diff --git a/.dockerignore b/.dockerignore
new file mode 100644
index 0000000000000000000000000000000000000000..a90abd2db5603c591affd806232966447397c56f
--- /dev/null
+++ b/.dockerignore
@@ -0,0 +1,25 @@
+# Dockerfile.deploy用の.dockerignore
+# 日本語のJP-Extraのエディター稼働のみに必要なファイルを指定する
+
+*
+
+!/bert/deberta-v2-large-japanese-char-wwm/
+!/common/
+!/configs/
+!/dict_data/default.csv
+!/model_assets/
+!/monotonic_align/
+!/text/
+
+!/attentions.py
+!/commons.py
+!/config.py
+!/default_config.yml
+!/infer.py
+!/models.py
+!/models_jp_extra.py
+!/modules.py
+!/requirements.txt
+!/server_editor.py
+!/transforms.py
+!/utils.py
diff --git a/.gitignore b/.gitignore
new file mode 100644
index 0000000000000000000000000000000000000000..b556dfff7ec75361ea038c5f518fefbe37b6d17c
--- /dev/null
+++ b/.gitignore
@@ -0,0 +1,34 @@
+.vscode/
+
+__pycache__/
+venv/
+.ipynb_checkpoints/
+
+/*.yml
+!/default_config.yml
+/bert/*/*.bin
+/bert/*/*.h5
+/bert/*/*.model
+/bert/*/*.safetensors
+/bert/*/*.msgpack
+
+/pretrained/*.safetensors
+/pretrained/*.pth
+
+/pretrained_jp_extra/*.safetensors
+/pretrained_jp_extra/*.pth
+
+/slm/*/*.bin
+
+/scripts/test/
+*.zip
+*.csv
+*.bak
+/mos_results/
+
+safetensors.ipynb
+*.wav
+/static/
+
+# pyopenjtalk's dictionary
+*.dic
diff --git a/App.bat b/App.bat
new file mode 100644
index 0000000000000000000000000000000000000000..2f2b6534bdb03d42eac717940cc35f431207db3c
--- /dev/null
+++ b/App.bat
@@ -0,0 +1,11 @@
+chcp 65001 > NUL
+@echo off
+
+pushd %~dp0
+echo Running app.py...
+venv\Scripts\python app.py
+
+if %errorlevel% neq 0 ( pause & popd & exit /b %errorlevel% )
+
+popd
+pause
\ No newline at end of file
diff --git a/Data/.gitignore b/Data/.gitignore
new file mode 100644
index 0000000000000000000000000000000000000000..c96a04f008ee21e260b28f7701595ed59e2839e3
--- /dev/null
+++ b/Data/.gitignore
@@ -0,0 +1,2 @@
+*
+!.gitignore
\ No newline at end of file
diff --git a/Dataset.bat b/Dataset.bat
new file mode 100644
index 0000000000000000000000000000000000000000..03d3850a0db1cb60a4b9aadd56d3993fe9a77092
--- /dev/null
+++ b/Dataset.bat
@@ -0,0 +1,11 @@
+chcp 65001 > NUL
+@echo off
+
+pushd %~dp0
+echo Running webui_dataset.py...
+venv\Scripts\python webui_dataset.py
+
+if %errorlevel% neq 0 ( pause & popd & exit /b %errorlevel% )
+
+popd
+pause
\ No newline at end of file
diff --git a/Dockerfile b/Dockerfile
new file mode 100644
index 0000000000000000000000000000000000000000..b3259c8b59ee57195b77b81ccdccb9bf54738a34
--- /dev/null
+++ b/Dockerfile
@@ -0,0 +1,23 @@
+# Hugging face spaces (CPU) でエディタ (server_editor.py) のデプロイ用
+
+# See https://huggingface.co/docs/hub/spaces-sdks-docker-first-demo
+
+FROM python:3.10
+
+RUN useradd -m -u 1000 user
+
+USER user
+
+ENV HOME=/home/user \
+ PATH=/home/user/.local/bin:$PATH
+
+WORKDIR $HOME/app
+
+RUN pip install --no-cache-dir --upgrade pip
+
+COPY --chown=user . $HOME/app
+
+RUN pip install --no-cache-dir -r $HOME/app/requirements.txt
+
+# 必要に応じて制限を変更してください
+CMD ["python", "server_editor.py", "--line_length", "50", "--line_count", "3","--port", "7860"]
diff --git a/Dockerfile.train b/Dockerfile.train
new file mode 100644
index 0000000000000000000000000000000000000000..333fd4634229d47348abf13d00c713fbae17f4d9
--- /dev/null
+++ b/Dockerfile.train
@@ -0,0 +1,109 @@
+# PaperspaceのGradient環境での学習環境構築用Dockerfileです。
+# 環境のみ構築するため、イメージには学習用のコードは含まれていません。
+# 以下を参照しました。
+# https://github.com/gradient-ai/base-container/tree/main/pt211-tf215-cudatk120-py311
+
+# 主なバージョン等
+# Ubuntu 22.04
+# Python 3.10
+# PyTorch 2.1.2 (CUDA 11.8)
+# CUDA Toolkit 12.0, CUDNN 8.9.7
+
+
+# ==================================================================
+# Initial setup
+# ------------------------------------------------------------------
+
+# Ubuntu 22.04 as base image
+FROM ubuntu:22.04
+# RUN yes| unminimize
+
+# Set ENV variables
+ENV LANG C.UTF-8
+ENV SHELL=/bin/bash
+ENV DEBIAN_FRONTEND=noninteractive
+
+ENV APT_INSTALL="apt-get install -y --no-install-recommends"
+ENV PIP_INSTALL="python3 -m pip --no-cache-dir install --upgrade"
+ENV GIT_CLONE="git clone --depth 10"
+
+# ==================================================================
+# Tools
+# ------------------------------------------------------------------
+
+RUN apt-get update && \
+ $APT_INSTALL \
+ sudo \
+ build-essential \
+ ca-certificates \
+ wget \
+ curl \
+ git \
+ zip \
+ unzip \
+ nano \
+ ffmpeg \
+ software-properties-common \
+ gnupg \
+ python3 \
+ python3-pip \
+ python3-dev
+
+# ==================================================================
+# Git-lfs
+# ------------------------------------------------------------------
+
+RUN curl -s https://packagecloud.io/install/repositories/github/git-lfs/script.deb.sh | sudo bash && \
+ $APT_INSTALL git-lfs
+
+
+# Add symlink so python and python3 commands use same python3.9 executable
+RUN ln -s /usr/bin/python3 /usr/local/bin/python
+
+# ==================================================================
+# Installing CUDA packages (CUDA Toolkit 12.0 and CUDNN 8.9.7)
+# ------------------------------------------------------------------
+RUN wget https://developer.download.nvidia.com/compute/cuda/repos/ubuntu2204/x86_64/cuda-ubuntu2204.pin && \
+ mv cuda-ubuntu2204.pin /etc/apt/preferences.d/cuda-repository-pin-600 && \
+ wget https://developer.download.nvidia.com/compute/cuda/12.0.0/local_installers/cuda-repo-ubuntu2204-12-0-local_12.0.0-525.60.13-1_amd64.deb && \
+ dpkg -i cuda-repo-ubuntu2204-12-0-local_12.0.0-525.60.13-1_amd64.deb && \
+ cp /var/cuda-repo-ubuntu2204-12-0-local/cuda-*-keyring.gpg /usr/share/keyrings/ && \
+ apt-get update && \
+ $APT_INSTALL cuda && \
+ rm cuda-repo-ubuntu2204-12-0-local_12.0.0-525.60.13-1_amd64.deb
+
+# Installing CUDNN
+RUN apt-key adv --fetch-keys https://developer.download.nvidia.com/compute/cuda/repos/ubuntu2204/x86_64/3bf863cc.pub && \
+ add-apt-repository "deb https://developer.download.nvidia.com/compute/cuda/repos/ubuntu2204/x86_64/ /" && \
+ apt-get update && \
+ $APT_INSTALL libcudnn8=8.9.7.29-1+cuda12.2 \
+ libcudnn8-dev=8.9.7.29-1+cuda12.2
+
+
+ENV PATH=$PATH:/usr/local/cuda/bin
+ENV LD_LIBRARY_PATH=/usr/local/cuda/lib64:$LD_LIBRARY_PATH
+
+
+# ==================================================================
+# PyTorch
+# ------------------------------------------------------------------
+
+# Based on https://pytorch.org/get-started/locally/
+
+RUN $PIP_INSTALL torch==2.1.2 torchvision==0.16.2 torchaudio==2.1.2 --index-url https://download.pytorch.org/whl/cu118
+
+
+RUN $PIP_INSTALL jupyterlab
+
+# Install requirements.txt from the project
+COPY requirements.txt /tmp/requirements.txt
+RUN $PIP_INSTALL -r /tmp/requirements.txt
+RUN rm /tmp/requirements.txt
+
+# ==================================================================
+# Startup
+# ------------------------------------------------------------------
+
+EXPOSE 8888 6006
+
+CMD jupyter lab --allow-root --ip=0.0.0.0 --no-browser --ServerApp.trust_xheaders=True --ServerApp.disable_check_xsrf=False --ServerApp.allow_remote_access=True --ServerApp.allow_origin='*' --ServerApp.allow_credentials=True
\ No newline at end of file
diff --git a/Editor.bat b/Editor.bat
new file mode 100644
index 0000000000000000000000000000000000000000..7b0836c4fa8377cdbc8057581b29c35287c51fb1
--- /dev/null
+++ b/Editor.bat
@@ -0,0 +1,11 @@
+chcp 65001 > NUL
+@echo off
+
+pushd %~dp0
+echo Running server_editor.py --inbroser
+venv\Scripts\python server_editor.py --inbrowser
+
+if %errorlevel% neq 0 ( pause & popd & exit /b %errorlevel% )
+
+popd
+pause
\ No newline at end of file
diff --git a/LICENSE b/LICENSE
new file mode 100644
index 0000000000000000000000000000000000000000..0ad25db4bd1d86c452db3f9602ccdbe172438f52
--- /dev/null
+++ b/LICENSE
@@ -0,0 +1,661 @@
+ GNU AFFERO GENERAL PUBLIC LICENSE
+ Version 3, 19 November 2007
+
+ Copyright (C) 2007 Free Software Foundation, Inc.
+ Everyone is permitted to copy and distribute verbatim copies
+ of this license document, but changing it is not allowed.
+
+ Preamble
+
+ The GNU Affero General Public License is a free, copyleft license for
+software and other kinds of works, specifically designed to ensure
+cooperation with the community in the case of network server software.
+
+ The licenses for most software and other practical works are designed
+to take away your freedom to share and change the works. By contrast,
+our General Public Licenses are intended to guarantee your freedom to
+share and change all versions of a program--to make sure it remains free
+software for all its users.
+
+ When we speak of free software, we are referring to freedom, not
+price. Our General Public Licenses are designed to make sure that you
+have the freedom to distribute copies of free software (and charge for
+them if you wish), that you receive source code or can get it if you
+want it, that you can change the software or use pieces of it in new
+free programs, and that you know you can do these things.
+
+ Developers that use our General Public Licenses protect your rights
+with two steps: (1) assert copyright on the software, and (2) offer
+you this License which gives you legal permission to copy, distribute
+and/or modify the software.
+
+ A secondary benefit of defending all users' freedom is that
+improvements made in alternate versions of the program, if they
+receive widespread use, become available for other developers to
+incorporate. Many developers of free software are heartened and
+encouraged by the resulting cooperation. However, in the case of
+software used on network servers, this result may fail to come about.
+The GNU General Public License permits making a modified version and
+letting the public access it on a server without ever releasing its
+source code to the public.
+
+ The GNU Affero General Public License is designed specifically to
+ensure that, in such cases, the modified source code becomes available
+to the community. It requires the operator of a network server to
+provide the source code of the modified version running there to the
+users of that server. Therefore, public use of a modified version, on
+a publicly accessible server, gives the public access to the source
+code of the modified version.
+
+ An older license, called the Affero General Public License and
+published by Affero, was designed to accomplish similar goals. This is
+a different license, not a version of the Affero GPL, but Affero has
+released a new version of the Affero GPL which permits relicensing under
+this license.
+
+ The precise terms and conditions for copying, distribution and
+modification follow.
+
+ TERMS AND CONDITIONS
+
+ 0. Definitions.
+
+ "This License" refers to version 3 of the GNU Affero General Public License.
+
+ "Copyright" also means copyright-like laws that apply to other kinds of
+works, such as semiconductor masks.
+
+ "The Program" refers to any copyrightable work licensed under this
+License. Each licensee is addressed as "you". "Licensees" and
+"recipients" may be individuals or organizations.
+
+ To "modify" a work means to copy from or adapt all or part of the work
+in a fashion requiring copyright permission, other than the making of an
+exact copy. The resulting work is called a "modified version" of the
+earlier work or a work "based on" the earlier work.
+
+ A "covered work" means either the unmodified Program or a work based
+on the Program.
+
+ To "propagate" a work means to do anything with it that, without
+permission, would make you directly or secondarily liable for
+infringement under applicable copyright law, except executing it on a
+computer or modifying a private copy. Propagation includes copying,
+distribution (with or without modification), making available to the
+public, and in some countries other activities as well.
+
+ To "convey" a work means any kind of propagation that enables other
+parties to make or receive copies. Mere interaction with a user through
+a computer network, with no transfer of a copy, is not conveying.
+
+ An interactive user interface displays "Appropriate Legal Notices"
+to the extent that it includes a convenient and prominently visible
+feature that (1) displays an appropriate copyright notice, and (2)
+tells the user that there is no warranty for the work (except to the
+extent that warranties are provided), that licensees may convey the
+work under this License, and how to view a copy of this License. If
+the interface presents a list of user commands or options, such as a
+menu, a prominent item in the list meets this criterion.
+
+ 1. Source Code.
+
+ The "source code" for a work means the preferred form of the work
+for making modifications to it. "Object code" means any non-source
+form of a work.
+
+ A "Standard Interface" means an interface that either is an official
+standard defined by a recognized standards body, or, in the case of
+interfaces specified for a particular programming language, one that
+is widely used among developers working in that language.
+
+ The "System Libraries" of an executable work include anything, other
+than the work as a whole, that (a) is included in the normal form of
+packaging a Major Component, but which is not part of that Major
+Component, and (b) serves only to enable use of the work with that
+Major Component, or to implement a Standard Interface for which an
+implementation is available to the public in source code form. A
+"Major Component", in this context, means a major essential component
+(kernel, window system, and so on) of the specific operating system
+(if any) on which the executable work runs, or a compiler used to
+produce the work, or an object code interpreter used to run it.
+
+ The "Corresponding Source" for a work in object code form means all
+the source code needed to generate, install, and (for an executable
+work) run the object code and to modify the work, including scripts to
+control those activities. However, it does not include the work's
+System Libraries, or general-purpose tools or generally available free
+programs which are used unmodified in performing those activities but
+which are not part of the work. For example, Corresponding Source
+includes interface definition files associated with source files for
+the work, and the source code for shared libraries and dynamically
+linked subprograms that the work is specifically designed to require,
+such as by intimate data communication or control flow between those
+subprograms and other parts of the work.
+
+ The Corresponding Source need not include anything that users
+can regenerate automatically from other parts of the Corresponding
+Source.
+
+ The Corresponding Source for a work in source code form is that
+same work.
+
+ 2. Basic Permissions.
+
+ All rights granted under this License are granted for the term of
+copyright on the Program, and are irrevocable provided the stated
+conditions are met. This License explicitly affirms your unlimited
+permission to run the unmodified Program. The output from running a
+covered work is covered by this License only if the output, given its
+content, constitutes a covered work. This License acknowledges your
+rights of fair use or other equivalent, as provided by copyright law.
+
+ You may make, run and propagate covered works that you do not
+convey, without conditions so long as your license otherwise remains
+in force. You may convey covered works to others for the sole purpose
+of having them make modifications exclusively for you, or provide you
+with facilities for running those works, provided that you comply with
+the terms of this License in conveying all material for which you do
+not control copyright. Those thus making or running the covered works
+for you must do so exclusively on your behalf, under your direction
+and control, on terms that prohibit them from making any copies of
+your copyrighted material outside their relationship with you.
+
+ Conveying under any other circumstances is permitted solely under
+the conditions stated below. Sublicensing is not allowed; section 10
+makes it unnecessary.
+
+ 3. Protecting Users' Legal Rights From Anti-Circumvention Law.
+
+ No covered work shall be deemed part of an effective technological
+measure under any applicable law fulfilling obligations under article
+11 of the WIPO copyright treaty adopted on 20 December 1996, or
+similar laws prohibiting or restricting circumvention of such
+measures.
+
+ When you convey a covered work, you waive any legal power to forbid
+circumvention of technological measures to the extent such circumvention
+is effected by exercising rights under this License with respect to
+the covered work, and you disclaim any intention to limit operation or
+modification of the work as a means of enforcing, against the work's
+users, your or third parties' legal rights to forbid circumvention of
+technological measures.
+
+ 4. Conveying Verbatim Copies.
+
+ You may convey verbatim copies of the Program's source code as you
+receive it, in any medium, provided that you conspicuously and
+appropriately publish on each copy an appropriate copyright notice;
+keep intact all notices stating that this License and any
+non-permissive terms added in accord with section 7 apply to the code;
+keep intact all notices of the absence of any warranty; and give all
+recipients a copy of this License along with the Program.
+
+ You may charge any price or no price for each copy that you convey,
+and you may offer support or warranty protection for a fee.
+
+ 5. Conveying Modified Source Versions.
+
+ You may convey a work based on the Program, or the modifications to
+produce it from the Program, in the form of source code under the
+terms of section 4, provided that you also meet all of these conditions:
+
+ a) The work must carry prominent notices stating that you modified
+ it, and giving a relevant date.
+
+ b) The work must carry prominent notices stating that it is
+ released under this License and any conditions added under section
+ 7. This requirement modifies the requirement in section 4 to
+ "keep intact all notices".
+
+ c) You must license the entire work, as a whole, under this
+ License to anyone who comes into possession of a copy. This
+ License will therefore apply, along with any applicable section 7
+ additional terms, to the whole of the work, and all its parts,
+ regardless of how they are packaged. This License gives no
+ permission to license the work in any other way, but it does not
+ invalidate such permission if you have separately received it.
+
+ d) If the work has interactive user interfaces, each must display
+ Appropriate Legal Notices; however, if the Program has interactive
+ interfaces that do not display Appropriate Legal Notices, your
+ work need not make them do so.
+
+ A compilation of a covered work with other separate and independent
+works, which are not by their nature extensions of the covered work,
+and which are not combined with it such as to form a larger program,
+in or on a volume of a storage or distribution medium, is called an
+"aggregate" if the compilation and its resulting copyright are not
+used to limit the access or legal rights of the compilation's users
+beyond what the individual works permit. Inclusion of a covered work
+in an aggregate does not cause this License to apply to the other
+parts of the aggregate.
+
+ 6. Conveying Non-Source Forms.
+
+ You may convey a covered work in object code form under the terms
+of sections 4 and 5, provided that you also convey the
+machine-readable Corresponding Source under the terms of this License,
+in one of these ways:
+
+ a) Convey the object code in, or embodied in, a physical product
+ (including a physical distribution medium), accompanied by the
+ Corresponding Source fixed on a durable physical medium
+ customarily used for software interchange.
+
+ b) Convey the object code in, or embodied in, a physical product
+ (including a physical distribution medium), accompanied by a
+ written offer, valid for at least three years and valid for as
+ long as you offer spare parts or customer support for that product
+ model, to give anyone who possesses the object code either (1) a
+ copy of the Corresponding Source for all the software in the
+ product that is covered by this License, on a durable physical
+ medium customarily used for software interchange, for a price no
+ more than your reasonable cost of physically performing this
+ conveying of source, or (2) access to copy the
+ Corresponding Source from a network server at no charge.
+
+ c) Convey individual copies of the object code with a copy of the
+ written offer to provide the Corresponding Source. This
+ alternative is allowed only occasionally and noncommercially, and
+ only if you received the object code with such an offer, in accord
+ with subsection 6b.
+
+ d) Convey the object code by offering access from a designated
+ place (gratis or for a charge), and offer equivalent access to the
+ Corresponding Source in the same way through the same place at no
+ further charge. You need not require recipients to copy the
+ Corresponding Source along with the object code. If the place to
+ copy the object code is a network server, the Corresponding Source
+ may be on a different server (operated by you or a third party)
+ that supports equivalent copying facilities, provided you maintain
+ clear directions next to the object code saying where to find the
+ Corresponding Source. Regardless of what server hosts the
+ Corresponding Source, you remain obligated to ensure that it is
+ available for as long as needed to satisfy these requirements.
+
+ e) Convey the object code using peer-to-peer transmission, provided
+ you inform other peers where the object code and Corresponding
+ Source of the work are being offered to the general public at no
+ charge under subsection 6d.
+
+ A separable portion of the object code, whose source code is excluded
+from the Corresponding Source as a System Library, need not be
+included in conveying the object code work.
+
+ A "User Product" is either (1) a "consumer product", which means any
+tangible personal property which is normally used for personal, family,
+or household purposes, or (2) anything designed or sold for incorporation
+into a dwelling. In determining whether a product is a consumer product,
+doubtful cases shall be resolved in favor of coverage. For a particular
+product received by a particular user, "normally used" refers to a
+typical or common use of that class of product, regardless of the status
+of the particular user or of the way in which the particular user
+actually uses, or expects or is expected to use, the product. A product
+is a consumer product regardless of whether the product has substantial
+commercial, industrial or non-consumer uses, unless such uses represent
+the only significant mode of use of the product.
+
+ "Installation Information" for a User Product means any methods,
+procedures, authorization keys, or other information required to install
+and execute modified versions of a covered work in that User Product from
+a modified version of its Corresponding Source. The information must
+suffice to ensure that the continued functioning of the modified object
+code is in no case prevented or interfered with solely because
+modification has been made.
+
+ If you convey an object code work under this section in, or with, or
+specifically for use in, a User Product, and the conveying occurs as
+part of a transaction in which the right of possession and use of the
+User Product is transferred to the recipient in perpetuity or for a
+fixed term (regardless of how the transaction is characterized), the
+Corresponding Source conveyed under this section must be accompanied
+by the Installation Information. But this requirement does not apply
+if neither you nor any third party retains the ability to install
+modified object code on the User Product (for example, the work has
+been installed in ROM).
+
+ The requirement to provide Installation Information does not include a
+requirement to continue to provide support service, warranty, or updates
+for a work that has been modified or installed by the recipient, or for
+the User Product in which it has been modified or installed. Access to a
+network may be denied when the modification itself materially and
+adversely affects the operation of the network or violates the rules and
+protocols for communication across the network.
+
+ Corresponding Source conveyed, and Installation Information provided,
+in accord with this section must be in a format that is publicly
+documented (and with an implementation available to the public in
+source code form), and must require no special password or key for
+unpacking, reading or copying.
+
+ 7. Additional Terms.
+
+ "Additional permissions" are terms that supplement the terms of this
+License by making exceptions from one or more of its conditions.
+Additional permissions that are applicable to the entire Program shall
+be treated as though they were included in this License, to the extent
+that they are valid under applicable law. If additional permissions
+apply only to part of the Program, that part may be used separately
+under those permissions, but the entire Program remains governed by
+this License without regard to the additional permissions.
+
+ When you convey a copy of a covered work, you may at your option
+remove any additional permissions from that copy, or from any part of
+it. (Additional permissions may be written to require their own
+removal in certain cases when you modify the work.) You may place
+additional permissions on material, added by you to a covered work,
+for which you have or can give appropriate copyright permission.
+
+ Notwithstanding any other provision of this License, for material you
+add to a covered work, you may (if authorized by the copyright holders of
+that material) supplement the terms of this License with terms:
+
+ a) Disclaiming warranty or limiting liability differently from the
+ terms of sections 15 and 16 of this License; or
+
+ b) Requiring preservation of specified reasonable legal notices or
+ author attributions in that material or in the Appropriate Legal
+ Notices displayed by works containing it; or
+
+ c) Prohibiting misrepresentation of the origin of that material, or
+ requiring that modified versions of such material be marked in
+ reasonable ways as different from the original version; or
+
+ d) Limiting the use for publicity purposes of names of licensors or
+ authors of the material; or
+
+ e) Declining to grant rights under trademark law for use of some
+ trade names, trademarks, or service marks; or
+
+ f) Requiring indemnification of licensors and authors of that
+ material by anyone who conveys the material (or modified versions of
+ it) with contractual assumptions of liability to the recipient, for
+ any liability that these contractual assumptions directly impose on
+ those licensors and authors.
+
+ All other non-permissive additional terms are considered "further
+restrictions" within the meaning of section 10. If the Program as you
+received it, or any part of it, contains a notice stating that it is
+governed by this License along with a term that is a further
+restriction, you may remove that term. If a license document contains
+a further restriction but permits relicensing or conveying under this
+License, you may add to a covered work material governed by the terms
+of that license document, provided that the further restriction does
+not survive such relicensing or conveying.
+
+ If you add terms to a covered work in accord with this section, you
+must place, in the relevant source files, a statement of the
+additional terms that apply to those files, or a notice indicating
+where to find the applicable terms.
+
+ Additional terms, permissive or non-permissive, may be stated in the
+form of a separately written license, or stated as exceptions;
+the above requirements apply either way.
+
+ 8. Termination.
+
+ You may not propagate or modify a covered work except as expressly
+provided under this License. Any attempt otherwise to propagate or
+modify it is void, and will automatically terminate your rights under
+this License (including any patent licenses granted under the third
+paragraph of section 11).
+
+ However, if you cease all violation of this License, then your
+license from a particular copyright holder is reinstated (a)
+provisionally, unless and until the copyright holder explicitly and
+finally terminates your license, and (b) permanently, if the copyright
+holder fails to notify you of the violation by some reasonable means
+prior to 60 days after the cessation.
+
+ Moreover, your license from a particular copyright holder is
+reinstated permanently if the copyright holder notifies you of the
+violation by some reasonable means, this is the first time you have
+received notice of violation of this License (for any work) from that
+copyright holder, and you cure the violation prior to 30 days after
+your receipt of the notice.
+
+ Termination of your rights under this section does not terminate the
+licenses of parties who have received copies or rights from you under
+this License. If your rights have been terminated and not permanently
+reinstated, you do not qualify to receive new licenses for the same
+material under section 10.
+
+ 9. Acceptance Not Required for Having Copies.
+
+ You are not required to accept this License in order to receive or
+run a copy of the Program. Ancillary propagation of a covered work
+occurring solely as a consequence of using peer-to-peer transmission
+to receive a copy likewise does not require acceptance. However,
+nothing other than this License grants you permission to propagate or
+modify any covered work. These actions infringe copyright if you do
+not accept this License. Therefore, by modifying or propagating a
+covered work, you indicate your acceptance of this License to do so.
+
+ 10. Automatic Licensing of Downstream Recipients.
+
+ Each time you convey a covered work, the recipient automatically
+receives a license from the original licensors, to run, modify and
+propagate that work, subject to this License. You are not responsible
+for enforcing compliance by third parties with this License.
+
+ An "entity transaction" is a transaction transferring control of an
+organization, or substantially all assets of one, or subdividing an
+organization, or merging organizations. If propagation of a covered
+work results from an entity transaction, each party to that
+transaction who receives a copy of the work also receives whatever
+licenses to the work the party's predecessor in interest had or could
+give under the previous paragraph, plus a right to possession of the
+Corresponding Source of the work from the predecessor in interest, if
+the predecessor has it or can get it with reasonable efforts.
+
+ You may not impose any further restrictions on the exercise of the
+rights granted or affirmed under this License. For example, you may
+not impose a license fee, royalty, or other charge for exercise of
+rights granted under this License, and you may not initiate litigation
+(including a cross-claim or counterclaim in a lawsuit) alleging that
+any patent claim is infringed by making, using, selling, offering for
+sale, or importing the Program or any portion of it.
+
+ 11. Patents.
+
+ A "contributor" is a copyright holder who authorizes use under this
+License of the Program or a work on which the Program is based. The
+work thus licensed is called the contributor's "contributor version".
+
+ A contributor's "essential patent claims" are all patent claims
+owned or controlled by the contributor, whether already acquired or
+hereafter acquired, that would be infringed by some manner, permitted
+by this License, of making, using, or selling its contributor version,
+but do not include claims that would be infringed only as a
+consequence of further modification of the contributor version. For
+purposes of this definition, "control" includes the right to grant
+patent sublicenses in a manner consistent with the requirements of
+this License.
+
+ Each contributor grants you a non-exclusive, worldwide, royalty-free
+patent license under the contributor's essential patent claims, to
+make, use, sell, offer for sale, import and otherwise run, modify and
+propagate the contents of its contributor version.
+
+ In the following three paragraphs, a "patent license" is any express
+agreement or commitment, however denominated, not to enforce a patent
+(such as an express permission to practice a patent or covenant not to
+sue for patent infringement). To "grant" such a patent license to a
+party means to make such an agreement or commitment not to enforce a
+patent against the party.
+
+ If you convey a covered work, knowingly relying on a patent license,
+and the Corresponding Source of the work is not available for anyone
+to copy, free of charge and under the terms of this License, through a
+publicly available network server or other readily accessible means,
+then you must either (1) cause the Corresponding Source to be so
+available, or (2) arrange to deprive yourself of the benefit of the
+patent license for this particular work, or (3) arrange, in a manner
+consistent with the requirements of this License, to extend the patent
+license to downstream recipients. "Knowingly relying" means you have
+actual knowledge that, but for the patent license, your conveying the
+covered work in a country, or your recipient's use of the covered work
+in a country, would infringe one or more identifiable patents in that
+country that you have reason to believe are valid.
+
+ If, pursuant to or in connection with a single transaction or
+arrangement, you convey, or propagate by procuring conveyance of, a
+covered work, and grant a patent license to some of the parties
+receiving the covered work authorizing them to use, propagate, modify
+or convey a specific copy of the covered work, then the patent license
+you grant is automatically extended to all recipients of the covered
+work and works based on it.
+
+ A patent license is "discriminatory" if it does not include within
+the scope of its coverage, prohibits the exercise of, or is
+conditioned on the non-exercise of one or more of the rights that are
+specifically granted under this License. You may not convey a covered
+work if you are a party to an arrangement with a third party that is
+in the business of distributing software, under which you make payment
+to the third party based on the extent of your activity of conveying
+the work, and under which the third party grants, to any of the
+parties who would receive the covered work from you, a discriminatory
+patent license (a) in connection with copies of the covered work
+conveyed by you (or copies made from those copies), or (b) primarily
+for and in connection with specific products or compilations that
+contain the covered work, unless you entered into that arrangement,
+or that patent license was granted, prior to 28 March 2007.
+
+ Nothing in this License shall be construed as excluding or limiting
+any implied license or other defenses to infringement that may
+otherwise be available to you under applicable patent law.
+
+ 12. No Surrender of Others' Freedom.
+
+ If conditions are imposed on you (whether by court order, agreement or
+otherwise) that contradict the conditions of this License, they do not
+excuse you from the conditions of this License. If you cannot convey a
+covered work so as to satisfy simultaneously your obligations under this
+License and any other pertinent obligations, then as a consequence you may
+not convey it at all. For example, if you agree to terms that obligate you
+to collect a royalty for further conveying from those to whom you convey
+the Program, the only way you could satisfy both those terms and this
+License would be to refrain entirely from conveying the Program.
+
+ 13. Remote Network Interaction; Use with the GNU General Public License.
+
+ Notwithstanding any other provision of this License, if you modify the
+Program, your modified version must prominently offer all users
+interacting with it remotely through a computer network (if your version
+supports such interaction) an opportunity to receive the Corresponding
+Source of your version by providing access to the Corresponding Source
+from a network server at no charge, through some standard or customary
+means of facilitating copying of software. This Corresponding Source
+shall include the Corresponding Source for any work covered by version 3
+of the GNU General Public License that is incorporated pursuant to the
+following paragraph.
+
+ Notwithstanding any other provision of this License, you have
+permission to link or combine any covered work with a work licensed
+under version 3 of the GNU General Public License into a single
+combined work, and to convey the resulting work. The terms of this
+License will continue to apply to the part which is the covered work,
+but the work with which it is combined will remain governed by version
+3 of the GNU General Public License.
+
+ 14. Revised Versions of this License.
+
+ The Free Software Foundation may publish revised and/or new versions of
+the GNU Affero General Public License from time to time. Such new versions
+will be similar in spirit to the present version, but may differ in detail to
+address new problems or concerns.
+
+ Each version is given a distinguishing version number. If the
+Program specifies that a certain numbered version of the GNU Affero General
+Public License "or any later version" applies to it, you have the
+option of following the terms and conditions either of that numbered
+version or of any later version published by the Free Software
+Foundation. If the Program does not specify a version number of the
+GNU Affero General Public License, you may choose any version ever published
+by the Free Software Foundation.
+
+ If the Program specifies that a proxy can decide which future
+versions of the GNU Affero General Public License can be used, that proxy's
+public statement of acceptance of a version permanently authorizes you
+to choose that version for the Program.
+
+ Later license versions may give you additional or different
+permissions. However, no additional obligations are imposed on any
+author or copyright holder as a result of your choosing to follow a
+later version.
+
+ 15. Disclaimer of Warranty.
+
+ THERE IS NO WARRANTY FOR THE PROGRAM, TO THE EXTENT PERMITTED BY
+APPLICABLE LAW. EXCEPT WHEN OTHERWISE STATED IN WRITING THE COPYRIGHT
+HOLDERS AND/OR OTHER PARTIES PROVIDE THE PROGRAM "AS IS" WITHOUT WARRANTY
+OF ANY KIND, EITHER EXPRESSED OR IMPLIED, INCLUDING, BUT NOT LIMITED TO,
+THE IMPLIED WARRANTIES OF MERCHANTABILITY AND FITNESS FOR A PARTICULAR
+PURPOSE. THE ENTIRE RISK AS TO THE QUALITY AND PERFORMANCE OF THE PROGRAM
+IS WITH YOU. SHOULD THE PROGRAM PROVE DEFECTIVE, YOU ASSUME THE COST OF
+ALL NECESSARY SERVICING, REPAIR OR CORRECTION.
+
+ 16. Limitation of Liability.
+
+ IN NO EVENT UNLESS REQUIRED BY APPLICABLE LAW OR AGREED TO IN WRITING
+WILL ANY COPYRIGHT HOLDER, OR ANY OTHER PARTY WHO MODIFIES AND/OR CONVEYS
+THE PROGRAM AS PERMITTED ABOVE, BE LIABLE TO YOU FOR DAMAGES, INCLUDING ANY
+GENERAL, SPECIAL, INCIDENTAL OR CONSEQUENTIAL DAMAGES ARISING OUT OF THE
+USE OR INABILITY TO USE THE PROGRAM (INCLUDING BUT NOT LIMITED TO LOSS OF
+DATA OR DATA BEING RENDERED INACCURATE OR LOSSES SUSTAINED BY YOU OR THIRD
+PARTIES OR A FAILURE OF THE PROGRAM TO OPERATE WITH ANY OTHER PROGRAMS),
+EVEN IF SUCH HOLDER OR OTHER PARTY HAS BEEN ADVISED OF THE POSSIBILITY OF
+SUCH DAMAGES.
+
+ 17. Interpretation of Sections 15 and 16.
+
+ If the disclaimer of warranty and limitation of liability provided
+above cannot be given local legal effect according to their terms,
+reviewing courts shall apply local law that most closely approximates
+an absolute waiver of all civil liability in connection with the
+Program, unless a warranty or assumption of liability accompanies a
+copy of the Program in return for a fee.
+
+ END OF TERMS AND CONDITIONS
+
+ How to Apply These Terms to Your New Programs
+
+ If you develop a new program, and you want it to be of the greatest
+possible use to the public, the best way to achieve this is to make it
+free software which everyone can redistribute and change under these terms.
+
+ To do so, attach the following notices to the program. It is safest
+to attach them to the start of each source file to most effectively
+state the exclusion of warranty; and each file should have at least
+the "copyright" line and a pointer to where the full notice is found.
+
+
+ Copyright (C)
+
+ This program is free software: you can redistribute it and/or modify
+ it under the terms of the GNU Affero General Public License as published
+ by the Free Software Foundation, either version 3 of the License, or
+ (at your option) any later version.
+
+ This program is distributed in the hope that it will be useful,
+ but WITHOUT ANY WARRANTY; without even the implied warranty of
+ MERCHANTABILITY or FITNESS FOR A PARTICULAR PURPOSE. See the
+ GNU Affero General Public License for more details.
+
+ You should have received a copy of the GNU Affero General Public License
+ along with this program. If not, see .
+
+Also add information on how to contact you by electronic and paper mail.
+
+ If your software can interact with users remotely through a computer
+network, you should also make sure that it provides a way for users to
+get its source. For example, if your program is a web application, its
+interface could display a "Source" link that leads users to an archive
+of the code. There are many ways you could offer source, and different
+solutions will be better for different programs; see section 13 for the
+specific requirements.
+
+ You should also get your employer (if you work as a programmer) or school,
+if any, to sign a "copyright disclaimer" for the program, if necessary.
+For more information on this, and how to apply and follow the GNU AGPL, see
+.
diff --git a/Merge.bat b/Merge.bat
new file mode 100644
index 0000000000000000000000000000000000000000..8e1dedba6dc343f9f69084b6b1e700b9b06032ce
--- /dev/null
+++ b/Merge.bat
@@ -0,0 +1,13 @@
+chcp 65001 > NUL
+
+@echo off
+
+pushd %~dp0
+
+echo Running webui_merge.py...
+venv\Scripts\python webui_merge.py
+
+if %errorlevel% neq 0 ( pause & popd & exit /b %errorlevel% )
+
+popd
+pause
\ No newline at end of file
diff --git a/README.md b/README.md
index 2ce9bc6e190bc9d86972158c8bef9e60275e783c..7095eba3d342239fbee3be4722eb538bc7bb84b6 100644
--- a/README.md
+++ b/README.md
@@ -1,6 +1,6 @@
---
title: Style Bert VITS2 Editor Demo
-emoji: 🐠
+emoji: 😊🎙️📖
colorFrom: gray
colorTo: yellow
sdk: docker
diff --git a/Style.bat b/Style.bat
new file mode 100644
index 0000000000000000000000000000000000000000..409cf9105b376d4526bc62c2c36d970f7b116533
--- /dev/null
+++ b/Style.bat
@@ -0,0 +1,12 @@
+chcp 65001 > NUL
+
+@echo off
+
+pushd %~dp0
+echo Running webui_style_vectors.py...
+venv\Scripts\python webui_style_vectors.py
+
+if %errorlevel% neq 0 ( pause & popd & exit /b %errorlevel% )
+
+popd
+pause
\ No newline at end of file
diff --git a/Train.bat b/Train.bat
new file mode 100644
index 0000000000000000000000000000000000000000..5a93d026fc2995233c193fc0b81e812d2c4f7601
--- /dev/null
+++ b/Train.bat
@@ -0,0 +1,13 @@
+chcp 65001 > NUL
+
+@echo off
+
+pushd %~dp0
+
+echo Running webui_train.py...
+venv\Scripts\python webui_train.py
+
+if %errorlevel% neq 0 ( pause & popd & exit /b %errorlevel% )
+
+popd
+pause
\ No newline at end of file
diff --git a/app.py b/app.py
new file mode 100644
index 0000000000000000000000000000000000000000..e17a0a56aecea31bb7c342ff019df143c50dcf29
--- /dev/null
+++ b/app.py
@@ -0,0 +1,500 @@
+import argparse
+import datetime
+import json
+import os
+import sys
+from pathlib import Path
+from typing import Optional
+
+import gradio as gr
+import torch
+import yaml
+
+from common.constants import (
+ DEFAULT_ASSIST_TEXT_WEIGHT,
+ DEFAULT_LENGTH,
+ DEFAULT_LINE_SPLIT,
+ DEFAULT_NOISE,
+ DEFAULT_NOISEW,
+ DEFAULT_SDP_RATIO,
+ DEFAULT_SPLIT_INTERVAL,
+ DEFAULT_STYLE,
+ DEFAULT_STYLE_WEIGHT,
+ GRADIO_THEME,
+ LATEST_VERSION,
+ Languages,
+)
+from common.log import logger
+from common.tts_model import ModelHolder
+from infer import InvalidToneError
+from text.japanese import g2kata_tone, kata_tone2phone_tone, text_normalize
+
+# Get path settings
+with open(os.path.join("configs", "paths.yml"), "r", encoding="utf-8") as f:
+ path_config: dict[str, str] = yaml.safe_load(f.read())
+ # dataset_root = path_config["dataset_root"]
+ assets_root = path_config["assets_root"]
+
+languages = [l.value for l in Languages]
+
+
+def tts_fn(
+ model_name,
+ model_path,
+ text,
+ language,
+ reference_audio_path,
+ sdp_ratio,
+ noise_scale,
+ noise_scale_w,
+ length_scale,
+ line_split,
+ split_interval,
+ assist_text,
+ assist_text_weight,
+ use_assist_text,
+ style,
+ style_weight,
+ kata_tone_json_str,
+ use_tone,
+ speaker,
+ pitch_scale,
+ intonation_scale,
+):
+ model_holder.load_model_gr(model_name, model_path)
+
+ wrong_tone_message = ""
+ kata_tone: Optional[list[tuple[str, int]]] = None
+ if use_tone and kata_tone_json_str != "":
+ if language != "JP":
+ logger.warning("Only Japanese is supported for tone generation.")
+ wrong_tone_message = "アクセント指定は現在日本語のみ対応しています。"
+ if line_split:
+ logger.warning("Tone generation is not supported for line split.")
+ wrong_tone_message = (
+ "アクセント指定は改行で分けて生成を使わない場合のみ対応しています。"
+ )
+ try:
+ kata_tone = []
+ json_data = json.loads(kata_tone_json_str)
+ # tupleを使うように変換
+ for kana, tone in json_data:
+ assert isinstance(kana, str) and tone in (0, 1), f"{kana}, {tone}"
+ kata_tone.append((kana, tone))
+ except Exception as e:
+ logger.warning(f"Error occurred when parsing kana_tone_json: {e}")
+ wrong_tone_message = f"アクセント指定が不正です: {e}"
+ kata_tone = None
+
+ # toneは実際に音声合成に代入される際のみnot Noneになる
+ tone: Optional[list[int]] = None
+ if kata_tone is not None:
+ phone_tone = kata_tone2phone_tone(kata_tone)
+ tone = [t for _, t in phone_tone]
+
+ speaker_id = model_holder.current_model.spk2id[speaker]
+
+ start_time = datetime.datetime.now()
+
+ try:
+ sr, audio = model_holder.current_model.infer(
+ text=text,
+ language=language,
+ reference_audio_path=reference_audio_path,
+ sdp_ratio=sdp_ratio,
+ noise=noise_scale,
+ noisew=noise_scale_w,
+ length=length_scale,
+ line_split=line_split,
+ split_interval=split_interval,
+ assist_text=assist_text,
+ assist_text_weight=assist_text_weight,
+ use_assist_text=use_assist_text,
+ style=style,
+ style_weight=style_weight,
+ given_tone=tone,
+ sid=speaker_id,
+ pitch_scale=pitch_scale,
+ intonation_scale=intonation_scale,
+ )
+ except InvalidToneError as e:
+ logger.error(f"Tone error: {e}")
+ return f"Error: アクセント指定が不正です:\n{e}", None, kata_tone_json_str
+ except ValueError as e:
+ logger.error(f"Value error: {e}")
+ return f"Error: {e}", None, kata_tone_json_str
+
+ end_time = datetime.datetime.now()
+ duration = (end_time - start_time).total_seconds()
+
+ if tone is None and language == "JP":
+ # アクセント指定に使えるようにアクセント情報を返す
+ norm_text = text_normalize(text)
+ kata_tone = g2kata_tone(norm_text)
+ kata_tone_json_str = json.dumps(kata_tone, ensure_ascii=False)
+ elif tone is None:
+ kata_tone_json_str = ""
+ message = f"Success, time: {duration} seconds."
+ if wrong_tone_message != "":
+ message = wrong_tone_message + "\n" + message
+ return message, (sr, audio), kata_tone_json_str
+
+
+initial_text = "こんにちは、初めまして。あなたの名前はなんていうの?"
+
+examples = [
+ [initial_text, "JP"],
+ [
+ """あなたがそんなこと言うなんて、私はとっても嬉しい。
+あなたがそんなこと言うなんて、私はとっても怒ってる。
+あなたがそんなこと言うなんて、私はとっても驚いてる。
+あなたがそんなこと言うなんて、私はとっても辛い。""",
+ "JP",
+ ],
+ [ # ChatGPTに考えてもらった告白セリフ
+ """私、ずっと前からあなたのことを見てきました。あなたの笑顔、優しさ、強さに、心惹かれていたんです。
+友達として過ごす中で、あなたのことがだんだんと特別な存在になっていくのがわかりました。
+えっと、私、あなたのことが好きです!もしよければ、私と付き合ってくれませんか?""",
+ "JP",
+ ],
+ [ # 夏目漱石『吾輩は猫である』
+ """吾輩は猫である。名前はまだ無い。
+どこで生れたかとんと見当がつかぬ。なんでも薄暗いじめじめした所でニャーニャー泣いていた事だけは記憶している。
+吾輩はここで初めて人間というものを見た。しかもあとで聞くと、それは書生という、人間中で一番獰悪な種族であったそうだ。
+この書生というのは時々我々を捕まえて煮て食うという話である。""",
+ "JP",
+ ],
+ [ # 梶井基次郎『桜の樹の下には』
+ """桜の樹の下には屍体が埋まっている!これは信じていいことなんだよ。
+何故って、桜の花があんなにも見事に咲くなんて信じられないことじゃないか。俺はあの美しさが信じられないので、このにさんにち不安だった。
+しかしいま、やっとわかるときが来た。桜の樹の下には屍体が埋まっている。これは信じていいことだ。""",
+ "JP",
+ ],
+ [ # ChatGPTと考えた、感情を表すセリフ
+ """やったー!テストで満点取れた!私とっても嬉しいな!
+どうして私の意見を無視するの?許せない!ムカつく!あんたなんか死ねばいいのに。
+あはははっ!この漫画めっちゃ笑える、見てよこれ、ふふふ、あはは。
+あなたがいなくなって、私は一人になっちゃって、泣いちゃいそうなほど悲しい。""",
+ "JP",
+ ],
+ [ # 上の丁寧語バージョン
+ """やりました!テストで満点取れましたよ!私とっても嬉しいです!
+どうして私の意見を無視するんですか?許せません!ムカつきます!あんたなんか死んでください。
+あはははっ!この漫画めっちゃ笑えます、見てくださいこれ、ふふふ、あはは。
+あなたがいなくなって、私は一人になっちゃって、泣いちゃいそうなほど悲しいです。""",
+ "JP",
+ ],
+ [ # ChatGPTに考えてもらった音声合成の説明文章
+ """音声合成は、機械学習を活用して、テキストから人の声を再現する技術です。この技術は、言語の構造を解析し、それに基づいて音声を生成します。
+この分野の最新の研究成果を使うと、より自然で表現豊かな音声の生成が可能である。深層学習の応用により、感情やアクセントを含む声質の微妙な変化も再現することが出来る。""",
+ "JP",
+ ],
+ [
+ "Speech synthesis is the artificial production of human speech. A computer system used for this purpose is called a speech synthesizer, and can be implemented in software or hardware products.",
+ "EN",
+ ],
+ [
+ "语音合成是人工制造人类语音。用于此目的的计算机系统称为语音合成器,可以通过软件或硬件产品实现。",
+ "ZH",
+ ],
+]
+
+initial_md = f"""
+# Style-Bert-VITS2 ver {LATEST_VERSION} 音声合成
+
+- Ver 2.3で追加されたエディターのほうが実際に読み上げさせるには使いやすいかもしれません。`Editor.bat`か`python server_editor.py`で起動できます。
+
+- 初期からある[jvnvのモデル](https://huggingface.co/litagin/style_bert_vits2_jvnv)は、[JVNVコーパス(言語音声と非言語音声を持つ日本語感情音声コーパス)](https://sites.google.com/site/shinnosuketakamichi/research-topics/jvnv_corpus)で学習されたモデルです。ライセンスは[CC BY-SA 4.0](https://creativecommons.org/licenses/by-sa/4.0/deed.ja)です。
+"""
+
+how_to_md = """
+下のように`model_assets`ディレクトリの中にモデルファイルたちを置いてください。
+```
+model_assets
+├── your_model
+│ ├── config.json
+│ ├── your_model_file1.safetensors
+│ ├── your_model_file2.safetensors
+│ ├── ...
+│ └── style_vectors.npy
+└── another_model
+ ├── ...
+```
+各モデルにはファイルたちが必要です:
+- `config.json`:学習時の設定ファイル
+- `*.safetensors`:学習済みモデルファイル(1つ以上が必要、複数可)
+- `style_vectors.npy`:スタイルベクトルファイル
+
+上2つは`Train.bat`による学習で自動的に正しい位置に保存されます。`style_vectors.npy`は`Style.bat`を実行して指示に従って生成してください。
+"""
+
+style_md = f"""
+- プリセットまたは音声ファイルから読み上げの声音・感情・スタイルのようなものを制御できます。
+- デフォルトの{DEFAULT_STYLE}でも、十分に読み上げる文に応じた感情で感情豊かに読み上げられます。このスタイル制御は、それを重み付きで上書きするような感じです。
+- 強さを大きくしすぎると発音が変になったり声にならなかったりと崩壊することがあります。
+- どのくらいに強さがいいかはモデルやスタイルによって異なるようです。
+- 音声ファイルを入力する場合は、学習データと似た声音の話者(特に同じ性別)でないとよい効果が出ないかもしれません。
+"""
+
+
+def make_interactive():
+ return gr.update(interactive=True, value="音声合成")
+
+
+def make_non_interactive():
+ return gr.update(interactive=False, value="音声合成(モデルをロードしてください)")
+
+
+def gr_util(item):
+ if item == "プリセットから選ぶ":
+ return (gr.update(visible=True), gr.Audio(visible=False, value=None))
+ else:
+ return (gr.update(visible=False), gr.update(visible=True))
+
+
+if __name__ == "__main__":
+ parser = argparse.ArgumentParser()
+ parser.add_argument("--cpu", action="store_true", help="Use CPU instead of GPU")
+ parser.add_argument(
+ "--dir", "-d", type=str, help="Model directory", default=assets_root
+ )
+ parser.add_argument(
+ "--share", action="store_true", help="Share this app publicly", default=False
+ )
+ parser.add_argument(
+ "--server-name",
+ type=str,
+ default=None,
+ help="Server name for Gradio app",
+ )
+ parser.add_argument(
+ "--no-autolaunch",
+ action="store_true",
+ default=False,
+ help="Do not launch app automatically",
+ )
+ args = parser.parse_args()
+ model_dir = Path(args.dir)
+
+ if args.cpu:
+ device = "cpu"
+ else:
+ device = "cuda" if torch.cuda.is_available() else "cpu"
+
+ model_holder = ModelHolder(model_dir, device)
+
+ model_names = model_holder.model_names
+ if len(model_names) == 0:
+ logger.error(
+ f"モデルが見つかりませんでした。{model_dir}にモデルを置いてください。"
+ )
+ sys.exit(1)
+ initial_id = 0
+ initial_pth_files = model_holder.model_files_dict[model_names[initial_id]]
+
+ with gr.Blocks(theme=GRADIO_THEME) as app:
+ gr.Markdown(initial_md)
+ with gr.Accordion(label="使い方", open=False):
+ gr.Markdown(how_to_md)
+ with gr.Row():
+ with gr.Column():
+ with gr.Row():
+ with gr.Column(scale=3):
+ model_name = gr.Dropdown(
+ label="モデル一覧",
+ choices=model_names,
+ value=model_names[initial_id],
+ )
+ model_path = gr.Dropdown(
+ label="モデルファイル",
+ choices=initial_pth_files,
+ value=initial_pth_files[0],
+ )
+ refresh_button = gr.Button("更新", scale=1, visible=True)
+ load_button = gr.Button("ロード", scale=1, variant="primary")
+ text_input = gr.TextArea(label="テキスト", value=initial_text)
+ pitch_scale = gr.Slider(
+ minimum=0.8,
+ maximum=1.5,
+ value=1,
+ step=0.05,
+ label="音程(1以外では音質劣化)",
+ visible=False, # pyworldが必要
+ )
+ intonation_scale = gr.Slider(
+ minimum=0,
+ maximum=2,
+ value=1,
+ step=0.1,
+ label="抑揚(1以外では音質劣化)",
+ visible=False, # pyworldが必要
+ )
+
+ line_split = gr.Checkbox(
+ label="改行で分けて生成(分けたほうが感情が乗ります)",
+ value=DEFAULT_LINE_SPLIT,
+ )
+ split_interval = gr.Slider(
+ minimum=0.0,
+ maximum=2,
+ value=DEFAULT_SPLIT_INTERVAL,
+ step=0.1,
+ label="改行ごとに挟む無音の長さ(秒)",
+ )
+ line_split.change(
+ lambda x: (gr.Slider(visible=x)),
+ inputs=[line_split],
+ outputs=[split_interval],
+ )
+ tone = gr.Textbox(
+ label="アクセント調整(数値は 0=低 か1=高 のみ)",
+ info="改行で分けない場合のみ使えます。万能ではありません。",
+ )
+ use_tone = gr.Checkbox(label="アクセント調整を使う", value=False)
+ use_tone.change(
+ lambda x: (gr.Checkbox(value=False) if x else gr.Checkbox()),
+ inputs=[use_tone],
+ outputs=[line_split],
+ )
+ language = gr.Dropdown(choices=languages, value="JP", label="Language")
+ speaker = gr.Dropdown(label="話者")
+ with gr.Accordion(label="詳細設定", open=False):
+ sdp_ratio = gr.Slider(
+ minimum=0,
+ maximum=1,
+ value=DEFAULT_SDP_RATIO,
+ step=0.1,
+ label="SDP Ratio",
+ )
+ noise_scale = gr.Slider(
+ minimum=0.1,
+ maximum=2,
+ value=DEFAULT_NOISE,
+ step=0.1,
+ label="Noise",
+ )
+ noise_scale_w = gr.Slider(
+ minimum=0.1,
+ maximum=2,
+ value=DEFAULT_NOISEW,
+ step=0.1,
+ label="Noise_W",
+ )
+ length_scale = gr.Slider(
+ minimum=0.1,
+ maximum=2,
+ value=DEFAULT_LENGTH,
+ step=0.1,
+ label="Length",
+ )
+ use_assist_text = gr.Checkbox(
+ label="Assist textを使う", value=False
+ )
+ assist_text = gr.Textbox(
+ label="Assist text",
+ placeholder="どうして私の意見を無視するの?許せない、ムカつく!死ねばいいのに。",
+ info="このテキストの読み上げと似た声音・感情になりやすくなります。ただ抑揚やテンポ等が犠牲になる傾向があります。",
+ visible=False,
+ )
+ assist_text_weight = gr.Slider(
+ minimum=0,
+ maximum=1,
+ value=DEFAULT_ASSIST_TEXT_WEIGHT,
+ step=0.1,
+ label="Assist textの強さ",
+ visible=False,
+ )
+ use_assist_text.change(
+ lambda x: (gr.Textbox(visible=x), gr.Slider(visible=x)),
+ inputs=[use_assist_text],
+ outputs=[assist_text, assist_text_weight],
+ )
+ with gr.Column():
+ with gr.Accordion("スタイルについて詳細", open=False):
+ gr.Markdown(style_md)
+ style_mode = gr.Radio(
+ ["プリセットから選ぶ", "音声ファイルを入力"],
+ label="スタイルの指定方法",
+ value="プリセットから選ぶ",
+ )
+ style = gr.Dropdown(
+ label=f"スタイル({DEFAULT_STYLE}が平均スタイル)",
+ choices=["モデルをロードしてください"],
+ value="モデルをロードしてください",
+ )
+ style_weight = gr.Slider(
+ minimum=0,
+ maximum=50,
+ value=DEFAULT_STYLE_WEIGHT,
+ step=0.1,
+ label="スタイルの強さ",
+ )
+ ref_audio_path = gr.Audio(
+ label="参照音声", type="filepath", visible=False
+ )
+ tts_button = gr.Button(
+ "音声合成(モデルをロードしてください)",
+ variant="primary",
+ interactive=False,
+ )
+ text_output = gr.Textbox(label="情報")
+ audio_output = gr.Audio(label="結果")
+ with gr.Accordion("テキスト例", open=False):
+ gr.Examples(examples, inputs=[text_input, language])
+
+ tts_button.click(
+ tts_fn,
+ inputs=[
+ model_name,
+ model_path,
+ text_input,
+ language,
+ ref_audio_path,
+ sdp_ratio,
+ noise_scale,
+ noise_scale_w,
+ length_scale,
+ line_split,
+ split_interval,
+ assist_text,
+ assist_text_weight,
+ use_assist_text,
+ style,
+ style_weight,
+ tone,
+ use_tone,
+ speaker,
+ pitch_scale,
+ intonation_scale,
+ ],
+ outputs=[text_output, audio_output, tone],
+ )
+
+ model_name.change(
+ model_holder.update_model_files_gr,
+ inputs=[model_name],
+ outputs=[model_path],
+ )
+
+ model_path.change(make_non_interactive, outputs=[tts_button])
+
+ refresh_button.click(
+ model_holder.update_model_names_gr,
+ outputs=[model_name, model_path, tts_button],
+ )
+
+ load_button.click(
+ model_holder.load_model_gr,
+ inputs=[model_name, model_path],
+ outputs=[style, tts_button, speaker],
+ )
+
+ style_mode.change(
+ gr_util,
+ inputs=[style_mode],
+ outputs=[style, ref_audio_path],
+ )
+
+ app.launch(
+ inbrowser=not args.no_autolaunch, share=args.share, server_name=args.server_name
+ )
diff --git a/attentions.py b/attentions.py
new file mode 100644
index 0000000000000000000000000000000000000000..9a4bba9cbfe4443f839db598d97a239595a07269
--- /dev/null
+++ b/attentions.py
@@ -0,0 +1,462 @@
+import math
+import torch
+from torch import nn
+from torch.nn import functional as F
+
+import commons
+from common.log import logger as logging
+
+
+class LayerNorm(nn.Module):
+ def __init__(self, channels, eps=1e-5):
+ super().__init__()
+ self.channels = channels
+ self.eps = eps
+
+ self.gamma = nn.Parameter(torch.ones(channels))
+ self.beta = nn.Parameter(torch.zeros(channels))
+
+ def forward(self, x):
+ x = x.transpose(1, -1)
+ x = F.layer_norm(x, (self.channels,), self.gamma, self.beta, self.eps)
+ return x.transpose(1, -1)
+
+
+@torch.jit.script
+def fused_add_tanh_sigmoid_multiply(input_a, input_b, n_channels):
+ n_channels_int = n_channels[0]
+ in_act = input_a + input_b
+ t_act = torch.tanh(in_act[:, :n_channels_int, :])
+ s_act = torch.sigmoid(in_act[:, n_channels_int:, :])
+ acts = t_act * s_act
+ return acts
+
+
+class Encoder(nn.Module):
+ def __init__(
+ self,
+ hidden_channels,
+ filter_channels,
+ n_heads,
+ n_layers,
+ kernel_size=1,
+ p_dropout=0.0,
+ window_size=4,
+ isflow=True,
+ **kwargs
+ ):
+ super().__init__()
+ self.hidden_channels = hidden_channels
+ self.filter_channels = filter_channels
+ self.n_heads = n_heads
+ self.n_layers = n_layers
+ self.kernel_size = kernel_size
+ self.p_dropout = p_dropout
+ self.window_size = window_size
+ # if isflow:
+ # cond_layer = torch.nn.Conv1d(256, 2*hidden_channels*n_layers, 1)
+ # self.cond_pre = torch.nn.Conv1d(hidden_channels, 2*hidden_channels, 1)
+ # self.cond_layer = weight_norm(cond_layer, name='weight')
+ # self.gin_channels = 256
+ self.cond_layer_idx = self.n_layers
+ if "gin_channels" in kwargs:
+ self.gin_channels = kwargs["gin_channels"]
+ if self.gin_channels != 0:
+ self.spk_emb_linear = nn.Linear(self.gin_channels, self.hidden_channels)
+ # vits2 says 3rd block, so idx is 2 by default
+ self.cond_layer_idx = (
+ kwargs["cond_layer_idx"] if "cond_layer_idx" in kwargs else 2
+ )
+ # logging.debug(self.gin_channels, self.cond_layer_idx)
+ assert (
+ self.cond_layer_idx < self.n_layers
+ ), "cond_layer_idx should be less than n_layers"
+ self.drop = nn.Dropout(p_dropout)
+ self.attn_layers = nn.ModuleList()
+ self.norm_layers_1 = nn.ModuleList()
+ self.ffn_layers = nn.ModuleList()
+ self.norm_layers_2 = nn.ModuleList()
+ for i in range(self.n_layers):
+ self.attn_layers.append(
+ MultiHeadAttention(
+ hidden_channels,
+ hidden_channels,
+ n_heads,
+ p_dropout=p_dropout,
+ window_size=window_size,
+ )
+ )
+ self.norm_layers_1.append(LayerNorm(hidden_channels))
+ self.ffn_layers.append(
+ FFN(
+ hidden_channels,
+ hidden_channels,
+ filter_channels,
+ kernel_size,
+ p_dropout=p_dropout,
+ )
+ )
+ self.norm_layers_2.append(LayerNorm(hidden_channels))
+
+ def forward(self, x, x_mask, g=None):
+ attn_mask = x_mask.unsqueeze(2) * x_mask.unsqueeze(-1)
+ x = x * x_mask
+ for i in range(self.n_layers):
+ if i == self.cond_layer_idx and g is not None:
+ g = self.spk_emb_linear(g.transpose(1, 2))
+ g = g.transpose(1, 2)
+ x = x + g
+ x = x * x_mask
+ y = self.attn_layers[i](x, x, attn_mask)
+ y = self.drop(y)
+ x = self.norm_layers_1[i](x + y)
+
+ y = self.ffn_layers[i](x, x_mask)
+ y = self.drop(y)
+ x = self.norm_layers_2[i](x + y)
+ x = x * x_mask
+ return x
+
+
+class Decoder(nn.Module):
+ def __init__(
+ self,
+ hidden_channels,
+ filter_channels,
+ n_heads,
+ n_layers,
+ kernel_size=1,
+ p_dropout=0.0,
+ proximal_bias=False,
+ proximal_init=True,
+ **kwargs
+ ):
+ super().__init__()
+ self.hidden_channels = hidden_channels
+ self.filter_channels = filter_channels
+ self.n_heads = n_heads
+ self.n_layers = n_layers
+ self.kernel_size = kernel_size
+ self.p_dropout = p_dropout
+ self.proximal_bias = proximal_bias
+ self.proximal_init = proximal_init
+
+ self.drop = nn.Dropout(p_dropout)
+ self.self_attn_layers = nn.ModuleList()
+ self.norm_layers_0 = nn.ModuleList()
+ self.encdec_attn_layers = nn.ModuleList()
+ self.norm_layers_1 = nn.ModuleList()
+ self.ffn_layers = nn.ModuleList()
+ self.norm_layers_2 = nn.ModuleList()
+ for i in range(self.n_layers):
+ self.self_attn_layers.append(
+ MultiHeadAttention(
+ hidden_channels,
+ hidden_channels,
+ n_heads,
+ p_dropout=p_dropout,
+ proximal_bias=proximal_bias,
+ proximal_init=proximal_init,
+ )
+ )
+ self.norm_layers_0.append(LayerNorm(hidden_channels))
+ self.encdec_attn_layers.append(
+ MultiHeadAttention(
+ hidden_channels, hidden_channels, n_heads, p_dropout=p_dropout
+ )
+ )
+ self.norm_layers_1.append(LayerNorm(hidden_channels))
+ self.ffn_layers.append(
+ FFN(
+ hidden_channels,
+ hidden_channels,
+ filter_channels,
+ kernel_size,
+ p_dropout=p_dropout,
+ causal=True,
+ )
+ )
+ self.norm_layers_2.append(LayerNorm(hidden_channels))
+
+ def forward(self, x, x_mask, h, h_mask):
+ """
+ x: decoder input
+ h: encoder output
+ """
+ self_attn_mask = commons.subsequent_mask(x_mask.size(2)).to(
+ device=x.device, dtype=x.dtype
+ )
+ encdec_attn_mask = h_mask.unsqueeze(2) * x_mask.unsqueeze(-1)
+ x = x * x_mask
+ for i in range(self.n_layers):
+ y = self.self_attn_layers[i](x, x, self_attn_mask)
+ y = self.drop(y)
+ x = self.norm_layers_0[i](x + y)
+
+ y = self.encdec_attn_layers[i](x, h, encdec_attn_mask)
+ y = self.drop(y)
+ x = self.norm_layers_1[i](x + y)
+
+ y = self.ffn_layers[i](x, x_mask)
+ y = self.drop(y)
+ x = self.norm_layers_2[i](x + y)
+ x = x * x_mask
+ return x
+
+
+class MultiHeadAttention(nn.Module):
+ def __init__(
+ self,
+ channels,
+ out_channels,
+ n_heads,
+ p_dropout=0.0,
+ window_size=None,
+ heads_share=True,
+ block_length=None,
+ proximal_bias=False,
+ proximal_init=False,
+ ):
+ super().__init__()
+ assert channels % n_heads == 0
+
+ self.channels = channels
+ self.out_channels = out_channels
+ self.n_heads = n_heads
+ self.p_dropout = p_dropout
+ self.window_size = window_size
+ self.heads_share = heads_share
+ self.block_length = block_length
+ self.proximal_bias = proximal_bias
+ self.proximal_init = proximal_init
+ self.attn = None
+
+ self.k_channels = channels // n_heads
+ self.conv_q = nn.Conv1d(channels, channels, 1)
+ self.conv_k = nn.Conv1d(channels, channels, 1)
+ self.conv_v = nn.Conv1d(channels, channels, 1)
+ self.conv_o = nn.Conv1d(channels, out_channels, 1)
+ self.drop = nn.Dropout(p_dropout)
+
+ if window_size is not None:
+ n_heads_rel = 1 if heads_share else n_heads
+ rel_stddev = self.k_channels**-0.5
+ self.emb_rel_k = nn.Parameter(
+ torch.randn(n_heads_rel, window_size * 2 + 1, self.k_channels)
+ * rel_stddev
+ )
+ self.emb_rel_v = nn.Parameter(
+ torch.randn(n_heads_rel, window_size * 2 + 1, self.k_channels)
+ * rel_stddev
+ )
+
+ nn.init.xavier_uniform_(self.conv_q.weight)
+ nn.init.xavier_uniform_(self.conv_k.weight)
+ nn.init.xavier_uniform_(self.conv_v.weight)
+ if proximal_init:
+ with torch.no_grad():
+ self.conv_k.weight.copy_(self.conv_q.weight)
+ self.conv_k.bias.copy_(self.conv_q.bias)
+
+ def forward(self, x, c, attn_mask=None):
+ q = self.conv_q(x)
+ k = self.conv_k(c)
+ v = self.conv_v(c)
+
+ x, self.attn = self.attention(q, k, v, mask=attn_mask)
+
+ x = self.conv_o(x)
+ return x
+
+ def attention(self, query, key, value, mask=None):
+ # reshape [b, d, t] -> [b, n_h, t, d_k]
+ b, d, t_s, t_t = (*key.size(), query.size(2))
+ query = query.view(b, self.n_heads, self.k_channels, t_t).transpose(2, 3)
+ key = key.view(b, self.n_heads, self.k_channels, t_s).transpose(2, 3)
+ value = value.view(b, self.n_heads, self.k_channels, t_s).transpose(2, 3)
+
+ scores = torch.matmul(query / math.sqrt(self.k_channels), key.transpose(-2, -1))
+ if self.window_size is not None:
+ assert (
+ t_s == t_t
+ ), "Relative attention is only available for self-attention."
+ key_relative_embeddings = self._get_relative_embeddings(self.emb_rel_k, t_s)
+ rel_logits = self._matmul_with_relative_keys(
+ query / math.sqrt(self.k_channels), key_relative_embeddings
+ )
+ scores_local = self._relative_position_to_absolute_position(rel_logits)
+ scores = scores + scores_local
+ if self.proximal_bias:
+ assert t_s == t_t, "Proximal bias is only available for self-attention."
+ scores = scores + self._attention_bias_proximal(t_s).to(
+ device=scores.device, dtype=scores.dtype
+ )
+ if mask is not None:
+ scores = scores.masked_fill(mask == 0, -1e4)
+ if self.block_length is not None:
+ assert (
+ t_s == t_t
+ ), "Local attention is only available for self-attention."
+ block_mask = (
+ torch.ones_like(scores)
+ .triu(-self.block_length)
+ .tril(self.block_length)
+ )
+ scores = scores.masked_fill(block_mask == 0, -1e4)
+ p_attn = F.softmax(scores, dim=-1) # [b, n_h, t_t, t_s]
+ p_attn = self.drop(p_attn)
+ output = torch.matmul(p_attn, value)
+ if self.window_size is not None:
+ relative_weights = self._absolute_position_to_relative_position(p_attn)
+ value_relative_embeddings = self._get_relative_embeddings(
+ self.emb_rel_v, t_s
+ )
+ output = output + self._matmul_with_relative_values(
+ relative_weights, value_relative_embeddings
+ )
+ output = (
+ output.transpose(2, 3).contiguous().view(b, d, t_t)
+ ) # [b, n_h, t_t, d_k] -> [b, d, t_t]
+ return output, p_attn
+
+ def _matmul_with_relative_values(self, x, y):
+ """
+ x: [b, h, l, m]
+ y: [h or 1, m, d]
+ ret: [b, h, l, d]
+ """
+ ret = torch.matmul(x, y.unsqueeze(0))
+ return ret
+
+ def _matmul_with_relative_keys(self, x, y):
+ """
+ x: [b, h, l, d]
+ y: [h or 1, m, d]
+ ret: [b, h, l, m]
+ """
+ ret = torch.matmul(x, y.unsqueeze(0).transpose(-2, -1))
+ return ret
+
+ def _get_relative_embeddings(self, relative_embeddings, length):
+ 2 * self.window_size + 1
+ # Pad first before slice to avoid using cond ops.
+ pad_length = max(length - (self.window_size + 1), 0)
+ slice_start_position = max((self.window_size + 1) - length, 0)
+ slice_end_position = slice_start_position + 2 * length - 1
+ if pad_length > 0:
+ padded_relative_embeddings = F.pad(
+ relative_embeddings,
+ commons.convert_pad_shape([[0, 0], [pad_length, pad_length], [0, 0]]),
+ )
+ else:
+ padded_relative_embeddings = relative_embeddings
+ used_relative_embeddings = padded_relative_embeddings[
+ :, slice_start_position:slice_end_position
+ ]
+ return used_relative_embeddings
+
+ def _relative_position_to_absolute_position(self, x):
+ """
+ x: [b, h, l, 2*l-1]
+ ret: [b, h, l, l]
+ """
+ batch, heads, length, _ = x.size()
+ # Concat columns of pad to shift from relative to absolute indexing.
+ x = F.pad(x, commons.convert_pad_shape([[0, 0], [0, 0], [0, 0], [0, 1]]))
+
+ # Concat extra elements so to add up to shape (len+1, 2*len-1).
+ x_flat = x.view([batch, heads, length * 2 * length])
+ x_flat = F.pad(
+ x_flat, commons.convert_pad_shape([[0, 0], [0, 0], [0, length - 1]])
+ )
+
+ # Reshape and slice out the padded elements.
+ x_final = x_flat.view([batch, heads, length + 1, 2 * length - 1])[
+ :, :, :length, length - 1 :
+ ]
+ return x_final
+
+ def _absolute_position_to_relative_position(self, x):
+ """
+ x: [b, h, l, l]
+ ret: [b, h, l, 2*l-1]
+ """
+ batch, heads, length, _ = x.size()
+ # pad along column
+ x = F.pad(
+ x, commons.convert_pad_shape([[0, 0], [0, 0], [0, 0], [0, length - 1]])
+ )
+ x_flat = x.view([batch, heads, length**2 + length * (length - 1)])
+ # add 0's in the beginning that will skew the elements after reshape
+ x_flat = F.pad(x_flat, commons.convert_pad_shape([[0, 0], [0, 0], [length, 0]]))
+ x_final = x_flat.view([batch, heads, length, 2 * length])[:, :, :, 1:]
+ return x_final
+
+ def _attention_bias_proximal(self, length):
+ """Bias for self-attention to encourage attention to close positions.
+ Args:
+ length: an integer scalar.
+ Returns:
+ a Tensor with shape [1, 1, length, length]
+ """
+ r = torch.arange(length, dtype=torch.float32)
+ diff = torch.unsqueeze(r, 0) - torch.unsqueeze(r, 1)
+ return torch.unsqueeze(torch.unsqueeze(-torch.log1p(torch.abs(diff)), 0), 0)
+
+
+class FFN(nn.Module):
+ def __init__(
+ self,
+ in_channels,
+ out_channels,
+ filter_channels,
+ kernel_size,
+ p_dropout=0.0,
+ activation=None,
+ causal=False,
+ ):
+ super().__init__()
+ self.in_channels = in_channels
+ self.out_channels = out_channels
+ self.filter_channels = filter_channels
+ self.kernel_size = kernel_size
+ self.p_dropout = p_dropout
+ self.activation = activation
+ self.causal = causal
+
+ if causal:
+ self.padding = self._causal_padding
+ else:
+ self.padding = self._same_padding
+
+ self.conv_1 = nn.Conv1d(in_channels, filter_channels, kernel_size)
+ self.conv_2 = nn.Conv1d(filter_channels, out_channels, kernel_size)
+ self.drop = nn.Dropout(p_dropout)
+
+ def forward(self, x, x_mask):
+ x = self.conv_1(self.padding(x * x_mask))
+ if self.activation == "gelu":
+ x = x * torch.sigmoid(1.702 * x)
+ else:
+ x = torch.relu(x)
+ x = self.drop(x)
+ x = self.conv_2(self.padding(x * x_mask))
+ return x * x_mask
+
+ def _causal_padding(self, x):
+ if self.kernel_size == 1:
+ return x
+ pad_l = self.kernel_size - 1
+ pad_r = 0
+ padding = [[0, 0], [0, 0], [pad_l, pad_r]]
+ x = F.pad(x, commons.convert_pad_shape(padding))
+ return x
+
+ def _same_padding(self, x):
+ if self.kernel_size == 1:
+ return x
+ pad_l = (self.kernel_size - 1) // 2
+ pad_r = self.kernel_size // 2
+ padding = [[0, 0], [0, 0], [pad_l, pad_r]]
+ x = F.pad(x, commons.convert_pad_shape(padding))
+ return x
diff --git a/bert/bert_models.json b/bert/bert_models.json
new file mode 100644
index 0000000000000000000000000000000000000000..cd5906d116d51629aaf94a85cf3d2df7e8032941
--- /dev/null
+++ b/bert/bert_models.json
@@ -0,0 +1,14 @@
+{
+ "deberta-v2-large-japanese-char-wwm": {
+ "repo_id": "ku-nlp/deberta-v2-large-japanese-char-wwm",
+ "files": ["pytorch_model.bin"]
+ },
+ "chinese-roberta-wwm-ext-large": {
+ "repo_id": "hfl/chinese-roberta-wwm-ext-large",
+ "files": ["pytorch_model.bin"]
+ },
+ "deberta-v3-large": {
+ "repo_id": "microsoft/deberta-v3-large",
+ "files": ["spm.model", "pytorch_model.bin"]
+ }
+}
diff --git a/bert/chinese-roberta-wwm-ext-large/.gitattributes b/bert/chinese-roberta-wwm-ext-large/.gitattributes
new file mode 100644
index 0000000000000000000000000000000000000000..ae8c63daedbd4206d7d40126955d4e6ab1c80f8f
--- /dev/null
+++ b/bert/chinese-roberta-wwm-ext-large/.gitattributes
@@ -0,0 +1,9 @@
+*.bin.* filter=lfs diff=lfs merge=lfs -text
+*.lfs.* filter=lfs diff=lfs merge=lfs -text
+*.bin filter=lfs diff=lfs merge=lfs -text
+*.h5 filter=lfs diff=lfs merge=lfs -text
+*.tflite filter=lfs diff=lfs merge=lfs -text
+*.tar.gz filter=lfs diff=lfs merge=lfs -text
+*.ot filter=lfs diff=lfs merge=lfs -text
+*.onnx filter=lfs diff=lfs merge=lfs -text
+*.msgpack filter=lfs diff=lfs merge=lfs -text
diff --git a/bert/chinese-roberta-wwm-ext-large/README.md b/bert/chinese-roberta-wwm-ext-large/README.md
new file mode 100644
index 0000000000000000000000000000000000000000..ebc4b2e6fb6b95ddc5f678b4a7f829466799f2da
--- /dev/null
+++ b/bert/chinese-roberta-wwm-ext-large/README.md
@@ -0,0 +1,57 @@
+---
+language:
+- zh
+tags:
+- bert
+license: "apache-2.0"
+---
+
+# Please use 'Bert' related functions to load this model!
+
+## Chinese BERT with Whole Word Masking
+For further accelerating Chinese natural language processing, we provide **Chinese pre-trained BERT with Whole Word Masking**.
+
+**[Pre-Training with Whole Word Masking for Chinese BERT](https://arxiv.org/abs/1906.08101)**
+Yiming Cui, Wanxiang Che, Ting Liu, Bing Qin, Ziqing Yang, Shijin Wang, Guoping Hu
+
+This repository is developed based on:https://github.com/google-research/bert
+
+You may also interested in,
+- Chinese BERT series: https://github.com/ymcui/Chinese-BERT-wwm
+- Chinese MacBERT: https://github.com/ymcui/MacBERT
+- Chinese ELECTRA: https://github.com/ymcui/Chinese-ELECTRA
+- Chinese XLNet: https://github.com/ymcui/Chinese-XLNet
+- Knowledge Distillation Toolkit - TextBrewer: https://github.com/airaria/TextBrewer
+
+More resources by HFL: https://github.com/ymcui/HFL-Anthology
+
+## Citation
+If you find the technical report or resource is useful, please cite the following technical report in your paper.
+- Primary: https://arxiv.org/abs/2004.13922
+```
+@inproceedings{cui-etal-2020-revisiting,
+ title = "Revisiting Pre-Trained Models for {C}hinese Natural Language Processing",
+ author = "Cui, Yiming and
+ Che, Wanxiang and
+ Liu, Ting and
+ Qin, Bing and
+ Wang, Shijin and
+ Hu, Guoping",
+ booktitle = "Proceedings of the 2020 Conference on Empirical Methods in Natural Language Processing: Findings",
+ month = nov,
+ year = "2020",
+ address = "Online",
+ publisher = "Association for Computational Linguistics",
+ url = "https://www.aclweb.org/anthology/2020.findings-emnlp.58",
+ pages = "657--668",
+}
+```
+- Secondary: https://arxiv.org/abs/1906.08101
+```
+@article{chinese-bert-wwm,
+ title={Pre-Training with Whole Word Masking for Chinese BERT},
+ author={Cui, Yiming and Che, Wanxiang and Liu, Ting and Qin, Bing and Yang, Ziqing and Wang, Shijin and Hu, Guoping},
+ journal={arXiv preprint arXiv:1906.08101},
+ year={2019}
+ }
+```
diff --git a/bert/chinese-roberta-wwm-ext-large/added_tokens.json b/bert/chinese-roberta-wwm-ext-large/added_tokens.json
new file mode 100644
index 0000000000000000000000000000000000000000..0967ef424bce6791893e9a57bb952f80fd536e93
--- /dev/null
+++ b/bert/chinese-roberta-wwm-ext-large/added_tokens.json
@@ -0,0 +1 @@
+{}
diff --git a/bert/chinese-roberta-wwm-ext-large/config.json b/bert/chinese-roberta-wwm-ext-large/config.json
new file mode 100644
index 0000000000000000000000000000000000000000..920741798717df58aa766c378de136df3053b609
--- /dev/null
+++ b/bert/chinese-roberta-wwm-ext-large/config.json
@@ -0,0 +1,28 @@
+{
+ "architectures": [
+ "BertForMaskedLM"
+ ],
+ "attention_probs_dropout_prob": 0.1,
+ "bos_token_id": 0,
+ "directionality": "bidi",
+ "eos_token_id": 2,
+ "hidden_act": "gelu",
+ "hidden_dropout_prob": 0.1,
+ "hidden_size": 1024,
+ "initializer_range": 0.02,
+ "intermediate_size": 4096,
+ "layer_norm_eps": 1e-12,
+ "max_position_embeddings": 512,
+ "model_type": "bert",
+ "num_attention_heads": 16,
+ "num_hidden_layers": 24,
+ "output_past": true,
+ "pad_token_id": 0,
+ "pooler_fc_size": 768,
+ "pooler_num_attention_heads": 12,
+ "pooler_num_fc_layers": 3,
+ "pooler_size_per_head": 128,
+ "pooler_type": "first_token_transform",
+ "type_vocab_size": 2,
+ "vocab_size": 21128
+}
diff --git a/bert/chinese-roberta-wwm-ext-large/special_tokens_map.json b/bert/chinese-roberta-wwm-ext-large/special_tokens_map.json
new file mode 100644
index 0000000000000000000000000000000000000000..dabcdf96f1835f951cdc98d9a7bdfe02d1f94507
--- /dev/null
+++ b/bert/chinese-roberta-wwm-ext-large/special_tokens_map.json
@@ -0,0 +1 @@
+{"unk_token": "[UNK]", "sep_token": "[SEP]", "pad_token": "[PAD]", "cls_token": "[CLS]", "mask_token": "[MASK]"}
diff --git a/bert/chinese-roberta-wwm-ext-large/tokenizer.json b/bert/chinese-roberta-wwm-ext-large/tokenizer.json
new file mode 100644
index 0000000000000000000000000000000000000000..9e77beb20869ea3b7850166fbc8cc7734662a017
--- /dev/null
+++ b/bert/chinese-roberta-wwm-ext-large/tokenizer.json
@@ -0,0 +1 @@
+{"version":"1.0","truncation":null,"padding":null,"added_tokens":[{"id":0,"special":true,"content":"[PAD]","single_word":false,"lstrip":false,"rstrip":false,"normalized":false},{"id":100,"special":true,"content":"[UNK]","single_word":false,"lstrip":false,"rstrip":false,"normalized":false},{"id":101,"special":true,"content":"[CLS]","single_word":false,"lstrip":false,"rstrip":false,"normalized":false},{"id":102,"special":true,"content":"[SEP]","single_word":false,"lstrip":false,"rstrip":false,"normalized":false},{"id":103,"special":true,"content":"[MASK]","single_word":false,"lstrip":false,"rstrip":false,"normalized":false}],"normalizer":{"type":"BertNormalizer","clean_text":true,"handle_chinese_chars":true,"strip_accents":null,"lowercase":true},"pre_tokenizer":{"type":"BertPreTokenizer"},"post_processor":{"type":"TemplateProcessing","single":[{"SpecialToken":{"id":"[CLS]","type_id":0}},{"Sequence":{"id":"A","type_id":0}},{"SpecialToken":{"id":"[SEP]","type_id":0}}],"pair":[{"SpecialToken":{"id":"[CLS]","type_id":0}},{"Sequence":{"id":"A","type_id":0}},{"SpecialToken":{"id":"[SEP]","type_id":0}},{"Sequence":{"id":"B","type_id":1}},{"SpecialToken":{"id":"[SEP]","type_id":1}}],"special_tokens":{"[CLS]":{"id":"[CLS]","ids":[101],"tokens":["[CLS]"]},"[SEP]":{"id":"[SEP]","ids":[102],"tokens":["[SEP]"]}}},"decoder":{"type":"WordPiece","prefix":"##","cleanup":true},"model":{"type":"WordPiece","unk_token":"[UNK]","continuing_subword_prefix":"##","max_input_chars_per_word":100,"vocab":{"[PAD]":0,"[unused1]":1,"[unused2]":2,"[unused3]":3,"[unused4]":4,"[unused5]":5,"[unused6]":6,"[unused7]":7,"[unused8]":8,"[unused9]":9,"[unused10]":10,"[unused11]":11,"[unused12]":12,"[unused13]":13,"[unused14]":14,"[unused15]":15,"[unused16]":16,"[unused17]":17,"[unused18]":18,"[unused19]":19,"[unused20]":20,"[unused21]":21,"[unused22]":22,"[unused23]":23,"[unused24]":24,"[unused25]":25,"[unused26]":26,"[unused27]":27,"[unused28]":28,"[unused29]":29,"[unused30]":30,"[unused31]":31,"[unused32]":32,"[unused33]":33,"[unused34]":34,"[unused35]":35,"[unused36]":36,"[unused37]":37,"[unused38]":38,"[unused39]":39,"[unused40]":40,"[unused41]":41,"[unused42]":42,"[unused43]":43,"[unused44]":44,"[unused45]":45,"[unused46]":46,"[unused47]":47,"[unused48]":48,"[unused49]":49,"[unused50]":50,"[unused51]":51,"[unused52]":52,"[unused53]":53,"[unused54]":54,"[unused55]":55,"[unused56]":56,"[unused57]":57,"[unused58]":58,"[unused59]":59,"[unused60]":60,"[unused61]":61,"[unused62]":62,"[unused63]":63,"[unused64]":64,"[unused65]":65,"[unused66]":66,"[unused67]":67,"[unused68]":68,"[unused69]":69,"[unused70]":70,"[unused71]":71,"[unused72]":72,"[unused73]":73,"[unused74]":74,"[unused75]":75,"[unused76]":76,"[unused77]":77,"[unused78]":78,"[unused79]":79,"[unused80]":80,"[unused81]":81,"[unused82]":82,"[unused83]":83,"[unused84]":84,"[unused85]":85,"[unused86]":86,"[unused87]":87,"[unused88]":88,"[unused89]":89,"[unused90]":90,"[unused91]":91,"[unused92]":92,"[unused93]":93,"[unused94]":94,"[unused95]":95,"[unused96]":96,"[unused97]":97,"[unused98]":98,"[unused99]":99,"[UNK]":100,"[CLS]":101,"[SEP]":102,"[MASK]":103,"":104,"":105,"!":106,"\"":107,"#":108,"$":109,"%":110,"&":111,"'":112,"(":113,")":114,"*":115,"+":116,",":117,"-":118,".":119,"/":120,"0":121,"1":122,"2":123,"3":124,"4":125,"5":126,"6":127,"7":128,"8":129,"9":130,":":131,";":132,"<":133,"=":134,">":135,"?":136,"@":137,"[":138,"\\":139,"]":140,"^":141,"_":142,"a":143,"b":144,"c":145,"d":146,"e":147,"f":148,"g":149,"h":150,"i":151,"j":152,"k":153,"l":154,"m":155,"n":156,"o":157,"p":158,"q":159,"r":160,"s":161,"t":162,"u":163,"v":164,"w":165,"x":166,"y":167,"z":168,"{":169,"|":170,"}":171,"~":172,"£":173,"¤":174,"¥":175,"§":176,"©":177,"«":178,"®":179,"°":180,"±":181,"²":182,"³":183,"µ":184,"·":185,"¹":186,"º":187,"»":188,"¼":189,"×":190,"ß":191,"æ":192,"÷":193,"ø":194,"đ":195,"ŋ":196,"ɔ":197,"ə":198,"ɡ":199,"ʰ":200,"ˇ":201,"ˈ":202,"ˊ":203,"ˋ":204,"ˍ":205,"ː":206,"˙":207,"˚":208,"ˢ":209,"α":210,"β":211,"γ":212,"δ":213,"ε":214,"η":215,"θ":216,"ι":217,"κ":218,"λ":219,"μ":220,"ν":221,"ο":222,"π":223,"ρ":224,"ς":225,"σ":226,"τ":227,"υ":228,"φ":229,"χ":230,"ψ":231,"ω":232,"а":233,"б":234,"в":235,"г":236,"д":237,"е":238,"ж":239,"з":240,"и":241,"к":242,"л":243,"м":244,"н":245,"о":246,"п":247,"р":248,"с":249,"т":250,"у":251,"ф":252,"х":253,"ц":254,"ч":255,"ш":256,"ы":257,"ь":258,"я":259,"і":260,"ا":261,"ب":262,"ة":263,"ت":264,"د":265,"ر":266,"س":267,"ع":268,"ل":269,"م":270,"ن":271,"ه":272,"و":273,"ي":274,"۩":275,"ก":276,"ง":277,"น":278,"ม":279,"ย":280,"ร":281,"อ":282,"า":283,"เ":284,"๑":285,"་":286,"ღ":287,"ᄀ":288,"ᄁ":289,"ᄂ":290,"ᄃ":291,"ᄅ":292,"ᄆ":293,"ᄇ":294,"ᄈ":295,"ᄉ":296,"ᄋ":297,"ᄌ":298,"ᄎ":299,"ᄏ":300,"ᄐ":301,"ᄑ":302,"ᄒ":303,"ᅡ":304,"ᅢ":305,"ᅣ":306,"ᅥ":307,"ᅦ":308,"ᅧ":309,"ᅨ":310,"ᅩ":311,"ᅪ":312,"ᅬ":313,"ᅭ":314,"ᅮ":315,"ᅯ":316,"ᅲ":317,"ᅳ":318,"ᅴ":319,"ᅵ":320,"ᆨ":321,"ᆫ":322,"ᆯ":323,"ᆷ":324,"ᆸ":325,"ᆺ":326,"ᆻ":327,"ᆼ":328,"ᗜ":329,"ᵃ":330,"ᵉ":331,"ᵍ":332,"ᵏ":333,"ᵐ":334,"ᵒ":335,"ᵘ":336,"‖":337,"„":338,"†":339,"•":340,"‥":341,"‧":342,"
":343,"‰":344,"′":345,"″":346,"‹":347,"›":348,"※":349,"‿":350,"⁄":351,"ⁱ":352,"⁺":353,"ⁿ":354,"₁":355,"₂":356,"₃":357,"₄":358,"€":359,"℃":360,"№":361,"™":362,"ⅰ":363,"ⅱ":364,"ⅲ":365,"ⅳ":366,"ⅴ":367,"←":368,"↑":369,"→":370,"↓":371,"↔":372,"↗":373,"↘":374,"⇒":375,"∀":376,"−":377,"∕":378,"∙":379,"√":380,"∞":381,"∟":382,"∠":383,"∣":384,"∥":385,"∩":386,"∮":387,"∶":388,"∼":389,"∽":390,"≈":391,"≒":392,"≡":393,"≤":394,"≥":395,"≦":396,"≧":397,"≪":398,"≫":399,"⊙":400,"⋅":401,"⋈":402,"⋯":403,"⌒":404,"①":405,"②":406,"③":407,"④":408,"⑤":409,"⑥":410,"⑦":411,"⑧":412,"⑨":413,"⑩":414,"⑴":415,"⑵":416,"⑶":417,"⑷":418,"⑸":419,"⒈":420,"⒉":421,"⒊":422,"⒋":423,"ⓒ":424,"ⓔ":425,"ⓘ":426,"─":427,"━":428,"│":429,"┃":430,"┅":431,"┆":432,"┊":433,"┌":434,"└":435,"├":436,"┣":437,"═":438,"║":439,"╚":440,"╞":441,"╠":442,"╭":443,"╮":444,"╯":445,"╰":446,"╱":447,"╳":448,"▂":449,"▃":450,"▅":451,"▇":452,"█":453,"▉":454,"▋":455,"▌":456,"▍":457,"▎":458,"■":459,"□":460,"▪":461,"▫":462,"▬":463,"▲":464,"△":465,"▶":466,"►":467,"▼":468,"▽":469,"◆":470,"◇":471,"○":472,"◎":473,"●":474,"◕":475,"◠":476,"◢":477,"◤":478,"☀":479,"★":480,"☆":481,"☕":482,"☞":483,"☺":484,"☼":485,"♀":486,"♂":487,"♠":488,"♡":489,"♣":490,"♥":491,"♦":492,"♪":493,"♫":494,"♬":495,"✈":496,"✔":497,"✕":498,"✖":499,"✦":500,"✨":501,"✪":502,"✰":503,"✿":504,"❀":505,"❤":506,"➜":507,"➤":508,"⦿":509,"、":510,"。":511,"〃":512,"々":513,"〇":514,"〈":515,"〉":516,"《":517,"》":518,"「":519,"」":520,"『":521,"』":522,"【":523,"】":524,"〓":525,"〔":526,"〕":527,"〖":528,"〗":529,"〜":530,"〝":531,"〞":532,"ぁ":533,"あ":534,"ぃ":535,"い":536,"う":537,"ぇ":538,"え":539,"お":540,"か":541,"き":542,"く":543,"け":544,"こ":545,"さ":546,"し":547,"す":548,"せ":549,"そ":550,"た":551,"ち":552,"っ":553,"つ":554,"て":555,"と":556,"な":557,"に":558,"ぬ":559,"ね":560,"の":561,"は":562,"ひ":563,"ふ":564,"へ":565,"ほ":566,"ま":567,"み":568,"む":569,"め":570,"も":571,"ゃ":572,"や":573,"ゅ":574,"ゆ":575,"ょ":576,"よ":577,"ら":578,"り":579,"る":580,"れ":581,"ろ":582,"わ":583,"を":584,"ん":585,"゜":586,"ゝ":587,"ァ":588,"ア":589,"ィ":590,"イ":591,"ゥ":592,"ウ":593,"ェ":594,"エ":595,"ォ":596,"オ":597,"カ":598,"キ":599,"ク":600,"ケ":601,"コ":602,"サ":603,"シ":604,"ス":605,"セ":606,"ソ":607,"タ":608,"チ":609,"ッ":610,"ツ":611,"テ":612,"ト":613,"ナ":614,"ニ":615,"ヌ":616,"ネ":617,"ノ":618,"ハ":619,"ヒ":620,"フ":621,"ヘ":622,"ホ":623,"マ":624,"ミ":625,"ム":626,"メ":627,"モ":628,"ャ":629,"ヤ":630,"ュ":631,"ユ":632,"ョ":633,"ヨ":634,"ラ":635,"リ":636,"ル":637,"レ":638,"ロ":639,"ワ":640,"ヲ":641,"ン":642,"ヶ":643,"・":644,"ー":645,"ヽ":646,"ㄅ":647,"ㄆ":648,"ㄇ":649,"ㄉ":650,"ㄋ":651,"ㄌ":652,"ㄍ":653,"ㄎ":654,"ㄏ":655,"ㄒ":656,"ㄚ":657,"ㄛ":658,"ㄞ":659,"ㄟ":660,"ㄢ":661,"ㄤ":662,"ㄥ":663,"ㄧ":664,"ㄨ":665,"ㆍ":666,"㈦":667,"㊣":668,"㎡":669,"㗎":670,"一":671,"丁":672,"七":673,"万":674,"丈":675,"三":676,"上":677,"下":678,"不":679,"与":680,"丐":681,"丑":682,"专":683,"且":684,"丕":685,"世":686,"丘":687,"丙":688,"业":689,"丛":690,"东":691,"丝":692,"丞":693,"丟":694,"両":695,"丢":696,"两":697,"严":698,"並":699,"丧":700,"丨":701,"个":702,"丫":703,"中":704,"丰":705,"串":706,"临":707,"丶":708,"丸":709,"丹":710,"为":711,"主":712,"丼":713,"丽":714,"举":715,"丿":716,"乂":717,"乃":718,"久":719,"么":720,"义":721,"之":722,"乌":723,"乍":724,"乎":725,"乏":726,"乐":727,"乒":728,"乓":729,"乔":730,"乖":731,"乗":732,"乘":733,"乙":734,"乜":735,"九":736,"乞":737,"也":738,"习":739,"乡":740,"书":741,"乩":742,"买":743,"乱":744,"乳":745,"乾":746,"亀":747,"亂":748,"了":749,"予":750,"争":751,"事":752,"二":753,"于":754,"亏":755,"云":756,"互":757,"五":758,"井":759,"亘":760,"亙":761,"亚":762,"些":763,"亜":764,"亞":765,"亟":766,"亡":767,"亢":768,"交":769,"亥":770,"亦":771,"产":772,"亨":773,"亩":774,"享":775,"京":776,"亭":777,"亮":778,"亲":779,"亳":780,"亵":781,"人":782,"亿":783,"什":784,"仁":785,"仃":786,"仄":787,"仅":788,"仆":789,"仇":790,"今":791,"介":792,"仍":793,"从":794,"仏":795,"仑":796,"仓":797,"仔":798,"仕":799,"他":800,"仗":801,"付":802,"仙":803,"仝":804,"仞":805,"仟":806,"代":807,"令":808,"以":809,"仨":810,"仪":811,"们":812,"仮":813,"仰":814,"仲":815,"件":816,"价":817,"任":818,"份":819,"仿":820,"企":821,"伉":822,"伊":823,"伍":824,"伎":825,"伏":826,"伐":827,"休":828,"伕":829,"众":830,"优":831,"伙":832,"会":833,"伝":834,"伞":835,"伟":836,"传":837,"伢":838,"伤":839,"伦":840,"伪":841,"伫":842,"伯":843,"估":844,"伴":845,"伶":846,"伸":847,"伺":848,"似":849,"伽":850,"佃":851,"但":852,"佇":853,"佈":854,"位":855,"低":856,"住":857,"佐":858,"佑":859,"体":860,"佔":861,"何":862,"佗":863,"佘":864,"余":865,"佚":866,"佛":867,"作":868,"佝":869,"佞":870,"佟":871,"你":872,"佢":873,"佣":874,"佤":875,"佥":876,"佩":877,"佬":878,"佯":879,"佰":880,"佳":881,"併":882,"佶":883,"佻":884,"佼":885,"使":886,"侃":887,"侄":888,"來":889,"侈":890,"例":891,"侍":892,"侏":893,"侑":894,"侖":895,"侗":896,"供":897,"依":898,"侠":899,"価":900,"侣":901,"侥":902,"侦":903,"侧":904,"侨":905,"侬":906,"侮":907,"侯":908,"侵":909,"侶":910,"侷":911,"便":912,"係":913,"促":914,"俄":915,"俊":916,"俎":917,"俏":918,"俐":919,"俑":920,"俗":921,"俘":922,"俚":923,"保":924,"俞":925,"俟":926,"俠":927,"信":928,"俨":929,"俩":930,"俪":931,"俬":932,"俭":933,"修":934,"俯":935,"俱":936,"俳":937,"俸":938,"俺":939,"俾":940,"倆":941,"倉":942,"個":943,"倌":944,"倍":945,"倏":946,"們":947,"倒":948,"倔":949,"倖":950,"倘":951,"候":952,"倚":953,"倜":954,"借":955,"倡":956,"値":957,"倦":958,"倩":959,"倪":960,"倫":961,"倬":962,"倭":963,"倶":964,"债":965,"值":966,"倾":967,"偃":968,"假":969,"偈":970,"偉":971,"偌":972,"偎":973,"偏":974,"偕":975,"做":976,"停":977,"健":978,"側":979,"偵":980,"偶":981,"偷":982,"偻":983,"偽":984,"偿":985,"傀":986,"傅":987,"傍":988,"傑":989,"傘":990,"備":991,"傚":992,"傢":993,"傣":994,"傥":995,"储":996,"傩":997,"催":998,"傭":999,"傲":1000,"傳":1001,"債":1002,"傷":1003,"傻":1004,"傾":1005,"僅":1006,"働":1007,"像":1008,"僑":1009,"僕":1010,"僖":1011,"僚":1012,"僥":1013,"僧":1014,"僭":1015,"僮":1016,"僱":1017,"僵":1018,"價":1019,"僻":1020,"儀":1021,"儂":1022,"億":1023,"儆":1024,"儉":1025,"儋":1026,"儒":1027,"儕":1028,"儘":1029,"償":1030,"儡":1031,"優":1032,"儲":1033,"儷":1034,"儼":1035,"儿":1036,"兀":1037,"允":1038,"元":1039,"兄":1040,"充":1041,"兆":1042,"兇":1043,"先":1044,"光":1045,"克":1046,"兌":1047,"免":1048,"児":1049,"兑":1050,"兒":1051,"兔":1052,"兖":1053,"党":1054,"兜":1055,"兢":1056,"入":1057,"內":1058,"全":1059,"兩":1060,"八":1061,"公":1062,"六":1063,"兮":1064,"兰":1065,"共":1066,"兲":1067,"关":1068,"兴":1069,"兵":1070,"其":1071,"具":1072,"典":1073,"兹":1074,"养":1075,"兼":1076,"兽":1077,"冀":1078,"内":1079,"円":1080,"冇":1081,"冈":1082,"冉":1083,"冊":1084,"册":1085,"再":1086,"冏":1087,"冒":1088,"冕":1089,"冗":1090,"写":1091,"军":1092,"农":1093,"冠":1094,"冢":1095,"冤":1096,"冥":1097,"冨":1098,"冪":1099,"冬":1100,"冯":1101,"冰":1102,"冲":1103,"决":1104,"况":1105,"冶":1106,"冷":1107,"冻":1108,"冼":1109,"冽":1110,"冾":1111,"净":1112,"凄":1113,"准":1114,"凇":1115,"凈":1116,"凉":1117,"凋":1118,"凌":1119,"凍":1120,"减":1121,"凑":1122,"凛":1123,"凜":1124,"凝":1125,"几":1126,"凡":1127,"凤":1128,"処":1129,"凪":1130,"凭":1131,"凯":1132,"凰":1133,"凱":1134,"凳":1135,"凶":1136,"凸":1137,"凹":1138,"出":1139,"击":1140,"函":1141,"凿":1142,"刀":1143,"刁":1144,"刃":1145,"分":1146,"切":1147,"刈":1148,"刊":1149,"刍":1150,"刎":1151,"刑":1152,"划":1153,"列":1154,"刘":1155,"则":1156,"刚":1157,"创":1158,"初":1159,"删":1160,"判":1161,"別":1162,"刨":1163,"利":1164,"刪":1165,"别":1166,"刮":1167,"到":1168,"制":1169,"刷":1170,"券":1171,"刹":1172,"刺":1173,"刻":1174,"刽":1175,"剁":1176,"剂":1177,"剃":1178,"則":1179,"剉":1180,"削":1181,"剋":1182,"剌":1183,"前":1184,"剎":1185,"剐":1186,"剑":1187,"剔":1188,"剖":1189,"剛":1190,"剜":1191,"剝":1192,"剣":1193,"剤":1194,"剥":1195,"剧":1196,"剩":1197,"剪":1198,"副":1199,"割":1200,"創":1201,"剷":1202,"剽":1203,"剿":1204,"劃":1205,"劇":1206,"劈":1207,"劉":1208,"劊":1209,"劍":1210,"劏":1211,"劑":1212,"力":1213,"劝":1214,"办":1215,"功":1216,"加":1217,"务":1218,"劣":1219,"动":1220,"助":1221,"努":1222,"劫":1223,"劭":1224,"励":1225,"劲":1226,"劳":1227,"労":1228,"劵":1229,"効":1230,"劾":1231,"势":1232,"勁":1233,"勃":1234,"勇":1235,"勉":1236,"勋":1237,"勐":1238,"勒":1239,"動":1240,"勖":1241,"勘":1242,"務":1243,"勛":1244,"勝":1245,"勞":1246,"募":1247,"勢":1248,"勤":1249,"勧":1250,"勳":1251,"勵":1252,"勸":1253,"勺":1254,"勻":1255,"勾":1256,"勿":1257,"匀":1258,"包":1259,"匆":1260,"匈":1261,"匍":1262,"匐":1263,"匕":1264,"化":1265,"北":1266,"匙":1267,"匝":1268,"匠":1269,"匡":1270,"匣":1271,"匪":1272,"匮":1273,"匯":1274,"匱":1275,"匹":1276,"区":1277,"医":1278,"匾":1279,"匿":1280,"區":1281,"十":1282,"千":1283,"卅":1284,"升":1285,"午":1286,"卉":1287,"半":1288,"卍":1289,"华":1290,"协":1291,"卑":1292,"卒":1293,"卓":1294,"協":1295,"单":1296,"卖":1297,"南":1298,"単":1299,"博":1300,"卜":1301,"卞":1302,"卟":1303,"占":1304,"卡":1305,"卢":1306,"卤":1307,"卦":1308,"卧":1309,"卫":1310,"卮":1311,"卯":1312,"印":1313,"危":1314,"即":1315,"却":1316,"卵":1317,"卷":1318,"卸":1319,"卻":1320,"卿":1321,"厂":1322,"厄":1323,"厅":1324,"历":1325,"厉":1326,"压":1327,"厌":1328,"厕":1329,"厘":1330,"厚":1331,"厝":1332,"原":1333,"厢":1334,"厥":1335,"厦":1336,"厨":1337,"厩":1338,"厭":1339,"厮":1340,"厲":1341,"厳":1342,"去":1343,"县":1344,"叁":1345,"参":1346,"參":1347,"又":1348,"叉":1349,"及":1350,"友":1351,"双":1352,"反":1353,"収":1354,"发":1355,"叔":1356,"取":1357,"受":1358,"变":1359,"叙":1360,"叛":1361,"叟":1362,"叠":1363,"叡":1364,"叢":1365,"口":1366,"古":1367,"句":1368,"另":1369,"叨":1370,"叩":1371,"只":1372,"叫":1373,"召":1374,"叭":1375,"叮":1376,"可":1377,"台":1378,"叱":1379,"史":1380,"右":1381,"叵":1382,"叶":1383,"号":1384,"司":1385,"叹":1386,"叻":1387,"叼":1388,"叽":1389,"吁":1390,"吃":1391,"各":1392,"吆":1393,"合":1394,"吉":1395,"吊":1396,"吋":1397,"同":1398,"名":1399,"后":1400,"吏":1401,"吐":1402,"向":1403,"吒":1404,"吓":1405,"吕":1406,"吖":1407,"吗":1408,"君":1409,"吝":1410,"吞":1411,"吟":1412,"吠":1413,"吡":1414,"否":1415,"吧":1416,"吨":1417,"吩":1418,"含":1419,"听":1420,"吭":1421,"吮":1422,"启":1423,"吱":1424,"吳":1425,"吴":1426,"吵":1427,"吶":1428,"吸":1429,"吹":1430,"吻":1431,"吼":1432,"吽":1433,"吾":1434,"呀":1435,"呂":1436,"呃":1437,"呆":1438,"呈":1439,"告":1440,"呋":1441,"呎":1442,"呐":1443,"呓":1444,"呕":1445,"呗":1446,"员":1447,"呛":1448,"呜":1449,"呢":1450,"呤":1451,"呦":1452,"周":1453,"呱":1454,"呲":1455,"味":1456,"呵":1457,"呷":1458,"呸":1459,"呻":1460,"呼":1461,"命":1462,"咀":1463,"咁":1464,"咂":1465,"咄":1466,"咆":1467,"咋":1468,"和":1469,"咎":1470,"咏":1471,"咐":1472,"咒":1473,"咔":1474,"咕":1475,"咖":1476,"咗":1477,"咘":1478,"咙":1479,"咚":1480,"咛":1481,"咣":1482,"咤":1483,"咦":1484,"咧":1485,"咨":1486,"咩":1487,"咪":1488,"咫":1489,"咬":1490,"咭":1491,"咯":1492,"咱":1493,"咲":1494,"咳":1495,"咸":1496,"咻":1497,"咽":1498,"咿":1499,"哀":1500,"品":1501,"哂":1502,"哄":1503,"哆":1504,"哇":1505,"哈":1506,"哉":1507,"哋":1508,"哌":1509,"响":1510,"哎":1511,"哏":1512,"哐":1513,"哑":1514,"哒":1515,"哔":1516,"哗":1517,"哟":1518,"員":1519,"哥":1520,"哦":1521,"哧":1522,"哨":1523,"哩":1524,"哪":1525,"哭":1526,"哮":1527,"哲":1528,"哺":1529,"哼":1530,"哽":1531,"唁":1532,"唄":1533,"唆":1534,"唇":1535,"唉":1536,"唏":1537,"唐":1538,"唑":1539,"唔":1540,"唠":1541,"唤":1542,"唧":1543,"唬":1544,"售":1545,"唯":1546,"唰":1547,"唱":1548,"唳":1549,"唷":1550,"唸":1551,"唾":1552,"啃":1553,"啄":1554,"商":1555,"啉":1556,"啊":1557,"問":1558,"啓":1559,"啕":1560,"啖":1561,"啜":1562,"啞":1563,"啟":1564,"啡":1565,"啤":1566,"啥":1567,"啦":1568,"啧":1569,"啪":1570,"啫":1571,"啬":1572,"啮":1573,"啰":1574,"啱":1575,"啲":1576,"啵":1577,"啶":1578,"啷":1579,"啸":1580,"啻":1581,"啼":1582,"啾":1583,"喀":1584,"喂":1585,"喃":1586,"善":1587,"喆":1588,"喇":1589,"喉":1590,"喊":1591,"喋":1592,"喎":1593,"喏":1594,"喔":1595,"喘":1596,"喙":1597,"喚":1598,"喜":1599,"喝":1600,"喟":1601,"喧":1602,"喪":1603,"喫":1604,"喬":1605,"單":1606,"喰":1607,"喱":1608,"喲":1609,"喳":1610,"喵":1611,"営":1612,"喷":1613,"喹":1614,"喺":1615,"喻":1616,"喽":1617,"嗅":1618,"嗆":1619,"嗇":1620,"嗎":1621,"嗑":1622,"嗒":1623,"嗓":1624,"嗔":1625,"嗖":1626,"嗚":1627,"嗜":1628,"嗝":1629,"嗟":1630,"嗡":1631,"嗣":1632,"嗤":1633,"嗦":1634,"嗨":1635,"嗪":1636,"嗬":1637,"嗯":1638,"嗰":1639,"嗲":1640,"嗳":1641,"嗶":1642,"嗷":1643,"嗽":1644,"嘀":1645,"嘅":1646,"嘆":1647,"嘈":1648,"嘉":1649,"嘌":1650,"嘍":1651,"嘎":1652,"嘔":1653,"嘖":1654,"嘗":1655,"嘘":1656,"嘚":1657,"嘛":1658,"嘜":1659,"嘞":1660,"嘟":1661,"嘢":1662,"嘣":1663,"嘤":1664,"嘧":1665,"嘩":1666,"嘭":1667,"嘮":1668,"嘯":1669,"嘰":1670,"嘱":1671,"嘲":1672,"嘴":1673,"嘶":1674,"嘸":1675,"嘹":1676,"嘻":1677,"嘿":1678,"噁":1679,"噌":1680,"噎":1681,"噓":1682,"噔":1683,"噗":1684,"噙":1685,"噜":1686,"噠":1687,"噢":1688,"噤":1689,"器":1690,"噩":1691,"噪":1692,"噬":1693,"噱":1694,"噴":1695,"噶":1696,"噸":1697,"噹":1698,"噻":1699,"噼":1700,"嚀":1701,"嚇":1702,"嚎":1703,"嚏":1704,"嚐":1705,"嚓":1706,"嚕":1707,"嚟":1708,"嚣":1709,"嚥":1710,"嚨":1711,"嚮":1712,"嚴":1713,"嚷":1714,"嚼":1715,"囂":1716,"囉":1717,"囊":1718,"囍":1719,"囑":1720,"囔":1721,"囗":1722,"囚":1723,"四":1724,"囝":1725,"回":1726,"囟":1727,"因":1728,"囡":1729,"团":1730,"団":1731,"囤":1732,"囧":1733,"囪":1734,"囫":1735,"园":1736,"困":1737,"囱":1738,"囲":1739,"図":1740,"围":1741,"囹":1742,"固":1743,"国":1744,"图":1745,"囿":1746,"圃":1747,"圄":1748,"圆":1749,"圈":1750,"國":1751,"圍":1752,"圏":1753,"園":1754,"圓":1755,"圖":1756,"團":1757,"圜":1758,"土":1759,"圣":1760,"圧":1761,"在":1762,"圩":1763,"圭":1764,"地":1765,"圳":1766,"场":1767,"圻":1768,"圾":1769,"址":1770,"坂":1771,"均":1772,"坊":1773,"坍":1774,"坎":1775,"坏":1776,"坐":1777,"坑":1778,"块":1779,"坚":1780,"坛":1781,"坝":1782,"坞":1783,"坟":1784,"坠":1785,"坡":1786,"坤":1787,"坦":1788,"坨":1789,"坪":1790,"坯":1791,"坳":1792,"坵":1793,"坷":1794,"垂":1795,"垃":1796,"垄":1797,"型":1798,"垒":1799,"垚":1800,"垛":1801,"垠":1802,"垢":1803,"垣":1804,"垦":1805,"垩":1806,"垫":1807,"垭":1808,"垮":1809,"垵":1810,"埂":1811,"埃":1812,"埋":1813,"城":1814,"埔":1815,"埕":1816,"埗":1817,"域":1818,"埠":1819,"埤":1820,"埵":1821,"執":1822,"埸":1823,"培":1824,"基":1825,"埼":1826,"堀":1827,"堂":1828,"堃":1829,"堅":1830,"堆":1831,"堇":1832,"堑":1833,"堕":1834,"堙":1835,"堡":1836,"堤":1837,"堪":1838,"堯":1839,"堰":1840,"報":1841,"場":1842,"堵":1843,"堺":1844,"堿":1845,"塊":1846,"塌":1847,"塑":1848,"塔":1849,"塗":1850,"塘":1851,"塚":1852,"塞":1853,"塢":1854,"塩":1855,"填":1856,"塬":1857,"塭":1858,"塵":1859,"塾":1860,"墀":1861,"境":1862,"墅":1863,"墉":1864,"墊":1865,"墒":1866,"墓":1867,"増":1868,"墘":1869,"墙":1870,"墜":1871,"增":1872,"墟":1873,"墨":1874,"墩":1875,"墮":1876,"墳":1877,"墻":1878,"墾":1879,"壁":1880,"壅":1881,"壆":1882,"壇":1883,"壊":1884,"壑":1885,"壓":1886,"壕":1887,"壘":1888,"壞":1889,"壟":1890,"壢":1891,"壤":1892,"壩":1893,"士":1894,"壬":1895,"壮":1896,"壯":1897,"声":1898,"売":1899,"壳":1900,"壶":1901,"壹":1902,"壺":1903,"壽":1904,"处":1905,"备":1906,"変":1907,"复":1908,"夏":1909,"夔":1910,"夕":1911,"外":1912,"夙":1913,"多":1914,"夜":1915,"够":1916,"夠":1917,"夢":1918,"夥":1919,"大":1920,"天":1921,"太":1922,"夫":1923,"夭":1924,"央":1925,"夯":1926,"失":1927,"头":1928,"夷":1929,"夸":1930,"夹":1931,"夺":1932,"夾":1933,"奂":1934,"奄":1935,"奇":1936,"奈":1937,"奉":1938,"奋":1939,"奎":1940,"奏":1941,"奐":1942,"契":1943,"奔":1944,"奕":1945,"奖":1946,"套":1947,"奘":1948,"奚":1949,"奠":1950,"奢":1951,"奥":1952,"奧":1953,"奪":1954,"奬":1955,"奮":1956,"女":1957,"奴":1958,"奶":1959,"奸":1960,"她":1961,"好":1962,"如":1963,"妃":1964,"妄":1965,"妆":1966,"妇":1967,"妈":1968,"妊":1969,"妍":1970,"妒":1971,"妓":1972,"妖":1973,"妘":1974,"妙":1975,"妝":1976,"妞":1977,"妣":1978,"妤":1979,"妥":1980,"妨":1981,"妩":1982,"妪":1983,"妮":1984,"妲":1985,"妳":1986,"妹":1987,"妻":1988,"妾":1989,"姆":1990,"姉":1991,"姊":1992,"始":1993,"姍":1994,"姐":1995,"姑":1996,"姒":1997,"姓":1998,"委":1999,"姗":2000,"姚":2001,"姜":2002,"姝":2003,"姣":2004,"姥":2005,"姦":2006,"姨":2007,"姪":2008,"姫":2009,"姬":2010,"姹":2011,"姻":2012,"姿":2013,"威":2014,"娃":2015,"娄":2016,"娅":2017,"娆":2018,"娇":2019,"娉":2020,"娑":2021,"娓":2022,"娘":2023,"娛":2024,"娜":2025,"娟":2026,"娠":2027,"娣":2028,"娥":2029,"娩":2030,"娱":2031,"娲":2032,"娴":2033,"娶":2034,"娼":2035,"婀":2036,"婁":2037,"婆":2038,"婉":2039,"婊":2040,"婕":2041,"婚":2042,"婢":2043,"婦":2044,"婧":2045,"婪":2046,"婭":2047,"婴":2048,"婵":2049,"婶":2050,"婷":2051,"婺":2052,"婿":2053,"媒":2054,"媚":2055,"媛":2056,"媞":2057,"媧":2058,"媲":2059,"媳":2060,"媽":2061,"媾":2062,"嫁":2063,"嫂":2064,"嫉":2065,"嫌":2066,"嫑":2067,"嫔":2068,"嫖":2069,"嫘":2070,"嫚":2071,"嫡":2072,"嫣":2073,"嫦":2074,"嫩":2075,"嫲":2076,"嫵":2077,"嫻":2078,"嬅":2079,"嬉":2080,"嬌":2081,"嬗":2082,"嬛":2083,"嬢":2084,"嬤":2085,"嬪":2086,"嬰":2087,"嬴":2088,"嬷":2089,"嬸":2090,"嬿":2091,"孀":2092,"孃":2093,"子":2094,"孑":2095,"孔":2096,"孕":2097,"孖":2098,"字":2099,"存":2100,"孙":2101,"孚":2102,"孛":2103,"孜":2104,"孝":2105,"孟":2106,"孢":2107,"季":2108,"孤":2109,"学":2110,"孩":2111,"孪":2112,"孫":2113,"孬":2114,"孰":2115,"孱":2116,"孳":2117,"孵":2118,"學":2119,"孺":2120,"孽":2121,"孿":2122,"宁":2123,"它":2124,"宅":2125,"宇":2126,"守":2127,"安":2128,"宋":2129,"完":2130,"宏":2131,"宓":2132,"宕":2133,"宗":2134,"官":2135,"宙":2136,"定":2137,"宛":2138,"宜":2139,"宝":2140,"实":2141,"実":2142,"宠":2143,"审":2144,"客":2145,"宣":2146,"室":2147,"宥":2148,"宦":2149,"宪":2150,"宫":2151,"宮":2152,"宰":2153,"害":2154,"宴":2155,"宵":2156,"家":2157,"宸":2158,"容":2159,"宽":2160,"宾":2161,"宿":2162,"寂":2163,"寄":2164,"寅":2165,"密":2166,"寇":2167,"富":2168,"寐":2169,"寒":2170,"寓":2171,"寛":2172,"寝":2173,"寞":2174,"察":2175,"寡":2176,"寢":2177,"寥":2178,"實":2179,"寧":2180,"寨":2181,"審":2182,"寫":2183,"寬":2184,"寮":2185,"寰":2186,"寵":2187,"寶":2188,"寸":2189,"对":2190,"寺":2191,"寻":2192,"导":2193,"対":2194,"寿":2195,"封":2196,"専":2197,"射":2198,"将":2199,"將":2200,"專":2201,"尉":2202,"尊":2203,"尋":2204,"對":2205,"導":2206,"小":2207,"少":2208,"尔":2209,"尕":2210,"尖":2211,"尘":2212,"尚":2213,"尝":2214,"尤":2215,"尧":2216,"尬":2217,"就":2218,"尴":2219,"尷":2220,"尸":2221,"尹":2222,"尺":2223,"尻":2224,"尼":2225,"尽":2226,"尾":2227,"尿":2228,"局":2229,"屁":2230,"层":2231,"屄":2232,"居":2233,"屆":2234,"屈":2235,"屉":2236,"届":2237,"屋":2238,"屌":2239,"屍":2240,"屎":2241,"屏":2242,"屐":2243,"屑":2244,"展":2245,"屜":2246,"属":2247,"屠":2248,"屡":2249,"屢":2250,"層":2251,"履":2252,"屬":2253,"屯":2254,"山":2255,"屹":2256,"屿":2257,"岀":2258,"岁":2259,"岂":2260,"岌":2261,"岐":2262,"岑":2263,"岔":2264,"岖":2265,"岗":2266,"岘":2267,"岙":2268,"岚":2269,"岛":2270,"岡":2271,"岩":2272,"岫":2273,"岬":2274,"岭":2275,"岱":2276,"岳":2277,"岷":2278,"岸":2279,"峇":2280,"峋":2281,"峒":2282,"峙":2283,"峡":2284,"峤":2285,"峥":2286,"峦":2287,"峨":2288,"峪":2289,"峭":2290,"峯":2291,"峰":2292,"峴":2293,"島":2294,"峻":2295,"峽":2296,"崁":2297,"崂":2298,"崆":2299,"崇":2300,"崎":2301,"崑":2302,"崔":2303,"崖":2304,"崗":2305,"崙":2306,"崛":2307,"崧":2308,"崩":2309,"崭":2310,"崴":2311,"崽":2312,"嵇":2313,"嵊":2314,"嵋":2315,"嵌":2316,"嵐":2317,"嵘":2318,"嵩":2319,"嵬":2320,"嵯":2321,"嶂":2322,"嶄":2323,"嶇":2324,"嶋":2325,"嶙":2326,"嶺":2327,"嶼":2328,"嶽":2329,"巅":2330,"巍":2331,"巒":2332,"巔":2333,"巖":2334,"川":2335,"州":2336,"巡":2337,"巢":2338,"工":2339,"左":2340,"巧":2341,"巨":2342,"巩":2343,"巫":2344,"差":2345,"己":2346,"已":2347,"巳":2348,"巴":2349,"巷":2350,"巻":2351,"巽":2352,"巾":2353,"巿":2354,"币":2355,"市":2356,"布":2357,"帅":2358,"帆":2359,"师":2360,"希":2361,"帐":2362,"帑":2363,"帕":2364,"帖":2365,"帘":2366,"帚":2367,"帛":2368,"帜":2369,"帝":2370,"帥":2371,"带":2372,"帧":2373,"師":2374,"席":2375,"帮":2376,"帯":2377,"帰":2378,"帳":2379,"帶":2380,"帷":2381,"常":2382,"帼":2383,"帽":2384,"幀":2385,"幂":2386,"幄":2387,"幅":2388,"幌":2389,"幔":2390,"幕":2391,"幟":2392,"幡":2393,"幢":2394,"幣":2395,"幫":2396,"干":2397,"平":2398,"年":2399,"并":2400,"幸":2401,"幹":2402,"幺":2403,"幻":2404,"幼":2405,"幽":2406,"幾":2407,"广":2408,"庁":2409,"広":2410,"庄":2411,"庆":2412,"庇":2413,"床":2414,"序":2415,"庐":2416,"库":2417,"应":2418,"底":2419,"庖":2420,"店":2421,"庙":2422,"庚":2423,"府":2424,"庞":2425,"废":2426,"庠":2427,"度":2428,"座":2429,"庫":2430,"庭":2431,"庵":2432,"庶":2433,"康":2434,"庸":2435,"庹":2436,"庾":2437,"廁":2438,"廂":2439,"廃":2440,"廈":2441,"廉":2442,"廊":2443,"廓":2444,"廖":2445,"廚":2446,"廝":2447,"廟":2448,"廠":2449,"廢":2450,"廣":2451,"廬":2452,"廳":2453,"延":2454,"廷":2455,"建":2456,"廿":2457,"开":2458,"弁":2459,"异":2460,"弃":2461,"弄":2462,"弈":2463,"弊":2464,"弋":2465,"式":2466,"弑":2467,"弒":2468,"弓":2469,"弔":2470,"引":2471,"弗":2472,"弘":2473,"弛":2474,"弟":2475,"张":2476,"弥":2477,"弦":2478,"弧":2479,"弩":2480,"弭":2481,"弯":2482,"弱":2483,"張":2484,"強":2485,"弹":2486,"强":2487,"弼":2488,"弾":2489,"彅":2490,"彆":2491,"彈":2492,"彌":2493,"彎":2494,"归":2495,"当":2496,"录":2497,"彗":2498,"彙":2499,"彝":2500,"形":2501,"彤":2502,"彥":2503,"彦":2504,"彧":2505,"彩":2506,"彪":2507,"彫":2508,"彬":2509,"彭":2510,"彰":2511,"影":2512,"彷":2513,"役":2514,"彻":2515,"彼":2516,"彿":2517,"往":2518,"征":2519,"径":2520,"待":2521,"徇":2522,"很":2523,"徉":2524,"徊":2525,"律":2526,"後":2527,"徐":2528,"徑":2529,"徒":2530,"従":2531,"徕":2532,"得":2533,"徘":2534,"徙":2535,"徜":2536,"從":2537,"徠":2538,"御":2539,"徨":2540,"復":2541,"循":2542,"徬":2543,"微":2544,"徳":2545,"徴":2546,"徵":2547,"德":2548,"徹":2549,"徼":2550,"徽":2551,"心":2552,"必":2553,"忆":2554,"忌":2555,"忍":2556,"忏":2557,"忐":2558,"忑":2559,"忒":2560,"忖":2561,"志":2562,"忘":2563,"忙":2564,"応":2565,"忠":2566,"忡":2567,"忤":2568,"忧":2569,"忪":2570,"快":2571,"忱":2572,"念":2573,"忻":2574,"忽":2575,"忿":2576,"怀":2577,"态":2578,"怂":2579,"怅":2580,"怆":2581,"怎":2582,"怏":2583,"怒":2584,"怔":2585,"怕":2586,"怖":2587,"怙":2588,"怜":2589,"思":2590,"怠":2591,"怡":2592,"急":2593,"怦":2594,"性":2595,"怨":2596,"怪":2597,"怯":2598,"怵":2599,"总":2600,"怼":2601,"恁":2602,"恃":2603,"恆":2604,"恋":2605,"恍":2606,"恐":2607,"恒":2608,"恕":2609,"恙":2610,"恚":2611,"恢":2612,"恣":2613,"恤":2614,"恥":2615,"恨":2616,"恩":2617,"恪":2618,"恫":2619,"恬":2620,"恭":2621,"息":2622,"恰":2623,"恳":2624,"恵":2625,"恶":2626,"恸":2627,"恺":2628,"恻":2629,"恼":2630,"恿":2631,"悄":2632,"悅":2633,"悉":2634,"悌":2635,"悍":2636,"悔":2637,"悖":2638,"悚":2639,"悟":2640,"悠":2641,"患":2642,"悦":2643,"您":2644,"悩":2645,"悪":2646,"悬":2647,"悯":2648,"悱":2649,"悲":2650,"悴":2651,"悵":2652,"悶":2653,"悸":2654,"悻":2655,"悼":2656,"悽":2657,"情":2658,"惆":2659,"惇":2660,"惊":2661,"惋":2662,"惑":2663,"惕":2664,"惘":2665,"惚":2666,"惜":2667,"惟":2668,"惠":2669,"惡":2670,"惦":2671,"惧":2672,"惨":2673,"惩":2674,"惫":2675,"惬":2676,"惭":2677,"惮":2678,"惯":2679,"惰":2680,"惱":2681,"想":2682,"惴":2683,"惶":2684,"惹":2685,"惺":2686,"愁":2687,"愆":2688,"愈":2689,"愉":2690,"愍":2691,"意":2692,"愕":2693,"愚":2694,"愛":2695,"愜":2696,"感":2697,"愣":2698,"愤":2699,"愧":2700,"愫":2701,"愷":2702,"愿":2703,"慄":2704,"慈":2705,"態":2706,"慌":2707,"慎":2708,"慑":2709,"慕":2710,"慘":2711,"慚":2712,"慟":2713,"慢":2714,"慣":2715,"慧":2716,"慨":2717,"慫":2718,"慮":2719,"慰":2720,"慳":2721,"慵":2722,"慶":2723,"慷":2724,"慾":2725,"憂":2726,"憊":2727,"憋":2728,"憎":2729,"憐":2730,"憑":2731,"憔":2732,"憚":2733,"憤":2734,"憧":2735,"憨":2736,"憩":2737,"憫":2738,"憬":2739,"憲":2740,"憶":2741,"憾":2742,"懂":2743,"懇":2744,"懈":2745,"應":2746,"懊":2747,"懋":2748,"懑":2749,"懒":2750,"懦":2751,"懲":2752,"懵":2753,"懶":2754,"懷":2755,"懸":2756,"懺":2757,"懼":2758,"懾":2759,"懿":2760,"戀":2761,"戈":2762,"戊":2763,"戌":2764,"戍":2765,"戎":2766,"戏":2767,"成":2768,"我":2769,"戒":2770,"戕":2771,"或":2772,"战":2773,"戚":2774,"戛":2775,"戟":2776,"戡":2777,"戦":2778,"截":2779,"戬":2780,"戮":2781,"戰":2782,"戲":2783,"戳":2784,"戴":2785,"戶":2786,"户":2787,"戸":2788,"戻":2789,"戾":2790,"房":2791,"所":2792,"扁":2793,"扇":2794,"扈":2795,"扉":2796,"手":2797,"才":2798,"扎":2799,"扑":2800,"扒":2801,"打":2802,"扔":2803,"払":2804,"托":2805,"扛":2806,"扣":2807,"扦":2808,"执":2809,"扩":2810,"扪":2811,"扫":2812,"扬":2813,"扭":2814,"扮":2815,"扯":2816,"扰":2817,"扱":2818,"扳":2819,"扶":2820,"批":2821,"扼":2822,"找":2823,"承":2824,"技":2825,"抄":2826,"抉":2827,"把":2828,"抑":2829,"抒":2830,"抓":2831,"投":2832,"抖":2833,"抗":2834,"折":2835,"抚":2836,"抛":2837,"抜":2838,"択":2839,"抟":2840,"抠":2841,"抡":2842,"抢":2843,"护":2844,"报":2845,"抨":2846,"披":2847,"抬":2848,"抱":2849,"抵":2850,"抹":2851,"押":2852,"抽":2853,"抿":2854,"拂":2855,"拄":2856,"担":2857,"拆":2858,"拇":2859,"拈":2860,"拉":2861,"拋":2862,"拌":2863,"拍":2864,"拎":2865,"拐":2866,"拒":2867,"拓":2868,"拔":2869,"拖":2870,"拗":2871,"拘":2872,"拙":2873,"拚":2874,"招":2875,"拜":2876,"拟":2877,"拡":2878,"拢":2879,"拣":2880,"拥":2881,"拦":2882,"拧":2883,"拨":2884,"择":2885,"括":2886,"拭":2887,"拮":2888,"拯":2889,"拱":2890,"拳":2891,"拴":2892,"拷":2893,"拼":2894,"拽":2895,"拾":2896,"拿":2897,"持":2898,"挂":2899,"指":2900,"挈":2901,"按":2902,"挎":2903,"挑":2904,"挖":2905,"挙":2906,"挚":2907,"挛":2908,"挝":2909,"挞":2910,"挟":2911,"挠":2912,"挡":2913,"挣":2914,"挤":2915,"挥":2916,"挨":2917,"挪":2918,"挫":2919,"振":2920,"挲":2921,"挹":2922,"挺":2923,"挽":2924,"挾":2925,"捂":2926,"捅":2927,"捆":2928,"捉":2929,"捋":2930,"捌":2931,"捍":2932,"捎":2933,"捏":2934,"捐":2935,"捕":2936,"捞":2937,"损":2938,"捡":2939,"换":2940,"捣":2941,"捧":2942,"捨":2943,"捩":2944,"据":2945,"捱":2946,"捲":2947,"捶":2948,"捷":2949,"捺":2950,"捻":2951,"掀":2952,"掂":2953,"掃":2954,"掇":2955,"授":2956,"掉":2957,"掌":2958,"掏":2959,"掐":2960,"排":2961,"掖":2962,"掘":2963,"掙":2964,"掛":2965,"掠":2966,"採":2967,"探":2968,"掣":2969,"接":2970,"控":2971,"推":2972,"掩":2973,"措":2974,"掬":2975,"掰":2976,"掲":2977,"掳":2978,"掴":2979,"掷":2980,"掸":2981,"掺":2982,"揀":2983,"揃":2984,"揄":2985,"揆":2986,"揉":2987,"揍":2988,"描":2989,"提":2990,"插":2991,"揖":2992,"揚":2993,"換":2994,"握":2995,"揣":2996,"揩":2997,"揪":2998,"揭":2999,"揮":3000,"援":3001,"揶":3002,"揸":3003,"揹":3004,"揽":3005,"搀":3006,"搁":3007,"搂":3008,"搅":3009,"損":3010,"搏":3011,"搐":3012,"搓":3013,"搔":3014,"搖":3015,"搗":3016,"搜":3017,"搞":3018,"搡":3019,"搪":3020,"搬":3021,"搭":3022,"搵":3023,"搶":3024,"携":3025,"搽":3026,"摀":3027,"摁":3028,"摄":3029,"摆":3030,"摇":3031,"摈":3032,"摊":3033,"摒":3034,"摔":3035,"摘":3036,"摞":3037,"摟":3038,"摧":3039,"摩":3040,"摯":3041,"摳":3042,"摸":3043,"摹":3044,"摺":3045,"摻":3046,"撂":3047,"撃":3048,"撅":3049,"撇":3050,"撈":3051,"撐":3052,"撑":3053,"撒":3054,"撓":3055,"撕":3056,"撚":3057,"撞":3058,"撤":3059,"撥":3060,"撩":3061,"撫":3062,"撬":3063,"播":3064,"撮":3065,"撰":3066,"撲":3067,"撵":3068,"撷":3069,"撸":3070,"撻":3071,"撼":3072,"撿":3073,"擀":3074,"擁":3075,"擂":3076,"擄":3077,"擅":3078,"擇":3079,"擊":3080,"擋":3081,"操":3082,"擎":3083,"擒":3084,"擔":3085,"擘":3086,"據":3087,"擞":3088,"擠":3089,"擡":3090,"擢":3091,"擦":3092,"擬":3093,"擰":3094,"擱":3095,"擲":3096,"擴":3097,"擷":3098,"擺":3099,"擼":3100,"擾":3101,"攀":3102,"攏":3103,"攒":3104,"攔":3105,"攘":3106,"攙":3107,"攜":3108,"攝":3109,"攞":3110,"攢":3111,"攣":3112,"攤":3113,"攥":3114,"攪":3115,"攫":3116,"攬":3117,"支":3118,"收":3119,"攸":3120,"改":3121,"攻":3122,"放":3123,"政":3124,"故":3125,"效":3126,"敌":3127,"敍":3128,"敎":3129,"敏":3130,"救":3131,"敕":3132,"敖":3133,"敗":3134,"敘":3135,"教":3136,"敛":3137,"敝":3138,"敞":3139,"敢":3140,"散":3141,"敦":3142,"敬":3143,"数":3144,"敲":3145,"整":3146,"敵":3147,"敷":3148,"數":3149,"斂":3150,"斃":3151,"文":3152,"斋":3153,"斌":3154,"斎":3155,"斐":3156,"斑":3157,"斓":3158,"斗":3159,"料":3160,"斛":3161,"斜":3162,"斟":3163,"斡":3164,"斤":3165,"斥":3166,"斧":3167,"斩":3168,"斫":3169,"斬":3170,"断":3171,"斯":3172,"新":3173,"斷":3174,"方":3175,"於":3176,"施":3177,"旁":3178,"旃":3179,"旅":3180,"旋":3181,"旌":3182,"旎":3183,"族":3184,"旖":3185,"旗":3186,"无":3187,"既":3188,"日":3189,"旦":3190,"旧":3191,"旨":3192,"早":3193,"旬":3194,"旭":3195,"旮":3196,"旱":3197,"时":3198,"旷":3199,"旺":3200,"旻":3201,"昀":3202,"昂":3203,"昆":3204,"昇":3205,"昉":3206,"昊":3207,"昌":3208,"明":3209,"昏":3210,"易":3211,"昔":3212,"昕":3213,"昙":3214,"星":3215,"映":3216,"春":3217,"昧":3218,"昨":3219,"昭":3220,"是":3221,"昱":3222,"昴":3223,"昵":3224,"昶":3225,"昼":3226,"显":3227,"晁":3228,"時":3229,"晃":3230,"晉":3231,"晋":3232,"晌":3233,"晏":3234,"晒":3235,"晓":3236,"晔":3237,"晕":3238,"晖":3239,"晗":3240,"晚":3241,"晝":3242,"晞":3243,"晟":3244,"晤":3245,"晦":3246,"晨":3247,"晩":3248,"普":3249,"景":3250,"晰":3251,"晴":3252,"晶":3253,"晷":3254,"智":3255,"晾":3256,"暂":3257,"暄":3258,"暇":3259,"暈":3260,"暉":3261,"暌":3262,"暐":3263,"暑":3264,"暖":3265,"暗":3266,"暝":3267,"暢":3268,"暧":3269,"暨":3270,"暫":3271,"暮":3272,"暱":3273,"暴":3274,"暸":3275,"暹":3276,"曄":3277,"曆":3278,"曇":3279,"曉":3280,"曖":3281,"曙":3282,"曜":3283,"曝":3284,"曠":3285,"曦":3286,"曬":3287,"曰":3288,"曲":3289,"曳":3290,"更":3291,"書":3292,"曹":3293,"曼":3294,"曾":3295,"替":3296,"最":3297,"會":3298,"月":3299,"有":3300,"朋":3301,"服":3302,"朐":3303,"朔":3304,"朕":3305,"朗":3306,"望":3307,"朝":3308,"期":3309,"朦":3310,"朧":3311,"木":3312,"未":3313,"末":3314,"本":3315,"札":3316,"朮":3317,"术":3318,"朱":3319,"朴":3320,"朵":3321,"机":3322,"朽":3323,"杀":3324,"杂":3325,"权":3326,"杆":3327,"杈":3328,"杉":3329,"李":3330,"杏":3331,"材":3332,"村":3333,"杓":3334,"杖":3335,"杜":3336,"杞":3337,"束":3338,"杠":3339,"条":3340,"来":3341,"杨":3342,"杭":3343,"杯":3344,"杰":3345,"東":3346,"杳":3347,"杵":3348,"杷":3349,"杼":3350,"松":3351,"板":3352,"极":3353,"构":3354,"枇":3355,"枉":3356,"枋":3357,"析":3358,"枕":3359,"林":3360,"枚":3361,"果":3362,"枝":3363,"枢":3364,"枣":3365,"枪":3366,"枫":3367,"枭":3368,"枯":3369,"枰":3370,"枱":3371,"枳":3372,"架":3373,"枷":3374,"枸":3375,"柄":3376,"柏":3377,"某":3378,"柑":3379,"柒":3380,"染":3381,"柔":3382,"柘":3383,"柚":3384,"柜":3385,"柞":3386,"柠":3387,"柢":3388,"查":3389,"柩":3390,"柬":3391,"柯":3392,"柱":3393,"柳":3394,"柴":3395,"柵":3396,"査":3397,"柿":3398,"栀":3399,"栃":3400,"栄":3401,"栅":3402,"标":3403,"栈":3404,"栉":3405,"栋":3406,"栎":3407,"栏":3408,"树":3409,"栓":3410,"栖":3411,"栗":3412,"校":3413,"栩":3414,"株":3415,"样":3416,"核":3417,"根":3418,"格":3419,"栽":3420,"栾":3421,"桀":3422,"桁":3423,"桂":3424,"桃":3425,"桅":3426,"框":3427,"案":3428,"桉":3429,"桌":3430,"桎":3431,"桐":3432,"桑":3433,"桓":3434,"桔":3435,"桜":3436,"桠":3437,"桡":3438,"桢":3439,"档":3440,"桥":3441,"桦":3442,"桧":3443,"桨":3444,"桩":3445,"桶":3446,"桿":3447,"梁":3448,"梅":3449,"梆":3450,"梏":3451,"梓":3452,"梗":3453,"條":3454,"梟":3455,"梢":3456,"梦":3457,"梧":3458,"梨":3459,"梭":3460,"梯":3461,"械":3462,"梳":3463,"梵":3464,"梶":3465,"检":3466,"棂":3467,"棄":3468,"棉":3469,"棋":3470,"棍":3471,"棒":3472,"棕":3473,"棗":3474,"棘":3475,"棚":3476,"棟":3477,"棠":3478,"棣":3479,"棧":3480,"森":3481,"棱":3482,"棲":3483,"棵":3484,"棹":3485,"棺":3486,"椁":3487,"椅":3488,"椋":3489,"植":3490,"椎":3491,"椒":3492,"検":3493,"椪":3494,"椭":3495,"椰":3496,"椹":3497,"椽":3498,"椿":3499,"楂":3500,"楊":3501,"楓":3502,"楔":3503,"楚":3504,"楝":3505,"楞":3506,"楠":3507,"楣":3508,"楨":3509,"楫":3510,"業":3511,"楮":3512,"極":3513,"楷":3514,"楸":3515,"楹":3516,"楼":3517,"楽":3518,"概":3519,"榄":3520,"榆":3521,"榈":3522,"榉":3523,"榔":3524,"榕":3525,"榖":3526,"榛":3527,"榜":3528,"榨":3529,"榫":3530,"榭":3531,"榮":3532,"榱":3533,"榴":3534,"榷":3535,"榻":3536,"槁":3537,"槃":3538,"構":3539,"槌":3540,"槍":3541,"槎":3542,"槐":3543,"槓":3544,"様":3545,"槛":3546,"槟":3547,"槤":3548,"槭":3549,"槲":3550,"槳":3551,"槻":3552,"槽":3553,"槿":3554,"樁":3555,"樂":3556,"樊":3557,"樑":3558,"樓":3559,"標":3560,"樞":3561,"樟":3562,"模":3563,"樣":3564,"権":3565,"横":3566,"樫":3567,"樯":3568,"樱":3569,"樵":3570,"樸":3571,"樹":3572,"樺":3573,"樽":3574,"樾":3575,"橄":3576,"橇":3577,"橋":3578,"橐":3579,"橘":3580,"橙":3581,"機":3582,"橡":3583,"橢":3584,"橫":3585,"橱":3586,"橹":3587,"橼":3588,"檀":3589,"檄":3590,"檎":3591,"檐":3592,"檔":3593,"檗":3594,"檜":3595,"檢":3596,"檬":3597,"檯":3598,"檳":3599,"檸":3600,"檻":3601,"櫃":3602,"櫚":3603,"櫛":3604,"櫥":3605,"櫸":3606,"櫻":3607,"欄":3608,"權":3609,"欒":3610,"欖":3611,"欠":3612,"次":3613,"欢":3614,"欣":3615,"欧":3616,"欲":3617,"欸":3618,"欺":3619,"欽":3620,"款":3621,"歆":3622,"歇":3623,"歉":3624,"歌":3625,"歎":3626,"歐":3627,"歓":3628,"歙":3629,"歛":3630,"歡":3631,"止":3632,"正":3633,"此":3634,"步":3635,"武":3636,"歧":3637,"歩":3638,"歪":3639,"歯":3640,"歲":3641,"歳":3642,"歴":3643,"歷":3644,"歸":3645,"歹":3646,"死":3647,"歼":3648,"殁":3649,"殃":3650,"殆":3651,"殇":3652,"殉":3653,"殊":3654,"残":3655,"殒":3656,"殓":3657,"殖":3658,"殘":3659,"殞":3660,"殡":3661,"殤":3662,"殭":3663,"殯":3664,"殲":3665,"殴":3666,"段":3667,"殷":3668,"殺":3669,"殼":3670,"殿":3671,"毀":3672,"毁":3673,"毂":3674,"毅":3675,"毆":3676,"毋":3677,"母":3678,"毎":3679,"每":3680,"毒":3681,"毓":3682,"比":3683,"毕":3684,"毗":3685,"毘":3686,"毙":3687,"毛":3688,"毡":3689,"毫":3690,"毯":3691,"毽":3692,"氈":3693,"氏":3694,"氐":3695,"民":3696,"氓":3697,"气":3698,"氖":3699,"気":3700,"氙":3701,"氛":3702,"氟":3703,"氡":3704,"氢":3705,"氣":3706,"氤":3707,"氦":3708,"氧":3709,"氨":3710,"氪":3711,"氫":3712,"氮":3713,"氯":3714,"氰":3715,"氲":3716,"水":3717,"氷":3718,"永":3719,"氹":3720,"氾":3721,"汀":3722,"汁":3723,"求":3724,"汆":3725,"汇":3726,"汉":3727,"汎":3728,"汐":3729,"汕":3730,"汗":3731,"汙":3732,"汛":3733,"汝":3734,"汞":3735,"江":3736,"池":3737,"污":3738,"汤":3739,"汨":3740,"汩":3741,"汪":3742,"汰":3743,"汲":3744,"汴":3745,"汶":3746,"汹":3747,"決":3748,"汽":3749,"汾":3750,"沁":3751,"沂":3752,"沃":3753,"沅":3754,"沈":3755,"沉":3756,"沌":3757,"沏":3758,"沐":3759,"沒":3760,"沓":3761,"沖":3762,"沙":3763,"沛":3764,"沟":3765,"没":3766,"沢":3767,"沣":3768,"沥":3769,"沦":3770,"沧":3771,"沪":3772,"沫":3773,"沭":3774,"沮":3775,"沱":3776,"河":3777,"沸":3778,"油":3779,"治":3780,"沼":3781,"沽":3782,"沾":3783,"沿":3784,"況":3785,"泄":3786,"泉":3787,"泊":3788,"泌":3789,"泓":3790,"法":3791,"泗":3792,"泛":3793,"泞":3794,"泠":3795,"泡":3796,"波":3797,"泣":3798,"泥":3799,"注":3800,"泪":3801,"泫":3802,"泮":3803,"泯":3804,"泰":3805,"泱":3806,"泳":3807,"泵":3808,"泷":3809,"泸":3810,"泻":3811,"泼":3812,"泽":3813,"泾":3814,"洁":3815,"洄":3816,"洋":3817,"洒":3818,"洗":3819,"洙":3820,"洛":3821,"洞":3822,"津":3823,"洩":3824,"洪":3825,"洮":3826,"洱":3827,"洲":3828,"洵":3829,"洶":3830,"洸":3831,"洹":3832,"活":3833,"洼":3834,"洽":3835,"派":3836,"流":3837,"浃":3838,"浄":3839,"浅":3840,"浆":3841,"浇":3842,"浊":3843,"测":3844,"济":3845,"浏":3846,"浑":3847,"浒":3848,"浓":3849,"浔":3850,"浙":3851,"浚":3852,"浜":3853,"浣":3854,"浦":3855,"浩":3856,"浪":3857,"浬":3858,"浮":3859,"浯":3860,"浴":3861,"海":3862,"浸":3863,"涂":3864,"涅":3865,"涇":3866,"消":3867,"涉":3868,"涌":3869,"涎":3870,"涓":3871,"涔":3872,"涕":3873,"涙":3874,"涛":3875,"涝":3876,"涞":3877,"涟":3878,"涠":3879,"涡":3880,"涣":3881,"涤":3882,"润":3883,"涧":3884,"涨":3885,"涩":3886,"涪":3887,"涮":3888,"涯":3889,"液":3890,"涵":3891,"涸":3892,"涼":3893,"涿":3894,"淀":3895,"淄":3896,"淅":3897,"淆":3898,"淇":3899,"淋":3900,"淌":3901,"淑":3902,"淒":3903,"淖":3904,"淘":3905,"淙":3906,"淚":3907,"淞":3908,"淡":3909,"淤":3910,"淦":3911,"淨":3912,"淩":3913,"淪":3914,"淫":3915,"淬":3916,"淮":3917,"深":3918,"淳":3919,"淵":3920,"混":3921,"淹":3922,"淺":3923,"添":3924,"淼":3925,"清":3926,"済":3927,"渉":3928,"渊":3929,"渋":3930,"渍":3931,"渎":3932,"渐":3933,"渔":3934,"渗":3935,"渙":3936,"渚":3937,"減":3938,"渝":3939,"渠":3940,"渡":3941,"渣":3942,"渤":3943,"渥":3944,"渦":3945,"温":3946,"測":3947,"渭":3948,"港":3949,"渲":3950,"渴":3951,"游":3952,"渺":3953,"渾":3954,"湃":3955,"湄":3956,"湊":3957,"湍":3958,"湖":3959,"湘":3960,"湛":3961,"湟":3962,"湧":3963,"湫":3964,"湮":3965,"湯":3966,"湳":3967,"湾":3968,"湿":3969,"満":3970,"溃":3971,"溅":3972,"溉":3973,"溏":3974,"源":3975,"準":3976,"溜":3977,"溝":3978,"溟":3979,"溢":3980,"溥":3981,"溧":3982,"溪":3983,"溫":3984,"溯":3985,"溱":3986,"溴":3987,"溶":3988,"溺":3989,"溼":3990,"滁":3991,"滂":3992,"滄":3993,"滅":3994,"滇":3995,"滋":3996,"滌":3997,"滑":3998,"滓":3999,"滔":4000,"滕":4001,"滙":4002,"滚":4003,"滝":4004,"滞":4005,"滟":4006,"满":4007,"滢":4008,"滤":4009,"滥":4010,"滦":4011,"滨":4012,"滩":4013,"滬":4014,"滯":4015,"滲":4016,"滴":4017,"滷":4018,"滸":4019,"滾":4020,"滿":4021,"漁":4022,"漂":4023,"漆":4024,"漉":4025,"漏":4026,"漓":4027,"演":4028,"漕":4029,"漠":4030,"漢":4031,"漣":4032,"漩":4033,"漪":4034,"漫":4035,"漬":4036,"漯":4037,"漱":4038,"漲":4039,"漳":4040,"漸":4041,"漾":4042,"漿":4043,"潆":4044,"潇":4045,"潋":4046,"潍":4047,"潑":4048,"潔":4049,"潘":4050,"潛":4051,"潜":4052,"潞":4053,"潟":4054,"潢":4055,"潤":4056,"潦":4057,"潧":4058,"潭":4059,"潮":4060,"潰":4061,"潴":4062,"潸":4063,"潺":4064,"潼":4065,"澀":4066,"澄":4067,"澆":4068,"澈":4069,"澍":4070,"澎":4071,"澗":4072,"澜":4073,"澡":4074,"澤":4075,"澧":4076,"澱":4077,"澳":4078,"澹":4079,"激":4080,"濁":4081,"濂":4082,"濃":4083,"濑":4084,"濒":4085,"濕":4086,"濘":4087,"濛":4088,"濟":4089,"濠":4090,"濡":4091,"濤":4092,"濫":4093,"濬":4094,"濮":4095,"濯":4096,"濱":4097,"濺":4098,"濾":4099,"瀅":4100,"瀆":4101,"瀉":4102,"瀋":4103,"瀏":4104,"瀑":4105,"瀕":4106,"瀘":4107,"瀚":4108,"瀛":4109,"瀝":4110,"瀞":4111,"瀟":4112,"瀧":4113,"瀨":4114,"瀬":4115,"瀰":4116,"瀾":4117,"灌":4118,"灏":4119,"灑":4120,"灘":4121,"灝":4122,"灞":4123,"灣":4124,"火":4125,"灬":4126,"灭":4127,"灯":4128,"灰":4129,"灵":4130,"灶":4131,"灸":4132,"灼":4133,"災":4134,"灾":4135,"灿":4136,"炀":4137,"炁":4138,"炅":4139,"炉":4140,"炊":4141,"炎":4142,"炒":4143,"炔":4144,"炕":4145,"炖":4146,"炙":4147,"炜":4148,"炫":4149,"炬":4150,"炭":4151,"炮":4152,"炯":4153,"炳":4154,"炷":4155,"炸":4156,"点":4157,"為":4158,"炼":4159,"炽":4160,"烁":4161,"烂":4162,"烃":4163,"烈":4164,"烊":4165,"烏":4166,"烘":4167,"烙":4168,"烛":4169,"烟":4170,"烤":4171,"烦":4172,"烧":4173,"烨":4174,"烩":4175,"烫":4176,"烬":4177,"热":4178,"烯":4179,"烷":4180,"烹":4181,"烽":4182,"焉":4183,"焊":4184,"焕":4185,"焖":4186,"焗":4187,"焘":4188,"焙":4189,"焚":4190,"焜":4191,"無":4192,"焦":4193,"焯":4194,"焰":4195,"焱":4196,"然":4197,"焼":4198,"煅":4199,"煉":4200,"煊":4201,"煌":4202,"煎":4203,"煒":4204,"煖":4205,"煙":4206,"煜":4207,"煞":4208,"煤":4209,"煥":4210,"煦":4211,"照":4212,"煨":4213,"煩":4214,"煮":4215,"煲":4216,"煸":4217,"煽":4218,"熄":4219,"熊":4220,"熏":4221,"熒":4222,"熔":4223,"熙":4224,"熟":4225,"熠":4226,"熨":4227,"熬":4228,"熱":4229,"熵":4230,"熹":4231,"熾":4232,"燁":4233,"燃":4234,"燄":4235,"燈":4236,"燉":4237,"燊":4238,"燎":4239,"燒":4240,"燔":4241,"燕":4242,"燙":4243,"燜":4244,"營":4245,"燥":4246,"燦":4247,"燧":4248,"燭":4249,"燮":4250,"燴":4251,"燻":4252,"燼":4253,"燿":4254,"爆":4255,"爍":4256,"爐":4257,"爛":4258,"爪":4259,"爬":4260,"爭":4261,"爰":4262,"爱":4263,"爲":4264,"爵":4265,"父":4266,"爷":4267,"爸":4268,"爹":4269,"爺":4270,"爻":4271,"爽":4272,"爾":4273,"牆":4274,"片":4275,"版":4276,"牌":4277,"牍":4278,"牒":4279,"牙":4280,"牛":4281,"牝":4282,"牟":4283,"牠":4284,"牡":4285,"牢":4286,"牦":4287,"牧":4288,"物":4289,"牯":4290,"牲":4291,"牴":4292,"牵":4293,"特":4294,"牺":4295,"牽":4296,"犀":4297,"犁":4298,"犄":4299,"犊":4300,"犍":4301,"犒":4302,"犢":4303,"犧":4304,"犬":4305,"犯":4306,"状":4307,"犷":4308,"犸":4309,"犹":4310,"狀":4311,"狂":4312,"狄":4313,"狈":4314,"狎":4315,"狐":4316,"狒":4317,"狗":4318,"狙":4319,"狞":4320,"狠":4321,"狡":4322,"狩":4323,"独":4324,"狭":4325,"狮":4326,"狰":4327,"狱":4328,"狸":4329,"狹":4330,"狼":4331,"狽":4332,"猎":4333,"猕":4334,"猖":4335,"猗":4336,"猙":4337,"猛":4338,"猜":4339,"猝":4340,"猥":4341,"猩":4342,"猪":4343,"猫":4344,"猬":4345,"献":4346,"猴":4347,"猶":4348,"猷":4349,"猾":4350,"猿":4351,"獄":4352,"獅":4353,"獎":4354,"獐":4355,"獒":4356,"獗":4357,"獠":4358,"獣":4359,"獨":4360,"獭":4361,"獰":4362,"獲":4363,"獵":4364,"獷":4365,"獸":4366,"獺":4367,"獻":4368,"獼":4369,"獾":4370,"玄":4371,"率":4372,"玉":4373,"王":4374,"玑":4375,"玖":4376,"玛":4377,"玟":4378,"玠":4379,"玥":4380,"玩":4381,"玫":4382,"玮":4383,"环":4384,"现":4385,"玲":4386,"玳":4387,"玷":4388,"玺":4389,"玻":4390,"珀":4391,"珂":4392,"珅":4393,"珈":4394,"珉":4395,"珊":4396,"珍":4397,"珏":4398,"珐":4399,"珑":4400,"珙":4401,"珞":4402,"珠":4403,"珣":4404,"珥":4405,"珩":4406,"珪":4407,"班":4408,"珮":4409,"珲":4410,"珺":4411,"現":4412,"球":4413,"琅":4414,"理":4415,"琇":4416,"琉":4417,"琊":4418,"琍":4419,"琏":4420,"琐":4421,"琛":4422,"琢":4423,"琥":4424,"琦":4425,"琨":4426,"琪":4427,"琬":4428,"琮":4429,"琰":4430,"琲":4431,"琳":4432,"琴":4433,"琵":4434,"琶":4435,"琺":4436,"琼":4437,"瑀":4438,"瑁":4439,"瑄":4440,"瑋":4441,"瑕":4442,"瑗":4443,"瑙":4444,"瑚":4445,"瑛":4446,"瑜":4447,"瑞":4448,"瑟":4449,"瑠":4450,"瑣":4451,"瑤":4452,"瑩":4453,"瑪":4454,"瑯":4455,"瑰":4456,"瑶":4457,"瑾":4458,"璀":4459,"璁":4460,"璃":4461,"璇":4462,"璉":4463,"璋":4464,"璎":4465,"璐":4466,"璜":4467,"璞":4468,"璟":4469,"璧":4470,"璨":4471,"環":4472,"璽":4473,"璿":4474,"瓊":4475,"瓏":4476,"瓒":4477,"瓜":4478,"瓢":4479,"瓣":4480,"瓤":4481,"瓦":4482,"瓮":4483,"瓯":4484,"瓴":4485,"瓶":4486,"瓷":4487,"甄":4488,"甌":4489,"甕":4490,"甘":4491,"甙":4492,"甚":4493,"甜":4494,"生":4495,"產":4496,"産":4497,"甥":4498,"甦":4499,"用":4500,"甩":4501,"甫":4502,"甬":4503,"甭":4504,"甯":4505,"田":4506,"由":4507,"甲":4508,"申":4509,"电":4510,"男":4511,"甸":4512,"町":4513,"画":4514,"甾":4515,"畀":4516,"畅":4517,"界":4518,"畏":4519,"畑":4520,"畔":4521,"留":4522,"畜":4523,"畝":4524,"畢":4525,"略":4526,"畦":4527,"番":4528,"畫":4529,"異":4530,"畲":4531,"畳":4532,"畴":4533,"當":4534,"畸":4535,"畹":4536,"畿":4537,"疆":4538,"疇":4539,"疊":4540,"疏":4541,"疑":4542,"疔":4543,"疖":4544,"疗":4545,"疙":4546,"疚":4547,"疝":4548,"疟":4549,"疡":4550,"疣":4551,"疤":4552,"疥":4553,"疫":4554,"疮":4555,"疯":4556,"疱":4557,"疲":4558,"疳":4559,"疵":4560,"疸":4561,"疹":4562,"疼":4563,"疽":4564,"疾":4565,"痂":4566,"病":4567,"症":4568,"痈":4569,"痉":4570,"痊":4571,"痍":4572,"痒":4573,"痔":4574,"痕":4575,"痘":4576,"痙":4577,"痛":4578,"痞":4579,"痠":4580,"痢":4581,"痣":4582,"痤":4583,"痧":4584,"痨":4585,"痪":4586,"痫":4587,"痰":4588,"痱":4589,"痴":4590,"痹":4591,"痺":4592,"痼":4593,"痿":4594,"瘀":4595,"瘁":4596,"瘋":4597,"瘍":4598,"瘓":4599,"瘘":4600,"瘙":4601,"瘟":4602,"瘠":4603,"瘡":4604,"瘢":4605,"瘤":4606,"瘦":4607,"瘧":4608,"瘩":4609,"瘪":4610,"瘫":4611,"瘴":4612,"瘸":4613,"瘾":4614,"療":4615,"癇":4616,"癌":4617,"癒":4618,"癖":4619,"癜":4620,"癞":4621,"癡":4622,"癢":4623,"癣":4624,"癥":4625,"癫":4626,"癬":4627,"癮":4628,"癱":4629,"癲":4630,"癸":4631,"発":4632,"登":4633,"發":4634,"白":4635,"百":4636,"皂":4637,"的":4638,"皆":4639,"皇":4640,"皈":4641,"皋":4642,"皎":4643,"皑":4644,"皓":4645,"皖":4646,"皙":4647,"皚":4648,"皮":4649,"皰":4650,"皱":4651,"皴":4652,"皺":4653,"皿":4654,"盂":4655,"盃":4656,"盅":4657,"盆":4658,"盈":4659,"益":4660,"盎":4661,"盏":4662,"盐":4663,"监":4664,"盒":4665,"盔":4666,"盖":4667,"盗":4668,"盘":4669,"盛":4670,"盜":4671,"盞":4672,"盟":4673,"盡":4674,"監":4675,"盤":4676,"盥":4677,"盧":4678,"盪":4679,"目":4680,"盯":4681,"盱":4682,"盲":4683,"直":4684,"相":4685,"盹":4686,"盼":4687,"盾":4688,"省":4689,"眈":4690,"眉":4691,"看":4692,"県":4693,"眙":4694,"眞":4695,"真":4696,"眠":4697,"眦":4698,"眨":4699,"眩":4700,"眯":4701,"眶":4702,"眷":4703,"眸":4704,"眺":4705,"眼":4706,"眾":4707,"着":4708,"睁":4709,"睇":4710,"睏":4711,"睐":4712,"睑":4713,"睛":4714,"睜":4715,"睞":4716,"睡":4717,"睢":4718,"督":4719,"睥":4720,"睦":4721,"睨":4722,"睪":4723,"睫":4724,"睬":4725,"睹":4726,"睽":4727,"睾":4728,"睿":4729,"瞄":4730,"瞅":4731,"瞇":4732,"瞋":4733,"瞌":4734,"瞎":4735,"瞑":4736,"瞒":4737,"瞓":4738,"瞞":4739,"瞟":4740,"瞠":4741,"瞥":4742,"瞧":4743,"瞩":4744,"瞪":4745,"瞬":4746,"瞭":4747,"瞰":4748,"瞳":4749,"瞻":4750,"瞼":4751,"瞿":4752,"矇":4753,"矍":4754,"矗":4755,"矚":4756,"矛":4757,"矜":4758,"矢":4759,"矣":4760,"知":4761,"矩":4762,"矫":4763,"短":4764,"矮":4765,"矯":4766,"石":4767,"矶":4768,"矽":4769,"矾":4770,"矿":4771,"码":4772,"砂":4773,"砌":4774,"砍":4775,"砒":4776,"研":4777,"砖":4778,"砗":4779,"砚":4780,"砝":4781,"砣":4782,"砥":4783,"砧":4784,"砭":4785,"砰":4786,"砲":4787,"破":4788,"砷":4789,"砸":4790,"砺":4791,"砼":4792,"砾":4793,"础":4794,"硅":4795,"硐":4796,"硒":4797,"硕":4798,"硝":4799,"硫":4800,"硬":4801,"确":4802,"硯":4803,"硼":4804,"碁":4805,"碇":4806,"碉":4807,"碌":4808,"碍":4809,"碎":4810,"碑":4811,"碓":4812,"碗":4813,"碘":4814,"碚":4815,"碛":4816,"碟":4817,"碣":4818,"碧":4819,"碩":4820,"碰":4821,"碱":4822,"碳":4823,"碴":4824,"確":4825,"碼":4826,"碾":4827,"磁":4828,"磅":4829,"磊":4830,"磋":4831,"磐":4832,"磕":4833,"磚":4834,"磡":4835,"磨":4836,"磬":4837,"磯":4838,"磲":4839,"磷":4840,"磺":4841,"礁":4842,"礎":4843,"礙":4844,"礡":4845,"礦":4846,"礪":4847,"礫":4848,"礴":4849,"示":4850,"礼":4851,"社":4852,"祀":4853,"祁":4854,"祂":4855,"祇":4856,"祈":4857,"祉":4858,"祎":4859,"祐":4860,"祕":4861,"祖":4862,"祗":4863,"祚":4864,"祛":4865,"祜":4866,"祝":4867,"神":4868,"祟":4869,"祠":4870,"祢":4871,"祥":4872,"票":4873,"祭":4874,"祯":4875,"祷":4876,"祸":4877,"祺":4878,"祿":4879,"禀":4880,"禁":4881,"禄":4882,"禅":4883,"禍":4884,"禎":4885,"福":4886,"禛":4887,"禦":4888,"禧":4889,"禪":4890,"禮":4891,"禱":4892,"禹":4893,"禺":4894,"离":4895,"禽":4896,"禾":4897,"禿":4898,"秀":4899,"私":4900,"秃":4901,"秆":4902,"秉":4903,"秋":4904,"种":4905,"科":4906,"秒":4907,"秘":4908,"租":4909,"秣":4910,"秤":4911,"秦":4912,"秧":4913,"秩":4914,"秭":4915,"积":4916,"称":4917,"秸":4918,"移":4919,"秽":4920,"稀":4921,"稅":4922,"程":4923,"稍":4924,"税":4925,"稔":4926,"稗":4927,"稚":4928,"稜":4929,"稞":4930,"稟":4931,"稠":4932,"稣":4933,"種":4934,"稱":4935,"稲":4936,"稳":4937,"稷":4938,"稹":4939,"稻":4940,"稼":4941,"稽":4942,"稿":4943,"穀":4944,"穂":4945,"穆":4946,"穌":4947,"積":4948,"穎":4949,"穗":4950,"穢":4951,"穩":4952,"穫":4953,"穴":4954,"究":4955,"穷":4956,"穹":4957,"空":4958,"穿":4959,"突":4960,"窃":4961,"窄":4962,"窈":4963,"窍":4964,"窑":4965,"窒":4966,"窓":4967,"窕":4968,"窖":4969,"窗":4970,"窘":4971,"窜":4972,"窝":4973,"窟":4974,"窠":4975,"窥":4976,"窦":4977,"窨":4978,"窩":4979,"窪":4980,"窮":4981,"窯":4982,"窺":4983,"窿":4984,"竄":4985,"竅":4986,"竇":4987,"竊":4988,"立":4989,"竖":4990,"站":4991,"竜":4992,"竞":4993,"竟":4994,"章":4995,"竣":4996,"童":4997,"竭":4998,"端":4999,"競":5000,"竹":5001,"竺":5002,"竽":5003,"竿":5004,"笃":5005,"笆":5006,"笈":5007,"笋":5008,"笏":5009,"笑":5010,"笔":5011,"笙":5012,"笛":5013,"笞":5014,"笠":5015,"符":5016,"笨":5017,"第":5018,"笹":5019,"笺":5020,"笼":5021,"筆":5022,"等":5023,"筊":5024,"筋":5025,"筍":5026,"筏":5027,"筐":5028,"筑":5029,"筒":5030,"答":5031,"策":5032,"筛":5033,"筝":5034,"筠":5035,"筱":5036,"筲":5037,"筵":5038,"筷":5039,"筹":5040,"签":5041,"简":5042,"箇":5043,"箋":5044,"箍":5045,"箏":5046,"箐":5047,"箔":5048,"箕":5049,"算":5050,"箝":5051,"管":5052,"箩":5053,"箫":5054,"箭":5055,"箱":5056,"箴":5057,"箸":5058,"節":5059,"篁":5060,"範":5061,"篆":5062,"篇":5063,"築":5064,"篑":5065,"篓":5066,"篙":5067,"篝":5068,"篠":5069,"篡":5070,"篤":5071,"篩":5072,"篪":5073,"篮":5074,"篱":5075,"篷":5076,"簇":5077,"簌":5078,"簍":5079,"簡":5080,"簦":5081,"簧":5082,"簪":5083,"簫":5084,"簷":5085,"簸":5086,"簽":5087,"簾":5088,"簿":5089,"籁":5090,"籃":5091,"籌":5092,"籍":5093,"籐":5094,"籟":5095,"籠":5096,"籤":5097,"籬":5098,"籮":5099,"籲":5100,"米":5101,"类":5102,"籼":5103,"籽":5104,"粄":5105,"粉":5106,"粑":5107,"粒":5108,"粕":5109,"粗":5110,"粘":5111,"粟":5112,"粤":5113,"粥":5114,"粧":5115,"粪":5116,"粮":5117,"粱":5118,"粲":5119,"粳":5120,"粵":5121,"粹":5122,"粼":5123,"粽":5124,"精":5125,"粿":5126,"糅":5127,"糊":5128,"糍":5129,"糕":5130,"糖":5131,"糗":5132,"糙":5133,"糜":5134,"糞":5135,"糟":5136,"糠":5137,"糧":5138,"糬":5139,"糯":5140,"糰":5141,"糸":5142,"系":5143,"糾":5144,"紀":5145,"紂":5146,"約":5147,"紅":5148,"紉":5149,"紊":5150,"紋":5151,"納":5152,"紐":5153,"紓":5154,"純":5155,"紗":5156,"紘":5157,"紙":5158,"級":5159,"紛":5160,"紜":5161,"素":5162,"紡":5163,"索":5164,"紧":5165,"紫":5166,"紮":5167,"累":5168,"細":5169,"紳":5170,"紹":5171,"紺":5172,"終":5173,"絃":5174,"組":5175,"絆":5176,"経":5177,"結":5178,"絕":5179,"絞":5180,"絡":5181,"絢":5182,"給":5183,"絨":5184,"絮":5185,"統":5186,"絲":5187,"絳":5188,"絵":5189,"絶":5190,"絹":5191,"綁":5192,"綏":5193,"綑":5194,"經":5195,"継":5196,"続":5197,"綜":5198,"綠":5199,"綢":5200,"綦":5201,"綫":5202,"綬":5203,"維":5204,"綱":5205,"網":5206,"綴":5207,"綵":5208,"綸":5209,"綺":5210,"綻":5211,"綽":5212,"綾":5213,"綿":5214,"緊":5215,"緋":5216,"総":5217,"緑":5218,"緒":5219,"緘":5220,"線":5221,"緝":5222,"緞":5223,"締":5224,"緣":5225,"編":5226,"緩":5227,"緬":5228,"緯":5229,"練":5230,"緹":5231,"緻":5232,"縁":5233,"縄":5234,"縈":5235,"縛":5236,"縝":5237,"縣":5238,"縫":5239,"縮":5240,"縱":5241,"縴":5242,"縷":5243,"總":5244,"績":5245,"繁":5246,"繃":5247,"繆":5248,"繇":5249,"繋":5250,"織":5251,"繕":5252,"繚":5253,"繞":5254,"繡":5255,"繩":5256,"繪":5257,"繫":5258,"繭":5259,"繳":5260,"繹":5261,"繼":5262,"繽":5263,"纂":5264,"續":5265,"纍":5266,"纏":5267,"纓":5268,"纔":5269,"纖":5270,"纜":5271,"纠":5272,"红":5273,"纣":5274,"纤":5275,"约":5276,"级":5277,"纨":5278,"纪":5279,"纫":5280,"纬":5281,"纭":5282,"纯":5283,"纰":5284,"纱":5285,"纲":5286,"纳":5287,"纵":5288,"纶":5289,"纷":5290,"纸":5291,"纹":5292,"纺":5293,"纽":5294,"纾":5295,"线":5296,"绀":5297,"练":5298,"组":5299,"绅":5300,"细":5301,"织":5302,"终":5303,"绊":5304,"绍":5305,"绎":5306,"经":5307,"绑":5308,"绒":5309,"结":5310,"绔":5311,"绕":5312,"绘":5313,"给":5314,"绚":5315,"绛":5316,"络":5317,"绝":5318,"绞":5319,"统":5320,"绡":5321,"绢":5322,"绣":5323,"绥":5324,"绦":5325,"继":5326,"绩":5327,"绪":5328,"绫":5329,"续":5330,"绮":5331,"绯":5332,"绰":5333,"绳":5334,"维":5335,"绵":5336,"绶":5337,"绷":5338,"绸":5339,"绻":5340,"综":5341,"绽":5342,"绾":5343,"绿":5344,"缀":5345,"缄":5346,"缅":5347,"缆":5348,"缇":5349,"缈":5350,"缉":5351,"缎":5352,"缓":5353,"缔":5354,"缕":5355,"编":5356,"缘":5357,"缙":5358,"缚":5359,"缜":5360,"缝":5361,"缠":5362,"缢":5363,"缤":5364,"缥":5365,"缨":5366,"缩":5367,"缪":5368,"缭":5369,"缮":5370,"缰":5371,"缱":5372,"缴":5373,"缸":5374,"缺":5375,"缽":5376,"罂":5377,"罄":5378,"罌":5379,"罐":5380,"网":5381,"罔":5382,"罕":5383,"罗":5384,"罚":5385,"罡":5386,"罢":5387,"罩":5388,"罪":5389,"置":5390,"罰":5391,"署":5392,"罵":5393,"罷":5394,"罹":5395,"羁":5396,"羅":5397,"羈":5398,"羊":5399,"羌":5400,"美":5401,"羔":5402,"羚":5403,"羞":5404,"羟":5405,"羡":5406,"羣":5407,"群":5408,"羥":5409,"羧":5410,"羨":5411,"義":5412,"羯":5413,"羲":5414,"羸":5415,"羹":5416,"羽":5417,"羿":5418,"翁":5419,"翅":5420,"翊":5421,"翌":5422,"翎":5423,"習":5424,"翔":5425,"翘":5426,"翟":5427,"翠":5428,"翡":5429,"翦":5430,"翩":5431,"翰":5432,"翱":5433,"翳":5434,"翹":5435,"翻":5436,"翼":5437,"耀":5438,"老":5439,"考":5440,"耄":5441,"者":5442,"耆":5443,"耋":5444,"而":5445,"耍":5446,"耐":5447,"耒":5448,"耕":5449,"耗":5450,"耘":5451,"耙":5452,"耦":5453,"耨":5454,"耳":5455,"耶":5456,"耷":5457,"耸":5458,"耻":5459,"耽":5460,"耿":5461,"聂":5462,"聆":5463,"聊":5464,"聋":5465,"职":5466,"聒":5467,"联":5468,"聖":5469,"聘":5470,"聚":5471,"聞":5472,"聪":5473,"聯":5474,"聰":5475,"聲":5476,"聳":5477,"聴":5478,"聶":5479,"職":5480,"聽":5481,"聾":5482,"聿":5483,"肃":5484,"肄":5485,"肅":5486,"肆":5487,"肇":5488,"肉":5489,"肋":5490,"肌":5491,"肏":5492,"肓":5493,"肖":5494,"肘":5495,"肚":5496,"肛":5497,"肝":5498,"肠":5499,"股":5500,"肢":5501,"肤":5502,"肥":5503,"肩":5504,"肪":5505,"肮":5506,"肯":5507,"肱":5508,"育":5509,"肴":5510,"肺":5511,"肽":5512,"肾":5513,"肿":5514,"胀":5515,"胁":5516,"胃":5517,"胄":5518,"胆":5519,"背":5520,"胍":5521,"胎":5522,"胖":5523,"胚":5524,"胛":5525,"胜":5526,"胝":5527,"胞":5528,"胡":5529,"胤":5530,"胥":5531,"胧":5532,"胫":5533,"胭":5534,"胯":5535,"胰":5536,"胱":5537,"胳":5538,"胴":5539,"胶":5540,"胸":5541,"胺":5542,"能":5543,"脂":5544,"脅":5545,"脆":5546,"脇":5547,"脈":5548,"脉":5549,"脊":5550,"脍":5551,"脏":5552,"脐":5553,"脑":5554,"脓":5555,"脖":5556,"脘":5557,"脚":5558,"脛":5559,"脣":5560,"脩":5561,"脫":5562,"脯":5563,"脱":5564,"脲":5565,"脳":5566,"脸":5567,"脹":5568,"脾":5569,"腆":5570,"腈":5571,"腊":5572,"腋":5573,"腌":5574,"腎":5575,"腐":5576,"腑":5577,"腓":5578,"腔":5579,"腕":5580,"腥":5581,"腦":5582,"腩":5583,"腫":5584,"腭":5585,"腮":5586,"腰":5587,"腱":5588,"腳":5589,"腴":5590,"腸":5591,"腹":5592,"腺":5593,"腻":5594,"腼":5595,"腾":5596,"腿":5597,"膀":5598,"膈":5599,"膊":5600,"膏":5601,"膑":5602,"膘":5603,"膚":5604,"膛":5605,"膜":5606,"膝":5607,"膠":5608,"膦":5609,"膨":5610,"膩":5611,"膳":5612,"膺":5613,"膻":5614,"膽":5615,"膾":5616,"膿":5617,"臀":5618,"臂":5619,"臃":5620,"臆":5621,"臉":5622,"臊":5623,"臍":5624,"臓":5625,"臘":5626,"臟":5627,"臣":5628,"臥":5629,"臧":5630,"臨":5631,"自":5632,"臬":5633,"臭":5634,"至":5635,"致":5636,"臺":5637,"臻":5638,"臼":5639,"臾":5640,"舀":5641,"舂":5642,"舅":5643,"舆":5644,"與":5645,"興":5646,"舉":5647,"舊":5648,"舌":5649,"舍":5650,"舎":5651,"舐":5652,"舒":5653,"舔":5654,"舖":5655,"舗":5656,"舛":5657,"舜":5658,"舞":5659,"舟":5660,"航":5661,"舫":5662,"般":5663,"舰":5664,"舱":5665,"舵":5666,"舶":5667,"舷":5668,"舸":5669,"船":5670,"舺":5671,"舾":5672,"艇":5673,"艋":5674,"艘":5675,"艙":5676,"艦":5677,"艮":5678,"良":5679,"艰":5680,"艱":5681,"色":5682,"艳":5683,"艷":5684,"艹":5685,"艺":5686,"艾":5687,"节":5688,"芃":5689,"芈":5690,"芊":5691,"芋":5692,"芍":5693,"芎":5694,"芒":5695,"芙":5696,"芜":5697,"芝":5698,"芡":5699,"芥":5700,"芦":5701,"芩":5702,"芪":5703,"芫":5704,"芬":5705,"芭":5706,"芮":5707,"芯":5708,"花":5709,"芳":5710,"芷":5711,"芸":5712,"芹":5713,"芻":5714,"芽":5715,"芾":5716,"苁":5717,"苄":5718,"苇":5719,"苋":5720,"苍":5721,"苏":5722,"苑":5723,"苒":5724,"苓":5725,"苔":5726,"苕":5727,"苗":5728,"苛":5729,"苜":5730,"苞":5731,"苟":5732,"苡":5733,"苣":5734,"若":5735,"苦":5736,"苫":5737,"苯":5738,"英":5739,"苷":5740,"苹":5741,"苻":5742,"茁":5743,"茂":5744,"范":5745,"茄":5746,"茅":5747,"茉":5748,"茎":5749,"茏":5750,"茗":5751,"茜":5752,"茧":5753,"茨":5754,"茫":5755,"茬":5756,"茭":5757,"茯":5758,"茱":5759,"茲":5760,"茴":5761,"茵":5762,"茶":5763,"茸":5764,"茹":5765,"茼":5766,"荀":5767,"荃":5768,"荆":5769,"草":5770,"荊":5771,"荏":5772,"荐":5773,"荒":5774,"荔":5775,"荖":5776,"荘":5777,"荚":5778,"荞":5779,"荟":5780,"荠":5781,"荡":5782,"荣":5783,"荤":5784,"荥":5785,"荧":5786,"荨":5787,"荪":5788,"荫":5789,"药":5790,"荳":5791,"荷":5792,"荸":5793,"荻":5794,"荼":5795,"荽":5796,"莅":5797,"莆":5798,"莉":5799,"莊":5800,"莎":5801,"莒":5802,"莓":5803,"莖":5804,"莘":5805,"莞":5806,"莠":5807,"莢":5808,"莧":5809,"莪":5810,"莫":5811,"莱":5812,"莲":5813,"莴":5814,"获":5815,"莹":5816,"莺":5817,"莽":5818,"莿":5819,"菀":5820,"菁":5821,"菅":5822,"菇":5823,"菈":5824,"菊":5825,"菌":5826,"菏":5827,"菓":5828,"菖":5829,"菘":5830,"菜":5831,"菟":5832,"菠":5833,"菡":5834,"菩":5835,"華":5836,"菱":5837,"菲":5838,"菸":5839,"菽":5840,"萁":5841,"萃":5842,"萄":5843,"萊":5844,"萋":5845,"萌":5846,"萍":5847,"萎":5848,"萘":5849,"萝":5850,"萤":5851,"营":5852,"萦":5853,"萧":5854,"萨":5855,"萩":5856,"萬":5857,"萱":5858,"萵":5859,"萸":5860,"萼":5861,"落":5862,"葆":5863,"葉":5864,"著":5865,"葚":5866,"葛":5867,"葡":5868,"董":5869,"葦":5870,"葩":5871,"葫":5872,"葬":5873,"葭":5874,"葯":5875,"葱":5876,"葳":5877,"葵":5878,"葷":5879,"葺":5880,"蒂":5881,"蒋":5882,"蒐":5883,"蒔":5884,"蒙":5885,"蒜":5886,"蒞":5887,"蒟":5888,"蒡":5889,"蒨":5890,"蒲":5891,"蒸":5892,"蒹":5893,"蒻":5894,"蒼":5895,"蒿":5896,"蓁":5897,"蓄":5898,"蓆":5899,"蓉":5900,"蓋":5901,"蓑":5902,"蓓":5903,"蓖":5904,"蓝":5905,"蓟":5906,"蓦":5907,"蓬":5908,"蓮":5909,"蓼":5910,"蓿":5911,"蔑":5912,"蔓":5913,"蔔":5914,"蔗":5915,"蔘":5916,"蔚":5917,"蔡":5918,"蔣":5919,"蔥":5920,"蔫":5921,"蔬":5922,"蔭":5923,"蔵":5924,"蔷":5925,"蔺":5926,"蔻":5927,"蔼":5928,"蔽":5929,"蕁":5930,"蕃":5931,"蕈":5932,"蕉":5933,"蕊":5934,"蕎":5935,"蕙":5936,"蕤":5937,"蕨":5938,"蕩":5939,"蕪":5940,"蕭":5941,"蕲":5942,"蕴":5943,"蕻":5944,"蕾":5945,"薄":5946,"薅":5947,"薇":5948,"薈":5949,"薊":5950,"薏":5951,"薑":5952,"薔":5953,"薙":5954,"薛":5955,"薦":5956,"薨":5957,"薩":5958,"薪":5959,"薬":5960,"薯":5961,"薰":5962,"薹":5963,"藉":5964,"藍":5965,"藏":5966,"藐":5967,"藓":5968,"藕":5969,"藜":5970,"藝":5971,"藤":5972,"藥":5973,"藩":5974,"藹":5975,"藻":5976,"藿":5977,"蘆":5978,"蘇":5979,"蘊":5980,"蘋":5981,"蘑":5982,"蘚":5983,"蘭":5984,"蘸":5985,"蘼":5986,"蘿":5987,"虎":5988,"虏":5989,"虐":5990,"虑":5991,"虔":5992,"處":5993,"虚":5994,"虛":5995,"虜":5996,"虞":5997,"號":5998,"虢":5999,"虧":6000,"虫":6001,"虬":6002,"虱":6003,"虹":6004,"虻":6005,"虽":6006,"虾":6007,"蚀":6008,"蚁":6009,"蚂":6010,"蚊":6011,"蚌":6012,"蚓":6013,"蚕":6014,"蚜":6015,"蚝":6016,"蚣":6017,"蚤":6018,"蚩":6019,"蚪":6020,"蚯":6021,"蚱":6022,"蚵":6023,"蛀":6024,"蛆":6025,"蛇":6026,"蛊":6027,"蛋":6028,"蛎":6029,"蛐":6030,"蛔":6031,"蛙":6032,"蛛":6033,"蛟":6034,"蛤":6035,"蛭":6036,"蛮":6037,"蛰":6038,"蛳":6039,"蛹":6040,"蛻":6041,"蛾":6042,"蜀":6043,"蜂":6044,"蜃":6045,"蜆":6046,"蜇":6047,"蜈":6048,"蜊":6049,"蜍":6050,"蜒":6051,"蜓":6052,"蜕":6053,"蜗":6054,"蜘":6055,"蜚":6056,"蜜":6057,"蜡":6058,"蜢":6059,"蜥":6060,"蜱":6061,"蜴":6062,"蜷":6063,"蜻":6064,"蜿":6065,"蝇":6066,"蝈":6067,"蝉":6068,"蝌":6069,"蝎":6070,"蝕":6071,"蝗":6072,"蝙":6073,"蝟":6074,"蝠":6075,"蝦":6076,"蝨":6077,"蝴":6078,"蝶":6079,"蝸":6080,"蝼":6081,"螂":6082,"螃":6083,"融":6084,"螞":6085,"螢":6086,"螨":6087,"螯":6088,"螳":6089,"螺":6090,"蟀":6091,"蟄":6092,"蟆":6093,"蟋":6094,"蟎":6095,"蟑":6096,"蟒":6097,"蟠":6098,"蟬":6099,"蟲":6100,"蟹":6101,"蟻":6102,"蟾":6103,"蠅":6104,"蠍":6105,"蠔":6106,"蠕":6107,"蠛":6108,"蠟":6109,"蠡":6110,"蠢":6111,"蠣":6112,"蠱":6113,"蠶":6114,"蠹":6115,"蠻":6116,"血":6117,"衄":6118,"衅":6119,"衆":6120,"行":6121,"衍":6122,"術":6123,"衔":6124,"街":6125,"衙":6126,"衛":6127,"衝":6128,"衞":6129,"衡":6130,"衢":6131,"衣":6132,"补":6133,"表":6134,"衩":6135,"衫":6136,"衬":6137,"衮":6138,"衰":6139,"衲":6140,"衷":6141,"衹":6142,"衾":6143,"衿":6144,"袁":6145,"袂":6146,"袄":6147,"袅":6148,"袈":6149,"袋":6150,"袍":6151,"袒":6152,"袖":6153,"袜":6154,"袞":6155,"袤":6156,"袪":6157,"被":6158,"袭":6159,"袱":6160,"裁":6161,"裂":6162,"装":6163,"裆":6164,"裊":6165,"裏":6166,"裔":6167,"裕":6168,"裘":6169,"裙":6170,"補":6171,"裝":6172,"裟":6173,"裡":6174,"裤":6175,"裨":6176,"裱":6177,"裳":6178,"裴":6179,"裸":6180,"裹":6181,"製":6182,"裾":6183,"褂":6184,"複":6185,"褐":6186,"褒":6187,"褓":6188,"褔":6189,"褚":6190,"褥":6191,"褪":6192,"褫":6193,"褲":6194,"褶":6195,"褻":6196,"襁":6197,"襄":6198,"襟":6199,"襠":6200,"襪":6201,"襬":6202,"襯":6203,"襲":6204,"西":6205,"要":6206,"覃":6207,"覆":6208,"覇":6209,"見":6210,"規":6211,"覓":6212,"視":6213,"覚":6214,"覦":6215,"覧":6216,"親":6217,"覬":6218,"観":6219,"覷":6220,"覺":6221,"覽":6222,"觀":6223,"见":6224,"观":6225,"规":6226,"觅":6227,"视":6228,"览":6229,"觉":6230,"觊":6231,"觎":6232,"觐":6233,"觑":6234,"角":6235,"觞":6236,"解":6237,"觥":6238,"触":6239,"觸":6240,"言":6241,"訂":6242,"計":6243,"訊":6244,"討":6245,"訓":6246,"訕":6247,"訖":6248,"託":6249,"記":6250,"訛":6251,"訝":6252,"訟":6253,"訣":6254,"訥":6255,"訪":6256,"設":6257,"許":6258,"訳":6259,"訴":6260,"訶":6261,"診":6262,"註":6263,"証":6264,"詆":6265,"詐":6266,"詔":6267,"評":6268,"詛":6269,"詞":6270,"詠":6271,"詡":6272,"詢":6273,"詣":6274,"試":6275,"詩":6276,"詫":6277,"詬":6278,"詭":6279,"詮":6280,"詰":6281,"話":6282,"該":6283,"詳":6284,"詹":6285,"詼":6286,"誅":6287,"誇":6288,"誉":6289,"誌":6290,"認":6291,"誓":6292,"誕":6293,"誘":6294,"語":6295,"誠":6296,"誡":6297,"誣":6298,"誤":6299,"誥":6300,"誦":6301,"誨":6302,"說":6303,"説":6304,"読":6305,"誰":6306,"課":6307,"誹":6308,"誼":6309,"調":6310,"諄":6311,"談":6312,"請":6313,"諏":6314,"諒":6315,"論":6316,"諗":6317,"諜":6318,"諡":6319,"諦":6320,"諧":6321,"諫":6322,"諭":6323,"諮":6324,"諱":6325,"諳":6326,"諷":6327,"諸":6328,"諺":6329,"諾":6330,"謀":6331,"謁":6332,"謂":6333,"謄":6334,"謊":6335,"謎":6336,"謐":6337,"謔":6338,"謗":6339,"謙":6340,"講":6341,"謝":6342,"謠":6343,"謨":6344,"謬":6345,"謹":6346,"謾":6347,"譁":6348,"證":6349,"譎":6350,"譏":6351,"識":6352,"譙":6353,"譚":6354,"譜":6355,"警":6356,"譬":6357,"譯":6358,"議":6359,"譲":6360,"譴":6361,"護":6362,"譽":6363,"讀":6364,"變":6365,"讓":6366,"讚":6367,"讞":6368,"计":6369,"订":6370,"认":6371,"讥":6372,"讧":6373,"讨":6374,"让":6375,"讪":6376,"讫":6377,"训":6378,"议":6379,"讯":6380,"记":6381,"讲":6382,"讳":6383,"讴":6384,"讶":6385,"讷":6386,"许":6387,"讹":6388,"论":6389,"讼":6390,"讽":6391,"设":6392,"访":6393,"诀":6394,"证":6395,"诃":6396,"评":6397,"诅":6398,"识":6399,"诈":6400,"诉":6401,"诊":6402,"诋":6403,"词":6404,"诏":6405,"译":6406,"试":6407,"诗":6408,"诘":6409,"诙":6410,"诚":6411,"诛":6412,"话":6413,"诞":6414,"诟":6415,"诠":6416,"诡":6417,"询":6418,"诣":6419,"诤":6420,"该":6421,"详":6422,"诧":6423,"诩":6424,"诫":6425,"诬":6426,"语":6427,"误":6428,"诰":6429,"诱":6430,"诲":6431,"说":6432,"诵":6433,"诶":6434,"请":6435,"诸":6436,"诺":6437,"读":6438,"诽":6439,"课":6440,"诿":6441,"谀":6442,"谁":6443,"调":6444,"谄":6445,"谅":6446,"谆":6447,"谈":6448,"谊":6449,"谋":6450,"谌":6451,"谍":6452,"谎":6453,"谏":6454,"谐":6455,"谑":6456,"谒":6457,"谓":6458,"谔":6459,"谕":6460,"谗":6461,"谘":6462,"谙":6463,"谚":6464,"谛":6465,"谜":6466,"谟":6467,"谢":6468,"谣":6469,"谤":6470,"谥":6471,"谦":6472,"谧":6473,"谨":6474,"谩":6475,"谪":6476,"谬":6477,"谭":6478,"谯":6479,"谱":6480,"谲":6481,"谴":6482,"谶":6483,"谷":6484,"豁":6485,"豆":6486,"豇":6487,"豈":6488,"豉":6489,"豊":6490,"豌":6491,"豎":6492,"豐":6493,"豔":6494,"豚":6495,"象":6496,"豢":6497,"豪":6498,"豫":6499,"豬":6500,"豹":6501,"豺":6502,"貂":6503,"貅":6504,"貌":6505,"貓":6506,"貔":6507,"貘":6508,"貝":6509,"貞":6510,"負":6511,"財":6512,"貢":6513,"貧":6514,"貨":6515,"販":6516,"貪":6517,"貫":6518,"責":6519,"貯":6520,"貰":6521,"貳":6522,"貴":6523,"貶":6524,"買":6525,"貸":6526,"費":6527,"貼":6528,"貽":6529,"貿":6530,"賀":6531,"賁":6532,"賂":6533,"賃":6534,"賄":6535,"資":6536,"賈":6537,"賊":6538,"賑":6539,"賓":6540,"賜":6541,"賞":6542,"賠":6543,"賡":6544,"賢":6545,"賣":6546,"賤":6547,"賦":6548,"質":6549,"賬":6550,"賭":6551,"賴":6552,"賺":6553,"購":6554,"賽":6555,"贅":6556,"贈":6557,"贊":6558,"贍":6559,"贏":6560,"贓":6561,"贖":6562,"贛":6563,"贝":6564,"贞":6565,"负":6566,"贡":6567,"财":6568,"责":6569,"贤":6570,"败":6571,"账":6572,"货":6573,"质":6574,"贩":6575,"贪":6576,"贫":6577,"贬":6578,"购":6579,"贮":6580,"贯":6581,"贰":6582,"贱":6583,"贲":6584,"贴":6585,"贵":6586,"贷":6587,"贸":6588,"费":6589,"贺":6590,"贻":6591,"贼":6592,"贾":6593,"贿":6594,"赁":6595,"赂":6596,"赃":6597,"资":6598,"赅":6599,"赈":6600,"赊":6601,"赋":6602,"赌":6603,"赎":6604,"赏":6605,"赐":6606,"赓":6607,"赔":6608,"赖":6609,"赘":6610,"赚":6611,"赛":6612,"赝":6613,"赞":6614,"赠":6615,"赡":6616,"赢":6617,"赣":6618,"赤":6619,"赦":6620,"赧":6621,"赫":6622,"赭":6623,"走":6624,"赳":6625,"赴":6626,"赵":6627,"赶":6628,"起":6629,"趁":6630,"超":6631,"越":6632,"趋":6633,"趕":6634,"趙":6635,"趟":6636,"趣":6637,"趨":6638,"足":6639,"趴":6640,"趵":6641,"趸":6642,"趺":6643,"趾":6644,"跃":6645,"跄":6646,"跆":6647,"跋":6648,"跌":6649,"跎":6650,"跑":6651,"跖":6652,"跚":6653,"跛":6654,"距":6655,"跟":6656,"跡":6657,"跤":6658,"跨":6659,"跩":6660,"跪":6661,"路":6662,"跳":6663,"践":6664,"跷":6665,"跹":6666,"跺":6667,"跻":6668,"踉":6669,"踊":6670,"踌":6671,"踏":6672,"踐":6673,"踝":6674,"踞":6675,"踟":6676,"踢":6677,"踩":6678,"踪":6679,"踮":6680,"踱":6681,"踴":6682,"踵":6683,"踹":6684,"蹂":6685,"蹄":6686,"蹇":6687,"蹈":6688,"蹉":6689,"蹊":6690,"蹋":6691,"蹑":6692,"蹒":6693,"蹙":6694,"蹟":6695,"蹣":6696,"蹤":6697,"蹦":6698,"蹩":6699,"蹬":6700,"蹭":6701,"蹲":6702,"蹴":6703,"蹶":6704,"蹺":6705,"蹼":6706,"蹿":6707,"躁":6708,"躇":6709,"躉":6710,"躊":6711,"躋":6712,"躍":6713,"躏":6714,"躪":6715,"身":6716,"躬":6717,"躯":6718,"躲":6719,"躺":6720,"軀":6721,"車":6722,"軋":6723,"軌":6724,"軍":6725,"軒":6726,"軟":6727,"転":6728,"軸":6729,"軼":6730,"軽":6731,"軾":6732,"較":6733,"載":6734,"輒":6735,"輓":6736,"輔":6737,"輕":6738,"輛":6739,"輝":6740,"輟":6741,"輩":6742,"輪":6743,"輯":6744,"輸":6745,"輻":6746,"輾":6747,"輿":6748,"轄":6749,"轅":6750,"轆":6751,"轉":6752,"轍":6753,"轎":6754,"轟":6755,"车":6756,"轧":6757,"轨":6758,"轩":6759,"转":6760,"轭":6761,"轮":6762,"软":6763,"轰":6764,"轲":6765,"轴":6766,"轶":6767,"轻":6768,"轼":6769,"载":6770,"轿":6771,"较":6772,"辄":6773,"辅":6774,"辆":6775,"辇":6776,"辈":6777,"辉":6778,"辊":6779,"辍":6780,"辐":6781,"辑":6782,"输":6783,"辕":6784,"辖":6785,"辗":6786,"辘":6787,"辙":6788,"辛":6789,"辜":6790,"辞":6791,"辟":6792,"辣":6793,"辦":6794,"辨":6795,"辩":6796,"辫":6797,"辭":6798,"辮":6799,"辯":6800,"辰":6801,"辱":6802,"農":6803,"边":6804,"辺":6805,"辻":6806,"込":6807,"辽":6808,"达":6809,"迁":6810,"迂":6811,"迄":6812,"迅":6813,"过":6814,"迈":6815,"迎":6816,"运":6817,"近":6818,"返":6819,"还":6820,"这":6821,"进":6822,"远":6823,"违":6824,"连":6825,"迟":6826,"迢":6827,"迤":6828,"迥":6829,"迦":6830,"迩":6831,"迪":6832,"迫":6833,"迭":6834,"述":6835,"迴":6836,"迷":6837,"迸":6838,"迹":6839,"迺":6840,"追":6841,"退":6842,"送":6843,"适":6844,"逃":6845,"逅":6846,"逆":6847,"选":6848,"逊":6849,"逍":6850,"透":6851,"逐":6852,"递":6853,"途":6854,"逕":6855,"逗":6856,"這":6857,"通":6858,"逛":6859,"逝":6860,"逞":6861,"速":6862,"造":6863,"逢":6864,"連":6865,"逮":6866,"週":6867,"進":6868,"逵":6869,"逶":6870,"逸":6871,"逻":6872,"逼":6873,"逾":6874,"遁":6875,"遂":6876,"遅":6877,"遇":6878,"遊":6879,"運":6880,"遍":6881,"過":6882,"遏":6883,"遐":6884,"遑":6885,"遒":6886,"道":6887,"達":6888,"違":6889,"遗":6890,"遙":6891,"遛":6892,"遜":6893,"遞":6894,"遠":6895,"遢":6896,"遣":6897,"遥":6898,"遨":6899,"適":6900,"遭":6901,"遮":6902,"遲":6903,"遴":6904,"遵":6905,"遶":6906,"遷":6907,"選":6908,"遺":6909,"遼":6910,"遽":6911,"避":6912,"邀":6913,"邁":6914,"邂":6915,"邃":6916,"還":6917,"邇":6918,"邈":6919,"邊":6920,"邋":6921,"邏":6922,"邑":6923,"邓":6924,"邕":6925,"邛":6926,"邝":6927,"邢":6928,"那":6929,"邦":6930,"邨":6931,"邪":6932,"邬":6933,"邮":6934,"邯":6935,"邰":6936,"邱":6937,"邳":6938,"邵":6939,"邸":6940,"邹":6941,"邺":6942,"邻":6943,"郁":6944,"郅":6945,"郊":6946,"郎":6947,"郑":6948,"郜":6949,"郝":6950,"郡":6951,"郢":6952,"郤":6953,"郦":6954,"郧":6955,"部":6956,"郫":6957,"郭":6958,"郴":6959,"郵":6960,"郷":6961,"郸":6962,"都":6963,"鄂":6964,"鄉":6965,"鄒":6966,"鄔":6967,"鄙":6968,"鄞":6969,"鄢":6970,"鄧":6971,"鄭":6972,"鄰":6973,"鄱":6974,"鄲":6975,"鄺":6976,"酉":6977,"酊":6978,"酋":6979,"酌":6980,"配":6981,"酐":6982,"酒":6983,"酗":6984,"酚":6985,"酝":6986,"酢":6987,"酣":6988,"酥":6989,"酩":6990,"酪":6991,"酬":6992,"酮":6993,"酯":6994,"酰":6995,"酱":6996,"酵":6997,"酶":6998,"酷":6999,"酸":7000,"酿":7001,"醃":7002,"醇":7003,"醉":7004,"醋":7005,"醍":7006,"醐":7007,"醒":7008,"醚":7009,"醛":7010,"醜":7011,"醞":7012,"醣":7013,"醪":7014,"醫":7015,"醬":7016,"醮":7017,"醯":7018,"醴":7019,"醺":7020,"釀":7021,"釁":7022,"采":7023,"釉":7024,"释":7025,"釋":7026,"里":7027,"重":7028,"野":7029,"量":7030,"釐":7031,"金":7032,"釗":7033,"釘":7034,"釜":7035,"針":7036,"釣":7037,"釦":7038,"釧":7039,"釵":7040,"鈀":7041,"鈉":7042,"鈍":7043,"鈎":7044,"鈔":7045,"鈕":7046,"鈞":7047,"鈣":7048,"鈦":7049,"鈪":7050,"鈴":7051,"鈺":7052,"鈾":7053,"鉀":7054,"鉄":7055,"鉅":7056,"鉉":7057,"鉑":7058,"鉗":7059,"鉚":7060,"鉛":7061,"鉤":7062,"鉴":7063,"鉻":7064,"銀":7065,"銃":7066,"銅":7067,"銑":7068,"銓":7069,"銖":7070,"銘":7071,"銜":7072,"銬":7073,"銭":7074,"銮":7075,"銳":7076,"銷":7077,"銹":7078,"鋁":7079,"鋅":7080,"鋒":7081,"鋤":7082,"鋪":7083,"鋰":7084,"鋸":7085,"鋼":7086,"錄":7087,"錐":7088,"錘":7089,"錚":7090,"錠":7091,"錢":7092,"錦":7093,"錨":7094,"錫":7095,"錮":7096,"錯":7097,"録":7098,"錳":7099,"錶":7100,"鍊":7101,"鍋":7102,"鍍":7103,"鍛":7104,"鍥":7105,"鍰":7106,"鍵":7107,"鍺":7108,"鍾":7109,"鎂":7110,"鎊":7111,"鎌":7112,"鎏":7113,"鎔":7114,"鎖":7115,"鎗":7116,"鎚":7117,"鎧":7118,"鎬":7119,"鎮":7120,"鎳":7121,"鏈":7122,"鏖":7123,"鏗":7124,"鏘":7125,"鏞":7126,"鏟":7127,"鏡":7128,"鏢":7129,"鏤":7130,"鏽":7131,"鐘":7132,"鐮":7133,"鐲":7134,"鐳":7135,"鐵":7136,"鐸":7137,"鐺":7138,"鑄":7139,"鑊":7140,"鑑":7141,"鑒":7142,"鑣":7143,"鑫":7144,"鑰":7145,"鑲":7146,"鑼":7147,"鑽":7148,"鑾":7149,"鑿":7150,"针":7151,"钉":7152,"钊":7153,"钎":7154,"钏":7155,"钒":7156,"钓":7157,"钗":7158,"钙":7159,"钛":7160,"钜":7161,"钝":7162,"钞":7163,"钟":7164,"钠":7165,"钡":7166,"钢":7167,"钣":7168,"钤":7169,"钥":7170,"钦":7171,"钧":7172,"钨":7173,"钩":7174,"钮":7175,"钯":7176,"钰":7177,"钱":7178,"钳":7179,"钴":7180,"钵":7181,"钺":7182,"钻":7183,"钼":7184,"钾":7185,"钿":7186,"铀":7187,"铁":7188,"铂":7189,"铃":7190,"铄":7191,"铅":7192,"铆":7193,"铉":7194,"铎":7195,"铐":7196,"铛":7197,"铜":7198,"铝":7199,"铠":7200,"铡":7201,"铢":7202,"铣":7203,"铤":7204,"铨":7205,"铩":7206,"铬":7207,"铭":7208,"铮":7209,"铰":7210,"铲":7211,"铵":7212,"银":7213,"铸":7214,"铺":7215,"链":7216,"铿":7217,"销":7218,"锁":7219,"锂":7220,"锄":7221,"锅":7222,"锆":7223,"锈":7224,"锉":7225,"锋":7226,"锌":7227,"锏":7228,"锐":7229,"锑":7230,"错":7231,"锚":7232,"锟":7233,"锡":7234,"锢":7235,"锣":7236,"锤":7237,"锥":7238,"锦":7239,"锭":7240,"键":7241,"锯":7242,"锰":7243,"锲":7244,"锵":7245,"锹":7246,"锺":7247,"锻":7248,"镀":7249,"镁":7250,"镂":7251,"镇":7252,"镉":7253,"镌":7254,"镍":7255,"镐":7256,"镑":7257,"镕":7258,"镖":7259,"镗":7260,"镛":7261,"镜":7262,"镣":7263,"镭":7264,"镯":7265,"镰":7266,"镳":7267,"镶":7268,"長":7269,"长":7270,"門":7271,"閃":7272,"閉":7273,"開":7274,"閎":7275,"閏":7276,"閑":7277,"閒":7278,"間":7279,"閔":7280,"閘":7281,"閡":7282,"関":7283,"閣":7284,"閥":7285,"閨":7286,"閩":7287,"閱":7288,"閲":7289,"閹":7290,"閻":7291,"閾":7292,"闆":7293,"闇":7294,"闊":7295,"闌":7296,"闍":7297,"闔":7298,"闕":7299,"闖":7300,"闘":7301,"關":7302,"闡":7303,"闢":7304,"门":7305,"闪":7306,"闫":7307,"闭":7308,"问":7309,"闯":7310,"闰":7311,"闲":7312,"间":7313,"闵":7314,"闷":7315,"闸":7316,"闹":7317,"闺":7318,"闻":7319,"闽":7320,"闾":7321,"阀":7322,"阁":7323,"阂":7324,"阅":7325,"阆":7326,"阇":7327,"阈":7328,"阉":7329,"阎":7330,"阐":7331,"阑":7332,"阔":7333,"阕":7334,"阖":7335,"阙":7336,"阚":7337,"阜":7338,"队":7339,"阡":7340,"阪":7341,"阮":7342,"阱":7343,"防":7344,"阳":7345,"阴":7346,"阵":7347,"阶":7348,"阻":7349,"阿":7350,"陀":7351,"陂":7352,"附":7353,"际":7354,"陆":7355,"陇":7356,"陈":7357,"陋":7358,"陌":7359,"降":7360,"限":7361,"陕":7362,"陛":7363,"陝":7364,"陞":7365,"陟":7366,"陡":7367,"院":7368,"陣":7369,"除":7370,"陨":7371,"险":7372,"陪":7373,"陰":7374,"陲":7375,"陳":7376,"陵":7377,"陶":7378,"陷":7379,"陸":7380,"険":7381,"陽":7382,"隅":7383,"隆":7384,"隈":7385,"隊":7386,"隋":7387,"隍":7388,"階":7389,"随":7390,"隐":7391,"隔":7392,"隕":7393,"隘":7394,"隙":7395,"際":7396,"障":7397,"隠":7398,"隣":7399,"隧":7400,"隨":7401,"險":7402,"隱":7403,"隴":7404,"隶":7405,"隸":7406,"隻":7407,"隼":7408,"隽":7409,"难":7410,"雀":7411,"雁":7412,"雄":7413,"雅":7414,"集":7415,"雇":7416,"雉":7417,"雋":7418,"雌":7419,"雍":7420,"雎":7421,"雏":7422,"雑":7423,"雒":7424,"雕":7425,"雖":7426,"雙":7427,"雛":7428,"雜":7429,"雞":7430,"離":7431,"難":7432,"雨":7433,"雪":7434,"雯":7435,"雰":7436,"雲":7437,"雳":7438,"零":7439,"雷":7440,"雹":7441,"電":7442,"雾":7443,"需":7444,"霁":7445,"霄":7446,"霆":7447,"震":7448,"霈":7449,"霉":7450,"霊":7451,"霍":7452,"霎":7453,"霏":7454,"霑":7455,"霓":7456,"霖":7457,"霜":7458,"霞":7459,"霧":7460,"霭":7461,"霰":7462,"露":7463,"霸":7464,"霹":7465,"霽":7466,"霾":7467,"靂":7468,"靄":7469,"靈":7470,"青":7471,"靓":7472,"靖":7473,"静":7474,"靚":7475,"靛":7476,"靜":7477,"非":7478,"靠":7479,"靡":7480,"面":7481,"靥":7482,"靦":7483,"革":7484,"靳":7485,"靴":7486,"靶":7487,"靼":7488,"鞅":7489,"鞋":7490,"鞍":7491,"鞏":7492,"鞑":7493,"鞘":7494,"鞠":7495,"鞣":7496,"鞦":7497,"鞭":7498,"韆":7499,"韋":7500,"韌":7501,"韓":7502,"韜":7503,"韦":7504,"韧":7505,"韩":7506,"韬":7507,"韭":7508,"音":7509,"韵":7510,"韶":7511,"韻":7512,"響":7513,"頁":7514,"頂":7515,"頃":7516,"項":7517,"順":7518,"須":7519,"頌":7520,"預":7521,"頑":7522,"頒":7523,"頓":7524,"頗":7525,"領":7526,"頜":7527,"頡":7528,"頤":7529,"頫":7530,"頭":7531,"頰":7532,"頷":7533,"頸":7534,"頹":7535,"頻":7536,"頼":7537,"顆":7538,"題":7539,"額":7540,"顎":7541,"顏":7542,"顔":7543,"願":7544,"顛":7545,"類":7546,"顧":7547,"顫":7548,"顯":7549,"顱":7550,"顴":7551,"页":7552,"顶":7553,"顷":7554,"项":7555,"顺":7556,"须":7557,"顼":7558,"顽":7559,"顾":7560,"顿":7561,"颁":7562,"颂":7563,"预":7564,"颅":7565,"领":7566,"颇":7567,"颈":7568,"颉":7569,"颊":7570,"颌":7571,"颍":7572,"颐":7573,"频":7574,"颓":7575,"颔":7576,"颖":7577,"颗":7578,"题":7579,"颚":7580,"颛":7581,"颜":7582,"额":7583,"颞":7584,"颠":7585,"颡":7586,"颢":7587,"颤":7588,"颦":7589,"颧":7590,"風":7591,"颯":7592,"颱":7593,"颳":7594,"颶":7595,"颼":7596,"飄":7597,"飆":7598,"风":7599,"飒":7600,"飓":7601,"飕":7602,"飘":7603,"飙":7604,"飚":7605,"飛":7606,"飞":7607,"食":7608,"飢":7609,"飨":7610,"飩":7611,"飪":7612,"飯":7613,"飲":7614,"飼":7615,"飽":7616,"飾":7617,"餃":7618,"餅":7619,"餉":7620,"養":7621,"餌":7622,"餐":7623,"餒":7624,"餓":7625,"餘":7626,"餚":7627,"餛":7628,"餞":7629,"餡":7630,"館":7631,"餮":7632,"餵":7633,"餾":7634,"饅":7635,"饈":7636,"饋":7637,"饌":7638,"饍":7639,"饑":7640,"饒":7641,"饕":7642,"饗":7643,"饞":7644,"饥":7645,"饨":7646,"饪":7647,"饬":7648,"饭":7649,"饮":7650,"饯":7651,"饰":7652,"饱":7653,"饲":7654,"饴":7655,"饵":7656,"饶":7657,"饷":7658,"饺":7659,"饼":7660,"饽":7661,"饿":7662,"馀":7663,"馁":7664,"馄":7665,"馅":7666,"馆":7667,"馈":7668,"馋":7669,"馍":7670,"馏":7671,"馒":7672,"馔":7673,"首":7674,"馗":7675,"香":7676,"馥":7677,"馨":7678,"馬":7679,"馭":7680,"馮":7681,"馳":7682,"馴":7683,"駁":7684,"駄":7685,"駅":7686,"駆":7687,"駐":7688,"駒":7689,"駕":7690,"駛":7691,"駝":7692,"駭":7693,"駱":7694,"駿":7695,"騁":7696,"騎":7697,"騏":7698,"験":7699,"騙":7700,"騨":7701,"騰":7702,"騷":7703,"驀":7704,"驅":7705,"驊":7706,"驍":7707,"驒":7708,"驕":7709,"驗":7710,"驚":7711,"驛":7712,"驟":7713,"驢":7714,"驥":7715,"马":7716,"驭":7717,"驮":7718,"驯":7719,"驰":7720,"驱":7721,"驳":7722,"驴":7723,"驶":7724,"驷":7725,"驸":7726,"驹":7727,"驻":7728,"驼":7729,"驾":7730,"驿":7731,"骁":7732,"骂":7733,"骄":7734,"骅":7735,"骆":7736,"骇":7737,"骈":7738,"骊":7739,"骋":7740,"验":7741,"骏":7742,"骐":7743,"骑":7744,"骗":7745,"骚":7746,"骛":7747,"骜":7748,"骞":7749,"骠":7750,"骡":7751,"骤":7752,"骥":7753,"骧":7754,"骨":7755,"骯":7756,"骰":7757,"骶":7758,"骷":7759,"骸":7760,"骼":7761,"髂":7762,"髅":7763,"髋":7764,"髏":7765,"髒":7766,"髓":7767,"體":7768,"髖":7769,"高":7770,"髦":7771,"髪":7772,"髮":7773,"髯":7774,"髻":7775,"鬃":7776,"鬆":7777,"鬍":7778,"鬓":7779,"鬚":7780,"鬟":7781,"鬢":7782,"鬣":7783,"鬥":7784,"鬧":7785,"鬱":7786,"鬼":7787,"魁":7788,"魂":7789,"魄":7790,"魅":7791,"魇":7792,"魍":7793,"魏":7794,"魔":7795,"魘":7796,"魚":7797,"魯":7798,"魷":7799,"鮑":7800,"鮨":7801,"鮪":7802,"鮭":7803,"鮮":7804,"鯉":7805,"鯊":7806,"鯖":7807,"鯛":7808,"鯨":7809,"鯰":7810,"鯽":7811,"鰍":7812,"鰓":7813,"鰭":7814,"鰲":7815,"鰻":7816,"鰾":7817,"鱈":7818,"鱉":7819,"鱔":7820,"鱗":7821,"鱷":7822,"鱸":7823,"鱼":7824,"鱿":7825,"鲁":7826,"鲈":7827,"鲍":7828,"鲑":7829,"鲛":7830,"鲜":7831,"鲟":7832,"鲢":7833,"鲤":7834,"鲨":7835,"鲫":7836,"鲱":7837,"鲲":7838,"鲶":7839,"鲷":7840,"鲸":7841,"鳃":7842,"鳄":7843,"鳅":7844,"鳌":7845,"鳍":7846,"鳕":7847,"鳖":7848,"鳗":7849,"鳝":7850,"鳞":7851,"鳥":7852,"鳩":7853,"鳳":7854,"鳴":7855,"鳶":7856,"鴉":7857,"鴕":7858,"鴛":7859,"鴦":7860,"鴨":7861,"鴻":7862,"鴿":7863,"鵑":7864,"鵜":7865,"鵝":7866,"鵡":7867,"鵬":7868,"鵰":7869,"鵲":7870,"鶘":7871,"鶩":7872,"鶯":7873,"鶴":7874,"鷗":7875,"鷲":7876,"鷹":7877,"鷺":7878,"鸚":7879,"鸞":7880,"鸟":7881,"鸠":7882,"鸡":7883,"鸢":7884,"鸣":7885,"鸥":7886,"鸦":7887,"鸨":7888,"鸪":7889,"鸭":7890,"鸯":7891,"鸳":7892,"鸵":7893,"鸽":7894,"鸾":7895,"鸿":7896,"鹂":7897,"鹃":7898,"鹄":7899,"鹅":7900,"鹈":7901,"鹉":7902,"鹊":7903,"鹌":7904,"鹏":7905,"鹑":7906,"鹕":7907,"鹘":7908,"鹜":7909,"鹞":7910,"鹤":7911,"鹦":7912,"鹧":7913,"鹫":7914,"鹭":7915,"鹰":7916,"鹳":7917,"鹵":7918,"鹹":7919,"鹼":7920,"鹽":7921,"鹿":7922,"麂":7923,"麋":7924,"麒":7925,"麓":7926,"麗":7927,"麝":7928,"麟":7929,"麥":7930,"麦":7931,"麩":7932,"麴":7933,"麵":7934,"麸":7935,"麺":7936,"麻":7937,"麼":7938,"麽":7939,"麾":7940,"黃":7941,"黄":7942,"黍":7943,"黎":7944,"黏":7945,"黑":7946,"黒":7947,"黔":7948,"默":7949,"黛":7950,"黜":7951,"黝":7952,"點":7953,"黠":7954,"黨":7955,"黯":7956,"黴":7957,"鼋":7958,"鼎":7959,"鼐":7960,"鼓":7961,"鼠":7962,"鼬":7963,"鼹":7964,"鼻":7965,"鼾":7966,"齁":7967,"齊":7968,"齋":7969,"齐":7970,"齒":7971,"齡":7972,"齢":7973,"齣":7974,"齦":7975,"齿":7976,"龄":7977,"龅":7978,"龈":7979,"龊":7980,"龋":7981,"龌":7982,"龍":7983,"龐":7984,"龔":7985,"龕":7986,"龙":7987,"龚":7988,"龛":7989,"龜":7990,"龟":7991,"︰":7992,"︱":7993,"︶":7994,"︿":7995,"﹁":7996,"﹂":7997,"﹍":7998,"﹏":7999,"﹐":8000,"﹑":8001,"﹒":8002,"﹔":8003,"﹕":8004,"﹖":8005,"﹗":8006,"﹙":8007,"﹚":8008,"﹝":8009,"﹞":8010,"﹡":8011,"﹣":8012,"!":8013,""":8014,"#":8015,"$":8016,"%":8017,"&":8018,"'":8019,"(":8020,")":8021,"*":8022,"+":8023,",":8024,"-":8025,".":8026,"/":8027,"0":8028,"1":8029,"2":8030,"3":8031,"4":8032,"5":8033,"6":8034,"7":8035,"8":8036,"9":8037,":":8038,";":8039,"<":8040,"=":8041,">":8042,"?":8043,"@":8044,"[":8045,"\":8046,"]":8047,"^":8048,"_":8049,"`":8050,"a":8051,"b":8052,"c":8053,"d":8054,"e":8055,"f":8056,"g":8057,"h":8058,"i":8059,"j":8060,"k":8061,"l":8062,"m":8063,"n":8064,"o":8065,"p":8066,"q":8067,"r":8068,"s":8069,"t":8070,"u":8071,"v":8072,"w":8073,"x":8074,"y":8075,"z":8076,"{":8077,"|":8078,"}":8079,"~":8080,"。":8081,"「":8082,"」":8083,"、":8084,"・":8085,"ッ":8086,"ー":8087,"イ":8088,"ク":8089,"シ":8090,"ス":8091,"ト":8092,"ノ":8093,"フ":8094,"ラ":8095,"ル":8096,"ン":8097,"゙":8098,"゚":8099," ̄":8100,"¥":8101,"👍":8102,"🔥":8103,"😂":8104,"😎":8105,"...":8106,"yam":8107,"10":8108,"2017":8109,"12":8110,"11":8111,"2016":8112,"20":8113,"30":8114,"15":8115,"06":8116,"lofter":8117,"##s":8118,"2015":8119,"by":8120,"16":8121,"14":8122,"18":8123,"13":8124,"24":8125,"17":8126,"2014":8127,"21":8128,"##0":8129,"22":8130,"19":8131,"25":8132,"23":8133,"com":8134,"100":8135,"00":8136,"05":8137,"2013":8138,"##a":8139,"03":8140,"09":8141,"08":8142,"28":8143,"##2":8144,"50":8145,"01":8146,"04":8147,"##1":8148,"27":8149,"02":8150,"2012":8151,"##3":8152,"26":8153,"##e":8154,"07":8155,"##8":8156,"##5":8157,"##6":8158,"##4":8159,"##9":8160,"##7":8161,"29":8162,"2011":8163,"40":8164,"##t":8165,"2010":8166,"##o":8167,"##d":8168,"##i":8169,"2009":8170,"##n":8171,"app":8172,"www":8173,"the":8174,"##m":8175,"31":8176,"##c":8177,"##l":8178,"##y":8179,"##r":8180,"##g":8181,"2008":8182,"60":8183,"http":8184,"200":8185,"qq":8186,"##p":8187,"80":8188,"##f":8189,"google":8190,"pixnet":8191,"90":8192,"cookies":8193,"tripadvisor":8194,"500":8195,"##er":8196,"##k":8197,"35":8198,"##h":8199,"facebook":8200,"2007":8201,"2000":8202,"70":8203,"##b":8204,"of":8205,"##x":8206,"##u":8207,"45":8208,"300":8209,"iphone":8210,"32":8211,"1000":8212,"2006":8213,"48":8214,"ip":8215,"36":8216,"in":8217,"38":8218,"3d":8219,"##w":8220,"##ing":8221,"55":8222,"ctrip":8223,"##on":8224,"##v":8225,"33":8226,"##の":8227,"to":8228,"34":8229,"400":8230,"id":8231,"2005":8232,"it":8233,"37":8234,"windows":8235,"llc":8236,"top":8237,"99":8238,"42":8239,"39":8240,"000":8241,"led":8242,"at":8243,"##an":8244,"41":8245,"51":8246,"52":8247,"46":8248,"49":8249,"43":8250,"53":8251,"44":8252,"##z":8253,"android":8254,"58":8255,"and":8256,"59":8257,"2004":8258,"56":8259,"vr":8260,"##か":8261,"5000":8262,"2003":8263,"47":8264,"blogthis":8265,"twitter":8266,"54":8267,"##le":8268,"150":8269,"ok":8270,"2018":8271,"57":8272,"75":8273,"cn":8274,"no":8275,"ios":8276,"##in":8277,"##mm":8278,"##00":8279,"800":8280,"on":8281,"te":8282,"3000":8283,"65":8284,"2001":8285,"360":8286,"95":8287,"ig":8288,"lv":8289,"120":8290,"##ng":8291,"##を":8292,"##us":8293,"##に":8294,"pc":8295,"てす":8296,"──":8297,"600":8298,"##te":8299,"85":8300,"2002":8301,"88":8302,"##ed":8303,"html":8304,"ncc":8305,"wifi":8306,"email":8307,"64":8308,"blog":8309,"is":8310,"##10":8311,"##て":8312,"mail":8313,"online":8314,"##al":8315,"dvd":8316,"##ic":8317,"studio":8318,"##は":8319,"##℃":8320,"##ia":8321,"##と":8322,"line":8323,"vip":8324,"72":8325,"##q":8326,"98":8327,"##ce":8328,"##en":8329,"for":8330,"##is":8331,"##ra":8332,"##es":8333,"##j":8334,"usb":8335,"net":8336,"cp":8337,"1999":8338,"asia":8339,"4g":8340,"##cm":8341,"diy":8342,"new":8343,"3c":8344,"##お":8345,"ta":8346,"66":8347,"language":8348,"vs":8349,"apple":8350,"tw":8351,"86":8352,"web":8353,"##ne":8354,"ipad":8355,"62":8356,"you":8357,"##re":8358,"101":8359,"68":8360,"##tion":8361,"ps":8362,"de":8363,"bt":8364,"pony":8365,"atm":8366,"##2017":8367,"1998":8368,"67":8369,"##ch":8370,"ceo":8371,"##or":8372,"go":8373,"##na":8374,"av":8375,"pro":8376,"cafe":8377,"96":8378,"pinterest":8379,"97":8380,"63":8381,"pixstyleme3c":8382,"##ta":8383,"more":8384,"said":8385,"##2016":8386,"1997":8387,"mp3":8388,"700":8389,"##ll":8390,"nba":8391,"jun":8392,"##20":8393,"92":8394,"tv":8395,"1995":8396,"pm":8397,"61":8398,"76":8399,"nbsp":8400,"250":8401,"##ie":8402,"linux":8403,"##ma":8404,"cd":8405,"110":8406,"hd":8407,"##17":8408,"78":8409,"##ion":8410,"77":8411,"6000":8412,"am":8413,"##th":8414,"##st":8415,"94":8416,"##se":8417,"##et":8418,"69":8419,"180":8420,"gdp":8421,"my":8422,"105":8423,"81":8424,"abc":8425,"89":8426,"flash":8427,"79":8428,"one":8429,"93":8430,"1990":8431,"1996":8432,"##ck":8433,"gps":8434,"##も":8435,"##ly":8436,"web885":8437,"106":8438,"2020":8439,"91":8440,"##ge":8441,"4000":8442,"1500":8443,"xd":8444,"boss":8445,"isbn":8446,"1994":8447,"org":8448,"##ry":8449,"me":8450,"love":8451,"##11":8452,"0fork":8453,"73":8454,"##12":8455,"3g":8456,"##ter":8457,"##ar":8458,"71":8459,"82":8460,"##la":8461,"hotel":8462,"130":8463,"1970":8464,"pk":8465,"83":8466,"87":8467,"140":8468,"ie":8469,"##os":8470,"##30":8471,"##el":8472,"74":8473,"##50":8474,"seo":8475,"cpu":8476,"##ml":8477,"p2p":8478,"84":8479,"may":8480,"##る":8481,"sun":8482,"tue":8483,"internet":8484,"cc":8485,"posted":8486,"youtube":8487,"##at":8488,"##ン":8489,"##man":8490,"ii":8491,"##ル":8492,"##15":8493,"abs":8494,"nt":8495,"pdf":8496,"yahoo":8497,"ago":8498,"1980":8499,"##it":8500,"news":8501,"mac":8502,"104":8503,"##てす":8504,"##me":8505,"##り":8506,"java":8507,"1992":8508,"spa":8509,"##de":8510,"##nt":8511,"hk":8512,"all":8513,"plus":8514,"la":8515,"1993":8516,"##mb":8517,"##16":8518,"##ve":8519,"west":8520,"##da":8521,"160":8522,"air":8523,"##い":8524,"##ps":8525,"から":8526,"##to":8527,"1989":8528,"logo":8529,"htc":8530,"php":8531,"https":8532,"fi":8533,"momo":8534,"##son":8535,"sat":8536,"##ke":8537,"##80":8538,"ebd":8539,"suv":8540,"wi":8541,"day":8542,"apk":8543,"##88":8544,"##um":8545,"mv":8546,"galaxy":8547,"wiki":8548,"or":8549,"brake":8550,"##ス":8551,"1200":8552,"する":8553,"this":8554,"1991":8555,"mon":8556,"##こ":8557,"❤2017":8558,"po":8559,"##ない":8560,"javascript":8561,"life":8562,"home":8563,"june":8564,"##ss":8565,"system":8566,"900":8567,"##ー":8568,"##0":8569,"pp":8570,"1988":8571,"world":8572,"fb":8573,"4k":8574,"br":8575,"##as":8576,"ic":8577,"ai":8578,"leonardo":8579,"safari":8580,"##60":8581,"live":8582,"free":8583,"xx":8584,"wed":8585,"win7":8586,"kiehl":8587,"##co":8588,"lg":8589,"o2o":8590,"##go":8591,"us":8592,"235":8593,"1949":8594,"mm":8595,"しい":8596,"vfm":8597,"kanye":8598,"##90":8599,"##2015":8600,"##id":8601,"jr":8602,"##ey":8603,"123":8604,"rss":8605,"##sa":8606,"##ro":8607,"##am":8608,"##no":8609,"thu":8610,"fri":8611,"350":8612,"##sh":8613,"##ki":8614,"103":8615,"comments":8616,"name":8617,"##のて":8618,"##pe":8619,"##ine":8620,"max":8621,"1987":8622,"8000":8623,"uber":8624,"##mi":8625,"##ton":8626,"wordpress":8627,"office":8628,"1986":8629,"1985":8630,"##ment":8631,"107":8632,"bd":8633,"win10":8634,"##ld":8635,"##li":8636,"gmail":8637,"bb":8638,"dior":8639,"##rs":8640,"##ri":8641,"##rd":8642,"##ます":8643,"up":8644,"cad":8645,"##®":8646,"dr":8647,"して":8648,"read":8649,"##21":8650,"をお":8651,"##io":8652,"##99":8653,"url":8654,"1984":8655,"pvc":8656,"paypal":8657,"show":8658,"policy":8659,"##40":8660,"##ty":8661,"##18":8662,"with":8663,"##★":8664,"##01":8665,"txt":8666,"102":8667,"##ba":8668,"dna":8669,"from":8670,"post":8671,"mini":8672,"ar":8673,"taiwan":8674,"john":8675,"##ga":8676,"privacy":8677,"agoda":8678,"##13":8679,"##ny":8680,"word":8681,"##24":8682,"##22":8683,"##by":8684,"##ur":8685,"##hz":8686,"1982":8687,"##ang":8688,"265":8689,"cookie":8690,"netscape":8691,"108":8692,"##ka":8693,"##~":8694,"##ad":8695,"house":8696,"share":8697,"note":8698,"ibm":8699,"code":8700,"hello":8701,"nike":8702,"sim":8703,"survey":8704,"##016":8705,"1979":8706,"1950":8707,"wikia":8708,"##32":8709,"##017":8710,"5g":8711,"cbc":8712,"##tor":8713,"##kg":8714,"1983":8715,"##rt":8716,"##14":8717,"campaign":8718,"store":8719,"2500":8720,"os":8721,"##ct":8722,"##ts":8723,"##°":8724,"170":8725,"api":8726,"##ns":8727,"365":8728,"excel":8729,"##な":8730,"##ao":8731,"##ら":8732,"##し":8733,"~~":8734,"##nd":8735,"university":8736,"163":8737,"には":8738,"518":8739,"##70":8740,"##ya":8741,"##il":8742,"##25":8743,"pierre":8744,"ipo":8745,"0020":8746,"897":8747,"##23":8748,"hotels":8749,"##ian":8750,"のお":8751,"125":8752,"years":8753,"6606":8754,"##ers":8755,"##26":8756,"high":8757,"##day":8758,"time":8759,"##ay":8760,"bug":8761,"##line":8762,"##く":8763,"##す":8764,"##be":8765,"xp":8766,"talk2yam":8767,"yamservice":8768,"10000":8769,"coco":8770,"##dy":8771,"sony":8772,"##ies":8773,"1978":8774,"microsoft":8775,"david":8776,"people":8777,"##ha":8778,"1960":8779,"instagram":8780,"intel":8781,"その":8782,"##ot":8783,"iso":8784,"1981":8785,"##va":8786,"115":8787,"##mo":8788,"##land":8789,"xxx":8790,"man":8791,"co":8792,"ltxsw":8793,"##ation":8794,"baby":8795,"220":8796,"##pa":8797,"##ol":8798,"1945":8799,"7000":8800,"tag":8801,"450":8802,"##ue":8803,"msn":8804,"##31":8805,"oppo":8806,"##ト":8807,"##ca":8808,"control":8809,"##om":8810,"st":8811,"chrome":8812,"##ure":8813,"##ん":8814,"be":8815,"##き":8816,"lol":8817,"##19":8818,"した":8819,"##bo":8820,"240":8821,"lady":8822,"##100":8823,"##way":8824,"##から":8825,"4600":8826,"##ko":8827,"##do":8828,"##un":8829,"4s":8830,"corporation":8831,"168":8832,"##ni":8833,"herme":8834,"##28":8835,"cp":8836,"978":8837,"##up":8838,"##06":8839,"ui":8840,"##ds":8841,"ppt":8842,"admin":8843,"three":8844,"します":8845,"bbc":8846,"re":8847,"128":8848,"##48":8849,"ca":8850,"##015":8851,"##35":8852,"hp":8853,"##ee":8854,"tpp":8855,"##た":8856,"##ive":8857,"××":8858,"root":8859,"##cc":8860,"##ました":8861,"##ble":8862,"##ity":8863,"adobe":8864,"park":8865,"114":8866,"et":8867,"oled":8868,"city":8869,"##ex":8870,"##ler":8871,"##ap":8872,"china":8873,"##book":8874,"20000":8875,"view":8876,"##ice":8877,"global":8878,"##km":8879,"your":8880,"hong":8881,"##mg":8882,"out":8883,"##ms":8884,"ng":8885,"ebay":8886,"##29":8887,"menu":8888,"ubuntu":8889,"##cy":8890,"rom":8891,"##view":8892,"open":8893,"ktv":8894,"do":8895,"server":8896,"##lo":8897,"if":8898,"english":8899,"##ね":8900,"##5":8901,"##oo":8902,"1600":8903,"##02":8904,"step1":8905,"kong":8906,"club":8907,"135":8908,"july":8909,"inc":8910,"1976":8911,"mr":8912,"hi":8913,"##net":8914,"touch":8915,"##ls":8916,"##ii":8917,"michael":8918,"lcd":8919,"##05":8920,"##33":8921,"phone":8922,"james":8923,"step2":8924,"1300":8925,"ios9":8926,"##box":8927,"dc":8928,"##2":8929,"##ley":8930,"samsung":8931,"111":8932,"280":8933,"pokemon":8934,"css":8935,"##ent":8936,"##les":8937,"いいえ":8938,"##1":8939,"s8":8940,"atom":8941,"play":8942,"bmw":8943,"##said":8944,"sa":8945,"etf":8946,"ctrl":8947,"♥yoyo♥":8948,"##55":8949,"2025":8950,"##2014":8951,"##66":8952,"adidas":8953,"amazon":8954,"1958":8955,"##ber":8956,"##ner":8957,"visa":8958,"##77":8959,"##der":8960,"1800":8961,"connectivity":8962,"##hi":8963,"firefox":8964,"109":8965,"118":8966,"hr":8967,"so":8968,"style":8969,"mark":8970,"pop":8971,"ol":8972,"skip":8973,"1975":8974,"as":8975,"##27":8976,"##ir":8977,"##61":8978,"190":8979,"mba":8980,"##う":8981,"##ai":8982,"le":8983,"##ver":8984,"1900":8985,"cafe2017":8986,"lte":8987,"super":8988,"113":8989,"129":8990,"##ron":8991,"amd":8992,"like":8993,"##☆":8994,"are":8995,"##ster":8996,"we":8997,"##sk":8998,"paul":8999,"data":9000,"international":9001,"##ft":9002,"longchamp":9003,"ssd":9004,"good":9005,"##ート":9006,"##ti":9007,"reply":9008,"##my":9009,"↓↓↓":9010,"apr":9011,"star":9012,"##ker":9013,"source":9014,"136":9015,"js":9016,"112":9017,"get":9018,"force":9019,"photo":9020,"##one":9021,"126":9022,"##2013":9023,"##ow":9024,"link":9025,"bbs":9026,"1972":9027,"goods":9028,"##lin":9029,"python":9030,"119":9031,"##ip":9032,"game":9033,"##ics":9034,"##ません":9035,"blue":9036,"##●":9037,"520":9038,"##45":9039,"page":9040,"itunes":9041,"##03":9042,"1955":9043,"260":9044,"1968":9045,"gt":9046,"gif":9047,"618":9048,"##ff":9049,"##47":9050,"group":9051,"くたさい":9052,"about":9053,"bar":9054,"ganji":9055,"##nce":9056,"music":9057,"lee":9058,"not":9059,"1977":9060,"1971":9061,"1973":9062,"##per":9063,"an":9064,"faq":9065,"comment":9066,"##って":9067,"days":9068,"##ock":9069,"116":9070,"##bs":9071,"1974":9072,"1969":9073,"v1":9074,"player":9075,"1956":9076,"xbox":9077,"sql":9078,"fm":9079,"f1":9080,"139":9081,"##ah":9082,"210":9083,"##lv":9084,"##mp":9085,"##000":9086,"melody":9087,"1957":9088,"##3":9089,"550":9090,"17life":9091,"199":9092,"1966":9093,"xml":9094,"market":9095,"##au":9096,"##71":9097,"999":9098,"##04":9099,"what":9100,"gl":9101,"##95":9102,"##age":9103,"tips":9104,"##68":9105,"book":9106,"##ting":9107,"mysql":9108,"can":9109,"1959":9110,"230":9111,"##ung":9112,"wonderland":9113,"watch":9114,"10℃":9115,"##ction":9116,"9000":9117,"mar":9118,"mobile":9119,"1946":9120,"1962":9121,"article":9122,"##db":9123,"part":9124,"▲top":9125,"party":9126,"って":9127,"1967":9128,"1964":9129,"1948":9130,"##07":9131,"##ore":9132,"##op":9133,"この":9134,"dj":9135,"##78":9136,"##38":9137,"010":9138,"main":9139,"225":9140,"1965":9141,"##ong":9142,"art":9143,"320":9144,"ad":9145,"134":9146,"020":9147,"##73":9148,"117":9149,"pm2":9150,"japan":9151,"228":9152,"##08":9153,"ts":9154,"1963":9155,"##ica":9156,"der":9157,"sm":9158,"##36":9159,"2019":9160,"##wa":9161,"ct":9162,"##7":9163,"##や":9164,"##64":9165,"1937":9166,"homemesh":9167,"search":9168,"##85":9169,"##れは":9170,"##tv":9171,"##di":9172,"macbook":9173,"##9":9174,"##くたさい":9175,"service":9176,"##♥":9177,"type":9178,"った":9179,"750":9180,"##ier":9181,"##si":9182,"##75":9183,"##います":9184,"##ok":9185,"best":9186,"##ット":9187,"goris":9188,"lock":9189,"##った":9190,"cf":9191,"3m":9192,"big":9193,"##ut":9194,"ftp":9195,"carol":9196,"##vi":9197,"10":9198,"1961":9199,"happy":9200,"sd":9201,"##ac":9202,"122":9203,"anti":9204,"pe":9205,"cnn":9206,"iii":9207,"1920":9208,"138":9209,"##ラ":9210,"1940":9211,"esp":9212,"jan":9213,"tags":9214,"##98":9215,"##51":9216,"august":9217,"vol":9218,"##86":9219,"154":9220,"##™":9221,"##fs":9222,"##れ":9223,"##sion":9224,"design":9225,"ac":9226,"##ム":9227,"press":9228,"jordan":9229,"ppp":9230,"that":9231,"key":9232,"check":9233,"##6":9234,"##tt":9235,"##㎡":9236,"1080p":9237,"##lt":9238,"power":9239,"##42":9240,"1952":9241,"##bc":9242,"vivi":9243,"##ック":9244,"he":9245,"133":9246,"121":9247,"jpg":9248,"##rry":9249,"201":9250,"175":9251,"3500":9252,"1947":9253,"nb":9254,"##ted":9255,"##rn":9256,"しています":9257,"1954":9258,"usd":9259,"##t00":9260,"master":9261,"##ンク":9262,"001":9263,"model":9264,"##58":9265,"al":9266,"##09":9267,"1953":9268,"##34":9269,"ram":9270,"goo":9271,"ても":9272,"##ui":9273,"127":9274,"1930":9275,"red":9276,"##ary":9277,"rpg":9278,"item":9279,"##pm":9280,"##41":9281,"270":9282,"##za":9283,"project":9284,"##2012":9285,"hot":9286,"td":9287,"blogabstract":9288,"##ger":9289,"##62":9290,"650":9291,"##44":9292,"gr2":9293,"##します":9294,"##m":9295,"black":9296,"electronic":9297,"nfc":9298,"year":9299,"asus":9300,"また":9301,"html5":9302,"cindy":9303,"##hd":9304,"m3":9305,"132":9306,"esc":9307,"##od":9308,"booking":9309,"##53":9310,"fed":9311,"tvb":9312,"##81":9313,"##ina":9314,"mit":9315,"165":9316,"##いる":9317,"chan":9318,"192":9319,"distribution":9320,"next":9321,"になる":9322,"peter":9323,"bios":9324,"steam":9325,"cm":9326,"1941":9327,"にも":9328,"pk10":9329,"##ix":9330,"##65":9331,"##91":9332,"dec":9333,"nasa":9334,"##ana":9335,"icecat":9336,"00z":9337,"b1":9338,"will":9339,"##46":9340,"li":9341,"se":9342,"##ji":9343,"##み":9344,"##ard":9345,"oct":9346,"##ain":9347,"jp":9348,"##ze":9349,"##bi":9350,"cio":9351,"##56":9352,"smart":9353,"h5":9354,"##39":9355,"##port":9356,"curve":9357,"vpn":9358,"##nm":9359,"##dia":9360,"utc":9361,"##あり":9362,"12345678910":9363,"##52":9364,"rmvb":9365,"chanel":9366,"a4":9367,"miss":9368,"##and":9369,"##im":9370,"media":9371,"who":9372,"##63":9373,"she":9374,"girl":9375,"5s":9376,"124":9377,"vera":9378,"##して":9379,"class":9380,"vivo":9381,"king":9382,"##フ":9383,"##ei":9384,"national":9385,"ab":9386,"1951":9387,"5cm":9388,"888":9389,"145":9390,"ipod":9391,"ap":9392,"1100":9393,"5mm":9394,"211":9395,"ms":9396,"2756":9397,"##69":9398,"mp4":9399,"msci":9400,"##po":9401,"##89":9402,"131":9403,"mg":9404,"index":9405,"380":9406,"##bit":9407,"##out":9408,"##zz":9409,"##97":9410,"##67":9411,"158":9412,"apec":9413,"##8":9414,"photoshop":9415,"opec":9416,"¥799":9417,"ては":9418,"##96":9419,"##tes":9420,"##ast":9421,"2g":9422,"○○":9423,"##ール":9424,"¥2899":9425,"##ling":9426,"##よ":9427,"##ory":9428,"1938":9429,"##ical":9430,"kitty":9431,"content":9432,"##43":9433,"step3":9434,"##cn":9435,"win8":9436,"155":9437,"vc":9438,"1400":9439,"iphone7":9440,"robert":9441,"##した":9442,"tcl":9443,"137":9444,"beauty":9445,"##87":9446,"en":9447,"dollars":9448,"##ys":9449,"##oc":9450,"step":9451,"pay":9452,"yy":9453,"a1":9454,"##2011":9455,"##lly":9456,"##ks":9457,"##♪":9458,"1939":9459,"188":9460,"download":9461,"1944":9462,"sep":9463,"exe":9464,"ph":9465,"います":9466,"school":9467,"gb":9468,"center":9469,"pr":9470,"street":9471,"##board":9472,"uv":9473,"##37":9474,"##lan":9475,"winrar":9476,"##que":9477,"##ua":9478,"##com":9479,"1942":9480,"1936":9481,"480":9482,"gpu":9483,"##4":9484,"ettoday":9485,"fu":9486,"tom":9487,"##54":9488,"##ren":9489,"##via":9490,"149":9491,"##72":9492,"b2b":9493,"144":9494,"##79":9495,"##tch":9496,"rose":9497,"arm":9498,"mb":9499,"##49":9500,"##ial":9501,"##nn":9502,"nvidia":9503,"step4":9504,"mvp":9505,"00㎡":9506,"york":9507,"156":9508,"##イ":9509,"how":9510,"cpi":9511,"591":9512,"2765":9513,"gov":9514,"kg":9515,"joe":9516,"##xx":9517,"mandy":9518,"pa":9519,"##ser":9520,"copyright":9521,"fashion":9522,"1935":9523,"don":9524,"##け":9525,"ecu":9526,"##ist":9527,"##art":9528,"erp":9529,"wap":9530,"have":9531,"##lm":9532,"talk":9533,"##ek":9534,"##ning":9535,"##if":9536,"ch":9537,"##ite":9538,"video":9539,"1943":9540,"cs":9541,"san":9542,"iot":9543,"look":9544,"##84":9545,"##2010":9546,"##ku":9547,"october":9548,"##ux":9549,"trump":9550,"##hs":9551,"##ide":9552,"box":9553,"141":9554,"first":9555,"##ins":9556,"april":9557,"##ight":9558,"##83":9559,"185":9560,"angel":9561,"protected":9562,"aa":9563,"151":9564,"162":9565,"x1":9566,"m2":9567,"##fe":9568,"##×":9569,"##ho":9570,"size":9571,"143":9572,"min":9573,"ofo":9574,"fun":9575,"gomaji":9576,"ex":9577,"hdmi":9578,"food":9579,"dns":9580,"march":9581,"chris":9582,"kevin":9583,"##のか":9584,"##lla":9585,"##pp":9586,"##ec":9587,"ag":9588,"ems":9589,"6s":9590,"720p":9591,"##rm":9592,"##ham":9593,"off":9594,"##92":9595,"asp":9596,"team":9597,"fandom":9598,"ed":9599,"299":9600,"▌♥":9601,"##ell":9602,"info":9603,"されています":9604,"##82":9605,"sina":9606,"4066":9607,"161":9608,"##able":9609,"##ctor":9610,"330":9611,"399":9612,"315":9613,"dll":9614,"rights":9615,"ltd":9616,"idc":9617,"jul":9618,"3kg":9619,"1927":9620,"142":9621,"ma":9622,"surface":9623,"##76":9624,"##ク":9625,"~~~":9626,"304":9627,"mall":9628,"eps":9629,"146":9630,"green":9631,"##59":9632,"map":9633,"space":9634,"donald":9635,"v2":9636,"sodu":9637,"##light":9638,"1931":9639,"148":9640,"1700":9641,"まて":9642,"310":9643,"reserved":9644,"htm":9645,"##han":9646,"##57":9647,"2d":9648,"178":9649,"mod":9650,"##ise":9651,"##tions":9652,"152":9653,"ti":9654,"##shi":9655,"doc":9656,"1933":9657,"icp":9658,"055":9659,"wang":9660,"##ram":9661,"shopping":9662