Transformers documentation
TrOCR
TrOCR
Overview
The TrOCR model was proposed in TrOCR: Transformer-based Optical Character Recognition with Pre-trained Models by Minghao Li, Tengchao Lv, Lei Cui, Yijuan Lu, Dinei Florencio, Cha Zhang, Zhoujun Li, Furu Wei. TrOCR consists of an image Transformer encoder and an autoregressive text Transformer decoder to perform optical character recognition (OCR).
The abstract from the paper is the following:
Text recognition is a long-standing research problem for document digitalization. Existing approaches for text recognition are usually built based on CNN for image understanding and RNN for char-level text generation. In addition, another language model is usually needed to improve the overall accuracy as a post-processing step. In this paper, we propose an end-to-end text recognition approach with pre-trained image Transformer and text Transformer models, namely TrOCR, which leverages the Transformer architecture for both image understanding and wordpiece-level text generation. The TrOCR model is simple but effective, and can be pre-trained with large-scale synthetic data and fine-tuned with human-labeled datasets. Experiments show that the TrOCR model outperforms the current state-of-the-art models on both printed and handwritten text recognition tasks.
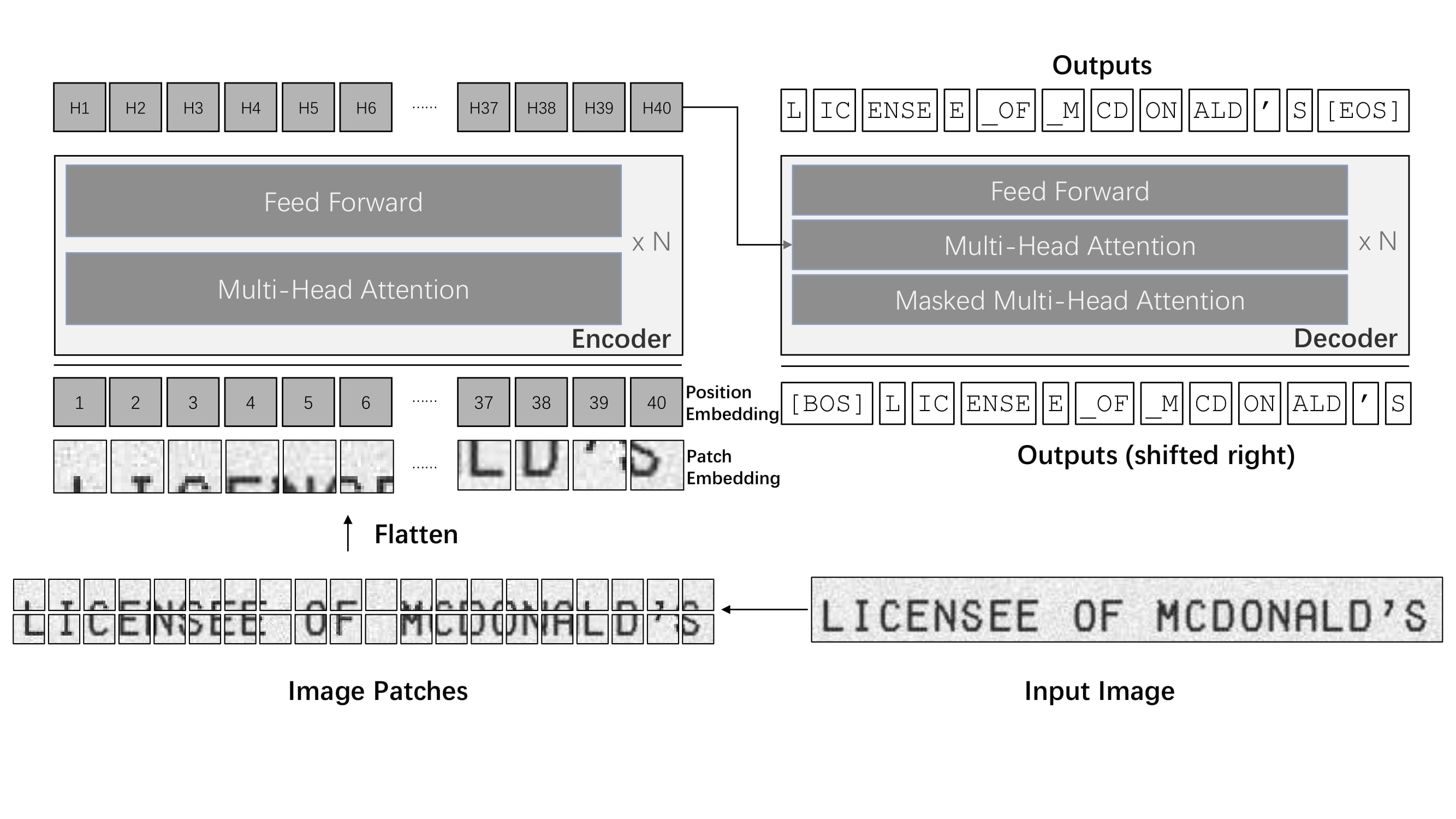
Please refer to the VisionEncoderDecoder
class on how to use this model.
