to pre-tokenize a corpus and caching it for using it later in the pre-training of a RoBERTa model. I always get the following warning:
```
Dataset text downloaded and prepared to /gpfswork/rech/project/user/.cache/hf-datasets/text/default-1850886023af0077/0.0.0/acc32f2f2ef863c93c2f30c52f7df6cc9053a1c2230b8d7da0d210404683ca08. Subsequent calls will reuse this data.
Parameter 'function'=<function encode_dataset.<locals>.<lambda> at 0x14a92157b280> of the transform datasets.arrow_dataset.Dataset.filter@2.0.1 couldn't be hashed properly, a random hash was used instead. Make sure your transforms and parameters are serializable with pickle or dill for the dataset fingerprinting and caching to work. If you reuse this transform, the caching mechanism will consider it to be different from the previous calls and recompute everything. This warning is only showed once. Subsequent hashing failures won't be showed.
```
And when I launch the pre-training the pre-tokenized corpus is not found and it is tokenized again, which makes me waste precious GPU hours.
For me, the workaround was downgrading `dill` and `multiprocess` to the following versions:
```
dill 0.3.4
multiprocess 0.70.12.2
``` | ## Describe the bug
I am trying to tokenize a dataset with spaCy. I found that no matter what I do, the spaCy language object (`nlp`) prevents `datasets` from pickling correctly - or so the warning says - even though manually pickling is no issue. It should not be an issue either, since spaCy objects are picklable.
## Steps to reproduce the bug
Here is a [colab](https://colab.research.google.com/drive/1gt75LCBIzsmBMvvipEOvWulvyZseBiA7?usp=sharing) but for some reason I cannot reproduce it there. That may have to do with logging/tqdm on Colab, or with running things in notebooks. I tried below code on Windows and Ubuntu as a Python script and getting the same issue (warning below).
```python
import pickle
from datasets import load_dataset
import spacy
class Processor:
def __init__(self):
self.nlp = spacy.load("en_core_web_sm", disable=["tagger", "parser", "ner", "lemmatizer"])
@staticmethod
def collate(batch):
return [d["en"] for d in batch]
def parse(self, batch):
batch = batch["translation"]
return {"translation_tok": [{"en_tok": " ".join([t.text for t in doc])} for doc in self.nlp.pipe(self.collate(batch))]}
def process(self):
ds = load_dataset("wmt16", "de-en", split="train[:10%]")
ds = ds.map(self.parse, batched=True, num_proc=6)
if __name__ == '__main__':
pr = Processor()
# succeeds
with open("temp.pkl", "wb") as f:
pickle.dump(pr, f)
print("Successfully pickled!")
pr.process()
```
---
Here is a small change that includes `Hasher.hash` that shows that the hasher cannot seem to successfully pickle parts form the NLP object.
```python
from datasets.fingerprint import Hasher
import pickle
from datasets import load_dataset
import spacy
class Processor:
def __init__(self):
self.nlp = spacy.load("en_core_web_sm", disable=["tagger", "parser", "ner", "lemmatizer"])
@staticmethod
def collate(batch):
return [d["en"] for d in batch]
def parse(self, batch):
batch = batch["translation"]
return {"translation_tok": [{"en_tok": " ".join([t.text for t in doc])} for doc in self.nlp.pipe(self.collate(batch))]}
def process(self):
ds = load_dataset("wmt16", "de-en", split="train[:10]")
return ds.map(self.parse, batched=True)
if __name__ == '__main__':
pr = Processor()
# succeeds
with open("temp.pkl", "wb") as f:
pickle.dump(pr, f)
print("Successfully pickled class instance!")
# succeeds
with open("temp.pkl", "wb") as f:
pickle.dump(pr.nlp, f)
print("Successfully pickled nlp!")
# fails
print(Hasher.hash(pr.nlp))
pr.process()
```
## Expected results
This to be picklable, working (fingerprinted), and no warning.
## Actual results
In the first snippet, I get this warning
Parameter 'function'=<function Processor.parse at 0x7f44982247a0> of the transform datasets.arrow_dataset.Dataset._map_single couldn't be hashed properly, a random hash was used instead. Make sure your transforms and parameters are serializable with pickle or dill for the dataset fingerprinting and caching to work. If you reuse this transform, the caching mechanism will consider it to be different from the previous calls and recompute everything. This warning is only showed once. Subsequent hashing failures won't be showed.
In the second, I get this traceback which directs to the `Hasher.hash` line.
```
Traceback (most recent call last):
File " \Python\Python36\lib\pickle.py", line 918, in save_global
obj2, parent = _getattribute(module, name)
File " \Python\Python36\lib\pickle.py", line 266, in _getattribute
.format(name, obj))
AttributeError: Can't get local attribute 'add_codes.<locals>.ErrorsWithCodes' on <function add_codes at 0x00000296FF606EA0>
During handling of the above exception, another exception occurred:
Traceback (most recent call last):
File " scratch_4.py", line 40, in <module>
print(Hasher.hash(pr.nlp))
File " \lib\site-packages\datasets\fingerprint.py", line 191, in hash
return cls.hash_default(value)
File " \lib\site-packages\datasets\fingerprint.py", line 184, in hash_default
return cls.hash_bytes(dumps(value))
File " \lib\site-packages\datasets\utils\py_utils.py", line 345, in dumps
dump(obj, file)
File " \lib\site-packages\datasets\utils\py_utils.py", line 320, in dump
Pickler(file, recurse=True).dump(obj)
File " \lib\site-packages\dill\_dill.py", line 498, in dump
StockPickler.dump(self, obj)
File " \Python\Python36\lib\pickle.py", line 409, in dump
self.save(obj)
File " \Python\Python36\lib\pickle.py", line 521, in save
self.save_reduce(obj=obj, *rv)
File " \Python\Python36\lib\pickle.py", line 634, in save_reduce
save(state)
File " \Python\Python36\lib\pickle.py", line 476, in save
f(self, obj) # Call unbound method with explicit self
File " \lib\site-packages\dill\_dill.py", line 990, in save_module_dict
StockPickler.save_dict(pickler, obj)
File " \Python\Python36\lib\pickle.py", line 821, in save_dict
self._batch_setitems(obj.items())
File " \Python\Python36\lib\pickle.py", line 847, in _batch_setitems
save(v)
File " \Python\Python36\lib\pickle.py", line 476, in save
f(self, obj) # Call unbound method with explicit self
File " \Python\Python36\lib\pickle.py", line 781, in save_list
self._batch_appends(obj)
File " \Python\Python36\lib\pickle.py", line 805, in _batch_appends
save(x)
File " \Python\Python36\lib\pickle.py", line 476, in save
f(self, obj) # Call unbound method with explicit self
File " \Python\Python36\lib\pickle.py", line 736, in save_tuple
save(element)
File " \Python\Python36\lib\pickle.py", line 521, in save
self.save_reduce(obj=obj, *rv)
File " \Python\Python36\lib\pickle.py", line 634, in save_reduce
save(state)
File " \Python\Python36\lib\pickle.py", line 476, in save
f(self, obj) # Call unbound method with explicit self
File " \Python\Python36\lib\pickle.py", line 736, in save_tuple
save(element)
File " \Python\Python36\lib\pickle.py", line 476, in save
f(self, obj) # Call unbound method with explicit self
File " \lib\site-packages\dill\_dill.py", line 990, in save_module_dict
StockPickler.save_dict(pickler, obj)
File " \Python\Python36\lib\pickle.py", line 821, in save_dict
self._batch_setitems(obj.items())
File " \Python\Python36\lib\pickle.py", line 847, in _batch_setitems
save(v)
File " \Python\Python36\lib\pickle.py", line 476, in save
f(self, obj) # Call unbound method with explicit self
File " \lib\site-packages\dill\_dill.py", line 1176, in save_instancemethod0
pickler.save_reduce(MethodType, (obj.__func__, obj.__self__), obj=obj)
File " \Python\Python36\lib\pickle.py", line 610, in save_reduce
save(args)
File " \Python\Python36\lib\pickle.py", line 476, in save
f(self, obj) # Call unbound method with explicit self
File " \Python\Python36\lib\pickle.py", line 736, in save_tuple
save(element)
File " \Python\Python36\lib\pickle.py", line 476, in save
f(self, obj) # Call unbound method with explicit self
File " \lib\site-packages\datasets\utils\py_utils.py", line 523, in save_function
obj=obj,
File " \Python\Python36\lib\pickle.py", line 610, in save_reduce
save(args)
File " \Python\Python36\lib\pickle.py", line 476, in save
f(self, obj) # Call unbound method with explicit self
File " \Python\Python36\lib\pickle.py", line 751, in save_tuple
save(element)
File " \Python\Python36\lib\pickle.py", line 476, in save
f(self, obj) # Call unbound method with explicit self
File " \lib\site-packages\dill\_dill.py", line 990, in save_module_dict
StockPickler.save_dict(pickler, obj)
File " \Python\Python36\lib\pickle.py", line 821, in save_dict
self._batch_setitems(obj.items())
File " \Python\Python36\lib\pickle.py", line 847, in _batch_setitems
save(v)
File " \Python\Python36\lib\pickle.py", line 521, in save
self.save_reduce(obj=obj, *rv)
File " \Python\Python36\lib\pickle.py", line 605, in save_reduce
save(cls)
File " \Python\Python36\lib\pickle.py", line 476, in save
f(self, obj) # Call unbound method with explicit self
File " \lib\site-packages\dill\_dill.py", line 1439, in save_type
StockPickler.save_global(pickler, obj, name=name)
File " \Python\Python36\lib\pickle.py", line 922, in save_global
(obj, module_name, name))
_pickle.PicklingError: Can't pickle <class 'spacy.errors.add_codes.<locals>.ErrorsWithCodes'>: it's not found as spacy.errors.add_codes.<locals>.ErrorsWithCodes
```
## Environment info
Tried on both Linux and Windows
- `datasets` version: 1.14.0
- Platform: Windows-10-10.0.19041-SP0 + Python 3.7.9; Linux-5.11.0-38-generic-x86_64-with-Ubuntu-20.04-focal + Python 3.7.12
- PyArrow version: 6.0.0
| 167 | "Property couldn't be hashed properly" even though fully picklable
## Describe the bug
I am trying to tokenize a dataset with spaCy. I found that no matter what I do, the spaCy language object (`nlp`) prevents `datasets` from pickling correctly - or so the warning says - even though manually pickling is no issue. It should not be an issue either, since spaCy objects are picklable.
## Steps to reproduce the bug
Here is a [colab](https://colab.research.google.com/drive/1gt75LCBIzsmBMvvipEOvWulvyZseBiA7?usp=sharing) but for some reason I cannot reproduce it there. That may have to do with logging/tqdm on Colab, or with running things in notebooks. I tried below code on Windows and Ubuntu as a Python script and getting the same issue (warning below).
```python
import pickle
from datasets import load_dataset
import spacy
class Processor:
def __init__(self):
self.nlp = spacy.load("en_core_web_sm", disable=["tagger", "parser", "ner", "lemmatizer"])
@staticmethod
def collate(batch):
return [d["en"] for d in batch]
def parse(self, batch):
batch = batch["translation"]
return {"translation_tok": [{"en_tok": " ".join([t.text for t in doc])} for doc in self.nlp.pipe(self.collate(batch))]}
def process(self):
ds = load_dataset("wmt16", "de-en", split="train[:10%]")
ds = ds.map(self.parse, batched=True, num_proc=6)
if __name__ == '__main__':
pr = Processor()
# succeeds
with open("temp.pkl", "wb") as f:
pickle.dump(pr, f)
print("Successfully pickled!")
pr.process()
```
---
Here is a small change that includes `Hasher.hash` that shows that the hasher cannot seem to successfully pickle parts form the NLP object.
```python
from datasets.fingerprint import Hasher
import pickle
from datasets import load_dataset
import spacy
class Processor:
def __init__(self):
self.nlp = spacy.load("en_core_web_sm", disable=["tagger", "parser", "ner", "lemmatizer"])
@staticmethod
def collate(batch):
return [d["en"] for d in batch]
def parse(self, batch):
batch = batch["translation"]
return {"translation_tok": [{"en_tok": " ".join([t.text for t in doc])} for doc in self.nlp.pipe(self.collate(batch))]}
def process(self):
ds = load_dataset("wmt16", "de-en", split="train[:10]")
return ds.map(self.parse, batched=True)
if __name__ == '__main__':
pr = Processor()
# succeeds
with open("temp.pkl", "wb") as f:
pickle.dump(pr, f)
print("Successfully pickled class instance!")
# succeeds
with open("temp.pkl", "wb") as f:
pickle.dump(pr.nlp, f)
print("Successfully pickled nlp!")
# fails
print(Hasher.hash(pr.nlp))
pr.process()
```
## Expected results
This to be picklable, working (fingerprinted), and no warning.
## Actual results
In the first snippet, I get this warning
Parameter 'function'=<function Processor.parse at 0x7f44982247a0> of the transform datasets.arrow_dataset.Dataset._map_single couldn't be hashed properly, a random hash was used instead. Make sure your transforms and parameters are serializable with pickle or dill for the dataset fingerprinting and caching to work. If you reuse this transform, the caching mechanism will consider it to be different from the previous calls and recompute everything. This warning is only showed once. Subsequent hashing failures won't be showed.
In the second, I get this traceback which directs to the `Hasher.hash` line.
```
Traceback (most recent call last):
File " \Python\Python36\lib\pickle.py", line 918, in save_global
obj2, parent = _getattribute(module, name)
File " \Python\Python36\lib\pickle.py", line 266, in _getattribute
.format(name, obj))
AttributeError: Can't get local attribute 'add_codes.<locals>.ErrorsWithCodes' on <function add_codes at 0x00000296FF606EA0>
During handling of the above exception, another exception occurred:
Traceback (most recent call last):
File " scratch_4.py", line 40, in <module>
print(Hasher.hash(pr.nlp))
File " \lib\site-packages\datasets\fingerprint.py", line 191, in hash
return cls.hash_default(value)
File " \lib\site-packages\datasets\fingerprint.py", line 184, in hash_default
return cls.hash_bytes(dumps(value))
File " \lib\site-packages\datasets\utils\py_utils.py", line 345, in dumps
dump(obj, file)
File " \lib\site-packages\datasets\utils\py_utils.py", line 320, in dump
Pickler(file, recurse=True).dump(obj)
File " \lib\site-packages\dill\_dill.py", line 498, in dump
StockPickler.dump(self, obj)
File " \Python\Python36\lib\pickle.py", line 409, in dump
self.save(obj)
File " \Python\Python36\lib\pickle.py", line 521, in save
self.save_reduce(obj=obj, *rv)
File " \Python\Python36\lib\pickle.py", line 634, in save_reduce
save(state)
File " \Python\Python36\lib\pickle.py", line 476, in save
f(self, obj) # Call unbound method with explicit self
File " \lib\site-packages\dill\_dill.py", line 990, in save_module_dict
StockPickler.save_dict(pickler, obj)
File " \Python\Python36\lib\pickle.py", line 821, in save_dict
self._batch_setitems(obj.items())
File " \Python\Python36\lib\pickle.py", line 847, in _batch_setitems
save(v)
File " \Python\Python36\lib\pickle.py", line 476, in save
f(self, obj) # Call unbound method with explicit self
File " \Python\Python36\lib\pickle.py", line 781, in save_list
self._batch_appends(obj)
File " \Python\Python36\lib\pickle.py", line 805, in _batch_appends
save(x)
File " \Python\Python36\lib\pickle.py", line 476, in save
f(self, obj) # Call unbound method with explicit self
File " \Python\Python36\lib\pickle.py", line 736, in save_tuple
save(element)
File " \Python\Python36\lib\pickle.py", line 521, in save
self.save_reduce(obj=obj, *rv)
File " \Python\Python36\lib\pickle.py", line 634, in save_reduce
save(state)
File " \Python\Python36\lib\pickle.py", line 476, in save
f(self, obj) # Call unbound method with explicit self
File " \Python\Python36\lib\pickle.py", line 736, in save_tuple
save(element)
File " \Python\Python36\lib\pickle.py", line 476, in save
f(self, obj) # Call unbound method with explicit self
File " \lib\site-packages\dill\_dill.py", line 990, in save_module_dict
StockPickler.save_dict(pickler, obj)
File " \Python\Python36\lib\pickle.py", line 821, in save_dict
self._batch_setitems(obj.items())
File " \Python\Python36\lib\pickle.py", line 847, in _batch_setitems
save(v)
File " \Python\Python36\lib\pickle.py", line 476, in save
f(self, obj) # Call unbound method with explicit self
File " \lib\site-packages\dill\_dill.py", line 1176, in save_instancemethod0
pickler.save_reduce(MethodType, (obj.__func__, obj.__self__), obj=obj)
File " \Python\Python36\lib\pickle.py", line 610, in save_reduce
save(args)
File " \Python\Python36\lib\pickle.py", line 476, in save
f(self, obj) # Call unbound method with explicit self
File " \Python\Python36\lib\pickle.py", line 736, in save_tuple
save(element)
File " \Python\Python36\lib\pickle.py", line 476, in save
f(self, obj) # Call unbound method with explicit self
File " \lib\site-packages\datasets\utils\py_utils.py", line 523, in save_function
obj=obj,
File " \Python\Python36\lib\pickle.py", line 610, in save_reduce
save(args)
File " \Python\Python36\lib\pickle.py", line 476, in save
f(self, obj) # Call unbound method with explicit self
File " \Python\Python36\lib\pickle.py", line 751, in save_tuple
save(element)
File " \Python\Python36\lib\pickle.py", line 476, in save
f(self, obj) # Call unbound method with explicit self
File " \lib\site-packages\dill\_dill.py", line 990, in save_module_dict
StockPickler.save_dict(pickler, obj)
File " \Python\Python36\lib\pickle.py", line 821, in save_dict
self._batch_setitems(obj.items())
File " \Python\Python36\lib\pickle.py", line 847, in _batch_setitems
save(v)
File " \Python\Python36\lib\pickle.py", line 521, in save
self.save_reduce(obj=obj, *rv)
File " \Python\Python36\lib\pickle.py", line 605, in save_reduce
save(cls)
File " \Python\Python36\lib\pickle.py", line 476, in save
f(self, obj) # Call unbound method with explicit self
File " \lib\site-packages\dill\_dill.py", line 1439, in save_type
StockPickler.save_global(pickler, obj, name=name)
File " \Python\Python36\lib\pickle.py", line 922, in save_global
(obj, module_name, name))
_pickle.PicklingError: Can't pickle <class 'spacy.errors.add_codes.<locals>.ErrorsWithCodes'>: it's not found as spacy.errors.add_codes.<locals>.ErrorsWithCodes
```
## Environment info
Tried on both Linux and Windows
- `datasets` version: 1.14.0
- Platform: Windows-10-10.0.19041-SP0 + Python 3.7.9; Linux-5.11.0-38-generic-x86_64-with-Ubuntu-20.04-focal + Python 3.7.12
- PyArrow version: 6.0.0
I've been having an issue that might be related to this when trying to pre-tokenize a corpus and caching it for using it later in the pre-training of a RoBERTa model. I always get the following warning:
```
Dataset text downloaded and prepared to /gpfswork/rech/project/user/.cache/hf-datasets/text/default-1850886023af0077/0.0.0/acc32f2f2ef863c93c2f30c52f7df6cc9053a1c2230b8d7da0d210404683ca08. Subsequent calls will reuse this data.
Parameter 'function'=<function encode_dataset.<locals>.<lambda> at 0x14a92157b280> of the transform datasets.arrow_dataset.Dataset.filter@2.0.1 couldn't be hashed properly, a random hash was used instead. Make sure your transforms and parameters are serializable with pickle or dill for the dataset fingerprinting and caching to work. If you reuse this transform, the caching mechanism will consider it to be different from the previous calls and recompute everything. This warning is only showed once. Subsequent hashing failures won't be showed.
```
And when I launch the pre-training the pre-tokenized corpus is not found and it is tokenized again, which makes me waste precious GPU hours.
For me, the workaround was downgrading `dill` and `multiprocess` to the following versions:
```
dill 0.3.4
multiprocess 0.70.12.2
``` |
https://github.com/huggingface/datasets/issues/3178 | "Property couldn't be hashed properly" even though fully picklable | > Hi ! If your function is not picklable, then the fingerprint of the resulting dataset can't be computed. The fingerprint is a hash that is used by the cache to reload previously computed datasets: the dataset file is named `cache-<fingerprint>.arrow` in your dataset's cache directory.
>
> As a workaround you can set the fingerprint that is going to be used by the cache:
>
> ```python
> result = my_dataset.map(func, new_fingerprint=new_fingerprint)
> ```
>
> Any future call to `map` with the same `new_fingerprint` will reload the result from the cache.
>
> **Be careful using this though: if you change your `func`, be sure to change the `new_fingerprint` as well.**
Is the argument `new_fingerprint` available for datasetDict ? I can only use it on arrow datasets but might be useful to generalize it to DatasetDict's map as well ? @lhoestq | ## Describe the bug
I am trying to tokenize a dataset with spaCy. I found that no matter what I do, the spaCy language object (`nlp`) prevents `datasets` from pickling correctly - or so the warning says - even though manually pickling is no issue. It should not be an issue either, since spaCy objects are picklable.
## Steps to reproduce the bug
Here is a [colab](https://colab.research.google.com/drive/1gt75LCBIzsmBMvvipEOvWulvyZseBiA7?usp=sharing) but for some reason I cannot reproduce it there. That may have to do with logging/tqdm on Colab, or with running things in notebooks. I tried below code on Windows and Ubuntu as a Python script and getting the same issue (warning below).
```python
import pickle
from datasets import load_dataset
import spacy
class Processor:
def __init__(self):
self.nlp = spacy.load("en_core_web_sm", disable=["tagger", "parser", "ner", "lemmatizer"])
@staticmethod
def collate(batch):
return [d["en"] for d in batch]
def parse(self, batch):
batch = batch["translation"]
return {"translation_tok": [{"en_tok": " ".join([t.text for t in doc])} for doc in self.nlp.pipe(self.collate(batch))]}
def process(self):
ds = load_dataset("wmt16", "de-en", split="train[:10%]")
ds = ds.map(self.parse, batched=True, num_proc=6)
if __name__ == '__main__':
pr = Processor()
# succeeds
with open("temp.pkl", "wb") as f:
pickle.dump(pr, f)
print("Successfully pickled!")
pr.process()
```
---
Here is a small change that includes `Hasher.hash` that shows that the hasher cannot seem to successfully pickle parts form the NLP object.
```python
from datasets.fingerprint import Hasher
import pickle
from datasets import load_dataset
import spacy
class Processor:
def __init__(self):
self.nlp = spacy.load("en_core_web_sm", disable=["tagger", "parser", "ner", "lemmatizer"])
@staticmethod
def collate(batch):
return [d["en"] for d in batch]
def parse(self, batch):
batch = batch["translation"]
return {"translation_tok": [{"en_tok": " ".join([t.text for t in doc])} for doc in self.nlp.pipe(self.collate(batch))]}
def process(self):
ds = load_dataset("wmt16", "de-en", split="train[:10]")
return ds.map(self.parse, batched=True)
if __name__ == '__main__':
pr = Processor()
# succeeds
with open("temp.pkl", "wb") as f:
pickle.dump(pr, f)
print("Successfully pickled class instance!")
# succeeds
with open("temp.pkl", "wb") as f:
pickle.dump(pr.nlp, f)
print("Successfully pickled nlp!")
# fails
print(Hasher.hash(pr.nlp))
pr.process()
```
## Expected results
This to be picklable, working (fingerprinted), and no warning.
## Actual results
In the first snippet, I get this warning
Parameter 'function'=<function Processor.parse at 0x7f44982247a0> of the transform datasets.arrow_dataset.Dataset._map_single couldn't be hashed properly, a random hash was used instead. Make sure your transforms and parameters are serializable with pickle or dill for the dataset fingerprinting and caching to work. If you reuse this transform, the caching mechanism will consider it to be different from the previous calls and recompute everything. This warning is only showed once. Subsequent hashing failures won't be showed.
In the second, I get this traceback which directs to the `Hasher.hash` line.
```
Traceback (most recent call last):
File " \Python\Python36\lib\pickle.py", line 918, in save_global
obj2, parent = _getattribute(module, name)
File " \Python\Python36\lib\pickle.py", line 266, in _getattribute
.format(name, obj))
AttributeError: Can't get local attribute 'add_codes.<locals>.ErrorsWithCodes' on <function add_codes at 0x00000296FF606EA0>
During handling of the above exception, another exception occurred:
Traceback (most recent call last):
File " scratch_4.py", line 40, in <module>
print(Hasher.hash(pr.nlp))
File " \lib\site-packages\datasets\fingerprint.py", line 191, in hash
return cls.hash_default(value)
File " \lib\site-packages\datasets\fingerprint.py", line 184, in hash_default
return cls.hash_bytes(dumps(value))
File " \lib\site-packages\datasets\utils\py_utils.py", line 345, in dumps
dump(obj, file)
File " \lib\site-packages\datasets\utils\py_utils.py", line 320, in dump
Pickler(file, recurse=True).dump(obj)
File " \lib\site-packages\dill\_dill.py", line 498, in dump
StockPickler.dump(self, obj)
File " \Python\Python36\lib\pickle.py", line 409, in dump
self.save(obj)
File " \Python\Python36\lib\pickle.py", line 521, in save
self.save_reduce(obj=obj, *rv)
File " \Python\Python36\lib\pickle.py", line 634, in save_reduce
save(state)
File " \Python\Python36\lib\pickle.py", line 476, in save
f(self, obj) # Call unbound method with explicit self
File " \lib\site-packages\dill\_dill.py", line 990, in save_module_dict
StockPickler.save_dict(pickler, obj)
File " \Python\Python36\lib\pickle.py", line 821, in save_dict
self._batch_setitems(obj.items())
File " \Python\Python36\lib\pickle.py", line 847, in _batch_setitems
save(v)
File " \Python\Python36\lib\pickle.py", line 476, in save
f(self, obj) # Call unbound method with explicit self
File " \Python\Python36\lib\pickle.py", line 781, in save_list
self._batch_appends(obj)
File " \Python\Python36\lib\pickle.py", line 805, in _batch_appends
save(x)
File " \Python\Python36\lib\pickle.py", line 476, in save
f(self, obj) # Call unbound method with explicit self
File " \Python\Python36\lib\pickle.py", line 736, in save_tuple
save(element)
File " \Python\Python36\lib\pickle.py", line 521, in save
self.save_reduce(obj=obj, *rv)
File " \Python\Python36\lib\pickle.py", line 634, in save_reduce
save(state)
File " \Python\Python36\lib\pickle.py", line 476, in save
f(self, obj) # Call unbound method with explicit self
File " \Python\Python36\lib\pickle.py", line 736, in save_tuple
save(element)
File " \Python\Python36\lib\pickle.py", line 476, in save
f(self, obj) # Call unbound method with explicit self
File " \lib\site-packages\dill\_dill.py", line 990, in save_module_dict
StockPickler.save_dict(pickler, obj)
File " \Python\Python36\lib\pickle.py", line 821, in save_dict
self._batch_setitems(obj.items())
File " \Python\Python36\lib\pickle.py", line 847, in _batch_setitems
save(v)
File " \Python\Python36\lib\pickle.py", line 476, in save
f(self, obj) # Call unbound method with explicit self
File " \lib\site-packages\dill\_dill.py", line 1176, in save_instancemethod0
pickler.save_reduce(MethodType, (obj.__func__, obj.__self__), obj=obj)
File " \Python\Python36\lib\pickle.py", line 610, in save_reduce
save(args)
File " \Python\Python36\lib\pickle.py", line 476, in save
f(self, obj) # Call unbound method with explicit self
File " \Python\Python36\lib\pickle.py", line 736, in save_tuple
save(element)
File " \Python\Python36\lib\pickle.py", line 476, in save
f(self, obj) # Call unbound method with explicit self
File " \lib\site-packages\datasets\utils\py_utils.py", line 523, in save_function
obj=obj,
File " \Python\Python36\lib\pickle.py", line 610, in save_reduce
save(args)
File " \Python\Python36\lib\pickle.py", line 476, in save
f(self, obj) # Call unbound method with explicit self
File " \Python\Python36\lib\pickle.py", line 751, in save_tuple
save(element)
File " \Python\Python36\lib\pickle.py", line 476, in save
f(self, obj) # Call unbound method with explicit self
File " \lib\site-packages\dill\_dill.py", line 990, in save_module_dict
StockPickler.save_dict(pickler, obj)
File " \Python\Python36\lib\pickle.py", line 821, in save_dict
self._batch_setitems(obj.items())
File " \Python\Python36\lib\pickle.py", line 847, in _batch_setitems
save(v)
File " \Python\Python36\lib\pickle.py", line 521, in save
self.save_reduce(obj=obj, *rv)
File " \Python\Python36\lib\pickle.py", line 605, in save_reduce
save(cls)
File " \Python\Python36\lib\pickle.py", line 476, in save
f(self, obj) # Call unbound method with explicit self
File " \lib\site-packages\dill\_dill.py", line 1439, in save_type
StockPickler.save_global(pickler, obj, name=name)
File " \Python\Python36\lib\pickle.py", line 922, in save_global
(obj, module_name, name))
_pickle.PicklingError: Can't pickle <class 'spacy.errors.add_codes.<locals>.ErrorsWithCodes'>: it's not found as spacy.errors.add_codes.<locals>.ErrorsWithCodes
```
## Environment info
Tried on both Linux and Windows
- `datasets` version: 1.14.0
- Platform: Windows-10-10.0.19041-SP0 + Python 3.7.9; Linux-5.11.0-38-generic-x86_64-with-Ubuntu-20.04-focal + Python 3.7.12
- PyArrow version: 6.0.0
| 143 | "Property couldn't be hashed properly" even though fully picklable
## Describe the bug
I am trying to tokenize a dataset with spaCy. I found that no matter what I do, the spaCy language object (`nlp`) prevents `datasets` from pickling correctly - or so the warning says - even though manually pickling is no issue. It should not be an issue either, since spaCy objects are picklable.
## Steps to reproduce the bug
Here is a [colab](https://colab.research.google.com/drive/1gt75LCBIzsmBMvvipEOvWulvyZseBiA7?usp=sharing) but for some reason I cannot reproduce it there. That may have to do with logging/tqdm on Colab, or with running things in notebooks. I tried below code on Windows and Ubuntu as a Python script and getting the same issue (warning below).
```python
import pickle
from datasets import load_dataset
import spacy
class Processor:
def __init__(self):
self.nlp = spacy.load("en_core_web_sm", disable=["tagger", "parser", "ner", "lemmatizer"])
@staticmethod
def collate(batch):
return [d["en"] for d in batch]
def parse(self, batch):
batch = batch["translation"]
return {"translation_tok": [{"en_tok": " ".join([t.text for t in doc])} for doc in self.nlp.pipe(self.collate(batch))]}
def process(self):
ds = load_dataset("wmt16", "de-en", split="train[:10%]")
ds = ds.map(self.parse, batched=True, num_proc=6)
if __name__ == '__main__':
pr = Processor()
# succeeds
with open("temp.pkl", "wb") as f:
pickle.dump(pr, f)
print("Successfully pickled!")
pr.process()
```
---
Here is a small change that includes `Hasher.hash` that shows that the hasher cannot seem to successfully pickle parts form the NLP object.
```python
from datasets.fingerprint import Hasher
import pickle
from datasets import load_dataset
import spacy
class Processor:
def __init__(self):
self.nlp = spacy.load("en_core_web_sm", disable=["tagger", "parser", "ner", "lemmatizer"])
@staticmethod
def collate(batch):
return [d["en"] for d in batch]
def parse(self, batch):
batch = batch["translation"]
return {"translation_tok": [{"en_tok": " ".join([t.text for t in doc])} for doc in self.nlp.pipe(self.collate(batch))]}
def process(self):
ds = load_dataset("wmt16", "de-en", split="train[:10]")
return ds.map(self.parse, batched=True)
if __name__ == '__main__':
pr = Processor()
# succeeds
with open("temp.pkl", "wb") as f:
pickle.dump(pr, f)
print("Successfully pickled class instance!")
# succeeds
with open("temp.pkl", "wb") as f:
pickle.dump(pr.nlp, f)
print("Successfully pickled nlp!")
# fails
print(Hasher.hash(pr.nlp))
pr.process()
```
## Expected results
This to be picklable, working (fingerprinted), and no warning.
## Actual results
In the first snippet, I get this warning
Parameter 'function'=<function Processor.parse at 0x7f44982247a0> of the transform datasets.arrow_dataset.Dataset._map_single couldn't be hashed properly, a random hash was used instead. Make sure your transforms and parameters are serializable with pickle or dill for the dataset fingerprinting and caching to work. If you reuse this transform, the caching mechanism will consider it to be different from the previous calls and recompute everything. This warning is only showed once. Subsequent hashing failures won't be showed.
In the second, I get this traceback which directs to the `Hasher.hash` line.
```
Traceback (most recent call last):
File " \Python\Python36\lib\pickle.py", line 918, in save_global
obj2, parent = _getattribute(module, name)
File " \Python\Python36\lib\pickle.py", line 266, in _getattribute
.format(name, obj))
AttributeError: Can't get local attribute 'add_codes.<locals>.ErrorsWithCodes' on <function add_codes at 0x00000296FF606EA0>
During handling of the above exception, another exception occurred:
Traceback (most recent call last):
File " scratch_4.py", line 40, in <module>
print(Hasher.hash(pr.nlp))
File " \lib\site-packages\datasets\fingerprint.py", line 191, in hash
return cls.hash_default(value)
File " \lib\site-packages\datasets\fingerprint.py", line 184, in hash_default
return cls.hash_bytes(dumps(value))
File " \lib\site-packages\datasets\utils\py_utils.py", line 345, in dumps
dump(obj, file)
File " \lib\site-packages\datasets\utils\py_utils.py", line 320, in dump
Pickler(file, recurse=True).dump(obj)
File " \lib\site-packages\dill\_dill.py", line 498, in dump
StockPickler.dump(self, obj)
File " \Python\Python36\lib\pickle.py", line 409, in dump
self.save(obj)
File " \Python\Python36\lib\pickle.py", line 521, in save
self.save_reduce(obj=obj, *rv)
File " \Python\Python36\lib\pickle.py", line 634, in save_reduce
save(state)
File " \Python\Python36\lib\pickle.py", line 476, in save
f(self, obj) # Call unbound method with explicit self
File " \lib\site-packages\dill\_dill.py", line 990, in save_module_dict
StockPickler.save_dict(pickler, obj)
File " \Python\Python36\lib\pickle.py", line 821, in save_dict
self._batch_setitems(obj.items())
File " \Python\Python36\lib\pickle.py", line 847, in _batch_setitems
save(v)
File " \Python\Python36\lib\pickle.py", line 476, in save
f(self, obj) # Call unbound method with explicit self
File " \Python\Python36\lib\pickle.py", line 781, in save_list
self._batch_appends(obj)
File " \Python\Python36\lib\pickle.py", line 805, in _batch_appends
save(x)
File " \Python\Python36\lib\pickle.py", line 476, in save
f(self, obj) # Call unbound method with explicit self
File " \Python\Python36\lib\pickle.py", line 736, in save_tuple
save(element)
File " \Python\Python36\lib\pickle.py", line 521, in save
self.save_reduce(obj=obj, *rv)
File " \Python\Python36\lib\pickle.py", line 634, in save_reduce
save(state)
File " \Python\Python36\lib\pickle.py", line 476, in save
f(self, obj) # Call unbound method with explicit self
File " \Python\Python36\lib\pickle.py", line 736, in save_tuple
save(element)
File " \Python\Python36\lib\pickle.py", line 476, in save
f(self, obj) # Call unbound method with explicit self
File " \lib\site-packages\dill\_dill.py", line 990, in save_module_dict
StockPickler.save_dict(pickler, obj)
File " \Python\Python36\lib\pickle.py", line 821, in save_dict
self._batch_setitems(obj.items())
File " \Python\Python36\lib\pickle.py", line 847, in _batch_setitems
save(v)
File " \Python\Python36\lib\pickle.py", line 476, in save
f(self, obj) # Call unbound method with explicit self
File " \lib\site-packages\dill\_dill.py", line 1176, in save_instancemethod0
pickler.save_reduce(MethodType, (obj.__func__, obj.__self__), obj=obj)
File " \Python\Python36\lib\pickle.py", line 610, in save_reduce
save(args)
File " \Python\Python36\lib\pickle.py", line 476, in save
f(self, obj) # Call unbound method with explicit self
File " \Python\Python36\lib\pickle.py", line 736, in save_tuple
save(element)
File " \Python\Python36\lib\pickle.py", line 476, in save
f(self, obj) # Call unbound method with explicit self
File " \lib\site-packages\datasets\utils\py_utils.py", line 523, in save_function
obj=obj,
File " \Python\Python36\lib\pickle.py", line 610, in save_reduce
save(args)
File " \Python\Python36\lib\pickle.py", line 476, in save
f(self, obj) # Call unbound method with explicit self
File " \Python\Python36\lib\pickle.py", line 751, in save_tuple
save(element)
File " \Python\Python36\lib\pickle.py", line 476, in save
f(self, obj) # Call unbound method with explicit self
File " \lib\site-packages\dill\_dill.py", line 990, in save_module_dict
StockPickler.save_dict(pickler, obj)
File " \Python\Python36\lib\pickle.py", line 821, in save_dict
self._batch_setitems(obj.items())
File " \Python\Python36\lib\pickle.py", line 847, in _batch_setitems
save(v)
File " \Python\Python36\lib\pickle.py", line 521, in save
self.save_reduce(obj=obj, *rv)
File " \Python\Python36\lib\pickle.py", line 605, in save_reduce
save(cls)
File " \Python\Python36\lib\pickle.py", line 476, in save
f(self, obj) # Call unbound method with explicit self
File " \lib\site-packages\dill\_dill.py", line 1439, in save_type
StockPickler.save_global(pickler, obj, name=name)
File " \Python\Python36\lib\pickle.py", line 922, in save_global
(obj, module_name, name))
_pickle.PicklingError: Can't pickle <class 'spacy.errors.add_codes.<locals>.ErrorsWithCodes'>: it's not found as spacy.errors.add_codes.<locals>.ErrorsWithCodes
```
## Environment info
Tried on both Linux and Windows
- `datasets` version: 1.14.0
- Platform: Windows-10-10.0.19041-SP0 + Python 3.7.9; Linux-5.11.0-38-generic-x86_64-with-Ubuntu-20.04-focal + Python 3.7.12
- PyArrow version: 6.0.0
> Hi ! If your function is not picklable, then the fingerprint of the resulting dataset can't be computed. The fingerprint is a hash that is used by the cache to reload previously computed datasets: the dataset file is named `cache-<fingerprint>.arrow` in your dataset's cache directory.
>
> As a workaround you can set the fingerprint that is going to be used by the cache:
>
> ```python
> result = my_dataset.map(func, new_fingerprint=new_fingerprint)
> ```
>
> Any future call to `map` with the same `new_fingerprint` will reload the result from the cache.
>
> **Be careful using this though: if you change your `func`, be sure to change the `new_fingerprint` as well.**
Is the argument `new_fingerprint` available for datasetDict ? I can only use it on arrow datasets but might be useful to generalize it to DatasetDict's map as well ? @lhoestq |
https://github.com/huggingface/datasets/issues/3178 | "Property couldn't be hashed properly" even though fully picklable | > I've been having an issue that might be related to this when trying to pre-tokenize a corpus and caching it for using it later in the pre-training of a RoBERTa model. I always get the following warning:
>
> ```
> Dataset text downloaded and prepared to /gpfswork/rech/project/user/.cache/hf-datasets/text/default-1850886023af0077/0.0.0/acc32f2f2ef863c93c2f30c52f7df6cc9053a1c2230b8d7da0d210404683ca08. Subsequent calls will reuse this data.
> Parameter 'function'=<function encode_dataset.<locals>.<lambda> at 0x14a92157b280> of the transform datasets.arrow_dataset.Dataset.filter@2.0.1 couldn't be hashed properly, a random hash was used instead. Make sure your transforms and parameters are serializable with pickle or dill for the dataset fingerprinting and caching to work. If you reuse this transform, the caching mechanism will consider it to be different from the previous calls and recompute everything. This warning is only showed once. Subsequent hashing failures won't be showed.
> ```
>
> And when I launch the pre-training the pre-tokenized corpus is not found and it is tokenized again, which makes me waste precious GPU hours.
>
> For me, the workaround was downgrading `dill` and `multiprocess` to the following versions:
>
> ```
> dill 0.3.4
> multiprocess 0.70.12.2
> ```
This worked for me - thanks! | ## Describe the bug
I am trying to tokenize a dataset with spaCy. I found that no matter what I do, the spaCy language object (`nlp`) prevents `datasets` from pickling correctly - or so the warning says - even though manually pickling is no issue. It should not be an issue either, since spaCy objects are picklable.
## Steps to reproduce the bug
Here is a [colab](https://colab.research.google.com/drive/1gt75LCBIzsmBMvvipEOvWulvyZseBiA7?usp=sharing) but for some reason I cannot reproduce it there. That may have to do with logging/tqdm on Colab, or with running things in notebooks. I tried below code on Windows and Ubuntu as a Python script and getting the same issue (warning below).
```python
import pickle
from datasets import load_dataset
import spacy
class Processor:
def __init__(self):
self.nlp = spacy.load("en_core_web_sm", disable=["tagger", "parser", "ner", "lemmatizer"])
@staticmethod
def collate(batch):
return [d["en"] for d in batch]
def parse(self, batch):
batch = batch["translation"]
return {"translation_tok": [{"en_tok": " ".join([t.text for t in doc])} for doc in self.nlp.pipe(self.collate(batch))]}
def process(self):
ds = load_dataset("wmt16", "de-en", split="train[:10%]")
ds = ds.map(self.parse, batched=True, num_proc=6)
if __name__ == '__main__':
pr = Processor()
# succeeds
with open("temp.pkl", "wb") as f:
pickle.dump(pr, f)
print("Successfully pickled!")
pr.process()
```
---
Here is a small change that includes `Hasher.hash` that shows that the hasher cannot seem to successfully pickle parts form the NLP object.
```python
from datasets.fingerprint import Hasher
import pickle
from datasets import load_dataset
import spacy
class Processor:
def __init__(self):
self.nlp = spacy.load("en_core_web_sm", disable=["tagger", "parser", "ner", "lemmatizer"])
@staticmethod
def collate(batch):
return [d["en"] for d in batch]
def parse(self, batch):
batch = batch["translation"]
return {"translation_tok": [{"en_tok": " ".join([t.text for t in doc])} for doc in self.nlp.pipe(self.collate(batch))]}
def process(self):
ds = load_dataset("wmt16", "de-en", split="train[:10]")
return ds.map(self.parse, batched=True)
if __name__ == '__main__':
pr = Processor()
# succeeds
with open("temp.pkl", "wb") as f:
pickle.dump(pr, f)
print("Successfully pickled class instance!")
# succeeds
with open("temp.pkl", "wb") as f:
pickle.dump(pr.nlp, f)
print("Successfully pickled nlp!")
# fails
print(Hasher.hash(pr.nlp))
pr.process()
```
## Expected results
This to be picklable, working (fingerprinted), and no warning.
## Actual results
In the first snippet, I get this warning
Parameter 'function'=<function Processor.parse at 0x7f44982247a0> of the transform datasets.arrow_dataset.Dataset._map_single couldn't be hashed properly, a random hash was used instead. Make sure your transforms and parameters are serializable with pickle or dill for the dataset fingerprinting and caching to work. If you reuse this transform, the caching mechanism will consider it to be different from the previous calls and recompute everything. This warning is only showed once. Subsequent hashing failures won't be showed.
In the second, I get this traceback which directs to the `Hasher.hash` line.
```
Traceback (most recent call last):
File " \Python\Python36\lib\pickle.py", line 918, in save_global
obj2, parent = _getattribute(module, name)
File " \Python\Python36\lib\pickle.py", line 266, in _getattribute
.format(name, obj))
AttributeError: Can't get local attribute 'add_codes.<locals>.ErrorsWithCodes' on <function add_codes at 0x00000296FF606EA0>
During handling of the above exception, another exception occurred:
Traceback (most recent call last):
File " scratch_4.py", line 40, in <module>
print(Hasher.hash(pr.nlp))
File " \lib\site-packages\datasets\fingerprint.py", line 191, in hash
return cls.hash_default(value)
File " \lib\site-packages\datasets\fingerprint.py", line 184, in hash_default
return cls.hash_bytes(dumps(value))
File " \lib\site-packages\datasets\utils\py_utils.py", line 345, in dumps
dump(obj, file)
File " \lib\site-packages\datasets\utils\py_utils.py", line 320, in dump
Pickler(file, recurse=True).dump(obj)
File " \lib\site-packages\dill\_dill.py", line 498, in dump
StockPickler.dump(self, obj)
File " \Python\Python36\lib\pickle.py", line 409, in dump
self.save(obj)
File " \Python\Python36\lib\pickle.py", line 521, in save
self.save_reduce(obj=obj, *rv)
File " \Python\Python36\lib\pickle.py", line 634, in save_reduce
save(state)
File " \Python\Python36\lib\pickle.py", line 476, in save
f(self, obj) # Call unbound method with explicit self
File " \lib\site-packages\dill\_dill.py", line 990, in save_module_dict
StockPickler.save_dict(pickler, obj)
File " \Python\Python36\lib\pickle.py", line 821, in save_dict
self._batch_setitems(obj.items())
File " \Python\Python36\lib\pickle.py", line 847, in _batch_setitems
save(v)
File " \Python\Python36\lib\pickle.py", line 476, in save
f(self, obj) # Call unbound method with explicit self
File " \Python\Python36\lib\pickle.py", line 781, in save_list
self._batch_appends(obj)
File " \Python\Python36\lib\pickle.py", line 805, in _batch_appends
save(x)
File " \Python\Python36\lib\pickle.py", line 476, in save
f(self, obj) # Call unbound method with explicit self
File " \Python\Python36\lib\pickle.py", line 736, in save_tuple
save(element)
File " \Python\Python36\lib\pickle.py", line 521, in save
self.save_reduce(obj=obj, *rv)
File " \Python\Python36\lib\pickle.py", line 634, in save_reduce
save(state)
File " \Python\Python36\lib\pickle.py", line 476, in save
f(self, obj) # Call unbound method with explicit self
File " \Python\Python36\lib\pickle.py", line 736, in save_tuple
save(element)
File " \Python\Python36\lib\pickle.py", line 476, in save
f(self, obj) # Call unbound method with explicit self
File " \lib\site-packages\dill\_dill.py", line 990, in save_module_dict
StockPickler.save_dict(pickler, obj)
File " \Python\Python36\lib\pickle.py", line 821, in save_dict
self._batch_setitems(obj.items())
File " \Python\Python36\lib\pickle.py", line 847, in _batch_setitems
save(v)
File " \Python\Python36\lib\pickle.py", line 476, in save
f(self, obj) # Call unbound method with explicit self
File " \lib\site-packages\dill\_dill.py", line 1176, in save_instancemethod0
pickler.save_reduce(MethodType, (obj.__func__, obj.__self__), obj=obj)
File " \Python\Python36\lib\pickle.py", line 610, in save_reduce
save(args)
File " \Python\Python36\lib\pickle.py", line 476, in save
f(self, obj) # Call unbound method with explicit self
File " \Python\Python36\lib\pickle.py", line 736, in save_tuple
save(element)
File " \Python\Python36\lib\pickle.py", line 476, in save
f(self, obj) # Call unbound method with explicit self
File " \lib\site-packages\datasets\utils\py_utils.py", line 523, in save_function
obj=obj,
File " \Python\Python36\lib\pickle.py", line 610, in save_reduce
save(args)
File " \Python\Python36\lib\pickle.py", line 476, in save
f(self, obj) # Call unbound method with explicit self
File " \Python\Python36\lib\pickle.py", line 751, in save_tuple
save(element)
File " \Python\Python36\lib\pickle.py", line 476, in save
f(self, obj) # Call unbound method with explicit self
File " \lib\site-packages\dill\_dill.py", line 990, in save_module_dict
StockPickler.save_dict(pickler, obj)
File " \Python\Python36\lib\pickle.py", line 821, in save_dict
self._batch_setitems(obj.items())
File " \Python\Python36\lib\pickle.py", line 847, in _batch_setitems
save(v)
File " \Python\Python36\lib\pickle.py", line 521, in save
self.save_reduce(obj=obj, *rv)
File " \Python\Python36\lib\pickle.py", line 605, in save_reduce
save(cls)
File " \Python\Python36\lib\pickle.py", line 476, in save
f(self, obj) # Call unbound method with explicit self
File " \lib\site-packages\dill\_dill.py", line 1439, in save_type
StockPickler.save_global(pickler, obj, name=name)
File " \Python\Python36\lib\pickle.py", line 922, in save_global
(obj, module_name, name))
_pickle.PicklingError: Can't pickle <class 'spacy.errors.add_codes.<locals>.ErrorsWithCodes'>: it's not found as spacy.errors.add_codes.<locals>.ErrorsWithCodes
```
## Environment info
Tried on both Linux and Windows
- `datasets` version: 1.14.0
- Platform: Windows-10-10.0.19041-SP0 + Python 3.7.9; Linux-5.11.0-38-generic-x86_64-with-Ubuntu-20.04-focal + Python 3.7.12
- PyArrow version: 6.0.0
| 188 | "Property couldn't be hashed properly" even though fully picklable
## Describe the bug
I am trying to tokenize a dataset with spaCy. I found that no matter what I do, the spaCy language object (`nlp`) prevents `datasets` from pickling correctly - or so the warning says - even though manually pickling is no issue. It should not be an issue either, since spaCy objects are picklable.
## Steps to reproduce the bug
Here is a [colab](https://colab.research.google.com/drive/1gt75LCBIzsmBMvvipEOvWulvyZseBiA7?usp=sharing) but for some reason I cannot reproduce it there. That may have to do with logging/tqdm on Colab, or with running things in notebooks. I tried below code on Windows and Ubuntu as a Python script and getting the same issue (warning below).
```python
import pickle
from datasets import load_dataset
import spacy
class Processor:
def __init__(self):
self.nlp = spacy.load("en_core_web_sm", disable=["tagger", "parser", "ner", "lemmatizer"])
@staticmethod
def collate(batch):
return [d["en"] for d in batch]
def parse(self, batch):
batch = batch["translation"]
return {"translation_tok": [{"en_tok": " ".join([t.text for t in doc])} for doc in self.nlp.pipe(self.collate(batch))]}
def process(self):
ds = load_dataset("wmt16", "de-en", split="train[:10%]")
ds = ds.map(self.parse, batched=True, num_proc=6)
if __name__ == '__main__':
pr = Processor()
# succeeds
with open("temp.pkl", "wb") as f:
pickle.dump(pr, f)
print("Successfully pickled!")
pr.process()
```
---
Here is a small change that includes `Hasher.hash` that shows that the hasher cannot seem to successfully pickle parts form the NLP object.
```python
from datasets.fingerprint import Hasher
import pickle
from datasets import load_dataset
import spacy
class Processor:
def __init__(self):
self.nlp = spacy.load("en_core_web_sm", disable=["tagger", "parser", "ner", "lemmatizer"])
@staticmethod
def collate(batch):
return [d["en"] for d in batch]
def parse(self, batch):
batch = batch["translation"]
return {"translation_tok": [{"en_tok": " ".join([t.text for t in doc])} for doc in self.nlp.pipe(self.collate(batch))]}
def process(self):
ds = load_dataset("wmt16", "de-en", split="train[:10]")
return ds.map(self.parse, batched=True)
if __name__ == '__main__':
pr = Processor()
# succeeds
with open("temp.pkl", "wb") as f:
pickle.dump(pr, f)
print("Successfully pickled class instance!")
# succeeds
with open("temp.pkl", "wb") as f:
pickle.dump(pr.nlp, f)
print("Successfully pickled nlp!")
# fails
print(Hasher.hash(pr.nlp))
pr.process()
```
## Expected results
This to be picklable, working (fingerprinted), and no warning.
## Actual results
In the first snippet, I get this warning
Parameter 'function'=<function Processor.parse at 0x7f44982247a0> of the transform datasets.arrow_dataset.Dataset._map_single couldn't be hashed properly, a random hash was used instead. Make sure your transforms and parameters are serializable with pickle or dill for the dataset fingerprinting and caching to work. If you reuse this transform, the caching mechanism will consider it to be different from the previous calls and recompute everything. This warning is only showed once. Subsequent hashing failures won't be showed.
In the second, I get this traceback which directs to the `Hasher.hash` line.
```
Traceback (most recent call last):
File " \Python\Python36\lib\pickle.py", line 918, in save_global
obj2, parent = _getattribute(module, name)
File " \Python\Python36\lib\pickle.py", line 266, in _getattribute
.format(name, obj))
AttributeError: Can't get local attribute 'add_codes.<locals>.ErrorsWithCodes' on <function add_codes at 0x00000296FF606EA0>
During handling of the above exception, another exception occurred:
Traceback (most recent call last):
File " scratch_4.py", line 40, in <module>
print(Hasher.hash(pr.nlp))
File " \lib\site-packages\datasets\fingerprint.py", line 191, in hash
return cls.hash_default(value)
File " \lib\site-packages\datasets\fingerprint.py", line 184, in hash_default
return cls.hash_bytes(dumps(value))
File " \lib\site-packages\datasets\utils\py_utils.py", line 345, in dumps
dump(obj, file)
File " \lib\site-packages\datasets\utils\py_utils.py", line 320, in dump
Pickler(file, recurse=True).dump(obj)
File " \lib\site-packages\dill\_dill.py", line 498, in dump
StockPickler.dump(self, obj)
File " \Python\Python36\lib\pickle.py", line 409, in dump
self.save(obj)
File " \Python\Python36\lib\pickle.py", line 521, in save
self.save_reduce(obj=obj, *rv)
File " \Python\Python36\lib\pickle.py", line 634, in save_reduce
save(state)
File " \Python\Python36\lib\pickle.py", line 476, in save
f(self, obj) # Call unbound method with explicit self
File " \lib\site-packages\dill\_dill.py", line 990, in save_module_dict
StockPickler.save_dict(pickler, obj)
File " \Python\Python36\lib\pickle.py", line 821, in save_dict
self._batch_setitems(obj.items())
File " \Python\Python36\lib\pickle.py", line 847, in _batch_setitems
save(v)
File " \Python\Python36\lib\pickle.py", line 476, in save
f(self, obj) # Call unbound method with explicit self
File " \Python\Python36\lib\pickle.py", line 781, in save_list
self._batch_appends(obj)
File " \Python\Python36\lib\pickle.py", line 805, in _batch_appends
save(x)
File " \Python\Python36\lib\pickle.py", line 476, in save
f(self, obj) # Call unbound method with explicit self
File " \Python\Python36\lib\pickle.py", line 736, in save_tuple
save(element)
File " \Python\Python36\lib\pickle.py", line 521, in save
self.save_reduce(obj=obj, *rv)
File " \Python\Python36\lib\pickle.py", line 634, in save_reduce
save(state)
File " \Python\Python36\lib\pickle.py", line 476, in save
f(self, obj) # Call unbound method with explicit self
File " \Python\Python36\lib\pickle.py", line 736, in save_tuple
save(element)
File " \Python\Python36\lib\pickle.py", line 476, in save
f(self, obj) # Call unbound method with explicit self
File " \lib\site-packages\dill\_dill.py", line 990, in save_module_dict
StockPickler.save_dict(pickler, obj)
File " \Python\Python36\lib\pickle.py", line 821, in save_dict
self._batch_setitems(obj.items())
File " \Python\Python36\lib\pickle.py", line 847, in _batch_setitems
save(v)
File " \Python\Python36\lib\pickle.py", line 476, in save
f(self, obj) # Call unbound method with explicit self
File " \lib\site-packages\dill\_dill.py", line 1176, in save_instancemethod0
pickler.save_reduce(MethodType, (obj.__func__, obj.__self__), obj=obj)
File " \Python\Python36\lib\pickle.py", line 610, in save_reduce
save(args)
File " \Python\Python36\lib\pickle.py", line 476, in save
f(self, obj) # Call unbound method with explicit self
File " \Python\Python36\lib\pickle.py", line 736, in save_tuple
save(element)
File " \Python\Python36\lib\pickle.py", line 476, in save
f(self, obj) # Call unbound method with explicit self
File " \lib\site-packages\datasets\utils\py_utils.py", line 523, in save_function
obj=obj,
File " \Python\Python36\lib\pickle.py", line 610, in save_reduce
save(args)
File " \Python\Python36\lib\pickle.py", line 476, in save
f(self, obj) # Call unbound method with explicit self
File " \Python\Python36\lib\pickle.py", line 751, in save_tuple
save(element)
File " \Python\Python36\lib\pickle.py", line 476, in save
f(self, obj) # Call unbound method with explicit self
File " \lib\site-packages\dill\_dill.py", line 990, in save_module_dict
StockPickler.save_dict(pickler, obj)
File " \Python\Python36\lib\pickle.py", line 821, in save_dict
self._batch_setitems(obj.items())
File " \Python\Python36\lib\pickle.py", line 847, in _batch_setitems
save(v)
File " \Python\Python36\lib\pickle.py", line 521, in save
self.save_reduce(obj=obj, *rv)
File " \Python\Python36\lib\pickle.py", line 605, in save_reduce
save(cls)
File " \Python\Python36\lib\pickle.py", line 476, in save
f(self, obj) # Call unbound method with explicit self
File " \lib\site-packages\dill\_dill.py", line 1439, in save_type
StockPickler.save_global(pickler, obj, name=name)
File " \Python\Python36\lib\pickle.py", line 922, in save_global
(obj, module_name, name))
_pickle.PicklingError: Can't pickle <class 'spacy.errors.add_codes.<locals>.ErrorsWithCodes'>: it's not found as spacy.errors.add_codes.<locals>.ErrorsWithCodes
```
## Environment info
Tried on both Linux and Windows
- `datasets` version: 1.14.0
- Platform: Windows-10-10.0.19041-SP0 + Python 3.7.9; Linux-5.11.0-38-generic-x86_64-with-Ubuntu-20.04-focal + Python 3.7.12
- PyArrow version: 6.0.0
> I've been having an issue that might be related to this when trying to pre-tokenize a corpus and caching it for using it later in the pre-training of a RoBERTa model. I always get the following warning:
>
> ```
> Dataset text downloaded and prepared to /gpfswork/rech/project/user/.cache/hf-datasets/text/default-1850886023af0077/0.0.0/acc32f2f2ef863c93c2f30c52f7df6cc9053a1c2230b8d7da0d210404683ca08. Subsequent calls will reuse this data.
> Parameter 'function'=<function encode_dataset.<locals>.<lambda> at 0x14a92157b280> of the transform datasets.arrow_dataset.Dataset.filter@2.0.1 couldn't be hashed properly, a random hash was used instead. Make sure your transforms and parameters are serializable with pickle or dill for the dataset fingerprinting and caching to work. If you reuse this transform, the caching mechanism will consider it to be different from the previous calls and recompute everything. This warning is only showed once. Subsequent hashing failures won't be showed.
> ```
>
> And when I launch the pre-training the pre-tokenized corpus is not found and it is tokenized again, which makes me waste precious GPU hours.
>
> For me, the workaround was downgrading `dill` and `multiprocess` to the following versions:
>
> ```
> dill 0.3.4
> multiprocess 0.70.12.2
> ```
This worked for me - thanks! |
https://github.com/huggingface/datasets/issues/3178 | "Property couldn't be hashed properly" even though fully picklable | I see this has just been closed - it seems quite relevant to another tokenizer I have been trying to use, the `vinai/phobert` family of tokenizers
https://huggingface.co/vinai/phobert-base
https://huggingface.co/vinai/phobert-large
I ran into an issue where a large dataset took several hours to tokenize, the process hung, and I was unable to use the cached version of the tokenized data:
https://discuss.huggingface.co/t/cache-parallelize-long-tokenization-step/25791/3
I don't see any way to specify the hash of the tokenizer or the fingerprint of the tokenized data to use, so is the tokenized dataset basically lost at this point? Is there a good way to avoid this happening again if I retokenize the data?
| ## Describe the bug
I am trying to tokenize a dataset with spaCy. I found that no matter what I do, the spaCy language object (`nlp`) prevents `datasets` from pickling correctly - or so the warning says - even though manually pickling is no issue. It should not be an issue either, since spaCy objects are picklable.
## Steps to reproduce the bug
Here is a [colab](https://colab.research.google.com/drive/1gt75LCBIzsmBMvvipEOvWulvyZseBiA7?usp=sharing) but for some reason I cannot reproduce it there. That may have to do with logging/tqdm on Colab, or with running things in notebooks. I tried below code on Windows and Ubuntu as a Python script and getting the same issue (warning below).
```python
import pickle
from datasets import load_dataset
import spacy
class Processor:
def __init__(self):
self.nlp = spacy.load("en_core_web_sm", disable=["tagger", "parser", "ner", "lemmatizer"])
@staticmethod
def collate(batch):
return [d["en"] for d in batch]
def parse(self, batch):
batch = batch["translation"]
return {"translation_tok": [{"en_tok": " ".join([t.text for t in doc])} for doc in self.nlp.pipe(self.collate(batch))]}
def process(self):
ds = load_dataset("wmt16", "de-en", split="train[:10%]")
ds = ds.map(self.parse, batched=True, num_proc=6)
if __name__ == '__main__':
pr = Processor()
# succeeds
with open("temp.pkl", "wb") as f:
pickle.dump(pr, f)
print("Successfully pickled!")
pr.process()
```
---
Here is a small change that includes `Hasher.hash` that shows that the hasher cannot seem to successfully pickle parts form the NLP object.
```python
from datasets.fingerprint import Hasher
import pickle
from datasets import load_dataset
import spacy
class Processor:
def __init__(self):
self.nlp = spacy.load("en_core_web_sm", disable=["tagger", "parser", "ner", "lemmatizer"])
@staticmethod
def collate(batch):
return [d["en"] for d in batch]
def parse(self, batch):
batch = batch["translation"]
return {"translation_tok": [{"en_tok": " ".join([t.text for t in doc])} for doc in self.nlp.pipe(self.collate(batch))]}
def process(self):
ds = load_dataset("wmt16", "de-en", split="train[:10]")
return ds.map(self.parse, batched=True)
if __name__ == '__main__':
pr = Processor()
# succeeds
with open("temp.pkl", "wb") as f:
pickle.dump(pr, f)
print("Successfully pickled class instance!")
# succeeds
with open("temp.pkl", "wb") as f:
pickle.dump(pr.nlp, f)
print("Successfully pickled nlp!")
# fails
print(Hasher.hash(pr.nlp))
pr.process()
```
## Expected results
This to be picklable, working (fingerprinted), and no warning.
## Actual results
In the first snippet, I get this warning
Parameter 'function'=<function Processor.parse at 0x7f44982247a0> of the transform datasets.arrow_dataset.Dataset._map_single couldn't be hashed properly, a random hash was used instead. Make sure your transforms and parameters are serializable with pickle or dill for the dataset fingerprinting and caching to work. If you reuse this transform, the caching mechanism will consider it to be different from the previous calls and recompute everything. This warning is only showed once. Subsequent hashing failures won't be showed.
In the second, I get this traceback which directs to the `Hasher.hash` line.
```
Traceback (most recent call last):
File " \Python\Python36\lib\pickle.py", line 918, in save_global
obj2, parent = _getattribute(module, name)
File " \Python\Python36\lib\pickle.py", line 266, in _getattribute
.format(name, obj))
AttributeError: Can't get local attribute 'add_codes.<locals>.ErrorsWithCodes' on <function add_codes at 0x00000296FF606EA0>
During handling of the above exception, another exception occurred:
Traceback (most recent call last):
File " scratch_4.py", line 40, in <module>
print(Hasher.hash(pr.nlp))
File " \lib\site-packages\datasets\fingerprint.py", line 191, in hash
return cls.hash_default(value)
File " \lib\site-packages\datasets\fingerprint.py", line 184, in hash_default
return cls.hash_bytes(dumps(value))
File " \lib\site-packages\datasets\utils\py_utils.py", line 345, in dumps
dump(obj, file)
File " \lib\site-packages\datasets\utils\py_utils.py", line 320, in dump
Pickler(file, recurse=True).dump(obj)
File " \lib\site-packages\dill\_dill.py", line 498, in dump
StockPickler.dump(self, obj)
File " \Python\Python36\lib\pickle.py", line 409, in dump
self.save(obj)
File " \Python\Python36\lib\pickle.py", line 521, in save
self.save_reduce(obj=obj, *rv)
File " \Python\Python36\lib\pickle.py", line 634, in save_reduce
save(state)
File " \Python\Python36\lib\pickle.py", line 476, in save
f(self, obj) # Call unbound method with explicit self
File " \lib\site-packages\dill\_dill.py", line 990, in save_module_dict
StockPickler.save_dict(pickler, obj)
File " \Python\Python36\lib\pickle.py", line 821, in save_dict
self._batch_setitems(obj.items())
File " \Python\Python36\lib\pickle.py", line 847, in _batch_setitems
save(v)
File " \Python\Python36\lib\pickle.py", line 476, in save
f(self, obj) # Call unbound method with explicit self
File " \Python\Python36\lib\pickle.py", line 781, in save_list
self._batch_appends(obj)
File " \Python\Python36\lib\pickle.py", line 805, in _batch_appends
save(x)
File " \Python\Python36\lib\pickle.py", line 476, in save
f(self, obj) # Call unbound method with explicit self
File " \Python\Python36\lib\pickle.py", line 736, in save_tuple
save(element)
File " \Python\Python36\lib\pickle.py", line 521, in save
self.save_reduce(obj=obj, *rv)
File " \Python\Python36\lib\pickle.py", line 634, in save_reduce
save(state)
File " \Python\Python36\lib\pickle.py", line 476, in save
f(self, obj) # Call unbound method with explicit self
File " \Python\Python36\lib\pickle.py", line 736, in save_tuple
save(element)
File " \Python\Python36\lib\pickle.py", line 476, in save
f(self, obj) # Call unbound method with explicit self
File " \lib\site-packages\dill\_dill.py", line 990, in save_module_dict
StockPickler.save_dict(pickler, obj)
File " \Python\Python36\lib\pickle.py", line 821, in save_dict
self._batch_setitems(obj.items())
File " \Python\Python36\lib\pickle.py", line 847, in _batch_setitems
save(v)
File " \Python\Python36\lib\pickle.py", line 476, in save
f(self, obj) # Call unbound method with explicit self
File " \lib\site-packages\dill\_dill.py", line 1176, in save_instancemethod0
pickler.save_reduce(MethodType, (obj.__func__, obj.__self__), obj=obj)
File " \Python\Python36\lib\pickle.py", line 610, in save_reduce
save(args)
File " \Python\Python36\lib\pickle.py", line 476, in save
f(self, obj) # Call unbound method with explicit self
File " \Python\Python36\lib\pickle.py", line 736, in save_tuple
save(element)
File " \Python\Python36\lib\pickle.py", line 476, in save
f(self, obj) # Call unbound method with explicit self
File " \lib\site-packages\datasets\utils\py_utils.py", line 523, in save_function
obj=obj,
File " \Python\Python36\lib\pickle.py", line 610, in save_reduce
save(args)
File " \Python\Python36\lib\pickle.py", line 476, in save
f(self, obj) # Call unbound method with explicit self
File " \Python\Python36\lib\pickle.py", line 751, in save_tuple
save(element)
File " \Python\Python36\lib\pickle.py", line 476, in save
f(self, obj) # Call unbound method with explicit self
File " \lib\site-packages\dill\_dill.py", line 990, in save_module_dict
StockPickler.save_dict(pickler, obj)
File " \Python\Python36\lib\pickle.py", line 821, in save_dict
self._batch_setitems(obj.items())
File " \Python\Python36\lib\pickle.py", line 847, in _batch_setitems
save(v)
File " \Python\Python36\lib\pickle.py", line 521, in save
self.save_reduce(obj=obj, *rv)
File " \Python\Python36\lib\pickle.py", line 605, in save_reduce
save(cls)
File " \Python\Python36\lib\pickle.py", line 476, in save
f(self, obj) # Call unbound method with explicit self
File " \lib\site-packages\dill\_dill.py", line 1439, in save_type
StockPickler.save_global(pickler, obj, name=name)
File " \Python\Python36\lib\pickle.py", line 922, in save_global
(obj, module_name, name))
_pickle.PicklingError: Can't pickle <class 'spacy.errors.add_codes.<locals>.ErrorsWithCodes'>: it's not found as spacy.errors.add_codes.<locals>.ErrorsWithCodes
```
## Environment info
Tried on both Linux and Windows
- `datasets` version: 1.14.0
- Platform: Windows-10-10.0.19041-SP0 + Python 3.7.9; Linux-5.11.0-38-generic-x86_64-with-Ubuntu-20.04-focal + Python 3.7.12
- PyArrow version: 6.0.0
| 105 | "Property couldn't be hashed properly" even though fully picklable
## Describe the bug
I am trying to tokenize a dataset with spaCy. I found that no matter what I do, the spaCy language object (`nlp`) prevents `datasets` from pickling correctly - or so the warning says - even though manually pickling is no issue. It should not be an issue either, since spaCy objects are picklable.
## Steps to reproduce the bug
Here is a [colab](https://colab.research.google.com/drive/1gt75LCBIzsmBMvvipEOvWulvyZseBiA7?usp=sharing) but for some reason I cannot reproduce it there. That may have to do with logging/tqdm on Colab, or with running things in notebooks. I tried below code on Windows and Ubuntu as a Python script and getting the same issue (warning below).
```python
import pickle
from datasets import load_dataset
import spacy
class Processor:
def __init__(self):
self.nlp = spacy.load("en_core_web_sm", disable=["tagger", "parser", "ner", "lemmatizer"])
@staticmethod
def collate(batch):
return [d["en"] for d in batch]
def parse(self, batch):
batch = batch["translation"]
return {"translation_tok": [{"en_tok": " ".join([t.text for t in doc])} for doc in self.nlp.pipe(self.collate(batch))]}
def process(self):
ds = load_dataset("wmt16", "de-en", split="train[:10%]")
ds = ds.map(self.parse, batched=True, num_proc=6)
if __name__ == '__main__':
pr = Processor()
# succeeds
with open("temp.pkl", "wb") as f:
pickle.dump(pr, f)
print("Successfully pickled!")
pr.process()
```
---
Here is a small change that includes `Hasher.hash` that shows that the hasher cannot seem to successfully pickle parts form the NLP object.
```python
from datasets.fingerprint import Hasher
import pickle
from datasets import load_dataset
import spacy
class Processor:
def __init__(self):
self.nlp = spacy.load("en_core_web_sm", disable=["tagger", "parser", "ner", "lemmatizer"])
@staticmethod
def collate(batch):
return [d["en"] for d in batch]
def parse(self, batch):
batch = batch["translation"]
return {"translation_tok": [{"en_tok": " ".join([t.text for t in doc])} for doc in self.nlp.pipe(self.collate(batch))]}
def process(self):
ds = load_dataset("wmt16", "de-en", split="train[:10]")
return ds.map(self.parse, batched=True)
if __name__ == '__main__':
pr = Processor()
# succeeds
with open("temp.pkl", "wb") as f:
pickle.dump(pr, f)
print("Successfully pickled class instance!")
# succeeds
with open("temp.pkl", "wb") as f:
pickle.dump(pr.nlp, f)
print("Successfully pickled nlp!")
# fails
print(Hasher.hash(pr.nlp))
pr.process()
```
## Expected results
This to be picklable, working (fingerprinted), and no warning.
## Actual results
In the first snippet, I get this warning
Parameter 'function'=<function Processor.parse at 0x7f44982247a0> of the transform datasets.arrow_dataset.Dataset._map_single couldn't be hashed properly, a random hash was used instead. Make sure your transforms and parameters are serializable with pickle or dill for the dataset fingerprinting and caching to work. If you reuse this transform, the caching mechanism will consider it to be different from the previous calls and recompute everything. This warning is only showed once. Subsequent hashing failures won't be showed.
In the second, I get this traceback which directs to the `Hasher.hash` line.
