import gradio as gr
import ffmpeg
from pathlib import Path
import os
import ast
import json
import base64
import requests
import moviepy.editor as mp
from PIL import Image, ImageSequence
import cv2
API_URL = "https://api-inference.huggingface.co/models/facebook/wav2vec2-base-960h"
HF_TOKEN = os.environ["HF_TOKEN"]
headers = {"Authorization": f"Bearer {HF_TOKEN}"}
def generate_transcripts(in_video): #generate_gifs(in_video, gif_transcript):
print("********* Inside generate_transcripts() **********")
#convert video to audio
print(f" input video is : {in_video}")
#sample
video_path = Path("./ShiaLaBeouf.mp4")
audio_memory, _ = ffmpeg.input(in_video).output('-', format="wav", ac=1, ar='16k').overwrite_output().global_args('-loglevel', 'quiet').run(capture_stdout=True)
#audio_memory, _ = ffmpeg.input(video_path).output('-', format="wav", ac=1, ar='16k').overwrite_output().global_args('-loglevel', 'quiet').run(capture_stdout=True)
#Getting transcripts using wav2Vec2 huggingface hosted accelerated inference
#sending audio file in request along with stride and chunk length information
model_response = query_api(audio_memory)
#model response has both - transcripts as well as character timestamps or chunks
print(f"model_response is : {model_response}")
transcription = model_response["text"].lower()
chnk = model_response["chunks"]
#creating lists from chunks to consume downstream easily
timestamps = [[chunk["text"].lower(), chunk["timestamp"][0], chunk["timestamp"][1]]
for chunk in chnk]
#getting words and word timestamps
words, words_timestamp = get_word_timestamps(timestamps)
print(f"Total words in the audio transcript is:{len(words)}, transcript word list is :{words}, type of words is :{type(words)} ")
print(f"Total Word timestamps derived fromcharacter timestamp are :{len(words_timestamp)}, Word timestamps are :{words_timestamp}")
return transcription, words, words_timestamp
def generate_gifs(in_video, gif_transcript, words, words_timestamp):
print("********* Inside generate_gifs() **********")
#creating list from input gif transcript
#gif = "don't let your dreams be dreams"
gif = gif_transcript
#gif = gif_transcript
giflist = gif.split()
#getting gif indexes from the generator
# Converting string to list
words = ast.literal_eval(words)
words_timestamp = ast.literal_eval(words_timestamp)
print(f"words is :{words}")
print(f"type of words is :{type(words)}")
print(f"length of words is :{len(words)}")
print(f"giflist is :{giflist}")
giflist_indxs = list(list(get_gif_word_indexes(words, giflist))[0])
print(f"giflist_indxs is : {giflist_indxs}")
#getting start and end timestamps for a gif video
start_seconds, end_seconds = get_gif_timestamps(giflist_indxs, words_timestamp)
print(f"start_seconds, end_seconds are : ({start_seconds}, {end_seconds})")
#generated .gif image
gif_out, vid_out = gen_moviepy_gif(in_video, start_seconds, end_seconds)
return gif_out
#calling the hosted model
def query_api(audio_bytes: bytes):
"""
Query for Huggingface Inference API for Automatic Speech Recognition task
"""
print("********* Inside query_api() **********")
payload = json.dumps({
"inputs": base64.b64encode(audio_bytes).decode("utf-8"),
"parameters": {
"return_timestamps": "char",
"chunk_length_s": 10,
"stride_length_s": [4, 2]
},
"options": {"use_gpu": False}