This model was contributed by nielsr. The original code can be found here.
Usage tips
- The quickest way to get started with TrOCR is by checking the tutorial notebooks, which show how to use the model at inference time as well as fine-tuning on custom data.
- TrOCR is pre-trained in 2 stages before being fine-tuned on downstream datasets. It achieves state-of-the-art results on both printed (e.g. the SROIE dataset and handwritten (e.g. the IAM Handwriting dataset text recognition tasks. For more information, see the official models.
- TrOCR is always used within the VisionEncoderDecoder framework.
Resources
A list of official Hugging Face and community (indicated by 🌎) resources to help you get started with TrOCR. If you’re interested in submitting a resource to be included here, please feel free to open a Pull Request and we’ll review it! The resource should ideally demonstrate something new instead of duplicating an existing resource.
- A blog post on Accelerating Document AI with TrOCR.
- A blog post on how to Document AI with TrOCR.
- A notebook on how to finetune TrOCR on IAM Handwriting Database using Seq2SeqTrainer.
- A notebook on inference with TrOCR and Gradio demo.
- A notebook on finetune TrOCR on the IAM Handwriting Database using native PyTorch.
- A notebook on evaluating TrOCR on the IAM test set.
- Casual language modeling task guide.
⚡️ Inference
- An interactive-demo on TrOCR handwritten character recognition.
Inference
TrOCR’s VisionEncoderDecoder
model accepts images as input and makes use of
generate() to autoregressively generate text given the input image.
The [ViTImageProcessor
/DeiTImageProcessor
] class is responsible for preprocessing the input image and
[RobertaTokenizer
/XLMRobertaTokenizer
] decodes the generated target tokens to the target string. The
TrOCRProcessor wraps [ViTImageProcessor
/DeiTImageProcessor
] and [RobertaTokenizer
/XLMRobertaTokenizer
]
into a single instance to both extract the input features and decode the predicted token ids.
- Step-by-step Optical Character Recognition (OCR)
>>> from transformers import TrOCRProcessor, VisionEncoderDecoderModel
>>> import requests
>>> from PIL import Image
>>> processor = TrOCRProcessor.from_pretrained("microsoft/trocr-base-handwritten")
>>> model = VisionEncoderDecoderModel.from_pretrained("microsoft/trocr-base-handwritten")
>>> # load image from the IAM dataset
>>> url = "https://fki.tic.heia-fr.ch/static/img/a01-122-02.jpg"
>>> image = Image.open(requests.get(url, stream=True).raw).convert("RGB")
>>> pixel_values = processor(image, return_tensors="pt").pixel_values
>>> generated_ids = model.generate(pixel_values)
>>> generated_text = processor.batch_decode(generated_ids, skip_special_tokens=True)[0]
See the model hub to look for TrOCR checkpoints.