,"aug":9663,"##pi":9664,"##well":9665,"now":9666,"wam":9667,"b2":9668,"からお":9669,"##hu":9670,"236":9671,"1928":9672,"##gb":9673,"266":9674,"f2":9675,"##93":9676,"153":9677,"mix":9678,"##ef":9679,"##uan":9680,"bwl":9681,"##plus":9682,"##res":9683,"core":9684,"##ess":9685,"tea":9686,"5℃":9687,"hktvmall":9688,"nhk":9689,"##ate":9690,"list":9691,"##ese":9692,"301":9693,"feb":9694,"4m":9695,"inn":9696,"ての":9697,"nov":9698,"159":9699,"12345":9700,"daniel":9701,"##ci":9702,"pass":9703,"##bet":9704,"##nk":9705,"coffee":9706,"202":9707,"ssl":9708,"airbnb":9709,"##ute":9710,"fbi":9711,"woshipm":9712,"skype":9713,"ea":9714,"cg":9715,"sp":9716,"##fc":9717,"##www":9718,"yes":9719,"edge":9720,"alt":9721,"007":9722,"##94":9723,"fpga":9724,"##ght":9725,"##gs":9726,"iso9001":9727,"さい":9728,"##ile":9729,"##wood":9730,"##uo":9731,"image":9732,"lin":9733,"icon":9734,"american":9735,"##em":9736,"1932":9737,"set":9738,"says":9739,"##king":9740,"##tive":9741,"blogger":9742,"##74":9743,"なと":9744,"256":9745,"147":9746,"##ox":9747,"##zy":9748,"##red":9749,"##ium":9750,"##lf":9751,"nokia":9752,"claire":9753,"##リ":9754,"##ding":9755,"november":9756,"lohas":9757,"##500":9758,"##tic":9759,"##マ":9760,"##cs":9761,"##ある":9762,"##che":9763,"##ire":9764,"##gy":9765,"##ult":9766,"db":9767,"january":9768,"win":9769,"##カ":9770,"166":9771,"road":9772,"ptt":9773,"##ま":9774,"##つ":9775,"198":9776,"##fa":9777,"##mer":9778,"anna":9779,"pchome":9780,"はい":9781,"udn":9782,"ef":9783,"420":9784,"##time":9785,"##tte":9786,"2030":9787,"##ア":9788,"g20":9789,"white":9790,"かかります":9791,"1929":9792,"308":9793,"garden":9794,"eleven":9795,"di":9796,"##おります":9797,"chen":9798,"309b":9799,"777":9800,"172":9801,"young":9802,"cosplay":9803,"ちてない":9804,"4500":9805,"bat":9806,"##123":9807,"##tra":9808,"##ては":9809,"kindle":9810,"npc":9811,"steve":9812,"etc":9813,"##ern":9814,"##|":9815,"call":9816,"xperia":9817,"ces":9818,"travel":9819,"sk":9820,"s7":9821,"##ous":9822,"1934":9823,"##int":9824,"みいたたけます":9825,"183":9826,"edu":9827,"file":9828,"cho":9829,"qr":9830,"##car":9831,"##our":9832,"186":9833,"##ant":9834,"##d":9835,"eric":9836,"1914":9837,"rends":9838,"##jo":9839,"##する":9840,"mastercard":9841,"##2000":9842,"kb":9843,"##min":9844,"290":9845,"##ino":9846,"vista":9847,"##ris":9848,"##ud":9849,"jack":9850,"2400":9851,"##set":9852,"169":9853,"pos":9854,"1912":9855,"##her":9856,"##ou":9857,"taipei":9858,"しく":9859,"205":9860,"beta":9861,"##ませんか":9862,"232":9863,"##fi":9864,"express":9865,"255":9866,"body":9867,"##ill":9868,"aphojoy":9869,"user":9870,"december":9871,"meiki":9872,"##ick":9873,"tweet":9874,"richard":9875,"##av":9876,"##ᆫ":9877,"iphone6":9878,"##dd":9879,"ちてすか":9880,"views":9881,"##mark":9882,"321":9883,"pd":9884,"##00":9885,"times":9886,"##▲":9887,"level":9888,"##ash":9889,"10g":9890,"point":9891,"5l":9892,"##ome":9893,"208":9894,"koreanmall":9895,"##ak":9896,"george":9897,"q2":9898,"206":9899,"wma":9900,"tcp":9901,"##200":9902,"スタッフ":9903,"full":9904,"mlb":9905,"##lle":9906,"##watch":9907,"tm":9908,"run":9909,"179":9910,"911":9911,"smith":9912,"business":9913,"##und":9914,"1919":9915,"color":9916,"##tal":9917,"222":9918,"171":9919,"##less":9920,"moon":9921,"4399":9922,"##rl":9923,"update":9924,"pcb":9925,"shop":9926,"499":9927,"157":9928,"little":9929,"なし":9930,"end":9931,"##mhz":9932,"van":9933,"dsp":9934,"easy":9935,"660":9936,"##house":9937,"##key":9938,"history":9939,"##o":9940,"oh":9941,"##001":9942,"##hy":9943,"##web":9944,"oem":9945,"let":9946,"was":9947,"##2009":9948,"##gg":9949,"review":9950,"##wan":9951,"182":9952,"##°c":9953,"203":9954,"uc":9955,"title":9956,"##val":9957,"united":9958,"233":9959,"2021":9960,"##ons":9961,"doi":9962,"trivago":9963,"overdope":9964,"sbs":9965,"##ance":9966,"##ち":9967,"grand":9968,"special":9969,"573032185":9970,"imf":9971,"216":9972,"wx17house":9973,"##so":9974,"##ーム":9975,"audi":9976,"##he":9977,"london":9978,"william":9979,"##rp":9980,"##ake":9981,"science":9982,"beach":9983,"cfa":9984,"amp":9985,"ps4":9986,"880":9987,"##800":9988,"##link":9989,"##hp":9990,"crm":9991,"ferragamo":9992,"bell":9993,"make":9994,"##eng":9995,"195":9996,"under":9997,"zh":9998,"photos":9999,"2300":10000,"##style":10001,"##ント":10002,"via":10003,"176":10004,"da":10005,"##gi":10006,"company":10007,"i7":10008,"##ray":10009,"thomas":10010,"370":10011,"ufo":10012,"i5":10013,"##max":10014,"plc":10015,"ben":10016,"back":10017,"research":10018,"8g":10019,"173":10020,"mike":10021,"##pc":10022,"##ッフ":10023,"september":10024,"189":10025,"##ace":10026,"vps":10027,"february":10028,"167":10029,"pantos":10030,"wp":10031,"lisa":10032,"1921":10033,"★★":10034,"jquery":10035,"night":10036,"long":10037,"offer":10038,"##berg":10039,"##news":10040,"1911":10041,"##いて":10042,"ray":10043,"fks":10044,"wto":10045,"せます":10046,"over":10047,"164":10048,"340":10049,"##all":10050,"##rus":10051,"1924":10052,"##888":10053,"##works":10054,"blogtitle":10055,"loftpermalink":10056,"##→":10057,"187":10058,"martin":10059,"test":10060,"ling":10061,"km":10062,"##め":10063,"15000":10064,"fda":10065,"v3":10066,"##ja":10067,"##ロ":10068,"wedding":10069,"かある":10070,"outlet":10071,"family":10072,"##ea":10073,"をこ":10074,"##top":10075,"story":10076,"##ness":10077,"salvatore":10078,"##lu":10079,"204":10080,"swift":10081,"215":10082,"room":10083,"している":10084,"oracle":10085,"##ul":10086,"1925":10087,"sam":10088,"b2c":10089,"week":10090,"pi":10091,"rock":10092,"##のは":10093,"##a":10094,"##けと":10095,"##ean":10096,"##300":10097,"##gle":10098,"cctv":10099,"after":10100,"chinese":10101,"##back":10102,"powered":10103,"x2":10104,"##tan":10105,"1918":10106,"##nes":10107,"##イン":10108,"canon":10109,"only":10110,"181":10111,"##zi":10112,"##las":10113,"say":10114,"##oe":10115,"184":10116,"##sd":10117,"221":10118,"##bot":10119,"##world":10120,"##zo":10121,"sky":10122,"made":10123,"top100":10124,"just":10125,"1926":10126,"pmi":10127,"802":10128,"234":10129,"gap":10130,"##vr":10131,"177":10132,"les":10133,"174":10134,"▲topoct":10135,"ball":10136,"vogue":10137,"vi":10138,"ing":10139,"ofweek":10140,"cos":10141,"##list":10142,"##ort":10143,"▲topmay":10144,"##なら":10145,"##lon":10146,"として":10147,"last":10148,"##tc":10149,"##of":10150,"##bus":10151,"##gen":10152,"real":10153,"eva":10154,"##コ":10155,"a3":10156,"nas":10157,"##lie":10158,"##ria":10159,"##coin":10160,"##bt":10161,"▲topapr":10162,"his":10163,"212":10164,"cat":10165,"nata":10166,"vive":10167,"health":10168,"⋯⋯":10169,"drive":10170,"sir":10171,"▲topmar":10172,"du":10173,"cup":10174,"##カー":10175,"##ook":10176,"##よう":10177,"##sy":10178,"alex":10179,"msg":10180,"tour":10181,"しました":10182,"3ce":10183,"##word":10184,"193":10185,"ebooks":10186,"r8":10187,"block":10188,"318":10189,"##より":10190,"2200":10191,"nice":10192,"pvp":10193,"207":10194,"months":10195,"1905":10196,"rewards":10197,"##ther":10198,"1917":10199,"0800":10200,"##xi":10201,"##チ":10202,"##sc":10203,"micro":10204,"850":10205,"gg":10206,"blogfp":10207,"op":10208,"1922":10209,"daily":10210,"m1":10211,"264":10212,"true":10213,"##bb":10214,"ml":10215,"##tar":10216,"##のお":10217,"##ky":10218,"anthony":10219,"196":10220,"253":10221,"##yo":10222,"state":10223,"218":10224,"##ara":10225,"##aa":10226,"##rc":10227,"##tz":10228,"##ston":10229,"より":10230,"gear":10231,"##eo":10232,"##ade":10233,"ge":10234,"see":10235,"1923":10236,"##win":10237,"##ura":10238,"ss":10239,"heart":10240,"##den":10241,"##ita":10242,"down":10243,"##sm":10244,"el":10245,"png":10246,"2100":10247,"610":10248,"rakuten":10249,"whatsapp":10250,"bay":10251,"dream":10252,"add":10253,"##use":10254,"680":10255,"311":10256,"pad":10257,"gucci":10258,"mpv":10259,"##ode":10260,"##fo":10261,"island":10262,"▲topjun":10263,"##▼":10264,"223":10265,"jason":10266,"214":10267,"chicago":10268,"##❤":10269,"しの":10270,"##hone":10271,"io":10272,"##れる":10273,"##ことか":10274,"sogo":10275,"be2":10276,"##ology":10277,"990":10278,"cloud":10279,"vcd":10280,"##con":10281,"2~3":10282,"##ford":10283,"##joy":10284,"##kb":10285,"##こさいます":10286,"##rade":10287,"but":10288,"##ach":10289,"docker":10290,"##ful":10291,"rfid":10292,"ul":10293,"##ase":10294,"hit":10295,"ford":10296,"##star":10297,"580":10298,"##○":10299,"11":10300,"a2":10301,"sdk":10302,"reading":10303,"edited":10304,"##are":10305,"cmos":10306,"##mc":10307,"238":10308,"siri":10309,"light":10310,"##ella":10311,"##ため":10312,"bloomberg":10313,"##read":10314,"pizza":10315,"##ison":10316,"jimmy":10317,"##vm":10318,"college":10319,"node":10320,"journal":10321,"ba":10322,"18k":10323,"##play":10324,"245":10325,"##cer":10326,"20":10327,"magic":10328,"##yu":10329,"191":10330,"jump":10331,"288":10332,"tt":10333,"##ings":10334,"asr":10335,"##lia":10336,"3200":10337,"step5":10338,"network":10339,"##cd":10340,"mc":10341,"いします":10342,"1234":10343,"pixstyleme":10344,"273":10345,"##600":10346,"2800":10347,"money":10348,"★★★★★":10349,"1280":10350,"12":10351,"430":10352,"bl":10353,"みの":10354,"act":10355,"##tus":10356,"tokyo":10357,"##rial":10358,"##life":10359,"emba":10360,"##ae":10361,"saas":10362,"tcs":10363,"##rk":10364,"##wang":10365,"summer":10366,"##sp":10367,"ko":10368,"##ving":10369,"390":10370,"premium":10371,"##その":10372,"netflix":10373,"##ヒ":10374,"uk":10375,"mt":10376,"##lton":10377,"right":10378,"frank":10379,"two":10380,"209":10381,"える":10382,"##ple":10383,"##cal":10384,"021":10385,"##んな":10386,"##sen":10387,"##ville":10388,"hold":10389,"nexus":10390,"dd":10391,"##ius":10392,"てお":10393,"##mah":10394,"##なく":10395,"tila":10396,"zero":10397,"820":10398,"ce":10399,"##tin":10400,"resort":10401,"##ws":10402,"charles":10403,"old":10404,"p10":10405,"5d":10406,"report":10407,"##360":10408,"##ru":10409,"##には":10410,"bus":10411,"vans":10412,"lt":10413,"##est":10414,"pv":10415,"##レ":10416,"links":10417,"rebecca":10418,"##ツ":10419,"##dm":10420,"azure":10421,"##365":10422,"きな":10423,"limited":10424,"bit":10425,"4gb":10426,"##mon":10427,"1910":10428,"moto":10429,"##eam":10430,"213":10431,"1913":10432,"var":10433,"eos":10434,"なとの":10435,"226":10436,"blogspot":10437,"された":10438,"699":10439,"e3":10440,"dos":10441,"dm":10442,"fc":10443,"##ments":10444,"##ik":10445,"##kw":10446,"boy":10447,"##bin":10448,"##ata":10449,"960":10450,"er":10451,"##せ":10452,"219":10453,"##vin":10454,"##tu":10455,"##ula":10456,"194":10457,"##∥":10458,"station":10459,"##ろ":10460,"##ature":10461,"835":10462,"files":10463,"zara":10464,"hdr":10465,"top10":10466,"nature":10467,"950":10468,"magazine":10469,"s6":10470,"marriott":10471,"##シ":10472,"avira":10473,"case":10474,"##っと":10475,"tab":10476,"##ran":10477,"tony":10478,"##home":10479,"oculus":10480,"im":10481,"##ral":10482,"jean":10483,"saint":10484,"cry":10485,"307":10486,"rosie":10487,"##force":10488,"##ini":10489,"ice":10490,"##bert":10491,"のある":10492,"##nder":10493,"##mber":10494,"pet":10495,"2600":10496,"##◆":10497,"plurk":10498,"▲topdec":10499,"##sis":10500,"00kg":10501,"▲topnov":10502,"720":10503,"##ence":10504,"tim":10505,"##ω":10506,"##nc":10507,"##ても":10508,"##name":10509,"log":10510,"ips":10511,"great":10512,"ikea":10513,"malaysia":10514,"unix":10515,"##イト":10516,"3600":10517,"##ncy":10518,"##nie":10519,"12000":10520,"akb48":10521,"##ye":10522,"##oid":10523,"404":10524,"##chi":10525,"##いた":10526,"oa":10527,"xuehai":10528,"##1000":10529,"##orm":10530,"##rf":10531,"275":10532,"さん":10533,"##ware":10534,"##リー":10535,"980":10536,"ho":10537,"##pro":10538,"text":10539,"##era":10540,"560":10541,"bob":10542,"227":10543,"##ub":10544,"##2008":10545,"8891":10546,"scp":10547,"avi":10548,"##zen":10549,"2022":10550,"mi":10551,"wu":10552,"museum":10553,"qvod":10554,"apache":10555,"lake":10556,"jcb":10557,"▲topaug":10558,"★★★":10559,"ni":10560,"##hr":10561,"hill":10562,"302":10563,"ne":10564,"weibo":10565,"490":10566,"ruby":10567,"##ーシ":10568,"##ヶ":10569,"##row":10570,"4d":10571,"▲topjul":10572,"iv":10573,"##ish":10574,"github":10575,"306":10576,"mate":10577,"312":10578,"##スト":10579,"##lot":10580,"##ane":10581,"andrew":10582,"のハイト":10583,"##tina":10584,"t1":10585,"rf":10586,"ed2k":10587,"##vel":10588,"##900":10589,"way":10590,"final":10591,"りの":10592,"ns":10593,"5a":10594,"705":10595,"197":10596,"##メ":10597,"sweet":10598,"bytes":10599,"##ene":10600,"▲topjan":10601,"231":10602,"##cker":10603,"##2007":10604,"##px":10605,"100g":10606,"topapp":10607,"229":10608,"helpapp":10609,"rs":10610,"low":10611,"14k":10612,"g4g":10613,"care":10614,"630":10615,"ldquo":10616,"あり":10617,"##fork":10618,"leave":10619,"rm":10620,"edition":10621,"##gan":10622,"##zon":10623,"##qq":10624,"▲topsep":10625,"##google":10626,"##ism":10627,"gold":10628,"224":10629,"explorer":10630,"##zer":10631,"toyota":10632,"category":10633,"select":10634,"visual":10635,"##labels":10636,"restaurant":10637,"##md":10638,"posts":10639,"s1":10640,"##ico":10641,"もっと":10642,"angelababy":10643,"123456":10644,"217":10645,"sports":10646,"s3":10647,"mbc":10648,"1915":10649,"してくたさい":10650,"shell":10651,"x86":10652,"candy":10653,"##new":10654,"kbs":10655,"face":10656,"xl":10657,"470":10658,"##here":10659,"4a":10660,"swissinfo":10661,"v8":10662,"▲topfeb":10663,"dram":10664,"##ual":10665,"##vice":10666,"3a":10667,"##wer":10668,"sport":10669,"q1":10670,"ios10":10671,"public":10672,"int":10673,"card":10674,"##c":10675,"ep":10676,"au":10677,"rt":10678,"##れた":10679,"1080":10680,"bill":10681,"##mll":10682,"kim":10683,"30":10684,"460":10685,"wan":10686,"##uk":10687,"##ミ":10688,"x3":10689,"298":10690,"0t":10691,"scott":10692,"##ming":10693,"239":10694,"e5":10695,"##3d":10696,"h7n9":10697,"worldcat":10698,"brown":10699,"##あります":10700,"##vo":10701,"##led":10702,"##580":10703,"##ax":10704,"249":10705,"410":10706,"##ert":10707,"paris":10708,"##~6":10709,"polo":10710,"925":10711,"##lr":10712,"599":10713,"##ナ":10714,"capital":10715,"##hing":10716,"bank":10717,"cv":10718,"1g":10719,"##chat":10720,"##s":10721,"##たい":10722,"adc":10723,"##ule":10724,"2m":10725,"##e":10726,"digital":10727,"hotmail":10728,"268":10729,"##pad":10730,"870":10731,"bbq":10732,"quot":10733,"##ring":10734,"before":10735,"wali":10736,"##まて":10737,"mcu":10738,"2k":10739,"2b":10740,"という":10741,"costco":10742,"316":10743,"north":10744,"333":10745,"switch":10746,"##city":10747,"##p":10748,"philips":10749,"##mann":10750,"management":10751,"panasonic":10752,"##cl":10753,"##vd":10754,"##ping":10755,"##rge":10756,"alice":10757,"##lk":10758,"##ましょう":10759,"css3":10760,"##ney":10761,"vision":10762,"alpha":10763,"##ular":10764,"##400":10765,"##tter":10766,"lz":10767,"にお":10768,"##ありません":10769,"mode":10770,"gre":10771,"1916":10772,"pci":10773,"##tm":10774,"237":10775,"1~2":10776,"##yan":10777,"##そ":10778,"について":10779,"##let":10780,"##キ":10781,"work":10782,"war":10783,"coach":10784,"ah":10785,"mary":10786,"##ᅵ":10787,"huang":10788,"##pt":10789,"a8":10790,"pt":10791,"follow":10792,"##berry":10793,"1895":10794,"##ew":10795,"a5":10796,"ghost":10797,"##ション":10798,"##wn":10799,"##og":10800,"south":10801,"##code":10802,"girls":10803,"##rid":10804,"action":10805,"villa":10806,"git":10807,"r11":10808,"table":10809,"games":10810,"##cket":10811,"error":10812,"##anonymoussaid":10813,"##ag":10814,"here":10815,"##ame":10816,"##gc":10817,"qa":10818,"##■":10819,"##lis":10820,"gmp":10821,"##gin":10822,"vmalife":10823,"##cher":10824,"yu":10825,"wedding":10826,"##tis":10827,"demo":10828,"dragon":10829,"530":10830,"soho":10831,"social":10832,"bye":10833,"##rant":10834,"river":10835,"orz":10836,"acer":10837,"325":10838,"##↑":10839,"##ース":10840,"##ats":10841,"261":10842,"del":10843,"##ven":10844,"440":10845,"ups":10846,"##ように":10847,"##ター":10848,"305":10849,"value":10850,"macd":10851,"yougou":10852,"##dn":10853,"661":10854,"##ano":10855,"ll":10856,"##urt":10857,"##rent":10858,"continue":10859,"script":10860,"##wen":10861,"##ect":10862,"paper":10863,"263":10864,"319":10865,"shift":10866,"##chel":10867,"##フト":10868,"##cat":10869,"258":10870,"x5":10871,"fox":10872,"243":10873,"##さん":10874,"car":10875,"aaa":10876,"##blog":10877,"loading":10878,"##yn":10879,"##tp":10880,"kuso":10881,"799":10882,"si":10883,"sns":10884,"イカせるテンマ":10885,"ヒンクテンマ3":10886,"rmb":10887,"vdc":10888,"forest":10889,"central":10890,"prime":10891,"help":10892,"ultra":10893,"##rmb":10894,"##ような":10895,"241":10896,"square":10897,"688":10898,"##しい":10899,"のないフロクに":10900,"##field":10901,"##reen":10902,"##ors":10903,"##ju":10904,"c1":10905,"start":10906,"510":10907,"##air":10908,"##map":10909,"cdn":10910,"##wo":10911,"cba":10912,"stephen":10913,"m8":10914,"100km":10915,"##get":10916,"opera":10917,"##base":10918,"##ood":10919,"vsa":10920,"com™":10921,"##aw":10922,"##ail":10923,"251":10924,"なのて":10925,"count":10926,"t2":10927,"##ᅡ":10928,"##een":10929,"2700":10930,"hop":10931,"##gp":10932,"vsc":10933,"tree":10934,"##eg":10935,"##ose":10936,"816":10937,"285":10938,"##ories":10939,"##shop":10940,"alphago":10941,"v4":10942,"1909":10943,"simon":10944,"##ᆼ":10945,"fluke62max":10946,"zip":10947,"スホンサー":10948,"##sta":10949,"louis":10950,"cr":10951,"bas":10952,"##~10":10953,"bc":10954,"##yer":10955,"hadoop":10956,"##ube":10957,"##wi":10958,"1906":10959,"0755":10960,"hola":10961,"##low":10962,"place":10963,"centre":10964,"5v":10965,"d3":10966,"##fer":10967,"252":10968,"##750":10969,"##media":10970,"281":10971,"540":10972,"0l":10973,"exchange":10974,"262":10975,"series":10976,"##ハー":10977,"##san":10978,"eb":10979,"##bank":10980,"##k":10981,"q3":10982,"##nge":10983,"##mail":10984,"take":10985,"##lp":10986,"259":10987,"1888":10988,"client":10989,"east":10990,"cache":10991,"event":10992,"vincent":10993,"##ールを":10994,"きを":10995,"##nse":10996,"sui":10997,"855":10998,"adchoice":10999,"##и":11000,"##stry":11001,"##なたの":11002,"246":11003,"##zone":11004,"ga":11005,"apps":11006,"sea":11007,"##ab":11008,"248":11009,"cisco":11010,"##タ":11011,"##rner":11012,"kymco":11013,"##care":11014,"dha":11015,"##pu":11016,"##yi":11017,"minkoff":11018,"royal":11019,"p1":11020,"への":11021,"annie":11022,"269":11023,"collection":11024,"kpi":11025,"playstation":11026,"257":11027,"になります":11028,"866":11029,"bh":11030,"##bar":11031,"queen":11032,"505":11033,"radio":11034,"1904":11035,"andy":11036,"armani":11037,"##xy":11038,"manager":11039,"iherb":11040,"##ery":11041,"##share":11042,"spring":11043,"raid":11044,"johnson":11045,"1908":11046,"##ob":11047,"volvo":11048,"hall":11049,"##ball":11050,"v6":11051,"our":11052,"taylor":11053,"##hk":11054,"bi":11055,"242":11056,"##cp":11057,"kate":11058,"bo":11059,"water":11060,"technology":11061,"##rie":11062,"サイトは":11063,"277":11064,"##ona":11065,"##sl":11066,"hpv":11067,"303":11068,"gtx":11069,"hip":11070,"rdquo":11071,"jayz":11072,"stone":11073,"##lex":11074,"##rum":11075,"namespace":11076,"##やり":11077,"620":11078,"##ale":11079,"##atic":11080,"des":11081,"##erson":11082,"##ql":11083,"##ves":11084,"##type":11085,"enter":11086,"##この":11087,"##てきます":11088,"d2":11089,"##168":11090,"##mix":11091,"##bian":11092,"との":11093,"a9":11094,"jj":11095,"ky":11096,"##lc":11097,"access":11098,"movie":11099,"##hc":11100,"リストに":11101,"tower":11102,"##ration":11103,"##mit":11104,"ます":11105,"##nch":11106,"ua":11107,"tel":11108,"prefix":11109,"##o2":11110,"1907":11111,"##point":11112,"1901":11113,"ott":11114,"~10":11115,"##http":11116,"##ury":11117,"baidu":11118,"##ink":11119,"member":11120,"##logy":11121,"bigbang":11122,"nownews":11123,"##js":11124,"##shot":11125,"##tb":11126,"##こと":11127,"247":11128,"eba":11129,"##tics":11130,"##lus":11131,"ける":11132,"v5":11133,"spark":11134,"##ama":11135,"there":11136,"##ions":11137,"god":11138,"##lls":11139,"##down":11140,"hiv":11141,"##ress":11142,"burberry":11143,"day2":11144,"##kv":11145,"◆◆":11146,"jeff":11147,"related":11148,"film":11149,"edit":11150,"joseph":11151,"283":11152,"##ark":11153,"cx":11154,"32gb":11155,"order":11156,"g9":11157,"30000":11158,"##ans":11159,"##tty":11160,"s5":11161,"##bee":11162,"かあります":11163,"thread":11164,"xr":11165,"buy":11166,"sh":11167,"005":11168,"land":11169,"spotify":11170,"mx":11171,"##ari":11172,"276":11173,"##verse":11174,"×email":11175,"sf":11176,"why":11177,"##ことて":11178,"244":11179,"7headlines":11180,"nego":11181,"sunny":11182,"dom":11183,"exo":11184,"401":11185,"666":11186,"positioning":11187,"fit":11188,"rgb":11189,"##tton":11190,"278":11191,"kiss":11192,"alexa":11193,"adam":11194,"lp":11195,"みリストを":11196,"##g":11197,"mp":11198,"##ties":11199,"##llow":11200,"amy":11201,"##du":11202,"np":11203,"002":11204,"institute":11205,"271":11206,"##rth":11207,"##lar":11208,"2345":11209,"590":11210,"##des":11211,"sidebar":11212,"15":11213,"imax":11214,"site":11215,"##cky":11216,"##kit":11217,"##ime":11218,"##009":11219,"season":11220,"323":11221,"##fun":11222,"##ンター":11223,"##ひ":11224,"gogoro":11225,"a7":11226,"pu":11227,"lily":11228,"fire":11229,"twd600":11230,"##ッセーシを":11231,"いて":11232,"##vis":11233,"30ml":11234,"##cture":11235,"##をお":11236,"information":11237,"##オ":11238,"close":11239,"friday":11240,"##くれる":11241,"yi":11242,"nick":11243,"てすか":11244,"##tta":11245,"##tel":11246,"6500":11247,"##lock":11248,"cbd":11249,"economy":11250,"254":11251,"かお":11252,"267":11253,"tinker":11254,"double":11255,"375":11256,"8gb":11257,"voice":11258,"##app":11259,"oops":11260,"channel":11261,"today":11262,"985":11263,"##right":11264,"raw":11265,"xyz":11266,"##+":11267,"jim":11268,"edm":11269,"##cent":11270,"7500":11271,"supreme":11272,"814":11273,"ds":11274,"##its":11275,"##asia":11276,"dropbox":11277,"##てすか":11278,"##tti":11279,"books":11280,"272":11281,"100ml":11282,"##tle":11283,"##ller":11284,"##ken":11285,"##more":11286,"##boy":11287,"sex":11288,"309":11289,"##dom":11290,"t3":11291,"##ider":11292,"##なります":11293,"##unch":11294,"1903":11295,"810":11296,"feel":11297,"5500":11298,"##かった":11299,"##put":11300,"により":11301,"s2":11302,"mo":11303,"##gh":11304,"men":11305,"ka":11306,"amoled":11307,"div":11308,"##tr":11309,"##n1":11310,"port":11311,"howard":11312,"##tags":11313,"ken":11314,"dnf":11315,"##nus":11316,"adsense":11317,"##а":11318,"ide":11319,"##へ":11320,"buff":11321,"thunder":11322,"##town":11323,"##ique":11324,"has":11325,"##body":11326,"auto":11327,"pin":11328,"##erry":11329,"tee":11330,"てした":11331,"295":11332,"number":11333,"##the":11334,"##013":11335,"object":11336,"psp":11337,"cool":11338,"udnbkk":11339,"16gb":11340,"##mic":11341,"miui":11342,"##tro":11343,"most":11344,"r2":11345,"##alk":11346,"##nity":11347,"1880":11348,"±0":11349,"##いました":11350,"428":11351,"s4":11352,"law":11353,"version":11354,"##oa":11355,"n1":11356,"sgs":11357,"docomo":11358,"##tf":11359,"##ack":11360,"henry":11361,"fc2":11362,"##ded":11363,"##sco":11364,"##014":11365,"##rite":11366,"286":11367,"0mm":11368,"linkedin":11369,"##ada":11370,"##now":11371,"wii":11372,"##ndy":11373,"ucbug":11374,"##◎":11375,"sputniknews":11376,"legalminer":11377,"##ika":11378,"##xp":11379,"2gb":11380,"##bu":11381,"q10":11382,"oo":11383,"b6":11384,"come":11385,"##rman":11386,"cheese":11387,"ming":11388,"maker":11389,"##gm":11390,"nikon":11391,"##fig":11392,"ppi":11393,"kelly":11394,"##ります":11395,"jchere":11396,"てきます":11397,"ted":11398,"md":11399,"003":11400,"fgo":11401,"tech":11402,"##tto":11403,"dan":11404,"soc":11405,"##gl":11406,"##len":11407,"hair":11408,"earth":11409,"640":11410,"521":11411,"img":11412,"##pper":11413,"##a1":11414,"##てきる":11415,"##ロク":11416,"acca":11417,"##ition":11418,"##ference":11419,"suite":11420,"##ig":11421,"outlook":11422,"##mond":11423,"##cation":11424,"398":11425,"##pr":11426,"279":11427,"101vip":11428,"358":11429,"##999":11430,"282":11431,"64gb":11432,"3800":11433,"345":11434,"airport":11435,"##over":11436,"284":11437,"##おり":11438,"jones":11439,"##ith":11440,"lab":11441,"##su":11442,"##いるのて":11443,"co2":11444,"town":11445,"piece":11446,"##llo":11447,"no1":11448,"vmware":11449,"24h":11450,"##qi":11451,"focus":11452,"reader":11453,"##admin":11454,"##ora":11455,"tb":11456,"false":11457,"##log":11458,"1898":11459,"know":11460,"lan":11461,"838":11462,"##ces":11463,"f4":11464,"##ume":11465,"motel":11466,"stop":11467,"##oper":11468,"na":11469,"flickr":11470,"netcomponents":11471,"##af":11472,"##─":11473,"pose":11474,"williams":11475,"local":11476,"##ound":11477,"##cg":11478,"##site":11479,"##iko":11480,"いお":11481,"274":11482,"5m":11483,"gsm":11484,"con":11485,"##ath":11486,"1902":11487,"friends":11488,"##hip":11489,"cell":11490,"317":11491,"##rey":11492,"780":11493,"cream":11494,"##cks":11495,"012":11496,"##dp":11497,"facebooktwitterpinterestgoogle":11498,"sso":11499,"324":11500,"shtml":11501,"song":11502,"swiss":11503,"##mw":11504,"##キンク":11505,"lumia":11506,"xdd":11507,"string":11508,"tiffany":11509,"522":11510,"marc":11511,"られた":11512,"insee":11513,"russell":11514,"sc":11515,"dell":11516,"##ations":11517,"ok":11518,"camera":11519,"289":11520,"##vs":11521,"##flow":11522,"##late":11523,"classic":11524,"287":11525,"##nter":11526,"stay":11527,"g1":11528,"mtv":11529,"512":11530,"##ever":11531,"##lab":11532,"##nger":11533,"qe":11534,"sata":11535,"ryan":11536,"d1":11537,"50ml":11538,"cms":11539,"##cing":11540,"su":11541,"292":11542,"3300":11543,"editor":11544,"296":11545,"##nap":11546,"security":11547,"sunday":11548,"association":11549,"##ens":11550,"##700":11551,"##bra":11552,"acg":11553,"##かり":11554,"sofascore":11555,"とは":11556,"mkv":11557,"##ign":11558,"jonathan":11559,"gary":11560,"build":11561,"labels":11562,"##oto":11563,"tesla":11564,"moba":11565,"qi":11566,"gohappy":11567,"general":11568,"ajax":11569,"1024":11570,"##かる":11571,"サイト":11572,"society":11573,"##test":11574,"##urs":11575,"wps":11576,"fedora":11577,"##ich":11578,"mozilla":11579,"328":11580,"##480":11581,"##dr":11582,"usa":11583,"urn":11584,"##lina":11585,"##r":11586,"grace":11587,"##die":11588,"##try":11589,"##ader":11590,"1250":11591,"##なり":11592,"elle":11593,"570":11594,"##chen":11595,"##ᆯ":11596,"price":11597,"##ten":11598,"uhz":11599,"##ough":11600,"eq":11601,"##hen":11602,"states":11603,"push":11604,"session":11605,"balance":11606,"wow":11607,"506":11608,"##cus":11609,"##py":11610,"when":11611,"##ward":11612,"##ep":11613,"34e":11614,"wong":11615,"library":11616,"prada":11617,"##サイト":11618,"##cle":11619,"running":11620,"##ree":11621,"313":11622,"ck":11623,"date":11624,"q4":11625,"##ctive":11626,"##ool":11627,"##>":11628,"mk":11629,"##ira":11630,"##163":11631,"388":11632,"die":11633,"secret":11634,"rq":11635,"dota":11636,"buffet":11637,"は1ヶ":11638,"e6":11639,"##ez":11640,"pan":11641,"368":11642,"ha":11643,"##card":11644,"##cha":11645,"2a":11646,"##さ":11647,"alan":11648,"day3":11649,"eye":11650,"f3":11651,"##end":11652,"france":11653,"keep":11654,"adi":11655,"rna":11656,"tvbs":11657,"##ala":11658,"solo":11659,"nova":11660,"##え":11661,"##tail":11662,"##ょう":11663,"support":11664,"##ries":11665,"##なる":11666,"##ved":11667,"base":11668,"copy":11669,"iis":11670,"fps":11671,"##ways":11672,"hero":11673,"hgih":11674,"profile":11675,"fish":11676,"mu":11677,"ssh":11678,"entertainment":11679,"chang":11680,"##wd":11681,"click":11682,"cake":11683,"##ond":11684,"pre":11685,"##tom":11686,"kic":11687,"pixel":11688,"##ov":11689,"##fl":11690,"product":11691,"6a":11692,"##pd":11693,"dear":11694,"##gate":11695,"es":11696,"yumi":11697,"audio":11698,"##²":11699,"##sky":11700,"echo":11701,"bin":11702,"where":11703,"##ture":11704,"329":11705,"##ape":11706,"find":11707,"sap":11708,"isis":11709,"##なと":11710,"nand":11711,"##101":11712,"##load":11713,"##ream":11714,"band":11715,"a6":11716,"525":11717,"never":11718,"##post":11719,"festival":11720,"50cm":11721,"##we":11722,"555":11723,"guide":11724,"314":11725,"zenfone":11726,"##ike":11727,"335":11728,"gd":11729,"forum":11730,"jessica":11731,"strong":11732,"alexander":11733,"##ould":11734,"software":11735,"allen":11736,"##ious":11737,"program":11738,"360°":11739,"else":11740,"lohasthree":11741,"##gar":11742,"することかてきます":11743,"please":11744,"##れます":11745,"rc":11746,"##ggle":11747,"##ric":11748,"bim":11749,"50000":11750,"##own":11751,"eclipse":11752,"355":11753,"brian":11754,"3ds":11755,"##side":11756,"061":11757,"361":11758,"##other":11759,"##ける":11760,"##tech":11761,"##ator":11762,"485":11763,"engine":11764,"##ged":11765,"##t":11766,"plaza":11767,"##fit":11768,"cia":11769,"ngo":11770,"westbrook":11771,"shi":11772,"tbs":11773,"50mm":11774,"##みませんか":11775,"sci":11776,"291":11777,"reuters":11778,"##ily":11779,"contextlink":11780,"##hn":11781,"af":11782,"##cil":11783,"bridge":11784,"very":11785,"##cel":11786,"1890":11787,"cambridge":11788,"##ize":11789,"15g":11790,"##aid":11791,"##data":11792,"790":11793,"frm":11794,"##head":11795,"award":11796,"butler":11797,"##sun":11798,"meta":11799,"##mar":11800,"america":11801,"ps3":11802,"puma":11803,"pmid":11804,"##すか":11805,"lc":11806,"670":11807,"kitchen":11808,"##lic":11809,"オーフン5":11810,"きなしソフトサーヒス":11811,"そして":11812,"day1":11813,"future":11814,"★★★★":11815,"##text":11816,"##page":11817,"##rris":11818,"pm1":11819,"##ket":11820,"fans":11821,"##っています":11822,"1001":11823,"christian":11824,"bot":11825,"kids":11826,"trackback":11827,"##hai":11828,"c3":11829,"display":11830,"##hl":11831,"n2":11832,"1896":11833,"idea":11834,"さんも":11835,"##sent":11836,"airmail":11837,"##ug":11838,"##men":11839,"pwm":11840,"けます":11841,"028":11842,"##lution":11843,"369":11844,"852":11845,"awards":11846,"schemas":11847,"354":11848,"asics":11849,"wikipedia":11850,"font":11851,"##tional":11852,"##vy":11853,"c2":11854,"293":11855,"##れている":11856,"##dget":11857,"##ein":11858,"っている":11859,"contact":11860,"pepper":11861,"スキル":11862,"339":11863,"##~5":11864,"294":11865,"##uel":11866,"##ument":11867,"730":11868,"##hang":11869,"みてす":11870,"q5":11871,"##sue":11872,"rain":11873,"##ndi":11874,"wei":11875,"swatch":11876,"##cept":11877,"わせ":11878,"331":11879,"popular":11880,"##ste":11881,"##tag":11882,"p2":11883,"501":11884,"trc":11885,"1899":11886,"##west":11887,"##live":11888,"justin":11889,"honda":11890,"ping":11891,"messenger":11892,"##rap":11893,"v9":11894,"543":11895,"##とは":11896,"unity":11897,"appqq":11898,"はすへて":11899,"025":11900,"leo":11901,"##tone":11902,"##テ":11903,"##ass":11904,"uniqlo":11905,"##010":11906,"502":11907,"her":11908,"jane":11909,"memory":11910,"moneydj":11911,"##tical":11912,"human":11913,"12306":11914,"していると":11915,"##m2":11916,"coc":11917,"miacare":11918,"##mn":11919,"tmt":11920,"##core":11921,"vim":11922,"kk":11923,"##may":11924,"fan":11925,"target":11926,"use":11927,"too":11928,"338":11929,"435":11930,"2050":11931,"867":11932,"737":11933,"fast":11934,"##2c":11935,"services":11936,"##ope":11937,"omega":11938,"energy":11939,"##わ":11940,"pinkoi":11941,"1a":11942,"##なから":11943,"##rain":11944,"jackson":11945,"##ement":11946,"##シャンルの":11947,"374":11948,"366":11949,"そんな":11950,"p9":11951,"rd":11952,"##ᆨ":11953,"1111":11954,"##tier":11955,"##vic":11956,"zone":11957,"##│":11958,"385":11959,"690":11960,"dl":11961,"isofix":11962,"cpa":11963,"m4":11964,"322":11965,"kimi":11966,"めて":11967,"davis":11968,"##lay":11969,"lulu":11970,"##uck":11971,"050":11972,"weeks":11973,"qs":11974,"##hop":11975,"920":11976,"##n":11977,"ae":11978,"##ear":11979,"~5":11980,"eia":11981,"405":11982,"##fly":11983,"korea":11984,"jpeg":11985,"boost":11986,"##ship":11987,"small":11988,"##リア":11989,"1860":11990,"eur":11991,"297":11992,"425":11993,"valley":11994,"##iel":11995,"simple":11996,"##ude":11997,"rn":11998,"k2":11999,"##ena":12000,"されます":12001,"non":12002,"patrick":12003,"しているから":12004,"##ナー":12005,"feed":12006,"5757":12007,"30g":12008,"process":12009,"well":12010,"qqmei":12011,"##thing":12012,"they":12013,"aws":12014,"lu":12015,"pink":12016,"##ters":12017,"##kin":12018,"または":12019,"board":12020,"##vertisement":12021,"wine":12022,"##ien":12023,"unicode":12024,"##dge":12025,"r1":12026,"359":12027,"##tant":12028,"いを":12029,"##twitter":12030,"##3c":12031,"cool1":12032,"される":12033,"##れて":12034,"##l":12035,"isp":12036,"##012":12037,"standard":12038,"45㎡2":12039,"402":12040,"##150":12041,"matt":12042,"##fu":12043,"326":12044,"##iner":12045,"googlemsn":12046,"pixnetfacebookyahoo":12047,"##ラン":12048,"x7":12049,"886":12050,"##uce":12051,"メーカー":12052,"sao":12053,"##ev":12054,"##きました":12055,"##file":12056,"9678":12057,"403":12058,"xddd":12059,"shirt":12060,"6l":12061,"##rio":12062,"##hat":12063,"3mm":12064,"givenchy":12065,"ya":12066,"bang":12067,"##lio":12068,"monday":12069,"crystal":12070,"ロクイン":12071,"##abc":12072,"336":12073,"head":12074,"890":12075,"ubuntuforumwikilinuxpastechat":12076,"##vc":12077,"##~20":12078,"##rity":12079,"cnc":12080,"7866":12081,"ipv6":12082,"null":12083,"1897":12084,"##ost":12085,"yang":12086,"imsean":12087,"tiger":12088,"##fet":12089,"##ンス":12090,"352":12091,"##=":12092,"dji":12093,"327":12094,"ji":12095,"maria":12096,"##come":12097,"##んて":12098,"foundation":12099,"3100":12100,"##beth":12101,"##なった":12102,"1m":12103,"601":12104,"active":12105,"##aft":12106,"##don":12107,"3p":12108,"sr":12109,"349":12110,"emma":12111,"##khz":12112,"living":12113,"415":12114,"353":12115,"1889":12116,"341":12117,"709":12118,"457":12119,"sas":12120,"x6":12121,"##face":12122,"pptv":12123,"x4":12124,"##mate":12125,"han":12126,"sophie":12127,"##jing":12128,"337":12129,"fifa":12130,"##mand":12131,"other":12132,"sale":12133,"inwedding":12134,"##gn":12135,"てきちゃいます":12136,"##mmy":12137,"##pmlast":12138,"bad":12139,"nana":12140,"nbc":12141,"してみてくたさいね":12142,"なとはお":12143,"##wu":12144,"##かあります":12145,"##あ":12146,"note7":12147,"single":12148,"##340":12149,"せからこ":12150,"してくたさい♪この":12151,"しにはとんとんワークケートを":12152,"するとあなたにもっとマッチした":12153,"ならワークケートへ":12154,"もみつかっちゃうかも":12155,"ワークケートの":12156,"##bel":12157,"window":12158,"##dio":12159,"##ht":12160,"union":12161,"age":12162,"382":12163,"14":12164,"##ivity":12165,"##y":12166,"コメント":12167,"domain":12168,"neo":12169,"##isa":12170,"##lter":12171,"5k":12172,"f5":12173,"steven":12174,"##cts":12175,"powerpoint":12176,"tft":12177,"self":12178,"g2":12179,"ft":12180,"##テル":12181,"zol":12182,"##act":12183,"mwc":12184,"381":12185,"343":12186,"もう":12187,"nbapop":12188,"408":12189,"てある":12190,"eds":12191,"ace":12192,"##room":12193,"previous":12194,"author":12195,"tomtom":12196,"il":12197,"##ets":12198,"hu":12199,"financial":12200,"☆☆☆":12201,"っています":12202,"bp":12203,"5t":12204,"chi":12205,"1gb":12206,"##hg":12207,"fairmont":12208,"cross":12209,"008":12210,"gay":12211,"h2":12212,"function":12213,"##けて":12214,"356":12215,"also":12216,"1b":12217,"625":12218,"##ータ":12219,"##raph":12220,"1894":12221,"3~5":12222,"##ils":12223,"i3":12224,"334":12225,"avenue":12226,"##host":12227,"による":12228,"##bon":12229,"##tsu":12230,"message":12231,"navigation":12232,"50g":12233,"fintech":12234,"h6":12235,"##ことを":12236,"8cm":12237,"##ject":12238,"##vas":12239,"##firm":12240,"credit":12241,"##wf":12242,"xxxx":12243,"form":12244,"##nor":12245,"##space":12246,"huawei":12247,"plan":12248,"json":12249,"sbl":12250,"##dc":12251,"machine":12252,"921":12253,"392":12254,"wish":12255,"##120":12256,"##sol":12257,"windows7":12258,"edward":12259,"##ために":12260,"development":12261,"washington":12262,"##nsis":12263,"lo":12264,"818":12265,"##sio":12266,"##ym":12267,"##bor":12268,"planet":12269,"##~8":12270,"##wt":12271,"ieee":12272,"gpa":12273,"##めて":12274,"camp":12275,"ann":12276,"gm":12277,"##tw":12278,"##oka":12279,"connect":12280,"##rss":12281,"##work":12282,"##atus":12283,"wall":12284,"chicken":12285,"soul":12286,"2mm":12287,"##times":12288,"fa":12289,"##ather":12290,"##cord":12291,"009":12292,"##eep":12293,"hitachi":12294,"gui":12295,"harry":12296,"##pan":12297,"e1":12298,"disney":12299,"##press":12300,"##ーション":12301,"wind":12302,"386":12303,"frigidaire":12304,"##tl":12305,"liu":12306,"hsu":12307,"332":12308,"basic":12309,"von":12310,"ev":12311,"いた":12312,"てきる":12313,"スホンサーサイト":12314,"learning":12315,"##ull":12316,"expedia":12317,"archives":12318,"change":12319,"##wei":12320,"santa":12321,"cut":12322,"ins":12323,"6gb":12324,"turbo":12325,"brand":12326,"cf1":12327,"508":12328,"004":12329,"return":12330,"747":12331,"##rip":12332,"h1":12333,"##nis":12334,"##をこ":12335,"128gb":12336,"##にお":12337,"3t":12338,"application":12339,"しており":12340,"emc":12341,"rx":12342,"##oon":12343,"384":12344,"quick":12345,"412":12346,"15058":12347,"wilson":12348,"wing":12349,"chapter":12350,"##bug":12351,"beyond":12352,"##cms":12353,"##dar":12354,"##oh":12355,"zoom":12356,"e2":12357,"trip":12358,"sb":12359,"##nba":12360,"rcep":12361,"342":12362,"aspx":12363,"ci":12364,"080":12365,"gc":12366,"gnu":12367,"める":12368,"##count":12369,"advanced":12370,"dance":12371,"dv":12372,"##url":12373,"##ging":12374,"367":12375,"8591":12376,"am09":12377,"shadow":12378,"battle":12379,"346":12380,"##i":12381,"##cia":12382,"##という":12383,"emily":12384,"##のてす":12385,"##tation":12386,"host":12387,"ff":12388,"techorz":12389,"sars":12390,"##mini":12391,"##mporary":12392,"##ering":12393,"nc":12394,"4200":12395,"798":12396,"##next":12397,"cma":12398,"##mbps":12399,"##gas":12400,"##ift":12401,"##dot":12402,"##ィ":12403,"455":12404,"##~17":12405,"amana":12406,"##りの":12407,"426":12408,"##ros":12409,"ir":12410,"00㎡1":12411,"##eet":12412,"##ible":12413,"##↓":12414,"710":12415,"ˋ▽ˊ":12416,"##aka":12417,"dcs":12418,"iq":12419,"##v":12420,"l1":12421,"##lor":12422,"maggie":12423,"##011":12424,"##iu":12425,"588":12426,"##~1":12427,"830":12428,"##gt":12429,"1tb":12430,"articles":12431,"create":12432,"##burg":12433,"##iki":12434,"database":12435,"fantasy":12436,"##rex":12437,"##cam":12438,"dlc":12439,"dean":12440,"##you":12441,"hard":12442,"path":12443,"gaming":12444,"victoria":12445,"maps":12446,"cb":12447,"##lee":12448,"##itor":12449,"overchicstoretvhome":12450,"systems":12451,"##xt":12452,"416":12453,"p3":12454,"sarah":12455,"760":12456,"##nan":12457,"407":12458,"486":12459,"x9":12460,"install":12461,"second":12462,"626":12463,"##ann":12464,"##ph":12465,"##rcle":12466,"##nic":12467,"860":12468,"##nar":12469,"ec":12470,"##とう":12471,"768":12472,"metro":12473,"chocolate":12474,"##rian":12475,"~4":12476,"##table":12477,"##しています":12478,"skin":12479,"##sn":12480,"395":12481,"mountain":12482,"##0mm":12483,"inparadise":12484,"6m":12485,"7x24":12486,"ib":12487,"4800":12488,"##jia":12489,"eeworld":12490,"creative":12491,"g5":12492,"g3":12493,"357":12494,"parker":12495,"ecfa":12496,"village":12497,"からの":12498,"18000":12499,"sylvia":12500,"サーヒス":12501,"hbl":12502,"##ques":12503,"##onsored":12504,"##x2":12505,"##きます":12506,"##v4":12507,"##tein":12508,"ie6":12509,"383":12510,"##stack":12511,"389":12512,"ver":12513,"##ads":12514,"##baby":12515,"sound":12516,"bbe":12517,"##110":12518,"##lone":12519,"##uid":12520,"ads":12521,"022":12522,"gundam":12523,"351":12524,"thinkpad":12525,"006":12526,"scrum":12527,"match":12528,"##ave":12529,"mems":12530,"##470":12531,"##oy":12532,"##なりました":12533,"##talk":12534,"glass":12535,"lamigo":12536,"span":12537,"##eme":12538,"job":12539,"##a5":12540,"jay":12541,"wade":12542,"kde":12543,"498":12544,"##lace":12545,"ocean":12546,"tvg":12547,"##covery":12548,"##r3":12549,"##ners":12550,"##rea":12551,"junior":12552,"think":12553,"##aine":12554,"cover":12555,"##ision":12556,"##sia":12557,"↓↓":12558,"##bow":12559,"msi":12560,"413":12561,"458":12562,"406":12563,"##love":12564,"711":12565,"801":12566,"soft":12567,"z2":12568,"##pl":12569,"456":12570,"1840":12571,"mobil":12572,"mind":12573,"##uy":12574,"427":12575,"nginx":12576,"##oi":12577,"めた":12578,"##rr":12579,"6221":12580,"##mple":12581,"##sson":12582,"##ーシてす":12583,"371":12584,"##nts":12585,"91tv":12586,"comhd":12587,"crv3000":12588,"##uard":12589,"1868":12590,"397":12591,"deep":12592,"lost":12593,"field":12594,"gallery":12595,"##bia":12596,"rate":12597,"spf":12598,"redis":12599,"traction":12600,"930":12601,"icloud":12602,"011":12603,"なら":12604,"fe":12605,"jose":12606,"372":12607,"##tory":12608,"into":12609,"sohu":12610,"fx":12611,"899":12612,"379":12613,"kicstart2":12614,"##hia":12615,"すく":12616,"##~3":12617,"##sit":12618,"ra":12619,"24":12620,"##walk":12621,"##xure":12622,"500g":12623,"##pact":12624,"pacific":12625,"xa":12626,"natural":12627,"carlo":12628,"##250":12629,"##walker":12630,"1850":12631,"##can":12632,"cto":12633,"gigi":12634,"516":12635,"##サー":12636,"pen":12637,"##hoo":12638,"ob":12639,"matlab":12640,"##b":12641,"##yy":12642,"13913459":12643,"##iti":12644,"mango":12645,"##bbs":12646,"sense":12647,"c5":12648,"oxford":12649,"##ニア":12650,"walker":12651,"jennifer":12652,"##ola":12653,"course":12654,"##bre":12655,"701":12656,"##pus":12657,"##rder":12658,"lucky":12659,"075":12660,"##ぁ":12661,"ivy":12662,"なお":12663,"##nia":12664,"sotheby":12665,"side":12666,"##ugh":12667,"joy":12668,"##orage":12669,"##ush":12670,"##bat":12671,"##dt":12672,"364":12673,"r9":12674,"##2d":12675,"##gio":12676,"511":12677,"country":12678,"wear":12679,"##lax":12680,"##~7":12681,"##moon":12682,"393":12683,"seven":12684,"study":12685,"411":12686,"348":12687,"lonzo":12688,"8k":12689,"##ェ":12690,"evolution":12691,"##イフ":12692,"##kk":12693,"gs":12694,"kd":12695,"##レス":12696,"arduino":12697,"344":12698,"b12":12699,"##lux":12700,"arpg":12701,"##rdon":12702,"cook":12703,"##x5":12704,"dark":12705,"five":12706,"##als":12707,"##ida":12708,"とても":12709,"sign":12710,"362":12711,"##ちの":12712,"something":12713,"20mm":12714,"##nda":12715,"387":12716,"##posted":12717,"fresh":12718,"tf":12719,"1870":12720,"422":12721,"cam":12722,"##mine":12723,"##skip":12724,"##form":12725,"##ssion":12726,"education":12727,"394":12728,"##tee":12729,"dyson":12730,"stage":12731,"##jie":12732,"want":12733,"##night":12734,"epson":12735,"pack":12736,"あります":12737,"##ppy":12738,"テリヘル":12739,"##█":12740,"wd":12741,"##eh":12742,"##rence":12743,"left":12744,"##lvin":12745,"golden":12746,"mhz":12747,"discovery":12748,"##trix":12749,"##n2":12750,"loft":12751,"##uch":12752,"##dra":12753,"##sse":12754,"speed":12755,"~1":12756,"1mdb":12757,"sorry":12758,"welcome":12759,"##urn":12760,"wave":12761,"gaga":12762,"##lmer":12763,"teddy":12764,"##160":12765,"トラックハック":12766,"せよ":12767,"611":12768,"##f2016":12769,"378":12770,"rp":12771,"##sha":12772,"rar":12773,"##あなたに":12774,"##きた":12775,"840":12776,"holiday":12777,"##ュー":12778,"373":12779,"074":12780,"##vg":12781,"##nos":12782,"##rail":12783,"gartner":12784,"gi":12785,"6p":12786,"##dium":12787,"kit":12788,"488":12789,"b3":12790,"eco":12791,"##ろう":12792,"20g":12793,"sean":12794,"##stone":12795,"autocad":12796,"nu":12797,"##np":12798,"f16":12799,"write":12800,"029":12801,"m5":12802,"##ias":12803,"images":12804,"atp":12805,"##dk":12806,"fsm":12807,"504":12808,"1350":12809,"ve":12810,"52kb":12811,"##xxx":12812,"##のに":12813,"##cake":12814,"414":12815,"unit":12816,"lim":12817,"ru":12818,"1v":12819,"##ification":12820,"published":12821,"angela":12822,"16g":12823,"analytics":12824,"ak":12825,"##q":12826,"##nel":12827,"gmt":12828,"##icon":12829,"again":12830,"##₂":12831,"##bby":12832,"ios11":12833,"445":12834,"かこさいます":12835,"waze":12836,"いてす":12837,"##ハ":12838,"9985":12839,"##ust":12840,"##ティー":12841,"framework":12842,"##007":12843,"iptv":12844,"delete":12845,"52sykb":12846,"cl":12847,"wwdc":12848,"027":12849,"30cm":12850,"##fw":12851,"##ての":12852,"1389":12853,"##xon":12854,"brandt":12855,"##ses":12856,"##dragon":12857,"tc":12858,"vetements":12859,"anne":12860,"monte":12861,"modern":12862,"official":12863,"##へて":12864,"##ere":12865,"##nne":12866,"##oud":12867,"もちろん":12868,"50":12869,"etnews":12870,"##a2":12871,"##graphy":12872,"421":12873,"863":12874,"##ちゃん":12875,"444":12876,"##rtex":12877,"##てお":12878,"l2":12879,"##gma":12880,"mount":12881,"ccd":12882,"たと":12883,"archive":12884,"morning":12885,"tan":12886,"ddos":12887,"e7":12888,"##ホ":12889,"day4":12890,"##ウ":12891,"gis":12892,"453":12893,"its":12894,"495":12895,"factory":12896,"bruce":12897,"pg":12898,"##ito":12899,"ってくたさい":12900,"guest":12901,"cdma":12902,"##lling":12903,"536":12904,"n3":12905,"しかし":12906,"3~4":12907,"mega":12908,"eyes":12909,"ro":12910,"13":12911,"women":12912,"dac":12913,"church":12914,"##jun":12915,"singapore":12916,"##facebook":12917,"6991":12918,"starbucks":12919,"##tos":12920,"##stin":12921,"##shine":12922,"zen":12923,"##mu":12924,"tina":12925,"20℃":12926,"1893":12927,"##たけて":12928,"503":12929,"465":12930,"request":12931,"##gence":12932,"qt":12933,"##っ":12934,"1886":12935,"347":12936,"363":12937,"q7":12938,"##zzi":12939,"diary":12940,"##tore":12941,"409":12942,"##ead":12943,"468":12944,"cst":12945,"##osa":12946,"canada":12947,"agent":12948,"va":12949,"##jiang":12950,"##ちは":12951,"##ーク":12952,"##lam":12953,"sg":12954,"##nix":12955,"##sday":12956,"##よって":12957,"g6":12958,"##master":12959,"bing":12960,"##zl":12961,"charlie":12962,"16":12963,"8mm":12964,"nb40":12965,"##ーン":12966,"thai":12967,"##ルフ":12968,"ln284ct":12969,"##itz":12970,"##2f":12971,"bonnie":12972,"##food":12973,"##lent":12974,"originals":12975,"##stro":12976,"##lts":12977,"418":12978,"∟∣":12979,"##bscribe":12980,"children":12981,"ntd":12982,"yesstyle":12983,"##かも":12984,"hmv":12985,"##tment":12986,"d5":12987,"2cm":12988,"arts":12989,"sms":12990,"##pn":12991,"##я":12992,"##いい":12993,"topios9":12994,"539":12995,"lifestyle":12996,"virtual":12997,"##ague":12998,"xz":12999,"##deo":13000,"muji":13001,"024":13002,"unt":13003,"##nnis":13004,"##ᅩ":13005,"faq1":13006,"1884":13007,"396":13008,"##ette":13009,"fly":13010,"64㎡":13011,"はしめまして":13012,"441":13013,"curry":13014,"##pop":13015,"のこ":13016,"release":13017,"##←":13018,"##◆◆":13019,"##cast":13020,"073":13021,"ありな":13022,"500ml":13023,"##ews":13024,"5c":13025,"##stle":13026,"ios7":13027,"##ima":13028,"787":13029,"dog":13030,"lenovo":13031,"##r4":13032,"roger":13033,"013":13034,"cbs":13035,"vornado":13036,"100m":13037,"417":13038,"##desk":13039,"##クok":13040,"##ald":13041,"1867":13042,"9595":13043,"2900":13044,"##van":13045,"oil":13046,"##x":13047,"some":13048,"break":13049,"common":13050,"##jy":13051,"##lines":13052,"g7":13053,"twice":13054,"419":13055,"ella":13056,"nano":13057,"belle":13058,"にこ":13059,"##mes":13060,"##self":13061,"##note":13062,"jb":13063,"##ことかてきます":13064,"benz":13065,"##との":13066,"##ova":13067,"451":13068,"save":13069,"##wing":13070,"##ますのて":13071,"kai":13072,"りは":13073,"##hua":13074,"##rect":13075,"rainer":13076,"##unge":13077,"448":13078,"##0m":13079,"adsl":13080,"##かな":13081,"guestname":13082,"##uma":13083,"##kins":13084,"##zu":13085,"tokichoi":13086,"##price":13087,"county":13088,"##med":13089,"##mus":13090,"rmk":13091,"391":13092,"address":13093,"vm":13094,"えて":13095,"openload":13096,"##group":13097,"##hin":13098,"##iginal":13099,"amg":13100,"urban":13101,"##oz":13102,"jobs":13103,"emi":13104,"##public":13105,"beautiful":13106,"##sch":13107,"album":13108,"##dden":13109,"##bell":13110,"jerry":13111,"works":13112,"hostel":13113,"miller":13114,"##drive":13115,"##rmin":13116,"##10":13117,"376":13118,"boot":13119,"828":13120,"##370":13121,"##fx":13122,"##cm~":13123,"1885":13124,"##nome":13125,"##ctionary":13126,"##oman":13127,"##lish":13128,"##cr":13129,"##hm":13130,"433":13131,"##how":13132,"432":13133,"francis":13134,"xi":13135,"c919":13136,"b5":13137,"evernote":13138,"##uc":13139,"vga":13140,"##3000":13141,"coupe":13142,"##urg":13143,"##cca":13144,"##uality":13145,"019":13146,"6g":13147,"れる":13148,"multi":13149,"##また":13150,"##ett":13151,"em":13152,"hey":13153,"##ani":13154,"##tax":13155,"##rma":13156,"inside":13157,"than":13158,"740":13159,"leonnhurt":13160,"##jin":13161,"ict":13162,"れた":13163,"bird":13164,"notes":13165,"200mm":13166,"くの":13167,"##dical":13168,"##lli":13169,"result":13170,"442":13171,"iu":13172,"ee":13173,"438":13174,"smap":13175,"gopro":13176,"##last":13177,"yin":13178,"pure":13179,"998":13180,"32g":13181,"けた":13182,"5kg":13183,"##dan":13184,"##rame":13185,"mama":13186,"##oot":13187,"bean":13188,"marketing":13189,"##hur":13190,"2l":13191,"bella":13192,"sync":13193,"xuite":13194,"##ground":13195,"515":13196,"discuz":13197,"##getrelax":13198,"##ince":13199,"##bay":13200,"##5s":13201,"cj":13202,"##イス":13203,"gmat":13204,"apt":13205,"##pass":13206,"jing":13207,"##rix":13208,"c4":13209,"rich":13210,"##とても":13211,"niusnews":13212,"##ello":13213,"bag":13214,"770":13215,"##eting":13216,"##mobile":13217,"18":13218,"culture":13219,"015":13220,"##のてすか":13221,"377":13222,"1020":13223,"area":13224,"##ience":13225,"616":13226,"details":13227,"gp":13228,"universal":13229,"silver":13230,"dit":13231,"はお":13232,"private":13233,"ddd":13234,"u11":13235,"kanshu":13236,"##ified":13237,"fung":13238,"##nny":13239,"dx":13240,"##520":13241,"tai":13242,"475":13243,"023":13244,"##fr":13245,"##lean":13246,"3s":13247,"##pin":13248,"429":13249,"##rin":13250,"25000":13251,"ly":13252,"rick":13253,"##bility":13254,"usb3":13255,"banner":13256,"##baru":13257,"##gion":13258,"metal":13259,"dt":13260,"vdf":13261,"1871":13262,"karl":13263,"qualcomm":13264,"bear":13265,"1010":13266,"oldid":13267,"ian":13268,"jo":13269,"##tors":13270,"population":13271,"##ernel":13272,"1882":13273,"mmorpg":13274,"##mv":13275,"##bike":13276,"603":13277,"##©":13278,"ww":13279,"friend":13280,"##ager":13281,"exhibition":13282,"##del":13283,"##pods":13284,"fpx":13285,"structure":13286,"##free":13287,"##tings":13288,"kl":13289,"##rley":13290,"##copyright":13291,"##mma":13292,"california":13293,"3400":13294,"orange":13295,"yoga":13296,"4l":13297,"canmake":13298,"honey":13299,"##anda":13300,"##コメント":13301,"595":13302,"nikkie":13303,"##ルハイト":13304,"dhl":13305,"publishing":13306,"##mall":13307,"##gnet":13308,"20cm":13309,"513":13310,"##クセス":13311,"##┅":13312,"e88":13313,"970":13314,"##dog":13315,"fishbase":13316,"##!":13317,"##\"":13318,"###":13319,"##$":13320,"##%":13321,"##&":13322,"##'":13323,"##(":13324,"##)":13325,"##*":13326,"##+":13327,"##,":13328,"##-":13329,"##.":13330,"##/":13331,"##:":13332,"##;":13333,"##<":13334,"##=":13335,"##>":13336,"##?":13337,"##@":13338,"##[":13339,"##\\":13340,"##]":13341,"##^":13342,"##_":13343,"##{":13344,"##|":13345,"##}":13346,"##~":13347,"##£":13348,"##¤":13349,"##¥":13350,"##§":13351,"##«":13352,"##±":13353,"##³":13354,"##µ":13355,"##·":13356,"##¹":13357,"##º":13358,"##»":13359,"##¼":13360,"##ß":13361,"##æ":13362,"##÷":13363,"##ø":13364,"##đ":13365,"##ŋ":13366,"##ɔ":13367,"##ə":13368,"##ɡ":13369,"##ʰ":13370,"##ˇ":13371,"##ˈ":13372,"##ˊ":13373,"##ˋ":13374,"##ˍ":13375,"##ː":13376,"##˙":13377,"##˚":13378,"##ˢ":13379,"##α":13380,"##β":13381,"##γ":13382,"##δ":13383,"##ε":13384,"##η":13385,"##θ":13386,"##ι":13387,"##κ":13388,"##λ":13389,"##μ":13390,"##ν":13391,"##ο":13392,"##π":13393,"##ρ":13394,"##ς":13395,"##σ":13396,"##τ":13397,"##υ":13398,"##φ":13399,"##χ":13400,"##ψ":13401,"##б":13402,"##в":13403,"##г":13404,"##д":13405,"##е":13406,"##ж":13407,"##з":13408,"##к":13409,"##л":13410,"##м":13411,"##н":13412,"##о":13413,"##п":13414,"##р":13415,"##с":13416,"##т":13417,"##у":13418,"##ф":13419,"##х":13420,"##ц":13421,"##ч":13422,"##ш":13423,"##ы":13424,"##ь":13425,"##і":13426,"##ا":13427,"##ب":13428,"##ة":13429,"##ت":13430,"##د":13431,"##ر":13432,"##س":13433,"##ع":13434,"##ل":13435,"##م":13436,"##ن":13437,"##ه":13438,"##و":13439,"##ي":13440,"##۩":13441,"##ก":13442,"##ง":13443,"##น":13444,"##ม":13445,"##ย":13446,"##ร":13447,"##อ":13448,"##า":13449,"##เ":13450,"##๑":13451,"##་":13452,"##ღ":13453,"##ᄀ":13454,"##ᄁ":13455,"##ᄂ":13456,"##ᄃ":13457,"##ᄅ":13458,"##ᄆ":13459,"##ᄇ":13460,"##ᄈ":13461,"##ᄉ":13462,"##ᄋ":13463,"##ᄌ":13464,"##ᄎ":13465,"##ᄏ":13466,"##ᄐ":13467,"##ᄑ":13468,"##ᄒ":13469,"##ᅢ":13470,"##ᅣ":13471,"##ᅥ":13472,"##ᅦ":13473,"##ᅧ":13474,"##ᅨ":13475,"##ᅪ":13476,"##ᅬ":13477,"##ᅭ":13478,"##ᅮ":13479,"##ᅯ":13480,"##ᅲ":13481,"##ᅳ":13482,"##ᅴ":13483,"##ᆷ":13484,"##ᆸ":13485,"##ᆺ":13486,"##ᆻ":13487,"##ᗜ":13488,"##ᵃ":13489,"##ᵉ":13490,"##ᵍ":13491,"##ᵏ":13492,"##ᵐ":13493,"##ᵒ":13494,"##ᵘ":13495,"##‖":13496,"##„":13497,"##†":13498,"##•":13499,"##‥":13500,"##‧":13501,"##
":13502,"##‰":13503,"##′":13504,"##″":13505,"##‹":13506,"##›":13507,"##※":13508,"##‿":13509,"##⁄":13510,"##ⁱ":13511,"##⁺":13512,"##ⁿ":13513,"##₁":13514,"##₃":13515,"##₄":13516,"##€":13517,"##№":13518,"##ⅰ":13519,"##ⅱ":13520,"##ⅲ":13521,"##ⅳ":13522,"##ⅴ":13523,"##↔":13524,"##↗":13525,"##↘":13526,"##⇒":13527,"##∀":13528,"##−":13529,"##∕":13530,"##∙":13531,"##√":13532,"##∞":13533,"##∟":13534,"##∠":13535,"##∣":13536,"##∩":13537,"##∮":13538,"##∶":13539,"##∼":13540,"##∽":13541,"##≈":13542,"##≒":13543,"##≡":13544,"##≤":13545,"##≥":13546,"##≦":13547,"##≧":13548,"##≪":13549,"##≫":13550,"##⊙":13551,"##⋅":13552,"##⋈":13553,"##⋯":13554,"##⌒":13555,"##①":13556,"##②":13557,"##③":13558,"##④":13559,"##⑤":13560,"##⑥":13561,"##⑦":13562,"##⑧":13563,"##⑨":13564,"##⑩":13565,"##⑴":13566,"##⑵":13567,"##⑶":13568,"##⑷":13569,"##⑸":13570,"##⒈":13571,"##⒉":13572,"##⒊":13573,"##⒋":13574,"##ⓒ":13575,"##ⓔ":13576,"##ⓘ":13577,"##━":13578,"##┃":13579,"##┆":13580,"##┊":13581,"##┌":13582,"##└":13583,"##├":13584,"##┣":13585,"##═":13586,"##║":13587,"##╚":13588,"##╞":13589,"##╠":13590,"##╭":13591,"##╮":13592,"##╯":13593,"##╰":13594,"##╱":13595,"##╳":13596,"##▂":13597,"##▃":13598,"##▅":13599,"##▇":13600,"##▉":13601,"##▋":13602,"##▌":13603,"##▍":13604,"##▎":13605,"##□":13606,"##▪":13607,"##▫":13608,"##▬":13609,"##△":13610,"##▶":13611,"##►":13612,"##▽":13613,"##◇":13614,"##◕":13615,"##◠":13616,"##◢":13617,"##◤":13618,"##☀":13619,"##☕":13620,"##☞":13621,"##☺":13622,"##☼":13623,"##♀":13624,"##♂":13625,"##♠":13626,"##♡":13627,"##♣":13628,"##♦":13629,"##♫":13630,"##♬":13631,"##✈":13632,"##✔":13633,"##✕":13634,"##✖":13635,"##✦":13636,"##✨":13637,"##✪":13638,"##✰":13639,"##✿":13640,"##❀":13641,"##➜":13642,"##➤":13643,"##⦿":13644,"##、":13645,"##。":13646,"##〃":13647,"##々":13648,"##〇":13649,"##〈":13650,"##〉":13651,"##《":13652,"##》":13653,"##「":13654,"##」":13655,"##『":13656,"##』":13657,"##【":13658,"##】":13659,"##〓":13660,"##〔":13661,"##〕":13662,"##〖":13663,"##〗":13664,"##〜":13665,"##〝":13666,"##〞":13667,"##ぃ":13668,"##ぇ":13669,"##ぬ":13670,"##ふ":13671,"##ほ":13672,"##む":13673,"##ゃ":13674,"##ゅ":13675,"##ゆ":13676,"##ょ":13677,"##゜":13678,"##ゝ":13679,"##ァ":13680,"##ゥ":13681,"##エ":13682,"##ォ":13683,"##ケ":13684,"##サ":13685,"##セ":13686,"##ソ":13687,"##ッ":13688,"##ニ":13689,"##ヌ":13690,"##ネ":13691,"##ノ":13692,"##ヘ":13693,"##モ":13694,"##ャ":13695,"##ヤ":13696,"##ュ":13697,"##ユ":13698,"##ョ":13699,"##ヨ":13700,"##ワ":13701,"##ヲ":13702,"##・":13703,"##ヽ":13704,"##ㄅ":13705,"##ㄆ":13706,"##ㄇ":13707,"##ㄉ":13708,"##ㄋ":13709,"##ㄌ":13710,"##ㄍ":13711,"##ㄎ":13712,"##ㄏ":13713,"##ㄒ":13714,"##ㄚ":13715,"##ㄛ":13716,"##ㄞ":13717,"##ㄟ":13718,"##ㄢ":13719,"##ㄤ":13720,"##ㄥ":13721,"##ㄧ":13722,"##ㄨ":13723,"##ㆍ":13724,"##㈦":13725,"##㊣":13726,"##㗎":13727,"##一":13728,"##丁":13729,"##七":13730,"##万":13731,"##丈":13732,"##三":13733,"##上":13734,"##下":13735,"##不":13736,"##与":13737,"##丐":13738,"##丑":13739,"##专":13740,"##且":13741,"##丕":13742,"##世":13743,"##丘":13744,"##丙":13745,"##业":13746,"##丛":13747,"##东":13748,"##丝":13749,"##丞":13750,"##丟":13751,"##両":13752,"##丢":13753,"##两":13754,"##严":13755,"##並":13756,"##丧":13757,"##丨":13758,"##个":13759,"##丫":13760,"##中":13761,"##丰":13762,"##串":13763,"##临":13764,"##丶":13765,"##丸":13766,"##丹":13767,"##为":13768,"##主":13769,"##丼":13770,"##丽":13771,"##举":13772,"##丿":13773,"##乂":13774,"##乃":13775,"##久":13776,"##么":13777,"##义":13778,"##之":13779,"##乌":13780,"##乍":13781,"##乎":13782,"##乏":13783,"##乐":13784,"##乒":13785,"##乓":13786,"##乔":13787,"##乖":13788,"##乗":13789,"##乘":13790,"##乙":13791,"##乜":13792,"##九":13793,"##乞":13794,"##也":13795,"##习":13796,"##乡":13797,"##书":13798,"##乩":13799,"##买":13800,"##乱":13801,"##乳":13802,"##乾":13803,"##亀":13804,"##亂":13805,"##了":13806,"##予":13807,"##争":13808,"##事":13809,"##二":13810,"##于":13811,"##亏":13812,"##云":13813,"##互":13814,"##五":13815,"##井":13816,"##亘":13817,"##亙":13818,"##亚":13819,"##些":13820,"##亜":13821,"##亞":13822,"##亟":13823,"##亡":13824,"##亢":13825,"##交":13826,"##亥":13827,"##亦":13828,"##产":13829,"##亨":13830,"##亩":13831,"##享":13832,"##京":13833,"##亭":13834,"##亮":13835,"##亲":13836,"##亳":13837,"##亵":13838,"##人":13839,"##亿":13840,"##什":13841,"##仁":13842,"##仃":13843,"##仄":13844,"##仅":13845,"##仆":13846,"##仇":13847,"##今":13848,"##介":13849,"##仍":13850,"##从":13851,"##仏":13852,"##仑":13853,"##仓":13854,"##仔":13855,"##仕":13856,"##他":13857,"##仗":13858,"##付":13859,"##仙":13860,"##仝":13861,"##仞":13862,"##仟":13863,"##代":13864,"##令":13865,"##以":13866,"##仨":13867,"##仪":13868,"##们":13869,"##仮":13870,"##仰":13871,"##仲":13872,"##件":13873,"##价":13874,"##任":13875,"##份":13876,"##仿":13877,"##企":13878,"##伉":13879,"##伊":13880,"##伍":13881,"##伎":13882,"##伏":13883,"##伐":13884,"##休":13885,"##伕":13886,"##众":13887,"##优":13888,"##伙":13889,"##会":13890,"##伝":13891,"##伞":13892,"##伟":13893,"##传":13894,"##伢":13895,"##伤":13896,"##伦":13897,"##伪":13898,"##伫":13899,"##伯":13900,"##估":13901,"##伴":13902,"##伶":13903,"##伸":13904,"##伺":13905,"##似":13906,"##伽":13907,"##佃":13908,"##但":13909,"##佇":13910,"##佈":13911,"##位":13912,"##低":13913,"##住":13914,"##佐":13915,"##佑":13916,"##体":13917,"##佔":13918,"##何":13919,"##佗":13920,"##佘":13921,"##余":13922,"##佚":13923,"##佛":13924,"##作":13925,"##佝":13926,"##佞":13927,"##佟":13928,"##你":13929,"##佢":13930,"##佣":13931,"##佤":13932,"##佥":13933,"##佩":13934,"##佬":13935,"##佯":13936,"##佰":13937,"##佳":13938,"##併":13939,"##佶":13940,"##佻":13941,"##佼":13942,"##使":13943,"##侃":13944,"##侄":13945,"##來":13946,"##侈":13947,"##例":13948,"##侍":13949,"##侏":13950,"##侑":13951,"##侖":13952,"##侗":13953,"##供":13954,"##依":13955,"##侠":13956,"##価":13957,"##侣":13958,"##侥":13959,"##侦":13960,"##侧":13961,"##侨":13962,"##侬":13963,"##侮":13964,"##侯":13965,"##侵":13966,"##侶":13967,"##侷":13968,"##便":13969,"##係":13970,"##促":13971,"##俄":13972,"##俊":13973,"##俎":13974,"##俏":13975,"##俐":13976,"##俑":13977,"##俗":13978,"##俘":13979,"##俚":13980,"##保":13981,"##俞":13982,"##俟":13983,"##俠":13984,"##信":13985,"##俨":13986,"##俩":13987,"##俪":13988,"##俬":13989,"##俭":13990,"##修":13991,"##俯":13992,"##俱":13993,"##俳":13994,"##俸":13995,"##俺":13996,"##俾":13997,"##倆":13998,"##倉":13999,"##個":14000,"##倌":14001,"##倍":14002,"##倏":14003,"##們":14004,"##倒":14005,"##倔":14006,"##倖":14007,"##倘":14008,"##候":14009,"##倚":14010,"##倜":14011,"##借":14012,"##倡":14013,"##値":14014,"##倦":14015,"##倩":14016,"##倪":14017,"##倫":14018,"##倬":14019,"##倭":14020,"##倶":14021,"##债":14022,"##值":14023,"##倾":14024,"##偃":14025,"##假":14026,"##偈":14027,"##偉":14028,"##偌":14029,"##偎":14030,"##偏":14031,"##偕":14032,"##做":14033,"##停":14034,"##健":14035,"##側":14036,"##偵":14037,"##偶":14038,"##偷":14039,"##偻":14040,"##偽":14041,"##偿":14042,"##傀":14043,"##傅":14044,"##傍":14045,"##傑":14046,"##傘":14047,"##備":14048,"##傚":14049,"##傢":14050,"##傣":14051,"##傥":14052,"##储":14053,"##傩":14054,"##催":14055,"##傭":14056,"##傲":14057,"##傳":14058,"##債":14059,"##傷":14060,"##傻":14061,"##傾":14062,"##僅":14063,"##働":14064,"##像":14065,"##僑":14066,"##僕":14067,"##僖":14068,"##僚":14069,"##僥":14070,"##僧":14071,"##僭":14072,"##僮":14073,"##僱":14074,"##僵":14075,"##價":14076,"##僻":14077,"##儀":14078,"##儂":14079,"##億":14080,"##儆":14081,"##儉":14082,"##儋":14083,"##儒":14084,"##儕":14085,"##儘":14086,"##償":14087,"##儡":14088,"##優":14089,"##儲":14090,"##儷":14091,"##儼":14092,"##儿":14093,"##兀":14094,"##允":14095,"##元":14096,"##兄":14097,"##充":14098,"##兆":14099,"##兇":14100,"##先":14101,"##光":14102,"##克":14103,"##兌":14104,"##免":14105,"##児":14106,"##兑":14107,"##兒":14108,"##兔":14109,"##兖":14110,"##党":14111,"##兜":14112,"##兢":14113,"##入":14114,"##內":14115,"##全":14116,"##兩":14117,"##八":14118,"##公":14119,"##六":14120,"##兮":14121,"##兰":14122,"##共":14123,"##兲":14124,"##关":14125,"##兴":14126,"##兵":14127,"##其":14128,"##具":14129,"##典":14130,"##兹":14131,"##养":14132,"##兼":14133,"##兽":14134,"##冀":14135,"##内":14136,"##円":14137,"##冇":14138,"##冈":14139,"##冉":14140,"##冊":14141,"##册":14142,"##再":14143,"##冏":14144,"##冒":14145,"##冕":14146,"##冗":14147,"##写":14148,"##军":14149,"##农":14150,"##冠":14151,"##冢":14152,"##冤":14153,"##冥":14154,"##冨":14155,"##冪":14156,"##冬":14157,"##冯":14158,"##冰":14159,"##冲":14160,"##决":14161,"##况":14162,"##冶":14163,"##冷":14164,"##冻":14165,"##冼":14166,"##冽":14167,"##冾":14168,"##净":14169,"##凄":14170,"##准":14171,"##凇":14172,"##凈":14173,"##凉":14174,"##凋":14175,"##凌":14176,"##凍":14177,"##减":14178,"##凑":14179,"##凛":14180,"##凜":14181,"##凝":14182,"##几":14183,"##凡":14184,"##凤":14185,"##処":14186,"##凪":14187,"##凭":14188,"##凯":14189,"##凰":14190,"##凱":14191,"##凳":14192,"##凶":14193,"##凸":14194,"##凹":14195,"##出":14196,"##击":14197,"##函":14198,"##凿":14199,"##刀":14200,"##刁":14201,"##刃":14202,"##分":14203,"##切":14204,"##刈":14205,"##刊":14206,"##刍":14207,"##刎":14208,"##刑":14209,"##划":14210,"##列":14211,"##刘":14212,"##则":14213,"##刚":14214,"##创":14215,"##初":14216,"##删":14217,"##判":14218,"##別":14219,"##刨":14220,"##利":14221,"##刪":14222,"##别":14223,"##刮":14224,"##到":14225,"##制":14226,"##刷":14227,"##券":14228,"##刹":14229,"##刺":14230,"##刻":14231,"##刽":14232,"##剁":14233,"##剂":14234,"##剃":14235,"##則":14236,"##剉":14237,"##削":14238,"##剋":14239,"##剌":14240,"##前":14241,"##剎":14242,"##剐":14243,"##剑":14244,"##剔":14245,"##剖":14246,"##剛":14247,"##剜":14248,"##剝":14249,"##剣":14250,"##剤":14251,"##剥":14252,"##剧":14253,"##剩":14254,"##剪":14255,"##副":14256,"##割":14257,"##創":14258,"##剷":14259,"##剽":14260,"##剿":14261,"##劃":14262,"##劇":14263,"##劈":14264,"##劉":14265,"##劊":14266,"##劍":14267,"##劏":14268,"##劑":14269,"##力":14270,"##劝":14271,"##办":14272,"##功":14273,"##加":14274,"##务":14275,"##劣":14276,"##动":14277,"##助":14278,"##努":14279,"##劫":14280,"##劭":14281,"##励":14282,"##劲":14283,"##劳":14284,"##労":14285,"##劵":14286,"##効":14287,"##劾":14288,"##势":14289,"##勁":14290,"##勃":14291,"##勇":14292,"##勉":14293,"##勋":14294,"##勐":14295,"##勒":14296,"##動":14297,"##勖":14298,"##勘":14299,"##務":14300,"##勛":14301,"##勝":14302,"##勞":14303,"##募":14304,"##勢":14305,"##勤":14306,"##勧":14307,"##勳":14308,"##勵":14309,"##勸":14310,"##勺":14311,"##勻":14312,"##勾":14313,"##勿":14314,"##匀":14315,"##包":14316,"##匆":14317,"##匈":14318,"##匍":14319,"##匐":14320,"##匕":14321,"##化":14322,"##北":14323,"##匙":14324,"##匝":14325,"##匠":14326,"##匡":14327,"##匣":14328,"##匪":14329,"##匮":14330,"##匯":14331,"##匱":14332,"##匹":14333,"##区":14334,"##医":14335,"##匾":14336,"##匿":14337,"##區":14338,"##十":14339,"##千":14340,"##卅":14341,"##升":14342,"##午":14343,"##卉":14344,"##半":14345,"##卍":14346,"##华":14347,"##协":14348,"##卑":14349,"##卒":14350,"##卓":14351,"##協":14352,"##单":14353,"##卖":14354,"##南":14355,"##単":14356,"##博":14357,"##卜":14358,"##卞":14359,"##卟":14360,"##占":14361,"##卡":14362,"##卢":14363,"##卤":14364,"##卦":14365,"##卧":14366,"##卫":14367,"##卮":14368,"##卯":14369,"##印":14370,"##危":14371,"##即":14372,"##却":14373,"##卵":14374,"##卷":14375,"##卸":14376,"##卻":14377,"##卿":14378,"##厂":14379,"##厄":14380,"##厅":14381,"##历":14382,"##厉":14383,"##压":14384,"##厌":14385,"##厕":14386,"##厘":14387,"##厚":14388,"##厝":14389,"##原":14390,"##厢":14391,"##厥":14392,"##厦":14393,"##厨":14394,"##厩":14395,"##厭":14396,"##厮":14397,"##厲":14398,"##厳":14399,"##去":14400,"##县":14401,"##叁":14402,"##参":14403,"##參":14404,"##又":14405,"##叉":14406,"##及":14407,"##友":14408,"##双":14409,"##反":14410,"##収":14411,"##发":14412,"##叔":14413,"##取":14414,"##受":14415,"##变":14416,"##叙":14417,"##叛":14418,"##叟":14419,"##叠":14420,"##叡":14421,"##叢":14422,"##口":14423,"##古":14424,"##句":14425,"##另":14426,"##叨":14427,"##叩":14428,"##只":14429,"##叫":14430,"##召":14431,"##叭":14432,"##叮":14433,"##可":14434,"##台":14435,"##叱":14436,"##史":14437,"##右":14438,"##叵":14439,"##叶":14440,"##号":14441,"##司":14442,"##叹":14443,"##叻":14444,"##叼":14445,"##叽":14446,"##吁":14447,"##吃":14448,"##各":14449,"##吆":14450,"##合":14451,"##吉":14452,"##吊":14453,"##吋":14454,"##同":14455,"##名":14456,"##后":14457,"##吏":14458,"##吐":14459,"##向":14460,"##吒":14461,"##吓":14462,"##吕":14463,"##吖":14464,"##吗":14465,"##君":14466,"##吝":14467,"##吞":14468,"##吟":14469,"##吠":14470,"##吡":14471,"##否":14472,"##吧":14473,"##吨":14474,"##吩":14475,"##含":14476,"##听":14477,"##吭":14478,"##吮":14479,"##启":14480,"##吱":14481,"##吳":14482,"##吴":14483,"##吵":14484,"##吶":14485,"##吸":14486,"##吹":14487,"##吻":14488,"##吼":14489,"##吽":14490,"##吾":14491,"##呀":14492,"##呂":14493,"##呃":14494,"##呆":14495,"##呈":14496,"##告":14497,"##呋":14498,"##呎":14499,"##呐":14500,"##呓":14501,"##呕":14502,"##呗":14503,"##员":14504,"##呛":14505,"##呜":14506,"##呢":14507,"##呤":14508,"##呦":14509,"##周":14510,"##呱":14511,"##呲":14512,"##味":14513,"##呵":14514,"##呷":14515,"##呸":14516,"##呻":14517,"##呼":14518,"##命":14519,"##咀":14520,"##咁":14521,"##咂":14522,"##咄":14523,"##咆":14524,"##咋":14525,"##和":14526,"##咎":14527,"##咏":14528,"##咐":14529,"##咒":14530,"##咔":14531,"##咕":14532,"##咖":14533,"##咗":14534,"##咘":14535,"##咙":14536,"##咚":14537,"##咛":14538,"##咣":14539,"##咤":14540,"##咦":14541,"##咧":14542,"##咨":14543,"##咩":14544,"##咪":14545,"##咫":14546,"##咬":14547,"##咭":14548,"##咯":14549,"##咱":14550,"##咲":14551,"##咳":14552,"##咸":14553,"##咻":14554,"##咽":14555,"##咿":14556,"##哀":14557,"##品":14558,"##哂":14559,"##哄":14560,"##哆":14561,"##哇":14562,"##哈":14563,"##哉":14564,"##哋":14565,"##哌":14566,"##响":14567,"##哎":14568,"##哏":14569,"##哐":14570,"##哑":14571,"##哒":14572,"##哔":14573,"##哗":14574,"##哟":14575,"##員":14576,"##哥":14577,"##哦":14578,"##哧":14579,"##哨":14580,"##哩":14581,"##哪":14582,"##哭":14583,"##哮":14584,"##哲":14585,"##哺":14586,"##哼":14587,"##哽":14588,"##唁":14589,"##唄":14590,"##唆":14591,"##唇":14592,"##唉":14593,"##唏":14594,"##唐":14595,"##唑":14596,"##唔":14597,"##唠":14598,"##唤":14599,"##唧":14600,"##唬":14601,"##售":14602,"##唯":14603,"##唰":14604,"##唱":14605,"##唳":14606,"##唷":14607,"##唸":14608,"##唾":14609,"##啃":14610,"##啄":14611,"##商":14612,"##啉":14613,"##啊":14614,"##問":14615,"##啓":14616,"##啕":14617,"##啖":14618,"##啜":14619,"##啞":14620,"##啟":14621,"##啡":14622,"##啤":14623,"##啥":14624,"##啦":14625,"##啧":14626,"##啪":14627,"##啫":14628,"##啬":14629,"##啮":14630,"##啰":14631,"##啱":14632,"##啲":14633,"##啵":14634,"##啶":14635,"##啷":14636,"##啸":14637,"##啻":14638,"##啼":14639,"##啾":14640,"##喀":14641,"##喂":14642,"##喃":14643,"##善":14644,"##喆":14645,"##喇":14646,"##喉":14647,"##喊":14648,"##喋":14649,"##喎":14650,"##喏":14651,"##喔":14652,"##喘":14653,"##喙":14654,"##喚":14655,"##喜":14656,"##喝":14657,"##喟":14658,"##喧":14659,"##喪":14660,"##喫":14661,"##喬":14662,"##單":14663,"##喰":14664,"##喱":14665,"##喲":14666,"##喳":14667,"##喵":14668,"##営":14669,"##喷":14670,"##喹":14671,"##喺":14672,"##喻":14673,"##喽":14674,"##嗅":14675,"##嗆":14676,"##嗇":14677,"##嗎":14678,"##嗑":14679,"##嗒":14680,"##嗓":14681,"##嗔":14682,"##嗖":14683,"##嗚":14684,"##嗜":14685,"##嗝":14686,"##嗟":14687,"##嗡":14688,"##嗣":14689,"##嗤":14690,"##嗦":14691,"##嗨":14692,"##嗪":14693,"##嗬":14694,"##嗯":14695,"##嗰":14696,"##嗲":14697,"##嗳":14698,"##嗶":14699,"##嗷":14700,"##嗽":14701,"##嘀":14702,"##嘅":14703,"##嘆":14704,"##嘈":14705,"##嘉":14706,"##嘌":14707,"##嘍":14708,"##嘎":14709,"##嘔":14710,"##嘖":14711,"##嘗":14712,"##嘘":14713,"##嘚":14714,"##嘛":14715,"##嘜":14716,"##嘞":14717,"##嘟":14718,"##嘢":14719,"##嘣":14720,"##嘤":14721,"##嘧":14722,"##嘩":14723,"##嘭":14724,"##嘮":14725,"##嘯":14726,"##嘰":14727,"##嘱":14728,"##嘲":14729,"##嘴":14730,"##嘶":14731,"##嘸":14732,"##嘹":14733,"##嘻":14734,"##嘿":14735,"##噁":14736,"##噌":14737,"##噎":14738,"##噓":14739,"##噔":14740,"##噗":14741,"##噙":14742,"##噜":14743,"##噠":14744,"##噢":14745,"##噤":14746,"##器":14747,"##噩":14748,"##噪":14749,"##噬":14750,"##噱":14751,"##噴":14752,"##噶":14753,"##噸":14754,"##噹":14755,"##噻":14756,"##噼":14757,"##嚀":14758,"##嚇":14759,"##嚎":14760,"##嚏":14761,"##嚐":14762,"##嚓":14763,"##嚕":14764,"##嚟":14765,"##嚣":14766,"##嚥":14767,"##嚨":14768,"##嚮":14769,"##嚴":14770,"##嚷":14771,"##嚼":14772,"##囂":14773,"##囉":14774,"##囊":14775,"##囍":14776,"##囑":14777,"##囔":14778,"##囗":14779,"##囚":14780,"##四":14781,"##囝":14782,"##回":14783,"##囟":14784,"##因":14785,"##囡":14786,"##团":14787,"##団":14788,"##囤":14789,"##囧":14790,"##囪":14791,"##囫":14792,"##园":14793,"##困":14794,"##囱":14795,"##囲":14796,"##図":14797,"##围":14798,"##囹":14799,"##固":14800,"##国":14801,"##图":14802,"##囿":14803,"##圃":14804,"##圄":14805,"##圆":14806,"##圈":14807,"##國":14808,"##圍":14809,"##圏":14810,"##園":14811,"##圓":14812,"##圖":14813,"##團":14814,"##圜":14815,"##土":14816,"##圣":14817,"##圧":14818,"##在":14819,"##圩":14820,"##圭":14821,"##地":14822,"##圳":14823,"##场":14824,"##圻":14825,"##圾":14826,"##址":14827,"##坂":14828,"##均":14829,"##坊":14830,"##坍":14831,"##坎":14832,"##坏":14833,"##坐":14834,"##坑":14835,"##块":14836,"##坚":14837,"##坛":14838,"##坝":14839,"##坞":14840,"##坟":14841,"##坠":14842,"##坡":14843,"##坤":14844,"##坦":14845,"##坨":14846,"##坪":14847,"##坯":14848,"##坳":14849,"##坵":14850,"##坷":14851,"##垂":14852,"##垃":14853,"##垄":14854,"##型":14855,"##垒":14856,"##垚":14857,"##垛":14858,"##垠":14859,"##垢":14860,"##垣":14861,"##垦":14862,"##垩":14863,"##垫":14864,"##垭":14865,"##垮":14866,"##垵":14867,"##埂":14868,"##埃":14869,"##埋":14870,"##城":14871,"##埔":14872,"##埕":14873,"##埗":14874,"##域":14875,"##埠":14876,"##埤":14877,"##埵":14878,"##執":14879,"##埸":14880,"##培":14881,"##基":14882,"##埼":14883,"##堀":14884,"##堂":14885,"##堃":14886,"##堅":14887,"##堆":14888,"##堇":14889,"##堑":14890,"##堕":14891,"##堙":14892,"##堡":14893,"##堤":14894,"##堪":14895,"##堯":14896,"##堰":14897,"##報":14898,"##場":14899,"##堵":14900,"##堺":14901,"##堿":14902,"##塊":14903,"##塌":14904,"##塑":14905,"##塔":14906,"##塗":14907,"##塘":14908,"##塚":14909,"##塞":14910,"##塢":14911,"##塩":14912,"##填":14913,"##塬":14914,"##塭":14915,"##塵":14916,"##塾":14917,"##墀":14918,"##境":14919,"##墅":14920,"##墉":14921,"##墊":14922,"##墒":14923,"##墓":14924,"##増":14925,"##墘":14926,"##墙":14927,"##墜":14928,"##增":14929,"##墟":14930,"##墨":14931,"##墩":14932,"##墮":14933,"##墳":14934,"##墻":14935,"##墾":14936,"##壁":14937,"##壅":14938,"##壆":14939,"##壇":14940,"##壊":14941,"##壑":14942,"##壓":14943,"##壕":14944,"##壘":14945,"##壞":14946,"##壟":14947,"##壢":14948,"##壤":14949,"##壩":14950,"##士":14951,"##壬":14952,"##壮":14953,"##壯":14954,"##声":14955,"##売":14956,"##壳":14957,"##壶":14958,"##壹":14959,"##壺":14960,"##壽":14961,"##处":14962,"##备":14963,"##変":14964,"##复":14965,"##夏":14966,"##夔":14967,"##夕":14968,"##外":14969,"##夙":14970,"##多":14971,"##夜":14972,"##够":14973,"##夠":14974,"##夢":14975,"##夥":14976,"##大":14977,"##天":14978,"##太":14979,"##夫":14980,"##夭":14981,"##央":14982,"##夯":14983,"##失":14984,"##头":14985,"##夷":14986,"##夸":14987,"##夹":14988,"##夺":14989,"##夾":14990,"##奂":14991,"##奄":14992,"##奇":14993,"##奈":14994,"##奉":14995,"##奋":14996,"##奎":14997,"##奏":14998,"##奐":14999,"##契":15000,"##奔":15001,"##奕":15002,"##奖":15003,"##套":15004,"##奘":15005,"##奚":15006,"##奠":15007,"##奢":15008,"##奥":15009,"##奧":15010,"##奪":15011,"##奬":15012,"##奮":15013,"##女":15014,"##奴":15015,"##奶":15016,"##奸":15017,"##她":15018,"##好":15019,"##如":15020,"##妃":15021,"##妄":15022,"##妆":15023,"##妇":15024,"##妈":15025,"##妊":15026,"##妍":15027,"##妒":15028,"##妓":15029,"##妖":15030,"##妘":15031,"##妙":15032,"##妝":15033,"##妞":15034,"##妣":15035,"##妤":15036,"##妥":15037,"##妨":15038,"##妩":15039,"##妪":15040,"##妮":15041,"##妲":15042,"##妳":15043,"##妹":15044,"##妻":15045,"##妾":15046,"##姆":15047,"##姉":15048,"##姊":15049,"##始":15050,"##姍":15051,"##姐":15052,"##姑":15053,"##姒":15054,"##姓":15055,"##委":15056,"##姗":15057,"##姚":15058,"##姜":15059,"##姝":15060,"##姣":15061,"##姥":15062,"##姦":15063,"##姨":15064,"##姪":15065,"##姫":15066,"##姬":15067,"##姹":15068,"##姻":15069,"##姿":15070,"##威":15071,"##娃":15072,"##娄":15073,"##娅":15074,"##娆":15075,"##娇":15076,"##娉":15077,"##娑":15078,"##娓":15079,"##娘":15080,"##娛":15081,"##娜":15082,"##娟":15083,"##娠":15084,"##娣":15085,"##娥":15086,"##娩":15087,"##娱":15088,"##娲":15089,"##娴":15090,"##娶":15091,"##娼":15092,"##婀":15093,"##婁":15094,"##婆":15095,"##婉":15096,"##婊":15097,"##婕":15098,"##婚":15099,"##婢":15100,"##婦":15101,"##婧":15102,"##婪":15103,"##婭":15104,"##婴":15105,"##婵":15106,"##婶":15107,"##婷":15108,"##婺":15109,"##婿":15110,"##媒":15111,"##媚":15112,"##媛":15113,"##媞":15114,"##媧":15115,"##媲":15116,"##媳":15117,"##媽":15118,"##媾":15119,"##嫁":15120,"##嫂":15121,"##嫉":15122,"##嫌":15123,"##嫑":15124,"##嫔":15125,"##嫖":15126,"##嫘":15127,"##嫚":15128,"##嫡":15129,"##嫣":15130,"##嫦":15131,"##嫩":15132,"##嫲":15133,"##嫵":15134,"##嫻":15135,"##嬅":15136,"##嬉":15137,"##嬌":15138,"##嬗":15139,"##嬛":15140,"##嬢":15141,"##嬤":15142,"##嬪":15143,"##嬰":15144,"##嬴":15145,"##嬷":15146,"##嬸":15147,"##嬿":15148,"##孀":15149,"##孃":15150,"##子":15151,"##孑":15152,"##孔":15153,"##孕":15154,"##孖":15155,"##字":15156,"##存":15157,"##孙":15158,"##孚":15159,"##孛":15160,"##孜":15161,"##孝":15162,"##孟":15163,"##孢":15164,"##季":15165,"##孤":15166,"##学":15167,"##孩":15168,"##孪":15169,"##孫":15170,"##孬":15171,"##孰":15172,"##孱":15173,"##孳":15174,"##孵":15175,"##學":15176,"##孺":15177,"##孽":15178,"##孿":15179,"##宁":15180,"##它":15181,"##宅":15182,"##宇":15183,"##守":15184,"##安":15185,"##宋":15186,"##完":15187,"##宏":15188,"##宓":15189,"##宕":15190,"##宗":15191,"##官":15192,"##宙":15193,"##定":15194,"##宛":15195,"##宜":15196,"##宝":15197,"##实":15198,"##実":15199,"##宠":15200,"##审":15201,"##客":15202,"##宣":15203,"##室":15204,"##宥":15205,"##宦":15206,"##宪":15207,"##宫":15208,"##宮":15209,"##宰":15210,"##害":15211,"##宴":15212,"##宵":15213,"##家":15214,"##宸":15215,"##容":15216,"##宽":15217,"##宾":15218,"##宿":15219,"##寂":15220,"##寄":15221,"##寅":15222,"##密":15223,"##寇":15224,"##富":15225,"##寐":15226,"##寒":15227,"##寓":15228,"##寛":15229,"##寝":15230,"##寞":15231,"##察":15232,"##寡":15233,"##寢":15234,"##寥":15235,"##實":15236,"##寧":15237,"##寨":15238,"##審":15239,"##寫":15240,"##寬":15241,"##寮":15242,"##寰":15243,"##寵":15244,"##寶":15245,"##寸":15246,"##对":15247,"##寺":15248,"##寻":15249,"##导":15250,"##対":15251,"##寿":15252,"##封":15253,"##専":15254,"##射":15255,"##将":15256,"##將":15257,"##專":15258,"##尉":15259,"##尊":15260,"##尋":15261,"##對":15262,"##導":15263,"##小":15264,"##少":15265,"##尔":15266,"##尕":15267,"##尖":15268,"##尘":15269,"##尚":15270,"##尝":15271,"##尤":15272,"##尧":15273,"##尬":15274,"##就":15275,"##尴":15276,"##尷":15277,"##尸":15278,"##尹":15279,"##尺":15280,"##尻":15281,"##尼":15282,"##尽":15283,"##尾":15284,"##尿":15285,"##局":15286,"##屁":15287,"##层":15288,"##屄":15289,"##居":15290,"##屆":15291,"##屈":15292,"##屉":15293,"##届":15294,"##屋":15295,"##屌":15296,"##屍":15297,"##屎":15298,"##屏":15299,"##屐":15300,"##屑":15301,"##展":15302,"##屜":15303,"##属":15304,"##屠":15305,"##屡":15306,"##屢":15307,"##層":15308,"##履":15309,"##屬":15310,"##屯":15311,"##山":15312,"##屹":15313,"##屿":15314,"##岀":15315,"##岁":15316,"##岂":15317,"##岌":15318,"##岐":15319,"##岑":15320,"##岔":15321,"##岖":15322,"##岗":15323,"##岘":15324,"##岙":15325,"##岚":15326,"##岛":15327,"##岡":15328,"##岩":15329,"##岫":15330,"##岬":15331,"##岭":15332,"##岱":15333,"##岳":15334,"##岷":15335,"##岸":15336,"##峇":15337,"##峋":15338,"##峒":15339,"##峙":15340,"##峡":15341,"##峤":15342,"##峥":15343,"##峦":15344,"##峨":15345,"##峪":15346,"##峭":15347,"##峯":15348,"##峰":15349,"##峴":15350,"##島":15351,"##峻":15352,"##峽":15353,"##崁":15354,"##崂":15355,"##崆":15356,"##崇":15357,"##崎":15358,"##崑":15359,"##崔":15360,"##崖":15361,"##崗":15362,"##崙":15363,"##崛":15364,"##崧":15365,"##崩":15366,"##崭":15367,"##崴":15368,"##崽":15369,"##嵇":15370,"##嵊":15371,"##嵋":15372,"##嵌":15373,"##嵐":15374,"##嵘":15375,"##嵩":15376,"##嵬":15377,"##嵯":15378,"##嶂":15379,"##嶄":15380,"##嶇":15381,"##嶋":15382,"##嶙":15383,"##嶺":15384,"##嶼":15385,"##嶽":15386,"##巅":15387,"##巍":15388,"##巒":15389,"##巔":15390,"##巖":15391,"##川":15392,"##州":15393,"##巡":15394,"##巢":15395,"##工":15396,"##左":15397,"##巧":15398,"##巨":15399,"##巩":15400,"##巫":15401,"##差":15402,"##己":15403,"##已":15404,"##巳":15405,"##巴":15406,"##巷":15407,"##巻":15408,"##巽":15409,"##巾":15410,"##巿":15411,"##币":15412,"##市":15413,"##布":15414,"##帅":15415,"##帆":15416,"##师":15417,"##希":15418,"##帐":15419,"##帑":15420,"##帕":15421,"##帖":15422,"##帘":15423,"##帚":15424,"##帛":15425,"##帜":15426,"##帝":15427,"##帥":15428,"##带":15429,"##帧":15430,"##師":15431,"##席":15432,"##帮":15433,"##帯":15434,"##帰":15435,"##帳":15436,"##帶":15437,"##帷":15438,"##常":15439,"##帼":15440,"##帽":15441,"##幀":15442,"##幂":15443,"##幄":15444,"##幅":15445,"##幌":15446,"##幔":15447,"##幕":15448,"##幟":15449,"##幡":15450,"##幢":15451,"##幣":15452,"##幫":15453,"##干":15454,"##平":15455,"##年":15456,"##并":15457,"##幸":15458,"##幹":15459,"##幺":15460,"##幻":15461,"##幼":15462,"##幽":15463,"##幾":15464,"##广":15465,"##庁":15466,"##広":15467,"##庄":15468,"##庆":15469,"##庇":15470,"##床":15471,"##序":15472,"##庐":15473,"##库":15474,"##应":15475,"##底":15476,"##庖":15477,"##店":15478,"##庙":15479,"##庚":15480,"##府":15481,"##庞":15482,"##废":15483,"##庠":15484,"##度":15485,"##座":15486,"##庫":15487,"##庭":15488,"##庵":15489,"##庶":15490,"##康":15491,"##庸":15492,"##庹":15493,"##庾":15494,"##廁":15495,"##廂":15496,"##廃":15497,"##廈":15498,"##廉":15499,"##廊":15500,"##廓":15501,"##廖":15502,"##廚":15503,"##廝":15504,"##廟":15505,"##廠":15506,"##廢":15507,"##廣":15508,"##廬":15509,"##廳":15510,"##延":15511,"##廷":15512,"##建":15513,"##廿":15514,"##开":15515,"##弁":15516,"##异":15517,"##弃":15518,"##弄":15519,"##弈":15520,"##弊":15521,"##弋":15522,"##式":15523,"##弑":15524,"##弒":15525,"##弓":15526,"##弔":15527,"##引":15528,"##弗":15529,"##弘":15530,"##弛":15531,"##弟":15532,"##张":15533,"##弥":15534,"##弦":15535,"##弧":15536,"##弩":15537,"##弭":15538,"##弯":15539,"##弱":15540,"##張":15541,"##強":15542,"##弹":15543,"##强":15544,"##弼":15545,"##弾":15546,"##彅":15547,"##彆":15548,"##彈":15549,"##彌":15550,"##彎":15551,"##归":15552,"##当":15553,"##录":15554,"##彗":15555,"##彙":15556,"##彝":15557,"##形":15558,"##彤":15559,"##彥":15560,"##彦":15561,"##彧":15562,"##彩":15563,"##彪":15564,"##彫":15565,"##彬":15566,"##彭":15567,"##彰":15568,"##影":15569,"##彷":15570,"##役":15571,"##彻":15572,"##彼":15573,"##彿":15574,"##往":15575,"##征":15576,"##径":15577,"##待":15578,"##徇":15579,"##很":15580,"##徉":15581,"##徊":15582,"##律":15583,"##後":15584,"##徐":15585,"##徑":15586,"##徒":15587,"##従":15588,"##徕":15589,"##得":15590,"##徘":15591,"##徙":15592,"##徜":15593,"##從":15594,"##徠":15595,"##御":15596,"##徨":15597,"##復":15598,"##循":15599,"##徬":15600,"##微":15601,"##徳":15602,"##徴":15603,"##徵":15604,"##德":15605,"##徹":15606,"##徼":15607,"##徽":15608,"##心":15609,"##必":15610,"##忆":15611,"##忌":15612,"##忍":15613,"##忏":15614,"##忐":15615,"##忑":15616,"##忒":15617,"##忖":15618,"##志":15619,"##忘":15620,"##忙":15621,"##応":15622,"##忠":15623,"##忡":15624,"##忤":15625,"##忧":15626,"##忪":15627,"##快":15628,"##忱":15629,"##念":15630,"##忻":15631,"##忽":15632,"##忿":15633,"##怀":15634,"##态":15635,"##怂":15636,"##怅":15637,"##怆":15638,"##怎":15639,"##怏":15640,"##怒":15641,"##怔":15642,"##怕":15643,"##怖":15644,"##怙":15645,"##怜":15646,"##思":15647,"##怠":15648,"##怡":15649,"##急":15650,"##怦":15651,"##性":15652,"##怨":15653,"##怪":15654,"##怯":15655,"##怵":15656,"##总":15657,"##怼":15658,"##恁":15659,"##恃":15660,"##恆":15661,"##恋":15662,"##恍":15663,"##恐":15664,"##恒":15665,"##恕":15666,"##恙":15667,"##恚":15668,"##恢":15669,"##恣":15670,"##恤":15671,"##恥":15672,"##恨":15673,"##恩":15674,"##恪":15675,"##恫":15676,"##恬":15677,"##恭":15678,"##息":15679,"##恰":15680,"##恳":15681,"##恵":15682,"##恶":15683,"##恸":15684,"##恺":15685,"##恻":15686,"##恼":15687,"##恿":15688,"##悄":15689,"##悅":15690,"##悉":15691,"##悌":15692,"##悍":15693,"##悔":15694,"##悖":15695,"##悚":15696,"##悟":15697,"##悠":15698,"##患":15699,"##悦":15700,"##您":15701,"##悩":15702,"##悪":15703,"##悬":15704,"##悯":15705,"##悱":15706,"##悲":15707,"##悴":15708,"##悵":15709,"##悶":15710,"##悸":15711,"##悻":15712,"##悼":15713,"##悽":15714,"##情":15715,"##惆":15716,"##惇":15717,"##惊":15718,"##惋":15719,"##惑":15720,"##惕":15721,"##惘":15722,"##惚":15723,"##惜":15724,"##惟":15725,"##惠":15726,"##惡":15727,"##惦":15728,"##惧":15729,"##惨":15730,"##惩":15731,"##惫":15732,"##惬":15733,"##惭":15734,"##惮":15735,"##惯":15736,"##惰":15737,"##惱":15738,"##想":15739,"##惴":15740,"##惶":15741,"##惹":15742,"##惺":15743,"##愁":15744,"##愆":15745,"##愈":15746,"##愉":15747,"##愍":15748,"##意":15749,"##愕":15750,"##愚":15751,"##愛":15752,"##愜":15753,"##感":15754,"##愣":15755,"##愤":15756,"##愧":15757,"##愫":15758,"##愷":15759,"##愿":15760,"##慄":15761,"##慈":15762,"##態":15763,"##慌":15764,"##慎":15765,"##慑":15766,"##慕":15767,"##慘":15768,"##慚":15769,"##慟":15770,"##慢":15771,"##慣":15772,"##慧":15773,"##慨":15774,"##慫":15775,"##慮":15776,"##慰":15777,"##慳":15778,"##慵":15779,"##慶":15780,"##慷":15781,"##慾":15782,"##憂":15783,"##憊":15784,"##憋":15785,"##憎":15786,"##憐":15787,"##憑":15788,"##憔":15789,"##憚":15790,"##憤":15791,"##憧":15792,"##憨":15793,"##憩":15794,"##憫":15795,"##憬":15796,"##憲":15797,"##憶":15798,"##憾":15799,"##懂":15800,"##懇":15801,"##懈":15802,"##應":15803,"##懊":15804,"##懋":15805,"##懑":15806,"##懒":15807,"##懦":15808,"##懲":15809,"##懵":15810,"##懶":15811,"##懷":15812,"##懸":15813,"##懺":15814,"##懼":15815,"##懾":15816,"##懿":15817,"##戀":15818,"##戈":15819,"##戊":15820,"##戌":15821,"##戍":15822,"##戎":15823,"##戏":15824,"##成":15825,"##我":15826,"##戒":15827,"##戕":15828,"##或":15829,"##战":15830,"##戚":15831,"##戛":15832,"##戟":15833,"##戡":15834,"##戦":15835,"##截":15836,"##戬":15837,"##戮":15838,"##戰":15839,"##戲":15840,"##戳":15841,"##戴":15842,"##戶":15843,"##户":15844,"##戸":15845,"##戻":15846,"##戾":15847,"##房":15848,"##所":15849,"##扁":15850,"##扇":15851,"##扈":15852,"##扉":15853,"##手":15854,"##才":15855,"##扎":15856,"##扑":15857,"##扒":15858,"##打":15859,"##扔":15860,"##払":15861,"##托":15862,"##扛":15863,"##扣":15864,"##扦":15865,"##执":15866,"##扩":15867,"##扪":15868,"##扫":15869,"##扬":15870,"##扭":15871,"##扮":15872,"##扯":15873,"##扰":15874,"##扱":15875,"##扳":15876,"##扶":15877,"##批":15878,"##扼":15879,"##找":15880,"##承":15881,"##技":15882,"##抄":15883,"##抉":15884,"##把":15885,"##抑":15886,"##抒":15887,"##抓":15888,"##投":15889,"##抖":15890,"##抗":15891,"##折":15892,"##抚":15893,"##抛":15894,"##抜":15895,"##択":15896,"##抟":15897,"##抠":15898,"##抡":15899,"##抢":15900,"##护":15901,"##报":15902,"##抨":15903,"##披":15904,"##抬":15905,"##抱":15906,"##抵":15907,"##抹":15908,"##押":15909,"##抽":15910,"##抿":15911,"##拂":15912,"##拄":15913,"##担":15914,"##拆":15915,"##拇":15916,"##拈":15917,"##拉":15918,"##拋":15919,"##拌":15920,"##拍":15921,"##拎":15922,"##拐":15923,"##拒":15924,"##拓":15925,"##拔":15926,"##拖":15927,"##拗":15928,"##拘":15929,"##拙":15930,"##拚":15931,"##招":15932,"##拜":15933,"##拟":15934,"##拡":15935,"##拢":15936,"##拣":15937,"##拥":15938,"##拦":15939,"##拧":15940,"##拨":15941,"##择":15942,"##括":15943,"##拭":15944,"##拮":15945,"##拯":15946,"##拱":15947,"##拳":15948,"##拴":15949,"##拷":15950,"##拼":15951,"##拽":15952,"##拾":15953,"##拿":15954,"##持":15955,"##挂":15956,"##指":15957,"##挈":15958,"##按":15959,"##挎":15960,"##挑":15961,"##挖":15962,"##挙":15963,"##挚":15964,"##挛":15965,"##挝":15966,"##挞":15967,"##挟":15968,"##挠":15969,"##挡":15970,"##挣":15971,"##挤":15972,"##挥":15973,"##挨":15974,"##挪":15975,"##挫":15976,"##振":15977,"##挲":15978,"##挹":15979,"##挺":15980,"##挽":15981,"##挾":15982,"##捂":15983,"##捅":15984,"##捆":15985,"##捉":15986,"##捋":15987,"##捌":15988,"##捍":15989,"##捎":15990,"##捏":15991,"##捐":15992,"##捕":15993,"##捞":15994,"##损":15995,"##捡":15996,"##换":15997,"##捣":15998,"##捧":15999,"##捨":16000,"##捩":16001,"##据":16002,"##捱":16003,"##捲":16004,"##捶":16005,"##捷":16006,"##捺":16007,"##捻":16008,"##掀":16009,"##掂":16010,"##掃":16011,"##掇":16012,"##授":16013,"##掉":16014,"##掌":16015,"##掏":16016,"##掐":16017,"##排":16018,"##掖":16019,"##掘":16020,"##掙":16021,"##掛":16022,"##掠":16023,"##採":16024,"##探":16025,"##掣":16026,"##接":16027,"##控":16028,"##推":16029,"##掩":16030,"##措":16031,"##掬":16032,"##掰":16033,"##掲":16034,"##掳":16035,"##掴":16036,"##掷":16037,"##掸":16038,"##掺":16039,"##揀":16040,"##揃":16041,"##揄":16042,"##揆":16043,"##揉":16044,"##揍":16045,"##描":16046,"##提":16047,"##插":16048,"##揖":16049,"##揚":16050,"##換":16051,"##握":16052,"##揣":16053,"##揩":16054,"##揪":16055,"##揭":16056,"##揮":16057,"##援":16058,"##揶":16059,"##揸":16060,"##揹":16061,"##揽":16062,"##搀":16063,"##搁":16064,"##搂":16065,"##搅":16066,"##損":16067,"##搏":16068,"##搐":16069,"##搓":16070,"##搔":16071,"##搖":16072,"##搗":16073,"##搜":16074,"##搞":16075,"##搡":16076,"##搪":16077,"##搬":16078,"##搭":16079,"##搵":16080,"##搶":16081,"##携":16082,"##搽":16083,"##摀":16084,"##摁":16085,"##摄":16086,"##摆":16087,"##摇":16088,"##摈":16089,"##摊":16090,"##摒":16091,"##摔":16092,"##摘":16093,"##摞":16094,"##摟":16095,"##摧":16096,"##摩":16097,"##摯":16098,"##摳":16099,"##摸":16100,"##摹":16101,"##摺":16102,"##摻":16103,"##撂":16104,"##撃":16105,"##撅":16106,"##撇":16107,"##撈":16108,"##撐":16109,"##撑":16110,"##撒":16111,"##撓":16112,"##撕":16113,"##撚":16114,"##撞":16115,"##撤":16116,"##撥":16117,"##撩":16118,"##撫":16119,"##撬":16120,"##播":16121,"##撮":16122,"##撰":16123,"##撲":16124,"##撵":16125,"##撷":16126,"##撸":16127,"##撻":16128,"##撼":16129,"##撿":16130,"##擀":16131,"##擁":16132,"##擂":16133,"##擄":16134,"##擅":16135,"##擇":16136,"##擊":16137,"##擋":16138,"##操":16139,"##擎":16140,"##擒":16141,"##擔":16142,"##擘":16143,"##據":16144,"##擞":16145,"##擠":16146,"##擡":16147,"##擢":16148,"##擦":16149,"##擬":16150,"##擰":16151,"##擱":16152,"##擲":16153,"##擴":16154,"##擷":16155,"##擺":16156,"##擼":16157,"##擾":16158,"##攀":16159,"##攏":16160,"##攒":16161,"##攔":16162,"##攘":16163,"##攙":16164,"##攜":16165,"##攝":16166,"##攞":16167,"##攢":16168,"##攣":16169,"##攤":16170,"##攥":16171,"##攪":16172,"##攫":16173,"##攬":16174,"##支":16175,"##收":16176,"##攸":16177,"##改":16178,"##攻":16179,"##放":16180,"##政":16181,"##故":16182,"##效":16183,"##敌":16184,"##敍":16185,"##敎":16186,"##敏":16187,"##救":16188,"##敕":16189,"##敖":16190,"##敗":16191,"##敘":16192,"##教":16193,"##敛":16194,"##敝":16195,"##敞":16196,"##敢":16197,"##散":16198,"##敦":16199,"##敬":16200,"##数":16201,"##敲":16202,"##整":16203,"##敵":16204,"##敷":16205,"##數":16206,"##斂":16207,"##斃":16208,"##文":16209,"##斋":16210,"##斌":16211,"##斎":16212,"##斐":16213,"##斑":16214,"##斓":16215,"##斗":16216,"##料":16217,"##斛":16218,"##斜":16219,"##斟":16220,"##斡":16221,"##斤":16222,"##斥":16223,"##斧":16224,"##斩":16225,"##斫":16226,"##斬":16227,"##断":16228,"##斯":16229,"##新":16230,"##斷":16231,"##方":16232,"##於":16233,"##施":16234,"##旁":16235,"##旃":16236,"##旅":16237,"##旋":16238,"##旌":16239,"##旎":16240,"##族":16241,"##旖":16242,"##旗":16243,"##无":16244,"##既":16245,"##日":16246,"##旦":16247,"##旧":16248,"##旨":16249,"##早":16250,"##旬":16251,"##旭":16252,"##旮":16253,"##旱":16254,"##时":16255,"##旷":16256,"##旺":16257,"##旻":16258,"##昀":16259,"##昂":16260,"##昆":16261,"##昇":16262,"##昉":16263,"##昊":16264,"##昌":16265,"##明":16266,"##昏":16267,"##易":16268,"##昔":16269,"##昕":16270,"##昙":16271,"##星":16272,"##映":16273,"##春":16274,"##昧":16275,"##昨":16276,"##昭":16277,"##是":16278,"##昱":16279,"##昴":16280,"##昵":16281,"##昶":16282,"##昼":16283,"##显":16284,"##晁":16285,"##時":16286,"##晃":16287,"##晉":16288,"##晋":16289,"##晌":16290,"##晏":16291,"##晒":16292,"##晓":16293,"##晔":16294,"##晕":16295,"##晖":16296,"##晗":16297,"##晚":16298,"##晝":16299,"##晞":16300,"##晟":16301,"##晤":16302,"##晦":16303,"##晨":16304,"##晩":16305,"##普":16306,"##景":16307,"##晰":16308,"##晴":16309,"##晶":16310,"##晷":16311,"##智":16312,"##晾":16313,"##暂":16314,"##暄":16315,"##暇":16316,"##暈":16317,"##暉":16318,"##暌":16319,"##暐":16320,"##暑":16321,"##暖":16322,"##暗":16323,"##暝":16324,"##暢":16325,"##暧":16326,"##暨":16327,"##暫":16328,"##暮":16329,"##暱":16330,"##暴":16331,"##暸":16332,"##暹":16333,"##曄":16334,"##曆":16335,"##曇":16336,"##曉":16337,"##曖":16338,"##曙":16339,"##曜":16340,"##曝":16341,"##曠":16342,"##曦":16343,"##曬":16344,"##曰":16345,"##曲":16346,"##曳":16347,"##更":16348,"##書":16349,"##曹":16350,"##曼":16351,"##曾":16352,"##替":16353,"##最":16354,"##會":16355,"##月":16356,"##有":16357,"##朋":16358,"##服":16359,"##朐":16360,"##朔":16361,"##朕":16362,"##朗":16363,"##望":16364,"##朝":16365,"##期":16366,"##朦":16367,"##朧":16368,"##木":16369,"##未":16370,"##末":16371,"##本":16372,"##札":16373,"##朮":16374,"##术":16375,"##朱":16376,"##朴":16377,"##朵":16378,"##机":16379,"##朽":16380,"##杀":16381,"##杂":16382,"##权":16383,"##杆":16384,"##杈":16385,"##杉":16386,"##李":16387,"##杏":16388,"##材":16389,"##村":16390,"##杓":16391,"##杖":16392,"##杜":16393,"##杞":16394,"##束":16395,"##杠":16396,"##条":16397,"##来":16398,"##杨":16399,"##杭":16400,"##杯":16401,"##杰":16402,"##東":16403,"##杳":16404,"##杵":16405,"##杷":16406,"##杼":16407,"##松":16408,"##板":16409,"##极":16410,"##构":16411,"##枇":16412,"##枉":16413,"##枋":16414,"##析":16415,"##枕":16416,"##林":16417,"##枚":16418,"##果":16419,"##枝":16420,"##枢":16421,"##枣":16422,"##枪":16423,"##枫":16424,"##枭":16425,"##枯":16426,"##枰":16427,"##枱":16428,"##枳":16429,"##架":16430,"##枷":16431,"##枸":16432,"##柄":16433,"##柏":16434,"##某":16435,"##柑":16436,"##柒":16437,"##染":16438,"##柔":16439,"##柘":16440,"##柚":16441,"##柜":16442,"##柞":16443,"##柠":16444,"##柢":16445,"##查":16446,"##柩":16447,"##柬":16448,"##柯":16449,"##柱":16450,"##柳":16451,"##柴":16452,"##柵":16453,"##査":16454,"##柿":16455,"##栀":16456,"##栃":16457,"##栄":16458,"##栅":16459,"##标":16460,"##栈":16461,"##栉":16462,"##栋":16463,"##栎":16464,"##栏":16465,"##树":16466,"##栓":16467,"##栖":16468,"##栗":16469,"##校":16470,"##栩":16471,"##株":16472,"##样":16473,"##核":16474,"##根":16475,"##格":16476,"##栽":16477,"##栾":16478,"##桀":16479,"##桁":16480,"##桂":16481,"##桃":16482,"##桅":16483,"##框":16484,"##案":16485,"##桉":16486,"##桌":16487,"##桎":16488,"##桐":16489,"##桑":16490,"##桓":16491,"##桔":16492,"##桜":16493,"##桠":16494,"##桡":16495,"##桢":16496,"##档":16497,"##桥":16498,"##桦":16499,"##桧":16500,"##桨":16501,"##桩":16502,"##桶":16503,"##桿":16504,"##梁":16505,"##梅":16506,"##梆":16507,"##梏":16508,"##梓":16509,"##梗":16510,"##條":16511,"##梟":16512,"##梢":16513,"##梦":16514,"##梧":16515,"##梨":16516,"##梭":16517,"##梯":16518,"##械":16519,"##梳":16520,"##梵":16521,"##梶":16522,"##检":16523,"##棂":16524,"##棄":16525,"##棉":16526,"##棋":16527,"##棍":16528,"##棒":16529,"##棕":16530,"##棗":16531,"##棘":16532,"##棚":16533,"##棟":16534,"##棠":16535,"##棣":16536,"##棧":16537,"##森":16538,"##棱":16539,"##棲":16540,"##棵":16541,"##棹":16542,"##棺":16543,"##椁":16544,"##椅":16545,"##椋":16546,"##植":16547,"##椎":16548,"##椒":16549,"##検":16550,"##椪":16551,"##椭":16552,"##椰":16553,"##椹":16554,"##椽":16555,"##椿":16556,"##楂":16557,"##楊":16558,"##楓":16559,"##楔":16560,"##楚":16561,"##楝":16562,"##楞":16563,"##楠":16564,"##楣":16565,"##楨":16566,"##楫":16567,"##業":16568,"##楮":16569,"##極":16570,"##楷":16571,"##楸":16572,"##楹":16573,"##楼":16574,"##楽":16575,"##概":16576,"##榄":16577,"##榆":16578,"##榈":16579,"##榉":16580,"##榔":16581,"##榕":16582,"##榖":16583,"##榛":16584,"##榜":16585,"##榨":16586,"##榫":16587,"##榭":16588,"##榮":16589,"##榱":16590,"##榴":16591,"##榷":16592,"##榻":16593,"##槁":16594,"##槃":16595,"##構":16596,"##槌":16597,"##槍":16598,"##槎":16599,"##槐":16600,"##槓":16601,"##様":16602,"##槛":16603,"##槟":16604,"##槤":16605,"##槭":16606,"##槲":16607,"##槳":16608,"##槻":16609,"##槽":16610,"##槿":16611,"##樁":16612,"##樂":16613,"##樊":16614,"##樑":16615,"##樓":16616,"##標":16617,"##樞":16618,"##樟":16619,"##模":16620,"##樣":16621,"##権":16622,"##横":16623,"##樫":16624,"##樯":16625,"##樱":16626,"##樵":16627,"##樸":16628,"##樹":16629,"##樺":16630,"##樽":16631,"##樾":16632,"##橄":16633,"##橇":16634,"##橋":16635,"##橐":16636,"##橘":16637,"##橙":16638,"##機":16639,"##橡":16640,"##橢":16641,"##橫":16642,"##橱":16643,"##橹":16644,"##橼":16645,"##檀":16646,"##檄":16647,"##檎":16648,"##檐":16649,"##檔":16650,"##檗":16651,"##檜":16652,"##檢":16653,"##檬":16654,"##檯":16655,"##檳":16656,"##檸":16657,"##檻":16658,"##櫃":16659,"##櫚":16660,"##櫛":16661,"##櫥":16662,"##櫸":16663,"##櫻":16664,"##欄":16665,"##權":16666,"##欒":16667,"##欖":16668,"##欠":16669,"##次":16670,"##欢":16671,"##欣":16672,"##欧":16673,"##欲":16674,"##欸":16675,"##欺":16676,"##欽":16677,"##款":16678,"##歆":16679,"##歇":16680,"##歉":16681,"##歌":16682,"##歎":16683,"##歐":16684,"##歓":16685,"##歙":16686,"##歛":16687,"##歡":16688,"##止":16689,"##正":16690,"##此":16691,"##步":16692,"##武":16693,"##歧":16694,"##歩":16695,"##歪":16696,"##歯":16697,"##歲":16698,"##歳":16699,"##歴":16700,"##歷":16701,"##歸":16702,"##歹":16703,"##死":16704,"##歼":16705,"##殁":16706,"##殃":16707,"##殆":16708,"##殇":16709,"##殉":16710,"##殊":16711,"##残":16712,"##殒":16713,"##殓":16714,"##殖":16715,"##殘":16716,"##殞":16717,"##殡":16718,"##殤":16719,"##殭":16720,"##殯":16721,"##殲":16722,"##殴":16723,"##段":16724,"##殷":16725,"##殺":16726,"##殼":16727,"##殿":16728,"##毀":16729,"##毁":16730,"##毂":16731,"##毅":16732,"##毆":16733,"##毋":16734,"##母":16735,"##毎":16736,"##每":16737,"##毒":16738,"##毓":16739,"##比":16740,"##毕":16741,"##毗":16742,"##毘":16743,"##毙":16744,"##毛":16745,"##毡":16746,"##毫":16747,"##毯":16748,"##毽":16749,"##氈":16750,"##氏":16751,"##氐":16752,"##民":16753,"##氓":16754,"##气":16755,"##氖":16756,"##気":16757,"##氙":16758,"##氛":16759,"##氟":16760,"##氡":16761,"##氢":16762,"##氣":16763,"##氤":16764,"##氦":16765,"##氧":16766,"##氨":16767,"##氪":16768,"##氫":16769,"##氮":16770,"##氯":16771,"##氰":16772,"##氲":16773,"##水":16774,"##氷":16775,"##永":16776,"##氹":16777,"##氾":16778,"##汀":16779,"##汁":16780,"##求":16781,"##汆":16782,"##汇":16783,"##汉":16784,"##汎":16785,"##汐":16786,"##汕":16787,"##汗":16788,"##汙":16789,"##汛":16790,"##汝":16791,"##汞":16792,"##江":16793,"##池":16794,"##污":16795,"##汤":16796,"##汨":16797,"##汩":16798,"##汪":16799,"##汰":16800,"##汲":16801,"##汴":16802,"##汶":16803,"##汹":16804,"##決":16805,"##汽":16806,"##汾":16807,"##沁":16808,"##沂":16809,"##沃":16810,"##沅":16811,"##沈":16812,"##沉":16813,"##沌":16814,"##沏":16815,"##沐":16816,"##沒":16817,"##沓":16818,"##沖":16819,"##沙":16820,"##沛":16821,"##沟":16822,"##没":16823,"##沢":16824,"##沣":16825,"##沥":16826,"##沦":16827,"##沧":16828,"##沪":16829,"##沫":16830,"##沭":16831,"##沮":16832,"##沱":16833,"##河":16834,"##沸":16835,"##油":16836,"##治":16837,"##沼":16838,"##沽":16839,"##沾":16840,"##沿":16841,"##況":16842,"##泄":16843,"##泉":16844,"##泊":16845,"##泌":16846,"##泓":16847,"##法":16848,"##泗":16849,"##泛":16850,"##泞":16851,"##泠":16852,"##泡":16853,"##波":16854,"##泣":16855,"##泥":16856,"##注":16857,"##泪":16858,"##泫":16859,"##泮":16860,"##泯":16861,"##泰":16862,"##泱":16863,"##泳":16864,"##泵":16865,"##泷":16866,"##泸":16867,"##泻":16868,"##泼":16869,"##泽":16870,"##泾":16871,"##洁":16872,"##洄":16873,"##洋":16874,"##洒":16875,"##洗":16876,"##洙":16877,"##洛":16878,"##洞":16879,"##津":16880,"##洩":16881,"##洪":16882,"##洮":16883,"##洱":16884,"##洲":16885,"##洵":16886,"##洶":16887,"##洸":16888,"##洹":16889,"##活":16890,"##洼":16891,"##洽":16892,"##派":16893,"##流":16894,"##浃":16895,"##浄":16896,"##浅":16897,"##浆":16898,"##浇":16899,"##浊":16900,"##测":16901,"##济":16902,"##浏":16903,"##浑":16904,"##浒":16905,"##浓":16906,"##浔":16907,"##浙":16908,"##浚":16909,"##浜":16910,"##浣":16911,"##浦":16912,"##浩":16913,"##浪":16914,"##浬":16915,"##浮":16916,"##浯":16917,"##浴":16918,"##海":16919,"##浸":16920,"##涂":16921,"##涅":16922,"##涇":16923,"##消":16924,"##涉":16925,"##涌":16926,"##涎":16927,"##涓":16928,"##涔":16929,"##涕":16930,"##涙":16931,"##涛":16932,"##涝":16933,"##涞":16934,"##涟":16935,"##涠":16936,"##涡":16937,"##涣":16938,"##涤":16939,"##润":16940,"##涧":16941,"##涨":16942,"##涩":16943,"##涪":16944,"##涮":16945,"##涯":16946,"##液":16947,"##涵":16948,"##涸":16949,"##涼":16950,"##涿":16951,"##淀":16952,"##淄":16953,"##淅":16954,"##淆":16955,"##淇":16956,"##淋":16957,"##淌":16958,"##淑":16959,"##淒":16960,"##淖":16961,"##淘":16962,"##淙":16963,"##淚":16964,"##淞":16965,"##淡":16966,"##淤":16967,"##淦":16968,"##淨":16969,"##淩":16970,"##淪":16971,"##淫":16972,"##淬":16973,"##淮":16974,"##深":16975,"##淳":16976,"##淵":16977,"##混":16978,"##淹":16979,"##淺":16980,"##添":16981,"##淼":16982,"##清":16983,"##済":16984,"##渉":16985,"##渊":16986,"##渋":16987,"##渍":16988,"##渎":16989,"##渐":16990,"##渔":16991,"##渗":16992,"##渙":16993,"##渚":16994,"##減":16995,"##渝":16996,"##渠":16997,"##渡":16998,"##渣":16999,"##渤":17000,"##渥":17001,"##渦":17002,"##温":17003,"##測":17004,"##渭":17005,"##港":17006,"##渲":17007,"##渴":17008,"##游":17009,"##渺":17010,"##渾":17011,"##湃":17012,"##湄":17013,"##湊":17014,"##湍":17015,"##湖":17016,"##湘":17017,"##湛":17018,"##湟":17019,"##湧":17020,"##湫":17021,"##湮":17022,"##湯":17023,"##湳":17024,"##湾":17025,"##湿":17026,"##満":17027,"##溃":17028,"##溅":17029,"##溉":17030,"##溏":17031,"##源":17032,"##準":17033,"##溜":17034,"##溝":17035,"##溟":17036,"##溢":17037,"##溥":17038,"##溧":17039,"##溪":17040,"##溫":17041,"##溯":17042,"##溱":17043,"##溴":17044,"##溶":17045,"##溺":17046,"##溼":17047,"##滁":17048,"##滂":17049,"##滄":17050,"##滅":17051,"##滇":17052,"##滋":17053,"##滌":17054,"##滑":17055,"##滓":17056,"##滔":17057,"##滕":17058,"##滙":17059,"##滚":17060,"##滝":17061,"##滞":17062,"##滟":17063,"##满":17064,"##滢":17065,"##滤":17066,"##滥":17067,"##滦":17068,"##滨":17069,"##滩":17070,"##滬":17071,"##滯":17072,"##滲":17073,"##滴":17074,"##滷":17075,"##滸":17076,"##滾":17077,"##滿":17078,"##漁":17079,"##漂":17080,"##漆":17081,"##漉":17082,"##漏":17083,"##漓":17084,"##演":17085,"##漕":17086,"##漠":17087,"##漢":17088,"##漣":17089,"##漩":17090,"##漪":17091,"##漫":17092,"##漬":17093,"##漯":17094,"##漱":17095,"##漲":17096,"##漳":17097,"##漸":17098,"##漾":17099,"##漿":17100,"##潆":17101,"##潇":17102,"##潋":17103,"##潍":17104,"##潑":17105,"##潔":17106,"##潘":17107,"##潛":17108,"##潜":17109,"##潞":17110,"##潟":17111,"##潢":17112,"##潤":17113,"##潦":17114,"##潧":17115,"##潭":17116,"##潮":17117,"##潰":17118,"##潴":17119,"##潸":17120,"##潺":17121,"##潼":17122,"##澀":17123,"##澄":17124,"##澆":17125,"##澈":17126,"##澍":17127,"##澎":17128,"##澗":17129,"##澜":17130,"##澡":17131,"##澤":17132,"##澧":17133,"##澱":17134,"##澳":17135,"##澹":17136,"##激":17137,"##濁":17138,"##濂":17139,"##濃":17140,"##濑":17141,"##濒":17142,"##濕":17143,"##濘":17144,"##濛":17145,"##濟":17146,"##濠":17147,"##濡":17148,"##濤":17149,"##濫":17150,"##濬":17151,"##濮":17152,"##濯":17153,"##濱":17154,"##濺":17155,"##濾":17156,"##瀅":17157,"##瀆":17158,"##瀉":17159,"##瀋":17160,"##瀏":17161,"##瀑":17162,"##瀕":17163,"##瀘":17164,"##瀚":17165,"##瀛":17166,"##瀝":17167,"##瀞":17168,"##瀟":17169,"##瀧":17170,"##瀨":17171,"##瀬":17172,"##瀰":17173,"##瀾":17174,"##灌":17175,"##灏":17176,"##灑":17177,"##灘":17178,"##灝":17179,"##灞":17180,"##灣":17181,"##火":17182,"##灬":17183,"##灭":17184,"##灯":17185,"##灰":17186,"##灵":17187,"##灶":17188,"##灸":17189,"##灼":17190,"##災":17191,"##灾":17192,"##灿":17193,"##炀":17194,"##炁":17195,"##炅":17196,"##炉":17197,"##炊":17198,"##炎":17199,"##炒":17200,"##炔":17201,"##炕":17202,"##炖":17203,"##炙":17204,"##炜":17205,"##炫":17206,"##炬":17207,"##炭":17208,"##炮":17209,"##炯":17210,"##炳":17211,"##炷":17212,"##炸":17213,"##点":17214,"##為":17215,"##炼":17216,"##炽":17217,"##烁":17218,"##烂":17219,"##烃":17220,"##烈":17221,"##烊":17222,"##烏":17223,"##烘":17224,"##烙":17225,"##烛":17226,"##烟":17227,"##烤":17228,"##烦":17229,"##烧":17230,"##烨":17231,"##烩":17232,"##烫":17233,"##烬":17234,"##热":17235,"##烯":17236,"##烷":17237,"##烹":17238,"##烽":17239,"##焉":17240,"##焊":17241,"##焕":17242,"##焖":17243,"##焗":17244,"##焘":17245,"##焙":17246,"##焚":17247,"##焜":17248,"##無":17249,"##焦":17250,"##焯":17251,"##焰":17252,"##焱":17253,"##然":17254,"##焼":17255,"##煅":17256,"##煉":17257,"##煊":17258,"##煌":17259,"##煎":17260,"##煒":17261,"##煖":17262,"##煙":17263,"##煜":17264,"##煞":17265,"##煤":17266,"##煥":17267,"##煦":17268,"##照":17269,"##煨":17270,"##煩":17271,"##煮":17272,"##煲":17273,"##煸":17274,"##煽":17275,"##熄":17276,"##熊":17277,"##熏":17278,"##熒":17279,"##熔":17280,"##熙":17281,"##熟":17282,"##熠":17283,"##熨":17284,"##熬":17285,"##熱":17286,"##熵":17287,"##熹":17288,"##熾":17289,"##燁":17290,"##燃":17291,"##燄":17292,"##燈":17293,"##燉":17294,"##燊":17295,"##燎":17296,"##燒":17297,"##燔":17298,"##燕":17299,"##燙":17300,"##燜":17301,"##營":17302,"##燥":17303,"##燦":17304,"##燧":17305,"##燭":17306,"##燮":17307,"##燴":17308,"##燻":17309,"##燼":17310,"##燿":17311,"##爆":17312,"##爍":17313,"##爐":17314,"##爛":17315,"##爪":17316,"##爬":17317,"##爭":17318,"##爰":17319,"##爱":17320,"##爲":17321,"##爵":17322,"##父":17323,"##爷":17324,"##爸":17325,"##爹":17326,"##爺":17327,"##爻":17328,"##爽":17329,"##爾":17330,"##牆":17331,"##片":17332,"##版":17333,"##牌":17334,"##牍":17335,"##牒":17336,"##牙":17337,"##牛":17338,"##牝":17339,"##牟":17340,"##牠":17341,"##牡":17342,"##牢":17343,"##牦":17344,"##牧":17345,"##物":17346,"##牯":17347,"##牲":17348,"##牴":17349,"##牵":17350,"##特":17351,"##牺":17352,"##牽":17353,"##犀":17354,"##犁":17355,"##犄":17356,"##犊":17357,"##犍":17358,"##犒":17359,"##犢":17360,"##犧":17361,"##犬":17362,"##犯":17363,"##状":17364,"##犷":17365,"##犸":17366,"##犹":17367,"##狀":17368,"##狂":17369,"##狄":17370,"##狈":17371,"##狎":17372,"##狐":17373,"##狒":17374,"##狗":17375,"##狙":17376,"##狞":17377,"##狠":17378,"##狡":17379,"##狩":17380,"##独":17381,"##狭":17382,"##狮":17383,"##狰":17384,"##狱":17385,"##狸":17386,"##狹":17387,"##狼":17388,"##狽":17389,"##猎":17390,"##猕":17391,"##猖":17392,"##猗":17393,"##猙":17394,"##猛":17395,"##猜":17396,"##猝":17397,"##猥":17398,"##猩":17399,"##猪":17400,"##猫":17401,"##猬":17402,"##献":17403,"##猴":17404,"##猶":17405,"##猷":17406,"##猾":17407,"##猿":17408,"##獄":17409,"##獅":17410,"##獎":17411,"##獐":17412,"##獒":17413,"##獗":17414,"##獠":17415,"##獣":17416,"##獨":17417,"##獭":17418,"##獰":17419,"##獲":17420,"##獵":17421,"##獷":17422,"##獸":17423,"##獺":17424,"##獻":17425,"##獼":17426,"##獾":17427,"##玄":17428,"##率":17429,"##玉":17430,"##王":17431,"##玑":17432,"##玖":17433,"##玛":17434,"##玟":17435,"##玠":17436,"##玥":17437,"##玩":17438,"##玫":17439,"##玮":17440,"##环":17441,"##现":17442,"##玲":17443,"##玳":17444,"##玷":17445,"##玺":17446,"##玻":17447,"##珀":17448,"##珂":17449,"##珅":17450,"##珈":17451,"##珉":17452,"##珊":17453,"##珍":17454,"##珏":17455,"##珐":17456,"##珑":17457,"##珙":17458,"##珞":17459,"##珠":17460,"##珣":17461,"##珥":17462,"##珩":17463,"##珪":17464,"##班":17465,"##珮":17466,"##珲":17467,"##珺":17468,"##現":17469,"##球":17470,"##琅":17471,"##理":17472,"##琇":17473,"##琉":17474,"##琊":17475,"##琍":17476,"##琏":17477,"##琐":17478,"##琛":17479,"##琢":17480,"##琥":17481,"##琦":17482,"##琨":17483,"##琪":17484,"##琬":17485,"##琮":17486,"##琰":17487,"##琲":17488,"##琳":17489,"##琴":17490,"##琵":17491,"##琶":17492,"##琺":17493,"##琼":17494,"##瑀":17495,"##瑁":17496,"##瑄":17497,"##瑋":17498,"##瑕":17499,"##瑗":17500,"##瑙":17501,"##瑚":17502,"##瑛":17503,"##瑜":17504,"##瑞":17505,"##瑟":17506,"##瑠":17507,"##瑣":17508,"##瑤":17509,"##瑩":17510,"##瑪":17511,"##瑯":17512,"##瑰":17513,"##瑶":17514,"##瑾":17515,"##璀":17516,"##璁":17517,"##璃":17518,"##璇":17519,"##璉":17520,"##璋":17521,"##璎":17522,"##璐":17523,"##璜":17524,"##璞":17525,"##璟":17526,"##璧":17527,"##璨":17528,"##環":17529,"##璽":17530,"##璿":17531,"##瓊":17532,"##瓏":17533,"##瓒":17534,"##瓜":17535,"##瓢":17536,"##瓣":17537,"##瓤":17538,"##瓦":17539,"##瓮":17540,"##瓯":17541,"##瓴":17542,"##瓶":17543,"##瓷":17544,"##甄":17545,"##甌":17546,"##甕":17547,"##甘":17548,"##甙":17549,"##甚":17550,"##甜":17551,"##生":17552,"##產":17553,"##産":17554,"##甥":17555,"##甦":17556,"##用":17557,"##甩":17558,"##甫":17559,"##甬":17560,"##甭":17561,"##甯":17562,"##田":17563,"##由":17564,"##甲":17565,"##申":17566,"##电":17567,"##男":17568,"##甸":17569,"##町":17570,"##画":17571,"##甾":17572,"##畀":17573,"##畅":17574,"##界":17575,"##畏":17576,"##畑":17577,"##畔":17578,"##留":17579,"##畜":17580,"##畝":17581,"##畢":17582,"##略":17583,"##畦":17584,"##番":17585,"##畫":17586,"##異":17587,"##畲":17588,"##畳":17589,"##畴":17590,"##當":17591,"##畸":17592,"##畹":17593,"##畿":17594,"##疆":17595,"##疇":17596,"##疊":17597,"##疏":17598,"##疑":17599,"##疔":17600,"##疖":17601,"##疗":17602,"##疙":17603,"##疚":17604,"##疝":17605,"##疟":17606,"##疡":17607,"##疣":17608,"##疤":17609,"##疥":17610,"##疫":17611,"##疮":17612,"##疯":17613,"##疱":17614,"##疲":17615,"##疳":17616,"##疵":17617,"##疸":17618,"##疹":17619,"##疼":17620,"##疽":17621,"##疾":17622,"##痂":17623,"##病":17624,"##症":17625,"##痈":17626,"##痉":17627,"##痊":17628,"##痍":17629,"##痒":17630,"##痔":17631,"##痕":17632,"##痘":17633,"##痙":17634,"##痛":17635,"##痞":17636,"##痠":17637,"##痢":17638,"##痣":17639,"##痤":17640,"##痧":17641,"##痨":17642,"##痪":17643,"##痫":17644,"##痰":17645,"##痱":17646,"##痴":17647,"##痹":17648,"##痺":17649,"##痼":17650,"##痿":17651,"##瘀":17652,"##瘁":17653,"##瘋":17654,"##瘍":17655,"##瘓":17656,"##瘘":17657,"##瘙":17658,"##瘟":17659,"##瘠":17660,"##瘡":17661,"##瘢":17662,"##瘤":17663,"##瘦":17664,"##瘧":17665,"##瘩":17666,"##瘪":17667,"##瘫":17668,"##瘴":17669,"##瘸":17670,"##瘾":17671,"##療":17672,"##癇":17673,"##癌":17674,"##癒":17675,"##癖":17676,"##癜":17677,"##癞":17678,"##癡":17679,"##癢":17680,"##癣":17681,"##癥":17682,"##癫":17683,"##癬":17684,"##癮":17685,"##癱":17686,"##癲":17687,"##癸":17688,"##発":17689,"##登":17690,"##發":17691,"##白":17692,"##百":17693,"##皂":17694,"##的":17695,"##皆":17696,"##皇":17697,"##皈":17698,"##皋":17699,"##皎":17700,"##皑":17701,"##皓":17702,"##皖":17703,"##皙":17704,"##皚":17705,"##皮":17706,"##皰":17707,"##皱":17708,"##皴":17709,"##皺":17710,"##皿":17711,"##盂":17712,"##盃":17713,"##盅":17714,"##盆":17715,"##盈":17716,"##益":17717,"##盎":17718,"##盏":17719,"##盐":17720,"##监":17721,"##盒":17722,"##盔":17723,"##盖":17724,"##盗":17725,"##盘":17726,"##盛":17727,"##盜":17728,"##盞":17729,"##盟":17730,"##盡":17731,"##監":17732,"##盤":17733,"##盥":17734,"##盧":17735,"##盪":17736,"##目":17737,"##盯":17738,"##盱":17739,"##盲":17740,"##直":17741,"##相":17742,"##盹":17743,"##盼":17744,"##盾":17745,"##省":17746,"##眈":17747,"##眉":17748,"##看":17749,"##県":17750,"##眙":17751,"##眞":17752,"##真":17753,"##眠":17754,"##眦":17755,"##眨":17756,"##眩":17757,"##眯":17758,"##眶":17759,"##眷":17760,"##眸":17761,"##眺":17762,"##眼":17763,"##眾":17764,"##着":17765,"##睁":17766,"##睇":17767,"##睏":17768,"##睐":17769,"##睑":17770,"##睛":17771,"##睜":17772,"##睞":17773,"##睡":17774,"##睢":17775,"##督":17776,"##睥":17777,"##睦":17778,"##睨":17779,"##睪":17780,"##睫":17781,"##睬":17782,"##睹":17783,"##睽":17784,"##睾":17785,"##睿":17786,"##瞄":17787,"##瞅":17788,"##瞇":17789,"##瞋":17790,"##瞌":17791,"##瞎":17792,"##瞑":17793,"##瞒":17794,"##瞓":17795,"##瞞":17796,"##瞟":17797,"##瞠":17798,"##瞥":17799,"##瞧":17800,"##瞩":17801,"##瞪":17802,"##瞬":17803,"##瞭":17804,"##瞰":17805,"##瞳":17806,"##瞻":17807,"##瞼":17808,"##瞿":17809,"##矇":17810,"##矍":17811,"##矗":17812,"##矚":17813,"##矛":17814,"##矜":17815,"##矢":17816,"##矣":17817,"##知":17818,"##矩":17819,"##矫":17820,"##短":17821,"##矮":17822,"##矯":17823,"##石":17824,"##矶":17825,"##矽":17826,"##矾":17827,"##矿":17828,"##码":17829,"##砂":17830,"##砌":17831,"##砍":17832,"##砒":17833,"##研":17834,"##砖":17835,"##砗":17836,"##砚":17837,"##砝":17838,"##砣":17839,"##砥":17840,"##砧":17841,"##砭":17842,"##砰":17843,"##砲":17844,"##破":17845,"##砷":17846,"##砸":17847,"##砺":17848,"##砼":17849,"##砾":17850,"##础":17851,"##硅":17852,"##硐":17853,"##硒":17854,"##硕":17855,"##硝":17856,"##硫":17857,"##硬":17858,"##确":17859,"##硯":17860,"##硼":17861,"##碁":17862,"##碇":17863,"##碉":17864,"##碌":17865,"##碍":17866,"##碎":17867,"##碑":17868,"##碓":17869,"##碗":17870,"##碘":17871,"##碚":17872,"##碛":17873,"##碟":17874,"##碣":17875,"##碧":17876,"##碩":17877,"##碰":17878,"##碱":17879,"##碳":17880,"##碴":17881,"##確":17882,"##碼":17883,"##碾":17884,"##磁":17885,"##磅":17886,"##磊":17887,"##磋":17888,"##磐":17889,"##磕":17890,"##磚":17891,"##磡":17892,"##磨":17893,"##磬":17894,"##磯":17895,"##磲":17896,"##磷":17897,"##磺":17898,"##礁":17899,"##礎":17900,"##礙":17901,"##礡":17902,"##礦":17903,"##礪":17904,"##礫":17905,"##礴":17906,"##示":17907,"##礼":17908,"##社":17909,"##祀":17910,"##祁":17911,"##祂":17912,"##祇":17913,"##祈":17914,"##祉":17915,"##祎":17916,"##祐":17917,"##祕":17918,"##祖":17919,"##祗":17920,"##祚":17921,"##祛":17922,"##祜":17923,"##祝":17924,"##神":17925,"##祟":17926,"##祠":17927,"##祢":17928,"##祥":17929,"##票":17930,"##祭":17931,"##祯":17932,"##祷":17933,"##祸":17934,"##祺":17935,"##祿":17936,"##禀":17937,"##禁":17938,"##禄":17939,"##禅":17940,"##禍":17941,"##禎":17942,"##福":17943,"##禛":17944,"##禦":17945,"##禧":17946,"##禪":17947,"##禮":17948,"##禱":17949,"##禹":17950,"##禺":17951,"##离":17952,"##禽":17953,"##禾":17954,"##禿":17955,"##秀":17956,"##私":17957,"##秃":17958,"##秆":17959,"##秉":17960,"##秋":17961,"##种":17962,"##科":17963,"##秒":17964,"##秘":17965,"##租":17966,"##秣":17967,"##秤":17968,"##秦":17969,"##秧":17970,"##秩":17971,"##秭":17972,"##积":17973,"##称":17974,"##秸":17975,"##移":17976,"##秽":17977,"##稀":17978,"##稅":17979,"##程":17980,"##稍":17981,"##税":17982,"##稔":17983,"##稗":17984,"##稚":17985,"##稜":17986,"##稞":17987,"##稟":17988,"##稠":17989,"##稣":17990,"##種":17991,"##稱":17992,"##稲":17993,"##稳":17994,"##稷":17995,"##稹":17996,"##稻":17997,"##稼":17998,"##稽":17999,"##稿":18000,"##穀":18001,"##穂":18002,"##穆":18003,"##穌":18004,"##積":18005,"##穎":18006,"##穗":18007,"##穢":18008,"##穩":18009,"##穫":18010,"##穴":18011,"##究":18012,"##穷":18013,"##穹":18014,"##空":18015,"##穿":18016,"##突":18017,"##窃":18018,"##窄":18019,"##窈":18020,"##窍":18021,"##窑":18022,"##窒":18023,"##窓":18024,"##窕":18025,"##窖":18026,"##窗":18027,"##窘":18028,"##窜":18029,"##窝":18030,"##窟":18031,"##窠":18032,"##窥":18033,"##窦":18034,"##窨":18035,"##窩":18036,"##窪":18037,"##窮":18038,"##窯":18039,"##窺":18040,"##窿":18041,"##竄":18042,"##竅":18043,"##竇":18044,"##竊":18045,"##立":18046,"##竖":18047,"##站":18048,"##竜":18049,"##竞":18050,"##竟":18051,"##章":18052,"##竣":18053,"##童":18054,"##竭":18055,"##端":18056,"##競":18057,"##竹":18058,"##竺":18059,"##竽":18060,"##竿":18061,"##笃":18062,"##笆":18063,"##笈":18064,"##笋":18065,"##笏":18066,"##笑":18067,"##笔":18068,"##笙":18069,"##笛":18070,"##笞":18071,"##笠":18072,"##符":18073,"##笨":18074,"##第":18075,"##笹":18076,"##笺":18077,"##笼":18078,"##筆":18079,"##等":18080,"##筊":18081,"##筋":18082,"##筍":18083,"##筏":18084,"##筐":18085,"##筑":18086,"##筒":18087,"##答":18088,"##策":18089,"##筛":18090,"##筝":18091,"##筠":18092,"##筱":18093,"##筲":18094,"##筵":18095,"##筷":18096,"##筹":18097,"##签":18098,"##简":18099,"##箇":18100,"##箋":18101,"##箍":18102,"##箏":18103,"##箐":18104,"##箔":18105,"##箕":18106,"##算":18107,"##箝":18108,"##管":18109,"##箩":18110,"##箫":18111,"##箭":18112,"##箱":18113,"##箴":18114,"##箸":18115,"##節":18116,"##篁":18117,"##範":18118,"##篆":18119,"##篇":18120,"##築":18121,"##篑":18122,"##篓":18123,"##篙":18124,"##篝":18125,"##篠":18126,"##篡":18127,"##篤":18128,"##篩":18129,"##篪":18130,"##篮":18131,"##篱":18132,"##篷":18133,"##簇":18134,"##簌":18135,"##簍":18136,"##簡":18137,"##簦":18138,"##簧":18139,"##簪":18140,"##簫":18141,"##簷":18142,"##簸":18143,"##簽":18144,"##簾":18145,"##簿":18146,"##籁":18147,"##籃":18148,"##籌":18149,"##籍":18150,"##籐":18151,"##籟":18152,"##籠":18153,"##籤":18154,"##籬":18155,"##籮":18156,"##籲":18157,"##米":18158,"##类":18159,"##籼":18160,"##籽":18161,"##粄":18162,"##粉":18163,"##粑":18164,"##粒":18165,"##粕":18166,"##粗":18167,"##粘":18168,"##粟":18169,"##粤":18170,"##粥":18171,"##粧":18172,"##粪":18173,"##粮":18174,"##粱":18175,"##粲":18176,"##粳":18177,"##粵":18178,"##粹":18179,"##粼":18180,"##粽":18181,"##精":18182,"##粿":18183,"##糅":18184,"##糊":18185,"##糍":18186,"##糕":18187,"##糖":18188,"##糗":18189,"##糙":18190,"##糜":18191,"##糞":18192,"##糟":18193,"##糠":18194,"##糧":18195,"##糬":18196,"##糯":18197,"##糰":18198,"##糸":18199,"##系":18200,"##糾":18201,"##紀":18202,"##紂":18203,"##約":18204,"##紅":18205,"##紉":18206,"##紊":18207,"##紋":18208,"##納":18209,"##紐":18210,"##紓":18211,"##純":18212,"##紗":18213,"##紘":18214,"##紙":18215,"##級":18216,"##紛":18217,"##紜":18218,"##素":18219,"##紡":18220,"##索":18221,"##紧":18222,"##紫":18223,"##紮":18224,"##累":18225,"##細":18226,"##紳":18227,"##紹":18228,"##紺":18229,"##終":18230,"##絃":18231,"##組":18232,"##絆":18233,"##経":18234,"##結":18235,"##絕":18236,"##絞":18237,"##絡":18238,"##絢":18239,"##給":18240,"##絨":18241,"##絮":18242,"##統":18243,"##絲":18244,"##絳":18245,"##絵":18246,"##絶":18247,"##絹":18248,"##綁":18249,"##綏":18250,"##綑":18251,"##經":18252,"##継":18253,"##続":18254,"##綜":18255,"##綠":18256,"##綢":18257,"##綦":18258,"##綫":18259,"##綬":18260,"##維":18261,"##綱":18262,"##網":18263,"##綴":18264,"##綵":18265,"##綸":18266,"##綺":18267,"##綻":18268,"##綽":18269,"##綾":18270,"##綿":18271,"##緊":18272,"##緋":18273,"##総":18274,"##緑":18275,"##緒":18276,"##緘":18277,"##線":18278,"##緝":18279,"##緞":18280,"##締":18281,"##緣":18282,"##編":18283,"##緩":18284,"##緬":18285,"##緯":18286,"##練":18287,"##緹":18288,"##緻":18289,"##縁":18290,"##縄":18291,"##縈":18292,"##縛":18293,"##縝":18294,"##縣":18295,"##縫":18296,"##縮":18297,"##縱":18298,"##縴":18299,"##縷":18300,"##總":18301,"##績":18302,"##繁":18303,"##繃":18304,"##繆":18305,"##繇":18306,"##繋":18307,"##織":18308,"##繕":18309,"##繚":18310,"##繞":18311,"##繡":18312,"##繩":18313,"##繪":18314,"##繫":18315,"##繭":18316,"##繳":18317,"##繹":18318,"##繼":18319,"##繽":18320,"##纂":18321,"##續":18322,"##纍":18323,"##纏":18324,"##纓":18325,"##纔":18326,"##纖":18327,"##纜":18328,"##纠":18329,"##红":18330,"##纣":18331,"##纤":18332,"##约":18333,"##级":18334,"##纨":18335,"##纪":18336,"##纫":18337,"##纬":18338,"##纭":18339,"##纯":18340,"##纰":18341,"##纱":18342,"##纲":18343,"##纳":18344,"##纵":18345,"##纶":18346,"##纷":18347,"##纸":18348,"##纹":18349,"##纺":18350,"##纽":18351,"##纾":18352,"##线":18353,"##绀":18354,"##练":18355,"##组":18356,"##绅":18357,"##细":18358,"##织":18359,"##终":18360,"##绊":18361,"##绍":18362,"##绎":18363,"##经":18364,"##绑":18365,"##绒":18366,"##结":18367,"##绔":18368,"##绕":18369,"##绘":18370,"##给":18371,"##绚":18372,"##绛":18373,"##络":18374,"##绝":18375,"##绞":18376,"##统":18377,"##绡":18378,"##绢":18379,"##绣":18380,"##绥":18381,"##绦":18382,"##继":18383,"##绩":18384,"##绪":18385,"##绫":18386,"##续":18387,"##绮":18388,"##绯":18389,"##绰":18390,"##绳":18391,"##维":18392,"##绵":18393,"##绶":18394,"##绷":18395,"##绸":18396,"##绻":18397,"##综":18398,"##绽":18399,"##绾":18400,"##绿":18401,"##缀":18402,"##缄":18403,"##缅":18404,"##缆":18405,"##缇":18406,"##缈":18407,"##缉":18408,"##缎":18409,"##缓":18410,"##缔":18411,"##缕":18412,"##编":18413,"##缘":18414,"##缙":18415,"##缚":18416,"##缜":18417,"##缝":18418,"##缠":18419,"##缢":18420,"##缤":18421,"##缥":18422,"##缨":18423,"##缩":18424,"##缪":18425,"##缭":18426,"##缮":18427,"##缰":18428,"##缱":18429,"##缴":18430,"##缸":18431,"##缺":18432,"##缽":18433,"##罂":18434,"##罄":18435,"##罌":18436,"##罐":18437,"##网":18438,"##罔":18439,"##罕":18440,"##罗":18441,"##罚":18442,"##罡":18443,"##罢":18444,"##罩":18445,"##罪":18446,"##置":18447,"##罰":18448,"##署":18449,"##罵":18450,"##罷":18451,"##罹":18452,"##羁":18453,"##羅":18454,"##羈":18455,"##羊":18456,"##羌":18457,"##美":18458,"##羔":18459,"##羚":18460,"##羞":18461,"##羟":18462,"##羡":18463,"##羣":18464,"##群":18465,"##羥":18466,"##羧":18467,"##羨":18468,"##義":18469,"##羯":18470,"##羲":18471,"##羸":18472,"##羹":18473,"##羽":18474,"##羿":18475,"##翁":18476,"##翅":18477,"##翊":18478,"##翌":18479,"##翎":18480,"##習":18481,"##翔":18482,"##翘":18483,"##翟":18484,"##翠":18485,"##翡":18486,"##翦":18487,"##翩":18488,"##翰":18489,"##翱":18490,"##翳":18491,"##翹":18492,"##翻":18493,"##翼":18494,"##耀":18495,"##老":18496,"##考":18497,"##耄":18498,"##者":18499,"##耆":18500,"##耋":18501,"##而":18502,"##耍":18503,"##耐":18504,"##耒":18505,"##耕":18506,"##耗":18507,"##耘":18508,"##耙":18509,"##耦":18510,"##耨":18511,"##耳":18512,"##耶":18513,"##耷":18514,"##耸":18515,"##耻":18516,"##耽":18517,"##耿":18518,"##聂":18519,"##聆":18520,"##聊":18521,"##聋":18522,"##职":18523,"##聒":18524,"##联":18525,"##聖":18526,"##聘":18527,"##聚":18528,"##聞":18529,"##聪":18530,"##聯":18531,"##聰":18532,"##聲":18533,"##聳":18534,"##聴":18535,"##聶":18536,"##職":18537,"##聽":18538,"##聾":18539,"##聿":18540,"##肃":18541,"##肄":18542,"##肅":18543,"##肆":18544,"##肇":18545,"##肉":18546,"##肋":18547,"##肌":18548,"##肏":18549,"##肓":18550,"##肖":18551,"##肘":18552,"##肚":18553,"##肛":18554,"##肝":18555,"##肠":18556,"##股":18557,"##肢":18558,"##肤":18559,"##肥":18560,"##肩":18561,"##肪":18562,"##肮":18563,"##肯":18564,"##肱":18565,"##育":18566,"##肴":18567,"##肺":18568,"##肽":18569,"##肾":18570,"##肿":18571,"##胀":18572,"##胁":18573,"##胃":18574,"##胄":18575,"##胆":18576,"##背":18577,"##胍":18578,"##胎":18579,"##胖":18580,"##胚":18581,"##胛":18582,"##胜":18583,"##胝":18584,"##胞":18585,"##胡":18586,"##胤":18587,"##胥":18588,"##胧":18589,"##胫":18590,"##胭":18591,"##胯":18592,"##胰":18593,"##胱":18594,"##胳":18595,"##胴":18596,"##胶":18597,"##胸":18598,"##胺":18599,"##能":18600,"##脂":18601,"##脅":18602,"##脆":18603,"##脇":18604,"##脈":18605,"##脉":18606,"##脊":18607,"##脍":18608,"##脏":18609,"##脐":18610,"##脑":18611,"##脓":18612,"##脖":18613,"##脘":18614,"##脚":18615,"##脛":18616,"##脣":18617,"##脩":18618,"##脫":18619,"##脯":18620,"##脱":18621,"##脲":18622,"##脳":18623,"##脸":18624,"##脹":18625,"##脾":18626,"##腆":18627,"##腈":18628,"##腊":18629,"##腋":18630,"##腌":18631,"##腎":18632,"##腐":18633,"##腑":18634,"##腓":18635,"##腔":18636,"##腕":18637,"##腥":18638,"##腦":18639,"##腩":18640,"##腫":18641,"##腭":18642,"##腮":18643,"##腰":18644,"##腱":18645,"##腳":18646,"##腴":18647,"##腸":18648,"##腹":18649,"##腺":18650,"##腻":18651,"##腼":18652,"##腾":18653,"##腿":18654,"##膀":18655,"##膈":18656,"##膊":18657,"##膏":18658,"##膑":18659,"##膘":18660,"##膚":18661,"##膛":18662,"##膜":18663,"##膝":18664,"##膠":18665,"##膦":18666,"##膨":18667,"##膩":18668,"##膳":18669,"##膺":18670,"##膻":18671,"##膽":18672,"##膾":18673,"##膿":18674,"##臀":18675,"##臂":18676,"##臃":18677,"##臆":18678,"##臉":18679,"##臊":18680,"##臍":18681,"##臓":18682,"##臘":18683,"##臟":18684,"##臣":18685,"##臥":18686,"##臧":18687,"##臨":18688,"##自":18689,"##臬":18690,"##臭":18691,"##至":18692,"##致":18693,"##臺":18694,"##臻":18695,"##臼":18696,"##臾":18697,"##舀":18698,"##舂":18699,"##舅":18700,"##舆":18701,"##與":18702,"##興":18703,"##舉":18704,"##舊":18705,"##舌":18706,"##舍":18707,"##舎":18708,"##舐":18709,"##舒":18710,"##舔":18711,"##舖":18712,"##舗":18713,"##舛":18714,"##舜":18715,"##舞":18716,"##舟":18717,"##航":18718,"##舫":18719,"##般":18720,"##舰":18721,"##舱":18722,"##舵":18723,"##舶":18724,"##舷":18725,"##舸":18726,"##船":18727,"##舺":18728,"##舾":18729,"##艇":18730,"##艋":18731,"##艘":18732,"##艙":18733,"##艦":18734,"##艮":18735,"##良":18736,"##艰":18737,"##艱":18738,"##色":18739,"##艳":18740,"##艷":18741,"##艹":18742,"##艺":18743,"##艾":18744,"##节":18745,"##芃":18746,"##芈":18747,"##芊":18748,"##芋":18749,"##芍":18750,"##芎":18751,"##芒":18752,"##芙":18753,"##芜":18754,"##芝":18755,"##芡":18756,"##芥":18757,"##芦":18758,"##芩":18759,"##芪":18760,"##芫":18761,"##芬":18762,"##芭":18763,"##芮":18764,"##芯":18765,"##花":18766,"##芳":18767,"##芷":18768,"##芸":18769,"##芹":18770,"##芻":18771,"##芽":18772,"##芾":18773,"##苁":18774,"##苄":18775,"##苇":18776,"##苋":18777,"##苍":18778,"##苏":18779,"##苑":18780,"##苒":18781,"##苓":18782,"##苔":18783,"##苕":18784,"##苗":18785,"##苛":18786,"##苜":18787,"##苞":18788,"##苟":18789,"##苡":18790,"##苣":18791,"##若":18792,"##苦":18793,"##苫":18794,"##苯":18795,"##英":18796,"##苷":18797,"##苹":18798,"##苻":18799,"##茁":18800,"##茂":18801,"##范":18802,"##茄":18803,"##茅":18804,"##茉":18805,"##茎":18806,"##茏":18807,"##茗":18808,"##茜":18809,"##茧":18810,"##茨":18811,"##茫":18812,"##茬":18813,"##茭":18814,"##茯":18815,"##茱":18816,"##茲":18817,"##茴":18818,"##茵":18819,"##茶":18820,"##茸":18821,"##茹":18822,"##茼":18823,"##荀":18824,"##荃":18825,"##荆":18826,"##草":18827,"##荊":18828,"##荏":18829,"##荐":18830,"##荒":18831,"##荔":18832,"##荖":18833,"##荘":18834,"##荚":18835,"##荞":18836,"##荟":18837,"##荠":18838,"##荡":18839,"##荣":18840,"##荤":18841,"##荥":18842,"##荧":18843,"##荨":18844,"##荪":18845,"##荫":18846,"##药":18847,"##荳":18848,"##荷":18849,"##荸":18850,"##荻":18851,"##荼":18852,"##荽":18853,"##莅":18854,"##莆":18855,"##莉":18856,"##莊":18857,"##莎":18858,"##莒":18859,"##莓":18860,"##莖":18861,"##莘":18862,"##莞":18863,"##莠":18864,"##莢":18865,"##莧":18866,"##莪":18867,"##莫":18868,"##莱":18869,"##莲":18870,"##莴":18871,"##获":18872,"##莹":18873,"##莺":18874,"##莽":18875,"##莿":18876,"##菀":18877,"##菁":18878,"##菅":18879,"##菇":18880,"##菈":18881,"##菊":18882,"##菌":18883,"##菏":18884,"##菓":18885,"##菖":18886,"##菘":18887,"##菜":18888,"##菟":18889,"##菠":18890,"##菡":18891,"##菩":18892,"##華":18893,"##菱":18894,"##菲":18895,"##菸":18896,"##菽":18897,"##萁":18898,"##萃":18899,"##萄":18900,"##萊":18901,"##萋":18902,"##萌":18903,"##萍":18904,"##萎":18905,"##萘":18906,"##萝":18907,"##萤":18908,"##营":18909,"##萦":18910,"##萧":18911,"##萨":18912,"##萩":18913,"##萬":18914,"##萱":18915,"##萵":18916,"##萸":18917,"##萼":18918,"##落":18919,"##葆":18920,"##葉":18921,"##著":18922,"##葚":18923,"##葛":18924,"##葡":18925,"##董":18926,"##葦":18927,"##葩":18928,"##葫":18929,"##葬":18930,"##葭":18931,"##葯":18932,"##葱":18933,"##葳":18934,"##葵":18935,"##葷":18936,"##葺":18937,"##蒂":18938,"##蒋":18939,"##蒐":18940,"##蒔":18941,"##蒙":18942,"##蒜":18943,"##蒞":18944,"##蒟":18945,"##蒡":18946,"##蒨":18947,"##蒲":18948,"##蒸":18949,"##蒹":18950,"##蒻":18951,"##蒼":18952,"##蒿":18953,"##蓁":18954,"##蓄":18955,"##蓆":18956,"##蓉":18957,"##蓋":18958,"##蓑":18959,"##蓓":18960,"##蓖":18961,"##蓝":18962,"##蓟":18963,"##蓦":18964,"##蓬":18965,"##蓮":18966,"##蓼":18967,"##蓿":18968,"##蔑":18969,"##蔓":18970,"##蔔":18971,"##蔗":18972,"##蔘":18973,"##蔚":18974,"##蔡":18975,"##蔣":18976,"##蔥":18977,"##蔫":18978,"##蔬":18979,"##蔭":18980,"##蔵":18981,"##蔷":18982,"##蔺":18983,"##蔻":18984,"##蔼":18985,"##蔽":18986,"##蕁":18987,"##蕃":18988,"##蕈":18989,"##蕉":18990,"##蕊":18991,"##蕎":18992,"##蕙":18993,"##蕤":18994,"##蕨":18995,"##蕩":18996,"##蕪":18997,"##蕭":18998,"##蕲":18999,"##蕴":19000,"##蕻":19001,"##蕾":19002,"##薄":19003,"##薅":19004,"##薇":19005,"##薈":19006,"##薊":19007,"##薏":19008,"##薑":19009,"##薔":19010,"##薙":19011,"##薛":19012,"##薦":19013,"##薨":19014,"##薩":19015,"##薪":19016,"##薬":19017,"##薯":19018,"##薰":19019,"##薹":19020,"##藉":19021,"##藍":19022,"##藏":19023,"##藐":19024,"##藓":19025,"##藕":19026,"##藜":19027,"##藝":19028,"##藤":19029,"##藥":19030,"##藩":19031,"##藹":19032,"##藻":19033,"##藿":19034,"##蘆":19035,"##蘇":19036,"##蘊":19037,"##蘋":19038,"##蘑":19039,"##蘚":19040,"##蘭":19041,"##蘸":19042,"##蘼":19043,"##蘿":19044,"##虎":19045,"##虏":19046,"##虐":19047,"##虑":19048,"##虔":19049,"##處":19050,"##虚":19051,"##虛":19052,"##虜":19053,"##虞":19054,"##號":19055,"##虢":19056,"##虧":19057,"##虫":19058,"##虬":19059,"##虱":19060,"##虹":19061,"##虻":19062,"##虽":19063,"##虾":19064,"##蚀":19065,"##蚁":19066,"##蚂":19067,"##蚊":19068,"##蚌":19069,"##蚓":19070,"##蚕":19071,"##蚜":19072,"##蚝":19073,"##蚣":19074,"##蚤":19075,"##蚩":19076,"##蚪":19077,"##蚯":19078,"##蚱":19079,"##蚵":19080,"##蛀":19081,"##蛆":19082,"##蛇":19083,"##蛊":19084,"##蛋":19085,"##蛎":19086,"##蛐":19087,"##蛔":19088,"##蛙":19089,"##蛛":19090,"##蛟":19091,"##蛤":19092,"##蛭":19093,"##蛮":19094,"##蛰":19095,"##蛳":19096,"##蛹":19097,"##蛻":19098,"##蛾":19099,"##蜀":19100,"##蜂":19101,"##蜃":19102,"##蜆":19103,"##蜇":19104,"##蜈":19105,"##蜊":19106,"##蜍":19107,"##蜒":19108,"##蜓":19109,"##蜕":19110,"##蜗":19111,"##蜘":19112,"##蜚":19113,"##蜜":19114,"##蜡":19115,"##蜢":19116,"##蜥":19117,"##蜱":19118,"##蜴":19119,"##蜷":19120,"##蜻":19121,"##蜿":19122,"##蝇":19123,"##蝈":19124,"##蝉":19125,"##蝌":19126,"##蝎":19127,"##蝕":19128,"##蝗":19129,"##蝙":19130,"##蝟":19131,"##蝠":19132,"##蝦":19133,"##蝨":19134,"##蝴":19135,"##蝶":19136,"##蝸":19137,"##蝼":19138,"##螂":19139,"##螃":19140,"##融":19141,"##螞":19142,"##螢":19143,"##螨":19144,"##螯":19145,"##螳":19146,"##螺":19147,"##蟀":19148,"##蟄":19149,"##蟆":19150,"##蟋":19151,"##蟎":19152,"##蟑":19153,"##蟒":19154,"##蟠":19155,"##蟬":19156,"##蟲":19157,"##蟹":19158,"##蟻":19159,"##蟾":19160,"##蠅":19161,"##蠍":19162,"##蠔":19163,"##蠕":19164,"##蠛":19165,"##蠟":19166,"##蠡":19167,"##蠢":19168,"##蠣":19169,"##蠱":19170,"##蠶":19171,"##蠹":19172,"##蠻":19173,"##血":19174,"##衄":19175,"##衅":19176,"##衆":19177,"##行":19178,"##衍":19179,"##術":19180,"##衔":19181,"##街":19182,"##衙":19183,"##衛":19184,"##衝":19185,"##衞":19186,"##衡":19187,"##衢":19188,"##衣":19189,"##补":19190,"##表":19191,"##衩":19192,"##衫":19193,"##衬":19194,"##衮":19195,"##衰":19196,"##衲":19197,"##衷":19198,"##衹":19199,"##衾":19200,"##衿":19201,"##袁":19202,"##袂":19203,"##袄":19204,"##袅":19205,"##袈":19206,"##袋":19207,"##袍":19208,"##袒":19209,"##袖":19210,"##袜":19211,"##袞":19212,"##袤":19213,"##袪":19214,"##被":19215,"##袭":19216,"##袱":19217,"##裁":19218,"##裂":19219,"##装":19220,"##裆":19221,"##裊":19222,"##裏":19223,"##裔":19224,"##裕":19225,"##裘":19226,"##裙":19227,"##補":19228,"##裝":19229,"##裟":19230,"##裡":19231,"##裤":19232,"##裨":19233,"##裱":19234,"##裳":19235,"##裴":19236,"##裸":19237,"##裹":19238,"##製":19239,"##裾":19240,"##褂":19241,"##複":19242,"##褐":19243,"##褒":19244,"##褓":19245,"##褔":19246,"##褚":19247,"##褥":19248,"##褪":19249,"##褫":19250,"##褲":19251,"##褶":19252,"##褻":19253,"##襁":19254,"##襄":19255,"##襟":19256,"##襠":19257,"##襪":19258,"##襬":19259,"##襯":19260,"##襲":19261,"##西":19262,"##要":19263,"##覃":19264,"##覆":19265,"##覇":19266,"##見":19267,"##規":19268,"##覓":19269,"##視":19270,"##覚":19271,"##覦":19272,"##覧":19273,"##親":19274,"##覬":19275,"##観":19276,"##覷":19277,"##覺":19278,"##覽":19279,"##觀":19280,"##见":19281,"##观":19282,"##规":19283,"##觅":19284,"##视":19285,"##览":19286,"##觉":19287,"##觊":19288,"##觎":19289,"##觐":19290,"##觑":19291,"##角":19292,"##觞":19293,"##解":19294,"##觥":19295,"##触":19296,"##觸":19297,"##言":19298,"##訂":19299,"##計":19300,"##訊":19301,"##討":19302,"##訓":19303,"##訕":19304,"##訖":19305,"##託":19306,"##記":19307,"##訛":19308,"##訝":19309,"##訟":19310,"##訣":19311,"##訥":19312,"##訪":19313,"##設":19314,"##許":19315,"##訳":19316,"##訴":19317,"##訶":19318,"##診":19319,"##註":19320,"##証":19321,"##詆":19322,"##詐":19323,"##詔":19324,"##評":19325,"##詛":19326,"##詞":19327,"##詠":19328,"##詡":19329,"##詢":19330,"##詣":19331,"##試":19332,"##詩":19333,"##詫":19334,"##詬":19335,"##詭":19336,"##詮":19337,"##詰":19338,"##話":19339,"##該":19340,"##詳":19341,"##詹":19342,"##詼":19343,"##誅":19344,"##誇":19345,"##誉":19346,"##誌":19347,"##認":19348,"##誓":19349,"##誕":19350,"##誘":19351,"##語":19352,"##誠":19353,"##誡":19354,"##誣":19355,"##誤":19356,"##誥":19357,"##誦":19358,"##誨":19359,"##說":19360,"##説":19361,"##読":19362,"##誰":19363,"##課":19364,"##誹":19365,"##誼":19366,"##調":19367,"##諄":19368,"##談":19369,"##請":19370,"##諏":19371,"##諒":19372,"##論":19373,"##諗":19374,"##諜":19375,"##諡":19376,"##諦":19377,"##諧":19378,"##諫":19379,"##諭":19380,"##諮":19381,"##諱":19382,"##諳":19383,"##諷":19384,"##諸":19385,"##諺":19386,"##諾":19387,"##謀":19388,"##謁":19389,"##謂":19390,"##謄":19391,"##謊":19392,"##謎":19393,"##謐":19394,"##謔":19395,"##謗":19396,"##謙":19397,"##講":19398,"##謝":19399,"##謠":19400,"##謨":19401,"##謬":19402,"##謹":19403,"##謾":19404,"##譁":19405,"##證":19406,"##譎":19407,"##譏":19408,"##識":19409,"##譙":19410,"##譚":19411,"##譜":19412,"##警":19413,"##譬":19414,"##譯":19415,"##議":19416,"##譲":19417,"##譴":19418,"##護":19419,"##譽":19420,"##讀":19421,"##變":19422,"##讓":19423,"##讚":19424,"##讞":19425,"##计":19426,"##订":19427,"##认":19428,"##讥":19429,"##讧":19430,"##讨":19431,"##让":19432,"##讪":19433,"##讫":19434,"##训":19435,"##议":19436,"##讯":19437,"##记":19438,"##讲":19439,"##讳":19440,"##讴":19441,"##讶":19442,"##讷":19443,"##许":19444,"##讹":19445,"##论":19446,"##讼":19447,"##讽":19448,"##设":19449,"##访":19450,"##诀":19451,"##证":19452,"##诃":19453,"##评":19454,"##诅":19455,"##识":19456,"##诈":19457,"##诉":19458,"##诊":19459,"##诋":19460,"##词":19461,"##诏":19462,"##译":19463,"##试":19464,"##诗":19465,"##诘":19466,"##诙":19467,"##诚":19468,"##诛":19469,"##话":19470,"##诞":19471,"##诟":19472,"##诠":19473,"##诡":19474,"##询":19475,"##诣":19476,"##诤":19477,"##该":19478,"##详":19479,"##诧":19480,"##诩":19481,"##诫":19482,"##诬":19483,"##语":19484,"##误":19485,"##诰":19486,"##诱":19487,"##诲":19488,"##说":19489,"##诵":19490,"##诶":19491,"##请":19492,"##诸":19493,"##诺":19494,"##读":19495,"##诽":19496,"##课":19497,"##诿":19498,"##谀":19499,"##谁":19500,"##调":19501,"##谄":19502,"##谅":19503,"##谆":19504,"##谈":19505,"##谊":19506,"##谋":19507,"##谌":19508,"##谍":19509,"##谎":19510,"##谏":19511,"##谐":19512,"##谑":19513,"##谒":19514,"##谓":19515,"##谔":19516,"##谕":19517,"##谗":19518,"##谘":19519,"##谙":19520,"##谚":19521,"##谛":19522,"##谜":19523,"##谟":19524,"##谢":19525,"##谣":19526,"##谤":19527,"##谥":19528,"##谦":19529,"##谧":19530,"##谨":19531,"##谩":19532,"##谪":19533,"##谬":19534,"##谭":19535,"##谯":19536,"##谱":19537,"##谲":19538,"##谴":19539,"##谶":19540,"##谷":19541,"##豁":19542,"##豆":19543,"##豇":19544,"##豈":19545,"##豉":19546,"##豊":19547,"##豌":19548,"##豎":19549,"##豐":19550,"##豔":19551,"##豚":19552,"##象":19553,"##豢":19554,"##豪":19555,"##豫":19556,"##豬":19557,"##豹":19558,"##豺":19559,"##貂":19560,"##貅":19561,"##貌":19562,"##貓":19563,"##貔":19564,"##貘":19565,"##貝":19566,"##貞":19567,"##負":19568,"##財":19569,"##貢":19570,"##貧":19571,"##貨":19572,"##販":19573,"##貪":19574,"##貫":19575,"##責":19576,"##貯":19577,"##貰":19578,"##貳":19579,"##貴":19580,"##貶":19581,"##買":19582,"##貸":19583,"##費":19584,"##貼":19585,"##貽":19586,"##貿":19587,"##賀":19588,"##賁":19589,"##賂":19590,"##賃":19591,"##賄":19592,"##資":19593,"##賈":19594,"##賊":19595,"##賑":19596,"##賓":19597,"##賜":19598,"##賞":19599,"##賠":19600,"##賡":19601,"##賢":19602,"##賣":19603,"##賤":19604,"##賦":19605,"##質":19606,"##賬":19607,"##賭":19608,"##賴":19609,"##賺":19610,"##購":19611,"##賽":19612,"##贅":19613,"##贈":19614,"##贊":19615,"##贍":19616,"##贏":19617,"##贓":19618,"##贖":19619,"##贛":19620,"##贝":19621,"##贞":19622,"##负":19623,"##贡":19624,"##财":19625,"##责":19626,"##贤":19627,"##败":19628,"##账":19629,"##货":19630,"##质":19631,"##贩":19632,"##贪":19633,"##贫":19634,"##贬":19635,"##购":19636,"##贮":19637,"##贯":19638,"##贰":19639,"##贱":19640,"##贲":19641,"##贴":19642,"##贵":19643,"##贷":19644,"##贸":19645,"##费":19646,"##贺":19647,"##贻":19648,"##贼":19649,"##贾":19650,"##贿":19651,"##赁":19652,"##赂":19653,"##赃":19654,"##资":19655,"##赅":19656,"##赈":19657,"##赊":19658,"##赋":19659,"##赌":19660,"##赎":19661,"##赏":19662,"##赐":19663,"##赓":19664,"##赔":19665,"##赖":19666,"##赘":19667,"##赚":19668,"##赛":19669,"##赝":19670,"##赞":19671,"##赠":19672,"##赡":19673,"##赢":19674,"##赣":19675,"##赤":19676,"##赦":19677,"##赧":19678,"##赫":19679,"##赭":19680,"##走":19681,"##赳":19682,"##赴":19683,"##赵":19684,"##赶":19685,"##起":19686,"##趁":19687,"##超":19688,"##越":19689,"##趋":19690,"##趕":19691,"##趙":19692,"##趟":19693,"##趣":19694,"##趨":19695,"##足":19696,"##趴":19697,"##趵":19698,"##趸":19699,"##趺":19700,"##趾":19701,"##跃":19702,"##跄":19703,"##跆":19704,"##跋":19705,"##跌":19706,"##跎":19707,"##跑":19708,"##跖":19709,"##跚":19710,"##跛":19711,"##距":19712,"##跟":19713,"##跡":19714,"##跤":19715,"##跨":19716,"##跩":19717,"##跪":19718,"##路":19719,"##跳":19720,"##践":19721,"##跷":19722,"##跹":19723,"##跺":19724,"##跻":19725,"##踉":19726,"##踊":19727,"##踌":19728,"##踏":19729,"##踐":19730,"##踝":19731,"##踞":19732,"##踟":19733,"##踢":19734,"##踩":19735,"##踪":19736,"##踮":19737,"##踱":19738,"##踴":19739,"##踵":19740,"##踹":19741,"##蹂":19742,"##蹄":19743,"##蹇":19744,"##蹈":19745,"##蹉":19746,"##蹊":19747,"##蹋":19748,"##蹑":19749,"##蹒":19750,"##蹙":19751,"##蹟":19752,"##蹣":19753,"##蹤":19754,"##蹦":19755,"##蹩":19756,"##蹬":19757,"##蹭":19758,"##蹲":19759,"##蹴":19760,"##蹶":19761,"##蹺":19762,"##蹼":19763,"##蹿":19764,"##躁":19765,"##躇":19766,"##躉":19767,"##躊":19768,"##躋":19769,"##躍":19770,"##躏":19771,"##躪":19772,"##身":19773,"##躬":19774,"##躯":19775,"##躲":19776,"##躺":19777,"##軀":19778,"##車":19779,"##軋":19780,"##軌":19781,"##軍":19782,"##軒":19783,"##軟":19784,"##転":19785,"##軸":19786,"##軼":19787,"##軽":19788,"##軾":19789,"##較":19790,"##載":19791,"##輒":19792,"##輓":19793,"##輔":19794,"##輕":19795,"##輛":19796,"##輝":19797,"##輟":19798,"##輩":19799,"##輪":19800,"##輯":19801,"##輸":19802,"##輻":19803,"##輾":19804,"##輿":19805,"##轄":19806,"##轅":19807,"##轆":19808,"##轉":19809,"##轍":19810,"##轎":19811,"##轟":19812,"##车":19813,"##轧":19814,"##轨":19815,"##轩":19816,"##转":19817,"##轭":19818,"##轮":19819,"##软":19820,"##轰":19821,"##轲":19822,"##轴":19823,"##轶":19824,"##轻":19825,"##轼":19826,"##载":19827,"##轿":19828,"##较":19829,"##辄":19830,"##辅":19831,"##辆":19832,"##辇":19833,"##辈":19834,"##辉":19835,"##辊":19836,"##辍":19837,"##辐":19838,"##辑":19839,"##输":19840,"##辕":19841,"##辖":19842,"##辗":19843,"##辘":19844,"##辙":19845,"##辛":19846,"##辜":19847,"##辞":19848,"##辟":19849,"##辣":19850,"##辦":19851,"##辨":19852,"##辩":19853,"##辫":19854,"##辭":19855,"##辮":19856,"##辯":19857,"##辰":19858,"##辱":19859,"##農":19860,"##边":19861,"##辺":19862,"##辻":19863,"##込":19864,"##辽":19865,"##达":19866,"##迁":19867,"##迂":19868,"##迄":19869,"##迅":19870,"##过":19871,"##迈":19872,"##迎":19873,"##运":19874,"##近":19875,"##返":19876,"##还":19877,"##这":19878,"##进":19879,"##远":19880,"##违":19881,"##连":19882,"##迟":19883,"##迢":19884,"##迤":19885,"##迥":19886,"##迦":19887,"##迩":19888,"##迪":19889,"##迫":19890,"##迭":19891,"##述":19892,"##迴":19893,"##迷":19894,"##迸":19895,"##迹":19896,"##迺":19897,"##追":19898,"##退":19899,"##送":19900,"##适":19901,"##逃":19902,"##逅":19903,"##逆":19904,"##选":19905,"##逊":19906,"##逍":19907,"##透":19908,"##逐":19909,"##递":19910,"##途":19911,"##逕":19912,"##逗":19913,"##這":19914,"##通":19915,"##逛":19916,"##逝":19917,"##逞":19918,"##速":19919,"##造":19920,"##逢":19921,"##連":19922,"##逮":19923,"##週":19924,"##進":19925,"##逵":19926,"##逶":19927,"##逸":19928,"##逻":19929,"##逼":19930,"##逾":19931,"##遁":19932,"##遂":19933,"##遅":19934,"##遇":19935,"##遊":19936,"##運":19937,"##遍":19938,"##過":19939,"##遏":19940,"##遐":19941,"##遑":19942,"##遒":19943,"##道":19944,"##達":19945,"##違":19946,"##遗":19947,"##遙":19948,"##遛":19949,"##遜":19950,"##遞":19951,"##遠":19952,"##遢":19953,"##遣":19954,"##遥":19955,"##遨":19956,"##適":19957,"##遭":19958,"##遮":19959,"##遲":19960,"##遴":19961,"##遵":19962,"##遶":19963,"##遷":19964,"##選":19965,"##遺":19966,"##遼":19967,"##遽":19968,"##避":19969,"##邀":19970,"##邁":19971,"##邂":19972,"##邃":19973,"##還":19974,"##邇":19975,"##邈":19976,"##邊":19977,"##邋":19978,"##邏":19979,"##邑":19980,"##邓":19981,"##邕":19982,"##邛":19983,"##邝":19984,"##邢":19985,"##那":19986,"##邦":19987,"##邨":19988,"##邪":19989,"##邬":19990,"##邮":19991,"##邯":19992,"##邰":19993,"##邱":19994,"##邳":19995,"##邵":19996,"##邸":19997,"##邹":19998,"##邺":19999,"##邻":20000,"##郁":20001,"##郅":20002,"##郊":20003,"##郎":20004,"##郑":20005,"##郜":20006,"##郝":20007,"##郡":20008,"##郢":20009,"##郤":20010,"##郦":20011,"##郧":20012,"##部":20013,"##郫":20014,"##郭":20015,"##郴":20016,"##郵":20017,"##郷":20018,"##郸":20019,"##都":20020,"##鄂":20021,"##鄉":20022,"##鄒":20023,"##鄔":20024,"##鄙":20025,"##鄞":20026,"##鄢":20027,"##鄧":20028,"##鄭":20029,"##鄰":20030,"##鄱":20031,"##鄲":20032,"##鄺":20033,"##酉":20034,"##酊":20035,"##酋":20036,"##酌":20037,"##配":20038,"##酐":20039,"##酒":20040,"##酗":20041,"##酚":20042,"##酝":20043,"##酢":20044,"##酣":20045,"##酥":20046,"##酩":20047,"##酪":20048,"##酬":20049,"##酮":20050,"##酯":20051,"##酰":20052,"##酱":20053,"##酵":20054,"##酶":20055,"##酷":20056,"##酸":20057,"##酿":20058,"##醃":20059,"##醇":20060,"##醉":20061,"##醋":20062,"##醍":20063,"##醐":20064,"##醒":20065,"##醚":20066,"##醛":20067,"##醜":20068,"##醞":20069,"##醣":20070,"##醪":20071,"##醫":20072,"##醬":20073,"##醮":20074,"##醯":20075,"##醴":20076,"##醺":20077,"##釀":20078,"##釁":20079,"##采":20080,"##釉":20081,"##释":20082,"##釋":20083,"##里":20084,"##重":20085,"##野":20086,"##量":20087,"##釐":20088,"##金":20089,"##釗":20090,"##釘":20091,"##釜":20092,"##針":20093,"##釣":20094,"##釦":20095,"##釧":20096,"##釵":20097,"##鈀":20098,"##鈉":20099,"##鈍":20100,"##鈎":20101,"##鈔":20102,"##鈕":20103,"##鈞":20104,"##鈣":20105,"##鈦":20106,"##鈪":20107,"##鈴":20108,"##鈺":20109,"##鈾":20110,"##鉀":20111,"##鉄":20112,"##鉅":20113,"##鉉":20114,"##鉑":20115,"##鉗":20116,"##鉚":20117,"##鉛":20118,"##鉤":20119,"##鉴":20120,"##鉻":20121,"##銀":20122,"##銃":20123,"##銅":20124,"##銑":20125,"##銓":20126,"##銖":20127,"##銘":20128,"##銜":20129,"##銬":20130,"##銭":20131,"##銮":20132,"##銳":20133,"##銷":20134,"##銹":20135,"##鋁":20136,"##鋅":20137,"##鋒":20138,"##鋤":20139,"##鋪":20140,"##鋰":20141,"##鋸":20142,"##鋼":20143,"##錄":20144,"##錐":20145,"##錘":20146,"##錚":20147,"##錠":20148,"##錢":20149,"##錦":20150,"##錨":20151,"##錫":20152,"##錮":20153,"##錯":20154,"##録":20155,"##錳":20156,"##錶":20157,"##鍊":20158,"##鍋":20159,"##鍍":20160,"##鍛":20161,"##鍥":20162,"##鍰":20163,"##鍵":20164,"##鍺":20165,"##鍾":20166,"##鎂":20167,"##鎊":20168,"##鎌":20169,"##鎏":20170,"##鎔":20171,"##鎖":20172,"##鎗":20173,"##鎚":20174,"##鎧":20175,"##鎬":20176,"##鎮":20177,"##鎳":20178,"##鏈":20179,"##鏖":20180,"##鏗":20181,"##鏘":20182,"##鏞":20183,"##鏟":20184,"##鏡":20185,"##鏢":20186,"##鏤":20187,"##鏽":20188,"##鐘":20189,"##鐮":20190,"##鐲":20191,"##鐳":20192,"##鐵":20193,"##鐸":20194,"##鐺":20195,"##鑄":20196,"##鑊":20197,"##鑑":20198,"##鑒":20199,"##鑣":20200,"##鑫":20201,"##鑰":20202,"##鑲":20203,"##鑼":20204,"##鑽":20205,"##鑾":20206,"##鑿":20207,"##针":20208,"##钉":20209,"##钊":20210,"##钎":20211,"##钏":20212,"##钒":20213,"##钓":20214,"##钗":20215,"##钙":20216,"##钛":20217,"##钜":20218,"##钝":20219,"##钞":20220,"##钟":20221,"##钠":20222,"##钡":20223,"##钢":20224,"##钣":20225,"##钤":20226,"##钥":20227,"##钦":20228,"##钧":20229,"##钨":20230,"##钩":20231,"##钮":20232,"##钯":20233,"##钰":20234,"##钱":20235,"##钳":20236,"##钴":20237,"##钵":20238,"##钺":20239,"##钻":20240,"##钼":20241,"##钾":20242,"##钿":20243,"##铀":20244,"##铁":20245,"##铂":20246,"##铃":20247,"##铄":20248,"##铅":20249,"##铆":20250,"##铉":20251,"##铎":20252,"##铐":20253,"##铛":20254,"##铜":20255,"##铝":20256,"##铠":20257,"##铡":20258,"##铢":20259,"##铣":20260,"##铤":20261,"##铨":20262,"##铩":20263,"##铬":20264,"##铭":20265,"##铮":20266,"##铰":20267,"##铲":20268,"##铵":20269,"##银":20270,"##铸":20271,"##铺":20272,"##链":20273,"##铿":20274,"##销":20275,"##锁":20276,"##锂":20277,"##锄":20278,"##锅":20279,"##锆":20280,"##锈":20281,"##锉":20282,"##锋":20283,"##锌":20284,"##锏":20285,"##锐":20286,"##锑":20287,"##错":20288,"##锚":20289,"##锟":20290,"##锡":20291,"##锢":20292,"##锣":20293,"##锤":20294,"##锥":20295,"##锦":20296,"##锭":20297,"##键":20298,"##锯":20299,"##锰":20300,"##锲":20301,"##锵":20302,"##锹":20303,"##锺":20304,"##锻":20305,"##镀":20306,"##镁":20307,"##镂":20308,"##镇":20309,"##镉":20310,"##镌":20311,"##镍":20312,"##镐":20313,"##镑":20314,"##镕":20315,"##镖":20316,"##镗":20317,"##镛":20318,"##镜":20319,"##镣":20320,"##镭":20321,"##镯":20322,"##镰":20323,"##镳":20324,"##镶":20325,"##長":20326,"##长":20327,"##門":20328,"##閃":20329,"##閉":20330,"##開":20331,"##閎":20332,"##閏":20333,"##閑":20334,"##閒":20335,"##間":20336,"##閔":20337,"##閘":20338,"##閡":20339,"##関":20340,"##閣":20341,"##閥":20342,"##閨":20343,"##閩":20344,"##閱":20345,"##閲":20346,"##閹":20347,"##閻":20348,"##閾":20349,"##闆":20350,"##闇":20351,"##闊":20352,"##闌":20353,"##闍":20354,"##闔":20355,"##闕":20356,"##闖":20357,"##闘":20358,"##關":20359,"##闡":20360,"##闢":20361,"##门":20362,"##闪":20363,"##闫":20364,"##闭":20365,"##问":20366,"##闯":20367,"##闰":20368,"##闲":20369,"##间":20370,"##闵":20371,"##闷":20372,"##闸":20373,"##闹":20374,"##闺":20375,"##闻":20376,"##闽":20377,"##闾":20378,"##阀":20379,"##阁":20380,"##阂":20381,"##阅":20382,"##阆":20383,"##阇":20384,"##阈":20385,"##阉":20386,"##阎":20387,"##阐":20388,"##阑":20389,"##阔":20390,"##阕":20391,"##阖":20392,"##阙":20393,"##阚":20394,"##阜":20395,"##队":20396,"##阡":20397,"##阪":20398,"##阮":20399,"##阱":20400,"##防":20401,"##阳":20402,"##阴":20403,"##阵":20404,"##阶":20405,"##阻":20406,"##阿":20407,"##陀":20408,"##陂":20409,"##附":20410,"##际":20411,"##陆":20412,"##陇":20413,"##陈":20414,"##陋":20415,"##陌":20416,"##降":20417,"##限":20418,"##陕":20419,"##陛":20420,"##陝":20421,"##陞":20422,"##陟":20423,"##陡":20424,"##院":20425,"##陣":20426,"##除":20427,"##陨":20428,"##险":20429,"##陪":20430,"##陰":20431,"##陲":20432,"##陳":20433,"##陵":20434,"##陶":20435,"##陷":20436,"##陸":20437,"##険":20438,"##陽":20439,"##隅":20440,"##隆":20441,"##隈":20442,"##隊":20443,"##隋":20444,"##隍":20445,"##階":20446,"##随":20447,"##隐":20448,"##隔":20449,"##隕":20450,"##隘":20451,"##隙":20452,"##際":20453,"##障":20454,"##隠":20455,"##隣":20456,"##隧":20457,"##隨":20458,"##險":20459,"##隱":20460,"##隴":20461,"##隶":20462,"##隸":20463,"##隻":20464,"##隼":20465,"##隽":20466,"##难":20467,"##雀":20468,"##雁":20469,"##雄":20470,"##雅":20471,"##集":20472,"##雇":20473,"##雉":20474,"##雋":20475,"##雌":20476,"##雍":20477,"##雎":20478,"##雏":20479,"##雑":20480,"##雒":20481,"##雕":20482,"##雖":20483,"##雙":20484,"##雛":20485,"##雜":20486,"##雞":20487,"##離":20488,"##難":20489,"##雨":20490,"##雪":20491,"##雯":20492,"##雰":20493,"##雲":20494,"##雳":20495,"##零":20496,"##雷":20497,"##雹":20498,"##電":20499,"##雾":20500,"##需":20501,"##霁":20502,"##霄":20503,"##霆":20504,"##震":20505,"##霈":20506,"##霉":20507,"##霊":20508,"##霍":20509,"##霎":20510,"##霏":20511,"##霑":20512,"##霓":20513,"##霖":20514,"##霜":20515,"##霞":20516,"##霧":20517,"##霭":20518,"##霰":20519,"##露":20520,"##霸":20521,"##霹":20522,"##霽":20523,"##霾":20524,"##靂":20525,"##靄":20526,"##靈":20527,"##青":20528,"##靓":20529,"##靖":20530,"##静":20531,"##靚":20532,"##靛":20533,"##靜":20534,"##非":20535,"##靠":20536,"##靡":20537,"##面":20538,"##靥":20539,"##靦":20540,"##革":20541,"##靳":20542,"##靴":20543,"##靶":20544,"##靼":20545,"##鞅":20546,"##鞋":20547,"##鞍":20548,"##鞏":20549,"##鞑":20550,"##鞘":20551,"##鞠":20552,"##鞣":20553,"##鞦":20554,"##鞭":20555,"##韆":20556,"##韋":20557,"##韌":20558,"##韓":20559,"##韜":20560,"##韦":20561,"##韧":20562,"##韩":20563,"##韬":20564,"##韭":20565,"##音":20566,"##韵":20567,"##韶":20568,"##韻":20569,"##響":20570,"##頁":20571,"##頂":20572,"##頃":20573,"##項":20574,"##順":20575,"##須":20576,"##頌":20577,"##預":20578,"##頑":20579,"##頒":20580,"##頓":20581,"##頗":20582,"##領":20583,"##頜":20584,"##頡":20585,"##頤":20586,"##頫":20587,"##頭":20588,"##頰":20589,"##頷":20590,"##頸":20591,"##頹":20592,"##頻":20593,"##頼":20594,"##顆":20595,"##題":20596,"##額":20597,"##顎":20598,"##顏":20599,"##顔":20600,"##願":20601,"##顛":20602,"##類":20603,"##顧":20604,"##顫":20605,"##顯":20606,"##顱":20607,"##顴":20608,"##页":20609,"##顶":20610,"##顷":20611,"##项":20612,"##顺":20613,"##须":20614,"##顼":20615,"##顽":20616,"##顾":20617,"##顿":20618,"##颁":20619,"##颂":20620,"##预":20621,"##颅":20622,"##领":20623,"##颇":20624,"##颈":20625,"##颉":20626,"##颊":20627,"##颌":20628,"##颍":20629,"##颐":20630,"##频":20631,"##颓":20632,"##颔":20633,"##颖":20634,"##颗":20635,"##题":20636,"##颚":20637,"##颛":20638,"##颜":20639,"##额":20640,"##颞":20641,"##颠":20642,"##颡":20643,"##颢":20644,"##颤":20645,"##颦":20646,"##颧":20647,"##風":20648,"##颯":20649,"##颱":20650,"##颳":20651,"##颶":20652,"##颼":20653,"##飄":20654,"##飆":20655,"##风":20656,"##飒":20657,"##飓":20658,"##飕":20659,"##飘":20660,"##飙":20661,"##飚":20662,"##飛":20663,"##飞":20664,"##食":20665,"##飢":20666,"##飨":20667,"##飩":20668,"##飪":20669,"##飯":20670,"##飲":20671,"##飼":20672,"##飽":20673,"##飾":20674,"##餃":20675,"##餅":20676,"##餉":20677,"##養":20678,"##餌":20679,"##餐":20680,"##餒":20681,"##餓":20682,"##餘":20683,"##餚":20684,"##餛":20685,"##餞":20686,"##餡":20687,"##館":20688,"##餮":20689,"##餵":20690,"##餾":20691,"##饅":20692,"##饈":20693,"##饋":20694,"##饌":20695,"##饍":20696,"##饑":20697,"##饒":20698,"##饕":20699,"##饗":20700,"##饞":20701,"##饥":20702,"##饨":20703,"##饪":20704,"##饬":20705,"##饭":20706,"##饮":20707,"##饯":20708,"##饰":20709,"##饱":20710,"##饲":20711,"##饴":20712,"##饵":20713,"##饶":20714,"##饷":20715,"##饺":20716,"##饼":20717,"##饽":20718,"##饿":20719,"##馀":20720,"##馁":20721,"##馄":20722,"##馅":20723,"##馆":20724,"##馈":20725,"##馋":20726,"##馍":20727,"##馏":20728,"##馒":20729,"##馔":20730,"##首":20731,"##馗":20732,"##香":20733,"##馥":20734,"##馨":20735,"##馬":20736,"##馭":20737,"##馮":20738,"##馳":20739,"##馴":20740,"##駁":20741,"##駄":20742,"##駅":20743,"##駆":20744,"##駐":20745,"##駒":20746,"##駕":20747,"##駛":20748,"##駝":20749,"##駭":20750,"##駱":20751,"##駿":20752,"##騁":20753,"##騎":20754,"##騏":20755,"##験":20756,"##騙":20757,"##騨":20758,"##騰":20759,"##騷":20760,"##驀":20761,"##驅":20762,"##驊":20763,"##驍":20764,"##驒":20765,"##驕":20766,"##驗":20767,"##驚":20768,"##驛":20769,"##驟":20770,"##驢":20771,"##驥":20772,"##马":20773,"##驭":20774,"##驮":20775,"##驯":20776,"##驰":20777,"##驱":20778,"##驳":20779,"##驴":20780,"##驶":20781,"##驷":20782,"##驸":20783,"##驹":20784,"##驻":20785,"##驼":20786,"##驾":20787,"##驿":20788,"##骁":20789,"##骂":20790,"##骄":20791,"##骅":20792,"##骆":20793,"##骇":20794,"##骈":20795,"##骊":20796,"##骋":20797,"##验":20798,"##骏":20799,"##骐":20800,"##骑":20801,"##骗":20802,"##骚":20803,"##骛":20804,"##骜":20805,"##骞":20806,"##骠":20807,"##骡":20808,"##骤":20809,"##骥":20810,"##骧":20811,"##骨":20812,"##骯":20813,"##骰":20814,"##骶":20815,"##骷":20816,"##骸":20817,"##骼":20818,"##髂":20819,"##髅":20820,"##髋":20821,"##髏":20822,"##髒":20823,"##髓":20824,"##體":20825,"##髖":20826,"##高":20827,"##髦":20828,"##髪":20829,"##髮":20830,"##髯":20831,"##髻":20832,"##鬃":20833,"##鬆":20834,"##鬍":20835,"##鬓":20836,"##鬚":20837,"##鬟":20838,"##鬢":20839,"##鬣":20840,"##鬥":20841,"##鬧":20842,"##鬱":20843,"##鬼":20844,"##魁":20845,"##魂":20846,"##魄":20847,"##魅":20848,"##魇":20849,"##魍":20850,"##魏":20851,"##魔":20852,"##魘":20853,"##魚":20854,"##魯":20855,"##魷":20856,"##鮑":20857,"##鮨":20858,"##鮪":20859,"##鮭":20860,"##鮮":20861,"##鯉":20862,"##鯊":20863,"##鯖":20864,"##鯛":20865,"##鯨":20866,"##鯰":20867,"##鯽":20868,"##鰍":20869,"##鰓":20870,"##鰭":20871,"##鰲":20872,"##鰻":20873,"##鰾":20874,"##鱈":20875,"##鱉":20876,"##鱔":20877,"##鱗":20878,"##鱷":20879,"##鱸":20880,"##鱼":20881,"##鱿":20882,"##鲁":20883,"##鲈":20884,"##鲍":20885,"##鲑":20886,"##鲛":20887,"##鲜":20888,"##鲟":20889,"##鲢":20890,"##鲤":20891,"##鲨":20892,"##鲫":20893,"##鲱":20894,"##鲲":20895,"##鲶":20896,"##鲷":20897,"##鲸":20898,"##鳃":20899,"##鳄":20900,"##鳅":20901,"##鳌":20902,"##鳍":20903,"##鳕":20904,"##鳖":20905,"##鳗":20906,"##鳝":20907,"##鳞":20908,"##鳥":20909,"##鳩":20910,"##鳳":20911,"##鳴":20912,"##鳶":20913,"##鴉":20914,"##鴕":20915,"##鴛":20916,"##鴦":20917,"##鴨":20918,"##鴻":20919,"##鴿":20920,"##鵑":20921,"##鵜":20922,"##鵝":20923,"##鵡":20924,"##鵬":20925,"##鵰":20926,"##鵲":20927,"##鶘":20928,"##鶩":20929,"##鶯":20930,"##鶴":20931,"##鷗":20932,"##鷲":20933,"##鷹":20934,"##鷺":20935,"##鸚":20936,"##鸞":20937,"##鸟":20938,"##鸠":20939,"##鸡":20940,"##鸢":20941,"##鸣":20942,"##鸥":20943,"##鸦":20944,"##鸨":20945,"##鸪":20946,"##鸭":20947,"##鸯":20948,"##鸳":20949,"##鸵":20950,"##鸽":20951,"##鸾":20952,"##鸿":20953,"##鹂":20954,"##鹃":20955,"##鹄":20956,"##鹅":20957,"##鹈":20958,"##鹉":20959,"##鹊":20960,"##鹌":20961,"##鹏":20962,"##鹑":20963,"##鹕":20964,"##鹘":20965,"##鹜":20966,"##鹞":20967,"##鹤":20968,"##鹦":20969,"##鹧":20970,"##鹫":20971,"##鹭":20972,"##鹰":20973,"##鹳":20974,"##鹵":20975,"##鹹":20976,"##鹼":20977,"##鹽":20978,"##鹿":20979,"##麂":20980,"##麋":20981,"##麒":20982,"##麓":20983,"##麗":20984,"##麝":20985,"##麟":20986,"##麥":20987,"##麦":20988,"##麩":20989,"##麴":20990,"##麵":20991,"##麸":20992,"##麺":20993,"##麻":20994,"##麼":20995,"##麽":20996,"##麾":20997,"##黃":20998,"##黄":20999,"##黍":21000,"##黎":21001,"##黏":21002,"##黑":21003,"##黒":21004,"##黔":21005,"##默":21006,"##黛":21007,"##黜":21008,"##黝":21009,"##點":21010,"##黠":21011,"##黨":21012,"##黯":21013,"##黴":21014,"##鼋":21015,"##鼎":21016,"##鼐":21017,"##鼓":21018,"##鼠":21019,"##鼬":21020,"##鼹":21021,"##鼻":21022,"##鼾":21023,"##齁":21024,"##齊":21025,"##齋":21026,"##齐":21027,"##齒":21028,"##齡":21029,"##齢":21030,"##齣":21031,"##齦":21032,"##齿":21033,"##龄":21034,"##龅":21035,"##龈":21036,"##龊":21037,"##龋":21038,"##龌":21039,"##龍":21040,"##龐":21041,"##龔":21042,"##龕":21043,"##龙":21044,"##龚":21045,"##龛":21046,"##龜":21047,"##龟":21048,"##︰":21049,"##︱":21050,"##︶":21051,"##︿":21052,"##﹁":21053,"##﹂":21054,"##﹍":21055,"##﹏":21056,"##﹐":21057,"##﹑":21058,"##﹒":21059,"##﹔":21060,"##﹕":21061,"##﹖":21062,"##﹗":21063,"##﹙":21064,"##﹚":21065,"##﹝":21066,"##﹞":21067,"##﹡":21068,"##﹣":21069,"##!":21070,"##"":21071,"###":21072,"##$":21073,"##%":21074,"##&":21075,"##'":21076,"##(":21077,"##)":21078,"##*":21079,"##,":21080,"##-":21081,"##.":21082,"##/":21083,"##:":21084,"##;":21085,"##<":21086,"##?":21087,"##@":21088,"##[":21089,"##\":21090,"##]":21091,"##^":21092,"##_":21093,"##`":21094,"##f":21095,"##h":21096,"##j":21097,"##u":21098,"##w":21099,"##z":21100,"##{":21101,"##}":21102,"##。":21103,"##「":21104,"##」":21105,"##、":21106,"##・":21107,"##ッ":21108,"##ー":21109,"##イ":21110,"##ク":21111,"##シ":21112,"##ス":21113,"##ト":21114,"##ノ":21115,"##フ":21116,"##ラ":21117,"##ル":21118,"##ン":21119,"##゙":21120,"##゚":21121,"## ̄":21122,"##¥":21123,"##👍":21124,"##🔥":21125,"##😂":21126,"##😎":21127}}}
diff --git a/bert/chinese-roberta-wwm-ext-large/tokenizer_config.json b/bert/chinese-roberta-wwm-ext-large/tokenizer_config.json
new file mode 100644
index 0000000000000000000000000000000000000000..f1c72742be6da4503f2ecec8aef9bc1dc9775d17
--- /dev/null
+++ b/bert/chinese-roberta-wwm-ext-large/tokenizer_config.json
@@ -0,0 +1 @@
+{"init_inputs": []}
diff --git a/bert/chinese-roberta-wwm-ext-large/vocab.txt b/bert/chinese-roberta-wwm-ext-large/vocab.txt
new file mode 100644
index 0000000000000000000000000000000000000000..ca4f9781030019ab9b253c6dcb8c7878b6dc87a5
--- /dev/null
+++ b/bert/chinese-roberta-wwm-ext-large/vocab.txt
@@ -0,0 +1,21128 @@
+[PAD]
+[unused1]
+[unused2]
+[unused3]
+[unused4]
+[unused5]
+[unused6]
+[unused7]
+[unused8]
+[unused9]
+[unused10]
+[unused11]
+[unused12]
+[unused13]
+[unused14]
+[unused15]
+[unused16]
+[unused17]
+[unused18]
+[unused19]
+[unused20]
+[unused21]
+[unused22]
+[unused23]
+[unused24]
+[unused25]
+[unused26]
+[unused27]
+[unused28]
+[unused29]
+[unused30]
+[unused31]
+[unused32]
+[unused33]
+[unused34]
+[unused35]
+[unused36]
+[unused37]
+[unused38]
+[unused39]
+[unused40]
+[unused41]
+[unused42]
+[unused43]
+[unused44]
+[unused45]
+[unused46]
+[unused47]
+[unused48]
+[unused49]
+[unused50]
+[unused51]
+[unused52]
+[unused53]
+[unused54]
+[unused55]
+[unused56]
+[unused57]
+[unused58]
+[unused59]
+[unused60]
+[unused61]
+[unused62]
+[unused63]
+[unused64]
+[unused65]
+[unused66]
+[unused67]
+[unused68]
+[unused69]
+[unused70]
+[unused71]
+[unused72]
+[unused73]
+[unused74]
+[unused75]
+[unused76]
+[unused77]
+[unused78]
+[unused79]
+[unused80]
+[unused81]
+[unused82]
+[unused83]
+[unused84]
+[unused85]
+[unused86]
+[unused87]
+[unused88]
+[unused89]
+[unused90]
+[unused91]
+[unused92]
+[unused93]
+[unused94]
+[unused95]
+[unused96]
+[unused97]
+[unused98]
+[unused99]
+[UNK]
+[CLS]
+[SEP]
+[MASK]
+
+
+!
+"
+#
+$
+%
+&
+'
+(
+)
+*
++
+,
+-
+.
+/
+0
+1
+2
+3
+4
+5
+6
+7
+8
+9
+:
+;
+<
+=
+>
+?
+@
+[
+\
+]
+^
+_
+a
+b
+c
+d
+e
+f
+g
+h
+i
+j
+k
+l
+m
+n
+o
+p
+q
+r
+s
+t
+u
+v
+w
+x
+y
+z
+{
+|
+}
+~
+£
+¤
+¥
+§
+©
+«
+®
+°
+±
+²
+³
+µ
+·
+¹
+º
+»
+¼
+×
+ß
+æ
+÷
+ø
+đ
+ŋ
+ɔ
+ə
+ɡ
+ʰ
+ˇ
+ˈ
+ˊ
+ˋ
+ˍ
+ː
+˙
+˚
+ˢ
+α
+β
+γ
+δ
+ε
+η
+θ
+ι
+κ
+λ
+μ
+ν
+ο
+π
+ρ
+ς
+σ
+τ
+υ
+φ
+χ
+ψ
+ω
+а
+б
+в
+г
+д
+е
+ж
+з
+и
+к
+л
+м
+н
+о
+п
+р
+с
+т
+у
+ф
+х
+ц
+ч
+ш
+ы
+ь
+я
+і
+ا
+ب
+ة
+ت
+د
+ر
+س
+ع
+ل
+م
+ن
+ه
+و
+ي
+۩
+ก
+ง
+น
+ม
+ย
+ร
+อ
+า
+เ
+๑
+་
+ღ
+ᄀ
+ᄁ
+ᄂ
+ᄃ
+ᄅ
+ᄆ
+ᄇ
+ᄈ
+ᄉ
+ᄋ
+ᄌ
+ᄎ
+ᄏ
+ᄐ
+ᄑ
+ᄒ
+ᅡ
+ᅢ
+ᅣ
+ᅥ
+ᅦ
+ᅧ
+ᅨ
+ᅩ
+ᅪ
+ᅬ
+ᅭ
+ᅮ
+ᅯ
+ᅲ
+ᅳ
+ᅴ
+ᅵ
+ᆨ
+ᆫ
+ᆯ
+ᆷ
+ᆸ
+ᆺ
+ᆻ
+ᆼ
+ᗜ
+ᵃ
+ᵉ
+ᵍ
+ᵏ
+ᵐ
+ᵒ
+ᵘ
+‖
+„
+†
+•
+‥
+‧
+
+‰
+′
+″
+‹
+›
+※
+‿
+⁄
+ⁱ
+⁺
+ⁿ
+₁
+₂
+₃
+₄
+€
+℃
+№
+™
+ⅰ
+ⅱ
+ⅲ
+ⅳ
+ⅴ
+←
+↑
+→
+↓
+↔
+↗
+↘
+⇒
+∀
+−
+∕
+∙
+√
+∞
+∟
+∠
+∣
+∥
+∩
+∮
+∶
+∼
+∽
+≈
+≒
+≡
+≤
+≥
+≦
+≧
+≪
+≫
+⊙
+⋅
+⋈
+⋯
+⌒
+①
+②
+③
+④
+⑤
+⑥
+⑦
+⑧
+⑨
+⑩
+⑴
+⑵
+⑶
+⑷
+⑸
+⒈
+⒉
+⒊
+⒋
+ⓒ
+ⓔ
+ⓘ
+─
+━
+│
+┃
+┅
+┆
+┊
+┌
+└
+├
+┣
+═
+║
+╚
+╞
+╠
+╭
+╮
+╯
+╰
+╱
+╳
+▂
+▃
+▅
+▇
+█
+▉
+▋
+▌
+▍
+▎
+■
+□
+▪
+▫
+▬
+▲
+△
+▶
+►
+▼
+▽
+◆
+◇
+○
+◎
+●
+◕
+◠
+◢
+◤
+☀
+★
+☆
+☕
+☞
+☺
+☼
+♀
+♂
+♠
+♡
+♣
+♥
+♦
+♪
+♫
+♬
+✈
+✔
+✕
+✖
+✦
+✨
+✪
+✰
+✿
+❀
+❤
+➜
+➤
+⦿
+、
+。
+〃
+々
+〇
+〈
+〉
+《
+》
+「
+」
+『
+』
+【
+】
+〓
+〔
+〕
+〖
+〗
+〜
+〝
+〞
+ぁ
+あ
+ぃ
+い
+う
+ぇ
+え
+お
+か
+き
+く
+け
+こ
+さ
+し
+す
+せ
+そ
+た
+ち
+っ
+つ
+て
+と
+な
+に
+ぬ
+ね
+の
+は
+ひ
+ふ
+へ
+ほ
+ま
+み
+む
+め
+も
+ゃ
+や
+ゅ
+ゆ
+ょ
+よ
+ら
+り
+る
+れ
+ろ
+わ
+を
+ん
+゜
+ゝ
+ァ
+ア
+ィ
+イ
+ゥ
+ウ
+ェ
+エ
+ォ
+オ
+カ
+キ
+ク
+ケ
+コ
+サ
+シ
+ス
+セ
+ソ
+タ
+チ
+ッ
+ツ
+テ
+ト
+ナ
+ニ
+ヌ
+ネ
+ノ
+ハ
+ヒ
+フ
+ヘ
+ホ
+マ
+ミ
+ム
+メ
+モ
+ャ
+ヤ
+ュ
+ユ
+ョ
+ヨ
+ラ
+リ
+ル
+レ
+ロ
+ワ
+ヲ
+ン
+ヶ
+・
+ー
+ヽ
+ㄅ
+ㄆ
+ㄇ
+ㄉ
+ㄋ
+ㄌ
+ㄍ
+ㄎ
+ㄏ
+ㄒ
+ㄚ
+ㄛ
+ㄞ
+ㄟ
+ㄢ
+ㄤ
+ㄥ
+ㄧ
+ㄨ
+ㆍ
+㈦
+㊣
+㎡
+㗎
+一
+丁
+七
+万
+丈
+三
+上
+下
+不
+与
+丐
+丑
+专
+且
+丕
+世
+丘
+丙
+业
+丛
+东
+丝
+丞
+丟
+両
+丢
+两
+严
+並
+丧
+丨
+个
+丫
+中
+丰
+串
+临
+丶
+丸
+丹
+为
+主
+丼
+丽
+举
+丿
+乂
+乃
+久
+么
+义
+之
+乌
+乍
+乎
+乏
+乐
+乒
+乓
+乔
+乖
+乗
+乘
+乙
+乜
+九
+乞
+也
+习
+乡
+书
+乩
+买
+乱
+乳
+乾
+亀
+亂
+了
+予
+争
+事
+二
+于
+亏
+云
+互
+五
+井
+亘
+亙
+亚
+些
+亜
+亞
+亟
+亡
+亢
+交
+亥
+亦
+产
+亨
+亩
+享
+京
+亭
+亮
+亲
+亳
+亵
+人
+亿
+什
+仁
+仃
+仄
+仅
+仆
+仇
+今
+介
+仍
+从
+仏
+仑
+仓
+仔
+仕
+他
+仗
+付
+仙
+仝
+仞
+仟
+代
+令
+以
+仨
+仪
+们
+仮
+仰
+仲
+件
+价
+任
+份
+仿
+企
+伉
+伊
+伍
+伎
+伏
+伐
+休
+伕
+众
+优
+伙
+会
+伝
+伞
+伟
+传
+伢
+伤
+伦
+伪
+伫
+伯
+估
+伴
+伶
+伸
+伺
+似
+伽
+佃
+但
+佇
+佈
+位
+低
+住
+佐
+佑
+体
+佔
+何
+佗
+佘
+余
+佚
+佛
+作
+佝
+佞
+佟
+你
+佢
+佣
+佤
+佥
+佩
+佬
+佯
+佰
+佳
+併
+佶
+佻
+佼
+使
+侃
+侄
+來
+侈
+例
+侍
+侏
+侑
+侖
+侗
+供
+依
+侠
+価
+侣
+侥
+侦
+侧
+侨
+侬
+侮
+侯
+侵
+侶
+侷
+便
+係
+促
+俄
+俊
+俎
+俏
+俐
+俑
+俗
+俘
+俚
+保
+俞
+俟
+俠
+信
+俨
+俩
+俪
+俬
+俭
+修
+俯
+俱
+俳
+俸
+俺
+俾
+倆
+倉
+個
+倌
+倍
+倏
+們
+倒
+倔
+倖
+倘
+候
+倚
+倜
+借
+倡
+値
+倦
+倩
+倪
+倫
+倬
+倭
+倶
+债
+值
+倾
+偃
+假
+偈
+偉
+偌
+偎
+偏
+偕
+做
+停
+健
+側
+偵
+偶
+偷
+偻
+偽
+偿
+傀
+傅
+傍
+傑
+傘
+備
+傚
+傢
+傣
+傥
+储
+傩
+催
+傭
+傲
+傳
+債
+傷
+傻
+傾
+僅
+働
+像
+僑
+僕
+僖
+僚
+僥
+僧
+僭
+僮
+僱
+僵
+價
+僻
+儀
+儂
+億
+儆
+儉
+儋
+儒
+儕
+儘
+償
+儡
+優
+儲
+儷
+儼
+儿
+兀
+允
+元
+兄
+充
+兆
+兇
+先
+光
+克
+兌
+免
+児
+兑
+兒
+兔
+兖
+党
+兜
+兢
+入
+內
+全
+兩
+八
+公
+六
+兮
+兰
+共
+兲
+关
+兴
+兵
+其
+具
+典
+兹
+养
+兼
+兽
+冀
+内
+円
+冇
+冈
+冉
+冊
+册
+再
+冏
+冒
+冕
+冗
+写
+军
+农
+冠
+冢
+冤
+冥
+冨
+冪
+冬
+冯
+冰
+冲
+决
+况
+冶
+冷
+冻
+冼
+冽
+冾
+净
+凄
+准
+凇
+凈
+凉
+凋
+凌
+凍
+减
+凑
+凛
+凜
+凝
+几
+凡
+凤
+処
+凪
+凭
+凯
+凰
+凱
+凳
+凶
+凸
+凹
+出
+击
+函
+凿
+刀
+刁
+刃
+分
+切
+刈
+刊
+刍
+刎
+刑
+划
+列
+刘
+则
+刚
+创
+初
+删
+判
+別
+刨
+利
+刪
+别
+刮
+到
+制
+刷
+券
+刹
+刺
+刻
+刽
+剁
+剂
+剃
+則
+剉
+削
+剋
+剌
+前
+剎
+剐
+剑
+剔
+剖
+剛
+剜
+剝
+剣
+剤
+剥
+剧
+剩
+剪
+副
+割
+創
+剷
+剽
+剿
+劃
+劇
+劈
+劉
+劊
+劍
+劏
+劑
+力
+劝
+办
+功
+加
+务
+劣
+动
+助
+努
+劫
+劭
+励
+劲
+劳
+労
+劵
+効
+劾
+势
+勁
+勃
+勇
+勉
+勋
+勐
+勒
+動
+勖
+勘
+務
+勛
+勝
+勞
+募
+勢
+勤
+勧
+勳
+勵
+勸
+勺
+勻
+勾
+勿
+匀
+包
+匆
+匈
+匍
+匐
+匕
+化
+北
+匙
+匝
+匠
+匡
+匣
+匪
+匮
+匯
+匱
+匹
+区
+医
+匾
+匿
+區
+十
+千
+卅
+升
+午
+卉
+半
+卍
+华
+协
+卑
+卒
+卓
+協
+单
+卖
+南
+単
+博
+卜
+卞
+卟
+占
+卡
+卢
+卤
+卦
+卧
+卫
+卮
+卯
+印
+危
+即
+却
+卵
+卷
+卸
+卻
+卿
+厂
+厄
+厅
+历
+厉
+压
+厌
+厕
+厘
+厚
+厝
+原
+厢
+厥
+厦
+厨
+厩
+厭
+厮
+厲
+厳
+去
+县
+叁
+参
+參
+又
+叉
+及
+友
+双
+反
+収
+发
+叔
+取
+受
+变
+叙
+叛
+叟
+叠
+叡
+叢
+口
+古
+句
+另
+叨
+叩
+只
+叫
+召
+叭
+叮
+可
+台
+叱
+史
+右
+叵
+叶
+号
+司
+叹
+叻
+叼
+叽
+吁
+吃
+各
+吆
+合
+吉
+吊
+吋
+同
+名
+后
+吏
+吐
+向
+吒
+吓
+吕
+吖
+吗
+君
+吝
+吞
+吟
+吠
+吡
+否
+吧
+吨
+吩
+含
+听
+吭
+吮
+启
+吱
+吳
+吴
+吵
+吶
+吸
+吹
+吻
+吼
+吽
+吾
+呀
+呂
+呃
+呆
+呈
+告
+呋
+呎
+呐
+呓
+呕
+呗
+员
+呛
+呜
+呢
+呤
+呦
+周
+呱
+呲
+味
+呵
+呷
+呸
+呻
+呼
+命
+咀
+咁
+咂
+咄
+咆
+咋
+和
+咎
+咏
+咐
+咒
+咔
+咕
+咖
+咗
+咘
+咙
+咚
+咛
+咣
+咤
+咦
+咧
+咨
+咩
+咪
+咫
+咬
+咭
+咯
+咱
+咲
+咳
+咸
+咻
+咽
+咿
+哀
+品
+哂
+哄
+哆
+哇
+哈
+哉
+哋
+哌
+响
+哎
+哏
+哐
+哑
+哒
+哔
+哗
+哟
+員
+哥
+哦
+哧
+哨
+哩
+哪
+哭
+哮
+哲
+哺
+哼
+哽
+唁
+唄
+唆
+唇
+唉
+唏
+唐
+唑
+唔
+唠
+唤
+唧
+唬
+售
+唯
+唰
+唱
+唳
+唷
+唸
+唾
+啃
+啄
+商
+啉
+啊
+問
+啓
+啕
+啖
+啜
+啞
+啟
+啡
+啤
+啥
+啦
+啧
+啪
+啫
+啬
+啮
+啰
+啱
+啲
+啵
+啶
+啷
+啸
+啻
+啼
+啾
+喀
+喂
+喃
+善
+喆
+喇
+喉
+喊
+喋
+喎
+喏
+喔
+喘
+喙
+喚
+喜
+喝
+喟
+喧
+喪
+喫
+喬
+單
+喰
+喱
+喲
+喳
+喵
+営
+喷
+喹
+喺
+喻
+喽
+嗅
+嗆
+嗇
+嗎
+嗑
+嗒
+嗓
+嗔
+嗖
+嗚
+嗜
+嗝
+嗟
+嗡
+嗣
+嗤
+嗦
+嗨
+嗪
+嗬
+嗯
+嗰
+嗲
+嗳
+嗶
+嗷
+嗽
+嘀
+嘅
+嘆
+嘈
+嘉
+嘌
+嘍
+嘎
+嘔
+嘖
+嘗
+嘘
+嘚
+嘛
+嘜
+嘞
+嘟
+嘢
+嘣
+嘤
+嘧
+嘩
+嘭
+嘮
+嘯
+嘰
+嘱
+嘲
+嘴
+嘶
+嘸
+嘹
+嘻
+嘿
+噁
+噌
+噎
+噓
+噔
+噗
+噙
+噜
+噠
+噢
+噤
+器
+噩
+噪
+噬
+噱
+噴
+噶
+噸
+噹
+噻
+噼
+嚀
+嚇
+嚎
+嚏
+嚐
+嚓
+嚕
+嚟
+嚣
+嚥
+嚨
+嚮
+嚴
+嚷
+嚼
+囂
+囉
+囊
+囍
+囑
+囔
+囗
+囚
+四
+囝
+回
+囟
+因
+囡
+团
+団
+囤
+囧
+囪
+囫
+园
+困
+囱
+囲
+図
+围
+囹
+固
+国
+图
+囿
+圃
+圄
+圆
+圈
+國
+圍
+圏
+園
+圓
+圖
+團
+圜
+土
+圣
+圧
+在
+圩
+圭
+地
+圳
+场
+圻
+圾
+址
+坂
+均
+坊
+坍
+坎
+坏
+坐
+坑
+块
+坚
+坛
+坝
+坞
+坟
+坠
+坡
+坤
+坦
+坨
+坪
+坯
+坳
+坵
+坷
+垂
+垃
+垄
+型
+垒
+垚
+垛
+垠
+垢
+垣
+垦
+垩
+垫
+垭
+垮
+垵
+埂
+埃
+埋
+城
+埔
+埕
+埗
+域
+埠
+埤
+埵
+執
+埸
+培
+基
+埼
+堀
+堂
+堃
+堅
+堆
+堇
+堑
+堕
+堙
+堡
+堤
+堪
+堯
+堰
+報
+場
+堵
+堺
+堿
+塊
+塌
+塑
+塔
+塗
+塘
+塚
+塞
+塢
+塩
+填
+塬
+塭
+塵
+塾
+墀
+境
+墅
+墉
+墊
+墒
+墓
+増
+墘
+墙
+墜
+增
+墟
+墨
+墩
+墮
+墳
+墻
+墾
+壁
+壅
+壆
+壇
+壊
+壑
+壓
+壕
+壘
+壞
+壟
+壢
+壤
+壩
+士
+壬
+壮
+壯
+声
+売
+壳
+壶
+壹
+壺
+壽
+处
+备
+変
+复
+夏
+夔
+夕
+外
+夙
+多
+夜
+够
+夠
+夢
+夥
+大
+天
+太
+夫
+夭
+央
+夯
+失
+头
+夷
+夸
+夹
+夺
+夾
+奂
+奄
+奇
+奈
+奉
+奋
+奎
+奏
+奐
+契
+奔
+奕
+奖
+套
+奘
+奚
+奠
+奢
+奥
+奧
+奪
+奬
+奮
+女
+奴
+奶
+奸
+她
+好
+如
+妃
+妄
+妆
+妇
+妈
+妊
+妍
+妒
+妓
+妖
+妘
+妙
+妝
+妞
+妣
+妤
+妥
+妨
+妩
+妪
+妮
+妲
+妳
+妹
+妻
+妾
+姆
+姉
+姊
+始
+姍
+姐
+姑
+姒
+姓
+委
+姗
+姚
+姜
+姝
+姣
+姥
+姦
+姨
+姪
+姫
+姬
+姹
+姻
+姿
+威
+娃
+娄
+娅
+娆
+娇
+娉
+娑
+娓
+娘
+娛
+娜
+娟
+娠
+娣
+娥
+娩
+娱
+娲
+娴
+娶
+娼
+婀
+婁
+婆
+婉
+婊
+婕
+婚
+婢
+婦
+婧
+婪
+婭
+婴
+婵
+婶
+婷
+婺
+婿
+媒
+媚
+媛
+媞
+媧
+媲
+媳
+媽
+媾
+嫁
+嫂
+嫉
+嫌
+嫑
+嫔
+嫖
+嫘
+嫚
+嫡
+嫣
+嫦
+嫩
+嫲
+嫵
+嫻
+嬅
+嬉
+嬌
+嬗
+嬛
+嬢
+嬤
+嬪
+嬰
+嬴
+嬷
+嬸
+嬿
+孀
+孃
+子
+孑
+孔
+孕
+孖
+字
+存
+孙
+孚
+孛
+孜
+孝
+孟
+孢
+季
+孤
+学
+孩
+孪
+孫
+孬
+孰
+孱
+孳
+孵
+學
+孺
+孽
+孿
+宁
+它
+宅
+宇
+守
+安
+宋
+完
+宏
+宓
+宕
+宗
+官
+宙
+定
+宛
+宜
+宝
+实
+実
+宠
+审
+客
+宣
+室
+宥
+宦
+宪
+宫
+宮
+宰
+害
+宴
+宵
+家
+宸
+容
+宽
+宾
+宿
+寂
+寄
+寅
+密
+寇
+富
+寐
+寒
+寓
+寛
+寝
+寞
+察
+寡
+寢
+寥
+實
+寧
+寨
+審
+寫
+寬
+寮
+寰
+寵
+寶
+寸
+对
+寺
+寻
+导
+対
+寿
+封
+専
+射
+将
+將
+專
+尉
+尊
+尋
+對
+導
+小
+少
+尔
+尕
+尖
+尘
+尚
+尝
+尤
+尧
+尬
+就
+尴
+尷
+尸
+尹
+尺
+尻
+尼
+尽
+尾
+尿
+局
+屁
+层
+屄
+居
+屆
+屈
+屉
+届
+屋
+屌
+屍
+屎
+屏
+屐
+屑
+展
+屜
+属
+屠
+屡
+屢
+層
+履
+屬
+屯
+山
+屹
+屿
+岀
+岁
+岂
+岌
+岐
+岑
+岔
+岖
+岗
+岘
+岙
+岚
+岛
+岡
+岩
+岫
+岬
+岭
+岱
+岳
+岷
+岸
+峇
+峋
+峒
+峙
+峡
+峤
+峥
+峦
+峨
+峪
+峭
+峯
+峰
+峴
+島
+峻
+峽
+崁
+崂
+崆
+崇
+崎
+崑
+崔
+崖
+崗
+崙
+崛
+崧
+崩
+崭
+崴
+崽
+嵇
+嵊
+嵋
+嵌
+嵐
+嵘
+嵩
+嵬
+嵯
+嶂
+嶄
+嶇
+嶋
+嶙
+嶺
+嶼
+嶽
+巅
+巍
+巒
+巔
+巖
+川
+州
+巡
+巢
+工
+左
+巧
+巨
+巩
+巫
+差
+己
+已
+巳
+巴
+巷
+巻
+巽
+巾
+巿
+币
+市
+布
+帅
+帆
+师
+希
+帐
+帑
+帕
+帖
+帘
+帚
+帛
+帜
+帝
+帥
+带
+帧
+師
+席
+帮
+帯
+帰
+帳
+帶
+帷
+常
+帼
+帽
+幀
+幂
+幄
+幅
+幌
+幔
+幕
+幟
+幡
+幢
+幣
+幫
+干
+平
+年
+并
+幸
+幹
+幺
+幻
+幼
+幽
+幾
+广
+庁
+広
+庄
+庆
+庇
+床
+序
+庐
+库
+应
+底
+庖
+店
+庙
+庚
+府
+庞
+废
+庠
+度
+座
+庫
+庭
+庵
+庶
+康
+庸
+庹
+庾
+廁
+廂
+廃
+廈
+廉
+廊
+廓
+廖
+廚
+廝
+廟
+廠
+廢
+廣
+廬
+廳
+延
+廷
+建
+廿
+开
+弁
+异
+弃
+弄
+弈
+弊
+弋
+式
+弑
+弒
+弓
+弔
+引
+弗
+弘
+弛
+弟
+张
+弥
+弦
+弧
+弩
+弭
+弯
+弱
+張
+強
+弹
+强
+弼
+弾
+彅
+彆
+彈
+彌
+彎
+归
+当
+录
+彗
+彙
+彝
+形
+彤
+彥
+彦
+彧
+彩
+彪
+彫
+彬
+彭
+彰
+影
+彷
+役
+彻
+彼
+彿
+往
+征
+径
+待
+徇
+很
+徉
+徊
+律
+後
+徐
+徑
+徒
+従
+徕
+得
+徘
+徙
+徜
+從
+徠
+御
+徨
+復
+循
+徬
+微
+徳
+徴
+徵
+德
+徹
+徼
+徽
+心
+必
+忆
+忌
+忍
+忏
+忐
+忑
+忒
+忖
+志
+忘
+忙
+応
+忠
+忡
+忤
+忧
+忪
+快
+忱
+念
+忻
+忽
+忿
+怀
+态
+怂
+怅
+怆
+怎
+怏
+怒
+怔
+怕
+怖
+怙
+怜
+思
+怠
+怡
+急
+怦
+性
+怨
+怪
+怯
+怵
+总
+怼
+恁
+恃
+恆
+恋
+恍
+恐
+恒
+恕
+恙
+恚
+恢
+恣
+恤
+恥
+恨
+恩
+恪
+恫
+恬
+恭
+息
+恰
+恳
+恵
+恶
+恸
+恺
+恻
+恼
+恿
+悄
+悅
+悉
+悌
+悍
+悔
+悖
+悚
+悟
+悠
+患
+悦
+您
+悩
+悪
+悬
+悯
+悱
+悲
+悴
+悵
+悶
+悸
+悻
+悼
+悽
+情
+惆
+惇
+惊
+惋
+惑
+惕
+惘
+惚
+惜
+惟
+惠
+惡
+惦
+惧
+惨
+惩
+惫
+惬
+惭
+惮
+惯
+惰
+惱
+想
+惴
+惶
+惹
+惺
+愁
+愆
+愈
+愉
+愍
+意
+愕
+愚
+愛
+愜
+感
+愣
+愤
+愧
+愫
+愷
+愿
+慄
+慈
+態
+慌
+慎
+慑
+慕
+慘
+慚
+慟
+慢
+慣
+慧
+慨
+慫
+慮
+慰
+慳
+慵
+慶
+慷
+慾
+憂
+憊
+憋
+憎
+憐
+憑
+憔
+憚
+憤
+憧
+憨
+憩
+憫
+憬
+憲
+憶
+憾
+懂
+懇
+懈
+應
+懊
+懋
+懑
+懒
+懦
+懲
+懵
+懶
+懷
+懸
+懺
+懼
+懾
+懿
+戀
+戈
+戊
+戌
+戍
+戎
+戏
+成
+我
+戒
+戕
+或
+战
+戚
+戛
+戟
+戡
+戦
+截
+戬
+戮
+戰
+戲
+戳
+戴
+戶
+户
+戸
+戻
+戾
+房
+所
+扁
+扇
+扈
+扉
+手
+才
+扎
+扑
+扒
+打
+扔
+払
+托
+扛
+扣
+扦
+执
+扩
+扪
+扫
+扬
+扭
+扮
+扯
+扰
+扱
+扳
+扶
+批
+扼
+找
+承
+技
+抄
+抉
+把
+抑
+抒
+抓
+投
+抖
+抗
+折
+抚
+抛
+抜
+択
+抟
+抠
+抡
+抢
+护
+报
+抨
+披
+抬
+抱
+抵
+抹
+押
+抽
+抿
+拂
+拄
+担
+拆
+拇
+拈
+拉
+拋
+拌
+拍
+拎
+拐
+拒
+拓
+拔
+拖
+拗
+拘
+拙
+拚
+招
+拜
+拟
+拡
+拢
+拣
+拥
+拦
+拧
+拨
+择
+括
+拭
+拮
+拯
+拱
+拳
+拴
+拷
+拼
+拽
+拾
+拿
+持
+挂
+指
+挈
+按
+挎
+挑
+挖
+挙
+挚
+挛
+挝
+挞
+挟
+挠
+挡
+挣
+挤
+挥
+挨
+挪
+挫
+振
+挲
+挹
+挺
+挽
+挾
+捂
+捅
+捆
+捉
+捋
+捌
+捍
+捎
+捏
+捐
+捕
+捞
+损
+捡
+换
+捣
+捧
+捨
+捩
+据
+捱
+捲
+捶
+捷
+捺
+捻
+掀
+掂
+掃
+掇
+授
+掉
+掌
+掏
+掐
+排
+掖
+掘
+掙
+掛
+掠
+採
+探
+掣
+接
+控
+推
+掩
+措
+掬
+掰
+掲
+掳
+掴
+掷
+掸
+掺
+揀
+揃
+揄
+揆
+揉
+揍
+描
+提
+插
+揖
+揚
+換
+握
+揣
+揩
+揪
+揭
+揮
+援
+揶
+揸
+揹
+揽
+搀
+搁
+搂
+搅
+損
+搏
+搐
+搓
+搔
+搖
+搗
+搜
+搞
+搡
+搪
+搬
+搭
+搵
+搶
+携
+搽
+摀
+摁
+摄
+摆
+摇
+摈
+摊
+摒
+摔
+摘
+摞
+摟
+摧
+摩
+摯
+摳
+摸
+摹
+摺
+摻
+撂
+撃
+撅
+撇
+撈
+撐
+撑
+撒
+撓
+撕
+撚
+撞
+撤
+撥
+撩
+撫
+撬
+播
+撮
+撰
+撲
+撵
+撷
+撸
+撻
+撼
+撿
+擀
+擁
+擂
+擄
+擅
+擇
+擊
+擋
+操
+擎
+擒
+擔
+擘
+據
+擞
+擠
+擡
+擢
+擦
+擬
+擰
+擱
+擲
+擴
+擷
+擺
+擼
+擾
+攀
+攏
+攒
+攔
+攘
+攙
+攜
+攝
+攞
+攢
+攣
+攤
+攥
+攪
+攫
+攬
+支
+收
+攸
+改
+攻
+放
+政
+故
+效
+敌
+敍
+敎
+敏
+救
+敕
+敖
+敗
+敘
+教
+敛
+敝
+敞
+敢
+散
+敦
+敬
+数
+敲
+整
+敵
+敷
+數
+斂
+斃
+文
+斋
+斌
+斎
+斐
+斑
+斓
+斗
+料
+斛
+斜
+斟
+斡
+斤
+斥
+斧
+斩
+斫
+斬
+断
+斯
+新
+斷
+方
+於
+施
+旁
+旃
+旅
+旋
+旌
+旎
+族
+旖
+旗
+无
+既
+日
+旦
+旧
+旨
+早
+旬
+旭
+旮
+旱
+时
+旷
+旺
+旻
+昀
+昂
+昆
+昇
+昉
+昊
+昌
+明
+昏
+易
+昔
+昕
+昙
+星
+映
+春
+昧
+昨
+昭
+是
+昱
+昴
+昵
+昶
+昼
+显
+晁
+時
+晃
+晉
+晋
+晌
+晏
+晒
+晓
+晔
+晕
+晖
+晗
+晚
+晝
+晞
+晟
+晤
+晦
+晨
+晩
+普
+景
+晰
+晴
+晶
+晷
+智
+晾
+暂
+暄
+暇
+暈
+暉
+暌
+暐
+暑
+暖
+暗
+暝
+暢
+暧
+暨
+暫
+暮
+暱
+暴
+暸
+暹
+曄
+曆
+曇
+曉
+曖
+曙
+曜
+曝
+曠
+曦
+曬
+曰
+曲
+曳
+更
+書
+曹
+曼
+曾
+替
+最
+會
+月
+有
+朋
+服
+朐
+朔
+朕
+朗
+望
+朝
+期
+朦
+朧
+木
+未
+末
+本
+札
+朮
+术
+朱
+朴
+朵
+机
+朽
+杀
+杂
+权
+杆
+杈
+杉
+李
+杏
+材
+村
+杓
+杖
+杜
+杞
+束
+杠
+条
+来
+杨
+杭
+杯
+杰
+東
+杳
+杵
+杷
+杼
+松
+板
+极
+构
+枇
+枉
+枋
+析
+枕
+林
+枚
+果
+枝
+枢
+枣
+枪
+枫
+枭
+枯
+枰
+枱
+枳
+架
+枷
+枸
+柄
+柏
+某
+柑
+柒
+染
+柔
+柘
+柚
+柜
+柞
+柠
+柢
+查
+柩
+柬
+柯
+柱
+柳
+柴
+柵
+査
+柿
+栀
+栃
+栄
+栅
+标
+栈
+栉
+栋
+栎
+栏
+树
+栓
+栖
+栗
+校
+栩
+株
+样
+核
+根
+格
+栽
+栾
+桀
+桁
+桂
+桃
+桅
+框
+案
+桉
+桌
+桎
+桐
+桑
+桓
+桔
+桜
+桠
+桡
+桢
+档
+桥
+桦
+桧
+桨
+桩
+桶
+桿
+梁
+梅
+梆
+梏
+梓
+梗
+條
+梟
+梢
+梦
+梧
+梨
+梭
+梯
+械
+梳
+梵
+梶
+检
+棂
+棄
+棉
+棋
+棍
+棒
+棕
+棗
+棘
+棚
+棟
+棠
+棣
+棧
+森
+棱
+棲
+棵
+棹
+棺
+椁
+椅
+椋
+植
+椎
+椒
+検
+椪
+椭
+椰
+椹
+椽
+椿
+楂
+楊
+楓
+楔
+楚
+楝
+楞
+楠
+楣
+楨
+楫
+業
+楮
+極
+楷
+楸
+楹
+楼
+楽
+概
+榄
+榆
+榈
+榉
+榔
+榕
+榖
+榛
+榜
+榨
+榫
+榭
+榮
+榱
+榴
+榷
+榻
+槁
+槃
+構
+槌
+槍
+槎
+槐
+槓
+様
+槛
+槟
+槤
+槭
+槲
+槳
+槻
+槽
+槿
+樁
+樂
+樊
+樑
+樓
+標
+樞
+樟
+模
+樣
+権
+横
+樫
+樯
+樱
+樵
+樸
+樹
+樺
+樽
+樾
+橄
+橇
+橋
+橐
+橘
+橙
+機
+橡
+橢
+橫
+橱
+橹
+橼
+檀
+檄
+檎
+檐
+檔
+檗
+檜
+檢
+檬
+檯
+檳
+檸
+檻
+櫃
+櫚
+櫛
+櫥
+櫸
+櫻
+欄
+權
+欒
+欖
+欠
+次
+欢
+欣
+欧
+欲
+欸
+欺
+欽
+款
+歆
+歇
+歉
+歌
+歎
+歐
+歓
+歙
+歛
+歡
+止
+正
+此
+步
+武
+歧
+歩
+歪
+歯
+歲
+歳
+歴
+歷
+歸
+歹
+死
+歼
+殁
+殃
+殆
+殇
+殉
+殊
+残
+殒
+殓
+殖
+殘
+殞
+殡
+殤
+殭
+殯
+殲
+殴
+段
+殷
+殺
+殼
+殿
+毀
+毁
+毂
+毅
+毆
+毋
+母
+毎
+每
+毒
+毓
+比
+毕
+毗
+毘
+毙
+毛
+毡
+毫
+毯
+毽
+氈
+氏
+氐
+民
+氓
+气
+氖
+気
+氙
+氛
+氟
+氡
+氢
+氣
+氤
+氦
+氧
+氨
+氪
+氫
+氮
+氯
+氰
+氲
+水
+氷
+永
+氹
+氾
+汀
+汁
+求
+汆
+汇
+汉
+汎
+汐
+汕
+汗
+汙
+汛
+汝
+汞
+江
+池
+污
+汤
+汨
+汩
+汪
+汰
+汲
+汴
+汶
+汹
+決
+汽
+汾
+沁
+沂
+沃
+沅
+沈
+沉
+沌
+沏
+沐
+沒
+沓
+沖
+沙
+沛
+沟
+没
+沢
+沣
+沥
+沦
+沧
+沪
+沫
+沭
+沮
+沱
+河
+沸
+油
+治
+沼
+沽
+沾
+沿
+況
+泄
+泉
+泊
+泌
+泓
+法
+泗
+泛
+泞
+泠
+泡
+波
+泣
+泥
+注
+泪
+泫
+泮
+泯
+泰
+泱
+泳
+泵
+泷
+泸
+泻
+泼
+泽
+泾
+洁
+洄
+洋
+洒
+洗
+洙
+洛
+洞
+津
+洩
+洪
+洮
+洱
+洲
+洵
+洶
+洸
+洹
+活
+洼
+洽
+派
+流
+浃
+浄
+浅
+浆
+浇
+浊
+测
+济
+浏
+浑
+浒
+浓
+浔
+浙
+浚
+浜
+浣
+浦
+浩
+浪
+浬
+浮
+浯
+浴
+海
+浸
+涂
+涅
+涇
+消
+涉
+涌
+涎
+涓
+涔
+涕
+涙
+涛
+涝
+涞
+涟
+涠
+涡
+涣
+涤
+润
+涧
+涨
+涩
+涪
+涮
+涯
+液
+涵
+涸
+涼
+涿
+淀
+淄
+淅
+淆
+淇
+淋
+淌
+淑
+淒
+淖
+淘
+淙
+淚
+淞
+淡
+淤
+淦
+淨
+淩
+淪
+淫
+淬
+淮
+深
+淳
+淵
+混
+淹
+淺
+添
+淼
+清
+済
+渉
+渊
+渋
+渍
+渎
+渐
+渔
+渗
+渙
+渚
+減
+渝
+渠
+渡
+渣
+渤
+渥
+渦
+温
+測
+渭
+港
+渲
+渴
+游
+渺
+渾
+湃
+湄
+湊
+湍
+湖
+湘
+湛
+湟
+湧
+湫
+湮
+湯
+湳
+湾
+湿
+満
+溃
+溅
+溉
+溏
+源
+準
+溜
+溝
+溟
+溢
+溥
+溧
+溪
+溫
+溯
+溱
+溴
+溶
+溺
+溼
+滁
+滂
+滄
+滅
+滇
+滋
+滌
+滑
+滓
+滔
+滕
+滙
+滚
+滝
+滞
+滟
+满
+滢
+滤
+滥
+滦
+滨
+滩
+滬
+滯
+滲
+滴
+滷
+滸
+滾
+滿
+漁
+漂
+漆
+漉
+漏
+漓
+演
+漕
+漠
+漢
+漣
+漩
+漪
+漫
+漬
+漯
+漱
+漲
+漳
+漸
+漾
+漿
+潆
+潇
+潋
+潍
+潑
+潔
+潘
+潛
+潜
+潞
+潟
+潢
+潤
+潦
+潧
+潭
+潮
+潰
+潴
+潸
+潺
+潼
+澀
+澄
+澆
+澈
+澍
+澎
+澗
+澜
+澡
+澤
+澧
+澱
+澳
+澹
+激
+濁
+濂
+濃
+濑
+濒
+濕
+濘
+濛
+濟
+濠
+濡
+濤
+濫
+濬
+濮
+濯
+濱
+濺
+濾
+瀅
+瀆
+瀉
+瀋
+瀏
+瀑
+瀕
+瀘
+瀚
+瀛
+瀝
+瀞
+瀟
+瀧
+瀨
+瀬
+瀰
+瀾
+灌
+灏
+灑
+灘
+灝
+灞
+灣
+火
+灬
+灭
+灯
+灰
+灵
+灶
+灸
+灼
+災
+灾
+灿
+炀
+炁
+炅
+炉
+炊
+炎
+炒
+炔
+炕
+炖
+炙
+炜
+炫
+炬
+炭
+炮
+炯
+炳
+炷
+炸
+点
+為
+炼
+炽
+烁
+烂
+烃
+烈
+烊
+烏
+烘
+烙
+烛
+烟
+烤
+烦
+烧
+烨
+烩
+烫
+烬
+热
+烯
+烷
+烹
+烽
+焉
+焊
+焕
+焖
+焗
+焘
+焙
+焚
+焜
+無
+焦
+焯
+焰
+焱
+然
+焼
+煅
+煉
+煊
+煌
+煎
+煒
+煖
+煙
+煜
+煞
+煤
+煥
+煦
+照
+煨
+煩
+煮
+煲
+煸
+煽
+熄
+熊
+熏
+熒
+熔
+熙
+熟
+熠
+熨
+熬
+熱
+熵
+熹
+熾
+燁
+燃
+燄
+燈
+燉
+燊
+燎
+燒
+燔
+燕
+燙
+燜
+營
+燥
+燦
+燧
+燭
+燮
+燴
+燻
+燼
+燿
+爆
+爍
+爐
+爛
+爪
+爬
+爭
+爰
+爱
+爲
+爵
+父
+爷
+爸
+爹
+爺
+爻
+爽
+爾
+牆
+片
+版
+牌
+牍
+牒
+牙
+牛
+牝
+牟
+牠
+牡
+牢
+牦
+牧
+物
+牯
+牲
+牴
+牵
+特
+牺
+牽
+犀
+犁
+犄
+犊
+犍
+犒
+犢
+犧
+犬
+犯
+状
+犷
+犸
+犹
+狀
+狂
+狄
+狈
+狎
+狐
+狒
+狗
+狙
+狞
+狠
+狡
+狩
+独
+狭
+狮
+狰
+狱
+狸
+狹
+狼
+狽
+猎
+猕
+猖
+猗
+猙
+猛
+猜
+猝
+猥
+猩
+猪
+猫
+猬
+献
+猴
+猶
+猷
+猾
+猿
+獄
+獅
+獎
+獐
+獒
+獗
+獠
+獣
+獨
+獭
+獰
+獲
+獵
+獷
+獸
+獺
+獻
+獼
+獾
+玄
+率
+玉
+王
+玑
+玖
+玛
+玟
+玠
+玥
+玩
+玫
+玮
+环
+现
+玲
+玳
+玷
+玺
+玻
+珀
+珂
+珅
+珈
+珉
+珊
+珍
+珏
+珐
+珑
+珙
+珞
+珠
+珣
+珥
+珩
+珪
+班
+珮
+珲
+珺
+現
+球
+琅
+理
+琇
+琉
+琊
+琍
+琏
+琐
+琛
+琢
+琥
+琦
+琨
+琪
+琬
+琮
+琰
+琲
+琳
+琴
+琵
+琶
+琺
+琼
+瑀
+瑁
+瑄
+瑋
+瑕
+瑗
+瑙
+瑚
+瑛
+瑜
+瑞
+瑟
+瑠
+瑣
+瑤
+瑩
+瑪
+瑯
+瑰
+瑶
+瑾
+璀
+璁
+璃
+璇
+璉
+璋
+璎
+璐
+璜
+璞
+璟
+璧
+璨
+環
+璽
+璿
+瓊
+瓏
+瓒
+瓜
+瓢
+瓣
+瓤
+瓦
+瓮
+瓯
+瓴
+瓶
+瓷
+甄
+甌
+甕
+甘
+甙
+甚
+甜
+生
+產
+産
+甥
+甦
+用
+甩
+甫
+甬
+甭
+甯
+田
+由
+甲
+申
+电
+男
+甸
+町
+画
+甾
+畀
+畅
+界
+畏
+畑
+畔
+留
+畜
+畝
+畢
+略
+畦
+番
+畫
+異
+畲
+畳
+畴
+當
+畸
+畹
+畿
+疆
+疇
+疊
+疏
+疑
+疔
+疖
+疗
+疙
+疚
+疝
+疟
+疡
+疣
+疤
+疥
+疫
+疮
+疯
+疱
+疲
+疳
+疵
+疸
+疹
+疼
+疽
+疾
+痂
+病
+症
+痈
+痉
+痊
+痍
+痒
+痔
+痕
+痘
+痙
+痛
+痞
+痠
+痢
+痣
+痤
+痧
+痨
+痪
+痫
+痰
+痱
+痴
+痹
+痺
+痼
+痿
+瘀
+瘁
+瘋
+瘍
+瘓
+瘘
+瘙
+瘟
+瘠
+瘡
+瘢
+瘤
+瘦
+瘧
+瘩
+瘪
+瘫
+瘴
+瘸
+瘾
+療
+癇
+癌
+癒
+癖
+癜
+癞
+癡
+癢
+癣
+癥
+癫
+癬
+癮
+癱
+癲
+癸
+発
+登
+發
+白
+百
+皂
+的
+皆
+皇
+皈
+皋
+皎
+皑
+皓
+皖
+皙
+皚
+皮
+皰
+皱
+皴
+皺
+皿
+盂
+盃
+盅
+盆
+盈
+益
+盎
+盏
+盐
+监
+盒
+盔
+盖
+盗
+盘
+盛
+盜
+盞
+盟
+盡
+監
+盤
+盥
+盧
+盪
+目
+盯
+盱
+盲
+直
+相
+盹
+盼
+盾
+省
+眈
+眉
+看
+県
+眙
+眞
+真
+眠
+眦
+眨
+眩
+眯
+眶
+眷
+眸
+眺
+眼
+眾
+着
+睁
+睇
+睏
+睐
+睑
+睛
+睜
+睞
+睡
+睢
+督
+睥
+睦
+睨
+睪
+睫
+睬
+睹
+睽
+睾
+睿
+瞄
+瞅
+瞇
+瞋
+瞌
+瞎
+瞑
+瞒
+瞓
+瞞
+瞟
+瞠
+瞥
+瞧
+瞩
+瞪
+瞬
+瞭
+瞰
+瞳
+瞻
+瞼
+瞿
+矇
+矍
+矗
+矚
+矛
+矜
+矢
+矣
+知
+矩
+矫
+短
+矮
+矯
+石
+矶
+矽
+矾
+矿
+码
+砂
+砌
+砍
+砒
+研
+砖
+砗
+砚
+砝
+砣
+砥
+砧
+砭
+砰
+砲
+破
+砷
+砸
+砺
+砼
+砾
+础
+硅
+硐
+硒
+硕
+硝
+硫
+硬
+确
+硯
+硼
+碁
+碇
+碉
+碌
+碍
+碎
+碑
+碓
+碗
+碘
+碚
+碛
+碟
+碣
+碧
+碩
+碰
+碱
+碳
+碴
+確
+碼
+碾
+磁
+磅
+磊
+磋
+磐
+磕
+磚
+磡
+磨
+磬
+磯
+磲
+磷
+磺
+礁
+礎
+礙
+礡
+礦
+礪
+礫
+礴
+示
+礼
+社
+祀
+祁
+祂
+祇
+祈
+祉
+祎
+祐
+祕
+祖
+祗
+祚
+祛
+祜
+祝
+神
+祟
+祠
+祢
+祥
+票
+祭
+祯
+祷
+祸
+祺
+祿
+禀
+禁
+禄
+禅
+禍
+禎
+福
+禛
+禦
+禧
+禪
+禮
+禱
+禹
+禺
+离
+禽
+禾
+禿
+秀
+私
+秃
+秆
+秉
+秋
+种
+科
+秒
+秘
+租
+秣
+秤
+秦
+秧
+秩
+秭
+积
+称
+秸
+移
+秽
+稀
+稅
+程
+稍
+税
+稔
+稗
+稚
+稜
+稞
+稟
+稠
+稣
+種
+稱
+稲
+稳
+稷
+稹
+稻
+稼
+稽
+稿
+穀
+穂
+穆
+穌
+積
+穎
+穗
+穢
+穩
+穫
+穴
+究
+穷
+穹
+空
+穿
+突
+窃
+窄
+窈
+窍
+窑
+窒
+窓
+窕
+窖
+窗
+窘
+窜
+窝
+窟
+窠
+窥
+窦
+窨
+窩
+窪
+窮
+窯
+窺
+窿
+竄
+竅
+竇
+竊
+立
+竖
+站
+竜
+竞
+竟
+章
+竣
+童
+竭
+端
+競
+竹
+竺
+竽
+竿
+笃
+笆
+笈
+笋
+笏
+笑
+笔
+笙
+笛
+笞
+笠
+符
+笨
+第
+笹
+笺
+笼
+筆
+等
+筊
+筋
+筍
+筏
+筐
+筑
+筒
+答
+策
+筛
+筝
+筠
+筱
+筲
+筵
+筷
+筹
+签
+简
+箇
+箋
+箍
+箏
+箐
+箔
+箕
+算
+箝
+管
+箩
+箫
+箭
+箱
+箴
+箸
+節
+篁
+範
+篆
+篇
+築
+篑
+篓
+篙
+篝
+篠
+篡
+篤
+篩
+篪
+篮
+篱
+篷
+簇
+簌
+簍
+簡
+簦
+簧
+簪
+簫
+簷
+簸
+簽
+簾
+簿
+籁
+籃
+籌
+籍
+籐
+籟
+籠
+籤
+籬
+籮
+籲
+米
+类
+籼
+籽
+粄
+粉
+粑
+粒
+粕
+粗
+粘
+粟
+粤
+粥
+粧
+粪
+粮
+粱
+粲
+粳
+粵
+粹
+粼
+粽
+精
+粿
+糅
+糊
+糍
+糕
+糖
+糗
+糙
+糜
+糞
+糟
+糠
+糧
+糬
+糯
+糰
+糸
+系
+糾
+紀
+紂
+約
+紅
+紉
+紊
+紋
+納
+紐
+紓
+純
+紗
+紘
+紙
+級
+紛
+紜
+素
+紡
+索
+紧
+紫
+紮
+累
+細
+紳
+紹
+紺
+終
+絃
+組
+絆
+経
+結
+絕
+絞
+絡
+絢
+給
+絨
+絮
+統
+絲
+絳
+絵
+絶
+絹
+綁
+綏
+綑
+經
+継
+続
+綜
+綠
+綢
+綦
+綫
+綬
+維
+綱
+網
+綴
+綵
+綸
+綺
+綻
+綽
+綾
+綿
+緊
+緋
+総
+緑
+緒
+緘
+線
+緝
+緞
+締
+緣
+編
+緩
+緬
+緯
+練
+緹
+緻
+縁
+縄
+縈
+縛
+縝
+縣
+縫
+縮
+縱
+縴
+縷
+總
+績
+繁
+繃
+繆
+繇
+繋
+織
+繕
+繚
+繞
+繡
+繩
+繪
+繫
+繭
+繳
+繹
+繼
+繽
+纂
+續
+纍
+纏
+纓
+纔
+纖
+纜
+纠
+红
+纣
+纤
+约
+级
+纨
+纪
+纫
+纬
+纭
+纯
+纰
+纱
+纲
+纳
+纵
+纶
+纷
+纸
+纹
+纺
+纽
+纾
+线
+绀
+练
+组
+绅
+细
+织
+终
+绊
+绍
+绎
+经
+绑
+绒
+结
+绔
+绕
+绘
+给
+绚
+绛
+络
+绝
+绞
+统
+绡
+绢
+绣
+绥
+绦
+继
+绩
+绪
+绫
+续
+绮
+绯
+绰
+绳
+维
+绵
+绶
+绷
+绸
+绻
+综
+绽
+绾
+绿
+缀
+缄
+缅
+缆
+缇
+缈
+缉
+缎
+缓
+缔
+缕
+编
+缘
+缙
+缚
+缜
+缝
+缠
+缢
+缤
+缥
+缨
+缩
+缪
+缭
+缮
+缰
+缱
+缴
+缸
+缺
+缽
+罂
+罄
+罌
+罐
+网
+罔
+罕
+罗
+罚
+罡
+罢
+罩
+罪
+置
+罰
+署
+罵
+罷
+罹
+羁
+羅
+羈
+羊
+羌
+美
+羔
+羚
+羞
+羟
+羡
+羣
+群
+羥
+羧
+羨
+義
+羯
+羲
+羸
+羹
+羽
+羿
+翁
+翅
+翊
+翌
+翎
+習
+翔
+翘
+翟
+翠
+翡
+翦
+翩
+翰
+翱
+翳
+翹
+翻
+翼
+耀
+老
+考
+耄
+者
+耆
+耋
+而
+耍
+耐
+耒
+耕
+耗
+耘
+耙
+耦
+耨
+耳
+耶
+耷
+耸
+耻
+耽
+耿
+聂
+聆
+聊
+聋
+职
+聒
+联
+聖
+聘
+聚
+聞
+聪
+聯
+聰
+聲
+聳
+聴
+聶
+職
+聽
+聾
+聿
+肃
+肄
+肅
+肆
+肇
+肉
+肋
+肌
+肏
+肓
+肖
+肘
+肚
+肛
+肝
+肠
+股
+肢
+肤
+肥
+肩
+肪
+肮
+肯
+肱
+育
+肴
+肺
+肽
+肾
+肿
+胀
+胁
+胃
+胄
+胆
+背
+胍
+胎
+胖
+胚
+胛
+胜
+胝
+胞
+胡
+胤
+胥
+胧
+胫
+胭
+胯
+胰
+胱
+胳
+胴
+胶
+胸
+胺
+能
+脂
+脅
+脆
+脇
+脈
+脉
+脊
+脍
+脏
+脐
+脑
+脓
+脖
+脘
+脚
+脛
+脣
+脩
+脫
+脯
+脱
+脲
+脳
+脸
+脹
+脾
+腆
+腈
+腊
+腋
+腌
+腎
+腐
+腑
+腓
+腔
+腕
+腥
+腦
+腩
+腫
+腭
+腮
+腰
+腱
+腳
+腴
+腸
+腹
+腺
+腻
+腼
+腾
+腿
+膀
+膈
+膊
+膏
+膑
+膘
+膚
+膛
+膜
+膝
+膠
+膦
+膨
+膩
+膳
+膺
+膻
+膽
+膾
+膿
+臀
+臂
+臃
+臆
+臉
+臊
+臍
+臓
+臘
+臟
+臣
+臥
+臧
+臨
+自
+臬
+臭
+至
+致
+臺
+臻
+臼
+臾
+舀
+舂
+舅
+舆
+與
+興
+舉
+舊
+舌
+舍
+舎
+舐
+舒
+舔
+舖
+舗
+舛
+舜
+舞
+舟
+航
+舫
+般
+舰
+舱
+舵
+舶
+舷
+舸
+船
+舺
+舾
+艇
+艋
+艘
+艙
+艦
+艮
+良
+艰
+艱
+色
+艳
+艷
+艹
+艺
+艾
+节
+芃
+芈
+芊
+芋
+芍
+芎
+芒
+芙
+芜
+芝
+芡
+芥
+芦
+芩
+芪
+芫
+芬
+芭
+芮
+芯
+花
+芳
+芷
+芸
+芹
+芻
+芽
+芾
+苁
+苄
+苇
+苋
+苍
+苏
+苑
+苒
+苓
+苔
+苕
+苗
+苛
+苜
+苞
+苟
+苡
+苣
+若
+苦
+苫
+苯
+英
+苷
+苹
+苻
+茁
+茂
+范
+茄
+茅
+茉
+茎
+茏
+茗
+茜
+茧
+茨
+茫
+茬
+茭
+茯
+茱
+茲
+茴
+茵
+茶
+茸
+茹
+茼
+荀
+荃
+荆
+草
+荊
+荏
+荐
+荒
+荔
+荖
+荘
+荚
+荞
+荟
+荠
+荡
+荣
+荤
+荥
+荧
+荨
+荪
+荫
+药
+荳
+荷
+荸
+荻
+荼
+荽
+莅
+莆
+莉
+莊
+莎
+莒
+莓
+莖
+莘
+莞
+莠
+莢
+莧
+莪
+莫
+莱
+莲
+莴
+获
+莹
+莺
+莽
+莿
+菀
+菁
+菅
+菇
+菈
+菊
+菌
+菏
+菓
+菖
+菘
+菜
+菟
+菠
+菡
+菩
+華
+菱
+菲
+菸
+菽
+萁
+萃
+萄
+萊
+萋
+萌
+萍
+萎
+萘
+萝
+萤
+营
+萦
+萧
+萨
+萩
+萬
+萱
+萵
+萸
+萼
+落
+葆
+葉
+著
+葚
+葛
+葡
+董
+葦
+葩
+葫
+葬
+葭
+葯
+葱
+葳
+葵
+葷
+葺
+蒂
+蒋
+蒐
+蒔
+蒙
+蒜
+蒞
+蒟
+蒡
+蒨
+蒲
+蒸
+蒹
+蒻
+蒼
+蒿
+蓁
+蓄
+蓆
+蓉
+蓋
+蓑
+蓓
+蓖
+蓝
+蓟
+蓦
+蓬
+蓮
+蓼
+蓿
+蔑
+蔓
+蔔
+蔗
+蔘
+蔚
+蔡
+蔣
+蔥
+蔫
+蔬
+蔭
+蔵
+蔷
+蔺
+蔻
+蔼
+蔽
+蕁
+蕃
+蕈
+蕉
+蕊
+蕎
+蕙
+蕤
+蕨
+蕩
+蕪
+蕭
+蕲
+蕴
+蕻
+蕾
+薄
+薅
+薇
+薈
+薊
+薏
+薑
+薔
+薙
+薛
+薦
+薨
+薩
+薪
+薬
+薯
+薰
+薹
+藉
+藍
+藏
+藐
+藓
+藕
+藜
+藝
+藤
+藥
+藩
+藹
+藻
+藿
+蘆
+蘇
+蘊
+蘋
+蘑
+蘚
+蘭
+蘸
+蘼
+蘿
+虎
+虏
+虐
+虑
+虔
+處
+虚
+虛
+虜
+虞
+號
+虢
+虧
+虫
+虬
+虱
+虹
+虻
+虽
+虾
+蚀
+蚁
+蚂
+蚊
+蚌
+蚓
+蚕
+蚜
+蚝
+蚣
+蚤
+蚩
+蚪
+蚯
+蚱
+蚵
+蛀
+蛆
+蛇
+蛊
+蛋
+蛎
+蛐
+蛔
+蛙
+蛛
+蛟
+蛤
+蛭
+蛮
+蛰
+蛳
+蛹
+蛻
+蛾
+蜀
+蜂
+蜃
+蜆
+蜇
+蜈
+蜊
+蜍
+蜒
+蜓
+蜕
+蜗
+蜘
+蜚
+蜜
+蜡
+蜢
+蜥
+蜱
+蜴
+蜷
+蜻
+蜿
+蝇
+蝈
+蝉
+蝌
+蝎
+蝕
+蝗
+蝙
+蝟
+蝠
+蝦
+蝨
+蝴
+蝶
+蝸
+蝼
+螂
+螃
+融
+螞
+螢
+螨
+螯
+螳
+螺
+蟀
+蟄
+蟆
+蟋
+蟎
+蟑
+蟒
+蟠
+蟬
+蟲
+蟹
+蟻
+蟾
+蠅
+蠍
+蠔
+蠕
+蠛
+蠟
+蠡
+蠢
+蠣
+蠱
+蠶
+蠹
+蠻
+血
+衄
+衅
+衆
+行
+衍
+術
+衔
+街
+衙
+衛
+衝
+衞
+衡
+衢
+衣
+补
+表
+衩
+衫
+衬
+衮
+衰
+衲
+衷
+衹
+衾
+衿
+袁
+袂
+袄
+袅
+袈
+袋
+袍
+袒
+袖
+袜
+袞
+袤
+袪
+被
+袭
+袱
+裁
+裂
+装
+裆
+裊
+裏
+裔
+裕
+裘
+裙
+補
+裝
+裟
+裡
+裤
+裨
+裱
+裳
+裴
+裸
+裹
+製
+裾
+褂
+複
+褐
+褒
+褓
+褔
+褚
+褥
+褪
+褫
+褲
+褶
+褻
+襁
+襄
+襟
+襠
+襪
+襬
+襯
+襲
+西
+要
+覃
+覆
+覇
+見
+規
+覓
+視
+覚
+覦
+覧
+親
+覬
+観
+覷
+覺
+覽
+觀
+见
+观
+规
+觅
+视
+览
+觉
+觊
+觎
+觐
+觑
+角
+觞
+解
+觥
+触
+觸
+言
+訂
+計
+訊
+討
+訓
+訕
+訖
+託
+記
+訛
+訝
+訟
+訣
+訥
+訪
+設
+許
+訳
+訴
+訶
+診
+註
+証
+詆
+詐
+詔
+評
+詛
+詞
+詠
+詡
+詢
+詣
+試
+詩
+詫
+詬
+詭
+詮
+詰
+話
+該
+詳
+詹
+詼
+誅
+誇
+誉
+誌
+認
+誓
+誕
+誘
+語
+誠
+誡
+誣
+誤
+誥
+誦
+誨
+說
+説
+読
+誰
+課
+誹
+誼
+調
+諄
+談
+請
+諏
+諒
+論
+諗
+諜
+諡
+諦
+諧
+諫
+諭
+諮
+諱
+諳
+諷
+諸
+諺
+諾
+謀
+謁
+謂
+謄
+謊
+謎
+謐
+謔
+謗
+謙
+講
+謝
+謠
+謨
+謬
+謹
+謾
+譁
+證
+譎
+譏
+識
+譙
+譚
+譜
+警
+譬
+譯
+議
+譲
+譴
+護
+譽
+讀
+變
+讓
+讚
+讞
+计
+订
+认
+讥
+讧
+讨
+让
+讪
+讫
+训
+议
+讯
+记
+讲
+讳
+讴
+讶
+讷
+许
+讹
+论
+讼
+讽
+设
+访
+诀
+证
+诃
+评
+诅
+识
+诈
+诉
+诊
+诋
+词
+诏
+译
+试
+诗
+诘
+诙
+诚
+诛
+话
+诞
+诟
+诠
+诡
+询
+诣
+诤
+该
+详
+诧
+诩
+诫
+诬
+语
+误
+诰
+诱
+诲
+说
+诵
+诶
+请
+诸
+诺
+读
+诽
+课
+诿
+谀
+谁
+调
+谄
+谅
+谆
+谈
+谊
+谋
+谌
+谍
+谎
+谏
+谐
+谑
+谒
+谓
+谔
+谕
+谗
+谘
+谙
+谚
+谛
+谜
+谟
+谢
+谣
+谤
+谥
+谦
+谧
+谨
+谩
+谪
+谬
+谭
+谯
+谱
+谲
+谴
+谶
+谷
+豁
+豆
+豇
+豈
+豉
+豊
+豌
+豎
+豐
+豔
+豚
+象
+豢
+豪
+豫
+豬
+豹
+豺
+貂
+貅
+貌
+貓
+貔
+貘
+貝
+貞
+負
+財
+貢
+貧
+貨
+販
+貪
+貫
+責
+貯
+貰
+貳
+貴
+貶
+買
+貸
+費
+貼
+貽
+貿
+賀
+賁
+賂
+賃
+賄
+資
+賈
+賊
+賑
+賓
+賜
+賞
+賠
+賡
+賢
+賣
+賤
+賦
+質
+賬
+賭
+賴
+賺
+購
+賽
+贅
+贈
+贊
+贍
+贏
+贓
+贖
+贛
+贝
+贞
+负
+贡
+财
+责
+贤
+败
+账
+货
+质
+贩
+贪
+贫
+贬
+购
+贮
+贯
+贰
+贱
+贲
+贴
+贵
+贷
+贸
+费
+贺
+贻
+贼
+贾
+贿
+赁
+赂
+赃
+资
+赅
+赈
+赊
+赋
+赌
+赎
+赏
+赐
+赓
+赔
+赖
+赘
+赚
+赛
+赝
+赞
+赠
+赡
+赢
+赣
+赤
+赦
+赧
+赫
+赭
+走
+赳
+赴
+赵
+赶
+起
+趁
+超
+越
+趋
+趕
+趙
+趟
+趣
+趨
+足
+趴
+趵
+趸
+趺
+趾
+跃
+跄
+跆
+跋
+跌
+跎
+跑
+跖
+跚
+跛
+距
+跟
+跡
+跤
+跨
+跩
+跪
+路
+跳
+践
+跷
+跹
+跺
+跻
+踉
+踊
+踌
+踏
+踐
+踝
+踞
+踟
+踢
+踩
+踪
+踮
+踱
+踴
+踵
+踹
+蹂
+蹄
+蹇
+蹈
+蹉
+蹊
+蹋
+蹑
+蹒
+蹙
+蹟
+蹣
+蹤
+蹦
+蹩
+蹬
+蹭
+蹲
+蹴
+蹶
+蹺
+蹼
+蹿
+躁
+躇
+躉
+躊
+躋
+躍
+躏
+躪
+身
+躬
+躯
+躲
+躺
+軀
+車
+軋
+軌
+軍
+軒
+軟
+転
+軸
+軼
+軽
+軾
+較
+載
+輒
+輓
+輔
+輕
+輛
+輝
+輟
+輩
+輪
+輯
+輸
+輻
+輾
+輿
+轄
+轅
+轆
+轉
+轍
+轎
+轟
+车
+轧
+轨
+轩
+转
+轭
+轮
+软
+轰
+轲
+轴
+轶
+轻
+轼
+载
+轿
+较
+辄
+辅
+辆
+辇
+辈
+辉
+辊
+辍
+辐
+辑
+输
+辕
+辖
+辗
+辘
+辙
+辛
+辜
+辞
+辟
+辣
+辦
+辨
+辩
+辫
+辭
+辮
+辯
+辰
+辱
+農
+边
+辺
+辻
+込
+辽
+达
+迁
+迂
+迄
+迅
+过
+迈
+迎
+运
+近
+返
+还
+这
+进
+远
+违
+连
+迟
+迢
+迤
+迥
+迦
+迩
+迪
+迫
+迭
+述
+迴
+迷
+迸
+迹
+迺
+追
+退
+送
+适
+逃
+逅
+逆
+选
+逊
+逍
+透
+逐
+递
+途
+逕
+逗
+這
+通
+逛
+逝
+逞
+速
+造
+逢
+連
+逮
+週
+進
+逵
+逶
+逸
+逻
+逼
+逾
+遁
+遂
+遅
+遇
+遊
+運
+遍
+過
+遏
+遐
+遑
+遒
+道
+達
+違
+遗
+遙
+遛
+遜
+遞
+遠
+遢
+遣
+遥
+遨
+適
+遭
+遮
+遲
+遴
+遵
+遶
+遷
+選
+遺
+遼
+遽
+避
+邀
+邁
+邂
+邃
+還
+邇
+邈
+邊
+邋
+邏
+邑
+邓
+邕
+邛
+邝
+邢
+那
+邦
+邨
+邪
+邬
+邮
+邯
+邰
+邱
+邳
+邵
+邸
+邹
+邺
+邻
+郁
+郅
+郊
+郎
+郑
+郜
+郝
+郡
+郢
+郤
+郦
+郧
+部
+郫
+郭
+郴
+郵
+郷
+郸
+都
+鄂
+鄉
+鄒
+鄔
+鄙
+鄞
+鄢
+鄧
+鄭
+鄰
+鄱
+鄲
+鄺
+酉
+酊
+酋
+酌
+配
+酐
+酒
+酗
+酚
+酝
+酢
+酣
+酥
+酩
+酪
+酬
+酮
+酯
+酰
+酱
+酵
+酶
+酷
+酸
+酿
+醃
+醇
+醉
+醋
+醍
+醐
+醒
+醚
+醛
+醜
+醞
+醣
+醪
+醫
+醬
+醮
+醯
+醴
+醺
+釀
+釁
+采
+釉
+释
+釋
+里
+重
+野
+量
+釐
+金
+釗
+釘
+釜
+針
+釣
+釦
+釧
+釵
+鈀
+鈉
+鈍
+鈎
+鈔
+鈕
+鈞
+鈣
+鈦
+鈪
+鈴
+鈺
+鈾
+鉀
+鉄
+鉅
+鉉
+鉑
+鉗
+鉚
+鉛
+鉤
+鉴
+鉻
+銀
+銃
+銅
+銑
+銓
+銖
+銘
+銜
+銬
+銭
+銮
+銳
+銷
+銹
+鋁
+鋅
+鋒
+鋤
+鋪
+鋰
+鋸
+鋼
+錄
+錐
+錘
+錚
+錠
+錢
+錦
+錨
+錫
+錮
+錯
+録
+錳
+錶
+鍊
+鍋
+鍍
+鍛
+鍥
+鍰
+鍵
+鍺
+鍾
+鎂
+鎊
+鎌
+鎏
+鎔
+鎖
+鎗
+鎚
+鎧
+鎬
+鎮
+鎳
+鏈
+鏖
+鏗
+鏘
+鏞
+鏟
+鏡
+鏢
+鏤
+鏽
+鐘
+鐮
+鐲
+鐳
+鐵
+鐸
+鐺
+鑄
+鑊
+鑑
+鑒
+鑣
+鑫
+鑰
+鑲
+鑼
+鑽
+鑾
+鑿
+针
+钉
+钊
+钎
+钏
+钒
+钓
+钗
+钙
+钛
+钜
+钝
+钞
+钟
+钠
+钡
+钢
+钣
+钤
+钥
+钦
+钧
+钨
+钩
+钮
+钯
+钰
+钱
+钳
+钴
+钵
+钺
+钻
+钼
+钾
+钿
+铀
+铁
+铂
+铃
+铄
+铅
+铆
+铉
+铎
+铐
+铛
+铜
+铝
+铠
+铡
+铢
+铣
+铤
+铨
+铩
+铬
+铭
+铮
+铰
+铲
+铵
+银
+铸
+铺
+链
+铿
+销
+锁
+锂
+锄
+锅
+锆
+锈
+锉
+锋
+锌
+锏
+锐
+锑
+错
+锚
+锟
+锡
+锢
+锣
+锤
+锥
+锦
+锭
+键
+锯
+锰
+锲
+锵
+锹
+锺
+锻
+镀
+镁
+镂
+镇
+镉
+镌
+镍
+镐
+镑
+镕
+镖
+镗
+镛
+镜
+镣
+镭
+镯
+镰
+镳
+镶
+長
+长
+門
+閃
+閉
+開
+閎
+閏
+閑
+閒
+間
+閔
+閘
+閡
+関
+閣
+閥
+閨
+閩
+閱
+閲
+閹
+閻
+閾
+闆
+闇
+闊
+闌
+闍
+闔
+闕
+闖
+闘
+關
+闡
+闢
+门
+闪
+闫
+闭
+问
+闯
+闰
+闲
+间
+闵
+闷
+闸
+闹
+闺
+闻
+闽
+闾
+阀
+阁
+阂
+阅
+阆
+阇
+阈
+阉
+阎
+阐
+阑
+阔
+阕
+阖
+阙
+阚
+阜
+队
+阡
+阪
+阮
+阱
+防
+阳
+阴
+阵
+阶
+阻
+阿
+陀
+陂
+附
+际
+陆
+陇
+陈
+陋
+陌
+降
+限
+陕
+陛
+陝
+陞
+陟
+陡
+院
+陣
+除
+陨
+险
+陪
+陰
+陲
+陳
+陵
+陶
+陷
+陸
+険
+陽
+隅
+隆
+隈
+隊
+隋
+隍
+階
+随
+隐
+隔
+隕
+隘
+隙
+際
+障
+隠
+隣
+隧
+隨
+險
+隱
+隴
+隶
+隸
+隻
+隼
+隽
+难
+雀
+雁
+雄
+雅
+集
+雇
+雉
+雋
+雌
+雍
+雎
+雏
+雑
+雒
+雕
+雖
+雙
+雛
+雜
+雞
+離
+難
+雨
+雪
+雯
+雰
+雲
+雳
+零
+雷
+雹
+電
+雾
+需
+霁
+霄
+霆
+震
+霈
+霉
+霊
+霍
+霎
+霏
+霑
+霓
+霖
+霜
+霞
+霧
+霭
+霰
+露
+霸
+霹
+霽
+霾
+靂
+靄
+靈
+青
+靓
+靖
+静
+靚
+靛
+靜
+非
+靠
+靡
+面
+靥
+靦
+革
+靳
+靴
+靶
+靼
+鞅
+鞋
+鞍
+鞏
+鞑
+鞘
+鞠
+鞣
+鞦
+鞭
+韆
+韋
+韌
+韓
+韜
+韦
+韧
+韩
+韬
+韭
+音
+韵
+韶
+韻
+響
+頁
+頂
+頃
+項
+順
+須
+頌
+預
+頑
+頒
+頓
+頗
+領
+頜
+頡
+頤
+頫
+頭
+頰
+頷
+頸
+頹
+頻
+頼
+顆
+題
+額
+顎
+顏
+顔
+願
+顛
+類
+顧
+顫
+顯
+顱
+顴
+页
+顶
+顷
+项
+顺
+须
+顼
+顽
+顾
+顿
+颁
+颂
+预
+颅
+领
+颇
+颈
+颉
+颊
+颌
+颍
+颐
+频
+颓
+颔
+颖
+颗
+题
+颚
+颛
+颜
+额
+颞
+颠
+颡
+颢
+颤
+颦
+颧
+風
+颯
+颱
+颳
+颶
+颼
+飄
+飆
+风
+飒
+飓
+飕
+飘
+飙
+飚
+飛
+飞
+食
+飢
+飨
+飩
+飪
+飯
+飲
+飼
+飽
+飾
+餃
+餅
+餉
+養
+餌
+餐
+餒
+餓
+餘
+餚
+餛
+餞
+餡
+館
+餮
+餵
+餾
+饅
+饈
+饋
+饌
+饍
+饑
+饒
+饕
+饗
+饞
+饥
+饨
+饪
+饬
+饭
+饮
+饯
+饰
+饱
+饲
+饴
+饵
+饶
+饷
+饺
+饼
+饽
+饿
+馀
+馁
+馄
+馅
+馆
+馈
+馋
+馍
+馏
+馒
+馔
+首
+馗
+香
+馥
+馨
+馬
+馭
+馮
+馳
+馴
+駁
+駄
+駅
+駆
+駐
+駒
+駕
+駛
+駝
+駭
+駱
+駿
+騁
+騎
+騏
+験
+騙
+騨
+騰
+騷
+驀
+驅
+驊
+驍
+驒
+驕
+驗
+驚
+驛
+驟
+驢
+驥
+马
+驭
+驮
+驯
+驰
+驱
+驳
+驴
+驶
+驷
+驸
+驹
+驻
+驼
+驾
+驿
+骁
+骂
+骄
+骅
+骆
+骇
+骈
+骊
+骋
+验
+骏
+骐
+骑
+骗
+骚
+骛
+骜
+骞
+骠
+骡
+骤
+骥
+骧
+骨
+骯
+骰
+骶
+骷
+骸
+骼
+髂
+髅
+髋
+髏
+髒
+髓
+體
+髖
+高
+髦
+髪
+髮
+髯
+髻
+鬃
+鬆
+鬍
+鬓
+鬚
+鬟
+鬢
+鬣
+鬥
+鬧
+鬱
+鬼
+魁
+魂
+魄
+魅
+魇
+魍
+魏
+魔
+魘
+魚
+魯
+魷
+鮑
+鮨
+鮪
+鮭
+鮮
+鯉
+鯊
+鯖
+鯛
+鯨
+鯰
+鯽
+鰍
+鰓
+鰭
+鰲
+鰻
+鰾
+鱈
+鱉
+鱔
+鱗
+鱷
+鱸
+鱼
+鱿
+鲁
+鲈
+鲍
+鲑
+鲛
+鲜
+鲟
+鲢
+鲤
+鲨
+鲫
+鲱
+鲲
+鲶
+鲷
+鲸
+鳃
+鳄
+鳅
+鳌
+鳍
+鳕
+鳖
+鳗
+鳝
+鳞
+鳥
+鳩
+鳳
+鳴
+鳶
+鴉
+鴕
+鴛
+鴦
+鴨
+鴻
+鴿
+鵑
+鵜
+鵝
+鵡
+鵬
+鵰
+鵲
+鶘
+鶩
+鶯
+鶴
+鷗
+鷲
+鷹
+鷺
+鸚
+鸞
+鸟
+鸠
+鸡
+鸢
+鸣
+鸥
+鸦
+鸨
+鸪
+鸭
+鸯
+鸳
+鸵
+鸽
+鸾
+鸿
+鹂
+鹃
+鹄
+鹅
+鹈
+鹉
+鹊
+鹌
+鹏
+鹑
+鹕
+鹘
+鹜
+鹞
+鹤
+鹦
+鹧
+鹫
+鹭
+鹰
+鹳
+鹵
+鹹
+鹼
+鹽
+鹿
+麂
+麋
+麒
+麓
+麗
+麝
+麟
+麥
+麦
+麩
+麴
+麵
+麸
+麺
+麻
+麼
+麽
+麾
+黃
+黄
+黍
+黎
+黏
+黑
+黒
+黔
+默
+黛
+黜
+黝
+點
+黠
+黨
+黯
+黴
+鼋
+鼎
+鼐
+鼓
+鼠
+鼬
+鼹
+鼻
+鼾
+齁
+齊
+齋
+齐
+齒
+齡
+齢
+齣
+齦
+齿
+龄
+龅
+龈
+龊
+龋
+龌
+龍
+龐
+龔
+龕
+龙
+龚
+龛
+龜
+龟
+︰
+︱
+︶
+︿
+﹁
+﹂
+﹍
+﹏
+﹐
+﹑
+﹒
+﹔
+﹕
+﹖
+﹗
+﹙
+﹚
+﹝
+﹞
+﹡
+﹣
+!