}).encode("utf-8")
response = requests.request(
"POST", API_URL, headers=headers, data=payload)
json_reponse = json.loads(response.content.decode("utf-8"))
print(f"json_reponse is :{json_reponse}")
return json_reponse
#getting word timestamps from character timestamps
def get_word_timestamps(timestamps):
words, word = [], []
letter_timestamp, word_timestamp, words_timestamp = [], [], []
for idx,entry in enumerate(timestamps):
word.append(entry[0])
letter_timestamp.append(entry[1])
if entry[0] == ' ':
words.append(''.join(word))
word_timestamp.append(letter_timestamp[0])
word_timestamp.append(timestamps[idx-1][2])
words_timestamp.append(word_timestamp)
word, word_timestamp, letter_timestamp = [], [], []
words = [word.strip() for word in words]
return words, words_timestamp
#getting index of gif words in main transcript
def get_gif_word_indexes(total_words_list, gif_words_list):
if not gif_words_list:
return
# just optimization
COUNT=0
lengthgif_words_list = len(gif_words_list)
firstgif_words_list = gif_words_list[0]
print(f"total_words_list is :{total_words_list}")
print(f"length of total_words_list is :{len(total_words_list)}")
print(f"gif_words_list is :{gif_words_list}")
print(f"length of gif_words_list is :{len(gif_words_list)}")
for idx, item in enumerate(total_words_list):
COUNT+=1
if item == firstgif_words_list:
if total_words_list[idx:idx+lengthgif_words_list] == gif_words_list:
print(f"value of tuple is : {tuple(range(idx, idx+lengthgif_words_list))}")
yield tuple(range(idx, idx+lengthgif_words_list))
#getting start and end timestamps for gif transcript
def get_gif_timestamps(giflist_indxs, words_timestamp):
print(f"******** Inside get_gif_timestamps() **********")
min_idx = min(giflist_indxs)
max_idx = max(giflist_indxs)
print(f"min_idx is :{min_idx}")
print(f"max_idx is :{max_idx}")
gif_words_timestamp = words_timestamp[min_idx : max_idx+1]
print(f"words_timestamp is :{words_timestamp}")
print(f"gif_words_timestamp is :{gif_words_timestamp}")
start_seconds, end_seconds = gif_words_timestamp[0][0], gif_words_timestamp[-1][-1]
print(f"start_seconds, end_seconds are :{start_seconds},{end_seconds}")
return start_seconds, end_seconds
#extracting the video and building and serving a .gif image
def gen_moviepy_gif(in_video, start_seconds, end_seconds):
print("******** inside moviepy_gif () ***************")
#sample
video_path = "./ShiaLaBeouf.mp4"
video = mp.VideoFileClip(in_video)
#video = mp.VideoFileClip(video_path)
final_clip = video.subclip(start_seconds, end_seconds)
#writing to RAM
final_clip.write_gif("gifimage.gif") #, program='ffmpeg', tempfiles=True, fps=15, fuzz=3)
final_clip.write_videofile("gifimage.mp4")
final_clip.close()
#reading in a variable
gif_img = mp.VideoFileClip("gifimage.gif")
#gif_vid = mp.VideoFileClip("gifimage.mp4")
#im = Image.open("gifimage.gif")
#vid_cap = cv2.VideoCapture('gifimage.mp4')
return "gifimage.gif", "gifimage.mp4" #im, gif_img, gif_vid, vid_cap, #"gifimage.mp4"
sample_video = ['./ShiaLaBeouf.mp4']
sample_vid = gr.Video(label='Video file') #for displaying the example
examples = gr.components.Dataset(components=[sample_vid], samples=[sample_video], type='values')
demo = gr.Blocks()
with demo:
gr.Markdown("""# **Create Any GIF From Your Favorite Videos!** """)
gr.Markdown("""