```
Traceback (most recent call last):
File " \Python\Python36\lib\pickle.py", line 918, in save_global
obj2, parent = _getattribute(module, name)
File " \Python\Python36\lib\pickle.py", line 266, in _getattribute
.format(name, obj))
AttributeError: Can't get local attribute 'add_codes.<locals>.ErrorsWithCodes' on <function add_codes at 0x00000296FF606EA0>
During handling of the above exception, another exception occurred:
Traceback (most recent call last):
File " scratch_4.py", line 40, in <module>
print(Hasher.hash(pr.nlp))
File " \lib\site-packages\datasets\fingerprint.py", line 191, in hash
return cls.hash_default(value)
File " \lib\site-packages\datasets\fingerprint.py", line 184, in hash_default
return cls.hash_bytes(dumps(value))
File " \lib\site-packages\datasets\utils\py_utils.py", line 345, in dumps
dump(obj, file)
File " \lib\site-packages\datasets\utils\py_utils.py", line 320, in dump
Pickler(file, recurse=True).dump(obj)
File " \lib\site-packages\dill\_dill.py", line 498, in dump
StockPickler.dump(self, obj)
File " \Python\Python36\lib\pickle.py", line 409, in dump
self.save(obj)
File " \Python\Python36\lib\pickle.py", line 521, in save
self.save_reduce(obj=obj, *rv)
File " \Python\Python36\lib\pickle.py", line 634, in save_reduce
save(state)
File " \Python\Python36\lib\pickle.py", line 476, in save
f(self, obj) # Call unbound method with explicit self
File " \lib\site-packages\dill\_dill.py", line 990, in save_module_dict
StockPickler.save_dict(pickler, obj)
File " \Python\Python36\lib\pickle.py", line 821, in save_dict
self._batch_setitems(obj.items())
File " \Python\Python36\lib\pickle.py", line 847, in _batch_setitems
save(v)
File " \Python\Python36\lib\pickle.py", line 476, in save
f(self, obj) # Call unbound method with explicit self
File " \Python\Python36\lib\pickle.py", line 781, in save_list
self._batch_appends(obj)
File " \Python\Python36\lib\pickle.py", line 805, in _batch_appends
save(x)
File " \Python\Python36\lib\pickle.py", line 476, in save
f(self, obj) # Call unbound method with explicit self
File " \Python\Python36\lib\pickle.py", line 736, in save_tuple
save(element)
File " \Python\Python36\lib\pickle.py", line 521, in save
self.save_reduce(obj=obj, *rv)
File " \Python\Python36\lib\pickle.py", line 634, in save_reduce
save(state)
File " \Python\Python36\lib\pickle.py", line 476, in save
f(self, obj) # Call unbound method with explicit self
File " \Python\Python36\lib\pickle.py", line 736, in save_tuple
save(element)
File " \Python\Python36\lib\pickle.py", line 476, in save
f(self, obj) # Call unbound method with explicit self
File " \lib\site-packages\dill\_dill.py", line 990, in save_module_dict
StockPickler.save_dict(pickler, obj)
File " \Python\Python36\lib\pickle.py", line 821, in save_dict
self._batch_setitems(obj.items())
File " \Python\Python36\lib\pickle.py", line 847, in _batch_setitems
save(v)
File " \Python\Python36\lib\pickle.py", line 476, in save
f(self, obj) # Call unbound method with explicit self
File " \lib\site-packages\dill\_dill.py", line 1176, in save_instancemethod0
pickler.save_reduce(MethodType, (obj.__func__, obj.__self__), obj=obj)
File " \Python\Python36\lib\pickle.py", line 610, in save_reduce
save(args)
File " \Python\Python36\lib\pickle.py", line 476, in save
f(self, obj) # Call unbound method with explicit self
File " \Python\Python36\lib\pickle.py", line 736, in save_tuple
save(element)
File " \Python\Python36\lib\pickle.py", line 476, in save
f(self, obj) # Call unbound method with explicit self
File " \lib\site-packages\datasets\utils\py_utils.py", line 523, in save_function
obj=obj,
File " \Python\Python36\lib\pickle.py", line 610, in save_reduce
save(args)
File " \Python\Python36\lib\pickle.py", line 476, in save
f(self, obj) # Call unbound method with explicit self
File " \Python\Python36\lib\pickle.py", line 751, in save_tuple
save(element)
File " \Python\Python36\lib\pickle.py", line 476, in save
f(self, obj) # Call unbound method with explicit self
File " \lib\site-packages\dill\_dill.py", line 990, in save_module_dict
StockPickler.save_dict(pickler, obj)
File " \Python\Python36\lib\pickle.py", line 821, in save_dict
self._batch_setitems(obj.items())
File " \Python\Python36\lib\pickle.py", line 847, in _batch_setitems
save(v)
File " \Python\Python36\lib\pickle.py", line 521, in save
self.save_reduce(obj=obj, *rv)
File " \Python\Python36\lib\pickle.py", line 605, in save_reduce
save(cls)
File " \Python\Python36\lib\pickle.py", line 476, in save
f(self, obj) # Call unbound method with explicit self
File " \lib\site-packages\dill\_dill.py", line 1439, in save_type
StockPickler.save_global(pickler, obj, name=name)
File " \Python\Python36\lib\pickle.py", line 922, in save_global
(obj, module_name, name))
_pickle.PicklingError: Can't pickle <class 'spacy.errors.add_codes.<locals>.ErrorsWithCodes'>: it's not found as spacy.errors.add_codes.<locals>.ErrorsWithCodes
```
## Environment info
Tried on both Linux and Windows
- `datasets` version: 1.14.0
- Platform: Windows-10-10.0.19041-SP0 + Python 3.7.9; Linux-5.11.0-38-generic-x86_64-with-Ubuntu-20.04-focal + Python 3.7.12
- PyArrow version: 6.0.0
I see this has just been closed - it seems quite relevant to another tokenizer I have been trying to use, the `vinai/phobert` family of tokenizers
https://huggingface.co/vinai/phobert-base
https://huggingface.co/vinai/phobert-large
I ran into an issue where a large dataset took several hours to tokenize, the process hung, and I was unable to use the cached version of the tokenized data:
https://discuss.huggingface.co/t/cache-parallelize-long-tokenization-step/25791/3
I don't see any way to specify the hash of the tokenizer or the fingerprint of the tokenized data to use, so is the tokenized dataset basically lost at this point? Is there a good way to avoid this happening again if I retokenize the data?
|
https://github.com/huggingface/datasets/issues/3178 | "Property couldn't be hashed properly" even though fully picklable | In your case it looks like the job failed before caching the data - maybe one of the processes crashed | ## Describe the bug
I am trying to tokenize a dataset with spaCy. I found that no matter what I do, the spaCy language object (`nlp`) prevents `datasets` from pickling correctly - or so the warning says - even though manually pickling is no issue. It should not be an issue either, since spaCy objects are picklable.
## Steps to reproduce the bug
Here is a [colab](https://colab.research.google.com/drive/1gt75LCBIzsmBMvvipEOvWulvyZseBiA7?usp=sharing) but for some reason I cannot reproduce it there. That may have to do with logging/tqdm on Colab, or with running things in notebooks. I tried below code on Windows and Ubuntu as a Python script and getting the same issue (warning below).
```python
import pickle
from datasets import load_dataset
import spacy
class Processor:
def __init__(self):
self.nlp = spacy.load("en_core_web_sm", disable=["tagger", "parser", "ner", "lemmatizer"])
@staticmethod
def collate(batch):
return [d["en"] for d in batch]
def parse(self, batch):
batch = batch["translation"]
return {"translation_tok": [{"en_tok": " ".join([t.text for t in doc])} for doc in self.nlp.pipe(self.collate(batch))]}
def process(self):
ds = load_dataset("wmt16", "de-en", split="train[:10%]")
ds = ds.map(self.parse, batched=True, num_proc=6)
if __name__ == '__main__':
pr = Processor()
# succeeds
with open("temp.pkl", "wb") as f:
pickle.dump(pr, f)
print("Successfully pickled!")
pr.process()
```
---
Here is a small change that includes `Hasher.hash` that shows that the hasher cannot seem to successfully pickle parts form the NLP object.
```python
from datasets.fingerprint import Hasher
import pickle
from datasets import load_dataset
import spacy
class Processor:
def __init__(self):
self.nlp = spacy.load("en_core_web_sm", disable=["tagger", "parser", "ner", "lemmatizer"])
@staticmethod
def collate(batch):
return [d["en"] for d in batch]
def parse(self, batch):
batch = batch["translation"]
return {"translation_tok": [{"en_tok": " ".join([t.text for t in doc])} for doc in self.nlp.pipe(self.collate(batch))]}
def process(self):
ds = load_dataset("wmt16", "de-en", split="train[:10]")
return ds.map(self.parse, batched=True)
if __name__ == '__main__':
pr = Processor()
# succeeds
with open("temp.pkl", "wb") as f:
pickle.dump(pr, f)
print("Successfully pickled class instance!")
# succeeds
with open("temp.pkl", "wb") as f:
pickle.dump(pr.nlp, f)
print("Successfully pickled nlp!")
# fails
print(Hasher.hash(pr.nlp))
pr.process()
```
## Expected results
This to be picklable, working (fingerprinted), and no warning.
## Actual results
In the first snippet, I get this warning
Parameter 'function'=<function Processor.parse at 0x7f44982247a0> of the transform datasets.arrow_dataset.Dataset._map_single couldn't be hashed properly, a random hash was used instead. Make sure your transforms and parameters are serializable with pickle or dill for the dataset fingerprinting and caching to work. If you reuse this transform, the caching mechanism will consider it to be different from the previous calls and recompute everything. This warning is only showed once. Subsequent hashing failures won't be showed.
In the second, I get this traceback which directs to the `Hasher.hash` line.
```
Traceback (most recent call last):
File " \Python\Python36\lib\pickle.py", line 918, in save_global
obj2, parent = _getattribute(module, name)
File " \Python\Python36\lib\pickle.py", line 266, in _getattribute
.format(name, obj))
AttributeError: Can't get local attribute 'add_codes.<locals>.ErrorsWithCodes' on <function add_codes at 0x00000296FF606EA0>
During handling of the above exception, another exception occurred:
Traceback (most recent call last):
File " scratch_4.py", line 40, in <module>
print(Hasher.hash(pr.nlp))
File " \lib\site-packages\datasets\fingerprint.py", line 191, in hash
return cls.hash_default(value)
File " \lib\site-packages\datasets\fingerprint.py", line 184, in hash_default
return cls.hash_bytes(dumps(value))
File " \lib\site-packages\datasets\utils\py_utils.py", line 345, in dumps
dump(obj, file)
File " \lib\site-packages\datasets\utils\py_utils.py", line 320, in dump
Pickler(file, recurse=True).dump(obj)
File " \lib\site-packages\dill\_dill.py", line 498, in dump
StockPickler.dump(self, obj)
File " \Python\Python36\lib\pickle.py", line 409, in dump
self.save(obj)
File " \Python\Python36\lib\pickle.py", line 521, in save
self.save_reduce(obj=obj, *rv)
File " \Python\Python36\lib\pickle.py", line 634, in save_reduce
save(state)
File " \Python\Python36\lib\pickle.py", line 476, in save
f(self, obj) # Call unbound method with explicit self
File " \lib\site-packages\dill\_dill.py", line 990, in save_module_dict
StockPickler.save_dict(pickler, obj)
File " \Python\Python36\lib\pickle.py", line 821, in save_dict
self._batch_setitems(obj.items())
File " \Python\Python36\lib\pickle.py", line 847, in _batch_setitems
save(v)
File " \Python\Python36\lib\pickle.py", line 476, in save
f(self, obj) # Call unbound method with explicit self
File " \Python\Python36\lib\pickle.py", line 781, in save_list
self._batch_appends(obj)
File " \Python\Python36\lib\pickle.py", line 805, in _batch_appends
save(x)
File " \Python\Python36\lib\pickle.py", line 476, in save
f(self, obj) # Call unbound method with explicit self
File " \Python\Python36\lib\pickle.py", line 736, in save_tuple
save(element)
File " \Python\Python36\lib\pickle.py", line 521, in save
self.save_reduce(obj=obj, *rv)
File " \Python\Python36\lib\pickle.py", line 634, in save_reduce
save(state)
File " \Python\Python36\lib\pickle.py", line 476, in save
f(self, obj) # Call unbound method with explicit self
File " \Python\Python36\lib\pickle.py", line 736, in save_tuple
save(element)
File " \Python\Python36\lib\pickle.py", line 476, in save
f(self, obj) # Call unbound method with explicit self
File " \lib\site-packages\dill\_dill.py", line 990, in save_module_dict
StockPickler.save_dict(pickler, obj)
File " \Python\Python36\lib\pickle.py", line 821, in save_dict
self._batch_setitems(obj.items())
File " \Python\Python36\lib\pickle.py", line 847, in _batch_setitems
save(v)
File " \Python\Python36\lib\pickle.py", line 476, in save
f(self, obj) # Call unbound method with explicit self
File " \lib\site-packages\dill\_dill.py", line 1176, in save_instancemethod0
pickler.save_reduce(MethodType, (obj.__func__, obj.__self__), obj=obj)
File " \Python\Python36\lib\pickle.py", line 610, in save_reduce
save(args)
File " \Python\Python36\lib\pickle.py", line 476, in save
f(self, obj) # Call unbound method with explicit self
File " \Python\Python36\lib\pickle.py", line 736, in save_tuple
save(element)
File " \Python\Python36\lib\pickle.py", line 476, in save
f(self, obj) # Call unbound method with explicit self
File " \lib\site-packages\datasets\utils\py_utils.py", line 523, in save_function
obj=obj,
File " \Python\Python36\lib\pickle.py", line 610, in save_reduce
save(args)
File " \Python\Python36\lib\pickle.py", line 476, in save
f(self, obj) # Call unbound method with explicit self
File " \Python\Python36\lib\pickle.py", line 751, in save_tuple
save(element)
File " \Python\Python36\lib\pickle.py", line 476, in save
f(self, obj) # Call unbound method with explicit self
File " \lib\site-packages\dill\_dill.py", line 990, in save_module_dict
StockPickler.save_dict(pickler, obj)
File " \Python\Python36\lib\pickle.py", line 821, in save_dict
self._batch_setitems(obj.items())
File " \Python\Python36\lib\pickle.py", line 847, in _batch_setitems
save(v)
File " \Python\Python36\lib\pickle.py", line 521, in save
self.save_reduce(obj=obj, *rv)
File " \Python\Python36\lib\pickle.py", line 605, in save_reduce
save(cls)
File " \Python\Python36\lib\pickle.py", line 476, in save
f(self, obj) # Call unbound method with explicit self
File " \lib\site-packages\dill\_dill.py", line 1439, in save_type
StockPickler.save_global(pickler, obj, name=name)
File " \Python\Python36\lib\pickle.py", line 922, in save_global
(obj, module_name, name))
_pickle.PicklingError: Can't pickle <class 'spacy.errors.add_codes.<locals>.ErrorsWithCodes'>: it's not found as spacy.errors.add_codes.<locals>.ErrorsWithCodes
```
## Environment info
Tried on both Linux and Windows
- `datasets` version: 1.14.0
- Platform: Windows-10-10.0.19041-SP0 + Python 3.7.9; Linux-5.11.0-38-generic-x86_64-with-Ubuntu-20.04-focal + Python 3.7.12
- PyArrow version: 6.0.0
| 20 | "Property couldn't be hashed properly" even though fully picklable
## Describe the bug
I am trying to tokenize a dataset with spaCy. I found that no matter what I do, the spaCy language object (`nlp`) prevents `datasets` from pickling correctly - or so the warning says - even though manually pickling is no issue. It should not be an issue either, since spaCy objects are picklable.
## Steps to reproduce the bug
Here is a [colab](https://colab.research.google.com/drive/1gt75LCBIzsmBMvvipEOvWulvyZseBiA7?usp=sharing) but for some reason I cannot reproduce it there. That may have to do with logging/tqdm on Colab, or with running things in notebooks. I tried below code on Windows and Ubuntu as a Python script and getting the same issue (warning below).
```python
import pickle
from datasets import load_dataset
import spacy
class Processor:
def __init__(self):
self.nlp = spacy.load("en_core_web_sm", disable=["tagger", "parser", "ner", "lemmatizer"])
@staticmethod
def collate(batch):
return [d["en"] for d in batch]
def parse(self, batch):
batch = batch["translation"]
return {"translation_tok": [{"en_tok": " ".join([t.text for t in doc])} for doc in self.nlp.pipe(self.collate(batch))]}
def process(self):
ds = load_dataset("wmt16", "de-en", split="train[:10%]")
ds = ds.map(self.parse, batched=True, num_proc=6)
if __name__ == '__main__':
pr = Processor()
# succeeds
with open("temp.pkl", "wb") as f:
pickle.dump(pr, f)
print("Successfully pickled!")
pr.process()
```
---
Here is a small change that includes `Hasher.hash` that shows that the hasher cannot seem to successfully pickle parts form the NLP object.
```python
from datasets.fingerprint import Hasher
import pickle
from datasets import load_dataset
import spacy
class Processor:
def __init__(self):
self.nlp = spacy.load("en_core_web_sm", disable=["tagger", "parser", "ner", "lemmatizer"])
@staticmethod
def collate(batch):
return [d["en"] for d in batch]
def parse(self, batch):
batch = batch["translation"]
return {"translation_tok": [{"en_tok": " ".join([t.text for t in doc])} for doc in self.nlp.pipe(self.collate(batch))]}
def process(self):
ds = load_dataset("wmt16", "de-en", split="train[:10]")
return ds.map(self.parse, batched=True)
if __name__ == '__main__':
pr = Processor()
# succeeds
with open("temp.pkl", "wb") as f:
pickle.dump(pr, f)
print("Successfully pickled class instance!")
# succeeds
with open("temp.pkl", "wb") as f:
pickle.dump(pr.nlp, f)
print("Successfully pickled nlp!")
# fails
print(Hasher.hash(pr.nlp))
pr.process()
```
## Expected results
This to be picklable, working (fingerprinted), and no warning.
## Actual results
In the first snippet, I get this warning
Parameter 'function'=<function Processor.parse at 0x7f44982247a0> of the transform datasets.arrow_dataset.Dataset._map_single couldn't be hashed properly, a random hash was used instead. Make sure your transforms and parameters are serializable with pickle or dill for the dataset fingerprinting and caching to work. If you reuse this transform, the caching mechanism will consider it to be different from the previous calls and recompute everything. This warning is only showed once. Subsequent hashing failures won't be showed.
In the second, I get this traceback which directs to the `Hasher.hash` line.
```
Traceback (most recent call last):
File " \Python\Python36\lib\pickle.py", line 918, in save_global
obj2, parent = _getattribute(module, name)
File " \Python\Python36\lib\pickle.py", line 266, in _getattribute
.format(name, obj))
AttributeError: Can't get local attribute 'add_codes.<locals>.ErrorsWithCodes' on <function add_codes at 0x00000296FF606EA0>
During handling of the above exception, another exception occurred:
Traceback (most recent call last):
File " scratch_4.py", line 40, in <module>
print(Hasher.hash(pr.nlp))
File " \lib\site-packages\datasets\fingerprint.py", line 191, in hash
return cls.hash_default(value)
File " \lib\site-packages\datasets\fingerprint.py", line 184, in hash_default
return cls.hash_bytes(dumps(value))
File " \lib\site-packages\datasets\utils\py_utils.py", line 345, in dumps
dump(obj, file)
File " \lib\site-packages\datasets\utils\py_utils.py", line 320, in dump
Pickler(file, recurse=True).dump(obj)
File " \lib\site-packages\dill\_dill.py", line 498, in dump
StockPickler.dump(self, obj)
File " \Python\Python36\lib\pickle.py", line 409, in dump
self.save(obj)
File " \Python\Python36\lib\pickle.py", line 521, in save
self.save_reduce(obj=obj, *rv)
File " \Python\Python36\lib\pickle.py", line 634, in save_reduce
save(state)
File " \Python\Python36\lib\pickle.py", line 476, in save
f(self, obj) # Call unbound method with explicit self
File " \lib\site-packages\dill\_dill.py", line 990, in save_module_dict
StockPickler.save_dict(pickler, obj)
File " \Python\Python36\lib\pickle.py", line 821, in save_dict
self._batch_setitems(obj.items())
File " \Python\Python36\lib\pickle.py", line 847, in _batch_setitems
save(v)
File " \Python\Python36\lib\pickle.py", line 476, in save
f(self, obj) # Call unbound method with explicit self
File " \Python\Python36\lib\pickle.py", line 781, in save_list
self._batch_appends(obj)
File " \Python\Python36\lib\pickle.py", line 805, in _batch_appends
save(x)
File " \Python\Python36\lib\pickle.py", line 476, in save
f(self, obj) # Call unbound method with explicit self
File " \Python\Python36\lib\pickle.py", line 736, in save_tuple
save(element)
File " \Python\Python36\lib\pickle.py", line 521, in save
self.save_reduce(obj=obj, *rv)
File " \Python\Python36\lib\pickle.py", line 634, in save_reduce
save(state)
File " \Python\Python36\lib\pickle.py", line 476, in save
f(self, obj) # Call unbound method with explicit self
File " \Python\Python36\lib\pickle.py", line 736, in save_tuple
save(element)
File " \Python\Python36\lib\pickle.py", line 476, in save
f(self, obj) # Call unbound method with explicit self
File " \lib\site-packages\dill\_dill.py", line 990, in save_module_dict
StockPickler.save_dict(pickler, obj)
File " \Python\Python36\lib\pickle.py", line 821, in save_dict
self._batch_setitems(obj.items())
File " \Python\Python36\lib\pickle.py", line 847, in _batch_setitems
save(v)
File " \Python\Python36\lib\pickle.py", line 476, in save
f(self, obj) # Call unbound method with explicit self
File " \lib\site-packages\dill\_dill.py", line 1176, in save_instancemethod0
pickler.save_reduce(MethodType, (obj.__func__, obj.__self__), obj=obj)
File " \Python\Python36\lib\pickle.py", line 610, in save_reduce
save(args)
File " \Python\Python36\lib\pickle.py", line 476, in save
f(self, obj) # Call unbound method with explicit self
File " \Python\Python36\lib\pickle.py", line 736, in save_tuple
save(element)
File " \Python\Python36\lib\pickle.py", line 476, in save
f(self, obj) # Call unbound method with explicit self
File " \lib\site-packages\datasets\utils\py_utils.py", line 523, in save_function
obj=obj,
File " \Python\Python36\lib\pickle.py", line 610, in save_reduce
save(args)
File " \Python\Python36\lib\pickle.py", line 476, in save
f(self, obj) # Call unbound method with explicit self
File " \Python\Python36\lib\pickle.py", line 751, in save_tuple
save(element)
File " \Python\Python36\lib\pickle.py", line 476, in save
f(self, obj) # Call unbound method with explicit self
File " \lib\site-packages\dill\_dill.py", line 990, in save_module_dict
StockPickler.save_dict(pickler, obj)
File " \Python\Python36\lib\pickle.py", line 821, in save_dict
self._batch_setitems(obj.items())
File " \Python\Python36\lib\pickle.py", line 847, in _batch_setitems
save(v)
File " \Python\Python36\lib\pickle.py", line 521, in save
self.save_reduce(obj=obj, *rv)
File " \Python\Python36\lib\pickle.py", line 605, in save_reduce
save(cls)
File " \Python\Python36\lib\pickle.py", line 476, in save
f(self, obj) # Call unbound method with explicit self
File " \lib\site-packages\dill\_dill.py", line 1439, in save_type
StockPickler.save_global(pickler, obj, name=name)
File " \Python\Python36\lib\pickle.py", line 922, in save_global
(obj, module_name, name))
_pickle.PicklingError: Can't pickle <class 'spacy.errors.add_codes.<locals>.ErrorsWithCodes'>: it's not found as spacy.errors.add_codes.<locals>.ErrorsWithCodes
```
## Environment info
Tried on both Linux and Windows
- `datasets` version: 1.14.0
- Platform: Windows-10-10.0.19041-SP0 + Python 3.7.9; Linux-5.11.0-38-generic-x86_64-with-Ubuntu-20.04-focal + Python 3.7.12
- PyArrow version: 6.0.0
In your case it looks like the job failed before caching the data - maybe one of the processes crashed |
https://github.com/huggingface/datasets/issues/3178 | "Property couldn't be hashed properly" even though fully picklable | Interesting. Thanks for the observation. Any suggestions on how to start tracking that down? Perhaps run it singlethreaded and see if it crashes? | ## Describe the bug
I am trying to tokenize a dataset with spaCy. I found that no matter what I do, the spaCy language object (`nlp`) prevents `datasets` from pickling correctly - or so the warning says - even though manually pickling is no issue. It should not be an issue either, since spaCy objects are picklable.
## Steps to reproduce the bug
Here is a [colab](https://colab.research.google.com/drive/1gt75LCBIzsmBMvvipEOvWulvyZseBiA7?usp=sharing) but for some reason I cannot reproduce it there. That may have to do with logging/tqdm on Colab, or with running things in notebooks. I tried below code on Windows and Ubuntu as a Python script and getting the same issue (warning below).
```python
import pickle
from datasets import load_dataset
import spacy
class Processor:
def __init__(self):
self.nlp = spacy.load("en_core_web_sm", disable=["tagger", "parser", "ner", "lemmatizer"])
@staticmethod
def collate(batch):
return [d["en"] for d in batch]
def parse(self, batch):
batch = batch["translation"]
return {"translation_tok": [{"en_tok": " ".join([t.text for t in doc])} for doc in self.nlp.pipe(self.collate(batch))]}
def process(self):
ds = load_dataset("wmt16", "de-en", split="train[:10%]")
ds = ds.map(self.parse, batched=True, num_proc=6)
if __name__ == '__main__':
pr = Processor()
# succeeds
with open("temp.pkl", "wb") as f:
pickle.dump(pr, f)
print("Successfully pickled!")
pr.process()
```
---
Here is a small change that includes `Hasher.hash` that shows that the hasher cannot seem to successfully pickle parts form the NLP object.
```python
from datasets.fingerprint import Hasher
import pickle
from datasets import load_dataset
import spacy
class Processor:
def __init__(self):
self.nlp = spacy.load("en_core_web_sm", disable=["tagger", "parser", "ner", "lemmatizer"])
@staticmethod
def collate(batch):
return [d["en"] for d in batch]
def parse(self, batch):
batch = batch["translation"]
return {"translation_tok": [{"en_tok": " ".join([t.text for t in doc])} for doc in self.nlp.pipe(self.collate(batch))]}
def process(self):
ds = load_dataset("wmt16", "de-en", split="train[:10]")
return ds.map(self.parse, batched=True)
if __name__ == '__main__':
pr = Processor()
# succeeds
with open("temp.pkl", "wb") as f:
pickle.dump(pr, f)
print("Successfully pickled class instance!")
# succeeds
with open("temp.pkl", "wb") as f:
pickle.dump(pr.nlp, f)
print("Successfully pickled nlp!")
# fails
print(Hasher.hash(pr.nlp))
pr.process()
```
## Expected results
This to be picklable, working (fingerprinted), and no warning.
## Actual results
In the first snippet, I get this warning
Parameter 'function'=<function Processor.parse at 0x7f44982247a0> of the transform datasets.arrow_dataset.Dataset._map_single couldn't be hashed properly, a random hash was used instead. Make sure your transforms and parameters are serializable with pickle or dill for the dataset fingerprinting and caching to work. If you reuse this transform, the caching mechanism will consider it to be different from the previous calls and recompute everything. This warning is only showed once. Subsequent hashing failures won't be showed.
In the second, I get this traceback which directs to the `Hasher.hash` line.
```
Traceback (most recent call last):
File " \Python\Python36\lib\pickle.py", line 918, in save_global
obj2, parent = _getattribute(module, name)
File " \Python\Python36\lib\pickle.py", line 266, in _getattribute
.format(name, obj))
AttributeError: Can't get local attribute 'add_codes.<locals>.ErrorsWithCodes' on <function add_codes at 0x00000296FF606EA0>
During handling of the above exception, another exception occurred:
Traceback (most recent call last):
File " scratch_4.py", line 40, in <module>
print(Hasher.hash(pr.nlp))
File " \lib\site-packages\datasets\fingerprint.py", line 191, in hash
return cls.hash_default(value)
File " \lib\site-packages\datasets\fingerprint.py", line 184, in hash_default
return cls.hash_bytes(dumps(value))
File " \lib\site-packages\datasets\utils\py_utils.py", line 345, in dumps
dump(obj, file)
File " \lib\site-packages\datasets\utils\py_utils.py", line 320, in dump
Pickler(file, recurse=True).dump(obj)
File " \lib\site-packages\dill\_dill.py", line 498, in dump
StockPickler.dump(self, obj)
File " \Python\Python36\lib\pickle.py", line 409, in dump
self.save(obj)
File " \Python\Python36\lib\pickle.py", line 521, in save
self.save_reduce(obj=obj, *rv)
File " \Python\Python36\lib\pickle.py", line 634, in save_reduce
save(state)
File " \Python\Python36\lib\pickle.py", line 476, in save
f(self, obj) # Call unbound method with explicit self
File " \lib\site-packages\dill\_dill.py", line 990, in save_module_dict
StockPickler.save_dict(pickler, obj)
File " \Python\Python36\lib\pickle.py", line 821, in save_dict
self._batch_setitems(obj.items())
File " \Python\Python36\lib\pickle.py", line 847, in _batch_setitems
save(v)
File " \Python\Python36\lib\pickle.py", line 476, in save
f(self, obj) # Call unbound method with explicit self
File " \Python\Python36\lib\pickle.py", line 781, in save_list
self._batch_appends(obj)
File " \Python\Python36\lib\pickle.py", line 805, in _batch_appends
save(x)
File " \Python\Python36\lib\pickle.py", line 476, in save
f(self, obj) # Call unbound method with explicit self
File " \Python\Python36\lib\pickle.py", line 736, in save_tuple
save(element)
File " \Python\Python36\lib\pickle.py", line 521, in save
self.save_reduce(obj=obj, *rv)
File " \Python\Python36\lib\pickle.py", line 634, in save_reduce
save(state)
File " \Python\Python36\lib\pickle.py", line 476, in save
f(self, obj) # Call unbound method with explicit self
File " \Python\Python36\lib\pickle.py", line 736, in save_tuple
save(element)
File " \Python\Python36\lib\pickle.py", line 476, in save
f(self, obj) # Call unbound method with explicit self
File " \lib\site-packages\dill\_dill.py", line 990, in save_module_dict
StockPickler.save_dict(pickler, obj)
File " \Python\Python36\lib\pickle.py", line 821, in save_dict
self._batch_setitems(obj.items())
File " \Python\Python36\lib\pickle.py", line 847, in _batch_setitems
save(v)
File " \Python\Python36\lib\pickle.py", line 476, in save
f(self, obj) # Call unbound method with explicit self
File " \lib\site-packages\dill\_dill.py", line 1176, in save_instancemethod0
pickler.save_reduce(MethodType, (obj.__func__, obj.__self__), obj=obj)
File " \Python\Python36\lib\pickle.py", line 610, in save_reduce
save(args)
File " \Python\Python36\lib\pickle.py", line 476, in save
f(self, obj) # Call unbound method with explicit self
File " \Python\Python36\lib\pickle.py", line 736, in save_tuple
save(element)
File " \Python\Python36\lib\pickle.py", line 476, in save
f(self, obj) # Call unbound method with explicit self
File " \lib\site-packages\datasets\utils\py_utils.py", line 523, in save_function
obj=obj,
File " \Python\Python36\lib\pickle.py", line 610, in save_reduce
save(args)
File " \Python\Python36\lib\pickle.py", line 476, in save
f(self, obj) # Call unbound method with explicit self
File " \Python\Python36\lib\pickle.py", line 751, in save_tuple
save(element)
File " \Python\Python36\lib\pickle.py", line 476, in save
f(self, obj) # Call unbound method with explicit self
File " \lib\site-packages\dill\_dill.py", line 990, in save_module_dict
StockPickler.save_dict(pickler, obj)
File " \Python\Python36\lib\pickle.py", line 821, in save_dict
self._batch_setitems(obj.items())
File " \Python\Python36\lib\pickle.py", line 847, in _batch_setitems
save(v)
File " \Python\Python36\lib\pickle.py", line 521, in save
self.save_reduce(obj=obj, *rv)
File " \Python\Python36\lib\pickle.py", line 605, in save_reduce
save(cls)
File " \Python\Python36\lib\pickle.py", line 476, in save
f(self, obj) # Call unbound method with explicit self
File " \lib\site-packages\dill\_dill.py", line 1439, in save_type
StockPickler.save_global(pickler, obj, name=name)
File " \Python\Python36\lib\pickle.py", line 922, in save_global
(obj, module_name, name))
_pickle.PicklingError: Can't pickle <class 'spacy.errors.add_codes.<locals>.ErrorsWithCodes'>: it's not found as spacy.errors.add_codes.<locals>.ErrorsWithCodes
```
## Environment info
Tried on both Linux and Windows
- `datasets` version: 1.14.0
- Platform: Windows-10-10.0.19041-SP0 + Python 3.7.9; Linux-5.11.0-38-generic-x86_64-with-Ubuntu-20.04-focal + Python 3.7.12
- PyArrow version: 6.0.0
| 23 | "Property couldn't be hashed properly" even though fully picklable
## Describe the bug
I am trying to tokenize a dataset with spaCy. I found that no matter what I do, the spaCy language object (`nlp`) prevents `datasets` from pickling correctly - or so the warning says - even though manually pickling is no issue. It should not be an issue either, since spaCy objects are picklable.
## Steps to reproduce the bug
Here is a [colab](https://colab.research.google.com/drive/1gt75LCBIzsmBMvvipEOvWulvyZseBiA7?usp=sharing) but for some reason I cannot reproduce it there. That may have to do with logging/tqdm on Colab, or with running things in notebooks. I tried below code on Windows and Ubuntu as a Python script and getting the same issue (warning below).
```python
import pickle
from datasets import load_dataset
import spacy
class Processor:
def __init__(self):
self.nlp = spacy.load("en_core_web_sm", disable=["tagger", "parser", "ner", "lemmatizer"])
@staticmethod
def collate(batch):
return [d["en"] for d in batch]
def parse(self, batch):
batch = batch["translation"]
return {"translation_tok": [{"en_tok": " ".join([t.text for t in doc])} for doc in self.nlp.pipe(self.collate(batch))]}
def process(self):
ds = load_dataset("wmt16", "de-en", split="train[:10%]")
ds = ds.map(self.parse, batched=True, num_proc=6)
if __name__ == '__main__':
pr = Processor()
# succeeds
with open("temp.pkl", "wb") as f:
pickle.dump(pr, f)
print("Successfully pickled!")
pr.process()
```
---
Here is a small change that includes `Hasher.hash` that shows that the hasher cannot seem to successfully pickle parts form the NLP object.
```python
from datasets.fingerprint import Hasher
import pickle
from datasets import load_dataset
import spacy
class Processor:
def __init__(self):
self.nlp = spacy.load("en_core_web_sm", disable=["tagger", "parser", "ner", "lemmatizer"])
@staticmethod
def collate(batch):
return [d["en"] for d in batch]
def parse(self, batch):
batch = batch["translation"]
return {"translation_tok": [{"en_tok": " ".join([t.text for t in doc])} for doc in self.nlp.pipe(self.collate(batch))]}
def process(self):
ds = load_dataset("wmt16", "de-en", split="train[:10]")
return ds.map(self.parse, batched=True)
if __name__ == '__main__':
pr = Processor()
# succeeds
with open("temp.pkl", "wb") as f:
pickle.dump(pr, f)
print("Successfully pickled class instance!")
# succeeds
with open("temp.pkl", "wb") as f:
pickle.dump(pr.nlp, f)
print("Successfully pickled nlp!")
# fails
print(Hasher.hash(pr.nlp))
pr.process()
```
## Expected results
This to be picklable, working (fingerprinted), and no warning.
## Actual results
In the first snippet, I get this warning
Parameter 'function'=<function Processor.parse at 0x7f44982247a0> of the transform datasets.arrow_dataset.Dataset._map_single couldn't be hashed properly, a random hash was used instead. Make sure your transforms and parameters are serializable with pickle or dill for the dataset fingerprinting and caching to work. If you reuse this transform, the caching mechanism will consider it to be different from the previous calls and recompute everything. This warning is only showed once. Subsequent hashing failures won't be showed.
In the second, I get this traceback which directs to the `Hasher.hash` line.
```
Traceback (most recent call last):
File " \Python\Python36\lib\pickle.py", line 918, in save_global
obj2, parent = _getattribute(module, name)
File " \Python\Python36\lib\pickle.py", line 266, in _getattribute
.format(name, obj))
AttributeError: Can't get local attribute 'add_codes.<locals>.ErrorsWithCodes' on <function add_codes at 0x00000296FF606EA0>
During handling of the above exception, another exception occurred:
Traceback (most recent call last):
File " scratch_4.py", line 40, in <module>
print(Hasher.hash(pr.nlp))
File " \lib\site-packages\datasets\fingerprint.py", line 191, in hash
return cls.hash_default(value)
File " \lib\site-packages\datasets\fingerprint.py", line 184, in hash_default
return cls.hash_bytes(dumps(value))
File " \lib\site-packages\datasets\utils\py_utils.py", line 345, in dumps
dump(obj, file)
File " \lib\site-packages\datasets\utils\py_utils.py", line 320, in dump
Pickler(file, recurse=True).dump(obj)
File " \lib\site-packages\dill\_dill.py", line 498, in dump
StockPickler.dump(self, obj)
File " \Python\Python36\lib\pickle.py", line 409, in dump
self.save(obj)
File " \Python\Python36\lib\pickle.py", line 521, in save
self.save_reduce(obj=obj, *rv)
File " \Python\Python36\lib\pickle.py", line 634, in save_reduce
save(state)
File " \Python\Python36\lib\pickle.py", line 476, in save
f(self, obj) # Call unbound method with explicit self
File " \lib\site-packages\dill\_dill.py", line 990, in save_module_dict
StockPickler.save_dict(pickler, obj)
File " \Python\Python36\lib\pickle.py", line 821, in save_dict
self._batch_setitems(obj.items())
File " \Python\Python36\lib\pickle.py", line 847, in _batch_setitems
save(v)
File " \Python\Python36\lib\pickle.py", line 476, in save
f(self, obj) # Call unbound method with explicit self
File " \Python\Python36\lib\pickle.py", line 781, in save_list
self._batch_appends(obj)
File " \Python\Python36\lib\pickle.py", line 805, in _batch_appends
save(x)
File " \Python\Python36\lib\pickle.py", line 476, in save
f(self, obj) # Call unbound method with explicit self
File " \Python\Python36\lib\pickle.py", line 736, in save_tuple
save(element)
File " \Python\Python36\lib\pickle.py", line 521, in save
self.save_reduce(obj=obj, *rv)
File " \Python\Python36\lib\pickle.py", line 634, in save_reduce
save(state)
File " \Python\Python36\lib\pickle.py", line 476, in save
f(self, obj) # Call unbound method with explicit self
File " \Python\Python36\lib\pickle.py", line 736, in save_tuple
save(element)
File " \Python\Python36\lib\pickle.py", line 476, in save
f(self, obj) # Call unbound method with explicit self
File " \lib\site-packages\dill\_dill.py", line 990, in save_module_dict
StockPickler.save_dict(pickler, obj)
File " \Python\Python36\lib\pickle.py", line 821, in save_dict
self._batch_setitems(obj.items())
File " \Python\Python36\lib\pickle.py", line 847, in _batch_setitems
save(v)
File " \Python\Python36\lib\pickle.py", line 476, in save
f(self, obj) # Call unbound method with explicit self
File " \lib\site-packages\dill\_dill.py", line 1176, in save_instancemethod0
pickler.save_reduce(MethodType, (obj.__func__, obj.__self__), obj=obj)
File " \Python\Python36\lib\pickle.py", line 610, in save_reduce
save(args)
File " \Python\Python36\lib\pickle.py", line 476, in save
f(self, obj) # Call unbound method with explicit self
File " \Python\Python36\lib\pickle.py", line 736, in save_tuple
save(element)
File " \Python\Python36\lib\pickle.py", line 476, in save
f(self, obj) # Call unbound method with explicit self
File " \lib\site-packages\datasets\utils\py_utils.py", line 523, in save_function
obj=obj,
File " \Python\Python36\lib\pickle.py", line 610, in save_reduce
save(args)
File " \Python\Python36\lib\pickle.py", line 476, in save
f(self, obj) # Call unbound method with explicit self
File " \Python\Python36\lib\pickle.py", line 751, in save_tuple
save(element)
File " \Python\Python36\lib\pickle.py", line 476, in save
f(self, obj) # Call unbound method with explicit self
File " \lib\site-packages\dill\_dill.py", line 990, in save_module_dict
StockPickler.save_dict(pickler, obj)
File " \Python\Python36\lib\pickle.py", line 821, in save_dict
self._batch_setitems(obj.items())
File " \Python\Python36\lib\pickle.py", line 847, in _batch_setitems
save(v)
File " \Python\Python36\lib\pickle.py", line 521, in save
self.save_reduce(obj=obj, *rv)
File " \Python\Python36\lib\pickle.py", line 605, in save_reduce
save(cls)
File " \Python\Python36\lib\pickle.py", line 476, in save
f(self, obj) # Call unbound method with explicit self
File " \lib\site-packages\dill\_dill.py", line 1439, in save_type
StockPickler.save_global(pickler, obj, name=name)
File " \Python\Python36\lib\pickle.py", line 922, in save_global
(obj, module_name, name))
_pickle.PicklingError: Can't pickle <class 'spacy.errors.add_codes.<locals>.ErrorsWithCodes'>: it's not found as spacy.errors.add_codes.<locals>.ErrorsWithCodes
```
## Environment info
Tried on both Linux and Windows
- `datasets` version: 1.14.0
- Platform: Windows-10-10.0.19041-SP0 + Python 3.7.9; Linux-5.11.0-38-generic-x86_64-with-Ubuntu-20.04-focal + Python 3.7.12
- PyArrow version: 6.0.0
Interesting. Thanks for the observation. Any suggestions on how to start tracking that down? Perhaps run it singlethreaded and see if it crashes? |
https://github.com/huggingface/datasets/issues/3178 | "Property couldn't be hashed properly" even though fully picklable | You can monitor your RAM and disk space in case a process dies from OOM or disk full, and when it hangs you can check how many processes are running. IIRC there are other start methods for multiprocessing in python that may show an error message if a process dies.
Running on a single process can also help debugging this indeed | ## Describe the bug
I am trying to tokenize a dataset with spaCy. I found that no matter what I do, the spaCy language object (`nlp`) prevents `datasets` from pickling correctly - or so the warning says - even though manually pickling is no issue. It should not be an issue either, since spaCy objects are picklable.
## Steps to reproduce the bug
Here is a [colab](https://colab.research.google.com/drive/1gt75LCBIzsmBMvvipEOvWulvyZseBiA7?usp=sharing) but for some reason I cannot reproduce it there. That may have to do with logging/tqdm on Colab, or with running things in notebooks. I tried below code on Windows and Ubuntu as a Python script and getting the same issue (warning below).
```python
import pickle
from datasets import load_dataset
import spacy
class Processor:
def __init__(self):
self.nlp = spacy.load("en_core_web_sm", disable=["tagger", "parser", "ner", "lemmatizer"])
@staticmethod
def collate(batch):
return [d["en"] for d in batch]
def parse(self, batch):
batch = batch["translation"]
return {"translation_tok": [{"en_tok": " ".join([t.text for t in doc])} for doc in self.nlp.pipe(self.collate(batch))]}
def process(self):
ds = load_dataset("wmt16", "de-en", split="train[:10%]")
ds = ds.map(self.parse, batched=True, num_proc=6)
if __name__ == '__main__':
pr = Processor()
# succeeds
with open("temp.pkl", "wb") as f:
pickle.dump(pr, f)
print("Successfully pickled!")
pr.process()
```
---
Here is a small change that includes `Hasher.hash` that shows that the hasher cannot seem to successfully pickle parts form the NLP object.
```python
from datasets.fingerprint import Hasher
import pickle
from datasets import load_dataset
import spacy
class Processor:
def __init__(self):
self.nlp = spacy.load("en_core_web_sm", disable=["tagger", "parser", "ner", "lemmatizer"])
@staticmethod
def collate(batch):
return [d["en"] for d in batch]
def parse(self, batch):
batch = batch["translation"]
return {"translation_tok": [{"en_tok": " ".join([t.text for t in doc])} for doc in self.nlp.pipe(self.collate(batch))]}
def process(self):
ds = load_dataset("wmt16", "de-en", split="train[:10]")
return ds.map(self.parse, batched=True)
if __name__ == '__main__':
pr = Processor()
# succeeds
with open("temp.pkl", "wb") as f:
pickle.dump(pr, f)
print("Successfully pickled class instance!")
# succeeds
with open("temp.pkl", "wb") as f:
pickle.dump(pr.nlp, f)
print("Successfully pickled nlp!")
# fails
print(Hasher.hash(pr.nlp))
pr.process()
```
## Expected results
This to be picklable, working (fingerprinted), and no warning.
## Actual results
In the first snippet, I get this warning
Parameter 'function'=<function Processor.parse at 0x7f44982247a0> of the transform datasets.arrow_dataset.Dataset._map_single couldn't be hashed properly, a random hash was used instead. Make sure your transforms and parameters are serializable with pickle or dill for the dataset fingerprinting and caching to work. If you reuse this transform, the caching mechanism will consider it to be different from the previous calls and recompute everything. This warning is only showed once. Subsequent hashing failures won't be showed.
In the second, I get this traceback which directs to the `Hasher.hash` line.
```
Traceback (most recent call last):
File " \Python\Python36\lib\pickle.py", line 918, in save_global
obj2, parent = _getattribute(module, name)
File " \Python\Python36\lib\pickle.py", line 266, in _getattribute
.format(name, obj))
AttributeError: Can't get local attribute 'add_codes.<locals>.ErrorsWithCodes' on <function add_codes at 0x00000296FF606EA0>
During handling of the above exception, another exception occurred:
Traceback (most recent call last):
File " scratch_4.py", line 40, in <module>
print(Hasher.hash(pr.nlp))
File " \lib\site-packages\datasets\fingerprint.py", line 191, in hash
return cls.hash_default(value)
File " \lib\site-packages\datasets\fingerprint.py", line 184, in hash_default
return cls.hash_bytes(dumps(value))
File " \lib\site-packages\datasets\utils\py_utils.py", line 345, in dumps
dump(obj, file)
File " \lib\site-packages\datasets\utils\py_utils.py", line 320, in dump
Pickler(file, recurse=True).dump(obj)
File " \lib\site-packages\dill\_dill.py", line 498, in dump
StockPickler.dump(self, obj)
File " \Python\Python36\lib\pickle.py", line 409, in dump
self.save(obj)
File " \Python\Python36\lib\pickle.py", line 521, in save
self.save_reduce(obj=obj, *rv)
File " \Python\Python36\lib\pickle.py", line 634, in save_reduce
save(state)
File " \Python\Python36\lib\pickle.py", line 476, in save
f(self, obj) # Call unbound method with explicit self
File " \lib\site-packages\dill\_dill.py", line 990, in save_module_dict
StockPickler.save_dict(pickler, obj)
File " \Python\Python36\lib\pickle.py", line 821, in save_dict
self._batch_setitems(obj.items())
File " \Python\Python36\lib\pickle.py", line 847, in _batch_setitems
save(v)
File " \Python\Python36\lib\pickle.py", line 476, in save
f(self, obj) # Call unbound method with explicit self
File " \Python\Python36\lib\pickle.py", line 781, in save_list
self._batch_appends(obj)
File " \Python\Python36\lib\pickle.py", line 805, in _batch_appends
save(x)
File " \Python\Python36\lib\pickle.py", line 476, in save
f(self, obj) # Call unbound method with explicit self
File " \Python\Python36\lib\pickle.py", line 736, in save_tuple
save(element)
File " \Python\Python36\lib\pickle.py", line 521, in save
self.save_reduce(obj=obj, *rv)
File " \Python\Python36\lib\pickle.py", line 634, in save_reduce
save(state)
File " \Python\Python36\lib\pickle.py", line 476, in save
f(self, obj) # Call unbound method with explicit self
File " \Python\Python36\lib\pickle.py", line 736, in save_tuple
save(element)
File " \Python\Python36\lib\pickle.py", line 476, in save
f(self, obj) # Call unbound method with explicit self
File " \lib\site-packages\dill\_dill.py", line 990, in save_module_dict
StockPickler.save_dict(pickler, obj)
File " \Python\Python36\lib\pickle.py", line 821, in save_dict
self._batch_setitems(obj.items())
File " \Python\Python36\lib\pickle.py", line 847, in _batch_setitems
save(v)
File " \Python\Python36\lib\pickle.py", line 476, in save
f(self, obj) # Call unbound method with explicit self
File " \lib\site-packages\dill\_dill.py", line 1176, in save_instancemethod0
pickler.save_reduce(MethodType, (obj.__func__, obj.__self__), obj=obj)
File " \Python\Python36\lib\pickle.py", line 610, in save_reduce
save(args)
File " \Python\Python36\lib\pickle.py", line 476, in save
f(self, obj) # Call unbound method with explicit self
File " \Python\Python36\lib\pickle.py", line 736, in save_tuple
save(element)
File " \Python\Python36\lib\pickle.py", line 476, in save
f(self, obj) # Call unbound method with explicit self
File " \lib\site-packages\datasets\utils\py_utils.py", line 523, in save_function
obj=obj,
File " \Python\Python36\lib\pickle.py", line 610, in save_reduce
save(args)
File " \Python\Python36\lib\pickle.py", line 476, in save
f(self, obj) # Call unbound method with explicit self
File " \Python\Python36\lib\pickle.py", line 751, in save_tuple
save(element)
File " \Python\Python36\lib\pickle.py", line 476, in save
f(self, obj) # Call unbound method with explicit self
File " \lib\site-packages\dill\_dill.py", line 990, in save_module_dict
StockPickler.save_dict(pickler, obj)
File " \Python\Python36\lib\pickle.py", line 821, in save_dict
self._batch_setitems(obj.items())
File " \Python\Python36\lib\pickle.py", line 847, in _batch_setitems
save(v)
File " \Python\Python36\lib\pickle.py", line 521, in save
self.save_reduce(obj=obj, *rv)
File " \Python\Python36\lib\pickle.py", line 605, in save_reduce
save(cls)
File " \Python\Python36\lib\pickle.py", line 476, in save
f(self, obj) # Call unbound method with explicit self
File " \lib\site-packages\dill\_dill.py", line 1439, in save_type
StockPickler.save_global(pickler, obj, name=name)
File " \Python\Python36\lib\pickle.py", line 922, in save_global
(obj, module_name, name))
_pickle.PicklingError: Can't pickle <class 'spacy.errors.add_codes.<locals>.ErrorsWithCodes'>: it's not found as spacy.errors.add_codes.<locals>.ErrorsWithCodes
```
## Environment info
Tried on both Linux and Windows
- `datasets` version: 1.14.0
- Platform: Windows-10-10.0.19041-SP0 + Python 3.7.9; Linux-5.11.0-38-generic-x86_64-with-Ubuntu-20.04-focal + Python 3.7.12
- PyArrow version: 6.0.0
| 61 | "Property couldn't be hashed properly" even though fully picklable
## Describe the bug
I am trying to tokenize a dataset with spaCy. I found that no matter what I do, the spaCy language object (`nlp`) prevents `datasets` from pickling correctly - or so the warning says - even though manually pickling is no issue. It should not be an issue either, since spaCy objects are picklable.
## Steps to reproduce the bug
Here is a [colab](https://colab.research.google.com/drive/1gt75LCBIzsmBMvvipEOvWulvyZseBiA7?usp=sharing) but for some reason I cannot reproduce it there. That may have to do with logging/tqdm on Colab, or with running things in notebooks. I tried below code on Windows and Ubuntu as a Python script and getting the same issue (warning below).
```python
import pickle
from datasets import load_dataset
import spacy
class Processor:
def __init__(self):
self.nlp = spacy.load("en_core_web_sm", disable=["tagger", "parser", "ner", "lemmatizer"])
@staticmethod
def collate(batch):
return [d["en"] for d in batch]
def parse(self, batch):
batch = batch["translation"]
return {"translation_tok": [{"en_tok": " ".join([t.text for t in doc])} for doc in self.nlp.pipe(self.collate(batch))]}
def process(self):
ds = load_dataset("wmt16", "de-en", split="train[:10%]")
ds = ds.map(self.parse, batched=True, num_proc=6)
if __name__ == '__main__':
pr = Processor()
# succeeds
with open("temp.pkl", "wb") as f:
pickle.dump(pr, f)
print("Successfully pickled!")
pr.process()
```
---
Here is a small change that includes `Hasher.hash` that shows that the hasher cannot seem to successfully pickle parts form the NLP object.
```python
from datasets.fingerprint import Hasher
import pickle
from datasets import load_dataset
import spacy
class Processor:
def __init__(self):
self.nlp = spacy.load("en_core_web_sm", disable=["tagger", "parser", "ner", "lemmatizer"])
@staticmethod
def collate(batch):
return [d["en"] for d in batch]
def parse(self, batch):
batch = batch["translation"]
return {"translation_tok": [{"en_tok": " ".join([t.text for t in doc])} for doc in self.nlp.pipe(self.collate(batch))]}
def process(self):
ds = load_dataset("wmt16", "de-en", split="train[:10]")
return ds.map(self.parse, batched=True)
if __name__ == '__main__':
pr = Processor()
# succeeds
with open("temp.pkl", "wb") as f:
pickle.dump(pr, f)
print("Successfully pickled class instance!")
# succeeds
with open("temp.pkl", "wb") as f:
pickle.dump(pr.nlp, f)
print("Successfully pickled nlp!")
# fails
print(Hasher.hash(pr.nlp))
pr.process()
```
## Expected results
This to be picklable, working (fingerprinted), and no warning.
## Actual results
In the first snippet, I get this warning
Parameter 'function'=<function Processor.parse at 0x7f44982247a0> of the transform datasets.arrow_dataset.Dataset._map_single couldn't be hashed properly, a random hash was used instead. Make sure your transforms and parameters are serializable with pickle or dill for the dataset fingerprinting and caching to work. If you reuse this transform, the caching mechanism will consider it to be different from the previous calls and recompute everything. This warning is only showed once. Subsequent hashing failures won't be showed.
In the second, I get this traceback which directs to the `Hasher.hash` line.
```
Traceback (most recent call last):
File " \Python\Python36\lib\pickle.py", line 918, in save_global
obj2, parent = _getattribute(module, name)
File " \Python\Python36\lib\pickle.py", line 266, in _getattribute
.format(name, obj))
AttributeError: Can't get local attribute 'add_codes.<locals>.ErrorsWithCodes' on <function add_codes at 0x00000296FF606EA0>
During handling of the above exception, another exception occurred:
Traceback (most recent call last):
File " scratch_4.py", line 40, in <module>
print(Hasher.hash(pr.nlp))
File " \lib\site-packages\datasets\fingerprint.py", line 191, in hash
return cls.hash_default(value)
File " \lib\site-packages\datasets\fingerprint.py", line 184, in hash_default
return cls.hash_bytes(dumps(value))
File " \lib\site-packages\datasets\utils\py_utils.py", line 345, in dumps
dump(obj, file)
File " \lib\site-packages\datasets\utils\py_utils.py", line 320, in dump
Pickler(file, recurse=True).dump(obj)
File " \lib\site-packages\dill\_dill.py", line 498, in dump
StockPickler.dump(self, obj)
File " \Python\Python36\lib\pickle.py", line 409, in dump
self.save(obj)
File " \Python\Python36\lib\pickle.py", line 521, in save
self.save_reduce(obj=obj, *rv)
File " \Python\Python36\lib\pickle.py", line 634, in save_reduce
save(state)
File " \Python\Python36\lib\pickle.py", line 476, in save
f(self, obj) # Call unbound method with explicit self
File " \lib\site-packages\dill\_dill.py", line 990, in save_module_dict
StockPickler.save_dict(pickler, obj)
File " \Python\Python36\lib\pickle.py", line 821, in save_dict
self._batch_setitems(obj.items())
File " \Python\Python36\lib\pickle.py", line 847, in _batch_setitems
save(v)
File " \Python\Python36\lib\pickle.py", line 476, in save
f(self, obj) # Call unbound method with explicit self
File " \Python\Python36\lib\pickle.py", line 781, in save_list
self._batch_appends(obj)
File " \Python\Python36\lib\pickle.py", line 805, in _batch_appends
save(x)
File " \Python\Python36\lib\pickle.py", line 476, in save
f(self, obj) # Call unbound method with explicit self
File " \Python\Python36\lib\pickle.py", line 736, in save_tuple
save(element)
File " \Python\Python36\lib\pickle.py", line 521, in save
self.save_reduce(obj=obj, *rv)
File " \Python\Python36\lib\pickle.py", line 634, in save_reduce
save(state)
File " \Python\Python36\lib\pickle.py", line 476, in save
f(self, obj) # Call unbound method with explicit self
File " \Python\Python36\lib\pickle.py", line 736, in save_tuple
save(element)
File " \Python\Python36\lib\pickle.py", line 476, in save
f(self, obj) # Call unbound method with explicit self
File " \lib\site-packages\dill\_dill.py", line 990, in save_module_dict
StockPickler.save_dict(pickler, obj)
File " \Python\Python36\lib\pickle.py", line 821, in save_dict
self._batch_setitems(obj.items())
File " \Python\Python36\lib\pickle.py", line 847, in _batch_setitems
save(v)
File " \Python\Python36\lib\pickle.py", line 476, in save
f(self, obj) # Call unbound method with explicit self
File " \lib\site-packages\dill\_dill.py", line 1176, in save_instancemethod0
pickler.save_reduce(MethodType, (obj.__func__, obj.__self__), obj=obj)
File " \Python\Python36\lib\pickle.py", line 610, in save_reduce
save(args)
File " \Python\Python36\lib\pickle.py", line 476, in save
f(self, obj) # Call unbound method with explicit self
File " \Python\Python36\lib\pickle.py", line 736, in save_tuple
save(element)
File " \Python\Python36\lib\pickle.py", line 476, in save
f(self, obj) # Call unbound method with explicit self
File " \lib\site-packages\datasets\utils\py_utils.py", line 523, in save_function
obj=obj,
File " \Python\Python36\lib\pickle.py", line 610, in save_reduce
save(args)
File " \Python\Python36\lib\pickle.py", line 476, in save
f(self, obj) # Call unbound method with explicit self
File " \Python\Python36\lib\pickle.py", line 751, in save_tuple
save(element)
File " \Python\Python36\lib\pickle.py", line 476, in save
f(self, obj) # Call unbound method with explicit self
File " \lib\site-packages\dill\_dill.py", line 990, in save_module_dict
StockPickler.save_dict(pickler, obj)
File " \Python\Python36\lib\pickle.py", line 821, in save_dict
self._batch_setitems(obj.items())
File " \Python\Python36\lib\pickle.py", line 847, in _batch_setitems
save(v)
File " \Python\Python36\lib\pickle.py", line 521, in save
self.save_reduce(obj=obj, *rv)
File " \Python\Python36\lib\pickle.py", line 605, in save_reduce
save(cls)
File " \Python\Python36\lib\pickle.py", line 476, in save
f(self, obj) # Call unbound method with explicit self
File " \lib\site-packages\dill\_dill.py", line 1439, in save_type
StockPickler.save_global(pickler, obj, name=name)
File " \Python\Python36\lib\pickle.py", line 922, in save_global
(obj, module_name, name))
_pickle.PicklingError: Can't pickle <class 'spacy.errors.add_codes.<locals>.ErrorsWithCodes'>: it's not found as spacy.errors.add_codes.<locals>.ErrorsWithCodes
```
## Environment info
Tried on both Linux and Windows
- `datasets` version: 1.14.0
- Platform: Windows-10-10.0.19041-SP0 + Python 3.7.9; Linux-5.11.0-38-generic-x86_64-with-Ubuntu-20.04-focal + Python 3.7.12
- PyArrow version: 6.0.0
You can monitor your RAM and disk space in case a process dies from OOM or disk full, and when it hangs you can check how many processes are running. IIRC there are other start methods for multiprocessing in python that may show an error message if a process dies.
Running on a single process can also help debugging this indeed |
https://github.com/huggingface/datasets/issues/3178 | "Property couldn't be hashed properly" even though fully picklable | Hi @tung-msol could you open a new issue and share the error you got and the map function you used ? | ## Describe the bug
I am trying to tokenize a dataset with spaCy. I found that no matter what I do, the spaCy language object (`nlp`) prevents `datasets` from pickling correctly - or so the warning says - even though manually pickling is no issue. It should not be an issue either, since spaCy objects are picklable.
## Steps to reproduce the bug
Here is a [colab](https://colab.research.google.com/drive/1gt75LCBIzsmBMvvipEOvWulvyZseBiA7?usp=sharing) but for some reason I cannot reproduce it there. That may have to do with logging/tqdm on Colab, or with running things in notebooks. I tried below code on Windows and Ubuntu as a Python script and getting the same issue (warning below).
```python
import pickle
from datasets import load_dataset
import spacy
class Processor:
def __init__(self):
self.nlp = spacy.load("en_core_web_sm", disable=["tagger", "parser", "ner", "lemmatizer"])
@staticmethod
def collate(batch):
return [d["en"] for d in batch]
def parse(self, batch):
batch = batch["translation"]
return {"translation_tok": [{"en_tok": " ".join([t.text for t in doc])} for doc in self.nlp.pipe(self.collate(batch))]}
def process(self):
ds = load_dataset("wmt16", "de-en", split="train[:10%]")
ds = ds.map(self.parse, batched=True, num_proc=6)
if __name__ == '__main__':
pr = Processor()
# succeeds
with open("temp.pkl", "wb") as f:
pickle.dump(pr, f)
print("Successfully pickled!")
pr.process()
```
---
Here is a small change that includes `Hasher.hash` that shows that the hasher cannot seem to successfully pickle parts form the NLP object.
```python
from datasets.fingerprint import Hasher
import pickle
from datasets import load_dataset
import spacy
class Processor:
def __init__(self):
self.nlp = spacy.load("en_core_web_sm", disable=["tagger", "parser", "ner", "lemmatizer"])
@staticmethod
def collate(batch):
return [d["en"] for d in batch]
def parse(self, batch):
batch = batch["translation"]
return {"translation_tok": [{"en_tok": " ".join([t.text for t in doc])} for doc in self.nlp.pipe(self.collate(batch))]}
def process(self):
ds = load_dataset("wmt16", "de-en", split="train[:10]")
return ds.map(self.parse, batched=True)
if __name__ == '__main__':
pr = Processor()
# succeeds
with open("temp.pkl", "wb") as f:
pickle.dump(pr, f)
print("Successfully pickled class instance!")
# succeeds
with open("temp.pkl", "wb") as f:
pickle.dump(pr.nlp, f)
print("Successfully pickled nlp!")
# fails
print(Hasher.hash(pr.nlp))
pr.process()
```
## Expected results
This to be picklable, working (fingerprinted), and no warning.
## Actual results
In the first snippet, I get this warning
Parameter 'function'=<function Processor.parse at 0x7f44982247a0> of the transform datasets.arrow_dataset.Dataset._map_single couldn't be hashed properly, a random hash was used instead. Make sure your transforms and parameters are serializable with pickle or dill for the dataset fingerprinting and caching to work. If you reuse this transform, the caching mechanism will consider it to be different from the previous calls and recompute everything. This warning is only showed once. Subsequent hashing failures won't be showed.
In the second, I get this traceback which directs to the `Hasher.hash` line.
```
Traceback (most recent call last):
File " \Python\Python36\lib\pickle.py", line 918, in save_global
obj2, parent = _getattribute(module, name)
File " \Python\Python36\lib\pickle.py", line 266, in _getattribute
.format(name, obj))
AttributeError: Can't get local attribute 'add_codes.<locals>.ErrorsWithCodes' on <function add_codes at 0x00000296FF606EA0>
During handling of the above exception, another exception occurred:
Traceback (most recent call last):
File " scratch_4.py", line 40, in <module>
print(Hasher.hash(pr.nlp))
File " \lib\site-packages\datasets\fingerprint.py", line 191, in hash
return cls.hash_default(value)
File " \lib\site-packages\datasets\fingerprint.py", line 184, in hash_default
return cls.hash_bytes(dumps(value))
File " \lib\site-packages\datasets\utils\py_utils.py", line 345, in dumps
dump(obj, file)
File " \lib\site-packages\datasets\utils\py_utils.py", line 320, in dump
Pickler(file, recurse=True).dump(obj)
File " \lib\site-packages\dill\_dill.py", line 498, in dump
StockPickler.dump(self, obj)
File " \Python\Python36\lib\pickle.py", line 409, in dump
self.save(obj)
File " \Python\Python36\lib\pickle.py", line 521, in save
self.save_reduce(obj=obj, *rv)
File " \Python\Python36\lib\pickle.py", line 634, in save_reduce
save(state)
File " \Python\Python36\lib\pickle.py", line 476, in save
f(self, obj) # Call unbound method with explicit self
File " \lib\site-packages\dill\_dill.py", line 990, in save_module_dict
StockPickler.save_dict(pickler, obj)
File " \Python\Python36\lib\pickle.py", line 821, in save_dict
self._batch_setitems(obj.items())
File " \Python\Python36\lib\pickle.py", line 847, in _batch_setitems
save(v)
File " \Python\Python36\lib\pickle.py", line 476, in save
f(self, obj) # Call unbound method with explicit self
File " \Python\Python36\lib\pickle.py", line 781, in save_list
self._batch_appends(obj)
File " \Python\Python36\lib\pickle.py", line 805, in _batch_appends
save(x)
File " \Python\Python36\lib\pickle.py", line 476, in save
f(self, obj) # Call unbound method with explicit self
File " \Python\Python36\lib\pickle.py", line 736, in save_tuple
save(element)
File " \Python\Python36\lib\pickle.py", line 521, in save
self.save_reduce(obj=obj, *rv)
File " \Python\Python36\lib\pickle.py", line 634, in save_reduce
save(state)
File " \Python\Python36\lib\pickle.py", line 476, in save
f(self, obj) # Call unbound method with explicit self
File " \Python\Python36\lib\pickle.py", line 736, in save_tuple
save(element)
File " \Python\Python36\lib\pickle.py", line 476, in save
f(self, obj) # Call unbound method with explicit self
File " \lib\site-packages\dill\_dill.py", line 990, in save_module_dict
StockPickler.save_dict(pickler, obj)
File " \Python\Python36\lib\pickle.py", line 821, in save_dict
self._batch_setitems(obj.items())
File " \Python\Python36\lib\pickle.py", line 847, in _batch_setitems
save(v)
File " \Python\Python36\lib\pickle.py", line 476, in save
f(self, obj) # Call unbound method with explicit self
File " \lib\site-packages\dill\_dill.py", line 1176, in save_instancemethod0
pickler.save_reduce(MethodType, (obj.__func__, obj.__self__), obj=obj)
File " \Python\Python36\lib\pickle.py", line 610, in save_reduce
save(args)
File " \Python\Python36\lib\pickle.py", line 476, in save
f(self, obj) # Call unbound method with explicit self
File " \Python\Python36\lib\pickle.py", line 736, in save_tuple
save(element)
File " \Python\Python36\lib\pickle.py", line 476, in save
f(self, obj) # Call unbound method with explicit self
File " \lib\site-packages\datasets\utils\py_utils.py", line 523, in save_function
obj=obj,
File " \Python\Python36\lib\pickle.py", line 610, in save_reduce
save(args)
File " \Python\Python36\lib\pickle.py", line 476, in save
f(self, obj) # Call unbound method with explicit self
File " \Python\Python36\lib\pickle.py", line 751, in save_tuple
save(element)
File " \Python\Python36\lib\pickle.py", line 476, in save
f(self, obj) # Call unbound method with explicit self
File " \lib\site-packages\dill\_dill.py", line 990, in save_module_dict
StockPickler.save_dict(pickler, obj)
File " \Python\Python36\lib\pickle.py", line 821, in save_dict
self._batch_setitems(obj.items())
File " \Python\Python36\lib\pickle.py", line 847, in _batch_setitems
save(v)
File " \Python\Python36\lib\pickle.py", line 521, in save
self.save_reduce(obj=obj, *rv)
File " \Python\Python36\lib\pickle.py", line 605, in save_reduce
save(cls)
File " \Python\Python36\lib\pickle.py", line 476, in save
f(self, obj) # Call unbound method with explicit self
File " \lib\site-packages\dill\_dill.py", line 1439, in save_type
StockPickler.save_global(pickler, obj, name=name)
File " \Python\Python36\lib\pickle.py", line 922, in save_global
(obj, module_name, name))
_pickle.PicklingError: Can't pickle <class 'spacy.errors.add_codes.<locals>.ErrorsWithCodes'>: it's not found as spacy.errors.add_codes.<locals>.ErrorsWithCodes
```
## Environment info
Tried on both Linux and Windows
- `datasets` version: 1.14.0
- Platform: Windows-10-10.0.19041-SP0 + Python 3.7.9; Linux-5.11.0-38-generic-x86_64-with-Ubuntu-20.04-focal + Python 3.7.12
- PyArrow version: 6.0.0
| 21 | "Property couldn't be hashed properly" even though fully picklable
## Describe the bug
I am trying to tokenize a dataset with spaCy. I found that no matter what I do, the spaCy language object (`nlp`) prevents `datasets` from pickling correctly - or so the warning says - even though manually pickling is no issue. It should not be an issue either, since spaCy objects are picklable.
## Steps to reproduce the bug
Here is a [colab](https://colab.research.google.com/drive/1gt75LCBIzsmBMvvipEOvWulvyZseBiA7?usp=sharing) but for some reason I cannot reproduce it there. That may have to do with logging/tqdm on Colab, or with running things in notebooks. I tried below code on Windows and Ubuntu as a Python script and getting the same issue (warning below).
```python
import pickle
from datasets import load_dataset
import spacy
class Processor:
def __init__(self):
self.nlp = spacy.load("en_core_web_sm", disable=["tagger", "parser", "ner", "lemmatizer"])
@staticmethod
def collate(batch):
return [d["en"] for d in batch]
def parse(self, batch):
batch = batch["translation"]
return {"translation_tok": [{"en_tok": " ".join([t.text for t in doc])} for doc in self.nlp.pipe(self.collate(batch))]}
def process(self):
ds = load_dataset("wmt16", "de-en", split="train[:10%]")
ds = ds.map(self.parse, batched=True, num_proc=6)
if __name__ == '__main__':
pr = Processor()
# succeeds
with open("temp.pkl", "wb") as f:
pickle.dump(pr, f)
print("Successfully pickled!")
pr.process()
```
---
Here is a small change that includes `Hasher.hash` that shows that the hasher cannot seem to successfully pickle parts form the NLP object.
```python
from datasets.fingerprint import Hasher
import pickle
from datasets import load_dataset
import spacy
class Processor:
def __init__(self):
self.nlp = spacy.load("en_core_web_sm", disable=["tagger", "parser", "ner", "lemmatizer"])
@staticmethod
def collate(batch):
return [d["en"] for d in batch]
def parse(self, batch):
batch = batch["translation"]
return {"translation_tok": [{"en_tok": " ".join([t.text for t in doc])} for doc in self.nlp.pipe(self.collate(batch))]}
def process(self):
ds = load_dataset("wmt16", "de-en", split="train[:10]")
return ds.map(self.parse, batched=True)
if __name__ == '__main__':
pr = Processor()
# succeeds
with open("temp.pkl", "wb") as f:
pickle.dump(pr, f)
print("Successfully pickled class instance!")
# succeeds
with open("temp.pkl", "wb") as f:
pickle.dump(pr.nlp, f)
print("Successfully pickled nlp!")
# fails
print(Hasher.hash(pr.nlp))
pr.process()
```
## Expected results
This to be picklable, working (fingerprinted), and no warning.
## Actual results
In the first snippet, I get this warning
Parameter 'function'=<function Processor.parse at 0x7f44982247a0> of the transform datasets.arrow_dataset.Dataset._map_single couldn't be hashed properly, a random hash was used instead. Make sure your transforms and parameters are serializable with pickle or dill for the dataset fingerprinting and caching to work. If you reuse this transform, the caching mechanism will consider it to be different from the previous calls and recompute everything. This warning is only showed once. Subsequent hashing failures won't be showed.
In the second, I get this traceback which directs to the `Hasher.hash` line.
