Process audio data¶
🤗 Datasets supports an datasets.Audio
feature, enabling users to load and process raw audio files for training. This guide will show you how to:
Load your own custom audio dataset.
Resample audio files.
Use
datasets.Dataset.map()
with audio files.
Installation¶
The datasets.Audio
feature is an experimental feature and should be installed as an extra dependency in 🤗 Datasets. Install the datasets.Audio
feature with pip:
>>> pip install datasets[audio]
Users should also install torchaudio and librosa, two common libraries used by 🤗 Datasets for handling audio data.
>>> pip install librosa
>>> pip install torchaudio
Important
torchaudio’s sox_io
backend supports decoding mp3
files. Unfortunately, the sox_io
backend is only available on Linux/macOS, and is not supported by Windows.
Then you can load an audio dataset the same way you would load a text dataset. For example, load the Common Voice dataset with the Turkish configuration:
>>> from datasets import load_dataset, load_metric, Audio
>>> common_voice = load_dataset("common_voice", "tr", split="train")
Audio datasets¶
Audio datasets commonly have an audio
and path
or file
column.
audio
is the actual audio file that is loaded and resampled on-the-fly upon calling it.
>>> common_voice[0]["audio"]
{'array': array([ 0.0000000e+00, 0.0000000e+00, 0.0000000e+00, ...,
-8.8930130e-05, -3.8027763e-05, -2.9146671e-05], dtype=float32),
'path': '/root/.cache/huggingface/datasets/downloads/extracted/05be0c29807a73c9b099873d2f5975dae6d05e9f7d577458a2466ecb9a2b0c6b/cv-corpus-6.1-2020-12-11/tr/clips/common_voice_tr_21921195.mp3',
'sampling_rate': 48000}
When you access an audio file, it is automatically decoded and resampled. Generally, you should query an audio file like: common_voice[0]["audio"]
. If you query an audio file with common_voice["audio"][0]
instead, all the audio files in your dataset will be decoded and resampled. This process can take a long time if you have a large dataset.
path
or file
is an absolute path to an audio file.
>>> common_voice[0]["path"]
/root/.cache/huggingface/datasets/downloads/extracted/05be0c29807a73c9b099873d2f5975dae6d05e9f7d577458a2466ecb9a2b0c6b/cv-corpus-6.1-2020-12-11/tr/clips/common_voice_tr_21921195.mp3
The path
is useful if you want to load your own audio dataset. In this case, provide a column of audio file paths to datasets.Dataset.cast_column()
:
>>> my_audio_dataset = my_audio_dataset.cast_column("paths_to_my_audio_files", Audio())
Resample¶
Some models expect the audio data to have a certain sampling rate due to how the model was pretrained. For example, the XLSR-Wav2Vec2 model expects the input to have a sampling rate of 16kHz, but an audio file from the Common Voice dataset has a sampling rate of 48kHz. You can use datasets.Dataset.cast_column()
to downsample the sampling rate to 16kHz:
>>> common_voice = common_voice.cast_column("audio", Audio(sampling_rate=16_000))