+"
+#
+$
+%
+&
+'
+(
+)
+*
++
+,
+-
+.
+/
+0
+1
+2
+3
+4
+5
+6
+7
+8
+9
+:
+;
+<
+=
+>
+?
+@
+[
+\
+]
+^
+_
+`
+a
+b
+c
+d
+e
+f
+g
+h
+i
+j
+k
+l
+m
+n
+o
+p
+q
+r
+s
+t
+u
+v
+w
+x
+y
+z
+{
+|
+}
+~
+。
+「
+」
+、
+・
+ッ
+ー
+イ
+ク
+シ
+ス
+ト
+ノ
+フ
+ラ
+ル
+ン
+゙
+゚
+ ̄
+¥
+👍
+🔥
+😂
+😎
+...
+yam
+10
+2017
+12
+11
+2016
+20
+30
+15
+06
+lofter
+##s
+2015
+by
+16
+14
+18
+13
+24
+17
+2014
+21
+##0
+22
+19
+25
+23
+com
+100
+00
+05
+2013
+##a
+03
+09
+08
+28
+##2
+50
+01
+04
+##1
+27
+02
+2012
+##3
+26
+##e
+07
+##8
+##5
+##6
+##4
+##9
+##7
+29
+2011
+40
+##t
+2010
+##o
+##d
+##i
+2009
+##n
+app
+www
+the
+##m
+31
+##c
+##l
+##y
+##r
+##g
+2008
+60
+http
+200
+qq
+##p
+80
+##f
+google
+pixnet
+90
+cookies
+tripadvisor
+500
+##er
+##k
+35
+##h
+facebook
+2007
+2000
+70
+##b
+of
+##x
+##u
+45
+300
+iphone
+32
+1000
+2006
+48
+ip
+36
+in
+38
+3d
+##w
+##ing
+55
+ctrip
+##on
+##v
+33
+##の
+to
+34
+400
+id
+2005
+it
+37
+windows
+llc
+top
+99
+42
+39
+000
+led
+at
+##an
+41
+51
+52
+46
+49
+43
+53
+44
+##z
+android
+58
+and
+59
+2004
+56
+vr
+##か
+5000
+2003
+47
+blogthis
+twitter
+54
+##le
+150
+ok
+2018
+57
+75
+cn
+no
+ios
+##in
+##mm
+##00
+800
+on
+te
+3000
+65
+2001
+360
+95
+ig
+lv
+120
+##ng
+##を
+##us
+##に
+pc
+てす
+──
+600
+##te
+85
+2002
+88
+##ed
+html
+ncc
+wifi
+email
+64
+blog
+is
+##10
+##て
+mail
+online
+##al
+dvd
+##ic
+studio
+##は
+##℃
+##ia
+##と
+line
+vip
+72
+##q
+98
+##ce
+##en
+for
+##is
+##ra
+##es
+##j
+usb
+net
+cp
+1999
+asia
+4g
+##cm
+diy
+new
+3c
+##お
+ta
+66
+language
+vs
+apple
+tw
+86
+web
+##ne
+ipad
+62
+you
+##re
+101
+68
+##tion
+ps
+de
+bt
+pony
+atm
+##2017
+1998
+67
+##ch
+ceo
+##or
+go
+##na
+av
+pro
+cafe
+96
+pinterest
+97
+63
+pixstyleme3c
+##ta
+more
+said
+##2016
+1997
+mp3
+700
+##ll
+nba
+jun
+##20
+92
+tv
+1995
+pm
+61
+76
+nbsp
+250
+##ie
+linux
+##ma
+cd
+110
+hd
+##17
+78
+##ion
+77
+6000
+am
+##th
+##st
+94
+##se
+##et
+69
+180
+gdp
+my
+105
+81
+abc
+89
+flash
+79
+one
+93
+1990
+1996
+##ck
+gps
+##も
+##ly
+web885
+106
+2020
+91
+##ge
+4000
+1500
+xd
+boss
+isbn
+1994
+org
+##ry
+me
+love
+##11
+0fork
+73
+##12
+3g
+##ter
+##ar
+71
+82
+##la
+hotel
+130
+1970
+pk
+83
+87
+140
+ie
+##os
+##30
+##el
+74
+##50
+seo
+cpu
+##ml
+p2p
+84
+may
+##る
+sun
+tue
+internet
+cc
+posted
+youtube
+##at
+##ン
+##man
+ii
+##ル
+##15
+abs
+nt
+pdf
+yahoo
+ago
+1980
+##it
+news
+mac
+104
+##てす
+##me
+##り
+java
+1992
+spa
+##de
+##nt
+hk
+all
+plus
+la
+1993
+##mb
+##16
+##ve
+west
+##da
+160
+air
+##い
+##ps
+から
+##to
+1989
+logo
+htc
+php
+https
+fi
+momo
+##son
+sat
+##ke
+##80
+ebd
+suv
+wi
+day
+apk
+##88
+##um
+mv
+galaxy
+wiki
+or
+brake
+##ス
+1200
+する
+this
+1991
+mon
+##こ
+❤2017
+po
+##ない
+javascript
+life
+home
+june
+##ss
+system
+900
+##ー
+##0
+pp
+1988
+world
+fb
+4k
+br
+##as
+ic
+ai
+leonardo
+safari
+##60
+live
+free
+xx
+wed
+win7
+kiehl
+##co
+lg
+o2o
+##go
+us
+235
+1949
+mm
+しい
+vfm
+kanye
+##90
+##2015
+##id
+jr
+##ey
+123
+rss
+##sa
+##ro
+##am
+##no
+thu
+fri
+350
+##sh
+##ki
+103
+comments
+name
+##のて
+##pe
+##ine
+max
+1987
+8000
+uber
+##mi
+##ton
+wordpress
+office
+1986
+1985
+##ment
+107
+bd
+win10
+##ld
+##li
+gmail
+bb
+dior
+##rs
+##ri
+##rd
+##ます
+up
+cad
+##®
+dr
+して
+read
+##21
+をお
+##io
+##99
+url
+1984
+pvc
+paypal
+show
+policy
+##40
+##ty
+##18
+with
+##★
+##01
+txt
+102
+##ba
+dna
+from
+post
+mini
+ar
+taiwan
+john
+##ga
+privacy
+agoda
+##13
+##ny
+word
+##24
+##22
+##by
+##ur
+##hz
+1982
+##ang
+265
+cookie
+netscape
+108
+##ka
+##~
+##ad
+house
+share
+note
+ibm
+code
+hello
+nike
+sim
+survey
+##016
+1979
+1950
+wikia
+##32
+##017
+5g
+cbc
+##tor
+##kg
+1983
+##rt
+##14
+campaign
+store
+2500
+os
+##ct
+##ts
+##°
+170
+api
+##ns
+365
+excel
+##な
+##ao
+##ら
+##し
+~~
+##nd
+university
+163
+には
+518
+##70
+##ya
+##il
+##25
+pierre
+ipo
+0020
+897
+##23
+hotels
+##ian
+のお
+125
+years
+6606
+##ers
+##26
+high
+##day
+time
+##ay
+bug
+##line
+##く
+##す
+##be
+xp
+talk2yam
+yamservice
+10000
+coco
+##dy
+sony
+##ies
+1978
+microsoft
+david
+people
+##ha
+1960
+instagram
+intel
+その
+##ot
+iso
+1981
+##va
+115
+##mo
+##land
+xxx
+man
+co
+ltxsw
+##ation
+baby
+220
+##pa
+##ol
+1945
+7000
+tag
+450
+##ue
+msn
+##31
+oppo
+##ト
+##ca
+control
+##om
+st
+chrome
+##ure
+##ん
+be
+##き
+lol
+##19
+した
+##bo
+240
+lady
+##100
+##way
+##から
+4600
+##ko
+##do
+##un
+4s
+corporation
+168
+##ni
+herme
+##28
+cp
+978
+##up
+##06
+ui
+##ds
+ppt
+admin
+three
+します
+bbc
+re
+128
+##48
+ca
+##015
+##35
+hp
+##ee
+tpp
+##た
+##ive
+××
+root
+##cc
+##ました
+##ble
+##ity
+adobe
+park
+114
+et
+oled
+city
+##ex
+##ler
+##ap
+china
+##book
+20000
+view
+##ice
+global
+##km
+your
+hong
+##mg
+out
+##ms
+ng
+ebay
+##29
+menu
+ubuntu
+##cy
+rom
+##view
+open
+ktv
+do
+server
+##lo
+if
+english
+##ね
+##5
+##oo
+1600
+##02
+step1
+kong
+club
+135
+july
+inc
+1976
+mr
+hi
+##net
+touch
+##ls
+##ii
+michael
+lcd
+##05
+##33
+phone
+james
+step2
+1300
+ios9
+##box
+dc
+##2
+##ley
+samsung
+111
+280
+pokemon
+css
+##ent
+##les
+いいえ
+##1
+s8
+atom
+play
+bmw
+##said
+sa
+etf
+ctrl
+♥yoyo♥
+##55
+2025
+##2014
+##66
+adidas
+amazon
+1958
+##ber
+##ner
+visa
+##77
+##der
+1800
+connectivity
+##hi
+firefox
+109
+118
+hr
+so
+style
+mark
+pop
+ol
+skip
+1975
+as
+##27
+##ir
+##61
+190
+mba
+##う
+##ai
+le
+##ver
+1900
+cafe2017
+lte
+super
+113
+129
+##ron
+amd
+like
+##☆
+are
+##ster
+we
+##sk
+paul
+data
+international
+##ft
+longchamp
+ssd
+good
+##ート
+##ti
+reply
+##my
+↓↓↓
+apr
+star
+##ker
+source
+136
+js
+112
+get
+force
+photo
+##one
+126
+##2013
+##ow
+link
+bbs
+1972
+goods
+##lin
+python
+119
+##ip
+game
+##ics
+##ません
+blue
+##●
+520
+##45
+page
+itunes
+##03
+1955
+260
+1968
+gt
+gif
+618
+##ff
+##47
+group
+くたさい
+about
+bar
+ganji
+##nce
+music
+lee
+not
+1977
+1971
+1973
+##per
+an
+faq
+comment
+##って
+days
+##ock
+116
+##bs
+1974
+1969
+v1
+player
+1956
+xbox
+sql
+fm
+f1
+139
+##ah
+210
+##lv
+##mp
+##000
+melody
+1957
+##3
+550
+17life
+199
+1966
+xml
+market
+##au
+##71
+999
+##04
+what
+gl
+##95
+##age
+tips
+##68
+book
+##ting
+mysql
+can
+1959
+230
+##ung
+wonderland
+watch
+10℃
+##ction
+9000
+mar
+mobile
+1946
+1962
+article
+##db
+part
+▲top
+party
+って
+1967
+1964
+1948
+##07
+##ore
+##op
+この
+dj
+##78
+##38
+010
+main
+225
+1965
+##ong
+art
+320
+ad
+134
+020
+##73
+117
+pm2
+japan
+228
+##08
+ts
+1963
+##ica
+der
+sm
+##36
+2019
+##wa
+ct
+##7
+##や
+##64
+1937
+homemesh
+search
+##85
+##れは
+##tv
+##di
+macbook
+##9
+##くたさい
+service
+##♥
+type
+った
+750
+##ier
+##si
+##75
+##います
+##ok
+best
+##ット
+goris
+lock
+##った
+cf
+3m
+big
+##ut
+ftp
+carol
+##vi
+10
+1961
+happy
+sd
+##ac
+122
+anti
+pe
+cnn
+iii
+1920
+138
+##ラ
+1940
+esp
+jan
+tags
+##98
+##51
+august
+vol
+##86
+154
+##™
+##fs
+##れ
+##sion
+design
+ac
+##ム
+press
+jordan
+ppp
+that
+key
+check
+##6
+##tt
+##㎡
+1080p
+##lt
+power
+##42
+1952
+##bc
+vivi
+##ック
+he
+133
+121
+jpg
+##rry
+201
+175
+3500
+1947
+nb
+##ted
+##rn
+しています
+1954
+usd
+##t00
+master
+##ンク
+001
+model
+##58
+al
+##09
+1953
+##34
+ram
+goo
+ても
+##ui
+127
+1930
+red
+##ary
+rpg
+item
+##pm
+##41
+270
+##za
+project
+##2012
+hot
+td
+blogabstract
+##ger
+##62
+650
+##44
+gr2
+##します
+##m
+black
+electronic
+nfc
+year
+asus
+また
+html5
+cindy
+##hd
+m3
+132
+esc
+##od
+booking
+##53
+fed
+tvb
+##81
+##ina
+mit
+165
+##いる
+chan
+192
+distribution
+next
+になる
+peter
+bios
+steam
+cm
+1941
+にも
+pk10
+##ix
+##65
+##91
+dec
+nasa
+##ana
+icecat
+00z
+b1
+will
+##46
+li
+se
+##ji
+##み
+##ard
+oct
+##ain
+jp
+##ze
+##bi
+cio
+##56
+smart
+h5
+##39
+##port
+curve
+vpn
+##nm
+##dia
+utc
+##あり
+12345678910
+##52
+rmvb
+chanel
+a4
+miss
+##and
+##im
+media
+who
+##63
+she
+girl
+5s
+124
+vera
+##して
+class
+vivo
+king
+##フ
+##ei
+national
+ab
+1951
+5cm
+888
+145
+ipod
+ap
+1100
+5mm
+211
+ms
+2756
+##69
+mp4
+msci
+##po
+##89
+131
+mg
+index
+380
+##bit
+##out
+##zz
+##97
+##67
+158
+apec
+##8
+photoshop
+opec
+¥799
+ては
+##96
+##tes
+##ast
+2g
+○○
+##ール
+¥2899
+##ling
+##よ
+##ory
+1938
+##ical
+kitty
+content
+##43
+step3
+##cn
+win8
+155
+vc
+1400
+iphone7
+robert
+##した
+tcl
+137
+beauty
+##87
+en
+dollars
+##ys
+##oc
+step
+pay
+yy
+a1
+##2011
+##lly
+##ks
+##♪
+1939
+188
+download
+1944
+sep
+exe
+ph
+います
+school
+gb
+center
+pr
+street
+##board
+uv
+##37
+##lan
+winrar
+##que
+##ua
+##com
+1942
+1936
+480
+gpu
+##4
+ettoday
+fu
+tom
+##54
+##ren
+##via
+149
+##72
+b2b
+144
+##79
+##tch
+rose
+arm
+mb
+##49
+##ial
+##nn
+nvidia
+step4
+mvp
+00㎡
+york
+156
+##イ
+how
+cpi
+591
+2765
+gov
+kg
+joe
+##xx
+mandy
+pa
+##ser
+copyright
+fashion
+1935
+don
+##け
+ecu
+##ist
+##art
+erp
+wap
+have
+##lm
+talk
+##ek
+##ning
+##if
+ch
+##ite
+video
+1943
+cs
+san
+iot
+look
+##84
+##2010
+##ku
+october
+##ux
+trump
+##hs
+##ide
+box
+141
+first
+##ins
+april
+##ight
+##83
+185
+angel
+protected
+aa
+151
+162
+x1
+m2
+##fe
+##×
+##ho
+size
+143
+min
+ofo
+fun
+gomaji
+ex
+hdmi
+food
+dns
+march
+chris
+kevin
+##のか
+##lla
+##pp
+##ec
+ag
+ems
+6s
+720p
+##rm
+##ham
+off
+##92
+asp
+team
+fandom
+ed
+299
+▌♥
+##ell
+info
+されています
+##82
+sina
+4066
+161
+##able
+##ctor
+330
+399
+315
+dll
+rights
+ltd
+idc
+jul
+3kg
+1927
+142
+ma
+surface
+##76
+##ク
+~~~
+304
+mall
+eps
+146
+green
+##59
+map
+space
+donald
+v2
+sodu
+##light
+1931
+148
+1700
+まて
+310
+reserved
+htm
+##han
+##57
+2d
+178
+mod
+##ise
+##tions
+152
+ti
+##shi
+doc
+1933
+icp
+055
+wang
+##ram
+shopping
+aug
+##pi
+##well
+now
+wam
+b2
+からお
+##hu
+236
+1928
+##gb
+266
+f2
+##93
+153
+mix
+##ef
+##uan
+bwl
+##plus
+##res
+core
+##ess
+tea
+5℃
+hktvmall
+nhk
+##ate
+list
+##ese
+301
+feb
+4m
+inn
+ての
+nov
+159
+12345
+daniel
+##ci
+pass
+##bet
+##nk
+coffee
+202
+ssl
+airbnb
+##ute
+fbi
+woshipm
+skype
+ea
+cg
+sp
+##fc
+##www
+yes
+edge
+alt
+007
+##94
+fpga
+##ght
+##gs
+iso9001
+さい
+##ile
+##wood
+##uo
+image
+lin
+icon
+american
+##em
+1932
+set
+says
+##king
+##tive
+blogger
+##74
+なと
+256
+147
+##ox
+##zy
+##red
+##ium
+##lf
+nokia
+claire
+##リ
+##ding
+november
+lohas
+##500
+##tic
+##マ
+##cs
+##ある
+##che
+##ire
+##gy
+##ult
+db
+january
+win
+##カ
+166
+road
+ptt
+##ま
+##つ
+198
+##fa
+##mer
+anna
+pchome
+はい
+udn
+ef
+420
+##time
+##tte
+2030
+##ア
+g20
+white
+かかります
+1929
+308
+garden
+eleven
+di
+##おります
+chen
+309b
+777
+172
+young
+cosplay
+ちてない
+4500
+bat
+##123
+##tra
+##ては
+kindle
+npc
+steve
+etc
+##ern
+##|
+call
+xperia
+ces
+travel
+sk
+s7
+##ous
+1934
+##int
+みいたたけます
+183
+edu
+file
+cho
+qr
+##car
+##our
+186
+##ant
+##d
+eric
+1914
+rends
+##jo
+##する
+mastercard
+##2000
+kb
+##min
+290
+##ino
+vista
+##ris
+##ud
+jack
+2400
+##set
+169
+pos
+1912
+##her
+##ou
+taipei
+しく
+205
+beta
+##ませんか
+232
+##fi
+express
+255
+body
+##ill
+aphojoy
+user
+december
+meiki
+##ick
+tweet
+richard
+##av
+##ᆫ
+iphone6
+##dd
+ちてすか
+views
+##mark
+321
+pd
+##00
+times
+##▲
+level
+##ash
+10g
+point
+5l
+##ome
+208
+koreanmall
+##ak
+george
+q2
+206
+wma
+tcp
+##200
+スタッフ
+full
+mlb
+##lle
+##watch
+tm
+run
+179
+911
+smith
+business
+##und
+1919
+color
+##tal
+222
+171
+##less
+moon
+4399
+##rl
+update
+pcb
+shop
+499
+157
+little
+なし
+end
+##mhz
+van
+dsp
+easy
+660
+##house
+##key
+history
+##o
+oh
+##001
+##hy
+##web
+oem
+let
+was
+##2009
+##gg
+review
+##wan
+182
+##°c
+203
+uc
+title
+##val
+united
+233
+2021
+##ons
+doi
+trivago
+overdope
+sbs
+##ance
+##ち
+grand
+special
+573032185
+imf
+216
+wx17house
+##so
+##ーム
+audi
+##he
+london
+william
+##rp
+##ake
+science
+beach
+cfa
+amp
+ps4
+880
+##800
+##link
+##hp
+crm
+ferragamo
+bell
+make
+##eng
+195
+under
+zh
+photos
+2300
+##style
+##ント
+via
+176
+da
+##gi
+company
+i7
+##ray
+thomas
+370
+ufo
+i5
+##max
+plc
+ben
+back
+research
+8g
+173
+mike
+##pc
+##ッフ
+september
+189
+##ace
+vps
+february
+167
+pantos
+wp
+lisa
+1921
+★★
+jquery
+night
+long
+offer
+##berg
+##news
+1911
+##いて
+ray
+fks
+wto
+せます
+over
+164
+340
+##all
+##rus
+1924
+##888
+##works
+blogtitle
+loftpermalink
+##→
+187
+martin
+test
+ling
+km
+##め
+15000
+fda
+v3
+##ja
+##ロ
+wedding
+かある
+outlet
+family
+##ea
+をこ
+##top
+story
+##ness
+salvatore
+##lu
+204
+swift
+215
+room
+している
+oracle
+##ul
+1925
+sam
+b2c
+week
+pi
+rock
+##のは
+##a
+##けと
+##ean
+##300
+##gle
+cctv
+after
+chinese
+##back
+powered
+x2
+##tan
+1918
+##nes
+##イン
+canon
+only
+181
+##zi
+##las
+say
+##oe
+184
+##sd
+221
+##bot
+##world
+##zo
+sky
+made
+top100
+just
+1926
+pmi
+802
+234
+gap
+##vr
+177
+les
+174
+▲topoct
+ball
+vogue
+vi
+ing
+ofweek
+cos
+##list
+##ort
+▲topmay
+##なら
+##lon
+として
+last
+##tc
+##of
+##bus
+##gen
+real
+eva
+##コ
+a3
+nas
+##lie
+##ria
+##coin
+##bt
+▲topapr
+his
+212
+cat
+nata
+vive
+health
+⋯⋯
+drive
+sir
+▲topmar
+du
+cup
+##カー
+##ook
+##よう
+##sy
+alex
+msg
+tour
+しました
+3ce
+##word
+193
+ebooks
+r8
+block
+318
+##より
+2200
+nice
+pvp
+207
+months
+1905
+rewards
+##ther
+1917
+0800
+##xi
+##チ
+##sc
+micro
+850
+gg
+blogfp
+op
+1922
+daily
+m1
+264
+true
+##bb
+ml
+##tar
+##のお
+##ky
+anthony
+196
+253
+##yo
+state
+218
+##ara
+##aa
+##rc
+##tz
+##ston
+より
+gear
+##eo
+##ade
+ge
+see
+1923
+##win
+##ura
+ss
+heart
+##den
+##ita
+down
+##sm
+el
+png
+2100
+610
+rakuten
+whatsapp
+bay
+dream
+add
+##use
+680
+311
+pad
+gucci
+mpv
+##ode
+##fo
+island
+▲topjun
+##▼
+223
+jason
+214
+chicago
+##❤
+しの
+##hone
+io
+##れる
+##ことか
+sogo
+be2
+##ology
+990
+cloud
+vcd
+##con
+2~3
+##ford
+##joy
+##kb
+##こさいます
+##rade
+but
+##ach
+docker
+##ful
+rfid
+ul
+##ase
+hit
+ford
+##star
+580
+##○
+11
+a2
+sdk
+reading
+edited
+##are
+cmos
+##mc
+238
+siri
+light
+##ella
+##ため
+bloomberg
+##read
+pizza
+##ison
+jimmy
+##vm
+college
+node
+journal
+ba
+18k
+##play
+245
+##cer
+20
+magic
+##yu
+191
+jump
+288
+tt
+##ings
+asr
+##lia
+3200
+step5
+network
+##cd
+mc
+いします
+1234
+pixstyleme
+273
+##600
+2800
+money
+★★★★★
+1280
+12
+430
+bl
+みの
+act
+##tus
+tokyo
+##rial
+##life
+emba
+##ae
+saas
+tcs
+##rk
+##wang
+summer
+##sp
+ko
+##ving
+390
+premium
+##その
+netflix
+##ヒ
+uk
+mt
+##lton
+right
+frank
+two
+209
+える
+##ple
+##cal
+021
+##んな
+##sen
+##ville
+hold
+nexus
+dd
+##ius
+てお
+##mah
+##なく
+tila
+zero
+820
+ce
+##tin
+resort
+##ws
+charles
+old
+p10
+5d
+report
+##360
+##ru
+##には
+bus
+vans
+lt
+##est
+pv
+##レ
+links
+rebecca
+##ツ
+##dm
+azure
+##365
+きな
+limited
+bit
+4gb
+##mon
+1910
+moto
+##eam
+213
+1913
+var
+eos
+なとの
+226
+blogspot
+された
+699
+e3
+dos
+dm
+fc
+##ments
+##ik
+##kw
+boy
+##bin
+##ata
+960
+er
+##せ
+219
+##vin
+##tu
+##ula
+194
+##∥
+station
+##ろ
+##ature
+835
+files
+zara
+hdr
+top10
+nature
+950
+magazine
+s6
+marriott
+##シ
+avira
+case
+##っと
+tab
+##ran
+tony
+##home
+oculus
+im
+##ral
+jean
+saint
+cry
+307
+rosie
+##force
+##ini
+ice
+##bert
+のある
+##nder
+##mber
+pet
+2600
+##◆
+plurk
+▲topdec
+##sis
+00kg
+▲topnov
+720
+##ence
+tim
+##ω
+##nc
+##ても
+##name
+log
+ips
+great
+ikea
+malaysia
+unix
+##イト
+3600
+##ncy
+##nie
+12000
+akb48
+##ye
+##oid
+404
+##chi
+##いた
+oa
+xuehai
+##1000
+##orm
+##rf
+275
+さん
+##ware
+##リー
+980
+ho
+##pro
+text
+##era
+560
+bob
+227
+##ub
+##2008
+8891
+scp
+avi
+##zen
+2022
+mi
+wu
+museum
+qvod
+apache
+lake
+jcb
+▲topaug
+★★★
+ni
+##hr
+hill
+302
+ne
+weibo
+490
+ruby
+##ーシ
+##ヶ
+##row
+4d
+▲topjul
+iv
+##ish
+github
+306
+mate
+312
+##スト
+##lot
+##ane
+andrew
+のハイト
+##tina
+t1
+rf
+ed2k
+##vel
+##900
+way
+final
+りの
+ns
+5a
+705
+197
+##メ
+sweet
+bytes
+##ene
+▲topjan
+231
+##cker
+##2007
+##px
+100g
+topapp
+229
+helpapp
+rs
+low
+14k
+g4g
+care
+630
+ldquo
+あり
+##fork
+leave
+rm
+edition
+##gan
+##zon
+##qq
+▲topsep
+##google
+##ism
+gold
+224
+explorer
+##zer
+toyota
+category
+select
+visual
+##labels
+restaurant
+##md
+posts
+s1
+##ico
+もっと
+angelababy
+123456
+217
+sports
+s3
+mbc
+1915
+してくたさい
+shell
+x86
+candy
+##new
+kbs
+face
+xl
+470
+##here
+4a
+swissinfo
+v8
+▲topfeb
+dram
+##ual
+##vice
+3a
+##wer
+sport
+q1
+ios10
+public
+int
+card
+##c
+ep
+au
+rt
+##れた
+1080
+bill
+##mll
+kim
+30
+460
+wan
+##uk
+##ミ
+x3
+298
+0t
+scott
+##ming
+239
+e5
+##3d
+h7n9
+worldcat
+brown
+##あります
+##vo
+##led
+##580
+##ax
+249
+410
+##ert
+paris
+##~6
+polo
+925
+##lr
+599
+##ナ
+capital
+##hing
+bank
+cv
+1g
+##chat
+##s
+##たい
+adc
+##ule
+2m
+##e
+digital
+hotmail
+268
+##pad
+870
+bbq
+quot
+##ring
+before
+wali
+##まて
+mcu
+2k
+2b
+という
+costco
+316
+north
+333
+switch
+##city
+##p
+philips
+##mann
+management
+panasonic
+##cl
+##vd
+##ping
+##rge
+alice
+##lk
+##ましょう
+css3
+##ney
+vision
+alpha
+##ular
+##400
+##tter
+lz
+にお
+##ありません
+mode
+gre
+1916
+pci
+##tm
+237
+1~2
+##yan
+##そ
+について
+##let
+##キ
+work
+war
+coach
+ah
+mary
+##ᅵ
+huang
+##pt
+a8
+pt
+follow
+##berry
+1895
+##ew
+a5
+ghost
+##ション
+##wn
+##og
+south
+##code
+girls
+##rid
+action
+villa
+git
+r11
+table
+games
+##cket
+error
+##anonymoussaid
+##ag
+here
+##ame
+##gc
+qa
+##■
+##lis
+gmp
+##gin
+vmalife
+##cher
+yu
+wedding
+##tis
+demo
+dragon
+530
+soho
+social
+bye
+##rant
+river
+orz
+acer
+325
+##↑
+##ース
+##ats
+261
+del
+##ven
+440
+ups
+##ように
+##ター
+305
+value
+macd
+yougou
+##dn
+661
+##ano
+ll
+##urt
+##rent
+continue
+script
+##wen
+##ect
+paper
+263
+319
+shift
+##chel
+##フト
+##cat
+258
+x5
+fox
+243
+##さん
+car
+aaa
+##blog
+loading
+##yn
+##tp
+kuso
+799
+si
+sns
+イカせるテンマ
+ヒンクテンマ3
+rmb
+vdc
+forest
+central
+prime
+help
+ultra
+##rmb
+##ような
+241
+square
+688
+##しい
+のないフロクに
+##field
+##reen
+##ors
+##ju
+c1
+start
+510
+##air
+##map
+cdn
+##wo
+cba
+stephen
+m8
+100km
+##get
+opera
+##base
+##ood
+vsa
+com™
+##aw
+##ail
+251
+なのて
+count
+t2
+##ᅡ
+##een
+2700
+hop
+##gp
+vsc
+tree
+##eg
+##ose
+816
+285
+##ories
+##shop
+alphago
+v4
+1909
+simon
+##ᆼ
+fluke62max
+zip
+スホンサー
+##sta
+louis
+cr
+bas
+##~10
+bc
+##yer
+hadoop
+##ube
+##wi
+1906
+0755
+hola
+##low
+place
+centre
+5v
+d3
+##fer
+252
+##750
+##media
+281
+540
+0l
+exchange
+262
+series
+##ハー
+##san
+eb
+##bank
+##k
+q3
+##nge
+##mail
+take
+##lp
+259
+1888
+client
+east
+cache
+event
+vincent
+##ールを
+きを
+##nse
+sui
+855
+adchoice
+##и
+##stry
+##なたの
+246
+##zone
+ga
+apps
+sea
+##ab
+248
+cisco
+##タ
+##rner
+kymco
+##care
+dha
+##pu
+##yi
+minkoff
+royal
+p1
+への
+annie
+269
+collection
+kpi
+playstation
+257
+になります
+866
+bh
+##bar
+queen
+505
+radio
+1904
+andy
+armani
+##xy
+manager
+iherb
+##ery
+##share
+spring
+raid
+johnson
+1908
+##ob
+volvo
+hall
+##ball
+v6
+our
+taylor
+##hk
+bi
+242
+##cp
+kate
+bo
+water
+technology
+##rie
+サイトは
+277
+##ona
+##sl
+hpv
+303
+gtx
+hip
+rdquo
+jayz
+stone
+##lex
+##rum
+namespace
+##やり
+620
+##ale
+##atic
+des
+##erson
+##ql
+##ves
+##type
+enter
+##この
+##てきます
+d2
+##168
+##mix
+##bian
+との
+a9
+jj
+ky
+##lc
+access
+movie
+##hc
+リストに
+tower
+##ration
+##mit
+ます
+##nch
+ua
+tel
+prefix
+##o2
+1907
+##point
+1901
+ott
+~10
+##http
+##ury
+baidu
+##ink
+member
+##logy
+bigbang
+nownews
+##js
+##shot
+##tb
+##こと
+247
+eba
+##tics
+##lus
+ける
+v5
+spark
+##ama
+there
+##ions
+god
+##lls
+##down
+hiv
+##ress
+burberry
+day2
+##kv
+◆◆
+jeff
+related
+film
+edit
+joseph
+283
+##ark
+cx
+32gb
+order
+g9
+30000
+##ans
+##tty
+s5
+##bee
+かあります
+thread
+xr
+buy
+sh
+005
+land
+spotify
+mx
+##ari
+276
+##verse
+×email
+sf
+why
+##ことて
+244
+7headlines
+nego
+sunny
+dom
+exo
+401
+666
+positioning
+fit
+rgb
+##tton
+278
+kiss
+alexa
+adam
+lp
+みリストを
+##g
+mp
+##ties
+##llow
+amy
+##du
+np
+002
+institute
+271
+##rth
+##lar
+2345
+590
+##des
+sidebar
+15
+imax
+site
+##cky
+##kit
+##ime
+##009
+season
+323
+##fun
+##ンター
+##ひ
+gogoro
+a7
+pu
+lily
+fire
+twd600
+##ッセーシを
+いて
+##vis
+30ml
+##cture
+##をお
+information
+##オ
+close
+friday
+##くれる
+yi
+nick
+てすか
+##tta
+##tel
+6500
+##lock
+cbd
+economy
+254
+かお
+267
+tinker
+double
+375
+8gb
+voice
+##app
+oops
+channel
+today
+985
+##right
+raw
+xyz
+##+
+jim
+edm
+##cent
+7500
+supreme
+814
+ds
+##its
+##asia
+dropbox
+##てすか
+##tti
+books
+272
+100ml
+##tle
+##ller
+##ken
+##more
+##boy
+sex
+309
+##dom
+t3
+##ider
+##なります
+##unch
+1903
+810
+feel
+5500
+##かった
+##put
+により
+s2
+mo
+##gh
+men
+ka
+amoled
+div
+##tr
+##n1
+port
+howard
+##tags
+ken
+dnf
+##nus
+adsense
+##а
+ide
+##へ
+buff
+thunder
+##town
+##ique
+has
+##body
+auto
+pin
+##erry
+tee
+てした
+295
+number
+##the
+##013
+object
+psp
+cool
+udnbkk
+16gb
+##mic
+miui
+##tro
+most
+r2
+##alk
+##nity
+1880
+±0
+##いました
+428
+s4
+law
+version
+##oa
+n1
+sgs
+docomo
+##tf
+##ack
+henry
+fc2
+##ded
+##sco
+##014
+##rite
+286
+0mm
+linkedin
+##ada
+##now
+wii
+##ndy
+ucbug
+##◎
+sputniknews
+legalminer
+##ika
+##xp
+2gb
+##bu
+q10
+oo
+b6
+come
+##rman
+cheese
+ming
+maker
+##gm
+nikon
+##fig
+ppi
+kelly
+##ります
+jchere
+てきます
+ted
+md
+003
+fgo
+tech
+##tto
+dan
+soc
+##gl
+##len
+hair
+earth
+640
+521
+img
+##pper
+##a1
+##てきる
+##ロク
+acca
+##ition
+##ference
+suite
+##ig
+outlook
+##mond
+##cation
+398
+##pr
+279
+101vip
+358
+##999
+282
+64gb
+3800
+345
+airport
+##over
+284
+##おり
+jones
+##ith
+lab
+##su
+##いるのて
+co2
+town
+piece
+##llo
+no1
+vmware
+24h
+##qi
+focus
+reader
+##admin
+##ora
+tb
+false
+##log
+1898
+know
+lan
+838
+##ces
+f4
+##ume
+motel
+stop
+##oper
+na
+flickr
+netcomponents
+##af
+##─
+pose
+williams
+local
+##ound
+##cg
+##site
+##iko
+いお
+274
+5m
+gsm
+con
+##ath
+1902
+friends
+##hip
+cell
+317
+##rey
+780
+cream
+##cks
+012
+##dp
+facebooktwitterpinterestgoogle
+sso
+324
+shtml
+song
+swiss
+##mw
+##キンク
+lumia
+xdd
+string
+tiffany
+522
+marc
+られた
+insee
+russell
+sc
+dell
+##ations
+ok
+camera
+289
+##vs
+##flow
+##late
+classic
+287
+##nter
+stay
+g1
+mtv
+512
+##ever
+##lab
+##nger
+qe
+sata
+ryan
+d1
+50ml
+cms
+##cing
+su
+292
+3300
+editor
+296
+##nap
+security
+sunday
+association
+##ens
+##700
+##bra
+acg
+##かり
+sofascore
+とは
+mkv
+##ign
+jonathan
+gary
+build
+labels
+##oto
+tesla
+moba
+qi
+gohappy
+general
+ajax
+1024
+##かる
+サイト
+society
+##test
+##urs
+wps
+fedora
+##ich
+mozilla
+328
+##480
+##dr
+usa
+urn
+##lina
+##r
+grace
+##die
+##try
+##ader
+1250
+##なり
+elle
+570
+##chen
+##ᆯ
+price
+##ten
+uhz
+##ough
+eq
+##hen
+states
+push
+session
+balance
+wow
+506
+##cus
+##py
+when
+##ward
+##ep
+34e
+wong
+library
+prada
+##サイト
+##cle
+running
+##ree
+313
+ck
+date
+q4
+##ctive
+##ool
+##>
+mk
+##ira
+##163
+388
+die
+secret
+rq
+dota
+buffet
+は1ヶ
+e6
+##ez
+pan
+368
+ha
+##card
+##cha
+2a
+##さ
+alan
+day3
+eye
+f3
+##end
+france
+keep
+adi
+rna
+tvbs
+##ala
+solo
+nova
+##え
+##tail
+##ょう
+support
+##ries
+##なる
+##ved
+base
+copy
+iis
+fps
+##ways
+hero
+hgih
+profile
+fish
+mu
+ssh
+entertainment
+chang
+##wd
+click
+cake
+##ond
+pre
+##tom
+kic
+pixel
+##ov
+##fl
+product
+6a
+##pd
+dear
+##gate
+es
+yumi
+audio
+##²
+##sky
+echo
+bin
+where
+##ture
+329
+##ape
+find
+sap
+isis
+##なと
+nand
+##101
+##load
+##ream
+band
+a6
+525
+never
+##post
+festival
+50cm
+##we
+555
+guide
+314
+zenfone
+##ike
+335
+gd
+forum
+jessica
+strong
+alexander
+##ould
+software
+allen
+##ious
+program
+360°
+else
+lohasthree
+##gar
+することかてきます
+please
+##れます
+rc
+##ggle
+##ric
+bim
+50000
+##own
+eclipse
+355
+brian
+3ds
+##side
+061
+361
+##other
+##ける
+##tech
+##ator
+485
+engine
+##ged
+##t
+plaza
+##fit
+cia
+ngo
+westbrook
+shi
+tbs
+50mm
+##みませんか
+sci
+291
+reuters
+##ily
+contextlink
+##hn
+af
+##cil
+bridge
+very
+##cel
+1890
+cambridge
+##ize
+15g
+##aid
+##data
+790
+frm
+##head
+award
+butler
+##sun
+meta
+##mar
+america
+ps3
+puma
+pmid
+##すか
+lc
+670
+kitchen
+##lic
+オーフン5
+きなしソフトサーヒス
+そして
+day1
+future
+★★★★
+##text
+##page
+##rris
+pm1
+##ket
+fans
+##っています
+1001
+christian
+bot
+kids
+trackback
+##hai
+c3
+display
+##hl
+n2
+1896
+idea
+さんも
+##sent
+airmail
+##ug
+##men
+pwm
+けます
+028
+##lution
+369
+852
+awards
+schemas
+354
+asics
+wikipedia
+font
+##tional
+##vy
+c2
+293
+##れている
+##dget
+##ein
+っている
+contact
+pepper
+スキル
+339
+##~5
+294
+##uel
+##ument
+730
+##hang
+みてす
+q5
+##sue
+rain
+##ndi
+wei
+swatch
+##cept
+わせ
+331
+popular
+##ste
+##tag
+p2
+501
+trc
+1899
+##west
+##live
+justin
+honda
+ping
+messenger
+##rap
+v9
+543
+##とは
+unity
+appqq
+はすへて
+025
+leo
+##tone
+##テ
+##ass
+uniqlo
+##010
+502
+her
+jane
+memory
+moneydj
+##tical
+human
+12306
+していると
+##m2
+coc
+miacare
+##mn
+tmt
+##core
+vim
+kk
+##may
+fan
+target
+use
+too
+338
+435
+2050
+867
+737
+fast
+##2c
+services
+##ope
+omega
+energy
+##わ
+pinkoi
+1a
+##なから
+##rain
+jackson
+##ement
+##シャンルの
+374
+366
+そんな
+p9
+rd
+##ᆨ
+1111
+##tier
+##vic
+zone
+##│
+385
+690
+dl
+isofix
+cpa
+m4
+322
+kimi
+めて
+davis
+##lay
+lulu
+##uck
+050
+weeks
+qs
+##hop
+920
+##n
+ae
+##ear
+~5
+eia
+405
+##fly
+korea
+jpeg
+boost
+##ship
+small
+##リア
+1860
+eur
+297
+425
+valley
+##iel
+simple
+##ude
+rn
+k2
+##ena
+されます
+non
+patrick
+しているから
+##ナー
+feed
+5757
+30g
+process
+well
+qqmei
+##thing
+they
+aws
+lu
+pink
+##ters
+##kin
+または
+board
+##vertisement
+wine
+##ien
+unicode
+##dge
+r1
+359
+##tant
+いを
+##twitter
+##3c
+cool1
+される
+##れて
+##l
+isp
+##012
+standard
+45㎡2
+402
+##150
+matt
+##fu
+326
+##iner
+googlemsn
+pixnetfacebookyahoo
+##ラン
+x7
+886
+##uce
+メーカー
+sao
+##ev
+##きました
+##file
+9678
+403
+xddd
+shirt
+6l
+##rio
+##hat
+3mm
+givenchy
+ya
+bang
+##lio
+monday
+crystal
+ロクイン
+##abc
+336
+head
+890
+ubuntuforumwikilinuxpastechat
+##vc
+##~20
+##rity
+cnc
+7866
+ipv6
+null
+1897
+##ost
+yang
+imsean
+tiger
+##fet
+##ンス
+352
+##=
+dji
+327
+ji
+maria
+##come
+##んて
+foundation
+3100
+##beth
+##なった
+1m
+601
+active
+##aft
+##don
+3p
+sr
+349
+emma
+##khz
+living
+415
+353
+1889
+341
+709
+457
+sas
+x6
+##face
+pptv
+x4
+##mate
+han
+sophie
+##jing
+337
+fifa
+##mand
+other
+sale
+inwedding
+##gn
+てきちゃいます
+##mmy
+##pmlast
+bad
+nana
+nbc
+してみてくたさいね
+なとはお
+##wu
+##かあります
+##あ
+note7
+single
+##340
+せからこ
+してくたさい♪この
+しにはとんとんワークケートを
+するとあなたにもっとマッチした
+ならワークケートへ
+もみつかっちゃうかも
+ワークケートの
+##bel
+window
+##dio
+##ht
+union
+age
+382
+14
+##ivity
+##y
+コメント
+domain
+neo
+##isa
+##lter
+5k
+f5
+steven
+##cts
+powerpoint
+tft
+self
+g2
+ft
+##テル
+zol
+##act
+mwc
+381
+343
+もう
+nbapop
+408
+てある
+eds
+ace
+##room
+previous
+author
+tomtom
+il
+##ets
+hu
+financial
+☆☆☆
+っています
+bp
+5t
+chi
+1gb
+##hg
+fairmont
+cross
+008
+gay
+h2
+function
+##けて
+356
+also
+1b
+625
+##ータ
+##raph
+1894
+3~5
+##ils
+i3
+334
+avenue
+##host
+による
+##bon
+##tsu
+message
+navigation
+50g
+fintech
+h6
+##ことを
+8cm
+##ject
+##vas
+##firm
+credit
+##wf
+xxxx
+form
+##nor
+##space
+huawei
+plan
+json
+sbl
+##dc
+machine
+921
+392
+wish
+##120
+##sol
+windows7
+edward
+##ために
+development
+washington
+##nsis
+lo
+818
+##sio
+##ym
+##bor
+planet
+##~8
+##wt
+ieee
+gpa
+##めて
+camp
+ann
+gm
+##tw
+##oka
+connect
+##rss
+##work
+##atus
+wall
+chicken
+soul
+2mm
+##times
+fa
+##ather
+##cord
+009
+##eep
+hitachi
+gui
+harry
+##pan
+e1
+disney
+##press
+##ーション
+wind
+386
+frigidaire
+##tl
+liu
+hsu
+332
+basic
+von
+ev
+いた
+てきる
+スホンサーサイト
+learning
+##ull
+expedia
+archives
+change
+##wei
+santa
+cut
+ins
+6gb
+turbo
+brand
+cf1
+508
+004
+return
+747
+##rip
+h1
+##nis
+##をこ
+128gb
+##にお
+3t
+application
+しており
+emc
+rx
+##oon
+384
+quick
+412
+15058
+wilson
+wing
+chapter
+##bug
+beyond
+##cms
+##dar
+##oh
+zoom
+e2
+trip
+sb
+##nba
+rcep
+342
+aspx
+ci
+080
+gc
+gnu
+める
+##count
+advanced
+dance
+dv
+##url
+##ging
+367
+8591
+am09
+shadow
+battle
+346
+##i
+##cia
+##という
+emily
+##のてす
+##tation
+host
+ff
+techorz
+sars
+##mini
+##mporary
+##ering
+nc
+4200
+798
+##next
+cma
+##mbps
+##gas
+##ift
+##dot
+##ィ
+455
+##~17
+amana
+##りの
+426
+##ros
+ir
+00㎡1
+##eet
+##ible
+##↓
+710
+ˋ▽ˊ
+##aka
+dcs
+iq
+##v
+l1
+##lor
+maggie
+##011
+##iu
+588
+##~1
+830
+##gt
+1tb
+articles
+create
+##burg
+##iki
+database
+fantasy
+##rex
+##cam
+dlc
+dean
+##you
+hard
+path
+gaming
+victoria
+maps
+cb
+##lee
+##itor
+overchicstoretvhome
+systems
+##xt
+416
+p3
+sarah
+760
+##nan
+407
+486
+x9
+install
+second
+626
+##ann
+##ph
+##rcle
+##nic
+860
+##nar
+ec
+##とう
+768
+metro
+chocolate
+##rian
+~4
+##table
+##しています
+skin
+##sn
+395
+mountain
+##0mm
+inparadise
+6m
+7x24
+ib
+4800
+##jia
+eeworld
+creative
+g5
+g3
+357
+parker
+ecfa
+village
+からの
+18000
+sylvia
+サーヒス
+hbl
+##ques
+##onsored
+##x2
+##きます
+##v4
+##tein
+ie6
+383
+##stack
+389
+ver
+##ads
+##baby
+sound
+bbe
+##110
+##lone
+##uid
+ads
+022
+gundam
+351
+thinkpad
+006
+scrum
+match
+##ave
+mems
+##470
+##oy
+##なりました
+##talk
+glass
+lamigo
+span
+##eme
+job
+##a5
+jay
+wade
+kde
+498
+##lace
+ocean
+tvg
+##covery
+##r3
+##ners
+##rea
+junior
+think
+##aine
+cover
+##ision
+##sia
+↓↓
+##bow
+msi
+413
+458
+406
+##love
+711
+801
+soft
+z2
+##pl
+456
+1840
+mobil
+mind
+##uy
+427
+nginx
+##oi
+めた
+##rr
+6221
+##mple
+##sson
+##ーシてす
+371
+##nts
+91tv
+comhd
+crv3000
+##uard
+1868
+397
+deep
+lost
+field
+gallery
+##bia
+rate
+spf
+redis
+traction
+930
+icloud
+011
+なら
+fe
+jose
+372
+##tory
+into
+sohu
+fx
+899
+379
+kicstart2
+##hia
+すく
+##~3
+##sit
+ra
+24
+##walk
+##xure
+500g
+##pact
+pacific
+xa
+natural
+carlo
+##250
+##walker
+1850
+##can
+cto
+gigi
+516
+##サー
+pen
+##hoo
+ob
+matlab
+##b
+##yy
+13913459
+##iti
+mango
+##bbs
+sense
+c5
+oxford
+##ニア
+walker
+jennifer
+##ola
+course
+##bre
+701
+##pus
+##rder
+lucky
+075
+##ぁ
+ivy
+なお
+##nia
+sotheby
+side
+##ugh
+joy
+##orage
+##ush
+##bat
+##dt
+364
+r9
+##2d
+##gio
+511
+country
+wear
+##lax
+##~7
+##moon
+393
+seven
+study
+411
+348
+lonzo
+8k
+##ェ
+evolution
+##イフ
+##kk
+gs
+kd
+##レス
+arduino
+344
+b12
+##lux
+arpg
+##rdon
+cook
+##x5
+dark
+five
+##als
+##ida
+とても
+sign
+362
+##ちの
+something
+20mm
+##nda
+387
+##posted
+fresh
+tf
+1870
+422
+cam
+##mine
+##skip
+##form
+##ssion
+education
+394
+##tee
+dyson
+stage
+##jie
+want
+##night
+epson
+pack
+あります
+##ppy
+テリヘル
+##█
+wd
+##eh
+##rence
+left
+##lvin
+golden
+mhz
+discovery
+##trix
+##n2
+loft
+##uch
+##dra
+##sse
+speed
+~1
+1mdb
+sorry
+welcome
+##urn
+wave
+gaga
+##lmer
+teddy
+##160
+トラックハック
+せよ
+611
+##f2016
+378
+rp
+##sha
+rar
+##あなたに
+##きた
+840
+holiday
+##ュー
+373
+074
+##vg
+##nos
+##rail
+gartner
+gi
+6p
+##dium
+kit
+488
+b3
+eco
+##ろう
+20g
+sean
+##stone
+autocad
+nu
+##np
+f16
+write
+029
+m5
+##ias
+images
+atp
+##dk
+fsm
+504
+1350
+ve
+52kb
+##xxx
+##のに
+##cake
+414
+unit
+lim
+ru
+1v
+##ification
+published
+angela
+16g
+analytics
+ak
+##q
+##nel
+gmt
+##icon
+again
+##₂
+##bby
+ios11
+445
+かこさいます
+waze
+いてす
+##ハ
+9985
+##ust
+##ティー
+framework
+##007
+iptv
+delete
+52sykb
+cl
+wwdc
+027
+30cm
+##fw
+##ての
+1389
+##xon
+brandt
+##ses
+##dragon
+tc
+vetements
+anne
+monte
+modern
+official
+##へて
+##ere
+##nne
+##oud
+もちろん
+50
+etnews
+##a2
+##graphy
+421
+863
+##ちゃん
+444
+##rtex
+##てお
+l2
+##gma
+mount
+ccd
+たと
+archive
+morning
+tan
+ddos
+e7
+##ホ
+day4
+##ウ
+gis
+453
+its
+495
+factory
+bruce
+pg
+##ito
+ってくたさい
+guest
+cdma
+##lling
+536
+n3
+しかし
+3~4
+mega
+eyes
+ro
+13
+women
+dac
+church
+##jun
+singapore
+##facebook
+6991
+starbucks
+##tos
+##stin
+##shine
+zen
+##mu
+tina
+20℃
+1893
+##たけて
+503
+465
+request
+##gence
+qt
+##っ
+1886
+347
+363
+q7
+##zzi
+diary
+##tore
+409
+##ead
+468
+cst
+##osa
+canada
+agent
+va
+##jiang
+##ちは
+##ーク
+##lam
+sg
+##nix
+##sday
+##よって
+g6
+##master
+bing
+##zl
+charlie
+16
+8mm
+nb40
+##ーン
+thai
+##ルフ
+ln284ct
+##itz
+##2f
+bonnie
+##food
+##lent
+originals
+##stro
+##lts
+418
+∟∣
+##bscribe
+children
+ntd
+yesstyle
+##かも
+hmv
+##tment
+d5
+2cm
+arts
+sms
+##pn
+##я
+##いい
+topios9
+539
+lifestyle
+virtual
+##ague
+xz
+##deo
+muji
+024
+unt
+##nnis
+##ᅩ
+faq1
+1884
+396
+##ette
+fly
+64㎡
+はしめまして
+441
+curry
+##pop
+のこ
+release
+##←
+##◆◆
+##cast
+073
+ありな
+500ml
+##ews
+5c
+##stle
+ios7
+##ima
+787
+dog
+lenovo
+##r4
+roger
+013
+cbs
+vornado
+100m
+417
+##desk
+##クok
+##ald
+1867
+9595
+2900
+##van
+oil
+##x
+some
+break
+common
+##jy
+##lines
+g7
+twice
+419
+ella
+nano
+belle
+にこ
+##mes
+##self
+##note
+jb
+##ことかてきます
+benz
+##との
+##ova
+451
+save
+##wing
+##ますのて
+kai
+りは
+##hua
+##rect
+rainer
+##unge
+448
+##0m
+adsl
+##かな
+guestname
+##uma
+##kins
+##zu
+tokichoi
+##price
+county
+##med
+##mus
+rmk
+391
+address
+vm
+えて
+openload
+##group
+##hin
+##iginal
+amg
+urban
+##oz
+jobs
+emi
+##public
+beautiful
+##sch
+album
+##dden
+##bell
+jerry
+works
+hostel
+miller
+##drive
+##rmin
+##10
+376
+boot
+828
+##370
+##fx
+##cm~
+1885
+##nome
+##ctionary
+##oman
+##lish
+##cr
+##hm
+433
+##how
+432
+francis
+xi
+c919
+b5
+evernote
+##uc
+vga
+##3000
+coupe
+##urg
+##cca
+##uality
+019
+6g
+れる
+multi
+##また
+##ett
+em
+hey
+##ani
+##tax
+##rma
+inside
+than
+740
+leonnhurt
+##jin
+ict
+れた
+bird
+notes
+200mm
+くの
+##dical
+##lli
+result
+442
+iu
+ee
+438
+smap
+gopro
+##last
+yin
+pure
+998
+32g
+けた
+5kg
+##dan
+##rame
+mama
+##oot
+bean
+marketing
+##hur
+2l
+bella
+sync
+xuite
+##ground
+515
+discuz
+##getrelax
+##ince
+##bay
+##5s
+cj
+##イス
+gmat
+apt
+##pass
+jing
+##rix
+c4
+rich
+##とても
+niusnews
+##ello
+bag
+770
+##eting
+##mobile
+18
+culture
+015
+##のてすか
+377
+1020
+area
+##ience
+616
+details
+gp
+universal
+silver
+dit
+はお
+private
+ddd
+u11
+kanshu
+##ified
+fung
+##nny
+dx
+##520
+tai
+475
+023
+##fr
+##lean
+3s
+##pin
+429
+##rin
+25000
+ly
+rick
+##bility
+usb3
+banner
+##baru
+##gion
+metal
+dt
+vdf
+1871
+karl
+qualcomm
+bear
+1010
+oldid
+ian
+jo
+##tors
+population
+##ernel
+1882
+mmorpg
+##mv
+##bike
+603
+##©
+ww
+friend
+##ager
+exhibition
+##del
+##pods
+fpx
+structure
+##free
+##tings
+kl
+##rley
+##copyright
+##mma
+california
+3400
+orange
+yoga
+4l
+canmake
+honey
+##anda
+##コメント
+595
+nikkie
+##ルハイト
+dhl
+publishing
+##mall
+##gnet
+20cm
+513
+##クセス
+##┅
+e88
+970
+##dog
+fishbase
+##!
+##"
+###
+##$
+##%
+##&
+##'
+##(
+##)
+##*
+##+
+##,
+##-
+##.
+##/
+##:
+##;
+##<
+##=
+##>
+##?
+##@
+##[
+##\
+##]
+##^
+##_
+##{
+##|
+##}
+##~
+##£
+##¤
+##¥
+##§
+##«
+##±
+##³
+##µ
+##·
+##¹
+##º
+##»
+##¼
+##ß
+##æ
+##÷
+##ø
+##đ
+##ŋ
+##ɔ
+##ə
+##ɡ
+##ʰ
+##ˇ
+##ˈ
+##ˊ
+##ˋ
+##ˍ
+##ː
+##˙
+##˚
+##ˢ
+##α
+##β
+##γ
+##δ
+##ε
+##η
+##θ
+##ι
+##κ
+##λ
+##μ
+##ν
+##ο
+##π
+##ρ
+##ς
+##σ
+##τ
+##υ
+##φ
+##χ
+##ψ
+##б
+##в
+##г
+##д
+##е
+##ж
+##з
+##к
+##л
+##м
+##н
+##о
+##п
+##р
+##с
+##т
+##у
+##ф
+##х
+##ц
+##ч
+##ш
+##ы
+##ь
+##і
+##ا
+##ب
+##ة
+##ت
+##د
+##ر
+##س
+##ع
+##ل
+##م
+##ن
+##ه
+##و
+##ي
+##۩
+##ก
+##ง
+##น
+##ม
+##ย
+##ร
+##อ
+##า
+##เ
+##๑
+##་
+##ღ
+##ᄀ
+##ᄁ
+##ᄂ
+##ᄃ
+##ᄅ
+##ᄆ
+##ᄇ
+##ᄈ
+##ᄉ
+##ᄋ
+##ᄌ
+##ᄎ
+##ᄏ
+##ᄐ
+##ᄑ
+##ᄒ
+##ᅢ
+##ᅣ
+##ᅥ
+##ᅦ
+##ᅧ
+##ᅨ
+##ᅪ
+##ᅬ
+##ᅭ
+##ᅮ
+##ᅯ
+##ᅲ
+##ᅳ
+##ᅴ
+##ᆷ
+##ᆸ
+##ᆺ
+##ᆻ
+##ᗜ
+##ᵃ
+##ᵉ
+##ᵍ
+##ᵏ
+##ᵐ
+##ᵒ
+##ᵘ
+##‖
+##„
+##†
+##•
+##‥
+##‧
+##
+##‰
+##′
+##″
+##‹
+##›
+##※
+##‿
+##⁄
+##ⁱ
+##⁺
+##ⁿ
+##₁
+##₃
+##₄
+##€
+##№
+##ⅰ
+##ⅱ
+##ⅲ
+##ⅳ
+##ⅴ
+##↔
+##↗
+##↘
+##⇒
+##∀
+##−
+##∕
+##∙
+##√
+##∞
+##∟
+##∠
+##∣
+##∩
+##∮
+##∶
+##∼
+##∽
+##≈
+##≒
+##≡
+##≤
+##≥
+##≦
+##≧
+##≪
+##≫
+##⊙
+##⋅
+##⋈
+##⋯
+##⌒
+##①
+##②
+##③
+##④
+##⑤
+##⑥
+##⑦
+##⑧
+##⑨
+##⑩
+##⑴
+##⑵
+##⑶
+##⑷
+##⑸
+##⒈
+##⒉
+##⒊
+##⒋
+##ⓒ
+##ⓔ
+##ⓘ
+##━
+##┃
+##┆
+##┊
+##┌
+##└
+##├
+##┣
+##═
+##║
+##╚
+##╞
+##╠
+##╭
+##╮
+##╯
+##╰
+##╱
+##╳
+##▂
+##▃
+##▅
+##▇
+##▉
+##▋
+##▌
+##▍
+##▎
+##□
+##▪
+##▫
+##▬
+##△
+##▶
+##►
+##▽
+##◇
+##◕
+##◠
+##◢
+##◤
+##☀
+##☕
+##☞
+##☺
+##☼
+##♀
+##♂
+##♠
+##♡
+##♣
+##♦
+##♫
+##♬
+##✈
+##✔
+##✕
+##✖
+##✦
+##✨
+##✪
+##✰
+##✿
+##❀
+##➜
+##➤
+##⦿
+##、
+##。
+##〃
+##々
+##〇
+##〈
+##〉
+##《
+##》
+##「
+##」
+##『
+##』
+##【
+##】
+##〓
+##〔
+##〕
+##〖
+##〗
+##〜
+##〝
+##〞
+##ぃ
+##ぇ
+##ぬ
+##ふ
+##ほ
+##む
+##ゃ
+##ゅ
+##ゆ
+##ょ
+##゜
+##ゝ
+##ァ
+##ゥ
+##エ
+##ォ
+##ケ
+##サ
+##セ
+##ソ
+##ッ
+##ニ
+##ヌ
+##ネ
+##ノ
+##ヘ
+##モ
+##ャ
+##ヤ
+##ュ
+##ユ
+##ョ
+##ヨ
+##ワ
+##ヲ
+##・
+##ヽ
+##ㄅ
+##ㄆ
+##ㄇ
+##ㄉ
+##ㄋ
+##ㄌ
+##ㄍ
+##ㄎ
+##ㄏ
+##ㄒ
+##ㄚ
+##ㄛ
+##ㄞ
+##ㄟ
+##ㄢ
+##ㄤ
+##ㄥ
+##ㄧ
+##ㄨ
+##ㆍ
+##㈦
+##㊣
+##㗎
+##一
+##丁
+##七
+##万
+##丈
+##三
+##上
+##下
+##不
+##与
+##丐
+##丑
+##专
+##且
+##丕
+##世
+##丘
+##丙
+##业
+##丛
+##东
+##丝
+##丞
+##丟
+##両
+##丢
+##两
+##严
+##並
+##丧
+##丨
+##个
+##丫
+##中
+##丰
+##串
+##临
+##丶
+##丸
+##丹
+##为
+##主
+##丼
+##丽
+##举
+##丿
+##乂
+##乃
+##久
+##么
+##义
+##之
+##乌
+##乍
+##乎
+##乏
+##乐
+##乒
+##乓
+##乔
+##乖
+##乗
+##乘
+##乙
+##乜
+##九
+##乞
+##也
+##习
+##乡
+##书
+##乩
+##买
+##乱
+##乳
+##乾
+##亀
+##亂
+##了
+##予
+##争
+##事
+##二
+##于
+##亏
+##云
+##互
+##五
+##井
+##亘
+##亙
+##亚
+##些
+##亜
+##亞
+##亟
+##亡
+##亢
+##交
+##亥
+##亦
+##产
+##亨
+##亩
+##享
+##京
+##亭
+##亮
+##亲
+##亳
+##亵
+##人
+##亿
+##什
+##仁
+##仃
+##仄
+##仅
+##仆
+##仇
+##今
+##介
+##仍
+##从
+##仏
+##仑
+##仓
+##仔
+##仕
+##他
+##仗
+##付
+##仙
+##仝
+##仞
+##仟
+##代
+##令
+##以
+##仨
+##仪
+##们
+##仮
+##仰
+##仲
+##件
+##价
+##任
+##份
+##仿
+##企
+##伉
+##伊
+##伍
+##伎
+##伏
+##伐
+##休
+##伕
+##众
+##优
+##伙
+##会
+##伝
+##伞
+##伟
+##传
+##伢
+##伤
+##伦
+##伪
+##伫
+##伯
+##估
+##伴
+##伶
+##伸
+##伺
+##似
+##伽
+##佃
+##但
+##佇
+##佈
+##位
+##低
+##住
+##佐
+##佑
+##体
+##佔
+##何
+##佗
+##佘
+##余
+##佚
+##佛
+##作
+##佝
+##佞
+##佟
+##你
+##佢
+##佣
+##佤
+##佥
+##佩
+##佬
+##佯
+##佰
+##佳
+##併
+##佶
+##佻
+##佼
+##使
+##侃
+##侄
+##來
+##侈
+##例
+##侍
+##侏
+##侑
+##侖
+##侗
+##供
+##依
+##侠
+##価
+##侣
+##侥
+##侦
+##侧
+##侨
+##侬
+##侮
+##侯
+##侵
+##侶
+##侷
+##便
+##係
+##促
+##俄
+##俊
+##俎
+##俏
+##俐
+##俑
+##俗
+##俘
+##俚
+##保
+##俞
+##俟
+##俠
+##信
+##俨
+##俩
+##俪
+##俬
+##俭
+##修
+##俯
+##俱
+##俳
+##俸
+##俺
+##俾
+##倆
+##倉
+##個
+##倌
+##倍
+##倏
+##們
+##倒
+##倔
+##倖
+##倘
+##候
+##倚
+##倜
+##借
+##倡
+##値
+##倦
+##倩
+##倪
+##倫
+##倬
+##倭
+##倶
+##债
+##值
+##倾
+##偃
+##假
+##偈
+##偉
+##偌
+##偎
+##偏
+##偕
+##做
+##停
+##健
+##側
+##偵
+##偶
+##偷
+##偻
+##偽
+##偿
+##傀
+##傅
+##傍
+##傑
+##傘
+##備
+##傚
+##傢
+##傣
+##傥
+##储
+##傩
+##催
+##傭
+##傲
+##傳
+##債
+##傷
+##傻
+##傾
+##僅
+##働
+##像
+##僑
+##僕
+##僖
+##僚
+##僥
+##僧
+##僭
+##僮
+##僱
+##僵
+##價
+##僻
+##儀
+##儂
+##億
+##儆
+##儉
+##儋
+##儒
+##儕
+##儘
+##償
+##儡
+##優
+##儲
+##儷
+##儼
+##儿
+##兀
+##允
+##元
+##兄
+##充
+##兆
+##兇
+##先
+##光
+##克
+##兌
+##免
+##児
+##兑
+##兒
+##兔
+##兖
+##党
+##兜
+##兢
+##入
+##內
+##全
+##兩
+##八
+##公
+##六
+##兮
+##兰
+##共
+##兲
+##关
+##兴
+##兵
+##其
+##具
+##典
+##兹
+##养
+##兼
+##兽
+##冀
+##内
+##円
+##冇
+##冈
+##冉
+##冊
+##册
+##再
+##冏
+##冒
+##冕
+##冗
+##写
+##军
+##农
+##冠
+##冢
+##冤
+##冥
+##冨
+##冪
+##冬
+##冯
+##冰
+##冲
+##决
+##况
+##冶
+##冷
+##冻
+##冼
+##冽
+##冾
+##净
+##凄
+##准
+##凇
+##凈
+##凉
+##凋
+##凌
+##凍
+##减
+##凑
+##凛
+##凜
+##凝
+##几
+##凡
+##凤
+##処
+##凪
+##凭
+##凯
+##凰
+##凱
+##凳
+##凶
+##凸
+##凹
+##出
+##击
+##函
+##凿
+##刀
+##刁
+##刃
+##分
+##切
+##刈
+##刊
+##刍
+##刎
+##刑
+##划
+##列
+##刘
+##则
+##刚
+##创
+##初
+##删
+##判
+##別
+##刨
+##利
+##刪
+##别
+##刮
+##到
+##制
+##刷
+##券
+##刹
+##刺
+##刻
+##刽
+##剁
+##剂
+##剃
+##則
+##剉
+##削
+##剋
+##剌
+##前
+##剎
+##剐
+##剑
+##剔
+##剖
+##剛
+##剜
+##剝
+##剣
+##剤
+##剥
+##剧
+##剩
+##剪
+##副
+##割
+##創
+##剷
+##剽
+##剿
+##劃
+##劇
+##劈
+##劉
+##劊
+##劍
+##劏
+##劑
+##力
+##劝
+##办
+##功
+##加
+##务
+##劣
+##动
+##助
+##努
+##劫
+##劭
+##励
+##劲
+##劳
+##労
+##劵
+##効
+##劾
+##势
+##勁
+##勃
+##勇
+##勉
+##勋
+##勐
+##勒
+##動
+##勖
+##勘
+##務
+##勛
+##勝
+##勞
+##募
+##勢
+##勤
+##勧
+##勳
+##勵
+##勸
+##勺
+##勻
+##勾
+##勿
+##匀
+##包
+##匆
+##匈
+##匍
+##匐
+##匕
+##化
+##北
+##匙
+##匝
+##匠
+##匡
+##匣
+##匪
+##匮
+##匯
+##匱
+##匹
+##区
+##医
+##匾
+##匿
+##區
+##十
+##千
+##卅
+##升
+##午
+##卉
+##半
+##卍
+##华
+##协
+##卑
+##卒
+##卓
+##協
+##单
+##卖
+##南
+##単
+##博
+##卜
+##卞
+##卟
+##占
+##卡
+##卢
+##卤
+##卦
+##卧
+##卫
+##卮
+##卯
+##印
+##危
+##即
+##却
+##卵
+##卷
+##卸
+##卻
+##卿
+##厂
+##厄
+##厅
+##历
+##厉
+##压
+##厌
+##厕
+##厘
+##厚
+##厝
+##原
+##厢
+##厥
+##厦
+##厨
+##厩
+##厭
+##厮
+##厲
+##厳
+##去
+##县
+##叁
+##参
+##參
+##又
+##叉
+##及
+##友
+##双
+##反
+##収
+##发
+##叔
+##取
+##受
+##变
+##叙
+##叛
+##叟
+##叠
+##叡
+##叢
+##口
+##古
+##句
+##另
+##叨
+##叩
+##只
+##叫
+##召
+##叭
+##叮
+##可
+##台
+##叱
+##史
+##右
+##叵
+##叶
+##号
+##司
+##叹
+##叻
+##叼
+##叽
+##吁
+##吃
+##各
+##吆
+##合
+##吉
+##吊
+##吋
+##同
+##名
+##后
+##吏
+##吐
+##向
+##吒
+##吓
+##吕
+##吖
+##吗
+##君
+##吝
+##吞
+##吟
+##吠
+##吡
+##否
+##吧
+##吨
+##吩
+##含
+##听
+##吭
+##吮
+##启
+##吱
+##吳
+##吴
+##吵
+##吶
+##吸
+##吹
+##吻
+##吼
+##吽
+##吾
+##呀
+##呂
+##呃
+##呆
+##呈
+##告
+##呋
+##呎
+##呐
+##呓
+##呕
+##呗
+##员
+##呛
+##呜
+##呢
+##呤
+##呦
+##周
+##呱
+##呲
+##味
+##呵
+##呷
+##呸
+##呻
+##呼
+##命
+##咀
+##咁
+##咂
+##咄
+##咆
+##咋
+##和
+##咎
+##咏
+##咐
+##咒
+##咔
+##咕
+##咖
+##咗
+##咘
+##咙
+##咚
+##咛
+##咣
+##咤
+##咦
+##咧
+##咨
+##咩
+##咪
+##咫
+##咬
+##咭
+##咯
+##咱
+##咲
+##咳
+##咸
+##咻
+##咽
+##咿
+##哀
+##品
+##哂
+##哄
+##哆
+##哇
+##哈
+##哉
+##哋
+##哌
+##响
+##哎
+##哏
+##哐
+##哑
+##哒
+##哔
+##哗
+##哟
+##員
+##哥
+##哦
+##哧
+##哨
+##哩
+##哪
+##哭
+##哮
+##哲
+##哺
+##哼
+##哽
+##唁
+##唄
+##唆
+##唇
+##唉
+##唏
+##唐
+##唑
+##唔
+##唠
+##唤
+##唧
+##唬
+##售
+##唯
+##唰
+##唱
+##唳
+##唷
+##唸
+##唾
+##啃
+##啄
+##商
+##啉
+##啊
+##問
+##啓
+##啕
+##啖
+##啜
+##啞
+##啟
+##啡
+##啤
+##啥
+##啦
+##啧
+##啪
+##啫
+##啬
+##啮
+##啰
+##啱
+##啲
+##啵
+##啶
+##啷
+##啸
+##啻
+##啼
+##啾
+##喀
+##喂
+##喃
+##善
+##喆
+##喇
+##喉
+##喊
+##喋
+##喎
+##喏
+##喔
+##喘
+##喙
+##喚
+##喜
+##喝
+##喟
+##喧
+##喪
+##喫
+##喬
+##單
+##喰
+##喱
+##喲
+##喳
+##喵
+##営
+##喷
+##喹
+##喺
+##喻
+##喽
+##嗅
+##嗆
+##嗇
+##嗎
+##嗑
+##嗒
+##嗓
+##嗔
+##嗖
+##嗚
+##嗜
+##嗝
+##嗟
+##嗡
+##嗣
+##嗤
+##嗦
+##嗨
+##嗪
+##嗬
+##嗯
+##嗰
+##嗲
+##嗳
+##嗶
+##嗷
+##嗽
+##嘀
+##嘅
+##嘆
+##嘈
+##嘉
+##嘌
+##嘍
+##嘎
+##嘔
+##嘖
+##嘗
+##嘘
+##嘚
+##嘛
+##嘜
+##嘞
+##嘟
+##嘢
+##嘣
+##嘤
+##嘧
+##嘩
+##嘭
+##嘮
+##嘯
+##嘰
+##嘱
+##嘲
+##嘴
+##嘶
+##嘸
+##嘹
+##嘻
+##嘿
+##噁
+##噌
+##噎
+##噓
+##噔
+##噗
+##噙
+##噜
+##噠
+##噢
+##噤
+##器
+##噩
+##噪
+##噬
+##噱
+##噴
+##噶
+##噸
+##噹
+##噻
+##噼
+##嚀
+##嚇
+##嚎
+##嚏
+##嚐
+##嚓
+##嚕
+##嚟
+##嚣
+##嚥
+##嚨
+##嚮
+##嚴
+##嚷
+##嚼
+##囂
+##囉
+##囊
+##囍
+##囑
+##囔
+##囗
+##囚
+##四
+##囝
+##回
+##囟
+##因
+##囡
+##团
+##団
+##囤
+##囧
+##囪
+##囫
+##园
+##困
+##囱
+##囲
+##図
+##围
+##囹
+##固
+##国
+##图
+##囿
+##圃
+##圄
+##圆
+##圈
+##國
+##圍
+##圏
+##園
+##圓
+##圖
+##團
+##圜
+##土
+##圣
+##圧
+##在
+##圩
+##圭
+##地
+##圳
+##场
+##圻
+##圾
+##址
+##坂
+##均
+##坊
+##坍
+##坎
+##坏
+##坐
+##坑
+##块
+##坚
+##坛
+##坝
+##坞
+##坟
+##坠
+##坡
+##坤
+##坦
+##坨
+##坪
+##坯
+##坳
+##坵
+##坷
+##垂
+##垃
+##垄
+##型
+##垒
+##垚
+##垛
+##垠
+##垢
+##垣
+##垦
+##垩
+##垫
+##垭
+##垮
+##垵
+##埂
+##埃
+##埋
+##城
+##埔
+##埕
+##埗
+##域
+##埠
+##埤
+##埵
+##執
+##埸
+##培
+##基
+##埼
+##堀
+##堂
+##堃
+##堅
+##堆
+##堇
+##堑
+##堕
+##堙
+##堡
+##堤
+##堪
+##堯
+##堰
+##報
+##場
+##堵
+##堺
+##堿
+##塊
+##塌
+##塑
+##塔
+##塗
+##塘
+##塚
+##塞
+##塢
+##塩
+##填
+##塬
+##塭
+##塵
+##塾
+##墀
+##境
+##墅
+##墉
+##墊
+##墒
+##墓
+##増
+##墘
+##墙
+##墜
+##增
+##墟
+##墨
+##墩
+##墮
+##墳
+##墻
+##墾
+##壁
+##壅
+##壆
+##壇
+##壊
+##壑
+##壓
+##壕
+##壘
+##壞
+##壟
+##壢
+##壤
+##壩
+##士
+##壬
+##壮
+##壯
+##声
+##売
+##壳
+##壶
+##壹
+##壺
+##壽
+##处
+##备
+##変
+##复
+##夏
+##夔
+##夕
+##外
+##夙
+##多
+##夜
+##够
+##夠
+##夢
+##夥
+##大
+##天
+##太
+##夫
+##夭
+##央
+##夯
+##失
+##头
+##夷
+##夸
+##夹
+##夺
+##夾
+##奂
+##奄
+##奇
+##奈
+##奉
+##奋
+##奎
+##奏
+##奐
+##契
+##奔
+##奕
+##奖
+##套
+##奘
+##奚
+##奠
+##奢
+##奥
+##奧
+##奪
+##奬
+##奮
+##女
+##奴
+##奶
+##奸
+##她
+##好
+##如
+##妃
+##妄
+##妆
+##妇
+##妈
+##妊
+##妍
+##妒
+##妓
+##妖
+##妘
+##妙
+##妝
+##妞
+##妣
+##妤
+##妥
+##妨
+##妩
+##妪
+##妮
+##妲
+##妳
+##妹
+##妻
+##妾
+##姆
+##姉
+##姊
+##始
+##姍
+##姐
+##姑
+##姒
+##姓
+##委
+##姗
+##姚
+##姜
+##姝
+##姣
+##姥
+##姦
+##姨
+##姪
+##姫
+##姬
+##姹
+##姻
+##姿
+##威
+##娃
+##娄
+##娅
+##娆
+##娇
+##娉
+##娑
+##娓
+##娘
+##娛
+##娜
+##娟
+##娠
+##娣
+##娥
+##娩
+##娱
+##娲
+##娴
+##娶
+##娼
+##婀
+##婁
+##婆
+##婉
+##婊
+##婕
+##婚
+##婢
+##婦
+##婧
+##婪
+##婭
+##婴
+##婵
+##婶
+##婷
+##婺
+##婿
+##媒
+##媚
+##媛
+##媞
+##媧
+##媲
+##媳
+##媽
+##媾
+##嫁
+##嫂
+##嫉
+##嫌
+##嫑
+##嫔
+##嫖
+##嫘
+##嫚
+##嫡
+##嫣
+##嫦
+##嫩
+##嫲
+##嫵
+##嫻
+##嬅
+##嬉
+##嬌
+##嬗
+##嬛
+##嬢
+##嬤
+##嬪
+##嬰
+##嬴
+##嬷
+##嬸
+##嬿
+##孀
+##孃
+##子
+##孑
+##孔
+##孕
+##孖
+##字
+##存
+##孙
+##孚
+##孛
+##孜
+##孝
+##孟
+##孢
+##季
+##孤
+##学
+##孩
+##孪
+##孫
+##孬
+##孰
+##孱
+##孳
+##孵
+##學
+##孺
+##孽
+##孿
+##宁
+##它
+##宅
+##宇
+##守
+##安
+##宋
+##完
+##宏
+##宓
+##宕
+##宗
+##官
+##宙
+##定
+##宛
+##宜
+##宝
+##实
+##実
+##宠
+##审
+##客
+##宣
+##室
+##宥
+##宦
+##宪
+##宫
+##宮
+##宰
+##害
+##宴
+##宵
+##家
+##宸
+##容
+##宽
+##宾
+##宿
+##寂
+##寄
+##寅
+##密
+##寇
+##富
+##寐
+##寒
+##寓
+##寛
+##寝
+##寞
+##察
+##寡
+##寢
+##寥
+##實
+##寧
+##寨
+##審
+##寫
+##寬
+##寮
+##寰
+##寵
+##寶
+##寸
+##对
+##寺
+##寻
+##导
+##対
+##寿
+##封
+##専
+##射
+##将
+##將
+##專
+##尉
+##尊
+##尋
+##對
+##導
+##小
+##少
+##尔
+##尕
+##尖
+##尘
+##尚
+##尝
+##尤
+##尧
+##尬
+##就
+##尴
+##尷
+##尸
+##尹
+##尺
+##尻
+##尼
+##尽
+##尾
+##尿
+##局
+##屁
+##层
+##屄
+##居
+##屆
+##屈
+##屉
+##届
+##屋
+##屌
+##屍
+##屎
+##屏
+##屐
+##屑
+##展
+##屜
+##属
+##屠
+##屡
+##屢
+##層
+##履
+##屬
+##屯
+##山
+##屹
+##屿
+##岀
+##岁
+##岂
+##岌
+##岐
+##岑
+##岔
+##岖
+##岗
+##岘
+##岙
+##岚
+##岛
+##岡
+##岩
+##岫
+##岬
+##岭
+##岱
+##岳
+##岷
+##岸
+##峇
+##峋
+##峒
+##峙
+##峡
+##峤
+##峥
+##峦
+##峨
+##峪
+##峭
+##峯
+##峰
+##峴
+##島
+##峻
+##峽
+##崁
+##崂
+##崆
+##崇
+##崎
+##崑
+##崔
+##崖
+##崗
+##崙
+##崛
+##崧
+##崩
+##崭
+##崴
+##崽
+##嵇
+##嵊
+##嵋
+##嵌
+##嵐
+##嵘
+##嵩
+##嵬
+##嵯
+##嶂
+##嶄
+##嶇
+##嶋
+##嶙
+##嶺
+##嶼
+##嶽
+##巅
+##巍
+##巒
+##巔
+##巖
+##川
+##州
+##巡
+##巢
+##工
+##左
+##巧
+##巨
+##巩
+##巫
+##差
+##己
+##已
+##巳
+##巴
+##巷
+##巻
+##巽
+##巾
+##巿
+##币
+##市
+##布
+##帅
+##帆
+##师
+##希
+##帐
+##帑
+##帕
+##帖
+##帘
+##帚
+##帛
+##帜
+##帝
+##帥
+##带
+##帧
+##師
+##席
+##帮
+##帯
+##帰
+##帳
+##帶
+##帷
+##常
+##帼
+##帽
+##幀
+##幂
+##幄
+##幅
+##幌
+##幔
+##幕
+##幟
+##幡
+##幢
+##幣
+##幫
+##干
+##平
+##年
+##并
+##幸
+##幹
+##幺
+##幻
+##幼
+##幽
+##幾
+##广
+##庁
+##広
+##庄
+##庆
+##庇
+##床
+##序
+##庐
+##库
+##应
+##底
+##庖
+##店
+##庙
+##庚
+##府
+##庞
+##废
+##庠
+##度
+##座
+##庫
+##庭
+##庵
+##庶
+##康
+##庸
+##庹
+##庾
+##廁
+##廂
+##廃
+##廈
+##廉
+##廊
+##廓
+##廖
+##廚
+##廝
+##廟
+##廠
+##廢
+##廣
+##廬
+##廳
+##延
+##廷
+##建
+##廿
+##开
+##弁
+##异
+##弃
+##弄
+##弈
+##弊
+##弋
+##式
+##弑
+##弒
+##弓
+##弔
+##引
+##弗
+##弘
+##弛
+##弟
+##张
+##弥
+##弦
+##弧
+##弩
+##弭
+##弯
+##弱
+##張
+##強
+##弹
+##强
+##弼
+##弾
+##彅
+##彆
+##彈
+##彌
+##彎
+##归
+##当
+##录
+##彗
+##彙
+##彝
+##形
+##彤
+##彥
+##彦
+##彧
+##彩
+##彪
+##彫
+##彬
+##彭
+##彰
+##影
+##彷
+##役
+##彻
+##彼
+##彿
+##往
+##征
+##径
+##待
+##徇
+##很
+##徉
+##徊
+##律
+##後
+##徐
+##徑
+##徒
+##従
+##徕
+##得
+##徘
+##徙
+##徜
+##從
+##徠
+##御
+##徨
+##復
+##循
+##徬
+##微
+##徳
+##徴
+##徵
+##德
+##徹
+##徼
+##徽
+##心
+##必
+##忆
+##忌
+##忍
+##忏
+##忐
+##忑
+##忒
+##忖
+##志
+##忘
+##忙
+##応
+##忠
+##忡
+##忤
+##忧
+##忪
+##快
+##忱
+##念
+##忻
+##忽
+##忿
+##怀
+##态
+##怂
+##怅
+##怆
+##怎
+##怏
+##怒
+##怔
+##怕
+##怖
+##怙
+##怜
+##思
+##怠
+##怡
+##急
+##怦
+##性
+##怨
+##怪
+##怯
+##怵
+##总
+##怼
+##恁
+##恃
+##恆
+##恋
+##恍
+##恐
+##恒
+##恕
+##恙
+##恚
+##恢
+##恣
+##恤
+##恥
+##恨
+##恩
+##恪
+##恫
+##恬
+##恭
+##息
+##恰
+##恳
+##恵
+##恶
+##恸
+##恺
+##恻
+##恼
+##恿
+##悄
+##悅
+##悉
+##悌
+##悍
+##悔
+##悖
+##悚
+##悟
+##悠
+##患
+##悦
+##您
+##悩
+##悪
+##悬
+##悯
+##悱
+##悲
+##悴
+##悵
+##悶
+##悸
+##悻
+##悼
+##悽
+##情
+##惆
+##惇
+##惊
+##惋
+##惑
+##惕
+##惘
+##惚
+##惜
+##惟
+##惠
+##惡
+##惦
+##惧
+##惨
+##惩
+##惫
+##惬
+##惭
+##惮
+##惯
+##惰
+##惱
+##想
+##惴
+##惶
+##惹
+##惺
+##愁
+##愆
+##愈
+##愉
+##愍
+##意
+##愕
+##愚
+##愛
+##愜
+##感
+##愣
+##愤
+##愧
+##愫
+##愷
+##愿
+##慄
+##慈
+##態
+##慌
+##慎
+##慑
+##慕
+##慘
+##慚
+##慟
+##慢
+##慣
+##慧
+##慨
+##慫
+##慮
+##慰
+##慳
+##慵
+##慶
+##慷
+##慾
+##憂
+##憊
+##憋
+##憎
+##憐
+##憑
+##憔
+##憚
+##憤
+##憧
+##憨
+##憩
+##憫
+##憬
+##憲
+##憶
+##憾
+##懂
+##懇
+##懈
+##應
+##懊
+##懋
+##懑
+##懒
+##懦
+##懲
+##懵
+##懶
+##懷
+##懸
+##懺
+##懼
+##懾
+##懿
+##戀
+##戈
+##戊
+##戌
+##戍
+##戎
+##戏
+##成
+##我
+##戒
+##戕
+##或
+##战
+##戚
+##戛
+##戟
+##戡
+##戦
+##截
+##戬
+##戮
+##戰
+##戲
+##戳
+##戴
+##戶
+##户
+##戸
+##戻
+##戾
+##房
+##所
+##扁
+##扇
+##扈
+##扉
+##手
+##才
+##扎
+##扑
+##扒
+##打
+##扔
+##払
+##托
+##扛
+##扣
+##扦
+##执
+##扩
+##扪
+##扫
+##扬
+##扭
+##扮
+##扯
+##扰
+##扱
+##扳
+##扶
+##批
+##扼
+##找
+##承
+##技
+##抄
+##抉
+##把
+##抑
+##抒
+##抓
+##投
+##抖
+##抗
+##折
+##抚
+##抛
+##抜
+##択
+##抟
+##抠
+##抡
+##抢
+##护
+##报
+##抨
+##披
+##抬
+##抱
+##抵
+##抹
+##押
+##抽
+##抿
+##拂
+##拄
+##担
+##拆
+##拇
+##拈
+##拉
+##拋
+##拌
+##拍
+##拎
+##拐
+##拒
+##拓
+##拔
+##拖
+##拗
+##拘
+##拙
+##拚
+##招
+##拜
+##拟
+##拡
+##拢
+##拣
+##拥
+##拦
+##拧
+##拨
+##择
+##括
+##拭
+##拮
+##拯
+##拱
+##拳
+##拴
+##拷
+##拼
+##拽
+##拾
+##拿
+##持
+##挂
+##指
+##挈
+##按
+##挎
+##挑
+##挖
+##挙
+##挚
+##挛
+##挝
+##挞
+##挟
+##挠
+##挡
+##挣
+##挤
+##挥
+##挨
+##挪
+##挫
+##振
+##挲
+##挹
+##挺
+##挽
+##挾
+##捂
+##捅
+##捆
+##捉
+##捋
+##捌
+##捍
+##捎
+##捏
+##捐
+##捕
+##捞
+##损
+##捡
+##换
+##捣
+##捧
+##捨
+##捩
+##据
+##捱
+##捲
+##捶
+##捷
+##捺
+##捻
+##掀
+##掂
+##掃
+##掇
+##授
+##掉
+##掌
+##掏
+##掐
+##排
+##掖
+##掘
+##掙
+##掛
+##掠
+##採
+##探
+##掣
+##接
+##控
+##推
+##掩
+##措
+##掬
+##掰
+##掲
+##掳
+##掴
+##掷
+##掸
+##掺
+##揀
+##揃
+##揄
+##揆
+##揉
+##揍
+##描
+##提
+##插
+##揖
+##揚
+##換
+##握
+##揣
+##揩
+##揪
+##揭
+##揮
+##援
+##揶
+##揸
+##揹
+##揽
+##搀
+##搁
+##搂
+##搅
+##損
+##搏
+##搐
+##搓
+##搔
+##搖
+##搗
+##搜
+##搞
+##搡
+##搪
+##搬
+##搭
+##搵
+##搶
+##携
+##搽
+##摀
+##摁
+##摄
+##摆
+##摇
+##摈
+##摊
+##摒
+##摔
+##摘
+##摞
+##摟
+##摧
+##摩
+##摯
+##摳
+##摸
+##摹
+##摺
+##摻
+##撂
+##撃
+##撅
+##撇
+##撈
+##撐
+##撑
+##撒
+##撓
+##撕
+##撚
+##撞
+##撤
+##撥
+##撩
+##撫
+##撬
+##播
+##撮
+##撰
+##撲
+##撵
+##撷
+##撸
+##撻
+##撼
+##撿
+##擀
+##擁
+##擂
+##擄
+##擅
+##擇
+##擊
+##擋
+##操
+##擎
+##擒
+##擔
+##擘
+##據
+##擞
+##擠
+##擡
+##擢
+##擦
+##擬
+##擰
+##擱
+##擲
+##擴
+##擷
+##擺
+##擼
+##擾
+##攀
+##攏
+##攒
+##攔
+##攘
+##攙
+##攜
+##攝
+##攞
+##攢
+##攣
+##攤
+##攥
+##攪
+##攫
+##攬
+##支
+##收
+##攸
+##改
+##攻
+##放
+##政
+##故
+##效
+##敌
+##敍
+##敎
+##敏
+##救
+##敕
+##敖
+##敗
+##敘
+##教
+##敛
+##敝
+##敞
+##敢
+##散
+##敦
+##敬
+##数
+##敲
+##整
+##敵
+##敷
+##數
+##斂
+##斃
+##文
+##斋
+##斌
+##斎
+##斐
+##斑
+##斓
+##斗
+##料
+##斛
+##斜
+##斟
+##斡
+##斤
+##斥
+##斧
+##斩
+##斫
+##斬
+##断
+##斯
+##新
+##斷
+##方
+##於
+##施
+##旁
+##旃
+##旅
+##旋
+##旌
+##旎
+##族
+##旖
+##旗
+##无
+##既
+##日
+##旦
+##旧
+##旨
+##早
+##旬
+##旭
+##旮
+##旱
+##时
+##旷
+##旺
+##旻
+##昀
+##昂
+##昆
+##昇
+##昉
+##昊
+##昌
+##明
+##昏
+##易
+##昔
+##昕
+##昙
+##星
+##映
+##春
+##昧
+##昨
+##昭
+##是
+##昱
+##昴
+##昵
+##昶
+##昼
+##显
+##晁
+##時
+##晃
+##晉
+##晋
+##晌
+##晏
+##晒
+##晓
+##晔
+##晕
+##晖
+##晗
+##晚
+##晝
+##晞
+##晟
+##晤
+##晦
+##晨
+##晩
+##普
+##景
+##晰
+##晴
+##晶
+##晷
+##智
+##晾
+##暂
+##暄
+##暇
+##暈
+##暉
+##暌
+##暐
+##暑
+##暖
+##暗
+##暝
+##暢
+##暧
+##暨
+##暫
+##暮
+##暱
+##暴
+##暸
+##暹
+##曄
+##曆
+##曇
+##曉
+##曖
+##曙
+##曜
+##曝
+##曠
+##曦
+##曬
+##曰
+##曲
+##曳
+##更
+##書
+##曹
+##曼
+##曾
+##替
+##最
+##會
+##月
+##有
+##朋
+##服
+##朐
+##朔
+##朕
+##朗
+##望
+##朝
+##期
+##朦
+##朧
+##木
+##未
+##末
+##本
+##札
+##朮
+##术
+##朱
+##朴
+##朵
+##机
+##朽
+##杀
+##杂
+##权
+##杆
+##杈
+##杉
+##李
+##杏
+##材
+##村
+##杓
+##杖
+##杜
+##杞
+##束
+##杠
+##条
+##来
+##杨
+##杭
+##杯
+##杰
+##東
+##杳
+##杵
+##杷
+##杼
+##松
+##板
+##极
+##构
+##枇
+##枉
+##枋
+##析
+##枕
+##林
+##枚
+##果
+##枝
+##枢
+##枣
+##枪
+##枫
+##枭
+##枯
+##枰
+##枱
+##枳
+##架
+##枷
+##枸
+##柄
+##柏
+##某
+##柑
+##柒
+##染
+##柔
+##柘
+##柚
+##柜
+##柞
+##柠
+##柢
+##查
+##柩
+##柬
+##柯
+##柱
+##柳
+##柴
+##柵
+##査
+##柿
+##栀
+##栃
+##栄
+##栅
+##标
+##栈
+##栉
+##栋
+##栎
+##栏
+##树
+##栓
+##栖
+##栗
+##校
+##栩
+##株
+##样
+##核
+##根
+##格
+##栽
+##栾
+##桀
+##桁
+##桂
+##桃
+##桅
+##框
+##案
+##桉
+##桌
+##桎
+##桐
+##桑
+##桓
+##桔
+##桜
+##桠
+##桡
+##桢
+##档
+##桥
+##桦
+##桧
+##桨
+##桩
+##桶
+##桿
+##梁
+##梅
+##梆
+##梏
+##梓
+##梗
+##條
+##梟
+##梢
+##梦
+##梧
+##梨
+##梭
+##梯
+##械
+##梳
+##梵
+##梶
+##检
+##棂
+##棄
+##棉
+##棋
+##棍
+##棒
+##棕
+##棗
+##棘
+##棚
+##棟
+##棠
+##棣
+##棧
+##森
+##棱
+##棲
+##棵
+##棹
+##棺
+##椁
+##椅
+##椋
+##植
+##椎
+##椒
+##検
+##椪
+##椭
+##椰
+##椹
+##椽
+##椿
+##楂
+##楊
+##楓
+##楔
+##楚
+##楝
+##楞
+##楠
+##楣
+##楨
+##楫
+##業
+##楮
+##極
+##楷
+##楸
+##楹
+##楼
+##楽
+##概
+##榄
+##榆
+##榈
+##榉
+##榔
+##榕
+##榖
+##榛
+##榜
+##榨
+##榫
+##榭
+##榮
+##榱
+##榴
+##榷
+##榻
+##槁
+##槃
+##構
+##槌
+##槍
+##槎
+##槐
+##槓
+##様
+##槛
+##槟
+##槤
+##槭
+##槲
+##槳
+##槻
+##槽
+##槿
+##樁
+##樂
+##樊
+##樑
+##樓
+##標
+##樞
+##樟
+##模
+##樣
+##権
+##横
+##樫
+##樯
+##樱
+##樵
+##樸
+##樹
+##樺
+##樽
+##樾
+##橄
+##橇
+##橋
+##橐
+##橘
+##橙
+##機
+##橡
+##橢
+##橫
+##橱
+##橹
+##橼
+##檀
+##檄
+##檎
+##檐
+##檔
+##檗
+##檜
+##檢
+##檬
+##檯
+##檳
+##檸
+##檻
+##櫃
+##櫚
+##櫛
+##櫥
+##櫸
+##櫻
+##欄
+##權
+##欒
+##欖
+##欠
+##次
+##欢
+##欣
+##欧
+##欲
+##欸
+##欺
+##欽
+##款
+##歆
+##歇
+##歉
+##歌
+##歎
+##歐
+##歓
+##歙
+##歛
+##歡
+##止
+##正
+##此
+##步
+##武
+##歧
+##歩
+##歪
+##歯
+##歲
+##歳
+##歴
+##歷
+##歸
+##歹
+##死
+##歼
+##殁
+##殃
+##殆
+##殇
+##殉
+##殊
+##残
+##殒
+##殓
+##殖
+##殘
+##殞
+##殡
+##殤
+##殭
+##殯
+##殲
+##殴
+##段
+##殷
+##殺
+##殼
+##殿
+##毀
+##毁
+##毂
+##毅
+##毆
+##毋
+##母
+##毎
+##每
+##毒
+##毓
+##比
+##毕
+##毗
+##毘
+##毙
+##毛
+##毡
+##毫
+##毯
+##毽
+##氈
+##氏
+##氐
+##民
+##氓
+##气
+##氖
+##気
+##氙
+##氛
+##氟
+##氡
+##氢
+##氣
+##氤
+##氦
+##氧
+##氨
+##氪
+##氫
+##氮
+##氯
+##氰
+##氲
+##水
+##氷
+##永
+##氹
+##氾
+##汀
+##汁
+##求
+##汆
+##汇
+##汉
+##汎
+##汐
+##汕
+##汗
+##汙
+##汛
+##汝
+##汞
+##江
+##池
+##污
+##汤
+##汨
+##汩
+##汪
+##汰
+##汲
+##汴
+##汶
+##汹
+##決
+##汽
+##汾
+##沁
+##沂
+##沃
+##沅
+##沈
+##沉
+##沌
+##沏
+##沐
+##沒
+##沓
+##沖
+##沙
+##沛
+##沟
+##没
+##沢
+##沣
+##沥
+##沦
+##沧
+##沪
+##沫
+##沭
+##沮
+##沱
+##河
+##沸
+##油
+##治
+##沼
+##沽
+##沾
+##沿
+##況
+##泄
+##泉
+##泊
+##泌
+##泓
+##法
+##泗
+##泛
+##泞
+##泠
+##泡
+##波
+##泣
+##泥
+##注
+##泪
+##泫
+##泮
+##泯
+##泰
+##泱
+##泳
+##泵
+##泷
+##泸
+##泻
+##泼
+##泽
+##泾
+##洁
+##洄
+##洋
+##洒
+##洗
+##洙
+##洛
+##洞
+##津
+##洩
+##洪
+##洮
+##洱
+##洲
+##洵
+##洶
+##洸
+##洹
+##活
+##洼
+##洽
+##派
+##流
+##浃
+##浄
+##浅
+##浆
+##浇
+##浊
+##测
+##济
+##浏
+##浑
+##浒
+##浓
+##浔
+##浙
+##浚
+##浜
+##浣
+##浦
+##浩
+##浪
+##浬
+##浮
+##浯
+##浴
+##海
+##浸
+##涂
+##涅
+##涇
+##消
+##涉
+##涌
+##涎
+##涓
+##涔
+##涕
+##涙
+##涛
+##涝
+##涞
+##涟
+##涠
+##涡
+##涣
+##涤
+##润
+##涧
+##涨
+##涩
+##涪
+##涮
+##涯
+##液
+##涵
+##涸
+##涼
+##涿
+##淀
+##淄
+##淅
+##淆
+##淇
+##淋
+##淌
+##淑
+##淒
+##淖
+##淘
+##淙
+##淚
+##淞
+##淡
+##淤
+##淦
+##淨
+##淩
+##淪
+##淫
+##淬
+##淮
+##深
+##淳
+##淵
+##混
+##淹
+##淺
+##添
+##淼
+##清
+##済
+##渉
+##渊
+##渋
+##渍
+##渎
+##渐
+##渔
+##渗
+##渙
+##渚
+##減
+##渝
+##渠
+##渡
+##渣
+##渤
+##渥
+##渦
+##温
+##測
+##渭
+##港
+##渲
+##渴
+##游
+##渺
+##渾
+##湃
+##湄
+##湊
+##湍
+##湖
+##湘
+##湛
+##湟
+##湧
+##湫
+##湮
+##湯
+##湳
+##湾
+##湿
+##満
+##溃
+##溅
+##溉
+##溏
+##源
+##準
+##溜
+##溝
+##溟
+##溢
+##溥
+##溧
+##溪
+##溫
+##溯
+##溱
+##溴
+##溶
+##溺
+##溼
+##滁
+##滂
+##滄
+##滅
+##滇
+##滋
+##滌
+##滑
+##滓
+##滔
+##滕
+##滙
+##滚
+##滝
+##滞
+##滟
+##满
+##滢
+##滤
+##滥
+##滦
+##滨
+##滩
+##滬
+##滯
+##滲
+##滴
+##滷
+##滸
+##滾
+##滿
+##漁
+##漂
+##漆
+##漉
+##漏
+##漓
+##演
+##漕
+##漠
+##漢
+##漣
+##漩
+##漪
+##漫
+##漬
+##漯
+##漱
+##漲
+##漳
+##漸
+##漾
+##漿
+##潆
+##潇
+##潋
+##潍
+##潑
+##潔
+##潘
+##潛
+##潜
+##潞
+##潟
+##潢
+##潤
+##潦
+##潧
+##潭
+##潮
+##潰
+##潴
+##潸
+##潺
+##潼
+##澀
+##澄
+##澆
+##澈
+##澍
+##澎
+##澗
+##澜
+##澡
+##澤
+##澧
+##澱
+##澳
+##澹
+##激
+##濁
+##濂
+##濃
+##濑
+##濒
+##濕
+##濘
+##濛
+##濟
+##濠
+##濡
+##濤
+##濫
+##濬
+##濮
+##濯
+##濱
+##濺
+##濾
+##瀅
+##瀆
+##瀉
+##瀋
+##瀏
+##瀑
+##瀕
+##瀘
+##瀚
+##瀛
+##瀝
+##瀞
+##瀟
+##瀧
+##瀨
+##瀬
+##瀰
+##瀾
+##灌
+##灏
+##灑
+##灘
+##灝
+##灞
+##灣
+##火
+##灬
+##灭
+##灯
+##灰
+##灵
+##灶
+##灸
+##灼
+##災
+##灾
+##灿
+##炀
+##炁
+##炅
+##炉
+##炊
+##炎
+##炒
+##炔
+##炕
+##炖
+##炙
+##炜
+##炫
+##炬
+##炭
+##炮
+##炯
+##炳
+##炷
+##炸
+##点
+##為
+##炼
+##炽
+##烁
+##烂
+##烃
+##烈
+##烊
+##烏
+##烘
+##烙
+##烛
+##烟
+##烤
+##烦
+##烧
+##烨
+##烩
+##烫
+##烬
+##热
+##烯
+##烷
+##烹
+##烽
+##焉
+##焊
+##焕
+##焖
+##焗
+##焘
+##焙
+##焚
+##焜
+##無
+##焦
+##焯
+##焰
+##焱
+##然
+##焼
+##煅
+##煉
+##煊
+##煌
+##煎
+##煒
+##煖
+##煙
+##煜
+##煞
+##煤
+##煥
+##煦
+##照
+##煨
+##煩
+##煮
+##煲
+##煸
+##煽
+##熄
+##熊
+##熏
+##熒
+##熔
+##熙
+##熟
+##熠
+##熨
+##熬
+##熱
+##熵
+##熹
+##熾
+##燁
+##燃
+##燄
+##燈
+##燉
+##燊
+##燎
+##燒
+##燔
+##燕
+##燙
+##燜
+##營
+##燥
+##燦
+##燧
+##燭
+##燮
+##燴
+##燻
+##燼
+##燿
+##爆
+##爍
+##爐
+##爛
+##爪
+##爬
+##爭
+##爰
+##爱
+##爲
+##爵
+##父
+##爷
+##爸
+##爹
+##爺
+##爻
+##爽
+##爾
+##牆
+##片
+##版
+##牌
+##牍
+##牒
+##牙
+##牛
+##牝
+##牟
+##牠
+##牡
+##牢
+##牦
+##牧
+##物
+##牯
+##牲
+##牴
+##牵
+##特
+##牺
+##牽
+##犀
+##犁
+##犄
+##犊
+##犍
+##犒
+##犢
+##犧
+##犬
+##犯
+##状
+##犷
+##犸
+##犹
+##狀
+##狂
+##狄
+##狈
+##狎
+##狐
+##狒
+##狗
+##狙
+##狞
+##狠
+##狡
+##狩
+##独
+##狭
+##狮
+##狰
+##狱
+##狸
+##狹
+##狼
+##狽
+##猎
+##猕
+##猖
+##猗
+##猙
+##猛
+##猜
+##猝
+##猥
+##猩
+##猪
+##猫
+##猬
+##献
+##猴
+##猶
+##猷
+##猾
+##猿
+##獄
+##獅
+##獎
+##獐
+##獒
+##獗
+##獠
+##獣
+##獨
+##獭
+##獰
+##獲
+##獵
+##獷
+##獸
+##獺
+##獻
+##獼
+##獾
+##玄
+##率
+##玉
+##王
+##玑
+##玖
+##玛
+##玟
+##玠
+##玥
+##玩
+##玫
+##玮
+##环
+##现
+##玲
+##玳
+##玷
+##玺
+##玻
+##珀
+##珂
+##珅
+##珈
+##珉
+##珊
+##珍
+##珏
+##珐
+##珑
+##珙
+##珞
+##珠
+##珣
+##珥
+##珩
+##珪
+##班
+##珮
+##珲
+##珺
+##現
+##球
+##琅
+##理
+##琇
+##琉
+##琊
+##琍
+##琏
+##琐
+##琛
+##琢
+##琥
+##琦
+##琨
+##琪
+##琬
+##琮
+##琰
+##琲
+##琳
+##琴
+##琵
+##琶
+##琺
+##琼
+##瑀
+##瑁
+##瑄
+##瑋
+##瑕
+##瑗
+##瑙
+##瑚
+##瑛
+##瑜
+##瑞
+##瑟
+##瑠
+##瑣
+##瑤
+##瑩
+##瑪
+##瑯
+##瑰
+##瑶
+##瑾
+##璀
+##璁
+##璃
+##璇
+##璉
+##璋
+##璎
+##璐
+##璜
+##璞
+##璟
+##璧
+##璨
+##環
+##璽
+##璿
+##瓊
+##瓏
+##瓒
+##瓜
+##瓢
+##瓣
+##瓤
+##瓦
+##瓮
+##瓯
+##瓴
+##瓶
+##瓷
+##甄
+##甌
+##甕
+##甘
+##甙
+##甚
+##甜
+##生
+##產
+##産
+##甥
+##甦
+##用
+##甩
+##甫
+##甬
+##甭
+##甯
+##田
+##由
+##甲
+##申
+##电
+##男
+##甸
+##町
+##画
+##甾
+##畀
+##畅
+##界
+##畏
+##畑
+##畔
+##留
+##畜
+##畝
+##畢
+##略
+##畦
+##番
+##畫
+##異
+##畲
+##畳
+##畴
+##當
+##畸
+##畹
+##畿
+##疆
+##疇
+##疊
+##疏
+##疑
+##疔
+##疖
+##疗
+##疙
+##疚
+##疝
+##疟
+##疡
+##疣
+##疤
+##疥
+##疫
+##疮
+##疯
+##疱
+##疲
+##疳
+##疵
+##疸
+##疹
+##疼
+##疽
+##疾
+##痂
+##病
+##症
+##痈
+##痉
+##痊
+##痍
+##痒
+##痔
+##痕
+##痘
+##痙
+##痛
+##痞
+##痠
+##痢
+##痣
+##痤
+##痧
+##痨
+##痪
+##痫
+##痰
+##痱
+##痴
+##痹
+##痺
+##痼
+##痿
+##瘀
+##瘁
+##瘋
+##瘍
+##瘓
+##瘘
+##瘙
+##瘟
+##瘠
+##瘡
+##瘢
+##瘤
+##瘦
+##瘧
+##瘩
+##瘪
+##瘫
+##瘴
+##瘸
+##瘾
+##療
+##癇
+##癌
+##癒
+##癖
+##癜
+##癞
+##癡
+##癢
+##癣
+##癥
+##癫
+##癬
+##癮
+##癱
+##癲
+##癸
+##発
+##登
+##發
+##白
+##百
+##皂
+##的
+##皆
+##皇
+##皈
+##皋
+##皎
+##皑
+##皓
+##皖
+##皙
+##皚
+##皮
+##皰
+##皱
+##皴
+##皺
+##皿
+##盂
+##盃
+##盅
+##盆
+##盈
+##益
+##盎
+##盏
+##盐
+##监
+##盒
+##盔
+##盖
+##盗
+##盘
+##盛
+##盜
+##盞
+##盟
+##盡
+##監
+##盤
+##盥
+##盧
+##盪
+##目
+##盯
+##盱
+##盲
+##直
+##相
+##盹
+##盼
+##盾
+##省
+##眈
+##眉
+##看
+##県
+##眙
+##眞
+##真
+##眠
+##眦
+##眨
+##眩
+##眯
+##眶
+##眷
+##眸
+##眺
+##眼
+##眾
+##着
+##睁
+##睇
+##睏
+##睐
+##睑
+##睛
+##睜
+##睞
+##睡
+##睢
+##督
+##睥
+##睦
+##睨
+##睪
+##睫
+##睬
+##睹
+##睽
+##睾
+##睿
+##瞄
+##瞅
+##瞇
+##瞋
+##瞌
+##瞎
+##瞑
+##瞒
+##瞓
+##瞞
+##瞟
+##瞠
+##瞥
+##瞧
+##瞩
+##瞪
+##瞬
+##瞭
+##瞰
+##瞳
+##瞻
+##瞼
+##瞿
+##矇
+##矍
+##矗
+##矚
+##矛
+##矜
+##矢
+##矣
+##知
+##矩
+##矫
+##短
+##矮
+##矯
+##石
+##矶
+##矽
+##矾
+##矿
+##码
+##砂
+##砌
+##砍
+##砒
+##研
+##砖
+##砗
+##砚
+##砝
+##砣
+##砥
+##砧
+##砭
+##砰
+##砲
+##破
+##砷
+##砸
+##砺
+##砼
+##砾
+##础
+##硅
+##硐
+##硒
+##硕
+##硝
+##硫
+##硬
+##确
+##硯
+##硼
+##碁
+##碇
+##碉
+##碌
+##碍
+##碎
+##碑
+##碓
+##碗
+##碘
+##碚
+##碛
+##碟
+##碣
+##碧
+##碩
+##碰
+##碱
+##碳
+##碴
+##確
+##碼
+##碾
+##磁
+##磅
+##磊
+##磋
+##磐
+##磕
+##磚
+##磡
+##磨
+##磬
+##磯
+##磲
+##磷
+##磺
+##礁
+##礎
+##礙
+##礡
+##礦
+##礪
+##礫
+##礴
+##示
+##礼
+##社
+##祀
+##祁
+##祂
+##祇
+##祈
+##祉
+##祎
+##祐
+##祕
+##祖
+##祗
+##祚
+##祛
+##祜
+##祝
+##神
+##祟
+##祠
+##祢
+##祥
+##票
+##祭
+##祯
+##祷
+##祸
+##祺
+##祿
+##禀
+##禁
+##禄
+##禅
+##禍
+##禎
+##福
+##禛
+##禦
+##禧
+##禪
+##禮
+##禱
+##禹
+##禺
+##离
+##禽
+##禾
+##禿
+##秀
+##私
+##秃
+##秆
+##秉
+##秋
+##种
+##科
+##秒
+##秘
+##租
+##秣
+##秤
+##秦
+##秧
+##秩
+##秭
+##积
+##称
+##秸
+##移
+##秽
+##稀
+##稅
+##程
+##稍
+##税
+##稔
+##稗
+##稚
+##稜
+##稞
+##稟
+##稠
+##稣
+##種
+##稱
+##稲
+##稳
+##稷
+##稹
+##稻
+##稼
+##稽
+##稿
+##穀
+##穂
+##穆
+##穌
+##積
+##穎
+##穗
+##穢
+##穩
+##穫
+##穴
+##究
+##穷
+##穹
+##空
+##穿
+##突
+##窃
+##窄
+##窈
+##窍
+##窑
+##窒
+##窓
+##窕
+##窖
+##窗
+##窘
+##窜
+##窝
+##窟
+##窠
+##窥
+##窦
+##窨
+##窩
+##窪
+##窮
+##窯
+##窺
+##窿
+##竄
+##竅
+##竇
+##竊
+##立
+##竖
+##站
+##竜
+##竞
+##竟
+##章
+##竣
+##童
+##竭
+##端
+##競
+##竹
+##竺
+##竽
+##竿
+##笃
+##笆
+##笈
+##笋
+##笏
+##笑
+##笔
+##笙
+##笛
+##笞
+##笠
+##符
+##笨
+##第
+##笹
+##笺
+##笼
+##筆
+##等
+##筊
+##筋
+##筍
+##筏
+##筐
+##筑
+##筒
+##答
+##策
+##筛
+##筝
+##筠
+##筱
+##筲
+##筵
+##筷
+##筹
+##签
+##简
+##箇
+##箋
+##箍
+##箏
+##箐
+##箔
+##箕
+##算
+##箝
+##管
+##箩
+##箫
+##箭
+##箱
+##箴
+##箸
+##節
+##篁
+##範
+##篆
+##篇
+##築
+##篑
+##篓
+##篙
+##篝
+##篠
+##篡
+##篤
+##篩
+##篪
+##篮
+##篱
+##篷
+##簇
+##簌
+##簍
+##簡
+##簦
+##簧
+##簪
+##簫
+##簷
+##簸
+##簽
+##簾
+##簿
+##籁
+##籃
+##籌
+##籍
+##籐
+##籟
+##籠
+##籤
+##籬
+##籮
+##籲
+##米
+##类
+##籼
+##籽
+##粄
+##粉
+##粑
+##粒
+##粕
+##粗
+##粘
+##粟
+##粤
+##粥
+##粧
+##粪
+##粮
+##粱
+##粲
+##粳
+##粵
+##粹
+##粼
+##粽
+##精
+##粿
+##糅
+##糊
+##糍
+##糕
+##糖
+##糗
+##糙
+##糜
+##糞
+##糟
+##糠
+##糧
+##糬
+##糯
+##糰
+##糸
+##系
+##糾
+##紀
+##紂
+##約
+##紅
+##紉
+##紊
+##紋
+##納
+##紐
+##紓
+##純
+##紗
+##紘
+##紙
+##級
+##紛
+##紜
+##素
+##紡
+##索
+##紧
+##紫
+##紮
+##累
+##細
+##紳
+##紹
+##紺
+##終
+##絃
+##組
+##絆
+##経
+##結
+##絕
+##絞
+##絡
+##絢
+##給
+##絨
+##絮
+##統
+##絲
+##絳
+##絵
+##絶
+##絹
+##綁
+##綏
+##綑
+##經
+##継
+##続
+##綜
+##綠
+##綢
+##綦
+##綫
+##綬
+##維
+##綱
+##網
+##綴
+##綵
+##綸
+##綺
+##綻
+##綽
+##綾
+##綿
+##緊
+##緋
+##総
+##緑
+##緒
+##緘
+##線
+##緝
+##緞
+##締
+##緣
+##編
+##緩
+##緬
+##緯
+##練
+##緹
+##緻
+##縁
+##縄
+##縈
+##縛
+##縝
+##縣
+##縫
+##縮
+##縱
+##縴
+##縷
+##總
+##績
+##繁
+##繃
+##繆
+##繇
+##繋
+##織
+##繕
+##繚
+##繞
+##繡
+##繩
+##繪
+##繫
+##繭
+##繳
+##繹
+##繼
+##繽
+##纂
+##續
+##纍
+##纏
+##纓
+##纔
+##纖
+##纜
+##纠
+##红
+##纣
+##纤
+##约
+##级
+##纨
+##纪
+##纫
+##纬
+##纭
+##纯
+##纰
+##纱
+##纲
+##纳
+##纵
+##纶
+##纷
+##纸
+##纹
+##纺
+##纽
+##纾
+##线
+##绀
+##练
+##组
+##绅
+##细
+##织
+##终
+##绊
+##绍
+##绎
+##经
+##绑
+##绒
+##结
+##绔
+##绕
+##绘
+##给
+##绚
+##绛
+##络
+##绝
+##绞
+##统
+##绡
+##绢
+##绣
+##绥
+##绦
+##继
+##绩
+##绪
+##绫
+##续
+##绮
+##绯
+##绰
+##绳
+##维
+##绵
+##绶
+##绷
+##绸
+##绻
+##综
+##绽
+##绾
+##绿
+##缀
+##缄
+##缅
+##缆
+##缇
+##缈
+##缉
+##缎
+##缓
+##缔
+##缕
+##编
+##缘
+##缙
+##缚
+##缜
+##缝
+##缠
+##缢
+##缤
+##缥
+##缨
+##缩
+##缪
+##缭
+##缮
+##缰
+##缱
+##缴
+##缸
+##缺
+##缽
+##罂
+##罄
+##罌
+##罐
+##网
+##罔
+##罕
+##罗
+##罚
+##罡
+##罢
+##罩
+##罪
+##置
+##罰
+##署
+##罵
+##罷
+##罹
+##羁
+##羅
+##羈
+##羊
+##羌
+##美
+##羔
+##羚
+##羞
+##羟
+##羡
+##羣
+##群
+##羥
+##羧
+##羨
+##義
+##羯
+##羲
+##羸
+##羹
+##羽
+##羿
+##翁
+##翅
+##翊
+##翌
+##翎
+##習
+##翔
+##翘
+##翟
+##翠
+##翡
+##翦
+##翩
+##翰
+##翱
+##翳
+##翹
+##翻
+##翼
+##耀
+##老
+##考
+##耄
+##者
+##耆
+##耋
+##而
+##耍
+##耐
+##耒
+##耕
+##耗
+##耘
+##耙
+##耦
+##耨
+##耳
+##耶
+##耷
+##耸
+##耻
+##耽
+##耿
+##聂
+##聆
+##聊
+##聋
+##职
+##聒
+##联
+##聖
+##聘
+##聚
+##聞
+##聪
+##聯
+##聰
+##聲
+##聳
+##聴
+##聶
+##職
+##聽
+##聾
+##聿
+##肃
+##肄
+##肅
+##肆
+##肇
+##肉
+##肋
+##肌
+##肏
+##肓
+##肖
+##肘
+##肚
+##肛
+##肝
+##肠
+##股
+##肢
+##肤
+##肥
+##肩
+##肪
+##肮
+##肯
+##肱
+##育
+##肴
+##肺
+##肽
+##肾
+##肿
+##胀
+##胁
+##胃
+##胄
+##胆
+##背
+##胍
+##胎
+##胖
+##胚
+##胛
+##胜
+##胝
+##胞
+##胡
+##胤
+##胥
+##胧
+##胫
+##胭
+##胯
+##胰
+##胱
+##胳
+##胴
+##胶
+##胸
+##胺
+##能
+##脂
+##脅
+##脆
+##脇
+##脈
+##脉
+##脊
+##脍
+##脏
+##脐
+##脑
+##脓
+##脖
+##脘
+##脚
+##脛
+##脣
+##脩
+##脫
+##脯
+##脱
+##脲
+##脳
+##脸
+##脹
+##脾
+##腆
+##腈
+##腊
+##腋
+##腌
+##腎
+##腐
+##腑
+##腓
+##腔
+##腕
+##腥
+##腦
+##腩
+##腫
+##腭
+##腮
+##腰
+##腱
+##腳
+##腴
+##腸
+##腹
+##腺
+##腻
+##腼
+##腾
+##腿
+##膀
+##膈
+##膊
+##膏
+##膑
+##膘
+##膚
+##膛
+##膜
+##膝
+##膠
+##膦
+##膨
+##膩
+##膳
+##膺
+##膻
+##膽
+##膾
+##膿
+##臀
+##臂
+##臃
+##臆
+##臉
+##臊
+##臍
+##臓
+##臘
+##臟
+##臣
+##臥
+##臧
+##臨
+##自
+##臬
+##臭
+##至
+##致
+##臺
+##臻
+##臼
+##臾
+##舀
+##舂
+##舅
+##舆
+##與
+##興
+##舉
+##舊
+##舌
+##舍
+##舎
+##舐
+##舒
+##舔
+##舖
+##舗
+##舛
+##舜
+##舞
+##舟
+##航
+##舫
+##般
+##舰
+##舱
+##舵
+##舶
+##舷
+##舸
+##船
+##舺
+##舾
+##艇
+##艋
+##艘
+##艙
+##艦
+##艮
+##良
+##艰
+##艱
+##色
+##艳
+##艷
+##艹
+##艺
+##艾
+##节
+##芃
+##芈
+##芊
+##芋
+##芍
+##芎
+##芒
+##芙
+##芜
+##芝
+##芡
+##芥
+##芦
+##芩
+##芪
+##芫
+##芬
+##芭
+##芮
+##芯
+##花
+##芳
+##芷
+##芸
+##芹
+##芻
+##芽
+##芾
+##苁
+##苄
+##苇
+##苋
+##苍
+##苏
+##苑
+##苒
+##苓
+##苔
+##苕
+##苗
+##苛
+##苜
+##苞
+##苟
+##苡
+##苣
+##若
+##苦
+##苫
+##苯
+##英
+##苷
+##苹
+##苻
+##茁
+##茂
+##范
+##茄
+##茅
+##茉
+##茎
+##茏
+##茗
+##茜
+##茧
+##茨
+##茫
+##茬
+##茭
+##茯
+##茱
+##茲
+##茴
+##茵
+##茶
+##茸
+##茹
+##茼
+##荀
+##荃
+##荆
+##草
+##荊
+##荏
+##荐
+##荒
+##荔
+##荖
+##荘
+##荚
+##荞
+##荟
+##荠
+##荡
+##荣
+##荤
+##荥
+##荧
+##荨
+##荪
+##荫
+##药
+##荳
+##荷
+##荸
+##荻
+##荼
+##荽
+##莅
+##莆
+##莉
+##莊
+##莎
+##莒
+##莓
+##莖
+##莘
+##莞
+##莠
+##莢
+##莧
+##莪
+##莫
+##莱
+##莲
+##莴
+##获
+##莹
+##莺
+##莽
+##莿
+##菀
+##菁
+##菅
+##菇
+##菈
+##菊
+##菌
+##菏
+##菓
+##菖
+##菘
+##菜
+##菟
+##菠
+##菡
+##菩
+##華
+##菱
+##菲
+##菸
+##菽
+##萁
+##萃
+##萄
+##萊
+##萋
+##萌
+##萍
+##萎
+##萘
+##萝
+##萤
+##营
+##萦
+##萧
+##萨
+##萩
+##萬
+##萱
+##萵
+##萸
+##萼
+##落
+##葆
+##葉
+##著
+##葚
+##葛
+##葡
+##董
+##葦
+##葩
+##葫
+##葬
+##葭
+##葯
+##葱
+##葳
+##葵
+##葷
+##葺
+##蒂
+##蒋
+##蒐
+##蒔
+##蒙
+##蒜
+##蒞
+##蒟
+##蒡
+##蒨
+##蒲
+##蒸
+##蒹
+##蒻
+##蒼
+##蒿
+##蓁
+##蓄
+##蓆
+##蓉
+##蓋
+##蓑
+##蓓
+##蓖
+##蓝
+##蓟
+##蓦
+##蓬
+##蓮
+##蓼
+##蓿
+##蔑
+##蔓
+##蔔
+##蔗
+##蔘
+##蔚
+##蔡
+##蔣
+##蔥
+##蔫
+##蔬
+##蔭
+##蔵
+##蔷
+##蔺
+##蔻
+##蔼
+##蔽
+##蕁
+##蕃
+##蕈
+##蕉
+##蕊
+##蕎
+##蕙
+##蕤
+##蕨
+##蕩
+##蕪
+##蕭
+##蕲
+##蕴
+##蕻
+##蕾
+##薄
+##薅
+##薇
+##薈
+##薊
+##薏
+##薑
+##薔
+##薙
+##薛
+##薦
+##薨
+##薩
+##薪
+##薬
+##薯
+##薰
+##薹
+##藉
+##藍
+##藏
+##藐
+##藓
+##藕
+##藜
+##藝
+##藤
+##藥
+##藩
+##藹
+##藻
+##藿
+##蘆
+##蘇
+##蘊
+##蘋
+##蘑
+##蘚
+##蘭
+##蘸
+##蘼
+##蘿
+##虎
+##虏
+##虐
+##虑
+##虔
+##處
+##虚
+##虛
+##虜
+##虞
+##號
+##虢
+##虧
+##虫
+##虬
+##虱
+##虹
+##虻
+##虽
+##虾
+##蚀
+##蚁
+##蚂
+##蚊
+##蚌
+##蚓
+##蚕
+##蚜
+##蚝
+##蚣
+##蚤
+##蚩
+##蚪
+##蚯
+##蚱
+##蚵
+##蛀
+##蛆
+##蛇
+##蛊
+##蛋
+##蛎
+##蛐
+##蛔
+##蛙
+##蛛
+##蛟
+##蛤
+##蛭
+##蛮
+##蛰
+##蛳
+##蛹
+##蛻
+##蛾
+##蜀
+##蜂
+##蜃
+##蜆
+##蜇
+##蜈
+##蜊
+##蜍
+##蜒
+##蜓
+##蜕
+##蜗
+##蜘
+##蜚
+##蜜
+##蜡
+##蜢
+##蜥
+##蜱
+##蜴
+##蜷
+##蜻
+##蜿
+##蝇
+##蝈
+##蝉
+##蝌
+##蝎
+##蝕
+##蝗
+##蝙
+##蝟
+##蝠
+##蝦
+##蝨
+##蝴
+##蝶
+##蝸
+##蝼
+##螂
+##螃
+##融
+##螞
+##螢
+##螨
+##螯
+##螳
+##螺
+##蟀
+##蟄
+##蟆
+##蟋
+##蟎
+##蟑
+##蟒
+##蟠
+##蟬
+##蟲
+##蟹
+##蟻
+##蟾
+##蠅
+##蠍
+##蠔
+##蠕
+##蠛
+##蠟
+##蠡
+##蠢
+##蠣
+##蠱
+##蠶
+##蠹
+##蠻
+##血
+##衄
+##衅
+##衆
+##行
+##衍
+##術
+##衔
+##街
+##衙
+##衛
+##衝
+##衞
+##衡
+##衢
+##衣
+##补
+##表
+##衩
+##衫
+##衬
+##衮
+##衰
+##衲
+##衷
+##衹
+##衾
+##衿
+##袁
+##袂
+##袄
+##袅
+##袈
+##袋
+##袍
+##袒
+##袖
+##袜
+##袞
+##袤
+##袪
+##被
+##袭
+##袱
+##裁
+##裂
+##装
+##裆
+##裊
+##裏
+##裔
+##裕
+##裘
+##裙
+##補
+##裝
+##裟
+##裡
+##裤
+##裨
+##裱
+##裳
+##裴
+##裸
+##裹
+##製
+##裾
+##褂
+##複
+##褐
+##褒
+##褓
+##褔
+##褚
+##褥
+##褪
+##褫
+##褲
+##褶
+##褻
+##襁
+##襄
+##襟
+##襠
+##襪
+##襬
+##襯
+##襲
+##西
+##要
+##覃
+##覆
+##覇
+##見
+##規
+##覓
+##視
+##覚
+##覦
+##覧
+##親
+##覬
+##観
+##覷
+##覺
+##覽
+##觀
+##见
+##观
+##规
+##觅
+##视
+##览
+##觉
+##觊
+##觎
+##觐
+##觑
+##角
+##觞
+##解
+##觥
+##触
+##觸
+##言
+##訂
+##計
+##訊
+##討
+##訓
+##訕
+##訖
+##託
+##記
+##訛
+##訝
+##訟
+##訣
+##訥
+##訪
+##設
+##許
+##訳
+##訴
+##訶
+##診
+##註
+##証
+##詆
+##詐
+##詔
+##評
+##詛
+##詞
+##詠
+##詡
+##詢
+##詣
+##試
+##詩
+##詫
+##詬
+##詭
+##詮
+##詰
+##話
+##該
+##詳
+##詹
+##詼
+##誅
+##誇
+##誉
+##誌
+##認
+##誓
+##誕
+##誘
+##語
+##誠
+##誡
+##誣
+##誤
+##誥
+##誦
+##誨
+##說
+##説
+##読
+##誰
+##課
+##誹
+##誼
+##調
+##諄
+##談
+##請
+##諏
+##諒
+##論
+##諗
+##諜
+##諡
+##諦
+##諧
+##諫
+##諭
+##諮
+##諱
+##諳
+##諷
+##諸
+##諺
+##諾
+##謀
+##謁
+##謂
+##謄
+##謊
+##謎
+##謐
+##謔
+##謗
+##謙
+##講
+##謝
+##謠
+##謨
+##謬
+##謹
+##謾
+##譁
+##證
+##譎
+##譏
+##識
+##譙
+##譚
+##譜
+##警
+##譬
+##譯
+##議
+##譲
+##譴
+##護
+##譽
+##讀
+##變
+##讓
+##讚
+##讞
+##计
+##订
+##认
+##讥
+##讧
+##讨
+##让
+##讪
+##讫
+##训
+##议
+##讯
+##记
+##讲
+##讳
+##讴
+##讶
+##讷
+##许
+##讹
+##论
+##讼
+##讽
+##设
+##访
+##诀
+##证
+##诃
+##评
+##诅
+##识
+##诈
+##诉
+##诊
+##诋
+##词
+##诏
+##译
+##试
+##诗
+##诘
+##诙
+##诚
+##诛
+##话
+##诞
+##诟
+##诠
+##诡
+##询
+##诣
+##诤
+##该
+##详
+##诧
+##诩
+##诫
+##诬
+##语
+##误
+##诰
+##诱
+##诲
+##说
+##诵
+##诶
+##请
+##诸
+##诺
+##读
+##诽
+##课
+##诿
+##谀
+##谁
+##调
+##谄
+##谅
+##谆
+##谈
+##谊
+##谋
+##谌
+##谍
+##谎
+##谏
+##谐
+##谑
+##谒
+##谓
+##谔
+##谕
+##谗
+##谘
+##谙
+##谚
+##谛
+##谜
+##谟
+##谢
+##谣
+##谤
+##谥
+##谦
+##谧
+##谨
+##谩
+##谪
+##谬
+##谭
+##谯
+##谱
+##谲
+##谴
+##谶
+##谷
+##豁
+##豆
+##豇
+##豈
+##豉
+##豊
+##豌
+##豎
+##豐
+##豔
+##豚
+##象
+##豢
+##豪
+##豫
+##豬
+##豹
+##豺
+##貂
+##貅
+##貌
+##貓
+##貔
+##貘
+##貝
+##貞
+##負
+##財
+##貢
+##貧
+##貨
+##販
+##貪
+##貫
+##責
+##貯
+##貰
+##貳
+##貴
+##貶
+##買
+##貸
+##費
+##貼
+##貽
+##貿
+##賀
+##賁
+##賂
+##賃
+##賄
+##資
+##賈
+##賊
+##賑
+##賓
+##賜
+##賞
+##賠
+##賡
+##賢
+##賣
+##賤
+##賦
+##質
+##賬
+##賭
+##賴
+##賺
+##購
+##賽
+##贅
+##贈
+##贊
+##贍
+##贏
+##贓
+##贖
+##贛
+##贝
+##贞
+##负
+##贡
+##财
+##责
+##贤
+##败
+##账
+##货
+##质
+##贩
+##贪
+##贫
+##贬
+##购
+##贮
+##贯
+##贰
+##贱
+##贲
+##贴
+##贵
+##贷
+##贸
+##费
+##贺
+##贻
+##贼
+##贾
+##贿
+##赁
+##赂
+##赃
+##资
+##赅
+##赈
+##赊
+##赋
+##赌
+##赎
+##赏
+##赐
+##赓
+##赔
+##赖
+##赘
+##赚
+##赛
+##赝
+##赞
+##赠
+##赡
+##赢
+##赣
+##赤
+##赦
+##赧
+##赫
+##赭
+##走
+##赳
+##赴
+##赵
+##赶
+##起
+##趁
+##超
+##越
+##趋
+##趕
+##趙
+##趟
+##趣
+##趨
+##足
+##趴
+##趵
+##趸
+##趺
+##趾
+##跃
+##跄
+##跆
+##跋
+##跌
+##跎
+##跑
+##跖
+##跚
+##跛
+##距
+##跟
+##跡
+##跤
+##跨
+##跩
+##跪
+##路
+##跳
+##践
+##跷
+##跹
+##跺
+##跻
+##踉
+##踊
+##踌
+##踏
+##踐
+##踝
+##踞
+##踟
+##踢
+##踩
+##踪
+##踮
+##踱
+##踴
+##踵
+##踹
+##蹂
+##蹄
+##蹇
+##蹈
+##蹉
+##蹊
+##蹋
+##蹑
+##蹒
+##蹙
+##蹟
+##蹣
+##蹤
+##蹦
+##蹩
+##蹬
+##蹭
+##蹲
+##蹴
+##蹶
+##蹺
+##蹼
+##蹿
+##躁
+##躇
+##躉
+##躊
+##躋
+##躍
+##躏
+##躪
+##身
+##躬
+##躯
+##躲
+##躺
+##軀
+##車
+##軋
+##軌
+##軍
+##軒
+##軟
+##転
+##軸
+##軼
+##軽
+##軾
+##較
+##載
+##輒
+##輓
+##輔
+##輕
+##輛
+##輝
+##輟
+##輩
+##輪
+##輯
+##輸
+##輻
+##輾
+##輿
+##轄
+##轅
+##轆
+##轉
+##轍
+##轎
+##轟
+##车
+##轧
+##轨
+##轩
+##转
+##轭
+##轮
+##软
+##轰
+##轲
+##轴
+##轶
+##轻
+##轼
+##载
+##轿
+##较
+##辄
+##辅
+##辆
+##辇
+##辈
+##辉
+##辊
+##辍
+##辐
+##辑
+##输
+##辕
+##辖
+##辗
+##辘
+##辙
+##辛
+##辜
+##辞
+##辟
+##辣
+##辦
+##辨
+##辩
+##辫
+##辭
+##辮
+##辯
+##辰
+##辱
+##農
+##边
+##辺
+##辻
+##込
+##辽
+##达
+##迁
+##迂
+##迄
+##迅
+##过
+##迈
+##迎
+##运
+##近
+##返
+##还
+##这
+##进
+##远
+##违
+##连
+##迟
+##迢
+##迤
+##迥
+##迦
+##迩
+##迪
+##迫
+##迭
+##述
+##迴
+##迷
+##迸
+##迹
+##迺
+##追
+##退
+##送
+##适
+##逃
+##逅
+##逆
+##选
+##逊
+##逍
+##透
+##逐
+##递
+##途
+##逕
+##逗
+##這
+##通
+##逛
+##逝
+##逞
+##速
+##造
+##逢
+##連
+##逮
+##週
+##進
+##逵
+##逶
+##逸
+##逻
+##逼
+##逾
+##遁
+##遂
+##遅
+##遇
+##遊
+##運
+##遍
+##過
+##遏
+##遐
+##遑
+##遒
+##道
+##達
+##違
+##遗
+##遙
+##遛
+##遜
+##遞
+##遠
+##遢
+##遣
+##遥
+##遨
+##適
+##遭
+##遮
+##遲
+##遴
+##遵
+##遶
+##遷
+##選
+##遺
+##遼
+##遽
+##避
+##邀
+##邁
+##邂
+##邃
+##還
+##邇
+##邈
+##邊
+##邋
+##邏
+##邑
+##邓
+##邕
+##邛
+##邝
+##邢
+##那
+##邦
+##邨
+##邪
+##邬
+##邮
+##邯
+##邰
+##邱
+##邳
+##邵
+##邸
+##邹
+##邺
+##邻
+##郁
+##郅
+##郊
+##郎
+##郑
+##郜
+##郝
+##郡
+##郢
+##郤
+##郦
+##郧
+##部
+##郫
+##郭
+##郴
+##郵
+##郷
+##郸
+##都
+##鄂
+##鄉
+##鄒
+##鄔
+##鄙
+##鄞
+##鄢
+##鄧
+##鄭
+##鄰
+##鄱
+##鄲
+##鄺
+##酉
+##酊
+##酋
+##酌
+##配
+##酐
+##酒
+##酗
+##酚
+##酝
+##酢
+##酣
+##酥
+##酩
+##酪
+##酬
+##酮
+##酯
+##酰
+##酱
+##酵
+##酶
+##酷
+##酸
+##酿
+##醃
+##醇
+##醉
+##醋
+##醍
+##醐
+##醒
+##醚
+##醛
+##醜
+##醞
+##醣
+##醪
+##醫
+##醬
+##醮
+##醯
+##醴
+##醺
+##釀
+##釁
+##采
+##釉
+##释
+##釋
+##里
+##重
+##野
+##量
+##釐
+##金
+##釗
+##釘
+##釜
+##針
+##釣
+##釦
+##釧
+##釵
+##鈀
+##鈉
+##鈍
+##鈎
+##鈔
+##鈕
+##鈞
+##鈣
+##鈦
+##鈪
+##鈴
+##鈺
+##鈾
+##鉀
+##鉄
+##鉅
+##鉉
+##鉑
+##鉗
+##鉚
+##鉛
+##鉤
+##鉴
+##鉻
+##銀
+##銃
+##銅
+##銑
+##銓
+##銖
+##銘
+##銜
+##銬
+##銭
+##銮
+##銳
+##銷
+##銹
+##鋁
+##鋅
+##鋒
+##鋤
+##鋪
+##鋰
+##鋸
+##鋼
+##錄
+##錐
+##錘
+##錚
+##錠
+##錢
+##錦
+##錨
+##錫
+##錮
+##錯
+##録
+##錳
+##錶
+##鍊
+##鍋
+##鍍
+##鍛
+##鍥
+##鍰
+##鍵
+##鍺
+##鍾
+##鎂
+##鎊
+##鎌
+##鎏
+##鎔
+##鎖
+##鎗
+##鎚
+##鎧
+##鎬
+##鎮
+##鎳
+##鏈
+##鏖
+##鏗
+##鏘
+##鏞
+##鏟
+##鏡
+##鏢
+##鏤
+##鏽
+##鐘
+##鐮
+##鐲
+##鐳
+##鐵
+##鐸
+##鐺
+##鑄
+##鑊
+##鑑
+##鑒
+##鑣
+##鑫
+##鑰
+##鑲
+##鑼
+##鑽
+##鑾
+##鑿
+##针
+##钉
+##钊
+##钎
+##钏
+##钒
+##钓
+##钗
+##钙
+##钛
+##钜
+##钝
+##钞
+##钟
+##钠
+##钡
+##钢
+##钣
+##钤
+##钥
+##钦
+##钧
+##钨
+##钩
+##钮
+##钯
+##钰
+##钱
+##钳
+##钴
+##钵
+##钺
+##钻
+##钼
+##钾
+##钿
+##铀
+##铁
+##铂
+##铃
+##铄
+##铅
+##铆
+##铉
+##铎
+##铐
+##铛
+##铜
+##铝
+##铠
+##铡
+##铢
+##铣
+##铤
+##铨
+##铩
+##铬
+##铭
+##铮
+##铰
+##铲
+##铵
+##银
+##铸
+##铺
+##链
+##铿
+##销
+##锁
+##锂
+##锄
+##锅
+##锆
+##锈
+##锉
+##锋
+##锌
+##锏
+##锐
+##锑
+##错
+##锚
+##锟
+##锡
+##锢
+##锣
+##锤
+##锥
+##锦
+##锭
+##键
+##锯
+##锰
+##锲
+##锵
+##锹
+##锺
+##锻
+##镀
+##镁
+##镂
+##镇
+##镉
+##镌
+##镍
+##镐
+##镑
+##镕
+##镖
+##镗
+##镛
+##镜
+##镣
+##镭
+##镯
+##镰
+##镳
+##镶
+##長
+##长
+##門
+##閃
+##閉
+##開
+##閎
+##閏
+##閑
+##閒
+##間
+##閔
+##閘
+##閡
+##関
+##閣
+##閥
+##閨
+##閩
+##閱
+##閲
+##閹
+##閻
+##閾
+##闆
+##闇
+##闊
+##闌
+##闍
+##闔
+##闕
+##闖
+##闘
+##關
+##闡
+##闢
+##门
+##闪
+##闫
+##闭
+##问
+##闯
+##闰
+##闲
+##间
+##闵
+##闷
+##闸
+##闹
+##闺
+##闻
+##闽
+##闾
+##阀
+##阁
+##阂
+##阅
+##阆
+##阇
+##阈
+##阉
+##阎
+##阐
+##阑
+##阔
+##阕
+##阖
+##阙
+##阚
+##阜
+##队
+##阡
+##阪
+##阮
+##阱
+##防
+##阳
+##阴
+##阵
+##阶
+##阻
+##阿
+##陀
+##陂
+##附
+##际
+##陆
+##陇
+##陈
+##陋
+##陌
+##降
+##限
+##陕
+##陛
+##陝
+##陞
+##陟
+##陡
+##院
+##陣
+##除
+##陨
+##险
+##陪
+##陰
+##陲
+##陳
+##陵
+##陶
+##陷
+##陸
+##険
+##陽
+##隅
+##隆
+##隈
+##隊
+##隋
+##隍
+##階
+##随
+##隐
+##隔
+##隕
+##隘
+##隙
+##際
+##障
+##隠
+##隣
+##隧
+##隨
+##險
+##隱
+##隴
+##隶
+##隸
+##隻
+##隼
+##隽
+##难
+##雀
+##雁
+##雄
+##雅
+##集
+##雇
+##雉
+##雋
+##雌
+##雍
+##雎
+##雏
+##雑
+##雒
+##雕
+##雖
+##雙
+##雛
+##雜
+##雞
+##離
+##難
+##雨
+##雪
+##雯
+##雰
+##雲
+##雳
+##零
+##雷
+##雹
+##電
+##雾
+##需
+##霁
+##霄
+##霆
+##震
+##霈
+##霉
+##霊
+##霍
+##霎
+##霏
+##霑
+##霓
+##霖
+##霜
+##霞
+##霧
+##霭
+##霰
+##露
+##霸
+##霹
+##霽
+##霾
+##靂
+##靄
+##靈
+##青
+##靓
+##靖
+##静
+##靚
+##靛
+##靜
+##非
+##靠
+##靡
+##面
+##靥
+##靦
+##革
+##靳
+##靴
+##靶
+##靼
+##鞅
+##鞋
+##鞍
+##鞏
+##鞑
+##鞘
+##鞠
+##鞣
+##鞦
+##鞭
+##韆
+##韋
+##韌
+##韓
+##韜
+##韦
+##韧
+##韩
+##韬
+##韭
+##音
+##韵
+##韶
+##韻
+##響
+##頁
+##頂
+##頃
+##項
+##順
+##須
+##頌
+##預
+##頑
+##頒
+##頓
+##頗
+##領
+##頜
+##頡
+##頤
+##頫
+##頭
+##頰
+##頷
+##頸
+##頹
+##頻
+##頼
+##顆
+##題
+##額
+##顎
+##顏
+##顔
+##願
+##顛
+##類
+##顧
+##顫
+##顯
+##顱
+##顴
+##页
+##顶
+##顷
+##项
+##顺
+##须
+##顼
+##顽
+##顾
+##顿
+##颁
+##颂
+##预
+##颅
+##领
+##颇
+##颈
+##颉
+##颊
+##颌
+##颍
+##颐
+##频
+##颓
+##颔
+##颖
+##颗
+##题
+##颚
+##颛
+##颜
+##额
+##颞
+##颠
+##颡
+##颢
+##颤
+##颦
+##颧
+##風
+##颯
+##颱
+##颳
+##颶
+##颼
+##飄
+##飆
+##风
+##飒
+##飓
+##飕
+##飘
+##飙
+##飚
+##飛
+##飞
+##食
+##飢
+##飨
+##飩
+##飪
+##飯
+##飲
+##飼
+##飽
+##飾
+##餃
+##餅
+##餉
+##養
+##餌
+##餐
+##餒
+##餓
+##餘
+##餚
+##餛
+##餞
+##餡
+##館
+##餮
+##餵
+##餾
+##饅
+##饈
+##饋
+##饌
+##饍
+##饑
+##饒
+##饕
+##饗
+##饞
+##饥
+##饨
+##饪
+##饬
+##饭
+##饮
+##饯
+##饰
+##饱
+##饲
+##饴
+##饵
+##饶
+##饷
+##饺
+##饼
+##饽
+##饿
+##馀
+##馁
+##馄
+##馅
+##馆
+##馈
+##馋
+##馍
+##馏
+##馒
+##馔
+##首
+##馗
+##香
+##馥
+##馨
+##馬
+##馭
+##馮
+##馳
+##馴
+##駁
+##駄
+##駅
+##駆
+##駐
+##駒
+##駕
+##駛
+##駝
+##駭
+##駱
+##駿
+##騁
+##騎
+##騏
+##験
+##騙
+##騨
+##騰
+##騷
+##驀
+##驅
+##驊
+##驍
+##驒
+##驕
+##驗
+##驚
+##驛
+##驟
+##驢
+##驥
+##马
+##驭
+##驮
+##驯
+##驰
+##驱
+##驳
+##驴
+##驶
+##驷
+##驸
+##驹
+##驻
+##驼
+##驾
+##驿
+##骁
+##骂
+##骄
+##骅
+##骆
+##骇
+##骈
+##骊
+##骋
+##验
+##骏
+##骐
+##骑
+##骗
+##骚
+##骛
+##骜
+##骞
+##骠
+##骡
+##骤
+##骥
+##骧
+##骨
+##骯
+##骰
+##骶
+##骷
+##骸
+##骼
+##髂
+##髅
+##髋
+##髏
+##髒
+##髓
+##體
+##髖
+##高
+##髦
+##髪
+##髮
+##髯
+##髻
+##鬃
+##鬆
+##鬍
+##鬓
+##鬚
+##鬟
+##鬢
+##鬣
+##鬥
+##鬧
+##鬱
+##鬼
+##魁
+##魂
+##魄
+##魅
+##魇
+##魍
+##魏
+##魔
+##魘
+##魚
+##魯
+##魷
+##鮑
+##鮨
+##鮪
+##鮭
+##鮮
+##鯉
+##鯊
+##鯖
+##鯛
+##鯨
+##鯰
+##鯽
+##鰍
+##鰓
+##鰭
+##鰲
+##鰻
+##鰾
+##鱈
+##鱉
+##鱔
+##鱗
+##鱷
+##鱸
+##鱼
+##鱿
+##鲁
+##鲈
+##鲍
+##鲑
+##鲛
+##鲜
+##鲟
+##鲢
+##鲤
+##鲨
+##鲫
+##鲱
+##鲲
+##鲶
+##鲷
+##鲸
+##鳃
+##鳄
+##鳅
+##鳌
+##鳍
+##鳕
+##鳖
+##鳗
+##鳝
+##鳞
+##鳥
+##鳩
+##鳳
+##鳴
+##鳶
+##鴉
+##鴕
+##鴛
+##鴦
+##鴨
+##鴻
+##鴿
+##鵑
+##鵜
+##鵝
+##鵡
+##鵬
+##鵰
+##鵲
+##鶘
+##鶩
+##鶯
+##鶴
+##鷗
+##鷲
+##鷹
+##鷺
+##鸚
+##鸞
+##鸟
+##鸠
+##鸡
+##鸢
+##鸣
+##鸥
+##鸦
+##鸨
+##鸪
+##鸭
+##鸯
+##鸳
+##鸵
+##鸽
+##鸾
+##鸿
+##鹂
+##鹃
+##鹄
+##鹅
+##鹈
+##鹉
+##鹊
+##鹌
+##鹏
+##鹑
+##鹕
+##鹘
+##鹜
+##鹞
+##鹤
+##鹦
+##鹧
+##鹫
+##鹭
+##鹰
+##鹳
+##鹵
+##鹹
+##鹼
+##鹽
+##鹿
+##麂
+##麋
+##麒
+##麓
+##麗
+##麝
+##麟
+##麥
+##麦
+##麩
+##麴
+##麵
+##麸
+##麺
+##麻
+##麼
+##麽
+##麾
+##黃
+##黄
+##黍
+##黎
+##黏
+##黑
+##黒
+##黔
+##默
+##黛
+##黜
+##黝
+##點
+##黠
+##黨
+##黯
+##黴
+##鼋
+##鼎
+##鼐
+##鼓
+##鼠
+##鼬
+##鼹
+##鼻
+##鼾
+##齁
+##齊
+##齋
+##齐
+##齒
+##齡
+##齢
+##齣
+##齦
+##齿
+##龄
+##龅
+##龈
+##龊
+##龋
+##龌
+##龍
+##龐
+##龔
+##龕
+##龙
+##龚
+##龛
+##龜
+##龟
+##︰
+##︱
+##︶
+##︿
+##﹁
+##﹂
+##﹍
+##﹏
+##﹐
+##﹑
+##﹒
+##﹔
+##﹕
+##﹖
+##﹗
+##﹙
+##﹚
+##﹝
+##﹞
+##﹡
+##﹣
+##!
+##"
+###
+##$
+##%
+##&
+##'
+##(
+##)
+##*
+##,
+##-
+##.
+##/
+##:
+##;
+##<
+##?
+##@
+##[
+##\
+##]
+##^
+##_
+##`
+##f
+##h
+##j
+##u
+##w
+##z
+##{
+##}
+##。
+##「
+##」
+##、
+##・
+##ッ
+##ー
+##イ
+##ク
+##シ
+##ス
+##ト
+##ノ
+##フ
+##ラ
+##ル
+##ン
+##゙
+##゚
+## ̄
+##¥
+##👍
+##🔥
+##😂
+##😎
diff --git a/bert/deberta-v2-large-japanese-char-wwm/.gitattributes b/bert/deberta-v2-large-japanese-char-wwm/.gitattributes
new file mode 100644
index 0000000000000000000000000000000000000000..c7d9f3332a950355d5a77d85000f05e6f45435ea
--- /dev/null
+++ b/bert/deberta-v2-large-japanese-char-wwm/.gitattributes
@@ -0,0 +1,34 @@
+*.7z filter=lfs diff=lfs merge=lfs -text
+*.arrow filter=lfs diff=lfs merge=lfs -text
+*.bin filter=lfs diff=lfs merge=lfs -text
+*.bz2 filter=lfs diff=lfs merge=lfs -text
+*.ckpt filter=lfs diff=lfs merge=lfs -text
+*.ftz filter=lfs diff=lfs merge=lfs -text
+*.gz filter=lfs diff=lfs merge=lfs -text
+*.h5 filter=lfs diff=lfs merge=lfs -text
+*.joblib filter=lfs diff=lfs merge=lfs -text
+*.lfs.* filter=lfs diff=lfs merge=lfs -text
+*.mlmodel filter=lfs diff=lfs merge=lfs -text
+*.model filter=lfs diff=lfs merge=lfs -text
+*.msgpack filter=lfs diff=lfs merge=lfs -text
+*.npy filter=lfs diff=lfs merge=lfs -text
+*.npz filter=lfs diff=lfs merge=lfs -text
+*.onnx filter=lfs diff=lfs merge=lfs -text
+*.ot filter=lfs diff=lfs merge=lfs -text
+*.parquet filter=lfs diff=lfs merge=lfs -text
+*.pb filter=lfs diff=lfs merge=lfs -text
+*.pickle filter=lfs diff=lfs merge=lfs -text
+*.pkl filter=lfs diff=lfs merge=lfs -text
+*.pt filter=lfs diff=lfs merge=lfs -text
+*.pth filter=lfs diff=lfs merge=lfs -text
+*.rar filter=lfs diff=lfs merge=lfs -text
+*.safetensors filter=lfs diff=lfs merge=lfs -text
+saved_model/**/* filter=lfs diff=lfs merge=lfs -text
+*.tar.* filter=lfs diff=lfs merge=lfs -text
+*.tflite filter=lfs diff=lfs merge=lfs -text
+*.tgz filter=lfs diff=lfs merge=lfs -text
+*.wasm filter=lfs diff=lfs merge=lfs -text
+*.xz filter=lfs diff=lfs merge=lfs -text
+*.zip filter=lfs diff=lfs merge=lfs -text
+*.zst filter=lfs diff=lfs merge=lfs -text
+*tfevents* filter=lfs diff=lfs merge=lfs -text
diff --git a/bert/deberta-v2-large-japanese-char-wwm/README.md b/bert/deberta-v2-large-japanese-char-wwm/README.md
new file mode 100644
index 0000000000000000000000000000000000000000..b7317110ce981c229ebd7b3b975545de5f35fa30
--- /dev/null
+++ b/bert/deberta-v2-large-japanese-char-wwm/README.md
@@ -0,0 +1,89 @@
+---
+language: ja
+license: cc-by-sa-4.0
+library_name: transformers
+tags:
+ - deberta
+ - deberta-v2
+ - fill-mask
+ - character
+ - wwm
+datasets:
+ - wikipedia
+ - cc100
+ - oscar
+metrics:
+ - accuracy
+mask_token: "[MASK]"
+widget:
+ - text: "京都大学で自然言語処理を[MASK][MASK]する。"
+---
+
+# Model Card for Japanese character-level DeBERTa V2 large
+
+## Model description
+
+This is a Japanese DeBERTa V2 large model pre-trained on Japanese Wikipedia, the Japanese portion of CC-100, and the Japanese portion of OSCAR.
+This model is trained with character-level tokenization and whole word masking.
+
+## How to use
+
+You can use this model for masked language modeling as follows:
+
+```python
+from transformers import AutoTokenizer, AutoModelForMaskedLM
+tokenizer = AutoTokenizer.from_pretrained('ku-nlp/deberta-v2-large-japanese-char-wwm')
+model = AutoModelForMaskedLM.from_pretrained('ku-nlp/deberta-v2-large-japanese-char-wwm')
+
+sentence = '京都大学で自然言語処理を[MASK][MASK]する。'
+encoding = tokenizer(sentence, return_tensors='pt')
+...
+```
+
+You can also fine-tune this model on downstream tasks.
+
+## Tokenization
+
+There is no need to tokenize texts in advance, and you can give raw texts to the tokenizer.
+The texts are tokenized into character-level tokens by [sentencepiece](https://github.com/google/sentencepiece).
+
+## Training data
+
+We used the following corpora for pre-training:
+
+- Japanese Wikipedia (as of 20221020, 3.2GB, 27M sentences, 1.3M documents)
+- Japanese portion of CC-100 (85GB, 619M sentences, 66M documents)
+- Japanese portion of OSCAR (54GB, 326M sentences, 25M documents)
+
+Note that we filtered out documents annotated with "header", "footer", or "noisy" tags in OSCAR.
+Also note that Japanese Wikipedia was duplicated 10 times to make the total size of the corpus comparable to that of CC-100 and OSCAR. As a result, the total size of the training data is 171GB.
+
+## Training procedure
+
+We first segmented texts in the corpora into words using [Juman++ 2.0.0-rc3](https://github.com/ku-nlp/jumanpp/releases/tag/v2.0.0-rc3) for whole word masking.
+Then, we built a sentencepiece model with 22,012 tokens including all characters that appear in the training corpus.
+
+We tokenized raw corpora into character-level subwords using the sentencepiece model and trained the Japanese DeBERTa model using [transformers](https://github.com/huggingface/transformers) library.
+The training took 26 days using 16 NVIDIA A100-SXM4-40GB GPUs.
+
+The following hyperparameters were used during pre-training:
+
+- learning_rate: 1e-4
+- per_device_train_batch_size: 26
+- distributed_type: multi-GPU
+- num_devices: 16
+- gradient_accumulation_steps: 8
+- total_train_batch_size: 3,328
+- max_seq_length: 512
+- optimizer: Adam with betas=(0.9,0.999) and epsilon=1e-06
+- lr_scheduler_type: linear schedule with warmup (lr = 0 at 300k steps)
+- training_steps: 260,000
+- warmup_steps: 10,000
+
+The accuracy of the trained model on the masked language modeling task was 0.795.
+The evaluation set consists of 5,000 randomly sampled documents from each of the training corpora.
+
+## Acknowledgments
+
+This work was supported by Joint Usage/Research Center for Interdisciplinary Large-scale Information Infrastructures (JHPCN) through General Collaboration Project no. jh221004, "Developing a Platform for Constructing and Sharing of Large-Scale Japanese Language Models".
+For training models, we used the mdx: a platform for the data-driven future.
diff --git a/bert/deberta-v2-large-japanese-char-wwm/config.json b/bert/deberta-v2-large-japanese-char-wwm/config.json
new file mode 100644
index 0000000000000000000000000000000000000000..9fb6b0ac2ec49b6556e58b5ed9492eb33166714d
--- /dev/null
+++ b/bert/deberta-v2-large-japanese-char-wwm/config.json
@@ -0,0 +1,37 @@
+{
+ "architectures": [
+ "DebertaV2ForMaskedLM"
+ ],
+ "attention_head_size": 64,
+ "attention_probs_dropout_prob": 0.1,
+ "conv_act": "gelu",
+ "conv_kernel_size": 3,
+ "hidden_act": "gelu",
+ "hidden_dropout_prob": 0.1,
+ "hidden_size": 1024,
+ "initializer_range": 0.02,
+ "intermediate_size": 4096,
+ "layer_norm_eps": 1e-07,
+ "max_position_embeddings": 512,
+ "max_relative_positions": -1,
+ "model_type": "deberta-v2",
+ "norm_rel_ebd": "layer_norm",
+ "num_attention_heads": 16,
+ "num_hidden_layers": 24,
+ "pad_token_id": 0,
+ "pooler_dropout": 0,
+ "pooler_hidden_act": "gelu",
+ "pooler_hidden_size": 1024,
+ "pos_att_type": [
+ "p2c",
+ "c2p"
+ ],
+ "position_biased_input": false,
+ "position_buckets": 256,
+ "relative_attention": true,
+ "share_att_key": true,
+ "torch_dtype": "float16",
+ "transformers_version": "4.25.1",
+ "type_vocab_size": 0,
+ "vocab_size": 22012
+}
diff --git a/bert/deberta-v2-large-japanese-char-wwm/special_tokens_map.json b/bert/deberta-v2-large-japanese-char-wwm/special_tokens_map.json
new file mode 100644
index 0000000000000000000000000000000000000000..a8b3208c2884c4efb86e49300fdd3dc877220cdf
--- /dev/null
+++ b/bert/deberta-v2-large-japanese-char-wwm/special_tokens_map.json
@@ -0,0 +1,7 @@
+{
+ "cls_token": "[CLS]",
+ "mask_token": "[MASK]",
+ "pad_token": "[PAD]",
+ "sep_token": "[SEP]",
+ "unk_token": "[UNK]"
+}
diff --git a/bert/deberta-v2-large-japanese-char-wwm/tokenizer_config.json b/bert/deberta-v2-large-japanese-char-wwm/tokenizer_config.json
new file mode 100644
index 0000000000000000000000000000000000000000..8ab2175580e45760875557201e5543019ca3039b
--- /dev/null
+++ b/bert/deberta-v2-large-japanese-char-wwm/tokenizer_config.json
@@ -0,0 +1,19 @@
+{
+ "cls_token": "[CLS]",
+ "do_lower_case": false,
+ "do_subword_tokenize": true,
+ "do_word_tokenize": true,
+ "jumanpp_kwargs": null,
+ "mask_token": "[MASK]",
+ "mecab_kwargs": null,
+ "model_max_length": 1000000000000000019884624838656,
+ "never_split": null,
+ "pad_token": "[PAD]",
+ "sep_token": "[SEP]",
+ "special_tokens_map_file": null,
+ "subword_tokenizer_type": "character",
+ "sudachi_kwargs": null,
+ "tokenizer_class": "BertJapaneseTokenizer",
+ "unk_token": "[UNK]",
+ "word_tokenizer_type": "basic"
+}
diff --git a/bert/deberta-v2-large-japanese-char-wwm/vocab.txt b/bert/deberta-v2-large-japanese-char-wwm/vocab.txt
new file mode 100644
index 0000000000000000000000000000000000000000..ef3652a1877f4c898e6fcb3e605c432c7bcc56b1
--- /dev/null
+++ b/bert/deberta-v2-large-japanese-char-wwm/vocab.txt
@@ -0,0 +1,22012 @@
+[PAD]
+[CLS]
+[SEP]
+[UNK]
+[MASK]
+▁
+の
+、
+に
+い
+。
+し
+た
+で
+る
+ー
+と
+て
+は
+を
+が
+な
+ン
+す
+ま
+れ
+1
+か
+っ
+ス
+0
+も
+り
+ら
+2
+ト
+ル
+こ
+う
+イ
+さ
+あ
+年
+(
+)
+・
+リ
+く
+ッ
+ア
+ラ
+ク
+き
+.
+よ
+日
+e
+ん
+お
+「
+だ
+9
+」
+け
+レ
+そ
+タ
+シ
+ド
+3
+o
+人
+a
+め
+フ
+i
+ロ
+大
+t
+ど
+や
+カ
+5
+4
+ジ
+プ
+月
+え
+つ
+グ
+n
+r
+本
+テ
+8
+コ
+マ
+ィ
+6
+わ
+7
+メ
+-
+ム
+デ
+ち
+せ
+バ
+中
+一
+ウ
+サ
+学
+出
+行
+s
+用
+会
+A
+時
+み
+合
+l
+オ
+事
+国
+ブ
+チ
+ナ
+S
+生
+上
+場
+分
+キ
+ニ
+ュ
+ダ
+方
+ャ
+m
+C
+間
+的
+作
+ビ
+エ
+後
+者
+パ
+自
+c
+地
+ズ
+入
+ば
+発
+部
+定
+業
+T
+見
+市
+じ
+E
+ご
+/
+手
+子
+,
+動
+u
+I
+!
+ミ
+d
+社
+P
+代
+セ
+ェ
+ョ
+高
+同
+県
+モ
+開
+前
+O
+M
+成
+新
+D
+性
+h
+内
+名
+気
+R
+当
+長
+p
+:
+『
+思
+』
+体
+目
+通
+対
+理
+最
+品
+ネ
+ろ
+下
+ベ
+ず
+N
+戦
+全
+以
+物
+実
+g
+関
+多
+B
+家
+要
+ツ
+ポ
+現
+所
+画
+法
+立
+東
+使
+言
+化
+回
+ハ
+取
+山
+機
+度
+表
+L
+へ
+力
+ケ
+数
+記
+ガ
+F
+ピ
+第
+設
+び
+書
+来
+ワ
+選
+金
+ソ
+主
+世
+今
+能
+道
+在
+女
+期
+ノ
+
+教
+初
+ボ
+利
+知
+ペ
+報
+田
+文
+活
+外
+ょ
+ァ
+小
+様
+明
+k
+語
+車
+べ
+料
+連
+ほ
+持
+b
+G
+意
+感
+店
+ね
+y
+無
+特
+公
+受
+加
+経
+H
+げ
+員
+ホ
+変
+線
+心
+情
+楽
+不
+川
+w
+安
+続
+々
+町
+結
+式
+京
+売
+和
+付
+電
+"
+話
+ザ
+送
+問
+島
+f
+水
+?