```
Traceback (most recent call last):
File " \Python\Python36\lib\pickle.py", line 918, in save_global
obj2, parent = _getattribute(module, name)
File " \Python\Python36\lib\pickle.py", line 266, in _getattribute
.format(name, obj))
AttributeError: Can't get local attribute 'add_codes.<locals>.ErrorsWithCodes' on <function add_codes at 0x00000296FF606EA0>
During handling of the above exception, another exception occurred:
Traceback (most recent call last):
File " scratch_4.py", line 40, in <module>
print(Hasher.hash(pr.nlp))
File " \lib\site-packages\datasets\fingerprint.py", line 191, in hash
return cls.hash_default(value)
File " \lib\site-packages\datasets\fingerprint.py", line 184, in hash_default
return cls.hash_bytes(dumps(value))
File " \lib\site-packages\datasets\utils\py_utils.py", line 345, in dumps
dump(obj, file)
File " \lib\site-packages\datasets\utils\py_utils.py", line 320, in dump
Pickler(file, recurse=True).dump(obj)
File " \lib\site-packages\dill\_dill.py", line 498, in dump
StockPickler.dump(self, obj)
File " \Python\Python36\lib\pickle.py", line 409, in dump
self.save(obj)
File " \Python\Python36\lib\pickle.py", line 521, in save
self.save_reduce(obj=obj, *rv)
File " \Python\Python36\lib\pickle.py", line 634, in save_reduce
save(state)
File " \Python\Python36\lib\pickle.py", line 476, in save
f(self, obj) # Call unbound method with explicit self
File " \lib\site-packages\dill\_dill.py", line 990, in save_module_dict
StockPickler.save_dict(pickler, obj)
File " \Python\Python36\lib\pickle.py", line 821, in save_dict
self._batch_setitems(obj.items())
File " \Python\Python36\lib\pickle.py", line 847, in _batch_setitems
save(v)
File " \Python\Python36\lib\pickle.py", line 476, in save
f(self, obj) # Call unbound method with explicit self
File " \Python\Python36\lib\pickle.py", line 781, in save_list
self._batch_appends(obj)
File " \Python\Python36\lib\pickle.py", line 805, in _batch_appends
save(x)
File " \Python\Python36\lib\pickle.py", line 476, in save
f(self, obj) # Call unbound method with explicit self
File " \Python\Python36\lib\pickle.py", line 736, in save_tuple
save(element)
File " \Python\Python36\lib\pickle.py", line 521, in save
self.save_reduce(obj=obj, *rv)
File " \Python\Python36\lib\pickle.py", line 634, in save_reduce
save(state)
File " \Python\Python36\lib\pickle.py", line 476, in save
f(self, obj) # Call unbound method with explicit self
File " \Python\Python36\lib\pickle.py", line 736, in save_tuple
save(element)
File " \Python\Python36\lib\pickle.py", line 476, in save
f(self, obj) # Call unbound method with explicit self
File " \lib\site-packages\dill\_dill.py", line 990, in save_module_dict
StockPickler.save_dict(pickler, obj)
File " \Python\Python36\lib\pickle.py", line 821, in save_dict
self._batch_setitems(obj.items())
File " \Python\Python36\lib\pickle.py", line 847, in _batch_setitems
save(v)
File " \Python\Python36\lib\pickle.py", line 476, in save
f(self, obj) # Call unbound method with explicit self
File " \lib\site-packages\dill\_dill.py", line 1176, in save_instancemethod0
pickler.save_reduce(MethodType, (obj.__func__, obj.__self__), obj=obj)
File " \Python\Python36\lib\pickle.py", line 610, in save_reduce
save(args)
File " \Python\Python36\lib\pickle.py", line 476, in save
f(self, obj) # Call unbound method with explicit self
File " \Python\Python36\lib\pickle.py", line 736, in save_tuple
save(element)
File " \Python\Python36\lib\pickle.py", line 476, in save
f(self, obj) # Call unbound method with explicit self
File " \lib\site-packages\datasets\utils\py_utils.py", line 523, in save_function
obj=obj,
File " \Python\Python36\lib\pickle.py", line 610, in save_reduce
save(args)
File " \Python\Python36\lib\pickle.py", line 476, in save
f(self, obj) # Call unbound method with explicit self
File " \Python\Python36\lib\pickle.py", line 751, in save_tuple
save(element)
File " \Python\Python36\lib\pickle.py", line 476, in save
f(self, obj) # Call unbound method with explicit self
File " \lib\site-packages\dill\_dill.py", line 990, in save_module_dict
StockPickler.save_dict(pickler, obj)
File " \Python\Python36\lib\pickle.py", line 821, in save_dict
self._batch_setitems(obj.items())
File " \Python\Python36\lib\pickle.py", line 847, in _batch_setitems
save(v)
File " \Python\Python36\lib\pickle.py", line 521, in save
self.save_reduce(obj=obj, *rv)
File " \Python\Python36\lib\pickle.py", line 605, in save_reduce
save(cls)
File " \Python\Python36\lib\pickle.py", line 476, in save
f(self, obj) # Call unbound method with explicit self
File " \lib\site-packages\dill\_dill.py", line 1439, in save_type
StockPickler.save_global(pickler, obj, name=name)
File " \Python\Python36\lib\pickle.py", line 922, in save_global
(obj, module_name, name))
_pickle.PicklingError: Can't pickle <class 'spacy.errors.add_codes.<locals>.ErrorsWithCodes'>: it's not found as spacy.errors.add_codes.<locals>.ErrorsWithCodes
```
## Environment info
Tried on both Linux and Windows
- `datasets` version: 1.14.0
- Platform: Windows-10-10.0.19041-SP0 + Python 3.7.9; Linux-5.11.0-38-generic-x86_64-with-Ubuntu-20.04-focal + Python 3.7.12
- PyArrow version: 6.0.0
Hi @tung-msol could you open a new issue and share the error you got and the map function you used ? |
https://github.com/huggingface/datasets/issues/3177 | More control over TQDM when using map/filter with multiple processes | Hi,
It's hard to provide an API that would cover all use-cases with tqdm in this project.
However, you can make it work by defining a custom decorator (a bit hacky tho) as follows:
```python
import datasets
def progress_only_on_rank_0(func):
def wrapper(*args, **kwargs):
rank = kwargs.get("rank")
disable_tqdm = kwargs.get("disable_tqdm", False)
disable_tqdm = True if rank is not None and rank > 0 else disable_tqdm
kwargs["disable_tqdm"] = disable_tqdm
return func(*args, **kwargs)
return wrapper
datasets.Dataset._map_single = progress_only_on_rank_0(datasets.Dataset._map_single)
```
EDIT: Ups, closed by accident.
Thanks for the provided links. `Trainer` requires this for training in multi-node distributed setting. However, `Dataset.map` doesn't support that yet.
Do you have an API for this in mind? `Dataset.map` is already bloated with the arguments, so IMO it's not a good idea to add a new arg there.
| It would help with the clutter in my terminal if tqdm is only shown for rank 0 when using `num_proces>0` in the map and filter methods of datasets.
```python
dataset.map(lambda examples: tokenize(examples["text"]), batched=True, num_proc=6)
```
The above snippet leads to a lot of TQDM bars and depending on your terminal, these will not overwrite but keep pushing each other down.
```
#0: 0%| | 0/13 [00:00<?, ?ba/s]
#1: 0%| | 0/13 [00:00<?, ?ba/s]
#2: 0%| | 0/13 [00:00<?, ?ba/s]
#3: 0%| | 0/13 [00:00<?, ?ba/s]
#4: 0%| | 0/13 [00:00<?, ?ba/s]
#5: 0%| | 0/13 [00:00<?, ?ba/s]
#0: 8%| | 1/13 [00:00<?, ?ba/s]
#1: 8%| | 1/13 [00:00<?, ?ba/s]
...
```
Instead, it would be welcome if we had the option to only show the progress of rank 0. | 129 | More control over TQDM when using map/filter with multiple processes
It would help with the clutter in my terminal if tqdm is only shown for rank 0 when using `num_proces>0` in the map and filter methods of datasets.
```python
dataset.map(lambda examples: tokenize(examples["text"]), batched=True, num_proc=6)
```
The above snippet leads to a lot of TQDM bars and depending on your terminal, these will not overwrite but keep pushing each other down.
```
#0: 0%| | 0/13 [00:00<?, ?ba/s]
#1: 0%| | 0/13 [00:00<?, ?ba/s]
#2: 0%| | 0/13 [00:00<?, ?ba/s]
#3: 0%| | 0/13 [00:00<?, ?ba/s]
#4: 0%| | 0/13 [00:00<?, ?ba/s]
#5: 0%| | 0/13 [00:00<?, ?ba/s]
#0: 8%| | 1/13 [00:00<?, ?ba/s]
#1: 8%| | 1/13 [00:00<?, ?ba/s]
...
```
Instead, it would be welcome if we had the option to only show the progress of rank 0.
Hi,
It's hard to provide an API that would cover all use-cases with tqdm in this project.
However, you can make it work by defining a custom decorator (a bit hacky tho) as follows:
```python
import datasets
def progress_only_on_rank_0(func):
def wrapper(*args, **kwargs):
rank = kwargs.get("rank")
disable_tqdm = kwargs.get("disable_tqdm", False)
disable_tqdm = True if rank is not None and rank > 0 else disable_tqdm
kwargs["disable_tqdm"] = disable_tqdm
return func(*args, **kwargs)
return wrapper
datasets.Dataset._map_single = progress_only_on_rank_0(datasets.Dataset._map_single)
```
EDIT: Ups, closed by accident.
Thanks for the provided links. `Trainer` requires this for training in multi-node distributed setting. However, `Dataset.map` doesn't support that yet.
Do you have an API for this in mind? `Dataset.map` is already bloated with the arguments, so IMO it's not a good idea to add a new arg there.
|
https://github.com/huggingface/datasets/issues/3177 | More control over TQDM when using map/filter with multiple processes | Inspiration may be found at `transformers`.
https://github.com/huggingface/transformers/blob/4a394cf53f05e73ab9bbb4b179a40236a5ffe45a/src/transformers/trainer.py#L1231-L1233
To get unique IDs for each worker, see https://stackoverflow.com/a/10192611/1150683 | It would help with the clutter in my terminal if tqdm is only shown for rank 0 when using `num_proces>0` in the map and filter methods of datasets.
```python
dataset.map(lambda examples: tokenize(examples["text"]), batched=True, num_proc=6)
```
The above snippet leads to a lot of TQDM bars and depending on your terminal, these will not overwrite but keep pushing each other down.
```
#0: 0%| | 0/13 [00:00<?, ?ba/s]
#1: 0%| | 0/13 [00:00<?, ?ba/s]
#2: 0%| | 0/13 [00:00<?, ?ba/s]
#3: 0%| | 0/13 [00:00<?, ?ba/s]
#4: 0%| | 0/13 [00:00<?, ?ba/s]
#5: 0%| | 0/13 [00:00<?, ?ba/s]
#0: 8%| | 1/13 [00:00<?, ?ba/s]
#1: 8%| | 1/13 [00:00<?, ?ba/s]
...
```
Instead, it would be welcome if we had the option to only show the progress of rank 0. | 16 | More control over TQDM when using map/filter with multiple processes
It would help with the clutter in my terminal if tqdm is only shown for rank 0 when using `num_proces>0` in the map and filter methods of datasets.
```python
dataset.map(lambda examples: tokenize(examples["text"]), batched=True, num_proc=6)
```
The above snippet leads to a lot of TQDM bars and depending on your terminal, these will not overwrite but keep pushing each other down.
```
#0: 0%| | 0/13 [00:00<?, ?ba/s]
#1: 0%| | 0/13 [00:00<?, ?ba/s]
#2: 0%| | 0/13 [00:00<?, ?ba/s]
#3: 0%| | 0/13 [00:00<?, ?ba/s]
#4: 0%| | 0/13 [00:00<?, ?ba/s]
#5: 0%| | 0/13 [00:00<?, ?ba/s]
#0: 8%| | 1/13 [00:00<?, ?ba/s]
#1: 8%| | 1/13 [00:00<?, ?ba/s]
...
```
Instead, it would be welcome if we had the option to only show the progress of rank 0.
Inspiration may be found at `transformers`.
https://github.com/huggingface/transformers/blob/4a394cf53f05e73ab9bbb4b179a40236a5ffe45a/src/transformers/trainer.py#L1231-L1233
To get unique IDs for each worker, see https://stackoverflow.com/a/10192611/1150683 |
https://github.com/huggingface/datasets/issues/3172 | `SystemError 15` thrown in `Dataset.__del__` when using `Dataset.map()` with `num_proc>1` | NB: even if the error is raised, the dataset is successfully cached. So restarting the script after every `map()` allows to ultimately run the whole preprocessing. But this prevents to realistically run the code over multiple nodes. | ## Describe the bug
I use `datasets.map` to preprocess some data in my application. The error `SystemError 15` is thrown at the end of the execution of `Dataset.map()` (only with `num_proc>1`. Traceback included bellow.
The exception is raised only when the code runs within a specific context. Despite ~10h spent investigating this issue, I have failed to isolate the bug, so let me describe my setup.
In my project, `Dataset` is wrapped into a `LightningDataModule` and the data is preprocessed when calling `LightningDataModule.setup()`. Calling `.setup()` in an isolated script works fine (even when wrapped with `hydra.main()`). However, when calling `.setup()` within the experiment script (depends on `pytorch_lightning`), the script crashes and `SystemError 15`.
I could avoid throwing this error by modifying ` Dataset.__del__()` (see bellow), but I believe this only moves the problem somewhere else. I am completely stuck with this issue, any hint would be welcome.
```python
class Dataset()
...
def __del__(self):
if hasattr(self, "_data"):
_ = self._data # <- ugly trick that allows avoiding the issue.
del self._data
if hasattr(self, "_indices"):
del self._indices
```
## Steps to reproduce the bug
```python
# Unfortunately I couldn't isolate the bug.
```
## Expected results
Calling `Dataset.map()` without throwing an exception. Or at least raising a more detailed exception/traceback.
## Actual results
```
Exception ignored in: <function Dataset.__del__ at 0x7f7cec179160>███████████████████████████████████████████████████| 5/5 [00:05<00:00, 1.17ba/s]
Traceback (most recent call last):
File ".../python3.8/site-packages/datasets/arrow_dataset.py", line 906, in __del__
del self._data
File ".../python3.8/site-packages/ray/worker.py", line 1033, in sigterm_handler
sys.exit(signum)
SystemExit: 15
```
## Environment info
Tested on 2 environments:
**Environment 1.**
- `datasets` version: 1.14.0
- Platform: macOS-10.16-x86_64-i386-64bit
- Python version: 3.8.8
- PyArrow version: 6.0.0
**Environment 2.**
- `datasets` version: 1.14.0
- Platform: Linux-4.18.0-305.19.1.el8_4.x86_64-x86_64-with-glibc2.28
- Python version: 3.9.7
- PyArrow version: 6.0.0
| 37 | `SystemError 15` thrown in `Dataset.__del__` when using `Dataset.map()` with `num_proc>1`
## Describe the bug
I use `datasets.map` to preprocess some data in my application. The error `SystemError 15` is thrown at the end of the execution of `Dataset.map()` (only with `num_proc>1`. Traceback included bellow.
The exception is raised only when the code runs within a specific context. Despite ~10h spent investigating this issue, I have failed to isolate the bug, so let me describe my setup.
In my project, `Dataset` is wrapped into a `LightningDataModule` and the data is preprocessed when calling `LightningDataModule.setup()`. Calling `.setup()` in an isolated script works fine (even when wrapped with `hydra.main()`). However, when calling `.setup()` within the experiment script (depends on `pytorch_lightning`), the script crashes and `SystemError 15`.
I could avoid throwing this error by modifying ` Dataset.__del__()` (see bellow), but I believe this only moves the problem somewhere else. I am completely stuck with this issue, any hint would be welcome.
```python
class Dataset()
...
def __del__(self):
if hasattr(self, "_data"):
_ = self._data # <- ugly trick that allows avoiding the issue.
del self._data
if hasattr(self, "_indices"):
del self._indices
```
## Steps to reproduce the bug
```python
# Unfortunately I couldn't isolate the bug.
```
## Expected results
Calling `Dataset.map()` without throwing an exception. Or at least raising a more detailed exception/traceback.
## Actual results
```
Exception ignored in: <function Dataset.__del__ at 0x7f7cec179160>███████████████████████████████████████████████████| 5/5 [00:05<00:00, 1.17ba/s]
Traceback (most recent call last):
File ".../python3.8/site-packages/datasets/arrow_dataset.py", line 906, in __del__
del self._data
File ".../python3.8/site-packages/ray/worker.py", line 1033, in sigterm_handler
sys.exit(signum)
SystemExit: 15
```
## Environment info
Tested on 2 environments:
**Environment 1.**
- `datasets` version: 1.14.0
- Platform: macOS-10.16-x86_64-i386-64bit
- Python version: 3.8.8
- PyArrow version: 6.0.0
**Environment 2.**
- `datasets` version: 1.14.0
- Platform: Linux-4.18.0-305.19.1.el8_4.x86_64-x86_64-with-glibc2.28
- Python version: 3.9.7
- PyArrow version: 6.0.0
NB: even if the error is raised, the dataset is successfully cached. So restarting the script after every `map()` allows to ultimately run the whole preprocessing. But this prevents to realistically run the code over multiple nodes. |
https://github.com/huggingface/datasets/issues/3172 | `SystemError 15` thrown in `Dataset.__del__` when using `Dataset.map()` with `num_proc>1` | Hi,
It's not easy to debug the problem without the script. I may be wrong since I'm not very familiar with PyTorch Lightning, but shouldn't you preprocess the data in the `prepare_data` function of `LightningDataModule` and not in the `setup` function.
As you can't modify the module state in `prepare_data` (according to the docs), use the `cache_file_name` argument in `Dataset.map` there, and reload the processed data in `setup` with `Dataset.from_file(cache_file_name)`. If `num_proc>1`, check the docs on the `suffix_template` argument of `Dataset.map` to get an idea what the final `cache_file_names` are going to be.
Let me know if this helps. | ## Describe the bug
I use `datasets.map` to preprocess some data in my application. The error `SystemError 15` is thrown at the end of the execution of `Dataset.map()` (only with `num_proc>1`. Traceback included bellow.
The exception is raised only when the code runs within a specific context. Despite ~10h spent investigating this issue, I have failed to isolate the bug, so let me describe my setup.
In my project, `Dataset` is wrapped into a `LightningDataModule` and the data is preprocessed when calling `LightningDataModule.setup()`. Calling `.setup()` in an isolated script works fine (even when wrapped with `hydra.main()`). However, when calling `.setup()` within the experiment script (depends on `pytorch_lightning`), the script crashes and `SystemError 15`.
I could avoid throwing this error by modifying ` Dataset.__del__()` (see bellow), but I believe this only moves the problem somewhere else. I am completely stuck with this issue, any hint would be welcome.
```python
class Dataset()
...
def __del__(self):
if hasattr(self, "_data"):
_ = self._data # <- ugly trick that allows avoiding the issue.
del self._data
if hasattr(self, "_indices"):
del self._indices
```
## Steps to reproduce the bug
```python
# Unfortunately I couldn't isolate the bug.
```
## Expected results
Calling `Dataset.map()` without throwing an exception. Or at least raising a more detailed exception/traceback.
## Actual results
```
Exception ignored in: <function Dataset.__del__ at 0x7f7cec179160>███████████████████████████████████████████████████| 5/5 [00:05<00:00, 1.17ba/s]
Traceback (most recent call last):
File ".../python3.8/site-packages/datasets/arrow_dataset.py", line 906, in __del__
del self._data
File ".../python3.8/site-packages/ray/worker.py", line 1033, in sigterm_handler
sys.exit(signum)
SystemExit: 15
```
## Environment info
Tested on 2 environments:
**Environment 1.**
- `datasets` version: 1.14.0
- Platform: macOS-10.16-x86_64-i386-64bit
- Python version: 3.8.8
- PyArrow version: 6.0.0
**Environment 2.**
- `datasets` version: 1.14.0
- Platform: Linux-4.18.0-305.19.1.el8_4.x86_64-x86_64-with-glibc2.28
- Python version: 3.9.7
- PyArrow version: 6.0.0
| 99 | `SystemError 15` thrown in `Dataset.__del__` when using `Dataset.map()` with `num_proc>1`
## Describe the bug
I use `datasets.map` to preprocess some data in my application. The error `SystemError 15` is thrown at the end of the execution of `Dataset.map()` (only with `num_proc>1`. Traceback included bellow.
The exception is raised only when the code runs within a specific context. Despite ~10h spent investigating this issue, I have failed to isolate the bug, so let me describe my setup.
In my project, `Dataset` is wrapped into a `LightningDataModule` and the data is preprocessed when calling `LightningDataModule.setup()`. Calling `.setup()` in an isolated script works fine (even when wrapped with `hydra.main()`). However, when calling `.setup()` within the experiment script (depends on `pytorch_lightning`), the script crashes and `SystemError 15`.
I could avoid throwing this error by modifying ` Dataset.__del__()` (see bellow), but I believe this only moves the problem somewhere else. I am completely stuck with this issue, any hint would be welcome.
```python
class Dataset()
...
def __del__(self):
if hasattr(self, "_data"):
_ = self._data # <- ugly trick that allows avoiding the issue.
del self._data
if hasattr(self, "_indices"):
del self._indices
```
## Steps to reproduce the bug
```python
# Unfortunately I couldn't isolate the bug.
```
## Expected results
Calling `Dataset.map()` without throwing an exception. Or at least raising a more detailed exception/traceback.
## Actual results
```
Exception ignored in: <function Dataset.__del__ at 0x7f7cec179160>███████████████████████████████████████████████████| 5/5 [00:05<00:00, 1.17ba/s]
Traceback (most recent call last):
File ".../python3.8/site-packages/datasets/arrow_dataset.py", line 906, in __del__
del self._data
File ".../python3.8/site-packages/ray/worker.py", line 1033, in sigterm_handler
sys.exit(signum)
SystemExit: 15
```
## Environment info
Tested on 2 environments:
**Environment 1.**
- `datasets` version: 1.14.0
- Platform: macOS-10.16-x86_64-i386-64bit
- Python version: 3.8.8
- PyArrow version: 6.0.0
**Environment 2.**
- `datasets` version: 1.14.0
- Platform: Linux-4.18.0-305.19.1.el8_4.x86_64-x86_64-with-glibc2.28
- Python version: 3.9.7
- PyArrow version: 6.0.0
Hi,
It's not easy to debug the problem without the script. I may be wrong since I'm not very familiar with PyTorch Lightning, but shouldn't you preprocess the data in the `prepare_data` function of `LightningDataModule` and not in the `setup` function.
As you can't modify the module state in `prepare_data` (according to the docs), use the `cache_file_name` argument in `Dataset.map` there, and reload the processed data in `setup` with `Dataset.from_file(cache_file_name)`. If `num_proc>1`, check the docs on the `suffix_template` argument of `Dataset.map` to get an idea what the final `cache_file_names` are going to be.
Let me know if this helps. |
https://github.com/huggingface/datasets/issues/3172 | `SystemError 15` thrown in `Dataset.__del__` when using `Dataset.map()` with `num_proc>1` | Hi @mariosasko, thank you for the hint, that helped me to move forward with that issue.
I did a major refactoring of my project to disentangle my `LightningDataModule` and `Dataset`. Just FYI, it looks like:
```python
class Builder():
def __call__() -> DatasetDict:
# load and preprocess the data
return dataset
class DataModule(LightningDataModule):
def prepare_data():
self.builder()
def setup():
self.dataset = self.builder()
```
Unfortunately, the entanglement between `LightningDataModule` and `Dataset` was not the issue.
The culprit was `hydra` and a slight adjustment of the structure of my project solved this issue. The problematic project structure was:
```
src/
| - cli.py
| - training/
| -experiment.py
# code in experiment.py
def run_experiment(config):
# preprocess data and run
# code in cli.py
@hydra.main(...)
def run(config):
return run_experiment(config)
```
Moving `run()` from `clip.py` to `training.experiment.py` solved the issue with `SystemError 15`. No idea why.
Even if the traceback was referring to `Dataset.__del__`, the problem does not seem to be primarily related to `datasets`, so I will close this issue. Thank you for your help! | ## Describe the bug
I use `datasets.map` to preprocess some data in my application. The error `SystemError 15` is thrown at the end of the execution of `Dataset.map()` (only with `num_proc>1`. Traceback included bellow.
The exception is raised only when the code runs within a specific context. Despite ~10h spent investigating this issue, I have failed to isolate the bug, so let me describe my setup.
In my project, `Dataset` is wrapped into a `LightningDataModule` and the data is preprocessed when calling `LightningDataModule.setup()`. Calling `.setup()` in an isolated script works fine (even when wrapped with `hydra.main()`). However, when calling `.setup()` within the experiment script (depends on `pytorch_lightning`), the script crashes and `SystemError 15`.
I could avoid throwing this error by modifying ` Dataset.__del__()` (see bellow), but I believe this only moves the problem somewhere else. I am completely stuck with this issue, any hint would be welcome.
```python
class Dataset()
...
def __del__(self):
if hasattr(self, "_data"):
_ = self._data # <- ugly trick that allows avoiding the issue.
del self._data
if hasattr(self, "_indices"):
del self._indices
```
## Steps to reproduce the bug
```python
# Unfortunately I couldn't isolate the bug.
```
## Expected results
Calling `Dataset.map()` without throwing an exception. Or at least raising a more detailed exception/traceback.
## Actual results
```
Exception ignored in: <function Dataset.__del__ at 0x7f7cec179160>███████████████████████████████████████████████████| 5/5 [00:05<00:00, 1.17ba/s]
Traceback (most recent call last):
File ".../python3.8/site-packages/datasets/arrow_dataset.py", line 906, in __del__
del self._data
File ".../python3.8/site-packages/ray/worker.py", line 1033, in sigterm_handler
sys.exit(signum)
SystemExit: 15
```
## Environment info
Tested on 2 environments:
**Environment 1.**
- `datasets` version: 1.14.0
- Platform: macOS-10.16-x86_64-i386-64bit
- Python version: 3.8.8
- PyArrow version: 6.0.0
**Environment 2.**
- `datasets` version: 1.14.0
- Platform: Linux-4.18.0-305.19.1.el8_4.x86_64-x86_64-with-glibc2.28
- Python version: 3.9.7
- PyArrow version: 6.0.0
| 170 | `SystemError 15` thrown in `Dataset.__del__` when using `Dataset.map()` with `num_proc>1`
## Describe the bug
I use `datasets.map` to preprocess some data in my application. The error `SystemError 15` is thrown at the end of the execution of `Dataset.map()` (only with `num_proc>1`. Traceback included bellow.
The exception is raised only when the code runs within a specific context. Despite ~10h spent investigating this issue, I have failed to isolate the bug, so let me describe my setup.
In my project, `Dataset` is wrapped into a `LightningDataModule` and the data is preprocessed when calling `LightningDataModule.setup()`. Calling `.setup()` in an isolated script works fine (even when wrapped with `hydra.main()`). However, when calling `.setup()` within the experiment script (depends on `pytorch_lightning`), the script crashes and `SystemError 15`.
I could avoid throwing this error by modifying ` Dataset.__del__()` (see bellow), but I believe this only moves the problem somewhere else. I am completely stuck with this issue, any hint would be welcome.
```python
class Dataset()
...
def __del__(self):
if hasattr(self, "_data"):
_ = self._data # <- ugly trick that allows avoiding the issue.
del self._data
if hasattr(self, "_indices"):
del self._indices
```
## Steps to reproduce the bug
```python
# Unfortunately I couldn't isolate the bug.
```
## Expected results
Calling `Dataset.map()` without throwing an exception. Or at least raising a more detailed exception/traceback.
## Actual results
```
Exception ignored in: <function Dataset.__del__ at 0x7f7cec179160>███████████████████████████████████████████████████| 5/5 [00:05<00:00, 1.17ba/s]
Traceback (most recent call last):
File ".../python3.8/site-packages/datasets/arrow_dataset.py", line 906, in __del__
del self._data
File ".../python3.8/site-packages/ray/worker.py", line 1033, in sigterm_handler
sys.exit(signum)
SystemExit: 15
```
## Environment info
Tested on 2 environments:
**Environment 1.**
- `datasets` version: 1.14.0
- Platform: macOS-10.16-x86_64-i386-64bit
- Python version: 3.8.8
- PyArrow version: 6.0.0
**Environment 2.**
- `datasets` version: 1.14.0
- Platform: Linux-4.18.0-305.19.1.el8_4.x86_64-x86_64-with-glibc2.28
- Python version: 3.9.7
- PyArrow version: 6.0.0
Hi @mariosasko, thank you for the hint, that helped me to move forward with that issue.
I did a major refactoring of my project to disentangle my `LightningDataModule` and `Dataset`. Just FYI, it looks like:
```python
class Builder():
def __call__() -> DatasetDict:
# load and preprocess the data
return dataset
class DataModule(LightningDataModule):
def prepare_data():
self.builder()
def setup():
self.dataset = self.builder()
```
Unfortunately, the entanglement between `LightningDataModule` and `Dataset` was not the issue.
The culprit was `hydra` and a slight adjustment of the structure of my project solved this issue. The problematic project structure was:
```
src/
| - cli.py
| - training/
| -experiment.py
# code in experiment.py
def run_experiment(config):
# preprocess data and run
# code in cli.py
@hydra.main(...)
def run(config):
return run_experiment(config)
```
Moving `run()` from `clip.py` to `training.experiment.py` solved the issue with `SystemError 15`. No idea why.
Even if the traceback was referring to `Dataset.__del__`, the problem does not seem to be primarily related to `datasets`, so I will close this issue. Thank you for your help! |
https://github.com/huggingface/datasets/issues/3172 | `SystemError 15` thrown in `Dataset.__del__` when using `Dataset.map()` with `num_proc>1` | Please allow me to revive this discussion, as I have an extremely similar issue. Instead of an error, my datasets functions simply aren't caching properly. My setup is almost the same as yours, with hydra to configure my experiment parameters.
@vlievin Could you confirm if your code correctly loads the cache? If so, do you have any public code that I can reference for comparison?
I will post a full example with hydra that illustrates this problem in a little bit, probably on another thread. | ## Describe the bug
I use `datasets.map` to preprocess some data in my application. The error `SystemError 15` is thrown at the end of the execution of `Dataset.map()` (only with `num_proc>1`. Traceback included bellow.
The exception is raised only when the code runs within a specific context. Despite ~10h spent investigating this issue, I have failed to isolate the bug, so let me describe my setup.
In my project, `Dataset` is wrapped into a `LightningDataModule` and the data is preprocessed when calling `LightningDataModule.setup()`. Calling `.setup()` in an isolated script works fine (even when wrapped with `hydra.main()`). However, when calling `.setup()` within the experiment script (depends on `pytorch_lightning`), the script crashes and `SystemError 15`.
I could avoid throwing this error by modifying ` Dataset.__del__()` (see bellow), but I believe this only moves the problem somewhere else. I am completely stuck with this issue, any hint would be welcome.
```python
class Dataset()
...
def __del__(self):
if hasattr(self, "_data"):
_ = self._data # <- ugly trick that allows avoiding the issue.
del self._data
if hasattr(self, "_indices"):
del self._indices
```
## Steps to reproduce the bug
```python
# Unfortunately I couldn't isolate the bug.
```
## Expected results
Calling `Dataset.map()` without throwing an exception. Or at least raising a more detailed exception/traceback.
## Actual results
```
Exception ignored in: <function Dataset.__del__ at 0x7f7cec179160>███████████████████████████████████████████████████| 5/5 [00:05<00:00, 1.17ba/s]
Traceback (most recent call last):
File ".../python3.8/site-packages/datasets/arrow_dataset.py", line 906, in __del__
del self._data
File ".../python3.8/site-packages/ray/worker.py", line 1033, in sigterm_handler
sys.exit(signum)
SystemExit: 15
```
## Environment info
Tested on 2 environments:
**Environment 1.**
- `datasets` version: 1.14.0
- Platform: macOS-10.16-x86_64-i386-64bit
- Python version: 3.8.8
- PyArrow version: 6.0.0
**Environment 2.**
- `datasets` version: 1.14.0
- Platform: Linux-4.18.0-305.19.1.el8_4.x86_64-x86_64-with-glibc2.28
- Python version: 3.9.7
- PyArrow version: 6.0.0
| 85 | `SystemError 15` thrown in `Dataset.__del__` when using `Dataset.map()` with `num_proc>1`
## Describe the bug
I use `datasets.map` to preprocess some data in my application. The error `SystemError 15` is thrown at the end of the execution of `Dataset.map()` (only with `num_proc>1`. Traceback included bellow.
The exception is raised only when the code runs within a specific context. Despite ~10h spent investigating this issue, I have failed to isolate the bug, so let me describe my setup.
In my project, `Dataset` is wrapped into a `LightningDataModule` and the data is preprocessed when calling `LightningDataModule.setup()`. Calling `.setup()` in an isolated script works fine (even when wrapped with `hydra.main()`). However, when calling `.setup()` within the experiment script (depends on `pytorch_lightning`), the script crashes and `SystemError 15`.
I could avoid throwing this error by modifying ` Dataset.__del__()` (see bellow), but I believe this only moves the problem somewhere else. I am completely stuck with this issue, any hint would be welcome.
```python
class Dataset()
...
def __del__(self):
if hasattr(self, "_data"):
_ = self._data # <- ugly trick that allows avoiding the issue.
del self._data
if hasattr(self, "_indices"):
del self._indices
```
## Steps to reproduce the bug
```python
# Unfortunately I couldn't isolate the bug.
```
## Expected results
Calling `Dataset.map()` without throwing an exception. Or at least raising a more detailed exception/traceback.
## Actual results
```
Exception ignored in: <function Dataset.__del__ at 0x7f7cec179160>███████████████████████████████████████████████████| 5/5 [00:05<00:00, 1.17ba/s]
Traceback (most recent call last):
File ".../python3.8/site-packages/datasets/arrow_dataset.py", line 906, in __del__
del self._data
File ".../python3.8/site-packages/ray/worker.py", line 1033, in sigterm_handler
sys.exit(signum)
SystemExit: 15
```
## Environment info
Tested on 2 environments:
**Environment 1.**
- `datasets` version: 1.14.0
- Platform: macOS-10.16-x86_64-i386-64bit
- Python version: 3.8.8
- PyArrow version: 6.0.0
**Environment 2.**
- `datasets` version: 1.14.0
- Platform: Linux-4.18.0-305.19.1.el8_4.x86_64-x86_64-with-glibc2.28
- Python version: 3.9.7
- PyArrow version: 6.0.0
Please allow me to revive this discussion, as I have an extremely similar issue. Instead of an error, my datasets functions simply aren't caching properly. My setup is almost the same as yours, with hydra to configure my experiment parameters.
@vlievin Could you confirm if your code correctly loads the cache? If so, do you have any public code that I can reference for comparison?
I will post a full example with hydra that illustrates this problem in a little bit, probably on another thread. |
https://github.com/huggingface/datasets/issues/3172 | `SystemError 15` thrown in `Dataset.__del__` when using `Dataset.map()` with `num_proc>1` | Hello @mariomeissner, very sorry for the late reply, I hope you have found a solution to your problem!
I don't have public code at the moment. I have not experienced any other issue with hydra, even if I don't understand why changing the location of the definition of `run()` fixed the problem.
Overall, I don't have issue with caching anymore, even when
1. using custom fingerprints using the argument `new_fingerprint
2. when using `num_proc>1` | ## Describe the bug
I use `datasets.map` to preprocess some data in my application. The error `SystemError 15` is thrown at the end of the execution of `Dataset.map()` (only with `num_proc>1`. Traceback included bellow.
The exception is raised only when the code runs within a specific context. Despite ~10h spent investigating this issue, I have failed to isolate the bug, so let me describe my setup.
In my project, `Dataset` is wrapped into a `LightningDataModule` and the data is preprocessed when calling `LightningDataModule.setup()`. Calling `.setup()` in an isolated script works fine (even when wrapped with `hydra.main()`). However, when calling `.setup()` within the experiment script (depends on `pytorch_lightning`), the script crashes and `SystemError 15`.
I could avoid throwing this error by modifying ` Dataset.__del__()` (see bellow), but I believe this only moves the problem somewhere else. I am completely stuck with this issue, any hint would be welcome.
```python
class Dataset()
...
def __del__(self):
if hasattr(self, "_data"):
_ = self._data # <- ugly trick that allows avoiding the issue.
del self._data
if hasattr(self, "_indices"):
del self._indices
```
## Steps to reproduce the bug
```python
# Unfortunately I couldn't isolate the bug.
```
## Expected results
Calling `Dataset.map()` without throwing an exception. Or at least raising a more detailed exception/traceback.
## Actual results
```
Exception ignored in: <function Dataset.__del__ at 0x7f7cec179160>███████████████████████████████████████████████████| 5/5 [00:05<00:00, 1.17ba/s]
Traceback (most recent call last):
File ".../python3.8/site-packages/datasets/arrow_dataset.py", line 906, in __del__
del self._data
File ".../python3.8/site-packages/ray/worker.py", line 1033, in sigterm_handler
sys.exit(signum)
SystemExit: 15
```
## Environment info
Tested on 2 environments:
**Environment 1.**
- `datasets` version: 1.14.0
- Platform: macOS-10.16-x86_64-i386-64bit
- Python version: 3.8.8
- PyArrow version: 6.0.0
**Environment 2.**
- `datasets` version: 1.14.0
- Platform: Linux-4.18.0-305.19.1.el8_4.x86_64-x86_64-with-glibc2.28
- Python version: 3.9.7
- PyArrow version: 6.0.0
| 74 | `SystemError 15` thrown in `Dataset.__del__` when using `Dataset.map()` with `num_proc>1`
## Describe the bug
I use `datasets.map` to preprocess some data in my application. The error `SystemError 15` is thrown at the end of the execution of `Dataset.map()` (only with `num_proc>1`. Traceback included bellow.
The exception is raised only when the code runs within a specific context. Despite ~10h spent investigating this issue, I have failed to isolate the bug, so let me describe my setup.
In my project, `Dataset` is wrapped into a `LightningDataModule` and the data is preprocessed when calling `LightningDataModule.setup()`. Calling `.setup()` in an isolated script works fine (even when wrapped with `hydra.main()`). However, when calling `.setup()` within the experiment script (depends on `pytorch_lightning`), the script crashes and `SystemError 15`.
I could avoid throwing this error by modifying ` Dataset.__del__()` (see bellow), but I believe this only moves the problem somewhere else. I am completely stuck with this issue, any hint would be welcome.
```python
class Dataset()
...
def __del__(self):
if hasattr(self, "_data"):
_ = self._data # <- ugly trick that allows avoiding the issue.
del self._data
if hasattr(self, "_indices"):
del self._indices
```
## Steps to reproduce the bug
```python
# Unfortunately I couldn't isolate the bug.
```
## Expected results
Calling `Dataset.map()` without throwing an exception. Or at least raising a more detailed exception/traceback.
## Actual results
```
Exception ignored in: <function Dataset.__del__ at 0x7f7cec179160>███████████████████████████████████████████████████| 5/5 [00:05<00:00, 1.17ba/s]
Traceback (most recent call last):
File ".../python3.8/site-packages/datasets/arrow_dataset.py", line 906, in __del__
del self._data
File ".../python3.8/site-packages/ray/worker.py", line 1033, in sigterm_handler
sys.exit(signum)
SystemExit: 15
```
## Environment info
Tested on 2 environments:
**Environment 1.**
- `datasets` version: 1.14.0
- Platform: macOS-10.16-x86_64-i386-64bit
- Python version: 3.8.8
- PyArrow version: 6.0.0
**Environment 2.**
- `datasets` version: 1.14.0
- Platform: Linux-4.18.0-305.19.1.el8_4.x86_64-x86_64-with-glibc2.28
- Python version: 3.9.7
- PyArrow version: 6.0.0
Hello @mariomeissner, very sorry for the late reply, I hope you have found a solution to your problem!
I don't have public code at the moment. I have not experienced any other issue with hydra, even if I don't understand why changing the location of the definition of `run()` fixed the problem.
Overall, I don't have issue with caching anymore, even when
1. using custom fingerprints using the argument `new_fingerprint
2. when using `num_proc>1` |
https://github.com/huggingface/datasets/issues/3172 | `SystemError 15` thrown in `Dataset.__del__` when using `Dataset.map()` with `num_proc>1` | I solved my issue by turning the map callable into a class static method, like they do in `lightning-transformers`. Very strange... | ## Describe the bug
I use `datasets.map` to preprocess some data in my application. The error `SystemError 15` is thrown at the end of the execution of `Dataset.map()` (only with `num_proc>1`. Traceback included bellow.
The exception is raised only when the code runs within a specific context. Despite ~10h spent investigating this issue, I have failed to isolate the bug, so let me describe my setup.
In my project, `Dataset` is wrapped into a `LightningDataModule` and the data is preprocessed when calling `LightningDataModule.setup()`. Calling `.setup()` in an isolated script works fine (even when wrapped with `hydra.main()`). However, when calling `.setup()` within the experiment script (depends on `pytorch_lightning`), the script crashes and `SystemError 15`.
I could avoid throwing this error by modifying ` Dataset.__del__()` (see bellow), but I believe this only moves the problem somewhere else. I am completely stuck with this issue, any hint would be welcome.
```python
class Dataset()
...
def __del__(self):
if hasattr(self, "_data"):
_ = self._data # <- ugly trick that allows avoiding the issue.
del self._data
if hasattr(self, "_indices"):
del self._indices
```
## Steps to reproduce the bug
```python
# Unfortunately I couldn't isolate the bug.
```
## Expected results
Calling `Dataset.map()` without throwing an exception. Or at least raising a more detailed exception/traceback.
## Actual results
```
Exception ignored in: <function Dataset.__del__ at 0x7f7cec179160>███████████████████████████████████████████████████| 5/5 [00:05<00:00, 1.17ba/s]
Traceback (most recent call last):
File ".../python3.8/site-packages/datasets/arrow_dataset.py", line 906, in __del__
del self._data
File ".../python3.8/site-packages/ray/worker.py", line 1033, in sigterm_handler
sys.exit(signum)
SystemExit: 15
```
## Environment info
Tested on 2 environments:
**Environment 1.**
- `datasets` version: 1.14.0
- Platform: macOS-10.16-x86_64-i386-64bit
- Python version: 3.8.8
- PyArrow version: 6.0.0
**Environment 2.**
- `datasets` version: 1.14.0
- Platform: Linux-4.18.0-305.19.1.el8_4.x86_64-x86_64-with-glibc2.28
- Python version: 3.9.7
- PyArrow version: 6.0.0
| 21 | `SystemError 15` thrown in `Dataset.__del__` when using `Dataset.map()` with `num_proc>1`
## Describe the bug
I use `datasets.map` to preprocess some data in my application. The error `SystemError 15` is thrown at the end of the execution of `Dataset.map()` (only with `num_proc>1`. Traceback included bellow.
The exception is raised only when the code runs within a specific context. Despite ~10h spent investigating this issue, I have failed to isolate the bug, so let me describe my setup.
In my project, `Dataset` is wrapped into a `LightningDataModule` and the data is preprocessed when calling `LightningDataModule.setup()`. Calling `.setup()` in an isolated script works fine (even when wrapped with `hydra.main()`). However, when calling `.setup()` within the experiment script (depends on `pytorch_lightning`), the script crashes and `SystemError 15`.
I could avoid throwing this error by modifying ` Dataset.__del__()` (see bellow), but I believe this only moves the problem somewhere else. I am completely stuck with this issue, any hint would be welcome.
```python
class Dataset()
...
def __del__(self):
if hasattr(self, "_data"):
_ = self._data # <- ugly trick that allows avoiding the issue.
del self._data
if hasattr(self, "_indices"):
del self._indices
```
## Steps to reproduce the bug
```python
# Unfortunately I couldn't isolate the bug.
```
## Expected results
Calling `Dataset.map()` without throwing an exception. Or at least raising a more detailed exception/traceback.
## Actual results
```
Exception ignored in: <function Dataset.__del__ at 0x7f7cec179160>███████████████████████████████████████████████████| 5/5 [00:05<00:00, 1.17ba/s]
Traceback (most recent call last):
File ".../python3.8/site-packages/datasets/arrow_dataset.py", line 906, in __del__
del self._data
File ".../python3.8/site-packages/ray/worker.py", line 1033, in sigterm_handler
sys.exit(signum)
SystemExit: 15
```
## Environment info
Tested on 2 environments:
**Environment 1.**
- `datasets` version: 1.14.0
- Platform: macOS-10.16-x86_64-i386-64bit
- Python version: 3.8.8
- PyArrow version: 6.0.0
**Environment 2.**
- `datasets` version: 1.14.0
- Platform: Linux-4.18.0-305.19.1.el8_4.x86_64-x86_64-with-glibc2.28
- Python version: 3.9.7
- PyArrow version: 6.0.0
I solved my issue by turning the map callable into a class static method, like they do in `lightning-transformers`. Very strange... |
https://github.com/huggingface/datasets/issues/3172 | `SystemError 15` thrown in `Dataset.__del__` when using `Dataset.map()` with `num_proc>1` | I have this issue with datasets v2.5.2 with Python 3.8.10 on Ubuntu 20.04.4 LTS. It does not occur when num_proc=1. When num_proc>1, it intermittently occurs and will cause process to hang. As previously mentioned, it occurs even when datasets have been previously cached. I have tried wrapping logic in a static class as suggested with @mariomeissner with no improvement. | ## Describe the bug
I use `datasets.map` to preprocess some data in my application. The error `SystemError 15` is thrown at the end of the execution of `Dataset.map()` (only with `num_proc>1`. Traceback included bellow.
The exception is raised only when the code runs within a specific context. Despite ~10h spent investigating this issue, I have failed to isolate the bug, so let me describe my setup.
In my project, `Dataset` is wrapped into a `LightningDataModule` and the data is preprocessed when calling `LightningDataModule.setup()`. Calling `.setup()` in an isolated script works fine (even when wrapped with `hydra.main()`). However, when calling `.setup()` within the experiment script (depends on `pytorch_lightning`), the script crashes and `SystemError 15`.
I could avoid throwing this error by modifying ` Dataset.__del__()` (see bellow), but I believe this only moves the problem somewhere else. I am completely stuck with this issue, any hint would be welcome.
```python
class Dataset()
...
def __del__(self):
if hasattr(self, "_data"):
_ = self._data # <- ugly trick that allows avoiding the issue.
del self._data
if hasattr(self, "_indices"):
del self._indices
```
## Steps to reproduce the bug
```python
# Unfortunately I couldn't isolate the bug.
```
## Expected results
Calling `Dataset.map()` without throwing an exception. Or at least raising a more detailed exception/traceback.
## Actual results
```
Exception ignored in: <function Dataset.__del__ at 0x7f7cec179160>███████████████████████████████████████████████████| 5/5 [00:05<00:00, 1.17ba/s]
Traceback (most recent call last):
File ".../python3.8/site-packages/datasets/arrow_dataset.py", line 906, in __del__
del self._data
File ".../python3.8/site-packages/ray/worker.py", line 1033, in sigterm_handler
sys.exit(signum)
SystemExit: 15
```
## Environment info
Tested on 2 environments:
**Environment 1.**
- `datasets` version: 1.14.0
- Platform: macOS-10.16-x86_64-i386-64bit
- Python version: 3.8.8
- PyArrow version: 6.0.0
**Environment 2.**
- `datasets` version: 1.14.0
- Platform: Linux-4.18.0-305.19.1.el8_4.x86_64-x86_64-with-glibc2.28
- Python version: 3.9.7
- PyArrow version: 6.0.0
| 59 | `SystemError 15` thrown in `Dataset.__del__` when using `Dataset.map()` with `num_proc>1`
## Describe the bug
I use `datasets.map` to preprocess some data in my application. The error `SystemError 15` is thrown at the end of the execution of `Dataset.map()` (only with `num_proc>1`. Traceback included bellow.
The exception is raised only when the code runs within a specific context. Despite ~10h spent investigating this issue, I have failed to isolate the bug, so let me describe my setup.
In my project, `Dataset` is wrapped into a `LightningDataModule` and the data is preprocessed when calling `LightningDataModule.setup()`. Calling `.setup()` in an isolated script works fine (even when wrapped with `hydra.main()`). However, when calling `.setup()` within the experiment script (depends on `pytorch_lightning`), the script crashes and `SystemError 15`.
I could avoid throwing this error by modifying ` Dataset.__del__()` (see bellow), but I believe this only moves the problem somewhere else. I am completely stuck with this issue, any hint would be welcome.
```python
class Dataset()
...
def __del__(self):
if hasattr(self, "_data"):
_ = self._data # <- ugly trick that allows avoiding the issue.
del self._data
if hasattr(self, "_indices"):
del self._indices
```
## Steps to reproduce the bug
```python
# Unfortunately I couldn't isolate the bug.
```
## Expected results
Calling `Dataset.map()` without throwing an exception. Or at least raising a more detailed exception/traceback.
## Actual results
```
Exception ignored in: <function Dataset.__del__ at 0x7f7cec179160>███████████████████████████████████████████████████| 5/5 [00:05<00:00, 1.17ba/s]
Traceback (most recent call last):
File ".../python3.8/site-packages/datasets/arrow_dataset.py", line 906, in __del__
del self._data
File ".../python3.8/site-packages/ray/worker.py", line 1033, in sigterm_handler
sys.exit(signum)
SystemExit: 15
```
## Environment info
Tested on 2 environments:
**Environment 1.**
- `datasets` version: 1.14.0
- Platform: macOS-10.16-x86_64-i386-64bit
- Python version: 3.8.8
- PyArrow version: 6.0.0
**Environment 2.**
- `datasets` version: 1.14.0
- Platform: Linux-4.18.0-305.19.1.el8_4.x86_64-x86_64-with-glibc2.28
- Python version: 3.9.7
- PyArrow version: 6.0.0
I have this issue with datasets v2.5.2 with Python 3.8.10 on Ubuntu 20.04.4 LTS. It does not occur when num_proc=1. When num_proc>1, it intermittently occurs and will cause process to hang. As previously mentioned, it occurs even when datasets have been previously cached. I have tried wrapping logic in a static class as suggested with @mariomeissner with no improvement. |
https://github.com/huggingface/datasets/issues/3172 | `SystemError 15` thrown in `Dataset.__del__` when using `Dataset.map()` with `num_proc>1` | Can someone provide a reproducer to help us debug this (e.g., a `hydra` repo with dummy model and data)? | ## Describe the bug
I use `datasets.map` to preprocess some data in my application. The error `SystemError 15` is thrown at the end of the execution of `Dataset.map()` (only with `num_proc>1`. Traceback included bellow.
The exception is raised only when the code runs within a specific context. Despite ~10h spent investigating this issue, I have failed to isolate the bug, so let me describe my setup.
In my project, `Dataset` is wrapped into a `LightningDataModule` and the data is preprocessed when calling `LightningDataModule.setup()`. Calling `.setup()` in an isolated script works fine (even when wrapped with `hydra.main()`). However, when calling `.setup()` within the experiment script (depends on `pytorch_lightning`), the script crashes and `SystemError 15`.
I could avoid throwing this error by modifying ` Dataset.__del__()` (see bellow), but I believe this only moves the problem somewhere else. I am completely stuck with this issue, any hint would be welcome.
```python
class Dataset()
...
def __del__(self):
if hasattr(self, "_data"):
_ = self._data # <- ugly trick that allows avoiding the issue.
del self._data
if hasattr(self, "_indices"):
del self._indices
```
## Steps to reproduce the bug
```python
# Unfortunately I couldn't isolate the bug.
```
## Expected results
Calling `Dataset.map()` without throwing an exception. Or at least raising a more detailed exception/traceback.
## Actual results
```
Exception ignored in: <function Dataset.__del__ at 0x7f7cec179160>███████████████████████████████████████████████████| 5/5 [00:05<00:00, 1.17ba/s]
Traceback (most recent call last):
File ".../python3.8/site-packages/datasets/arrow_dataset.py", line 906, in __del__
del self._data
File ".../python3.8/site-packages/ray/worker.py", line 1033, in sigterm_handler
sys.exit(signum)
SystemExit: 15
```
## Environment info
Tested on 2 environments:
**Environment 1.**
- `datasets` version: 1.14.0
- Platform: macOS-10.16-x86_64-i386-64bit
- Python version: 3.8.8
- PyArrow version: 6.0.0
**Environment 2.**
- `datasets` version: 1.14.0
- Platform: Linux-4.18.0-305.19.1.el8_4.x86_64-x86_64-with-glibc2.28
- Python version: 3.9.7
- PyArrow version: 6.0.0
| 19 | `SystemError 15` thrown in `Dataset.__del__` when using `Dataset.map()` with `num_proc>1`
## Describe the bug
I use `datasets.map` to preprocess some data in my application. The error `SystemError 15` is thrown at the end of the execution of `Dataset.map()` (only with `num_proc>1`. Traceback included bellow.
The exception is raised only when the code runs within a specific context. Despite ~10h spent investigating this issue, I have failed to isolate the bug, so let me describe my setup.
In my project, `Dataset` is wrapped into a `LightningDataModule` and the data is preprocessed when calling `LightningDataModule.setup()`. Calling `.setup()` in an isolated script works fine (even when wrapped with `hydra.main()`). However, when calling `.setup()` within the experiment script (depends on `pytorch_lightning`), the script crashes and `SystemError 15`.
I could avoid throwing this error by modifying ` Dataset.__del__()` (see bellow), but I believe this only moves the problem somewhere else. I am completely stuck with this issue, any hint would be welcome.
```python
class Dataset()
...
def __del__(self):
if hasattr(self, "_data"):
_ = self._data # <- ugly trick that allows avoiding the issue.
del self._data
if hasattr(self, "_indices"):
del self._indices
```
## Steps to reproduce the bug
```python
# Unfortunately I couldn't isolate the bug.
```
## Expected results
Calling `Dataset.map()` without throwing an exception. Or at least raising a more detailed exception/traceback.
## Actual results
```
Exception ignored in: <function Dataset.__del__ at 0x7f7cec179160>███████████████████████████████████████████████████| 5/5 [00:05<00:00, 1.17ba/s]
Traceback (most recent call last):
File ".../python3.8/site-packages/datasets/arrow_dataset.py", line 906, in __del__
del self._data
File ".../python3.8/site-packages/ray/worker.py", line 1033, in sigterm_handler
sys.exit(signum)
SystemExit: 15
```
## Environment info
Tested on 2 environments:
**Environment 1.**
- `datasets` version: 1.14.0
- Platform: macOS-10.16-x86_64-i386-64bit
- Python version: 3.8.8
- PyArrow version: 6.0.0
**Environment 2.**
- `datasets` version: 1.14.0
- Platform: Linux-4.18.0-305.19.1.el8_4.x86_64-x86_64-with-glibc2.28
- Python version: 3.9.7
- PyArrow version: 6.0.0
Can someone provide a reproducer to help us debug this (e.g., a `hydra` repo with dummy model and data)? |
https://github.com/huggingface/datasets/issues/3171 | Raise exceptions instead of using assertions for control flow | Adding the remaining tasks for this issue to help new code contributors.
$ cd src/datasets && ack assert -lc
- [x] commands/convert.py:1
- [x] arrow_reader.py:3
- [x] load.py:7
- [x] utils/py_utils.py:2
- [x] features/features.py:9
- [x] arrow_writer.py:7
- [x] search.py:6
- [x] table.py:1
- [x] metric.py:3
- [x] tasks/image_classification.py:1
- [x] arrow_dataset.py:17
- [x] fingerprint.py:6
- [x] io/json.py:1
- [x] io/csv.py:1 | Motivated by https://github.com/huggingface/transformers/issues/12789 in Transformers, one welcoming change would be replacing assertions with proper exceptions. The only type of assertions we should keep are those used as sanity checks.
Currently, there is a total of 87 files with the `assert` statements (located under `datasets` and `src/datasets`), so when working on this, to manage the PR size, only modify 4-5 files at most before submitting a PR. | 61 | Raise exceptions instead of using assertions for control flow
Motivated by https://github.com/huggingface/transformers/issues/12789 in Transformers, one welcoming change would be replacing assertions with proper exceptions. The only type of assertions we should keep are those used as sanity checks.
Currently, there is a total of 87 files with the `assert` statements (located under `datasets` and `src/datasets`), so when working on this, to manage the PR size, only modify 4-5 files at most before submitting a PR.
Adding the remaining tasks for this issue to help new code contributors.
$ cd src/datasets && ack assert -lc
- [x] commands/convert.py:1
- [x] arrow_reader.py:3
- [x] load.py:7
- [x] utils/py_utils.py:2
- [x] features/features.py:9
- [x] arrow_writer.py:7
- [x] search.py:6
- [x] table.py:1
- [x] metric.py:3
- [x] tasks/image_classification.py:1
- [x] arrow_dataset.py:17
- [x] fingerprint.py:6
- [x] io/json.py:1
- [x] io/csv.py:1 |
https://github.com/huggingface/datasets/issues/3171 | Raise exceptions instead of using assertions for control flow | Hi all,
I am interested in taking up `fingerprint.py`, `search.py`, `arrow_writer.py` and `metric.py`. Will raise a PR soon! | Motivated by https://github.com/huggingface/transformers/issues/12789 in Transformers, one welcoming change would be replacing assertions with proper exceptions. The only type of assertions we should keep are those used as sanity checks.
Currently, there is a total of 87 files with the `assert` statements (located under `datasets` and `src/datasets`), so when working on this, to manage the PR size, only modify 4-5 files at most before submitting a PR. | 18 | Raise exceptions instead of using assertions for control flow
Motivated by https://github.com/huggingface/transformers/issues/12789 in Transformers, one welcoming change would be replacing assertions with proper exceptions. The only type of assertions we should keep are those used as sanity checks.
Currently, there is a total of 87 files with the `assert` statements (located under `datasets` and `src/datasets`), so when working on this, to manage the PR size, only modify 4-5 files at most before submitting a PR.
Hi all,
I am interested in taking up `fingerprint.py`, `search.py`, `arrow_writer.py` and `metric.py`. Will raise a PR soon! |
https://github.com/huggingface/datasets/issues/3168 | OpenSLR/83 is empty | Hi @tyrius02, thanks for reporting. I see you self-assigned this issue: are you working on this? | ## Describe the bug
As the summary says, openslr / SLR83 / train is empty.
The dataset returned after loading indicates there are **zero** rows. The correct number should be **17877**.
## Steps to reproduce the bug
```python
import datasets
datasets.load_dataset('openslr', 'SLR83')
```
## Expected results
```
DatasetDict({
train: Dataset({
features: ['path', 'audio', 'sentence'],
num_rows: 17877
})
})
```
## Actual results
```
DatasetDict({
train: Dataset({
features: ['path', 'audio', 'sentence'],
num_rows: 0
})
})
```
## Environment info
<!-- You can run the command `datasets-cli env` and copy-and-paste its output below. -->
- `datasets` version: 1.14.1.dev0 (master HEAD)
- Platform: Ubuntu 20.04
- Python version: 3.7.10
- PyArrow version: 3.0.0
| 16 | OpenSLR/83 is empty
## Describe the bug
As the summary says, openslr / SLR83 / train is empty.
The dataset returned after loading indicates there are **zero** rows. The correct number should be **17877**.
## Steps to reproduce the bug
```python
import datasets
datasets.load_dataset('openslr', 'SLR83')
```
## Expected results
```
DatasetDict({
train: Dataset({
features: ['path', 'audio', 'sentence'],
num_rows: 17877
})
})
```
## Actual results
```
DatasetDict({
train: Dataset({
features: ['path', 'audio', 'sentence'],
num_rows: 0
})
})
```
## Environment info
<!-- You can run the command `datasets-cli env` and copy-and-paste its output below. -->
- `datasets` version: 1.14.1.dev0 (master HEAD)
- Platform: Ubuntu 20.04
- Python version: 3.7.10
- PyArrow version: 3.0.0
Hi @tyrius02, thanks for reporting. I see you self-assigned this issue: are you working on this? |
https://github.com/huggingface/datasets/issues/3168 | OpenSLR/83 is empty | @albertvillanova Yes. Figured I introduced the broken config, I should fix it too.
I've got it working, but I'm struggling with one of the tests. I've started a PR so I/we can work through it. | ## Describe the bug
As the summary says, openslr / SLR83 / train is empty.
The dataset returned after loading indicates there are **zero** rows. The correct number should be **17877**.
## Steps to reproduce the bug
```python
import datasets
datasets.load_dataset('openslr', 'SLR83')
```
## Expected results
```
DatasetDict({
train: Dataset({
features: ['path', 'audio', 'sentence'],
num_rows: 17877
})
})
```
## Actual results
```
DatasetDict({
train: Dataset({
features: ['path', 'audio', 'sentence'],
num_rows: 0
})
})
```
## Environment info
<!-- You can run the command `datasets-cli env` and copy-and-paste its output below. -->
- `datasets` version: 1.14.1.dev0 (master HEAD)
- Platform: Ubuntu 20.04
- Python version: 3.7.10
- PyArrow version: 3.0.0
| 35 | OpenSLR/83 is empty
## Describe the bug
As the summary says, openslr / SLR83 / train is empty.
The dataset returned after loading indicates there are **zero** rows. The correct number should be **17877**.
## Steps to reproduce the bug
```python
import datasets
datasets.load_dataset('openslr', 'SLR83')
```
## Expected results
```
DatasetDict({
train: Dataset({
features: ['path', 'audio', 'sentence'],
num_rows: 17877
})
})
```
## Actual results
```
DatasetDict({
train: Dataset({
features: ['path', 'audio', 'sentence'],
num_rows: 0
})
})
```
## Environment info
<!-- You can run the command `datasets-cli env` and copy-and-paste its output below. -->
- `datasets` version: 1.14.1.dev0 (master HEAD)
- Platform: Ubuntu 20.04
- Python version: 3.7.10
- PyArrow version: 3.0.0
@albertvillanova Yes. Figured I introduced the broken config, I should fix it too.
I've got it working, but I'm struggling with one of the tests. I've started a PR so I/we can work through it. |
https://github.com/huggingface/datasets/issues/3167 | bookcorpusopen no longer works | I tried with the latest changes from #3280 on google colab and it worked fine :)
We'll do a new release soon, in the meantime you can use the updated version with:
```python
load_dataset("bookcorpusopen", revision="master")
``` | ## Describe the bug
When using the latest version of datasets (1.14.0), I cannot use the `bookcorpusopen` dataset. The process blocks always around `9924 examples [00:06, 1439.61 examples/s]` when preparing the dataset. I also noticed that after half an hour the process is automatically killed because of the RAM usage (the machine has 1TB of RAM...).
This did not happen with 1.4.1.
I tried also `rm -rf ~/.cache/huggingface` but did not help.
Changing python version between 3.7, 3.8 and 3.9 did not help too.
## Steps to reproduce the bug
```python
import datasets
d = datasets.load_dataset('bookcorpusopen')
```
## Expected results
A clear and concise description of the expected results.
## Actual results
Specify the actual results or traceback.
## Environment info
<!-- You can run the command `datasets-cli env` and copy-and-paste its output below. -->
- `datasets` version: 1.14.0
- Platform: Linux-5.4.0-1054-aws-x86_64-with-glibc2.27
- Python version: 3.9.7
- PyArrow version: 4.0.1
| 36 | bookcorpusopen no longer works
## Describe the bug
When using the latest version of datasets (1.14.0), I cannot use the `bookcorpusopen` dataset. The process blocks always around `9924 examples [00:06, 1439.61 examples/s]` when preparing the dataset. I also noticed that after half an hour the process is automatically killed because of the RAM usage (the machine has 1TB of RAM...).
This did not happen with 1.4.1.
I tried also `rm -rf ~/.cache/huggingface` but did not help.
Changing python version between 3.7, 3.8 and 3.9 did not help too.
## Steps to reproduce the bug
```python
import datasets
d = datasets.load_dataset('bookcorpusopen')
```
## Expected results
A clear and concise description of the expected results.
## Actual results
Specify the actual results or traceback.
## Environment info
<!-- You can run the command `datasets-cli env` and copy-and-paste its output below. -->
- `datasets` version: 1.14.0
- Platform: Linux-5.4.0-1054-aws-x86_64-with-glibc2.27
- Python version: 3.9.7
- PyArrow version: 4.0.1
I tried with the latest changes from #3280 on google colab and it worked fine :)
We'll do a new release soon, in the meantime you can use the updated version with:
```python
load_dataset("bookcorpusopen", revision="master")
``` |
https://github.com/huggingface/datasets/issues/3164 | Add raw data files to the Hub with GitHub LFS for canonical dataset | Hi @zlucia, I would actually suggest hosting the dataset as a huggingface.co-hosted dataset.
The only difference with a "canonical"/legacy dataset is that it's nested under an organization (here `stanford` or `stanfordnlp` for instance – completely up to you) but then you can upload your data using git-lfs (unlike "canonical" datasets where we don't host the data)
Let me know if this fits your use case!
cc'ing @osanseviero @lhoestq and rest of the team 🤗 | I'm interested in sharing the CaseHOLD dataset (https://arxiv.org/abs/2104.08671) as a canonical dataset on the HuggingFace Hub and would like to add the raw data files to the Hub with GitHub LFS, since it seems like a more sustainable long term storage solution, compared to other storage solutions available to my team. From what I can tell, this option is not immediately supported if one follows the sharing steps detailed here: [https://huggingface.co/docs/datasets/share_dataset.html#sharing-a-canonical-dataset](https://huggingface.co/docs/datasets/share_dataset.html#sharing-a-canonical-dataset), since GitHub LFS is not supported for public forks. Is there a way to request this? Thanks! | 74 | Add raw data files to the Hub with GitHub LFS for canonical dataset
I'm interested in sharing the CaseHOLD dataset (https://arxiv.org/abs/2104.08671) as a canonical dataset on the HuggingFace Hub and would like to add the raw data files to the Hub with GitHub LFS, since it seems like a more sustainable long term storage solution, compared to other storage solutions available to my team. From what I can tell, this option is not immediately supported if one follows the sharing steps detailed here: [https://huggingface.co/docs/datasets/share_dataset.html#sharing-a-canonical-dataset](https://huggingface.co/docs/datasets/share_dataset.html#sharing-a-canonical-dataset), since GitHub LFS is not supported for public forks. Is there a way to request this? Thanks!
Hi @zlucia, I would actually suggest hosting the dataset as a huggingface.co-hosted dataset.
The only difference with a "canonical"/legacy dataset is that it's nested under an organization (here `stanford` or `stanfordnlp` for instance – completely up to you) but then you can upload your data using git-lfs (unlike "canonical" datasets where we don't host the data)
Let me know if this fits your use case!
cc'ing @osanseviero @lhoestq and rest of the team 🤗 |
https://github.com/huggingface/datasets/issues/3164 | Add raw data files to the Hub with GitHub LFS for canonical dataset | Hi @zlucia,
As @julien-c pointed out, the way to store/host raw data files in our Hub is by using what we call "community" datasets:
- either at your personal namespace: `load_dataset("zlucia/casehold")`
- or at an organization namespace: for example, if you create the organization `reglab`, then `load_dataset("reglab/casehold")`
Please note that "canonical" datasets do not normally store/host their raw data at our Hub, but in a third-party server. For "canonical" datasets, we just host the "loading script", that is, a Python script that downloads the raw data from a third-party server, creates the HuggingFace dataset from it and caches it locally.
In order to create an organization namespace in our Hub, please follow this link: https://huggingface.co/organizations/new
There are already many organizations at our Hub (complete list here: https://huggingface.co/organizations), such as:
- Stanford CRFM: https://huggingface.co/stanford-crfm
- Stanford NLP: https://huggingface.co/stanfordnlp
- Stanford CS329S: Machine Learning Systems Design: https://huggingface.co/stanford-cs329s
Also note that you in your organization namespace:
- you can add any number of members
- you can store both raw datasets and models, and those can be immediately accessed using `datasets` and `transformers`
Once you have created an organization, these are the steps to upload/host a raw dataset:
- The no-code procedure: https://huggingface.co/docs/datasets/upload_dataset.html
- Using the command line (terminal): https://huggingface.co/docs/datasets/share.html#add-a-community-dataset
Please, feel free to ping me if you have any further questions or need help.
| I'm interested in sharing the CaseHOLD dataset (https://arxiv.org/abs/2104.08671) as a canonical dataset on the HuggingFace Hub and would like to add the raw data files to the Hub with GitHub LFS, since it seems like a more sustainable long term storage solution, compared to other storage solutions available to my team. From what I can tell, this option is not immediately supported if one follows the sharing steps detailed here: [https://huggingface.co/docs/datasets/share_dataset.html#sharing-a-canonical-dataset](https://huggingface.co/docs/datasets/share_dataset.html#sharing-a-canonical-dataset), since GitHub LFS is not supported for public forks. Is there a way to request this? Thanks! | 222 | Add raw data files to the Hub with GitHub LFS for canonical dataset
I'm interested in sharing the CaseHOLD dataset (https://arxiv.org/abs/2104.08671) as a canonical dataset on the HuggingFace Hub and would like to add the raw data files to the Hub with GitHub LFS, since it seems like a more sustainable long term storage solution, compared to other storage solutions available to my team. From what I can tell, this option is not immediately supported if one follows the sharing steps detailed here: [https://huggingface.co/docs/datasets/share_dataset.html#sharing-a-canonical-dataset](https://huggingface.co/docs/datasets/share_dataset.html#sharing-a-canonical-dataset), since GitHub LFS is not supported for public forks. Is there a way to request this? Thanks!
Hi @zlucia,
As @julien-c pointed out, the way to store/host raw data files in our Hub is by using what we call "community" datasets:
- either at your personal namespace: `load_dataset("zlucia/casehold")`
- or at an organization namespace: for example, if you create the organization `reglab`, then `load_dataset("reglab/casehold")`
Please note that "canonical" datasets do not normally store/host their raw data at our Hub, but in a third-party server. For "canonical" datasets, we just host the "loading script", that is, a Python script that downloads the raw data from a third-party server, creates the HuggingFace dataset from it and caches it locally.
In order to create an organization namespace in our Hub, please follow this link: https://huggingface.co/organizations/new
There are already many organizations at our Hub (complete list here: https://huggingface.co/organizations), such as:
- Stanford CRFM: https://huggingface.co/stanford-crfm
- Stanford NLP: https://huggingface.co/stanfordnlp
- Stanford CS329S: Machine Learning Systems Design: https://huggingface.co/stanford-cs329s
Also note that you in your organization namespace:
- you can add any number of members
- you can store both raw datasets and models, and those can be immediately accessed using `datasets` and `transformers`
Once you have created an organization, these are the steps to upload/host a raw dataset:
- The no-code procedure: https://huggingface.co/docs/datasets/upload_dataset.html
- Using the command line (terminal): https://huggingface.co/docs/datasets/share.html#add-a-community-dataset
Please, feel free to ping me if you have any further questions or need help.
|
https://github.com/huggingface/datasets/issues/3164 | Add raw data files to the Hub with GitHub LFS for canonical dataset | Ah I see, I think I was unclear whether there were benefits to uploading a canonical dataset vs. a community provided dataset. Thanks for clarifying. I'll see if we want to create an organization namespace and otherwise, will upload the dataset under my personal namespace. | I'm interested in sharing the CaseHOLD dataset (https://arxiv.org/abs/2104.08671) as a canonical dataset on the HuggingFace Hub and would like to add the raw data files to the Hub with GitHub LFS, since it seems like a more sustainable long term storage solution, compared to other storage solutions available to my team. From what I can tell, this option is not immediately supported if one follows the sharing steps detailed here: [https://huggingface.co/docs/datasets/share_dataset.html#sharing-a-canonical-dataset](https://huggingface.co/docs/datasets/share_dataset.html#sharing-a-canonical-dataset), since GitHub LFS is not supported for public forks. Is there a way to request this? Thanks! | 45 | Add raw data files to the Hub with GitHub LFS for canonical dataset
I'm interested in sharing the CaseHOLD dataset (https://arxiv.org/abs/2104.08671) as a canonical dataset on the HuggingFace Hub and would like to add the raw data files to the Hub with GitHub LFS, since it seems like a more sustainable long term storage solution, compared to other storage solutions available to my team. From what I can tell, this option is not immediately supported if one follows the sharing steps detailed here: [https://huggingface.co/docs/datasets/share_dataset.html#sharing-a-canonical-dataset](https://huggingface.co/docs/datasets/share_dataset.html#sharing-a-canonical-dataset), since GitHub LFS is not supported for public forks. Is there a way to request this? Thanks!
Ah I see, I think I was unclear whether there were benefits to uploading a canonical dataset vs. a community provided dataset. Thanks for clarifying. I'll see if we want to create an organization namespace and otherwise, will upload the dataset under my personal namespace. |
https://github.com/huggingface/datasets/issues/3162 | `datasets-cli test` should work with datasets without scripts | > It would be really useful to be able to run `datasets-cli test`for datasets that don't have scripts attached to them (whether the datasets are private or not).
>
> I wasn't able to run the script for a private test dataset that I had created on the hub (https://huggingface.co/datasets/huggingface/DataMeasurementsTest/tree/main) -- although @lhoestq came to save the day!
why don't you try to share that info with people, so you can also save some days. | It would be really useful to be able to run `datasets-cli test`for datasets that don't have scripts attached to them (whether the datasets are private or not).
