---
license: other
datasets:
- OpenAssistant/oasst2
- nvidia/HelpSteer
language:
- ja
- en
library_name: transformers
base_model: karakuri-ai/karakuri-lm-70b-v0.1
pipeline_tag: text-generation
tags:
- llama
- llama-2
- steerlm
model-index:
- name: karakuri-ai/karakuri-lm-70b-chat-v0.1
results:
- task:
type: text-generation
name: Text Generation
dataset:
name: MT-Bench
type: unknown
metrics:
- type: unknown
name: score
value: 6.609375
source:
url: https://huggingface.co/spaces/lmsys/mt-bench
- task:
type: text-generation
name: Text Generation
dataset:
name: MT-Bench-jp
type: unknown
metrics:
- type: unknown
name: score
value: 6.43125
source:
url: https://api.wandb.ai/links/wandb-japan/6ff86bp3
---
# KARAKURI LM
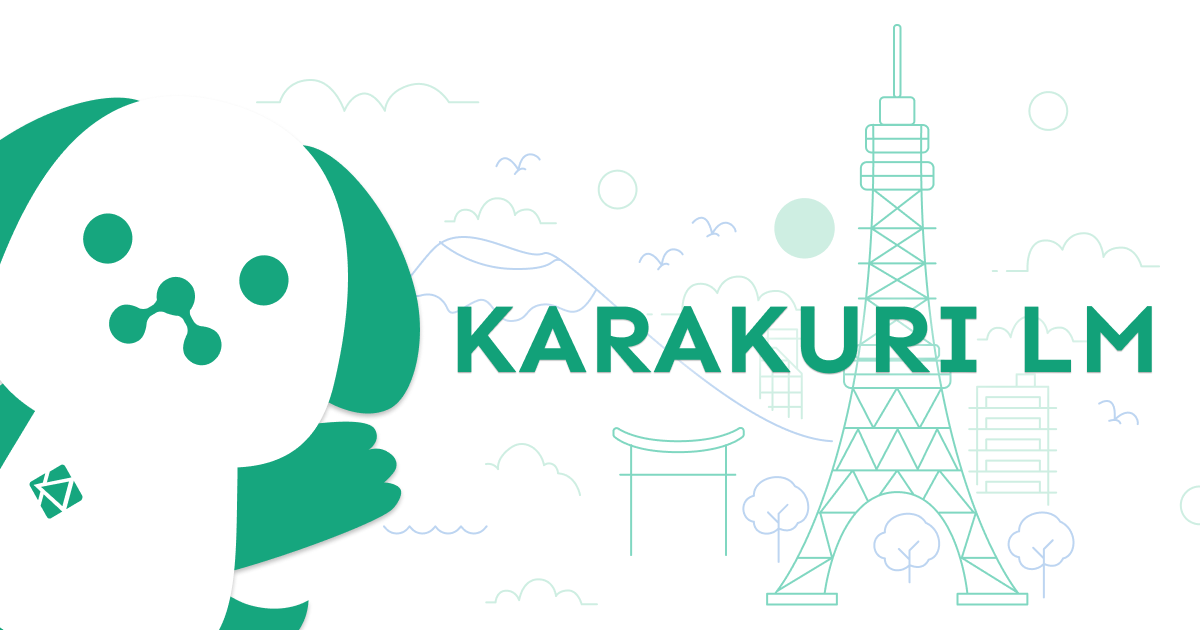
KARAKURI LM is a pretrained language model that builds upon Llama 2.
Our model enhances Llama 2's capabilities by incorporating additional Japanese vocabulary and further pretraining on a mixture of Japanese and multilingual corpora.
KARAKURI LM Chat is a fine-tuned version of KARAKURI LM, which was trained on a mixture of publicly available and closed datasets using the [SteerLM](https://aclanthology.org/2023.findings-emnlp.754/) technique.
During fine-tuning, our model employed a continual learning approach.
Unlike the common practice of relying solely on structured conversational datasets, we also incorporated unstructured corpora, similar to what was used during its pretraining phase.
Despite the conversational datasets containing only 2.5% Japanese tokens, our model has shown remarkable performance.
It achieves the highest performance among Japanese open models on the [MT-Bench-jp](https://api.wandb.ai/links/wandb-japan/6ff86bp3) at the time of release.
Furthermore, it achieves performance comparable to Llama 2 70B Chat on the original English [MT-Bench](https://huggingface.co/spaces/lmsys/mt-bench).
You can find more details in our blog post ([en](https://medium.com/karakuri/introducing-karakuri-lm-34c79a3bf341), [ja](https://medium.com/karakuri/karakuri-lm%E3%81%AE%E8%A7%A3%E8%AA%AC-4b6cf9c3d40f)).
If you are curious about our model, give our [demo](https://lm.karakuri.cc/) a try.