### Now you can get your own unlimited supply of cool GIFs and reactions from the videos you most like..
Some cool sample .gif images generated using this Space -
Sample GIF 1 |
Sample GIF 2 |
Sample GIF 3 |
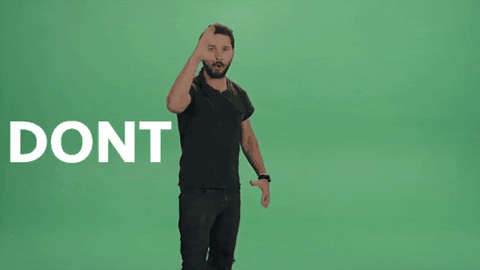 |
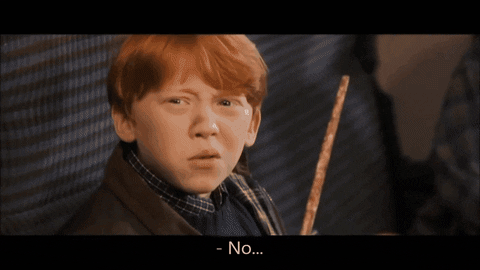 |
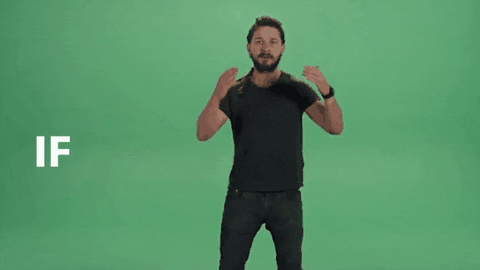 |
**Motivation and background:** In this Gradio-Space cum Blog, I will be taking you through my efforts in reproducing the brilliant app [Edit Video By Editing Text](https://huggingface.co/spaces/radames/edit-video-by-editing-text) by [@radames](https://huggingface.co/radames). My valule-adds are -
- A permanent supply for your own new GIFs
- This Space written in the form of a Notebook or a Blog if I may, to help someone understand how they can too build this kind of an app.
**How To Use:** 1. Upload a video or simply click on the Shia LaBeouf's sample provided here.
2. Then click on 'Generate transcripts' button and first textbox will display the extract Transcript from the audio associated with your sample.
3. Clip the text from transcript or type manually in the second Textbox provided.
4. A .Gif image will get generated on the right hand side of animated Shia Labeouf!
Hopee you have fun using this 😀
""")
with gr.Row():
#for incoming video
input_video = gr.Video(label="Upload a Video", visible=True)
#to generate and display transcriptions for input video
text_transcript = gr.Textbox(label="Transcripts", lines = 10, interactive = True )
#Just to move dgata between function hence keeping visible false
text_words = gr.Textbox(visible=False)
text_wordstimestamps = gr.Textbox(visible=False)
#to copy paste required gif transcript / or to populate by itslef on pressing the button
text_gif_transcript = gr.Textbox(label="Transcripts", placeholder="Copy paste transcripts here to create GIF image" , lines = 3, interactive = True )
def load_gif_text(text):
print("****** inside load_gif_text() ******")
print("text for gif is : ", text)
return text
text_transcript.change(load_gif_text, text_transcript, text_gif_transcript )
out_gif = gr.Image(label="Generated GIF image")
with gr.Row():
button_transcript = gr.Button("Generate transcripts")
button_gifs = gr.Button("Create Gif")
with gr.Row():
#to render video example on mouse hover/click
examples.render()
#to load sample video into input_video upon clicking on it
def load_examples(video):
print("****** inside load_example() ******")
print("in_video is : ", video[0])
return video[0]
examples.click(load_examples, examples, input_video)
with gr.Row():
gr.Markdown(""" I will start with a short note on my understanding of Radames's app and tools used in it -
- His is a supercool and handy proof of concept of a simple video editor where you can edit a video by playing with its audio transcriptions (ASR pipeline output).
- Both of our apps uses **Huggingface's [Automatic Speech Recognition Pipeline]**(https://huggingface.co/tasks/automatic-speech-recognition) build over **Wav2Vec2** model which internally uses CTC to improve predictions. The pipeline allows you to predict text transcriptions along with the timestamps for every characters and pauses that are there in the audio text.
- His app uses FFmpeg library to a good extent to clip and merge videos. FFmpeg is an open-source library for video handling consisting of a suite of functions for handling video, audio, and other multimedia files. My app uses FFmpeg as well as Moviepy to do the bulk of video+audio processing.
