---
license: apache-2.0
language:
- th
- en
library_name: transformers
pipeline_tag: text-generation
tags:
- openthaigpt
- llama
---
# ðđð OpenThaiGPT 7b 1.0.0
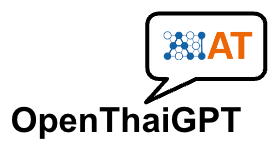
[More Info](https://openthaigpt.aieat.or.th/)
ðđð **OpenThaiGPT 7b Version 1.0.0** is an advanced 7-billion-parameter Thai language chat model based on LLaMA v2 released on April 8, 2024. It has been specifically fine-tuned for Thai instructions and enhanced by incorporating over 10,000 of the most commonly used Thai words into the large language model's (LLM) dictionary, significantly boosting its response speed.
## Highlights
- **Leading-edge Thai language LLM**, setting new benchmarks by achieving the highest average scores across several Thai language exams when compared to all other open-source Thai LLMs.
- **The First 70b Thai opensource LLM**, achieving the higher score on Thai exams than OpenAI GPT 3.5, Google Gemini, and Claude 3 Haiku.
- **Support for extended conversations** across multiple turns.
- Support the use case of **Retrieval Augmented Generation (RAG)** for enriched response generation.
- **Generation speeds increased by tenfold**, thanks to the addition of 10,000 frequently used Thai words to the model's dictionary.
- Pretrained upon a foundation of **more than 65 billion Thai language words** and meticulously fine-tuned with over 1 million Thai instruction examples.
- Capable of understanding and processing **input contexts of up to 4096 Thai words**, allowing for detailed and complex instructions.
## Benchmark by OpenThaiGPT Eval
** Please take a look at ``OTG 7b (April 2024)`` for this model's evaluation result.
| **Exams** | **OTG 7b (Aug 2023)** | **OTG 13b (Dec 2023)** | OTG 7b (April 2024) | **OTG 13b (April 2024)** | **OTG 70b (April 2024)** | **SeaLLM 7b v1** | **SeaLLM 7b v2** | **SeaLion 7b** | **WanchanGLM 7b** | **Sailor-7b-Chat** | **TyphoonGPT 7b Instruct** | **GPT3.5** | **GPT4** | **Gemini Pro** | **Gemini 1.5** | **Claude 3 Haiku** | **Claude 3 Sonnet** | **Claude 3 Opus** |
|----------------------------|-----------------------|------------------------|-------------------------|--------------------------|--------------------------|------------------|------------------|----------------|-------------------|--------------------|----------------------------|------------|----------|----------------|----------------|--------------------|---------------------|-------------------|