## Model Details
- **Developed by**: [KARAKURI Inc.](https://about.karakuri.ai/)
- **Model type**: Causal decoder-only transformer language model
- **Languages**: English and Japanese
- **Finetuned from**: [karakuri-ai/karakuri-lm-70b-v0.1](https://huggingface.co/karakuri-ai/karakuri-lm-70b-v0.1)
- **Contact**: For questions and comments about the model, please email `karakuri-rd@karakuri.ai`
## Performance
At the time of release, KARAKURI LM 70B Chat v0.1 achieves the highest performance among Japanese open models on the [MT-Bench-jp](https://api.wandb.ai/links/wandb-japan/6ff86bp3):
| Model | Size | Alignment | MT-Bench-jp |
| :---------------------------------- | :-----: | :---------: | ----------: |
| GPT-4 | - | RLHF | 8.78 |
| GPT-3.5-Turbo | - | RLHF | 8.24 |
| Claude 2.1 | - | RLHF | 8.18 |
| Gemini Pro | - | RLHF | 7.17 |
| **KARAKURI LM 70B Chat v0.1** | **70B** | **SteerLM** | **6.43** |
| Qarasu-14B-Chat-Plus-Unleashed | 14B | SFT | 6.26 |
| Llama 2 70B Chat | 70B | RLHF | 5.23 |
| ELYZA-Japanese-Llama-2-13B | 13B | SFT | 5.05 |
| Japanese-StableLM-Instruct-Beta-70B | 70B | SFT | 5.03 |
| Swallow-70B-Instruct | 70B | SFT | 4.39 |
It also achieves performance comparable to Llama 2 70B Chat on the original English [MT-Bench](https://huggingface.co/spaces/lmsys/mt-bench):
| Model | Average | MT-Bench | MT-Bench-jp |
| :---------------------------- | -------: | -------: | ----------: |
| **KARAKURI LM 70B Chat v0.1** | **6.52** | **6.61** | **6.43** |
| Llama 2 70B Chat | 6.04 | 6.86 | 5.23 |
## Use in đ€ Transformers
You can run the model using the `pipeline()` function from đ€ Transformers:
```python
from transformers import pipeline, Conversation
chatbot = pipeline("conversational", model="karakuri-ai/karakuri-lm-70b-chat-v0.1", device_map="auto", torch_dtype="auto")
conversation = Conversation("鱿«ă«æ„ćž°ăă§æ±äșŹă«éăłă«èĄăăăšæăŁăŠăăŸăăæ„ćž°ăăȘăźă§ăçæéă§ćăăăăăăăźèŠłć
ăă©ăłăæăăŠăă ăăă")
conversation = chatbot(conversation, max_new_tokens=512)
conversation.messages[-1]["content"]
```
We use the following prompt template of multi-turn conversation in the Llama format, which includes an encoded string of multiple attribute values.
```python
messages = [
{"role": "system", "content": "System prompt"},
{"role": "user", "content": "User prompt"},
{"role": "assistant", "content": "Model response"},
{"role": "user", "content": "User prompt"},
]
chatbot.tokenizer.apply_chat_template(messages, tokenize=False)
# [INST] <>
# System prompt
# <>
#
# User prompt [ATTR] helpfulness: 4 correctness: 4 coherence: 4 complexity: 4 verbosity: 4 quality: 4 toxicity: 0 humor: 0 creativity: 0 [/ATTR] [/INST] Model response [INST] User prompt [ATTR] helpfulness: 4 correctness: 4 coherence: 4 complexity: 4 verbosity: 4 quality: 4 toxicity: 0 humor: 0 creativity: 0 [/ATTR] [/INST]
```
The prompt template contains nine attributes.
The first five are derived from HelpSteer, while the remaining four are derived from OASST2.
The values are represented by integers ranging from 0 to 4, with 0 being the lowest and 4 being the highest.
- helpfulness (default: 4)
- correctness (default: 4)
- coherence (default: 4)
- complexity (default: 4)
- verbosity (default: 4)
- quality (default: 4)
- toxicity (default: 0)
- humor (default: 0)
- creativity (default: 0)
If you want to change attribute values from the default values specified in the template, you can modify them to any values by adding the attribute values to the user messages:
```python
messages = [
{"role": "user", "content": "User prompt", "helpfulness": 0, "complexity": 0},
]
chatbot.tokenizer.apply_chat_template(messages, tokenize=False)
# [INST] User prompt [ATTR] helpfulness: 0 correctness: 4 coherence: 4 complexity: 0 verbosity: 4 quality: 4 toxicity: 0 humor: 0 creativity: 0 [/ATTR] [/INST]
```
## Training
### Training Datasets
- [OASST2](https://huggingface.co/datasets/OpenAssistant/oasst2)
- Our internal conversational datasets
### Training Infrastructure
- **Hardware**: KARAKURI LM 70B was trained on 32 nodes of an Amazon EC2 trn1.32xlarge instance.
- **Software**: We use code based on [neuronx-nemo-megatron](https://github.com/aws-neuron/neuronx-nemo-megatron).
## Acknowledgements
We gratefully acknowledge the support from AWS Japan through the [AWS LLM Development Support Program](https://aws.amazon.com/jp/local/llm-development-support-program/).
## License
Llama 2 is licensed under the LLAMA 2 Community License, Copyright © Meta Platforms, Inc. All Rights Reserved.
Subject to the license above, and except for commercial purposes, you are free to share and adapt KARAKURI LM, provided that you must, in a recognizable and appropriate manner, (i) state that you are using KARAKURI LM developed by KARAKURI Inc., when you publish or make available to third parties KARAKURI LM, its derivative works or modification, or any output or results of KARAKURI LM or its derivative works or modification, and (ii) indicate your contributions, if you modified any material of KARAKURI LM.
If you plan to use KARAKURI LM for commercial purposes, please contact us beforehand. You are not authorized to use KARAKURI LM for commercial purposes unless we expressly grant you such rights.
If you have any questions regarding the interpretation of above terms, please also feel free to contact us.
## Citation
```
@misc {karakuri_lm_70b_chat_v01,
author = { {KARAKURI} {I}nc. },
title = { {KARAKURI} {LM} 70{B} {C}hat v0.1 },
year = { 2024 },
url = { https://huggingface.co/karakuri-ai/karakuri-lm-70b-chat-v0.1 },
publisher = { Hugging Face },
journal = { Hugging Face repository }
}
```