Let me now briefly take you through the code and process involved in building this app *step by step* 😉 lol -
- Firstly, I have used ffmpeg to extract audio from video (this code line is directly from Radames's above app) -
```
audio_memory, _ = ffmpeg.input(video_path).output('-', format="wav", ac=1, ar='16k').overwrite_output().global_args('-loglevel', 'quiet').run(capture_stdout=True)
```
- Then I am calling the ASR model as a service, using the Accelerated Inference API. Below is the code snippet for doing so -
```
def query(in_audio):
payload = json.dumps({ "inputs": base64.b64encode(in_audio).decode("utf-8"),
"parameters": {
"return_timestamps": "char",
"chunk_length_s": 10,
"stride_length_s": [4, 2]
},
"options": {"use_gpu": False}
}).encode("utf-8")
response = requests.request("POST", API_URL, data=payload)
json_response = json.loads(response.content.decode("utf-8"))
return json_response
```
- The transcript thus generated might have some words which are not correctly interpreted, for example, *tomorrow* is translated as 'to morrow', *hard at it* is translated as 'hot ati' and so on. However this won't hinder in the use-case I am demoing here, so we let's move on.
> do it just do it don't let your dreams be dreams yesterday you said to morrow so just do it make you dreams can't yro just do it some people dream of success while you're going to wake up and work hot ati nothing is impossible you should get to the point where any one else would quit and you're luck in a stop there no what are you waiting for do et jot do it just you can just do it if you're tired is starting over stop giving up
- The other output generated by this ASR pipeline is a list of character timestamps dictionaries, look at the below sample to get an idea -
```
{'text': 'D', 'timestamp': [2.36, 2.38]},
{'text': 'O', 'timestamp': [2.52, 2.56]},
{'text': ' ', 'timestamp': [2.68, 2.72]},
{'text': 'I', 'timestamp': [2.84, 2.86]},
{'text': 'T', 'timestamp': [2.88, 2.92]},
{'text': ' ', 'timestamp': [2.94, 2.98]},
{'text': 'J', 'timestamp': [4.48, 4.52]},
```
- Next, using these character timestamps I have extracted word timestamps (by taking the start_timestamp of the first letters and the en_timestamp of the last letter in any give word.
- Further when a *sub-transcript* is provided for the producing the GIF, I calculated the start and end timestamp for the whole group of words.
- I have then used *moviepy* library to extract / concat videos into smaller clips and also to save the final processed videofile as a.GIF image.
```
import moviepy.editor as mp
video = mp.VideoFileClip(video_path)
final_clip = video.subclip(start_seconds, end_seconds)
#writing to RAM
final_clip.write_gif("gifimage.gif") #, program='ffmpeg', tempfiles=True, fps=15, fuzz=3)
final_clip.write_videofile("gifimage.mp4")
final_clip.close()
```
While working on apps for [Gradio Blocks Party](https://huggingface.co/Gradio-Blocks) I have gained a good amount of knowledge about Gradio and Huggingface APIs and infrastructure. Such events help you to revise and develop your understanding of various ML applications and tools. For example, couple apps I have recently worked on included concepts like Question Answering, Sentence Trnasformers, Summarization, Image Generation, LLMs, Prompt Engineering, and now ASR and Video processing.
I absolutely enjoy building on Spaces, and I believe Spaces is much more than just a platform to showcase your ML demos. I suppose it has the potential to act like an ML Product Sandbox with the benefit of now having entire Huggingface infrastructure behind it. I believe Spaces can become some sort of a playground for future ML products and ideas. All of this is extremely exciting and I hope for the best outcome.
Thanks for reading so far, I will see you at my next Space demo. Keep learning and sharing.
My last two Gradio Blocks Party apps can be found here -
- [Gradio-Blocks/GPTJ6B_Poetry_LatentDiff_Illustration](https://huggingface.co/spaces/Gradio-Blocks/GPTJ6B_Poetry_LatentDiff_Illustration), and
- [Gradio-Blocks/Ask_Questions_To_YouTube_Videos](https://huggingface.co/spaces/Gradio-Blocks/Ask_Questions_To_YouTube_Videos)
""")
button_transcript.click(generate_transcripts, input_video, [text_transcript, text_words, text_wordstimestamps ])
button_gifs.click(generate_gifs, [input_video, text_gif_transcript, text_words, text_wordstimestamps], out_gif )
demo.launch(debug=True)