I wasn't able to run the script for a private test dataset that I had created on the hub (https://huggingface.co/datasets/huggingface/DataMeasurementsTest/tree/main) -- although @lhoestq came to save the day!
| 75 | `datasets-cli test` should work with datasets without scripts
It would be really useful to be able to run `datasets-cli test`for datasets that don't have scripts attached to them (whether the datasets are private or not).
I wasn't able to run the script for a private test dataset that I had created on the hub (https://huggingface.co/datasets/huggingface/DataMeasurementsTest/tree/main) -- although @lhoestq came to save the day!
> It would be really useful to be able to run `datasets-cli test`for datasets that don't have scripts attached to them (whether the datasets are private or not).
>
> I wasn't able to run the script for a private test dataset that I had created on the hub (https://huggingface.co/datasets/huggingface/DataMeasurementsTest/tree/main) -- although @lhoestq came to save the day!
why don't you try to share that info with people, so you can also save some days. |
https://github.com/huggingface/datasets/issues/3162 | `datasets-cli test` should work with datasets without scripts | Hi ! You can run the command if you download the repository
```
git clone https://huggingface.co/datasets/huggingface/DataMeasurementsTest
```
and run the command
```
datasets-cli test DataMeasurementsTest/DataMeasurementsTest.py
```
(though on my side it doesn't manage to download the data since the dataset is private ^^) | It would be really useful to be able to run `datasets-cli test`for datasets that don't have scripts attached to them (whether the datasets are private or not).
I wasn't able to run the script for a private test dataset that I had created on the hub (https://huggingface.co/datasets/huggingface/DataMeasurementsTest/tree/main) -- although @lhoestq came to save the day!
| 43 | `datasets-cli test` should work with datasets without scripts
It would be really useful to be able to run `datasets-cli test`for datasets that don't have scripts attached to them (whether the datasets are private or not).
I wasn't able to run the script for a private test dataset that I had created on the hub (https://huggingface.co/datasets/huggingface/DataMeasurementsTest/tree/main) -- although @lhoestq came to save the day!
Hi ! You can run the command if you download the repository
```
git clone https://huggingface.co/datasets/huggingface/DataMeasurementsTest
```
and run the command
```
datasets-cli test DataMeasurementsTest/DataMeasurementsTest.py
```
(though on my side it doesn't manage to download the data since the dataset is private ^^) |
https://github.com/huggingface/datasets/issues/3162 | `datasets-cli test` should work with datasets without scripts | > Hi ! You can run the command if you download the repository
>
> ```
> git clone https://huggingface.co/datasets/huggingface/DataMeasurementsTest
> ```
>
> and run the command
>
> ```
> datasets-cli test DataMeasurementsTest/DataMeasurementsTest.py
> ```
>
> (though on my side it doesn't manage to download the data since the dataset is private ^^)
Hi! Thanks for the info.
git cannot find the repository. Do you know if they have depreciated these tests and created a new one? | It would be really useful to be able to run `datasets-cli test`for datasets that don't have scripts attached to them (whether the datasets are private or not).
I wasn't able to run the script for a private test dataset that I had created on the hub (https://huggingface.co/datasets/huggingface/DataMeasurementsTest/tree/main) -- although @lhoestq came to save the day!
| 80 | `datasets-cli test` should work with datasets without scripts
It would be really useful to be able to run `datasets-cli test`for datasets that don't have scripts attached to them (whether the datasets are private or not).
I wasn't able to run the script for a private test dataset that I had created on the hub (https://huggingface.co/datasets/huggingface/DataMeasurementsTest/tree/main) -- although @lhoestq came to save the day!
> Hi ! You can run the command if you download the repository
>
> ```
> git clone https://huggingface.co/datasets/huggingface/DataMeasurementsTest
> ```
>
> and run the command
>
> ```
> datasets-cli test DataMeasurementsTest/DataMeasurementsTest.py
> ```
>
> (though on my side it doesn't manage to download the data since the dataset is private ^^)
Hi! Thanks for the info.
git cannot find the repository. Do you know if they have depreciated these tests and created a new one? |
https://github.com/huggingface/datasets/issues/3162 | `datasets-cli test` should work with datasets without scripts | I think it's become private, but feel free to try with any other dataset like `lhoestq/test` for example at `https://huggingface.co/datasets/lhoestq/test` | It would be really useful to be able to run `datasets-cli test`for datasets that don't have scripts attached to them (whether the datasets are private or not).
I wasn't able to run the script for a private test dataset that I had created on the hub (https://huggingface.co/datasets/huggingface/DataMeasurementsTest/tree/main) -- although @lhoestq came to save the day!
| 20 | `datasets-cli test` should work with datasets without scripts
It would be really useful to be able to run `datasets-cli test`for datasets that don't have scripts attached to them (whether the datasets are private or not).
I wasn't able to run the script for a private test dataset that I had created on the hub (https://huggingface.co/datasets/huggingface/DataMeasurementsTest/tree/main) -- although @lhoestq came to save the day!
I think it's become private, but feel free to try with any other dataset like `lhoestq/test` for example at `https://huggingface.co/datasets/lhoestq/test` |
https://github.com/huggingface/datasets/issues/3162 | `datasets-cli test` should work with datasets without scripts | > I think it's become private, but feel free to try with any other dataset like `lhoestq/test` for example at `https://huggingface.co/datasets/lhoestq/test`
your example repo and this page `https://huggingface.co/docs/datasets/add_dataset.html` helped me to solve.. thanks a lot | It would be really useful to be able to run `datasets-cli test`for datasets that don't have scripts attached to them (whether the datasets are private or not).
I wasn't able to run the script for a private test dataset that I had created on the hub (https://huggingface.co/datasets/huggingface/DataMeasurementsTest/tree/main) -- although @lhoestq came to save the day!
| 35 | `datasets-cli test` should work with datasets without scripts
It would be really useful to be able to run `datasets-cli test`for datasets that don't have scripts attached to them (whether the datasets are private or not).
I wasn't able to run the script for a private test dataset that I had created on the hub (https://huggingface.co/datasets/huggingface/DataMeasurementsTest/tree/main) -- although @lhoestq came to save the day!
> I think it's become private, but feel free to try with any other dataset like `lhoestq/test` for example at `https://huggingface.co/datasets/lhoestq/test`
your example repo and this page `https://huggingface.co/docs/datasets/add_dataset.html` helped me to solve.. thanks a lot |
https://github.com/huggingface/datasets/issues/3155 | Illegal instruction (core dumped) at datasets import | It seems to be an issue with how conda-forge is building the binaries. It works on some machines, but not a machine with AMD Opteron 8384 processors. | ## Describe the bug
I install datasets using conda and when I import datasets I get: "Illegal instruction (core dumped)"
## Steps to reproduce the bug
```
conda create --prefix path/to/env
conda activate path/to/env
conda install -c huggingface -c conda-forge datasets
# exits with output "Illegal instruction (core dumped)"
python -m datasets
```
## Environment info
When I run "datasets-cli env", I also get "Illegal instruction (core dumped)"
If I run the following commands:
```
conda create --prefix path/to/another/new/env
conda activate path/to/another/new/env
conda install -c huggingface transformers
transformers-cli env
```
Then I get:
- `transformers` version: 4.11.3
- Platform: Linux-5.4.0-67-generic-x86_64-with-glibc2.17
- Python version: 3.8.12
- PyTorch version (GPU?): not installed (NA)
- Tensorflow version (GPU?): not installed (NA)
- Flax version (CPU?/GPU?/TPU?): not installed (NA)
- Jax version: not installed
- JaxLib version: not installed
- Using GPU in script?: No
- Using distributed or parallel set-up in script?: No
Let me know what additional information you need in order to debug this issue. Thanks in advance! | 27 | Illegal instruction (core dumped) at datasets import
## Describe the bug
I install datasets using conda and when I import datasets I get: "Illegal instruction (core dumped)"
## Steps to reproduce the bug
```
conda create --prefix path/to/env
conda activate path/to/env
conda install -c huggingface -c conda-forge datasets
# exits with output "Illegal instruction (core dumped)"
python -m datasets
```
## Environment info
When I run "datasets-cli env", I also get "Illegal instruction (core dumped)"
If I run the following commands:
```
conda create --prefix path/to/another/new/env
conda activate path/to/another/new/env
conda install -c huggingface transformers
transformers-cli env
```
Then I get:
- `transformers` version: 4.11.3
- Platform: Linux-5.4.0-67-generic-x86_64-with-glibc2.17
- Python version: 3.8.12
- PyTorch version (GPU?): not installed (NA)
- Tensorflow version (GPU?): not installed (NA)
- Flax version (CPU?/GPU?/TPU?): not installed (NA)
- Jax version: not installed
- JaxLib version: not installed
- Using GPU in script?: No
- Using distributed or parallel set-up in script?: No
Let me know what additional information you need in order to debug this issue. Thanks in advance!
It seems to be an issue with how conda-forge is building the binaries. It works on some machines, but not a machine with AMD Opteron 8384 processors. |
https://github.com/huggingface/datasets/issues/3154 | Sacrebleu unexpected behaviour/requirement for data format | Hi @BramVanroy!
Good question. This project relies on PyArrow (tables) to store data too big to fit in RAM. In the case of metrics, this means that the number of predictions and references has to match to form a table.
That's why your example throws an error even though it matches the schema:
```python
refs = [
['The dog bit the man.', 'It was not unexpected.', 'The man bit him first.'],
['The dog had bit the man.', 'No one was surprised.', 'The man had bitten the dog.'],
] # len(refs) = 2
hyps = ['The dog bit the man.', "It wasn't surprising.", 'The man had just bitten him.'] # len(hyps) = 3
```
Instead, it should be:
```python
refs = [
['The dog bit the man.', 'The dog had bit the man.'],
['It was not unexpected.', 'No one was surprised.'],
['The man bit him first.', 'The man had bitten the dog.'],
] # len(refs) = 3
hyps = ['The dog bit the man.', "It wasn't surprising.", 'The man had just bitten him.'] # len(hyps) = 3
```
However, `sacreblue` works with the format that's described in your example, hence this part:
https://github.com/huggingface/datasets/blob/87c71b9c29a40958973004910f97e4892559dfed/metrics/sacrebleu/sacrebleu.py#L94-L99
Hope you get an idea! | ## Describe the bug
When comparing with the original `sacrebleu` implementation, the `datasets` implementation does some strange things that I do not quite understand. This issue was triggered when I was trying to implement TER and found the datasets implementation of BLEU [here](https://github.com/huggingface/datasets/pull/3153).
In the below snippet, the original sacrebleu snippet works just fine whereas the datasets implementation throws an error.
## Steps to reproduce the bug
```python
import sacrebleu
import datasets
refs = [
['The dog bit the man.', 'It was not unexpected.', 'The man bit him first.'],
['The dog had bit the man.', 'No one was surprised.', 'The man had bitten the dog.'],
]
hyps = ['The dog bit the man.', "It wasn't surprising.", 'The man had just bitten him.']
expected_bleu = 48.530827
ds_bleu = datasets.load_metric("sacrebleu")
bleu_score_sb = sacrebleu.corpus_bleu(hyps, refs).score
print(bleu_score_sb, expected_bleu)
# works: 48.5308...
bleu_score_ds = ds_bleu.compute(predictions=hyps, references=refs)["score"]
print(bleu_score_ds, expected_bleu)
# ValueError: Predictions and/or references don't match the expected format.
```
This seems to be related to how datasets forces the features format here:
https://github.com/huggingface/datasets/blob/87c71b9c29a40958973004910f97e4892559dfed/metrics/sacrebleu/sacrebleu.py#L94-L99
and then manipulates the references during the compute stage here
https://github.com/huggingface/datasets/blob/87c71b9c29a40958973004910f97e4892559dfed/metrics/sacrebleu/sacrebleu.py#L119-L122
I do not quite understand why that is required since sacrebleu handles argument parsing quite well [by itself](https://github.com/mjpost/sacrebleu/blob/2787185dd0f8d224c72ee5a831d163c2ac711a47/sacrebleu/metrics/base.py#L229).
## Actual results
Traceback (most recent call last):
File "C:\Users\bramv\AppData\Roaming\JetBrains\PyCharm2020.3\scratches\scratch_23.py", line 23, in <module>
bleu_score_ds = ds_bleu.compute(predictions=hyps, references=refs)["score"]
File "C:\dev\python\datasets\src\datasets\metric.py", line 392, in compute
self.add_batch(predictions=predictions, references=references)
File "C:\dev\python\datasets\src\datasets\metric.py", line 439, in add_batch
raise ValueError(
ValueError: Predictions and/or references don't match the expected format.
Expected format: {'predictions': Value(dtype='string', id='sequence'), 'references': Sequence(feature=Value(dtype='string', id='sequence'), length=-1, id='references')},
Input predictions: ['The dog bit the man.', "It wasn't surprising.", 'The man had just bitten him.'],
Input references: [['The dog bit the man.', 'It was not unexpected.', 'The man bit him first.'], ['The dog had bit the man.', 'No one was surprised.', 'The man had bitten the dog.']]
## Environment info
- `datasets` version: 1.14.1.dev0
- Platform: Windows-10-10.0.19041-SP0
- Python version: 3.9.2
- PyArrow version: 4.0.1
| 197 | Sacrebleu unexpected behaviour/requirement for data format
## Describe the bug
When comparing with the original `sacrebleu` implementation, the `datasets` implementation does some strange things that I do not quite understand. This issue was triggered when I was trying to implement TER and found the datasets implementation of BLEU [here](https://github.com/huggingface/datasets/pull/3153).
In the below snippet, the original sacrebleu snippet works just fine whereas the datasets implementation throws an error.
## Steps to reproduce the bug
```python
import sacrebleu
import datasets
refs = [
['The dog bit the man.', 'It was not unexpected.', 'The man bit him first.'],
['The dog had bit the man.', 'No one was surprised.', 'The man had bitten the dog.'],
]
hyps = ['The dog bit the man.', "It wasn't surprising.", 'The man had just bitten him.']
expected_bleu = 48.530827
ds_bleu = datasets.load_metric("sacrebleu")
bleu_score_sb = sacrebleu.corpus_bleu(hyps, refs).score
print(bleu_score_sb, expected_bleu)
# works: 48.5308...
bleu_score_ds = ds_bleu.compute(predictions=hyps, references=refs)["score"]
print(bleu_score_ds, expected_bleu)
# ValueError: Predictions and/or references don't match the expected format.
```
This seems to be related to how datasets forces the features format here:
https://github.com/huggingface/datasets/blob/87c71b9c29a40958973004910f97e4892559dfed/metrics/sacrebleu/sacrebleu.py#L94-L99
and then manipulates the references during the compute stage here
https://github.com/huggingface/datasets/blob/87c71b9c29a40958973004910f97e4892559dfed/metrics/sacrebleu/sacrebleu.py#L119-L122
I do not quite understand why that is required since sacrebleu handles argument parsing quite well [by itself](https://github.com/mjpost/sacrebleu/blob/2787185dd0f8d224c72ee5a831d163c2ac711a47/sacrebleu/metrics/base.py#L229).
## Actual results
Traceback (most recent call last):
File "C:\Users\bramv\AppData\Roaming\JetBrains\PyCharm2020.3\scratches\scratch_23.py", line 23, in <module>
bleu_score_ds = ds_bleu.compute(predictions=hyps, references=refs)["score"]
File "C:\dev\python\datasets\src\datasets\metric.py", line 392, in compute
self.add_batch(predictions=predictions, references=references)
File "C:\dev\python\datasets\src\datasets\metric.py", line 439, in add_batch
raise ValueError(
ValueError: Predictions and/or references don't match the expected format.
Expected format: {'predictions': Value(dtype='string', id='sequence'), 'references': Sequence(feature=Value(dtype='string', id='sequence'), length=-1, id='references')},
Input predictions: ['The dog bit the man.', "It wasn't surprising.", 'The man had just bitten him.'],
Input references: [['The dog bit the man.', 'It was not unexpected.', 'The man bit him first.'], ['The dog had bit the man.', 'No one was surprised.', 'The man had bitten the dog.']]
## Environment info
- `datasets` version: 1.14.1.dev0
- Platform: Windows-10-10.0.19041-SP0
- Python version: 3.9.2
- PyArrow version: 4.0.1
Hi @BramVanroy!
Good question. This project relies on PyArrow (tables) to store data too big to fit in RAM. In the case of metrics, this means that the number of predictions and references has to match to form a table.
That's why your example throws an error even though it matches the schema:
```python
refs = [
['The dog bit the man.', 'It was not unexpected.', 'The man bit him first.'],
['The dog had bit the man.', 'No one was surprised.', 'The man had bitten the dog.'],
] # len(refs) = 2
hyps = ['The dog bit the man.', "It wasn't surprising.", 'The man had just bitten him.'] # len(hyps) = 3
```
Instead, it should be:
```python
refs = [
['The dog bit the man.', 'The dog had bit the man.'],
['It was not unexpected.', 'No one was surprised.'],
['The man bit him first.', 'The man had bitten the dog.'],
] # len(refs) = 3
hyps = ['The dog bit the man.', "It wasn't surprising.", 'The man had just bitten him.'] # len(hyps) = 3
```
However, `sacreblue` works with the format that's described in your example, hence this part:
https://github.com/huggingface/datasets/blob/87c71b9c29a40958973004910f97e4892559dfed/metrics/sacrebleu/sacrebleu.py#L94-L99
Hope you get an idea! |
https://github.com/huggingface/datasets/issues/3154 | Sacrebleu unexpected behaviour/requirement for data format | Thanks, that makes sense. It is a bit unfortunate because it may be confusing to users since the input format is suddenly different than what they may expect from the underlying library/metric. But it is understandable due to how `datasets` works! | ## Describe the bug
When comparing with the original `sacrebleu` implementation, the `datasets` implementation does some strange things that I do not quite understand. This issue was triggered when I was trying to implement TER and found the datasets implementation of BLEU [here](https://github.com/huggingface/datasets/pull/3153).
In the below snippet, the original sacrebleu snippet works just fine whereas the datasets implementation throws an error.
## Steps to reproduce the bug
```python
import sacrebleu
import datasets
refs = [
['The dog bit the man.', 'It was not unexpected.', 'The man bit him first.'],
['The dog had bit the man.', 'No one was surprised.', 'The man had bitten the dog.'],
]
hyps = ['The dog bit the man.', "It wasn't surprising.", 'The man had just bitten him.']
expected_bleu = 48.530827
ds_bleu = datasets.load_metric("sacrebleu")
bleu_score_sb = sacrebleu.corpus_bleu(hyps, refs).score
print(bleu_score_sb, expected_bleu)
# works: 48.5308...
bleu_score_ds = ds_bleu.compute(predictions=hyps, references=refs)["score"]
print(bleu_score_ds, expected_bleu)
# ValueError: Predictions and/or references don't match the expected format.
```
This seems to be related to how datasets forces the features format here:
https://github.com/huggingface/datasets/blob/87c71b9c29a40958973004910f97e4892559dfed/metrics/sacrebleu/sacrebleu.py#L94-L99
and then manipulates the references during the compute stage here
https://github.com/huggingface/datasets/blob/87c71b9c29a40958973004910f97e4892559dfed/metrics/sacrebleu/sacrebleu.py#L119-L122
I do not quite understand why that is required since sacrebleu handles argument parsing quite well [by itself](https://github.com/mjpost/sacrebleu/blob/2787185dd0f8d224c72ee5a831d163c2ac711a47/sacrebleu/metrics/base.py#L229).
## Actual results
Traceback (most recent call last):
File "C:\Users\bramv\AppData\Roaming\JetBrains\PyCharm2020.3\scratches\scratch_23.py", line 23, in <module>
bleu_score_ds = ds_bleu.compute(predictions=hyps, references=refs)["score"]
File "C:\dev\python\datasets\src\datasets\metric.py", line 392, in compute
self.add_batch(predictions=predictions, references=references)
File "C:\dev\python\datasets\src\datasets\metric.py", line 439, in add_batch
raise ValueError(
ValueError: Predictions and/or references don't match the expected format.
Expected format: {'predictions': Value(dtype='string', id='sequence'), 'references': Sequence(feature=Value(dtype='string', id='sequence'), length=-1, id='references')},
Input predictions: ['The dog bit the man.', "It wasn't surprising.", 'The man had just bitten him.'],
Input references: [['The dog bit the man.', 'It was not unexpected.', 'The man bit him first.'], ['The dog had bit the man.', 'No one was surprised.', 'The man had bitten the dog.']]
## Environment info
- `datasets` version: 1.14.1.dev0
- Platform: Windows-10-10.0.19041-SP0
- Python version: 3.9.2
- PyArrow version: 4.0.1
| 41 | Sacrebleu unexpected behaviour/requirement for data format
## Describe the bug
When comparing with the original `sacrebleu` implementation, the `datasets` implementation does some strange things that I do not quite understand. This issue was triggered when I was trying to implement TER and found the datasets implementation of BLEU [here](https://github.com/huggingface/datasets/pull/3153).
In the below snippet, the original sacrebleu snippet works just fine whereas the datasets implementation throws an error.
## Steps to reproduce the bug
```python
import sacrebleu
import datasets
refs = [
['The dog bit the man.', 'It was not unexpected.', 'The man bit him first.'],
['The dog had bit the man.', 'No one was surprised.', 'The man had bitten the dog.'],
]
hyps = ['The dog bit the man.', "It wasn't surprising.", 'The man had just bitten him.']
expected_bleu = 48.530827
ds_bleu = datasets.load_metric("sacrebleu")
bleu_score_sb = sacrebleu.corpus_bleu(hyps, refs).score
print(bleu_score_sb, expected_bleu)
# works: 48.5308...
bleu_score_ds = ds_bleu.compute(predictions=hyps, references=refs)["score"]
print(bleu_score_ds, expected_bleu)
# ValueError: Predictions and/or references don't match the expected format.
```
This seems to be related to how datasets forces the features format here:
https://github.com/huggingface/datasets/blob/87c71b9c29a40958973004910f97e4892559dfed/metrics/sacrebleu/sacrebleu.py#L94-L99
and then manipulates the references during the compute stage here
https://github.com/huggingface/datasets/blob/87c71b9c29a40958973004910f97e4892559dfed/metrics/sacrebleu/sacrebleu.py#L119-L122
I do not quite understand why that is required since sacrebleu handles argument parsing quite well [by itself](https://github.com/mjpost/sacrebleu/blob/2787185dd0f8d224c72ee5a831d163c2ac711a47/sacrebleu/metrics/base.py#L229).
## Actual results
Traceback (most recent call last):
File "C:\Users\bramv\AppData\Roaming\JetBrains\PyCharm2020.3\scratches\scratch_23.py", line 23, in <module>
bleu_score_ds = ds_bleu.compute(predictions=hyps, references=refs)["score"]
File "C:\dev\python\datasets\src\datasets\metric.py", line 392, in compute
self.add_batch(predictions=predictions, references=references)
File "C:\dev\python\datasets\src\datasets\metric.py", line 439, in add_batch
raise ValueError(
ValueError: Predictions and/or references don't match the expected format.
Expected format: {'predictions': Value(dtype='string', id='sequence'), 'references': Sequence(feature=Value(dtype='string', id='sequence'), length=-1, id='references')},
Input predictions: ['The dog bit the man.', "It wasn't surprising.", 'The man had just bitten him.'],
Input references: [['The dog bit the man.', 'It was not unexpected.', 'The man bit him first.'], ['The dog had bit the man.', 'No one was surprised.', 'The man had bitten the dog.']]
## Environment info
- `datasets` version: 1.14.1.dev0
- Platform: Windows-10-10.0.19041-SP0
- Python version: 3.9.2
- PyArrow version: 4.0.1
Thanks, that makes sense. It is a bit unfortunate because it may be confusing to users since the input format is suddenly different than what they may expect from the underlying library/metric. But it is understandable due to how `datasets` works! |
https://github.com/huggingface/datasets/issues/3148 | Streaming with num_workers != 0 | I can confirm that I was able to reproduce the bug. This seems odd given that #3423 reports duplicate data retrieval when `num_workers` and `streaming` are used together, which is obviously different from what is reported here. | ## Describe the bug
When using dataset streaming with pytorch DataLoader, the setting num_workers to anything other than 0 causes the code to freeze forever before yielding the first batch.
The code owner is likely @lhoestq
## Steps to reproduce the bug
For your convenience, we've prepped a colab notebook that reproduces the bug
https://colab.research.google.com/drive/1Mgl0oTZSNIE3UeGl_oX9wPCOIxRg19h1?usp=sharing
```python
!pip install datasets==1.14.0
should_freeze_forever = True
# ^-- set this to True in order to freeze forever, set to False in order to work normally
import torch
from datasets import load_dataset
data = load_dataset("oscar", "unshuffled_deduplicated_bn", split="train", streaming=True)
data = data.map(lambda x: {"text": x["text"], "orig": f"oscar[{x['id']}]"}, batched=True)
data = data.shuffle(100, seed=1337)
data = data.with_format("torch")
loader = torch.utils.data.DataLoader(data, batch_size=2, num_workers=2 if should_freeze_forever else 0)
# v-- the code should freeze forever at this line
for i, row in enumerate(loader):
print(row)
if i > 10: break
print("DONE!")
```
## Expected results
The code should not freeze forever with num_workers=2
## Actual results
The code freezes forever with num_workers=2
## Environment info
- `datasets` version: 1.14.0 (also found in previous versions)
- Platform: google colab (also locally)
- Python version: 3.7, (also 3.8)
- PyArrow version: 3.0.0
| 37 | Streaming with num_workers != 0
## Describe the bug
When using dataset streaming with pytorch DataLoader, the setting num_workers to anything other than 0 causes the code to freeze forever before yielding the first batch.
The code owner is likely @lhoestq
## Steps to reproduce the bug
For your convenience, we've prepped a colab notebook that reproduces the bug
https://colab.research.google.com/drive/1Mgl0oTZSNIE3UeGl_oX9wPCOIxRg19h1?usp=sharing
```python
!pip install datasets==1.14.0
should_freeze_forever = True
# ^-- set this to True in order to freeze forever, set to False in order to work normally
import torch
from datasets import load_dataset
data = load_dataset("oscar", "unshuffled_deduplicated_bn", split="train", streaming=True)
data = data.map(lambda x: {"text": x["text"], "orig": f"oscar[{x['id']}]"}, batched=True)
data = data.shuffle(100, seed=1337)
data = data.with_format("torch")
loader = torch.utils.data.DataLoader(data, batch_size=2, num_workers=2 if should_freeze_forever else 0)
# v-- the code should freeze forever at this line
for i, row in enumerate(loader):
print(row)
if i > 10: break
print("DONE!")
```
## Expected results
The code should not freeze forever with num_workers=2
## Actual results
The code freezes forever with num_workers=2
## Environment info
- `datasets` version: 1.14.0 (also found in previous versions)
- Platform: google colab (also locally)
- Python version: 3.7, (also 3.8)
- PyArrow version: 3.0.0
I can confirm that I was able to reproduce the bug. This seems odd given that #3423 reports duplicate data retrieval when `num_workers` and `streaming` are used together, which is obviously different from what is reported here. |
https://github.com/huggingface/datasets/issues/3148 | Streaming with num_workers != 0 | Any update? A possible solution is to have multiple arrow files as shards, and handle them like what webdatasets does.
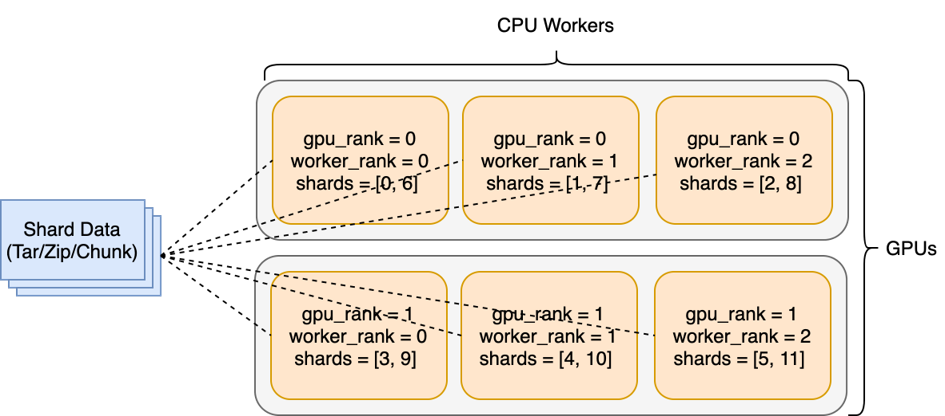
Pytorch's new dataset RFC is supporting sharding now, which may helps avoid duplicate data under streaming mode. (https://github.com/pytorch/pytorch/blob/master/torch/utils/data/datapipes/iter/grouping.py#L13)
| ## Describe the bug
When using dataset streaming with pytorch DataLoader, the setting num_workers to anything other than 0 causes the code to freeze forever before yielding the first batch.
The code owner is likely @lhoestq
## Steps to reproduce the bug
For your convenience, we've prepped a colab notebook that reproduces the bug
https://colab.research.google.com/drive/1Mgl0oTZSNIE3UeGl_oX9wPCOIxRg19h1?usp=sharing
```python
!pip install datasets==1.14.0
should_freeze_forever = True
# ^-- set this to True in order to freeze forever, set to False in order to work normally
import torch
from datasets import load_dataset
data = load_dataset("oscar", "unshuffled_deduplicated_bn", split="train", streaming=True)
data = data.map(lambda x: {"text": x["text"], "orig": f"oscar[{x['id']}]"}, batched=True)
data = data.shuffle(100, seed=1337)
data = data.with_format("torch")
loader = torch.utils.data.DataLoader(data, batch_size=2, num_workers=2 if should_freeze_forever else 0)
# v-- the code should freeze forever at this line
for i, row in enumerate(loader):
print(row)
if i > 10: break
print("DONE!")
```
## Expected results
The code should not freeze forever with num_workers=2
## Actual results
The code freezes forever with num_workers=2
## Environment info
- `datasets` version: 1.14.0 (also found in previous versions)
- Platform: google colab (also locally)
- Python version: 3.7, (also 3.8)
- PyArrow version: 3.0.0
| 39 | Streaming with num_workers != 0
## Describe the bug
When using dataset streaming with pytorch DataLoader, the setting num_workers to anything other than 0 causes the code to freeze forever before yielding the first batch.
The code owner is likely @lhoestq
## Steps to reproduce the bug
For your convenience, we've prepped a colab notebook that reproduces the bug
https://colab.research.google.com/drive/1Mgl0oTZSNIE3UeGl_oX9wPCOIxRg19h1?usp=sharing
```python
!pip install datasets==1.14.0
should_freeze_forever = True
# ^-- set this to True in order to freeze forever, set to False in order to work normally
import torch
from datasets import load_dataset
data = load_dataset("oscar", "unshuffled_deduplicated_bn", split="train", streaming=True)
data = data.map(lambda x: {"text": x["text"], "orig": f"oscar[{x['id']}]"}, batched=True)
data = data.shuffle(100, seed=1337)
data = data.with_format("torch")
loader = torch.utils.data.DataLoader(data, batch_size=2, num_workers=2 if should_freeze_forever else 0)
# v-- the code should freeze forever at this line
for i, row in enumerate(loader):
print(row)
if i > 10: break
print("DONE!")
```
## Expected results
The code should not freeze forever with num_workers=2
## Actual results
The code freezes forever with num_workers=2
## Environment info
- `datasets` version: 1.14.0 (also found in previous versions)
- Platform: google colab (also locally)
- Python version: 3.7, (also 3.8)
- PyArrow version: 3.0.0
Any update? A possible solution is to have multiple arrow files as shards, and handle them like what webdatasets does.
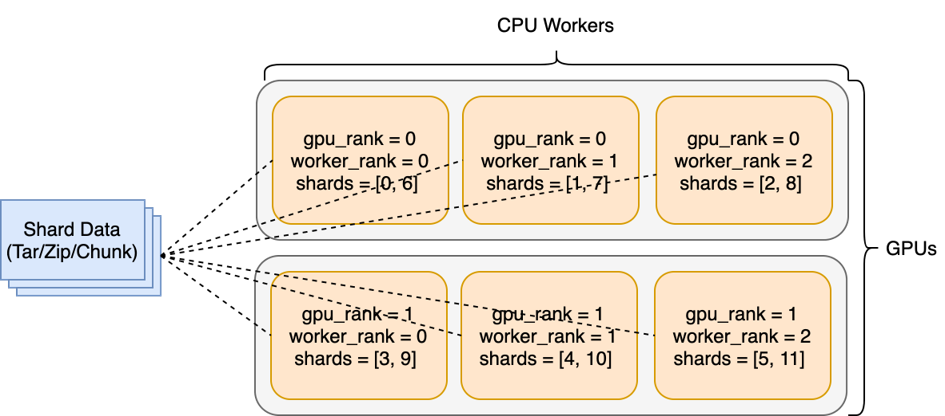
Pytorch's new dataset RFC is supporting sharding now, which may helps avoid duplicate data under streaming mode. (https://github.com/pytorch/pytorch/blob/master/torch/utils/data/datapipes/iter/grouping.py#L13)
|
https://github.com/huggingface/datasets/issues/3148 | Streaming with num_workers != 0 | Hi ! Thanks for the insights :) Note that in streaming mode there're usually no arrow files. The data are streamed from TAR, ZIP, text, etc. files directly from the web. Though for sharded datasets we can definitely adopt a similar strategy ! | ## Describe the bug
When using dataset streaming with pytorch DataLoader, the setting num_workers to anything other than 0 causes the code to freeze forever before yielding the first batch.
The code owner is likely @lhoestq
## Steps to reproduce the bug
For your convenience, we've prepped a colab notebook that reproduces the bug
https://colab.research.google.com/drive/1Mgl0oTZSNIE3UeGl_oX9wPCOIxRg19h1?usp=sharing
```python
!pip install datasets==1.14.0
should_freeze_forever = True
# ^-- set this to True in order to freeze forever, set to False in order to work normally
import torch
from datasets import load_dataset
data = load_dataset("oscar", "unshuffled_deduplicated_bn", split="train", streaming=True)
data = data.map(lambda x: {"text": x["text"], "orig": f"oscar[{x['id']}]"}, batched=True)
data = data.shuffle(100, seed=1337)
data = data.with_format("torch")
loader = torch.utils.data.DataLoader(data, batch_size=2, num_workers=2 if should_freeze_forever else 0)
# v-- the code should freeze forever at this line
for i, row in enumerate(loader):
print(row)
if i > 10: break
print("DONE!")
```
## Expected results
The code should not freeze forever with num_workers=2
## Actual results
The code freezes forever with num_workers=2
## Environment info
- `datasets` version: 1.14.0 (also found in previous versions)
- Platform: google colab (also locally)
- Python version: 3.7, (also 3.8)
- PyArrow version: 3.0.0
| 43 | Streaming with num_workers != 0
## Describe the bug
When using dataset streaming with pytorch DataLoader, the setting num_workers to anything other than 0 causes the code to freeze forever before yielding the first batch.
The code owner is likely @lhoestq
## Steps to reproduce the bug
For your convenience, we've prepped a colab notebook that reproduces the bug
https://colab.research.google.com/drive/1Mgl0oTZSNIE3UeGl_oX9wPCOIxRg19h1?usp=sharing
```python
!pip install datasets==1.14.0
should_freeze_forever = True
# ^-- set this to True in order to freeze forever, set to False in order to work normally
import torch
from datasets import load_dataset
data = load_dataset("oscar", "unshuffled_deduplicated_bn", split="train", streaming=True)
data = data.map(lambda x: {"text": x["text"], "orig": f"oscar[{x['id']}]"}, batched=True)
data = data.shuffle(100, seed=1337)
data = data.with_format("torch")
loader = torch.utils.data.DataLoader(data, batch_size=2, num_workers=2 if should_freeze_forever else 0)
# v-- the code should freeze forever at this line
for i, row in enumerate(loader):
print(row)
if i > 10: break
print("DONE!")
```
## Expected results
The code should not freeze forever with num_workers=2
## Actual results
The code freezes forever with num_workers=2
## Environment info
- `datasets` version: 1.14.0 (also found in previous versions)
- Platform: google colab (also locally)
- Python version: 3.7, (also 3.8)
- PyArrow version: 3.0.0
Hi ! Thanks for the insights :) Note that in streaming mode there're usually no arrow files. The data are streamed from TAR, ZIP, text, etc. files directly from the web. Though for sharded datasets we can definitely adopt a similar strategy ! |
https://github.com/huggingface/datasets/issues/3145 | [when Image type will exist] provide a way to get the data as binary + filename | @severo I'll keep that in mind.
You can track progress on the Image feature in #3163 (still in the early stage). | **Is your feature request related to a problem? Please describe.**
When a dataset cell contains a value of type Image (be it from a remote URL, an Array2D/3D, or any other way to represent images), I want to be able to write the image to the disk, with the correct filename, and optionally to know its mimetype, in order to serve it on the web.
Note: this issue would apply exactly the same for the `Audio` type.
**Describe the solution you'd like**
If a "cell" has the type `Image`, provide a way to get the binary content of the file, and the filename, eg as:
```python
filename: str
data: bytes
```
**Describe alternatives you've considered**
A way to write the cell to the disk (passing a local directory), and then return the pathname, filename, and mimetype.
| 21 | [when Image type will exist] provide a way to get the data as binary + filename
**Is your feature request related to a problem? Please describe.**
When a dataset cell contains a value of type Image (be it from a remote URL, an Array2D/3D, or any other way to represent images), I want to be able to write the image to the disk, with the correct filename, and optionally to know its mimetype, in order to serve it on the web.
Note: this issue would apply exactly the same for the `Audio` type.
**Describe the solution you'd like**
If a "cell" has the type `Image`, provide a way to get the binary content of the file, and the filename, eg as:
```python
filename: str
data: bytes
```
**Describe alternatives you've considered**
A way to write the cell to the disk (passing a local directory), and then return the pathname, filename, and mimetype.
@severo I'll keep that in mind.
You can track progress on the Image feature in #3163 (still in the early stage). |
https://github.com/huggingface/datasets/issues/3145 | [when Image type will exist] provide a way to get the data as binary + filename | Hi ! As discussed with @severo offline it looks like the dataset viewer already supports reading PIL images, so maybe the dataset viewer doesn't need to disable decoding after all | **Is your feature request related to a problem? Please describe.**
When a dataset cell contains a value of type Image (be it from a remote URL, an Array2D/3D, or any other way to represent images), I want to be able to write the image to the disk, with the correct filename, and optionally to know its mimetype, in order to serve it on the web.
Note: this issue would apply exactly the same for the `Audio` type.
**Describe the solution you'd like**
If a "cell" has the type `Image`, provide a way to get the binary content of the file, and the filename, eg as:
```python
filename: str
data: bytes
```
**Describe alternatives you've considered**
A way to write the cell to the disk (passing a local directory), and then return the pathname, filename, and mimetype.
| 30 | [when Image type will exist] provide a way to get the data as binary + filename
**Is your feature request related to a problem? Please describe.**
When a dataset cell contains a value of type Image (be it from a remote URL, an Array2D/3D, or any other way to represent images), I want to be able to write the image to the disk, with the correct filename, and optionally to know its mimetype, in order to serve it on the web.
Note: this issue would apply exactly the same for the `Audio` type.
**Describe the solution you'd like**
If a "cell" has the type `Image`, provide a way to get the binary content of the file, and the filename, eg as:
```python
filename: str
data: bytes
```
**Describe alternatives you've considered**
A way to write the cell to the disk (passing a local directory), and then return the pathname, filename, and mimetype.
Hi ! As discussed with @severo offline it looks like the dataset viewer already supports reading PIL images, so maybe the dataset viewer doesn't need to disable decoding after all |
https://github.com/huggingface/datasets/issues/3142 | Provide a way to write a streamed dataset to the disk | Yes, I agree this feature is much needed. We could do something similar to what TF does (https://www.tensorflow.org/api_docs/python/tf/data/Dataset#cache).
Ideally, if the entire streamed dataset is consumed/cached, the generated cache should be reusable for the Arrow dataset. | **Is your feature request related to a problem? Please describe.**
The streaming mode allows to get the 100 first rows of a dataset very quickly. But it does not cache the answer, so a posterior call to get the same 100 rows will send a request to the server again and again.
**Describe the solution you'd like**
Provide a way to write the streamed rows of a dataset on the disk, and to load from it later.
**Describe alternatives you've considered**
Provide a third mode: `lazy`, which would use the local cache for the data that have already been fetched previously, and use streaming to get the rest of the requested data.
| 36 | Provide a way to write a streamed dataset to the disk
**Is your feature request related to a problem? Please describe.**
The streaming mode allows to get the 100 first rows of a dataset very quickly. But it does not cache the answer, so a posterior call to get the same 100 rows will send a request to the server again and again.
**Describe the solution you'd like**
Provide a way to write the streamed rows of a dataset on the disk, and to load from it later.
**Describe alternatives you've considered**
Provide a third mode: `lazy`, which would use the local cache for the data that have already been fetched previously, and use streaming to get the rest of the requested data.
Yes, I agree this feature is much needed. We could do something similar to what TF does (https://www.tensorflow.org/api_docs/python/tf/data/Dataset#cache).
Ideally, if the entire streamed dataset is consumed/cached, the generated cache should be reusable for the Arrow dataset. |
https://github.com/huggingface/datasets/issues/3135 | Make inspect.get_dataset_config_names always return a non-empty list of configs | Hi @severo, I guess this issue requests not only to be able to access the configuration name (by using `inspect.get_dataset_config_names`), but the configuration itself as well (I mean you use the name to get the configuration afterwards, maybe using `builder_cls.builder_configs`), is this right? | **Is your feature request related to a problem? Please describe.**
Currently, some datasets have a configuration, while others don't. It would be simpler for the user to always have configuration names to refer to
**Describe the solution you'd like**
In that sense inspect.get_dataset_config_names should always return at least one configuration name, be it `default` or `Check___region_1` (for community datasets like `Check/region_1`).
https://github.com/huggingface/datasets/blob/c5747a5e1dde2670b7f2ca6e79e2ffd99dff85af/src/datasets/inspect.py#L161
| 43 | Make inspect.get_dataset_config_names always return a non-empty list of configs
**Is your feature request related to a problem? Please describe.**
Currently, some datasets have a configuration, while others don't. It would be simpler for the user to always have configuration names to refer to
**Describe the solution you'd like**
In that sense inspect.get_dataset_config_names should always return at least one configuration name, be it `default` or `Check___region_1` (for community datasets like `Check/region_1`).
https://github.com/huggingface/datasets/blob/c5747a5e1dde2670b7f2ca6e79e2ffd99dff85af/src/datasets/inspect.py#L161
Hi @severo, I guess this issue requests not only to be able to access the configuration name (by using `inspect.get_dataset_config_names`), but the configuration itself as well (I mean you use the name to get the configuration afterwards, maybe using `builder_cls.builder_configs`), is this right? |
https://github.com/huggingface/datasets/issues/3135 | Make inspect.get_dataset_config_names always return a non-empty list of configs | Yes, maybe the issue could be reformulated. As a user, I want to avoid having to manage special cases:
- I want to be able to get the names of a dataset's configs, and use them in the rest of the API (get the data, get the split names, etc).
- I don't want to have to manage datasets with named configs (`glue`) differently from datasets without named configs (`acronym_identification`, `Check/region_1`) | **Is your feature request related to a problem? Please describe.**
Currently, some datasets have a configuration, while others don't. It would be simpler for the user to always have configuration names to refer to
**Describe the solution you'd like**
In that sense inspect.get_dataset_config_names should always return at least one configuration name, be it `default` or `Check___region_1` (for community datasets like `Check/region_1`).
https://github.com/huggingface/datasets/blob/c5747a5e1dde2670b7f2ca6e79e2ffd99dff85af/src/datasets/inspect.py#L161
| 71 | Make inspect.get_dataset_config_names always return a non-empty list of configs
**Is your feature request related to a problem? Please describe.**
Currently, some datasets have a configuration, while others don't. It would be simpler for the user to always have configuration names to refer to
**Describe the solution you'd like**
In that sense inspect.get_dataset_config_names should always return at least one configuration name, be it `default` or `Check___region_1` (for community datasets like `Check/region_1`).
https://github.com/huggingface/datasets/blob/c5747a5e1dde2670b7f2ca6e79e2ffd99dff85af/src/datasets/inspect.py#L161
Yes, maybe the issue could be reformulated. As a user, I want to avoid having to manage special cases:
- I want to be able to get the names of a dataset's configs, and use them in the rest of the API (get the data, get the split names, etc).
- I don't want to have to manage datasets with named configs (`glue`) differently from datasets without named configs (`acronym_identification`, `Check/region_1`) |
https://github.com/huggingface/datasets/issues/3134 | Couldn't reach https://raw.githubusercontent.com/huggingface/datasets/1.11.0/metrics/rouge/rouge.py | Hi,
Did you try to run the code multiple times (GitHub URLs can be down sometimes for various reasons)? I can access `https://raw.githubusercontent.com/huggingface/datasets/1.11.0/metrics/rouge/rouge.py`, so this code is working without an error on my side.
Additionally, can you please run the `datasets-cli env` command because it seems to me that you are using the `datasets` version different from `1.12.1`? | datasets version: 1.12.1
`metric = datasets.load_metric('rouge')`
The error:
> ConnectionError Traceback (most recent call last)
> <ipython-input-3-dd10a0c5212f> in <module>
> ----> 1 metric = datasets.load_metric('rouge')
>
> /usr/local/lib/python3.6/dist-packages/datasets/load.py in load_metric(path, config_name, process_id, num_process, cache_dir, experiment_id, keep_in_memory, download_config, download_mode, script_version, **metric_init_kwargs)
> 613 download_config=download_config,
> 614 download_mode=download_mode,
> --> 615 dataset=False,
> 616 )
> 617 metric_cls = import_main_class(module_path, dataset=False)
>
> /usr/local/lib/python3.6/dist-packages/datasets/load.py in prepare_module(path, script_version, download_config, download_mode, dataset, force_local_path, dynamic_modules_path, return_resolved_file_path, **download_kwargs)
> 328 file_path = hf_github_url(path=path, name=name, dataset=dataset, version=script_version)
> 329 try:
> --> 330 local_path = cached_path(file_path, download_config=download_config)
> 331 except FileNotFoundError:
> 332 if script_version is not None:
>
> /usr/local/lib/python3.6/dist-packages/datasets/utils/file_utils.py in cached_path(url_or_filename, download_config, **download_kwargs)
> 296 use_etag=download_config.use_etag,
> 297 max_retries=download_config.max_retries,
> --> 298 use_auth_token=download_config.use_auth_token,
> 299 )
> 300 elif os.path.exists(url_or_filename):
>
> /usr/local/lib/python3.6/dist-packages/datasets/utils/file_utils.py in get_from_cache(url, cache_dir, force_download, proxies, etag_timeout, resume_download, user_agent, local_files_only, use_etag, max_retries, use_auth_token)
> 603 raise FileNotFoundError("Couldn't find file at {}".format(url))
> 604 _raise_if_offline_mode_is_enabled(f"Tried to reach {url}")
> --> 605 raise ConnectionError("Couldn't reach {}".format(url))
> 606
> 607 # Try a second time
>
> ConnectionError: Couldn't reach https://raw.githubusercontent.com/huggingface/datasets/1.11.0/metrics/rouge/rouge.py
Is there any remedy to solve the connection issue ? | 58 | Couldn't reach https://raw.githubusercontent.com/huggingface/datasets/1.11.0/metrics/rouge/rouge.py
datasets version: 1.12.1
`metric = datasets.load_metric('rouge')`
The error:
> ConnectionError Traceback (most recent call last)
> <ipython-input-3-dd10a0c5212f> in <module>
> ----> 1 metric = datasets.load_metric('rouge')
>
> /usr/local/lib/python3.6/dist-packages/datasets/load.py in load_metric(path, config_name, process_id, num_process, cache_dir, experiment_id, keep_in_memory, download_config, download_mode, script_version, **metric_init_kwargs)
> 613 download_config=download_config,
> 614 download_mode=download_mode,
> --> 615 dataset=False,
> 616 )
> 617 metric_cls = import_main_class(module_path, dataset=False)
>
> /usr/local/lib/python3.6/dist-packages/datasets/load.py in prepare_module(path, script_version, download_config, download_mode, dataset, force_local_path, dynamic_modules_path, return_resolved_file_path, **download_kwargs)
> 328 file_path = hf_github_url(path=path, name=name, dataset=dataset, version=script_version)
> 329 try:
> --> 330 local_path = cached_path(file_path, download_config=download_config)
> 331 except FileNotFoundError:
> 332 if script_version is not None:
>
> /usr/local/lib/python3.6/dist-packages/datasets/utils/file_utils.py in cached_path(url_or_filename, download_config, **download_kwargs)
> 296 use_etag=download_config.use_etag,
> 297 max_retries=download_config.max_retries,
> --> 298 use_auth_token=download_config.use_auth_token,
> 299 )
> 300 elif os.path.exists(url_or_filename):
>
> /usr/local/lib/python3.6/dist-packages/datasets/utils/file_utils.py in get_from_cache(url, cache_dir, force_download, proxies, etag_timeout, resume_download, user_agent, local_files_only, use_etag, max_retries, use_auth_token)
> 603 raise FileNotFoundError("Couldn't find file at {}".format(url))
> 604 _raise_if_offline_mode_is_enabled(f"Tried to reach {url}")
> --> 605 raise ConnectionError("Couldn't reach {}".format(url))
> 606
> 607 # Try a second time
>
> ConnectionError: Couldn't reach https://raw.githubusercontent.com/huggingface/datasets/1.11.0/metrics/rouge/rouge.py
Is there any remedy to solve the connection issue ?
Hi,
Did you try to run the code multiple times (GitHub URLs can be down sometimes for various reasons)? I can access `https://raw.githubusercontent.com/huggingface/datasets/1.11.0/metrics/rouge/rouge.py`, so this code is working without an error on my side.
Additionally, can you please run the `datasets-cli env` command because it seems to me that you are using the `datasets` version different from `1.12.1`? |
https://github.com/huggingface/datasets/issues/3134 | Couldn't reach https://raw.githubusercontent.com/huggingface/datasets/1.11.0/metrics/rouge/rouge.py | Same issue when running `metric = datasets.load_metric("accuracy")`.
Error info is:
```
metric = datasets.load_metric("accuracy")
Traceback (most recent call last):
File "<ipython-input-2-d25db38b26c5>", line 1, in <module>
metric = datasets.load_metric("accuracy")
File "D:\anaconda3\lib\site-packages\datasets\load.py", line 610, in load_metric
module_path, _ = prepare_module(
File "D:\anaconda3\lib\site-packages\datasets\load.py", line 330, in prepare_module
local_path = cached_path(file_path, download_config=download_config)
File "D:\anaconda3\lib\site-packages\datasets\utils\file_utils.py", line 288, in cached_path
output_path = get_from_cache(
File "D:\anaconda3\lib\site-packages\datasets\utils\file_utils.py", line 605, in get_from_cache
raise ConnectionError("Couldn't reach {}".format(url))
ConnectionError: Couldn't reach https://raw.githubusercontent.com/huggingface/datasets/1.11.0/metrics/accuracy/accuracy.py
```
My `datasets-cli env` result is as follows:
- `datasets` version: 1.11.0
- Platform: Windows-10-10.0.19041-SP0
- Python version: 3.8.8
- PyArrow version: 6.0.0
@yananchen1989 did you find a way to solve this? | datasets version: 1.12.1
`metric = datasets.load_metric('rouge')`
The error:
> ConnectionError Traceback (most recent call last)
> <ipython-input-3-dd10a0c5212f> in <module>
> ----> 1 metric = datasets.load_metric('rouge')
>
> /usr/local/lib/python3.6/dist-packages/datasets/load.py in load_metric(path, config_name, process_id, num_process, cache_dir, experiment_id, keep_in_memory, download_config, download_mode, script_version, **metric_init_kwargs)
> 613 download_config=download_config,
> 614 download_mode=download_mode,
> --> 615 dataset=False,
> 616 )
> 617 metric_cls = import_main_class(module_path, dataset=False)
>
> /usr/local/lib/python3.6/dist-packages/datasets/load.py in prepare_module(path, script_version, download_config, download_mode, dataset, force_local_path, dynamic_modules_path, return_resolved_file_path, **download_kwargs)
> 328 file_path = hf_github_url(path=path, name=name, dataset=dataset, version=script_version)
> 329 try:
> --> 330 local_path = cached_path(file_path, download_config=download_config)
> 331 except FileNotFoundError:
> 332 if script_version is not None:
>
> /usr/local/lib/python3.6/dist-packages/datasets/utils/file_utils.py in cached_path(url_or_filename, download_config, **download_kwargs)
> 296 use_etag=download_config.use_etag,
> 297 max_retries=download_config.max_retries,
> --> 298 use_auth_token=download_config.use_auth_token,
> 299 )
> 300 elif os.path.exists(url_or_filename):
>
> /usr/local/lib/python3.6/dist-packages/datasets/utils/file_utils.py in get_from_cache(url, cache_dir, force_download, proxies, etag_timeout, resume_download, user_agent, local_files_only, use_etag, max_retries, use_auth_token)
> 603 raise FileNotFoundError("Couldn't find file at {}".format(url))
> 604 _raise_if_offline_mode_is_enabled(f"Tried to reach {url}")
> --> 605 raise ConnectionError("Couldn't reach {}".format(url))
> 606
> 607 # Try a second time
>
> ConnectionError: Couldn't reach https://raw.githubusercontent.com/huggingface/datasets/1.11.0/metrics/rouge/rouge.py
Is there any remedy to solve the connection issue ? | 103 | Couldn't reach https://raw.githubusercontent.com/huggingface/datasets/1.11.0/metrics/rouge/rouge.py
datasets version: 1.12.1
`metric = datasets.load_metric('rouge')`
The error:
> ConnectionError Traceback (most recent call last)
> <ipython-input-3-dd10a0c5212f> in <module>
> ----> 1 metric = datasets.load_metric('rouge')
>
> /usr/local/lib/python3.6/dist-packages/datasets/load.py in load_metric(path, config_name, process_id, num_process, cache_dir, experiment_id, keep_in_memory, download_config, download_mode, script_version, **metric_init_kwargs)
> 613 download_config=download_config,
> 614 download_mode=download_mode,
> --> 615 dataset=False,
> 616 )
> 617 metric_cls = import_main_class(module_path, dataset=False)
>
> /usr/local/lib/python3.6/dist-packages/datasets/load.py in prepare_module(path, script_version, download_config, download_mode, dataset, force_local_path, dynamic_modules_path, return_resolved_file_path, **download_kwargs)
> 328 file_path = hf_github_url(path=path, name=name, dataset=dataset, version=script_version)
> 329 try:
> --> 330 local_path = cached_path(file_path, download_config=download_config)
> 331 except FileNotFoundError:
> 332 if script_version is not None:
>
> /usr/local/lib/python3.6/dist-packages/datasets/utils/file_utils.py in cached_path(url_or_filename, download_config, **download_kwargs)
> 296 use_etag=download_config.use_etag,
> 297 max_retries=download_config.max_retries,
> --> 298 use_auth_token=download_config.use_auth_token,
> 299 )
> 300 elif os.path.exists(url_or_filename):
>
> /usr/local/lib/python3.6/dist-packages/datasets/utils/file_utils.py in get_from_cache(url, cache_dir, force_download, proxies, etag_timeout, resume_download, user_agent, local_files_only, use_etag, max_retries, use_auth_token)
> 603 raise FileNotFoundError("Couldn't find file at {}".format(url))
> 604 _raise_if_offline_mode_is_enabled(f"Tried to reach {url}")
> --> 605 raise ConnectionError("Couldn't reach {}".format(url))
> 606
> 607 # Try a second time
>
> ConnectionError: Couldn't reach https://raw.githubusercontent.com/huggingface/datasets/1.11.0/metrics/rouge/rouge.py
Is there any remedy to solve the connection issue ?
Same issue when running `metric = datasets.load_metric("accuracy")`.
Error info is:
```
metric = datasets.load_metric("accuracy")
Traceback (most recent call last):
File "<ipython-input-2-d25db38b26c5>", line 1, in <module>
metric = datasets.load_metric("accuracy")
File "D:\anaconda3\lib\site-packages\datasets\load.py", line 610, in load_metric
module_path, _ = prepare_module(
File "D:\anaconda3\lib\site-packages\datasets\load.py", line 330, in prepare_module
local_path = cached_path(file_path, download_config=download_config)
File "D:\anaconda3\lib\site-packages\datasets\utils\file_utils.py", line 288, in cached_path
output_path = get_from_cache(
File "D:\anaconda3\lib\site-packages\datasets\utils\file_utils.py", line 605, in get_from_cache
raise ConnectionError("Couldn't reach {}".format(url))
ConnectionError: Couldn't reach https://raw.githubusercontent.com/huggingface/datasets/1.11.0/metrics/accuracy/accuracy.py
```
My `datasets-cli env` result is as follows:
- `datasets` version: 1.11.0
- Platform: Windows-10-10.0.19041-SP0
- Python version: 3.8.8
- PyArrow version: 6.0.0
@yananchen1989 did you find a way to solve this? |
https://github.com/huggingface/datasets/issues/3134 | Couldn't reach https://raw.githubusercontent.com/huggingface/datasets/1.11.0/metrics/rouge/rouge.py | It seems to be able to solve this issue by adding the equivalent `accuracy.py` locally.
change `metric = datasets.load_metric("accuracy")` to `metric = datasets.load_metric(path = "./accuracy.py")`.
Copy `accuracy.py` from browser at [accuracy.py](https://raw.githubusercontent.com/huggingface/datasets/1.11.0/metrics/accuracy/accuracy.py) | datasets version: 1.12.1
`metric = datasets.load_metric('rouge')`
The error:
> ConnectionError Traceback (most recent call last)
> <ipython-input-3-dd10a0c5212f> in <module>
> ----> 1 metric = datasets.load_metric('rouge')
>
> /usr/local/lib/python3.6/dist-packages/datasets/load.py in load_metric(path, config_name, process_id, num_process, cache_dir, experiment_id, keep_in_memory, download_config, download_mode, script_version, **metric_init_kwargs)
> 613 download_config=download_config,
> 614 download_mode=download_mode,
> --> 615 dataset=False,
> 616 )
> 617 metric_cls = import_main_class(module_path, dataset=False)
>
> /usr/local/lib/python3.6/dist-packages/datasets/load.py in prepare_module(path, script_version, download_config, download_mode, dataset, force_local_path, dynamic_modules_path, return_resolved_file_path, **download_kwargs)
> 328 file_path = hf_github_url(path=path, name=name, dataset=dataset, version=script_version)
> 329 try:
> --> 330 local_path = cached_path(file_path, download_config=download_config)
> 331 except FileNotFoundError:
> 332 if script_version is not None:
>
> /usr/local/lib/python3.6/dist-packages/datasets/utils/file_utils.py in cached_path(url_or_filename, download_config, **download_kwargs)
> 296 use_etag=download_config.use_etag,
> 297 max_retries=download_config.max_retries,
> --> 298 use_auth_token=download_config.use_auth_token,
> 299 )
> 300 elif os.path.exists(url_or_filename):
>
> /usr/local/lib/python3.6/dist-packages/datasets/utils/file_utils.py in get_from_cache(url, cache_dir, force_download, proxies, etag_timeout, resume_download, user_agent, local_files_only, use_etag, max_retries, use_auth_token)
> 603 raise FileNotFoundError("Couldn't find file at {}".format(url))
> 604 _raise_if_offline_mode_is_enabled(f"Tried to reach {url}")
> --> 605 raise ConnectionError("Couldn't reach {}".format(url))
> 606
> 607 # Try a second time
>
> ConnectionError: Couldn't reach https://raw.githubusercontent.com/huggingface/datasets/1.11.0/metrics/rouge/rouge.py
Is there any remedy to solve the connection issue ? | 31 | Couldn't reach https://raw.githubusercontent.com/huggingface/datasets/1.11.0/metrics/rouge/rouge.py
datasets version: 1.12.1
`metric = datasets.load_metric('rouge')`
The error:
> ConnectionError Traceback (most recent call last)
> <ipython-input-3-dd10a0c5212f> in <module>
> ----> 1 metric = datasets.load_metric('rouge')
>
> /usr/local/lib/python3.6/dist-packages/datasets/load.py in load_metric(path, config_name, process_id, num_process, cache_dir, experiment_id, keep_in_memory, download_config, download_mode, script_version, **metric_init_kwargs)
> 613 download_config=download_config,
> 614 download_mode=download_mode,
> --> 615 dataset=False,
> 616 )
> 617 metric_cls = import_main_class(module_path, dataset=False)
>
> /usr/local/lib/python3.6/dist-packages/datasets/load.py in prepare_module(path, script_version, download_config, download_mode, dataset, force_local_path, dynamic_modules_path, return_resolved_file_path, **download_kwargs)
> 328 file_path = hf_github_url(path=path, name=name, dataset=dataset, version=script_version)
> 329 try:
> --> 330 local_path = cached_path(file_path, download_config=download_config)
> 331 except FileNotFoundError:
> 332 if script_version is not None:
>
> /usr/local/lib/python3.6/dist-packages/datasets/utils/file_utils.py in cached_path(url_or_filename, download_config, **download_kwargs)
> 296 use_etag=download_config.use_etag,
> 297 max_retries=download_config.max_retries,
> --> 298 use_auth_token=download_config.use_auth_token,
> 299 )
> 300 elif os.path.exists(url_or_filename):
>
> /usr/local/lib/python3.6/dist-packages/datasets/utils/file_utils.py in get_from_cache(url, cache_dir, force_download, proxies, etag_timeout, resume_download, user_agent, local_files_only, use_etag, max_retries, use_auth_token)
> 603 raise FileNotFoundError("Couldn't find file at {}".format(url))
> 604 _raise_if_offline_mode_is_enabled(f"Tried to reach {url}")
> --> 605 raise ConnectionError("Couldn't reach {}".format(url))
> 606
> 607 # Try a second time
>
> ConnectionError: Couldn't reach https://raw.githubusercontent.com/huggingface/datasets/1.11.0/metrics/rouge/rouge.py
Is there any remedy to solve the connection issue ?
It seems to be able to solve this issue by adding the equivalent `accuracy.py` locally.
change `metric = datasets.load_metric("accuracy")` to `metric = datasets.load_metric(path = "./accuracy.py")`.
Copy `accuracy.py` from browser at [accuracy.py](https://raw.githubusercontent.com/huggingface/datasets/1.11.0/metrics/accuracy/accuracy.py) |
https://github.com/huggingface/datasets/issues/3134 | Couldn't reach https://raw.githubusercontent.com/huggingface/datasets/1.11.0/metrics/rouge/rouge.py | > It seems to be able to solve this issue by adding the equivalent `accuracy.py` locally. change `metric = datasets.load_metric("accuracy")` to `metric = datasets.load_metric(path = "./accuracy.py")`. Copy `accuracy.py` from browser at [accuracy.py](https://raw.githubusercontent.com/huggingface/datasets/1.11.0/metrics/accuracy/accuracy.py)
This is really a good way | datasets version: 1.12.1
`metric = datasets.load_metric('rouge')`
The error:
> ConnectionError Traceback (most recent call last)
> <ipython-input-3-dd10a0c5212f> in <module>
> ----> 1 metric = datasets.load_metric('rouge')
>
> /usr/local/lib/python3.6/dist-packages/datasets/load.py in load_metric(path, config_name, process_id, num_process, cache_dir, experiment_id, keep_in_memory, download_config, download_mode, script_version, **metric_init_kwargs)
> 613 download_config=download_config,
> 614 download_mode=download_mode,
> --> 615 dataset=False,
> 616 )
> 617 metric_cls = import_main_class(module_path, dataset=False)
>
> /usr/local/lib/python3.6/dist-packages/datasets/load.py in prepare_module(path, script_version, download_config, download_mode, dataset, force_local_path, dynamic_modules_path, return_resolved_file_path, **download_kwargs)
> 328 file_path = hf_github_url(path=path, name=name, dataset=dataset, version=script_version)
> 329 try:
> --> 330 local_path = cached_path(file_path, download_config=download_config)
> 331 except FileNotFoundError:
> 332 if script_version is not None:
>
> /usr/local/lib/python3.6/dist-packages/datasets/utils/file_utils.py in cached_path(url_or_filename, download_config, **download_kwargs)
> 296 use_etag=download_config.use_etag,
> 297 max_retries=download_config.max_retries,
> --> 298 use_auth_token=download_config.use_auth_token,
> 299 )
> 300 elif os.path.exists(url_or_filename):
>
> /usr/local/lib/python3.6/dist-packages/datasets/utils/file_utils.py in get_from_cache(url, cache_dir, force_download, proxies, etag_timeout, resume_download, user_agent, local_files_only, use_etag, max_retries, use_auth_token)
> 603 raise FileNotFoundError("Couldn't find file at {}".format(url))
> 604 _raise_if_offline_mode_is_enabled(f"Tried to reach {url}")
> --> 605 raise ConnectionError("Couldn't reach {}".format(url))
> 606
> 607 # Try a second time
>
> ConnectionError: Couldn't reach https://raw.githubusercontent.com/huggingface/datasets/1.11.0/metrics/rouge/rouge.py
Is there any remedy to solve the connection issue ? | 38 | Couldn't reach https://raw.githubusercontent.com/huggingface/datasets/1.11.0/metrics/rouge/rouge.py
datasets version: 1.12.1
`metric = datasets.load_metric('rouge')`
The error:
> ConnectionError Traceback (most recent call last)
> <ipython-input-3-dd10a0c5212f> in <module>
> ----> 1 metric = datasets.load_metric('rouge')
>
> /usr/local/lib/python3.6/dist-packages/datasets/load.py in load_metric(path, config_name, process_id, num_process, cache_dir, experiment_id, keep_in_memory, download_config, download_mode, script_version, **metric_init_kwargs)
> 613 download_config=download_config,
> 614 download_mode=download_mode,
> --> 615 dataset=False,
> 616 )
> 617 metric_cls = import_main_class(module_path, dataset=False)
>
> /usr/local/lib/python3.6/dist-packages/datasets/load.py in prepare_module(path, script_version, download_config, download_mode, dataset, force_local_path, dynamic_modules_path, return_resolved_file_path, **download_kwargs)
> 328 file_path = hf_github_url(path=path, name=name, dataset=dataset, version=script_version)
> 329 try:
> --> 330 local_path = cached_path(file_path, download_config=download_config)
> 331 except FileNotFoundError:
> 332 if script_version is not None:
>
> /usr/local/lib/python3.6/dist-packages/datasets/utils/file_utils.py in cached_path(url_or_filename, download_config, **download_kwargs)
> 296 use_etag=download_config.use_etag,
> 297 max_retries=download_config.max_retries,
> --> 298 use_auth_token=download_config.use_auth_token,
> 299 )
> 300 elif os.path.exists(url_or_filename):
>
> /usr/local/lib/python3.6/dist-packages/datasets/utils/file_utils.py in get_from_cache(url, cache_dir, force_download, proxies, etag_timeout, resume_download, user_agent, local_files_only, use_etag, max_retries, use_auth_token)
> 603 raise FileNotFoundError("Couldn't find file at {}".format(url))
> 604 _raise_if_offline_mode_is_enabled(f"Tried to reach {url}")
> --> 605 raise ConnectionError("Couldn't reach {}".format(url))
> 606
> 607 # Try a second time
>
> ConnectionError: Couldn't reach https://raw.githubusercontent.com/huggingface/datasets/1.11.0/metrics/rouge/rouge.py
Is there any remedy to solve the connection issue ?
> It seems to be able to solve this issue by adding the equivalent `accuracy.py` locally. change `metric = datasets.load_metric("accuracy")` to `metric = datasets.load_metric(path = "./accuracy.py")`. Copy `accuracy.py` from browser at [accuracy.py](https://raw.githubusercontent.com/huggingface/datasets/1.11.0/metrics/accuracy/accuracy.py)
This is really a good way |
https://github.com/huggingface/datasets/issues/3127 | datasets-cli: convertion of a tfds dataset to a huggingface one. | Hi,
the MNIST dataset is already available on the Hub. You can use it as follows:
```python
import datasets
dataset_dict = datasets.load_dataset("mnist")
```
As for the conversion of TFDS datasets to HF datasets, we will be working on it in the coming months, so stay tuned. | ### Discussed in https://github.com/huggingface/datasets/discussions/3079
<div type='discussions-op-text'>
<sup>Originally posted by **vitalyshalumov** October 14, 2021</sup>
I'm trying to convert a tfds dataset to a huggingface one.
I've tried:
1. datasets-cli convert --tfds_path ~/tensorflow_datasets/mnist/3.0.1/ --datasets_directory ~/.cache/huggingface/datasets/mnist/3.0.1/
2. datasets-cli convert --tfds_path ~/tensorflow_datasets/mnist/3.0.1/ --datasets_directory ~/.cache/huggingface/datasets/
and other permutations.
The script appears to be running and finishing without an error but when looking in the huggingface/datasets/ folder nothing is created.
</div> | 46 | datasets-cli: convertion of a tfds dataset to a huggingface one.
### Discussed in https://github.com/huggingface/datasets/discussions/3079
<div type='discussions-op-text'>
<sup>Originally posted by **vitalyshalumov** October 14, 2021</sup>
I'm trying to convert a tfds dataset to a huggingface one.
I've tried:
1. datasets-cli convert --tfds_path ~/tensorflow_datasets/mnist/3.0.1/ --datasets_directory ~/.cache/huggingface/datasets/mnist/3.0.1/
2. datasets-cli convert --tfds_path ~/tensorflow_datasets/mnist/3.0.1/ --datasets_directory ~/.cache/huggingface/datasets/
and other permutations.
The script appears to be running and finishing without an error but when looking in the huggingface/datasets/ folder nothing is created.
</div>
Hi,
the MNIST dataset is already available on the Hub. You can use it as follows:
```python
import datasets
dataset_dict = datasets.load_dataset("mnist")
```
As for the conversion of TFDS datasets to HF datasets, we will be working on it in the coming months, so stay tuned. |
https://github.com/huggingface/datasets/issues/3126 | "arabic_billion_words" dataset does not create the full dataset | Thanks for reporting, @vitalyshalumov.
Apparently the script to parse the data has a bug, and does not generate the entire dataset.
I'm fixing it. | ## Describe the bug
When running:
raw_dataset = load_dataset('arabic_billion_words','Alittihad')
the correct dataset file is pulled from the url.
But, the generated dataset includes just a small portion of the data included in the file.
This is true for all other portions of the "arabic_billion_words" dataset ('Almasryalyoum',.....)
## Steps to reproduce the bug
```python
# Sample code to reproduce the bug
raw_dataset = load_dataset('arabic_billion_words','Alittihad')
#The screen message
Downloading and preparing dataset arabic_billion_words/Alittihad (download: 332.13 MiB, generated: 20.62 MiB, post-processed: Unknown size, total: 352.74 MiB)
## Expected results
over 100K sentences
## Actual results
only 11K sentences
## Environment info
<!-- You can run the command `datasets-cli env` and copy-and-paste its output below. -->
- `datasets` version: 1.14.0
- Platform: Linux-5.8.0-63-generic-x86_64-with-glibc2.29
- Python version: 3.8.10
- PyArrow version: 4.0.1
| 24 | "arabic_billion_words" dataset does not create the full dataset
## Describe the bug
When running:
raw_dataset = load_dataset('arabic_billion_words','Alittihad')
the correct dataset file is pulled from the url.
But, the generated dataset includes just a small portion of the data included in the file.
This is true for all other portions of the "arabic_billion_words" dataset ('Almasryalyoum',.....)
## Steps to reproduce the bug
```python
# Sample code to reproduce the bug
raw_dataset = load_dataset('arabic_billion_words','Alittihad')
#The screen message
Downloading and preparing dataset arabic_billion_words/Alittihad (download: 332.13 MiB, generated: 20.62 MiB, post-processed: Unknown size, total: 352.74 MiB)
## Expected results
over 100K sentences
## Actual results
only 11K sentences
## Environment info
<!-- You can run the command `datasets-cli env` and copy-and-paste its output below. -->
- `datasets` version: 1.14.0
- Platform: Linux-5.8.0-63-generic-x86_64-with-glibc2.29
- Python version: 3.8.10
- PyArrow version: 4.0.1
Thanks for reporting, @vitalyshalumov.
Apparently the script to parse the data has a bug, and does not generate the entire dataset.