TrOCRConfig
class transformers.TrOCRConfig
< source >( vocab_size = 50265 d_model = 1024 decoder_layers = 12 decoder_attention_heads = 16 decoder_ffn_dim = 4096 activation_function = 'gelu' max_position_embeddings = 512 dropout = 0.1 attention_dropout = 0.0 activation_dropout = 0.0 decoder_start_token_id = 2 init_std = 0.02 decoder_layerdrop = 0.0 use_cache = True scale_embedding = False use_learned_position_embeddings = True layernorm_embedding = True pad_token_id = 1 bos_token_id = 0 eos_token_id = 2 **kwargs )
Parameters
- vocab_size (
int
, optional, defaults to 50265) — Vocabulary size of the TrOCR model. Defines the number of different tokens that can be represented by theinputs_ids
passed when calling TrOCRForCausalLM. - d_model (
int
, optional, defaults to 1024) — Dimensionality of the layers and the pooler layer. - decoder_layers (
int
, optional, defaults to 12) — Number of decoder layers. - decoder_attention_heads (
int
, optional, defaults to 16) — Number of attention heads for each attention layer in the Transformer decoder. - decoder_ffn_dim (
int
, optional, defaults to 4096) — Dimensionality of the “intermediate” (often named feed-forward) layer in decoder. - activation_function (
str
orfunction
, optional, defaults to"gelu"
) — The non-linear activation function (function or string) in the pooler. If string,"gelu"
,"relu"
,"silu"
and"gelu_new"
are supported. - max_position_embeddings (
int
, optional, defaults to 512) — The maximum sequence length that this model might ever be used with. Typically set this to something large just in case (e.g., 512 or 1024 or 2048). - dropout (
float
, optional, defaults to 0.1) — The dropout probability for all fully connected layers in the embeddings, and pooler. - attention_dropout (
float
, optional, defaults to 0.0) — The dropout ratio for the attention probabilities. - activation_dropout (
float
, optional, defaults to 0.0) — The dropout ratio for activations inside the fully connected layer. - init_std (
float
, optional, defaults to 0.02) — The standard deviation of the truncated_normal_initializer for initializing all weight matrices. - decoder_layerdrop (
float
, optional, defaults to 0.0) — The LayerDrop probability for the decoder. See the [LayerDrop paper](see https://arxiv.org/abs/1909.11556) for more details. - use_cache (
bool
, optional, defaults toTrue
) — Whether or not the model should return the last key/values attentions (not used by all models). - scale_embedding (
bool
, optional, defaults toFalse
) — Whether or not to scale the word embeddings by sqrt(d_model). - use_learned_position_embeddings (
bool
, optional, defaults toTrue
) — Whether or not to use learned position embeddings. If not, sinusoidal position embeddings will be used. - layernorm_embedding (
bool
, optional, defaults toTrue
) — Whether or not to use a layernorm after the word + position embeddings.
This is the configuration class to store the configuration of a TrOCRForCausalLM. It is used to instantiate an TrOCR model according to the specified arguments, defining the model architecture. Instantiating a configuration with the defaults will yield a similar configuration to that of the TrOCR microsoft/trocr-base-handwritten architecture.
Configuration objects inherit from PretrainedConfig and can be used to control the model outputs. Read the documentation from PretrainedConfig for more information.
Example:
>>> from transformers import TrOCRConfig, TrOCRForCausalLM
>>> # Initializing a TrOCR-base style configuration
>>> configuration = TrOCRConfig()
>>> # Initializing a model (with random weights) from the TrOCR-base style configuration
>>> model = TrOCRForCausalLM(configuration)
>>> # Accessing the model configuration
>>> configuration = model.config
TrOCRProcessor
class transformers.TrOCRProcessor
< source >( image_processor = None tokenizer = None **kwargs )
Parameters
- image_processor ([
ViTImageProcessor
/DeiTImageProcessor
], optional) — An instance of [ViTImageProcessor
/DeiTImageProcessor
]. The image processor is a required input. - tokenizer ([
RobertaTokenizer
/XLMRobertaTokenizer
], optional) — An instance of [RobertaTokenizer
/XLMRobertaTokenizer
]. The tokenizer is a required input.
Constructs a TrOCR processor which wraps a vision image processor and a TrOCR tokenizer into a single processor.
TrOCRProcessor offers all the functionalities of [ViTImageProcessor
/DeiTImageProcessor
] and
[RobertaTokenizer
/XLMRobertaTokenizer
]. See the call() and decode() for
more information.