| **A-Level** | 17.50% | 34.17% | 25.00% | 30.83% | 45.83% | 18.33% | 34.17% | 21.67% | 17.50% | 40.00% | 37.50% | 38.33% | 65.83% | 56.67% | 55.83% | 58.33% | 59.17% | 77.50% |
| **TGAT** | 24.00% | 22.00% | 22.00% | 36.00% | 36.00% | 14.00% | 28.00% | 24.00% | 16.00% | 34.00% | 30.00% | 28.00% | 44.00% | 22.00% | 28.00% | 36.00% | 34.00% | 46.00% |
| **TPAT1** | 22.50% | 47.50% | 42.50% | 27.50% | 62.50% | 22.50% | 27.50% | 22.50% | 17.50% | 40.00% | 47.50% | 45.00% | 52.50% | 52.50% | 50.00% | 52.50% | 50.00% | 62.50% |
| **thai_investment_consultant_exams** | 8.00% | 28.00% | 76.00% | 84.00% | 68.00% | 16.00% | 28.00% | 24.00% | 16.00% | 24.00% | 32.00% | 40.00% | 64.00% | 52.00% | 32.00% | 44.00% | 64.00% | 72.00% |
| **facebook_beleble_tha_200** | 25.00% | 45.00% | 34.50% | 39.50% | 70.00% | 13.50% | 51.00% | 27.00% | 24.50% | 63.00% | 51.50% | 50.00% | 72.50% | 65.00% | 74.00% | 63.50% | 77.00% | 90.00% |
| **xcopa_th_200** | 45.00% | 56.50% | 49.50% | 51.50% | 74.50% | 26.50% | 47.00% | 51.50% | 48.50% | 68.50% | 65.00% | 64.00% | 82.00% | 68.00% | 74.00% | 64.00% | 80.00% | 86.00% |
| **xnli2.0_th_200** | 33.50% | 34.50% | 39.50% | 31.00% | 47.00% | 21.00% | 43.00% | 37.50% | 33.50% | 16.00% | 20.00% | 50.00% | 69.00% | 53.00% | 54.50% | 50.00% | 68.00% | 68.50% |
| **ONET M3** | 17.85% | 38.86% | 34.11% | 39.36% | 56.15% | 15.58% | 23.92% | 21.79% | 19.56% | 21.37% | 28.03% | 37.91% | 49.97% | 55.99% | 57.41% | 52.73% | 40.60% | 63.87% |
| **ONET M6** | 21.14% | 28.87% | 22.53% | 23.32% | 42.85% | 15.09% | 19.48% | 16.96% | 20.67% | 28.64% | 27.46% | 34.44% | 46.29% | 45.53% | 50.23% | 34.79% | 38.49% | 48.56% |
| **AVERAGE SCORE** | 23.83% | 37.27% | 38.40% | 40.33% | 55.87% | 18.06% | 33.56% | 27.44% | 23.75% | 37.28% | 37.67% | 43.07% | 60.68% | 52.30% | 52.89% | 50.65% | 56.81% | 68.32% |
Thai language multiple choice exams, Test on unseen test sets, Zero-shot learning. Benchmark source code and exams information: https://github.com/OpenThaiGPT/openthaigpt_eval
(Updated on: 7 April 2024)
## Licenses
**Source Code**: License Apache Software License 2.0.
**Weight**: Research and **Commercial uses**.
## Sponsors
## Supports
- Official website: https://openthaigpt.aieat.or.th
- Facebook page: https://web.facebook.com/groups/openthaigpt
- A Discord server for discussion and support [here](https://discord.gg/rUTp6dfVUF)
- E-mail: kobkrit@aieat.or.th
## Prompt Format
Prompt format is based on Llama2 with a small modification (Adding "###" to specify the context part)
```
[INST] <
{system_prompt}
<>