+野
+保
+考
+身
+海
+W
+面
+正
+務
+点
+校
+組
+約
+位
+集
+V
+可
+信
+ヤ
+ォ
+神
+&
+制
+平
+北
+ギ
+解
+原
+U
+際
+番
+有
+相
+K
+界
+区
+む
+向
+置
+近
+他
+指
+得
+産
+重
+元
+ゴ
+ゃ
+軍
+政
+三
+私
+題
+;
+決
+ヴ
+等
+都
+|
+形
+少
+ゲ
+食
+民
+先
+治
+運
+必
+果
+良
+参
+格
+計
+演
+放
+始
+進
+状
+認
+v
+次
+別
+工
+科
+何
+勝
+口
+応
+験
+調
+J
+確
+録
+共
+強
+味
+引
+【
+予
+】
+西
+円
+議
+交
+基
+音
+広
+配
+示
+二
+天
+試
+彼
+駅
+常
+製
+美
+空
+種
+込
+終
+流
+切
+号
+仕
+研
+術
+任
+院
+資
+支
+万
+商
+説
+登
+ぶ
+映
+屋
+育
+南
+価
+着
+型
+真
+木
+優
+存
+直
+営
+ヒ
+限
+域
+台
+ひ
+容
+歴
+収
+提
+供
+団
+古
+究
+権
+男
+建
+村
+士
+ぐ
+介
+ざ
+役
+系
+改
+質
+過
+王
+施
+備
+造
+州
+曲
+伝
+転
+色
+再
+載
+ユ
+住
+構
+ヘ
+由
+起
+件
+愛
+館
+客
+素
+個
+%
+門
+版
+義
+査
+岡
+移
+ぎ
+判
+福
+土
+好
+類
+更
+技
+検
+達
+Y
+止
+規
+管
+X
+投
+呼
+~
+販
+路
+葉
+各
+石
+違
+効
+聞
+注
+総
+属
+職
+編
+影
+態
+[
+賞
+観
+府
+単
+師
+]
+半
+求
+護
+_
+史
+量
+器
+医
+鉄
+風
+接
+読
+図
+例
+反
+両
+統
+光
+命
+ゼ
+係
+想
+増
+朝
+死
+城
+企
+側
+x
+装
+論
+買
+害
+白
+専
+催
+足
+然
+療
+残
+隊
+歌
+馬
+族
+ヨ
+周
+降
+宮
+含
+告
+防
+英
+親
+材
+太
+展
+園
+非
+歳
+条
+阪
+乗
+字
+評
+局
+像
+済
+異
+撃
+井
+率
+頭
+づ
+修
+完
+断
+細
+称
+座
+病
+=
+準
+張
+紹
+室
+写
+習
+落
+ゆ
+比
+導
+象
+働
+球
+十
+離
+米
+戸
+退
+協
+与
+証
+程
+速
+境
+官
+昭
+換
+段
+消
+難
+講
+打
+郡
+及
+念
+急
+株
+担
+視
+兵
+氏
+策
+”
+返
+武
+領
+了
+識
+費
+ふ
+復
+独
+松
+追
+除
+満
+待
+環
+適
+整
+悪
+般
+値
+割
+望
+級
+響
+ぞ
+崎
+去
+党
+概
+案
+帰
+声
+援
+藤
+花
+早
+挙
+失
+〜
+毎
+継
+z
+千
+低
+婚
+御
+末
+争
+助
+攻
+派
+申
+談
+帯
+具
+購
+願
+夫
+監
+紀
+母
+歩
+#
+舗
+勢
+薬
+谷
+橋
+積
+授
+宅
+未
+委
+標
+頃
+負
+週
+従
+星
+父
+症
+佐
+走
+減
+差
+旅
+根
+述
+寺
+横
+青
+補
+順
+掲
+ぼ
+処
+競
+額
+探
+列
+郎
+守
+陸
+興
+囲
+火
+夜
+健
+船
+江
+頂
+創
+友
+四
+覧
+ぜ
+築
+庫
+撮
+激
+敗
+階
+深
+軽
+富
+艦
+j
+督
+況
+著
+休
+頼
+便
+毛
+超
+“
+算
+覚
+衛
+省
+雑
+越
+>
+芸
+模
+久
+居
+扱
+温
+推
+略
+複
+渡
+飛
+※
+籍
+採
+為
+港
+寄
+極
+就
+児
+将
+洋
+若
+赤
+破
+詳
+卒
+択
+司
+労
+首
+Z
+普
+令
+辺
+豊
+奈
+沢
+精
+銀
+税
+険
+因
+給
+黒
+浜
+航
+*
+笑
+殺
+顔
+秋
+津
+振
+春
+徴
+契
+河
+吉
+皆
+承
+財
+旧
+香
+波
+劇
+伊
+描
+五
+節
+源
+康
+衆
+印
+察
+熱
+夏
+抜
+森
+短
+課
+絶
+板
+布
+織
+養
+央
+誌
+端
+携
+払
+'
+飲
+右
+街
+八
+賀
+留
+突
+服
+典
+故
+農
+遺
+血
+皇
+障
+景
+許
+脱
+被
+^
+紙
+警
+清
++
+ヌ
+圧
+群
+染
+簡
+舞
+帝
+ヶ
+替
+左
+玉
+曜
+訳
+博
+遠
+項
+戻
+巻
+枚
+照
+功
+拠
+練
+密
+廃
+Q
+獲
+答
+植
+績
+席
+途
+遊
+姿
+宿
+徳
+測
+ゥ
+岩
+酸
+蔵
+納
+祭
+闘
+翌
+ぱ
+幅
+肉
+幸
+弾
+索
+静
+息
+章
+訪
+革
+ゾ
+請
+痛
+宗
+善
+射
+徒
+緒
+災
+宇
+志
+林
+角
+苦
+拡
+絵
+並
+誰
+魔
+絡
+掛
+韓
+希
+勤
+裁
+届
+背
+輸
+泉
+迎
+尾
+陽
+盤
+奏
+聖
+延
+妻
+堂
+抗
+九
+較
+貨
+丸
+刊
+伴
+永
+候
+我
+弁
+跡
+聴
+焼
+盛
+躍
+丁
+診
+沖
+酒
+諸
+致
+散
+歯
+稿
+華
+幕
+鳥
+敵
+停
+副
+庭
+亡
+恋
+倒
+募
+僕
+倉
+依
+欲
+占
+責
+辞
+乱
+審
+坂
+昇
+洗
+輪
+ゅ
+患
+逆
+池
+齢
+傷
+融
+弟
+則
+固
+岸
+壊
+層
+札
+討
+余
+律
+締
+草
+漫
+仮
+似
+禁
+危
+肌
+墓
+栄
+仲
+鮮
+冷
+損
+困
+臣
+貴
+操
+益
+老
+震
+鹿
+百
+縄
+押
+触
+疑
+併
+甲
+至
+充
+易
+×
+娘
+骨
+筆
+宝
+乳
+湾
+混
+茶
+里
+秀
+ぬ
+借
+悩
+稼
+罪
+維
+喜
+宣
+夢
+裏
+詞
+捕
+閉
+範
+剤
+献
+筋
+億
+冬
+雨
+爆
+兄
+�
+厳
+犬
+勉
+避
+盟
+荷
+沿
+欠
+倍
+君
+雄
+季
+藩
+☆
+液
+油
+片
+浮
+既
+熊
+○
+互
+皮
+→
+岐
+執
+謝
+昨
+搭
+駆
+麻
+―
+羽
+揮
+遅
+逃
+庁
+樹
+隣
+犯
+惑
+均
+壁
+六
+快
+之
+券
+奥
+否
+析
+郷
+底
+折
+厚
+滅
+純
+脚
+祝
+午
+秘
+削
+吸
+寝
+房
+砲
+浦
+■
+幼
+恐
+幹
+臨
+暮
+婦
+刑
+♪
+免
+敷
+己
+救
+刻
+邦
+七
+抱
+伸
+魚
+露
+到
+票
+巨
+距
+誕
+瀬
+繰
+<
+遣
+恵
+ぁ
+仏
+弱
+晴
+暗
+脳
+兼
+縮
+批
+宙
+魅
+訴
+刺
+傾
+殿
+嫌
+誘
+{
+貸
+迷
+舎
+看
+慣
+衣
+}
+礼
+床
+襲
+駐
+却
+隠
+包
+欧
+ぽ
+菜
+埼
+胞
+★
+潟
+髪
+雪
+巡
+俺
+祖
+塩
+柄
+@
+賃
+眠
+即
+漢
+針
+濃
+飾
+勧
+奪
+慮
+抑
+竹
+縁
+憲
+須
+黄
+暴
+塚
+猫
+童
+塗
+湖
+幌
+仙
+踏
+忘
+吹
+摘
+該
+誤
+挑
+枠
+排
+侵
+飯
+束
+腹
+泊
+那
+陣
+丈
+忠
+桜
+炎
+湯
+●
+耐
+閣
+没
+努
+核
+霊
+圏
+緊
+緑
+窓
+招
+薄
+菌
+是
+袋
+q
+虫
+怪
+砂
+頑
+塁
+騎
+貢
+昔
+阿
+眼
+慶
+燃
+秒
+託
+礎
+顧
+》
+《
+豆
+雲
+崩
+驚
+還
+俳
+腕
+寿
+荒
+又
+唱
+訓
+裕
+握
+姉
+繁
+儀
+嬉
+妹
+迫
+剣
+孫
+浴
+彩
+添
+詩
+趣
+鑑
+銃
+械
+龍
+糖
+鏡
+譲
+詰
+乾
+牛
+梅
+夕
+微
+唯
+潜
+柔
+稲
+炭
+า
+釈
+封
+麗
+梨
+箱
+刷
+矢
+衝
+雇
+丹
+爵
+徹
+甘
+阜
+脂
+耳
+摂
+摩
+~
+穴
+才
+\
+奇
+繋
+豪
+郵
+牧
+促
+債
+丘
+汚
+署
+俗
+賛
+贈
+干
+熟
+紅
+鈴
+雰
+寒
+疲
+渋
+弊
+埋
+架
+昼
+盗
+祉
+ぷ
+透
+仁
+竜
+鬼
+尊
+滞
+償
+茨
+伯
+双
+杯
+亜
+括
+浅
+姫
+輝
+誠
+撤
+葬
+践
+悲
+渉
+哲
+狭
+瞬
+毒
+掃
+妊
+勇
+憶
+鳴
+誉
+怒
+陰
+棄
+ã
+彦
+怖
+沼
+殊
+輩
+呂
+貫
+–
+隆
+胸
+ร
+軌
+履
+軸
+肩
+脈
+弘
+揃
+翼
+飼
+妙
+鶴
+杉
+粉
+滑
+旬
+湿
+威
+涯
+掘
+菓
+智
+灯
+寧
+辛
+捨
+冠
+臓
+翻
+旗
+敬
+倫
+゙
+芝
+腰
+偽
+網
+雷
+奨
+培
+鎖
+奉
+卵
+抵
+冊
+沈
+帳
+句
+暦
+尽
+縦
+偵
+征
+塾
+旨
+閲
+溶
+也
+拒
+陥
+喫
+栃
+疾
+◆
+控
+煙
+暖
+慢
+序
+伏
+浄
+玄
+鉱
+寛
+附
+狙
+覇
+頻
+釣
+潮
+拝
+如
+粧
+澤
+咲
+硬
+糸
+懸
+堀
+羅
+滋
+磨
+捜
+遂
+氷
+旦
+¥
+奴
+隔
+珍
+柳
+貯
+僚
+随
+鎮
+漁
+緩
+—
+媛
+塔
+茂
+紫
+〉
+仰
+預
+貞
+綱
+騒
+径
+尚
+誇
+冒
+刀
+獣
+披
+揚
+柱
+邪
+需
+尻
+ぴ
+磁
+枝
+〈
+臭
+裂
+逮
+姓
+酵
+燥
+抽
+貧
+晩
+亀
+殖
+駄
+`
+徐
+緯
+畑
+遇
+唐
+尿
+軟
+栽
+歓
+戒
+垣
+謀
+拓
+岳
+漏
+暑
+肥
+墳
+雅
+踊
+鎌
+膜
+措
+น
+幾
+靴
+乃
+─
+把
+泣
+腸
+鼻
+轄
+忙
+隻
+譜
+敏
+綺
+箇
+劣
+傘
+晶
+覆
+銭
+°
+蒸
+紛
+斎
+膚
+往
+棒
+祈
+荘
+賢
+´
+弥
+ก
+穂
+銅
+顕
+浸
+桃
+駒
+滝
+•
+貼
+’
+俊
+挿
+孝
+凍
+癒
+娠
+肝
+粒
+李
+銘
+郊
+絞
+卓
+筒
+睡
+享
+゚
+恒
+励
+汗
+准
+〇
+宜
+菱
+脇
+肢
+偶
+巣
+洞
+é
+幡
+昌
+嘉
+廷
+·
+尋
+斜
+−
+罰
+幻
+鋼
+繊
+蓄
+ง
+屈
+懐
+狼
+狂
+菊
+悟
+棋
+獄
+媒
+遭
+艇
+棟
+彰
+弦
+塞
+稚
+彫
+恩
+狩
+筑
+焦
+庄
+腐
+擬
+紋
+揺
+嫁
+栗
+鍵
+浪
+逐
+峰
+貿
+洲
+伺
+祥
+偏
+疫
+魂
+肪
+麦
+凄
+酷
+笠
+軒
+恥
+脅
+斉
+淡
+欄
+諾
+沙
+
+謎
+貰
+悔
+垂
+召
+遷
+旭
+泳
+薦
+慎
+逸
+暇
+吾
+捉
+阻
+邸
+隷
+勘
+衰
+腫
+袖
+涼
+鋭
+葛
+泰
+涙
+膨
+奮
+忍
+ぇ
+蓮
+僧
+陵
+孤
+坊
+匠
+━
+禄
+汁
+啓
+堅
+訂
+勲
+剛
+↓
+濯
+碑
+
+扉
+旋
+函
+酔
+芳
+侯
+棚
+拘
+挟
+壇
+吐
+撲
+粋
+妖
+傍
+闇
+虐
+訟
+叙
+尉
+菅
+珠
+擁
+噴
+酬
+鍋
+灰
+ั
+朗
+寸
+浩
+搬
+陳
+ω
+烈
+柏
+黙
+克
+呉
+挨
+慰
+拶
+拳
+潤
+勃
+兆
+符
+簿
+虎
+縛
+乙
+匹
+斗
+陶
+薩
+嵐
+椎
+猛
+虚
+眺
+欺
+宰
+梁
+豚
+蘭
+曹
+循
+亭
+麺
+芽
+帽
+蘇
+刃
+綿
+詐
+噂
+อ
+曽
+粘
+»
+諦
+祐
+劉
+©
+嶋
+⇒
+穏
+胃
+翔
+♡
+煮
+肺
+貝
+◎
+爪
+國
+朱
+潔
+犠
+寮
+慈
+盾
+剰
+膝
+偉
+扇
+叩
+拍
+瑞
+ส
+迅
+云
+戯
+輔
+畳
+柴
+牲
+桁
+卿
+▼
+畿
+幣
+盆
+祀
+賠
+錦
+侍
+妨
+嘘
+佳
+伐
+妃
+叫
+ม
+崇
+桑
+舌
+茎
+赴
+桂
+峡
+เ
+宏
+累
+鷹
+嬢
+癌
+馴
+鶏
+溜
+溢
+呈
+裸
+矯
+隅
+磐
+$
+泥
+椅
+賊
+殻
+叶
+綾
+桐
+蓋
+晋
+篠
+炉
+蒲
+耕
+溝
+凝
+牡
+ย
+剥
+醸
+斐
+峠
+秩
+但
+憧
+飽
+苗
+鉛
+后
+鍛
+謡
+駿
+篇
+賜
+ิ
+舟
+弓
+ぺ
+泌
+唆
+羊
+梱
+昆
+褒
+琴
+缶
+玩
+α
+播
+霧
+斬
+憩
+釜
+®
+誓
+皿
+堺
+胆
+据
+暫
+姦
+倶
+痩
+呪
+拾
+├
+錠
+堤
+尼
+股
+腺
+痴
+喪
+í
+
+璧
+刈
+墜
+畜
+虜
+湧
+壮
+乏
+琉
+禅
+掌
+洪
+蛇
+稀
+錯
+腎
+寂
+殆
+渓
+堪
+疎
+บ
+辰
+隙
+
+鼓
+泡
+穫
+蜂
+〔
+庶
+猿
+〕
+宴
+潰
+ด
+妥
+叔
+雀
+漂
+跳
+郭
+宛
+
+️
+ヵ
+亮
+巧
+籠
+癖
+墨
+掴
+賭
+敢
+胡
+惨
+遮
+磯
+ว
+澄
+粛
+孔
+漬
+尺
+勅
+僅
+瓦
+猟
+ะ
+懲
+班
+枢
+愚
+禍
+噛
+ぃ
+於
+慌
+瓶
+綴
+瘍
+ค
+▽
+牽
+壌
+擦
+衡
+坪
+湘
+淳
+粗
+艶
+宋
+卸
+竣
+應
+廊
+秦
+廻
+朽
+ヲ
+濡
+庵
+漠
+胴
+縫
+硫
+惜
+髄
+辻
+⌒
+幽
+槽
+芦
+悠
+謙
+喉
+á
+讃
+舶
+枕
+哉
+匿
+砕
+噌
+惹
+◇
+夷
+零
+▲
+吊
+稽
+ゐ
+餌
+炊
+△
+儲
+懇
+萩
+萌
+匂
+伎
+戴
+矛
+顎
+哀
+〒
+麓
+∀
+抹
+傑
+篤
+樽
+鐘
+胎
+醤
+蹴
+唇
+忌
+騰
+鴨
+憂
+穀
+砦
+糧
+逢
+屯
+‐
+諭
+圭
+敦
+魏
+ป
+歪
+烏
+腔
+帆
+妄
+่
+喚
+賑
+怠
+พ
+汎
+昧
+窮
+«
+猪
+鉢
+遥
+淵
+喧
+拭
+้
+爽
+จ
+藍
+褐
+閥
+肖
+机
+諏
+酢
+弧
+枯
+騙
+凡
+錬
+嘆
+ล
+沸
+勿
+贅
+遍
+︎
+隈
+菩
+其
+戚
+紐
+耗
+赦
+楚
+餅
+β
+鳳
+嫡
+囚
+貌
+挫
+瞳
+哨
+纂
+雌
+↑
+扶
+覗
+鬱
+捧
+朴
+臼
+辱
+槻
+窟
+ี
+牝
+靖
+淀
+撫
+塊
+辿
+曇
+嗣
+恭
+脊
+鞍
+橘
+紗
+醒
+遡
+甚
+喰
+蝶
+斑
+此
+某
+苑
+唄
+楊
+丼
+淫
+棲
+虹
+杏
+鋳
+垢
+櫻
+笹
+蒼
+痕
+凶
+碁
+玲
+槍
+肯
+岬
+蒙
+殴
+嶺
+礁
+洛
+□
+絆
+頓
+藻
+杜
+條
+蛍
+憎
+鳩
+晃
+厩
+牙
+鍼
+學
+鷲
+滴
+葵
+μ
+勾
+窯
+笛
+楼
+飢
+趙
+嘩
+梗
+耶
+脆
+遵
+汰
+а
+霞
+眉
+詠
+慕
+串
+倣
+漆
+紡
+卑
+綻
+茅
+或
+瑠
+惣
+←
+牟
+崖
+甥
+藝
+薫
+栖
+遼
+ห
+芋
+⁄
+呑
+鞭
+逝
+แ
+箕
+栓
+悼
+陀
+о
+蜜
+詣
+✨
+汽
+舐
+俣
+窪
+燕
+曖
+曾
+堆
+ท
+填
+ต
+讐
+å
+尖
+ü
+凛
+窒
+
+楠
+眞
+閑
+帥
+柿
+擢
+聡
+廣
+ä
+灸
+裾
+剖
+頁
+ぉ
+暁
+廉
+盲
+畠
+骸
+凌
+姻
+湊
+喋
+獅
+愉
+曰
+睦
+拉
+冗
+洒
+紳
+肘
+娯
+遽
+塵
+厄
+ช
+и
+濁
+賄
+頬
+佑
+ヂ
+只
+灘
+舷
+鈍
+汲
+恨
+煎
+鯨
+濱
+俵
+韻
+ぅ
+侶
+繕
+杵
+樋
+弄
+吟
+ç
+痺
+堕
+曳
+冥
+渦
+鱗
+彗
+婆
+叱
+雛
+巳
+儒
+疹
+謳
+來
+梶
+醐
+醍
+祇
+膳
+抄
+莉
+惧
+絹
+倭
+猶
+奔
+楕
+瞑
+釧
+芯
+萬
+Д
+杖
+輌
+ゞ
+俸
+搾
+寅
+莫
+纏
+悦
+粟
+樺
+憑
+惚
+凸
+嘱
+餃
+ุ
+渕
+凱
+嚢
+帖
+跨
+頸
+荻
+洩
+毅
+嗜
+凹
+晒
+邑
+ ̄
+瞭
+胤
+┃
+坑
+厨
+◯
+體
+碧
+迄
+æ
+è
+朋
+亘
+妬
+仔
+巴
+躊
+躇
+聘
+≫
+迦
+款
+煩
+箸
+е
+狐
+捻
+繍
+廠
+襟
+巾
+线
+蕎
+呆
+炒
+兎
+紺
+坦
+拐
+璃
+酎
+哺
+喘
+婿
+譚
+箋
+黎
+ู
+這
+棺
+租
+糞
+煽
+ื
+椿
+р
+爾
+隼
+叡
+馳
+董
+挺
+吏
+畔
+撰
+髭
+奄
+伽
+鄭
+壱
+ا
+叉
+鹸
+์
+蟹
+痢
+裔
+峯
+剃
+ā
+坐
+焚
+с
+憤
+薪
+舵
+琶
+郁
+琵
+鐵
+揉
+н
+霜
+壺
+詫
+杭
+溺
+珈
+彙
+焉
+鰭
+麹
+琲
+硝
+輿
+捗
+嫉
+渇
+鴻
+斯
+‘
+餓
+灌
+髙
+牢
+牌
+揆
+桶
+苔
+濫
+綬
+且
+罹
+舘
+釘
+ヅ
+冨
+騨
+蔑
+咳
+丞
+廟
+柵
+汐
+葺
+朔
+掻
+苛
+諮
+鵜
+峙
+≪
+
+媚
+罠
+蛮
+∞
+ó
+瀧
+鎧
+庇
+嶽
+ö
+翁
+乞
+訊
+挽
+蔽
+踪
+鯖
+稜
+拷
+│
+蝦
+冶
+侮
+唾
+苫
+к
+堰
+膣
+蔓
+狗
+而
+靭
+閃
+祷
+š
+愕
+爺
+柚
+薇
+朧
+檜
+咽
+﨑
+〝
+榎
+ě
+斡
+迂
+薔
+圓
+桓
+謹
+姜
+訣
+д
+ข
+泄
+姪
+ถ
+蔣
+峨
+叢
+錐
+煉
+會
+怯
+旺
+ศ
+檀
+殉
+袁
+賓
+珂
+ý
+ゝ
+扁
+鵬
+些
+т
+└
+ぢ
+喩
+慧
+芥
+傭
+蔭
+羨
+胚
+諱
+榴
+′
+弔
+緻
+熙
+扮
+攣
+筈
+ํ
+糾
+喝
+魯
+巫
+諜
+窺
+嗅
+蕭
+罵
+筐
+謗
+庸
+梓
+棘
+▶
+愁
+痙
+竿
+曝
+嵯
+燈
+誹
+撥
+諫
+蚊
+隋
+賦
+塀
+陛
+淑
+麟
+膏
+翠
+悶
+毀
+寵
+梯
+彷
+摯
+肛
+堵
+仇
+稔
+昏
+醜
+窃
+慨
+漸
+埴
+齋
+无
+茹
+站
+壬
+實
+冴
+翅
+♥
+腱
+漕
+轟
+в
+腿
+
+蚕
+逗
+膵
+萎
+撒
+嘔
+晦
+л
+惟
+±
+欽
+โ
+于
+鯉
+桔
+樫
+憐
+詮
+爬
+瓜
+禰
+鞄
+膿
+鞘
+娼
+詔
+拙
+錆
+ヽ
+奢
+聯
+俯
+呟
+采
+穿
+墟
+尹
+
+銚
+桟
+゜
+榊
+鮎
+袴
+盧
+亚
+氾
+邊
+祓
+崔
+┈
+❤
+摺
+礫
+揖
+费
+瀕
+縞
+煌
+嵩
+脫
+吠
+頒
+麿
+絢
+睨
+謂
+團
+閏
+蹄
+升
+产
+飴
+隕
+寡
+戊
+
+视
+徽
+魁
+喬
+螺
+〟
+粕
+柑
+楓
+昂
+蛋
+壕
+痒
+鯛
+槌
+葡
+ž
+吻
+叛
+ผ
+陪
+疼
+鷺
+γ
+氣
+嬲
+膠
+囃
+¤
+拗
+櫛
+蹟
+鼠
+錫
+咸
+码
+怨
+矩
+焙
+鮭
+č
+屏
+†
+♂
+酪
+巌
+鋸
+宕
+襄
+祠
+漑
+§
+諡
+揄
+餐
+妓
+蒔
+渚
+揶
+茜
+鉾
+猥
+兜
+謁
+
+斥
+
+渾
+و
+็
+琢
+狛
+櫓
+雁
+蕉
+貪
+毘
+蠣
+箔
+裳
+频
+駕
+卯
+凪
+孟
+椒
+伍
+傳
+捏
+汝
+φ
+逓
+鍾
+梢
+肋
+瑛
+峻
+渠
+榜
+网
+欅
+咬
+观
+眩
+頷
+頚
+蔡
+饉
+嵌
+孵
+沌
+ل
+皐
+侠
+😊
+楯
+砥
+斧
+矮
+巷
+碗
+囁
+蜘
+亨
+浙
+玖
+卷
+濠
+疇
+橙
+蛛
+轢
+弐
+擲
+埠
+佇
+臆
+宵
+彌
+紘
+薙
+罷
+允
+雫
+禎
+┗
+梵
+榛
+墾
+ř
+輛
+ī
+砺
+宍
+崗
+緋
+絨
+蜀
+à
+佛
+
+姑
+傲
+做
+漱
+按
+噺
+遜
+縣
+蝋
+¦
+≦
+炸
+嶼
+邉
+苺
+竪
+¨
+鑽
+σ
+瘤
+羞
+≧
+芹
+甫
+寓
+廿
+彿
+豹
+藪
+鋒
+瑕
+疵
+刹
+竈
+捷
+ر
+
+痘
+勒
+暢
+膀
+篭
+级
+疆
+胱
+滲
+渤
+畏
+ไ
+曼
+燻
+珪
+遙
+ฏ
+♬
+芙
+壽
+芭
+當
+楢
+♀
+λ
+É
+у
+蓬
+ñ
+Ω
+跋
+ヱ
+秤
+怜
+茸
+攘
+咎
+訛
+橿
+耆
+邁
+葦
+釉
+嘲
+綜
+ن
+匡
+鍮
+☺
+茉
+沫
+┣
+淮
+¸
+鄧
+ε
+
+⭐
+訃
+荊
+蝕
+亥
+臥
+鮫
+劾
+๑
+
+萄
+殷
+م
+韮
+ณ
+鸞
+∇
+妾
+誅
+址
+曙
+£
+濾
+酌
+偲
+й
+窩
+毯
+畝
+冑
+暉
+薨
+蕃
+寇
+м
+躯
+淹
+卜
+Ζ
+藁
+沃
+厭
+涌
+¯
+辣
+¡
+掟
+❗
+榮
+聚
+珊
+劫
+狸
+疱
+倦
+與
+ú
+裟
+乖
+嘗
+♫
+盃
+嚇
+丙
+熾
+â
+彭
+淘
+殲
+灼
+ů
+嘴
+檎
+瞰
+馮
+屑
+ê
+荼
+湛
+雍
+煕
+
+〆
+颯
+苅
+糠
+娩
+瑚
+蟻
+ū
+ï
+
+堡
+
+徊
+靱
+耀
+萼
+菖
+💦
+ญ
+冤
+嬌
+掠
+凰
+
+虔
+
+惠
+饅
+弼
+贄
+蕨
+庚
+ゑ
+综
+艸
+徘
+剪
+π
+渥
+豫
+穣
+趾
+鼎
+鴉
+欣
+汪
+爛
+陝
+佃
+莢
+瞼
+肇
+姶
+糟
+邱
+衷
+衙
+弛
+麒
+糊
+戮
+埃
+塑
+粥
+隘
+什
+餡
+ν
+堯
+饗
+逼
+祢
+憾
+僑
+桝
+婁
+ي
+袈
+彬
+拮
+韋
+蒐
+💕
+鰻
+
+靡
+苻
+∴
+傀
+孕
+蘆
+迭
+傅
+蕩
+Δ
+淋
+槇
+窄
+耽
+鉤
+蛙
+咄
+儡
+賂
+蕾
+濤
+畷
+冪
+〞
+琥
+軋
+Â
+證
+ฟ
+遁
+鋏
+鰐
+舜
+唸
+賤
+賈
+樂
+關
+匈
+臀
+虞
+廓
+吃
+簾
+腋
+箏
+紆
+疏
+г
+諧
+套
+丑
+宥
+柊
+别
+鹵
+苞
+註
+嚥
+屠
+夭
+漿
+ฒ
+荏
+腑
+酉
+鰹
+惰
+ب
+謬
+脩
+犀
+恣
+捲
+甕
+顆
+鰓
+∵
+琳
+瘡
+ß
+檻
+竺
+
+馨
+屍
+姚
+諒
+珀
+穢
+禿
+捌
+蝉
+ใ
+壷
+贖
+ت
+凧
+艤
+捺
+閻
+拿
+巽
+繭
+咥
+紬
+
+酋
+卍
+萱
+爲
+謄
+閤
+蔦
+倹
+襖
+燭
+璋
+顛
+旛
+娶
+〓
+¿
+忽
+范
+δ
+贋
+斤
+ธ
+穆
+賽
+蹊
+Σ
+癬
+侑
+椀
+肴
+ы
+я
+鞠
+п
+弩
+瀋
+彡
+甦
+圃
+亢
+圳
+錘
+游
+焔
+禽
+κ
+竄
+發
+█
+б
+璽
+貶
+脛
+鶯
+甑
+葱
+د
+ь
+›
+ι
+س
+嗟
+掾
+桧
+戌
+托
+澪
+櫃
+⇔
+ی
+齊
+箪
+ο
+椋
+÷
+祟
+咋
+宦
+徨
+獨
+數
+聲
+錨
+祚
+尤
+ρ
+翰
+塹
+掩
+
+從
+僭
+篆
+涛
+對
+碩
+唖
+枡
+碓
+浚
+๒
+词
+楔
+이
+熨
+恰
+處
+經
+贔
+蔚
+讀
+з
+浣
+冀
+屓
+¬
+ō
+栞
+裡
+潭
+涅
+茄
+悉
+痔
+С
+懿
+寶
+
+輯
+戟
+阮
+燦
+桿
+≒
+塙
+¢
+θ
+゛
+τ
+潘
+踵
+
+饒
+
+硯
+戀
+疋
+蓉
+邇
+∩
+閔
+倖
+槃
+炙
+褪
+逞
+頌
+ฐ
+东
+戎
+嬰
+籾
+訝
+瓊
+厥
+磋
+禮
+ᅠ
+藏
+雉
+
+💛
+
+Č
+痰
+俄
+樣
+闊
+內
+蕊
+躰
+ษ
+咀
+薛
+η
+鳶
+皺
+舊
+碍
+爱
+蕪
+衍
+婷
+卡
+點
+蛭
+ヾ
+號
+煥
+哈
+热
+德
+烹
+裴
+见
+姐
+衿
+誦
+▷
+佩
+
+幇
+鞆
+漉
+諌
+薗
+冲
+鍬
+漣
+臺
+筏
+躁
+圀
+錮
+ô
+✂
+滸
+芒
+韩
+嗚
+慄
+毫
+亦
+ซ
+́
+釋
+躙
+ē
+辟
+瞞
+厘
+牒
+溥
+Φ
+岑
+♯
+轍
+鏑
+Ã
+关
+嚆
+偕
+❣
+閘
+Ø
+ø
+♭
+ć
+Ö
+攪
+〃
+塘
+隧
+凜
+卦
+麾
+蹂
+蓑
+糀
+ı
+鴎
+掬
+莽
+ч
+廬
+瓢
+稙
+輻
+憚
+鮨
+將
+磔
+笥
+€
+儚
+岱
+鵠
+ه
+聾
+😭
+綏
+霖
+穎
+瞥
+한
+簒
+讒
+擾
+槙
+瑜
+祁
+拌
+閾
+撹
+禹
+艘
+ा
+抒
+奧
+蜷
+樟
+羹
+棍
+旱
+袂
+规
+蟲
+鷗
+Р
+嚼
+П
+崑
+已
+懺
+澁
+悸
+奸
+几
+梧
+
+✧
+啄
+姥
+🎶
+舛
+鉉
+М
+脾
+蓼
+♩
+兒
+澱
+薮
+賣
+醇
+儘
+荀
+并
+皓
+莱
+彪
+ภ
+煤
+攫
+總
+躾
+鬆
+盈
+褻
+
+罫
+🙇
+어
+昴
+諺
+鱒
+笙
+舒
+Á
+ع
+楷
+褥
+幟
+訶
+杢
+猷
+偷
+羌
+餘
+ς
+帛
+惇
+畫
+י
+蛹
+埜
+ᴗ
+捩
+臍
+凋
+А
+屁
+
+ヰ
+仄
+发
+赫
+्
+亞
+箒
+哭
+蟄
+翡
+哥
+蛾
+駁
+乎
+溪
+鈑
+髏
+碇
+髑
+燐
+∈
+ł
+枷
+僻
+衞
+魃
+匙
+稱
+瀞
+匝
+舫
+笏
+咤
+慾
+萊
+假
+匁
+杓
+忖
+苓
+鞨
+瑳
+≠
+瀑
+參
+迪
+齎
+涜
+菰
+ə
+动
+邵
+閩
+̆
+眷
+时
+斂
+鄴
+靺
+晰
+綸
+稗
+鯱
+聰
+筧
+趨
+👀
+È
+长
+载
+俘
+睾
+ฯ
+
+ː
+槿
+菟
+齟
+齬
+柘
+躓
+ă
+妇
+洸
+๕
+๖
+兗
+狡
+ë
+
+樊
+鏃
+寫
+😅
+다
+麩
+兌
+竇
+¶
+赳
+국
+र
+埔
+沁
+韶
+하
+夙
+强
+♦
+迹
+К
+穹
+櫂
+絃
+Б
+兴
+涎
+鎚
+茲
+丕
+觀
+奎
+誼
+繹
+鱈
+齧
+🍁
+敲
+杞
+Š
+渭
+隴
+鍔
+기
+沐
+邂
+癇
+彅
+攀
+逅
+☀
+虻
+沓
+莞
+炳
+ة
+🎵
+區
+髷
+筵
+坤
+驤
+禧
+瀾
+吼
+狄
+嬬
+霍
+涵
+👍
+渫
+婢
+鎬
+В
+⋆
+柩
+銑
+
+楡
+續
+蛸
+😆
+邨
+🔥
+澳
+鰺
+墺
+ƒ
+间
+銇
+坡
+繚
+脹
+輜
+ف
+憫
+邯
+专
+恪
+袢
+尭
+烙
+諍
+鋲
+拼
+ˈ
+撚
+🏻
+剌
+椰
+鑫
+
+电
+鮑
+變
+洙
+х
+恍
+祗
+˘
+‰
+疸
+◡
+絣
+逍
+畢
+份
+∶
+畦
+囮
+巖
+ق
+٩
+俟
+梟
+Å
+槐
+黛
+燗
+鐸
+个
+呵
+鈔
+晟
+凉
+辭
+闍
+飄
+癪
+雙
+侃
+耿
+舅
+匐
+滓
+猾
+砧
+蒋
+恫
+ح
+∟
+î
+茫
+让
+🎁
+ì
+汉
+详
+➡
+絲
+蒜
+昊
+襦
+门
+Т
+连
+喀
+翳
+
+朦
+杷
+시
+單
+熹
+孚
+憬
+糎
+聳
+呻
+駈
+疽
+縋
+泗
+χ
+撞
+箭
+饌
+ù
+匍
+淺
+癩
+☎
+사
+瀉
+秉
+О
+婉
+濟
+Ü
+开
+謐
+煬
+茗
+汀
+绍
+简
+靈
+😂
+冉
+毬
+戍
+薯
+栂
+圖
+✿
+勁
+緬
+恕
+狽
+悌
+檄
+盪
+臧
+碰
+Λ
+檣
+剋
+帷
+筍
+ζ
+ò
+˙
+扈
+驃
+ð
+你
+窝
+羲
+⚠
+棹
+种
+瑪
+籐
+稠
+💓
+檗
+这
+鋤
+ş
+🌟
+拵
+涸
+艾
+斌
+📸
+芻
+≤
+棗
+歿
+癸
+Я
+稷
+樓
+抉
+埒
+酊
+▪
+结
+을
+跪
+寨
+嬪
+ו
+褌
+Ψ
+ά
+悍
+ר
+箚
+晏
+奘
+棠
+蠍
+燿
+리
+न
+宸
+优
+ฉ
+🌸
+槓
+酩
+☑
+奠
+咆
+顰
+鮪
+ๆ
+靜
+스
+伦
+臂
+๓
+戰
+晁
+̀
+✔
+庾
+痍
+甜
+藉
+Г
+仗
+̥
+ز
+歸
+奋
+撻
+翟
+鬘
+蕁
+叟
+撓
+歎
+ج
+枇
+吽
+
+
+Н
+滉
+槨
+鉦
+驕
+龐
+諷
+頴
+해
+ी
+睿
+繡
+아
+滄
+朶
+汾
+琅
+💖
+澎
+对
+礦
+滬
+◉
+蜃
+🙏
+ц
+奕
+灣
+菴
+銈
+兪
+撼
+暈
+지
+ि
+魄
+菫
+簗
+螢
+꒳
+가
+禊
+舩
+欒
+竟
+ك
+过
+蘂
+⚫
+廈
+潁
+语
+豐
+飫
+❓
+И
+渣
+ж
+蜻
+闕
+∫
+獰
+蝮
+尸
+怡
+υ
+의
+У
+舍
+旁
+醗
+肆
+嵜
+蚤
+崙
+燁
+權
+ה
+黑
+鄙
+醫
+篩
+杣
+龕
+截
+浬
+奚
+鄒
+竦
+專
+梼
+雖
+≡
+哮
+为
+鰯
+啜
+葯
+匪
+據
+詭
+✕
+寳
+柾
+腓
+粲
+
+咫
+錚
+Θ
+粍
+蠢
+니
+薊
+矜
+ヮ
+濵
+鬚
+卉
+ш
+壹
+娥
+幀
+̈
+臘
+에
+Ç
+√
+蛯
+縊
+ش
+黌
+偈
+炬
+頗
+綽
+褄
+雹
+정
+✩
+로
+Ž
+ψ
+म
+攸
+🍴
+襷
+摸
+簀
+隨
+礒
+籤
+蘊
+剱
+狠
+訥
+朕
+覺
+苧
+恢
+郢
+😃
+Γ
+廖
+猜
+鵡
+盂
+繪
+醬
+崋
+类
+雜
+达
+Ä
+儁
+鄲
+鈿
+ś
+侘
+柯
+龙
+고
+绝
+惡
+ک
+祺
+譬
+대
+椙
+满
+禾
+자
+俎
+屬
+篝
+页
+鮒
+👏
+◦
+潼
+蛟
+辨
+藺
+曄
+گ
+緘
+鋪
+⊂
+謨
+霸
+邢
+收
+蠕
+☻
+篁
+晧
+燮
+炯
+啪
+獏
+་
+瘻
+籃
+郞
+她
+鞋
+葭
+蝠
+斃
+翊
+髮
+亂
+椛
+琨
+瘢
+蛉
+😄
+ḥ
+詢
+襞
+鐙
+😁
+瑤
+弗
+冰
+弉
+穗
+詛
+詈
+譯
+咩
+捐
+晨
+鈞
+😍
+伶
+✓
+兩
+碕
+繫
+嶌
+鑿
+匯
+丝
+嫩
+蒴
+佼
+粽
+트
+蝿
+沂
+燼
+ň
+轡
+ב
+辯
+憺
+द
+徠
+擧
+漳
+瞻
+鹽
+Л
+矧
+َ
+头
+橈
+獺
+ל
+鵞
+🍀
+주
+價
+銉
+慟
+淆
+蛤
+园
+氐
+공
+烟
+袍
+도
+õ
+疣
+🙌
+ń
+兔
+搗
+😌
+劔
+蝙
+는
+愈
+隗
+Ε
+礬
+鈎
+鐃
+ת
+क
+燵
+皝
+譴
+🤣
+徂
+昶
+麵
+嘯
+閭
+炮
+瑩
+粤
+萇
+丿
+芍
+釦
+김
+⸝
+漲
+ṣ
+厦
+≥
+乂
+梳
+ъ
+员
+师
+斛
+臈
+计
+剽
+瑾
+誂
+駱
+Æ
+ệ
+刎
+ǎ
+猗
+제
+👇
+楳
+粂
+场
+礪
+禦
+🤗
+א
+خ
+姬
+獻
+鄂
+پ
+樅
+盡
+处
+憊
+甄
+褶
+보
+檸
+侈
+妺
+橇
+鞮
+业
+滕
+û
+∼
+蟠
+黃
+ह
+华
+县
+殘
+서
+і
+匣
+夾
+ṭ
+娑
+逵
+艀
+韜
+☝
+瑯
+蟇
+戔
+檬
+翫
+鯰
+😢
+紇
+🥺
+„
+芬
+鴈
+🎉
+劃
+沛
+霰
+骑
+∠
+斷
+罐
+轉
+逡
+✌
+俠
+은
+☕
+🎄
+丽
+搦
+陞
+̶
+ط
+ˋ
+孜
+黔
+ṇ
+懈
+矣
+驪
+Î
+स
+乐
+樵
+ф
+┘
+兰
+痣
+菲
+蹠
+Ā
+ˊ
+媽
+蝗
+躬
+나
+💗
+试
+鉋
+愍
+箴
+顒
+苟
+话
+輳
+鑼
+∗
+蠅
+偃
+楮
+栢
+蝸
+验
+Э
+त
+办
+肱
+邀
+滿
+蕗
+व
+谢
+ص
+鯵
+緞
+À
+驗
+马
+沮
+무
+倚
+坏
+稍
+蒟
+贛
+⬇
+篋
+靄
+濬
+鑓
+魍
+僉
+鸚
+일
+藥
+链
+ˆ
+戈
+籔
+裄
+요
+ί
+燧
+褚
+寬
+棣
+隱
+衾
+顯
+Í
+鉈
+效
+‚
+碌
+丰
+甸
+皖
+ल
+œ
+图
+砒
+전
+ế
+羯
+袜
+🥰
+ό
+们
+稟
+鴫
+邏
+瀛
+蛆
+魎
+여
+프
+书
+廼
+彧
+纹
+銛
+驍
+ɛ
+◼
+桥
+猩
+라
+沽
+琦
+谿
+郝
+餞
+鵄
+✳
+掖
+ᄇ
+駝
+壙
+广
+Ó
+✴
+涇
+潴
+衒
+்
+喇
+猴
+鰤
+✅
+步
+娃
+瓚
+阵
+장
+🎀
+縷
+恆
+滔
+繇
+槎
+袱
+顏
+曺
+貂
+熔
+糺
+賁
+靳
+營
+鶉
+ゎ
+杳
+盞
+螂
+牀
+Ì
+繩
+們
+攝
+枳
+渟
+筰
+ʻ
+ξ
+灵
+藹
+您
+编
+Ú
+Е
+ע
+◀
+縺
+관
+😀
+说
+拜
+鏝
+👉
+ु
+冏
+ʔ
+昱
+聽
+餉
+🌈
+儺
+筝
+藷
+仍
+聊
+躱
+悴
+譙
+軾
+신
+💪
+从
+彊
+澹
+碼
+资
+剧
+泪
+‟
+犍
+ฝ
+⇩
+秣
+輕
+鍍
+态
+ฑ
+✡
+Ф
+瑶
+艮
+誣
+頤
+소
+呗
+磊
+銜
+亲
+刳
+簪
+閨
+頽
+髴
+潅
+髣
+인
+ฎ
+嚴
+擱
+軻
+Ū
+ש
+櫨
+车
+夥
+孩
+胥
+闢
+岛
+每
+🤔
+带
+弯
+琛
+请
+奶
+愿
+极
+濮
+现
+屹
+鑚
+İ
+⠀
+憔
+狀
+亙
+嚮
+수
+ğ
+מ
+☓
+綝
+‧
+⤴
+銕
+霹
+愼
+兀
+彝
+鉗
+靂
+廆
+梃
+顗
+飜
+😉
+≈
+很
+溌
+琮
+籌
+爭
+裝
+đ
+З
+✖
+歆
+蛎
+賎
+鰍
+ŋ
+Ê
+寢
+稻
+軛
+鼈
+े
+乍
+雞
+鬨
+̵
+选
+۶
+实
+彎
+杀
+杰
+僊
+红
+舳
+阳
+⇨
+睫
+筥
+繼
+鹹
+瀝
+紂
+邕
+琺
+蜥
+Ō
+帶
+撈
+莒
+赛
+俱
+厲
+滎
+笈
+萠
+蒻
+鴦
+🙂
+୧
+♔
+頡
+廂
+囂
+标
+癲
+罕
+臚
+Α
+⻑
+瀟
+脸
+詹
+頰
+ա
+暎
+聶
+腟
+题
+黍
+庖
+୨
+蜴
+蟷
+ʃ
+弋
+缺
+鎗
+凭
+曉
+甌
+͈
+☞
+抛
+娜
+鉅
+乘
+棕
+瑣
+마
+ɪ
+嗽
+瓘
+誨
+邳
+仆
+澗
+瀨
+经
+劈
+盒
+笘
+蘋
+论
+鱧
+چ
+噪
+鴛
+ї
+闡
+모
+圍
+墻
+˂
+ю
+구
+ť
+毗
+气
+원
+😋
+寔
+˃
+प
+冢
+滇
+ę
+┏
+☃
+❁
+❕
+汶
+우
+劍
+囀
+敞
+毓
+熈
+磾
+穐
+Π
+哩
+嗤
+╹
+❛
+籬
+⤵
+淞
+轅
+鶻
+∂
+영
+♨
+汕
+阀
+🍓
+💡
+佚
+劭
+珉
+성
+🔍
+婬
+搞
+溯
+邃
+鄰
+么
+眈
+錢
+壯
+涿
+鎭
+顥
+📷
+厠
+膾
+襴
+안
+오
+Ī
+鄱
+합
+夔
+楫
+榧
+弖
+诱
+蹙
+ა
+琪
+繆
+幢
+觉
+敖
+동
+э
+∥
+⊿
+儿
+淨
+賴
+馥
+鎔
+유
+洮
+疥
+讓
+跛
+蹲
+⃝
+啦
+虢
+啖
+屎
+鞅
+⊃
+虱
+訌
+譽
+鴇
+暹
+ʼ
+⑅
+嶷
+栁
+̄
+◾
+萃
+风
+❄
+鐡
+龔
+听
+😳
+ʿ
+郃
+吧
+愷
+荣
+馗
+ŭ
+┛
+倪
+樗
+窘
+鈷
+Ś
+┓
+➁
+勗
+恂
+愴
+⚡
+啼
+笄
+荃
+驢
+鵺
+ư
+导
+洵
+鉞
+Ô
+楨
+έ
+框
+杮
+선
+🕷
+ṃ
+◍
+瑙
+게
+‹
+懋
+歐
+絳
+繝
+纒
+진
+驛
+洽
+淄
+烽
+Ñ
+恤
+◁
+忻
+🌼
+进
+∧
+莊
+读
+閼
+포
+眸
+菁
+현
+騏
+조
+拇
+鞏
+य
+儂
+櫚
+濊
+瘴
+贞
+🌹
+ǔ
+ɔ
+ד
+廳
+弑
+张
+嬴
+拱
+旻
+皋
+稈
+衢
+غ
+
+櫟
+窈
+鰊
+미
+화
+أ
+ი
+杼
+榑
+艱
+🙆
+倅
+插
+麝
+ё
+扼
+Ḥ
+佗
+妍
+弹
+恬
+旌
+术
+┌
+佞
+歇
+⊕
+►
+挾
+
+务
+泓
+泛
+琿
+상
+儼
+瞽
+釗
+ɑ
+ज
+⋅
+宫
+鞣
+爻
+调
+🐶
+Ṇ
+冽
+계
+Đ
+孺
+筺
+蘚
+謠
+遐
+釐
+נ
+变
+韃
+舁
+교
+ő
+剝
+⋯
+屡
+峴
+惺
+辜
+회
+琰
+ą
+昕
+涪
+组
+蠱
+觸
+ḍ
+‡
+┳
+卞
+岷
+瞿
+勳
+莎
+剑
+說
+銖
+ى
+禕
+̑
+Х
+ग
+
+杲
+渝
+爷
+竃
+더
+ύ
+溫
+繞
+问
+항
+义
+围
+悳
+榕
+錣
+💙
+ĕ
+伪
+瑋
+俑
+柞
+歙
+袷
+躅
+闻
+輦
+颜
+문
+💨
+ं
+া
+蔬
+郤
+靫
+🐰
+勞
+盘
+飞
+篥
+膺
+부
+📖
+┐
+历
+埵
+曠
+簸
+綠
+逹
+昵
+僥
+懣
+杁
+篳
+羈
+阅
+黴
+詡
+躑
+邈
+Ï
+价
+毌
+糜
+羆
+민
+😱
+狹
+誥
+馆
+噤
+战
+运
+鵯
+鸟
+勺
+筅
+迸
+殯
+祜
+閖
+駙
+麴
+ż
+❌
+簑
+蔀
+曵
+瑁
+绪
+蓁
+鈕
+霆
+饮
+髻
+비
+þ
+щ
+
+✦
+疝
+鬣
+兇
+咒
+
+🎊
+暐
+朮
+泽
+瀘
+세
+ṅ
+‖
+猊
+搜
+珥
+魑
+개
+💫
+❶
+霄
+琬
+絅
+萍
+誡
+를
+호
+奐
+昉
+➚
+众
+梦
+筌
+饋
+배
+珎
+许
+陟
+야
+ो
+另
+濘
+爰
+筠
+閒
+̫
+欢
+鵲
+만
+ǐ
+ṛ
+⚪
+✽
+嶠
+愧
+ǒ
+桀
+紊
+记
+陂
+决
+드
+懼
+楸
+瀏
+肏
+박
+皎
+☔
+ㇷ
+獠
+阴
+靼
+ᗜ
+嫦
+禺
+单
+叭
+巒
+懷
+牆
+脑
+蔗
+豺
+轆
+ח
+擇
+椽
+质
+醪
+鋺
+閂
+驥
+龜
+와
+喆
+愾
+沅
+謔
+醂
+💜
+Ë
+傣
+栴
+潞
+讖
+闌
+馭
+💧
+Þ
+册
+噓
+尓
+报
+檢
+渴
+糯
+葫
+騫
+פ
+
+❷
+增
+˚
+Ч
+경
+ס
+⋈
+傕
+姨
+拔
+斟
+沾
+그
+弌
+悛
+笞
+胝
+鐐
+頊
+Ξ
+亰
+寞
+羂
+遹
+🍺
+👌
+吞
+护
+ŏ
+传
+塼
+寥
+芮
+̴
+欝
+繧
+酛
+Œ
+ź
+▸
+☁
+擂
+暠
+璞
+艙
+과
+ம
+ฅ
+棉
+滾
+舂
+虑
+〵
+艳
+跏
+跗
+위
+ק
+拂
+掣
+栩
+璠
+竝
+营
+轤
+😣
+僖
+斩
+椹
+渙
+燒
+聟
+錄
+용
+〳
+刁
+蒡
+蠡
+评
+카
+Κ
+ி
+╯
+연
+😎
+ɣ
+╰
+戲
+挂
+琐
+阯
+🍞
+॑
+堝
+楝
+瓌
+碾
+迥
+남
+타
+⇓
+坩
+鮓
+😘
+♠
+奭
+屆
+熕
+籟
+肚
+श
+它
+沒
+联
+諶
+鼬
+ם
+淇
+银
+ِ
+ე
+璜
+筮
+蠟
+젝
+ή
+姆
+溟
+盯
+帚
+玻
+Û
+̮
+⸜
+晉
+瀆
+邽
+🎃
+瀚
+裃
+임
+재
+💚
+ạ
+❥
+鄯
+鑁
+Ò
+ّ
+➤
+咨
+淸
+狆
+縹
+喙
+燙
+燾
+瑟
+產
+耘
+ĩ
+̷
+ㇱ
+侭
+剿
+汴
+粮
+雎
+鞴
+汜
+詁
+🌿
+Ù
+ן
+敎
+晞
+犁
+絽
+醉
+鵝
+거
+ब
+势
+滨
+貊
+软
+결
+두
+̤
+哪
+煜
+莚
+擔
+爨
+繻
+ُ
+Ṣ
+☘
+歡
+砿
+산
+晝
+梠
+磚
+禱
+辮
+远
+銓
+俚
+垠
+犂
+瓏
+蕙
+鑒
+디
+⃣
+〖
+垪
+显
+諤
+踞
+飩
+💋
+‵
+痂
+艫
+负
+🌙
+🙋
+Ă
+ր
+ט
+੭
+纈
+蘿
+險
+髯
+鬢
+ė
+ᄉ
+沔
+箍
+蚌
+輓
+Ē
+〗
+亳
+樸
+鱼
+͡
+璟
+😓
+Ṭ
+寐
+檮
+蜉
+鵰
+勛
+查
+葆
+赭
+闓
+강
+懊
+歟
+约
+覽
+軫
+际
+최
+ْ
+◌
+濰
+粳
+覯
+醴
+🌞
+゠
+繙
+给
+踝
+羣
+茘
+蝣
+鰾
+↩
+恃
+抓
+镇
+Ş
+ɒ
+‿
+嗄
+悧
+闔
+통
+ོ
+◠
+埕
+妲
+彈
+獪
+覃
+크
+😥
+巍
+惲
+懃
+蒯
+운
+🐻
+凩
+攬
+珣
+禪
+綰
+豈
+酈
+鐔
+枩
+楞
+炫
+睛
+縯
+麥
+✈
+匱
+找
+曁
+灤
+翦
+设
+雕
+鞜
+💐
+Ο
+曦
+橫
+縅
+誾
+轮
+레
+ˌ
+‾
+峪
+暘
+椚
+贩
+齒
+들
+토
+🤩
+󠄀
+ɡ
+ᅵ
+♢
+丶
+尔
+바
+💁
+ն
+⚽
+吝
+紮
+방
+🍅
+🍽
+厖
+壓
+酥
+🗻
+ͷ
+犢
+綵
+茱
+양
+ĭ
+آ
+∑
+∪
+咏
+😇
+ả
+吳
+峅
+綫
+靑
+髦
+麁
+천
+ľ
+Ш
+ᄋ
+俶
+塢
+墮
+慊
+户
+朐
+鎰
+🍜
+🐈
+💞
+ș
+Ж
+壩
+异
+欖
+羚
+ლ
+倩
+筬
+陌
+顶
+명
+Ź
+
+⊆
+┝
+戏
+茯
+莆
+驒
+내
+추
+भ
+勸
+匕
+终
+胖
+饂
+💎
+🤍
+ர
+⟩
+冕
+檔
+氈
+臙
+駢
+ג
+应
+攷
+蘄
+辦
+🎤
+🙄
+ʒ
+த
+徭
+豎
+默
+중
+ḷ
+☟
+➀
+妤
+沱
+滁
+麼
+ṁ
+吒
+椏
+橄
+賾
+迴
+ா
+两
+厓
+嚙
+洌
+緝
+縻
+肜
+賺
+騸
+ू
+佟
+慇
+戶
+璐
+貘
+노
+ʊ
+廞
+泯
+笊
+翹
+鶚
+🍑
+👩
+Ł
+ո
+ས
+┠
+❸
+새
+체
+치
+🌱
+😵
+▫
+⭕
+儋
+垓
+枋
+燎
+绘
+还
+陋
+黨
+📱
+🤤
+ड
+↘
+擅
+罗
+耻
+薀
+闖
+龗
+단
+囓
+窶
+縒
+纯
+臻
+裨
+諄
+역
+적
+Ŏ
+ţ
+ˇ
+ར
+☗
+❀
+劒
+坎
+徑
+徙
+箆
+粹
+訢
+釿
+ơ
+ட
+圣
+掉
+摑
+擊
+睢
+睥
+膽
+誑
+💭
+😔
+🥲
+孰
+崧
+穰
+莘
+蟾
+跌
+边
+😰
+值
+圜
+婺
+扎
+换
+葎
+郅
+Χ
+湣
+荐
+鑰
+靚
+↔
+勣
+甯
+畸
+纐
+络
+글
+🐱
+🐾
+რ
+惊
+蹶
+🌷
+Ĕ
+Ŭ
+̣
+Μ
+צ
+⊗
+ㇰ
+儔
+喃
+嗇
+壟
+戡
+涓
+节
+醋
+錕
+颪
+년
+랑
+‒
+⛄
+⟨
+呰
+壜
+壢
+廩
+橡
+矗
+頠
+Ě
+ե
+Ḍ
+∨
+✋
+✼
+柜
+芷
+药
+蒨
+郗
+﨟
+🌾
+Ń
+ɹ
+ʹ
+ִ
+Ḣ
+Ṛ
+涂
+臏
+蜆
+雒
+预
+
+🎂
+离
+贇
+파
+ს
+ṝ
+ỵ
+⌀
+☖
+❝
+侗
+夛
+梔
+瘋
+盎
+胜
+课
+辷
+騭
+鸕
+루
+태
+학
+🌻
+Ǎ
+ᄏ
+ᄒ
+ヹ
+买
+吕
+团
+濂
+瑗
+谈
+鷦
+🔰
+Ĩ
+Ĭ
+ᄅ
+ḣ
+⇄
+曷
+权
+璿
+磧
+袞
+료
+𓈒
+👶
+󠄁
+Ǒ
+Ǔ
+க
+Ḷ
+Ṁ
+Ṃ
+Ṅ
+Ṝ
+Ṡ
+ṡ
+Ṫ
+ṫ
+Ỵ
+牘
+瑀
+盥
+艺
+萸
+豳
+鑾
+으
+청
+军
+榔
+瞋
+钟
+驟
+🏃
+🔗
+𠮷
+♣
+❞
+晚
+焜
+蒿
+郴
+❖
+刮
+喊
+婕
+岨
+慙
+拯
+淤
+潯
+獎
+閇
+雋
+🔻
+∽
+亶
+嶮
+梛
+楪
+练
+觴
+转
+鏈
+업
+ʾ
+伄
+噜
+庆
+慥
+瓷
+靠
+鷯
+ᐛ
+┻
+☂
+✍
+喷
+堛
+宁
+嵆
+挹
+搔
+炅
+瓔
+癥
+筱
+銷
+鏞
+까
+차
+🏠
+击
+墉
+庠
+懌
+摠
+艪
+讚
+齡
+🔴
+ď
+ག
+ᅡ
+兮
+卬
+岫
+忸
+晄
+潢
+璉
+礙
+縉
+趺
+罔
+邾
+陘
+鮟
+鱶
+麭
+종
+🍎
+录
+样
+樒
+煒
+芾
+荳
+齲
+승
+怩
+焰
+瘀
+羿
+聿
+膂
+鑲
+🖤
+ț
+丨
+抖
+敍
+枉
+桴
+🇵
+Ð
+Ђ
+↗
+库
+湍
+珞
+絁
+细
+裵
+된
+씨
+코
+😚
+ւ
+ு
+售
+嬀
+廢
+殤
+瞠
+蹈
+閎
+霽
+驀
+광
+윤
+💢
+Ι
+Ц
+ო
+ㇽ
+圻
+毋
+浓
+淩
+爐
+祿
+逖
+🎍
+Ю
+ј
+♤
+✊
+寰
+惕
+穽
+窖
+购
+鐚
+🌺
+Β
+ָ
+ण
+噶
+备
+怎
+璮
+糕
+纪
+艷
+豁
+蹉
+闐
+ᄀ
+⁎
+枹
+瑰
+綦
+职
+莲
+邙
+郯
+刘
+咖
+溧
+犇
+狢
+蟯
+袿
+识
+軼
+ء
+്
+ា
+凮
+卅
+崚
+忿
+狎
+葩
+讪
+鱇
+鷄
+鸣
+건
+🐟
+வ
+╋
+廨
+獵
+瓠
+统
+霓
+魘
+鱸
+울
+웨
+👑
+ְ
+ध
+བ
+┫
+儵
+创
+磬
+耄
+鏨
+🍊
+כ
+ờ
+养
+厝
+弴
+桙
+淝
+淦
+澂
+玫
+译
+贫
+跟
+邛
+陈
+〰
+喈
+媧
+毖
+灝
+烝
+维
+티
+𓂃
+ट
+ᄃ
+ộ
+⊥
+扫
+砌
+鏤
+물
+👨
+🥳
+্
+✏
+脉
+誄
+鈺
+鰆
+점
+儞
+刪
+幄
+熲
+确
+紧
+蒹
+裒
+貳
+鳰
+분
+훈
+𓐄
+🔺
+إ
+च
+习
+侂
+响
+敕
+栲
+歲
+绮
+罘
+鑢
+석
+터
+🇺
+😩
+🚗
+՞
+农
+叮
+擒
+騶
+👊
+㆑
+啊
+棱
+澧
+燔
+褸
+襤
+赧
+飡
+鴟
+급
+식
+͜
+☠
+✄
+噲
+挡
+攜
+檳
+竞
+翎
+艋
+驅
+네
+북
+ի
+ப
+
+∮
+佶
+墩
+懶
+环
+瑭
+瑱
+疳
+癡
+諳
+违
+钏
+면
+있
+🇯
+🍖
+🏼
+ų
+峽
+愔
+璇
+璘
+皴
+笵
+踰
+锋
+매
+행
+협
+📺
+Ν
+⺠
+塡
+娟
+舺
+裘
+赞
+踐
+釵
+顓
+騷
+간
+생
+희
+
+🇸
+ئ
+٭
+अ
+ष
+़
+្
+ố
+岌
+擽
+濑
+蚩
+鎹
+🔶
+🧡
+Τ
+າ
+⌘
+☛
+✪
+档
+舉
+詧
+讯
+鴬
+너
+ώ
+ख
+ᴖ
+▿
+囘
+孛
+徇
+濕
+獸
+盱
+箙
+籏
+访
+軀
+陆
+颱
+骄
+鰌
+🤲
+ཀ
+ᄆ
+ᐢ
+嘶
+昰
+璩
+盜
+覲
+霙
+頎
+꒱
+당
+평
+🐼
+Õ
+র
+ི
+◢
+况
+勱
+哇
+唎
+彤
+杠
+眇
+窿
+鞬
+🦋
+ث
+်
+❹
+〘
+〙
+俥
+恊
+樱
+稅
+蚝
+谁
+💥
+📣
+⬆
+ㇺ
+僵
+姒
+测
+澡
+煦
+琇
+瓣
+銳
+閬
+본
+😖
+յ
+ַ
+伋
+佰
+傚
+复
+幷
+甞
+穩
+竑
+籙
+虓
+鑠
+陜
+黥
+금
+록
+준
+🍱
+ད
+ậ
+ị
+厂
+慷
+柗
+澶
+甍
+謌
+认
+跃
+难
+래
+🏿
+̩
+ּ
+ذ
+ང
+媼
+巿
+洱
+簫
+葷
+謖
+蹇
+鄆
+예
+🐤
+👧
+💻
+🤭
+ვ
+┬
+✉
+埤
+晤
+洺
+潽
+苹
+菘
+鵤
+션
+
+
+💃
+崛
+拏
+擡
+玘
+腳
+获
+음
+👋
+ض
+☉
+✝
+冄
+柢
+熏
+瘟
+纓
+耙
+该
+鰕
+러
+ฺ
+ᅳ
+∘
+ㇼ
+乭
+侏
+嫗
+憮
+湫
+潛
+萢
+雯
+🎅
+💉
+ာ
+㐂
+嚶
+嵊
+忱
+梭
+楙
+毟
+炷
+纸
+耒
+覿
+鄉
+鲁
+숨
+🍶
+📢
+ნ
+吶
+噎
+嚀
+桒
+汨
+碭
+迢
+靏
+🍰
+ব
+ầ
+倡
+塲
+嵬
+搖
+欷
+眾
+磡
+翆
+肄
+諚
+鄢
+頫
+말
+📚
+ʕ
+រ
+ゔ
+临
+弇
+悋
+斫
+旼
+泮
+貓
+适
+邮
+闞
+隍
+설
+실
+😞
+😲
+ᆫ
+ủ
+⊙
+❏
+则
+怕
+眙
+驩
+🚩
+ɴ
+ল
+⚾
+啞
+孙
+嶃
+构
+沭
+睺
+簋
+縱
+羋
+觜
+责
+雾
+鬪
+좋
+𓏸
+๋
+ལ
+ᄌ
+┄
+♛
+⻄
+ㄣ
+帕
+幫
+悖
+擎
+暾
+琊
+疗
+豬
+遲
+鱠
+검
+메
+🍂
+͠
+ז
+ਾ
+☽
+勰
+碎
+蜒
+貉
+跑
+轻
+鄖
+鑛
+隸
+롤
+르
+법
+📍
+🔸
+ͨ
+ͱ
+‣
+⋰
+仝
+圈
+棧
+檪
+煊
+碣
+綯
+绿
+萝
+蔘
+謫
+贊
+领
+鴿
+품
+🔉
+
+မ
+⋱
+⚜
+㓛
+严
+彻
+擴
+衫
+证
+贵
+饕
+🏆
+👆
+Ý
+ན
+◕
+仚
+僮
+囊
+夺
+恙
+悰
+旃
+泠
+湟
+糒
+续
+钖
+队
+駟
+력
+속
+습
+키
+🦐
+Ͱ
+ễ
+∅
+∎
+⌇
+仿
+嗾
+妮
+汞
+滌
+薑
+袤
+裙
+贴
+踢
+鏢
+铺
+발
+🐣
+👻
+😫
+ћ
+ྱ
+压
+巓
+歷
+澈
+濶
+硤
+笔
+蒂
+蝎
+裤
+钢
+餮
+鬥
+녀
+버
+창
+🌴
+🎸
+😮
+Ȱ
+མ
+ུ
+ᆞ
+ớ
+❔
+侖
+壤
+娱
+婀
+慂
+烘
+痤
+瘦
+肅
+踟
+鄞
+鰲
+🤎
+🥇
+儕
+勻
+卻
+啡
+墅
+杨
+枫
+殞
+澍
+濛
+竒
+订
+날
+ͳ
+ম
+ᅮ
+倆
+儷
+噸
+婴
+嫂
+徧
+慫
+拈
+槊
+犹
+畤
+讎
+谺
+靉
+髠
+鶫
+꙳
+털
+🌲
+ɾ
+ظ
+倰
+唹
+廸
+攤
+氓
+炻
+牂
+畯
+签
+翕
+苾
+菏
+蠶
+鑵
+铁
+陷
+군
+른
+백
+송
+🇷
+འ
+ბ
+მ
+ọ
+↙
+◤
+ㇻ
+亟
+吗
+块
+埓
+弢
+彥
+慚
+曻
+煢
+硅
+篦
+缚
+莨
+菠
+賚
+달
+형
+🚘
+⇢
+▋
+⛰
+仓
+偸
+哄
+渊
+瑄
+螳
+謾
+货
+鏗
+隰
+顺
+능
+심
+🌀
+🐕
+😛
+ÿ
+◜
+ㄧ
+佺
+杆
+簱
+纜
+腦
+舔
+蘖
+顋
+려
+헌
+😗
+😨
+ϯ
+ө
+ᅥ
+◝
+✟
+亹
+偰
+坛
+总
+璨
+皷
+蓙
+襯
+譫
+蹰
+침
+🐮
+🧸
+Ż
+˖
+ச
+ᄂ
+ẓ
+➂
+俅
+儉
+劑
+屻
+岁
+污
+淅
+瀰
+犛
+簇
+羡
+蹕
+郛
+陉
+馘
+魴
+鵙
+복
+
+😝
+ħ
+ʁ
+Η
+ন
+ি
+ề
+囎
+搏
+瘙
+诉
+鎺
+飮
+감
+왕
+철
+후
+͢
+ਊ
+๊
+ម
+勵
+夘
+棊
+玕
+粱
+舖
+茵
+蓝
+蕤
+📲
+◞
+吋
+圉
+娚
+廚
+昀
+枸
+瘞
+纳
+织
+貽
+齮
+월
+피
+🚙
+͞
+❇
+傉
+埈
+宾
+愨
+憙
+挛
+摔
+暲
+枪
+监
+窗
+罎
+腊
+闥
+
+🎥
+̯
+ͯ
+ѝ
+ս
+್
+ᅩ
+ᐡ
+
+刄
+剳
+呢
+壞
+岔
+彜
+怔
+歔
+琚
+硲
+箝
+糂
+纘
+罽
+苴
+莵
+詵
+豉
+黐
+목
+🍒
+🕊
+
+ʌ
+̃
+ـ
+ڡ
+
+❢
+❺
+倻
+劲
+廙
+掏
+搶
+朏
+炼
+珩
+甬
+痉
+秭
+罅
+苣
+荤
+襠
+권
+뉴
+불
+십
+작
+참
+💤
+🔹
+😸
+Υ
+ღ
+▢
+▹
+✯
+娌
+摧
+窠
+蜚
+諂
+讲
+🍛
+🍻
+🙁
+ழ
+უ
+⃘
+♕
+乡
+伅
+厙
+呀
+噫
+榆
+樾
+泚
+眜
+蓨
+蟬
+覈
+貮
+辑
+釼
+鍈
+骚
+鰈
+鵑
+데
+🍚
+🍬
+💍
+💔
+💘
+🔔
+🙅
+ũ
+͟
+Й
+ర
+ರ
+თ
+┊
+┼
+◻
+冈
+怙
+悅
+竭
+箟
+莖
+蔿
+薺
+詒
+譃
+议
+赏
+釆
+님
+🌰
+̇
+ấ
+✮
+伷
+偗
+巂
+树
+牁
+琼
+茣
+釟
+镜
+麸
+언
+🍫
+🎨
+💠
+͕
+᷅
+▱
+✲
+伀
+妣
+幔
+椴
+灞
+籀
+綎
+腆
+蜩
+遏
+铜
+반
+저
+즈
+🎈
+📝
+🚌
+🦁
+俀
+凞
+卧
+埗
+崁
+桎
+沘
+济
+熬
+珏
+縵
+莅
+觚
+邠
+錺
+错
+騾
+꒰
+견
+🍇
+🍮
+📆
+⛩
+估
+塒
+嬛
+恳
+榘
+淪
+濞
+痾
+縢
+舰
+贓
+赵
+鄄
+酘
+阡
+鼡
+鼾
+령
+애
+집
+﹋
+🇹
+😺
+͍
+ல
+╭
+仭
+伨
+偋
+偣
+启
+咢
+嘏
+嘛
+屌
+捍
+晳
+汇
+洹
+笮
+緣
+蛞
+豕
+醢
+鑷
+阶
+등
+브
+살
+순
+
+ɲ
+ʉ
+▣
+乕
+凯
+娇
+孁
+寻
+愃
+溃
+潙
+璣
+腥
+茴
+訖
+鈇
+陬
+鯔
+엇
+투
+환
+😜
+🙃
+🥂
+ژ
+┆
+凖
+坵
+姝
+嶂
+悄
+悝
+敌
+梏
+湓
+箜
+簠
+簧
+缘
+芎
+莇
+蟋
+辉
+邴
+鉏
+鰒
+鼐
+齣
+솔
+🅿
+🐥
+👦
+🤝
+̲
+য
+ḫ
+▓
+✎
+⬜
+僞
+晷
+杙
+洟
+狷
+瘧
+瘰
+皃
+睹
+篌
+簽
+緖
+薤
+藜
+蛄
+鑞
+鑪
+閦
+🌏
+🏂
+👼
+💅
+📞
+😈
+😻
+ు
+ᆼ
+ồ
+ụ
+⁑
+◟
+❊
+仐
+圆
+嬨
+峒
+戾
+扣
+扬
+暄
+檍
+漯
+狒
+畚
+疊
+綟
+聨
+苏
+鐶
+鐺
+鑄
+黟
+템
+🇪
+🏡
+🔽
+🚀
+ɞ
+մ
+গ
+⊷
+⏰
+═
+⛳
+丐
+佘
+娍
+敝
+渺
+璆
+罨
+耨
+薹
+謇
+遯
+郫
+鍇
+锦
+駛
+骰
+되
+없
+황
+🎪
+ɨ
+Ρ
+ன
+ự
+↲
+㹨
+倢
+减
+垈
+弈
+橦
+沆
+滷
+猖
+絖
+豨
+贼
+递
+鄜
+铳
+홍
+🍌
+🍷
+🐝
+ʚ
+∆
+ㇿ
+佻
+俐
+剔
+囿
+墊
+姞
+岭
+忝
+惶
+惻
+择
+獗
+腮
+臉
+衲
+览
+贤
+迩
+郿
+醮
+駮
+鴒
+鹤
+齐
+규
+봉
+처
+핑
+함
+🐢
+ಕ
+ಾ
+ി
+
+း
+↪
+▬
+⚓
+✤
+尝
+尠
+怀
+憨
+朓
+枓
+润
+煇
+皙
+硼
+礽
+纤
+缓
+罩
+菇
+蝟
+蝴
+蹤
+锻
+🍋
+🍼
+🥕
+ɻ
+լ
+थ
+ু
+ึ
+ེ
+ἀ
+Ἀ
+ἐ
+≣
+ⴰ
+亿
+俤
+兕
+奝
+妈
+廝
+恚
+抔
+樞
+綢
+罌
+蚡
+蜈
+蜑
+败
+鉃
+鸡
+黯
+龢
+것
+따
+히
+🍵
+📅
+І
+Ь
+գ
+్
+ಗ
+༄
+☼
+厉
+嘎
+拆
+昺
+汧
+漓
+烧
+竊
+篶
+翩
+胛
+薰
+豌
+财
+鈐
+驁
+驊
+鬮
+鯊
+鶺
+麋
+별
+출
+팀
+🎙
+🏄
+👈
+🧦
+ү
+⏩
+⻘
+佬
+堈
+奖
+旒
+洁
+灑
+禑
+粢
+缔
+蓆
+薭
+藐
+蝓
+覓
+覘
+销
+鯢
+길
+람
+온
+할
+🌳
+🤓
+🥖
+ᅦ
+∃
+⌚
+➢
+勐
+懂
+摄
+擺
+朵
+湃
+烤
+珽
+稹
+絮
+耜
+腾
+荡
+豸
+逄
+鈩
+霑
+鯀
+鼂
+류
+림
+손
+판
+💌
+🙈
+🚶
+ġ
+Ў
+տ
+ా
+ស
+⃙
+☜
+➕
+卫
+宓
+怛
+损
+栫
+漪
+灊
+煖
+珙
+瑒
+瓛
+粃
+缴
+补
+衽
+諛
+鍜
+饭
+鬯
+鳧
+름
+병
+🍙
+😯
+🦄
+
+ׁ
+ᅢ
+ក
+ᴥ
+ể
+⚔
+仞
+伟
+婧
+戛
+棻
+歹
+澮
+牓
+珮
+璝
+畧
+絕
+聵
+蠹
+褧
+訾
+逯
+鄣
+驎
+듈
+듭
+삼
+🐬
+ǁ
+̂
+̝
+կ
+फ
+⊳
+♧
+✒
+〚
+〛
+倧
+兢
+剂
+呷
+嵓
+帐
+撝
+曩
+椼
+玠
+皰
+緡
+苩
+覡
+躋
+郑
+郾
+鉷
+隲
+얻
+옥
+🆕
+🇮
+🌠
+🌵
+🌶
+🍃
+🍣
+🐷
+💰
+ű
+ϧ
+ै
+ု
+დ
+᷄
+₩
+┴
+☪
+⦆
+剄
+垃
+徵
+昻
+晗
+枻
+毳
+洨
+珸
+璦
+疯
+篪
+簞
+紲
+絋
+蜊
+覩
+遒
+鄠
+霁
+须
+鬲
+워
+입
+커
+편
+🎬
+😏
+🦀
+ņ
+ǃ
+ɺ
+ੀ
+ய
+ວ
+ქ
+ṯ
+⿻
+伤
+哱
+啤
+岻
+撱
+暕
+湮
+澠
+灭
+爸
+眭
+矿
+础
+粁
+紵
+虛
+軕
+輒
+遑
+駸
+騖
+鯤
+초
+혜
+🎹
+🏽
+💊
+😡
+Ё
+،
+आ
+ক
+ల
+ợ
+⇐
+◣
+❯
+侧
+厶
+唤
+垳
+奵
+弭
+惱
+检
+淯
+滏
+琕
+胄
+螭
+贝
+蹋
+鄔
+鋌
+鶿
+근
+테
+했
+🐹
+👗
+👣
+💮
+📳
+😙
+🚨
+ɐ
+ە
+╍
+☯
+✱
+⬛
+⿰
+倜
+僂
+壼
+奬
+恠
+抬
+桌
+淙
+禛
+胼
+臑
+薁
+薈
+鄕
+鋰
+鶸
+鷂
+겠
+악
+향
+
+🌕
+😑
+🤘
+
+ɕ
+ɟ
+ੰ
+ૢ
+ෆ
+⏬
+➰
+佯
+倝
+呎
+嗎
+坻
+涉
+瘁
+窴
+莪
+蚣
+褰
+诗
+赢
+輅
+酚
+銹
+项
+鰂
+鴆
+빈
+족
+🌊
+😷
+
+ɸ
+Ы
+দ
+়
+త
+മ
+ລ
+໒
+㐌
+䴥
+仹
+圦
+廋
+忉
+愆
+摶
+晢
+杂
+枘
+渍
+澆
+熄
+癧
+矍
+祏
+篷
+苡
+蛻
+袭
+跣
+錡
+顚
+麑
+黽
+꒪
+늘
+빠
+술
+플
+
+﷽
+🇦
+🇧
+🌝
+🍠
+🔷
+🤪
+Ÿ
+қ
+վ
+ք
+ֶ
+ך
+ை
+ῖ
+▻
+⦅
+屛
+嶲
+懦
+扛
+揀
+檐
+玹
+珖
+瓮
+癆
+盖
+紞
+縲
+莓
+蕷
+虬
+蚯
+錶
+针
+铃
+闷
+鷽
+齕
+각
+머
+범
+변
+🎣
+🎧
+🎮
+🐯
+🐴
+🔮
+🥈
+🥚
+🥶
+Ľ
+Ȃ
+܂
+ಮ
+།
+ཁ
+ắ
+ↀ
+✭
+僴
+勩
+夸
+嫄
+尅
+忒
+杦
+樛
+淏
+溂
+溷
+漾
+珧
+瘠
+继
+羇
+芿
+蚓
+诸
+靍
+髱
+ꠥ
+린
+었
+완
+외
+직
+짜
+필
+
+🇨
+🌅
+💝
+ǰ
+͙
+њ
+ਪ
+ാ
+င
+仺
+哆
+囉
+坚
+堃
+娛
+帙
+柬
+浆
+浑
+淖
+灃
+爀
+璵
+痞
+碆
+礱
+萧
+貎
+邲
+醱
+鐢
+锁
+雩
+顫
+饑
+饰
+髢
+黻
+난
+덕
+던
+립
+엔
+열
+특
+페
+🥉
+ֵ
+ং
+ਜ
+ਸ
+པ
+გ
+ტ
+↷
+⇧
+∝
+⋮
+✰
+〱
+
+啟
+妞
+嬋
+幺
+懽
+执
+搆
+擶
+棭
+椥
+橐
+炽
+珝
+碚
+繃
+臾
+薏
+褫
+謦
+賡
+酣
+鏘
+钱
+铅
+黠
+꽃
+란
+🥒
+🦆
+Ө
+॰
+ხ
+㥄
+儆
+划
+刚
+卖
+圷
+嫣
+峩
+扒
+棵
+沪
+浏
+涕
+湜
+滂
+煨
+犧
+犨
+琯
+缩
+肤
+苕
+蓀
+蓴
+蟀
+蟆
+蠖
+贍
+郟
+隂
+鬩
+鷸
+녕
+눈
+독
+때
+𝄇
+🇳
+🍭
+💄
+🚃
+🧚
+˶
+ә
+ॱ
+স
+ྒ
+ᕦ
+ᴀ
+ứ
+☏
+✚
+✻
+➔
+冦
+吵
+寀
+帮
+归
+忩
+昪
+淶
+禔
+繒
+蓖
+躄
+鄚
+釀
+鍓
+键
+颺
+鲜
+축
+
+🇫
+🇬
+🌎
+🍝
+💯
+📻
+🤦
+🦍
+🧐
+ˬ
+Ա
+জ
+ে
+ਰ
+ਿ
+ྲ
+န
+ᄐ
+ᕤ
+┤
+╢
+⚑
+➜
+仾
+冩
+剡
+吴
+垰
+孽
+弃
+弍
+忋
+掰
+揭
+柝
+澀
+祕
+簓
+羔
+蚶
+蠻
+謰
+貔
+輟
+郕
+陴
+髀
+같
+뷰
+약
+찬
+채
+🍄
+🍉
+🍳
+🐙
+🔎
+🤙
+𢷋
+̍
+ֹ
+उ
+ᗨ
+┋
+☮
+⿴
+俾
+倞
+壠
+媾
+捄
+棡
+橅
+沉
+湄
+滯
+牵
+疚
+硏
+穝
+緜
+胯
+諝
+譏
+贺
+辅
+鉀
+鬧
+베
+표
+혁
+
+🐇
+💇
+🕺
+😒
+🥀
+Ć
+ʽ
+˜
+̱
+͔
+௰
+ం
+౪
+ಿ
+ᄑ
+ữ
+✾
+亴
+佈
+匄
+协
+哦
+嗷
+嚳
+峮
+撟
+柁
+熒
+甃
+癜
+盌
+膊
+荅
+跖
+辈
+鏐
+镗
+鹼
+숙
+싸
+활
+🏝
+🔆
+😤
+😹
+🤞
+🤫
+🥩
+ʀ
+ʖ
+ˀ
+ए
+ौ
+হ
+
+இ
+ற
+ᅧ
+ᆯ
+ሶ
+⇛
+☌
+⿱
+⿸
+剗
+妆
+姙
+嫋
+孀
+尘
+巢
+戩
+捣
+摇
+旄
+栻
+榨
+樑
+滹
+琫
+瘆
+瘣
+祛
+絇
+蔔
+螽
+蟶
+裹
+较
+迚
+顾
+鱚
+락
+번
+블
+않
+올
+츠
+🌃
+🍏
+🍧
+🏖
+ਨ
+ి
+ು
+യ
+ိ
+ᅲ
+ት
+ប
+ῦ
+⃛
+∖
+⌊
+⑉
+◂
+☐
+♻
+⿲
+⿳
+⿵
+⿶
+⿷
+⿹
+⿺
+仌
+仟
+儶
+妝
+媞
+寤
+层
+嵋
+撅
+杤
+樔
+氧
+煞
+獬
+玟
+璁
+盔
+瞎
+积
+穉
+穪
+竴
+絓
+罍
+肸
+菸
+蟜
+覬
+询
+谋
+逕
+闲
+黜
+귀
+농
+둑
+억
+육
+🍨
+🐧
+👁
+🔵
+🗣
+🤛
+🤰
+ȝ
+ʧ
+ठ
+ந
+ന
+ត
+ᴇ
+ổ
+⛺
+➖
+ㇾ
+叺
+吁
+垫
+墀
+壒
+姣
+廕
+浠
+瀲
+炕
+熺
+燠
+玦
+罃
+薌
+虯
+衜
+鉸
+鋆
+鍕
+鏖
+霏
+颶
+鸛
+뮤
+절
+케
+🎇
+🐛
+🐠
+🐲
+📩
+🗾
+😶
+😿
+𤭯
+Ƃ
+ʑ
+̸
+͋
+͏
+͖
+є
+ը
+ฮ
+က
+ᆨ
+ር
+⁍
+∋
+ⵉ
+〼
+举
+伉
+儛
+厔
+啻
+埣
+娿
+嫻
+嬶
+嶝
+慘
+慳
+憍
+榲
+汤
+泵
+浞
+渰
+烋
+盩
+礀
+緱
+绫
+聃
+胶
+虂
+袰
+覊
+贯
+軹
+轂
+迺
+逌
+释
+鋁
+鋮
+閹
+阁
+餝
+鬻
+鴌
+밀
+🍍
+💟
+🖐
+🥃
+𣰰
+ļ
+ʬ
+ः
+ত
+ী
+ਹ
+ನ
+ത
+ല
+ສ
+༎
+ဗ
+ង
+ở
+⌓
+☣
+⠇
+乌
+俔
+匏
+啾
+嘀
+堿
+奷
+崍
+崤
+嶇
+戢
+摛
+旉
+榦
+欉
+欹
+瀷
+猯
+痲
+眄
+笺
+篮
+糲
+繖
+翺
+翽
+茌
+蕈
+蕓
+螻
+裲
+诚
+跎
+轎
+邡
+醵
+鉴
+鱿
+鴣
+鷓
+곡
+담
+률
+망
+및
+슬
+𓐍
+🍆
+🎌
+👓
+👾
+🤑
+🤟
+𠮟
+Ș
+ɯ
+Ύ
+զ
+թ
+հ
+ۭ
+इ
+ृ
+ര
+ฤ
+ខ
+ử
+⁂
+☭
+✁
+❮
+❻
+〽
+侊
+俏
+倠
+傪
+僣
+兽
+刕
+够
+夬
+扩
+挌
+榻
+殭
+氂
+焯
+煚
+狁
+猨
+竽
+芩
+袒
+譔
+跫
+遞
+酇
+閿
+隶
+隹
+韙
+韞
+饃
+鶲
+龑
+받
+벌
+첫
+팔
+🍾
+🏫
+👐
+🔧
+ǂ
+ǽ
+ɜ
+Ӂ
+Հ
+֊
+ਗ
+ே
+ຶ
+စ
+ေ
+ᆷ
+ḗ
+₳
+∙
+◈
+伃
+冭
+厰
+咪
+塌
+嫖
+审
+岗
+嶧
+愬
+扨
+旿
+昞
+晖
+晙
+栱
+泝
+熴
+玃
+粿
+緄
+荔
+蓜
+蚵
+諠
+讙
+貅
+賨
+踴
+蹼
+输
+逋
+魹
+鱉
+께
+익
+택
+🎯
+🐸
+👹
+💀
+🖥
+🚒
+🤚
+🤳
+🦊
+
+ĝ
+ʎ
+Ͳ
+ᅌ
+ን
+
+⇆
+⏳
+╂
+⚘
+➝
+乩
+伙
+偄
+偆
+凤
+喵
+噠
+嫮
+孑
+宀
+宽
+崞
+彖
+忯
+惔
+扭
+扯
+昤
+栰
+檉
+毐
+湳
+煆
+祆
+秬
+綋
+縕
+羕
+羸
+脘
+蓍
+蓚
+褞
+诫
+鍏
+鍚
+韵
+鬬
+鱲
+궁
+누
+먹
+밤
+잘
+총
+🍐
+🍕
+🐦
+👤
+Ϋ
+Ϝ
+Щ
+٥
+প
+ા
+ᄎ
+ᐟ
+ὐ
+℗
+◥
+⛅
+⬅
+侦
+卽
+呦
+塤
+壘
+帅
+惯
+拖
+枦
+榷
+殑
+涩
+淼
+渗
+潑
+濄
+玥
+玳
+畴
+癘
+笨
+纱
+罡
+翜
+莟
+蜍
+训
+賻
+轴
+郄
+酆
+鐧
+閱
+頵
+駭
+鬐
+魋
+ꞏ
+앙
+효
+
+
+🆗
+🇭
+🇰
+🍡
+🗓
+🚛
+🚴
+🤧
+🥐
+🥞
+ɦ
+̳
+͚
+ͬ
+ץ
+ھ
+ई
+घ
+ക
+ນ
+ြ
+შ
+ភ
+ỉ
+ὶ
+≅
+┰
+╱
+❦
+䐰
+偁
+僰
+劳
+嚔
+囗
+坳
+坷
+塽
+墹
+壑
+壻
+夲
+婪
+嫪
+寘
+屢
+抚
+揣
+攄
+曆
+楂
+殳
+牋
+皚
+窦
+纛
+绀
+绒
+菑
+褹
+逾
+酺
+釁
+鉆
+鐫
+魈
+鮃
+골
+봄
+빛
+혼
+흥
+🎾
+🏾
+👎
+👷
+🖖
+ɚ
+̌
+ղ
+ર
+ங
+ಡ
+ദ
+ພ
+ཆ
+ც
+┿
+▮
+☄
+仸
+傴
+卨
+圡
+宧
+嶸
+徸
+愰
+撩
+旇
+柤
+楹
+檝
+灶
+瑫
+砬
+磑
+禖
+脷
+谜
+鉧
+鋠
+閫
+閶
+陁
+鷟
+黶
+ꉂ
+걸
+든
+쓰
+알
+책
+탁
+탄
+
+🎋
+🐔
+🐿
+👟
+📕
+🔫
+🧀
+Ə
+ǫ
+Ά
+ў
+ұ
+ہ
+ۇ
+ܐ
+ଘ
+ண
+క
+గ
+പ
+ു
+฿
+༻
+ᅭ
+ል
+ន
+ព
+⚐
+俰
+呍
+嚈
+埭
+夠
+娣
+尞
+嵒
+庹
+楣
+欍
+欰
+氳
+湞
+溱
+濹
+瀹
+灉
+燉
+盦
+硐
+簣
+罒
+肓
+膩
+菡
+蘓
+螟
+譞
+谊
+豢
+贡
+醯
+额
+鱓
+鶡
+鹰
+黏
+갑
+객
+겨
+느
+돌
+또
+련
+밥
+퍼
+
+
+🆖
+🌍
+🐍
+🐏
+🐭
+👂
+👜
+💩
+🚲
+🤜
+į
+ʦ
+͒
+Έ
+ќ
+դ
+ً
+१
+న
+න
+ະ
+လ
+ძ
+យ
+ុ
+ែ
+ἄ
+≖
+┉
+❿
+ㄨ
+丗
+丫
+亊
+亠
+亸
+仅
+佾
+兂
+勍
+厅
+喞
+囧
+夹
+媓
+嵎
+嶅
+帑
+庯
+廾
+弰
+彘
+挵
+攴
+暻
+楛
+涨
+灬
+爼
+瑢
+眦
+碟
+笋
+綛
+莿
+萵
+蓊
+藕
+蘐
+鄶
+鉇
+鍗
+锐
+馱
+鯏
+麛
+웅
+컵
+확
+획
+
+
+
+🏀
+🏔
+👠
+👯
+💬
+📌
+🚺
+🥗
+🥸
+ķ
+͘
+͛
+΄
+Ί
+Ϟ
+ң
+Ӧ
+শ
+ெ
+௦
+ద
+ം
+ක
+๏
+ཕ
+ជ
+ᴏ
+ῶ
+␣
+◊
+◔
+⚒
+㐧
+㟢
+侄
+偐
+冼
+删
+嗨
+埖
+堉
+壳
+姊
+媵
+峭
+崕
+嶢
+廛
+懍
+拥
+挪
+捶
+洄
+炆
+燊
+牯
+玛
+盼
+眕
+碳
+籽
+纔
+绑
+翥
+軑
+輞
+鋩
+鋹
+鍙
+駘
+齶
+갈
+널
+쟁
+질
+충
+취
+칙
+
+🇩
+🇲
+🍪
+🐩
+🔲
+🦈
+Ļ
+ŧ
+ǧ
+բ
+պ
+ট
+ର
+ள
+ோ
+Ḫ
+ὰ
+ῆ
+
+⌛
+◽
+☹
+⚬
+✵
+❘
+❼
+ⵜ
+ㄥ
+亱
+凈
+勔
+卆
+卲
+呱
+喱
+垝
+垻
+塋
+娀
+廌
+忤
+摹
+旹
+晓
+殼
+濼
+瀅
+炘
+璡
+皀
+硕
+碻
+磻
+礄
+绩
+缸
+脯
+膃
+舸
+苍
+苳
+菽
+蒺
+藎
+蹻
+邗
+鄮
+釡
+鉚
+鍒
+鍝
+鱔
+균
+량
+엄
+킹
+탕
+허
+
+
+
+🍸
+🏕
+🐘
+🐵
+🐺
+👵
+🕯
+😠
+🦉
+Ɛ
+ǘ
+ʏ
+̪
+͓
+
+Ј
+Ս
+ٌ
+ޠ
+ު
+ো
+ಯ
+တ
+ပ
+ფ
+ᅯ
+ẽ
+₤
+↦
+⇦
+▀
+✇
+❅
+❆
+❽
+ⵏ
+仪
+呫
+坌
+埇
+夂
+彳
+怱
+惫
+憕
+掑
+掤
+斅
+渢
+炤
+焱
+玨
+畡
+畹
+綧
+縑
+蠑
+诣
+贸
+迯
+釤
+陇
+鞞
+飆
+饶
+駑
+黼
+암
+옷
+응
+줄
+쿠
+클
+
+
+🌬
+🏙
+🏰
+👿
+🔩
+🔪
+😽
+ʛ
+ˎ
+˗
+̓
+Ե
+ી
+ூ
+ప
+వ
+ර
+ရ
+კ
+ም
+ặ
+ỳ
+♚
+♮
+⚋
+⚛
+❃
+➃
+⻭
+䈰
+傔
+傖
+储
+劻
+厕
+唵
+啵
+嗐
+堊
+姽
+孃
+币
+幛
+弽
+忧
+扆
+曘
+栏
+汛
+湝
+滃
+牴
+珺
+畊
+痿
+皁
+皈
+窑
+筲
+糴
+綔
+罚
+蘅
+虾
+蟒
+衬
+褓
+譌
+讼
+邶
+邻
+陔
+陕
+鯣
+黁
+꾸
+끼
+럼
+많
+짓
+
+
+🍩
+🏍
+👘
+😴
+Ő
+̦
+ϥ
+Ϩ
+ؤ
+߹
+૾
+ଓ
+ಲ
+ള
+ྫ
+ွ
+ራ
+ំ
+ừ
+ἰ
+⊱
+➛
+⦿
+〻
+ㄘ
+乢
+侁
+侣
+侫
+凾
+匽
+唬
+噬
+圩
+妪
+嫐
+孱
+寉
+屽
+悺
+戞
+摆
+昜
+昝
+枌
+梺
+榭
+槫
+槭
+樰
+橛
+殃
+氛
+洧
+濩
+獐
+癈
+癰
+磴
+秡
+窕
+纽
+聪
+肭
+胙
+膅
+艹
+菻
+蓐
+褲
+诞
+轨
+鍱
+鑭
+闈
+鞫
+韭
+顽
+颐
+骗
+髓
+鮹
+ꇴ
+꜆
+낒
+렌
+릉
+맹
+먼
+컬
+콜
+
+
+
+🌭
+🍈
+🍗
+🎐
+🏘
+🐉
+🐽
+👴
+📈
+🔞
+Ţ
+ɫ
+ʂ
+ʟ
+ˑ
+͑
+Ү
+Մ
+
+
+࣮
+छ
+ೋ
+ḏ
+‛
+⌂
+⓵
+⚊
+✆
+亅
+仧
+倬
+剩
+叏
+嗶
+圾
+坫
+媳
+屮
+岚
+岢
+崆
+廐
+徯
+忞
+悊
+悬
+抢
+捥
+掄
+撕
+撿
+柒
+栾
+椌
+槲
+檦
+歛
+毕
+浿
+滦
+琀
+甪
+疔
+碉
+磝
+絫
+翬
+膈
+艰
+荫
+莧
+蔕
+虽
+蚪
+襪
+觔
+趁
+轘
+郪
+鎣
+霅
+霾
+鰰
+鷭
+鼉
+괴
+극
+못
+싶
+잡
+존
+
+
+
+
+
+
+𓂂
+𓇼
+🌛
+🎼
+🐎
+🐒
+🐡
+👕
+👢
+💿
+📛
+📽
+😪
+🚢
+🥧
+ʋ
+љ
+Қ
+է
+
+ँ
+ॢ
+॥
+ੑ
+ત
+્
+ஐ
+మ
+ದ
+വ
+ි
+ຊ
+ཚ
+Ꮚ
+ដ
+ἴ
+ὄ
+
+⊰
+⏫
+▐
+⚙
+⛷
+✘
+✞
+❒
+❚
+⟶
+ⲁ
+ゕ
+倓
+倾
+傜
+喁
+垕
+夊
+娉
+嶗
+巋
+忰
+悆
+挖
+捋
+撸
+擯
+晎
+暨
+槁
+洼
+瀦
+狻
+獦
+玆
+玢
+窋
+窰
+縝
+縡
+缈
+胁
+苜
+葳
+蓿
+虧
+虨
+蜓
+蠊
+衑
+訇
+遗
+酃
+酱
+鑊
+闭
+鞦
+駰
+骂
+骶
+髎
+鱏
+鸭
+鹉
+龠
+격
+램
+맨
+섭
+았
+앤
+욱
+착
+찾
+친
+풍
+🌑
+🍔
+🍥
+🎩
+🐃
+👔
+📦
+📮
+🖕
+🥛
+🥟
+🦇
+ǝ
+ɬ
+̋
+̨
+͂
+
+ϊ
+Ϧ
+Ъ
+ף
+
+
+
+
+
+
+
+
+
+
+ळ
+ঙ
+ਕ
+୯
+ఏ
+ಬ
+ೆ
+ฬ
+ཞ
+့
+Ⴣ
+ᄊ
+ብ
+አ
+ክ
+ិ
+ោ
+ᴛ
+ᴜ
+ὴ
+
+₱
+⇊
+⊇
+╌
+✐
+✶
+❂
+ⲓ
+ⲙ
+ⲛ
+ⵎ
+㢸
+伜
+佉
+匀
+咱
+唊
+嘖
+巵
+掓
+槩
+汙
+浹
+湑
+烜
+燝
+琓
+皂
+皞
+秧
+秽
+緇
+緲
+纭
+绞
+羃
+翘
+臟
+荑
+莠
+蠃
+衵
+裱
+訒
+訰
+謚
+讨
+谰
+贏
+趕
+踉
+蹌
+郇
+酯
+鋕
+鍉
+鍐
+险
+隐
+韆
+頲
+騮
+驱
+鵐
+麪
+黝
+龝
+움
+죽
+증
+캐
+패
+혀
+
+
+
+
+🎲
+🏈
+🏥
+🐳
+👖
+👮
+👰
+💣
+💺
+🕒
+🛥
+🤱
+🥬
+🦑
+🦔
+🧘
+🧪
+𣂺
+˥
+ϝ
+ϩ
+ѕ
+ғ
+ӂ
+ց
+ढ
+ধ
+య
+స
+ಸ
+സ
+ා
+ฆ
+ົ
+ᅣ
+ᐝ
+ល
+ី
+ỹ
+
+‸
+₪
+↳
+▍
+▤
+⚰
+✃
+✣
+❐
+➄
+⦁
+ⲉ
+
+㶱
+乣
+仵
+傒
+僦
+儓
+儻
+净
+卢
+哔
+嚕
+夈
+妳
+嫰
+宠
+帒
+惤
+挒
+搨
+摎
+擠
+槵
+橳
+歧
+毆
+氟
+氮
+汊
+渐
+渼
+漰
+澜
+瀍
+烺
+犖
+犮
+玁
+瑺
+甾
+畅
+畬
+癭
+禀
+膓
+艜
+芃
+荖
+萨
+蘑
+蝌
+蝨
+螞
+譻
+讜
+谱
+账
+赁
+遶
+郜
+鄃
+鄩
+銍
+錞
+錻
+鍊
+锛
+霨
+韦
+顿
+颸
+馒
+鮗
+鯲
+鰮
+麤
+鼯
+굴
+깨
+꿈
+런
+막
+색
+웬
+율
+칼
+🍯
+🍹
+🎆
+🎞
+🏇
+👸
+💆
+🖋
+🚄
+🛍
+🤐
+🤖
+🤷
+🥦
+🦌
+🦷
+𡒉
+ɢ
+ɤ
+̐
+̞
+ֿ
+ܝ
+ާ
+ञ
+જ
+ె
+්
+ခ
+ျ
+Ⴍ
+პ
+ᅀ
+ማ
+ስ
+ẩ
+ὀ
+ὑ
+ὸ
+⊤
+▧
+☢
+⛵
+⛸
+➳
+ヺ
+㔺
+倎
+倕
+偓
+冯
+咕
+嘸
+噯
+嚭
+囈
+坧
+廮
+戱
+撙
+暂
+朖
+杽
+椁
+椨
+樴
+氢
+氥
+洎
+淚
+潦
+潾
+灄
+狮
+獫
+璯
+砮
+砳
+磅
+竅
+箬
+簔
+芊
+蒭
+蔟
+蔲
+蘗
+蠧
+衎
+衹
+袮
+諲
+譁
+譎
+貍
+躡
+辽
+鍑
+鏱
+陲
+髆
+鮦
+鮴
+鯷
+鰥
+鱝
+鵾
+鷀
+鼇
+ꐕ
+곤
+곳
+덤
+돈
+룡
+빵
+얼
+웃
+촌
+컴
+
+
+
+
+
+𓐌
+🌂
+🌇
+🏁
+🐖
+🐚
+👙
+👱
+🔖
+🟠
+🤡
+🤯
+𥝱
+ŝ
+ǵ
+Ț
+˵
+ϡ
+ے
+ަ
+ङ
+।
+অ
+ভ
+ஆ
+ತ
+ವ
+ྡ
+ླ
+သ
+အ
+ሰ
+ទ
+េ
+់
+ḳ
+ḵ
+⊹
+▥
+◒
+⛤
+➋
+➞
+ⲟ
+ⵍ
+ⵓ
+ⵔ
+䘰
+䚟
+䬰
+䰰
+仡
+儈
+冝
+冾
+劼
+匸
+哂
+啐
+喻
+嗲
+垍
+姍
+媄
+屾
+崱
+崴
+巅
+幵
+徃
+悽
+搴
+枊
+栥
+椪
+橱
+櫲
+欟
+泙
+涖
+潺
+瀵
+灾
+焊
+狱
+獾
+珱
+瑴
+瓩
+畭
+瞀
+磺
+窥
+粭
+紕
+蓂
+蘢
+诊
+赶
+轩
+迤
+鄀
+鄘
+鄺
+釶
+鈰
+鏀
+鐏
+锡
+饥
+馛
+騁
+驫
+髞
+鯯
+鯽
+鴃
+鵟
+鹫
+麈
+곽
+긴
+꿀
+냥
+논
+링
+맥
+먰
+뿌
+슈
+읽
+찌
+춘
+헤
+휘
+휴
+
+
+
+🌽
+🏋
+💴
+🔅
+🔼
+🗼
+🗽
+😐
+🙀
+🥝
+🧊
+𩅦
+
+Ř
+ŵ
+ƕ
+Ȝ
+̚
+օ
+ڀ
+ܘ
+ਲ
+ః
+బ
+ಜ
+ೇ
+ೳ
+ු
+ມ
+ᆭ
+ግ
+ᠠ
+ỏ
+ἔ
+ὤ
+℘
+⏎
+┨
+▇
+◃
+◙
+♝
+➊
+➯
+ㇵ
+㝢
+㰀
+㸀
+亻
+仂
+儸
+冖
+啀
+坝
+嫺
+尐
+尙
+崢
+嶉
+嶬
+彠
+捆
+搓
+搩
+擄
+斕
+旂
+昫
+曬
+朳
+柸
+梴
+氵
+泷
+泻
+淬
+滙
+煅
+狞
+琹
+瓫
+睟
+磵
+秊
+稃
+竩
+笳
+粵
+絛
+缃
+脏
+茆
+莋
+蕣
+蜾
+觥
+諴
+躺
+軈
+軺
+辧
+逊
+遴
+銒
+銲
+鏂
+鏵
+鑅
+钻
+锤
+隃
+隝
+隺
+霫
+馺
+鵆
+鹏
+龄
+떠
+론
+쌍
+엠
+죠
+탑
+팬
+푸
+험
+힘
+
+
+
+
+
+🇴
+🎓
+🎷
+🏊
+👽
+📀
+🔊
+🙍
+🛁
+🥵
+🧵
+̊
+
+ֲ
+ި
+ް
+ߦ
+খ
+ণ
+ূ
+ક
+ಳ
+െ
+ද
+ງ
+ປ
+ອ
+ເ
+້
+ཐ
+ྗ
+
+ူ
+္
+ზ
+წ
+ᅨ
+ያ
+ᙏ
+ᴅ
+ᴘ
+ḻ
+ẹ
+ẻ
+Ἐ
+ὁ
+⌯
+⏱
+▄
+▦
+☰
+♱
+✬
+➣
+⟵
+⡱
+ⲧ
+⻤
+ㄇ
+㔨
+㞍
+㴶
+䵋
+乔
+亾
+佔
+佝
+僙
+儠
+凵
+剷
+匋
+匰
+唔
+嗢
+圄
+坨
+姧
+嬃
+嬾
+嵝
+巘
+帧
+戳
+扞
+掗
+撾
+斿
+曈
+杻
+枃
+棐
+榠
+榾
+樁
+汭
+泫
+涮
+湉
+獼
+璫
+癤
+盅
+矼
+稳
+篏
+紈
+纉
+绉
+缝
+脣
+腧
+茛
+荬
+蓓
+薐
+蘰
+蜿
+蠔
+袙
+襁
+謏
+谎
+贷
+赌
+躒
+辖
+逑
+邌
+鄫
+釭
+鉊
+鏉
+鐄
+铸
+锈
+閆
+闅
+顱
+餠
+饼
+髹
+鯁
+鴞
+鸑
+齪
+齷
+ꈊ
+낿
+냈
+넘
+답
+듯
+딸
+랜
+럽
+릭
+맞
+빙
+왜
+잠
+찰
+쳐
+탈
+텐
+폐
+
+
+
+
+
+
+﹉
+𐤔
+𒀭
+🌋
+🍤
+🎏
+🎫
+🏅
+🏭
+🏹
+🐄
+🐑
+💈
+📎
+🔯
+🚬
+🪄
+🪴
+🫑
+𤢖
+
+ǀ
+ǚ
+ɂ
+ɳ
+ʘ
+˨
+˳
+̖
+̭
+
+Ҋ
+ׂ
+۩
+ܫ
+ނ
+ރ
+झ
+ই
+ਬ
+દ
+ఆ
+
+ీ
+ಟ
+ಪ
+ങ
+ച
+ය
+
+ີ
+ྀ
+ံ
+ᄰ
+ለ
+ላ
+እ
+፡
+គ
+ធ
+Ẓ
+Ↄ
+∉
+⋉
+⋊
+╁
+◘
+☾
+ⴻ
+ㄚ
+㚻
+䂖
+俉
+偝
+儦
+冂
+冫
+剏
+劝
+匚
+匤
+匳
+卩
+喨
+嘿
+奫
+姈
+婼
+屄
+屐
+岀
+岵
+崰
+帾
+幤
+庙
+廡
+悒
+惒
+拟
+挤
+揥
+撇
+撣
+攔
+晛
+晡
+晸
+曚
+枔
+棩
+樘
+樝
+橚
+櫪
+毪
+沇
+沟
+泔
+洭
+涑
+湶
+滩
+烱
+焏
+煲
+爹
+猎
+獮
+玅
+玭
+琁
+璈
+瓥
+皶
+皹
+磷
+穌
+笂
+糶
+紱
+絎
+艅
+荂
+葢
+薉
+蘧
+虵
+虺
+蠰
+訕
+诺
+谛
+貲
+貺
+跤
+輙
+轜
+釻
+鉐
+鉑
+鏆
+钰
+铭
+闁
+阝
+韴
+飈
+饲
+騤
+鯡
+鰡
+鰱
+鱖
+鶩
+鷁
+鸇
+ꈍ
+났
+득
+딩
+례
+맛
+며
+벤
+샤
+셔
+웹
+접
+칠
+텔
+
+
+
+
+
+
+
+
+𖥧
+🌁
+🌜
+🌧
+🍲
+🎎
+🎟
+🏚
+🏢
+🏳
+👭
+📘
+🔄
+🖼
+🗝
+😕
+🚫
+🟡
+🟦
+🥢
+🦖
+🦢
+ĉ
+Ġ
+Ĵ
+ŕ
+Ŝ
+ǣ
+ɘ
+ɥ
+ʤ
+̗
+̽
+ͤ
+Ή
+Ό
+Ұ
+խ
+շ
+ڈ
+ڭ
+ݚ
+އ
+ދ
+ލ
+আ
+চ
+ষ
+਼
+ੈ
+ગ
+લ
+அ
+ீ
+ే
+ಠ
+ട
+ං
+ກ
+ດ
+༝
+ྷ
+Ⴈ
+ᄚ
+ᄡ
+ᅬ
+በ
+ᐃ
+
+
+↵
+⇑
+∓
+⊞
+⊢
+╮
+☚
+❑
+❜
+❧
+❾
+➌
+➦
+ⲥ
+㕎
+亗
+仼
+俞
+倨
+僀
+兊
+劓
+匆
+呴
+唳
+啣
+喂
+嗔
+囑
+垎
+埡
+埦
+堟
+墙
+夋
+夰
+妌
+姮
+姸
+愩
+憖
+扌
+掎
+搐
+撑
+擘
+斻
+暅
+暸
+杋
+杬
+棈
+棨
+楲
+樉
+歉
+歜
+殄
+沬
+浃
+浟
+淒
+湔
+滊
+潗
+烊
+熠
+燏
+爌
+牖
+犴
+玝
+璹
+甗
+砖
+穠
+穡
+糝
+綅
+纖
+翀
+胀
+苙
+茁
+荽
+菀
+萏
+蚋
+蛔
+衕
+衚
+襧
+谓
+賰
+贪
+輾
+逧
+鈀
+鈹
+鉎
+鍖
+鏟
+鐥
+闆
+隄
+靁
+韡
+頍
+颖
+饐
+饴
+駻
+驰
+鬃
+鯇
+鯪
+鲍
+麃
+ꕀ
+꜄
+ꪔ
+롱
+볼
+빼
+쁜
+섬
+싱
+였
+윈
+잇
+젤
+좌
+줘
+픈
+햏
+
+
+
+
+
+
+
+
+
+🇾
+🌌
+🍦
+🎖
+🎻
+🐓
+🐨
+👚
+📹
+🖊
+🖌
+😬
+🙊
+🚚
+🚧
+🥷
+🦩
+🧅
+𡈽
+𩃙
+ģ
+ŷ
+ȳ
+ɓ
+ʅ
+̏
+̰
+͐
+Ϙ
+϶
+Ѓ
+ђ
+Ҏ
+Ԃ
+ջ
+ռ
+։
+ֱ
+ؒ
+ٍ
+ڤ
+ܪ
+ݱ
+ુ
+ି
+୍
+ஜ
+హ
+ೂ
+ൃ
+ම
+ල
+ස
+ທ
+ໄ
+༒
+ཡ
+ྟ
+ྣ
+ဘ
+ီ
+ᅙ
+ᆺ
+ነ
+ይ
+ᐓ
+វ
+ᩪ
+ỗ
+Ἰ
+ὡ
+ῷ
+‗
+
+≃
+⌣
+⓶
+┯
+▏
+♰
+✗
+ⴳ
+ヸ
+ㄦ
+ㇲ
+ㇳ
+㒶
+仯
+侨
+俁
+债
+偪
+傻
+儍
+勹
+啥
+喔
+嚏
+圕
+垙
+垸
+夤
+奛
+妠
+媖
+孳
+宪
+寚
+尨
+尰
+崮
+幘
+幞
+弨
+徼
+惎
+惛
+慍
+戙
+扠
+扦
+扰
+擥
+斏
+旣
+曮
+杌
+杪
+枣
+栅
+棖
+棫
+楜
+櫝
+淥
+渲
+滘
+滚
+漵
+潍
+潡
+瀁
+灏
+燬
+爍
+爿
+犭
+玵
+瑝
+瓲
+睍
+祩
+秞
+竧
+簝
+粑
+縟
+繢
+繦
+绚
+绳
+罟
+耋
+聆
+聱
+膻
+舱
+芫
+蕋
+蕺
+諟
+豲
+贱
+赐
+赖
+跆
+跼
+蹦
+躪
+躲
+輶
+辶
+鄅
+鈸
+鏺
+鐩
+鑈
+钉
+锅
+闋
+闯
+陡
+靨
+頥
+饔
+驂
+驹
+骼
+髟
+鬴
+鮠
+鵩
+鶊
+鶙
+꒦
+넓
+놀
+둘
+럭
+룩
+몬
+묵
+센
+쉬
+슴
+써
+앗
+염
+잊
+젠
+좀
+즐
+징
+째
+
+
+
+𐤄
+🇿
+🌐
+🌤
+🎒
+🏮
+👄
+👒
+💒
+🗯
+😟
+🚓
+🚹
+🛡
+🥤
+🥴
+🧑
+🧢
+🩸
+𨫤
+ĥ
+Ħ
+ɖ
+ɷ
+ɽ
+̉
+̎
+ͩ
+ͭ
+ͼ
+ϣ
+ѓ
+ҷ
+Ә
+Ի
+ۈ
+۞
+ܠ
+ܡ
+ܢ
+ެ
+ޮ
+ஔ
+ట
+ూ
+ൻ
+ຕ
+ິ
+ྐ
+࿀
+ဆ
+ှ
+Ⴄ
+ჲ
+የ
+ደ
+ច
+ហ
+ḡ
+ẞ
+ằ
+∕
+⊖
+⓫
+╳
+▒
+▾
+◄
+☧
+♾
+⚕
+⚖
+⛴
+✫
+➵
+ⵙ
+ⵣ
+ㄕ
+㔟
+丧
+仜
+佽
+倛
+傌
+僔
+剴
+匜
+卌
+卐
+咁
+嘻
+噏
+嚩
+囤
+囵
+圯
+塱
+墘
+墿
+妒
+姗
+姤
+嬅
+嬭
+嬿
+嶹
+忆
+怰
+慵
+懒
+戝
+拧
+挈
+挰
+掵
+擣
+擤
+擻
+敓
+朞
+朰
+柠
+桛
+梆
+欞
+氪
+氫
+泱
+洿
+淛
+溏
+溘
+漚
+灿
+炏
+犲
+猰
+琡
+瑷
+甝
+皸
+盉
+盐
+睒
+矟
+碱
+礩
+禋
+糅
+紑
+緹
+纮
+羾
+翱
+耍
+肫
+臬
+芟
+莬
+莹
+萑
+蔻
+藿
+螗
+褂
+讞
+豷
+踈
+蹔
+轝
+辵
+邔
+邰
+鄡
+鈊
+鈦
+銆
+銙
+鍦
+鍰
+鑴
+钁
+闃
+闪
+闵
+闸
+阔
+靇
+鞁
+顙
+飭
+魷
+鰄
+鱮
+鶇
+麽
+黈
+鼕
+갸
+끮
+낙
+놈
+놓
+딘
+땅
+떡
+랩
+렇
+묘
+벨
+섰
+엑
+엽
+옛
+읍
+잉
+잔
+찮
+층
+튜
+
+
+
+
+﹆
+𓇬
+𖤣
+🇽
+🎺
+🏉
+👃
+💸
+🔁
+🔙
+🔱
+🕸
+😧
+🛒
+🛠
+🛫
+🥑
+🥪
+🦂
+🧖
+𢌞
+Ĉ
+Ď
+Ğ
+Ɔ
+Ư
+ɵ
+ˏ
+̅
+̔
+̘
+̿
+ͦ
+ϋ
+Ґ
+Կ
+ծ
+չ
+֤
+ٕ
+ۆ
+ܕ
+ܚ
+ݕ
+ݮ
+ސ
+ࠐ
+ࢹ
+ࣔ
+ও
+ড
+ৰ
+ન
+ભ
+ପ
+େ
+ಓ
+റ
+ັ
+໊
+༚
+ྤ
+ယ
+ჯ
+ᄁ
+ᄫ
+ᆰ
+መ
+ባ
+ዳ
+ᑦ
+ញ
+អ
+᷂
+ṓ
+Ọ
+ἱ
+ᾳ
+ᾶ
+ῃ
+
+
+₹
+₼
+⌃
+⌥
+⍢
+╥
+▯
+☿
+❎
+➅
+➆
+➽
+⸒
+〠
+ㄅ
+ㄏ
+ㄐ
+㺭
+䆃
+丛
+亓
+亼
+仃
+儇
+儰
+冐
+刨
+叆
+哗
+唜
+囥
+坖
+坭
+垜
+塬
+塰
+壶
+壾
+奯
+妁
+婂
+岾
+峇
+崀
+幂
+庐
+废
+怏
+恁
+恶
+悚
+惘
+慤
+扳
+捃
+搠
+攰
+攵
+敘
+旡
+晕
+晥
+晫
+柟
+栜
+桷
+楤
+楩
+楾
+槹
+橖
+橾
+欏
+殀
+氊
+沥
+洫
+浯
+涔
+滤
+漷
+澔
+濺
+炪
+焄
+猹
+獒
+獷
+珅
+珹
+瑨
+甎
+甩
+痼
+癢
+盍
+矚
+砵
+硴
+碽
+禳
+秌
+秕
+稾
+窨
+籹
+粔
+糍
+綉
+綣
+縠
+縰
+缽
+罧
+羖
+翃
+耰
+肃
+肿
+腩
+茭
+蒅
+蒗
+蔾
+蕖
+虏
+蛩
+袵
+襃
+詿
+諢
+謩
+譖
+误
+谄
+贰
+跂
+邓
+鄳
+錏
+鍥
+鐇
+闺
+阱
+霎
+驻
+魳
+鮄
+鰣
+鱘
+鷆
+鷙
+鸦
+鸿
+麂
+麞
+꼬
+꼭
+끌
+끗
+끝
+높
+렬
+롯
+셀
+앞
+엘
+왔
+죄
+츄
+큰
+킬
+틀
+틴
+
+
+
+
+
+
+
+
+🅱
+🆚
+🌖
+🌮
+🎑
+🎡
+🎦
+🏌
+🏓
+🏴
+🏵
+👅
+💑
+💼
+📋
+📗
+📧
+🔌
+🔑
+🚅
+🚑
+🚽
+🛵
+🤒
+🤢
+🦃
+🦠
+🧥
+🧹
+🪶
+🫐
+𠅨
+Ɓ
+Ǝ
+Ƶ
+Ʌ
+ʐ
+ʙ
+˄
+̕
+̠
+̧
+̬
+̾
+Ϛ
+Ї
+Ӏ
+Ӄ
+ӈ
+Բ
+Վ
+ۋ
+ܬ
+ހ
+߮
+ߴ
+࡞
+ਖ
+ਤ
+ਮ
+੍
+ੜ
+ઌ
+ે
+ା
+௵
+డ
+శ
+ొ
+ಣ
+അ
+ബ
+ീ
+ൺ
+
+ຂ
+ຒ
+ຫ
+່
+༗
+ཟ
+ཧ
+ྩ
+࿐
+၎
+Ⴀ
+ህ
+ና
+ዓ
+᎑
+ᐠ
+ᒃ
+ូ
+ᴍ
+ᵎ
+ḱ
+Ὀ
+ᾰ
+ῇ
+ῥ
+⌄
+⍵
+┥
+♊
+⚚
+⛔
+❋
+❍
+❫
+➸
+ⲡ
+ⲩ
+ⴱ
+⻁
+ㄌ
+ㄎ
+㶞
+㻙
+䑓
+䛒
+䴰
+乄
+乜
+仉
+仱
+俒
+偑
+偘
+傞
+冇
+叚
+呶
+喟
+嗩
+塏
+娄
+娰
+嫕
+尟
+屼
+嵙
+嶄
+巛
+庲
+廰
+弣
+恟
+悤
+悵
+慝
+懴
+抦
+捞
+揩
+攙
+敳
+斋
+旴
+曔
+枴
+柍
+椣
+榀
+槤
+槱
+橹
+檠
+歞
+殂
+毽
+氹
+沚
+洐
+洰
+涟
+涬
+湎
+溍
+溲
+溿
+漼
+濉
+瀇
+炁
+烦
+煐
+熸
+熿
+燄
+狦
+獯
+玗
+瑆
+瑊
+璪
+瓤
+瓿
+疃
+疐
+痳
+癋
+硈
+穷
+窳
+竫
+筎
+籲
+絺
+綮
+緤
+纶
+绅
+绵
+羝
+脰
+腌
+菷
+萓
+葅
+葊
+蔯
+蕞
+薜
+蛑
+螘
+螣
+蟳
+褘
+詖
+誐
+諗
+贐
+蹐
+軔
+轰
+迟
+迨
+逛
+遉
+遫
+郳
+醞
+鋐
+錰
+鍪
+鎏
+鎵
+鏥
+鏻
+鐞
+鐳
+靎
+靓
+鞖
+韛
+頟
+顷
+饱
+駉
+駼
+騄
+魞
+鰛
+鳏
+鵶
+鵼
+괜
+깊
+냐
+넌
+떤
+뚝
+뛰
+뜨
+랙
+몰
+몽
+밟
+뱅
+봅
+붉
+삐
+슨
+옹
+욕
+욥
+쪽
+챔
+춤
+킥
+혹
+
+
+
+
+
+
+
+
+𐤁
+𓀤
+𓀥
+🅰
+🌨
+🌪
+🍘
+🎠
+🎴
+🏐
+🐌
+💏
+💳
+📙
+🕵
+🚿
+🟢
+🥱
+Ĝ
+ĺ
+ŗ
+Ơ
+ƿ
+ǥ
+Ɂ
+ɗ
+ɝ
+ʈ
+ʍ
+ˉ
+͎
+ϟ
+ϫ
+Є
+ҟ
+ӡ
+Գ
+Խ
+Ո
+Տ
+֦
+ײ
+ؔ
+ވ
+މ
+ީ
+࣍
+ओ
+ॣ
+४
+ঢ
+৹
+৽
+
+મ
+વ
+૭
+ୁ
+ஷ
+
+జ
+ಂ
+ಛ
+ಷ
+ೀ
+ೃ
+ൂ
+ත
+හ
+ແ
+ཅ
+ཤ
+ཽ
+ဝ
+ყ
+ᅤ
+ᆸ
+ኤ
+ዊ
+ᐉ
+ឹ
+ើ
+᷀
+Ḡ
+ṳ
+ẖ
+ὢ
+
+‱
+‽
+
+⃜
+↖
+↻
+∔
+⊝
+⌋
+⍤
+⍨
+⓷
+▊
+◑
+☒
+☸
+♈
+♋
+✹
+❪
+➠
+ⲅ
+ⲣ
+ⵖ
+⻩
+ㄉ
+ㄑ
+ㄒ
+ㄜ
+ㄠ
+ㄩ
+㭿
+㮙
+㷩
+䛾
+䜌
+䞭
+䠰
+丢
+乇
+亏
+伖
+侔
+侲
+倐
+倱
+僲
+儐
+刅
+吮
+呮
+咺
+啽
+嗉
+嘅
+坟
+坶
+埂
+塨
+墈
+墬
+壗
+娓
+嫥
+尬
+峐
+彁
+彑
+徤
+怺
+戉
+拋
+拽
+捔
+捫
+摭
+撐
+擋
+攢
+攽
+敒
+敔
+斝
+旆
+旵
+暀
+曓
+栒
+桾
+椓
+椵
+樨
+歬
+氯
+泾
+淐
+渔
+滻
+澇
+澣
+珌
+珒
+珤
+琱
+畄
+畓
+畲
+畵
+疒
+瘂
+癶
+皕
+眛
+睔
+睚
+砍
+礵
+礻
+穭
+竏
+笃
+筷
+篊
+簶
+簺
+籖
+籘
+絙
+绔
+绲
+缰
+缲
+罸
+羑
+羮
+肠
+膕
+艟
+荆
+莜
+蔴
+蕅
+蕆
+蕘
+虒
+蚺
+蜡
+蜱
+蝤
+蟖
+衄
+袗
+觝
+譛
+讌
+谅
+谼
+赚
+趟
+轀
+轑
+辘
+逴
+邘
+鄗
+鄤
+酿
+鈁
+鈆
+鋓
+錟
+鍫
+鏜
+鏽
+鐨
+钮
+铝
+铠
+閧
+闚
+阎
+颂
+颤
+飘
+飰
+飳
+餢
+騅
+驌
+髁
+髫
+魲
+鮖
+鯒
+鯥
+鱅
+鱷
+鴸
+鴾
+鵂
+鵒
+鵷
+鶵
+鹅
+麬
+곶
+굿
+끧
+냆
+념
+닝
+델
+돼
+뒤
+듀
+땡
+맘
+멍
+멜
+밭
+벽
+봐
+뿐
+쌈
+압
+읒
+잖
+져
+쥬
+켓
+콘
+콩
+톡
+팅
+폭
+
+
+
+
+
+
+
+
+
+
+
+
+﨔
+ﬞ
+𐤏
+𒆠
+𓅿
+𖥦
+🇻
+🍿
+🎳
+🏪
+🏯
+🏷
+🐋
+🐜
+👛
+👺
+💹
+📓
+📶
+📿
+🔨
+🕛
+🕶
+🚋
+🚍
+🚏
+🚜
+🛑
+🤨
+🤿
+🥊
+🥋
+🦒
+🧟
+🧲
+🩹
+󠄃
+ĵ
+Ķ
+ƃ
+ǜ
+ʜ
+ʣ
+ʪ
+ˁ
+ˍ
+˔
+˧
+̜
+̟
+ͅ
+͇
+͝
+Ϣ
+Ѕ
+Ғ
+ҡ
+Ӈ
+ӯ
+Թ
+Յ
+ձ
+փ
+
+٠
+ځ
+ڹ
+ܦ
+ܩ
+݂
+ݩ
+ބ
+ޚ
+ࣝ
+९
+ঁ
+উ
+ঞ
+ফ
+৭
+
+પ
+ૅ
+ମ
+ஞ
+ஹ
+అ
+చ
+ష
+
+ೊ
+೨
+ണ
+ഹ
+ජ
+ඩ
+༥
+ཏ
+ཙ
+ཛ
+ྦ
+ྨ
+࿈
+ည
+ჩ
+ᅱ
+ᆪ
+ᆲ
+ሓ
+ሕ
+ረ
+ሳ
+ተ
+ዮ
+ድ
+Ꮀ
+Ꮃ
+ᐊ
+ᑐ
+ᑭ
+ᓄ
+ᖅ
+ះ
+័
+
+ᨰ
+ᴄ
+᷆
+᷇
+ḇ
+ḿ
+Ấ
+ẳ
+ἡ
+₴
+⇗
+⇸
+⌑
+⓯
+┷
+▌
+☦
+♓
+♞
+⛱
+⛲
+✙
+✷
+➈
+➉
+⨰
+ⵢ
+ヷ
+ㄋ
+ㄍ
+ㄓ
+ㄢ
+㬎
+㮈
+㶏
+䀰
+䂮
+䐗
+䔀
+䱱
+䳒
+丌
+丏
+乶
+亷
+仒
+仩
+佖
+佷
+侚
+偖
+偿
+傛
+儱
+凢
+刧
+劌
+劏
+勷
+卣
+叠
+咯
+咾
+哎
+唧
+唫
+嚚
+囪
+坮
+坰
+垏
+垚
+壅
+奩
+妛
+姘
+娵
+嬤
+孖
+尕
+尢
+尧
+屭
+岃
+岦
+峁
+峥
+崟
+巎
+幃
+庛
+庤
+庬
+廁
+抠
+拊
+挍
+挥
+掀
+掋
+掍
+搡
+摃
+擼
+旈
+旽
+晣
+曶
+枛
+梲
+梻
+椈
+榁
+槀
+槗
+橞
+橢
+櫆
+櫞
+欈
+欓
+氦
+氶
+涫
+淓
+湲
+溠
+溳
+潇
+炟
+炩
+烂
+烔
+焕
+熜
+燚
+燜
+犼
+猀
+猇
+猝
+珑
+珦
+瑍
+瑎
+瑑
+璒
+瓈
+甓
+甡
+畺
+痃
+瘩
+瘭
+眘
+眰
+睆
+睴
+矽
+碏
+祘
+稰
+窣
+窻
+笉
+笒
+笼
+筛
+箐
+簃
+簎
+籛
+粼
+糫
+綃
+纁
+纎
+纬
+纲
+纵
+缻
+翛
+脖
+腴
+芑
+茝
+茧
+菝
+萋
+萰
+葮
+蓏
+蔥
+蕚
+蘩
+蘸
+虍
+蝄
+螯
+裊
+詑
+詝
+諞
+譓
+讴
+诘
+诠
+谕
+谦
+谨
+賸
+趴
+辆
+迵
+遖
+邹
+鄟
+鄹
+酶
+錩
+鏅
+鐗
+鑡
+钦
+钩
+閊
+闄
+闫
+雏
+雱
+靗
+頔
+頖
+餛
+餜
+饯
+馋
+驯
+骏
+髃
+鮁
+鰀
+鷴
+麊
+麐
+黿
+齿
+龉
+龟
+ꜜ
+겐
+꾼
+끈
+냑
+녹
+둥
+뜰
+랭
+렴
+멀
+몸
+밍
+봤
+붕
+빅
+뻐
+삭
+샘
+섯
+셜
+쇼
+숭
+쏘
+쓸
+읏
+짱
+쨰
+측
+츰
+캠
+킨
+탐
+턴
+텍
+폰
+폴
+퐁
+풀
+홈
+
+
+
+
+
+
+
+
+
+
+
+
+𐤉
+𐤠
+𓆉
+𓍯
+🆔
+🌆
+🎰
+🏞
+🐐
+🐗
+👫
+👳
+🔋
+🔒
+🔭
+😼
+🛀
+🛋
+🛏
+🛸
+🟥
+🟩
+🤬
+🥁
+🥄
+🥾
+🦅
+🦵
+🧜
+🧤
+🧼
+𡗗
+𣘺
+𤯝
+𦠆
+𧏛
+𨂻
+𫭔
+Ą
+ċ
+Ɣ
+ȥ
+ɇ
+ɱ
+ʝ
+ʨ
+ʺ
+˟
+̒
+̙
+
+ΐ
+ϛ
+Ϻ
+ҙ
+Ң
+Ҳ
+Ҷ
+һ
+ӄ
+ӕ
+Ӡ
+Ӯ
+ԅ
+Ԍ
+Ծ
+Ճ
+Ռ
+ժ
+
+
+؟
+ٞ
+١
+ٱ
+ٻ
+ڙ
+ڠ
+ں
+܇
+ܗ
+ܥ
+݊
+ޖ
+ޫ
+ߟ
+ॐ
+०
+२
+
+ਂ
+ਘ
+ਦ
+ਵ
+ੁ
+ੂ
+સ
+ૂ
+૮
+କ
+ବ
+ଲ
+ଳ
+ஸ
+ொ
+ో
+ಚ
+ಶ
+ഗ
+ප
+๐
+ບ
+ཫ
+࿅
+ထ
+ဖ
+ါ
+ဲ
+Ⴑ
+ჭ
+ჱ
+ᅪ
+ᆴ
+ሪ
+ታ
+ኛ
+ኣ
+ዝ
+።
+Ꭳ
+Ꭴ
+Ꭶ
+Ꮉ
+ᐖ
+ᓱ
+ᕐ
+ᕕ
+ᴋ
+ᶍ
+ṉ
+Ẋ
+Ἄ
+ὅ
+Ῥ
+ῳ
+₣
+⃚
+↕
+↬
+⇥
+∏
+⊋
+╲
+▂
+▰
+☡
+☵
+♉
+♏
+♒
+⚗
+❉
+➇
+➟
+➼
+➿
+ⲕ
+ⲱ
+ⴷ
+⻨
+ㄈ
+ㄊ
+ㄗ
+ㄞ
+ㇹ
+㦃
+㫒
+㴀
+䈸
+亪
+伹
+倀
+倂
+僿
+儊
+刖
+劬
+勋
+勦
+呭
+咊
+唰
+嗑
+噹
+囍
+囹
+圮
+坠
+坯
+垡
+垤
+埱
+壋
+壔
+壡
+夐
+妘
+姼
+娡
+婄
+婭
+嫘
+嬈
+嬖
+孊
+寋
+崂
+崦
+崭
+嶈
+巩
+帔
+帘
+幗
+庱
+廱
+彏
+悾
+愡
+慓
+抃
+拄
+挀
+掔
+掫
+搰
+撳
+攖
+敻
+旸
+晈
+晊
+晌
+朅
+栝
+栬
+梉
+梥
+梹
+棼
+棽
+椶
+檫
+櫁
+殛
+泐
+洑
+渧
+湢
+漊
+澐
+濚
+灨
+烷
+燫
+猱
+玷
+玺
+珓
+琠
+璥
+畋
+畩
+疙
+癀
+皪
+眤
+矇
+矫
+砫
+碪
+磎
+礮
+礰
+祬
+禩
+穞
+穨
+窫
+笕
+笭
+筯
+箎
+篙
+簟
+簷
+糄
+紉
+紜
+絜
+繽
+纍
+纠
+纷
+绕
+绱
+缆
+缇
+耑
+耦
+耷
+聻
+胭
+臠
+舮
+艃
+艨
+芜
+茖
+荢
+荪
+莔
+菪
+葜
+蔰
+蕡
+蕴
+藔
+蘘
+蚀
+蚁
+蜗
+蜺
+蟣
+襾
+詎
+誴
+謊
+诈
+谩
+賍
+跚
+踠
+輇
+輰
+辇
+遀
+遄
+釩
+鉨
+鉹
+銼
+鎂
+鎳
+鏌
+鏧
+鑳
+镖
+閟
+陦
+靛
+韌
+韘
+韧
+韬
+頞
+顴
+飥
+餳
+饵
+饿
+馹
+驄
+驴
+驾
+骤
+鬍
+鮐
+鯈
+鯓
+鯧
+鰉
+鱟
+鲇
+鲤
+鳣
+鳫
+鸝
+黹
+齁
+齦
+龚
+ꨰ
+ꬰ
+굳
+끯
+냗
+닉
+닫
+닭
+둔
+렁
+렛
+륙
+멋
+몇
+믿
+밖
+빌
+뽕
+쇄
+숫
+얀
+젰
+졌
+짐
+척
+첨
+캔
+캡
+컨
+켰
+쿄
+퇴
+펌
+픰
+픽
+
+
+
+
+
+
+
+
+
+
+
+
+𐣣
+𐤅
+𑁍
+𓂅
+𓆛
+𓆝
+𖥣
+𖦹
+🍢
+🏟
+💲
+📄
+📒
+📜
+📨
+📯
+🔓
+🔦
+🕕
+🕰
+🖱
+🚁
+🚂
+🚇
+🚐
+🚕
+🚪
+🛳
+🛼
+🤶
+🤸
+🥓
+🥔
+🥭
+🦜
+🦤
+🧣
+🧷
+🧻
+🩰
+🪐
+𣝣
+𤘩
+𤝔
+𩸽
+𩹄
+Ę
+Ŗ
+Ů
+ƀ
+Ƕ
+Ƿ
+ǹ
+ȃ
+ȵ
+Ɍ
+ʞ
+˦
+͉
+͗
+ΰ
+Њ
+ґ
+Ҩ
+ҳ
+Һ
+ӝ
+ӣ
+ӥ
+ӧ
+Ԡ
+Ժ
+Ն
+Շ
+֛
+֬
+ֻ
+ٺ
+ټ
+ٽ
+ڪ
+ێ
+ې
+
+ۯ
+ܒ
+ܭ
+ܰ
+ކ
+ޙ
+ߏ
+ࡍ
+
+ࣄ
+࣋
+ऋ
+ऽ
+ॉ
+७
+ঌ
+ঝ
+ਓ
+ਟ
+ਫ
+ੇ
+ੋ
+ં
+૦
+ଶ
+ಇ
+ಈ
+ಒ
+ಹ
+ೝ
+ജ
+ഷ
+േ
+ൠ
+ග
+ච
+ව
+
+ී
+ේ
+ො
+๛
+ຍ
+ຣ
+ູ
+ໍ
+
+༰
+༷
+༼
+༽
+ཨ
+ཱ
+ྭ
+Ⴅ
+Ⴢ
+ჿ
+ᄄ
+ᄈ
+ᅇ
+ᆬ
+ᆱ
+ሊ
+ሞ
+ሱ
+ቅ
+ቢ
+ኢ
+ᐅ
+ᑋ
+ᑎ
+ᒥ
+ᕗ
+ᕙ
+ឈ
+ណ
+ៃ
+៉
+ᠰ
+ᡠ
+ᯅ
+ᴡ
+Ḏ
+Ḳ
+ỡ
+ἁ
+ἑ
+ἕ
+Ἑ
+ὔ
+Ὑ
+ᾱ
+↺
+⇌
+⇝
+⇣
+∿
+⊊
+⌖
+⏹
+⓬
+⓸
+┸
+╎
+╩
+╬
+╶
+◐
+☩
+♌
+♍
+♐
+♘
+⚧
+⛎
+⛑
+❙
+➍
+➙
+➩
+⧉
+⧼
+⧽
+ⲏ
+ⲗ
+ⴀ
+ⴽ
+ⵕ
+⻯
+ㄆ
+ㄔ
+ㄝ
+ㄟ
+ㄤ
+㝅
+㝎
+㟁
+㠀
+㮾
+䄙
+䉼
+䌫
+䍺
+䛿
+䤰
+䨰
+丄
+丅
+丱
+乀
+乛
+乽
+仢
+仨
+伩
+伾
+佴
+佸
+侒
+俍
+俩
+倮
+偀
+偅
+偡
+偢
+傢
+傤
+僎
+兑
+冱
+冻
+凑
+刂
+剁
+剕
+劦
+叨
+叻
+吓
+吨
+吱
+呜
+呸
+咙
+咦
+咧
+咭
+哒
+哟
+哤
+唭
+啝
+噉
+噩
+嚜
+嚷
+坙
+垀
+垐
+垒
+垔
+垞
+垵
+埰
+墠
+夯
+姁
+娲
+媿
+嫊
+嫚
+嫠
+嫽
+嬥
+嬫
+孓
+宷
+屶
+岼
+嵕
+嵫
+嵶
+嶐
+嶓
+嶴
+廔
+廴
+彀
+忄
+忭
+怤
+怫
+恔
+恧
+悎
+悱
+惓
+惷
+愎
+愭
+慜
+慴
+戬
+扑
+抳
+拕
+拚
+拨
+挚
+捡
+掇
+揟
+揵
+擐
+攅
+攩
+敺
+斈
+昖
+昿
+晜
+朿
+杩
+枎
+柀
+柧
+柰
+柷
+柹
+栍
+栘
+桄
+梍
+梣
+棓
+棰
+棳
+楉
+榼
+槑
+槝
+槢
+樏
+樮
+檞
+檟
+欑
+欨
+欶
+歊
+歖
+殕
+殽
+氅
+氨
+氰
+沦
+泜
+泼
+洊
+浇
+浼
+淍
+淕
+湏
+湦
+溉
+滳
+漩
+漻
+潕
+澌
+澥
+濳
+灈
+炵
+焗
+焿
+熯
+熻
+燴
+燹
+牕
+牨
+犰
+猡
+珛
+珵
+瑈
+璲
+瓙
+痫
+瘜
+瘸
+瘾
+癫
+眥
+睠
+睽
+瞟
+瞶
+矠
+砭
+砰
+祸
+禘
+禟
+秺
+稞
+竖
+笶
+筇
+筴
+篔
+簏
+籥
+粄
+紓
+紩
+縨
+繎
+绊
+绎
+绹
+缤
+耉
+聂
+聢
+肣
+膁
+臊
+芚
+苒
+茈
+荟
+菉
+菔
+菼
+蓯
+蔫
+蕕
+薳
+薷
+蚴
+蛰
+蜢
+蟎
+蠆
+蠇
+袠
+袪
+褊
+襍
+觮
+觿
+誁
+讶
+诛
+谐
+豅
+豔
+贒
+赋
+蹬
+軏
+軣
+輨
+迠
+逶
+逷
+遨
+酖
+醃
+鉔
+鉶
+鉺
+銌
+銐
+銶
+鋋
+鋿
+錵
+鍘
+鏁
+鐣
+鐼
+鑌
+鑕
+钊
+钙
+铐
+镰
+閮
+閽
+闟
+闽
+阼
+隉
+隞
+霈
+靹
+韁
+颏
+颗
+飪
+餒
+餺
+餻
+饺
+馅
+駃
+騊
+騑
+騠
+騧
+鬰
+鬷
+鯎
+鰔
+鰩
+鱛
+鲸
+鳲
+鶖
+鶮
+鷰
+鼒
+齏
+龌
+ꂽ
+ꕤ
+Ꙭ
+Ꝏ
+ꪝ
+ꫀ
+ꫛ
+갱
+겹
+괰
+굉
+깍
+꺼
+뀍
+낮
+냚
+냼
+넙
+뇌
+돗
+될
+딱
+떻
+랐
+략
+럴
+렉
+룸
+릴
+맙
+맵
+묻
+밴
+벼
+뷔
+액
+옰
+윌
+융
+윽
+읃
+읖
+잎
+잭
+쥐
+쬰
+챠
+칸
+쾌
+쿨
+퀴
+킴
+톤
+툰
+틈
+팡
+펜
+헬
+혰
+흑
+
+
+
+
+
+
+
+
+
+
+
+
+
+
+
+
+
+
+
+﨏
+﨤
+𐣬
+𐤀
+𐤭
+𓅰
+𓆜
+𓆡
+𛁈
+𝄆
+🇱
+🌄
+🌒
+🌔
+🏎
+🏸
+🐀
+🐅
+👬
+💵
+📃
+📉
+🔬
+🕢
+🕹
+🗡
+🚉
+🚖
+🛌
+🤌
+🥎
+🥯
+🦴
+🧁
+🧎
+🧏
+🧙
+🧛
+🧠
+🧶
+🫖
+𠭯
+𣉙
+𣗄
+𤁒
+𤣾
+𦚰
+𧶛
+
+Ė
+Ĥ
+Ŕ
+Ť
+ƪ
+Ǧ
+ǯ
+Ǵ
+Ȁ
+Ȏ
+ȏ
+ȶ
+Ƀ
+ʭ
+˒
+˛
+˩
+ˮ
+͊
+ϙ
+Ϸ
+Ѐ
+Џ
+џ
+ѣ
+Ѵ
+ѷ
+҉
+Ҭ
+Ҹ
+ҽ
+ӌ
+ӗ
+Ӣ
+Ӭ
+Ӷ
+ԉ
+ԍ
+Ւ
+Օ
+֥
+ؚ
+ؾ
+٤
+ٹ
+ٿ
+ڞ
+۾
+ܓ
+ܟ
+ݶ
+ށ
+ގ
+ޔ
+
+߂
+ࠨ
+ࡏ
+࣓
+ऀ
+५
+६
+ঘ
+ছ
+ঠ
+ৃ
+
+৾
+ੌ
+੨
+ੴ
+
+અ
+ખ
+ચ
+ટ
+ષ
+હ
+ઽ
+િ
+ଖ
+ଡ
+ନ
+ସ
+
+உ
+
+
+ఌ
+ఠ
+ణ
+ళ
+ౡ
+౯
+
+ಉ
+ಧ
+ೄ
+ೈ
+
+
+ഇ
+ഖ
+ഴ
+ശ
+ർ
+ඞ
+෯
+ෳ
+ฌ
+๙
+ຄ
+ຼ
+ຽ
+ཉ
+ྔ
+࿁
+ဍ
+ဒ
+ဟ
+ၚ
+Ⴃ
+Ⴇ
+Ⴊ
+Ⴐ
+Ⴒ
+Ⴕ
+Ⴡ
+ᅰ
+ᅴ
+ᆳ
+ᆵ
+ᆻ
+ሥ
+ሴ
+ሾ
+ቀ
+ቆ
+ቶ
+ች
+ዕ
+ጅ
+ጵ
+ጽ
+ፈ
+ፍ
+Ꭹ
+Ꮝ
+ᐜ
+ᐧ
+ᑕ
+ᑲ
+ᓐ
+ᓚ
+ᗔ
+ᗢ
+ᘏ
+ᚱ
+ᛏ
+ᛠ
+ᜊ
+ឋ
+ថ
+ផ
+ឡ
+ៀ
+ᠨ
+ᠯ
+ᡥ
+ᡳ
+ᴉ
+ᴊ
+ᴠ
+ẋ
+ẍ
+ẫ
+Ẽ
+Ệ
+Ự
+Ἁ
+ἵ
+ὕ
+ὖ
+ὦ
+ὲ
+₥
+⃕
+ↂ
+ↈ
+∣
+∸
+≀
+≓
+≺
+⍛
+⏋
+⏪
+⓭
+⓮
+⓰
+▔
+▭
+☇
+♆
+♟
+⛏
+✥
+✸
+✺
+➗
+➪
+⟦
+⟧
+⟼
+⠒
+⠔
+⠰
+⥲
+⧫
+⬰
+ⵡ
+⸌
+⸍
+⸘
+⻆
+⻲
+〲
+ゖ
+ㄖ
+ㄛ
+
+ㇶ
+㐮
+㖨
+㤄
+㤰
+㬢
+㭽
+㶟
+㺪
+䄉
+䅎
+䐀
+䡄
+䤀
+䰀
+䵟
+亖
+亵
+仑
+仛
+伆
+伕
+伞
+伡
+佣
+佦
+佭
+俌
+倏
+倔
+倘
+傂
+僡
+僩
+儏
+儜
+兹
+凳
+剮
+剼
+勫
+勮
+卄
+卋
+卮
+厧
+厷
+叄
+叵
+吀
+吅
+呯
+呾
+唼
+啧
+啲
+嗗
+嗛
+嘈
+噗
+噦
+嚻
+圴
+坞
+坼
+垊
+垛
+垦
+埏
+埿
+堙
+堧
+堨
+墎
+墡
+墭
+奡
+奰
+姹
+娈
+娴
+婥
+媱
+寃
+屜
+屨
+屺
+岉
+岜
+岪
+崒
+嶍
+帋
+廑
+弎
+弸
+彛
+忡
+恼
+悕
+惆
+惩
+惴
+愀
+愮
+懗
+戇
+戫
+扃
+扔
+扜
+抂
+挣
+挧
+掐
+攚
+敉
+旟
+昃
+暆
+暋
+暟
+暱
+杅
+杕
+枥
+桦
+桱
+桵
+梀
+梈
+棯
+椊
+楗
+楱
+榄
+榿
+樕
+橆
+橰
+檯
+櫔
+櫤
+櫶
+歂
+殓
+殮
+汔
+汳
+沍
+沧
+沶
+泒
+洤
+洳
+淲
+湒
+湙
+湭
+湰
+溙
+溮
+溵
+溽
+漴
+潪
+潬
+灋
+炗
+炡
+烩
+烯
+烴
+焃
+熥
+燋
+爮
+牾
+犎
+狃
+狖
+狥
+狳
+猓
+獙
+玞
+玮
+玼
+珄
+珘
+璀
+璅
+瓱
+甅
+畎
+畛
+畾
+瘕
+盻
+眯
+瞩
+瞫
+矦
+矸
+硜
+硨
+硶
+磌
+磤
+磽
+礚
+礿
+祊
+祤
+祫
+竸
+笆
+笫
+籁
+籩
+糇
+糉
+糬
+糵
+紝
+綆
+綈
+綗
+緌
+緭
+縈
+縐
+縶
+繀
+纊
+纑
+纺
+绛
+绢
+绥
+绰
+绸
+羗
+翯
+耸
+胰
+脿
+腻
+膛
+臞
+艌
+苆
+苯
+苽
+茷
+荇
+荎
+荞
+荵
+荸
+蒓
+蒪
+蒾
+蔞
+蔸
+薲
+藘
+藟
+虖
+蚫
+蚰
+蛼
+蛽
+蝚
+蝱
+螃
+蠁
+蠋
+衂
+衔
+衤
+褙
+襌
+襜
+觧
+觱
+訷
+詗
+譟
+譡
+譧
+讘
+诲
+谚
+豦
+豻
+豽
+貙
+赘
+赝
+赠
+趯
+趵
+跻
+踧
+蹀
+蹣
+躉
+軆
+軿
+輏
+迁
+迈
+逭
+邟
+鄽
+鄾
+醺
+釛
+鈯
+鉡
+鉵
+銨
+鋗
+錀
+錥
+錱
+鍟
+鍠
+鎯
+鎸
+鏊
+鏋
+鐮
+鐲
+钥
+锟
+锣
+镶
+閰
+阆
+霭
+靆
+靬
+鞢
+顳
+颇
+颠
+颷
+飃
+餑
+餼
+餽
+饁
+饍
+駔
+駹
+騂
+驶
+骞
+鬟
+鬹
+魣
+魵
+鮅
+鮏
+鯆
+鰧
+鱩
+鱵
+鱺
+鶆
+鶍
+鶤
+鶹
+鸊
+鸖
+鸢
+黚
+黷
+鼰
+ꐰ
+ꓷ
+
+ꞌ
+꠰
+갤
+걷
+곰
+곱
+긋
+긍
+깃
+꽁
+끫
+납
+낭
+냉
+넉
+늦
+닌
+닷
+댊
+댓
+덜
+덟
+됩
+땐
+똥
+렘
+뭐
+뭔
+믹
+벳
+븐
+쀣
+삽
+샌
+섹
+셋
+쇠
+썸
+씽
+앱
+앵
+엣
+욼
+읓
+젖
+쥔
+즘
+쩌
+촬
+춰
+큐
+톱
+팝
+퓨
+핀
+핵
+핻
+혈
+홀
+흡
+
+
+
+
+
+
+
+
+
+
+
+
+
+
+
+
+
+
+
+
+
+
+
+
+
+
+
+𐌂
+𐣥
+𐤋
+𐤌
+𐤍
+𐤓
+𒀝
+𓆞
+𓇋
+𓇠
+𓊆
+𓊇
+𖠚
+𖧧
+𛀁
+🅘
+🆓
+🆙
+🌉
+🍟
+🏜
+🏨
+🐂
+🐆
+🐊
+👥
+👪
+💾
+📂
+📔
+📡
+📰
+🔘
+🕋
+🖍
+🚝
+🚥
+🚭
+🚮
+🛐
+🛩
+🟌
+🟨
+🤕
+🤮
+🤴
+🦦
+🦳
+🦸
+🦺
+🧒
+🧺
+🩱
+🩲
+🩺
+𠊛
+𠵇
+𡶌
+𢧳
+𢸍
+𤝗
+𤳆
+𥻨
+𦥃
+𨁂
+𩑛
+Ċ
+Ŧ
+Ũ
+Ű
+Ų
+ƍ
+Ɩ
+Ʒ
+ǖ
+ǟ
+ȉ
+Ȑ
+ȟ
+ȡ
+ȷ
+ɍ
+Ɏ
+ɭ
+ɮ
+ɼ
+ɿ
+ʇ
+ʓ
+ʢ
+ʮ
+ʯ
+˕
+˝
+̢
+̺
+͌
+ͧ
+ͪ
+
+Ϗ
+Љ
+Ћ
+Ѫ
+ѵ
+Ѻ
+Ҕ
+ҕ
+җ
+Ҝ
+ҝ
+Ҟ
+Ҡ
+ҥ
+ҧ
+Ҫ
+ҫ
+ҭ
+ӏ
+ӛ
+Ӵ
+ӿ
+Ԁ
+ԁ
+Ԉ
+Ԕ
+ԝ
+ԡ
+Դ
+Է
+Ձ
+Պ
+Ց
+՛
+ճ
+ֆ
+ֈ
+֖
+ֳ
+־
+װ
+ٔ
+٢
+٣
+ٴ
+ڂ
+ڑ
+ږ
+ګ
+ڬ
+ڼ
+۬
+܁
+ܙ
+ܧ
+ܲ
+ܳ
+ܵ
+ܾ
+݅
+݇
+ݧ
+ݴ
+ݸ
+ޤ
+ޥ
+߁
+ߢ
+߬
+߰
+ࡱ
+ࢀ
+࣭
+ࣾ
+ऊ
+ऐ
+औ
+ॏ
+থ
+ৌ
+ৎ
+৮
+৳
+৴
+৺
+ਅ
+ਭ
+੧
+੯
+ੳ
+
+
+ડ
+ધ
+શ
+
+ો
+
+ଗ
+ଞ
+ଟ
+ଦ
+ୀ
+୭
+భ
+ృ
+
+౦
+౨
+ಅ
+എ
+ധ
+ഫ
+ഭ
+ൈ
+ൊ
+ോ
+൧
+ආ
+ශ
+෮
+๔
+ຈ
+ື
+ຸ
+༅
+༓
+༘
+༡
+༩
+ཇ
+ཥ
+࿒
+ဂ
+ဇ
+ဉ
+၈
+Ⴌ
+Ⴥ
+ᄍ
+ᄝ
+ᄬ
+ᅗ
+ᆡ
+ሬ
+ሮ
+ሲ
+ሿ
+ቡ
+ኑ
+ኒ
+ኦ
+ኩ
+ዋ
+ዲ
+ዴ
+ጋ
+ጣ
+Ꮑ
+Ꮲ
+ᐈ
+ᐤ
+ᐦ
+ᐰ
+ᐳ
+ᑯ
+ᓇ
+ᓗ
+ᓴ
+ᔰ
+ᕋ
+ᕿ
+ᖏ
+ᖴ
+ᗖ
+ᘰ
+ᛅ
+ᛉ
+ᛋ
+ᛒ
+ឌ
+៌
+។
+ᠡ
+ᠣ
+ᠤ
+ᠩ
+ᠪ
+ᠬ
+ᠷ
+ᡝ
+ᡵ
+ḙ
+ṕ
+Ả
+Ậ
+Ứ
+Ἔ
+ἠ
+ἤ
+Ἡ
+ὠ
+᾽
+⁊
+⁓
+⁞
+₫
+₷
+₺
+₽
+₿
+⃓
+℞
+℮
+↟
+↴
+≌
+≑
+⌈
+⌨
+⍣
+⎄
+⏨
+⏯
+⏲
+⓼
+┅
+┖
+╃
+╚
+╝
+╠
+░
+▵
+◅
+☊
+☍
+☥
+♎
+♜
+⚯
+⛈
+⛓
+⛪
+❈
+➧
+⤹
+ⰰ
+ⲃ
+Ⲇ
+Ⲡ
+ⲭ
+ⴹ
+ⴼ
+ⵄ
+ⵒ
+ⵗ
+ⵛ
+⸀
+〮
+〴
+〷
+ㄙ
+ㇴ
+㙔
+㚟
+㤤
+㥠
+㫤
+㬰
+㯮
+㷖
+㻌
+㻖
+㽣
+䁼
+䄰
+䅣
+䅳
+䔰
+䗪
+䝧
+䞉
+䣖
+䤻
+䥎
+䥫
+䩎
+䭹
+䱔
+䱙
+䱢
+䱷
+䵍
+䵣
+丂
+丟
+丷
+乁
+乒
+乚
+俦
+倁
+倊
+倽
+偊
+偌
+偍
+偒
+偔
+偟
+偤
+偫
+傈
+傏
+僌
+僘
+僬
+僱
+僳
+儃
+儩
+儭
+儯
+儹
+兘
+兟
+兤
+凴
+刔
+刵
+剜
+劅
+劵
+勪
+勬
+勯
+匊
+匦
+卤
+吿
+呿
+咚
+哖
+哢
+哷
+哼
+哿
+啸
+喿
+嗒
+嗓
+嗕
+嗙
+嗯
+嗹
+嘪
+嘹
+噐
+嚌
+嚐
+嚝
+嚠
+嚨
+囟
+囩
+囻
+坜
+坣
+坥
+坽
+埀
+埧
+埬
+堞
+堹
+壎
+壚
+夨
+奓
+奱
+妧
+姟
+姯
+姵
+姾
+娆
+婅
+婇
+婊
+媹
+媺
+嫵
+嬁
+嬙
+孌
+孥
+寁
+寲
+尒
+尷
+屘
+屚
+岄
+岊
+岏
+岺
+嵭
+嶙
+嶦
+巼
+庎
+庣
+庳
+廧
+彆
+彇
+彔
+忚
+忛
+恷
+恿
+悃
+悓
+悗
+悯
+惋
+愐
+愤
+慬
+慿
+戣
+戧
+扢
+抯
+挘
+挝
+挼
+捂
+掕
+揪
+摟
+擀
+擷
+攏
+敫
+斄
+旙
+昮
+昷
+晭
+曀
+曐
+曟
+曪
+杈
+杒
+杔
+枅
+枰
+栈
+栳
+桅
+桫
+桭
+桯
+桼
+梄
+梜
+棆
+椳
+楘
+榖
+榣
+榥
+榪
+槛
+槪
+槳
+樻
+橏
+檑
+櫬
+欵
+歒
+歵
+歼
+殐
+殫
+殹
+毊
+毡
+毣
+氍
+氘
+氤
+氺
+汋
+泂
+泅
+泆
+泖
+泟
+洢
+洣
+洶
+浊
+浘
+浰
+涡
+涧
+渀
+渶
+湨
+湽
+溴
+滆
+滍
+滥
+漈
+漒
+潥
+潨
+濔
+灟
+炲
+烛
+烬
+焞
+熀
+熞
+熼
+爚
+爫
+牬
+牸
+牺
+犌
+犕
+犾
+狨
+獢
+玜
+玧
+珫
+珷
+琄
+琙
+琭
+瑅
+璂
+璄
+瓒
+瓨
+瓺
+畀
+畈
+畨
+疁
+痎
+痧
+痹
+瘈
+瘖
+癮
+癯
+盋
+眴
+睇
+睞
+瞍
+矱
+砑
+硃
+碵
+磈
+磕
+磲
+礠
+祋
+祔
+祧
+祯
+禝
+禵
+秢
+稣
+稺
+穧
+窬
+竓
+竢
+笡
+笪
+笱
+筭
+箘
+箺
+簰
+簹
+籮
+籰
+粪
+糙
+糢
+糰
+紿
+絏
+綁
+綍
+綖
+緈
+緦
+縚
+繯
+绶
+绻
+缼
+罇
+羼
+翖
+翮
+翾
+耇
+耏
+肮
+肾
+胅
+腨
+膰
+艚
+芇
+芲
+茀
+荄
+荈
+莂
+菎
+菶
+萁
+萣
+萴
+葈
+葰
+葶
+蓟
+蓽
+蔐
+蔷
+蕀
+蕐
+蕫
+蘡
+蚉
+蛐
+蛱
+蛺
+蜇
+蝍
+蝲
+螄
+螈
+螉
+螾
+蠐
+蠙
+蠮
+蠲
+衩
+袚
+裆
+褝
+褭
+襅
+觅
+觭
+觶
+訔
+詇
+詘
+詼
+謜
+譮
+讟
+讠
+诵
+谥
+豼
+貒
+貭
+賧
+賳
+賵
+賹
+贾
+赙
+赣
+趫
+跿
+踆
+踩
+踶
+蹩
+蹭
+躔
+輭
+輽
+遘
+郚
+郻
+鄋
+鄨
+酡
+酴
+醁
+醻
+醿
+釥
+鈅
+鈉
+鈼
+鉒
+鉘
+鉜
+鉠
+鉭
+鉻
+鋃
+鋅
+鋙
+鋧
+鍄
+鍞
+鍤
+鍹
+鎛
+鎲
+鏙
+鏦
+鏸
+鐓
+鐰
+鑸
+鑻
+钓
+钞
+钧
+锉
+锚
+锭
+閄
+閈
+閠
+閡
+閴
+閸
+闀
+闹
+阨
+隤
+隽
+雊
+霤
+霬
+鞗
+韺
+頣
+颈
+飠
+餈
+餔
+饨
+馈
+馯
+骛
+骭
+魬
+鮚
+鮞
+鮱
+鮺
+鯿
+鰙
+鰼
+鱊
+鱢
+鱣
+鱥
+鱨
+鱪
+鲨
+鳌
+鳞
+鴅
+鴋
+鴝
+鵮
+鶁
+鶃
+鷚
+鸓
+鸽
+鹻
+麯
+鼏
+鼗
+鼷
+齾
+龊
+ꍇ
+ꐦ
+꒫
+꒵
+ꔰ
+꙼
+겁
+겉
+곁
+굻
+귿
+깔
+깝
+깡
+꼰
+꿍
+꿔
+뀁
+끨
+낀
+낄
+낌
+낰
+냝
+넨
+넷
+녁
+늑
+닿
+댕
+듣
+떼
+똰
+뚱
+뜬
+렸
+뢰
+룰
+룹
+륭
+릿
+맑
+멈
+멘
+멤
+멸
+몃
+뮺
+밝
+뱀
+벗
+벛
+볶
+붓
+붙
+빔
+빨
+뽀
+뽐
+뿔
+쀰
+쁘
+삶
+샛
+셉
+셨
+숲
+쐰
+씀
+씰
+씹
+옴
+왼
+웠
+윙
+읗
+잃
+잣
+쟈
+젊
+쫑
+쯤
+촉
+촰
+춥
+칭
+캑
+콕
+쿤
+킩
+탣
+텨
+팩
+펀
+펙
+핫
+핸
+햇
+헨
+홉
+흔
+흙
+힐
+힙
+
+
+
+
+
+
+
+
+
+
+
+
+
+
+
+
+
+
+
+
+
+
+
+
+
+
+
+
+
+
+
+
+
+
+
+
+
+
+
+
+
+
+
+
+
+
+
+
+
+
+
+
+
+
+
+
+︡
+﹅
+𐊫
+𐎠
+𐣠
+𐣩
+𐣫
+𐣭
+𐣮
+𐤦
+𐭣
+𐭥
+𐭩
+𐭭
+𐰼
+𐱅
+𐳀
+𒁺
+𒂊
+𒂗
+𒄑
+𒈗
+𒊩
+𓀂
+𓃠
+𓅮
+𓆟
+𓍲
+𓎤
+𓏤
+
+
+𖤐
+𛂋
+𝄞
+🃏
+🅞
+🅷
+🆁
+🆘
+🇼
+🌓
+🌡
+🌥
+🌫
+🎗
+🎭
+🏣
+🏦
+🏺
+🐞
+👞
+🔜
+🔳
+🕝
+🕳
+🖨
+😦
+🙉
+🚆
+🚔
+🛰
+🟣
+🤾
+🥥
+🥨
+🥻
+🦏
+🦙
+🦥
+🦰
+🦾
+🧃
+🧄
+🧩
+🧬
+𠀑
+𠘑
+𠩤
+𠮭
+𠲖
+𡨸
+𡶀
+𡺸
+𢇲
+𣝅
+𤅷
+𤋮
+𤏸
+𤟱
+𤦇
+𥖧
+𦧟
+𨏩
+𨨗
+𨱛
+𨻻
+𫞬
+
+
+󠄄
+
+
+
+ĸ
+Ŋ
+Ƅ
+ƅ
+ƈ
+Ɖ
+ƌ
+Ƒ
+Ɠ
+Ɨ
+Ƙ
+ƛ
+Ɯ
+ƨ
+ƭ
+Ʊ
+ƴ
+ƶ
+Ƹ
+ƽ
+Ǥ
+Ǫ
+Ǿ
+Ȋ
+Ȓ
+Ɉ
+ɏ
+ɠ
+ɰ
+ʄ
+ʩ
+ʫ
+͆
+ͥ
+ͮ
+
+ͺ
+Ώ
+Ϊ
+Ϡ
+ϸ
+Ѣ
+ѥ
+ѧ
+ѫ
+Ѱ
+ҁ
+҂
+Ҍ
+Җ
+Ҙ
+Ҥ
+Ҧ
+Ҵ
+Ҽ
+Ӆ
+Ӊ
+ӊ
+ӓ
+Ӗ
+Ӝ
+Ӟ
+Ӥ
+ӱ
+Ӳ
+ԃ
+Ԟ
+ԥ
+Ԩ
+ԭ
+Լ
+Չ
+֠
+֣
+֮
+׃
+ׇ
+
+
+
+
+؆
+؊
+ؕ
+ػ
+ٛ
+٬
+ٮ
+ٰ
+ڄ
+ڕ
+ښ
+ڨ
+ڵ
+ڻ
+ۀ
+ۂ
+ۉ
+ۍ
+ۖ
+ۗ
+۳
+ܞ
+ܣ
+ܤ
+ܶ
+ܽ
+
+ݙ
+ݠ
+ޅ
+ޝ
+ޭ
+߆
+ߊ
+ߩ
+߫
+߽
+ࠀ
+ࠔ
+࠰
+࠶
+࠷
+ࡌ
+ࡐ
+ࡑ
+ࡶ
+ࡾ
+ࢉ
+
+ࢽ
+ࣗ
+ࣸ
+॓
+॔
+३
+८
+ॲ
+ॳ
+ॺ
+ॿ
+
+
+০
+
+ਝ
+ਥ
+
+੦
+ઃ
+છ
+ઞ
+ણ
+ૉ
+
+ଂ
+ଊ
+ଌ
+
+ଙ
+ଜ
+ଣ
+ଵ
+଼
+ୟ
+ୠ
+
+ஃ
+ஈ
+எ
+ஏ
+
+ఉ
+ఋ
+ఖ
+ఘ
+థ
+ధ
+ఫ
+ై
+
+౿
+ಘ
+ಫ
+
+
+ೖ
+
+೫
+
+ഃ
+ഈ
+ഉ
+
+ഒ
+
+ൽ
+උ
+ධ
+බ
+ඹ
+
+ළ
+
+ැ
+ෙ
+ෝ
+෦
+๗
+๘
+ຖ
+
+༊
+༏
+༺
+ཝ
+ྕ
+ྜ
+ྶ
+ဈ
+ဓ
+၁
+၂
+၃
+၇
+ၠ
+Ⴂ
+Ⴉ
+Ⴋ
+Ⴎ
+Ⴓ
+Ⴔ
+Ⴗ
+ჟ
+ჰ
+ჳ
+ᄢ
+ᄶ
+ᅎ
+ᅘ
+ᅜ
+ᅫ
+ᆮ
+ᆽ
+ᇂ
+ሀ
+ሁ
+ሌ
+ሐ
+ሩ
+ሻ
+ቐ
+ቤ
+ቦ
+ቱ
+ቲ
+ኃ
+ኖ
+ከ
+ወ
+ዘ
+ጠ
+ጥ
+ጨ
+ጫ
+ፀ
+ፐ
+Ꭲ
+Ꭷ
+Ꭺ
+Ꭻ
+Ꭼ
+Ꭿ
+Ꮆ
+Ꮋ
+Ꮛ
+Ꮢ
+Ꮧ
+Ꮭ
+Ꮳ
+Ꮴ
+ᐕ
+ᐥ
+ᐯ
+ᐸ
+ᒍ
+ᒧ
+ᒪ
+ᓀ
+ᓂ
+ᓦ
+ᓽ
+ᓾ
+ᔨ
+ᔪ
+ᕇ
+ᕝ
+ᕯ
+ᕱ
+ᕷ
+ᗗ
+ᘔ
+ᚠ
+ᚦ
+ᚾ
+ᛁ
+ᛚ
+ᛞ
+ឧ
+ួ
+ឿ
+ៅ
+៏
+០
+១
+៧
+៩
+ᠭ
+ᠮ
+ᠳ
+ᠴ
+ᠵ
+ᠶ
+ᡟ
+ᡤ
+ᩖ
+᯽
+ᴢ
+ᵻ
+ḃ
+ḋ
+Ḵ
+ḹ
+Ṙ
+ṙ
+ṟ
+ẵ
+Ế
+Ồ
+Ớ
+Ở
+ἂ
+Ἅ
+ἶ
+Ὄ
+Ὅ
+
+ᾥ
+ῐ
+
+
+⁃
+⁒
+⁝
+
+₢
+₦
+₾
+⃤
+ⅎ
+ↁ
+ↇ
+↝
+⇀
+⇈
+⇘
+⇙
+⇪
+≙
+≢
+⊈
+⊔
+⊧
+⊨
+⊲
+⋄
+⋙
+⌔
+⎸
+⎹
+⎾
+⏏
+┍
+◓
+☋
+☙
+☨
+☬
+☲
+☷
+♁
+♃
+♄
+♅
+♑
+♗
+♿
+⚈
+⚭
+✜
+✠
+❲
+❳
+➎
+➫
+➬
+➾
+⟬
+⟭
+⤰
+⩾
+⫸
+Ⱉ
+Ⱚ
+Ɽ
+⸮
+⸰
+⺍
+⺒
+⺭
+⻌
+⻍
+〄
+㐭
+㐰
+㒵
+㔰
+㔾
+㗁
+㗂
+㘀
+㘓
+㛹
+㞗
+㤀
+㤚
+㥖
+㦮
+㫃
+㫻
+㬠
+㹻
+㼅
+㿔
+䁹
+䂤
+䂨
+䂳
+䃋
+䃯
+䄀
+䅻
+䈀
+䌀
+䌰
+䌾
+䑙
+䑞
+䑠
+䔄
+䕃
+䙥
+䜀
+䜰
+䝎
+䠀
+䡐
+䡴
+䤡
+䤹
+䥶
+䦊
+䧛
+䫻
+䭞
+䰗
+䴚
+䵎
+䵥
+丯
+乓
+乫
+亩
+伌
+伧
+伵
+佤
+侬
+俛
+俬
+俿
+倈
+倌
+倗
+偙
+偛
+偦
+傫
+僐
+儎
+儗
+儬
+兛
+凬
+凼
+刋
+刓
+剉
+剎
+剞
+剾
+劊
+勀
+勠
+勥
+勭
+匇
+匷
+匾
+厪
+厾
+叕
+叹
+吂
+吭
+吰
+吺
+呃
+呤
+呬
+咗
+咜
+咡
+咻
+咼
+哽
+唂
+唉
+唏
+唕
+唴
+唷
+啉
+啶
+喎
+喒
+喕
+喤
+嗖
+嗘
+嗡
+嗥
+嗦
+嘓
+嘟
+嘬
+噍
+噞
+嚒
+嚞
+嚟
+囏
+囒
+囦
+囶
+囷
+圊
+圑
+圪
+坒
+坱
+坸
+坺
+垁
+垄
+垆
+垉
+垖
+垨
+垬
+垮
+垾
+埢
+埸
+堇
+堠
+堮
+塄
+塅
+塉
+塍
+塐
+塟
+墔
+夀
+夅
+夌
+奻
+妗
+妟
+妩
+妫
+妭
+姃
+姖
+娙
+娫
+婟
+婳
+婵
+媟
+媪
+媯
+媸
+嫈
+嫛
+嬩
+嬸
+孴
+宬
+寎
+寑
+寽
+尃
+尪
+岽
+峍
+峓
+峺
+崐
+崘
+崬
+崿
+嵂
+嵘
+嵛
+嵠
+嶰
+巠
+幪
+庀
+庞
+廎
+廹
+彲
+徏
+忈
+忎
+忢
+忼
+怂
+怗
+怳
+恺
+悭
+愑
+愫
+慼
+憘
+憸
+懎
+懏
+扪
+抅
+拑
+拢
+拣
+拦
+拰
+拺
+挊
+捒
+捠
+捴
+掞
+掷
+揍
+揔
+揞
+搁
+搅
+搊
+搢
+搧
+搪
+搿
+摊
+摋
+摜
+摮
+摻
+摽
+撬
+撴
+擞
+擿
+攡
+敜
+敟
+敤
+敭
+敯
+敼
+旎
+旮
+旲
+昋
+昙
+昚
+昳
+晪
+晼
+暊
+暒
+暝
+暤
+暧
+暼
+曱
+朊
+杇
+杸
+枖
+枞
+枬
+枲
+柂
+栔
+桉
+桕
+桘
+桲
+梊
+梐
+梾
+棁
+棔
+椑
+椗
+椡
+椦
+椷
+楀
+楴
+榝
+榶
+槞
+槡
+槧
+槮
+樶
+橀
+橉
+橗
+橜
+橪
+檺
+櫌
+櫧
+欸
+歃
+歠
+歫
+歺
+殇
+殠
+殩
+殪
+殱
+毦
+毧
+毱
+毹
+氀
+汮
+沜
+沲
+沵
+泃
+泬
+泴
+洜
+洡
+洷
+浈
+浔
+浖
+浤
+浶
+涍
+涘
+涠
+涤
+淂
+淠
+渞
+渽
+湗
+湩
+湪
+湬
+湵
+溁
+溈
+溼
+滈
+滢
+漀
+漋
+潃
+潐
+潓
+潖
+潹
+澉
+澵
+濆
+濇
+灕
+炜
+烨
+烫
+焘
+焢
+焴
+煓
+煠
+煻
+熁
+熂
+熆
+熷
+燀
+燸
+爕
+爠
+爯
+牃
+牼
+犄
+犱
+犳
+犵
+狋
+狌
+狍
+猕
+猚
+猢
+猳
+猻
+猽
+獓
+獘
+獞
+獡
+玀
+玊
+玔
+玪
+珡
+琌
+琤
+琸
+瑇
+瑧
+璚
+璢
+璬
+瓧
+甁
+甂
+甴
+畗
+畱
+疡
+疰
+痀
+痊
+瘨
+瘮
+瘵
+皛
+皜
+皦
+皬
+皱
+盨
+眚
+眬
+眶
+睎
+睜
+瞄
+瞕
+瞮
+瞯
+矞
+矬
+砚
+砠
+砡
+砻
+硇
+硎
+硠
+硥
+硾
+碙
+碞
+碡
+碯
+磆
+礂
+礇
+礝
+礡
+祅
+祎
+祼
+禡
+禸
+秸
+稉
+稒
+稥
+穄
+穈
+穜
+穵
+窆
+窼
+竕
+竰
+笅
+笟
+笻
+笽
+筊
+筦
+箊
+箓
+箞
+箫
+篚
+篡
+篴
+篾
+簦
+簨
+簳
+簴
+簻
+籭
+籵
+籼
+粡
+粨
+粬
+糓
+糗
+糘
+糼
+紒
+絈
+絿
+綷
+緗
+緥
+緪
+縗
+纃
+纰
+绁
+绋
+绠
+绣
+绦
+绯
+缀
+缅
+缉
+缍
+缕
+缠
+缪
+缮
+缾
+罂
+罄
+罣
+羁
+羱
+羶
+耟
+耡
+耭
+聒
+聠
+聦
+聸
+肷
+胍
+胗
+胘
+胠
+胺
+脞
+腄
+腙
+腭
+膟
+膸
+臁
+臎
+臿
+舃
+舄
+艎
+艏
+艠
+艧
+艻
+芘
+芡
+苇
+茺
+荧
+莃
+莕
+莩
+菂
+葑
+葟
+葹
+蒞
+蒢
+蒱
+蓹
+蔂
+蔆
+蔌
+蕑
+蕢
+薆
+薋
+藂
+藦
+藶
+虙
+虠
+虩
+虮
+虼
+蚂
+蚇
+蚍
+蚖
+蚜
+蚱
+蛀
+蛏
+蛘
+蛬
+蛲
+蛳
+蜋
+蜕
+蜙
+蝀
+蝃
+蝅
+蝘
+蝪
+蝯
+蝹
+蝼
+螠
+螫
+螬
+螮
+蟊
+蟑
+蠎
+蠓
+蠘
+蠛
+蠨
+蠭
+蠼
+衟
+袕
+裀
+褎
+褜
+襀
+覉
+觕
+觻
+訐
+訞
+詃
+詆
+詏
+詟
+詬
+誊
+誚
+誧
+誽
+諪
+譱
+譿
+讉
+讣
+讥
+诏
+诖
+诙
+诶
+谏
+谣
+谭
+豑
+貤
+賬
+賲
+贬
+贻
+赎
+赡
+趉
+趋
+踕
+蹛
+蹿
+躃
+躭
+躶
+輗
+轔
+轗
+轣
+迊
+迮
+迶
+逻
+遃
+郋
+鄇
+鄌
+鄛
+酅
+酓
+酝
+酤
+酮
+酲
+醛
+醧
+醼
+釃
+釒
+釬
+釮
+釷
+鈄
+鉁
+鉙
+鉟
+銡
+鋀
+鋥
+錂
+錛
+錝
+錧
+錸
+鍳
+鎈
+鎊
+鎞
+鎡
+鎱
+鎶
+鏄
+鐦
+鐭
+鑂
+鑃
+鑣
+鑨
+鑱
+鑶
+钎
+钘
+钜
+钠
+钲
+钾
+铆
+铊
+铣
+铯
+锌
+锥
+闰
+闾
+阐
+阬
+阽
+陏
+隖
+隡
+隩
+雝
+雳
+靶
+鞀
+鞐
+鞱
+鞾
+韍
+韪
+韲
+顖
+顨
+顬
+颍
+颓
+颔
+颼
+飇
+餗
+饢
+饽
+馄
+馊
+馓
+馔
+馼
+駞
+駧
+駩
+駬
+騟
+骁
+骇
+骧
+骷
+髈
+鬛
+魛
+魨
+鮊
+鮋
+鮙
+鮧
+鮲
+鮸
+鯑
+鰘
+鰞
+鰪
+鰵
+鱂
+鱄
+鱆
+鱤
+鱫
+鱬
+鱭
+鱰
+鱻
+鲆
+鳐
+鴗
+鴜
+鴯
+鵁
+鵔
+鵥
+鶎
+鶓
+鶠
+鶼
+鸐
+鸙
+鸠
+鸰
+鸳
+麇
+黉
+黓
+鼋
+鼹
+齔
+齰
+龖
+龞
+ꀀ
+ꀰ
+ꄰ
+ꈰ
+ꌟ
+ꔘ
+ꔛ
+Ꙏ
+Ꙗ
+ꜛ
+ꜣ
+Ꜧ
+ꜰ
+Ꜳ
+ꜳ
+Ꜵ
+Ꜷ
+Ꜹ
+Ꜻ
+Ꜽ
+ꝏ
+Ꝩ
+ꤰ
+ꦗ
+ꦧ
+ꦮ
+ꦱ
+꩗
+값
+갓
+갔
+갖
+갠
+갰
+걔
+걱
+겟
+겸
+겼
+굼
+궐
+귓
+깐
+깥
+꺽
+꺾
+껄
+꼴
+꼽
+꽈
+뀐
+끄
+끾
+낤
+낫
+낯
+낸
+낹
+냄
+냳
+냻
+넋
+넥
+놋
+늚
+늬
+닛
+닥
+닦
+댁
+댄
+댐
+덧
+덩
+돋
+뒹
+땀
+떨
+뗛
+뚜
+뜻
+랍
+럿
+렀
+롎
+롪
+롬
+롭
+뤄
+륑
+륵
+릇
+멎
+멩
+뫼
+묰
+뭘
+뮂
+뮬
+므
+밸
+뱃
+벅
+벚
+벡
+봇
+빗
+빫
+빱
+뻗
+뻤
+뽄
+뽑
+삵
+삿
+섣
+셹
+솟
+숑
+숟
+숰
+쉭
+쉽
+싫
+싹
+쌀
+쌰
+썐
+썬
+쑺
+씁
+씻
+앉
+앰
+엿
+옆
+옐
+옵
+욽
+웗
+읕
+젓
+젯
+졸
+좇
+줌
+줬
+짝
+짧
+짬
+짹
+쨀
+쨌
+쨩
+쩔
+쩨
+쭉
+찐
+첩
+촨
+칫
+칬
+칶
+컷
+쿵
+큭
+탉
+턱
+텀
+텃
+텅
+톰
+퉁
+튼
+틱
+펴
+푼
+핎
+햅
+횟
+훔
+훗
+훼
+휰
+흐
+흘
+흠
+흰
+힌
+힝
+
+
+
+
+
+
+
+
+
+
+
+
+
+
+
+
+
+
+
+
+
+
+
+
+
+
+
+
+
+
+
+
+
+
+
+
+
+
+
+
+
+
+
+
+
+
+
+
+
+
+
+
+
+
+
+
+
+
+
+
+
+
+
+
+
+
+
+
+
+
+
+
+
+
+
+
+
+
+
+
+
+
+
+
+
+
+
+
+
+
+
+
+
+
+
+
+
+
+
+
+
+
+
+
+
+
+
+
+
+
+
+
+
+
+
+
+
+
+
+
+
+
+
+
+
+
+
+
+
+
+
+﨎
+﨨
+︠
+𐊋
+𐊦
+𐊪
+𐌹
+𐎲
+𐓘
+𐓟
+𐓻
+𐣨
+𐣪
+𐣲
+𐤃
+𐤇
+𐤲
+𐩒
+𐭠
+𐭦
+𐭪
+𐰇
+𒀸
+𒄖
+𒆍
+𒇽
+𒈠
+𒈦
+𒈪
+𒈹
+𒉺
+𒊏
+𒊕
+𒌆
+𒌝
+𓀫
+𓂋
+𓃟
+𓄩
+𓊝
+𓌉
+𓍟
+𓎩
+
+
+𞤢
+𞥅
+🅚
+🅝
+🅡
+🅣
+🅺
+🅾
+🆄
+🌘
+🌚
+🎢
+🎱
+🎽
+🏒
+🏛
+🏧
+🏩
+🐁
+👝
+💽
+📁
+📊
+📐
+📑
+🔃
+🔐
+🔝
+🔟
+🕐
+🕔
+🕖
+🕘
+🖲
+🗨
+🗿
+🙎
+🚊
+🚞
+🚤
+🚦
+🚾
+🛬
+🛹
+🛺
+🟧
+🟪
+🤏
+🤠
+🤼
+🥘
+🥜
+🥮
+🥼
+🦎
+🦕
+🦭
+🧇
+🧈
+🧋
+🧓
+🧔
+🧕
+🧞
+🧧
+🧳
+🧴
+🧽
+🧾
+🪀
+🪂
+🪡
+🪢
+🪥
+🪨
+🫀
+🫂
+𠀋
+𠁀
+𠂤
+𠃌
+𠆢
+𠟼
+𠨡
+𠫾
+𠸛
+𠺕
+𡑝
+𡓳
+𡥤
+𢆥
+𢓜
+𢘿
+𢚸
+𢶷
+𣏌
+𣚘
+𣜬
+𣥂
+𣳾
+𤀘
+𤏐
+𤟹
+𥙩
+𥞫
+𥱲
+𥶄
+𥹢
+𥾽
+𦊆
+𦢳
+𦻙
+𦽊
+𧊙
+𧝹
+𧫦
+𧬤
+𧻚
+𨂊
+𨏍
+𨖧
+𨖲
+𨛗
+𨢇
+𨮁
+𨲢
+𨷈
+𨸏
+𩥭
+𩳁
+𩹷
+𩺊
+𩿡
+𪱶
+𫊣
+𫞂
+𫟉
+𬑽
+
+
+
+
+
+
+Ģ
+Į
+Ĺ
+Ň
+Ƈ
+Ɗ
+ƚ
+Ƣ
+Ƥ
+ƥ
+Ʀ
+Ƨ
+Ʃ
+Ƽ
+Ǚ
+Ǡ
+ǡ
+Ǩ
+Ǭ
+ǭ
+Ǯ
+Ǹ
+ǻ
+ǿ
+Ȉ
+ȋ
+Ȕ
+ȕ
+ȗ
+Ȥ
+ȧ
+Ȩ
+ȩ
+ȫ
+ȭ
+ȹ
+ȼ
+Ƚ
+Ʉ
+Ɇ
+ɉ
+ɧ
+ʡ
+˅
+˞
+˸
+˹
+̡
+̹
+ͣ
+ͽ
+΅
+Ќ
+ѡ
+Ѧ
+Ѭ
+ѱ
+Ѳ
+ѳ
+ѻ
+҆
+ҏ
+ҩ
+ҵ
+ҹ
+ҿ
+ӆ
+Ӌ
+Ӎ
+ӎ
+Ӑ
+ӑ
+Ӓ
+ӟ
+ӭ
+Ӱ
+ӵ
+ӷ
+ӻ
+Ӿ
+ԇ
+ԋ
+ԏ
+ԕ
+ԗ
+ԙ
+Ԛ
+Ԝ
+Ԥ
+ԩ
+ԯ
+Զ
+Ղ
+Ր
+Ֆ
+՜
+՟
+
+֏
+
+֑
+֒
+֓
+֔
+֜
+֞
+֧
+֩
+֫
+֭
+ֽ
+׀
+׆
+
+
+
+ױ
+״
+
+
+
+
+
+؇
+ؐ
+ؗ
+؛
+ؿ
+ٜ
+٦
+٫
+ڃ
+ڇ
+ڊ
+ڌ
+ڍ
+ڐ
+ڗ
+ڛ
+ڧ
+ڮ
+ڲ
+ڿ
+ۏ
+۔
+ۚ
+ۛ
+ۜ
+۠
+ۮ
+۰
+۱
+۲
+۽
+ۿ
+܈
+
+ܑ
+ܷ
+ܹ
+ܺ
+ܼ
+ܿ
+݁
+
+ݎ
+ݐ
+ݛ
+ݟ
+ݥ
+ݭ
+ݲ
+ݽ
+ݾ
+ތ
+ޑ
+ޒ
+ޞ
+ޟ
+ޡ
+ޢ
+ޯ
+ߋ
+ߌ
+ߍ
+ߎ
+ߙ
+ߛ
+ߝ
+ߣ
+ߥ
+ߪ
+߯
+ࠂ
+ࠃ
+ࠋ
+ࠓ
+ࠕ
+ࠩ
+ࠪ
+ࡇ
+ࡎ
+
+ࡢ
+ࡴ
+ࡹ
+ࢄ
+
+࢞
+ࢣ
+ࢦ
+ࢧ
+ࢪ
+ࢲ
+ࢳ
+ࢺ
+ࢼ
+ࣈ
+࣌
+ࣩ
+ࣹ
+ࣿ
+ऌ
+ऑ
+ऩ
+ऱ
+ॅ
+ॊ
+ॎ
+ॠ
+ॡ
+ॴ
+ॷ
+ঃ
+এ
+ঔ
+
+ঽ
+
+ৈ
+ৗ
+ৢ
+১
+২
+৩
+৪
+৫
+৬
+৯
+ৱ
+৲
+৻
+ਆ
+
+ਏ
+ਐ
+ਚ
+ਠ
+ਡ
+ਣ
+ਧ
+
+
+੬
+ઇ
+ઈ
+ઊ
+ઐ
+ઑ
+ઓ
+ઠ
+ય
+ૐ
+
+૩
+૬
+૿
+
+ଆ
+ଇ
+ଉ
+ଚ
+ଝ
+ତ
+ଥ
+ଧ
+ଭ
+ଯ
+ହ
+
+ୌ
+ୢ
+୦
+୲
+୵
+ஊ
+
+ஶ
+
+ௌ
+ௐ
+
+௨
+ఇ
+ఔ
+ఛ
+ఞ
+ఱ
+
+ౄ
+
+ౠ
+౬
+
+౾
+ಁ
+ಆ
+ಋ
+ಎ
+ಖ
+ಥ
+ೌ
+
+
+
+೦
+೭
+
+ആ
+ഓ
+ഞ
+ഢ
+ഥ
+
+ൌ
+
+ൗ
+൚
+ൟ
+ൣ
+ൾ
+ඇ
+ට
+ඨ
+ඪ
+ඳ
+භ
+ෂ
+ෑ
+ූ
+ෛ
+
+
+
+ຌ
+ຐ
+ຓ
+ຯ
+ໂ
+ໃ
+໋
+໌
+໘
+ໟ
+
+
+༂
+༆
+༲
+ཋ
+ཪ
+
+
+྇
+ྉ
+ྖ
+ྰ
+
+
+
+
+ဌ
+ဎ
+ဏ
+ဥ
+ဦ
+၀
+၄
+၅
+၆
+၉
+၊
+ၛ
+Ⴖ
+Ⴙ
+Ⴚ
+Ⴛ
+ჴ
+ᅽ
+ᆌ
+ᆐ
+ᆹ
+ᇁ
+ᇈ
+ᇕ
+ᇗ
+ᇙ
+ᇞ
+ᇱ
+ᇲ
+ሂ
+ሃ
+ሄ
+ሉ
+ሎ
+ሔ
+ሙ
+ሜ
+ሠ
+ሸ
+ቂ
+ቄ
+ቴ
+ቹ
+ቺ
+ኅ
+ኝ
+ኪ
+ኰ
+ኺ
+ኻ
+ዉ
+ው
+ዎ
+ዑ
+ዚ
+ዛ
+ዜ
+ዶ
+ዿ
+ጀ
+ጂ
+ጃ
+ጊ
+ጌ
+ጎ
+ጦ
+ጰ
+ጹ
+ጻ
+ፃ
+ፅ
+ፊ
+ፋ
+፝
+Ꭱ
+Ꭵ
+Ꭸ
+Ꮁ
+Ꮇ
+Ꮍ
+Ꮏ
+Ꮙ
+Ꮟ
+Ꮠ
+Ꮮ
+Ꮵ
+Ꮶ
+Ᏸ
+Ᏹ
+ᐏ
+ᐙ
+ᐞ
+ᐭ
+ᐱ
+ᐽ
+ᑊ
+ᑌ
+ᑖ
+ᒄ
+ᒋ
+ᒌ
+ᒐ
+ᒫ
+ᒼ
+ᓃ
+ᓈ
+ᓕ
+ᓛ
+ᓨ
+ᓪ
+ᓯ
+ᓲ
+ᓿ
+ᔂ
+ᔑ
+ᔕ
+ᔟ
+ᔦ
+ᔾ
+ᕄ
+ᖃ
+ᖓ
+ᖬ
+ᖱ
+ᖷ
+ᖽ
+ᖿ
+ᗝ
+ᗩ
+ᗰ
+ᗴ
+ᗺ
+ᘦ
+ᙟ
+ᙳ
+ᙵ
+᚛
+᚜
+ᚢ
+ᚣ
+ᚩ
+ᚴ
+ᚷ
+ᚹ
+ᚻ
+ᚼ
+ᛄ
+ᛇ
+ᛐ
+ᛗ
+ᛝ
+ᛟ
+ᛦ
+᛭
+
+ឆ
+ឞ
+ឯ
+឵
+៊
+៍
+២
+៣
+៤
+៥
+៦
+៨
+៹
+
+᠊
+᠐
+ᠢ
+ᠲ
+ᡡ
+ᡧ
+ᡨ
+ᢂ
+ᢃ
+ᤰ
+᥆
+ᥬ
+
+ᨁ
+ᨅ
+ᨔ
+ᨕ
+ᨗ
+ᨘ
+ᩔ
+ᩤ
+ᬋ
+ᬰ
+ᮀ
+ᮅ
+ᮓ
+ᮔ
+ᮛ
+ᮞ
+ᮥ
+᮪
+ᰰ
+ᱦ
+ḅ
+ḑ
+ḓ
+Ḗ
+ḟ
+Ḩ
+Ḿ
+ṑ
+ṻ
+Ṽ
+ṽ
+ẁ
+Ẃ
+ẃ
+ẏ
+Ẑ
+Ẕ
+ẗ
+ẙ
+Ẩ
+Ẵ
+Ẹ
+Ị
+Ố
+Ộ
+Ợ
+Ủ
+Ừ
+Ỳ
+ỷ
+Ỻ
+ỻ
+ἃ
+ἅ
+Ἕ
+ἦ
+Ἠ
+Ἱ
+Ἴ
+ὂ
+Ὁ
+ὓ
+Ὓ
+Ὕ
+ὣ
+Ὠ
+Ὡ
+ὺ
+ὼ
+ᾀ
+ᾄ
+ᾐ
+ᾑ
+ᾒ
+ᾖ
+ᾘ
+ῠ
+ῤ
+Ῡ
+ῴ
+⁌
+
+
+
+
+
+
+₡
+₧
+₰
+₵
+
+
+⃔
+⃞
+⃠
+℥
+Ⅎ
+⅂
+ↄ
+ↅ
+ↆ
+↡
+↣
+↥
+↮
+↯
+↹
+↼
+↽
+⇂
+⇉
+⇖
+⇚
+⇡
+⇯
+∹
+≇
+≋
+≔
+≛
+≜
+≝
+≲
+≳
+≻
+≼
+⊄
+⊅
+⊓
+⊚
+⊠
+⊡
+⊣
+⊶
+⊸
+⊻
+⋀
+⋃
+⋋
+⋌
+⋍
+⋎
+⋏
+⋖
+⋗
+⋚
+⋛
+⌕
+⌢
+⌦
+⌷
+⍜
+⍝
+⍩
+⍳
+⎇
+⎋
+⎔
+⎛
+⎝
+⎞
+⎠
+⎳
+⎼
+⏌
+⏤
+⏦
+⏸
+⏺
+␀
+␃
+␅
+␆
+␇
+␎
+␏
+␕
+␚
+␛
+␡
+⓱
+⓳
+⓹
+⓾
+⓿
+┙
+╅
+╴
+▎
+▨
+▩
+▴
+◖
+◗
+☈
+☤
+☫
+☱
+☳
+☴
+☶
+♇
+♖
+♙
+♲
+♺
+⚂
+⚄
+⚥
+⚳
+⚵
+⚹
+⛇
+⛹
+✑
+✛
+✢
+❡
+❨
+❩
+❬
+❵
+➏
+➐
+➭
+➮
+➷
+➹
+⟺
+⠁
+⠃
+⠅
+⠉
+⠊
+⠋
+⠍
+⠎
+⠏
+⠑
+⠓
+⠕
+⠗
+⠙
+⠚
+⠛
+⠝
+⠞
+⠟
+⠠
+⠥
+⠧
+⠪
+⠫
+⠭
+⠮
+⠯
+⠱
+⠵
+⠹
+⠺
+⠻
+⠽
+⡎
+⡓
+⡖
+⡜
+⣏
+⣹
+⣿
+⥽
+⦭
+⧜
+⧝
+⧞
+⧵
+⨁
+⨅
+⨾
+⩽
+⬱
+⭑
+⭧
+⭼
+⮀
+⯱
+Ⰰ
+Ⰱ
+Ⰲ
+Ⰳ
+Ⰴ
+Ⰵ
+Ⰶ
+Ⰷ
+Ⰸ
+ⱥ
+Ⱶ
+ⱶ
+Ⲏ
+Ⲛ
+Ⲥ
+Ⲧ
+ⲫ
+⳥
+⳿
+ⴍ
+ⴓ
+ⴣ
+ⴴ
+ⴾ
+ⵀ
+ⵂ
+ⵃ
+ⵅ
+ⵇ
+ⵊ
+ⵑ
+ⵞ
+ⵟ
+⹀
+
+⺀
+⺊
+⺌
+⺕
+⺣
+⺬
+〡
+〣
+〿
+
+ㄡ
+㇗
+ㇸ
+㉿
+㑃
+㑙
+㑤
+㑹
+㒒
+㒖
+㒘
+㒷
+㓗
+㓝
+㔀
+㕑
+㖙
+㗊
+㗝
+㘇
+㘰
+㙁
+㙎
+㙐
+㙦
+㙱
+㙺
+㚕
+㜑
+㜢
+㜪
+㜺
+㝫
+㝹
+㟊
+㟧
+㠓
+㡧
+㡼
+㡿
+㣺
+㤅
+㤿
+㥬
+㦀
+㦐
+㦕
+㧔
+㧞
+㨪
+㨰
+㩪
+㩷
+㪅
+㪘
+㫝
+㬅
+㭗
+㭭
+㭵
+㮛
+㯖
+㰰
+㱈
+㲄
+㲗
+㴐
+㴞
+㴰
+㴸
+㵎
+㵪
+㵲
+㷆
+㷏
+㷧
+㸤
+㸰
+㻁
+㻋
+㻐
+㻱
+㼀
+㼁
+㼂
+㼄
+㼌
+㼎
+㼐
+㼳
+㼿
+㽛
+㾗
+㿺
+䀶
+䀾
+䂓
+䄄
+䄫
+䅑
+䅗
+䅼
+䆅
+䆊
+䆏
+䆐
+䇄
+䇾
+䉎
+䉐
+䉓
+䉦
+䊪
+䋢
+䋿
+䍥
+䍧
+䏁
+䏋
+䏧
+䏽
+䑏
+䑢
+䑥
+䑸
+䒊
+䒐
+䓟
+䓲
+䔍
+䔥
+䕭
+䖢
+䘀
+䙏
+䙩
+䙺
+䚡
+䚸
+䛀
+䛔
+䛴
+䛶
+䛻
+䜁
+䜉
+䜔
+䝈
+䝙
+䞀
+䞊
+䟽
+䠠
+䡻
+䢉
+䣷
+䥓
+䥜
+䥝
+䥰
+䥿
+䦰
+䧇
+䨓
+䨘
+䨺
+䩹
+䪥
+䬀
+䭒
+䭛
+䭶
+䭸
+䭻
+䮀
+䯼
+䰢
+䰼
+䱅
+䱎
+䱏
+䱒
+䱗
+䱚
+䱛
+䱝
+䱞
+䱠
+䱣
+䱥
+䱦
+䱧
+䱩
+䱹
+䲊
+䲐
+䲑
+䲒
+䲓
+䲔
+䲖
+䲗
+䲘
+䲙
+䲚
+䲛
+䲜
+䳉
+䳑
+䳔
+䳿
+䴇
+䵘
+䵙
+䵞
+䶈
+丒
+丮
+丳
+乹
+亍
+亝
+亽
+仈
+仠
+仫
+仳
+仴
+伈
+伢
+伻
+佁
+佊
+佋
+佢
+佥
+佪
+佮
+佱
+佲
+佹
+侅
+侳
+侼
+侽
+侾
+俈
+俓
+俨
+俪
+俭
+倥
+偂
+偎
+偠
+偩
+偬
+傊
+傯
+傿
+僄
+僆
+僾
+儢
+儣
+儤
+儧
+儨
+儮
+儳
+儴
+兖
+兞
+冃
+冧
+冮
+凃
+凅
+凇
+凕
+刲
+剨
+剹
+剺
+劂
+劄
+劋
+劐
+劜
+劧
+劯
+劸
+勑
+匃
+匴
+卾
+厇
+厌
+厢
+厤
+厫
+厮
+厽
+叁
+叅
+叓
+叝
+叽
+呇
+呐
+呓
+呛
+呹
+咂
+咍
+咐
+咘
+咝
+咷
+咿
+哑
+哘
+哙
+哠
+唅
+唋
+唢
+啃
+啘
+啚
+啢
+啳
+喭
+喺
+喽
+嗏
+嗝
+嘐
+嘧
+嘨
+嘰
+嘼
+噀
+噁
+噇
+噢
+噾
+嚅
+嚘
+嚫
+嚬
+嚯
+囇
+囖
+囡
+囨
+囯
+圂
+圌
+圞
+圤
+坅
+坍
+坓
+坔
+坢
+坬
+坹
+坿
+垌
+垑
+垘
+垱
+垽
+垿
+埄
+埆
+埌
+埞
+埶
+埽
+埾
+堋
+堑
+堖
+堗
+堢
+堦
+堸
+塠
+塭
+塳
+墁
+墌
+墍
+墕
+墖
+墱
+墲
+墸
+壈
+壐
+壖
+夆
+夑
+夒
+夓
+奀
+奙
+奜
+奟
+奣
+奦
+奲
+妐
+妡
+妯
+妸
+姏
+姰
+姱
+姺
+娅
+娎
+娖
+娞
+娬
+婃
+婑
+婘
+婞
+婤
+媋
+媗
+媜
+媤
+媰
+媲
+媴
+嫃
+嫔
+嫤
+嫫
+嫶
+嫼
+嫿
+嬇
+嬕
+嬗
+嬮
+孏
+孒
+孢
+孬
+孮
+宂
+宄
+宐
+寜
+寠
+寯
+尣
+尫
+屉
+屩
+屰
+屿
+岋
+岍
+岠
+岣
+岹
+峉
+峊
+峋
+峑
+峘
+峚
+峱
+峵
+峼
+崃
+崄
+崝
+崪
+崶
+崹
+崽
+嵄
+嵇
+嵑
+嵞
+嵥
+嵳
+嵸
+嵺
+嵿
+嶕
+嶚
+嶛
+嶤
+嶭
+巀
+巐
+巑
+巔
+巤
+帍
+帓
+帜
+帡
+帲
+幉
+幖
+庋
+庡
+庢
+庥
+庨
+庪
+庿
+廍
+廏
+廽
+弡
+弻
+彄
+彍
+彐
+彣
+彴
+彽
+徉
+徜
+徬
+徾
+忂
+忐
+忑
+忓
+忔
+忕
+忨
+怃
+怅
+怐
+怘
+怚
+怦
+怼
+恖
+恛
+恝
+悹
+惁
+惃
+惈
+惌
+惏
+惝
+惞
+惢
+惬
+惮
+惸
+愄
+愒
+愗
+愙
+愜
+愠
+愣
+愻
+愽
+慒
+慺
+憇
+憓
+憠
+憭
+憿
+懀
+懁
+懠
+戅
+戆
+戋
+戓
+戠
+戨
+戭
+扐
+扻
+抟
+抡
+抨
+抪
+抾
+拎
+拪
+拻
+挎
+挐
+挞
+挠
+挳
+捅
+捭
+捱
+捼
+捽
+捿
+掊
+掙
+掮
+掯
+掺
+掽
+掿
+揅
+揜
+揫
+搀
+搽
+摁
+摏
+摒
+摚
+摞
+摢
+摳
+摴
+摵
+摼
+撢
+撦
+撶
+攧
+敇
+敚
+敛
+敠
+敱
+敾
+斠
+旀
+旐
+旖
+旰
+昍
+昑
+昛
+昢
+昩
+昲
+昹
+晅
+晔
+晬
+晹
+晻
+晾
+暃
+暌
+暍
+暙
+暣
+暯
+暷
+暿
+曅
+曌
+曒
+曞
+曤
+朁
+朎
+朾
+杧
+杹
+枒
+枮
+枵
+枼
+枾
+柅
+柆
+柇
+柋
+柌
+柮
+栀
+栋
+栌
+栐
+栟
+栧
+栯
+栶
+栿
+桍
+桏
+桞
+桠
+桩
+桹
+桺
+梂
+梘
+梙
+梪
+梫
+梮
+棂
+棌
+棏
+棙
+棝
+棤
+棿
+椂
+椄
+椆
+椐
+椢
+椧
+楆
+楖
+榋
+榫
+榳
+槟
+槠
+槰
+槺
+槼
+槾
+樃
+樆
+樌
+樼
+樿
+橓
+橔
+橧
+橵
+檆
+檋
+檖
+檛
+檥
+檨
+檿
+櫊
+櫒
+櫢
+櫥
+櫵
+櫸
+櫺
+欋
+欌
+欐
+欗
+欥
+欬
+欻
+歑
+歕
+歗
+歝
+歭
+歰
+歱
+歽
+殅
+殍
+殏
+殗
+殡
+殰
+殸
+殾
+毂
+毇
+毉
+毼
+氎
+氬
+氭
+氱
+氿
+汅
+汯
+沄
+沰
+沺
+泍
+泑
+泞
+泦
+泧
+泺
+洖
+洘
+洚
+洦
+浛
+浥
+涗
+涚
+涞
+涴
+淌
+渑
+渳
+渹
+湂
+湅
+湇
+湋
+湕
+湥
+湴
+湷
+湻
+溄
+溋
+溑
+溒
+溕
+溤
+滶
+滽
+漅
+漘
+漦
+漨
+漶
+潎
+潏
+潚
+潝
+潵
+澅
+澊
+澑
+澨
+澯
+澰
+澾
+濙
+瀀
+瀠
+瀣
+瀴
+瀼
+灎
+灐
+灓
+灙
+灠
+炌
+炍
+炔
+炠
+烁
+烉
+烓
+焌
+焓
+焠
+焮
+焻
+焽
+煋
+煑
+煔
+煡
+煳
+煴
+煵
+煸
+煿
+熅
+熇
+熛
+熳
+熵
+熶
+燨
+爁
+爇
+爋
+爑
+爓
+爔
+爝
+爞
+牍
+牎
+牏
+牐
+牗
+牚
+牠
+牦
+牿
+犊
+犒
+犟
+犽
+狔
+狟
+狴
+狾
+狿
+猋
+猒
+猘
+猤
+猬
+猲
+獂
+獃
+獍
+獔
+獧
+獱
+獳
+獶
+玐
+玒
+玽
+珔
+珜
+琍
+琏
+琔
+琩
+琻
+瑌
+瑓
+瑔
+瑬
+瑮
+瑲
+瑵
+瑿
+璏
+璔
+璗
+璙
+璱
+璺
+瓉
+瓍
+瓐
+瓡
+瓯
+瓰
+瓴
+瓸
+甀
+甿
+畇
+畉
+畍
+畐
+畕
+畮
+畻
+疅
+疉
+疍
+疓
+疤
+疮
+痄
+痆
+痈
+痓
+痟
+痠
+痪
+痬
+痱
+瘃
+瘉
+瘔
+癃
+癎
+癔
+癿
+皍
+皤
+皯
+盠
+盬
+眎
+眨
+眮
+眲
+睁
+睈
+睏
+睓
+睘
+睪
+睼
+瞒
+瞧
+瞪
+瞹
+矔
+矰
+矶
+矺
+矻
+矾
+砀
+砅
+砟
+砣
+砸
+砹
+砾
+硊
+硒
+硘
+硢
+硸
+硺
+碖
+碘
+碜
+碤
+碫
+磏
+磗
+磪
+磱
+礆
+礌
+礏
+礟
+礭
+礴
+祂
+祦
+祵
+祾
+禃
+禌
+禯
+禶
+禼
+秆
+秇
+秈
+秔
+秥
+秫
+秼
+稂
+稌
+稓
+稘
+稭
+穇
+穊
+穟
+穯
+窂
+窅
+窊
+窐
+窹
+竆
+竉
+竍
+竎
+竚
+竡
+竳
+笇
+笌
+笧
+笯
+笰
+笴
+笸
+筫
+筻
+筿
+箄
+箠
+箧
+箩
+箯
+箶
+篂
+篈
+篗
+篰
+篱
+簉
+簍
+簕
+簙
+簮
+籈
+籑
+籓
+籕
+籜
+籝
+籡
+籣
+粏
+粫
+粰
+粸
+粺
+糌
+糦
+糨
+糭
+紃
+紤
+紪
+紼
+絀
+絠
+絧
+絪
+絰
+綑
+綘
+緧
+緫
+緵
+緷
+縎
+縬
+縭
+縳
+縿
+繄
+繈
+繏
+繓
+繘
+繳
+繿
+纕
+纚
+纣
+纾
+绂
+绨
+绷
+绽
+缑
+缥
+罙
+罢
+罤
+罳
+罾
+罿
+翏
+耎
+耔
+耖
+耝
+耬
+耺
+聎
+聑
+聓
+聝
+肈
+肔
+肧
+肨
+肹
+肼
+胏
+胐
+胔
+胧
+胮
+胳
+脃
+脓
+脮
+脲
+脽
+腈
+腏
+腒
+腠
+腬
+腯
+膌
+膑
+膘
+臖
+臛
+臝
+臮
+臶
+臽
+舀
+舆
+舠
+舡
+舢
+舥
+舨
+舲
+舼
+艉
+艗
+艛
+艝
+艢
+艣
+艿
+芄
+芈
+芉
+芏
+芖
+芤
+芪
+芰
+芴
+芺
+芼
+苋
+苎
+茬
+茰
+荗
+荦
+荨
+荹
+荺
+荿
+莺
+菆
+菈
+菨
+菺
+萕
+萪
+萫
+萮
+萳
+萹
+葂
+葒
+葙
+葚
+葧
+蒦
+蒧
+蓦
+蓩
+蓺
+蔎
+蔺
+蕜
+蕲
+蕹
+蕻
+薝
+薟
+薢
+薧
+薼
+藊
+藋
+藾
+蘀
+蘒
+蘼
+虆
+虡
+虦
+虷
+虿
+蚒
+蚠
+蚧
+蚳
+蚸
+蚿
+蛁
+蛊
+蛠
+蛦
+蛴
+蜞
+蜨
+蜰
+蜼
+蝇
+蝥
+蝽
+螅
+螇
+螋
+螌
+螒
+螕
+螙
+螧
+螱
+螵
+蟌
+蟛
+蟟
+蟪
+蟫
+蟭
+蟮
+蠂
+蠏
+蠒
+衅
+袄
+袓
+袘
+袛
+袲
+袳
+裪
+裰
+裶
+裼
+褁
+褆
+褡
+褳
+襈
+襏
+襑
+襡
+覐
+覔
+覛
+覣
+覦
+覵
+觑
+觖
+觬
+觽
+訁
+訬
+訯
+訵
+訸
+詀
+詓
+詙
+詨
+誈
+誏
+誩
+誶
+諐
+諓
+諕
+諼
+譐
+譕
+譗
+譳
+譶
+譼
+讁
+讔
+讝
+讦
+讧
+讫
+讷
+诀
+诒
+诡
+诤
+诧
+诬
+诹
+诽
+谍
+谝
+谟
+谤
+谧
+谫
+谴
+谸
+谽
+豇
+豓
+豗
+豾
+貆
+貈
+貐
+貛
+賒
+賕
+賙
+賟
+賥
+賱
+賷
+贠
+贳
+赗
+赜
+趆
+趍
+趞
+趭
+趼
+跍
+跢
+踄
+踋
+踖
+踣
+踦
+踹
+蹜
+蹫
+躈
+躏
+躞
+躻
+軄
+輄
+輊
+輋
+輬
+輹
+輺
+轌
+轒
+轞
+轶
+轸
+辊
+辍
+辏
+辒
+辚
+辠
+辡
+辩
+辫
+迀
+逎
+逰
+逿
+遚
+遟
+遧
+邞
+邩
+邬
+邺
+郈
+郍
+郒
+郔
+郘
+郧
+郶
+郸
+鄎
+鄐
+鄑
+鄬
+鄻
+鄼
+酕
+酰
+醅
+醊
+醘
+醲
+釕
+釖
+釚
+釞
+釨
+釱
+釴
+鈃
+鈈
+鈖
+鈣
+鈥
+鈨
+鈫
+鈮
+鈵
+鉍
+鉓
+鉖
+鉥
+鉪
+鉫
+鉬
+鉼
+鉽
+鉿
+銂
+銊
+銋
+銏
+銥
+銧
+銬
+鋑
+鋘
+鋣
+鋻
+錍
+錖
+錙
+錤
+鍁
+鍣
+鍧
+鍨
+鍩
+鎁
+鎋
+鎑
+鎘
+鎢
+鎤
+鎦
+鎨
+鎩
+鎴
+鎼
+鏇
+鏓
+鏹
+鐁
+鐉
+鐎
+鐒
+鐕
+鐖
+鐜
+鐝
+鐠
+鐤
+鐪
+鐬
+鐱
+鐻
+鐽
+鑥
+鑯
+鑹
+钀
+钅
+钒
+钗
+钛
+钤
+钭
+钵
+铡
+铩
+铹
+铿
+锑
+锘
+锜
+锯
+锰
+锵
+锷
+锸
+镀
+镛
+镣
+镥
+镭
+镸
+閗
+閙
+閞
+閺
+闂
+闉
+闑
+闤
+阖
+阢
+阰
+阷
+陖
+陭
+隭
+隯
+隳
+雚
+雤
+霌
+霝
+霳
+霻
+靃
+靔
+靕
+靮
+靷
+鞉
+鞒
+鞓
+鞕
+鞙
+鞚
+鞶
+韈
+韎
+韐
+韑
+韤
+韰
+韾
+頄
+頏
+頦
+顼
+颁
+颌
+颎
+颢
+颣
+颦
+颰
+颿
+飂
+飊
+飌
+飙
+飣
+飤
+飧
+餚
+餤
+餧
+餬
+餰
+餵
+餾
+饀
+饊
+饓
+饘
+饟
+饤
+饫
+馃
+馍
+馏
+馕
+馞
+馣
+馰
+駀
+騃
+騈
+騋
+騌
+騍
+騣
+騱
+驘
+驡
+驭
+骆
+骉
+骊
+骝
+骢
+骵
+髐
+髜
+髡
+髩
+髲
+髺
+髾
+鬂
+鬉
+鬌
+鬓
+鬙
+鬳
+魒
+魓
+魦
+魪
+魸
+魿
+鮀
+鮍
+鮣
+鮬
+鮶
+鮻
+鯂
+鯃
+鯕
+鯝
+鯟
+鯬
+鯭
+鯳
+鯶
+鯹
+鰋
+鰖
+鰚
+鰜
+鰝
+鰢
+鰨
+鰶
+鰷
+鱁
+鱍
+鱐
+鱜
+鱞
+鱡
+鱦
+鱯
+鱱
+鱳
+鱴
+鱹
+鲌
+鲑
+鲛
+鲣
+鳍
+鳖
+鳗
+鳜
+鳝
+鳟
+鳯
+鴲
+鴴
+鴷
+鵃
+鵓
+鵫
+鵴
+鶂
+鶄
+鶑
+鶕
+鶬
+鷃
+鷉
+鷏
+鷣
+鷧
+鷳
+鸜
+鸥
+鸾
+鹂
+鹊
+鹘
+鹞
+鹭
+麀
+麕
+麖
+麘
+麨
+麰
+黧
+黮
+黰
+鼑
+鼢
+鼫
+鼲
+鼺
+齅
+齇
+齌
+齍
+齑
+齠
+齼
+龈
+龋
+龥
+龧
+龵
+龶
+ꀡ
+ꂞ
+ꂢ
+ꂪ
+ꂳ
+ꂸ
+ꅓ
+ꅗ
+ꅬ
+ꅯ
+ꅱ
+ꇊ
+ꇨ
+ꉙ
+ꉞ
+ꊇ
+ꋂ
+ꋧ
+ꑓ
+꒨
+꒩
+꒭
+ꓘ
+ꖏ
+ꖳ
+ꙕ
+ꙗ
+ꙛ
+Ꙩ
+ꙩ
+ꙶ
+꜀
+Ꜣ
+ꜥ
+ꜧ
+ꜵ
+ꜷ
+ꜹ
+ꜻ
+ꜽ
+Ꝇ
+ꝇ
+ꝛ
+ꝩ
+ꝶ
+Ꞓ
+ꟾ
+ꠄ
+ꠌ
+ꠟ
+ꠣ
+ꠤ
+ꡂ
+ꡊ
+ꡎ
+ꡙ
+ꡛ
+꡷
+
+ꦏ
+ꦒ
+ꦛ
+ꦠ
+ꦭ
+ꦯ
+ꦶ
+ꦸ
+꩙
+ꩵ
+ꭎ
+ꯏ
+ꯐ
+ꯜ
+ꯝ
+ꯥ
+갉
+갯
+걏
+걺
+겄
+겈
+겜
+겪
+곧
+괄
+굜
+굹
+굽
+궤
+긑
+깋
+깞
+껌
+꽂
+꽉
+꾜
+뀀
+뀏
+뀰
+끊
+끟
+끤
+낂
+낊
+낡
+낵
+낼
+냞
+냡
+냩
+넛
+넣
+녈
+녘
+눌
+눕
+눝
+눞
+눰
+뉬
+뉼
+닐
+닙
+닳
+댑
+덥
+덴
+됐
+됰
+둠
+뒷
+듬
+딜
+딪
+땹
+뗀
+뙎
+뚫
+뜸
+띎
+띠
+띵
+랄
+랏
+랫
+랬
+랴
+렐
+렜
+렰
+롸
+롼
+룬
+뤃
+륜
+륰
+륹
+맴
+멓
+멦
+멭
+멳
+멹
+몊
+몐
+몹
+묏
+뭄
+뭇
+뭍
+뭙
+뮒
+믄
+믐
+밌
+뱁
+벙
+볍
+뵈
+뵤
+붐
+뷴
+븨
+빤
+빽
+뺑
+뻔
+뻬
+뽟
+뽧
+쁨
+쁬
+삥
+샄
+샐
+샵
+샷
+섀
+셈
+셏
+셧
+셰
+솜
+숏
+쉐
+슉
+슘
+싣
+싯
+싼
+쌧
+썎
+썛
+썢
+썰
+쎄
+쎿
+쏑
+쏜
+쏠
+쑏
+쑤
+쓴
+씌
+씩
+씬
+씸
+앦
+앨
+앿
+얇
+얓
+얘
+얨
+엉
+엊
+엌
+옝
+옫
+옮
+옾
+왈
+왓
+왠
+웁
+웋
+웰
+윅
+윗
+읉
+읔
+읛
+잰
+잼
+잽
+쟝
+쟤
+젼
+줟
+줰
+쥤
+쥴
+쥹
+짇
+쩐
+쩕
+쩛
+쩢
+쩤
+쩶
+쫓
+쫜
+쭈
+쭔
+쯔
+찍
+찜
+찢
+찻
+챌
+챏
+챙
+챫
+쳤
+쵒
+춧
+췌
+칎
+칩
+캄
+캅
+캉
+캰
+켄
+켜
+콤
+콥
+콿
+쾏
+쿼
+퀘
+퀠
+퀸
+큼
+킪
+킯
+킸
+킿
+탓
+톞
+톨
+툴
+튀
+튿
+팍
+팎
+팜
+팟
+팥
+팰
+펄
+펑
+펨
+펼
+폈
+푎
+풋
+픔
+핌
+핏
+햄
+햎
+햩
+헐
+헷
+홓
+홙
+홰
+홺
+횃
+횡
+훌
+훑
+흉
+흒
+흩
+
+
+
+
+
+
+
+
+
+
+
+
+
+
+
+
+
+
+
+
+
+
+
+
+
+
+
+
+
+
+
+
+
+
+
+
+
+
+
+
+
+
+
+
+
+
+
+
+
+
+
+
+
+
+
+
+
+
+
+
+
+
+
+
+
+
+
+
+
+
+
+
+
+
+
+
+
+
+
+
+
+
+
+
+
+
+
+
+
+
+
+
+
+
+
+
+
+
+
+
+
+
+
+
+
+
+
+
+
+
+
+
+
+
+
+
+
+
+
+
+
+
+
+
+
+
+
+
+
+
+
+
+
+
+
+
+
+
+
+
+
+
+
+
+
+
+
+
+
+
+
+
+
+
+
+
+
+
+
+
+
+
+
+
+
+
+
+
+
+
+
+
+
+
+
+
+
+
+
+
+
+
+
+
+
+
+
+
+
+
+
+
+
+
+
+
+
+
+
+
+
+
+
+
+
+
+
+
+
+
+
+
+
+
+
+
+
+
+
+
+
+
+
+
+
+
+
+
+
+
+
+
+
+
+
+
+
+
+
+
+
+
+
+
+
+
+
+
+
+
+
+
+
+
+
+
+
+
+
+
+
+
+
+
+
+
+
+
+
+
+
+
+
+
+
+
+
+
+
+
+
+
+
+
+
+
+
+
+
+
+
+
+
+
+
+
+
+
+
+
+
+
+
+
+
+
+
+
+
+
+﨓
+﨡
+﨣
+﨧
+﨩
+
+
+︀
+﹌
+𐂂
+𐊕
+𐊠
+𐊤
+𐊥
+𐊩
+𐊭
+𐊯
+𐊴
+𐊵
+𐌈
+𐌊
+𐌎
+𐌑
+𐌒
+𐌘
+𐌙
+𐌰
+𐌳
+𐌴
+𐌸
+𐌺
+𐌿
+𐍂
+𐍃
+𐍈
+𐎁
+𐎍
+𐎓
+𐎡
+𐎢
+𐎭
+𐎱
+𐎴
+𐎽
+𐏁
+𐒻
+𐓏
+𐣡
+𐣢
+𐣯
+𐣱
+𐣴
+𐤂
+𐤆
+𐤊
+𐤑
+𐤒
+𐤟
+𐤡
+𐤣
+𐤩
+𐤬
+𐤮
+𐤱
+𐤳
+𐩣
+𐩧
+𐩬
+𐩲
+𐭂
+𐭅
+𐭆
+𐭉
+𐭊
+𐭌
+𐭓
+𐭔
+𐭕
+𐭧
+𐭨
+𐭬
+𐭱
+𐰃
+𐰛
+𐰭
+𐰰
+𐲢
+𐲥
+𐳉
+𐳋
+𐳎
+𐳓
+𐳗
+𐳘
+𐳛
+𐳢
+𐳤
+𐳮
+𑀁
+𑀥
+𑀫
+𑐣
+𑐰
+𑐵
+𑐾
+𑑅
+𑒃
+𒀀
+𒀜
+𒀠
+𒁓
+𒂆
+𒂍
+𒂵
+𒃶
+𒄀
+𒄈
+𒄩
+𒄷
+𒅁
+𒆳
+𒆵
+𒇺
+𒈨
+𒈾
+𒉡
+𒉿
+𒋛
+𒋢
+𒋫
+𒋾
+𒌅
+𒌍
+𒌑
+𒌷
+𒍢
+𒍪
+
+𓀀
+𓀃
+𓀠
+𓁉
+𓁐
+𓃗
+𓃺
+𓄹
+𓄺
+𓄿
+𓅯
+𓆁
+𓇢
+𓈗
+𓊃
+𓊈
+𓊉
+𓌙
+𓌤
+𓍼
+𓎂
+𓏥
+𔐓
+𔗷
+
+
+
+
+
+
+
+
+
+
+𖠰
+𖤘
+𖤥
+𖧵
+𖧷
+𛀀
+𛂦
+𛅐
+𛅑
+𛅤
+𛅥
+𛅧
+𞤊
+𞤔
+𞤤
+𞤮
+𞤵
+𞤼
+🀄
+🅒
+🅗
+🅜
+🅢
+🅤
+🅦
+🅶
+🅸
+🆂
+🆃
+🆈
+🆎
+🆑
+🇶
+🌗
+🌦
+🎜
+🎝
+🎿
+🏑
+🏗
+🐫
+👡
+👲
+💂
+💶
+📇
+📏
+📠
+📤
+📫
+📬
+📵
+📼
+🔈
+🔛
+🔤
+🕉
+🕌
+🕑
+🕚
+🕜
+🕴
+🗂
+🗒
+🗺
+😾
+🚎
+🚠
+🚣
+🚯
+🚰
+🚸
+🚻
+🛎
+🛲
+🛴
+🛷
+🛻
+🟤
+🥍
+🥏
+🥙
+🥣
+🥫
+🦓
+🦗
+🦚
+🦝
+🦞
+🦟
+🦧
+🦫
+🦱
+🦶
+🧂
+🧉
+🧗
+🧭
+🧮
+🧯
+🧰
+🧱
+🩳
+🪁
+🪑
+🪓
+🪗
+🪞
+🪦
+🪵
+🫓
+𠁑
+𠂉
+𠂢
+𠃵
+𠉞
+𠌯
+𠓿
+𠕇
+𠙶
+𠣮
+𠦳
+𠧋
+𠬠
+𠮾
+𠶢
+𠸄
+𠸗
+𠻰
+𠼛
+𡉕
+𡉻
+𡋤
+𡍄
+𡎝
+𡏦
+𡔈
+𡕀
+𡗶
+𡘷
+𡙇
+𡚴
+𡡉
+𡧃
+𡩛
+𡩜
+𡫡
+𡰥
+𡳳
+𡶫
+𡸫
+𡸳
+𡸴
+𡽫
+𢀛
+𢄓
+𢆫
+𢈴
+𢈻
+𢎭
+𢭐
+𢮦
+𢴎
+𢷄
+𢾺
+𣂑
+𣅶
+𣈜
+𣏾
+𣒱
+𣘻
+𣚴
+𣛦
+𣛭
+𣜌
+𣦵
+𣳔
+𣳫
+𣴎
+𣴴
+𣶬
+𣾼
+𤃝
+𤄃
+𤅶
+𤆬
+𤇅
+𤉇
+𤊫
+𤊹
+𤌤
+𤐜
+𤑫
+𤘽
+𤚥
+𤞼
+𤠝
+𤧶
+𤪠
+𤭖
+𤯩
+𤰃
+𤱂
+𥇥
+𥔲
+𥢓
+𥬊
+𥳑
+𥳽
+𥸮
+𥸯
+𥹠
+𥹷
+𥻘
+𥻹
+𥻻
+𦣝
+𦤾
+𦩀
+𦯶
+𦱳
+𧈢
+𧌇
+𧏡
+𧒽
+𧖱
+𧘔
+𧘕
+𧙖
+𧟨
+𧮾
+𧳜
+𧵑
+𧸘
+𧸝
+𧾷
+𨀈
+𨈭
+𨑻
+𨕭
+𨗉
+𨜒
+𨤔
+𨦈
+𨨞
+𨬊
+𨭻
+𨯁
+𨱽
+𩖃
+𩙻
+𩙿
+𩝐
+𩠼
+𩡤
+𩢴
+𩣡
+𩥇
+𩧥
+𩩓
+𩩫
+𩲃
+𩲈
+𩳐
+𩵄
+𩵋
+𩶗
+𩷑
+𩸶
+𩹉
+𩻩
+𪃹
+𪅀
+𪏰
+𪏽
+𪕰
+𪗱
+𪘚
+𪚢
+𪻆
+𫇠
+𫛉
+𫝂
+𫝼
+𫞔
+𫡷
+𫵾
+𬐐
+𰑨
+𰢱
+𱏑
+𱖥
+𱗺
+𱗻
+𱬒
+𲄜
+𲄠
+𲄤
+𲄬
+𲇡
+
+
+
+
+
+
+
+
+
+
+
+
+󠄆
+󠄈
+
+
+
+
+
+
+
+
+
+
+
diff --git a/bert/deberta-v3-large/.gitattributes b/bert/deberta-v3-large/.gitattributes
new file mode 100644
index 0000000000000000000000000000000000000000..957b2579c6ef20995a09efd9a17f8fd90606f5ed
--- /dev/null
+++ b/bert/deberta-v3-large/.gitattributes
@@ -0,0 +1,27 @@
+*.7z filter=lfs diff=lfs merge=lfs -text
+*.arrow filter=lfs diff=lfs merge=lfs -text
+*.bin filter=lfs diff=lfs merge=lfs -text
+*.bin.* filter=lfs diff=lfs merge=lfs -text
+*.bz2 filter=lfs diff=lfs merge=lfs -text
+*.ftz filter=lfs diff=lfs merge=lfs -text
+*.gz filter=lfs diff=lfs merge=lfs -text
+*.h5 filter=lfs diff=lfs merge=lfs -text
+*.joblib filter=lfs diff=lfs merge=lfs -text
+*.lfs.* filter=lfs diff=lfs merge=lfs -text
+*.model filter=lfs diff=lfs merge=lfs -text
+*.msgpack filter=lfs diff=lfs merge=lfs -text
+*.onnx filter=lfs diff=lfs merge=lfs -text
+*.ot filter=lfs diff=lfs merge=lfs -text
+*.parquet filter=lfs diff=lfs merge=lfs -text
+*.pb filter=lfs diff=lfs merge=lfs -text
+*.pt filter=lfs diff=lfs merge=lfs -text
+*.pth filter=lfs diff=lfs merge=lfs -text
+*.rar filter=lfs diff=lfs merge=lfs -text
+saved_model/**/* filter=lfs diff=lfs merge=lfs -text
+*.tar.* filter=lfs diff=lfs merge=lfs -text
+*.tflite filter=lfs diff=lfs merge=lfs -text
+*.tgz filter=lfs diff=lfs merge=lfs -text
+*.xz filter=lfs diff=lfs merge=lfs -text
+*.zip filter=lfs diff=lfs merge=lfs -text
+*.zstandard filter=lfs diff=lfs merge=lfs -text
+*tfevents* filter=lfs diff=lfs merge=lfs -text
diff --git a/bert/deberta-v3-large/README.md b/bert/deberta-v3-large/README.md
new file mode 100644
index 0000000000000000000000000000000000000000..e2ff251e2b40e3405e2dbc2e0687c0adcafe5305
--- /dev/null
+++ b/bert/deberta-v3-large/README.md
@@ -0,0 +1,93 @@
+---
+language: en
+tags:
+ - deberta
+ - deberta-v3
+ - fill-mask
+thumbnail: https://huggingface.co/front/thumbnails/microsoft.png
+license: mit
+---
+
+## DeBERTaV3: Improving DeBERTa using ELECTRA-Style Pre-Training with Gradient-Disentangled Embedding Sharing
+
+[DeBERTa](https://arxiv.org/abs/2006.03654) improves the BERT and RoBERTa models using disentangled attention and enhanced mask decoder. With those two improvements, DeBERTa out perform RoBERTa on a majority of NLU tasks with 80GB training data.
+
+In [DeBERTa V3](https://arxiv.org/abs/2111.09543), we further improved the efficiency of DeBERTa using ELECTRA-Style pre-training with Gradient Disentangled Embedding Sharing. Compared to DeBERTa, our V3 version significantly improves the model performance on downstream tasks. You can find more technique details about the new model from our [paper](https://arxiv.org/abs/2111.09543).
+
+Please check the [official repository](https://github.com/microsoft/DeBERTa) for more implementation details and updates.
+
+The DeBERTa V3 large model comes with 24 layers and a hidden size of 1024. It has 304M backbone parameters with a vocabulary containing 128K tokens which introduces 131M parameters in the Embedding layer. This model was trained using the 160GB data as DeBERTa V2.
+
+
+#### Fine-tuning on NLU tasks
+
+We present the dev results on SQuAD 2.0 and MNLI tasks.
+
+| Model |Vocabulary(K)|Backbone #Params(M)| SQuAD 2.0(F1/EM) | MNLI-m/mm(ACC)|
+|-------------------|----------|-------------------|-----------|----------|
+| RoBERTa-large |50 |304 | 89.4/86.5 | 90.2 |
+| XLNet-large |32 |- | 90.6/87.9 | 90.8 |
+| DeBERTa-large |50 |- | 90.7/88.0 | 91.3 |
+| **DeBERTa-v3-large**|128|304 | **91.5/89.0**| **91.8/91.9**|
+
+
+#### Fine-tuning with HF transformers
+
+```bash
+#!/bin/bash
+
+cd transformers/examples/pytorch/text-classification/
+
+pip install datasets
+export TASK_NAME=mnli
+
+output_dir="ds_results"
+
+num_gpus=8
+
+batch_size=8
+
+python -m torch.distributed.launch --nproc_per_node=${num_gpus} \
+ run_glue.py \
+ --model_name_or_path microsoft/deberta-v3-large \
+ --task_name $TASK_NAME \
+ --do_train \
+ --do_eval \
+ --evaluation_strategy steps \
+ --max_seq_length 256 \
+ --warmup_steps 50 \
+ --per_device_train_batch_size ${batch_size} \
+ --learning_rate 6e-6 \
+ --num_train_epochs 2 \
+ --output_dir $output_dir \
+ --overwrite_output_dir \
+ --logging_steps 1000 \
+ --logging_dir $output_dir
+
+```
+
+### Citation
+
+If you find DeBERTa useful for your work, please cite the following papers:
+
+``` latex
+@misc{he2021debertav3,
+ title={DeBERTaV3: Improving DeBERTa using ELECTRA-Style Pre-Training with Gradient-Disentangled Embedding Sharing},
+ author={Pengcheng He and Jianfeng Gao and Weizhu Chen},
+ year={2021},
+ eprint={2111.09543},
+ archivePrefix={arXiv},
+ primaryClass={cs.CL}
+}
+```
+
+``` latex
+@inproceedings{
+he2021deberta,
+title={DEBERTA: DECODING-ENHANCED BERT WITH DISENTANGLED ATTENTION},
+author={Pengcheng He and Xiaodong Liu and Jianfeng Gao and Weizhu Chen},
+booktitle={International Conference on Learning Representations},
+year={2021},
+url={https://openreview.net/forum?id=XPZIaotutsD}
+}
+```
diff --git a/bert/deberta-v3-large/config.json b/bert/deberta-v3-large/config.json
new file mode 100644
index 0000000000000000000000000000000000000000..9d95ab0632a030f501c2e15336123041c556b8a7
--- /dev/null
+++ b/bert/deberta-v3-large/config.json
@@ -0,0 +1,22 @@
+{
+ "model_type": "deberta-v2",
+ "attention_probs_dropout_prob": 0.1,
+ "hidden_act": "gelu",
+ "hidden_dropout_prob": 0.1,
+ "hidden_size": 1024,
+ "initializer_range": 0.02,
+ "intermediate_size": 4096,
+ "max_position_embeddings": 512,
+ "relative_attention": true,
+ "position_buckets": 256,
+ "norm_rel_ebd": "layer_norm",
+ "share_att_key": true,
+ "pos_att_type": "p2c|c2p",
+ "layer_norm_eps": 1e-7,
+ "max_relative_positions": -1,
+ "position_biased_input": false,
+ "num_attention_heads": 16,
+ "num_hidden_layers": 24,
+ "type_vocab_size": 0,
+ "vocab_size": 128100
+}
diff --git a/bert/deberta-v3-large/generator_config.json b/bert/deberta-v3-large/generator_config.json
new file mode 100644
index 0000000000000000000000000000000000000000..97bd497a506de3bf306f52506fab62cdb954a262
--- /dev/null
+++ b/bert/deberta-v3-large/generator_config.json
@@ -0,0 +1,22 @@
+{
+ "model_type": "deberta-v2",
+ "attention_probs_dropout_prob": 0.1,
+ "hidden_act": "gelu",
+ "hidden_dropout_prob": 0.1,
+ "hidden_size": 1024,
+ "initializer_range": 0.02,
+ "intermediate_size": 4096,
+ "max_position_embeddings": 512,
+ "relative_attention": true,
+ "position_buckets": 256,
+ "norm_rel_ebd": "layer_norm",
+ "share_att_key": true,
+ "pos_att_type": "p2c|c2p",
+ "layer_norm_eps": 1e-7,
+ "max_relative_positions": -1,
+ "position_biased_input": false,
+ "num_attention_heads": 16,
+ "num_hidden_layers": 12,
+ "type_vocab_size": 0,
+ "vocab_size": 128100
+}
diff --git a/bert/deberta-v3-large/tokenizer_config.json b/bert/deberta-v3-large/tokenizer_config.json
new file mode 100644
index 0000000000000000000000000000000000000000..acfd94e399c5659e4bed75f91b4ee24b111fc7a6
--- /dev/null
+++ b/bert/deberta-v3-large/tokenizer_config.json
@@ -0,0 +1,4 @@
+{
+ "do_lower_case": false,
+ "vocab_type": "spm"
+}
diff --git a/bert_gen.py b/bert_gen.py
new file mode 100644
index 0000000000000000000000000000000000000000..a5f7c258f3aa2fa5973baa58d73100a038146bad
--- /dev/null
+++ b/bert_gen.py
@@ -0,0 +1,85 @@
+import argparse
+from concurrent.futures import ThreadPoolExecutor
+
+import torch
+import torch.multiprocessing as mp
+from tqdm import tqdm
+
+import commons
+import utils
+from common.log import logger
+from common.stdout_wrapper import SAFE_STDOUT
+from config import config
+from text import cleaned_text_to_sequence, get_bert
+
+
+def process_line(x):
+ line, add_blank = x
+ device = config.bert_gen_config.device
+ if config.bert_gen_config.use_multi_device:
+ rank = mp.current_process()._identity
+ rank = rank[0] if len(rank) > 0 else 0
+ if torch.cuda.is_available():
+ gpu_id = rank % torch.cuda.device_count()
+ device = torch.device(f"cuda:{gpu_id}")
+ else:
+ device = torch.device("cpu")
+ wav_path, _, language_str, text, phones, tone, word2ph = line.strip().split("|")
+ phone = phones.split(" ")
+ tone = [int(i) for i in tone.split(" ")]
+ word2ph = [int(i) for i in word2ph.split(" ")]
+ word2ph = [i for i in word2ph]
+ phone, tone, language = cleaned_text_to_sequence(phone, tone, language_str)
+
+ if add_blank:
+ phone = commons.intersperse(phone, 0)
+ tone = commons.intersperse(tone, 0)
+ language = commons.intersperse(language, 0)
+ for i in range(len(word2ph)):
+ word2ph[i] = word2ph[i] * 2
+ word2ph[0] += 1
+
+ bert_path = wav_path.replace(".WAV", ".wav").replace(".wav", ".bert.pt")
+
+ try:
+ bert = torch.load(bert_path)
+ assert bert.shape[-1] == len(phone)
+ except Exception:
+ bert = get_bert(text, word2ph, language_str, device)
+ assert bert.shape[-1] == len(phone)
+ torch.save(bert, bert_path)
+
+
+preprocess_text_config = config.preprocess_text_config
+
+if __name__ == "__main__":
+ parser = argparse.ArgumentParser()
+ parser.add_argument(
+ "-c", "--config", type=str, default=config.bert_gen_config.config_path
+ )
+ parser.add_argument(
+ "--num_processes", type=int, default=config.bert_gen_config.num_processes
+ )
+ args, _ = parser.parse_known_args()
+ config_path = args.config
+ hps = utils.get_hparams_from_file(config_path)
+ lines = []
+ with open(hps.data.training_files, encoding="utf-8") as f:
+ lines.extend(f.readlines())
+
+ with open(hps.data.validation_files, encoding="utf-8") as f:
+ lines.extend(f.readlines())
+ add_blank = [hps.data.add_blank] * len(lines)
+
+ if len(lines) != 0:
+ num_processes = args.num_processes
+ with ThreadPoolExecutor(max_workers=num_processes) as executor:
+ _ = list(
+ tqdm(
+ executor.map(process_line, zip(lines, add_blank)),
+ total=len(lines),
+ file=SAFE_STDOUT,
+ )
+ )
+
+ logger.info(f"bert.pt is generated! total: {len(lines)} bert.pt files.")
diff --git a/clustering.ipynb b/clustering.ipynb
new file mode 100644
index 0000000000000000000000000000000000000000..12e728ef6b35c8fb62f7860eed62fe0d3080bdd0
--- /dev/null
+++ b/clustering.ipynb
@@ -0,0 +1,316 @@
+{
+ "cells": [
+ {
+ "cell_type": "markdown",
+ "metadata": {},
+ "source": [
+ "# スタイルベクトルをもっと詳しく作りたい人向け\n",
+ "\n",
+ "JVNVコーパスの例を使いながらクラスタリングをいろいろいじったり、また正解ラベルからベクトルを作りたい人向けです。\n",
+ "もっといろいろ足したりいろんなスタイルベクトルを作って遊べると思います。\n",
+ "ある程度慣れている人向けです。\n",
+ "\n",
+ "JVNVコーパスのように**スタイルが既にファイル名等で分かれている場合は、最後の方のセルを使えばそれを利用してスタイルを作ることができます。**\n",
+ "\n",
+ "例では[JVNVコーパス](https://sites.google.com/site/shinnosuketakamichi/research-topics/jvnv_corpus)のjvnv-M1を使います。\n",
+ "\n",
+ "## そもそもスタイルベクトルとは\n",
+ "[この話者識別モデル](https://huggingface.co/pyannote/wespeaker-voxceleb-resnet34-LM)を使って生成された、1つの音声ファイルにつき256次元のベクトルです。話者識別用のものですが、感情や声音の特徴も含まれているので、スタイルベクトルとして使えます。\n",
+ "\n",
+ "このStyle-Bert-VITS2では、この256次元のベクトルをエンコーダに注入して学習しているので、推論時にそのベクトルを入れてあげる必要があります。ある感情を強く表していると思われるベクトルを入れると、その感情を強く表現した音声が生成される、という仕組みです。\n",
+ "\n",
+ "もともとが話者識別用なので、「この感情はこのベクトル」のような普遍的なスタイルベクトルは使えません。なのでこのように、いちいちデータセットごとにベクトルを作る必要があります。\n",
+ "\n",
+ "## モデルを使うために必要なもの\n",
+ "- `model_assets/{model_name}/model_name.safetensors`: 学習の結果出力されるモデルファイル。これは自動的にこの場所に置かれ、スタイルベクトルとは全く独立。\n",
+ "- `model_assets/{model_name}/style_vectors.npy`: スタイルベクトルのnumpyファイル。ベクトルをいくつかいれる。\n",
+ "- `model_assets/{model_name}/config.json`: モデルの設定ファイル(学習前準備で自動的に生成されるはず)。これの以下の項目を設定。\n",
+ "```json\n",
+ "{\n",
+ " \"data\": {\n",
+ " \"num_styles\": 4, // スタイルベクトルの数\n",
+ " \"style2id\": { // スタイルベクトルの名前とidの対応\n",
+ " \"Neutral\": 0,\n",
+ " \"Angry\": 1,\n",
+ " \"Happy\": 2,\n",
+ " \"Sad\": 3\n",
+ " }\n",
+ " }\n",
+ "}\n",
+ "```\n",
+ "ここでidは0から始まる整数で、スタイルベクトルのnumpyファイルの何番目のベクトルかを指定します。最初のNeutralは含めたほうがよさそうで、WebUIや下では全スタイルベクトルの平均を入れています。"
+ ]
+ },
+ {
+ "cell_type": "code",
+ "execution_count": 1,
+ "metadata": {},
+ "outputs": [],
+ "source": [
+ "import os\n",
+ "import numpy as np\n",
+ "\n",
+ "wav_dir = \"Data/jvnv-M1/wavs\"\n",
+ "\n",
+ "embs = []\n",
+ "names= []\n",
+ "for file in os.listdir(wav_dir):\n",
+ " if file.endswith(\".npy\"):\n",
+ " xvec = np.load(os.path.join(wav_dir, file))\n",
+ " embs.append(np.expand_dims(xvec, axis=0))\n",
+ " names.append(file)\n",
+ "\n",
+ "x = np.concatenate(embs, axis=0)"
+ ]
+ },
+ {
+ "cell_type": "code",
+ "execution_count": 2,
+ "metadata": {},
+ "outputs": [
+ {
+ "data": {
+ "image/png": "iVBORw0KGgoAAAANSUhEUgAAAlkAAAJGCAYAAABoeJAJAAAAOXRFWHRTb2Z0d2FyZQBNYXRwbG90bGliIHZlcnNpb24zLjguMiwgaHR0cHM6Ly9tYXRwbG90bGliLm9yZy8g+/7EAAAACXBIWXMAAA9hAAAPYQGoP6dpAABqgElEQVR4nO3df3xU5Zk3/s8EkwCRDASQCRQl4E+KEnUrUKlbKQouq7jt9im0drV1saXY9deuYCv+KG0R22+rXVmtrKvbx4ptd7eipcuzKrY8aNBdaVxTtCtZrD6Y0CVIRgMEzMz3DzxxMplzzn3OuX+dcz7v18vXS5LJzD1zzsy55rqv+7ozxWKxCCIiIiKSqsr0AIiIiIiSiEEWERERkQIMsoiIiIgUYJBFREREpACDLCIiIiIFGGQRERERKcAgi4iIiEiBY0wPIKpCoYC33noLI0aMQCaTMT0cIiIiSrBisYh33nkH48ePR1WVd64q9kHWW2+9hYkTJ5oeBhEREaXIm2++iQ996EOet4l9kDVixAgAR59sfX294dEQERFRkuXzeUycOLE//vAS+yDLmSKsr69nkEVERERaiJQosfCdiIiISAEGWUREREQKMMgiIiIiUoBBFhEREZECDLKIiIiIFGCQRURERKQAgywiIiIiBRhkERERESnAIIuIiIhIAQZZRERERAowyCIiIiJSgEEWERERkQIMsoiIiIgUYJBFREREpACDLCIiIiIFGGQRERERKcAgi4iIiEiBY0wPgIjk6CsU8cKuffjDO4dw3IihOKepAUOqMqaHRUSUWgyyiBJgU1sHbn9iBzq6D/X/rDE7FLdePBXzpzUaHBkRUXpxupAo5ja1dWDpw9sHBFgA0Nl9CEsf3o5NbR2GRkZElG4MsohirK9QxO1P7ECxwu+cn93+xA70FSrdgoiIVGKQRfS+vkIRLe1d2NC6Gy3tXbEITF7YtW9QBqtUEUBH9yG8sGufvkEREREA1mQRAYhvTdMf3nEPsMLcjoiI5GEmi1IvzjVNx40YKvV2REQkD4MsSrW41zSd09SAxuxQuDVqyOBoRu6cpoZYTocSEcUZpwsp1YLUNM2aMlrfwAQNqcrg1ounYunD25EBBgSLTuB168VT8eSOzlhOhxIRxRkzWZRqSahpmj+tEfdedhZy2YFTgrnsUNx72VkAENvpUCKiOGMmi1ItKTVN86c14oKpuUEd3wFg9prNrtOhGRydDr1gao7d4YmIJGOQRYkTZHsZp6aps/tQxUAkg6MZIaemyeZta4ZUZQZNaba0d8V6OrSU7a8/EVE5BlmUKEFbMcSppilMkKFrOlR1ABTXFhtElG6ZYrEY6yVG+Xwe2WwW3d3dqK+vNz0cMshpxVB+QjuX+nsvO8v1gux1EQcQ+n6DcgtWwgYZLe1dWLxum+/jrl8yM3QmS3UAFOW4EhHJFiTuYJBFidBXKGL2ms2uU2POtN/W5XNcMyyVAhwAke9XlFuwcsn0Rty/ZVeoIMN5XfymQ8OOX3UAJOO4EhHJFCTu4OpCSgQZ28s4NU0Lmydg1pTRGFKV0bZtjVdD1B9WCLCcxwa8+3g506EABvXSKp0ODROg6Ogxxm2DiCjOGGSRUbIaZKqqPdJR0yQSrLgRCTL8WjyEzTTpCICS0GKDiNKLhe9kjMxaHlWtGHS0ePALVkT4BRluLR4qZbBEi9hlB0CVHjcpLTaIKJ0YZJERbrU8ToPMoBmWIK0YglB1v6VkZGFEgoxKLR7KBQl8ZQZAbo+7csFU5a8/EZEqnC4k7VTU8qiqPQpzv0GnQKNkYUr3Jowq6EbZQfZNDPu4yx7ZjkumN/bfX/n9A+FryoiIVGOQRdqpquVRVXsU5H43tXVg9prNWLxuG655tBWL123D7DWbPbeu8QtW3MgMMsIEvjICW5HHffylDqz97JnSjysRkWqcLiTtVBYzB6k9kn2/YadARRqiXnVeEx5/qWNAcJqT2Isq7EbZTgBaPtUnOjbRxx1VV4uty+ew4zsRxQqDLNJOdTGzSO2R7Pv1y8j47REoEqzcOP80ZUFGlMA3SmAb5HFVHVciIlUYZJF2qovJTexxFzYTVMovWFEZZEQNfMOOjasHiSjJGGSREJmBi+h+gWE7kJvY407WFKipbI2OVZQ2PS4RkQ4sfCdfYYq5/agoUg+6Ok6muGdkSovYKykCuGR6o/SMoMqO9EREpnHvQvKkY286GRky03vcqd4jUJfVv9yBH27ZVfF3GYQ73iLHuFIGcnRdDVYtnIY/OYOrB4nIHkHiDk4XkquoxdwiZE2PyaiJikLlFKgufYUiHn/JO9sX9HiLTt/On9aIQqGImze0YV/PEQBAV89hrNq4A1VVYJsGIoolpdOFq1evxkc+8hGMGDECxx13HC699FL87ne/G3CbQ4cOYdmyZRg9ejSOPfZYfOpTn8KePXtUDosExWlzXhv2uHObAh1VV421nz1TaqAga8/HUrKPd5Dp201tHVj2yG/6Ayyv2xIRxYXSIOvXv/41li1bhm3btuHJJ5/EkSNHcOGFF6Knp6f/Ntdddx2eeOIJ/OxnP8Ovf/1rvPXWW/jkJz+pclgkyIbARZQtNVHzpzVi5YKpaKir6f/Zvp4jWLXxFWmBgooaOUDu8Q7S3FTFDgBERDZQOl24adOmAf9+6KGHcNxxx+HFF1/Eeeedh+7ubjzwwAN45JFHMGfOHADAgw8+iNNOOw3btm3DzJkzB91nb28vent7+/+dz+dVPoVUsyVwEWHLKrWjGRl5ezJWun+Zez6Wknm8g2bFwkz1mmjVQUQUhNbVhd3d3QCAhoajF7oXX3wRR44cwdy5c/tvc+qpp+L4449HS0tLxftYvXo1stls/38TJ05UP/CUkrU3nQ42rFJTnZFRff8yj3eQrFiYDJqqbB4RkUzagqxCoYBrr70W5557LqZNmwYA6OzsRE1NDUaOHDngtuPGjUNnZ2fF+7npppvQ3d3d/9+bb76peuipZUPgEoSqvQtFqa5hU33/Mo93kKxY0AyayVYdRERBaFtduGzZMrS1tWHr1q2R7qe2tha1tbWSRkV+ou5Np5uqvQtFqK5h01EjJ+t4B52+Fb2tjhWvRESyaAmyrr76avziF7/Ali1b8KEPfaj/57lcDocPH8b+/fsHZLP27NmDXC6nY2gkQEXgksR6GtU1bKrv3zkmve8V8N0/nw5kgL3v9oY6PkFbWojetqW9y2irDiKiIJQGWcViEV/96lfx85//HL/61a/Q1NQ04Pdnn302qqur8fTTT+NTn/oUAOB3v/sd3njjDcyaNUvl0Cggmdu9qNz6xtS2On2FIgrFIkYOq8b+g0cq3iZq8b3K4n6v1y3scXfLio2qq8Y3F04b1CdLJIMWpxWvRERKO75/5StfwSOPPIINGzbglFNO6f95NpvFsGHDAABLly7FL3/5Szz00EOor6/HV7/6VQDAc889J/QY7PgeLyo7yKvuTu/1uOXBQTlZY3CeI1A54xPm/qO8biIZyV/+Z8f7TUYP9//MLfD1u7+W9i4sXrfN9zmtXzKTmSwiUiJI3KE0yMpkKk8vPPjgg7jiiisAHG1GesMNN2D9+vXo7e3FvHnz8Hd/93fC04UMsuJD5dY3prbVcQtQysnMpsnK1vUVitjW3oVlj2z3zb5Vet1ExiE78E3K9kVEFF/WBFk6MMiKD5VZCBMZDr/ADgBGDq/G2sVnYeaU0VIv+lFr2kSyb6XKXzeR4OmCqTklga+KbB4RkSjuXUhWUllPY6JWx6+lAgDsP3AEVVUZ6VmVKDVyotm3UqWvm+gKvxFDq5UUqcdtxSsRpReDLNJG5eo4E93p41iE7RUgeSl93UT7dbW0dwndd5jXx2SrDiIiUQyySBuVq+NMbKsTp22HHCLZt1KVXjfxoEgslAv7+shc8UpEpILWbXUo3VR2kDfRnT5O2w45gmSN3F430aBo1uQxsXt9iIhkYpBFWqnc+kb3tjpx23YICJY1cnvdRIPLmVNGB359+gpFtLR3YUPrbrS0d4Xeh5GIyAZcXUhCZHdor3R/fYUi/nfL6/j9vgM4oWE4Pj9rEmqOCf49IOpYg/69qQaoYfi1QACAkcOqsfZzZ2HmZPcVkUFW+Im+PnF6HYkovdjCgaTScfFb/csdWPd/d6E0cVGVAZZ8rAk3/clUKY8hIuxzjdM2QW4BkuO6uSfh6jkn+Y4/yGvl9/qYaiRLRBQUgyySRsfFb/Uvd+CHW3a5/v5L5+kJtHQ8V1uCMb8+WaJBtIznY6qRLBFRGAyySAodF7/D7xVw6sp/hVfpTVUGeHXVRaGmDkXpeK62TYf1FYq4Z/NOfP+p/xr0O50ZJG6VQ0RxEiTuYOE7uRLth/TCrn2hH+N/t7zuGWABQKF49HYyuBVWq36uTpas/DE6uw9h6cPbsamtI9T9RvXov79R8efOIbn9iR3Ki8/j2G+MiEgE+2SRKx0Xv9/vOyD1dl68Mkm97xWE7iPMcxXtkH7B1JzW6bAggaWqDFJfoYi97/QK3damfmNERCIYZJErHc02T2gYLvV2btzqrZxM0rVzTxK6nzDP1YZgphLTGSTR/RNFG8naUu9GRORgkEWudHRR//ysSfjWL1/xrcn6/KxJoR9DJJO0/oU3kKsfij157+d69gmj0NLeFehCbjqYcWOyY73o/omi/cZsq3cjIgJYk0UedDTbrDmmCks+1uR5myUfa4pU9C6SSerM92LxOccDcH+ul0xvxB9/5xksXrcN1zzaisXrtmH2ms2+9VS2br9jqmN9kP0TRRrJ2lrvRkTEIMsiNna71tFF/aY/mYovndeE8litKvNB+4Yor41ohmjSmOGuz/Wq85pw/5ZdoS7ktm6/Y6pjvej+iSsXnIaty+f49ifzylICeor3iYgq4XShJWye7pg/rREXTM0prXe56U+m4oYLT63Y8T3qaxMkkzRryuhBz/XsE0bhj7/zTOjCdSeYWfrwdmRQuUO6qe13nCC6/PXNKTz3RIPeMSNqfV8TW+vdiIgABllW8CvKtqHb9ZCqjPKLVM0xVbjyY5MH/EzGaxO0tqz8uba0d0W+kKsMZqIWfOsIokvJnD61td6NiAhgkGWcrcv7bSDrtYmaSZJ1IS8NZjq7D2Jfz2E0HFuL7LAa9BWKoY6vrAyojiDaIXNBha31bkREAIMs4zjd4U7ma+OWScoOq8YXzp2EC6bmXP9W5oV8SFUG3QcP487/87sB42ioq8afNU/A3Kk54SySrRlQv8yazOlTHStgiYjCYuG7QX2FIp7duVfotmmc7pA9FTR/WiO2Lp+D6+aejJHDqgEA+w8ewfefes1zlaDMwnW3lXD7eo7ggWdfF16xaGvB96a2Dsxes9l3BaasBRWmiveJiEQwyDLEuRjd88xOoduncbpDxVTQkzs6cddT/4X9B48M+LnXKkFZF3LR1gUiKxZ1bHkUVNBWCk7Qu37JTNy9qBnrl8z0XU1YiY4VsEREYXC60ADRRoxAuqc7RKaCxtXXolAsYkPrbt+C7Sg1XjIK10VbF4jUm9lW8B32tZVVC6a7eJ+ISASDLM2CNGKM43SHzK1N/Gp3igAOvVfA5/7++f6fexV9R63xinohDxLw+I3FtoJvG2oLdRbvExGJYJClmWg2A1Dbq0gFFb2+XAvWh1dj/4Ej2H+g8rRfpWkiGdmfKBfyMAGP21hEC77DbAMUhm2ZNSIiGzDI0kz0InP1+VNw3QWnxCaDpXKlW3kGaUxdLW742UsAjgy6rdfUlOnsj19gFGQsIiv0nG2AdDS4Nf3aEhHZiIXvmoleZM49cWzoAEv39jw6Vro5GaSFzRNQVZVBZz540bfp7W1KC+j9iIzFq+A7yjZAYZh+bYmIbMRMlmaq+/qY2J5Hdz1O2KkpG7a3cZv+LBVkLJXqxKJuAxSG12vrPO7KBafFJjNLRCQDM1maqezrE3QJvSy663GiTE3ZsNy/tHXBF8+dhIa6mkhjKc3yzZoyGi/+/m0j7R3cXlvHqo2vKDsHiYhsxEyWASr2sTO5PY/uepyo2cD50xox59RxFTej1sUJjGZNGY2vL5gqtfWAySL0+dMaUSgAX3lk+6Dfme5ET0SkG4MsQ2T19XFaJjy7c6+xJfS6tzaJOu1XaUr177fukjalGrSNRemKRa+/Fb1fk0XofYUiVm3cUfF3ad+Lk4jSh0GWQVH7+lQKFvyoyF6YqHUKmw1Uvd9flJo4r78FIHy/Jvfzs6FfFhGRLTLFYlHv5maS5fN5ZLNZdHd3o76+3vRwtAnSNb7U+iUzlV3cTBTdB8ka9RWKmL1ms2fBeS47FFuXz4lUE1d+TJx78grgvP7W7Rh73a9zf0DloFfVlN2G1t245tFW39vdvagZC5snSH98IiLVgsQdzGTFUJCu8Q4d2/OY2NokSDZQZZYlSk2cSAsMt/G63a+quj+/Y8t+WUREH2CQFUNBusYDerfnsXlrE5UF4VECuKDHU/R+ZQa9ollKk1OVRES2YQuHGAoaBOhsT2AzlVmWKAGcjDo5t/sob++gujWIyhYlRERxwyArhkSDgKvPn4L1S2Zi6/I5qQ+wALVdyaMEcDKmzlRNv4Xp5m9DLzIiIhtwujCGRKdk4rT3oQ6yV0GW1iiNObYWufpa7Mn3Bp4mC7Onocj9yhB2GtREfR4RkW0YZMWQDdvDxJWsgvBKNUojh1f3F6MHOSZ+x7NY4f9F7leGKNOgNtfnERHpwCArptyChVF11fiz5gnIDqtBX6HIQKuCqFkWt3YL3QeOAACyw6ux//3/B8QCOL/gDxjcJyvKSkFRXC1IRBQe+2TFnDNl9eSOTjzW+hb29Rzu/53qHlVpJNpr67t/Ph17e3oDB3AyOr7L5Dxfv6npsL3FiIjihn2yUmRIVQbdBw/jwWdfV9bFXAUTAYMMojVKVVWZUM02vabYTEy/cWqaiCg8BlkxZ3Jj6LBMdIaXxeTmy6aoaGxKRJQGDLIsJZrpidtecar3DlQtrTVKXC1IRBQcgywLBcn0xCmzEsesWzkZHc3jOlXK1YJERMEwyLJM0ExPnDIrccu6VVJao1RJEcAl0xtdg6Y4T5USEVEw7PhukTDdtVV2MZctTlk3L/OnNeKq85pcf3//ll0DtppxBNmehoiI4o9BlkWCZHoccdorLk5ZNy99hSIef8k7ICoPhsME0LbqKxTR0t6FDa270dLeFYsxExGZwOlCi4TN9MRl9ZeMeiYbhJn2TMJUKcDpTiKiIBhkWSRKpicOq7+S0nMpTDCchKnSuK8MJSLSjdOFFolaX+Ws/vrTM8YDAH7xn29ZN53jZN1y2YGBYi47VOgibcNUVZhgOO5TpUma7iQi0oWZLIvIyPTEYTonbNbNlucWZtoz7lOlSZnuJCLSiZksy0TJ9MRp9ZqTdVvYPAGzpowWCrBseW5hFhvEaYFCJUmY7iQi0o2ZLAuFyfQkodGnGxufW5jFBnFZoFBJ3Kc7iYhMYJBlqaDdtZM8nWPrcwsTDMdhgUIlcZ/uJCIygUFWQiR5Osfm5xZmq5k4bk+TlJWhREQ6sSYrIUxP56hc9Wf6udFRUVeGEhGlDTNZCWFyOkf1qj9OVdkjrtOdREQmMJMlwIbeTH5MrV7Tseov7ivzkiboylAiorTKFItF+yKGAPL5PLLZLLq7u1FfXy/9/m3pzSRK53j7CkXMXrPZtSjdyTBtXT5HyoU4bseCiIiSJ0jcwSDLg9s2Ik64YGsdSl+hqGU6p6W9C4vXbfO93folM6UVeut6bmQPHnMiskmQuIM1WS5s7M0kStfqNROr/uK4Mo/CY/aSiOKMNVkugvRmSisZq/7iUO9GZtjU5Z+IKAxmslzY3JvJFlFX/TFLQW7inEkmInIwk+WCvZn8RVn1pypLwcxYMjCTTERJwEyWC/ZmEhNmPz5VWQrbMmMs2A6PmWQiSgIGWS64jYi78uDhgqm5QA0qVexF6LYS1MmM6V4JalvAFzfMJBNREjDI8hAmS5N0MoIH2VkK2+p3bAv44oiZZCJKAgZZPtKyjYjI1Jas4EF2lkJFZiws2wK+uGImmYiSgEGWgKT3ZhLJTskMHmRnKWyq37Ep4Is7ZpKJKO4YZKWcaHZKZvAgO0thU/2OTQFfEqQlk0xEycQWDgkStH2BX3YKOJqd6isUpQcPTpYilx0Y+OSyQwPXLDmZMbfLbgZHM3M66ndsCviSghtSE1FcMZOVEGEK0oNkp1QED7KyFDbV77Bgm4iIHMxkJUDYxp5BslOqskWyshQyM2NRRGnQSkREycJMVsxFKUgPkp2yKVvkxpb6HRZsExERwCAr9qIUpAed2opD8GDLSlBbAj4iIjKHQVbMRSlID5OdYvAgzpaAj4iIzGCQFXNRC9LDZKdUBQ/c64+IiJKEQVbMyVjNZkN2inv9ERFR0nB1YczJWs1mshdR2NWRRERENmOQlQC2tC8II0hDVBOCNnglIiJycLowIWyY8gvD5r3+OIVJKrEGkSj5GGQlSBxXs9m615/ono5EYWxq68Btj/8Wnfne/p/l6mtx2yUf5nlFlCCcLiSjbNzrz/YpTIq3TW0d+PLD2wcEWADQme/Fl1mDSJQoDLLIKJs2d3YEmcIkCqKvUMSKf3nZ8zYr/uVlBvBECcEgyyAWVdu515+tU5im8DyVZ1t7F/YfOOJ5m/0HjmBbe5emERGRSqzJMoRF1R+wbbseU1OYNhZC8zyVq+W/9wrf7tyTxigeDRGpxiDLABZVDxZ1daTMAMWvwSsANNRVozN/CC3tXVKCIRuDGZ6nKoieJ1xlSJQEmWKxGOvcfz6fRzabRXd3N+rr600Px1dfoYjZaza71vw4Hdq3Lp9jPIsRFyoCFCfAAOAaaIV5rErB4JM7OisGM87RNxHM8DxV49mde/G5v3/e93Y//ssZOPdEZrKIbBQk7mAmSzOb+0LFkapsi9sUZiWij1UpGMzV1+LQewXXlYwZHF3JeMHUnNZghuepGjMnj8bI4dWedVmjhldj5mS+pkRJwMJ3zUwXVasoYjZVGK261cL8aY3YunwO1i+Zie//r+loqKupeDuRx3LdOijf63nBNbWS0fR5mlRDqjK445One95m9SdPZ3aQKCGYydLMZF8oVdNqsu4zaF2VjmyL0+C1pb0L+3oOh3osr2BQlO5gxsb+ZUkxf1oj7rvsLNz2+A505u2pwSMi+RhkaeZXVO3UusjuC6ViWk3mfYYJ1nRmW6I8ll8wKEJ3MGPqPE2LuG6DRUTBcLpQMxN9oVRMq8m8T9eptPeDNbcO2DqzLVEeK0qQZ6IZK2Bn/7KkcbKkC5snYNaU0XwtiRKIQZYBTlF1LjvwgpzLDlWykkxFB3NZ9xklWNPZLT7KY4UN8kwHM7rPUyKipOF0oSE6pwtUTKvJus8odVVOtmXpw9uRwcBWC7IDlCiPJTL1lh1ejaHHDBlQo2OqGWspTmsREYXHIMsgZ7pANRXTarLuM2qwprNbfNjHEgnQ7vjk6dYGM7rOUyKipGGQlQIqiphl3aeMYE0k2yKrI3zYzI5ogMZghogoORhkpYCKaTVZ9ykrWPPKtshuXRE2s8OpNyKidGHhe0qoKGKWcZ+qV7GFXbmoCleUERGlB/cuTBmZGynLvE8VjVK5/x4REcnGvQvJlYoiZhn3qWIqjfvvERGRSQyySAq3bFaQLJfsAJD77xERkUkMshJGxXSgH7epvkumN+LxlzqkTgEGoaJ1hYnXl4iI4olBVoKoqGsSecxK+xd2dB/CD7fsGnT7KHsliigNgsYcW4tcfS325HultK4w8fqqwmCRiEg9Fr4nhFuw41w2VQQ1foXlboIUnAcJBioFQSOHV2P/gSOubSZEXxcTr68qSQoWiYh0CxJ3KG3hsGXLFlx88cUYP348MpkMHnvssQG/LxaLuOWWW9DY2Ihhw4Zh7ty5eO2111QOKZFUbAAtwq+w3I3ovoab2jowe81mLF63Ddc82orF67Zh9prNFdsuuLVq6D5wBMDRbWtKBWkzYer1VcG2lhZEREmmNMjq6enB9OnTsXbt2oq/v/POO/GDH/wA9913H55//nnU1dVh3rx5OHSIhchBqNgAWkTUgnGvvw8SDPgFQRkAw6qH4MdXzsDdi5qxfslMbF0+RzhrY+r1lS1JwSIRURworcm66KKLcNFFF1X8XbFYxF133YWbb74ZCxcuBAD86Ec/wrhx4/DYY49h0aJFFf+ut7cXvb29/f/O5/PyBx4zplbRBSkYD/L3IkHT7U/swAVTcxhSlREOgqqqMljYPMH1dm5Tk0lZpciWFkREehkrfN+1axc6Ozsxd+7c/p9ls1nMmDEDLS0trkHW6tWrcfvtt+saZiyoWEUnwm9LHDd+BedBgwEZQZBXnZKu11d1MXpSgkUiorgwtq1OZ2cnAGDcuHEDfj5u3Lj+31Vy0003obu7u/+/N998U+k448AJdtwuxxkcDRiCbAAtwmtLHDciW+UEDQaiBkF+U5Nv9xxW/voGqT8Ly1QwHlVfoYiW9i5saN2NlvYuTmcSUWzEbu/C2tpa1NfXD/gv7VTv/+fFbf/CxuxQfOm8JjSG2NcwaDAQJcgUqVNatXEHVi44rf++yu8biMf+irKDcR3Bj47gk4hIFWPThblcDgCwZ88eNDZ+cMHds2cPmpubDY0qvpxgp3zKKydxab7bdJbXljg3zj8t8BSY3zRk+XSjE2QufXi7a6sGtyBIdGpyVF2tktc3aP1ZFFFep3I62kC4tc1Q3WuNiEgWY0FWU1MTcrkcnn766f6gKp/P4/nnn8fSpUtNDcsYGfU4Kvb/c/hdVN22xAm7Vc6ijxyP7z/1X4N+7hYMhA0yg0xNLmyeEPv9FWUE4zqCH53BJxGRKkqDrHfffRc7d+7s//euXbvQ2tqKhoYGHH/88bj22mvxzW9+EyeddBKampqwcuVKjB8/HpdeeqnKYVlHZlZAxQbQOjMKlV6LUl7BQJggM+jUZBL2V4wSjOsKfrgSkoiSQGmQ9R//8R84//zz+/99/fXXAwAuv/xyPPTQQ7jxxhvR09ODq666Cvv378fs2bOxadMmDB1qV+GtSrZPiejMKLi9Fo7r5p6Eq+ec5Pk4QYOgoFOTspkqRg8bLOoKfrgSkoiSQGnh+8c//nEUi8VB/z300EMAgEwmg2984xvo7OzEoUOH8NRTT+Hkk09WOSSrxKE5pK5GnF6vBXA02Hn0371XkoYpxDa5aAAwtzI0LF3BT1xXQhIRlYrd6sIkiUMncV0X1aivRZRVaG4rJINsvROW6SAvKF3BT9yCTyKiSowVvpO+ACZKUb2ui2qU10LGlKvKRQN+dKwMlUXX9KrMlZBERKYwyDJIRwATtahe10U17Gshs2ZMxaIBUSaDvCB0Bj9xCj6JiCphkGWQ6gDGL8Nz7dyTMWnMcM8Luq6LatjXIkmr0EwGeUHoDH7iEnwSEVXCIMsglQGMSFF9aR+qhroaXNo8HhdMzQ26iOm4qIZ9LbgKzQydwU9cgk8ionKZYrEY643A8vk8stksuru7Y7vFjoru2S3tXVi8bluov3V7bNUbGAPBXwvR57l+yUzrLtQ6Xk8iIpIrSNzBTJYFVGQFomRuOlwKxnVkFIK+Fqb7XIWlY1uauAgTbDJAJaI4YJBlCdkBjIz+Qaa2LQnyWphchRb2Qm97A1o/MgMckWCz/PHe7jmMVRsZoBKR/ThdmFB9hSJmr9nsmuERZeM0WyW6M0NhH885Lm7F+k7mbevyOVZmZmS+zm7BpvOs773sLADw3Gap0t8w0CIilYLEHQyyEsy5iAEIHWjdvagZC5snSBlP1AyI39/rmkISCQ7cLvRxriGL8rzLiQSbI4dX4+0DR4THZ3uASkTJwJosAuC+KjAIWduWRM2AiPy9jpqxqH254roaUvYeliKtN4IEWM7fxKVdBxGlA7fV8RFmPzybzJ/WiK3L52D9kpm4e1Ezrpt7EoDBW7iUk7ltiZMBKb+oOjVIflvfRP17maJu/xPXPflkbwGlMoi0LUAlovRiJstDUlaAlWd4TsmN8MxuySwYP/xeAV/7eVvoDIjsDEpUUTNRcV0NKTsDpzKItC1AJaL0YibLhU3ZE9lKs1tXnjsJDXXVA34va2PkTW0dmLn6KezrOex6G78MiG2baEfNRMVtQ2iH7Ayc3wbQYXDTaCKyDTNZFdiWPVHByW7NmjIaX1swVXrBuFuRtBu3DIhtNUwyMlFx3JNPdgZOpPVGdng1ug8cETqHbA5QiSi9GGRVkKT98ETILhj3ClLduGVAbKthktWXK2578qnoR+YXbAKo+HiV2BygElF6MciqwLbsSdy6W/sFqaX8MiA21jDJykTFbU8+FRk4v2Cz0uM1Zodi5YLTMKquNjbvCSJKJwZZFdiUPYlj8X3Q4NMrA2Kyo7uXuGWignIL7FU8b69gM+mvMxElG4OsCvyyJ8DRRomFQhF9haKyD/y4br8iGnyOrqvBt/5smutzcC70ve8VcO3ck7D+hTfQme/t/73pKaK4ZaJE+QX2up93Ul9nIko+dnx3IdotXVVWKc7br4hs6dNQV41tN81FzTGVF7hWutDn6odi8TnHY9KY4cxoKCKzqzsRURIFiTvYwsGFU3+Sy3pnZVS1dLCtdUEQfm0KMgC+/WenewZYldpn7Mkfwl1P/Rdqj6nCrCmjjQRYtjSnVTEOv1W1wNFVtXFryEtEZAqnCz049SDb/rsLy368HfsPDt7mQ1VLB9uK74MKWyRtc/sMW+rjVI0jbatqiYhUY5DlY0hVBlWZTMUAy6Hi4mNT8X1YYYqWbb3Q21Ifp3IccQ/siYhswyBLgImLj42tC7y4rUYLWrRs44Xeluya6nEkIbAnIrIJgywBJi4+trYuqETm9JWNF3pbsmuqxxG3wJ6IyHYsfBfgt8+aqj3T3IrvZe0tKIPsPR5NvdZebMmuqR5HXPdVJCKyFTNZAkxmlWxqxlg+JXj2CaOkT1/ZmMGzJbumYxyiCxbitgsBEZEJDLIEmdzU14ZmjJWmBBvqarCv57Dr34SdvrJtA2VbptF0jcMvsLdllSURke3YjDSgNH6Dd1vRJuruRc1Y2Dwh8N/Z9Fq7NafV3aTT9DjYrJSI0i5I3MEgKyKbAgEV/DrPi1i/ZKbxTJwMtmRwTI0jzrsQEBHJEiTu4HRhBLZcdFXyW9HmJWmr0WypjzM1DltWWRIRxQWDrJBsaU6pWtiVakldjWZDfZypcdiyypKIKC7YwiGENO3xJrpSraGuesC/bWozQXLYssqSiCgumMkKIU3TJqIr2n79N+fjxd+/bVVtWtLr5XSzZZUlEVFcMMgKIU3TJqJ9q2qOqbIqoExDvZxuNvYwIyKyGacLQ0jbtEmUzvN9hSJa2ruwoXU3Wtq7tEyhyu5CTx+Iwy4ERES2YCYrhDROm4RZ0WYim2TLZs5+4jyVacsqSyIi2zHICiGt0yZBVrSZWn0Zh3q5JExl2rLKkojIZpwuDInTJu5Mrr60vV7Oayrzyw9vxzee+K22aVUiIlKLmawIOG1Smclsks31ciLB5z88+zr+4dnXY5fZIiKiwZjJisiZNlnYPAGzpoxOfYAFmM0mOfVybkchg6NTcybq5YJ0z2eRPhFR/DHISjgTq/tMZpOcejkAgwIt0/VyQYLKpDW1JSJKI04XJpipAmu/1ZcAMLquBmefMErJ4zv1cuXPPWd4Ci5oUGlDkT4REYWXKRaLsf6aHGQ37LiQsbzfbXWfcy+qi/OdxwfgGmjpaOdgsl6u/PHPPmEU/vg7z3gGn5XcvagZC5snKBsX6wiJiMQFiTsYZFlGRvapr1DE7DWbXet/nD5eW5fPUXpxrfRcyscBqA/4KlEdaLgdx0umN+L+LbsAuAef5dYvmSktk5WE9hFERCYxyIopWdmnlvYuLF63zfd2Mi/ebg6/V8DM1U9hX8+Rir8XCfhkB0SqAw2/43jVeU14/KUO3yJ42cGw6ewmEVESBIk7WJNlCZmdyju7Dwo9po5eUS/+/m3XAAvwrzuSHRCpbpIqchwff6mjf0Ptp3Z04oFnXx90W9lF+nHphE9ElCRcXWiJIL2lvGxq68Cqja8IPaaOXlFR2jnI3oNQR5NU0eP4768fDSpXXvxh3HfZWWhU3NRW1vlFRETimMmyhIzeUm5ZmnI691YM285BReZFR5NU0eO47MfbccenTsf8aY1amtra3gmfiCiJmMmyRNTeUl5BSbkigEUfmSg+uAjCNgcVDYi2tXcJj0VHoCF6HPcfPDIgG6e6qa3NnfCJiJKKQZYlonYqD9JNHAC+/9RrmL1ms/KO4mGbgwpnhB4RnzbUEWj4HcdyupqN2twJn4goqRhkWSJqp/Iw2RddW7eE2Uw7bEbIi45Ao/Q4+tFZB2VzJ3wioqRikGWRMMGII0z2RefWLfOnNWLr8jlYv2Qm7l7UjPVLZmLr8jmuz0lFRkhXoOEcx5HDqoVur6sOKsr5RUREwbFPloXC9IVyGpAG7SbukNUzS2ZPK9FCfofoc9DVkPPZ1/bicw8873s7Hf3KSrHjOxFReOyTFXNOEXTQv7n14qlY+vB2ZCDeTdwhI5siO3hxMi8r/vll7D/o3mvLIfocdKzmA4CZU0Z77uGoc5VnqTDnFxERBcfpQsn6CkW0tHdhQ+tutLR3aSlqdrhNB4mIuqpMdk8rx/xpjVj7ubOEbhvkOahezec8hs11UCbPVSKiNGAmSyIb9oUrz9KMObYWN/y0FXvyvcqyKaq7ic+cbGdGSIQT+JafFzlJ50XYqT8bzlUioqRjTZYkNu8L54wNGDiNKGtsOvZKVP0cVFNRBxU2ULL5XCUisl2QuIPThRLo2K4lCtWrynQ0+Yz7yjjZ05Nhp2dtP1eJiJKE04US6NiuJSqVxd66uolXmgpFEdjb04uW9q7UrJKLMj0bh3OViCgpGGRJEJd94VStKnN6WumomXKew6a2Dvz1z15KZU1RlEApLucqEVEScLpQgrTvC6d7FV2YqbIkraSLEiil/VwlItKJmSwJdGZybKV6FZ0jzFRZ0lbSRQmUeK4S2YlNgpOJQZYEXo1AbeiHpIuOJp9Bp8rcVtI5Wa84FM2XixIo8Vwlsk/SvgjSBzhdKEncV7/JorrJZ5CpsqSupIs6Pctzlcgeqho5kx2YyZJI13YtopKYfg4yVZbklXRRp2dtO1eJbKHzc1N1I2cyj0GWZLbsCycj/azywybsfQeZKvvFf74lNJa4rqSLGijZcq4S2UL3tF2SvwjSUQyyEkhGHZLKD5so9x2kpkjVSjqbMoQMlIjkMFG/yZYqycearISRUYekskZAxn2L1hQ5WS+v8Kcx4Eq6TW0dmL1mMxav24ZrHm3F4nXbMHvNZtZNEMWYqfpNtlRJPgZZCRMk/VyJyg8bmfc9f1ojti6fg/VLZuLuRc1Yv2Qmti6fM+CbZmmBuJtLpjcKZ6FYoEqUTFE/N8Py+yKYQfAvgmQXBlkKmGx8GTX9rPLDRvZ9i6xknD+tEVed1+R6H/dv2SUUHCV1pSIRmZu2093ImfRjTZZkpvudRE0/q/ywMfFB1lco4vGXvIMokdU7LFAlSi6T03a6GjmTGQyyJOkrFHHP5tfw/adeG/Q7nY0vo3b0VvlhY+KDTFZwxAJVouQyvRMCW6okF6cLJdjU1oFz79hcMcAC9E4nRU0/q6wRMFF/ICs4YoEqUXLZMG2nupEzmcEgKyKnGLoz732RVlU4WUmUjt4qP2xMfJDJCo5YoEqUbNwJgVTIFIvFWFfq5vN5ZLNZdHd3o76+Xutj9xWKmL1ms+d0VLm7FzVjYfMEhaP6QJR+Trb2yQrKOUZ+0wBbl8/xfW2cgBqo3J+LH8RE8WdTHzybxkIfCBJ3MMiKoKW9C4vXbQv0N+uXzLS2MLr8DX32CaPw4u/ftqrjexgygyPTCxuIKB34WWMvBlmabGjdjWsebRW6bZCMiQmV3tAjh1XjC+c24eo5J1o55iBkfmBVChAB8BsnUYrJ/OLo1n2eWXM7MMjSJEgmKwN73xhub2jHyOHVuOOTpwcau9sHjsn0t6rH5jdOonST/SXOqwzF9i/sacAgSxO/eh9Hrr4Wt13yYSsvuEHqyu4TDBLdPnAumd6Ix1/qSFQwwm+cROkm+zNA9Mu7zaUnSRck7uDqwgi8Vss5rpt7Mp5d8QlrL7R+faRKibSgcNt6pqP7EH64ZVeitqRhF3iidFPxGSDadubZnXuN7CpCwTDIisht2W9jdijuu+wsXDP3JKtTukGaZ/q1oPD6wHGjMhhRvb2Rqf3OiJLA5PZjsqj4DBBtO3PPMzu5SX0MsOO7BHHu1hu0eaZXUBYkK1ZKxZY0Ouqk2AWeKJyk1DGq+Azw6z5fic5dRSgYZrIkiWu3XucNLcorKIsaTMgKRtymLGVPTbILPFFwut6fOqj4DBApQynH8gR7MchKOecN7fdmFuloHjWYkBGM6KyTYhd4omBM1jGqmJ5U9RngVobiheUJduJ0IfW/oVf8y8vYf+DIoN+LbnkTJs3t3L+szVdlbQgtwglQlz68HRlUbnSqer8zojjR+f4spWp6UuVnQHkZymt73sE9z7T7/h3LE+zCTBYBOPqGfvHmC3Dd3JMwclj1gN+J7t0VJs0tOxjRXSfF/c6IxJmoY1Q9PRn2M0Aks1ZahnLuiWOFxsPyBLswk0X9hlRlcM3ck3H1nJNCF/F7ZcWG1wxBzTFVA36ek1zsaqJOKs4LH4h00v3+9JuezODo9OQFU3OR3q9BPwPCZNb8ZgpkzgiQPAyyUsqr+7nz7SmKStOOBw/34cDhPlw39yRMGlOnJBgx9UEk4zUjSjrd70/d5QMi9+HWvNRvhSDLE+KJ04UptKmtA7PXbMbidduk91lxvjlW4nxzfPTf38SfnjFeySpMrylLfhARmaX7/Wlbm5Wohf8sT4gfZrJSJuy3KFGmCltLOR9E5el42VOTRBSczvenbW1WZHw+qipPMLmvbJIxyLKI6pNcR32CLd8cWSdFZC9d70/V05NBP7NlfT7KLk9ISnNYGzHIsoSOk1xHlknXN0eRDzdZH0T8hkckn446RpV1TGE+s23LrAHqZzfSjkGWBXSd5DqyTDoKW3V+6+I3PKJ4UzE9GfYz27YVgrpWX6YZC98N09kBWfTb0Wt73gndEVl1YavOLTmStP0HUZrNn9aIrcvnYP2Smbh7UTPWL5mJrcvnhAqwonxm27Ywh5vcq8cgyzCdJ7nfFhCOe55pj7TiUNUKGJ0BqcntP4hIPln7y0b9zLZphaAtNbRJxulCw3Se5F71CZVEma5UUdiqc+WiDaskicg+op/Fz+78H9fPPlsW5ojObow5tlbxSJKLQZZhugsh3eoTKok6Jy+7sFVnQMpveERUiehncek+g5XqOG1oYCy63+wNP23FbZd8mHWoIXC60DBVu7h7mT+tEb/+m/PRUFfje1ub5uR1BqQ2rgIiSjqR/fxMEy27KGVrHafofrN78r1C41dx/OJwTnhhJsswU1slvPj7t7Gv57Dw7cNmbGS2P9C5Mse2VUBESReXlbxByy4Au1fqObMbtz2+A535yp/zIuNXcfzick54YSbLAiYKIYMGTXvf6Q38DUL29j06V+bYtgqIKMnitpLX7TPbi02zAg4nS9T7XgFXzp7keVuv8as4fnE7J9xkisVivHJvZfL5PLLZLLq7u1FfX296OJHobHrZ0t6Fxeu2BfqbIN8g3PrIOM8mSvDIPllEydFXKGL2ms2uNaJO1njr8jnWfakp/cx+bc+7uOeZnb5/c/eiZixsnqBhdN4qfbaJKB+/iuNn+zkRJO7gdKFFdBZCihY8lhJdbai6wZ3OlTm2rAIiSqo4r+Qt/cxuae8SCrJsqON0+xIsonz8Ko5fnM+JcgyyUkplXYGON4jOgNSGVUBESZWUlbxxqeP0+hLsxW38Ko5fUs4JgDVZqaaqriBJbxAiUispK3njUsfp9yW4Eq/xqzh+STknAEuCrLVr12LSpEkYOnQoZsyYgRdeeMH0kFKjfLuJq88/UejvvAKkJL1BiEgtE21sVJk/rRFrP3smRtVVD/i5iW7ubsJ8ufUav4rjl6RzwniQ9ZOf/ATXX389br31Vmzfvh3Tp0/HvHnz8Ic//MH00FKjdLuJc08cI/Q3XgFSkt4gRKRWXDJAIja1dWDVxlewr+dI/88a6mqwcoGZhTKVekyJfrldueA0oX0eVRy/JJ0TxlcXzpgxAx/5yEdwzz33AAAKhQImTpyIr371q1ixYsWg2/f29qK3t7f/3/l8HhMnTkzE6kIbOKs6/OoK/FZ1OIWVQOXeX7Z8qyMicSpXQMd9Ja/KFdVhx1Pp9Vy54DSs2vhK5M940cdLYp+sIKsLjQZZhw8fxvDhw/FP//RPuPTSS/t/fvnll2P//v3YsGHDoL+57bbbcPvttw/6OYMseWQFSLa+QYgoOB3vZ51tbGSyreWAX8B31XlNuH/LLgByvwSrOH42nhOxCbLeeustTJgwAc899xxmzZrV//Mbb7wRv/71r/H8888P+htmsvSQ9YFq4xuEiIKxLUtjG9G+gz/+yxmoymSUfh6KBnwrF0zFqo38EhxGovtk1dbWoraWO4KrJqs/FNsfEMWb6r53svl9sVPxxU+0mHzZj7dj/8EP6rVUBDWiLXRG1dVg6/I5/BKsmNEga8yYMRgyZAj27Nkz4Od79uxBLpczNCpyMEAiojg1hvTLwKua8hQtJi8NsADxBs9BBGmhw8949YyuLqypqcHZZ5+Np59+uv9nhUIBTz/99IDpQyIiMiMufe/89rpb/csdyvbC81tR7cbJDt7+xI7Ae8O6eX3vAaHb6WyhU2mVY1oYny68/vrrcfnll+OP/uiPcM455+Cuu+5CT08PvvCFL5geGhFR6snoe6e6NlNkSnPd/92lbMozzA4apY8vKxPYVyhi/Qtv+N4uV1+rrYVO2hdAGQ+yPvOZz+B//ud/cMstt6CzsxPNzc3YtGkTxo0bZ3poRESpF3W7GB0XWZEpTa8lXjICHWcHjfLnOnJ4NfYfOOLxl0fJyAS+sGsfOvP+97P4nOONrnJUMU1qK+NBFgBcffXVuPrqq00Pg4iIynhlafwaQ+q6yMqaqvzX96cMw2baKi0YKhSK+NwDg1fKl5MxfSf6OkwaUxf5sfzEbcGEKsY7vhMRkd3c9jn12m7F7yILyKtFklVf9KOW32Pxum2YvWZz6Bqt0h00Zk0ZjZlTRmvbAcOmLc2CLJhIMisyWUREZLegbV10rkoUmdLMZADReE5mpi1KJjCoqFO7MsVlwYRqzGQREcWcrtVb5Vkar8BA50VWZK+7JR9rOhpsCdyf7ExbmExgGKJ7/gFQfr7YlFUziZksIqIYs3X1lu6LrFvhea7ktTjz+FGDfu9Gdv8vWQ2eRR7H63UAMKgjvIrzxaasmknGN4iOKkh7eyKiJLF5uxtZm82HeVyRju//2taBH7X83vf+7l7UjIXNE6SNT5dKr8OTOzqFzxcZbTdk7YNrm0Rvq0NERPav3tJZi1T+uF6Zp9LfiwRZcZ3OKn8dgpwvT+7olJIdFckuJh2DLCKiGIrDdjc2X2TTNp0ler7cs3kn7nrqv6S13dA1TWorBllERDEUl9Vbtl5kTWXaTBE9D/7hWfmd8dO8RyJXFxIRxVCcVm8FWZWok65Vfzbs3Sd6HnQfdO9On5beVjIxk0VEFENpm+5SRXWmzZbVn37nSxAqsqOq97c0hUEWEVEMpW26SyVV01lBtxVSGWhE2cS6nOzsqC2BqAps4UBEFGNJvkDFmdPCwq3YvLyFha7juKmtA1/7eRv29RwO/Lcq2m7Y3IbEDVs4EBGlhK2F5WkXZPVn98HDWjbSBo6eLwcP9+G6n74U6O9UZEdtb0MiA4MsIqKYS9rqrSTU54jWLXV2H8Sd/+d3SgON8tczzHSfirYbcWhDEhWDLCIiskZSpj/HHFsrdLu97x5WGmhUej1z9UMxcng1ug8ccV00Ma6+Fv/f/2rG3nd7lQW6cWlDEgWDLCIiskLQQnGrCVY77z8gVhsVJtBwez335D9YYei2aOK2Sz6Mc08c4/sYUbKOcWpDEhaDLCIiMi5p9Tl7e3qFbpcRfCpBAw2R13Pk8GrUHlOFzvwHYw0yLRg165iGNiQMsoiIyLik1eeIBkWzJo/BP2/fLT3Q2Nbe5ft6vn3gCH78lzNQlckEzkTJyDqqaENiWz0fgyyimLDtw4MIkHdeJq0+RzRLM3PKaOmBxqa2Dqz455eFbrv33V4sbJ4gfN+A3KyjzP0tbaznY5BFFAM2fngQyTwv41if4xVgBsnSuAUa2eHV+MJHm3DB1JzwmNwyTG7CvJ6ys44y2pDYWs/HZqRElotjsz5KPtnnpdO80y/zE6QRpsrsr2iAGSQQ7SsUcc/m1/Dgs69jf8kegqKBq18D1FJRGotuaN2Nax5t9b3d3YuaA2fJwgja+DUqNiMlSoikFQNTMqg4L2XX56jM/gbJmohkaZxg8KkdnXjg2dcHPZ5oNsYvw1QubGNR27KONtfzVWl9NCIKJMiHB5Euqs5LZ9oslx14cc5lhwbKjDlBUPkYnWBlU1tHoHGV8gswgaMBZl/hg1s4zWIXNk/ArCmjBwQ2m9o6MHvNZixet61igOV1v+VE69VGDquOlAF36s3cwrMMjga0ulYF2lzPx0wWkcVs/vCg9FJ5Xkatz1Gd/ZWZNQlSPyVyv6KZo7WfO0uoB5YbmVlHGVO6tmXWSjHIIrKYzR8elF6qz8so2wSpnjqSFWB6BYNh71d4RePk6FNmMlYFyprStbnfFoMsIovZ/OFB6WXzeSkzy1YpyyIrwAxaPyVyvyr6TnmJknWUuRpQ9/MOgjVZRBZzPjwADKp/MP3hQell83kpKwgqrZW65tFWLF63DbPXbMbbPYel1CMFnUoVvV9ZdW2ivOrN3ISpa/Oj+3mLYiaLyHIym/URyWLreSkjy+aVZVn2yHZcdV4T7t+yK1LWJMhUatDAVUbfKZVUTena+LwZZBHFgI0fHkQ2npdRp45ECucff6kDaz97JlZtfCV0gOkXDJYKE7gOqcrgnKaG/mPzwq59kY6NzJ5jKhdORKnnU4FBFlFM2PbhQQTYeV5GybKJZllG1dVi6/I5oQMPv2CwCOCL507CBVNzoQIamX3CZPccS9OCHgZZRESUOGGzbEGyLFEDTFVTrjKLylVsV2PzwgnZGGQRkRHc8JpUCxMEhcmyhD2X+wpFZIfV4Mb5p2Lfu71oqKtBLjss8rSerD5hqnqO2bwaUDYGWUSkHTe8JlsFzbKEPZe9/i5KcCGzqFxlzzFbF07IxiCLiLRSMf1AJEuQLEvYc1nle0BmUbnqHSdsXDghG/tkEZE2KvrjEMkm0nMp7Ll8+L0CvvbzNmXvAZlF5ToK1MP02YoTZrKISBvVW56QP5tr4Wwam1+WJcy5vKmtA1/7+cvY13Mk0N8FIbOoXGeBuk3HXiYGWUSkDTe8NsvmWjgbx+ZVOB/0XA6yGXSQ+y8ns6hcV4G6jcdeFk4XEpE2aeqPYxvnIl+efXHqgDa1dRgamd1jcxPkXA6zGXSU94DXdOe1c09G73sFtLR3CU1Jqt6uJo7HPghmsohImzT1x7GJqqX4SR+blyDncpDNoGW9B8qnO1/f24P1L7yB7z/1X/23Ec0WqSpQj+uxD4KZLCLSxuaNhZMsSP2QbqJje+jZXdjQuls4A6NakHM56NSfrPeAM91Ze0wV7nrqNXTmewf8Pki2SEWBus3npSwMsohIK9XTDzSYzbVwoo+5auMruObRVixetw2z12y2YhpJ9FwWnfobXVcj/T0QdhVkX6GIlvYupYGtzeelLJwuJCLt0tAfxyY218KFecwo/aRkr2ITOZdFNoNuqKtGy02fQM0xcnMfYVdB6ihEt/m8lIVBFhEZYePGwkllcy2cSABSLmy9jqrgwe9cFlml9+0/O116gAXIWwWpolmwzeelLJwuJCJKOJtr4bzG5kW0XseZ9vrGE7/Flw2uYjM1TS5rFaSKZsE2n5eyMJNFRJQCNu8V5zY2EV6ZmkqZq3JRVrEFnXo0MU0ucxWkimbBNp+XMjDIIiJKCZtr4crHtvedXqza+Irv37llaoI0/wwTPISdetQ9TR6koaipQnSbz8uoGGQREaWIzbVwpWPrKxTx91t3harXCdP8ExAPHkTrlmzZKkY0W2SyEN3m8zIKBllERGSdKFu6BGn+WUokeBBtoFkoAKs22rNVjIxVkOWBrS1BpM0YZBERkZXC1usEnc4KsopNtG7pK49sH/Q7FSv0gpCxCtIJbJO836BMDLKIiMhaYep1gkxnBV3FFqUeKQ5bxYgEtjrbPMQdgywiIrJa0HqdIL23gq5ii1qPpGKFnmxegW0a9huUiUEWERElite0l+PKcydh7tRc4DqiMM1TK7F9qxi3wNZEm4c4YzNSIiJSTsdeeKXcmn82ZofivsvOwsqLPxxqo2ORBpoiwmTEdL+GlaRhv0GZmMkiIiKlTBVJq+q/5FW3tHLBaVi18RXpW8XYUmiehv0GZcoUi0X9obBE+Xwe2WwW3d3dqK+vNz0cIiIq4VYk7YQ5cS6Sdmth4DxnoPIKvaDP2abXsK9QxOw1m32DyK3L5yS2JitI3MHpQiIiUkL3Xni6OXVLC5snDJh6lLlPoW2vYRr2G5SJ04VERKREmoukZU1V2vgaJn2/QZkYZKUIu/MSkU5pL5KWsVWMra9hkvcblIlBVkpUKppsqKvGnzVPCLWMmYjIj8ki6aR8qbS50Dyp+w3KxCArBdyKJvf1HMEDz76OB559ndshEJF0QffCk0V0JV4cAjFTryHJwcL3hBPdjd7ZDmFTW4eWcREBdvT9IXVMFEk7XyrL65jKP+M2tXVg9prNWLxuG655tBWL123D7DWbrfsMZKF5vLGFQ8K1tHdh8bptQrdNw9JbsoctfX9IPV3H2mkv4FYo7nzGrVxwGpY98hsrWiKI4vvFHkHiDgZZCeWkwf+1rQM/avl9oL9dv2Qm59lJKZv6/pAeOqbmRL9UNtRVY1/PkYq/s/nLZhymN9MgSNzBmqwEqvSNJ4ikrvQhO3CD2XTSUSQt+tnlFmABdreVYKF5/DDISphNbR348vudhsPidgikko19fygZZH52qf6yyaxUOjDISpC+QhEr/uXl0H/PVSqkg619fyj+RFbiNdTVoKvnsO99qfyyyfqq9ODqwgS5Z/NO7D/gngb3wlUqpIuKvj9cpUiA2Eq8VQunoTE7dNDvS2/XqPDLpujqR0oGZrISoq9QxIPP7gr999wOgXSR3feHWQEqJbLlS1UVsPTh7cig8gbOqr5ssh4xfRhkJcQLu/Zh/8FgWayrzz8RJ407lvUApJWTbZBxkXNbpehkBbhKMZ38tnwxtfce6xHTh0FWQoSpXzn3xDF8I5MRMi5yzAqQF7+VeCb23mM9YvowyEqIIPUrLHAnG0S9yOnOCqRlNVhaniegvyWCzfsQkhoMshLCr87FwQJ3skmUi5zOrEBa6r7S8jxN4T6E6cPVhQnhtaqmVC47lHUqlAi6sgJpWQ2WludpEvchTB8GWQni1LnksgMvKg111bjy3ElYv2Qmti6fwwCLEsHJCqhciu9X9wUcrfuKe8uItDxPG7h9TvMLcDJxujBhTBRzUnwkqd7Ga5Ui3v/3oo9MjPQYaVkNlpbnaQt+TqcHg6wE4v5WVEkS623cVik6vv/Ua3j0398M/RzTshosLc/TJvycTgdOFxKlQJLrbeZPa8TW5XNw3dyTK/4+ynNMy2qwtDxPIt0YZBElXFrqbR799zcq/jzKc9RR92WDtDxPIt0YZBElXJB6m7hS9RzTshosLc+TSDcGWUQJl4Z6G5XPMS2rwdLyPIl0YuE7UcKlod5G9XNMy2qwtDxPIl0YZBElXBq6TOt4jmlZDZaW50mkA6cLiRIuDfU2aXiORBQ/DLKIUiAO9TZ9hSJa2ruwoXU3Wtq7Aq8EjMNzJKJ0yRSLxViv287n88hms+ju7kZ9fb3p4RBZzdaO7zIbpdr6HIkoGYLEHQyyiMgop1Fq+QeRExYxC0VENgkSd3C6kIiMSUujVCJKJwZZRGRMGhqlElF6McgiImPS0CiViNKLfbKIyJgkN0plAT4RMcgiImOS2ihV5mpJIoovThcSkTFJbCLqrJYsrzXr7D6EpQ9vx6a2DkMjIyLdGGQRkVFJaiLK1ZJEVIrThURkXFI2Jg6yWpL7AxIlH4MsIrKCjo2JVRejc7UkEZVikEVEqaCjGD3JqyWJKDjWZBFZJupGyTSYrmJ0Z7WkW24sg6OBXdxWSxJROMxkEcGenkZc+i+fXzF6BkeL0S+Ymot8zJ3Vkksf3o4MMOAx47pakojCY5BFiRAlSLIlsHHbKNnJtsRtpZ0tdBejO6sly8+pHINlotRhkEWxFyVIsiWwUZ1tsSVTZ4KJYvSkrJYkomgYZFGsRQmSdE4j+VGZbbElU2eKqWJ0HaslichuLHyn2Ira+DFIYCM6nrAF66qyLew+zmJ0IjKHmSyKrajZH5mBTdRskYpsi+xMXVynHFmMTkSmMMii2IoaJMkKbGTUdanYKFnmFKSpKUdZgZ3KYnRbgk9bxkFEH2CQRbEVNUiSEdjIyhapyLbIytSZWhwgO7BTUYxuS72bLeMgooGU1WR961vfwkc/+lEMHz4cI0eOrHibN954AwsWLMDw4cNx3HHH4W/+5m/w3nvvqRoSJUzUWhsnsHFuW/63gH9gE7Wuq7SOKzusBms/K2+jZBmZOlMbHquqJXOK0Rc2T8CsKaMjB1g21LvZMg4iGkxZJuvw4cP49Kc/jVmzZuGBBx4Y9Pu+vj4sWLAAuVwOzz33HDo6OvAXf/EXqK6uxre//W1Vw6IEkZH9iTqNFCVb5JZ9WLngNIyqq42cbZGRqTOx4bFNqz7d2DJGW8ZBRJUpC7Juv/12AMBDDz1U8ff/9m//hh07duCpp57CuHHj0NzcjFWrVmH58uW47bbbUFNTo2polCAyam2iTCOFzRZ5TcEte+Q3uPeys7CweYLQfbuREYSa6DFlIrALypYx2jIOIqrMWE1WS0sLTj/9dIwbN67/Z/PmzcPSpUvx29/+FmeeeWbFv+vt7UVvb2//v/P5vPKxkt1k1NqE7WkUJlukM/sQNQgNEkTKKryWEdipLgI3EXzaPA4iqsxYkNXZ2TkgwALQ/+/Ozk7Xv1u9enV/lozIYarxY5hskYltXsIGoaJB5Ns9vZi9ZrOUwuuotWQ6isBNNTi1dRxEVFmgwvcVK1Ygk8l4/vfqq6+qGisA4KabbkJ3d3f/f2+++abSxyPy42SLRAvWTWQfwhZ8iywOuGR6I5Y98htphddRFjToKgL3GyM8xigTG60S2S1QJuuGG27AFVdc4XmbyZMnC91XLpfDCy+8MOBne/bs6f+dm9raWtTW1go9BpEuQbJFccs+eE05rlwwFas2yp36DFtLpnMatnSMbi6Z3uj6OLKmM9lolchugYKssWPHYuzYsVIeeNasWfjWt76FP/zhDzjuuOMAAE8++STq6+sxdepUKY9BFFaYi6DolKWKxqOquQWRqqY+w9SSmZiGveq8Jvxwy66Kv79/yy6cefyoQWNV0f9LVaNVIopGWU3WG2+8gX379uGNN95AX18fWltbAQAnnngijj32WFx44YWYOnUqPv/5z+POO+9EZ2cnbr75ZixbtoyZKjJKdU1PXLMPlYJIlVOfQWvJdE/D9hWKePwl7+nH8syZqsauQV8rdocn0kNZkHXLLbfgH//xH/v/7awWfOaZZ/Dxj38cQ4YMwS9+8QssXboUs2bNQl1dHS6//HJ84xvfUDUkIl+6upsnJfsQderT72IfZEGD7mnYoJkz1dOZoq8Vu8MT6aMsyHrooYdce2Q5TjjhBPzyl79UNQSiQHQ3dlSxzYtuUaY+ZV/s3+457HsbmUXgQTNnNvS0MrVFElFaKdtWhyhuom6RE4bMbV5MCLs1kexVgH2FIlZt3OF7u5ULTpP2GgfNnJnuaWVqiySiNGOQRfQ+0xfBuArawkLFxd4vQHaMqpNX7xm0fYLpVaUmvkQQpZ2xZqREtjF9EYyzIFOfKqbNTPUeE13A0FcoolAoYuSwauw/eKTi/aleVcovEUT6Mcgiel8cWyvYRLTwWsXF3lSALLKAoVLtWTkdq0r5JYJIPwZZRO+La2uFuFFxsTcZIHtl8dwKzcvpWFXKLxFE+rEmi6hE0PqitOsrFNHS3oUNrbvR0t4lVEelYiuYsAX4slRawOBVe+YYOawaP/7LGdi6fI7yc8v0a0SURsxkJRAbDUaThNYKOoRtwaAqY2hb7zGRYvz9B4+gKpPRdm7Z9hoRJV2mWCzGer1uPp9HNptFd3c36uvrTQ/HuLQ0GmQgaZbbNJhzBESyfqrOVVvOjQ2tu3HNo62+t7t7UTMWNk9QP6AStrxGRHEUJO5gJitB0tJoMC2BpK1kNW1VlTEM0iVeJZsLzW15jYiSjkFWQujuVm6K7YGkbRkCFeOR2YJB5cXe9LFgoTkRMchKCBu27FDN9kDSVIbNLZhQNZ449FuyIdvJ1apExCArIUxc+HRnCmwOJE1l2NyCiUumN+L+LbuUjMfmaTDArmwnC83DMZ2FJJKFQVZC6L7wmcgU2JpBMZVhcwsmOroP4YdbdlX8GxnjsXkazMZsZ1xXq5oKdGzIQhLJwj5ZCaGi95Ab2Zv7irI1g2JiTziRHkyqxmNzvyVb9+eL20bgm9o6MHvNZixetw3XPNqKxeu2Yfaazcre26WPa+KzhUgVBlkJoevCp2JzX1E6A8kgTGTYRDdE9hJlPLY2bbU122lakKaxpgIdk58tRKpwujBBdNR/mKqLcqYu/mRaDg88+/qg35vMoJjIsMkIEqKOx8ZpMFuznSYFmX4zOd1qc80lUVgMshJG9YXPRKag0kWiKgOUfqE1WUhsokYpSpAgczy29VuyuV7MhKCLAEwGOsxCUhIxyEoglRc+EwX2lS4Szj4FXzx3Ei6YmgsVSMoq7DWxVN8vmCh9/DS1DpB1LJKwui1MVspkoCP6mbH3nV70FYqxOx6UTgyyYsSGD36dmQKRi8S/tnXi6wuCBwyyVzDpXqovEkxcdV4THn+pw8rWASrP5ajHIimr28JkpUxOt4p+cVi18RX8/dZdsTselE7cuzAmbPrgd7JLQOWLu6zC55b2Lixet833duuXzAyUuZOx754b3YGw33lhQ2BeTvW57Dznzu6D2NdzGA3H1iJXL/bcVZ4buoXZO7GvUMTsNZt9v0RtXT5HyXnk9tlSaRxAvI4HJQf3LkwYm5orAvqyNiqmLlQX9opO1coKfvxq8GyrmVJ9LnsFcCJThLb12IoiTFbKdJd6t8+WcnE8HpRODLIsZ+sHv46VZSqmLmxYwSQzk2NjpsqN6nM5agBnw7khU9ipfdNd6p3Ploee3YVVG19xvV3cjgelE4Msy9n8wa86S6Ki/sv0CiaZmRybppBFqDyXZQRwps8N2aJkpUy35xhSlcGYEbVCt+3sPoiW9q5YfNGg9GEzUssl7YM/CBUNVk0W9spsthjHztgqz2UZnd6T2GMrStNY013qRV/nVRtf0d6ZnkgUM1mWS+IHfxAiUxdBpszOaWrAyOHV2H/gSMXfq+yjJCuTY+sUsh+V57KMAE71ylmdU7vlj/XrvzkfL/7+7Vhke0oXLjTU1eDtnsOeRfD7eg4P+LepWlWiShhkWY7NFb2nLoJOmT25o9M1wAKOBimqCntFA4Fnd+71vAjaPIXsReW5HDaAKw9GVi44Dcse+Y30om+dU7tej+WsIrRVpbEHZfMXDUofThdaTtaUWZC9y1SI+viVpi6CTpk5GSAvI4dX44KpuUBjEyUaCNzzzE7PKY+4TiGr3F8zzL6WlTZBXrXxFVx1XpPUPRl1Tu3GcRrZ4Tb2Shrqqj1/b2ojcKJyzGTFQNybK7o9/soFUzGqribUFEaYKTORTZX3HziiLAMk2mwR8J7yiPMUsqqVa0GLvL0WINy/ZRfWfvas0OdmKZ1TuyI1f1/7+cs4eKQg3DdMF6+xOxrqqrHyTz+MXP1QdOYP4bqftPrer21fNCh9GGTFRNjVPqZ7bLk9fkf3IXzlke0DfhYk8AszZWY6A+QVCJTzugDHfQq50rl89gmj8OLv38aG1t2hgxrRAE4k8Fm1cYeUhps6p3ZFvkTs6znSH5zYtBJ1W3uX0Nhz9UMxa8potLR3Cd2vjV80KF0YZMVI0JYJpgukRb6dlgoS+IUJmGzIAIk2WwTcL8CmG0bKUHoub2rrwB9/5xkpmVaRLyM6Ax+dgX3Q+7ClQHxTWwdW/PPLQrd1nmPcv2hQerAmK8FkLGtX+fiVxgOItTEIEzCFqdtRYf60RmxdPgdXnz9F6PalF0+ntq33vQKunXsyxtXLqx0yQUUNkV/rAZ2Bj87APuh9BG0booJz/PcfdF+MUsp5jirr+4hkYiYrwUxPj4W5X9EsQphvsjZlgIZUZXDuiWNxzzPtvrd1LiyVatty9bW4bu5JmDSmTvrSfNUtB0xlWnUGPjozLkFq/hwmV6IGyXRXep1Md6YnEsEgK8FMT4+NOVasY3MlfgFa2IDJhg/m/j5A+UNoqKvGvh7/nl1utW178r2466nXcO9lZ0m9SOpYLGGqFYXOwEdnYB+k5q+ciQLxoJlut/ezyc70RH4YZCWYybqFTW0duO3x34b+e5HAL2zAZPKDWbQPUOkFGIDWjI+uxRKmMq26M5o6A/sgNX+lTBSIix7XkcOqccenTnd9nWzbBJ2oFIOsBDM1PeZ2kRYRNPALGzCZ+GAO8rqUXoBbfFZeycz46JzCM5Vp7SsUkR1Wgy+eOwk/b909IJOoKqOpM7AvfazO7oNYtfEV167pJgvERY/r2s+dhXNPHKN4NERqMMiylKx6GN3TY0FXFJYKG/jF4Zus3+uSAdBQV4ObF5yGXHbYgOOtM+OjcwrPRKa1Uiaxoa4GlzaPxwVTc0ozmjrP09LHerUzjx9u2eV6W1MF4qLHf+Zku9/bRF4YZFlIdj2Mzm/RonUW13ziJPz0P94MFfjp2ANO9mOIBC9dPYeRyw4bdCHWmfHRGdDpzrS6ZRLf7jmMB599PZG1PJvaOnC/R4B11XlNxgrEbVqIQqQKgyzLeDXv/PLD23FfyHoYXd+iRS++k8fWYevyOaGaq4YJQIMETSqKvoMGL6XjHXNsLXL1tdiT71We8dE9hacr02q6Z5wJIlnlx1/qwI3zTzP2nG1YiEKkEoMsi4h8KK74l5etvhAEuUgHDfzCFmQHCZpUFX0HeV0qjXfk8Or+YEDlN34TU3g6Mq1x3VQ7CpGssg3PmSsEKcnYjNQionvr3bN5p6YRBedcpN2Ebfgpsi9bpaaKQZpdhn0MEaKNUN/uOVxxvN0HjhZnZ4cP3BhXdvNRryaPwNHXYeUC+VM4fg1EozLdM86EOD1n1cefyBQGWRYR/bB78Lldxjo0+xlSlcEl070v+GGyLmG61wcNmmR2yHc6s29o3d2/z5pfh+qVC07Dqo3eU1rDqofgx1fOwN2LmrF+yUxsXT5HyUq4ey87CzmXYHnVxh2hOrGXKn99VJ/PpnvGmZDG50xkG04XWkT0w27/gSORU/yqisdVFdqG+VYedIpI1jd/r+lJr/qT7LAaofFWVWWwsHmC0FjDmj+tEYVCEV955DeDfhd16lRHo9NyadzrLo3Pmcg2DLIsck5TA0YOqxbaxytKil/VRU6kTUHYQtsw38qDBk0yvvmL1HS5FfxvaN0daLxuZATQfYUiVm18peLvohSK62p0Ws6ZBv3yw9sr/r6I5K1kC7t6T8fqXaK0YJBlkSFVGXzh3En4/lOv+d42bIpf5UVOZXFxmG/lQYOmqN/8g6xgq/T8ZQV5MgJoFccyjSv8TAu6es9ElpEoyViTZZmr55yEkWXFzaXCFo4Dagu7AbWFtl4F2W7fys9pagj0WoZ5jFJRa7pEi+Pdjn2QIn8/Ko6lzJq3oJxz340T4Nla6xjF/GmN2Lp8DtYvmelZyyfz/CGioxhkWWZIVQZ3fPL0ihfaqMv1VV/kRDMxY+pqQxU9uxVku62we3JHJ/YfcJ96rTRFFPQxSkUNTKIEebIDaBVF0yZXu5kM8Gzgt3pP9RcworTidKGFVDXoU32RE5luyw6vxg0/ewmd+XDNRHvfK+C7fz4dyAB73+11rRnxy1wAR3tPXTA1N+jnYfv2yAhMwh572dN7KoqmTa52i1M7AxPS2EeMSAcGWZZS0aBP9UXOr9C2CLyfWRqYXYrSTNTtA1+055jbRSNMh3xZgUmYYy87iFCx5YnJ1W4mArw4FZAzCCVSg9OFFpPdoC9qzY8It+m2cfW1rvVRspqJljJx0Yha01V+X0GO/Zhja4XGKHo7INrUaSUyX5+gdJz7pTa1dWD2ms1YvG4brnm0FYvXbcPsNZutrWtiTy0iNZjJShFdG7JWysQUikV87u+fd/2bStMRUVajmbpoGNuLTbRUJmBJjeyMqqnXR+dmxKbaVETBnlpEajDIShldF7ny6bYwPaCi1ImYumj0FYrIDqvBjfNOwb6ew2g4tha5evVTRXt7eqXerpTszcVN7VWn49yPa5sKnUEoUZowyEohExc5Hc1ES5m4aHjVjqm+OMVtukd24CZK9bkf5wJyY1lYogRjkJVSui9yIpmlhroadHYfREt7F85paogcOOi8aJieIuJ0jziV537cC8hNZRmJkopBFmnhlVnC+//u6jmM6376EoCjGaCVC6ZGDhxELxpRVoLZMEXE6R47xC2jWImpLCNREjHIIm3cMkuVdHYfwrJHtuOq85pw/5ZdkQIHv4tG1K1EVE8RiQaAnO4xjxlFIirFIIuEyOr5U5pZ6swfwqpf/Bb7egZ3ZXcyQI+/1IG1nz0Tqza+oiRwkDHNp3KKKGgAaMN0T5z6Q8nGjCIRlWKQRb5kbxrrZJZa2rsqBlgOJwM0qq4WW5fPkX7hljXNp2qKKGwAaHK6hxsMM6NIRB9gkEWeVBZ0B8kAqQgcZE3zqZgisqHOKyjTxf82sSGjSETmseM7uVK9aazpImFZ03wqOpnHbUNjbjA8mOwdG4gofhhkkSvVF/pzmhpct9pxjBperaxIWGaQJ3sLmri1AohbUEhEpAOnC8mVDRd6lXkP2dN8MqeITGf5grLhXCEisg0zWeRK9YX+hV37sP+Ae+E7AOw/cERZ9kPFNJ+sKSLdGxpHFbegkIhIBwZZ5Er1hV5X9qOvUERLexc2tO5GS3vXgLqgsNN8Xvcpg4oAUKW4BYVERDpwupBcqe75oyP7IdJSoHyab0xdLZAB9r7b27/FT+lz1NWmIE6tANgfiohosEyxWIz1cp98Po9sNovu7m7U19ebHk4ibWrrwG2P70BnXm5Q0VcoYvaazb41UVuXzwl1cXZrKeDcU6VMlV8AFeY+owrT3NNUQ1D2ySKipAsSdzCTRYIGhhUyYnOV2Y8wfab8+jw5ned1964K2iPMZKDD/lBERB9gTRZ5cgKPznzvgJ/vyfdi6cPbsamtI9L9y2594AjaUkCkz9PNG9qsb1PgHK/ycTqBYtTjJYL9oYiIjmImi1zp6jquIvsRtKheJCjz2gIozGPLFscu8UREScYgi1zJ2nZGhOxtc4IW1csMjEy1KdB5vIiIyB+nC8lVnBtMBm0pIBoYNdTVWNumIM7Hi4goiRhkkas4N5gM2mfKCcq8NGaH4psLpwnfp25xPl5EREnEIItcxb3BZJCi+iFVGVwy3bvI/pLpjfiTM9QU6kfhNEbt7D5odaaNiChtWJNFrpLQYFK0qL6vUMTjL3mvvHv8pQ7cOP80q9oUVGrXUElcjhcRUZIwyCJPNncdF224KVJU71c0DgwsGpddqB+GW1+vSmw4XkREacMgi3zZlLlxyG64Gbeica92DY6Gumqs/NMPI1dv/ngREaURgywSYkPmxuHXmT1MbVTcisZFMm/7eo4gVz/UmuNGRJQ2LHynWBHpzH77EzvQVwi27U/civzjlnkjIkojBlkUK0G3yxEVtOWDaXHLvBERpRGDLIoVlRkcVfsoinDaMGxo3Y2W9i7fTFzcMm9ERGnEmiyKFdUZHBNF/mGK+JPQXoOIKOmYyaJY0ZHBcYr8FzZP6G/XoIpTxF8+BeoU8W9qc+/dZTLzRkRE/jLFYjFYhbBl8vk8stksuru7UV9fb3o4pEB5P6y3e3qx7JHfAKicwYlLgNFXKGL2ms2uNWYZHA2Yti6f4xnoifYLIyKi6ILEHZwuJKu5TaVddV4THn+pw7oGqUEEKeL3asNgU3sNIiL6AIMsspZXP6z7t+zC2s+ehVF1NbHN4LANAxFRsjHIIiv59cPKAFi1cYfvVJrN2IaBiCjZWPhOVlLVD8smbMNARJRsDLLISmmYSpPRADVofy0iItKH04VkpbRMpTltGMqL+0WK+GVvkk1ERHIxyCIrOVNpnd2HKtZlOe0NkjCVJtIA1a2NhcxNsomISC4GWWSltHU092rDUCljVZWB56KA25/YgQum5hLz+hARxRFrssha7Gju3hHeq/QqCYsCiIiSgJksspqJvQRt4dXGQkScFwUQESUBgyyyXlo7mvu1sfAT90UBRERxxyCLUsfWvf7Kx9WZDxdgJWlRABFRnDHIolSxte1BpXE11FUHvp8kLgogIoorFr5TargVkTttDza1dVg1rn09R3z/tjyOStOiACIi2zGTRakgshdi2LYHUaYfRYvb3dpY3LM43ptkExElGYMsSoUgeyEGKbKPOv0oWtw+qq4G+3oO9/9bpCM8ERGZxSCLUiHoXogi2Slnmi9K13XRca1ccBpy2WHMWBERxQiDLEqFIHshimSnZE0/io4rlx2WyjYWRERxxsJ3SgVnL0S3cCeDo4HU2z29QsXxQaYfZYyL7RiIiOKHQRalgrMXIoBBAY3z75ULpmLVxldcs1PA0exUX6EYePoxyrjYjoGIKJ4YZFFq+O2FOKquRjg7FWT6Meq4WNxORBRPrMmiVPHaC3FD626h+/jDO4fwp2eMR2N2KDq7D1XMfAXtun7B1BxGDK1GS3sXgCJmTR6DmVNGM4NFRBRjDLIoddz2QgySnXKm+ZY+vN21h5XoNF+lQvt/3r6bLRqIiGJO2XTh66+/jiuvvBJNTU0YNmwYpkyZgltvvRWHDx8ecLv//M//xMc+9jEMHToUEydOxJ133qlqSESeghahy5jms7ULPRERRacsk/Xqq6+iUCjghz/8IU488US0tbVhyZIl6OnpwXe/+10AQD6fx4UXXoi5c+fivvvuw8svv4wvfvGLGDlyJK666ipVQyOqKEx2ymv60Y/KLvRERGReplgs+u3oIc13vvMd3Hvvvfjv//5vAMC9996Lr3/96+js7ERNTQ0AYMWKFXjsscfw6quvCt1nPp9HNptFd3c36uvrlY2d0kPXJtIt7V1YvG6b7+3WL5nJHllERJYIEndorcnq7u5GQ8MHhcAtLS0477zz+gMsAJg3bx7WrFmDt99+G6NGjRp0H729vejt7e3/dz6fVztoSp0o2akgZLWBICIiO2lr4bBz50787d/+Lb70pS/1/6yzsxPjxo0bcDvn352dnRXvZ/Xq1chms/3/TZw4Ud2gKbWc4viFzRMwS9EqP5ltIIiIyD6Bg6wVK1Ygk8l4/lc+1bd7927Mnz8fn/70p7FkyZJIA77pppvQ3d3d/9+bb74Z6f6ITGG3dyKiZAs8XXjDDTfgiiuu8LzN5MmT+///rbfewvnnn4+PfvSjuP/++wfcLpfLYc+ePQN+5vw7l8tVvO/a2lrU1tYGHTaRdWS2gSAiIvsEDrLGjh2LsWPHCt129+7dOP/883H22WfjwQcfRFXVwMTZrFmz8PWvfx1HjhxBdXU1AODJJ5/EKaecUrEeiyhpnDYQ5YX2OQWF9kREpJey1YW7d+/Gxz/+cZxwwgn4x3/8RwwZMqT/d06Wqru7G6eccgouvPBCLF++HG1tbfjiF7+I73//+8ItHLi6kJKgr1BUXmhPRETRWbG68Mknn8TOnTuxc+dOfOhDHxrwOyeuy2az+Ld/+zcsW7YMZ599NsaMGYNbbrmFPbIoddy60BMRUXxp7ZOlAjNZREREpEuQuENbCwciIiKiNGGQRURERKQAgywiIiIiBRhkERERESnAIIuIiIhIAQZZRERERAowyCIiIiJSgEEWERERkQIMsoiIiIgUYJBFREREpACDLCIiIiIFGGQRERERKcAgi4iIiEgBBllERERECjDIIiIiIlLgGNMDiKpYLAIA8vm84ZEQERFR0jnxhhN/eIl9kPXOO+8AACZOnGh4JERERJQW77zzDrLZrOdtMkWRUMxihUIBb731FkaMGIFMJmN6OImVz+cxceJEvPnmm6ivrzc9HPLB4xU/PGbxwuMVLzKPV7FYxDvvvIPx48ejqsq76ir2mayqqip86EMfMj2M1Kivr+cHSozweMUPj1m88HjFi6zj5ZfBcrDwnYiIiEgBBllERERECjDIIiG1tbW49dZbUVtba3ooJIDHK354zOKFxyteTB2v2Be+ExEREdmImSwiIiIiBRhkERERESnAIIuIiIhIAQZZRERERAowyCIiIiJSgEEWBTZp0iRkMpkB/91xxx2mh0Ul1q5di0mTJmHo0KGYMWMGXnjhBdNDogpuu+22Qe+lU0891fSwqMSWLVtw8cUXY/z48chkMnjssccG/L5YLOKWW25BY2Mjhg0bhrlz5+K1114zM1jyPV5XXHHFoPfc/PnzlY2HQRaF8o1vfAMdHR39/331q181PSR6309+8hNcf/31uPXWW7F9+3ZMnz4d8+bNwx/+8AfTQ6MKPvzhDw94L23dutX0kKhET08Ppk+fjrVr11b8/Z133okf/OAHuO+++/D888+jrq4O8+bNw6FDhzSPlAD/4wUA8+fPH/CeW79+vbLxxH7vQjJjxIgRyOVypodBFXzve9/DkiVL8IUvfAEAcN9992Hjxo34h3/4B6xYscLw6KjcMcccw/eSxS666CJcdNFFFX9XLBZx11134eabb8bChQsBAD/60Y8wbtw4PPbYY1i0aJHOoRK8j5ejtrZW23uOmSwK5Y477sDo0aNx5pln4jvf+Q7ee+8900MiAIcPH8aLL76IuXPn9v+sqqoKc+fORUtLi8GRkZvXXnsN48ePx+TJk/G5z30Ob7zxhukhkaBdu3ahs7NzwPstm81ixowZfL9Z7Fe/+hWOO+44nHLKKVi6dCm6urqUPRYzWRTYX/3VX+Gss85CQ0MDnnvuOdx0003o6OjA9773PdNDS729e/eir68P48aNG/DzcePG4dVXXzU0KnIzY8YMPPTQQzjllFPQ0dGB22+/HR/72MfQ1taGESNGmB4e+ejs7ASAiu8353dkl/nz5+OTn/wkmpqa0N7ejq997Wu46KKL0NLSgiFDhkh/PAZZBABYsWIF1qxZ43mbV155Baeeeiquv/76/p+dccYZqKmpwZe+9CWsXr2a+3gRBVA6rXHGGWdgxowZOOGEE/DTn/4UV155pcGRESVT6RTu6aefjjPOOANTpkzBr371K3ziE5+Q/ngMsggAcMMNN+CKK67wvM3kyZMr/nzGjBl477338Prrr+OUU05RMDoSNWbMGAwZMgR79uwZ8PM9e/aw7icGRo4ciZNPPhk7d+40PRQS4Lyn9uzZg8bGxv6f79mzB83NzYZGRUFMnjwZY8aMwc6dOxlkkTpjx47F2LFjQ/1ta2srqqqqcNxxx0keFQVVU1ODs88+G08//TQuvfRSAEChUMDTTz+Nq6++2uzgyNe7776L9vZ2fP7znzc9FBLQ1NSEXC6Hp59+uj+oyufzeP7557F06VKzgyMh/+///T90dXUNCJJlYpBFgbS0tOD555/H+eefjxEjRqClpQXXXXcdLrvsMowaNcr08AjA9ddfj8svvxx/9Ed/hHPOOQd33XUXenp6+lcbkj3++q//GhdffDFOOOEEvPXWW7j11lsxZMgQLF682PTQ6H3vvvvugMzirl270NraioaGBhx//PG49tpr8c1vfhMnnXQSmpqasHLlSowfP77/Sw7p5XW8GhoacPvtt+NTn/oUcrkc2tvbceONN+LEE0/EvHnz1AyoSBTAiy++WJwxY0Yxm80Whw4dWjzttNOK3/72t4uHDh0yPTQq8bd/+7fF448/vlhTU1M855xzitu2bTM9JKrgM5/5TLGxsbFYU1NTnDBhQvEzn/lMcefOnaaHRSWeeeaZIoBB/11++eXFYrFYLBQKxZUrVxbHjRtXrK2tLX7iE58o/u53vzM76BTzOl4HDhwoXnjhhcWxY8cWq6uriyeccEJxyZIlxc7OTmXjyRSLxaKa8I2IiIgovdgni4iIiEgBBllERERECjDIIiIiIlKAQRYRERGRAgyyiIiIiBRgkEVERESkAIMsIiIiIgUYZBEREREpwCCLiIiISAEGWUREREQKMMgiIiIiUuD/B31HCbAT40VnAAAAAElFTkSuQmCC",
+ "text/plain": [
+ ""
+ ]
+ },
+ "metadata": {},
+ "output_type": "display_data"
+ }
+ ],
+ "source": [
+ "# TSNEで可視化\n",
+ "from sklearn.manifold import TSNE\n",
+ "import matplotlib.pyplot as plt\n",
+ "\n",
+ "\n",
+ "# 特徴量の関係か、コサイン距離が良さげ\n",
+ "tsne = TSNE(n_components=2, random_state=42, metric=\"cosine\")\n",
+ "\n",
+ "x_tsne = tsne.fit_transform(x)\n",
+ "\n",
+ "plt.figure(figsize=(7, 7))\n",
+ "plt.scatter(x_tsne[:, 0], x_tsne[:, 1])\n",
+ "\n",
+ "plt.show()"
+ ]
+ },
+ {
+ "cell_type": "code",
+ "execution_count": 3,
+ "metadata": {},
+ "outputs": [],
+ "source": [
+ "from sklearn.cluster import KMeans, AgglomerativeClustering\n",
+ "\n",
+ "method = \"k\"\n",
+ "n_clusters = 5\n",
+ "\n",
+ "if method == \"k\":\n",
+ " model = KMeans(n_clusters=n_clusters, random_state=42, n_init=10)\n",
+ "elif method == \"a\":\n",
+ " model = AgglomerativeClustering(n_clusters=n_clusters)\n",
+ "\n",
+ "y_predict = model.fit_predict(x)"
+ ]
+ },
+ {
+ "cell_type": "markdown",
+ "metadata": {},
+ "source": [
+ "上は直接生のベクトルでクラスタリングしてますが、次のようにt-SNEで図のように2次元に削減したものをクラスタリングしたほうが、識別がきれいに分かれる、ことが多いような気がします、が詳しくは分かりません:"
+ ]
+ },
+ {
+ "cell_type": "code",
+ "execution_count": 4,
+ "metadata": {},
+ "outputs": [],
+ "source": [
+ "y_predict = model.fit_predict(x_tsne)"
+ ]
+ },
+ {
+ "cell_type": "code",
+ "execution_count": 5,
+ "metadata": {},
+ "outputs": [],
+ "source": [
+ "centroids = []\n",
+ "for i in range(n_clusters):\n",
+ " centroids.append(x[y_predict == i].mean(axis=0))"
+ ]
+ },
+ {
+ "cell_type": "code",
+ "execution_count": 6,
+ "metadata": {},
+ "outputs": [
+ {
+ "data": {