I'm fixing it. |
https://github.com/huggingface/datasets/issues/3123 | Segmentation fault when loading datasets from file | Hi ! I created an issue on Arrow's JIRA after making a minimum reproducible example
https://issues.apache.org/jira/browse/ARROW-14439
```python
import io
import pyarrow.json as paj
batch = b'{"a": [], "b": 1}\n{"b": 1}'
block_size = 12
paj.read_json(
io.BytesIO(batch), read_options=paj.ReadOptions(block_size=block_size)
)
```
I don't see a way to workaround this properly now without hurting the performance of the JSON loader significantly though | ## Describe the bug
Custom dataset loading sometimes segfaults and kills the process if chunks contain a variety of features/
## Steps to reproduce the bug
Download an example file:
```
wget https://gist.githubusercontent.com/TevenLeScao/11e2184394b3fa47d693de2550942c6b/raw/4232704d08fbfcaf93e5b51def9e5051507651ad/tiny_kelm.jsonl
```
Then in Python:
```
import datasets
tiny_kelm = datasets.load_dataset("json", data_files="tiny_kelm.jsonl", chunksize=100000)
```
## Expected results
a `tiny_kelm` functional dataset
## Actual results
☠️ `Segmentation fault (core dumped)` ☠️
## Environment info
- `datasets` version: 1.14.0
- Platform: Linux-5.11.0-38-generic-x86_64-with-glibc2.29
- Python version: 3.8.10
- PyArrow version: 5.0.0 | 58 | Segmentation fault when loading datasets from file
## Describe the bug
Custom dataset loading sometimes segfaults and kills the process if chunks contain a variety of features/
## Steps to reproduce the bug
Download an example file:
```
wget https://gist.githubusercontent.com/TevenLeScao/11e2184394b3fa47d693de2550942c6b/raw/4232704d08fbfcaf93e5b51def9e5051507651ad/tiny_kelm.jsonl
```
Then in Python:
```
import datasets
tiny_kelm = datasets.load_dataset("json", data_files="tiny_kelm.jsonl", chunksize=100000)
```
## Expected results
a `tiny_kelm` functional dataset
## Actual results
☠️ `Segmentation fault (core dumped)` ☠️
## Environment info
- `datasets` version: 1.14.0
- Platform: Linux-5.11.0-38-generic-x86_64-with-glibc2.29
- Python version: 3.8.10
- PyArrow version: 5.0.0
Hi ! I created an issue on Arrow's JIRA after making a minimum reproducible example
https://issues.apache.org/jira/browse/ARROW-14439
```python
import io
import pyarrow.json as paj
batch = b'{"a": [], "b": 1}\n{"b": 1}'
block_size = 12
paj.read_json(
io.BytesIO(batch), read_options=paj.ReadOptions(block_size=block_size)
)
```
I don't see a way to workaround this properly now without hurting the performance of the JSON loader significantly though |
https://github.com/huggingface/datasets/issues/3123 | Segmentation fault when loading datasets from file | The issue has been fixed in pyarrow 6.0.0, please update pyarrow :)
The issue was due to missing fields in the JSON data of type list. Now it's working fine and missing list fields are replaced with empty lists | ## Describe the bug
Custom dataset loading sometimes segfaults and kills the process if chunks contain a variety of features/
## Steps to reproduce the bug
Download an example file:
```
wget https://gist.githubusercontent.com/TevenLeScao/11e2184394b3fa47d693de2550942c6b/raw/4232704d08fbfcaf93e5b51def9e5051507651ad/tiny_kelm.jsonl
```
Then in Python:
```
import datasets
tiny_kelm = datasets.load_dataset("json", data_files="tiny_kelm.jsonl", chunksize=100000)
```
## Expected results
a `tiny_kelm` functional dataset
## Actual results
☠️ `Segmentation fault (core dumped)` ☠️
## Environment info
- `datasets` version: 1.14.0
- Platform: Linux-5.11.0-38-generic-x86_64-with-glibc2.29
- Python version: 3.8.10
- PyArrow version: 5.0.0 | 39 | Segmentation fault when loading datasets from file
## Describe the bug
Custom dataset loading sometimes segfaults and kills the process if chunks contain a variety of features/
## Steps to reproduce the bug
Download an example file:
```
wget https://gist.githubusercontent.com/TevenLeScao/11e2184394b3fa47d693de2550942c6b/raw/4232704d08fbfcaf93e5b51def9e5051507651ad/tiny_kelm.jsonl
```
Then in Python:
```
import datasets
tiny_kelm = datasets.load_dataset("json", data_files="tiny_kelm.jsonl", chunksize=100000)
```
## Expected results
a `tiny_kelm` functional dataset
## Actual results
☠️ `Segmentation fault (core dumped)` ☠️
## Environment info
- `datasets` version: 1.14.0
- Platform: Linux-5.11.0-38-generic-x86_64-with-glibc2.29
- Python version: 3.8.10
- PyArrow version: 5.0.0
The issue has been fixed in pyarrow 6.0.0, please update pyarrow :)
The issue was due to missing fields in the JSON data of type list. Now it's working fine and missing list fields are replaced with empty lists |
https://github.com/huggingface/datasets/issues/3122 | OSError with a custom dataset loading script | Hi,
there is a difference in how the `data_dir` is zipped between the `classla/janes_tag` and the `classla/reldi_hr` dataset. After unzipping, for the former, the data files (`*.conllup`) are in the root directory (root -> data files), and for the latter, they are inside the `data` directory (root -> `data` -> data files).
This can be fixed by removing the `os.path.join` call in https://huggingface.co/datasets/classla/janes_tag/blob/main/janes_tag.py#L86
Let me know if this works for you. | ## Describe the bug
I am getting an OS error when trying to load the newly uploaded dataset classla/janes_tag. What puzzles me is that I have already uploaded a very similar dataset - classla/reldi_hr - with no issues. The loading scripts for the two datasets are almost identical and they have the same directory structure, yet I am only getting an error with janes_tag.
## Steps to reproduce the bug
```python
dataset = datasets.load_dataset('classla/janes_tag', split='validation')
```
## Expected results
Dataset correctly loaded.
## Actual results
Traceback (most recent call last):
File "C:/mypath/test.py", line 91, in <module>
load_and_print('janes_tag')
File "C:/mypath/test.py", line 32, in load_and_print
dataset = datasets.load_dataset('classla/{}'.format(ds_name), split='validation')
File "C:\mypath\venv\lib\site-packages\datasets\load.py", line 1632, in load_dataset
use_auth_token=use_auth_token,
File "C:\mypath\venv\lib\site-packages\datasets\builder.py", line 608, in download_and_prepare
dl_manager=dl_manager, verify_infos=verify_infos, **download_and_prepare_kwargs
File "C:\mypath\venv\lib\site-packages\datasets\builder.py", line 704, in _download_and_prepare
) from None
OSError: Cannot find data file.
Original error:
[Errno 2] No such file or directory: 'C:\\mypath\\.cache\\huggingface\\datasets\\downloads\\2c9996e44bdc5af9c89bffb9e6d7a3e42fdb2f56bacab45de13b20f3032ea7ca\\data\\train_all.conllup'
## Environment info
<!-- You can run the command `datasets-cli env` and copy-and-paste its output below. -->
- `datasets` version: 1.14.0
- Platform: Windows-10-10.0.19041-SP0
- Python version: 3.7.5
- PyArrow version: 3.0.0
| 71 | OSError with a custom dataset loading script
## Describe the bug
I am getting an OS error when trying to load the newly uploaded dataset classla/janes_tag. What puzzles me is that I have already uploaded a very similar dataset - classla/reldi_hr - with no issues. The loading scripts for the two datasets are almost identical and they have the same directory structure, yet I am only getting an error with janes_tag.
## Steps to reproduce the bug
```python
dataset = datasets.load_dataset('classla/janes_tag', split='validation')
```
## Expected results
Dataset correctly loaded.
## Actual results
Traceback (most recent call last):
File "C:/mypath/test.py", line 91, in <module>
load_and_print('janes_tag')
File "C:/mypath/test.py", line 32, in load_and_print
dataset = datasets.load_dataset('classla/{}'.format(ds_name), split='validation')
File "C:\mypath\venv\lib\site-packages\datasets\load.py", line 1632, in load_dataset
use_auth_token=use_auth_token,
File "C:\mypath\venv\lib\site-packages\datasets\builder.py", line 608, in download_and_prepare
dl_manager=dl_manager, verify_infos=verify_infos, **download_and_prepare_kwargs
File "C:\mypath\venv\lib\site-packages\datasets\builder.py", line 704, in _download_and_prepare
) from None
OSError: Cannot find data file.
Original error:
[Errno 2] No such file or directory: 'C:\\mypath\\.cache\\huggingface\\datasets\\downloads\\2c9996e44bdc5af9c89bffb9e6d7a3e42fdb2f56bacab45de13b20f3032ea7ca\\data\\train_all.conllup'
## Environment info
<!-- You can run the command `datasets-cli env` and copy-and-paste its output below. -->
- `datasets` version: 1.14.0
- Platform: Windows-10-10.0.19041-SP0
- Python version: 3.7.5
- PyArrow version: 3.0.0
Hi,
there is a difference in how the `data_dir` is zipped between the `classla/janes_tag` and the `classla/reldi_hr` dataset. After unzipping, for the former, the data files (`*.conllup`) are in the root directory (root -> data files), and for the latter, they are inside the `data` directory (root -> `data` -> data files).
This can be fixed by removing the `os.path.join` call in https://huggingface.co/datasets/classla/janes_tag/blob/main/janes_tag.py#L86
Let me know if this works for you. |
https://github.com/huggingface/datasets/issues/3122 | OSError with a custom dataset loading script | Hi Mario,
I had already tried that before, but it didn't work. I have now recreated the `classla/janes_tag` zip file so that it also contains the `data` directory, but I am still getting the same error. | ## Describe the bug
I am getting an OS error when trying to load the newly uploaded dataset classla/janes_tag. What puzzles me is that I have already uploaded a very similar dataset - classla/reldi_hr - with no issues. The loading scripts for the two datasets are almost identical and they have the same directory structure, yet I am only getting an error with janes_tag.
## Steps to reproduce the bug
```python
dataset = datasets.load_dataset('classla/janes_tag', split='validation')
```
## Expected results
Dataset correctly loaded.
## Actual results
Traceback (most recent call last):
File "C:/mypath/test.py", line 91, in <module>
load_and_print('janes_tag')
File "C:/mypath/test.py", line 32, in load_and_print
dataset = datasets.load_dataset('classla/{}'.format(ds_name), split='validation')
File "C:\mypath\venv\lib\site-packages\datasets\load.py", line 1632, in load_dataset
use_auth_token=use_auth_token,
File "C:\mypath\venv\lib\site-packages\datasets\builder.py", line 608, in download_and_prepare
dl_manager=dl_manager, verify_infos=verify_infos, **download_and_prepare_kwargs
File "C:\mypath\venv\lib\site-packages\datasets\builder.py", line 704, in _download_and_prepare
) from None
OSError: Cannot find data file.
Original error:
[Errno 2] No such file or directory: 'C:\\mypath\\.cache\\huggingface\\datasets\\downloads\\2c9996e44bdc5af9c89bffb9e6d7a3e42fdb2f56bacab45de13b20f3032ea7ca\\data\\train_all.conllup'
## Environment info
<!-- You can run the command `datasets-cli env` and copy-and-paste its output below. -->
- `datasets` version: 1.14.0
- Platform: Windows-10-10.0.19041-SP0
- Python version: 3.7.5
- PyArrow version: 3.0.0
| 36 | OSError with a custom dataset loading script
## Describe the bug
I am getting an OS error when trying to load the newly uploaded dataset classla/janes_tag. What puzzles me is that I have already uploaded a very similar dataset - classla/reldi_hr - with no issues. The loading scripts for the two datasets are almost identical and they have the same directory structure, yet I am only getting an error with janes_tag.
## Steps to reproduce the bug
```python
dataset = datasets.load_dataset('classla/janes_tag', split='validation')
```
## Expected results
Dataset correctly loaded.
## Actual results
Traceback (most recent call last):
File "C:/mypath/test.py", line 91, in <module>
load_and_print('janes_tag')
File "C:/mypath/test.py", line 32, in load_and_print
dataset = datasets.load_dataset('classla/{}'.format(ds_name), split='validation')
File "C:\mypath\venv\lib\site-packages\datasets\load.py", line 1632, in load_dataset
use_auth_token=use_auth_token,
File "C:\mypath\venv\lib\site-packages\datasets\builder.py", line 608, in download_and_prepare
dl_manager=dl_manager, verify_infos=verify_infos, **download_and_prepare_kwargs
File "C:\mypath\venv\lib\site-packages\datasets\builder.py", line 704, in _download_and_prepare
) from None
OSError: Cannot find data file.
Original error:
[Errno 2] No such file or directory: 'C:\\mypath\\.cache\\huggingface\\datasets\\downloads\\2c9996e44bdc5af9c89bffb9e6d7a3e42fdb2f56bacab45de13b20f3032ea7ca\\data\\train_all.conllup'
## Environment info
<!-- You can run the command `datasets-cli env` and copy-and-paste its output below. -->
- `datasets` version: 1.14.0
- Platform: Windows-10-10.0.19041-SP0
- Python version: 3.7.5
- PyArrow version: 3.0.0
Hi Mario,
I had already tried that before, but it didn't work. I have now recreated the `classla/janes_tag` zip file so that it also contains the `data` directory, but I am still getting the same error. |
https://github.com/huggingface/datasets/issues/3122 | OSError with a custom dataset loading script | Hi,
I just tried to download the `classla/janes_tag` dataset, and this time the zip file is extracted correctly. However, the script is now throwing the IndexError, probably due to a bug in the `_generate_examples`.
Let me know if you are still getting the same error. | ## Describe the bug
I am getting an OS error when trying to load the newly uploaded dataset classla/janes_tag. What puzzles me is that I have already uploaded a very similar dataset - classla/reldi_hr - with no issues. The loading scripts for the two datasets are almost identical and they have the same directory structure, yet I am only getting an error with janes_tag.
## Steps to reproduce the bug
```python
dataset = datasets.load_dataset('classla/janes_tag', split='validation')
```
## Expected results
Dataset correctly loaded.
## Actual results
Traceback (most recent call last):
File "C:/mypath/test.py", line 91, in <module>
load_and_print('janes_tag')
File "C:/mypath/test.py", line 32, in load_and_print
dataset = datasets.load_dataset('classla/{}'.format(ds_name), split='validation')
File "C:\mypath\venv\lib\site-packages\datasets\load.py", line 1632, in load_dataset
use_auth_token=use_auth_token,
File "C:\mypath\venv\lib\site-packages\datasets\builder.py", line 608, in download_and_prepare
dl_manager=dl_manager, verify_infos=verify_infos, **download_and_prepare_kwargs
File "C:\mypath\venv\lib\site-packages\datasets\builder.py", line 704, in _download_and_prepare
) from None
OSError: Cannot find data file.
Original error:
[Errno 2] No such file or directory: 'C:\\mypath\\.cache\\huggingface\\datasets\\downloads\\2c9996e44bdc5af9c89bffb9e6d7a3e42fdb2f56bacab45de13b20f3032ea7ca\\data\\train_all.conllup'
## Environment info
<!-- You can run the command `datasets-cli env` and copy-and-paste its output below. -->
- `datasets` version: 1.14.0
- Platform: Windows-10-10.0.19041-SP0
- Python version: 3.7.5
- PyArrow version: 3.0.0
| 45 | OSError with a custom dataset loading script
## Describe the bug
I am getting an OS error when trying to load the newly uploaded dataset classla/janes_tag. What puzzles me is that I have already uploaded a very similar dataset - classla/reldi_hr - with no issues. The loading scripts for the two datasets are almost identical and they have the same directory structure, yet I am only getting an error with janes_tag.
## Steps to reproduce the bug
```python
dataset = datasets.load_dataset('classla/janes_tag', split='validation')
```
## Expected results
Dataset correctly loaded.
## Actual results
Traceback (most recent call last):
File "C:/mypath/test.py", line 91, in <module>
load_and_print('janes_tag')
File "C:/mypath/test.py", line 32, in load_and_print
dataset = datasets.load_dataset('classla/{}'.format(ds_name), split='validation')
File "C:\mypath\venv\lib\site-packages\datasets\load.py", line 1632, in load_dataset
use_auth_token=use_auth_token,
File "C:\mypath\venv\lib\site-packages\datasets\builder.py", line 608, in download_and_prepare
dl_manager=dl_manager, verify_infos=verify_infos, **download_and_prepare_kwargs
File "C:\mypath\venv\lib\site-packages\datasets\builder.py", line 704, in _download_and_prepare
) from None
OSError: Cannot find data file.
Original error:
[Errno 2] No such file or directory: 'C:\\mypath\\.cache\\huggingface\\datasets\\downloads\\2c9996e44bdc5af9c89bffb9e6d7a3e42fdb2f56bacab45de13b20f3032ea7ca\\data\\train_all.conllup'
## Environment info
<!-- You can run the command `datasets-cli env` and copy-and-paste its output below. -->
- `datasets` version: 1.14.0
- Platform: Windows-10-10.0.19041-SP0
- Python version: 3.7.5
- PyArrow version: 3.0.0
Hi,
I just tried to download the `classla/janes_tag` dataset, and this time the zip file is extracted correctly. However, the script is now throwing the IndexError, probably due to a bug in the `_generate_examples`.
Let me know if you are still getting the same error. |
https://github.com/huggingface/datasets/issues/3122 | OSError with a custom dataset loading script | Hi,
could you try to download the dataset with a different `cache_dir` like so:
```python
import datasets
dataset = datasets.load_dataset('classla/janes_tag', split='validation', cache_dir="path/to/different/cache/dir")
```
If this works, then most likely the cached extracted data is causing issues. This data is stored at `~/.cache/huggingface/datasets/downloads/extracted` and needs to be deleted, and then it should work (you can easily locate the directory with the path given in the `OSError` message). Additionally, I'd suggest you to update `datasets` to the newest version with:
```
pip install -U datasets
``` | ## Describe the bug
I am getting an OS error when trying to load the newly uploaded dataset classla/janes_tag. What puzzles me is that I have already uploaded a very similar dataset - classla/reldi_hr - with no issues. The loading scripts for the two datasets are almost identical and they have the same directory structure, yet I am only getting an error with janes_tag.
## Steps to reproduce the bug
```python
dataset = datasets.load_dataset('classla/janes_tag', split='validation')
```
## Expected results
Dataset correctly loaded.
## Actual results
Traceback (most recent call last):
File "C:/mypath/test.py", line 91, in <module>
load_and_print('janes_tag')
File "C:/mypath/test.py", line 32, in load_and_print
dataset = datasets.load_dataset('classla/{}'.format(ds_name), split='validation')
File "C:\mypath\venv\lib\site-packages\datasets\load.py", line 1632, in load_dataset
use_auth_token=use_auth_token,
File "C:\mypath\venv\lib\site-packages\datasets\builder.py", line 608, in download_and_prepare
dl_manager=dl_manager, verify_infos=verify_infos, **download_and_prepare_kwargs
File "C:\mypath\venv\lib\site-packages\datasets\builder.py", line 704, in _download_and_prepare
) from None
OSError: Cannot find data file.
Original error:
[Errno 2] No such file or directory: 'C:\\mypath\\.cache\\huggingface\\datasets\\downloads\\2c9996e44bdc5af9c89bffb9e6d7a3e42fdb2f56bacab45de13b20f3032ea7ca\\data\\train_all.conllup'
## Environment info
<!-- You can run the command `datasets-cli env` and copy-and-paste its output below. -->
- `datasets` version: 1.14.0
- Platform: Windows-10-10.0.19041-SP0
- Python version: 3.7.5
- PyArrow version: 3.0.0
| 84 | OSError with a custom dataset loading script
## Describe the bug
I am getting an OS error when trying to load the newly uploaded dataset classla/janes_tag. What puzzles me is that I have already uploaded a very similar dataset - classla/reldi_hr - with no issues. The loading scripts for the two datasets are almost identical and they have the same directory structure, yet I am only getting an error with janes_tag.
## Steps to reproduce the bug
```python
dataset = datasets.load_dataset('classla/janes_tag', split='validation')
```
## Expected results
Dataset correctly loaded.
## Actual results
Traceback (most recent call last):
File "C:/mypath/test.py", line 91, in <module>
load_and_print('janes_tag')
File "C:/mypath/test.py", line 32, in load_and_print
dataset = datasets.load_dataset('classla/{}'.format(ds_name), split='validation')
File "C:\mypath\venv\lib\site-packages\datasets\load.py", line 1632, in load_dataset
use_auth_token=use_auth_token,
File "C:\mypath\venv\lib\site-packages\datasets\builder.py", line 608, in download_and_prepare
dl_manager=dl_manager, verify_infos=verify_infos, **download_and_prepare_kwargs
File "C:\mypath\venv\lib\site-packages\datasets\builder.py", line 704, in _download_and_prepare
) from None
OSError: Cannot find data file.
Original error:
[Errno 2] No such file or directory: 'C:\\mypath\\.cache\\huggingface\\datasets\\downloads\\2c9996e44bdc5af9c89bffb9e6d7a3e42fdb2f56bacab45de13b20f3032ea7ca\\data\\train_all.conllup'
## Environment info
<!-- You can run the command `datasets-cli env` and copy-and-paste its output below. -->
- `datasets` version: 1.14.0
- Platform: Windows-10-10.0.19041-SP0
- Python version: 3.7.5
- PyArrow version: 3.0.0
Hi,
could you try to download the dataset with a different `cache_dir` like so:
```python
import datasets
dataset = datasets.load_dataset('classla/janes_tag', split='validation', cache_dir="path/to/different/cache/dir")
```
If this works, then most likely the cached extracted data is causing issues. This data is stored at `~/.cache/huggingface/datasets/downloads/extracted` and needs to be deleted, and then it should work (you can easily locate the directory with the path given in the `OSError` message). Additionally, I'd suggest you to update `datasets` to the newest version with:
```
pip install -U datasets
``` |
https://github.com/huggingface/datasets/issues/3122 | OSError with a custom dataset loading script | Thank you, deleting the `~/.cache/huggingface/datasets/downloads/extracted` directory helped. However, I am still having problems.
There was indeed a bug in the script that was throwing an `IndexError`, which I have now corrected (added the condition to skip the lines starting with '# text') and it is working locally, but still throws an error when I try to load the dataset from HuggingFace. I literally copied and pasted the `_generate_examples` function and ran it on the `dev_all.conllup` file, which I even re-downloaded from the repository to be certain that the files are exactly the same. I also deleted everything again just in case, but it didn't help. The code works locally, but throws an `IndexError` when loading from `datasets.` | ## Describe the bug
I am getting an OS error when trying to load the newly uploaded dataset classla/janes_tag. What puzzles me is that I have already uploaded a very similar dataset - classla/reldi_hr - with no issues. The loading scripts for the two datasets are almost identical and they have the same directory structure, yet I am only getting an error with janes_tag.
## Steps to reproduce the bug
```python
dataset = datasets.load_dataset('classla/janes_tag', split='validation')
```
## Expected results
Dataset correctly loaded.
## Actual results
Traceback (most recent call last):
File "C:/mypath/test.py", line 91, in <module>
load_and_print('janes_tag')
File "C:/mypath/test.py", line 32, in load_and_print
dataset = datasets.load_dataset('classla/{}'.format(ds_name), split='validation')
File "C:\mypath\venv\lib\site-packages\datasets\load.py", line 1632, in load_dataset
use_auth_token=use_auth_token,
File "C:\mypath\venv\lib\site-packages\datasets\builder.py", line 608, in download_and_prepare
dl_manager=dl_manager, verify_infos=verify_infos, **download_and_prepare_kwargs
File "C:\mypath\venv\lib\site-packages\datasets\builder.py", line 704, in _download_and_prepare
) from None
OSError: Cannot find data file.
Original error:
[Errno 2] No such file or directory: 'C:\\mypath\\.cache\\huggingface\\datasets\\downloads\\2c9996e44bdc5af9c89bffb9e6d7a3e42fdb2f56bacab45de13b20f3032ea7ca\\data\\train_all.conllup'
## Environment info
<!-- You can run the command `datasets-cli env` and copy-and-paste its output below. -->
- `datasets` version: 1.14.0
- Platform: Windows-10-10.0.19041-SP0
- Python version: 3.7.5
- PyArrow version: 3.0.0
| 117 | OSError with a custom dataset loading script
## Describe the bug
I am getting an OS error when trying to load the newly uploaded dataset classla/janes_tag. What puzzles me is that I have already uploaded a very similar dataset - classla/reldi_hr - with no issues. The loading scripts for the two datasets are almost identical and they have the same directory structure, yet I am only getting an error with janes_tag.
## Steps to reproduce the bug
```python
dataset = datasets.load_dataset('classla/janes_tag', split='validation')
```
## Expected results
Dataset correctly loaded.
## Actual results
Traceback (most recent call last):
File "C:/mypath/test.py", line 91, in <module>
load_and_print('janes_tag')
File "C:/mypath/test.py", line 32, in load_and_print
dataset = datasets.load_dataset('classla/{}'.format(ds_name), split='validation')
File "C:\mypath\venv\lib\site-packages\datasets\load.py", line 1632, in load_dataset
use_auth_token=use_auth_token,
File "C:\mypath\venv\lib\site-packages\datasets\builder.py", line 608, in download_and_prepare
dl_manager=dl_manager, verify_infos=verify_infos, **download_and_prepare_kwargs
File "C:\mypath\venv\lib\site-packages\datasets\builder.py", line 704, in _download_and_prepare
) from None
OSError: Cannot find data file.
Original error:
[Errno 2] No such file or directory: 'C:\\mypath\\.cache\\huggingface\\datasets\\downloads\\2c9996e44bdc5af9c89bffb9e6d7a3e42fdb2f56bacab45de13b20f3032ea7ca\\data\\train_all.conllup'
## Environment info
<!-- You can run the command `datasets-cli env` and copy-and-paste its output below. -->
- `datasets` version: 1.14.0
- Platform: Windows-10-10.0.19041-SP0
- Python version: 3.7.5
- PyArrow version: 3.0.0
Thank you, deleting the `~/.cache/huggingface/datasets/downloads/extracted` directory helped. However, I am still having problems.
There was indeed a bug in the script that was throwing an `IndexError`, which I have now corrected (added the condition to skip the lines starting with '# text') and it is working locally, but still throws an error when I try to load the dataset from HuggingFace. I literally copied and pasted the `_generate_examples` function and ran it on the `dev_all.conllup` file, which I even re-downloaded from the repository to be certain that the files are exactly the same. I also deleted everything again just in case, but it didn't help. The code works locally, but throws an `IndexError` when loading from `datasets.` |
https://github.com/huggingface/datasets/issues/3122 | OSError with a custom dataset loading script | Hi,
Did some investigation.
To fix the dataset script on the Hub, append the following labels to the `names` list of the `upos_tags` field:
```'INTJ NOUN', 'AUX PRON', 'PART ADV', 'PRON ADP', 'INTJ INTJ', 'VERB NOUN', 'NOUN AUX'```.
This step is required to avoid an error due to missing labels in the following step which is:
```python
load_dataset("classla/janes_tag", split="validation", download_mode="force_redownload")
```
This will generate and cache the dataset, so specifying `download_mode` will not be required anymore unless you update the script/data on the Hub. | ## Describe the bug
I am getting an OS error when trying to load the newly uploaded dataset classla/janes_tag. What puzzles me is that I have already uploaded a very similar dataset - classla/reldi_hr - with no issues. The loading scripts for the two datasets are almost identical and they have the same directory structure, yet I am only getting an error with janes_tag.
## Steps to reproduce the bug
```python
dataset = datasets.load_dataset('classla/janes_tag', split='validation')
```
## Expected results
Dataset correctly loaded.
## Actual results
Traceback (most recent call last):
File "C:/mypath/test.py", line 91, in <module>
load_and_print('janes_tag')
File "C:/mypath/test.py", line 32, in load_and_print
dataset = datasets.load_dataset('classla/{}'.format(ds_name), split='validation')
File "C:\mypath\venv\lib\site-packages\datasets\load.py", line 1632, in load_dataset
use_auth_token=use_auth_token,
File "C:\mypath\venv\lib\site-packages\datasets\builder.py", line 608, in download_and_prepare
dl_manager=dl_manager, verify_infos=verify_infos, **download_and_prepare_kwargs
File "C:\mypath\venv\lib\site-packages\datasets\builder.py", line 704, in _download_and_prepare
) from None
OSError: Cannot find data file.
Original error:
[Errno 2] No such file or directory: 'C:\\mypath\\.cache\\huggingface\\datasets\\downloads\\2c9996e44bdc5af9c89bffb9e6d7a3e42fdb2f56bacab45de13b20f3032ea7ca\\data\\train_all.conllup'
## Environment info
<!-- You can run the command `datasets-cli env` and copy-and-paste its output below. -->
- `datasets` version: 1.14.0
- Platform: Windows-10-10.0.19041-SP0
- Python version: 3.7.5
- PyArrow version: 3.0.0
| 84 | OSError with a custom dataset loading script
## Describe the bug
I am getting an OS error when trying to load the newly uploaded dataset classla/janes_tag. What puzzles me is that I have already uploaded a very similar dataset - classla/reldi_hr - with no issues. The loading scripts for the two datasets are almost identical and they have the same directory structure, yet I am only getting an error with janes_tag.
## Steps to reproduce the bug
```python
dataset = datasets.load_dataset('classla/janes_tag', split='validation')
```
## Expected results
Dataset correctly loaded.
## Actual results
Traceback (most recent call last):
File "C:/mypath/test.py", line 91, in <module>
load_and_print('janes_tag')
File "C:/mypath/test.py", line 32, in load_and_print
dataset = datasets.load_dataset('classla/{}'.format(ds_name), split='validation')
File "C:\mypath\venv\lib\site-packages\datasets\load.py", line 1632, in load_dataset
use_auth_token=use_auth_token,
File "C:\mypath\venv\lib\site-packages\datasets\builder.py", line 608, in download_and_prepare
dl_manager=dl_manager, verify_infos=verify_infos, **download_and_prepare_kwargs
File "C:\mypath\venv\lib\site-packages\datasets\builder.py", line 704, in _download_and_prepare
) from None
OSError: Cannot find data file.
Original error:
[Errno 2] No such file or directory: 'C:\\mypath\\.cache\\huggingface\\datasets\\downloads\\2c9996e44bdc5af9c89bffb9e6d7a3e42fdb2f56bacab45de13b20f3032ea7ca\\data\\train_all.conllup'
## Environment info
<!-- You can run the command `datasets-cli env` and copy-and-paste its output below. -->
- `datasets` version: 1.14.0
- Platform: Windows-10-10.0.19041-SP0
- Python version: 3.7.5
- PyArrow version: 3.0.0
Hi,
Did some investigation.
To fix the dataset script on the Hub, append the following labels to the `names` list of the `upos_tags` field:
```'INTJ NOUN', 'AUX PRON', 'PART ADV', 'PRON ADP', 'INTJ INTJ', 'VERB NOUN', 'NOUN AUX'```.
This step is required to avoid an error due to missing labels in the following step which is:
```python
load_dataset("classla/janes_tag", split="validation", download_mode="force_redownload")
```
This will generate and cache the dataset, so specifying `download_mode` will not be required anymore unless you update the script/data on the Hub. |
https://github.com/huggingface/datasets/issues/3119 | Add OpenSLR 83 - Crowdsourced high-quality UK and Ireland English Dialect speech | Ugh. The index files for SLR83 are CSV, not TSV. I need to add logic to process these index files. | ## Adding a Dataset
- **Name:** *openslr**
- **Description:** *Data set which contains male and female recordings of English from various dialects of the UK and Ireland.*
- **Paper:** *https://www.openslr.org/resources/83/about.html*
- **Data:** *Eleven separate data files can be found via https://www.openslr.org/resources/83/*
- **Motivation:** *Increase english ASR data with UK and Irish dialects*
Instructions to add a new dataset can be found [here](https://github.com/huggingface/datasets/blob/master/ADD_NEW_DATASET.md).
The *openslr* dataset already exists, this will add additional subset, *SLR83*. | 20 | Add OpenSLR 83 - Crowdsourced high-quality UK and Ireland English Dialect speech
## Adding a Dataset
- **Name:** *openslr**
- **Description:** *Data set which contains male and female recordings of English from various dialects of the UK and Ireland.*
- **Paper:** *https://www.openslr.org/resources/83/about.html*
- **Data:** *Eleven separate data files can be found via https://www.openslr.org/resources/83/*
- **Motivation:** *Increase english ASR data with UK and Irish dialects*
Instructions to add a new dataset can be found [here](https://github.com/huggingface/datasets/blob/master/ADD_NEW_DATASET.md).
The *openslr* dataset already exists, this will add additional subset, *SLR83*.
Ugh. The index files for SLR83 are CSV, not TSV. I need to add logic to process these index files. |
https://github.com/huggingface/datasets/issues/3114 | load_from_disk in DatasetsDict/Dataset not working with PyArrowHDFS wrapper implementing fsspec.spec.AbstractFileSystem | Hi ! Can you try again with pyarrow 6.0.0 ? I think it includes some changes regarding filesystems compatibility with fsspec. | ## Describe the bug
Passing a PyArrowHDFS implementation of fsspec.spec.AbstractFileSystem (in the `fs` param required by `load_from_disk` methods in `DatasetDict` (in datasets_dict.py) and `Dataset` (in arrow_dataset.py) results in an error when calling the download method in the `fs` parameter.
## Steps to reproduce the bug
The documentation for the `fs` parameter states:
```
fs (:class:`~filesystems.S3FileSystem` or ``fsspec.spec.AbstractFileSystem``, optional, default ``None``):
Instance of the remote filesystem used to download the files from.
```
`PyArrowHDFS` from [fsspec](https://filesystem-spec.readthedocs.io/en/latest/_modules/fsspec/implementations/hdfs.html) implements `fsspec.spec.AbstractFileSystem`. However, when using it as shown below, I get an error.
```python
from fsspec.implementations.hdfs import PyArrowHDFS
...
transformed_corpus_path = "/user/my_user/clickbait/transformed_ds/"
fs = PyArrowHDFS(host, port, user, kerb_ticket=kerb_ticket)
dss = DatasetDict.load_from_disk(transformed_corpus_path, fs, True)
```
## Expected results
Previous to load from disk, I have managed to successfully store in HDFS the data and meta-information of a DatasetDict by doing:
```python
transformed_corpus_path = "/user/my_user/clickbait/transformed_ds/"
fs = PyArrowHDFS(host, port, user, kerb_ticket=kerb_ticket)
my_datasets.save_to_disk(transformed_corpus_path, fs=fs)
```
As I have 3 datasets in the DatasetDict named `my_datasets`, the previous Python code creates the following contents in HDFS:
```sh
$ hadoop fs -ls "/user/my_user/clickbait/transformed_ds/"
Found 4 items
-rw------- 3 my_user users 43 2021-10-19 03:08 /user/my_user/clickbait/transformed_ds/dataset_dict.json
drwx------ - my_user users 0 2021-10-19 03:08 /user/my_user/clickbait/transformed_ds/test
drwx------ - my_user users 0 2021-10-19 03:08 /user/my_user/clickbait/transformed_ds/train
drwx------ - my_user users 0 2021-10-19 03:08 /user/my_user/clickbait/transformed_ds/validation
```
I would expect to recover on `dss` the Arrow-backed datasets I previously saved in HDFS calling the `save_to_disk` method on the `DatasetDict` object when invoking `DatasetDict.load_from_disk(...)` as described above.
## Actual results
However, when trying to recover the saved datasets, I get this error:
```
...
File "/home/fperez/dev/neuromancer/neuromancer/corpus.py", line 186, in load_transformed_corpus_from_disk
dss = DatasetDict.load_from_disk(transformed_corpus_path, fs, True)
File "/home/fperez/anaconda3/envs/neuromancer/lib/python3.9/site-packages/datasets/dataset_dict.py", line 748, in load_from_disk
dataset_dict[k] = Dataset.load_from_disk(dataset_dict_split_path, fs, keep_in_memory=keep_in_memory)
File "/home/fperez/anaconda3/envs/neuromancer/lib/python3.9/site-packages/datasets/arrow_dataset.py", line 1048, in load_from_disk
fs.download(src_dataset_path, dataset_path.as_posix(), recursive=True)
File "pyarrow/_hdfsio.pyx", line 438, in pyarrow._hdfsio.HadoopFileSystem.download
TypeError: download() got an unexpected keyword argument 'recursive'
```
Examining the [signature of the download method in pyarrow 5.0.0](https://github.com/apache/arrow/blob/54d2bd89c99df72fa091b025452f85dd5d88e3cf/python/pyarrow/_hdfsio.pyx#L438) we can see that there's no download parameter:
```python
def download(self, path, stream, buffer_size=None):
with self.open(path, 'rb') as f:
f.download(stream, buffer_size=buffer_size)
```
## Environment info
- `datasets` version: 1.13.3
- Platform: Linux-3.10.0-1160.15.2.el7.x86_64-x86_64-with-glibc2.33
- Python version: 3.9.7
- PyArrow version: 5.0.0
| 21 | load_from_disk in DatasetsDict/Dataset not working with PyArrowHDFS wrapper implementing fsspec.spec.AbstractFileSystem
## Describe the bug
Passing a PyArrowHDFS implementation of fsspec.spec.AbstractFileSystem (in the `fs` param required by `load_from_disk` methods in `DatasetDict` (in datasets_dict.py) and `Dataset` (in arrow_dataset.py) results in an error when calling the download method in the `fs` parameter.
## Steps to reproduce the bug
The documentation for the `fs` parameter states:
```
fs (:class:`~filesystems.S3FileSystem` or ``fsspec.spec.AbstractFileSystem``, optional, default ``None``):
Instance of the remote filesystem used to download the files from.
```
`PyArrowHDFS` from [fsspec](https://filesystem-spec.readthedocs.io/en/latest/_modules/fsspec/implementations/hdfs.html) implements `fsspec.spec.AbstractFileSystem`. However, when using it as shown below, I get an error.
```python
from fsspec.implementations.hdfs import PyArrowHDFS
...
transformed_corpus_path = "/user/my_user/clickbait/transformed_ds/"
fs = PyArrowHDFS(host, port, user, kerb_ticket=kerb_ticket)
dss = DatasetDict.load_from_disk(transformed_corpus_path, fs, True)
```
## Expected results
Previous to load from disk, I have managed to successfully store in HDFS the data and meta-information of a DatasetDict by doing:
```python
transformed_corpus_path = "/user/my_user/clickbait/transformed_ds/"
fs = PyArrowHDFS(host, port, user, kerb_ticket=kerb_ticket)
my_datasets.save_to_disk(transformed_corpus_path, fs=fs)
```
As I have 3 datasets in the DatasetDict named `my_datasets`, the previous Python code creates the following contents in HDFS:
```sh
$ hadoop fs -ls "/user/my_user/clickbait/transformed_ds/"
Found 4 items
-rw------- 3 my_user users 43 2021-10-19 03:08 /user/my_user/clickbait/transformed_ds/dataset_dict.json
drwx------ - my_user users 0 2021-10-19 03:08 /user/my_user/clickbait/transformed_ds/test
drwx------ - my_user users 0 2021-10-19 03:08 /user/my_user/clickbait/transformed_ds/train
drwx------ - my_user users 0 2021-10-19 03:08 /user/my_user/clickbait/transformed_ds/validation
```
I would expect to recover on `dss` the Arrow-backed datasets I previously saved in HDFS calling the `save_to_disk` method on the `DatasetDict` object when invoking `DatasetDict.load_from_disk(...)` as described above.
## Actual results
However, when trying to recover the saved datasets, I get this error:
```
...
File "/home/fperez/dev/neuromancer/neuromancer/corpus.py", line 186, in load_transformed_corpus_from_disk
dss = DatasetDict.load_from_disk(transformed_corpus_path, fs, True)
File "/home/fperez/anaconda3/envs/neuromancer/lib/python3.9/site-packages/datasets/dataset_dict.py", line 748, in load_from_disk
dataset_dict[k] = Dataset.load_from_disk(dataset_dict_split_path, fs, keep_in_memory=keep_in_memory)
File "/home/fperez/anaconda3/envs/neuromancer/lib/python3.9/site-packages/datasets/arrow_dataset.py", line 1048, in load_from_disk
fs.download(src_dataset_path, dataset_path.as_posix(), recursive=True)
File "pyarrow/_hdfsio.pyx", line 438, in pyarrow._hdfsio.HadoopFileSystem.download
TypeError: download() got an unexpected keyword argument 'recursive'
```
Examining the [signature of the download method in pyarrow 5.0.0](https://github.com/apache/arrow/blob/54d2bd89c99df72fa091b025452f85dd5d88e3cf/python/pyarrow/_hdfsio.pyx#L438) we can see that there's no download parameter:
```python
def download(self, path, stream, buffer_size=None):
with self.open(path, 'rb') as f:
f.download(stream, buffer_size=buffer_size)
```
## Environment info
- `datasets` version: 1.13.3
- Platform: Linux-3.10.0-1160.15.2.el7.x86_64-x86_64-with-glibc2.33
- Python version: 3.9.7
- PyArrow version: 5.0.0
Hi ! Can you try again with pyarrow 6.0.0 ? I think it includes some changes regarding filesystems compatibility with fsspec. |
https://github.com/huggingface/datasets/issues/3114 | load_from_disk in DatasetsDict/Dataset not working with PyArrowHDFS wrapper implementing fsspec.spec.AbstractFileSystem | Hi @lhoestq! I ended up using `fsspec.implementations.arrow.HadoopFileSystem` which doesn't have the problem I described with pyarrow 5.0.0.
I'll try again with `PyArrowHDFS` once I update arrow to 6.0.0.
Thanks! | ## Describe the bug
Passing a PyArrowHDFS implementation of fsspec.spec.AbstractFileSystem (in the `fs` param required by `load_from_disk` methods in `DatasetDict` (in datasets_dict.py) and `Dataset` (in arrow_dataset.py) results in an error when calling the download method in the `fs` parameter.
## Steps to reproduce the bug
The documentation for the `fs` parameter states:
```
fs (:class:`~filesystems.S3FileSystem` or ``fsspec.spec.AbstractFileSystem``, optional, default ``None``):
Instance of the remote filesystem used to download the files from.
```
`PyArrowHDFS` from [fsspec](https://filesystem-spec.readthedocs.io/en/latest/_modules/fsspec/implementations/hdfs.html) implements `fsspec.spec.AbstractFileSystem`. However, when using it as shown below, I get an error.
```python
from fsspec.implementations.hdfs import PyArrowHDFS
...
transformed_corpus_path = "/user/my_user/clickbait/transformed_ds/"
fs = PyArrowHDFS(host, port, user, kerb_ticket=kerb_ticket)
dss = DatasetDict.load_from_disk(transformed_corpus_path, fs, True)
```
## Expected results
Previous to load from disk, I have managed to successfully store in HDFS the data and meta-information of a DatasetDict by doing:
```python
transformed_corpus_path = "/user/my_user/clickbait/transformed_ds/"
fs = PyArrowHDFS(host, port, user, kerb_ticket=kerb_ticket)
my_datasets.save_to_disk(transformed_corpus_path, fs=fs)
```
As I have 3 datasets in the DatasetDict named `my_datasets`, the previous Python code creates the following contents in HDFS:
```sh
$ hadoop fs -ls "/user/my_user/clickbait/transformed_ds/"
Found 4 items
-rw------- 3 my_user users 43 2021-10-19 03:08 /user/my_user/clickbait/transformed_ds/dataset_dict.json
drwx------ - my_user users 0 2021-10-19 03:08 /user/my_user/clickbait/transformed_ds/test
drwx------ - my_user users 0 2021-10-19 03:08 /user/my_user/clickbait/transformed_ds/train
drwx------ - my_user users 0 2021-10-19 03:08 /user/my_user/clickbait/transformed_ds/validation
```
I would expect to recover on `dss` the Arrow-backed datasets I previously saved in HDFS calling the `save_to_disk` method on the `DatasetDict` object when invoking `DatasetDict.load_from_disk(...)` as described above.
## Actual results
However, when trying to recover the saved datasets, I get this error:
```
...
File "/home/fperez/dev/neuromancer/neuromancer/corpus.py", line 186, in load_transformed_corpus_from_disk
dss = DatasetDict.load_from_disk(transformed_corpus_path, fs, True)
File "/home/fperez/anaconda3/envs/neuromancer/lib/python3.9/site-packages/datasets/dataset_dict.py", line 748, in load_from_disk
dataset_dict[k] = Dataset.load_from_disk(dataset_dict_split_path, fs, keep_in_memory=keep_in_memory)
File "/home/fperez/anaconda3/envs/neuromancer/lib/python3.9/site-packages/datasets/arrow_dataset.py", line 1048, in load_from_disk
fs.download(src_dataset_path, dataset_path.as_posix(), recursive=True)
File "pyarrow/_hdfsio.pyx", line 438, in pyarrow._hdfsio.HadoopFileSystem.download
TypeError: download() got an unexpected keyword argument 'recursive'
```
Examining the [signature of the download method in pyarrow 5.0.0](https://github.com/apache/arrow/blob/54d2bd89c99df72fa091b025452f85dd5d88e3cf/python/pyarrow/_hdfsio.pyx#L438) we can see that there's no download parameter:
```python
def download(self, path, stream, buffer_size=None):
with self.open(path, 'rb') as f:
f.download(stream, buffer_size=buffer_size)
```
## Environment info
- `datasets` version: 1.13.3
- Platform: Linux-3.10.0-1160.15.2.el7.x86_64-x86_64-with-glibc2.33
- Python version: 3.9.7
- PyArrow version: 5.0.0
| 29 | load_from_disk in DatasetsDict/Dataset not working with PyArrowHDFS wrapper implementing fsspec.spec.AbstractFileSystem
## Describe the bug
Passing a PyArrowHDFS implementation of fsspec.spec.AbstractFileSystem (in the `fs` param required by `load_from_disk` methods in `DatasetDict` (in datasets_dict.py) and `Dataset` (in arrow_dataset.py) results in an error when calling the download method in the `fs` parameter.
## Steps to reproduce the bug
The documentation for the `fs` parameter states:
```
fs (:class:`~filesystems.S3FileSystem` or ``fsspec.spec.AbstractFileSystem``, optional, default ``None``):
Instance of the remote filesystem used to download the files from.
```
`PyArrowHDFS` from [fsspec](https://filesystem-spec.readthedocs.io/en/latest/_modules/fsspec/implementations/hdfs.html) implements `fsspec.spec.AbstractFileSystem`. However, when using it as shown below, I get an error.
```python
from fsspec.implementations.hdfs import PyArrowHDFS
...
transformed_corpus_path = "/user/my_user/clickbait/transformed_ds/"
fs = PyArrowHDFS(host, port, user, kerb_ticket=kerb_ticket)
dss = DatasetDict.load_from_disk(transformed_corpus_path, fs, True)
```
## Expected results
Previous to load from disk, I have managed to successfully store in HDFS the data and meta-information of a DatasetDict by doing:
```python
transformed_corpus_path = "/user/my_user/clickbait/transformed_ds/"
fs = PyArrowHDFS(host, port, user, kerb_ticket=kerb_ticket)
my_datasets.save_to_disk(transformed_corpus_path, fs=fs)
```
As I have 3 datasets in the DatasetDict named `my_datasets`, the previous Python code creates the following contents in HDFS:
```sh
$ hadoop fs -ls "/user/my_user/clickbait/transformed_ds/"
Found 4 items
-rw------- 3 my_user users 43 2021-10-19 03:08 /user/my_user/clickbait/transformed_ds/dataset_dict.json
drwx------ - my_user users 0 2021-10-19 03:08 /user/my_user/clickbait/transformed_ds/test
drwx------ - my_user users 0 2021-10-19 03:08 /user/my_user/clickbait/transformed_ds/train
drwx------ - my_user users 0 2021-10-19 03:08 /user/my_user/clickbait/transformed_ds/validation
```
I would expect to recover on `dss` the Arrow-backed datasets I previously saved in HDFS calling the `save_to_disk` method on the `DatasetDict` object when invoking `DatasetDict.load_from_disk(...)` as described above.
## Actual results
However, when trying to recover the saved datasets, I get this error:
```
...
File "/home/fperez/dev/neuromancer/neuromancer/corpus.py", line 186, in load_transformed_corpus_from_disk
dss = DatasetDict.load_from_disk(transformed_corpus_path, fs, True)
File "/home/fperez/anaconda3/envs/neuromancer/lib/python3.9/site-packages/datasets/dataset_dict.py", line 748, in load_from_disk
dataset_dict[k] = Dataset.load_from_disk(dataset_dict_split_path, fs, keep_in_memory=keep_in_memory)
File "/home/fperez/anaconda3/envs/neuromancer/lib/python3.9/site-packages/datasets/arrow_dataset.py", line 1048, in load_from_disk
fs.download(src_dataset_path, dataset_path.as_posix(), recursive=True)
File "pyarrow/_hdfsio.pyx", line 438, in pyarrow._hdfsio.HadoopFileSystem.download
TypeError: download() got an unexpected keyword argument 'recursive'
```
Examining the [signature of the download method in pyarrow 5.0.0](https://github.com/apache/arrow/blob/54d2bd89c99df72fa091b025452f85dd5d88e3cf/python/pyarrow/_hdfsio.pyx#L438) we can see that there's no download parameter:
```python
def download(self, path, stream, buffer_size=None):
with self.open(path, 'rb') as f:
f.download(stream, buffer_size=buffer_size)
```
## Environment info
- `datasets` version: 1.13.3
- Platform: Linux-3.10.0-1160.15.2.el7.x86_64-x86_64-with-glibc2.33
- Python version: 3.9.7
- PyArrow version: 5.0.0
Hi @lhoestq! I ended up using `fsspec.implementations.arrow.HadoopFileSystem` which doesn't have the problem I described with pyarrow 5.0.0.
I'll try again with `PyArrowHDFS` once I update arrow to 6.0.0.
Thanks! |
https://github.com/huggingface/datasets/issues/3113 | Loading Data from HDF files | I would also like this support or something similar. Geospatial datasets come in netcdf which is derived from hdf5, or zarr. I've gotten zarr stores to work with datasets and streaming, but it takes awhile to convert the data to zarr if it's not stored in that natively. | **Is your feature request related to a problem? Please describe.**
More often than not I come along big HDF datasets, and currently there is no straight forward way to feed them to a dataset.
**Describe the solution you'd like**
I would love to see a `from_h5` method that gets an interface implemented by the user on how items are extracted from dataset (in case of multiple datasets containing elements like arrays and metadata and etc).
**Describe alternatives you've considered**
Currently I manually load hdf files using `h5py` and implement PyTorch dataset interface. For small h5 files I load them into a pandas dataframe and use `from_pandas` function in the `datasets` package to load them, but for big datasets this is not feasible.
**Additional context**
HDF files are widespread throughout different domains and are one of the go to's for many researchers/scientists/engineers who work with numerical data. Given `datasets`' usecases have outgrown NLP use cases, it will make a lot of sense focusing on things like supporting HDF files.
| 48 | Loading Data from HDF files
**Is your feature request related to a problem? Please describe.**
More often than not I come along big HDF datasets, and currently there is no straight forward way to feed them to a dataset.
**Describe the solution you'd like**
I would love to see a `from_h5` method that gets an interface implemented by the user on how items are extracted from dataset (in case of multiple datasets containing elements like arrays and metadata and etc).
**Describe alternatives you've considered**
Currently I manually load hdf files using `h5py` and implement PyTorch dataset interface. For small h5 files I load them into a pandas dataframe and use `from_pandas` function in the `datasets` package to load them, but for big datasets this is not feasible.
**Additional context**
HDF files are widespread throughout different domains and are one of the go to's for many researchers/scientists/engineers who work with numerical data. Given `datasets`' usecases have outgrown NLP use cases, it will make a lot of sense focusing on things like supporting HDF files.
I would also like this support or something similar. Geospatial datasets come in netcdf which is derived from hdf5, or zarr. I've gotten zarr stores to work with datasets and streaming, but it takes awhile to convert the data to zarr if it's not stored in that natively. |
https://github.com/huggingface/datasets/issues/3113 | Loading Data from HDF files | @mariosasko , I would like to contribute on this "good second issue" . Is there anything in the works for this Issue or can I go ahead ?
| **Is your feature request related to a problem? Please describe.**
More often than not I come along big HDF datasets, and currently there is no straight forward way to feed them to a dataset.
**Describe the solution you'd like**
I would love to see a `from_h5` method that gets an interface implemented by the user on how items are extracted from dataset (in case of multiple datasets containing elements like arrays and metadata and etc).
**Describe alternatives you've considered**
Currently I manually load hdf files using `h5py` and implement PyTorch dataset interface. For small h5 files I load them into a pandas dataframe and use `from_pandas` function in the `datasets` package to load them, but for big datasets this is not feasible.
**Additional context**
HDF files are widespread throughout different domains and are one of the go to's for many researchers/scientists/engineers who work with numerical data. Given `datasets`' usecases have outgrown NLP use cases, it will make a lot of sense focusing on things like supporting HDF files.
| 28 | Loading Data from HDF files
**Is your feature request related to a problem? Please describe.**
More often than not I come along big HDF datasets, and currently there is no straight forward way to feed them to a dataset.
**Describe the solution you'd like**
I would love to see a `from_h5` method that gets an interface implemented by the user on how items are extracted from dataset (in case of multiple datasets containing elements like arrays and metadata and etc).
**Describe alternatives you've considered**
Currently I manually load hdf files using `h5py` and implement PyTorch dataset interface. For small h5 files I load them into a pandas dataframe and use `from_pandas` function in the `datasets` package to load them, but for big datasets this is not feasible.
**Additional context**
HDF files are widespread throughout different domains and are one of the go to's for many researchers/scientists/engineers who work with numerical data. Given `datasets`' usecases have outgrown NLP use cases, it will make a lot of sense focusing on things like supporting HDF files.
@mariosasko , I would like to contribute on this "good second issue" . Is there anything in the works for this Issue or can I go ahead ?
|
https://github.com/huggingface/datasets/issues/3113 | Loading Data from HDF files | Hi @VijayKalmath! As far as I know, nobody is working on it, so feel free to take over. Also, before you start, I suggest you comment `#self-assign` on this issue to assign it to yourself. | **Is your feature request related to a problem? Please describe.**
More often than not I come along big HDF datasets, and currently there is no straight forward way to feed them to a dataset.
**Describe the solution you'd like**
I would love to see a `from_h5` method that gets an interface implemented by the user on how items are extracted from dataset (in case of multiple datasets containing elements like arrays and metadata and etc).
**Describe alternatives you've considered**
Currently I manually load hdf files using `h5py` and implement PyTorch dataset interface. For small h5 files I load them into a pandas dataframe and use `from_pandas` function in the `datasets` package to load them, but for big datasets this is not feasible.
**Additional context**
HDF files are widespread throughout different domains and are one of the go to's for many researchers/scientists/engineers who work with numerical data. Given `datasets`' usecases have outgrown NLP use cases, it will make a lot of sense focusing on things like supporting HDF files.
| 35 | Loading Data from HDF files
**Is your feature request related to a problem? Please describe.**
More often than not I come along big HDF datasets, and currently there is no straight forward way to feed them to a dataset.
**Describe the solution you'd like**
I would love to see a `from_h5` method that gets an interface implemented by the user on how items are extracted from dataset (in case of multiple datasets containing elements like arrays and metadata and etc).
**Describe alternatives you've considered**
Currently I manually load hdf files using `h5py` and implement PyTorch dataset interface. For small h5 files I load them into a pandas dataframe and use `from_pandas` function in the `datasets` package to load them, but for big datasets this is not feasible.
**Additional context**
HDF files are widespread throughout different domains and are one of the go to's for many researchers/scientists/engineers who work with numerical data. Given `datasets`' usecases have outgrown NLP use cases, it will make a lot of sense focusing on things like supporting HDF files.
Hi @VijayKalmath! As far as I know, nobody is working on it, so feel free to take over. Also, before you start, I suggest you comment `#self-assign` on this issue to assign it to yourself. |
https://github.com/huggingface/datasets/issues/3112 | OverflowError: There was an overflow in the <class 'pyarrow.lib.ListArray'>. Try to reduce writer_batch_size to have batches smaller than 2GB | I am very unsure on why you tagged me here. I am not a maintainer of the Datasets library and have no idea how to help you. | ## Describe the bug
Despite having batches way under 2Gb when running `datasets.map()`, after processing correctly the data of the first batch without fuss and irrespective of writer_batch_size (say 2,4,8,16,32,64 and 128 in my case), it returns the following error :
> OverflowError: There was an overflow in the <class 'pyarrow.lib.ListArray'>. Try to reduce writer_batch_size to have batches smaller than 2GB
Note that I always run `batch_size=writer_batch_size` :
## Steps to reproduce the bug
```python
datasets.map(lambda example : {"column_name" : function(arguments)}, batched=False, remove_columns = datasets.column_names, batch_size=batch_size, writer_batch_size=batch_size, disable_nullable=True, num_proc=None, desc="blablabla")
```
## Introspecting CUDA memory during bug
Placed within `function(arguments)` the following statement to introspect memory usage, merely a little over 1/4 of 2Gb
`print(torch.cuda.memory_summary(device=device, abbreviated=False))`
> |===========================================================================|
| PyTorch CUDA memory summary, device ID 0 |
|---------------------------------------------------------------------------|
| CUDA OOMs: 0 | cudaMalloc retries: 0 |
|===========================================================================|
| Metric | Cur Usage | Peak Usage | Tot Alloc | Tot Freed |
|---------------------------------------------------------------------------|
| Allocated memory | 541418 KB | 545725 KB | 555695 KB | 14276 KB |
| from large pool | 540672 KB | 544431 KB | 544431 KB | 3759 KB |
| from small pool | 746 KB | 1714 KB | 11264 KB | 10517 KB |
|---------------------------------------------------------------------------|
| Active memory | 541418 KB | 545725 KB | 555695 KB | 14276 KB |
| from large pool | 540672 KB | 544431 KB | 544431 KB | 3759 KB |
| from small pool | 746 KB | 1714 KB | 11264 KB | 10517 KB |
|---------------------------------------------------------------------------|
| GPU reserved memory | 598016 KB | 598016 KB | 598016 KB | 0 B |
| from large pool | 595968 KB | 595968 KB | 595968 KB | 0 B |
| from small pool | 2048 KB | 2048 KB | 2048 KB | 0 B |
|---------------------------------------------------------------------------|
| Non-releasable memory | 36117 KB | 52292 KB | 274275 KB | 238158 KB |
| from large pool | 34816 KB | 51537 KB | 261713 KB | 226897 KB |
| from small pool | 1301 KB | 2045 KB | 12562 KB | 11261 KB |
|---------------------------------------------------------------------------|
| Allocations | 198 | 224 | 478 | 280 |
| from large pool | 74 | 75 | 75 | 1 |
| from small pool | 124 | 150 | 403 | 279 |
|---------------------------------------------------------------------------|
| Active allocs | 198 | 224 | 478 | 280 |
| from large pool | 74 | 75 | 75 | 1 |
| from small pool | 124 | 150 | 403 | 279 |
|---------------------------------------------------------------------------|
| GPU reserved segments | 21 | 21 | 21 | 0 |
| from large pool | 20 | 20 | 20 | 0 |
| from small pool | 1 | 1 | 1 | 0 |
|---------------------------------------------------------------------------|
| Non-releasable allocs | 18 | 23 | 166 | 148 |
| from large pool | 17 | 18 | 19 | 2 |
| from small pool | 1 | 6 | 147 | 146 |
|===========================================================================|
## Expected results
Efficiently process the datasets and write it down to disk.
## Actual results
--------------------------------------------------------------------------
OverflowError Traceback (most recent call last)
~\anaconda3\envs\xxx\lib\site-packages\datasets\arrow_dataset.py in _map_single(self, function, with_indices, input_columns, batched, batch_size, drop_last_batch, remove_columns, keep_in_memory, load_from_cache_file, cache_file_name, writer_batch_size, features, disable_nullable, fn_kwargs, new_fingerprint, rank, offset, disable_tqdm, desc, cache_only)
2390 else:
-> 2391 writer.write(example)
2392 else:
~\anaconda3\envs\xxx\lib\site-packages\datasets\arrow_writer.py in write(self, example, key, writer_batch_size)
367
--> 368 self.write_examples_on_file()
369
~\anaconda3\envs\xxx\lib\site-packages\datasets\arrow_writer.py in write_examples_on_file(self)
316 if not isinstance(pa_array[0], pa.lib.FloatScalar):
--> 317 raise OverflowError(
318 "There was an overflow in the {}. Try to reduce writer_batch_size to have batches smaller than 2GB".format(
OverflowError: There was an overflow in the <class 'pyarrow.lib.ListArray'>. Try to reduce writer_batch_size to have batches smaller than 2GB
During handling of the above exception, another exception occurred:
OverflowError Traceback (most recent call last)
~\AppData\Local\Temp/ipykernel_16268/2456940807.py in <module>
3 #tracker = OfflineEmissionsTracker(country_iso_code="FRA", project_name='xxx'+time_stamp,output_dir='./codecarbon')
4 #tracker.start()
----> 5 process_datasets(source_datasets_paths, dataset_dir, LM_tokenizer, LMhead_model, datasets_selection=['wikipedia'], from_scratch=True,
6 clean_sentences=False, negative_sampling=False, translate=False, tokenize=False, generate_embeddings=True, concatenate_embeddings=False,
7 max_sample=10000, padding='do_not_pad', truncation=True, cpu_batch_size=1000, gpu_batch_size=2, cpu_writer_batch_size=1000, gpu_writer_batch_size=2, disable_nullable=True, num_proc=None) #
~\xxx\xxx.py in process_datasets(source_datasets_paths, dataset_dir, LM_tokenizer, LMhead_model, datasets_selection, from_scratch, clean_sentences, translate, negative_sampling, tokenize, generate_embeddings, concatenate_embeddings, max_sample, padding, truncation, cpu_batch_size, gpu_batch_size, cpu_writer_batch_size, gpu_writer_batch_size, disable_nullable, num_proc)
481 for column in tqdm(dataset.column_names, desc=f'Processing column', leave=False):
482 if "xxx_" in column:
--> 483 dataset = dataset.map(lambda example :
484 {"embeddings_"+str(column).replace("translated_",""):function(input_ids=example[column],
485 token_type_ids=example[column.replace("input_ids","token_type_ids")],
~\anaconda3\envs\xxx\lib\site-packages\datasets\arrow_dataset.py in map(self, function, with_indices, input_columns, batched, batch_size, drop_last_batch, remove_columns, keep_in_memory, load_from_cache_file, cache_file_name, writer_batch_size, features, disable_nullable, fn_kwargs, num_proc, suffix_template, new_fingerprint, desc)
2034
2035 if num_proc is None or num_proc == 1:
-> 2036 return self._map_single(
2037 function=function,
2038 with_indices=with_indices,
~\anaconda3\envs\xxx\lib\site-packages\datasets\arrow_dataset.py in wrapper(*args, **kwargs)
501 self: "Dataset" = kwargs.pop("self")
502 # apply actual function
--> 503 out: Union["Dataset", "DatasetDict"] = func(self, *args, **kwargs)
504 datasets: List["Dataset"] = list(out.values()) if isinstance(out, dict) else [out]
505 for dataset in datasets:
~\anaconda3\envs\xxx\lib\site-packages\datasets\arrow_dataset.py in wrapper(*args, **kwargs)
468 }
469 # apply actual function
--> 470 out: Union["Dataset", "DatasetDict"] = func(self, *args, **kwargs)
471 datasets: List["Dataset"] = list(out.values()) if isinstance(out, dict) else [out]
472 # re-apply format to the output
~\anaconda3\envs\xxx\lib\site-packages\datasets\fingerprint.py in wrapper(*args, **kwargs)
404 # Call actual function
405
--> 406 out = func(self, *args, **kwargs)
407
408 # Update fingerprint of in-place transforms + update in-place history of transforms
~\anaconda3\envs\xxx\lib\site-packages\datasets\arrow_dataset.py in _map_single(self, function, with_indices, input_columns, batched, batch_size, drop_last_batch, remove_columns, keep_in_memory, load_from_cache_file, cache_file_name, writer_batch_size, features, disable_nullable, fn_kwargs, new_fingerprint, rank, offset, disable_tqdm, desc, cache_only)
2425 if update_data:
2426 if writer is not None:
-> 2427 writer.finalize()
2428 if tmp_file is not None:
2429 tmp_file.close()
~\anaconda3\envs\xxx\lib\site-packages\datasets\arrow_writer.py in finalize(self, close_stream)
440 # Re-intializing to empty list for next batch
441 self.hkey_record = []
--> 442 self.write_examples_on_file()
443 if self.pa_writer is None:
444 if self._schema is not None:
~\anaconda3\envs\xxx\lib\site-packages\datasets\arrow_writer.py in write_examples_on_file(self)
315 # This check fails with FloatArrays with nans, which is not what we want, so account for that:
316 if not isinstance(pa_array[0], pa.lib.FloatScalar):
--> 317 raise OverflowError(
318 "There was an overflow in the {}. Try to reduce writer_batch_size to have batches smaller than 2GB".format(
319 type(pa_array)
OverflowError: There was an overflow in the <class 'pyarrow.lib.ListArray'>. Try to reduce writer_batch_size to have batches smaller than 2GB
## Environment info
<!-- You can run the command `datasets-cli env` and copy-and-paste its output below. -->
- `datasets` version: 1.13.3
- Platform: Windows-10-10.0.19042-SP0
- Python version: 3.8.11
- PyArrow version: 3.0.0
##Next steps
Testing on Linux.
@albertvillanova
| 27 | OverflowError: There was an overflow in the <class 'pyarrow.lib.ListArray'>. Try to reduce writer_batch_size to have batches smaller than 2GB
## Describe the bug
Despite having batches way under 2Gb when running `datasets.map()`, after processing correctly the data of the first batch without fuss and irrespective of writer_batch_size (say 2,4,8,16,32,64 and 128 in my case), it returns the following error :
> OverflowError: There was an overflow in the <class 'pyarrow.lib.ListArray'>. Try to reduce writer_batch_size to have batches smaller than 2GB
Note that I always run `batch_size=writer_batch_size` :
## Steps to reproduce the bug
```python
datasets.map(lambda example : {"column_name" : function(arguments)}, batched=False, remove_columns = datasets.column_names, batch_size=batch_size, writer_batch_size=batch_size, disable_nullable=True, num_proc=None, desc="blablabla")
```
## Introspecting CUDA memory during bug
Placed within `function(arguments)` the following statement to introspect memory usage, merely a little over 1/4 of 2Gb
`print(torch.cuda.memory_summary(device=device, abbreviated=False))`
> |===========================================================================|
| PyTorch CUDA memory summary, device ID 0 |
|---------------------------------------------------------------------------|
| CUDA OOMs: 0 | cudaMalloc retries: 0 |
|===========================================================================|
| Metric | Cur Usage | Peak Usage | Tot Alloc | Tot Freed |
|---------------------------------------------------------------------------|
| Allocated memory | 541418 KB | 545725 KB | 555695 KB | 14276 KB |
| from large pool | 540672 KB | 544431 KB | 544431 KB | 3759 KB |
| from small pool | 746 KB | 1714 KB | 11264 KB | 10517 KB |
|---------------------------------------------------------------------------|
| Active memory | 541418 KB | 545725 KB | 555695 KB | 14276 KB |
| from large pool | 540672 KB | 544431 KB | 544431 KB | 3759 KB |
| from small pool | 746 KB | 1714 KB | 11264 KB | 10517 KB |
|---------------------------------------------------------------------------|
| GPU reserved memory | 598016 KB | 598016 KB | 598016 KB | 0 B |
| from large pool | 595968 KB | 595968 KB | 595968 KB | 0 B |
| from small pool | 2048 KB | 2048 KB | 2048 KB | 0 B |
|---------------------------------------------------------------------------|
| Non-releasable memory | 36117 KB | 52292 KB | 274275 KB | 238158 KB |
| from large pool | 34816 KB | 51537 KB | 261713 KB | 226897 KB |
| from small pool | 1301 KB | 2045 KB | 12562 KB | 11261 KB |
|---------------------------------------------------------------------------|
| Allocations | 198 | 224 | 478 | 280 |
| from large pool | 74 | 75 | 75 | 1 |
| from small pool | 124 | 150 | 403 | 279 |
|---------------------------------------------------------------------------|
| Active allocs | 198 | 224 | 478 | 280 |
| from large pool | 74 | 75 | 75 | 1 |
| from small pool | 124 | 150 | 403 | 279 |
|---------------------------------------------------------------------------|
| GPU reserved segments | 21 | 21 | 21 | 0 |
| from large pool | 20 | 20 | 20 | 0 |
| from small pool | 1 | 1 | 1 | 0 |
|---------------------------------------------------------------------------|
| Non-releasable allocs | 18 | 23 | 166 | 148 |
| from large pool | 17 | 18 | 19 | 2 |
| from small pool | 1 | 6 | 147 | 146 |
|===========================================================================|
## Expected results
Efficiently process the datasets and write it down to disk.
## Actual results
--------------------------------------------------------------------------
OverflowError Traceback (most recent call last)
~\anaconda3\envs\xxx\lib\site-packages\datasets\arrow_dataset.py in _map_single(self, function, with_indices, input_columns, batched, batch_size, drop_last_batch, remove_columns, keep_in_memory, load_from_cache_file, cache_file_name, writer_batch_size, features, disable_nullable, fn_kwargs, new_fingerprint, rank, offset, disable_tqdm, desc, cache_only)
2390 else:
-> 2391 writer.write(example)
2392 else:
~\anaconda3\envs\xxx\lib\site-packages\datasets\arrow_writer.py in write(self, example, key, writer_batch_size)
367
--> 368 self.write_examples_on_file()
369
~\anaconda3\envs\xxx\lib\site-packages\datasets\arrow_writer.py in write_examples_on_file(self)
316 if not isinstance(pa_array[0], pa.lib.FloatScalar):
--> 317 raise OverflowError(
318 "There was an overflow in the {}. Try to reduce writer_batch_size to have batches smaller than 2GB".format(
OverflowError: There was an overflow in the <class 'pyarrow.lib.ListArray'>. Try to reduce writer_batch_size to have batches smaller than 2GB
During handling of the above exception, another exception occurred:
OverflowError Traceback (most recent call last)
~\AppData\Local\Temp/ipykernel_16268/2456940807.py in <module>
3 #tracker = OfflineEmissionsTracker(country_iso_code="FRA", project_name='xxx'+time_stamp,output_dir='./codecarbon')
4 #tracker.start()
----> 5 process_datasets(source_datasets_paths, dataset_dir, LM_tokenizer, LMhead_model, datasets_selection=['wikipedia'], from_scratch=True,
6 clean_sentences=False, negative_sampling=False, translate=False, tokenize=False, generate_embeddings=True, concatenate_embeddings=False,
7 max_sample=10000, padding='do_not_pad', truncation=True, cpu_batch_size=1000, gpu_batch_size=2, cpu_writer_batch_size=1000, gpu_writer_batch_size=2, disable_nullable=True, num_proc=None) #
~\xxx\xxx.py in process_datasets(source_datasets_paths, dataset_dir, LM_tokenizer, LMhead_model, datasets_selection, from_scratch, clean_sentences, translate, negative_sampling, tokenize, generate_embeddings, concatenate_embeddings, max_sample, padding, truncation, cpu_batch_size, gpu_batch_size, cpu_writer_batch_size, gpu_writer_batch_size, disable_nullable, num_proc)
481 for column in tqdm(dataset.column_names, desc=f'Processing column', leave=False):
482 if "xxx_" in column:
--> 483 dataset = dataset.map(lambda example :
484 {"embeddings_"+str(column).replace("translated_",""):function(input_ids=example[column],
485 token_type_ids=example[column.replace("input_ids","token_type_ids")],
~\anaconda3\envs\xxx\lib\site-packages\datasets\arrow_dataset.py in map(self, function, with_indices, input_columns, batched, batch_size, drop_last_batch, remove_columns, keep_in_memory, load_from_cache_file, cache_file_name, writer_batch_size, features, disable_nullable, fn_kwargs, num_proc, suffix_template, new_fingerprint, desc)
2034
2035 if num_proc is None or num_proc == 1:
-> 2036 return self._map_single(
2037 function=function,
2038 with_indices=with_indices,
~\anaconda3\envs\xxx\lib\site-packages\datasets\arrow_dataset.py in wrapper(*args, **kwargs)
501 self: "Dataset" = kwargs.pop("self")
502 # apply actual function
--> 503 out: Union["Dataset", "DatasetDict"] = func(self, *args, **kwargs)
504 datasets: List["Dataset"] = list(out.values()) if isinstance(out, dict) else [out]
505 for dataset in datasets:
~\anaconda3\envs\xxx\lib\site-packages\datasets\arrow_dataset.py in wrapper(*args, **kwargs)
468 }
469 # apply actual function
--> 470 out: Union["Dataset", "DatasetDict"] = func(self, *args, **kwargs)
471 datasets: List["Dataset"] = list(out.values()) if isinstance(out, dict) else [out]
472 # re-apply format to the output
~\anaconda3\envs\xxx\lib\site-packages\datasets\fingerprint.py in wrapper(*args, **kwargs)
404 # Call actual function
405
--> 406 out = func(self, *args, **kwargs)
407
408 # Update fingerprint of in-place transforms + update in-place history of transforms
~\anaconda3\envs\xxx\lib\site-packages\datasets\arrow_dataset.py in _map_single(self, function, with_indices, input_columns, batched, batch_size, drop_last_batch, remove_columns, keep_in_memory, load_from_cache_file, cache_file_name, writer_batch_size, features, disable_nullable, fn_kwargs, new_fingerprint, rank, offset, disable_tqdm, desc, cache_only)
2425 if update_data:
2426 if writer is not None:
-> 2427 writer.finalize()
2428 if tmp_file is not None:
2429 tmp_file.close()
~\anaconda3\envs\xxx\lib\site-packages\datasets\arrow_writer.py in finalize(self, close_stream)
440 # Re-intializing to empty list for next batch
441 self.hkey_record = []
--> 442 self.write_examples_on_file()
443 if self.pa_writer is None:
444 if self._schema is not None:
~\anaconda3\envs\xxx\lib\site-packages\datasets\arrow_writer.py in write_examples_on_file(self)
315 # This check fails with FloatArrays with nans, which is not what we want, so account for that:
316 if not isinstance(pa_array[0], pa.lib.FloatScalar):
--> 317 raise OverflowError(
318 "There was an overflow in the {}. Try to reduce writer_batch_size to have batches smaller than 2GB".format(
319 type(pa_array)
OverflowError: There was an overflow in the <class 'pyarrow.lib.ListArray'>. Try to reduce writer_batch_size to have batches smaller than 2GB
## Environment info
<!-- You can run the command `datasets-cli env` and copy-and-paste its output below. -->
- `datasets` version: 1.13.3
- Platform: Windows-10-10.0.19042-SP0
- Python version: 3.8.11
- PyArrow version: 3.0.0
##Next steps
Testing on Linux.