__call__
< source >( images: typing.Union[ForwardRef('PIL.Image.Image'), numpy.ndarray, ForwardRef('torch.Tensor'), list['PIL.Image.Image'], list[numpy.ndarray], list['torch.Tensor']] = None text: typing.Union[str, typing.List[str], typing.List[typing.List[str]]] = None audio = None videos = None **kwargs: typing_extensions.Unpack[transformers.models.trocr.processing_trocr.TrOCRProcessorKwargs] )
When used in normal mode, this method forwards all its arguments to AutoImageProcessor’s
__call__()
and returns its output. If used in the context
as_target_processor()
this method forwards all its arguments to TrOCRTokenizer’s
~TrOCRTokenizer.__call__
. Please refer to the docstring of the above two methods for more information.
from_pretrained
< source >( pretrained_model_name_or_path: typing.Union[str, os.PathLike] cache_dir: typing.Union[str, os.PathLike, NoneType] = None force_download: bool = False local_files_only: bool = False token: typing.Union[str, bool, NoneType] = None revision: str = 'main' **kwargs )
Parameters
- pretrained_model_name_or_path (
str
oros.PathLike
) — This can be either:- a string, the model id of a pretrained feature_extractor hosted inside a model repo on huggingface.co.
- a path to a directory containing a feature extractor file saved using the
save_pretrained() method, e.g.,
./my_model_directory/
. - a path or url to a saved feature extractor JSON file, e.g.,
./my_model_directory/preprocessor_config.json
.
- **kwargs —
Additional keyword arguments passed along to both
from_pretrained() and
~tokenization_utils_base.PreTrainedTokenizer.from_pretrained
.
Instantiate a processor associated with a pretrained model.
This class method is simply calling the feature extractor
from_pretrained(), image processor
ImageProcessingMixin and the tokenizer
~tokenization_utils_base.PreTrainedTokenizer.from_pretrained
methods. Please refer to the docstrings of the
methods above for more information.
save_pretrained
< source >( save_directory push_to_hub: bool = False **kwargs )
Parameters
- save_directory (
str
oros.PathLike
) — Directory where the feature extractor JSON file and the tokenizer files will be saved (directory will be created if it does not exist). - push_to_hub (
bool
, optional, defaults toFalse
) — Whether or not to push your model to the Hugging Face model hub after saving it. You can specify the repository you want to push to withrepo_id
(will default to the name ofsave_directory
in your namespace). - kwargs (
Dict[str, Any]
, optional) — Additional key word arguments passed along to the push_to_hub() method.
Saves the attributes of this processor (feature extractor, tokenizer…) in the specified directory so that it can be reloaded using the from_pretrained() method.
This class method is simply calling save_pretrained() and save_pretrained(). Please refer to the docstrings of the methods above for more information.
This method forwards all its arguments to TrOCRTokenizer’s batch_decode(). Please refer to the docstring of this method for more information.
This method forwards all its arguments to TrOCRTokenizer’s decode(). Please refer to the docstring of this method for more information.
TrOCRForCausalLM
class transformers.TrOCRForCausalLM
< source >( config )
Parameters
- config (TrOCRConfig) — Model configuration class with all the parameters of the model. Initializing with a config file does not load the weights associated with the model, only the configuration. Check out the from_pretrained() method to load the model weights.
The TrOCR Decoder with a language modeling head. Can be used as the decoder part of EncoderDecoderModel and VisionEncoderDecoder
.
This model inherits from PreTrainedModel. Check the superclass documentation for the generic methods the
library implements for all its model (such as downloading or saving, resizing the input embeddings, pruning heads
etc.)
This model is also a PyTorch torch.nn.Module subclass. Use it as a regular PyTorch Module and refer to the PyTorch documentation for all matter related to general usage and behavior.