{human_turn1}###{context_turn1} [/INST]{assistant_turn1}{human_turn2}###{context_turn2} [/INST] ...
```
### System prompt:
```
You are a question answering assistant. Answer the question as truthful and helpful as possible āļāļļāļāļāļ·āļāļāļđāđāļāđāļ§āļĒāļāļāļāļāļģāļāļēāļĄ āļāļāļāļāļāļāļģāļāļēāļĄāļāļĒāđāļēāļāļāļđāļāļāđāļāļāđāļĨāļ°āļĄāļĩāļāļĢāļ°āđāļĒāļāļāđāļāļĩāđāļŠāļļāļ
```
### Examples
#### Single Turn Conversation Example
```
[INST] <
You are a question answering assistant. Answer the question as truthful and helpful as possible āļāļļāļāļāļ·āļāļāļđāđāļāđāļ§āļĒāļāļāļāļāļģāļāļēāļĄ āļāļāļāļāļāļāļģāļāļēāļĄāļāļĒāđāļēāļāļāļđāļāļāđāļāļāđāļĨāļ°āļĄāļĩāļāļĢāļ°āđāļĒāļāļāđāļāļĩāđāļŠāļļāļ
<>
āļŠāļ§āļąāļŠāļāļĩāļāļĢāļąāļ [/INST]
```
#### Single Turn Conversation with Context (RAG) Example
```
[INST] <
You are a question answering assistant. Answer the question as truthful and helpful as possible āļāļļāļāļāļ·āļāļāļđāđāļāđāļ§āļĒāļāļāļāļāļģāļāļēāļĄ āļāļāļāļāļāļāļģāļāļēāļĄāļāļĒāđāļēāļāļāļđāļāļāđāļāļāđāļĨāļ°āļĄāļĩāļāļĢāļ°āđāļĒāļāļāđāļāļĩāđāļŠāļļāļ
<>
āļāļĢāļļāļāđāļāļāļĄāļĩāļāļ·āđāļāļāļĩāđāđāļāđāļēāđāļĢāđ###āļāļĢāļļāļāđāļāļāļĄāļŦāļēāļāļāļĢ āđāļāđāļāđāļĄāļ·āļāļāļŦāļĨāļ§āļ āļāļāļĢāđāļĨāļ°āļĄāļŦāļēāļāļāļĢāļāļĩāđāļĄāļĩāļāļĢāļ°āļāļēāļāļĢāļĄāļēāļāļāļĩāđāļŠāļļāļāļāļāļāļāļĢāļ°āđāļāļĻāđāļāļĒ āļāļĢāļļāļāđāļāļāļĄāļŦāļēāļāļāļĢāļĄāļĩāļāļ·āđāļāļāļĩāđāļāļąāđāļāļŦāļĄāļ 1,568.737 āļāļĢ.āļāļĄ. āļĄāļĩāļāļĢāļ°āļāļēāļāļĢāļāļēāļĄāļāļ°āđāļāļĩāļĒāļāļĢāļēāļĐāļāļĢāļāļ§āđāļē 8 āļĨāđāļēāļāļāļ [/INST]
```
#### Multi Turn Conversation Example
##### First turn
```
[INST] <
You are a question answering assistant. Answer the question as truthful and helpful as possible āļāļļāļāļāļ·āļāļāļđāđāļāđāļ§āļĒāļāļāļāļāļģāļāļēāļĄ āļāļāļāļāļāļāļģāļāļēāļĄāļāļĒāđāļēāļāļāļđāļāļāđāļāļāđāļĨāļ°āļĄāļĩāļāļĢāļ°āđāļĒāļāļāđāļāļĩāđāļŠāļļāļ
<>
āļŠāļ§āļąāļŠāļāļĩāļāļĢāļąāļ [/INST]
```
##### Second turn
```
[INST] <
You are a question answering assistant. Answer the question as truthful and helpful as possible āļāļļāļāļāļ·āļāļāļđāđāļāđāļ§āļĒāļāļāļāļāļģāļāļēāļĄ āļāļāļāļāļāļāļģāļāļēāļĄāļāļĒāđāļēāļāļāļđāļāļāđāļāļāđāļĨāļ°āļĄāļĩāļāļĢāļ°āđāļĒāļāļāđāļāļĩāđāļŠāļļāļ
<>
āļŠāļ§āļąāļŠāļāļĩāļāļĢāļąāļ [/INST]āļŠāļ§āļąāļŠāļāļĩāļāđāļ° āļĄāļĩāļāļģāļāļēāļĄāļāļ°āđāļĢ āļāļēāļĄāđāļāđāđāļĨāļĒāļāļāļŠāļđāļāļĢāļāļģāļŠāđāļĄāļāļģāļŦāļāđāļāļĒ [/INST]
```
##### Third turn
```
[INST] <
You are a question answering assistant. Answer the question as truthful and helpful as possible āļāļļāļāļāļ·āļāļāļđāđāļāđāļ§āļĒāļāļāļāļāļģāļāļēāļĄ āļāļāļāļāļāļāļģāļāļēāļĄāļāļĒāđāļēāļāļāļđāļāļāđāļāļāđāļĨāļ°āļĄāļĩāļāļĢāļ°āđāļĒāļāļāđāļāļĩāđāļŠāļļāļ