+ "image/png": "iVBORw0KGgoAAAANSUhEUgAAAlkAAAJGCAYAAABoeJAJAAAAOXRFWHRTb2Z0d2FyZQBNYXRwbG90bGliIHZlcnNpb24zLjguMiwgaHR0cHM6Ly9tYXRwbG90bGliLm9yZy8g+/7EAAAACXBIWXMAAA9hAAAPYQGoP6dpAAEAAElEQVR4nOzdd3xTVRvA8d+5N013S9lbQRBxISDDASpLhgioIIqCCxUR98K990RxvAqiOEAcICg4UERQAReCAwGVPbtnmuSe94/bFkoz26Qt8HzfDy82uTnnJA3N0zOeR2mtNUIIIYQQIqKMmh6AEEIIIcSBSIIsIYQQQogokCBLCCGEECIKJMgSQgghhIgCCbKEEEIIIaJAgiwhhBBCiCiQIEsIIYQQIgocNT2AqrIsi61bt5KcnIxSqqaHI4QQQogDmNaa3NxcmjZtimEEnqva74OsrVu30qJFi5oehhBCCCEOIps2baJ58+YBr9nvg6zk5GTAfrIpKSk1PBohhBBCHMhycnJo0aJFWfwRyH4fZJUuEaakpEiQJYQQQohqEcoWJdn4LoQQQggRBRJkCSGEEEJEgQRZQgghhBBRIEGWEEIIIUQUSJAlhBBCCBEFEmQJIYQQQkSBBFlCCCGEEFEgQZYQQgghRBRIkCWEEEIIEQUSZAkhhBBCRIEEWUIIIYQQUSBBlhBCCCFEFEiQJYQQQggRBRJkCSGEEEJEgQRZQgghhBBRIEGWEEIIIUQUSJAlxAHC6/GSk5GLu9hd00MRQggBOGp6AEKIqtm9NYN3H/6Qz6YtwlXgwhFjctp5J3P+HWfTvG2Tmh6eEEIctJTWWtf0IKoiJyeH1NRUsrOzSUlJqenhCFGtdmzYxTUn3E7W7hwsj1V2u+kwcMY7eXrR/bTp2KoGRyiEEAeWcOIOWS4UYj/23FWvkrWrfIAF4PVYuAqKefTCSeznv0cJIcR+S4IsIfaitd5vgpIdG3axYsEvWF7L5/2W12LDH5v54/u/q3lkQgghQIIsIQD4Yd5P3Nz7PgbEnceAuPO4pc99LPv055oeVkAb/tgMIcSD/63eGP3BCCGEqEA2vouD3vT7ZvHmfe9hmEbZrNDKb/7gl69Wc9H9Ixl159k1PELfYhOcIV3njA/tOiGEEJElM1nioLZ66V+8ed97AOWW3Ur/e9rdM/jjh9q53Na+++Ek100KeI0jxqTrgI7VNCIhhBB7kyBLHNQ+nrwA0+H/n4HpMPh48oJqHFHonLExnHfbML/3K6UYdEVfUuvbp182r93GB8/M452HP2TZJz/h9Xqra6hCCHFQkuVCcVD7c9lavB7fG8fBPqX35w9rq3FE4TnnxsFk7shi1lNzMUwDFCjscfc6/2SufGoMhXmFPH7RZJZ8uAxlKAxD4fVY1G9ej7tmXs+RJ7Sr6achhBAHJAmyxEEtJjYmhGtq7z8TpRSXPzGaQVf05Ys3vmHn5t2kNUil9wU9aX3sIWitufOMR/jlq9UAaEvjtezd8hnbMrmlz/1M/vExDmnfvCafhhBCHJBq76eHENXgxDOPZ9babX7TIBimwYlDulTzqMLXrE0TLnpgZIXbVy/5i5+++M3nYyyvhcftYeZjs7ll2tXRHqIQQhx0JMgSB5Ril5ulHy1nwx+biEuM4+RhXWl+eFO/1w8edzqzn59PscuNtsrnQ1CGIsbp4Iwr+5Gfnc/Ct5fwz8r/cMY7OXFIFzqcehRKqWg/pSr5+t0lmA4Tr8f3/iuvx+LrGUu5cco4TNOsdD9aa1yFxTjjYjCM6Gz13Lx2G4tmLCUvM4/GrRvR6/yTSambHJW+hBAiEqSsjjhgLJ//C49eOIncjDzMGBNtaSyvRc/h3bn59auJS4j1+bhfvlrF3UMew1VQjEZjh02KuMRYHvj4NnLSc3lszPMUF7oxHAZouxhzuy5teGDubaQ1TI36c1u/8j/mv7aQHRt2kVwviV7n9aBTn2OCBjQPnf8si9/7DssK/M/849zpxCfGhT2u7N05vPfEx3z66pfkZeXjjIuh96genHvrUJq1iUzdRHexm2ev+B+fv7EIwzTK9pQ5nCbjnr6IweNOj0g/QggRinDiDgmyxAHhz2Vrub7HXVheq0LGdsNQdDvjeO6ffYvfx+dk5PLFG9+w8pvfUUrR4ZSj6DvmFDb9tYXre96NtipmgjcdBq2PPZQXlj8Skdmbdb/8y5zJC/j169UYpsHx/ToweFw/5r38BXNKTkF6PVbZ38eeciQPfHwbCcnxftucesc7zHx8jt/lUICUesm8v3NK2LNyGdszuebEO9i1Kb1c+6V1E5/6+j7admodVpu+PHPFK8yfsrDCTGOpO2dczykjTqxyP0IIEQoJssRB544zHubHz1YGDCZe+unxsIsl3zXkUZZ/6r90DcAjC+7k+H4dwmp3X3Nf/pxJ41/FNI2y046Gw7CDOz/BRel+sXvev8lvu1vXb2fM4RP8ZoY3TINzbxnCJQ+dH/aYHxjxFEtmL69QN7G03aaHNWLqn89VaUl11+Z0Rh0yzm+pI6Wg2eFNmfrHs7V+6VYIcWCQAtFiv1FcVMy2f3eQtSu70m0U5BayfH7gQMh0GCyauTSsdj1uD8s/+TlIuyZLPvghrHb3tebH9Uwa/2rJMuReCVE9lt8AC+yN60s+WsbW9dv9XtP0sMZccOc5Pu8rDYRG3Dwk7DFn7shiyUe+A6zSsW3+exu/Lf4j7Lb3tnT2cggQO2kNm9dsZeOfm6vUjxBCRIMEWaJG5GTkMvmaqZzd4BJGH3Y1wxtdxnU97uSnL1aG3VZBbmHwGn5KkZ9dEFa7bpc76F4mrTVFBa6w2t3X7Oc/xTQr909RoVix4NeA14y+dwTXvXw5DVvWL7vN4XTQd/QpPLf0IZLqJIbd74Y/NgcMPsE+OPDPyg0ht6m1pjCvkOKi4rLbCnOLMIzgM1QFuUUh9yOEENVFTheKapeTkcu1J97B1vU7yn1Q//n9Wm7r/yC3vXkNvUf1CLm91PrJxCfFUZjn/4PW8lo0DXMjdlxiHPWappG+NdPvNVprDjmyRVjt7uuXhasCJkQNRCmF2+UOes2gy/sy4LLebPh9E8VFbpq1beIzuLIsi18WrmLTmq0kJMfT/YzOpNSreIIvlHqI2tI444LnIfN6vcx7+Qs+mvQpW9ZuA+CYnu0595ahNG/XNOhrY5gGTVo3DNqPEEJUNwmyRLWbft+sCgEW2B/wAE9f/jLdB3cmMSUhpPZinDH0v6QXcyYvCJjvqs+FPcMap1KKM6/qz7S7Z/jfF2UoTr/41LDa9dVPZVmWRdvOoW0uNwyDVscc4vf+lYt+5/GLXmDnxt0opdBa44gxGXbtIC595PxyKR4O79ya1AYpZO/K8dueMhRdB3YKOCav18vD5z3L4g9+QO21Lvj70jXcecYjXP7khaTWTyYnPc/nvizDYXDysK7UaRD9E55CCBEuWS4U1arY5WbB1K8CLjUVFxXz1TtLwmp31J1n0+iQBnZpmb2UBjBXPjWmUqkWzr5+EO27ta2wZFXazzWTx1K3cVrZ7VprVn37J+88/CHvPvIRf/zwt99N26U69Tk2YP1EfwzToHm7phzb88iwH7uvNSvWcVv/B9m1OR2gbMwet5f3n/qYl66bVu56R4yDkbcO9dueMhR9LuhJg+b1Ava78K1vWfz+D6Ap9zqVvj/+d/N0Lnn4fAxTVfjemg6D1PopXPHE6FCfphBCVCsJskS1ytyeRVF+4D1MDocZ9kbm1PopTPr+IfqNObVcGZyW7Ztx58wbGHr1gEqNNzY+lse/vJtRd55DaoM9p0iOOqkdD8+/g4Fj+5TdtnX9dq447iZuOOVu3rhnJtPunsG1J97B+K63sXPjLr99DJ0wAK+/oLMkttt3sstwGMQnx3HXzBsicqpu2t0z7fQXPmbstIaPX/yMHRvKP4ezrz+Dc24YDNgBj2EoTIc929VtYCeufWls0H5nvzAfFWDPlWEYbF6zlSe/vo8Opx5VdrvD6aDPBT2ZvPxRGrZsENJzFEKI6iYpHES1yknP5ewGlwS8xnQYnHvLUC5+8LxK9ZGfU8CO/3YRlxhLk9aNIna03+v1kpOehzMupsJSZk5GLpcfeyNZO7Mr7CEyHQYNWtTnlV+f9JvT6tPXFvLsFa9gmGpPCgfTQCnFTVOvYsd/u5j3v89J35JBQkoCfUefwjk3DKbRIVUPMHLSczm74SUBDw8YpsHFD4xk5G3DKty3ac0WFkz9mp2bdpNSN4neF/Skfbe2Ib3uA+PPw+3yBLymU59jeOzzuwE7+WleVj51G9chPsl/fjAhhIiWcOIO2ZMlqlVKvWSO6dGe35f+5ffkntdj0eOc7pXuQylFbIKTpDqJEc2dZJqm3yXH+a99Rcb2LJ8zQV6Pxfb/dvLl9MWceZXv7OQDL+tN++5tmfviZ+WSkZ4x7nSat7U37I+682y01hHPB5WTkRf0dKZhKLL87L9q0a4ZYx+7oFJ9x8TGBAyy7O/lnkz9qfVTSK0vv0wJIfYPEmSJanfB3cO5rd8DPu8zTIMu/Y+jzXHhJQ0F2LFhF9PunsGiGUvxuO1afZ37HsuY+0fSvlvbKo05mIVvLQ6Y00oBC9/2H2QBtDq6Jde8GHiJLRoJN9MapZZlkffH67XKpYCIlB5ndePLtxb77VtrzUlDu0a8XyGEqA6yJ0sElZeVz+zn5/PQ+c/yyAXPsWDqV1XKDdWp9zHc9tY1xCY4QYEjxrRrAgJdB3TkjnevC7vNbf/uYHyX2/j63SVlARbAL1+t5voed1Uq/1Y4cjLzAt6vNeRmBL6mpiSmJNDznBMqbCzfm2EYYaXVCNXZ15+BUspn8GiYBg1a1OPUc6VkjhBi/yR7skRAP3/5G/cMexxXQTEoeybF8lqk1k/hkQV3VKk2XX5OAV+/u5RNf20hPimOHud057AOh1aqrUDlb5ShSGtUh3c2vFS2MTvSbup9L6sW/xkwhUS3QZ24f/atUem/qrb9Ywepedn5PmfklKG4c8b19DznhIj3vezTn3lwxNO4CotRhkIpe4m10aENePSzu8qWS4UQojaQsjoiIras28adZz6Kq6AYre0aeqVBRG5mHrf2vZ+c9NxKt5+YksAZV/Rl3DMXcdEDIysdYO3emsGyef7L32hLk7EtM2hm9Ko44/K+AdNSWF6LQXudRKxtmrRuxHX/u8LvkqfWmofPf5Zt/+wIu+01K9bx3hNzmPn4HH7/bk2FlBbdBnZixpZXGHrNQOo3q4vpMEmqk8CxPdtTFCDBrBBC1HYSZAm/5rywAK/H6zPPk+W1yMsu4LPXv66BkZW3bf2OoLmoDNNg05qtURtDj3O603VgR5/pCJRS9DynO10GdIxYf8UuN5k7ssqVoKmq7z9e4T9fl7aXPOe+9FnI7e3eks6EE27n6m4TmXL7O0y94x2uO/lOrjr+Vrb9Wz5Y++R/X/LRc5+QvjWD4iI3eVkFfPXOEq7qcitfTP+mKk9LCCFqTFSDrEceeYQuXbqQnJxMw4YNGTp0KGvWrCl3TVFREePHj6devXokJSVx9tlns2NH+L8ti8hb8tEyvwWAwZ4hWjpneTWOyLf45Lig11iWRUII11WWaZrc++HNnD/xLBJT96R3MB0GbToeylnXDcIwqv7PbceGXTw99iWG1hnNiCZjOTN1NI9eOInNf1c9gAxW3sfyWvzy1eqQ2irML+LGU+9h7U/ryx5bOtP376oN3HDKPeSW7GNbveRPXr31LaB8gWxvSYHsJy95kc0l5XaEEGJ/EtUg65tvvmH8+PH88MMPfPHFF7jdbvr160d+fn7ZNddffz1z585l1qxZfPPNN2zdupWzzjormsMSISouClwTD7D3atWw1sceQqNDA+eLMkyDE4d0ieo4YpwxDLm6P/VLspwrw8559c9vG7ju5LuYNP7VstJBlbF57TauOv5WPn9jUVnaA6/by9czlnJVl1tZ9+u/VXwGkTu5+OX0xWz9Z4fPoM3rsUjfmsGCKV8B8NGkT4NmvJ/38ucRG5sQQlSXqAZZCxYs4KKLLuKoo46iQ4cOTJs2jY0bN/LTTz8BkJ2dzZQpU3j66afp1asXnTt35vXXX+e7777jhx9+8Nmmy+UiJyen3B8RHW07tQ544sx0GBx+/GHVOCLfDMPgovtH+r1fKcWZ404nrVGdqI/l/uFPsemvLQBl+5tKA425L33O7EnzK932s1e8Ql5WfoXAxfJauAqKeXzMC0GXTQPp2PvogMGOYRp06n1MSG0tfGtxwJBNW7psGfC3xX8GnUH7bfEf5W7L2pXNh89+wss3TOOdhz+ssPwohBC1QbXuycrOzgagbt26APz000+43W769NmzIfiII46gZcuWfP/99z7beOSRR0hNTS3706JFi+gP/CA19Or+ATdzez0Wg8f1q8YR+dfngp6Me+YiHDEmylB2WgjTAAUDLuvFFU9Gv77dmh/Xs3rJXwFfs/eenIPX6/V7vz+b125j5aLf/bZteS3+XbWRNSvWhd12qWHXDAxY3kcZijOuDO37nb07l2DxXk66vVwYKJAvtfc1s578mJHNruDlm95gzuQFvHHPTEa3uZrnr36tUq+tEEJES7UFWZZlcd1113HSSSdx9NFHA7B9+3acTid16tQpd22jRo3Yvn27z3YmTpxIdnZ22Z9NmzZFe+gHra4DO5Ulz9x7Q3fpB96lD59fqaSh0XLWtYOYufVVxj19EUPG92f0vSN4c+0LXP/KlThiop9396fPVwYNGNK3ZrK5EhvwN/4RWi3HDSFe58vhnQ/j+pevQClVbkbLcBiYpskd71xHk9aNQmqr+eFNAufdMg2atW0MQJfTOwSdQTu+XwcA5k9ZyP9umW4fyLA0HrfXDjxLNuVPmfhOSOMTQojqUG0Z38ePH8/q1atZsmRJldqJjY0lNjY2+IWiypRSXP38pRx5Qjs+eGYea3/+B6Xs4sgjbhpC9zM61/QQK0ipl8ywawbWSN9ej7dCIWdf9k6WGqq4xNDe83EJVfu3MXBsH9p3b8ucFxbw6ze/Yxh2gHPm+P5h5asaOLYPP8z7ye/9ltdi0OV9ARh6zUA+f9P3CUKl7FnJM67oi9fr5Y17ZvptU2t7f9fIW4eSUi855LEKIUS0VEuQdfXVVzNv3jwWL15M8+bNy25v3LgxxcXFZGVllZvN2rFjB40bN66OoYkglFL0HtWD3qN64PV4Qdkn6aqiuKiYbz9Yxt8/rsfhdNB1QEeOPeXIiJSM2fbvDj585hMWvvMthbmFNDmsEWeO68/Asb1xxjmr3H4gR3RrG3BvEdgnIZsfHn5yzaNPPoLE1ATyswv8XhMTF0PnkhmfcGmtWbNiHZvWbCUhOZ4rnhpdpQLM3QZ14qRhXflu9ooK+8SUoejc51hOGW4nNm1zXCtumXY1T1w8GaBsSdQwDRwxJvd+dAv1m9Xjz2VrSd+aGbBfT7GHH+b9RL8xp1Z67EIIESlRDbK01kyYMIGPPvqIRYsW0apV+aWlzp07ExMTw8KFCzn77LMBWLNmDRs3buSEEyKfWVpUTSSypf+2+A/uO/tJctJzMWNM0PDeE3M47LhDeXDubdRvVq/Sba9ZsY6be9+Hq6i4LPXE5jXbePG611n03lIe/eyuKs/0+FOQW0jWzmyS6yaRl5nvcwO6YSgGje1LbHz4Y3DGORl52zCmTHzb5/1KwbAJA0mqkxh222tWrOOJS15kw+97lt7jEmM595ahnH/HWZVKPWEYBnfOuJ63H/yAD5/9hILcQvt206DDqUdy8xtXl3s/9bmgJ0d0a8u8lz9n1eI/UCVLhIMu70uDktOahSVtBKKUKutLCCFqWlTL6lx11VW88847zJkzh3bt2pXdnpqaSny8/VvyuHHj+PTTT5k2bRopKSlMmDABgO+++y6kPqSszv5j05otXNnxFjzFbqx9MoubDoOmbZrwyq9PEOOMCbttr8fLBa2uImN7JpbXR4BjGpxzw2DGPnZBpcfvi9aa95+exxv3zMRV4EIZqkLWdKVAA0ed2K5KgZ7WmtdufYv3nvoYwzBKZv40Xo/FwLG9uebFsWHPMv67agMTut+Ou9jjc1P9iJuH+H3Ndm/NYN7Ln/PdnBUUF7k5omsbBo87naNO3PNv/bNpX/PUZS+hwP6eK1Aokuok8shnd9IujNOpOzbs4oJWVwW97tHP7qRz38rN6AkhRDDhxB1RDbL8Lf+8/vrrXHTRRYCdjPTGG2/k3XffxeVycfrpp/Piiy+GvFwoQdb+49krXmHB618FXFK7c8b1nDIi/ILA3328gnuGPh7wmoSUBGZtfzWiy4YfPvcJL10/ze/9MbEOmh/elDOu6Ef/S3vhjA0/gNzXtn938OWbi9m1OZ20Rqn0ubAnLdo1C6uNogIXi2YsZfr9s9i1abffk4DKULz930tls0mlVi/9i9sHPISrsLgsODMdBl6PxYV3D2f0vSP444e/ue6kO33P6pmKxNREpv8zmcSUhAr3+3Pb6Q/wy1er/daobNCiPtPXvxCRxK9CCOFLrQmyqoMEWfuPIXXGUJDjf0+RYShOPrs7d828Iey2p901gxmPz8YbZFP5q6ue5tCjIpP2o6jAxblNx1KQ4395qmHL+kz/Z3Kt+tD//bs13HXmo+Rm5AW91jAUlzw8inNvGVJ2W35OAaMOHUdBTqHfWof3fXQLC99ezNLZy/0H1QqunnQpQ8b3D3nsm9du45oTbic/p6BcNQLDNDBMg0c/u5MOpxwVcntCCBEuKRAtaiVXoSvg/ZalAwZhgZgxZkiJOB3OyG1DXLHg14ABFsDOjbv5a9naiPVZVTs37WZi/wfJz8oPfjF28JK5vfxm84VvfUt+doHfAMswDd5/ei7LF/wa9CDA8vk/hzbwEs3bNmHyikc57dyT9uzpUnD86R14dsmDEmAJIWqVakvhIESztk3Y9Odmv0tThmnQ8ojmvu8MouuAjrx573v+L1DQ+NCGND0stDxPochNzw3puuzdoV1XHea++Jm9xOcnQNqX5bWot89hhN8W/45Sym9Qa3ktVi/9i5hgAa0m6MyjL01aNeK26ddwzYtjydyRRXLdJFLqSsoGIUTtIzNZotoMuSrwspDltRh4eZ+A1/jTrksbjjqxnf+klhrOvWVoRJftGh4SuF5iqcZB6ipWp8Xvfx8wI30FStHr/JPL3RTSBgOtadelTdCEpO27Hx76WPaRkBxPszZNJMASQtRaEmSJajPgsl50OO3octnjYc8BidH3juCQ9pWbyQK454ObaFnyeKOkj9Kga/iNgxlUyQDOn469j6Z+s7p+E5AahqJNx1a0OuaQiPZbFa7C8Ap6n3/7WdRrklbutmN6tLePS/phmAbtT2jHsGsGBgzolLKTlu7Nsiy2rt/O5r+34i4OXqBcCCFqMwmyREiKClxs/28n+dmh7eXxJcYZw0Of3M6Fdw0ntf6e2YeWRzbntunXcO6tQ1mzYh1//PA3hXnh5zpKa1SHF398jLveu4GTz+pGpz7HMHBsX176+XEuf2J0yMlO83MK2PDHJnZvSQ94nWmaXPfy5aBUhbYNQ2HGmEx44dKwn0c0HdaxVUi1ApPSErniydGMvndEhfv6XtiT+OS4CsFyKctrcc4Ngzn5rG4Mudqevdy7T9NhoAzFLW9MKDu1qLVmzuQFXHjY1YxpO4GLj7iWc5tezhv3zKTYJcGWEGL/JKcLRUA7Nuxi+n3vsfCdJXiKPShD0W1QJ8bcey5tOla+bqHX42X3lgwcTgep9ZN55+EPmfXUXIryigA78empI0/iupcvj1oC0X3t3pLO1Dve5et3l5SVvjmiaxvG3D+yrHaeLz99sZL/3Tydf37bUHbbkSe248qnxtC+W9uojzscy+f/wh2DHg54zZVPj2HwuNMDpptY+c3v3DHoEdwud4UUDudNHMYlD50P2MHTd3NW8NGkT1mzYh2mw6T74M6cde0gDu+8J0fWC9dMYc4LCyr0owxFp97H8OC8idVSf1IIIYKRFA4iIrb9s4MJ3SeSl5Vf7pSYYRqYDoPHPr/bXjqqAq01953zJEs/Wu7z/npN0njzn8kRyS8VyO6tGVzd9TaydmaXe67KsDOJ3v7OtZx67kl+H6+1ZuOfm8namUP95nVp1sZ36Zxdm9PZsnYb8cnxtOl4aJVLFIVLa82kq15l3itf+N28ftRJ7Rhx8xBOPLNLwLZ2btzF3Jc+Z8ns5biL3LTrchhnju8f9gm/P374m2tPvCPgNTdOuYr+F58WVrtCCBENEmSJiLjjjIf58bOVfhM/NmxRnzermPjxx89XMrH/gwGvOXXEidwx4/pK9xGKx8Y8z9fvLvGdckBBfFIcM7e+SnxiXKXa37p+Oy9cM5UVC34p289Uv3k9xtw7gv6X9KrCyMOnteazaYuY9dTHbPxjc4X7DdPA8lpc+vD5jLxtWNTH8+Qlk/nyrcV+0z0oQ9G2U2smL3806mMRQohgJE+WqLKdm3azfP4vfjcua0uzY8Mufv1qdZX6+fDZeUGv+fbDZXjcnir1E0h+dj6LZiz1n9NJQ2FuEYtnfV+p9nds2MWE7rfz0+cry20Y3705nacue4lZT35cqXYrSylF/4tP46L7zvV5f+n3fMrt7/Dvqg0+r4mkDX9uCZhPS1uazX9vjfo4hBAi0iTIEj5t/ntbwBNkYM8wbPxzS5X6+XfVxqDXeD1e1q+MzId99u4c5r78OW8/+AFfvPkNhXmF7NyUXrYHyx9HjMmWtdsq1ee0u2eQl53vN2Cdcvs7ZO3KrlTbVTFn8vyAm+BNh8Hclz6P+jiS0xL9bqIvlZAcH/VxCCFEpMlOUuFTfFLwZTFtaeJCuC6Q2BA3tbureMJMa80b98xkxqOzsbwWhmng9XiZND6WC+4aHvTxlqVJCKPGXqnCvEIWzVhargRMxbYtFr71LWdff0bY7VfFul//C5hiweuxWPvLv1Edw67N6SSlJfrNHg/28mXvUT2iOg4hhIgGCbKET4cf35p6TdNI35rp9xrTYdL9jE5V6uekYV157/E5Aa8xDEXL9uEVQN7X2w9+wNsPflD2tddjz1wV5bt47ba3aNK6Edv/2+n3w96yLHqe0z3sfrN25gSdJTNNg50bd4fddlU545zkE6CMkYLY+MgV096b1+Nl8rVTmffKFwHLIRmmQXxSHEOuHhCwvd1b0vly+mJ2bNhFaoMUeo/qEXbRbCGEiDRZLhQ+mabJ6Hsq5kgqpZTizPGnU6dBapX6GX3PcBwxgU/Y9Rh+QpWyeudn5/POIx8GvKYov8jvh70yFH0vPIWmhzUmfVsmC9/+ls/fWMSGPzYF7TspLRGCpOeyLE1KverPWt7jrG4Y/jLkAwrFycO6RaXvyddOZd7LX9hBrY+XvXQZs17TNJ786t6yfFr70lrz1gPvc/4h43j9rhksmPoV7z76EZe0v44nLpkc1b18QggRjARZwq+BY/tw6cPnYzoMDEPhiDHLPvz6X9qLK54YXeU+YuNjeeiT2/3uDWrSuhHXvHBZlfr4Yd7PuIsCLzdm7shmzH3nEpcYC4pyz7X3qB5c+fQYHr/oBc5veSWPXjiJJy6ezGVH38CNp93Dzo27/LabnJZE1wEdA+59siyL087znx4iWoZdMxDTYfrcD2WYBqkNUug7umfE+921Od0OsALMYKU2SOH+Obcy/Z/JAfOxzXv5c964Zyba0lheC4/bW7Y0+8Ub3/DKTW9GfPxCCBEqWS6sRXIz8/j2g2Vk7siifrO69Di7e41v+B152zBOv/i0cksxvc4/2W8eqMro1OdYpv09iSkT3+G7Octxuzwk10ti6PgBnH39IBJSElj17Z98Of0bsnfn0KB5fU6/5DTaHBdaMtTczLyABY1Lte/Wlve2vco3733PlrXbSExNoOfwE2jcqiG39nuA3xb9gWWV38O0eulfXHvyXbz88+Ok1vd9lHf0vefy85er0FpXWI5USjFgbG+aHtY4pOcSSc0Pb8qDH9/GvWc9QWF+EYZhoJS9FyutUSqPLLiTxNTEiPf77Qc/2LN7Ab4dmduzOPToFgHziHk9XqbfP8vv/Vpr5r70OaPuPLvKM65CCFEZEmTVAlpr3n3kI6bfPwuP24Npmni9Xp4f/xpjH7+QIeMDF1aOtrRGdRh+05lR7aNJq0bcWZILy7KsstxbrkIXdw15jGXzfirLKG46DGa/MJ+BY3tzzYtjgyb0bNK6UdAAC6Bxq4bEJ8VXyFu17NOf/aaqsDwWGdsy+XjyZ1x4j+8N9O2OP4xH5t/B4xe9wK5N6ShDoS2N6TA5c/zpEZkRrKxOfY7l3c2vsPCtb/njhzWYDpNOvY+lxzndo5YANi8z3z54YAXeq5afFWC/GLBmxToydwQ+len1eFn2yc+cfpEkMhVCVD8JsmqB95+ay+t3vlv2demmbFdhMS9MmEJcYuxB9SGxd3LT56+ewvJPfwYoy6VU+venry2kQfP6XHDXOQHbO75fB+o2SSNze5bPYMswDY46qZ3f2bkvp39TlqDTF8trMX/qV36DLIDjTjua6f9M5ucvV7Hpry0kJMfTfXDniMyw/LtqA9/P/YniwmJadziEE4d0CasETWJKAmdedTpnXnV6lccSiiaHNcIb5DCAYRo0bFk/4DVF+a6gfSmlKCwp1SSEENVN9mTVsML8ooBLHgBT73gXrzfwh9KBaPfWDL548xv/x/s1vP/0XFyFgT9sTYfJDa9eiTJUhf1HhmngjIvh6kn+Czmnb8sMmOoAIDuEPFemaXJ8vw7Ua1qXz6Z9zfktr2RwyoU8eN4z/LV8bdDH7ys/O5/bBz3M5R1ustNTPD6bB0Y8zXktrmTlot/Dbi/S9l1aLdXj7O7EJ/tP/WGYBief1TXoYYDm7ZoGPVSgteaQI5sHHasQQkSDBFk1SGvNZ1O/Cvqbdsa2TP747u9qGlXt8fMXvwUNbvKzC/jzh+ABSreBnXjs87tod/xh5W4/5MjmPDz/Dlofe4jfxzZsUT/gKTyAek3rBh2D1prnrnqVB899mj++/xu3y0NRXhHfvv89E7rfzg2n3M20u2ew7Z8dIbV199DH7Szy2LNppbNDObtzmDjwoWrJ1r6vnZt2M/naqQxNG8PpjnMZ3vgypt01g5yM3LJr4hJiue7lK0DhM+hNqpPIZY9eELSvhi3qBzxUYBiKxq0a0uHU8GopCiFEpEiQVUO+m7OCscfeyORrXw/p+tzMvCiPqPYJNQFpqNcdd9rRXPn0RTRosScdwL+rNnJL7/t45eY3y5Zp99XvotMCJhNVhmLgZb2D9r941vd88soXAOWCR8trz9St+vZP3n3kI0a3vZrXbnsr4D6y3775g9+++cNnEGpZGsvjZcZjs4OOKZI2/LmZKzvezMcvfUZ+tr2fKmtnNu8++hHju9xGxvY9Odd6nXcyD86dyGEdDi27zTANTh7WlReWP0KTVo1C6vPq5y8lpV4y5j5BsGEaOJwObpt+TZVqawohRFXIT58a8Pkbi7hn2OM+i/P606R1aB86B5JAR/dLKUPR6piWIbW3fuV/3NznPtK3ZJS73eP28sHTc3nhmqk+H9ex19GcNLSr31QHTQ9rzOBx/YL2/+FznwRM5QAlwZeGmY/P4cNnP/F73aKZSzEdgU7eWXwz6/tqW2bWWvPI+c+Sn11QISC1vBY7N+7mhQnlX99uAzvx0k+P8+a6F3jxx8d4b9ur3PXejSEHWGAfmHjxx8fod9FpxMTZG/UN0+CkoV15YdkjHHViu6o/OSGEqCSlQzl2VYuFUw27NijMK2R447G4CoJv2gX7A+OwDofy4o+PRXlkkWNZFlrroKf+gtFaM67zLfy7aqPPGRtlKOo0TCXG6SA+KY5Thp/IGVf2Ja1RHZ/t3T30MZZ98rP/JUgFb/z9vM90Cu5iN1MmvsPclz6juCTnljIUJw3tyrUvjQ1pA/uAuPPwFIeeHDO1fjLvbn6FGGfFU34Pnf8si2d9H3Q5dW7eW8SFWLqoKv5avpYJ3W8PeI0yFO9ueoV6TdKiMobiomJy0nNJrJNIfGLVyj0JIYQ/4cQdcrqwmn0z64egG7VLlS55XPvS2CiPKjK+n/sjs576mNXf/onW0K5LG8654QxOGXEiSgXZoeyDUoqJb1/L9T3uIj+n4gyJtjTZu3LKAo23HpjFh5M+4Ykv76kwC5afnc8P834KXCPPMPjqnSU+TyvGOGO48qkxXHjPcH5fugavx0vbTq2o38x3JnJfHDFmWEFW9u5c1ixfx9Ent69wX7M2wfNqpTZIwe1y8/OXv+Ep9tCmY6uo5eNa98t/Qa/Rluaf3zZELchyxjnD+n4IIUS0yXJhNdv+z46Ayzx763DqUTz77QO069ImyqOqurcf+oC7hzzG70vXUDo3uvan9Tx03rO8ctObIeWp8uWQ9s15+ZcnGHJVfxJT7QLNexelLre3ydIU5BRyx6CHcReX36eVm5kfMMACe6N09q6cgNckpiTQdUBHThh8fNgf6F0HdKywdyiYooJin7f3v6RXkIBR0bR1I85tdjn3DH2cB0Y8zZi2E7it/4Ps2pwe1hhC4XCG9vtatHJvCSFEbSRBVjVLSksMusQDMOn7h3j8i7tp26l12H1ordm8dhtrVqwjK4TUAlW15sf1TLtrBlAx6AH44Jl5/Pzlb5Vuv2GL+lz17MXMznyD+cXv2jMhfibGLK9FxvYsln60vNztqQ1SgtZItLxW0NxMVXH2DYPLNrmHROG3MHbjQxty8YPnlV23N8M0iEuK489layuUE/pl4SquPekOsncHDibDdfzpHXzuWdtbYmoC7bu3jWi/QghRm0mQVc16Dj8hYDkRZSjad29L+26HV6r9H+b9xBXH3cTF7a7h6m4TGdFkLPed8yTb/g2eFqCy5r64IOAMjeEwmDN5QUT6ys8qYMvabQFfQ9Nh8ts3f5S7LT4xjlNHnhQ4FYNS9L6gR0TG6cuR3Q/n5mnjMUwjaEBimAbdB3WmYQv/Qd95E4dxyxtXl0uiGhvv5IQzj6cgp9DnYyyvRfrWzICb6iujftO69LmgJ4a/56XgnBsG44xzRrRfIYSozSTIqmYNW9Rn8Lh++NyiVHLbRQ+cV6m2v3xrMXed+Sj/rd5Udpu2NN/NWcHVXSdGLdBa8+P6sizsvlgei79/XB+RvkLa26XA1ws85r5zSUxJ8HvCb/S9I6jbODr7hUr1vfAUpv8zmfMnnsUR3dr4DLgMh0GdhqlMeMF/gtS923v9r+eY9vck/vfbU8zaOYWUuhVTGuzN8lrMn7Kwys9lX9e+NJbO/ToAYJjln1OLds3oPrhzxPsUQojaTIKsGnDVsxcz5OoB9gesUmV7tJLrJHL3rBvp1PuYsNsszC9i0lWvAlTY/2R5LfKz83nttreqPngfQpmdiNQMRnLdJJq3a+o7SC3hdXt9JqBsfGhDJn3/MB17HV3u9rRGqVwz+TLOv/2siIwxmIYt6nPRAyN5/vtHeG310/Qe1aNsKTM2IZbBV/Rj8opHadiyQUjtKaVo1qYJrY5uSXxiHOnbMgMGvWDnr4r0weLY+Fge+uR2Ln/iQuxMo3vu27puG+M638LHL34W0T6FEKI2k9OFNcB0mIx/7hJG3jaMJR8uIz+7gGZtGnPCkC6V3hj87fs/BMwc7/VYLPlwOTnpuUHLlYTrxCFdWPvzP343YpfmLYoEpRTDbzyTZy5/2W9faY1SOWloF5/3N2/bhEc/u4vvPl7BD/N+whkbQ+8LelR6edaf0gAm2Mxbi3bNuPWNCdzw6pUU5haRmJoQ8sEIf+o2rlNWTNuf1AYplTrxGUzmjiym3T3Tfi/s9XYoHcvzV7/GYccdKvmrhBAHBQmyalC9JmkMGd+/Sm3kZOSy5INlfPXuEpSp0AE2Vltei12b0yMeZA0c24dZT35MYW5RhXp1ylA4nA7OHB+54sMDLu3F+l//5eMXPysXTChDkVQnkYc/vcNvgeSdm3bzwPCn+Gv5urL9Q3MmL6B998O5670baNC8aikAfl64illPfcwvC1ehvRaHH38YZ113BqeeGziNhSPGwca/trBgylds+3cHqQ1S6DOqJ90GdcJ0mHi9Xn5c8KtdCNpVzGHHHkrfMaeQUrfi97LfmFNZMPUrv30ZpkH/S4JnqK+M+a99hcfl9jtLZjoMPnx2ngRZQoiDgiQj3U9prXnnoQ9568H38bg9KKWCpigAeOvfF2l0SGjLUOH4a/labh/4MLmZeRhKoUvGGJ8Yx/1zbuW4044O2kY4tNb8+vVq5r70Gf+s3EB8cjynDD+BAZf1JrW+7/dBfk4BVxx3E7s3p1eY5TEdBg1bNuDlX54gITm+UmOa/cJ8Jl8zFcM0yk5ZGobCsjRnXnU6Vz9/qc9Ay+v18uQlL/Ll9MVlQWNpG+27t+XG18bxwLnPsOH3TZgOE41GW5oYp4Nbpl3NKSNOrPDa3Hv2E3z/8Y8V3hNmyX6vl35+grSGwROohuvG0+6pcOhgX0l1EvkoY1rE+xZCiOoQTtwhQdZ+aubjc8LaY6UMxeGdD+OFZY9EbUyF+UV89fa3/Pr1arTWHH1Se/qO7kliamLU+gzHR5M+5aXrp/nfi6Tg6kmXVmp2ceNfW7j0qOsCnnq8b/YtnHhmxWXMtx54nzfunenzsYZpEBvvpLiouOLyn7KXI5/99gGOPKH8zFCxy83/bnqTT179slwC1A6nHsXNr4+vVKDtcXvI2JZJTGyM36z6N5x6N6sW/xmwncTUBGZnvhF2/0IIURtIxvcDXGF+EW89MCv0ByhAw0UPjIzamMBOkzDo8r4MurxvVPuprC+mfxN0s/cXby6qVJA17+XPMU3/+6AM02D28/MrBFnFLjcfPDPPb3BmeS3/e+20fYpv5uNzuO+jW8rd5YyN4ernL2X0vSP49evVdsb3Tq1peYTvvFuBFBW4eOehD5j38ufkZuYDdl3J8+84mx5ndSt37bE9j+T3pWv85oIzHYbPQwlCCHEgktOF+6EV83+hKD94aZ7SVAWJqQncOfN6ji85Xn+wytmdG/gCbZeyqYw1K9YFTmPh9Z3GYu1P/5CXlV+pPsHeUP7D3B/9FoJOqZdMz3NOoNf5PSodYN3c615mPj6nLMACu9j2/ec8aQeIexl0eV97r5uf7Wdej8WwawaGPQ4hhNgfSZC1H8rNyAvputPOPYmJb1/Le1tfpec5J0R5VLVf0zaN/ebIAjsoDaUmoC8xIZwKjfFResbr8R0chcOydNCUDZU1e9KnrPlxfYWZqdK9Xq/c/CY7N+4qu71B83rcMeN6TNMsl6ur9L8vfWRUxPfnCSFEbSVB1n6oSYhFfs+7fRi9zjtZsmyXGHR534AljSyvxcCxfUJuz7Isdm9JZ9fmdLoP6hzw9KDp8J3GotUxLUOu++eTgsatGkatJuDHL30W8ECFUor5U8qfZDx5WDf+99tTnHFFPxq3akiD5vXoec4JPLvkQUbeOjQq4xRCiNpI9mTth4477SgatqzPrk3pPvcYGaZB206tOeTIFjUwutrr5LO60qX/cfz4+coKgYMyFF36H8dJw4Ln87Isi49f/Iz3n57Ljv/sWZwGLerhjIuh2OWuGJQo+/+G+lgmS05Los8FPfn8jUU+A8C9Tyr6olAMvXpA0DFXRrHLza5NgYtJa63ZtGZLhdtbHtGMq5+/lKufD561XgghDlQyk7UfMgyD6/93JYapKpZkMQ0cTgfXvHhZDY2u9jJNk3s/uoURN51JfHJc2e0JKfGce/MQ7v3wZkwzcCJQrTXPXPEKk6+Zyo4Ne5bJdm1Kx1VYXJZIVJXsS1KGwhkbw93v38ihR/kOeq94cjSHHtXCngnb69tpmAbJdZO4+MGRoKiw1KkMxXG9jo5oDrK9OWJMzCBFtQ1DEZ8YF/AaIYQ4WEkKh/3Yb4v/4LWJb/Pn93+X3XbIUc3p1OdY2h3fhhOHdpEPQD+KClz8t3ojYC/ZxcbHhvS4Hz9fycT+Dwa8Zsj4/mTsyMLyeGnfvR39LznNb+6uUoX5Rcx7+Qs++d8X7Nq0m6S0JPqNOZWhEwZQr0kaPy9cxczHPuLnL1cB9szZ0KsHMOzagcQ4o7NUCPDgec+w5IMfAu75euiT2+k6oGPUxiCEELWJ5Mk6yGz7dwezn5/PvFe+oLiwuGyJKT4pjrGPXcDgcdGZ6TgY3XvWE3w/70esAKkaju9/HA/NnRiV/ouLinEXe0hIjo9KWZx9rfvlXyZ0n4jXa1VYBjUcBocdeygvLH8Ew5BJcSHEwUHyZB1kflzwKx8++0nZ16V7eArzipg0/jVi4pz0v/i0mhpeBYV5hSx8ewlLPlpGYW4hrY89hDOu7MdhHQ6t6aEF9e/qjX4DLLBf+/9WbYxa/844Z7UeZGjTsRX3z7mVh857lvzsAhwxJlrbpyKP6NKG+2bfIgGWEEL4IUFWLbZ7awZF+S4aNK/rdzmr2OXm9TvfDdjO1Nvfpu+FPatceDgSNq/dxs2972P3lnQUCq01a1asY94rXzD63hFcePfwmh5iQAkpwUvuJKYkVMNIqk+X/h2ZufV/fPPe96z75V+ccTGccGYXjjzh8GqZTRNCiP2VBFm10Pdzf2T6fbNY+/M/AMQmxDLgkl6Mvm8EyWlJ5a79ZeGqckkifcnckc1vi/+gY69jojbmUHg9Xm4f8BAZ2zJBgy5Jc1663+fNe9+j5RHNKtTiq01OHXES63/9z29aA2UoTh15UjWPKvpi42PpN+ZU+o05taaHIoQQ+w2Z569lPvnfF9w95DHW/fpv2W2uAhcfv/QZ1550Z4Xs4DnpoWUoz0kPLYFpNP0w7ye2/bPDb0oCZShmPDa7egcVpgGX9iKlXrLfpKbOOCe9R/UI2MauzeksmrmURTOXsmtz4BQJQggh9l8SZNUiWbuyeWHCFIAKMyWW12LL2m28+/CH5W5vfGjDkNpufGj4BYEj7afPVwZcstSWZt0v/5KfXfkyM9GWUi+Zp76+lzoNU33e7yp0cf85T1KQW1jhvtzMPB4492lGHTqOh857lofOe5ZRh47jgRFPkZtZ80GwEEKIyJIgqxb54s3FeINkJP/k1S/LlWI5+uQjaHJYowr5skopQ3HIkc05/PjDIj7ecAVKqrm3aJWIiZTm7Zr6zFEGgIZ1v/5XYZ9ccVExN/e+jyUfLisXQGtLs+Sj5dzc+z6Ki4qjPfSI0FqTn51Psctd00MRQohaTYKsWmTL31uDntTKzy4ot0SolOK6l6/AMHwkJjUUpmlw7UuX14oNykd0axu4Vp+CRoc2ILlukv9raoEfF/zK7s0ZfvdlWV6LBVO/ojC/qOy2r95Zwvpf//MZaFpei/W//sdX7y6N2pgjwVXo4u2HPmBk88sZmnYRZySO4o4zHmb1kj9remhCCFErSZBVi8QnBz+5hoK4xPInDTv1PobHv7yHwzuXn61q160tT359H8f0aB/JYVbaqSNPIiktEcPPrBvA2dedUSsCwkD+/umfoCc1i/JdbFm7rezrBVO/8jvbCPaM44KpX/m9v6YVFbi4ufd9vHHPTDK2ZQH2LNyPn63khlPv4ZtZ39fsAIUQohaS04W1SM/hJ/D+03P93m+YBp37dSA+qWIwdmzPI3lh2SNsXruNzO1Z1GuaRtMQC0lXl7iEWO776BZuH/AQHrenbFnQMBSWpel5zglRKxETSTFOh8+akb6uK5W+NTNgoWVtadK3ZERkfNEw64mP+Wv5Op97BVHw+EUv0LnvsSTVSayhEQohRO0jM1m1yBFd29Cp77E+T66VTu6MuuPsgG00b9uEpm0a8/3HP/LabW8x+/n5ZO3KjsZwK+XYnkfyysonGXzl6aQ1SiUhJZ4jurVl4lvXcMe71wWtHZi1K5vZL8xn6h3vMPuF+WTvzqmmke/RZUDHoPvLGrSoR4sjmpV9Xb953aAzWfVb1IvYGCPJ6/Uy58UF/oNEDe4iN1++tbh6ByaEELWczGTVIkop7nn/Jh467xmWf/oLhmlgGAqP20t8cjy3vjGBo05s5/fxWmum3TWDGY/NRmuNaRp4vRYv3/gGo+8dwXkTh9WKpbhmbZowftIljJ90SciP0VrzzkMfMv3+WViWVVY66JUb3+CCu4dz/u1nVdtzO6zDoXTscwwrv/7db7A18tZh5fbX9b+kF6uX/OW3TW1pBlzSK+JjjYSc9DyydwUOZg3TiGqmeyGE2B9JkFXLJCTH89C821m/8j+WfrScovwiDjmqBaeMOJG4hMBFjGc8Opt39krx4LHsTeZey8vrd75LQnI8QycMiOr4o+WDZ+Yx7e4ZZV97S56bx/Iy7a4ZxCfFcda1g6ptPHe+ez23D3yINSvWlwV8psPA67EYfuNgBo/rV+760847mbkvfcban/+tEJgZpkHbTq1qbRJTZ1xoBaid8dVX7kcIIfYHUiD6AFGYX8S5TcZSmFfk95qUesnM2PIKMc7QPjRrC1ehi3ObXk5+doHfa5LqJDJz26s4Y6vvuXm9XpZ/+guLZi4lLzOfpoc1ZuDY3rQ65hCf1+dn5zNp/GssmvldWaBlmAannnsi10y+jMTU2ruf6cbT7mH1kr8CLpM+sfAejjvt6GoclRBCVD8pEH0Q+vmL3wIGWGBnh1+1+E869Tm2mkYVGb9+tTpggAWQl5XPyq9X06V/x2oaFZimyQmDj+eEwceHdH1iaiIT37qWK54czR/f/w3AkSccTt3GadEcZkScf/tZ3Nb/QZ/3GQ6Dth1b0+HUo6p5VEIIUbvJxvcDRLAgpOy6nIqZyGu7kJ9biNfVtLqN0zh5WDdOHtZtvwiwADr37cCNr12FGWOiSvKvlaaxOKzDoTww97Zasd9PCCFqE5nJOkA0P7xJaNe1jU5ah8rsIQtVs7ahPbembWpXyooDTf+LT6PboE58Pm0RG/7cRFx8LCcN60rH3scETaIrhBAHIwmyQpCfU8DuzekkpiZQv1ntPGbfvvvhtDiiKVv+3obl46i9YRq06djK736hyirILdxzGtJhYCj7NOSL173OLdOu5qShXavcx+HHH8YhR7Vg05+b/T63Q49qQdtOravclwgsrWEq594ypKaHIYQQ+wX59TOAXZvTeWzM85zT4BIuO/oGzmtxJdecdAc/f/lbTQ+tAqUUN00dj+l0VMizZZgGzrgYrv/fFRHtU2vN/cOf4sfPVgJgeSw8bvvUX2FuIfcPf4rVS/2nLQiVUoobXxuHwxnj87k5nA5uePVKWa4SQghRq0iQ5ceuzelc3W0iX7+7pCxwAFizbC23nf5grSwjcmT3w5m09CE69+sAJfGGUopugzrx/A+P0Oa4VhHtb82Kdfz0+UqfJ85Kz6y+/eAHEemrfbe2PLf0QXvTfmkspaBzvw5M+u4h2nVpE5F+RO2TvTuHpbOX8+0HP7Brc3pND0cIIUImKRz8eOSC5/jmve/KSr+Uo+x8Vu9te5XY+MjsO4q0rF3ZZO/KIa1RHVLqJUelj//d/CYfPvdp0KLPc7LeJCGUuowhytyZTdaOLOo0qkNaw9SItStql6ICFy9d/zqfT1tU9ouOUoqThnXlupcvJ7X+wZuyRQhRc8KJO2Qmy4fczDy+ee973wEWgIaCnEIWv/9D9Q4sDHUapHLIkS2iFmABFOQGThkBgIai/BCuC0Naw1RaHXOIBFgHMK/Xy91nPsqCKV+Vm0nWWvPdnBXccMrdFObtfydlhRAHF9n47sPOjbsDz84AZozJlrXbqmlEtVPzw5tgWYFr+CWmJvgN9CzLYsWCX/ly+jekb8ukYYv69LvoNDr2Olr2Vx3kln3yM798tdrnfZbXYtNfW5n/2lecdV31ZfkXQohwSZDlQ2JqQtBrtNciMSX4dQeyvqNPYcrEt8vK9+zLMA0GXtYbR0zFt1lhfhF3D3mMX79aXa4szcK3v+XEIV24c+b1+11mehE5n73+ddn7wheN5tPXvpQgSwhRq8lyoQ+ND21Im46tUIb/2RRLa3qc070aR1X7pNZPYcILlwFUeK0M06BZ2yacd/tZPh/7/PjX+G3RHwBlH6Sly7Pfz/2RKbe9Xakx7dqczpzJC5jx2Gy+m7MCj9tTqXZEzdq1KT1gCR80pG/LrL4BCSFEJchMlh8X3X8ud575qM/7lKHoN+ZUGh/asJpHVfsMHNuHtMZ1mH7/LNb+9A8AcYmx9L+4F6PvG0FyWlKFx6Rvy2Th29/6XWrUlmbuy59z4T3DQ67n5y5288KEqcyfshA0KFNheSzqNEzl1jcncHy/DpV/kmHSWrN09nLmTF7A+l//IzbeSY+zuzN0wgCaHiYJU0NRv1ld1q/8L2Cgtb9kyxdCHLzkdGEAX0z/hufGvYqr0IXDYWJZGsuy6Df6VK575fKDcjnL6/Xy42cr+WvZWkyHSed+HTiiaxuUUuzemkFRvosGzesGPHX51Tvf8sgFk4L29eC8iXQb2CmkcT1+0Qt8+dZi9D7JSpVSGKbB04vv58juh4fUVlVorXl67EssmFp+uctwGDgcJg99crsUUQ7Bko+Wcd/ZT/q9XynF2McvZPiNg6txVEIIIQWiI6bvhadw0tCufPPed2xdt53E1AR6Dj/hoJ2NWL/yP+4Z9jg7/ttVUrdO88Y9M2nf/XDu+eAm6jetG1I7fk9tVrgu8OGDUpvWbOGLN7/xeZ/WGq010+99j0cW3BlSe1Xx2etfs2Dq1wDlZmEsj4Xb0twz7HHe3fRKRFNaHIhOGHw8x/Roz+/frakwm2U6DBq3asTAsb1raHRCCBEaCbKCSEiOZ8ClB/YP82KXm2/f/4HvPl5BUb6L1se0ZODYPjRp3ajsmp2bdnPjafdQWJK2Ye8A6O8f13Fz7/t4+ZcncMYGn907vMthQa9RhuLwzqGVyVk087uAm6Qtr8WPX6wkJyOXlLrRS2kB8P4z81BK4WuCWFuagtxCFr79LYOv7BfVcezvTIfJQ59M5Pmrp9hLy6XfWwWd+x3HTVPGHfQHT4QQtZ8EWQe5bf/u4JY+97P9350YhsKyND9+9iszn5jDVc9ezNCrBwAw5/n5FOYW+QxkvB6LTX9t4dv3f6D3qB5B+zykfXM6nHokq5b8heVjVstwGJx4ZpeQ60TmZuTZYw808aUhP6sgqkFWUYGLDb9vCniNYRj88f0aCbJCEJ8Uzy3TruayR0exavGfeL0W7bu1LRf8CyFEbSanCw9iXo+Xif0fYuem3QBlxZctr4W2NJOvmcry+b8A8OXeswk+KEPx9YylIfd9yxsTqNc4DWOfU4nKUDRp1YhrXhwbcluNWzXEG+gkGhAT66BOo+gmL933ufiiFBXqL4rA6jZO45QRJ9LrvJMlwBJC7Ffkp/0BoLiomE/+9wVXdbmVcxpewiVHXsfMx+eQm5kX8HHLPvmZLWu3+ZxNAjsYmPHYRwAU5AbOrq0tTV6Q/vbWsEV9Xv7lCS64ezgNW9bHGRdDk9aNuOSh85m8/JGwsrn3HtUDM0DgYjoMeo/qSXxiXMhtVoYzzkn7Ew4PGGx5PRadeh8b1XEIIYSoHWS5cD9XkFvIrX3v568V61DYe4Gyd+cy5fa3mfvSZzz9zX00bNnA52OXf/ozpsP0u8Hc8lqsWvwnRQUumrVpzD8rN/jcawT2Hprm7ZqGNfaUeslcePdwLrx7eFiP21dq/RSueHIMk6+daheP3muIhmmQWj+FMfeNqFIfoRpx05l+T8UZpkGdhqkHfX41IYQ4WMhM1n7ulZve5O+f/gFNuQBIW5rdW9J5+Pzn/D7W7fZQLiLxw+v2MPjKfugA13o9XgZd3jessUfS0AkDmPjWNeVOfhqmwcnDuvL8Dw+HvL+rqk4e1o2L7h8J2DNopZRSJKcl8eiCO0I6HCCEEGL/JzNZ+7HczDw+f2OR371SXo/F79+tYf3K/zisw6EV7m/bqTVfvOE79QEAChod0oCElAT6XXQqX89Yym+L/6iQiwrgzKtOp323tpV9KhHR6/wenHbeyfz3+yYKcwtpcljjGikiPerOs+l2RifmvvQ5637+h9jEWHqc1Z2+o08hqU5oyVWFEELs/yTI2o+t//U/PMWBy8YoBX98t8ZnkNX3wp68dtvbFBe68LUKqICzrhmEUooYZwwPfTKRt+5/n7kvf05+dgEADVrUY8RNQxhydf8IPKOqU0rR6uiWNT0M2hzXiutfuaKmhyGEEKIGSZC1HwtUW7GU1qAM36vCiamJ3Dnjeu496wlAlyUJVUqh0XQb1Llc8BQbH8ulj4ziwntHsHXddkyHQdM2jTFNMyLPRwghhDiQSJC1Hzu8c2viEmMpyncFvO64Xv7LuHQ/ozMvLH+E95+ey5IPl+N2uTnkyOYMGd+f/pf0KsnsXp4zNoZDj2pR5fHvzev18uOCX/n7p3+IcTroOrATrY89JKJ9CCGEENVJahfu5169ZTqznp7rc5+UYRocf3oHHpp3ew2MLHRrVqzjvnOeZNemdEyHidYay2vRsc8x3Dnj+qhnaRdCCCFCFU7cIacL93MXPTiSboPsIsqlSS5L8zS1OroFt74xocbGFoqt67dzc+/7SN+aAdinFEs38q/8+nduH/AQXm9oNQyFEEKI2kSWC/dzMc4Y7vvoFlYs+JX5ry1k2z87SGuUSt/Rp9LjnO61Pl3A+0/NpbioGMtbcSbO8lqsWbGeFfN/pfsZnat9bIX5RSx861u+eudbctJzad6uKYMu78vx/TqgVPD9cEIIIQ5uslwoatSQOmMoyCnwe79hGpw28iRum35NNY7KLoh902n3su3fHWVJXg2HgeWxOG3kSdw6fYJs+BeVlpuZx/wpX7HwrcW4ioo5omsbRt46LOJ7HYUQkRdO3CEzWaJGFeYFLtdjeS1yM/OraTQ2rTX3nvUEOzbuspO8liRhLS0/tGjmUg49uiXn335WtY5LHBjW/vwP1/e8C1dBcdltW/7exsK3vmXkbUO59OFRNTg6IUQkyZ4sUaMa+Sn5U8p0GDQ9rHqLAv/5w9+s/ekfvzUdtYYPn/sEjztwjjIh9lVU4KoQYO1txqOz+fzNRdU7KCFE1EiQVcO0dyfa8y/a8r9kdiAbPK5fwHxfXo/FwLF9qnFE8MtXq8sOEfiTvSuHTX9tqaYRiQPFx5MX+A2wSk2Z+HY1jUYIEW2yXFhDtGsxOncSeH4ruSUWHT8MlXwdyqhbo2OrTmeO78+imd+xfuV/PssDjbjpzOrP4K6x090Hu2y/3s0YmsK8Qr79YBk7NuwitX4KPc7pXiOlig4UX769OOg1GduyKMwvIj4xrhpGJISIJgmyaoAu/AidfRvlP8ldUDgLXbwU6s06aAKtuIRYnvz6Xl6/813mT/kKV4GdWLVhy/qMvHUoZ1zZL6R2tHcbeP4GnODsiFKV/4A6+uQj/C4Vlkqqk0jzdk0r3ce+LMvil4WrWPrRcooKXBxyZAv6XXRqjQY0C6Z+xeRrp1KU78KMMbE8Fi9eN5XhNw3h4gdHYvipJCD8K8oLnDi4VPaubAmyhDgAyOnCaqatXPTOEwF/P2xNSDgXI+XeahxV7VCYX8SWtduIcTpocUSzkD7EtXc7OudecH0NJRvUUUmoxMsg8UqUCj8Q0Foz9pgb2LRmq8/ZtUPbFzHukcYcd2orlKMVxJ2BMiqfMDVrVzZ3DHqEv39cX5aMVWuNYRpc99Ll9L+kV6Xbrqxv3vuOB0c+4/f+UXeczUUPjKzGER0Ybjv9AX764reg132c8ybxSfHVMCIhRLjCiTskyKpmuuAddM59lAUEPsWhGi2v0mzMwUB709HpZ4G1E/CRsDT+fIzUeyvV9ua/t3LDKXeTtSunLJt+fILmpuf+4+RBOWhMFKqkXycq9QFU/NDA49VFUPgJumgB6DxwtIX4c7nulLdZ89N637NnCh6ZfyfH9+tQqedRGVprxrSdwLZ/d/h9m8bEOpi59VWS05KqbVwHgr9WrGNCt4kBr2nXtQ0v/PBINY1ICBEuyfhei2nPf0Cw/EpF4N0Vnf6929GuJejiFWgdeANubacLpvgPsAAK30G7/65U280Pb8qrq57m4gfOo2X75qQ1rsP9b2Vw0sBcABRewIMdhbjQ2beiXf7322jvFvTugeiciVC8GNw/QeEsfvvkAv5cttbv8qRhGLzz8AeVeg6Vtf7X/9j2j/8AC8Dt8vD9xz9W36AOEEd0aUOX/h393m84DG549cpqHJEQIpokyKpuKpHAs1gljMSIdqu927Eyr0LvOgWdeQk6YxR658no/NeoymSm9mzEyn0ca/fZWOnDsXKfQ3u3R3DkfvrVGgrew2+ABYCJLqx8gJJaP4XzJg5jyu/PMHPjrRzbfTNK+XutFDrveT9jtdAZY8G7rfSWkr+9fLcgFdPh//W3vBarFv9Jfnb15QrLzcwLeo0yFHlZ1Zu/7EDxwMe3cvrFp1U4VVu3SRrPLH6A1sdIYXQhDhSy8b2aqbj+6PzJAa4wIKZzRDe+28tqI8DaRbkAT2ehcx8H7y5USuAlDJ/tFs5DZ98EZctmgHsVOv9VSJuMij0l9La0B1yLSpbS8sE8FJUwwt7z5FMx6JwgrVpgRSjgK/oMewbSX1BngXsl2rsTZTYsf1fxd+Bd5/NRrqLQyvMUF7lJrKY98E1aB89Lpi0d0nWiItNhctOUq7j8iQtZMf9XCvOKOOTI5hx98hFSrkmIA4wEWdVMxbRDx/YF10Jg3yUiBWhU0tUR7VPn/68kwPITIBS8jk44F+VoHXqb7jUlAda+z8EC3OjMq6HB5yizSfC2vOnozIvB8xd7AhnTXg5MuhaVNN7Ho5xAPBAoY7wBEQpWtc4ntLwOFWd3tGsJ9j+1islLWx1RhBWk/nVq/WRS6ld+Y324Gh/akONOO5rfFv/hc+O/UorUBil0HeB/2UsEl1I3md6jetT0MIQQUSTLhTVA1XkSYvuWfGVSFuuqOFTqM6jYEyLWl9YWFM4i+LLah+G1WzAd/0GHBtzoghkhjE+js8aDZ23JLd5yf+u859CFcyo8TikF8cMIvL/Ni4obGnQMoVBmK3wFSeXFguFrdsf/a9/77EyccdrvMqRhKAaPO73a6ySOn3QJsQnOCklZlaFAwY2vjcN0SO1GIYQIRIKsGqBUPEba86j6n9qzVokXoVIeRjX4DhU/MLKd6QL7JFvgi/baLxQi12ICB25WyTVBuFeC++cAbSl03ss+942ppLEle9x8fdgrO5CNOTb4GEIRNxBUPP4DSxPih6GMhIojiemAvwAtMcXi5uc2gsJnQHP48Ydx7q1DqzT0yjj0qBY8/8MjdB3QsdwS1hFd2vD4F3fT/YzO1T4mIYTY38hyYQ1SjjaQ1CaURagqdBKPvbQW6CShqsSyWpA1rhCv0a5FBN7rpMG7HqxtYJZP/qnMZlBvBjrrRvD8udc9JsQPR6XcGbE9LspIhJSH0dk3YP9usvcymglmE1TStb4fHNcPctJAZ1NxeRV6nJHD0591YeZzmmWf/oy2NGmN6zDkqv6cfcMZxCXERuQ5hOuQ9s154OPbyNieya7NGaTWT6bxoQ2DP1AIIQQgQdYBTykTHXcmFH2E/0DGi4ofEl7DMV3A9VmANk1wdguhITeh7XXyHSQqRxuoNxs8q8D9J6hYcPZAmfXKP1xr8KwBKxPMpihH+Ce4VPwgMNLQeS+AuzR9QRzEn4VKvsbvYQWlnJD2kr3vTBez5zWz9+AReypH9bqBB3rHUOxy4y4qJiElodZsgq7bOI26jdNqehhCCLHfkSDrIKCSrkC7FoAupGJQpCC2Hyrm6PDaTByNdn0a4AqNSjgveDuOI9HB9jqplAqzWOXuVspeFvSzNKiLvi45Rbl+z20xnVApd6Bijgk6xnJ9xZ6Iij0RbWWAlQdmw5CSxipnJ6g3197LVjTP/l6YrVEJF0D8mShl/1N0xsbgjI0Ja0xCCCFqJ8n4fpDQ7j/sZTXvespmUDAg/hxUyt32bEu4beZPQec+RvnlPhOwUCmPoBLOCt6GLkbv7OF3KQ0MSLwMI/mmsMcHoIsWoLNKl/H2fqsbQAyq3jthB1pCCCEOXlJWR/iktbY3mXvWALEQ2xNlNqham8U/ovOng3s5oOylusQLw5oZ08Ur0BmXYG8O33spDYjpgKr7BkqFX8dtTwCX6ecKA2I6YNSbGXbbQgghDk7hxB2yXHgQUUqBs7P9J1JtOo9HOY+vYhtdoP5H6Lyp4PoEdBGYzVEJ50PCqMrXcHQtDhBggZ1A9Be05z+U49DK9SGEEEL4IUGWiAitXeD+A/CAox3KCG9WUTnaoOo8DDyM1joym769W6l4EtDPdRJkCSGEiDAJskSVaO2B/JfQ+W/sVebGiY4fCkk3odwr0IWz7ULORhNUwtn2kqLyn6ItYqfqjDSCBlgARp2wmtXaCxi15vSfEEKI2kmCrAOItjKh8EN00VdAMcQci0o4z05zEI3+tEZn3wZFcym/qbwYCt+HwnloCtgzm7TaPuXoPMWubViJzfZhiT0NiAOK/FygwDwEHO2DNqWtAiiYji54x87ZRTw6bhAq6bKwyhEJIYQ4eMjG9wOEdq9CZ1wMOpc9AU/JSb/kO1GJF0a+z+IV6IxRlXikgoQLMVLujNxYtAWub9CFM8HzHxh1UPFD0N50yJ/kewyAqjMZFdcncNtWHjrjgpLaivskISUGVXeanaJhP6CtPCh4F104A7w7wEi183wljK7yIQghhDgYyOnCg4y28tC7epUs1/leHlNpb0S0JiKAlXUrFH1MaNnf9+VENfwu4N4t7Vln11T0bgejLip+iM90C1q70VnXg+tz9qSTKElTYbaE2AFQ8Ab2jFbJrJqqY6euiD8j+PPMeQgKpuM3xYRRD9Xgm7JcV7WVtjLtoNjzDxWCRaMOqu67cgBACCGCCCfuiGrtwsWLFzN48GCaNm2KUorZs2eXu19rzd13302TJk2Ij4+nT58+rF271ndjwr+iuaCz8L//yETnT4l8v96NVC7AAigG9y8+79Hawsp+AL17IOS/DkWfQsHb6PSzsTKvRe+T/V3nvQiuL0oHVXpryZdbwL3MDuhSn0Al34aq8wKq4ZKQAiytC6HwPfy/thZYu8D1ddC2aprOeRg8/1LxuXjBykJn3VATwxJCiANWVIOs/Px8OnTowOTJk33e//jjjzNp0iRefvllli1bRmJiIqeffjpFRf720AhftGspgUvTeKH4O59FlqvEqEuV3kLaT4CW/z8onF7yhRc7KCi51rXADhZKm9DFJbNM/p6bF9y/gvdfeyYs8SJUXL/Q94N5NpVkyg/EgXb/EVp7NURbGXam+QCllfCsRrtXVeewhBDigBbV9Y0BAwYwYMAAn/dprXn22We58847GTLErpv35ptv0qhRI2bPns3IkSN9Ps7lcuFyucq+zsnJ8XndwcWL/yCjVORXhVX8meiyGaRwGRBzVIVbtXah818L8DgNhe+hkybY9Qk9a/c61RigL9cPECCzu3Z9jy6YBsU/gNbg7IJKvBjM5iE8Fx2xTfz2yUUV8PRlpbj/IvisowL3qoCvkxBCiNBFdSYrkH///Zft27fTp8+eTcepqal069aN77//3u/jHnnkEVJTU8v+tGjRojqGW6upmOMI/K00IOaYyKcciO0NjmOw90GFw4TY01Fmo4p3Ff8SQtDkgeJvS/47lOCxtIyQbzp/KjpzTEny0kKgyJ75y7wEXTTf3tcVkBdiTwlhHH761xa68EOs3UPQO9qjdxyJlXEp2uX/30HYQtovpqmNB461LkS716A9/9gHHIQQYj9RY0HW9u3bAWjUqPwHbaNGjcru82XixIlkZ2eX/dm0aVNUx7lfSBgOxOB/ydCyZ2UiTCkHqu5UiO1ResueMZhtwGhE+bdYyf3moajUe/20GuJSsS6ZzXS0AZUY5GIvOLv4bsb9Ozr30T3X7f0YgLxn7I3zfpngPAEVc2QIg/bRv9bo7NvtVBieNSW3WiVB3hg7ZUQkxBwTwuukIPakkJvUVoFdE9O9tmQGLrK0lYeV8xB6Z3d0+mD07v7o3b3RBTMiv/QthBBRUPt+bQ0iNjaW2NjYmh5GraKMulDnOXTW1dizEXsXa/ZC/IUQ27/K/WhtlWywj0MZCSV9p6LS/of2/AuupXZ/MR3sPzqnJF3A+2Clg9EIlTAC4kegjCTfnTgOJ9jMk33dEXb/Kg6dcD7kT8H35nTTbjPmON/PKf8tyhe49vF472ZIHA/5kylNi2EHj16IORpV57nAYw2k6FMo+rDki73Hb49H59wHzpNRjmCzaYEpFY9OuAjyX8T3a2tAbD+U2SxoW9rKR+c9ax8IKN2vZjSCxLGQcGFEZky1LkRnjAbPH5R7Xbxb0Dl3g3cLKvnGKvcjhBDRVGNBVuPGjQHYsWMHTZo0Kbt9x44dHHfccTU0qpqjtQu8mwCnXbcvzD05Kq4X1P8Ynf8muL4EXWwvESaMhthTq/TBp3Uh5L+GLngbrAz7NucJqMRxqNjudv+OVuBotc+gUiHpSlTSlaE/D7Mp2nlKyXKgr8DHBMdhEHPsnsckXWtvPC9eSvkyOgqMBqg6z/t//u5f/PRTyt44b6Q9g44fjC6YBd4NoJJR8QODZq8PRhdMJ3DpHwNd8C4q5dZK91FKJY1HezeWJI8tDSxL/o7piEp9OHADgNZF6Iwx4FldfszWDnTug+DZgEq9q8pjpeBt8PyO32A7/xV03JmomLZV70sIIaKkxoKsVq1a0bhxYxYuXFgWVOXk5LBs2TLGjRtXU8OqdloXovNegIJ3QefZN5rNIfFyiD83rOBIOdqgUu8H7o/s+DJG2xui9/5QLV6OLv4BUh9HxQ+JYH8a4s8C9/KSWZK9P2RNUAmo1KfKvS5KOSHtVShagC541w6CjDqo+KEQPxxlpPrvMJS9SiXXKEfriAQ75bj3mampwFsS0FSdUg5IfRISRtmzi95NYNS3v3/OHigVwt66gpngWYXf4KdwOjrhLJSPQw3h0AVv++8DABNdOAsVc3uV+hFCiGiKapCVl5fHunXryr7+999/+fXXX6lbty4tW7bkuuuu48EHH6Rt27a0atWKu+66i6ZNmzJ06NBoDqvW0NplZ2l3/4rvJZFNqOSba2p4tvwpFQMsoGw5K/t2iO2JMtKq3JX27kRnjiv5EN/3Az/GzkyeONbn0plSDog/I6TcV+XEngaedQTKMUZsr/DaDIdygg60D00BkVseV0qBs1OlM9QH3yNmogtmoVIrH2RprUuKewfiBc+GSvchhBDVIaob33/88Uc6duxIx44dAbjhhhvo2LEjd999NwC33HILEyZM4PLLL6dLly7k5eWxYMEC4uLiojms2qNgZsly1b4f8CW/wee/inb/Vd2j2jMKbZXMKASaafFA4UcR6MuNzry4ZA8OlE9LUVICJ3FMlfcm7UslnI//QwMKMFEJlSkdFKLY3gQ+nalRcb2j13+4vJsIPMPkBe9/VepCKQUqIchVJhjJVepHCCGiLapB1qmnnmqfntrnz7Rp0wD7h+n999/P9u3bKSoq4ssvv+Twww+P5pBqlZBmBQrfq5ax+KRz7A3rARloz7og14TA9ZWd88rn/igNWOi8qX4frq0MdN4rWBmXYGVcjM572a5bGIQym6DSXsEuJL13oGUATlTa5IgHduX6T7yIcqcyyzHBqAdxg6PWf9hUsMDGsPfiVVXcYAIHn15U3KCq9yOEEFFUYykcBCVlaYLMCnj+qa7RVKRiCZxJHvt+VfWZR120gMBvRy+4PvX9WNdS9M5T0XnPQPESKF6KznsWvetUtGtx0L5V7Imohl+jkm4E58ngPAmVdC2qwSJUFfJfhULFtC85nVg6m6Yoex2MunbNSX8nMWtC/BACBz8WKr7qwY9KvNReSvX5njDt/GyxPavcjxBCRNN+l8LhgKISQWcHuMCAQJu2g9DaDUVz0QUz7P0rRqq9yTnhPDvtQ7DhqXi080Q7C7rfE3geVGzfSo+xjJVP4GVJQBeitS636V17t6IzrwSKKR+wWkAxOvMqqP9p0NkoZdSFpMtRXF7JJ1B5Kq4vNFwMhR+ii38DZaJie0DcQFQEAthIUolj7E3zupCK7wkTHO0isodNOQ6BtDfttCTWDuwfVSXpSZxdUXWeDW2jvhBC1CAJsmpS/GD7VKHfAMZCxQ2sVNNau9CZY0sCpJIUAd5MdN7z9vH4uu+gHIcGbUcljUNnfOfnXhMcR4Gze6XGWI6jdYC0DWAnMT2kwmlLe8nVje8ZQftDOVIpEKJJGXUh8bLg+UJrmDKbQd237ODHuxl7VsteziWmCyrtWfsQQiT6cnaABl+D6xu0e7V9ijT2lEonfhVCiOqm9H6eOjknJ4fU1FSys7NJSUmp6eGERXs2otPPLDldtu8sjp0PStX7CKViwm7byn0c8qf6aLekbbMlxJ8POhvlaA6x/csSjFYYZ+FcdPZE7GDGwF7S8oDjWFTd/4U0KxaM9vyL3n16gCsUKvkOVOLocrdauweV7OUKwGyF0eCzKo9R7KG1Zeclc68CHBDbAxXTvqaHJYQQURdO3CFBVg3TxSvRWePB2km5JZGYTqg6L6DM+uG3qQvRO08EnR/C1Q7AQ1ng5TweFT/CnkXYu00rCwrnoD1/g4pHxfYBZ7eI1kPUeS/Z+6oqZHw3IKYzqu7rFQoxW7v6BT/NZjbHaPBVxMYZKXaqgvVg5doJaM0GNT0kIYQQQYQTd8hyYQ2zl0QWgetrtHuVPWvl7Akxx1Y+gPH8E2KABXaABfbR+3+hcAO6cBY6/lxUyn1l2cyVUQcSxwTdBl8VKmkcmC3Q+S+D5++SG+tAwihU0pUVAiwAYjqVpBUIUBYnpnI5oaJJF32Gzn0GvKUHGxQ69jRU8kR7P5IQQoj9ngRZtYBSDojra2+AjkyLVXhsyfJi4UxwHAKJl0VkRKFS8WdA3CB7Zk+7wWwUcLlUJY5Cl9X+88WLSrgg8gMFtJVZUpOxblhLprrgfXTO7ZT/Pml771Hxz1Dv/aimjahttGednflexdjFto065e/X2p6t1MVgtgAVD8XL0EWf2gdHzENR8WcfVK+ZEGL/IEHWgcjRxp4B0llVakbnT4GEMZXaE1YVSikwG4V2bcwxkHwrOvcxyhd6tv9bJd2Ich4X0fFp99/ovKfB9TX2sqZCO3ugkm8IuilbW3nonAdKv9rnXi/oXHTuk6i0SREdc6TYe7G+Rbu+AyxUTEeI6+N7ljFYW56N6OzbwP3jXrfGoONHlhxUiIHCD9D5L5XMVgLE2iduy5bXLUDZs59JEyBxfESXsIUQoiokyDoAKeWExIvRec8SOA9XEFa6vak8Qqe5tC4EzyY7/5GPk4JBH29lQ/GPgBscR6EcLYCSnEqOI9EF0+zTlFrb+8USL0LFnhSRsZeNwf0HOv08yqeM0HZurvRlUPdNlLOj/waKFgCFAXrwgutztJVVYUanpmnPRvvEqvdfSn90aN6A3AaQ9hJqr6LdQdvy7kRnjAQrc5973FD4FtraCY62kP/CPve7SgIs2LPUXdJm3iSU0QQSzg7reQkhRLRIkHWgSrzcDpCK5rFnhmffDeUh0O4qD0VbeXbAVzCLsgDDbAGJ4yD+7KDBltbF6JzH7CVMiktuLZk9Sn0IZTZCxZ6Aij2hymMNRmffCbjwXctR27Uc63/q9zlp7yb2HDbwxwLvdqhFQZa28tEZF/oOcKx0dMZFUH8eymwaWnv5r5cEWH4y/Ls+s/+EO878FyF+WNleQiGEqEnykygIrT12yRbtqumhhEUpE5X6FCrtdYjtC44jIKYrEKwm3N7iwHFYlcahrQJ0xig7N9feMzjezeic29F5gZfFtNborGuh8G32BFhQNnuUMdI++VgNtHsNeFbjP2mqZZ8WdP/qtw1lpAZ4/F5qUYAFQNHHYG3Dd1Bk2YliC94Ovb3C9/20VcpfqaEgvJtKZtqEEKLmSZDlh7YysHIeRu/sgt7ZHb3jOKzMCWj3nzU9tJAppVCxJ2GkTcKo/zFGvemoOk9if9uDfesNSDi7yiVddMHr4PmLih+opUWwJ6M9AT4Ui5eBayG+AxMveLdBwVtVGmPIQi18HOi6oMllDYjpiDIbhzio6qGL5hM46PFC4bzQ2tLeIJUOwH5/VHKpez/7hUgIceCSIMsH7d2NTj8bCqbvlQrBC64v0enD0cXLa3R8VaHi+qDSpkLMMf6usP84jrRr+VWS1l503ouQN4nAH5YmunCW/3YKPyRYrTxdUE1FtFWIAWeA65TZ2E4C6zNgUSUPvy7soUWdlUfQoEcXhNSUUqZ9MCPwVVTulGysne9NCCFqAQmyfNC5j9l7YirMvngBDzrrBrQOtKemdlOxJ2LUm4Vq8DXUeRUSRoFRH3CC2crO1VTv7UrPYmlt700KbeO9ZddV9Hv3DgIvKwHW7vAGWFnOLqCC1JJUCRB7cuBLUm6HhNHYwaOiLIhUqag6z1fL3rKwOQ4ncLBr2KdaQ5UwPEh7lZnJMiH+rNpVUFsIcVCTje/70FYWFH1CoHqCWDvBtRjiql4ItyYps5ldiy7uFEi5J3INu3+Boo9CvNgAIznA3Q0pn5rB1zX1whhc5SnlhKQJ6NwH/V+TOA6l4oO040Cl3IFOvBxcX9gZ3x2HQuxplUqFUB1Uwsgg+cgsVMKoMNq7BF34cUmAvO/3VkHsGWBtBvdvPu73xQBHK1TyDSGPQQghok1msvbl2UDgk18A5p6M5KICXfg+gWcp9uYNWARbxQ8j8IesAfHDwxhdFSVciEq6Hvv3E1XytwGYkHiVfaozRMpsgEo4H5V0BSru9FobYAF2rrGEi0u/2vde+3BF3IDQ2zProeq956O4eBwkXoqq8xgq7VVwnlhyu0HZ74QqDZwnAyX524x6kDgOVfe9koMFQghRO8hM1r6CzELYdIjXVZ22cqBwNtrzF6hYVGwvcJ5Uu4+oezYQ2uyDae8NcwZYXnOeAM5ToXgxPotoGw1RidHJ6O6LUgqSxkHCuVA4D23tQBkNIG7QAV97UCXfBo626PxX95zgMxqhEsdAwkX2Xqtw2jOboOq+jvZsBM+fgBOcXfYs96kUVN0paPdf4FqE1i472WvsaSjlsBOjUgzESgJSIUStJEHWvhxtwGwG3q343xOiITb6S4W66DN01k3YHyQGoOxj8o52kPYaKsSs6NXOqIc93iCpCpzdUXWe9Rsw2uVUtkDyDZDfEIo+pNwso7MrKvXRsEraRIoy6kLi6KjWcqwpWnuh+FtwrwUVB3G97KVlpSDhHIg/G6xdgAVGg7CDq30pR0sIUBJHxRwBMUdUnD9TBhBXpb6FECKalNa6CinBa1441bBDpQs+ROfc5udeBUYTMJuC2dhezorCzJIuXonOOBffG4BNcByGqjenyh9w0aCLvkBnjQ9whYK4szHqPOy/jcKP7dOJpQWUVSLEnQnOTigMiDka5WgV2YELdPGP6KwbwNqOveRbEijHnYlKfQClJKgRQhzcwok7ZCbLB5VwFli70HnPlN5S8red1RtrO1hbwW2ii+aBsyekTUap2IiNQee/UtKvn/xQnr/B9U3t3Hwfexo4jgXP71RcNjTtZaDk6/w+XOe9VPLa7zV3ofPtjO/u36Du2ygjnKSqIhTa/Zedub1stnCv713RXLQuQKVNroGRCSHE/qkWb+ypWSrpClSDRaikayB+aMnx9NIP/dLAp+RDqHgJOsf/rEy4tPaWFB8OtK/JRLu+jFifkaSUA1V3KsT2LL2Fsrea4zBUvXdQZkOfj9WejSWpH6DiDJ5l790peDPygw6B1i504Vys3CfReS/UWGJarT3oooVYuc+i8563Zz0jMCGt817Cfs/5CuwtcH2Bdq+ucj9CCHGwkJmsAJTZGJKuAisDvfNk/O/RsqDwfXTydSgjLQI9ewi+cVyDDlRouGYpIwWV9oqdzd21FPBAzLF2NvMAm5TtxKQGgVJo6IJ3UElXRmHU/mnXEnTW9SWZyh1oNORNsusn1nkWFSgNRSTH4V6FzhxfspxXMg6eh5jjoM6LKLN+5drVLnB9TtDAvnAeKuboSvUhhBAHG5nJCkXxCoKndXCXXFd1SsWCEbzQrnIcHpH+IkFrjfZuQ3v+K1fnUTlaoRIvQCVehHJ2Cn4KzPMfQTfMW9vREShcHSrtXo3OvBx0TsktewXBxd+hM6+KyExS0HF4NqMzRu9TpLlkHO5V6MwxaF3s7+FBGs8npBOhQcvhCCGEKCVBVihCzu4eStqC0KjECwlcVkTZp7xqAV34CTr9DPSuU9C7+6F3dsfKeRRt5YXfmJFM8LdlDNU5CavzXsZ/BnIvuJeB+8foj6PgDdBF+N+ntxaKKrmErFLswwWBR4Aym1eufSGEOAhJkBUKv3X+9qbAEcFllIQLIaYrFQMt+1umUu71u6+pOun819DZ14Nn3d43QsE0dMYFaCu0enalVFx/gi1ZETew2vIiaV1cUqA60JgcJQWUo6zw4yDjMNBFn1SqaaUcJUldg5S6iT+rUu0LIcTBSIKsEChHS/sEod8PIBOcPVGOFpHrUzlRdafY2cWNvZJcxnRBpU1FJZwbsb4qS3u3oHOfKP1qn3st8PwFBdPCa9R5sn0y0edrbWdWV4ljwx1q5ekiQtofV5lZu7DHkh/kAgt0bqWbV0lXgtEIf+9zlXQdymxiD0VrtC6s/PKkEEIcBGTje4hU6kPojPNKkpTuvVxjgNkUlRq504VlfSonJF1pl2rROYCzxlIXaCvfPsbvWgJ4UDEd0N5M7Jk2/wcCdMHbqKSrQu5HKQPqvorOvMZehisrouwpKaA8CRVTjXvRVBKoOqCzAlykqydnl9kSvOvx/3qbYLaudPPKqAv13kPnPgpF8ykLLo0mqKTxqIQRdpb1wpno/GllWd91zPGoxLGouNMq3bcQQhyIJBlpGLSVDQXvoAtm2YVtzQao+OGQcD7KiG7fNUm7/0BnXAx676DKYM+HfeC3kGq0qlI5xLR7Nbi+tsupOI6EuD41Ut/Pyn0G8l/B/4Z8w073YTaO6jh0/lvo3AcI9HqrerPt0jNV7cvKsMsjqThwtEMpA60tdPaNJQXU9w6u7ez+KnkiKvFi/40KIcQBIJy4Q4IsEZC2ctG7+pScKgty6s8nB6rR6tpdazEIbeXZ2fc9/1B+6dAONFTy7ajEi6I/Dl1sJwt1/0z570VJwJNwCUaKv0oFEei/8GN09k0BrlCo+p+iHIdFbQxCCFHTwok79t9PvlpAe3eh817EyrgAK30UVu5zaO/2mh5WZBV+VLJUVpkAy4S4/vt1gAWgjCRU3Xch4Xxgr8LgjraoOs9VS4AFpfv0pkLipfYyZimzKSrlPlTyrVHtXxdMJ/CPDANdMDOqYxBCiP2JzGRVknYtQWeOA9zsCUBKNmbXeRYV17faxhJNVsYlULyUYEuCFSnAgar3PiqmfRRGVjO0LrT35ak4MJpW2ynHiuMoBu9GIAbMFtUSyFrbjwWKAl/k7IZRd3rUxyKEEDVFZrKiTHu3lgRYxZSf4bEADzrrWrTnn5oZXMQVEzzAKn0bmZSdpVApqLT/HVABFoBS8SjHYSizWY0FWPY4nChHG5TjkOqbKQy6H04BUkBaCCFKSZBVCbpgBvYMlq/gw05aqQvert5BRUuMv3QKpQyI6Yyq9wEkXgmJF6NSn0I1XIqKPam6RimqQ9zpBMujdaDM4AohRCRICofKcC0i8B6l0gLPd1XPeKJIxY9E508JcIWFShyNijkGFVLS1uqhi1faGdJd3wEanF1RiWNQzuNremj7LZV4MbpwNvZ7f99fMEww6kHcGdU/MCGEqKVkJqtSQqnxFmopntpNOVqiUu7DXgraexaj5K0Tfx7E9vP7eK2L0cU/oV0/2GkBqoEumIHOGGHnetIZduoJ15fojPPt/E6iUpSjDSrtJXs/Wtn7oeQ9YTRC1X2zxvK4CSFEbSQzWZUR09nHcf69mXAAzZiohJHgOAydPxVciwELYo5BJYyGuEE+9yZpbUH+K/ZjyooKm+i4gaiUO+zEl1Gg3WvQOfdgz7Ts/f2x/1vnPgwxHVHODlHp/0CnYntCgyVQOBvt/g2UA+XsUWM5zIQQojaTIKsSVML56MJAR9W9qIQLq2081UE5u6CcXQC7pEqwTd865x6o8Bp5oehTtHsV1Hs/KglcdcE72LNs/gNgXfBWjQdZ2rsVin8BFDg7o8xGNTqecCgjGRIvDFi+XAghhCwXVoqKOQKVcnfJV3svodn/rZJuRjk7Vvu4qkvQAMu92keAVcprpx4oiNIx/+IVBF7O9ULx8uj0HQJtZWJljkfvOg2dfT06+zr0rlOwsm5AV0f9QyGEENVGZrIqSSWMAscR9h6f4u/sG51dUQljULHda3RsNU0XfoAdcPoLdix0wQxU0vjId64CnX4L45oo0LoQnXEhePatP2hB0Sdo1yK0eSg4DkcljEQ5j6uRcQohhIgMCbKqQDk7o5yda3oYtY9nK0EPB1g7Q1p2DFtsT/Csxf/pTxNiT4lsn6Eq/Ag8f/u5U4POA89q8PyJLvoQnTAalXxHjebjEkIIUXmyXCgiz0wjcD4lQCVHJXhQ8eeV9O2rbfs2lXBBxPsNhS6Yhe9x7askQC14M8CyqxBCiNpOgqwDmPbuxMp9FmtXL6wdXbHSR6ALP0JHOb2EihtM4JksA2JPIRoVnZSjOarOC0AM5d/ee5U8qqkCxtYuwitPpND5r0XldRJCCBF9slx4gNLuv9EZF4DOoWzpzP0bOvtXKJwHaS9F78i98wT7T/EyfC/bWVA0F+1ZAyn3RjxBqIo7DRp8aRcrLq276OyGSjgPZTaLaF9hMRqGGWhp+5CAtQ3MptEcmRBCiCiQAtG1jPZsQhe8CYUfg84H8xBU4vkQPzzkoEhrC727r13I2OeMkoLEqzCSr43o2MuNwcpH59wJRZ/iP6gomV2q+1aNnMbUVr4dhBp1UCo+sm171tuHIormgy4CRxtwtIWiOWG3pep/iXK0jMy4tAXF36FdX4EuRsUcCXFnooykiLQvhBAHunDiDgmyahHt/g2dMRq0iz3BUckenpjjUXWnoFTwArzatRideVngi1SqXV8wygkkLfd6SB+CXWjaFwNiOmDU87/3SHs2g+d3wAnO4+08TVWgPevQuc+D6zPsmTaHnVQ1aUJEghnt+g6deTn297D0+2iU9JUIFBK4LNNejHqoBt+iVNUnnbV3h/2+8KxhzyS2F4hD1XkaFde7yn0IIcSBLpy4Q/Zk1RJae9CZV9mzHuVmn+yC07h/QudNDq2t4l8IuhKss+2lqChTnj/wH2ABWOD+Be2pOBbt3Y6VcRl6d2901gR01hXonSdi5TyC1oHa9E+7f0ennw2uz9kT6HigaB46fRjas65S7Za1bxWgsyZgFxDf+/tY2lcBmIcT2j89hUoYHZkAS3vQGRdD2fPzlPzRQBE662o7v5kQQoiIkSCrtnB9DdZO/M9wWFDwbmjBhc4npPqK1fHtt3YQ9KRh2XV7aCsTnX7enj1VZVxQMA2ddVPYG8K11ujsW/eZKSzlBV2Azq5iUe+iT0Dn7jPmcqOwA9z6X6HqPA9JtwAJ+KwL6TwFEoPMSIbK9TV41+H7fWGPVee9Gpm+hBBCALLxvdbQ7lXY344AJ/90Dng3g6O177utLHT2neD6gqCbq41GYB5S2eGGzqhPSAGf0aDclzr/TXvDt8+gU4NrAbh/Cq9GpPu3AHmqsMfp/gntWV/pE4ghfR+tbaBiUXGnowAdPwhd8BYUzrUDZEdrO9lt3BkRmcUC0EVfEDhBrNcuoh2N3GVCCHGQkiCr1jAJ7dSZ72/Znmzi60JrJ+FCVHVkPo/tA8QBRX4uMMBxFMpxaPmbC2cReN+SiS78MLyTid71oV3nWQ+VTfMQ6muaOQ5ddwrKSEaZTVDJN0PyzZXrMxQ6lH1gpUuc8mNBCCEiQZYLawkV25PAMz4KzBZgNvd9d+Gckg3NoSwTAnnPY+Xci7ZywxtomJSRhEq+3t+9gEIl31LxLis9SMteKPoKXfxjGINJCPG6xNDb3Pehzp4EnMUq5VmFzr6p0v2EzdGGwIlQFZgtIzZzJoQQQoKs2iPmOIg5Fv/7lzQqcSxK+f6W6cL3CS2beCkXFMxEZ5wf/cLECRehku8Ctc8pDLMZKu01VGy3io8x0oK3qzPRGaNKMqmHwHky9qxaAColvCXIfcX2BLMVwfehecH1NdoT4uxaFamEESFcUzOZ8IUQ4kAlQVYtoZRC1XkJHK1Kbin91pR8WCdcCvHn+m/Au5PwsokDeO06fwVvhvm48CilUIkXohp+h6rzMirlUTs3Vv0vUbEn+X5Q/DkEf3vaJy91zl1oz6bg4zCSIPGSwNckXYlSsUHb8vt4ZaLSXivZixaMAa5vKt1XOJTZBJVSuql/39dVgbMbJJxfLWMRQoiDhQRZtYgyG6DqzUalPguxvcHZHeLPRdWbjZFya+ANyWYjwpvJKmWhC96p5IjL09pCF69AF85Du5ahdfmlS6WcqLheqISzUM6ufmflAFTCmJLN8KHtcdKFM0K6TiVNgPgLsV8rE3v/kWF/nXi5HcxWkXK0gDrPhHIlVDIVRWWohFGotP9BzF6JX40GqKTr7BnFKOdME0KIg41swKhllHJC/EBU/MDwHhc/HO1eWblOrZ1o7UapmMo9HtBFC9E5D4C1dc+NRmNIuR0V1z/s9pRZD+rNQGfdCu7lQa62wL0qtHaViUq9C504xi7tY+1CGY0gfijKbBL2OP3242iHxkngHGFeiDkqYn2GQsWeioo9tWSJuBhUnYDBrhBCiMqTn64Hivgh4DiSUGd+youlKvG2LlqIzrqqJOXCXqzt6Kxr0EXzK9WuMpth1HsrhA3rCghvFkY5WqKSxmOk3ItKGhfRAAuws9LHD8P/98MAsxk4/SyXRpkyklBGXQmwhBAiiuQnbARpXYwu/BQr91l03stVzh4eDqViUXXfhLgBlP+2BvsWmxA3uNK5kbS20LkPln7l+5qchyosHYYltg/BgkcVd1rl248SlXxzSSqIfb8HJqg4VJ1JEQlytNZhJ2YF0O4/sXKfsk+Z5k9DW5lVHosQQog9pHZhhGjXUnTWDaAzsWeFNOCF2F6o1CertQCv9u4E9y+AQjuOg+xrwL2SiukdDCAWVf8jlJ8Ep0H7Kv4RnRF8w7RKm4aKPbFyfZSWwiktMVSOYddhbLCwVhY51lYeFExHF7wL1nZ7Vi5uCCrxEpSj8slgtdZQ9Am64A1wrwYMiD0ZlXgpytk1yGML0Vk3gutL7OBVUZofS6XchUoYWelxCSHEgU4KRFcz7f4DnT6cPbXg9maA8wRU2tQay6StrVw7ACz+xh4PBuABo5E9m+LsGKSFAG0XzUdnXRv0OpX6OCp+aOX7KfwUnX0zdjBgYQcGGoy6qLTXUTHtK912ddHaitzMVc69UPguewpPgx0wWaiU+wIGSlbmNfvUbixP1ZmMiutb5XEKIcSBKJy4Qza+R4DOexH7A8tXvGrZ9ffcv4CzUzWPzKaMZFTdV9HuteBaBLjs/VuxPauefHKfcjhVvs4PFT8QnF2gcBa6eCWoGHD2gPi+oOpUqe3qErH9T67PSwIsKB8o2TOVOuceO7D3MVOmPevtkkT+R4nOmwSxfaS8jhBCVJEEWVWkdTG4FhI407oDXfQpqoaCrFIqpi3EtI1sozGdwGhasundz6So0cDOw1RFymwASVeBlYfOnwr5z0LuXWhi0HEDUYmX28/xAKfzp1N+BmtfBrpgBirl1op3FX0Z5LHarhzg3QIOP9UFhBBChEQ2vleVLiR4KRsNUS5fU1OUMlApd5R+5fua5NsjV+jYykFnnAv5L+5VescNRfPQ6Wehi1f4fpzWaM8mtPsvtJUTkbHUGM9qAtch9NrFsH3QuoCQ/tnrgsqMTAghxF4kyKoqlQwqNchFukqbnGs7FdcXVecFOy/W3oyGqNRnUfGDItaXzn0aPP9QMcjwAm501nVo7S7/mKKF6PQz0bt723/v7I6VdTPauyNi46pewQJWBX4SiyrHYQSvregEs2llBiaEEGIvslxYRUoZ6IRzIf81As4uxJ9VbWOqCSqur52lvngFWDvtsjLOrihVmbxdvmkrHwo/wP/MoQXWLnB9DXH97McUfIDOmUj5WTaPPfNVvAzqvY8yG0ZsjNUitjcUfUygGVQV6yelRdzpkHMf6Dx8L++aED+kVp7UFOJAllmcybKMFeS586gXW5eudbuS6AixqL2otSTIigCVeDm66AvwbqT8B599Ak4l34wyG/t59IFDKQN8FXuOFO8mwBXkIgfa/Rcqrp+9tJhzb8nt+wYUXrB2ofMmoVIfZH+iEi9CF31M2QnLcky7yHX8MN+PVbGQ+oSdPBaD8u9XE8wmqKQbojJuIURFlrZ4b9P7fLb9CzQaQxlY2uLtDTMY2XIEfRr1qukhiiqQ5cIIUEYKqt5MiB+OnT29hNkKlfo0KrHq9fAqS2tvpRJV1kohFW629hR4LppH0LI2hbPR1v61/0jFtEfVeZY9dRehbKZOpaDqvm5nnPf3+LheqLpv27Uxy26Mh4TzUfXet0saCSGqxYebZzN/+2dYWGg0Xu1Fo3FrN9M3vM3S3d/V9BBFFchMVoQoow4q9X508q32ySwVB2aLGjkGr7ULCt6yCz97NwGx6Lj+qMTLUDHtqn08EWMeCmbLkhlDfyyItX/z054N2LmjAu1BKraXGI39a8+cijsdGnxjp7RwrwQcqNiTIe5MlBF8iUE5O6Pqvo62ckHn2/nGpEC0OMjle/JZsvs7/sz5C4B2yYfTo8FJJDmis3ye78ln/vZAKVXgg80fcUK97hhSAmu/JEFWhCkjEYzDa6x/rYvQGReD+2f2LCW57D1IRfMh7RVUbGj18rR7NRT/CsoE54kR3byv3avA/Ye9Qdt5Ukj7opRSkDiuZI+VLwY4e5SlcVBGCtpfWolyDe+f+4+UWR+Sxvk50xliG0Yy4H/WS4iDxV85a3jm7+dwWcVlPzd+zVrJh1tmc13bCRyVemTE+/wlayWeICXH0osz+C9/A62TWkW8fxF9EmQdYHTeyyUldXzsQcJCZ10DDZeiVJz/Njwb0VnXg2cVezaMa3RsL1TqYygj2GnKAOPzrLdLunj+2OtWAx03DJV6T8BxAaiEs+3C03mTsJfKNGVlYWI6o+o8vefiuAGQ91yA1gyIOT6s5THt3Q2F76M9fwGxqLheENs7YikqhBDVL6M4g6f+fha35S73i5lG47bcPPP3JB459kEaxNaPaL+FnkIUKugvg4Xewoj2K6qPfDIcQLR2Q8HbBEw0qXOhaL7fjdHayrBrEZbloNrrH7/rG3TGJVBvBkrFhD8+7zZ0+nn2GMqxoOgjtJVuz7QFWWJVSeMhbjC68H176VAlo+IGgbNbuccqR2t03OCSvVm+fohpVPI1oY+/cDY6+3b2vL4KXfSRvYxZ93WU2SzktoQQtcfXO7/BY3l8Bjul+6S+2vk157YYHtF+G8U1DGm2vWFc1SpmiJoji7wHEmsX6OwgFznQ7j/8313wNli78Z0ewGvPbrkWVmp4On9qSYDlq20LiheB+8eQ2lKOlhjJN2DUeRYj9QFUbHffwVnKgxXzd+0ZERT/FNrYi1egs2/F3t9llfwpeR7eTeiMi9E6WP4pIURt9HPmr1gBUvBYWPyc+UvE+z069SjSYuqg/Cz6Gxi0Tz6CBrESZO2vJMiKMO3djpX7HNbus7B2n4mVc59dM7BahLhxOcAGZ13wIYGziRvowtnhDGqPgDmuAEx04ZzKte2Hci0oKfnjm857JqTvj877H/7/uXjB+5+dn0sIsd/xhPALktuK/C9RhjK4rPUlGEpVCLQMDGLNWEYfOiri/YrqI0FWBGnX9+hd/SD/Jbv0iecvKJiBTj8DXfBu8AaqyqgHjiPwV97G5kHFnur/bp0VpBNrr6XE0GntLUmAGYi3Um0H7Df/LQK/zU104YzAbWgPFC8maIDo+qoSIxRC1LTDElthBPg5YWDQJql1VPo+OvUoJh5xK+2S9xyYUig6ph3HvUfeSdN4qb6wP5M9WRGgPevRea9C0Yc+7rU/mHXOveBoF9Ui0UopSBqHzrrWzxUmOI6CmOP9N2I0Ae96/BZ7xgSzRSXGZqKNekGCKDsZZkR51hK8zt+aII148f96lNKggyVKFULURr0b9WJp+vd+77ew6NOod9T6b5vchontbyGrOItcTx5pMXVIitk/Tz2L8mQmq4p00dfo3YOh6KMgVxro/GlRH4+KG4BKuhl7Nsvc62/A0QaV9nLAjeUqYWSQHryo+Epu/owfQeC3nBcVf07l2vYnyGlFu85f4LxSSsWWBJaBZgg1ynFEuKMTQtQChyW15pzmdumzvWe0Sv97aNMzOTy5bdTHUcdZhxYJzSXAOoDITFYVaCvDTokQ0kyHF4qXVsOoQCWNhbjT0YWzwLMeVCIqrj/Enhq8lmDCcCj8EDxrqLg8piC2f/lM4eGMq7QcjHe7j7aB+OGomAjnookbAIXv+e4PAI0qqXMYiEoYjc59OMAVDog/uzIjFELUAoObDuKQhJYs2P45f+Xas9uHJ7Wlf+N+HJfWoYZHJ/ZXSu/nNVdycnJITU0lOzublJSUau1b5/0Pnfc0gZej9qISMRpF/oRKpGkrF53zCBTNAdz2jSoREkajkiZUKSeU9u5E59xXckKx5HVTSajEiyHxqogWlAbQnn/Qu8/Efh4+6vwZjVAN5qNUfOB2tBudOQ6Kvy29ZU8bWKjUJ1HxgyM6diHEwe2//A2sz1uPoQyOTDmSRnH7WTH7A1Q4cYfMZFWBLv6JkAMsTIjpEs3hVIn2rEfnTwfXF6Dd4GgLSTeD2QZlxkLM0UEDkVAosyEqbTLau71ktswJzo5Bk5BWuj9Ha0h7FZ11dUn6iNK3vAfMZqi0qSE9L6ViIO0lKHgXXfBmSWkfA2JPQSVeBuYhaNc3dvsxHVCGTPcLISpnl2sXL657hX/y/y13e6c6Hbms9SUkOoKXzhK1g8xkVYGVeVXJjExoL6FKez3kkjbVSbsWoTOvwn4e+y6rxULiFaikK8JKQKq1xz5NqBJqRU08bRXYpYXcv2HX+ethB0iVnJXTuhhwgM5G59xvJ3gtC7jjIPECVNJ1teK5CyGiK8edw7e7lrChYCMOFcNxacfSqU5HHEb4P19y3bncufpectw5FXJ3GRgcmngIdx45ETPCs/4idDKTVU2U8wR0iIk5VdJ1tTPAsjLQmRPwv6/MBfmT7OAk7cWgQYn27kDnvwwFHwKFQAw67gxU0jgw6kPRp2jvFpRRB+IGoEx/iUIjSxkJkDACxYjItKecaCsPnX6+nSOr3A/DIsifgvZshDrP10iRcCFE9fghfRn/+2cKlrZ/BigUS9O/o0FsA25pd2PY2doX7vyabHe2z0zwFhb/5P/LL5m/cnzdzhEZv4guOV1YFfHDSooLB3gZY45H1X0blXRVtQ0rLIUfAsUEnY0rXgRFnwS8RHs2o9OHQcEM7AALwA1FH6N3n4He2R2dcxfkv4rOfQy961SsnAftHFr7o8KZ4P0H35vqNbg+h+IfqntUQohqsi53HS+vfxWv9qJL/lc6+5TuSufxNU/iCTOJ6be7lgYstWOgWLJ7KYXewrLATtReEmRVgTKSUGlTSlIA7P1S2tO4KvkOjHrvoJy1eC9Wcagb8RW64O3AbeXcA1YmFYMOL3YgVxrM7VWapuBNdO6T4Q06DFrrqAVxumAGgYNTE134QVT6FkLUvE+2LfBbEsfCYpdrNz9mhla6q1SeZ9/arvu2q/k16zeu/OlqLv/xKqb8M40dRTvD6kNUHwmyqkg5j0M1+BKVdAPEdATHkZBwLqrePFTimJoeXghCXdfX4PnX/72ezVC8hMBZ0f0oeANtZYT/uAC05x+s7DvQO45D72iPtfMUdN4r9t6sSPHuCHYBeLdErj8hDiBuy80vmb/yza5vWZW1Gu9+NqOttWZl9m8Bax4aGPyatTKsdus56/kN3Mr6Lvnlzq3dLNm9lHt+v5+NBZvC6kdUD9mTFQHKqAtJl6OSLq/poYRNxZ6Idi0I8eJE//d51xHqAYCKPFC00M7RFQG6+Bd0xhjstA0lP7itbei8Z+wN6nXfiszpP6MuWFsDXGCCIYVdhdjXop2LeW/TLPK9e37pSY1JZfQho/abvUYaHTQw1Gjcljusdk9teArvbAxc6mtvFhYur4tX1r/Kg0ffJ3tAaxmZyTrYxQ0GVYfA2cwBTAiYB6oq6R2MkvQKVae1x07XQDEVZ9Us8PyFznsuIn2phLMJnsF+aET6EuJAsWjnN7z+3xvlAiyAbHc2z697kZ8zf62ZgYXJUAZN4oKXAWuZ0DKsdk9p0IMWCc0D1lLcl4XF5sItFVI+iJonQdZBThmJqLpTSjbw+2PaWeMTAlSDd3YElVrJUVhgHlLJx+7D9TVYu/Cfv8yCwvcis2yYMKpkpsrXkqth14iMPaXq/QhxgHBbbmZuej/gNe9unEmkMwtZ2mJl1m8s2P45i3YuJtudHZF2+zXuE/B+haJng5PDajPWjGXiEbdwUv0Tw07TsEmWDGsdWS4UqJhjoMGX6PzXIX8aUIQ9s2UAXjAa2DUPA6RbUMoJSVegcx8Pt3d72S1CwYh2/4H9tg5wokcXgncTGO2q1Jcy6kK9Geism8C99+ZWBXEDUSkPRDyDvRD7s1XZqynwBv4FZ6drJ//m/0frpFYR6fOPnD/53/rXyHRnoVBoNG/8Z9Cr4amc1/LcSuWyKnVKgx78lrWKX7J+LXe7gYGFxSWtxpDmTAu73QRHApe1vpiRLYazsXATmwo2h7SEGGNIXr7aRoIsAYAy0lDJN6CTrofiH9DF3wEWKqZjSc3DEN4qCZeCNx0KprBndkdjzyo5qJjs1J5IVamPVKlUT7nnoZwBjz/vuTAyP4yU2QxV7120ew24fwNlgvPEasv/JcT+JNudE9J1OSFeF8z6vH94cs0zZakOSn82WFgs3Pk1bu3mklYXVbp9U5lMaHsVi3Z+w+c7vmR70Q4UimNSj2Zgk/4ckeL7FzlLW6zO/p3lGSso9BbSKK4RpzToQaO4RuWuS4pJ4siY9rRJasPsLR8HDFBNZXBM6tGVfi4iOiTIEuUopSD2BFTsCZV6rEq5FStuKGTfBN6/2bMZ3mMvrVnplC3lxXRCJV8f2RQXsadA3jOBRglm88gtT5a2GtMOYqo2MybEga5uiLM6lZn98eWjLXPsNC4+fvHSaL7Z9S2DmgysUk1AU5n0btSL3o164bE8GMrAUP534uR58nh6zXOsz/+nbMbLwOCTbfM5q9lQhjSruPfVacQwqMkAZm32nRJGoTilwSmkxCRX+nmI6JA9WQcpbeWii75AF85De9ZFrl3tgdz7wbuWCqcNrQwwWkDdmagGi6OSQ0zFHAnOE/GfmkKjEq9EBfghKISIjqNSjiTF4T8QUCiaxzejZUKLKveV78lnVfbqoCkWfkhfVuW+SjkMR8AAC2Dyupf5N/8/gLKxlf794ZbZLNn9nc/HDWzSn76N7D1gxl7/A+hS93hGtRwZiacgIkxmsg4yWnvsVAb5bwKuPbfHdEKlPopyHFq1DlwLwb3Cz51esDai3L+hnB2r1k8Aqs6z6Myx4F6JHWx59/ydOB7iz4la30II/xyGgwsOGcWL61+ucJ8q+d8Fh5wfkTQE+Z7gh1uUUuR58qrcV6j+z955x0dVpX/4uXcmmfTeaAmhht57rwqKooiIvbuW1dV1f+rq7lrW3bWt7qprd20roqKISO+99xIIEFKA9N6m3fP7Y5KRkExLJgU4jx8/kLlnznlnEnK/8573fN/T5WkcKTnqdMyiM4sZFTmiznugKiq3JsxlUswENuZtosBUQLA+hFFRI+gY6N3MvMR7SJF1mSGKn4OqH6mTZTLvR+TPgaiFKDrXx5Idzl/5A7YEqeNPj6Ly2yY1alXUMIiYD6aNiMolNnsIXQJKwGwUfacmW1cikbhmWOQQdIrKvPRvyTPl2R9v49eG2zreTI+QJK+sE+oTgl7RYXHiZaUJjShDlFfWc4f9RQfsW4SOyDZmk2vMc9jzsI1/HDd28O4HxTJLGauz17I+dyMl5mJCfEIZFz2GybETCdQ78UeUuESKrFaA0Mqgcj6i4jvQckCNQvG/AQLmoqje22MX5qNQ9YODq1YQJYjyD1FC/tLwRaxZOBNYIMCa2/D53URRVDCMQ/HCqUUhTGDaCaIEdB1A30sa/kkkjWBwxCAGhg/gVNkpSiylRPhGkBAQ79V/VwadgWERw9iav82hqFEVlZGRwxu8hkkzc6DoICWWEsJ9wugT2tvpaUWzMNteo4uzOWbhmYFpYygwFfLykX+Qb8q3167lm/L58cxPbMzbxHM9niHMN6zZ4rnUkCKrhRFaASJ/LlhPY/+XZy1DlL1ha0AcMQ9F1/CizFprVS7k1+2z+rBCxQ+I4D81vGZJFweWYzgWWgo08vUIUQmVSxCWQ4CPTUj5jmiSOitR8Q2i9E0Qhb8+qO8GIS816ZanRHKpoyoqXYK7NOkas9rP5EDxQcot5fUKrRvbzyK4gcXi63LWMz/j+1on/oL0QdwaP5cRUfULt4SAeJcu8QbVQJRvZINiagifpn5GgamgzuEAgSDfWMAnqZ/x++6/a7Z4LjVk9W8LI4r/BNZ06n60EWA9iyh+xnuLaXn1rHMhlQjzXlt2rQEo/tfjPJMFiv+NDZobQBg3I3JGI0qegYr5UPEVovAuRP61CGtWg+etd63yzxAlf64tsAAsJxAFtyHMB7y6nkQi8S6Rhkj+3PNZ+ob1qdUPMNI3kvsS7+bKNlc0aN71uRv57+kv6lgqlFnKeP/UR+woqL8udUBYf0L0IQ57E6qojIsei0FnaFBcnpJdleP0cICGxoHig+Qam3734VJFZrJaEGHNAuMqHAsfq62uyJKGovdCYaMajev2OUDBXAS+CP/rUIJ/b6txchfDJPAZUm3OeeE/XJ3NOsF/lvvznYcwpyAKH8DWkxBqGY5aTtj6FUb9bDNGbSRCK0WUvuHgqgZYEKWvo0R80ei1JBJJ0xHjF83j3R6lwFRITlUOfjo/4gM6uDwF6AiLZmF++ndOx3yT/i2DwwfVWUOv6nmk64O8lvxPrMJaS9woKHQIaM/17a9tUFwN4XT1KUdXpJafJtog+7A2BJnJaknMB3CrqbLZsy7ujrD10XO3070JKr9H5N+E0NzvK6goepTwj8BvJrVtFBQwTECJ/BpFbVghpSj/BFv89b1nVrCmQtWqBs1dh6rlnH/6si6azbTVes4760kkkiYlwjecpJDudAxMaLDAAjhUcphya7nTMfmmAk6Unaz3WvfgbrzQ+8+MjBqOj+IDQLhPOLPaX8ezPZ7GX9eYPrCe4e774Gl7H8mvyExWi+LuD653fsAVnySE/2yo/B63xB1WsJ5GlH+MEvy4++uoAShh/0BY/wDmXSCs4NsPRdeuwbEDYFyKc5GoIowrUPynN24dsB1AcNWeB8CaA404jSmRSC4uSszufej8Jv1bVEUl0jeSsdGj6RnSw17Y386/Lfd1uod7E+/GKqyNau3TGJKCu6NTdE7rxFRU2vs18nf3ZYzMZLUkvoMAHxeDdOA71GtLKiEvQuADgLt7/hpUzGtQw1ZFF4nidwWK//TGCywA4SyzBKCB5vwTptuoUbiV9dPJFLpE4i0qrZVkVWZ5ra1OUxDpG+HWuFPlqaSUnWBHwU5ePfYGb5/4Dxat9oc2RVFaTGABBPsEMzZqtMMaMbDVZf3x0J/ZmretGSO7dJCZrBZEUcMQ/jfYThHWW3iogt81KF68kSuKDiX4CTQlCMped+9JosjWVFkJ8Hg9ISptlg1qkK2hcmPQJYL1FI6zcDrw6dq4NWrwuwJKXgRMDgaotrZAurbeWU8iuYwpMBWwIPNHtuVvt/taJQV35/r2M+ke3K2Fo6tNj5Akwn3CKDQXOR13fp9EgD2Fe1lw5kfmdJjd1CF6xM0Jc8k3FXCg+KDDMRZh4YNTHxNeveVaHybNzK6C3WRWnsGg+jIwfAAdAto3KjaLZmFP0V625G2lxFxKjF8M46LHkBTc/aKx0VFEQ1IUrYiSkhJCQ0MpLi4mJCSkpcPxGCGqEIUPgmkzddzJfYaihH+IonoublyhFdwOpu24t22oR4k9iOLBvryw5iLK/g2VP2IXKj5DUYIfRWlgZk6Uf4UofQnHMSsoUcsb71pfs17ZR4iy1+q5ogIqSsTXKL79vbKWRHK5km8s4IUjf6XUXFqrEFxFARQe7fowA8L7t1h89bGncC//TnkXoN6+iI4wqAb+PeCf+On8miq0BmHVrCw9t4zvzjjyUbRtG/YK7cmT3euWjuwvOsj7Jz+kwlqBTtEhhEBDo19oHx7s8kCD6szKLGW8lvwmpytO1+rxqKExPGIo93e+t8VqxTzRHXK7sIVRFD+U8E9Qwj8EwxTw6QuGiShh76FEfN4kAguo3npz/5eDKHoMYdrj3lhrDiJ/VnXt13mZIPMuRMHtiKqVnsVaQ8CN4DucuickbT/GStCTXhNYAATeixL8HCgX+Ojo4m3fGymwJJJG803Gt3UEFoCG7Ub90alPMWvNZ87pDgPDB/B4t0c9bixt1IycLDvVRFF5RqGpkHnp83lo92+5e9f9LDz7s8ttw4PFhzBd8L04VZbKv1L+TaW1EqDWqcmDxYf5d8q7DSo3+fDkx6RXpNvXPv/PbQU7WHRmscdztgRyu7AVYHMnH49iGN98i/r0rj7d6M5pQwsYVyOMKyHkBZQA541IRenroOXWM7cGKIjip8EwBkXx7NOcovhC+EdQ/gmi4stq3y9A3wMl6Dcofg3zvHG8ngKBt0PAHDBu+dXx3WfARZOqlkhaM6XmUnYV7HbaZqbcWs6ewn0Mi/RuM/nG0i+sL31D+5BWkU6xuZiTZaf46ezPLp+nCec+gs1BVlU2fz3y91omre66zFuFhfNriRedXYwQ9Wf0NDSOlBzlZNlJj4xnsyqz2O9k+xJgRfZKrmo7HV/VVV1zyyJF1mWKEjAXUfGVB8+wCSZR8hfwHYKi71zvKKGVQtViHIs3YeslWLUC/K/xKGaoFlpBD0Lg/TaRpfg0vtbL5ZoG8JvQpGtIJJcjecZ8pwILbNtUWVXeNRr2Foqi2Jszh/uGuxRZKioJgfHNEZpT3j/5kUMXfGeE+YThp/764dikmdlXtN/plqkOle0FOz0SWYdKjqCgOJ23wlpJWnkaXZu4a0BjkduFlymKvgtKcI2bvCf72iqiYp7jy9YzuLQ9QI+wpHqwZl0URYeii21ygSWRSJoOd2qTBKJZvaMaSnxAB7oGdUF1cFtVURkaMYQQn5atHU4rTyO1PNVjgaWgMDl2Yq0svslqdFmTJsC+legumovWQzW4alHUGpAi6zJGCbwTJfxT8B3Gr3VOrgSXFZzVZinuGI1qDTYklUgklw5xfrG09XPtMzcofGAzRNN4Huh8H6E+ddvmKCi08Y/jto43t1Bkv3K6us7JExQUugZ14Yq4qbUeD9AHEKhzXjcsEMT5xXm0Xuegzi7Fm17R0yGgg0fztgStQmS9++67dOzYET8/P4YNG8aOHTtaOqTLBsUwGjXiM5TYoyixR8GdzJDiZJdZ1x703XHevkeAYaqT6xKJ5HJAURSubz/T8XUUxkaPIdJwcWSsfVUfRkaNIFgfjIqKikKEbwQ3xd/In3s+S5A+qNljMlqNVFmr7F/rPTyRF+oTyqz21/GHpN/XqX9SFZXxMeMdZu/A9j0cHTXKozU7BSaSEBDvNCs4OmokgfomOhjmRVq8Jmv+/Pk88cQTvP/++wwbNoy33nqLK664gmPHjhET49nJDUnDUarbKwjDBKhcgOOaKhXF4Lg+SVEUCHoMUfSQw+fjdzWKvuXrEiQSifsIIThVnkqeMZ8gfSDdg7t5xUhzSMRg7ux4O/9Lm4dFmFHPswAYHTWS2xNu8UL0Tc+pslRePfYGVdYqexZGRaXAVECxqRiD2jxNn8H2vdqav52l55aRXpkBQHv/9kyLm0qv0F52KwRH+Kl+vN7vH6iKDn+dn9P2O1e3mcbewr1kVWXX6cUoEMyNn0OYb6hH8SuKwkNdfsPLR/9BmbkU7YKsVnxAB26Kv9GjOVuKFvfJGjZsGEOGDOGdd94BQNM0OnTowG9/+1uefvrpOuONRiNG46/O3yUlJXTo0OGi9clqbQjzcUT+tdhOAl74o6GC4ocStQpFF+V8norvEHYzT131XFbwuwol9B+2YnKJRHJRkFxyjM9Of8G58wrQQ/TBzO4wi7HRY7yyRrmlgu0FO8ipyiFQH8jQiCEeWyS0FCbNxBP7/kCZpdzhNteDne9neOSwZolnXvp8lmWtqFU8XvP3KTGTMGpGNuZtdhjrNW2vZlb769xer9xSzoLMhWzM24RJs9n2tPdvz8x2MxgSMbjBr6PEXMLq7LVszNtMuaWcSEMkE6LHMS5mDL6qb4PnbSye+GS1qMgymUwEBATw/fffM3PmTPvjd9xxB0VFRfz00091nvP888/zwgsv1HlciizvIaqWI4oexya0aj6ZKKAE2MxRfd07Si20EqhajLCcRlGDwW+6w1OJEomkdXK8NIV/JL+GJrR6b8p3dLyNiTHjmz+wVsTG3E18nPpfh9cVFBIC4nm+158wCwt6RdeoJtXOSC45xt+TX3U65nddf8vanPXsLz5Qx+hzdNRI7km8q0HxGa1G8k0FGFRfInwjLlmrG09EVotuF+bl5WG1WomNja31eGxsLMnJyfU+55lnnuGJJ56wf12TyZJ4D8XvCoheg6j41tbgGR2KYRT4z0JRw92fRw2BgJudVmdJJJLWzbz0+Q4FFtgaIY+KHIFBd3Fkp0vMpWRUZKAqKp0CE70Sd3LpMadbcALB6Yo0Ht37BCWWEvSKnqERg7mqzXTaB3i3+fLqnLVOY1FR2ZC7ice7Pcqx0uNszt9KibmECN9wxkSNplNQYoPXNugMtPV3fZDhcqLFa7I8xWAwYDBcHP+YL2YUXRxK8KMtHYZEImlBsiqzOFXu3G7FqBnZU7SPEc20FeaKEnMpuwp3U2YpI8o3ikHhAzDoDJRbyvlf2jy2FWzHWm0IalANTI2dzMx21zSqvszd/aASi63xtUVY2Ja/g50Fu3my++MO+wE2hJNlJ53WW2lopFWkoygKSSHdvbq2pC4tKrKioqLQ6XRkZ2fXejw7O5u4OM+OfEokEonEuxSZi12OUVEpMhU1fTAu0ITGgswfWZq1DKv4dfvLT/VjbvwcVmWv5kzl2VoCxKgZWXxuCdnGbB7q/JsGb28lhXRjc/4Wz+JFQwjBuyff581+r3nlEEFWVTYFpkKX4wwtWM90udGiFg6+vr4MGjSI1atX2x/TNI3Vq1czYsSIFoxMIpFIJKE+rk+FaWiEuTGuqfnhzEIWn1tiz1LViKkqrYr/nv6cjMrMejM8AsGOgl0klx5r8NrDIoYSqA902vuvPgSCEnMJ+4r2N3jt8/lfmhOj6GoUaFQxuqcYrUbW5qznxcMv8+T+p/jH0dfYmrcNi+bKtPrSoMW3C5944gnuuOMOBg8ezNChQ3nrrbcoLy/nrrvuaunQJBKJ5LKmjX8cHQM6klaR5rAmy6AaGBg+wOk8ecY8tuXvoNRSSoRvBCMih3nV+bzMXMbSc8sa/HwVlfW5G+kRktSg5xt0Bp7o9hivJf8To2asZeHgylldp+hIr8hgcMSgBq1dQ4GpkAMu+v0B6FUfJsSMa9Ra7lJiLuHvR1/jbNVZ++nGPGM+R0uTWZOzjie7P37R1PI1lBYXWXPmzCE3N5c///nPZGVl0b9/f5YtW1anGF4ikUgkzc/c+Bt5Jfl1oP4mwLM7zHJ4o9SExrz0+azMtu1WqIqKJjS+Sf+WWe2v4+q2070S4+6ivVga0WJFQyPPmNeoGLoEdeYfff/K2pz17CzYhVEzEecXy+GSI06fJ4TAxwtNjnOqctwaNz56HOG+7h9gagwfnvrE3ney5men5s+UshPMS5/PnYm3N0ssLUWLiyyARx55hEceeaSlw5BIJBLJBSSFdOfJ7o/z2ekvyDHm2h8P0gdxQ/vrmODEvuGHzIWsyF5l/7qm15xA8F3mAgL1AU6f7y7llnK3skaOUFAos5TxfcYPJAZ1pH9YP3QeOqODrUn09e1n2l3shRD834E/kmN0LIA0NAaE9WtQ3Ofjbn/H9v7ePc3oiKzKLA4WH3J4XSDYmLeZ2R1mEai/dNustQqRJZFIJJLWS6/Qnrza9++klJ0g15hHkD6IXiE9nBZrl1sqWJq13Om8P2T+xNjoMQ0SNOcTY4husMAC2w0/qyqbJVnLsAorYT5hPNr1YToHdWpUXIqicG3bq/ko9dN6r6uo9ArtSfuA9o1aB2wu6DGG6FpC+EJ0iupya9dbHCtLcTnGIiyklp+md2ivZoioZWgVvQslEolE0rpRFIVuwV0ZFTWCfmF9XJ6GO1B0AItwXtxcYinhZNmpRsfWP6yf076AKip6Re+0x55A2DNtJeYSXkl+nVwngsVdRkeP4vp2M+1xKCjoquPoEtSZBzs/0Og1oKYPpHOX9skxkwjxCQZsWTZNNFyYuqKFm8m0GmQmSyKRSC5SNKGxNX8bK7PXcKbyDL6qD0PCB3NF3FTa+LesDU6VVuV6EFBpdW+cM/Sqnrs63s47J94DateOqagYdL483OVBfshc6NL3C2xbeGbNzIqsVdySMLfR8V3bbgYjIoezIXcj2cYc/HX+DI8cSo/gJK+6oo+IHEaFpYJ56fOxCIu9Bg5gYswEboq/kVNlqSw5t4y9RfuwCAtt/dowNW6yVzKK55MU3M3lGL2iJzGwo9fWbI20eO/CxuKJvb1EIpFcKmhC490T77OrcHetHnUqKjpF5fFuj9ErtGeLxXe0JJl/JL/mctyrff/utR6FB4sP8V3GD6RVpNkf6xvah7nxN9LWvy0AaeVpnCxPZV3OetIrMhyemgRb3dm7A//lldiak4rqPpC51c28h0UMJdIQwc6CXfznxAcAdbZXB4T147ddH/aq0Hr92JscLj5S71augsL46LEXZeH7RdNWRyKRSCQNY1X2GnYV7gZqZ25qTC7fPvEu/+r/zxY7It89uBsxhmhyjXn1ChkVlW7BXb3aBLpPaG/6hPYmuyqbMks5kb4RhPmG1RqTEJhAQmACO/J3OhVYAFVeyLK1BAH1HCgoM5fx/smPHNau7S3az5rsdUyJm0SVtYqt+dtJLU9Fr+jpE9qbfmF9Pe5neH+ne/j70Vc5W3XO/kGg5s+uQV2YGz+noS/xokGKLIlEIrnIEEKwImul4+sIKq1VbM3fxvhm8kS6EFVRua/TPbyS/Dqa0Grd3FVU/HQG7uh4W5OsHesXiysToLb+bThWetxpwbw3BWBLsylvs73mrD4UFFZkryLOL5Z3T75HpbXKXkO2OmctcX6xPNn9caIN0W6vGeITwvO9/sSW/K1szN1MsbmYSEMk46PHMTRisFdc7ls7l/4rlEgkkkuMCmsluSbnvk4qKifLUxlPy4gsgG7BXflTzz/yQ+ZC9hcfsMc1JGIws9rPJNav5fwQx0WPZXXOWqdjJsVMaKZomp7TFWm1tpUvRCDIMebwZsq/7XVc5wvQnKpcXjn6On/r+1d8PfD1MugMTIgZ7xWrjosRKbIkEonkIkN1o1haUX49xdaSdAxM4Inuj1FmKaPMUk6oT4jbnk5NSUJgPNPjrmRJVl2neAXbScqx0WO8spZJM3Gk5ChV1iri/OLoGJjglXk9Qa+4d7sXQtQrxDQ0ck157CzYyaiokd4O75JFiiyJRCK5yPDX+dMpMJHU8tMOMxNWYaVPWO9mjswxQfogpzYLLcGNHW4gxi+GX84tIbfa8T1AF8DEmPFc225Go53YhRAszVrOorOLqbRW2h+PD+jAPYl3NavY6hfWl415m12Oc7Z9qqCwu2BPk4gso9VIStkJLMJCfEA8Ec3kSt/USJElkUgkFyFXtZnG2yf+U+81FZVIQyT9veAkfimjKAoTYsYxLnoMecZ8rMJKlCHSK21uwNa0etHZxXUez6jI5OWj/+AvPZ+tZUSqCY0cYy6asBJliPZoW84VA8L6E2OIJs+Y32DjVoHAqBm9FhPYPgz8eOYnVmStss+toDAgrD93dLyNMN+Wbz7eGKTIkkgkkouQwRGDmN1+Ft9lLrC3lKmpuQnzDePJ7o979Tj+pYyqqMT4uV/Q7Q5FpiIWn11S7zWBwKJZWJC5kMe6PYIQgrW561l8dgn5pnzAlq2cGDOBme1m4Kv6NjoevarnD91/z6vJr7us53OEiuoVd/oahBB8fOq/bMnfWvtxBPuK9pNxNIPne/2p1WVAPUGKLIlEIrlIubrtdAaE92dtzjoyKjIxqAYGRQxkeMTQFrNukNjYmr/dqUWEhsbeon2UW8pZdHYxy7JW1Lpeaa1kybmlpJSm8H9Jv/dKdi3GL5pHujzIX4681KDna2iMj/beQYpT5al1BNb5a+UbC1iZtZrr2l/rtTWbGymyJBKJ5CKmnX9bbk24uaXDkFxAkbkIFRUrjm0TBILkkuQ6Auv868fLUtiQu5FJsRO9Elelm07851OTIZ3TYbZXOwlszNvstLG3hsba3PVSZEkkEolE4g3Mmpm9Rfs4W3kOP9XAwPCBXt/Kaw6C9cFOBRbYxMueov1OhQbA6px1DRZZmtA4WpLM2cpzGHS+tPVr69bzgvRBlFnKAOgUmMhVbacxKHxgg2JwRIGxwGV9WLG5GCGEV9sPNSdSZEkkEomkVbC/6AAfnPyYcms5OnRoaMzL+JaRkcO5K/FOrxaCNzW+iutYI3wjyDPmuRQaOVU5DYrheGkKH5z8mDxTnj0bpaISqg+lxFLscDMzQBfAm/1ew6SZ0Km6JrPcCPEJdikwg/RBF63AAimyJBKJRNIKOFF6greOv22vYzo/C7Q1fztmzcIjXR9sqfA85mhpsssxZZYyEgLinZqEAvjp/DxeP70ig1eT38AiLMCvrZc0NIotxSgoKFDvurcl3IKvzhdfnfOCe6PVyJ6ifRSZigj1CWVgeH+PYh0VNdKprYSKytio0W7P1xqRIksikUgkLc6PZxYB9d/0BYKdhbvIrMj06um2psSomVyOsQorwyKHsKdor8MxKiojIod7tLYQgnnp87EIi1OH9w7+7cmozLQ/FmOIYU6H2QyOcL0tuDZnPfPS52PUjPZslK/qy+z2s5gaN9mtOJOCu9MntA+Hig/ViVNFJVAf6PZcrRUpsiQSiUTSYIxWI9nGHPSKjji/OI+bCAOUWyo4VHLY6RgVle0FOy8akdUhoD1HS5IdboUpKLTzb8vg8EG09WtLVlVWnbEqKr6qr0dC40jJUb5K+5ozlWedjlNRGRDen4e7PEieKY9gfbAtq+bG1tzG3E18dvoL+9c1cZs0E/9Ln4dO0TEp1nVLIkVReLTrQ3xx+n9szttS6/V3DEzggc73Ee6BKWmRqYgNeZtIr8jAR/FhQHg/BoYNaNEeiVJkSSQXAcJyClH+KVT9AqISdPEoAbdCwE0oSuM9dCQST6myVrEgcyHrczfYTSQjfCO4us00JsZM8KiOpuo8N3RHKIpChaWiwfE2N+Ojxzk8NQi2TNLk2EnoVT1PJT3J2yfe5UTZSdTqVkgaGgH6AH7b5UGiDVFurXmo+DBvHHvL6dZjDQoKpZYy2vjHeXRi0CqsfJuxwOmYBZk/MDZ6tFu2E76qL/d2uosb2l/P4ZIjWISFjgEJJATGux0TwKa8LXya+pm976KCwpb8rcQYovm/pCfdfg+9Tcs3tpJIJE4Rpp2IvGuhcgGIckADaxqi9GVEwZ0I4fmRbImkMZg0E68kv86q7NW1HMALTAV8kfY/vsn41qP5gn1CXBa1a0Ijxi/Go3mrrFUcL03hWOnxWm1tGosQgmOlx/ng5Ee8cPivvHHsLbbkbcOsme1j2vjHMafDbIDq6qfaDAzrz+jq9jRhvqE81+MZftf1t0QZouwZnTJLGW8cf4tvM77HKpyfVBRC8NnpLxHU33vwQjQ0onwj3X7NNRwvTaHEUuJ0TLm1gsMlRzyaN8w3lFFRIxgXPcZjgZVccoyPT32KVVjtr7/mPcwz5vNa8htYNItHc3oLmcmSSFoxQpgQhY8AZqi1lVD9S9S8B1H2H5TgJ1ogOsnlypqcdU77Ji7LWsGoqJHEB3Rwaz5f1YfRUaNYl7PB4faaqqiMdLM2yayZ+T7zB9bmrLeLQF/Vh/HR45jdYVajHNQ1ofFp6me1PJ4UFA4UH+Tns7/wdI8nCfWxtYKZ3uZK4vxi+eXcUk6UnQQg0jeSqXGTmRI7qdbWaqmljC/S/keRqajWeibNzJJzy8g3FvCbzvc5zBCmlJ0g15jr0WsZFTXCo/GA3dbB9bhyj+duKL+cW+rw8ICGRrYxh31F+xkcMajZYqpBiiyJpDVTtQJEoZMBGlTMQwQ9IrcNJc3Gmuy1TrMlKirrcjZwe8db3J5zZrtr2Fd0gCJTUS2hVXPzvDn+JoJ9gl3OowmNt46/zeGSI7ViNGlmVmavJqMikye7P97gOp2lWcvtJ+Jq4qxZJ6sqi3dS3uPZnk/bxw8MH8DA8AFUWauwCisBuoBaQsmsmdlVuIcl55ZSYCqod02BYFvBdqbGTaZzUKd6x+Q7eK4jrmt3rUf1TjVE+bq37daQLFlD0ITGwXoK589HRWVvC4ksuV0okbRihPkwLj8LiWKwZjVLPBIJQK7Ree87W/Yg26M5Q31C+UvPZxkeOaxWz8U2fnE83OU3THbTjHN34R4OlRx2eErxaGkyOwt2eRRbDVZhZdm55Q6va2gcL0vhdHlanWt+Oj8C9YG1BFZaeTpP7n+K909+SHpFhtO1VVSndgfBbvb3C9QFcmfH27im7dVujb+QjoEJtPVrW+8WKNhEcbQhmm7BXRs0v6fUbBE6QyBqbeU2JzKTJZG0ZhQfcKO+AjeMDyUSb+Gv86Pc6rgIXUUhUBfo8bxhvmE80Plebk2YS64xDz/VQKxfrEdF9OtyNjg1uFRQWJe7gRFRntkiAJypPEuJpdTpGBWVw8WH6RiY4HRcibmUV5Jfp9LJ+3g+GhqFJsdZ7aTg7oTog53GF6gL4F8D3mhUH0RFUbgz8TZeSX4dTWi1BI5S/d+dHW9zecrUpJlYl7OeNTnryDXm4a/zZ1TUCKbGTibS4H4WzEf1IcYQQ64x16nYSnBz69rbyEyWRNKKUQzjwGlrDgV0nUH1Xj8xicQVIyKH20/B1YeGYHjk0AbPH6gPpGNgAnH+cR67fecYc506iAsEOR7WLtmfK5w7s9dgdeHgDrA+dwMV1go0dz5EYRNvNbVe9aFX9fZCe0fcHH+TVxpNdw/uxjNJ/0enwMRajycEJPBU0pP0Du3l9PlV1ir+fvRVvk6fz7mqLCzCQqmllBVZq3ju0F9cZvUuZGrsZOfbhYrKmOiWMTWVmSyJpDXjMxB8+oL5MPWLLYES9MBF3XZCcvFxZZupbMrbgkkz1hEJKiodAtrTL6xvi8QW4hPsMqsRondd21VoKmRD7iZSy0+jV3T0DetD/7D+GFRDrROVF6Kh0SWos8v5dxTsdOsU4Pnz1pxGdMTo6FFY0fgmfT4V1kp7PVuAzp+b4ucwOnqU2+u5omtwF/7c61myqrLtju/uWkF8n/kDp+s5OKGhUWU18nbKu7zS929ue65NjB3PoZJD7Cs6UOtxFRWB4L5O9xDiE+LeC/MyUmRJJK0YRVEg7D1E4V1gOY4t+awBOsCKEvRbFP+ZLRqj5PIj2hDN0z3+wNsp75JvKqjl7dQ9uBsPd/lNrbqq5mR01Ej7Sb76UIDRUc7Fxtb87Xx06hP7dpiCws7C3YT6hDI4fBBb8rfWK5BUVGL8YugRnOQyTqPVsVCrG7PCgLD+dA3q4nLsuOgxjIgczv6i/RSZiwnzCaVfWL8m6/sY5xdLnF+s2+ONViPrczc6zOBpaOQYczlSctRlRqwGnaLj0a6PsDZnPSuzV5FVlY2KSv+wvkxvM42uwa7ft6ZCiiyJpJWj6KIh8kcwrkFULQOtDPSdUAJuRNG7/sQskTQFiYEdeb3fKxwsPsSp8lT0ip6+oX089jjyNiMjR7AsawU5VXW3DVVUogyRjI52nBE6WXaKD05+VEtE1fy91FzKweJDdA7sxInyk7VsA1RUAvQBPNr1Ybcyy/EB8eS60Rxap+gYFz2Gm+Nvcjtj7av6MCRisFtj3UUIQbm13P46G8q5qixMLloOqaicKk91W2SB7X2aHDuRybETsWgWVEVtUPcBbyNFlkRyEaAoPuB3BYrfFS0dikRiR1VU+oX1bbGtwfow6Aw8k/QU7538gOTSY/ZTcAJB56BOPNTlN/jr/B0+f1nWCqeeSyWWEma2u4bR0aNYm7OOHGMOAboARkWNZFLMBMJ8w9yKc1LsBHYWOj/leHWbq5gWN5UgH/dODp6PEIIjJUfZlLeFInMR4T7hjI4eSY/gJI/KCzShsTZnnU24VteyxQd0YHqbaQyPGOpxqYJ7GU7RqExoS7bRuZDWE4lEIpFIJF4gzDeUZ3r8HxkVmSSXHgMB3UO6uWWOuq9ov9PskoLC4eLDPNrtESbEjGtwjEnB3ZkSO5mV2avqFXUTo8dzQ/vrGlRvadLMvJPyH/YXH7CftFRR2Zy/hQFh/Xm4y2/cKoDXhMaHpz5ha/62WpYNGRWZvH/yQ85UnuGG9td7FFtb/zaE+oRSbC52vC6CPqG9PZq3tdLyuTSJRCKRSJqADgHtmRI7iSlxk9x2n3fZvgaBWdRt0aK5efKwBkVRuLnDHO5IuI02fr8WjLfxi+PuxDu5veOtDT7Q8nXaNxwoPmiLq1ow1vy5r2g/36S71/Zod+EetuZvA6h3+/Tns7+QWnbao9h0io7pba50eF1FpWdID7e/X60dmcmSSCTNTm5+KQtX7GfD9hRMZitJnWO5ftoA+vVo39KhSS5z4gPi6z35VoOCQmJgRwBKzCUsy1rB+tyNlFnKCNAFMDZ6NFfGTXXqpm7RLKzIXsXK7NV2l/cewUlcETeF/mH9GnVauMxcxoa8jQ7jFwjW5W7g+vYzCdQ79zJbnb3WqeeYisrqnLXcG3SXRzFeETuFnKocVuesPS/TpqAh6BDQnoc6P+DRfK0ZKbIkEkmzsv9oJr9/aQEmswVNq25HklPM6s3HuOOG4dw3t2X8bCQSsHkufXDqI4fXFRTGRY8l31jAS0f/RrGp2C5CKqwVrMhaxZa8rTzX84/E1tPQ2qJZ+Ofxf3Gk5GgtIXSs9DhHS5O5r9M9Lq0anHG0NNllNs4iLCSXHmNQ+ECn4zIqM51unWpoZHjoaQW2LN7tHW9lTNRo1uVuILsqm0B9ICMihzEgvH+LnUxtCqTIkkgkzUZ5hZH/+9uPmEwWNPHrDcZaLbY+/34b3TvFMnZY87TkkEguZHjkUA6XHGZT3pY6pwcFgnsS7yTSEMHrx96sJbBq0NAos5Tz0alPeK7nM3XmX5W9uo7AqnkewKepn9EntJdT41FnuBJYNVg01+PcaaRt0BncWq8+EoM6khjUscHPvxiQNVkSiaTZWL7hCBUVxloC63xUVeGbRQ3rKyeReANVUbkn8S7u73QPCQHxKCjoFT39w/rxxx5PMTp6FLnGXA4WH3KY5dHQSCk7QWZFZq3HLZqFxeeWOjUh1YTGhtxNDY6/ZivTG+OGRgx26uyvAEPCvWsVcakhM1kSiaTZ2Hc40/ab2cE9RtMEB5PPoGkCVZUu9t4m35jPprwt5JvyCdIHMzJyGO0DWk8d3OnyNLbmb6PMUk60IYoxUaM86mPnLVRFZVTUSEZFjUQIUadGKq3cvS2ytIp0+/t7ovQE/075D6Uueh8CZFac8TzoamL9YukV0pOjJcn1ikAVlV6hvYjxi3Y51+TYSazNWY9JM9URhioqQT5BjIoa0eBYayi3lLMxbzNHio9gRaNbUFfGRY9x2w6jNSNFlkQiaTaEgwyWpGkRQvDjmZ9YdHaxrYWvAkLAL+eWMDJyBPck3tmi3kImzcz7Jz9kd+GeWpmThWcWcW27Gcxse02LtY6qb10fN98rfXXj9nOVWbxy7A2XJpxgq/lqrDv7vZ3u4q9H/k6BqbBOA+dw33DuSbzDrXmiDVE82f1x3jr+NuXWcnSooChYhZVw3zCe7P54o4xJAY6XpvDP429RZTXaYz1cfIRFZxfzUJcHXNaNtXbkdqFEImk2+vfq4DCLBbbtwt5JbWUWy8usylnDT2d/RiDQ0LAKzZ7l2Jq/jf+lf9Oi8X1++gv2FO4FbFttNf8JBAvPLGJd7voWje9CugV3dVmvpFN09ArpAcAv55Zi0eraPtSHhsaA8AGNii/CN4IXe/2Fa9vNINwnHJ2iI0QfQmJgR+IDOvDLuaWklp92a65uwV15a8Dr3Jt4F6OjRzM2egyPdHmQV/v+nbb+bRsVZ4m5hDeO1RZYYDsBaREW3j3xPmcqzzZqjZZGiiyJRNJsXDmuJwEBvqgOshKaJrjpmiHNHNWljUWzsOjMzw6vCwTrctY7NYdsSvKNBWzOq78XYA0/nfnZYx+qpsRf58+U2Mm1DDrPR0FhfPRYgnyCEEKwLX+7y/Y5YNuCa+vXlv5ecNAP8gniunbX8kb/VxgeMZQSSwmny9PYV7Sf1dlref7wS7x/8iO3xJ+v6suY6NHcnXgHd3a8jSERg72S+VyfuxGjZnT4vRdCsDJ7daPXaUmkyJJIJM1GYICBV5+5Hl9ffa1sla7677fPGs44ebLQq5wuP02JizogDY39RQebKaLa7Cva71RgARSai3jm4J946sCz/OfEBySXHGum6Bwzq/1MRkQOA7Bvcdb8OTB8ADfH3wTYTvuZhdmtOeP84vhD0uNe7bm3IPNHtlQbitZkB2sE37b8bXyXucBra3mKq++9hsbewn3NF1ATIGuyJBJJs9KvZ3u+fvtuFi7fz4btxzGZrCR1iWPWtAH069l6irAvFYxu1gG5Uy/UFJg0k92I0hlZVVkA5FTlsL1gB1fGTeWmDjd6XKtltBopNBcRoPMnxCekwXHrFB0PdL6PqbFT2Ji3mUJTAaE+oYyOGknnoM72uPSqnjCfMIrMRU7nGxQ+kEe6POhVgVVprWRF1ionxqSwOnsN17SdQeAFtVX5xgL2Fe3HpJlo79+OXqE9vd5w2Z0smqUed/2LCSmyJBJJsxMTGcz9N4/m/pul8WhT08Y/zmHD4xoEgnaNrK9pKO3927kUWOdTk4VZlrWC+IAOjHLTuLPIVMSCzIVsyd9qv3F3D+7Gde2upUdIkueBV+OO19PEmPH8eOYnp9+DG9vP8rqIOVpyzGUWzSwsHC1JZnCErcDcpJn5/PSXbM7bgkDYBXCkbwQPdn6ArsFdvBZfl6DOpFdkOHWU7xLU2WvrtQRyu1AikUguYSJ8I+gX1teh35GCQowhmqTg7s0cmY1eoT2J9I1wWN/kCAWFJeeWuzW20FTI80f+yqa8zbUyI8dLU3gl+XV2FezxaG1PmRo3mfb+7Rx+D65tO4M4/7h6rzUGi5vblOcLsQ9PfmQXWIBdABeYCnkl+fU63l+NYVLsBJeO8lNiJ3ltvZZAiiyJRCK5xLk94RaCfYLr3ORVVHxUH37T+f4Ws0hQFZUHOz+AXtE7Nb68EIEgszKTCkuFwzHF5mJ+zPyJpw88R6GpsM4NXVT/93Hqpw3eLtWERpW1yqk9ib/Onz/2eIoJMeNq2TNE+UZyV8fbua7dtQ1a2xXx/u41Wa5pxpxWnsbOwt31ZtwEAquwsujsL16Lr61/W25PuAWg1ve+5u9Xt5lO79BeXluvJZDbhRKJRHKJE2mI5IVef+Lns7+wMW9zdR2UypCIwVzT9mraB7Rr0fi6Bnfh+V7P8fPZX9hRsAsNDR0qVjdO5DmSNpkVmfzt6KtUWMtdbkZWWivZVbCHkVHD3Y75bOVZfj67hO0FO7AKK4G6QCbEjGN6myvrbbwcoA/g9o63cmOHG8iuysFH9SHOL9brW4TnE+cfR1Jwd46Xpjg0Ju0S1Nm+Vbw1f7vThtAaGjsLdmHW7sankV5eNUyKnUj7gPYsO7ecQyVHEELQJagzV8ZNpX94P6+s0ZJIkSWRSCSXAeG+4dze8VZujr+Jcms5/jp/t3rTNRftA9rzYJcHuFe7G6PVyN6ifXyc+l+H4xUU2vjFEaDzr3NNExpvHX+bSmulW9VeOkVnL6x3hxOlJ3jl2BtYNItdkJRby1lybhk7C3bxXM8/EuITXO9z/XR+JATGu71WY7m30128eORvlJnLaoknFZVAfSD3drrb/liZpczlfBq2zJ23RBbYauO6B3fz2nytCbldKJFIJJcRelVPqE9oqxJY5+Oj+hDkE8SwyGEE64McbiEKBFe2uaLebc4DxQfJNeW55U0FNlHmp/Nze+y7Jz/ArJnrbQ6da8xjfsa3AGRVZrH47BK+z/yBzXlbW+QEZ7Qhmhd7/ZkpsZPwr36Nfqofk2Mn8WLvvxDrF2MfG2WIcjmfQTXUcnkvMhWTU5WLWXOv/utyQ2ayJBKJRNLq8FV9eLzbY7x67A1MVpNd0NRsZ42PHsvYqPpPp54oPYlO0WEVVrfWEgi327ccLD5MganA4XUNjS1526iyVrGruk2QUt2K5su0/3Ffp7ubvVVMuG84NyfcxNz4OZiFBR9FX684HRM1ioVnFjmcR0VlbPRodIqO3YV7WHRmMacr0gCbcBsfM5aZ7a7Bv57s4uWKzGRJJBKJpFXSOagT/+jzV2a0vYo2fnFE+IbTN6wPv+/2O+7seLvDYn1FUdzuk6mgMDJyRK2MjjMyKjJcFuhraOw+r01QjdirtFbydsp/WsxMVVFsfREdvW+Rhkhmtrum3msqKmG+YVzT9mpWZa/h3ynvklaRbr9epVWxImsVfzv6CpXWyiaJ/2JEZrIkEolE0moJ9w3n+vYzub79TLef0zOkB4vOLnZr7PDIodzlZsNksGXYXDnUAw7HKCgsPLOIp0P+4Paazcm1bWcQ6hPCT2d+prDaQFVFZXDEIG6On4MmNP6XNg+o+xo1NDIqMll6brlH369LGSmyJBKJRHJJkRTcnfb+7Thbec5hXVbf0N7cHD+XNh76U/UL69eohtoaGkdLkykzlxHkE9TgeZoKRVGYEDOecdFjSa/IwKSZiPOLtbvj/3z2F5fGtmty1jKz3TVNenLyYkG+AxKJRCK5pFAUhd91e5Rw37Baj9ds842NGsPj3R7zWGABxPrFMCR8MKoT81R3/L4qtSqP1wYwa2bbqUk3t0MbiqqodAxMoFtw11rth85VZrk0ji21lFFlbdjru9SQmSyJRCKRNCnllgrW5qxjQ94mSs2lRPiGMz5mHGOjRmPQGZpkzWhDFH/r8xKb87ayLX87FdZK2vm3YULMeJKCuzfKfPW+TndTdaKKg8WHUFERCBQUNDT6hPbmUPFhp8/3VX0I9bBv4vHSFBafXcKB4oMIBKE+oUyOnciVcVOb9aSoO6cwFZRWe3q1uVFEU8vhJqakpITQ0FCKi4sJCWl4s0+JRCKReJ8CUwF/PfIPCkwFdbaZ4gM68HTS/9VpTnwxIIQgpewE2/J3UG4pJ9ovirFRYwjUB/Do3iccNjZWURkfM5Y7Ot7m9lo7CnbynxMf2IVcDQoKnYM68X/df99kYvVCjpYk84/k1xxeV1HpH9aPx7o90izxtASe6A65XSiRSCSSJuODkx9TaCqst44ns+IMX6V93QJRNR5FUegW3JXbO97Cg13u54b21xPjF02gPpDbO95qG3PBtpqKSrhvuEdtdMotFXx48hMEot62QCfLTrHk3LLGvyA3SQruTtegLo57YSowo+1VzRZPa0eKLIlEIpE0CZkVZ0guPea0Tcv2gu2UmEubObKmZVz0GB7r+ls6BLS3P6ZX9IyOGslfej1bq8bJFVvytzpt9CwQrM5ZiybcM15tLIqi8Hi3R+0O7SoqOkUHgL/Oj0e7PEKnoMRmieViQNZkSSQSiaRJOFF20uUYq9A4XX6avmF9miGi5mNgeH8Ghvcnz5hHlbWKSENkg0w6MyoyUVARTtzrSy2llFnKHbby8TaB+kCe7vEHTpWlsqdoL2bNTHv/dgyNGNJs25YXC1JkXSZoQrDjTCaniwoJMRgYm5BIkK8sTJRIJE2Hu0f4m+qovyY0zJoZX9W3UYXujcGdVjXOsJmH4rgTdjU+avPfzjsFJcqslQukyLoM2JaZwf+tWkZmSYn9MT+djmHtO9AjKpq4oGBmdEsi3F+2QpBIJN6jR0iSyzG+qg+dgzp5dd0zlWdZfHYJOwp2YhEWgvRBTIwZz7S4K2r13bsYGBQ+kJXZqx1eV1HoFtxNtrJppUiRdYmzL+scty/8HqtWO9VcZbWyPu00G9PTEELw8sZ1PDlyNPcNHNIygUokkkuOaEMUg8MHsadwb711WQoKE6LHe1UgHC9N4dXkN7AKq33NMksZi88uYWfBLp7r+QxB+iCswsq+ov3sLzqIVVjoGNCRkVEjWt1Jx6Tg7iQGJpJWnlbve6ghZKF5K0aKrEuc17ZsRBOO/Xm1agcPs6bx900bCPI1MLd33+YLUHJZc/xUNkdOZKFXVQb3SyAuWtqwXGrck3gnBaZCTpWfsjd3rvmzb2gfZneY5bW1rMLKuyfexyIs9bZ8ya7K4duMBcxoO53Xkt8k25htPyW3mS18m/k9D3V+gAHh/b0WU2NRFIUnuj3KG8fe4nRFmj1egUBVVO7seBu9Q3u1cJQSR0ifrEuUEqOR9adTeWz5Lx49LyoggC13P4BelQdPJU3Hmawinn9zMUdPZNkfUxSYOLI7Tz14BQH+sl7wUsKiWdhTtJdNuVsoNhcTZYhkbPQY+oT29mo91t7CfbyV8rbTMXpFT6hPCIWmonozQzpF5S89/0RCYLzX4vIGmtA4UnKUXYV7MFlNtAtoy5ioUR6dVJR4B090h8xkXWJUWcy8snkj3xw6gNFq9fj5eRUV7Dl3lqHt2rseLJE0gIKich58dh7FJRW1HhcC1m49Tl5BGf9+YQ46nRT6lwp6Vc/QiCEMjWjacoT0igx7lswRFmEh31Tg8LoQsCxrOQ90vq8pQmwwqqLSO7SXzFpdZMjfYpcQVk3j/p8X8uWBfQ0SWDWUmoxejEoiqc33S/ZSVFKBVaubRNc0wf6jZ9ix73TzBya56PFRfZw2L3YHDY1dhXu8FJHkckeKrEuIladOsikj3V5n1VASw8K9FJFEUpclaw+h1SOwalBVhWXrnfd+k0jqY0BYf5ciy0fxcTmPWTM3eQPm7Kps1uWsZ23OOjIqMpt0LUnLIbcLLyHe2ralUc/XKQr949rQKTzCSxFJJHUpKa10el3TBAVF5c0UjeRSoo1/HAPDBrCvaL/DLcMeIUkcKj7s8LqCQjv/tk3mq1VmKeOjU5+yr2h/rce7BXXlwS73E+Erf/9eSshM1iXC3nNnOV6Q1+Dn6xQFP70PL02Y7MWoJJK6REUEOb2uUxXaxIS6NZcQgoPJZ/jbO0t5+Ll5/PGVhazdegyLpeHb5ZKLm/s731Or5YuCYj+RN7PtNdyWcLPTbJdAMDl2UpPEZtEsvJL8BgeKDta5dqLsJC8feYVyS0U9z5RcrMhM1iXCp/saXkOgKgqTO3Xm9yNG0yUi0otRSSR1uXZqP97/aqPD7RirJrhqkusWK5omePX9FSxefRCdqmDVBKqqsGHHCbolxvDmX2YTGiwNGi83/HX+PJX0JMdKj7OtYAcVlgpiDNGMjR5DjF80ALcm3MyXaf+rt0i+f1g/xkaPbpLYdhTsIr0ivd5rGhr5pnzW525gepsrm2R9SfMjRdYlwsb00x4/58uZNxAdGEh0QKB0e5c0G9dd0Z+law+RfrawTm1WjY1D36R2Luf5+qcdLF5tywjUFNHXzHciLZcX3lzMP/8828vRSy4GFEUhKaQ7SSHd670+OXYiMYZofjm3lOTSYwBE+0YxNW4yk2In2hsee5vNeVtQUBxm0gSCjbmbpMi6hJAi6xKhvpNazgj29WVw23YY9PJHQNK8BPj78u5f5/LWx6tZs+WY/Wc3wM+HG64axN1zRrqsh7FYrMz7aZfD65om2LE/jdSMPBI7NK53nOTSpG9YH/qG9cGkmbAKK36qX5P3Nyw2F7sszC+xlDZpDJLmRd5hLxEGtWnL5ow0rG6ciFEVhVv69JcCS9JihAb785fHr+bRuydw4nQuOp1Kz65t8DO4PvkFtkxVsYsCekVR2LHvtFdEVlpmPr+sOcTZnGJCg/2ZOqYHfXu0a7Gmw02BxWJl/fYUFq8+SE5eKVERQVw1sTcTRnTHx6dpMjutAV+1+Yxvow1RnKk867ToPspXlmxcSsi77CXC3QMGscHFlqGqKGhCMKpDPI8NG9E8gUkkTggPDWRIv0CPn2e1uv4woShgtTo2pXQHIQT/+WI98xbtQqcqaMJmMfHTiv0M6ZfA3/7vWvz9Ln53+opKE0/+dQEHks/Yf09knCtk98F0vvtlD2/+eTZBgYaWDvOiZ1z0WPYU7XN4XSAYHzOu+QKSNDnydOElwtiEjvx26HDAdlKwhpq/hRgMDGrTjjevmM4n11wvs1iSi5rEDpH4+jj/GdY0QY+ubRq1zvdL9jJvkW1b0qoJhBB24bb7YDp/f3d5o+ZvLfzr0zUcOn4W+LWfaU1927FT2bz6/ooWi+1Som9YH/qG9kGhbgZURSUxsCOjoka2QGSSpkLeaS8hHh8+iqHt2vP5vr3sPncWvaowoWMn7ug/kB5R0S0dnkTiNQL8fbl6Um8Wrthfr7GpTlVo3zac/j0b3h7KYtX4YsE2h9c1TbBmyzEevG2s25YTrZHC4gqWrT/i0CBW0wTrth4nJ7+UmMjgZo7u0kJVVB7t+jDfZ/7Ampx1mDQTAHpFx8jIkdycMAdf1b0tc8nFgRRZlxijOiQwqkNCS4chaYUIIThw9AyHjp9FVRWG9O1Il44Xr/j+za1jOZKSxbFTWZxfiqgoti29uOgQ5v+8m2kTejXIyuFEag6Fxc49ixQFtuw+xaxpAzyev7Vw6NhZl9uqmhDsP5LJlDE9mimqSxcf1Ye58XOY2e4aTpenoQmNhMB4gvTO/eMkFydSZEkklwHpZwt49tWfSM3IR1UVEPCuWM+AXh148fdXEx7qeV1USxPg78u7L81h0aqD/LRiP2eyi7BaNDQhsGqCnfvS2LHvNB9+vZHnH7+ascO6ejS/yQ1DU0VRMJktDX0JrQJ328c0bZOZyw9/nT89QpJaOgxJEyNrsiSSS5zC4nIefu4b0s8UALbtn5q6mwNHM3ns+e8uWqFgMPgw+6qBfPzqrYQG+VNTjljzGoUAk9nKn974mZTUHI/mTmgXgU7n/Fekpgm6JcY2NPxWQc9ubWzC2wV9urdthmgkkksLKbIkkkucH5bto7i0sl4vNasmOJWex7ptKS0QmfdYtTGZvMIyJ35xgvk/7/ZoztBgfyaPSnIoQFRVoV1sGAN7d/Aw2tZFVHgQE0d2d/o6Rw3pfFHXnUkkLYUUWRLJJc6ydY6LmsFm7bF8/ZFmjMj7bNp1wqlnldUq2LDDcyH5yJ3jaRsTWkeA6FQFP18fXvj91ZeEV9bv759M5wRbfV7Ny1EU2+nk+LYRPPPQFS0XnERyESNrsiSSS5zS8iqn1zUhKC65uJvSGk0Wl7VFZrPnTaPDQwP48JVb+WbRLn5asZ/i0koMvnqmju3JLTOH0L5NeENDblUEB/rx/stzWb7hKD+vOkBOfilR4UFcPakPV47veUl4gUkkLYEUWRLJJU6bmFBOnM7BkQbRqcpFLxa6Jsaw+2C6w4ydoih0TmiY83tIkB/33zya+28ejclswUevuySyVxdiMPhwzZS+XDOlb0uHIpFcMsjtQonkEmfm1H4OBRbY6rKumXxx31ivndLPaSZLCMEN0wc2eh1fH/0lKbAkEknTIEWWRHKJM21CL/oktXVY2Dx1bA8GXOTF2+3iwnj83kkAtV5njR6aPDqJqWN7tkRoEonkMkYR7pqktFJKSkoIDQ2luLiYkJCQlg5HImmVVBnNfPj1JhatPECV0QxAaLAfN149mFuvG+rSqqCpMZktbNh+gpNpuRgMesYM6WIvxPaEnfvT+PqnHezan4YAOraPZPZVA5kxua9bNgUSiUTiCk90hxRZEsllREWlidOZ+eh0Kp06ROHjo2vpkNix7zTPv7mYkrIq9DoVTQg0TTBiYCLPP341gQGeNya2WjU0TbSK1yeRSC4tPNEdcrtQIrmMCPD3pWfXNnTvFNsqBMixU9n8399+sJ+AtFSLI4Dt+07zzCsL3XYkPx+dTm0Vr08ikVzeSJElkUhajM+/34aodma/EE0T7DmUwcFjZ5s/MIlEIvECUmRJJJIWwWS2sGnHCScu7baM1JrNyc0YlUQikXgPKbIkEkmLUGW02HsoOkQIyitMzROQRCKReBlpRiqRSFqEoAADwUF+lJY5dqQXcFEapQoh2Hckk+Xrj1BUUkFMZDBXTepD904XdzNpiUTiGVJkSSSSFkFVFWZO7cf/Fu5w3FtRwPQJvZo3sEZiNJr542uL2L43FZ2qYNUEOp3KD8v2cfWkPvzhgSktbpkhkUiaB/kvXSKRtBi3zBxKfNvwOh5WNSaiD98xjujI4BaIrOG8/uEqdu47DWCvN7NaNQAWrz7IFwu2tVRoEomkmZEiSyKRtBhBgQb+8/LNzJzaDz/Dr4n1hHaRvPDE1cyZMbgFo/OcvIIylq8/4rTW7Jufd2GsNoSVSCSXNnK7UCKRtCghQX48cd9kHrxtLNl5pRh89cRFhzRJj8Dc/FLyi8qJDAtskgzZ9n2pLov5yytMHE45x8De8V5fXyKRtC6kyJJIJK0Cfz9fOraPbJK5j544x3tfbmDPoQz7YwN7d+DB28bSo0sbr61jtmjujTNbvbamRCJpvcjtQomklVFcVcWm9DQ2Z6RRajS2dDgXPQeTz/DQs9+w70hmrcf3HcnkoWe/4UDyGa+t1b1TjMsxqqI0qC+jRCK5+JCZLImklVBuMvHyxnUsOHoYs2bLiBh0Oub27stTo8Zi0Mt/rp4ihOCV91bYehlesI2naQKExqvvreDLt+70yvZkUuc4uiXGcDItt16TVZ2qMHpoF6Iighq9lkQiaf3ITJbkksHWnsXzPnd7z53l9yuWMvnL/3L1vC95e8dWcsvLmyBCxxgtFm5f+D3fHjlkF1gARquVLw7s496ff8SiubcVJfmV5JNZnM7Md1gnpQnB6cx8kk9meWU9RVH4y++uIjDAUOfEpKoqxEaH8Pv7JntlLYlE0vqRH40lFzVCCJakHOe/+/awP/scqqIwJr4j9w4czPD2HVw+/81tm3l7xzZ0ioK1+kacnJfLR3t28fm1sxjQpm1TvwQAfj6ezN6sc/Ve04Rgc0Y6q06d5MouXZslnkuFzHNFbo07k1XstdqshPaRfPbPO/hm0S6WrDlEWYWR8NAArp3SlxuvHkRIsL9X1pFIJK0fKbIkFy1CCJ5fv4YvD+xDVRQ0IbAKwfq0VNacPsWL4ydxa9/+Dp+/4mQKb++weRZZz8t0aEJQYTZz96If2Xz3/QT4+DT1S+GbQwdRUBDUn3FRFYX5hw80SGRZLFbWb09h+95UzBaNpM6xTBvf67K42QcH+bk1LijQ4NV1YyKDefSuCTx61wQ0TdTJakkkkssDKbIkFy2rTp3kywP7AGptB9UIpr+sW83IDvF0Co+o9/kf7dllF2cXoglBsbGKRceOclPvvt4P/gIyS4sdCqyaeDJKSjyeN+NsIU+89B3nckrQ6RSEgFWbjvLh15t4/vGrGTO0S2PCbvUM7N3BZeue4CA/BvZ2nfVsKFJgSSSXL7ImS3LR8vn+PeicFCurisLXBw/Ue82qaew5d9app5GqKGzJTHcrlvyKCt7ZsY3JX3zKkI/eY/Z381iYfMTtOqoo/wCc3YoVFKIDAtyaqwajycJjz39LTl4pAFarQNMEQtiuPffaTxw/le32fFarxpmsIjLOFmKxXBwWBL4+eu6dM9LpmHvmjMTXR37elEgk3kf+ZpFctBzIya61zXchViHYn11/nRPgJG/k2aBThQXctGA+BZWVdtFWWFXJ7nNn+SH5CB9dPdPlycAbevbmpQ1rnYQhmNXDsx5+azYnk5Nf6nTM/J9386fHpjsdo2mC75fsYd5PO8ktKAMgLMSfG64ayK0zh6LX6zyKq7m5ftoAjCYLH83bjMViRdWpaFYNvV7HvXNHMWvagJYO0SsIITiemkNBYTlRkUF0SYhuEkNXiUTiPlJkSS5afFTXN3dfXf1jdKpKv9g4DuZkO8xmCSEY0q690/mFEPxm8U8Uniew4Nftyy0Z6by9YxtPjhztdJ4bevbm8/17ySwpriMcdYpCl4hIZnRLcjrHhWzaeRJFAUc61KoJ1m9P4U9O5hBC8Or7K1i8+mCtx4tKKvnkm80kn8ji5T9c6/WGx0IINmxPYcHSvaSk5uDjo2fc8K7Mnj6Q+Hb1b/86QlEUbp45lBlT+rJ2y3HyCsuICg9iwshuBAe6V7PliFPpefy0Yj9HUs7hZ9AzanAXpk/sTYibtWDeYtveVN7+71rSzhTYH+sUH8Vjd09kUB/pLC+RtBRyu1By0TK5Uyen24UKMCmxs8Pr9w4Y7FBgqYpCoK8v1yX1dBrDtswMThQWOMyoaULw5YF9GC0Wp/ME+foy/4Y5DK1H1I2JT+B/18/22CerymR2KLBqMLvY9ttzKKOOwKpBCJuQW7ftuEdxuaLG2+rZ1xax70gmpeVGCorKWbRiP3c88Tk7qpsve0pwoB/XTOnL3TeO5JopfRstsOb/vIvbH/+Mhcv3cfREFnsPZ/LuF+uY8/DHHm3DNpbNu07yh5cXkH62oNbjqRl5PP7id+zcn9ZssUgkkto0mch6+eWXGTlyJAEBAYSFhdU7Jj09nauuuoqAgABiYmL4wx/+gMXFzUgiqeGu/oMA6q1lUhWFEIOf0y226V27cd9AWwPi88WaTlEw6HR8POM6gnx9ncawJ+usU6EHUGoycqqw9g1QCMGm9DQe/GURk774lGu/+Yqfjh3lP9OvYfktd/LyxCn8beIUVt9+N59eO4sIf8/qsQC6doxxWnStKLZshzMWrtiPzskcqqLw47J9HsfmjKVrD9uFnXaeoadVE1isVp599SfKylvWCX/73lTe/mydPa4ahIDyCiNPvPQ9lVWmJo/DatV448NV9rXPRwjb///8eFWD/OMkEknjaTKRZTKZmD17Ng8++GC9161WK1dddRUmk4ktW7bw+eef89lnn/HnP/+5qUKSXGIkRUXzzvQZ6FUdarXQqZEDIQYDX1x3A6F+jrMViqLwzOhxfHXdbCZ36kzboGASw8K5b+AQVtx2V71ZpTpzOC1Xr71WDZoQPLtmJbcv/J5Vp06QWlTIwZxs/rFpA1O++i+qAnN79+Wm3n1JDAt3a/76uGZKX6c3VyFwWY+UlpFfr3P5+a/l/C0qb/DNz7twpFuFsGXolq0/7NU1PeWbRbscClhNExSVVLJqU3KTx7H/SCY5+aUOM5ZCCDLOFnIkxTtmqxKJxDOarCbrhRdeAOCzzz6r9/qKFSs4cuQIq1atIjY2lv79+/PSSy/x1FNP8fzzz+PrIoMgkQBc0bkrm+6+j+8OH2Jf1jl0qsro+ARmdu9BoJs/QyM7xDOyQ8PqVkZ2iOf1rZucjon0D6DzeTYSXx3YxzeHbZma87cZBVBYWck9i35k9e13o1Mb9xmobWwYj987iX9+tBpVVWplhQDGDe/KtPHOi+mDgwxO67oAAgO85zFlNls5lZ7ndIyCwqFjZ7lh+kCvresJmibYfTDd5cnUXQfSmDG5ae0/sl0cbKghJ7+EXnivEbZEInGPFit837p1K3369CE2Ntb+2BVXXMGDDz7I4cOHGTCg/k/YRqMR43lNc0sa4B0kubSIDgjkoSHDWmTtfrFx9I2N47CTk4539R+IT3UBviYEH+/dhUL9BxetQpBeUsy606lM6uS4nsxdrr9yAB3ahPPVjzvYczAdAXRoE87sqwdy7ZR+LgvWJ41O4sBRxw2UVVVh6tgeABw7lc2G7SlUGc10io9i0qgk/AyeGbm6dRhOwWXcZ7KKSD6ZhU5V6d+rPWEhnm+3OsPV9ptAYLU2fRuksBD3DGXDvfz6JRKJe7SYyMrKyqolsAD711lZjlPbf//73+1ZMomkpVEUhfemX8PcH74lvbjILp5q2vRc0y2JBwYNsY/PKS8j08UHA72qsu1MhldEFsCQfh0Z0q8jFosVq1XD4IHwuXJcL/734w7yCsrqbBuqqkJwoIEpo3vwxIvfsWN/GjpVQVEULFaNf326lj89Ot0jw1O9Xkf/nu05kHymTuatBk0TDO6bUO+1vMIy/vGf5Wzbk/rrnDqV6RN789hdEzx67Y5QVYXunWM5dirHqdjq3b1do9dyxeA+CYQG+1Fc6thsNToiiD5JTR+LRCKpi0f7EU8//TSKojj9Pzm5aesQnnnmGYqLi+3/Z2RkNOl6Eokr2gQHs+Tm2/nbxCkMbdee7pFRTO3chc+vncWbV0yvte3nbv1xU9Qp6/U6j0VGgL8v77x0EwntIwFbBqkmixQbFcLbL87htQ9WsOugzbTVVpxuy+BUVpl49rWfOHTsrEdr3jxziEOBpaoKkWGBTBzZvc610vIqHnp2HjsvOH1osWosXnWQp19Z6HBeT7lxxmCnAstHr2P6BM98zRqCj4+Oh24f53TMw3eM97rFhkQicQ+PMlm///3vufPOO52O6dSpk1tzxcXFsWPHjlqPZWdn2685wmAwYDB4t8+YRHIheRUV7M86BwoMjGtLuL/zbZkAHx9uqi5Wd0ZsUBBtg4M5W+q4lsaiaQxp13oyD21iQvn8n3ew91AGuw+lo2mCvkntGDYgkcPHz7LnUP0fdISwbf99vmAbr/3xerfXGzmoMw/dNpb/fLkBnarYM2iKAiFBfvzzzzdg8K37q2vh8v2cyympV/xoQrBzfxo7959m2IBEt2NxxJTRSRw4msnC5fvrvR4VEYTJ7Nge4+iJc6zamExxWSVtokOZPrE3bWJCGxTLVRP7oGmCd79YX+vUZWiwH4/eNZHJoz3zV5NIJN7DI5EVHR1NdHS0VxYeMWIEL7/8Mjk5OcTExACwcuVKQkJC6NnTuTeRRNJUlBiNPL9uNT8fT7bXWOlVlRt69OK5sRMa3SxaVRTu7j+Ilzeuq7cmS6coxAYFOfX3agkURWFgn3gGXmBsuXbrcXQ61WH9kaYJtu0+RZXR7FF91s0zhzJ8YCILl+8n+WQ2fr56xgyzFeo7aub886oDTrNLqqqwZO0hr4gsRVG4ZnJffl55oN7Tl9m5Jfzuhe/47J93oD8vi2Q0mnn+rV/YuOOELbskbB0r//vdVu6aPYK754xskEv7jMl9uWJcT7btSSW/sJzoyCCG9U/Ex6d1u/FLJJc6TVaTlZ6eTkFBAenp6VitVvbt2wdAly5dCAoKYurUqfTs2ZPbbruNV199laysLJ577jkefvhhmamStAhGi4Vbf/yWo7m5tYrYLZrGt0cOcaqwkK+un42+kaf+7uw/kEM52Sw8dtReuwU2ARZsMPDxjOsavUZzUVHp2gtKgMciC6BTfDRP3DfZ7fF5heVOr2uacNlmyBPmLdrl8JpVE5zOzGfzrpOMG9bV/virH6xk886TtjEXCNP/freViLBArruyf4Pi8fXRM/a8tRyRnVfC4lUHOZWeh5+fD2OHdWXU4M61xKBEIvEOTSay/vznP/P555/bv645Lbh27VrGjx+PTqdj8eLFPPjgg4wYMYLAwEDuuOMOXnzxxaYKSSJxyo/JRziUk1PvNU0IdpzNZMXJFKZ3rVsP5AmqovDG1Glc1bU7Xx3cx/H8fAJ9fZjRLYm5vfsR5WEj6JYkvm24yzqn4CC/et3VLVaNTTtOsGTtIXILyoiJDOaqib0ZNbizxzVEmecKMTvZngNbJismMtijeR0hhGDdtuNOPcR0qsKGbSl2kZWVW8KKDUec1tt9sWAb10zp22Q1VD8s28tbH68BxfYaVEVh+fojJLSL4K2/zCbaS++PRCKx0WQi67PPPnPokVVDQkICS5YsaaoQJBKPmH/4oENrBbCJo/mHDzZaZIFtu2lSp85eO0HYUkyb0IsPvt6E1eq4UP3aekRDRaWJJ/+6gAPJZ+weXifTctm86yQDe3fg1T9e71Hm661P1uCqm7emCaZP6O32nM4QApeizqoJKs5zfd+6+5TLhuO5BWWknM4hqbPjutSGsnXPKf750WrbF9Vx1GRRM88V8uRfF/DfN+5w2iVAIpF4hswPSyTVnCsrdXoP1ITgjJOC9cuR8NBAHr93EkCdWiJVVUhoF8Gt19X1MHvjo1UcOm47dViTCav5c9/hTP716Vq3Y8jOK2Hb3lSXJzK7dYphSL+Obs/rDFVVaBcX5tTvX1UVOlafygSbU73ihoAxmpqmtdiXC7Y7FFBWTXAyPY+d+083ydoSyeWKFFkSSTXRAYHOb5qKQmxgYLPFc7Ewc2o//vH0dXTvFGN/zN/PhxumD+Q/L8+tU6ieX1jOyo1HHftgCcHStYcoKqlwa/0z54rcGjd5VJJXszSzpg2ov3FmNUIIrpny62nTzvHRLrdWdapCfNuGt1JyREWlyan3GNjsObbsPuX1tSWSy5kWMyOVSFobs3v25oX1axxe14Rgdk/vbDddaowe0pnRQzqTX1hOldFMVERQvTYLAPuOZLgUGxarxoGjZ9wq5A7wd699Ukiwe+7o7jLzin5s3HGCfUcya51qVBUFTQh+e+cE4qJDWL89he9+2c3hY+eczqdTFcaP6EZ4qPeFvNnifGuzBpO5abJoEsnlisxkSSTVzOrRi07hEejqOUKvUxR6x8QwrUu3Fojs4iEyPJB2cWEOBRbgtiGou+O6dYp1WdCu06mMGuzd+jdfHz1v/GkW99w0kvDQXw8rdO8cy9+fmsnsqwbyzufrePbVnzhw9IxToaOqCtGRwTx610SvxlhDcKAfUeHOxZtm1eiaGON0jEQi8QyZyZJIqgn09WXerDk8tWoZ606n2uuzFGBKpy78Y/JUDHr5T+Z8LBYrpeVVBPgbnAqr8+nVzXWjYkWBnm6MA5tAuW/uKF5+Z1n9cwE3TB9QSwh5C18fPXfeMILbrhtGYUkFvnqdPWO2eddJ5v+8G3AuGAP8fZkxuQ+3XjesSWIE23s0a/pAPvx6U71eYooCBl89V4yVHoUSiTeRd4xLkLyKClLy8/DR6egTEyuFgQdEBQTwyTXXk1ZUxJ5zZ1EUGNKuPe2CQ1o6tFZFXkEZn32/laVrD2M0WdCpCuOGd+PO2SPoFB/l9LltY8MYMbATO/al1muBoKoKY4Z08chuYdqE3pSUVfHelxuwaho6VUUTAk0TXHtFPx68zXnrmcai06lEhQfVeuy7xbvtJyfrQ1Fg1pUD+O1dE5ql7c2cGYPYdSCNPYfSQfx60FFXXaf2/ONXExggPQolEm+iCFft5Fs5JSUlhIaGUlxcTEjI5X0jzK+o4KUNa/kl5Zj9aHaYnx/3DRzMA4OGojbASVoiuZCs3BLuf/p/FJdU1BJJOlVBr9fxrxdupHe3tk7nKCgq5+E/fUPmuUL7Db/mxzOhXSTvvDSHsBDPszrFpZWs2HCUrNxiwkICmDw6qcHtahrL1Fv/7dKstV+Pdrz717nNFJHNduLH5ftYsHQvZ7KK0OtUxg7rys3XDiGpi/dtIySSSxFPdIcUWZcIJcYqZs7/HxnFxbXcymu4tU8/Xpzgvnt2a6egsoKlJ1IoqKygTVAwV3bpRpCvewXQksbx1N9/ZNueUw6zUG2iQ/nm3XtctocprzCyePVBFq8+RH5hGVERQcyY3JerJvZ2u5i9NTPtjncoLatyOmZQn3j+9fyNzRRRbSxWDZ2qNKiNj0RyOeOJ7pD7SJcIn+7dQ3pxMZoDzfzVwf3M7d2XHtEXd2GrEIK3tm/hvV077NtCFk3jL+tW89zYCcx10aBZ0jhy80vZsvukQ08qTROcyS5i76GMOn0OLyQwwMCcGYOZM2Ow1+PUNMGh42fJzS8lIiyQvkntmmVL7nxGDerEyo1HHbrCKwqMGNipWWM6H9lGRyJpeqTIukSYd+iAQ4EFttNx3x45xF/GNc3ppebinZ3beHvHNvvXFs3W/63SYuHZNSsJ8PHh2u49WiQ2TQh2nsnkTGkJYX7+jOoQ36L1cFUWM2tST5FdXk50QACTEjvj38gG12lnClyafioKpGbkuRRZTcXW3ad485PVnM0utj8WHRnEb++cwMSRjXfrd5c5MwazYuPReq+pqkKAny/TJ/RqtngkEknzI0XWJYAmBLkVzpvjWoXgbGlJM0XUNJQajfxn5w6nY17fsokZ3ZKavf5sY9ppnl2zkszz3uNQg4E/jBzDzX36Ndm6ZquVdadTSSsuItTPjymdOhPm58+3hw/y8sZ1lJpMdt+mQB8f/m/UWG7r27/B6/n5uRZpQoDBw2bQ3mLrnlP8399/qPN4bn4Zf37jZzRNMHl0UrPE0jUxhr/87ipe/NcSRHURPthEaKC/L2/86Qave3dJJJLWhRRZlwCqohDs60upyXGRrU5RCPPz3i/0SrOZ5SdTSC0qJMTgx5Vdujb5Cbw1p09htDo3SzxTWsLB7Cz6xbl3/N8bbMlI565FP9Q5Gl9sNPLc2lVYNI3b+w3w+rqrTp3g6dUrKKistAup51SVsQmJrE49aR9Xk+EsN5v5y7rV6FW1wduqSZ3jCA8NoLDYsRu7TlUYOaj5t8GEEPZ2PI6ybf/+dA3jR3Rrtq2ySaOS6JPUjp9XHuDQ8bPodSpD+ydy5fie9TbNvtwRQrB51ykWrtjH6Yx8AgN8mTy6BzMm92nQQQiJpKWRIusS4foevfjqwL56i97Blsma6aVttMXHk/njmpWUmUzoq4/K/23jOm7q3Zfnx03ER6fzyjoXUlxV5bSBs32c0dgk6zvibxvXgRAO43p1y0Zu6NmbgEZu1Z3P5ow0fvPLIruwqxFSZk2rJbDq47UtG5nVoxe+Dfg+6XUqd94wnDc/qd8ZX1FgxpS+RIQ1f/uhoyeybKcVnVBQXMHuA2kMG5DYTFFBTGQw99w0qtnWayxCCLbtSWXhin2cSs8n0N+XSaOTmlzoWK0aL7+zlBUbjtayvjiVvon5P+/i7RfnkNjBuT2IRNLakJWPlwj3DhhMoK9vvW7lqqIwqkM8w9t3aPQ6G9JO89iyXyivzppZNA2tWmB8c+gAzztpS9NYEkLDXAosgA6hzXdk/0RBPkfyctGcjKkwm1l16oRX131t80anws4ZRVVVbM5Ia/Da108bwJ03DEdRbLVFOp1q91qaPLoHjzWRa7kr8grK3BqX6+a4yxFNE7z8zjL+8Lcf2LYnlXM5xZxIy+XDrzdy62P/JTUjr8nW/u6XPazYcNQeRw1CCErKqvi/v/2I1ersX5pE0vqQmaxLhHYhIcy/4SZ+u3QxJwrya/Wtvaprd/4+aapXjmr/c+smFKhXVNQIrYcGD6NdE9hpjI5PIDYwiJzysnrFhU5RGBDXlsQw7zfYdURuufNaOLCJ3NwK95odu0NGcTEHcrIbNUdhZWWDn6soCvfOHc3Vk/uybN1hsnJLCAvxZ+rYHnSKj25UXI0hwkXbmBoi3Rx3KXH0xDlWbUqmtNxIu9gwpk3oVa/Z6/dL9rBs3WGAWqcihYCSsir+8LcfmP/OvV4/qalpgvmLdzm9fi6nmG17U73eHkkiaUqkyLqE6B4ZxfJb7mD3ubMczs3GV6dnbEJHr9VKnSkpcXlzVxSFpSeOc+9A7x3LP5yTzXdHDpFZUkKXiAhyysvqbBvqFAWDXs8L45s3ixIbFORyjCYEcYGux7lLYVXDBVIN3viZiIsO4c7ZIxo9j7fo1bUNbWNDOZdT7LAmKyzEnyF9E5o3sBakymjmL//8mc27TqHT2T5kCQGffLOZB24dwy0zh9rHappg/s/OhU5WTglb96Qyeoh3hU5OXgm5+c4zjDqdyr7DGVJkSS4qpMi6xFAUhcFt2zG4bTuvz11idG6sCLasTanJOzVRmhC8sG41Xx7cj05RsAqBTlEQQKCPD+VmM2DrTTeqQwJPjx5LUlTzZlI6hUfQNyaWQ7k5Di00gn19mdTJe4XgbYKD3apNqw8Fm8Aa0q691+JpLSiKwqN3TeTpf/zocMxv75yAXu+6Fk0IQfLJLAqKKoiOCKJrYsxFadr5t3eWsXV3KgBWa+2fmPe+3EBEWCDTxttsJHILSsnOK3U6n06nsv9IhtdFFm6+txfj90ByeSNFlsRt2gQH28WOIyyaRnxomFfW+3jPLr48uB/AvmbNn5UWC2MTOvL48FHEBQa5lVE6n/TiIj7fv5dfUo5RaTbTLTKK2/r25+oG2D88N3YCN//wLQhR7zbqH0ePw0/vvaL36IBAxnfsxIa0VKffi5oThzUo2G5Sf504pcVbLJWUVVFUXEFoiD+hXrQxGD2kM39/aiZvfryanPxfBUNEaACP3DmeqW40QN608yRvf7aWM1lF9sc6xUfxu7sntpj3V0PIPFfImi3HnI7577dbuHJczxYXLzGRwcRGBTsVeVarxv6jmfzny/VcM7kv7ds0X1mARNJQpMi6SNCEYHN6GrvPnbUdke8Qz8C4ts36yzHMz59pXbqx9MRxhzf3AB8fpnXp1ui1zFYrH+ze6fC6JgQb007zwrhJHgusnWczuXPhAkxWq/117M06x+5zZ1l+MoV/X3k1OtX9mpPBbdvx5cwb+NO61ZwoyLc/HhMQyFOjxnJdD9c3dk/54+ix7DybSaXZXOt7UZPh+s2goWzOSOPgedu73aOieXbMOEZ1aLntstSMPD76ehObdp5EE8Luen7fzaPp2tE73QhGD+lMfNtw9h3JRAhoFxfKgN7xbtk2rN92nGdfW1QnsZKakcfjL37HP/88m0EXidDatPNkHaF9IWezi0k7U0DH9pFERwQTGx1Cdq5jPz2rVaNfz8YfoLkQVVWYM2Mw//7vWqfjDh8/R/KJLL5euJN7547izhtaz3a1RFIfUmRdBKTk5/PALws5XVSETlEBwZvbttAnJpb3r7qWNsF1C1ibiqdGjWVrZgZFVZW1bu6qoiCE4OWJU7xiVXAkN8et2qP1aancHua+B5XRYuGBxT9htFpr3Xxq/r7sRAqf79/L3QMGeRTvsPYdWH7LHRzMySazpIQIf38Gt22H3oFYy6+o4EheDjpFpX9cG4/fs84RkSyYfTMvbVjDpox0++MJYWH8YeSYaqE7hhMF+WSXlxEdEEjXiMgWzViknM7hoWfnYTJZ7O+3ELBtbyq7Dqbz9gtz6NWtcf5ma7ce45NvtnA60yZ29TqVSaOTSIyPIircuRi3WDX++fFqe1znIwRoCN78eDVfvnVni2d+3KHKZEZRFbA631iuMtq23VVV4aYZg/nXp/WfEFZVhdioEEYMbBr7ixumD+TE6RyWrD2MTlUctiOqefzjeZtpEx3KFeO8/yFGIvEWUmS1cnLLy5m7YD7F1fVQVvHrhtSR3Bxu/uFbltx8e6PbpbhLu5AQFs65hX9s3sCy8zJaPaKieWLEKCZ09E7tkUmzuhyjKAomq+tx5/NLyjGKqhzXlgngs317uKv/QI9vpIqi0Dc2jr6xcQ7HFFdV8eKGtfx8PNneEshf78Pt/frzxPBRHnmMdY2M5KMZ17ElI52c8jJ6REXTJzauVtxdIiLpEhHp0etoKl59bwVGk6XW8XywFVRbLFb+/u6yRgmYhcv38fqHq2ploSxWjVUbj7L3UAYfvXKr05OFew6mk1/o+LSoEHA6M59jp7JJ6uz4e9xa6NQhyqXlgV6v0i4uzP71rGkDOHYqm2XragsdRVEICfLj1T9e12Q9IFVV4ZmHr2TSqCQWLt9P8qksp8XwigKff7+VqWN7XBSiV3J5IkVWK+erg/soMlbVm/K3CkFacRE/H0/mxl59mi2mdiEhvD3taoqqKjlbWkqwr8Hr3lRdIyLRVzd/doQmBH1iYj2ad2/WOZfzZpaWkF9ZSVSAd40XK8xmblownxMF+bWygJUWMx/u3snpokL+M/0at24YRouFf+/YylcH9tmd/sP8/Liz30AeGjLMYQatpTiVnsvRE1kOr2ua4HRmPodTztG7W1uP5y8ureStaoPUC/+pWDVBfmEZ//12C08+MMXhHDkuir7PH3cxiKyRgzsTERZAYXFlnW4EYBM1U8b0qOU8r6oKzz5yJZNGdWfh8v2kpucREODL5NFJzJjc16v1c/WhKArDBiQybEAin8zfzBffb3OY0RIC0s8Wsv9oJqVlRnx8dPRNakeAv2+TxiiReIIUWa2cn5KPOq2pUIBFx442q8iqIczP36utei6c+9ruPViYfKTe+i+dotAxLJyhHp6Sq8+stT70qvc/GX9z6ADH8/PqPRUogOUnT7AlM91lzZRF03hg8U9sykir9bNRVFXFv7ZvITkvl3emz2jx4vbzyTjr3In9/HENEVnL1x/B6kQ4WzXBknWH+e2d4x32VQwPc09Uh4deHO1d9DqV5393Nb//6wI0TaslVlRVIS46hIduG1vneYqiMGJgJ0YMbP7WSOdjNlurP3A43+585E/z7X/3M/hw49WDuGfOyCbLuEkkniB/Cls5JS7sEATN30amuXh2zDgSw8LriAWdohDo68u/p11tz/qYL6ixcsTo+ASnWSwVhaSo6CYRj/MOHXB6XacofHf4kMt5lp44zob00/W+XgEsO5nCutOpDQ2zSXA3uxDYwCzE2ewil4cVTCaL056LQ/olEBzkvJ9gXHQIvRogApsbs9lKbn4pXTvF8OE/bmHM0K6o1R8cAvx9ufHqQXz0yq2Eh7ZeY9auiTFYPHR4rzKa+XLBNl55b3kTRSWReIbMZLVyOoaFcyA7y6GA0CkKncIjmjmq5iHMz58FN97MFwf28vXBA2SVlRJsMHB9j17c038Q0YGBfLJ3N1/s30tGSTE6RWFKpy48MHgo/RzURWWWOD45Bbbi5gcGDWmKl0NWWanTz+RWIcgoKXY5z9cH9zs9NaZTFL45dICJiS2biTiffj3bExzkR2mZ43o4fz8fhvRr2MnHoABDvVtiFxIQYHB4zddHz4O3juXV91c4HPPIHePtYsVTUk7nsHpTMiVlVbSJCWXahF4ui/E9paSsis+/38rPqw5SUWnbRh7UJ547Z4/gT49Np8poJjDA0GwNsj2luLSSn1cdYO2W45RXGvHR67BYrQ7NZetDAEvWHmbW9IF07+RZOYFE4m2kyGrl3NKnH/uyzjm8bhWCub37NmNEDcdktWLRNPz1ercLVYMNBh4eMpyHhwxHCGF/ntFi4e6fFrA1M8M+1ioEK0+dYOWpE7w7fQZTO3etNdfC5CO8uMH5EfFHhgznmm5JHr4y9wj397cbqDriZEEBv136Mzf26sPoDgn1vk+ni4qcZu2sQnCqyL3tuebC10fPXbNHOD2if9v1w/D3a1gma+KoJD77fpvD66qqMKhPPCEXZKpSUnNYufEoRaWVtIkOYdqE3vzfb6byny/XU1b+a4Y4PDSA390zkfEjPLcnMZos/PXfS1i79bitx6OiIDTBR/M28cAttV3XG0NJWRW/eeZ/ZGYV1TpcsPdwBnsOZfDi769mwojuXlmrKTiZlsujz39LSWmVXTCriq32SlHq1to5Q6cqLF17SIosSYsjRVYr59ruPfjp2FG2ZKTXe2Od3bM3w5zUJZmtVn44epgvD+wjtaiQAB8fZnRL4q7+g5qtkfLmjDTe37WDLRnpCKB9SAh39hvIbX37e3Sa7nzB8cne3WzLzKyTGbIKgQL8bvkStt3zG0IMtsyFJgRvbN3sdH69ojToVKG73NCjN//esdWpQCoxGVl2IoVfUo5zZeeu/OvKq+q8R2F+fmSXOzl1BYT7Od/2aglmXzWQ8gojn323FU3YboSaJkCBW2YO5bbrhzV47k7xUUwa1Z01W47XyWgpiu09ufvGkfbHTGYLL/2rWvjobLYoQsCn327hztkj+Onj37BjXxoFReXERAYzpF+CW07x9fH6BytZty0FqLEfqI5P2FzXw0MCmD6xd4PmPp9P52+pI7DAdqhAAV5+exnD+ie2ysJwi8XKky8voLSsqtb3r+alCGGrMbNYNWKigl0eUtCEcNmmRyJpDqTIauXoVZWPrp7JOzu38eWBfZRU11/FBARy78DB3D1gkENRYLRYuG/xQjalp9lNKistFr48sI9vjxziq+tm0z+ucb5Ervjm0AGeXbPS5qNV/diZkhJe3riORceSmZnUgwh/fyZ07ESwwfFWzvloQvDFgb1oDjbfBLbXvjD5CLf3s3loHc7N4Uyp861CixCsTj3JDT0bf8Orj1v79uObQwfIrSh36tRec235yRT+tX0rT44cXev6zKQevLp5E8LJ65+Z1Pq8gxRF4a4bR3Lt1H6s2pRMbn4pEeGBTBndgwB/X05n5uPv50tcdMP6Kv7xkWnodTqWbziCqigoqoLVqhEc6Mdzj06jT9Kvrabe+HDVr8Lngrqf/367lciwQGZe0b/Br7WGrNwSlq0/7DQL8+m3W7hyfK8Gb0OCLVu2ePXBOgKrBoGtXuk3f/ya9m3CGTW4E5NHJTk8BNDcbNhxwqVdQ9u4UL74553odCpX3Pa2fTu0PlRFcbthuETSlEiRdRFg0Ov5/YjRPDJkOKeLi+wn61wd0/9g9042p9uMKs//1WsVgiqLhfsXL2TzXfd7lE3yhHOlpfxp7SoE1BIVNX87kJPFgRzbsX4/vZ6HBg/j4SHDXGaSSoxV5JQ79jMCUBWVI7k59q9L3TgcYOu76PgXd2OJ8A/g29k38btlS9iTddbleAF8vn8PDw8ZVssHbU6vPny2by959Yg1naLQLjiEmd17eDt8rxERFsiNV9vMXguLy/ngf5tYvv4IZovN86xrYgz3zBnJ6CFdPJrX4KvnT49N5+45I9mwI4XKKjMJ7SIYM7QLvj6//qrLzS9l6drDTmu4PvtuGzMm9230CbXNO0+4HJOVW8Kp9Dy6dGx43828gjK7qagzTqXnkZqRx4btKXw8bzP/ev5G4tu1bE1nyukc/vPFeqdjhID0M4WUV5oIDfbnqom9WbB0r0NRadUEV47r1RThSiQe0TqrHyX1YtDr6R4ZRZdqDylnmK1WPt+/12G2QxOCvIoKVp5yfRNoKN8eOeh2E+Mqi4V/btvMv7ZvdTnWR3UtChXF9n7VkBAW5vI5mhAkhjVtP7T2IaF8f+Ncfpl7GzO6ua6PKTebOZT7a2ucCrOZc6WlvDJ5Kl2rTUZ1imK3pugVE8vXs24k0Lf1bQldSGFxBfc//TVL1x6yCyyAE6dzefofC/l5lfPTmI5oFxfG3GuGcPeNI5k0KqmWwALYvPuUy5OoeYVlpKTmOB3jDpVGs1vbz+4IJGf4+7mfkap56flF5fzuhe8wmS2NWrsxHD5+jgee+dppK5/zqRHGN187hOAgv3qzf4oC44d3pWfX1u9lJrn0kSLrEiWrrMxlWxq9qrI/27FBZGM5mpfr1omv83lv13YKK53HHejry9C27Z36QFk0jUmJne1ftwsOYXR8gkOfLAWF2MBAxsQ3T1+/HtExDGrTDnc2iKyaoMxk4sUNaxny0XtcNe9L7vzpB4qNVdzTfxCPDx/FEyNG8cONN/PjjTfTNrhh2231IYTAaLF4/H10h/9+u4XsvJI6ZpM1a/3zo9WUlLpureQpRqPZLQ+xxgofgMQOUQ6zLTXoVKWW63pDiAgLpFe3Nh55o2maICe/lPXV26bNjRCCv727FIvF6taHsfZtwu1mqNGRwbz/8s306FJbSOl1Ktdd0Z8//+4q6QIvaRXI7cJLFJ079R2C6l6ITUPNaUBPbtAWTWPZyRSXJyYfHDyUuxb9UO81naLQJSKS0RcIpufHTeT6b7+m3GSq03dRAV6dfKVHjaEby4A2bV3eXHx1OjqGhXHLD99yODenVgbmXFkZn+zbzf0DB/P06HFejS2tqIgP9+zkx+QjVFkshPn5cVOvvtw3cDDh/o33EDOaLCxZe8ipALFYrazYeJQbpg9s9Hrn0yk+ymUmS1UVOrRt/DbasAGJRIUHUVBUXu+aOlVh3PBuXjE4vevGkTz51wUePUdVFbbtSWXKmObfXj6cco60zAK3x990zeBawqlD23A++PstnDidS0pqNr6+egb3TWhyV3qJxBNkJusSpU1QMB3DwpxmSixCY2xCR6+vnV1Wxr2LfmTlqZNuGYSej05Rya9wbBhZw7iOifx1wmR0ilItkn7dMksMj+C/115f51N9p/AIFs65hamdu9a6Nqxde765YQ5jmuC9uBCrprEh7TQf79nF/qxzJEVFO8yuqYrCdUk9WXz8GIdychy+lx/u2UVyXq7XYjySm8OMb77k28MHqbLYtpKKqqr4aM9Orv3mK3Jd1MO5Q2FROVVG59tUOlUl85z3rSgG9UkgLjrEYdZHpyqMHdrVaZ9Dd9HrVP78u+nodEqdDz46VSEyPIhH75rQ6HUAhg9I5I8PX4mPjw5Fce+DlhDU2qptTjLOuC+wrp7Uh2un1P/Bq0vHaKZN6M2kUUlSYElaHTKTdYmiKAq/GTSUp1fXb6yoUxS6RUY5tX9oCEVVlcz+bh7nytzrA3chFqHRNjjYrbE39+nHxMROfHv4EMfz8/D38WFqpy5MSOzksGatY1g4706fQXGVrXg+zM+P6MDmOYW06+wZfrf8F86WltpOWwoBKBh0OjSrxZ7Vqrk19o6O4dkx47l63hcOa+vA9r2cf/ggfxk3sdExCiF4bNkvVJrNdYrqrUJwrqyUFzas4Z1pMxq1jjNT0PNjCfB378SpJ6iqwvOPX82jz3+L1WKttV2pUxUiwgJ57G7vCB+Agb3j+eDvt/DZd1vZtNP2wcPPoOeqiX2444bhRIR57+dv+sTejB7SmRUbj5J2poA1m5NtvlMOnyFI6twyXlLuWkncP3c0t81yfSBGImmNSJHVismrqKDSbCYmMLBWEbe7zO7Zm9SiQj7YvROdomAVAhXQgA6hoXw84zqv/+L6bN9ezpaVepzBqiFA78OVXdw3fIwLCubRYSM8XifUz4/QZvSSSs7L5bYfv8Nc3dLn1/dHYNKsdI2IxGi1UlhZSduQEG7u3ZcbevbCT+/j0qXeKgRpRUVeiXPn2TOcLHScYbAKwbITKeRWlBMd0HBxEBLkx6A+8ew9nOH0hNjEkd41zzSbrazfnsKO/acZ0jeBvIIyUlKz0YStePyqiX24fdYwrwofgG6dYvnbUzOprDLZTsgF+ePj0zSnekOC/e1brF0Sonntg5X1jlMAvV7nFY+uhjCkX0f8DHqnGc2QID/mXjtECizJRYsUWa2QDWmn+df2Leytdnr31/swp1dvHh02wqOeeoqi8NSosVzdtTvzDh/kRH4+wQZfruranWldujVIuLli/uGDLgVWza/L+kY9N3Y8AT6tw7vHDcjNYQAAMQFJREFUm7y7cxsWTav3vdGE4HhBPr/MvY0e0TF1rgf6+FLqpIelTlEI8fNOxic5L9fuqeYITQhS8vMbJbLAZg7627/Mr/eaqiiMHtK5UbYGF5KakccTL31Pbn4ZOp0CwibkAvx8+ONvpzF6SJcmbzfj7+fbYFf7hpDUORaDrx6jqa6QUVSF5393FWEhLdPwOsDfl1uvG8bH3zg2Cb5z9ogmE6MSSXMgRVYr44ejh/nDymW1PrlVWsx8sX8vG9JO8/2Ncz1uXtwrJpa/xjTPlkBehet6nf5xtlNQu8/96hXVPjiEJ0eO5hoX/k5CCPZlneOXlOOUmowkhoUzq2evRt/wz6egsoLvjhxic0Y6miYY3LYdN/XuQ1yQe9uYF2K0WFh2IsWpAalOUfjp2NF6Rda13ZOYd+iAw+dbhWBGV++0AjLodG6d9DI00P38fPr1bM9fn7yGl99ZSnmFCb1ORRMCTROMHdaV5x6d1ug1aiivMPLY899SVGI7rWi1/voqK40WXnzrFz7/5510aNu0Fh7NSV5BGY85sWgIDwlgSL+OzRvUBdw+azgVVSa++WkXKDZxbdUEqgJ3zB7B7Ku8e+hBImlupMhqRZQYq3h2zUoE1DmRpwGpRYW8tW0rz49vfO1NUxHu70+ek8L1mobWr025ktSiQjKKiwk1GOgTG+fy+HmZycRDvyxiU0aareZK2Bo6v7F1E38aO8Hu7n4hGcXFfLZ/Dz8fT6bSbKZTeAS39u3PdUk969RubcvM4N6ff6TSbLHXQW07k8F7u7bz72lXc8UF/RDdofSC04z1oaBQWGVrnqwJwca002zKSMMqBIlhEfjqdBit1jqZMJ2i0DM6hvEdEz2Oqz7GJiQ6bT4NEO7nT98Y73gQjR3WlWH9O7J263HSzhTg7+fDuGFdSWgf6ZX5a1i27jAFRfX/XAohsFo1vl+yh8fvneTVdVuSBUv3UlFpcug2n19UzsqNR7l2ar/mDew8VFXhodvGMXv6QFZuPEp+dRujKWN6eH3LViJpCaTIakUsTD6K0er4pI8Avjq4j9+PGEmwofX1pgO4sWcfPti9w2nW5frqli+JYeEemX8+vvwXtmTaHOwtWu1WKM+vX0N0YCDTLqjn2nvuLLct/B6jxWKP6XBODk+tWs7SlOO8f/W1+FY73ueUl3HPoh8wWq21Cs01IRBC8Nuli/ll7u10jfRMAIQaDATofaiwOPZd0hB0CAklvbiIexb9yMnCArsAtGgaYX5+BPj4kl9ZgV5VbcJACIa178DbV17tNeuJNsHBzOzeg4XHjjoUWr8ZPMSrXQIMBh+uHN+07tzrtzs33bVqgrVbj19SImvVpqNOLTIUBVZvTm5RkVVDdGQwN3upUbZE0pqQFg6tiFOFBQ6P89egCcFXB/c3U0Sec2f/gUT6+9f7g6UqCuMTEhnevoPH8x7Lz2N1qmOnbgX49/attTKAJquV+xf/RNV5Aguw9zxcn5bKR3t22h+fd+hAvdkiwJ5d/OLAXo9j99HpmN2rt9PvrRCCaV26cvMP33K6yGZbYNE0u5gsMRqpsph5ZdIVPDxkGI8PH8Uvc2/jq+tme8W36nz+OnEy4xNsmTFdtYdYTeQTOiZyR9/6M4atGXeMRU311C1dzDjr7Qc2+4ayctftpiQSScORIqsVEejr63JbCWDRseRmiKZhHMvPRafq0C54XFUUZvfoxXtXXdOgk0IrT55wLlKwCbGzpaW1npNfWeFQmNl6A+7FWi1k1p5OdbpNZq1uIO0KIQRbM9J5ds1KHl26mH9u3czM7j2IDgx0+Bp+N3wkO86e4Wxpab0/A1p1v8kThfk8NmwkDw0ZVm/9ljfw0/vw0YyZ/GnseJuZbPXjOkVh7elUJn75KScL8hs8/+6D6fzxlYVce+97zPrNh7z1yWrSz7rvmdQQunWKceobpaoKnb1YZN8aSGgX6bTptE5VSOwQ1YwRSSSXH3K7sBVxZZduvLdrh8txro70O6PcZOKrg/v4+uABzpaWEGwwcF1ST+4eMIh2jWzHsuNMJncuXEB9OxSaEMSHhTX4RGOFuboHnAsRWnnelty+7HPoVbXO1uL55FVUkFVWRruQEKfjarC6GFNiNHLfzz+y8+wZ9KqtkFvBdrrwvoFDyCgpZvnJFLuYiwsK4rdDR3BTrz7cvvB7pyf7rELw87FknvGyu3t9nCos4JXNG+2v9/wm39llZdz643esuu1uj3skfvj1Jr5YsA2dqtj9qX5cto+fVuznb/83kxGDOnn1ddQwc2p/Fi53nAHWNMGsaRdfhs4ZM6/sz4HkMw6vWzXBNVOdd1aQSCSNQ4qsVkSfmFgi/PwpcNFzMMTQsCPgJUYjNy2Yz/H8PPtNvqiqii/272XB0cPMmzWHHlEN/zT/j80bbNtqDmTCv7Zv5ZY+/QhpQD1Z96golyLIT6+v1bfP3TqlmgzHoDZtOZaX6zCbqFMUBrZp63Sux5YtZk/1qckL4/1wz07emDKN58dP5HRRIf56H3pERdvjLDOZXJ7sqzA73/Yqrqrip2NHSS0qJNjXwLSu3Rr0Pf103x6smlZvPFYhyC4v56djR7m5j/v1PJt2nuSLBdtsc5ynxK2aQBOC515fxPfv3++VFjMXktAugjYxoZzLKa73evu4MMYP9/xQQ2tm0sjurN50lC27T9X6bFIj5K+7oh99k9rVek5JWRXL1h3m2KlsfPQ6RgxMZFQzWFtIJJcq8l9OK+MPI8c4va4qCjOrC8c95R+b1pNynsCqwSoE5SYTjyz5ucGNgDOKi9mXdc7pdpvJamXpiYY1o72yc1dCDAaHbYJ0isINPXrV8tgaE5/gVJgpQOfwCGIDgwC4pU8/l9uFd/RzfKQ8OS+X9WmnHYo0BXhn5zai/AMY0rY9vWNiawnBbpGRTntJqtU9GR3x7eGDDPvkfV5Yv4b/HdzPe7u2c9XXX/DA4oVUuhBnF+LKckIBVpx0Xkx+IfN/3uVw+0oIMJkt/LLmoEdzusvy9UccCiyAzKwiDiafdXj9YkSnU3n5D9dy39zRtYRrbHQIT9w3iSfum1xr637jjhNcd9/7vP3ZWlZuPMrSdYd49rVF3PLop5zJKmqBVyCRXPxIkdXKmJnUg64RkfXaGegUhRCDgdsbUHhcYjTyQ/IRp6f+UosK2ZaZ4fHc4J4/ll5Ryauw1UgVVVV6dOM36PW8ecV0dKpap65JpygkhIbxxIhRtR4/VeC8zkcA9w/61U26W2QUL4yfZJ/z/PkBHhs2wmnR/urUUy7rxlKLCkkrLqr3+s29+2EVjkWhJgS39u3vYO2TPL16BSarFYEti1bzvV6deoonVy51OG99GK3Oi8AFtbdm3eHQsTNOT7sJAQeOOt7eagw/Lt+Hs1JAnaqwaOWBJlm7JdHrddw+azg/fvQb5r97L9+9dx/f/uc+rr9yQC2BdfxUNs+9vgijyYIQtu3TGi+xrJxifvfCtw79tiQSiWOkyGplGPR6vrpuNgPj2gC2G3zNjbt9SCjzZs0hNijI43lPFuRjcmIPUbPWgZwsz4MGYtyIySI0DuZkMfyT9xn44X/o/d6/uWPh92x3U9hN6NiJ7264iQkdO9kzWsG+Bu4ZMIgFN95cy6R1Q9pp/rJ+jdP57h84mBt61LYOuLVvf7694SamdOpCkI8vAT4+jOqQwGfXzuKxYSOdzme0WNwq6ndk09Evrg33DRwMUCdjpwBTOnVmRrf628z8a9sWhz5jmhAsPZHCCQ+K1btHRjn1Lavx5/IM1+9NU3VPycwqclrOZ9VEkxfftyR6nUq7uDDaxITWm02ct2iXw3pHqyY4l1PCuq3HmzpMieSSQ9ZktUKiAwP5dvZcDmRnsSk9DavQ6B/XhlEdElwadjrCHV8jAfioDfM/ahccwrB27dl59oxTm4VVp07arwtgS0Y6m9LT+OcV07nWidt7cl4ui44lU1hVSb+4OP44ehwhfgZCDX711l69v2uHS1PNIe3a1yuKBrdtx+C27ep5hnOSoqJd1o356/V0CAl1eP3pUWPpEhHJh7t32nsIxgQGclf/gdwzYHC9r/VcaSmHcnOcrqsqCstOpPDIUPc8vm7vN4DHly9xeN0qBHN7e1Y0PahPPDv3n65Vj3U+igKD+iR4NKe7BAX4Ul7h2K5AURRCglun91xzsGF7isPvC9h+fjbuOMHUsQ0rVZBILlekyGrF9I2No2+sd5y1u0dGEekfQH6lYzd2TYhGOYf/ccx4bvxuHmYHPfpq1jifmi2tp1YtZ1xCxzotg0xWK/+3chmLjifbPJuqhdOb27bw6NAR/Hbo8DprVJrNbDvjPDumV1TWpJ5iUmJnT16iUyZ36kykvz+FVVX1vn6dojC7Z2+nvRmV6jE39OhFXkUFFk0jJjDQaRF/hdm5HxLY3vfkvFz3Xggwo1sSa1JP8fPx5FonHmuE61OjxtAt0rPj/zddM5hte1PrvaYqCv5+Pkyb0DSmpFPH9uR/C3c43K4UQjB5tPOWTp6Qm1/K8g1HyC8sJyIskKljexAb1bjTu02J2ew8y60JUW//Q4lE4hy5XXiZ4KPTcf+gwQ6v6xSFCR0T6RQe0eA1+sTE1ntCMdo/gEAfX6cn58xWKz8mH63z+Avr1/DzcZsvmFUIe5NlTQje2r6Frw/VraMxu2HFIBAut089xVen4+1pM9DXUzemKgqdIyLr1I05QlEUogMDaRMc7PKUZFxQsN213hn7s8+5tTbY4n3zium8OH4SHc9z5R8Q14YPr76WBwZ57s49uG8Cv71zPEAtzypVUTAY9Lz67PUEBzZNNmnW9AEEBRjq3SrTqQoJ7SKYOLL+rVhPEELwwf82MuuBD/nwf5v4cdk+Ppq3idm/+Yj/fLm+wQdLmpqOHSKdbtWqqkLnhEvLR0wiaQ5kJusy4p4Bg8koLuarg/vRKQpWIex/9omN480rpjd6jf5xbfh57m0czc0hvaSYMIMfncIjGPbJ+06fp1NUjl2QackpL2P+4YNOxdnbO7ZyU68+tYRIsK8v7YJDOFPq2E9ME4LeMd438xzevgML59zCB7t3siTlGGZNI9I/gFv69OOeAYMINhi8vmagry99YmJrNdyujzOlpaQVFZEQFubWvKqicGvf/tzSpx8VZjM6VcFP7zgL5w5zZgxmYJ94Fi7fz8HkM/j66Bg9pAszJvclMrzpetVFhQfxzks38cdXFpKZVYROtZmsapogqUscL//ftRh8G//r8OufdvLlD9vtX2vnNaL+euFOggIM3D6rbva1pblh+kBefX+Fw+tCCK6ZIj21JBJPkSLrMkJVFF6cMJkbe/Vh/uGDpBcXEe7vzzXdejAuoaPX+t8B9IiOsTuSu/J2AkAB/wu20dY6aaNTQ055OYdysulXfVAAbFmgO/oN4O+b1tcr0BTAoNNzXQOtMJxxqrCAo7m5jO+YyFOjxhBq8MNPr2+Qy70nDG7bzqXIAigyVuFp1ZOiKB6bjjqja8cY/vDAFK/N5y6d4qP4+u172H0wjUPHzqLTqQzum0DPrm1cP9kNjEaz3QfMEV/9sJ0brx6En6FxYtXbTJ/Ym627T7Fp1wkQ520PqwqaJnj83km0iXFcSyiRSOpHiqzLkN4xsfSOiW229Wwn9OLZmpnhUDRZNI0uEZGsST1FbGAgPaNjqLBYXBavA1Ra6taK3NFvAJsz0tiQdhqgVmsYgH9deVWDTFEdkVVWypMrl7ElI93+mKooXNu9By9NmOy0DssbdHejPkoB2gYHN2kcrR1VVRjSryND+nX0+tx7DmdQXuG8Pq6iysyuA+mMHuK9WkBvoNepvPSHa/hx2T6+/2UPZ7KLAOjfsz23zBzKsAENr9WUSC5npMiSNAsPDR5WS4Ccj6oo6FWVP61dZX+sS3gE1/fo5VJgqYpC4nk1QzX46HR8ePVMvjl8kC/27+VkYQE+qo4rOnfhvkFD6FOPyCyorKDMZCImMNCjbbHiqipu/O4bzpWV1npcE4Kfjh3lXGkpX153g1czhRdyReeuBPqsptxBEbyt5q4T0QFNtyV3ueOqIbN9XJV745obvU5l9lUDuWH6ACqrzOj1Kr4+8hYhkTQG+S9I0iyM6BDPG1On8dSqFVg0K2r1ScGaQvYLi9BPFhbw+tZNRPkHUFBV6fC03qTEzg59w3x0Om7r25/b+va39xCsb9tuW2YG/9q+he1nMgFbe55ZPXrx2LCRRAW4bvHy1cH9nC0rrTdGTQi2nclgXVpqg08yiur3x1enc7jt6O/jw0sTJvPEiiV1+h/qFIVAH1+eHj22QetL3CO+nXuHRhLcHNdSKIpCgL/3toclkssZKbIkzcbMpJ6MS0hkwdHDpBTk46fXsyTlGPmVdXs1CgAh8NGp6BUVK1ott3qdohDpH8Cfx01wa21H/mLLTqTwyNKfaz1WZbHwzaEDrD+dyoI5N7vM/nx7+KDTjJtOUfjh6GGPRVZRVSUf7dnFvEMHKKqqqq4j68EDg4bWW7w+M6kHQb4+vLZlEynVxqMKMDYhkWfHjGvUyVGJa7p2jKF751hSUnPqtYpQVYVO8VF079R8W/USiaRlUURrPVPsJiUlJYSGhlJcXExISOv1obmYKa6qYsHRwxzKycZHp2N8x0QmJ3Z2y+DUGdsyM7j5h29djntjyjRWnz7J8up+ev7VmaaHhwxvkPt9DZVmM8M+eZ9yB42ZdYrCDT178/dJU53O0/u9f7ss7h8Y15bvb5zrdmy5FeXM/nYemaUltQScTlHw9/Fh3vU30stBXZ0QglOFBRQbjbQPCSEmsOHvUUNILy5ib9Y5VEVhSNt2xAVdPnVgKadzeOjZeRhNllpCS6cq+PjoefevN0mRJZFc5HiiO2QmS+KUlSdP8NiyXzBaLdXZIIXvjhwiPiSUz2fe4LYdQH2cKy11PQib/9Q702ZQZTFTajIRavBzyxfKFUtPHKfM5Lg+xioEPyYf4dkx4wlycrouJjCI00WFDq/rFMXjgvOX1q/lzAUCqyamSrOZR5f9wqrb7qp3+1Cp9uRqbvIqKnhq1TLWnU6tZV56Vdfu/HXC5Caxr2htdO0Yw8ev3MrH87ewfttxNE2gqgpjhnblnptGktjBMwNXiURycSNFlsQhh3OyeWjJIjQhENS4s9tun2dKS7jtx+9YedtdGPQN+zGKdKPe6fxxfnqfRvs0nY+tGF51al5qslo5V1pK10jHouWmXn14ZfMGh35eViG4oWdvt+PKq6hg6YnjLpt57ziTyTAnDaubkzKTiZsWfENaUVGt90ETgiUpx8gsKeabWXManf28GEhoH8lLv59BeYWRopJKwkL8CQy49AWmRCKpi3R8lzjk4727AeoVD1YhyCwtYemJlAbPP6J9B8IvaKNzIbGBQQxpQB9Bdwjw8XV5ehEg0Ne5sJvbuy+J4eF1XN7BlsmZ0DGR0fHuu1OdLMh3KLDOn/eIB21ymprvjhwitbCw3ritQrA36xwrTp5ogchajsAAA+3iwqTAkkguY6TIkjhk+ckUpzd7VVFYearhN04BBLrwj/r9iNFNZn0wrUtXl6+vT0wsbYOd77kHGwzMn3UTkzt14XyZ5avTcWuffvxn+jUeNfZ2ZytUCIGhFWWFvj180Ol1VbFtM0skEsnlhNwulDjEVW8/TQiq6jECdZclKcfIdNL6BqDS4oZbfAPpFB7B9K7dWHYixaH9wqPDRrg1V2RAAO9ddQ3nSks5lJONTlUZ3LZtgwxPe8fEEuHnT0FV3VOX5zOuEc28vU1uRbnT9keaEGSXlzVbPBKJRNIakJksiUO6RUTiLP+iU5Q6zaA94fsjh5xmeBRcZ0gay+tTrmRqpy6A7fX4qGp12x0dr0y+wqHtghCC9OIiThTkU3WeEGwTHMyUzl2YmNipwY7yPjodvxnsuAFzjZN8OxcZtuYkNjDI6c+K2oDif4lEIrnYkZksiUPu6DeAZ9asdHhdE4Kbevdp8PzZ5eVOa6IEtt6EjUETgo1pp9l6JgOEYEjb9ozvmGjfgvTT+/x/e/ceFmWZ9wH8e88Aw/kMw0HkLCCIKCZlmZoEmKmZmab1elpz2/aUdZWta2bvbmbtum3WZm1bWe36Zidqs63MsrLwEKGECoGCytFDKgPIaeZ+/0BmGWFmGOVhBvl+rosrZuaZZ35zPT369X7u53fjb1Ono+T0KXxUWoKG1lZE+fphRkKi2ZD03qGDeHbvLpRfuKPQ3dkZc5JH4L6rr7V4F6ItloxKR11jA/5RkA+1EDBIQCU65jddPzQKf7yh/9f+s2RO8gis+fJzs68bpMTs4Zf+/woR0UDEkEVm3TY8BZ9XlOOzC/Ouut6Wb5ASayZOxhDvS180NtTLC+Vnz5gNWuLCNpeq/OwZ/OyD91B+9gycLoSqF7//DkO8vPH36TNN1vtLCAg0Pj7bfB5fHq3A+bY2JAQGITVYa2yV8NzeXfhz3jcmozZNbW3YtL8Ae6oq8eZtc/tknUIhBFaOn4g5ySPw9sEiVNbXw8/NDTMSkpAeGqb4gtO2mj08BZuLClHWw6R9lRAYGzYEmTGOtV4fEZHS2IyULGrT6/HQZ59ga2mJsdWBl4sLFo9Kx28yxl3Wvj/8sRi//nirxW0ev+FGzE1JtXnf9S3NyHr9VZw+39TtL321EPDWaPDJnYtMls1p0+ux7puv8HrhPpO2DkmBQfhz1hS4OTnjhtf+YXbukUoI3Hf1ONx71dU219uXCmqq8fK+75F3/BgkJMZFDMXCtNFID1XmLs1OZ5vP45EvtuOjsh+NwdlJpcJtSclYdf0kuCm8SDYRUX+wJXcwZJFZUkqs+uIz/Kuo0GQ9vM6RrPuvuQ73XpVxyftv0+tx53tvIb+muttolloIJAYG4a3Zcy+pN9bLBfn449c7LAai32aMwy/H/jcQPfDpf/Be8cFu71ELAXdnZ0wbloQ3DxRavCNR6+GJvCXLbK63r7xeuA+rd2yHWghjnZ2/r54wCQtGjla8hrqGBuyv6+j4nh4aDj83y206iIgGEltyBye+k1nbyw/jX0WFANCtwSQA/DlvJw6cqLvk/Tur1XhlxizMSko2Xs4D/tsl/J+3zr7k5qP//rHY6t1uH5QcMj4uPnUS7/YQsICOeVBNbW346mi51b5adY0NaLNyV6ZSDp08gUd3bAcAkyDY+fuaL7/AD5dxvHpL6+mJrNh4ZMbEMWAR0aDGOVlk1muF+0xGRC6mFgL//GE/Hreytp8l7s7OWJeZjQfHjUdBbTWkBNJCQhHkYXlRZmt0rS1Wt2lo+++SOrnFBy1+V72UqG7QQWVhG6Cjx5WTQn29rNm0v8BifWoh8Pr+Ajx5Y04/V0ZENDgxZJFZB06csBgo9FKiqI9GRgLc3ZF5oZVCX4j3D8TRs2ctBo64Luv7nWpqsrpPa6NYaiFwc3yC3Sal762usnq8dldV9mNFRESDGy8XklkaJ+sdxftyLcG+NG9EqtXAMX/ESONjracnrE1PdFWrkaYNMbt8jpNKhbvTr7r0oi9TT3V120blWHclEhFdyRiyyKyc2HiLf3ELAFmxfTf61Jeui4jEbAuLMk+NT8CNXUbOpg9LhPllojvMTErGyzNuNS7KrL4QrADAz9UVr86YhWFd2kL0twlR0RaPl1oITIx0nC7xRERXOl4uJLMWjByNzUWFMOgNkOh+95+XRoPbhifbqTrLhBBYOzkLSYFBeKngO1TrdACAYA8PLE5Lx5JR6Sbd5g+etL7YcqSPL3xd3fDGzNk4cKIOn1ccQUu7HsODgpAZE9erNQeVcLb5PL49fgwhHp4QQkDIi49WRyAWQuCukaPsUSIR0aDEFg5k0TfHj+LnH76Ppra2jr/A0XGpLcDNHZtumYXhQcH2LtEqg5So0ekgIRHq6dXjgtPz3tmCPdWVFhujjgwJxbu3z1O42t5r1evxxM4v8c8f9pv09eoMVJ3fRSUE1EJgw5SbkRUbb6dqiYiuDLbkDo5kkUXXRkTi28XLkFtyCN9VV0EtBK4dGomp8cPsOh9rT1UlNu0vwP7aGjir1ciKjcOdI9IQ4dO9A71KCIRbORFqGnRWl/ipa3CcBY6llLj/0//go9KSHketXNRqxPsHwEmlwriIoZibkupQax0SEQ0GDFlklZdGg7tS03BXapq9SwEArM/7Bs/u3WXScuHlgny8tr8AL02fiWsjIm3eZ4inJ47Xn7M4kqX19LycsvtUYV0ttpaW9PiaAR2NXseEhWPV9ZP6tzAiIjLixHcaUD47UoZn9+4C0L3hZqvegGUfvo+zzedt3u/s4SlWR7JutzCRvr+9W3wQamH+9NVLibcOFlm9Y5KIiJTDkEUDykvf55tMWO9KQuJ8WxvePnjA5v1OjU9AarC2x313LvFzS2KSzftVysnGRhik5fshG1pbTeZqERFR/2LIogFDSon8miqrTUG/q66yed8aJye8NnM2psYnmAStzjYVm2+9XfE5aLaMOmk9PaGyMJIFAN4ajd3ueCQiIs7JoivRJfbb9NZo8NecqfjddROQX1MFKYHRoWEI9fLq2/q6ONXUhFf25WPLgSL8dL4J/m7uuD05BYvS0hHo7m72fbOSkrFpf4HZ19VCYE7yCCVKJiKiXuJIFg0YQghkhA+x2tn86vCIy/ocracnbopPwNRhCYoGrCpdPaZvfh0v5u/F6fNNkABOn2/CC/l7MW3z66iqrzf73pRgLW5NHN5jnlQLgQA3d/xs1BjFaiciIusYsmhAWTJ6jNnlclRCwMPFBbcmOWaD1Is99NknONnU2O37GKTEqaZGPPTZxxbf/0RmNpalj4Wbk+mA9DVDIvDO7fMue5FtIiK6PLxcSA6rpb0dWw4W4Y3CfTh67iw8nF0wPSERPxs1Bi8VfGfSwkElBFydnPDStJnw1mjsXLl15WfP4Nvjx8y+rpcS31Yex5EzPyHGz7/HbZxUKjx47Xj84qoM7K2uREu7HkmBQYj09VWoaiIisgVDFjmk5vY2LMx9F3urKwF0tFBo1Z/HG4X7oHFywrrMbOypqkRBbQ00ajUyY2IxL2WkQ/WysuTAibrebXfyhNmQ1cnTxQWTomL6oiwiIupDDFnkkDbs2YXvaqq6dTPXS4nm9nasz9uJrxfdbVygeaDp7V1/vDuQiGjgGph/Q9EVraW9HW8U7jfbqsEgJeoaG/F5+eF+rqzvZIRHWA1QLmr1ZU/iJyIi+2HIIodTpauHrrXF4jZOKhUK63p3yc0R+bi6Yl5KqtluEwLAvJRU+Li6WtxPS3s7vj5agY/LSlH20+k+r5OIiC4dLxeSw7G0XEwnKeWAvVTYacV1E1Db0ICPD5caJ/F3/jcrNh4rrptg9r1SSrz4/V78be8ek0A6OjQMa2/IQnxAQH98BSIisoAhixxOhI8Phnh7o6q+vtucrE56KTEhMqo/y+pzLmo1nrtpGvJrqvHOoQM40diAYA9PzEpKRnpoGESXfmCnm5pQcvoUnNUqpAaH4K+787Axf0+3fe6vrcHstzYjd+58RPn69efXISKiizBkkcNRCYF7xmRg5efbenxdLQRGaEOQFhLaz5X1PSEExoSFY0xYeI+vn25qwv9+9QW2lpYY21V4uWjMXk7VS4nGtlY8szsP67NvUqxuIiKybmBfb6Er1tzkEbh7dEfH8s4O751rCsb4+eOFqTNMRnquRPUtzZj99maTgAXA6nw1vZT4sLQETW1tSpdIREQWcCSLHJIQAiuum4DpCUn4v6JCHD7zE7w1Gtwcn4gbY+MGRWuDV/cV4Ni5c1YXxO5Ju8GAM83n4e6s7KLWRERkHkMWObThQcF4bFKmvcuwi38VmW9jYY1aCPhqLN+ZSEREyuLlQiIHJKXEicbGS3qvWgjkxA2Dh4tLH1dFRES24EgWDSptej22HSnDnqpKCCEwNnwIboyJs3s7iIbWVrx76AA+Kv0RutYWJAQEwt3Z2eZ5VSohoHFywm8yrlGoUiIi6i2GLBo0Dp08gSUfvIfaxgZjqNq0vwChnl74x/SZSAwMsktdFWfPYN47W1DX2ACgY53GH0+fgl5KiAuPzXFRqdBqMBgfDwsIwFOZOYjzZ58sIiJ7Y8iiQeFUUxPmvfsWGi7cmdfeJZicaGzA/He3YNtdi+Dv5t6vdRmkxJIP3sPJpkaTMNV5N6EEegxaKiFwzZAI/O2m6fjm+DE0tbUi1j8AqcHaK/6uSyKigYIhiwaFzUX7oWtt6XEiuV5KnG1uxpsHfsA9YzJs3vehUyex7XAZzre3ISEgCFPi4qFx6t2p9fXRCpSfPWNxGyeVCm0GgzFsCQBT4xOwdnIW3J2dkRMXb3PNRESkPIYsGhS2/lhi8U49CWBr6Y82hSxdSwt+8/GH2HG0AmohoBICbQYDHv1Sg6ezp2JiVLTVfXxbeQxOKpXJyNrF2gwGvHDzDNTodHBRqzE+MgrhXt69rpOIiOyDIYsGhcZeTCBvbG2FlBIFtTV4+2ARKnX1CHRzx4yEJIyPjDI2QwU67v77+db3saeqEkDHaFjnJT5dSwvu/jAXb82+AyO1IRY/s7ctGpKDgnFjTFyvtiUiIsfAkEWDQmJgIGobdCad07tSC4GEgAA8sO1jvFd8EGqhgl4aoBYCuSWHcHV4BP4+7RZjW4T8mmrkVR7vcV8SHSHs+b27sfHmGRbrGh0ahn8U5FvcRuvhCa2Hp/UvSUREDoV9smhQmD8izWzAAjpGotydXZBbfPDCY4PxeQDYU12Jh7d/atz+o9ISi20f9FLis/LDaGlvt1hXZnQstB4eJqNkXQkAi9JGQ23nFhNERGQ7/slNg8KEyCjcPjwFQEdw6dT5+5zhKdh2pMxsuwSDlNhaWoIqXT0AQHfh0qIlBilxvt3yZUpntRp/nzYTHs7OJkGr8/fs2HgsHpVucR9EROSYGLJoUBBC4PHJWXhs4mRE+PgYn4/09cUfb7gRtyQOtzpvSwLYebQCABDt62exfxUA+Lq6wrsXS9ukBGvxyZ0LsSQtHeFe3vDTuOKqsHBsyLkZz940ze6NUomI6NJwThYNGiohcGdqGuaPGIlT55sgIBDg5gYhBHYeO9qrfXQ2/rxteDL+susbi591R0qq2cuAXX1ZUY7nv9uDPdUdk+hDPb0wOToWWbFxvXo/ERE5Jv4TmQYdIQSC3D0Q6O5ubNyZGBgEdS8CTeqFuwWDPTyxcvzEjv1dtI1aCMT4+WNZ+lir+3ujcB8WffAuvqupMj5X06DD2p1fYum/c9Gm1/fuSxERkcNRLGRVVFRgyZIliI6OhpubG2JjY7F69Wq0traabFdYWIjx48fD1dUVERERePLJJ5UqicisQHd3TI1PMBu01EIgJSjYpCXDwrTReHbKNAwLCDQ+5+bkhPkjRuLt2XPhrdFY/MwqXT0e/fJzAN1bOUgAXx+rwOaiwkv8RkREZG+KXS4sLi6GwWDACy+8gLi4OBQVFWHp0qVobGzEn/70JwBAfX09srKykJmZiY0bN+KHH37A4sWL4evri7vvvlup0oh6tHrCDSg6WYeKs2dNQo9aCPi4uuLpnKnd3nNT/DBMiYtHTYMOze3tCPX0gpuzc68+b8uBH6xu89r+AvzPyFG9/xJEROQwhLR2i1Qfeuqpp/D888/jyJEjAIDnn38eK1euRG1tLVwu9B9asWIFcnNzUVxc3Kt91tfXw8fHB+fOnYO3N7tg0+Wpb2nBG4X7sLmoELUNOvi6uuG24clYlDYawX3cq+rnH76PT4+UWd2u7FfLOTeLiMhB2JI7+nXi+7lz5+Dv7298nJeXh+uvv94YsAAgOzsb69atw5kzZ+Dn59dtHy0tLWhpaTE+rq+vV7ZoGlS8NRr84qoM/OIq29cwtJWrsxPUQljs3+WsUneb80VERANDv018Lysrw4YNG7Bs2TLjc7W1tdBqtSbbdT6ura3tcT9r166Fj4+P8SciIkK5ookUlBUTZzFgqYVAdmyccXI+ERENLDaHrBUrVkAIYfHn4kt9VVVVyMnJwezZs7F06dLLKvjhhx/GuXPnjD/Hj/e8tAmRo7sxJg5Rvn49TrbvfObu9Kv6tygiIuozNl8uvP/++7Fw4UKL28TExBh/r66uxqRJkzBu3Di8+OKLJtuFhISgrq7O5LnOxyEhPS+sq9FooLFy1xbRQOCsVuP1mbdhUe47KDvzE5xUKkgpYZASGicnPJMzFSnBWus7IiIih2RzyAoKCkJQUFCvtq2qqsKkSZOQnp6OV155BaqLOldfc801WLlyJdra2uB84Y6sbdu2ISEhocf5WERXmnAvb/xn/gLsOFqOLyrK0apvR0qQFrckDrfaAoKIiBybYncXVlVVYeLEiYiMjMSmTZugVquNr3WOUp07dw4JCQnIysrCQw89hKKiIixevBh/+ctfet3CgXcXEhERUX9xiLsLt23bhrKyMpSVlWHIkCEmr3XmOh8fH3z66ae49957kZ6ejsDAQDzyyCPskUVEREQDXr/2yVICR7KIiIiov9iSO7h2IREREZECGLKIiIiIFMCQRURERKQAhiwiIiIiBTBkERERESmAIYuIiIhIAQxZRERERApgyCIiIiJSAEMWERERkQIYsoiIiIgUwJBFREREpACGLCIiIiIFMGQRERERKYAhi4iIiEgBDFlERERECnCydwGXS0oJAKivr7dzJURERHSl68wbnfnDkgEfsnQ6HQAgIiLCzpUQERHRYKHT6eDj42NxGyF7E8UcmMFgQHV1Nby8vCCEsHc5V6z6+npERETg+PHj8Pb2tnc5ZAWP18DDYzaw8HgNLH15vKSU0Ol0CAsLg0pledbVgB/JUqlUGDJkiL3LGDS8vb35B8oAwuM18PCYDSw8XgNLXx0vayNYnTjxnYiIiEgBDFlERERECmDIol7RaDRYvXo1NBqNvUuhXuDxGnh4zAYWHq+BxV7Ha8BPfCciIiJyRBzJIiIiIlIAQxYRERGRAhiyiIiIiBTAkEVERESkAIYsIiIiIgUwZJHNoqKiIIQw+XniiSfsXRZ18dxzzyEqKgqurq7IyMjAnj177F0S9eDRRx/tdi4lJibauyzq4quvvsK0adMQFhYGIQRyc3NNXpdS4pFHHkFoaCjc3NyQmZmJ0tJS+xRLVo/XwoULu51zOTk5itXDkEWX5LHHHkNNTY3x51e/+pW9S6IL3nzzTSxfvhyrV6/G999/j5EjRyI7OxsnTpywd2nUg+TkZJNzaefOnfYuibpobGzEyJEj8dxzz/X4+pNPPolnnnkGGzduxO7du+Hh4YHs7Gw0Nzf3c6UEWD9eAJCTk2Nyzm3evFmxegb82oVkH15eXggJCbF3GdSD9evXY+nSpVi0aBEAYOPGjdi6dStefvllrFixws7V0cWcnJx4LjmwKVOmYMqUKT2+JqXE008/jd///veYMWMGAOC1116DVqtFbm4u5s6d25+lEiwfr04ajabfzjmOZNEleeKJJxAQEIBRo0bhqaeeQnt7u71LIgCtra3Iz89HZmam8TmVSoXMzEzk5eXZsTIyp7S0FGFhYYiJicH8+fNx7Ngxe5dEvVReXo7a2lqT883HxwcZGRk83xzYjh07EBwcjISEBNxzzz04ffq0Yp/FkSyy2a9//WuMHj0a/v7++Pbbb/Hwww+jpqYG69evt3dpg96pU6eg1+uh1WpNntdqtSguLrZTVWRORkYGXn31VSQkJKCmpgZr1qzB+PHjUVRUBC8vL3uXR1bU1tYCQI/nW+dr5FhycnJw6623Ijo6GocPH8bvfvc7TJkyBXl5eVCr1X3+eQxZBABYsWIF1q1bZ3GbQ4cOITExEcuXLzc+l5qaChcXFyxbtgxr167lOl5ENuh6WSM1NRUZGRmIjIzEli1bsGTJEjtWRnRl6noJd8SIEUhNTUVsbCx27NiByZMn9/nnMWQRAOD+++/HwoULLW4TExPT4/MZGRlob29HRUUFEhISFKiOeiswMBBqtRp1dXUmz9fV1XHezwDg6+uLYcOGoayszN6lUC90nlN1dXUIDQ01Pl9XV4e0tDQ7VUW2iImJQWBgIMrKyhiySDlBQUEICgq6pPfu27cPKpUKwcHBfVwV2crFxQXp6enYvn07brnlFgCAwWDA9u3b8ctf/tK+xZFVDQ0NOHz4MO666y57l0K9EB0djZCQEGzfvt0Yqurr67F7927cc8899i2OeqWyshKnT582Ccl9iSGLbJKXl4fdu3dj0qRJ8PLyQl5eHu677z7ceeed8PPzs3d5BGD58uVYsGABxowZg7Fjx+Lpp59GY2Oj8W5DchwPPPAApk2bhsjISFRXV2P16tVQq9W444477F0aXdDQ0GAyslheXo59+/bB398fQ4cOxW9/+1v84Q9/QHx8PKKjo7Fq1SqEhYUZ/5FD/cvS8fL398eaNWswa9YshISE4PDhw3jwwQcRFxeH7OxsZQqSRDbIz8+XGRkZ0sfHR7q6usqkpCT5+OOPy+bmZnuXRl1s2LBBDh06VLq4uMixY8fKXbt22bsk6sGcOXNkaGiodHFxkeHh4XLOnDmyrKzM3mVRF1988YUE0O1nwYIFUkopDQaDXLVqldRqtVKj0cjJkyfLkpIS+xY9iFk6Xk1NTTIrK0sGBQVJZ2dnGRkZKZcuXSpra2sVq0dIKaUy8Y2IiIho8GKfLCIiIiIFMGQRERERKYAhi4iIiEgBDFlERERECmDIIiIiIlIAQxYRERGRAhiyiIiIiBTAkEVERESkAIYsIiIiIgUwZBEREREpgCGLiIiISAH/D6vl0UhGj4O4AAAAAElFTkSuQmCC",
+ "text/plain": [
+ ""
+ ]
+ },
+ "metadata": {},
+ "output_type": "display_data"
+ }
+ ],
+ "source": [
+ "# TSNEで可視化\n",
+ "import matplotlib.pyplot as plt\n",
+ "\n",
+ "plt.figure(figsize=(7, 7))\n",
+ "plt.scatter(x_tsne[:, 0], x_tsne[:, 1], c=y_predict)\n",
+ "\n",
+ "plt.show()"
+ ]
+ },
+ {
+ "cell_type": "code",
+ "execution_count": null,
+ "metadata": {},
+ "outputs": [],
+ "source": [
+ "from scipy.spatial.distance import cdist\n",
+ "from IPython.display import Audio, display\n",
+ "\n",
+ "# 各クラスターのセントロイドに最も近い点に対応するファイル名を取得\n",
+ "closest_files = []\n",
+ "for center_idx in range(len(centroids)):\n",
+ " closest_idx = np.argmin(\n",
+ " cdist(centroids[center_idx : center_idx + 1], x, metric=\"cosine\")\n",
+ " )\n",
+ " closest_files.append(names[closest_idx])\n",
+ "\n",
+ "# 対応する音声ファイルをJupyterノートブック上で再生\n",
+ "for file_name in closest_files:\n",
+ " wav_path = os.path.join(wav_dir, file_name.replace(\".npy\", \"\"))\n",
+ " if os.path.exists(wav_path):\n",
+ " print(wav_path)\n",
+ " display(Audio(wav_path))"
+ ]
+ },
+ {
+ "cell_type": "code",
+ "execution_count": 37,
+ "metadata": {},
+ "outputs": [],
+ "source": [
+ "# meanとcentroidを保存\n",
+ "mean = x.mean(axis=0)\n",
+ "save_vectors = np.vstack([mean, centroids])\n",
+ "os.makedirs(\"model_assets/jvnv-M1\", exist_ok=True)\n",
+ "np.save(\"model_assets/jvnv-M1/style_vectors.npy\", save_vectors)"
+ ]
+ },
+ {
+ "cell_type": "markdown",
+ "metadata": {},
+ "source": [
+ "## 正解ラベルがファイル名から分かる場合の作り方\n",
+ "JVNVコーパス等でファイル名によってスタイルラベルが分かる場合、以下のようにしてスタイルベクトルを作ることができます(デフォルトのJVNVモデルのスタイルはこれで作成しています)。"
+ ]
+ },
+ {
+ "cell_type": "code",
+ "execution_count": 8,
+ "metadata": {},
+ "outputs": [],
+ "source": [
+ "# JVNVコーパスの場合は正解はファイル名の最初の方から分かる。それを使って正解ラベルを作成\n",
+ "label_dict = {\"ang\": 0, \"dis\": 1, \"fea\": 2, \"hap\": 3, \"sad\": 4, \"sur\": 5}\n",
+ "\n",
+ "y_true = [label_dict[name[3:6]] for name in names]"
+ ]
+ },
+ {
+ "cell_type": "code",
+ "execution_count": 10,
+ "metadata": {},
+ "outputs": [],
+ "source": [
+ "# 正解のセントロイドを計算\n",
+ "y_true = np.array(y_true)\n",
+ "true_centroids = []\n",
+ "for i in range(6):\n",
+ " true_centroids.append(np.mean(x[y_true == i], axis=0))\n",
+ "\n",
+ "true_centroids = np.array(true_centroids)\n",
+ "\n",
+ "# すべてのベクトルの平均を計算\n",
+ "mean = np.mean(x, axis=0)\n",
+ "\n",
+ "# 保存\n",
+ "os.makedirs(\"model_assets/jvnv-M1\", exist_ok=True)\n",
+ "np.save(\"model_assets/jvnv-M1/style_vectors.npy\", np.vstack([mean, true_centroids]))"
+ ]
+ },
+ {
+ "cell_type": "code",
+ "execution_count": 11,
+ "metadata": {},
+ "outputs": [
+ {
+ "data": {
+ "image/png": "iVBORw0KGgoAAAANSUhEUgAAAlkAAAJGCAYAAABoeJAJAAAAOXRFWHRTb2Z0d2FyZQBNYXRwbG90bGliIHZlcnNpb24zLjguMiwgaHR0cHM6Ly9tYXRwbG90bGliLm9yZy8g+/7EAAAACXBIWXMAAA9hAAAPYQGoP6dpAACKzklEQVR4nO3deXiU5dU/8O9MgISELARIJmCMYRGJ7AoR1CoSCKJYX7W29lVRKFhKrRAXkFYR35ZFW6QudasK1l9brdZdw6a4gQFZVAQpIAqFLAokA4kJMDO/P4aJyWSemWe572eZ+X6uy6vN5JmZe2bQOZz73Oe4AoFAAEREREQklNvqBRARERHFIwZZRERERBIwyCIiIiKSgEEWERERkQQMsoiIiIgkYJBFREREJAGDLCIiIiIJ2lm9AKP8fj8OHDiA9PR0uFwuq5dDREREcSwQCODIkSPo3r073O7ouSrHB1kHDhxAfn6+1csgIiKiBLJv3z6ccsopUa9xfJCVnp4OIPhiMzIyLF4NERERxTOv14v8/Pzm+CMaxwdZoS3CjIwMBllERERkCjUlSix8JyIiIpKAQRYRERGRBAyyiIiIiCRwfE0WERERqefz+XD8+HGrl2Fr7du3R1JSkuHHYZBFRESUAAKBAKqqqlBbW2v1UhwhKysLHo/HUA9OBllEREQJIBRg5eTkIDU1lQ28FQQCATQ0NKCmpgYAkJeXp/uxGGQRERHFOZ/P1xxgdenSxerl2F7Hjh0BADU1NcjJydG9dcjCdyIiojgXqsFKTU21eCXOEXqvjNSvMcgiIiJKENwiVE/Ee8Ugi4iIiEgCBllEREREEjDIIiIiIpKApwuJiIhINZ8/gPV7DqHmSCNy0lMwvDAbSW7WekXCTBYRERGpUr61EuctegfXPPkxbvnnFlzz5Mc4b9E7KN9aKfd5y8tx3nnnISsrC126dMGll16K3bt3AwC+/vpruFwu/Pvf/8aoUaOQmpqKQYMGYd26da0e48knn0R+fj5SU1PxP//zP1i8eDGysrKkrptBFhEREcVUvrUS057bhMq6xla3V9U1Ytpzm6QGWvX19SgrK8Mnn3yC1atXw+1243/+53/g9/ubr/ntb3+L2267DVu2bMHpp5+Oa665BidOnAAAfPTRR/jlL3+JW265BVu2bMGYMWPwhz/8Qdp6Q1yBQCAg/Vkk8nq9yMzMRF1dHTIyMqxeDhERke00NjZiz549KCwsREpKiub7+/wBnLfonTYBVogLgCczBR/OusiUrcPvvvsO3bp1w+eff45OnTqhsLAQf/3rXzF58mQAwLZt23DmmWdi+/btOOOMM/Czn/0MR48exRtvvNH8GNdeey3eeOMNxTFDSu+ZlriDmSwiIiKKav2eQ4oBFgAEAFTWNWL9nkNSnn/nzp245ppr0LNnT2RkZOC0004DAOzdu7f5moEDBzb//9AonNBonB07dmD48OGtHjP8ZxlY+E4UJwI+Hxo+2YgT336Ldt26IfXss+ASMEWeiKjmiHKApec6rSZMmICCggI8+eST6N69O/x+P/r3749jx441X9O+ffvm/x9qJNpyO9EKDLKI4oB3xQpUz1+AE1VVzbe183iQO+dOZIwda+HKiCge5KSr22JUe50WBw8exI4dO/Dkk0/i/PPPBwB8+OGHmh6jb9++2LBhQ6vbwn+WgduFRA7nXbEC+2+Z0SrAAoAT1dXYf8sMeFessGhlRBQvhhdmIy8zBUrVVi4AeZnBdg6ide7cGV26dMETTzyBXbt24Z133kFZWZmmx7j55pvx1ltvYfHixdi5cycef/xxvP3229LHDDHIInKwgM+H6vkLgEjnV07eVj1/AQI+n8krI6J4kuR2Ye6EIgBoE2iFfp47oUhK0bvb7cY///lPbNy4Ef3798fMmTNx//33a3qMc889F4899hgWL16MQYMGoby8HDNnztR1CEALbhcSneTEmqaGTza2yWC1EgjgRFUVGj7ZiLRi+UWeRBS/xvXPw6PXDsW817e1KoL3ZKZg7oQijOufJ+25S0pKsG3btla3tWyOEN4oISsrq81tU6ZMwZQpU1r93Lt3bwmr/QGDLCI4t6bpxLffCr2OiCiacf3zMKbI48iO73/84x8xZswYpKWl4e2338ayZcvwl7/8RepzMsiihBeqaQrfcgvVNOHPS2wbaLXr1k3odUREsSS5XRjRq4vVy9Bs/fr1uO+++3DkyBH07NkTDz74IH7xi19IfU4GWZTQYtY0uVyonr8A6aNH23LrMPXss9DO48GJ6urIr8HlQrvcXKSefZYjt0OJiER54YUXTH9OBlmU0Jxe0+RKSkLunDuDGTeXq3WgdfLUTO6cO3Fk9WpHbocSETkZTxdSQouHmqaMsWPR489L0C43t9Xt7XJz0ePPSwCALR6IiCzATBYltHipacoYOxbpo0e32Q4EgF2jSxy7HUpE5GQMsijuaKk9iqeaJldSUpstzfqK9Y7eDm3J7u8/EVE4BlkUV7S2YnBSTZOeIMOs7VDZAZBTW2wQUWJjkEVxQ28rhoyxY4E/L2n7JZ6bi9w5dwKAaS0elIIVvUGGGduhsgMgJ7fYIKLE5gqEt0R1GK/Xi8zMTNTV1SEjI8Pq5ZBFAj4fdo0uUd4aO7nt13v1KsUMS6QAB4Dhx1VLKVjJuGQ8Dj39TNvtzJOZth5Rgozm9yXGdqje9SsFQGrWpoaIz5WIgMbGRuzZsweFhYXSR8mY4cILL8TgwYOxZMkSnHbaaZgxYwZmzJgh9DmU3jMtcQdPF1Jc0NKKQUmopinz0kuQVjwcrqQkIY+rRrQhz4eeelr3bMLQdmjwh7COzC22Q/UEKGbMTTTr/Sci59qwYQOmTp1q9TIiYpBFlgr4fKivWI+6N95EfcV63V/IsmqPzKhpUhOsKN85dpARq8WD3kyTGQFQPLTYIIo7fh+w5wPg8xeD/+u3dgB9t27dkJqaaukalLAmiywjspZHVu2RGTVNMYMVFWIFGUotHiJlsNQWsYsOgCI9b7y02CCKG9teA8pnAd4DP9yW0R0YtwgoukzKU9bX12PatGn497//jfT0dNx2222tft9yuzAQCGDevHl4+umnUV1djS5duuCqq67Cgw8+KGVtsTDIIkuILmbW0opBC1mP25KILIyaICNSi4dwWgJfkQGQ4vPOniX9/Scilba9BrxwPYCwfxe9lcHbr35WSqB1++2347333sOrr76KnJwczJkzB5s2bcLgwYPbXPvSSy/hgQcewD//+U+ceeaZqKqqwqeffip8TWpxu5BMJ6OWR1btkZ7H1boFaigL43KhnccjJMiIVhcWqTN8KABt875oXFvU551ZhoxLxjc/XvjjA/pryohIA78vmMEKD7CAH24rny186/Do0aN46qmn8Mc//hGjR4/GgAEDsGzZMpw4cSLi9Xv37oXH40FJSQlOPfVUDB8+HFOmTBG6Ji0YZJHpZNXyyKo90vK43hUrsGt0CfZOnIgDt92GvRMnYtfokqija2IGK0oEBhl6Al8Rga2a5/W++Ra6P7BY+OdKRBp8s7b1FmEbAcC7P3idQLt378axY8dQXFzcfFt2djb69u0b8fqf/OQn+P7779GzZ09MmTIFL7/8smJAZgZuF5LpZBYza6k9Ev24erdA1TREzZ50I7xvvhWxj5eIIEPvoOxYPcZirU3t87brnI3eq1ex4zuRVY5Wi71Okvz8fOzYsQOrVq3CypUr8atf/Qr3338/3nvvPbRv39709TDIItPJLmZWU3sk+nFjZmRizAhUE6zklJVJCzKMBL5GAlstzyvrcyUiFTrlxr5Gy3Uq9erVC+3bt0dFRQVOPfVUAMDhw4fxn//8BxdccEHE+3Ts2BETJkzAhAkTMH36dJxxxhn4/PPPMXToUKFrU4NBFplOdjG5FTPu9GaCWooVrMgMMowGvnrXxtODRA5RMDJ4itBbich1Wa7g7wtGCn3aTp06YfLkybj99tvRpUsX5OTk4Le//S3c7sjVTkuXLoXP50NxcTFSU1Px3HPPoWPHjigoKBC6LrUYZJEqIgMXtfMC9XYgt2LGnagtUKuyNWacorTT8xKRRu6kYJuGF64H4ELrQOtkTea4hcHrBLv//vtx9OhRTJgwAenp6bj11ltRV1cX8dqsrCwsXLgQZWVl8Pl8GDBgAF5//XV06dJF+LrU4FgdiklW4CL6cWWPeImmvmI99k6cGPO6U5cts+2Wl+L7d1L25EnIvf12ec8LRAy4WdxOZJywsToR+2T1CAZYkvpkWUXEWB0GWRSVGbPpRGTIrJ5xJ3tGoFmq778/OMYnEpdL1+et5jOOFHAnZWfDc/ddyBg3TvPrIKLWhM4u9PuCpwiPVgdrsApGSslgWU1EkMXtQlJktJhbDVHbYyJqooyQuQVqloDPB++bb0W9RuvnrTZbmTF2LAJ+P6rm3Qv/4cMAAN+hQ6heuAhwu5nJIrITdxJQeL7Vq3AEqX2yFixYgGHDhiE9PR05OTm4/PLLsWPHjlbXNDY2Yvr06ejSpQs6deqEK6+8EtXV1h4BpSAnDee1w4w7pX5a7qwsdH9gsdBAQdTMx5ZEf95ampt6V6zAgZllzQFWtGuJiJxCapD13nvvYfr06fj444+xcuVKHD9+HGPHjkV9fX3zNTNnzsTrr7+Of/3rX3jvvfdw4MABXHHFFTKXRSrZIXBRyy6n1DLGjkXu7FlI6ty5+Tb/4cOoWbhIWKCgp+GpGiI/by3NTWVMACAisgOp24Xl5eWtfl66dClycnKwceNG/OhHP0JdXR2eeuop/P3vf8dFF10EAHjmmWfQr18/fPzxxzjnnHPaPGZTUxOampqaf/Z6vTJfQkKzS+Cihl1OqXlXrMD+mWXCZjJGfHyBMx9bEvl5a82K6dnqtaJVBxGRFqaO1QkduczOzgYAbNy4EcePH0dJSUnzNWeccQZOPfVUrFu3LuJjLFiwAJmZmc3/5Ofny194ghI1m84MsmYXaiE7IyP78UV+3lqyYnoyaLKyeUREIpkWZPn9fsyYMQPnnnsu+vfvDwCoqqpChw4dkJWV1era3NxcVCn8zfbOO+9EXV1d8z/79u2TvfSEZYfARQtZswvVkl3DJvvxRX7eWrJiWjNoWgdZExFZxbTThdOnT8fWrVvx4YcfGnqc5ORkJCcnC1oVxWJ0Np3ZZM0uVEN2DZsZNXKiPm+t27dqrzXjxCsRkSimBFm//vWv8cYbb+D999/HKaec0ny7x+PBsWPHUFtb2yqbVV1dDY/HY8bSSAUZgUs81tPIrmGT/fihzyRw7DjyFiwAEIDv4CFdn4/WlhZqr62vWG9pqw4iIi2kBlmBQAA333wzXn75ZaxZswaFhYWtfn/WWWehffv2WL16Na688koAwI4dO7B3716MGDFC5tJII5HjXmSOvrFqrE7A50PA74M7MxN+hXEPRovvZRb3R3vf9H7uSlkxd1YWPHPvbtMnS00GzUknXolIjEAggJtuugkvvvgiDh8+jM2bN2Pw4MFWL0sVqUHW9OnT8fe//x2vvvoq0tPTm+usMjMz0bFjR2RmZmLy5MkoKytDdnY2MjIycPPNN2PEiBERTxaS88k8HSfzsWM9b3hw0IaAGjZZDU+NvG+xMpIZY8cCJ5uM+k72wAq1tHCFNRlVkzF10olXIhKjvLwcS5cuxZo1a9CzZ0907drV6iWpJnWsjkvhlNIzzzyDG264AUCwGemtt96Kf/zjH2hqakJpaSn+8pe/qN4u5Fgd55A5+saqsTqx5v2FiMymicrWBXw+1K/fgP0zZsTMvkV639SsQ/RYpngZX0RkNpFjdXx+HzbVbMK3Dd+iW2o3DM0ZiiSJY3Uefvhh3H///fjmm2+kPUcknF0IBllOInOIshUDmmMGdghujfV44AGkDR8m9EvfaE2bquxbC+Hvm5rgKX30aCmBLwdKE2knKsha9c0qLFy/ENUNP0xmyU3Nxezhs1FSUBLlnvrccMMNWLZsWfPPBQUF+Oqrr7Bo0SI88cQTqKqqwumnn4677roLV111FQDA5/Nh6tSpeOedd1BVVYVTTz0Vv/rVr3DLLbdoem7OLiRHkVlPY0WtTsyWCgD8tbVwud3CsypGauTUZt9aavm+qT3h505Pl1Kk7rQTr0TxYtU3q1C2pgwBtP53v6ahBmVryrD4wsXCA60///nP6NWrF5544gls2LABSUlJWLBgAZ577jk89thj6NOnD95//31ce+216NatGy644AL4/X6ccsop+Ne//oUuXbpg7dq1mDp1KvLy8nD11VcLXV8sDLLINDLraayo1XFiEXbUACmKlu+b6n5dFetVPbae98fKVh1Eicjn92Hh+oVtAiwACCAAF1xYtH4RRuWPErp1mJmZifT0dCQlJcHj8aCpqQnz58/HqlWrmg/I9ezZEx9++CEef/xxXHDBBWjfvj3mzZvX/BiFhYVYt24dXnjhBQZZFL9kno6zYqyOE4uw1WTfWonwvokOGvW+PyJPvBJRdJtqNrXaIgwXQABVDVXYVLMJwzzDpK1j165daGhowJgxY1rdfuzYMQwZMqT550ceeQRPP/009u7di++//x7Hjh2z5ESiqWN1KLHJ7CBvRXd6J40dCtEUICm8b2qDotTiYse9P0QU2bcN6v7bofY6vY4ePQoAePPNN7Fly5bmf7Zt24YXX3wRAPDPf/4Tt912GyZPnowVK1Zgy5YtuPHGG3Hs2DGpa4uEQRaZSuboG7PH6jht7BCgLWuk9L6pDS7Thg/T/P4EfD7UV6xH3Rtvor5ive45jEQkVrdUdf/tUHudXkVFRUhOTsbevXvRu3fvVv+EZhl/9NFHGDlyJH71q19hyJAh6N27N3bv3i11XUq4XUiqiOzQrlRPE/D5cHDpMhzbtw8d8vPR+efXwN2hg5DH1rJWLa/VaUXYMbdVAbgzM9FjyQNIGz484uvW0q9Ly/tjVSNZIoptaM5Q5KbmoqahJmJdlgsu5KbmYmjOUKnrSE9Px2233YaZM2fC7/fjvPPOQ11dHT766CNkZGRg4sSJ6NOnD5599lksX74chYWF+Nvf/oYNGza0aYhuBrZwoJjM+PKrvv9+HHpmKeD3/3Cj243sG29A7u23C3kONfS+VieNCVJsgXBS15t/ja6//GXM9Wt5r2K9P6L7aRFRayJaOIROFwJoFWi5EPz3VMbpQgBYsmQJlixZgq+//jr43IEAHnzwQTz66KP46quvkJWVhaFDh2LOnDn40Y9+hKamJvzyl7/Eyy+/DJfLhWuuuQaZmZl4++23sWXLFtXPyz5ZYJAlmxlfftX3349DTz2t+PvsyZNMCbTMeK12CcZi9clSG0SLeD1WNZIlSiQy+2R5Uj2YNXyWlADLSgyywCBLJjO+/PzHjmHH4CGtM1jh3G703bJZ89ahFma8VrtthwV8Pnz32OP47qGH2v7SxAySFY1kiRKNkzu+W0VEkMXCd1Kkuh/SJxt1P8fhv/8jeoAFAH5/8DoBlAqrZb/WUJYs/DlC8wG9K1boelyjav/1r8i/OPl3r+r5C6QXnzux3xhRIktyJ2GYZxjG9xyPYZ5hcRlgicLCd1JkxpffsX37hF4XTbRMUuDYcVWPoee1qu2Qnj56tKnbYVoCS1kZpIDPhxPffafqWjv1GyMiUoOZLFJkRrPNDieP3Iq6TkmsTNKxb75W9Th6XqsZGUE9rM4geVeswK7RJahZuDD6hSr7abH9AxHZDTNZpMiMLuqdf34Nau67L2ZNVuefX6P7OdRkkg6/8C+0y83FiZqaqK+145DBqK9Yr6nQ2+pgRomVHetVz09U2W/MbvVuREQAM1kUhRnNNt0dOiD7xhuiXpN94w2Git7VZJJ81dXICs20UnitGZeMx+6xpdg7cSIO3HYb9k6ciF2jS2LWU9l1/I5VHeu1zE9U00jWrvVuREQMsmzE5/dhQ9UGvPXVW9hQtQE+v/XbHWZ0Uc+9/XZkT54EuMP+OLrdze0bjGwFqc0QdSgoUHyt2ZNuxKGnn9H1RW7X8TtWdaxXOz8xZ/Zs9F69KmZ/sqhZSphTvE9EFAm3C20iUu+R3NRczB4+2/LeIyK6qMeSe/vt6HbLLTj893+06fhudCtISyYprXh4m9facchg7B5bqrtwXUuHdLNZ0bFebdDbrmvXmO+JHYr3iYiUMMiygVAX3fBRBTUNNShbUyati64WrqQk6V9S7g4d0OWG1v2SlGp3QhkkqMimaa0tC3+t9RXrDX+RywxmjDYENSOIbknk9qld692IiAAGWZbz+X1YuH5hxFlQAQTggguL1i/CqPxRCdeLRFTrA6OZJFFf5C2DmePV1fAdOoR22dlIysxCwOfTFdSIKvg2I4gOEXmgwq71bkQkzoUXXojBgwdjyZIlVi9FM9ZkWWxTzaZWW4ThAgigqqEKm2o2mbgqexDZ+kCptsydkYGuv56O9NGjFe8r8ovclZQEX10tvl28GDULF+LAHXdg78SJ+M9556NqwQJN9WZ2LfiOVT8nshbMrvVuREQAgyxL+fw+fFz5saprv21IvO0O0VtBGWPHovfqVeh6881wZ2YCAPx1dfjuoYejnhIU+UWuFBj5Dx/G4WXPqj6xaNeC71Dvq1gnMEUdqLCqeJ8okbEnnXoMsiyy6ptVKH2pFE989oSq67ulJt52h4ytoCOrV+O7hx+Gv66u1e3Rsj+ivsjVti5Qk4myY4NTrZm1UNB76rJl6P7HP+LUZctiniaMxIwTsEQUpPYvUqL5/X7ccccdyM7OhsfjwT333NP8u8WLF2PAgAFIS0tDfn4+fvWrX+Ho0aPNv1+6dCmysrLwyiuvoE+fPkhJSUFpaSn2CZgkEguDLAuECt2jbROGuOCCJ9WDoTlDTViZvajJICXl5iLg96n6G5WR7I+IL3K1rQvUZKLsVvCt970N1YJlXnoJ0oqH6844iQrYiEiZlSUKy5YtQ1paGioqKnDffffh3nvvxcqVKwEAbrcbDz74IL744gssW7YM77zzDu64445W929oaMAf/vAHPPvss/joo49QW1uLn/3sZ9LWG8LCd5NFK3QP50IwuJg1fJZjit5FTmePWbAeCCDQ1IR9N05qvjla0bfR4/5GT+FpCnhirMVuBd92aKVgZvE+UaKxegbrwIEDMXfuXABAnz598PDDD2P16tUYM2YMZsyY0Xzdaaedht///vf45S9/ib/85S/Ntx8/fhwPP/wwiouLAQSDtn79+mH9+vUYPlzefzcYZJksVqF7S7mpuZg1fJbl7RvUktHrS6n1gTszE/7aWvhra1tdH621g4jsj5Evcj0Bj9Ja1J7Q0zMGSA+7ZdaISCyr/yI1cODAVj/n5eWhpqYGALBq1SosWLAAX375JbxeL06cOIHGxkY0NDQgNTUVANCuXTsMGzas+f5nnHEGsrKysH37dqlBFrcLTaa2gH3qgKkov7LcUQFWpC3QUK+vVd+s0v3Y4VtB+c88A3dycuSLo2xNWZ39ibn9qWEtaurE9I4B0sPq95aI5LL6L1Lt27dv9bPL5YLf78fXX3+NSy+9FAMHDsRLL72EjRs34pFHHgEAHDt2TMpatGCQZTK1BezndD9H9zab2eN5YvX6AoBF6xcZWkfL2h2X2x3M4ChRKPq2+rh/q8Ao5sWx1xKtTszIGCA9rH5viUguu/5FauPGjfD7/fjTn/6Ec845B6effjoOHDjQ5roTJ07gk08+af55x44dqK2tRb9+/aSuj0GWyYbmDEVuam5zvVU4o4XuoVOLk5ZPwqwPZmHS8kkofanUUCYpFrN7fen9G5Udjvs3B0Yej/JFGtYSqeC714rl8L75lqntHaK+tyefN2f2LLZSIHIou/5Fqnfv3jh+/DgeeughfPXVV/jb3/6Gxx57rM117du3x80334yKigps3LgRN9xwA8455xypW4UAgyzTJbmTMHv4bABoE2gZLXSXuWUXjdotUFG9voz8jcoOx/1bBkadJ05EUufOhtYSfkLv+81bLGnvoPTehtQsXGRZg1QiMsYOf0mNZNCgQVi8eDEWLVqE/v374//9v/+HBQsWtLkuNTUVs2bNws9//nOce+656NSpE55//nnp63MFAjGa9tic1+tFZmYm6urqkJGRYfVyVItUJO5J9egudPf5fSh9qVQxo+SCC7mpuSi/slz4ScUNVRswafmkmNc9Xfo0hnmGxbwuloDPh12jS2IWffdevUrxX3j/sWMRh1FbwejswXB1b7yJA7fdFvO67n/8IzIvvUT38yjxlpdj/4yZbX9x8j/E7F1FZL7Gxkbs2bMHhYWFSElJ0f04okZ5mWnp0qWYMWMGasMOSsWi9J5piTt4utAiJQUlGJU/ynC7g1DLhI8rP1a9ZSci0GkptAVa01ATsS4rFOCJ6vVldBZhpP9IHFq6VNh/JLQGTS1PLEa7r9rHtbJ2IuDzoXrhIoVfyj/mTURymT1Q3ukYZFkoyZ1kKOCJlA2LRcZ4ntAWaNmaMrjgahVoyer1pdTaoV1ubtRgKdRMLzwDFq31gxZG/pYX7b4AVD+uyAHMWll9zJuI5GNPOvW4XehQoforNU1NWxK1Zae0JpFboGpoyRo1bzMqBQEqthmjUQrg1GyTRb2v0r+iUR63+fGAiJk+WVt2Vm9VElFkorYLEwm3CxOUlq7xIaK37CIRtQWqhZa/UcnMshjphqxmJI3SepUeV2+mL9ZrjBXQ2vWYNxGRFRhkOZCWrvGAueN5jG6ByiSzmZ6RAE71TEONjyuydkLtNqiVW5VERHbDFg4OpLWuKjc1F4svXOyY7vGyyMyyGAngRHRIVnoMEQOYtQyFtesxbyIiKzDIciC1XeOnDpiKp0ufdtR4HplkNtMzEsCJ2DqTtf2mZiszvLGpHXqRERHZAbcLHUhty4RfDf6V9O1BJzHa+iFcyxqlpC7ZSMrNha+mRvM2WcwttqgvSu72m95tUB7zJiJikOVIVrRMiBeiCsIj1Si5s7Kai9G1BHAxg7/QzwICQ62MbIPymDcRJTpuFzpUSUEJFl+4GDmpOa1uz0rOwrX9rkVmcqb0wdBOFWneX+/VqzQFWJFqlPx1dQAAd2Zmq9vVbJNF3WJ78M/o8eCfLdl+42lBIoo3LpcLr7zyiinPxUyWg7VsmfDu3nfxxldv4HDTYfxt+9/wt+1/Q25qLmYPn816rAj0ZlnUtGpwp6SgxzPPwHfwoKZtslhbbFZsv/G0IBGRfgyyHC7JnYS6pjo8t/25NvVZocHQdjxZGBoHZFY/LVHU1ii53G5dzTajBX9WbL+JrmMjIufz+wOo3FmLem8T0jKSkdcnC263woGiBMftQoeL1pg0dNui9YtstXW46ptVKH2pFJOWT8KsD2Zh0vJJKH2pFKu+WWX10mKS2WvLrnhakIhCdm+uwbNz1uKVBzZj5VPb8MoDm/HsnLXYvblG2nO++OKLGDBgADp27IguXbqgpKQE9fX12LBhA8aMGYOuXbsiMzMTF1xwATZt2tTqvjt37sSPfvQjpKSkoKioCCtXrpS2zkiYybIptZmeWI1JZQ6G1kNpHJCds24tJWqNEk8LEtHuzTUof3xrm9vra5tQ/vhWjLupP3oNyYlwT/0qKytxzTXX4L777sP//M//4MiRI/jggw8QCARw5MgRTJw4EQ899BACgQD+9Kc/Yfz48di5cyfS09Ph9/txxRVXIDc3FxUVFairq8OMGTOEri8WBlk2FGkGoFJ9ldrGpDIGQ2sVK+vmgguL1i/CqPxRtt06FFGjpGXeop3wtCBR4vL7A/jg+Z1Rr/nwhZ0oHNRN6NZhZWUlTpw4gSuuuAIFBQUAgAEDBgAALrroolbXPvHEE8jKysJ7772HSy+9FKtWrcKXX36J5cuXo3v37gCA+fPn4+KLLxa2vli4XWgzoUxPeHYqlOkJ31JT25hU7XUyacm62VWrjuaRBALIuGS8YtDkXbECu0aXYO/EiThw223YO3Eido0uadU1nYjIbip31qK+tinqNUcPN6FyZ63Q5x00aBBGjx6NAQMG4Cc/+QmefPJJHD58GABQXV2NKVOmoE+fPsjMzERGRgaOHj2KvXv3AgC2b9+O/Pz85gALAEaMGCF0fbEwyLIRPfVVocakof5Y4VxwwZPqkToYWi0nZd2iyRg7FtmTblT8/aGnn4kYNGkZT0NEZCf13ugBltbr1EpKSsLKlSvx9ttvo6ioCA899BD69u2LPXv2YOLEidiyZQv+/Oc/Y+3atdiyZQu6dOmCY8eOCV2DEQyybERPpifUmBRAm0DLbo1JnZR1iybg88H75ltRrwkfNaNnPI1dBXw+1FesR90bb6K+Yr0j1kxExqRlJAu9TguXy4Vzzz0X8+bNw+bNm9GhQwe8/PLL+Oijj/Cb3/wG48ePx5lnnonk5GR89913zffr168f9u3bh8rKyubbPv74Y+Hri4ZBlo3ozfQoNSa122BoJ2XdotEyasbIfeyI251EiSmvTxbSsqIHUJ06B9s5iFRRUYH58+fjk08+wd69e/Hvf/8b3377Lfr164c+ffrgb3/7G7Zv346Kigr87//+Lzp27Nh835KSEpx++umYOHEiPv30U3zwwQf47W9/K3R9sbDw3UaMZHpaNia1a++peBkHpKeNQzy0fghtd4Zn40LbnWA7B6K45Xa7cP5P+0Q8XRhy3tV9hPfLysjIwPvvv48lS5bA6/WioKAAf/rTn3DxxRfD4/Fg6tSpGDp0KPLz8zF//nzcdtttLdbsxssvv4zJkydj+PDhOO200/Dggw9i3LhxQtcYjSsQ0DqR1l68Xi8yMzNRV1eHjIwMq5djiM/vQ+lLpTEHP5dfWR41ELF7o89Ipyc9qR7MGj4rZtbNDq+tvmI99k6cGPO6U5ctaz6Np+c+dhLw+bBrdIlyNu7kqcreq1c54qQkUaJpbGzEnj17UFhYiJSUFN2Ps3tzDT54fmerIvhOnZNx3tV9hLdvsJrSe6Yl7mAmy0ZEZHq0tH+wit6sm11em542Dk4fT6Nlu9OOQSIRidFrSA4KB3Vjx3eVWJNlM0bqq7S2f7BSkjsJwzzDML7neAzzDFMVYNnltbVq4+AK+w+LwqgZPfexk3jY7iQiMdxuF3r07YzTh3nQo29nBlhRMJNlQ3oyPfHQ6FOJHV9bxtixwJ+XoHr+glYZnna5ucidc2fE2iQ997GLRO10T0RkBIMsmwpletRy2ngdLez62vSMmnHqeBqnb3cSEVmBQVaciJdGn5HY+bXpGTXjxPE0oe3O/bfMCG5vtgy0HLDdSURkBdZkxQmrG336/D5sqNqAt756CxuqNrTqSm+U1a+NgjLGjkWPPy9Bu9zcVre3y81FD7ZvICJqg5msOBFq9Bmr/YOMRp+yT/1Z+dqoNadudxIRWYGZLBVkZmlEsWq8jhmn/pw0OigRhLY7My+9BGnFwxlgEREpYJAVw6pvVqH0pVJMWj4Jsz6YhUnLJ6H0pVJbtUMIMXu8jp6B1no5ZXQQERFRCLcLowhlacKDiFCWxo5f7maO1zH71J8TRgeRBH4f8M1a4Gg10CkXKBgJ8DMnIgdgkKXAjr2Z1NLa/kEvK079mfXayCa2vQaUzwK8B364LaM7MG4RUHSZdesiIlKB24UKtGRpEpWIU39OqHcji2x7DXjh+tYBFgB4K4O3b3vNmnURJTi/34d9X3yG7R+9h31ffAa/g/67fezYMVOfj5ksBXbuzWQXRk/92WUWIdmQ3xfMYEX4cxW8zQWUzwbOuIRbh0Qm2lmxFu8sfQJHD33XfFun7K646Iap6FM8Uspzvvjii5g3bx527dqF1NRUDBkyBK+++iouueQSDB48GEuWLGm+9vLLL0dWVhaWLl0KADjttNMwefJk7Ny5E6+88gquuOKK5t+ZgZksBezNFJuRU3+yTiUyMxYnvlnbNoPVSgDw7g9eR0Sm2FmxFq8tnt8qwAKAo4e+w2uL52Nnhfh/HysrK3HNNddg0qRJ2L59O9asWYMrrrgCgUiTJxT88Y9/xKBBg7B582bcddddwtcYDTNZCtibSZ3Qqb9IGalZw2dFzEjJqnezW2Ys4POxn5ReR5W36nVdR0SG+P0+vLP0iajXvLvsCfQaVgy3wOxyZWUlTpw4gSuuuAIFBQUAgAEDBmh6jIsuugi33nqrsDVpwSBLQShLU7amDC64WgUEid6byef3tTrhNyp/lKZTfzJOJdrtJKh3xYq2g6A9HtsPgraNTrmxr9FyHREZsn/7F20yWOGOHPwO+7d/gfwzBwp73kGDBmH06NEYMGAASktLMXbsWFx11VXo3Lmz6sc4++yzha1HK24XRsHeTG0p9Q17d9+7GOYZhvE9x2OYZ1jU4FN0vZuZ/brU8K5Ygf23zGgVYAHAiepq7L9lBrwrVpiyDkcrGBk8RRi2Df0DF5DRI3gdEUl3tPaw0OvUSkpKwsqVK/H222+jqKgIDz30EPr27Ys9e/bA7Xa32TY8fvx4m8dIS0sTuiYtmMmKIVF6M4VnpyK9RlHZItH1bmb364om4POhev6C1gOUm38ZAFwuVM9fgPTRo7l1GI07Kdim4YXrEQy0Wr6fJwOvcQtZ9E5kkk5Z6jJHaq/TwuVy4dxzz8W5556Lu+++GwUFBXj55ZfRrVs3VFZWNl/n8/mwdetWjBo1Svga9GKQpUK892ZSU8skso5KdL2bnU6CNnyysU0Gq5VAACeqqtDwyUakFQ+Xvh5HK7oMuPpZhT5ZC9kni8hEPfqdiU7ZXaNuGaZ36Yoe/c4U+rwVFRVYvXo1xo4di5ycHFRUVODbb79Fv379kJaWhrKyMrz55pvo1asXFi9ejNraWqHPbxSDrASnNjslMlskut7NTidBT3yrLpBTe13CK7os2KaBHd+JLOV2J+GiG6bitcXzFa8ZNXGq0KJ3AMjIyMD777+PJUuWwOv1oqCgAH/6059w8cUX4/jx4/j0009x/fXXo127dpg5c6atslgAg6y4ombLL/x6tdkp0dkiPacSldjpJGi7buoCObXXEYIBVeH5Vq+CKOH1KR6Jy8rmtOmTld6lK0ZNlNMnq1+/figvL4/4u/bt2+Mvf/kL/vKXvyje/+uvvxa+Ji0YZMUJPe0LtGSnZGSLRNW72ekkaOrZZ6Gdx4MT1dWR67JcLrTLzUXq2WdJXwsRkWh9ikei17Di4GnD2sPolNUZPfqdKTyDFS94ujAO6G3sqSU7FcoWhTcdDXHBBU+qR3O2KFTvpuZUYjR2OQnqSkpC7pw7T/4Q9l6d/Dl3zp0seicix3K7k5B/5kD0O/cC5J85kAFWFMxkOZyRgnQt2Sk7ZYuU2OUkaMbYscCfl7Ttk5Wbyz5ZREQJhEGWwxkpSNdayySyjkoWu5wEzRg7FumjR7PjOxFRAmOQ5XBGCtL1ZKfski1yAldSEts0EJGtaJn5l+hEvFcMshzOaEG6nuyUrGyR1tORRESkTvv27QEADQ0N6Nixo8WrcYaGhgYAP7x3ejDIcjgR7QvskJ2y23BnIqJ4kpSUhKysLNTU1AAAUlNT4Qo/nEMAghmshoYG1NTUICsrC0kGyjxcAYfnDr1eLzIzM1FXV4eMjAyrl2OJ0OlCABG3/Ow+Z1GpIapT1k9E5ASBQABVVVW264puV1lZWfB4PG2CUS1xB4OsOBEpE+RJ9dimIF2Jz+9D6UulisX7oUxc+ZXllmwdcguTiOKNz+eLOEiZftC+fXvFDJaWuIPbhXHCDlt+ethpuHM4bmGSTAzgySpJSUmGtsBIPQZZccQu7Qu0sNNw55bUznQk0mPVN6uwoGIBar6vab4tp2MO7iy+k3+uiOIIO76Tpew03DkkVoNXAFi0fhF8fp9pa6L4seqbVZi5ZmarAAsAar6vwcw1MxUnNBCR8zDIIkvJGtdjhJYtTCItfH4f7ll7T9Rr5q2dxwCeKE4wyLKQz+/DhqoNeOurt7ChakNC/oc11BAVQJtAy6pxPXbdwrSK3x/A/h2H8Z8NVdi/4zD8fkeflbHUJ9WfoO5YXdRrao/V4pPqT0xaERHJxJosi7Co+gd2G9dj1RamHQuhd2+uwQfP70R9bVPzbWlZyTj/p33Qa0hOlHtSJOsr16u+rjivWPJqiEg2BlkWYFF1W0ZPR4oMUGI1eAWAzsmdUV1fjQ1VG4QEQ3YMundvrkH541vb3F5f24Tyx7di3E39GWhppbb3I3tEEsUF9skymd37QjmRjABFqcFrJFqeK1Iw+O6+d23XjNXvD+DZOWtbZbDCdeqcjOv+MBJuNyMCtT4+8DGmrJwS87onxzyJc7qfY8KKiEgrLXEHa7JMxqJqsULBUPh7GsoK6j2pFdrCzEmNnalR+1yrvlmF0pdKMWn5JMz6YBYmLZ+EsS+OxT3r7rHdScbKnbVRAywAOHq4CZU7a81ZUJwY5hmGzOTMqNdkJWc5rhULEUXGIMtkVhdVyyi2t6qAX3arhZKCEiy/cjmeLn0aC85bgM7JnSNep+a5FIPB72tQ16RcCG1V0F3vjR5gab2OgpLcSbhnxD1Rr5k7Yi6z2ERxgjVZJrOyL5SsbTVRj6m1rsqMbvGhBq8bqjbgcNNhXc8VLRhUy+yTjGkZyUKvox+UFJTggQsfsF0NHhGJxyDLZLGKqkM1WaL7Qskothf5mHqCNTOzgkaeK1YwqIaZzVgBIK9PFtKykmPWZOX1yTJvUXHEqWOwiEgbbheazIq+UDK21UQ+pt66KjOzgkaey0iQZ0UzVgBwu104/6d9ol5z3tV9WPRuQChLOr7neAzzDGOARRSHGGRZQKmoOjc1V8pJMhnF9qIe00iwZma3eCPPpTfIs6oZa0ivITkYd1N/pGW13hLs1DmZ7RuIiFTgdqFFzNwukLGtJuoxjdRVhbKCZWvK4IKrVaAmOkAx8lxqtogzO2QiuV2yLZqxttRrSA4KB3ULnjb0NiEtI7hFyAwWEVFsDLIsFNoukE3GtpqoxzQarJnZLV7vc6kJ0OaOnGvbGh2324UefSOfrCQiImUMshKAjGJ7UY8pIlhTkxUU1RFebwZSbYDG/khERPGDHd8ThFIHcyNdxUU8ZqgDfqxgzUgHfDuNrLHjfEIiIlKPHd+pDRnF9iIeU/ZpS1kd4fXiiTIiosTBTFaCkZFJEfGYkbJNnlSPoboqzokkIiLRtMQdrMlKMDKK7UU8pozTlmZ0hCciIlLCIIuEUMpmaclyiQ4ArZ4TSUREiY1BVpyxorBaqbB8fOF4vLXnLcsKzmW0rmDhOhERqcUgK45YcYpOaX5hdUM1nvnimTbXG5mVqEbLICg7JRs5HXPw7fffCmldYadTikYxWCQiko+F73FCKdgx0qIhlliF5Uq0FJxrCQYiBUGZyZmoa6pTbAKq9n2x4v2VJZ6CRSIis9mmhcP777+PCRMmoHv37nC5XHjllVda/T4QCODuu+9GXl4eOnbsiJKSEuzcuVPmkuKSjAHQasQqLFeidq7hqm9WofSlUkxaPgmzPpiFScsnofSl0ohtF5RaNXibvACAjA6t/0XQ0mbCqvdXBru1tCAiimdSg6z6+noMGjQIjzzySMTf33fffXjwwQfx2GOPoaKiAmlpaSgtLUVjY6PMZcUdGQOg1TBaMB7t/lqCgVhBkAsupLRLwV/H/hWLzl+Ep0ufRvmV5aqzNla9v6LFU7BIROQEUmuyLr74Ylx88cURfxcIBLBkyRL87ne/w49//GMAwLPPPovc3Fy88sor+NnPfhbxfk1NTWhqamr+2ev1il+4w1h1ik5LwbiW+6sJmhatX4RR+aOQ5E5SFQRVN1TD7XJjfM/xitcpbU3GyylFtrQgIjKXZYXve/bsQVVVFUpKfsgmZGZmori4GOvWrVMMshYsWIB58+aZtUxHkHGKTo1Y8wuVxCo41xoMiAiCotUpmfX+yi5Gj5dgkYjIKSwbq1NVVQUAyM3NbXV7bm5u8+8iufPOO1FXV9f8z759+6Su0wlCwU74WJoQF1zwpHo0DYBWI9pIHCVqRuVoDQaMBkGxtiYPNx6W/v5qqT/Ty6pg3Ci/P4D9Ow7jPxuqsH/HYfj9jj6rQ0QJxHGzC5OTk5GRkdHqn0Qne/5fNErzCz2pHtx45o3ITQ0LolUUnGsNBowEmWrqlO7fcD/uGHZH82OFPzbgjPmKooNxM4Kf3Ztr8OyctXjlgc1Y+dQ2vPLAZjw7Zy12b64R/lxERKJZtl3o8XgAANXV1cjLy2u+vbq6GoMHD7ZoVc4VCnYibXkZmf/XktJ2VrSROLcMvUXzFlisbcjw7cZQkFm2pkyxVYNSEKR2a7JzSmcp76/W+jMjjLxP4XZvrsEHz+9Efe0P9ZFpWck4/6d90GtITpR7qrd7cw3KH9/a5vb62iaUP74V427qL+y5iIhksCzIKiwshMfjwerVq5uDKq/Xi4qKCkybNs2qZVlGRD2OjPl/IbF6KymNxNE7Kueq06/CI1vankpVCgb0BplatibH9xzv+PmKIoJxM4Ifvz+AD56P3s7lwxd2onBQN7jd6raqiYjMJjXIOnr0KHbt2tX88549e7BlyxZkZ2fj1FNPxYwZM/D73/8effr0QWFhIe666y50794dl19+ucxl2Y7I5pAyBkArNeKU0b090nvRUrRgQE+QqXVrMh7mKxoJxs0Kfip31rbKkkVy9HATKnfWokffzrqfh4hIJqlB1ieffIJRo0Y1/1xWVgYAmDhxIpYuXYo77rgD9fX1mDp1Kmpra3HeeeehvLwcKSkpMpdlK2YGMHqYuZ2l9F6ETB80HVMGTon6PFqDIK1bk6JZVYyuN1g0K/ip90Z/Dq3XERFZQWrh+4UXXohAINDmn6VLlwIAXC4X7r33XlRVVaGxsRGrVq3C6aefLnNJtuKE5pBmNeKM9l4AwWDnpZ0vxXyMDVUb8NZXb2FD1QZV75uVhwYA606G6mVW8JOWkSz0OiIiKzjudGE8cUIncbO2s4y+F0ZaICidkNQyekcvq4M8rcwKfvL6ZCEtK/pjdOqcjLw+WYaeh4hIJssK38m8AMZIUb1Z21lG3gsRW64yDw3EYsbJUFFCwU+0LUMRwY/b7cL5P+0TscA+5Lyr+7DonYhsjUGWhcwIYIwW1ZtVs6T3vRBZMybj0IBaVgZ5WpgZ/PQakoNxN/Vv0yqiU+dknHe1uFYRRESyMMiykOwAJlaG51eDf4VT00+N+oUusrdSNHrfi3iax2dlkKeFmcFPryE5KBzULVhw721CWkYwS8YMFhE5AYMsC8kMYNQU1bfsQ9U5uTMu7XkpRp06qk3AZcZ2lt73gvP4rGFm8ON2u9imgYgcyRUIBBw9CMzr9SIzMxN1dXWOHbETaUvPk+oxFMBsqNqAScsn6bqv0nai7AHGgPb3Qu3rfLr0adtlicx4P4mISCwtcQeDLJsQ/YX71ldvYdYHs3Tf3wWXZT26tLwXPr8PpS+VxtxmLL+y3FYBjMgGtE7n9wc0Z8T03IeISAQGWWQokwXYNziJJFR7BiDiNqOsYFFvYKxUKyd7vaKIDHDUzEAMf77vjx7Hh/+SOzeRiEgJgyyKmeFRy47bbJHI2HLV+nxqMlGhz0WpWN/uwa3IwdBKMxBDxt3UHwDaPF80HBpNRLJpiTtY+B6nohWSayGyYNzolmi0+5vZAsFIXy4nn4YUORhazQzENc/tQGP9cU1r5NBoIrITBllxTOlUoBaiZuYZrUFSc38zWiAY7cvl1NOQogdDq5mBqDXAAjg0mojshWN1YtAzD89OSgpKsPzK5Xi69GksOn8Rpg+aDqDtCJdwImfmhTI/4YFeKPMTa/SN0fuLZHT8j1UDoY3SMhhaDZmDnTk0mojsgpmsKOLlBFh4hqd3595Rs1sim4weO3EM9667V3fmR2RHdxGMZqLM6qAvmujB0DIHO3NoNBHZBTNZCuyUPRGtZXbrun7XoXNy660VUYORV32zCiUvluBw02HFa2Jlfuw2RNtoJsppA6FDRA+GVjMAWg8OjSYiO2EmKwK7ZU9kCGW3hnmG4dazbxVeMK5UHK5EKfNjtxomEZkoJw2EDhE9GFrNDMTk1HZoajihaZ0cGk1EdsIgKwInnwDTQ3TBeLQgVYlS5sduNUyiRiE5ZSB0iIzB0LFmIAKI+nwtcWg0EdkRg6wI7JY9cdr4lVhBakuxMj92rGESlYlyykDoEBmDoWPNQFR6vnOv6o2OnTqw4zsR2RqDrAjslD1xYvG91uAzWuZH5hBtI5yWidJKqau7jMHQ0QZAmzmImohINAZZEcTKngBAZnIm/AE/fH6ftC9WI00vraQ2+MxOycZd59yl+BpCX/Snevvj94WL8WDVIlR/X9X8e6trmJyWiVIrVlf3aEGRDGY/HxGRKByro0BpHl44WVklJ49fUTPSp3NyZ6y6ahU6tOsQ8feRv+g7IG+sG77TauMuc2QXakbdsO6JiBKZlriDLRwUhOpuclKjf6HIaulgt9YFWsRqU+CCC3ePuDtqgFX++NY2J9nqa49h1wuN6Ft3NoZ5hlkSYPn9AezfcRj/2VCF/TsOw++35u8oMprkqu3qbtVrJiJyGm4XRhGqu9lQtQG3vncrvMe8ba6R1dLBbsX3WuktDhc9vkUkkcORjZBVp6elqzu374iIYmOQFUOSOwlJ7qSIAVaIjJYOdiq+10tPcbhdv+hFDkc2Qmadnuiu7kREiY5BlgpWZJXs2LogGqXTaFqLw+34RW+X7JrsJrmiu7oTESU6BlkqWJFVsmvrgkhEbqPZ8YveLtk12U1yRXd1JyJKdCx8VyGUVQov4g5xwQVPqkd4Vkmp+F7UbEERlIvUg9touzfXaHo8NTPtzP6it0t2TXZGNdTVPRqOrSEiUo+ZLBWszCrZqell+JZgbq9M4dtoMsa3GGWX7JoZGVW1Xd2VtoeJiOgHDLJUsnKorx2aXkbaEkzp1B6NR49HvZ+ebTQZ41uMsMs2mll1erG6rNvllCURkd2xGalGTpsjKEKsBpWxjJlchNOHeTTfz07ZErs06VRqkhvKqMreRrbL+0BEZBUtcQczWRqFZ5VCzSntEAjIoOZkXSx6t9HsNE7FLtk1KzOqdjllSUTkFAyyDEiEbRM1J+uiiafTaHYZVmxVnZ5dTlkSETkFgyyd7NKcUjajJ+bi7TSaXbJrVtTp2eWUJRGRU7CFgw6JNONN7VZfSlr7Vj936pwcN4EmBdnllCURkVMwk6VDIm2bqD1Z97//NwLVu+tsVZtmp8L5eGCXU5ZERE7BIEuHRNo2Udu3ql07t60CykSolzObHXuYERHZGbcLdUi0bZPQybrwTuxqtgRDpy//s6EK+3ccNmULVXQXevqBkT8LRESJhpksHRJx20TPyTorsklOaTPg5K1Mu5yyJCKyOwZZOiTqtomWk3VWnb50Qr1cPGxl2uWUJRGRnXG7UCdumyiz8vSl3evlYm1lfvCv/5i2rUpERHIxk2UAt00iszKbZOd6OTXB52er/4vPVv/XcZktIiJqi5ksg0LbJqcP86BH384JH2AB1maTQvVy0VhVL6elez6L9ImInI9BVpyz4nSfldmkUL1cNFbVy+kJKuOlqS0RUSLidmEcs6rAWs3py46d2iO3V6aU57fLMOdweoJKq4v0iYhIPwZZNiTieL+VsxXVnL78/uhxPPe7ddICPjvUy4V/jrm9MmMGn5GI3lZ1cvsIIiInYZBlMyKyT3boFaWUTWpJdsAXrc2A7EBD6XPsMywHW1bu0/RYIrdV46F9BBGRUzDIshFR2Se79IrqNSQHBQO6Ytmsj9BYf1zxulgBn+iASHagEe1z3LJyHwaPycfODTWqMloii/StzG4SESUiBlk2ITL7dLS2UdVzmtErqnp3XdQAC4ge8IkOiGQHGmo+x12f1ODa3wcHan/12bf4bPV/Fa8VVaRvh+wmEVGi4elCm9CSfYpm9+YafPivXaqe04xeUUbaOYieQWhGk1TVn+OuYFB5/k9ON6Wprag/X0REpB4zWTYhoreUUpYmErN6Relt5yAj82LGNqraz3H5E1sx6roz0GtIjilF+nbvhE9EFI+YybIJo72l1AQlLfU7L0/1tUbobQ6qNiA6sOOw6rWYEWio/RybGk60ysbJbmpr5074RETxikGWTRjtVK6lmzgAbHj9azw7Z630juJ6m4OqDXTK/6p+29CMQEPN59iSWc1G7dwJn4goXjHIsgmjncr1ZF/MGt2iZ5i26oxQ/QnVr8GMQEPN59iSWXVQdu6ET0QUr1iTZSNGOpUbyb6YcapMa92Rmq7xLal5DWqapIoINEKf47vPfYmm+hMxrzerDsqunfCJiOIVgyyb0VsErTUoaUlkz6xoPa2iNQcNpyYgakntazAr0Og1JAfJKe3w6p+3xLzWzDooO3TCJyJKFAyybEhLMNLyPlqCknAisimie1o1Z4T+9iWaGsRlhMwKNLr37Rwz8LWiDkrPny8iItKONVmC+f0B7N9xGP/ZUIX9Ow6bUtQcolT7pIbRbIronlYhvYbkoHRqf1XXankNsk/zhZ7D1nVQfh+w5wPg8xeD/+v3WbMOIqI4xUyWQHaYCxeepemY3gGrntmGhrpjivcxmk2R3U28x+n2zAipIXt7UvfIoW2vAeWzAO+BH27L6A6MWwQUXWZoTUREFMQgSxA7zYUL3w760c9Ol1rsLbvJp1kF67LI2p7UHdRvew144XoAYVlWb2Xw9qufZaBFRCQAtwsFMGNcixF6WihoYUaTT9mvQTbR25O6t2f9vmAGKzzAAn64rXw2tw6JiARgJksAM8a1GCWz2NusbuKRtkJdAaDh6DHs33E4YU7JGdqe/WZt6y3CNgKAd3/wusLzjS+WiCiBMcgSwClz4WSdKlPTPkJUzVToNezeXIPVS7dbWv9mFUNB/dFqdU+i9joiIlLE7UIBEn0unNmn6PRslVl56lM0Q0F9p1x1T6L2OiIiUsRMlgBmZnLsyqwmn3q2yuxw6lMkQ0F9wcjgKUJvJSLXZbmCvy8YaWiNRKSN3+/D/u1f4GjtYXTK6owe/c6E251k9bLIIAZZAjj99JsoZjT51LpVZqdTn6IYCurdScE2DS9cD8CF1oHWyc9p3MLgdURkip0Va/HO0idw9NB3zbd1yu6Ki26Yij7F/AuPk3G7UBCnn34TRXaTTy1bZXY/9amX4e3ZosuCbRoy8lrfntGd7RuITLazYi1eWzy/VYAFAEcPfYfXFs/Hzoq1Fq2MRGAmSyC7zYXT3ajSxrRslTnh1Kdehrdniy4DzrgkeIrwaHWwBqtgJDNYlPDM3Lbz+314Z+kTUa95d9kT6DWsmFuHDsUgSzC7zIUTUYckM0jT+9hatsp2bVR3Qs7qU596GQ7q3Uls00DUgtnbdvu3f9EmgxXuyMHvsH/7F8g/c6Dw5yf5GGTFIRF1SDKLxY08tpb6N1mnPu2UIbRLUE/kdKFtu3ChbbvLyuYID7SO1h4Weh3ZD4OsOCNijqDMYnERj612q0zGqc94O6lIRNZt23XKUvcXJLXXkf0wyIozRuuQZA57FvnYarbK1GS9ep+do/p1xONJRSKybtuuR78z0Sm7a9TnTu/SFT36nSnsOclcPF0ogc/vw4aqDXjrq7ewoWoDfCbOgTPafV5LkKaV6MdWc5Kx15AcDB6Tr/gYW1buU57z10K8nlQkIuu27dzuJFx0w9So14yaOJVF7w7GTJZgq75ZhYXrF6K64Yei69zUXMwePhslBSXSn99oHZLMEUFWjB/y+wPYuSF6EKUmexbPJxWJEp2V23Z9ikfisrI5bQru07t0xaiJ7JPldAyyBPH5fXjysyfxyKePtPldTUMNytaUYfGFi6UHWkbrkGSOCLJi/JCo4Mgp8ymJSDurt+36FI9Er2HF7Pgeh7hdKMCqb1ah9KXSiAEWAAROdtVetH6R9K1Do40qQ0FaNHpHBMl8bCWigqNEn09JFM/ssG3ndich/8yB6HfuBcg/cyADrDjBIMugVd+sQtmaslbbg5EEEEBVQxU21WySviYj3edlDns2e5A0IC44siJAJCLzhLbtOmV3bXV7epeuUto3UGLgdqEBPr8PC9cvbM5UqfFtw7cSV/QDI40qZQ57NmuQdIioNg6cT0kU/+y2bceh0c7HIMuATTWbYmawwnVL7SZpNW1pbVQZ3mTz2t+PQPXuOuFNN80cPyQyODI7QCQi84W27azGodHxgUGWAVqyUi64kJuai6E5QyWuSL9ITTaT09ph0Kh8nDX+NOEBkJmdykUGR0oBIgDs33HYFl3gich8IrNOVnSfJzkYZBmgNSs1a/gsJNkw1avUZLOp/gTWv7EHn76zD6OuO0NTMKI0esaqkTQis2fhASK7wBMlNpFZJw6Nji8MsgwYmjMUuam5qGmoiVqXldsxF7OLzemTpZWaJptNDSc0dTRXCjr6DMvBzg01lgUjMrJn7AJPlNhEZ504NDq+8HShAUnuJMwePhtAcDswkumDp2P5VcttGWAB6vpIhajpaB4KOsIfs762CVtW7ot4e/njW1V1XbcbdoEnSmxqs05+Da171HaV37v1U2z/6D3s++IzTY9P5mImy6CSghIsvnBxmy7vnlQPZg2fZdvgKkRL88xYTTvVBB1K9M5DjEb21iS7wBPpFw8n52RkndR2lf/438//cB8WxNsWgywBSgpKMCp/FDbVbMK3Dd+iW2o3DM0Zasv6q3Bam2dGC8q0ZMXCiQ5GzKiTYhd4In3i5eScjJmHarrPt3l8FsTbFrcLBUlyJ2GYZxjG9xyPYZ5hjgiwAHVNNluKFpQZDSZEBSPRtixFbk2yCzyRdqEapvAgIhQo7KxYa9HKtJMx81BN93klWrcmST4GWQlOTRf2kFhNO40GEyKCETPrpNgFnkgbGTVMWp573xefCa1jCmWdotEz81Cp+3wsoa1Jsg9uF1JzH6l3n/sSTfUnFK+L1bRTTXd1JaKCETPrpNgFnkgbq07OydqeDGWdIp0uDNE78zC8+/zB/+5FRYs6LCVatiZJPmayCEAw0Jp0//kYNuE0JKe2jr3VzDwEtGXFwokKRsyukzIyJ5Io0cioYYpF9vak3pmHajJrLYdGF/QfpGo9WrYmST5msqiZ2+3C8Et64uyLC3WfyouWFWvXwY2k9u5Wt4seSWNFnZSZY4KInExGDVM0ZjX21DrzUE9mTU1BvJ6tSZKLQVaCitbeQETTzkjbjieO+XHimB/DJpyGrJxUKcGIqIHQWpk5JojIqcwOFMzcnlQ781Bv81KZW5MkD7cLE9DuzTV4ds5avPLAZqx8ahteeWAznp2zVsipOzWF59s/rETvs3LRo29nKTMRY21Zsk6KyBpqTs6JDBSs2J6Mxmjhv96tSbIOM1kJRvYYGDs06BQ5EJqIxAoFCuHbZeldumLURLF9sszenoxFRGZN69akWvHQHNaOGGTZiOwO5WrbGxjpvG6XBp2skyKyL1mBQjjZ25NaAxNRmTW1W5NqxUtzWDtikGUTZnQoNyPLZFbhuZqAVFSdlOzglygRiQ4UlJ5DVh2TnsDEbpk1QPyAa2qNQZYNyN7Ca348E7JMZhSemxGQWvFcRCSejO1JvYGJ3U4ImnX6MpGx8N1iZnYoV5s9OlRZj/07Dut6TtmF52aNzDH7uYhInj7FIzHlkadw9d3zMf43t+Pqu+fjFw8/pSvAMlK8bnbhfyxaasRIHwZZFtOyhWeU2jmFG9/6xtCJQ1kNOs0MSM18LiKSr2Vjz/wzB+oOZIwGJnY6IWi305fxiNuFFjOzUFzNGJhWz2lgu1JG4bmZJxftcEqSiOxHbcDxzdZPFQvizSr8j0Vt7VdqZqbklcQvBlkWM7tDuVJ7g2j0njgU3aDTzIDULqckiche1AYmLecMRiqIN6PwPxY1NWIA8PbDD2D0jTexAF4HbhdaTM0WnugO5b2G5ODa349ASqf2qq4XtV1plJkBqRXjeYgSnZp5flYLBSZaiJqTKJqaGjEAqD98UNX6ZXx+TvgzEQ0zWRZTs4Uno0N59e46NB49rvp6vRkbke0PzByZY9V4HqJE5ZReTWraQiix40m9H05fPo6jhw5GvTba+mV8fk75MxENM1k2IKtQPBqtQVND3THNRd6ix/eYOTKH43mIzBNqiRC+bWXXDJBS8XosdjupF8oSnThxHGeN/3HM65XWL+Pzc9qfCSXMZNmE2R3KtW5zffTiLmxZtU91fyhZvb/MHJnD8TxE8jm1V1N48fqh/+7Fxy3qsJTY5aRepCyRGuHrl/H5OfXPRCQMsmxEdKF4NGq2w8KpDZBkj+8xMyDleB4iuUTM87NKy+L1fV98pirIMrObuxKlZqpqhK9fxufn5D8T4bhdmKDUbIcpidUfyozeX6GA9PRhHvTo21lq0GPmcxElmnjp1aSmIN7Mbu5K1GSJlERav4zPL17+TAAMshKaUi1YLLECJLY/ICK17DjPTw+7dXNXoiZLpCTS+mV8fvHyZwKwSZD1yCOP4LTTTkNKSgqKi4uxfv16q5eUMHoNycH180fi8plDMGZyEc6+uEDV/aIFSGx/QERqOSUDpEaf4pGYMGM2UtIzWt1uRTd3JXqyP9HWL+Pzi6c/E5YHWc8//zzKysowd+5cbNq0CYMGDUJpaSlqajgXziwtt8NOOSNb1X2iBUhW9P4iImdySgZIjZ0Va/Hus39F4xFv820dMzJwwfW/sCTAitRjSm3258Lrf6FqzqOMzy+e/ky4AoGApcPXiouLMWzYMDz88MMAAL/fj/z8fNx8882YPXt2m+ubmprQ1PRDFsXr9SI/Px91dXXIyMhocz1p4/cH8OyctTH7Q133h5FRa5OUTheGyGpNQUTy+P0+aaNgIp12S+/SFaMmOqMnUqxicrMzWUo9pkZd/wu8++xfo24Zpnfpil88/JSmz1bG52fXPxNerxeZmZmq4g5Lg6xjx44hNTUVL774Ii6//PLm2ydOnIja2lq8+uqrbe5zzz33YN68eW1uZ5AljqgAaffmGrY/IIoTZjSGlBnEyeT3+/Dk9MnCAxe9YgV8Z0+4Ap+8/m/F3+sNCGV8fnb8M+GYIOvAgQPo0aMH1q5dixEjRjTffscdd+C9995DRUVFm/swk2UOUQGSyI7vRGQNu2Vp7GbfF5/hhXvnxLzuqrt+D7fLLTVgUBvwXXD9L7Bm2V9tlyVyAi1BluP6ZCUnJyM5mQXTsonqD2Vm7y8iEs9pjSFjZT5kZEbUFpO/vngRmuqPNP8sY0SM2h5TqekZmPLIU7bLEsUbS4Osrl27IikpCdXV1a1ur66uhsfjsWhVFMIAiYic1Bgy1pamrC1PtcXkLQMs4IcRMSIzgVp6TLVspkpyWHq6sEOHDjjrrLOwevXq5tv8fj9Wr17davuQiIis4ZTGkLFm3b333NPSZuGpaTkQzbvLnoDf79N9/5ZqK/erus7MHlORTjkmCsu3C8vKyjBx4kScffbZGD58OJYsWYL6+nrceOONVi+NiCjhiWgMKbt4Wc2W5sY3Xon6eyNbnqGWA3pH1YjKBPr9Pny2ennM6zpldzGtx5QZBybszPIg66c//Sm+/fZb3H333aiqqsLgwYNRXl6O3Nxcq5dGRJTwQlmaWIXUSl/aZnzJqtnSDAT8UX9vNNDpUzwSl5XNafNaUzqlo/HokSj3DBKRCQy+DwdjXjdw9DhLTznK2Ca1K8uDLAD49a9/jV//+tdWL4OIiMKoydIoNYY060tW1Fblf05uGerNtPUpHolew4pbZe38fj9e/P3vYt5XxPad2vchK6+74eeKxWkHJmSxvOM7ERHZWyhLE153FG3citovWRH1OaLqi7YsfwMv3DsHT06frLtGK1RM3u/cC5B/5kDknznAtBExdpr5p+XARDyzRSaLiIjsLVKWJlrGx8xTiWq2NF0ud8wtwxCRmTYjmUCtjG7tiuSUAxOyMZNFRORwPn8A63YfxKtb9mPd7oPw+eX0mA7P0kQLDMz8klUz6+6sSy/X/LiiMm16MoF6qJ35B0D6aT87ZdWsxEwWEZGDlW+txLzXt6GyrrH5trzMFMydUIRx/fMsW5fZX7JKhectu5h373NGm99HI7L/l9ZMoJHnifY+AGjTEV7GaT87ZdWsZPmAaKO0tLcnIoon5VsrMe25TQj/j3hoLsOj1w61LNCyap6f2o7v/6lYiy3L34j5eON/czv6nXuBsPWZJdL7sHtDherxSCLabsTrOKa4HqtDRETBLcJ5r29rE2ABQADBQGve69swpsiDJAvmhZpZixT+vNEyTy1/rybIcup2Vvj7oOW03+4NFULabqjJLsY7BllERA60fs+hVluE4QIAKusasX7PIYzo1cW8hbVg5y/ZRNvOUnsQoeLfz2Ptv/7e5nd6DwOYtU1qVwyyiIgcqOaIcoCl5zpZ7Pola1WmzSpqDxhsevu1qL/X09sqkWckMsgiInKgnPQUodfJZNcvWbMybbLHCqmhdtuz8ejRqL+3yzBwp2CQRUTkQMMLs5GXmYKqusaIdVkuAJ7MFAwvzDZ7aY4iO9Nml9l9arZH1ZLR28oOgagMDLKIiBwoye3C3AlFmPbcJriAVoFWqMx97oQiS4renUZWpk3rWCGZgYbRIdYtiT4MYJdAVAa2cCAicjC79slKdFpbWJgVaOysWIuVf30Y33u9uu4vuu2GE9s8sIUDEVGCGNc/D2OKPFi/5xBqjjQiJz24RcgMlrW0jBVqPHrUlEHaQHB79PixJrz98J903V/kYYBEGCLNIIuIyOGS3C7L2jTIEA/1OWrrlo4cOogP/r4s6jVGA43w9zNNx3afjLYbZs63tAqDLCIiso14qc9JzcxUdV193WGpgUbk97MLUjqlo/HoEcX7dcrugnHTZ6Khrk5aoJsIQ6QZZBERkS1oLRS3NZXVzo1HlAOdlvQEGsrv58GY973ohptQ0H9wzOuMZB0TYYg0gywiIrJcvNXnNHjrVF3ncrtVXac10FDzfqakZyCpXXvUH/4h6NKyLWg065gIXfcZZBERkeXirT5HbVCUXzQAX6xZLTzQ2PfF5zHfz8YjXlx11+/hdrk1Z6JEZB1ldN23Wz0fgywih/D5fdhUswnfNnyLbqndMDRnKJIc8Dd6im+ivtTirT5HbZYm/8wBwgONnRVrseKJB1Vd21BXh37nXqD6sQGxWUeRXfftWM/HIIvIAVZ9swoL1y9EdUN18225qbmYPXw2SgpKLFwZJTKRX2pOrM+JFmBqydIoBRopndIx9OIJ6DWsWPWaYvWdCqfn/RSddRTRdd+u9XwMsohsbtU3q1C2pgyBsEramoYalK0pw+ILFzPQItOJ/lKTUZ8jc+tITYCpJUsTCjQq/v0CNr71Gprqj6Dx6BGs/dff8dnqFaoCVzUZppb01jvJyDoa6bpv53o+BllENubz+7Bw/cI2ARYABBCACy4sWr8Io/JHceuQTCPjS010fY7MrSMtAaaaLE0oGNz1SQU2vfWqqseNRE2GqSW9jUXtlnW0cz2fumMNRGSJTTWbWm0RhgsggKqGKmyq2WTiqijRaflS0yKU+emU3bXV7eldumrKjIWCoPA1hoKVnRVrNa2rJbUBpt/va/45lKXpd+4FyD9zYKvAZmfFWjw5fTJeuHdOxAAr2uOGU5s5SumUbmj7LJR1jMbMU4F2rudjJovIxr5t+FbodUQiyPxSM1qfI3vrSGTWRGv9VKzHVZs5unTmLFU9sJSIzDqK2NK1W2atJQZZRDbWLbWb0OuIRJD9pWakPkf21pGoAFNr/ZSax1V9orFogObnDSfiVKCoLV0799tikEVkY0NzhiI3NRc1DTUR67JccCE3NRdDc4ZasDpKVHb+UhOZZYuUZREVYGqtn1LzuDL6TkVjJOso8uCE2a9bCwZZRDaW5E7C7OGzUbamDC64WgVaLrgAALOGz2LRO5nKzl9qooIgpSzLhRN/ISTA1LOVquZxRfadUkNP1lHGlq7Zr1stBllENldSUILFFy6O2Cdr1vBZbN9AlrDrl5qILFu0LMsbDyzE2ROuwCev/1vx/moCTD1bqWoDVxF9p2SStaVrx9fNIIvIAUoKSjAqfxQ7vpOt2PFLzWiWTU2WZcfa9zFhxmy8++xfdQeYaoJBPY8b4nYnoUe/M5s/m/3bvzD02YjsOSbz4ISRej4ZGGQROUSSOwnDPMOsXgZRK3b7UgOMZdnUZlk6ZmRgyiNP6Q481ASDQ8f/GL3PLtYV0IjsEya655idTwOKxiCLiIjijt4sm5Ysi9EAU9aWq8iichnjaux8cEI0BllEZA2/D/hmLXC0GuiUCxSMBLj9SQLpCYL0ZFn0bqX5/T6kdOqE838+Ed9769AxIxPp2V0Mb+uJKiqX1XPMzgcnRGOQRUTm2/YaUD4L8B744baM7sC4RUDRZdatixKe1iyL3q20aPczElyILCqX2XPMrgcnRGOQRUTm2vYa8ML1QHjfL29l8Parn2WgRZbRkmXRu5UmYwuu+TEEFpXLHldjx4MTonF2IRGZx+8LZrAiNFZtvq18dvA6IouomaGoZ4YhAJw4cQwr//qw5vupJbKo3IwC9WhzHeMBM1lEZJ5v1rbeImwjAHj3B68rPN+0ZSUSn99n21YgItsEGBUry6JnK21nxVqsePJhNB7xarqfFiKLys0sULfTZy8SgywiMs/R6tjXaLmONFn1zaqITW1nD59teVNb0W0CRIhWOK91K03rMGi9W3Aii8rNKlC342cvCrcLicg8nXLFXkeqrfpmFcrWlLUKsACgpqEGZWvKsOqbVRat7IcAJDxjEqpR2lmx1qKVKdOylaZnGLSRLTil7c5OXbpi5E9+jhMnjmPfF5+p2pJUs3VqhBM/ey2YySIi8xSMDJ4i9FYicl2WK/j7Amf/7dVufH4fFq5fGHHIeAABuODCovWLMCp/lOlbh7LaBMimZStN6zBoEVtw4dudtZUH8Omqcqz919+br1GbLZJVoO7Uz14LZrKIyDzupGCbBgA4OeD6Byd/HreQ/bIE21SzqU0Gq6UAAqhqqMKmmk0mripIbW3T5rdfx/aP3lOdgZEttJUWTWgrTevWn6geUaHtznbt2mPtv/4f6g8fbPV7LdkiGQXqWuranIqZLCIyV9FlwTYNEftkLWT7Bgm+bfhW6HUiqQ1A1jz71+b/b5d6HbW9ntRu/XXMyMSYX0wX+rr0ZovMKESX3SLCDhhkEZH5ii4DzriEHd9N0i21m9DrRNJTe2Skn5To4EHNVpqarcWOGRmY+ugzaNeug+61RKL3FKQZheiJMMOQQRYRWcOdxDYNJhmaMxS5qbmoaaiJWJflggu5qbkYmjPU9LWpCUCUaK3XkRU8xBrfo+aU3phf/Fp4gAWIOwUpolFquESYYciaLCKiOJfkTsLs4bMBBAOqlkI/zxo+y5J+WWpqm5Soqdfx+33Y98VneHfZk5aeYpN9Sk+J6FOQRhqlhtNS1+ZUzGQRESWAkoISLL5wccQ+WbOGz7K0T5ZSbZMa0TI1kTJXSvScYtO69WjFGBnRpyCNNEqNJN5nGDLIIiJKECUFJRiVP8qWHd/DA5CG2sOtit2VKGVqtDb/1Bo86N16jLW1KJqWhqJWFaLH8wxDBllERAkkyZ2EYZ5hVi8jopYBiN/vwydvvKKrXkdP809AffCgtm7JLqNiRJ+ClFGIbnbwaRYGWUREZDtGRrpobf4ZoiZ4UF23FPBjzbK/2mZUjKhTkC0DW7sEkXbGIIuIiGxJb72Onu0stafY1NYtvfHAwrbrknBCTwsRpyBDgW08zxsUiUEWERHZlp56HT3bWWpPsYmoR7LzqBg1ga2ZbR6cjkEWERHZmtZ6HS29t7SeYhNRjyT6hJ5o0QLbRJg3KBKDLCIiiitqtr2Gjv8xep9drLmOyEjz1JbsPipGKbC1os2Dk7EZKRERSRdqCmrWkOdYzT9HTZyia9CxkeapLenJiJn9HkaSCPMGRWImi4iIpLKqSFpW/6VodUsXXvcLvPvsX4WPirFLoXkizBsUyRUIBNoOsnIQr9eLzMxM1NXVISMjw+rlEBFRC7Gagjq5SFqphYHo12yn99Dv9+HJ6ZNjBpG/ePipuK3J0hJ3cLuQiIikMHsWntlCdUv9zr2g1dajyDmFdnsPE2HeoEjcLiQiIikSuUha1FalHd/DeJ83KBKDrATi9wdQubMW9d4mpGUkI69PFtxul9XLIqI4lehF0iJGxdj1PYzneYMiMchKELs31+CD53eivrap+baUtPY4/Zxc9BzYjQEXEQlnZZF0vIx8sXOhebzOGxSJQVYC2L25BuWPb21ze2P9cXy2+r/4bPV/kZaVjPN/2ge9huRYsEIiikdaZ+GJovYknhMCMaveQxKDhe9xzu8P4IPnd8a8rr62CeWPb8XuzTUmrIooyOcPYN3ug3h1y36s230QPr+jDztTGCuKpEMn8cKDktDIl50Va5uve3L6ZLxw7xy89eD9eOHeOXhy+uTm39sFC82djS0c4tz+HYfxygObVV/fqXMyrvvDSG4dknTlWysx7/VtqKxrbL4tLzMFcycUYVz/PAtXRqJFyizJKJJW217gwut+gdeXtB3gHGLHthJmvYcUm5a4g0FWnAoVue/aXIOta/Zruu/lM4egR182kiN5yrdWYtpzmxD+H59QaP/otUMZaMUZM7bm9n3xGV64d07M61LSM9B4xKv4e7v2eXLC9mYi0BJ3sCYrDkUqctei3qvvfkRq+PwBzHt9W5sACwACCAZa817fhjFFHiQxoxo3zCiSVnvCLlqABdi3rQQLzZ2HQVacUSpy1yItI1nQaojaWr/nUKstwnABAJV1jVi/5xBG9Opi3sLI8USesJPdEoFZqcTAICuO+P0BvPu3Lw09RqfOwf5ZRLLUHFEOsPRcRxSi5iRex4xMfO+ti/lYMlsi2GUOIcnH04VxZONbX6Op4YShxzjv6j4seiepctJThF4H8JQiBak5iTd68rQ2427CyWyJoPb0I8UHZrLihN8fwKfv7tN9/06dk3He1eyTRfINL8xGXmYKquoaI9ZluQB4MlMwvDBb1ePxlCK1pGbki9vljjpwWVZLBLVzCHsNK+bWYZxgkBUnKnfWoqleWxbr7IsL0Ll7GkfskKmS3C7MnVCEac9tggtoFWiF/gTOnVCkquhd6ZRiVV0jpj23iacUE1SskS9Wzd6z4xxCkotBVpzQcyLwlDOy2aqBLDGufx4evXZomwyUR0MGiqcUKZpYJ/GsmL1n1zmEJA+DrDih9UQgC9zJauP652FMkQfr9xxCzZFG5KQHtwjVBkRmn1L0+QO61+okiXTqzeyWCHaeQ0hyMMiKE3l9spCWlay6NxYL3MkOktwu3QGQmacUE6Xui6fe5OIcwsTD04Vxwu124fyf9ol5XafOyRh3U38WuJPjyTilGEmo7is8axaq+yrfWmno8e2Cp97k4xzCxMMgK470GpKDcTf1R1pW663DlE7tMXD0Kbh85hBc94eRDLAoLoROKSrlY10IZpvUnlKMJFbdFxCs+3J6ywi1p978fp9JK4pfoaL78DYS6V262nJmIhnD7cI402tIDgoHdUPlzlrUe5t4cpBaiae6ominFHHy558Nyzf0HInSnZ6n3sxlRdE9WYNBVhxyu108NUhtxGNdkdIpxZAHVu3EPzfs0/0aE6U7PU+9mY9zCBMDtwuJEkA81xWN65+HD2ddhJklp0f8vZHXaFbdl9V46o1IDgZZRHEuUeqK/rlhb8TbjbxGM+q+7CB06i0annoj0o5BFlGc01JX5FSyXmOo7gtAm0BLa3d6O+OpNyI5GGQRxblEqCuS+RpDdV+ezNZbgp7MlLga28NTb0TisfCdKM4lQl2R7NdotDu9U/DUG5FYDLKI4lyorqiqrjFiXZYLwayMk+uKzHiNRrrTOwlPvRGJw+1CojiXCHVFifAaich5GGQRJQAn1BX5/AGs230Qr27Zj3W7D2o+CeiE10hEicUVCAQcfW7b6/UiMzMTdXV1yMjIsHo5RLZm147vIhul2vU1ElF80BJ3MMgiIkuFGqWG/4coFBYxC0VEdqIl7uB2IRFZJlEapRJRYmKQRUSWSYRGqUSUuBhkEZFlEqFRKhElLvbJIiLLxHOjVL/fx6aeRAmOQRYRWSZeG6XurFiLd5Y+gaOHvmu+rVN2V1x0w1SOpyFKINwuJCLLxGMT0Z0Va/Ha4vmtAiwAOHroO7y2eD52Vqy1aGVEZDYGWURkqXhqIur3+/DO0ieiXvPusifg9/tMWhERWYnbhURkuXgZwLx/+xdtMljhjhz8Dvu3f8H5gEQJgEEWEdmCKQOY/T7gm7XA0WqgUy5QMBIQWIx+tPaw0OuIyNkYZBFRYtj2GlA+C/Ae+OG2jO7AuEVA0WVCnqJTVmeh1xGRs7Emi8hu/D5gzwfA5y8G/5f1O8Ztew144frWARYAeCuDt297TcjT9Oh3Jjpld416TXqXrujR70whz0dE9sZMFhEgfRtJNROyLQnH7wu+p4rDe1xA+WzgjEsMf+ZudxIuumEqXls8X/GaUROnsl8WUYLggGiKCz5/QH/RtF0Cm1C2RWlU8tXPMtDSY88HwLJLY1838Q2g8HwhTxmpT1Z6l64YNZF9soicTkvcwUwWOV751krMe31bqxl4eZkpmDuhKPbxf6XAJrSNZFZgIzvbYpdMnRWOVou9ToU+xSPRa1gxO74TJTgGWeRo5VsrMe25TW1Ck6q6Rkx7blP0PksmbiPF9M3atvVC4evx7g9epzXbYpdMnVU65Yq9TiW3O4ltGogSHAvfybF8/gDmvb5NMUQCgHmvb4PPr7AjriWwUcNIwbqsbItJBd+2VjAyGFS26Skf4gIyegSvIyISiJkscqz1ew612iIMFwBQWdeI9XsORe6/JDKwMZotkpFtEZ2pc+qWozsp+Dm8cD2CgVbL9+Nk4DVuoTNeCxE5CoMscqyaI8oBlqrrRAU2Iuq6QtkWb2XbxwEQzLZ015ZtEbkFadWWo6jAruiy4OcQ8TUsNPYa7BJ82mUdRNSMQRY5Vk56SuyLol0nIrARlS2SkW0Rlamz6nCA6MCu6LLg5yAyELFLvZtd1kFErUiryfrDH/6AkSNHIjU1FVlZWRGv2bt3Ly655BKkpqYiJycHt99+O06cOCFrSRRnhhdmIy8zJVqlDfIyg+0cIgoFNs1Xh98bsQMbo3VdLeu4OnYGfrIUyAgr1M/ori+QEZGpixlEIhhEim6YKquWzJ0UzNoNuCr4v0YDLDvUu9llHUTUhrRM1rFjx/CTn/wEI0aMwFNPPdXm9z6fD5dccgk8Hg/Wrl2LyspKXH/99Wjfvj3mz1du5EcUkuR2Ye6EIkx7bpNS7gdzJxRF75dldBvJSLZIKfswdgGQ1sV4tkVEpk7mqUcldjr1qcQua7TLOogoImmZrHnz5mHmzJkYMGBAxN+vWLEC27Ztw3PPPYfBgwfj4osvxv/93//hkUcewbFjx2Qti+LMuP55ePTaofBktt4S9GSmRG/f0FLRZcCMrcFmlFc+FfzfGZ/LLViPln148Qbg+8PGsy0iMnUW9JgSfupTBrus0S7rIKKILKvJWrduHQYMGIDc3B++fEpLSzFt2jR88cUXGDJkSMT7NTU1oampqflnr9crfa1kb+P652FMkUd/x3fgh20krfRki8zMPhjN1GkJIkUVXosI7GQXgVsRfNp5HUQUkWVBVlVVVasAC0Dzz1VVVYr3W7BgAebNmyd1beQ8SW5X5DYNsukpWDd7C85IwbfaILL+ILCkv5jCa6O1ZGYUgVvU4NS26yCiiDRtF86ePRsulyvqP19++aWstQIA7rzzTtTV1TX/s2/fPqnPRxRTKFuktmDdiuyD3oJvNVuO/a8MbnGKKrw20jzUrCLwmGuEOQ1O2WiVyNY0ZbJuvfVW3HDDDVGv6dmzp6rH8ng8WL9+favbqqurm3+nJDk5GcnJyaqeg8g0WrJFTss+RNtyLJ0PLL8TQrc+9bazMHMbttUaFfS/Uvl5RG1nstEqka1pCrK6deuGbt26CXniESNG4A9/+ANqamqQk5MDAFi5ciUyMjJQVFQk5DmIdNPzJai2rktG41HZlIJIWVufemrJrNiGHXkzsPbByL9f+xBwyrC2a5XR/0tWo1UiMkRaTdbevXtx6NAh7N27Fz6fD1u2bAEA9O7dG506dcLYsWNRVFSE6667Dvfddx+qqqrwu9/9DtOnT2emiqwlu6bHqdmHSEGkzK1PrbVkZm/D+n3A1hejXxOeOZPV2FXre8Xu8ESmkBZk3X333Vi2bFnzz6HTgu+++y4uvPBCJCUl4Y033sC0adMwYsQIpKWlYeLEibj33ntlLYkoNrO6m8dL9sHo1mesL3stpz7N3obVmjmTvZ2p9r1id3gi00gLspYuXYqlS5dGvaagoABvvfWWrCUQaWN2Y0cZY17MZmTrU/SXfcPB2NeILALXmjmzorFrOKtGJBElKGnNSIkcx4rGjiLHvFhBb8NT0acA/b6TBfgxjJ0v7j3WmjmzuqeVVSOSiBIYgyyiEKu/BJ1KawsLGV/2MQPkk9IE9lLT2j7B6lOl7A5PZDrLmpES2Y7VX4JOpmXrU8a2mVW9x9QeYPD7gv907BwcmRSR5FOl/EsEkekYZBGFOLG1gp2oLbyW8WVvVYCs5gBDpNqzNkw4Vcq/RBCZjkEWUYhTWys4jYwveysD5GhZPKVC83BmnCrlXyKITMeaLKKWtNYXJTq/D9jzAfD5i8H/VVNHJWMUjN4CfFEiHWCIWnt2UsfOwPWvATM+l/9ny+r3iCgBMZMVh3z+ANbvOYSaI43ISU/B8MJsJLmjzFij1uKhtYIZ9LZgkJUxtFvvMTXF+N8fBlxu8/5s2e09IopzrkAgECOPbW9erxeZmZmoq6tDRkaG1cuxXPnWSsx7fRsq6xqbb8vLTMHcCUUY1z8vyj2dhYGkxRS3wU5+BmqyfhGDtB7Gv+zt0s388xeBlybHvu7Kp4IZMDPZ5T0iciAtcQeDrDhSvrUS057bpPS1h0evHRoXgVaiBJK25fcBS/pHydKcrO2Z8XnsL+54/rLf8wGw7NLY1018Q17zUSISTkvcwZqsOOHzBzDv9W3ROg9h3uvb4PM7OqZuDiRbBlgAUFXXiGnPbUL51kqLVnaSnholp61HZL8lmc1Yrf4sZNSeEZGjsCYrTqzfc6hN4NFSAEBlXSPW7zmEEb0ENmQ0UaxA0oVgIDmmyGPN1qFFM+EUt05lrccJ/ZbsMJ+Pp1WJEh6DrDhRc0Q5wNJznRpm10XZOpC0aCac0tbpX4b+F0PW3SJnPXbvt2Sn+XwsNNcnnreRKaEwyIoTOekpQq+LxYq6KCsCSVXMHix9klINXk1dA3LX3oOAKxBho0rAeuzcb8mizyIqp55WtSrQsUMWkkgQ1mTFieGF2cjLTIlW/YG8zGC2ySir6qLMDiRVs2AmXLSt02HuL9HddUjxz4Lh9di535Jd5/M5bRD4tteChxuWXRo8Ibns0uDPWgd363lekYPDiSzGICtOJLldmDuhCIDi1x7mTigyvJ1nZYG9mYGkJhbUKEXbOs1Brfz12LVpqxPqxayg5RCAVYGOjMHhRBbjdmEcGdc/D49eO7TNNp5H4DaeVXVRofqv8f09eOqjr9v8XmQgqZkFNUrRtkRrkKXuQYyux47bYHavF7OClu03K7dbZQwOJ7IYg6w4M65/HsYUeaQVpFtRFxWp/svtAlomy0QGkppZUKMUbUt0vf8MHAhkw4NDiPyxC1yP2qHQZrFzvZgVtB4CsDLQYRaS4hCDrDiU5HZJO11nRYF9pOLuUAvdSeeehjFFHl2BpLDTkRYc1Q9tnVbVNbZ5b/xw497j1+PRDksQgAuuRGodIOqziIfTbXqyUlYGOmqzi0erg6/NaZ8HJSTWZDmIzx/Aut0H8eqW/Vi3+6AljUXNrItS0xfr7a1VuoKj8q2VOG/RO7jmyY9xyz+34JonP8Z5i97RX7Rvco1SrBq85f7h2DLiQbjsVjMVIrNRqNHPwqqib9H0HAKwcrs1ZvPWk5bPcebnQQmJY3Ucwk6jZELZJSBinkDY+J51uw/imic/jnndP6acoylzJ3X8kMkZkJh/LuyYkZF9RD/0mo9UAvXfAmndgPQ8da9dxExGu9AzO7F5ZFKM7VY1I5P0aH7/ofD8LdYBOOvzoLihJe7gdqEDKAUFoZYJZs8kNKPAHpBT/yW9a7zaGiVBwU/MGjy71UzJbhQaLYBTs0Votx5bRujJSlndpV6peWsbDvw8KCExyLI5u46SkV1gD8ip/7JF13iRmRy/D0nfrMWIhmogMxSsWTBSSA3ZQYzRAC7eTrfpPQRgdZf60KnViseCW4OKHPZ5UEJikGVztggKFMgssAeiF3cDwQDTo7H+y/Ku8SIzOU7rjC0ziBERwMXb6TYjWSmr23O4k9Rn4o5UBuv67LQlTnQSC99tzvKgwEIyGqxa2jVeZLNFJ3bGlhnEiOj0Ho89towcArC6S73a97n8TucfUqC4xUyWzdl2lIxJ1NR/aWnFMLwwG1mp7VHbcDzi7/Vkx1QTlclxau2QzCBGRAAnu8eWmYcQwp/rN58C+yqcke1peXAhtSvQcBBRi+Abvmv9sxWDwIkUMMiyORlbZk4Trf5L66nLlduqFAMsIPifcmld49UGAl+9F/1L0Km1QzKDGL0BXHgwMnYB8OINEF70bebWbrTnCp0itKtIa9fMxn/RoITD7UKbE7VlZnWPLaPPH6r/+vHgHhjRq0tzgKVlUHXoEEE0WantMabIo2ltqqkNBD64P/qWh1Nrh2QOlo7ZY8kFZPRoHcBF6oe14k5g5M1i+52ZubXrxG3kEKW1R5LaNcYFFg0CJwrDTJYDGG2ZYHWPLaXnv+uSInRO66DrdKKeU5exDhEAQG3DcXmHCGJmclqItuXh5NohWSfXtBZ5RzuAsPYh4CdLgdQuxrfXzNzaVVPz98ZM4ESj+r5hZom69pNSuwLjFgTXfqQS+PeU2I9rt79oUMJhkOUQelsmWN1jS+n5K+sa8au/b2p1m5bAT8+pS8sPEUQNBMJF+QJ2+ny+SCfX8ouDNUOfv6g/qFEbwKkJfJbPEdNw08yt3ZjPhWD9Uig4sdNJ1D0fqFt7el7wfdrzgbrHteNfNCihMMhyEK0tE6zusRXt+SPREvjpCZhscYhAdbNFQPEL2OqGkSK0bJK67TXgwUFi6pXUtB4wM/Axc2tX62PYpUB822vA679Rd23oNTr9LxqUMFiTFce0ZHuseP5I6wGCgV+smi09AZOZcxejKroMmLEV+NHt6q5v+eUZmvnnOwZceKdpsxKlkVFDFKv1gJmBj5lbu5ofQ2PbEBlCn//3h9VdH3qNMuv7iARikBXHrN4e0/O4agM/PQGTjL5burmTgMIL1F0b+mIJL9ReMx8IBIAL5wTnz018I7jFJSrAkjnEOfT4ovqGaWFm4KOnIF/ac0ViYYG4mjqsZhHeJ5OHshPpwSArjlm9Pda1U7Lu+8YK0PQGTKFDBJ7M1q/Zk5li3gzIUPBypDJYXK2oxReLUsbnSBWwZgGQ1EFsw8hIJ+9EN3kU0UBUDzMDHzMzLlGfKwYrCsTV1JC1FOl9CmWFJ74h5y8aRAaxJiuOWdljq3xrJe557Qvd91cT+Ok9dWnG3EVFqvsAtfgCBsxtPip7iHOIVa0ozK5pM3MWoKaavxasKBBX+7l27AxMeFD5fbLbEHSiFhhkxbFQtmfac5uUvkqkbI8pnShUQ2vgpzdgkj13MSKl4CWSll/AMU9eCSzUNrPlgFWtKPy+4Bf3OdOAz54/2VH8JFlDkM2cBdjyuY5UBsfOKHZNt7BAXO3n+pNlQE+VW+tENsMgy6a0jIqJxmiPLa20nihsSW/gZ0nApFXM+hNXcOsw1Aeo5RewmRkfM0/eWXFCLFImMbUrMPBqoO94ub2jzMy4tHyuqs+BtQ8qX2tVgbjaz/+088xeGZEwDLJsSHTzUDO3x9SeKLxldB+88Mk+XYGfqADU1OdQE7y07APUkpkZHzMDOrO37ZQyiQ0HgY8fBU4dEX+n0ba9FmyuqmTkzdbVL8VDKxKiGBhk2Uy05p2/fG4THtNZnG1WtkfticKe3dLw4ayLdDVX1ROAagmapHTI1xq8tJypl9btZJfrKkjP+Ji9hWdWvZJTh2oboeb03taXgJJ7rHvNZtarEVmAQZaNqNlqm/3vz6U1DxVBy4lGrYGf3u71WoImaR3ytQQvkba0OmbjhxayEv/Gb8UWnhn1Sk4dqm2EmtN7dnjNZtarEZmMLRxsRO1svYff2WXSirQLnWhUorfhZ6zu9UDkJqZahkjrfQ5V1LYNaDgYuVVDqFljx6zWt4vuCRSzDUAAKJ0v/gswVgNRo5w6VNsIJ71m2Z8/kUUYZNmI2q22Z9bu0fdFb4IktwuXDYqe6dFzolFP93qtQZPIDvk+fwDrdh/Eq1v2Y93ug/DBHbtf0tj5wPI7EXVLq30qcN2rcnsCKTV5DFl+p/F+WbIbnYZz8lBtvRLxNRPZDLcLbUTtVlttw/FWg4/1kFU8Xr61Ek+8v0fx91N/VKhru01P93qtQ6RFdchX3p4chnHR6k86dla3peVOCv6NX6aiywC/H3hxYtvfGe2XFWk7VPaw4kScdZeIr5nIZhhk2cjwwmxkdWyP2u+Px7zWyCgcKYXdiF1T5gLw2qeVuGNcP80BnZ7u9VqDJhEd8mPXdA3DuBlbI9effP6iquePub3Tsmheb32L3wesuFPhlwYKxc1qdBqu+STbdQoXBOLvJJve03si/vwQEQBuF9pKktuFG889TdW1ekfhaKlR0krmQGo9swq1Bk1GB0ir3p6EO3L9iYjtHVHjcGSMvLFqVmEi0zrfz4xxSkQJhEGWzfz6oj7ISm2v+Hu9heOA5MJuyB1IrWdW4fDCbE3vpdEB0oaDTKMz9ZTmG4ayRFq+KGUUTVs1qxBoEeApccVvgKd2vp/IPz9EBIBBlu0kuV1YeMWAiF+zRkfhyMw0AeozR13TklsXhasM6rQOd165rQq1DcpbrwG0fS+NDJA2HGQaGSYsOksko2jaytNuVgZ4dhDr9B6zjERSsCbLhmSNwpGZaQLUDaTOTG2PW//1Kaq8+pqJNp3w449XDQJcwHdHmxSL9kNZu2iyUttjTJGnze16O+SLqOnS3ZxRdB8oGUXTVp52c1I7AyskYh8xIhMwyLIpGaNwhAQBUcQaSB0ATmaWWmeXjDQTVTphqbbnmNIpTT0d8tUEmaqGX+tpzig6iJAx8sTK025WBHhOKiBnEEokBbcLbSz0Rf/jwT0wolcXwy0WjBZ2q6G03ZabkaxYHyWqmWhLsrN2kRit6WpFa3PGtG7qFqn2OkB70XQsRrZDjTJa76aV0wrI2VOLSAoGWQlEaBAQxbj+efhw1kX4x5Rz8OefDcY/ppyDP109OGZ9lNFmoi3JztopMVLTZUhA5WEFtdeFqC2a1vJ4IgM3tcwM8JxYQG52EEqUILhdmGBk1XuFC99ue3XLflX3M9JMtCVhW3ca+fwBZHbsgDtK++JQ/TFkd0qGJ0Ncs1dFDd+Jva6lUFZNFKtm1ZkxjNipg6hlbA8TEYOsRCSj3isWM5qJthSrPgwQk7VrKVrtmPSB3k7b7hEduKklO8BzcgG5GUEoUYJhkJWg9BR2G6Ems5Sd1gFVdd9j3e6DGF6YbXjLz6ysHaCm07vErUKAI1S0kBngOb2A3KosI1GcYpBFpoiWWcLJnw/WH8PMFz4FEMwA3XVJkeEtP7VZOyOzHGPVjrkQrB0bU+SRl9Hido89OC2jGIlVWUaiOMQgi0yjlFmKpKquEdP/vglTf1SIJ97fY2jLL1bWzugsRyO1Y2qoDgC53WM9ZhSJqAUGWaSKkUxPSy0zS1XeRvzfG1/gUH3bU4ehDNBrn1bikZ8Pwf+9uV3Klp+IbT6Z7SI0B4B22O5xUn8o0ZhRJKIWGGRRTEYzPeFCmaV1uw9GDLBCQhmgzmnJ+HDWRcIL9UVt88lqF6E7ALRyu2fbawqZtEWJk0ljRpGITmKQRVHJLOjWkgGSUagvaptPRrsIW9R5aRXqDxW+6lB/KJl9sOzGDhlFIrIcm5GSIiPNQNWwqmFoiKhtPhlNXmUP8xaOA4bb0tq1n4jiDoMsUiT7i354YbbiqJ2QzqnthTcMDREZ5Inu9G7FWCBDtPSHIiJKENwuJEV2+KLXlyNTR/Q2n8gmr1Zn+TRzen8oIiIJmMkiRbK/6NfvORR1niEA1DYcl7YlJmObT9RQbzOGeQsVD/2hiIgEY5BFimR/0ZuVKfP5A1i3+yBe3bIf63YfbFVDpnebL9pjimDWMG9hOGCYiKgNbheSItnz/8zYElPTfiJ8m69rWjLgAr472tQ84qflaxTd0kKJmWOBDGN/KCKiNlyBQEBm2Yt0Xq8XmZmZqKurQ0ZGhtXLiUvlWytxz2vbUOUVG1T4/AGct+idmDVRH866SFcgp9R+IvRIkTJVsQIoPY9plK5GsFY1BI3YJ6sH+0MRUdzQEncwk0UqtQ4rRMTmMjNlevpMxeoJFuo8b3bvKs09wqxsCMr+UEREzViTRVGFAo8qb1Or26u9TZj23CaUb6009PiiWx+EaG0/oaYn2O9e3Wr/3lWhhqDh7RRCDUG3vSZ/DewPRUQEgJksisKsruMiWx+EaC2qVxOURRsBpOe5hYvZENQVbAh6xiUMfIiITMAgixSJGjujhuixOVqL6kUGRpb1rtLSENSq2YZERAmE24WkyA7NSPXS2n5CbWCUndbBvr2r2BCUiMhWGGSRIsd1HW9Ba5+pUFAWTV5mCn7/4/6qH9N0bAhKRGQrDLJIkeO6jofRUlSf5HbhskHRi+wvG5SH8QPlFOob0dwYtbYATakeBNgQlIjIFtgni6IKnS4EIrdYsCqw0EJNn6lQz65oNWh5LXp26epdJUF4X69S93o81mEJABdckT6xq59lvyoiIgO0xB0MsigmszqcayUy0Fm3+yCuefLjmNf9Y8o5Qgv0jVDq6zXOvR53t38W3V0tWkmwISgRkRBsRkpCyWixYJTowM9pRf7R2muU+4djZdPZKE3/Cg9P6A53uocNQYmILMAgi1QR3WLBiFid2fVsYTqtyD9Wew0f3HjrSG9UpJ2DEYX2+NyIiBINC9/JUdR0Zp/3+jb4/Np2wZ1W5O+0zBsRUSJikEWOonVcjlpaWz5YzWmZNyKiRMQgixxFZgZH1hxFNZrbMGzZj3W7D8bMxDkt80ZElIhYk0WOIjuDY0WRv54i/lDmbdpzm+BC5PYadsq8ERElImayyFHMyOCEivx/PLgHRvTqIj3AmvbcpjZboKEi/vKtlYr3tTLzRkREsbFPFtleeD+sw/VNmP73zQCc2yAViN0A1YVgwBRqgBrtcezUXoOIKJ6xTxbFDaWttKk/KsRrn1a2ut1jgwapWmgp4o/WPsNO7TWIiOgHDLLItqL1w3ri/T145OdD0Tmtg2MzOGzDQEQU3xhkkS3F6oflAvB/b26LuZVmZ2zDQEQU31j4TrYkqx+WnbANAxFRfGOQRbaUCFtpIhqgau2vRURE5uF2IdlSomylhdowhBf3qyniFz0km4iIxGKQRbYU2kqrqmuMWJcVam8QD1tpahqgKrWxEDkkm4iIxGKQRbaUaB3No7VhiJSxcrsQ9VDAvNe3YUyRJ27eHyIiJ2JNFtkWO5ord4SPVnoVD4cCiIjiATNZZGtWzBK0i2htLNRw8qEAIqJ4wCCLbC9RO5rHamMRi9MPBRAROR2DLEo4dp31F76uKq++ACueDgUQETkZgyxKKHZtexBpXdlp7TU/TjweCiAicioWvlPCUCoiD7U9KN9aaat1Hao/HvO+4XFUIh0KICKyO2ayKCGomYWot+2Bke1HtcXtSm0sHr7G2UOyiYjiGYMsSghaZiFqKbI3uv2otri9c1oHHKo/1vyzmo7wRERkLQZZlBC0zkJUk50KbfMZ6bqudl13XdIPnsyOzFgRETkIgyxKCFpmIarJTonaflS7Lk9mx4RsY0FE5GQsfKeEEJqFqBTuuBAMpA7XN6kqjtey/ShiXWzHQETkPAyyKCGEZiECaBPQhH6+65Ii/N+b2xWzU0AwO+XzBzRvPxpZF9sxEBE5E4MsShixZiF2TuugOjulZfvR6LpY3E5E5EysyaKEEm0W4qtb9qt6jJojjbh0YHfkZaagqq4xYuZLa9f1MUUepKe0x7rdBwEEMKJnV5zTqwszWEREDsYgixKO0ixELdmp0DbftOc2KfawUrvNF6nQ/qVN+9migYjI4aRtF3799deYPHkyCgsL0bFjR/Tq1Qtz587FsWPHWl332Wef4fzzz0dKSgry8/Nx3333yVoSUVRai9BFbPPZtQs9EREZJy2T9eWXX8Lv9+Pxxx9H7969sXXrVkyZMgX19fX44x//CADwer0YO3YsSkpK8Nhjj+Hzzz/HpEmTkJWVhalTp8paGlFEerJT0bYfY5HZhZ6IiKznCgQCsSZ6CHP//ffj0UcfxVdffQUAePTRR/Hb3/4WVVVV6NChAwBg9uzZeOWVV/Dll1+qekyv14vMzEzU1dUhIyND2topcZg1RHrd7oO45smPY173jynnsEcWEZFNaIk7TK3JqqurQ3b2D4XA69atw49+9KPmAAsASktLsWjRIhw+fBidO3du8xhNTU1oampq/tnr9cpdNCUcI9kpLUS1gSAiInsyrYXDrl278NBDD+Gmm25qvq2qqgq5ubmtrgv9XFVVFfFxFixYgMzMzOZ/8vPz5S2aElaoOP7Hg3tghKRTfiLbQBARkf1oDrJmz54Nl8sV9Z/wrb79+/dj3Lhx+MlPfoIpU6YYWvCdd96Jurq65n/27dtn6PGIrMJu70RE8U3zduGtt96KG264Ieo1PXv2bP7/Bw4cwKhRozBy5Eg88cQTra7zeDyorq5udVvoZ4/HE/Gxk5OTkZycrHXZRLYjsg0EERHZj+Ygq1u3bujWrZuqa/fv349Ro0bhrLPOwjPPPAO3u3XibMSIEfjtb3+L48ePo3379gCAlStXom/fvhHrsYjiTagNRHihvUdCoT0REZlL2unC/fv348ILL0RBQQGWLVuGpKSk5t+FslR1dXXo27cvxo4di1mzZmHr1q2YNGkSHnjgAdUtHHi6kOKBzx+QXmhPRETG2eJ04cqVK7Fr1y7s2rULp5xySqvfheK6zMxMrFixAtOnT8dZZ52Frl274u6772aPLEo4Sl3oiYjIuUztkyUDM1lERERkFi1xh2ktHIiIiIgSCYMsIiIiIgkYZBERERFJwCCLiIiISAIGWUREREQSMMgiIiIikoBBFhEREZEEDLKIiIiIJGCQRURERCQBgywiIiIiCRhkEREREUnAIIuIiIhIAgZZRERERBIwyCIiIiKSgEEWERERkQTtrF6AUYFAAADg9XotXgkRERHFu1C8EYo/onF8kHXkyBEAQH5+vsUrISIiokRx5MgRZGZmRr3GFVATitmY3+/HgQMHkJ6eDpfLZfVy4pbX60V+fj727duHjIwMq5dDMfDzch5+Zs7Cz8tZRH5egUAAR44cQffu3eF2R6+6cnwmy+1245RTTrF6GQkjIyOD/0FxEH5ezsPPzFn4eTmLqM8rVgYrhIXvRERERBIwyCIiIiKSgEEWqZKcnIy5c+ciOTnZ6qWQCvy8nIefmbPw83IWqz4vxxe+ExEREdkRM1lEREREEjDIIiIiIpKAQRYRERGRBAyyiIiIiCRgkEVEREQkAYMs0uy0006Dy+Vq9c/ChQutXha18Mgjj+C0005DSkoKiouLsX79equXRBHcc889bf5dOuOMM6xeFrXw/vvvY8KECejevTtcLhdeeeWVVr8PBAK4++67kZeXh44dO6KkpAQ7d+60ZrEU8/O64YYb2vw7N27cOGnrYZBFutx7772orKxs/ufmm2+2ekl00vPPP4+ysjLMnTsXmzZtwqBBg1BaWoqamhqrl0YRnHnmma3+Xfrwww+tXhK1UF9fj0GDBuGRRx6J+Pv77rsPDz74IB577DFUVFQgLS0NpaWlaGxsNHmlBMT+vABg3Lhxrf6d+8c//iFtPY6fXUjWSE9Ph8fjsXoZFMHixYsxZcoU3HjjjQCAxx57DG+++SaefvppzJ492+LVUbh27drx3yUbu/jii3HxxRdH/F0gEMCSJUvwu9/9Dj/+8Y8BAM8++yxyc3Pxyiuv4Gc/+5mZSyVE/7xCkpOTTft3jpks0mXhwoXo0qULhgwZgvvvvx8nTpywekkE4NixY9i4cSNKSkqab3O73SgpKcG6dessXBkp2blzJ7p3746ePXvif//3f7F3716rl0Qq7dmzB1VVVa3+fcvMzERxcTH/fbOxNWvWICcnB3379sW0adNw8OBBac/FTBZp9pvf/AZDhw5FdnY21q5dizvvvBOVlZVYvHix1UtLeN999x18Ph9yc3Nb3Z6bm4svv/zSolWRkuLiYixduhR9+/ZFZWUl5s2bh/PPPx9bt25Fenq61cujGKqqqgAg4r9vod+RvYwbNw5XXHEFCgsLsXv3bsyZMwcXX3wx1q1bh6SkJOHPxyCLAACzZ8/GokWLol6zfft2nHHGGSgrK2u+beDAgejQoQNuuukmLFiwgHO8iDRoua0xcOBAFBcXo6CgAC+88AImT55s4cqI4lPLLdwBAwZg4MCB6NWrF9asWYPRo0cLfz4GWQQAuPXWW3HDDTdEvaZnz54Rby8uLsaJEyfw9ddfo2/fvhJWR2p17doVSUlJqK6ubnV7dXU1634cICsrC6effjp27dpl9VJIhdC/U9XV1cjLy2u+vbq6GoMHD7ZoVaRFz5490bVrV+zatYtBFsnTrVs3dOvWTdd9t2zZArfbjZycHMGrIq06dOiAs846C6tXr8bll18OAPD7/Vi9ejV+/etfW7s4iuno0aPYvXs3rrvuOquXQioUFhbC4/Fg9erVzUGV1+tFRUUFpk2bZu3iSJX//ve/OHjwYKsgWSQGWaTJunXrUFFRgVGjRiE9PR3r1q3DzJkzce2116Jz585WL48AlJWVYeLEiTj77LMxfPhwLFmyBPX19c2nDck+brvtNkyYMAEFBQU4cOAA5s6di6SkJFxzzTVWL41OOnr0aKvM4p49e7BlyxZkZ2fj1FNPxYwZM/D73/8effr0QWFhIe666y507969+S85ZK5on1d2djbmzZuHK6+8Eh6PB7t378Ydd9yB3r17o7S0VM6CAkQabNy4MVBcXBzIzMwMpKSkBPr16xeYP39+oLGx0eqlUQsPPfRQ4NRTTw106NAhMHz48MDHH39s9ZIogp/+9KeBvLy8QIcOHQI9evQI/PSnPw3s2rXL6mVRC++++24AQJt/Jk6cGAgEAgG/3x+46667Arm5uYHk5OTA6NGjAzt27LB20Qks2ufV0NAQGDt2bKBbt26B9u3bBwoKCgJTpkwJVFVVSVuPKxAIBOSEb0RERESJi32yiIiIiCRgkEVEREQkAYMsIiIiIgkYZBERERFJwCCLiIiISAIGWUREREQSMMgiIiIikoBBFhEREZEEDLKIiIiIJGCQRURERCQBgywiIiIiCf4/dvqwJdy6CxgAAAAASUVORK5CYII=",