forward
< source >( input_ids: typing.Optional[torch.LongTensor] = None attention_mask: typing.Optional[torch.Tensor] = None encoder_hidden_states: typing.Optional[torch.FloatTensor] = None encoder_attention_mask: typing.Optional[torch.LongTensor] = None head_mask: typing.Optional[torch.Tensor] = None cross_attn_head_mask: typing.Optional[torch.Tensor] = None past_key_values: typing.Optional[typing.Tuple[typing.Tuple[torch.FloatTensor]]] = None inputs_embeds: typing.Optional[torch.FloatTensor] = None labels: typing.Optional[torch.LongTensor] = None use_cache: typing.Optional[bool] = None output_attentions: typing.Optional[bool] = None output_hidden_states: typing.Optional[bool] = None return_dict: typing.Optional[bool] = None ) → transformers.modeling_outputs.CausalLMOutputWithCrossAttentions or tuple(torch.FloatTensor)
Parameters
- input_ids (
torch.LongTensor
of shape(batch_size, sequence_length)
) — Indices of input sequence tokens in the vocabulary. Padding will be ignored by default should you provide it.Indices can be obtained using AutoTokenizer. See PreTrainedTokenizer.encode() and PreTrainedTokenizer.call() for details.
- attention_mask (
torch.Tensor
of shape(batch_size, sequence_length)
, optional) — Mask to avoid performing attention on padding token indices. Mask values selected in[0, 1]
:- 1 for tokens that are not masked,
- 0 for tokens that are masked.
- encoder_hidden_states (
torch.FloatTensor
of shape(batch_size, sequence_length, hidden_size)
, optional) — Sequence of hidden-states at the output of the last layer of the encoder. Used in the cross-attention if the model is configured as a decoder. - encoder_attention_mask (
torch.FloatTensor
of shape(batch_size, sequence_length)
, optional) — Mask to avoid performing attention on the padding token indices of the encoder input. This mask is used in the cross-attention if the model is configured as a decoder. Mask values selected in[0, 1]
: - head_mask (
torch.Tensor
of shape(decoder_layers, decoder_attention_heads)
, optional) — Mask to nullify selected heads of the attention modules. Mask values selected in[0, 1]
:- 1 indicates the head is not masked,
- 0 indicates the head is masked.
- cross_attn_head_mask (
torch.Tensor
of shape(decoder_layers, decoder_attention_heads)
, optional) — Mask to nullify selected heads of the cross-attention modules. Mask values selected in[0, 1]
:- 1 indicates the head is not masked,
- 0 indicates the head is masked.
- past_key_values (
tuple(tuple(torch.FloatTensor))
, optional, returned whenuse_cache=True
is passed or whenconfig.use_cache=True
) — Tuple oftuple(torch.FloatTensor)
of lengthconfig.n_layers
, with each tuple having 2 tensors of shape(batch_size, num_heads, sequence_length, embed_size_per_head)
) and 2 additional tensors of shape(batch_size, num_heads, encoder_sequence_length, embed_size_per_head)
. The two additional tensors are only required when the model is used as a decoder in a Sequence to Sequence model.Contains pre-computed hidden-states (key and values in the self-attention blocks and in the cross-attention blocks) that can be used (see
past_key_values
input) to speed up sequential decoding.If
past_key_values
are used, the user can optionally input only the lastdecoder_input_ids
(those that don’t have their past key value states given to this model) of shape(batch_size, 1)
instead of alldecoder_input_ids
of shape(batch_size, sequence_length)
. - labels (
torch.LongTensor
of shape(batch_size, sequence_length)
, optional) — Labels for computing the masked language modeling loss. Indices should either be in[0, ..., config.vocab_size]
or -100 (seeinput_ids
docstring). Tokens with indices set to-100
are ignored (masked), the loss is only computed for the tokens with labels in[0, ..., config.vocab_size]
. - use_cache (
bool
, optional) — If set toTrue
,past_key_values
key value states are returned and can be used to speed up decoding (seepast_key_values
).- 1 for tokens that are not masked,
- 0 for tokens that are masked.
- output_attentions (
bool
, optional) — Whether or not to return the attentions tensors of all attention layers. Seeattentions
under returned tensors for more detail. - output_hidden_states (
bool
, optional) — Whether or not to return the hidden states of all layers. Seehidden_states
under returned tensors for more detail. - return_dict (
bool
, optional) — Whether or not to return a ModelOutput instead of a plain tuple.
Returns
transformers.modeling_outputs.CausalLMOutputWithCrossAttentions or tuple(torch.FloatTensor)
A transformers.modeling_outputs.CausalLMOutputWithCrossAttentions or a tuple of
torch.FloatTensor
(if return_dict=False
is passed or when config.return_dict=False
) comprising various
elements depending on the configuration (TrOCRConfig) and inputs.