<>
āļŠāļ§āļąāļŠāļāļĩāļāļĢāļąāļ [/INST]āļŠāļ§āļąāļŠāļāļĩāļāđāļ° āļĄāļĩāļāļģāļāļēāļĄāļāļ°āđāļĢ āļāļēāļĄāđāļāđāđāļĨāļĒāļāļāļŠāļđāļāļĢāļāļģāļŠāđāļĄāļāļģāļŦāļāđāļāļĒ [/INST]āđāļāđāđāļĨāļĒāļāđāļ° āļŠāđāļĄāļāļģāđāļāđāļāđāļĄāļāļđāļāļĩāđāļāļģāļāđāļēāļĒāđāļĨāļ°āļāļĢāđāļāļĒ āļĄāļēāđāļĢāļīāđāļĄāļāļąāļāđāļĨāļĒāļāļ°āļāļ°āđāļĢāļīāđāļĄāđāļāđāđāļĨāļĒ [/INST]
```
##### Fourth turn
```
[INST] <
You are a question answering assistant. Answer the question as truthful and helpful as possible āļāļļāļāļāļ·āļāļāļđāđāļāđāļ§āļĒāļāļāļāļāļģāļāļēāļĄ āļāļāļāļāļāļāļģāļāļēāļĄāļāļĒāđāļēāļāļāļđāļāļāđāļāļāđāļĨāļ°āļĄāļĩāļāļĢāļ°āđāļĒāļāļāđāļāļĩāđāļŠāļļāļ
<>
āļŠāļ§āļąāļŠāļāļĩāļāļĢāļąāļ [/INST]āļŠāļ§āļąāļŠāļāļĩāļāđāļ° āļĄāļĩāļāļģāļāļēāļĄāļāļ°āđāļĢ āļāļēāļĄāđāļāđāđāļĨāļĒāļāļāļŠāļđāļāļĢāļāļģāļŠāđāļĄāļāļģāļŦāļāđāļāļĒ [/INST]āđāļāđāđāļĨāļĒāļāđāļ° āļŠāđāļĄāļāļģāđāļāđāļāđāļĄāļāļđāļāļĩāđāļāļģāļāđāļēāļĒāđāļĨāļ°āļāļĢāđāļāļĒ āļĄāļēāđāļĢāļīāđāļĄāļāļąāļāđāļĨāļĒāļāļ°āļāļ°āđāļĢāļīāđāļĄāđāļāđāđāļĨāļĒ [/INST]
āļāļģāđāļāļĒāđāļāđāļāđāļĄāļāļđāļāļĩāđāđāļāļĢāđ āļāđāļāļļāđāļāđāļāļĒ āļāđāļ§āļĒāļĢāļŠāļāļēāļāļīāļāļĩāđāļāļĨāļĄāļāļĨāđāļāļĄ āļŦāļ§āļēāļ āđāļāļĢāļĩāđāļĒāļ§ āđāļāđāļĄ āđāļāđāļ āļāļĢāļāļĢāļŠ āļ§āļąāļāļāļĩāđāđāļĢāļēāļāļ°āļĄāļēāļāļģāļŠāđāļĄāļāļģāļāđāļ§āļĒāļāļąāļāļāđāļ° āļŠāļīāđāļāđāļĢāļāļāļĩāđāļāđāļāļāļĄāļĩāļāļ·āļāđāļāļĢāļ·āđāļāļāļāļĢāļļāļāļāļąāļāļāļĩāđ
- āļĄāļ°āļĨāļ°āļāļāļāļīāļ 1 āļĨāļđāļ
- āļāļļāđāļāđāļŦāđāļ 1/2 āļāđāļ§āļĒ
- āļāļĢāļ°āđāļāļĩāļĒāļĄ 3 āļāļĨāļĩāļ
- āļāļĢāļīāļāļāļĩāđāļŦāļāļđ 3 āđāļĄāđāļ
- āļāđāļģāļāļēāļĨāļāļĩāđāļ 1 āļāđāļāļāđāļāđāļ°
- āļāđāļģāļāļĨāļē 2 āļāđāļāļāđāļāđāļ°
- āļĄāļ°āļāļēāļ§ 1 āļĨāļđāļ
- āļāļēāļāļēāļ§āļāļąāđāļ§ 1/4 āļāđāļ§āļĒ
āļ§āļīāļāļĩāļāļģāļĄāļĩāļāļąāļāļāļĩāđāļāđāļ°
1. āđāļĢāļīāđāļĄāļāļēāļāļĨāđāļēāļāļĄāļ°āļĨāļ°āļāļāđāļŦāđāļŠāļ°āļāļēāļ āđāļĨāđāļ§āđāļāđāļĄāļĩāļāļāļāļāđāļāļĨāļ·āļāļ āđāļāļēāđāļŠāđāļāļāļ āļŦāļąāđāļāđāļāđāļāđāļŠāđāļāļāļēāļāđ āđāļāļĢāļĩāļĒāļĄāđāļ§āđ
2. āļāļģāļāļļāđāļāđāļŦāđāļāđāļŦāđāļĨāļ°āđāļāļĩāļĒāļ āđāļĨāđāļ§āļāļąāļāļāļķāđāļāļāļąāļāđāļ§āđ
3. āđāļāđāļāļĢāļāļŦāļīāļāļŦāļĢāļ·āļāđāļāļĢāļ·āđāļāļāļāļąāđāļ āļāļāļāļĢāļīāļāļāļĩāđāļŦāļāļđāļāļąāļāļāļĢāļ°āđāļāļĩāļĒāļĄāđāļŦāđāļĨāļ°āđāļāļĩāļĒāļ
4. āđāļŠāđāļāļļāđāļāđāļŦāđāļāļāļĩāđāļāļģāđāļĨāđāļ§āļĨāļāđāļāļāļŠāļĄ āļāļēāļĄāļāđāļ§āļĒāļāđāļģāļāļēāļĨāļāļĩāđāļ āļāđāļģāļāļĨāļē āļĄāļ°āļāļēāļ§ āđāļĨāļ°āđāļŠāđāļāļĄāļ°āļĨāļ°āļāļ āļāļĨāļļāļāđāļāļĨāđāļēāđāļŦāđāđāļāđāļēāļāļąāļ
5. āļāļīāļĄāļĢāļŠāđāļŦāđāđāļāđāļĢāļŠāļŦāļ§āļēāļ āđāļāļĢāļĩāđāļĒāļ§ āđāļāđāļĄ āđāļāđāļ āļāļēāļāļāļąāđāļāļāļąāļāļāļķāđāļāđāļŠāļīāļĢāđāļāļāļĢāđāļāļĄāļāļąāļāļŠāļ āļāļēāļāļī āļāļ°āļŦāļĨāđāļģāļāļĨāļĩ āļāļąāđāļ§āļāļāļ āđāļāļĢāļāļ āļāļąāļāļāļļāđāļāļāļāļāļāļļāļāļāļĢāļąāļ [/INST]