@albertvillanova
I am very unsure on why you tagged me here. I am not a maintainer of the Datasets library and have no idea how to help you. |
https://github.com/huggingface/datasets/issues/3112 | OverflowError: There was an overflow in the <class 'pyarrow.lib.ListArray'>. Try to reduce writer_batch_size to have batches smaller than 2GB | Ok got it, tensor full of NaNs, cf.
~\anaconda3\envs\xxx\lib\site-packages\datasets\arrow_writer.py in write_examples_on_file(self)
315 # This check fails with FloatArrays with nans, which is not what we want, so account for that: | ## Describe the bug
Despite having batches way under 2Gb when running `datasets.map()`, after processing correctly the data of the first batch without fuss and irrespective of writer_batch_size (say 2,4,8,16,32,64 and 128 in my case), it returns the following error :
> OverflowError: There was an overflow in the <class 'pyarrow.lib.ListArray'>. Try to reduce writer_batch_size to have batches smaller than 2GB
Note that I always run `batch_size=writer_batch_size` :
## Steps to reproduce the bug
```python
datasets.map(lambda example : {"column_name" : function(arguments)}, batched=False, remove_columns = datasets.column_names, batch_size=batch_size, writer_batch_size=batch_size, disable_nullable=True, num_proc=None, desc="blablabla")
```
## Introspecting CUDA memory during bug
Placed within `function(arguments)` the following statement to introspect memory usage, merely a little over 1/4 of 2Gb
`print(torch.cuda.memory_summary(device=device, abbreviated=False))`
> |===========================================================================|
| PyTorch CUDA memory summary, device ID 0 |
|---------------------------------------------------------------------------|
| CUDA OOMs: 0 | cudaMalloc retries: 0 |
|===========================================================================|
| Metric | Cur Usage | Peak Usage | Tot Alloc | Tot Freed |
|---------------------------------------------------------------------------|
| Allocated memory | 541418 KB | 545725 KB | 555695 KB | 14276 KB |
| from large pool | 540672 KB | 544431 KB | 544431 KB | 3759 KB |
| from small pool | 746 KB | 1714 KB | 11264 KB | 10517 KB |
|---------------------------------------------------------------------------|
| Active memory | 541418 KB | 545725 KB | 555695 KB | 14276 KB |
| from large pool | 540672 KB | 544431 KB | 544431 KB | 3759 KB |
| from small pool | 746 KB | 1714 KB | 11264 KB | 10517 KB |
|---------------------------------------------------------------------------|
| GPU reserved memory | 598016 KB | 598016 KB | 598016 KB | 0 B |
| from large pool | 595968 KB | 595968 KB | 595968 KB | 0 B |
| from small pool | 2048 KB | 2048 KB | 2048 KB | 0 B |
|---------------------------------------------------------------------------|
| Non-releasable memory | 36117 KB | 52292 KB | 274275 KB | 238158 KB |
| from large pool | 34816 KB | 51537 KB | 261713 KB | 226897 KB |
| from small pool | 1301 KB | 2045 KB | 12562 KB | 11261 KB |
|---------------------------------------------------------------------------|
| Allocations | 198 | 224 | 478 | 280 |
| from large pool | 74 | 75 | 75 | 1 |
| from small pool | 124 | 150 | 403 | 279 |
|---------------------------------------------------------------------------|
| Active allocs | 198 | 224 | 478 | 280 |
| from large pool | 74 | 75 | 75 | 1 |
| from small pool | 124 | 150 | 403 | 279 |
|---------------------------------------------------------------------------|
| GPU reserved segments | 21 | 21 | 21 | 0 |
| from large pool | 20 | 20 | 20 | 0 |
| from small pool | 1 | 1 | 1 | 0 |
|---------------------------------------------------------------------------|
| Non-releasable allocs | 18 | 23 | 166 | 148 |
| from large pool | 17 | 18 | 19 | 2 |
| from small pool | 1 | 6 | 147 | 146 |
|===========================================================================|
## Expected results
Efficiently process the datasets and write it down to disk.
## Actual results
--------------------------------------------------------------------------
OverflowError Traceback (most recent call last)
~\anaconda3\envs\xxx\lib\site-packages\datasets\arrow_dataset.py in _map_single(self, function, with_indices, input_columns, batched, batch_size, drop_last_batch, remove_columns, keep_in_memory, load_from_cache_file, cache_file_name, writer_batch_size, features, disable_nullable, fn_kwargs, new_fingerprint, rank, offset, disable_tqdm, desc, cache_only)
2390 else:
-> 2391 writer.write(example)
2392 else:
~\anaconda3\envs\xxx\lib\site-packages\datasets\arrow_writer.py in write(self, example, key, writer_batch_size)
367
--> 368 self.write_examples_on_file()
369
~\anaconda3\envs\xxx\lib\site-packages\datasets\arrow_writer.py in write_examples_on_file(self)
316 if not isinstance(pa_array[0], pa.lib.FloatScalar):
--> 317 raise OverflowError(
318 "There was an overflow in the {}. Try to reduce writer_batch_size to have batches smaller than 2GB".format(
OverflowError: There was an overflow in the <class 'pyarrow.lib.ListArray'>. Try to reduce writer_batch_size to have batches smaller than 2GB
During handling of the above exception, another exception occurred:
OverflowError Traceback (most recent call last)
~\AppData\Local\Temp/ipykernel_16268/2456940807.py in <module>
3 #tracker = OfflineEmissionsTracker(country_iso_code="FRA", project_name='xxx'+time_stamp,output_dir='./codecarbon')
4 #tracker.start()
----> 5 process_datasets(source_datasets_paths, dataset_dir, LM_tokenizer, LMhead_model, datasets_selection=['wikipedia'], from_scratch=True,
6 clean_sentences=False, negative_sampling=False, translate=False, tokenize=False, generate_embeddings=True, concatenate_embeddings=False,
7 max_sample=10000, padding='do_not_pad', truncation=True, cpu_batch_size=1000, gpu_batch_size=2, cpu_writer_batch_size=1000, gpu_writer_batch_size=2, disable_nullable=True, num_proc=None) #
~\xxx\xxx.py in process_datasets(source_datasets_paths, dataset_dir, LM_tokenizer, LMhead_model, datasets_selection, from_scratch, clean_sentences, translate, negative_sampling, tokenize, generate_embeddings, concatenate_embeddings, max_sample, padding, truncation, cpu_batch_size, gpu_batch_size, cpu_writer_batch_size, gpu_writer_batch_size, disable_nullable, num_proc)
481 for column in tqdm(dataset.column_names, desc=f'Processing column', leave=False):
482 if "xxx_" in column:
--> 483 dataset = dataset.map(lambda example :
484 {"embeddings_"+str(column).replace("translated_",""):function(input_ids=example[column],
485 token_type_ids=example[column.replace("input_ids","token_type_ids")],
~\anaconda3\envs\xxx\lib\site-packages\datasets\arrow_dataset.py in map(self, function, with_indices, input_columns, batched, batch_size, drop_last_batch, remove_columns, keep_in_memory, load_from_cache_file, cache_file_name, writer_batch_size, features, disable_nullable, fn_kwargs, num_proc, suffix_template, new_fingerprint, desc)
2034
2035 if num_proc is None or num_proc == 1:
-> 2036 return self._map_single(
2037 function=function,
2038 with_indices=with_indices,
~\anaconda3\envs\xxx\lib\site-packages\datasets\arrow_dataset.py in wrapper(*args, **kwargs)
501 self: "Dataset" = kwargs.pop("self")
502 # apply actual function
--> 503 out: Union["Dataset", "DatasetDict"] = func(self, *args, **kwargs)
504 datasets: List["Dataset"] = list(out.values()) if isinstance(out, dict) else [out]
505 for dataset in datasets:
~\anaconda3\envs\xxx\lib\site-packages\datasets\arrow_dataset.py in wrapper(*args, **kwargs)
468 }
469 # apply actual function
--> 470 out: Union["Dataset", "DatasetDict"] = func(self, *args, **kwargs)
471 datasets: List["Dataset"] = list(out.values()) if isinstance(out, dict) else [out]
472 # re-apply format to the output
~\anaconda3\envs\xxx\lib\site-packages\datasets\fingerprint.py in wrapper(*args, **kwargs)
404 # Call actual function
405
--> 406 out = func(self, *args, **kwargs)
407
408 # Update fingerprint of in-place transforms + update in-place history of transforms
~\anaconda3\envs\xxx\lib\site-packages\datasets\arrow_dataset.py in _map_single(self, function, with_indices, input_columns, batched, batch_size, drop_last_batch, remove_columns, keep_in_memory, load_from_cache_file, cache_file_name, writer_batch_size, features, disable_nullable, fn_kwargs, new_fingerprint, rank, offset, disable_tqdm, desc, cache_only)
2425 if update_data:
2426 if writer is not None:
-> 2427 writer.finalize()
2428 if tmp_file is not None:
2429 tmp_file.close()
~\anaconda3\envs\xxx\lib\site-packages\datasets\arrow_writer.py in finalize(self, close_stream)
440 # Re-intializing to empty list for next batch
441 self.hkey_record = []
--> 442 self.write_examples_on_file()
443 if self.pa_writer is None:
444 if self._schema is not None:
~\anaconda3\envs\xxx\lib\site-packages\datasets\arrow_writer.py in write_examples_on_file(self)
315 # This check fails with FloatArrays with nans, which is not what we want, so account for that:
316 if not isinstance(pa_array[0], pa.lib.FloatScalar):
--> 317 raise OverflowError(
318 "There was an overflow in the {}. Try to reduce writer_batch_size to have batches smaller than 2GB".format(
319 type(pa_array)
OverflowError: There was an overflow in the <class 'pyarrow.lib.ListArray'>. Try to reduce writer_batch_size to have batches smaller than 2GB
## Environment info
<!-- You can run the command `datasets-cli env` and copy-and-paste its output below. -->
- `datasets` version: 1.13.3
- Platform: Windows-10-10.0.19042-SP0
- Python version: 3.8.11
- PyArrow version: 3.0.0
##Next steps
Testing on Linux.
@albertvillanova
| 30 | OverflowError: There was an overflow in the <class 'pyarrow.lib.ListArray'>. Try to reduce writer_batch_size to have batches smaller than 2GB
## Describe the bug
Despite having batches way under 2Gb when running `datasets.map()`, after processing correctly the data of the first batch without fuss and irrespective of writer_batch_size (say 2,4,8,16,32,64 and 128 in my case), it returns the following error :
> OverflowError: There was an overflow in the <class 'pyarrow.lib.ListArray'>. Try to reduce writer_batch_size to have batches smaller than 2GB
Note that I always run `batch_size=writer_batch_size` :
## Steps to reproduce the bug
```python
datasets.map(lambda example : {"column_name" : function(arguments)}, batched=False, remove_columns = datasets.column_names, batch_size=batch_size, writer_batch_size=batch_size, disable_nullable=True, num_proc=None, desc="blablabla")
```
## Introspecting CUDA memory during bug
Placed within `function(arguments)` the following statement to introspect memory usage, merely a little over 1/4 of 2Gb
`print(torch.cuda.memory_summary(device=device, abbreviated=False))`
> |===========================================================================|
| PyTorch CUDA memory summary, device ID 0 |
|---------------------------------------------------------------------------|
| CUDA OOMs: 0 | cudaMalloc retries: 0 |
|===========================================================================|
| Metric | Cur Usage | Peak Usage | Tot Alloc | Tot Freed |
|---------------------------------------------------------------------------|
| Allocated memory | 541418 KB | 545725 KB | 555695 KB | 14276 KB |
| from large pool | 540672 KB | 544431 KB | 544431 KB | 3759 KB |
| from small pool | 746 KB | 1714 KB | 11264 KB | 10517 KB |
|---------------------------------------------------------------------------|
| Active memory | 541418 KB | 545725 KB | 555695 KB | 14276 KB |
| from large pool | 540672 KB | 544431 KB | 544431 KB | 3759 KB |
| from small pool | 746 KB | 1714 KB | 11264 KB | 10517 KB |
|---------------------------------------------------------------------------|
| GPU reserved memory | 598016 KB | 598016 KB | 598016 KB | 0 B |
| from large pool | 595968 KB | 595968 KB | 595968 KB | 0 B |
| from small pool | 2048 KB | 2048 KB | 2048 KB | 0 B |
|---------------------------------------------------------------------------|
| Non-releasable memory | 36117 KB | 52292 KB | 274275 KB | 238158 KB |
| from large pool | 34816 KB | 51537 KB | 261713 KB | 226897 KB |
| from small pool | 1301 KB | 2045 KB | 12562 KB | 11261 KB |
|---------------------------------------------------------------------------|
| Allocations | 198 | 224 | 478 | 280 |
| from large pool | 74 | 75 | 75 | 1 |
| from small pool | 124 | 150 | 403 | 279 |
|---------------------------------------------------------------------------|
| Active allocs | 198 | 224 | 478 | 280 |
| from large pool | 74 | 75 | 75 | 1 |
| from small pool | 124 | 150 | 403 | 279 |
|---------------------------------------------------------------------------|
| GPU reserved segments | 21 | 21 | 21 | 0 |
| from large pool | 20 | 20 | 20 | 0 |
| from small pool | 1 | 1 | 1 | 0 |
|---------------------------------------------------------------------------|
| Non-releasable allocs | 18 | 23 | 166 | 148 |
| from large pool | 17 | 18 | 19 | 2 |
| from small pool | 1 | 6 | 147 | 146 |
|===========================================================================|
## Expected results
Efficiently process the datasets and write it down to disk.
## Actual results
--------------------------------------------------------------------------
OverflowError Traceback (most recent call last)
~\anaconda3\envs\xxx\lib\site-packages\datasets\arrow_dataset.py in _map_single(self, function, with_indices, input_columns, batched, batch_size, drop_last_batch, remove_columns, keep_in_memory, load_from_cache_file, cache_file_name, writer_batch_size, features, disable_nullable, fn_kwargs, new_fingerprint, rank, offset, disable_tqdm, desc, cache_only)
2390 else:
-> 2391 writer.write(example)
2392 else:
~\anaconda3\envs\xxx\lib\site-packages\datasets\arrow_writer.py in write(self, example, key, writer_batch_size)
367
--> 368 self.write_examples_on_file()
369
~\anaconda3\envs\xxx\lib\site-packages\datasets\arrow_writer.py in write_examples_on_file(self)
316 if not isinstance(pa_array[0], pa.lib.FloatScalar):
--> 317 raise OverflowError(
318 "There was an overflow in the {}. Try to reduce writer_batch_size to have batches smaller than 2GB".format(
OverflowError: There was an overflow in the <class 'pyarrow.lib.ListArray'>. Try to reduce writer_batch_size to have batches smaller than 2GB
During handling of the above exception, another exception occurred:
OverflowError Traceback (most recent call last)
~\AppData\Local\Temp/ipykernel_16268/2456940807.py in <module>
3 #tracker = OfflineEmissionsTracker(country_iso_code="FRA", project_name='xxx'+time_stamp,output_dir='./codecarbon')
4 #tracker.start()
----> 5 process_datasets(source_datasets_paths, dataset_dir, LM_tokenizer, LMhead_model, datasets_selection=['wikipedia'], from_scratch=True,
6 clean_sentences=False, negative_sampling=False, translate=False, tokenize=False, generate_embeddings=True, concatenate_embeddings=False,
7 max_sample=10000, padding='do_not_pad', truncation=True, cpu_batch_size=1000, gpu_batch_size=2, cpu_writer_batch_size=1000, gpu_writer_batch_size=2, disable_nullable=True, num_proc=None) #
~\xxx\xxx.py in process_datasets(source_datasets_paths, dataset_dir, LM_tokenizer, LMhead_model, datasets_selection, from_scratch, clean_sentences, translate, negative_sampling, tokenize, generate_embeddings, concatenate_embeddings, max_sample, padding, truncation, cpu_batch_size, gpu_batch_size, cpu_writer_batch_size, gpu_writer_batch_size, disable_nullable, num_proc)
481 for column in tqdm(dataset.column_names, desc=f'Processing column', leave=False):
482 if "xxx_" in column:
--> 483 dataset = dataset.map(lambda example :
484 {"embeddings_"+str(column).replace("translated_",""):function(input_ids=example[column],
485 token_type_ids=example[column.replace("input_ids","token_type_ids")],
~\anaconda3\envs\xxx\lib\site-packages\datasets\arrow_dataset.py in map(self, function, with_indices, input_columns, batched, batch_size, drop_last_batch, remove_columns, keep_in_memory, load_from_cache_file, cache_file_name, writer_batch_size, features, disable_nullable, fn_kwargs, num_proc, suffix_template, new_fingerprint, desc)
2034
2035 if num_proc is None or num_proc == 1:
-> 2036 return self._map_single(
2037 function=function,
2038 with_indices=with_indices,
~\anaconda3\envs\xxx\lib\site-packages\datasets\arrow_dataset.py in wrapper(*args, **kwargs)
501 self: "Dataset" = kwargs.pop("self")
502 # apply actual function
--> 503 out: Union["Dataset", "DatasetDict"] = func(self, *args, **kwargs)
504 datasets: List["Dataset"] = list(out.values()) if isinstance(out, dict) else [out]
505 for dataset in datasets:
~\anaconda3\envs\xxx\lib\site-packages\datasets\arrow_dataset.py in wrapper(*args, **kwargs)
468 }
469 # apply actual function
--> 470 out: Union["Dataset", "DatasetDict"] = func(self, *args, **kwargs)
471 datasets: List["Dataset"] = list(out.values()) if isinstance(out, dict) else [out]
472 # re-apply format to the output
~\anaconda3\envs\xxx\lib\site-packages\datasets\fingerprint.py in wrapper(*args, **kwargs)
404 # Call actual function
405
--> 406 out = func(self, *args, **kwargs)
407
408 # Update fingerprint of in-place transforms + update in-place history of transforms
~\anaconda3\envs\xxx\lib\site-packages\datasets\arrow_dataset.py in _map_single(self, function, with_indices, input_columns, batched, batch_size, drop_last_batch, remove_columns, keep_in_memory, load_from_cache_file, cache_file_name, writer_batch_size, features, disable_nullable, fn_kwargs, new_fingerprint, rank, offset, disable_tqdm, desc, cache_only)
2425 if update_data:
2426 if writer is not None:
-> 2427 writer.finalize()
2428 if tmp_file is not None:
2429 tmp_file.close()
~\anaconda3\envs\xxx\lib\site-packages\datasets\arrow_writer.py in finalize(self, close_stream)
440 # Re-intializing to empty list for next batch
441 self.hkey_record = []
--> 442 self.write_examples_on_file()
443 if self.pa_writer is None:
444 if self._schema is not None:
~\anaconda3\envs\xxx\lib\site-packages\datasets\arrow_writer.py in write_examples_on_file(self)
315 # This check fails with FloatArrays with nans, which is not what we want, so account for that:
316 if not isinstance(pa_array[0], pa.lib.FloatScalar):
--> 317 raise OverflowError(
318 "There was an overflow in the {}. Try to reduce writer_batch_size to have batches smaller than 2GB".format(
319 type(pa_array)
OverflowError: There was an overflow in the <class 'pyarrow.lib.ListArray'>. Try to reduce writer_batch_size to have batches smaller than 2GB
## Environment info
<!-- You can run the command `datasets-cli env` and copy-and-paste its output below. -->
- `datasets` version: 1.13.3
- Platform: Windows-10-10.0.19042-SP0
- Python version: 3.8.11
- PyArrow version: 3.0.0
##Next steps
Testing on Linux.
@albertvillanova
Ok got it, tensor full of NaNs, cf.
~\anaconda3\envs\xxx\lib\site-packages\datasets\arrow_writer.py in write_examples_on_file(self)
315 # This check fails with FloatArrays with nans, which is not what we want, so account for that: |
https://github.com/huggingface/datasets/issues/3099 | AttributeError: module 'huggingface_hub.hf_api' has no attribute 'DatasetInfo' | Hi @JTWang2000, thanks for reporting.
However, I cannot reproduce your reported bug:
```python
>>> from datasets import load_dataset
>>> dataset = load_dataset("sst", "default")
>>> dataset
DatasetDict({
train: Dataset({
features: ['sentence', 'label', 'tokens', 'tree'],
num_rows: 8544
})
validation: Dataset({
features: ['sentence', 'label', 'tokens', 'tree'],
num_rows: 1101
})
test: Dataset({
features: ['sentence', 'label', 'tokens', 'tree'],
num_rows: 2210
})
})
```
Maybe, the cause is that you have a quite old version of `huggingface_hub`. Could you please try to update it and confirm if the problem persists?
```
pip install -U huggingface_hub
``` | ## Describe the bug
When using `pip install datasets`
or use `conda install -c huggingface -c conda-forge datasets`
cannot install datasets
## Steps to reproduce the bug
```python
from datasets import load_dataset
dataset = load_dataset("sst", "default")
```
## Actual results
---------------------------------------------------------------------------
AttributeError Traceback (most recent call last)
<ipython-input-5-fbe7981e6e21> in <module>
1 import torch
2 import transformers
----> 3 from datasets import load_dataset
4
5 dataset = load_dataset("sst", "default")
~/miniforge3/envs/actor/lib/python3.8/site-packages/datasets/__init__.py in <module>
35 from .arrow_reader import ArrowReader, ReadInstruction
36 from .arrow_writer import ArrowWriter
---> 37 from .builder import ArrowBasedBuilder, BeamBasedBuilder, BuilderConfig, DatasetBuilder, GeneratorBasedBuilder
38 from .combine import interleave_datasets
39 from .dataset_dict import DatasetDict, IterableDatasetDict
~/miniforge3/envs/actor/lib/python3.8/site-packages/datasets/builder.py in <module>
42 )
43 from .arrow_writer import ArrowWriter, BeamWriter
---> 44 from .data_files import DataFilesDict, _sanitize_patterns
45 from .dataset_dict import DatasetDict, IterableDatasetDict
46 from .fingerprint import Hasher
~/miniforge3/envs/actor/lib/python3.8/site-packages/datasets/data_files.py in <module>
118
119 def _exec_patterns_in_dataset_repository(
--> 120 dataset_info: huggingface_hub.hf_api.DatasetInfo,
121 patterns: List[str],
122 allowed_extensions: Optional[list] = None,
AttributeError: module 'huggingface_hub.hf_api' has no attribute 'DatasetInfo'
## Environment info
<!-- You can run the command `datasets-cli env` and copy-and-paste its output below. -->
- `datasets` version: 1.13.3
- Platform: macOS-11.3.1-arm64-arm-64bit
- Python version: 3.8.10
- PyArrow version: 5.0.0
| 90 | AttributeError: module 'huggingface_hub.hf_api' has no attribute 'DatasetInfo'
## Describe the bug
When using `pip install datasets`
or use `conda install -c huggingface -c conda-forge datasets`
cannot install datasets
## Steps to reproduce the bug
```python
from datasets import load_dataset
dataset = load_dataset("sst", "default")
```
## Actual results
---------------------------------------------------------------------------
AttributeError Traceback (most recent call last)
<ipython-input-5-fbe7981e6e21> in <module>
1 import torch
2 import transformers
----> 3 from datasets import load_dataset
4
5 dataset = load_dataset("sst", "default")
~/miniforge3/envs/actor/lib/python3.8/site-packages/datasets/__init__.py in <module>
35 from .arrow_reader import ArrowReader, ReadInstruction
36 from .arrow_writer import ArrowWriter
---> 37 from .builder import ArrowBasedBuilder, BeamBasedBuilder, BuilderConfig, DatasetBuilder, GeneratorBasedBuilder
38 from .combine import interleave_datasets
39 from .dataset_dict import DatasetDict, IterableDatasetDict
~/miniforge3/envs/actor/lib/python3.8/site-packages/datasets/builder.py in <module>
42 )
43 from .arrow_writer import ArrowWriter, BeamWriter
---> 44 from .data_files import DataFilesDict, _sanitize_patterns
45 from .dataset_dict import DatasetDict, IterableDatasetDict
46 from .fingerprint import Hasher
~/miniforge3/envs/actor/lib/python3.8/site-packages/datasets/data_files.py in <module>
118
119 def _exec_patterns_in_dataset_repository(
--> 120 dataset_info: huggingface_hub.hf_api.DatasetInfo,
121 patterns: List[str],
122 allowed_extensions: Optional[list] = None,
AttributeError: module 'huggingface_hub.hf_api' has no attribute 'DatasetInfo'
## Environment info
<!-- You can run the command `datasets-cli env` and copy-and-paste its output below. -->
- `datasets` version: 1.13.3
- Platform: macOS-11.3.1-arm64-arm-64bit
- Python version: 3.8.10
- PyArrow version: 5.0.0
Hi @JTWang2000, thanks for reporting.
However, I cannot reproduce your reported bug:
```python
>>> from datasets import load_dataset
>>> dataset = load_dataset("sst", "default")
>>> dataset
DatasetDict({
train: Dataset({
features: ['sentence', 'label', 'tokens', 'tree'],
num_rows: 8544
})
validation: Dataset({
features: ['sentence', 'label', 'tokens', 'tree'],
num_rows: 1101
})
test: Dataset({
features: ['sentence', 'label', 'tokens', 'tree'],
num_rows: 2210
})
})
```
Maybe, the cause is that you have a quite old version of `huggingface_hub`. Could you please try to update it and confirm if the problem persists?
```
pip install -U huggingface_hub
``` |
https://github.com/huggingface/datasets/issues/3099 | AttributeError: module 'huggingface_hub.hf_api' has no attribute 'DatasetInfo' | Im facing the same issue. I did run the upgrade command but that doesnt seem to resolve the issue | ## Describe the bug
When using `pip install datasets`
or use `conda install -c huggingface -c conda-forge datasets`
cannot install datasets
## Steps to reproduce the bug
```python
from datasets import load_dataset
dataset = load_dataset("sst", "default")
```
## Actual results
---------------------------------------------------------------------------
AttributeError Traceback (most recent call last)
<ipython-input-5-fbe7981e6e21> in <module>
1 import torch
2 import transformers
----> 3 from datasets import load_dataset
4
5 dataset = load_dataset("sst", "default")
~/miniforge3/envs/actor/lib/python3.8/site-packages/datasets/__init__.py in <module>
35 from .arrow_reader import ArrowReader, ReadInstruction
36 from .arrow_writer import ArrowWriter
---> 37 from .builder import ArrowBasedBuilder, BeamBasedBuilder, BuilderConfig, DatasetBuilder, GeneratorBasedBuilder
38 from .combine import interleave_datasets
39 from .dataset_dict import DatasetDict, IterableDatasetDict
~/miniforge3/envs/actor/lib/python3.8/site-packages/datasets/builder.py in <module>
42 )
43 from .arrow_writer import ArrowWriter, BeamWriter
---> 44 from .data_files import DataFilesDict, _sanitize_patterns
45 from .dataset_dict import DatasetDict, IterableDatasetDict
46 from .fingerprint import Hasher
~/miniforge3/envs/actor/lib/python3.8/site-packages/datasets/data_files.py in <module>
118
119 def _exec_patterns_in_dataset_repository(
--> 120 dataset_info: huggingface_hub.hf_api.DatasetInfo,
121 patterns: List[str],
122 allowed_extensions: Optional[list] = None,
AttributeError: module 'huggingface_hub.hf_api' has no attribute 'DatasetInfo'
## Environment info
<!-- You can run the command `datasets-cli env` and copy-and-paste its output below. -->
- `datasets` version: 1.13.3
- Platform: macOS-11.3.1-arm64-arm-64bit
- Python version: 3.8.10
- PyArrow version: 5.0.0
| 19 | AttributeError: module 'huggingface_hub.hf_api' has no attribute 'DatasetInfo'
## Describe the bug
When using `pip install datasets`
or use `conda install -c huggingface -c conda-forge datasets`
cannot install datasets
## Steps to reproduce the bug
```python
from datasets import load_dataset
dataset = load_dataset("sst", "default")
```
## Actual results
---------------------------------------------------------------------------
AttributeError Traceback (most recent call last)
<ipython-input-5-fbe7981e6e21> in <module>
1 import torch
2 import transformers
----> 3 from datasets import load_dataset
4
5 dataset = load_dataset("sst", "default")
~/miniforge3/envs/actor/lib/python3.8/site-packages/datasets/__init__.py in <module>
35 from .arrow_reader import ArrowReader, ReadInstruction
36 from .arrow_writer import ArrowWriter
---> 37 from .builder import ArrowBasedBuilder, BeamBasedBuilder, BuilderConfig, DatasetBuilder, GeneratorBasedBuilder
38 from .combine import interleave_datasets
39 from .dataset_dict import DatasetDict, IterableDatasetDict
~/miniforge3/envs/actor/lib/python3.8/site-packages/datasets/builder.py in <module>
42 )
43 from .arrow_writer import ArrowWriter, BeamWriter
---> 44 from .data_files import DataFilesDict, _sanitize_patterns
45 from .dataset_dict import DatasetDict, IterableDatasetDict
46 from .fingerprint import Hasher
~/miniforge3/envs/actor/lib/python3.8/site-packages/datasets/data_files.py in <module>
118
119 def _exec_patterns_in_dataset_repository(
--> 120 dataset_info: huggingface_hub.hf_api.DatasetInfo,
121 patterns: List[str],
122 allowed_extensions: Optional[list] = None,
AttributeError: module 'huggingface_hub.hf_api' has no attribute 'DatasetInfo'
## Environment info
<!-- You can run the command `datasets-cli env` and copy-and-paste its output below. -->
- `datasets` version: 1.13.3
- Platform: macOS-11.3.1-arm64-arm-64bit
- Python version: 3.8.10
- PyArrow version: 5.0.0
Im facing the same issue. I did run the upgrade command but that doesnt seem to resolve the issue |
https://github.com/huggingface/datasets/issues/3099 | AttributeError: module 'huggingface_hub.hf_api' has no attribute 'DatasetInfo' | Hi @aneeshjain, could you please specify which `huggingface_hub` version you are using?
Besides that, please run `datasets-cli env` and copy-and-paste its output below. | ## Describe the bug
When using `pip install datasets`
or use `conda install -c huggingface -c conda-forge datasets`
cannot install datasets
## Steps to reproduce the bug
```python
from datasets import load_dataset
dataset = load_dataset("sst", "default")
```
## Actual results
---------------------------------------------------------------------------
AttributeError Traceback (most recent call last)
<ipython-input-5-fbe7981e6e21> in <module>
1 import torch
2 import transformers
----> 3 from datasets import load_dataset
4
5 dataset = load_dataset("sst", "default")
~/miniforge3/envs/actor/lib/python3.8/site-packages/datasets/__init__.py in <module>
35 from .arrow_reader import ArrowReader, ReadInstruction
36 from .arrow_writer import ArrowWriter
---> 37 from .builder import ArrowBasedBuilder, BeamBasedBuilder, BuilderConfig, DatasetBuilder, GeneratorBasedBuilder
38 from .combine import interleave_datasets
39 from .dataset_dict import DatasetDict, IterableDatasetDict
~/miniforge3/envs/actor/lib/python3.8/site-packages/datasets/builder.py in <module>
42 )
43 from .arrow_writer import ArrowWriter, BeamWriter
---> 44 from .data_files import DataFilesDict, _sanitize_patterns
45 from .dataset_dict import DatasetDict, IterableDatasetDict
46 from .fingerprint import Hasher
~/miniforge3/envs/actor/lib/python3.8/site-packages/datasets/data_files.py in <module>
118
119 def _exec_patterns_in_dataset_repository(
--> 120 dataset_info: huggingface_hub.hf_api.DatasetInfo,
121 patterns: List[str],
122 allowed_extensions: Optional[list] = None,
AttributeError: module 'huggingface_hub.hf_api' has no attribute 'DatasetInfo'
## Environment info
<!-- You can run the command `datasets-cli env` and copy-and-paste its output below. -->
- `datasets` version: 1.13.3
- Platform: macOS-11.3.1-arm64-arm-64bit
- Python version: 3.8.10
- PyArrow version: 5.0.0
| 23 | AttributeError: module 'huggingface_hub.hf_api' has no attribute 'DatasetInfo'
## Describe the bug
When using `pip install datasets`
or use `conda install -c huggingface -c conda-forge datasets`
cannot install datasets
## Steps to reproduce the bug
```python
from datasets import load_dataset
dataset = load_dataset("sst", "default")
```
## Actual results
---------------------------------------------------------------------------
AttributeError Traceback (most recent call last)
<ipython-input-5-fbe7981e6e21> in <module>
1 import torch
2 import transformers
----> 3 from datasets import load_dataset
4
5 dataset = load_dataset("sst", "default")
~/miniforge3/envs/actor/lib/python3.8/site-packages/datasets/__init__.py in <module>
35 from .arrow_reader import ArrowReader, ReadInstruction
36 from .arrow_writer import ArrowWriter
---> 37 from .builder import ArrowBasedBuilder, BeamBasedBuilder, BuilderConfig, DatasetBuilder, GeneratorBasedBuilder
38 from .combine import interleave_datasets
39 from .dataset_dict import DatasetDict, IterableDatasetDict
~/miniforge3/envs/actor/lib/python3.8/site-packages/datasets/builder.py in <module>
42 )
43 from .arrow_writer import ArrowWriter, BeamWriter
---> 44 from .data_files import DataFilesDict, _sanitize_patterns
45 from .dataset_dict import DatasetDict, IterableDatasetDict
46 from .fingerprint import Hasher
~/miniforge3/envs/actor/lib/python3.8/site-packages/datasets/data_files.py in <module>
118
119 def _exec_patterns_in_dataset_repository(
--> 120 dataset_info: huggingface_hub.hf_api.DatasetInfo,
121 patterns: List[str],
122 allowed_extensions: Optional[list] = None,
AttributeError: module 'huggingface_hub.hf_api' has no attribute 'DatasetInfo'
## Environment info
<!-- You can run the command `datasets-cli env` and copy-and-paste its output below. -->
- `datasets` version: 1.13.3
- Platform: macOS-11.3.1-arm64-arm-64bit
- Python version: 3.8.10
- PyArrow version: 5.0.0
Hi @aneeshjain, could you please specify which `huggingface_hub` version you are using?
Besides that, please run `datasets-cli env` and copy-and-paste its output below. |
https://github.com/huggingface/datasets/issues/3099 | AttributeError: module 'huggingface_hub.hf_api' has no attribute 'DatasetInfo' | The problem seems to be with the latest version of `datasets`. After running `pip install -U datasets huggingface_hub`, I get the following:
```bash
python -c "import huggingface_hub; print(f'hbvers={huggingface_hub.__version__}'); import datasets; print(f'dvers={datasets.__version__}')"
hbvers=0.0.8
Traceback (most recent call last):
File "<string>", line 1, in <module>
File "/opt/conda/lib/python3.6/site-packages/datasets/__init__.py", line 37, in <module>
from .builder import ArrowBasedBuilder, BeamBasedBuilder, BuilderConfig, DatasetBuilder, GeneratorBasedBuilder
File "/opt/conda/lib/python3.6/site-packages/datasets/builder.py", line 44, in <module>
from .data_files import DataFilesDict, _sanitize_patterns
File "/opt/conda/lib/python3.6/site-packages/datasets/data_files.py", line 122, in <module>
allowed_extensions: Optional[list] = None,
AttributeError: module 'huggingface_hub.hf_api' has no attribute 'DatasetInfo'
````
Note that pip reports the latest `datasets` version as
```bash
pip show datasets
Name: datasets
Version: 1.14.0
```
However, if I downgrade datasets with `pip install datasets==1.11.0`, things now work
```bash
python -c "import huggingface_hub; print(f'hbvers={huggingface_hub.__version__}'); import datasets; print(f'dvers={datasets.__version__}')"
hbvers=0.0.8
dvers=1.11.0
```` | ## Describe the bug
When using `pip install datasets`
or use `conda install -c huggingface -c conda-forge datasets`
cannot install datasets
## Steps to reproduce the bug
```python
from datasets import load_dataset
dataset = load_dataset("sst", "default")
```
## Actual results
---------------------------------------------------------------------------
AttributeError Traceback (most recent call last)
<ipython-input-5-fbe7981e6e21> in <module>
1 import torch
2 import transformers
----> 3 from datasets import load_dataset
4
5 dataset = load_dataset("sst", "default")
~/miniforge3/envs/actor/lib/python3.8/site-packages/datasets/__init__.py in <module>
35 from .arrow_reader import ArrowReader, ReadInstruction
36 from .arrow_writer import ArrowWriter
---> 37 from .builder import ArrowBasedBuilder, BeamBasedBuilder, BuilderConfig, DatasetBuilder, GeneratorBasedBuilder
38 from .combine import interleave_datasets
39 from .dataset_dict import DatasetDict, IterableDatasetDict
~/miniforge3/envs/actor/lib/python3.8/site-packages/datasets/builder.py in <module>
42 )
43 from .arrow_writer import ArrowWriter, BeamWriter
---> 44 from .data_files import DataFilesDict, _sanitize_patterns
45 from .dataset_dict import DatasetDict, IterableDatasetDict
46 from .fingerprint import Hasher
~/miniforge3/envs/actor/lib/python3.8/site-packages/datasets/data_files.py in <module>
118
119 def _exec_patterns_in_dataset_repository(
--> 120 dataset_info: huggingface_hub.hf_api.DatasetInfo,
121 patterns: List[str],
122 allowed_extensions: Optional[list] = None,
AttributeError: module 'huggingface_hub.hf_api' has no attribute 'DatasetInfo'
## Environment info
<!-- You can run the command `datasets-cli env` and copy-and-paste its output below. -->
- `datasets` version: 1.13.3
- Platform: macOS-11.3.1-arm64-arm-64bit
- Python version: 3.8.10
- PyArrow version: 5.0.0
| 128 | AttributeError: module 'huggingface_hub.hf_api' has no attribute 'DatasetInfo'
## Describe the bug
When using `pip install datasets`
or use `conda install -c huggingface -c conda-forge datasets`
cannot install datasets
## Steps to reproduce the bug
```python
from datasets import load_dataset
dataset = load_dataset("sst", "default")
```
## Actual results
---------------------------------------------------------------------------
AttributeError Traceback (most recent call last)
<ipython-input-5-fbe7981e6e21> in <module>
1 import torch
2 import transformers
----> 3 from datasets import load_dataset
4
5 dataset = load_dataset("sst", "default")
~/miniforge3/envs/actor/lib/python3.8/site-packages/datasets/__init__.py in <module>
35 from .arrow_reader import ArrowReader, ReadInstruction
36 from .arrow_writer import ArrowWriter
---> 37 from .builder import ArrowBasedBuilder, BeamBasedBuilder, BuilderConfig, DatasetBuilder, GeneratorBasedBuilder
38 from .combine import interleave_datasets
39 from .dataset_dict import DatasetDict, IterableDatasetDict
~/miniforge3/envs/actor/lib/python3.8/site-packages/datasets/builder.py in <module>
42 )
43 from .arrow_writer import ArrowWriter, BeamWriter
---> 44 from .data_files import DataFilesDict, _sanitize_patterns
45 from .dataset_dict import DatasetDict, IterableDatasetDict
46 from .fingerprint import Hasher
~/miniforge3/envs/actor/lib/python3.8/site-packages/datasets/data_files.py in <module>
118
119 def _exec_patterns_in_dataset_repository(
--> 120 dataset_info: huggingface_hub.hf_api.DatasetInfo,
121 patterns: List[str],
122 allowed_extensions: Optional[list] = None,
AttributeError: module 'huggingface_hub.hf_api' has no attribute 'DatasetInfo'
## Environment info
<!-- You can run the command `datasets-cli env` and copy-and-paste its output below. -->
- `datasets` version: 1.13.3
- Platform: macOS-11.3.1-arm64-arm-64bit
- Python version: 3.8.10
- PyArrow version: 5.0.0
The problem seems to be with the latest version of `datasets`. After running `pip install -U datasets huggingface_hub`, I get the following:
```bash
python -c "import huggingface_hub; print(f'hbvers={huggingface_hub.__version__}'); import datasets; print(f'dvers={datasets.__version__}')"
hbvers=0.0.8
Traceback (most recent call last):
File "<string>", line 1, in <module>
File "/opt/conda/lib/python3.6/site-packages/datasets/__init__.py", line 37, in <module>
from .builder import ArrowBasedBuilder, BeamBasedBuilder, BuilderConfig, DatasetBuilder, GeneratorBasedBuilder
File "/opt/conda/lib/python3.6/site-packages/datasets/builder.py", line 44, in <module>
from .data_files import DataFilesDict, _sanitize_patterns
File "/opt/conda/lib/python3.6/site-packages/datasets/data_files.py", line 122, in <module>
allowed_extensions: Optional[list] = None,
AttributeError: module 'huggingface_hub.hf_api' has no attribute 'DatasetInfo'
````
Note that pip reports the latest `datasets` version as
```bash
pip show datasets
Name: datasets
Version: 1.14.0
```
However, if I downgrade datasets with `pip install datasets==1.11.0`, things now work
```bash
python -c "import huggingface_hub; print(f'hbvers={huggingface_hub.__version__}'); import datasets; print(f'dvers={datasets.__version__}')"
hbvers=0.0.8
dvers=1.11.0
```` |
https://github.com/huggingface/datasets/issues/3099 | AttributeError: module 'huggingface_hub.hf_api' has no attribute 'DatasetInfo' | > Hi @JTWang2000, thanks for reporting.
>
> However, I cannot reproduce your reported bug:
>
> ```python
> >>> from datasets import load_dataset
>
> >>> dataset = load_dataset("sst", "default")
> >>> dataset
> DatasetDict({
> train: Dataset({
> features: ['sentence', 'label', 'tokens', 'tree'],
> num_rows: 8544
> })
> validation: Dataset({
> features: ['sentence', 'label', 'tokens', 'tree'],
> num_rows: 1101
> })
> test: Dataset({
> features: ['sentence', 'label', 'tokens', 'tree'],
> num_rows: 2210
> })
> })
> ```
>
> Maybe, the cause is that you have a quite old version of `huggingface_hub`. Could you please try to update it and confirm if the problem persists?
>
> ```
> pip install -U huggingface_hub
> ```
My problem solved after updating huggingface hub command. Thanks a lot and sorry for the late reply. | ## Describe the bug
When using `pip install datasets`
or use `conda install -c huggingface -c conda-forge datasets`
cannot install datasets
## Steps to reproduce the bug
```python
from datasets import load_dataset
dataset = load_dataset("sst", "default")
```
## Actual results
---------------------------------------------------------------------------
AttributeError Traceback (most recent call last)
<ipython-input-5-fbe7981e6e21> in <module>
1 import torch
2 import transformers
----> 3 from datasets import load_dataset
4
5 dataset = load_dataset("sst", "default")
~/miniforge3/envs/actor/lib/python3.8/site-packages/datasets/__init__.py in <module>
35 from .arrow_reader import ArrowReader, ReadInstruction
36 from .arrow_writer import ArrowWriter
---> 37 from .builder import ArrowBasedBuilder, BeamBasedBuilder, BuilderConfig, DatasetBuilder, GeneratorBasedBuilder
38 from .combine import interleave_datasets
39 from .dataset_dict import DatasetDict, IterableDatasetDict
~/miniforge3/envs/actor/lib/python3.8/site-packages/datasets/builder.py in <module>
42 )
43 from .arrow_writer import ArrowWriter, BeamWriter
---> 44 from .data_files import DataFilesDict, _sanitize_patterns
45 from .dataset_dict import DatasetDict, IterableDatasetDict
46 from .fingerprint import Hasher
~/miniforge3/envs/actor/lib/python3.8/site-packages/datasets/data_files.py in <module>
118
119 def _exec_patterns_in_dataset_repository(
--> 120 dataset_info: huggingface_hub.hf_api.DatasetInfo,
121 patterns: List[str],
122 allowed_extensions: Optional[list] = None,
AttributeError: module 'huggingface_hub.hf_api' has no attribute 'DatasetInfo'
## Environment info
<!-- You can run the command `datasets-cli env` and copy-and-paste its output below. -->
- `datasets` version: 1.13.3
- Platform: macOS-11.3.1-arm64-arm-64bit
- Python version: 3.8.10
- PyArrow version: 5.0.0
| 137 | AttributeError: module 'huggingface_hub.hf_api' has no attribute 'DatasetInfo'
## Describe the bug
When using `pip install datasets`
or use `conda install -c huggingface -c conda-forge datasets`
cannot install datasets
## Steps to reproduce the bug
```python
from datasets import load_dataset
dataset = load_dataset("sst", "default")
```
## Actual results
---------------------------------------------------------------------------
AttributeError Traceback (most recent call last)
<ipython-input-5-fbe7981e6e21> in <module>
1 import torch
2 import transformers
----> 3 from datasets import load_dataset
4
5 dataset = load_dataset("sst", "default")
~/miniforge3/envs/actor/lib/python3.8/site-packages/datasets/__init__.py in <module>
35 from .arrow_reader import ArrowReader, ReadInstruction
36 from .arrow_writer import ArrowWriter
---> 37 from .builder import ArrowBasedBuilder, BeamBasedBuilder, BuilderConfig, DatasetBuilder, GeneratorBasedBuilder
38 from .combine import interleave_datasets
39 from .dataset_dict import DatasetDict, IterableDatasetDict
~/miniforge3/envs/actor/lib/python3.8/site-packages/datasets/builder.py in <module>
42 )
43 from .arrow_writer import ArrowWriter, BeamWriter
---> 44 from .data_files import DataFilesDict, _sanitize_patterns
45 from .dataset_dict import DatasetDict, IterableDatasetDict
46 from .fingerprint import Hasher
~/miniforge3/envs/actor/lib/python3.8/site-packages/datasets/data_files.py in <module>
118
119 def _exec_patterns_in_dataset_repository(
--> 120 dataset_info: huggingface_hub.hf_api.DatasetInfo,
121 patterns: List[str],
122 allowed_extensions: Optional[list] = None,
AttributeError: module 'huggingface_hub.hf_api' has no attribute 'DatasetInfo'
## Environment info
<!-- You can run the command `datasets-cli env` and copy-and-paste its output below. -->
- `datasets` version: 1.13.3
- Platform: macOS-11.3.1-arm64-arm-64bit
- Python version: 3.8.10
- PyArrow version: 5.0.0
> Hi @JTWang2000, thanks for reporting.
>
> However, I cannot reproduce your reported bug:
>
> ```python
> >>> from datasets import load_dataset
>
> >>> dataset = load_dataset("sst", "default")
> >>> dataset
> DatasetDict({
> train: Dataset({
> features: ['sentence', 'label', 'tokens', 'tree'],
> num_rows: 8544
> })
> validation: Dataset({
> features: ['sentence', 'label', 'tokens', 'tree'],
> num_rows: 1101
> })
> test: Dataset({
> features: ['sentence', 'label', 'tokens', 'tree'],
> num_rows: 2210
> })
> })
> ```
>
> Maybe, the cause is that you have a quite old version of `huggingface_hub`. Could you please try to update it and confirm if the problem persists?
>
> ```
> pip install -U huggingface_hub
> ```
My problem solved after updating huggingface hub command. Thanks a lot and sorry for the late reply. |
https://github.com/huggingface/datasets/issues/3099 | AttributeError: module 'huggingface_hub.hf_api' has no attribute 'DatasetInfo' | @tjruwase, please note that versions of `datsets` and `huggingface_hub` must be compatible one with each other:
- In `datasets` version `1.11.0`, the requirement on `huggingface_hub` was: `huggingface_hub<0.1.0`
https://github.com/huggingface/datasets/blob/2cc00f372a96133e701275eec4d6b26d15257289/setup.py#L90
- Therefore, your installed `huggingface_hub` version `0.0.8` was compatible
- In `datasets` version `1.12.0`, the requirement on `huggingface_hub` was: `huggingface_hub>=0.0.14,<0.1.0`
https://github.com/huggingface/datasets/blob/6c766f9115d686182d76b1b937cb27e099c45d68/setup.py#L104
- Therefore, your installed `huggingface_hub` version `0.0.8` was no longer compatible
- Currently, in `datasets` version `1.15.1`, the requirement on `huggingface_hub` is: `huggingface_hub>=0.1.0,<1.0.0`
https://github.com/huggingface/datasets/blob/018100679d21cf27136f0eccb1c50e3a9c968ce2/setup.py#L102
@JTWang2000, thanks for your answer. I close this issue then. | ## Describe the bug
When using `pip install datasets`
or use `conda install -c huggingface -c conda-forge datasets`
cannot install datasets
## Steps to reproduce the bug
```python
from datasets import load_dataset
dataset = load_dataset("sst", "default")
```
## Actual results
---------------------------------------------------------------------------
AttributeError Traceback (most recent call last)
<ipython-input-5-fbe7981e6e21> in <module>
1 import torch
2 import transformers
----> 3 from datasets import load_dataset
4
5 dataset = load_dataset("sst", "default")
~/miniforge3/envs/actor/lib/python3.8/site-packages/datasets/__init__.py in <module>
35 from .arrow_reader import ArrowReader, ReadInstruction
36 from .arrow_writer import ArrowWriter
---> 37 from .builder import ArrowBasedBuilder, BeamBasedBuilder, BuilderConfig, DatasetBuilder, GeneratorBasedBuilder
38 from .combine import interleave_datasets
39 from .dataset_dict import DatasetDict, IterableDatasetDict
~/miniforge3/envs/actor/lib/python3.8/site-packages/datasets/builder.py in <module>
42 )
43 from .arrow_writer import ArrowWriter, BeamWriter
---> 44 from .data_files import DataFilesDict, _sanitize_patterns
45 from .dataset_dict import DatasetDict, IterableDatasetDict
46 from .fingerprint import Hasher
~/miniforge3/envs/actor/lib/python3.8/site-packages/datasets/data_files.py in <module>
118
119 def _exec_patterns_in_dataset_repository(
--> 120 dataset_info: huggingface_hub.hf_api.DatasetInfo,
121 patterns: List[str],
122 allowed_extensions: Optional[list] = None,
AttributeError: module 'huggingface_hub.hf_api' has no attribute 'DatasetInfo'
## Environment info
<!-- You can run the command `datasets-cli env` and copy-and-paste its output below. -->
- `datasets` version: 1.13.3
- Platform: macOS-11.3.1-arm64-arm-64bit
- Python version: 3.8.10
- PyArrow version: 5.0.0
| 83 | AttributeError: module 'huggingface_hub.hf_api' has no attribute 'DatasetInfo'
## Describe the bug
When using `pip install datasets`
or use `conda install -c huggingface -c conda-forge datasets`
cannot install datasets
## Steps to reproduce the bug
```python
from datasets import load_dataset
dataset = load_dataset("sst", "default")
```
## Actual results
---------------------------------------------------------------------------
AttributeError Traceback (most recent call last)
<ipython-input-5-fbe7981e6e21> in <module>
1 import torch
2 import transformers
----> 3 from datasets import load_dataset
4
5 dataset = load_dataset("sst", "default")
~/miniforge3/envs/actor/lib/python3.8/site-packages/datasets/__init__.py in <module>
35 from .arrow_reader import ArrowReader, ReadInstruction
36 from .arrow_writer import ArrowWriter
---> 37 from .builder import ArrowBasedBuilder, BeamBasedBuilder, BuilderConfig, DatasetBuilder, GeneratorBasedBuilder
38 from .combine import interleave_datasets
39 from .dataset_dict import DatasetDict, IterableDatasetDict
~/miniforge3/envs/actor/lib/python3.8/site-packages/datasets/builder.py in <module>
42 )
43 from .arrow_writer import ArrowWriter, BeamWriter
---> 44 from .data_files import DataFilesDict, _sanitize_patterns
45 from .dataset_dict import DatasetDict, IterableDatasetDict
46 from .fingerprint import Hasher
~/miniforge3/envs/actor/lib/python3.8/site-packages/datasets/data_files.py in <module>
118
119 def _exec_patterns_in_dataset_repository(
--> 120 dataset_info: huggingface_hub.hf_api.DatasetInfo,
121 patterns: List[str],
122 allowed_extensions: Optional[list] = None,
AttributeError: module 'huggingface_hub.hf_api' has no attribute 'DatasetInfo'
## Environment info
<!-- You can run the command `datasets-cli env` and copy-and-paste its output below. -->
- `datasets` version: 1.13.3
- Platform: macOS-11.3.1-arm64-arm-64bit
- Python version: 3.8.10
- PyArrow version: 5.0.0
@tjruwase, please note that versions of `datsets` and `huggingface_hub` must be compatible one with each other:
- In `datasets` version `1.11.0`, the requirement on `huggingface_hub` was: `huggingface_hub<0.1.0`
https://github.com/huggingface/datasets/blob/2cc00f372a96133e701275eec4d6b26d15257289/setup.py#L90
- Therefore, your installed `huggingface_hub` version `0.0.8` was compatible
- In `datasets` version `1.12.0`, the requirement on `huggingface_hub` was: `huggingface_hub>=0.0.14,<0.1.0`
https://github.com/huggingface/datasets/blob/6c766f9115d686182d76b1b937cb27e099c45d68/setup.py#L104
- Therefore, your installed `huggingface_hub` version `0.0.8` was no longer compatible
- Currently, in `datasets` version `1.15.1`, the requirement on `huggingface_hub` is: `huggingface_hub>=0.1.0,<1.0.0`
https://github.com/huggingface/datasets/blob/018100679d21cf27136f0eccb1c50e3a9c968ce2/setup.py#L102
@JTWang2000, thanks for your answer. I close this issue then. |
https://github.com/huggingface/datasets/issues/3095 | `cast_column` makes audio decoding fail | Thanks for reporting, @patrickvonplaten.
I think the issue is related to mp3 resampling, not to `cast_column`.
You can check that `cast_column` works OK with non-mp3 audio files:
```python
from datasets import load_dataset
import datasets
ds = load_dataset("arabic_speech_corpus", split="train")
ds = ds.cast_column("audio", datasets.features.Audio(sampling_rate=16_000))
print(ds[0]["audio"])
```
I'm fixing it. | ## Describe the bug
After changing the sampling rate automatic decoding fails.
## Steps to reproduce the bug
```python
from datasets import load_dataset
import datasets
ds = load_dataset("common_voice", "ab", split="train")
ds = ds.cast_column("audio", datasets.features.Audio(sampling_rate=16_000))
print(ds[0]["audio"]) # <- this fails currently
```
yields:
```
TypeError: forward() takes 2 positional arguments but 4 were given
```
## Expected results
no failure
## Actual results
Specify the actual results or traceback.
## Environment info
<!-- You can run the command `datasets-cli env` and copy-and-paste its output below. -->
Copy-and-paste the text below in your GitHub issue.
- `datasets` version: 1.13.2 (master)
- Platform: Linux-5.11.0-1019-aws-x86_64-with-glibc2.29
- Python version: 3.8.10
- PyArrow version: 5.0.0
| 47 | `cast_column` makes audio decoding fail
## Describe the bug
After changing the sampling rate automatic decoding fails.
## Steps to reproduce the bug
```python
from datasets import load_dataset
import datasets
ds = load_dataset("common_voice", "ab", split="train")
ds = ds.cast_column("audio", datasets.features.Audio(sampling_rate=16_000))
print(ds[0]["audio"]) # <- this fails currently
```
yields:
```
TypeError: forward() takes 2 positional arguments but 4 were given
```
## Expected results
no failure
## Actual results
Specify the actual results or traceback.
## Environment info
<!-- You can run the command `datasets-cli env` and copy-and-paste its output below. -->
Copy-and-paste the text below in your GitHub issue.
- `datasets` version: 1.13.2 (master)
- Platform: Linux-5.11.0-1019-aws-x86_64-with-glibc2.29
- Python version: 3.8.10
- PyArrow version: 5.0.0
Thanks for reporting, @patrickvonplaten.
I think the issue is related to mp3 resampling, not to `cast_column`.
You can check that `cast_column` works OK with non-mp3 audio files:
```python
from datasets import load_dataset
import datasets
ds = load_dataset("arabic_speech_corpus", split="train")
ds = ds.cast_column("audio", datasets.features.Audio(sampling_rate=16_000))
print(ds[0]["audio"])
```
I'm fixing it. |
https://github.com/huggingface/datasets/issues/3094 | Support loading a dataset from SQLite files | for reference Kaggle has a good number of open source datasets stored in sqlite
Alternatively a tutorial or tool on how to convert from sqlite to parquet would be cool too | As requested by @julien-c, we could eventually support loading a dataset from SQLite files, like it is the case for JSON/CSV files. | 31 | Support loading a dataset from SQLite files
As requested by @julien-c, we could eventually support loading a dataset from SQLite files, like it is the case for JSON/CSV files.
for reference Kaggle has a good number of open source datasets stored in sqlite
Alternatively a tutorial or tool on how to convert from sqlite to parquet would be cool too |
https://github.com/huggingface/datasets/issues/3094 | Support loading a dataset from SQLite files | Hello, could we leverage [`pandas.read_sql`](https://pandas.pydata.org/docs/reference/api/pandas.read_sql.html) for this?
This would be basically the same as [`CSVBuilder`](https://github.com/huggingface/datasets/blob/7380140accf522a4363bb56c0b77a4190f49bed6/src/datasets/packaged_modules/csv/csv.py#L127)
, but uses `pandas.read_sql(..., chunksize=1)` instead of `pandas.read_csv(..., iterator=True)`
I'm happy to work on this :)
self-assign | As requested by @julien-c, we could eventually support loading a dataset from SQLite files, like it is the case for JSON/CSV files. | 32 | Support loading a dataset from SQLite files
As requested by @julien-c, we could eventually support loading a dataset from SQLite files, like it is the case for JSON/CSV files.
Hello, could we leverage [`pandas.read_sql`](https://pandas.pydata.org/docs/reference/api/pandas.read_sql.html) for this?
This would be basically the same as [`CSVBuilder`](https://github.com/huggingface/datasets/blob/7380140accf522a4363bb56c0b77a4190f49bed6/src/datasets/packaged_modules/csv/csv.py#L127)
, but uses `pandas.read_sql(..., chunksize=1)` instead of `pandas.read_csv(..., iterator=True)`
I'm happy to work on this :)
self-assign |
https://github.com/huggingface/datasets/issues/3093 | Error loading json dataset with multiple splits if keys in nested dicts have a different order | Hi,
even Pandas, which is less strict compared to PyArrow when it comes to reading JSON, doesn't support different orderings:
```python
import io
import pandas as pd
s = """
{"a": {"c": 8, "b": 5}}
{"a": {"b": 7, "c": 6}}
"""
buffer = io.StringIO(s)
df = pd.read_json(buffer, lines=True)
print(df.shape[0]) # 0
```
So we can't even fall back to Pandas in such cases.
It seems the only option is a script that recursively re-orders fields to enforce deterministic order:
```python
with open("train.json", "r") as fin:
with open("train_reordered.json", "w") as fout:
for line in fin:
obj_jsonl = json.loads(line.strip())
fout.write(json.dumps(obj_jsonl, sort_keys=True) + "\n")
``` | ## Describe the bug
Loading a json dataset with multiple splits that have nested dicts with keys in different order results in the error below.
If the keys in the nested dicts always have the same order or even if you just load a single split in which the nested dicts don't have the same order, everything works fine.
## Steps to reproduce the bug
Create two json files:
train.json
```
{"a": {"c": 8, "b": 5}}
{"a": {"b": 7, "c": 6}}
```
test.json
```
{"a": {"b": 1, "c": 2}}
{"a": {"b": 3, "c": 4}}
```
```python
from datasets import load_dataset
# Loading the files individually works (even though the keys in train.json don't have the same order)
load_dataset('json', data_files={"test": "test.json"})
load_dataset('json', data_files={"train": "train.json"})
# Loading both splits fails
load_dataset('json', data_files={"train": "train.json", "test": "test.json"})
```
## Expected results
Loading both splits should not give an error whether the nested dicts are have the same order or not.
## Actual results
```
>>> load_dataset('json', data_files={"train": "train.json", "test": "test.json"})
Using custom data configuration default-f1bc76fd07398c4c
Downloading and preparing dataset json/default to /home/dthulke/.cache/huggingface/datasets/json/default-f1bc76fd07398c4c/0.0.0/c2d554c3377ea79c7664b93dc65d0803b45e3279000f993c7bfd18937fd7f426...
100%|█████████████████████████████████████████████████████████████████████████████████████████████████████████████████████████████████████████████████████████████████████████████████████████████████████████████████████████████████████████████████████████████████████████████████████████| 2/2 [00:00<00:00, 8839.42it/s]
100%|██████████████████████████████████████████████████████████████████████████████████████████████████████████████████████████████████████████████████████████████████████████████████████████████████████████████████████████████████████████████████████████████████████████████████████████| 2/2 [00:00<00:00, 477.82it/s]
Traceback (most recent call last):
File "<stdin>", line 1, in <module>
File "/home/dthulke/venvs/venv_torch_transformers/lib/python3.6/site-packages/datasets/load.py", line 1632, in load_dataset
use_auth_token=use_auth_token,
File "/home/dthulke/venvs/venv_torch_transformers/lib/python3.6/site-packages/datasets/builder.py", line 608, in download_and_prepare
dl_manager=dl_manager, verify_infos=verify_infos, **download_and_prepare_kwargs
File "/home/dthulke/venvs/venv_torch_transformers/lib/python3.6/site-packages/datasets/builder.py", line 697, in _download_and_prepare
self._prepare_split(split_generator, **prepare_split_kwargs)
File "/home/dthulke/venvs/venv_torch_transformers/lib/python3.6/site-packages/datasets/builder.py", line 1159, in _prepare_split
writer.write_table(table)
File "/home/dthulke/venvs/venv_torch_transformers/lib/python3.6/site-packages/datasets/arrow_writer.py", line 428, in write_table
pa_table = pa.Table.from_arrays([pa_table[name] for name in self._schema.names], schema=self._schema)
File "pyarrow/table.pxi", line 1596, in pyarrow.lib.Table.from_arrays
File "pyarrow/table.pxi", line 592, in pyarrow.lib._sanitize_arrays
File "pyarrow/array.pxi", line 329, in pyarrow.lib.asarray
File "pyarrow/table.pxi", line 277, in pyarrow.lib.ChunkedArray.cast
File "/home/dthulke/venvs/venv_torch_transformers/lib/python3.6/site-packages/pyarrow/compute.py", line 297, in cast
return call_function("cast", [arr], options)
File "pyarrow/_compute.pyx", line 527, in pyarrow._compute.call_function
File "pyarrow/_compute.pyx", line 337, in pyarrow._compute.Function.call
File "pyarrow/error.pxi", line 143, in pyarrow.lib.pyarrow_internal_check_status
File "pyarrow/error.pxi", line 120, in pyarrow.lib.check_status
pyarrow.lib.ArrowNotImplementedError: Unsupported cast from struct<b: int64, c: int64> to struct using function cast_struct
```
## Environment info
- `datasets` version: 1.13.2
- Platform: Linux-4.15.0-147-generic-x86_64-with-Ubuntu-18.04-bionic
- Python version: 3.6.9
- PyArrow version: 5.0.0
| 102 | Error loading json dataset with multiple splits if keys in nested dicts have a different order
## Describe the bug
Loading a json dataset with multiple splits that have nested dicts with keys in different order results in the error below.
If the keys in the nested dicts always have the same order or even if you just load a single split in which the nested dicts don't have the same order, everything works fine.
## Steps to reproduce the bug
Create two json files:
train.json
```
{"a": {"c": 8, "b": 5}}
{"a": {"b": 7, "c": 6}}
```
test.json
```
{"a": {"b": 1, "c": 2}}
{"a": {"b": 3, "c": 4}}
```
```python
from datasets import load_dataset
# Loading the files individually works (even though the keys in train.json don't have the same order)
load_dataset('json', data_files={"test": "test.json"})
load_dataset('json', data_files={"train": "train.json"})
# Loading both splits fails
load_dataset('json', data_files={"train": "train.json", "test": "test.json"})
```
## Expected results
Loading both splits should not give an error whether the nested dicts are have the same order or not.
## Actual results
```
>>> load_dataset('json', data_files={"train": "train.json", "test": "test.json"})
Using custom data configuration default-f1bc76fd07398c4c
Downloading and preparing dataset json/default to /home/dthulke/.cache/huggingface/datasets/json/default-f1bc76fd07398c4c/0.0.0/c2d554c3377ea79c7664b93dc65d0803b45e3279000f993c7bfd18937fd7f426...
100%|█████████████████████████████████████████████████████████████████████████████████████████████████████████████████████████████████████████████████████████████████████████████████████████████████████████████████████████████████████████████████████████████████████████████████████████| 2/2 [00:00<00:00, 8839.42it/s]
100%|██████████████████████████████████████████████████████████████████████████████████████████████████████████████████████████████████████████████████████████████████████████████████████████████████████████████████████████████████████████████████████████████████████████████████████████| 2/2 [00:00<00:00, 477.82it/s]
Traceback (most recent call last):
File "<stdin>", line 1, in <module>
File "/home/dthulke/venvs/venv_torch_transformers/lib/python3.6/site-packages/datasets/load.py", line 1632, in load_dataset
use_auth_token=use_auth_token,
File "/home/dthulke/venvs/venv_torch_transformers/lib/python3.6/site-packages/datasets/builder.py", line 608, in download_and_prepare
dl_manager=dl_manager, verify_infos=verify_infos, **download_and_prepare_kwargs
File "/home/dthulke/venvs/venv_torch_transformers/lib/python3.6/site-packages/datasets/builder.py", line 697, in _download_and_prepare
self._prepare_split(split_generator, **prepare_split_kwargs)
File "/home/dthulke/venvs/venv_torch_transformers/lib/python3.6/site-packages/datasets/builder.py", line 1159, in _prepare_split
writer.write_table(table)
File "/home/dthulke/venvs/venv_torch_transformers/lib/python3.6/site-packages/datasets/arrow_writer.py", line 428, in write_table
pa_table = pa.Table.from_arrays([pa_table[name] for name in self._schema.names], schema=self._schema)
File "pyarrow/table.pxi", line 1596, in pyarrow.lib.Table.from_arrays
File "pyarrow/table.pxi", line 592, in pyarrow.lib._sanitize_arrays
File "pyarrow/array.pxi", line 329, in pyarrow.lib.asarray
File "pyarrow/table.pxi", line 277, in pyarrow.lib.ChunkedArray.cast
File "/home/dthulke/venvs/venv_torch_transformers/lib/python3.6/site-packages/pyarrow/compute.py", line 297, in cast
return call_function("cast", [arr], options)
File "pyarrow/_compute.pyx", line 527, in pyarrow._compute.call_function
File "pyarrow/_compute.pyx", line 337, in pyarrow._compute.Function.call
File "pyarrow/error.pxi", line 143, in pyarrow.lib.pyarrow_internal_check_status
File "pyarrow/error.pxi", line 120, in pyarrow.lib.check_status
pyarrow.lib.ArrowNotImplementedError: Unsupported cast from struct<b: int64, c: int64> to struct using function cast_struct
```
## Environment info
- `datasets` version: 1.13.2
- Platform: Linux-4.15.0-147-generic-x86_64-with-Ubuntu-18.04-bionic
- Python version: 3.6.9
- PyArrow version: 5.0.0
Hi,
even Pandas, which is less strict compared to PyArrow when it comes to reading JSON, doesn't support different orderings:
```python
import io
import pandas as pd
s = """
{"a": {"c": 8, "b": 5}}
{"a": {"b": 7, "c": 6}}
"""
buffer = io.StringIO(s)
df = pd.read_json(buffer, lines=True)
print(df.shape[0]) # 0
```
So we can't even fall back to Pandas in such cases.
It seems the only option is a script that recursively re-orders fields to enforce deterministic order:
```python
with open("train.json", "r") as fin:
with open("train_reordered.json", "w") as fout:
for line in fin:
obj_jsonl = json.loads(line.strip())
fout.write(json.dumps(obj_jsonl, sort_keys=True) + "\n")
``` |
https://github.com/huggingface/datasets/issues/3091 | `blog_authorship_corpus` is broken | Hi @fdtomasi, thanks for reporting.
You are right: the original host data URL does no longer exist.
I've contacted the authors of the dataset to ask them if they host this dataset in another URL. | ## Describe the bug
The dataset `blog_authorship_corpus` is broken.
By bypassing the checksum checks, the loading does not return any error but the resulting dataset is empty.
I suspect it is because the data download url is broken (http://www.cs.biu.ac.il/~koppel/blogs/blogs.zip).
## Steps to reproduce the bug
```python
from datasets import load_dataset
ds = load_dataset("blog_authorship_corpus", split="train", download_mode='force_redownload')
```
## Expected results
No error.
## Actual results
```
---------------------------------------------------------------------------
NonMatchingChecksumError Traceback (most recent call last)
/tmp/ipykernel_5237/1729238701.py in <module>
2 ds = load_dataset(
3 "blog_authorship_corpus", split="train",
----> 4 download_mode='force_redownload'
5 )
/opt/conda/lib/python3.7/site-packages/datasets/load.py in load_dataset(path, name, data_dir, data_files, split, cache_dir, features, download_config, download_mode, ignore_verifications, keep_in_memory, save_infos, script_version, use_auth_token, task, streaming, **config_kwargs)
1115 ignore_verifications=ignore_verifications,
1116 try_from_hf_gcs=try_from_hf_gcs,
-> 1117 use_auth_token=use_auth_token,
1118 )
1119
/opt/conda/lib/python3.7/site-packages/datasets/builder.py in download_and_prepare(self, download_config, download_mode, ignore_verifications, try_from_hf_gcs, dl_manager, base_path, use_auth_token, **download_and_prepare_kwargs)
635 if not downloaded_from_gcs:
636 self._download_and_prepare(
--> 637 dl_manager=dl_manager, verify_infos=verify_infos, **download_and_prepare_kwargs
638 )
639 # Sync info
/opt/conda/lib/python3.7/site-packages/datasets/builder.py in _download_and_prepare(self, dl_manager, verify_infos, **prepare_split_kwargs)
707 if verify_infos:
708 verify_checksums(
--> 709 self.info.download_checksums, dl_manager.get_recorded_sizes_checksums(), "dataset source files"
710 )
711
/opt/conda/lib/python3.7/site-packages/datasets/utils/info_utils.py in verify_checksums(expected_checksums, recorded_checksums, verification_name)
38 if len(bad_urls) > 0:
39 error_msg = "Checksums didn't match" + for_verification_name + ":\n"
---> 40 raise NonMatchingChecksumError(error_msg + str(bad_urls))
41 logger.info("All the checksums matched successfully" + for_verification_name)
42
NonMatchingChecksumError: Checksums didn't match for dataset source files:
['http://www.cs.biu.ac.il/~koppel/blogs/blogs.zip']
```
## Environment info
- `datasets` version: 1.13.2
- Platform: Linux-4.19.0-18-cloud-amd64-x86_64-with-debian-10.11
- Python version: 3.7.10
- PyArrow version: 5.0.0 | 35 | `blog_authorship_corpus` is broken
## Describe the bug
The dataset `blog_authorship_corpus` is broken.