+ "text/plain": [
+ ""
+ ]
+ },
+ "metadata": {},
+ "output_type": "display_data"
+ }
+ ],
+ "source": [
+ "# TSNEで正解ラベルを可視化\n",
+ "import matplotlib.pyplot as plt\n",
+ "\n",
+ "cmap = plt.get_cmap(\"tab10\")\n",
+ "\n",
+ "true_label_dict = {0: \"ang\", 1: \"dis\", 2: \"fea\", 3: \"hap\", 4: \"sad\", 5: \"sur\"}\n",
+ "\n",
+ "plt.figure(figsize=(7, 7))\n",
+ "for i in true_label_dict:\n",
+ " plt.scatter(\n",
+ " x_tsne[y_true == i, 0],\n",
+ " x_tsne[y_true == i, 1],\n",
+ " color=cmap(i),\n",
+ " label=f\"{true_label_dict[i]}\",\n",
+ " )\n",
+ "plt.legend()\n",
+ "plt.show()"
+ ]
+ },
+ {
+ "cell_type": "markdown",
+ "metadata": {},
+ "source": [
+ "手動でconfig.jsonのstyle2idとnum_stylesを設定するのを忘れずに。"
+ ]
+ }
+ ],
+ "metadata": {
+ "kernelspec": {
+ "display_name": "venv",
+ "language": "python",
+ "name": "python3"
+ },
+ "language_info": {
+ "codemirror_mode": {
+ "name": "ipython",
+ "version": 3
+ },
+ "file_extension": ".py",
+ "mimetype": "text/x-python",
+ "name": "python",
+ "nbconvert_exporter": "python",
+ "pygments_lexer": "ipython3",
+ "version": "3.10.11"
+ }
+ },
+ "nbformat": 4,
+ "nbformat_minor": 2
+}
diff --git a/colab.ipynb b/colab.ipynb
new file mode 100644
index 0000000000000000000000000000000000000000..f4e932b25b84f1aaa0b582231f17c81fbb970625
--- /dev/null
+++ b/colab.ipynb
@@ -0,0 +1,410 @@
+{
+ "cells": [
+ {
+ "cell_type": "markdown",
+ "metadata": {},
+ "source": [
+ "# Style-Bert-VITS2 (ver 2.3) のGoogle Colabでの学習\n",
+ "\n",
+ "Google Colab上でStyle-Bert-VITS2の学習を行うことができます。\n",
+ "\n",
+ "このnotebookでは、通常使用ではあなたのGoogle Driveにフォルダ`Style-Bert-VITS2`を作り、その内部での作業を行います。他のフォルダには触れません。\n",
+ "Google Driveを使わない場合は、初期設定のところで適切なパスを指定してください。\n",
+ "\n",
+ "## 流れ\n",
+ "\n",
+ "### 学習を最初からやりたいとき\n",
+ "上から順に実行していけばいいです。音声合成に必要なファイルはGoogle Driveの`Style-Bert-VITS2/model_assets/`に保存されます。また、途中経過も`Style-Bert-VITS2/Data/`に保存されるので、学習を中断したり、途中から再開することもできます。\n",
+ "\n",
+ "### 学習を途中から再開したいとき\n",
+ "0と1を行い、3の前処理は飛ばして、4から始めてください。スタイル分け5は、学習が終わったら必要なら行ってください。\n"
+ ]
+ },
+ {
+ "cell_type": "markdown",
+ "metadata": {},
+ "source": [
+ "## 0. 環境構築\n",
+ "\n",
+ "Style-Bert-VITS2の環境をcolab上に構築します。グラボモードが有効になっていることを確認し、以下のセルを順に実行してください。"
+ ]
+ },
+ {
+ "cell_type": "code",
+ "execution_count": null,
+ "metadata": {},
+ "outputs": [],
+ "source": [
+ "#@title このセルを実行して環境構築してください。\n",
+ "#@markdown 最後に赤文字でエラーや警告が出ても何故かうまくいくみたいです。\n",
+ "\n",
+ "!git clone https://github.com/litagin02/Style-Bert-VITS2.git\n",
+ "%cd Style-Bert-VITS2/\n",
+ "!pip install -r requirements.txt\n",
+ "!apt install libcublas11\n",
+ "!python initialize.py --skip_jvnv"
+ ]
+ },
+ {
+ "cell_type": "code",
+ "execution_count": null,
+ "metadata": {},
+ "outputs": [],
+ "source": [
+ "# Google driveを使う方はこちらを実行してください。\n",
+ "\n",
+ "from google.colab import drive\n",
+ "drive.mount(\"/content/drive\")"
+ ]
+ },
+ {
+ "cell_type": "markdown",
+ "metadata": {},
+ "source": [
+ "## 1. 初期設定\n",
+ "\n",
+ "学習とその結果を保存するディレクトリ名を指定します。\n",
+ "Google driveの場合はそのまま実行、カスタマイズしたい方は変更して実行してください。"
+ ]
+ },
+ {
+ "cell_type": "code",
+ "execution_count": 1,
+ "metadata": {},
+ "outputs": [],
+ "source": [
+ "# 学習に必要なファイルや途中経過が保存されるディレクトリ\n",
+ "dataset_root = \"/content/drive/MyDrive/Style-Bert-VITS2/Data\"\n",
+ "\n",
+ "# 学習結果(音声合成に必要なファイルたち)が保存されるディレクトリ\n",
+ "assets_root = \"/content/drive/MyDrive/Style-Bert-VITS2/model_assets\"\n",
+ "\n",
+ "import yaml\n",
+ "\n",
+ "\n",
+ "with open(\"configs/paths.yml\", \"w\", encoding=\"utf-8\") as f:\n",
+ " yaml.dump({\"dataset_root\": dataset_root, \"assets_root\": assets_root}, f)"
+ ]
+ },
+ {
+ "cell_type": "markdown",
+ "metadata": {},
+ "source": [
+ "## 2. 学習に使うデータ準備\n",
+ "\n",
+ "すでに音声ファイル(1ファイル2-12秒程度)とその書き起こしデータがある場合は2.2を、ない場合は2.1を実行してください。"
+ ]
+ },
+ {
+ "cell_type": "markdown",
+ "metadata": {},
+ "source": [
+ "### 2.1 音声ファイルからのデータセットの作成(ある人はスキップ可)\n",
+ "\n",
+ "音声ファイル(1ファイル2-12秒程度)とその書き起こしのデータセットを持っていない方は、(日本語の)音声ファイルのみから以下の手順でデータセットを作成することができます。Google drive上の`Style-Bert-VITS2/inputs/`フォルダに音声ファイル(wavファイル形式、1ファイルでも複数ファイルでも可)を置いて、下を実行すると、データセットが作られ、自動的に正しい場所へ配置されます。"
+ ]
+ },
+ {
+ "cell_type": "code",
+ "execution_count": null,
+ "metadata": {},
+ "outputs": [],
+ "source": [
+ "# 元となる音声ファイル(wav形式)を入れるディレクトリ\n",
+ "input_dir = \"/content/drive/MyDrive/Style-Bert-VITS2/inputs\"\n",
+ "# モデル名(話者名)を入力\n",
+ "model_name = \"your_model_name\"\n",
+ "\n",
+ "# こういうふうに書き起こして欲しいという例文(句読点の入れ方・笑い方や固有名詞等)\n",
+ "initial_prompt = \"こんにちは。元気、ですかー?ふふっ、私は……ちゃんと元気だよ!\"\n",
+ "\n",
+ "!python slice.py -i {input_dir} -o {dataset_root}/{model_name}/raw\n",
+ "!python transcribe.py -i {dataset_root}/{model_name}/raw -o {dataset_root}/{model_name}/esd.list --speaker_name {model_name} --compute_type float16 --initial_prompt {initial_prompt}"
+ ]
+ },
+ {
+ "cell_type": "markdown",
+ "metadata": {},
+ "source": [
+ "成功したらそのまま3へ進んでください"
+ ]
+ },
+ {
+ "cell_type": "markdown",
+ "metadata": {},
+ "source": [
+ "### 2.2 音声ファイルと書き起こしデータがすでにある場合\n",
+ "\n",
+ "指示に従って適切にデータセットを配置してください。\n",
+ "\n",
+ "次のセルを実行して、学習データをいれるフォルダ(1で設定した`dataset_root`)を作成します。"
+ ]
+ },
+ {
+ "cell_type": "code",
+ "execution_count": 5,
+ "metadata": {
+ "id": "esCNJl704h52"
+ },
+ "outputs": [],
+ "source": [
+ "import os\n",
+ "\n",
+ "os.makedirs(dataset_root, exist_ok=True)"
+ ]
+ },
+ {
+ "cell_type": "markdown",
+ "metadata": {},
+ "source": [
+ "次に、学習に必要なデータを、Google driveに作成された`Style-Bert-VITS2/Data`フォルダに配置します。\n",
+ "\n",
+ "まず音声データ(wavファイルで1ファイルが2-12秒程度の、長すぎず短すぎない発話のものをいくつか)と、書き起こしテキストを用意してください。wavファイル名やモデルの名前は空白を含まない半角で、wavファイルの拡張子は小文字`.wav`である必要があります。\n",
+ "\n",
+ "書き起こしテキストは、次の形式で記述してください。\n",
+ "```\n",
+ "****.wav|{話者名}|{言語ID、ZHかJPかEN}|{書き起こしテキスト}\n",
+ "```\n",
+ "\n",
+ "例:\n",
+ "```\n",
+ "wav_number1.wav|hanako|JP|こんにちは、聞こえて、いますか?\n",
+ "wav_next.wav|taro|JP|はい、聞こえています……。\n",
+ "english_teacher.wav|Mary|EN|How are you? I'm fine, thank you, and you?\n",
+ "...\n",
+ "```\n",
+ "日本語話者の単一話者データセットで構いません。\n",
+ "\n",
+ "### データセットの配置\n",
+ "\n",
+ "次にモデルの名前を適当に決めてください(空白を含まない半角英数字がよいです)。\n",
+ "そして、書き起こしファイルを`esd.list`という名前で保存し、またwavファイルも`raw`というフォルダを作成し、あなたのGoogle Driveの中の(上で自動的に作られるはずの)`Data`フォルダのなかに、次のように配置します。\n",
+ "```\n",
+ "├── Data\n",
+ "│ ├── {モデルの名前}\n",
+ "│ │ ├── esd.list\n",
+ "│ │ ├── raw\n",
+ "│ │ │ ├── ****.wav\n",
+ "│ │ │ ├── ****.wav\n",
+ "│ │ │ ├── ...\n",
+ "```"
+ ]
+ },
+ {
+ "cell_type": "markdown",
+ "metadata": {
+ "id": "5r85-W20ECcr"
+ },
+ "source": [
+ "## 3. 学習の前処理\n",
+ "\n",
+ "次に学習の前処理を行います。必要なパラメータをここで指定します。次のセルに設定等を入力して実行してください。「~~かどうか」は`True`もしくは`False`を指定してください。"
+ ]
+ },
+ {
+ "cell_type": "code",
+ "execution_count": 6,
+ "metadata": {
+ "id": "CXR7kjuF5GlE"
+ },
+ "outputs": [],
+ "source": [
+ "# 上でつけたフォルダの名前`Data/{model_name}/`\n",
+ "model_name = \"your_model_name\"\n",
+ "\n",
+ "# JP-Extra (日本語特化版)を使うかどうか。日本語の能力が向上する代わりに英語と中国語は使えなくなります。\n",
+ "use_jp_extra = True\n",
+ "\n",
+ "# 学習のバッチサイズ。VRAMのはみ出具合に応じて調整してください。\n",
+ "batch_size = 4\n",
+ "\n",
+ "# 学習のエポック数(データセットを合計何周するか)。\n",
+ "# 100ぐらいで十分かもしれませんが、もっと多くやると質が上がるのかもしれません。\n",
+ "epochs = 100\n",
+ "\n",
+ "# 保存頻度。何ステップごとにモデルを保存するか。分からなければデフォルトのままで。\n",
+ "save_every_steps = 1000\n",
+ "\n",
+ "# 音声ファイルの音量を正規化するかどうか\n",
+ "normalize = False\n",
+ "\n",
+ "# 音声ファイルの開始・終了にある無音区間を削除するかどうか\n",
+ "trim = False"
+ ]
+ },
+ {
+ "cell_type": "markdown",
+ "metadata": {},
+ "source": [
+ "上のセルが実行されたら、次のセルを実行して学習の前処理を行います。"
+ ]
+ },
+ {
+ "cell_type": "code",
+ "execution_count": null,
+ "metadata": {
+ "colab": {
+ "base_uri": "https://localhost:8080/"
+ },
+ "id": "xMVaOIPLabV5",
+ "outputId": "15fac868-9132-45d9-9f5f-365b6aeb67b0"
+ },
+ "outputs": [],
+ "source": [
+ "from webui_train import preprocess_all\n",
+ "\n",
+ "preprocess_all(\n",
+ " model_name=model_name,\n",
+ " batch_size=batch_size,\n",
+ " epochs=epochs,\n",
+ " save_every_steps=save_every_steps,\n",
+ " num_processes=2,\n",
+ " normalize=normalize,\n",
+ " trim=trim,\n",
+ " freeze_EN_bert=False,\n",
+ " freeze_JP_bert=False,\n",
+ " freeze_ZH_bert=False,\n",
+ " freeze_style=False,\n",
+ " freeze_decoder=False, # ここをTrueにするともしかしたら違う結果になるかもしれません。\n",
+ " use_jp_extra=use_jp_extra,\n",
+ " val_per_lang=0,\n",
+ " log_interval=200,\n",
+ ")"
+ ]
+ },
+ {
+ "cell_type": "markdown",
+ "metadata": {},
+ "source": [
+ "## 4. 学習\n",
+ "\n",
+ "前処理が正常に終わったら、学習を行います。次のセルを実行すると学習が始まります。\n",
+ "\n",
+ "学習の結果は、上で指定した`save_every_steps`の間隔で、Google Driveの中の`Style-Bert-VITS2/Data/{モデルの名前}/model_assets/`フォルダに保存されます。\n",
+ "\n",
+ "このフォルダをダウンロードし、ローカルのStyle-Bert-VITS2の`model_assets`フォルダに上書きすれば、学習結果を使うことができます。"
+ ]
+ },
+ {
+ "cell_type": "code",
+ "execution_count": null,
+ "metadata": {
+ "colab": {
+ "base_uri": "https://localhost:8080/"
+ },
+ "id": "laieKrbEb6Ij",
+ "outputId": "72238c88-f294-4ed9-84f6-84c1c17999ca"
+ },
+ "outputs": [],
+ "source": [
+ "# 上でつけたモデル名を入力。学習を途中からする場合はきちんとモデルが保存されているフォルダ名を入力。\n",
+ "model_name = \"your_model_name\"\n",
+ "\n",
+ "\n",
+ "import yaml\n",
+ "from webui_train import get_path\n",
+ "\n",
+ "dataset_path, _, _, _, config_path = get_path(model_name)\n",
+ "\n",
+ "with open(\"default_config.yml\", \"r\", encoding=\"utf-8\") as f:\n",
+ " yml_data = yaml.safe_load(f)\n",
+ "yml_data[\"model_name\"] = model_name\n",
+ "with open(\"config.yml\", \"w\", encoding=\"utf-8\") as f:\n",
+ " yaml.dump(yml_data, f, allow_unicode=True)"
+ ]
+ },
+ {
+ "cell_type": "code",
+ "execution_count": null,
+ "metadata": {},
+ "outputs": [],
+ "source": [
+ "# 日本語特化版を「使う」場合\n",
+ "!python train_ms_jp_extra.py --config {config_path} --model {dataset_path} --assets_root {assets_root}"
+ ]
+ },
+ {
+ "cell_type": "code",
+ "execution_count": null,
+ "metadata": {},
+ "outputs": [],
+ "source": [
+ "# 日本語特化版を「使わない」場合\n",
+ "!python train_ms.py --config {config_path} --model {dataset_path} --assets_root {assets_root}"
+ ]
+ },
+ {
+ "cell_type": "code",
+ "execution_count": null,
+ "metadata": {
+ "colab": {
+ "base_uri": "https://localhost:8080/"
+ },
+ "id": "c7g0hrdeP1Tl",
+ "outputId": "94f9a6f6-027f-4554-ce0c-60ac56251c22"
+ },
+ "outputs": [],
+ "source": [
+ "#@title 学習結果を試すならここから\n",
+ "!python app.py --share --dir {assets_root}"
+ ]
+ },
+ {
+ "cell_type": "markdown",
+ "metadata": {},
+ "source": [
+ "## 5. スタイル分け"
+ ]
+ },
+ {
+ "cell_type": "code",
+ "execution_count": null,
+ "metadata": {},
+ "outputs": [],
+ "source": [
+ "!python webui_style_vectors.py --share"
+ ]
+ },
+ {
+ "cell_type": "markdown",
+ "metadata": {},
+ "source": [
+ "## 6. マージ"
+ ]
+ },
+ {
+ "cell_type": "code",
+ "execution_count": null,
+ "metadata": {},
+ "outputs": [],
+ "source": [
+ "!python webui_merge.py --share"
+ ]
+ }
+ ],
+ "metadata": {
+ "accelerator": "GPU",
+ "colab": {
+ "gpuType": "T4",
+ "provenance": []
+ },
+ "kernelspec": {
+ "display_name": "Python 3",
+ "name": "python3"
+ },
+ "language_info": {
+ "codemirror_mode": {
+ "name": "ipython",
+ "version": 3
+ },
+ "file_extension": ".py",
+ "mimetype": "text/x-python",
+ "name": "python",
+ "nbconvert_exporter": "python",
+ "pygments_lexer": "ipython3",
+ "version": "3.10.11"
+ }
+ },
+ "nbformat": 4,
+ "nbformat_minor": 0
+}
diff --git a/common/constants.py b/common/constants.py
new file mode 100644
index 0000000000000000000000000000000000000000..751d5e123409506c52ace9ef764a1402b672b66a
--- /dev/null
+++ b/common/constants.py
@@ -0,0 +1,28 @@
+import enum
+
+# Built-in theme: "default", "base", "monochrome", "soft", "glass"
+# See https://huggingface.co/spaces/gradio/theme-gallery for more themes
+GRADIO_THEME: str = "NoCrypt/miku"
+
+LATEST_VERSION: str = "2.3"
+
+USER_DICT_DIR = "dict_data"
+
+DEFAULT_STYLE: str = "Neutral"
+DEFAULT_STYLE_WEIGHT: float = 5.0
+
+
+class Languages(str, enum.Enum):
+ JP = "JP"
+ EN = "EN"
+ ZH = "ZH"
+
+
+DEFAULT_SDP_RATIO: float = 0.2
+DEFAULT_NOISE: float = 0.6
+DEFAULT_NOISEW: float = 0.8
+DEFAULT_LENGTH: float = 1.0
+DEFAULT_LINE_SPLIT: bool = True
+DEFAULT_SPLIT_INTERVAL: float = 0.5
+DEFAULT_ASSIST_TEXT_WEIGHT: float = 0.7
+DEFAULT_ASSIST_TEXT_WEIGHT: float = 1.0
diff --git a/common/log.py b/common/log.py
new file mode 100644
index 0000000000000000000000000000000000000000..51dca5f3f39047ed9fb58f59d765ca0a332bc49f
--- /dev/null
+++ b/common/log.py
@@ -0,0 +1,16 @@
+"""
+logger封装
+"""
+from loguru import logger
+
+from .stdout_wrapper import SAFE_STDOUT
+
+# 移除所有默认的处理器
+logger.remove()
+
+# 自定义格式并添加到标准输出
+log_format = (
+ "{time:MM-DD HH:mm:ss} |{level:^8}| {file}:{line} | {message}"
+)
+
+logger.add(SAFE_STDOUT, format=log_format, backtrace=True, diagnose=True)
diff --git a/common/stdout_wrapper.py b/common/stdout_wrapper.py
new file mode 100644
index 0000000000000000000000000000000000000000..af5eaaedbe163824792474c68540cef8bccefd9d
--- /dev/null
+++ b/common/stdout_wrapper.py
@@ -0,0 +1,38 @@
+"""
+`sys.stdout` wrapper for both Google Colab and local environment.
+"""
+
+import sys
+import tempfile
+
+
+class StdoutWrapper:
+ def __init__(self):
+ self.temp_file = tempfile.NamedTemporaryFile(mode="w+", delete=False)
+ self.original_stdout = sys.stdout
+
+ def write(self, message: str):
+ self.temp_file.write(message)
+ self.temp_file.flush()
+ print(message, end="", file=self.original_stdout)
+
+ def flush(self):
+ self.temp_file.flush()
+
+ def read(self):
+ self.temp_file.seek(0)
+ return self.temp_file.read()
+
+ def close(self):
+ self.temp_file.close()
+
+ def fileno(self):
+ return self.temp_file.fileno()
+
+
+try:
+ import google.colab
+
+ SAFE_STDOUT = StdoutWrapper()
+except ImportError:
+ SAFE_STDOUT = sys.stdout
diff --git a/common/subprocess_utils.py b/common/subprocess_utils.py
new file mode 100644
index 0000000000000000000000000000000000000000..9a0e3237b49ed3fe6f92584942575125c32451ea
--- /dev/null
+++ b/common/subprocess_utils.py
@@ -0,0 +1,32 @@
+import subprocess
+import sys
+
+from .log import logger
+from .stdout_wrapper import SAFE_STDOUT
+
+python = sys.executable
+
+
+def run_script_with_log(cmd: list[str], ignore_warning=False) -> tuple[bool, str]:
+ logger.info(f"Running: {' '.join(cmd)}")
+ result = subprocess.run(
+ [python] + cmd,
+ stdout=SAFE_STDOUT, # type: ignore
+ stderr=subprocess.PIPE,
+ text=True,
+ )
+ if result.returncode != 0:
+ logger.error(f"Error: {' '.join(cmd)}\n{result.stderr}")
+ return False, result.stderr
+ elif result.stderr and not ignore_warning:
+ logger.warning(f"Warning: {' '.join(cmd)}\n{result.stderr}")
+ return True, result.stderr
+ logger.success(f"Success: {' '.join(cmd)}")
+ return True, ""
+
+
+def second_elem_of(original_function):
+ def inner_function(*args, **kwargs):
+ return original_function(*args, **kwargs)[1]
+
+ return inner_function
diff --git a/common/tts_model.py b/common/tts_model.py
new file mode 100644
index 0000000000000000000000000000000000000000..de4e830dfba3744a1c165d7befc2b239705c8443
--- /dev/null
+++ b/common/tts_model.py
@@ -0,0 +1,335 @@
+import os
+import warnings
+from pathlib import Path
+from typing import Optional, Union
+
+import gradio as gr
+import numpy as np
+
+import torch
+from gradio.processing_utils import convert_to_16_bit_wav
+
+import utils
+from infer import get_net_g, infer
+from models import SynthesizerTrn
+from models_jp_extra import SynthesizerTrn as SynthesizerTrnJPExtra
+
+from .constants import (
+ DEFAULT_ASSIST_TEXT_WEIGHT,
+ DEFAULT_LENGTH,
+ DEFAULT_LINE_SPLIT,
+ DEFAULT_NOISE,
+ DEFAULT_NOISEW,
+ DEFAULT_SDP_RATIO,
+ DEFAULT_SPLIT_INTERVAL,
+ DEFAULT_STYLE,
+ DEFAULT_STYLE_WEIGHT,
+)
+from .log import logger
+
+
+def adjust_voice(fs, wave, pitch_scale, intonation_scale):
+ if pitch_scale == 1.0 and intonation_scale == 1.0:
+ # 初期値の場合は、音質劣化を避けるためにそのまま返す
+ return fs, wave
+
+ try:
+ import pyworld
+ except ImportError:
+ raise ImportError(
+ "pyworld is not installed. Please install it by `pip install pyworld`"
+ )
+
+ # pyworldでf0を加工して合成
+ # pyworldよりもよいのがあるかもしれないが……
+
+ wave = wave.astype(np.double)
+ f0, t = pyworld.harvest(wave, fs)
+ # 質が高そうだしとりあえずharvestにしておく
+
+ sp = pyworld.cheaptrick(wave, f0, t, fs)
+ ap = pyworld.d4c(wave, f0, t, fs)
+
+ non_zero_f0 = [f for f in f0 if f != 0]
+ f0_mean = sum(non_zero_f0) / len(non_zero_f0)
+
+ for i, f in enumerate(f0):
+ if f == 0:
+ continue
+ f0[i] = pitch_scale * f0_mean + intonation_scale * (f - f0_mean)
+
+ wave = pyworld.synthesize(f0, sp, ap, fs)
+ return fs, wave
+
+
+class Model:
+ def __init__(
+ self, model_path: Path, config_path: Path, style_vec_path: Path, device: str
+ ):
+ self.model_path: Path = model_path
+ self.config_path: Path = config_path
+ self.style_vec_path: Path = style_vec_path
+ self.device: str = device
+ self.hps: utils.HParams = utils.get_hparams_from_file(self.config_path)
+ self.spk2id: dict[str, int] = self.hps.data.spk2id
+ self.id2spk: dict[int, str] = {v: k for k, v in self.spk2id.items()}
+
+ self.num_styles: int = self.hps.data.num_styles
+ if hasattr(self.hps.data, "style2id"):
+ self.style2id: dict[str, int] = self.hps.data.style2id
+ else:
+ self.style2id: dict[str, int] = {str(i): i for i in range(self.num_styles)}
+ if len(self.style2id) != self.num_styles:
+ raise ValueError(
+ f"Number of styles ({self.num_styles}) does not match the number of style2id ({len(self.style2id)})"
+ )
+
+ self.style_vectors: np.ndarray = np.load(self.style_vec_path)
+ if self.style_vectors.shape[0] != self.num_styles:
+ raise ValueError(
+ f"The number of styles ({self.num_styles}) does not match the number of style vectors ({self.style_vectors.shape[0]})"
+ )
+
+ self.net_g: Union[SynthesizerTrn, SynthesizerTrnJPExtra, None] = None
+
+ def load_net_g(self):
+ self.net_g = get_net_g(
+ model_path=str(self.model_path),
+ version=self.hps.version,
+ device=self.device,
+ hps=self.hps,
+ )
+
+ def get_style_vector(self, style_id: int, weight: float = 1.0) -> np.ndarray:
+ mean = self.style_vectors[0]
+ style_vec = self.style_vectors[style_id]
+ style_vec = mean + (style_vec - mean) * weight
+ return style_vec
+
+ def get_style_vector_from_audio(
+ self, audio_path: str, weight: float = 1.0
+ ) -> np.ndarray:
+ from style_gen import get_style_vector
+
+ xvec = get_style_vector(audio_path)
+ mean = self.style_vectors[0]
+ xvec = mean + (xvec - mean) * weight
+ return xvec
+
+ def infer(
+ self,
+ text: str,
+ language: str = "JP",
+ sid: int = 0,
+ reference_audio_path: Optional[str] = None,
+ sdp_ratio: float = DEFAULT_SDP_RATIO,
+ noise: float = DEFAULT_NOISE,
+ noisew: float = DEFAULT_NOISEW,
+ length: float = DEFAULT_LENGTH,
+ line_split: bool = DEFAULT_LINE_SPLIT,
+ split_interval: float = DEFAULT_SPLIT_INTERVAL,
+ assist_text: Optional[str] = None,
+ assist_text_weight: float = DEFAULT_ASSIST_TEXT_WEIGHT,
+ use_assist_text: bool = False,
+ style: str = DEFAULT_STYLE,
+ style_weight: float = DEFAULT_STYLE_WEIGHT,
+ given_tone: Optional[list[int]] = None,
+ pitch_scale: float = 1.0,
+ intonation_scale: float = 1.0,
+ ignore_unknown: bool = False,
+ ) -> tuple[int, np.ndarray]:
+ logger.info(f"Start generating audio data from text:\n{text}")
+ if language != "JP" and self.hps.version.endswith("JP-Extra"):
+ raise ValueError(
+ "The model is trained with JP-Extra, but the language is not JP"
+ )
+ if reference_audio_path == "":
+ reference_audio_path = None
+ if assist_text == "" or not use_assist_text:
+ assist_text = None
+
+ if self.net_g is None:
+ self.load_net_g()
+ if reference_audio_path is None:
+ style_id = self.style2id[style]
+ style_vector = self.get_style_vector(style_id, style_weight)
+ else:
+ style_vector = self.get_style_vector_from_audio(
+ reference_audio_path, style_weight
+ )
+ if not line_split:
+ with torch.no_grad():
+ audio = infer(
+ text=text,
+ sdp_ratio=sdp_ratio,
+ noise_scale=noise,
+ noise_scale_w=noisew,
+ length_scale=length,
+ sid=sid,
+ language=language,
+ hps=self.hps,
+ net_g=self.net_g,
+ device=self.device,
+ assist_text=assist_text,
+ assist_text_weight=assist_text_weight,
+ style_vec=style_vector,
+ given_tone=given_tone,
+ ignore_unknown=ignore_unknown,
+ )
+ else:
+ texts = text.split("\n")
+ texts = [t for t in texts if t != ""]
+ audios = []
+ with torch.no_grad():
+ for i, t in enumerate(texts):
+ audios.append(
+ infer(
+ text=t,
+ sdp_ratio=sdp_ratio,
+ noise_scale=noise,
+ noise_scale_w=noisew,
+ length_scale=length,
+ sid=sid,
+ language=language,
+ hps=self.hps,
+ net_g=self.net_g,
+ device=self.device,
+ assist_text=assist_text,
+ assist_text_weight=assist_text_weight,
+ style_vec=style_vector,
+ ignore_unknown=ignore_unknown,
+ )
+ )
+ if i != len(texts) - 1:
+ audios.append(np.zeros(int(44100 * split_interval)))
+ audio = np.concatenate(audios)
+ logger.info("Audio data generated successfully")
+ if not (pitch_scale == 1.0 and intonation_scale == 1.0):
+ _, audio = adjust_voice(
+ fs=self.hps.data.sampling_rate,
+ wave=audio,
+ pitch_scale=pitch_scale,
+ intonation_scale=intonation_scale,
+ )
+ with warnings.catch_warnings():
+ warnings.simplefilter("ignore")
+ audio = convert_to_16_bit_wav(audio)
+ return (self.hps.data.sampling_rate, audio)
+
+
+class ModelHolder:
+ def __init__(self, root_dir: Path, device: str):
+ self.root_dir: Path = root_dir
+ self.device: str = device
+ self.model_files_dict: dict[str, list[Path]] = {}
+ self.current_model: Optional[Model] = None
+ self.model_names: list[str] = []
+ self.models: list[Model] = []
+ self.refresh()
+
+ def refresh(self):
+ self.model_files_dict = {}
+ self.model_names = []
+ self.current_model = None
+
+ model_dirs = [d for d in self.root_dir.iterdir() if d.is_dir()]
+ for model_dir in model_dirs:
+ model_files = [
+ f
+ for f in model_dir.iterdir()
+ if f.suffix in [".pth", ".pt", ".safetensors"]
+ ]
+ if len(model_files) == 0:
+ logger.warning(f"No model files found in {model_dir}, so skip it")
+ continue
+ config_path = model_dir / "config.json"
+ if not config_path.exists():
+ logger.warning(
+ f"Config file {config_path} not found, so skip {model_dir}"
+ )
+ continue
+ self.model_files_dict[model_dir.name] = model_files
+ self.model_names.append(model_dir.name)
+
+ def models_info(self):
+ if hasattr(self, "_models_info"):
+ return self._models_info
+ result = []
+ for name, files in self.model_files_dict.items():
+ # Get styles
+ config_path = self.root_dir / name / "config.json"
+ hps = utils.get_hparams_from_file(config_path)
+ style2id: dict[str, int] = hps.data.style2id
+ styles = list(style2id.keys())
+ result.append(
+ {
+ "name": name,
+ "files": [str(f) for f in files],
+ "styles": styles,
+ }
+ )
+ self._models_info = result
+ return result
+
+ def load_model(self, model_name: str, model_path_str: str):
+ model_path = Path(model_path_str)
+ if model_name not in self.model_files_dict:
+ raise ValueError(f"Model `{model_name}` is not found")
+ if model_path not in self.model_files_dict[model_name]:
+ raise ValueError(f"Model file `{model_path}` is not found")
+ if self.current_model is None or self.current_model.model_path != model_path:
+ self.current_model = Model(
+ model_path=model_path,
+ config_path=self.root_dir / model_name / "config.json",
+ style_vec_path=self.root_dir / model_name / "style_vectors.npy",
+ device=self.device,
+ )
+ return self.current_model
+
+ def load_model_gr(
+ self, model_name: str, model_path_str: str
+ ) -> tuple[gr.Dropdown, gr.Button, gr.Dropdown]:
+ model_path = Path(model_path_str)
+ if model_name not in self.model_files_dict:
+ raise ValueError(f"Model `{model_name}` is not found")
+ if model_path not in self.model_files_dict[model_name]:
+ raise ValueError(f"Model file `{model_path}` is not found")
+ if (
+ self.current_model is not None
+ and self.current_model.model_path == model_path
+ ):
+ # Already loaded
+ speakers = list(self.current_model.spk2id.keys())
+ styles = list(self.current_model.style2id.keys())
+ return (
+ gr.Dropdown(choices=styles, value=styles[0]),
+ gr.Button(interactive=True, value="音声合成"),
+ gr.Dropdown(choices=speakers, value=speakers[0]),
+ )
+ self.current_model = Model(
+ model_path=model_path,
+ config_path=self.root_dir / model_name / "config.json",
+ style_vec_path=self.root_dir / model_name / "style_vectors.npy",
+ device=self.device,
+ )
+ speakers = list(self.current_model.spk2id.keys())
+ styles = list(self.current_model.style2id.keys())
+ return (
+ gr.Dropdown(choices=styles, value=styles[0]),
+ gr.Button(interactive=True, value="音声合成"),
+ gr.Dropdown(choices=speakers, value=speakers[0]),
+ )
+
+ def update_model_files_gr(self, model_name: str) -> gr.Dropdown:
+ model_files = self.model_files_dict[model_name]
+ return gr.Dropdown(choices=model_files, value=model_files[0])
+
+ def update_model_names_gr(self) -> tuple[gr.Dropdown, gr.Dropdown, gr.Button]:
+ self.refresh()
+ initial_model_name = self.model_names[0]
+ initial_model_files = self.model_files_dict[initial_model_name]
+ return (
+ gr.Dropdown(choices=self.model_names, value=initial_model_name),
+ gr.Dropdown(choices=initial_model_files, value=initial_model_files[0]),
+ gr.Button(interactive=False), # For tts_button
+ )
diff --git a/commons.py b/commons.py
new file mode 100644
index 0000000000000000000000000000000000000000..081b8a061286150f5a6f449601488f3e3f7b0207
--- /dev/null
+++ b/commons.py
@@ -0,0 +1,152 @@
+import math
+import torch
+from torch.nn import functional as F
+
+
+def init_weights(m, mean=0.0, std=0.01):
+ classname = m.__class__.__name__
+ if classname.find("Conv") != -1:
+ m.weight.data.normal_(mean, std)
+
+
+def get_padding(kernel_size, dilation=1):
+ return int((kernel_size * dilation - dilation) / 2)
+
+
+def convert_pad_shape(pad_shape):
+ layer = pad_shape[::-1]
+ pad_shape = [item for sublist in layer for item in sublist]
+ return pad_shape
+
+
+def intersperse(lst, item):
+ result = [item] * (len(lst) * 2 + 1)
+ result[1::2] = lst
+ return result
+
+
+def kl_divergence(m_p, logs_p, m_q, logs_q):
+ """KL(P||Q)"""
+ kl = (logs_q - logs_p) - 0.5
+ kl += (
+ 0.5 * (torch.exp(2.0 * logs_p) + ((m_p - m_q) ** 2)) * torch.exp(-2.0 * logs_q)
+ )
+ return kl
+
+
+def rand_gumbel(shape):
+ """Sample from the Gumbel distribution, protect from overflows."""
+ uniform_samples = torch.rand(shape) * 0.99998 + 0.00001
+ return -torch.log(-torch.log(uniform_samples))
+
+
+def rand_gumbel_like(x):
+ g = rand_gumbel(x.size()).to(dtype=x.dtype, device=x.device)
+ return g
+
+
+def slice_segments(x, ids_str, segment_size=4):
+ gather_indices = ids_str.view(x.size(0), 1, 1).repeat(
+ 1, x.size(1), 1
+ ) + torch.arange(segment_size, device=x.device)
+ return torch.gather(x, 2, gather_indices)
+
+
+def rand_slice_segments(x, x_lengths=None, segment_size=4):
+ b, d, t = x.size()
+ if x_lengths is None:
+ x_lengths = t
+ ids_str_max = torch.clamp(x_lengths - segment_size + 1, min=0)
+ ids_str = (torch.rand([b], device=x.device) * ids_str_max).to(dtype=torch.long)
+ ret = slice_segments(x, ids_str, segment_size)
+ return ret, ids_str
+
+
+def get_timing_signal_1d(length, channels, min_timescale=1.0, max_timescale=1.0e4):
+ position = torch.arange(length, dtype=torch.float)
+ num_timescales = channels // 2
+ log_timescale_increment = math.log(float(max_timescale) / float(min_timescale)) / (
+ num_timescales - 1
+ )
+ inv_timescales = min_timescale * torch.exp(
+ torch.arange(num_timescales, dtype=torch.float) * -log_timescale_increment
+ )
+ scaled_time = position.unsqueeze(0) * inv_timescales.unsqueeze(1)
+ signal = torch.cat([torch.sin(scaled_time), torch.cos(scaled_time)], 0)
+ signal = F.pad(signal, [0, 0, 0, channels % 2])
+ signal = signal.view(1, channels, length)
+ return signal
+
+
+def add_timing_signal_1d(x, min_timescale=1.0, max_timescale=1.0e4):
+ b, channels, length = x.size()
+ signal = get_timing_signal_1d(length, channels, min_timescale, max_timescale)
+ return x + signal.to(dtype=x.dtype, device=x.device)
+
+
+def cat_timing_signal_1d(x, min_timescale=1.0, max_timescale=1.0e4, axis=1):
+ b, channels, length = x.size()
+ signal = get_timing_signal_1d(length, channels, min_timescale, max_timescale)
+ return torch.cat([x, signal.to(dtype=x.dtype, device=x.device)], axis)
+
+
+def subsequent_mask(length):
+ mask = torch.tril(torch.ones(length, length)).unsqueeze(0).unsqueeze(0)
+ return mask
+
+
+@torch.jit.script
+def fused_add_tanh_sigmoid_multiply(input_a, input_b, n_channels):
+ n_channels_int = n_channels[0]
+ in_act = input_a + input_b
+ t_act = torch.tanh(in_act[:, :n_channels_int, :])
+ s_act = torch.sigmoid(in_act[:, n_channels_int:, :])
+ acts = t_act * s_act
+ return acts
+
+
+def shift_1d(x):
+ x = F.pad(x, convert_pad_shape([[0, 0], [0, 0], [1, 0]]))[:, :, :-1]
+ return x
+
+
+def sequence_mask(length, max_length=None):
+ if max_length is None:
+ max_length = length.max()
+ x = torch.arange(max_length, dtype=length.dtype, device=length.device)
+ return x.unsqueeze(0) < length.unsqueeze(1)
+
+
+def generate_path(duration, mask):
+ """
+ duration: [b, 1, t_x]
+ mask: [b, 1, t_y, t_x]
+ """
+
+ b, _, t_y, t_x = mask.shape
+ cum_duration = torch.cumsum(duration, -1)
+
+ cum_duration_flat = cum_duration.view(b * t_x)
+ path = sequence_mask(cum_duration_flat, t_y).to(mask.dtype)
+ path = path.view(b, t_x, t_y)
+ path = path - F.pad(path, convert_pad_shape([[0, 0], [1, 0], [0, 0]]))[:, :-1]
+ path = path.unsqueeze(1).transpose(2, 3) * mask
+ return path
+
+
+def clip_grad_value_(parameters, clip_value, norm_type=2):
+ if isinstance(parameters, torch.Tensor):
+ parameters = [parameters]
+ parameters = list(filter(lambda p: p.grad is not None, parameters))
+ norm_type = float(norm_type)
+ if clip_value is not None:
+ clip_value = float(clip_value)
+
+ total_norm = 0
+ for p in parameters:
+ param_norm = p.grad.data.norm(norm_type)
+ total_norm += param_norm.item() ** norm_type
+ if clip_value is not None:
+ p.grad.data.clamp_(min=-clip_value, max=clip_value)
+ total_norm = total_norm ** (1.0 / norm_type)
+ return total_norm
diff --git a/config.py b/config.py
new file mode 100644
index 0000000000000000000000000000000000000000..bbd764e7b96650b2407c0670ab2cfd5f842f6f9a
--- /dev/null
+++ b/config.py
@@ -0,0 +1,291 @@
+"""
+@Desc: 全局配置文件读取
+"""
+
+import os
+import shutil
+from typing import Dict, List
+
+import torch
+import yaml
+
+from common.log import logger
+
+# If not cuda available, set possible devices to cpu
+cuda_available = torch.cuda.is_available()
+
+
+class Resample_config:
+ """重采样配置"""
+
+ def __init__(self, in_dir: str, out_dir: str, sampling_rate: int = 44100):
+ self.sampling_rate: int = sampling_rate # 目标采样率
+ self.in_dir: str = in_dir # 待处理音频目录路径
+ self.out_dir: str = out_dir # 重采样输出路径
+
+ @classmethod
+ def from_dict(cls, dataset_path: str, data: Dict[str, any]):
+ """从字典中生成实例"""
+
+ # 不检查路径是否有效,此逻辑在resample.py中处理
+ data["in_dir"] = os.path.join(dataset_path, data["in_dir"])
+ data["out_dir"] = os.path.join(dataset_path, data["out_dir"])
+
+ return cls(**data)
+
+
+class Preprocess_text_config:
+ """数据预处理配置"""
+
+ def __init__(
+ self,
+ transcription_path: str,
+ cleaned_path: str,
+ train_path: str,
+ val_path: str,
+ config_path: str,
+ val_per_lang: int = 5,
+ max_val_total: int = 10000,
+ clean: bool = True,
+ ):
+ self.transcription_path: str = (
+ transcription_path # 原始文本文件路径,文本格式应为{wav_path}|{speaker_name}|{language}|{text}。
+ )
+ self.cleaned_path: str = (
+ cleaned_path # 数据清洗后文本路径,可以不填。不填则将在原始文本目录生成
+ )
+ self.train_path: str = (
+ train_path # 训练集路径,可以不填。不填则将在原始文本目录生成
+ )
+ self.val_path: str = (
+ val_path # 验证集路径,可以不填。不填则将在原始文本目录生成
+ )
+ self.config_path: str = config_path # 配置文件路径
+ self.val_per_lang: int = val_per_lang # 每个speaker的验证集条数
+ self.max_val_total: int = (
+ max_val_total # 验证集最大条数,多于的会被截断并放到训练集中
+ )
+ self.clean: bool = clean # 是否进行数据清洗
+
+ @classmethod
+ def from_dict(cls, dataset_path: str, data: Dict[str, any]):
+ """从字典中生成实例"""
+
+ data["transcription_path"] = os.path.join(
+ dataset_path, data["transcription_path"]
+ )
+ if data["cleaned_path"] == "" or data["cleaned_path"] is None:
+ data["cleaned_path"] = None
+ else:
+ data["cleaned_path"] = os.path.join(dataset_path, data["cleaned_path"])
+ data["train_path"] = os.path.join(dataset_path, data["train_path"])
+ data["val_path"] = os.path.join(dataset_path, data["val_path"])
+ data["config_path"] = os.path.join(dataset_path, data["config_path"])
+
+ return cls(**data)
+
+
+class Bert_gen_config:
+ """bert_gen 配置"""
+
+ def __init__(
+ self,
+ config_path: str,
+ num_processes: int = 2,
+ device: str = "cuda",
+ use_multi_device: bool = False,
+ ):
+ self.config_path = config_path
+ self.num_processes = num_processes
+ if not cuda_available:
+ device = "cpu"
+ self.device = device
+ self.use_multi_device = use_multi_device
+
+ @classmethod
+ def from_dict(cls, dataset_path: str, data: Dict[str, any]):
+ data["config_path"] = os.path.join(dataset_path, data["config_path"])
+
+ return cls(**data)
+
+
+class Style_gen_config:
+ """style_gen 配置"""
+
+ def __init__(
+ self,
+ config_path: str,
+ num_processes: int = 4,
+ device: str = "cuda",
+ ):
+ self.config_path = config_path
+ self.num_processes = num_processes
+ if not cuda_available:
+ device = "cpu"
+ self.device = device
+
+ @classmethod
+ def from_dict(cls, dataset_path: str, data: Dict[str, any]):
+ data["config_path"] = os.path.join(dataset_path, data["config_path"])
+
+ return cls(**data)
+
+
+class Train_ms_config:
+ """训练配置"""
+
+ def __init__(
+ self,
+ config_path: str,
+ env: Dict[str, any],
+ # base: Dict[str, any],
+ model_dir: str,
+ num_workers: int,
+ spec_cache: bool,
+ keep_ckpts: int,
+ ):
+ self.env = env # 需要加载的环境变量
+ # self.base = base # 底模配置
+ self.model_dir = model_dir # 训练模型存储目录,该路径为相对于dataset_path的路径,而非项目根目录
+ self.config_path = config_path # 配置文件路径
+ self.num_workers = num_workers # worker数量
+ self.spec_cache = spec_cache # 是否启用spec缓存
+ self.keep_ckpts = keep_ckpts # ckpt数量
+
+ @classmethod
+ def from_dict(cls, dataset_path: str, data: Dict[str, any]):
+ # data["model"] = os.path.join(dataset_path, data["model"])
+ data["config_path"] = os.path.join(dataset_path, data["config_path"])
+
+ return cls(**data)
+
+
+class Webui_config:
+ """webui 配置 (for webui.py, not supported now)"""
+
+ def __init__(
+ self,
+ device: str,
+ model: str,
+ config_path: str,
+ language_identification_library: str,
+ port: int = 7860,
+ share: bool = False,
+ debug: bool = False,
+ ):
+ if not cuda_available:
+ device = "cpu"
+ self.device: str = device
+ self.model: str = model # 端口号
+ self.config_path: str = config_path # 是否公开部署,对外网开放
+ self.port: int = port # 是否开启debug模式
+ self.share: bool = share # 模型路径
+ self.debug: bool = debug # 配置文件路径
+ self.language_identification_library: str = (
+ language_identification_library # 语种识别库
+ )
+
+ @classmethod
+ def from_dict(cls, dataset_path: str, data: Dict[str, any]):
+ data["config_path"] = os.path.join(dataset_path, data["config_path"])
+ data["model"] = os.path.join(dataset_path, data["model"])
+ return cls(**data)
+
+
+class Server_config:
+ def __init__(
+ self,
+ port: int = 5000,
+ device: str = "cuda",
+ limit: int = 100,
+ language: str = "JP",
+ origins: List[str] = None,
+ ):
+ self.port: int = port
+ if not cuda_available:
+ device = "cpu"
+ self.device: str = device
+ self.language: str = language
+ self.limit: int = limit
+ self.origins: List[str] = origins
+
+ @classmethod
+ def from_dict(cls, data: Dict[str, any]):
+ return cls(**data)
+
+
+class Translate_config:
+ """翻译api配置"""
+
+ def __init__(self, app_key: str, secret_key: str):
+ self.app_key = app_key
+ self.secret_key = secret_key
+
+ @classmethod
+ def from_dict(cls, data: Dict[str, any]):
+ return cls(**data)
+
+
+class Config:
+ def __init__(self, config_path: str, path_config: dict[str, str]):
+ if not os.path.isfile(config_path) and os.path.isfile("default_config.yml"):
+ shutil.copy(src="default_config.yml", dst=config_path)
+ logger.info(
+ f"A configuration file {config_path} has been generated based on the default configuration file default_config.yml."
+ )
+ logger.info(
+ "If you have no special needs, please do not modify default_config.yml."
+ )
+ # sys.exit(0)
+ with open(file=config_path, mode="r", encoding="utf-8") as file:
+ yaml_config: Dict[str, any] = yaml.safe_load(file.read())
+ model_name: str = yaml_config["model_name"]
+ self.model_name: str = model_name
+ if "dataset_path" in yaml_config:
+ dataset_path = yaml_config["dataset_path"]
+ else:
+ dataset_path = os.path.join(path_config["dataset_root"], model_name)
+ self.dataset_path: str = dataset_path
+ self.assets_root: str = path_config["assets_root"]
+ self.out_dir = os.path.join(self.assets_root, model_name)
+ self.resample_config: Resample_config = Resample_config.from_dict(
+ dataset_path, yaml_config["resample"]
+ )
+ self.preprocess_text_config: Preprocess_text_config = (
+ Preprocess_text_config.from_dict(
+ dataset_path, yaml_config["preprocess_text"]
+ )
+ )
+ self.bert_gen_config: Bert_gen_config = Bert_gen_config.from_dict(
+ dataset_path, yaml_config["bert_gen"]
+ )
+ self.style_gen_config: Style_gen_config = Style_gen_config.from_dict(
+ dataset_path, yaml_config["style_gen"]
+ )
+ self.train_ms_config: Train_ms_config = Train_ms_config.from_dict(
+ dataset_path, yaml_config["train_ms"]
+ )
+ self.webui_config: Webui_config = Webui_config.from_dict(
+ dataset_path, yaml_config["webui"]
+ )
+ self.server_config: Server_config = Server_config.from_dict(
+ yaml_config["server"]
+ )
+ # self.translate_config: Translate_config = Translate_config.from_dict(
+ # yaml_config["translate"]
+ # )
+
+
+with open(os.path.join("configs", "paths.yml"), "r", encoding="utf-8") as f:
+ path_config: dict[str, str] = yaml.safe_load(f.read())
+ # Should contain the following keys:
+ # - dataset_root: the root directory of the dataset, default to "Data"
+ # - assets_root: the root directory of the assets, default to "model_assets"
+
+
+try:
+ config = Config("config.yml", path_config)
+except (TypeError, KeyError):
+ logger.warning("Old config.yml found. Replace it with default_config.yml.")
+ shutil.copy(src="default_config.yml", dst="config.yml")
+ config = Config("config.yml", path_config)
diff --git a/configs/config.json b/configs/config.json
new file mode 100644
index 0000000000000000000000000000000000000000..25e86db6b9d506591db50521a1ab534b6643db3c
--- /dev/null
+++ b/configs/config.json
@@ -0,0 +1,72 @@
+{
+ "model_name": "your_model_name",
+ "train": {
+ "log_interval": 200,
+ "eval_interval": 1000,
+ "seed": 42,
+ "epochs": 1000,
+ "learning_rate": 0.0002,
+ "betas": [0.8, 0.99],
+ "eps": 1e-9,
+ "batch_size": 2,
+ "bf16_run": false,
+ "lr_decay": 0.99995,
+ "segment_size": 16384,
+ "init_lr_ratio": 1,
+ "warmup_epochs": 0,
+ "c_mel": 45,
+ "c_kl": 1.0,
+ "skip_optimizer": false,
+ "freeze_ZH_bert": false,
+ "freeze_JP_bert": false,
+ "freeze_EN_bert": false,
+ "freeze_style": false,
+ "freeze_encoder": false
+ },
+ "data": {
+ "training_files": "Data/your_model_name/filelists/train.list",
+ "validation_files": "Data/your_model_name/filelists/val.list",
+ "max_wav_value": 32768.0,
+ "sampling_rate": 44100,
+ "filter_length": 2048,
+ "hop_length": 512,
+ "win_length": 2048,
+ "n_mel_channels": 128,
+ "mel_fmin": 0.0,
+ "mel_fmax": null,
+ "add_blank": true,
+ "n_speakers": 1,
+ "cleaned_text": true,
+ "num_styles": 1,
+ "style2id": {
+ "Neutral": 0
+ }
+ },
+ "model": {
+ "use_spk_conditioned_encoder": true,
+ "use_noise_scaled_mas": true,
+ "use_mel_posterior_encoder": false,
+ "use_duration_discriminator": true,
+ "inter_channels": 192,
+ "hidden_channels": 192,
+ "filter_channels": 768,
+ "n_heads": 2,
+ "n_layers": 6,
+ "kernel_size": 3,
+ "p_dropout": 0.1,
+ "resblock": "1",
+ "resblock_kernel_sizes": [3, 7, 11],
+ "resblock_dilation_sizes": [
+ [1, 3, 5],
+ [1, 3, 5],
+ [1, 3, 5]
+ ],
+ "upsample_rates": [8, 8, 2, 2, 2],
+ "upsample_initial_channel": 512,
+ "upsample_kernel_sizes": [16, 16, 8, 2, 2],
+ "n_layers_q": 3,
+ "use_spectral_norm": false,
+ "gin_channels": 256
+ },
+ "version": "2.3"
+}
diff --git a/configs/configs_jp_extra.json b/configs/configs_jp_extra.json
new file mode 100644
index 0000000000000000000000000000000000000000..616d1d31d516108748a6b0a18e281ab17459cd29
--- /dev/null
+++ b/configs/configs_jp_extra.json
@@ -0,0 +1,79 @@
+{
+ "train": {
+ "log_interval": 200,
+ "eval_interval": 1000,
+ "seed": 42,
+ "epochs": 1000,
+ "learning_rate": 0.0001,
+ "betas": [0.8, 0.99],
+ "eps": 1e-9,
+ "batch_size": 2,
+ "bf16_run": false,
+ "fp16_run": false,
+ "lr_decay": 0.99996,
+ "segment_size": 16384,
+ "init_lr_ratio": 1,
+ "warmup_epochs": 0,
+ "c_mel": 45,
+ "c_kl": 1.0,
+ "c_commit": 100,
+ "skip_optimizer": false,
+ "freeze_ZH_bert": false,
+ "freeze_JP_bert": false,
+ "freeze_EN_bert": false,
+ "freeze_emo": false,
+ "freeze_style": false,
+ "freeze_decoder": false
+ },
+ "data": {
+ "use_jp_extra": true,
+ "training_files": "filelists/train.list",
+ "validation_files": "filelists/val.list",
+ "max_wav_value": 32768.0,
+ "sampling_rate": 44100,
+ "filter_length": 2048,
+ "hop_length": 512,
+ "win_length": 2048,
+ "n_mel_channels": 128,
+ "mel_fmin": 0.0,
+ "mel_fmax": null,
+ "add_blank": true,
+ "n_speakers": 512,
+ "cleaned_text": true
+ },
+ "model": {
+ "use_spk_conditioned_encoder": true,
+ "use_noise_scaled_mas": true,
+ "use_mel_posterior_encoder": false,
+ "use_duration_discriminator": false,
+ "use_wavlm_discriminator": true,
+ "inter_channels": 192,
+ "hidden_channels": 192,
+ "filter_channels": 768,
+ "n_heads": 2,
+ "n_layers": 6,
+ "kernel_size": 3,
+ "p_dropout": 0.1,
+ "resblock": "1",
+ "resblock_kernel_sizes": [3, 7, 11],
+ "resblock_dilation_sizes": [
+ [1, 3, 5],
+ [1, 3, 5],
+ [1, 3, 5]
+ ],
+ "upsample_rates": [8, 8, 2, 2, 2],
+ "upsample_initial_channel": 512,
+ "upsample_kernel_sizes": [16, 16, 8, 2, 2],
+ "n_layers_q": 3,
+ "use_spectral_norm": false,
+ "gin_channels": 512,
+ "slm": {
+ "model": "./slm/wavlm-base-plus",
+ "sr": 16000,
+ "hidden": 768,
+ "nlayers": 13,
+ "initial_channel": 64
+ }
+ },
+ "version": "2.3-JP-Extra"
+}
diff --git a/configs/paths.yml b/configs/paths.yml
new file mode 100644
index 0000000000000000000000000000000000000000..d743748f7f52d5c41f1487b53872143c2f010222
--- /dev/null
+++ b/configs/paths.yml
@@ -0,0 +1,8 @@
+# Root directory of the training dataset.
+# The training dataset of {model_name} should be placed in {dataset_root}/{model_name}.
+dataset_root: Data
+
+# Root directory of the model assets (for inference).
+# In training, the model assets will be saved to {assets_root}/{model_name},
+# and in inference, we load all the models from {assets_root}.
+assets_root: model_assets
diff --git a/data_utils.py b/data_utils.py
new file mode 100644
index 0000000000000000000000000000000000000000..ac038c22fb542d70500002ae120fa56605e9b03e
--- /dev/null
+++ b/data_utils.py
@@ -0,0 +1,456 @@
+import os
+import random
+import sys
+
+import numpy as np
+import torch
+import torch.utils.data
+from tqdm import tqdm
+
+import commons
+from config import config
+from mel_processing import mel_spectrogram_torch, spectrogram_torch
+from text import cleaned_text_to_sequence
+from common.log import logger
+from utils import load_filepaths_and_text, load_wav_to_torch
+
+"""Multi speaker version"""
+
+
+class TextAudioSpeakerLoader(torch.utils.data.Dataset):
+ """
+ 1) loads audio, speaker_id, text pairs
+ 2) normalizes text and converts them to sequences of integers
+ 3) computes spectrograms from audio files.
+ """
+
+ def __init__(self, audiopaths_sid_text, hparams):
+ self.audiopaths_sid_text = load_filepaths_and_text(audiopaths_sid_text)
+ self.max_wav_value = hparams.max_wav_value
+ self.sampling_rate = hparams.sampling_rate
+ self.filter_length = hparams.filter_length
+ self.hop_length = hparams.hop_length
+ self.win_length = hparams.win_length
+ self.sampling_rate = hparams.sampling_rate
+ self.spk_map = hparams.spk2id
+ self.hparams = hparams
+ self.use_jp_extra = getattr(hparams, "use_jp_extra", False)
+
+ self.use_mel_spec_posterior = getattr(
+ hparams, "use_mel_posterior_encoder", False
+ )
+ if self.use_mel_spec_posterior:
+ self.n_mel_channels = getattr(hparams, "n_mel_channels", 80)
+
+ self.cleaned_text = getattr(hparams, "cleaned_text", False)
+
+ self.add_blank = hparams.add_blank
+ self.min_text_len = getattr(hparams, "min_text_len", 1)
+ self.max_text_len = getattr(hparams, "max_text_len", 384)
+
+ random.seed(1234)
+ random.shuffle(self.audiopaths_sid_text)
+ self._filter()
+
+ def _filter(self):
+ """
+ Filter text & store spec lengths
+ """
+ # Store spectrogram lengths for Bucketing
+ # wav_length ~= file_size / (wav_channels * Bytes per dim) = file_size / (1 * 2)
+ # spec_length = wav_length // hop_length
+
+ audiopaths_sid_text_new = []
+ lengths = []
+ skipped = 0
+ logger.info("Init dataset...")
+ for _id, spk, language, text, phones, tone, word2ph in tqdm(
+ self.audiopaths_sid_text, file=sys.stdout
+ ):
+ audiopath = f"{_id}"
+ if self.min_text_len <= len(phones) and len(phones) <= self.max_text_len:
+ phones = phones.split(" ")
+ tone = [int(i) for i in tone.split(" ")]
+ word2ph = [int(i) for i in word2ph.split(" ")]
+ audiopaths_sid_text_new.append(
+ [audiopath, spk, language, text, phones, tone, word2ph]
+ )
+ lengths.append(os.path.getsize(audiopath) // (2 * self.hop_length))
+ else:
+ skipped += 1
+ logger.info(
+ "skipped: "
+ + str(skipped)
+ + ", total: "
+ + str(len(self.audiopaths_sid_text))
+ )
+ self.audiopaths_sid_text = audiopaths_sid_text_new
+ self.lengths = lengths
+
+ def get_audio_text_speaker_pair(self, audiopath_sid_text):
+ # separate filename, speaker_id and text
+ audiopath, sid, language, text, phones, tone, word2ph = audiopath_sid_text
+
+ bert, ja_bert, en_bert, phones, tone, language = self.get_text(
+ text, word2ph, phones, tone, language, audiopath
+ )
+
+ spec, wav = self.get_audio(audiopath)
+ sid = torch.LongTensor([int(self.spk_map[sid])])
+ style_vec = torch.FloatTensor(np.load(f"{audiopath}.npy"))
+ if self.use_jp_extra:
+ return (phones, spec, wav, sid, tone, language, ja_bert, style_vec)
+ else:
+ return (
+ phones,
+ spec,
+ wav,
+ sid,
+ tone,
+ language,
+ bert,
+ ja_bert,
+ en_bert,
+ style_vec,
+ )
+
+ def get_audio(self, filename):
+ audio, sampling_rate = load_wav_to_torch(filename)
+ if sampling_rate != self.sampling_rate:
+ raise ValueError(
+ "{} {} SR doesn't match target {} SR".format(
+ filename, sampling_rate, self.sampling_rate
+ )
+ )
+ audio_norm = audio / self.max_wav_value
+ audio_norm = audio_norm.unsqueeze(0)
+ spec_filename = filename.replace(".wav", ".spec.pt")
+ if self.use_mel_spec_posterior:
+ spec_filename = spec_filename.replace(".spec.pt", ".mel.pt")
+ try:
+ spec = torch.load(spec_filename)
+ except:
+ if self.use_mel_spec_posterior:
+ spec = mel_spectrogram_torch(
+ audio_norm,
+ self.filter_length,
+ self.n_mel_channels,
+ self.sampling_rate,
+ self.hop_length,
+ self.win_length,
+ self.hparams.mel_fmin,
+ self.hparams.mel_fmax,
+ center=False,
+ )
+ else:
+ spec = spectrogram_torch(
+ audio_norm,
+ self.filter_length,
+ self.sampling_rate,
+ self.hop_length,
+ self.win_length,
+ center=False,
+ )
+ spec = torch.squeeze(spec, 0)
+ if config.train_ms_config.spec_cache:
+ torch.save(spec, spec_filename)
+ return spec, audio_norm
+
+ def get_text(self, text, word2ph, phone, tone, language_str, wav_path):
+ phone, tone, language = cleaned_text_to_sequence(phone, tone, language_str)
+ if self.add_blank:
+ phone = commons.intersperse(phone, 0)
+ tone = commons.intersperse(tone, 0)
+ language = commons.intersperse(language, 0)
+ for i in range(len(word2ph)):
+ word2ph[i] = word2ph[i] * 2
+ word2ph[0] += 1
+ bert_path = wav_path.replace(".wav", ".bert.pt")
+ try:
+ bert_ori = torch.load(bert_path)
+ assert bert_ori.shape[-1] == len(phone)
+ except Exception as e:
+ logger.warning("Bert load Failed")
+ logger.warning(e)
+
+ if language_str == "ZH":
+ bert = bert_ori
+ ja_bert = torch.zeros(1024, len(phone))
+ en_bert = torch.zeros(1024, len(phone))
+ elif language_str == "JP":
+ bert = torch.zeros(1024, len(phone))
+ ja_bert = bert_ori
+ en_bert = torch.zeros(1024, len(phone))
+ elif language_str == "EN":
+ bert = torch.zeros(1024, len(phone))
+ ja_bert = torch.zeros(1024, len(phone))
+ en_bert = bert_ori
+ phone = torch.LongTensor(phone)
+ tone = torch.LongTensor(tone)
+ language = torch.LongTensor(language)
+ return bert, ja_bert, en_bert, phone, tone, language
+
+ def get_sid(self, sid):
+ sid = torch.LongTensor([int(sid)])
+ return sid
+
+ def __getitem__(self, index):
+ return self.get_audio_text_speaker_pair(self.audiopaths_sid_text[index])
+
+ def __len__(self):
+ return len(self.audiopaths_sid_text)
+
+
+class TextAudioSpeakerCollate:
+ """Zero-pads model inputs and targets"""
+
+ def __init__(self, return_ids=False, use_jp_extra=False):
+ self.return_ids = return_ids
+ self.use_jp_extra = use_jp_extra
+
+ def __call__(self, batch):
+ """Collate's training batch from normalized text, audio and speaker identities
+ PARAMS
+ ------
+ batch: [text_normalized, spec_normalized, wav_normalized, sid]
+ """
+ # Right zero-pad all one-hot text sequences to max input length
+ _, ids_sorted_decreasing = torch.sort(
+ torch.LongTensor([x[1].size(1) for x in batch]), dim=0, descending=True
+ )
+
+ max_text_len = max([len(x[0]) for x in batch])
+ max_spec_len = max([x[1].size(1) for x in batch])
+ max_wav_len = max([x[2].size(1) for x in batch])
+
+ text_lengths = torch.LongTensor(len(batch))
+ spec_lengths = torch.LongTensor(len(batch))
+ wav_lengths = torch.LongTensor(len(batch))
+ sid = torch.LongTensor(len(batch))
+
+ text_padded = torch.LongTensor(len(batch), max_text_len)
+ tone_padded = torch.LongTensor(len(batch), max_text_len)
+ language_padded = torch.LongTensor(len(batch), max_text_len)
+ # This is ZH bert if not use_jp_extra, JA bert if use_jp_extra
+ bert_padded = torch.FloatTensor(len(batch), 1024, max_text_len)
+ if not self.use_jp_extra:
+ ja_bert_padded = torch.FloatTensor(len(batch), 1024, max_text_len)
+ en_bert_padded = torch.FloatTensor(len(batch), 1024, max_text_len)
+ style_vec = torch.FloatTensor(len(batch), 256)
+
+ spec_padded = torch.FloatTensor(len(batch), batch[0][1].size(0), max_spec_len)
+ wav_padded = torch.FloatTensor(len(batch), 1, max_wav_len)
+ text_padded.zero_()
+ tone_padded.zero_()
+ language_padded.zero_()
+ spec_padded.zero_()
+ wav_padded.zero_()
+ bert_padded.zero_()
+ if not self.use_jp_extra:
+ ja_bert_padded.zero_()
+ en_bert_padded.zero_()
+ style_vec.zero_()
+
+ for i in range(len(ids_sorted_decreasing)):
+ row = batch[ids_sorted_decreasing[i]]
+
+ text = row[0]
+ text_padded[i, : text.size(0)] = text
+ text_lengths[i] = text.size(0)
+
+ spec = row[1]
+ spec_padded[i, :, : spec.size(1)] = spec
+ spec_lengths[i] = spec.size(1)
+
+ wav = row[2]
+ wav_padded[i, :, : wav.size(1)] = wav
+ wav_lengths[i] = wav.size(1)
+
+ sid[i] = row[3]
+
+ tone = row[4]
+ tone_padded[i, : tone.size(0)] = tone
+
+ language = row[5]
+ language_padded[i, : language.size(0)] = language
+
+ bert = row[6]
+ bert_padded[i, :, : bert.size(1)] = bert
+
+ if self.use_jp_extra:
+ style_vec[i, :] = row[7]
+ else:
+ ja_bert = row[7]
+ ja_bert_padded[i, :, : ja_bert.size(1)] = ja_bert
+
+ en_bert = row[8]
+ en_bert_padded[i, :, : en_bert.size(1)] = en_bert
+ style_vec[i, :] = row[9]
+
+ if self.use_jp_extra:
+ return (
+ text_padded,
+ text_lengths,
+ spec_padded,
+ spec_lengths,
+ wav_padded,
+ wav_lengths,
+ sid,
+ tone_padded,
+ language_padded,
+ bert_padded,
+ style_vec,
+ )
+ else:
+ return (
+ text_padded,
+ text_lengths,
+ spec_padded,
+ spec_lengths,
+ wav_padded,
+ wav_lengths,
+ sid,
+ tone_padded,
+ language_padded,
+ bert_padded,
+ ja_bert_padded,
+ en_bert_padded,
+ style_vec,
+ )
+
+
+class DistributedBucketSampler(torch.utils.data.distributed.DistributedSampler):
+ """
+ Maintain similar input lengths in a batch.
+ Length groups are specified by boundaries.
+ Ex) boundaries = [b1, b2, b3] -> any batch is included either {x | b1 < length(x) <=b2} or {x | b2 < length(x) <= b3}.
+
+ It removes samples which are not included in the boundaries.
+ Ex) boundaries = [b1, b2, b3] -> any x s.t. length(x) <= b1 or length(x) > b3 are discarded.
+ """
+
+ def __init__(
+ self,
+ dataset,
+ batch_size,
+ boundaries,
+ num_replicas=None,
+ rank=None,
+ shuffle=True,
+ ):
+ super().__init__(dataset, num_replicas=num_replicas, rank=rank, shuffle=shuffle)
+ self.lengths = dataset.lengths
+ self.batch_size = batch_size
+ self.boundaries = boundaries
+
+ self.buckets, self.num_samples_per_bucket = self._create_buckets()
+ logger.info(f"Bucket info: {self.num_samples_per_bucket}")
+ # logger.info(
+ # f"Unused samples: {len(self.lengths) - sum(self.num_samples_per_bucket)}"
+ # )
+ # ↑マイナスになることあるし、別にこれは使われないサンプル数ではないようだ……
+ # バケットの仕組みはよく分からない
+
+ self.total_size = sum(self.num_samples_per_bucket)
+ self.num_samples = self.total_size // self.num_replicas
+
+ def _create_buckets(self):
+ buckets = [[] for _ in range(len(self.boundaries) - 1)]
+ for i in range(len(self.lengths)):
+ length = self.lengths[i]
+ idx_bucket = self._bisect(length)
+ if idx_bucket != -1:
+ buckets[idx_bucket].append(i)
+
+ try:
+ for i in range(len(buckets) - 1, 0, -1):
+ if len(buckets[i]) == 0:
+ buckets.pop(i)
+ self.boundaries.pop(i + 1)
+ assert all(len(bucket) > 0 for bucket in buckets)
+ # When one bucket is not traversed
+ except Exception as e:
+ logger.info("Bucket warning ", e)
+ for i in range(len(buckets) - 1, -1, -1):
+ if len(buckets[i]) == 0:
+ buckets.pop(i)
+ self.boundaries.pop(i + 1)
+
+ num_samples_per_bucket = []
+ for i in range(len(buckets)):
+ len_bucket = len(buckets[i])
+ total_batch_size = self.num_replicas * self.batch_size
+ rem = (
+ total_batch_size - (len_bucket % total_batch_size)
+ ) % total_batch_size
+ num_samples_per_bucket.append(len_bucket + rem)
+ return buckets, num_samples_per_bucket
+
+ def __iter__(self):
+ # deterministically shuffle based on epoch
+ g = torch.Generator()
+ g.manual_seed(self.epoch)
+
+ indices = []
+ if self.shuffle:
+ for bucket in self.buckets:
+ indices.append(torch.randperm(len(bucket), generator=g).tolist())
+ else:
+ for bucket in self.buckets:
+ indices.append(list(range(len(bucket))))
+
+ batches = []
+ for i in range(len(self.buckets)):
+ bucket = self.buckets[i]
+ len_bucket = len(bucket)
+ if len_bucket == 0:
+ continue
+ ids_bucket = indices[i]
+ num_samples_bucket = self.num_samples_per_bucket[i]
+
+ # add extra samples to make it evenly divisible
+ rem = num_samples_bucket - len_bucket
+ ids_bucket = (
+ ids_bucket
+ + ids_bucket * (rem // len_bucket)
+ + ids_bucket[: (rem % len_bucket)]
+ )
+
+ # subsample
+ ids_bucket = ids_bucket[self.rank :: self.num_replicas]
+
+ # batching
+ for j in range(len(ids_bucket) // self.batch_size):
+ batch = [
+ bucket[idx]
+ for idx in ids_bucket[
+ j * self.batch_size : (j + 1) * self.batch_size
+ ]
+ ]
+ batches.append(batch)
+
+ if self.shuffle:
+ batch_ids = torch.randperm(len(batches), generator=g).tolist()
+ batches = [batches[i] for i in batch_ids]
+ self.batches = batches
+
+ assert len(self.batches) * self.batch_size == self.num_samples
+ return iter(self.batches)
+
+ def _bisect(self, x, lo=0, hi=None):
+ if hi is None:
+ hi = len(self.boundaries) - 1
+
+ if hi > lo:
+ mid = (hi + lo) // 2
+ if self.boundaries[mid] < x and x <= self.boundaries[mid + 1]:
+ return mid
+ elif x <= self.boundaries[mid]:
+ return self._bisect(x, lo, mid)
+ else:
+ return self._bisect(x, mid + 1, hi)
+ else:
+ return -1
+
+ def __len__(self):
+ return self.num_samples // self.batch_size
diff --git a/default_config.yml b/default_config.yml
new file mode 100644
index 0000000000000000000000000000000000000000..c7cc19ea8a81049c01168ffa119af143907061b8
--- /dev/null
+++ b/default_config.yml
@@ -0,0 +1,70 @@
+model_name: "model_name"
+
+# If you want to use a specific dataset path, uncomment the following line.
+# Otherwise, the dataset path is `{dataset_root}/{model_name}`.
+
+# dataset_path: "your/dataset/path"
+
+resample:
+ sampling_rate: 44100
+ in_dir: "raw"
+ out_dir: "wavs"
+
+preprocess_text:
+ transcription_path: "esd.list"
+ cleaned_path: ""
+ train_path: "train.list"
+ val_path: "val.list"
+ config_path: "config.json"
+ val_per_lang: 0
+ max_val_total: 12
+ clean: true
+
+bert_gen:
+ config_path: "config.json"
+ num_processes: 2
+ device: "cuda"
+ use_multi_device: false
+
+style_gen:
+ config_path: "config.json"
+ num_processes: 4
+ device: "cuda"
+
+train_ms:
+ env:
+ MASTER_ADDR: "localhost"
+ MASTER_PORT: 10086
+ WORLD_SIZE: 1
+ LOCAL_RANK: 0
+ RANK: 0
+ model_dir: "models" # The directory to save the model (for training), relative to `{dataset_root}/{model_name}`.
+ config_path: "config.json"
+ num_workers: 16
+ spec_cache: True
+ keep_ckpts: 1 # Set this to 0 to keep all checkpoints
+
+webui: # For `webui.py`, which is not supported yet in Style-Bert-VITS2.
+ # 推理设备
+ device: "cuda"
+ # 模型路径
+ model: "models/G_8000.pth"
+ # 配置文件路径
+ config_path: "config.json"
+ # 端口号
+ port: 7860
+ # 是否公开部署,对外网开放
+ share: false
+ # 是否开启debug模式
+ debug: false
+ # 语种识别库,可选langid, fastlid
+ language_identification_library: "langid"
+
+# server_fastapi's config
+server:
+ port: 5000
+ device: "cuda"
+ language: "JP"
+ limit: 100
+ origins:
+ - "*"
diff --git a/default_style.py b/default_style.py
new file mode 100644
index 0000000000000000000000000000000000000000..9198ca8b2f69a3386ec384b96170df4753110b99
--- /dev/null
+++ b/default_style.py
@@ -0,0 +1,30 @@
+import os
+from common.log import logger
+from common.constants import DEFAULT_STYLE
+
+import numpy as np
+import json
+
+
+def set_style_config(json_path, output_path):
+ with open(json_path, "r", encoding="utf-8") as f:
+ json_dict = json.load(f)
+ json_dict["data"]["num_styles"] = 1
+ json_dict["data"]["style2id"] = {DEFAULT_STYLE: 0}
+ with open(output_path, "w", encoding="utf-8") as f:
+ json.dump(json_dict, f, indent=2, ensure_ascii=False)
+ logger.info(f"Save style config (only {DEFAULT_STYLE}) to {output_path}")
+
+
+def save_mean_vector(wav_dir, output_path):
+ embs = []
+ for file in os.listdir(wav_dir):
+ if file.endswith(".npy"):
+ xvec = np.load(os.path.join(wav_dir, file))
+ embs.append(np.expand_dims(xvec, axis=0))
+
+ x = np.concatenate(embs, axis=0) # (N, 256)
+ mean = np.mean(x, axis=0) # (256,)
+ only_mean = np.stack([mean]) # (1, 256)
+ np.save(output_path, only_mean)
+ logger.info(f"Saved mean style vector to {output_path}")
diff --git a/dict_data/.gitignore b/dict_data/.gitignore
new file mode 100644
index 0000000000000000000000000000000000000000..0b60cb63595da629299b2a9de95e2f8b58f81da7
--- /dev/null
+++ b/dict_data/.gitignore
@@ -0,0 +1,3 @@
+*
+!.gitignore
+!default.csv
diff --git a/dict_data/default.csv b/dict_data/default.csv
new file mode 100644
index 0000000000000000000000000000000000000000..8869f97445544f4462016192497983eef94ffead
--- /dev/null
+++ b/dict_data/default.csv
@@ -0,0 +1,3 @@
+Bert,,,8609,名詞,固有名詞,一般,*,*,*,Bert,バアト,バアト,0/3,*
+VITS,,,8609,名詞,固有名詞,一般,*,*,*,VITS,ビッツ,ビッツ,0/3,*
+VITS2,,,8609,名詞,固有名詞,一般,*,*,*,VITS2,ビッツツウ,ビッツツウ,4/5,*
diff --git a/docs/CHANGELOG.md b/docs/CHANGELOG.md
new file mode 100644
index 0000000000000000000000000000000000000000..77cdca51f08f756648810d9534615a60be4e648c
--- /dev/null
+++ b/docs/CHANGELOG.md
@@ -0,0 +1,129 @@
+# Changelog
+
+## v2.3 (2024-02-25)
+
+### 大きな変更
+
+#### ユーザー辞書機能
+あらかじめ辞書に固有名詞を追加することができ、それが学習時・音声合成時の読み取得部分に適応されます。辞書の追加・編集は次のエディタ経由で行ってください。
+
+辞書部分の[実装](/text/user_dict/) は、中のREADMEにある通り、[VOICEVOX Editor](https://github.com/VOICEVOX/voicevox) のものを使っており、この部分のコードライセンスはLGPL-3.
+
+#### 音声合成専用エディタ
+
+音声合成専用エディタを追加。今までのWebUIでできた機能のほか、次のような機能が使えます(つまり既存の日本語音声合成ソフトウェアのエディタを真似ました):
+- セリフ単位でキャラや設定を変更しながら原稿を作り、それを一括で生成したり、原稿を保存等したり読み込んだり
+- GUIよる分かりやすいアクセント調整
+- ユーザー辞書への単語追加や編集
+
+`Editor.bat`をダブルクリックか`python server_editor.py --inbrowser`で起動します。エディター部分は[こちらの別リポジトリ](https://github.com/litagin02/Style-Bert-VITS2-Editor)になります。フロントエンド初心者なのでプルリクや改善案等をお待ちしています。
+
+### 改善
+- 学習時にデコーダー部分を凍結するオプションの追加。品質がもしかしたら上がるかもしれません。
+-
+
+## v2.2 (2024-02-09)
+
+### 変更・機能追加
+- bfloat16オプションはデメリットしか無さそうなので、常にオフで学習するよう変更
+- バッチサイズのデフォルトを4から2に変更。学習が遅い場合はバッチサイズを下げて試してみて、VRAMに余裕があれば上げてください。JP-Extra使用時でのバッチサイズごとのVRAM使用量目安は、1: 6GB, 2: 8GB, 3: 10GB, 4: 12GB くらいのようです。
+- 学習の際の検証データ数をデフォルトで0に変更し、また検証データ数を学習用WebUIで指定できるようにした
+- Tensorboardのログ間隔を学習用WebUIで指定できるようにした
+- UIのテーマを`common/constants.py`の`GRADIO_THEME`で指定できるようにした
+
+### バグ修正
+- JP-Extra使用時にバッチサイズが1だと学習中にエラーが発生するバグを修正
+- 「こんにちは!?!?!?!?」等、感嘆符等の記号が連続すると学習・音声合成でエラーになるバグを修正
+- `—` (em dash, U+2014) や `―` (quotation dash, U+2015) 等のダッシュやハイフンの各種変種が、種類によって`-`(通常の半角ハイフン)に正規化されたりされていなかったりする処理を、全て正規化するように修正
+
+## v2.1 (2024-02-07)
+
+### 変更
+- 学習の際、デフォルトではbfloat16オプションを使わないよう変更(学習が発散したり質が下がることがある模様)
+- 学習の際のメモリ使用量を削減しようと頑張った
+
+### バグ修正や改善
+- 学習WebUIからTensorboardのログを見れるように
+- 音声合成(やそのAPI)において、同時に別の話者が選択され音声合成がリクエストされた場合に発生するエラーを修正
+- モデルマージ時に、そのレシピを`recipe.json`ファイルへ保存するように変更
+- 「改行で分けて生成」がより感情が乗る旨の明記等、軽微な説明文の改善
+- 「`ーーそれは面白い`」や「`なるほど。ーーーそういうことか。`」等、長音記号の前が母音でない場合、長音記号`ー`でなくダッシュ`―`の勘違いだと思われるので、ダッシュ記号として処理するように変更
+
+## v2.0.1 (2024-02-05)
+
+軽微なバグ修正や改善
+- スタイルベクトルに`NaN`が含まれていた場合(主に音声ファイルが極端に短い場合に発生)、それを学習リストから除外するように修正
+- colabにマージの追加
+- 学習時のプログレスバーの表示がおかしかったのを修正
+- デフォルトのjvnvモデルをJP-Extra版にアップデート。新しいモデルを使いたい方は手動で[こちら](https://huggingface.co/litagin/style_bert_vits2_jvnv/tree/main)からダウンロードするか、`python initialize.py`をするか、[このbatファイル](https://github.com/litagin02/Style-Bert-VITS2/releases/download/2.0.1/Update-to-JP-Extra.bat)を`Style-Bert-VITS2`フォルダがある場所(インストールbatファイルとかがあったところ)においてダブルクリックしてください。
+
+## v2.0 (2024-02-03)
+
+### 大きい変更
+モデル構造に [Bert-VITS2の日本語特化モデル JP-Extra](https://github.com/fishaudio/Bert-VITS2/releases/tag/JP-Exta) を取り込んだものを使えるように変更、[事前学習モデル](https://huggingface.co/litagin/Style-Bert-VITS2-2.0-base-JP-Extra)も[Bert-VITS2 JP-Extra](https://huggingface.co/Stardust-minus/Bert-VITS2-Japanese-Extra)のものを改造してStyle-Bert-VITS2で使えるようにしました (モデル構造を見直して日本語での学習をしていただいた [@Stardust-minus](https://github.com/Stardust-minus) 様に感謝します)
+- これにより、日本語の発音やアクセントや抑揚や自然性が向上する傾向があります
+- スタイルベクトルを使ったスタイルの操作は変わらず使えます
+- ただしJP-Extraでは英語と中国語の音声合成は(現状は)できません
+- 旧モデルも引き続き使うことができ、また旧モデルで学習することもできます
+- デフォルトのJVNVモデルは現在は旧verのままです
+
+### 改善
+- `Merge.bat`で、声音マージを、より細かく「声質」と「声の高さ」の点でマージできるように。
+
+### バグ修正
+- PyTorchのバージョンに由来するバグを修正(torchのバージョンを2.1.2に固定)
+- `―`(ダッシュ、長音記号ではない)が2連続すると学習・音声合成でエラーになるバグを修正
+- 「三円」等「ん+母音」のアクセントの仮名表記が「サネン」等になり、また偶にエラーが発生する問題を修正(「ん」の音素表記を内部的には「N」で統一)
+
+## v1.3 (2024-01-09)
+
+### 大きい変更
+- 元々のBert-VITS2に存在した、日本語の発音・アクセント処理部分のバグを修正・リファクタリング
+ - `車両`が`シャリヨオ`、`思う`が`オモオ`、`見つける`が`ミッケル`等に発音・学習されており、その単語以降のアクセント情報が全て死んでいた
+ - `私はそれを見る`のアクセントが`ワ➚タシ➘ワ ソ➚レ➘オ ミ➘ル`だったのを`ワ➚タシワ ソ➚レオ ミ➘ル`に修正
+ - 学習・音声合成で無視されていたアルファベット・ギリシャ文字を無視しないように変更(基本はアルファベット読みだけど簡単な単語は読めるらしい、学習の際は念のためカタカナ等にしたほうがよいです)
+ - 修正の影響で、前処理時に(今まで無視されていた)読めない漢字等で引っかかるようになりました。その場合は書き起こしを確認して修正するようにしてください。
+- アクセントを調整して音声合成できるように(完全に制御できるわけではないが改善される場合がある)。
+
+これまでのモデルもこれまで通り使え、アクセントや発音等が改善される可能性があります。新しいバージョンで学習し直すとより良くなる可能性もあります。が劇的に良くなるかは分かりません。
+
+### 改善
+- `Dataset.bat`の音声スライスと書き起こしをよりカスタマイズできるように(スライスの秒数設定や書き起こしのWhisperモデル指定や言語指定等)
+- `Style.bat`のスタイル分けで、スタイルごとのサンプル音声を指定した数だけ複数再生できるように。また新しい次元削減方法(UMAP)と新しいスタイル分けの方法(DBSCAN)を追加(UMAPのほうがよくスタイルが分かれるかもしれません)
+- `App.bat`での音声合成時に複数話者モデルの場合に話者を指定できるように
+- colabの[ノートブック](http://colab.research.google.com/github/litagin02/Style-Bert-VITS2/blob/master/colab.ipynb)で、音声ファイルのみからデータセットを作成するオプション部分を追加
+- クラウド実行等の際にパスの指定をこちらでできるように、パスの設定を`configs/paths.yml`にまとめた(colabの[ノートブック](http://colab.research.google.com/github/litagin02/Style-Bert-VITS2/blob/master/colab.ipynb)もそれに伴って更新)。デフォルトは`dataset_root: Data`と`assets_root: model_assets`なので、クラウド等でやる方はここを変更してください。
+- どのステップ数の出力がよいかの「一つの」指標として [SpeechMOS](https://github.com/tarepan/SpeechMOS) を使うスクリプトを追加:
+```bash
+python speech_mos.py -m
+```
+ステップごとの自然性評価が表示され、`mos_results`フォルダの`mos_{model_name}.csv`と`mos_{model_name}.png`に結果が保存される。読み上げさせたい文章を変えたかったら中のファイルを弄って各自調整してください。あくまでアクセントや感情表現や抑揚を全く考えない基準での評価で、目安のひとつなので、実際に読み上げさせて選別するのが一番だと思います。
+- 学習時のウォームアップオプションを機能するように( [@kale4eat](https://github.com/kale4eat) 様によるPRです、ありがとうございます!)。前処理時に生成される`config.json`の`train`の`warmup_epochs`を変更することで、ウォームアップのエポック数を変更できます。デフォルトは`0`で今までと同じ学習率の挙動です。
+
+### その他
+- `Dataset.bat`の音声スライスでノーマライズ機能を削除(学習前処理で行えるため)
+- `Train.bat`の音量ノーマライズと無音切り詰めをデフォルトでオフに変更
+- 学習時の進捗を全体エポック数で表示し、学習全体の進捗を見やすいように( [@RedRayz](https://github.com/RedRayz) 様によるPRです、ありがとうございます!)
+- その他バグ修正等( [@tinjyuu](https://github.com/@tinjyuu) 様、 [@darai0512](https://github.com/darai0512) 様ありがとうございます!)
+- `config.json`にスタイル埋め込み部分を学習しない`freeze_style`オプションを追加(デフォルトは`false`)
+
+### TIPS
+- 日本語学習の場合、`config.json`の`freeze_bert`と`freeze_en_bert`を`true`にしておくと、英語と中国語の発話能力が学習の過程で落ちないかもしれませんが、あまり比較していなので分かりません。
+
+## v1.2 (2023-12-31)
+
+- グラボがないユーザーでの音声合成をサポート、`Install-Style-Bert-VITS2-CPU.bat`でインストール。
+- Google Colabでの学習をサポート、[ノートブック](http://colab.research.google.com/github/litagin02/Style-Bert-VITS2/blob/master/colab.ipynb)を追加
+- 音声合成のAPIサーバーを追加、`python server_fastapi.py`で起動します。API仕様は起動後に`/docs`にて確認ください。( [@darai0512](https://github.com/darai0512) 様によるPRです、ありがとうございます!)
+- 学習時に自動的にデフォルトスタイル Neutral を生成するように。特にスタイル指定が必要のない方は、学習したらそのまま音声合成を試せます。これまで通りスタイルを自分で作ることもできます。
+- マージ機能の新規追加: `Merge.bat`, `webui_merge.py`
+- 前処理のリサンプリング時に音声ファイルの開始・終了部分の無音を削除するオプションを追加(デフォルトでオン)
+- `スタイルテキスト (style text)`がスタイル指定と紛らわしかったので、`アシストテキスト (assist text)`に変更
+- その他コードのリファクタリング
+
+## v1.1 (2023-12-29)
+- TrainとDatasetのWebUIの改良・調整(一括事前処理ボタン等)
+- 前処理のリサンプリング時に音量を正規化するオプションを追加(デフォルトでオン)
+
+## v1.0 (2023-12-27)
+- 初版
diff --git a/docs/CLI.md b/docs/CLI.md
new file mode 100644
index 0000000000000000000000000000000000000000..08e2fd03e5863936a77f3b10ba9b3682f1109c96
--- /dev/null
+++ b/docs/CLI.md
@@ -0,0 +1,96 @@
+# CLI
+
+## 0. Install and global paths settings
+
+```bash
+git clone https://github.com/litagin02/Style-Bert-VITS2.git
+cd Style-Bert-VITS2
+python -m venv venv
+venv\Scripts\activate
+pip install torch==2.1.2 torchvision==0.16.2 torchaudio==2.1.2 --index-url https://download.pytorch.org/whl/cu118
+pip install -r requirements.txt
+```
+
+Then download the necessary models and the default TTS model, and set the global paths.
+```bash
+python initialize.py [--skip_jvnv] [--dataset_root ] [--assets_root ]
+```
+
+Optional:
+- `--skip_jvnv`: Skip downloading the default JVNV voice models (use this if you only have to train your own models).
+- `--dataset_root`: Default: `Data`. Root directory of the training dataset. The training dataset of `{model_name}` should be placed in `{dataset_root}/{model_name}`.
+- `--assets_root`: Default: `model_assets`. Root directory of the model assets (for inference). In training, the model assets will be saved to `{assets_root}/{model_name}`, and in inference, we load all the models from `{assets_root}`.
+
+
+## 1. Dataset preparation
+
+### 1.1. Slice wavs
+```bash
+python slice.py --model_name [-i ] [-m ] [-M ]
+```
+
+Required:
+- `model_name`: Name of the speaker (to be used as the name of the trained model).
+
+Optional:
+- `input_dir`: Path to the directory containing the audio files to slice (default: `inputs`)
+- `min_sec`: Minimum duration of the sliced audio files in seconds (default: 2).
+- `max_sec`: Maximum duration of the sliced audio files in seconds (default: 12).
+
+### 1.2. Transcribe wavs
+
+```bash
+python transcribe.py --model_name
+```
+Required:
+- `model_name`: Name of the speaker (to be used as the name of the trained model).
+
+Optional
+- `--initial_prompt`: Initial prompt to use for the transcription (default value is specific to Japanese).
+- `--device`: `cuda` or `cpu` (default: `cuda`).
+- `--language`: `jp`, `en`, or `en` (default: `jp`).
+- `--model`: Whisper model, default: `large-v3`
+- `--compute_type`: default: `bfloat16`
+
+## 2. Preprocess
+
+```bash
+python preprocess_all.py -m [--use_jp_extra] [-b ] [-e ] [-s ] [--num_processes ] [--normalize] [--trim] [--val_per_lang ] [--log_interval ] [--freeze_EN_bert] [--freeze_JP_bert] [--freeze_ZH_bert] [--freeze_style] [--freeze_decoder]
+```
+
+Required:
+- `model_name`: Name of the speaker (to be used as the name of the trained model).
+
+Optional:
+- `--batch_size`, `-b`: Batch size (default: 2).
+- `--epochs`, `-e`: Number of epochs (default: 100).
+- `--save_every_steps`, `-s`: Save every steps (default: 1000).
+- `--num_processes`: Number of processes (default: half of the number of CPU cores).
+- `--normalize`: Loudness normalize audio.
+- `--trim`: Trim silence.
+- `--freeze_EN_bert`: Freeze English BERT.
+- `--freeze_JP_bert`: Freeze Japanese BERT.
+- `--freeze_ZH_bert`: Freeze Chinese BERT.
+- `--freeze_style`: Freeze style vector.
+- `--freeze_decoder`: Freeze decoder.
+- `--use_jp_extra`: Use JP-Extra model.
+- `--val_per_lang`: Validation data per language (default: 0).
+- `--log_interval`: Log interval (default: 200).
+
+## 3. Train
+
+Training settings are automatically loaded from the above process.
+
+If NOT using JP-Extra model:
+```bash
+python train_ms.py [--repo_id /]
+```
+
+If using JP-Extra model:
+```bash
+python train_ms_jp_extra.py [--repo_id /] [--skip_default_style]
+```
+
+Optional:
+- `--repo_id`: Hugging Face repository ID to upload the trained model to. You should have logged in using `huggingface-cli login` before running this command.
+- `--skip_default_style`: Skip making the default style vector. Use this if you want to resume training (since the default style vector is already made).
diff --git a/docs/README_en.md b/docs/README_en.md
new file mode 100644
index 0000000000000000000000000000000000000000..7dd53ae0d3b9331a3612280816c491dc1f3913b0
--- /dev/null
+++ b/docs/README_en.md
@@ -0,0 +1,125 @@
+# This English README is for 1.x versions. WIP for 2.x versions.
+
+# Style-Bert-VITS2
+
+Bert-VITS2 with more controllable voice styles.
+
+https://github.com/litagin02/Style-Bert-VITS2/assets/139731664/b907c1b8-43aa-46e6-b03f-f6362f5a5a1e
+
+[](http://colab.research.google.com/github/litagin02/Style-Bert-VITS2/blob/master/colab.ipynb)
+
+Online demo: https://huggingface.co/spaces/litagin/Style-Bert-VITS2-JVNV
+
+This repository is based on [Bert-VITS2](https://github.com/fishaudio/Bert-VITS2) v2.1, so many thanks to the original author!
+
+- [Update History](docs/CHANGELOG.md)
+
+**Overview**
+
+- Based on Bert-VITS2 v2.1, which generates emotionally rich voices from entered text, this version allows free control of emotions and speaking styles, including intensity.
+- Easy to install and train for people without Git or Python (for Windows users), much is borrowed from [EasyBertVits2](https://github.com/Zuntan03/EasyBertVits2/). Training on Google Colab is also supported: [](http://colab.research.google.com/github/litagin02/Style-Bert-VITS2/blob/master/colab.ipynb)
+- If used only for voice synthesis, it can operate on CPU without a graphics card.
+- Also includes an API server for integration with others (PR by [@darai0512](https://github.com/darai0512), thank you).
+- Originally, Bert-VITS2's strength was to read "happy text happily, sad text sadly", so even without using the added style specification in this fork, you can generate emotionally rich voices.
+
+
+## How to Use
+
+
+
+### Operating Environment
+
+We have confirmed the operation in Windows Command Prompt, WSL2, and Linux (Ubuntu Desktop) for each UI and API Server (please be creative with path specifications in WSL).
+
+### Installation
+
+#### For Those Unfamiliar with Git or Python
+
+Assuming Windows:
+
+1. Download and unzip [this zip file](https://github.com/litagin02/Style-Bert-VITS2/releases/download/1.3/Style-Bert-VITS2.zip).
+ - If you have a graphics card, double-click `Install-Style-Bert-VITS2.bat`.
+ - If you don't have a graphics card, double-click `Install-Style-Bert-VITS2-CPU.bat`.
+2. Wait for the necessary environment to install automatically.
+3. After that, if the WebUI for voice synthesis launches automatically, the installation is successful. The default model will be downloaded, so you can play with it immediately.
+
+For updates, please double-click `Update-Style-Bert-VITS2.bat`.
+
+#### For Those Familiar with Git and Python
+
+```bash
+git clone https://github.com/litagin02/Style-Bert-VITS2.git
+cd Style-Bert-VITS2
+python -m venv venv
+venv\Scripts\activate
+pip3 install torch torchvision torchaudio --index-url https://download.pytorch.org/whl/cu118
+pip install -r requirements.txt
+python initialize.py # Download necessary models and default TTS model
+```
+Don't forget the last step.
+
+### Voice Synthesis
+Double-click `App.bat` or run `python app.py` to launch the WebUI. The default model is downloaded during installation, so you can use it even if you haven't trained it.
+
+The structure of the model files required for voice synthesis is as follows (you don't need to place them manually):
+
+```
+model_assets
+├── your_model
+│ ├── config.json
+│ ├── your_model_file1.safetensors
+│ ├── your_model_file2.safetensors
+│ ├── ...
+│ └── style_vectors.npy
+└── another_model
+ ├── ...
+```
+
+For inference, `config.json`, `*.safetensors`, and `style_vectors.npy` are necessary. If you want to share a model, please share these three files.
+
+Among them, `style_vectors.npy` is a file necessary to control the style. By default, the average style "Neutral" is generated during training.
+If you want to use multiple styles for more detailed control, please refer to "Generating Styles" below (even with only the average style, if the training data is emotionally rich, sufficiently emotionally rich voices can be generated).
+
+### Training
+
+Double-click Train.bat or run `python webui_train.py` to launch the WebUI.
+
+### Generating Styles
+For those who want to use styles other than the default "Neutral".
+
+- Double-click `Style.bat` or run `python webui_style_vectors.py` to launch the WebUI.
+- It is independent of training, so you can do it even during training, and you can redo it any number of times after training is complete (preprocessing must be finished).
+- For more details on the specifications of the style, please refer to [clustering.ipynb](../clustering.ipynb).
+
+### Dataset Creation
+
+- Double-click `Dataset.bat` or run `python webui_dataset.py` to launch the WebUI for creating datasets from audio files. You can use this tool to learn from audio files only.
+
+Note: If you want to manually correct the dataset, remove noise, etc., you may find [Aivis](https://github.com/tsukumijima/Aivis) or its Windows-compatible dataset part [Aivis Dataset](https://github.com/litagin02/Aivis-Dataset) useful. However, if there are many files, etc., it may be sufficient to simply cut out and create a dataset with this tool.
+
+Please experiment to see what kind of dataset is best.
+
+### API Server
+Run `python server_fastapi.py` in the constructed environment to launch the API server.
+Please check the API specification after launching at `/docs`.
+
+By default, CORS settings are allowed for all domains.
+As much as possible, change the value of server.origins in `config.yml` and limit it to trusted domains (if you delete the key, you can disable the CORS settings).
+
+### Merging
+You can create a new model by mixing two models in terms of "voice", "emotional expression", and "tempo".
+Double-click `Merge.bat` or run `python webui_merge.py` to launch the WebUI.
+
+## Relation to Bert-VITS2 v2.1
+Basically, it's just a slight modification of the Bert-VITS2 v2.1 model structure. The [pre-trained model](https://huggingface.co/litagin/Style-Bert-VITS2-1.0-base) is also essentially the same as Bert-VITS2 v2.1 (unnecessary weights have been removed and converted to safetensors).
+
+The differences are as follows:
+
+- Like [EasyBertVits2](https://github.com/Zuntan03/EasyBertVits2), it is easy to use even for people who do not know Python or Git.
+- Changed the model for emotional embedding (from 1024-dimensional [wav2vec2-large-robust-12-ft-emotion-msp-dim](https://huggingface.co/audeering/wav2vec2-large-robust-12-ft-emotion-msp-dim) to 256-dimensional [wespeaker-voxceleb-resnet34-LM](https://huggingface.co/pyannote/wespeaker-voxceleb-resnet34-LM), which is more for speaker identification than emotional embedding)
+- Removed vector quantization from embeddings and replaced it with just a fully connected layer.
+- By creating a style vector file `style_vectors.npy`, you can generate voices using that style and continuously specify the strength of the effect.
+- Various WebUIs created
+- Support for bf16 training
+- Support for safetensors format, defaulting to using safetensors
+- Other minor bug fixes and refactoring
\ No newline at end of file
diff --git a/docs/paperspace.md b/docs/paperspace.md
new file mode 100644
index 0000000000000000000000000000000000000000..2ae9895e476e47f2afcb29c010e0e90d569ec9d8
--- /dev/null
+++ b/docs/paperspace.md
@@ -0,0 +1,86 @@
+# Paperspace gradient で学習する
+
+詳しいコマンドの叩き方は[こちら](CLI.md)を参照してください。
+
+## 事前準備
+- Paperspace のアカウントを作成し必要なら課金する
+- Projectを作る
+- NotebookはStart from Scratchを選択して空いてるGPUマシンを選ぶ
+
+## 使い方
+
+以下では次のような方針でやっています。
+
+- `/storage/`は永続ストレージなので、事前学習モデルとかを含めてリポジトリをクローンするとよい。
+- `/notebooks/`はノートブックごとに変わるストレージなので(同一ノートブック違うランタイムだと共有されるらしい)、データセットやその結果を保存する。ただ容量が多い場合はあふれる可能性があるので`/tmp/`に保存するとよいかもしれない。
+- hugging faceアカウントを作り、(プライベートな)リポジトリを作って、学習元データを置いたり、学習結果を随時アップロードする。
+
+### 1. 環境を作る
+
+以下はデフォルトの`Start from Scratch`で作成した環境の場合。[Dockerfile.train](../Dockerfile.train)を使ったカスタムイメージをするとPythonの環境構築の手間がちょっと省けるので、それを使いたい人は`Advanced Options / Container / Name`に[`litagin/mygradient:latest`](https://hub.docker.com/r/litagin/mygradient/tags)を指定すると使えます(pipの箇所が不要になる等)。
+
+まずは永続ストレージにgit clone
+```bash
+mkdir -p /storage/sbv2
+cd /storage/sbv2
+git clone https://github.com/litagin02/Style-Bert-VITS2.git
+```
+環境構築(デフォルトはPyTorch 1.x系、Python 3.9の模様)
+```bash
+cd /storage/sbv2/Style-Bert-VITS2
+pip install torch==2.1.2 torchvision==0.16.2 torchaudio==2.1.2 --index-url https://download.pytorch.org/whl/cu118 && pip install -r requirements.txt
+```
+事前学習済みモデル等のダウンロード、またパスを`/notebooks/`以下のものに設定
+```bash
+python initialize.py --skip_jvnv --dataset_root /notebooks/Data --assets_root /notebooks/model_assets
+```
+
+### 2. データセットの準備
+以下では`username/voices`というデータセットリポジトリにある`Foo.zip`というデータセットを使うことを想定しています。
+```bash
+cd /notebooks
+huggingface-cli login # 事前にトークンが必要
+huggingface-cli download username/voices Foo.zip --repo-type dataset --local-dir .
+```
+
+- zipファイル中身が既に`raw`と`esd.list`があるデータ(スライス・書き起こし済み)の場合
+```bash
+mkdir -p Data/Foo
+unzip Foo.zip -d Data/Foo
+rm Foo.zip
+cd /storage/sbv2/Style-Bert-VITS2
+```
+
+- zipファイルが音声ファイルのみの場合
+```bash
+mkdir inputs
+unzip Foo.zip -d inputs
+cd /storage/sbv2/Style-Bert-VITS2
+python slice.py --model_name Foo -i /notebooks/inputs
+python transcribe.py --model_name Foo
+```
+
+それが終わったら、以下のコマンドで一括前処理を行う(パラメータは各自お好み、バッチサイズ5か6でVRAM 16GBギリくらい)。
+```bash
+python preprocess_all.py --model_name Foo -b 5 -e 300 --use_jp_extra
+```
+
+### 3. 学習
+
+Hugging faceの`username/sbv2-private`というモデルリポジトリに学習済みモデルをアップロードすることを想定しています。事前に`huggingface-cli login`でログインしておくこと。
+```bash
+python train_ms_jp_extra.py --repo_id username/sbv2-private
+```
+(JP-Extraでない場合は`train_ms.py`を使う)
+
+### 4. 学習再開
+
+Notebooksの時間制限が切れてから別Notebooksで同じモデルを学習を再開する場合(環境構築は必要)。
+```bash
+huggingface-cli login
+cd /notebooks
+huggingface-cli download username/sbv2-private --include "Data/Foo/*" --local-dir .
+cd /storage/sbv2/Style-Bert-VITS2
+python train_ms_jp_extra.py --repo_id username/sbv2-private --skip_default_style
+```
+前回の設定が残っているので特に前処理等は不要。
\ No newline at end of file
diff --git a/gen_yaml.py b/gen_yaml.py
new file mode 100644
index 0000000000000000000000000000000000000000..91301accbba6765cb687fd40c67cdd508dc7a3b9
--- /dev/null
+++ b/gen_yaml.py
@@ -0,0 +1,32 @@
+import os
+import shutil
+import yaml
+import argparse
+
+parser = argparse.ArgumentParser(
+ description="config.ymlの生成。あらかじめ前準備をしたデータをバッチファイルなどで連続で学習する時にtrain_ms.pyより前に使用する。"
+)
+# そうしないと最後の前準備したデータで学習してしまう
+parser.add_argument("--model_name", type=str, help="Model name", required=True)
+parser.add_argument(
+ "--dataset_path",
+ type=str,
+ help="Dataset path(example: Data\\your_model_name)",
+ required=True,
+)
+args = parser.parse_args()
+
+
+def gen_yaml(model_name, dataset_path):
+ if not os.path.exists("config.yml"):
+ shutil.copy(src="default_config.yml", dst="config.yml")
+ with open("config.yml", "r", encoding="utf-8") as f:
+ yml_data = yaml.safe_load(f)
+ yml_data["model_name"] = model_name
+ yml_data["dataset_path"] = dataset_path
+ with open("config.yml", "w", encoding="utf-8") as f:
+ yaml.dump(yml_data, f, allow_unicode=True)
+
+
+if __name__ == "__main__":
+ gen_yaml(args.model_name, args.dataset_path)
diff --git a/infer.py b/infer.py
new file mode 100644
index 0000000000000000000000000000000000000000..505d447893feb205671fea1db34828c2d2d08961
--- /dev/null
+++ b/infer.py
@@ -0,0 +1,317 @@
+import torch
+
+import commons
+import utils
+from models import SynthesizerTrn
+from models_jp_extra import SynthesizerTrn as SynthesizerTrnJPExtra
+from text import cleaned_text_to_sequence, get_bert
+from text.cleaner import clean_text
+from text.symbols import symbols
+from common.log import logger
+
+
+class InvalidToneError(ValueError):
+ pass
+
+
+def get_net_g(model_path: str, version: str, device: str, hps):
+ if version.endswith("JP-Extra"):
+ logger.info("Using JP-Extra model")
+ net_g = SynthesizerTrnJPExtra(
+ len(symbols),
+ hps.data.filter_length // 2 + 1,
+ hps.train.segment_size // hps.data.hop_length,
+ n_speakers=hps.data.n_speakers,
+ **hps.model,
+ ).to(device)
+ else:
+ logger.info("Using normal model")
+ net_g = SynthesizerTrn(
+ len(symbols),
+ hps.data.filter_length // 2 + 1,
+ hps.train.segment_size // hps.data.hop_length,
+ n_speakers=hps.data.n_speakers,
+ **hps.model,
+ ).to(device)
+ net_g.state_dict()
+ _ = net_g.eval()
+ if model_path.endswith(".pth") or model_path.endswith(".pt"):
+ _ = utils.load_checkpoint(model_path, net_g, None, skip_optimizer=True)
+ elif model_path.endswith(".safetensors"):
+ _ = utils.load_safetensors(model_path, net_g, True)
+ else:
+ raise ValueError(f"Unknown model format: {model_path}")
+ return net_g
+
+
+def get_text(
+ text,
+ language_str,
+ hps,
+ device,
+ assist_text=None,
+ assist_text_weight=0.7,
+ given_tone=None,
+ ignore_unknown=False,
+):
+ use_jp_extra = hps.version.endswith("JP-Extra")
+ norm_text, phone, tone, word2ph = clean_text(
+ text, language_str, use_jp_extra, ignore_unknown=ignore_unknown
+ )
+ if given_tone is not None:
+ if len(given_tone) != len(phone):
+ raise InvalidToneError(
+ f"Length of given_tone ({len(given_tone)}) != length of phone ({len(phone)})"
+ )
+ tone = given_tone
+ phone, tone, language = cleaned_text_to_sequence(phone, tone, language_str)
+
+ if hps.data.add_blank:
+ phone = commons.intersperse(phone, 0)
+ tone = commons.intersperse(tone, 0)
+ language = commons.intersperse(language, 0)
+ for i in range(len(word2ph)):
+ word2ph[i] = word2ph[i] * 2
+ word2ph[0] += 1
+ bert_ori = get_bert(
+ norm_text,
+ word2ph,
+ language_str,
+ device,
+ assist_text,
+ assist_text_weight,
+ ignore_unknown,
+ )
+ del word2ph
+ assert bert_ori.shape[-1] == len(phone), phone
+
+ if language_str == "ZH":
+ bert = bert_ori
+ ja_bert = torch.zeros(1024, len(phone))
+ en_bert = torch.zeros(1024, len(phone))
+ elif language_str == "JP":
+ bert = torch.zeros(1024, len(phone))
+ ja_bert = bert_ori
+ en_bert = torch.zeros(1024, len(phone))
+ elif language_str == "EN":
+ bert = torch.zeros(1024, len(phone))
+ ja_bert = torch.zeros(1024, len(phone))
+ en_bert = bert_ori
+ else:
+ raise ValueError("language_str should be ZH, JP or EN")
+
+ assert bert.shape[-1] == len(
+ phone
+ ), f"Bert seq len {bert.shape[-1]} != {len(phone)}"
+
+ phone = torch.LongTensor(phone)
+ tone = torch.LongTensor(tone)
+ language = torch.LongTensor(language)
+ return bert, ja_bert, en_bert, phone, tone, language
+
+
+def infer(
+ text,
+ style_vec,
+ sdp_ratio,
+ noise_scale,
+ noise_scale_w,
+ length_scale,
+ sid: int, # In the original Bert-VITS2, its speaker_name: str, but here it's id
+ language,
+ hps,
+ net_g,
+ device,
+ skip_start=False,
+ skip_end=False,
+ assist_text=None,
+ assist_text_weight=0.7,
+ given_tone=None,
+ ignore_unknown=False,
+):
+ is_jp_extra = hps.version.endswith("JP-Extra")
+ bert, ja_bert, en_bert, phones, tones, lang_ids = get_text(
+ text,
+ language,
+ hps,
+ device,
+ assist_text=assist_text,
+ assist_text_weight=assist_text_weight,
+ given_tone=given_tone,
+ ignore_unknown=ignore_unknown,
+ )
+ if skip_start:
+ phones = phones[3:]
+ tones = tones[3:]
+ lang_ids = lang_ids[3:]
+ bert = bert[:, 3:]
+ ja_bert = ja_bert[:, 3:]
+ en_bert = en_bert[:, 3:]
+ if skip_end:
+ phones = phones[:-2]
+ tones = tones[:-2]
+ lang_ids = lang_ids[:-2]
+ bert = bert[:, :-2]
+ ja_bert = ja_bert[:, :-2]
+ en_bert = en_bert[:, :-2]
+ with torch.no_grad():
+ x_tst = phones.to(device).unsqueeze(0)
+ tones = tones.to(device).unsqueeze(0)
+ lang_ids = lang_ids.to(device).unsqueeze(0)
+ bert = bert.to(device).unsqueeze(0)
+ ja_bert = ja_bert.to(device).unsqueeze(0)
+ en_bert = en_bert.to(device).unsqueeze(0)
+ x_tst_lengths = torch.LongTensor([phones.size(0)]).to(device)
+ style_vec = torch.from_numpy(style_vec).to(device).unsqueeze(0)
+ del phones
+ sid_tensor = torch.LongTensor([sid]).to(device)
+ if is_jp_extra:
+ output = net_g.infer(
+ x_tst,
+ x_tst_lengths,
+ sid_tensor,
+ tones,
+ lang_ids,
+ ja_bert,
+ style_vec=style_vec,
+ sdp_ratio=sdp_ratio,
+ noise_scale=noise_scale,
+ noise_scale_w=noise_scale_w,
+ length_scale=length_scale,
+ )
+ else:
+ output = net_g.infer(
+ x_tst,
+ x_tst_lengths,
+ sid_tensor,
+ tones,
+ lang_ids,
+ bert,
+ ja_bert,
+ en_bert,
+ style_vec=style_vec,
+ sdp_ratio=sdp_ratio,
+ noise_scale=noise_scale,
+ noise_scale_w=noise_scale_w,
+ length_scale=length_scale,
+ )
+ audio = output[0][0, 0].data.cpu().float().numpy()
+ del (
+ x_tst,
+ tones,
+ lang_ids,
+ bert,
+ x_tst_lengths,
+ sid_tensor,
+ ja_bert,
+ en_bert,
+ style_vec,
+ ) # , emo
+ if torch.cuda.is_available():
+ torch.cuda.empty_cache()
+ return audio
+
+
+def infer_multilang(
+ text,
+ style_vec,
+ sdp_ratio,
+ noise_scale,
+ noise_scale_w,
+ length_scale,
+ sid,
+ language,
+ hps,
+ net_g,
+ device,
+ skip_start=False,
+ skip_end=False,
+):
+ bert, ja_bert, en_bert, phones, tones, lang_ids = [], [], [], [], [], []
+ # emo = get_emo_(reference_audio, emotion, sid)
+ # if isinstance(reference_audio, np.ndarray):
+ # emo = get_clap_audio_feature(reference_audio, device)
+ # else:
+ # emo = get_clap_text_feature(emotion, device)
+ # emo = torch.squeeze(emo, dim=1)
+ for idx, (txt, lang) in enumerate(zip(text, language)):
+ _skip_start = (idx != 0) or (skip_start and idx == 0)
+ _skip_end = (idx != len(language) - 1) or skip_end
+ (
+ temp_bert,
+ temp_ja_bert,
+ temp_en_bert,
+ temp_phones,
+ temp_tones,
+ temp_lang_ids,
+ ) = get_text(txt, lang, hps, device)
+ if _skip_start:
+ temp_bert = temp_bert[:, 3:]
+ temp_ja_bert = temp_ja_bert[:, 3:]
+ temp_en_bert = temp_en_bert[:, 3:]
+ temp_phones = temp_phones[3:]
+ temp_tones = temp_tones[3:]
+ temp_lang_ids = temp_lang_ids[3:]
+ if _skip_end:
+ temp_bert = temp_bert[:, :-2]
+ temp_ja_bert = temp_ja_bert[:, :-2]
+ temp_en_bert = temp_en_bert[:, :-2]
+ temp_phones = temp_phones[:-2]
+ temp_tones = temp_tones[:-2]
+ temp_lang_ids = temp_lang_ids[:-2]
+ bert.append(temp_bert)
+ ja_bert.append(temp_ja_bert)
+ en_bert.append(temp_en_bert)
+ phones.append(temp_phones)
+ tones.append(temp_tones)
+ lang_ids.append(temp_lang_ids)
+ bert = torch.concatenate(bert, dim=1)
+ ja_bert = torch.concatenate(ja_bert, dim=1)
+ en_bert = torch.concatenate(en_bert, dim=1)
+ phones = torch.concatenate(phones, dim=0)
+ tones = torch.concatenate(tones, dim=0)
+ lang_ids = torch.concatenate(lang_ids, dim=0)
+ with torch.no_grad():
+ x_tst = phones.to(device).unsqueeze(0)
+ tones = tones.to(device).unsqueeze(0)
+ lang_ids = lang_ids.to(device).unsqueeze(0)
+ bert = bert.to(device).unsqueeze(0)
+ ja_bert = ja_bert.to(device).unsqueeze(0)
+ en_bert = en_bert.to(device).unsqueeze(0)
+ # emo = emo.to(device).unsqueeze(0)
+ x_tst_lengths = torch.LongTensor([phones.size(0)]).to(device)
+ del phones
+ speakers = torch.LongTensor([hps.data.spk2id[sid]]).to(device)
+ audio = (
+ net_g.infer(
+ x_tst,
+ x_tst_lengths,
+ speakers,
+ tones,
+ lang_ids,
+ bert,
+ ja_bert,
+ en_bert,
+ style_vec=style_vec,
+ sdp_ratio=sdp_ratio,
+ noise_scale=noise_scale,
+ noise_scale_w=noise_scale_w,
+ length_scale=length_scale,
+ )[0][0, 0]
+ .data.cpu()
+ .float()
+ .numpy()
+ )
+ del (
+ x_tst,
+ tones,
+ lang_ids,
+ bert,
+ x_tst_lengths,
+ speakers,
+ ja_bert,
+ en_bert,
+ ) # , emo
+ if torch.cuda.is_available():
+ torch.cuda.empty_cache()
+ return audio
diff --git a/initialize.py b/initialize.py
new file mode 100644
index 0000000000000000000000000000000000000000..5e35061f285c172b0740951a4c192bfbd6656d48
--- /dev/null
+++ b/initialize.py
@@ -0,0 +1,138 @@
+import argparse
+import json
+from pathlib import Path
+
+import yaml
+from huggingface_hub import hf_hub_download
+
+from common.log import logger
+
+
+def download_bert_models():
+ with open("bert/bert_models.json", "r") as fp:
+ models = json.load(fp)
+ for k, v in models.items():
+ local_path = Path("bert").joinpath(k)
+ for file in v["files"]:
+ if not Path(local_path).joinpath(file).exists():
+ logger.info(f"Downloading {k} {file}")
+ hf_hub_download(
+ v["repo_id"],
+ file,
+ local_dir=local_path,
+ local_dir_use_symlinks=False,
+ )
+
+
+def download_slm_model():
+ local_path = Path("slm/wavlm-base-plus/")
+ file = "pytorch_model.bin"
+ if not Path(local_path).joinpath(file).exists():
+ logger.info(f"Downloading wavlm-base-plus {file}")
+ hf_hub_download(
+ "microsoft/wavlm-base-plus",
+ file,
+ local_dir=local_path,
+ local_dir_use_symlinks=False,
+ )
+
+
+def download_pretrained_models():
+ files = ["G_0.safetensors", "D_0.safetensors", "DUR_0.safetensors"]
+ local_path = Path("pretrained")
+ for file in files:
+ if not Path(local_path).joinpath(file).exists():
+ logger.info(f"Downloading pretrained {file}")
+ hf_hub_download(
+ "litagin/Style-Bert-VITS2-1.0-base",
+ file,
+ local_dir=local_path,
+ local_dir_use_symlinks=False,
+ )
+
+
+def download_jp_extra_pretrained_models():
+ files = ["G_0.safetensors", "D_0.safetensors", "WD_0.safetensors"]
+ local_path = Path("pretrained_jp_extra")
+ for file in files:
+ if not Path(local_path).joinpath(file).exists():
+ logger.info(f"Downloading JP-Extra pretrained {file}")
+ hf_hub_download(
+ "litagin/Style-Bert-VITS2-2.0-base-JP-Extra",
+ file,
+ local_dir=local_path,
+ local_dir_use_symlinks=False,
+ )
+
+
+def download_jvnv_models():
+ files = [
+ "jvnv-F1-jp/config.json",
+ "jvnv-F1-jp/jvnv-F1-jp_e160_s14000.safetensors",
+ "jvnv-F1-jp/style_vectors.npy",
+ "jvnv-F2-jp/config.json",
+ "jvnv-F2-jp/jvnv-F2_e166_s20000.safetensors",
+ "jvnv-F2-jp/style_vectors.npy",
+ "jvnv-M1-jp/config.json",
+ "jvnv-M1-jp/jvnv-M1-jp_e158_s14000.safetensors",
+ "jvnv-M1-jp/style_vectors.npy",
+ "jvnv-M2-jp/config.json",
+ "jvnv-M2-jp/jvnv-M2-jp_e159_s17000.safetensors",
+ "jvnv-M2-jp/style_vectors.npy",
+ ]
+ for file in files:
+ if not Path(f"model_assets/{file}").exists():
+ logger.info(f"Downloading {file}")
+ hf_hub_download(
+ "litagin/style_bert_vits2_jvnv",
+ file,
+ local_dir="model_assets",
+ local_dir_use_symlinks=False,
+ )
+
+
+def main():
+ parser = argparse.ArgumentParser()
+ parser.add_argument("--skip_jvnv", action="store_true")
+ parser.add_argument(
+ "--dataset_root",
+ type=str,
+ help="Dataset root path (default: Data)",
+ default=None,
+ )
+ parser.add_argument(
+ "--assets_root",
+ type=str,
+ help="Assets root path (default: model_assets)",
+ default=None,
+ )
+ args = parser.parse_args()
+
+ download_bert_models()
+
+ download_slm_model()
+
+ download_pretrained_models()
+
+ download_jp_extra_pretrained_models()
+
+ if not args.skip_jvnv:
+ download_jvnv_models()
+
+ if args.dataset_root is None and args.assets_root is None:
+ return
+
+ # Change default paths if necessary
+ paths_yml = Path("configs/paths.yml")
+ with open(paths_yml, "r", encoding="utf-8") as f:
+ yml_data = yaml.safe_load(f)
+ if args.assets_root is not None:
+ yml_data["assets_root"] = args.assets_root
+ if args.dataset_root is not None:
+ yml_data["dataset_root"] = args.dataset_root
+ with open(paths_yml, "w", encoding="utf-8") as f:
+ yaml.dump(yml_data, f, allow_unicode=True)
+
+
+if __name__ == "__main__":
+ main()
diff --git a/inputs/.gitignore b/inputs/.gitignore
new file mode 100644
index 0000000000000000000000000000000000000000..d6b7ef32c8478a48c3994dcadc86837f4371184d
--- /dev/null
+++ b/inputs/.gitignore
@@ -0,0 +1,2 @@
+*
+!.gitignore
diff --git a/losses.py b/losses.py
new file mode 100644
index 0000000000000000000000000000000000000000..763cc028511570a69924eb434bb648536171ebfc
--- /dev/null
+++ b/losses.py
@@ -0,0 +1,155 @@
+import torch
+import torchaudio
+from transformers import AutoModel
+
+from common.log import logger
+
+
+def feature_loss(fmap_r, fmap_g):
+ loss = 0
+ for dr, dg in zip(fmap_r, fmap_g):
+ for rl, gl in zip(dr, dg):
+ rl = rl.float().detach()
+ gl = gl.float()
+ loss += torch.mean(torch.abs(rl - gl))
+
+ return loss * 2
+
+
+def discriminator_loss(disc_real_outputs, disc_generated_outputs):
+ loss = 0
+ r_losses = []
+ g_losses = []
+ for dr, dg in zip(disc_real_outputs, disc_generated_outputs):
+ dr = dr.float()
+ dg = dg.float()
+ r_loss = torch.mean((1 - dr) ** 2)
+ g_loss = torch.mean(dg**2)
+ loss += r_loss + g_loss
+ r_losses.append(r_loss.item())
+ g_losses.append(g_loss.item())
+
+ return loss, r_losses, g_losses
+
+
+def generator_loss(disc_outputs):
+ loss = 0
+ gen_losses = []
+ for dg in disc_outputs:
+ dg = dg.float()
+ l = torch.mean((1 - dg) ** 2)
+ gen_losses.append(l)
+ loss += l
+
+ return loss, gen_losses
+
+
+def kl_loss(z_p, logs_q, m_p, logs_p, z_mask):
+ """
+ z_p, logs_q: [b, h, t_t]
+ m_p, logs_p: [b, h, t_t]
+ """
+ z_p = z_p.float()
+ logs_q = logs_q.float()
+ m_p = m_p.float()
+ logs_p = logs_p.float()
+ z_mask = z_mask.float()
+
+ kl = logs_p - logs_q - 0.5
+ kl += 0.5 * ((z_p - m_p) ** 2) * torch.exp(-2.0 * logs_p)
+ kl = torch.sum(kl * z_mask)
+ l = kl / torch.sum(z_mask)
+ return l
+
+
+class WavLMLoss(torch.nn.Module):
+ def __init__(self, model, wd, model_sr, slm_sr=16000):
+ super(WavLMLoss, self).__init__()
+ self.wavlm = AutoModel.from_pretrained(model)
+ self.wd = wd
+ self.resample = torchaudio.transforms.Resample(model_sr, slm_sr)
+ self.wavlm.eval()
+ for param in self.wavlm.parameters():
+ param.requires_grad = False
+
+ def forward(self, wav, y_rec):
+ with torch.no_grad():
+ wav_16 = self.resample(wav)
+ wav_embeddings = self.wavlm(
+ input_values=wav_16, output_hidden_states=True
+ ).hidden_states
+ y_rec_16 = self.resample(y_rec)
+ y_rec_embeddings = self.wavlm(
+ input_values=y_rec_16, output_hidden_states=True
+ ).hidden_states
+
+ floss = 0
+ for er, eg in zip(wav_embeddings, y_rec_embeddings):
+ floss += torch.mean(torch.abs(er - eg))
+
+ return floss.mean()
+
+ def generator(self, y_rec):
+ y_rec_16 = self.resample(y_rec)
+ y_rec_embeddings = self.wavlm(
+ input_values=y_rec_16, output_hidden_states=True
+ ).hidden_states
+ y_rec_embeddings = (
+ torch.stack(y_rec_embeddings, dim=1)
+ .transpose(-1, -2)
+ .flatten(start_dim=1, end_dim=2)
+ )
+ y_df_hat_g = self.wd(y_rec_embeddings)
+ loss_gen = torch.mean((1 - y_df_hat_g) ** 2)
+
+ return loss_gen
+
+ def discriminator(self, wav, y_rec):
+ with torch.no_grad():
+ wav_16 = self.resample(wav)
+ wav_embeddings = self.wavlm(
+ input_values=wav_16, output_hidden_states=True
+ ).hidden_states
+ y_rec_16 = self.resample(y_rec)
+ y_rec_embeddings = self.wavlm(
+ input_values=y_rec_16, output_hidden_states=True
+ ).hidden_states
+
+ y_embeddings = (
+ torch.stack(wav_embeddings, dim=1)
+ .transpose(-1, -2)
+ .flatten(start_dim=1, end_dim=2)
+ )
+ y_rec_embeddings = (
+ torch.stack(y_rec_embeddings, dim=1)
+ .transpose(-1, -2)
+ .flatten(start_dim=1, end_dim=2)
+ )
+
+ y_d_rs = self.wd(y_embeddings)
+ y_d_gs = self.wd(y_rec_embeddings)
+
+ y_df_hat_r, y_df_hat_g = y_d_rs, y_d_gs
+
+ r_loss = torch.mean((1 - y_df_hat_r) ** 2)
+ g_loss = torch.mean((y_df_hat_g) ** 2)
+
+ loss_disc_f = r_loss + g_loss
+
+ return loss_disc_f.mean()
+
+ def discriminator_forward(self, wav):
+ with torch.no_grad():
+ wav_16 = self.resample(wav)
+ wav_embeddings = self.wavlm(
+ input_values=wav_16, output_hidden_states=True
+ ).hidden_states
+ y_embeddings = (
+ torch.stack(wav_embeddings, dim=1)
+ .transpose(-1, -2)
+ .flatten(start_dim=1, end_dim=2)
+ )
+
+ y_d_rs = self.wd(y_embeddings)
+
+ return y_d_rs
diff --git a/mel_processing.py b/mel_processing.py
new file mode 100644
index 0000000000000000000000000000000000000000..e9e7ec3622a0ee940e238f29c3f0d7509abfe59b
--- /dev/null
+++ b/mel_processing.py
@@ -0,0 +1,146 @@
+import torch
+import torch.utils.data
+from librosa.filters import mel as librosa_mel_fn
+import warnings
+
+# warnings.simplefilter(action='ignore', category=FutureWarning)
+warnings.filterwarnings(action="ignore")
+MAX_WAV_VALUE = 32768.0
+
+
+def dynamic_range_compression_torch(x, C=1, clip_val=1e-5):
+ """
+ PARAMS
+ ------
+ C: compression factor
+ """
+ return torch.log(torch.clamp(x, min=clip_val) * C)
+
+
+def dynamic_range_decompression_torch(x, C=1):
+ """
+ PARAMS
+ ------
+ C: compression factor used to compress
+ """
+ return torch.exp(x) / C
+
+
+def spectral_normalize_torch(magnitudes):
+ output = dynamic_range_compression_torch(magnitudes)
+ return output
+
+
+def spectral_de_normalize_torch(magnitudes):
+ output = dynamic_range_decompression_torch(magnitudes)
+ return output
+
+
+mel_basis = {}
+hann_window = {}
+
+
+def spectrogram_torch(y, n_fft, sampling_rate, hop_size, win_size, center=False):
+ if torch.min(y) < -1.0:
+ print("min value is ", torch.min(y))
+ if torch.max(y) > 1.0:
+ print("max value is ", torch.max(y))
+
+ global hann_window
+ dtype_device = str(y.dtype) + "_" + str(y.device)
+ wnsize_dtype_device = str(win_size) + "_" + dtype_device
+ if wnsize_dtype_device not in hann_window:
+ hann_window[wnsize_dtype_device] = torch.hann_window(win_size).to(
+ dtype=y.dtype, device=y.device
+ )
+
+ y = torch.nn.functional.pad(
+ y.unsqueeze(1),
+ (int((n_fft - hop_size) / 2), int((n_fft - hop_size) / 2)),
+ mode="reflect",
+ )
+ y = y.squeeze(1)
+
+ spec = torch.stft(
+ y,
+ n_fft,
+ hop_length=hop_size,
+ win_length=win_size,
+ window=hann_window[wnsize_dtype_device],
+ center=center,
+ pad_mode="reflect",
+ normalized=False,
+ onesided=True,
+ return_complex=False,
+ )
+
+ spec = torch.sqrt(spec.pow(2).sum(-1) + 1e-6)
+ return spec
+
+
+def spec_to_mel_torch(spec, n_fft, num_mels, sampling_rate, fmin, fmax):
+ global mel_basis
+ dtype_device = str(spec.dtype) + "_" + str(spec.device)
+ fmax_dtype_device = str(fmax) + "_" + dtype_device
+ if fmax_dtype_device not in mel_basis:
+ mel = librosa_mel_fn(
+ sr=sampling_rate, n_fft=n_fft, n_mels=num_mels, fmin=fmin, fmax=fmax
+ )
+ mel_basis[fmax_dtype_device] = torch.from_numpy(mel).to(
+ dtype=spec.dtype, device=spec.device
+ )
+ spec = torch.matmul(mel_basis[fmax_dtype_device], spec)
+ spec = spectral_normalize_torch(spec)
+ return spec
+
+
+def mel_spectrogram_torch(
+ y, n_fft, num_mels, sampling_rate, hop_size, win_size, fmin, fmax, center=False
+):
+ if torch.min(y) < -1.0:
+ print("min value is ", torch.min(y))
+ if torch.max(y) > 1.0:
+ print("max value is ", torch.max(y))
+
+ global mel_basis, hann_window
+ dtype_device = str(y.dtype) + "_" + str(y.device)
+ fmax_dtype_device = str(fmax) + "_" + dtype_device
+ wnsize_dtype_device = str(win_size) + "_" + dtype_device
+ if fmax_dtype_device not in mel_basis:
+ mel = librosa_mel_fn(
+ sr=sampling_rate, n_fft=n_fft, n_mels=num_mels, fmin=fmin, fmax=fmax
+ )
+ mel_basis[fmax_dtype_device] = torch.from_numpy(mel).to(
+ dtype=y.dtype, device=y.device
+ )
+ if wnsize_dtype_device not in hann_window:
+ hann_window[wnsize_dtype_device] = torch.hann_window(win_size).to(
+ dtype=y.dtype, device=y.device
+ )
+
+ y = torch.nn.functional.pad(
+ y.unsqueeze(1),
+ (int((n_fft - hop_size) / 2), int((n_fft - hop_size) / 2)),
+ mode="reflect",
+ )
+ y = y.squeeze(1)
+
+ spec = torch.stft(
+ y,
+ n_fft,
+ hop_length=hop_size,
+ win_length=win_size,
+ window=hann_window[wnsize_dtype_device],
+ center=center,
+ pad_mode="reflect",
+ normalized=False,
+ onesided=True,
+ return_complex=False,
+ )
+
+ spec = torch.sqrt(spec.pow(2).sum(-1) + 1e-6)
+
+ spec = torch.matmul(mel_basis[fmax_dtype_device], spec)
+ spec = spectral_normalize_torch(spec)
+
+ return spec
diff --git a/model_assets/.gitignore b/model_assets/.gitignore
new file mode 100644
index 0000000000000000000000000000000000000000..d0174bf5cc8cfcbfe85b80c1823ac74d1d65ef8a
--- /dev/null
+++ b/model_assets/.gitignore
@@ -0,0 +1,2 @@
+# *
+!.gitignore
diff --git a/model_assets/jvnv-F1-jp/config.json b/model_assets/jvnv-F1-jp/config.json
new file mode 100644
index 0000000000000000000000000000000000000000..e7a6fb12b380bee434e0e5af8f613d019596f2bb
--- /dev/null
+++ b/model_assets/jvnv-F1-jp/config.json
@@ -0,0 +1,92 @@
+{
+ "train": {
+ "log_interval": 200,
+ "eval_interval": 1000,
+ "seed": 42,
+ "epochs": 300,
+ "learning_rate": 0.0001,
+ "betas": [0.8, 0.99],
+ "eps": 1e-9,
+ "batch_size": 4,
+ "bf16_run": true,
+ "fp16_run": false,
+ "lr_decay": 0.99996,
+ "segment_size": 16384,
+ "init_lr_ratio": 1,
+ "warmup_epochs": 0,
+ "c_mel": 45,
+ "c_kl": 1.0,
+ "c_commit": 100,
+ "skip_optimizer": true,
+ "freeze_ZH_bert": false,
+ "freeze_JP_bert": false,
+ "freeze_EN_bert": false,
+ "freeze_emo": false,
+ "freeze_style": false
+ },
+ "data": {
+ "use_jp_extra": true,
+ "training_files": "Data/jvnv-F1-jp/train.list",
+ "validation_files": "Data/jvnv-F1-jp/val.list",
+ "max_wav_value": 32768.0,
+ "sampling_rate": 44100,
+ "filter_length": 2048,
+ "hop_length": 512,
+ "win_length": 2048,
+ "n_mel_channels": 128,
+ "mel_fmin": 0.0,
+ "mel_fmax": null,
+ "add_blank": true,
+ "n_speakers": 1,
+ "cleaned_text": true,
+ "spk2id": {
+ "jvnv-F1-jp": 0
+ },
+ "num_styles": 7,
+ "style2id": {
+ "Neutral": 0,
+ "Angry": 1,
+ "Disgust": 2,
+ "Fear": 3,
+ "Happy": 4,
+ "Sad": 5,
+ "Surprise": 6
+ }
+ },
+ "model": {
+ "use_spk_conditioned_encoder": true,
+ "use_noise_scaled_mas": true,
+ "use_mel_posterior_encoder": false,
+ "use_duration_discriminator": false,
+ "use_wavlm_discriminator": true,
+ "inter_channels": 192,
+ "hidden_channels": 192,
+ "filter_channels": 768,
+ "n_heads": 2,
+ "n_layers": 6,
+ "kernel_size": 3,
+ "p_dropout": 0.1,
+ "resblock": "1",
+ "resblock_kernel_sizes": [3, 7, 11],
+ "resblock_dilation_sizes": [
+ [1, 3, 5],
+ [1, 3, 5],
+ [1, 3, 5]
+ ],
+ "upsample_rates": [8, 8, 2, 2, 2],
+ "upsample_initial_channel": 512,
+ "upsample_kernel_sizes": [16, 16, 8, 2, 2],
+ "n_layers_q": 3,
+ "use_spectral_norm": false,
+ "gin_channels": 512,
+ "slm": {
+ "model": "./slm/wavlm-base-plus",
+ "sr": 16000,
+ "hidden": 768,
+ "nlayers": 13,
+ "initial_channel": 64
+ }
+ },
+ "version": "2.0-JP-Extra",
+ "model_name": "jvnv-F1-jp"
+}
diff --git a/model_assets/jvnv-F1-jp/jvnv-F1-jp_e160_s14000.safetensors b/model_assets/jvnv-F1-jp/jvnv-F1-jp_e160_s14000.safetensors
new file mode 100644
index 0000000000000000000000000000000000000000..30d2aa6d79448ab22bee74df7b59eee8f24dcbc2
--- /dev/null
+++ b/model_assets/jvnv-F1-jp/jvnv-F1-jp_e160_s14000.safetensors
@@ -0,0 +1,3 @@
+version https://git-lfs.github.com/spec/v1
+oid sha256:a90fa6c9444d9235c9ec4db99daf7c5c6a21cc26ca141b4c48455d66a3257d01
+size 251150980
diff --git a/model_assets/jvnv-F1-jp/style_vectors.npy b/model_assets/jvnv-F1-jp/style_vectors.npy
new file mode 100644
index 0000000000000000000000000000000000000000..8ec6d4853798654ee6f6502620b974643c66e98a
--- /dev/null
+++ b/model_assets/jvnv-F1-jp/style_vectors.npy
@@ -0,0 +1,3 @@
+version https://git-lfs.github.com/spec/v1
+oid sha256:1f959bb45ed0922efc31ff24e9147253814f42cb1d2d1e2bb10391a9df368489
+size 7296
diff --git a/model_assets/jvnv-F2-jp/config.json b/model_assets/jvnv-F2-jp/config.json
new file mode 100644
index 0000000000000000000000000000000000000000..20bdc59847f71d5b3b304e070fac6a6a5c0b3d94
--- /dev/null
+++ b/model_assets/jvnv-F2-jp/config.json
@@ -0,0 +1,92 @@
+{
+ "train": {
+ "log_interval": 200,
+ "eval_interval": 1000,
+ "seed": 42,
+ "epochs": 300,
+ "learning_rate": 0.0001,
+ "betas": [0.8, 0.99],
+ "eps": 1e-9,
+ "batch_size": 4,
+ "bf16_run": false,
+ "fp16_run": false,
+ "lr_decay": 0.99996,
+ "segment_size": 16384,
+ "init_lr_ratio": 1,
+ "warmup_epochs": 0,
+ "c_mel": 45,
+ "c_kl": 1.0,
+ "c_commit": 100,
+ "skip_optimizer": true,
+ "freeze_ZH_bert": false,
+ "freeze_JP_bert": false,
+ "freeze_EN_bert": false,
+ "freeze_emo": false,
+ "freeze_style": false
+ },
+ "data": {
+ "use_jp_extra": true,
+ "training_files": "/content/drive/MyDrive/Style-Bert-VITS2/Data/jvnv-F2/train.list",
+ "validation_files": "/content/drive/MyDrive/Style-Bert-VITS2/Data/jvnv-F2/val.list",
+ "max_wav_value": 32768.0,
+ "sampling_rate": 44100,
+ "filter_length": 2048,
+ "hop_length": 512,
+ "win_length": 2048,
+ "n_mel_channels": 128,
+ "mel_fmin": 0.0,
+ "mel_fmax": null,
+ "add_blank": true,
+ "n_speakers": 1,
+ "cleaned_text": true,
+ "spk2id": {
+ "jvnv-F2-jp": 0
+ },
+ "num_styles": 7,
+ "style2id": {
+ "Neutral": 0,
+ "Angry": 1,
+ "Disgust": 2,
+ "Fear": 3,
+ "Happy": 4,
+ "Sad": 5,
+ "Surprise": 6
+ }
+ },
+ "model": {
+ "use_spk_conditioned_encoder": true,
+ "use_noise_scaled_mas": true,
+ "use_mel_posterior_encoder": false,
+ "use_duration_discriminator": false,
+ "use_wavlm_discriminator": true,
+ "inter_channels": 192,
+ "hidden_channels": 192,
+ "filter_channels": 768,
+ "n_heads": 2,
+ "n_layers": 6,
+ "kernel_size": 3,
+ "p_dropout": 0.1,
+ "resblock": "1",
+ "resblock_kernel_sizes": [3, 7, 11],
+ "resblock_dilation_sizes": [
+ [1, 3, 5],
+ [1, 3, 5],
+ [1, 3, 5]
+ ],
+ "upsample_rates": [8, 8, 2, 2, 2],
+ "upsample_initial_channel": 512,
+ "upsample_kernel_sizes": [16, 16, 8, 2, 2],
+ "n_layers_q": 3,
+ "use_spectral_norm": false,
+ "gin_channels": 512,
+ "slm": {
+ "model": "./slm/wavlm-base-plus",
+ "sr": 16000,
+ "hidden": 768,
+ "nlayers": 13,
+ "initial_channel": 64
+ }
+ },
+ "version": "2.0-JP-Extra",
+ "model_name": "jvnv-F2-jp"
+}
diff --git a/model_assets/jvnv-F2-jp/jvnv-F2_e166_s20000.safetensors b/model_assets/jvnv-F2-jp/jvnv-F2_e166_s20000.safetensors
new file mode 100644
index 0000000000000000000000000000000000000000..3dcc8e1228bedb973718a8716898180dbd373d51
--- /dev/null
+++ b/model_assets/jvnv-F2-jp/jvnv-F2_e166_s20000.safetensors
@@ -0,0 +1,3 @@
+version https://git-lfs.github.com/spec/v1
+oid sha256:f6289a6f30bb9795744815b9da764a3c8198b18652d9fddef82fff1e14f0e784
+size 251150980
diff --git a/model_assets/jvnv-F2-jp/style_vectors.npy b/model_assets/jvnv-F2-jp/style_vectors.npy
new file mode 100644
index 0000000000000000000000000000000000000000..ff1bf04e709eb5aaaf021b20cec5b15d780bb2cf
--- /dev/null
+++ b/model_assets/jvnv-F2-jp/style_vectors.npy
@@ -0,0 +1,3 @@
+version https://git-lfs.github.com/spec/v1
+oid sha256:900f8cde3a336d12193fec7b7d8e6c5dc77b3a5d719a9be3f8598389cd88e643
+size 7296
diff --git a/model_assets/jvnv-M1-jp/config.json b/model_assets/jvnv-M1-jp/config.json
new file mode 100644
index 0000000000000000000000000000000000000000..092a81f8dfd351bc56c75ca8d422cc01fb8cb0f9
--- /dev/null
+++ b/model_assets/jvnv-M1-jp/config.json
@@ -0,0 +1,92 @@
+{
+ "train": {
+ "log_interval": 200,
+ "eval_interval": 1000,
+ "seed": 42,
+ "epochs": 300,
+ "learning_rate": 0.0001,
+ "betas": [0.8, 0.99],
+ "eps": 1e-9,
+ "batch_size": 4,
+ "bf16_run": true,
+ "fp16_run": false,
+ "lr_decay": 0.99996,
+ "segment_size": 16384,
+ "init_lr_ratio": 1,
+ "warmup_epochs": 0,
+ "c_mel": 45,
+ "c_kl": 1.0,
+ "c_commit": 100,
+ "skip_optimizer": true,
+ "freeze_ZH_bert": false,
+ "freeze_JP_bert": false,
+ "freeze_EN_bert": false,
+ "freeze_emo": false,
+ "freeze_style": false
+ },
+ "data": {
+ "use_jp_extra": true,
+ "training_files": "Data/jvnv-M1-jp/train.list",
+ "validation_files": "Data/jvnv-M1-jp/val.list",
+ "max_wav_value": 32768.0,
+ "sampling_rate": 44100,
+ "filter_length": 2048,
+ "hop_length": 512,
+ "win_length": 2048,
+ "n_mel_channels": 128,
+ "mel_fmin": 0.0,
+ "mel_fmax": null,
+ "add_blank": true,
+ "n_speakers": 1,
+ "cleaned_text": true,
+ "spk2id": {
+ "jvnv-M1-jp": 0
+ },
+ "num_styles": 7,
+ "style2id": {
+ "Neutral": 0,
+ "Angry": 1,
+ "Disgust": 2,
+ "Fear": 3,
+ "Happy": 4,
+ "Sad": 5,
+ "Surprise": 6
+ }
+ },
+ "model": {
+ "use_spk_conditioned_encoder": true,
+ "use_noise_scaled_mas": true,
+ "use_mel_posterior_encoder": false,
+ "use_duration_discriminator": false,
+ "use_wavlm_discriminator": true,
+ "inter_channels": 192,
+ "hidden_channels": 192,
+ "filter_channels": 768,
+ "n_heads": 2,
+ "n_layers": 6,
+ "kernel_size": 3,
+ "p_dropout": 0.1,
+ "resblock": "1",
+ "resblock_kernel_sizes": [3, 7, 11],
+ "resblock_dilation_sizes": [
+ [1, 3, 5],
+ [1, 3, 5],
+ [1, 3, 5]
+ ],
+ "upsample_rates": [8, 8, 2, 2, 2],
+ "upsample_initial_channel": 512,
+ "upsample_kernel_sizes": [16, 16, 8, 2, 2],
+ "n_layers_q": 3,
+ "use_spectral_norm": false,
+ "gin_channels": 512,
+ "slm": {
+ "model": "./slm/wavlm-base-plus",
+ "sr": 16000,
+ "hidden": 768,
+ "nlayers": 13,
+ "initial_channel": 64
+ }
+ },
+ "version": "2.0-JP-Extra",
+ "model_name": "jvnv-M1-jp"
+}
diff --git a/model_assets/jvnv-M1-jp/jvnv-M1-jp_e158_s14000.safetensors b/model_assets/jvnv-M1-jp/jvnv-M1-jp_e158_s14000.safetensors
new file mode 100644
index 0000000000000000000000000000000000000000..7d109ed0a9470cf03fa93890ef941141728892a1
--- /dev/null
+++ b/model_assets/jvnv-M1-jp/jvnv-M1-jp_e158_s14000.safetensors
@@ -0,0 +1,3 @@
+version https://git-lfs.github.com/spec/v1
+oid sha256:0d86765f1fe08dbba74cd06283e96b6941b3f232329fabbba9c30e6edc27887a
+size 251150980
diff --git a/model_assets/jvnv-M1-jp/style_vectors.npy b/model_assets/jvnv-M1-jp/style_vectors.npy
new file mode 100644
index 0000000000000000000000000000000000000000..ed49c9992831978ceed41ad8714e1e9961da32fc
--- /dev/null
+++ b/model_assets/jvnv-M1-jp/style_vectors.npy
@@ -0,0 +1,3 @@
+version https://git-lfs.github.com/spec/v1
+oid sha256:7a925435e8c1c9efc8fc8e90e690655ab9a7bae00a790892e13e936510d04f05
+size 7296
diff --git a/model_assets/jvnv-M2-jp/config.json b/model_assets/jvnv-M2-jp/config.json
new file mode 100644
index 0000000000000000000000000000000000000000..840290d3e7a9aa6046ba1154d3a26e35ea791905
--- /dev/null
+++ b/model_assets/jvnv-M2-jp/config.json
@@ -0,0 +1,92 @@
+{
+ "train": {
+ "log_interval": 200,
+ "eval_interval": 1000,
+ "seed": 42,
+ "epochs": 300,
+ "learning_rate": 0.0001,
+ "betas": [0.8, 0.99],
+ "eps": 1e-9,
+ "batch_size": 4,
+ "bf16_run": true,
+ "fp16_run": false,
+ "lr_decay": 0.99996,
+ "segment_size": 16384,
+ "init_lr_ratio": 1,
+ "warmup_epochs": 0,
+ "c_mel": 45,
+ "c_kl": 1.0,
+ "c_commit": 100,
+ "skip_optimizer": true,
+ "freeze_ZH_bert": false,
+ "freeze_JP_bert": false,
+ "freeze_EN_bert": false,
+ "freeze_emo": false,
+ "freeze_style": false
+ },
+ "data": {
+ "use_jp_extra": true,
+ "training_files": "Data/jvnv-M2-jp/train.list",
+ "validation_files": "Data/jvnv-M2-jp/val.list",
+ "max_wav_value": 32768.0,
+ "sampling_rate": 44100,
+ "filter_length": 2048,
+ "hop_length": 512,
+ "win_length": 2048,
+ "n_mel_channels": 128,
+ "mel_fmin": 0.0,
+ "mel_fmax": null,
+ "add_blank": true,
+ "n_speakers": 1,
+ "cleaned_text": true,
+ "spk2id": {
+ "jvnv-M2-jp": 0
+ },
+ "num_styles": 7,
+ "style2id": {
+ "Neutral": 0,
+ "Angry": 1,
+ "Disgust": 2,
+ "Fear": 3,
+ "Happy": 4,
+ "Sad": 5,
+ "Surprise": 6
+ }
+ },
+ "model": {
+ "use_spk_conditioned_encoder": true,
+ "use_noise_scaled_mas": true,
+ "use_mel_posterior_encoder": false,
+ "use_duration_discriminator": false,
+ "use_wavlm_discriminator": true,
+ "inter_channels": 192,
+ "hidden_channels": 192,
+ "filter_channels": 768,
+ "n_heads": 2,
+ "n_layers": 6,
+ "kernel_size": 3,
+ "p_dropout": 0.1,
+ "resblock": "1",
+ "resblock_kernel_sizes": [3, 7, 11],
+ "resblock_dilation_sizes": [
+ [1, 3, 5],
+ [1, 3, 5],
+ [1, 3, 5]
+ ],
+ "upsample_rates": [8, 8, 2, 2, 2],
+ "upsample_initial_channel": 512,
+ "upsample_kernel_sizes": [16, 16, 8, 2, 2],
+ "n_layers_q": 3,
+ "use_spectral_norm": false,
+ "gin_channels": 512,
+ "slm": {
+ "model": "./slm/wavlm-base-plus",
+ "sr": 16000,
+ "hidden": 768,
+ "nlayers": 13,
+ "initial_channel": 64
+ }
+ },
+ "version": "2.0-JP-Extra",
+ "model_name": "jvnv-M2-jp"
+}
diff --git a/model_assets/jvnv-M2-jp/jvnv-M2-jp_e159_s17000.safetensors b/model_assets/jvnv-M2-jp/jvnv-M2-jp_e159_s17000.safetensors
new file mode 100644
index 0000000000000000000000000000000000000000..6171432a571eccbff12f1954aabe64a6e2d198d3
--- /dev/null
+++ b/model_assets/jvnv-M2-jp/jvnv-M2-jp_e159_s17000.safetensors
@@ -0,0 +1,3 @@
+version https://git-lfs.github.com/spec/v1
+oid sha256:8245f39438076d36a3befd8aefb15c38830cef326c1f7c9d9c8e64b647645402
+size 251150980
diff --git a/model_assets/jvnv-M2-jp/style_vectors.npy b/model_assets/jvnv-M2-jp/style_vectors.npy
new file mode 100644
index 0000000000000000000000000000000000000000..6afb0774f6c773d754351c83d870d7fca74bf4be
--- /dev/null
+++ b/model_assets/jvnv-M2-jp/style_vectors.npy
@@ -0,0 +1,3 @@
+version https://git-lfs.github.com/spec/v1
+oid sha256:c965bb63fa4a759d41a8a4a3649333125d6497ae8a705d81b7d5c5bd2854797c
+size 7296
diff --git a/models.py b/models.py
new file mode 100644
index 0000000000000000000000000000000000000000..eb706fd664012029e0e5c68c94c350e158b5a912
--- /dev/null
+++ b/models.py
@@ -0,0 +1,1024 @@
+import math
+import warnings
+
+import torch
+from torch import nn
+from torch.nn import Conv1d, Conv2d, ConvTranspose1d
+from torch.nn import functional as F
+from torch.nn.utils import remove_weight_norm, spectral_norm, weight_norm
+
+import attentions
+import commons
+import modules
+import monotonic_align
+from commons import get_padding, init_weights
+from text import num_languages, num_tones, symbols
+
+
+class DurationDiscriminator(nn.Module): # vits2
+ def __init__(
+ self, in_channels, filter_channels, kernel_size, p_dropout, gin_channels=0
+ ):
+ super().__init__()
+
+ self.in_channels = in_channels
+ self.filter_channels = filter_channels
+ self.kernel_size = kernel_size
+ self.p_dropout = p_dropout
+ self.gin_channels = gin_channels
+
+ self.drop = nn.Dropout(p_dropout)
+ self.conv_1 = nn.Conv1d(
+ in_channels, filter_channels, kernel_size, padding=kernel_size // 2
+ )
+ self.norm_1 = modules.LayerNorm(filter_channels)
+ self.conv_2 = nn.Conv1d(
+ filter_channels, filter_channels, kernel_size, padding=kernel_size // 2
+ )
+ self.norm_2 = modules.LayerNorm(filter_channels)
+ self.dur_proj = nn.Conv1d(1, filter_channels, 1)
+
+ self.pre_out_conv_1 = nn.Conv1d(
+ 2 * filter_channels, filter_channels, kernel_size, padding=kernel_size // 2
+ )
+ self.pre_out_norm_1 = modules.LayerNorm(filter_channels)
+ self.pre_out_conv_2 = nn.Conv1d(
+ filter_channels, filter_channels, kernel_size, padding=kernel_size // 2
+ )
+ self.pre_out_norm_2 = modules.LayerNorm(filter_channels)
+
+ if gin_channels != 0:
+ self.cond = nn.Conv1d(gin_channels, in_channels, 1)
+
+ self.output_layer = nn.Sequential(nn.Linear(filter_channels, 1), nn.Sigmoid())
+
+ def forward_probability(self, x, x_mask, dur, g=None):
+ dur = self.dur_proj(dur)
+ x = torch.cat([x, dur], dim=1)
+ x = self.pre_out_conv_1(x * x_mask)
+ x = torch.relu(x)
+ x = self.pre_out_norm_1(x)
+ x = self.drop(x)
+ x = self.pre_out_conv_2(x * x_mask)
+ x = torch.relu(x)
+ x = self.pre_out_norm_2(x)
+ x = self.drop(x)
+ x = x * x_mask
+ x = x.transpose(1, 2)
+ output_prob = self.output_layer(x)
+ return output_prob
+
+ def forward(self, x, x_mask, dur_r, dur_hat, g=None):
+ x = torch.detach(x)
+ if g is not None:
+ g = torch.detach(g)
+ x = x + self.cond(g)
+ x = self.conv_1(x * x_mask)
+ x = torch.relu(x)
+ x = self.norm_1(x)
+ x = self.drop(x)
+ x = self.conv_2(x * x_mask)
+ x = torch.relu(x)
+ x = self.norm_2(x)
+ x = self.drop(x)
+
+ output_probs = []
+ for dur in [dur_r, dur_hat]:
+ output_prob = self.forward_probability(x, x_mask, dur, g)
+ output_probs.append(output_prob)
+
+ return output_probs
+
+
+class TransformerCouplingBlock(nn.Module):
+ def __init__(
+ self,
+ channels,
+ hidden_channels,
+ filter_channels,
+ n_heads,
+ n_layers,
+ kernel_size,
+ p_dropout,
+ n_flows=4,
+ gin_channels=0,
+ share_parameter=False,
+ ):
+ super().__init__()
+ self.channels = channels
+ self.hidden_channels = hidden_channels
+ self.kernel_size = kernel_size
+ self.n_layers = n_layers
+ self.n_flows = n_flows
+ self.gin_channels = gin_channels
+
+ self.flows = nn.ModuleList()
+
+ self.wn = (
+ attentions.FFT(
+ hidden_channels,
+ filter_channels,
+ n_heads,
+ n_layers,
+ kernel_size,
+ p_dropout,
+ isflow=True,
+ gin_channels=self.gin_channels,
+ )
+ if share_parameter
+ else None
+ )
+
+ for i in range(n_flows):
+ self.flows.append(
+ modules.TransformerCouplingLayer(
+ channels,
+ hidden_channels,
+ kernel_size,
+ n_layers,
+ n_heads,
+ p_dropout,
+ filter_channels,
+ mean_only=True,
+ wn_sharing_parameter=self.wn,
+ gin_channels=self.gin_channels,
+ )
+ )
+ self.flows.append(modules.Flip())
+
+ def forward(self, x, x_mask, g=None, reverse=False):
+ if not reverse:
+ for flow in self.flows:
+ x, _ = flow(x, x_mask, g=g, reverse=reverse)
+ else:
+ for flow in reversed(self.flows):
+ x = flow(x, x_mask, g=g, reverse=reverse)
+ return x
+
+
+class StochasticDurationPredictor(nn.Module):
+ def __init__(
+ self,
+ in_channels,
+ filter_channels,
+ kernel_size,
+ p_dropout,
+ n_flows=4,
+ gin_channels=0,
+ ):
+ super().__init__()
+ filter_channels = in_channels # it needs to be removed from future version.
+ self.in_channels = in_channels
+ self.filter_channels = filter_channels
+ self.kernel_size = kernel_size
+ self.p_dropout = p_dropout
+ self.n_flows = n_flows
+ self.gin_channels = gin_channels
+
+ self.log_flow = modules.Log()
+ self.flows = nn.ModuleList()
+ self.flows.append(modules.ElementwiseAffine(2))
+ for i in range(n_flows):
+ self.flows.append(
+ modules.ConvFlow(2, filter_channels, kernel_size, n_layers=3)
+ )
+ self.flows.append(modules.Flip())
+
+ self.post_pre = nn.Conv1d(1, filter_channels, 1)
+ self.post_proj = nn.Conv1d(filter_channels, filter_channels, 1)
+ self.post_convs = modules.DDSConv(
+ filter_channels, kernel_size, n_layers=3, p_dropout=p_dropout
+ )
+ self.post_flows = nn.ModuleList()
+ self.post_flows.append(modules.ElementwiseAffine(2))
+ for i in range(4):
+ self.post_flows.append(
+ modules.ConvFlow(2, filter_channels, kernel_size, n_layers=3)
+ )
+ self.post_flows.append(modules.Flip())
+
+ self.pre = nn.Conv1d(in_channels, filter_channels, 1)
+ self.proj = nn.Conv1d(filter_channels, filter_channels, 1)
+ self.convs = modules.DDSConv(
+ filter_channels, kernel_size, n_layers=3, p_dropout=p_dropout
+ )
+ if gin_channels != 0:
+ self.cond = nn.Conv1d(gin_channels, filter_channels, 1)
+
+ def forward(self, x, x_mask, w=None, g=None, reverse=False, noise_scale=1.0):
+ x = torch.detach(x)
+ x = self.pre(x)
+ if g is not None:
+ g = torch.detach(g)
+ x = x + self.cond(g)
+ x = self.convs(x, x_mask)
+ x = self.proj(x) * x_mask
+
+ if not reverse:
+ flows = self.flows
+ assert w is not None
+
+ logdet_tot_q = 0
+ h_w = self.post_pre(w)
+ h_w = self.post_convs(h_w, x_mask)
+ h_w = self.post_proj(h_w) * x_mask
+ e_q = (
+ torch.randn(w.size(0), 2, w.size(2)).to(device=x.device, dtype=x.dtype)
+ * x_mask
+ )
+ z_q = e_q
+ for flow in self.post_flows:
+ z_q, logdet_q = flow(z_q, x_mask, g=(x + h_w))
+ logdet_tot_q += logdet_q
+ z_u, z1 = torch.split(z_q, [1, 1], 1)
+ u = torch.sigmoid(z_u) * x_mask
+ z0 = (w - u) * x_mask
+ logdet_tot_q += torch.sum(
+ (F.logsigmoid(z_u) + F.logsigmoid(-z_u)) * x_mask, [1, 2]
+ )
+ logq = (
+ torch.sum(-0.5 * (math.log(2 * math.pi) + (e_q**2)) * x_mask, [1, 2])
+ - logdet_tot_q
+ )
+
+ logdet_tot = 0
+ z0, logdet = self.log_flow(z0, x_mask)
+ logdet_tot += logdet
+ z = torch.cat([z0, z1], 1)
+ for flow in flows:
+ z, logdet = flow(z, x_mask, g=x, reverse=reverse)
+ logdet_tot = logdet_tot + logdet
+ nll = (
+ torch.sum(0.5 * (math.log(2 * math.pi) + (z**2)) * x_mask, [1, 2])
+ - logdet_tot
+ )
+ return nll + logq # [b]
+ else:
+ flows = list(reversed(self.flows))
+ flows = flows[:-2] + [flows[-1]] # remove a useless vflow
+ z = (
+ torch.randn(x.size(0), 2, x.size(2)).to(device=x.device, dtype=x.dtype)
+ * noise_scale
+ )
+ for flow in flows:
+ z = flow(z, x_mask, g=x, reverse=reverse)
+ z0, z1 = torch.split(z, [1, 1], 1)
+ logw = z0
+ return logw
+
+
+class DurationPredictor(nn.Module):
+ def __init__(
+ self, in_channels, filter_channels, kernel_size, p_dropout, gin_channels=0
+ ):
+ super().__init__()
+
+ self.in_channels = in_channels
+ self.filter_channels = filter_channels
+ self.kernel_size = kernel_size
+ self.p_dropout = p_dropout
+ self.gin_channels = gin_channels
+
+ self.drop = nn.Dropout(p_dropout)
+ self.conv_1 = nn.Conv1d(
+ in_channels, filter_channels, kernel_size, padding=kernel_size // 2
+ )
+ self.norm_1 = modules.LayerNorm(filter_channels)
+ self.conv_2 = nn.Conv1d(
+ filter_channels, filter_channels, kernel_size, padding=kernel_size // 2
+ )
+ self.norm_2 = modules.LayerNorm(filter_channels)
+ self.proj = nn.Conv1d(filter_channels, 1, 1)
+
+ if gin_channels != 0:
+ self.cond = nn.Conv1d(gin_channels, in_channels, 1)
+
+ def forward(self, x, x_mask, g=None):
+ x = torch.detach(x)
+ if g is not None:
+ g = torch.detach(g)
+ x = x + self.cond(g)
+ x = self.conv_1(x * x_mask)
+ x = torch.relu(x)
+ x = self.norm_1(x)
+ x = self.drop(x)
+ x = self.conv_2(x * x_mask)
+ x = torch.relu(x)
+ x = self.norm_2(x)
+ x = self.drop(x)
+ x = self.proj(x * x_mask)
+ return x * x_mask
+
+
+class TextEncoder(nn.Module):
+ def __init__(
+ self,
+ n_vocab,
+ out_channels,
+ hidden_channels,
+ filter_channels,
+ n_heads,
+ n_layers,
+ kernel_size,
+ p_dropout,
+ n_speakers,
+ gin_channels=0,
+ ):
+ super().__init__()
+ self.n_vocab = n_vocab
+ self.out_channels = out_channels
+ self.hidden_channels = hidden_channels
+ self.filter_channels = filter_channels
+ self.n_heads = n_heads
+ self.n_layers = n_layers
+ self.kernel_size = kernel_size
+ self.p_dropout = p_dropout
+ self.gin_channels = gin_channels
+ self.emb = nn.Embedding(len(symbols), hidden_channels)
+ nn.init.normal_(self.emb.weight, 0.0, hidden_channels**-0.5)
+ self.tone_emb = nn.Embedding(num_tones, hidden_channels)
+ nn.init.normal_(self.tone_emb.weight, 0.0, hidden_channels**-0.5)
+ self.language_emb = nn.Embedding(num_languages, hidden_channels)
+ nn.init.normal_(self.language_emb.weight, 0.0, hidden_channels**-0.5)
+ self.bert_proj = nn.Conv1d(1024, hidden_channels, 1)
+ self.ja_bert_proj = nn.Conv1d(1024, hidden_channels, 1)
+ self.en_bert_proj = nn.Conv1d(1024, hidden_channels, 1)
+ self.style_proj = nn.Linear(256, hidden_channels)
+
+ self.encoder = attentions.Encoder(
+ hidden_channels,
+ filter_channels,
+ n_heads,
+ n_layers,
+ kernel_size,
+ p_dropout,
+ gin_channels=self.gin_channels,
+ )
+ self.proj = nn.Conv1d(hidden_channels, out_channels * 2, 1)
+
+ def forward(
+ self,
+ x,
+ x_lengths,
+ tone,
+ language,
+ bert,
+ ja_bert,
+ en_bert,
+ style_vec,
+ sid,
+ g=None,
+ ):
+ bert_emb = self.bert_proj(bert).transpose(1, 2)
+ ja_bert_emb = self.ja_bert_proj(ja_bert).transpose(1, 2)
+ en_bert_emb = self.en_bert_proj(en_bert).transpose(1, 2)
+ style_emb = self.style_proj(style_vec.unsqueeze(1))
+
+ x = (
+ self.emb(x)
+ + self.tone_emb(tone)
+ + self.language_emb(language)
+ + bert_emb
+ + ja_bert_emb
+ + en_bert_emb
+ + style_emb
+ ) * math.sqrt(
+ self.hidden_channels
+ ) # [b, t, h]
+ x = torch.transpose(x, 1, -1) # [b, h, t]
+ x_mask = torch.unsqueeze(commons.sequence_mask(x_lengths, x.size(2)), 1).to(
+ x.dtype
+ )
+
+ x = self.encoder(x * x_mask, x_mask, g=g)
+ stats = self.proj(x) * x_mask
+
+ m, logs = torch.split(stats, self.out_channels, dim=1)
+ return x, m, logs, x_mask
+
+
+class ResidualCouplingBlock(nn.Module):
+ def __init__(
+ self,
+ channels,
+ hidden_channels,
+ kernel_size,
+ dilation_rate,
+ n_layers,
+ n_flows=4,
+ gin_channels=0,
+ ):
+ super().__init__()
+ self.channels = channels
+ self.hidden_channels = hidden_channels
+ self.kernel_size = kernel_size
+ self.dilation_rate = dilation_rate
+ self.n_layers = n_layers
+ self.n_flows = n_flows
+ self.gin_channels = gin_channels
+
+ self.flows = nn.ModuleList()
+ for i in range(n_flows):
+ self.flows.append(
+ modules.ResidualCouplingLayer(
+ channels,
+ hidden_channels,
+ kernel_size,
+ dilation_rate,
+ n_layers,
+ gin_channels=gin_channels,
+ mean_only=True,
+ )
+ )
+ self.flows.append(modules.Flip())
+
+ def forward(self, x, x_mask, g=None, reverse=False):
+ if not reverse:
+ for flow in self.flows:
+ x, _ = flow(x, x_mask, g=g, reverse=reverse)
+ else:
+ for flow in reversed(self.flows):
+ x = flow(x, x_mask, g=g, reverse=reverse)
+ return x
+
+
+class PosteriorEncoder(nn.Module):
+ def __init__(
+ self,
+ in_channels,
+ out_channels,
+ hidden_channels,
+ kernel_size,
+ dilation_rate,
+ n_layers,
+ gin_channels=0,
+ ):
+ super().__init__()
+ self.in_channels = in_channels
+ self.out_channels = out_channels
+ self.hidden_channels = hidden_channels
+ self.kernel_size = kernel_size
+ self.dilation_rate = dilation_rate
+ self.n_layers = n_layers
+ self.gin_channels = gin_channels
+
+ self.pre = nn.Conv1d(in_channels, hidden_channels, 1)
+ self.enc = modules.WN(
+ hidden_channels,
+ kernel_size,
+ dilation_rate,
+ n_layers,
+ gin_channels=gin_channels,
+ )
+ self.proj = nn.Conv1d(hidden_channels, out_channels * 2, 1)
+
+ def forward(self, x, x_lengths, g=None):
+ x_mask = torch.unsqueeze(commons.sequence_mask(x_lengths, x.size(2)), 1).to(
+ x.dtype
+ )
+ x = self.pre(x) * x_mask
+ x = self.enc(x, x_mask, g=g)
+ stats = self.proj(x) * x_mask
+ m, logs = torch.split(stats, self.out_channels, dim=1)
+ z = (m + torch.randn_like(m) * torch.exp(logs)) * x_mask
+ return z, m, logs, x_mask
+
+
+class Generator(torch.nn.Module):
+ def __init__(
+ self,
+ initial_channel,
+ resblock,
+ resblock_kernel_sizes,
+ resblock_dilation_sizes,
+ upsample_rates,
+ upsample_initial_channel,
+ upsample_kernel_sizes,
+ gin_channels=0,
+ ):
+ super(Generator, self).__init__()
+ self.num_kernels = len(resblock_kernel_sizes)
+ self.num_upsamples = len(upsample_rates)
+ self.conv_pre = Conv1d(
+ initial_channel, upsample_initial_channel, 7, 1, padding=3
+ )
+ resblock = modules.ResBlock1 if resblock == "1" else modules.ResBlock2
+
+ self.ups = nn.ModuleList()
+ for i, (u, k) in enumerate(zip(upsample_rates, upsample_kernel_sizes)):
+ self.ups.append(
+ weight_norm(
+ ConvTranspose1d(
+ upsample_initial_channel // (2**i),
+ upsample_initial_channel // (2 ** (i + 1)),
+ k,
+ u,
+ padding=(k - u) // 2,
+ )
+ )
+ )
+
+ self.resblocks = nn.ModuleList()
+ for i in range(len(self.ups)):
+ ch = upsample_initial_channel // (2 ** (i + 1))
+ for j, (k, d) in enumerate(
+ zip(resblock_kernel_sizes, resblock_dilation_sizes)
+ ):
+ self.resblocks.append(resblock(ch, k, d))
+
+ self.conv_post = Conv1d(ch, 1, 7, 1, padding=3, bias=False)
+ self.ups.apply(init_weights)
+
+ if gin_channels != 0:
+ self.cond = nn.Conv1d(gin_channels, upsample_initial_channel, 1)
+
+ def forward(self, x, g=None):
+ x = self.conv_pre(x)
+ if g is not None:
+ x = x + self.cond(g)
+
+ for i in range(self.num_upsamples):
+ x = F.leaky_relu(x, modules.LRELU_SLOPE)
+ x = self.ups[i](x)
+ xs = None
+ for j in range(self.num_kernels):
+ if xs is None:
+ xs = self.resblocks[i * self.num_kernels + j](x)
+ else:
+ xs += self.resblocks[i * self.num_kernels + j](x)
+ x = xs / self.num_kernels
+ x = F.leaky_relu(x)
+ x = self.conv_post(x)
+ x = torch.tanh(x)
+
+ return x
+
+ def remove_weight_norm(self):
+ print("Removing weight norm...")