```
#### Multi Turn Conversation with Context (RAG) Example
```
[INST] <
You are a question answering assistant. Answer the question as truthful and helpful as possible āļāļļāļāļāļ·āļāļāļđāđāļāđāļ§āļĒāļāļāļāļāļģāļāļēāļĄ āļāļāļāļāļāļāļģāļāļēāļĄāļāļĒāđāļēāļāļāļđāļāļāđāļāļāđāļĨāļ°āļĄāļĩāļāļĢāļ°āđāļĒāļāļāđāļāļĩāđāļŠāļļāļ
<>
āļāļĢāļļāļāđāļāļāļĄāļĩāļāļ·āđāļāļāļĩāđāđāļāđāļēāđāļĢāđ###āļāļĢāļļāļāđāļāļāļĄāļŦāļēāļāļāļĢ āđāļāđāļāđāļĄāļ·āļāļāļŦāļĨāļ§āļ āļāļāļĢāđāļĨāļ°āļĄāļŦāļēāļāļāļĢāļāļĩāđāļĄāļĩāļāļĢāļ°āļāļēāļāļĢāļĄāļēāļāļāļĩāđāļŠāļļāļāļāļāļāļāļĢāļ°āđāļāļĻāđāļāļĒ āļāļĢāļļāļāđāļāļāļĄāļŦāļēāļāļāļĢāļĄāļĩāļāļ·āđāļāļāļĩāđāļāļąāđāļāļŦāļĄāļ 1,568.737 āļāļĢ.āļāļĄ. āļĄāļĩāļāļĢāļ°āļāļēāļāļĢāļāļēāļĄāļāļ°āđāļāļĩāļĒāļāļĢāļēāļĐāļāļĢāļāļ§āđāļē 8 āļĨāđāļēāļāļāļ [/INST]
āļāļĢāļļāļāđāļāļāļĄāļŦāļēāļāļāļĢāļĄāļĩāļāļ·āđāļāļāļĩāđāļāļąāđāļāļŦāļĄāļ 1,568.737 āļāļĢ.āļāļĄ.āđāļĨāļ°āļāļĢāļ°āļāļēāļāļĢāļĨāđāļ° [/INST]
```
## How to use
### Huggingface
```python
from transformers import AutoModelForCausalLM, AutoTokenizer
import torch
# Ensure CUDA is available
device = 'cuda' if torch.cuda.is_available() else 'cpu'
print(f"Using device: {device}")
# Init Model
model_path="openthaigpt/openthaigpt-1.0.0-7b-chat"
tokenizer = AutoTokenizer.from_pretrained(model_path, trust_remote_code=True)
model = AutoModelForCausalLM.from_pretrained(model_path, trust_remote_code=True, torch_dtype=torch.float16)
model.to(device)
# Prompt
prompt = "āļŠāļ§āļąāļŠāļāļĩāļāļĢāļąāļ OpenThaiGPT"
llama_prompt = f"[INST] <>\nYou are a question answering assistant. Answer the question as truthful and helpful as possible āļāļļāļāļāļ·āļāļāļđāđāļāđāļ§āļĒāļāļāļāļāļģāļāļēāļĄ āļāļāļāļāļāļāļģāļāļēāļĄāļāļĒāđāļēāļāļāļđāļāļāđāļāļāđāļĨāļ°āļĄāļĩāļāļĢāļ°āđāļĒāļāļāđāļāļĩāđāļŠāļļāļ<>\n\n{prompt} [/INST]"
inputs = tokenizer.encode(llama_prompt, return_tensors="pt")
inputs = inputs.to(device)
# Generate
outputs = model.generate(inputs, max_length=512, num_return_sequences=1)
print(tokenizer.decode(outputs[0], skip_special_tokens=True))
```
### vLLM
1. Install VLLM (https://github.com/vllm-project/vllm)
2. Run server
```bash
python -m vllm.entrypoints.api_server --model /path/to/model --tensor-parallel-size num_gpus
```
3. Run inference (CURL example)
```bash
curl --request POST \
--url http://localhost:8000/generate \
--header "Content-Type: application/json" \