By bypassing the checksum checks, the loading does not return any error but the resulting dataset is empty.
I suspect it is because the data download url is broken (http://www.cs.biu.ac.il/~koppel/blogs/blogs.zip).
## Steps to reproduce the bug
```python
from datasets import load_dataset
ds = load_dataset("blog_authorship_corpus", split="train", download_mode='force_redownload')
```
## Expected results
No error.
## Actual results
```
---------------------------------------------------------------------------
NonMatchingChecksumError Traceback (most recent call last)
/tmp/ipykernel_5237/1729238701.py in <module>
2 ds = load_dataset(
3 "blog_authorship_corpus", split="train",
----> 4 download_mode='force_redownload'
5 )
/opt/conda/lib/python3.7/site-packages/datasets/load.py in load_dataset(path, name, data_dir, data_files, split, cache_dir, features, download_config, download_mode, ignore_verifications, keep_in_memory, save_infos, script_version, use_auth_token, task, streaming, **config_kwargs)
1115 ignore_verifications=ignore_verifications,
1116 try_from_hf_gcs=try_from_hf_gcs,
-> 1117 use_auth_token=use_auth_token,
1118 )
1119
/opt/conda/lib/python3.7/site-packages/datasets/builder.py in download_and_prepare(self, download_config, download_mode, ignore_verifications, try_from_hf_gcs, dl_manager, base_path, use_auth_token, **download_and_prepare_kwargs)
635 if not downloaded_from_gcs:
636 self._download_and_prepare(
--> 637 dl_manager=dl_manager, verify_infos=verify_infos, **download_and_prepare_kwargs
638 )
639 # Sync info
/opt/conda/lib/python3.7/site-packages/datasets/builder.py in _download_and_prepare(self, dl_manager, verify_infos, **prepare_split_kwargs)
707 if verify_infos:
708 verify_checksums(
--> 709 self.info.download_checksums, dl_manager.get_recorded_sizes_checksums(), "dataset source files"
710 )
711
/opt/conda/lib/python3.7/site-packages/datasets/utils/info_utils.py in verify_checksums(expected_checksums, recorded_checksums, verification_name)
38 if len(bad_urls) > 0:
39 error_msg = "Checksums didn't match" + for_verification_name + ":\n"
---> 40 raise NonMatchingChecksumError(error_msg + str(bad_urls))
41 logger.info("All the checksums matched successfully" + for_verification_name)
42
NonMatchingChecksumError: Checksums didn't match for dataset source files:
['http://www.cs.biu.ac.il/~koppel/blogs/blogs.zip']
```
## Environment info
- `datasets` version: 1.13.2
- Platform: Linux-4.19.0-18-cloud-amd64-x86_64-with-debian-10.11
- Python version: 3.7.10
- PyArrow version: 5.0.0
Hi @fdtomasi, thanks for reporting.
You are right: the original host data URL does no longer exist.
I've contacted the authors of the dataset to ask them if they host this dataset in another URL. |
https://github.com/huggingface/datasets/issues/3091 | `blog_authorship_corpus` is broken | Hi, @fdtomasi, the URL is fixed.
The fix is already in our master branch and it will be accessible in our next release.
In the meantime, you can include the fix if you install the `datasets` library from the master branch:
```
pip install -U git+ssh://git@github.com/huggingface/datasets.git@master#egg=datasest
```
or
```
pip install -U git+https://github.com/huggingface/datasets.git@master#egg=datasets
``` | ## Describe the bug
The dataset `blog_authorship_corpus` is broken.
By bypassing the checksum checks, the loading does not return any error but the resulting dataset is empty.
I suspect it is because the data download url is broken (http://www.cs.biu.ac.il/~koppel/blogs/blogs.zip).
## Steps to reproduce the bug
```python
from datasets import load_dataset
ds = load_dataset("blog_authorship_corpus", split="train", download_mode='force_redownload')
```
## Expected results
No error.
## Actual results
```
---------------------------------------------------------------------------
NonMatchingChecksumError Traceback (most recent call last)
/tmp/ipykernel_5237/1729238701.py in <module>
2 ds = load_dataset(
3 "blog_authorship_corpus", split="train",
----> 4 download_mode='force_redownload'
5 )
/opt/conda/lib/python3.7/site-packages/datasets/load.py in load_dataset(path, name, data_dir, data_files, split, cache_dir, features, download_config, download_mode, ignore_verifications, keep_in_memory, save_infos, script_version, use_auth_token, task, streaming, **config_kwargs)
1115 ignore_verifications=ignore_verifications,
1116 try_from_hf_gcs=try_from_hf_gcs,
-> 1117 use_auth_token=use_auth_token,
1118 )
1119
/opt/conda/lib/python3.7/site-packages/datasets/builder.py in download_and_prepare(self, download_config, download_mode, ignore_verifications, try_from_hf_gcs, dl_manager, base_path, use_auth_token, **download_and_prepare_kwargs)
635 if not downloaded_from_gcs:
636 self._download_and_prepare(
--> 637 dl_manager=dl_manager, verify_infos=verify_infos, **download_and_prepare_kwargs
638 )
639 # Sync info
/opt/conda/lib/python3.7/site-packages/datasets/builder.py in _download_and_prepare(self, dl_manager, verify_infos, **prepare_split_kwargs)
707 if verify_infos:
708 verify_checksums(
--> 709 self.info.download_checksums, dl_manager.get_recorded_sizes_checksums(), "dataset source files"
710 )
711
/opt/conda/lib/python3.7/site-packages/datasets/utils/info_utils.py in verify_checksums(expected_checksums, recorded_checksums, verification_name)
38 if len(bad_urls) > 0:
39 error_msg = "Checksums didn't match" + for_verification_name + ":\n"
---> 40 raise NonMatchingChecksumError(error_msg + str(bad_urls))
41 logger.info("All the checksums matched successfully" + for_verification_name)
42
NonMatchingChecksumError: Checksums didn't match for dataset source files:
['http://www.cs.biu.ac.il/~koppel/blogs/blogs.zip']
```
## Environment info
- `datasets` version: 1.13.2
- Platform: Linux-4.19.0-18-cloud-amd64-x86_64-with-debian-10.11
- Python version: 3.7.10
- PyArrow version: 5.0.0 | 54 | `blog_authorship_corpus` is broken
## Describe the bug
The dataset `blog_authorship_corpus` is broken.
By bypassing the checksum checks, the loading does not return any error but the resulting dataset is empty.
I suspect it is because the data download url is broken (http://www.cs.biu.ac.il/~koppel/blogs/blogs.zip).
## Steps to reproduce the bug
```python
from datasets import load_dataset
ds = load_dataset("blog_authorship_corpus", split="train", download_mode='force_redownload')
```
## Expected results
No error.
## Actual results
```
---------------------------------------------------------------------------
NonMatchingChecksumError Traceback (most recent call last)
/tmp/ipykernel_5237/1729238701.py in <module>
2 ds = load_dataset(
3 "blog_authorship_corpus", split="train",
----> 4 download_mode='force_redownload'
5 )
/opt/conda/lib/python3.7/site-packages/datasets/load.py in load_dataset(path, name, data_dir, data_files, split, cache_dir, features, download_config, download_mode, ignore_verifications, keep_in_memory, save_infos, script_version, use_auth_token, task, streaming, **config_kwargs)
1115 ignore_verifications=ignore_verifications,
1116 try_from_hf_gcs=try_from_hf_gcs,
-> 1117 use_auth_token=use_auth_token,
1118 )
1119
/opt/conda/lib/python3.7/site-packages/datasets/builder.py in download_and_prepare(self, download_config, download_mode, ignore_verifications, try_from_hf_gcs, dl_manager, base_path, use_auth_token, **download_and_prepare_kwargs)
635 if not downloaded_from_gcs:
636 self._download_and_prepare(
--> 637 dl_manager=dl_manager, verify_infos=verify_infos, **download_and_prepare_kwargs
638 )
639 # Sync info
/opt/conda/lib/python3.7/site-packages/datasets/builder.py in _download_and_prepare(self, dl_manager, verify_infos, **prepare_split_kwargs)
707 if verify_infos:
708 verify_checksums(
--> 709 self.info.download_checksums, dl_manager.get_recorded_sizes_checksums(), "dataset source files"
710 )
711
/opt/conda/lib/python3.7/site-packages/datasets/utils/info_utils.py in verify_checksums(expected_checksums, recorded_checksums, verification_name)
38 if len(bad_urls) > 0:
39 error_msg = "Checksums didn't match" + for_verification_name + ":\n"
---> 40 raise NonMatchingChecksumError(error_msg + str(bad_urls))
41 logger.info("All the checksums matched successfully" + for_verification_name)
42
NonMatchingChecksumError: Checksums didn't match for dataset source files:
['http://www.cs.biu.ac.il/~koppel/blogs/blogs.zip']
```
## Environment info
- `datasets` version: 1.13.2
- Platform: Linux-4.19.0-18-cloud-amd64-x86_64-with-debian-10.11
- Python version: 3.7.10
- PyArrow version: 5.0.0
Hi, @fdtomasi, the URL is fixed.
The fix is already in our master branch and it will be accessible in our next release.
In the meantime, you can include the fix if you install the `datasets` library from the master branch:
```
pip install -U git+ssh://git@github.com/huggingface/datasets.git@master#egg=datasest
```
or
```
pip install -U git+https://github.com/huggingface/datasets.git@master#egg=datasets
``` |
https://github.com/huggingface/datasets/issues/3089 | JNLPBA Dataset | # Steps to reproduce
To reproduce:
```python
from datasets import load_dataset
dataset = load_dataset('jnlpba')
dataset['train'].features['ner_tags']
```
Output:
```python
Sequence(feature=ClassLabel(num_classes=3, names=['O', 'B', 'I'], names_file=None, id=None), length=-1, id=None)
```
| ## Describe the bug
A clear and concise description of what the bug is.
## Steps to reproduce the bug
```python
# Sample code to reproduce the bug
```
## Expected results
The dataset loading script for this dataset is incorrect. This is a biomedical dataset used for named entity recognition. The entities in the [script](https://github.com/huggingface/datasets/blob/master/datasets/jnlpba/jnlpba.py#L81-L83) are: O, B, and I. The correct entities from the original data file are:
['O',
'B-DNA',
'I-DNA',
'B-RNA',
'I-RNA',
'B-cell_line',
'I-cell_line',
'B-cell_type',
'I-cell_type',
'B-protein',
'I-protein']
## Actual results
The dataset loader script needs to include the following NER names:
['O',
'B-DNA',
'I-DNA',
'B-RNA',
'I-RNA',
'B-cell_line',
'I-cell_line',
'B-cell_type',
'I-cell_type',
'B-protein',
'I-protein']
And the [data](https://github.com/huggingface/datasets/blob/master/datasets/jnlpba/jnlpba.py#L46) that is being pulled has been modified from the original dataset and does not include the original NER tags.
## Environment info
<!-- You can run the command `datasets-cli env` and copy-and-paste its output below. -->
- `datasets` version:
- Platform:
- Python version:
- PyArrow version:
| 27 | JNLPBA Dataset
## Describe the bug
A clear and concise description of what the bug is.
## Steps to reproduce the bug
```python
# Sample code to reproduce the bug
```
## Expected results
The dataset loading script for this dataset is incorrect. This is a biomedical dataset used for named entity recognition. The entities in the [script](https://github.com/huggingface/datasets/blob/master/datasets/jnlpba/jnlpba.py#L81-L83) are: O, B, and I. The correct entities from the original data file are:
['O',
'B-DNA',
'I-DNA',
'B-RNA',
'I-RNA',
'B-cell_line',
'I-cell_line',
'B-cell_type',
'I-cell_type',
'B-protein',
'I-protein']
## Actual results
The dataset loader script needs to include the following NER names:
['O',
'B-DNA',
'I-DNA',
'B-RNA',
'I-RNA',
'B-cell_line',
'I-cell_line',
'B-cell_type',
'I-cell_type',
'B-protein',
'I-protein']
And the [data](https://github.com/huggingface/datasets/blob/master/datasets/jnlpba/jnlpba.py#L46) that is being pulled has been modified from the original dataset and does not include the original NER tags.
## Environment info
<!-- You can run the command `datasets-cli env` and copy-and-paste its output below. -->
- `datasets` version:
- Platform:
- Python version:
- PyArrow version:
# Steps to reproduce
To reproduce:
```python
from datasets import load_dataset
dataset = load_dataset('jnlpba')
dataset['train'].features['ner_tags']
```
Output:
```python
Sequence(feature=ClassLabel(num_classes=3, names=['O', 'B', 'I'], names_file=None, id=None), length=-1, id=None)
```
|
https://github.com/huggingface/datasets/issues/3089 | JNLPBA Dataset | Since I cannot create a branch here is the updated code:
```python
# coding=utf-8
# Copyright 2020 HuggingFace Datasets Authors.
#
# Licensed under the Apache License, Version 2.0 (the "License");
# you may not use this file except in compliance with the License.
# You may obtain a copy of the License at
#
# http://www.apache.org/licenses/LICENSE-2.0
#
# Unless required by applicable law or agreed to in writing, software
# distributed under the License is distributed on an "AS IS" BASIS,
# WITHOUT WARRANTIES OR CONDITIONS OF ANY KIND, either express or implied.
# See the License for the specific language governing permissions and
# limitations under the License.
# Lint as: python3
"""Introduction to the Bio-Entity Recognition Task at JNLPBA"""
import os
import datasets
logger = datasets.logging.get_logger(__name__)
_CITATION = """\
@inproceedings{kim2004introduction,
title={Introduction to the bio-entity recognition task at JNLPBA},
author={Kim, Jin-Dong and Ohta, Tomoko and Tsuruoka, Yoshimasa and Tateisi, Yuka and Collier, Nigel},
booktitle={Proceedings of the international joint workshop on natural language processing in biomedicine and its applications},
pages={70--75},
year={2004},
organization={Citeseer}
}
"""
_DESCRIPTION = """\
The data came from the GENIA version 3.02 corpus (Kim et al., 2003). This was formed from a controlled search
on MEDLINE using the MeSH terms human, blood cells and transcription factors. From this search 2,000 abstracts
were selected and hand annotated according to a small taxonomy of 48 classes based on a chemical classification.
Among the classes, 36 terminal classes were used to annotate the GENIA corpus.
"""
_HOMEPAGE = "http://www.geniaproject.org/shared-tasks/bionlp-jnlpba-shared-task-2004"
_TRAIN_URL = "http://www.nactem.ac.uk/GENIA/current/Shared-tasks/JNLPBA/Train/Genia4ERtraining.tar.gz"
_VAL_URL = 'http://www.nactem.ac.uk/GENIA/current/Shared-tasks/JNLPBA/Evaluation/Genia4ERtest.tar.gz'
_URLS = {
"train": _TRAIN_URL,
"val": _VAL_URL,
}
_TRAIN_DIRECTORY = "Genia4ERtraining"
_VAL_DIRECTORY = "Genia4ERtest"
_TRAIN_FILE = "Genia4ERtask1.iob2"
_VAL_FILE = "Genia4EReval1.iob2"
class JNLPBAConfig(datasets.BuilderConfig):
"""BuilderConfig for JNLPBA"""
def __init__(self, **kwargs):
"""BuilderConfig for JNLPBA.
Args:
**kwargs: keyword arguments forwarded to super.
"""
super(JNLPBAConfig, self).__init__(**kwargs)
class JNLPBA(datasets.GeneratorBasedBuilder):
"""JNLPBA dataset."""
BUILDER_CONFIGS = [
JNLPBAConfig(name="jnlpba", version=datasets.Version("1.0.0"), description="JNLPBA dataset"),
]
def _info(self):
return datasets.DatasetInfo(
description=_DESCRIPTION,
features=datasets.Features(
{
"id": datasets.Value("string"),
"tokens": datasets.Sequence(datasets.Value("string")),
"ner_tags": datasets.Sequence(
datasets.features.ClassLabel(
names=[
'O',
'B-DNA',
'I-DNA',
'B-RNA',
'I-RNA',
'B-cell_line',
'I-cell_line',
'B-cell_type',
'I-cell_type',
'B-protein',
'I-protein',
]
)
),
}
),
supervised_keys=None,
homepage=_HOMEPAGE,
citation=_CITATION,
)
def _split_generators(self, dl_manager):
downloaded_files = dl_manager.download_and_extract(_URLS)
return [
datasets.SplitGenerator(name=datasets.Split.TRAIN,
gen_kwargs={"filepath": os.path.join(downloaded_files['train'], _TRAIN_FILE)}),
datasets.SplitGenerator(name=datasets.Split.VALIDATION,
gen_kwargs={"filepath": os.path.join(downloaded_files['val'], _VAL_FILE)})
]
def _generate_examples(self, filepath):
logger.info("⏳ Generating examples from = %s", filepath)
with open(filepath, encoding="utf-8") as f:
guid = 0
tokens = []
ner_tags = []
for line in f:
if line.startswith('###'):
continue
if line == '' or line == '\n':
if tokens:
yield guid, {
"id": str(guid),
"tokens": tokens,
"ner_tags": ner_tags,
}
guid += 1
tokens = []
ner_tags = []
else:
# tokens are tab separated
splits = line.split("\t")
#print(splits)
#print(len(splits))
if len(splits) < 2:
splits = splits[0].split()
tokens.append(splits[0])
ner_tags.append(splits[1].rstrip())
# last example
yield guid, {
"id": str(guid),
"tokens": tokens,
"ner_tags": ner_tags,
}
``` | ## Describe the bug
A clear and concise description of what the bug is.
## Steps to reproduce the bug
```python
# Sample code to reproduce the bug
```
## Expected results
The dataset loading script for this dataset is incorrect. This is a biomedical dataset used for named entity recognition. The entities in the [script](https://github.com/huggingface/datasets/blob/master/datasets/jnlpba/jnlpba.py#L81-L83) are: O, B, and I. The correct entities from the original data file are:
['O',
'B-DNA',
'I-DNA',
'B-RNA',
'I-RNA',
'B-cell_line',
'I-cell_line',
'B-cell_type',
'I-cell_type',
'B-protein',
'I-protein']
## Actual results
The dataset loader script needs to include the following NER names:
['O',
'B-DNA',
'I-DNA',
'B-RNA',
'I-RNA',
'B-cell_line',
'I-cell_line',
'B-cell_type',
'I-cell_type',
'B-protein',
'I-protein']
And the [data](https://github.com/huggingface/datasets/blob/master/datasets/jnlpba/jnlpba.py#L46) that is being pulled has been modified from the original dataset and does not include the original NER tags.
## Environment info
<!-- You can run the command `datasets-cli env` and copy-and-paste its output below. -->
- `datasets` version:
- Platform:
- Python version:
- PyArrow version:
| 455 | JNLPBA Dataset
## Describe the bug
A clear and concise description of what the bug is.
## Steps to reproduce the bug
```python
# Sample code to reproduce the bug
```
## Expected results
The dataset loading script for this dataset is incorrect. This is a biomedical dataset used for named entity recognition. The entities in the [script](https://github.com/huggingface/datasets/blob/master/datasets/jnlpba/jnlpba.py#L81-L83) are: O, B, and I. The correct entities from the original data file are:
['O',
'B-DNA',
'I-DNA',
'B-RNA',
'I-RNA',
'B-cell_line',
'I-cell_line',
'B-cell_type',
'I-cell_type',
'B-protein',
'I-protein']
## Actual results
The dataset loader script needs to include the following NER names:
['O',
'B-DNA',
'I-DNA',
'B-RNA',
'I-RNA',
'B-cell_line',
'I-cell_line',
'B-cell_type',
'I-cell_type',
'B-protein',
'I-protein']
And the [data](https://github.com/huggingface/datasets/blob/master/datasets/jnlpba/jnlpba.py#L46) that is being pulled has been modified from the original dataset and does not include the original NER tags.
## Environment info
<!-- You can run the command `datasets-cli env` and copy-and-paste its output below. -->
- `datasets` version:
- Platform:
- Python version:
- PyArrow version:
Since I cannot create a branch here is the updated code:
```python
# coding=utf-8
# Copyright 2020 HuggingFace Datasets Authors.
#
# Licensed under the Apache License, Version 2.0 (the "License");
# you may not use this file except in compliance with the License.
# You may obtain a copy of the License at
#
# http://www.apache.org/licenses/LICENSE-2.0
#
# Unless required by applicable law or agreed to in writing, software
# distributed under the License is distributed on an "AS IS" BASIS,
# WITHOUT WARRANTIES OR CONDITIONS OF ANY KIND, either express or implied.
# See the License for the specific language governing permissions and
# limitations under the License.
# Lint as: python3
"""Introduction to the Bio-Entity Recognition Task at JNLPBA"""
import os
import datasets
logger = datasets.logging.get_logger(__name__)
_CITATION = """\
@inproceedings{kim2004introduction,
title={Introduction to the bio-entity recognition task at JNLPBA},
author={Kim, Jin-Dong and Ohta, Tomoko and Tsuruoka, Yoshimasa and Tateisi, Yuka and Collier, Nigel},
booktitle={Proceedings of the international joint workshop on natural language processing in biomedicine and its applications},
pages={70--75},
year={2004},
organization={Citeseer}
}
"""
_DESCRIPTION = """\
The data came from the GENIA version 3.02 corpus (Kim et al., 2003). This was formed from a controlled search
on MEDLINE using the MeSH terms human, blood cells and transcription factors. From this search 2,000 abstracts
were selected and hand annotated according to a small taxonomy of 48 classes based on a chemical classification.
Among the classes, 36 terminal classes were used to annotate the GENIA corpus.
"""
_HOMEPAGE = "http://www.geniaproject.org/shared-tasks/bionlp-jnlpba-shared-task-2004"
_TRAIN_URL = "http://www.nactem.ac.uk/GENIA/current/Shared-tasks/JNLPBA/Train/Genia4ERtraining.tar.gz"
_VAL_URL = 'http://www.nactem.ac.uk/GENIA/current/Shared-tasks/JNLPBA/Evaluation/Genia4ERtest.tar.gz'
_URLS = {
"train": _TRAIN_URL,
"val": _VAL_URL,
}
_TRAIN_DIRECTORY = "Genia4ERtraining"
_VAL_DIRECTORY = "Genia4ERtest"
_TRAIN_FILE = "Genia4ERtask1.iob2"
_VAL_FILE = "Genia4EReval1.iob2"
class JNLPBAConfig(datasets.BuilderConfig):
"""BuilderConfig for JNLPBA"""
def __init__(self, **kwargs):
"""BuilderConfig for JNLPBA.
Args:
**kwargs: keyword arguments forwarded to super.
"""
super(JNLPBAConfig, self).__init__(**kwargs)
class JNLPBA(datasets.GeneratorBasedBuilder):
"""JNLPBA dataset."""
BUILDER_CONFIGS = [
JNLPBAConfig(name="jnlpba", version=datasets.Version("1.0.0"), description="JNLPBA dataset"),
]
def _info(self):
return datasets.DatasetInfo(
description=_DESCRIPTION,
features=datasets.Features(
{
"id": datasets.Value("string"),
"tokens": datasets.Sequence(datasets.Value("string")),
"ner_tags": datasets.Sequence(
datasets.features.ClassLabel(
names=[
'O',
'B-DNA',
'I-DNA',
'B-RNA',
'I-RNA',
'B-cell_line',
'I-cell_line',
'B-cell_type',
'I-cell_type',
'B-protein',
'I-protein',
]
)
),
}
),
supervised_keys=None,
homepage=_HOMEPAGE,
citation=_CITATION,
)
def _split_generators(self, dl_manager):
downloaded_files = dl_manager.download_and_extract(_URLS)
return [
datasets.SplitGenerator(name=datasets.Split.TRAIN,
gen_kwargs={"filepath": os.path.join(downloaded_files['train'], _TRAIN_FILE)}),
datasets.SplitGenerator(name=datasets.Split.VALIDATION,
gen_kwargs={"filepath": os.path.join(downloaded_files['val'], _VAL_FILE)})
]
def _generate_examples(self, filepath):
logger.info("⏳ Generating examples from = %s", filepath)
with open(filepath, encoding="utf-8") as f:
guid = 0
tokens = []
ner_tags = []
for line in f:
if line.startswith('###'):
continue
if line == '' or line == '\n':
if tokens:
yield guid, {
"id": str(guid),
"tokens": tokens,
"ner_tags": ner_tags,
}
guid += 1
tokens = []
ner_tags = []
else:
# tokens are tab separated
splits = line.split("\t")
#print(splits)
#print(len(splits))
if len(splits) < 2:
splits = splits[0].split()
tokens.append(splits[0])
ner_tags.append(splits[1].rstrip())
# last example
yield guid, {
"id": str(guid),
"tokens": tokens,
"ner_tags": ner_tags,
}
``` |
https://github.com/huggingface/datasets/issues/3084 | VisibleDeprecationWarning when using `set_format("numpy")` | I just opened a PR and I verified that the code you provided doesn't show any deprecation warning :) | Code to reproduce:
```
from datasets import load_dataset
dataset = load_dataset("glue", "mnli")
from transformers import AutoTokenizer
tokenizer = AutoTokenizer.from_pretrained('distilbert-base-cased')
def tokenize_function(dataset):
return tokenizer(dataset['premise'])
tokenized_datasets = dataset.map(tokenize_function, batched=True, remove_columns=dataset['train'].features)
tokenized_datasets.set_format("numpy")
tokenized_datasets['train'][5:8]
```
Outputs:
```
python3.9/site-packages/datasets/formatting/formatting.py:167: VisibleDeprecationWarning: Creating an ndarray from ragged nested sequences (which is a list-or-tuple of lists-or-tuples-or ndarrays with different lengths or shapes) is deprecated. If you meant to do this, you must specify 'dtype=object' when creating the ndarray
return np.array(array, copy=False, **self.np_array_kwargs)
``` | 19 | VisibleDeprecationWarning when using `set_format("numpy")`
Code to reproduce:
```
from datasets import load_dataset
dataset = load_dataset("glue", "mnli")
from transformers import AutoTokenizer
tokenizer = AutoTokenizer.from_pretrained('distilbert-base-cased')
def tokenize_function(dataset):
return tokenizer(dataset['premise'])
tokenized_datasets = dataset.map(tokenize_function, batched=True, remove_columns=dataset['train'].features)
tokenized_datasets.set_format("numpy")
tokenized_datasets['train'][5:8]
```
Outputs:
```
python3.9/site-packages/datasets/formatting/formatting.py:167: VisibleDeprecationWarning: Creating an ndarray from ragged nested sequences (which is a list-or-tuple of lists-or-tuples-or ndarrays with different lengths or shapes) is deprecated. If you meant to do this, you must specify 'dtype=object' when creating the ndarray
return np.array(array, copy=False, **self.np_array_kwargs)
```
I just opened a PR and I verified that the code you provided doesn't show any deprecation warning :) |
https://github.com/huggingface/datasets/issues/3073 | Import error installing with ppc64le | This seems to be an issue with importing PyArrow so I posted the problem [here](https://issues.apache.org/jira/browse/ARROW-14323), and I'm closing this issue.
| ## Describe the bug
Installing the datasets library with a computer running with ppc64le seems to cause an issue when importing the datasets library.
```
python
Python 3.6.13 | packaged by conda-forge | (default, Sep 23 2021, 07:37:44)
[GCC 9.4.0] on linux
Type "help", "copyright", "credits" or "license" for more information.
>>> import datasets
Illegal instruction (core dumped)
```
Error when importing
`Illegal instruction (core dumped)`
## Steps to reproduce the bug
I get this error when installing the library by using conda. I can't install with pip I believe because pyarrow only has the ppc64le library on conda forge
```
conda create --name transformers_py36_v2 python=3.6
conda activate transformers_py36_v2
conda install datasets
```
## Tracebacks
conda create --name transformers_py36_v2 python=3.6
```
Collecting package metadata (current_repodata.json): done
Solving environment: done
==> WARNING: A newer version of conda exists. <==
current version: 4.9.2
latest version: 4.10.3
Please update conda by running
$ conda update -n base -c defaults conda
## Package Plan ##
environment location: /p/home/gerryc/.conda/envs/transformers_py36_v2
added / updated specs:
- python=3.6
The following NEW packages will be INSTALLED:
_libgcc_mutex conda-forge/linux-ppc64le::_libgcc_mutex-0.1-conda_forge
_openmp_mutex conda-forge/linux-ppc64le::_openmp_mutex-4.5-1_gnu
ca-certificates conda-forge/linux-ppc64le::ca-certificates-2021.10.8-h1084571_0
certifi pkgs/main/linux-ppc64le::certifi-2020.12.5-py36h6ffa863_0
ld_impl_linux-ppc~ conda-forge/linux-ppc64le::ld_impl_linux-ppc64le-2.36.1-ha35d02b_2
libffi conda-forge/linux-ppc64le::libffi-3.4.2-h3b9df90_4
libgcc-ng conda-forge/linux-ppc64le::libgcc-ng-11.2.0-h7698a5e_11
libgomp conda-forge/linux-ppc64le::libgomp-11.2.0-h7698a5e_11
libstdcxx-ng conda-forge/linux-ppc64le::libstdcxx-ng-11.2.0-habdf983_11
libzlib conda-forge/linux-ppc64le::libzlib-1.2.11-h339bb43_1013
ncurses conda-forge/linux-ppc64le::ncurses-6.2-hea85c5d_4
openssl conda-forge/linux-ppc64le::openssl-1.1.1l-h4e0d66e_0
pip conda-forge/noarch::pip-21.3-pyhd8ed1ab_0
python conda-forge/linux-ppc64le::python-3.6.13-h57873ef_2_cpython
readline conda-forge/linux-ppc64le::readline-8.1-h5c45dff_0
setuptools pkgs/main/linux-ppc64le::setuptools-58.0.4-py36h6ffa863_0
sqlite conda-forge/linux-ppc64le::sqlite-3.36.0-h4e2196e_2
tk conda-forge/linux-ppc64le::tk-8.6.11-h41c6715_1
wheel conda-forge/noarch::wheel-0.37.0-pyhd8ed1ab_1
xz conda-forge/linux-ppc64le::xz-5.2.5-h6eb9509_1
zlib conda-forge/linux-ppc64le::zlib-1.2.11-h339bb43_1013
Proceed ([y]/n)? y
Preparing transaction: done
Verifying transaction: done
Executing transaction: done
#
# To activate this environment, use
#
# $ conda activate transformers_py36_v2
#
# To deactivate an active environment, use
#
# $ conda deactivate
```
conda activate transformers_py36_v2
conda install datasets
```
Collecting package metadata (current_repodata.json): done
Solving environment: failed with initial frozen solve. Retrying with flexible solve.
Solving environment: failed with repodata from current_repodata.json, will retry with next repodata source.
Collecting package metadata (repodata.json): done
Solving environment: done
==> WARNING: A newer version of conda exists. <==
current version: 4.9.2
latest version: 4.10.3
Please update conda by running
$ conda update -n base -c defaults conda
## Package Plan ##
environment location: /p/home/gerryc/.conda/envs/transformers_py36_v2
added / updated specs:
- datasets
The following NEW packages will be INSTALLED:
abseil-cpp conda-forge/linux-ppc64le::abseil-cpp-20210324.2-h3b9df90_0
aiohttp conda-forge/linux-ppc64le::aiohttp-3.7.4.post0-py36hc33305d_0
arrow-cpp conda-forge/linux-ppc64le::arrow-cpp-5.0.0-py36hf9cf308_8_cpu
async-timeout conda-forge/noarch::async-timeout-3.0.1-py_1000
attrs conda-forge/noarch::attrs-21.2.0-pyhd8ed1ab_0
aws-c-cal conda-forge/linux-ppc64le::aws-c-cal-0.5.11-hb3fac3d_0
aws-c-common conda-forge/linux-ppc64le::aws-c-common-0.6.2-h4e0d66e_0
aws-c-event-stream conda-forge/linux-ppc64le::aws-c-event-stream-0.2.7-h76da5f2_13
aws-c-io conda-forge/linux-ppc64le::aws-c-io-0.10.5-hf6a6c7c_0
aws-checksums conda-forge/linux-ppc64le::aws-checksums-0.1.11-hfe76d68_7
aws-sdk-cpp conda-forge/linux-ppc64le::aws-sdk-cpp-1.8.186-h90855e8_3
brotlipy conda-forge/linux-ppc64le::brotlipy-0.7.0-py36hc33305d_1001
bzip2 conda-forge/linux-ppc64le::bzip2-1.0.8-h4e0d66e_4
c-ares conda-forge/linux-ppc64le::c-ares-1.17.2-h4e0d66e_0
cffi conda-forge/linux-ppc64le::cffi-1.14.6-py36h021ab3c_1
chardet conda-forge/linux-ppc64le::chardet-4.0.0-py36h270354c_1
colorama conda-forge/noarch::colorama-0.4.4-pyh9f0ad1d_0
cryptography conda-forge/linux-ppc64le::cryptography-3.4.7-py36hc71b123_0
dataclasses conda-forge/noarch::dataclasses-0.8-pyh787bdff_2
datasets conda-forge/noarch::datasets-1.12.1-pyhd8ed1ab_1
dill conda-forge/noarch::dill-0.3.4-pyhd8ed1ab_0
filelock conda-forge/noarch::filelock-3.3.0-pyhd8ed1ab_0
fsspec conda-forge/noarch::fsspec-2021.10.0-pyhd8ed1ab_0
gflags conda-forge/linux-ppc64le::gflags-2.2.2-hb209c28_1004
glog conda-forge/linux-ppc64le::glog-0.5.0-h4040248_0
grpc-cpp conda-forge/linux-ppc64le::grpc-cpp-1.40.0-h2bf711c_2
huggingface_hub conda-forge/noarch::huggingface_hub-0.0.19-pyhd8ed1ab_0
idna conda-forge/noarch::idna-2.10-pyh9f0ad1d_0
idna_ssl conda-forge/noarch::idna_ssl-1.0.0-0
importlib-metadata conda-forge/linux-ppc64le::importlib-metadata-4.8.1-py36h270354c_0
importlib_metadata conda-forge/noarch::importlib_metadata-4.8.1-hd8ed1ab_0
krb5 conda-forge/linux-ppc64le::krb5-1.19.2-haf43566_2
libblas conda-forge/linux-ppc64le::libblas-3.9.0-11_linuxppc64le_openblas
libbrotlicommon conda-forge/linux-ppc64le::libbrotlicommon-1.0.9-h4e0d66e_5
libbrotlidec conda-forge/linux-ppc64le::libbrotlidec-1.0.9-h4e0d66e_5
libbrotlienc conda-forge/linux-ppc64le::libbrotlienc-1.0.9-h4e0d66e_5
libcblas conda-forge/linux-ppc64le::libcblas-3.9.0-11_linuxppc64le_openblas
libcurl conda-forge/linux-ppc64le::libcurl-7.79.1-he415e40_1
libedit conda-forge/linux-ppc64le::libedit-3.1.20191231-h41a240f_2
libev conda-forge/linux-ppc64le::libev-4.33-h6eb9509_1
libevent conda-forge/linux-ppc64le::libevent-2.1.10-h97db324_4
libgfortran-ng conda-forge/linux-ppc64le::libgfortran-ng-11.2.0-hfdc3801_11
libgfortran5 conda-forge/linux-ppc64le::libgfortran5-11.2.0-he58fbb4_11
liblapack conda-forge/linux-ppc64le::liblapack-3.9.0-11_linuxppc64le_openblas
libnghttp2 conda-forge/linux-ppc64le::libnghttp2-1.43.0-h42039ad_1
libopenblas conda-forge/linux-ppc64le::libopenblas-0.3.17-pthreads_h486567c_1
libprotobuf conda-forge/linux-ppc64le::libprotobuf-3.18.1-h690f14c_0
libssh2 conda-forge/linux-ppc64le::libssh2-1.10.0-ha5a9321_2
libthrift conda-forge/linux-ppc64le::libthrift-0.15.0-h54f692e_1
libutf8proc conda-forge/linux-ppc64le::libutf8proc-2.6.1-h4e0d66e_0
lz4-c conda-forge/linux-ppc64le::lz4-c-1.9.3-h3b9df90_1
multidict conda-forge/linux-ppc64le::multidict-5.2.0-py36hc33305d_0
multiprocess conda-forge/linux-ppc64le::multiprocess-0.70.12.2-py36hc33305d_0
numpy conda-forge/linux-ppc64le::numpy-1.19.5-py36h86665d4_1
orc conda-forge/linux-ppc64le::orc-1.7.0-hae6b4bd_0
packaging conda-forge/noarch::packaging-21.0-pyhd8ed1ab_0
pandas conda-forge/linux-ppc64le::pandas-1.1.5-py36hab1a6e6_0
parquet-cpp conda-forge/noarch::parquet-cpp-1.5.1-2
pyarrow conda-forge/linux-ppc64le::pyarrow-5.0.0-py36h7a46c7e_8_cpu
pycparser conda-forge/noarch::pycparser-2.20-pyh9f0ad1d_2
pyopenssl conda-forge/noarch::pyopenssl-21.0.0-pyhd8ed1ab_0
pyparsing conda-forge/noarch::pyparsing-2.4.7-pyh9f0ad1d_0
pysocks conda-forge/linux-ppc64le::pysocks-1.7.1-py36h270354c_3
python-dateutil conda-forge/noarch::python-dateutil-2.8.2-pyhd8ed1ab_0
python-xxhash conda-forge/linux-ppc64le::python-xxhash-2.0.2-py36hc33305d_0
python_abi conda-forge/linux-ppc64le::python_abi-3.6-2_cp36m
pytz conda-forge/noarch::pytz-2021.3-pyhd8ed1ab_0
pyyaml conda-forge/linux-ppc64le::pyyaml-5.4.1-py36hc33305d_1
re2 conda-forge/linux-ppc64le::re2-2021.09.01-h3b9df90_0
requests conda-forge/noarch::requests-2.25.1-pyhd3deb0d_0
s2n conda-forge/linux-ppc64le::s2n-1.0.10-h97db324_0
six conda-forge/noarch::six-1.16.0-pyh6c4a22f_0
snappy conda-forge/linux-ppc64le::snappy-1.1.8-hb209c28_3
tqdm conda-forge/noarch::tqdm-4.62.3-pyhd8ed1ab_0
typing-extensions conda-forge/noarch::typing-extensions-3.10.0.2-hd8ed1ab_0
typing_extensions conda-forge/noarch::typing_extensions-3.10.0.2-pyha770c72_0
urllib3 conda-forge/noarch::urllib3-1.26.7-pyhd8ed1ab_0
xxhash conda-forge/linux-ppc64le::xxhash-0.8.0-h4e0d66e_3
yaml conda-forge/linux-ppc64le::yaml-0.2.5-h6eb9509_0
yarl conda-forge/linux-ppc64le::yarl-1.6.3-py36hc33305d_2
zipp conda-forge/noarch::zipp-3.6.0-pyhd8ed1ab_0
zstd conda-forge/linux-ppc64le::zstd-1.5.0-h65c4b1a_0
The following packages will be UPDATED:
certifi pkgs/main::certifi-2020.12.5-py36h6ff~ --> conda-forge::certifi-2021.5.30-py36h270354c_0
Proceed ([y]/n)? y
Preparing transaction: done
Verifying transaction: done
Executing transaction: done
```
## Environment info
<!-- You can run the command `datasets-cli env` and copy-and-paste its output below. -->
- `datasets` version: 1.12.1
- Platform: Red Hat Enterprise Linux 8.2 (Ootpa)
- Python version: 3.6
- PyArrow version: pyarrow - 5.0.0 - py36h7a46c7e_8_cpu - conda-forge
Any help would be appreciated! I've been struggling on installing datasets on this machine.
| 20 | Import error installing with ppc64le
## Describe the bug
Installing the datasets library with a computer running with ppc64le seems to cause an issue when importing the datasets library.
```
python
Python 3.6.13 | packaged by conda-forge | (default, Sep 23 2021, 07:37:44)
[GCC 9.4.0] on linux
Type "help", "copyright", "credits" or "license" for more information.
>>> import datasets
Illegal instruction (core dumped)
```
Error when importing
`Illegal instruction (core dumped)`
## Steps to reproduce the bug
I get this error when installing the library by using conda. I can't install with pip I believe because pyarrow only has the ppc64le library on conda forge
```
conda create --name transformers_py36_v2 python=3.6
conda activate transformers_py36_v2
conda install datasets
```
## Tracebacks
conda create --name transformers_py36_v2 python=3.6
```
Collecting package metadata (current_repodata.json): done
Solving environment: done
==> WARNING: A newer version of conda exists. <==
current version: 4.9.2
latest version: 4.10.3
Please update conda by running
$ conda update -n base -c defaults conda
## Package Plan ##
environment location: /p/home/gerryc/.conda/envs/transformers_py36_v2
added / updated specs:
- python=3.6
The following NEW packages will be INSTALLED:
_libgcc_mutex conda-forge/linux-ppc64le::_libgcc_mutex-0.1-conda_forge
_openmp_mutex conda-forge/linux-ppc64le::_openmp_mutex-4.5-1_gnu
ca-certificates conda-forge/linux-ppc64le::ca-certificates-2021.10.8-h1084571_0
certifi pkgs/main/linux-ppc64le::certifi-2020.12.5-py36h6ffa863_0
ld_impl_linux-ppc~ conda-forge/linux-ppc64le::ld_impl_linux-ppc64le-2.36.1-ha35d02b_2
libffi conda-forge/linux-ppc64le::libffi-3.4.2-h3b9df90_4
libgcc-ng conda-forge/linux-ppc64le::libgcc-ng-11.2.0-h7698a5e_11
libgomp conda-forge/linux-ppc64le::libgomp-11.2.0-h7698a5e_11
libstdcxx-ng conda-forge/linux-ppc64le::libstdcxx-ng-11.2.0-habdf983_11
libzlib conda-forge/linux-ppc64le::libzlib-1.2.11-h339bb43_1013
ncurses conda-forge/linux-ppc64le::ncurses-6.2-hea85c5d_4
openssl conda-forge/linux-ppc64le::openssl-1.1.1l-h4e0d66e_0
pip conda-forge/noarch::pip-21.3-pyhd8ed1ab_0
python conda-forge/linux-ppc64le::python-3.6.13-h57873ef_2_cpython
readline conda-forge/linux-ppc64le::readline-8.1-h5c45dff_0
setuptools pkgs/main/linux-ppc64le::setuptools-58.0.4-py36h6ffa863_0
sqlite conda-forge/linux-ppc64le::sqlite-3.36.0-h4e2196e_2
tk conda-forge/linux-ppc64le::tk-8.6.11-h41c6715_1
wheel conda-forge/noarch::wheel-0.37.0-pyhd8ed1ab_1
xz conda-forge/linux-ppc64le::xz-5.2.5-h6eb9509_1
zlib conda-forge/linux-ppc64le::zlib-1.2.11-h339bb43_1013
Proceed ([y]/n)? y
Preparing transaction: done
Verifying transaction: done
Executing transaction: done
#
# To activate this environment, use
#
# $ conda activate transformers_py36_v2
#
# To deactivate an active environment, use
#
# $ conda deactivate
```
conda activate transformers_py36_v2
conda install datasets
```
Collecting package metadata (current_repodata.json): done
Solving environment: failed with initial frozen solve. Retrying with flexible solve.
Solving environment: failed with repodata from current_repodata.json, will retry with next repodata source.
Collecting package metadata (repodata.json): done
Solving environment: done
==> WARNING: A newer version of conda exists. <==
current version: 4.9.2
latest version: 4.10.3
Please update conda by running
$ conda update -n base -c defaults conda
## Package Plan ##
environment location: /p/home/gerryc/.conda/envs/transformers_py36_v2
added / updated specs:
- datasets
The following NEW packages will be INSTALLED:
abseil-cpp conda-forge/linux-ppc64le::abseil-cpp-20210324.2-h3b9df90_0
aiohttp conda-forge/linux-ppc64le::aiohttp-3.7.4.post0-py36hc33305d_0
arrow-cpp conda-forge/linux-ppc64le::arrow-cpp-5.0.0-py36hf9cf308_8_cpu
async-timeout conda-forge/noarch::async-timeout-3.0.1-py_1000
attrs conda-forge/noarch::attrs-21.2.0-pyhd8ed1ab_0
aws-c-cal conda-forge/linux-ppc64le::aws-c-cal-0.5.11-hb3fac3d_0
aws-c-common conda-forge/linux-ppc64le::aws-c-common-0.6.2-h4e0d66e_0
aws-c-event-stream conda-forge/linux-ppc64le::aws-c-event-stream-0.2.7-h76da5f2_13
aws-c-io conda-forge/linux-ppc64le::aws-c-io-0.10.5-hf6a6c7c_0
aws-checksums conda-forge/linux-ppc64le::aws-checksums-0.1.11-hfe76d68_7
aws-sdk-cpp conda-forge/linux-ppc64le::aws-sdk-cpp-1.8.186-h90855e8_3
brotlipy conda-forge/linux-ppc64le::brotlipy-0.7.0-py36hc33305d_1001
bzip2 conda-forge/linux-ppc64le::bzip2-1.0.8-h4e0d66e_4
c-ares conda-forge/linux-ppc64le::c-ares-1.17.2-h4e0d66e_0
cffi conda-forge/linux-ppc64le::cffi-1.14.6-py36h021ab3c_1
chardet conda-forge/linux-ppc64le::chardet-4.0.0-py36h270354c_1
colorama conda-forge/noarch::colorama-0.4.4-pyh9f0ad1d_0
cryptography conda-forge/linux-ppc64le::cryptography-3.4.7-py36hc71b123_0
dataclasses conda-forge/noarch::dataclasses-0.8-pyh787bdff_2
datasets conda-forge/noarch::datasets-1.12.1-pyhd8ed1ab_1
dill conda-forge/noarch::dill-0.3.4-pyhd8ed1ab_0
filelock conda-forge/noarch::filelock-3.3.0-pyhd8ed1ab_0
fsspec conda-forge/noarch::fsspec-2021.10.0-pyhd8ed1ab_0
gflags conda-forge/linux-ppc64le::gflags-2.2.2-hb209c28_1004
glog conda-forge/linux-ppc64le::glog-0.5.0-h4040248_0
grpc-cpp conda-forge/linux-ppc64le::grpc-cpp-1.40.0-h2bf711c_2
huggingface_hub conda-forge/noarch::huggingface_hub-0.0.19-pyhd8ed1ab_0
idna conda-forge/noarch::idna-2.10-pyh9f0ad1d_0
idna_ssl conda-forge/noarch::idna_ssl-1.0.0-0
importlib-metadata conda-forge/linux-ppc64le::importlib-metadata-4.8.1-py36h270354c_0
importlib_metadata conda-forge/noarch::importlib_metadata-4.8.1-hd8ed1ab_0
krb5 conda-forge/linux-ppc64le::krb5-1.19.2-haf43566_2
libblas conda-forge/linux-ppc64le::libblas-3.9.0-11_linuxppc64le_openblas
libbrotlicommon conda-forge/linux-ppc64le::libbrotlicommon-1.0.9-h4e0d66e_5
libbrotlidec conda-forge/linux-ppc64le::libbrotlidec-1.0.9-h4e0d66e_5
libbrotlienc conda-forge/linux-ppc64le::libbrotlienc-1.0.9-h4e0d66e_5
libcblas conda-forge/linux-ppc64le::libcblas-3.9.0-11_linuxppc64le_openblas
libcurl conda-forge/linux-ppc64le::libcurl-7.79.1-he415e40_1
libedit conda-forge/linux-ppc64le::libedit-3.1.20191231-h41a240f_2
libev conda-forge/linux-ppc64le::libev-4.33-h6eb9509_1
libevent conda-forge/linux-ppc64le::libevent-2.1.10-h97db324_4
libgfortran-ng conda-forge/linux-ppc64le::libgfortran-ng-11.2.0-hfdc3801_11
libgfortran5 conda-forge/linux-ppc64le::libgfortran5-11.2.0-he58fbb4_11
liblapack conda-forge/linux-ppc64le::liblapack-3.9.0-11_linuxppc64le_openblas
libnghttp2 conda-forge/linux-ppc64le::libnghttp2-1.43.0-h42039ad_1
libopenblas conda-forge/linux-ppc64le::libopenblas-0.3.17-pthreads_h486567c_1
libprotobuf conda-forge/linux-ppc64le::libprotobuf-3.18.1-h690f14c_0
libssh2 conda-forge/linux-ppc64le::libssh2-1.10.0-ha5a9321_2
libthrift conda-forge/linux-ppc64le::libthrift-0.15.0-h54f692e_1
libutf8proc conda-forge/linux-ppc64le::libutf8proc-2.6.1-h4e0d66e_0
lz4-c conda-forge/linux-ppc64le::lz4-c-1.9.3-h3b9df90_1
multidict conda-forge/linux-ppc64le::multidict-5.2.0-py36hc33305d_0
multiprocess conda-forge/linux-ppc64le::multiprocess-0.70.12.2-py36hc33305d_0
numpy conda-forge/linux-ppc64le::numpy-1.19.5-py36h86665d4_1
orc conda-forge/linux-ppc64le::orc-1.7.0-hae6b4bd_0
packaging conda-forge/noarch::packaging-21.0-pyhd8ed1ab_0
pandas conda-forge/linux-ppc64le::pandas-1.1.5-py36hab1a6e6_0
parquet-cpp conda-forge/noarch::parquet-cpp-1.5.1-2
pyarrow conda-forge/linux-ppc64le::pyarrow-5.0.0-py36h7a46c7e_8_cpu
pycparser conda-forge/noarch::pycparser-2.20-pyh9f0ad1d_2
pyopenssl conda-forge/noarch::pyopenssl-21.0.0-pyhd8ed1ab_0
pyparsing conda-forge/noarch::pyparsing-2.4.7-pyh9f0ad1d_0
pysocks conda-forge/linux-ppc64le::pysocks-1.7.1-py36h270354c_3
python-dateutil conda-forge/noarch::python-dateutil-2.8.2-pyhd8ed1ab_0
python-xxhash conda-forge/linux-ppc64le::python-xxhash-2.0.2-py36hc33305d_0
python_abi conda-forge/linux-ppc64le::python_abi-3.6-2_cp36m
pytz conda-forge/noarch::pytz-2021.3-pyhd8ed1ab_0
pyyaml conda-forge/linux-ppc64le::pyyaml-5.4.1-py36hc33305d_1
re2 conda-forge/linux-ppc64le::re2-2021.09.01-h3b9df90_0
requests conda-forge/noarch::requests-2.25.1-pyhd3deb0d_0
s2n conda-forge/linux-ppc64le::s2n-1.0.10-h97db324_0
six conda-forge/noarch::six-1.16.0-pyh6c4a22f_0
snappy conda-forge/linux-ppc64le::snappy-1.1.8-hb209c28_3
tqdm conda-forge/noarch::tqdm-4.62.3-pyhd8ed1ab_0
typing-extensions conda-forge/noarch::typing-extensions-3.10.0.2-hd8ed1ab_0
typing_extensions conda-forge/noarch::typing_extensions-3.10.0.2-pyha770c72_0
urllib3 conda-forge/noarch::urllib3-1.26.7-pyhd8ed1ab_0
xxhash conda-forge/linux-ppc64le::xxhash-0.8.0-h4e0d66e_3
yaml conda-forge/linux-ppc64le::yaml-0.2.5-h6eb9509_0
yarl conda-forge/linux-ppc64le::yarl-1.6.3-py36hc33305d_2
zipp conda-forge/noarch::zipp-3.6.0-pyhd8ed1ab_0
zstd conda-forge/linux-ppc64le::zstd-1.5.0-h65c4b1a_0
The following packages will be UPDATED:
certifi pkgs/main::certifi-2020.12.5-py36h6ff~ --> conda-forge::certifi-2021.5.30-py36h270354c_0
Proceed ([y]/n)? y
Preparing transaction: done
Verifying transaction: done
Executing transaction: done
```
## Environment info
<!-- You can run the command `datasets-cli env` and copy-and-paste its output below. -->
- `datasets` version: 1.12.1
- Platform: Red Hat Enterprise Linux 8.2 (Ootpa)
- Python version: 3.6
- PyArrow version: pyarrow - 5.0.0 - py36h7a46c7e_8_cpu - conda-forge
Any help would be appreciated! I've been struggling on installing datasets on this machine.
This seems to be an issue with importing PyArrow so I posted the problem [here](https://issues.apache.org/jira/browse/ARROW-14323), and I'm closing this issue.
|
https://github.com/huggingface/datasets/issues/3071 | Custom plain text dataset, plain json dataset and plain csv dataset are remove from datasets template folder | Hi @zixiliuUSC,
As explained in the documentation (https://huggingface.co/docs/datasets/loading.html#json), we support loading any dataset in JSON (as well as CSV, text, Parquet) format:
```python
ds = load_dataset('json', data_files='my_file.json')
``` | ## Adding a Dataset
- **Name:** text, json, csv
- **Description:** I am developing a customized dataset loading script. The problem is mainly about my custom dataset is seperate into many files and I only find a dataset loading template in [https://github.com/huggingface/datasets/blob/1.2.1/datasets/json/json.py](https://github.com/huggingface/datasets/blob/1.2.1/datasets/json/json.py) that can handle my circumstance. I'm afraid these templates are too old to use. Could you re-add these three templates to current master branch?
| 28 | Custom plain text dataset, plain json dataset and plain csv dataset are remove from datasets template folder
## Adding a Dataset
- **Name:** text, json, csv
- **Description:** I am developing a customized dataset loading script. The problem is mainly about my custom dataset is seperate into many files and I only find a dataset loading template in [https://github.com/huggingface/datasets/blob/1.2.1/datasets/json/json.py](https://github.com/huggingface/datasets/blob/1.2.1/datasets/json/json.py) that can handle my circumstance. I'm afraid these templates are too old to use. Could you re-add these three templates to current master branch?
Hi @zixiliuUSC,
As explained in the documentation (https://huggingface.co/docs/datasets/loading.html#json), we support loading any dataset in JSON (as well as CSV, text, Parquet) format:
```python
ds = load_dataset('json', data_files='my_file.json')
``` |
https://github.com/huggingface/datasets/issues/3064 | Make `interleave_datasets` more robust | Hi ! Sorry for the late response
I agree `interleave_datasets` would benefit a lot from having more flexibility. If I understand correctly it would be nice to be able to define stopping strategies like `stop="first_exhausted"` (default) or `stop="all_exhausted"`. If you'd like to contribute this feature I'd be happy to give you some pointers :)
Also one can already set the max number of iterations per dataset by doing `dataset.take(n)` on the dataset that should only have `n` samples.
Regarding the `iter_cnt` counter, I think this requires a bit more thoughts, since we might have to be able to backpropagate the the counter if `map` or other transforms have been applied after `interleave_datasets`. | **Is your feature request related to a problem? Please describe.**
Right now there are few hiccups using `interleave_datasets`. Interleaved dataset iterates until the smallest dataset completes it's iterator. In this way larger datasets may not complete full epoch of iteration.
It creates new problems in calculation of epoch since there are no way to track which dataset from `interleave_datasets` completes how many epoch.
**Describe the solution you'd like**
For `interleave_datasets` module,
- [ ] Add a boolean argument `--stop-iter` in `interleave_datasets` that enables dataset to either iterate infinite amount of time or not. That means it should not return `StopIterator` exception in case `--stop-iter=False`.
- [ ] Internal list variable `iter_cnt` that explains how many times (in steps/epochs) each dataset iterates at a given point.
- [ ] Add an argument `--max-iter` (list type) that explain maximum amount of time each of the dataset can iterate. After complete `--max-iter` of one dataset, other dataset should continue sampling and when all the dataset finish their respective `--max-iter`, only then return `StopIterator`
Note: I'm new to `datasets` api. May be these features are already there in the datasets.
Since multitask training is the latest trends, I believe this feature would make the `datasets` api more popular.
@lhoestq | 112 | Make `interleave_datasets` more robust
**Is your feature request related to a problem? Please describe.**
Right now there are few hiccups using `interleave_datasets`. Interleaved dataset iterates until the smallest dataset completes it's iterator. In this way larger datasets may not complete full epoch of iteration.
It creates new problems in calculation of epoch since there are no way to track which dataset from `interleave_datasets` completes how many epoch.
**Describe the solution you'd like**
For `interleave_datasets` module,
- [ ] Add a boolean argument `--stop-iter` in `interleave_datasets` that enables dataset to either iterate infinite amount of time or not. That means it should not return `StopIterator` exception in case `--stop-iter=False`.
- [ ] Internal list variable `iter_cnt` that explains how many times (in steps/epochs) each dataset iterates at a given point.
- [ ] Add an argument `--max-iter` (list type) that explain maximum amount of time each of the dataset can iterate. After complete `--max-iter` of one dataset, other dataset should continue sampling and when all the dataset finish their respective `--max-iter`, only then return `StopIterator`
Note: I'm new to `datasets` api. May be these features are already there in the datasets.
Since multitask training is the latest trends, I believe this feature would make the `datasets` api more popular.
@lhoestq
Hi ! Sorry for the late response
I agree `interleave_datasets` would benefit a lot from having more flexibility. If I understand correctly it would be nice to be able to define stopping strategies like `stop="first_exhausted"` (default) or `stop="all_exhausted"`. If you'd like to contribute this feature I'd be happy to give you some pointers :)
Also one can already set the max number of iterations per dataset by doing `dataset.take(n)` on the dataset that should only have `n` samples.
Regarding the `iter_cnt` counter, I think this requires a bit more thoughts, since we might have to be able to backpropagate the the counter if `map` or other transforms have been applied after `interleave_datasets`. |
https://github.com/huggingface/datasets/issues/3064 | Make `interleave_datasets` more robust | @sbmaruf I just notice that (1)`interleave_datasets` only samples indices once and reuse for all epochs, and (2) it's limited by the smallest dataset. Do you figure out an alternative way to achieve the same purpose? | **Is your feature request related to a problem? Please describe.**
Right now there are few hiccups using `interleave_datasets`. Interleaved dataset iterates until the smallest dataset completes it's iterator. In this way larger datasets may not complete full epoch of iteration.
It creates new problems in calculation of epoch since there are no way to track which dataset from `interleave_datasets` completes how many epoch.
**Describe the solution you'd like**
For `interleave_datasets` module,
- [ ] Add a boolean argument `--stop-iter` in `interleave_datasets` that enables dataset to either iterate infinite amount of time or not. That means it should not return `StopIterator` exception in case `--stop-iter=False`.
- [ ] Internal list variable `iter_cnt` that explains how many times (in steps/epochs) each dataset iterates at a given point.
- [ ] Add an argument `--max-iter` (list type) that explain maximum amount of time each of the dataset can iterate. After complete `--max-iter` of one dataset, other dataset should continue sampling and when all the dataset finish their respective `--max-iter`, only then return `StopIterator`
Note: I'm new to `datasets` api. May be these features are already there in the datasets.
Since multitask training is the latest trends, I believe this feature would make the `datasets` api more popular.
@lhoestq | 35 | Make `interleave_datasets` more robust
**Is your feature request related to a problem? Please describe.**
Right now there are few hiccups using `interleave_datasets`. Interleaved dataset iterates until the smallest dataset completes it's iterator. In this way larger datasets may not complete full epoch of iteration.
It creates new problems in calculation of epoch since there are no way to track which dataset from `interleave_datasets` completes how many epoch.
**Describe the solution you'd like**
For `interleave_datasets` module,
- [ ] Add a boolean argument `--stop-iter` in `interleave_datasets` that enables dataset to either iterate infinite amount of time or not. That means it should not return `StopIterator` exception in case `--stop-iter=False`.
- [ ] Internal list variable `iter_cnt` that explains how many times (in steps/epochs) each dataset iterates at a given point.
- [ ] Add an argument `--max-iter` (list type) that explain maximum amount of time each of the dataset can iterate. After complete `--max-iter` of one dataset, other dataset should continue sampling and when all the dataset finish their respective `--max-iter`, only then return `StopIterator`
Note: I'm new to `datasets` api. May be these features are already there in the datasets.
Since multitask training is the latest trends, I believe this feature would make the `datasets` api more popular.
@lhoestq
@sbmaruf I just notice that (1)`interleave_datasets` only samples indices once and reuse for all epochs, and (2) it's limited by the smallest dataset. Do you figure out an alternative way to achieve the same purpose? |
https://github.com/huggingface/datasets/issues/3063 | Windows CI is unable to test streaming properly because of SSL issues | I think this problem is already fixed:
```python
In [4]: import fsspec
...:
...: url = "https://moon-staging.huggingface.co/datasets/__DUMMY_TRANSFORMERS_USER__/my-dataset-16242824690709/resolve/main/.gitattributes"
...:
...: fsspec.open(url).open()
Out[4]: <File-like object HTTPFileSystem, https://moon-staging.huggingface.co/datasets/__DUMMY_TRANSFORMERS_USER__/my-dataset-16242824690709/resolve/main/.gitattribu
``` | In https://github.com/huggingface/datasets/pull/3041 the windows tests were skipped because of SSL issues with moon-staging.huggingface.co:443
The issue appears only on windows with asyncio. On Linux it works. With requests it works as well. And with the production environment huggingface.co it also works.
to reproduce on windows:
```python
import fsspec
# use any URL to a file in a dataset repo
url = "https://moon-staging.huggingface.co/datasets/__DUMMY_TRANSFORMERS_USER__/my-dataset-16242824690709/resolve/main/.gitattributes"
fsspec.open(url).open()
```
raises
```python
FileNotFoundError: https://moon-staging.huggingface.co/datasets/__DUMMY_TRANSFORMERS_USER__/my-dataset-16242824690709/resolve/main/.gitattributes
```
because of
```python
aiohttp.client_exceptions.ClientConnectorCertificateError: Cannot connect to host moon-staging.huggingface.co:443 ssl:True [SSLCertVerificationError: (1, '[SSL: CERTIFICATE_VERIFY_FAILED] certificate verify failed: certificate has expired (_ssl.c:1131)')]
``` | 26 | Windows CI is unable to test streaming properly because of SSL issues
In https://github.com/huggingface/datasets/pull/3041 the windows tests were skipped because of SSL issues with moon-staging.huggingface.co:443
The issue appears only on windows with asyncio. On Linux it works. With requests it works as well. And with the production environment huggingface.co it also works.
to reproduce on windows:
```python
import fsspec
# use any URL to a file in a dataset repo
url = "https://moon-staging.huggingface.co/datasets/__DUMMY_TRANSFORMERS_USER__/my-dataset-16242824690709/resolve/main/.gitattributes"
fsspec.open(url).open()
```
raises
```python
FileNotFoundError: https://moon-staging.huggingface.co/datasets/__DUMMY_TRANSFORMERS_USER__/my-dataset-16242824690709/resolve/main/.gitattributes
```
because of
```python
aiohttp.client_exceptions.ClientConnectorCertificateError: Cannot connect to host moon-staging.huggingface.co:443 ssl:True [SSLCertVerificationError: (1, '[SSL: CERTIFICATE_VERIFY_FAILED] certificate verify failed: certificate has expired (_ssl.c:1131)')]
```
I think this problem is already fixed:
```python
In [4]: import fsspec
...:
...: url = "https://moon-staging.huggingface.co/datasets/__DUMMY_TRANSFORMERS_USER__/my-dataset-16242824690709/resolve/main/.gitattributes"
...:
...: fsspec.open(url).open()
Out[4]: <File-like object HTTPFileSystem, https://moon-staging.huggingface.co/datasets/__DUMMY_TRANSFORMERS_USER__/my-dataset-16242824690709/resolve/main/.gitattribu
``` |
https://github.com/huggingface/datasets/issues/3061 | Feature request : add leave=True to dataset.map to enable tqdm nested bars (and whilst we're at it couldn't we get a way to access directly tqdm underneath?) | @lhoestq, @albertvillanova can we have `**tqdm_kwargs` in `map`? If there are any fields that are important to our tqdm (like iterable or unit), we can pop them before initialising the tqdm object so as to avoid duplicity. | **A clear and concise description of what you want to happen.**
It would be so nice to be able to nest HuggingFace `Datasets.map() ` progress bars in the grander scheme of things and whilst we're at it why not other functions.
**Describe alternatives you've considered**
By the way is there not a way to directly interact with underlying tqdm module ? **kwargs-ish?
**Additional context**
Furthering tqdm integration #2374 and huggingface/transformers#11797 solutioned by huggingface/transformers#12226 provided with tqdm description as `desc=`
@sgugger @bhavitvyamalik | 37 | Feature request : add leave=True to dataset.map to enable tqdm nested bars (and whilst we're at it couldn't we get a way to access directly tqdm underneath?)
**A clear and concise description of what you want to happen.**
It would be so nice to be able to nest HuggingFace `Datasets.map() ` progress bars in the grander scheme of things and whilst we're at it why not other functions.
**Describe alternatives you've considered**
By the way is there not a way to directly interact with underlying tqdm module ? **kwargs-ish?
**Additional context**
Furthering tqdm integration #2374 and huggingface/transformers#11797 solutioned by huggingface/transformers#12226 provided with tqdm description as `desc=`
@sgugger @bhavitvyamalik
@lhoestq, @albertvillanova can we have `**tqdm_kwargs` in `map`? If there are any fields that are important to our tqdm (like iterable or unit), we can pop them before initialising the tqdm object so as to avoid duplicity. |
https://github.com/huggingface/datasets/issues/3061 | Feature request : add leave=True to dataset.map to enable tqdm nested bars (and whilst we're at it couldn't we get a way to access directly tqdm underneath?) | Hi ! Sounds like a good idea :)
Also I think it would be better to have this as an actual parameters instead of kwargs to make it clearer | **A clear and concise description of what you want to happen.**
It would be so nice to be able to nest HuggingFace `Datasets.map() ` progress bars in the grander scheme of things and whilst we're at it why not other functions.
**Describe alternatives you've considered**
By the way is there not a way to directly interact with underlying tqdm module ? **kwargs-ish?
**Additional context**
Furthering tqdm integration #2374 and huggingface/transformers#11797 solutioned by huggingface/transformers#12226 provided with tqdm description as `desc=`
@sgugger @bhavitvyamalik | 29 | Feature request : add leave=True to dataset.map to enable tqdm nested bars (and whilst we're at it couldn't we get a way to access directly tqdm underneath?)
**A clear and concise description of what you want to happen.**
It would be so nice to be able to nest HuggingFace `Datasets.map() ` progress bars in the grander scheme of things and whilst we're at it why not other functions.
**Describe alternatives you've considered**
By the way is there not a way to directly interact with underlying tqdm module ? **kwargs-ish?
**Additional context**
Furthering tqdm integration #2374 and huggingface/transformers#11797 solutioned by huggingface/transformers#12226 provided with tqdm description as `desc=`
@sgugger @bhavitvyamalik
Hi ! Sounds like a good idea :)
Also I think it would be better to have this as an actual parameters instead of kwargs to make it clearer |
https://github.com/huggingface/datasets/issues/3060 | load_dataset('openwebtext') yields "Compressed file ended before the end-of-stream marker was reached" | Hi @RylanSchaeffer, thanks for reporting.
I'm sorry, but I was not able to reproduce your problem.
Normally, the reason for this type of error is that, during your download of the data files, this was not fully complete.
Could you please try to load the dataset again but forcing its redownload? Please use:
```python
dataset = load_dataset("openwebtext", download_mode="FORCE_REDOWNLOAD")
```
Let me know if the problem persists. | ## Describe the bug
When I try `load_dataset('openwebtext')`, I receive a "EOFError: Compressed file ended before the end-of-stream marker was reached" error.
## Steps to reproduce the bug
```
from datasets import load_dataset
dataset = load_dataset('openwebtext')
```
## Expected results
I expect the `dataset` variable to be properly constructed.
## Actual results
```
File "/home/rschaef/CoCoSci-Language-Distillation/distillation_v2/ratchet_learning/tasks/base.py", line 37, in create_dataset
dataset_str,
File "/home/rschaef/CoCoSci-Language-Distillation/cocosci/lib/python3.6/site-packages/datasets/load.py", line 1117, in load_dataset
use_auth_token=use_auth_token,
File "/home/rschaef/CoCoSci-Language-Distillation/cocosci/lib/python3.6/site-packages/datasets/builder.py", line 637, in download_and_prepare
dl_manager=dl_manager, verify_infos=verify_infos, **download_and_prepare_kwargs
File "/home/rschaef/CoCoSci-Language-Distillation/cocosci/lib/python3.6/site-packages/datasets/builder.py", line 704, in _download_and_prepare
split_generators = self._split_generators(dl_manager, **split_generators_kwargs)
File "/home/rschaef/.cache/huggingface/modules/datasets_modules/datasets/openwebtext/85b3ae7051d2d72e7c5fdf6dfb462603aaa26e9ed506202bf3a24d261c6c40a1/openwebtext.py", line 61, in _split_generators
dl_dir = dl_manager.download_and_extract(_URL)
File "/home/rschaef/CoCoSci-Language-Distillation/cocosci/lib/python3.6/site-packages/datasets/utils/download_manager.py", line 284, in download_and_extract
return self.extract(self.download(url_or_urls))
File "/home/rschaef/CoCoSci-Language-Distillation/cocosci/lib/python3.6/site-packages/datasets/utils/download_manager.py", line 261, in extract
partial(cached_path, download_config=download_config), path_or_paths, num_proc=num_proc, disable_tqdm=False
File "/home/rschaef/CoCoSci-Language-Distillation/cocosci/lib/python3.6/site-packages/datasets/utils/py_utils.py", line 197, in map_nested
return function(data_struct)
File "/home/rschaef/CoCoSci-Language-Distillation/cocosci/lib/python3.6/site-packages/datasets/utils/file_utils.py", line 316, in cached_path
output_path, force_extract=download_config.force_extract
File "/home/rschaef/CoCoSci-Language-Distillation/cocosci/lib/python3.6/site-packages/datasets/utils/extract.py", line 40, in extract
self.extractor.extract(input_path, output_path, extractor=extractor)
File "/home/rschaef/CoCoSci-Language-Distillation/cocosci/lib/python3.6/site-packages/datasets/utils/extract.py", line 179, in extract
return extractor.extract(input_path, output_path)
File "/home/rschaef/CoCoSci-Language-Distillation/cocosci/lib/python3.6/site-packages/datasets/utils/extract.py", line 53, in extract
tar_file.extractall(output_path)
File "/usr/lib/python3.6/tarfile.py", line 2010, in extractall
numeric_owner=numeric_owner)
File "/usr/lib/python3.6/tarfile.py", line 2052, in extract
numeric_owner=numeric_owner)
File "/usr/lib/python3.6/tarfile.py", line 2122, in _extract_member
self.makefile(tarinfo, targetpath)
File "/usr/lib/python3.6/tarfile.py", line 2171, in makefile
copyfileobj(source, target, tarinfo.size, ReadError, bufsize)
File "/usr/lib/python3.6/tarfile.py", line 249, in copyfileobj
buf = src.read(bufsize)
File "/usr/lib/python3.6/lzma.py", line 200, in read
return self._buffer.read(size)
File "/usr/lib/python3.6/_compression.py", line 68, in readinto
data = self.read(len(byte_view))
File "/usr/lib/python3.6/_compression.py", line 99, in read
raise EOFError("Compressed file ended before the "
python-BaseException
EOFError: Compressed file ended before the end-of-stream marker was reached
```
## Environment info
- `datasets` version: 1.12.1
- Platform: Linux-4.4.0-173-generic-x86_64-with-Ubuntu-16.04-xenial
- Python version: 3.6.10
- PyArrow version: 5.0.0 | 66 | load_dataset('openwebtext') yields "Compressed file ended before the end-of-stream marker was reached"
## Describe the bug
When I try `load_dataset('openwebtext')`, I receive a "EOFError: Compressed file ended before the end-of-stream marker was reached" error.
## Steps to reproduce the bug
```
from datasets import load_dataset
dataset = load_dataset('openwebtext')
```
## Expected results
I expect the `dataset` variable to be properly constructed.