The next time you load the audio file, the datasets.Audio
feature will load and resample it to 16kHz:
>>> common_voice_train[0]["audio"]
{'array': array([ 0.0000000e+00, 0.0000000e+00, 0.0000000e+00, ...,
-7.4556941e-05, -1.4621433e-05, -5.7861507e-05], dtype=float32),
'path': '/root/.cache/huggingface/datasets/downloads/extracted/05be0c29807a73c9b099873d2f5975dae6d05e9f7d577458a2466ecb9a2b0c6b/cv-corpus-6.1-2020-12-11/tr/clips/common_voice_tr_21921195.mp3',
'sampling_rate': 16000}
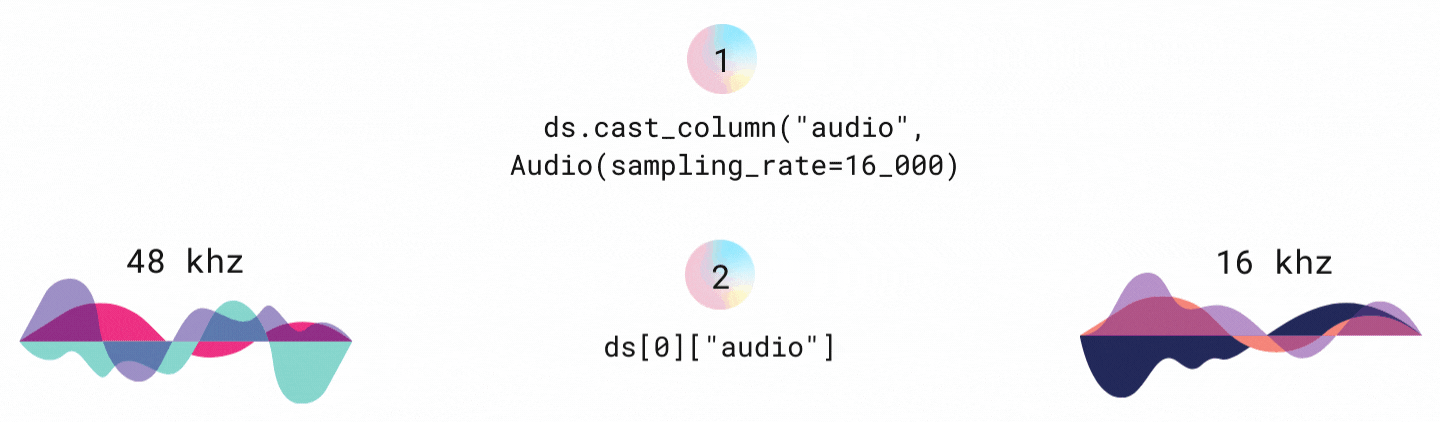
Map
¶
Just like text datasets, you can apply a preprocessing function over an entire dataset with datasets.Dataset.map()
, which is useful for preprocessing all of your audio data at once. Start with a speech recognition model of your choice, and load a processor
object that contains:
A feature extractor to convert the speech signal to the model’s input format. Every speech recognition model on the 🤗 Hub contains a predefined feature extractor that can be easily loaded with
AutoFeatureExtractor.from_pretrained(...)
.A tokenizer to convert the model’s output format to text. Fine-tuned speech recognition models, such as facebook/wav2vec2-base-960h, contain a predefined tokenizer that can be easily loaded with
AutoTokenizer.from_pretrained(...)
.For pretrained speech recognition models, such as facebook/wav2vec2-large-xlsr-53, a tokenizer needs to be created from the target text as explained here. The following example demonstrates how to load a feature extractor, tokenizer and processor for a pretrained speech recognition model:
>>> from transformers import AutoTokenizer, AutoFeatureExtractor, Wav2Vec2Processor
>>> model_checkpoint = "facebook/wav2vec2-large-xlsr-53"
>>> # after defining a vocab.json file you can instantiate a tokenizer object:
>>> tokenizer = AutoTokenizer("./vocab.json", unk_token="[UNK]", pad_token="[PAD]", word_delimiter_token="|")
>>> feature_extractor = AutoFeatureExtractor.from_pretrained(model_checkpoint)
>>> processor = Wav2Vec2Processor.from_pretrained(feature_extractor=feature_extractor, tokenizer=tokenizer)
For fine-tuned speech recognition models, you can simply load a predefined processor
object with:
>>> from transformers import Wav2Vec2Processor
>>> processor = Wav2Vec2Processor.from_pretrained("facebook/wav2vec2-base-960h")
Make sure to include the audio
key in your preprocessing function when you call datasets.Dataset.map()
so that you are actually resampling the audio data:
>>> def prepare_dataset(batch):
... audio = batch["audio"]
... batch["input_values"] = processor(audio["array"], sampling_rate=audio["sampling_rate"]).input_values[0]
... batch["input_length"] = len(batch["input_values"])
... with processor.as_target_processor():
... batch["labels"] = processor(batch["sentence"]).input_ids
... return batch
>>> common_voice_train = common_voice_train.map(prepare_dataset, remove_columns=common_voice_train.column_names)