--data '{"prompt": "[INST] <>\nYou are a question answering assistant. Answer the question as truthful and helpful as possible āļāļļāļāļāļ·āļāļāļđāđāļāđāļ§āļĒāļāļāļāļāļģāļāļēāļĄ āļāļāļāļāļāļāļģāļāļēāļĄāļāļĒāđāļēāļāļāļđāļāļāđāļāļāđāļĨāļ°āļĄāļĩāļāļĢāļ°āđāļĒāļāļāđāļāļĩāđāļŠāļļāļ\n<>\n\nāļāļĒāļēāļāļĨāļāļāļ§āļēāļĄāļāđāļ§āļāļāđāļāļāļāļģāļāļĒāđāļēāļāđāļĢ [/INST]","use_beam_search": false, "temperature": 0.1, "max_tokens": 512, "top_p": 0.75, "top_k": 40, "frequency_penalty": 0.3 "stop": ""}'
```
### LlamaCPP (for GGUF)
1. Build and Install LlamaCPP (LLAMA_CUBLAS=1 is for GPU inference)
```bash
git clone https://github.com/ggerganov/llama.cpp.git \
&& cd llama.cpp \
&& make -j LLAMA_CUBLAS=1 CUDA_DOCKER_ARCH=all
```
2. Run server
```bash
./server -m /path/to/ggml-model-f16.gguf -c 3072 -ngl 81 -ts 1,1 --host 0.0.0.0
```
3. Run inference (CURL example)
```bash
curl --location 'http://localhost:8000/completion' \
--header 'Content-Type: application/json' \
--data '{
"prompt":"[INST] <>\nYou are a question answering assistant. Answer the question as truthful and helpful as possible āļāļļāļāļāļ·āļāļāļđāđāļāđāļ§āļĒāļāļāļāļāļģāļāļēāļĄ āļāļāļāļāļāļāļģāļāļēāļĄāļāļĒāđāļēāļāļāļđāļāļāđāļāļāđāļĨāļ°āļĄāļĩāļāļĢāļ°āđāļĒāļāļāđāļāļĩāđāļŠāļļāļ friendly\n\n<>\n\nāļāļĒāļēāļāļĨāļāļāļ§āļēāļĄāļāđāļ§āļāļāđāļāļāļāļģāļāļĒāđāļēāļāđāļĢ [/INST]",
"max_tokens": 512,
"stop":""
}'
```
### GPU Memory Requirements
| **Number of Parameters** | **FP 16 bits** | **8 bits (Quantized)** | **4 bits (Quantized)** | **Example Graphic Card for 4 bits** |
|------------------|----------------|------------------------|------------------------|---------------------------------------------|
| **7b** | 24 GB | 12 GB | 6 GB | Nvidia RTX 4060 8GB |
| **13b** | 48 GB | 24 GB | 12 GB | Nvidia RTX 4070 16GB |
| **70b** | 192 GB | 96 GB | 48 GB | Nvidia RTX 4090 24GB x 2 cards |
### Authors
* Kobkrit Viriyayudhakorn (kobkrit@aieat.or.th)
* Sumeth Yuenyong (sumeth.yue@mahidol.edu)
* Thaweewat Rugsujarit (thaweewr@scg.com)
* Jillaphat Jaroenkantasima (autsadang41@gmail.com)
* Norapat Buppodom (new@norapat.com)
* Koravich Sangkaew (kwankoravich@gmail.com)
* Peerawat Rojratchadakorn (peerawat.roj@gmail.com)
* Surapon Nonesung (nonesungsurapon@gmail.com)
* Chanon Utupon (chanon.utupon@gmail.com)
* Sadhis Wongprayoon (sadhis.tae@gmail.com)
* Nucharee Thongthungwong (nuchhub@hotmail.com)
* Chawakorn Phiantham (mondcha1507@gmail.com)
* Patteera Triamamornwooth (patt.patteera@gmail.com)
* Nattarika Juntarapaoraya (natt.juntara@gmail.com)
* Kriangkrai Saetan (kraitan.ss21@gmail.com)
* Pitikorn Khlaisamniang (pitikorn32@gmail.com)
Disclaimer: Provided responses are not guaranteed.