## Actual results
```
File "/home/rschaef/CoCoSci-Language-Distillation/distillation_v2/ratchet_learning/tasks/base.py", line 37, in create_dataset
dataset_str,
File "/home/rschaef/CoCoSci-Language-Distillation/cocosci/lib/python3.6/site-packages/datasets/load.py", line 1117, in load_dataset
use_auth_token=use_auth_token,
File "/home/rschaef/CoCoSci-Language-Distillation/cocosci/lib/python3.6/site-packages/datasets/builder.py", line 637, in download_and_prepare
dl_manager=dl_manager, verify_infos=verify_infos, **download_and_prepare_kwargs
File "/home/rschaef/CoCoSci-Language-Distillation/cocosci/lib/python3.6/site-packages/datasets/builder.py", line 704, in _download_and_prepare
split_generators = self._split_generators(dl_manager, **split_generators_kwargs)
File "/home/rschaef/.cache/huggingface/modules/datasets_modules/datasets/openwebtext/85b3ae7051d2d72e7c5fdf6dfb462603aaa26e9ed506202bf3a24d261c6c40a1/openwebtext.py", line 61, in _split_generators
dl_dir = dl_manager.download_and_extract(_URL)
File "/home/rschaef/CoCoSci-Language-Distillation/cocosci/lib/python3.6/site-packages/datasets/utils/download_manager.py", line 284, in download_and_extract
return self.extract(self.download(url_or_urls))
File "/home/rschaef/CoCoSci-Language-Distillation/cocosci/lib/python3.6/site-packages/datasets/utils/download_manager.py", line 261, in extract
partial(cached_path, download_config=download_config), path_or_paths, num_proc=num_proc, disable_tqdm=False
File "/home/rschaef/CoCoSci-Language-Distillation/cocosci/lib/python3.6/site-packages/datasets/utils/py_utils.py", line 197, in map_nested
return function(data_struct)
File "/home/rschaef/CoCoSci-Language-Distillation/cocosci/lib/python3.6/site-packages/datasets/utils/file_utils.py", line 316, in cached_path
output_path, force_extract=download_config.force_extract
File "/home/rschaef/CoCoSci-Language-Distillation/cocosci/lib/python3.6/site-packages/datasets/utils/extract.py", line 40, in extract
self.extractor.extract(input_path, output_path, extractor=extractor)
File "/home/rschaef/CoCoSci-Language-Distillation/cocosci/lib/python3.6/site-packages/datasets/utils/extract.py", line 179, in extract
return extractor.extract(input_path, output_path)
File "/home/rschaef/CoCoSci-Language-Distillation/cocosci/lib/python3.6/site-packages/datasets/utils/extract.py", line 53, in extract
tar_file.extractall(output_path)
File "/usr/lib/python3.6/tarfile.py", line 2010, in extractall
numeric_owner=numeric_owner)
File "/usr/lib/python3.6/tarfile.py", line 2052, in extract
numeric_owner=numeric_owner)
File "/usr/lib/python3.6/tarfile.py", line 2122, in _extract_member
self.makefile(tarinfo, targetpath)
File "/usr/lib/python3.6/tarfile.py", line 2171, in makefile
copyfileobj(source, target, tarinfo.size, ReadError, bufsize)
File "/usr/lib/python3.6/tarfile.py", line 249, in copyfileobj
buf = src.read(bufsize)
File "/usr/lib/python3.6/lzma.py", line 200, in read
return self._buffer.read(size)
File "/usr/lib/python3.6/_compression.py", line 68, in readinto
data = self.read(len(byte_view))
File "/usr/lib/python3.6/_compression.py", line 99, in read
raise EOFError("Compressed file ended before the "
python-BaseException
EOFError: Compressed file ended before the end-of-stream marker was reached
```
## Environment info
- `datasets` version: 1.12.1
- Platform: Linux-4.4.0-173-generic-x86_64-with-Ubuntu-16.04-xenial
- Python version: 3.6.10
- PyArrow version: 5.0.0
Hi @RylanSchaeffer, thanks for reporting.
I'm sorry, but I was not able to reproduce your problem.
Normally, the reason for this type of error is that, during your download of the data files, this was not fully complete.
Could you please try to load the dataset again but forcing its redownload? Please use:
```python
dataset = load_dataset("openwebtext", download_mode="FORCE_REDOWNLOAD")
```
Let me know if the problem persists. |
https://github.com/huggingface/datasets/issues/3060 | load_dataset('openwebtext') yields "Compressed file ended before the end-of-stream marker was reached" | I close this issue for the moment. Feel free to re-open it again if the problem persists. | ## Describe the bug
When I try `load_dataset('openwebtext')`, I receive a "EOFError: Compressed file ended before the end-of-stream marker was reached" error.
## Steps to reproduce the bug
```
from datasets import load_dataset
dataset = load_dataset('openwebtext')
```
## Expected results
I expect the `dataset` variable to be properly constructed.
## Actual results
```
File "/home/rschaef/CoCoSci-Language-Distillation/distillation_v2/ratchet_learning/tasks/base.py", line 37, in create_dataset
dataset_str,
File "/home/rschaef/CoCoSci-Language-Distillation/cocosci/lib/python3.6/site-packages/datasets/load.py", line 1117, in load_dataset
use_auth_token=use_auth_token,
File "/home/rschaef/CoCoSci-Language-Distillation/cocosci/lib/python3.6/site-packages/datasets/builder.py", line 637, in download_and_prepare
dl_manager=dl_manager, verify_infos=verify_infos, **download_and_prepare_kwargs
File "/home/rschaef/CoCoSci-Language-Distillation/cocosci/lib/python3.6/site-packages/datasets/builder.py", line 704, in _download_and_prepare
split_generators = self._split_generators(dl_manager, **split_generators_kwargs)
File "/home/rschaef/.cache/huggingface/modules/datasets_modules/datasets/openwebtext/85b3ae7051d2d72e7c5fdf6dfb462603aaa26e9ed506202bf3a24d261c6c40a1/openwebtext.py", line 61, in _split_generators
dl_dir = dl_manager.download_and_extract(_URL)
File "/home/rschaef/CoCoSci-Language-Distillation/cocosci/lib/python3.6/site-packages/datasets/utils/download_manager.py", line 284, in download_and_extract
return self.extract(self.download(url_or_urls))
File "/home/rschaef/CoCoSci-Language-Distillation/cocosci/lib/python3.6/site-packages/datasets/utils/download_manager.py", line 261, in extract
partial(cached_path, download_config=download_config), path_or_paths, num_proc=num_proc, disable_tqdm=False
File "/home/rschaef/CoCoSci-Language-Distillation/cocosci/lib/python3.6/site-packages/datasets/utils/py_utils.py", line 197, in map_nested
return function(data_struct)
File "/home/rschaef/CoCoSci-Language-Distillation/cocosci/lib/python3.6/site-packages/datasets/utils/file_utils.py", line 316, in cached_path
output_path, force_extract=download_config.force_extract
File "/home/rschaef/CoCoSci-Language-Distillation/cocosci/lib/python3.6/site-packages/datasets/utils/extract.py", line 40, in extract
self.extractor.extract(input_path, output_path, extractor=extractor)
File "/home/rschaef/CoCoSci-Language-Distillation/cocosci/lib/python3.6/site-packages/datasets/utils/extract.py", line 179, in extract
return extractor.extract(input_path, output_path)
File "/home/rschaef/CoCoSci-Language-Distillation/cocosci/lib/python3.6/site-packages/datasets/utils/extract.py", line 53, in extract
tar_file.extractall(output_path)
File "/usr/lib/python3.6/tarfile.py", line 2010, in extractall
numeric_owner=numeric_owner)
File "/usr/lib/python3.6/tarfile.py", line 2052, in extract
numeric_owner=numeric_owner)
File "/usr/lib/python3.6/tarfile.py", line 2122, in _extract_member
self.makefile(tarinfo, targetpath)
File "/usr/lib/python3.6/tarfile.py", line 2171, in makefile
copyfileobj(source, target, tarinfo.size, ReadError, bufsize)
File "/usr/lib/python3.6/tarfile.py", line 249, in copyfileobj
buf = src.read(bufsize)
File "/usr/lib/python3.6/lzma.py", line 200, in read
return self._buffer.read(size)
File "/usr/lib/python3.6/_compression.py", line 68, in readinto
data = self.read(len(byte_view))
File "/usr/lib/python3.6/_compression.py", line 99, in read
raise EOFError("Compressed file ended before the "
python-BaseException
EOFError: Compressed file ended before the end-of-stream marker was reached
```
## Environment info
- `datasets` version: 1.12.1
- Platform: Linux-4.4.0-173-generic-x86_64-with-Ubuntu-16.04-xenial
- Python version: 3.6.10
- PyArrow version: 5.0.0 | 17 | load_dataset('openwebtext') yields "Compressed file ended before the end-of-stream marker was reached"
## Describe the bug
When I try `load_dataset('openwebtext')`, I receive a "EOFError: Compressed file ended before the end-of-stream marker was reached" error.
## Steps to reproduce the bug
```
from datasets import load_dataset
dataset = load_dataset('openwebtext')
```
## Expected results
I expect the `dataset` variable to be properly constructed.
## Actual results
```
File "/home/rschaef/CoCoSci-Language-Distillation/distillation_v2/ratchet_learning/tasks/base.py", line 37, in create_dataset
dataset_str,
File "/home/rschaef/CoCoSci-Language-Distillation/cocosci/lib/python3.6/site-packages/datasets/load.py", line 1117, in load_dataset
use_auth_token=use_auth_token,
File "/home/rschaef/CoCoSci-Language-Distillation/cocosci/lib/python3.6/site-packages/datasets/builder.py", line 637, in download_and_prepare
dl_manager=dl_manager, verify_infos=verify_infos, **download_and_prepare_kwargs
File "/home/rschaef/CoCoSci-Language-Distillation/cocosci/lib/python3.6/site-packages/datasets/builder.py", line 704, in _download_and_prepare
split_generators = self._split_generators(dl_manager, **split_generators_kwargs)
File "/home/rschaef/.cache/huggingface/modules/datasets_modules/datasets/openwebtext/85b3ae7051d2d72e7c5fdf6dfb462603aaa26e9ed506202bf3a24d261c6c40a1/openwebtext.py", line 61, in _split_generators
dl_dir = dl_manager.download_and_extract(_URL)
File "/home/rschaef/CoCoSci-Language-Distillation/cocosci/lib/python3.6/site-packages/datasets/utils/download_manager.py", line 284, in download_and_extract
return self.extract(self.download(url_or_urls))
File "/home/rschaef/CoCoSci-Language-Distillation/cocosci/lib/python3.6/site-packages/datasets/utils/download_manager.py", line 261, in extract
partial(cached_path, download_config=download_config), path_or_paths, num_proc=num_proc, disable_tqdm=False
File "/home/rschaef/CoCoSci-Language-Distillation/cocosci/lib/python3.6/site-packages/datasets/utils/py_utils.py", line 197, in map_nested
return function(data_struct)
File "/home/rschaef/CoCoSci-Language-Distillation/cocosci/lib/python3.6/site-packages/datasets/utils/file_utils.py", line 316, in cached_path
output_path, force_extract=download_config.force_extract
File "/home/rschaef/CoCoSci-Language-Distillation/cocosci/lib/python3.6/site-packages/datasets/utils/extract.py", line 40, in extract
self.extractor.extract(input_path, output_path, extractor=extractor)
File "/home/rschaef/CoCoSci-Language-Distillation/cocosci/lib/python3.6/site-packages/datasets/utils/extract.py", line 179, in extract
return extractor.extract(input_path, output_path)
File "/home/rschaef/CoCoSci-Language-Distillation/cocosci/lib/python3.6/site-packages/datasets/utils/extract.py", line 53, in extract
tar_file.extractall(output_path)
File "/usr/lib/python3.6/tarfile.py", line 2010, in extractall
numeric_owner=numeric_owner)
File "/usr/lib/python3.6/tarfile.py", line 2052, in extract
numeric_owner=numeric_owner)
File "/usr/lib/python3.6/tarfile.py", line 2122, in _extract_member
self.makefile(tarinfo, targetpath)
File "/usr/lib/python3.6/tarfile.py", line 2171, in makefile
copyfileobj(source, target, tarinfo.size, ReadError, bufsize)
File "/usr/lib/python3.6/tarfile.py", line 249, in copyfileobj
buf = src.read(bufsize)
File "/usr/lib/python3.6/lzma.py", line 200, in read
return self._buffer.read(size)
File "/usr/lib/python3.6/_compression.py", line 68, in readinto
data = self.read(len(byte_view))
File "/usr/lib/python3.6/_compression.py", line 99, in read
raise EOFError("Compressed file ended before the "
python-BaseException
EOFError: Compressed file ended before the end-of-stream marker was reached
```
## Environment info
- `datasets` version: 1.12.1
- Platform: Linux-4.4.0-173-generic-x86_64-with-Ubuntu-16.04-xenial
- Python version: 3.6.10
- PyArrow version: 5.0.0
I close this issue for the moment. Feel free to re-open it again if the problem persists. |
https://github.com/huggingface/datasets/issues/3058 | Dataset wikipedia and Bookcorpusopen cannot be fetched from dataloader. | Hi ! I think this issue is more related to the `transformers` project. Could you open an issue on https://github.com/huggingface/transformers ?
Anyway I think the issue could be that both wikipedia and bookcorpusopen have an additional "title" column, contrary to wikitext which only has a "text" column. After calling `load_dataset`, can you try doing `dataset = dataset.remove_columns("title")` ? | ## Describe the bug
I have used the previous version of `transformers` and `datasets`. The dataset `wikipedia` can be successfully used. Recently, I upgrade them to the newest version and find it raises errors. I also tried other datasets. The `wikitext` works and the `bookcorpusopen` raises the same errors as `wikipedia`.
## Steps to reproduce the bug
Run the `run_mlm_no_trainer.py` and the given script on this [link](https://github.com/huggingface/transformers/tree/master/examples/pytorch/language-modeling). Change the dataset from wikitext to wikipedia or bookcorpusopen. BTW, the library transformers is of version 4.11.3.
## Expected results
The data batchs are fetched from the data loader and train.
## Actual results
The first time to fetch data batch occurs error.
`Traceback (most recent call last):
File "/home/zyli/anaconda3/envs/LatestStacking/lib/python3.7/site-packages/transformers/tokenization_utils_base.py", line 705, in convert_to_tensors
tensor = as_tensor(value)
ValueError: too many dimensions 'str'
During handling of the above exception, another exception occurred:
Traceback (most recent call last):
File "src/original_run_mlm_no_trainer.py", line 528, in <module>
main()
File "src/original_run_mlm_no_trainer.py", line 488, in main
for step, batch in enumerate(train_dataloader):
File "/home/zyli/anaconda3/envs/LatestStacking/lib/python3.7/site-packages/accelerate/data_loader.py", line 303, in __iter__
for batch in super().__iter__():
File "/home/zyli/anaconda3/envs/LatestStacking/lib/python3.7/site-packages/torch/utils/data/dataloader.py", line 517, in __next__
data = self._next_data()
File "/home/zyli/anaconda3/envs/LatestStacking/lib/python3.7/site-packages/torch/utils/data/dataloader.py", line 557, in _next_data
data = self._dataset_fetcher.fetch(index) # may raise StopIteration
File "/home/zyli/anaconda3/envs/LatestStacking/lib/python3.7/site-packages/torch/utils/data/_utils/fetch.py", line 47, in fetch
return self.collate_fn(data)
File "/home/zyli/anaconda3/envs/LatestStacking/lib/python3.7/site-packages/transformers/data/data_collator.py", line 41, in __call__
return self.torch_call(features)
File "/home/zyli/anaconda3/envs/LatestStacking/lib/python3.7/site-packages/transformers/data/data_collator.py", line 671, in torch_call
batch = self.tokenizer.pad(examples, return_tensors="pt", pad_to_multiple_of=self.pad_to_multiple_of)
File "/home/zyli/anaconda3/envs/LatestStacking/lib/python3.7/site-packages/transformers/tokenization_utils_base.py", line 2774, in pad
return BatchEncoding(batch_outputs, tensor_type=return_tensors)
File "/home/zyli/anaconda3/envs/LatestStacking/lib/python3.7/site-packages/transformers/tokenization_utils_base.py", line 210, in __init__
self.convert_to_tensors(tensor_type=tensor_type, prepend_batch_axis=prepend_batch_axis)
File "/home/zyli/anaconda3/envs/LatestStacking/lib/python3.7/site-packages/transformers/tokenization_utils_base.py", line 722, in convert_to_tensors
"Unable to create tensor, you should probably activate truncation and/or padding "
ValueError: Unable to create tensor, you should probably activate truncation and/or padding with 'padding=True' 'truncation=True' to have batched tensors with the same length.
`
## Environment info
<!-- You can run the command `datasets-cli env` and copy-and-paste its output below. -->
- `datasets` version: 1.12.1
- Platform: Linux-5.8.0-59-generic-x86_64-with-debian-bullseye-sid
- Python version: 3.7.6
- PyArrow version: 5.0.0
| 58 | Dataset wikipedia and Bookcorpusopen cannot be fetched from dataloader.
## Describe the bug
I have used the previous version of `transformers` and `datasets`. The dataset `wikipedia` can be successfully used. Recently, I upgrade them to the newest version and find it raises errors. I also tried other datasets. The `wikitext` works and the `bookcorpusopen` raises the same errors as `wikipedia`.
## Steps to reproduce the bug
Run the `run_mlm_no_trainer.py` and the given script on this [link](https://github.com/huggingface/transformers/tree/master/examples/pytorch/language-modeling). Change the dataset from wikitext to wikipedia or bookcorpusopen. BTW, the library transformers is of version 4.11.3.
## Expected results
The data batchs are fetched from the data loader and train.
## Actual results
The first time to fetch data batch occurs error.
`Traceback (most recent call last):
File "/home/zyli/anaconda3/envs/LatestStacking/lib/python3.7/site-packages/transformers/tokenization_utils_base.py", line 705, in convert_to_tensors
tensor = as_tensor(value)
ValueError: too many dimensions 'str'
During handling of the above exception, another exception occurred:
Traceback (most recent call last):
File "src/original_run_mlm_no_trainer.py", line 528, in <module>
main()
File "src/original_run_mlm_no_trainer.py", line 488, in main
for step, batch in enumerate(train_dataloader):
File "/home/zyli/anaconda3/envs/LatestStacking/lib/python3.7/site-packages/accelerate/data_loader.py", line 303, in __iter__
for batch in super().__iter__():
File "/home/zyli/anaconda3/envs/LatestStacking/lib/python3.7/site-packages/torch/utils/data/dataloader.py", line 517, in __next__
data = self._next_data()
File "/home/zyli/anaconda3/envs/LatestStacking/lib/python3.7/site-packages/torch/utils/data/dataloader.py", line 557, in _next_data
data = self._dataset_fetcher.fetch(index) # may raise StopIteration
File "/home/zyli/anaconda3/envs/LatestStacking/lib/python3.7/site-packages/torch/utils/data/_utils/fetch.py", line 47, in fetch
return self.collate_fn(data)
File "/home/zyli/anaconda3/envs/LatestStacking/lib/python3.7/site-packages/transformers/data/data_collator.py", line 41, in __call__
return self.torch_call(features)
File "/home/zyli/anaconda3/envs/LatestStacking/lib/python3.7/site-packages/transformers/data/data_collator.py", line 671, in torch_call
batch = self.tokenizer.pad(examples, return_tensors="pt", pad_to_multiple_of=self.pad_to_multiple_of)
File "/home/zyli/anaconda3/envs/LatestStacking/lib/python3.7/site-packages/transformers/tokenization_utils_base.py", line 2774, in pad
return BatchEncoding(batch_outputs, tensor_type=return_tensors)
File "/home/zyli/anaconda3/envs/LatestStacking/lib/python3.7/site-packages/transformers/tokenization_utils_base.py", line 210, in __init__
self.convert_to_tensors(tensor_type=tensor_type, prepend_batch_axis=prepend_batch_axis)
File "/home/zyli/anaconda3/envs/LatestStacking/lib/python3.7/site-packages/transformers/tokenization_utils_base.py", line 722, in convert_to_tensors
"Unable to create tensor, you should probably activate truncation and/or padding "
ValueError: Unable to create tensor, you should probably activate truncation and/or padding with 'padding=True' 'truncation=True' to have batched tensors with the same length.
`
## Environment info
<!-- You can run the command `datasets-cli env` and copy-and-paste its output below. -->
- `datasets` version: 1.12.1
- Platform: Linux-5.8.0-59-generic-x86_64-with-debian-bullseye-sid
- Python version: 3.7.6
- PyArrow version: 5.0.0
Hi ! I think this issue is more related to the `transformers` project. Could you open an issue on https://github.com/huggingface/transformers ?
Anyway I think the issue could be that both wikipedia and bookcorpusopen have an additional "title" column, contrary to wikitext which only has a "text" column. After calling `load_dataset`, can you try doing `dataset = dataset.remove_columns("title")` ? |
https://github.com/huggingface/datasets/issues/3058 | Dataset wikipedia and Bookcorpusopen cannot be fetched from dataloader. | Removing the "title" column works! Thanks for your advice.
Maybe I should still create an issue to `transformers' to mark this solution? | ## Describe the bug
I have used the previous version of `transformers` and `datasets`. The dataset `wikipedia` can be successfully used. Recently, I upgrade them to the newest version and find it raises errors. I also tried other datasets. The `wikitext` works and the `bookcorpusopen` raises the same errors as `wikipedia`.
## Steps to reproduce the bug
Run the `run_mlm_no_trainer.py` and the given script on this [link](https://github.com/huggingface/transformers/tree/master/examples/pytorch/language-modeling). Change the dataset from wikitext to wikipedia or bookcorpusopen. BTW, the library transformers is of version 4.11.3.
## Expected results
The data batchs are fetched from the data loader and train.
## Actual results
The first time to fetch data batch occurs error.
`Traceback (most recent call last):
File "/home/zyli/anaconda3/envs/LatestStacking/lib/python3.7/site-packages/transformers/tokenization_utils_base.py", line 705, in convert_to_tensors
tensor = as_tensor(value)
ValueError: too many dimensions 'str'
During handling of the above exception, another exception occurred:
Traceback (most recent call last):
File "src/original_run_mlm_no_trainer.py", line 528, in <module>
main()
File "src/original_run_mlm_no_trainer.py", line 488, in main
for step, batch in enumerate(train_dataloader):
File "/home/zyli/anaconda3/envs/LatestStacking/lib/python3.7/site-packages/accelerate/data_loader.py", line 303, in __iter__
for batch in super().__iter__():
File "/home/zyli/anaconda3/envs/LatestStacking/lib/python3.7/site-packages/torch/utils/data/dataloader.py", line 517, in __next__
data = self._next_data()
File "/home/zyli/anaconda3/envs/LatestStacking/lib/python3.7/site-packages/torch/utils/data/dataloader.py", line 557, in _next_data
data = self._dataset_fetcher.fetch(index) # may raise StopIteration
File "/home/zyli/anaconda3/envs/LatestStacking/lib/python3.7/site-packages/torch/utils/data/_utils/fetch.py", line 47, in fetch
return self.collate_fn(data)
File "/home/zyli/anaconda3/envs/LatestStacking/lib/python3.7/site-packages/transformers/data/data_collator.py", line 41, in __call__
return self.torch_call(features)
File "/home/zyli/anaconda3/envs/LatestStacking/lib/python3.7/site-packages/transformers/data/data_collator.py", line 671, in torch_call
batch = self.tokenizer.pad(examples, return_tensors="pt", pad_to_multiple_of=self.pad_to_multiple_of)
File "/home/zyli/anaconda3/envs/LatestStacking/lib/python3.7/site-packages/transformers/tokenization_utils_base.py", line 2774, in pad
return BatchEncoding(batch_outputs, tensor_type=return_tensors)
File "/home/zyli/anaconda3/envs/LatestStacking/lib/python3.7/site-packages/transformers/tokenization_utils_base.py", line 210, in __init__
self.convert_to_tensors(tensor_type=tensor_type, prepend_batch_axis=prepend_batch_axis)
File "/home/zyli/anaconda3/envs/LatestStacking/lib/python3.7/site-packages/transformers/tokenization_utils_base.py", line 722, in convert_to_tensors
"Unable to create tensor, you should probably activate truncation and/or padding "
ValueError: Unable to create tensor, you should probably activate truncation and/or padding with 'padding=True' 'truncation=True' to have batched tensors with the same length.
`
## Environment info
<!-- You can run the command `datasets-cli env` and copy-and-paste its output below. -->
- `datasets` version: 1.12.1
- Platform: Linux-5.8.0-59-generic-x86_64-with-debian-bullseye-sid
- Python version: 3.7.6
- PyArrow version: 5.0.0
| 22 | Dataset wikipedia and Bookcorpusopen cannot be fetched from dataloader.
## Describe the bug
I have used the previous version of `transformers` and `datasets`. The dataset `wikipedia` can be successfully used. Recently, I upgrade them to the newest version and find it raises errors. I also tried other datasets. The `wikitext` works and the `bookcorpusopen` raises the same errors as `wikipedia`.
## Steps to reproduce the bug
Run the `run_mlm_no_trainer.py` and the given script on this [link](https://github.com/huggingface/transformers/tree/master/examples/pytorch/language-modeling). Change the dataset from wikitext to wikipedia or bookcorpusopen. BTW, the library transformers is of version 4.11.3.
## Expected results
The data batchs are fetched from the data loader and train.
## Actual results
The first time to fetch data batch occurs error.
`Traceback (most recent call last):
File "/home/zyli/anaconda3/envs/LatestStacking/lib/python3.7/site-packages/transformers/tokenization_utils_base.py", line 705, in convert_to_tensors
tensor = as_tensor(value)
ValueError: too many dimensions 'str'
During handling of the above exception, another exception occurred:
Traceback (most recent call last):
File "src/original_run_mlm_no_trainer.py", line 528, in <module>
main()
File "src/original_run_mlm_no_trainer.py", line 488, in main
for step, batch in enumerate(train_dataloader):
File "/home/zyli/anaconda3/envs/LatestStacking/lib/python3.7/site-packages/accelerate/data_loader.py", line 303, in __iter__
for batch in super().__iter__():
File "/home/zyli/anaconda3/envs/LatestStacking/lib/python3.7/site-packages/torch/utils/data/dataloader.py", line 517, in __next__
data = self._next_data()
File "/home/zyli/anaconda3/envs/LatestStacking/lib/python3.7/site-packages/torch/utils/data/dataloader.py", line 557, in _next_data
data = self._dataset_fetcher.fetch(index) # may raise StopIteration
File "/home/zyli/anaconda3/envs/LatestStacking/lib/python3.7/site-packages/torch/utils/data/_utils/fetch.py", line 47, in fetch
return self.collate_fn(data)
File "/home/zyli/anaconda3/envs/LatestStacking/lib/python3.7/site-packages/transformers/data/data_collator.py", line 41, in __call__
return self.torch_call(features)
File "/home/zyli/anaconda3/envs/LatestStacking/lib/python3.7/site-packages/transformers/data/data_collator.py", line 671, in torch_call
batch = self.tokenizer.pad(examples, return_tensors="pt", pad_to_multiple_of=self.pad_to_multiple_of)
File "/home/zyli/anaconda3/envs/LatestStacking/lib/python3.7/site-packages/transformers/tokenization_utils_base.py", line 2774, in pad
return BatchEncoding(batch_outputs, tensor_type=return_tensors)
File "/home/zyli/anaconda3/envs/LatestStacking/lib/python3.7/site-packages/transformers/tokenization_utils_base.py", line 210, in __init__
self.convert_to_tensors(tensor_type=tensor_type, prepend_batch_axis=prepend_batch_axis)
File "/home/zyli/anaconda3/envs/LatestStacking/lib/python3.7/site-packages/transformers/tokenization_utils_base.py", line 722, in convert_to_tensors
"Unable to create tensor, you should probably activate truncation and/or padding "
ValueError: Unable to create tensor, you should probably activate truncation and/or padding with 'padding=True' 'truncation=True' to have batched tensors with the same length.
`
## Environment info
<!-- You can run the command `datasets-cli env` and copy-and-paste its output below. -->
- `datasets` version: 1.12.1
- Platform: Linux-5.8.0-59-generic-x86_64-with-debian-bullseye-sid
- Python version: 3.7.6
- PyArrow version: 5.0.0
Removing the "title" column works! Thanks for your advice.
Maybe I should still create an issue to `transformers' to mark this solution? |
https://github.com/huggingface/datasets/issues/3057 | Error in per class precision computation | Hi @tidhamecha2, thanks for reporting.
Indeed, we fixed this issue just one week ago: #3008
The fix will be included in our next version release.
In the meantime, you can incorporate the fix by installing `datasets` from the master branch:
```
pip install -U git+ssh://git@github.com/huggingface/datasets.git@master#egg=datasest
```
or
```
pip install -U git+https://github.com/huggingface/datasets.git@master#egg=datasets
``` | ## Describe the bug
When trying to get the per class precision values by providing `average=None`, following error is thrown `ValueError: can only convert an array of size 1 to a Python scalar`
## Steps to reproduce the bug
```python
from datasets import load_dataset, load_metric
precision_metric = load_metric("precision")
predictions = [0, 2, 1, 0, 0, 1]
references = [0, 1, 2, 0, 1, 2]
results = precision_metric.compute(predictions=predictions, references=references, average=None)
```
## Expected results
` {'precision': array([0.66666667, 0. , 0. ])}`
as per https://github.com/huggingface/datasets/blob/master/metrics/precision/precision.py
## Actual results
```
output = self._compute(predictions=predictions, references=references, **kwargs)
File "~/.cache/huggingface/modules/datasets_modules/metrics/precision/94709a71c6fe37171ef49d3466fec24dee9a79846c9f176dff66a649e9811690/precision.py", line 110, in _compute
sample_weight=sample_weight,
ValueError: can only convert an array of size 1 to a Python scalar
```
## Environment info
<!-- You can run the command `datasets-cli env` and copy-and-paste its output below. -->
- `datasets` version: 1.12.1
- Platform: linux
- Python version: 3.6.9
- PyArrow version: 5.0.0
| 53 | Error in per class precision computation
## Describe the bug
When trying to get the per class precision values by providing `average=None`, following error is thrown `ValueError: can only convert an array of size 1 to a Python scalar`
## Steps to reproduce the bug
```python
from datasets import load_dataset, load_metric
precision_metric = load_metric("precision")
predictions = [0, 2, 1, 0, 0, 1]
references = [0, 1, 2, 0, 1, 2]
results = precision_metric.compute(predictions=predictions, references=references, average=None)
```
## Expected results
` {'precision': array([0.66666667, 0. , 0. ])}`
as per https://github.com/huggingface/datasets/blob/master/metrics/precision/precision.py
## Actual results
```
output = self._compute(predictions=predictions, references=references, **kwargs)
File "~/.cache/huggingface/modules/datasets_modules/metrics/precision/94709a71c6fe37171ef49d3466fec24dee9a79846c9f176dff66a649e9811690/precision.py", line 110, in _compute
sample_weight=sample_weight,
ValueError: can only convert an array of size 1 to a Python scalar
```
## Environment info
<!-- You can run the command `datasets-cli env` and copy-and-paste its output below. -->
- `datasets` version: 1.12.1
- Platform: linux
- Python version: 3.6.9
- PyArrow version: 5.0.0
Hi @tidhamecha2, thanks for reporting.
Indeed, we fixed this issue just one week ago: #3008
The fix will be included in our next version release.
In the meantime, you can incorporate the fix by installing `datasets` from the master branch:
```
pip install -U git+ssh://git@github.com/huggingface/datasets.git@master#egg=datasest
```
or
```
pip install -U git+https://github.com/huggingface/datasets.git@master#egg=datasets
``` |
https://github.com/huggingface/datasets/issues/3053 | load_dataset('the_pile_openwebtext2') produces ArrowInvalid, value too large to fit in C integer type | I was getting a similar error `pyarrow.lib.ArrowInvalid: Integer value 528 not in range: -128 to 127` - AFAICT, this is because the type specified for `reddit_scores` is `datasets.Sequence(datasets.Value("int8"))`, but the actual values can be well outside the max range for 8-bit integers.
I worked around this by downloading the `the_pile_openwebtext2.py` and editing it to use local files and drop reddit scores as a column (not needed for my purposes). | ## Describe the bug
When loading `the_pile_openwebtext2`, we get the error `pyarrow.lib.ArrowInvalid: Value 2111 too large to fit in C integer type`
## Steps to reproduce the bug
```python
import datasets
ds = datasets.load_dataset('the_pile_openwebtext2')
```
## Expected results
Should download the dataset, convert it to an arrow file, and return a working Dataset object.
## Actual results
The download works, but conversion to the arrow file fails as follows:
```
>>> ds = datasets.load_dataset('the_pile_openwebtext2')
Downloading and preparing dataset openwebtext2/plain_text (download: 27.33 GiB, generated: 63.86 GiB
, post-processed: Unknown size, total: 91.19 GiB) to /home/davidbau/.cache/huggingface/datasets/open
webtext2/plain_text/1.0.0/c48ec73ba3483bac673463f48f67e9a4fd8cb49a9d6ec4fb957f0b424b97cf25...
Traceback (most recent call last):
File "/home/davidbau/.conda/envs/tenv/lib/python3.9/site-packages/datasets/builder.py", line 1133,
in _prepare_split
writer.write(example, key)
File "/home/davidbau/.conda/envs/tenv/lib/python3.9/site-packages/datasets/arrow_writer.py", line
366, in write
self.write_examples_on_file()
File "/home/davidbau/.conda/envs/tenv/lib/python3.9/site-packages/datasets/arrow_writer.py", line
311, in write_examples_on_file
pa_array = pa.array(typed_sequence)
File "pyarrow/array.pxi", line 222, in pyarrow.lib.array
File "pyarrow/array.pxi", line 110, in pyarrow.lib._handle_arrow_array_protocol
File "/home/davidbau/.conda/envs/tenv/lib/python3.9/site-packages/datasets/arrow_writer.py", line
115, in __arrow_array__
out = pa.array(cast_to_python_objects(self.data, only_1d_for_numpy=True), type=type)
File "pyarrow/array.pxi", line 305, in pyarrow.lib.array
File "pyarrow/array.pxi", line 39, in pyarrow.lib._sequence_to_array
File "pyarrow/error.pxi", line 122, in pyarrow.lib.pyarrow_internal_check_status
File "pyarrow/error.pxi", line 84, in pyarrow.lib.check_status
pyarrow.lib.ArrowInvalid: Value 2111 too large to fit in C integer type
## Environment info
<!-- You can run the command `datasets-cli env` and copy-and-paste its output below. -->
- `datasets` version:
```
- Platform: Ubuntu 20.04
- Python version: python 3.9
- PyArrow version: 3.0.0
| 69 | load_dataset('the_pile_openwebtext2') produces ArrowInvalid, value too large to fit in C integer type
## Describe the bug
When loading `the_pile_openwebtext2`, we get the error `pyarrow.lib.ArrowInvalid: Value 2111 too large to fit in C integer type`
## Steps to reproduce the bug
```python
import datasets
ds = datasets.load_dataset('the_pile_openwebtext2')
```
## Expected results
Should download the dataset, convert it to an arrow file, and return a working Dataset object.
## Actual results
The download works, but conversion to the arrow file fails as follows:
```
>>> ds = datasets.load_dataset('the_pile_openwebtext2')
Downloading and preparing dataset openwebtext2/plain_text (download: 27.33 GiB, generated: 63.86 GiB
, post-processed: Unknown size, total: 91.19 GiB) to /home/davidbau/.cache/huggingface/datasets/open
webtext2/plain_text/1.0.0/c48ec73ba3483bac673463f48f67e9a4fd8cb49a9d6ec4fb957f0b424b97cf25...
Traceback (most recent call last):
File "/home/davidbau/.conda/envs/tenv/lib/python3.9/site-packages/datasets/builder.py", line 1133,
in _prepare_split
writer.write(example, key)
File "/home/davidbau/.conda/envs/tenv/lib/python3.9/site-packages/datasets/arrow_writer.py", line
366, in write
self.write_examples_on_file()
File "/home/davidbau/.conda/envs/tenv/lib/python3.9/site-packages/datasets/arrow_writer.py", line
311, in write_examples_on_file
pa_array = pa.array(typed_sequence)
File "pyarrow/array.pxi", line 222, in pyarrow.lib.array
File "pyarrow/array.pxi", line 110, in pyarrow.lib._handle_arrow_array_protocol
File "/home/davidbau/.conda/envs/tenv/lib/python3.9/site-packages/datasets/arrow_writer.py", line
115, in __arrow_array__
out = pa.array(cast_to_python_objects(self.data, only_1d_for_numpy=True), type=type)
File "pyarrow/array.pxi", line 305, in pyarrow.lib.array
File "pyarrow/array.pxi", line 39, in pyarrow.lib._sequence_to_array
File "pyarrow/error.pxi", line 122, in pyarrow.lib.pyarrow_internal_check_status
File "pyarrow/error.pxi", line 84, in pyarrow.lib.check_status
pyarrow.lib.ArrowInvalid: Value 2111 too large to fit in C integer type
## Environment info
<!-- You can run the command `datasets-cli env` and copy-and-paste its output below. -->
- `datasets` version:
```
- Platform: Ubuntu 20.04
- Python version: python 3.9
- PyArrow version: 3.0.0
I was getting a similar error `pyarrow.lib.ArrowInvalid: Integer value 528 not in range: -128 to 127` - AFAICT, this is because the type specified for `reddit_scores` is `datasets.Sequence(datasets.Value("int8"))`, but the actual values can be well outside the max range for 8-bit integers.
I worked around this by downloading the `the_pile_openwebtext2.py` and editing it to use local files and drop reddit scores as a column (not needed for my purposes). |
https://github.com/huggingface/datasets/issues/3052 | load_dataset cannot download the data and hangs on forever if cache dir specified | Issue was environment inconsistency, updating packages did the trick
`conda install -c huggingface -c conda-forge datasets`
> Collecting package metadata (current_repodata.json): done
> Solving environment: |
> The environment is inconsistent, please check the package plan carefully
> The following packages are causing the inconsistency:
>
> - conda-forge/noarch::datasets==1.12.1=pyhd8ed1ab_1
> - conda-forge/win-64::multiprocess==0.70.12.2=py38h294d835_0
> done
>
> Package Plan
>
> environment location: C:\xxx\anaconda3\envs\UnBias-94-1
>
> added / updated specs:
> - datasets
>
>
> The following NEW packages will be INSTALLED:
>
> dill conda-forge/noarch::dill-0.3.4-pyhd8ed1ab_0
>
> The following packages will be UPDATED:
>
> ca-certificates pkgs/main::ca-certificates-2021.9.30-~ --> conda-forge::ca-certificates-2021.10.8-h5b45459_0
> certifi pkgs/main::certifi-2021.5.30-py38haa9~ --> conda-forge::certifi-2021.10.8-py38haa244fe_0
>
> The following packages will be SUPERSEDED by a higher-priority channel:
> | ## Describe the bug
After updating datasets, a code that ran just fine for ages began to fail. Specifying _datasets.load_dataset_'s _cache_dir_ optional argument on Windows 10 machine results in data download to hang on forever. Same call without cache_dir works just fine. Surprisingly exact same code just runs perfectly fine on Linux docker instance running in cloud.
Unfortunately I updated Windows also at the same time and I can't remember which version of datasets was running in my conda environment prior to the update otherwise I would have tried both to check this out. :(
## Steps to reproduce the bug
```python
# Sample code to reproduce the bug
```
cache_dir = 'c:/data/datasets'
dataset = load_dataset('wikipedia', '20200501.en', split='train',cache_dir=cache_dir)
```
Note that exact same code without specifying _cache_dir_ argument works perfectly fine.
```
cache_dir = 'c:/data/datasets'
dataset = load_dataset('wikipedia', '20200501.en', split='train')
```
## Expected results
Downloads the dataset and cache is handled in the _cache_dir_ directory
## Actual results
Data download keeps hanging on forever, **NO TRACEBACK**!
## Environment info
- `datasets` version: 1.12.1
- Platform: Windows-10-10.0.19042-SP0
- Python version: 3.8.11
- PyArrow version: 3.0.0
| 118 | load_dataset cannot download the data and hangs on forever if cache dir specified
## Describe the bug
After updating datasets, a code that ran just fine for ages began to fail. Specifying _datasets.load_dataset_'s _cache_dir_ optional argument on Windows 10 machine results in data download to hang on forever. Same call without cache_dir works just fine. Surprisingly exact same code just runs perfectly fine on Linux docker instance running in cloud.
Unfortunately I updated Windows also at the same time and I can't remember which version of datasets was running in my conda environment prior to the update otherwise I would have tried both to check this out. :(
## Steps to reproduce the bug
```python
# Sample code to reproduce the bug
```
cache_dir = 'c:/data/datasets'
dataset = load_dataset('wikipedia', '20200501.en', split='train',cache_dir=cache_dir)
```
Note that exact same code without specifying _cache_dir_ argument works perfectly fine.
```
cache_dir = 'c:/data/datasets'
dataset = load_dataset('wikipedia', '20200501.en', split='train')
```
## Expected results
Downloads the dataset and cache is handled in the _cache_dir_ directory
## Actual results
Data download keeps hanging on forever, **NO TRACEBACK**!
## Environment info
- `datasets` version: 1.12.1
- Platform: Windows-10-10.0.19042-SP0
- Python version: 3.8.11
- PyArrow version: 3.0.0
Issue was environment inconsistency, updating packages did the trick
`conda install -c huggingface -c conda-forge datasets`
> Collecting package metadata (current_repodata.json): done
> Solving environment: |
> The environment is inconsistent, please check the package plan carefully
> The following packages are causing the inconsistency:
>
> - conda-forge/noarch::datasets==1.12.1=pyhd8ed1ab_1
> - conda-forge/win-64::multiprocess==0.70.12.2=py38h294d835_0
> done
>
> Package Plan
>
> environment location: C:\xxx\anaconda3\envs\UnBias-94-1
>
> added / updated specs:
> - datasets
>
>
> The following NEW packages will be INSTALLED:
>
> dill conda-forge/noarch::dill-0.3.4-pyhd8ed1ab_0
>
> The following packages will be UPDATED:
>
> ca-certificates pkgs/main::ca-certificates-2021.9.30-~ --> conda-forge::ca-certificates-2021.10.8-h5b45459_0
> certifi pkgs/main::certifi-2021.5.30-py38haa9~ --> conda-forge::certifi-2021.10.8-py38haa244fe_0
>
> The following packages will be SUPERSEDED by a higher-priority channel:
> |
https://github.com/huggingface/datasets/issues/3051 | Non-Matching Checksum Error with crd3 dataset | I got the same error for another dataset (`multi_woz_v22`):
```
datasets.utils.info_utils.NonMatchingChecksumError: Checksums didn't match for dataset source files:
['https://github.com/budzianowski/multiwoz/raw/master/data/MultiWOZ_2.2/dialog_acts.json', 'https://github.com/budzianowski/multiwoz/raw/master/data/MultiWOZ_2.2/test/dialogues_001.json']
``` | ## Describe the bug
When I try loading the crd3 dataset (https://huggingface.co/datasets/crd3), an error is thrown.
## Steps to reproduce the bug
```python
dataset = load_dataset('crd3', split='train')
```
## Expected results
I expect no error to be thrown.
## Actual results
A non-matching checksum error is thrown.
```
datasets.utils.info_utils.NonMatchingChecksumError: Checksums didn't match for dataset source files:
['https://github.com/RevanthRameshkumar/CRD3/archive/master.zip']
```
## Environment info
- `datasets` version: 1.12.1
- Platform: Linux-4.4.0-173-generic-x86_64-with-Ubuntu-16.04-xenial
- Python version: 3.6.10
- PyArrow version: 5.0.0
| 21 | Non-Matching Checksum Error with crd3 dataset
## Describe the bug
When I try loading the crd3 dataset (https://huggingface.co/datasets/crd3), an error is thrown.
## Steps to reproduce the bug
```python
dataset = load_dataset('crd3', split='train')
```
## Expected results
I expect no error to be thrown.
## Actual results
A non-matching checksum error is thrown.
```
datasets.utils.info_utils.NonMatchingChecksumError: Checksums didn't match for dataset source files:
['https://github.com/RevanthRameshkumar/CRD3/archive/master.zip']
```
## Environment info
- `datasets` version: 1.12.1
- Platform: Linux-4.4.0-173-generic-x86_64-with-Ubuntu-16.04-xenial
- Python version: 3.6.10
- PyArrow version: 5.0.0
I got the same error for another dataset (`multi_woz_v22`):
```
datasets.utils.info_utils.NonMatchingChecksumError: Checksums didn't match for dataset source files:
['https://github.com/budzianowski/multiwoz/raw/master/data/MultiWOZ_2.2/dialog_acts.json', 'https://github.com/budzianowski/multiwoz/raw/master/data/MultiWOZ_2.2/test/dialogues_001.json']
``` |
https://github.com/huggingface/datasets/issues/3051 | Non-Matching Checksum Error with crd3 dataset | I'm seeing the same issue as @RylanSchaeffer:
Python 3.7.11, macOs 11.4
datasets==1.14.0
fails on:
```python
dataset = datasets.load_dataset("multi_woz_v22")
``` | ## Describe the bug
When I try loading the crd3 dataset (https://huggingface.co/datasets/crd3), an error is thrown.
## Steps to reproduce the bug
```python
dataset = load_dataset('crd3', split='train')
```
## Expected results
I expect no error to be thrown.
## Actual results
A non-matching checksum error is thrown.
```
datasets.utils.info_utils.NonMatchingChecksumError: Checksums didn't match for dataset source files:
['https://github.com/RevanthRameshkumar/CRD3/archive/master.zip']
```
## Environment info
- `datasets` version: 1.12.1
- Platform: Linux-4.4.0-173-generic-x86_64-with-Ubuntu-16.04-xenial
- Python version: 3.6.10
- PyArrow version: 5.0.0
| 19 | Non-Matching Checksum Error with crd3 dataset
## Describe the bug
When I try loading the crd3 dataset (https://huggingface.co/datasets/crd3), an error is thrown.
## Steps to reproduce the bug
```python
dataset = load_dataset('crd3', split='train')
```
## Expected results
I expect no error to be thrown.
## Actual results
A non-matching checksum error is thrown.
```
datasets.utils.info_utils.NonMatchingChecksumError: Checksums didn't match for dataset source files:
['https://github.com/RevanthRameshkumar/CRD3/archive/master.zip']
```
## Environment info
- `datasets` version: 1.12.1
- Platform: Linux-4.4.0-173-generic-x86_64-with-Ubuntu-16.04-xenial
- Python version: 3.6.10
- PyArrow version: 5.0.0
I'm seeing the same issue as @RylanSchaeffer:
Python 3.7.11, macOs 11.4
datasets==1.14.0
fails on:
```python
dataset = datasets.load_dataset("multi_woz_v22")
``` |
https://github.com/huggingface/datasets/issues/3048 | Identify which shard data belongs to | Independently of this I think it raises the need to allow multiprocessing during streaming so that we get samples from multiple shards in one batch. | **Is your feature request related to a problem? Please describe.**
I'm training on a large dataset made of multiple sub-datasets.
During training I can observe some jumps in loss which may correspond to different shards.
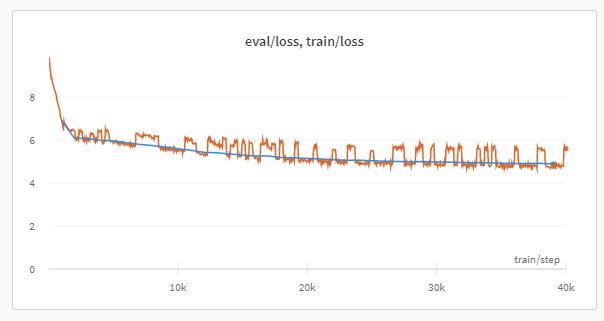
My suspicion is that either:
* some of the sub-datasets are harder for the model than others
* some of the sub-datasets are not formatted properly
I'd like to identify which shards correspond to those jumps.
**Describe the solution you'd like**
It would be nice to have a key associated to each data sample or data batch containing details on where the data comes from (shard idx + item idx within the shard).
This should be supported both in local and streaming mode.
**Describe alternatives you've considered**
A fix would be for me to add myself details (shard id, sample id) as part of each data sample.
The inconvenient is that it requires users to process/reupload every dataset when they need this feature. | 25 | Identify which shard data belongs to
**Is your feature request related to a problem? Please describe.**
I'm training on a large dataset made of multiple sub-datasets.
During training I can observe some jumps in loss which may correspond to different shards.
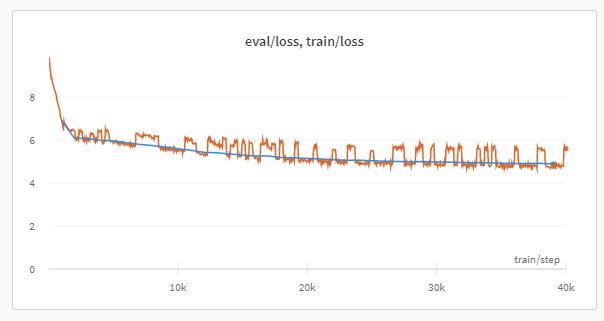
My suspicion is that either:
* some of the sub-datasets are harder for the model than others
* some of the sub-datasets are not formatted properly
I'd like to identify which shards correspond to those jumps.
**Describe the solution you'd like**
It would be nice to have a key associated to each data sample or data batch containing details on where the data comes from (shard idx + item idx within the shard).
This should be supported both in local and streaming mode.
**Describe alternatives you've considered**
A fix would be for me to add myself details (shard id, sample id) as part of each data sample.
The inconvenient is that it requires users to process/reupload every dataset when they need this feature.
Independently of this I think it raises the need to allow multiprocessing during streaming so that we get samples from multiple shards in one batch. |
https://github.com/huggingface/datasets/issues/3044 | Inconsistent caching behaviour when using `Dataset.map()` with a `new_fingerprint` and `num_proc>1` | Following the discussion in #3045 if would be nice to have a way to let users have a nice experience with caching even if the function is not hashable.
Currently a workaround is to make the function picklable. This can be done by implementing a callable class instead, that can be pickled using by implementing a custom `__getstate__` method for example.
However it sounds pretty complicated for a simple thing. Maybe one idea would be to have something similar to streamlit: they allow users to register the hashing of their own objects.
See the documentation about their `hash_funcs` here: https://docs.streamlit.io/library/advanced-features/caching#the-hash_funcs-parameter
Here is the example they give:
```python
class FileReference:
def __init__(self, filename):
self.filename = filename
def hash_file_reference(file_reference):
filename = file_reference.filename
return (filename, os.path.getmtime(filename))
@st.cache(hash_funcs={FileReference: hash_file_reference})
def func(file_reference):
...
``` | ## Describe the bug
Caching does not work when using `Dataset.map()` with:
1. a function that cannot be deterministically fingerprinted
2. `num_proc>1`
3. using a custom fingerprint set with the argument `new_fingerprint`.
This means that the dataset will be mapped with the function for each and every call, which does not happen if `num_proc==1`. In that case (`num_proc==1`) subsequent calls will load the transformed dataset from the cache, which is the expected behaviour. The example can easily be translated into a unit test.
I have a fix and will submit a pull request asap.
## Steps to reproduce the bug
```python
import hashlib
import json
import os
from typing import Dict, Any
import numpy as np
from datasets import load_dataset, Dataset
Batch = Dict[str, Any]
filename = 'example.json'
class Transformation():
"""A transformation with a random state that cannot be fingerprinted"""
def __init__(self):
self.state = np.random.random()
def __call__(self, batch: Batch) -> Batch:
batch['x'] = [np.random.random() for _ in batch['x']]
return batch
def generate_dataset():
"""generate a simple dataset"""
rgn = np.random.RandomState(24)
data = {
'data': [{'x': float(y), 'y': -float(y)} for y in
rgn.random(size=(1000,))]}
if not os.path.exists(filename):
with open(filename, 'w') as f:
f.write(json.dumps(data))
return filename
def process_dataset_with_cache(num_proc=1, remove_cache=False,
cache_expected_to_exist=False):
# load the generated dataset
dset: Dataset = next(
iter(load_dataset('json', data_files=filename, field='data').values()))
new_fingerprint = hashlib.md5("static-id".encode("utf8")).hexdigest()
# get the expected cached path
cache_path = dset._get_cache_file_path(new_fingerprint)
if remove_cache and os.path.exists(cache_path):
os.remove(cache_path)
# check that the cache exists, and print a statement
# if was actually expected to exist
cache_exist = os.path.exists(cache_path)
print(f"> cache file exists={cache_exist}")
if cache_expected_to_exist and not cache_exist:
print("=== Cache does not exist! ====")
# apply the transformation with the new fingerprint
dset = dset.map(
Transformation(),
batched=True,
num_proc=num_proc,
new_fingerprint=new_fingerprint,
desc="mapping dataset with transformation")
generate_dataset()
for num_proc in [1, 2]:
print(f"# num_proc={num_proc}, first pass")
# first pass to generate the cache (always create a new cache here)
process_dataset_with_cache(remove_cache=True,
num_proc=num_proc,
cache_expected_to_exist=False)
print(f"# num_proc={num_proc}, second pass")
# second pass, expects the cache to exist
process_dataset_with_cache(remove_cache=False,
num_proc=num_proc,
cache_expected_to_exist=True)
os.remove(filename)
```
## Expected results
In the above python example, with `num_proc=2`, the **cache file should exist in the second call** of `process_dataset_with_cache` ("=== Cache does not exist! ====" should not be printed).
When the cache is successfully created, `map()` is called only one time.
## Actual results
In the above python example, with `num_proc=2`, the **cache does not exist in the second call** of `process_dataset_with_cache` (this results in printing "=== Cache does not exist! ====").
Because the cache doesn't exist, the `map()` method is executed a second time and the dataset is not loaded from the cache.
## Environment info
- `datasets` version: 1.12.1
- Platform: macOS-10.16-x86_64-i386-64bit
- Python version: 3.8.8
- PyArrow version: 5.0.0
| 129 | Inconsistent caching behaviour when using `Dataset.map()` with a `new_fingerprint` and `num_proc>1`
## Describe the bug
Caching does not work when using `Dataset.map()` with:
1. a function that cannot be deterministically fingerprinted
2. `num_proc>1`
3. using a custom fingerprint set with the argument `new_fingerprint`.
This means that the dataset will be mapped with the function for each and every call, which does not happen if `num_proc==1`. In that case (`num_proc==1`) subsequent calls will load the transformed dataset from the cache, which is the expected behaviour. The example can easily be translated into a unit test.
I have a fix and will submit a pull request asap.
## Steps to reproduce the bug
```python
import hashlib
import json
import os
from typing import Dict, Any
import numpy as np
from datasets import load_dataset, Dataset
Batch = Dict[str, Any]
filename = 'example.json'
class Transformation():
"""A transformation with a random state that cannot be fingerprinted"""
def __init__(self):
self.state = np.random.random()
def __call__(self, batch: Batch) -> Batch:
batch['x'] = [np.random.random() for _ in batch['x']]
return batch
def generate_dataset():
"""generate a simple dataset"""
rgn = np.random.RandomState(24)
data = {
'data': [{'x': float(y), 'y': -float(y)} for y in
rgn.random(size=(1000,))]}
if not os.path.exists(filename):
with open(filename, 'w') as f:
f.write(json.dumps(data))
return filename
def process_dataset_with_cache(num_proc=1, remove_cache=False,
cache_expected_to_exist=False):
# load the generated dataset
dset: Dataset = next(
iter(load_dataset('json', data_files=filename, field='data').values()))
new_fingerprint = hashlib.md5("static-id".encode("utf8")).hexdigest()
# get the expected cached path
cache_path = dset._get_cache_file_path(new_fingerprint)
if remove_cache and os.path.exists(cache_path):
os.remove(cache_path)
# check that the cache exists, and print a statement
# if was actually expected to exist
cache_exist = os.path.exists(cache_path)
print(f"> cache file exists={cache_exist}")
if cache_expected_to_exist and not cache_exist:
print("=== Cache does not exist! ====")
# apply the transformation with the new fingerprint
dset = dset.map(
Transformation(),
batched=True,
num_proc=num_proc,
new_fingerprint=new_fingerprint,
desc="mapping dataset with transformation")
generate_dataset()
for num_proc in [1, 2]:
print(f"# num_proc={num_proc}, first pass")
# first pass to generate the cache (always create a new cache here)
process_dataset_with_cache(remove_cache=True,
num_proc=num_proc,
cache_expected_to_exist=False)
print(f"# num_proc={num_proc}, second pass")
# second pass, expects the cache to exist
process_dataset_with_cache(remove_cache=False,
num_proc=num_proc,
cache_expected_to_exist=True)
os.remove(filename)
```
## Expected results
In the above python example, with `num_proc=2`, the **cache file should exist in the second call** of `process_dataset_with_cache` ("=== Cache does not exist! ====" should not be printed).
When the cache is successfully created, `map()` is called only one time.
## Actual results
In the above python example, with `num_proc=2`, the **cache does not exist in the second call** of `process_dataset_with_cache` (this results in printing "=== Cache does not exist! ====").
Because the cache doesn't exist, the `map()` method is executed a second time and the dataset is not loaded from the cache.
## Environment info
- `datasets` version: 1.12.1
- Platform: macOS-10.16-x86_64-i386-64bit
- Python version: 3.8.8
- PyArrow version: 5.0.0
Following the discussion in #3045 if would be nice to have a way to let users have a nice experience with caching even if the function is not hashable.
Currently a workaround is to make the function picklable. This can be done by implementing a callable class instead, that can be pickled using by implementing a custom `__getstate__` method for example.
However it sounds pretty complicated for a simple thing. Maybe one idea would be to have something similar to streamlit: they allow users to register the hashing of their own objects.
See the documentation about their `hash_funcs` here: https://docs.streamlit.io/library/advanced-features/caching#the-hash_funcs-parameter
Here is the example they give:
```python
class FileReference:
def __init__(self, filename):
self.filename = filename
def hash_file_reference(file_reference):
filename = file_reference.filename
return (filename, os.path.getmtime(filename))
@st.cache(hash_funcs={FileReference: hash_file_reference})
def func(file_reference):
...
``` |
https://github.com/huggingface/datasets/issues/3044 | Inconsistent caching behaviour when using `Dataset.map()` with a `new_fingerprint` and `num_proc>1` | My solution was to generate a custom hash, and use the hash as a `new_fingerprint` argument to the `map()` method to enable caching. This works, but is quite hacky.
@lhoestq, this approach is very neat, this would make the whole caching mechanic more explicit. I don't have so much time to look into this right now, but I might give it a try in the future. | ## Describe the bug
Caching does not work when using `Dataset.map()` with:
1. a function that cannot be deterministically fingerprinted
2. `num_proc>1`
3. using a custom fingerprint set with the argument `new_fingerprint`.
This means that the dataset will be mapped with the function for each and every call, which does not happen if `num_proc==1`. In that case (`num_proc==1`) subsequent calls will load the transformed dataset from the cache, which is the expected behaviour. The example can easily be translated into a unit test.
I have a fix and will submit a pull request asap.
## Steps to reproduce the bug
```python
import hashlib
import json
import os
from typing import Dict, Any
import numpy as np
from datasets import load_dataset, Dataset
Batch = Dict[str, Any]
filename = 'example.json'
class Transformation():
"""A transformation with a random state that cannot be fingerprinted"""
def __init__(self):
self.state = np.random.random()
def __call__(self, batch: Batch) -> Batch:
batch['x'] = [np.random.random() for _ in batch['x']]
return batch
def generate_dataset():
"""generate a simple dataset"""
rgn = np.random.RandomState(24)
data = {
'data': [{'x': float(y), 'y': -float(y)} for y in
rgn.random(size=(1000,))]}
if not os.path.exists(filename):
with open(filename, 'w') as f:
f.write(json.dumps(data))
return filename
def process_dataset_with_cache(num_proc=1, remove_cache=False,
cache_expected_to_exist=False):
# load the generated dataset
dset: Dataset = next(
iter(load_dataset('json', data_files=filename, field='data').values()))
new_fingerprint = hashlib.md5("static-id".encode("utf8")).hexdigest()
# get the expected cached path
cache_path = dset._get_cache_file_path(new_fingerprint)
if remove_cache and os.path.exists(cache_path):
os.remove(cache_path)
# check that the cache exists, and print a statement
# if was actually expected to exist
cache_exist = os.path.exists(cache_path)
print(f"> cache file exists={cache_exist}")
if cache_expected_to_exist and not cache_exist:
print("=== Cache does not exist! ====")
# apply the transformation with the new fingerprint
dset = dset.map(
Transformation(),
batched=True,
num_proc=num_proc,
new_fingerprint=new_fingerprint,
desc="mapping dataset with transformation")
generate_dataset()
for num_proc in [1, 2]:
print(f"# num_proc={num_proc}, first pass")
# first pass to generate the cache (always create a new cache here)
process_dataset_with_cache(remove_cache=True,
num_proc=num_proc,
cache_expected_to_exist=False)
print(f"# num_proc={num_proc}, second pass")
# second pass, expects the cache to exist
process_dataset_with_cache(remove_cache=False,
num_proc=num_proc,
cache_expected_to_exist=True)
os.remove(filename)
```
## Expected results
In the above python example, with `num_proc=2`, the **cache file should exist in the second call** of `process_dataset_with_cache` ("=== Cache does not exist! ====" should not be printed).
When the cache is successfully created, `map()` is called only one time.
## Actual results
In the above python example, with `num_proc=2`, the **cache does not exist in the second call** of `process_dataset_with_cache` (this results in printing "=== Cache does not exist! ====").
Because the cache doesn't exist, the `map()` method is executed a second time and the dataset is not loaded from the cache.
## Environment info
- `datasets` version: 1.12.1
- Platform: macOS-10.16-x86_64-i386-64bit
- Python version: 3.8.8
- PyArrow version: 5.0.0
| 66 | Inconsistent caching behaviour when using `Dataset.map()` with a `new_fingerprint` and `num_proc>1`
## Describe the bug
Caching does not work when using `Dataset.map()` with:
1. a function that cannot be deterministically fingerprinted
2. `num_proc>1`
3. using a custom fingerprint set with the argument `new_fingerprint`.
This means that the dataset will be mapped with the function for each and every call, which does not happen if `num_proc==1`. In that case (`num_proc==1`) subsequent calls will load the transformed dataset from the cache, which is the expected behaviour. The example can easily be translated into a unit test.
I have a fix and will submit a pull request asap.
## Steps to reproduce the bug
```python
import hashlib
import json
import os
from typing import Dict, Any
import numpy as np
from datasets import load_dataset, Dataset
Batch = Dict[str, Any]
filename = 'example.json'
class Transformation():
"""A transformation with a random state that cannot be fingerprinted"""
def __init__(self):
self.state = np.random.random()
def __call__(self, batch: Batch) -> Batch:
batch['x'] = [np.random.random() for _ in batch['x']]
return batch
def generate_dataset():
"""generate a simple dataset"""
rgn = np.random.RandomState(24)
data = {
'data': [{'x': float(y), 'y': -float(y)} for y in
rgn.random(size=(1000,))]}
if not os.path.exists(filename):
with open(filename, 'w') as f:
f.write(json.dumps(data))
return filename
def process_dataset_with_cache(num_proc=1, remove_cache=False,
cache_expected_to_exist=False):
# load the generated dataset
dset: Dataset = next(
iter(load_dataset('json', data_files=filename, field='data').values()))
new_fingerprint = hashlib.md5("static-id".encode("utf8")).hexdigest()
# get the expected cached path
cache_path = dset._get_cache_file_path(new_fingerprint)
if remove_cache and os.path.exists(cache_path):
os.remove(cache_path)
# check that the cache exists, and print a statement
# if was actually expected to exist
cache_exist = os.path.exists(cache_path)
print(f"> cache file exists={cache_exist}")
if cache_expected_to_exist and not cache_exist:
print("=== Cache does not exist! ====")
# apply the transformation with the new fingerprint
dset = dset.map(
Transformation(),
batched=True,
num_proc=num_proc,
new_fingerprint=new_fingerprint,
desc="mapping dataset with transformation")
generate_dataset()
for num_proc in [1, 2]:
print(f"# num_proc={num_proc}, first pass")
# first pass to generate the cache (always create a new cache here)
process_dataset_with_cache(remove_cache=True,
num_proc=num_proc,
cache_expected_to_exist=False)
print(f"# num_proc={num_proc}, second pass")
# second pass, expects the cache to exist
process_dataset_with_cache(remove_cache=False,
num_proc=num_proc,
cache_expected_to_exist=True)
os.remove(filename)
```
## Expected results
In the above python example, with `num_proc=2`, the **cache file should exist in the second call** of `process_dataset_with_cache` ("=== Cache does not exist! ====" should not be printed).
When the cache is successfully created, `map()` is called only one time.
## Actual results
In the above python example, with `num_proc=2`, the **cache does not exist in the second call** of `process_dataset_with_cache` (this results in printing "=== Cache does not exist! ====").
Because the cache doesn't exist, the `map()` method is executed a second time and the dataset is not loaded from the cache.
## Environment info
- `datasets` version: 1.12.1
- Platform: macOS-10.16-x86_64-i386-64bit
- Python version: 3.8.8
- PyArrow version: 5.0.0
My solution was to generate a custom hash, and use the hash as a `new_fingerprint` argument to the `map()` method to enable caching. This works, but is quite hacky.
@lhoestq, this approach is very neat, this would make the whole caching mechanic more explicit. I don't have so much time to look into this right now, but I might give it a try in the future. |
https://github.com/huggingface/datasets/issues/3044 | Inconsistent caching behaviour when using `Dataset.map()` with a `new_fingerprint` and `num_proc>1` | Almost a year later and I'm in a similar boat. Using custom fingerprints and when using multiprocessing the cached datasets are saved with a template at the end of the filename (something like "000001_of_000008" for every process of num_proc). So if in the next time you run the script you set num_proc to a different number, the cache cannot be used.
Is there any way to get around this? I am processing a huge dataset so I do the processing on one machine and then transfer the processed data to another in its cache dir but currently that's not possible due to num_proc mismatch. | ## Describe the bug
Caching does not work when using `Dataset.map()` with:
1. a function that cannot be deterministically fingerprinted
2. `num_proc>1`
3. using a custom fingerprint set with the argument `new_fingerprint`.
This means that the dataset will be mapped with the function for each and every call, which does not happen if `num_proc==1`. In that case (`num_proc==1`) subsequent calls will load the transformed dataset from the cache, which is the expected behaviour. The example can easily be translated into a unit test.
I have a fix and will submit a pull request asap.
## Steps to reproduce the bug
```python
import hashlib
import json
import os
from typing import Dict, Any
import numpy as np
from datasets import load_dataset, Dataset
Batch = Dict[str, Any]
filename = 'example.json'
class Transformation():
"""A transformation with a random state that cannot be fingerprinted"""
def __init__(self):
self.state = np.random.random()
def __call__(self, batch: Batch) -> Batch:
batch['x'] = [np.random.random() for _ in batch['x']]
return batch
def generate_dataset():
"""generate a simple dataset"""
rgn = np.random.RandomState(24)
data = {
'data': [{'x': float(y), 'y': -float(y)} for y in
rgn.random(size=(1000,))]}
if not os.path.exists(filename):
with open(filename, 'w') as f:
f.write(json.dumps(data))
return filename
def process_dataset_with_cache(num_proc=1, remove_cache=False,
cache_expected_to_exist=False):
# load the generated dataset
dset: Dataset = next(
iter(load_dataset('json', data_files=filename, field='data').values()))
new_fingerprint = hashlib.md5("static-id".encode("utf8")).hexdigest()
# get the expected cached path
cache_path = dset._get_cache_file_path(new_fingerprint)
if remove_cache and os.path.exists(cache_path):
os.remove(cache_path)
# check that the cache exists, and print a statement
# if was actually expected to exist
cache_exist = os.path.exists(cache_path)
print(f"> cache file exists={cache_exist}")
if cache_expected_to_exist and not cache_exist:
print("=== Cache does not exist! ====")
# apply the transformation with the new fingerprint
dset = dset.map(
Transformation(),
batched=True,
num_proc=num_proc,
new_fingerprint=new_fingerprint,
desc="mapping dataset with transformation")
generate_dataset()
for num_proc in [1, 2]:
print(f"# num_proc={num_proc}, first pass")
# first pass to generate the cache (always create a new cache here)
process_dataset_with_cache(remove_cache=True,
num_proc=num_proc,
cache_expected_to_exist=False)
print(f"# num_proc={num_proc}, second pass")
# second pass, expects the cache to exist
process_dataset_with_cache(remove_cache=False,
num_proc=num_proc,
cache_expected_to_exist=True)
os.remove(filename)
```
## Expected results
In the above python example, with `num_proc=2`, the **cache file should exist in the second call** of `process_dataset_with_cache` ("=== Cache does not exist! ====" should not be printed).
When the cache is successfully created, `map()` is called only one time.
## Actual results
In the above python example, with `num_proc=2`, the **cache does not exist in the second call** of `process_dataset_with_cache` (this results in printing "=== Cache does not exist! ====").
Because the cache doesn't exist, the `map()` method is executed a second time and the dataset is not loaded from the cache.
## Environment info
- `datasets` version: 1.12.1
- Platform: macOS-10.16-x86_64-i386-64bit
- Python version: 3.8.8
- PyArrow version: 5.0.0
| 104 | Inconsistent caching behaviour when using `Dataset.map()` with a `new_fingerprint` and `num_proc>1`
## Describe the bug
Caching does not work when using `Dataset.map()` with:
1. a function that cannot be deterministically fingerprinted
2. `num_proc>1`
3. using a custom fingerprint set with the argument `new_fingerprint`.
This means that the dataset will be mapped with the function for each and every call, which does not happen if `num_proc==1`. In that case (`num_proc==1`) subsequent calls will load the transformed dataset from the cache, which is the expected behaviour. The example can easily be translated into a unit test.
I have a fix and will submit a pull request asap.
## Steps to reproduce the bug
```python
import hashlib
import json
import os
from typing import Dict, Any
import numpy as np
from datasets import load_dataset, Dataset
Batch = Dict[str, Any]
filename = 'example.json'
class Transformation():
"""A transformation with a random state that cannot be fingerprinted"""
def __init__(self):
self.state = np.random.random()
def __call__(self, batch: Batch) -> Batch:
batch['x'] = [np.random.random() for _ in batch['x']]
return batch
def generate_dataset():
"""generate a simple dataset"""
rgn = np.random.RandomState(24)
data = {
'data': [{'x': float(y), 'y': -float(y)} for y in
rgn.random(size=(1000,))]}
if not os.path.exists(filename):
with open(filename, 'w') as f:
f.write(json.dumps(data))
return filename
def process_dataset_with_cache(num_proc=1, remove_cache=False,
cache_expected_to_exist=False):
# load the generated dataset
dset: Dataset = next(
iter(load_dataset('json', data_files=filename, field='data').values()))
new_fingerprint = hashlib.md5("static-id".encode("utf8")).hexdigest()
# get the expected cached path
cache_path = dset._get_cache_file_path(new_fingerprint)
if remove_cache and os.path.exists(cache_path):
os.remove(cache_path)
# check that the cache exists, and print a statement
# if was actually expected to exist
cache_exist = os.path.exists(cache_path)
print(f"> cache file exists={cache_exist}")
if cache_expected_to_exist and not cache_exist:
print("=== Cache does not exist! ====")
# apply the transformation with the new fingerprint
dset = dset.map(
Transformation(),
batched=True,
num_proc=num_proc,
new_fingerprint=new_fingerprint,
desc="mapping dataset with transformation")
generate_dataset()
for num_proc in [1, 2]:
print(f"# num_proc={num_proc}, first pass")
# first pass to generate the cache (always create a new cache here)
process_dataset_with_cache(remove_cache=True,
num_proc=num_proc,
cache_expected_to_exist=False)
print(f"# num_proc={num_proc}, second pass")
# second pass, expects the cache to exist
process_dataset_with_cache(remove_cache=False,
num_proc=num_proc,
cache_expected_to_exist=True)
os.remove(filename)
```
## Expected results
In the above python example, with `num_proc=2`, the **cache file should exist in the second call** of `process_dataset_with_cache` ("=== Cache does not exist! ====" should not be printed).
When the cache is successfully created, `map()` is called only one time.
## Actual results
In the above python example, with `num_proc=2`, the **cache does not exist in the second call** of `process_dataset_with_cache` (this results in printing "=== Cache does not exist! ====").
Because the cache doesn't exist, the `map()` method is executed a second time and the dataset is not loaded from the cache.
## Environment info
- `datasets` version: 1.12.1
- Platform: macOS-10.16-x86_64-i386-64bit
- Python version: 3.8.8
- PyArrow version: 5.0.0
Almost a year later and I'm in a similar boat. Using custom fingerprints and when using multiprocessing the cached datasets are saved with a template at the end of the filename (something like "000001_of_000008" for every process of num_proc). So if in the next time you run the script you set num_proc to a different number, the cache cannot be used.
Is there any way to get around this? I am processing a huge dataset so I do the processing on one machine and then transfer the processed data to another in its cache dir but currently that's not possible due to num_proc mismatch. |