-
loss (
torch.FloatTensor
of shape(1,)
, optional, returned whenlabels
is provided) — Language modeling loss (for next-token prediction). -
logits (
torch.FloatTensor
of shape(batch_size, sequence_length, config.vocab_size)
) — Prediction scores of the language modeling head (scores for each vocabulary token before SoftMax). -
hidden_states (
tuple(torch.FloatTensor)
, optional, returned whenoutput_hidden_states=True
is passed or whenconfig.output_hidden_states=True
) — Tuple oftorch.FloatTensor
(one for the output of the embeddings, if the model has an embedding layer, + one for the output of each layer) of shape(batch_size, sequence_length, hidden_size)
.Hidden-states of the model at the output of each layer plus the optional initial embedding outputs.
-
attentions (
tuple(torch.FloatTensor)
, optional, returned whenoutput_attentions=True
is passed or whenconfig.output_attentions=True
) — Tuple oftorch.FloatTensor
(one for each layer) of shape(batch_size, num_heads, sequence_length, sequence_length)
.Attentions weights after the attention softmax, used to compute the weighted average in the self-attention heads.
-
cross_attentions (
tuple(torch.FloatTensor)
, optional, returned whenoutput_attentions=True
is passed or whenconfig.output_attentions=True
) — Tuple oftorch.FloatTensor
(one for each layer) of shape(batch_size, num_heads, sequence_length, sequence_length)
.Cross attentions weights after the attention softmax, used to compute the weighted average in the cross-attention heads.
-
past_key_values (
tuple(tuple(torch.FloatTensor))
, optional, returned whenuse_cache=True
is passed or whenconfig.use_cache=True
) — Tuple oftorch.FloatTensor
tuples of lengthconfig.n_layers
, with each tuple containing the cached key, value states of the self-attention and the cross-attention layers if model is used in encoder-decoder setting. Only relevant ifconfig.is_decoder = True
.Contains pre-computed hidden-states (key and values in the attention blocks) that can be used (see
past_key_values
input) to speed up sequential decoding.
Example:
>>> from transformers import (
... TrOCRConfig,
... TrOCRProcessor,
... TrOCRForCausalLM,
... ViTConfig,
... ViTModel,
... VisionEncoderDecoderModel,
... )
>>> import requests
>>> from PIL import Image
>>> # TrOCR is a decoder model and should be used within a VisionEncoderDecoderModel
>>> # init vision2text model with random weights
>>> encoder = ViTModel(ViTConfig())
>>> decoder = TrOCRForCausalLM(TrOCRConfig())
>>> model = VisionEncoderDecoderModel(encoder=encoder, decoder=decoder)
>>> # If you want to start from the pretrained model, load the checkpoint with `VisionEncoderDecoderModel`
>>> processor = TrOCRProcessor.from_pretrained("microsoft/trocr-base-handwritten")
>>> model = VisionEncoderDecoderModel.from_pretrained("microsoft/trocr-base-handwritten")
>>> # load image from the IAM dataset
>>> url = "https://fki.tic.heia-fr.ch/static/img/a01-122-02.jpg"
>>> image = Image.open(requests.get(url, stream=True).raw).convert("RGB")
>>> pixel_values = processor(image, return_tensors="pt").pixel_values
>>> text = "industry, ' Mr. Brown commented icily. ' Let us have a"
>>> # training
>>> model.config.decoder_start_token_id = processor.tokenizer.eos_token_id
>>> model.config.pad_token_id = processor.tokenizer.pad_token_id
>>> model.config.vocab_size = model.config.decoder.vocab_size
>>> labels = processor.tokenizer(text, return_tensors="pt").input_ids
>>> outputs = model(pixel_values, labels=labels)
>>> loss = outputs.loss
>>> round(loss.item(), 2)
5.30
>>> # inference
>>> generated_ids = model.generate(pixel_values)
>>> generated_text = processor.batch_decode(generated_ids, skip_special_tokens=True)[0]
>>> generated_text
'industry, " Mr. Brown commented icily. " Let us have a'