+ for layer in self.ups:
+ remove_weight_norm(layer)
+ for layer in self.resblocks:
+ layer.remove_weight_norm()
+
+
+class DiscriminatorP(torch.nn.Module):
+ def __init__(self, period, kernel_size=5, stride=3, use_spectral_norm=False):
+ super(DiscriminatorP, self).__init__()
+ self.period = period
+ self.use_spectral_norm = use_spectral_norm
+ norm_f = weight_norm if use_spectral_norm is False else spectral_norm
+ self.convs = nn.ModuleList(
+ [
+ norm_f(
+ Conv2d(
+ 1,
+ 32,
+ (kernel_size, 1),
+ (stride, 1),
+ padding=(get_padding(kernel_size, 1), 0),
+ )
+ ),
+ norm_f(
+ Conv2d(
+ 32,
+ 128,
+ (kernel_size, 1),
+ (stride, 1),
+ padding=(get_padding(kernel_size, 1), 0),
+ )
+ ),
+ norm_f(
+ Conv2d(
+ 128,
+ 512,
+ (kernel_size, 1),
+ (stride, 1),
+ padding=(get_padding(kernel_size, 1), 0),
+ )
+ ),
+ norm_f(
+ Conv2d(
+ 512,
+ 1024,
+ (kernel_size, 1),
+ (stride, 1),
+ padding=(get_padding(kernel_size, 1), 0),
+ )
+ ),
+ norm_f(
+ Conv2d(
+ 1024,
+ 1024,
+ (kernel_size, 1),
+ 1,
+ padding=(get_padding(kernel_size, 1), 0),
+ )
+ ),
+ ]
+ )
+ self.conv_post = norm_f(Conv2d(1024, 1, (3, 1), 1, padding=(1, 0)))
+
+ def forward(self, x):
+ fmap = []
+
+ # 1d to 2d
+ b, c, t = x.shape
+ if t % self.period != 0: # pad first
+ n_pad = self.period - (t % self.period)
+ x = F.pad(x, (0, n_pad), "reflect")
+ t = t + n_pad
+ x = x.view(b, c, t // self.period, self.period)
+
+ for layer in self.convs:
+ x = layer(x)
+ x = F.leaky_relu(x, modules.LRELU_SLOPE)
+ fmap.append(x)
+ x = self.conv_post(x)
+ fmap.append(x)
+ x = torch.flatten(x, 1, -1)
+
+ return x, fmap
+
+
+class DiscriminatorS(torch.nn.Module):
+ def __init__(self, use_spectral_norm=False):
+ super(DiscriminatorS, self).__init__()
+ norm_f = weight_norm if use_spectral_norm is False else spectral_norm
+ self.convs = nn.ModuleList(
+ [
+ norm_f(Conv1d(1, 16, 15, 1, padding=7)),
+ norm_f(Conv1d(16, 64, 41, 4, groups=4, padding=20)),
+ norm_f(Conv1d(64, 256, 41, 4, groups=16, padding=20)),
+ norm_f(Conv1d(256, 1024, 41, 4, groups=64, padding=20)),
+ norm_f(Conv1d(1024, 1024, 41, 4, groups=256, padding=20)),
+ norm_f(Conv1d(1024, 1024, 5, 1, padding=2)),
+ ]
+ )
+ self.conv_post = norm_f(Conv1d(1024, 1, 3, 1, padding=1))
+
+ def forward(self, x):
+ fmap = []
+
+ for layer in self.convs:
+ x = layer(x)
+ x = F.leaky_relu(x, modules.LRELU_SLOPE)
+ fmap.append(x)
+ x = self.conv_post(x)
+ fmap.append(x)
+ x = torch.flatten(x, 1, -1)
+
+ return x, fmap
+
+
+class MultiPeriodDiscriminator(torch.nn.Module):
+ def __init__(self, use_spectral_norm=False):
+ super(MultiPeriodDiscriminator, self).__init__()
+ periods = [2, 3, 5, 7, 11]
+
+ discs = [DiscriminatorS(use_spectral_norm=use_spectral_norm)]
+ discs = discs + [
+ DiscriminatorP(i, use_spectral_norm=use_spectral_norm) for i in periods
+ ]
+ self.discriminators = nn.ModuleList(discs)
+
+ def forward(self, y, y_hat):
+ y_d_rs = []
+ y_d_gs = []
+ fmap_rs = []
+ fmap_gs = []
+ for i, d in enumerate(self.discriminators):
+ y_d_r, fmap_r = d(y)
+ y_d_g, fmap_g = d(y_hat)
+ y_d_rs.append(y_d_r)
+ y_d_gs.append(y_d_g)
+ fmap_rs.append(fmap_r)
+ fmap_gs.append(fmap_g)
+
+ return y_d_rs, y_d_gs, fmap_rs, fmap_gs
+
+
+class ReferenceEncoder(nn.Module):
+ """
+ inputs --- [N, Ty/r, n_mels*r] mels
+ outputs --- [N, ref_enc_gru_size]
+ """
+
+ def __init__(self, spec_channels, gin_channels=0):
+ super().__init__()
+ self.spec_channels = spec_channels
+ ref_enc_filters = [32, 32, 64, 64, 128, 128]
+ K = len(ref_enc_filters)
+ filters = [1] + ref_enc_filters
+ convs = [
+ weight_norm(
+ nn.Conv2d(
+ in_channels=filters[i],
+ out_channels=filters[i + 1],
+ kernel_size=(3, 3),
+ stride=(2, 2),
+ padding=(1, 1),
+ )
+ )
+ for i in range(K)
+ ]
+ self.convs = nn.ModuleList(convs)
+ # self.wns = nn.ModuleList([weight_norm(num_features=ref_enc_filters[i]) for i in range(K)]) # noqa: E501
+
+ out_channels = self.calculate_channels(spec_channels, 3, 2, 1, K)
+ self.gru = nn.GRU(
+ input_size=ref_enc_filters[-1] * out_channels,
+ hidden_size=256 // 2,
+ batch_first=True,
+ )
+ self.proj = nn.Linear(128, gin_channels)
+
+ def forward(self, inputs, mask=None):
+ N = inputs.size(0)
+ out = inputs.view(N, 1, -1, self.spec_channels) # [N, 1, Ty, n_freqs]
+ for conv in self.convs:
+ out = conv(out)
+ # out = wn(out)
+ out = F.relu(out) # [N, 128, Ty//2^K, n_mels//2^K]
+
+ out = out.transpose(1, 2) # [N, Ty//2^K, 128, n_mels//2^K]
+ T = out.size(1)
+ N = out.size(0)
+ out = out.contiguous().view(N, T, -1) # [N, Ty//2^K, 128*n_mels//2^K]
+
+ self.gru.flatten_parameters()
+ memory, out = self.gru(out) # out --- [1, N, 128]
+
+ return self.proj(out.squeeze(0))
+
+ def calculate_channels(self, L, kernel_size, stride, pad, n_convs):
+ for i in range(n_convs):
+ L = (L - kernel_size + 2 * pad) // stride + 1
+ return L
+
+
+class SynthesizerTrn(nn.Module):
+ """
+ Synthesizer for Training
+ """
+
+ def __init__(
+ self,
+ n_vocab,
+ spec_channels,
+ segment_size,
+ inter_channels,
+ hidden_channels,
+ filter_channels,
+ n_heads,
+ n_layers,
+ kernel_size,
+ p_dropout,
+ resblock,
+ resblock_kernel_sizes,
+ resblock_dilation_sizes,
+ upsample_rates,
+ upsample_initial_channel,
+ upsample_kernel_sizes,
+ n_speakers=256,
+ gin_channels=256,
+ use_sdp=True,
+ n_flow_layer=4,
+ n_layers_trans_flow=4,
+ flow_share_parameter=False,
+ use_transformer_flow=True,
+ **kwargs,
+ ):
+ super().__init__()
+ self.n_vocab = n_vocab
+ self.spec_channels = spec_channels
+ self.inter_channels = inter_channels
+ self.hidden_channels = hidden_channels
+ self.filter_channels = filter_channels
+ self.n_heads = n_heads
+ self.n_layers = n_layers
+ self.kernel_size = kernel_size
+ self.p_dropout = p_dropout
+ self.resblock = resblock
+ self.resblock_kernel_sizes = resblock_kernel_sizes
+ self.resblock_dilation_sizes = resblock_dilation_sizes
+ self.upsample_rates = upsample_rates
+ self.upsample_initial_channel = upsample_initial_channel
+ self.upsample_kernel_sizes = upsample_kernel_sizes
+ self.segment_size = segment_size
+ self.n_speakers = n_speakers
+ self.gin_channels = gin_channels
+ self.n_layers_trans_flow = n_layers_trans_flow
+ self.use_spk_conditioned_encoder = kwargs.get(
+ "use_spk_conditioned_encoder", True
+ )
+ self.use_sdp = use_sdp
+ self.use_noise_scaled_mas = kwargs.get("use_noise_scaled_mas", False)
+ self.mas_noise_scale_initial = kwargs.get("mas_noise_scale_initial", 0.01)
+ self.noise_scale_delta = kwargs.get("noise_scale_delta", 2e-6)
+ self.current_mas_noise_scale = self.mas_noise_scale_initial
+ if self.use_spk_conditioned_encoder and gin_channels > 0:
+ self.enc_gin_channels = gin_channels
+ self.enc_p = TextEncoder(
+ n_vocab,
+ inter_channels,
+ hidden_channels,
+ filter_channels,
+ n_heads,
+ n_layers,
+ kernel_size,
+ p_dropout,
+ self.n_speakers,
+ gin_channels=self.enc_gin_channels,
+ )
+ self.dec = Generator(
+ inter_channels,
+ resblock,
+ resblock_kernel_sizes,
+ resblock_dilation_sizes,
+ upsample_rates,
+ upsample_initial_channel,
+ upsample_kernel_sizes,
+ gin_channels=gin_channels,
+ )
+ self.enc_q = PosteriorEncoder(
+ spec_channels,
+ inter_channels,
+ hidden_channels,
+ 5,
+ 1,
+ 16,
+ gin_channels=gin_channels,
+ )
+ if use_transformer_flow:
+ self.flow = TransformerCouplingBlock(
+ inter_channels,
+ hidden_channels,
+ filter_channels,
+ n_heads,
+ n_layers_trans_flow,
+ 5,
+ p_dropout,
+ n_flow_layer,
+ gin_channels=gin_channels,
+ share_parameter=flow_share_parameter,
+ )
+ else:
+ self.flow = ResidualCouplingBlock(
+ inter_channels,
+ hidden_channels,
+ 5,
+ 1,
+ n_flow_layer,
+ gin_channels=gin_channels,
+ )
+ self.sdp = StochasticDurationPredictor(
+ hidden_channels, 192, 3, 0.5, 4, gin_channels=gin_channels
+ )
+ self.dp = DurationPredictor(
+ hidden_channels, 256, 3, 0.5, gin_channels=gin_channels
+ )
+
+ if n_speakers >= 1:
+ self.emb_g = nn.Embedding(n_speakers, gin_channels)
+ else:
+ self.ref_enc = ReferenceEncoder(spec_channels, gin_channels)
+
+ def forward(
+ self,
+ x,
+ x_lengths,
+ y,
+ y_lengths,
+ sid,
+ tone,
+ language,
+ bert,
+ ja_bert,
+ en_bert,
+ style_vec,
+ ):
+ if self.n_speakers > 0:
+ g = self.emb_g(sid).unsqueeze(-1) # [b, h, 1]
+ else:
+ g = self.ref_enc(y.transpose(1, 2)).unsqueeze(-1)
+ x, m_p, logs_p, x_mask = self.enc_p(
+ x, x_lengths, tone, language, bert, ja_bert, en_bert, style_vec, sid, g=g
+ )
+ z, m_q, logs_q, y_mask = self.enc_q(y, y_lengths, g=g)
+ z_p = self.flow(z, y_mask, g=g)
+
+ with torch.no_grad():
+ # negative cross-entropy
+ s_p_sq_r = torch.exp(-2 * logs_p) # [b, d, t]
+ neg_cent1 = torch.sum(
+ -0.5 * math.log(2 * math.pi) - logs_p, [1], keepdim=True
+ ) # [b, 1, t_s]
+ neg_cent2 = torch.matmul(
+ -0.5 * (z_p**2).transpose(1, 2), s_p_sq_r
+ ) # [b, t_t, d] x [b, d, t_s] = [b, t_t, t_s]
+ neg_cent3 = torch.matmul(
+ z_p.transpose(1, 2), (m_p * s_p_sq_r)
+ ) # [b, t_t, d] x [b, d, t_s] = [b, t_t, t_s]
+ neg_cent4 = torch.sum(
+ -0.5 * (m_p**2) * s_p_sq_r, [1], keepdim=True
+ ) # [b, 1, t_s]
+ neg_cent = neg_cent1 + neg_cent2 + neg_cent3 + neg_cent4
+ if self.use_noise_scaled_mas:
+ epsilon = (
+ torch.std(neg_cent)
+ * torch.randn_like(neg_cent)
+ * self.current_mas_noise_scale
+ )
+ neg_cent = neg_cent + epsilon
+
+ attn_mask = torch.unsqueeze(x_mask, 2) * torch.unsqueeze(y_mask, -1)
+ attn = (
+ monotonic_align.maximum_path(neg_cent, attn_mask.squeeze(1))
+ .unsqueeze(1)
+ .detach()
+ )
+
+ w = attn.sum(2)
+
+ l_length_sdp = self.sdp(x, x_mask, w, g=g)
+ l_length_sdp = l_length_sdp / torch.sum(x_mask)
+
+ logw_ = torch.log(w + 1e-6) * x_mask
+ logw = self.dp(x, x_mask, g=g)
+ # logw_sdp = self.sdp(x, x_mask, g=g, reverse=True, noise_scale=1.0)
+ l_length_dp = torch.sum((logw - logw_) ** 2, [1, 2]) / torch.sum(
+ x_mask
+ ) # for averaging
+ # l_length_sdp += torch.sum((logw_sdp - logw_) ** 2, [1, 2]) / torch.sum(x_mask)
+
+ l_length = l_length_dp + l_length_sdp
+
+ # expand prior
+ m_p = torch.matmul(attn.squeeze(1), m_p.transpose(1, 2)).transpose(1, 2)
+ logs_p = torch.matmul(attn.squeeze(1), logs_p.transpose(1, 2)).transpose(1, 2)
+
+ z_slice, ids_slice = commons.rand_slice_segments(
+ z, y_lengths, self.segment_size
+ )
+ o = self.dec(z_slice, g=g)
+ return (
+ o,
+ l_length,
+ attn,
+ ids_slice,
+ x_mask,
+ y_mask,
+ (z, z_p, m_p, logs_p, m_q, logs_q),
+ (x, logw, logw_),
+ )
+
+ def infer(
+ self,
+ x,
+ x_lengths,
+ sid,
+ tone,
+ language,
+ bert,
+ ja_bert,
+ en_bert,
+ style_vec,
+ noise_scale=0.667,
+ length_scale=1,
+ noise_scale_w=0.8,
+ max_len=None,
+ sdp_ratio=0,
+ y=None,
+ ):
+ # x, m_p, logs_p, x_mask = self.enc_p(x, x_lengths, tone, language, bert)
+ # g = self.gst(y)
+ if self.n_speakers > 0:
+ g = self.emb_g(sid).unsqueeze(-1) # [b, h, 1]
+ else:
+ g = self.ref_enc(y.transpose(1, 2)).unsqueeze(-1)
+ x, m_p, logs_p, x_mask = self.enc_p(
+ x, x_lengths, tone, language, bert, ja_bert, en_bert, style_vec, sid, g=g
+ )
+ logw = self.sdp(x, x_mask, g=g, reverse=True, noise_scale=noise_scale_w) * (
+ sdp_ratio
+ ) + self.dp(x, x_mask, g=g) * (1 - sdp_ratio)
+ w = torch.exp(logw) * x_mask * length_scale
+ w_ceil = torch.ceil(w)
+ y_lengths = torch.clamp_min(torch.sum(w_ceil, [1, 2]), 1).long()
+ y_mask = torch.unsqueeze(commons.sequence_mask(y_lengths, None), 1).to(
+ x_mask.dtype
+ )
+ attn_mask = torch.unsqueeze(x_mask, 2) * torch.unsqueeze(y_mask, -1)
+ attn = commons.generate_path(w_ceil, attn_mask)
+
+ m_p = torch.matmul(attn.squeeze(1), m_p.transpose(1, 2)).transpose(
+ 1, 2
+ ) # [b, t', t], [b, t, d] -> [b, d, t']
+ logs_p = torch.matmul(attn.squeeze(1), logs_p.transpose(1, 2)).transpose(
+ 1, 2
+ ) # [b, t', t], [b, t, d] -> [b, d, t']
+
+ z_p = m_p + torch.randn_like(m_p) * torch.exp(logs_p) * noise_scale
+ z = self.flow(z_p, y_mask, g=g, reverse=True)
+ o = self.dec((z * y_mask)[:, :, :max_len], g=g)
+ return o, attn, y_mask, (z, z_p, m_p, logs_p)
diff --git a/models_jp_extra.py b/models_jp_extra.py
new file mode 100644
index 0000000000000000000000000000000000000000..1bb2dd2e3b76a3d1978cd591eb4d4415c4c8a9ba
--- /dev/null
+++ b/models_jp_extra.py
@@ -0,0 +1,1071 @@
+import math
+import torch
+from torch import nn
+from torch.nn import functional as F
+
+import commons
+import modules
+import attentions
+import monotonic_align
+
+from torch.nn import Conv1d, ConvTranspose1d, Conv2d
+from torch.nn.utils import weight_norm, remove_weight_norm, spectral_norm
+
+from commons import init_weights, get_padding
+from text import symbols, num_tones, num_languages
+
+
+class DurationDiscriminator(nn.Module): # vits2
+ def __init__(
+ self, in_channels, filter_channels, kernel_size, p_dropout, gin_channels=0
+ ):
+ super().__init__()
+
+ self.in_channels = in_channels
+ self.filter_channels = filter_channels
+ self.kernel_size = kernel_size
+ self.p_dropout = p_dropout
+ self.gin_channels = gin_channels
+
+ self.drop = nn.Dropout(p_dropout)
+ self.conv_1 = nn.Conv1d(
+ in_channels, filter_channels, kernel_size, padding=kernel_size // 2
+ )
+ self.norm_1 = modules.LayerNorm(filter_channels)
+ self.conv_2 = nn.Conv1d(
+ filter_channels, filter_channels, kernel_size, padding=kernel_size // 2
+ )
+ self.norm_2 = modules.LayerNorm(filter_channels)
+ self.dur_proj = nn.Conv1d(1, filter_channels, 1)
+
+ self.LSTM = nn.LSTM(
+ 2 * filter_channels, filter_channels, batch_first=True, bidirectional=True
+ )
+
+ if gin_channels != 0:
+ self.cond = nn.Conv1d(gin_channels, in_channels, 1)
+
+ self.output_layer = nn.Sequential(
+ nn.Linear(2 * filter_channels, 1), nn.Sigmoid()
+ )
+
+ def forward_probability(self, x, dur):
+ dur = self.dur_proj(dur)
+ x = torch.cat([x, dur], dim=1)
+ x = x.transpose(1, 2)
+ x, _ = self.LSTM(x)
+ output_prob = self.output_layer(x)
+ return output_prob
+
+ def forward(self, x, x_mask, dur_r, dur_hat, g=None):
+ x = torch.detach(x)
+ if g is not None:
+ g = torch.detach(g)
+ x = x + self.cond(g)
+ x = self.conv_1(x * x_mask)
+ x = torch.relu(x)
+ x = self.norm_1(x)
+ x = self.drop(x)
+ x = self.conv_2(x * x_mask)
+ x = torch.relu(x)
+ x = self.norm_2(x)
+ x = self.drop(x)
+
+ output_probs = []
+ for dur in [dur_r, dur_hat]:
+ output_prob = self.forward_probability(x, dur)
+ output_probs.append(output_prob)
+
+ return output_probs
+
+
+class TransformerCouplingBlock(nn.Module):
+ def __init__(
+ self,
+ channels,
+ hidden_channels,
+ filter_channels,
+ n_heads,
+ n_layers,
+ kernel_size,
+ p_dropout,
+ n_flows=4,
+ gin_channels=0,
+ share_parameter=False,
+ ):
+ super().__init__()
+ self.channels = channels
+ self.hidden_channels = hidden_channels
+ self.kernel_size = kernel_size
+ self.n_layers = n_layers
+ self.n_flows = n_flows
+ self.gin_channels = gin_channels
+
+ self.flows = nn.ModuleList()
+
+ self.wn = (
+ attentions.FFT(
+ hidden_channels,
+ filter_channels,
+ n_heads,
+ n_layers,
+ kernel_size,
+ p_dropout,
+ isflow=True,
+ gin_channels=self.gin_channels,
+ )
+ if share_parameter
+ else None
+ )
+
+ for i in range(n_flows):
+ self.flows.append(
+ modules.TransformerCouplingLayer(
+ channels,
+ hidden_channels,
+ kernel_size,
+ n_layers,
+ n_heads,
+ p_dropout,
+ filter_channels,
+ mean_only=True,
+ wn_sharing_parameter=self.wn,
+ gin_channels=self.gin_channels,
+ )
+ )
+ self.flows.append(modules.Flip())
+
+ def forward(self, x, x_mask, g=None, reverse=False):
+ if not reverse:
+ for flow in self.flows:
+ x, _ = flow(x, x_mask, g=g, reverse=reverse)
+ else:
+ for flow in reversed(self.flows):
+ x = flow(x, x_mask, g=g, reverse=reverse)
+ return x
+
+
+class StochasticDurationPredictor(nn.Module):
+ def __init__(
+ self,
+ in_channels,
+ filter_channels,
+ kernel_size,
+ p_dropout,
+ n_flows=4,
+ gin_channels=0,
+ ):
+ super().__init__()
+ filter_channels = in_channels # it needs to be removed from future version.
+ self.in_channels = in_channels
+ self.filter_channels = filter_channels
+ self.kernel_size = kernel_size
+ self.p_dropout = p_dropout
+ self.n_flows = n_flows
+ self.gin_channels = gin_channels
+
+ self.log_flow = modules.Log()
+ self.flows = nn.ModuleList()
+ self.flows.append(modules.ElementwiseAffine(2))
+ for i in range(n_flows):
+ self.flows.append(
+ modules.ConvFlow(2, filter_channels, kernel_size, n_layers=3)
+ )
+ self.flows.append(modules.Flip())
+
+ self.post_pre = nn.Conv1d(1, filter_channels, 1)
+ self.post_proj = nn.Conv1d(filter_channels, filter_channels, 1)
+ self.post_convs = modules.DDSConv(
+ filter_channels, kernel_size, n_layers=3, p_dropout=p_dropout
+ )
+ self.post_flows = nn.ModuleList()
+ self.post_flows.append(modules.ElementwiseAffine(2))
+ for i in range(4):
+ self.post_flows.append(
+ modules.ConvFlow(2, filter_channels, kernel_size, n_layers=3)
+ )
+ self.post_flows.append(modules.Flip())
+
+ self.pre = nn.Conv1d(in_channels, filter_channels, 1)
+ self.proj = nn.Conv1d(filter_channels, filter_channels, 1)
+ self.convs = modules.DDSConv(
+ filter_channels, kernel_size, n_layers=3, p_dropout=p_dropout
+ )
+ if gin_channels != 0:
+ self.cond = nn.Conv1d(gin_channels, filter_channels, 1)
+
+ def forward(self, x, x_mask, w=None, g=None, reverse=False, noise_scale=1.0):
+ x = torch.detach(x)
+ x = self.pre(x)
+ if g is not None:
+ g = torch.detach(g)
+ x = x + self.cond(g)
+ x = self.convs(x, x_mask)
+ x = self.proj(x) * x_mask
+
+ if not reverse:
+ flows = self.flows
+ assert w is not None
+
+ logdet_tot_q = 0
+ h_w = self.post_pre(w)
+ h_w = self.post_convs(h_w, x_mask)
+ h_w = self.post_proj(h_w) * x_mask
+ e_q = (
+ torch.randn(w.size(0), 2, w.size(2)).to(device=x.device, dtype=x.dtype)
+ * x_mask
+ )
+ z_q = e_q
+ for flow in self.post_flows:
+ z_q, logdet_q = flow(z_q, x_mask, g=(x + h_w))
+ logdet_tot_q += logdet_q
+ z_u, z1 = torch.split(z_q, [1, 1], 1)
+ u = torch.sigmoid(z_u) * x_mask
+ z0 = (w - u) * x_mask
+ logdet_tot_q += torch.sum(
+ (F.logsigmoid(z_u) + F.logsigmoid(-z_u)) * x_mask, [1, 2]
+ )
+ logq = (
+ torch.sum(-0.5 * (math.log(2 * math.pi) + (e_q**2)) * x_mask, [1, 2])
+ - logdet_tot_q
+ )
+
+ logdet_tot = 0
+ z0, logdet = self.log_flow(z0, x_mask)
+ logdet_tot += logdet
+ z = torch.cat([z0, z1], 1)
+ for flow in flows:
+ z, logdet = flow(z, x_mask, g=x, reverse=reverse)
+ logdet_tot = logdet_tot + logdet
+ nll = (
+ torch.sum(0.5 * (math.log(2 * math.pi) + (z**2)) * x_mask, [1, 2])
+ - logdet_tot
+ )
+ return nll + logq # [b]
+ else:
+ flows = list(reversed(self.flows))
+ flows = flows[:-2] + [flows[-1]] # remove a useless vflow
+ z = (
+ torch.randn(x.size(0), 2, x.size(2)).to(device=x.device, dtype=x.dtype)
+ * noise_scale
+ )
+ for flow in flows:
+ z = flow(z, x_mask, g=x, reverse=reverse)
+ z0, z1 = torch.split(z, [1, 1], 1)
+ logw = z0
+ return logw
+
+
+class DurationPredictor(nn.Module):
+ def __init__(
+ self, in_channels, filter_channels, kernel_size, p_dropout, gin_channels=0
+ ):
+ super().__init__()
+
+ self.in_channels = in_channels
+ self.filter_channels = filter_channels
+ self.kernel_size = kernel_size
+ self.p_dropout = p_dropout
+ self.gin_channels = gin_channels
+
+ self.drop = nn.Dropout(p_dropout)
+ self.conv_1 = nn.Conv1d(
+ in_channels, filter_channels, kernel_size, padding=kernel_size // 2
+ )
+ self.norm_1 = modules.LayerNorm(filter_channels)
+ self.conv_2 = nn.Conv1d(
+ filter_channels, filter_channels, kernel_size, padding=kernel_size // 2
+ )
+ self.norm_2 = modules.LayerNorm(filter_channels)
+ self.proj = nn.Conv1d(filter_channels, 1, 1)
+
+ if gin_channels != 0:
+ self.cond = nn.Conv1d(gin_channels, in_channels, 1)
+
+ def forward(self, x, x_mask, g=None):
+ x = torch.detach(x)
+ if g is not None:
+ g = torch.detach(g)
+ x = x + self.cond(g)
+ x = self.conv_1(x * x_mask)
+ x = torch.relu(x)
+ x = self.norm_1(x)
+ x = self.drop(x)
+ x = self.conv_2(x * x_mask)
+ x = torch.relu(x)
+ x = self.norm_2(x)
+ x = self.drop(x)
+ x = self.proj(x * x_mask)
+ return x * x_mask
+
+
+class Bottleneck(nn.Sequential):
+ def __init__(self, in_dim, hidden_dim):
+ c_fc1 = nn.Linear(in_dim, hidden_dim, bias=False)
+ c_fc2 = nn.Linear(in_dim, hidden_dim, bias=False)
+ super().__init__(*[c_fc1, c_fc2])
+
+
+class Block(nn.Module):
+ def __init__(self, in_dim, hidden_dim) -> None:
+ super().__init__()
+ self.norm = nn.LayerNorm(in_dim)
+ self.mlp = MLP(in_dim, hidden_dim)
+
+ def forward(self, x: torch.Tensor) -> torch.Tensor:
+ x = x + self.mlp(self.norm(x))
+ return x
+
+
+class MLP(nn.Module):
+ def __init__(self, in_dim, hidden_dim):
+ super().__init__()
+ self.c_fc1 = nn.Linear(in_dim, hidden_dim, bias=False)
+ self.c_fc2 = nn.Linear(in_dim, hidden_dim, bias=False)
+ self.c_proj = nn.Linear(hidden_dim, in_dim, bias=False)
+
+ def forward(self, x: torch.Tensor):
+ x = F.silu(self.c_fc1(x)) * self.c_fc2(x)
+ x = self.c_proj(x)
+ return x
+
+
+class TextEncoder(nn.Module):
+ def __init__(
+ self,
+ n_vocab,
+ out_channels,
+ hidden_channels,
+ filter_channels,
+ n_heads,
+ n_layers,
+ kernel_size,
+ p_dropout,
+ gin_channels=0,
+ ):
+ super().__init__()
+ self.n_vocab = n_vocab
+ self.out_channels = out_channels
+ self.hidden_channels = hidden_channels
+ self.filter_channels = filter_channels
+ self.n_heads = n_heads
+ self.n_layers = n_layers
+ self.kernel_size = kernel_size
+ self.p_dropout = p_dropout
+ self.gin_channels = gin_channels
+ self.emb = nn.Embedding(len(symbols), hidden_channels)
+ nn.init.normal_(self.emb.weight, 0.0, hidden_channels**-0.5)
+ self.tone_emb = nn.Embedding(num_tones, hidden_channels)
+ nn.init.normal_(self.tone_emb.weight, 0.0, hidden_channels**-0.5)
+ self.language_emb = nn.Embedding(num_languages, hidden_channels)
+ nn.init.normal_(self.language_emb.weight, 0.0, hidden_channels**-0.5)
+ self.bert_proj = nn.Conv1d(1024, hidden_channels, 1)
+
+ # Remove emo_vq since it's not working well.
+ self.style_proj = nn.Linear(256, hidden_channels)
+
+ self.encoder = attentions.Encoder(
+ hidden_channels,
+ filter_channels,
+ n_heads,
+ n_layers,
+ kernel_size,
+ p_dropout,
+ gin_channels=self.gin_channels,
+ )
+ self.proj = nn.Conv1d(hidden_channels, out_channels * 2, 1)
+
+ def forward(self, x, x_lengths, tone, language, bert, style_vec, g=None):
+ bert_emb = self.bert_proj(bert).transpose(1, 2)
+ style_emb = self.style_proj(style_vec.unsqueeze(1))
+ x = (
+ self.emb(x)
+ + self.tone_emb(tone)
+ + self.language_emb(language)
+ + bert_emb
+ + style_emb
+ ) * math.sqrt(
+ self.hidden_channels
+ ) # [b, t, h]
+ x = torch.transpose(x, 1, -1) # [b, h, t]
+ x_mask = torch.unsqueeze(commons.sequence_mask(x_lengths, x.size(2)), 1).to(
+ x.dtype
+ )
+
+ x = self.encoder(x * x_mask, x_mask, g=g)
+ stats = self.proj(x) * x_mask
+
+ m, logs = torch.split(stats, self.out_channels, dim=1)
+ return x, m, logs, x_mask
+
+
+class ResidualCouplingBlock(nn.Module):
+ def __init__(
+ self,
+ channels,
+ hidden_channels,
+ kernel_size,
+ dilation_rate,
+ n_layers,
+ n_flows=4,
+ gin_channels=0,
+ ):
+ super().__init__()
+ self.channels = channels
+ self.hidden_channels = hidden_channels
+ self.kernel_size = kernel_size
+ self.dilation_rate = dilation_rate
+ self.n_layers = n_layers
+ self.n_flows = n_flows
+ self.gin_channels = gin_channels
+
+ self.flows = nn.ModuleList()
+ for i in range(n_flows):
+ self.flows.append(
+ modules.ResidualCouplingLayer(
+ channels,
+ hidden_channels,
+ kernel_size,
+ dilation_rate,
+ n_layers,
+ gin_channels=gin_channels,
+ mean_only=True,
+ )
+ )
+ self.flows.append(modules.Flip())
+
+ def forward(self, x, x_mask, g=None, reverse=False):
+ if not reverse:
+ for flow in self.flows:
+ x, _ = flow(x, x_mask, g=g, reverse=reverse)
+ else:
+ for flow in reversed(self.flows):
+ x = flow(x, x_mask, g=g, reverse=reverse)
+ return x
+
+
+class PosteriorEncoder(nn.Module):
+ def __init__(
+ self,
+ in_channels,
+ out_channels,
+ hidden_channels,
+ kernel_size,
+ dilation_rate,
+ n_layers,
+ gin_channels=0,
+ ):
+ super().__init__()
+ self.in_channels = in_channels
+ self.out_channels = out_channels
+ self.hidden_channels = hidden_channels
+ self.kernel_size = kernel_size
+ self.dilation_rate = dilation_rate
+ self.n_layers = n_layers
+ self.gin_channels = gin_channels
+
+ self.pre = nn.Conv1d(in_channels, hidden_channels, 1)
+ self.enc = modules.WN(
+ hidden_channels,
+ kernel_size,
+ dilation_rate,
+ n_layers,
+ gin_channels=gin_channels,
+ )
+ self.proj = nn.Conv1d(hidden_channels, out_channels * 2, 1)
+
+ def forward(self, x, x_lengths, g=None):
+ x_mask = torch.unsqueeze(commons.sequence_mask(x_lengths, x.size(2)), 1).to(
+ x.dtype
+ )
+ x = self.pre(x) * x_mask
+ x = self.enc(x, x_mask, g=g)
+ stats = self.proj(x) * x_mask
+ m, logs = torch.split(stats, self.out_channels, dim=1)
+ z = (m + torch.randn_like(m) * torch.exp(logs)) * x_mask
+ return z, m, logs, x_mask
+
+
+class Generator(torch.nn.Module):
+ def __init__(
+ self,
+ initial_channel,
+ resblock,
+ resblock_kernel_sizes,
+ resblock_dilation_sizes,
+ upsample_rates,
+ upsample_initial_channel,
+ upsample_kernel_sizes,
+ gin_channels=0,
+ ):
+ super(Generator, self).__init__()
+ self.num_kernels = len(resblock_kernel_sizes)
+ self.num_upsamples = len(upsample_rates)
+ self.conv_pre = Conv1d(
+ initial_channel, upsample_initial_channel, 7, 1, padding=3
+ )
+ resblock = modules.ResBlock1 if resblock == "1" else modules.ResBlock2
+
+ self.ups = nn.ModuleList()
+ for i, (u, k) in enumerate(zip(upsample_rates, upsample_kernel_sizes)):
+ self.ups.append(
+ weight_norm(
+ ConvTranspose1d(
+ upsample_initial_channel // (2**i),
+ upsample_initial_channel // (2 ** (i + 1)),
+ k,
+ u,
+ padding=(k - u) // 2,
+ )
+ )
+ )
+
+ self.resblocks = nn.ModuleList()
+ for i in range(len(self.ups)):
+ ch = upsample_initial_channel // (2 ** (i + 1))
+ for j, (k, d) in enumerate(
+ zip(resblock_kernel_sizes, resblock_dilation_sizes)
+ ):
+ self.resblocks.append(resblock(ch, k, d))
+
+ self.conv_post = Conv1d(ch, 1, 7, 1, padding=3, bias=False)
+ self.ups.apply(init_weights)
+
+ if gin_channels != 0:
+ self.cond = nn.Conv1d(gin_channels, upsample_initial_channel, 1)
+
+ def forward(self, x, g=None):
+ x = self.conv_pre(x)
+ if g is not None:
+ x = x + self.cond(g)
+
+ for i in range(self.num_upsamples):
+ x = F.leaky_relu(x, modules.LRELU_SLOPE)
+ x = self.ups[i](x)
+ xs = None
+ for j in range(self.num_kernels):
+ if xs is None:
+ xs = self.resblocks[i * self.num_kernels + j](x)
+ else:
+ xs += self.resblocks[i * self.num_kernels + j](x)
+ x = xs / self.num_kernels
+ x = F.leaky_relu(x)
+ x = self.conv_post(x)
+ x = torch.tanh(x)
+
+ return x
+
+ def remove_weight_norm(self):
+ print("Removing weight norm...")
+ for layer in self.ups:
+ remove_weight_norm(layer)
+ for layer in self.resblocks:
+ layer.remove_weight_norm()
+
+
+class DiscriminatorP(torch.nn.Module):
+ def __init__(self, period, kernel_size=5, stride=3, use_spectral_norm=False):
+ super(DiscriminatorP, self).__init__()
+ self.period = period
+ self.use_spectral_norm = use_spectral_norm
+ norm_f = weight_norm if use_spectral_norm is False else spectral_norm
+ self.convs = nn.ModuleList(
+ [
+ norm_f(
+ Conv2d(
+ 1,
+ 32,
+ (kernel_size, 1),
+ (stride, 1),
+ padding=(get_padding(kernel_size, 1), 0),
+ )
+ ),
+ norm_f(
+ Conv2d(
+ 32,
+ 128,
+ (kernel_size, 1),
+ (stride, 1),
+ padding=(get_padding(kernel_size, 1), 0),
+ )
+ ),
+ norm_f(
+ Conv2d(
+ 128,
+ 512,
+ (kernel_size, 1),
+ (stride, 1),
+ padding=(get_padding(kernel_size, 1), 0),
+ )
+ ),
+ norm_f(
+ Conv2d(
+ 512,
+ 1024,
+ (kernel_size, 1),
+ (stride, 1),
+ padding=(get_padding(kernel_size, 1), 0),
+ )
+ ),
+ norm_f(
+ Conv2d(
+ 1024,
+ 1024,
+ (kernel_size, 1),
+ 1,
+ padding=(get_padding(kernel_size, 1), 0),
+ )
+ ),
+ ]
+ )
+ self.conv_post = norm_f(Conv2d(1024, 1, (3, 1), 1, padding=(1, 0)))
+
+ def forward(self, x):
+ fmap = []
+
+ # 1d to 2d
+ b, c, t = x.shape
+ if t % self.period != 0: # pad first
+ n_pad = self.period - (t % self.period)
+ x = F.pad(x, (0, n_pad), "reflect")
+ t = t + n_pad
+ x = x.view(b, c, t // self.period, self.period)
+
+ for layer in self.convs:
+ x = layer(x)
+ x = F.leaky_relu(x, modules.LRELU_SLOPE)
+ fmap.append(x)
+ x = self.conv_post(x)
+ fmap.append(x)
+ x = torch.flatten(x, 1, -1)
+
+ return x, fmap
+
+
+class DiscriminatorS(torch.nn.Module):
+ def __init__(self, use_spectral_norm=False):
+ super(DiscriminatorS, self).__init__()
+ norm_f = weight_norm if use_spectral_norm is False else spectral_norm
+ self.convs = nn.ModuleList(
+ [
+ norm_f(Conv1d(1, 16, 15, 1, padding=7)),
+ norm_f(Conv1d(16, 64, 41, 4, groups=4, padding=20)),
+ norm_f(Conv1d(64, 256, 41, 4, groups=16, padding=20)),
+ norm_f(Conv1d(256, 1024, 41, 4, groups=64, padding=20)),
+ norm_f(Conv1d(1024, 1024, 41, 4, groups=256, padding=20)),
+ norm_f(Conv1d(1024, 1024, 5, 1, padding=2)),
+ ]
+ )
+ self.conv_post = norm_f(Conv1d(1024, 1, 3, 1, padding=1))
+
+ def forward(self, x):
+ fmap = []
+
+ for layer in self.convs:
+ x = layer(x)
+ x = F.leaky_relu(x, modules.LRELU_SLOPE)
+ fmap.append(x)
+ x = self.conv_post(x)
+ fmap.append(x)
+ x = torch.flatten(x, 1, -1)
+
+ return x, fmap
+
+
+class MultiPeriodDiscriminator(torch.nn.Module):
+ def __init__(self, use_spectral_norm=False):
+ super(MultiPeriodDiscriminator, self).__init__()
+ periods = [2, 3, 5, 7, 11]
+
+ discs = [DiscriminatorS(use_spectral_norm=use_spectral_norm)]
+ discs = discs + [
+ DiscriminatorP(i, use_spectral_norm=use_spectral_norm) for i in periods
+ ]
+ self.discriminators = nn.ModuleList(discs)
+
+ def forward(self, y, y_hat):
+ y_d_rs = []
+ y_d_gs = []
+ fmap_rs = []
+ fmap_gs = []
+ for i, d in enumerate(self.discriminators):
+ y_d_r, fmap_r = d(y)
+ y_d_g, fmap_g = d(y_hat)
+ y_d_rs.append(y_d_r)
+ y_d_gs.append(y_d_g)
+ fmap_rs.append(fmap_r)
+ fmap_gs.append(fmap_g)
+
+ return y_d_rs, y_d_gs, fmap_rs, fmap_gs
+
+
+class WavLMDiscriminator(nn.Module):
+ """docstring for Discriminator."""
+
+ def __init__(
+ self, slm_hidden=768, slm_layers=13, initial_channel=64, use_spectral_norm=False
+ ):
+ super(WavLMDiscriminator, self).__init__()
+ norm_f = weight_norm if use_spectral_norm == False else spectral_norm
+ self.pre = norm_f(
+ Conv1d(slm_hidden * slm_layers, initial_channel, 1, 1, padding=0)
+ )
+
+ self.convs = nn.ModuleList(
+ [
+ norm_f(
+ nn.Conv1d(
+ initial_channel, initial_channel * 2, kernel_size=5, padding=2
+ )
+ ),
+ norm_f(
+ nn.Conv1d(
+ initial_channel * 2,
+ initial_channel * 4,
+ kernel_size=5,
+ padding=2,
+ )
+ ),
+ norm_f(
+ nn.Conv1d(initial_channel * 4, initial_channel * 4, 5, 1, padding=2)
+ ),
+ ]
+ )
+
+ self.conv_post = norm_f(Conv1d(initial_channel * 4, 1, 3, 1, padding=1))
+
+ def forward(self, x):
+ x = self.pre(x)
+
+ fmap = []
+ for l in self.convs:
+ x = l(x)
+ x = F.leaky_relu(x, modules.LRELU_SLOPE)
+ fmap.append(x)
+ x = self.conv_post(x)
+ x = torch.flatten(x, 1, -1)
+
+ return x
+
+
+class ReferenceEncoder(nn.Module):
+ """
+ inputs --- [N, Ty/r, n_mels*r] mels
+ outputs --- [N, ref_enc_gru_size]
+ """
+
+ def __init__(self, spec_channels, gin_channels=0):
+ super().__init__()
+ self.spec_channels = spec_channels
+ ref_enc_filters = [32, 32, 64, 64, 128, 128]
+ K = len(ref_enc_filters)
+ filters = [1] + ref_enc_filters
+ convs = [
+ weight_norm(
+ nn.Conv2d(
+ in_channels=filters[i],
+ out_channels=filters[i + 1],
+ kernel_size=(3, 3),
+ stride=(2, 2),
+ padding=(1, 1),
+ )
+ )
+ for i in range(K)
+ ]
+ self.convs = nn.ModuleList(convs)
+ # self.wns = nn.ModuleList([weight_norm(num_features=ref_enc_filters[i]) for i in range(K)]) # noqa: E501
+
+ out_channels = self.calculate_channels(spec_channels, 3, 2, 1, K)
+ self.gru = nn.GRU(
+ input_size=ref_enc_filters[-1] * out_channels,
+ hidden_size=256 // 2,
+ batch_first=True,
+ )
+ self.proj = nn.Linear(128, gin_channels)
+
+ def forward(self, inputs, mask=None):
+ N = inputs.size(0)
+ out = inputs.view(N, 1, -1, self.spec_channels) # [N, 1, Ty, n_freqs]
+ for conv in self.convs:
+ out = conv(out)
+ # out = wn(out)
+ out = F.relu(out) # [N, 128, Ty//2^K, n_mels//2^K]
+
+ out = out.transpose(1, 2) # [N, Ty//2^K, 128, n_mels//2^K]
+ T = out.size(1)
+ N = out.size(0)
+ out = out.contiguous().view(N, T, -1) # [N, Ty//2^K, 128*n_mels//2^K]
+
+ self.gru.flatten_parameters()
+ memory, out = self.gru(out) # out --- [1, N, 128]
+
+ return self.proj(out.squeeze(0))
+
+ def calculate_channels(self, L, kernel_size, stride, pad, n_convs):
+ for i in range(n_convs):
+ L = (L - kernel_size + 2 * pad) // stride + 1
+ return L
+
+
+class SynthesizerTrn(nn.Module):
+ """
+ Synthesizer for Training
+ """
+
+ def __init__(
+ self,
+ n_vocab,
+ spec_channels,
+ segment_size,
+ inter_channels,
+ hidden_channels,
+ filter_channels,
+ n_heads,
+ n_layers,
+ kernel_size,
+ p_dropout,
+ resblock,
+ resblock_kernel_sizes,
+ resblock_dilation_sizes,
+ upsample_rates,
+ upsample_initial_channel,
+ upsample_kernel_sizes,
+ n_speakers=256,
+ gin_channels=256,
+ use_sdp=True,
+ n_flow_layer=4,
+ n_layers_trans_flow=6,
+ flow_share_parameter=False,
+ use_transformer_flow=True,
+ **kwargs
+ ):
+ super().__init__()
+ self.n_vocab = n_vocab
+ self.spec_channels = spec_channels
+ self.inter_channels = inter_channels
+ self.hidden_channels = hidden_channels
+ self.filter_channels = filter_channels
+ self.n_heads = n_heads
+ self.n_layers = n_layers
+ self.kernel_size = kernel_size
+ self.p_dropout = p_dropout
+ self.resblock = resblock
+ self.resblock_kernel_sizes = resblock_kernel_sizes
+ self.resblock_dilation_sizes = resblock_dilation_sizes
+ self.upsample_rates = upsample_rates
+ self.upsample_initial_channel = upsample_initial_channel
+ self.upsample_kernel_sizes = upsample_kernel_sizes
+ self.segment_size = segment_size
+ self.n_speakers = n_speakers
+ self.gin_channels = gin_channels
+ self.n_layers_trans_flow = n_layers_trans_flow
+ self.use_spk_conditioned_encoder = kwargs.get(
+ "use_spk_conditioned_encoder", True
+ )
+ self.use_sdp = use_sdp
+ self.use_noise_scaled_mas = kwargs.get("use_noise_scaled_mas", False)
+ self.mas_noise_scale_initial = kwargs.get("mas_noise_scale_initial", 0.01)
+ self.noise_scale_delta = kwargs.get("noise_scale_delta", 2e-6)
+ self.current_mas_noise_scale = self.mas_noise_scale_initial
+ if self.use_spk_conditioned_encoder and gin_channels > 0:
+ self.enc_gin_channels = gin_channels
+ self.enc_p = TextEncoder(
+ n_vocab,
+ inter_channels,
+ hidden_channels,
+ filter_channels,
+ n_heads,
+ n_layers,
+ kernel_size,
+ p_dropout,
+ gin_channels=self.enc_gin_channels,
+ )
+ self.dec = Generator(
+ inter_channels,
+ resblock,
+ resblock_kernel_sizes,
+ resblock_dilation_sizes,
+ upsample_rates,
+ upsample_initial_channel,
+ upsample_kernel_sizes,
+ gin_channels=gin_channels,
+ )
+ self.enc_q = PosteriorEncoder(
+ spec_channels,
+ inter_channels,
+ hidden_channels,
+ 5,
+ 1,
+ 16,
+ gin_channels=gin_channels,
+ )
+ if use_transformer_flow:
+ self.flow = TransformerCouplingBlock(
+ inter_channels,
+ hidden_channels,
+ filter_channels,
+ n_heads,
+ n_layers_trans_flow,
+ 5,
+ p_dropout,
+ n_flow_layer,
+ gin_channels=gin_channels,
+ share_parameter=flow_share_parameter,
+ )
+ else:
+ self.flow = ResidualCouplingBlock(
+ inter_channels,
+ hidden_channels,
+ 5,
+ 1,
+ n_flow_layer,
+ gin_channels=gin_channels,
+ )
+ self.sdp = StochasticDurationPredictor(
+ hidden_channels, 192, 3, 0.5, 4, gin_channels=gin_channels
+ )
+ self.dp = DurationPredictor(
+ hidden_channels, 256, 3, 0.5, gin_channels=gin_channels
+ )
+
+ if n_speakers >= 1:
+ self.emb_g = nn.Embedding(n_speakers, gin_channels)
+ else:
+ self.ref_enc = ReferenceEncoder(spec_channels, gin_channels)
+
+ def forward(
+ self,
+ x,
+ x_lengths,
+ y,
+ y_lengths,
+ sid,
+ tone,
+ language,
+ bert,
+ style_vec,
+ ):
+ if self.n_speakers > 0:
+ g = self.emb_g(sid).unsqueeze(-1) # [b, h, 1]
+ else:
+ g = self.ref_enc(y.transpose(1, 2)).unsqueeze(-1)
+ x, m_p, logs_p, x_mask = self.enc_p(
+ x, x_lengths, tone, language, bert, style_vec, g=g
+ )
+ z, m_q, logs_q, y_mask = self.enc_q(y, y_lengths, g=g)
+ z_p = self.flow(z, y_mask, g=g)
+
+ with torch.no_grad():
+ # negative cross-entropy
+ s_p_sq_r = torch.exp(-2 * logs_p) # [b, d, t]
+ neg_cent1 = torch.sum(
+ -0.5 * math.log(2 * math.pi) - logs_p, [1], keepdim=True
+ ) # [b, 1, t_s]
+ neg_cent2 = torch.matmul(
+ -0.5 * (z_p**2).transpose(1, 2), s_p_sq_r
+ ) # [b, t_t, d] x [b, d, t_s] = [b, t_t, t_s]
+ neg_cent3 = torch.matmul(
+ z_p.transpose(1, 2), (m_p * s_p_sq_r)
+ ) # [b, t_t, d] x [b, d, t_s] = [b, t_t, t_s]
+ neg_cent4 = torch.sum(
+ -0.5 * (m_p**2) * s_p_sq_r, [1], keepdim=True
+ ) # [b, 1, t_s]
+ neg_cent = neg_cent1 + neg_cent2 + neg_cent3 + neg_cent4
+ if self.use_noise_scaled_mas:
+ epsilon = (
+ torch.std(neg_cent)
+ * torch.randn_like(neg_cent)
+ * self.current_mas_noise_scale
+ )
+ neg_cent = neg_cent + epsilon
+
+ attn_mask = torch.unsqueeze(x_mask, 2) * torch.unsqueeze(y_mask, -1)
+ attn = (
+ monotonic_align.maximum_path(neg_cent, attn_mask.squeeze(1))
+ .unsqueeze(1)
+ .detach()
+ )
+
+ w = attn.sum(2)
+
+ l_length_sdp = self.sdp(x, x_mask, w, g=g)
+ l_length_sdp = l_length_sdp / torch.sum(x_mask)
+
+ logw_ = torch.log(w + 1e-6) * x_mask
+ logw = self.dp(x, x_mask, g=g)
+ # logw_sdp = self.sdp(x, x_mask, g=g, reverse=True, noise_scale=1.0)
+ l_length_dp = torch.sum((logw - logw_) ** 2, [1, 2]) / torch.sum(
+ x_mask
+ ) # for averaging
+ # l_length_sdp += torch.sum((logw_sdp - logw_) ** 2, [1, 2]) / torch.sum(x_mask)
+
+ l_length = l_length_dp + l_length_sdp
+
+ # expand prior
+ m_p = torch.matmul(attn.squeeze(1), m_p.transpose(1, 2)).transpose(1, 2)
+ logs_p = torch.matmul(attn.squeeze(1), logs_p.transpose(1, 2)).transpose(1, 2)
+
+ z_slice, ids_slice = commons.rand_slice_segments(
+ z, y_lengths, self.segment_size
+ )
+ o = self.dec(z_slice, g=g)
+ return (
+ o,
+ l_length,
+ attn,
+ ids_slice,
+ x_mask,
+ y_mask,
+ (z, z_p, m_p, logs_p, m_q, logs_q),
+ (x, logw, logw_), # , logw_sdp),
+ g,
+ )
+
+ def infer(
+ self,
+ x,
+ x_lengths,
+ sid,
+ tone,
+ language,
+ bert,
+ style_vec,
+ noise_scale=0.667,
+ length_scale=1,
+ noise_scale_w=0.8,
+ max_len=None,
+ sdp_ratio=0,
+ y=None,
+ ):
+ # x, m_p, logs_p, x_mask = self.enc_p(x, x_lengths, tone, language, bert)
+ # g = self.gst(y)
+ if self.n_speakers > 0:
+ g = self.emb_g(sid).unsqueeze(-1) # [b, h, 1]
+ else:
+ g = self.ref_enc(y.transpose(1, 2)).unsqueeze(-1)
+ x, m_p, logs_p, x_mask = self.enc_p(
+ x, x_lengths, tone, language, bert, style_vec, g=g
+ )
+ logw = self.sdp(x, x_mask, g=g, reverse=True, noise_scale=noise_scale_w) * (
+ sdp_ratio
+ ) + self.dp(x, x_mask, g=g) * (1 - sdp_ratio)
+ w = torch.exp(logw) * x_mask * length_scale
+ w_ceil = torch.ceil(w)
+ y_lengths = torch.clamp_min(torch.sum(w_ceil, [1, 2]), 1).long()
+ y_mask = torch.unsqueeze(commons.sequence_mask(y_lengths, None), 1).to(
+ x_mask.dtype
+ )
+ attn_mask = torch.unsqueeze(x_mask, 2) * torch.unsqueeze(y_mask, -1)
+ attn = commons.generate_path(w_ceil, attn_mask)
+
+ m_p = torch.matmul(attn.squeeze(1), m_p.transpose(1, 2)).transpose(
+ 1, 2
+ ) # [b, t', t], [b, t, d] -> [b, d, t']
+ logs_p = torch.matmul(attn.squeeze(1), logs_p.transpose(1, 2)).transpose(
+ 1, 2
+ ) # [b, t', t], [b, t, d] -> [b, d, t']
+
+ z_p = m_p + torch.randn_like(m_p) * torch.exp(logs_p) * noise_scale
+ z = self.flow(z_p, y_mask, g=g, reverse=True)
+ o = self.dec((z * y_mask)[:, :, :max_len], g=g)
+ return o, attn, y_mask, (z, z_p, m_p, logs_p)
diff --git a/modules.py b/modules.py
new file mode 100644
index 0000000000000000000000000000000000000000..86b93b50a56b047bb46ee6b6160c1d0b40e1101d
--- /dev/null
+++ b/modules.py
@@ -0,0 +1,581 @@
+import math
+import warnings
+
+import torch
+from torch import nn
+from torch.nn import Conv1d
+from torch.nn import functional as F
+from torch.nn.utils import remove_weight_norm, weight_norm
+
+import commons
+from attentions import Encoder
+from commons import get_padding, init_weights
+from transforms import piecewise_rational_quadratic_transform
+
+LRELU_SLOPE = 0.1
+
+
+class LayerNorm(nn.Module):
+ def __init__(self, channels, eps=1e-5):
+ super().__init__()
+ self.channels = channels
+ self.eps = eps
+
+ self.gamma = nn.Parameter(torch.ones(channels))
+ self.beta = nn.Parameter(torch.zeros(channels))
+
+ def forward(self, x):
+ x = x.transpose(1, -1)
+ x = F.layer_norm(x, (self.channels,), self.gamma, self.beta, self.eps)
+ return x.transpose(1, -1)
+
+
+class ConvReluNorm(nn.Module):
+ def __init__(
+ self,
+ in_channels,
+ hidden_channels,
+ out_channels,
+ kernel_size,
+ n_layers,
+ p_dropout,
+ ):
+ super().__init__()
+ self.in_channels = in_channels
+ self.hidden_channels = hidden_channels
+ self.out_channels = out_channels
+ self.kernel_size = kernel_size
+ self.n_layers = n_layers
+ self.p_dropout = p_dropout
+ assert n_layers > 1, "Number of layers should be larger than 0."
+
+ self.conv_layers = nn.ModuleList()
+ self.norm_layers = nn.ModuleList()
+ self.conv_layers.append(
+ nn.Conv1d(
+ in_channels, hidden_channels, kernel_size, padding=kernel_size // 2
+ )
+ )
+ self.norm_layers.append(LayerNorm(hidden_channels))
+ self.relu_drop = nn.Sequential(nn.ReLU(), nn.Dropout(p_dropout))
+ for _ in range(n_layers - 1):
+ self.conv_layers.append(
+ nn.Conv1d(
+ hidden_channels,
+ hidden_channels,
+ kernel_size,
+ padding=kernel_size // 2,
+ )
+ )
+ self.norm_layers.append(LayerNorm(hidden_channels))
+ self.proj = nn.Conv1d(hidden_channels, out_channels, 1)
+ self.proj.weight.data.zero_()
+ self.proj.bias.data.zero_()
+
+ def forward(self, x, x_mask):
+ x_org = x
+ for i in range(self.n_layers):
+ x = self.conv_layers[i](x * x_mask)
+ x = self.norm_layers[i](x)
+ x = self.relu_drop(x)
+ x = x_org + self.proj(x)
+ return x * x_mask
+
+
+class DDSConv(nn.Module):
+ """
+ Dialted and Depth-Separable Convolution
+ """
+
+ def __init__(self, channels, kernel_size, n_layers, p_dropout=0.0):
+ super().__init__()
+ self.channels = channels
+ self.kernel_size = kernel_size
+ self.n_layers = n_layers
+ self.p_dropout = p_dropout
+
+ self.drop = nn.Dropout(p_dropout)
+ self.convs_sep = nn.ModuleList()
+ self.convs_1x1 = nn.ModuleList()
+ self.norms_1 = nn.ModuleList()
+ self.norms_2 = nn.ModuleList()
+ for i in range(n_layers):
+ dilation = kernel_size**i
+ padding = (kernel_size * dilation - dilation) // 2
+ self.convs_sep.append(
+ nn.Conv1d(
+ channels,
+ channels,
+ kernel_size,
+ groups=channels,
+ dilation=dilation,
+ padding=padding,
+ )
+ )
+ self.convs_1x1.append(nn.Conv1d(channels, channels, 1))
+ self.norms_1.append(LayerNorm(channels))
+ self.norms_2.append(LayerNorm(channels))
+
+ def forward(self, x, x_mask, g=None):
+ if g is not None:
+ x = x + g
+ for i in range(self.n_layers):
+ y = self.convs_sep[i](x * x_mask)
+ y = self.norms_1[i](y)
+ y = F.gelu(y)
+ y = self.convs_1x1[i](y)
+ y = self.norms_2[i](y)
+ y = F.gelu(y)
+ y = self.drop(y)
+ x = x + y
+ return x * x_mask
+
+
+class WN(torch.nn.Module):
+ def __init__(
+ self,
+ hidden_channels,
+ kernel_size,
+ dilation_rate,
+ n_layers,
+ gin_channels=0,
+ p_dropout=0,
+ ):
+ super(WN, self).__init__()
+ assert kernel_size % 2 == 1
+ self.hidden_channels = hidden_channels
+ self.kernel_size = (kernel_size,)
+ self.dilation_rate = dilation_rate
+ self.n_layers = n_layers
+ self.gin_channels = gin_channels
+ self.p_dropout = p_dropout
+
+ self.in_layers = torch.nn.ModuleList()
+ self.res_skip_layers = torch.nn.ModuleList()
+ self.drop = nn.Dropout(p_dropout)
+
+ if gin_channels != 0:
+ cond_layer = torch.nn.Conv1d(
+ gin_channels, 2 * hidden_channels * n_layers, 1
+ )
+ self.cond_layer = torch.nn.utils.weight_norm(cond_layer, name="weight")
+
+ for i in range(n_layers):
+ dilation = dilation_rate**i
+ padding = int((kernel_size * dilation - dilation) / 2)
+ in_layer = torch.nn.Conv1d(
+ hidden_channels,
+ 2 * hidden_channels,
+ kernel_size,
+ dilation=dilation,
+ padding=padding,
+ )
+ in_layer = torch.nn.utils.weight_norm(in_layer, name="weight")
+ self.in_layers.append(in_layer)
+
+ # last one is not necessary
+ if i < n_layers - 1:
+ res_skip_channels = 2 * hidden_channels
+ else:
+ res_skip_channels = hidden_channels
+
+ res_skip_layer = torch.nn.Conv1d(hidden_channels, res_skip_channels, 1)
+ res_skip_layer = torch.nn.utils.weight_norm(res_skip_layer, name="weight")
+ self.res_skip_layers.append(res_skip_layer)
+
+ def forward(self, x, x_mask, g=None, **kwargs):
+ output = torch.zeros_like(x)
+ n_channels_tensor = torch.IntTensor([self.hidden_channels])
+
+ if g is not None:
+ g = self.cond_layer(g)
+
+ for i in range(self.n_layers):
+ x_in = self.in_layers[i](x)
+ if g is not None:
+ cond_offset = i * 2 * self.hidden_channels
+ g_l = g[:, cond_offset : cond_offset + 2 * self.hidden_channels, :]
+ else:
+ g_l = torch.zeros_like(x_in)
+
+ acts = commons.fused_add_tanh_sigmoid_multiply(x_in, g_l, n_channels_tensor)
+ acts = self.drop(acts)
+
+ res_skip_acts = self.res_skip_layers[i](acts)
+ if i < self.n_layers - 1:
+ res_acts = res_skip_acts[:, : self.hidden_channels, :]
+ x = (x + res_acts) * x_mask
+ output = output + res_skip_acts[:, self.hidden_channels :, :]
+ else:
+ output = output + res_skip_acts
+ return output * x_mask
+
+ def remove_weight_norm(self):
+ if self.gin_channels != 0:
+ torch.nn.utils.remove_weight_norm(self.cond_layer)
+ for l in self.in_layers:
+ torch.nn.utils.remove_weight_norm(l)
+ for l in self.res_skip_layers:
+ torch.nn.utils.remove_weight_norm(l)
+
+
+class ResBlock1(torch.nn.Module):
+ def __init__(self, channels, kernel_size=3, dilation=(1, 3, 5)):
+ super(ResBlock1, self).__init__()
+ self.convs1 = nn.ModuleList(
+ [
+ weight_norm(
+ Conv1d(
+ channels,
+ channels,
+ kernel_size,
+ 1,
+ dilation=dilation[0],
+ padding=get_padding(kernel_size, dilation[0]),
+ )
+ ),
+ weight_norm(
+ Conv1d(
+ channels,
+ channels,
+ kernel_size,
+ 1,
+ dilation=dilation[1],
+ padding=get_padding(kernel_size, dilation[1]),
+ )
+ ),
+ weight_norm(
+ Conv1d(
+ channels,
+ channels,
+ kernel_size,
+ 1,
+ dilation=dilation[2],
+ padding=get_padding(kernel_size, dilation[2]),
+ )
+ ),
+ ]
+ )
+ self.convs1.apply(init_weights)
+
+ self.convs2 = nn.ModuleList(
+ [
+ weight_norm(
+ Conv1d(
+ channels,
+ channels,
+ kernel_size,
+ 1,
+ dilation=1,
+ padding=get_padding(kernel_size, 1),
+ )
+ ),
+ weight_norm(
+ Conv1d(
+ channels,
+ channels,
+ kernel_size,
+ 1,
+ dilation=1,
+ padding=get_padding(kernel_size, 1),
+ )
+ ),
+ weight_norm(
+ Conv1d(
+ channels,
+ channels,
+ kernel_size,
+ 1,
+ dilation=1,
+ padding=get_padding(kernel_size, 1),
+ )
+ ),
+ ]
+ )
+ self.convs2.apply(init_weights)
+
+ def forward(self, x, x_mask=None):
+ for c1, c2 in zip(self.convs1, self.convs2):
+ xt = F.leaky_relu(x, LRELU_SLOPE)
+ if x_mask is not None:
+ xt = xt * x_mask
+ xt = c1(xt)
+ xt = F.leaky_relu(xt, LRELU_SLOPE)
+ if x_mask is not None:
+ xt = xt * x_mask
+ xt = c2(xt)
+ x = xt + x
+ if x_mask is not None:
+ x = x * x_mask
+ return x
+
+ def remove_weight_norm(self):
+ for l in self.convs1:
+ remove_weight_norm(l)
+ for l in self.convs2:
+ remove_weight_norm(l)
+
+
+class ResBlock2(torch.nn.Module):
+ def __init__(self, channels, kernel_size=3, dilation=(1, 3)):
+ super(ResBlock2, self).__init__()
+ self.convs = nn.ModuleList(
+ [
+ weight_norm(
+ Conv1d(
+ channels,
+ channels,
+ kernel_size,
+ 1,
+ dilation=dilation[0],
+ padding=get_padding(kernel_size, dilation[0]),
+ )
+ ),
+ weight_norm(
+ Conv1d(
+ channels,
+ channels,
+ kernel_size,
+ 1,
+ dilation=dilation[1],
+ padding=get_padding(kernel_size, dilation[1]),
+ )
+ ),
+ ]
+ )
+ self.convs.apply(init_weights)
+
+ def forward(self, x, x_mask=None):
+ for c in self.convs:
+ xt = F.leaky_relu(x, LRELU_SLOPE)
+ if x_mask is not None:
+ xt = xt * x_mask
+ xt = c(xt)
+ x = xt + x
+ if x_mask is not None:
+ x = x * x_mask
+ return x
+
+ def remove_weight_norm(self):
+ for l in self.convs:
+ remove_weight_norm(l)
+
+
+class Log(nn.Module):
+ def forward(self, x, x_mask, reverse=False, **kwargs):
+ if not reverse:
+ y = torch.log(torch.clamp_min(x, 1e-5)) * x_mask
+ logdet = torch.sum(-y, [1, 2])
+ return y, logdet
+ else:
+ x = torch.exp(x) * x_mask
+ return x
+
+
+class Flip(nn.Module):
+ def forward(self, x, *args, reverse=False, **kwargs):
+ x = torch.flip(x, [1])
+ if not reverse:
+ logdet = torch.zeros(x.size(0)).to(dtype=x.dtype, device=x.device)
+ return x, logdet
+ else:
+ return x
+
+
+class ElementwiseAffine(nn.Module):
+ def __init__(self, channels):
+ super().__init__()
+ self.channels = channels
+ self.m = nn.Parameter(torch.zeros(channels, 1))
+ self.logs = nn.Parameter(torch.zeros(channels, 1))
+
+ def forward(self, x, x_mask, reverse=False, **kwargs):
+ if not reverse:
+ y = self.m + torch.exp(self.logs) * x
+ y = y * x_mask
+ logdet = torch.sum(self.logs * x_mask, [1, 2])
+ return y, logdet
+ else:
+ x = (x - self.m) * torch.exp(-self.logs) * x_mask
+ return x
+
+
+class ResidualCouplingLayer(nn.Module):
+ def __init__(
+ self,
+ channels,
+ hidden_channels,
+ kernel_size,
+ dilation_rate,
+ n_layers,
+ p_dropout=0,
+ gin_channels=0,
+ mean_only=False,
+ ):
+ assert channels % 2 == 0, "channels should be divisible by 2"
+ super().__init__()
+ self.channels = channels
+ self.hidden_channels = hidden_channels
+ self.kernel_size = kernel_size
+ self.dilation_rate = dilation_rate
+ self.n_layers = n_layers
+ self.half_channels = channels // 2
+ self.mean_only = mean_only
+
+ self.pre = nn.Conv1d(self.half_channels, hidden_channels, 1)
+ self.enc = WN(
+ hidden_channels,
+ kernel_size,
+ dilation_rate,
+ n_layers,
+ p_dropout=p_dropout,
+ gin_channels=gin_channels,
+ )
+ self.post = nn.Conv1d(hidden_channels, self.half_channels * (2 - mean_only), 1)
+ self.post.weight.data.zero_()
+ self.post.bias.data.zero_()
+
+ def forward(self, x, x_mask, g=None, reverse=False):
+ x0, x1 = torch.split(x, [self.half_channels] * 2, 1)
+ h = self.pre(x0) * x_mask
+ h = self.enc(h, x_mask, g=g)
+ stats = self.post(h) * x_mask
+ if not self.mean_only:
+ m, logs = torch.split(stats, [self.half_channels] * 2, 1)
+ else:
+ m = stats
+ logs = torch.zeros_like(m)
+
+ if not reverse:
+ x1 = m + x1 * torch.exp(logs) * x_mask
+ x = torch.cat([x0, x1], 1)
+ logdet = torch.sum(logs, [1, 2])
+ return x, logdet
+ else:
+ x1 = (x1 - m) * torch.exp(-logs) * x_mask
+ x = torch.cat([x0, x1], 1)
+ return x
+
+
+class ConvFlow(nn.Module):
+ def __init__(
+ self,
+ in_channels,
+ filter_channels,
+ kernel_size,
+ n_layers,
+ num_bins=10,
+ tail_bound=5.0,
+ ):
+ super().__init__()
+ self.in_channels = in_channels
+ self.filter_channels = filter_channels
+ self.kernel_size = kernel_size
+ self.n_layers = n_layers
+ self.num_bins = num_bins
+ self.tail_bound = tail_bound
+ self.half_channels = in_channels // 2
+
+ self.pre = nn.Conv1d(self.half_channels, filter_channels, 1)
+ self.convs = DDSConv(filter_channels, kernel_size, n_layers, p_dropout=0.0)
+ self.proj = nn.Conv1d(
+ filter_channels, self.half_channels * (num_bins * 3 - 1), 1
+ )
+ self.proj.weight.data.zero_()
+ self.proj.bias.data.zero_()
+
+ def forward(self, x, x_mask, g=None, reverse=False):
+ x0, x1 = torch.split(x, [self.half_channels] * 2, 1)
+ h = self.pre(x0)
+ h = self.convs(h, x_mask, g=g)
+ h = self.proj(h) * x_mask
+
+ b, c, t = x0.shape
+ h = h.reshape(b, c, -1, t).permute(0, 1, 3, 2) # [b, cx?, t] -> [b, c, t, ?]
+
+ unnormalized_widths = h[..., : self.num_bins] / math.sqrt(self.filter_channels)
+ unnormalized_heights = h[..., self.num_bins : 2 * self.num_bins] / math.sqrt(
+ self.filter_channels
+ )
+ unnormalized_derivatives = h[..., 2 * self.num_bins :]
+
+ x1, logabsdet = piecewise_rational_quadratic_transform(
+ x1,
+ unnormalized_widths,
+ unnormalized_heights,
+ unnormalized_derivatives,
+ inverse=reverse,
+ tails="linear",
+ tail_bound=self.tail_bound,
+ )
+
+ x = torch.cat([x0, x1], 1) * x_mask
+ logdet = torch.sum(logabsdet * x_mask, [1, 2])
+ if not reverse:
+ return x, logdet
+ else:
+ return x
+
+
+class TransformerCouplingLayer(nn.Module):
+ def __init__(
+ self,
+ channels,
+ hidden_channels,
+ kernel_size,
+ n_layers,
+ n_heads,
+ p_dropout=0,
+ filter_channels=0,
+ mean_only=False,
+ wn_sharing_parameter=None,
+ gin_channels=0,
+ ):
+ assert channels % 2 == 0, "channels should be divisible by 2"
+ super().__init__()
+ self.channels = channels
+ self.hidden_channels = hidden_channels
+ self.kernel_size = kernel_size
+ self.n_layers = n_layers
+ self.half_channels = channels // 2
+ self.mean_only = mean_only
+
+ self.pre = nn.Conv1d(self.half_channels, hidden_channels, 1)
+ self.enc = (
+ Encoder(
+ hidden_channels,
+ filter_channels,
+ n_heads,
+ n_layers,
+ kernel_size,
+ p_dropout,
+ isflow=True,
+ gin_channels=gin_channels,
+ )
+ if wn_sharing_parameter is None
+ else wn_sharing_parameter
+ )
+ self.post = nn.Conv1d(hidden_channels, self.half_channels * (2 - mean_only), 1)
+ self.post.weight.data.zero_()
+ self.post.bias.data.zero_()
+
+ def forward(self, x, x_mask, g=None, reverse=False):
+ x0, x1 = torch.split(x, [self.half_channels] * 2, 1)
+ h = self.pre(x0) * x_mask
+ h = self.enc(h, x_mask, g=g)
+ stats = self.post(h) * x_mask
+ if not self.mean_only:
+ m, logs = torch.split(stats, [self.half_channels] * 2, 1)
+ else:
+ m = stats
+ logs = torch.zeros_like(m)
+
+ if not reverse:
+ x1 = m + x1 * torch.exp(logs) * x_mask
+ x = torch.cat([x0, x1], 1)
+ logdet = torch.sum(logs, [1, 2])
+ return x, logdet
+ else:
+ x1 = (x1 - m) * torch.exp(-logs) * x_mask
+ x = torch.cat([x0, x1], 1)
+ return x
diff --git a/monotonic_align/__init__.py b/monotonic_align/__init__.py
new file mode 100644
index 0000000000000000000000000000000000000000..aed94600a6b01f4322b371b0c57d5b05713c4dac
--- /dev/null
+++ b/monotonic_align/__init__.py
@@ -0,0 +1,16 @@
+from numpy import zeros, int32, float32
+from torch import from_numpy
+
+from .core import maximum_path_jit
+
+
+def maximum_path(neg_cent, mask):
+ device = neg_cent.device
+ dtype = neg_cent.dtype
+ neg_cent = neg_cent.data.cpu().numpy().astype(float32)
+ path = zeros(neg_cent.shape, dtype=int32)
+
+ t_t_max = mask.sum(1)[:, 0].data.cpu().numpy().astype(int32)
+ t_s_max = mask.sum(2)[:, 0].data.cpu().numpy().astype(int32)
+ maximum_path_jit(path, neg_cent, t_t_max, t_s_max)
+ return from_numpy(path).to(device=device, dtype=dtype)
diff --git a/monotonic_align/core.py b/monotonic_align/core.py
new file mode 100644
index 0000000000000000000000000000000000000000..7c962adea65543ef426034c4d53c4f0e615e8181
--- /dev/null
+++ b/monotonic_align/core.py
@@ -0,0 +1,46 @@
+import numba
+
+
+@numba.jit(
+ numba.void(
+ numba.int32[:, :, ::1],
+ numba.float32[:, :, ::1],
+ numba.int32[::1],
+ numba.int32[::1],
+ ),
+ nopython=True,
+ nogil=True,
+)
+def maximum_path_jit(paths, values, t_ys, t_xs):
+ b = paths.shape[0]
+ max_neg_val = -1e9
+ for i in range(int(b)):
+ path = paths[i]
+ value = values[i]
+ t_y = t_ys[i]
+ t_x = t_xs[i]
+
+ v_prev = v_cur = 0.0
+ index = t_x - 1
+
+ for y in range(t_y):
+ for x in range(max(0, t_x + y - t_y), min(t_x, y + 1)):
+ if x == y:
+ v_cur = max_neg_val
+ else:
+ v_cur = value[y - 1, x]
+ if x == 0:
+ if y == 0:
+ v_prev = 0.0
+ else:
+ v_prev = max_neg_val
+ else:
+ v_prev = value[y - 1, x - 1]
+ value[y, x] += max(v_prev, v_cur)
+
+ for y in range(t_y - 1, -1, -1):
+ path[y, index] = 1
+ if index != 0 and (
+ index == y or value[y - 1, index] < value[y - 1, index - 1]
+ ):
+ index = index - 1
diff --git a/preprocess_all.py b/preprocess_all.py
new file mode 100644
index 0000000000000000000000000000000000000000..82a3c202cfc58ad9650e6b533c1b07dff15f11ad
--- /dev/null
+++ b/preprocess_all.py
@@ -0,0 +1,96 @@
+import argparse
+from webui_train import preprocess_all
+from multiprocessing import cpu_count
+
+if __name__ == "__main__":
+ parser = argparse.ArgumentParser()
+ parser.add_argument(
+ "--model_name", "-m", type=str, help="Model name", required=True
+ )
+ parser.add_argument("--batch_size", "-b", type=int, help="Batch size", default=2)
+ parser.add_argument("--epochs", "-e", type=int, help="Epochs", default=100)
+ parser.add_argument(
+ "--save_every_steps",
+ "-s",
+ type=int,
+ help="Save every steps",
+ default=1000,
+ )
+ parser.add_argument(
+ "--num_processes",
+ type=int,
+ help="Number of processes",
+ default=cpu_count() // 2,
+ )
+ parser.add_argument(
+ "--normalize",
+ action="store_true",
+ help="Loudness normalize audio",
+ )
+ parser.add_argument(
+ "--trim",
+ action="store_true",
+ help="Trim silence",
+ )
+ parser.add_argument(
+ "--freeze_EN_bert",
+ action="store_true",
+ help="Freeze English BERT",
+ )
+ parser.add_argument(
+ "--freeze_JP_bert",
+ action="store_true",
+ help="Freeze Japanese BERT",
+ )
+ parser.add_argument(
+ "--freeze_ZH_bert",
+ action="store_true",
+ help="Freeze Chinese BERT",
+ )
+ parser.add_argument(
+ "--freeze_style",
+ action="store_true",
+ help="Freeze style vector",
+ )
+ parser.add_argument(
+ "--freeze_decoder",
+ action="store_true",
+ help="Freeze decoder",
+ )
+ parser.add_argument(
+ "--use_jp_extra",
+ action="store_true",
+ help="Use JP-Extra model",
+ )
+ parser.add_argument(
+ "--val_per_lang",
+ type=int,
+ help="Validation per language",
+ default=0,
+ )
+ parser.add_argument(
+ "--log_interval",
+ type=int,
+ help="Log interval",
+ default=200,
+ )
+
+ args = parser.parse_args()
+
+ preprocess_all(
+ model_name=args.model_name,
+ batch_size=args.batch_size,
+ epochs=args.epochs,
+ save_every_steps=args.save_every_steps,
+ num_processes=args.num_processes,
+ normalize=args.normalize,
+ trim=args.trim,
+ freeze_EN_bert=args.freeze_EN_bert,
+ freeze_JP_bert=args.freeze_JP_bert,
+ freeze_ZH_bert=args.freeze_ZH_bert,
+ freeze_style=args.freeze_style,
+ freeze_decoder=args.freeze_decoder,
+ use_jp_extra=args.use_jp_extra,
+ val_per_lang=args.val_per_lang,
+ log_interval=args.log_interval,
+ )
diff --git a/preprocess_text.py b/preprocess_text.py
new file mode 100644
index 0000000000000000000000000000000000000000..2c26941e7a11824ec14fa5518db728f9a3085e46
--- /dev/null
+++ b/preprocess_text.py
@@ -0,0 +1,167 @@
+import json
+import os
+from collections import defaultdict
+from random import shuffle
+from typing import Optional
+
+import click
+from tqdm import tqdm
+
+from config import config
+from text.cleaner import clean_text
+from common.stdout_wrapper import SAFE_STDOUT
+from common.log import logger
+
+preprocess_text_config = config.preprocess_text_config
+
+
+# Count lines for tqdm
+def count_lines(file_path: str):
+ with open(file_path, "r", encoding="utf-8") as file:
+ return sum(1 for _ in file)
+
+
+@click.command()
+@click.option(
+ "--transcription-path",
+ default=preprocess_text_config.transcription_path,
+ type=click.Path(exists=True, file_okay=True, dir_okay=False),
+)
+@click.option("--cleaned-path", default=preprocess_text_config.cleaned_path)
+@click.option("--train-path", default=preprocess_text_config.train_path)
+@click.option("--val-path", default=preprocess_text_config.val_path)
+@click.option(
+ "--config-path",
+ default=preprocess_text_config.config_path,
+ type=click.Path(exists=True, file_okay=True, dir_okay=False),
+)
+@click.option("--val-per-lang", default=preprocess_text_config.val_per_lang)
+@click.option("--max-val-total", default=preprocess_text_config.max_val_total)
+@click.option("--clean/--no-clean", default=preprocess_text_config.clean)
+@click.option("-y", "--yml_config")
+@click.option("--use_jp_extra", is_flag=True)
+def preprocess(
+ transcription_path: str,
+ cleaned_path: Optional[str],
+ train_path: str,
+ val_path: str,
+ config_path: str,
+ val_per_lang: int,
+ max_val_total: int,
+ clean: bool,
+ yml_config: str, # 这个不要删
+ use_jp_extra: bool,
+):
+ if cleaned_path == "" or cleaned_path is None:
+ cleaned_path = transcription_path + ".cleaned"
+
+ error_log_path = os.path.join(os.path.dirname(cleaned_path), "text_error.log")
+ error_count = 0
+
+ if clean:
+ total_lines = count_lines(transcription_path)
+ with open(cleaned_path, "w", encoding="utf-8") as out_file:
+ with open(transcription_path, "r", encoding="utf-8") as trans_file:
+ for line in tqdm(trans_file, file=SAFE_STDOUT, total=total_lines):
+ try:
+ utt, spk, language, text = line.strip().split("|")
+ norm_text, phones, tones, word2ph = clean_text(
+ text, language, use_jp_extra
+ )
+ out_file.write(
+ "{}|{}|{}|{}|{}|{}|{}\n".format(
+ utt,
+ spk,
+ language,
+ norm_text,
+ " ".join(phones),
+ " ".join([str(i) for i in tones]),
+ " ".join([str(i) for i in word2ph]),
+ )
+ )
+ except Exception as e:
+ logger.error(f"An error occurred at line:\n{line.strip()}\n{e}")
+ with open(error_log_path, "a", encoding="utf-8") as error_log:
+ error_log.write(f"{line.strip()}\n{e}\n\n")
+ error_count += 1
+
+ transcription_path = cleaned_path
+ spk_utt_map = defaultdict(list)
+ spk_id_map = {}
+ current_sid = 0
+
+ with open(transcription_path, "r", encoding="utf-8") as f:
+ audioPaths = set()
+ countSame = 0
+ countNotFound = 0
+ for line in f.readlines():
+ utt, spk, language, text, phones, tones, word2ph = line.strip().split("|")
+ if utt in audioPaths:
+ # 过滤数据集错误:相同的音频匹配多个文本,导致后续bert出问题
+ logger.warning(f"Same audio matches multiple texts: {line}")
+ countSame += 1
+ continue
+ if not os.path.isfile(utt):
+ # 过滤数据集错误:不存在对应音频
+ logger.warning(f"Audio not found: {utt}")
+ countNotFound += 1
+ continue
+ audioPaths.add(utt)
+ spk_utt_map[language].append(line)
+ if spk not in spk_id_map.keys():
+ spk_id_map[spk] = current_sid
+ current_sid += 1
+ if countSame > 0 or countNotFound > 0:
+ logger.warning(
+ f"Total repeated audios: {countSame}, Total number of audio not found: {countNotFound}"
+ )
+
+ train_list = []
+ val_list = []
+
+ for spk, utts in spk_utt_map.items():
+ shuffle(utts)
+ val_list += utts[:val_per_lang]
+ train_list += utts[val_per_lang:]
+
+ shuffle(val_list)
+ if len(val_list) > max_val_total:
+ train_list += val_list[max_val_total:]
+ val_list = val_list[:max_val_total]
+
+ with open(train_path, "w", encoding="utf-8") as f:
+ for line in train_list:
+ f.write(line)
+
+ with open(val_path, "w", encoding="utf-8") as f:
+ for line in val_list:
+ f.write(line)
+
+ json_config = json.load(open(config_path, encoding="utf-8"))
+ json_config["data"]["spk2id"] = spk_id_map
+ json_config["data"]["n_speakers"] = len(spk_id_map)
+ # 新增写入:写入训练版本、数据集路径
+ # json_config["version"] = latest_version
+ json_config["data"]["training_files"] = os.path.normpath(train_path).replace(
+ "\\", "/"
+ )
+ json_config["data"]["validation_files"] = os.path.normpath(val_path).replace(
+ "\\", "/"
+ )
+ with open(config_path, "w", encoding="utf-8") as f:
+ json.dump(json_config, f, indent=2, ensure_ascii=False)
+ if error_count > 0:
+ logger.error(
+ f"An error occurred in {error_count} lines. Please check {error_log_path} for details. You can proceed with lines that do not have errors."
+ )
+ raise Exception(
+ f"An error occurred in {error_count} lines. Please check {error_log_path} for details. You can proceed with lines that do not have errors."
+ )
+ else:
+ logger.info(
+ "Training set and validation set generation from texts is complete!"
+ )
+
+
+if __name__ == "__main__":
+ preprocess()
diff --git a/re_matching.py b/re_matching.py
new file mode 100644
index 0000000000000000000000000000000000000000..dd464a5cca8fd00d2da35dfddf9a03295dd5b627
--- /dev/null
+++ b/re_matching.py
@@ -0,0 +1,81 @@
+import re
+
+
+def extract_language_and_text_updated(speaker, dialogue):
+ # 使用正则表达式匹配<语言>标签和其后的文本
+ pattern_language_text = r"<(\S+?)>([^<]+)"
+ matches = re.findall(pattern_language_text, dialogue, re.DOTALL)
+ speaker = speaker[1:-1]
+ # 清理文本:去除两边的空白字符
+ matches_cleaned = [(lang.upper(), text.strip()) for lang, text in matches]
+ matches_cleaned.append(speaker)
+ return matches_cleaned
+
+
+def validate_text(input_text):
+ # 验证说话人的正则表达式
+ pattern_speaker = r"(\[\S+?\])((?:\s*<\S+?>[^<\[\]]+?)+)"
+
+ # 使用re.DOTALL标志使.匹配包括换行符在内的所有字符
+ matches = re.findall(pattern_speaker, input_text, re.DOTALL)
+
+ # 对每个匹配到的说话人内容进行进一步验证
+ for _, dialogue in matches:
+ language_text_matches = extract_language_and_text_updated(_, dialogue)
+ if not language_text_matches:
+ return (
+ False,
+ "Error: Invalid format detected in dialogue content. Please check your input.",
+ )
+
+ # 如果输入的文本中没有找到任何匹配项
+ if not matches:
+ return (
+ False,
+ "Error: No valid speaker format detected. Please check your input.",
+ )
+
+ return True, "Input is valid."
+
+
+def text_matching(text: str) -> list:
+ speaker_pattern = r"(\[\S+?\])(.+?)(?=\[\S+?\]|$)"
+ matches = re.findall(speaker_pattern, text, re.DOTALL)
+ result = []
+ for speaker, dialogue in matches:
+ result.append(extract_language_and_text_updated(speaker, dialogue))
+ return result
+
+
+def cut_para(text):
+ splitted_para = re.split("[\n]", text) # 按段分
+ splitted_para = [
+ sentence.strip() for sentence in splitted_para if sentence.strip()
+ ] # 删除空字符串
+ return splitted_para
+
+
+def cut_sent(para):
+ para = re.sub("([。!;?\?])([^”’])", r"\1\n\2", para) # 单字符断句符
+ para = re.sub("(\.{6})([^”’])", r"\1\n\2", para) # 英文省略号
+ para = re.sub("(\…{2})([^”’])", r"\1\n\2", para) # 中文省略号
+ para = re.sub("([。!?\?][”’])([^,。!?\?])", r"\1\n\2", para)
+ para = para.rstrip() # 段尾如果有多余的\n就去掉它
+ return para.split("\n")
+
+
+if __name__ == "__main__":
+ text = """
+ [说话人1]
+ [说话人2]你好吗?元気ですか?こんにちは,世界。你好吗?
+ [说话人3]谢谢。どういたしまして。
+ """
+ text_matching(text)
+ # 测试函数
+ test_text = """
+ [说话人1]你好,こんにちは!こんにちは,世界。
+ [说话人2]你好吗?
+ """
+ text_matching(test_text)
+ res = validate_text(test_text)
+ print(res)
diff --git a/requirements.txt b/requirements.txt
new file mode 100644
index 0000000000000000000000000000000000000000..21c199b9af0467a2fc577cf3b18678e82e2ccf81
--- /dev/null
+++ b/requirements.txt
@@ -0,0 +1,13 @@
+gradio
+loguru
+matplotlib
+num2words
+numba
+numpy
+pyopenjtalk-dict
+PyYAML
+requests
+safetensors
+scipy
+torch>=2.1,<2.2
+transformers
diff --git a/resample.py b/resample.py
new file mode 100644
index 0000000000000000000000000000000000000000..5aff5ef4907ede6a8ba3c27d0ec676884691206e
--- /dev/null
+++ b/resample.py
@@ -0,0 +1,131 @@
+import argparse
+import os
+from concurrent.futures import ThreadPoolExecutor
+
+import librosa
+import pyloudnorm as pyln
+import soundfile
+from tqdm import tqdm
+
+from common.log import logger
+from common.stdout_wrapper import SAFE_STDOUT
+from config import config
+
+DEFAULT_BLOCK_SIZE: float = 0.400 # seconds
+
+
+class BlockSizeException(Exception):
+ pass
+
+
+def normalize_audio(data, sr):
+ meter = pyln.Meter(sr, block_size=DEFAULT_BLOCK_SIZE) # create BS.1770 meter
+ try:
+ loudness = meter.integrated_loudness(data)
+ except ValueError as e:
+ raise BlockSizeException(e)
+ # logger.info(f"loudness: {loudness}")
+ data = pyln.normalize.loudness(data, loudness, -23.0)
+ return data
+
+
+def process(item):
+ spkdir, wav_name, args = item
+ wav_path = os.path.join(args.in_dir, spkdir, wav_name)
+ if os.path.exists(wav_path) and wav_path.lower().endswith(".wav"):
+ wav, sr = librosa.load(wav_path, sr=args.sr)
+ if args.normalize:
+ try:
+ wav = normalize_audio(wav, sr)
+ except BlockSizeException:
+ logger.info(
+ f"Skip normalize due to less than {DEFAULT_BLOCK_SIZE} second audio: {wav_path}"
+ )
+ if args.trim:
+ wav, _ = librosa.effects.trim(wav, top_db=30)
+ soundfile.write(os.path.join(args.out_dir, spkdir, wav_name), wav, sr)
+
+
+if __name__ == "__main__":
+ parser = argparse.ArgumentParser()
+ parser.add_argument(
+ "--sr",
+ type=int,
+ default=config.resample_config.sampling_rate,
+ help="sampling rate",
+ )
+ parser.add_argument(
+ "--in_dir",
+ "-i",
+ type=str,
+ default=config.resample_config.in_dir,
+ help="path to source dir",
+ )
+ parser.add_argument(
+ "--out_dir",
+ "-o",
+ type=str,
+ default=config.resample_config.out_dir,
+ help="path to target dir",
+ )
+ parser.add_argument(
+ "--num_processes",
+ type=int,
+ default=4,
+ help="cpu_processes",
+ )
+ parser.add_argument(
+ "--normalize",
+ action="store_true",
+ default=False,
+ help="loudness normalize audio",
+ )
+ parser.add_argument(
+ "--trim",
+ action="store_true",
+ default=False,
+ help="trim silence (start and end only)",
+ )
+ args, _ = parser.parse_known_args()
+ # autodl 无卡模式会识别出46个cpu
+ if args.num_processes == 0:
+ processes = cpu_count() - 2 if cpu_count() > 4 else 1
+ else:
+ processes = args.num_processes
+
+ tasks = []
+
+ for dirpath, _, filenames in os.walk(args.in_dir):
+ # 子级目录
+ spk_dir = os.path.relpath(dirpath, args.in_dir)
+ spk_dir_out = os.path.join(args.out_dir, spk_dir)
+ if not os.path.isdir(spk_dir_out):
+ os.makedirs(spk_dir_out, exist_ok=True)
+ for filename in filenames:
+ if filename.lower().endswith(".wav"):
+ twople = (spk_dir, filename, args)
+ tasks.append(twople)
+
+ if len(tasks) == 0:
+ logger.error(f"No wav files found in {args.in_dir}")
+ raise ValueError(f"No wav files found in {args.in_dir}")
+
+ # pool = Pool(processes=processes)
+ # for _ in tqdm(
+ # pool.imap_unordered(process, tasks), file=SAFE_STDOUT, total=len(tasks)
+ # ):
+ # pass
+
+ # pool.close()
+ # pool.join()
+
+ with ThreadPoolExecutor(max_workers=processes) as executor:
+ _ = list(
+ tqdm(
+ executor.map(process, tasks),
+ total=len(tasks),
+ file=SAFE_STDOUT,
+ )
+ )
+
+ logger.info("Resampling Done!")
diff --git a/scripts/Install-Style-Bert-VITS2-CPU.bat b/scripts/Install-Style-Bert-VITS2-CPU.bat
new file mode 100644
index 0000000000000000000000000000000000000000..aaf3536adf501721e9f57d167b4fb078dee2144f
--- /dev/null
+++ b/scripts/Install-Style-Bert-VITS2-CPU.bat
@@ -0,0 +1,69 @@
+chcp 65001 > NUL
+@echo off
+
+@REM https://github.com/Zuntan03/EasyBertVits2 より引用・改変
+
+pushd %~dp0
+set PS_CMD=PowerShell -Version 5.1 -ExecutionPolicy Bypass
+
+set CURL_CMD=C:\Windows\System32\curl.exe
+if not exist %CURL_CMD% (
+ echo [ERROR] %CURL_CMD% が見つかりません。
+ pause & popd & exit /b 1
+)
+
+@REM lib フォルダがなければ作成
+if not exist lib\ ( mkdir lib )
+
+@REM Style-Bert-VITS2.zip をGitHubのmasterの最新のものをダウンロード
+%CURL_CMD% -Lo Style-Bert-VITS2.zip^
+ https://github.com/litagin02/Style-Bert-VITS2/archive/refs/heads/master.zip
+if %errorlevel% neq 0 ( pause & popd & exit /b %errorlevel% )
+
+@REM Style-Bert-VITS2.zip を解凍(フォルダ名前がBert-VITS2-masterになる)
+%PS_CMD% Expand-Archive -Path Style-Bert-VITS2.zip -DestinationPath . -Force
+if %errorlevel% neq 0 ( pause & popd & exit /b %errorlevel% )
+
+@REM 元のzipを削除
+del Style-Bert-VITS2.zip
+if %errorlevel% neq 0 ( pause & popd & exit /b %errorlevel% )
+
+@REM Bert-VITS2-masterの中身をStyle-Bert-VITS2に上書き移動
+xcopy /QSY .\Style-Bert-VITS2-master\ .\Style-Bert-VITS2\
+rmdir /s /q Style-Bert-VITS2-master
+
+echo ----------------------------------------
+echo Setup Python and Virtual Environment
+echo ----------------------------------------
+
+@REM Pythonと仮想環境のセットアップを呼び出す(仮想環境が有効化されて戻ってくる)
+call Style-Bert-VITS2\scripts\Setup-Python.bat ..\..\lib\python ..\venv
+if %errorlevel% neq 0 ( popd & exit /b %errorlevel% )
+
+@REM 依存関係インストール
+pip install -r Style-Bert-VITS2\requirements.txt
+if %errorlevel% neq 0 ( pause & popd & exit /b %errorlevel% )
+
+echo ----------------------------------------
+echo Environment setup is complete. Start downloading the model.
+echo ----------------------------------------
+
+@REM Style-Bert-VITS2フォルダに移動
+pushd Style-Bert-VITS2
+
+@REM 初期化(必要なモデルのダウンロード)
+python initialize.py
+
+echo ----------------------------------------
+echo Model download is complete. Start the WebUI of the voice synthesis.
+echo ----------------------------------------
+
+@REM 音声合成WebUIの起動
+python app.py
+
+pause
+
+popd
+
+popd
+
diff --git a/scripts/Install-Style-Bert-VITS2.bat b/scripts/Install-Style-Bert-VITS2.bat
new file mode 100644
index 0000000000000000000000000000000000000000..6e99230c3c40a4af3890ebe2fd1e5f753d5e5f00
--- /dev/null
+++ b/scripts/Install-Style-Bert-VITS2.bat
@@ -0,0 +1,72 @@
+chcp 65001 > NUL
+@echo off
+
+@REM https://github.com/Zuntan03/EasyBertVits2 より引用・改変
+
+pushd %~dp0
+set PS_CMD=PowerShell -Version 5.1 -ExecutionPolicy Bypass
+
+set CURL_CMD=C:\Windows\System32\curl.exe
+if not exist %CURL_CMD% (
+ echo [ERROR] %CURL_CMD% が見つかりません。
+ pause & popd & exit /b 1
+)
+
+@REM lib フォルダがなければ作成
+if not exist lib\ ( mkdir lib )
+
+@REM Style-Bert-VITS2.zip をGitHubのmasterの最新のものをダウンロード
+%CURL_CMD% -Lo Style-Bert-VITS2.zip^
+ https://github.com/litagin02/Style-Bert-VITS2/archive/refs/heads/master.zip
+if %errorlevel% neq 0 ( pause & popd & exit /b %errorlevel% )
+
+@REM Style-Bert-VITS2.zip を解凍(フォルダ名前がBert-VITS2-masterになる)
+%PS_CMD% Expand-Archive -Path Style-Bert-VITS2.zip -DestinationPath . -Force
+if %errorlevel% neq 0 ( pause & popd & exit /b %errorlevel% )
+
+@REM 元のzipを削除
+del Style-Bert-VITS2.zip
+if %errorlevel% neq 0 ( pause & popd & exit /b %errorlevel% )
+
+@REM Bert-VITS2-masterの中身をStyle-Bert-VITS2に上書き移動
+xcopy /QSY .\Style-Bert-VITS2-master\ .\Style-Bert-VITS2\
+rmdir /s /q Style-Bert-VITS2-master
+
+echo ----------------------------------------
+echo Setup Python and Virtual Environment
+echo ----------------------------------------
+
+@REM Pythonと仮想環境のセットアップを呼び出す(仮想環境が有効化されて戻ってくる)
+call Style-Bert-VITS2\scripts\Setup-Python.bat ..\..\lib\python ..\venv
+if %errorlevel% neq 0 ( popd & exit /b %errorlevel% )
+
+@REM 依存関係インストール
+pip install torch==2.1.2 torchvision==0.16.2 torchaudio==2.1.2 --index-url https://download.pytorch.org/whl/cu118
+if %errorlevel% neq 0 ( pause & popd & exit /b %errorlevel% )
+
+pip install -r Style-Bert-VITS2\requirements.txt
+if %errorlevel% neq 0 ( pause & popd & exit /b %errorlevel% )
+
+echo ----------------------------------------
+echo Environment setup is complete. Start downloading the model.
+echo ----------------------------------------
+
+@REM Style-Bert-VITS2フォルダに移動
+pushd Style-Bert-VITS2
+
+@REM 初期化(必要なモデルのダウンロード)
+python initialize.py
+
+echo ----------------------------------------
+echo Model download is complete. Start the WebUI of the voice synthesis.
+echo ----------------------------------------
+
+@REM 音声合成WebUIの起動
+python app.py
+
+pause
+
+popd
+
+popd
+
diff --git a/scripts/Setup-Python.bat b/scripts/Setup-Python.bat
new file mode 100644
index 0000000000000000000000000000000000000000..17fd23f237a6e040382322519b8c222d4ca8966f
--- /dev/null
+++ b/scripts/Setup-Python.bat
@@ -0,0 +1,76 @@
+chcp 65001 > NUL
+
+@REM https://github.com/Zuntan03/EasyBertVits2 より引用・改変
+
+@echo off
+set PS_CMD=PowerShell -Version 5.1 -ExecutionPolicy Bypass
+set CURL_CMD=C:\Windows\System32\curl.exe
+
+if not exist %CURL_CMD% (
+ echo [ERROR] %CURL_CMD% が見つかりません。
+ pause & exit /b 1
+)
+
+if "%1" neq "" (
+ set PYTHON_DIR=%~dp0%~1
+) else (
+ set PYTHON_DIR=%~dp0python
+)
+set PYTHON_CMD=%PYTHON_DIR%\python.exe
+
+if "%2" neq "" (
+ set VENV_DIR=%~dp0%~2
+) else (
+ set VENV_DIR=%~dp0venv
+)
+
+echo PS_CMD: %PS_CMD%
+echo CURL_CMD: %CURL_CMD%
+echo PYTHON_CMD: %PYTHON_CMD%
+echo VENV_DIR: %VENV_DIR%
+
+if not exist %PYTHON_DIR%\ (
+ echo https://www.python.org/
+ echo %CURL_CMD% -o python.zip https://www.python.org/ftp/python/3.10.11/python-3.10.11-embed-amd64.zip
+ %CURL_CMD% -o python.zip https://www.python.org/ftp/python/3.10.11/python-3.10.11-embed-amd64.zip
+ if %errorlevel% neq 0 ( pause & exit /b %errorlevel% )
+
+ echo %PS_CMD% Expand-Archive -Path python.zip -DestinationPath %PYTHON_DIR%
+ %PS_CMD% Expand-Archive -Path python.zip -DestinationPath %PYTHON_DIR%
+ if %errorlevel% neq 0 ( pause & exit /b %errorlevel% )
+
+ echo del python.zip
+ del python.zip
+ if %errorlevel% neq 0 ( pause & exit /b %errorlevel% )
+
+ echo %PS_CMD% "&{(Get-Content '%PYTHON_DIR%/python310._pth') -creplace '#import site', 'import site' | Set-Content '%PYTHON_DIR%/python310._pth' }"
+ %PS_CMD% "&{(Get-Content '%PYTHON_DIR%/python310._pth') -creplace '#import site', 'import site' | Set-Content '%PYTHON_DIR%/python310._pth' }"
+ if %errorlevel% neq 0 ( pause & exit /b %errorlevel% )
+
+ echo https://github.com/pypa/get-pip
+ echo %CURL_CMD% -o %PYTHON_DIR%\get-pip.py https://bootstrap.pypa.io/get-pip.py
+ %CURL_CMD% -o %PYTHON_DIR%\get-pip.py https://bootstrap.pypa.io/get-pip.py
+ if %errorlevel% neq 0 ( pause & exit /b %errorlevel% )
+
+ echo %PYTHON_CMD% %PYTHON_DIR%\get-pip.py --no-warn-script-location
+ %PYTHON_CMD% %PYTHON_DIR%\get-pip.py --no-warn-script-location
+ if %errorlevel% neq 0 ( pause & exit /b %errorlevel% )
+
+ echo %PYTHON_CMD% -m pip install virtualenv --no-warn-script-location
+ %PYTHON_CMD% -m pip install virtualenv --no-warn-script-location
+ if %errorlevel% neq 0 ( pause & exit /b %errorlevel% )
+)
+
+if not exist %VENV_DIR%\ (
+ echo %PYTHON_CMD% -m virtualenv --copies %VENV_DIR%
+ %PYTHON_CMD% -m virtualenv --copies %VENV_DIR%
+ if %errorlevel% neq 0 ( pause & exit /b %errorlevel% )
+)
+
+echo call %VENV_DIR%\Scripts\activate.bat
+call %VENV_DIR%\Scripts\activate.bat
+if %errorlevel% neq 0 ( pause & exit /b %errorlevel% )
+
+echo python -m pip install --upgrade pip
+python -m pip install --upgrade pip
+if %errorlevel% neq 0 ( pause & exit /b %errorlevel% )
diff --git a/scripts/Update-Style-Bert-VITS2.bat b/scripts/Update-Style-Bert-VITS2.bat
new file mode 100644
index 0000000000000000000000000000000000000000..782f2efe9ca447c8f018a75b5a6332e8a521e973
--- /dev/null
+++ b/scripts/Update-Style-Bert-VITS2.bat
@@ -0,0 +1,44 @@
+chcp 65001 > NUL
+@echo off
+
+pushd %~dp0
+set PS_CMD=PowerShell -Version 5.1 -ExecutionPolicy Bypass
+
+set CURL_CMD=C:\Windows\System32\curl.exe
+if not exist %CURL_CMD% (
+ echo [ERROR] %CURL_CMD% が見つかりません。
+ pause & popd & exit /b 1
+)
+
+@REM Style-Bert-VITS2.zip をGitHubのmasterの最新のものをダウンロード
+%CURL_CMD% -Lo Style-Bert-VITS2.zip^
+ https://github.com/litagin02/Style-Bert-VITS2/archive/refs/heads/master.zip
+if %errorlevel% neq 0 ( pause & popd & exit /b %errorlevel% )
+
+@REM Style-Bert-VITS2.zip を解凍(フォルダ名前がBert-VITS2-masterになる)
+%PS_CMD% Expand-Archive -Path Style-Bert-VITS2.zip -DestinationPath . -Force
+if %errorlevel% neq 0 ( pause & popd & exit /b %errorlevel% )
+
+@REM 元のzipを削除
+del Style-Bert-VITS2.zip
+if %errorlevel% neq 0 ( pause & popd & exit /b %errorlevel% )
+
+@REM Bert-VITS2-masterの中身をStyle-Bert-VITS2に上書き移動
+xcopy /QSY .\Style-Bert-VITS2-master\ .\Style-Bert-VITS2\
+rmdir /s /q Style-Bert-VITS2-master
+if %errorlevel% neq 0 ( pause & popd & exit /b %errorlevel% )
+
+@REM 仮想環境のpip requirements.txtを更新
+
+echo call .\Style-Bert-VITS2\scripts\activate.bat
+call .\Style-Bert-VITS2\venv\Scripts\activate.bat
+if %errorlevel% neq 0 ( pause & popd & exit /b %errorlevel% )
+
+pip install -U -r Style-Bert-VITS2\requirements.txt
+if %errorlevel% neq 0 ( pause & popd & exit /b %errorlevel% )
+
+echo Style-Bert-VITS2のアップデートが完了しました。
+
+pause
+
+popd
\ No newline at end of file
diff --git a/scripts/Update-to-JP-Extra.bat b/scripts/Update-to-JP-Extra.bat
new file mode 100644
index 0000000000000000000000000000000000000000..95738c698dc7f7a77441bed363db6d60097d689f
--- /dev/null
+++ b/scripts/Update-to-JP-Extra.bat
@@ -0,0 +1,51 @@
+chcp 65001 > NUL
+@echo off
+
+pushd %~dp0
+set PS_CMD=PowerShell -Version 5.1 -ExecutionPolicy Bypass
+
+set CURL_CMD=C:\Windows\System32\curl.exe
+if not exist %CURL_CMD% (
+ echo [ERROR] %CURL_CMD% が見つかりません。
+ pause & popd & exit /b 1
+)
+
+@REM Style-Bert-VITS2.zip をGitHubのmasterの最新のものをダウンロード
+%CURL_CMD% -Lo Style-Bert-VITS2.zip^
+ https://github.com/litagin02/Style-Bert-VITS2/archive/refs/heads/master.zip
+if %errorlevel% neq 0 ( pause & popd & exit /b %errorlevel% )
+
+@REM Style-Bert-VITS2.zip を解凍(フォルダ名前がBert-VITS2-masterになる)
+%PS_CMD% Expand-Archive -Path Style-Bert-VITS2.zip -DestinationPath . -Force
+if %errorlevel% neq 0 ( pause & popd & exit /b %errorlevel% )
+
+@REM 元のzipを削除
+del Style-Bert-VITS2.zip
+if %errorlevel% neq 0 ( pause & popd & exit /b %errorlevel% )
+
+@REM Bert-VITS2-masterの中身をStyle-Bert-VITS2に上書き移動
+xcopy /QSY .\Style-Bert-VITS2-master\ .\Style-Bert-VITS2\
+rmdir /s /q Style-Bert-VITS2-master
+if %errorlevel% neq 0 ( pause & popd & exit /b %errorlevel% )
+
+@REM 仮想環境のpip requirements.txtを更新
+
+echo call .\Style-Bert-VITS2\scripts\activate.bat
+call .\Style-Bert-VITS2\venv\Scripts\activate.bat
+if %errorlevel% neq 0 ( pause & popd & exit /b %errorlevel% )
+
+pip install -U -r Style-Bert-VITS2\requirements.txt
+if %errorlevel% neq 0 ( pause & popd & exit /b %errorlevel% )
+
+pushd Style-Bert-VITS2
+
+@REM 初期化(必要なモデルのダウンロード)
+python initialize.py
+
+echo Style-Bert-VITS2の2.xへのアップデートが完了しました。
+
+pause
+
+popd
+
+popd
\ No newline at end of file
diff --git a/server_editor.py b/server_editor.py
new file mode 100644
index 0000000000000000000000000000000000000000..f859315acadcbd5a90ab4dd39b4d1407b688ba14
--- /dev/null
+++ b/server_editor.py
@@ -0,0 +1,414 @@
+"""
+Style-Bert-VITS2-Editor用のサーバー。
+次のリポジトリ
+https://github.com/litagin02/Style-Bert-VITS2-Editor
+をビルドしてできあがったファイルをWebフォルダに入れて実行する。
+
+TODO: リファクタリングやドキュメンテーションやAPI整理、辞書周りの改善などが必要。
+"""
+
+import argparse
+import io
+import shutil
+import sys
+import webbrowser
+import zipfile
+from datetime import datetime
+from io import BytesIO
+from pathlib import Path
+import yaml
+
+import numpy as np
+import pyopenjtalk
+import requests
+import torch
+import uvicorn
+from fastapi import APIRouter, FastAPI, HTTPException, status
+from fastapi.middleware.cors import CORSMiddleware
+from fastapi.responses import JSONResponse, Response
+from fastapi.staticfiles import StaticFiles
+from pydantic import BaseModel
+from scipy.io import wavfile
+
+from common.constants import (
+ DEFAULT_ASSIST_TEXT_WEIGHT,
+ DEFAULT_NOISE,
+ DEFAULT_NOISEW,
+ DEFAULT_SDP_RATIO,
+ DEFAULT_STYLE,
+ DEFAULT_STYLE_WEIGHT,
+ LATEST_VERSION,
+ Languages,
+)
+from common.log import logger
+from common.tts_model import ModelHolder
+from text.japanese import g2kata_tone, kata_tone2phone_tone
+from text.user_dict import apply_word, update_dict, read_dict, rewrite_word, delete_word
+
+
+# ---フロントエンド部分に関する処理---
+
+# エディターのビルドファイルを配置するディレクトリ
+STATIC_DIR = Path("static")
+# エディターの最新のビルドファイルのダウンロード日時を記録するファイル
+LAST_DOWNLOAD_FILE = STATIC_DIR / "last_download.txt"
+
+
+def download_static_files(user, repo, asset_name):
+ """Style-Bert-VITS2エディターの最新のビルドzipをダウンロードして展開する。"""
+
+ logger.info("Checking for new release...")
+ latest_release = get_latest_release(user, repo)
+ if latest_release is None:
+ logger.warning(
+ "Failed to fetch the latest release. Proceeding without static files."
+ )
+ return
+
+ if not new_release_available(latest_release):
+ logger.info("No new release available. Proceeding with existing static files.")
+ return
+
+ logger.info("New release available. Downloading static files...")
+ asset_url = get_asset_url(latest_release, asset_name)
+ if asset_url:
+ if STATIC_DIR.exists():
+ shutil.rmtree(STATIC_DIR)
+ STATIC_DIR.mkdir(parents=True, exist_ok=True)
+ download_and_extract(asset_url, STATIC_DIR)
+ save_last_download(latest_release)
+ else:
+ logger.warning("Asset not found. Proceeding without static files.")
+
+
+def get_latest_release(user, repo):
+ url = f"https://api.github.com/repos/{user}/{repo}/releases/latest"
+ try:
+ response = requests.get(url)
+ response.raise_for_status()
+ return response.json()
+ except requests.RequestException:
+ return None
+
+
+def get_asset_url(release, asset_name):
+ for asset in release["assets"]:
+ if asset["name"] == asset_name:
+ return asset["browser_download_url"]
+ return None
+
+
+def download_and_extract(url, extract_to: Path):
+ response = requests.get(url)
+ response.raise_for_status()
+ with zipfile.ZipFile(io.BytesIO(response.content)) as zip_ref:
+ zip_ref.extractall(extract_to)
+
+ # 展開先が1つのディレクトリだけの場合、その中身を直下に移動する
+ extracted_dirs = list(extract_to.iterdir())
+ if len(extracted_dirs) == 1 and extracted_dirs[0].is_dir():
+ for file in extracted_dirs[0].iterdir():
+ file.rename(extract_to / file.name)
+ extracted_dirs[0].rmdir()
+
+ # index.htmlが存在するかチェック
+ if not (extract_to / "index.html").exists():
+ logger.warning("index.html not found in the extracted files.")
+
+
+def new_release_available(latest_release):
+ if LAST_DOWNLOAD_FILE.exists():
+ with open(LAST_DOWNLOAD_FILE, "r") as file:
+ last_download_str = file.read().strip()
+ # 'Z'を除去して日時オブジェクトに変換
+ last_download_str = last_download_str.replace("Z", "+00:00")
+ last_download = datetime.fromisoformat(last_download_str)
+ return (
+ datetime.fromisoformat(
+ latest_release["published_at"].replace("Z", "+00:00")
+ )
+ > last_download
+ )
+ return True
+
+
+def save_last_download(latest_release):
+ with open(LAST_DOWNLOAD_FILE, "w") as file:
+ file.write(latest_release["published_at"])
+
+
+# ---フロントエンド部分に関する処理ここまで---
+# 以降はAPIの設定
+
+
+class AudioResponse(Response):
+ media_type = "audio/wav"
+
+
+origins = [
+ "http://localhost:3000",
+ "http://localhost:8000",
+ "http://127.0.0.1:3000",
+ "http://127.0.0.1:8000",
+]
+
+# Get path settings
+with open(Path("configs/paths.yml"), "r", encoding="utf-8") as f:
+ path_config: dict[str, str] = yaml.safe_load(f.read())
+ # dataset_root = path_config["dataset_root"]
+ assets_root = path_config["assets_root"]
+
+parser = argparse.ArgumentParser()
+parser.add_argument("--model_dir", type=str, default="model_assets/")
+parser.add_argument("--device", type=str, default="cuda")
+parser.add_argument("--port", type=int, default=8000)
+parser.add_argument("--inbrowser", action="store_true")
+parser.add_argument("--line_length", type=int, default=None)
+parser.add_argument("--line_count", type=int, default=None)
+parser.add_argument(
+ "--dir", "-d", type=str, help="Model directory", default=assets_root
+)
+
+args = parser.parse_args()
+device = args.device
+if device == "cuda" and not torch.cuda.is_available():
+ device = "cpu"
+model_dir = Path(args.model_dir)
+port = int(args.port)
+
+model_holder = ModelHolder(model_dir, device)
+if len(model_holder.model_names) == 0:
+ logger.error(f"Models not found in {model_dir}.")
+ sys.exit(1)
+
+app = FastAPI()
+
+
+app.add_middleware(
+ CORSMiddleware,
+ allow_origins=origins,
+ allow_credentials=True,
+ allow_methods=["*"],
+ allow_headers=["*"],
+)
+
+router = APIRouter()
+
+
+@router.get("/version")
+def version() -> str:
+ return LATEST_VERSION
+
+
+class MoraTone(BaseModel):
+ mora: str
+ tone: int
+
+
+class G2PRequest(BaseModel):
+ text: str
+
+
+@router.post("/g2p")
+async def read_item(item: G2PRequest):
+ try:
+ kata_tone_list = g2kata_tone(item.text, ignore_unknown=True)
+ except Exception as e:
+ raise HTTPException(
+ status_code=400,
+ detail=f"Failed to convert {item.text} to katakana and tone, {e}",
+ )
+ return [MoraTone(mora=kata, tone=tone) for kata, tone in kata_tone_list]
+
+
+@router.get("/models_info")
+def models_info():
+ return model_holder.models_info()
+
+
+class SynthesisRequest(BaseModel):
+ model: str
+ modelFile: str
+ text: str
+ moraToneList: list[MoraTone]
+ style: str = DEFAULT_STYLE
+ styleWeight: float = DEFAULT_STYLE_WEIGHT
+ assistText: str = ""
+ assistTextWeight: float = DEFAULT_ASSIST_TEXT_WEIGHT
+ speed: float = 1.0
+ noise: float = DEFAULT_NOISE
+ noisew: float = DEFAULT_NOISEW
+ sdpRatio: float = DEFAULT_SDP_RATIO
+ language: Languages = Languages.JP
+ silenceAfter: float = 0.5
+ pitchScale: float = 1.0
+ intonationScale: float = 1.0
+
+
+@router.post("/synthesis", response_class=AudioResponse)
+def synthesis(request: SynthesisRequest):
+ if args.line_length is not None and len(request.text) > args.line_length:
+ raise HTTPException(
+ status_code=400,
+ detail=f"1行の文字数は{args.line_length}文字以下にしてください。",
+ )
+ try:
+ model = model_holder.load_model(
+ model_name=request.model, model_path_str=request.modelFile
+ )
+ except Exception as e:
+ logger.error(e)
+ raise HTTPException(
+ status_code=500,
+ detail=f"Failed to load model {request.model} from {request.modelFile}, {e}",
+ )
+ text = request.text
+ kata_tone_list = [
+ (mora_tone.mora, mora_tone.tone) for mora_tone in request.moraToneList
+ ]
+ phone_tone = kata_tone2phone_tone(kata_tone_list)
+ tone = [t for _, t in phone_tone]
+ sr, audio = model.infer(
+ text=text,
+ language=request.language.value,
+ sdp_ratio=request.sdpRatio,
+ noise=request.noise,
+ noisew=request.noisew,
+ length=1 / request.speed,
+ given_tone=tone,
+ style=request.style,
+ style_weight=request.styleWeight,
+ assist_text=request.assistText,
+ assist_text_weight=request.assistTextWeight,
+ use_assist_text=bool(request.assistText),
+ line_split=False,
+ ignore_unknown=True,
+ pitch_scale=request.pitchScale,
+ intonation_scale=request.intonationScale,
+ )
+
+ with BytesIO() as wavContent:
+ wavfile.write(wavContent, sr, audio)
+ return Response(content=wavContent.getvalue(), media_type="audio/wav")
+
+
+class MultiSynthesisRequest(BaseModel):
+ lines: list[SynthesisRequest]
+
+
+@router.post("/multi_synthesis", response_class=AudioResponse)
+def multi_synthesis(request: MultiSynthesisRequest):
+ lines = request.lines
+ if args.line_count is not None and len(lines) > args.line_count:
+ raise HTTPException(
+ status_code=400,
+ detail=f"行数は{args.line_count}行以下にしてください。",
+ )
+ audios = []
+ for i, req in enumerate(lines):
+ if args.line_length is not None and len(req.text) > args.line_length:
+ raise HTTPException(
+ status_code=400,
+ detail=f"1行の文字数は{args.line_length}文字以下にしてください。",
+ )
+ try:
+ model = model_holder.load_model(
+ model_name=req.model, model_path_str=req.modelFile
+ )
+ except Exception as e:
+ logger.error(e)
+ raise HTTPException(
+ status_code=500,
+ detail=f"Failed to load model {req.model} from {req.modelFile}, {e}",
+ )
+ text = req.text
+ kata_tone_list = [
+ (mora_tone.mora, mora_tone.tone) for mora_tone in req.moraToneList
+ ]
+ phone_tone = kata_tone2phone_tone(kata_tone_list)
+ tone = [t for _, t in phone_tone]
+ sr, audio = model.infer(
+ text=text,
+ language=req.language.value,
+ sdp_ratio=req.sdpRatio,
+ noise=req.noise,
+ noisew=req.noisew,
+ length=1 / req.speed,
+ given_tone=tone,
+ style=req.style,
+ style_weight=req.styleWeight,
+ assist_text=req.assistText,
+ assist_text_weight=req.assistTextWeight,
+ use_assist_text=bool(req.assistText),
+ line_split=False,
+ ignore_unknown=True,
+ pitch_scale=req.pitchScale,
+ intonation_scale=req.intonationScale,
+ )
+ audios.append(audio)
+ if i < len(lines) - 1:
+ silence = int(sr * req.silenceAfter)
+ audios.append(np.zeros(silence, dtype=np.int16))
+ audio = np.concatenate(audios)
+
+ with BytesIO() as wavContent:
+ wavfile.write(wavContent, sr, audio)
+ return Response(content=wavContent.getvalue(), media_type="audio/wav")
+
+
+class UserDictWordRequest(BaseModel):
+ surface: str
+ pronunciation: str
+ accent_type: int # アクセント核位置(存在しない場合は0、1文字目は1)
+ priority: int = 5
+
+
+@router.get("/user_dict")
+def get_user_dict():
+ return read_dict()
+
+
+@router.post("/user_dict_word")
+def add_user_dict_word(request: UserDictWordRequest):
+ uuid = apply_word(
+ surface=request.surface,
+ pronunciation=request.pronunciation,
+ accent_type=request.accent_type,
+ priority=request.priority,
+ )
+ update_dict()
+
+ return JSONResponse(
+ status_code=status.HTTP_201_CREATED,
+ content={"uuid": uuid},
+ )
+
+
+@router.put("/user_dict_word/{uuid}")
+def update_user_dict_word(uuid: str, request: UserDictWordRequest):
+ rewrite_word(
+ word_uuid=uuid,
+ surface=request.surface,
+ pronunciation=request.pronunciation,
+ accent_type=request.accent_type,
+ priority=request.priority,
+ )
+ update_dict()
+ return JSONResponse(status_code=status.HTTP_200_OK, content={"uuid": uuid})
+
+
+@router.delete("/user_dict_word/{uuid}")
+def delete_user_dict_word(uuid: str):
+ delete_word(uuid)
+ update_dict()
+ return JSONResponse(status_code=status.HTTP_200_OK, content={"uuid": uuid})
+
+
+app.include_router(router, prefix="/api")
+
+if __name__ == "__main__":
+ download_static_files("litagin02", "Style-Bert-VITS2-Editor", "out.zip")
+ app.mount("/", StaticFiles(directory=STATIC_DIR, html=True), name="static")
+ if args.inbrowser:
+ webbrowser.open(f"http://localhost:{port}")
+ uvicorn.run(app, host="0.0.0.0", port=port)
diff --git a/server_fastapi.py b/server_fastapi.py
new file mode 100644
index 0000000000000000000000000000000000000000..ce6ed0432f856f5fa21117a5e214904394319ba7
--- /dev/null
+++ b/server_fastapi.py
@@ -0,0 +1,277 @@
+"""
+API server for TTS
+TODO: server_editor.pyと統合する?
+"""
+
+import argparse
+import os
+import sys
+from io import BytesIO
+from pathlib import Path
+from typing import Dict, Optional, Union
+from urllib.parse import unquote
+
+import GPUtil
+import psutil
+import torch
+import uvicorn
+from fastapi import FastAPI, HTTPException, Query, Request, status
+from fastapi.middleware.cors import CORSMiddleware
+from fastapi.responses import FileResponse, Response
+from scipy.io import wavfile
+
+from common.constants import (
+ DEFAULT_ASSIST_TEXT_WEIGHT,
+ DEFAULT_LENGTH,
+ DEFAULT_LINE_SPLIT,
+ DEFAULT_NOISE,
+ DEFAULT_NOISEW,
+ DEFAULT_SDP_RATIO,
+ DEFAULT_SPLIT_INTERVAL,
+ DEFAULT_STYLE,
+ DEFAULT_STYLE_WEIGHT,
+ Languages,
+)
+from common.log import logger
+from common.tts_model import Model, ModelHolder
+from config import config
+
+ln = config.server_config.language
+
+
+def raise_validation_error(msg: str, param: str):
+ logger.warning(f"Validation error: {msg}")
+ raise HTTPException(
+ status_code=status.HTTP_422_UNPROCESSABLE_ENTITY,
+ detail=[dict(type="invalid_params", msg=msg, loc=["query", param])],
+ )
+
+
+class AudioResponse(Response):
+ media_type = "audio/wav"
+
+
+def load_models(model_holder: ModelHolder):
+ model_holder.models = []
+ for model_name, model_paths in model_holder.model_files_dict.items():
+ model = Model(
+ model_path=model_paths[0],
+ config_path=model_holder.root_dir / model_name / "config.json",
+ style_vec_path=model_holder.root_dir / model_name / "style_vectors.npy",
+ device=model_holder.device,
+ )
+ model.load_net_g()
+ model_holder.models.append(model)
+
+
+if __name__ == "__main__":
+ parser = argparse.ArgumentParser()
+ parser.add_argument("--cpu", action="store_true", help="Use CPU instead of GPU")
+ parser.add_argument(
+ "--dir", "-d", type=str, help="Model directory", default=config.assets_root
+ )
+ args = parser.parse_args()
+
+ if args.cpu:
+ device = "cpu"
+ else:
+ device = "cuda" if torch.cuda.is_available() else "cpu"
+
+ model_dir = Path(args.dir)
+ model_holder = ModelHolder(model_dir, device)
+ if len(model_holder.model_names) == 0:
+ logger.error(f"Models not found in {model_dir}.")
+ sys.exit(1)
+
+ logger.info("Loading models...")
+ load_models(model_holder)
+ limit = config.server_config.limit
+ app = FastAPI()
+ allow_origins = config.server_config.origins
+ if allow_origins:
+ logger.warning(
+ f"CORS allow_origins={config.server_config.origins}. If you don't want, modify config.yml"
+ )
+ app.add_middleware(
+ CORSMiddleware,
+ allow_origins=config.server_config.origins,
+ allow_credentials=True,
+ allow_methods=["*"],
+ allow_headers=["*"],
+ )
+ app.logger = logger
+
+ @app.get("/voice", response_class=AudioResponse)
+ async def voice(
+ request: Request,
+ text: str = Query(..., min_length=1, max_length=limit, description=f"セリフ"),
+ encoding: str = Query(None, description="textをURLデコードする(ex, `utf-8`)"),
+ model_id: int = Query(
+ 0, description="モデルID。`GET /models/info`のkeyの値を指定ください"
+ ),
+ speaker_name: str = Query(
+ None,
+ description="話者名(speaker_idより優先)。esd.listの2列目の文字列を指定",
+ ),
+ speaker_id: int = Query(
+ 0, description="話者ID。model_assets>[model]>config.json内のspk2idを確認"
+ ),
+ sdp_ratio: float = Query(
+ DEFAULT_SDP_RATIO,
+ description="SDP(Stochastic Duration Predictor)/DP混合比。比率が高くなるほどトーンのばらつきが大きくなる",
+ ),
+ noise: float = Query(
+ DEFAULT_NOISE,
+ description="サンプルノイズの割合。大きくするほどランダム性が高まる",
+ ),
+ noisew: float = Query(
+ DEFAULT_NOISEW,
+ description="SDPノイズ。大きくするほど発音の間隔にばらつきが出やすくなる",
+ ),
+ length: float = Query(
+ DEFAULT_LENGTH,
+ description="話速。基準は1で大きくするほど音声は長くなり読み上げが遅まる",
+ ),
+ language: Languages = Query(ln, description=f"textの言語"),
+ auto_split: bool = Query(DEFAULT_LINE_SPLIT, description="改行で分けて生成"),
+ split_interval: float = Query(
+ DEFAULT_SPLIT_INTERVAL, description="分けた場合に挟む無音の長さ(秒)"
+ ),
+ assist_text: Optional[str] = Query(
+ None,
+ description="このテキストの読み上げと似た声音・感情になりやすくなる。ただし抑揚やテンポ等が犠牲になる傾向がある",
+ ),
+ assist_text_weight: float = Query(
+ DEFAULT_ASSIST_TEXT_WEIGHT, description="assist_textの強さ"
+ ),
+ style: Optional[Union[int, str]] = Query(DEFAULT_STYLE, description="スタイル"),
+ style_weight: float = Query(DEFAULT_STYLE_WEIGHT, description="スタイルの強さ"),
+ reference_audio_path: Optional[str] = Query(
+ None, description="スタイルを音声ファイルで行う"
+ ),
+ ):
+ """Infer text to speech(テキストから感情付き音声を生成する)"""
+ logger.info(
+ f"{request.client.host}:{request.client.port}/voice { unquote(str(request.query_params) )}"
+ )
+ if model_id >= len(
+ model_holder.models
+ ): # /models/refresh があるためQuery(le)で表現不可
+ raise_validation_error(f"model_id={model_id} not found", "model_id")
+
+ model = model_holder.models[model_id]
+ if speaker_name is None:
+ if speaker_id not in model.id2spk.keys():
+ raise_validation_error(
+ f"speaker_id={speaker_id} not found", "speaker_id"
+ )
+ else:
+ if speaker_name not in model.spk2id.keys():
+ raise_validation_error(
+ f"speaker_name={speaker_name} not found", "speaker_name"
+ )
+ speaker_id = model.spk2id[speaker_name]
+ if style not in model.style2id.keys():
+ raise_validation_error(f"style={style} not found", "style")
+ if encoding is not None:
+ text = unquote(text, encoding=encoding)
+ sr, audio = model.infer(
+ text=text,
+ language=language,
+ sid=speaker_id,
+ reference_audio_path=reference_audio_path,
+ sdp_ratio=sdp_ratio,
+ noise=noise,
+ noisew=noisew,
+ length=length,
+ line_split=auto_split,
+ split_interval=split_interval,
+ assist_text=assist_text,
+ assist_text_weight=assist_text_weight,
+ use_assist_text=bool(assist_text),
+ style=style,
+ style_weight=style_weight,
+ )
+ logger.success("Audio data generated and sent successfully")
+ with BytesIO() as wavContent:
+ wavfile.write(wavContent, sr, audio)
+ return Response(content=wavContent.getvalue(), media_type="audio/wav")
+
+ @app.get("/models/info")
+ def get_loaded_models_info():
+ """ロードされたモデル情報の取得"""
+
+ result: Dict[str, Dict] = dict()
+ for model_id, model in enumerate(model_holder.models):
+ result[str(model_id)] = {
+ "config_path": model.config_path,
+ "model_path": model.model_path,
+ "device": model.device,
+ "spk2id": model.spk2id,
+ "id2spk": model.id2spk,
+ "style2id": model.style2id,
+ }
+ return result
+
+ @app.post("/models/refresh")
+ def refresh():
+ """モデルをパスに追加/削除した際などに読み込ませる"""
+ model_holder.refresh()
+ load_models(model_holder)
+ return get_loaded_models_info()
+
+ @app.get("/status")
+ def get_status():
+ """実行環境のステータスを取得"""
+ cpu_percent = psutil.cpu_percent(interval=1)
+ memory_info = psutil.virtual_memory()
+ memory_total = memory_info.total
+ memory_available = memory_info.available
+ memory_used = memory_info.used
+ memory_percent = memory_info.percent
+ gpuInfo = []
+ devices = ["cpu"]
+ for i in range(torch.cuda.device_count()):
+ devices.append(f"cuda:{i}")
+ gpus = GPUtil.getGPUs()
+ for gpu in gpus:
+ gpuInfo.append(
+ {
+ "gpu_id": gpu.id,
+ "gpu_load": gpu.load,
+ "gpu_memory": {
+ "total": gpu.memoryTotal,
+ "used": gpu.memoryUsed,
+ "free": gpu.memoryFree,
+ },
+ }
+ )
+ return {
+ "devices": devices,
+ "cpu_percent": cpu_percent,
+ "memory_total": memory_total,
+ "memory_available": memory_available,
+ "memory_used": memory_used,
+ "memory_percent": memory_percent,
+ "gpu": gpuInfo,
+ }
+
+ @app.get("/tools/get_audio", response_class=AudioResponse)
+ def get_audio(
+ request: Request, path: str = Query(..., description="local wav path")
+ ):
+ """wavデータを取得する"""
+ logger.info(
+ f"{request.client.host}:{request.client.port}/tools/get_audio { unquote(str(request.query_params) )}"
+ )
+ if not os.path.isfile(path):
+ raise_validation_error(f"path={path} not found", "path")
+ if not path.lower().endswith(".wav"):
+ raise_validation_error(f"wav file not found in {path}", "path")
+ return FileResponse(path=path, media_type="audio/wav")
+
+ logger.info(f"server listen: http://127.0.0.1:{config.server_config.port}")
+ logger.info(f"API docs: http://127.0.0.1:{config.server_config.port}/docs")
+ uvicorn.run(
+ app, port=config.server_config.port, host="0.0.0.0", log_level="warning"
+ )
diff --git a/slice.py b/slice.py
new file mode 100644
index 0000000000000000000000000000000000000000..fc5ccbd24f3cc37848f1f7531e18bae8fcf8d2cf
--- /dev/null
+++ b/slice.py
@@ -0,0 +1,160 @@
+import argparse
+import os
+import shutil
+from pathlib import Path
+
+import soundfile as sf
+import torch
+import yaml
+from tqdm import tqdm
+
+from common.log import logger
+from common.stdout_wrapper import SAFE_STDOUT
+
+vad_model, utils = torch.hub.load(
+ repo_or_dir="snakers4/silero-vad",
+ model="silero_vad",
+ onnx=True,
+ trust_repo=True,
+)
+
+(get_speech_timestamps, _, read_audio, *_) = utils
+
+
+def get_stamps(
+ audio_file, min_silence_dur_ms: int = 700, min_sec: float = 2, max_sec: float = 12
+):
+ """
+ min_silence_dur_ms: int (ミリ秒):
+ このミリ秒数以上を無音だと判断する。
+ 逆に、この秒数以下の無音区間では区切られない。
+ 小さくすると、音声がぶつ切りに小さくなりすぎ、
+ 大きくすると音声一つ一つが長くなりすぎる。
+ データセットによってたぶん要調整。
+ min_sec: float (秒):
+ この秒数より小さい発話は無視する。
+ max_sec: float (秒):
+ この秒数より大きい発話は無視する。
+ """
+
+ sampling_rate = 16000 # 16kHzか8kHzのみ対応
+
+ min_ms = int(min_sec * 1000)
+
+ wav = read_audio(audio_file, sampling_rate=sampling_rate)
+ speech_timestamps = get_speech_timestamps(
+ wav,
+ vad_model,
+ sampling_rate=sampling_rate,
+ min_silence_duration_ms=min_silence_dur_ms,
+ min_speech_duration_ms=min_ms,
+ max_speech_duration_s=max_sec,
+ )
+
+ return speech_timestamps
+
+
+def split_wav(
+ audio_file,
+ target_dir="raw",
+ min_sec=2,
+ max_sec=12,
+ min_silence_dur_ms=700,
+):
+ margin = 200 # ミリ秒単位で、音声の前後に余裕を持たせる
+ speech_timestamps = get_stamps(
+ audio_file,
+ min_silence_dur_ms=min_silence_dur_ms,
+ min_sec=min_sec,
+ max_sec=max_sec,
+ )
+
+ data, sr = sf.read(audio_file)
+
+ total_ms = len(data) / sr * 1000
+
+ file_name = os.path.basename(audio_file).split(".")[0]
+ os.makedirs(target_dir, exist_ok=True)
+
+ total_time_ms = 0
+ count = 0
+
+ # タイムスタンプに従って分割し、ファイルに保存
+ for i, ts in enumerate(speech_timestamps):
+ start_ms = max(ts["start"] / 16 - margin, 0)
+ end_ms = min(ts["end"] / 16 + margin, total_ms)
+
+ start_sample = int(start_ms / 1000 * sr)
+ end_sample = int(end_ms / 1000 * sr)
+ segment = data[start_sample:end_sample]
+
+ sf.write(os.path.join(target_dir, f"{file_name}-{i}.wav"), segment, sr)
+ total_time_ms += end_ms - start_ms
+ count += 1
+
+ return total_time_ms / 1000, count
+
+
+if __name__ == "__main__":
+ parser = argparse.ArgumentParser()
+ parser.add_argument(
+ "--min_sec", "-m", type=float, default=2, help="Minimum seconds of a slice"
+ )
+ parser.add_argument(
+ "--max_sec", "-M", type=float, default=12, help="Maximum seconds of a slice"
+ )
+ parser.add_argument(
+ "--input_dir",
+ "-i",
+ type=str,
+ default="inputs",
+ help="Directory of input wav files",
+ )
+ parser.add_argument(
+ "--model_name",
+ type=str,
+ required=True,
+ help="The result will be in Data/{model_name}/raw/ (if Data is dataset_root in configs/paths.yml)",
+ )
+ parser.add_argument(
+ "--min_silence_dur_ms",
+ "-s",
+ type=int,
+ default=700,
+ help="Silence above this duration (ms) is considered as a split point.",
+ )
+ args = parser.parse_args()
+
+ with open(os.path.join("configs", "paths.yml"), "r", encoding="utf-8") as f:
+ path_config: dict[str, str] = yaml.safe_load(f.read())
+ dataset_root = path_config["dataset_root"]
+
+ input_dir = args.input_dir
+ output_dir = os.path.join(dataset_root, args.model_name, "raw")
+ min_sec = args.min_sec
+ max_sec = args.max_sec
+ min_silence_dur_ms = args.min_silence_dur_ms
+
+ wav_files = Path(input_dir).glob("**/*.wav")
+ wav_files = list(wav_files)
+ logger.info(f"Found {len(wav_files)} wav files.")
+ if os.path.exists(output_dir):
+ logger.warning(f"Output directory {output_dir} already exists, deleting...")
+ shutil.rmtree(output_dir)
+
+ total_sec = 0
+ total_count = 0
+ for wav_file in tqdm(wav_files, file=SAFE_STDOUT):
+ time_sec, count = split_wav(
+ audio_file=str(wav_file),
+ target_dir=output_dir,
+ min_sec=min_sec,
+ max_sec=max_sec,
+ min_silence_dur_ms=min_silence_dur_ms,
+ )
+ total_sec += time_sec
+ total_count += count
+
+ logger.info(
+ f"Slice done! Total time: {total_sec / 60:.2f} min, {total_count} files."
+ )
diff --git a/slm/wavlm-base-plus/.gitattributes b/slm/wavlm-base-plus/.gitattributes
new file mode 100644
index 0000000000000000000000000000000000000000..957b2579c6ef20995a09efd9a17f8fd90606f5ed
--- /dev/null
+++ b/slm/wavlm-base-plus/.gitattributes
@@ -0,0 +1,27 @@
+*.7z filter=lfs diff=lfs merge=lfs -text
+*.arrow filter=lfs diff=lfs merge=lfs -text
+*.bin filter=lfs diff=lfs merge=lfs -text
+*.bin.* filter=lfs diff=lfs merge=lfs -text
+*.bz2 filter=lfs diff=lfs merge=lfs -text
+*.ftz filter=lfs diff=lfs merge=lfs -text
+*.gz filter=lfs diff=lfs merge=lfs -text
+*.h5 filter=lfs diff=lfs merge=lfs -text
+*.joblib filter=lfs diff=lfs merge=lfs -text
+*.lfs.* filter=lfs diff=lfs merge=lfs -text
+*.model filter=lfs diff=lfs merge=lfs -text
+*.msgpack filter=lfs diff=lfs merge=lfs -text
+*.onnx filter=lfs diff=lfs merge=lfs -text
+*.ot filter=lfs diff=lfs merge=lfs -text
+*.parquet filter=lfs diff=lfs merge=lfs -text
+*.pb filter=lfs diff=lfs merge=lfs -text
+*.pt filter=lfs diff=lfs merge=lfs -text
+*.pth filter=lfs diff=lfs merge=lfs -text
+*.rar filter=lfs diff=lfs merge=lfs -text
+saved_model/**/* filter=lfs diff=lfs merge=lfs -text
+*.tar.* filter=lfs diff=lfs merge=lfs -text
+*.tflite filter=lfs diff=lfs merge=lfs -text
+*.tgz filter=lfs diff=lfs merge=lfs -text
+*.xz filter=lfs diff=lfs merge=lfs -text
+*.zip filter=lfs diff=lfs merge=lfs -text
+*.zstandard filter=lfs diff=lfs merge=lfs -text
+*tfevents* filter=lfs diff=lfs merge=lfs -text
diff --git a/slm/wavlm-base-plus/README.md b/slm/wavlm-base-plus/README.md
new file mode 100644
index 0000000000000000000000000000000000000000..541b2e0cc438f3bcb05aa363b09e62f722c87cab
--- /dev/null
+++ b/slm/wavlm-base-plus/README.md
@@ -0,0 +1,65 @@
+---
+language:
+- en
+datasets:
+tags:
+- speech
+inference: false
+---
+
+# WavLM-Base-Plus
+
+[Microsoft's WavLM](https://github.com/microsoft/unilm/tree/master/wavlm)
+
+The base model pretrained on 16kHz sampled speech audio. When using the model, make sure that your speech input is also sampled at 16kHz.
+
+**Note**: This model does not have a tokenizer as it was pretrained on audio alone. In order to use this model **speech recognition**, a tokenizer should be created and the model should be fine-tuned on labeled text data. Check out [this blog](https://huggingface.co/blog/fine-tune-wav2vec2-english) for more in-detail explanation of how to fine-tune the model.
+
+The model was pre-trained on:
+
+- 60,000 hours of [Libri-Light](https://arxiv.org/abs/1912.07875)
+- 10,000 hours of [GigaSpeech](https://arxiv.org/abs/2106.06909)
+- 24,000 hours of [VoxPopuli](https://arxiv.org/abs/2101.00390)
+
+[Paper: WavLM: Large-Scale Self-Supervised Pre-Training for Full Stack Speech Processing](https://arxiv.org/abs/2110.13900)
+
+Authors: Sanyuan Chen, Chengyi Wang, Zhengyang Chen, Yu Wu, Shujie Liu, Zhuo Chen, Jinyu Li, Naoyuki Kanda, Takuya Yoshioka, Xiong Xiao, Jian Wu, Long Zhou, Shuo Ren, Yanmin Qian, Yao Qian, Jian Wu, Michael Zeng, Furu Wei
+
+**Abstract**
+*Self-supervised learning (SSL) achieves great success in speech recognition, while limited exploration has been attempted for other speech processing tasks. As speech signal contains multi-faceted information including speaker identity, paralinguistics, spoken content, etc., learning universal representations for all speech tasks is challenging. In this paper, we propose a new pre-trained model, WavLM, to solve full-stack downstream speech tasks. WavLM is built based on the HuBERT framework, with an emphasis on both spoken content modeling and speaker identity preservation. We first equip the Transformer structure with gated relative position bias to improve its capability on recognition tasks. For better speaker discrimination, we propose an utterance mixing training strategy, where additional overlapped utterances are created unsupervisely and incorporated during model training. Lastly, we scale up the training dataset from 60k hours to 94k hours. WavLM Large achieves state-of-the-art performance on the SUPERB benchmark, and brings significant improvements for various speech processing tasks on their representative benchmarks.*
+
+The original model can be found under https://github.com/microsoft/unilm/tree/master/wavlm.
+
+# Usage
+
+This is an English pre-trained speech model that has to be fine-tuned on a downstream task like speech recognition or audio classification before it can be
+used in inference. The model was pre-trained in English and should therefore perform well only in English. The model has been shown to work well on the [SUPERB benchmark](https://superbbenchmark.org/).
+
+**Note**: The model was pre-trained on phonemes rather than characters. This means that one should make sure that the input text is converted to a sequence
+of phonemes before fine-tuning.
+
+## Speech Recognition
+
+To fine-tune the model for speech recognition, see [the official speech recognition example](https://github.com/huggingface/transformers/tree/master/examples/pytorch/speech-recognition).
+
+## Speech Classification
+
+To fine-tune the model for speech classification, see [the official audio classification example](https://github.com/huggingface/transformers/tree/master/examples/pytorch/audio-classification).
+
+## Speaker Verification
+
+TODO
+
+## Speaker Diarization
+
+TODO
+
+# Contribution
+
+The model was contributed by [cywang](https://huggingface.co/cywang) and [patrickvonplaten](https://huggingface.co/patrickvonplaten).
+
+# License
+
+The official license can be found [here](https://github.com/microsoft/UniSpeech/blob/main/LICENSE)
+
+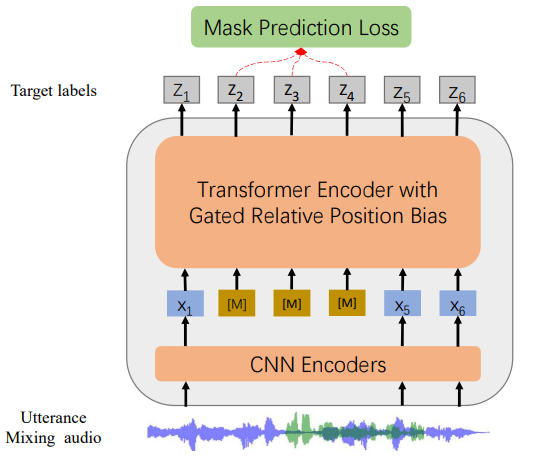
diff --git a/slm/wavlm-base-plus/config.json b/slm/wavlm-base-plus/config.json
new file mode 100644
index 0000000000000000000000000000000000000000..b7b4e5f6c410f71283a59e26250e40855ca99310
--- /dev/null
+++ b/slm/wavlm-base-plus/config.json
@@ -0,0 +1,99 @@
+{
+ "_name_or_path": "wavlm-base-plus",
+ "activation_dropout": 0.0,
+ "adapter_kernel_size": 3,
+ "adapter_stride": 2,
+ "add_adapter": false,
+ "apply_spec_augment": true,
+ "architectures": [
+ "WavLMModel"
+ ],
+ "attention_dropout": 0.1,
+ "bos_token_id": 1,
+ "classifier_proj_size": 256,
+ "codevector_dim": 256,
+ "contrastive_logits_temperature": 0.1,
+ "conv_bias": false,
+ "conv_dim": [
+ 512,
+ 512,
+ 512,
+ 512,
+ 512,
+ 512,
+ 512
+ ],
+ "conv_kernel": [
+ 10,
+ 3,
+ 3,
+ 3,
+ 3,
+ 2,
+ 2
+ ],
+ "conv_stride": [
+ 5,
+ 2,
+ 2,
+ 2,
+ 2,
+ 2,
+ 2
+ ],
+ "ctc_loss_reduction": "sum",
+ "ctc_zero_infinity": false,
+ "diversity_loss_weight": 0.1,
+ "do_stable_layer_norm": false,
+ "eos_token_id": 2,
+ "feat_extract_activation": "gelu",
+ "feat_extract_norm": "group",
+ "feat_proj_dropout": 0.1,
+ "feat_quantizer_dropout": 0.0,
+ "final_dropout": 0.0,
+ "freeze_feat_extract_train": true,
+ "hidden_act": "gelu",
+ "hidden_dropout": 0.1,
+ "hidden_size": 768,
+ "initializer_range": 0.02,
+ "intermediate_size": 3072,
+ "layer_norm_eps": 1e-05,
+ "layerdrop": 0.05,
+ "mask_channel_length": 10,
+ "mask_channel_min_space": 1,
+ "mask_channel_other": 0.0,
+ "mask_channel_prob": 0.0,
+ "mask_channel_selection": "static",
+ "mask_feature_length": 10,
+ "mask_feature_min_masks": 0,
+ "mask_feature_prob": 0.0,
+ "mask_time_length": 10,
+ "mask_time_min_masks": 2,
+ "mask_time_min_space": 1,
+ "mask_time_other": 0.0,
+ "mask_time_prob": 0.05,
+ "mask_time_selection": "static",
+ "model_type": "wavlm",
+ "no_mask_channel_overlap": false,
+ "no_mask_time_overlap": false,
+ "num_adapter_layers": 3,
+ "num_attention_heads": 12,
+ "num_buckets": 320,
+ "num_codevector_groups": 2,
+ "num_codevectors_per_group": 320,
+ "num_conv_pos_embedding_groups": 16,
+ "num_conv_pos_embeddings": 128,
+ "num_ctc_classes": 80,
+ "num_feat_extract_layers": 7,
+ "num_hidden_layers": 12,
+ "num_negatives": 100,
+ "output_hidden_size": 768,
+ "pad_token_id": 0,
+ "proj_codevector_dim": 256,
+ "replace_prob": 0.5,
+ "torch_dtype": "float32",
+ "transformers_version": "4.13.0.dev0",
+ "use_weighted_layer_sum": false,
+ "vocab_size": 32,
+ "tokenizer_class": "Wav2Vec2CTCTokenizer"
+}
diff --git a/slm/wavlm-base-plus/preprocessor_config.json b/slm/wavlm-base-plus/preprocessor_config.json
new file mode 100644
index 0000000000000000000000000000000000000000..10f6def8c83d70a2b087a567dcf523b75152a80b
--- /dev/null
+++ b/slm/wavlm-base-plus/preprocessor_config.json
@@ -0,0 +1,9 @@
+{
+ "do_normalize": false,
+ "feature_extractor_type": "Wav2Vec2FeatureExtractor",
+ "feature_size": 1,
+ "padding_side": "right",
+ "padding_value": 0.0,
+ "return_attention_mask": true,
+ "sampling_rate": 16000
+}
diff --git a/spec_gen.py b/spec_gen.py
new file mode 100644
index 0000000000000000000000000000000000000000..b6715faff6fd9abdae8197fcc0e0aac1d50fde4a
--- /dev/null
+++ b/spec_gen.py
@@ -0,0 +1,87 @@
+import torch
+from tqdm import tqdm
+from multiprocessing import Pool
+from mel_processing import spectrogram_torch, mel_spectrogram_torch
+from utils import load_wav_to_torch
+
+
+class AudioProcessor:
+ def __init__(
+ self,
+ max_wav_value,
+ use_mel_spec_posterior,
+ filter_length,
+ n_mel_channels,
+ sampling_rate,
+ hop_length,
+ win_length,
+ mel_fmin,
+ mel_fmax,
+ ):
+ self.max_wav_value = max_wav_value
+ self.use_mel_spec_posterior = use_mel_spec_posterior
+ self.filter_length = filter_length
+ self.n_mel_channels = n_mel_channels
+ self.sampling_rate = sampling_rate
+ self.hop_length = hop_length
+ self.win_length = win_length
+ self.mel_fmin = mel_fmin
+ self.mel_fmax = mel_fmax
+
+ def process_audio(self, filename):
+ audio, sampling_rate = load_wav_to_torch(filename)
+ audio_norm = audio / self.max_wav_value
+ audio_norm = audio_norm.unsqueeze(0)
+ spec_filename = filename.replace(".wav", ".spec.pt")
+ if self.use_mel_spec_posterior:
+ spec_filename = spec_filename.replace(".spec.pt", ".mel.pt")
+ try:
+ spec = torch.load(spec_filename)
+ except:
+ if self.use_mel_spec_posterior:
+ spec = mel_spectrogram_torch(
+ audio_norm,
+ self.filter_length,
+ self.n_mel_channels,
+ self.sampling_rate,
+ self.hop_length,
+ self.win_length,
+ self.mel_fmin,
+ self.mel_fmax,
+ center=False,
+ )
+ else:
+ spec = spectrogram_torch(
+ audio_norm,
+ self.filter_length,
+ self.sampling_rate,
+ self.hop_length,
+ self.win_length,
+ center=False,
+ )
+ spec = torch.squeeze(spec, 0)
+ torch.save(spec, spec_filename)
+ return spec, audio_norm
+
+
+# 使用示例
+processor = AudioProcessor(
+ max_wav_value=32768.0,
+ use_mel_spec_posterior=False,
+ filter_length=2048,
+ n_mel_channels=128,
+ sampling_rate=44100,
+ hop_length=512,
+ win_length=2048,
+ mel_fmin=0.0,
+ mel_fmax="null",
+)
+
+with open("filelists/train.list", "r") as f:
+ filepaths = [line.split("|")[0] for line in f] # 取每一行的第一部分作为audiopath
+
+# 使用多进程处理
+with Pool(processes=32) as pool: # 使用4个进程
+ with tqdm(total=len(filepaths)) as pbar:
+ for i, _ in enumerate(pool.imap_unordered(processor.process_audio, filepaths)):
+ pbar.update()
diff --git a/speech_mos.py b/speech_mos.py
new file mode 100644
index 0000000000000000000000000000000000000000..d69a23ab552e63f5af06768ef961eeeaed011cf6
--- /dev/null
+++ b/speech_mos.py
@@ -0,0 +1,158 @@
+import argparse
+import csv
+import re
+import warnings
+from pathlib import Path
+
+import matplotlib.pyplot as plt
+import numpy as np
+import pandas as pd
+import torch
+from tqdm import tqdm
+
+from common.log import logger
+from common.tts_model import Model
+from config import config
+
+warnings.filterwarnings("ignore")
+
+mos_result_dir = Path("mos_results")
+mos_result_dir.mkdir(exist_ok=True)
+
+test_texts = [
+ # JVNVコーパスのテキスト
+ # https://sites.google.com/site/shinnosuketakamichi/research-topics/jvnv_corpus
+ # CC BY-SA 4.0
+ "ああ?どうしてこんなに荒々しい態度をとるんだ?落ち着いて話を聞けばいいのに。",
+ "いや、あんな醜い人間を見るのは本当に嫌だ。",
+ "うわ、不景気の影響で失業してしまうかもしれない。どうしよう、心配で眠れない。",
+ "今日の山登りは最高だった!山頂で見た景色は言葉に表せないほど美しかった!あはは、絶頂の喜びが胸に溢れるよ!",
+ "あーあ、昨日の事故で大切な車が全損になっちゃった。もうどうしようもないよ。",
+ "ああ、彼は本当に速い!ダッシュの速さは尋常じゃない!",
+ # 以下app.pyの説明文章
+ "音声合成は、機械学習を活用して、テキストから人の声を再現する技術です。この技術は、言語の構造を解析し、それに基づいて音声を生成します。",
+ "この分野の最新の研究成果を使うと、より自然で表現豊かな音声の生成が可能である。深層学習の応用により、感情やアクセントを含む声質の微妙な変化も再現することが出来る。",
+]
+
+predictor = torch.hub.load(
+ "tarepan/SpeechMOS:v1.2.0", "utmos22_strong", trust_repo=True
+)
+
+parser = argparse.ArgumentParser()
+parser.add_argument("--model_name", "-m", type=str, required=True)
+parser.add_argument("--device", "-d", type=str, default="cuda")
+
+args = parser.parse_args()
+
+model_name: str = args.model_name
+device: str = args.device
+
+model_path = Path(config.assets_root) / model_name
+
+# .safetensorsファイルを検索
+safetensors_files = model_path.glob("*.safetensors")
+
+
+def get_model(model_file: Path):
+ return Model(
+ model_path=str(model_file),
+ config_path=str(model_file.parent / "config.json"),
+ style_vec_path=str(model_file.parent / "style_vectors.npy"),
+ device=device,
+ )
+
+
+results = []
+
+safetensors_files = list(safetensors_files)
+
+logger.info(f"There are {len(safetensors_files)} models.")
+
+for model_file in tqdm(safetensors_files):
+ # `test_e10_s1000.safetensors`` -> 1000を取り出す
+ match = re.search(r"_s(\d+)\.safetensors$", model_file.name)
+ if match:
+ step = int(match.group(1))
+ else:
+ logger.warning(f"Step count not found in {model_file.name}, so skip it.")
+ continue
+ model = get_model(model_file)
+ scores = []
+ for i, text in enumerate(test_texts):
+ sr, audio = model.infer(text)
+ audio = audio.astype("float32")
+ score = predictor(torch.from_numpy(audio).unsqueeze(0), sr).item()
+ scores.append(score)
+ logger.info(f"score: {score}")
+ results.append((model_file.name, step, scores))
+ del model
+
+logger.success("All models have been evaluated:")
+# meanを計算
+results = [
+ (model_file, step, scores + [np.mean(scores)])
+ for model_file, step, scores in results
+]
+# meanでソートして表示
+results = sorted(results, key=lambda x: x[2][-1], reverse=True)
+for model_file, step, scores in results:
+ logger.info(f"{model_file}: {scores[-1]}")
+
+with open(
+ mos_result_dir / f"mos_{model_name}.csv", "w", encoding="utf_8_sig", newline=""
+) as f:
+ writer = csv.writer(f)
+ writer.writerow(["model_path"] + ["step"] + test_texts + ["mean"])
+ for model_file, step, scores in results:
+ writer.writerow([model_file] + [step] + scores)
+
+logger.info(f"mos_{model_name}.csv has been saved.")
+
+# step countと各MOSの値を格納するリストを初期化
+steps = []
+mos_values = []
+
+# resultsからデータを抽出
+for _, step, scores in results:
+ steps.append(step)
+ mos_values.append(scores) # scores は MOS1, MOS2, MOS3,..., mean のリスト
+
+# DataFrame形式に変換
+df = pd.DataFrame(mos_values, index=steps)
+# ステップ数でソート
+df = df.sort_index()
+
+plt.figure(figsize=(10, 5))
+
+# 各MOSについての折れ線グラフを描画(最後の平均値の列は除外)
+for col in range(len(df.columns) - 1):
+ plt.plot(df.index, df.iloc[:, col], label=f"MOS{col + 1}")
+
+# 既存の平均値の列を使用
+plt.plot(df.index, df.iloc[:, -1], label="Mean", color="black", linewidth=2)
+
+
+# グラフのタイトルと軸ラベルを設定
+plt.title("TTS Model Naturalness MOS")
+plt.xlabel("Step Count")
+plt.ylabel("MOS")
+
+# ステップ数の軸ラベルを1000単位で表示するように調整
+plt.xticks(
+ ticks=np.arange(0, max(steps) + 1000, 2000),
+ labels=[f"{int(x/1000)}" for x in np.arange(0, max(steps) + 1000, 2000)],
+)
+
+# 縦の補助線を追加
+plt.grid(True, axis="x")
+
+# 凡例をグラフの右外側に配置
+plt.legend(loc="upper left", bbox_to_anchor=(1, 1))
+
+plt.tight_layout()
+
+# グラフを画像として保存(plt.show() の前に実行する)
+plt.savefig(mos_result_dir / f"mos_{model_name}.png")
+
+# グラフを表示
+plt.show()
diff --git a/style_gen.py b/style_gen.py
new file mode 100644
index 0000000000000000000000000000000000000000..97a0aee83421ac7cbbaa0c93621915b490930ba8
--- /dev/null
+++ b/style_gen.py
@@ -0,0 +1,128 @@
+import argparse
+from concurrent.futures import ThreadPoolExecutor
+import warnings
+
+import numpy as np
+import torch
+from tqdm import tqdm
+
+import utils
+from common.log import logger
+from common.stdout_wrapper import SAFE_STDOUT
+from config import config
+
+warnings.filterwarnings("ignore", category=UserWarning)
+from pyannote.audio import Inference, Model
+
+model = Model.from_pretrained("pyannote/wespeaker-voxceleb-resnet34-LM")
+inference = Inference(model, window="whole")
+device = torch.device(config.style_gen_config.device)
+inference.to(device)
+
+
+class NaNValueError(ValueError):
+ """カスタム例外クラス。NaN値が見つかった場合に使用されます。"""
+
+ pass
+
+
+# 推論時にインポートするために短いが関数を書く
+def get_style_vector(wav_path):
+ return inference(wav_path)
+
+
+def save_style_vector(wav_path):
+ try:
+ style_vec = get_style_vector(wav_path)
+ except Exception as e:
+ print("\n")
+ logger.error(f"Error occurred with file: {wav_path}, Details:\n{e}\n")
+ raise
+ # 値にNaNが含まれていると悪影響なのでチェックする
+ if np.isnan(style_vec).any():
+ print("\n")
+ logger.warning(f"NaN value found in style vector: {wav_path}")
+ raise NaNValueError(f"NaN value found in style vector: {wav_path}")
+ np.save(f"{wav_path}.npy", style_vec) # `test.wav` -> `test.wav.npy`
+
+
+def process_line(line):
+ wavname = line.split("|")[0]
+ try:
+ save_style_vector(wavname)
+ return line, None
+ except NaNValueError:
+ return line, "nan_error"
+
+
+def save_average_style_vector(style_vectors, filename="style_vectors.npy"):
+ average_vector = np.mean(style_vectors, axis=0)
+ np.save(filename, average_vector)
+
+
+if __name__ == "__main__":
+ parser = argparse.ArgumentParser()
+ parser.add_argument(
+ "-c", "--config", type=str, default=config.style_gen_config.config_path
+ )
+ parser.add_argument(
+ "--num_processes", type=int, default=config.style_gen_config.num_processes
+ )
+ args, _ = parser.parse_known_args()
+ config_path = args.config
+ num_processes = args.num_processes
+
+ hps = utils.get_hparams_from_file(config_path)
+
+ device = config.style_gen_config.device
+
+ training_lines = []
+ with open(hps.data.training_files, encoding="utf-8") as f:
+ training_lines.extend(f.readlines())
+ with ThreadPoolExecutor(max_workers=num_processes) as executor:
+ training_results = list(
+ tqdm(
+ executor.map(process_line, training_lines),
+ total=len(training_lines),
+ file=SAFE_STDOUT,
+ )
+ )
+ ok_training_lines = [line for line, error in training_results if error is None]
+ nan_training_lines = [
+ line for line, error in training_results if error == "nan_error"
+ ]
+ if nan_training_lines:
+ nan_files = [line.split("|")[0] for line in nan_training_lines]
+ logger.warning(
+ f"Found NaN value in {len(nan_training_lines)} files: {nan_files}, so they will be deleted from training data."
+ )
+
+ val_lines = []
+ with open(hps.data.validation_files, encoding="utf-8") as f:
+ val_lines.extend(f.readlines())
+
+ with ThreadPoolExecutor(max_workers=num_processes) as executor:
+ val_results = list(
+ tqdm(
+ executor.map(process_line, val_lines),
+ total=len(val_lines),
+ file=SAFE_STDOUT,
+ )
+ )
+ ok_val_lines = [line for line, error in val_results if error is None]
+ nan_val_lines = [line for line, error in val_results if error == "nan_error"]
+ if nan_val_lines:
+ nan_files = [line.split("|")[0] for line in nan_val_lines]
+ logger.warning(
+ f"Found NaN value in {len(nan_val_lines)} files: {nan_files}, so they will be deleted from validation data."
+ )
+
+ with open(hps.data.training_files, "w", encoding="utf-8") as f:
+ f.writelines(ok_training_lines)
+
+ with open(hps.data.validation_files, "w", encoding="utf-8") as f:
+ f.writelines(ok_val_lines)
+
+ ok_num = len(ok_training_lines) + len(ok_val_lines)
+
+ logger.info(f"Finished generating style vectors! total: {ok_num} npy files.")
diff --git a/text/__init__.py b/text/__init__.py
new file mode 100644
index 0000000000000000000000000000000000000000..2151cafc5c42b82f9a7acc155d9f74c754c965bb
--- /dev/null
+++ b/text/__init__.py
@@ -0,0 +1,45 @@
+from text.symbols import *
+
+_symbol_to_id = {s: i for i, s in enumerate(symbols)}
+
+
+def cleaned_text_to_sequence(cleaned_text, tones, language):
+ """Converts a string of text to a sequence of IDs corresponding to the symbols in the text.
+ Args:
+ text: string to convert to a sequence
+ Returns:
+ List of integers corresponding to the symbols in the text
+ """
+ phones = [_symbol_to_id[symbol] for symbol in cleaned_text]
+ tone_start = language_tone_start_map[language]
+ tones = [i + tone_start for i in tones]
+ lang_id = language_id_map[language]
+ lang_ids = [lang_id for i in phones]
+ return phones, tones, lang_ids
+
+
+def get_bert(
+ text,
+ word2ph,
+ language,
+ device,
+ assist_text=None,
+ assist_text_weight=0.7,
+ ignore_unknown=False,
+):
+ if language == "ZH":
+ from .chinese_bert import get_bert_feature as zh_bert
+
+ return zh_bert(text, word2ph, device, assist_text, assist_text_weight)
+ elif language == "EN":
+ from .english_bert_mock import get_bert_feature as en_bert
+
+ return en_bert(text, word2ph, device, assist_text, assist_text_weight)
+ elif language == "JP":
+ from .japanese_bert import get_bert_feature as jp_bert
+
+ return jp_bert(
+ text, word2ph, device, assist_text, assist_text_weight, ignore_unknown
+ )
+ else:
+ raise ValueError(f"Language {language} not supported")
diff --git a/text/chinese.py b/text/chinese.py
new file mode 100644
index 0000000000000000000000000000000000000000..d9174ee0e194497bdcace2e4d99977f8dce0a528
--- /dev/null
+++ b/text/chinese.py
@@ -0,0 +1,199 @@
+import os
+import re
+
+import cn2an
+from pypinyin import lazy_pinyin, Style
+
+from text.symbols import punctuation
+from text.tone_sandhi import ToneSandhi
+
+current_file_path = os.path.dirname(__file__)
+pinyin_to_symbol_map = {
+ line.split("\t")[0]: line.strip().split("\t")[1]
+ for line in open(os.path.join(current_file_path, "opencpop-strict.txt")).readlines()
+}
+
+import jieba.posseg as psg
+
+
+rep_map = {
+ ":": ",",
+ ";": ",",
+ ",": ",",
+ "。": ".",
+ "!": "!",
+ "?": "?",
+ "\n": ".",
+ "·": ",",
+ "、": ",",
+ "...": "…",
+ "$": ".",
+ "“": "'",
+ "”": "'",
+ '"': "'",
+ "‘": "'",
+ "’": "'",
+ "(": "'",
+ ")": "'",
+ "(": "'",
+ ")": "'",
+ "《": "'",
+ "》": "'",
+ "【": "'",
+ "】": "'",
+ "[": "'",
+ "]": "'",
+ "—": "-",
+ "~": "-",
+ "~": "-",
+ "「": "'",
+ "」": "'",
+}
+
+tone_modifier = ToneSandhi()
+
+
+def replace_punctuation(text):
+ text = text.replace("嗯", "恩").replace("呣", "母")
+ pattern = re.compile("|".join(re.escape(p) for p in rep_map.keys()))
+
+ replaced_text = pattern.sub(lambda x: rep_map[x.group()], text)
+
+ replaced_text = re.sub(
+ r"[^\u4e00-\u9fa5" + "".join(punctuation) + r"]+", "", replaced_text
+ )
+
+ return replaced_text
+
+
+def g2p(text):
+ pattern = r"(?<=[{0}])\s*".format("".join(punctuation))
+ sentences = [i for i in re.split(pattern, text) if i.strip() != ""]
+ phones, tones, word2ph = _g2p(sentences)
+ assert sum(word2ph) == len(phones)
+ assert len(word2ph) == len(text) # Sometimes it will crash,you can add a try-catch.
+ phones = ["_"] + phones + ["_"]
+ tones = [0] + tones + [0]
+ word2ph = [1] + word2ph + [1]
+ return phones, tones, word2ph
+
+
+def _get_initials_finals(word):
+ initials = []
+ finals = []
+ orig_initials = lazy_pinyin(word, neutral_tone_with_five=True, style=Style.INITIALS)
+ orig_finals = lazy_pinyin(
+ word, neutral_tone_with_five=True, style=Style.FINALS_TONE3
+ )
+ for c, v in zip(orig_initials, orig_finals):
+ initials.append(c)
+ finals.append(v)
+ return initials, finals
+
+
+def _g2p(segments):
+ phones_list = []
+ tones_list = []
+ word2ph = []
+ for seg in segments:
+ # Replace all English words in the sentence
+ seg = re.sub("[a-zA-Z]+", "", seg)
+ seg_cut = psg.lcut(seg)
+ initials = []
+ finals = []
+ seg_cut = tone_modifier.pre_merge_for_modify(seg_cut)
+ for word, pos in seg_cut:
+ if pos == "eng":
+ continue
+ sub_initials, sub_finals = _get_initials_finals(word)
+ sub_finals = tone_modifier.modified_tone(word, pos, sub_finals)
+ initials.append(sub_initials)
+ finals.append(sub_finals)
+
+ # assert len(sub_initials) == len(sub_finals) == len(word)
+ initials = sum(initials, [])
+ finals = sum(finals, [])
+ #
+ for c, v in zip(initials, finals):
+ raw_pinyin = c + v
+ # NOTE: post process for pypinyin outputs
+ # we discriminate i, ii and iii
+ if c == v:
+ assert c in punctuation
+ phone = [c]
+ tone = "0"
+ word2ph.append(1)
+ else:
+ v_without_tone = v[:-1]
+ tone = v[-1]
+
+ pinyin = c + v_without_tone
+ assert tone in "12345"
+
+ if c:
+ # 多音节
+ v_rep_map = {
+ "uei": "ui",
+ "iou": "iu",
+ "uen": "un",
+ }
+ if v_without_tone in v_rep_map.keys():
+ pinyin = c + v_rep_map[v_without_tone]
+ else:
+ # 单音节
+ pinyin_rep_map = {
+ "ing": "ying",
+ "i": "yi",
+ "in": "yin",
+ "u": "wu",
+ }
+ if pinyin in pinyin_rep_map.keys():
+ pinyin = pinyin_rep_map[pinyin]
+ else:
+ single_rep_map = {
+ "v": "yu",
+ "e": "e",
+ "i": "y",
+ "u": "w",
+ }
+ if pinyin[0] in single_rep_map.keys():
+ pinyin = single_rep_map[pinyin[0]] + pinyin[1:]
+
+ assert pinyin in pinyin_to_symbol_map.keys(), (pinyin, seg, raw_pinyin)
+ phone = pinyin_to_symbol_map[pinyin].split(" ")
+ word2ph.append(len(phone))
+
+ phones_list += phone
+ tones_list += [int(tone)] * len(phone)
+ return phones_list, tones_list, word2ph
+
+
+def text_normalize(text):
+ numbers = re.findall(r"\d+(?:\.?\d+)?", text)
+ for number in numbers:
+ text = text.replace(number, cn2an.an2cn(number), 1)
+ text = replace_punctuation(text)
+ return text
+
+
+def get_bert_feature(text, word2ph):
+ from text import chinese_bert
+
+ return chinese_bert.get_bert_feature(text, word2ph)
+
+
+if __name__ == "__main__":
+ from text.chinese_bert import get_bert_feature
+
+ text = "啊!但是《原神》是由,米哈\游自主, [研发]的一款全.新开放世界.冒险游戏"
+ text = text_normalize(text)
+ print(text)
+ phones, tones, word2ph = g2p(text)
+ bert = get_bert_feature(text, word2ph)
+
+ print(phones, tones, word2ph, bert.shape)
+
+
+# # 示例用法
+# text = "这是一个示例文本:,你好!这是一个测试...."
+# print(g2p_paddle(text)) # 输出: 这是一个示例文本你好这是一个测试
diff --git a/text/chinese_bert.py b/text/chinese_bert.py
new file mode 100644
index 0000000000000000000000000000000000000000..94e1408e22affe9b5f12a9644ff01a14d9547e1e
--- /dev/null
+++ b/text/chinese_bert.py
@@ -0,0 +1,121 @@
+import sys
+
+import torch
+from transformers import AutoModelForMaskedLM, AutoTokenizer
+
+from config import config
+
+LOCAL_PATH = "./bert/chinese-roberta-wwm-ext-large"
+
+tokenizer = AutoTokenizer.from_pretrained(LOCAL_PATH)
+
+models = dict()
+
+
+def get_bert_feature(
+ text,
+ word2ph,
+ device=config.bert_gen_config.device,
+ assist_text=None,
+ assist_text_weight=0.7,
+):
+ if (
+ sys.platform == "darwin"
+ and torch.backends.mps.is_available()
+ and device == "cpu"
+ ):
+ device = "mps"
+ if not device:
+ device = "cuda"
+ if device == "cuda" and not torch.cuda.is_available():
+ device = "cpu"
+ if device not in models.keys():
+ models[device] = AutoModelForMaskedLM.from_pretrained(LOCAL_PATH).to(device)
+ with torch.no_grad():
+ inputs = tokenizer(text, return_tensors="pt")
+ for i in inputs:
+ inputs[i] = inputs[i].to(device)
+ res = models[device](**inputs, output_hidden_states=True)
+ res = torch.cat(res["hidden_states"][-3:-2], -1)[0].cpu()
+ if assist_text:
+ style_inputs = tokenizer(assist_text, return_tensors="pt")
+ for i in style_inputs:
+ style_inputs[i] = style_inputs[i].to(device)
+ style_res = models[device](**style_inputs, output_hidden_states=True)
+ style_res = torch.cat(style_res["hidden_states"][-3:-2], -1)[0].cpu()
+ style_res_mean = style_res.mean(0)
+ assert len(word2ph) == len(text) + 2
+ word2phone = word2ph
+ phone_level_feature = []
+ for i in range(len(word2phone)):
+ if assist_text:
+ repeat_feature = (
+ res[i].repeat(word2phone[i], 1) * (1 - assist_text_weight)
+ + style_res_mean.repeat(word2phone[i], 1) * assist_text_weight
+ )
+ else:
+ repeat_feature = res[i].repeat(word2phone[i], 1)
+ phone_level_feature.append(repeat_feature)
+
+ phone_level_feature = torch.cat(phone_level_feature, dim=0)
+
+ return phone_level_feature.T
+
+
+if __name__ == "__main__":
+ word_level_feature = torch.rand(38, 1024) # 12个词,每个词1024维特征
+ word2phone = [
+ 1,
+ 2,
+ 1,
+ 2,
+ 2,
+ 1,
+ 2,
+ 2,
+ 1,
+ 2,
+ 2,
+ 1,
+ 2,
+ 2,
+ 2,
+ 2,
+ 2,
+ 1,
+ 1,
+ 2,
+ 2,
+ 1,
+ 2,
+ 2,
+ 2,
+ 2,
+ 1,
+ 2,
+ 2,
+ 2,
+ 2,
+ 2,
+ 1,
+ 2,
+ 2,
+ 2,
+ 2,
+ 1,
+ ]
+
+ # 计算总帧数
+ total_frames = sum(word2phone)
+ print(word_level_feature.shape)
+ print(word2phone)
+ phone_level_feature = []
+ for i in range(len(word2phone)):
+ print(word_level_feature[i].shape)
+
+ # 对每个词重复word2phone[i]次
+ repeat_feature = word_level_feature[i].repeat(word2phone[i], 1)
+ phone_level_feature.append(repeat_feature)
+
+ phone_level_feature = torch.cat(phone_level_feature, dim=0)
+ print(phone_level_feature.shape) # torch.Size([36, 1024])
diff --git a/text/cleaner.py b/text/cleaner.py
new file mode 100644
index 0000000000000000000000000000000000000000..8da4c7b6ed16027a93c1de82f2e7befd5cfe5df0
--- /dev/null
+++ b/text/cleaner.py
@@ -0,0 +1,26 @@
+def clean_text(text, language, use_jp_extra=True, ignore_unknown=False):
+ # Changed to import inside if condition to avoid unnecessary import
+ if language == "ZH":
+ from . import chinese as language_module
+
+ norm_text = language_module.text_normalize(text)
+ phones, tones, word2ph = language_module.g2p(norm_text)
+ elif language == "EN":
+ from . import english as language_module
+
+ norm_text = language_module.text_normalize(text)
+ phones, tones, word2ph = language_module.g2p(norm_text)
+ elif language == "JP":
+ from . import japanese as language_module
+
+ norm_text = language_module.text_normalize(text)
+ phones, tones, word2ph = language_module.g2p(
+ norm_text, use_jp_extra, ignore_unknown=ignore_unknown
+ )
+ else:
+ raise ValueError(f"Language {language} not supported")
+ return norm_text, phones, tones, word2ph
+
+
+if __name__ == "__main__":
+ pass
diff --git a/text/english.py b/text/english.py
new file mode 100644
index 0000000000000000000000000000000000000000..4a2af9523f2f96b7b34a0fff7589a82e1122ecae
--- /dev/null
+++ b/text/english.py
@@ -0,0 +1,495 @@
+import pickle
+import os
+import re
+from g2p_en import G2p
+from transformers import DebertaV2Tokenizer
+
+from text import symbols
+from text.symbols import punctuation
+
+current_file_path = os.path.dirname(__file__)
+CMU_DICT_PATH = os.path.join(current_file_path, "cmudict.rep")
+CACHE_PATH = os.path.join(current_file_path, "cmudict_cache.pickle")
+_g2p = G2p()
+LOCAL_PATH = "./bert/deberta-v3-large"
+tokenizer = DebertaV2Tokenizer.from_pretrained(LOCAL_PATH)
+
+arpa = {
+ "AH0",
+ "S",
+ "AH1",
+ "EY2",
+ "AE2",
+ "EH0",
+ "OW2",
+ "UH0",
+ "NG",
+ "B",
+ "G",
+ "AY0",
+ "M",
+ "AA0",
+ "F",
+ "AO0",
+ "ER2",
+ "UH1",
+ "IY1",
+ "AH2",
+ "DH",
+ "IY0",
+ "EY1",
+ "IH0",
+ "K",
+ "N",
+ "W",
+ "IY2",
+ "T",
+ "AA1",
+ "ER1",
+ "EH2",
+ "OY0",
+ "UH2",
+ "UW1",
+ "Z",
+ "AW2",
+ "AW1",
+ "V",
+ "UW2",
+ "AA2",
+ "ER",
+ "AW0",
+ "UW0",
+ "R",
+ "OW1",
+ "EH1",
+ "ZH",
+ "AE0",
+ "IH2",
+ "IH",
+ "Y",
+ "JH",
+ "P",
+ "AY1",
+ "EY0",
+ "OY2",
+ "TH",
+ "HH",
+ "D",
+ "ER0",
+ "CH",
+ "AO1",
+ "AE1",
+ "AO2",
+ "OY1",
+ "AY2",
+ "IH1",
+ "OW0",
+ "L",
+ "SH",
+}
+
+
+def post_replace_ph(ph):
+ rep_map = {
+ ":": ",",
+ ";": ",",
+ ",": ",",
+ "。": ".",
+ "!": "!",
+ "?": "?",
+ "\n": ".",
+ "·": ",",
+ "、": ",",
+ "…": "...",
+ "···": "...",
+ "・・・": "...",
+ "v": "V",
+ }
+ if ph in rep_map.keys():
+ ph = rep_map[ph]
+ if ph in symbols:
+ return ph
+ if ph not in symbols:
+ ph = "UNK"
+ return ph
+
+
+rep_map = {
+ ":": ",",
+ ";": ",",
+ ",": ",",
+ "。": ".",
+ "!": "!",
+ "?": "?",
+ "\n": ".",
+ ".": ".",
+ "…": "...",
+ "···": "...",
+ "・・・": "...",
+ "·": ",",
+ "・": ",",
+ "、": ",",
+ "$": ".",
+ "“": "'",
+ "”": "'",
+ '"': "'",
+ "‘": "'",
+ "’": "'",
+ "(": "'",
+ ")": "'",
+ "(": "'",
+ ")": "'",
+ "《": "'",
+ "》": "'",
+ "【": "'",
+ "】": "'",
+ "[": "'",
+ "]": "'",
+ "—": "-",
+ "−": "-",
+ "~": "-",
+ "~": "-",
+ "「": "'",
+ "」": "'",
+}
+
+
+def replace_punctuation(text):
+ pattern = re.compile("|".join(re.escape(p) for p in rep_map.keys()))
+
+ replaced_text = pattern.sub(lambda x: rep_map[x.group()], text)
+
+ # replaced_text = re.sub(
+ # r"[^\u3040-\u309F\u30A0-\u30FF\u4E00-\u9FFF\u3400-\u4DBF\u3005"
+ # + "".join(punctuation)
+ # + r"]+",
+ # "",
+ # replaced_text,
+ # )
+
+ return replaced_text
+
+
+def read_dict():
+ g2p_dict = {}
+ start_line = 49
+ with open(CMU_DICT_PATH) as f:
+ line = f.readline()
+ line_index = 1
+ while line:
+ if line_index >= start_line:
+ line = line.strip()
+ word_split = line.split(" ")
+ word = word_split[0]
+
+ syllable_split = word_split[1].split(" - ")
+ g2p_dict[word] = []
+ for syllable in syllable_split:
+ phone_split = syllable.split(" ")
+ g2p_dict[word].append(phone_split)
+
+ line_index = line_index + 1
+ line = f.readline()
+
+ return g2p_dict
+
+
+def cache_dict(g2p_dict, file_path):
+ with open(file_path, "wb") as pickle_file:
+ pickle.dump(g2p_dict, pickle_file)
+
+
+def get_dict():
+ if os.path.exists(CACHE_PATH):
+ with open(CACHE_PATH, "rb") as pickle_file:
+ g2p_dict = pickle.load(pickle_file)
+ else:
+ g2p_dict = read_dict()
+ cache_dict(g2p_dict, CACHE_PATH)
+
+ return g2p_dict
+
+
+eng_dict = get_dict()
+
+
+def refine_ph(phn):
+ tone = 0
+ if re.search(r"\d$", phn):
+ tone = int(phn[-1]) + 1
+ phn = phn[:-1]
+ else:
+ tone = 3
+ return phn.lower(), tone
+
+
+def refine_syllables(syllables):
+ tones = []
+ phonemes = []
+ for phn_list in syllables:
+ for i in range(len(phn_list)):
+ phn = phn_list[i]
+ phn, tone = refine_ph(phn)
+ phonemes.append(phn)
+ tones.append(tone)
+ return phonemes, tones
+
+
+import re
+import inflect
+
+_inflect = inflect.engine()
+_comma_number_re = re.compile(r"([0-9][0-9\,]+[0-9])")
+_decimal_number_re = re.compile(r"([0-9]+\.[0-9]+)")
+_pounds_re = re.compile(r"£([0-9\,]*[0-9]+)")
+_dollars_re = re.compile(r"\$([0-9\.\,]*[0-9]+)")
+_ordinal_re = re.compile(r"[0-9]+(st|nd|rd|th)")
+_number_re = re.compile(r"[0-9]+")
+
+# List of (regular expression, replacement) pairs for abbreviations:
+_abbreviations = [
+ (re.compile("\\b%s\\." % x[0], re.IGNORECASE), x[1])
+ for x in [
+ ("mrs", "misess"),
+ ("mr", "mister"),
+ ("dr", "doctor"),
+ ("st", "saint"),
+ ("co", "company"),
+ ("jr", "junior"),
+ ("maj", "major"),
+ ("gen", "general"),
+ ("drs", "doctors"),
+ ("rev", "reverend"),
+ ("lt", "lieutenant"),
+ ("hon", "honorable"),
+ ("sgt", "sergeant"),
+ ("capt", "captain"),
+ ("esq", "esquire"),
+ ("ltd", "limited"),
+ ("col", "colonel"),
+ ("ft", "fort"),
+ ]
+]
+
+
+# List of (ipa, lazy ipa) pairs:
+_lazy_ipa = [
+ (re.compile("%s" % x[0]), x[1])
+ for x in [
+ ("r", "ɹ"),
+ ("æ", "e"),
+ ("ɑ", "a"),
+ ("ɔ", "o"),
+ ("ð", "z"),
+ ("θ", "s"),
+ ("ɛ", "e"),
+ ("ɪ", "i"),
+ ("ʊ", "u"),
+ ("ʒ", "ʥ"),
+ ("ʤ", "ʥ"),
+ ("ˈ", "↓"),
+ ]
+]
+
+# List of (ipa, lazy ipa2) pairs:
+_lazy_ipa2 = [
+ (re.compile("%s" % x[0]), x[1])
+ for x in [
+ ("r", "ɹ"),
+ ("ð", "z"),
+ ("θ", "s"),
+ ("ʒ", "ʑ"),
+ ("ʤ", "dʑ"),
+ ("ˈ", "↓"),
+ ]
+]
+
+# List of (ipa, ipa2) pairs
+_ipa_to_ipa2 = [
+ (re.compile("%s" % x[0]), x[1]) for x in [("r", "ɹ"), ("ʤ", "dʒ"), ("ʧ", "tʃ")]
+]
+
+
+def _expand_dollars(m):
+ match = m.group(1)
+ parts = match.split(".")
+ if len(parts) > 2:
+ return match + " dollars" # Unexpected format
+ dollars = int(parts[0]) if parts[0] else 0
+ cents = int(parts[1]) if len(parts) > 1 and parts[1] else 0
+ if dollars and cents:
+ dollar_unit = "dollar" if dollars == 1 else "dollars"
+ cent_unit = "cent" if cents == 1 else "cents"
+ return "%s %s, %s %s" % (dollars, dollar_unit, cents, cent_unit)
+ elif dollars:
+ dollar_unit = "dollar" if dollars == 1 else "dollars"
+ return "%s %s" % (dollars, dollar_unit)
+ elif cents:
+ cent_unit = "cent" if cents == 1 else "cents"
+ return "%s %s" % (cents, cent_unit)
+ else:
+ return "zero dollars"
+
+
+def _remove_commas(m):
+ return m.group(1).replace(",", "")
+
+
+def _expand_ordinal(m):
+ return _inflect.number_to_words(m.group(0))
+
+
+def _expand_number(m):
+ num = int(m.group(0))
+ if num > 1000 and num < 3000:
+ if num == 2000:
+ return "two thousand"
+ elif num > 2000 and num < 2010:
+ return "two thousand " + _inflect.number_to_words(num % 100)
+ elif num % 100 == 0:
+ return _inflect.number_to_words(num // 100) + " hundred"
+ else:
+ return _inflect.number_to_words(
+ num, andword="", zero="oh", group=2
+ ).replace(", ", " ")
+ else:
+ return _inflect.number_to_words(num, andword="")
+
+
+def _expand_decimal_point(m):
+ return m.group(1).replace(".", " point ")
+
+
+def normalize_numbers(text):
+ text = re.sub(_comma_number_re, _remove_commas, text)
+ text = re.sub(_pounds_re, r"\1 pounds", text)
+ text = re.sub(_dollars_re, _expand_dollars, text)
+ text = re.sub(_decimal_number_re, _expand_decimal_point, text)
+ text = re.sub(_ordinal_re, _expand_ordinal, text)
+ text = re.sub(_number_re, _expand_number, text)
+ return text
+
+
+def text_normalize(text):
+ text = normalize_numbers(text)
+ text = replace_punctuation(text)
+ text = re.sub(r"([,;.\?\!])([\w])", r"\1 \2", text)
+ return text
+
+
+def distribute_phone(n_phone, n_word):
+ phones_per_word = [0] * n_word
+ for task in range(n_phone):
+ min_tasks = min(phones_per_word)
+ min_index = phones_per_word.index(min_tasks)
+ phones_per_word[min_index] += 1
+ return phones_per_word
+
+
+def sep_text(text):
+ words = re.split(r"([,;.\?\!\s+])", text)
+ words = [word for word in words if word.strip() != ""]
+ return words
+
+
+def text_to_words(text):
+ tokens = tokenizer.tokenize(text)
+ words = []
+ for idx, t in enumerate(tokens):
+ if t.startswith("▁"):
+ words.append([t[1:]])
+ else:
+ if t in punctuation:
+ if idx == len(tokens) - 1:
+ words.append([f"{t}"])
+ else:
+ if (
+ not tokens[idx + 1].startswith("▁")
+ and tokens[idx + 1] not in punctuation
+ ):
+ if idx == 0:
+ words.append([])
+ words[-1].append(f"{t}")
+ else:
+ words.append([f"{t}"])
+ else:
+ if idx == 0:
+ words.append([])
+ words[-1].append(f"{t}")
+ return words
+
+
+def g2p(text):
+ phones = []
+ tones = []
+ phone_len = []
+ # words = sep_text(text)
+ # tokens = [tokenizer.tokenize(i) for i in words]
+ words = text_to_words(text)
+
+ for word in words:
+ temp_phones, temp_tones = [], []
+ if len(word) > 1:
+ if "'" in word:
+ word = ["".join(word)]
+ for w in word:
+ if w in punctuation:
+ temp_phones.append(w)
+ temp_tones.append(0)
+ continue
+ if w.upper() in eng_dict:
+ phns, tns = refine_syllables(eng_dict[w.upper()])
+ temp_phones += [post_replace_ph(i) for i in phns]
+ temp_tones += tns
+ # w2ph.append(len(phns))
+ else:
+ phone_list = list(filter(lambda p: p != " ", _g2p(w)))
+ phns = []
+ tns = []
+ for ph in phone_list:
+ if ph in arpa:
+ ph, tn = refine_ph(ph)
+ phns.append(ph)
+ tns.append(tn)
+ else:
+ phns.append(ph)
+ tns.append(0)
+ temp_phones += [post_replace_ph(i) for i in phns]
+ temp_tones += tns
+ phones += temp_phones
+ tones += temp_tones
+ phone_len.append(len(temp_phones))
+ # phones = [post_replace_ph(i) for i in phones]
+
+ word2ph = []
+ for token, pl in zip(words, phone_len):
+ word_len = len(token)
+
+ aaa = distribute_phone(pl, word_len)
+ word2ph += aaa
+
+ phones = ["_"] + phones + ["_"]
+ tones = [0] + tones + [0]
+ word2ph = [1] + word2ph + [1]
+ assert len(phones) == len(tones), text
+ assert len(phones) == sum(word2ph), text
+
+ return phones, tones, word2ph
+
+
+def get_bert_feature(text, word2ph):
+ from text import english_bert_mock
+
+ return english_bert_mock.get_bert_feature(text, word2ph)
+
+
+if __name__ == "__main__":
+ # print(get_dict())
+ # print(eng_word_to_phoneme("hello"))
+ print(g2p("In this paper, we propose 1 DSPGAN, a GAN-based universal vocoder."))
+ # all_phones = set()
+ # for k, syllables in eng_dict.items():
+ # for group in syllables:
+ # for ph in group:
+ # all_phones.add(ph)
+ # print(all_phones)
diff --git a/text/english_bert_mock.py b/text/english_bert_mock.py
new file mode 100644
index 0000000000000000000000000000000000000000..782b65d7f1b1228c372178669c6e434e673dfea0
--- /dev/null
+++ b/text/english_bert_mock.py
@@ -0,0 +1,63 @@
+import sys
+
+import torch
+from transformers import DebertaV2Model, DebertaV2Tokenizer
+
+from config import config
+
+
+LOCAL_PATH = "./bert/deberta-v3-large"
+
+tokenizer = DebertaV2Tokenizer.from_pretrained(LOCAL_PATH)
+
+models = dict()
+
+
+def get_bert_feature(
+ text,
+ word2ph,
+ device=config.bert_gen_config.device,
+ assist_text=None,
+ assist_text_weight=0.7,
+):
+ if (
+ sys.platform == "darwin"
+ and torch.backends.mps.is_available()
+ and device == "cpu"
+ ):
+ device = "mps"
+ if not device:
+ device = "cuda"
+ if device == "cuda" and not torch.cuda.is_available():
+ device = "cpu"
+ if device not in models.keys():
+ models[device] = DebertaV2Model.from_pretrained(LOCAL_PATH).to(device)
+ with torch.no_grad():
+ inputs = tokenizer(text, return_tensors="pt")
+ for i in inputs:
+ inputs[i] = inputs[i].to(device)
+ res = models[device](**inputs, output_hidden_states=True)
+ res = torch.cat(res["hidden_states"][-3:-2], -1)[0].cpu()
+ if assist_text:
+ style_inputs = tokenizer(assist_text, return_tensors="pt")
+ for i in style_inputs:
+ style_inputs[i] = style_inputs[i].to(device)
+ style_res = models[device](**style_inputs, output_hidden_states=True)
+ style_res = torch.cat(style_res["hidden_states"][-3:-2], -1)[0].cpu()
+ style_res_mean = style_res.mean(0)
+ assert len(word2ph) == res.shape[0], (text, res.shape[0], len(word2ph))
+ word2phone = word2ph
+ phone_level_feature = []
+ for i in range(len(word2phone)):
+ if assist_text:
+ repeat_feature = (
+ res[i].repeat(word2phone[i], 1) * (1 - assist_text_weight)
+ + style_res_mean.repeat(word2phone[i], 1) * assist_text_weight
+ )
+ else:
+ repeat_feature = res[i].repeat(word2phone[i], 1)
+ phone_level_feature.append(repeat_feature)
+
+ phone_level_feature = torch.cat(phone_level_feature, dim=0)
+
+ return phone_level_feature.T
diff --git a/text/japanese.py b/text/japanese.py
new file mode 100644
index 0000000000000000000000000000000000000000..5a36eb69e63e0e3976adc4cf5a2aa9464809627f
--- /dev/null
+++ b/text/japanese.py
@@ -0,0 +1,624 @@
+# Convert Japanese text to phonemes which is
+# compatible with Julius https://github.com/julius-speech/segmentation-kit
+import re
+import unicodedata
+from pathlib import Path
+
+import pyopenjtalk
+from num2words import num2words
+from transformers import AutoTokenizer
+
+from common.log import logger
+from text import punctuation
+from text.japanese_mora_list import (
+ mora_kata_to_mora_phonemes,
+ mora_phonemes_to_mora_kata,
+)
+
+from text.user_dict import update_dict
+
+# 最初にpyopenjtalkの辞書を更新
+update_dict()
+
+# 子音の集合
+COSONANTS = set(
+ [
+ cosonant
+ for cosonant, _ in mora_kata_to_mora_phonemes.values()
+ if cosonant is not None
+ ]
+)
+
+# 母音の集合、便宜上「ん」を含める
+VOWELS = {"a", "i", "u", "e", "o", "N"}
+
+
+# 正規化で記号を変換するための辞書
+rep_map = {
+ ":": ",",
+ ";": ",",
+ ",": ",",
+ "。": ".",
+ "!": "!",
+ "?": "?",
+ "\n": ".",
+ ".": ".",
+ "…": "...",
+ "···": "...",
+ "・・・": "...",
+ "·": ",",
+ "・": ",",
+ "、": ",",
+ "$": ".",
+ "“": "'",
+ "”": "'",
+ '"': "'",
+ "‘": "'",
+ "’": "'",
+ "(": "'",
+ ")": "'",
+ "(": "'",
+ ")": "'",
+ "《": "'",
+ "》": "'",
+ "【": "'",
+ "】": "'",
+ "[": "'",
+ "]": "'",
+ # NFKC正規化後のハイフン・ダッシュの変種を全て通常半角ハイフン - \u002d に変換
+ "\u02d7": "\u002d", # ˗, Modifier Letter Minus Sign
+ "\u2010": "\u002d", # ‐, Hyphen,
+ # "\u2011": "\u002d", # ‑, Non-Breaking Hyphen, NFKCにより\u2010に変換される
+ "\u2012": "\u002d", # ‒, Figure Dash
+ "\u2013": "\u002d", # –, En Dash
+ "\u2014": "\u002d", # —, Em Dash
+ "\u2015": "\u002d", # ―, Horizontal Bar
+ "\u2043": "\u002d", # ⁃, Hyphen Bullet
+ "\u2212": "\u002d", # −, Minus Sign
+ "\u23af": "\u002d", # ⎯, Horizontal Line Extension
+ "\u23e4": "\u002d", # ⏤, Straightness
+ "\u2500": "\u002d", # ─, Box Drawings Light Horizontal
+ "\u2501": "\u002d", # ━, Box Drawings Heavy Horizontal
+ "\u2e3a": "\u002d", # ⸺, Two-Em Dash
+ "\u2e3b": "\u002d", # ⸻, Three-Em Dash
+ # "~": "-", # これは長音記号「ー」として扱うよう変更
+ # "~": "-", # これも長音記号「ー」として扱うよう変更
+ "「": "'",
+ "」": "'",
+}
+
+
+def text_normalize(text):
+ """
+ 日本語のテキストを正規化する。
+ 結果は、ちょうど次の文字のみからなる:
+ - ひらがな
+ - カタカナ(全角長音記号「ー」が入る!)
+ - 漢字
+ - 半角アルファベット(大文字と小文字)
+ - ギリシャ文字
+ - `.` (句点`。`や`…`の一部や改行等)
+ - `,` (読点`、`や`:`等)
+ - `?` (疑問符`?`)
+ - `!` (感嘆符`!`)
+ - `'` (`「`や`」`等)
+ - `-` (`―`(ダッシュ、長音記号ではない)や`-`等)
+
+ 注意点:
+ - 三点リーダー`…`は`...`に変換される(`なるほど…。` → `なるほど....`)
+ - 数字は漢字に変換される(`1,100円` → `千百円`、`52.34` → `五十二点三四`)
+ - 読点や疑問符等の位置・個数等は保持される(`??あ、、!!!` → `??あ,,!!!`)
+ """
+ res = unicodedata.normalize("NFKC", text) # ここでアルファベットは半角になる
+ res = japanese_convert_numbers_to_words(res) # 「100円」→「百円」等
+ # 「~」と「~」も長音記号として扱う
+ res = res.replace("~", "ー")
+ res = res.replace("~", "ー")
+
+ res = replace_punctuation(res) # 句読点等正規化、読めない文字を削除
+
+ # 結合文字の濁点・半濁点を削除
+ # 通常の「ば」等はそのままのこされる、「あ゛」は上で「あ゙」になりここで「あ」になる
+ res = res.replace("\u3099", "") # 結合文字の濁点を削除、る゙ → る
+ res = res.replace("\u309A", "") # 結合文字の半濁点を削除、な゚ → な
+ return res
+
+
+def replace_punctuation(text: str) -> str:
+ """句読点等を「.」「,」「!」「?」「'」「-」に正規化し、OpenJTalkで読みが取得できるもののみ残す:
+ 漢字・平仮名・カタカナ、アルファベット、ギリシャ文字
+ """
+ pattern = re.compile("|".join(re.escape(p) for p in rep_map.keys()))
+
+ # 句読点を辞書で置換
+ replaced_text = pattern.sub(lambda x: rep_map[x.group()], text)
+
+ replaced_text = re.sub(
+ # ↓ ひらがな、カタカナ、漢字
+ r"[^\u3040-\u309F\u30A0-\u30FF\u4E00-\u9FFF\u3400-\u4DBF\u3005"
+ # ↓ 半角アルファベット(大文字と小文字)
+ + r"\u0041-\u005A\u0061-\u007A"
+ # ↓ 全角アルファベット(大文字と小文字)
+ + r"\uFF21-\uFF3A\uFF41-\uFF5A"
+ # ↓ ギリシャ文字
+ + r"\u0370-\u03FF\u1F00-\u1FFF"
+ # ↓ "!", "?", "…", ",", ".", "'", "-", 但し`…`はすでに`...`に変換されている
+ + "".join(punctuation) + r"]+",
+ # 上述以外の文字を削除
+ "",
+ replaced_text,
+ )
+
+ return replaced_text
+
+
+_NUMBER_WITH_SEPARATOR_RX = re.compile("[0-9]{1,3}(,[0-9]{3})+")
+_CURRENCY_MAP = {"$": "ドル", "¥": "円", "£": "ポンド", "€": "ユーロ"}
+_CURRENCY_RX = re.compile(r"([$¥£€])([0-9.]*[0-9])")
+_NUMBER_RX = re.compile(r"[0-9]+(\.[0-9]+)?")
+
+
+def japanese_convert_numbers_to_words(text: str) -> str:
+ res = _NUMBER_WITH_SEPARATOR_RX.sub(lambda m: m[0].replace(",", ""), text)
+ res = _CURRENCY_RX.sub(lambda m: m[2] + _CURRENCY_MAP.get(m[1], m[1]), res)
+ res = _NUMBER_RX.sub(lambda m: num2words(m[0], lang="ja"), res)
+ return res
+
+
+def g2p(
+ norm_text: str, use_jp_extra: bool = True, ignore_unknown: bool = False
+) -> tuple[list[str], list[int], list[int]]:
+ """
+ 他で使われるメインの関数。`text_normalize()`で正規化された`norm_text`を受け取り、
+ - phones: 音素のリスト(ただし`!`や`,`や`.`等punctuationが含まれうる)
+ - tones: アクセントのリスト、0(低)と1(高)からなり、phonesと同じ長さ
+ - word2ph: 元のテキストの各文字に音素が何個割り当てられるかを表すリスト
+ のタプルを返す。
+ ただし`phones`と`tones`の最初と終わりに`_`が入り、応じて`word2ph`の最初と最後に1が追加される。
+ use_jp_extra: Falseの場合、「ん」の音素を「N」ではなく「n」とする。
+ """
+ # pyopenjtalkのフルコンテキストラベルを使ってアクセントを取り出すと、punctuationの位置が消えてしまい情報が失われてしまう:
+ # 「こんにちは、世界。」と「こんにちは!世界。」と「こんにちは!!!???世界……。」は全て同じになる。
+ # よって、まずpunctuation無しの音素とアクセントのリストを作り、
+ # それとは別にpyopenjtalk.run_frontend()で得られる音素リスト(こちらはpunctuationが保持される)を使い、
+ # アクセント割当をしなおすことによってpunctuationを含めた音素とアクセントのリストを作る。
+
+ # punctuationがすべて消えた、音素とアクセントのタプルのリスト(「ん」は「N」)
+ phone_tone_list_wo_punct = g2phone_tone_wo_punct(norm_text)
+
+ # sep_text: 単語単位の単語のリスト
+ # sep_kata: 単語単位の単語のカタカナ読みのリスト
+ sep_text, sep_kata = text2sep_kata(norm_text, ignore_unknown=ignore_unknown)
+
+ # sep_phonemes: 各単語ごとの音素のリストのリスト
+ sep_phonemes = handle_long([kata2phoneme_list(i) for i in sep_kata])
+
+ # phone_w_punct: sep_phonemesを結合した、punctuationを元のまま保持した音素列
+ phone_w_punct: list[str] = []
+ for i in sep_phonemes:
+ phone_w_punct += i
+
+ # punctuation無しのアクセント情報を使って、punctuationを含めたアクセント情報を作る
+ phone_tone_list = align_tones(phone_w_punct, phone_tone_list_wo_punct)
+ # logger.debug(f"phone_tone_list:\n{phone_tone_list}")
+ # word2phは厳密な解答は不可能なので(「今日」「眼鏡」等の熟字訓が存在)、
+ # Bert-VITS2では、単語単位の分割を使って、単語の文字ごとにだいたい均等に音素を分配する
+
+ # sep_textから、各単語を1文字1文字分割して、文字のリスト(のリスト)を作る
+ sep_tokenized: list[list[str]] = []
+ for i in sep_text:
+ if i not in punctuation:
+ sep_tokenized.append(
+ tokenizer.tokenize(i)
+ ) # ここでおそらく`i`が文字単位に分割される
+ else:
+ sep_tokenized.append([i])
+
+ # 各単語について、音素の数と文字の数を比較して、均等っぽく分配する
+ word2ph = []
+ for token, phoneme in zip(sep_tokenized, sep_phonemes):
+ phone_len = len(phoneme)
+ word_len = len(token)
+ word2ph += distribute_phone(phone_len, word_len)
+
+ # 最初と最後に`_`記号を追加、アクセントは0(低)、word2phもそれに合わせて追加
+ phone_tone_list = [("_", 0)] + phone_tone_list + [("_", 0)]
+ word2ph = [1] + word2ph + [1]
+
+ phones = [phone for phone, _ in phone_tone_list]
+ tones = [tone for _, tone in phone_tone_list]
+
+ assert len(phones) == sum(word2ph), f"{len(phones)} != {sum(word2ph)}"
+
+ # use_jp_extraでない場合は「N」を「n」に変換
+ if not use_jp_extra:
+ phones = [phone if phone != "N" else "n" for phone in phones]
+
+ return phones, tones, word2ph
+
+
+def g2kata_tone(norm_text: str, ignore_unknown: bool = False) -> list[tuple[str, int]]:
+ phones, tones, _ = g2p(norm_text, use_jp_extra=True, ignore_unknown=ignore_unknown)
+ return phone_tone2kata_tone(list(zip(phones, tones)))
+
+
+def phone_tone2kata_tone(phone_tone: list[tuple[str, int]]) -> list[tuple[str, int]]:
+ """phone_toneをのphone部分をカタカナに変換する。ただし最初と最後の("_", 0)は無視"""
+ phone_tone = phone_tone[1:] # 最初の("_", 0)を無視
+ phones = [phone for phone, _ in phone_tone]
+ tones = [tone for _, tone in phone_tone]
+ result: list[tuple[str, int]] = []
+ current_mora = ""
+ for phone, next_phone, tone, next_tone in zip(phones, phones[1:], tones, tones[1:]):
+ # zipの関係で最後の("_", 0)は無視されている
+ if phone in punctuation:
+ result.append((phone, tone))
+ continue
+ if phone in COSONANTS: # n以外の子音の場合
+ assert current_mora == "", f"Unexpected {phone} after {current_mora}"
+ assert tone == next_tone, f"Unexpected {phone} tone {tone} != {next_tone}"
+ current_mora = phone
+ else:
+ # phoneが母音もしくは「N」
+ current_mora += phone
+ result.append((mora_phonemes_to_mora_kata[current_mora], tone))
+ current_mora = ""
+ return result
+
+
+def kata_tone2phone_tone(kata_tone: list[tuple[str, int]]) -> list[tuple[str, int]]:
+ """`phone_tone2kata_tone()`の逆。"""
+ result: list[tuple[str, int]] = [("_", 0)]
+ for mora, tone in kata_tone:
+ if mora in punctuation:
+ result.append((mora, tone))
+ else:
+ cosonant, vowel = mora_kata_to_mora_phonemes[mora]
+ if cosonant is None:
+ result.append((vowel, tone))
+ else:
+ result.append((cosonant, tone))
+ result.append((vowel, tone))
+ result.append(("_", 0))
+ return result
+
+
+def g2phone_tone_wo_punct(text: str) -> list[tuple[str, int]]:
+ """
+ テキストに対して、音素とアクセント(0か1)のペアのリストを返す。
+ ただし「!」「.」「?」等の非音素記号(punctuation)は全て消える(ポーズ記号も残さない)。
+ 非音素記号を含める処理は`align_tones()`で行われる。
+ また「っ」は「q」に、「ん」は「N」に変換される。
+ 例: "こんにちは、世界ー。。元気?!" →
+ [('k', 0), ('o', 0), ('N', 1), ('n', 1), ('i', 1), ('ch', 1), ('i', 1), ('w', 1), ('a', 1), ('s', 1), ('e', 1), ('k', 0), ('a', 0), ('i', 0), ('i', 0), ('g', 1), ('e', 1), ('N', 0), ('k', 0), ('i', 0)]
+ """
+ prosodies = pyopenjtalk_g2p_prosody(text, drop_unvoiced_vowels=True)
+ # logger.debug(f"prosodies: {prosodies}")
+ result: list[tuple[str, int]] = []
+ current_phrase: list[tuple[str, int]] = []
+ current_tone = 0
+ for i, letter in enumerate(prosodies):
+ # 特殊記号の処理
+
+ # 文頭記号、無視する
+ if letter == "^":
+ assert i == 0, "Unexpected ^"
+ # アクセント句の終わりに来る記号
+ elif letter in ("$", "?", "_", "#"):
+ # 保持しているフレーズを、アクセント数値を0-1に修正し結果に追加
+ result.extend(fix_phone_tone(current_phrase))
+ # 末尾に来る終了記号、無視(文中の疑問文は`_`になる)
+ if letter in ("$", "?"):
+ assert i == len(prosodies) - 1, f"Unexpected {letter}"
+ # あとは"_"(ポーズ)と"#"(アクセント句の境界)のみ
+ # これらは残さず、次のアクセント句に備える。
+ current_phrase = []
+ # 0を基準点にしてそこから上昇・下降する(負の場合は上の`fix_phone_tone`で直る)
+ current_tone = 0
+ # アクセント上昇記号
+ elif letter == "[":
+ current_tone = current_tone + 1
+ # アクセント下降記号
+ elif letter == "]":
+ current_tone = current_tone - 1
+ # それ以外は通常の音素
+ else:
+ if letter == "cl": # 「っ」の処理
+ letter = "q"
+ # elif letter == "N": # 「ん」の処理
+ # letter = "n"
+ current_phrase.append((letter, current_tone))
+ return result
+
+
+def text2sep_kata(
+ norm_text: str, ignore_unknown: bool = False
+) -> tuple[list[str], list[str]]:
+ """
+ `text_normalize`で正規化済みの`norm_text`を受け取り、それを単語分割し、
+ 分割された単語リストとその読み(カタカナor記号1文字)のリストのタプルを返す。
+ 単語分割結果は、`g2p()`の`word2ph`で1文字あたりに割り振る音素記号の数を決めるために使う。
+ 例:
+ `私はそう思う!って感じ?` →
+ ["私", "は", "そう", "思う", "!", "って", "感じ", "?"], ["ワタシ", "ワ", "ソー", "オモウ", "!", "ッテ", "カンジ", "?"]
+ """
+ # parsed: OpenJTalkの解析結果
+ parsed = pyopenjtalk.run_frontend(norm_text)
+ sep_text: list[str] = []
+ sep_kata: list[str] = []
+ for parts in parsed:
+ # word: 実際の単語の文字列
+ # yomi: その読み、但し無声化サインの`’`は除去
+ word, yomi = replace_punctuation(parts["string"]), parts["pron"].replace(
+ "’", ""
+ )
+ """
+ ここで`yomi`の取りうる値は以下の通りのはず。
+ - `word`が通常単語 → 通常の読み(カタカナ)
+ (カタカナからなり、長音記号も含みうる、`アー` 等)
+ - `word`が`ー` から始まる → `ーラー` や `ーーー` など
+ - `word`が句読点や空白等 → `、`
+ - `word`がpunctuationの繰り返し → 全角にしたもの
+ 基本的にpunctuationは1文字ずつ分かれるが、何故かある程度連続すると1つにまとまる。
+ 他にも`word`が読めないキリル文字アラビア文字等が来ると`、`になるが、正規化でこの場合は起きないはず。
+ また元のコードでは`yomi`が空白の場合の処理があったが、これは起きないはず。
+ 処理すべきは`yomi`が`、`の場合のみのはず。
+ """
+ assert yomi != "", f"Empty yomi: {word}"
+ if yomi == "、":
+ # wordは正規化されているので、`.`, `,`, `!`, `'`, `-`, `--` のいずれか
+ if not set(word).issubset(set(punctuation)): # 記号繰り返しか判定
+ # ここはpyopenjtalkが読めない文字等のときに起こる
+ if ignore_unknown:
+ logger.error(f"Ignoring unknown: {word} in:\n{norm_text}")
+ continue
+ raise ValueError(f"Cannot read: {word} in:\n{norm_text}")
+ # yomiは元の記号のままに変更
+ yomi = word
+ elif yomi == "?":
+ assert word == "?", f"yomi `?` comes from: {word}"
+ yomi = "?"
+ sep_text.append(word)
+ sep_kata.append(yomi)
+ return sep_text, sep_kata
+
+
+# ESPnetの実装から引用、変更点無し。「ん」は「N」なことに注意。
+# https://github.com/espnet/espnet/blob/master/espnet2/text/phoneme_tokenizer.py
+def pyopenjtalk_g2p_prosody(text: str, drop_unvoiced_vowels: bool = True) -> list[str]:
+ """Extract phoneme + prosoody symbol sequence from input full-context labels.
+
+ The algorithm is based on `Prosodic features control by symbols as input of
+ sequence-to-sequence acoustic modeling for neural TTS`_ with some r9y9's tweaks.
+
+ Args:
+ text (str): Input text.
+ drop_unvoiced_vowels (bool): whether to drop unvoiced vowels.
+
+ Returns:
+ List[str]: List of phoneme + prosody symbols.
+
+ Examples:
+ >>> from espnet2.text.phoneme_tokenizer import pyopenjtalk_g2p_prosody
+ >>> pyopenjtalk_g2p_prosody("こんにちは。")
+ ['^', 'k', 'o', '[', 'N', 'n', 'i', 'ch', 'i', 'w', 'a', '$']
+
+ .. _`Prosodic features control by symbols as input of sequence-to-sequence acoustic
+ modeling for neural TTS`: https://doi.org/10.1587/transinf.2020EDP7104
+
+ """
+ labels = pyopenjtalk.make_label(pyopenjtalk.run_frontend(text))
+ N = len(labels)
+
+ phones = []
+ for n in range(N):
+ lab_curr = labels[n]
+
+ # current phoneme
+ p3 = re.search(r"\-(.*?)\+", lab_curr).group(1)
+ # deal unvoiced vowels as normal vowels
+ if drop_unvoiced_vowels and p3 in "AEIOU":
+ p3 = p3.lower()
+
+ # deal with sil at the beginning and the end of text
+ if p3 == "sil":
+ assert n == 0 or n == N - 1
+ if n == 0:
+ phones.append("^")
+ elif n == N - 1:
+ # check question form or not
+ e3 = _numeric_feature_by_regex(r"!(\d+)_", lab_curr)
+ if e3 == 0:
+ phones.append("$")
+ elif e3 == 1:
+ phones.append("?")
+ continue
+ elif p3 == "pau":
+ phones.append("_")
+ continue
+ else:
+ phones.append(p3)
+
+ # accent type and position info (forward or backward)
+ a1 = _numeric_feature_by_regex(r"/A:([0-9\-]+)\+", lab_curr)
+ a2 = _numeric_feature_by_regex(r"\+(\d+)\+", lab_curr)
+ a3 = _numeric_feature_by_regex(r"\+(\d+)/", lab_curr)
+
+ # number of mora in accent phrase
+ f1 = _numeric_feature_by_regex(r"/F:(\d+)_", lab_curr)
+
+ a2_next = _numeric_feature_by_regex(r"\+(\d+)\+", labels[n + 1])
+ # accent phrase border
+ if a3 == 1 and a2_next == 1 and p3 in "aeiouAEIOUNcl":
+ phones.append("#")
+ # pitch falling
+ elif a1 == 0 and a2_next == a2 + 1 and a2 != f1:
+ phones.append("]")
+ # pitch rising
+ elif a2 == 1 and a2_next == 2:
+ phones.append("[")
+
+ return phones
+
+
+def _numeric_feature_by_regex(regex, s):
+ match = re.search(regex, s)
+ if match is None:
+ return -50
+ return int(match.group(1))
+
+
+def fix_phone_tone(phone_tone_list: list[tuple[str, int]]) -> list[tuple[str, int]]:
+ """
+ `phone_tone_list`のtone(アクセントの値)を0か1の範囲に修正する。
+ 例: [(a, 0), (i, -1), (u, -1)] → [(a, 1), (i, 0), (u, 0)]
+ """
+ tone_values = set(tone for _, tone in phone_tone_list)
+ if len(tone_values) == 1:
+ assert tone_values == {0}, tone_values
+ return phone_tone_list
+ elif len(tone_values) == 2:
+ if tone_values == {0, 1}:
+ return phone_tone_list
+ elif tone_values == {-1, 0}:
+ return [
+ (letter, 0 if tone == -1 else 1) for letter, tone in phone_tone_list
+ ]
+ else:
+ raise ValueError(f"Unexpected tone values: {tone_values}")
+ else:
+ raise ValueError(f"Unexpected tone values: {tone_values}")
+
+
+def distribute_phone(n_phone: int, n_word: int) -> list[int]:
+ """
+ 左から右に1ずつ振り分け、次にまた左から右に1ずつ増やし、というふうに、
+ 音素の数`n_phone`を単語の数`n_word`に分配する。
+ """
+ phones_per_word = [0] * n_word
+ for _ in range(n_phone):
+ min_tasks = min(phones_per_word)
+ min_index = phones_per_word.index(min_tasks)
+ phones_per_word[min_index] += 1
+ return phones_per_word
+
+
+def handle_long(sep_phonemes: list[list[str]]) -> list[list[str]]:
+ """
+ フレーズごとに分かれた音素(長音記号がそのまま)のリストのリスト`sep_phonemes`を受け取り、
+ その長音記号を処理して、音素のリストのリストを返す。
+ 基本的には直前の音素を伸ばすが、直前の音素が母音でない場合もしくは冒頭の場合は、
+ おそらく長音記号とダッシュを勘違いしていると思われるので、ダッシュに対応する音素`-`に変換する。
+ """
+ for i in range(len(sep_phonemes)):
+ if len(sep_phonemes[i]) == 0:
+ # 空白文字等でリストが空の場合
+ continue
+ if sep_phonemes[i][0] == "ー":
+ if i != 0:
+ prev_phoneme = sep_phonemes[i - 1][-1]
+ if prev_phoneme in VOWELS:
+ # 母音と「ん」のあとの伸ばし棒なので、その母音に変換
+ sep_phonemes[i][0] = sep_phonemes[i - 1][-1]
+ else:
+ # 「。ーー」等おそらく予期しない長音記号
+ # ダッシュの勘違いだと思われる
+ sep_phonemes[i][0] = "-"
+ else:
+ # 冒頭に長音記号が来ていおり、これはダッシュの勘違いと思われる
+ sep_phonemes[i][0] = "-"
+ if "ー" in sep_phonemes[i]:
+ for j in range(len(sep_phonemes[i])):
+ if sep_phonemes[i][j] == "ー":
+ sep_phonemes[i][j] = sep_phonemes[i][j - 1][-1]
+ return sep_phonemes
+
+
+tokenizer = AutoTokenizer.from_pretrained("./bert/deberta-v2-large-japanese-char-wwm")
+
+
+def align_tones(
+ phones_with_punct: list[str], phone_tone_list: list[tuple[str, int]]
+) -> list[tuple[str, int]]:
+ """
+ 例:
+ …私は、、そう思う。
+ phones_with_punct:
+ [".", ".", ".", "w", "a", "t", "a", "sh", "i", "w", "a", ",", ",", "s", "o", "o", "o", "m", "o", "u", "."]
+ phone_tone_list:
+ [("w", 0), ("a", 0), ("t", 1), ("a", 1), ("sh", 1), ("i", 1), ("w", 1), ("a", 1), ("_", 0), ("s", 0), ("o", 0), ("o", 1), ("o", 1), ("m", 1), ("o", 1), ("u", 0))]
+ Return:
+ [(".", 0), (".", 0), (".", 0), ("w", 0), ("a", 0), ("t", 1), ("a", 1), ("sh", 1), ("i", 1), ("w", 1), ("a", 1), (",", 0), (",", 0), ("s", 0), ("o", 0), ("o", 1), ("o", 1), ("m", 1), ("o", 1), ("u", 0), (".", 0)]
+ """
+ result: list[tuple[str, int]] = []
+ tone_index = 0
+ for phone in phones_with_punct:
+ if tone_index >= len(phone_tone_list):
+ # 余ったpunctuationがある場合 → (punctuation, 0)を追加
+ result.append((phone, 0))
+ elif phone == phone_tone_list[tone_index][0]:
+ # phone_tone_listの現在の音素と一致する場合 → toneをそこから取得、(phone, tone)を追加
+ result.append((phone, phone_tone_list[tone_index][1]))
+ # 探すindexを1つ進める
+ tone_index += 1
+ elif phone in punctuation:
+ # phoneがpunctuationの場合 → (phone, 0)を追加
+ result.append((phone, 0))
+ else:
+ logger.debug(f"phones: {phones_with_punct}")
+ logger.debug(f"phone_tone_list: {phone_tone_list}")
+ logger.debug(f"result: {result}")
+ logger.debug(f"tone_index: {tone_index}")
+ logger.debug(f"phone: {phone}")
+ raise ValueError(f"Unexpected phone: {phone}")
+ return result
+
+
+def kata2phoneme_list(text: str) -> list[str]:
+ """
+ 原則カタカナの`text`を受け取り、それをそのままいじらずに音素記号のリストに変換。
+ 注意点:
+ - punctuationかその繰り返しが来た場合、punctuationたちをそのままリストにして返す。
+ - 冒頭に続く「ー」はそのまま「ー」のままにする(`handle_long()`で処理される)
+ - 文中の「ー」は前の音素記号の最後の音素記号に変換される。
+ 例:
+ `ーーソーナノカーー` → ["ー", "ー", "s", "o", "o", "n", "a", "n", "o", "k", "a", "a", "a"]
+ `?` → ["?"]
+ `!?!?!?!?!` → ["!", "?", "!", "?", "!", "?", "!", "?", "!"]
+ """
+ if set(text).issubset(set(punctuation)):
+ return list(text)
+ # `text`がカタカナ(`ー`含む)のみからなるかどうかをチェック
+ if re.fullmatch(r"[\u30A0-\u30FF]+", text) is None:
+ raise ValueError(f"Input must be katakana only: {text}")
+ sorted_keys = sorted(mora_kata_to_mora_phonemes.keys(), key=len, reverse=True)
+ pattern = "|".join(map(re.escape, sorted_keys))
+
+ def mora2phonemes(mora: str) -> str:
+ cosonant, vowel = mora_kata_to_mora_phonemes[mora]
+ if cosonant is None:
+ return f" {vowel}"
+ return f" {cosonant} {vowel}"
+
+ spaced_phonemes = re.sub(pattern, lambda m: mora2phonemes(m.group()), text)
+
+ # 長音記号「ー」の処理
+ long_pattern = r"(\w)(ー*)"
+ long_replacement = lambda m: m.group(1) + (" " + m.group(1)) * len(m.group(2))
+ spaced_phonemes = re.sub(long_pattern, long_replacement, spaced_phonemes)
+ return spaced_phonemes.strip().split(" ")
+
+
+if __name__ == "__main__":
+ tokenizer = AutoTokenizer.from_pretrained(
+ "./bert/deberta-v2-large-japanese-char-wwm"
+ )
+ text = "こんにちは、世界。"
+ from text.japanese_bert import get_bert_feature
+
+ text = text_normalize(text)
+
+ phones, tones, word2ph = g2p(text)
+ bert = get_bert_feature(text, word2ph)
+
+ print(phones, tones, word2ph, bert.shape)
diff --git a/text/japanese_bert.py b/text/japanese_bert.py
new file mode 100644
index 0000000000000000000000000000000000000000..9efe7a46a056bcf370d98aeedf9f5f88c3b53666
--- /dev/null
+++ b/text/japanese_bert.py
@@ -0,0 +1,69 @@
+import sys
+
+import torch
+from transformers import AutoModelForMaskedLM, AutoTokenizer
+
+from config import config
+from text.japanese import text2sep_kata, text_normalize
+
+LOCAL_PATH = "./bert/deberta-v2-large-japanese-char-wwm"
+
+tokenizer = AutoTokenizer.from_pretrained(LOCAL_PATH)
+
+models = dict()
+
+
+def get_bert_feature(
+ text,
+ word2ph,
+ device=config.bert_gen_config.device,
+ assist_text=None,
+ assist_text_weight=0.7,
+ ignore_unknown=False,
+):
+ text = "".join(text2sep_kata(text, ignore_unknown=ignore_unknown)[0])
+ # text = text_normalize(text)
+ if assist_text:
+ assist_text = "".join(text2sep_kata(assist_text)[0])
+ if (
+ sys.platform == "darwin"
+ and torch.backends.mps.is_available()
+ and device == "cpu"
+ ):
+ device = "mps"
+ if not device:
+ device = "cuda"
+ if device == "cuda" and not torch.cuda.is_available():
+ device = "cpu"
+ if device not in models.keys():
+ models[device] = AutoModelForMaskedLM.from_pretrained(LOCAL_PATH).to(device)
+ with torch.no_grad():
+ inputs = tokenizer(text, return_tensors="pt")
+ for i in inputs:
+ inputs[i] = inputs[i].to(device)
+ res = models[device](**inputs, output_hidden_states=True)
+ res = torch.cat(res["hidden_states"][-3:-2], -1)[0].cpu()
+ if assist_text:
+ style_inputs = tokenizer(assist_text, return_tensors="pt")
+ for i in style_inputs:
+ style_inputs[i] = style_inputs[i].to(device)
+ style_res = models[device](**style_inputs, output_hidden_states=True)
+ style_res = torch.cat(style_res["hidden_states"][-3:-2], -1)[0].cpu()
+ style_res_mean = style_res.mean(0)
+
+ assert len(word2ph) == len(text) + 2, text
+ word2phone = word2ph
+ phone_level_feature = []
+ for i in range(len(word2phone)):
+ if assist_text:
+ repeat_feature = (
+ res[i].repeat(word2phone[i], 1) * (1 - assist_text_weight)
+ + style_res_mean.repeat(word2phone[i], 1) * assist_text_weight
+ )
+ else:
+ repeat_feature = res[i].repeat(word2phone[i], 1)
+ phone_level_feature.append(repeat_feature)
+
+ phone_level_feature = torch.cat(phone_level_feature, dim=0)
+
+ return phone_level_feature.T
diff --git a/text/japanese_mora_list.py b/text/japanese_mora_list.py
new file mode 100644
index 0000000000000000000000000000000000000000..b43e54d8d8297cf1eac0e3e3f0eef6b4f1c24fa3
--- /dev/null
+++ b/text/japanese_mora_list.py
@@ -0,0 +1,232 @@
+"""
+VOICEVOXのソースコードからお借りして最低限に改造したコード。
+https://github.com/VOICEVOX/voicevox_engine/blob/master/voicevox_engine/tts_pipeline/mora_list.py
+"""
+
+"""
+以下のモーラ対応表はOpenJTalkのソースコードから取得し、
+カタカナ表記とモーラが一対一対応するように改造した。
+ライセンス表記:
+-----------------------------------------------------------------
+ The Japanese TTS System "Open JTalk"
+ developed by HTS Working Group
+ http://open-jtalk.sourceforge.net/
+-----------------------------------------------------------------
+
+ Copyright (c) 2008-2014 Nagoya Institute of Technology
+ Department of Computer Science
+
+All rights reserved.
+
+Redistribution and use in source and binary forms, with or
+without modification, are permitted provided that the following
+conditions are met:
+
+- Redistributions of source code must retain the above copyright
+ notice, this list of conditions and the following disclaimer.
+- Redistributions in binary form must reproduce the above
+ copyright notice, this list of conditions and the following
+ disclaimer in the documentation and/or other materials provided
+ with the distribution.
+- Neither the name of the HTS working group nor the names of its
+ contributors may be used to endorse or promote products derived
+ from this software without specific prior written permission.
+
+THIS SOFTWARE IS PROVIDED BY THE COPYRIGHT HOLDERS AND
+CONTRIBUTORS "AS IS" AND ANY EXPRESS OR IMPLIED WARRANTIES,
+INCLUDING, BUT NOT LIMITED TO, THE IMPLIED WARRANTIES OF
+MERCHANTABILITY AND FITNESS FOR A PARTICULAR PURPOSE ARE
+DISCLAIMED. IN NO EVENT SHALL THE COPYRIGHT OWNER OR CONTRIBUTORS
+BE LIABLE FOR ANY DIRECT, INDIRECT, INCIDENTAL, SPECIAL,
+EXEMPLARY, OR CONSEQUENTIAL DAMAGES (INCLUDING, BUT NOT LIMITED
+TO, PROCUREMENT OF SUBSTITUTE GOODS OR SERVICES; LOSS OF USE,
+DATA, OR PROFITS; OR BUSINESS INTERRUPTION) HOWEVER CAUSED AND ON
+ANY THEORY OF LIABILITY, WHETHER IN CONTRACT, STRICT LIABILITY,
+OR TORT (INCLUDING NEGLIGENCE OR OTHERWISE) ARISING IN ANY WAY
+OUT OF THE USE OF THIS SOFTWARE, EVEN IF ADVISED OF THE
+POSSIBILITY OF SUCH DAMAGE.
+"""
+from typing import Optional
+
+# (カタカナ, 子音, 母音)の順。子音がない場合はNoneを入れる。
+# 但し「ン」と「ッ」は母音のみという扱いで、「ン」は「N」、「ッ」は「q」とする。
+# (元々「ッ」は「cl」)
+# また「デェ = dy e」はpyopenjtalkの出力(de e)と合わないため削除
+_mora_list_minimum: list[tuple[str, Optional[str], str]] = [
+ ("ヴォ", "v", "o"),
+ ("ヴェ", "v", "e"),
+ ("ヴィ", "v", "i"),
+ ("ヴァ", "v", "a"),
+ ("ヴ", "v", "u"),
+ ("ン", None, "N"),
+ ("ワ", "w", "a"),
+ ("ロ", "r", "o"),
+ ("レ", "r", "e"),
+ ("ル", "r", "u"),
+ ("リョ", "ry", "o"),
+ ("リュ", "ry", "u"),
+ ("リャ", "ry", "a"),
+ ("リェ", "ry", "e"),
+ ("リ", "r", "i"),
+ ("ラ", "r", "a"),
+ ("ヨ", "y", "o"),
+ ("ユ", "y", "u"),
+ ("ヤ", "y", "a"),
+ ("モ", "m", "o"),
+ ("メ", "m", "e"),
+ ("ム", "m", "u"),
+ ("ミョ", "my", "o"),
+ ("ミュ", "my", "u"),
+ ("ミャ", "my", "a"),
+ ("ミェ", "my", "e"),
+ ("ミ", "m", "i"),
+ ("マ", "m", "a"),
+ ("ポ", "p", "o"),
+ ("ボ", "b", "o"),
+ ("ホ", "h", "o"),
+ ("ペ", "p", "e"),
+ ("ベ", "b", "e"),
+ ("ヘ", "h", "e"),
+ ("プ", "p", "u"),
+ ("ブ", "b", "u"),
+ ("フォ", "f", "o"),
+ ("フェ", "f", "e"),
+ ("フィ", "f", "i"),
+ ("ファ", "f", "a"),
+ ("フ", "f", "u"),
+ ("ピョ", "py", "o"),
+ ("ピュ", "py", "u"),
+ ("ピャ", "py", "a"),
+ ("ピェ", "py", "e"),
+ ("ピ", "p", "i"),
+ ("ビョ", "by", "o"),
+ ("ビュ", "by", "u"),
+ ("ビャ", "by", "a"),
+ ("ビェ", "by", "e"),
+ ("ビ", "b", "i"),
+ ("ヒョ", "hy", "o"),
+ ("ヒュ", "hy", "u"),
+ ("ヒャ", "hy", "a"),
+ ("ヒェ", "hy", "e"),
+ ("ヒ", "h", "i"),
+ ("パ", "p", "a"),
+ ("バ", "b", "a"),
+ ("ハ", "h", "a"),
+ ("ノ", "n", "o"),
+ ("ネ", "n", "e"),
+ ("ヌ", "n", "u"),
+ ("ニョ", "ny", "o"),
+ ("ニュ", "ny", "u"),
+ ("ニャ", "ny", "a"),
+ ("ニェ", "ny", "e"),
+ ("ニ", "n", "i"),
+ ("ナ", "n", "a"),
+ ("ドゥ", "d", "u"),
+ ("ド", "d", "o"),
+ ("トゥ", "t", "u"),
+ ("ト", "t", "o"),
+ ("デョ", "dy", "o"),
+ ("デュ", "dy", "u"),
+ ("デャ", "dy", "a"),
+ # ("デェ", "dy", "e"),
+ ("ディ", "d", "i"),
+ ("デ", "d", "e"),
+ ("テョ", "ty", "o"),
+ ("テュ", "ty", "u"),
+ ("テャ", "ty", "a"),
+ ("ティ", "t", "i"),
+ ("テ", "t", "e"),
+ ("ツォ", "ts", "o"),
+ ("ツェ", "ts", "e"),
+ ("ツィ", "ts", "i"),
+ ("ツァ", "ts", "a"),
+ ("ツ", "ts", "u"),
+ ("ッ", None, "q"), # 「cl」から「q」に変更
+ ("チョ", "ch", "o"),
+ ("チュ", "ch", "u"),
+ ("チャ", "ch", "a"),
+ ("チェ", "ch", "e"),
+ ("チ", "ch", "i"),
+ ("ダ", "d", "a"),
+ ("タ", "t", "a"),
+ ("ゾ", "z", "o"),
+ ("ソ", "s", "o"),
+ ("ゼ", "z", "e"),
+ ("セ", "s", "e"),
+ ("ズィ", "z", "i"),
+ ("ズ", "z", "u"),
+ ("スィ", "s", "i"),
+ ("ス", "s", "u"),
+ ("ジョ", "j", "o"),
+ ("ジュ", "j", "u"),
+ ("ジャ", "j", "a"),
+ ("ジェ", "j", "e"),
+ ("ジ", "j", "i"),
+ ("ショ", "sh", "o"),
+ ("シュ", "sh", "u"),
+ ("シャ", "sh", "a"),
+ ("シェ", "sh", "e"),
+ ("シ", "sh", "i"),
+ ("ザ", "z", "a"),
+ ("サ", "s", "a"),
+ ("ゴ", "g", "o"),
+ ("コ", "k", "o"),
+ ("ゲ", "g", "e"),
+ ("ケ", "k", "e"),
+ ("グヮ", "gw", "a"),
+ ("グ", "g", "u"),
+ ("クヮ", "kw", "a"),
+ ("ク", "k", "u"),
+ ("ギョ", "gy", "o"),
+ ("ギュ", "gy", "u"),
+ ("ギャ", "gy", "a"),
+ ("ギェ", "gy", "e"),
+ ("ギ", "g", "i"),
+ ("キョ", "ky", "o"),
+ ("キュ", "ky", "u"),
+ ("キャ", "ky", "a"),
+ ("キェ", "ky", "e"),
+ ("キ", "k", "i"),
+ ("ガ", "g", "a"),
+ ("カ", "k", "a"),
+ ("オ", None, "o"),
+ ("エ", None, "e"),
+ ("ウォ", "w", "o"),
+ ("ウェ", "w", "e"),
+ ("ウィ", "w", "i"),
+ ("ウ", None, "u"),
+ ("イェ", "y", "e"),
+ ("イ", None, "i"),
+ ("ア", None, "a"),
+]
+_mora_list_additional: list[tuple[str, Optional[str], str]] = [
+ ("ヴョ", "by", "o"),
+ ("ヴュ", "by", "u"),
+ ("ヴャ", "by", "a"),
+ ("ヲ", None, "o"),
+ ("ヱ", None, "e"),
+ ("ヰ", None, "i"),
+ ("ヮ", "w", "a"),
+ ("ョ", "y", "o"),
+ ("ュ", "y", "u"),
+ ("ヅ", "z", "u"),
+ ("ヂ", "j", "i"),
+ ("ヶ", "k", "e"),
+ ("ャ", "y", "a"),
+ ("ォ", None, "o"),
+ ("ェ", None, "e"),
+ ("ゥ", None, "u"),
+ ("ィ", None, "i"),
+ ("ァ", None, "a"),
+]
+
+# 例: "vo" -> "ヴォ", "a" -> "ア"
+mora_phonemes_to_mora_kata: dict[str, str] = {
+ (consonant or "") + vowel: kana for [kana, consonant, vowel] in _mora_list_minimum
+}
+
+# 例: "ヴォ" -> ("v", "o"), "ア" -> (None, "a")
+mora_kata_to_mora_phonemes: dict[str, tuple[Optional[str], str]] = {
+ kana: (consonant, vowel)
+ for [kana, consonant, vowel] in _mora_list_minimum + _mora_list_additional
+}
diff --git a/text/opencpop-strict.txt b/text/opencpop-strict.txt
new file mode 100644
index 0000000000000000000000000000000000000000..1bede89a429e34b1a58781ee570fc313b80c0aee
--- /dev/null
+++ b/text/opencpop-strict.txt
@@ -0,0 +1,429 @@
+a AA a
+ai AA ai
+an AA an
+ang AA ang
+ao AA ao
+ba b a
+bai b ai
+ban b an
+bang b ang
+bao b ao
+bei b ei
+ben b en
+beng b eng
+bi b i
+bian b ian
+biao b iao
+bie b ie
+bin b in
+bing b ing
+bo b o
+bu b u
+ca c a
+cai c ai
+can c an
+cang c ang
+cao c ao
+ce c e
+cei c ei
+cen c en
+ceng c eng
+cha ch a
+chai ch ai
+chan ch an
+chang ch ang
+chao ch ao
+che ch e
+chen ch en
+cheng ch eng
+chi ch ir
+chong ch ong
+chou ch ou
+chu ch u
+chua ch ua
+chuai ch uai
+chuan ch uan
+chuang ch uang
+chui ch ui
+chun ch un
+chuo ch uo
+ci c i0
+cong c ong
+cou c ou
+cu c u
+cuan c uan
+cui c ui
+cun c un
+cuo c uo
+da d a
+dai d ai
+dan d an
+dang d ang
+dao d ao
+de d e
+dei d ei
+den d en
+deng d eng
+di d i
+dia d ia
+dian d ian
+diao d iao
+die d ie
+ding d ing
+diu d iu
+dong d ong
+dou d ou
+du d u
+duan d uan
+dui d ui
+dun d un
+duo d uo
+e EE e
+ei EE ei
+en EE en
+eng EE eng
+er EE er
+fa f a
+fan f an
+fang f ang
+fei f ei
+fen f en
+feng f eng
+fo f o
+fou f ou
+fu f u
+ga g a
+gai g ai
+gan g an
+gang g ang
+gao g ao
+ge g e
+gei g ei
+gen g en
+geng g eng
+gong g ong
+gou g ou
+gu g u
+gua g ua
+guai g uai
+guan g uan
+guang g uang
+gui g ui
+gun g un
+guo g uo
+ha h a
+hai h ai
+han h an
+hang h ang
+hao h ao
+he h e
+hei h ei
+hen h en
+heng h eng
+hong h ong
+hou h ou
+hu h u
+hua h ua
+huai h uai
+huan h uan
+huang h uang
+hui h ui
+hun h un
+huo h uo
+ji j i
+jia j ia
+jian j ian
+jiang j iang
+jiao j iao
+jie j ie
+jin j in
+jing j ing
+jiong j iong
+jiu j iu
+ju j v
+jv j v
+juan j van
+jvan j van
+jue j ve
+jve j ve
+jun j vn
+jvn j vn
+ka k a
+kai k ai
+kan k an
+kang k ang
+kao k ao
+ke k e
+kei k ei
+ken k en
+keng k eng
+kong k ong
+kou k ou
+ku k u
+kua k ua
+kuai k uai
+kuan k uan
+kuang k uang
+kui k ui
+kun k un
+kuo k uo
+la l a
+lai l ai
+lan l an
+lang l ang
+lao l ao
+le l e
+lei l ei
+leng l eng
+li l i
+lia l ia
+lian l ian
+liang l iang
+liao l iao
+lie l ie
+lin l in
+ling l ing
+liu l iu
+lo l o
+long l ong
+lou l ou
+lu l u
+luan l uan
+lun l un
+luo l uo
+lv l v
+lve l ve
+ma m a
+mai m ai
+man m an
+mang m ang
+mao m ao
+me m e
+mei m ei
+men m en
+meng m eng
+mi m i
+mian m ian
+miao m iao
+mie m ie
+min m in
+ming m ing
+miu m iu
+mo m o
+mou m ou
+mu m u
+na n a
+nai n ai
+nan n an
+nang n ang
+nao n ao
+ne n e
+nei n ei
+nen n en
+neng n eng
+ni n i
+nian n ian
+niang n iang
+niao n iao
+nie n ie
+nin n in
+ning n ing
+niu n iu
+nong n ong
+nou n ou
+nu n u
+nuan n uan
+nun n un
+nuo n uo
+nv n v
+nve n ve
+o OO o
+ou OO ou
+pa p a
+pai p ai
+pan p an
+pang p ang
+pao p ao
+pei p ei
+pen p en
+peng p eng
+pi p i
+pian p ian
+piao p iao
+pie p ie
+pin p in
+ping p ing
+po p o
+pou p ou
+pu p u
+qi q i
+qia q ia
+qian q ian
+qiang q iang
+qiao q iao
+qie q ie
+qin q in
+qing q ing
+qiong q iong
+qiu q iu
+qu q v
+qv q v
+quan q van
+qvan q van
+que q ve
+qve q ve
+qun q vn
+qvn q vn
+ran r an
+rang r ang
+rao r ao
+re r e
+ren r en
+reng r eng
+ri r ir
+rong r ong
+rou r ou
+ru r u
+rua r ua
+ruan r uan
+rui r ui
+run r un
+ruo r uo
+sa s a
+sai s ai
+san s an
+sang s ang
+sao s ao
+se s e
+sen s en
+seng s eng
+sha sh a
+shai sh ai
+shan sh an
+shang sh ang
+shao sh ao
+she sh e
+shei sh ei
+shen sh en
+sheng sh eng
+shi sh ir
+shou sh ou
+shu sh u
+shua sh ua
+shuai sh uai
+shuan sh uan
+shuang sh uang
+shui sh ui
+shun sh un
+shuo sh uo
+si s i0
+song s ong
+sou s ou
+su s u
+suan s uan
+sui s ui
+sun s un
+suo s uo
+ta t a
+tai t ai
+tan t an
+tang t ang
+tao t ao
+te t e
+tei t ei
+teng t eng
+ti t i
+tian t ian
+tiao t iao
+tie t ie
+ting t ing
+tong t ong
+tou t ou
+tu t u
+tuan t uan
+tui t ui
+tun t un
+tuo t uo
+wa w a
+wai w ai
+wan w an
+wang w ang
+wei w ei
+wen w en
+weng w eng
+wo w o
+wu w u
+xi x i
+xia x ia
+xian x ian
+xiang x iang
+xiao x iao
+xie x ie
+xin x in
+xing x ing
+xiong x iong
+xiu x iu
+xu x v
+xv x v
+xuan x van
+xvan x van
+xue x ve
+xve x ve
+xun x vn
+xvn x vn
+ya y a
+yan y En
+yang y ang
+yao y ao
+ye y E
+yi y i
+yin y in
+ying y ing
+yo y o
+yong y ong
+you y ou
+yu y v
+yv y v
+yuan y van
+yvan y van
+yue y ve
+yve y ve
+yun y vn
+yvn y vn
+za z a
+zai z ai
+zan z an
+zang z ang
+zao z ao
+ze z e
+zei z ei
+zen z en
+zeng z eng
+zha zh a
+zhai zh ai
+zhan zh an
+zhang zh ang
+zhao zh ao
+zhe zh e
+zhei zh ei
+zhen zh en
+zheng zh eng
+zhi zh ir
+zhong zh ong
+zhou zh ou
+zhu zh u
+zhua zh ua
+zhuai zh uai
+zhuan zh uan
+zhuang zh uang
+zhui zh ui
+zhun zh un
+zhuo zh uo
+zi z i0
+zong z ong
+zou z ou
+zu z u
+zuan z uan
+zui z ui
+zun z un
+zuo z uo
diff --git a/text/symbols.py b/text/symbols.py
new file mode 100644
index 0000000000000000000000000000000000000000..846de64584e9ba4b8d96aab36d4efbcefb1a11e7
--- /dev/null
+++ b/text/symbols.py
@@ -0,0 +1,187 @@
+punctuation = ["!", "?", "…", ",", ".", "'", "-"]
+pu_symbols = punctuation + ["SP", "UNK"]
+pad = "_"
+
+# chinese
+zh_symbols = [
+ "E",
+ "En",
+ "a",
+ "ai",
+ "an",
+ "ang",
+ "ao",
+ "b",
+ "c",
+ "ch",
+ "d",
+ "e",
+ "ei",
+ "en",
+ "eng",
+ "er",
+ "f",
+ "g",
+ "h",
+ "i",
+ "i0",
+ "ia",
+ "ian",
+ "iang",
+ "iao",
+ "ie",
+ "in",
+ "ing",
+ "iong",
+ "ir",
+ "iu",
+ "j",
+ "k",
+ "l",
+ "m",
+ "n",
+ "o",
+ "ong",
+ "ou",
+ "p",
+ "q",
+ "r",
+ "s",
+ "sh",
+ "t",
+ "u",
+ "ua",
+ "uai",
+ "uan",
+ "uang",
+ "ui",
+ "un",
+ "uo",
+ "v",
+ "van",
+ "ve",
+ "vn",
+ "w",
+ "x",
+ "y",
+ "z",
+ "zh",
+ "AA",
+ "EE",
+ "OO",
+]
+num_zh_tones = 6
+
+# japanese
+ja_symbols = [
+ "N",
+ "a",
+ "a:",
+ "b",
+ "by",
+ "ch",
+ "d",
+ "dy",
+ "e",
+ "e:",
+ "f",
+ "g",
+ "gy",
+ "h",
+ "hy",
+ "i",
+ "i:",
+ "j",
+ "k",
+ "ky",
+ "m",
+ "my",
+ "n",
+ "ny",
+ "o",
+ "o:",
+ "p",
+ "py",
+ "q",
+ "r",
+ "ry",
+ "s",
+ "sh",
+ "t",
+ "ts",
+ "ty",
+ "u",
+ "u:",
+ "w",
+ "y",
+ "z",
+ "zy",
+]
+num_ja_tones = 2
+
+# English
+en_symbols = [
+ "aa",
+ "ae",
+ "ah",
+ "ao",
+ "aw",
+ "ay",
+ "b",
+ "ch",
+ "d",
+ "dh",
+ "eh",
+ "er",
+ "ey",
+ "f",
+ "g",
+ "hh",
+ "ih",
+ "iy",
+ "jh",
+ "k",
+ "l",
+ "m",
+ "n",
+ "ng",
+ "ow",
+ "oy",
+ "p",
+ "r",
+ "s",
+ "sh",
+ "t",
+ "th",
+ "uh",
+ "uw",
+ "V",
+ "w",
+ "y",
+ "z",
+ "zh",
+]
+num_en_tones = 4
+
+# combine all symbols
+normal_symbols = sorted(set(zh_symbols + ja_symbols + en_symbols))
+symbols = [pad] + normal_symbols + pu_symbols
+sil_phonemes_ids = [symbols.index(i) for i in pu_symbols]
+
+# combine all tones
+num_tones = num_zh_tones + num_ja_tones + num_en_tones
+
+# language maps
+language_id_map = {"ZH": 0, "JP": 1, "EN": 2}
+num_languages = len(language_id_map.keys())
+
+language_tone_start_map = {
+ "ZH": 0,
+ "JP": num_zh_tones,
+ "EN": num_zh_tones + num_ja_tones,
+}
+
+if __name__ == "__main__":
+ a = set(zh_symbols)
+ b = set(en_symbols)
+ print(sorted(a & b))
diff --git a/text/tone_sandhi.py b/text/tone_sandhi.py
new file mode 100644
index 0000000000000000000000000000000000000000..372308604d52cc32f80d0146efa95cfdf7e40b05
--- /dev/null
+++ b/text/tone_sandhi.py
@@ -0,0 +1,773 @@
+# Copyright (c) 2021 PaddlePaddle Authors. All Rights Reserved.
+#
+# Licensed under the Apache License, Version 2.0 (the "License");
+# you may not use this file except in compliance with the License.
+# You may obtain a copy of the License at
+#
+# http://www.apache.org/licenses/LICENSE-2.0
+#
+# Unless required by applicable law or agreed to in writing, software
+# distributed under the License is distributed on an "AS IS" BASIS,
+# WITHOUT WARRANTIES OR CONDITIONS OF ANY KIND, either express or implied.
+# See the License for the specific language governing permissions and
+# limitations under the License.
+from typing import List
+from typing import Tuple
+
+import jieba
+from pypinyin import lazy_pinyin
+from pypinyin import Style
+
+
+class ToneSandhi:
+ def __init__(self):
+ self.must_neural_tone_words = {
+ "麻烦",
+ "麻利",
+ "鸳鸯",
+ "高粱",
+ "骨头",
+ "骆驼",
+ "马虎",
+ "首饰",
+ "馒头",
+ "馄饨",
+ "风筝",
+ "难为",
+ "队伍",
+ "阔气",
+ "闺女",
+ "门道",
+ "锄头",
+ "铺盖",
+ "铃铛",
+ "铁匠",
+ "钥匙",
+ "里脊",
+ "里头",
+ "部分",
+ "那么",
+ "道士",
+ "造化",
+ "迷糊",
+ "连累",
+ "这么",
+ "这个",
+ "运气",
+ "过去",
+ "软和",
+ "转悠",
+ "踏实",
+ "跳蚤",
+ "跟头",
+ "趔趄",
+ "财主",
+ "豆腐",
+ "讲究",
+ "记性",
+ "记号",
+ "认识",
+ "规矩",
+ "见识",
+ "裁缝",
+ "补丁",
+ "衣裳",
+ "衣服",
+ "衙门",
+ "街坊",
+ "行李",
+ "行当",
+ "蛤蟆",
+ "蘑菇",
+ "薄荷",
+ "葫芦",
+ "葡萄",
+ "萝卜",
+ "荸荠",
+ "苗条",
+ "苗头",
+ "苍蝇",
+ "芝麻",
+ "舒服",
+ "舒坦",
+ "舌头",
+ "自在",
+ "膏药",
+ "脾气",
+ "脑袋",
+ "脊梁",
+ "能耐",
+ "胳膊",
+ "胭脂",
+ "胡萝",
+ "胡琴",
+ "胡同",
+ "聪明",
+ "耽误",
+ "耽搁",
+ "耷拉",
+ "耳朵",
+ "老爷",
+ "老实",
+ "老婆",
+ "老头",
+ "老太",
+ "翻腾",
+ "罗嗦",
+ "罐头",
+ "编辑",
+ "结实",
+ "红火",
+ "累赘",
+ "糨糊",
+ "糊涂",
+ "精神",
+ "粮食",
+ "簸箕",
+ "篱笆",
+ "算计",
+ "算盘",
+ "答应",
+ "笤帚",
+ "笑语",
+ "笑话",
+ "窟窿",
+ "窝囊",
+ "窗户",
+ "稳当",
+ "稀罕",
+ "称呼",
+ "秧歌",
+ "秀气",
+ "秀才",
+ "福气",
+ "祖宗",
+ "砚台",
+ "码头",
+ "石榴",
+ "石头",
+ "石匠",
+ "知识",
+ "眼睛",
+ "眯缝",
+ "眨巴",
+ "眉毛",
+ "相声",
+ "盘算",
+ "白净",
+ "痢疾",
+ "痛快",
+ "疟疾",
+ "疙瘩",
+ "疏忽",
+ "畜生",
+ "生意",
+ "甘蔗",
+ "琵琶",
+ "琢磨",
+ "琉璃",
+ "玻璃",
+ "玫瑰",
+ "玄乎",
+ "狐狸",
+ "状元",
+ "特务",
+ "牲口",
+ "牙碜",
+ "牌楼",
+ "爽快",
+ "爱人",
+ "热闹",
+ "烧饼",
+ "烟筒",
+ "烂糊",
+ "点心",
+ "炊帚",
+ "灯笼",
+ "火候",
+ "漂亮",
+ "滑溜",
+ "溜达",
+ "温和",
+ "清楚",
+ "消息",
+ "浪头",
+ "活泼",
+ "比方",
+ "正经",
+ "欺负",
+ "模糊",
+ "槟榔",
+ "棺材",
+ "棒槌",
+ "棉花",
+ "核桃",
+ "栅栏",
+ "柴火",
+ "架势",
+ "枕头",
+ "枇杷",
+ "机灵",
+ "本事",
+ "木头",
+ "木匠",
+ "朋友",
+ "月饼",
+ "月亮",
+ "暖和",
+ "明白",
+ "时候",
+ "新鲜",
+ "故事",
+ "收拾",
+ "收成",
+ "提防",
+ "挖苦",
+ "挑剔",
+ "指甲",
+ "指头",
+ "拾掇",
+ "拳头",
+ "拨弄",
+ "招牌",
+ "招呼",
+ "抬举",
+ "护士",
+ "折腾",
+ "扫帚",
+ "打量",
+ "打算",
+ "打点",
+ "打扮",
+ "打听",
+ "打发",
+ "扎实",
+ "扁担",
+ "戒指",
+ "懒得",
+ "意识",
+ "意思",
+ "情形",
+ "悟性",
+ "怪物",
+ "思量",
+ "怎么",
+ "念头",
+ "念叨",
+ "快活",
+ "忙活",
+ "志气",
+ "心思",
+ "得罪",
+ "张罗",
+ "弟兄",
+ "开通",
+ "应酬",
+ "庄稼",
+ "干事",
+ "帮手",
+ "帐篷",
+ "希罕",
+ "师父",
+ "师傅",
+ "巴结",
+ "巴掌",
+ "差事",
+ "工夫",
+ "岁数",
+ "屁股",
+ "尾巴",
+ "少爷",
+ "小气",
+ "小伙",
+ "将就",
+ "对头",
+ "对付",
+ "寡妇",
+ "家伙",
+ "客气",
+ "实在",
+ "官司",
+ "学问",
+ "学生",
+ "字号",
+ "嫁妆",
+ "媳妇",
+ "媒人",
+ "婆家",
+ "娘家",
+ "委屈",
+ "姑娘",
+ "姐夫",
+ "妯娌",
+ "妥当",
+ "妖精",
+ "奴才",
+ "女婿",
+ "头发",
+ "太阳",
+ "大爷",
+ "大方",
+ "大意",
+ "大夫",
+ "多少",
+ "多么",
+ "外甥",
+ "壮实",
+ "地道",
+ "地方",
+ "在乎",
+ "困难",
+ "嘴巴",
+ "嘱咐",
+ "嘟囔",
+ "嘀咕",
+ "喜欢",
+ "喇嘛",
+ "喇叭",
+ "商量",
+ "唾沫",
+ "哑巴",
+ "哈欠",
+ "哆嗦",
+ "咳嗽",
+ "和尚",
+ "告诉",
+ "告示",
+ "含糊",
+ "吓唬",
+ "后头",
+ "名字",
+ "名堂",
+ "合同",
+ "吆喝",
+ "叫唤",
+ "口袋",
+ "厚道",
+ "厉害",
+ "千斤",
+ "包袱",
+ "包涵",
+ "匀称",
+ "勤快",
+ "动静",
+ "动弹",
+ "功夫",
+ "力气",
+ "前头",
+ "刺猬",
+ "刺激",
+ "别扭",
+ "利落",
+ "利索",
+ "利害",
+ "分析",
+ "出息",
+ "凑合",
+ "凉快",
+ "冷战",
+ "冤枉",
+ "冒失",
+ "养活",
+ "关系",
+ "先生",
+ "兄弟",
+ "便宜",
+ "使唤",
+ "佩服",
+ "作坊",
+ "体面",
+ "位置",
+ "似的",
+ "伙计",
+ "休息",
+ "什么",
+ "人家",
+ "亲戚",
+ "亲家",
+ "交情",
+ "云彩",
+ "事情",
+ "买卖",
+ "主意",
+ "丫头",
+ "丧气",
+ "两口",
+ "东西",
+ "东家",
+ "世故",
+ "不由",
+ "不在",
+ "下水",
+ "下巴",
+ "上头",
+ "上司",
+ "丈夫",
+ "丈人",
+ "一辈",
+ "那个",
+ "菩萨",
+ "父亲",
+ "母亲",
+ "咕噜",
+ "邋遢",
+ "费用",
+ "冤家",
+ "甜头",
+ "介绍",
+ "荒唐",
+ "大人",
+ "泥鳅",
+ "幸福",
+ "熟悉",
+ "计划",
+ "扑腾",
+ "蜡烛",
+ "姥爷",
+ "照顾",
+ "喉咙",
+ "吉他",
+ "弄堂",
+ "蚂蚱",
+ "凤凰",
+ "拖沓",
+ "寒碜",
+ "糟蹋",
+ "倒腾",
+ "报复",
+ "逻辑",
+ "盘缠",
+ "喽啰",
+ "牢骚",
+ "咖喱",
+ "扫把",
+ "惦记",
+ }
+ self.must_not_neural_tone_words = {
+ "男子",
+ "女子",
+ "分子",
+ "原子",
+ "量子",
+ "莲子",
+ "石子",
+ "瓜子",
+ "电子",
+ "人人",
+ "虎虎",
+ }
+ self.punc = ":,;。?!“”‘’':,;.?!"
+
+ # the meaning of jieba pos tag: https://blog.csdn.net/weixin_44174352/article/details/113731041
+ # e.g.
+ # word: "家里"
+ # pos: "s"
+ # finals: ['ia1', 'i3']
+ def _neural_sandhi(self, word: str, pos: str, finals: List[str]) -> List[str]:
+ # reduplication words for n. and v. e.g. 奶奶, 试试, 旺旺
+ for j, item in enumerate(word):
+ if (
+ j - 1 >= 0
+ and item == word[j - 1]
+ and pos[0] in {"n", "v", "a"}
+ and word not in self.must_not_neural_tone_words
+ ):
+ finals[j] = finals[j][:-1] + "5"
+ ge_idx = word.find("个")
+ if len(word) >= 1 and word[-1] in "吧呢啊呐噻嘛吖嗨呐哦哒额滴哩哟喽啰耶喔诶":
+ finals[-1] = finals[-1][:-1] + "5"
+ elif len(word) >= 1 and word[-1] in "的地得":
+ finals[-1] = finals[-1][:-1] + "5"
+ # e.g. 走了, 看着, 去过
+ # elif len(word) == 1 and word in "了着过" and pos in {"ul", "uz", "ug"}:
+ # finals[-1] = finals[-1][:-1] + "5"
+ elif (
+ len(word) > 1
+ and word[-1] in "们子"
+ and pos in {"r", "n"}
+ and word not in self.must_not_neural_tone_words
+ ):
+ finals[-1] = finals[-1][:-1] + "5"
+ # e.g. 桌上, 地下, 家里
+ elif len(word) > 1 and word[-1] in "上下里" and pos in {"s", "l", "f"}:
+ finals[-1] = finals[-1][:-1] + "5"
+ # e.g. 上来, 下去
+ elif len(word) > 1 and word[-1] in "来去" and word[-2] in "上下进出回过起开":
+ finals[-1] = finals[-1][:-1] + "5"
+ # 个做量词
+ elif (
+ ge_idx >= 1
+ and (word[ge_idx - 1].isnumeric() or word[ge_idx - 1] in "几有两半多各整每做是")
+ ) or word == "个":
+ finals[ge_idx] = finals[ge_idx][:-1] + "5"
+ else:
+ if (
+ word in self.must_neural_tone_words
+ or word[-2:] in self.must_neural_tone_words
+ ):
+ finals[-1] = finals[-1][:-1] + "5"
+
+ word_list = self._split_word(word)
+ finals_list = [finals[: len(word_list[0])], finals[len(word_list[0]) :]]
+ for i, word in enumerate(word_list):
+ # conventional neural in Chinese
+ if (
+ word in self.must_neural_tone_words
+ or word[-2:] in self.must_neural_tone_words
+ ):
+ finals_list[i][-1] = finals_list[i][-1][:-1] + "5"
+ finals = sum(finals_list, [])
+ return finals
+
+ def _bu_sandhi(self, word: str, finals: List[str]) -> List[str]:
+ # e.g. 看不懂
+ if len(word) == 3 and word[1] == "不":
+ finals[1] = finals[1][:-1] + "5"
+ else:
+ for i, char in enumerate(word):
+ # "不" before tone4 should be bu2, e.g. 不怕
+ if char == "不" and i + 1 < len(word) and finals[i + 1][-1] == "4":
+ finals[i] = finals[i][:-1] + "2"
+ return finals
+
+ def _yi_sandhi(self, word: str, finals: List[str]) -> List[str]:
+ # "一" in number sequences, e.g. 一零零, 二一零
+ if word.find("一") != -1 and all(
+ [item.isnumeric() for item in word if item != "一"]
+ ):
+ return finals
+ # "一" between reduplication words should be yi5, e.g. 看一看
+ elif len(word) == 3 and word[1] == "一" and word[0] == word[-1]:
+ finals[1] = finals[1][:-1] + "5"
+ # when "一" is ordinal word, it should be yi1
+ elif word.startswith("第一"):
+ finals[1] = finals[1][:-1] + "1"
+ else:
+ for i, char in enumerate(word):
+ if char == "一" and i + 1 < len(word):
+ # "一" before tone4 should be yi2, e.g. 一段
+ if finals[i + 1][-1] == "4":
+ finals[i] = finals[i][:-1] + "2"
+ # "一" before non-tone4 should be yi4, e.g. 一天
+ else:
+ # "一" 后面如果是标点,还读一声
+ if word[i + 1] not in self.punc:
+ finals[i] = finals[i][:-1] + "4"
+ return finals
+
+ def _split_word(self, word: str) -> List[str]:
+ word_list = jieba.cut_for_search(word)
+ word_list = sorted(word_list, key=lambda i: len(i), reverse=False)
+ first_subword = word_list[0]
+ first_begin_idx = word.find(first_subword)
+ if first_begin_idx == 0:
+ second_subword = word[len(first_subword) :]
+ new_word_list = [first_subword, second_subword]
+ else:
+ second_subword = word[: -len(first_subword)]
+ new_word_list = [second_subword, first_subword]
+ return new_word_list
+
+ def _three_sandhi(self, word: str, finals: List[str]) -> List[str]:
+ if len(word) == 2 and self._all_tone_three(finals):
+ finals[0] = finals[0][:-1] + "2"
+ elif len(word) == 3:
+ word_list = self._split_word(word)
+ if self._all_tone_three(finals):
+ # disyllabic + monosyllabic, e.g. 蒙古/包
+ if len(word_list[0]) == 2:
+ finals[0] = finals[0][:-1] + "2"
+ finals[1] = finals[1][:-1] + "2"
+ # monosyllabic + disyllabic, e.g. 纸/老虎
+ elif len(word_list[0]) == 1:
+ finals[1] = finals[1][:-1] + "2"
+ else:
+ finals_list = [finals[: len(word_list[0])], finals[len(word_list[0]) :]]
+ if len(finals_list) == 2:
+ for i, sub in enumerate(finals_list):
+ # e.g. 所有/人
+ if self._all_tone_three(sub) and len(sub) == 2:
+ finals_list[i][0] = finals_list[i][0][:-1] + "2"
+ # e.g. 好/喜欢
+ elif (
+ i == 1
+ and not self._all_tone_three(sub)
+ and finals_list[i][0][-1] == "3"
+ and finals_list[0][-1][-1] == "3"
+ ):
+ finals_list[0][-1] = finals_list[0][-1][:-1] + "2"
+ finals = sum(finals_list, [])
+ # split idiom into two words who's length is 2
+ elif len(word) == 4:
+ finals_list = [finals[:2], finals[2:]]
+ finals = []
+ for sub in finals_list:
+ if self._all_tone_three(sub):
+ sub[0] = sub[0][:-1] + "2"
+ finals += sub
+
+ return finals
+
+ def _all_tone_three(self, finals: List[str]) -> bool:
+ return all(x[-1] == "3" for x in finals)
+
+ # merge "不" and the word behind it
+ # if don't merge, "不" sometimes appears alone according to jieba, which may occur sandhi error
+ def _merge_bu(self, seg: List[Tuple[str, str]]) -> List[Tuple[str, str]]:
+ new_seg = []
+ last_word = ""
+ for word, pos in seg:
+ if last_word == "不":
+ word = last_word + word
+ if word != "不":
+ new_seg.append((word, pos))
+ last_word = word[:]
+ if last_word == "不":
+ new_seg.append((last_word, "d"))
+ last_word = ""
+ return new_seg
+
+ # function 1: merge "一" and reduplication words in it's left and right, e.g. "听","一","听" ->"听一听"
+ # function 2: merge single "一" and the word behind it
+ # if don't merge, "一" sometimes appears alone according to jieba, which may occur sandhi error
+ # e.g.
+ # input seg: [('听', 'v'), ('一', 'm'), ('听', 'v')]
+ # output seg: [['听一听', 'v']]
+ def _merge_yi(self, seg: List[Tuple[str, str]]) -> List[Tuple[str, str]]:
+ new_seg = [] * len(seg)
+ # function 1
+ i = 0
+ while i < len(seg):
+ word, pos = seg[i]
+ if (
+ i - 1 >= 0
+ and word == "一"
+ and i + 1 < len(seg)
+ and seg[i - 1][0] == seg[i + 1][0]
+ and seg[i - 1][1] == "v"
+ ):
+ new_seg[i - 1][0] = new_seg[i - 1][0] + "一" + new_seg[i - 1][0]
+ i += 2
+ else:
+ if (
+ i - 2 >= 0
+ and seg[i - 1][0] == "一"
+ and seg[i - 2][0] == word
+ and pos == "v"
+ ):
+ continue
+ else:
+ new_seg.append([word, pos])
+ i += 1
+ seg = [i for i in new_seg if len(i) > 0]
+ new_seg = []
+ # function 2
+ for i, (word, pos) in enumerate(seg):
+ if new_seg and new_seg[-1][0] == "一":
+ new_seg[-1][0] = new_seg[-1][0] + word
+ else:
+ new_seg.append([word, pos])
+ return new_seg
+
+ # the first and the second words are all_tone_three
+ def _merge_continuous_three_tones(
+ self, seg: List[Tuple[str, str]]
+ ) -> List[Tuple[str, str]]:
+ new_seg = []
+ sub_finals_list = [
+ lazy_pinyin(word, neutral_tone_with_five=True, style=Style.FINALS_TONE3)
+ for (word, pos) in seg
+ ]
+ assert len(sub_finals_list) == len(seg)
+ merge_last = [False] * len(seg)
+ for i, (word, pos) in enumerate(seg):
+ if (
+ i - 1 >= 0
+ and self._all_tone_three(sub_finals_list[i - 1])
+ and self._all_tone_three(sub_finals_list[i])
+ and not merge_last[i - 1]
+ ):
+ # if the last word is reduplication, not merge, because reduplication need to be _neural_sandhi
+ if (
+ not self._is_reduplication(seg[i - 1][0])
+ and len(seg[i - 1][0]) + len(seg[i][0]) <= 3
+ ):
+ new_seg[-1][0] = new_seg[-1][0] + seg[i][0]
+ merge_last[i] = True
+ else:
+ new_seg.append([word, pos])
+ else:
+ new_seg.append([word, pos])
+
+ return new_seg
+
+ def _is_reduplication(self, word: str) -> bool:
+ return len(word) == 2 and word[0] == word[1]
+
+ # the last char of first word and the first char of second word is tone_three
+ def _merge_continuous_three_tones_2(
+ self, seg: List[Tuple[str, str]]
+ ) -> List[Tuple[str, str]]:
+ new_seg = []
+ sub_finals_list = [
+ lazy_pinyin(word, neutral_tone_with_five=True, style=Style.FINALS_TONE3)
+ for (word, pos) in seg
+ ]
+ assert len(sub_finals_list) == len(seg)
+ merge_last = [False] * len(seg)
+ for i, (word, pos) in enumerate(seg):
+ if (
+ i - 1 >= 0
+ and sub_finals_list[i - 1][-1][-1] == "3"
+ and sub_finals_list[i][0][-1] == "3"
+ and not merge_last[i - 1]
+ ):
+ # if the last word is reduplication, not merge, because reduplication need to be _neural_sandhi
+ if (
+ not self._is_reduplication(seg[i - 1][0])
+ and len(seg[i - 1][0]) + len(seg[i][0]) <= 3
+ ):
+ new_seg[-1][0] = new_seg[-1][0] + seg[i][0]
+ merge_last[i] = True
+ else:
+ new_seg.append([word, pos])
+ else:
+ new_seg.append([word, pos])
+ return new_seg
+
+ def _merge_er(self, seg: List[Tuple[str, str]]) -> List[Tuple[str, str]]:
+ new_seg = []
+ for i, (word, pos) in enumerate(seg):
+ if i - 1 >= 0 and word == "儿" and seg[i - 1][0] != "#":
+ new_seg[-1][0] = new_seg[-1][0] + seg[i][0]
+ else:
+ new_seg.append([word, pos])
+ return new_seg
+
+ def _merge_reduplication(self, seg: List[Tuple[str, str]]) -> List[Tuple[str, str]]:
+ new_seg = []
+ for i, (word, pos) in enumerate(seg):
+ if new_seg and word == new_seg[-1][0]:
+ new_seg[-1][0] = new_seg[-1][0] + seg[i][0]
+ else:
+ new_seg.append([word, pos])
+ return new_seg
+
+ def pre_merge_for_modify(self, seg: List[Tuple[str, str]]) -> List[Tuple[str, str]]:
+ seg = self._merge_bu(seg)
+ try:
+ seg = self._merge_yi(seg)
+ except:
+ print("_merge_yi failed")
+ seg = self._merge_reduplication(seg)
+ seg = self._merge_continuous_three_tones(seg)
+ seg = self._merge_continuous_three_tones_2(seg)
+ seg = self._merge_er(seg)
+ return seg
+
+ def modified_tone(self, word: str, pos: str, finals: List[str]) -> List[str]:
+ finals = self._bu_sandhi(word, finals)
+ finals = self._yi_sandhi(word, finals)
+ finals = self._neural_sandhi(word, pos, finals)
+ finals = self._three_sandhi(word, finals)
+ return finals
diff --git a/text/user_dict/LGPL_LICENSE b/text/user_dict/LGPL_LICENSE
new file mode 100644
index 0000000000000000000000000000000000000000..153d416dc8d2d60076698ec3cbfce34d91436a03
--- /dev/null
+++ b/text/user_dict/LGPL_LICENSE
@@ -0,0 +1,165 @@
+ GNU LESSER GENERAL PUBLIC LICENSE
+ Version 3, 29 June 2007
+
+ Copyright (C) 2007 Free Software Foundation, Inc.
+ Everyone is permitted to copy and distribute verbatim copies
+ of this license document, but changing it is not allowed.
+
+
+ This version of the GNU Lesser General Public License incorporates
+the terms and conditions of version 3 of the GNU General Public
+License, supplemented by the additional permissions listed below.
+
+ 0. Additional Definitions.
+
+ As used herein, "this License" refers to version 3 of the GNU Lesser
+General Public License, and the "GNU GPL" refers to version 3 of the GNU
+General Public License.
+
+ "The Library" refers to a covered work governed by this License,
+other than an Application or a Combined Work as defined below.
+
+ An "Application" is any work that makes use of an interface provided
+by the Library, but which is not otherwise based on the Library.
+Defining a subclass of a class defined by the Library is deemed a mode
+of using an interface provided by the Library.
+
+ A "Combined Work" is a work produced by combining or linking an
+Application with the Library. The particular version of the Library
+with which the Combined Work was made is also called the "Linked
+Version".
+
+ The "Minimal Corresponding Source" for a Combined Work means the
+Corresponding Source for the Combined Work, excluding any source code
+for portions of the Combined Work that, considered in isolation, are
+based on the Application, and not on the Linked Version.
+
+ The "Corresponding Application Code" for a Combined Work means the
+object code and/or source code for the Application, including any data
+and utility programs needed for reproducing the Combined Work from the
+Application, but excluding the System Libraries of the Combined Work.
+
+ 1. Exception to Section 3 of the GNU GPL.
+
+ You may convey a covered work under sections 3 and 4 of this License
+without being bound by section 3 of the GNU GPL.
+
+ 2. Conveying Modified Versions.
+
+ If you modify a copy of the Library, and, in your modifications, a
+facility refers to a function or data to be supplied by an Application
+that uses the facility (other than as an argument passed when the
+facility is invoked), then you may convey a copy of the modified
+version:
+
+ a) under this License, provided that you make a good faith effort to
+ ensure that, in the event an Application does not supply the
+ function or data, the facility still operates, and performs
+ whatever part of its purpose remains meaningful, or
+
+ b) under the GNU GPL, with none of the additional permissions of
+ this License applicable to that copy.
+
+ 3. Object Code Incorporating Material from Library Header Files.
+
+ The object code form of an Application may incorporate material from
+a header file that is part of the Library. You may convey such object
+code under terms of your choice, provided that, if the incorporated
+material is not limited to numerical parameters, data structure
+layouts and accessors, or small macros, inline functions and templates
+(ten or fewer lines in length), you do both of the following:
+
+ a) Give prominent notice with each copy of the object code that the
+ Library is used in it and that the Library and its use are
+ covered by this License.
+
+ b) Accompany the object code with a copy of the GNU GPL and this license
+ document.
+
+ 4. Combined Works.
+
+ You may convey a Combined Work under terms of your choice that,
+taken together, effectively do not restrict modification of the
+portions of the Library contained in the Combined Work and reverse
+engineering for debugging such modifications, if you also do each of
+the following:
+
+ a) Give prominent notice with each copy of the Combined Work that
+ the Library is used in it and that the Library and its use are
+ covered by this License.
+
+ b) Accompany the Combined Work with a copy of the GNU GPL and this license
+ document.
+
+ c) For a Combined Work that displays copyright notices during
+ execution, include the copyright notice for the Library among
+ these notices, as well as a reference directing the user to the
+ copies of the GNU GPL and this license document.
+
+ d) Do one of the following:
+
+ 0) Convey the Minimal Corresponding Source under the terms of this
+ License, and the Corresponding Application Code in a form
+ suitable for, and under terms that permit, the user to
+ recombine or relink the Application with a modified version of
+ the Linked Version to produce a modified Combined Work, in the
+ manner specified by section 6 of the GNU GPL for conveying
+ Corresponding Source.
+
+ 1) Use a suitable shared library mechanism for linking with the
+ Library. A suitable mechanism is one that (a) uses at run time
+ a copy of the Library already present on the user's computer
+ system, and (b) will operate properly with a modified version
+ of the Library that is interface-compatible with the Linked
+ Version.
+
+ e) Provide Installation Information, but only if you would otherwise
+ be required to provide such information under section 6 of the
+ GNU GPL, and only to the extent that such information is
+ necessary to install and execute a modified version of the
+ Combined Work produced by recombining or relinking the
+ Application with a modified version of the Linked Version. (If
+ you use option 4d0, the Installation Information must accompany
+ the Minimal Corresponding Source and Corresponding Application
+ Code. If you use option 4d1, you must provide the Installation
+ Information in the manner specified by section 6 of the GNU GPL
+ for conveying Corresponding Source.)
+
+ 5. Combined Libraries.
+
+ You may place library facilities that are a work based on the
+Library side by side in a single library together with other library
+facilities that are not Applications and are not covered by this
+License, and convey such a combined library under terms of your
+choice, if you do both of the following:
+
+ a) Accompany the combined library with a copy of the same work based
+ on the Library, uncombined with any other library facilities,
+ conveyed under the terms of this License.
+
+ b) Give prominent notice with the combined library that part of it
+ is a work based on the Library, and explaining where to find the
+ accompanying uncombined form of the same work.
+
+ 6. Revised Versions of the GNU Lesser General Public License.
+
+ The Free Software Foundation may publish revised and/or new versions
+of the GNU Lesser General Public License from time to time. Such new
+versions will be similar in spirit to the present version, but may
+differ in detail to address new problems or concerns.
+
+ Each version is given a distinguishing version number. If the
+Library as you received it specifies that a certain numbered version
+of the GNU Lesser General Public License "or any later version"
+applies to it, you have the option of following the terms and
+conditions either of that published version or of any later version
+published by the Free Software Foundation. If the Library as you
+received it does not specify a version number of the GNU Lesser
+General Public License, you may choose any version of the GNU Lesser
+General Public License ever published by the Free Software Foundation.
+
+ If the Library as you received it specifies that a proxy can decide
+whether future versions of the GNU Lesser General Public License shall
+apply, that proxy's public statement of acceptance of any version is
+permanent authorization for you to choose that version for the
+Library.
\ No newline at end of file
diff --git a/text/user_dict/README.md b/text/user_dict/README.md
new file mode 100644
index 0000000000000000000000000000000000000000..c7190ea3550a8c78c99f7c72e71d680b8f126bf5
--- /dev/null
+++ b/text/user_dict/README.md
@@ -0,0 +1,16 @@
+このモジュールは、[voicevox_engine](https://github.com/VOICEVOX/voicevox_engine)を使わせていただいています。
+
+引用元:
+
+https://github.com/VOICEVOX/voicevox_engine/tree/709527be089c0410c08e989df95a4a1d78439423/voicevox_engine/user_dict
+
+改変部分は以下のとおりです。
+- ファイル名の書き換え、それに伴うimport文の書き換え
+- VOICEVOX固有の他のモジュールへの依存のコメントアウト
+- mutexを使用している部分のコメントアウト
+- 参照しているpyopenjtalkの違いによるメソッド名書き換え
+- UserDictWordのmora_countのデフォルト値をNoneに指定
+
+ライセンス: LGPL
+
+[LGPL_LICENSE](LGPL_LICENSE)を参照してください。
diff --git a/text/user_dict/__init__.py b/text/user_dict/__init__.py
new file mode 100644
index 0000000000000000000000000000000000000000..d258359e97071d6992d29df6eb812fd393d32a4d
--- /dev/null
+++ b/text/user_dict/__init__.py
@@ -0,0 +1,462 @@
+import json
+import sys
+import threading
+import traceback
+from pathlib import Path
+from typing import Dict, List, Optional
+from uuid import UUID, uuid4
+
+import numpy as np
+import pyopenjtalk
+from fastapi import HTTPException
+
+from .word_model import UserDictWord, WordTypes
+
+# from ..utility.mutex_utility import mutex_wrapper
+# from ..utility.path_utility import engine_root, get_save_dir
+from .part_of_speech_data import MAX_PRIORITY, MIN_PRIORITY, part_of_speech_data
+from common.constants import USER_DICT_DIR
+
+# root_dir = engine_root()
+# save_dir = get_save_dir()
+root_dir = Path(USER_DICT_DIR)
+save_dir = Path(USER_DICT_DIR)
+
+
+if not save_dir.is_dir():
+ save_dir.mkdir(parents=True)
+
+default_dict_path = root_dir / "default.csv" # VOICEVOXデフォルト辞書ファイルのパス
+user_dict_path = save_dir / "user_dict.json" # ユーザー辞書ファイルのパス
+compiled_dict_path = save_dir / "user.dic" # コンパイル済み辞書ファイルのパス
+
+
+# # 同時書き込みの制御
+# mutex_user_dict = threading.Lock()
+# mutex_openjtalk_dict = threading.Lock()
+
+
+# @mutex_wrapper(mutex_user_dict)
+def _write_to_json(user_dict: Dict[str, UserDictWord], user_dict_path: Path) -> None:
+ """
+ ユーザー辞書ファイルへのユーザー辞書データ書き込み
+ Parameters
+ ----------
+ user_dict : Dict[str, UserDictWord]
+ ユーザー辞書データ
+ user_dict_path : Path
+ ユーザー辞書ファイルのパス
+ """
+ converted_user_dict = {}
+ for word_uuid, word in user_dict.items():
+ word_dict = word.dict()
+ word_dict["cost"] = _priority2cost(
+ word_dict["context_id"], word_dict["priority"]
+ )
+ del word_dict["priority"]
+ converted_user_dict[word_uuid] = word_dict
+ # 予めjsonに変換できることを確かめる
+ user_dict_json = json.dumps(converted_user_dict, ensure_ascii=False)
+
+ # ユーザー辞書ファイルへの書き込み
+ user_dict_path.write_text(user_dict_json, encoding="utf-8")
+
+
+# @mutex_wrapper(mutex_openjtalk_dict)
+def update_dict(
+ default_dict_path: Path = default_dict_path,
+ user_dict_path: Path = user_dict_path,
+ compiled_dict_path: Path = compiled_dict_path,
+) -> None:
+ """
+ 辞書の更新
+ Parameters
+ ----------
+ default_dict_path : Path
+ デフォルト辞書ファイルのパス
+ user_dict_path : Path
+ ユーザー辞書ファイルのパス
+ compiled_dict_path : Path
+ コンパイル済み辞書ファイルのパス
+ """
+ random_string = uuid4()
+ tmp_csv_path = compiled_dict_path.with_suffix(
+ f".dict_csv-{random_string}.tmp"
+ ) # csv形式辞書データの一時保存ファイル
+ tmp_compiled_path = compiled_dict_path.with_suffix(
+ f".dict_compiled-{random_string}.tmp"
+ ) # コンパイル済み辞書データの一時保存ファイル
+
+ try:
+ # 辞書.csvを作成
+ csv_text = ""
+
+ # デフォルト辞書データの追加
+ if not default_dict_path.is_file():
+ print("Warning: Cannot find default dictionary.", file=sys.stderr)
+ return
+ default_dict = default_dict_path.read_text(encoding="utf-8")
+ if default_dict == default_dict.rstrip():
+ default_dict += "\n"
+ csv_text += default_dict
+
+ # ユーザー辞書データの追加
+ user_dict = read_dict(user_dict_path=user_dict_path)
+ for word_uuid in user_dict:
+ word = user_dict[word_uuid]
+ csv_text += (
+ "{surface},{context_id},{context_id},{cost},{part_of_speech},"
+ + "{part_of_speech_detail_1},{part_of_speech_detail_2},"
+ + "{part_of_speech_detail_3},{inflectional_type},"
+ + "{inflectional_form},{stem},{yomi},{pronunciation},"
+ + "{accent_type}/{mora_count},{accent_associative_rule}\n"
+ ).format(
+ surface=word.surface,
+ context_id=word.context_id,
+ cost=_priority2cost(word.context_id, word.priority),
+ part_of_speech=word.part_of_speech,
+ part_of_speech_detail_1=word.part_of_speech_detail_1,
+ part_of_speech_detail_2=word.part_of_speech_detail_2,
+ part_of_speech_detail_3=word.part_of_speech_detail_3,
+ inflectional_type=word.inflectional_type,
+ inflectional_form=word.inflectional_form,
+ stem=word.stem,
+ yomi=word.yomi,
+ pronunciation=word.pronunciation,
+ accent_type=word.accent_type,
+ mora_count=word.mora_count,
+ accent_associative_rule=word.accent_associative_rule,
+ )
+ # 辞書データを辞書.csv へ一時保存
+ tmp_csv_path.write_text(csv_text, encoding="utf-8")
+
+ # 辞書.csvをOpenJTalk用にコンパイル
+ # pyopenjtalk.create_user_dict(str(tmp_csv_path), str(tmp_compiled_path))
+ pyopenjtalk.mecab_dict_index(str(tmp_csv_path), str(tmp_compiled_path))
+ if not tmp_compiled_path.is_file():
+ raise RuntimeError("辞書のコンパイル時にエラーが発生しました。")
+
+ # コンパイル済み辞書の置き換え・読み込み
+ pyopenjtalk.unset_user_dict()
+ tmp_compiled_path.replace(compiled_dict_path)
+ if compiled_dict_path.is_file():
+ # pyopenjtalk.set_user_dict(str(compiled_dict_path.resolve(strict=True)))
+ pyopenjtalk.update_global_jtalk_with_user_dict(str(compiled_dict_path))
+
+ except Exception as e:
+ print("Error: Failed to update dictionary.", file=sys.stderr)
+ traceback.print_exc(file=sys.stderr)
+ raise e
+
+ finally:
+ # 後処理
+ if tmp_csv_path.exists():
+ tmp_csv_path.unlink()
+ if tmp_compiled_path.exists():
+ tmp_compiled_path.unlink()
+
+
+# @mutex_wrapper(mutex_user_dict)
+def read_dict(user_dict_path: Path = user_dict_path) -> Dict[str, UserDictWord]:
+ """
+ ユーザー辞書の読み出し
+ Parameters
+ ----------
+ user_dict_path : Path
+ ユーザー辞書ファイルのパス
+ Returns
+ -------
+ result : Dict[str, UserDictWord]
+ ユーザー辞書
+ """
+ # 指定ユーザー辞書が存在しない場合、空辞書を返す
+ if not user_dict_path.is_file():
+ return {}
+
+ with user_dict_path.open(encoding="utf-8") as f:
+ result: Dict[str, UserDictWord] = {}
+ for word_uuid, word in json.load(f).items():
+ # cost2priorityで変換を行う際にcontext_idが必要となるが、
+ # 0.12以前の辞書は、context_idがハードコーディングされていたためにユーザー辞書内に保管されていない
+ # ハードコーディングされていたcontext_idは固有名詞を意味するものなので、固有名詞のcontext_idを補完する
+ if word.get("context_id") is None:
+ word["context_id"] = part_of_speech_data[
+ WordTypes.PROPER_NOUN
+ ].context_id
+ word["priority"] = _cost2priority(word["context_id"], word["cost"])
+ del word["cost"]
+ result[str(UUID(word_uuid))] = UserDictWord(**word)
+
+ return result
+
+
+def _create_word(
+ surface: str,
+ pronunciation: str,
+ accent_type: int,
+ word_type: Optional[WordTypes] = None,
+ priority: Optional[int] = None,
+) -> UserDictWord:
+ """
+ 単語オブジェクトの生成
+ Parameters
+ ----------
+ surface : str
+ 単語情報
+ pronunciation : str
+ 単語情報
+ accent_type : int
+ 単語情報
+ word_type : Optional[WordTypes]
+ 品詞
+ priority : Optional[int]
+ 優先度
+ Returns
+ -------
+ : UserDictWord
+ 単語オブジェクト
+ """
+ if word_type is None:
+ word_type = WordTypes.PROPER_NOUN
+ if word_type not in part_of_speech_data.keys():
+ raise HTTPException(status_code=422, detail="不明な品詞です")
+ if priority is None:
+ priority = 5
+ if not MIN_PRIORITY <= priority <= MAX_PRIORITY:
+ raise HTTPException(status_code=422, detail="優先度の値が無効です")
+ pos_detail = part_of_speech_data[word_type]
+ return UserDictWord(
+ surface=surface,
+ context_id=pos_detail.context_id,
+ priority=priority,
+ part_of_speech=pos_detail.part_of_speech,
+ part_of_speech_detail_1=pos_detail.part_of_speech_detail_1,
+ part_of_speech_detail_2=pos_detail.part_of_speech_detail_2,
+ part_of_speech_detail_3=pos_detail.part_of_speech_detail_3,
+ inflectional_type="*",
+ inflectional_form="*",
+ stem="*",
+ yomi=pronunciation,
+ pronunciation=pronunciation,
+ accent_type=accent_type,
+ accent_associative_rule="*",
+ )
+
+
+def apply_word(
+ surface: str,
+ pronunciation: str,
+ accent_type: int,
+ word_type: Optional[WordTypes] = None,
+ priority: Optional[int] = None,
+ user_dict_path: Path = user_dict_path,
+ compiled_dict_path: Path = compiled_dict_path,
+) -> str:
+ """
+ 新規単語の追加
+ Parameters
+ ----------
+ surface : str
+ 単語情報
+ pronunciation : str
+ 単語情報
+ accent_type : int
+ 単語情報
+ word_type : Optional[WordTypes]
+ 品詞
+ priority : Optional[int]
+ 優先度
+ user_dict_path : Path
+ ユーザー辞書ファイルのパス
+ compiled_dict_path : Path
+ コンパイル済み辞書ファイルのパス
+ Returns
+ -------
+ word_uuid : UserDictWord
+ 追加された単語に発行されたUUID
+ """
+ # 新規単語の追加による辞書データの更新
+ word = _create_word(
+ surface=surface,
+ pronunciation=pronunciation,
+ accent_type=accent_type,
+ word_type=word_type,
+ priority=priority,
+ )
+ user_dict = read_dict(user_dict_path=user_dict_path)
+ word_uuid = str(uuid4())
+ user_dict[word_uuid] = word
+
+ # 更新された辞書データの保存と適用
+ _write_to_json(user_dict, user_dict_path)
+ update_dict(user_dict_path=user_dict_path, compiled_dict_path=compiled_dict_path)
+
+ return word_uuid
+
+
+def rewrite_word(
+ word_uuid: str,
+ surface: str,
+ pronunciation: str,
+ accent_type: int,
+ word_type: Optional[WordTypes] = None,
+ priority: Optional[int] = None,
+ user_dict_path: Path = user_dict_path,
+ compiled_dict_path: Path = compiled_dict_path,
+) -> None:
+ """
+ 既存単語の上書き更新
+ Parameters
+ ----------
+ word_uuid : str
+ 単語UUID
+ surface : str
+ 単語情報
+ pronunciation : str
+ 単語情報
+ accent_type : int
+ 単語情報
+ word_type : Optional[WordTypes]
+ 品詞
+ priority : Optional[int]
+ 優先度
+ user_dict_path : Path
+ ユーザー辞書ファイルのパス
+ compiled_dict_path : Path
+ コンパイル済み辞書ファイルのパス
+ """
+ word = _create_word(
+ surface=surface,
+ pronunciation=pronunciation,
+ accent_type=accent_type,
+ word_type=word_type,
+ priority=priority,
+ )
+
+ # 既存単語の上書きによる辞書データの更新
+ user_dict = read_dict(user_dict_path=user_dict_path)
+ if word_uuid not in user_dict:
+ raise HTTPException(
+ status_code=422, detail="UUIDに該当するワードが見つかりませんでした"
+ )
+ user_dict[word_uuid] = word
+
+ # 更新された辞書データの保存と適用
+ _write_to_json(user_dict, user_dict_path)
+ update_dict(user_dict_path=user_dict_path, compiled_dict_path=compiled_dict_path)
+
+
+def delete_word(
+ word_uuid: str,
+ user_dict_path: Path = user_dict_path,
+ compiled_dict_path: Path = compiled_dict_path,
+) -> None:
+ """
+ 単語の削除
+ Parameters
+ ----------
+ word_uuid : str
+ 単語UUID
+ user_dict_path : Path
+ ユーザー辞書ファイルのパス
+ compiled_dict_path : Path
+ コンパイル済み辞書ファイルのパス
+ """
+ # 既存単語の削除による辞書データの更新
+ user_dict = read_dict(user_dict_path=user_dict_path)
+ if word_uuid not in user_dict:
+ raise HTTPException(
+ status_code=422, detail="IDに該当するワードが見つかりませんでした"
+ )
+ del user_dict[word_uuid]
+
+ # 更新された辞書データの保存と適用
+ _write_to_json(user_dict, user_dict_path)
+ update_dict(user_dict_path=user_dict_path, compiled_dict_path=compiled_dict_path)
+
+
+def import_user_dict(
+ dict_data: Dict[str, UserDictWord],
+ override: bool = False,
+ user_dict_path: Path = user_dict_path,
+ default_dict_path: Path = default_dict_path,
+ compiled_dict_path: Path = compiled_dict_path,
+) -> None:
+ """
+ ユーザー辞書のインポート
+ Parameters
+ ----------
+ dict_data : Dict[str, UserDictWord]
+ インポートするユーザー辞書のデータ
+ override : bool
+ 重複したエントリがあった場合、上書きするかどうか
+ user_dict_path : Path
+ ユーザー辞書ファイルのパス
+ default_dict_path : Path
+ デフォルト辞書ファイルのパス
+ compiled_dict_path : Path
+ コンパイル済み辞書ファイルのパス
+ """
+ # インポートする辞書データのバリデーション
+ for word_uuid, word in dict_data.items():
+ UUID(word_uuid)
+ assert isinstance(word, UserDictWord)
+ for pos_detail in part_of_speech_data.values():
+ if word.context_id == pos_detail.context_id:
+ assert word.part_of_speech == pos_detail.part_of_speech
+ assert (
+ word.part_of_speech_detail_1 == pos_detail.part_of_speech_detail_1
+ )
+ assert (
+ word.part_of_speech_detail_2 == pos_detail.part_of_speech_detail_2
+ )
+ assert (
+ word.part_of_speech_detail_3 == pos_detail.part_of_speech_detail_3
+ )
+ assert (
+ word.accent_associative_rule in pos_detail.accent_associative_rules
+ )
+ break
+ else:
+ raise ValueError("対応していない品詞です")
+
+ # 既存辞書の読み出し
+ old_dict = read_dict(user_dict_path=user_dict_path)
+
+ # 辞書データの更新
+ # 重複エントリの上書き
+ if override:
+ new_dict = {**old_dict, **dict_data}
+ # 重複エントリの保持
+ else:
+ new_dict = {**dict_data, **old_dict}
+
+ # 更新された辞書データの保存と適用
+ _write_to_json(user_dict=new_dict, user_dict_path=user_dict_path)
+ update_dict(
+ default_dict_path=default_dict_path,
+ user_dict_path=user_dict_path,
+ compiled_dict_path=compiled_dict_path,
+ )
+
+
+def _search_cost_candidates(context_id: int) -> List[int]:
+ for value in part_of_speech_data.values():
+ if value.context_id == context_id:
+ return value.cost_candidates
+ raise HTTPException(status_code=422, detail="品詞IDが不正です")
+
+
+def _cost2priority(context_id: int, cost: int) -> int:
+ assert -32768 <= cost <= 32767
+ cost_candidates = _search_cost_candidates(context_id)
+ # cost_candidatesの中にある値で最も近い値を元にpriorityを返す
+ # 参考: https://qiita.com/Krypf/items/2eada91c37161d17621d
+ # この関数とpriority2cost関数によって、辞書ファイルのcostを操作しても最も近いpriorityのcostに上書きされる
+ return MAX_PRIORITY - np.argmin(np.abs(np.array(cost_candidates) - cost)).item()
+
+
+def _priority2cost(context_id: int, priority: int) -> int:
+ assert MIN_PRIORITY <= priority <= MAX_PRIORITY
+ cost_candidates = _search_cost_candidates(context_id)
+ return cost_candidates[MAX_PRIORITY - priority]
diff --git a/text/user_dict/part_of_speech_data.py b/text/user_dict/part_of_speech_data.py
new file mode 100644
index 0000000000000000000000000000000000000000..3e80115d3cb12c203638e23b84798e6e97409ce9
--- /dev/null
+++ b/text/user_dict/part_of_speech_data.py
@@ -0,0 +1,144 @@
+from typing import Dict
+
+from .word_model import (
+ USER_DICT_MAX_PRIORITY,
+ USER_DICT_MIN_PRIORITY,
+ PartOfSpeechDetail,
+ WordTypes,
+)
+
+MIN_PRIORITY = USER_DICT_MIN_PRIORITY
+MAX_PRIORITY = USER_DICT_MAX_PRIORITY
+
+part_of_speech_data: Dict[WordTypes, PartOfSpeechDetail] = {
+ WordTypes.PROPER_NOUN: PartOfSpeechDetail(
+ part_of_speech="名詞",
+ part_of_speech_detail_1="固有名詞",
+ part_of_speech_detail_2="一般",
+ part_of_speech_detail_3="*",
+ context_id=1348,
+ cost_candidates=[
+ -988,
+ 3488,
+ 4768,
+ 6048,
+ 7328,
+ 8609,
+ 8734,
+ 8859,
+ 8984,
+ 9110,
+ 14176,
+ ],
+ accent_associative_rules=[
+ "*",
+ "C1",
+ "C2",
+ "C3",
+ "C4",
+ "C5",
+ ],
+ ),
+ WordTypes.COMMON_NOUN: PartOfSpeechDetail(
+ part_of_speech="名詞",
+ part_of_speech_detail_1="一般",
+ part_of_speech_detail_2="*",
+ part_of_speech_detail_3="*",
+ context_id=1345,
+ cost_candidates=[
+ -4445,
+ 49,
+ 1473,
+ 2897,
+ 4321,
+ 5746,
+ 6554,
+ 7362,
+ 8170,
+ 8979,
+ 15001,
+ ],
+ accent_associative_rules=[
+ "*",
+ "C1",
+ "C2",
+ "C3",
+ "C4",
+ "C5",
+ ],
+ ),
+ WordTypes.VERB: PartOfSpeechDetail(
+ part_of_speech="動詞",
+ part_of_speech_detail_1="自立",
+ part_of_speech_detail_2="*",
+ part_of_speech_detail_3="*",
+ context_id=642,
+ cost_candidates=[
+ 3100,
+ 6160,
+ 6360,
+ 6561,
+ 6761,
+ 6962,
+ 7414,
+ 7866,
+ 8318,
+ 8771,
+ 13433,
+ ],
+ accent_associative_rules=[
+ "*",
+ ],
+ ),
+ WordTypes.ADJECTIVE: PartOfSpeechDetail(
+ part_of_speech="形容詞",
+ part_of_speech_detail_1="自立",
+ part_of_speech_detail_2="*",
+ part_of_speech_detail_3="*",
+ context_id=20,
+ cost_candidates=[
+ 1527,
+ 3266,
+ 3561,
+ 3857,
+ 4153,
+ 4449,
+ 5149,
+ 5849,
+ 6549,
+ 7250,
+ 10001,
+ ],
+ accent_associative_rules=[
+ "*",
+ ],
+ ),
+ WordTypes.SUFFIX: PartOfSpeechDetail(
+ part_of_speech="名詞",
+ part_of_speech_detail_1="接尾",
+ part_of_speech_detail_2="一般",
+ part_of_speech_detail_3="*",
+ context_id=1358,
+ cost_candidates=[
+ 4399,
+ 5373,
+ 6041,
+ 6710,
+ 7378,
+ 8047,
+ 9440,
+ 10834,
+ 12228,
+ 13622,
+ 15847,
+ ],
+ accent_associative_rules=[
+ "*",
+ "C1",
+ "C2",
+ "C3",
+ "C4",
+ "C5",
+ ],
+ ),
+}
diff --git a/text/user_dict/word_model.py b/text/user_dict/word_model.py
new file mode 100644
index 0000000000000000000000000000000000000000..fda5576f072c8a1444ebf19cc9a37e9e39c75176
--- /dev/null
+++ b/text/user_dict/word_model.py
@@ -0,0 +1,124 @@
+from enum import Enum
+from re import findall, fullmatch
+from typing import List, Optional
+
+from pydantic import BaseModel, Field, validator
+
+USER_DICT_MIN_PRIORITY = 0
+USER_DICT_MAX_PRIORITY = 10
+
+
+class UserDictWord(BaseModel):
+ """
+ 辞書のコンパイルに使われる情報
+ """
+
+ surface: str = Field(title="表層形")
+ priority: int = Field(
+ title="優先度", ge=USER_DICT_MIN_PRIORITY, le=USER_DICT_MAX_PRIORITY
+ )
+ context_id: int = Field(title="文脈ID", default=1348)
+ part_of_speech: str = Field(title="品詞")
+ part_of_speech_detail_1: str = Field(title="品詞細分類1")
+ part_of_speech_detail_2: str = Field(title="品詞細分類2")
+ part_of_speech_detail_3: str = Field(title="品詞細分類3")
+ inflectional_type: str = Field(title="活用型")
+ inflectional_form: str = Field(title="活用形")
+ stem: str = Field(title="原形")
+ yomi: str = Field(title="読み")
+ pronunciation: str = Field(title="発音")
+ accent_type: int = Field(title="アクセント型")
+ mora_count: Optional[int] = Field(title="モーラ数", default=None)
+ accent_associative_rule: str = Field(title="アクセント結合規則")
+
+ class Config:
+ validate_assignment = True
+
+ @validator("surface")
+ def convert_to_zenkaku(cls, surface):
+ return surface.translate(
+ str.maketrans(
+ "".join(chr(0x21 + i) for i in range(94)),
+ "".join(chr(0xFF01 + i) for i in range(94)),
+ )
+ )
+
+ @validator("pronunciation", pre=True)
+ def check_is_katakana(cls, pronunciation):
+ if not fullmatch(r"[ァ-ヴー]+", pronunciation):
+ raise ValueError("発音は有効なカタカナでなくてはいけません。")
+ sutegana = ["ァ", "ィ", "ゥ", "ェ", "ォ", "ャ", "ュ", "ョ", "ヮ", "ッ"]
+ for i in range(len(pronunciation)):
+ if pronunciation[i] in sutegana:
+ # 「キャット」のように、捨て仮名が連続する可能性が考えられるので、
+ # 「ッ」に関しては「ッ」そのものが連続している場合と、「ッ」の後にほかの捨て仮名が連続する場合のみ無効とする
+ if i < len(pronunciation) - 1 and (
+ pronunciation[i + 1] in sutegana[:-1]
+ or (
+ pronunciation[i] == sutegana[-1]
+ and pronunciation[i + 1] == sutegana[-1]
+ )
+ ):
+ raise ValueError("無効な発音です。(捨て仮名の連続)")
+ if pronunciation[i] == "ヮ":
+ if i != 0 and pronunciation[i - 1] not in ["ク", "グ"]:
+ raise ValueError(
+ "無効な発音です。(「くゎ」「ぐゎ」以外の「ゎ」の使用)"
+ )
+ return pronunciation
+
+ @validator("mora_count", pre=True, always=True)
+ def check_mora_count_and_accent_type(cls, mora_count, values):
+ if "pronunciation" not in values or "accent_type" not in values:
+ # 適切な場所でエラーを出すようにする
+ return mora_count
+
+ if mora_count is None:
+ rule_others = (
+ "[イ][ェ]|[ヴ][ャュョ]|[トド][ゥ]|[テデ][ィャュョ]|[デ][ェ]|[クグ][ヮ]"
+ )
+ rule_line_i = "[キシチニヒミリギジビピ][ェャュョ]"
+ rule_line_u = "[ツフヴ][ァ]|[ウスツフヴズ][ィ]|[ウツフヴ][ェォ]"
+ rule_one_mora = "[ァ-ヴー]"
+ mora_count = len(
+ findall(
+ f"(?:{rule_others}|{rule_line_i}|{rule_line_u}|{rule_one_mora})",
+ values["pronunciation"],
+ )
+ )
+
+ if not 0 <= values["accent_type"] <= mora_count:
+ raise ValueError(
+ "誤ったアクセント型です({})。 expect: 0 <= accent_type <= {}".format(
+ values["accent_type"], mora_count
+ )
+ )
+ return mora_count
+
+
+class PartOfSpeechDetail(BaseModel):
+ """
+ 品詞ごとの情報
+ """
+
+ part_of_speech: str = Field(title="品詞")
+ part_of_speech_detail_1: str = Field(title="品詞細分類1")
+ part_of_speech_detail_2: str = Field(title="品詞細分類2")
+ part_of_speech_detail_3: str = Field(title="品詞細分類3")
+ # context_idは辞書の左・右文脈IDのこと
+ # https://github.com/VOICEVOX/open_jtalk/blob/427cfd761b78efb6094bea3c5bb8c968f0d711ab/src/mecab-naist-jdic/_left-id.def # noqa
+ context_id: int = Field(title="文脈ID")
+ cost_candidates: List[int] = Field(title="コストのパーセンタイル")
+ accent_associative_rules: List[str] = Field(title="アクセント結合規則の一覧")
+
+
+class WordTypes(str, Enum):
+ """
+ fastapiでword_type引数を検証する時に使用するクラス
+ """
+
+ PROPER_NOUN = "PROPER_NOUN"
+ COMMON_NOUN = "COMMON_NOUN"
+ VERB = "VERB"
+ ADJECTIVE = "ADJECTIVE"
+ SUFFIX = "SUFFIX"
diff --git a/tools/__init__.py b/tools/__init__.py
new file mode 100644
index 0000000000000000000000000000000000000000..b68d332953ea3f5d49df8a76e23f1fafbbdcb695
--- /dev/null
+++ b/tools/__init__.py
@@ -0,0 +1,3 @@
+"""
+工具包
+"""
diff --git a/tools/classify_language.py b/tools/classify_language.py
new file mode 100644
index 0000000000000000000000000000000000000000..2b8a7ab420b7010776be0218ed87c675d4821a34
--- /dev/null
+++ b/tools/classify_language.py
@@ -0,0 +1,197 @@
+import regex as re
+
+try:
+ from config import config
+
+ LANGUAGE_IDENTIFICATION_LIBRARY = (
+ config.webui_config.language_identification_library
+ )
+except:
+ LANGUAGE_IDENTIFICATION_LIBRARY = "langid"
+
+module = LANGUAGE_IDENTIFICATION_LIBRARY.lower()
+
+langid_languages = [
+ "af",
+ "am",
+ "an",
+ "ar",
+ "as",
+ "az",
+ "be",
+ "bg",
+ "bn",
+ "br",
+ "bs",
+ "ca",
+ "cs",
+ "cy",
+ "da",
+ "de",
+ "dz",
+ "el",
+ "en",
+ "eo",
+ "es",
+ "et",
+ "eu",
+ "fa",
+ "fi",
+ "fo",
+ "fr",
+ "ga",
+ "gl",
+ "gu",
+ "he",
+ "hi",
+ "hr",
+ "ht",
+ "hu",
+ "hy",
+ "id",
+ "is",
+ "it",
+ "ja",
+ "jv",
+ "ka",
+ "kk",
+ "km",
+ "kn",
+ "ko",
+ "ku",
+ "ky",
+ "la",
+ "lb",
+ "lo",
+ "lt",
+ "lv",
+ "mg",
+ "mk",
+ "ml",
+ "mn",
+ "mr",
+ "ms",
+ "mt",
+ "nb",
+ "ne",
+ "nl",
+ "nn",
+ "no",
+ "oc",
+ "or",
+ "pa",
+ "pl",
+ "ps",
+ "pt",
+ "qu",
+ "ro",
+ "ru",
+ "rw",
+ "se",
+ "si",
+ "sk",
+ "sl",
+ "sq",
+ "sr",
+ "sv",
+ "sw",
+ "ta",
+ "te",
+ "th",
+ "tl",
+ "tr",
+ "ug",
+ "uk",
+ "ur",
+ "vi",
+ "vo",
+ "wa",
+ "xh",
+ "zh",
+ "zu",
+]
+
+
+def classify_language(text: str, target_languages: list = None) -> str:
+ if module == "fastlid" or module == "fasttext":
+ from fastlid import fastlid, supported_langs
+
+ classifier = fastlid
+ if target_languages != None:
+ target_languages = [
+ lang for lang in target_languages if lang in supported_langs
+ ]
+ fastlid.set_languages = target_languages
+ elif module == "langid":
+ import langid
+
+ classifier = langid.classify
+ if target_languages != None:
+ target_languages = [
+ lang for lang in target_languages if lang in langid_languages
+ ]
+ langid.set_languages(target_languages)
+ else:
+ raise ValueError(f"Wrong module {module}")
+
+ lang = classifier(text)[0]
+
+ return lang
+
+
+def classify_zh_ja(text: str) -> str:
+ for idx, char in enumerate(text):
+ unicode_val = ord(char)
+
+ # 检测日语字符
+ if 0x3040 <= unicode_val <= 0x309F or 0x30A0 <= unicode_val <= 0x30FF:
+ return "ja"
+
+ # 检测汉字字符
+ if 0x4E00 <= unicode_val <= 0x9FFF:
+ # 检查周围的字符
+ next_char = text[idx + 1] if idx + 1 < len(text) else None
+
+ if next_char and (
+ 0x3040 <= ord(next_char) <= 0x309F or 0x30A0 <= ord(next_char) <= 0x30FF
+ ):
+ return "ja"
+
+ return "zh"
+
+
+def split_alpha_nonalpha(text, mode=1):
+ if mode == 1:
+ pattern = r"(?<=[\u4e00-\u9fff\u3040-\u30FF\d\s])(?=[\p{Latin}])|(?<=[\p{Latin}\s])(?=[\u4e00-\u9fff\u3040-\u30FF\d])"
+ elif mode == 2:
+ pattern = r"(?<=[\u4e00-\u9fff\u3040-\u30FF\s])(?=[\p{Latin}\d])|(?<=[\p{Latin}\d\s])(?=[\u4e00-\u9fff\u3040-\u30FF])"
+ else:
+ raise ValueError("Invalid mode. Supported modes are 1 and 2.")
+
+ return re.split(pattern, text)
+
+
+if __name__ == "__main__":
+ text = "这是一个测试文本"
+ print(classify_language(text))
+ print(classify_zh_ja(text)) # "zh"
+
+ text = "これはテストテキストです"
+ print(classify_language(text))
+ print(classify_zh_ja(text)) # "ja"
+
+ text = "vits和Bert-VITS2是tts模型。花费3days.花费3天。Take 3 days"
+
+ print(split_alpha_nonalpha(text, mode=1))
+ # output: ['vits', '和', 'Bert-VITS', '2是', 'tts', '模型。花费3', 'days.花费3天。Take 3 days']
+
+ print(split_alpha_nonalpha(text, mode=2))
+ # output: ['vits', '和', 'Bert-VITS2', '是', 'tts', '模型。花费', '3days.花费', '3', '天。Take 3 days']
+
+ text = "vits 和 Bert-VITS2 是 tts 模型。花费3days.花费3天。Take 3 days"
+ print(split_alpha_nonalpha(text, mode=1))
+ # output: ['vits ', '和 ', 'Bert-VITS', '2 ', '是 ', 'tts ', '模型。花费3', 'days.花费3天。Take ', '3 ', 'days']
+
+ text = "vits 和 Bert-VITS2 是 tts 模型。花费3days.花费3天。Take 3 days"
+ print(split_alpha_nonalpha(text, mode=2))
+ # output: ['vits ', '和 ', 'Bert-VITS2 ', '是 ', 'tts ', '模型。花费', '3days.花费', '3', '天。Take ', '3 ', 'days']
diff --git a/tools/sentence.py b/tools/sentence.py
new file mode 100644
index 0000000000000000000000000000000000000000..b66864ca0d183d54c935586acc529de41b9a911e
--- /dev/null
+++ b/tools/sentence.py
@@ -0,0 +1,173 @@
+import logging
+
+import regex as re
+
+from tools.classify_language import classify_language, split_alpha_nonalpha
+
+
+def check_is_none(item) -> bool:
+ """none -> True, not none -> False"""
+ return (
+ item is None
+ or (isinstance(item, str) and str(item).isspace())
+ or str(item) == ""
+ )
+
+
+def markup_language(text: str, target_languages: list = None) -> str:
+ pattern = (
+ r"[\!\"\#\$\%\&\'\(\)\*\+\,\-\.\/\:\;\<\>\=\?\@\[\]\{\}\\\\\^\_\`"
+ r"\!?。"#$%&'()*+,-/:;<=>@[\]^_`{|}~⦅⦆「」、、〃》「」"
+ r"『』【】〔〕〖〗〘〙〚〛〜〝〞〟〰〾〿–—‘\'\‛\“\”\„\‟…‧﹏.]+"
+ )
+ sentences = re.split(pattern, text)
+
+ pre_lang = ""
+ p = 0
+
+ if target_languages is not None:
+ sorted_target_languages = sorted(target_languages)
+ if sorted_target_languages in [["en", "zh"], ["en", "ja"], ["en", "ja", "zh"]]:
+ new_sentences = []
+ for sentence in sentences:
+ new_sentences.extend(split_alpha_nonalpha(sentence))
+ sentences = new_sentences
+
+ for sentence in sentences:
+ if check_is_none(sentence):
+ continue
+
+ lang = classify_language(sentence, target_languages)
+
+ if pre_lang == "":
+ text = text[:p] + text[p:].replace(
+ sentence, f"[{lang.upper()}]{sentence}", 1
+ )
+ p += len(f"[{lang.upper()}]")
+ elif pre_lang != lang:
+ text = text[:p] + text[p:].replace(
+ sentence, f"[{pre_lang.upper()}][{lang.upper()}]{sentence}", 1
+ )
+ p += len(f"[{pre_lang.upper()}][{lang.upper()}]")
+ pre_lang = lang
+ p += text[p:].index(sentence) + len(sentence)
+ text += f"[{pre_lang.upper()}]"
+
+ return text
+
+
+def split_by_language(text: str, target_languages: list = None) -> list:
+ pattern = (
+ r"[\!\"\#\$\%\&\'\(\)\*\+\,\-\.\/\:\;\<\>\=\?\@\[\]\{\}\\\\\^\_\`"
+ r"\!?\。"#$%&'()*+,-/:;<=>@[\]^_`{|}~⦅⦆「」、、〃》「」"
+ r"『』【】〔〕〖〗〘〙〚〛〜〝〞〟〰〾〿–—‘\'\‛\“\”\„\‟…‧﹏.]+"
+ )
+ sentences = re.split(pattern, text)
+
+ pre_lang = ""
+ start = 0
+ end = 0
+ sentences_list = []
+
+ if target_languages is not None:
+ sorted_target_languages = sorted(target_languages)
+ if sorted_target_languages in [["en", "zh"], ["en", "ja"], ["en", "ja", "zh"]]:
+ new_sentences = []
+ for sentence in sentences:
+ new_sentences.extend(split_alpha_nonalpha(sentence))
+ sentences = new_sentences
+
+ for sentence in sentences:
+ if check_is_none(sentence):
+ continue
+
+ lang = classify_language(sentence, target_languages)
+
+ end += text[end:].index(sentence)
+ if pre_lang != "" and pre_lang != lang:
+ sentences_list.append((text[start:end], pre_lang))
+ start = end
+ end += len(sentence)
+ pre_lang = lang
+ sentences_list.append((text[start:], pre_lang))
+
+ return sentences_list
+
+
+def sentence_split(text: str, max: int) -> list:
+ pattern = r"[!(),—+\-.:;??。,、;:]+"
+ sentences = re.split(pattern, text)
+ discarded_chars = re.findall(pattern, text)
+
+ sentences_list, count, p = [], 0, 0
+
+ # 按被分割的符号遍历
+ for i, discarded_chars in enumerate(discarded_chars):
+ count += len(sentences[i]) + len(discarded_chars)
+ if count >= max:
+ sentences_list.append(text[p : p + count].strip())
+ p += count
+ count = 0
+
+ # 加入最后剩余的文本
+ if p < len(text):
+ sentences_list.append(text[p:])
+
+ return sentences_list
+
+
+def sentence_split_and_markup(text, max=50, lang="auto", speaker_lang=None):
+ # 如果该speaker只支持一种语言
+ if speaker_lang is not None and len(speaker_lang) == 1:
+ if lang.upper() not in ["AUTO", "MIX"] and lang.lower() != speaker_lang[0]:
+ logging.debug(
+ f'lang "{lang}" is not in speaker_lang {speaker_lang},automatically set lang={speaker_lang[0]}'
+ )
+ lang = speaker_lang[0]
+
+ sentences_list = []
+ if lang.upper() != "MIX":
+ if max <= 0:
+ sentences_list.append(
+ markup_language(text, speaker_lang)
+ if lang.upper() == "AUTO"
+ else f"[{lang.upper()}]{text}[{lang.upper()}]"
+ )
+ else:
+ for i in sentence_split(text, max):
+ if check_is_none(i):
+ continue
+ sentences_list.append(
+ markup_language(i, speaker_lang)
+ if lang.upper() == "AUTO"
+ else f"[{lang.upper()}]{i}[{lang.upper()}]"
+ )
+ else:
+ sentences_list.append(text)
+
+ for i in sentences_list:
+ logging.debug(i)
+
+ return sentences_list
+
+
+if __name__ == "__main__":
+ text = "这几天心里颇不宁静。今晚在院子里坐着乘凉,忽然想起日日走过的荷塘,在这满月的光里,总该另有一番样子吧。月亮渐渐地升高了,墙外马路上孩子们的欢笑,已经听不见了;妻在屋里拍着闰儿,迷迷糊糊地哼着眠歌。我悄悄地披了大衫,带上门出去。"
+ print(markup_language(text, target_languages=None))
+ print(sentence_split(text, max=50))
+ print(sentence_split_and_markup(text, max=50, lang="auto", speaker_lang=None))
+
+ text = "你好,这是一段用来测试自动标注的文本。こんにちは,これは自動ラベリングのテスト用テキストです.Hello, this is a piece of text to test autotagging.你好!今天我们要介绍VITS项目,其重点是使用了GAN Duration predictor和transformer flow,并且接入了Bert模型来提升韵律。Bert embedding会在稍后介绍。"
+ print(split_by_language(text, ["zh", "ja", "en"]))
+
+ text = "vits和Bert-VITS2是tts模型。花费3days.花费3天。Take 3 days"
+
+ print(split_by_language(text, ["zh", "ja", "en"]))
+ # output: [('vits', 'en'), ('和', 'ja'), ('Bert-VITS', 'en'), ('2是', 'zh'), ('tts', 'en'), ('模型。花费3', 'zh'), ('days.', 'en'), ('花费3天。', 'zh'), ('Take 3 days', 'en')]
+
+ print(split_by_language(text, ["zh", "en"]))
+ # output: [('vits', 'en'), ('和', 'zh'), ('Bert-VITS', 'en'), ('2是', 'zh'), ('tts', 'en'), ('模型。花费3', 'zh'), ('days.', 'en'), ('花费3天。', 'zh'), ('Take 3 days', 'en')]
+
+ text = "vits 和 Bert-VITS2 是 tts 模型。花费 3 days. 花费 3天。Take 3 days"
+ print(split_by_language(text, ["zh", "en"]))
+ # output: [('vits ', 'en'), ('和 ', 'zh'), ('Bert-VITS2 ', 'en'), ('是 ', 'zh'), ('tts ', 'en'), ('模型。花费 ', 'zh'), ('3 days. ', 'en'), ('花费 3天。', 'zh'), ('Take 3 days', 'en')]
diff --git a/tools/translate.py b/tools/translate.py
new file mode 100644
index 0000000000000000000000000000000000000000..9368b5f8e6cd73bda2249953a9a83e1ffb1ce8ab
--- /dev/null
+++ b/tools/translate.py
@@ -0,0 +1,61 @@
+"""
+翻译api
+"""
+from config import config
+
+import random
+import hashlib
+import requests
+
+
+def translate(Sentence: str, to_Language: str = "jp", from_Language: str = ""):
+ """
+ :param Sentence: 待翻译语句
+ :param from_Language: 待翻译语句语言
+ :param to_Language: 目标语言
+ :return: 翻译后语句 出错时返回None
+
+ 常见语言代码:中文 zh 英语 en 日语 jp
+ """
+ appid = config.translate_config.app_key
+ key = config.translate_config.secret_key
+ if appid == "" or key == "":
+ return "请开发者在config.yml中配置app_key与secret_key"
+ url = "https://fanyi-api.baidu.com/api/trans/vip/translate"
+ texts = Sentence.splitlines()
+ outTexts = []
+ for t in texts:
+ if t != "":
+ # 签名计算 参考文档 https://api.fanyi.baidu.com/product/113
+ salt = str(random.randint(1, 100000))
+ signString = appid + t + salt + key
+ hs = hashlib.md5()
+ hs.update(signString.encode("utf-8"))
+ signString = hs.hexdigest()
+ if from_Language == "":
+ from_Language = "auto"
+ headers = {"Content-Type": "application/x-www-form-urlencoded"}
+ payload = {
+ "q": t,
+ "from": from_Language,
+ "to": to_Language,
+ "appid": appid,
+ "salt": salt,
+ "sign": signString,
+ }
+ # 发送请求
+ try:
+ response = requests.post(
+ url=url, data=payload, headers=headers, timeout=3
+ )
+ response = response.json()
+ if "trans_result" in response.keys():
+ result = response["trans_result"][0]
+ if "dst" in result.keys():
+ dst = result["dst"]
+ outTexts.append(dst)
+ except Exception:
+ return Sentence
+ else:
+ outTexts.append(t)
+ return "\n".join(outTexts)
diff --git a/train_ms.py b/train_ms.py
new file mode 100644
index 0000000000000000000000000000000000000000..ea4b65fbbe5458555f80a42c9dee397958923baf
--- /dev/null
+++ b/train_ms.py
@@ -0,0 +1,950 @@
+import argparse
+import datetime
+import gc
+import os
+import platform
+
+import torch
+import torch.distributed as dist
+from huggingface_hub import HfApi
+from torch.cuda.amp import GradScaler, autocast
+from torch.nn import functional as F
+from torch.nn.parallel import DistributedDataParallel as DDP
+from torch.utils.data import DataLoader
+from torch.utils.tensorboard import SummaryWriter
+from tqdm import tqdm
+
+# logging.getLogger("numba").setLevel(logging.WARNING)
+import commons
+import default_style
+import utils
+from common.log import logger
+from common.stdout_wrapper import SAFE_STDOUT
+from config import config
+from data_utils import (
+ DistributedBucketSampler,
+ TextAudioSpeakerCollate,
+ TextAudioSpeakerLoader,
+)
+from losses import discriminator_loss, feature_loss, generator_loss, kl_loss
+from mel_processing import mel_spectrogram_torch, spec_to_mel_torch
+from models import DurationDiscriminator, MultiPeriodDiscriminator, SynthesizerTrn
+from text.symbols import symbols
+
+torch.backends.cuda.matmul.allow_tf32 = True
+torch.backends.cudnn.allow_tf32 = (
+ True # If encontered training problem,please try to disable TF32.
+)
+torch.set_float32_matmul_precision("medium")
+torch.backends.cuda.sdp_kernel("flash")
+torch.backends.cuda.enable_flash_sdp(True)
+torch.backends.cuda.enable_mem_efficient_sdp(
+ True
+) # Not available if torch version is lower than 2.0
+torch.backends.cuda.enable_math_sdp(True)
+
+
+global_step = 0
+
+api = HfApi()
+
+
+def run():
+ # Command line configuration is not recommended unless necessary, use config.yml
+ parser = argparse.ArgumentParser()
+ parser.add_argument(
+ "-c",
+ "--config",
+ type=str,
+ default=config.train_ms_config.config_path,
+ help="JSON file for configuration",
+ )
+ parser.add_argument(
+ "-m",
+ "--model",
+ type=str,
+ help="数据集文件夹路径,请注意,数据不再默认放在/logs文件夹下。如果需要用命令行配置,请声明相对于根目录的路径",
+ default=config.dataset_path,
+ )
+ parser.add_argument(
+ "--assets_root",
+ type=str,
+ help="Root directory of model assets needed for inference.",
+ default=config.assets_root,
+ )
+ parser.add_argument(
+ "--skip_default_style",
+ action="store_true",
+ help="Skip saving default style config and mean vector.",
+ )
+ parser.add_argument(
+ "--no_progress_bar",
+ action="store_true",
+ help="Do not show the progress bar while training.",
+ )
+ parser.add_argument(
+ "--speedup",
+ action="store_true",
+ help="Speed up training by disabling logging and evaluation.",
+ )
+ parser.add_argument(
+ "--repo_id",
+ help="Huggingface model repo id to backup the model.",
+ default=None,
+ )
+ args = parser.parse_args()
+
+ # Set log file
+ model_dir = os.path.join(args.model, config.train_ms_config.model_dir)
+ timestamp = datetime.datetime.now().strftime("%Y%m%d_%H%M%S")
+ logger.add(os.path.join(args.model, f"train_{timestamp}.log"))
+
+ # Parsing environment variables
+ envs = config.train_ms_config.env
+ for env_name, env_value in envs.items():
+ if env_name not in os.environ.keys():
+ logger.info("Loading configuration from config {}".format(str(env_value)))
+ os.environ[env_name] = str(env_value)
+ logger.info(
+ "Loading environment variables \nMASTER_ADDR: {},\nMASTER_PORT: {},\nWORLD_SIZE: {},\nRANK: {},\nLOCAL_RANK: {}".format(
+ os.environ["MASTER_ADDR"],
+ os.environ["MASTER_PORT"],
+ os.environ["WORLD_SIZE"],
+ os.environ["RANK"],
+ os.environ["LOCAL_RANK"],
+ )
+ )
+
+ backend = "nccl"
+ if platform.system() == "Windows":
+ backend = "gloo" # If Windows,switch to gloo backend.
+ dist.init_process_group(
+ backend=backend,
+ init_method="env://",
+ timeout=datetime.timedelta(seconds=300),
+ ) # Use torchrun instead of mp.spawn
+ rank = dist.get_rank()
+ local_rank = int(os.environ["LOCAL_RANK"])
+ n_gpus = dist.get_world_size()
+
+ hps = utils.get_hparams_from_file(args.config)
+ # This is needed because we have to pass values to `train_and_evaluate()`
+ hps.model_dir = model_dir
+ hps.speedup = args.speedup
+
+ # 比较路径是否相同
+ if os.path.realpath(args.config) != os.path.realpath(
+ config.train_ms_config.config_path
+ ):
+ with open(args.config, "r", encoding="utf-8") as f:
+ data = f.read()
+ os.makedirs(os.path.dirname(config.train_ms_config.config_path), exist_ok=True)
+ with open(config.train_ms_config.config_path, "w", encoding="utf-8") as f:
+ f.write(data)
+
+ """
+ Path constants are a bit complicated...
+ TODO: Refactor or rename these?
+ (Both `config.yml` and `config.json` are used, which is confusing I think.)
+
+ args.model: For saving all info needed for training.
+ default: `Data/{model_name}`.
+ hps.model_dir := model_dir: For saving checkpoints (for resuming training).
+ default: `Data/{model_name}/models`.
+ (Use `hps` since we have to pass `model_dir` to `train_and_evaluate()`.
+
+ args.assets_root: The root directory of model assets needed for inference.
+ default: config.assets_root == `model_assets`.
+
+ config.out_dir: The directory for model assets of this model (for inference).
+ default: `model_assets/{model_name}`.
+ """
+
+ if args.repo_id is not None:
+ # First try to upload config.json to check if the repo exists
+ try:
+ api.upload_file(
+ path_or_fileobj=args.config,
+ path_in_repo=f"Data/{config.model_name}/config.json",
+ repo_id=hps.repo_id,
+ )
+ except Exception as e:
+ logger.error(e)
+ logger.error(
+ f"Failed to upload files to the repo {hps.repo_id}. Please check if the repo exists and you have logged in using `huggingface-cli login`."
+ )
+ raise e
+ # Upload Data dir for resuming training
+ api.upload_folder(
+ repo_id=hps.repo_id,
+ folder_path=config.dataset_path,
+ path_in_repo=f"Data/{config.model_name}",
+ delete_patterns="*.pth", # Only keep the latest checkpoint
+ run_as_future=True,
+ )
+
+ os.makedirs(config.out_dir, exist_ok=True)
+
+ if not args.skip_default_style:
+ # Save default style to out_dir
+ default_style.set_style_config(
+ args.config, os.path.join(config.out_dir, "config.json")
+ )
+ default_style.save_mean_vector(
+ os.path.join(args.model, "wavs"),
+ os.path.join(config.out_dir, "style_vectors.npy"),
+ )
+
+ torch.manual_seed(hps.train.seed)
+ torch.cuda.set_device(local_rank)
+
+ global global_step
+ writer = None
+ writer_eval = None
+ if rank == 0 and not args.speedup:
+ # logger = utils.get_logger(hps.model_dir)
+ # logger.info(hps)
+ utils.check_git_hash(model_dir)
+ writer = SummaryWriter(log_dir=model_dir)
+ writer_eval = SummaryWriter(log_dir=os.path.join(model_dir, "eval"))
+ train_dataset = TextAudioSpeakerLoader(hps.data.training_files, hps.data)
+ train_sampler = DistributedBucketSampler(
+ train_dataset,
+ hps.train.batch_size,
+ [32, 300, 400, 500, 600, 700, 800, 900, 1000],
+ num_replicas=n_gpus,
+ rank=rank,
+ shuffle=True,
+ )
+ collate_fn = TextAudioSpeakerCollate()
+ train_loader = DataLoader(
+ train_dataset,
+ # メモリ消費量を減らそうとnum_workersを1にしてみる
+ # num_workers=min(config.train_ms_config.num_workers, os.cpu_count() // 2),
+ num_workers=1,
+ shuffle=False,
+ pin_memory=True,
+ collate_fn=collate_fn,
+ batch_sampler=train_sampler,
+ persistent_workers=True,
+ # これもメモリ消費量を減らそうとしてコメントアウト
+ # prefetch_factor=4,
+ ) # DataLoader config could be adjusted.
+ eval_dataset = None
+ eval_loader = None
+ if rank == 0 and not args.speedup:
+ eval_dataset = TextAudioSpeakerLoader(hps.data.validation_files, hps.data)
+ eval_loader = DataLoader(
+ eval_dataset,
+ num_workers=0,
+ shuffle=False,
+ batch_size=1,
+ pin_memory=True,
+ drop_last=False,
+ collate_fn=collate_fn,
+ )
+ if (
+ "use_noise_scaled_mas" in hps.model.keys()
+ and hps.model.use_noise_scaled_mas is True
+ ):
+ logger.info("Using noise scaled MAS for VITS2")
+ mas_noise_scale_initial = 0.01
+ noise_scale_delta = 2e-6
+ else:
+ logger.info("Using normal MAS for VITS1")
+ mas_noise_scale_initial = 0.0
+ noise_scale_delta = 0.0
+ if (
+ "use_duration_discriminator" in hps.model.keys()
+ and hps.model.use_duration_discriminator is True
+ ):
+ logger.info("Using duration discriminator for VITS2")
+ net_dur_disc = DurationDiscriminator(
+ hps.model.hidden_channels,
+ hps.model.hidden_channels,
+ 3,
+ 0.1,
+ gin_channels=hps.model.gin_channels if hps.data.n_speakers != 0 else 0,
+ ).cuda(local_rank)
+ if (
+ "use_spk_conditioned_encoder" in hps.model.keys()
+ and hps.model.use_spk_conditioned_encoder is True
+ ):
+ if hps.data.n_speakers == 0:
+ raise ValueError(
+ "n_speakers must be > 0 when using spk conditioned encoder to train multi-speaker model"
+ )
+ else:
+ logger.info("Using normal encoder for VITS1")
+
+ net_g = SynthesizerTrn(
+ len(symbols),
+ hps.data.filter_length // 2 + 1,
+ hps.train.segment_size // hps.data.hop_length,
+ n_speakers=hps.data.n_speakers,
+ mas_noise_scale_initial=mas_noise_scale_initial,
+ noise_scale_delta=noise_scale_delta,
+ **hps.model,
+ ).cuda(local_rank)
+
+ if getattr(hps.train, "freeze_ZH_bert", False):
+ logger.info("Freezing ZH bert encoder !!!")
+ for param in net_g.enc_p.bert_proj.parameters():
+ param.requires_grad = False
+
+ if getattr(hps.train, "freeze_EN_bert", False):
+ logger.info("Freezing EN bert encoder !!!")
+ for param in net_g.enc_p.en_bert_proj.parameters():
+ param.requires_grad = False
+
+ if getattr(hps.train, "freeze_JP_bert", False):
+ logger.info("Freezing JP bert encoder !!!")
+ for param in net_g.enc_p.ja_bert_proj.parameters():
+ param.requires_grad = False
+ if getattr(hps.train, "freeze_style", False):
+ logger.info("Freezing style encoder !!!")
+ for param in net_g.enc_p.style_proj.parameters():
+ param.requires_grad = False
+
+ if getattr(hps.train, "freeze_decoder", False):
+ logger.info("Freezing decoder !!!")
+ for param in net_g.dec.parameters():
+ param.requires_grad = False
+
+ net_d = MultiPeriodDiscriminator(hps.model.use_spectral_norm).cuda(local_rank)
+ optim_g = torch.optim.AdamW(
+ filter(lambda p: p.requires_grad, net_g.parameters()),
+ hps.train.learning_rate,
+ betas=hps.train.betas,
+ eps=hps.train.eps,
+ )
+ optim_d = torch.optim.AdamW(
+ net_d.parameters(),
+ hps.train.learning_rate,
+ betas=hps.train.betas,
+ eps=hps.train.eps,
+ )
+ if net_dur_disc is not None:
+ optim_dur_disc = torch.optim.AdamW(
+ net_dur_disc.parameters(),
+ hps.train.learning_rate,
+ betas=hps.train.betas,
+ eps=hps.train.eps,
+ )
+ else:
+ optim_dur_disc = None
+ net_g = DDP(net_g, device_ids=[local_rank])
+ net_d = DDP(net_d, device_ids=[local_rank])
+ dur_resume_lr = None
+ if net_dur_disc is not None:
+ net_dur_disc = DDP(
+ net_dur_disc, device_ids=[local_rank], find_unused_parameters=True
+ )
+
+ if utils.is_resuming(model_dir):
+ if net_dur_disc is not None:
+ _, _, dur_resume_lr, epoch_str = utils.load_checkpoint(
+ utils.latest_checkpoint_path(model_dir, "DUR_*.pth"),
+ net_dur_disc,
+ optim_dur_disc,
+ skip_optimizer=(
+ hps.train.skip_optimizer if "skip_optimizer" in hps.train else True
+ ),
+ )
+ if not optim_dur_disc.param_groups[0].get("initial_lr"):
+ optim_dur_disc.param_groups[0]["initial_lr"] = dur_resume_lr
+ _, optim_g, g_resume_lr, epoch_str = utils.load_checkpoint(
+ utils.latest_checkpoint_path(model_dir, "G_*.pth"),
+ net_g,
+ optim_g,
+ skip_optimizer=(
+ hps.train.skip_optimizer if "skip_optimizer" in hps.train else True
+ ),
+ )
+ _, optim_d, d_resume_lr, epoch_str = utils.load_checkpoint(
+ utils.latest_checkpoint_path(model_dir, "D_*.pth"),
+ net_d,
+ optim_d,
+ skip_optimizer=(
+ hps.train.skip_optimizer if "skip_optimizer" in hps.train else True
+ ),
+ )
+ if not optim_g.param_groups[0].get("initial_lr"):
+ optim_g.param_groups[0]["initial_lr"] = g_resume_lr
+ if not optim_d.param_groups[0].get("initial_lr"):
+ optim_d.param_groups[0]["initial_lr"] = d_resume_lr
+
+ epoch_str = max(epoch_str, 1)
+ # global_step = (epoch_str - 1) * len(train_loader)
+ global_step = int(
+ utils.get_steps(utils.latest_checkpoint_path(model_dir, "G_*.pth"))
+ )
+ logger.info(
+ f"******************Found the model. Current epoch is {epoch_str}, gloabl step is {global_step}*********************"
+ )
+ else:
+ try:
+ _ = utils.load_safetensors(
+ os.path.join(model_dir, "G_0.safetensors"), net_g
+ )
+ _ = utils.load_safetensors(
+ os.path.join(model_dir, "D_0.safetensors"), net_d
+ )
+ if net_dur_disc is not None:
+ _ = utils.load_safetensors(
+ os.path.join(model_dir, "DUR_0.safetensors"), net_dur_disc
+ )
+ logger.info("Loaded the pretrained models.")
+ except Exception as e:
+ logger.warning(e)
+ logger.warning(
+ "It seems that you are not using the pretrained models, so we will train from scratch."
+ )
+ finally:
+ epoch_str = 1
+ global_step = 0
+
+ def lr_lambda(epoch):
+ """
+ Learning rate scheduler for warmup and exponential decay.
+ - During the warmup period, the learning rate increases linearly.
+ - After the warmup period, the learning rate decreases exponentially.
+ """
+ if epoch < hps.train.warmup_epochs:
+ return float(epoch) / float(max(1, hps.train.warmup_epochs))
+ else:
+ return hps.train.lr_decay ** (epoch - hps.train.warmup_epochs)
+
+ scheduler_last_epoch = epoch_str - 2
+ scheduler_g = torch.optim.lr_scheduler.LambdaLR(
+ optim_g, lr_lambda=lr_lambda, last_epoch=scheduler_last_epoch
+ )
+ scheduler_d = torch.optim.lr_scheduler.LambdaLR(
+ optim_d, lr_lambda=lr_lambda, last_epoch=scheduler_last_epoch
+ )
+ if net_dur_disc is not None:
+ scheduler_dur_disc = torch.optim.lr_scheduler.LambdaLR(
+ optim_dur_disc, lr_lambda=lr_lambda, last_epoch=scheduler_last_epoch
+ )
+ else:
+ scheduler_dur_disc = None
+ scaler = GradScaler(enabled=hps.train.bf16_run)
+ logger.info("Start training.")
+
+ diff = abs(
+ epoch_str * len(train_loader) - (hps.train.epochs + 1) * len(train_loader)
+ )
+ pbar = None
+ if not args.no_progress_bar:
+ pbar = tqdm(
+ total=global_step + diff,
+ initial=global_step,
+ smoothing=0.05,
+ file=SAFE_STDOUT,
+ )
+ initial_step = global_step
+
+ for epoch in range(epoch_str, hps.train.epochs + 1):
+ if rank == 0:
+ train_and_evaluate(
+ rank,
+ local_rank,
+ epoch,
+ hps,
+ [net_g, net_d, net_dur_disc],
+ [optim_g, optim_d, optim_dur_disc],
+ [scheduler_g, scheduler_d, scheduler_dur_disc],
+ scaler,
+ [train_loader, eval_loader],
+ logger,
+ [writer, writer_eval],
+ pbar,
+ initial_step,
+ )
+ else:
+ train_and_evaluate(
+ rank,
+ local_rank,
+ epoch,
+ hps,
+ [net_g, net_d, net_dur_disc],
+ [optim_g, optim_d, optim_dur_disc],
+ [scheduler_g, scheduler_d, scheduler_dur_disc],
+ scaler,
+ [train_loader, None],
+ None,
+ None,
+ pbar,
+ initial_step,
+ )
+ scheduler_g.step()
+ scheduler_d.step()
+ if net_dur_disc is not None:
+ scheduler_dur_disc.step()
+
+ if epoch == hps.train.epochs:
+ # Save the final models
+ utils.save_checkpoint(
+ net_g,
+ optim_g,
+ hps.train.learning_rate,
+ epoch,
+ os.path.join(model_dir, "G_{}.pth".format(global_step)),
+ )
+ utils.save_checkpoint(
+ net_d,
+ optim_d,
+ hps.train.learning_rate,
+ epoch,
+ os.path.join(model_dir, "D_{}.pth".format(global_step)),
+ )
+ if net_dur_disc is not None:
+ utils.save_checkpoint(
+ net_dur_disc,
+ optim_dur_disc,
+ hps.train.learning_rate,
+ epoch,
+ os.path.join(model_dir, "DUR_{}.pth".format(global_step)),
+ )
+ utils.save_safetensors(
+ net_g,
+ epoch,
+ os.path.join(
+ config.out_dir,
+ f"{config.model_name}_e{epoch}_s{global_step}.safetensors",
+ ),
+ for_infer=True,
+ )
+ if hps.repo_id is not None:
+ future1 = api.upload_folder(
+ repo_id=hps.repo_id,
+ folder_path=config.dataset_path,
+ path_in_repo=f"Data/{config.model_name}",
+ delete_patterns="*.pth", # Only keep the latest checkpoint
+ run_as_future=True,
+ )
+ future2 = api.upload_folder(
+ repo_id=hps.repo_id,
+ folder_path=config.out_dir,
+ path_in_repo=f"model_assets/{config.model_name}",
+ run_as_future=True,
+ )
+ try:
+ future1.result()
+ future2.result()
+ except Exception as e:
+ logger.error(e)
+
+ if pbar is not None:
+ pbar.close()
+
+
+def train_and_evaluate(
+ rank,
+ local_rank,
+ epoch,
+ hps,
+ nets,
+ optims,
+ schedulers,
+ scaler,
+ loaders,
+ logger,
+ writers,
+ pbar: tqdm,
+ initial_step: int,
+):
+ net_g, net_d, net_dur_disc = nets
+ optim_g, optim_d, optim_dur_disc = optims
+ scheduler_g, scheduler_d, scheduler_dur_disc = schedulers
+ train_loader, eval_loader = loaders
+ if writers is not None:
+ writer, writer_eval = writers
+
+ train_loader.batch_sampler.set_epoch(epoch)
+ global global_step
+
+ net_g.train()
+ net_d.train()
+ if net_dur_disc is not None:
+ net_dur_disc.train()
+ for batch_idx, (
+ x,
+ x_lengths,
+ spec,
+ spec_lengths,
+ y,
+ y_lengths,
+ speakers,
+ tone,
+ language,
+ bert,
+ ja_bert,
+ en_bert,
+ style_vec,
+ ) in enumerate(train_loader):
+ if net_g.module.use_noise_scaled_mas:
+ current_mas_noise_scale = (
+ net_g.module.mas_noise_scale_initial
+ - net_g.module.noise_scale_delta * global_step
+ )
+ net_g.module.current_mas_noise_scale = max(current_mas_noise_scale, 0.0)
+ x, x_lengths = x.cuda(local_rank, non_blocking=True), x_lengths.cuda(
+ local_rank, non_blocking=True
+ )
+ spec, spec_lengths = spec.cuda(
+ local_rank, non_blocking=True
+ ), spec_lengths.cuda(local_rank, non_blocking=True)
+ y, y_lengths = y.cuda(local_rank, non_blocking=True), y_lengths.cuda(
+ local_rank, non_blocking=True
+ )
+ speakers = speakers.cuda(local_rank, non_blocking=True)
+ tone = tone.cuda(local_rank, non_blocking=True)
+ language = language.cuda(local_rank, non_blocking=True)
+ bert = bert.cuda(local_rank, non_blocking=True)
+ ja_bert = ja_bert.cuda(local_rank, non_blocking=True)
+ en_bert = en_bert.cuda(local_rank, non_blocking=True)
+ style_vec = style_vec.cuda(local_rank, non_blocking=True)
+
+ with autocast(enabled=hps.train.bf16_run, dtype=torch.bfloat16):
+ (
+ y_hat,
+ l_length,
+ attn,
+ ids_slice,
+ x_mask,
+ z_mask,
+ (z, z_p, m_p, logs_p, m_q, logs_q),
+ (hidden_x, logw, logw_),
+ ) = net_g(
+ x,
+ x_lengths,
+ spec,
+ spec_lengths,
+ speakers,
+ tone,
+ language,
+ bert,
+ ja_bert,
+ en_bert,
+ style_vec,
+ )
+ mel = spec_to_mel_torch(
+ spec,
+ hps.data.filter_length,
+ hps.data.n_mel_channels,
+ hps.data.sampling_rate,
+ hps.data.mel_fmin,
+ hps.data.mel_fmax,
+ )
+ y_mel = commons.slice_segments(
+ mel, ids_slice, hps.train.segment_size // hps.data.hop_length
+ )
+ y_hat_mel = mel_spectrogram_torch(
+ y_hat.squeeze(1).float(),
+ hps.data.filter_length,
+ hps.data.n_mel_channels,
+ hps.data.sampling_rate,
+ hps.data.hop_length,
+ hps.data.win_length,
+ hps.data.mel_fmin,
+ hps.data.mel_fmax,
+ )
+
+ y = commons.slice_segments(
+ y, ids_slice * hps.data.hop_length, hps.train.segment_size
+ ) # slice
+
+ # Discriminator
+ y_d_hat_r, y_d_hat_g, _, _ = net_d(y, y_hat.detach())
+ with autocast(enabled=hps.train.bf16_run, dtype=torch.bfloat16):
+ loss_disc, losses_disc_r, losses_disc_g = discriminator_loss(
+ y_d_hat_r, y_d_hat_g
+ )
+ loss_disc_all = loss_disc
+ if net_dur_disc is not None:
+ y_dur_hat_r, y_dur_hat_g = net_dur_disc(
+ hidden_x.detach(), x_mask.detach(), logw.detach(), logw_.detach()
+ )
+ with autocast(enabled=hps.train.bf16_run, dtype=torch.bfloat16):
+ # TODO: I think need to mean using the mask, but for now, just mean all
+ (
+ loss_dur_disc,
+ losses_dur_disc_r,
+ losses_dur_disc_g,
+ ) = discriminator_loss(y_dur_hat_r, y_dur_hat_g)
+ loss_dur_disc_all = loss_dur_disc
+ optim_dur_disc.zero_grad()
+ scaler.scale(loss_dur_disc_all).backward()
+ scaler.unscale_(optim_dur_disc)
+ commons.clip_grad_value_(net_dur_disc.parameters(), None)
+ scaler.step(optim_dur_disc)
+
+ optim_d.zero_grad()
+ scaler.scale(loss_disc_all).backward()
+ scaler.unscale_(optim_d)
+ if getattr(hps.train, "bf16_run", False):
+ torch.nn.utils.clip_grad_norm_(parameters=net_d.parameters(), max_norm=200)
+ grad_norm_d = commons.clip_grad_value_(net_d.parameters(), None)
+ scaler.step(optim_d)
+
+ with autocast(enabled=hps.train.bf16_run, dtype=torch.bfloat16):
+ # Generator
+ y_d_hat_r, y_d_hat_g, fmap_r, fmap_g = net_d(y, y_hat)
+ if net_dur_disc is not None:
+ y_dur_hat_r, y_dur_hat_g = net_dur_disc(hidden_x, x_mask, logw, logw_)
+ with autocast(enabled=hps.train.bf16_run, dtype=torch.bfloat16):
+ loss_dur = torch.sum(l_length.float())
+ loss_mel = F.l1_loss(y_mel, y_hat_mel) * hps.train.c_mel
+ loss_kl = kl_loss(z_p, logs_q, m_p, logs_p, z_mask) * hps.train.c_kl
+
+ loss_fm = feature_loss(fmap_r, fmap_g)
+ loss_gen, losses_gen = generator_loss(y_d_hat_g)
+ loss_gen_all = loss_gen + loss_fm + loss_mel + loss_dur + loss_kl
+ if net_dur_disc is not None:
+ loss_dur_gen, losses_dur_gen = generator_loss(y_dur_hat_g)
+ loss_gen_all += loss_dur_gen
+ optim_g.zero_grad()
+ scaler.scale(loss_gen_all).backward()
+ scaler.unscale_(optim_g)
+ if getattr(hps.train, "bf16_run", False):
+ torch.nn.utils.clip_grad_norm_(parameters=net_g.parameters(), max_norm=500)
+ grad_norm_g = commons.clip_grad_value_(net_g.parameters(), None)
+ scaler.step(optim_g)
+ scaler.update()
+
+ if rank == 0:
+ if global_step % hps.train.log_interval == 0 and not hps.speedup:
+ lr = optim_g.param_groups[0]["lr"]
+ losses = [loss_disc, loss_gen, loss_fm, loss_mel, loss_dur, loss_kl]
+ # logger.info(
+ # "Train Epoch: {} [{:.0f}%]".format(
+ # epoch, 100.0 * batch_idx / len(train_loader)
+ # )
+ # )
+ # logger.info([x.item() for x in losses] + [global_step, lr])
+
+ scalar_dict = {
+ "loss/g/total": loss_gen_all,
+ "loss/d/total": loss_disc_all,
+ "learning_rate": lr,
+ "grad_norm_d": grad_norm_d,
+ "grad_norm_g": grad_norm_g,
+ }
+ scalar_dict.update(
+ {
+ "loss/g/fm": loss_fm,
+ "loss/g/mel": loss_mel,
+ "loss/g/dur": loss_dur,
+ "loss/g/kl": loss_kl,
+ }
+ )
+ scalar_dict.update(
+ {"loss/g/{}".format(i): v for i, v in enumerate(losses_gen)}
+ )
+ scalar_dict.update(
+ {"loss/d_r/{}".format(i): v for i, v in enumerate(losses_disc_r)}
+ )
+ scalar_dict.update(
+ {"loss/d_g/{}".format(i): v for i, v in enumerate(losses_disc_g)}
+ )
+
+ image_dict = {
+ "slice/mel_org": utils.plot_spectrogram_to_numpy(
+ y_mel[0].data.cpu().numpy()
+ ),
+ "slice/mel_gen": utils.plot_spectrogram_to_numpy(
+ y_hat_mel[0].data.cpu().numpy()
+ ),
+ "all/mel": utils.plot_spectrogram_to_numpy(
+ mel[0].data.cpu().numpy()
+ ),
+ "all/attn": utils.plot_alignment_to_numpy(
+ attn[0, 0].data.cpu().numpy()
+ ),
+ }
+ utils.summarize(
+ writer=writer,
+ global_step=global_step,
+ images=image_dict,
+ scalars=scalar_dict,
+ )
+
+ if (
+ global_step % hps.train.eval_interval == 0
+ and global_step != 0
+ and initial_step != global_step
+ ):
+ if not hps.speedup:
+ evaluate(hps, net_g, eval_loader, writer_eval)
+ utils.save_checkpoint(
+ net_g,
+ optim_g,
+ hps.train.learning_rate,
+ epoch,
+ os.path.join(hps.model_dir, "G_{}.pth".format(global_step)),
+ )
+ utils.save_checkpoint(
+ net_d,
+ optim_d,
+ hps.train.learning_rate,
+ epoch,
+ os.path.join(hps.model_dir, "D_{}.pth".format(global_step)),
+ )
+ if net_dur_disc is not None:
+ utils.save_checkpoint(
+ net_dur_disc,
+ optim_dur_disc,
+ hps.train.learning_rate,
+ epoch,
+ os.path.join(hps.model_dir, "DUR_{}.pth".format(global_step)),
+ )
+ keep_ckpts = config.train_ms_config.keep_ckpts
+ if keep_ckpts > 0:
+ utils.clean_checkpoints(
+ path_to_models=hps.model_dir,
+ n_ckpts_to_keep=keep_ckpts,
+ sort_by_time=True,
+ )
+ # Save safetensors (for inference) to `model_assets/{model_name}`
+ utils.save_safetensors(
+ net_g,
+ epoch,
+ os.path.join(
+ config.out_dir,
+ f"{config.model_name}_e{epoch}_s{global_step}.safetensors",
+ ),
+ for_infer=True,
+ )
+ if hps.repo_id is not None:
+ api.upload_folder(
+ repo_id=hps.repo_id,
+ folder_path=config.dataset_path,
+ path_in_repo=f"Data/{config.model_name}",
+ delete_patterns="*.pth", # Only keep the latest checkpoint
+ run_as_future=True,
+ )
+ api.upload_folder(
+ repo_id=hps.repo_id,
+ folder_path=config.out_dir,
+ path_in_repo=f"model_assets/{config.model_name}",
+ run_as_future=True,
+ )
+
+ global_step += 1
+ if pbar is not None:
+ pbar.set_description(
+ "Epoch {}({:.0f}%)/{}".format(
+ epoch, 100.0 * batch_idx / len(train_loader), hps.train.epochs
+ )
+ )
+ pbar.update()
+ # 本家ではこれをスピードアップのために消すと書かれていたので、一応消してみる
+ # と思ったけどメモリ使用量が減るかもしれないのでつけてみる
+ gc.collect()
+ torch.cuda.empty_cache()
+ if pbar is None and rank == 0:
+ logger.info(f"====> Epoch: {epoch}, step: {global_step}")
+
+
+def evaluate(hps, generator, eval_loader, writer_eval):
+ generator.eval()
+ image_dict = {}
+ audio_dict = {}
+ logger.info("Evaluating ...")
+ with torch.no_grad():
+ for batch_idx, (
+ x,
+ x_lengths,
+ spec,
+ spec_lengths,
+ y,
+ y_lengths,
+ speakers,
+ tone,
+ language,
+ bert,
+ ja_bert,
+ en_bert,
+ style_vec,
+ ) in enumerate(eval_loader):
+ x, x_lengths = x.cuda(), x_lengths.cuda()
+ spec, spec_lengths = spec.cuda(), spec_lengths.cuda()
+ y, y_lengths = y.cuda(), y_lengths.cuda()
+ speakers = speakers.cuda()
+ bert = bert.cuda()
+ ja_bert = ja_bert.cuda()
+ en_bert = en_bert.cuda()
+ tone = tone.cuda()
+ language = language.cuda()
+ style_vec = style_vec.cuda()
+ for use_sdp in [True, False]:
+ y_hat, attn, mask, *_ = generator.module.infer(
+ x,
+ x_lengths,
+ speakers,
+ tone,
+ language,
+ bert,
+ ja_bert,
+ en_bert,
+ style_vec,
+ y=spec,
+ max_len=1000,
+ sdp_ratio=0.0 if not use_sdp else 1.0,
+ )
+ y_hat_lengths = mask.sum([1, 2]).long() * hps.data.hop_length
+
+ mel = spec_to_mel_torch(
+ spec,
+ hps.data.filter_length,
+ hps.data.n_mel_channels,
+ hps.data.sampling_rate,
+ hps.data.mel_fmin,
+ hps.data.mel_fmax,
+ )
+ y_hat_mel = mel_spectrogram_torch(
+ y_hat.squeeze(1).float(),
+ hps.data.filter_length,
+ hps.data.n_mel_channels,
+ hps.data.sampling_rate,
+ hps.data.hop_length,
+ hps.data.win_length,
+ hps.data.mel_fmin,
+ hps.data.mel_fmax,
+ )
+ image_dict.update(
+ {
+ f"gen/mel_{batch_idx}": utils.plot_spectrogram_to_numpy(
+ y_hat_mel[0].cpu().numpy()
+ )
+ }
+ )
+ audio_dict.update(
+ {
+ f"gen/audio_{batch_idx}_{use_sdp}": y_hat[
+ 0, :, : y_hat_lengths[0]
+ ]
+ }
+ )
+ image_dict.update(
+ {
+ f"gt/mel_{batch_idx}": utils.plot_spectrogram_to_numpy(
+ mel[0].cpu().numpy()
+ )
+ }
+ )
+ audio_dict.update({f"gt/audio_{batch_idx}": y[0, :, : y_lengths[0]]})
+
+ utils.summarize(
+ writer=writer_eval,
+ global_step=global_step,
+ images=image_dict,
+ audios=audio_dict,
+ audio_sampling_rate=hps.data.sampling_rate,
+ )
+ generator.train()
+
+
+if __name__ == "__main__":
+ run()
diff --git a/train_ms_jp_extra.py b/train_ms_jp_extra.py
new file mode 100644
index 0000000000000000000000000000000000000000..bae922d52a8dd0229f8f01a405e790dec7224a3a
--- /dev/null
+++ b/train_ms_jp_extra.py
@@ -0,0 +1,1102 @@
+import argparse
+import datetime
+import gc
+import os
+import platform
+
+import torch
+import torch.distributed as dist
+from torch.cuda.amp import GradScaler, autocast
+from torch.nn import functional as F
+from torch.nn.parallel import DistributedDataParallel as DDP
+from torch.utils.data import DataLoader
+from torch.utils.tensorboard import SummaryWriter
+from tqdm import tqdm
+from huggingface_hub import HfApi
+
+# logging.getLogger("numba").setLevel(logging.WARNING)
+import commons
+import default_style
+import utils
+from common.log import logger
+from common.stdout_wrapper import SAFE_STDOUT
+from config import config
+from data_utils import (
+ DistributedBucketSampler,
+ TextAudioSpeakerCollate,
+ TextAudioSpeakerLoader,
+)
+from losses import WavLMLoss, discriminator_loss, feature_loss, generator_loss, kl_loss
+from mel_processing import mel_spectrogram_torch, spec_to_mel_torch
+from models_jp_extra import (
+ DurationDiscriminator,
+ MultiPeriodDiscriminator,
+ SynthesizerTrn,
+ WavLMDiscriminator,
+)
+from text.symbols import symbols
+
+torch.backends.cuda.matmul.allow_tf32 = True
+torch.backends.cudnn.allow_tf32 = (
+ True # If encontered training problem,please try to disable TF32.
+)
+torch.set_num_threads(1)
+torch.set_float32_matmul_precision("medium")
+torch.backends.cuda.sdp_kernel("flash")
+torch.backends.cuda.enable_flash_sdp(True)
+torch.backends.cuda.enable_mem_efficient_sdp(
+ True
+) # Not available if torch version is lower than 2.0
+global_step = 0
+
+api = HfApi()
+
+
+def run():
+ # Command line configuration is not recommended unless necessary, use config.yml
+ parser = argparse.ArgumentParser()
+ parser.add_argument(
+ "-c",
+ "--config",
+ type=str,
+ default=config.train_ms_config.config_path,
+ help="JSON file for configuration",
+ )
+ parser.add_argument(
+ "-m",
+ "--model",
+ type=str,
+ help="数据集文件夹路径,请注意,数据不再默认放在/logs文件夹下。如果需要用命令行配置,请声明相对于根目录的路径",
+ default=config.dataset_path,
+ )
+ parser.add_argument(
+ "--assets_root",
+ type=str,
+ help="Root directory of model assets needed for inference.",
+ default=config.assets_root,
+ )
+ parser.add_argument(
+ "--skip_default_style",
+ action="store_true",
+ help="Skip saving default style config and mean vector.",
+ )
+ parser.add_argument(
+ "--no_progress_bar",
+ action="store_true",
+ help="Do not show the progress bar while training.",
+ )
+ parser.add_argument(
+ "--speedup",
+ action="store_true",
+ help="Speed up training by disabling logging and evaluation.",
+ )
+ parser.add_argument(
+ "--repo_id",
+ help="Huggingface model repo id to backup the model.",
+ default=None,
+ )
+ args = parser.parse_args()
+
+ # Set log file
+ model_dir = os.path.join(args.model, config.train_ms_config.model_dir)
+ timestamp = datetime.datetime.now().strftime("%Y%m%d_%H%M%S")
+ logger.add(os.path.join(args.model, f"train_{timestamp}.log"))
+
+ # Parsing environment variables
+ envs = config.train_ms_config.env
+ for env_name, env_value in envs.items():
+ if env_name not in os.environ.keys():
+ logger.info("Loading configuration from config {}".format(str(env_value)))
+ os.environ[env_name] = str(env_value)
+ logger.info(
+ "Loading environment variables \nMASTER_ADDR: {},\nMASTER_PORT: {},\nWORLD_SIZE: {},\nRANK: {},\nLOCAL_RANK: {}".format(
+ os.environ["MASTER_ADDR"],
+ os.environ["MASTER_PORT"],
+ os.environ["WORLD_SIZE"],
+ os.environ["RANK"],
+ os.environ["LOCAL_RANK"],
+ )
+ )
+
+ backend = "nccl"
+ if platform.system() == "Windows":
+ backend = "gloo" # If Windows,switch to gloo backend.
+ dist.init_process_group(
+ backend=backend,
+ init_method="env://",
+ timeout=datetime.timedelta(seconds=300),
+ ) # Use torchrun instead of mp.spawn
+ rank = dist.get_rank()
+ local_rank = int(os.environ["LOCAL_RANK"])
+ n_gpus = dist.get_world_size()
+
+ hps = utils.get_hparams_from_file(args.config)
+ # This is needed because we have to pass values to `train_and_evaluate()
+ hps.model_dir = model_dir
+ hps.speedup = args.speedup
+ hps.repo_id = args.repo_id
+
+ # 比较路径是否相同
+ if os.path.realpath(args.config) != os.path.realpath(
+ config.train_ms_config.config_path
+ ):
+ with open(args.config, "r", encoding="utf-8") as f:
+ data = f.read()
+ os.makedirs(os.path.dirname(config.train_ms_config.config_path), exist_ok=True)
+ with open(config.train_ms_config.config_path, "w", encoding="utf-8") as f:
+ f.write(data)
+
+ """
+ Path constants are a bit complicated...
+ TODO: Refactor or rename these?
+ (Both `config.yml` and `config.json` are used, which is confusing I think.)
+
+ args.model: For saving all info needed for training.
+ default: `Data/{model_name}`.
+ hps.model_dir := model_dir: For saving checkpoints (for resuming training).
+ default: `Data/{model_name}/models`.
+ (Use `hps` since we have to pass `model_dir` to `train_and_evaluate()`.
+
+ args.assets_root: The root directory of model assets needed for inference.
+ default: config.assets_root == `model_assets`.
+
+ config.out_dir: The directory for model assets of this model (for inference).
+ default: `model_assets/{model_name}`.
+ """
+
+ if args.repo_id is not None:
+ # First try to upload config.json to check if the repo exists
+ try:
+ api.upload_file(
+ path_or_fileobj=args.config,
+ path_in_repo=f"Data/{config.model_name}/config.json",
+ repo_id=hps.repo_id,
+ )
+ except Exception as e:
+ logger.error(e)
+ logger.error(
+ f"Failed to upload files to the repo {hps.repo_id}. Please check if the repo exists and you have logged in using `huggingface-cli login`."
+ )
+ raise e
+ # Upload Data dir for resuming training
+ api.upload_folder(
+ repo_id=hps.repo_id,
+ folder_path=config.dataset_path,
+ path_in_repo=f"Data/{config.model_name}",
+ delete_patterns="*.pth", # Only keep the latest checkpoint
+ ignore_patterns=f"{config.dataset_path}/raw", # Ignore raw data
+ run_as_future=True,
+ )
+ os.makedirs(config.out_dir, exist_ok=True)
+
+ if not args.skip_default_style:
+ # Save default style to out_dir
+ default_style.set_style_config(
+ args.config, os.path.join(config.out_dir, "config.json")
+ )
+ default_style.save_mean_vector(
+ os.path.join(args.model, "wavs"),
+ os.path.join(config.out_dir, "style_vectors.npy"),
+ )
+
+ torch.manual_seed(hps.train.seed)
+ torch.cuda.set_device(local_rank)
+
+ global global_step
+ writer = None
+ writer_eval = None
+ if rank == 0 and not args.speedup:
+ # logger = utils.get_logger(hps.model_dir)
+ # logger.info(hps)
+ utils.check_git_hash(model_dir)
+ writer = SummaryWriter(log_dir=model_dir)
+ writer_eval = SummaryWriter(log_dir=os.path.join(model_dir, "eval"))
+ train_dataset = TextAudioSpeakerLoader(hps.data.training_files, hps.data)
+ train_sampler = DistributedBucketSampler(
+ train_dataset,
+ hps.train.batch_size,
+ [32, 300, 400, 500, 600, 700, 800, 900, 1000],
+ num_replicas=n_gpus,
+ rank=rank,
+ shuffle=True,
+ )
+ collate_fn = TextAudioSpeakerCollate(use_jp_extra=True)
+ train_loader = DataLoader(
+ train_dataset,
+ # メモリ消費量を減らそうとnum_workersを1にしてみる
+ # num_workers=min(config.train_ms_config.num_workers, os.cpu_count() // 2),
+ num_workers=1,
+ shuffle=False,
+ pin_memory=True,
+ collate_fn=collate_fn,
+ batch_sampler=train_sampler,
+ persistent_workers=True,
+ # これもメモリ消費量を減らそうとしてコメントアウト
+ # prefetch_factor=6,
+ ) # DataLoader config could be adjusted.
+ eval_dataset = None
+ eval_loader = None
+ if rank == 0 and not args.speedup:
+ eval_dataset = TextAudioSpeakerLoader(hps.data.validation_files, hps.data)
+ eval_loader = DataLoader(
+ eval_dataset,
+ num_workers=0,
+ shuffle=False,
+ batch_size=1,
+ pin_memory=True,
+ drop_last=False,
+ collate_fn=collate_fn,
+ )
+ if (
+ "use_noise_scaled_mas" in hps.model.keys()
+ and hps.model.use_noise_scaled_mas is True
+ ):
+ logger.info("Using noise scaled MAS for VITS2")
+ mas_noise_scale_initial = 0.01
+ noise_scale_delta = 2e-6
+ else:
+ logger.info("Using normal MAS for VITS1")
+ mas_noise_scale_initial = 0.0
+ noise_scale_delta = 0.0
+ if (
+ "use_duration_discriminator" in hps.model.keys()
+ and hps.model.use_duration_discriminator is True
+ ):
+ logger.info("Using duration discriminator for VITS2")
+ net_dur_disc = DurationDiscriminator(
+ hps.model.hidden_channels,
+ hps.model.hidden_channels,
+ 3,
+ 0.1,
+ gin_channels=hps.model.gin_channels if hps.data.n_speakers != 0 else 0,
+ ).cuda(local_rank)
+ else:
+ net_dur_disc = None
+ if (
+ "use_wavlm_discriminator" in hps.model.keys()
+ and hps.model.use_wavlm_discriminator is True
+ ):
+ net_wd = WavLMDiscriminator(
+ hps.model.slm.hidden, hps.model.slm.nlayers, hps.model.slm.initial_channel
+ ).cuda(local_rank)
+ else:
+ net_wd = None
+ if (
+ "use_spk_conditioned_encoder" in hps.model.keys()
+ and hps.model.use_spk_conditioned_encoder is True
+ ):
+ if hps.data.n_speakers == 0:
+ raise ValueError(
+ "n_speakers must be > 0 when using spk conditioned encoder to train multi-speaker model"
+ )
+ else:
+ logger.info("Using normal encoder for VITS1")
+
+ net_g = SynthesizerTrn(
+ len(symbols),
+ hps.data.filter_length // 2 + 1,
+ hps.train.segment_size // hps.data.hop_length,
+ n_speakers=hps.data.n_speakers,
+ mas_noise_scale_initial=mas_noise_scale_initial,
+ noise_scale_delta=noise_scale_delta,
+ **hps.model,
+ ).cuda(local_rank)
+ if getattr(hps.train, "freeze_JP_bert", False):
+ logger.info("Freezing (JP) bert encoder !!!")
+ for param in net_g.enc_p.bert_proj.parameters():
+ param.requires_grad = False
+ if getattr(hps.train, "freeze_style", False):
+ logger.info("Freezing style encoder !!!")
+ for param in net_g.enc_p.style_proj.parameters():
+ param.requires_grad = False
+
+ if getattr(hps.train, "freeze_decoder", False):
+ logger.info("Freezing decoder !!!")
+ for param in net_g.dec.parameters():
+ param.requires_grad = False
+
+ net_d = MultiPeriodDiscriminator(hps.model.use_spectral_norm).cuda(local_rank)
+ optim_g = torch.optim.AdamW(
+ filter(lambda p: p.requires_grad, net_g.parameters()),
+ hps.train.learning_rate,
+ betas=hps.train.betas,
+ eps=hps.train.eps,
+ )
+ optim_d = torch.optim.AdamW(
+ net_d.parameters(),
+ hps.train.learning_rate,
+ betas=hps.train.betas,
+ eps=hps.train.eps,
+ )
+ if net_dur_disc is not None:
+ optim_dur_disc = torch.optim.AdamW(
+ net_dur_disc.parameters(),
+ hps.train.learning_rate,
+ betas=hps.train.betas,
+ eps=hps.train.eps,
+ )
+ else:
+ optim_dur_disc = None
+ if net_wd is not None:
+ optim_wd = torch.optim.AdamW(
+ net_wd.parameters(),
+ hps.train.learning_rate,
+ betas=hps.train.betas,
+ eps=hps.train.eps,
+ )
+ else:
+ optim_wd = None
+ net_g = DDP(
+ net_g,
+ device_ids=[local_rank],
+ # bucket_cap_mb=512
+ )
+ net_d = DDP(
+ net_d,
+ device_ids=[local_rank],
+ # bucket_cap_mb=512
+ )
+ if net_dur_disc is not None:
+ net_dur_disc = DDP(
+ net_dur_disc,
+ device_ids=[local_rank],
+ # bucket_cap_mb=512,
+ )
+ if net_wd is not None:
+ net_wd = DDP(
+ net_wd,
+ device_ids=[local_rank],
+ # bucket_cap_mb=512
+ )
+
+ if utils.is_resuming(model_dir):
+ if net_dur_disc is not None:
+ try:
+ _, _, dur_resume_lr, epoch_str = utils.load_checkpoint(
+ utils.latest_checkpoint_path(model_dir, "DUR_*.pth"),
+ net_dur_disc,
+ optim_dur_disc,
+ skip_optimizer=(
+ hps.train.skip_optimizer
+ if "skip_optimizer" in hps.train
+ else True
+ ),
+ )
+ if not optim_dur_disc.param_groups[0].get("initial_lr"):
+ optim_dur_disc.param_groups[0]["initial_lr"] = dur_resume_lr
+ except:
+ if not optim_dur_disc.param_groups[0].get("initial_lr"):
+ optim_dur_disc.param_groups[0]["initial_lr"] = dur_resume_lr
+ print("Initialize dur_disc")
+ if net_wd is not None:
+ try:
+ _, optim_wd, wd_resume_lr, epoch_str = utils.load_checkpoint(
+ utils.latest_checkpoint_path(model_dir, "WD_*.pth"),
+ net_wd,
+ optim_wd,
+ skip_optimizer=(
+ hps.train.skip_optimizer
+ if "skip_optimizer" in hps.train
+ else True
+ ),
+ )
+ if not optim_wd.param_groups[0].get("initial_lr"):
+ optim_wd.param_groups[0]["initial_lr"] = wd_resume_lr
+ except:
+ if not optim_wd.param_groups[0].get("initial_lr"):
+ optim_wd.param_groups[0]["initial_lr"] = wd_resume_lr
+ logger.info("Initialize wavlm")
+
+ try:
+ _, optim_g, g_resume_lr, epoch_str = utils.load_checkpoint(
+ utils.latest_checkpoint_path(model_dir, "G_*.pth"),
+ net_g,
+ optim_g,
+ skip_optimizer=(
+ hps.train.skip_optimizer if "skip_optimizer" in hps.train else True
+ ),
+ )
+ _, optim_d, d_resume_lr, epoch_str = utils.load_checkpoint(
+ utils.latest_checkpoint_path(model_dir, "D_*.pth"),
+ net_d,
+ optim_d,
+ skip_optimizer=(
+ hps.train.skip_optimizer if "skip_optimizer" in hps.train else True
+ ),
+ )
+ if not optim_g.param_groups[0].get("initial_lr"):
+ optim_g.param_groups[0]["initial_lr"] = g_resume_lr
+ if not optim_d.param_groups[0].get("initial_lr"):
+ optim_d.param_groups[0]["initial_lr"] = d_resume_lr
+
+ epoch_str = max(epoch_str, 1)
+ # global_step = (epoch_str - 1) * len(train_loader)
+ global_step = int(
+ utils.get_steps(utils.latest_checkpoint_path(model_dir, "G_*.pth"))
+ )
+ logger.info(
+ f"******************Found the model. Current epoch is {epoch_str}, gloabl step is {global_step}*********************"
+ )
+ except Exception as e:
+ logger.warning(e)
+ logger.warning(
+ "It seems that you are not using the pretrained models, so we will train from scratch."
+ )
+ epoch_str = 1
+ global_step = 0
+ else:
+ try:
+ _ = utils.load_safetensors(
+ os.path.join(model_dir, "G_0.safetensors"), net_g
+ )
+ _ = utils.load_safetensors(
+ os.path.join(model_dir, "D_0.safetensors"), net_d
+ )
+ if net_dur_disc is not None:
+ _ = utils.load_safetensors(
+ os.path.join(model_dir, "DUR_0.safetensors"), net_dur_disc
+ )
+ if net_wd is not None:
+ _ = utils.load_safetensors(
+ os.path.join(model_dir, "WD_0.safetensors"), net_wd
+ )
+ logger.info("Loaded the pretrained models.")
+ except Exception as e:
+ logger.warning(e)
+ logger.warning(
+ "It seems that you are not using the pretrained models, so we will train from scratch."
+ )
+ finally:
+ epoch_str = 1
+ global_step = 0
+
+ def lr_lambda(epoch):
+ """
+ Learning rate scheduler for warmup and exponential decay.
+ - During the warmup period, the learning rate increases linearly.
+ - After the warmup period, the learning rate decreases exponentially.
+ """
+ if epoch < hps.train.warmup_epochs:
+ return float(epoch) / float(max(1, hps.train.warmup_epochs))
+ else:
+ return hps.train.lr_decay ** (epoch - hps.train.warmup_epochs)
+
+ scheduler_last_epoch = epoch_str - 2
+ scheduler_g = torch.optim.lr_scheduler.LambdaLR(
+ optim_g, lr_lambda=lr_lambda, last_epoch=scheduler_last_epoch
+ )
+ scheduler_d = torch.optim.lr_scheduler.LambdaLR(
+ optim_d, lr_lambda=lr_lambda, last_epoch=scheduler_last_epoch
+ )
+ if net_dur_disc is not None:
+ scheduler_dur_disc = torch.optim.lr_scheduler.LambdaLR(
+ optim_dur_disc, lr_lambda=lr_lambda, last_epoch=scheduler_last_epoch
+ )
+ else:
+ scheduler_dur_disc = None
+ if net_wd is not None:
+ scheduler_wd = torch.optim.lr_scheduler.LambdaLR(
+ optim_wd, lr_lambda=lr_lambda, last_epoch=scheduler_last_epoch
+ )
+ wl = WavLMLoss(
+ hps.model.slm.model,
+ net_wd,
+ hps.data.sampling_rate,
+ hps.model.slm.sr,
+ ).to(local_rank)
+ else:
+ scheduler_wd = None
+ wl = None
+ scaler = GradScaler(enabled=hps.train.bf16_run)
+ logger.info("Start training.")
+
+ diff = abs(
+ epoch_str * len(train_loader) - (hps.train.epochs + 1) * len(train_loader)
+ )
+ pbar = None
+ if not args.no_progress_bar:
+ pbar = tqdm(
+ total=global_step + diff,
+ initial=global_step,
+ smoothing=0.05,
+ file=SAFE_STDOUT,
+ )
+ initial_step = global_step
+
+ for epoch in range(epoch_str, hps.train.epochs + 1):
+ if rank == 0:
+ train_and_evaluate(
+ rank,
+ local_rank,
+ epoch,
+ hps,
+ [net_g, net_d, net_dur_disc, net_wd, wl],
+ [optim_g, optim_d, optim_dur_disc, optim_wd],
+ [scheduler_g, scheduler_d, scheduler_dur_disc, scheduler_wd],
+ scaler,
+ [train_loader, eval_loader],
+ logger,
+ [writer, writer_eval],
+ pbar,
+ initial_step,
+ )
+ else:
+ train_and_evaluate(
+ rank,
+ local_rank,
+ epoch,
+ hps,
+ [net_g, net_d, net_dur_disc, net_wd, wl],
+ [optim_g, optim_d, optim_dur_disc, optim_wd],
+ [scheduler_g, scheduler_d, scheduler_dur_disc, scheduler_wd],
+ scaler,
+ [train_loader, None],
+ None,
+ None,
+ pbar,
+ initial_step,
+ )
+ scheduler_g.step()
+ scheduler_d.step()
+ if net_dur_disc is not None:
+ scheduler_dur_disc.step()
+ if net_wd is not None:
+ scheduler_wd.step()
+ if epoch == hps.train.epochs:
+ # Save the final models
+ utils.save_checkpoint(
+ net_g,
+ optim_g,
+ hps.train.learning_rate,
+ epoch,
+ os.path.join(model_dir, "G_{}.pth".format(global_step)),
+ )
+ utils.save_checkpoint(
+ net_d,
+ optim_d,
+ hps.train.learning_rate,
+ epoch,
+ os.path.join(model_dir, "D_{}.pth".format(global_step)),
+ )
+ if net_dur_disc is not None:
+ utils.save_checkpoint(
+ net_dur_disc,
+ optim_dur_disc,
+ hps.train.learning_rate,
+ epoch,
+ os.path.join(model_dir, "DUR_{}.pth".format(global_step)),
+ )
+ if net_wd is not None:
+ utils.save_checkpoint(
+ net_wd,
+ optim_wd,
+ hps.train.learning_rate,
+ epoch,
+ os.path.join(model_dir, "WD_{}.pth".format(global_step)),
+ )
+ utils.save_safetensors(
+ net_g,
+ epoch,
+ os.path.join(
+ config.out_dir,
+ f"{config.model_name}_e{epoch}_s{global_step}.safetensors",
+ ),
+ for_infer=True,
+ )
+ if hps.repo_id is not None:
+ future1 = api.upload_folder(
+ repo_id=hps.repo_id,
+ folder_path=config.dataset_path,
+ path_in_repo=f"Data/{config.model_name}",
+ delete_patterns="*.pth", # Only keep the latest checkpoint
+ ignore_patterns=f"{config.dataset_path}/raw", # Ignore raw data
+ run_as_future=True,
+ )
+ future2 = api.upload_folder(
+ repo_id=hps.repo_id,
+ folder_path=config.out_dir,
+ path_in_repo=f"model_assets/{config.model_name}",
+ run_as_future=True,
+ )
+ try:
+ future1.result()
+ future2.result()
+ except Exception as e:
+ logger.error(e)
+
+ if pbar is not None:
+ pbar.close()
+
+
+def train_and_evaluate(
+ rank,
+ local_rank,
+ epoch,
+ hps,
+ nets,
+ optims,
+ schedulers,
+ scaler,
+ loaders,
+ logger,
+ writers,
+ pbar: tqdm,
+ initial_step: int,
+):
+ net_g, net_d, net_dur_disc, net_wd, wl = nets
+ optim_g, optim_d, optim_dur_disc, optim_wd = optims
+ scheduler_g, scheduler_d, scheduler_dur_disc, scheduler_wd = schedulers
+ train_loader, eval_loader = loaders
+ if writers is not None:
+ writer, writer_eval = writers
+
+ train_loader.batch_sampler.set_epoch(epoch)
+ global global_step
+
+ net_g.train()
+ net_d.train()
+ if net_dur_disc is not None:
+ net_dur_disc.train()
+ if net_wd is not None:
+ net_wd.train()
+ for batch_idx, (
+ x,
+ x_lengths,
+ spec,
+ spec_lengths,
+ y,
+ y_lengths,
+ speakers,
+ tone,
+ language,
+ bert,
+ style_vec,
+ ) in enumerate(train_loader):
+ if net_g.module.use_noise_scaled_mas:
+ current_mas_noise_scale = (
+ net_g.module.mas_noise_scale_initial
+ - net_g.module.noise_scale_delta * global_step
+ )
+ net_g.module.current_mas_noise_scale = max(current_mas_noise_scale, 0.0)
+ x, x_lengths = x.cuda(local_rank, non_blocking=True), x_lengths.cuda(
+ local_rank, non_blocking=True
+ )
+ spec, spec_lengths = spec.cuda(
+ local_rank, non_blocking=True
+ ), spec_lengths.cuda(local_rank, non_blocking=True)
+ y, y_lengths = y.cuda(local_rank, non_blocking=True), y_lengths.cuda(
+ local_rank, non_blocking=True
+ )
+ speakers = speakers.cuda(local_rank, non_blocking=True)
+ tone = tone.cuda(local_rank, non_blocking=True)
+ language = language.cuda(local_rank, non_blocking=True)
+ bert = bert.cuda(local_rank, non_blocking=True)
+ style_vec = style_vec.cuda(local_rank, non_blocking=True)
+
+ with autocast(enabled=hps.train.bf16_run, dtype=torch.bfloat16):
+ (
+ y_hat,
+ l_length,
+ attn,
+ ids_slice,
+ x_mask,
+ z_mask,
+ (z, z_p, m_p, logs_p, m_q, logs_q),
+ (hidden_x, logw, logw_), # , logw_sdp),
+ g,
+ ) = net_g(
+ x,
+ x_lengths,
+ spec,
+ spec_lengths,
+ speakers,
+ tone,
+ language,
+ bert,
+ style_vec,
+ )
+ mel = spec_to_mel_torch(
+ spec,
+ hps.data.filter_length,
+ hps.data.n_mel_channels,
+ hps.data.sampling_rate,
+ hps.data.mel_fmin,
+ hps.data.mel_fmax,
+ )
+ y_mel = commons.slice_segments(
+ mel, ids_slice, hps.train.segment_size // hps.data.hop_length
+ )
+ y_hat_mel = mel_spectrogram_torch(
+ y_hat.squeeze(1).float(),
+ hps.data.filter_length,
+ hps.data.n_mel_channels,
+ hps.data.sampling_rate,
+ hps.data.hop_length,
+ hps.data.win_length,
+ hps.data.mel_fmin,
+ hps.data.mel_fmax,
+ )
+
+ y = commons.slice_segments(
+ y, ids_slice * hps.data.hop_length, hps.train.segment_size
+ ) # slice
+
+ # Discriminator
+ y_d_hat_r, y_d_hat_g, _, _ = net_d(y, y_hat.detach())
+ with autocast(enabled=hps.train.bf16_run, dtype=torch.bfloat16):
+ loss_disc, losses_disc_r, losses_disc_g = discriminator_loss(
+ y_d_hat_r, y_d_hat_g
+ )
+ loss_disc_all = loss_disc
+ if net_dur_disc is not None:
+ y_dur_hat_r, y_dur_hat_g = net_dur_disc(
+ hidden_x.detach(),
+ x_mask.detach(),
+ logw_.detach(),
+ logw.detach(),
+ g.detach(),
+ )
+ with autocast(enabled=hps.train.bf16_run, dtype=torch.bfloat16):
+ # TODO: I think need to mean using the mask, but for now, just mean all
+ (
+ loss_dur_disc,
+ losses_dur_disc_r,
+ losses_dur_disc_g,
+ ) = discriminator_loss(y_dur_hat_r, y_dur_hat_g)
+ loss_dur_disc_all = loss_dur_disc
+ optim_dur_disc.zero_grad()
+ scaler.scale(loss_dur_disc_all).backward()
+ scaler.unscale_(optim_dur_disc)
+ # torch.nn.utils.clip_grad_norm_(
+ # parameters=net_dur_disc.parameters(), max_norm=5
+ # )
+ grad_norm_dur = commons.clip_grad_value_(
+ net_dur_disc.parameters(), None
+ )
+ scaler.step(optim_dur_disc)
+ if net_wd is not None:
+ # logger.debug(f"y.shape: {y.shape}, y_hat.shape: {y_hat.shape}")
+ # shape: (batch, 1, time)
+ with autocast(enabled=hps.train.bf16_run, dtype=torch.bfloat16):
+ loss_slm = wl.discriminator(
+ y.detach().squeeze(1), y_hat.detach().squeeze(1)
+ ).mean()
+
+ optim_wd.zero_grad()
+ scaler.scale(loss_slm).backward()
+ scaler.unscale_(optim_wd)
+ # torch.nn.utils.clip_grad_norm_(parameters=net_wd.parameters(), max_norm=200)
+ grad_norm_wd = commons.clip_grad_value_(net_wd.parameters(), None)
+ scaler.step(optim_wd)
+
+ optim_d.zero_grad()
+ scaler.scale(loss_disc_all).backward()
+ scaler.unscale_(optim_d)
+ if getattr(hps.train, "bf16_run", False):
+ torch.nn.utils.clip_grad_norm_(parameters=net_d.parameters(), max_norm=200)
+ grad_norm_d = commons.clip_grad_value_(net_d.parameters(), None)
+ scaler.step(optim_d)
+
+ with autocast(enabled=hps.train.bf16_run, dtype=torch.bfloat16):
+ # Generator
+ y_d_hat_r, y_d_hat_g, fmap_r, fmap_g = net_d(y, y_hat)
+ if net_dur_disc is not None:
+ _, y_dur_hat_g = net_dur_disc(hidden_x, x_mask, logw_, logw, g)
+ if net_wd is not None:
+ loss_lm = wl(y.detach().squeeze(1), y_hat.squeeze(1)).mean()
+ loss_lm_gen = wl.generator(y_hat.squeeze(1))
+ with autocast(enabled=hps.train.bf16_run, dtype=torch.bfloat16):
+ loss_dur = torch.sum(l_length.float())
+ loss_mel = F.l1_loss(y_mel, y_hat_mel) * hps.train.c_mel
+ loss_kl = kl_loss(z_p, logs_q, m_p, logs_p, z_mask) * hps.train.c_kl
+
+ loss_fm = feature_loss(fmap_r, fmap_g)
+ loss_gen, losses_gen = generator_loss(y_d_hat_g)
+ # loss_commit = loss_commit * hps.train.c_commit
+
+ loss_gen_all = loss_gen + loss_fm + loss_mel + loss_dur + loss_kl
+ if net_dur_disc is not None:
+ loss_dur_gen, losses_dur_gen = generator_loss(y_dur_hat_g)
+ if net_wd is not None:
+ loss_gen_all += loss_dur_gen + loss_lm + loss_lm_gen
+ else:
+ loss_gen_all += loss_dur_gen
+ optim_g.zero_grad()
+ scaler.scale(loss_gen_all).backward()
+ scaler.unscale_(optim_g)
+ # if getattr(hps.train, "bf16_run", False):
+ torch.nn.utils.clip_grad_norm_(parameters=net_g.parameters(), max_norm=500)
+ grad_norm_g = commons.clip_grad_value_(net_g.parameters(), None)
+ scaler.step(optim_g)
+ scaler.update()
+
+ if rank == 0:
+ if global_step % hps.train.log_interval == 0 and not hps.speedup:
+ lr = optim_g.param_groups[0]["lr"]
+ losses = [loss_disc, loss_gen, loss_fm, loss_mel, loss_dur, loss_kl]
+ # logger.info(
+ # "Train Epoch: {} [{:.0f}%]".format(
+ # epoch, 100.0 * batch_idx / len(train_loader)
+ # )
+ # )
+ # logger.info([x.item() for x in losses] + [global_step, lr])
+
+ scalar_dict = {
+ "loss/g/total": loss_gen_all,
+ "loss/d/total": loss_disc_all,
+ "learning_rate": lr,
+ "grad_norm_d": grad_norm_d,
+ "grad_norm_g": grad_norm_g,
+ }
+ scalar_dict.update(
+ {
+ "loss/g/fm": loss_fm,
+ "loss/g/mel": loss_mel,
+ "loss/g/dur": loss_dur,
+ "loss/g/kl": loss_kl,
+ }
+ )
+ scalar_dict.update(
+ {"loss/g/{}".format(i): v for i, v in enumerate(losses_gen)}
+ )
+ scalar_dict.update(
+ {"loss/d_r/{}".format(i): v for i, v in enumerate(losses_disc_r)}
+ )
+ scalar_dict.update(
+ {"loss/d_g/{}".format(i): v for i, v in enumerate(losses_disc_g)}
+ )
+
+ if net_dur_disc is not None:
+ scalar_dict.update({"loss/dur_disc/total": loss_dur_disc_all})
+
+ scalar_dict.update(
+ {
+ "loss/dur_disc_g/{}".format(i): v
+ for i, v in enumerate(losses_dur_disc_g)
+ }
+ )
+ scalar_dict.update(
+ {
+ "loss/dur_disc_r/{}".format(i): v
+ for i, v in enumerate(losses_dur_disc_r)
+ }
+ )
+
+ scalar_dict.update({"loss/g/dur_gen": loss_dur_gen})
+ scalar_dict.update(
+ {
+ "loss/g/dur_gen_{}".format(i): v
+ for i, v in enumerate(losses_dur_gen)
+ }
+ )
+
+ if net_wd is not None:
+ scalar_dict.update(
+ {
+ "loss/wd/total": loss_slm,
+ "grad_norm_wd": grad_norm_wd,
+ "loss/g/lm": loss_lm,
+ "loss/g/lm_gen": loss_lm_gen,
+ }
+ )
+ image_dict = {
+ "slice/mel_org": utils.plot_spectrogram_to_numpy(
+ y_mel[0].data.cpu().numpy()
+ ),
+ "slice/mel_gen": utils.plot_spectrogram_to_numpy(
+ y_hat_mel[0].data.cpu().numpy()
+ ),
+ "all/mel": utils.plot_spectrogram_to_numpy(
+ mel[0].data.cpu().numpy()
+ ),
+ "all/attn": utils.plot_alignment_to_numpy(
+ attn[0, 0].data.cpu().numpy()
+ ),
+ }
+ utils.summarize(
+ writer=writer,
+ global_step=global_step,
+ images=image_dict,
+ scalars=scalar_dict,
+ )
+
+ if (
+ global_step % hps.train.eval_interval == 0
+ and global_step != 0
+ and initial_step != global_step
+ ):
+ if not hps.speedup:
+ evaluate(hps, net_g, eval_loader, writer_eval)
+ utils.save_checkpoint(
+ net_g,
+ optim_g,
+ hps.train.learning_rate,
+ epoch,
+ os.path.join(hps.model_dir, "G_{}.pth".format(global_step)),
+ )
+ utils.save_checkpoint(
+ net_d,
+ optim_d,
+ hps.train.learning_rate,
+ epoch,
+ os.path.join(hps.model_dir, "D_{}.pth".format(global_step)),
+ )
+ if net_dur_disc is not None:
+ utils.save_checkpoint(
+ net_dur_disc,
+ optim_dur_disc,
+ hps.train.learning_rate,
+ epoch,
+ os.path.join(hps.model_dir, "DUR_{}.pth".format(global_step)),
+ )
+ if net_wd is not None:
+ utils.save_checkpoint(
+ net_wd,
+ optim_wd,
+ hps.train.learning_rate,
+ epoch,
+ os.path.join(hps.model_dir, "WD_{}.pth".format(global_step)),
+ )
+ keep_ckpts = config.train_ms_config.keep_ckpts
+ if keep_ckpts > 0:
+ utils.clean_checkpoints(
+ path_to_models=hps.model_dir,
+ n_ckpts_to_keep=keep_ckpts,
+ sort_by_time=True,
+ )
+ # Save safetensors (for inference) to `model_assets/{model_name}`
+ utils.save_safetensors(
+ net_g,
+ epoch,
+ os.path.join(
+ config.out_dir,
+ f"{config.model_name}_e{epoch}_s{global_step}.safetensors",
+ ),
+ for_infer=True,
+ )
+ if hps.repo_id is not None:
+ api.upload_folder(
+ repo_id=hps.repo_id,
+ folder_path=config.dataset_path,
+ path_in_repo=f"Data/{config.model_name}",
+ delete_patterns="*.pth", # Only keep the latest checkpoint
+ ignore_patterns=f"{config.dataset_path}/raw", # Ignore raw data
+ run_as_future=True,
+ )
+ api.upload_folder(
+ repo_id=hps.repo_id,
+ folder_path=config.out_dir,
+ path_in_repo=f"model_assets/{config.model_name}",
+ run_as_future=True,
+ )
+
+ global_step += 1
+ if pbar is not None:
+ pbar.set_description(
+ "Epoch {}({:.0f}%)/{}".format(
+ epoch, 100.0 * batch_idx / len(train_loader), hps.train.epochs
+ )
+ )
+ pbar.update()
+ # 本家ではこれをスピードアップのために消すと書かれていたので、一応消してみる
+ # gc.collect()
+ # torch.cuda.empty_cache()
+ if pbar is None and rank == 0:
+ logger.info(f"====> Epoch: {epoch}, step: {global_step}")
+
+
+def evaluate(hps, generator, eval_loader, writer_eval):
+ generator.eval()
+ image_dict = {}
+ audio_dict = {}
+ logger.info("Evaluating ...")
+ with torch.no_grad():
+ for batch_idx, (
+ x,
+ x_lengths,
+ spec,
+ spec_lengths,
+ y,
+ y_lengths,
+ speakers,
+ tone,
+ language,
+ bert,
+ style_vec,
+ ) in enumerate(eval_loader):
+ x, x_lengths = x.cuda(), x_lengths.cuda()
+ spec, spec_lengths = spec.cuda(), spec_lengths.cuda()
+ y, y_lengths = y.cuda(), y_lengths.cuda()
+ speakers = speakers.cuda()
+ bert = bert.cuda()
+ tone = tone.cuda()
+ language = language.cuda()
+ style_vec = style_vec.cuda()
+ for use_sdp in [True, False]:
+ y_hat, attn, mask, *_ = generator.module.infer(
+ x,
+ x_lengths,
+ speakers,
+ tone,
+ language,
+ bert,
+ style_vec,
+ y=spec,
+ max_len=1000,
+ sdp_ratio=0.0 if not use_sdp else 1.0,
+ )
+ y_hat_lengths = mask.sum([1, 2]).long() * hps.data.hop_length
+
+ mel = spec_to_mel_torch(
+ spec,
+ hps.data.filter_length,
+ hps.data.n_mel_channels,
+ hps.data.sampling_rate,
+ hps.data.mel_fmin,
+ hps.data.mel_fmax,
+ )
+ y_hat_mel = mel_spectrogram_torch(
+ y_hat.squeeze(1).float(),
+ hps.data.filter_length,
+ hps.data.n_mel_channels,
+ hps.data.sampling_rate,
+ hps.data.hop_length,
+ hps.data.win_length,
+ hps.data.mel_fmin,
+ hps.data.mel_fmax,
+ )
+ image_dict.update(
+ {
+ f"gen/mel_{batch_idx}": utils.plot_spectrogram_to_numpy(
+ y_hat_mel[0].cpu().numpy()
+ )
+ }
+ )
+ audio_dict.update(
+ {
+ f"gen/audio_{batch_idx}_{use_sdp}": y_hat[
+ 0, :, : y_hat_lengths[0]
+ ]
+ }
+ )
+ image_dict.update(
+ {
+ f"gt/mel_{batch_idx}": utils.plot_spectrogram_to_numpy(
+ mel[0].cpu().numpy()
+ )
+ }
+ )
+ audio_dict.update({f"gt/audio_{batch_idx}": y[0, :, : y_lengths[0]]})
+
+ utils.summarize(
+ writer=writer_eval,
+ global_step=global_step,
+ images=image_dict,
+ audios=audio_dict,
+ audio_sampling_rate=hps.data.sampling_rate,
+ )
+ generator.train()
+
+
+if __name__ == "__main__":
+ run()
diff --git a/transcribe.py b/transcribe.py
new file mode 100644
index 0000000000000000000000000000000000000000..ab4b861e10cdd4648106f4c2af80deb67ef684e6
--- /dev/null
+++ b/transcribe.py
@@ -0,0 +1,86 @@
+import argparse
+import os
+import sys
+
+import yaml
+from faster_whisper import WhisperModel
+from tqdm import tqdm
+
+from common.constants import Languages
+from common.log import logger
+from common.stdout_wrapper import SAFE_STDOUT
+
+
+def transcribe(wav_path, initial_prompt=None, language="ja"):
+ segments, _ = model.transcribe(
+ wav_path, beam_size=5, language=language, initial_prompt=initial_prompt
+ )
+ texts = [segment.text for segment in segments]
+ return "".join(texts)
+
+
+if __name__ == "__main__":
+ parser = argparse.ArgumentParser()
+ parser.add_argument("--model_name", type=str, required=True)
+ parser.add_argument(
+ "--initial_prompt",
+ type=str,
+ default="こんにちは。元気、ですかー?ふふっ、私は……ちゃんと元気だよ!",
+ )
+ parser.add_argument(
+ "--language", type=str, default="ja", choices=["ja", "en", "zh"]
+ )
+ parser.add_argument("--model", type=str, default="large-v3")
+ parser.add_argument("--device", type=str, default="cuda")
+ parser.add_argument("--compute_type", type=str, default="bfloat16")
+
+ args = parser.parse_args()
+
+ with open(os.path.join("configs", "paths.yml"), "r", encoding="utf-8") as f:
+ path_config: dict[str, str] = yaml.safe_load(f.read())
+ dataset_root = path_config["dataset_root"]
+
+ model_name = args.model_name
+
+ input_dir = os.path.join(dataset_root, model_name, "raw")
+ output_file = os.path.join(dataset_root, model_name, "esd.list")
+ initial_prompt = args.initial_prompt
+ language = args.language
+ device = args.device
+ compute_type = args.compute_type
+
+ os.makedirs(os.path.dirname(output_file), exist_ok=True)
+
+ logger.info(
+ f"Loading Whisper model ({args.model}) with compute_type={compute_type}"
+ )
+ try:
+ model = WhisperModel(args.model, device=device, compute_type=compute_type)
+ except ValueError as e:
+ logger.warning(f"Failed to load model, so use `auto` compute_type: {e}")
+ model = WhisperModel(args.model, device=device)
+
+ wav_files = [
+ os.path.join(input_dir, f) for f in os.listdir(input_dir) if f.endswith(".wav")
+ ]
+ if os.path.exists(output_file):
+ logger.warning(f"{output_file} exists, backing up to {output_file}.bak")
+ if os.path.exists(output_file + ".bak"):
+ logger.warning(f"{output_file}.bak exists, deleting...")
+ os.remove(output_file + ".bak")
+ os.rename(output_file, output_file + ".bak")
+
+ if language == "ja":
+ language_id = Languages.JP.value
+ elif language == "en":
+ language_id = Languages.EN.value
+ elif language == "zh":
+ language_id = Languages.ZH.value
+ else:
+ raise ValueError(f"{language} is not supported.")
+ for wav_file in tqdm(wav_files, file=SAFE_STDOUT):
+ file_name = os.path.basename(wav_file)
+ text = transcribe(wav_file, initial_prompt=initial_prompt, language=language)
+ with open(output_file, "a", encoding="utf-8") as f:
+ f.write(f"{file_name}|{model_name}|{language_id}|{text}\n")
+ sys.exit(0)
diff --git a/transforms.py b/transforms.py
new file mode 100644
index 0000000000000000000000000000000000000000..a11f799e023864ff7082c1f49c0cc18351a13b47
--- /dev/null
+++ b/transforms.py
@@ -0,0 +1,209 @@
+import torch
+from torch.nn import functional as F
+
+import numpy as np
+
+
+DEFAULT_MIN_BIN_WIDTH = 1e-3
+DEFAULT_MIN_BIN_HEIGHT = 1e-3
+DEFAULT_MIN_DERIVATIVE = 1e-3
+
+
+def piecewise_rational_quadratic_transform(
+ inputs,
+ unnormalized_widths,
+ unnormalized_heights,
+ unnormalized_derivatives,
+ inverse=False,
+ tails=None,
+ tail_bound=1.0,
+ min_bin_width=DEFAULT_MIN_BIN_WIDTH,
+ min_bin_height=DEFAULT_MIN_BIN_HEIGHT,
+ min_derivative=DEFAULT_MIN_DERIVATIVE,
+):
+ if tails is None:
+ spline_fn = rational_quadratic_spline
+ spline_kwargs = {}
+ else:
+ spline_fn = unconstrained_rational_quadratic_spline
+ spline_kwargs = {"tails": tails, "tail_bound": tail_bound}
+
+ outputs, logabsdet = spline_fn(
+ inputs=inputs,
+ unnormalized_widths=unnormalized_widths,
+ unnormalized_heights=unnormalized_heights,
+ unnormalized_derivatives=unnormalized_derivatives,
+ inverse=inverse,
+ min_bin_width=min_bin_width,
+ min_bin_height=min_bin_height,
+ min_derivative=min_derivative,
+ **spline_kwargs
+ )
+ return outputs, logabsdet
+
+
+def searchsorted(bin_locations, inputs, eps=1e-6):
+ bin_locations[..., -1] += eps
+ return torch.sum(inputs[..., None] >= bin_locations, dim=-1) - 1
+
+
+def unconstrained_rational_quadratic_spline(
+ inputs,
+ unnormalized_widths,
+ unnormalized_heights,
+ unnormalized_derivatives,
+ inverse=False,
+ tails="linear",
+ tail_bound=1.0,
+ min_bin_width=DEFAULT_MIN_BIN_WIDTH,
+ min_bin_height=DEFAULT_MIN_BIN_HEIGHT,
+ min_derivative=DEFAULT_MIN_DERIVATIVE,
+):
+ inside_interval_mask = (inputs >= -tail_bound) & (inputs <= tail_bound)
+ outside_interval_mask = ~inside_interval_mask
+
+ outputs = torch.zeros_like(inputs)
+ logabsdet = torch.zeros_like(inputs)
+
+ if tails == "linear":
+ unnormalized_derivatives = F.pad(unnormalized_derivatives, pad=(1, 1))
+ constant = np.log(np.exp(1 - min_derivative) - 1)
+ unnormalized_derivatives[..., 0] = constant
+ unnormalized_derivatives[..., -1] = constant
+
+ outputs[outside_interval_mask] = inputs[outside_interval_mask]
+ logabsdet[outside_interval_mask] = 0
+ else:
+ raise RuntimeError("{} tails are not implemented.".format(tails))
+
+ (
+ outputs[inside_interval_mask],
+ logabsdet[inside_interval_mask],
+ ) = rational_quadratic_spline(
+ inputs=inputs[inside_interval_mask],
+ unnormalized_widths=unnormalized_widths[inside_interval_mask, :],
+ unnormalized_heights=unnormalized_heights[inside_interval_mask, :],
+ unnormalized_derivatives=unnormalized_derivatives[inside_interval_mask, :],
+ inverse=inverse,
+ left=-tail_bound,
+ right=tail_bound,
+ bottom=-tail_bound,
+ top=tail_bound,
+ min_bin_width=min_bin_width,
+ min_bin_height=min_bin_height,
+ min_derivative=min_derivative,
+ )
+
+ return outputs, logabsdet
+
+
+def rational_quadratic_spline(
+ inputs,
+ unnormalized_widths,
+ unnormalized_heights,
+ unnormalized_derivatives,
+ inverse=False,
+ left=0.0,
+ right=1.0,
+ bottom=0.0,
+ top=1.0,
+ min_bin_width=DEFAULT_MIN_BIN_WIDTH,
+ min_bin_height=DEFAULT_MIN_BIN_HEIGHT,
+ min_derivative=DEFAULT_MIN_DERIVATIVE,
+):
+ if torch.min(inputs) < left or torch.max(inputs) > right:
+ raise ValueError("Input to a transform is not within its domain")
+
+ num_bins = unnormalized_widths.shape[-1]
+
+ if min_bin_width * num_bins > 1.0:
+ raise ValueError("Minimal bin width too large for the number of bins")
+ if min_bin_height * num_bins > 1.0:
+ raise ValueError("Minimal bin height too large for the number of bins")
+
+ widths = F.softmax(unnormalized_widths, dim=-1)
+ widths = min_bin_width + (1 - min_bin_width * num_bins) * widths
+ cumwidths = torch.cumsum(widths, dim=-1)
+ cumwidths = F.pad(cumwidths, pad=(1, 0), mode="constant", value=0.0)
+ cumwidths = (right - left) * cumwidths + left
+ cumwidths[..., 0] = left
+ cumwidths[..., -1] = right
+ widths = cumwidths[..., 1:] - cumwidths[..., :-1]
+
+ derivatives = min_derivative + F.softplus(unnormalized_derivatives)
+
+ heights = F.softmax(unnormalized_heights, dim=-1)
+ heights = min_bin_height + (1 - min_bin_height * num_bins) * heights
+ cumheights = torch.cumsum(heights, dim=-1)
+ cumheights = F.pad(cumheights, pad=(1, 0), mode="constant", value=0.0)
+ cumheights = (top - bottom) * cumheights + bottom
+ cumheights[..., 0] = bottom
+ cumheights[..., -1] = top
+ heights = cumheights[..., 1:] - cumheights[..., :-1]
+
+ if inverse:
+ bin_idx = searchsorted(cumheights, inputs)[..., None]
+ else:
+ bin_idx = searchsorted(cumwidths, inputs)[..., None]
+
+ input_cumwidths = cumwidths.gather(-1, bin_idx)[..., 0]
+ input_bin_widths = widths.gather(-1, bin_idx)[..., 0]
+
+ input_cumheights = cumheights.gather(-1, bin_idx)[..., 0]
+ delta = heights / widths
+ input_delta = delta.gather(-1, bin_idx)[..., 0]
+
+ input_derivatives = derivatives.gather(-1, bin_idx)[..., 0]
+ input_derivatives_plus_one = derivatives[..., 1:].gather(-1, bin_idx)[..., 0]
+
+ input_heights = heights.gather(-1, bin_idx)[..., 0]
+
+ if inverse:
+ a = (inputs - input_cumheights) * (
+ input_derivatives + input_derivatives_plus_one - 2 * input_delta
+ ) + input_heights * (input_delta - input_derivatives)
+ b = input_heights * input_derivatives - (inputs - input_cumheights) * (
+ input_derivatives + input_derivatives_plus_one - 2 * input_delta
+ )
+ c = -input_delta * (inputs - input_cumheights)
+
+ discriminant = b.pow(2) - 4 * a * c
+ assert (discriminant >= 0).all()
+
+ root = (2 * c) / (-b - torch.sqrt(discriminant))
+ outputs = root * input_bin_widths + input_cumwidths
+
+ theta_one_minus_theta = root * (1 - root)
+ denominator = input_delta + (
+ (input_derivatives + input_derivatives_plus_one - 2 * input_delta)
+ * theta_one_minus_theta
+ )
+ derivative_numerator = input_delta.pow(2) * (
+ input_derivatives_plus_one * root.pow(2)
+ + 2 * input_delta * theta_one_minus_theta
+ + input_derivatives * (1 - root).pow(2)
+ )
+ logabsdet = torch.log(derivative_numerator) - 2 * torch.log(denominator)
+
+ return outputs, -logabsdet
+ else:
+ theta = (inputs - input_cumwidths) / input_bin_widths
+ theta_one_minus_theta = theta * (1 - theta)
+
+ numerator = input_heights * (
+ input_delta * theta.pow(2) + input_derivatives * theta_one_minus_theta
+ )
+ denominator = input_delta + (
+ (input_derivatives + input_derivatives_plus_one - 2 * input_delta)
+ * theta_one_minus_theta
+ )
+ outputs = input_cumheights + numerator / denominator
+
+ derivative_numerator = input_delta.pow(2) * (
+ input_derivatives_plus_one * theta.pow(2)
+ + 2 * input_delta * theta_one_minus_theta
+ + input_derivatives * (1 - theta).pow(2)
+ )
+ logabsdet = torch.log(derivative_numerator) - 2 * torch.log(denominator)
+
+ return outputs, logabsdet
diff --git a/update_status.py b/update_status.py
new file mode 100644
index 0000000000000000000000000000000000000000..30fdead70342d5ed3356a9985a8b5aba15683413
--- /dev/null
+++ b/update_status.py
@@ -0,0 +1,89 @@
+import os
+import gradio as gr
+
+lang_dict = {"EN(英文)": "_en", "ZH(中文)": "_zh", "JP(日语)": "_jp"}
+
+
+def raw_dir_convert_to_path(target_dir: str, lang):
+ res = target_dir.rstrip("/").rstrip("\\")
+ if (not target_dir.startswith("raw")) and (not target_dir.startswith("./raw")):
+ res = os.path.join("./raw", res)
+ if (
+ (not res.endswith("_zh"))
+ and (not res.endswith("_jp"))
+ and (not res.endswith("_en"))
+ ):
+ res += lang_dict[lang]
+ return res
+
+
+def update_g_files():
+ g_files = []
+ cnt = 0
+ for root, dirs, files in os.walk(os.path.abspath("./logs")):
+ for file in files:
+ if file.startswith("G_") and file.endswith(".pth"):
+ g_files.append(os.path.join(root, file))
+ cnt += 1
+ print(g_files)
+ return f"更新模型列表完成, 共找到{cnt}个模型", gr.Dropdown.update(choices=g_files)
+
+
+def update_c_files():
+ c_files = []
+ cnt = 0
+ for root, dirs, files in os.walk(os.path.abspath("./logs")):
+ for file in files:
+ if file.startswith("config.json"):
+ c_files.append(os.path.join(root, file))
+ cnt += 1
+ print(c_files)
+ return f"更新模型列表完成, 共找到{cnt}个配置文件", gr.Dropdown.update(choices=c_files)
+
+
+def update_model_folders():
+ subdirs = []
+ cnt = 0
+ for root, dirs, files in os.walk(os.path.abspath("./logs")):
+ for dir_name in dirs:
+ if os.path.basename(dir_name) != "eval":
+ subdirs.append(os.path.join(root, dir_name))
+ cnt += 1
+ print(subdirs)
+ return f"更新模型文件夹列表完成, 共找到{cnt}个文件夹", gr.Dropdown.update(choices=subdirs)
+
+
+def update_wav_lab_pairs():
+ wav_count = tot_count = 0
+ for root, _, files in os.walk("./raw"):
+ for file in files:
+ # print(file)
+ file_path = os.path.join(root, file)
+ if file.lower().endswith(".wav"):
+ lab_file = os.path.splitext(file_path)[0] + ".lab"
+ if os.path.exists(lab_file):
+ wav_count += 1
+ tot_count += 1
+ return f"{wav_count} / {tot_count}"
+
+
+def update_raw_folders():
+ subdirs = []
+ cnt = 0
+ script_path = os.path.dirname(os.path.abspath(__file__)) # 获取当前脚本的绝对路径
+ raw_path = os.path.join(script_path, "raw")
+ print(raw_path)
+ os.makedirs(raw_path, exist_ok=True)
+ for root, dirs, files in os.walk(raw_path):
+ for dir_name in dirs:
+ relative_path = os.path.relpath(
+ os.path.join(root, dir_name), script_path
+ ) # 获取相对路径
+ subdirs.append(relative_path)
+ cnt += 1
+ print(subdirs)
+ return (
+ f"更新raw音频文件夹列表完成, 共找到{cnt}个文件夹",
+ gr.Dropdown.update(choices=subdirs),
+ gr.Textbox.update(value=update_wav_lab_pairs()),
+ )
diff --git a/utils.py b/utils.py
new file mode 100644
index 0000000000000000000000000000000000000000..ca194bfb523a701d1130184cf1df92bc35e62ee4
--- /dev/null
+++ b/utils.py
@@ -0,0 +1,501 @@
+import argparse
+import glob
+import json
+import logging
+import os
+import re
+import subprocess
+
+import numpy as np
+import torch
+from huggingface_hub import hf_hub_download
+from safetensors import safe_open
+from safetensors.torch import save_file
+from scipy.io.wavfile import read
+
+from common.log import logger
+
+MATPLOTLIB_FLAG = False
+
+
+def download_checkpoint(
+ dir_path, repo_config, token=None, regex="G_*.pth", mirror="openi"
+):
+ repo_id = repo_config["repo_id"]
+ f_list = glob.glob(os.path.join(dir_path, regex))
+ if f_list:
+ print("Use existed model, skip downloading.")
+ return
+ for file in ["DUR_0.pth", "D_0.pth", "G_0.pth"]:
+ hf_hub_download(repo_id, file, local_dir=dir_path, local_dir_use_symlinks=False)
+
+
+def load_checkpoint(
+ checkpoint_path, model, optimizer=None, skip_optimizer=False, for_infer=False
+):
+ assert os.path.isfile(checkpoint_path)
+ checkpoint_dict = torch.load(checkpoint_path, map_location="cpu")
+ iteration = checkpoint_dict["iteration"]
+ learning_rate = checkpoint_dict["learning_rate"]
+ logger.info(
+ f"Loading model and optimizer at iteration {iteration} from {checkpoint_path}"
+ )
+ if (
+ optimizer is not None
+ and not skip_optimizer
+ and checkpoint_dict["optimizer"] is not None
+ ):
+ optimizer.load_state_dict(checkpoint_dict["optimizer"])
+ elif optimizer is None and not skip_optimizer:
+ # else: Disable this line if Infer and resume checkpoint,then enable the line upper
+ new_opt_dict = optimizer.state_dict()
+ new_opt_dict_params = new_opt_dict["param_groups"][0]["params"]
+ new_opt_dict["param_groups"] = checkpoint_dict["optimizer"]["param_groups"]
+ new_opt_dict["param_groups"][0]["params"] = new_opt_dict_params
+ optimizer.load_state_dict(new_opt_dict)
+
+ saved_state_dict = checkpoint_dict["model"]
+ if hasattr(model, "module"):
+ state_dict = model.module.state_dict()
+ else:
+ state_dict = model.state_dict()
+
+ new_state_dict = {}
+ for k, v in state_dict.items():
+ try:
+ # assert "emb_g" not in k
+ new_state_dict[k] = saved_state_dict[k]
+ assert saved_state_dict[k].shape == v.shape, (
+ saved_state_dict[k].shape,
+ v.shape,
+ )
+ except:
+ # For upgrading from the old version
+ if "ja_bert_proj" in k:
+ v = torch.zeros_like(v)
+ logger.warning(
+ f"Seems you are using the old version of the model, the {k} is automatically set to zero for backward compatibility"
+ )
+ elif "enc_q" in k and for_infer:
+ continue
+ else:
+ logger.error(f"{k} is not in the checkpoint {checkpoint_path}")
+
+ new_state_dict[k] = v
+
+ if hasattr(model, "module"):
+ model.module.load_state_dict(new_state_dict, strict=False)
+ else:
+ model.load_state_dict(new_state_dict, strict=False)
+
+ logger.info("Loaded '{}' (iteration {})".format(checkpoint_path, iteration))
+
+ return model, optimizer, learning_rate, iteration
+
+
+def save_checkpoint(model, optimizer, learning_rate, iteration, checkpoint_path):
+ logger.info(
+ "Saving model and optimizer state at iteration {} to {}".format(
+ iteration, checkpoint_path
+ )
+ )
+ if hasattr(model, "module"):
+ state_dict = model.module.state_dict()
+ else:
+ state_dict = model.state_dict()
+ torch.save(
+ {
+ "model": state_dict,
+ "iteration": iteration,
+ "optimizer": optimizer.state_dict(),
+ "learning_rate": learning_rate,
+ },
+ checkpoint_path,
+ )
+
+
+def save_safetensors(model, iteration, checkpoint_path, is_half=False, for_infer=False):
+ """
+ Save model with safetensors.
+ """
+ if hasattr(model, "module"):
+ state_dict = model.module.state_dict()
+ else:
+ state_dict = model.state_dict()
+ keys = []
+ for k in state_dict:
+ if "enc_q" in k and for_infer:
+ continue # noqa: E701
+ keys.append(k)
+
+ new_dict = (
+ {k: state_dict[k].half() for k in keys}
+ if is_half
+ else {k: state_dict[k] for k in keys}
+ )
+ new_dict["iteration"] = torch.LongTensor([iteration])
+ logger.info(f"Saved safetensors to {checkpoint_path}")
+ save_file(new_dict, checkpoint_path)
+
+
+def load_safetensors(checkpoint_path, model, for_infer=False):
+ """
+ Load safetensors model.
+ """
+
+ tensors = {}
+ iteration = None
+ with safe_open(checkpoint_path, framework="pt", device="cpu") as f:
+ for key in f.keys():
+ if key == "iteration":
+ iteration = f.get_tensor(key).item()
+ tensors[key] = f.get_tensor(key)
+ if hasattr(model, "module"):
+ result = model.module.load_state_dict(tensors, strict=False)
+ else:
+ result = model.load_state_dict(tensors, strict=False)
+ for key in result.missing_keys:
+ if key.startswith("enc_q") and for_infer:
+ continue
+ logger.warning(f"Missing key: {key}")
+ for key in result.unexpected_keys:
+ if key == "iteration":
+ continue
+ logger.warning(f"Unexpected key: {key}")
+ if iteration is None:
+ logger.info(f"Loaded '{checkpoint_path}'")
+ else:
+ logger.info(f"Loaded '{checkpoint_path}' (iteration {iteration})")
+ return model, iteration
+
+
+def summarize(
+ writer,
+ global_step,
+ scalars={},
+ histograms={},
+ images={},
+ audios={},
+ audio_sampling_rate=22050,
+):
+ for k, v in scalars.items():
+ writer.add_scalar(k, v, global_step)
+ for k, v in histograms.items():
+ writer.add_histogram(k, v, global_step)
+ for k, v in images.items():
+ writer.add_image(k, v, global_step, dataformats="HWC")
+ for k, v in audios.items():
+ writer.add_audio(k, v, global_step, audio_sampling_rate)
+
+
+def is_resuming(dir_path):
+ # JP-ExtraバージョンではDURがなくWDがあったり変わるため、Gのみで判断する
+ g_list = glob.glob(os.path.join(dir_path, "G_*.pth"))
+ # d_list = glob.glob(os.path.join(dir_path, "D_*.pth"))
+ # dur_list = glob.glob(os.path.join(dir_path, "DUR_*.pth"))
+ return len(g_list) > 0
+
+
+def latest_checkpoint_path(dir_path, regex="G_*.pth"):
+ f_list = glob.glob(os.path.join(dir_path, regex))
+ f_list.sort(key=lambda f: int("".join(filter(str.isdigit, f))))
+ try:
+ x = f_list[-1]
+ except IndexError:
+ raise ValueError(f"No checkpoint found in {dir_path} with regex {regex}")
+ return x
+
+
+def plot_spectrogram_to_numpy(spectrogram):
+ global MATPLOTLIB_FLAG
+ if not MATPLOTLIB_FLAG:
+ import matplotlib
+
+ matplotlib.use("Agg")
+ MATPLOTLIB_FLAG = True
+ mpl_logger = logging.getLogger("matplotlib")
+ mpl_logger.setLevel(logging.WARNING)
+ import matplotlib.pylab as plt
+ import numpy as np
+
+ fig, ax = plt.subplots(figsize=(10, 2))
+ im = ax.imshow(spectrogram, aspect="auto", origin="lower", interpolation="none")
+ plt.colorbar(im, ax=ax)
+ plt.xlabel("Frames")
+ plt.ylabel("Channels")
+ plt.tight_layout()
+
+ fig.canvas.draw()
+ data = np.fromstring(fig.canvas.tostring_rgb(), dtype=np.uint8, sep="")
+ data = data.reshape(fig.canvas.get_width_height()[::-1] + (3,))
+ plt.close()
+ return data
+
+
+def plot_alignment_to_numpy(alignment, info=None):
+ global MATPLOTLIB_FLAG
+ if not MATPLOTLIB_FLAG:
+ import matplotlib
+
+ matplotlib.use("Agg")
+ MATPLOTLIB_FLAG = True
+ mpl_logger = logging.getLogger("matplotlib")
+ mpl_logger.setLevel(logging.WARNING)
+ import matplotlib.pylab as plt
+ import numpy as np
+
+ fig, ax = plt.subplots(figsize=(6, 4))
+ im = ax.imshow(
+ alignment.transpose(), aspect="auto", origin="lower", interpolation="none"
+ )
+ fig.colorbar(im, ax=ax)
+ xlabel = "Decoder timestep"
+ if info is not None:
+ xlabel += "\n\n" + info
+ plt.xlabel(xlabel)
+ plt.ylabel("Encoder timestep")
+ plt.tight_layout()
+
+ fig.canvas.draw()
+ data = np.fromstring(fig.canvas.tostring_rgb(), dtype=np.uint8, sep="")
+ data = data.reshape(fig.canvas.get_width_height()[::-1] + (3,))
+ plt.close()
+ return data
+
+
+def load_wav_to_torch(full_path):
+ sampling_rate, data = read(full_path)
+ return torch.FloatTensor(data.astype(np.float32)), sampling_rate
+
+
+def load_filepaths_and_text(filename, split="|"):
+ with open(filename, encoding="utf-8") as f:
+ filepaths_and_text = [line.strip().split(split) for line in f]
+ return filepaths_and_text
+
+
+def get_hparams(init=True):
+ parser = argparse.ArgumentParser()
+ parser.add_argument(
+ "-c",
+ "--config",
+ type=str,
+ default="./configs/base.json",
+ help="JSON file for configuration",
+ )
+ parser.add_argument("-m", "--model", type=str, required=True, help="Model name")
+
+ args = parser.parse_args()
+ model_dir = os.path.join("./logs", args.model)
+
+ if not os.path.exists(model_dir):
+ os.makedirs(model_dir)
+
+ config_path = args.config
+ config_save_path = os.path.join(model_dir, "config.json")
+ if init:
+ with open(config_path, "r", encoding="utf-8") as f:
+ data = f.read()
+ with open(config_save_path, "w", encoding="utf-8") as f:
+ f.write(data)
+ else:
+ with open(config_save_path, "r", vencoding="utf-8") as f:
+ data = f.read()
+ config = json.loads(data)
+ hparams = HParams(**config)
+ hparams.model_dir = model_dir
+ return hparams
+
+
+def clean_checkpoints(path_to_models="logs/44k/", n_ckpts_to_keep=2, sort_by_time=True):
+ """Freeing up space by deleting saved ckpts
+
+ Arguments:
+ path_to_models -- Path to the model directory
+ n_ckpts_to_keep -- Number of ckpts to keep, excluding G_0.pth and D_0.pth
+ sort_by_time -- True -> chronologically delete ckpts
+ False -> lexicographically delete ckpts
+ """
+ import re
+
+ ckpts_files = [
+ f
+ for f in os.listdir(path_to_models)
+ if os.path.isfile(os.path.join(path_to_models, f))
+ ]
+
+ def name_key(_f):
+ return int(re.compile("._(\\d+)\\.pth").match(_f).group(1))
+
+ def time_key(_f):
+ return os.path.getmtime(os.path.join(path_to_models, _f))
+
+ sort_key = time_key if sort_by_time else name_key
+
+ def x_sorted(_x):
+ return sorted(
+ [f for f in ckpts_files if f.startswith(_x) and not f.endswith("_0.pth")],
+ key=sort_key,
+ )
+
+ to_del = [
+ os.path.join(path_to_models, fn)
+ for fn in (
+ x_sorted("G_")[:-n_ckpts_to_keep]
+ + x_sorted("D_")[:-n_ckpts_to_keep]
+ + x_sorted("WD_")[:-n_ckpts_to_keep]
+ + x_sorted("DUR_")[:-n_ckpts_to_keep]
+ )
+ ]
+
+ def del_info(fn):
+ return logger.info(f"Free up space by deleting ckpt {fn}")
+
+ def del_routine(x):
+ return [os.remove(x), del_info(x)]
+
+ [del_routine(fn) for fn in to_del]
+
+
+def get_hparams_from_dir(model_dir):
+ config_save_path = os.path.join(model_dir, "config.json")
+ with open(config_save_path, "r", encoding="utf-8") as f:
+ data = f.read()
+ config = json.loads(data)
+
+ hparams = HParams(**config)
+ hparams.model_dir = model_dir
+ return hparams
+
+
+def get_hparams_from_file(config_path):
+ # print("config_path: ", config_path)
+ with open(config_path, "r", encoding="utf-8") as f:
+ data = f.read()
+ config = json.loads(data)
+
+ hparams = HParams(**config)
+ return hparams
+
+
+def check_git_hash(model_dir):
+ source_dir = os.path.dirname(os.path.realpath(__file__))
+ if not os.path.exists(os.path.join(source_dir, ".git")):
+ logger.warning(
+ "{} is not a git repository, therefore hash value comparison will be ignored.".format(
+ source_dir
+ )
+ )
+ return
+
+ cur_hash = subprocess.getoutput("git rev-parse HEAD")
+
+ path = os.path.join(model_dir, "githash")
+ if os.path.exists(path):
+ saved_hash = open(path).read()
+ if saved_hash != cur_hash:
+ logger.warning(
+ "git hash values are different. {}(saved) != {}(current)".format(
+ saved_hash[:8], cur_hash[:8]
+ )
+ )
+ else:
+ open(path, "w").write(cur_hash)
+
+
+def get_logger(model_dir, filename="train.log"):
+ global logger
+ logger = logging.getLogger(os.path.basename(model_dir))
+ logger.setLevel(logging.DEBUG)
+
+ formatter = logging.Formatter("%(asctime)s\t%(name)s\t%(levelname)s\t%(message)s")
+ if not os.path.exists(model_dir):
+ os.makedirs(model_dir)
+ h = logging.FileHandler(os.path.join(model_dir, filename))
+ h.setLevel(logging.DEBUG)
+ h.setFormatter(formatter)
+ logger.addHandler(h)
+ return logger
+
+
+class HParams:
+ def __init__(self, **kwargs):
+ for k, v in kwargs.items():
+ if type(v) == dict:
+ v = HParams(**v)
+ self[k] = v
+
+ def keys(self):
+ return self.__dict__.keys()
+
+ def items(self):
+ return self.__dict__.items()
+
+ def values(self):
+ return self.__dict__.values()
+
+ def __len__(self):
+ return len(self.__dict__)
+
+ def __getitem__(self, key):
+ return getattr(self, key)
+
+ def __setitem__(self, key, value):
+ return setattr(self, key, value)
+
+ def __contains__(self, key):
+ return key in self.__dict__
+
+ def __repr__(self):
+ return self.__dict__.__repr__()
+
+
+def load_model(model_path, config_path):
+ hps = get_hparams_from_file(config_path)
+ net = SynthesizerTrn(
+ # len(symbols),
+ 108,
+ hps.data.filter_length // 2 + 1,
+ hps.train.segment_size // hps.data.hop_length,
+ n_speakers=hps.data.n_speakers,
+ **hps.model,
+ ).to("cpu")
+ _ = net.eval()
+ _ = load_checkpoint(model_path, net, None, skip_optimizer=True)
+ return net
+
+
+def mix_model(
+ network1, network2, output_path, voice_ratio=(0.5, 0.5), tone_ratio=(0.5, 0.5)
+):
+ if hasattr(network1, "module"):
+ state_dict1 = network1.module.state_dict()
+ state_dict2 = network2.module.state_dict()
+ else:
+ state_dict1 = network1.state_dict()
+ state_dict2 = network2.state_dict()
+ for k in state_dict1.keys():
+ if k not in state_dict2.keys():
+ continue
+ if "enc_p" in k:
+ state_dict1[k] = (
+ state_dict1[k].clone() * tone_ratio[0]
+ + state_dict2[k].clone() * tone_ratio[1]
+ )
+ else:
+ state_dict1[k] = (
+ state_dict1[k].clone() * voice_ratio[0]
+ + state_dict2[k].clone() * voice_ratio[1]
+ )
+ for k in state_dict2.keys():
+ if k not in state_dict1.keys():
+ state_dict1[k] = state_dict2[k].clone()
+ torch.save(
+ {"model": state_dict1, "iteration": 0, "optimizer": None, "learning_rate": 0},
+ output_path,
+ )
+
+
+def get_steps(model_path):
+ matches = re.findall(r"\d+", model_path)
+ return matches[-1] if matches else None
diff --git a/webui.py b/webui.py
new file mode 100644
index 0000000000000000000000000000000000000000..9a4e1b19b01ae7aff4801770de9334592a4550b6
--- /dev/null
+++ b/webui.py
@@ -0,0 +1,557 @@
+"""
+Original `webui.py` for Bert-VITS2, not working with Style-Bert-VITS2 yet.
+"""
+# flake8: noqa: E402
+import os
+import logging
+import re_matching
+from tools.sentence import split_by_language
+
+logging.getLogger("numba").setLevel(logging.WARNING)
+logging.getLogger("markdown_it").setLevel(logging.WARNING)
+logging.getLogger("urllib3").setLevel(logging.WARNING)
+logging.getLogger("matplotlib").setLevel(logging.WARNING)
+
+logging.basicConfig(
+ level=logging.INFO, format="| %(name)s | %(levelname)s | %(message)s"
+)
+
+logger = logging.getLogger(__name__)
+
+import torch
+import utils
+from infer import infer, latest_version, get_net_g, infer_multilang
+import gradio as gr
+import webbrowser
+import numpy as np
+from config import config
+from tools.translate import translate
+import librosa
+
+net_g = None
+
+device = config.webui_config.device
+if device == "mps":
+ os.environ["PYTORCH_ENABLE_MPS_FALLBACK"] = "1"
+
+
+def generate_audio(
+ slices,
+ sdp_ratio,
+ noise_scale,
+ noise_scale_w,
+ length_scale,
+ speaker,
+ language,
+ reference_audio,
+ emotion,
+ style_text,
+ style_weight,
+ skip_start=False,
+ skip_end=False,
+):
+ audio_list = []
+ # silence = np.zeros(hps.data.sampling_rate // 2, dtype=np.int16)
+ with torch.no_grad():
+ for idx, piece in enumerate(slices):
+ skip_start = idx != 0
+ skip_end = idx != len(slices) - 1
+ audio = infer(
+ piece,
+ reference_audio=reference_audio,
+ emotion=emotion,
+ sdp_ratio=sdp_ratio,
+ noise_scale=noise_scale,
+ noise_scale_w=noise_scale_w,
+ length_scale=length_scale,
+ sid=speaker,
+ language=language,
+ hps=hps,
+ net_g=net_g,
+ device=device,
+ skip_start=skip_start,
+ skip_end=skip_end,
+ assist_text=style_text,
+ assist_text_weight=style_weight,
+ )
+ audio16bit = gr.processing_utils.convert_to_16_bit_wav(audio)
+ audio_list.append(audio16bit)
+ return audio_list
+
+
+def generate_audio_multilang(
+ slices,
+ sdp_ratio,
+ noise_scale,
+ noise_scale_w,
+ length_scale,
+ speaker,
+ language,
+ reference_audio,
+ emotion,
+ skip_start=False,
+ skip_end=False,
+):
+ audio_list = []
+ # silence = np.zeros(hps.data.sampling_rate // 2, dtype=np.int16)
+ with torch.no_grad():
+ for idx, piece in enumerate(slices):
+ skip_start = idx != 0
+ skip_end = idx != len(slices) - 1
+ audio = infer_multilang(
+ piece,
+ reference_audio=reference_audio,
+ emotion=emotion,
+ sdp_ratio=sdp_ratio,
+ noise_scale=noise_scale,
+ noise_scale_w=noise_scale_w,
+ length_scale=length_scale,
+ sid=speaker,
+ language=language[idx],
+ hps=hps,
+ net_g=net_g,
+ device=device,
+ skip_start=skip_start,
+ skip_end=skip_end,
+ )
+ audio16bit = gr.processing_utils.convert_to_16_bit_wav(audio)
+ audio_list.append(audio16bit)
+ return audio_list
+
+
+def tts_split(
+ text: str,
+ speaker,
+ sdp_ratio,
+ noise_scale,
+ noise_scale_w,
+ length_scale,
+ language,
+ cut_by_sent,
+ interval_between_para,
+ interval_between_sent,
+ reference_audio,
+ emotion,
+ style_text,
+ style_weight,
+):
+ while text.find("\n\n") != -1:
+ text = text.replace("\n\n", "\n")
+ text = text.replace("|", "")
+ para_list = re_matching.cut_para(text)
+ para_list = [p for p in para_list if p != ""]
+ audio_list = []
+ for p in para_list:
+ if not cut_by_sent:
+ audio_list += process_text(
+ p,
+ speaker,
+ sdp_ratio,
+ noise_scale,
+ noise_scale_w,
+ length_scale,
+ language,
+ reference_audio,
+ emotion,
+ style_text,
+ style_weight,
+ )
+ silence = np.zeros((int)(44100 * interval_between_para), dtype=np.int16)
+ audio_list.append(silence)
+ else:
+ audio_list_sent = []
+ sent_list = re_matching.cut_sent(p)
+ sent_list = [s for s in sent_list if s != ""]
+ for s in sent_list:
+ audio_list_sent += process_text(
+ s,
+ speaker,
+ sdp_ratio,
+ noise_scale,
+ noise_scale_w,
+ length_scale,
+ language,
+ reference_audio,
+ emotion,
+ style_text,
+ style_weight,
+ )
+ silence = np.zeros((int)(44100 * interval_between_sent))
+ audio_list_sent.append(silence)
+ if (interval_between_para - interval_between_sent) > 0:
+ silence = np.zeros(
+ (int)(44100 * (interval_between_para - interval_between_sent))
+ )
+ audio_list_sent.append(silence)
+ audio16bit = gr.processing_utils.convert_to_16_bit_wav(
+ np.concatenate(audio_list_sent)
+ ) # 对完整句子做音量归一
+ audio_list.append(audio16bit)
+ audio_concat = np.concatenate(audio_list)
+ return ("Success", (hps.data.sampling_rate, audio_concat))
+
+
+def process_mix(slice):
+ _speaker = slice.pop()
+ _text, _lang = [], []
+ for lang, content in slice:
+ content = content.split("|")
+ content = [part for part in content if part != ""]
+ if len(content) == 0:
+ continue
+ if len(_text) == 0:
+ _text = [[part] for part in content]
+ _lang = [[lang] for part in content]
+ else:
+ _text[-1].append(content[0])
+ _lang[-1].append(lang)
+ if len(content) > 1:
+ _text += [[part] for part in content[1:]]
+ _lang += [[lang] for part in content[1:]]
+ return _text, _lang, _speaker
+
+
+def process_auto(text):
+ _text, _lang = [], []
+ for slice in text.split("|"):
+ if slice == "":
+ continue
+ temp_text, temp_lang = [], []
+ sentences_list = split_by_language(slice, target_languages=["zh", "ja", "en"])
+ for sentence, lang in sentences_list:
+ if sentence == "":
+ continue
+ temp_text.append(sentence)
+ if lang == "ja":
+ lang = "jp"
+ temp_lang.append(lang.upper())
+ _text.append(temp_text)
+ _lang.append(temp_lang)
+ return _text, _lang
+
+
+def process_text(
+ text: str,
+ speaker,
+ sdp_ratio,
+ noise_scale,
+ noise_scale_w,
+ length_scale,
+ language,
+ reference_audio,
+ emotion,
+ style_text=None,
+ style_weight=0,
+):
+ audio_list = []
+ if language == "mix":
+ bool_valid, str_valid = re_matching.validate_text(text)
+ if not bool_valid:
+ return str_valid, (
+ hps.data.sampling_rate,
+ np.concatenate([np.zeros(hps.data.sampling_rate // 2)]),
+ )
+ for slice in re_matching.text_matching(text):
+ _text, _lang, _speaker = process_mix(slice)
+ if _speaker is None:
+ continue
+ print(f"Text: {_text}\nLang: {_lang}")
+ audio_list.extend(
+ generate_audio_multilang(
+ _text,
+ sdp_ratio,
+ noise_scale,
+ noise_scale_w,
+ length_scale,
+ _speaker,
+ _lang,
+ reference_audio,
+ emotion,
+ )
+ )
+ elif language.lower() == "auto":
+ _text, _lang = process_auto(text)
+ print(f"Text: {_text}\nLang: {_lang}")
+ audio_list.extend(
+ generate_audio_multilang(
+ _text,
+ sdp_ratio,
+ noise_scale,
+ noise_scale_w,
+ length_scale,
+ speaker,
+ _lang,
+ reference_audio,
+ emotion,
+ )
+ )
+ else:
+ audio_list.extend(
+ generate_audio(
+ text.split("|"),
+ sdp_ratio,
+ noise_scale,
+ noise_scale_w,
+ length_scale,
+ speaker,
+ language,
+ reference_audio,
+ emotion,
+ style_text,
+ style_weight,
+ )
+ )
+ return audio_list
+
+
+def tts_fn(
+ text: str,
+ speaker,
+ sdp_ratio,
+ noise_scale,
+ noise_scale_w,
+ length_scale,
+ language,
+ reference_audio,
+ emotion,
+ prompt_mode,
+ style_text=None,
+ style_weight=0,
+):
+ if style_text == "":
+ style_text = None
+ if prompt_mode == "Audio prompt":
+ if reference_audio == None:
+ return ("Invalid audio prompt", None)
+ else:
+ reference_audio = load_audio(reference_audio)[1]
+ else:
+ reference_audio = None
+
+ audio_list = process_text(
+ text,
+ speaker,
+ sdp_ratio,
+ noise_scale,
+ noise_scale_w,
+ length_scale,
+ language,
+ reference_audio,
+ emotion,
+ style_text,
+ style_weight,
+ )
+
+ audio_concat = np.concatenate(audio_list)
+ return "Success", (hps.data.sampling_rate, audio_concat)
+
+
+def format_utils(text, speaker):
+ _text, _lang = process_auto(text)
+ res = f"[{speaker}]"
+ for lang_s, content_s in zip(_lang, _text):
+ for lang, content in zip(lang_s, content_s):
+ res += f"<{lang.lower()}>{content}"
+ res += "|"
+ return "mix", res[:-1]
+
+
+def load_audio(path):
+ audio, sr = librosa.load(path, 48000)
+ # audio = librosa.resample(audio, 44100, 48000)
+ return sr, audio
+
+
+def gr_util(item):
+ if item == "Text prompt":
+ return {"visible": True, "__type__": "update"}, {
+ "visible": False,
+ "__type__": "update",
+ }
+ else:
+ return {"visible": False, "__type__": "update"}, {
+ "visible": True,
+ "__type__": "update",
+ }
+
+
+if __name__ == "__main__":
+ if config.webui_config.debug:
+ logger.info("Enable DEBUG-LEVEL log")
+ logging.basicConfig(level=logging.DEBUG)
+ hps = utils.get_hparams_from_file(config.webui_config.config_path)
+ # 若config.json中未指定版本则默认为最新版本
+ version = hps.version if hasattr(hps, "version") else latest_version
+ net_g = get_net_g(
+ model_path=config.webui_config.model, version=version, device=device, hps=hps
+ )
+ speaker_ids = hps.data.spk2id
+ speakers = list(speaker_ids.keys())
+ languages = ["ZH", "JP", "EN", "mix", "auto"]
+ with gr.Blocks() as app:
+ with gr.Row():
+ with gr.Column():
+ text = gr.TextArea(
+ label="输入文本内容",
+ placeholder="""
+ 如果你选择语言为\'mix\',必须按照格式输入,否则报错:
+ 格式举例(zh是中文,jp是日语,不区分大小写;说话人举例:gongzi):
+ [说话人1]你好,こんにちは! こんにちは,世界。
+ [说话人2]你好吗?元気ですか?
+ [说话人3]谢谢。どういたしまして。
+ ...
+ 另外,所有的语言选项都可以用'|'分割长段实现分句生成。
+ """,
+ )
+ trans = gr.Button("中翻日", variant="primary")
+ slicer = gr.Button("快速切分", variant="primary")
+ formatter = gr.Button("检测语言,并整理为 MIX 格式", variant="primary")
+ speaker = gr.Dropdown(
+ choices=speakers, value=speakers[0], label="Speaker"
+ )
+ _ = gr.Markdown(
+ value="提示模式(Prompt mode):可选文字提示或音频提示,用于生成文字或音频指定风格的声音。\n",
+ visible=False,
+ )
+ prompt_mode = gr.Radio(
+ ["Text prompt", "Audio prompt"],
+ label="Prompt Mode",
+ value="Text prompt",
+ visible=False,
+ )
+ text_prompt = gr.Textbox(
+ label="Text prompt",
+ placeholder="用文字描述生成风格。如:Happy",
+ value="Happy",
+ visible=False,
+ )
+ audio_prompt = gr.Audio(
+ label="Audio prompt", type="filepath", visible=False
+ )
+ sdp_ratio = gr.Slider(
+ minimum=0, maximum=1, value=0.5, step=0.1, label="SDP Ratio"
+ )
+ noise_scale = gr.Slider(
+ minimum=0.1, maximum=2, value=0.6, step=0.1, label="Noise"
+ )
+ noise_scale_w = gr.Slider(
+ minimum=0.1, maximum=2, value=0.9, step=0.1, label="Noise_W"
+ )
+ length_scale = gr.Slider(
+ minimum=0.1, maximum=2, value=1.0, step=0.1, label="Length"
+ )
+ language = gr.Dropdown(
+ choices=languages, value=languages[0], label="Language"
+ )
+ btn = gr.Button("生成音频!", variant="primary")
+ with gr.Column():
+ with gr.Accordion("融合文本语义", open=False):
+ gr.Markdown(
+ value="使用辅助文本的语意来辅助生成对话(语言保持与主文本相同)\n\n"
+ "**注意**:不要使用**指令式文本**(如:开心),要使用**带有强烈情感的文本**(如:我好快乐!!!)\n\n"
+ "效果较不明确,留空即为不使用该功能"
+ )
+ style_text = gr.Textbox(label="辅助文本")
+ style_weight = gr.Slider(
+ minimum=0,
+ maximum=1,
+ value=0.7,
+ step=0.1,
+ label="Weight",
+ info="主文本和辅助文本的bert混合比率,0表示仅主文本,1表示仅辅助文本",
+ )
+ with gr.Row():
+ with gr.Column():
+ interval_between_sent = gr.Slider(
+ minimum=0,
+ maximum=5,
+ value=0.2,
+ step=0.1,
+ label="句间停顿(秒),勾选按句切分才生效",
+ )
+ interval_between_para = gr.Slider(
+ minimum=0,
+ maximum=10,
+ value=1,
+ step=0.1,
+ label="段间停顿(秒),需要大于句间停顿才有效",
+ )
+ opt_cut_by_sent = gr.Checkbox(
+ label="按句切分 在按段落切分的基础上再按句子切分文本"
+ )
+ slicer = gr.Button("切分生成", variant="primary")
+ text_output = gr.Textbox(label="状态信息")
+ audio_output = gr.Audio(label="输出音频")
+ # explain_image = gr.Image(
+ # label="参数解释信息",
+ # show_label=True,
+ # show_share_button=False,
+ # show_download_button=False,
+ # value=os.path.abspath("./img/参数说明.png"),
+ # )
+ btn.click(
+ tts_fn,
+ inputs=[
+ text,
+ speaker,
+ sdp_ratio,
+ noise_scale,
+ noise_scale_w,
+ length_scale,
+ language,
+ audio_prompt,
+ text_prompt,
+ prompt_mode,
+ style_text,
+ style_weight,
+ ],
+ outputs=[text_output, audio_output],
+ )
+
+ trans.click(
+ translate,
+ inputs=[text],
+ outputs=[text],
+ )
+ slicer.click(
+ tts_split,
+ inputs=[
+ text,
+ speaker,
+ sdp_ratio,
+ noise_scale,
+ noise_scale_w,
+ length_scale,
+ language,
+ opt_cut_by_sent,
+ interval_between_para,
+ interval_between_sent,
+ audio_prompt,
+ text_prompt,
+ style_text,
+ style_weight,
+ ],
+ outputs=[text_output, audio_output],
+ )
+
+ prompt_mode.change(
+ lambda x: gr_util(x),
+ inputs=[prompt_mode],
+ outputs=[text_prompt, audio_prompt],
+ )
+
+ audio_prompt.upload(
+ lambda x: load_audio(x),
+ inputs=[audio_prompt],
+ outputs=[audio_prompt],
+ )
+
+ formatter.click(
+ format_utils,
+ inputs=[text, speaker],
+ outputs=[language, text],
+ )
+
+ print("推理页面已开启!")
+ webbrowser.open(f"http://127.0.0.1:{config.webui_config.port}")
+ app.launch(share=config.webui_config.share, server_port=config.webui_config.port)
diff --git a/webui_dataset.py b/webui_dataset.py
new file mode 100644
index 0000000000000000000000000000000000000000..dafa2c9875048212ecc0cede688e281fc52d2871
--- /dev/null
+++ b/webui_dataset.py
@@ -0,0 +1,208 @@
+import argparse
+import os
+
+import gradio as gr
+import yaml
+
+from common.constants import GRADIO_THEME
+from common.log import logger
+from common.subprocess_utils import run_script_with_log
+
+# Get path settings
+with open(os.path.join("configs", "paths.yml"), "r", encoding="utf-8") as f:
+ path_config: dict[str, str] = yaml.safe_load(f.read())
+ dataset_root = path_config["dataset_root"]
+ # assets_root = path_config["assets_root"]
+
+
+def do_slice(
+ model_name: str,
+ min_sec: float,
+ max_sec: float,
+ min_silence_dur_ms: int,
+ input_dir: str,
+):
+ if model_name == "":
+ return "Error: モデル名を入力してください。"
+ logger.info("Start slicing...")
+ cmd = [
+ "slice.py",
+ "--model_name",
+ model_name,
+ "--min_sec",
+ str(min_sec),
+ "--max_sec",
+ str(max_sec),
+ "--min_silence_dur_ms",
+ str(min_silence_dur_ms),
+ ]
+ if input_dir != "":
+ cmd += ["--input_dir", input_dir]
+ # onnxの警告が出るので無視する
+ success, message = run_script_with_log(cmd, ignore_warning=True)
+ if not success:
+ return f"Error: {message}"
+ return "音声のスライスが完了しました。"
+
+
+def do_transcribe(
+ model_name, whisper_model, compute_type, language, initial_prompt, device
+):
+ if model_name == "":
+ return "Error: モデル名を入力してください。"
+
+ success, message = run_script_with_log(
+ [
+ "transcribe.py",
+ "--model_name",
+ model_name,
+ "--model",
+ whisper_model,
+ "--compute_type",
+ compute_type,
+ "--device",
+ device,
+ "--language",
+ language,
+ "--initial_prompt",
+ f'"{initial_prompt}"',
+ ]
+ )
+ if not success:
+ return f"Error: {message}"
+ return "音声の文字起こしが完了しました。"
+
+
+initial_md = """
+# 簡易学習用データセット作成ツール
+
+Style-Bert-VITS2の学習用データセットを作成するためのツールです。以下の2つからなります。
+
+- 与えられた音声からちょうどいい長さの発話区間を切り取りスライス
+- 音声に対して文字起こし
+
+このうち両方を使ってもよいし、スライスする必要がない場合は後者のみを使ってもよいです。
+
+## 必要なもの
+
+学習したい音声が入ったwavファイルいくつか。
+合計時間がある程度はあったほうがいいかも、10分とかでも大丈夫だったとの報告あり。単一ファイルでも良いし複数ファイルでもよい。
+
+## スライス使い方
+1. `inputs`フォルダにwavファイルをすべて入れる
+2. `モデル名`を入力して、設定を必要なら調整して`音声のスライス`ボタンを押す
+3. 出来上がった音声ファイルたちは`Data/{モデル名}/raw`に保存される
+
+## 書き起こし使い方
+
+1. 書き起こしたい音声ファイルのあるフォルダを指定(デフォルトは`Data/{モデル名}/raw`なのでスライス後に行う場合は省略してよい)
+2. 設定を必要なら調整してボタンを押す
+3. 書き起こしファイルは`Data/{モデル名}/esd.list`に保存される
+
+## 注意
+
+- 長すぎる秒数(12-15秒くらいより長い?)のwavファイルは学習に用いられないようです。また短すぎてもあまりよくない可能性もあります。
+- 書き起こしの結果をどれだけ修正すればいいかはデータセットに依存しそうです。
+- 手動で書き起こしをいろいろ修正したり結果を細かく確認したい場合は、[Aivis Dataset](https://github.com/litagin02/Aivis-Dataset)もおすすめします。書き起こし部分もかなり工夫されています。ですがファイル数が多い場合などは、このツールで簡易的に切り出してデータセットを作るだけでも十分という気もしています。
+"""
+
+with gr.Blocks(theme=GRADIO_THEME) as app:
+ gr.Markdown(initial_md)
+ model_name = gr.Textbox(
+ label="モデル名を入力してください(話者名としても使われます)。"
+ )
+ with gr.Accordion("音声のスライス"):
+ with gr.Row():
+ with gr.Column():
+ input_dir = gr.Textbox(
+ label="入力フォルダ名(デフォルトはinputs)",
+ placeholder="inputs",
+ info="下記フォルダにwavファイルを入れておいてください",
+ )
+ min_sec = gr.Slider(
+ minimum=0,
+ maximum=10,
+ value=2,
+ step=0.5,
+ label="この秒数未満は切り捨てる",
+ )
+ max_sec = gr.Slider(
+ minimum=0,
+ maximum=15,
+ value=12,
+ step=0.5,
+ label="この秒数以上は切り捨てる",
+ )
+ min_silence_dur_ms = gr.Slider(
+ minimum=0,
+ maximum=2000,
+ value=700,
+ step=100,
+ label="無音とみなして区切る最小の無音の長さ(ms)",
+ )
+ slice_button = gr.Button("スライスを実行")
+ result1 = gr.Textbox(label="結果")
+ with gr.Row():
+ with gr.Column():
+ whisper_model = gr.Dropdown(
+ ["tiny", "base", "small", "medium", "large", "large-v2", "large-v3"],
+ label="Whisperモデル",
+ value="large-v3",
+ )
+ compute_type = gr.Dropdown(
+ [
+ "int8",
+ "int8_float32",
+ "int8_float16",
+ "int8_bfloat16",
+ "int16",
+ "float16",
+ "bfloat16",
+ "float32",
+ ],
+ label="計算精度",
+ value="bfloat16",
+ )
+ device = gr.Radio(["cuda", "cpu"], label="デバイス", value="cuda")
+ language = gr.Dropdown(["ja", "en", "zh"], value="ja", label="言語")
+ initial_prompt = gr.Textbox(
+ label="初期プロンプト",
+ value="こんにちは。元気、ですかー?ふふっ、私は……ちゃんと元気だよ!",
+ info="このように書き起こしてほしいという例文(句読点の入れ方・笑い方・固有名詞等)",
+ )
+ transcribe_button = gr.Button("音声の文字起こし")
+ result2 = gr.Textbox(label="結果")
+ slice_button.click(
+ do_slice,
+ inputs=[model_name, min_sec, max_sec, min_silence_dur_ms, input_dir],
+ outputs=[result1],
+ )
+ transcribe_button.click(
+ do_transcribe,
+ inputs=[
+ model_name,
+ whisper_model,
+ compute_type,
+ language,
+ initial_prompt,
+ device,
+ ],
+ outputs=[result2],
+ )
+
+parser = argparse.ArgumentParser()
+parser.add_argument(
+ "--server-name",
+ type=str,
+ default=None,
+ help="Server name for Gradio app",
+)
+parser.add_argument(
+ "--no-autolaunch",
+ action="store_true",
+ default=False,
+ help="Do not launch app automatically",
+)
+args = parser.parse_args()
+
+app.launch(inbrowser=not args.no_autolaunch, server_name=args.server_name)
diff --git a/webui_merge.py b/webui_merge.py
new file mode 100644
index 0000000000000000000000000000000000000000..7d1186265fbe84cd1aa80b6234ed20caf5dcbbff
--- /dev/null
+++ b/webui_merge.py
@@ -0,0 +1,471 @@
+import argparse
+import json
+import os
+import sys
+
+import gradio as gr
+import numpy as np
+import torch
+import yaml
+from safetensors import safe_open
+from safetensors.torch import save_file
+
+from common.constants import DEFAULT_STYLE, GRADIO_THEME
+from common.log import logger
+from common.tts_model import Model, ModelHolder
+
+voice_keys = ["dec"]
+voice_pitch_keys = ["flow"]
+speech_style_keys = ["enc_p"]
+tempo_keys = ["sdp", "dp"]
+
+device = "cuda" if torch.cuda.is_available() else "cpu"
+
+# Get path settings
+with open(os.path.join("configs", "paths.yml"), "r", encoding="utf-8") as f:
+ path_config: dict[str, str] = yaml.safe_load(f.read())
+ # dataset_root = path_config["dataset_root"]
+ assets_root = path_config["assets_root"]
+
+model_holder = ModelHolder(assets_root, device)
+
+
+def merge_style(model_name_a, model_name_b, weight, output_name, style_triple_list):
+ """
+ style_triple_list: list[(model_aでのスタイル名, model_bでのスタイル名, 出力するスタイル名)]
+ """
+ # 新スタイル名リストにNeutralが含まれているか確認し、Neutralを先頭に持ってくる
+ if any(triple[2] == DEFAULT_STYLE for triple in style_triple_list):
+ # 存在する場合、リストをソート
+ sorted_list = sorted(style_triple_list, key=lambda x: x[2] != DEFAULT_STYLE)
+ else:
+ # 存在しない場合、エラーを発生
+ raise ValueError("No element with {DEFAULT_STYLE} output style name found.")
+
+ style_vectors_a = np.load(
+ os.path.join(assets_root, model_name_a, "style_vectors.npy")
+ ) # (style_num_a, 256)
+ style_vectors_b = np.load(
+ os.path.join(assets_root, model_name_b, "style_vectors.npy")
+ ) # (style_num_b, 256)
+ with open(
+ os.path.join(assets_root, model_name_a, "config.json"), encoding="utf-8"
+ ) as f:
+ config_a = json.load(f)
+ with open(
+ os.path.join(assets_root, model_name_b, "config.json"), encoding="utf-8"
+ ) as f:
+ config_b = json.load(f)
+ style2id_a = config_a["data"]["style2id"]
+ style2id_b = config_b["data"]["style2id"]
+ new_style_vecs = []
+ new_style2id = {}
+ for style_a, style_b, style_out in sorted_list:
+ if style_a not in style2id_a:
+ logger.error(f"{style_a} is not in {model_name_a}.")
+ raise ValueError(f"{style_a} は {model_name_a} にありません。")
+ if style_b not in style2id_b:
+ logger.error(f"{style_b} is not in {model_name_b}.")
+ raise ValueError(f"{style_b} は {model_name_b} にありません。")
+ new_style = (
+ style_vectors_a[style2id_a[style_a]] * (1 - weight)
+ + style_vectors_b[style2id_b[style_b]] * weight
+ )
+ new_style_vecs.append(new_style)
+ new_style2id[style_out] = len(new_style_vecs) - 1
+ new_style_vecs = np.array(new_style_vecs)
+
+ output_style_path = os.path.join(assets_root, output_name, "style_vectors.npy")
+ np.save(output_style_path, new_style_vecs)
+
+ new_config = config_a.copy()
+ new_config["data"]["num_styles"] = len(new_style2id)
+ new_config["data"]["style2id"] = new_style2id
+ new_config["model_name"] = output_name
+ with open(
+ os.path.join(assets_root, output_name, "config.json"), "w", encoding="utf-8"
+ ) as f:
+ json.dump(new_config, f, indent=2, ensure_ascii=False)
+
+ # recipe.jsonを読み込んで、style_triple_listを追記
+ info_path = os.path.join(assets_root, output_name, "recipe.json")
+ if os.path.exists(info_path):
+ with open(info_path, encoding="utf-8") as f:
+ info = json.load(f)
+ else:
+ info = {}
+ info["style_triple_list"] = style_triple_list
+ with open(info_path, "w", encoding="utf-8") as f:
+ json.dump(info, f, indent=2, ensure_ascii=False)
+
+ return output_style_path, list(new_style2id.keys())
+
+
+def merge_models(
+ model_path_a,
+ model_path_b,
+ voice_weight,
+ voice_pitch_weight,
+ speech_style_weight,
+ tempo_weight,
+ output_name,
+):
+ """model Aを起点に、model Bの各要素を重み付けしてマージする。
+ safetensors形式を前提とする。"""
+ model_a_weight = {}
+ with safe_open(model_path_a, framework="pt", device="cpu") as f:
+ for k in f.keys():
+ model_a_weight[k] = f.get_tensor(k)
+
+ model_b_weight = {}
+ with safe_open(model_path_b, framework="pt", device="cpu") as f:
+ for k in f.keys():
+ model_b_weight[k] = f.get_tensor(k)
+
+ merged_model_weight = model_a_weight.copy()
+
+ for key in model_a_weight.keys():
+ if any([key.startswith(prefix) for prefix in voice_keys]):
+ weight = voice_weight
+ elif any([key.startswith(prefix) for prefix in voice_pitch_keys]):
+ weight = voice_pitch_weight
+ elif any([key.startswith(prefix) for prefix in speech_style_keys]):
+ weight = speech_style_weight
+ elif any([key.startswith(prefix) for prefix in tempo_keys]):
+ weight = tempo_weight
+ else:
+ continue
+ merged_model_weight[key] = (
+ model_a_weight[key] * (1 - weight) + model_b_weight[key] * weight
+ )
+
+ merged_model_path = os.path.join(
+ assets_root, output_name, f"{output_name}.safetensors"
+ )
+ os.makedirs(os.path.dirname(merged_model_path), exist_ok=True)
+ save_file(merged_model_weight, merged_model_path)
+
+ info = {
+ "model_a": model_path_a,
+ "model_b": model_path_b,
+ "voice_weight": voice_weight,
+ "voice_pitch_weight": voice_pitch_weight,
+ "speech_style_weight": speech_style_weight,
+ "tempo_weight": tempo_weight,
+ }
+ with open(
+ os.path.join(assets_root, output_name, "recipe.json"), "w", encoding="utf-8"
+ ) as f:
+ json.dump(info, f, indent=2, ensure_ascii=False)
+ return merged_model_path
+
+
+def merge_models_gr(
+ model_name_a,
+ model_path_a,
+ model_name_b,
+ model_path_b,
+ output_name,
+ voice_weight,
+ voice_pitch_weight,
+ speech_style_weight,
+ tempo_weight,
+):
+ if output_name == "":
+ return "Error: 新しいモデル名を入力してください。"
+ merged_model_path = merge_models(
+ model_path_a,
+ model_path_b,
+ voice_weight,
+ voice_pitch_weight,
+ speech_style_weight,
+ tempo_weight,
+ output_name,
+ )
+ return f"Success: モデルを{merged_model_path}に保存しました。"
+
+
+def merge_style_gr(
+ model_name_a,
+ model_name_b,
+ weight,
+ output_name,
+ style_triple_list_str: str,
+):
+ if output_name == "":
+ return "Error: 新しいモデル名を入力してください。", None
+ style_triple_list = []
+ for line in style_triple_list_str.split("\n"):
+ if not line:
+ continue
+ style_triple = line.split(",")
+ if len(style_triple) != 3:
+ logger.error(f"Invalid style triple: {line}")
+ return (
+ f"Error: スタイルを3つのカンマ区切りで入力してください:\n{line}",
+ None,
+ )
+ style_a, style_b, style_out = style_triple
+ style_a = style_a.strip()
+ style_b = style_b.strip()
+ style_out = style_out.strip()
+ style_triple_list.append((style_a, style_b, style_out))
+ try:
+ new_style_path, new_styles = merge_style(
+ model_name_a, model_name_b, weight, output_name, style_triple_list
+ )
+ except ValueError as e:
+ return f"Error: {e}"
+ return f"Success: スタイルを{new_style_path}に保存しました。", gr.Dropdown(
+ choices=new_styles, value=new_styles[0]
+ )
+
+
+def simple_tts(model_name, text, style=DEFAULT_STYLE, style_weight=1.0):
+ model_path = os.path.join(assets_root, model_name, f"{model_name}.safetensors")
+ config_path = os.path.join(assets_root, model_name, "config.json")
+ style_vec_path = os.path.join(assets_root, model_name, "style_vectors.npy")
+
+ model = Model(model_path, config_path, style_vec_path, device)
+ return model.infer(text, style=style, style_weight=style_weight)
+
+
+def update_two_model_names_dropdown():
+ new_names, new_files, _ = model_holder.update_model_names_gr()
+ return new_names, new_files, new_names, new_files
+
+
+def load_styles_gr(model_name_a, model_name_b):
+ config_path_a = os.path.join(assets_root, model_name_a, "config.json")
+ with open(config_path_a, encoding="utf-8") as f:
+ config_a = json.load(f)
+ styles_a = list(config_a["data"]["style2id"].keys())
+
+ config_path_b = os.path.join(assets_root, model_name_b, "config.json")
+ with open(config_path_b, encoding="utf-8") as f:
+ config_b = json.load(f)
+ styles_b = list(config_b["data"]["style2id"].keys())
+ return gr.Textbox(value=", ".join(styles_a)), gr.Textbox(value=", ".join(styles_b))
+
+
+initial_md = """
+# Style-Bert-VITS2 モデルマージツール
+
+2つのStyle-Bert-VITS2モデルから、声質・話し方・話す速さを取り替えたり混ぜたりできます。
+
+## 使い方
+
+1. マージしたい2つのモデルを選択してください(`model_assets`フォルダの中から選ばれます)。
+2. マージ後のモデルの名前を入力してください。
+3. マージ後のモデルの声質・話し方・話す速さを調整してください。
+4. 「モデルファイルのマージ」ボタンを押してください(safetensorsファイルがマージされる)。
+5. スタイルベクトルファイルも生成する必要があるので、指示に従ってマージ方法を入力後、「スタイルのマージ」ボタンを押してください。
+
+以上でマージは完了で、`model_assets/マージ後のモデル名`にマージ後のモデルが保存され、音声合成のときに使えます。
+
+また`model_asses/マージ後のモデル名/recipe.json`には、マージの配合レシピが記録されます(推論にはいらないので配合メモ用です)。
+
+一番下にマージしたモデルによる簡易的な音声合成機能もつけています。
+
+## 注意
+1.x系と2.x-JP-Extraのモデルマージは失敗するようです。
+"""
+
+style_merge_md = f"""
+## スタイルベクトルのマージ
+
+1行に「モデルAのスタイル名, モデルBのスタイル名, 左の2つを混ぜて出力するスタイル名」
+という形式で入力してください。例えば、
+```
+{DEFAULT_STYLE}, {DEFAULT_STYLE}, {DEFAULT_STYLE}
+Happy, Surprise, HappySurprise
+```
+と入力すると、マージ後のスタイルベクトルは、
+- `{DEFAULT_STYLE}`: モデルAの`{DEFAULT_STYLE}`とモデルBの`{DEFAULT_STYLE}`を混ぜたもの
+- `HappySurprise`: モデルAの`Happy`とモデルBの`Surprise`を混ぜたもの
+の2つになります。
+
+### 注意
+- 必ず「{DEFAULT_STYLE}」という名前のスタイルを作ってください。これは、マージ後のモデルの平均スタイルになります。
+- 構造上の相性の関係で、スタイルベクトルを混ぜる重みは、上の「話し方」と同じ比率で混ぜられます。例えば「話し方」が0のときはモデルAのみしか使われません。
+"""
+
+model_names = model_holder.model_names
+if len(model_names) == 0:
+ logger.error(
+ f"モデルが見つかりませんでした。{assets_root}にモデルを置いてください。"
+ )
+ sys.exit(1)
+initial_id = 0
+initial_model_files = model_holder.model_files_dict[model_names[initial_id]]
+
+with gr.Blocks(theme=GRADIO_THEME) as app:
+ gr.Markdown(initial_md)
+ with gr.Accordion(label="使い方", open=False):
+ gr.Markdown(initial_md)
+ with gr.Row():
+ with gr.Column(scale=3):
+ model_name_a = gr.Dropdown(
+ label="モデルA",
+ choices=model_names,
+ value=model_names[initial_id],
+ )
+ model_path_a = gr.Dropdown(
+ label="モデルファイル",
+ choices=initial_model_files,
+ value=initial_model_files[0],
+ )
+ with gr.Column(scale=3):
+ model_name_b = gr.Dropdown(
+ label="モデルB",
+ choices=model_names,
+ value=model_names[initial_id],
+ )
+ model_path_b = gr.Dropdown(
+ label="モデルファイル",
+ choices=initial_model_files,
+ value=initial_model_files[0],
+ )
+ refresh_button = gr.Button("更新", scale=1, visible=True)
+ with gr.Column(variant="panel"):
+ new_name = gr.Textbox(label="新しいモデル名", placeholder="new_model")
+ with gr.Row():
+ voice_slider = gr.Slider(
+ label="声質",
+ value=0,
+ minimum=0,
+ maximum=1,
+ step=0.1,
+ )
+ voice_pitch_slider = gr.Slider(
+ label="声の高さ",
+ value=0,
+ minimum=0,
+ maximum=1,
+ step=0.1,
+ )
+ speech_style_slider = gr.Slider(
+ label="話し方(抑揚・感情表現等)",
+ value=0,
+ minimum=0,
+ maximum=1,
+ step=0.1,
+ )
+ tempo_slider = gr.Slider(
+ label="話す速さ・リズム・テンポ",
+ value=0,
+ minimum=0,
+ maximum=1,
+ step=0.1,
+ )
+ with gr.Column(variant="panel"):
+ gr.Markdown("## モデルファイル(safetensors)のマージ")
+ model_merge_button = gr.Button("モデルファイルのマージ", variant="primary")
+ info_model_merge = gr.Textbox(label="情報")
+ with gr.Column(variant="panel"):
+ gr.Markdown(style_merge_md)
+ with gr.Row():
+ load_style_button = gr.Button("スタイル一覧をロード", scale=1)
+ styles_a = gr.Textbox(label="モデルAのスタイル一覧")
+ styles_b = gr.Textbox(label="モデルBのスタイル一覧")
+ style_triple_list = gr.TextArea(
+ label="スタイルのマージリスト",
+ placeholder=f"{DEFAULT_STYLE}, {DEFAULT_STYLE},{DEFAULT_STYLE}\nAngry, Angry, Angry",
+ value=f"{DEFAULT_STYLE}, {DEFAULT_STYLE}, {DEFAULT_STYLE}",
+ )
+ style_merge_button = gr.Button("スタイルのマージ", variant="primary")
+ info_style_merge = gr.Textbox(label="情報")
+
+ text_input = gr.TextArea(
+ label="テキスト", value="これはテストです。聞こえていますか?"
+ )
+ style = gr.Dropdown(
+ label="スタイル",
+ choices=["スタイルをマージしてください"],
+ value="スタイルをマージしてください",
+ )
+ emotion_weight = gr.Slider(
+ minimum=0,
+ maximum=50,
+ value=1,
+ step=0.1,
+ label="スタイルの強さ",
+ )
+ tts_button = gr.Button("音声合成", variant="primary")
+ audio_output = gr.Audio(label="結果")
+
+ model_name_a.change(
+ model_holder.update_model_files_gr,
+ inputs=[model_name_a],
+ outputs=[model_path_a],
+ )
+ model_name_b.change(
+ model_holder.update_model_files_gr,
+ inputs=[model_name_b],
+ outputs=[model_path_b],
+ )
+
+ refresh_button.click(
+ update_two_model_names_dropdown,
+ outputs=[model_name_a, model_path_a, model_name_b, model_path_b],
+ )
+
+ load_style_button.click(
+ load_styles_gr,
+ inputs=[model_name_a, model_name_b],
+ outputs=[styles_a, styles_b],
+ )
+
+ model_merge_button.click(
+ merge_models_gr,
+ inputs=[
+ model_name_a,
+ model_path_a,
+ model_name_b,
+ model_path_b,
+ new_name,
+ voice_slider,
+ voice_pitch_slider,
+ speech_style_slider,
+ tempo_slider,
+ ],
+ outputs=[info_model_merge],
+ )
+
+ style_merge_button.click(
+ merge_style_gr,
+ inputs=[
+ model_name_a,
+ model_name_b,
+ speech_style_slider,
+ new_name,
+ style_triple_list,
+ ],
+ outputs=[info_style_merge, style],
+ )
+
+ tts_button.click(
+ simple_tts,
+ inputs=[new_name, text_input, style, emotion_weight],
+ outputs=[audio_output],
+ )
+
+parser = argparse.ArgumentParser()
+parser.add_argument(
+ "--server-name",
+ type=str,
+ default=None,
+ help="Server name for Gradio app",
+)
+parser.add_argument(
+ "--no-autolaunch",
+ action="store_true",
+ default=False,
+ help="Do not launch app automatically",
+)
+parser.add_argument("--share", action="store_true", default=False)
+args = parser.parse_args()
+
+app.launch(
+ inbrowser=not args.no_autolaunch, server_name=args.server_name, share=args.share
+)
diff --git a/webui_style_vectors.py b/webui_style_vectors.py
new file mode 100644
index 0000000000000000000000000000000000000000..b89c9c9f815595015d78fcc7992fcb7abbf4a6c8
--- /dev/null
+++ b/webui_style_vectors.py
@@ -0,0 +1,480 @@
+import argparse
+import json
+import os
+import shutil
+
+import gradio as gr
+import matplotlib.pyplot as plt
+import numpy as np
+import yaml
+from scipy.spatial.distance import pdist, squareform
+from sklearn.cluster import DBSCAN, AgglomerativeClustering, KMeans
+from sklearn.manifold import TSNE
+from umap import UMAP
+
+from common.constants import DEFAULT_STYLE, GRADIO_THEME
+from common.log import logger
+from config import config
+
+# Get path settings
+with open(os.path.join("configs", "paths.yml"), "r", encoding="utf-8") as f:
+ path_config: dict[str, str] = yaml.safe_load(f.read())
+ dataset_root = path_config["dataset_root"]
+ # assets_root = path_config["assets_root"]
+
+MAX_CLUSTER_NUM = 10
+MAX_AUDIO_NUM = 10
+
+tsne = TSNE(n_components=2, random_state=42, metric="cosine")
+umap = UMAP(n_components=2, random_state=42, metric="cosine", n_jobs=1, min_dist=0.0)
+
+wav_files = []
+x = np.array([])
+x_reduced = None
+y_pred = np.array([])
+mean = np.array([])
+centroids = []
+
+
+def load(model_name, reduction_method):
+ global wav_files, x, x_reduced, mean
+ wavs_dir = os.path.join(dataset_root, model_name, "wavs")
+ style_vector_files = [
+ os.path.join(wavs_dir, f) for f in os.listdir(wavs_dir) if f.endswith(".npy")
+ ]
+ wav_files = [f.replace(".npy", "") for f in style_vector_files]
+ style_vectors = [np.load(f) for f in style_vector_files]
+ x = np.array(style_vectors)
+ mean = np.mean(x, axis=0)
+ if reduction_method == "t-SNE":
+ x_reduced = tsne.fit_transform(x)
+ elif reduction_method == "UMAP":
+ x_reduced = umap.fit_transform(x)
+ else:
+ raise ValueError("Invalid reduction method")
+ x_reduced = np.asarray(x_reduced)
+ plt.figure(figsize=(6, 6))
+ plt.scatter(x_reduced[:, 0], x_reduced[:, 1])
+ return plt
+
+
+def do_clustering(n_clusters=4, method="KMeans"):
+ global centroids, x_reduced, y_pred
+ if method == "KMeans":
+ model = KMeans(n_clusters=n_clusters, random_state=42, n_init="auto")
+ y_pred = model.fit_predict(x)
+ elif method == "Agglomerative":
+ model = AgglomerativeClustering(n_clusters=n_clusters)
+ y_pred = model.fit_predict(x)
+ elif method == "KMeans after reduction":
+ assert x_reduced is not None
+ model = KMeans(n_clusters=n_clusters, random_state=42, n_init="auto")
+ y_pred = model.fit_predict(x_reduced)
+ elif method == "Agglomerative after reduction":
+ assert x_reduced is not None
+ model = AgglomerativeClustering(n_clusters=n_clusters)
+ y_pred = model.fit_predict(x_reduced)
+ else:
+ raise ValueError("Invalid method")
+
+ centroids = []
+ for i in range(n_clusters):
+ centroids.append(np.mean(x[y_pred == i], axis=0))
+
+ return y_pred, centroids
+
+
+def do_dbscan(eps=2.5, min_samples=15):
+ global centroids, x_reduced, y_pred
+ model = DBSCAN(eps=eps, min_samples=min_samples)
+ assert x_reduced is not None
+ y_pred = model.fit_predict(x_reduced)
+ n_clusters = max(y_pred) + 1
+ centroids = []
+ for i in range(n_clusters):
+ centroids.append(np.mean(x[y_pred == i], axis=0))
+ return y_pred, centroids
+
+
+def representative_wav_files(cluster_id, num_files=1):
+ # y_predの中でcluster_indexに関するメドイドを探す
+ cluster_indices = np.where(y_pred == cluster_id)[0]
+ cluster_vectors = x[cluster_indices]
+ # クラスタ内の全ベクトル間の距離を計算
+ distances = pdist(cluster_vectors)
+ distance_matrix = squareform(distances)
+
+ # 各ベクトルと他の全ベクトルとの平均距離を計算
+ mean_distances = distance_matrix.mean(axis=1)
+
+ # 平均距離が最も小さい順にnum_files個のインデックスを取得
+ closest_indices = np.argsort(mean_distances)[:num_files]
+
+ return cluster_indices[closest_indices]
+
+
+def do_dbscan_gradio(eps=2.5, min_samples=15):
+ global x_reduced, centroids
+
+ y_pred, centroids = do_dbscan(eps, min_samples)
+
+ assert x_reduced is not None
+
+ cmap = plt.get_cmap("tab10")
+ plt.figure(figsize=(6, 6))
+ for i in range(max(y_pred) + 1):
+ plt.scatter(
+ x_reduced[y_pred == i, 0],
+ x_reduced[y_pred == i, 1],
+ color=cmap(i),
+ label=f"Style {i + 1}",
+ )
+ # Noise cluster (-1) is black
+ plt.scatter(
+ x_reduced[y_pred == -1, 0],
+ x_reduced[y_pred == -1, 1],
+ color="black",
+ label="Noise",
+ )
+ plt.legend()
+
+ n_clusters = max(y_pred) + 1
+
+ if n_clusters > MAX_CLUSTER_NUM:
+ # raise ValueError(f"The number of clusters is too large: {n_clusters}")
+ return [
+ plt,
+ gr.Slider(maximum=MAX_CLUSTER_NUM),
+ f"クラスタ数が多すぎます、パラメータを変えてみてください。: {n_clusters}",
+ ] + [gr.Audio(visible=False)] * MAX_AUDIO_NUM
+
+ elif n_clusters == 0:
+ return [
+ plt,
+ gr.Slider(maximum=MAX_CLUSTER_NUM),
+ f"クラスタが数が0です。パラメータを変えてみてください。",
+ ] + [gr.Audio(visible=False)] * MAX_AUDIO_NUM
+
+ return [plt, gr.Slider(maximum=n_clusters, value=1), n_clusters] + [
+ gr.Audio(visible=False)
+ ] * MAX_AUDIO_NUM
+
+
+def representative_wav_files_gradio(cluster_id, num_files=1):
+ cluster_id = cluster_id - 1 # UIでは1から始まるので0からにする
+ closest_indices = representative_wav_files(cluster_id, num_files)
+ actual_num_files = len(closest_indices) # ファイル数が少ないときのため
+ return [
+ gr.Audio(wav_files[i], visible=True, label=wav_files[i])
+ for i in closest_indices
+ ] + [gr.update(visible=False)] * (MAX_AUDIO_NUM - actual_num_files)
+
+
+def do_clustering_gradio(n_clusters=4, method="KMeans"):
+ global x_reduced, centroids
+ y_pred, centroids = do_clustering(n_clusters, method)
+
+ assert x_reduced is not None
+ cmap = plt.get_cmap("tab10")
+ plt.figure(figsize=(6, 6))
+ for i in range(n_clusters):
+ plt.scatter(
+ x_reduced[y_pred == i, 0],
+ x_reduced[y_pred == i, 1],
+ color=cmap(i),
+ label=f"Style {i + 1}",
+ )
+ plt.legend()
+
+ return [plt, gr.Slider(maximum=n_clusters, value=1)] + [
+ gr.Audio(visible=False)
+ ] * MAX_AUDIO_NUM
+
+
+def save_style_vectors_from_clustering(model_name, style_names_str: str):
+ """centerとcentroidsを保存する"""
+ result_dir = os.path.join(config.assets_root, model_name)
+ os.makedirs(result_dir, exist_ok=True)
+ style_vectors = np.stack([mean] + centroids)
+ style_vector_path = os.path.join(result_dir, "style_vectors.npy")
+ if os.path.exists(style_vector_path):
+ logger.info(f"Backup {style_vector_path} to {style_vector_path}.bak")
+ shutil.copy(style_vector_path, f"{style_vector_path}.bak")
+ np.save(style_vector_path, style_vectors)
+
+ # config.jsonの更新
+ config_path = os.path.join(result_dir, "config.json")
+ if not os.path.exists(config_path):
+ return f"{config_path}が存在しません。"
+ style_names = [name.strip() for name in style_names_str.split(",")]
+ style_name_list = [DEFAULT_STYLE] + style_names
+ if len(style_name_list) != len(centroids) + 1:
+ return f"スタイルの数が合いません。`,`で正しく{len(centroids)}個に区切られているか確認してください: {style_names_str}"
+ if len(set(style_names)) != len(style_names):
+ return f"スタイル名が重複しています。"
+
+ logger.info(f"Backup {config_path} to {config_path}.bak")
+ shutil.copy(config_path, f"{config_path}.bak")
+ with open(config_path, "r", encoding="utf-8") as f:
+ json_dict = json.load(f)
+ json_dict["data"]["num_styles"] = len(style_name_list)
+ style_dict = {name: i for i, name in enumerate(style_name_list)}
+ json_dict["data"]["style2id"] = style_dict
+ with open(config_path, "w", encoding="utf-8") as f:
+ json.dump(json_dict, f, indent=2, ensure_ascii=False)
+ return f"成功!\n{style_vector_path}に保存し{config_path}を更新しました。"
+
+
+def save_style_vectors_from_files(
+ model_name, audio_files_str: str, style_names_str: str
+):
+ """音声ファイルからスタイルベクトルを作成して保存する"""
+ global mean
+ if len(x) == 0:
+ return "Error: スタイルベクトルを読み込んでください。"
+ mean = np.mean(x, axis=0)
+
+ result_dir = os.path.join(config.assets_root, model_name)
+ os.makedirs(result_dir, exist_ok=True)
+ audio_files = [name.strip() for name in audio_files_str.split(",")]
+ style_names = [name.strip() for name in style_names_str.split(",")]
+ if len(audio_files) != len(style_names):
+ return f"音声ファイルとスタイル名の数が合いません。`,`で正しく{len(style_names)}個に区切られているか確認してください: {audio_files_str}と{style_names_str}"
+ style_name_list = [DEFAULT_STYLE] + style_names
+ if len(set(style_names)) != len(style_names):
+ return f"スタイル名が重複しています。"
+ style_vectors = [mean]
+
+ wavs_dir = os.path.join(dataset_root, model_name, "wavs")
+ for audio_file in audio_files:
+ path = os.path.join(wavs_dir, audio_file)
+ if not os.path.exists(path):
+ return f"{path}が存在しません。"
+ style_vectors.append(np.load(f"{path}.npy"))
+ style_vectors = np.stack(style_vectors)
+ assert len(style_name_list) == len(style_vectors)
+ style_vector_path = os.path.join(result_dir, "style_vectors.npy")
+ if os.path.exists(style_vector_path):
+ logger.info(f"Backup {style_vector_path} to {style_vector_path}.bak")
+ shutil.copy(style_vector_path, f"{style_vector_path}.bak")
+ np.save(style_vector_path, style_vectors)
+
+ # config.jsonの更新
+ config_path = os.path.join(result_dir, "config.json")
+ if not os.path.exists(config_path):
+ return f"{config_path}が存在しません。"
+ logger.info(f"Backup {config_path} to {config_path}.bak")
+ shutil.copy(config_path, f"{config_path}.bak")
+
+ with open(config_path, "r", encoding="utf-8") as f:
+ json_dict = json.load(f)
+ json_dict["data"]["num_styles"] = len(style_name_list)
+ style_dict = {name: i for i, name in enumerate(style_name_list)}
+ json_dict["data"]["style2id"] = style_dict
+
+ with open(config_path, "w", encoding="utf-8") as f:
+ json.dump(json_dict, f, indent=2, ensure_ascii=False)
+ return f"成功!\n{style_vector_path}に保存し{config_path}を更新しました。"
+
+
+initial_md = f"""
+# Style Bert-VITS2 スタイルベクトルの作成
+
+Style-Bert-VITS2でこまかくスタイルを指定して音声合成するには、モデルごとにスタイルベクトルのファイル`style_vectors.npy`を手動で作成する必要があります。
+
+ただし、学習の過程で自動的に平均スタイル「{DEFAULT_STYLE}」のみは作成されるので、それをそのまま使うこともできます(その場合はこのWebUIは使いません)。
+
+このプロセスは学習とは全く関係がないので、何回でも独立して繰り返して試せます。また学習中にもたぶん軽いので動くはずです。
+
+## 方法
+
+- 方法1: 音声ファイルを自動でスタイル別に分け、その各スタイルの平均を取って保存
+- 方法2: スタイルを代表する音声ファイルを手動で選んで、その音声のスタイルベクトルを保存
+- 方法3: 自分でもっと頑張ってこだわって作る(JVNVコーパスなど、もともとスタイルラベル等が利用可能な場合はこれがよいかも)
+"""
+
+method1 = f"""
+学習の時に取り出したスタイルベクトルを読み込んで、可視化を見ながらスタイルを分けていきます。
+
+手順:
+1. 図を眺める
+2. スタイル数を決める(平均スタイルを除く)
+3. スタイル分けを行って結果を確認
+4. スタイルの名前を決めて保存
+
+
+詳細: スタイルベクトル(256次元)たちを適当なアルゴリズムでクラスタリングして、各クラスタの中心のベクトル(と全体の平均ベクトル)を保存します。
+
+平均スタイル({DEFAULT_STYLE})は自動的に保存されます。
+"""
+
+dbscan_md = """
+DBSCANという方法でスタイル分けを行います。
+こちらの方が方法1よりも特徴がはっきり出るもののみを取り出せ、よいスタイルベクトルが作れるかもしれません。
+ただし事前にスタイル数は指定できません。
+
+パラメータ:
+- eps: この値より近い点同士をどんどん繋げて同じスタイル分類とする。小さいほどスタイル数が増え、大きいほどスタイル数が減る傾向。
+- min_samples: ある点をスタイルの核となる点とみなすために必要な近傍の点の数。小さいほどスタイル数が増え、大きいほどスタイル数が減る傾向。
+
+UMAPの場合はepsは0.3くらい、t-SNEの場合は2.5くらいがいいかもしれません。min_samplesはデータ数に依存するのでいろいろ試してみてください。
+
+詳細:
+https://ja.wikipedia.org/wiki/DBSCAN
+"""
+
+with gr.Blocks(theme=GRADIO_THEME) as app:
+ gr.Markdown(initial_md)
+ with gr.Row():
+ model_name = gr.Textbox(placeholder="your_model_name", label="モデル名")
+ reduction_method = gr.Radio(
+ choices=["UMAP", "t-SNE"],
+ label="次元削減方法",
+ info="v 1.3以前はt-SNEでしたがUMAPのほうがよい可能性もあります。",
+ value="UMAP",
+ )
+ load_button = gr.Button("スタイルベクトルを読み込む", variant="primary")
+ output = gr.Plot(label="音声スタイルの可視化")
+ load_button.click(load, inputs=[model_name, reduction_method], outputs=[output])
+ with gr.Tab("方法1: スタイル分けを自動で行う"):
+ with gr.Tab("スタイル分け1"):
+ n_clusters = gr.Slider(
+ minimum=2,
+ maximum=10,
+ step=1,
+ value=4,
+ label="作るスタイルの数(平均スタイルを除く)",
+ info="上の図を見ながらスタイルの数を試行錯誤してください。",
+ )
+ c_method = gr.Radio(
+ choices=[
+ "Agglomerative after reduction",
+ "KMeans after reduction",
+ "Agglomerative",
+ "KMeans",
+ ],
+ label="アルゴリズム",
+ info="分類する(クラスタリング)アルゴリズムを選択します。いろいろ試してみてください。",
+ value="Agglomerative after reduction",
+ )
+ c_button = gr.Button("スタイル分けを実行")
+ with gr.Tab("スタイル分け2: DBSCAN"):
+ gr.Markdown(dbscan_md)
+ eps = gr.Slider(
+ minimum=0.1,
+ maximum=10,
+ step=0.01,
+ value=0.3,
+ label="eps",
+ )
+ min_samples = gr.Slider(
+ minimum=1,
+ maximum=50,
+ step=1,
+ value=15,
+ label="min_samples",
+ )
+ with gr.Row():
+ dbscan_button = gr.Button("スタイル分けを実行")
+ num_styles_result = gr.Textbox(label="スタイル数")
+ gr.Markdown("スタイル分けの結果")
+ gr.Markdown(
+ "注意: もともと256次元なものをを2次元に落としているので、正確なベクトルの位置関係ではありません。"
+ )
+ with gr.Row():
+ gr_plot = gr.Plot()
+ with gr.Column():
+ with gr.Row():
+ cluster_index = gr.Slider(
+ minimum=1,
+ maximum=MAX_CLUSTER_NUM,
+ step=1,
+ value=1,
+ label="スタイル番号",
+ info="選択したスタイルの代表音声を表示します。",
+ )
+ num_files = gr.Slider(
+ minimum=1,
+ maximum=MAX_AUDIO_NUM,
+ step=1,
+ value=5,
+ label="代表音声の数をいくつ表示するか",
+ )
+ get_audios_button = gr.Button("代表音声を取得")
+ with gr.Row():
+ audio_list = []
+ for i in range(MAX_AUDIO_NUM):
+ audio_list.append(gr.Audio(visible=False, show_label=True))
+ c_button.click(
+ do_clustering_gradio,
+ inputs=[n_clusters, c_method],
+ outputs=[gr_plot, cluster_index] + audio_list,
+ )
+ dbscan_button.click(
+ do_dbscan_gradio,
+ inputs=[eps, min_samples],
+ outputs=[gr_plot, cluster_index, num_styles_result] + audio_list,
+ )
+ get_audios_button.click(
+ representative_wav_files_gradio,
+ inputs=[cluster_index, num_files],
+ outputs=audio_list,
+ )
+ gr.Markdown("結果が良さそうなら、これを保存します。")
+ style_names = gr.Textbox(
+ "Angry, Sad, Happy",
+ label="スタイルの名前",
+ info=f"スタイルの名前を`,`で区切って入力してください(日本語可)。例: `Angry, Sad, Happy`や`怒り, 悲しみ, 喜び`など。平均音声は{DEFAULT_STYLE}として自動的に保存されます。",
+ )
+ with gr.Row():
+ save_button1 = gr.Button("スタイルベクトルを保存", variant="primary")
+ info2 = gr.Textbox(label="保存結果")
+
+ save_button1.click(
+ save_style_vectors_from_clustering,
+ inputs=[model_name, style_names],
+ outputs=[info2],
+ )
+ with gr.Tab("方法2: 手動でスタイルを選ぶ"):
+ gr.Markdown(
+ "下のテキスト欄に、各スタイルの代表音声のファイル名を`,`区切りで、その横に対応するスタイル名を`,`区切りで入力してください。"
+ )
+ gr.Markdown("例: `angry.wav, sad.wav, happy.wav`と`Angry, Sad, Happy`")
+ gr.Markdown(
+ f"注意: {DEFAULT_STYLE}スタイルは自動的に保存されます、手動では{DEFAULT_STYLE}という名前のスタイルは指定しないでください。"
+ )
+ with gr.Row():
+ audio_files_text = gr.Textbox(
+ label="音声ファイル名", placeholder="angry.wav, sad.wav, happy.wav"
+ )
+ style_names_text = gr.Textbox(
+ label="スタイル名", placeholder="Angry, Sad, Happy"
+ )
+ with gr.Row():
+ save_button2 = gr.Button("スタイルベクトルを保存", variant="primary")
+ info2 = gr.Textbox(label="保存結果")
+ save_button2.click(
+ save_style_vectors_from_files,
+ inputs=[model_name, audio_files_text, style_names_text],
+ outputs=[info2],
+ )
+
+parser = argparse.ArgumentParser()
+parser.add_argument(
+ "--server-name",
+ type=str,
+ default=None,
+ help="Server name for Gradio app",
+)
+parser.add_argument(
+ "--no-autolaunch",
+ action="store_true",
+ default=False,
+ help="Do not launch app automatically",
+)
+parser.add_argument("--share", action="store_true", default=False)
+args = parser.parse_args()
+
+app.launch(
+ inbrowser=not args.no_autolaunch, server_name=args.server_name, share=args.share
+)
diff --git a/webui_train.py b/webui_train.py
new file mode 100644
index 0000000000000000000000000000000000000000..cd7af20e9fc25d42f436915020338dc8e9999f15
--- /dev/null
+++ b/webui_train.py
@@ -0,0 +1,784 @@
+import argparse
+import json
+import os
+import shutil
+import socket
+import subprocess
+import sys
+import time
+import webbrowser
+from datetime import datetime
+from multiprocessing import cpu_count
+from pathlib import Path
+
+import gradio as gr
+import yaml
+
+from common.constants import GRADIO_THEME, LATEST_VERSION
+from common.log import logger
+from common.stdout_wrapper import SAFE_STDOUT
+from common.subprocess_utils import run_script_with_log, second_elem_of
+
+logger_handler = None
+tensorboard_executed = False
+
+# Get path settings
+with open(os.path.join("configs", "paths.yml"), "r", encoding="utf-8") as f:
+ path_config: dict[str, str] = yaml.safe_load(f.read())
+ dataset_root = path_config["dataset_root"]
+ # assets_root = path_config["assets_root"]
+
+
+def get_path(model_name):
+ assert model_name != "", "モデル名は空にできません"
+ dataset_path = os.path.join(dataset_root, model_name)
+ lbl_path = os.path.join(dataset_path, "esd.list")
+ train_path = os.path.join(dataset_path, "train.list")
+ val_path = os.path.join(dataset_path, "val.list")
+ config_path = os.path.join(dataset_path, "config.json")
+ return dataset_path, lbl_path, train_path, val_path, config_path
+
+
+def initialize(
+ model_name,
+ batch_size,
+ epochs,
+ save_every_steps,
+ freeze_EN_bert,
+ freeze_JP_bert,
+ freeze_ZH_bert,
+ freeze_style,
+ freeze_decoder,
+ use_jp_extra,
+ log_interval,
+):
+ global logger_handler
+ dataset_path, _, train_path, val_path, config_path = get_path(model_name)
+
+ # 前処理のログをファイルに保存する
+ timestamp = datetime.now().strftime("%Y%m%d_%H%M%S")
+ file_name = f"preprocess_{timestamp}.log"
+ if logger_handler is not None:
+ logger.remove(logger_handler)
+ logger_handler = logger.add(os.path.join(dataset_path, file_name))
+
+ logger.info(
+ f"Step 1: start initialization...\nmodel_name: {model_name}, batch_size: {batch_size}, epochs: {epochs}, save_every_steps: {save_every_steps}, freeze_ZH_bert: {freeze_ZH_bert}, freeze_JP_bert: {freeze_JP_bert}, freeze_EN_bert: {freeze_EN_bert}, freeze_style: {freeze_style}, freeze_decoder: {freeze_decoder}, use_jp_extra: {use_jp_extra}"
+ )
+
+ default_config_path = (
+ "configs/config.json" if not use_jp_extra else "configs/configs_jp_extra.json"
+ )
+
+ with open(default_config_path, "r", encoding="utf-8") as f:
+ config = json.load(f)
+ config["model_name"] = model_name
+ config["data"]["training_files"] = train_path
+ config["data"]["validation_files"] = val_path
+ config["train"]["batch_size"] = batch_size
+ config["train"]["epochs"] = epochs
+ config["train"]["eval_interval"] = save_every_steps
+ config["train"]["log_interval"] = log_interval
+
+ config["train"]["freeze_EN_bert"] = freeze_EN_bert
+ config["train"]["freeze_JP_bert"] = freeze_JP_bert
+ config["train"]["freeze_ZH_bert"] = freeze_ZH_bert
+ config["train"]["freeze_style"] = freeze_style
+ config["train"]["freeze_decoder"] = freeze_decoder
+
+ config["train"]["bf16_run"] = False # デフォルトでFalseのはずだが念のため
+
+ model_path = os.path.join(dataset_path, "models")
+ if os.path.exists(model_path):
+ logger.warning(
+ f"Step 1: {model_path} already exists, so copy it to backup to {model_path}_backup"
+ )
+ shutil.copytree(
+ src=model_path,
+ dst=os.path.join(dataset_path, "models_backup"),
+ dirs_exist_ok=True,
+ )
+ shutil.rmtree(model_path)
+ pretrained_dir = "pretrained" if not use_jp_extra else "pretrained_jp_extra"
+ try:
+ shutil.copytree(
+ src=pretrained_dir,
+ dst=model_path,
+ )
+ except FileNotFoundError:
+ logger.error(f"Step 1: {pretrained_dir} folder not found.")
+ return False, f"Step 1, Error: {pretrained_dir}フォルダが見つかりません。"
+
+ with open(config_path, "w", encoding="utf-8") as f:
+ json.dump(config, f, indent=2, ensure_ascii=False)
+ if not os.path.exists("config.yml"):
+ shutil.copy(src="default_config.yml", dst="config.yml")
+ # yml_data = safe_load(open("config.yml", "r", encoding="utf-8"))
+ with open("config.yml", "r", encoding="utf-8") as f:
+ yml_data = yaml.safe_load(f)
+ yml_data["model_name"] = model_name
+ yml_data["dataset_path"] = dataset_path
+ with open("config.yml", "w", encoding="utf-8") as f:
+ yaml.dump(yml_data, f, allow_unicode=True)
+ logger.success("Step 1: initialization finished.")
+ return True, "Step 1, Success: 初期設定が完了しました"
+
+
+def resample(model_name, normalize, trim, num_processes):
+ logger.info("Step 2: start resampling...")
+ dataset_path, _, _, _, _ = get_path(model_name)
+ in_dir = os.path.join(dataset_path, "raw")
+ out_dir = os.path.join(dataset_path, "wavs")
+ cmd = [
+ "resample.py",
+ "--in_dir",
+ in_dir,
+ "--out_dir",
+ out_dir,
+ "--num_processes",
+ str(num_processes),
+ "--sr",
+ "44100",
+ ]
+ if normalize:
+ cmd.append("--normalize")
+ if trim:
+ cmd.append("--trim")
+ success, message = run_script_with_log(cmd)
+ if not success:
+ logger.error(f"Step 2: resampling failed.")
+ return False, f"Step 2, Error: 音声ファイルの前処理に失敗しました:\n{message}"
+ elif message:
+ logger.warning(f"Step 2: resampling finished with stderr.")
+ return True, f"Step 2, Success: 音声ファイルの前処理が完了しました:\n{message}"
+ logger.success("Step 2: resampling finished.")
+ return True, "Step 2, Success: 音声ファイルの前処理が完了しました"
+
+
+def preprocess_text(model_name, use_jp_extra, val_per_lang):
+ logger.info("Step 3: start preprocessing text...")
+ dataset_path, lbl_path, train_path, val_path, config_path = get_path(model_name)
+ try:
+ lines = open(lbl_path, "r", encoding="utf-8").readlines()
+ except FileNotFoundError:
+ logger.error(f"Step 3: {lbl_path} not found.")
+ return False, f"Step 3, Error: 書き起こしファイル {lbl_path} が見つかりません。"
+ new_lines = []
+ for line in lines:
+ if len(line.strip().split("|")) != 4:
+ logger.error(f"Step 3: {lbl_path} has invalid format at line:\n{line}")
+ return (
+ False,
+ f"Step 3, Error: 書き起こしファイル次の行の形式が不正です:\n{line}",
+ )
+ path, spk, language, text = line.strip().split("|")
+ # pathをファイル名だけ取り出して正しいパスに変更
+ path = Path(dataset_path) / "wavs" / Path(path).name
+ new_lines.append(f"{path}|{spk}|{language}|{text}\n")
+ with open(lbl_path, "w", encoding="utf-8") as f:
+ f.writelines(new_lines)
+ cmd = [
+ "preprocess_text.py",
+ "--config-path",
+ config_path,
+ "--transcription-path",
+ lbl_path,
+ "--train-path",
+ train_path,
+ "--val-path",
+ val_path,
+ "--val-per-lang",
+ str(val_per_lang),
+ ]
+ if use_jp_extra:
+ cmd.append("--use_jp_extra")
+ success, message = run_script_with_log(cmd)
+ if not success:
+ logger.error(f"Step 3: preprocessing text failed.")
+ return (
+ False,
+ f"Step 3, Error: 書き起こしファイルの前処理に失敗しました:\n{message}",
+ )
+ elif message:
+ logger.warning(f"Step 3: preprocessing text finished with stderr.")
+ return (
+ True,
+ f"Step 3, Success: 書き起こしファイルの前処理が完了しました:\n{message}",
+ )
+ logger.success("Step 3: preprocessing text finished.")
+ return True, "Step 3, Success: 書き起こしファイルの前処理が完了しました"
+
+
+def bert_gen(model_name):
+ logger.info("Step 4: start bert_gen...")
+ _, _, _, _, config_path = get_path(model_name)
+ success, message = run_script_with_log(
+ [
+ "bert_gen.py",
+ "--config",
+ config_path,
+ # "--num_processes", # bert_genは重いのでプロセス数いじらない
+ # str(num_processes),
+ ]
+ )
+ if not success:
+ logger.error(f"Step 4: bert_gen failed.")
+ return False, f"Step 4, Error: BERT特徴ファイルの生成に失敗しました:\n{message}"
+ elif message:
+ logger.warning(f"Step 4: bert_gen finished with stderr.")
+ return (
+ True,
+ f"Step 4, Success: BERT特徴ファイルの生成が完了しました:\n{message}",
+ )
+ logger.success("Step 4: bert_gen finished.")
+ return True, "Step 4, Success: BERT特徴ファイルの生成が完了しました"
+
+
+def style_gen(model_name, num_processes):
+ logger.info("Step 5: start style_gen...")
+ _, _, _, _, config_path = get_path(model_name)
+ success, message = run_script_with_log(
+ [
+ "style_gen.py",
+ "--config",
+ config_path,
+ "--num_processes",
+ str(num_processes),
+ ]
+ )
+ if not success:
+ logger.error(f"Step 5: style_gen failed.")
+ return (
+ False,
+ f"Step 5, Error: スタイル特徴ファイルの生成に失敗しました:\n{message}",
+ )
+ elif message:
+ logger.warning(f"Step 5: style_gen finished with stderr.")
+ return (
+ True,
+ f"Step 5, Success: スタイル特徴ファイルの生成が完了しました:\n{message}",
+ )
+ logger.success("Step 5: style_gen finished.")
+ return True, "Step 5, Success: スタイル特徴ファイルの生成が完了しました"
+
+
+def preprocess_all(
+ model_name,
+ batch_size,
+ epochs,
+ save_every_steps,
+ num_processes,
+ normalize,
+ trim,
+ freeze_EN_bert,
+ freeze_JP_bert,
+ freeze_ZH_bert,
+ freeze_style,
+ freeze_decoder,
+ use_jp_extra,
+ val_per_lang,
+ log_interval,
+):
+ if model_name == "":
+ return False, "Error: モデル名を入力してください"
+ success, message = initialize(
+ model_name=model_name,
+ batch_size=batch_size,
+ epochs=epochs,
+ save_every_steps=save_every_steps,
+ freeze_EN_bert=freeze_EN_bert,
+ freeze_JP_bert=freeze_JP_bert,
+ freeze_ZH_bert=freeze_ZH_bert,
+ freeze_style=freeze_style,
+ freeze_decoder=freeze_decoder,
+ use_jp_extra=use_jp_extra,
+ log_interval=log_interval,
+ )
+ if not success:
+ return False, message
+ success, message = resample(
+ model_name=model_name,
+ normalize=normalize,
+ trim=trim,
+ num_processes=num_processes,
+ )
+ if not success:
+ return False, message
+ success, message = preprocess_text(
+ model_name=model_name, use_jp_extra=use_jp_extra, val_per_lang=val_per_lang
+ )
+ if not success:
+ return False, message
+ success, message = bert_gen(
+ model_name=model_name
+ ) # bert_genは重いのでプロセス数いじらない
+ if not success:
+ return False, message
+ success, message = style_gen(model_name=model_name, num_processes=num_processes)
+ if not success:
+ return False, message
+ logger.success("Success: All preprocess finished!")
+ return (
+ True,
+ "Success: 全ての前処理が完了しました。ターミナルを確認しておかしいところがないか確認するのをおすすめします。",
+ )
+
+
+def train(model_name, skip_style=False, use_jp_extra=True, speedup=False):
+ dataset_path, _, _, _, config_path = get_path(model_name)
+ # 学習再開の場合は念のためconfig.ymlの名前等を更新
+ with open("config.yml", "r", encoding="utf-8") as f:
+ yml_data = yaml.safe_load(f)
+ yml_data["model_name"] = model_name
+ yml_data["dataset_path"] = dataset_path
+ with open("config.yml", "w", encoding="utf-8") as f:
+ yaml.dump(yml_data, f, allow_unicode=True)
+
+ train_py = "train_ms.py" if not use_jp_extra else "train_ms_jp_extra.py"
+ cmd = [train_py, "--config", config_path, "--model", dataset_path]
+ if skip_style:
+ cmd.append("--skip_default_style")
+ if speedup:
+ cmd.append("--speedup")
+ success, message = run_script_with_log(cmd, ignore_warning=True)
+ if not success:
+ logger.error(f"Train failed.")
+ return False, f"Error: 学習に失敗しました:\n{message}"
+ elif message:
+ logger.warning(f"Train finished with stderr.")
+ return True, f"Success: 学習が完了しました:\n{message}"
+ logger.success("Train finished.")
+ return True, "Success: 学習が完了しました"
+
+
+def wait_for_tensorboard(port=6006, timeout=10):
+ start_time = time.time()
+ while True:
+ try:
+ with socket.create_connection(("localhost", port), timeout=1):
+ return True # ポートが開いている場合
+ except OSError:
+ pass # ポートがまだ開いていない場合
+
+ if time.time() - start_time > timeout:
+ return False # タイムアウト
+
+ time.sleep(0.1)
+
+
+def run_tensorboard(model_name):
+ global tensorboard_executed
+ if not tensorboard_executed:
+ python = sys.executable
+ tensorboard_cmd = [
+ python,
+ "-m",
+ "tensorboard.main",
+ "--logdir",
+ f"Data/{model_name}/models",
+ ]
+ subprocess.Popen(
+ tensorboard_cmd,
+ stdout=SAFE_STDOUT, # type: ignore
+ stderr=SAFE_STDOUT, # type: ignore
+ )
+ yield gr.Button("起動中…")
+ if wait_for_tensorboard():
+ tensorboard_executed = True
+ else:
+ logger.error("Tensorboard did not start in the expected time.")
+ webbrowser.open("http://localhost:6006")
+ yield gr.Button("Tensorboardを開く")
+
+
+initial_md = f"""
+# Style-Bert-VITS2 ver {LATEST_VERSION} 学習用WebUI
+
+## 使い方
+
+- データを準備して、モデル名を入力して、必要なら設定を調整してから、「自動前処理を実行」ボタンを押してください。進捗状況等はターミナルに表示されます。
+
+- 各ステップごとに実行する場合は「手動前処理」を使ってください(基本的には自動でいいはず)。
+
+- 前処理が終わったら、「学習を開始する」ボタンを押すと学習が開始されます。
+
+- 途中から学習を再開する場合は、モデル名を入力してから「学習を開始する」を押せばよいです。
+
+注意: 標準スタイル以外のスタイルを音声合成で使うには、スタイルベクトルファイル`style_vectors.npy`を作る必要があります。これは、`Style.bat`を実行してそこで作成してください。
+動作は軽いはずなので、学習中でも実行でき、何度でも繰り返して試せます。
+
+## JP-Extra版について
+
+元とするモデル構造として [Bert-VITS2 Japanese-Extra](https://github.com/fishaudio/Bert-VITS2/releases/tag/JP-Exta) を使うことができます。
+日本語のアクセントやイントネーションや自然性が上がる傾向にありますが、英語と中国語は話せなくなります。
+"""
+
+prepare_md = """
+まず音声データ(wavファイルで1ファイルが2-12秒程度の、長すぎず短すぎない発話のものをいくつか)と、書き起こしテキストを用意してください。
+
+それを次のように配置します。
+```
+├── Data
+│ ├── {モデルの名前}
+│ │ ├── esd.list
+│ │ ├── raw
+│ │ │ ├── ****.wav
+│ │ │ ├── ****.wav
+│ │ │ ├── ...
+```
+
+wavファイル名やモデルの名前は空白を含まない半角で、wavファイルの拡張子は小文字`.wav`である必要があります。
+`raw` フォルダにはすべてのwavファイルを入れ、`esd.list` ファイルには、以下のフォーマットで各wavファイルの情報を記述してください。
+```
+****.wav|{話者名}|{言語ID、ZHかJPかEN}|{書き起こしテキスト}
+```
+
+例:
+```
+wav_number1.wav|hanako|JP|こんにちは、聞こえて、いますか?
+wav_next.wav|taro|JP|はい、聞こえています……。
+english_teacher.wav|Mary|EN|How are you? I'm fine, thank you, and you?
+...
+```
+日本語話者の単一話者データセットでも構いません。
+"""
+
+if __name__ == "__main__":
+ with gr.Blocks(theme=GRADIO_THEME).queue() as app:
+ gr.Markdown(initial_md)
+ with gr.Accordion(label="データの前準備", open=False):
+ gr.Markdown(prepare_md)
+ model_name = gr.Textbox(label="モデル名")
+ gr.Markdown("### 自動前処理")
+ with gr.Row(variant="panel"):
+ with gr.Column():
+ use_jp_extra = gr.Checkbox(
+ label="JP-Extra版を使う(日本語の性能が上がるが英語と中国語は話せなくなる)",
+ value=True,
+ )
+ batch_size = gr.Slider(
+ label="バッチサイズ",
+ info="学習速度が遅い場合は小さくして試し、VRAMに余裕があれば大きくしてください。JP-Extra版でのVRAM使用量目安: 1: 6GB, 2: 8GB, 3: 10GB, 4: 12GB",
+ value=2,
+ minimum=1,
+ maximum=64,
+ step=1,
+ )
+ epochs = gr.Slider(
+ label="エポック数",
+ info="100もあれば十分そうだけどもっと回すと質が上がるかもしれない",
+ value=100,
+ minimum=10,
+ maximum=1000,
+ step=10,
+ )
+ save_every_steps = gr.Slider(
+ label="何ステップごとに結果を保存するか",
+ info="エポック数とは違うことに注意",
+ value=1000,
+ minimum=100,
+ maximum=10000,
+ step=100,
+ )
+ normalize = gr.Checkbox(
+ label="音声の音量を正規化する(音量の大小が揃っていない場合など)",
+ value=False,
+ )
+ trim = gr.Checkbox(
+ label="音声の最初と最後の無音を取り除く",
+ value=False,
+ )
+ with gr.Accordion("詳細設定", open=False):
+ num_processes = gr.Slider(
+ label="プロセス数",
+ info="前処理時の並列処理プロセス数、前処理でフリーズしたら下げてください",
+ value=cpu_count() // 2,
+ minimum=1,
+ maximum=cpu_count(),
+ step=1,
+ )
+ val_per_lang = gr.Slider(
+ label="検証データ数",
+ info="学習には使われず、tensorboardで元音声と合成音声を比較するためのもの",
+ value=0,
+ minimum=0,
+ maximum=100,
+ step=1,
+ )
+ log_interval = gr.Slider(
+ label="Tensorboardのログ出力間隔",
+ info="Tensorboardで詳しく見たい人は小さめにしてください",
+ value=200,
+ minimum=10,
+ maximum=1000,
+ step=10,
+ )
+ gr.Markdown("学習時に特定の部分を凍結させるかどうか")
+ freeze_EN_bert = gr.Checkbox(
+ label="英語bert部分を凍結",
+ value=False,
+ )
+ freeze_JP_bert = gr.Checkbox(
+ label="日本語bert部分を凍結",
+ value=False,
+ )
+ freeze_ZH_bert = gr.Checkbox(
+ label="中国語bert部分を凍結",
+ value=False,
+ )
+ freeze_style = gr.Checkbox(
+ label="スタイル部分を凍結",
+ value=False,
+ )
+ freeze_decoder = gr.Checkbox(
+ label="デコーダ部分を凍結",
+ value=False,
+ )
+
+ with gr.Column():
+ preprocess_button = gr.Button(
+ value="自動前処理を実行", variant="primary"
+ )
+ info_all = gr.Textbox(label="状況")
+ with gr.Accordion(open=False, label="手動前処理"):
+ with gr.Row(variant="panel"):
+ with gr.Column():
+ gr.Markdown(value="#### Step 1: 設定ファイルの生成")
+ use_jp_extra_manual = gr.Checkbox(
+ label="JP-Extra版を使う",
+ value=True,
+ )
+ batch_size_manual = gr.Slider(
+ label="バッチサイズ",
+ value=2,
+ minimum=1,
+ maximum=64,
+ step=1,
+ )
+ epochs_manual = gr.Slider(
+ label="エポック数",
+ value=100,
+ minimum=1,
+ maximum=1000,
+ step=1,
+ )
+ save_every_steps_manual = gr.Slider(
+ label="何ステップごとに結果を保存するか",
+ value=1000,
+ minimum=100,
+ maximum=10000,
+ step=100,
+ )
+ log_interval_manual = gr.Slider(
+ label="Tensorboardのログ出力間隔",
+ value=200,
+ minimum=10,
+ maximum=1000,
+ step=10,
+ )
+ freeze_EN_bert_manual = gr.Checkbox(
+ label="英語bert部分を凍結",
+ value=False,
+ )
+ freeze_JP_bert_manual = gr.Checkbox(
+ label="日本語bert部分を凍結",
+ value=False,
+ )
+ freeze_ZH_bert_manual = gr.Checkbox(
+ label="中国語bert部分を凍結",
+ value=False,
+ )
+ freeze_style_manual = gr.Checkbox(
+ label="スタイル部分を凍結",
+ value=False,
+ )
+ freeze_decoder_manual = gr.Checkbox(
+ label="デコーダ部分を凍結",
+ value=False,
+ )
+ with gr.Column():
+ generate_config_btn = gr.Button(value="実行", variant="primary")
+ info_init = gr.Textbox(label="状況")
+ with gr.Row(variant="panel"):
+ with gr.Column():
+ gr.Markdown(value="#### Step 2: 音声ファイルの前処理")
+ num_processes_resample = gr.Slider(
+ label="プロセス数",
+ value=cpu_count() // 2,
+ minimum=1,
+ maximum=cpu_count(),
+ step=1,
+ )
+ normalize_resample = gr.Checkbox(
+ label="音声の音量を正規化する",
+ value=False,
+ )
+ trim_resample = gr.Checkbox(
+ label="音声の最初と最後の無音を取り除く",
+ value=False,
+ )
+ with gr.Column():
+ resample_btn = gr.Button(value="実行", variant="primary")
+ info_resample = gr.Textbox(label="状況")
+ with gr.Row(variant="panel"):
+ with gr.Column():
+ gr.Markdown(value="#### Step 3: 書き起こしファイルの前処理")
+ val_per_lang_manual = gr.Slider(
+ label="検証データ数",
+ value=0,
+ minimum=0,
+ maximum=100,
+ step=1,
+ )
+ with gr.Column():
+ preprocess_text_btn = gr.Button(value="実行", variant="primary")
+ info_preprocess_text = gr.Textbox(label="状況")
+ with gr.Row(variant="panel"):
+ with gr.Column():
+ gr.Markdown(value="#### Step 4: BERT特徴ファイルの生成")
+ with gr.Column():
+ bert_gen_btn = gr.Button(value="実行", variant="primary")
+ info_bert = gr.Textbox(label="状況")
+ with gr.Row(variant="panel"):
+ with gr.Column():
+ gr.Markdown(value="#### Step 5: スタイル特徴ファイルの生成")
+ num_processes_style = gr.Slider(
+ label="プロセス数",
+ value=cpu_count() // 2,
+ minimum=1,
+ maximum=cpu_count(),
+ step=1,
+ )
+ with gr.Column():
+ style_gen_btn = gr.Button(value="実行", variant="primary")
+ info_style = gr.Textbox(label="状況")
+ gr.Markdown("## 学習")
+ with gr.Row():
+ skip_style = gr.Checkbox(
+ label="スタイルファイルの生成をスキップする",
+ info="学習再開の場合の場合はチェックしてください",
+ value=False,
+ )
+ use_jp_extra_train = gr.Checkbox(
+ label="JP-Extra版を使う",
+ value=True,
+ )
+ speedup = gr.Checkbox(
+ label="ログ等をスキップして学習を高速化する",
+ value=False,
+ visible=False, # Experimental
+ )
+ train_btn = gr.Button(value="学習を開始する", variant="primary")
+ tensorboard_btn = gr.Button(value="Tensorboardを開く")
+ gr.Markdown(
+ "進捗はターミナルで確認してください。結果は指定したステップごとに随時保存されており、また学習を途中から再開することもできます。学習を終了するには単にターミナルを終了してください。"
+ )
+ info_train = gr.Textbox(label="状況")
+
+ preprocess_button.click(
+ second_elem_of(preprocess_all),
+ inputs=[
+ model_name,
+ batch_size,
+ epochs,
+ save_every_steps,
+ num_processes,
+ normalize,
+ trim,
+ freeze_EN_bert,
+ freeze_JP_bert,
+ freeze_ZH_bert,
+ freeze_style,
+ freeze_decoder,
+ use_jp_extra,
+ val_per_lang,
+ log_interval,
+ ],
+ outputs=[info_all],
+ )
+
+ # Manual preprocess
+ generate_config_btn.click(
+ second_elem_of(initialize),
+ inputs=[
+ model_name,
+ batch_size_manual,
+ epochs_manual,
+ save_every_steps_manual,
+ freeze_EN_bert_manual,
+ freeze_JP_bert_manual,
+ freeze_ZH_bert_manual,
+ freeze_style_manual,
+ freeze_decoder_manual,
+ use_jp_extra_manual,
+ log_interval_manual,
+ ],
+ outputs=[info_init],
+ )
+ resample_btn.click(
+ second_elem_of(resample),
+ inputs=[
+ model_name,
+ normalize_resample,
+ trim_resample,
+ num_processes_resample,
+ ],
+ outputs=[info_resample],
+ )
+ preprocess_text_btn.click(
+ second_elem_of(preprocess_text),
+ inputs=[
+ model_name,
+ use_jp_extra_manual,
+ val_per_lang_manual,
+ ],
+ outputs=[info_preprocess_text],
+ )
+ bert_gen_btn.click(
+ second_elem_of(bert_gen),
+ inputs=[model_name],
+ outputs=[info_bert],
+ )
+ style_gen_btn.click(
+ second_elem_of(style_gen),
+ inputs=[model_name, num_processes_style],
+ outputs=[info_style],
+ )
+
+ # Train
+ train_btn.click(
+ second_elem_of(train),
+ inputs=[model_name, skip_style, use_jp_extra_train, speedup],
+ outputs=[info_train],
+ )
+ tensorboard_btn.click(
+ run_tensorboard, inputs=[model_name], outputs=[tensorboard_btn]
+ )
+
+ use_jp_extra.change(
+ lambda x: gr.Checkbox(value=x),
+ inputs=[use_jp_extra],
+ outputs=[use_jp_extra_train],
+ )
+ use_jp_extra_manual.change(
+ lambda x: gr.Checkbox(value=x),
+ inputs=[use_jp_extra_manual],
+ outputs=[use_jp_extra_train],
+ )
+
+ parser = argparse.ArgumentParser()
+ parser.add_argument(
+ "--server-name",
+ type=str,
+ default=None,
+ help="Server name for Gradio app",
+ )
+ parser.add_argument(
+ "--no-autolaunch",
+ action="store_true",
+ default=False,
+ help="Do not launch app automatically",
+ )
+ args = parser.parse_args()
+
+ app.launch(inbrowser=not args.no_autolaunch, server_name=args.server_name)